A case study of scientific reasoning
- Published: December 1993
- Volume 23 , pages 199–207, ( 1993 )

Cite this article
- Campbell McRobbie 1 &
- Lyn English 1
147 Accesses
Explore all metrics
Concern is increasingly being expressed about the teaching of higher order thinking skills in schools and the levels of understanding of scientific concepts by students. Metaphors for the improvement of science education have included science as exploration and science as process skills for experimentation. As a result of a series of studies on how children relate evidence to their theories or beliefs, Kuhn (1993a) has suggested that changing the metaphor to science as argument may be a fruitful way to increase the development of higher order thinking skills and understanding in science instruction. This report is of a case study into the coordination of evidence and theories by a grade 7 primary school student. This student was not able to coordinate these elements in a way that would enable her to rationally consider evidence in relation to her theories. It appeared that the thinking skills associated with science as argument were similar for her in different domains of knowledge and context.
This is a preview of subscription content, log in via an institution to check access.
Access this article
Price includes VAT (Russian Federation)
Instant access to the full article PDF.
Rent this article via DeepDyve
Institutional subscriptions
Similar content being viewed by others
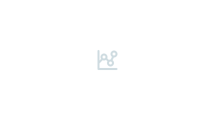
Science Teachers’ Views of Argument in Scientific Inquiry and Argument-Based Science Instruction
Aeran Choi, Elsun Seung & DaEun Kim
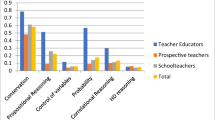
Scientific Reasoning Among Teachers and Teacher Trainees: the Case in Ethiopian Schools and Teacher Training Colleges
Dawit Asrat Getahun
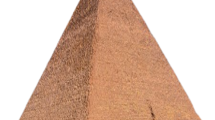
When Science Is Taught This Way, Students Become Critical Friends: Setting the Stage for Student Teachers
Paul Nnanyereugo Iwuanyanwu
Ash, A., Torrance, N., & Olson, D. (1993, April). The development of children's understanding of necessary and sufficient evidence. Paper presented at the annual conference of the American Educational Research Association, Atlanta, Georgia.
Carey, S. (1986). Cognitive science and science education. American Psychologist, 41 , 1123–1130.
Article Google Scholar
Chinn, C.A., & Brewer, W.F. (1993). The role of anomalous data in knowledge acquisition: A theoretical framework and implications for science instruction. Review of Educational Research, 63 (1), 1–49.
Google Scholar
Duschl, R. A., & Gitomer, D. H. (1991). Epistemological perspectives on conceptual change: Implications for educational practice. Journal of Research in Science Teaching, 28 , 839–858.
Galotti, K. M. (1989). Approaches to studying formal and everyday reasoning. Psychological Bulletin, 105 , 331–351.
Glaser, R. (1984). Education and thinking: The role of knowledge. American Psychologist, 39 (2), 93–104.
Holland, J.H., Holyoak, K.J., Nisbett, R.E., & Thagard, P.R. (1986). Induction: Processes inference, learning, and discovery , Cambridge, MA: The MIT Press.
Inhelder, B., & Piaget, J. (1958). The growth of logical thinking from childhood to adolescence . New York: Basic Books.
Klahr, D., & Dunbar, K. (1988). Dual space search during scientific reasoning. Cognitive Science, 12 , 1–48.
Kuhn, D. (1989). Children and adults as intuitive scientists. Psychological Review, 96 (4), 674–689.
Kuhn, D. (1993a). Connecting scientific and informal reasoning. Merrill-Palmer Quarterly, 39 , 74–103.
Kuhn, D. (1993b). Science as argument: Implications for teaching and learning scientific thinking. Science Education, 77 (3), 319–337.
Kuhn, D., Amsel, E., & O'Loughlin, M. (1988). The development of scientific thinking Skills . New York: Academic Press.
Kuhn, D., Schauble, L., & Garcia-Mila, M. (1992). Cross-domain development of scientific reasoning. Cognition and Instruction, 9 (4), 285–327.
Linn, M.C., & Songer, N.B. (1993). How do students make sense of science? Merrill-Palmer Quarterly, 39 , 47–73.
Mayer Committee. (1992). Employment-related key competencies: A proposal for consultation . Melbourne: Australian Education Council.
O'Brien, D. (1987). The development of conditional reasoning: An iffy proposition. In H. Reese (Ed.), Advances in Child Development and Behaviour (Vol. 20, pp. 61–90). Orlando, FL: Academic Press.
Reif, F., & Larkin, J.H. (1991). Cognition in scientific and everyday domains: Comparison and learning implications. Journal of Research in Science Teaching, 28 (9), 733–760.
Schauble, L. (1990). Belief revision in children: The role of prior knowledge and strategies for generating evidence. Journal of Experimental Child Psychology, 49 31–57.
Schauble, L., Klopfer, L., & Raghavan, K. (1991). Students' transition from an engineering model to a science model of experimentation. Journal of Research in Science Teaching, 28 , 859–882.
Sodian, B., Zaitchik, D., & Carey, S. (1991). Young children's differentiation of hypothetical beliefs from evidence. Child Development, 62 , 753–766.
Tobin, K., & Gallagher, J. (1987). What happens in high school science classrooms? Journal of Curriculum Studies, 19 , 549–560.
Download references
Author information
Authors and affiliations.
Centre for Mathematics and Science Education, Queensland University of Technology, Locked Bag 2, Red Hill, 4059, Brisbane, QLD
Campbell McRobbie ( Acting Director ) & Lyn English ( Associate Professor )
You can also search for this author in PubMed Google Scholar
Additional information
Specializations : science learning, scientific reasoning, learning environments, science teacher education.
Specializations : cognition, reasoning in science and mathermatics.
Rights and permissions
Reprints and permissions
About this article
McRobbie, C., English, L. A case study of scientific reasoning. Research in Science Education 23 , 199–207 (1993). https://doi.org/10.1007/BF02357061
Download citation
Issue Date : December 1993
DOI : https://doi.org/10.1007/BF02357061
Share this article
Anyone you share the following link with will be able to read this content:
Sorry, a shareable link is not currently available for this article.
Provided by the Springer Nature SharedIt content-sharing initiative
- Primary School
- Science Education
- Scientific Concept
- Thinking Skill
- Scientific Reasoning
- Find a journal
- Publish with us
- Track your research
Open Access is an initiative that aims to make scientific research freely available to all. To date our community has made over 100 million downloads. It’s based on principles of collaboration, unobstructed discovery, and, most importantly, scientific progression. As PhD students, we found it difficult to access the research we needed, so we decided to create a new Open Access publisher that levels the playing field for scientists across the world. How? By making research easy to access, and puts the academic needs of the researchers before the business interests of publishers.
We are a community of more than 103,000 authors and editors from 3,291 institutions spanning 160 countries, including Nobel Prize winners and some of the world’s most-cited researchers. Publishing on IntechOpen allows authors to earn citations and find new collaborators, meaning more people see your work not only from your own field of study, but from other related fields too.
Brief introduction to this section that descibes Open Access especially from an IntechOpen perspective
Want to get in touch? Contact our London head office or media team here
Our team is growing all the time, so we’re always on the lookout for smart people who want to help us reshape the world of scientific publishing.
Home > Books > Current Topics in Children's Learning and Cognition
The Emergence of Scientific Reasoning
Submitted: 31 March 2012 Published: 14 November 2012
DOI: 10.5772/53885
Cite this chapter
There are two ways to cite this chapter:
From the Edited Volume
Current Topics in Children's Learning and Cognition
Edited by Heidi Kloos, Bradley J. Morris and Joseph L. Amaral
To purchase hard copies of this book, please contact the representative in India: CBS Publishers & Distributors Pvt. Ltd. www.cbspd.com | [email protected]
Chapter metrics overview
4,944 Chapter Downloads
Impact of this chapter
Total Chapter Downloads on intechopen.com

Total Chapter Views on intechopen.com
Overall attention for this chapters
Author Information
Bradley j. morris.
- Kent State University, USA
Steve Croker
- Illinois State University, USA
Corinne Zimmerman
Amy m. masnick.
- Hofstra University, USA
*Address all correspondence to:
1. Introduction
Scientific reasoning encompasses the reasoning and problem-solving skills involved in generating, testing and revising hypotheses or theories, and in the case of fully developed skills, reflecting on the process of knowledge acquisition and knowledge change that results from such inquiry activities. Science, as a cultural institution, represents a “hallmark intellectual achievement of the human species” and these achievements are driven by both individual reasoning and collaborative cognition ( Feist, 2006 , p. ix).
Our goal in this chapter is to describe how young children build from their natural curiosity about their world to having the skills for systematically observing, predicting, and understanding that world. We suggest that scientific reasoning is a specific type of intentional information seeking, one that shares basic reasoning mechanisms and motivation with other types of information seeking ( Kuhn, 2011a ). For example, curiosity is a critical motivational component that underlies information seeking ( Jirout & Klahr, 2012 ), yet only in scientific reasoning is curiosity sated by deliberate data collection and formal analysis of evidence. In this way, scientific reasoning differs from other types of information seeking in that it requires additional cognitive resources as well as an integration of cultural tools. To that end, we provide an overview of how scientific reasoning emerges from the interaction between internal factors (e.g., cognitive and metacognitive development) and cultural and contextual factors.
The current state of empirical research on scientific reasoning presents seemingly contradictory conclusions. Young children are sometimes deemed “little scientists” because they appear to have abilities that are used in formal scientific reasoning (e.g., causal reasoning; Gopnik et al., 2004 ). At the same time, many studies show that older children (and sometimes adults) have difficulties with scientific reasoning. For example, children have difficulty in systematically designing controlled experiments, in drawing appropriate conclusions based on evidence, and in interpreting evidence (e.g., Croker, 2012 ; Chen & Klahr, 1999 ; Kuhn, 1989 ; Zimmerman, 2007 ).
