Information
- Author Services

Initiatives
You are accessing a machine-readable page. In order to be human-readable, please install an RSS reader.
All articles published by MDPI are made immediately available worldwide under an open access license. No special permission is required to reuse all or part of the article published by MDPI, including figures and tables. For articles published under an open access Creative Common CC BY license, any part of the article may be reused without permission provided that the original article is clearly cited. For more information, please refer to https://www.mdpi.com/openaccess .
Feature papers represent the most advanced research with significant potential for high impact in the field. A Feature Paper should be a substantial original Article that involves several techniques or approaches, provides an outlook for future research directions and describes possible research applications.
Feature papers are submitted upon individual invitation or recommendation by the scientific editors and must receive positive feedback from the reviewers.
Editor’s Choice articles are based on recommendations by the scientific editors of MDPI journals from around the world. Editors select a small number of articles recently published in the journal that they believe will be particularly interesting to readers, or important in the respective research area. The aim is to provide a snapshot of some of the most exciting work published in the various research areas of the journal.
Original Submission Date Received: .
- Active Journals
- Find a Journal
- Proceedings Series
- For Authors
- For Reviewers
- For Editors
- For Librarians
- For Publishers
- For Societies
- For Conference Organizers
- Open Access Policy
- Institutional Open Access Program
- Special Issues Guidelines
- Editorial Process
- Research and Publication Ethics
- Article Processing Charges
- Testimonials
- Preprints.org
- SciProfiles
- Encyclopedia
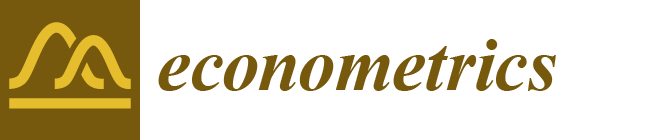
Article Menu
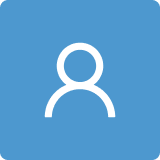
- Subscribe SciFeed
- Recommended Articles
- Google Scholar
- on Google Scholar
- Table of Contents
Find support for a specific problem in the support section of our website.
Please let us know what you think of our products and services.
Visit our dedicated information section to learn more about MDPI.
JSmol Viewer
Unit roots in economic and financial time series: a re-evaluation at the decision-based significance levels.

1. Introduction
2. decision-based level of significance for unit root tests, 2.1. decision-theoretic approach to unit root testing, 2.2. line of enlightened judgement and decision-based significance levels, 2.3. factors affecting the decision-based significance level, 3. calibration rules based on asymptotic local power.
- Model with a constant only ADF: α ^ * = 0.825 − 0.505 p + 0.028 k − 0.025 c − 0.002 X 0 * Phillips–Perron: α ^ * = 0.827 − 0.516 p + 0.029 k − 0.025 c − 0.002 X 0 * DF-GLS: α ^ * = 0.525 − 0.339 p + 0.018 k − 0.019 c + 0.025 X 0 * ERS-P: α ^ * = 0.509 − 0.309 p + 0.017 k − 0.018 c + 0.026 X 0 *
- Model with a constant and a linear trend ADF: α ^ * = 0.933 − 0.655 p + 0.037 k − 0.023 c − 0.001 X 0 * Phillips–Perron: α ^ * = 0.932 − 0.665 p + 0.037 k − 0.023 c − 0.001 X 0 * DF-GLS: α ^ * = 0.802 − 0.546 p + 0.031 k − 0.024 c + 0.017 X 0 * ERS-P: α ^ * = 0.793 − 0.541 p + 0.031 k − 0.023 c + 0.018 X 0 *
4. Re-Evaluation of Past Empirical Results
4.1. extended nelson–plosser data, 4.2. elliott–pesavento data, 4.3. rapach–weber data, 4.4. application of the calibration rules, 4.5. decision-based significance level under a specific loss function, 5. conclusions, supplementary materials, acknowledgments, author contributions, conflicts of interest, appendix a. further sensitivity analyses.
