- Skip to main content
- Skip to primary sidebar
- Skip to footer
- QuestionPro

- Solutions Industries Gaming Automotive Sports and events Education Government Travel & Hospitality Financial Services Healthcare Cannabis Technology Use Case NPS+ Communities Audience Contactless surveys Mobile LivePolls Member Experience GDPR Positive People Science 360 Feedback Surveys
- Resources Blog eBooks Survey Templates Case Studies Training Help center

Home Market Research Research Tools and Apps

Unit of Analysis: Definition, Types & Examples
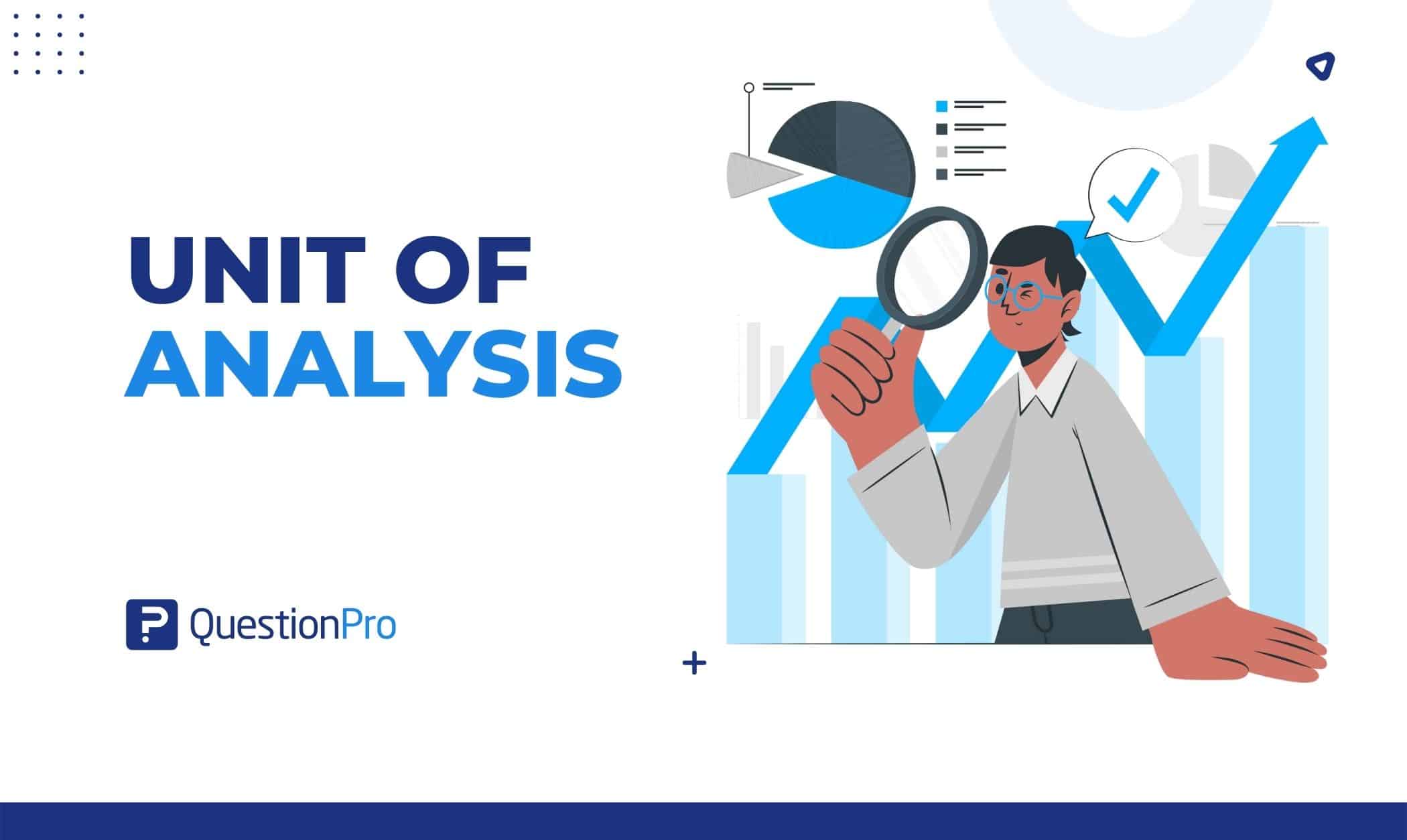
The unit of analysis is the people or things whose qualities will be measured. The unit of analysis is an essential part of a research project. It’s the main thing that a researcher looks at in his research.
A unit of analysis is the object about which you hope to have something to say at the end of your analysis, perhaps the major subject of your research.
In this blog post, we will explore and clarify the concept of the “unit of analysis,” including its definition, various types, and a concluding perspective on its significance.
What is a unit of analysis?
A unit of analysis is the thing you want to discuss after your research, probably what you would regard to be the primary emphasis of your research.
The researcher plans to comment on the primary topic or object in the research as a unit of analysis. The research question plays a significant role in determining it. The “who” or “what” that the researcher is interested in investigating is, to put it simply, the unit of analysis.
In his 2001 book Man, the State, and War, Waltz divides the world into three distinct spheres of study: the individual, the state, and war.
Understanding the reasoning behind the unit of analysis is vital. The likelihood of fruitful research increases if the rationale is understood. An individual, group, organization, nation, social phenomenon, etc., are a few examples.
LEARN ABOUT: Data Analytics Projects
Types of “unit of analysis”
In business research, there are almost unlimited types of possible analytical units. Data analytics and data analysis are closely related processes that involve extracting insights from data to make informed decisions. Even though the most typical unit of analysis is the individual, many research questions can be more precisely answered by looking at other types of units. Let’s find out,
1. Individual Level
The most prevalent unit of analysis in business research is the individual. These are the primary analytical units. The researcher may be interested in looking into:
- Employee actions
- Perceptions
- Attitudes or opinions.
Employees may come from wealthy or low-income families, as well as from rural or metropolitan areas.
A researcher might investigate if personnel from rural areas are more likely to arrive on time than those from urban areas. Additionally, he can check whether workers from rural areas who come from poorer families arrive on time compared to those from rural areas who come from wealthy families.
Each time, the individual (employee) serving as the analytical unit is discussed and explained. Employee analysis as a unit of analysis can shed light on issues in business, including customer and human resource behavior.
For example, employee work satisfaction and consumer purchasing patterns impact business, making research into these topics vital.
Psychologists typically concentrate on research on individuals. This research may significantly aid a firm’s success, as individuals’ knowledge and experiences reveal vital information. Thus, individuals are heavily utilized in business research.
2. Aggregates Level
Social science research does not usually focus on people. However, by combining individuals’ reactions, social scientists frequently describe and explain social interactions, communities, and groupings. Additionally, they research the collective of individuals, including communities, groups, and countries.
Aggregate levels can be divided into Groups (groups with an ad hoc structure) and Organizations (groups with a formal organization).
The following levels of the unit of analysis are made up of groups of people. A group is defined as two or more individuals who interact, share common traits, and feel connected to one another.
Many definitions also emphasize interdependence or objective resemblance (Turner, 1982; Platow, Grace, & Smithson, 2011) and those who identify as group members (Reicher, 1982) .
As a result, society and gangs serve as examples of groups. According to Webster’s Online Dictionary (2012), they can resemble some clubs but be far less formal.
Siblings, identical twins, family, and small group functioning are examples of studies with many units of analysis.
In such circumstances, a whole group might be compared to another. Families, gender-specific groups, pals, Facebook groups, and work departments can all be groups.
By analyzing groups, researchers can learn how they form and how age, experience, class, and gender affect them. When aggregated, an individual’s data describes the group they belong to.
Sociologists study groups like economists and businesspeople to form teams to complete projects. They continually research groups and group behavior.
Organizations
The next level of the unit of analysis is organizations, which are groups of people set up formally. Organizations could include businesses, religious groups, parts of the military, colleges, academic departments, supermarkets, business groups, and so on.
The social organization includes things like sexual composition, styles of leadership, organizational structure, systems of communication, and so on. (Susan & Wheelan, 2005; Chapais & Berman, 2004) . (Lim, Putnam, and Robert, 2010) say that well-known social organizations and religious institutions are among them.
Moody, White, and Douglas (2003) say social organizations are hierarchical. Hasmath, Hildebrandt, and Hsu (2016) say social organizations can take different forms. For example, they can be made by institutions like schools or governments.
Sociology, economics, political science, psychology, management, and organizational communication are some social science fields that study organizations (Douma & Schreuder, 2013) .
Organizations are different from groups in that they are more formal and have better organization. A researcher might want to study a company to generalize its results to the whole population of companies.
One way to look at an organization is by the number of employees, the net annual revenue, the net assets, the number of projects, and so on. He might want to know if big companies hire more or fewer women than small companies.
Organization researchers might be interested in how companies like Reliance, Amazon, and HCL affect our social and economic lives. People who work in business often study business organizations.
LEARN ABOUT: Data Management Framework
3. Social Level
The social level has 2 types,
Social Artifacts Level
Things are studied alongside humans. Social artifacts are human-made objects from diverse communities. Social artifacts are items, representations, assemblages, institutions, knowledge, and conceptual frameworks used to convey, interpret, or achieve a goal (IGI Global, 2017).
Cultural artifacts are anything humans generate that reveals their culture (Watts, 1981).
Social artifacts include books, newspapers, advertising, websites, technical devices, films, photographs, paintings, clothes, poems, jokes, students’ late excuses, scientific breakthroughs, furniture, machines, structures, etc. Infinite.
Humans build social objects for social behavior. As people or groups suggest a population in business research, each social object implies a class of items.
Same-class goods include business books, magazines, articles, and case studies. A business magazine’s quantity of articles, frequency, price, content, and editor in a research study may be characterized.
Then, a linked magazine’s population might be evaluated for description and explanation. Marx W. Wartofsky (1979) defined artifacts as primary artifacts utilized in production (like a camera), secondary artifacts connected to primary artifacts (like a camera user manual), and tertiary objects related to representations of secondary artifacts (like a camera user-manual sculpture).
The scientific study of an artifact reveals its creators and users. The artifact researcher may be interested in advertising, marketing, distribution, buying, etc.
Social Interaction Level
Social artifacts include social interaction. Such as:
- Eye contact with a coworker
- Buying something in a store
- Friendship decisions
- Road accidents
- Airline hijackings
- Professional counseling
- Whatsapp messaging
A researcher might study youthful employees’ smartphone addictions. Some addictions may involve social media, while others involve online games and movies that inhibit connection.
Smartphone addictions are examined as a societal phenomenon. Observation units are probably individuals (employees).
Anthropologists typically study social artifacts. They may be interested in the social order. A researcher who examines social interactions may be interested in how broader societal structures and factors impact daily behavior, festivals, and weddings.
LEARN ABOUT: Level of Analysis
Even though there is no perfect way to do research, it is generally agreed that researchers should try to find a unit of analysis that keeps the context needed to make sense of the data.
Researchers should consider the details of their research when deciding on the unit of analysis.
They should remember that consistent use of these units throughout the analysis process (from coding to developing categories and themes to interpreting the data) is essential to gaining insight from qualitative data and protecting the reliability of the results.
QuestionPro does much more than merely serve as survey software. We have a solution for every sector of the economy and every kind of issue. We also have systems for managing data, such as our research repository, Insights Hub.
LEARN MORE FREE TRIAL
MORE LIKE THIS
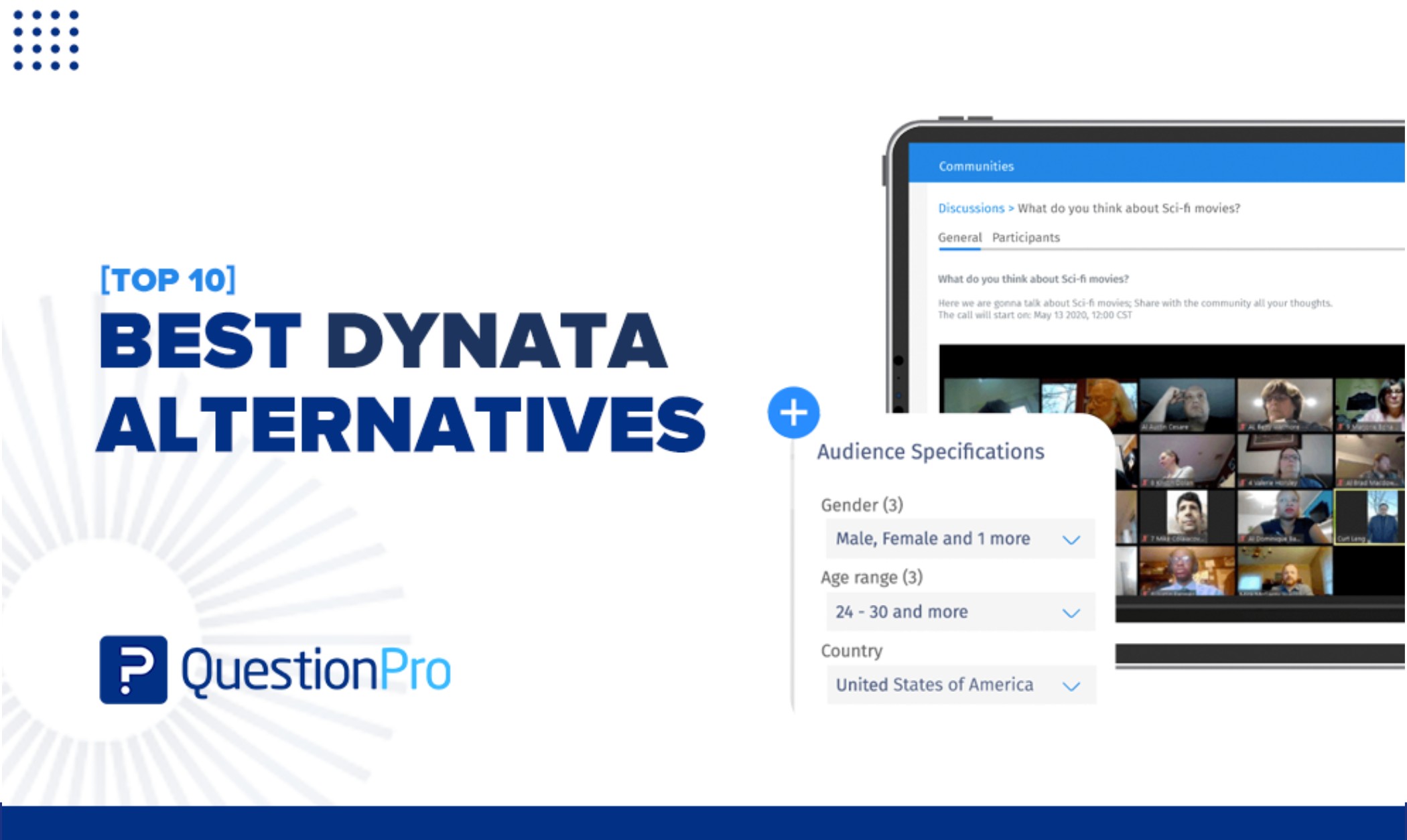
Top 10 Dynata Alternatives & Competitors
May 27, 2024
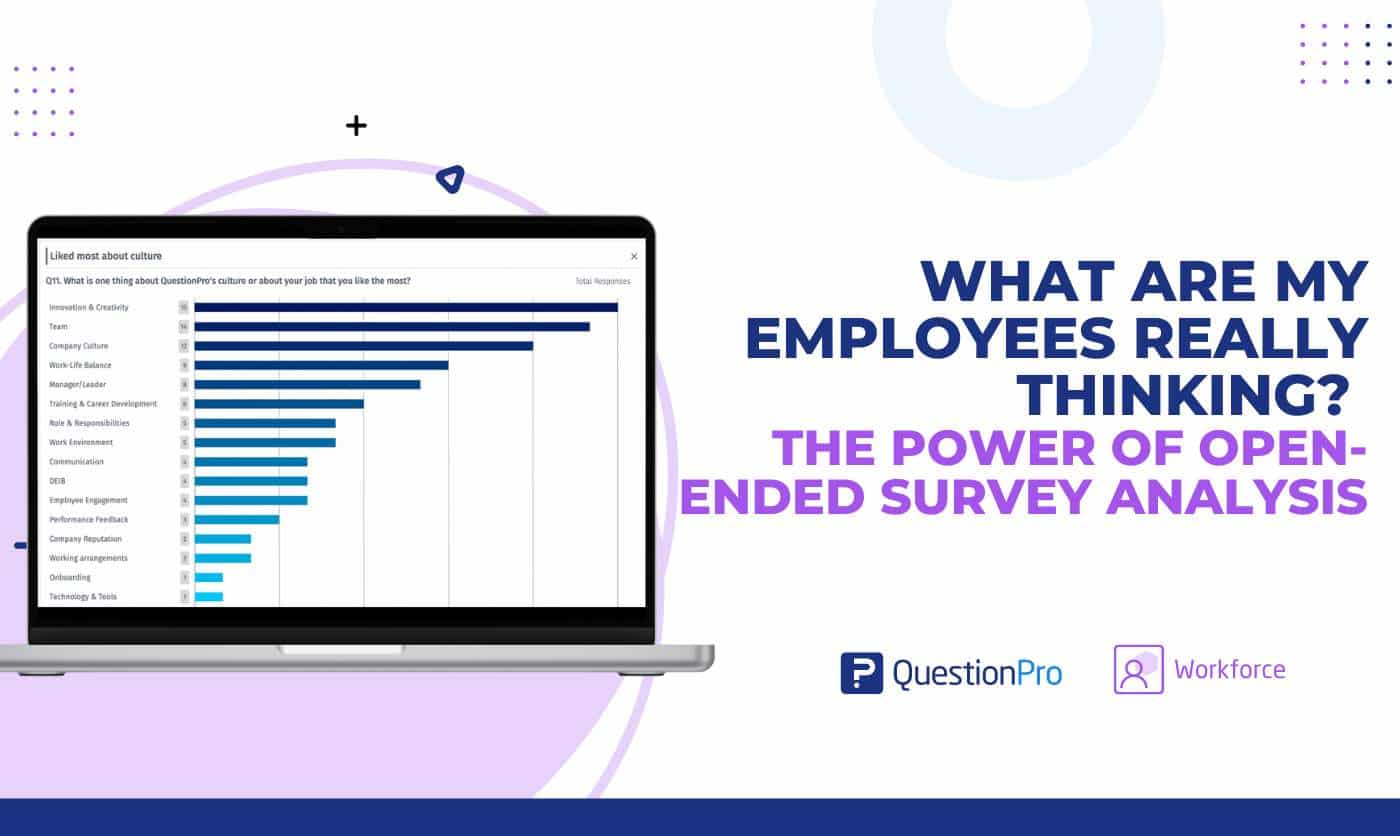
What Are My Employees Really Thinking? The Power of Open-ended Survey Analysis
May 24, 2024
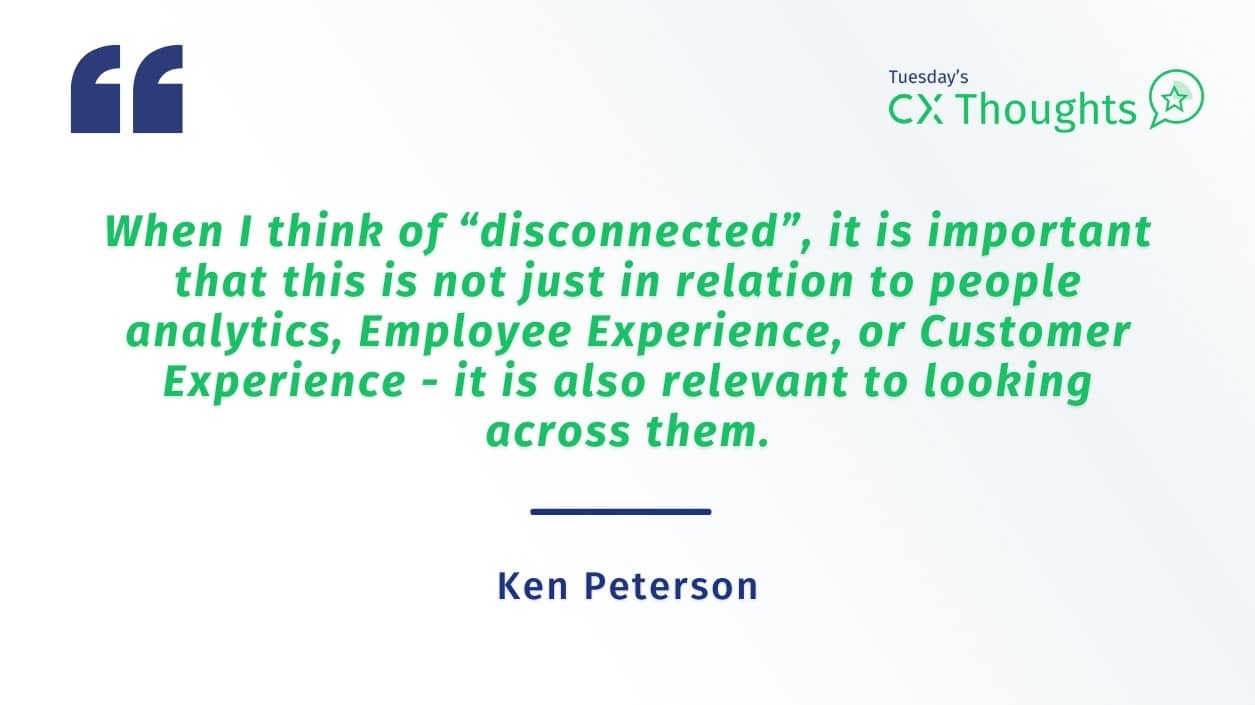
I Am Disconnected – Tuesday CX Thoughts
May 21, 2024
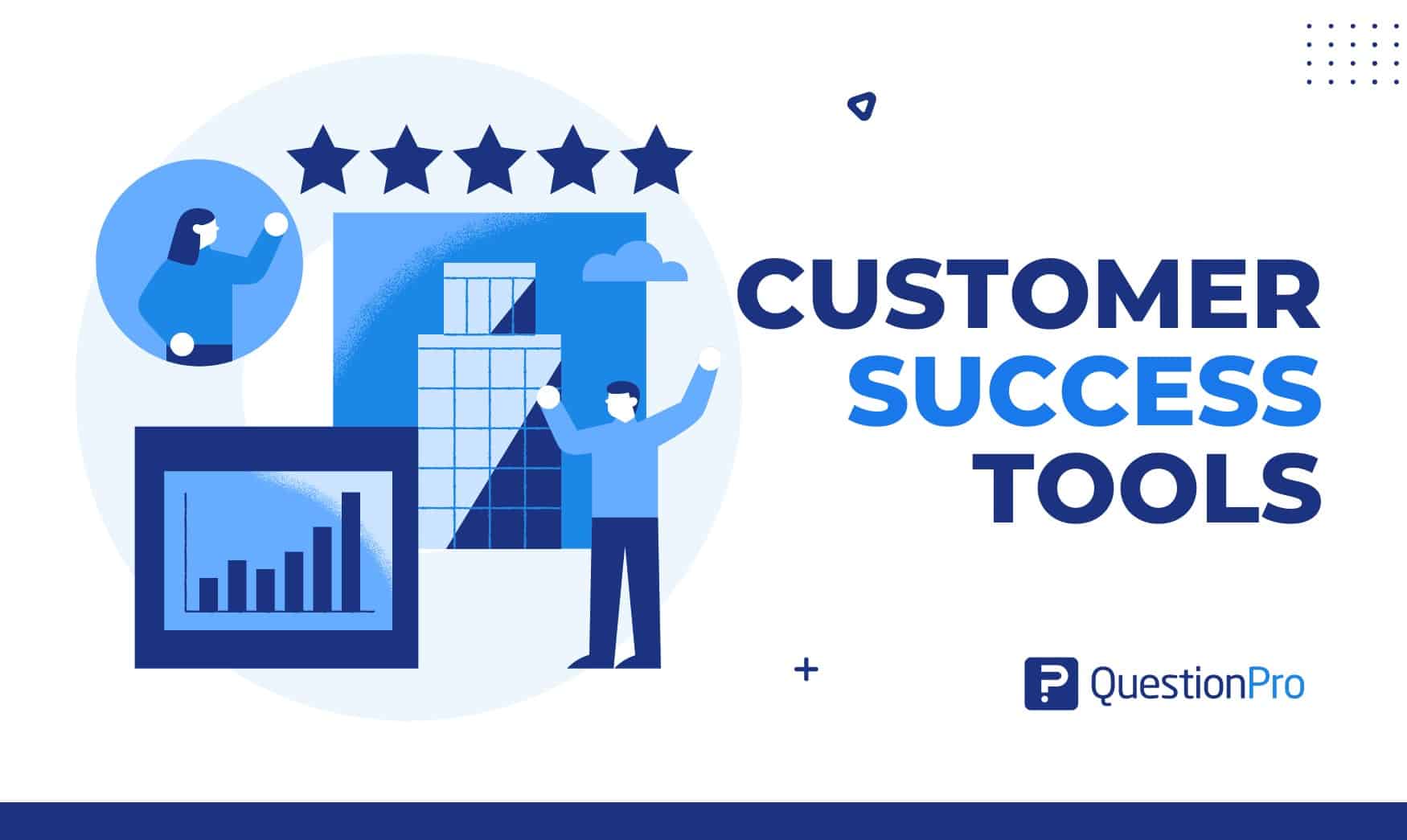
20 Best Customer Success Tools of 2024
May 20, 2024
Other categories
- Academic Research
- Artificial Intelligence
- Assessments
- Brand Awareness
- Case Studies
- Communities
- Consumer Insights
- Customer effort score
- Customer Engagement
- Customer Experience
- Customer Loyalty
- Customer Research
- Customer Satisfaction
- Employee Benefits
- Employee Engagement
- Employee Retention
- Friday Five
- General Data Protection Regulation
- Insights Hub
- Life@QuestionPro
- Market Research
- Mobile diaries
- Mobile Surveys
- New Features
- Online Communities
- Question Types
- Questionnaire
- QuestionPro Products
- Release Notes
- Research Tools and Apps
- Revenue at Risk
- Survey Templates
- Training Tips
- Uncategorized
- Video Learning Series
- What’s Coming Up
- Workforce Intelligence
Choosing the Right Unit of Analysis for Your Research Project
Table of content.
- Understanding the Unit of Analysis in Research
- Factors to Consider When Selecting the Right Unit of Analysis
- Common Mistakes to Avoid
A research project is like setting out on a voyage through uncharted territory; the unit of analysis is your compass, guiding every decision from methodology to interpretation.
It’s the beating heart of your data collection and the lens through which you view your findings. With deep-seated experience in research methodologies , our expertise recognizes that choosing an appropriate unit of analysis not only anchors your study but illuminates paths towards meaningful conclusions.
The right choice empowers researchers to extract patterns, answer pivotal questions, and offer insights into complex phenomena. But tread carefully—selecting an ill-suited unit can distort results or obscure significant relationships within data.
Remember this: A well-chosen unit of analysis acts as a beacon for accuracy and relevance throughout your scholarly inquiry. Continue reading to unlock the strategies for selecting this cornerstone of research design with precision—your project’s success depends on it.
Engage with us as we delve deeper into this critical aspect of research mastery.
Key Takeaways
- Your research questions and hypotheses drive the choice of your unit of analysis, shaping how you collect and interpret data.
- Avoid common mistakes like reductionism , which oversimplifies complex issues, and the ecological fallacy , where group-level findings are wrongly applied to individuals.
- Consider the availability and quality of data when selecting your unit of analysis to ensure your research is feasible and conclusions are valid.
- Differentiate between units of analysis (what you’re analyzing) and units of observation (what or who you’re observing) for clarity in your study.
- Ensure that your chosen unit aligns with both the theoretical framework and practical considerations such as time and resources.
The unit of analysis in research refers to the level at which data is collected and analyzed. It is essential for researchers to understand the different types of units of analysis, as well as their significance in shaping the research process and outcomes.
Definition and Importance
With resonio, the unit of analysis you choose lays the groundwork for your market research focus. Whether it’s individuals, organizations, or specific events, resonio’s platform facilitates targeted data collection and analysis to address your unique research questions. Our tool simplifies this selection process, ensuring that you can efficiently zero in on the most relevant unit for insightful and actionable results.
This crucial component serves as a navigational aid for your market research. The market research tool not only guides you in data collection but also in selecting the most effective sampling methods and approaches to hypothesis testing. Getting robust and reliable data, ensuring your research is both effective and straightforward.
Choosing the right unit of analysis is crucial, as it defines your research’s direction. resonio makes this easier, ensuring your choice aligns with your theoretical approach and data collection methods, thereby enhancing the validity and reliability of your results.
Additionally, resonio aids in steering clear of errors like reductionism and ecological fallacy, ensuring your conclusions match the data’s level of analysis
Difference between Unit of Analysis and Unit of Observation
Understanding the difference between the unit of analysis and observation is key. Let us clarify this distinction: the unit of analysis is what you’ll ultimately analyze, while the unit of observation is what you observe or measure during the study.
For example, in using resonio for educational research, individual test scores are the units of analysis, while the students providing these scores are the units of observation.
This distinction is essential as it clarifies the specific aspect under scrutiny and what will yield measurable data. It also emphasizes that researchers must carefully consider both elements to ensure their alignment with research questions and objectives .
Types of Units of Analysis: Individual, Aggregates, and Social
Choosing the right unit of analysis for a research project is critical. The types of units of analysis include individual, aggregates, and social.
- Individual: This type focuses on analyzing the attributes and characteristics of individual units, such as people or specific objects.
- Aggregates: Aggregates involve analyzing groups or collections of individual units, such as neighborhoods, organizations, or communities.
- Social: Social units of analysis emphasize analyzing broader social entities, such as cultures, societies, or institutions.
When selecting the right unit of analysis for a research project, researchers must consider various factors such as their research questions and hypotheses , data availability and quality, feasibility and practicality, as well as the theoretical framework and research design .
Each of these factors plays a crucial role in determining the most appropriate unit of analysis for the study.
Research Questions and Hypotheses
The research questions and hypotheses play a crucial role in determining the appropriate unit of analysis for a research project. They guide the researcher in identifying what exactly needs to be studied and analyzed, thereby influencing the selection of the most relevant unit of analysis.
The alignment between the research questions/hypotheses and the unit of analysis is essential to ensure that the study’s focus meets its intended objectives. Furthermore, clear research questions and hypotheses help define specific parameters for data collection and analysis, directly impacting which unit of analysis will best serve the study’s purpose.
It’s important to carefully consider how each research question or hypothesis relates to different potential units of analysis , as this connection will shape not only what you are studying but also how you will study it .
Data Availability and Quality
When considering the unit of analysis for a research project, researchers must take into account the availability and quality of data. The chosen unit of analysis should align with the available data sources to ensure that meaningful and accurate conclusions can be drawn.
Researchers need to evaluate whether the necessary data at the chosen level of analysis is accessible and reliable. Ensuring high-quality data will contribute to the validity and reliability of the study , enabling researchers to make sound interpretations and draw robust conclusions from their findings.
Choosing a unit of analysis without considering data availability and quality may lead to limitations in conducting thorough analysis or drawing valid conclusions. It is crucial for researchers to assess both factors before finalizing their selection, as it directly impacts the feasibility, accuracy, and rigor of their research project.
Feasibility and Practicality
When considering the feasibility and practicality of a unit of analysis for a research project, it is essential to assess the availability and quality of data related to the chosen unit.
Researchers should also evaluate whether the selected unit aligns with their theoretical framework and research design. The practical aspects such as time, resources, and potential challenges associated with analyzing the chosen unit must be thoroughly considered before finalizing the decision.
Moreover, it is crucial to ensure that the selected unit of analysis is feasible within the scope of the research questions and hypotheses. Additionally, researchers need to determine if the chosen unit can be effectively studied based on existing literature and sampling techniques utilized in similar studies.
By carefully evaluating these factors, researchers can make informed decisions regarding which unit of analysis will best suit their research goals.
Theoretical Framework and Research Design
The theoretical framework and research design establish the structure for a study based on existing theories and concepts. It guides the selection of the unit of analysis by providing a foundation for understanding how variables interact and influence one another.
Theoretical frameworks help to shape research questions , hypotheses, and data collection methods, ensuring that the chosen unit of analysis aligns with the study’s objectives. Research design serves as a blueprint outlining the procedures and techniques used to gather and analyze data, allowing researchers to make informed decisions regarding their unit of analysis while considering feasibility, practicality, and data availability .
Researchers often make the mistake of reductionism, where they oversimplify complex phenomena by focusing on one aspect. Another common mistake is the ecological fallacy, where conclusions about individual behavior are made based on group-level data.
Reductionism
Reductionism occurs when a researcher oversimplifies a complex phenomenon by analyzing it at too basic a level. This can lead to the loss of important nuances and details critical for understanding the broader context.
For instance, studying individual test scores without considering external factors like teaching quality or student motivation is reductionist. By focusing solely on one aspect, researchers miss out on comprehensive insights that may impact their findings.
In research projects, reductionism limits the depth of analysis and may result in skewed conclusions that don’t accurately reflect the real-world complexities. It’s essential for researchers to avoid reductionism by carefully selecting an appropriate unit of analysis that allows for a holistic understanding of the phenomenon under study.
Ecological Fallacy
The ecological fallacy involves making conclusions about individuals based on group-level data . This occurs when researchers mistakenly assume that relationships observed at the aggregate level also apply to individuals within that group.
For example, if a study finds a correlation between high levels of education and income at the city level, it doesn’t mean the same relationship applies to every individual within that city.
This fallacy can lead to erroneous generalizations and inaccurate assumptions about individuals based on broader trends. It is crucial for researchers to be mindful of this potential pitfall when selecting their unit of analysis, ensuring that their findings accurately represent the specific characteristics and behaviors of the individuals or entities under investigation.
Selecting the appropriate unit of analysis is critical for a research project’s success, shaping its focus and scope. Researchers must carefully align the chosen unit with their study objectives to ensure relevance.
The impact on findings and conclusions from this choice cannot be understated. Correctly choosing the unit of analysis can considerably influence the direction and outcomes of a research undertaking.
Robert Koch
I write about AI, SEO, Tech, and Innovation. Led by curiosity, I stay ahead of AI advancements. I aim for clarity and understand the necessity of change, taking guidance from Shaw: 'Progress is impossible without change,' and living by Welch's words: 'Change before you have to'.
- Privacy Overview
- Strictly Necessary Cookies
- Additional Cookies
This website uses cookies to provide you with the best user experience possible. Cookies are small text files that are cached when you visit a website to make the user experience more efficient. We are allowed to store cookies on your device if they are absolutely necessary for the operation of the site. For all other cookies we need your consent.
You can at any time change or withdraw your consent from the Cookie Declaration on our website. Find the link to your settings in our footer.
Find out more in our privacy policy about our use of cookies and how we process personal data.
Necessary cookies help make a website usable by enabling basic functions like page navigation and access to secure areas of the website. The website cannot properly without these cookies.
If you disable this cookie, we will not be able to save your preferences. This means that every time you visit this website you will need to enable or disable cookies again.
Any cookies that may not be particularly necessary for the website to function and is used specifically to collect user personal data via analytics, ads, other embedded contents are termed as additional cookies.
Please enable Strictly Necessary Cookies first so that we can save your preferences!
- Unit of Analysis: Definition, Types & Examples