In the following account, we suggest that despite the early emergence of many of the precursors of skilled scientific reasoning, its developmental trajectory is slow and requires instruction, support, and practice. In Section 2of the chapter, we discuss cognitive and metacognitive factors. We focus on two mechanisms that play a critical role in all cognitive processes (i.e., encoding and strategy acquisition/selection). Encoding involves attention to relevant information; it is foundational in all reasoning. Strategy use involves intentional approaches to seeking new knowledge and synthesizing existing knowledge. These two mechanisms are key components for any type of intentional information seeking yet follow a slightly different development trajectory in the development of scientific reasoning skills. We then discuss the analogous development of metacognitive awareness of what is being encoded, and metastrategic skills for choosing and deploying hypothesis testing and inference strategies. In Section 3, we describe the role of contextual factors such as direct and scaffolded instruction, and the cultural tools that support the development of the cognitive and metacognitive skills required for the emergence of scientific thinking.
2. The development of scientific reasoning
Effective scientific reasoning requires both deductive and inductive skills. Individuals must understand how to assess what is currently known or believed, develop testable questions, test hypotheses, and draw appropriate conclusions by coordinating empirical evidence and theory. Such reasoning also requires the ability to attend to information systematically and draw reasonable inferences from patterns that are observed. Further, it requires the ability to assess one’s reasoning at each stage in the process. Here, we describe some of the key issues in developing these cognitive and metacognitive scientific reasoning skills.
2.1. Cognitive processes and mechanisms
The main task for developmental researchers is to explain how children build on their intuitive curiosity about the world to become skilled scientific reasoners. Curiosity , defined as “the threshold of desired uncertainty in the environment that leads to exploratory behavior” ( Jirout & Klahr, 2012 , p. 150), will lead to information seeking. Information seeking activates a number of basic cognitive mechanisms that are used to extract (encode) information from the environment and then children (and adults) can act on this information in order to achieve a goal (i.e., use a strategy; Klahr, 2001; Kuhn, 2010 ). We turn our discussion to two such mechanisms and discuss how these mechanisms underlie the development of a specific type of information seeking: scientific reasoning.
A mechanistic account of the development of scientific reasoning includes information about the processes by which this change occurs, and how these processes lead to change over time (Klahr, 2001). Mechanisms can be described at varying levels (e.g., neurological, cognitive, interpersonal) and over different time scales. For example, neurological mechanisms (e.g., inhibition) operate at millisecond time scales (Burlea, Vidala, Tandonneta, & Hasbroucq, 2004) while learning mechanisms may operate over the course of minutes (e.g., inhibiting irrelevant information during problem solving; Becker, 2010 ). Many of the cognitive processes and mechanisms that account for learning and for problem solving across a variety of domains are important to the development of scientific reasoning skills and science knowledge acquisition. Many cognitive mechanisms have been identified as underlying scientific reasoning and other high-level cognition (e.g., analogy, statistical learning, categorization, imitation, inhibition; Goswami, 2008 ). However, due to space limitations we focus on what we argue are the two most critical mechanisms – encoding and strategy development –to illustrate the importance of individual level cognitive abilities.
2.1.1. Encoding
Encoding is the process of representing information and its context in memory as a result of attention to stimuli ( Chen, 2007 ; Siegler, 1989 ). As such, it is a central mechanism in scientific reasoning because we must represent information before we can reason about it, and the quality and process of representation can affect reasoning. Importantly, there are significant developmental changes in the ability to encode the relevant features that will lead to sound reasoning and problem solving ( Siegler, 1983 ; 1985 ). Encoding abilities improve with the acquisition of encoding strategies and with increases in children’s domain knowledge ( Siegler, 1989 ). Young children often encode irrelevant features due to limited domain knowledge (Gentner, Loewenstein, & Thompson, 2003). For example, when solving problems to make predictions about the state of a two-arm balance beam (i.e., tip left, tip right, or balance), children often erroneously encode distance to the fulcrum and amount of weight as a single factor, decreasing the likelihood of producing a correct solution (which requires weight and distance to be encoded and considered separately as causal factors, while recognizing non-causal factors such as color; Amsel, Goodman, Savoie, & Clark, 1996; Siegler, 1983 ). Increased domain knowledge helps children assess more effectively what information is and is not necessary to encode. Further, children’s encoding often improves with the acquisition of encoding strategies. For example, if a child is attempting to recall the location of an item in a complex environment, she may err in encoding only the features of the object itself without encoding its relative position. With experience, she may encode the relations between the target item and other objects (e.g., the star is in front of the box), a strategy known as cue learning. Encoding object position and relative position increases the likelihood of later recall and is an example of how encoding better information is more important than simply encoding more information ( Chen, 2007 ; Newcombe & Huttenlocher, 2000 ).
Effective encoding is dependent on directing attention to relevant information, which in turn leads to accurate representations that can guide reasoning. Across a variety of tasks, experts are more likely to attend to critical elements in problem solving, and less likely to attend to irrelevant information, compared to novices ( Gobet, 2005 ). Domain knowledge plays an important role in helping to guide attention to important features. Parents often direct a child’s attention to critical problem features during problem solving. For example, a parent may keep track of which items have been counted in order to help a child organize counting ( Saxe, Guberman, & Gearhart, 1987 ). Instructional interventions in which children were directed towards critical elements in problem solving improved their attention to these features ( Kloos & VanOrden, 2005 ). Although domain knowledge is helpful in directing attention to critical features, it may sometimes limit novel reasoning in a domain and limit the extent to which attention is paid to disconfirming evidence ( Li & Klahr, 2006 ). Finally, self-generated activity improves encoding. Self-generation of information from memory, rather than passive attention, is associated with more effective encoding because it recruits greater attentional resources than passive encoding ( Chi, 2009 ).
2.1.2. Strategy development
Strategies are sequences of procedural actions used to achieve a goal ( Siegler, 1996 ). In the context of scientific reasoning, strategies are the steps that guide children from their initial state (e.g., a question about the effects of weight and distance in balancing a scale) to a goal state (e.g., understanding the nature of the relationship between variables). We will briefly examine two components of strategy development: strategy acquisition and strategy selection . Strategies are particularly important in the development of scientific reasoning. Children often actively explore objects in a manner that is like hypothesis testing; however, these exploration strategies are not systematic investigations in which variables are manipulated and controlled as in formal hypothesis-testing strategies ( Klahr, 2000 ). The acquisition of increasingly optimal strategies for hypothesis testing, inference, and evidence evaluation leads to more effective scientific reasoning that allows children to construct more veridical knowledge.
New strategies are added to the repertoire of possible strategies through discovery, instruction, or other social interactions ( Chen, 2007 ; Gauvain, 2001 ; Siegler, 1996 ). There is evidence that children can discover strategies on their own ( Chen, 2007 ). Children often discover new strategies when they experience an insight into a new way of solving a familiar problem. For example, 10- and 11-year-olds discovered new strategies for evaluating causal relations between variables in a computerized task only after creating different cars (e.g., comparing the effects of engine size) and testing them ( Schauble, 1990 ). Similarly, when asked to determine the cause of a chemical reaction, children discovered new experimentation strategies only after several weeks ( Kuhn & Phelps, 1982 ). Over time, existing strategies may be modified to reduce time and complexity of implementation (e.g., eliminating redundant steps in a problem solving sequence; Klahr, 1984 ). For example, determining causal relations among variables requires more time when experimentation is unsystematic. In order to identify which variables resulted in the fastest car, children often constructed up to 25 cars, whereas an adult scientist identified the fastest car after constructing only seven cars ( Schauble, 1990 ).
Children also gain new strategies through social interaction, by being explicitly taught a strategy, imitating a strategy, or by collaborating in problem solving ( Gauvain, 2001 ). For example, when a parent asks a child questions about events in a photograph, the parent evokes memories of the event and helps to structure the child’s understanding of the depicted event, a process called conversational remembering ( Middleton, 1997 ). Conversational remembering improves children’s recall of events and often leads to children spontaneously using this strategy. Parent conversations about event structures improved children’s memory for these structures; for example, questions about a child’s day at school help to structure this event and improved recall ( Nelson, 1996 ). Children also learn new strategies by solving problems cooperatively with adults. In a sorting task, preschool children were more likely to improve their classification strategies after working with their mothers ( Freund, 1990 ). Further, children who worked with their parents on a hypothesis-testing task were more likely to identify causal variables than children who worked alone because parents helped children construct valid experiments, keep data records, and repeat experiments ( Gleason & Schauble, 2000 ).
Children also acquire strategies by interacting with an adult modeling a novel strategy. Middle-school children acquired a reading comprehension strategy (e.g., anticipating the ending of a story) after seeing it modeled by their teacher ( Palinscar, Brown, & Campione, 1993 ). Additionally, children can acquire new strategies from interactions with other children. Monitoring other children during problem solving improves a child’s understanding of the task and appears to improve how they evaluate their own performance ( Brownell & Carriger, 1991 ). Elementary school children who collaborated with other students to solve the balance-scale task outperformed students who worked alone ( Pine & Messer, 1998 ). Ten-year-olds working in dyads were more likely to discuss their strategies than children working alone and these discussions were associated with generating better hypotheses than children working alone ( Teasley, 1995 ).
More than one strategy may be useful for solving a problem, which requires a means to select among candidate strategies. One suggestion is that this process occurs by adaptive selection. In adaptive selection, strategies that match features of the problem are candidates for selection. One component of selection is that newer strategies tend to have a slightly higher priority for use when compared to older strategies ( Siegler, 1996 ). Successful selection is made on the basis of the effectiveness of the strategy and its cost (e.g., speed), and children tend to choose the fastest, most accurate strategy available (i.e., the most adaptive strategy).
Cognitive mechanisms provide the basic investigation and inferential tools used in scientific reasoning. The ability to reason about knowledge and the means for obtaining and evaluating knowledge provide powerful tools that augment children’s reasoning. Metacognitive abilities such as these may help explain some of the discrepancies between early scientific reasoning abilities and limitations in older children, as well as some of the developmental changes in encoding and strategy use.
2.2. Metacognitive and metastrategic processes
Sodian, Zaitchik, and Carey (1991 ) argue that two basic skills related to early metacognitive acquisitions are needed for scientific reasoning. First, children need to understand that inferences can be drawn from evidence. The theory of mind literature (e.g., Wellman, Cross, & Watson, 2001 ) suggests that it is not until the age of 4 that children understand that beliefs and knowledge are based on perceptual experience (i.e., evidence). As noted earlier, experimental work demonstrates that preschoolers can use evidence to make judgments about simple causal relationships (Gopnik, Sobel, Schulz, & Glymour, 2001; Schulz & Bonawitz, 2007; Schulz & Gopnik, 2004 ; Schulz, Gopnik,& Glymour, 2007). Similarly, several classic studies show that children as young as 6 can succeed in simple scientific reasoning tasks. Children between 6 and 9 can discriminate between a conclusive and an inclusive test of a simple hypothesis ( Sodian et al., 1991 ). Children as young as 5 can form a causal hypothesis based on a pattern of evidence, and even 4-year-olds seem to understand some of the principles of causal reasoning (Ruffman, Perner, Olson, & Doherty, 1993).