Introduction
A unit of analysis is the smallest level of analysis for a research project. It’s important to choose the right unit of analysis because it helps you make more accurate conclusions about your data.
What Is a Unit of Analysis?
A unit of analysis is the smallest element in a data set that can be used to identify and describe a phenomenon or the smallest unit that can be used to gather data about a subject. The unit of analysis will determine how you will define your variables, which are the things that you measure in your data.
If you want to understand why people buy a particular product, you should choose a unit of analysis that focuses on buying behavior. This means choosing a unit of analysis that is relevant to your research topic and question .
For example, if you want to study the needs of soldiers in a war zone, you will need to choose an appropriate unit of analysis for this study: soldiers or the war zone. In this case, choosing the right unit of analysis would be important because it could help you decide if your research design is appropriate for this particular subject and situation.
Why is Choosing the Right Unit of Analysis Important?
The unit of analysis is important because it helps you understand what you are trying to find out about your subject, and it also helps you to make decisions about how to proceed with your research.
Choosing the right unit of analysis is also important because it determines what information you’re going to use in your research. If you have a small sample, then you’ll have to choose whether or not to focus on the entire population or just a subset of it.
If you have a large sample, then you’ll be able to find out more about specific groups within your population. For example, if you want to understand why people buy certain types of products, then you should choose a unit of analysis that focuses on buying behavior.
This means choosing a unit of analysis that is relevant to your research topic and question.
Unit of Analysis vs Unit of Observation
Unit of analysis is a term used to refer to a particular part of a data set that can be analyzed. For example, in the case of a survey, the unit of analysis is an individual: the person who was selected to take part in the survey.
Unit of analysis is used in the social sciences to refer to the individuals or groups that have been studied. It can also be referred to as the unit of observation.
Unit of observation refers to a specific person or group in the study being observed by the researcher. An example would be a particular town, census tract, state, or other geographical location being studied by researchers conducting research on crime rates in that area.
Unit of analysis refers to the individual or group being studied by the researcher. An example would be an entire town being analyzed for crime rates over time.
Types of “Unit of Analysis”
The unit of analysis is a way to understand and study a phenomenon. There are four main types of unit of analysis: individuals, groups, artifacts (books, photos, newspapers), and geographical units (towns, census tracts, states).
- Individuals are the smallest level of analysis. For example, an individual may be a person or an animal. A group can be composed of individuals or a collection of people who interact with each other. For example, an individual might go to college with other individuals or a family might live together as roommates.
- An artifact is anything that can be studied using empirical methods—including books and photos but also any physical object like knives or phones.
- A geographical unit is smaller than an entire country but larger than just one city block or neighborhood; it may be smaller than just two houses but larger than just two houses in the same street.
- Social interactions include dyadic relations (such as friendships or romantic relationships) and divorces among many other things such as arrests.
Examples of Each Type of Unit of Analysis
- Individuals are the smallest unit of analysis. An individual is a person, animal, or thing. For example, an individual can be a person or a building.
- Artifacts are the next largest units of analysis. An artifact is something produced by human beings and is not alive. For example, a child’s toy is an artifact. Artifacts can include any material object that was produced by human activity and which has meaning to someone. Artifacts can be tangible or intangible and may be produced intentionally or accidentally.
- Geographical units are large geographic areas such as states, counties, provinces, etc. Geographical units may also refer to specific locations within these areas such as cities or townships.
- Social interaction refers to interactions between members of society (e.g., family members interacting with each other). Social interaction includes both formal interactions (such as attending school) and informal interactions (such as talking on the phone).
How Does a Social Scientist Choose a Unit of Analysis?
Social scientists choose a unit of analysis based on the purpose of their research, their research question, and the type of data they have. For example, if they are trying to understand the relationship between a person’s personality and their behavior, they would choose to study personality traits.
For example, if a researcher wanted to study the effects of legalizing marijuana on crime rates, they may choose to use administrative data from police departments. However, if they wanted to study how culture influences crime rates, they might use survey data from smaller groups of people who are further removed from the influence of culture (e.g., individuals living in different areas or countries).
Factors to Consider When Choosing a Unit of Analysis
The unit of analysis is the object or person that you are studying, and it determines what kind of data you are collecting and how you will analyze it.
Factors to consider when choosing a unit of analysis include:
- What is your purpose for studying this topic? Is it for a research paper or an article? If so, which type of paper do you want to write?
- What is the most appropriate unit for your study? If you are studying a specific event or period of time, this may be obvious. But if your focus is broader, such as all social sciences or all human development, then you need to determine how broad your scope should be before beginning any research process (see question one above) so that you know where to start in order for it to be effective (see question three below).
- How do other people define their units? This can be helpful when trying to understand what other people mean when they use certain terms like “social science” or “human development” because they may define those terms differently than what you would expect them to.
- The nature of the data collected. Is it quantitative or qualitative? If it’s qualitative, what kind of data is collected? How much time was spent observing each participant/examining their behavior?
- The scale used to measure variables. Is every variable measured on a one-to-one scale (like measurements between people)? Or do some variables only take on discrete values (like yes/no questions)?
The unit of analysis is the smallest part of a data set that you analyze. It’s important to remember that your data is made up of more than just one unit—you have lots of different units in your dataset, and each of those units has its own characteristics that you need to think about when you’re trying to analyze it.
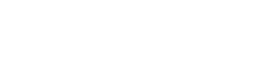
Connect to Formplus, Get Started Now - It's Free!
- Data Collection
- research questions
- unit of analysis
- Olayemi Jemimah Aransiola
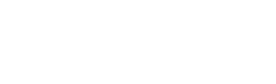
You may also like:
Research Summary: What Is It & How To Write One
Introduction A research summary is a requirement during academic research and sometimes you might need to prepare a research summary...

Projective Techniques In Surveys: Definition, Types & Pros & Cons
Introduction When you’re conducting a survey, you need to find out what people think about things. But how do you get an accurate and...
What is Field Research: Meaning, Examples, Pros & Cons
Introduction Field research is a method of research that deals with understanding and interpreting the social interactions of groups of...
The McNamara Fallacy: How Researchers Can Detect and to Avoid it.
Introduction The McNamara Fallacy is a common problem in research. It happens when researchers take a single piece of data as evidence...
Formplus - For Seamless Data Collection
Collect data the right way with a versatile data collection tool. try formplus and transform your work productivity today..

Community Blog
Keep up-to-date on postgraduate related issues with our quick reads written by students, postdocs, professors and industry leaders.
The Unit of Analysis Explained

- By DiscoverPhDs
- October 3, 2020
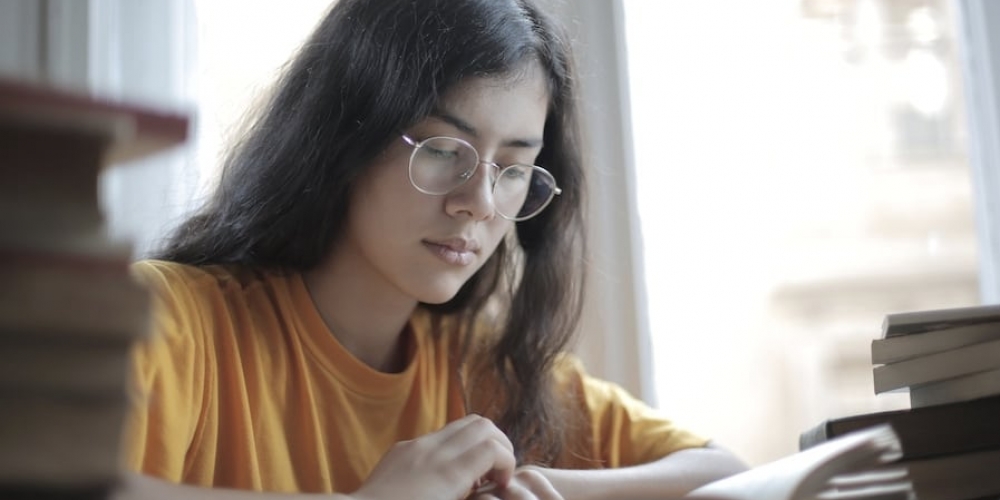
The unit of analysis refers to the main parameter that you’re investigating in your research project or study. Example of the different types of unit analysis that may be used in a project include:
- Individual people
- Groups of people
- Objects such as photographs, newspapers and books
- Geographical unit based on parameters such as cities or counties
- Social parameters such as births, deaths, divorces
The unit of analysis is named as such because the unit type is determined based on the actual data analysis that you perform in your project or study.
For example, if your research is based around data on exam grades for students at two different universities, then the unit of analysis is the data for the individual student due to each student having an exam score associated with them.
Conversely if your study is based on comparing noise level data between two different lecture halls full of students, then your unit of analysis here is the collective group of students in each hall rather than any data associated with an individual student.
In the same research study involving the same students, you may perform different types of analysis and this will be reflected by having different units of analysis. In the example of student exam scores, if you’re comparing individual exam grades then the unit of analysis is the individual student.
On the other hand, if you’re comparing the average exam grade between two universities, then the unit of analysis is now the group of students as you’re comparing the average of the group rather than individual exam grades.
These different levels of hierarchies of units of analysis can become complex with multiple levels. In fact, its complexity has led to a new field of statistical analysis that’s commonly known as hierarchical modelling.
As a researcher, you need to be clear on what your specific research questio n is. Based on this, you can define each data, observation or other variable and how they make up your dataset.
A clarity of your research question will help you identify your analysis units and the appropriate sample size needed to obtain a meaningful result (and is this a random sample/sampling unit or something else).
In developing your research method, you need to consider whether you’ll need any repeated observation of each measurement. You also need to consider whether you’re working with qualitative data/qualitative research or if this is quantitative content analysis.
The unit of analysis of your study is the specifically ‘who’ or what’ it is that your analysing – for example are you analysing the individual student, the group of students or even the whole university. You may have to consider a different unit of analysis based on the concept you’re considering, even if working with the same observation data set.

An abstract and introduction are the first two sections of your paper or thesis. This guide explains the differences between them and how to write them.

Scientific misconduct can be described as a deviation from the accepted standards of scientific research, study and publication ethics.

The scope and delimitations of a thesis, dissertation or paper define the topic and boundaries of a research problem – learn how to form them.
Join thousands of other students and stay up to date with the latest PhD programmes, funding opportunities and advice.
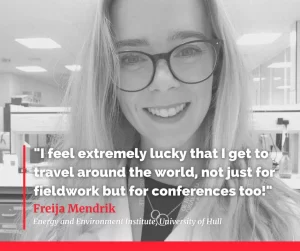
Browse PhDs Now

Statistical treatment of data is essential for all researchers, regardless of whether you’re a biologist, computer scientist or psychologist, but what exactly is it?
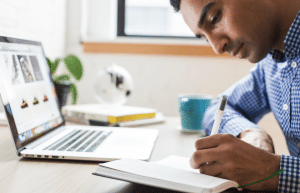
A well written figure legend will explain exactly what a figure means without having to refer to the main text. Our guide explains how to write one.

Kamal is a second year PhD student University of Toronto in the department of Chemistry. His research is focused on making hydrogen gas more affordable and easier to generate from water to use as a clean energy source.

Sara is currently in the 4th year of the Physics Doctoral Program at The Graduate Center of the City University of New York. Her research investigates quantum transport properties of 2D electron systems.
Join Thousands of Students
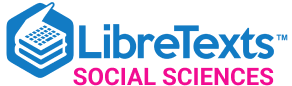
- school Campus Bookshelves
- menu_book Bookshelves
- perm_media Learning Objects
- login Login
- how_to_reg Request Instructor Account
- hub Instructor Commons
Margin Size
- Download Page (PDF)
- Download Full Book (PDF)
- Periodic Table
- Physics Constants
- Scientific Calculator
- Reference & Cite
- Tools expand_more
- Readability
selected template will load here
This action is not available.
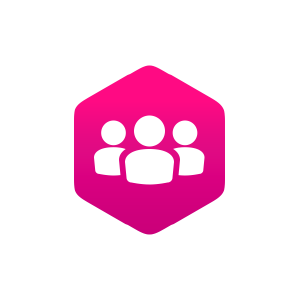
3.2: Unit of Analysis and Errors
- Last updated
- Save as PDF
- Page ID 124454

\( \newcommand{\vecs}[1]{\overset { \scriptstyle \rightharpoonup} {\mathbf{#1}} } \)
\( \newcommand{\vecd}[1]{\overset{-\!-\!\rightharpoonup}{\vphantom{a}\smash {#1}}} \)
\( \newcommand{\id}{\mathrm{id}}\) \( \newcommand{\Span}{\mathrm{span}}\)
( \newcommand{\kernel}{\mathrm{null}\,}\) \( \newcommand{\range}{\mathrm{range}\,}\)
\( \newcommand{\RealPart}{\mathrm{Re}}\) \( \newcommand{\ImaginaryPart}{\mathrm{Im}}\)
\( \newcommand{\Argument}{\mathrm{Arg}}\) \( \newcommand{\norm}[1]{\| #1 \|}\)
\( \newcommand{\inner}[2]{\langle #1, #2 \rangle}\)
\( \newcommand{\Span}{\mathrm{span}}\)
\( \newcommand{\id}{\mathrm{id}}\)
\( \newcommand{\kernel}{\mathrm{null}\,}\)
\( \newcommand{\range}{\mathrm{range}\,}\)
\( \newcommand{\RealPart}{\mathrm{Re}}\)
\( \newcommand{\ImaginaryPart}{\mathrm{Im}}\)
\( \newcommand{\Argument}{\mathrm{Arg}}\)
\( \newcommand{\norm}[1]{\| #1 \|}\)
\( \newcommand{\Span}{\mathrm{span}}\) \( \newcommand{\AA}{\unicode[.8,0]{x212B}}\)
\( \newcommand{\vectorA}[1]{\vec{#1}} % arrow\)
\( \newcommand{\vectorAt}[1]{\vec{\text{#1}}} % arrow\)
\( \newcommand{\vectorB}[1]{\overset { \scriptstyle \rightharpoonup} {\mathbf{#1}} } \)
\( \newcommand{\vectorC}[1]{\textbf{#1}} \)
\( \newcommand{\vectorD}[1]{\overrightarrow{#1}} \)
\( \newcommand{\vectorDt}[1]{\overrightarrow{\text{#1}}} \)
\( \newcommand{\vectE}[1]{\overset{-\!-\!\rightharpoonup}{\vphantom{a}\smash{\mathbf {#1}}}} \)
Learning Objective
- Define units of analysis and units of observation, and describe the two common errors people make when they confuse the two.
Units of Analysis
Another point to consider when designing a research project, and which might differ slightly in qualitative and quantitative studies, has to do with units of analysis. A unit of analysis is the entity that you wish to be able to say something about at the end of your study, probably what you’d consider to be the main focus of your study.
More specifically, your unit of analysis will be determined by your research question. For now, let’s go back to an example about students’ addictions to electronic gadgets. We’ll consider first how different kinds of research questions about this topic will yield different units of analysis. Then we’ll think about how those questions might be answered and with what kinds of data.
If we were to ask, “Which students are most likely to be addicted to their electronic gadgets?” our unit of analysis would be the individual . We might mail a survey to students on campus, and our aim would be to classify individuals according to their membership in certain social classes in order to see how membership in those classes correlated with gadget addiction. For example, we might find that majors in new media, men, and students with high socioeconomic status are all more likely than other students to become addicted to their electronic gadgets. Another possibility would be to ask, “How do students’ gadget addictions differ, and how are they similar?” In this case, we could conduct observations of addicted students and record when, where, why, and how they use their gadgets. In both cases, one using a survey and the other using observations, data are collected from individual students. The units of analysis for both would be individuals.
Another common unit of analysis in sociological inquiry is groups . Groups of course vary in size, and almost no group is too small or too large to be of interest to sociologists. Families, friendship groups, and street gangs make up some of the more common groups examined by sociologists. Employees in an organization, professionals in a particular domain (e.g., chefs, lawyers, sociologists), and members of clubs (e.g., Girl Scouts, Rotary, Red Hat Society) are all larger groups that sociologists might study. Finally, at the macro level, sociologists sometimes examine citizens of entire nations or residents of different continents or other regions.
A study of student addictions to their electronic gadgets at the group level might consider whether certain types of social clubs have more or fewer gadget-addicted members than other sorts of clubs. Perhaps we would find that clubs that emphasize physical fitness, such as the rugby club and the scuba club, have fewer gadget-addicted members than clubs that emphasize cerebral activity, such as the chess club and the sociology club. Our unit of analysis in this example is groups. If we had instead asked whether people who join cerebral clubs are more likely to be gadget-addicted than those who join social clubs, then our unit of analysis would have been individuals.
Organizations are yet another potential unit of analysis that social scientists might wish to say something about. As you may recall from other courses organizations include entities like corporations, colleges and universities, and even night clubs. At the organization level, a study of students’ electronic gadget addictions might ask, “How do different colleges address the problem of electronic gadget addiction?” In this case, our interest lies not in the experience of individual students but instead in the campus-to-campus differences in confronting gadget addictions. A researcher conducting a study of this type might examine schools’ written policies and procedures, which could be social interactions or artifacts. However, because he ultimately wishes to describe differences across campuses, the college would be his unit of analysis.
Of course, it would be silly in a textbook focused on social scientific research to neglect social interactions and artifacts as units of analysis. Social interactions are relevant when studying individual humans and looking at interactions between them such as arguments, emails, fights, dancing, etc. A researcher interested in students’ electronic gadget addictions could ask, “What do students talk about on social media platforms?" We could use social interactions on various social media websites to study this.
Another of analysis, artifacts , are product of social beings and their behavior such as books, pottery, etc. A researcher interested in students’ electronic gadget addictions could ask, "How are different phones interfaces structured to encourage additions?"
In sum, there are many potential units of analysis that a sociologist might examine, but some of the most common units include the following:
- Individuals
- Organizations
- Social interactions
Unit of Analysis Errors
One common error we see people make when it comes to both causality and units of analysis is something called the ecological fallacy . This occurs when claims about one lower-level unit of analysis are made based on data from some higher-level unit of analysis. In many cases, this occurs when claims are made about individuals, but only group-level data have been gathered. For example, we might want to understand whether electronic gadget addictions are more common on certain campuses than on others. Perhaps different campuses around the country have provided us with their campus percentage of gadget-addicted students, and we learn from these data that electronic gadget addictions are more common on campuses that have business programs than on campuses without them. We then conclude that business students are more likely than nonbusiness students to become addicted to their electronic gadgets. However, this would be an inappropriate conclusion to draw. Because we only have addiction rates by campus, we can only draw conclusions about campuses, not about the individual students on those campuses. Perhaps the sociology majors on the business campuses are the ones that caused the addiction rates on those campuses to be so high. The point is we simply don’t know because we only have campus-level data. By drawing conclusions about students when our data are about campuses, we run the risk of committing the ecological fallacy.
On the other hand, another mistake to be aware of is reductionism . Reductionism occurs when claims about some higher-level unit of analysis are made based on data from some lower-level unit of analysis. In this case, claims about groups are made based on individual-level data. An example of reductionism can be seen in some descriptions of the civil rights movement. On occasion, people have proclaimed that Rosa Parks started the civil rights movement in the United States by refusing to give up her seat to a white person while on a city bus in Montgomery, Alabama, in December 1955. Although it is true that Parks played an invaluable role in the movement, and that her act of civil disobedience gave others courage to stand up against racist policies, beliefs, and actions, to credit Parks with starting the movement is reductionist. Surely the confluence of many factors, from fights over legalized racial segregation to the Supreme Court’s historic decision to desegregate schools in 1954 to the creation of groups such as the Student Nonviolent Coordinating Committee (to name just a few), contributed to the rise and success of the American civil rights movement. In other words, the movement is attributable to many factors—some social, others political, others economic. Did Parks play a role? Of course she did—and a very important one at that. But did she cause the movement? To say yes would be reductionist.
It would be a mistake to conclude from the preceding discussion that researchers should avoid making any claims whatsoever about data or about relationships between variables. While it is important to be attentive to the possibility for error in causal reasoning about different levels of analysis, this warning should not prevent you from drawing well-reasoned analytic conclusions from your data. The point is to be cautious but not abandon entirely the social scientific quest to understand patterns of behavior.
KEY TAKEAWAYS
- A unit of analysis is the item you wish to be able to say something about at the end of your study.
- Ecological fallacy and reductionism are caused by generalizing to variables not of the same unit of analysis.
- Do a Google News search for the term ecological fallacy . Chances are good you’ll come across a number of news editorials using this term. Read a few of these editorials or articles, and print one out. Demonstrate your understanding of the term ecological fallacy by writing a short answer discussing whether the author of the article you printed out used the term correctly.
Units of Analysis
- Living reference work entry
- First Online: 11 November 2020
- Cite this living reference work entry
- Eric K. H. Chan 2
Complex data ; Level of analysis ; Nested data
In quality of life research, data are often structured in a nested or hierarchical manner (i.e., different units). Multilevel unit of analysis allows researchers to investigate the association and interaction between variables at different units or levels.
Quality of life (QoL) research has become more advanced. QoL research often involves investigating the relationships and interactions between individuals and the group to which they belong. Individuals often influence the group they belong to, and vice versa. For example, in cross-national happiness research, the population consists of countries and people within these countries. In this example, people are nested within countries. In this type of research, the population data are structured in a nested or hierarchical manner, and the sample data are sampled from the hierarchical or nested population. Researchers interested in the cross-national comparison of happiness...
This is a preview of subscription content, log in via an institution to check access.
Access this chapter
Institutional subscriptions
Hox, J. J. (2010). Multilevel analysis. Techniques and applications (2nd ed.). New York: Routledge.
Book Google Scholar
Raudenbush, S. W., & Bryk, A. S. (2002). Hierarchical linear models: Applications and data analysis methods (2nd ed.). Thousand Oaks: Sage.
Google Scholar
Zumbo, B. D., & Forer, B. (2011). Testing and measurement from a multilevel view: Psychometrics and validation. In J. Bovaird, K. Geisinger, & C. Buckendahl (Eds.), High stakes testing in education – science and practice in K-12 settings (Festschrift to Barbara Plake) . Washington, DC: American Psychological Press.
Download references
Author information
Authors and affiliations.
Measurement, Evaluation, and Research Methodology (MERM) Program, University of British Columbia, Vancouver, BC, Canada
Eric K. H. Chan
You can also search for this author in PubMed Google Scholar
Editor information
Editors and affiliations.
Dipartimento di Scienze Statistiche, Sapienza Università di Roma, Roma, Italy
Filomena Maggino
Section Editor information
Department of ECPS & Intitute of Applied Mathematics, University of British Columbia, Vancouver, BC, Canada
Bruno Zumbo
Rights and permissions
Reprints and permissions
Copyright information
© 2020 Springer Nature Switzerland AG
About this entry
Cite this entry.
Chan, E.K.H. (2020). Units of Analysis. In: Maggino, F. (eds) Encyclopedia of Quality of Life and Well-Being Research. Springer, Cham. https://doi.org/10.1007/978-3-319-69909-7_3107-2
Download citation
DOI : https://doi.org/10.1007/978-3-319-69909-7_3107-2
Received : 09 November 2019
Accepted : 02 December 2019
Published : 11 November 2020
Publisher Name : Springer, Cham
Print ISBN : 978-3-319-69909-7
Online ISBN : 978-3-319-69909-7
eBook Packages : Springer Reference Social Sciences Reference Module Humanities and Social Sciences Reference Module Business, Economics and Social Sciences
- Publish with us
Policies and ethics
- Find a journal
- Track your research
- Sign in to save searches and organize your favorite content.
- Not registered? Sign up
Recently viewed (0)
- Save Search
- Next Article
The Unit of Analysis in Field Research: Issues and Approaches to Design and Data Analysis
Click name to view affiliation
- Get Citation Alerts
Purchase article
Student 1 year online subscription, 1 year online subscription, student 2 year online subscription, 2 year online subscription.
By purchasing this content you agree and accept the terms and conditions
This paper addresses the appropriate unit of analysis in field research. We first discuss the issues related to this topic: (a) unit of measurement versus unit of analysis, (b) treatments and random assignment, (c) independence of observations, (d) moderating and control variables, and (e) correlational versus experimental research. We then present a model for determining the correct unit of analysis. In many instances, researchers should use class means or subgroup means, and this has implications for research design. In the third section, we discuss the related issues of (a) the burden of proof, (b) asking the right questions and getting the right answers, and (c) completing statistical analyses. How data are analyzed can affect the results, and researchers should consider these issues when planning their research.
* Stephen Silverman is with the Department of Kinesiology at the University of Illinois at Urbana-Champaign, Louise Freer Hall, 906 South Goodwin Ave., Urbana, IL61801. Melinda Solmon is with the Department of Kinesiology at the University of Maryland at College Park, College Park, MD 20742.
Journal of Teaching in Physical Education
Related Articles
Article metrics.
- Stephen Silverman
- Melinda Solmon
Google Scholar

© 2024 Human Kinetics
Powered by:
- [66.249.64.20|185.66.14.236]
- 185.66.14.236
Character limit 500 /500
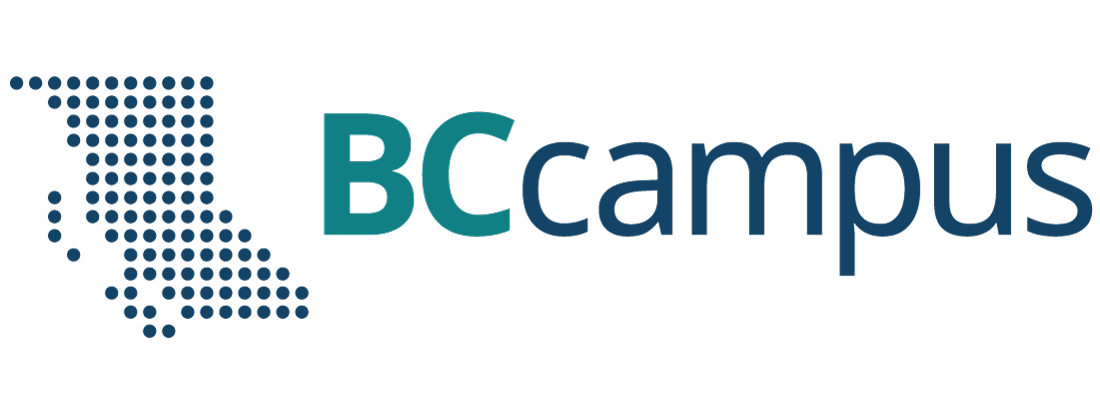
Want to create or adapt books like this? Learn more about how Pressbooks supports open publishing practices.
Chapter 4: Measurement and Units of Analysis
4.4 Units of Analysis and Units of Observation
Another point to consider when designing a research project, and which might differ slightly in qualitative and quantitative studies, has to do with units of analysis and units of observation. These two items concern what you, the researcher, actually observe in the course of your data collection and what you hope to be able to say about those observations. Table 3.1 provides a summary of the differences between units of analysis and observation.
Unit of Analysis
A unit of analysis is the entity that you wish to be able to say something about at the end of your study, probably what you would consider to be the main focus of your study.
Unit of Observation
A unit of observation is the item (or items) that you actually observe, measure, or collect in the course of trying to learn something about your unit of analysis. In a given study, the unit of observation might be the same as the unit of analysis, but that is not always the case. Further, units of analysis are not required to be the same as units of observation. What is required, however, is for researchers to be clear about how they define their units of analysis and observation, both to themselves and to their audiences. More specifically, your unit of analysis will be determined by your research question. Your unit of observation, on the other hand, is determined largely by the method of data collection that you use to answer that research question.
To demonstrate these differences, let us look at the topic of students’ addictions to their cell phones. We will consider first how different kinds of research questions about this topic will yield different units of analysis. Then we will think about how those questions might be answered and with what kinds of data. This leads us to a variety of units of observation.
If I were to ask, “Which students are most likely to be addicted to their cell phones?” our unit of analysis would be the individual. We might mail a survey to students on a university or college campus, with the aim to classify individuals according to their membership in certain social classes and, in turn, to see how membership in those classes correlates with addiction to cell phones. For example, we might find that students studying media, males, and students with high socioeconomic status are all more likely than other students to become addicted to their cell phones. Alternatively, we could ask, “How do students’ cell phone addictions differ and how are they similar? In this case, we could conduct observations of addicted students and record when, where, why, and how they use their cell phones. In both cases, one using a survey and the other using observations, data are collected from individual students. Thus, the unit of observation in both examples is the individual. But the units of analysis differ in the two studies. In the first one, our aim is to describe the characteristics of individuals. We may then make generalizations about the populations to which these individuals belong, but our unit of analysis is still the individual. In the second study, we will observe individuals in order to describe some social phenomenon, in this case, types of cell phone addictions. Consequently, our unit of analysis would be the social phenomenon.
Another common unit of analysis in sociological inquiry is groups. Groups, of course, vary in size, and almost no group is too small or too large to be of interest to sociologists. Families, friendship groups, and street gangs make up some of the more common micro-level groups examined by sociologists. Employees in an organization, professionals in a particular domain (e.g., chefs, lawyers, sociologists), and members of clubs (e.g., Girl Guides, Rotary, Red Hat Society) are all meso-level groups that sociologists might study. Finally, at the macro level, sociologists sometimes examine citizens of entire nations or residents of different continents or other regions.
A study of student addictions to their cell phones at the group level might consider whether certain types of social clubs have more or fewer cell phone-addicted members than other sorts of clubs. Perhaps we would find that clubs that emphasize physical fitness, such as the rugby club and the scuba club, have fewer cell phone-addicted members than clubs that emphasize cerebral activity, such as the chess club and the sociology club. Our unit of analysis in this example is groups. If we had instead asked whether people who join cerebral clubs are more likely to be cell phone-addicted than those who join social clubs, then our unit of analysis would have been individuals. In either case, however, our unit of observation would be individuals.
Organizations are yet another potential unit of analysis that social scientists might wish to say something about. Organizations include entities like corporations, colleges and universities, and even night clubs. At the organization level, a study of students’ cell phone addictions might ask, “How do different colleges address the problem of cell phone addiction?” In this case, our interest lies not in the experience of individual students but instead in the campus-to-campus differences in confronting cell phone addictions. A researcher conducting a study of this type might examine schools’ written policies and procedures, so his unit of observation would be documents. However, because he ultimately wishes to describe differences across campuses, the college would be his unit of analysis.
Social phenomena are also a potential unit of analysis. Many sociologists study a variety of social interactions and social problems that fall under this category. Examples include social problems like murder or rape; interactions such as counselling sessions, Facebook chatting, or wrestling; and other social phenomena such as voting and even cell phone use or misuse. A researcher interested in students’ cell phone addictions could ask, “What are the various types of cell phone addictions that exist among students?” Perhaps the researcher will discover that some addictions are primarily centred on social media such as chat rooms, Facebook, or texting, while other addictions centre on single-player games that discourage interaction with others. The resultant typology of cell phone addictions would tell us something about the social phenomenon (unit of analysis) being studied. As in several of the preceding examples, however, the unit of observation would likely be individual people.
Finally, a number of social scientists examine policies and principles, the last type of unit of analysis we will consider here. Studies that analyze policies and principles typically rely on documents as the unit of observation. Perhaps a researcher has been hired by a college to help it write an effective policy against cell phone use in the classroom. In this case, the researcher might gather all previously written policies from campuses all over the country, and compare policies at campuses where the use of cell phones in classroom is low to policies at campuses where the use of cell phones in the classroom is high.
In sum, there are many potential units of analysis that a sociologist might examine, but some of the most common units include the following:
- Individuals
- Organizations
- Social phenomena.
- Policies and principles.
Table 4.1 Units of analysis and units of observation: A hypothetical study of students’ addictions to cell phones.
Research Methods for the Social Sciences: An Introduction Copyright © 2020 by Valerie Sheppard is licensed under a Creative Commons Attribution-NonCommercial-ShareAlike 4.0 International License , except where otherwise noted.
Share This Book
Unit of analysis: definition, types, examples, and more
Last updated
16 April 2023
Reviewed by
Cathy Heath
Make research less tedious
Dovetail streamlines research to help you uncover and share actionable insights
- What is a unit of analysis?
A unit of analysis is an object of study within a research project. It is the smallest unit a researcher can use to identify and describe a phenomenon—the 'what' or 'who' the researcher wants to study.
For example, suppose a consultancy firm is hired to train the sales team in a solar company that is struggling to meet its targets. To evaluate their performance after the training, the unit of analysis would be the sales team—it's the main focus of the study.
Different methods, such as surveys , interviews, or sales data analysis, can be used to evaluate the sales team's performance and determine the effectiveness of the training.
- Units of observation vs. units of analysis
A unit of observation refers to the actual items or units being measured or collected during the research. In contrast, a unit of analysis is the entity that a researcher can comment on or make conclusions about at the end of the study.
In the example of the solar company sales team, the unit of observation would be the individual sales transactions or deals made by the sales team members. In contrast, the unit of analysis would be the sales team as a whole.
The firm may observe and collect data on individual sales transactions, but the ultimate conclusion would be based on the sales team's overall performance, as this is the entity that the firm is hired to improve.
In some studies, the unit of observation may be the same as the unit of analysis, but researchers need to define both clearly to themselves and their audiences.
- Unit of analysis types
Below are the main types of units of analysis:
Individuals – These are the smallest levels of analysis.
Groups – These are people who interact with each other.
Artifacts –These are material objects created by humans that a researcher can study using empirical methods.
Geographical units – These are smaller than a nation and range from a province to a neighborhood.
Social interactions – These are formal or informal interactions between society members.
- Importance of selecting the correct unit of analysis in research
Selecting the correct unit of analysis helps reveal more about the subject you are studying and how to continue with the research. It also helps determine the information you should use in the study. For instance, if a researcher has a large sample, the unit of analysis will help decide whether to focus on the whole population or a subset of it.
- Examples of a unit of analysis
Here are examples of a unit of analysis:
Individuals – A person, an animal, etc.
Groups – Gangs, roommates, etc.
Artifacts – Phones, photos, books, etc.
Geographical units – Provinces, counties, states, or specific areas such as neighborhoods, city blocks, or townships
Social interaction – Friendships, romantic relationships, etc.
- Factors to consider when selecting a unit of analysis
The main things to consider when choosing a unit of analysis are:
Research questions and hypotheses
Research questions can be descriptive if the study seeks to describe what exists or what is going on.
It can be relational if the study seeks to look at the relationship between variables. Or, it can be causal if the research aims at determining whether one or more variables affect or cause one or more outcome variables.
Your study's research question and hypothesis should guide you in choosing the correct unit of analysis.
Data availability and quality
Consider the nature of the data collected and the time spent observing each participant or studying their behavior. You should also consider the scale used to measure variables.
Some studies involve measuring every variable on a one-to-one scale, while others use variables with discrete values. All these influence the selection of a unit of analysis.
Feasibility and practicality
Look at your study and think about the unit of analysis that would be feasible and practical.