Second, according to Sodian et al. (1991 ), children need to understand that inference is itself a mechanism with which further knowledge can be acquired. Four-year-olds base their knowledge on perceptual experiences, whereas 6-year-olds understand that the testimony of others can also be used in making inferences ( Sodian & Wimmer, 1987 ). Other research suggests that children younger than 6 can make inferences based on testimony, but in very limited circumstances ( Koenig, Clément, & Harris, 2004 ). These findings may explain why, by the age of 6, children are able to succeed on simple causal reasoning, hypothesis testing, and evidence evaluation tasks.
Research with older children, however, has revealed that 8- to 12-year-olds have limitations in their abilities to (a) generate unconfounded experiments, (b) disconfirm hypotheses, (c) keep accurate and systematic records, and (d) evaluate evidence ( Klahr, Fay, & Dunbar, 1993 ; Kuhn, Garcia-Mila, Zohar, & Andersen, 1995; Schauble, 1990 , 1996 ; Zimmerman, Raghavan, & Sartoris, 2003 ). For example, Schauble (1990 ) presented children aged 9-11 with a computerized task in which they had to determine which of five factors affect the speed of racing cars. Children often varied several factors at once (only 22% of the experiments were classified as valid) and they often drew conclusions consistent with belief rather than the evidence generated. They used a positive test strategy, testing variables believed to influence speed (e.g., engine size) and not testing those believed to be non-causal (e.g., color). Some children recorded features without outcomes, or outcomes without features, but most wrote down nothing at all, relying on memory for details of experiments carried out over an eight-week period.
Although the performance differences between younger and older children may be interpreted as potentially contradictory, the differing cognitive and metacognitive demands of tasks used to study scientific reasoning at different ages may account for some of the disconnect in conclusions. Even though the simple tasks given to preschoolers and young children require them to understand evidence as a source of knowledge, such tasks require the cognitive abilities of induction and pattern recognition, but only limited metacognitive abilities. In contrast, the tasks used to study the development of scientific reasoning in older children (and adults) are more demanding and focused on hypothetico-deductive reasoning; they include more variables, involve more complex causal structures, require varying levels of domain knowledge, and are negotiated across much longer time scales. Moreover, the tasks given to older children and adults involve the acquisition, selection, and coordination of investigation strategies, combining background knowledge with empirical evidence. The results of investigation activities are then used in the acquisition, selection, and coordinationof evidence evaluation and inference strategies. With respect to encoding, increases in task complexity require attending to more information and making judgments about which features are relevant. This encoding happens in the context of prior knowledge and, in many cases, it is also necessary to inhibit prior knowledge (Zimmerman & Croker, in press).
Sodian and Bullock (2008 ) also argue that mature scientific reasoning involves the metastrategic process of being able to think explicitly about hypotheses and evidence, and that this skill is not fully mastered until adolescence at the very earliest. According to Amsel et al. (2008 ), metacognitive competence is important for hypothetical reasoning. These conclusions are consistent with Kuhn’s (1989 , 2005 , 2 011a ) argument that the defining feature of scientific thinking is the set of cognitive and metacognitive skills involved in differentiating and coordinating theory and evidence. Kuhn argues that the effective coordination of theory and evidence depends on three metacognitive abilities: (a) The ability to encode and represent evidence and theory separately, so that relations between them can be recognized; (b) the ability to treat theories as independent objects of thought (i.e., rather than a representation of “the way things are”); and (c) the ability to recognize that theories can be false, setting aside the acceptance of a theory so evidence can be assessed to determine the veridicality of a theory. When we consider these cognitive and metacognitive abilities in the larger social context, it is clear that skills that are highly valued by the scientific community may be at odds with the cultural and intuitive views of the individual reasoner ( Lemke, 2001 ). Thus, it often takes time for conceptual change to occur; evidence is not just evaluated in the context of the science investigation and science classroom, but within personal and community values. Conceptual change also takes place in the context of an individual’s personal epistemology, which can undergo developmental transitions (e.g., Sandoval, 2005 ).

2.2.1. Encoding and strategy use
Returning to the encoding and retrieval of information relevant to scientific reasoning tasks, many studies demonstrate that both children and adults are not always aware of their memory limitations while engaged in investigation tasks (e.g., Carey, Evans, Honda, Jay, & Unger, 1989; Dunbar & Klahr, 1989 ; Garcia-Mila & Andersen, 2007 ; Gleason & Schauble, 2000 ; Siegler & Liebert, 1975 ; Trafton & Trickett, 2001 ). Kanari and Millar (2004 ) found that children differentially recorded the results of experiments, depending on familiarity or strength of prior beliefs. For example, 10- to 14-year-olds recorded more data points when experimenting with unfamiliar items (e.g., using a force-meter to determine the factors affecting the force produced by the weight and surface area of boxes) than with familiar items (e.g., using a stopwatch to experiment with pendulums). Overall, children are less likely than adults to record experimental designs and outcomes, or to review notes they do keep, despite task demands that clearly necessitate a reliance on external memory aids.
Children are often asked to judge their memory abilities, and memory plays an important role in scientific reasoning. Children’s understanding of memory as a fallible process develops over middle childhood ( Jaswal & Dodson, 2009 ; Kreuzer, Leonard, & Flavell, 1975). Young children view all strategies on memory tasks as equally effective, whereas 8- to 10-year-olds start to discriminate between strategies, and 12-year-olds know which strategies work best ( Justice, 1986 ; Schneider, 1986 ). The development of metamemory continues through adolescence ( Schneider, 2008 ), so there may not be a particular age that memory and metamemory limitations are no longer a consideration for children and adolescents engaged in complex scientific reasoning tasks. However, it seems likely that metamemory limitations are more profound for children under 10-12 years.
Likewise, the acquisition of other metacognitive and metastrategic skills is a gradual process. Early strategies for coordinating theory and evidence are replaced with better ones, but there is not a stage-like change from using an older strategy to a newer one. Multiple strategies are concurrently available so the process of change is very much like Siegler’s (1996 ) overlapping waves model ( Kuhn et al., 1995 ). However, metastrategic competence does not appear to routinely develop in the absence of instruction. Kuhn and her colleagues have incorporated the use of specific practice opportunities and prompts to help children develop these types of competencies. For example, Kuhn, Black, Keselman, and Kaplan (2000) incorporated performance-level practice and metastrategic-level practice for sixth- to eighth-grade students. Performance-level exercise consisted of standard exploration of the task environment, whereas metalevel practice consisted of scenarios in which two individuals disagreed about the effect of a particular feature in a multivariable situation. Students then evaluated different strategies that could be used to resolve the disagreement. Such scenarios were provided twice a week during the course of ten weeks. Although no performance differences were found between the two types of practice with respect to the number of valid inferences, there were more sizeable differences in measures of understanding of task objectives and strategies (i.e., metastrategic understanding).
Similarly, Zohar and Peled (2008 ) focused instruction in the control-of-variables strategy (CVS) on metastrategic competence. Fifth-graders were given a computerized task in which they had to determine the effects of five variables on seed germination. Students in the control group were taught about seed germination, and students in the experimental group were given a metastrategic knowledge intervention over several sessions. The intervention consisted of describing CVS, discussing when it should be used, and discussing what features of a task indicate that CVS should be used. A second computerized task on potato growth was used to assess near transfer. A physical task in which participants had to determine which factors affect the distance a ball will roll was used to assess far transfer. The experimental group showed gains on both the strategic and the metastrategic level. The latter was measured by asking participants to explain what they had done. These gains were still apparent on the near and far transfer tasks when they were administered three months later. Moreover, low-academic achievers showed the largest gains. It is clear from these studies that although meta-level competencies may not develop routinely, they can certainly be learned via explicit instruction.
Metacognitive abilities are necessary precursors to sophisticated scientific thinking, and represent one of the ways in which children, adults, and professional scientists differ. In order for children’s behavior to go beyond demonstrating the correctness of one’s existing beliefs (e.g., Dunbar & Klahr, 1989 ) it is necessary for meta-level competencies to be developed and practiced ( Kuhn, 2005 ). With metacognitive control over the processes involved, children (and adults) can change what they believe based on evidence and, in doing so, are aware not only that they are changing a belief, but also know why they are changing a belief. Thus, sophisticated reasoning involves both the use of various strategies involved in hypothesis testing, induction, inference, and evidence evaluation, and a meta-level awareness of when, how, and why one should engage in these strategies.
3. Scientific reasoning in context
Much of the existing laboratory work on the development of scientific thinking has not overtly acknowledged the role of contextual factors. Although internal cognitive and metacognitive processes have been a primary focus of past work, and have helped us learn tremendously about the processes of scientific thinking, we argue that many of these studies focused on individual cognition have, in fact, included both social factors (in the form of, for example, collaborations with other students, or scaffolds by parents or teachers) and cultural tools that support scientific reasoning.
3.1. Instructional and peer support: The role of others in supporting cognitive development
Our goal in this section is to re-examine our two focal mechanisms (i.e., encoding and strategy) and show how the development of these cognitive acquisitions and metastrategic control of them are facilitated by both the social and physical environment.
3.1.1. Encoding
Children must learn to encode effectively, by knowing what information is critical to pay attention to. They do so in part with the aid of their teachers, parents, and peers. Once school begins, teachers play a clear role in children’s cognitive development. An ongoing debate in the field of science education concerns the relative value of having children learn and discover how the world works on their own (often called “discovery learning”) and having an instructor guide the learning more directly (often called “direct instruction”). Different researchers interpret these labels in divergent ways, which adds fuel to the debate (see e.g., Bonawitz et al., 2011 ; Hmelo-Silver, Duncan, & Chinn, 2007 ; Kirshner, Sweller, & Clark, 2006; Klahr, 2010 ; Mayer, 2004 ; Schmidt, Loyens, van Gog, & Paas, 2007 ). Regardless of definitions, though, this issue illustrates the core idea that learning takes place in a social context, with guidance that varies from minimal to didactic.