Theoretical framework and research design
The theoretical framework is crucial in research as it introduces and describes the theory explaining why the problem under research exists. As a structure that supports the theory of a study, it is a critical consideration when choosing the unit of analysis. Moreover, consider the overall strategy for collecting responses to your research questions.
- Common mistakes when choosing a unit of analysis
Below are common errors that occur when selecting a unit of analysis:
Reductionism
This error occurs when a researcher uses data from a lower-level unit of analysis to make claims about a higher-level unit of analysis. This includes using individual-level data to make claims about groups.
However, claiming that Rosa Parks started the movement would be reductionist. There are other factors behind the rise and success of the US civil rights movement. These include the Supreme Court’s historic decision to desegregate schools, protests over legalized racial segregation, and the formation of groups such as the Student Nonviolent Coordinating Committee (SNCC). In short, the movement is attributable to various political, social, and economic factors.
Ecological fallacy
This mistake occurs when researchers use data from a higher-level unit of analysis to make claims about one lower-level unit of analysis. It usually occurs when only group-level data is collected, but the researcher makes claims about individuals.
For instance, let's say a study seeks to understand whether addictions to electronic gadgets are more common in certain universities than others.
The researcher moves on and obtains data on the percentage of gadget-addicted students from different universities around the country. But looking at the data, the researcher notes that universities with engineering programs have more cases of gadget additions than campuses without the programs.
Concluding that engineering students are more likely to become addicted to their electronic gadgets would be inappropriate. The data available is only about gadget addiction rates by universities; thus, one can only make conclusions about institutions, not individual students at those universities.
Making claims about students while the data available is about the university puts the researcher at risk of committing an ecological fallacy.
- The lowdown
A unit of analysis is what you would consider the primary emphasis of your study. It is what you want to discuss after your study. Researchers should determine a unit of analysis that keeps the context required to make sense of the data. They should also keep the unit of analysis in mind throughout the analysis process to protect the reliability of the results.
What is the most common unit of analysis?
The individual is the most prevalent unit of analysis.
Can the unit of analysis and the unit of observation be one?
Some situations have the same unit of analysis and observation. For instance, let's say a tutor is hired to improve the oral French proficiency of a student who finds it difficult. A few months later, the tutor wants to evaluate the student's proficiency based on what they have taught them for the time period. In this case, the student is both the unit of analysis and the unit of observation.
Should you be using a customer insights hub?
Do you want to discover previous research faster?
Do you share your research findings with others?
Do you analyze research data?
Start for free today, add your research, and get to key insights faster
Editor’s picks
Last updated: 13 April 2023
Last updated: 14 February 2024
Last updated: 27 January 2024
Last updated: 18 April 2023
Last updated: 8 February 2023
Last updated: 23 January 2024
Last updated: 30 January 2024
Last updated: 7 February 2023
Last updated: 7 March 2023
Last updated: 18 May 2023
Last updated: 13 May 2024
Latest articles
Related topics, .css-je19u9{-webkit-align-items:flex-end;-webkit-box-align:flex-end;-ms-flex-align:flex-end;align-items:flex-end;display:-webkit-box;display:-webkit-flex;display:-ms-flexbox;display:flex;-webkit-flex-direction:row;-ms-flex-direction:row;flex-direction:row;-webkit-box-flex-wrap:wrap;-webkit-flex-wrap:wrap;-ms-flex-wrap:wrap;flex-wrap:wrap;-webkit-box-pack:center;-ms-flex-pack:center;-webkit-justify-content:center;justify-content:center;row-gap:0;text-align:center;max-width:671px;}@media (max-width: 1079px){.css-je19u9{max-width:400px;}.css-je19u9>span{white-space:pre;}}@media (max-width: 799px){.css-je19u9{max-width:400px;}.css-je19u9>span{white-space:pre;}} decide what to .css-1kiodld{max-height:56px;display:-webkit-box;display:-webkit-flex;display:-ms-flexbox;display:flex;-webkit-align-items:center;-webkit-box-align:center;-ms-flex-align:center;align-items:center;}@media (max-width: 1079px){.css-1kiodld{display:none;}} build next, decide what to build next.

Users report unexpectedly high data usage, especially during streaming sessions.

Users find it hard to navigate from the home page to relevant playlists in the app.

It would be great to have a sleep timer feature, especially for bedtime listening.

I need better filters to find the songs or artists I’m looking for.
Log in or sign up
Get started for free
Popular searches
- How to Get Participants For Your Study
- How to Do Segmentation?
- Conjoint Preference Share Simulator
- MaxDiff Analysis
- Likert Scales
- Reliability & Validity
Request consultation
Do you need support in running a pricing or product study? We can help you with agile consumer research and conjoint analysis.
Looking for an online survey platform?
Conjointly offers a great survey tool with multiple question types, randomisation blocks, and multilingual support. The Basic tier is always free.
Research Methods Knowledge Base
- Navigating the Knowledge Base
- Five Big Words
- Types of Research Questions
- Time in Research
- Types of Relationships
- Types of Data
Unit of Analysis
- Two Research Fallacies
- Philosophy of Research
- Ethics in Research
- Conceptualizing
- Evaluation Research
- Measurement
- Research Design
- Table of Contents
Fully-functional online survey tool with various question types, logic, randomisation, and reporting for unlimited number of surveys.
Completely free for academics and students .
One of the most important ideas in a research project is the unit of analysis . The unit of analysis is the major entity that you are analyzing in your study. For instance, any of the following could be a unit of analysis in a study:
- individuals
- artifacts (books, photos, newspapers)
- geographical units (town, census tract, state)
- social interactions (dyadic relations, divorces, arrests)
Why is it called the ‘unit of analysis’ and not something else (like, the unit of sampling)? Because it is the analysis you do in your study that determines what the unit is . For instance, if you are comparing the children in two classrooms on achievement test scores, the unit is the individual child because you have a score for each child. On the other hand, if you are comparing the two classes on classroom climate, your unit of analysis is the group, in this case the classroom, because you only have a classroom climate score for the class as a whole and not for each individual student. For different analyses in the same study you may have different units of analysis. If you decide to base an analysis on student scores, the individual is the unit. But you might decide to compare average classroom performance. In this case, since the data that goes into the analysis is the average itself (and not the individuals’ scores) the unit of analysis is actually the group. Even though you had data at the student level, you use aggregates in the analysis. In many areas of social research these hierarchies of analysis units have become particularly important and have spawned a whole area of statistical analysis sometimes referred to as hierarchical modeling . This is true in education, for instance, where we often compare classroom performance but collected achievement data at the individual student level.
Cookie Consent
Conjointly uses essential cookies to make our site work. We also use additional cookies in order to understand the usage of the site, gather audience analytics, and for remarketing purposes.
For more information on Conjointly's use of cookies, please read our Cookie Policy .
Which one are you?
I am new to conjointly, i am already using conjointly.
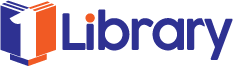
- No results found
Unit of Analysis
Chapter 5 research design and methodology, 5.6 unit of analysis.
As suggested by Yin (2009: 27), the case study research necessitates five essential components: ‘a study’s questions’, ‘its propositions, if any’, ‘its unit(s) of analysis’, ‘the logic
linking the data to the propositions’ and ‘the criteria for interpreting the findings’. Collis and Hussey (2009: 115) defined the unit of analysis as ‘the phenomenon under study, about which data is collected and analysed’. Therefore, as discussed in the previous section, for the purpose of studying MNC strategy concerning climate change adaptation, the research selected geographical locations and business sectors where climate change impacts are visible and well-understood.
According to the classification of unit of analysis by Piekkari and Welch (2011), the main unit/units of analysis for the research involves selected geographical locations and business sectors concerned about climate change adaptation strategies of MNCs. The classification proposed by Piekkari and Welch (2011) has four overlapping categories. These firstly involve ‘social units’ like ‘individual or individuals, a role, a group, an organisation, a community or social interactions’, secondly, ‘temporal units’ like ‘an episode or encounter, an event or a period of time’, thirdly, ‘geographical units’ like ‘countries, towns or states’ and fourthly ‘artefacts’ like ‘books, photos, newspapers or technological objects’ (Piekkari & Welch, 2011: 173). The research engages ‘geographical units’ such as Australia and Bangladesh, or ‘social units’ like an industry group, such as the agricultural seed business sector. However, these are not treated as the main unit/units of analysis of the research. Similar to the definition of Collis and Hussey (2009), Piekkari and Welch (2011: 173) also defined the unit of analysis as ‘the major entity analysed in the study’, which is a ‘context-specific choice’ on the basis of ‘the research questions, research propositions and research setting of the study’. From that perspective, according to the explanation provided by Yin (2009: 46), the research has multiple units of analysis, while following a ‘multiple-case (embedded) design’ due to the engagement of two countries and three business sectors.
The conceptual framework and research questions are concerned about MNCs’ strategy related to climate change adaptation. Therefore, after selecting geographical locations and business sectors, the research chose ten MNC subsidiaries of six MNCs (i.e., two subsidiaries of four companies and one subsidiary of two companies) from three sectors as the multiple units of analysis. Since the major focus of the research is on the agricultural seed business sector, data from four MNCs (i.e., SC1, SC2, BSS1, ASS1; see section 5.8.1 for details) from that sector are analysed. Of these, two MNCs have operations both in Bangladesh and Australia and four of their subsidiaries were chosen for the study (i.e., ASC1, BSC1, ASC2, BSC2). Of the two remaining MNCs, one operates in Australia with its headquarters in Christchurch, in New Zealand (i.e., ASS1), and the other operations in Bangladesh with its headquarters in Dhaka (i.e., BSS1). In addition, one MNC from each of the pharmaceutical and mining sectors with subsidiaries in both Bangladesh and Australia (a total of four subsidiaries) were chosen (i.e., APC1, BPC1, AMC1, BMC1). However, it should be noted that the choice of four subsidiaries of MNCs PC1 and MC1 from pharmaceutical and mining sectors as units of analysis is to address the validity of information primarily derived from MNCs within the agricultural seed business sector.
Information was also collected from other sources. The aim was to validate and provide reliable information about the units of analysis. It also assisted the researcher to comprehensively understand the influence of different factors which moderate the strategies of units of analysis of the research. The context and case under the ‘multiple-case (embedded) design’ for the research are also understood via the information collected from other sources.
Since the major focus of the research was on MNCs from the agricultural seed business sector, information was collected from three Australian and three Bangladeshi seed companies which do not have an international presence but have a strong domestic presence in Australia and Bangladesh. In addition, information from 18 sectoral experts, nine each from Australia and Bangladesh, on the agricultural seed business sector’s climate change adaptation was also analysed. Information from eight sectoral experts from Australia and Bangladesh on climate change adaptation of the pharmaceutical and mining sectors was analysed in relation to the units of analysis from these two sectors. Of these, four are from Australia and four from Bangladesh, two experts each from the each pharmaceutical and mining sectors. The mapping of information sources, along with the units of analysis (ten subsidiaries highlighted in red), is presented in tabular form in Table 5.1.
Table 5.1 Mapping of data sources of the research
TOTAL INTERVIEWS – 42
MNCs with subsidiaries in Australia and Bangladesh
MNCs operating either in Australia or in Bangladesh
Companies without any international presence
Expert opinions
Bangladesh Australia Bangladesh Australia Bangladesh Australia Bangladesh Australia
Agricultural Seed Business Sector – 30 Interviews
2 2 1 1 3 3 9 9
BSC1. ASC1. BSS1. ASS1. BSL1. ASL1. BSE1. ASE1.
BSC2. ASC2. BSL2. ASL2. BSE2. ASE2.
BSE3. ASE3. BSE4. ASE4. BSE5. ASE5. BSE6. ASE6. BSL3. ASL3. BSE7. ASE7. BSE8. ASE8. BSE9. ASE9.
Pharmaceutical Sector – 6 Interviews
1 1 0 0 0 0 2 2
BPC1. APC1. BPE1. APE1.
BPE2. APE2.
Mining Sector – 6 Interviews
BMC1. AMC1. BME1. AME1.
BME2. AME2.
- Environmental Idealism
- Corporate Environmental Responses under New Environmentalism
- Environmental Pragmatism
- Corporate Climate Change Adaptation Strategy
- Theory on Business Strategy
- Agricultural Seed Business Sector
- Cross-country Comparison
- Selecting Cases
- Unit of Analysis (You are here)
- Reliability and Validity
- Semi-structured Interviews
- Thematic Analysis and Coding
Related documents
Academia.edu no longer supports Internet Explorer.
To browse Academia.edu and the wider internet faster and more securely, please take a few seconds to upgrade your browser .
Enter the email address you signed up with and we'll email you a reset link.
- We're Hiring!
- Help Center
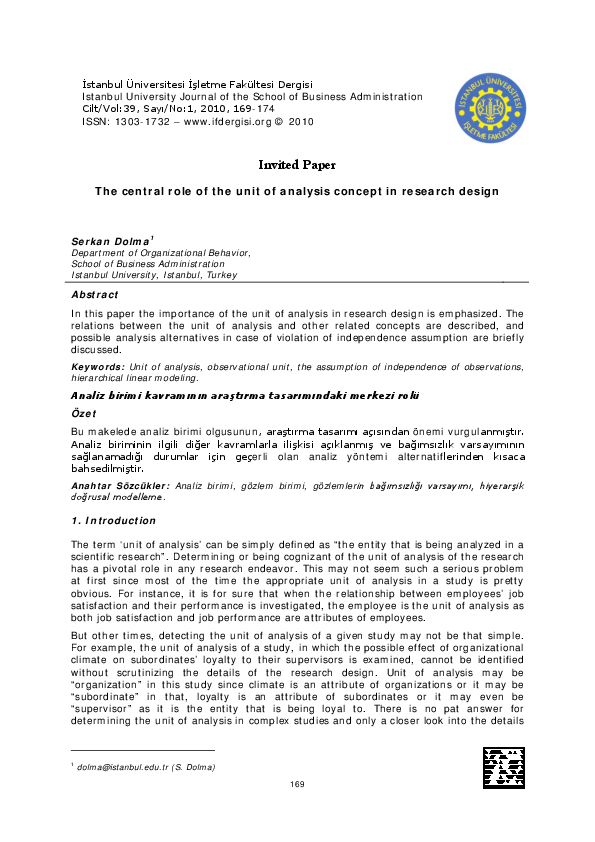
The central role of the unit of analysis concept in research design