Specifically, this debate is about the ideal role for adults in helping children to encode information. In direct instruction, there is a clear role for a teacher, often actively pointing out effective examples as compared to ineffective ones, or directly teaching a strategy to apply to new examples. And, indeed, there is evidence that more direct guidance to test variables systematically can help students in learning, particularly in the ability to apply their knowledge to new contexts (e.g., Klahr & Nigam, 2004 ; Lorch et al., 2010 ; Strand-Cary & Klahr, 2008 ). There is also evidence that scaffolded discovery learning can be effective (e.g., Alfieri, Brooks, Adrich, & Tenenbaum, 2011). Those who argue for discovery learning often do so because they note that pedagogical approaches commonly labeled as “discovery learning,” such as problem-based learning and inquiry learning, are in fact highly scaffolded, providing students with a structure in which to explore ( Alfieri et al., 2011 ; Hmelo-Silver et al., 2007 ; Schmidt et al., 2007 ). Even in microgenetic studies in which children are described as engaged in “self-directed learning,” researchers ask participants questions along that way that serve as prompts, hints, dialogue, and scaffolds that facilitate learning ( Klahr & Carver, 1995 ). What there appears to be little evidence for is “pure discovery learning” in which students are given little or no guidance and expected to discover rules of problem solving or other skills on their own ( Alfieri et al., 2011 ; Mayer, 2004 ). Thus, it is clear that formal education includes a critical role for a teacher to scaffold children’s scientific reasoning.
A common goal in science education is to correct the many misconceptions students bring to the classroom. Chinn and Malhotra (2002 ) examined the role of encoding evidence, interpreting evidence, generalization, and retention as possible impediments to correcting misconceptions. Over four experiments, they concluded that the key difficulty faced by children is in making accurate observations or properly encoding evidence that does not match prior beliefs. However, interventions involving an explanation of what scientists expected to happen (and why) were very effective in mediating conceptual change when encountering counterintuitive evidence. That is, with scaffolds, children made observations independent of theory, and changed their beliefs based on observed evidence. For example, the initial belief that a thermometer placed inside a sweater would display a higher temperature than a thermometer outside a sweater was revised after seeing evidence that disconfirmed this belief and hearing a scientist’s explanation that the temperature would be the same unless there was something warm inside the sweater. Instructional supports can play a crucial role in improving the encoding and observational skills required for reasoning about science.
In laboratory studies of reasoning, there is direct evidence of the role of adult scaffolding. Butler and Markman (2012a ) demonstrate that in complex tasks in which children need to find and use evidence, causal verbal framing (i.e., asking whether one event caused another) led young children to more effectively extract patterns from scenes they observed, which in turn led to more effective reasoning. In further work demonstrating the value of adult scaffolding in children’s encoding, Butler and Markman (2012b ) found that by age 4, children are much more likely to explore and make inductive inferences when adults intentionally try to teach something than when they are shown an “accidental” effect.
3.1.2. Strategy development and use
As discussed earlier in this chapter, learning which strategies are available and useful is a fundamental part of developing scientific thinking skills. Much research has looked at the role of adults in teaching strategies to children in both formal (i.e., school) and informal settings (e.g., museums, home; Fender & Crowley, 2007 ; Tenenbaum, Rappolt-Schlichtmann, & Zanger, 2004).
A central task in scientific reasoning involves the ability to design controlled experiments. Chen and Klahr (1999 ) found that directly instructing 7- to 10-year-old children in the strategies for designing unconfounded experiments led to learning in a short time frame. More impressively, the effectiveness of the training was shown seven months later, when older students given the strategy training were much better at correctly distinguishing confounded and unconfounded designs than those not explicitly trained in the strategy. In another study exploring the role of scaffolded strategy instruction, Kuhn and Dean (2005 ) worked with sixth graders on a task to evaluate the contribution of different factors to earthquake risk. All students given the suggestion to focus attention on just one variable were able to design unconfounded experiments, compared to only 11% in the control group given their typical science instruction. This ability to design unconfounded experiments increased the number of valid inferences in the intervention group, both immediately and three months later. Extended engagement alone resulted in minimal progress, confirming that even minor prompts and suggestions represent potentially powerful scaffolds. In yet another example, when taught to control variables either with or without metacognitive supports, 11-year-old children learned more when guided in thinking about how to approach each problem and evaluate the outcome ( Dejonckheere, Van de Keere, & Tallir, 2011 ). Slightly younger children did not benefit from the same manipulation, but 4- to 6-year-olds given an adapted version of the metacognitive instruction were able to reason more effectively about simpler physical science tasks than those who had no metacognitive supports (Dejonckheere, Van de Keere, & Mestdagh, 2010).
3.2. Cultural tools that support scientific reasoning
Clearly, even with the number of studies that have focused on individual cognition, a picture is beginning to emerge to illustrate the importance of social and cultural factors in the development of scientific reasoning. Many of the studies we describe highlight that even “controlled laboratory studies” are actually scientific reasoning in context. To illustrate, early work by Siegler and Liebert (1975 ) includes both an instructional context (a control condition plus two types of instruction: conceptual framework , and conceptual framework plus analogs ) and the role of cultural supports. In addition to traditional instruction about variables (factors, levels, tree diagrams), one type of instruction included practice with analogous problems. Moreover, 10- and 13-year-olds were provided with paper and pencil to keep track of their results. A key finding was that record keeping was an important mediating factor in success. Children who had the metacognitive awareness of memory limitations and therefore used the provided paper for record keeping were more successful at producing all possible combinations necessary to manipulate and isolate variables to test hypotheses.
3.2.1. Cultural resources to facilitate encoding and strategy use
The sociocultural perspective highlights the role that language, speech, symbols, signs, number systems, objects, and tools play in individual cognitive development ( Lemke, 2001 ). As highlighted in previous examples, adult and peer collaboration, dialogue, and other elements of the social environment are important mediators. In this section, we highlight some of the verbal, visual, and numerical elements of the physical context that support the emergence of scientific reasoning.
Most studies of scientific reasoning include some type of verbal and pictorial representation as an aid to reasoning. As encoding is the first step in solving problems and reasoning, the use of such supports reduces cognitive load. In studies of hypothesis testing strategies with children (e.g., Croker & Buchanan, 2011 ; Tschirgi, 1980 ), for example, multivariable situations are described both verbally and with the help of pictures that represent variables (e.g., type of beverage), levels of the variable (e.g., cola vs. milk), and hypothesis-testing strategies (see Figure 1 , panel A). In classic work by Kuhn, Amsel, and O’Loughlin (1988 ), a picture is provided that includes the outcomes (children depicted as healthy or sick) along with the levels of four dichotomous variables (e.g., orange/apple, baked potato/French fries, see Kuhn et al., 1988 , pp. 40-41). In fact, most studies that include children as participants provide pictorial supports (e.g., Ruffman et al., 1993 ; Koerber, Sodian, Thoermer, & Nett, 2005). Even at levels of increasing cognitive development and expertise, diagrams and visual aids are regularly used to support reasoning (e.g., Schunn & Dunbar, 1996 ; Trafton & Trickett, 2001 ; Veermans, van Joolingen, & de Jong, 2006).

Panel A illustrates the type of pictorial support that accompanies the verbal description of a hypothesis-testing task (from Croker & Buchanan, 2011 ). Panel B shows an example of a physical apparatus (from Triona & Klahr, 2007 ). Panel C shows a screenshot from an intelligent tutor designed to teach how to control variables in experimental design ( Siler & Klahr, 2012 ; see http://tedserver.psy.cmu.edu/demo/ted4.html, for a demonstration of the tutor).
Various elements of number and number systems are extremely important in science. Sophisticated scientific reasoning requires an understanding of data and the evaluation of numerical data. Early work on evidence evaluation (e.g., Shaklee, Holt, Elek, & Hall, 1988 ) included 2 x 2 contingency tables to examine the types of strategies children and adults used (e.g., comparing numbers in particular cells, the “sums of diagonals” strategy). Masnick and Morris (2008 ) used data tables to present evidence to be evaluated, and varied features of the presentation (e.g., sample size, variability of data). When asked to make decisions without the use of statistical tools, even third- and sixth-graders had rudimentary skills in detecting trends, overlapping data points, and the magnitude of differences. By sixth grade, participants had developing ideas about the importance of variability and the presence of outliers for drawing conclusions from numerical data.
Although language, symbols, and number systems are used as canonical examples of cultural tools and resources within the socio-cultural tradition ( Lemke, 2001 ), recent advances in computing and computer simulation are having a huge impact on the development and teaching of scientific reasoning. Although many studies have incorporated the use of physical systems ( Figure 1 , panel B) such as the canal task ( Gleason & Schauble, 2000 ), the ramps task (e.g., Masnick & Klahr, 2003 ), mixing chemicals ( Kuhn & Ho, 1980 ), and globes (Vosniadou, Skopeliti, & Ikospentaki, 2005), there is an increase in the use of interactive computer simulations (see Figure 1 , panel C). Simulations have been developed for electric circuits (Schauble, Glaser, Raghavan, & Reiner, 1992), genetics ( Echevarria, 2003 ), earthquakes ( Azmitia & Crowley, 2001 ), flooding risk ( Keselman, 2003 ), human memory ( Schunn & Anderson, 1999 ), and visual search (Métrailler, Reijnen, Kneser, & Opwis, 2008). Non-traditional science domains have also been used to develop inquiry skills. Examples include factors that affect TV enjoyment ( Kuhn et al., 1995 ), CD catalog sales ( Dean & Kuhn, 2007 ), athletic performance ( Lazonder, Wilhelm, & Van Lieburg, 2009 ), and shoe store sales ( Lazonder, Hagemans, & de Jong, 2010 ).
Computer simulations allow visualization of phenomena that are not directly observable in the classroom (e.g., atomic structure, planetary motion). Other advantages include that they are less prone to measurement error in apparatus set up, and that they can be programmed to record all actions taken (and their latencies). Moreover, many systems include a scaffolded method for participants to keep and consult records and notes. Importantly, there is evidence that simulated environments provide the same advantages as isomorphic “hands on” apparatus ( Klahr, Triona, & Williams, 2007 ; Triona & Klahr, 2007 ).
New lines of research are taking advantage of advances in computing and intelligent computer systems. Kuhn (2011b ) recently examined how to facilitate reasoning about multivariable causality, and the problems associated with the visualization of outcomes resulting from multiple causes (e.g., the causes for different cancer rates by geographical area). Participants had access to software that produces a visual display of data points that represent main effects and their interactions. Similarly, Klahr and colleagues (Siler, Mowery, Magaro, Willows, & Klahr, 2010 ) have developed an intelligent tutor to teach experimentation strategies (see Figure 1 , panel C). The use of intelligent tutors provides the unique opportunity of personally tailored learning and feedback experiences, dependent on each student’s pattern of errors. This immediate feedback can be particularly useful in helping develop metacognitive skills (e.g., Roll, Alaven, McLaren, & Koedinger, 2011) and facilitate effective student collaboration (Diziol, Walker, Rummel, & Koedinger, 2010).
Tweney, Doherty, and Mynatt (1981 ) noted some time ago that most tasks used to study scientific thinking were artificial because real investigations require aided cognition. However, as can be seen by several exemplars, even lab studies include support and assistance for many of the known cognitive limitations faced by both children and adults.
4. Summary and conclusions
Determining the developmental trajectory of scientific reasoning has been challenging, in part because scientific reasoning is not a unitary construct. Our goal was to outline how the investigation, evidence evaluation, and inference skills that constitute scientific reasoning emerge from intuitive information seeking via the interaction of individual and contextual factors. We describe the importance of (a) cognitive processes and mechanisms, (b) metacognitive and metastrategic skills, (c) the role of direct and scaffolded instruction, and (d) a context in which scientific activity is supported and which includes cultural tools (literacy, numeracy, technology) that facilitate the emergence of scientific reasoning. At the outset, we intended to keep section boundaries clean and neat. What was apparent to us, and may now be apparent to the reader, is that these elements are highly intertwined. It was difficult to discuss pure encoding in early childhood without noting the role that parents play. Likewise, it was difficult to discuss individual discovery of strategies, without noting such discovery takes place in the presence of peers, parents, and teachers. Similarly, discussing the teaching and learning of strategies is difficult without noting the role of cultural tools such as language, number, and symbol systems.
There is far more to a complete account of scientific reasoning than has been discussed here, including other cognitive mechanisms such as formal hypothesis testing, retrieval, and other reasoning processes. There are also relevant non-cognitive factors such as motivation, disposition, personality, argumentation skills, and personal epistemology, to name a few (see Feist, 2006 ). These additional considerations do not detract from our assertion that encoding and strategy use are critical to the development of scientific reasoning, and that we must consider cognitive and metacognitive skills within a social and physical context when seeking to understand the development of scientific reasoning. Scientific knowledge acquisition and, importantly, scientific knowledge change is the result of individual and social cognition that is mediated by education and cultural tools. The cultural institution of science has taken hundreds of years to develop. As individuals, we may start out with the curiosity and disposition to be little scientists, but it is a long journey from information seeking to skilled scientific reasoning, with the help of many scaffolds along the way.
Acknowledgements
All authors contributed equally to the manuscript. The authors thank Eric Amsel, Deanna Kuhn, and Jamie Jirout for comments on a previous version of this chapter.
© 2012 The Author(s). Licensee IntechOpen. This chapter is distributed under the terms of the Creative Commons Attribution 3.0 License , which permits unrestricted use, distribution, and reproduction in any medium, provided the original work is properly cited.
Continue reading from the same book
Edited by Heidi Kloos
Published: 14 November 2012
By Daisy A. Segovia and Angela M. Crossman
6815 downloads
By Joseph L. Amaral, Susan Collins, Kevin T. Bohache ...
4741 downloads
By Mieczyslaw Pokorski, Lukasz Borecki and Urszula Je...
3668 downloads

An official website of the United States government
The .gov means it’s official. Federal government websites often end in .gov or .mil. Before sharing sensitive information, make sure you’re on a federal government site.
The site is secure. The https:// ensures that you are connecting to the official website and that any information you provide is encrypted and transmitted securely.
- Publications
- Account settings
Preview improvements coming to the PMC website in October 2024. Learn More or Try it out now .
- Advanced Search
- Journal List
- Springer Nature - PMC COVID-19 Collection

A new framework for teaching scientific reasoning to students from application-oriented sciences
Krist vaesen.
Philosophy & Ethics, School of Innovation Sciences, Eindhoven University of Technology, P.O. Box 513, 5600 MB Eindhoven, The Netherlands
Wybo Houkes
Associated data.
Not applicable.
About three decades ago, the late Ronald Giere introduced a new framework for teaching scientific reasoning to science students. Giere’s framework presents a model-based alternative to the traditional statement approach—in which scientific inferences are reconstructed as explicit arguments, composed of (single-sentence) premises and a conclusion. Subsequent research in science education has shown that model-based approaches are particularly effective in teaching science students how to understand and evaluate scientific reasoning. One limitation of Giere’s framework, however, is that it covers only one type of scientific reasoning, namely the reasoning deployed in hypothesis-driven research practices. In this paper, we describe an extension of the framework. More specifically, we develop an additional model-based scheme that captures reasoning in application-oriented practices (which are very well represented in contemporary science). Our own teaching experience suggests that this extended framework is able to engage a wider audience than Giere’s original. With an eye on going beyond such anecdotal evidence, we invite our readers to test out the framework in their own teaching.
Introduction
The late Ronald Giere wrote a widely used textbook, entitled Understanding Scientific Reasoning , meant to introduce lower-division students to scientific reasoning. Throughout its four editions, the book was designed to impart to students the ability to understand and evaluate bits of scientific reasoning, as instantiated in popular press articles, semi-professional technical reports and scholarly publications. Given this aim, the book avoids in-depth historical reflection on the philosophy of science, or on the evaluative framework it adopts. Rather, in every edition, Giere simply introduces his framework, and then moves on to how it can be used.
Giere’s framework changed over time, though. In the first ( 1979 ) and second (1984) editions of the book, it fits the traditional statement approach, which Giere traces back to Mill’s A System of Logic ( 1843 ). This was in line, as he reported afterwards (Giere, 2001 ), with what he took to be the approach in the vast majority of textbooks in logic and reasoning. The statement approach assumes that
“the evaluation of any particular bit of reasoning is done by first reconstructing that reasoning as an explicit argument , with premises and a conclusion, and then examining the reconstructed argument to see if it exhibits the characteristic form of a good argument, whether deductive or inductive ” (Giere, 2001 , p. 21, italics added).
The basic aim of the statement approach is to determine whether one or more statements or linguistic expressions (viz., the conclusion of an explicit argument) are true or false or, at least, to determine whether it is reasonable to take the statements to be true or false on the basis of other statements. In the third (1991) and fourth (2005) editions, Giere abandons this approach in favour of a model-based approach. This reflects a then growing concern among philosophers of science that modern scientific claims simply do not translate well into statements, leading to ill-fitting or impoverished reconstructions. For instance, the behavior of complex systems such as coupled harmonic oscillators or of randomly breeding populations of predators and prey is typically represented by mathematical models; brain processes or processes within organizations are commonly represented by diagrams; and the study of turbulence and energy systems tends to be informed by the study of scale models. Even if one were to succeed in turning these different types of models into sets of linguistic expressions, it would, according to Giere, be pointless to assess the truth of such expressions: such expressions are true, by definition, of the models, but not of the world. Also, Giere contends that, since models are abstract objects, the relationship between model and world is not one of truth, but rather one of fit. Scientists are primarily engaged in assessing the fit between models and target systems in the real world, i.e., in assessing whether their models are sufficiently similar to target systems to study the behavior of the latter by means of the former.
Giere indicates that his model-based approach better resonates with students. This matches our own experience in teaching scientific reasoning. There is also more systematic evidence for the advantages of model-based approaches. For one, there is widespread consensus among researchers that model-based reasoning more accurately describes actual scientific cognition and practice than argumentative reasoning (Clement, 2008 ; Gilbert et al., 1998 ; Halloun, 2004 ; Justi & Gilbert, 1999 ; Passmore & Stewart, 2002 ; Taylor et al., 2003 ). Cognitive scientists even have proposed that human cognition in general (not just scientific cognition) is best described in terms of mental modelling (Johnson-Laird, 1983 , 2006 ; Nersessian, 2002 , 2008 ). Furthermore, in their typical science courses, students are introduced to theories by means of models rather than arguments. Model-based approaches, thus, tap into customary modes of thinking among science students and, accordingly, appear effective in science instruction (Böttcher & Meisert, 2011 ; Gobert & Clement, 1999 ; Gobert & Pallant, 2004 ; Gobert, 2005 ; Matthews, 2007 ). Finally, from a more evaluative perspective, statement approaches struggle to accommodate all the information that is relevant to evaluating a piece of scientific reasoning; model-based assessments fare much better in comparison. The principal object of analysis in a statement approach is a hypothesis, which is typically expressed in a single statement. In Giere’s framework, in contrast, the object of analysis is a model. Associated with a model is not just one or more hypotheses, but also crucial background information, such as auxiliary assumptions (i.e., assumptions that are assumed to hold but that are secondary to the hypotheses under investigation) and boundary conditions (i.e., the conditions that need to be satisfied for a proper empirical test of the model and its hypotheses). Additionally, in Giere’s framework a model is explicitly evaluated relative to competing models. Here, Giere does not distinguish between different types of models: he presents a framework that is meant to apply to mathematical models, scale models, and diagrams alike, focusing on their shared role in scientific reasoning.
In Section 2 , we will discuss Giere’s model-based framework in more detail, focusing on its role as an instrument to instruct students how to go about evaluating instances of scientific reasoning. In doing so, we will identify a serious limitation: the framework captures only one mode of reasoning, namely the reasoning employed in hypothesis-driven research. As teachers at a technical university, we have experienced that this makes Giere’s framework unsuitable for our particular audience. In the research practices which most of our students are educated in, hypothesis-testing is typically embedded in application-oriented epistemic activities. To capture this embedding and thus improve the usefulness to our audience, we developed an extension of Giere’s framework. Section 3 introduces this extended model-based framework for assessing application-oriented research. Section 4 discusses the wider applicability of our extended framework. Since much of contemporary science is application-oriented rather than hypothesis-driven, we submit that our framework will also benefit teachers that work outside the confines of a technical university.