2010, Journal of the School of Business Administration, …
Related Papers
Journal of General Management Research
Drsanjay Kumar
The main aim of the paper was to understand the different issues of unit of analysis in a business research. The qualitative approach was used to explore the different issues related to unit of analysis through in-depth review of literature. In this paper different levels of unit of analysis are described. The relation between the unit of analysis and unit of observation is explained. Faulty reasoning about the unit of analysis is discussed with suitable examples. The possible data analysis options are discussed taking into consideration the unit of analysis. This paper will be a good reference material for new researchers in understanding the unit of analysis for the planning and designing their research work at master's and doctorate level.
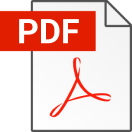
Niveda Subramanian
Anales De Psicologia
Carlos Santoyo
Joel Ashirwadam
Rekha Mudkanna
Symeou, L. & Lamprianou, J.
Iasonas Lamprianou , Loizos Symeou
mark vince agacite
Rahul Pilani
Lova Rakotoarison
RELATED PAPERS
Geoscientific Model Development Discussions
Turning Teaching Inside Out
Simone Davis
Ultima Decada
sandra manzano oyarzun
Scholarly and Research Communication
Michael Johnny
The Turkish Journal of Pediatrics
Dilsad Foto Ozdemir
Alzheimer's & Dementia
Gregory Jicha
Kannan Pandian
Journal of Statistical Mechanics: Theory and Experiment
Kimmo Kaski
如何购买贝德福特大学毕业证 bedfordhire毕业证学历证书学历学位认证原版一模一样
Indonesian Journal of Electrical Engineering and Computer Science
Satea Alnajjar
arXiv (Cornell University)
Metallurgical Transactions A
Dr. H. Muh. Arif, M. Ag.
Pharmaceuticals
Pablo Alarcón Guijo
UNSW毕业证成绩单 UNSW文凭证书
1比1仿制英国萨里大学 surrey毕业证学历证书GRE成绩单原版一模一样
Mahmood Dalhat
Lecture Notes in Computer Science
Marco Moreno
Norma Ducci
- We're Hiring!
- Help Center
- Find new research papers in:
- Health Sciences
- Earth Sciences
- Cognitive Science
- Mathematics
- Computer Science
- Academia ©2024
Thank you for visiting nature.com. You are using a browser version with limited support for CSS. To obtain the best experience, we recommend you use a more up to date browser (or turn off compatibility mode in Internet Explorer). In the meantime, to ensure continued support, we are displaying the site without styles and JavaScript.
- View all journals
- My Account Login
- Explore content
- About the journal
- Publish with us
- Sign up for alerts
- Open access
- Published: 21 November 2023
Connecting with fans in the digital age: an exploratory and comparative analysis of social media management in top football clubs
- Edgar Romero-Jara 1 ,
- Francesc Solanellas 2 ,
- Joshua Muñoz ORCID: orcid.org/0000-0001-6220-6328 2 &
- Samuel López-Carril ORCID: orcid.org/0000-0001-5278-057X 3
Humanities and Social Sciences Communications volume 10 , Article number: 858 ( 2023 ) Cite this article
- Business and management
- Cultural and media studies
In a globalised society, characterised by increasingly demanding markets and the accelerated growth of the digital approach, sports organisations face the challenge of connecting with fans, generating and maintaining audiences and communicating with stakeholders creatively and efficiently. Social media has become a fundamental tool, with engagement as a critical measurement element. However, despite its popularity and use, many questions about its application, measurement and real potential in the sports sector still need to be answered. Therefore, the main objective of this study is to carry out a descriptive and comparative analysis of the engagement generated through social media posts by elite football clubs in Europe, South America and North America. To this purpose, 19,745 Facebook, Twitter and Instagram posts were analysed, through the design, validation and application of an observation instrument, using content analysis techniques. The findings show evidence of a priority focus on “Marketing” and “Sports” type messages in terms of frequency, with high engagement rates. They were also showing a growing stream of “ESG” type messages, with a low posting frequency but engagement rates similar to “Marketing” and “Sport”. “Institutional” messages remain constant in all football clubs. “Commercial” messages still have growth potential in both regards, frequency and engaging fans, representing an opportunity for digital assets. Also, specific format combinations that generate greater engagement were identified: “text/image” and “text/videos” are the format combinations more used by football clubs on Facebook, Twitter and Instagram; however, resulting in different engagement rates. This study showed evidence of different social media management strategies adopted according to region, obtaining similar engagement rates. This research concludes with theoretical and practical applications that will be of interest to both academics and practitioners to maximise the potential of social media for fan engagement, social initiatives and as a marketing tool.
Similar content being viewed by others
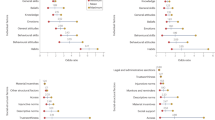
Determinants of behaviour and their efficacy as targets of behavioural change interventions
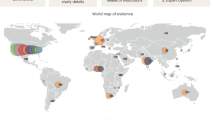
Toolbox of individual-level interventions against online misinformation
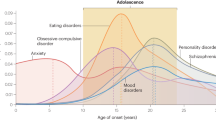
Mechanisms linking social media use to adolescent mental health vulnerability
Introduction.
In a context of booming technology and high organisational competitiveness (Ratten, 2020 ), digital tools have evolved from an essential add-on to crucial strategic and operational elements in sports organisations (Stegmann et al., 2021 ). Fans increasingly demand a connection with their favourite athletes and teams (Su et al., 2020 ) through digital channels such as social media, podcasts (Rohden et al., 2023 ), Esports (Cuesta-Valiño et al., 2022 ), among others. Today’s digitised world presents therefore, an opportunity for brands, sponsors, sports properties, and other stakeholders to interact in a complex and emotionally charged sector (Su et al., 2022 ) for fans from different age generations (Sheldon et al., 2021 ). Understanding and getting to know fans are at the forefront of every sports organisation’s objective.
Social media plays a fundamental role due to their ability to reach multiple audiences faster and generate a sense of connection with fans through a key measurement element: engagement (Doyle et al., 2022 ). Sports organisations, specifically football clubs, invest time, people and resources in managing social media to achieve their brand positioning and commercial and communication objectives (Anagnostopoulos et al., 2018 ; Maderer et al., 2018 ), with Facebook, Twitter and more recently, Instagram, being the most widely used (Abeza et al., 2019 ; Machado et al., 2020 ). However, the real potential of social media and its optimal use still poses many questions to be answered.
Although there are previous studies that have explored some aspects of social media in a sports context (e.g., Anagnostopoulos et al., 2018 ; Mastromartino and Naraine, 2022 ; Su et al., 2020 ), the potential impact and efficiency of content posted by football clubs on their social media channels remains unclear. For example, several studies point to various factors that contribute to fan engagement on social media depending on elements such as the type of content, the format used (e.g. photo, text or a combination of both) or the social media platform (see Einsle et al., 2023 ; Maderer et al., 2018 ; Su et al., 2020 ). This gap in the literature prompts a call to action from across the domains of sports marketing and sports management. Identifying the elements generated by football clubs on their official social media profiles can help them improve their marketing strategies and better support their fans. Based on this need and opportunity for management improvement, this study addresses the following research question:
RQ . What are the main characteristics of Facebook, Twitter, and Instagram posts from elite football clubs to understand the content type, format and social media platform that generate the highest engagement among social media consumers?
Grounded on the theoretical framework of relationship marketing, the main objective of this study is to carry out a descriptive and comparative analysis of the engagement generated through social media posts on Facebook, Twitter and Instagram by elite football clubs in Europe, South America and North America, using a categorisation approach developed from an existing model in the literature (see Solanellas et al., 2022 ), as well as the identification of key elements of high-impact social media posts. For this purpose, a new instrument was designed, validated and applied to analyse the use of social media as a marketing tool in sports management. By conducting this exploration, this paper contributes to the literature on sports marketing by identifying which social media and which types of content provoke the most interaction among fans. As a result, football team managers can gain a better understanding of how to target and personalise potential commercial and branding actions, thereby reinforcing the loyalty and commitment of fans to football clubs, and opening or consolidating new lines of action aligned with the strategic objectives of sport entities. Furthermore, the findings and conclusions presented in this study can assist sports managers in the decision-making process, as well as in planning, organising, directing, and effectively controlling social media platforms, thus enhancing engagement with fans in a digital environment.
The article is structured as follows. Firstly, the literature review presents the main theoretical and conceptual elements, focusing on social media and their relationship with marketing theory in sports and football. Secondly, the methodological aspects guiding the study’s process are detailed, including sample, instrument, research procedure, and data analysis. Thirdly, the study’s main results are presented. Fourth, the discussion section critically examines the findings in the context of existing literature, offering practical and theoretical implications for both academics and practitioners. Finally, the study concludes with the main conclusions and limitations.
Literature review
Social media and sports, a combination of great potential.
Social media is a collective term for media tools, platforms, and applications allowing consumers to connect, communicate, and collaborate (Williams and Chinn, 2010 ). They encourage interaction between users and the organisation and provide information from customers and the organisation faster than through conventional media (Kümpel et al., 2015 ; Shilbury et al., 2014 ). Furthermore, social media is considered a mass phenomenon due to its ability to transmit information in an agile and interactive way (Vivar, 2009 ), as well as a unique form of communication that transcends geographical and social boundaries through the instantaneous communication of information (Filo et al., 2015 ). Social Media is used in different sectors for marketing activities (Chen, 2023 ), brand equity and loyalty (Malarvizhi et al., 2022 ) to understand consumer´s behaviour, brand positioning, business revenue opportunities and social communication (Ramos et al., 2019 ). However, although the first studies about this phenomenon have been explored in the sports industry field, there is still a need for more evidence about its real potential, essential elements, and efficiency measurement in the sector.
Due to the high graphic, interactive and visual content of social media, their use in the sports industry, a sector of strong emotional influence, has become more relevant and pervasive in the last decade (Hull and Abeza, 2021 ), where the interest of the viewer has become crucial and increasingly demanding (Nisar et al. 2018 ). The differences that make the sports industry unique and particular are, among others: immediate results and changes (Davis and Hilbert, 2013 ) in addition to the fact that every decision is “in the spotlight” of the public (alluding to the complexity of fans, athletes, coaches, media and other stakeholders). Thus, athletes, teams and sports organisations have been using social media as part of their public relations and communication efforts (Filo et al., 2015 ; Pegoraro, 2010 ; Yan et al., 2019 ) to engage with their partners and fans (Zakerian et al., 2022 ), promoting interactions and increasing engagement with the sport product, as well as with the team in general (Abeza et al., 2019 ; Parganas and Anagnostopoulos, 2015 ).
The linking of social media within the integrated marketing communication process has changed communication strategies and consumer outreach, where marketing managers must include these tools when developing and executing their customer-focused promotional strategies (Lee and Kahle, 2016 ; Rehman et al., 2022 ). On the other hand, social media, directly and indirectly, impacts revenue generation and favours negotiation with sponsors due to their notoriety, visibility, and reach (Mastromartino and Naraine, 2022 ; Parganas and Anagnostopoulos, 2015 ). They are therefore considered a key tool for building and enhancing a brand’s reputation (Maderer et al., 2018 ) and an ideal platform to advertise and increase the visibility of a brand or company, as well as to interact with and analyse the actions of their fans and followers (Abeza et al., 2017 ; García-Fernández et al., 2015 ; Herrera-Torres et al., 2017 ).
Social media has also been used in sports education in recent years (Sanz-Labrador et al., 2021 ). Moreover, their application is increasingly common in construction and dissemination related to social responsibility (López-Carril and Anagnostopoulos, 2020 ; Sharpe et al., 2020 ). In this way, they have also become a key tool for interacting with fans, addressing a strengthened social approach, and gaining engagement from athletes, sponsors, and authorities (Einsle et al., 2023 ; Oviedo et al., 2014 ; Su et al., 2020 ). Beyond the digital environment, Cuesta-Valiño et al. ( 2021 ) pointed out the relevance of considering the emerging sustainable management approach to measure sports organisations’ goals. One of the most relevant challenges for this industry is to issue social media posts efficiently, using the proper formatting resources and at the right time, to generate the most significant possible impact and engagement.
Relationship marketing theory applied to social media in sports
The sports industry is a fast-growing and increasingly diverse market worldwide (Kim and Andrew, 2016 ). Football (soccer in North America) is one of the most popular sports worldwide as well as a cultural manifestation, characterised by its high emotional level and economic, political and social relevance (Bucher and Eckl, 2022 ; Petersen-Wagner and Ludvigsen, 2022 ). Only in Spain, the sports sector generates 3.3% of the Gross Domestic Product (GDP), of which 1.37% is produced through football (PWC, 2020 ).
Globalisation has demanded an adaptation at all levels due to the endless search for immediacy and access to information, where the business of sports is becoming more and more relationship-based and the importance of generating engagement (Einsle et al., 2023 ; Fried and Mumcu, 2017 ; García-Fernández et al., 2017 ) is one of the most relevant variables in generating loyalty in sports organisations (Loranca-Valle et al., 2021 ; Núñez-Barriopedro et al., 2021 ). Sports consumers are seen as “channels” through which sports products can be promoted (O’Shea and Alonso, 2011 ), and sports fans have become both the consumer and the advocates of the product. This is where relationship marketing theory helps us to better understand this phenomenon. As Abeza and Sanderson ( 2022 , p. 287) point out, relationship marketing theory “is based on the idea that a relationship between two parties creates additional value for those involved”. This theory is one of the most widely used to understand the phenomenon of social media in sports (Abeza and Sanderson, 2022 ) as highlighted by numerous authors who have used it in their studies (e.g., Abeza et al., 2017 , 2019 , 2020 ; Su et al., 2020 ; Williams and Chinn, 2010 ).
Merging the roots of relationship marketing theory (Möller and Halinen, 2000 ) and the particular characteristics of the sports sector, and taking into account the perspective of short-term transactions and immediate economic benefits (Abeza et al., 2017 ), social media represents opportunities for better knowledge about fans, more advanced consumer–organisation interaction, efficient fan engagement, efficient use of resources and agile evaluation of the relationship between fans and organisation (Abeza et al., 2019 , 2020 ). In view of this, and in line with Abeza and Sanderson ( 2022 ), social media thus becomes a channel through which to establish, maintain and cultivate long-term relationships beneficial to both parties (in our study, football clubs and fans).
Previous studies have addressed the use of specific social media in the context of sports, such as Facebook (Achen, 2019 ; Meng et al., 2015 ; Pegoraro et al., 2017 ; Waters et al., 2009 ), Twitter (Blaszka et al., 2012 ; Hambrick et al., 2010 ; Lovejoy and Saxton, 2012 ; Winand et al., 2019 ; Witkemper et al., 2012 ) and Instagram (Anagnostopoulos et al., 2018 ; Machado et al., 2020 ; Zakerian et al., 2022 ), because of the relevance in the use of these platforms in the sports sector. From another broader perspective, Solanellas et al. ( 2022 ) propose a practical analysis of multiple social media in sports organisations from a content categorisation point of view.
The results and contributions of the studies mentioned above, reveal the importance of further exploring the social media fan engagement phenomenon as a strategic perspective (Tafesse and Wien, 2018 ) and the added value that social media can generate in sports. In this sense, it is relevant for sports managers to know which techniques, methodologies and perspectives to use. Furthermore, as stated by Abeza and Sanderson ( 2022 ), it is necessary to go deeper into the theories behind its use. Taking these aspects into account, this work presents a new instrument of observation and measurement of social media posts by football organisations, as a basis for understanding and deepening the knowledge about the digital audience and its impact on the different objectives of the organisation. Thus, the study draws on relationship marketing theory to better understand how sports managers can make the most of the possibilities offered by social media to generate added value from the interaction between fans and football clubs. Particularly, the developed instrument focuses on the analysis of the type of content published by football clubs, categorising it into dimensions, as well as the engagement of the different publications according to the type of dimension to which they belong.
With a view to the implementation of the instrument, and to contribute to the literature related to the use of social media as a marketing tool in sports, this study analyses Facebook, Twitter and Instagram posts issued by elite football clubs from Europe, South America and North America, using a practical approach to content categorisation and taking the engagement factor as a key element for comparison.
Methodology
This study adopts an exploratory, descriptive, and comparative research design (Andrew et al., 2011 ) using the observational method and content analysis techniques. Content analysis involves the recounting and comparison of content, followed by the interpretation of the underlying context. It has been widely used in social media communication research, specifically in sports settings (e.g., Anagnostopoulos et al., 2018 ; Wang and Zhou, 2015 ; Winand et al., 2019 ), to interpret textual data through systematic classification, coding, and identifying themes or patterns (Hsieh and Shannon, 2005 ). First, exploratory studies are particularly useful when the phenomenon under investigation is in constant evolution (such as social media as a marketing tool), as well as when there are several factors and variables at play (Andrew et al., 2011 ). In this study, these are linked to the engagement that can be caused by the type of content or format used by elite football clubs on their social media accounts. Second, the descriptive aspect of the research design aims to describe and quantify the engagement levels in social media for the selected football clubs. By Collecting and analysing quantitative data on the interaction metrics, including likes, comments, shares, and follower counts, the study provided a comprehensive overview of the current state of engagement, and other variables, among the clubs, helping to build a foundation for further analysis and comparison. Lastly, the comparative aspect of the research design (Andrew et al., 2011 ) is valuable in this study because it enables a cross-regional analysis of three of the most traditional social media platforms. The study compared the engagement practices, elements, and strategies across three key regions of the football industry worldwide. Understanding potential differences can be useful for sports managers to design more optimised social media marketing strategies.
Considering the study design and observational method applied in this research (Anguera-Argilaga et al., 2011 ), a nonprobable sample design (see Battaglia, 2008 ) was established following several steps to make the following three decisions: (1) selection of football clubs, (2) social media platforms, and (3) period of time studied.
First, a geographical criterion was used to determine the origin of the football clubs under study. This criterion was based on a comprehensive and global perspective, considering factors such as historical significance, popularity, sporting achievements, and the modernisation of football worldwide. Based on these considerations, three regions were selected for analysis: Europe and South America, renowned for their broad global relevance and football tradition (e.g., the winning national teams of the 22 editions of the FIFA World Cup so far are from Europe and South America [Venkat, 2023 ]). Next, North America was chosen for its ascending market growth potential and global efforts to promote football. This is exemplified by upcoming milestones, such as the organisation of the FIFA World Cup 2026 in the United States, Mexico, and Canada, as well as the recent arrival of Lionel Messi into Major League Soccer (see Mizrahi, 2023 ). These three regions are governed by the three most influential regional football bodies of FIFA: Europe (UEFA), South America (CONMEBOL), and North America (CONCACAF). Second, to select the most relevant football clubs in these three regions, we followed some of the selection criteria set in similar studies (e.g., Anagnostopoulos et al., 2018 ; Maderer et al., 2018 ). Therefore, the rankings of four of the most influential football organisations or websites were considered: (1) the International Federation of Football History and Statistics (IFFHS) club ranking, (2) the Football World Rankings website, (3) the FIFA club and league ranking, and (4) the Transfermarkt player ranking website (of great relevance in the player transfer market). As a result of this process, 24 teams were pre-selected (9 from Europe, 9 from South America and 6 from North America) according to the objectives and the study design and the author’s agreement (Andrew et al., 2011 ; Anguera-Argilaga et al., 2011 ; Battaglia, 2008 ; Hernández-Sampieri et al., 2014 ). Finally, a random draw was made resulting in a selection of six teams from Europe, six from South America and four from North America (with a limit of two teams per league). This process resulted in the 16 teams whose use of social media is analysed in this study (see Table 1 ).
Following, social media to be analysed in the study were selected. It was noted in the literature that Facebook had been one of the first social media to be used by football clubs and other sports organisations, either to connect with fans or purely for informational purposes (Achen, 2019 ; Waters et al., 2009 ). Twitter and Instagram are also platforms that have become relevant, not only for marketers in sports but also in other sectors (Anagnostopoulos et al., 2018 ; Wang and Zhou, 2015 ). Although the use of Facebook, Twitter and Instagram as marketing tools for football clubs has been studied (e.g., Machado et al. 2020 ; Maderer et al. 2018 ; Nisar et al., 2018 ), there is a lack of literature comparing their potential engagement across a sample of teams from different geographic regions. Thus, it was deemed appropriate to select these three social media sources for our study.
Finally, the periods over which the publications were to be extracted were determined. Among other authors, Ashley and Tuten ( 2015 ) point out that, in a social media environment, two to four weeks are sufficient for a wide variety of posts to be made in a regular and cyclical context, excluding exceptional milestones or events that could have an extraordinary impact on engagement and that could bias regular reading. Therefore, 45 days for each club and each social media is set as an appropriate observation period.
Once the sample selection criteria had been defined, the links of all publications from the clubs selected in the study on the three social media were extracted through the Fanpage Karma software that allows data to be collected and interpreted (Lozano-Blasco et al., 2021 ). After prior data analysis, the final sample consisted of 19,745 publications, a very similar figure to that used in other related studies (e.g., Maderer et al., 2018 ; Yan et al., 2019 ).
Instrument and research procedure
Based on the review of the techniques and methodologies used to analyse the use of social media as a marketing tool for football clubs in previous studies, we proceeded to design and develop an observation and data collection instrument in a Microsoft Excel Spreadsheet (.xlsx format), taking as a starting point the model of content analysis proposed by Solanellas et al. ( 2022 ). Due to the nature of the study, the .xlsx data collection format was chosen for its flexibility, allowing for manual data collection and the application of the categorisation tool post-by-post. This format has been successfully used as a data collection tool in previous social media content analysis studies in football (e.g., López-Carril and Anagnostopoulos, 2020 ).
To ensure its rigour, the codebook was subsequently submitted for review to nine field experts. The selection of these experts was undertaken via judgmental nonprobability sampling, a method commonly employed in the literature due to the specialised and ever-evolving nature of the subject (Andrew et al., 2011 ). These individuals were chosen based on specific criteria, encompassing their professional roles in specialised, coordinating, managerial, or directorial positions tied to the digital domain. Moreover, their academic background, particularly in marketing, methodology, or digital tools, was considered. To ensure an extensive grasp of the subject matter, the chosen experts were required to have a minimum of five years of experience in the area and to be actively participating in their respective roles. This approach aimed to incorporate diverse viewpoints, offering insights from a spectrum of angles relevant to this research. As a result, the panel of experts was comprised of the following professionals: the Head of Digital from a prominent European professional football league (1), a Marketing Manager and an International Communications Manager from leading professional football clubs (2), Directors of digital marketing and branding agencies (2), professors specialising in marketing and sports management at Spanish universities (2), and the Vice-President of Sales along with the Head of Digital from sports business intelligence consultancies (2).
Semi-structured interviews were undertaken with these chosen experts to delve into pertinent aspects linked to the study. An interview guide was developed, following the methodological aspects indicated in specialised works in this field (see Andrew et al., 2011 ; Anguera-Argilaga et al., 2011 ). Furthermore, the interview guide encompassed critical aspects of social media management and relevant facets of football club management (e.g., post formats, observation timeframes, platforms for capturing and analysing social media posts), drawing upon the elements and variables derived from studies conducted by Parganas and Anagnostopoulos ( 2015 ) as well as Solanellas et al. ( 2022 ). Additionally, these interviews comprised discussions about the conception and execution of the observation tool, which was employed as a supplementary instrument for data collection. Further variables relevant to the research objectives were explored within these interviews.
The qualitative insights garnered from the experts’ conclusive remarks offered valuable suggestions that contributed to refining the study’s development and enhancing the observation tool. This iterative approach ensured the harmonisation of the tool with the research objectives and its effective alignment with the study’s research questions. After incorporating the modifications suggested in the experts’ evaluations, the study’s codebook adhered to the variables and categories illustrated in Table 2 .
The .xlsx instrument sheet was then pilot-tested. Seventy-five publications (25 from Facebook, 25 from Instagram and 25 from Twitter) from three different football clubs were randomly selected, conforming to a total sample of 225 publications. The data were collected in an observation sheet in .xlxs format for analysis purposes. During the analysis process, including the discussion of possible discrepancies in interpreting each publication as belonging to one or another of the dimensions of the study’s codebook, the authors decided that each publication would be classified only in one dimension, depending on the type of content that predominates in each post.
To measure the level of reliability and accuracy of the instrument (Andrew et al., 2011 ), the intra-observer reliability method was applied, incorporating 10–12 minute breaks every 40–45 min of observation. After 15 days, the same publications were re-coded using the same established protocol. The results of the coding provided a Kappa coefficient of 0.949, demonstrating a very high level of agreement and reliability, following the scale of Landis and Koch ( 1977 ).
To measure the reliability and accuracy of the instrument (Andrew et al. 2011 ), the intra-observer reliability method was applied. In the first stage, the data was collected and coded post-by-post by applying the xlsx. sheet, incorporating 10–12 minute breaks every 40–45 min of observation to ensure the quality of the data observed and collected. The same posts were re-coded using the same established protocol in the second stage. To ensure a more accurate application of the codebook and to avoid potential bias, a 15-day impasse was established between the two data collections. The coding results between the two stages provided a Kappa coefficient of 0.949, demonstrating a very high level of agreement and reliability, following the scale of Landis and Koch ( 1977 ).
Finally, based on the interaction data collected with the data collection instrument, the variable of engagement with the publications was calculated by adapting the formulas used by the Fanpage Karma ( 2022 ) and Rival IQ (Feehan, 2023 ) platforms (Fig. 1 ).
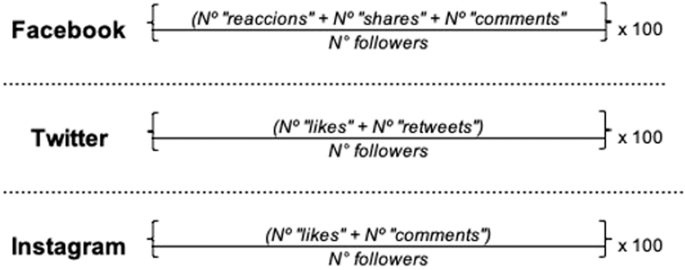
Adapted from Fanpage Karma ( 2022 ) and Rival IQ (Feehan, 2023 ) platforms.
Therefore, after the protocol and the .xlsx observation instrument sheet were tested and validated, the final procedure was established as follows: (a) social media posts from Facebook, Twitter and Instagram of the selected football clubs were extracted automatically using the FanPage Karma license and added to the .xlsx observation instrument sheet; (b) according to the Study Codebook (see Table 2 ) the data was collected and registered manually into the .xlsx observation instrument sheet by clicking the posts one by one; c) we proceeded to set up a database coding the variables from the data collected to perform the statistical analyses.
Data analysis
A descriptive analysis of the engagement generated by publications on social media and their content (dimensions and formats) on Facebook, Instagram and Twitter was carried out. To analyse the differences in engagement generated by the posts on each social media according to their content, we used the t-test for independent samples and the one-factor ANOVA. The significance value established is <0.05. A chi-square test and correspondence analysis were applied to identify and visualise points of association between the key variables. Data analysis was performed using the SPSS statistical package, version 27.0.
As shown in Table 3 , of the 19,745 posts observed and analysed, Twitter accounted for 64%, followed by Facebook at 22% and Instagram at 14%. However, from the point of view of engagement, Instagram reflects an average of 1.873, well above the other social media. Facebook follows it with 0.112 and Twitter with 0.045, showing an inverse behaviour to the number of posts made.
Frequency and engagement
In Fig. 2 , we can observe the strategy used by each club in terms of the frequency of posts on Facebook, Twitter and Instagram, as well as the levels of engagement obtained. On Facebook, the football clubs analysed posts at different frequencies. In Europe, we observe that the clubs with the highest frequency of posts are Liverpool FC and Manchester United FC, with n = 445 and n = 486, respectively. In contrast, the Spanish clubs (Real Madrid FC and FC Barcelona) have the lowest frequency of posts ( n = 195 and n = 118, respectively). On the other hand, beyond this difference in frequency, they have very similar engagement ratios.
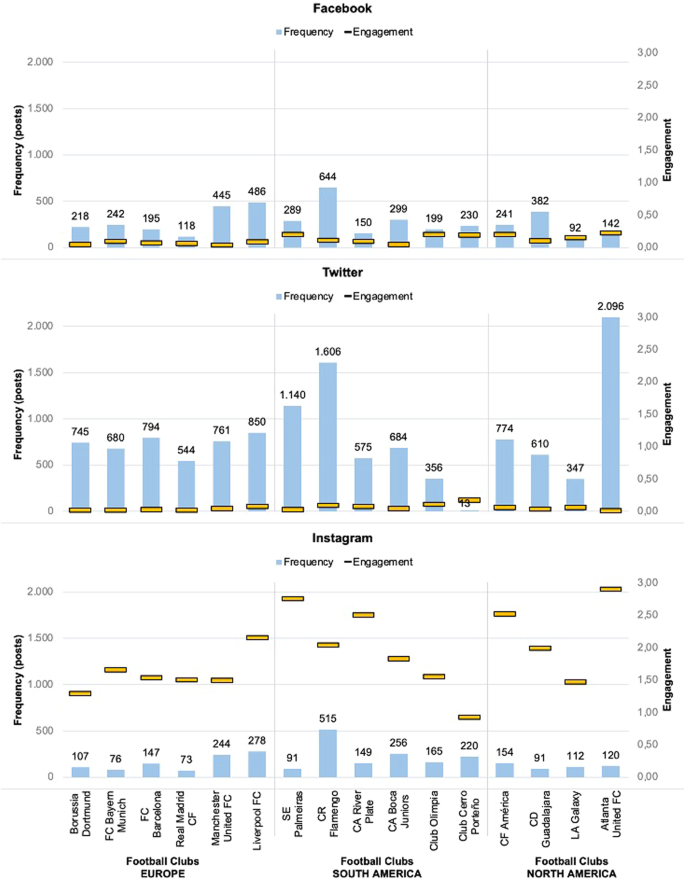
Frequency of posts and level of engagement generated on Facebook, Twitter and Instagram by the football clubs selected for this study (organised by regions).
The club with the highest frequency of publications is CR Flamengo from Brazil ( n = 644); however, SE Palmeiras, the other Brazilian club studied, despite registering fewer publications in the same period ( n = 289), shows much higher levels of engagement. SE Palmeiras (Brazil), Club Olimpia and Club Cerro Porteño (Paraguay), CF America (Mexico) and Atlanta United FC (USA) show the highest levels of engagement, with similar posting frequencies (between n = 142 and n = 241). On Twitter, the highest frequencies of posts were published compared to Facebook and Instagram, with CR Flamengo and Atlanta United FC being the clubs that posted the most ( n = 1606 and n = 2096, respectively). However, the levels of engagement identified show similar and homogeneous levels in the period analysed, regardless of the frequency of publications. On the other hand, the highest engagement levels were observed on Instagram, with a lower frequency of publications in all cases. Football clubs SE Palmeiras, CA River Plate, CF America and Atlanta United FC have the highest engagement values (2.5 and 3), with posting frequencies ranging from n = 91 to n = 154. European football clubs have very similar engagement ratios (around 1.00), while North American football clubs have different engagement values despite having similar posting frequencies ( n = 91 and n = 154).
Content dimensions of publications
As shown in Fig. 3 , we observe the dimensions proposed in this study, comparing the social media analysed and the engagement generated by each category. From this point of view, in terms of frequency, the “Marketing” and “Sport” dimensions are observed as the most used publication approaches by football clubs, followed by the “Institutional” dimension, “Commercial” and, finally, “ESG”. This order of frequency applies to Facebook, Twitter and Instagram.
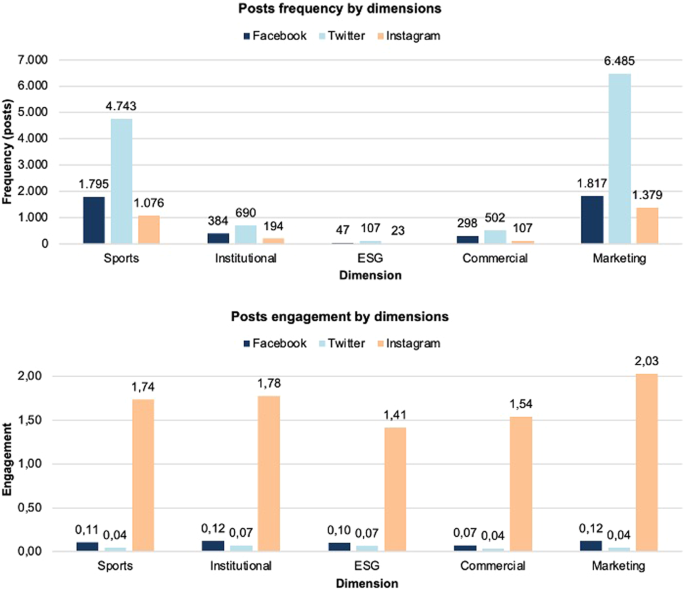
Categorisation in the posts’ dimensions and their relationship with the engagement generated by Facebook, Twitter and Instagram of the football clubs analysed.
In terms of engagement, the social media Instagram is the one that registers considerably higher values than the rest of the social media analysed, with the “Marketing” dimension generating the highest engagement (2.03). It is followed by the “Institutional” dimension (1.78) and the “Sports” dimension (1.74), closing with the “Commercial” and “ESG” dimensions, with values of 1.54 and 1.41, respectively. Facebook is the following social media that generates the highest engagement.