Giere’s framework
In Giere’s model-based alternative to the reconstructive statement approach, the primary purpose of observation, experimentation and scientific reasoning is to assess the fit between models and real-world target systems. Giere developed a representation of this decision process (see Fig. 1 , and the caption that accompanies it) to aid students in evaluating (popular) scientific reports; and here, we will use this representation together with an example to outline his framework.

Steps in analysing hypothesis-driven approaches; Step 1—Real world: identification of the target system, i.e., the aspect of the world that is to be captured by the model. Step 2—Model: development of a model, which is to be assessed for its fit with the target system. Step 3—Prediction: deduction of predictions from the model. Step 4—Data: data collection from the target system, in order to establish the (non-)agreement between data and predictions. Step 5/6—Negative/Positive evidence: evaluation of model-target fit, based on the (non-)agreement between data and prediction
Consider the following example, which is accessible for a broad audience. Epidemiologists might, as per Step 1 , identify an aspect of a real-world system that they want to better understand, for instance, the development over time of COVID-19 infections, recoveries and deaths. In Step 2 , they develop an epidemiological model that they hope adequately captures these trends. Figure 2 presents the graphical and mathematical expressions of one such model. The graph shows various independent variables, and their interactions, and how these drive the dependent variables, viz., number of individuals susceptible to infection (S), number of infected individuals (I), number of recovered individuals (R), number of deaths (D). The mathematical expressions summarize in which ways S, I, R and D are dependent on the independent variables.

Epidemiological model of COVID-19 (taken from Vega, 2020 ). The graph shows the (interactions among) independent variables, and how they affect the dependent variables (“Susceptible”, “Infected”, “Recovered” and “Deaths”). The equations (top-right) express these interdependencies in a mathematical form
In addition to this graphical representation and mathematical expression, the model comprises auxiliary assumptions and boundary conditions. As an example of the former, the model assumes that the strain that the pandemic puts on hospitals (Hospital Capacity Strain Index) is determined by the number of serious infection cases and the hospital capacity (expressed in number of beds), where the latter is held constant . The model thus ignores other factors that, arguably, affect the strain on hospitals, including, lower hospital capacity due to infections among or strain on hospital personnel, the duration and frequency of pandemic waves, additional care capacity through governmental or private investment, the occurrence of an additional pandemic or epidemic, and so forth. Another auxiliary assumption is that the model population is unstructured (e.g., in terms of contact clusters, age cohorts, and so forth). As to the model’s boundary conditions, the model will fit the target system to the extent that its initial conditions reflect the target system’s initial conditions (e.g., that population sizes in the model and target system are 100,000, that the fractions of the populations that are susceptible to infection are at 13%).
Ultimately, the epidemiologists wish to assess the fit between the real-world target (as identified in Step 1 ) and the model (as developed in Step 2 ). In order to do so, they, in Step 3 , derive testable hypotheses or predictions from the model. A couple of predictions are presented in Fig. 3 .

Number of infections over time, as predicted by the model of Vega ( 2020 ). The green line represents infections in a scenario without lockdown; the red and blue lines capture what would happen under different lockdown scenarios
Subsequently, in Step 4 , the predictions are empirically tested, using data from the real-world target system. Here the epidemiologists might use as evidence the infection records of the first wave of COVID-19. Finally, in Steps 5 and 6 , the agreement between this evidence and predictions (of Fig. 3 ) informs the epidemiologists’ evaluation of the fit between the model and real-world COVID-19 infection patterns. Negative evidence suggests a poor fit (Step 5); positive evidence, in the absence of plausible competing models, suggests a good fit (Step 6).
Having reconstructed the decision-making process of the epidemiologists along these lines, students are in a good position to evaluate it. They may formulate critical questions concerning the deduction of predictions from the model, and the inductive inferences in Step 5 and 6. Regarding the former, students should evaluate whether the predictions indeed follow from the model. Is it really the case that the prediction should hold, given the model’s auxiliary assumptions and boundary conditions? And is the prediction sufficiently surprising, precise and singular? As to the inductive inferences, to what extent are the epidemiologists’ conclusions derived in accordance with the decision tree of Steps 5 and 6? Are the epidemiologists concluding too much or too little? Do they sufficiently acknowledge remaining uncertainties, uncertainties resulting from, e.g., observational biases, low number of observations, deviations of observations from predictions, and the plausibility of competing models?
Giere’s and our own experience in using this framework confirms what research in science education has suggested about model-based approaches in general (Böttcher & Meisert, 2011 ; Gobert & Clement, 1999 ; Gobert & Pallant, 2004 ; Gobert, 2005 ; Matthews, 2007 ): many students find it relatively easy to internalize model-based frameworks (such as Giere’s) for evaluating reports of scientific findings. But, our own experience in teaching scientific reasoning to students in the specific context of a technical university indicates Giere’s framework doesn’t cater to everyone: for students from some programs (e.g., chemistry), internalizing the framework appears easier than for students from other programs (e.g., mechanical engineering); and in explaining the framework, we find it easier to evaluate relevant examples from one field of inquiry than from others.
This differentiation in comprehensibility of the framework brings to mind a conventional distinction between ‘fundamental’ and ‘applied’ fields of inquiry, where it is maintained that the former produce most of the theoretical knowledge that is utilized for practical problem-solving in the latter (e.g., Bunge, 1966 ). Since pitching this distinction at the level of fields or disciplines seems unsustainable (following criticisms reviewed in, e.g., Kant & Kerr, 2019 and Houkes & Meijers, 2021 ), we opt for a differentiation at the level of research practices instead.
This differentiation builds on Chang’s ( 2011 , 2012 ) practice-oriented view, which distinguishes several hierarchically ordered levels of analysis: mental and physical operations , which constitute epistemic activities that in turn make up a research practice . Here, research practices are characterized by their aim, while epistemic activities are rule-governed, routinized sets of operations that contribute to knowledge production in light of these aims. Lavoisier’s revolutionary way of doing chemistry, for example, can be understood as an innovative practice in its time, constituted by activities such as collecting gases, classifying compounds, and measuring weights, which comprise various operations.
In line with Chang’s practice-oriented analysis, hypothesis testing may be taken as an epistemic activity that is more central to some research practices—such as the episode from epidemiology that was reconstructed earlier in this section—than to others. Some practices are aimed at generating precisely the theoretical knowledge that may be gained through systematic hypothesis-testing; in other practices, however, the results of hypothesis testing are instrumental to more encompassing aims. Giere’s original framework may fit the mental model of students who have been primarily exposed to or educated in the former type of practices; but it is at best an incomplete fit for the latter, more encompassing type of practice. This diagnosis suggests a natural solution: to capture these more encompassing practices, we need to extend Giere’s framework.
A framework for assessing application-oriented research
Reconstruction of application-oriented scientific reasoning.
Research in the engineering sciences has received only limited attention from philosophers of science. Conventionally, it is understood as research that applies scientific methods and/or theories to the attainment of practical goals (Bunge, 1966 ). Consequently, also in the self-understanding and -presentation of many practitioners, these fields of inquiry go by labels such as ‘instrumental’ or ‘applied’ research, or are characterized in terms of ‘design’ rather than research. However, in-depth analyses of historical and contemporary episodes (e.g., Constant, 1999 ; Kroes, 1992 ; Vincenti, 1990 ) reveal that they involve more than merely deriving solutions to specific practical problems from fundamental theories. In particular, they can be understood as knowledge-producing activities in their own right. Some have claimed that application-oriented research involves a special type of ‘designerly’ or ‘engineering’ knowledge (as in, e.g., Cross, 1982 ). This knowledge has been characterized as ‘prescriptive’ (Meijers & Kroes, 2013 ), as consisting of ‘technical norms’ (Niiniluoto, 1993 ), or ‘technological rules’ (Houkes & Meijers, 2021 )—but it seems fair to say that these characterizations require further specification, in their content, scope and impact on differentiating application-oriented research (see, e.g., Kant & Kerr, 2019 ).
For our present purposes, we only need to assume that practices in the engineering sciences, in which most of our students are trained, involve epistemic activities such as hypothesis testing and are therefore genuinely knowledge-producing. Furthermore, we submit that the knowledge thus produced often has a wider scope than specific, ‘local’ practical problems (e.g., Adam et al. 2006 ; Wilholt, 2006 ), although they might be strongly geared towards solving such problems. Given this, we label such practices ‘application-oriented’. We submit that fields of inquiry such as mechanical engineering or fusion research frequently involve application-oriented practices, without thereby expressing commitment to any of the characterizations mentioned above. Still, the framework presented below is compatible with these characterizations: it can be supplemented with (suitably developed) analyses of, e.g., technical norms or prescriptive knowledge in application-oriented practices.
Figure 4 represents our application-oriented framework. Whereas Giere’s framework starts with a real-world phenomenon, which the researcher then wishes to capture with a suitable model, application-oriented approaches typically start with a model, which serves as a stand-in for a not-yet-existent target system (e.g., a software package, a novel protein). Or, whereas in hypothesis-driven approaches models are supposed to be descriptive of the world and to deliver theoretical knowledge, in application-oriented research, models intend to describe how the world might or should be and to yield practical knowledge. Our general assumption is that, in the epistemic activities captured by the application-oriented framework, researchers do not, without prior study, start building their real-world target system (or artifact for short). Rather, they first develop a model of the artifact (e.g., a blueprint, scale model, or computer model) and study the behavior of the model ( Model Phase in Fig. 4 ). Only if the model behaves as it should, the researcher takes the next step of actually producing and testing ( Artifact Phase in Fig. 4 ) the artifact for which the model served as a stand-in.

Steps in analysing application-oriented research. Problem Definition Phase: Step 0—Design specs: definition of the design specifications the artifact has to meet. Model Phase: Step 1—Model: development of model that acts as a stand-in for the artifact to be produced. Step 2—Model: derivation of predictions from the model, where predictions align with the design specs identified in Step 0. Step 3—Model data: collection of model data, and assessment of the (non-)agreement between model data and predictions. In case of agreement, and in case of reasonable analogy between model and artifact, Artifact Phase starts: Step 4—Artifact: developing artifact based on the model. Step 5: Deduction of predictions from the artifact, where predictions are identical to design specs of Step 0. Step 6—Artifact data: Collection of artifact data, and assessment of (non-)agreement between artifact data and predictions/design specs. The “New” symbols refer to procedures that are not shared with hypothesis-driven approaches
As can be seen in Fig. 4 , application-oriented research in fact involves a phase prior to model-building, denoted by “Problem definition phase”. In this phase ( Step 0 ), the design specs are determined, i.e., the properties or dispositions that the artifact ultimately ought to exhibit (e.g., the intended cost, functionalities and efficiency of a software program; the intended structure of a protein).