In the case of Facebook (see Supplementary Table S1 ), the findings show a significance of the engagement means between the “Commercial” and the “Sports” ( p = 0.000 < 0.05), “Institutional” ( p = 0.001 < 0.05) and “Marketing” type of the posts in Facebook.
On the other hand, Twitter (see Supplementary Table S2 ) is the one that generates the minor engagement, with very similar values between the different dimensions, despite being the one with the highest frequency of publications (Fig. 3 ). Unlike the previous dimensions, the “Institutional”, “ESG”, and “Commercial” dimensions are those with the highest engagement values (0.07), followed by the “Marketing” and “Sports” dimensions (both with 0.04). However, in this social media platform, the “Institutional” type of content is statistically significant with “Sports” ( p = 0.000 < 0.05), “Commercial” ( p = 0.000 < 0.05) and “Marketing” ( p = 0.000 < 0.05). Also, we can find significant engagement results between the “ESG” and the “Commercial” ( p = 0.033 < 0.05) dimensions.
On Instagram (see Supplementary Table S3 ), the “Marketing” dimension has the highest engagement value, as does the “Institutional” dimension (both with 0.12). It is followed by the “Sports” dimension (0.11), “ESG” (0.10) and finally, “Commercial” (0.07) (Fig. 3 ). Nevertheless, as difference of Facebook and Twitter, the findings show a strong relevance of “Marketing” dimensions posts (Supplementary Table S3 ), linked significantly with “Sports” ( p = 0.000 < 0.05), “Commercial” ( p = 0.000 < 0.05) and “Institutional” ( p = 0.002 < 0.05).
Types of formats in publications
Nine combinations of the most relevant formats have been identified in the publications analysed (Table 4 ), both in the frequency of use and engagement they generate.
On Facebook, the most frequent formats are “Text/Image” and “Text/Video” ( n = 2031 and n = 1265, respectively). However, the format with the highest engagement is “Image” (0.23), followed by “Text/Image” (0.13), “Text/Video” (0.12) and “Text/Link” (0.07). On Twitter, on the other hand, the “Text/Image” format is the most used ( n = 4412), “Text” ( n = 2499), “Text/Video” ( n = 2239) and “Image” ( n = 1534), with the “Text/Video” and “Text/Image” format combinations (0.07) registering the highest engagement. On Instagram, due to the nature of social media, the most frequent format is “Text/Image” ( n = 1986). In terms of engagement, the formats “Image” (2.20), “Text/Image” (1.95), “Text/Image/Polls” (1.93) and “Video” (1.84) have the highest values.
The correspondence analysis (Fig. 4 ) shows the degree of association between the variables and the categorisation dimensions proposed in this study in a relative position map. The chi-squared test yielded a result of 1027.65. The “Marketing” dimension shows a closer relationship with the “video” and “image” format resources. The “ESG” and “Institutional” content type shows an association with the “Image” and “Text” formats. The “Commercial” dimension, based on the characteristics of the categorisation, shows a relationship with the “Link” format as ideal points of association, considering the frequency and engagement analysed.
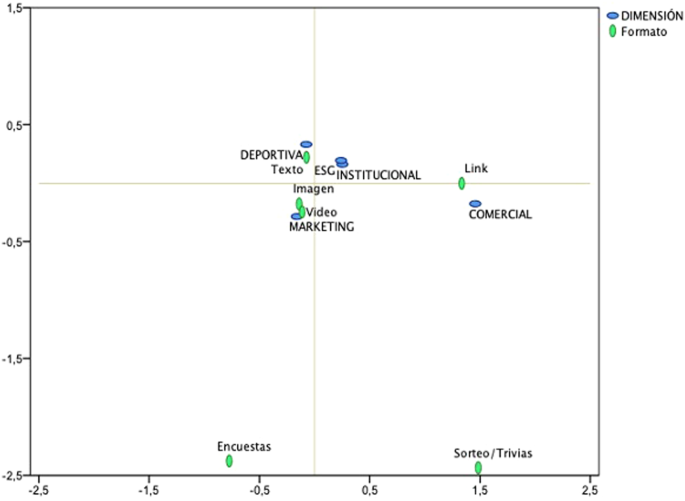
Correspondence analysis (dimensions and formats).
Nowadays, sports organisations and athletes use social media for communication purposes, brand positioning, visibility (Maderer et al., 2018 ; Winand et al., 2019 ; Zakerian et al., 2022 ) and even for potential business (Parganas and Anagnostopoulos, 2015 ), dedicating effort and resources. Previous studies reinforce the need to categorise the message delivered to understand this phenomenon according to the objective (Filo et al., 2015 ) and content analysis for effect (Meng et al., 2015 ). However, its optimal use still leaves many questions. The complexity of the market is evolving towards the need to understand the fan as a premise in a sector characterised by its high emotional charge. In the past, strategies focused on attracting and retaining fans. However, the current trend shows increased relevance in generating engagement (Oviedo et al., 2014 ) to generate links with fans. The sports industry, especially in the digital environment, is in an era where the goal is not just getting new followers and post social media content but interact and engage “to know the users better”.
First, this study provides evidence of relevant frequency-engagement relationships according to the dimensions of the study, depending on the type of social media used (Facebook, Twitter and Instagram). Regarding the dimensions of the content published, the posts related to “Marketing” and “Sport” are the most frequent due to the natural and traditional use of these tools as communicative, brand positioning and informative elements (Lee and Kahle, 2016 ; Rehman et al., 2022 ; Winand et al., 2019 ). This is attributable to the need for clubs to generate emotional content (such as videos or images of past iconic matches or campaigns involving athletes), on the one hand, and to broadcast messages alluding to sporting performance and results. Nevertheless, the findings show different engagement impacts not directly linked to the frequency of the posts but influenced by other elements, such as the social media platform, the dimension of the content and the format. The evidence shows there are specific content dimensions that statistically generate more engagement in each platform.
On Facebook, the most traditional platform football clubs use provides a more balanced frequency-engagement ratio, with a strong engagement with “commercial” content. This platform was one of the social media platforms that started monetising in other industries, characterised for its high brand impact, where the know-how and the platform interphase are more friendly to focus on this type of posts (and in some cases, to launch joint posts with brands). Even with the positive engagement impact of this platform, it is observed that efforts of this nature in the digital sphere are scarce in comparison to the rest, making this a relevant aspect in the spectrum of growth and an opportunity to explore, especially with the new assets that are appearing in the market and the growth of e-commerce.
On Twitter, on the other hand, the dimension that works best for engaging in “Institutional” is linked to “Sports”, “Marketing” and “Commercial” content, but not with “ESG”. However, the “ESG” linked with “Commercial” dimensions statically gets significantly more impact on this platform. The “ESG” dimension is emerging as this platform is used for promoting socio-political activities and promoting more altruistic purposes as previous authors as López-Carril and Anagnostopoulos ( 2020 ), and Sharpe et al. ( 2020 ) noted. This strategy shows a possible intention to use social media not only for marketing (communication) or sporting purposes but also as an element with socio-political aspects. The nature of Twitter as a microblogging site with the highest number of posts with the lower means of engagement, is more attractive for the audience looking for quick and summarised information because of its ability to increase the visibility and awareness of fans (Abeza et al., 2017 ). Sports managers can focus on this type of message for a potential higher engagement on Twitter.
In contrast, on Instagram, the focus is on “Marketing” content. This platform shows the lowest number of post frequency, with a high engagement means, attributable to the platform’s audio–visual formats and more interactive content, ratifying its growing popularity among users. As a fast-growing platform, there is a major link with “Sports”, “Institutional” and “Commercial” dimensions, which makes it an ideal platform for emotional content, easy to connect with brands, athletes, and sports properties, counting with a larger and more varied audience looking mainly, as the evidence suggests, for entertainment and club’s closeness perception. Therefore, like Anagnostopoulos et al. ( 2018 ), we recommend sports managers use Instagram for marketing purposes, considering the context as a relevant factor.
Finally, this study reveals the post format’s relevance as another key element. In this sense, on Facebook, the highest engagement values are generated by “Image” and “Text/Image” formats, as on Instagram and Twitter; however, in each social media platform, the frequencies generated by these records are different. In any case, the power of the image as valuable content in marketing stands out, as it has also been highlighted in previous studies (e.g., Anagnostopoulos et al., 2018 ; Doyle et al., 2022 ; Machado et al., 2020 ). Nevertheless, the results obtained regarding the engagement triggered by video format posts on Facebook, Twitter and Instagram are not as conclusive, as other studies have pointed out (e.g., Su et al., 2020 ). Probably because these social media are not focused on that format as other social media such as TikTok or YouTube may be. Regardless, based on the results obtained, it is necessary for sports managers and academics to continue to explore and make the appropriate combinations of the dimensions of content type categorised in this study, the publication format, as well as the social media used to channel them.
Theoretical implications
Built upon the framework of relationship marketing, this study brings theoretical value to the realms of sports marketing, sports management, and fan engagement, spanning across four distinct lines of action.
Firstly, the research introduces a novel theoretical approach to social media strategies by employing a 5-dimensional content categorisation system aligned with the strategic pillars of football organisations. Previous studies have predominantly approached the role of social media in sports reactively, primarily focusing on communication and branding aspects. In contrast, this study contributes to the literature by adopting a strategic perspective towards social media, establishing a linkage between the study dimensions and football club strategies. This foundation paves the way for future research to delve deeper into each proposed dimension, potentially identifying sub-groups and exploring them in greater detail. The proposed dimensions serve to systematically organise the primary facets of football organisations for digital context analysis, a realm of increasing importance within the sports industry. As such, this work marks a pioneering step towards a novel approach in this area of study.
Secondly, this study establishes a fresh frequency-engagement approach for social network management, dispelling the notion that post frequency directly correlates with generated engagement. In doing so, this work highlights additional pivotal factors beyond post frequency that influence engagement among users of football-related social media. This perspective is aligned with the ethos of Web 2.0, underscoring the significance of engaging and connecting with fans.
Thirdly, from a theoretical perspective, this study introduces an innovative analytical proposition focusing on prominent international football clubs. This innovation is realised through the calculation and translation of engagement ratios, facilitating cross-entity comparisons independent of geographical location and follower count. The instrument developed and applied in this study acts as a tool to identify valuable digital practices within the industry.
Finally, this study stands out by conducting simultaneous analyses of posts across three prominent social media platforms (Facebook, Twitter, and Instagram), adopting a distinctive multi-platform approach that is seldom observed in comparable studies which often focus on a single social media platform. Gaining insights into the effects of cross-platform and cross-format postings can empower sports managers to make strategic decisions with a comprehensive perspective.
Practical implications
This study introduces a novel practical tool designed for the computation of fan engagement across the Facebook, Twitter, and Instagram accounts of football clubs globally. Consequently, sports managers can employ this instrument to gain a more realistic comprehension of the performance of social media accounts belonging to clubs. Furthermore, the developed tool facilitates the assessment of fan engagement in relation to the content type being published. This capability can aid sports managers in fortifying the bond between clubs and their followers by generating heightened value through strategic social media initiatives.
It is important to note that sports managers should consider both internal factors (club tradition, organisational culture) and external factors (competition, fan behaviour, sports results) within the context of clubs. This consideration is essential for developing and planning optimal digital strategies and for generating the best possible engagement with the audience. This research furnishes empirical evidence for understanding, in a practical and actionable manner, the pivotal components of a social media post. This understanding permits the visualisation of optimal combinations of these elements, thereby increasing the likelihood of sports managers guiding the club toward success and fostering substantial user engagement. Therefore, football team managers can apply the findings of this study to plan, monitor, and evaluate the club’s social media content for increased engagement and “closeness” with digital fans. They can combine various formats based on individual post requirements to achieve the desired results. Additionally, football team managers can analyse club identity and overall strategies more practically and coherently, facilitating the planning and execution of more effective commercial, brand positioning, institutional, and other relevant digital goals, with engagement serving as a key metric.
Conclusions
Social media plays a key role in today’s sports management, especially in football clubs, due to its global reach and ability to interact and connect with fans in an industry of great popularity, emotional charge, and economic, political and social impact. This exploratory research grounded in relationship marketing theory provided a comparison of the engagement generated by elite football clubs under a unique categorisation proposal, derived and adapted from existing literature, which addresses dimensions linked to strategic areas of football organisations and takes into consideration key elements such as frequency and format combinations used to analyse the efficiency of posts on Facebook, Twitter and Instagram.
Based on the results obtained, three lines of action stand out. First, concerning the type of content of the post, the “Marketing” and “Sports” dimensions are the preferred categories for football clubs in terms of post frequency. Regarding the engagement rates, on Facebook, the “Commercial” dimension shows an opportunity for growth and development due to the good engagement impact and due to the technological boom and the emergence of new digital assets. On Twitter, the emerging “ESG” linked to “Commercial” perspective and the “Institutional” dimension gets a significant impact on Twitter. On Instagram, the “Marketing” dimension linked to “Sports”, “Institutional” and “Commercial”, makes this platform ideal for emotional and marketing purposes. Second, concerning social media sources, this study provides evidence that Instagram is the social media that generates the most engagement using the lowest frequency of posts, followed by Facebook and Twitter. There is no direct evidence that links the post’s frequency with the engagement generated. Finally, concerning the type of format of the post, the combination of formats that generates the most engagement in all cases is “Image”, “Text/Image”, and “Text/Video”.
In short, this research stimulates a practical reflection for professionals and academics on the exploration, analysis, and evaluation of the management of social media in football clubs, using the observation method and content analysis techniques, applying elements of reliability and scientific rigour. The results obtained in this study offer practical and managerial implications in sports management, fan engagement, digital marketing, and social media, among others, through a proposal for categorisation and unique variables, taking engagement and its influence within the context of analysis as the axis.
The above conclusions should be taken into consideration viewing a series of limitations of the study. Firstly, the sample is limited to one sport (football) and not a large number of football clubs from different regions of the world. Secondly, despite the high number of posts analysed, these are located over a short period of time, and it may be relevant to analyse the engagement of posts at different times of the season, as these can influence the type of content and the engagement of fans with the posts. Thirdly, the study is limited to analysing engagement on Facebook, Twitter and Instagram, leaving aside the analysis of the possibilities that other booming social media, such as TikTok or Twitch, are having in the field of marketing. Nevertheless, these limitations can be a starting point for future research lines including, among others: (a) to assess the application and feasibility of the technique for measuring social media engagement included in this work in other football organisations (e.g. leagues) or social media platforms (e.g., TikTok, Twitch); (b) to incorporate new variables of study (e.g., size of the social mass of sports clubs, financial budget, trophies won); (c) to conduct the study considering different phases of the sports season (e.g.; preseason, season, playoffs; postseason); (d) to analyse fan engagement relation of geographical regions to understand the digital user’s behaviours; (e) to conduct the study adding engagement prediction models in social media; and (f) to incorporate this model on an AI language to suggest and predict digital user engagement in a simulated context.
Data availability
The datasets generated and analysed during the current study are available from the corresponding author on reasonable request.
Abeza G, O’Reilly N, Finch D, Séguin B, Nadeau J (2020) The role of social media in the co-creation of value in relationship marketing: a multi-domain study. J Strateg Mark 28(6):472–493. https://doi.org/10.1080/0965254X.2018.1540496
Article Google Scholar
Abeza G, O’Reilly N, Seguin B (2019) Social media in relationship marketing: the perspective of professional sport managers in the MLB, NBA, NFL, and NHL. Commun Sport 7(1):80–109. https://doi.org/10.1177/2167479517740343
Abeza G, O’Reilly N, Seguin B, Nzindukiyimana O (2017) Social media as a relationship marketing tool in professional sport: a netnographical exploration. Int J Sport Commun 10(3):325–358. https://doi.org/10.1123/ijsc.2017-0041
Abeza G, Sanderson J (2022) Theory and social media in sport studies. Int J Sport Commun 15(4):284–292. https://doi.org/10.1123/ijsc.2022-0108
Achen RM (2019) Re-examining a model for measuring Facebook interaction and relationship quality. Sport Bus Manag 9(3):255–272. https://doi.org/10.1108/SBM-10-2018-0082
Anagnostopoulos C, Parganas P, Chadwick S, Fenton A (2018) Branding in pictures: using Instagram as a brand management tool in professional team sport organisations. Eur Sport Manag Q 18(4):413–438. https://doi.org/10.1080/16184742.2017.1410202
Andrew D, Pedersen P, McEvoy C (2011) Research methods and design in sport management. Human Kinetics
Anguera-Argilaga MT, Blanco-Villaseñor A, Hernández-Mendo A, Losada JL (2011) Observational designs: their suitability and application in sports psychology. Cuad Psicol Deporte 11:63–76
Google Scholar
Ashley C, Tuten T (2015) Creative strategies in social media marketing: an exploratory study of branded social content and consumer engagement. Psychol Mark 32(1):15–27. https://doi.org/10.1002/mar.20761
Battaglia MP (2008) Nonprobability sampling. In: Lavrakas PJ (ed) Encyclopedia of survey research methods. SAGE Publications, pp. 523–526
Blaszka M, Burch L, Frederick E, Clavio G, Walsh P (2012) #WorldSeries: an empirical examination of a Twitter hashtags during a major sport event. Int J Sport Commun 5:435–453. https://doi.org/10.1123/ijsc.5.4.435
Bucher B, Eckl J (2022) Football’s contribution to international order: the ludic and festive reproduction of international society by world societal actors. Int Theory 14(2):311–337. https://doi.org/10.1017/S1752971920000676
Chen Y (2023) Comparing content marketing strategies of digital brands using machine learning. Humanit Soc Sci Commun 10(1):57. https://doi.org/10.1057/s41599-023-01544-x
Cuesta-Valiño P, Gutiérrez-Rodríguez P, Loranca-Valle C (2022) Sponsorship image and value creation in E-sports. J Bus Res 145:198–209. https://doi.org/10.1016/j.jbusres.2022.02.084
Cuesta-Valiño P, Gutiérrez-Rodríguez P, Loranca-Valle C (2021) Sustainable management of sports federations: the indirect effects of perceived service on member’s loyalty. Sustainability 13(2):458. https://doi.org/10.3390/su13020458
Davis J, Hilbert JZ (2013) Sports marketing—creating long term value. Edward Elgar Publishing
Doyle JP, Su Y, Kunkel T (2022) Athlete branding via social media: examining the factors influencing consumer engagement on Instagram. Eur Sport Manag Q 22(4):506–526. https://doi.org/10.1080/16184742.2020.1806897
Einsle C-S, Escalera-Izquierdo G, García-Fernández J (2023) Social media hook sports events: a systematic review of engagement. Commun Soc 36(3):133–151. https://doi.org/10.15581/003.36.3.133-151
Fanpage Karma (2022) Metrics overview. https://academy.fanpagekarma.com/en/metrics/
Feehan B (2023) Social media industry benchmark report. Rival IQ. https://www.rivaliq.com/blog/social-media-industry-benchmark-report/
Filo K, Lock D, Karg A (2015) Sport and social media research: a review. Sport Manag Rev 18(2):166–181. https://doi.org/10.1016/j.smr.2014.11.001
Fried G, Mumcu C (2017) Sport analytics: a data-driven approach to sport business and management. Routledge
García-Fernández J, Elasri A, Pérez-Tur F, Triadó-Ivern X, Herrera-Torres L, Aparicio-Chueca P (2017) Social networks in fitness centres: the impact of fan engagement on annual turnover. J Phys Educ Sport 17:1068–1077. https://doi.org/10.7752/jpes.2017.03164
García-Fernández J, Fernández-Gavira J, Durán-Muñoz J, Vélez-Colon L (2015) La actividad en las redes sociales: un estudio de caso en la industria del fitness. Retos 28:44–48. https://doi.org/10.47197/retos.v0i28.34839
Hambrick M, Simmons J, Greenhalgh G, Greenwell C (2010) Understanding professional athletes’ use of Twitter. Int J Sport Commun 3(4):454–471. https://doi.org/10.1123/ijsc.3.4.454
Hernández-Sampieri R, Fernández-Collado C, Baptista-Lucio P (2014) Metodología de la investigación, 6th edn. McGraw-Hill
Herrera-Torres L, Pérez-Tur F, García-Fernández J, Fernández-Gavira J (2017) El uso de las redes sociales y el engagement de los clubes de la Liga Endesa ACB. Cuad Psicol Deporte 17(3):175–182
Hsieh H-F, Shannon ES (2005) Three approaches to qualitative content analysis. Nord J Digit Lit 15(9):1147–1288. https://doi.org/10.1177/1049732305276687
Hull K, Abeza G (2021) Introduction to social media in sport. In: Abeza G, O’Reilly N, Sanderson J, Frederick E (eds) Emerging issues and trends in sport business: vol. 2. Social media in sport theory and practice. World Scientific Publishing Company, pp. 1–28
Kim S, Andrew DPS (2016) Understanding sport organizations: the application of organization theory (2nd Edition). J Sport Manag 21(3):455–457. https://doi.org/10.1123/jsm.21.3.455
Kümpel AS, Karnowski V, Keyling T (2015) News sharing in social media: a review of current research on news sharing users, content, and networks. Soc Media Soc 1(2):1–14. https://doi.org/10.1177/2056305115610141
Landis JR, Koch GG (1977) The measurement of observer agreement for categorical data. Biometrics 33(1):159–174. https://doi.org/10.2307/2529310
Article CAS MATH PubMed Google Scholar
Lee C, Kahle L (2016) The linguistics of social media: communication of emotions and values in sport. Sport Mark Q 25(4):201–211
López-Carril S, Anagnostopoulos C (2020) COVID-19 and soccer teams on Instagram: the case of corporate social responsibility. Int J Sport Commun 13(3):447–457. https://doi.org/10.1123/ijsc.2020-0230
Loranca-Valle C, Cuesta-Valiño P, Núnez-Barriopedro E, Gutiérrez-Rodríguez P (2021) Management of loyalty and its main antecedents in sport organizations: a systematic analysis review. Front Psychol 12:783781. https://doi.org/10.3389/fpsyg.2021.783781
Article PubMed PubMed Central Google Scholar
Lovejoy K, Saxton GD (2012) Information, community, and action: how nonprofit organizations use social media. J Comput-Mediat Commun 17(3):337–353. https://doi.org/10.1111/j.1083-6101.2012.01576.x
Lozano-Blasco R, Quílez-Robres A, Delgado-Bujedo D, Latorre-Martínez MP (2021) YouTube’s growth in use among children 0–5 during COVID19: the occidental European case. Technol Soc 66:101648. https://doi.org/10.1016/j.techsoc.2021.101648
Machado JC, Martins CC, Ferreira FC, Silva SC, Duarte PA (2020) Motives to engage with sports brands on Facebook and Instagram—the case of a Portuguese football club. Int J Sports Mark Spons 21(2):325–349. https://doi.org/10.1108/IJSMS-06-2019-0066
Maderer D, Parganas P, Anagnostopoulos C (2018) Brand-image communication through social media: the case of European professional football clubs. Int J Sport Commun 11(3):319–338. https://doi.org/10.1123/ijsc.2018-0086
Malarvizhi CA, Al Mamun A, Jayashree S, Naznen F, Abir T (2022) Modelling the significance of social media marketing activities, brand equity and loyalty to predict consumers’ willingness to pay premium price for portable tech gadgets. Heliyon 8(8):e10145. https://doi.org/10.1016/j.heliyon.2022.e10145
Mastromartino B, Naraine ML (2022) (Dis)innovative digital strategy in professional sport: examining sponsor leveraging through social media. Int J Sports Mark Spons 23(5):934–949. https://doi.org/10.1108/IJSMS-02-2021-0032
Meng MD, Stavros C, Westberg K (2015) Engaging fans through social media: implications for team identification. Sport Bus Manag 5(3):199–217. https://doi.org/10.1108/SBM-06-2013-0013
Mizrahi I (2023) The Messi effect—how one single player will impact soccer in America. Forbes. https://www.forbes.com/sites/isaacmizrahi/2023/06/20/the-messi-effect--how-one-single-player-will-impact-soccer-in-america/?sh=45e4bda86ecf
Möller K, Halinen A (2000) Relationship marketing theory: its roots and direction. J Mark Manag 16(1–3):29–54. https://doi.org/10.1362/026725700785100460
Nisar TM, Prabhakar G, Patil PP (2018) Sports clubs’ use of social media to increase spectator interest. Int J Inf Manag 43:188–195. https://doi.org/10.1016/j.ijinfomgt.2018.08.003
Núñez-Barriopedro E, Cuesta-Valiño P, Gutiérrez-Rodríguez P, Ravina-Ripoll R (2021) How does happiness influence the loyalty of karate athletes? A model of structural equations from the constructs: consumer satisfaction, engagement, and meaningful. Front Psychol 12:653034. https://doi.org/10.3389/fpsyg.2021.653034
O’Shea M, Alonso AD (2011) Opportunity or obstacle? A preliminary study of professional sport organisations in the age of social media. Int J Sport Manag Mark 10(3-4):196–212. https://doi.org/10.1504/IJSMM.2011.044790
Oviedo MÁ, Muñoz M, Castellanos M, Sancho-Mejías M (2014) Metric proposal for customer engagement in Facebook. J Res Interact Mark 8(4):327–344. https://doi.org/10.1108/JRIM-05-2014-0028
Parganas P, Anagnostopoulos C (2015) Social media strategy in professional football: the case of Liverpool FC. Choregia 11(2):61–75. https://doi.org/10.4127/ch.2015.0102
Pegoraro A (2010) Look who’s talking-athletes on Twitter: a case study. Int J Sport Commun 3(4):501–514. https://doi.org/10.1123/ijsc.3.4.501
Pegoraro A, Scott O, Burch LM (2017) Strategic use of Facebook to build brand awareness. Int J Public Adm Digit Age 4(1):69–87. https://doi.org/10.4018/ijpada.2017010105
Petersen-Wagner R, Ludvigsen JAL (2022) Digital transformations in a platform society: a comparative analysis of European football leagues as YouTube complementors. Convergence 29(5):1330–1351. https://doi.org/10.1177/13548565221132705
PWC (2020) La industria deportiva aporta el 3,3% del PIB español y genera 414.000 puestos de trabajo. https://www.pwc.es/es/sala-prensa/notas-prensa/2020/industria-deportiva-pib-espanol.html
Ramos RF, Rita P, Moro S (2019) From institutional websites to social media and mobile applications: A usability perspective Eur Res Manag Bus. Econ 25:138–143. https://doi.org/10.1016/j.iedeen.2019.07.001
Ratten V (2020) Sport technology: a commentary. J High Technol Manag Res 31(1):100383. https://doi.org/10.1016/j.hitech.2020.100383
Rehman S ul, Gulzar R, Aslam W (2022) Developing the integrated marketing communication (IMC) through social media (SM): the modern marketing communication approach. SAGE Open 12(2) https://doi.org/10.1177/21582440221099936
Rohden SF, Tassinari G, Netto CF (2023) Listen as much as you want: the antecedents of the engagement of podcast consumers. Int J Internet Mark Advert 18(1):82–97. https://doi.org/10.1504/IJIMA.2023.128152
Sanz-Labrador I, Cuerdo-Mir M, Doncel-Pedrera LM (2021) The use of digital educational resources in times of COVID-19. Soc Media Soc 7(3) https://doi.org/10.1177/20563051211049246
Stegmann P, Nagel S, Ströbel T (2021) The digital transformation of value co-creation: a scoping review towards an agenda for sport marketing research. Eur Sport Manag Q 23(3):1221–1248. https://doi.org/10.1080/16184742.2021.1976241
Sharpe S, Mountifield C, Filo K (2020) The social media response from athletes and sport organizations to COVID-19: an altruistic tone. Int J Sport Commun 13(3):474–483. https://doi.org/10.1123/ijsc.2020-0220
Sheldon P, Antony MG, Ware LJ (2021) Baby Boomers’ use of Facebook and Instagram: uses and gratifications theory and contextual age indicators. Heliyon 7(4):e06670. https://doi.org/10.1016/j.heliyon.2021.e06670
Shilbury D, Westerbeek H, Quick S, Funk D, Karg A (2014) Strategic sport marketing. Sport Manag Rev 18(4):627–628. https://doi.org/10.1016/j.smr.2014.09.004
Solanellas F, Muñoz J, Romero-Jara E (2022) Redes sociales y el caso de las ligas deportivas durante el COVID-19. Movimento 28:e28049. https://doi.org/10.22456/1982-8918.123802
Su Y, Baker BJ, Doyle JP, Yan M (2020) Fan engagement in 15 seconds: Athletes’ relationship marketing during a pandemic via TikTok. Int J Sport Commun 13(3):436–446. https://doi.org/10.1123/ijsc.2020-0238
Su Y, Du J, Biscaia R, Inoue Y (2022) We are in this together: sport brand involvement and fans’ well-being. Eur Sport Manag Q 22(1):92–119. https://doi.org/10.1080/16184742.2021.1978519
Tafesse W, Wien A (2018) Implementing social media marketing strategically: an empirical assessment. J Mark Manag 34(9–10):732–749. https://doi.org/10.1080/0267257X.2018.1482365
Venkat R (2023) FIFA World Cup winners: why Brazilians are unique and Germany, Italy relentless—full roll of honour. International Olympic Committee. https://olympics.com/en/news/fifa-world-cup-winners-list-champions-record
Vivar JMF (2009) Nuevos modelos de comunicación, perfiles y tendencias en las redes sociales. Comunicar 16(33):73–81. https://doi.org/10.3916/c33-2009-02-007
Wang Y, Zhou S (2015) How do sports organizations use social media to build relationships? A content analysis of NBA clubs’ Twitter use. Int J Sport Commun 8(2):133–148. https://doi.org/10.1123/ijsc.2014-0083
Waters R, Burnett E, Lamm A, Lucas J (2009) Engaging stakeholders through Social networking: how nonprofit organizations are using Facebook. Public Relat Rev 35(2):102–106. https://doi.org/10.1016/j.pubrev.2009.01.006
Williams J, Chinn SJ (2010) Meeting relationship-marketing goals through social media: a conceptual model for sport marketers. Int J Sport Commun 3(4):422–437. https://doi.org/10.1123/ijsc.3.4.422
Winand M, Belot M, Merten S, Kolyperas D (2019) International sport federations’ social media communication: a content analysis of FIFA’s Twitter account. Int J Sport Commun 12(2):209–233. https://doi.org/10.1123/ijsc.2018-0173
Witkemper C, Lim C, Waldburger A (2012) Social media and sports marketing: examining the motivations and constraints of Twitter users. Sport Mark Q 21(3):170–183
Yan G, Watanabe NM, Shapiro SL, Naraine ML, Hull K (2019) Unfolding the Twitter scene of the 2017 UEFA Champions League Final: social media networks and power dynamics. Eur Sport Manag Q 19(4):419–436. https://doi.org/10.1080/16184742.2018.1517272
Zakerian A, Sarkoohi P, Ghafouri F, Keshkar S (2022) Developing a model for athletes’ personal brands on social networks (case study: Instagram). Int J Sport Manag Mark 22(1–2):142–160. https://doi.org/10.1504/IJSMM.2022.121253
Download references
Acknowledgements
The authors would like to acknowledge the experts who contributed their excellent technical knowledge and valuable inputs to the development of this work and the Fanpage Karma platform for providing the software licence to support this research. Edgar Romero-Jara would like to acknowledge the funding support of the pre-doctoral scholarship “National Academic Excellence Scholarship Programme Carlos Antonio López (BECAL)”, granted by the Government of Paraguay. Samuel López-Carril would like to acknowledge the funding support of the postdoctoral contract “Juan de la Cierva-formación 2021” (FJC2021-0477779-I), granted by the Spanish Ministry of Science and Innovation and by the European Union through the NextGenerationEU Funds (Plan de Recuperación, Transformación y Resilencia).
Author information
Authors and affiliations.
National Institute of Physical Education of Catalonia (INEFC), University of Barcelona (UB), Barcelona, Spain
Edgar Romero-Jara
Grup d’Investigació Social i Educativa de l’Activitat Física i de l’Esport (GISEAFE), National Institute of Physical Education of Catalonia (INEFC), University of Barcelona (UB), Barcelona, Spain
Francesc Solanellas & Joshua Muñoz
Universidad de Castilla–La Mancha (UCLM), Ciudad Real, Spain
Samuel López-Carril
You can also search for this author in PubMed Google Scholar
Contributions
ER-J (corresponding author) and FS: conception and design of the work. ER-J and JM: analysis and methodology. ER-J and SL-C: literature review, interpretation of data, drafting of the work. FS: supervised this work. All authors made substantial contributions, discussed the results, revised critically for important intellectual content, and approved the final version of the work.
Corresponding author
Correspondence to Edgar Romero-Jara .
Ethics declarations
Ethical statement.
This article does not contain any studies with human participants performed by any of the authors.
Informed consent
Competing interests.
The authors declare no competing interests.
Additional information
Publisher’s note Springer Nature remains neutral with regard to jurisdictional claims in published maps and institutional affiliations.
Supplementary information
Supplementary information, rights and permissions.
Open Access This article is licensed under a Creative Commons Attribution 4.0 International License, which permits use, sharing, adaptation, distribution and reproduction in any medium or format, as long as you give appropriate credit to the original author(s) and the source, provide a link to the Creative Commons license, and indicate if changes were made. The images or other third party material in this article are included in the article’s Creative Commons license, unless indicated otherwise in a credit line to the material. If material is not included in the article’s Creative Commons license and your intended use is not permitted by statutory regulation or exceeds the permitted use, you will need to obtain permission directly from the copyright holder. To view a copy of this license, visit http://creativecommons.org/licenses/by/4.0/ .
Reprints and permissions
About this article
Cite this article.
Romero-Jara, E., Solanellas, F., Muñoz, J. et al. Connecting with fans in the digital age: an exploratory and comparative analysis of social media management in top football clubs. Humanit Soc Sci Commun 10 , 858 (2023). https://doi.org/10.1057/s41599-023-02357-8
Download citation
Received : 18 April 2023
Accepted : 06 November 2023
Published : 21 November 2023
DOI : https://doi.org/10.1057/s41599-023-02357-8
Share this article
Anyone you share the following link with will be able to read this content:
Sorry, a shareable link is not currently available for this article.
Provided by the Springer Nature SharedIt content-sharing initiative
Quick links
- Explore articles by subject
- Guide to authors
- Editorial policies

COLUMBIA UNIVERSITY IN THE CITY OF NEW YORK

Dir, Budget & Financial Analysis
- Columbia University Medical Center
- Opening on: May 22 2024
- Job Type: Officer of Administration
- Bargaining Unit:
- Regular/Temporary: Regular
- End Date if Temporary:
- Hours Per Week: 35
- Standard Work Schedule:
- Salary Range: $180,000 - $200,000
Position Summary
The Director, Budget and Financial Analysis, plays a pivotal role in supporting campus budget operations for the Columbia University Irving Medical Center (CUIMC). Reporting to the Vice President of Budget and Planning, this position is responsible for developing and monitoring central administrative unit budgets, overseeing a combined annual operating budget of approximately $800M. The Director directly manages the operating budget for certain central administrative activities and serves as the primary budget liaison to central administrative units.
the support of three analysts is responsible for the review of school and department operating budgets and results, identification of potential issues and opportunities, and summarization of findings in executive format for senior leadership review.
Responsibilities
- Under the leadership of the Vice President, manages the CUIMC budget process for schools and academic departments, which have a combined annual operating budget of approximately $3.2B. With the assistance of a team of three analytical staff, the Director reviews annual budget submissions, quarterly estimate updates, and quarterly and year-end closes. Develops and maintains budget calendars and ensures that team activities are organized to ensure timely completion of deliverables. Coordinates the tracking of overall unit financial performance, inclusive of patient care, research, education, and administration activity. Identifies trends and potential financial risks. Collaborates with specialists in patient care finance and other areas to ensure robust and nuanced financial forecasts for all components of the budget.
- Develops solutions to optimize budget development, analysis, and reporting. Manages the central unit implementation of CUIMC standards for accounting and reporting, which ensures that revenues and expenses are allocated properly to individual programs so that profit & loss and other reports can be created accurately and efficiently. Offers training and technical advice on using university and campus financial systems and tools.
- Coordinates the preparation of summaries and variance analysis for senior management. Develops and reviews materials for senior campus executives, the University’s central budget office, and University executives and Trustees.
- Designs ad hoc analyses, often combining data from financial and other systems, to provide in-depth reports and forecasts as needed.
- Responsible for special projects as required. Performs related duties and responsibilities as assigned/requested. Gains familiarity with complementary budgetary/financial functions, such as the campus capital budget, to assist with necessary analysis if required and to understand the broader finance operation.
Minimum Qualifications
- Bachelor’s degree, as well as five-seven years of progressively comprehensive experience in budgeting and financial analysis
The successful candidate will be a self-starter with a well-developed knowledge of quantitative analysis and capable of working with minimal oversight to solve a problem or meet a need. Demonstrated financial analysis skills required, including the ability to prepare detailed financial reports, analyze variances and their drivers, and explain complex financial information. Attention to detail, plus the ability to work closely and collaboratively with a diverse group of administrators is essential. Expert-level computer proficiency (Microsoft Excel and Word) is required, including an in-depth knowledge of spreadsheet functions and pivot tables.
Preferred Qualifications
Experience in higher education, an academic medical center or hospital or in an equivalent environment preferred. MPA, MBA or comparable advanced degree, or equivalent experience, is preferred.
Equal Opportunity Employer / Disability / Veteran
Columbia University is committed to the hiring of qualified local residents.
Commitment to Diversity
Columbia university is dedicated to increasing diversity in its workforce, its student body, and its educational programs. achieving continued academic excellence and creating a vibrant university community require nothing less. in fulfilling its mission to advance diversity at the university, columbia seeks to hire, retain, and promote exceptionally talented individuals from diverse backgrounds. , share this job.
Thank you - we'll send an email shortly.
Other Recently Posted Jobs
Administrative Assistant
Practice coordinator.
Refer someone to this job

- ©2022 Columbia University
- Accessibility
- Administrator Log in
Wait! Before you go, are you interested in a career at Columbia University? Sign up here!
Thank you, for sharing your information. A member of our team will reach out to you soon!

This website uses cookies as well as similar tools and technologies to understand visitors' experiences. By continuing to use this website, you consent to Columbia University's usage of cookies and similar technologies, in accordance with the Columbia University Website Cookie Notice .
Log in using your username and password
- Search More Search for this keyword Advanced search
- Latest content
- Current issue
- BMJ Journals More You are viewing from: Google Indexer
You are here
- Online First
- Impact of NICE clinical guidelines for prevention and treatment of neonatal infections on antibiotic use in very preterm infants in England and Wales: an interrupted time series analysis
- Article Text
- Article info
- Citation Tools
- Rapid Responses
- Article metrics