The purpose of the Model Phase is developing one or more models that meet the researcher’s predefined specs. To the extent they do, and to the extent the models are an adequate analogue for the artifact yet to be built (see Section 3.2 ), the researcher moves to the Artifact Phase . Here one goes through the same building and testing cycle as in the Model Phase , but this time the object of analysis is the artifact rather than the model. Frameworks in design methodology, such as the ‘basic design cycle’ (Roozenburg & Eekels, 1995 ) and the ‘Function-Behavior-Structure’ model (Gero, 1990 ) also represent an iterative process of determining and satisfying design specs, but without bringing out the role of model-building and thus also without explicitly distinguishing the Model Phase and Artifact Phase .
Each of these cycles bears large similarity with the cycle in Giere’s framework. In the Model Phase , a model is developed ( Step 1 ) from which various predictions are derived ( Step 2 ). Arguably, the most salient predictions are those that pertain to the design specs identified in Step 0 , i.e., predictions concerning the extent to which the model will satisfy these specs. In order to assess this, one collects relevant data from the model ( Step 3 ), and evaluates whether the data agree with the predictions (i.e., design specs). If they don’t, one might reiterate the cycle; if they do, the artifact is built based on the model ( Step 4 ).
At Step 4 , one enters the Artifact Stage , characterized by a similar cycle: the artifact is produced ( Step 4 ); one formulates predictions about the artifact, viz., whether it exhibits the desired design specs ( Step 5) ; and one collects data that allow one to test these predictions of ( Step 6 ). In case the data agree with the design specs, the artifact is accepted; otherwise, it is adjusted or steps 1–5 are reiterated. Note that the design specs of the model ( Step 2 ) and the artifact ( Step 5 ) might be quantitatively or qualitatively different. While the latter might simply be taken from Step 0 , the former should fit the specific context of the model world.
To illustrate the application-oriented framework, consider another example from the biomedical sciences, one that also pertains to COVID-19. Let us assume that a researcher’s task is to design a novel protein that is able to bind to SARS-Cov-2. Given the known structure of the binding configuration of the virus, one can define, in the Problem Definition Phase , the structure that the new protein ought to have and the types of (energetic) conditions under which the protein needs to remain stable (Step 0). During the Model Phase , in Step 1, a computer model of a candidate protein is developed (see Fig. 5 ). Next, in Step 2, some testable predictions are derived concerning the candidate protein’s structure and stability. Tests of its structure and stability rely on model data (Step 3). Regarding stability, for instance, the researcher/computer needs to calculate the protein’s thermodynamic free energy; a configuration that has low thermodynamic free energy is more likely to effectively exhibit the requisite structure than a configuration that has high thermodynamic free energy.

A computer model for designing new proteins
In case there is agreement between model data and model predictions, and the analogy between model and artifact (real-world protein) is adequate (see below), the researcher moves to the Artifact Phase , and will actually produce the protein (Step 4), typically by means of gene manipulation techniques. Step (5) and (6) then carry out a test of the real-world protein’s compliance with the design specs (e.g., structure, stability under real-life conditions, etc.).
Evaluation of application-oriented scientific reasoning
Part of the value of a reconstruction along the lines of Fig. 4 lies in the critical questions to which it gives rise. It does so at four levels: the Problem Definition Phase , the Model Phase , the Artifact Phase , and at the level of the analogical inference connecting these last two phases. The evaluation of the Model Phase and the Artifact Phase are virtually identical to the evaluation involved in Giere’s hypothesis-driven framework; genuinely new evaluative steps (as indicated in Fig. 4 ) pertain to the Problem Definition Phase and the analogical inference that connects the Model and the Artifact Phases .
Problem definition phase (new evaluative step)
An application-oriented approach might fail as an epistemic activity well before any model or artifact is built. There are plenty of frameworks that may be used to judge the rationality of the researcher’s design specs. The researcher’s intended design specs might be—in terms of the well-known SMART-framework—insufficiently Specific, Measurable, Acceptable, Realistic or Time-related. Alternatively, students could assess the design specs along the lines of the evaluative framework of Edvardsson and Hansson ( 2005 ). This framework resembles the SMART framework, but is different in some of its details. According to it, design specs are rational, i.e., achievement-inducing, just in case they meet four criteria: precision, evaluability, approachability and motivity. Precision and evaluability are very similar to, respectively, the SMART criteria Specific and Measurable; and Approachability is a combination of SMART’s Realistic and Time-Related. Motivity, finally, refers to the degree to which a goal/design spec induces commitment in those involved in reaching it. Decision theory and the work of Millgram and Thagard ( 1996 ) suggest another evaluative criterion: the (in)coherence among design specs. Teachers might find still other frameworks useful; but in any case, the merit of our proposed teaching approach is that it forces students to reflect on the rationality of the problem definition phase.
Model phase
Given the strong parallel between Giere’s framework and our Model Phase, the latter can largely be evaluated according to Giere’s evaluation principles. Students first should determine whether the prediction of Step 2 indeed reasonably follows from the model. Is it feasible for the model to meet the design specs, given its auxiliary assumptions and boundary conditions? For instance, the researcher in the example might choose to redesign an existing protein rather than to model a protein from scratch. Accordingly, is it reasonable to think that the existing protein, given its structure and other properties (auxiliary assumptions), can ever be redesigned in such a way that it will behave as desired? Further, the prediction has to be assessed in terms of surprisingness, i.e., the degree to which the prediction goes beyond common sense; precision, i.e., the degree to which the prediction is specific rather than vague; and singularity, i.e., the degree to which the prediction is not a conjunction of predictions.
Next, students are to evaluate the (lack of) agreement between predicted design specs ( Step 2 ) and the data from Step 3 . Such evaluation involves the assessment of the quality of the data (e.g., number of observations, (in)variance of data across different conditions, deviations of data from predicted values), and informs the decision as to build a new model (in case of non-agreement) or to move to the Artifact Phase (in case of agreement). The latter decision is informed also by analogical reasoning.
Analogical reasoning (new evaluative step)
The model only forms a proper basis for the development of the artifact if the two are sufficiently similar in relevant respects. This gives rise to assessing an analogical inference of the following form: one observes that, in virtue of model’s properties a,b,c , the model meets design spec x ; accordingly, an artifact that, analogously to the model, has properties a,b,c , will probably also meet design spec x .
The strength of this inference depends on the extent and relevance of the similarities and dissimilarities between model and artifact. Students should first identify all relevant similarities and dissimilarities, where the criteria of relevance are set by the design specs. For instance, in order to justify the translation from model to real-world protein, a comparison between the surrounding environment of the model protein and the surrounding environment of the real-world protein is clearly relevant. Similarity in color, in contrast, says nothing about the real-world protein’s stability. Furthermore, students must assess the degree and number of these relevant similarities, and do the same for relevant dis similarities.
Finally, students need to identify other, independent models (e.g., scale model, other computer models) of the artifact to be produced, and assess the relevant (dis)similarities between these models and the artifact. It would strengthen the analogical inference if such existing models point in the same direction as the model under study. Conversely, their confidence in the analogical inference should decrease when other models that, in the relevant ways, are similar to the artifact do not satisfy the design specs.
Artifact phase
Assessment of the Artifact Phase largely follows the same procedure as the procedure described under the bullet Model Phase . Only the goals of the phases are different. Whereas the goal of the Model Phase is producing information that is relevant for further steps in the research process, the final step of the Artifact Phase simply ends the entire exercise. Ideally, the latter phase results in an artifact that meets the design specs identified in Step 0 .
Conclusion and discussion
We familiarize all our science students with both frameworks, for the simple reason that students typically encounter both types of research in their curriculum and later professional career. After all, parts of hypothesis-driven research are in fact application-oriented (e.g., design of experiments and equipment, tailor-making computer code), and parts of application-oriented research are hypothesis-driven (e.g., incorporation of hypothesis-driven theories into the design exercise). Further, in many disciplines both approaches peacefully coexist. As our examples of COVID-19 research show, the biomedical sciences comprise different research practices and corresponding epistemic activities; some of these are more hypothesis-driven, others more application-oriented.
Teaching the two frameworks together is also very efficient. There are a number of elements that recur in both approaches; learning about one approach facilitates learning about the other. Given such similarities, it is also easier for students to see and appreciate the crucial differences between the frameworks. These features are a great plus. Standard reconstructions of the research processes in the sciences and the engineering sciences give the impression that there is only limited overlap between the two (see, e.g., Fig. 6 ). We have seen, however, that application-oriented research relies on scientific tools, methods and modes of reasoning. Our framework makes this explicit.

Hill’s ( 1970 ) comparison of scientific reasoning (left) and reasoning in engineering sciences (Figure taken from Hughes, 2009 )
On a final note, our new framework has been mainly developed in response to perceived shortcomings for students at a technical university. There are, however, reasons to suppose that it could be deployed in courses for students from a wide range of disciplines. It has been noted by many that research in many disciplines has undergone a shift away from ‘fundamental’ issues to more ‘applied’ ones; that research efforts have become more interdisciplinary in response to financial and societal incentives; and that new fields of inquiry (e.g., biomedical research, education research, social and economic policy research, management science, intervention research, experimental design research), tend to be oriented towards particular areas of application. Many different interpretations have been given, for instance in terms of changing ‘modes’ of knowledge production (Gibbons et al., 1994 ); a ‘triple helix’ of private, public and academic partners (Etzkowitz & Leydesdorff, 2000 ); or a ‘commodification’ of academic research (Radder, 2010 ). So if we accept that it is taking place in some form, it entails that an increasing number of students would in the course of their educational programs be primarily exposed to application-oriented research practices and epistemic activities. Thus, if our experiences would generalize, philosophy of science teachers might well find that our extended version of Giere’s model-based framework is more comprehensible and useful for ever more students than Giere’s original version, let alone a statement-based approach. Surely, whether our experiences in fact generalize remains to be seen. In building on Giere's original framework, we have provisionally adopted his (implicit) assumption that different types of models – e.g., mathematical models, scale models, and diagrams – play sufficiently similar roles in scientific reasoning to be treated alike. A more differentiated approach may well be called for. Likewise, we have supposed that our framework for reconstructing application-oriented research is compatible with different proposals regarding the distinctive knowledge generated by such research (e,g., in the form of technical norms or other prescriptive knowledge). This supposition may well be incorrect, i.e., may turn out to gloss over distinctive features that would allow students from some programs to gain insight into research activities in their chosen disciplines. Such shortcomings can, however, be best identified in practice rather than discussed in the abstract. We therefore invite other teachers to more systematically study the merits and downsides of our application-oriented version of Giere's model-based framework.