- Mike Saunders 1 ,
- http://orcid.org/0000-0001-5668-4227 Shalini Ojha 2 , 3 ,
- http://orcid.org/0000-0003-3295-5891 Lisa Szatkowski 2
- 1 University of Nottingham School of Medicine , Nottingham , UK
- 2 Centre for Perinatal Research , University of Nottingham School of Medicine , Nottingham , UK
- 3 Neonatal Unit , University Hospitals of Derby and Burton NHS Foundation Trust , Derby , UK
- Correspondence to Dr Lisa Szatkowski, Centre for Perinatal Research, University of Nottingham School of Medicine, Nottingham, UK; Lisa.Szatkowski{at}nottingham.ac.uk
Objective To assess the impact of publication of UK National Institute for Health and Care Excellence (NICE) guidelines on the prevention and treatment of early-onset infections (EOIs) in neonates (clinical guideline 149 (CG149), published in 2012, and its 2021 update (NG195) on antibiotic use in very preterm infants.
Design Interrupted time series analysis using data from the National Neonatal Research Database.
Setting Neonatal units in England and Wales.
Participants Infants born at 22–31 weeks’ gestation from 1 January 2010 to 31 December 2022 and survived to discharge.
Interventions Publication of CG149 (August 2012) and NG195 (April 2021).
Main outcome measures Measures of antibiotic use, aggregated by month of birth: antibiotic use rate (AUR), the proportion of care days in receipt of at least one antibiotic; percentage of infants who received ≥1 day of antibiotics on days 1–3 for EOI and after day 3 for late-onset infection (LOI); percentage who received ≥1 prolonged antibiotic course ≥5 days for EOI and LOI.
Results 96% of infants received an antibiotic during inpatient stay. AUR declined at publication of CG149, without further impact at NG195 publication. There was no impact of CG149 on the underlying trend in infants receiving ≥1 day antibiotics for EOI or LOI, but post-NG195 the monthly trend began to decline for EOI (−0.20%, −0.26 to −0.14) and LOI (−0.23%, −0.33 to −0.12). Use of prolonged antibiotic courses for EOI and LOI declined at publication of CG149 and for LOI this trend accelerated post-NG195.
Conclusions Publications of NICE guidance were associated with reductions in antibiotic use; however neonatal antibiotic exposure remains extremely high.
- Neonatology
- Epidemiology
Data availability statement
Data may be obtained from a third party and are not publicly available. National Neonatal Research Database data may be obtained from a third party with relevant approvals.
This is an open access article distributed in accordance with the Creative Commons Attribution Non Commercial (CC BY-NC 4.0) license, which permits others to distribute, remix, adapt, build upon this work non-commercially, and license their derivative works on different terms, provided the original work is properly cited, appropriate credit is given, any changes made indicated, and the use is non-commercial. See: http://creativecommons.org/licenses/by-nc/4.0/ .
https://doi.org/10.1136/archdischild-2024-326983
Statistics from Altmetric.com
Request permissions.
If you wish to reuse any or all of this article please use the link below which will take you to the Copyright Clearance Center’s RightsLink service. You will be able to get a quick price and instant permission to reuse the content in many different ways.
WHAT IS ALREADY KNOWN ON THIS TOPIC
Antibiotics are very often prescribed to preterm infants, though irrational use is associated with harm. The National Institute for Health and Care Excellence published clinical guideline 149 (CG149) in 2012 and updated guidance in 2021 (NG195), with recommendations for neonatal antibiotic prescribing. The national impact of CG149 and NG195 on antibiotic use has not been studied.
WHAT THIS STUDY ADDS
Interrupted time series analysis quantified the impact of publication of CG149 and NG195 on measures of antibiotic initiation and prolonged use. Publication of CG149 was associated with decreases in the proportion of care days infants received at least one antibiotic and exposure to a prolonged antibiotic course, though with smaller or no change for extremely premature infants and those with bacterial infection or recorded indication(s) for antibiotics. Publication of NG195 was associated with decreases in antibiotic use for late onset infection. Over 95% of preterm infants studied were exposed to antibiotics.
HOW THIS STUDY MIGHT AFFECT RESEARCH, PRACTICE OR POLICY
This study finds that publication of national guidance CG149 and NG195 was associated with reductions in neonatal antibiotic use, possibly through more conservative and consistent antibiotic prescribing. However, antibiotic use remains extremely high among preterm infants. Further research is required to find evidence-based approaches to empiric antibiotic prescribing in newborn infants to identify reliable and safe means of optimising and safely reducing avoidable antibiotic exposure.
Introduction
Neonatal infection is common and burdensome. 1 2 Clinical manifestations can be non-specific and difficult to distinguish from other pathology. 3 Preterm infants have higher incidence and mortality of early-onset infection (EOI, within 72 hours of birth) and late-onset infection (LOI, ≥72 hours from birth) than term infants 2 4 5 and have prolonged hospital stays which carry risk of healthcare-associated infection.
Neonatal antibiotic use is empirical (given prior to confirmation of infection) and based on risk and clinical assessment, 4 as investigations may yield false negative results 3 and delay could risk deterioration. 6 Antibiotics are very commonly prescribed in neonatal units 7–9 and save lives but use risks drug toxicity, side effects 4 and antibiotic resistance. Prolonged antibiotic use is associated with harm, including necrotising enterocolitis (NEC), neurological injury, LOI, 8 10–12 invasive fungal diseases 13 and disruption of gut microbiota. 3
The National Institute for Health and Care Excellence (NICE) produces and publishes evidence-based clinical guidelines in the UK. Before 2012, there was no UK national guidance on preventing and treating neonatal infections. NICE published a consensus-based clinical guideline (CG149) in August 2012 2 with recommendations for intrapartum antibiotic prophylaxis, risk factors for and possible clinical signs of EOI, and indications for investigation and empirical treatment of EOI.
CG149 had potential limitations. Preterm birth after spontaneous labour with prolonged preterm rupture of membranes necessitated antibiotic use, meaning many premature babies receive antibiotics as default. Additionally, in practice a low threshold is used to manage maternal perinatal fever as sepsis 14 which obligated neonatal antibiotic prescribing. Clinical indicators of neonatal sepsis were non-specific, 1 based on low-quality evidence 2 and risked subjective interpretation. Additionally, antibiotic course length recommendations were unclear, the impact of intrapartum antibiotic prophylaxis on neonatal management was not described, and there were no recommendations for LOI.
New guidance (NG195) superseded CG149 in April 2021, 15 which introduced recommendations for managing LOI. The main recommendations in this revision were again introduced without any evidence-base or evaluation of impact of antibiotic exposure on babies. Based on evidence from the USA, NG195 permits the alternative of using the Kaiser Permanente neonatal sepsis risk calculator 16 (KP-SRC) to inform clinical decisions on EOI management. The KP-SRC, however, is only applicable to babies born at ≥34 weeks’ gestation and the recommendations for most preterm babies therefore remained consensus based.
It is not known whether the introduction of NICE CG149 and NG195 (summarised in online supplemental figure 1 ) altered antibiotic prescribing patterns. This is pertinent to ensure antibiotics are used wisely and prevent avoidable harms. This study aims to assess their impact on measures of antibiotic use in very and extremely preterm infants admitted to neonatal units in England and Wales.
Supplemental material
Data management and analysis were conducted using R V.4.3.2 17 and Stata V.18 (Stata, College Station, Texas, USA).
Data source and study population
We used data from the UK National Neonatal Research Database (NNRD), 18 derived from the electronic patient records of all infants admitted to NHS neonatal units in England and Wales, for very preterm infants (born at 28–31 weeks gestational age, GA) and extremely preterm infants (22–27 weeks GA) born from 1 January 2010 to 31 December 2022. Infants were excluded if missing data on sex, birth weight, final discharge destination, episodes of care; died; or were discharged for ongoing care. We also excluded infants with implausible birth weight for GA z-scores >4 SD above or below the mean, admitted >24 hours after birth or born with a lethal congenital anomaly ( online supplemental table 1 ).
Outcome measures
NNRD data on antibiotic exposure in the first 14 days are accurate. 18 Antibiotic use was identified by searching the NNRD daily drugs field for character strings matching antibiotic names with intravenous preparations ( online supplemental table 2 ), identified from the British National Formulary for Children (version September 2021). 19 Prophylactic, oral and topical preparations were excluded.
We calculated several measures of antibiotic use aggregated by month of birth. The antibiotic use rate (AUR) was the aggregate percentage of inpatient days in receipt of at least one antibiotic. We calculated the percentage of infants exposed to one or more days of antibiotics initiated day 1–3 (presumed to be for EOI) and initiated day 4 or later (presumed to be for LOI), with day of birth defined as day 1. We identified the percentage of infants who received a prolonged antibiotic course (≥5 consecutive days) for EOI or LOI. LOI antibiotic prescribing was defined using a ‘washout’ period of 2 days without antibiotics to exclude a continuing EOI antibiotic course. Finally, for the first day of antibiotic prescription for EOI and LOI, we identified which antibiotic(s) were prescribed.
Statistical methods
The study period is divided into three periods: (1) before CG149 publication (January 2010–July 2012); (2) between publication of CG149 and NG195 (August 2012–March 2021); (3) after NG195 publication (April 2021–December 2022). Characteristics of the study population by period were described.
We used segmented regression to investigate the impact of publications on the outcomes. 20 The regression models estimate (1) the monthly trend in each outcome in period 1; (2) any immediate absolute change in magnitude or trend in period 2 relative to period 1 and (3) any immediate absolute change in magnitude or trend in period 3 relative to period 2.
We built a parsimonious model for each outcome through backwards elimination of non-statistically significant variables, based on a significance level of 0.05. The autocorrelation function, partial autocorrelation function and Ljung-Box test demonstrated no significant autocorrelation of model residuals.
Subgroup analysis
We conducted prespecified subgroup analyses to investigate variations in impact defined by characteristics considered likely to influence antibiotic use: (1) very versus extremely preterm infants; (2) infants with versus without evidence of bacterial infection; (3) infants with versus without a recorded antibiotic indication(s) (based on presence of diagnosis codes for bacterial infection, isolation of an antibiotic-resistant organism or NEC). We identified characteristics for subgroup analyses 2 and 3 by searching for character strings in daily and episodic diagnoses records matching a list of diagnoses ( online supplemental table 3 ). We excluded diagnoses indicating risk of infection, suspected but unconfirmed infection and infections not specific to a bacterial cause.
Type(s) of antibiotic prescribed
We plotted the most frequently prescribed antibiotic monotherapy and combinations for EOI and LOI by birth year to illustrate changes over time. We present the top seven most frequently prescribed antibiotic(s) with the remainder categorised as ‘other’.
Data were available for 97 387 infants born at 22–31 weeks GA during the study period and admitted to neonatal units in England and Wales. After exclusions ( online supplemental table 4 ), 84 626 infants were included. Table 1 describes the population by time period.
- View inline
Description of characteristics of study population
On average, 546 infants were born each month, with relatively fewer per month in period 3. The proportion of infants first admitted to a neonatal intensive care unit increased over time but there were no clinically relevant differences in median GA, birth weight, birth weight for GA z-score and length of stay.
Table 2 describes overall use of antibiotics and indications for use. Almost all infants (96.0%, n=81 278) received at least one antibiotic during their stay. The percentage of infants who had a recorded antibiotic indication, evidence of bacterial infection or NEC declined over time, but there was little difference in the percentage with an antibiotic-resistant organism.
Antibiotic use and indications for use by study period
Table 3 shows the results of the interrupted time series analysis, and figures 1 and 2 show the fitted regression lines from the parsimonious models.
- Download figure
- Open in new tab
- Download powerpoint
Changes in the overall antibiotic use rate before and after the publication of CG149 and NG195. CG149, clinical guideline 149; NG195, NICE guideline 195.
Changes in the percentage of infants who received ≥1 day, and ≥1 prolonged course, of antibiotics for EOI (A and C) and LOI (B and D). CG149, clinical guideline 149; NG195, NICE guideline 195; EOI, early-onset infection; LOI, late-onset infection.
Month-on-month absolute percentage changes (with 95% CIs) in antibiotic use before and after the publication of CG149 and NG195
The median (IQR) AUR across the study period was 19.5% (18.5–20.2) of total care days per month. In period 1, AUR was increasing by 0.03% per month. This immediately declined by 1.02% in period 2 and the trend reversed, resulting in an absolute decrease of 0.02% per month, which did not change during period 3. Over the study period, the AUR declined from 20.5% to 17.5% of total care days, which equates to approximately 1000 fewer days of antibiotic use per month (approximately 2 fewer days of antibiotics per infant) on average.
Almost all (93.4%, n=79 006) infants received antibiotics for EOI. The prevalence was stable in periods 1 and 2, though declined in period 3 by 0.20% per month. Just over half (54.0%, n=45 684) received antibiotics for LOI. Prevalence was increasing by 0.02% per month in period 1 and did not change until period 3, where it declined by 0.23% per month.
Approximately one-third (35.7%, n=30 201) of infants received a prolonged EOI antibiotic course. The prevalence dropped by 1.87% in period 2 and continued to decline by 0.08% per month thereafter. Similarly, one-third (32.9%, n=27 796) were exposed to a prolonged LOI antibiotic course. Prevalence initially increased by 0.12% per month but immediately declined by 0.02% per month in period 2, accelerating to a decline of 0.14% per month in period 3.
Online supplemental table 5 and online supplemental figure 2 show the subgroup analyses. The number of infants born per month in some subgroups was small, resulting in greater monthly outcome variability and reduced power to detect small changes.
Extreme prematurity, bacterial infection and a recorded antibiotic indication were associated with a higher AUR and exposure to antibiotic(s) for ≥1 day, or ≥1 prolonged course, than the respective comparison subgroup.
Very premature infants observed a greater reduction in AUR, EOI antibiotic exposure and prolonged LOI exposure than extremely premature infants in period 2. During period 3, extremely premature babies observed greater reductions in AUR and EOI and LOI antibiotic exposure than very premature babies.
In period 2, the trend in AUR reduced for infants without bacterial infection, and EOI and LOI antibiotic use immediately reduced for infants with evidence of bacterial infection, but without a change in trend. Over the same period, infants without infection saw a temporary increase in EOI antibiotic use with a reduction in trend and no changes in LOI antibiotic use.
Figure 3 shows changes over time in the type of antibiotics prescribed on the first day of prescribing for EOI and LOI (full data in online supplemental table 6 ). For EOI, the percentage of infants receiving benzylpenicillin plus gentamicin increased from 72.3% in 2010 to 83.0% in 2014, remaining relatively stable since. Prescribing for LOI was more variable. Flucloxacillin plus gentamicin was the most frequent combination, received by 28.2% of infants, followed by cefotaxime and vancomycin (7.6%).
Type and combination of antibiotics prescribed on the first day of prescribing for EOI (A) and LOI (B), by year of birth. EOI, early-onset infection; LOI, late-onset infection.
We found that publication of NICE guidance in 2012 (CG149) and its update in 2021 (NG195) were associated with some reductions in antibiotic use for extremely and very preterm infants in England and Wales, resulting in more consistent antibiotic use, with defined course lengths. This may explain the temporary changes in antibiotic prescribing for LOI seen in 2012, with sustained decreasing trends only seen following the update of the guidance in 2021 when recommendations on antibiotic use for LOI were first introduced.
Choice of antibiotics for EOI has become increasingly consistent with NICE guidance. However, choice for LOI continues to vary substantially, perhaps due to omission of recommendations for LOI in 2012 and only a broad recommendation to use ‘a combination of narrow-spectrum antibiotics’ in 2021. 15
This is the first national evaluation of the impact of NICE guidelines on antibiotic use in preterms, though other studies have investigated the impact on term and near-term infants. 21 22 Almost all extremely and very preterm infants received antibiotics, reiterating that neonatal units are a priority setting to reduce avoidable antibiotic use.
For EOI antibiotic use in term and near-term infants, NICE now recommends an alternative approach, the KP-SRC. 16 While NICE standard recommendations are largely opinion based, the KP-SRC is based on a multivariable risk prediction model using data from large cohorts of infants born at ≥35 weeks’ gestation in California, USA. 16 The KP-SRC and other strategies recommended by the US American Academy of Paediatics, 23 similar to NICE, identify risk factors for EOI and clinical indicators of illness and need for repeat observations. The KP-SRC has been widely adopted in the UK and observational studies show large reductions in antibiotic use and laboratory testing compared with NICE 2012 (Goel 24 ) but there is a concern that more conservative use of antibiotics and fewer babies screened for EOI incurs a risk of missing a significant number of infants with infection. 25 Use of the KP-SRC has been subjected to numerous observational studies in the UK, but there is no randomised comparison with NICE 2021, although an ongoing trial is comparing a similar approach to NICE 2021 with the KP-SRC in the Netherlands. 26 It remains a strong priority to compare the approaches, to reduce antibiotic use without risk of delayed treatment leading to harm. Furthermore, the KP-SRC is designed for ≥35 weeks’ gestation and most premature infants, who are both at a higher risk of infection and disproportionately affected by antibiotic associated harm, are excluded. The only guidance in the UK for this population is the NICE recommendations, highlighting the need for developing an evidence-based approach to rationalise antibiotic use in this group.
Preterm infants often have clinical indicators of possible EOI as listed in the 2021 NICE guidance (eg, apnoea, feeding difficulty, temperature instability) due to prematurity and related comorbidities. Both iterations of NICE guidance combine recommendations for mature and preterm infants, overlooking the impact of prematurity on clinical presentation. Consequently, almost all very and extremely preterm infants receive antibiotics which may cause harm in the short term, such as increased risk of NEC, and via alterations in gut microbiome, impact long-term health of survivors of prematurity.
Interrupted time series analysis is the strongest quasi-experimental study design to evaluate the impact of national guidance over time. 20 There were few substantial changes in the study population case-mix over time, reducing the likelihood of confounding. This analysis assumed an instantaneous impact of guidance on antibiotic use, though this may not reflect reality. Anticipatory and lag effects were not assessed given the absence of literature to inform a defined time period of potential effect. It is also unclear to what extent NICE guidance is consistently implemented. An attempt to assess guidance compliance in did not specifically assess antibiotic prescribing. 27 Adherence to a similar guideline in the Netherlands was low, 28 especially non-prescribing. Similarly, German neonatal units also found gaps between national guidance and practice. 29
While our findings demonstrate a temporal association between guidance publication and antibiotic use, we cannot infer causality. This segmented regression approach assumes preintervention trends would have continued without intervention, which cannot be verified. Changes in trends may be alternatively explained by other contemporaneous changes in practice or interventions, such as local quality improvement projects.
From NNRD data, it is challenging to distinguish suspected from confirmed infection, and indications for prescribing are not recorded. Excluding infants who died, who may have had very high AURs which would have skewed the overall measure, likely under-reports the full extent of neonatal antibiotic prescribing.
Reducing avoidable antibiotic prescribing can prevent antibiotic-associated harm, antibiotic resistance and health system costs. 30 Future guidance must consider the impact of prematurity on presentation and treatment to avoid antibiotic prescribing in preterm infants in situations where risk exceeds benefit. We corroborate international findings of overall reductions in antibiotic use though neonatal antibiotic use remains extremely high. It will be essential to monitor the impact of NG195 on antibiotic prescribing and apply findings to guidance development.
Ethics statements
Patient consent for publication.
Not applicable.
Ethics approval
The NNRD has NHS Research Ethics Committee (REC) approval for compilation of electronic patient record data from individual neonatal units (ref: 16/LO/1930). Parents are able to withdraw consent for inclusion of their child’s data in the NNRD. Ethics approval for this study was granted by the Yorkshire & The Humber Leeds East REC (18/YH/0209), the South East Scotland REC01 (IRAS323099) and the Health Research Authority and Health and Care Research Wales (IRAS323099).
Acknowledgments
Electronic patient data recorded at participating neonatal units that collectively form the UK Neonatal Collaborative are transmitted to the Neonatal Data Analysis Unit to form the National Neonatal Research Database (NNRD). We are grateful to all the families that agreed to the inclusion of their baby’s data in the NNRD, the health professionals who recorded data and the Neonatal Data Analysis Unit team. This project is funded by the National Institute for Health and Care Research (NIHR) under its Research for Patient Benefit (RfPB) Programme (Grant Reference Number NIHR203590). The views expressed are those of the author(s) and not necessarily those of the NIHR or the Department of Health and Social Care.
- Caffrey Osvald E ,
- National Institute for Health and Care Excellence
- Klingenberg C ,
- Kornelisse RF ,
- Buonocore G , et al
- Mukhopadhyay S ,
- Sengupta S ,
- Kortsalioudaki C ,
- Buttery J , et al
- Vergnano S ,
- Kortsalioudaki C , et al
- Al-Turkait A ,
- Szatkowski L ,
- Choonara I , et al
- Roberts A , et al
- Bancalari A , et al
- Roberts A ,
- Sherlock R , et al
- Kuppala VS ,
- Meinzen-Derr J ,
- Morrow AL , et al
- Cotten CM ,
- McDonald S ,
- Stoll B , et al
- Fitzgerald DJ ,
- Knowles SJ ,
- Downey P , et al
- Kuzniewicz MW ,
- Puopolo KM ,
- Fischer A , et al
- R Core Team
- Battersby C ,
- Statnikov Y ,
- Santhakumaran S , et al
- Wagner AK ,
- Soumerai SB ,
- Zhang F , et al
- Mukherjee A ,
- Davidson L ,
- Anguvaa L , et al
- Macaskill L ,
- van Hasselt TJ , et al
- Benitz WE ,
- Zaoutis TE , et al
- Cannell S ,
- Davies G , et al
- Achten NB ,
- Klingenberg C , et al
- van der Weijden BM ,
- van der Weide MC ,
- Plötz FB , et al
- Ramalingaiah B ,
- Kennea N , et al
- Bekhof J , et al
- Goedicke-Fritz S ,
- Härtel C , et al
- Flannery DD ,
Supplementary materials
Supplementary data.
This web only file has been produced by the BMJ Publishing Group from an electronic file supplied by the author(s) and has not been edited for content.
- Data supplement 1
X @DrMikePSaunders, @shaliniojha7
Contributors MS designed and conceptualised the study, analysed and interpreted the data and drafted and revised the manuscript. LS designed and conceptualised the study, participated in analysis and interpretation of data, drafted and revised the manuscript, and is guarantor. SO designed and conceptualised the study, participated in interpretation of data and revised the manuscript.
Funding National Institute for Health and Care Research, Research for Patient Benefit Programme, NIHR203590.
Competing interests None declared.
Provenance and peer review Not commissioned; externally peer reviewed.
Supplemental material This content has been supplied by the author(s). It has not been vetted by BMJ Publishing Group Limited (BMJ) and may not have been peer-reviewed. Any opinions or recommendations discussed are solely those of the author(s) and are not endorsed by BMJ. BMJ disclaims all liability and responsibility arising from any reliance placed on the content. Where the content includes any translated material, BMJ does not warrant the accuracy and reliability of the translations (including but not limited to local regulations, clinical guidelines, terminology, drug names and drug dosages), and is not responsible for any error and/or omissions arising from translation and adaptation or otherwise.
Read the full text or download the PDF:
Research Design Review
A discussion of qualitative & quantitative research design, unit of analysis, qualitative data analysis: the unit of analysis.

As discussed in two earlier articles in Research Design Review (see “The Important Role of ‘Buckets’ in Qualitative Data Analysis” and “Finding Connections & Making Sense of Qualitative Data” ), the selection of the unit of analysis is one of the first steps in the qualitative data analysis process. The “unit of analysis” refers to the portion of content that will be the basis for decisions made during the development of codes. For example, in textual content analyses, the unit of analysis may be at the level of a word, a sentence (Milne & Adler, 1999), a paragraph, an article or chapter, an entire edition or volume, a complete response to an interview question, entire diaries from research participants, or some other level of text. The unit of analysis may not be defined by the content per se but rather by a characteristic of the content originator (e.g., person’s age), or the unit of analysis might be at the individual level with, for example, each participant in an in-depth interview (IDI) study treated as a case. Whatever the unit of analysis, the researcher will make coding decisions based on various elements of the content, including length, complexity, manifest meanings, and latent meanings based on such nebulous variables as the person’s tone or manner.
Deciding on the unit of analysis is a very important decision because it guides the development of codes as well as the coding process. If a weak unit of analysis is chosen, one of two outcomes may result: 1) If the unit chosen is too precise (i.e., at too much of a micro-level than what is actually needed), the researcher will set in motion Read Full Text
Share this:
- Click to share on Reddit (Opens in new window)
- Click to share on Twitter (Opens in new window)
- Click to share on LinkedIn (Opens in new window)
- Click to share on Facebook (Opens in new window)
- Click to share on Tumblr (Opens in new window)
- Click to email a link to a friend (Opens in new window)
- Click to print (Opens in new window)

- Already have a WordPress.com account? Log in now.
- Subscribe Subscribed
- Report this content
- View site in Reader
- Manage subscriptions
- Collapse this bar

IMAGES
VIDEO
COMMENTS
Units of Analysis and Methodologies for Qualitative Studies. Identifying & Planning Research. Jan 29, 2024. By Janet Salmons, PhD Manager, Sage Research Methods Community. Selecting the methodology is an essential piece of research design. This post is excerpted and adapted from Chapter 2 of Doing Qualitative Research Online (2022).
A unit of analysis is the thing you want to discuss after your research, probably what you would regard to be the primary emphasis of your research. The researcher plans to comment on the primary topic or object in the research as a unit of analysis. The research question plays a significant role in determining it.
Qualitative Data Analysis: The Unit of Analysis. The following is a modified excerpt from Applied Qualitative Research Design: A Total Quality Framework Approach (Roller & Lavrakas, 2015, pp. 262-263). As discussed in two earlier articles in Research DesignReview (see "The Important Role of 'Buckets' in Qualitative Data Analysis" and ...
The unit of analysis in research refers to the level at which data is collected and analyzed. It is essential for researchers to understand the different types of units of analysis, as well as their significance in shaping the research process and outcomes. ... The theoretical framework and research design establish the structure for a study ...
The unit of analysis is a way to understand and study a phenomenon. There are four main types of unit of analysis: individuals, groups, artifacts (books, photos, newspapers), and geographical units (towns, census tracts, states). Individuals are the smallest level of analysis. For example, an individual may be a person or an animal.
The unit of analysis refers to the main parameter that you're investigating in your research project or study. Example of the different types of unit analysis that may be used in a project include: Individual people. Groups of people. Objects such as photographs, newspapers and books. Geographical unit based on parameters such as cities or ...
The unit of analysis refers to the person, collective, or object that is the target of the investigation. Typical unit of analysis include individuals, groups, organizations, countries, technologies, objects, and such. For instance, if we are interested in studying people's shopping behavior, their learning outcomes, or their attitudes to new ...
This involves that the unit(s) of analysis is(/are) defined 'in part by the study's object, in part by the researcher's focus, in part by the audience of the research and in part by the research participants (as distinct from the research object)' (Matusov, 2007, p. 325). This opens an array of possibilities for analyses that take into ...
The rationale for using studies as the unit of analysis is two-fold: First, we can only include the same study sample once in a review. Including more than one article from the same study in a review, treating each article as a separate study, introduces bias into the review. That particular sample would be given undue weight in the synthesis ...
The unit of analysis is the entity that frames what is being looked at in a study, or is the entity being studied as a whole. In social science research, at the macro level, the most commonly referenced unit of analysis, considered to be a society is the state (polity) (i.e. country). At meso level, common units of observation include groups, organizations, and institutions, and at micro level ...
De Vaus (2001, p. 220) posits that the 'unit of analysis' in a case study research can be an individual, a family, a household, a community, an organisation, an event or even a decision. ... Further, a good research design channelises the energy and time of the researcher in the right direction, and keeps them focussed throughout the ...
A unit of analysis is the item you wish to be able to say something about at the end of your study while a unit of observation is the item that you actually observe. When researchers confuse their units of analysis and observation, they may be prone to committing either the ecological fallacy or reductionism.
The rationale for using studies as the unit of analysis is two-fold: First, we can only include the same study sample once in a review. Including more than one article from the same study in a review, treating each article as a separate study, introduces bias into the review. That particular sample would be given undue weight in the synthesis ...
The units of analysis for both would be individuals. Another common unit of analysis in sociological inquiry is groups. Groups of course vary in size, and almost no group is too small or too large to be of interest to sociologists. Families, friendship groups, and street gangs make up some of the more common groups examined by sociologists.
Multilevel unit of analysis allows researchers to investigate the association and interaction between variables at different units or levels. Quality of life (QoL) research has become more advanced. QoL research often involves investigating the relationships and interactions between individuals and the group to which they belong. Individuals ...
This paper addresses the appropriate unit of analysis in field research. We first discuss the issues related to this topic: (a) unit of measurement versus unit of analysis, (b) treatments and random assignment, (c) independence of observations, (d) moderating and control variables, and (e) correlational versus experimental research. We then present a model for determining the correct unit of ...
In sum, there are many potential units of analysis that a sociologist might examine, but some of the most common units include the following: Individuals; Groups; Organizations; Social phenomena. Policies and principles. Table 4.1 Units of analysis and units of observation: A hypothetical study of students' addictions to cell phones.
We would like to show you a description here but the site won't allow us.
The unit of analysis is the major entity that you are analyzing in your study. For instance, any of the following could be a unit of analysis in a study: individuals. groups. artifacts (books, photos, newspapers) geographical units (town, census tract, state) social interactions (dyadic relations, divorces, arrests)
Unit of Analysis. CHAPTER 5 Research Design and Methodology. As suggested by Yin (2009: 27), the case study research necessitates five essential components: 'a study's questions', 'its propositions, if any', 'its unit (s) of analysis', 'the logic. linking the data to the propositions' and 'the criteria for interpreting the ...
The relat ions bet ween t he unit of analysis and ot her r elat ed concept s are described, and possible analysis alt ernat ives in case of violat ion of independence assum pt ion are briefly discussed. Ke yw or ds: Unit of analysis, observat ional unit , t he assum pt ion of independence of observat ions, hierarchical linear m odeling.
The relations between the unit of analysis and other related concepts are described, and possible analysis alternatives in case of violation of independence assumption are briefly discussed. In this paper the importance of the unit of analysis in research design is emphasized. The relations between the unit of analysis and other related concepts are described, and possible analysis ...
Lastly, the comparative aspect of the research design (Andrew et al., 2011) is valuable in this study because it enables a cross-regional analysis of three of the most traditional social media ...
Job Type: Officer of Administration Bargaining Unit: Regular/Temporary: Regular End Date if Temporary: Hours Per Week: 35 Standard Work Schedule: Building: Salary Range: $180,000 - $200,000 The salary of the finalist selected for this role will be set based on a variety of factors, including but not limited to departmental budgets, qualifications, experience, education, licenses, specialty ...
Design Interrupted time series analysis using data from the National Neonatal Research Database. Setting Neonatal units in England and Wales. Participants Infants born at 22-31 weeks' gestation from 1 January 2010 to 31 December 2022 and survived to discharge. Interventions Publication of CG149 (August 2012) and NG195 (April 2021).
Comparative analyses with state-of-the-art models showcase the superior performance of our approach, with an accuracy improvement of 6% on MVSA-Single and 1.6% on MVSA-Multi. This research significantly contributes to optimizing SA in social media data by offering a versatile and potent framework for data management and analysis.
The "unit of analysis" refers to the portion of content that will be the basis for decisions made during the development of codes. For example, in textual content analyses, the unit of analysis may be at the level of a word, a sentence (Milne & Adler, 1999), a paragraph, an article or chapter, an entire edition or volume, a complete ...
Additionally, they reveal boundaries of the layer and column numbers for constructing the revolved body and highlight the impact of the aspect ratio and configuration of the waterbomb units on the formability of the revolved body. This method can provide insights for origami-based robot research and can be extended to model other origami patterns.
First, the weights of the information indicators of different links of the industrial equipment information traceability system were calculated using the EAHP hierarchical analysis method. The manufacturing link had the largest weight, with a value of 18.8%. Second, the system's functional module design is based on the weights.
The natural gas pipeline system (NGPS) is a complex network of units that is susceptible to potential random failures. Moreover, the environment in which the NGPS operates is characterized by complexity and uncertainty, including market volatility, natural disasters, and deliberate sabotage. While research on the NGPS has mainly focused on supply reliability through methods such as statistical ...