Data availability
Code availability, declarations.
This article belongs to the Topical Collection: Teaching philosophy of science to students from other disciplines
Guest Editors: Sara Green, Joeri Witteveen
Publisher's note
Springer Nature remains neutral with regard to jurisdictional claims in published maps and institutional affiliations.
- Adam M, Carrier M, Wilholt T. Moderate emergentism. Science and Public Policy. 2006; 33 :435–444. doi: 10.3152/147154306781778849. [ CrossRef ] [ Google Scholar ]
- Böttcher F, Meisert A. Argumentation in science education: A model-based framework. Science & Education. 2011; 20 :103–140. doi: 10.1007/s11191-010-9304-5. [ CrossRef ] [ Google Scholar ]
- Bunge M. Technology as applied science. Technology and Culture. 1966; 7 (3):329–347. doi: 10.2307/3101932. [ CrossRef ] [ Google Scholar ]
- Cross N. Designerly Ways of Knowing. Design Studies. 1982; 3 (4):221–227. doi: 10.1016/0142-694X(82)90040-0. [ CrossRef ] [ Google Scholar ]
- Chang H. The philosophical grammar of scientific practice. International Studies in the Philosophy of Science. 2011; 25 :205–221. doi: 10.1080/02698595.2011.605244. [ CrossRef ] [ Google Scholar ]
- Chang, H. (2012). Is Water H2O? Springer.
- Clement, J. J. (2008). Creative model construction in scientists and students: The role of imagery, analogy, and mental simulation . Springer.
- Constant, E. W. (1999). Reliable knowledge and unreliable stuff. Technology and Culture, 40 , 324–357.
- Edvardsson, K., & Hansson, S.O. (2005). When is a goal rational? Social Choice and Welfare, 24 , 2, 343–361.
- Etzkowitz H, Leydesdorff L. The dynamics of innovation. Research Policy. 2000; 29 :109–123. doi: 10.1016/S0048-7333(99)00055-4. [ CrossRef ] [ Google Scholar ]
- Gero JS. Design prototypes: A knowledge representation scheme for design. AI Magazine. 1990; 11 (4):26–36. [ Google Scholar ]
- Gibbons, M., Limoges, C., Nowotny, H., Schwartzman, S., Scott, P., & Trow, M. (1994). The New Production of Knowledge . SAGE.
- Giere, R.N. (1979, 1984, 1991, 2005). Understanding scientific reasoning (Eds. 1–4). Holt, Rinehart, and Winston.
- Giere, R. N. (2001). A new framework for teaching scientific reasoning. Argumentation, 15 (1), 21–33.
- Gilbert JK, Boulter C, Rutherford M. Models in explanations, part 1: Horses for courses? International Journal of Science Education. 1998; 20 (1):83–97. doi: 10.1080/0950069980200106. [ CrossRef ] [ Google Scholar ]
- Gobert JD, Clement JJ. Effect of student-generated diagram versus student-generated summaries on conceptual understanding of causal and dynamic knowledge in plate tectonics. Journal of Research in Science Teaching. 1999; 26 (1):39–53. doi: 10.1002/(SICI)1098-2736(199901)36:1<39::AID-TEA4>3.0.CO;2-I. [ CrossRef ] [ Google Scholar ]
- Gobert, J.D., & Pallant, A. (2004). Fostering students’ epistemologies of models via authentic model-based tasks. Journal of Science Education and Technology, 13 , 1.
- Gobert JD. The effects of different learning tasks on model-building in plate tectonics: Diagramming versus explaining. Journal of Geoscience Education. 2005; 53 (4):444–455. doi: 10.5408/1089-9995-53.4.444. [ CrossRef ] [ Google Scholar ]
- Halloun, I. A. (2004). Modeling theory in science education . Kluwer Academic Publishers.
- Hill, P. (1970). The Science of Engineering Design . Holt, Rinehart and Winston.
- Houkes, W., & Meijers, A.W.M. (2021). Engineering knowledge. Forthcoming in Vallor S (ed.), The Oxford Handbook of Philosophy of Technology . Oxford University.
- Hughes, J. (2009). Practical reasoning and engineering. In Meijers A (ed) Handbook of the Philosophy of Science. Volume 9: Philosophy of Technology and Engineering Sciences . Elsevier.
- Johnson-Laird, P. N. (1983). Mental models: Towards a cognitive science of language, inference, and consciousness . Cambridge University Press.
- Johnson-Laird, P.N. (2006). Mental models, sentential reasoning, and illusory inferences. In Held C, Knauff M, Vosgerau G, et al. (eds.) Mental models and the mind . Elsevier.
- Justi, R., & Gilbert, J. K. (1999). History and philosophy of science through models: The case of chemical kinetics. Science & Education, 8 , 287–307.
- Kant V, Kerr E. Taking stock of engineering epistemology: Multidisciplinary perspectives. Philosophy & Technology. 2019; 32 :685–726. doi: 10.1007/s13347-018-0331-5. [ CrossRef ] [ Google Scholar ]
- Kroes, P. A. (1992). On the role of design in engineering theories. In P. A. Kroes & M. Bakker (Eds.), Technological development and science in the industrial age (pp. 69–98). Kluwer.
- Matthews MR. Models in science and in science education: An introduction. Science & Education. 2007; 16 :647–652. doi: 10.1007/s11191-007-9089-3. [ CrossRef ] [ Google Scholar ]
- Meijers, A. W. M., & Kroes, P. A. (2013). Extending the scope of the theory of knowledge. In M. De Vries, S. O. Hansson, & A. W. M. Meijers (Eds.), Norms in Technology (pp. 15–34). Springer.
- Mill, J. S. (1843). A system of logic, ratiocinative and inductive, being a connected view of the principles of evidence, and the methods of scientific investigation . Harper & Brothers.
- Millgram, E., & Thagard, P. (1996). Deliberative coherence. Synthese, 108 , 63–88.
- Nersessian, N.J. (2002). The cognitive basis of model-based reasoning in science. In Carruthers P, Stich S, Siegal M (Eds.), The cognitive basis of science . Cambridge University Press.
- Nersessian, N. J. (2008). Creating scientific concepts . MIT Press.
- Niiniluoto I. The aim and structure of applied research. Erkenntnis. 1993; 38 :1–21. doi: 10.1007/BF01129020. [ CrossRef ] [ Google Scholar ]
- Passmore, C., & Stewart, J. (2002). A modeling approach to teaching evolutionary biology in high schools. Journal of Research in Science Teaching, 39 (3), 185–204.
- Radder, H. (Ed.) (2010). The commodification of scientific research . University of Pittsburgh Press.
- Roozenburg, N. F. M., & Eekels, J. (1995). Product design: Fundamentals and methods . Wiley.
- Taylor, I., Barker, M., & Jones, A. (2003). Promoting mental model building in astronomy education. International Journal of Science Education, 25 (10), 1205–1225.
- Vega, D.I. (2020). Lockdown, one, two, none, or smart. Modeling containing COVID-19 infection. A conceptual model. Science of the Total Environment 730: 138917. [ PMC free article ] [ PubMed ]
- Vincenti, W. G. (1990). What engineers know and how they know it . Johns Hopkins University Press.
- Wilholt T. Design rules: Industrial research and epistemic merit. Philosophy of Science. 2006; 73 (1):66–89. doi: 10.1086/510175. [ CrossRef ] [ Google Scholar ]

IMAGES
VIDEO
COMMENTS
Research in Science Education, 1993, 23, 199-207 A CASE STUDY OF SCIENTIFIC REASONING Campbell McRobbie & Lyn English Queensland University of Technology ABSTRACT Concern is increasingly being expressed about the teaching of higher order
In a study of undergraduate science students, advanced scientific reasoning was most often accompanied by accurate prior knowledge as well as sophisticated epistemological commitments; additionally, for students who had comparable levels of prior knowledge, skillful reasoning was associated with a strong epistemological commitment to the ...
The first desired outcome for this paper in the ‘hypothesis and theory’ category of this journal is to construct a qualitative theoretical framework or ‘field map’ of important scientific reasoning strategies for model construction, and major interconnections between them, on the basis of detailed case studies of scientific events.
The application of the LCTSR in the present case study is unique in that it explores the concept of scientific misconceptions concerning scientific reasoning skills. The addition of the confidence variable into the LCTSR establishes scientific misconceptions by exploring the correlation between correct responses to content-based statements ...
Open access peer-reviewed chapter. 1. Introduction. Scientific reasoning encompasses the reasoning and problem-solving skills involved in generating, testing and revising hypotheses or theories, and in the case of fully developed skills, reflecting on the process of knowledge acquisition and knowledge change that results from such inquiry activities.
Concern is increasingly being expressed about the teaching of higher order thinking skills in schools and the levels of understanding of scientific concepts by students. Metaphors for the improvement of science education have included science as exploration and science as process skills for experimentation. As a result of a series of studies on how children relate evidence to their theories or ...
The development of general scientific abilities is critical to enable students of science, technology, engineering, and mathematics (STEM) to successfully handle open-ended real-world tasks in future careers (1–6). Teaching goals in STEM education include fostering content knowledge and developing general scientific abilities. One such ability, scientific reasoning (7–9), is related to ...
Subsequent research in science education has shown that model-based approaches are particularly effective in teaching science students how to understand and evaluate scientific reasoning. One limitation of Giere’s framework, however, is that it covers only one type of scientific reasoning, namely the reasoning deployed in hypothesis-driven ...