Model with Constant Only | Model with Constant and Time Trend | |||||||
---|---|---|---|---|---|---|---|---|
c | c | |||||||
2.5 | 7.5 | 12.5 | 17.5 | 2.5 | 7.5 | 12.5 | 17.5 | |
X * = 1 | ||||||||
ADF | 0.58 | 0.38 | 0.22 | 0.13 | 0.60 | 0.46 | 0.31 | 0.22 |
DF–GLS | 0.40 | 0.17 | 0.09 | 0.05 | 0.56 | 0.41 | 0.20 | 0.14 |
X * = 3 | ||||||||
ADF | 0.59 | 0.38 | 0.22 | 0.13 | 0.59 | 0.46 | 0.31 | 0.21 |
DF–GLS | 0.41 | 0.21 | 0.14 | 0.09 | 0.56 | 0.41 | 0.23 | 0.17 |
X * = 5 | ||||||||
ADF | 0.59 | 0.38 | 0.22 | 0.12 | 0.60 | 0.46 | 0.30 | 0.21 |
DF–GLS | 0.44 | 0.28 | 0.21 | 0.17 | 0.55 | 0.44 | 0.28 | 0.22 |
p-Value | Sensitivity Analysis | |||
---|---|---|---|---|
ADF | DF–GLS | ADF | DF–GLS | |
Real GNP | 0.05 | 0.05 | H is rejected for all c values | H is rejected for all c values |
Nominal GNP | 0.58 | 0.49 | H is rejected when c = 2.5 | H is rejected when c = 2.5 |
Real per capital GNP | 0.04 | 0.06 | H is rejected for all c values | H is rejected for all c values |
Industrial Production | 0.26 | 0.27 | H is rejected when c = 2.5, 7.5, 12.5 | H is rejected only when c = 2.5, 7.5 (*) |
Employment | 0.18 | 0.04 | H is rejected for all c values | H is rejected for all c values |
Unemployment Rate | 0.01 | 0.01 | H is rejected for all c values | H is rejected for all c values |
GNP deflator | 0.70 | 0.73 | H is accepted for all c values | H is accepted for all c values |
Consumer Prices | 0.91 | 0.61 | H is accepted for all c values | H is accepted for all c values |
Wages | 0.53 | 0.37 | H is rejected when c = 2.5 | H is rejected when c = 2.5, 7.5 |
Real Wages | 0.75 | 0.51 | H is accepted for all c values | H is rejected when c = 2.5 |
Money Stock | 0.18 | 0.10 | H is rejected for all c values | H is rejected for all c values |
Velocity | 0.78 | 0.87 | H is accepted for all c values | H is accepted for all c values |
Interest Rate | 0.98 | 0.33 | H is accepted for all c values | H is rejected when c = 2.5, 7.5 |
Common Stock Prices | 0.64 | 0.63 | H is accepted for all c values | H is accepted for all c values |
- The real GNP and real per capita GNP are found to be trend-stationary for all c and X 0 * values, for both ADF and DF–GLS tests.
- The employment and money stock are found to be trend-stationary for all c and X 0 * values, for both ADF and DF–GLS tests.
- The nominal GNP is found to be trend-stationary only when c = 2.5. For other c values, it is found to be difference-stationary.
- Other nominal and price variables are found to be difference-stationary for all values of c and X 0 * values.
- The results are nearly the same as those reported in Section 4.1 of the paper, showing little sensitivity to the c and X 0 * values.
p-Value | Sensitivity Analysis | |||
---|---|---|---|---|
ADF | DF-GLS | ADF | DF-GLS | |
Austria | 0.414 | 0.225 | H is rejected when c = 2.5 | H is rejected when c = 2.5 (*) |
Belgium | 0.168 | 0.032 | H is rejected when c = 2.5, 7.5, 12.5 | H is rejected for all c values |
Canada | 0.629 | 0.503 | H is accepted for all c values | H is accepted for all c values |
Denmark | 0.116 | 0.020 | H is rejected for all c values | H is rejected for all c values |
Finland | 0.150 | 0.061 | H is rejected when c = 2.5, 7.5, 12.5 | H is rejected for all c values |
France | 0.306 | 0.047 | H is rejected when c = 2.5, 7.5 | H is rejected for all c values |
Germany | 0.288 | 0.043 | H is rejected when c = 2.5, 7.5 | H is rejected for all c values |
Italy | 0.301 | 0.047 | H is rejected when c = 2.5, 7.5 | H is rejected for all c values |
Japan | 0.185 | 0.207 | H is rejected when c = 2.5, 7.5, 12.5 | H is rejected when c = 2.5 (*) |
Netherlands | 0.401 | 0.082 | H is rejected when c = 2.5 | H is rejected for all c values |
Norway | 0.215 | 0.032 | H is rejected when c = 2.5, 7.5, 12.5 | H is rejected for all c values |
Spain | 0.319 | 0.130 | H is rejected when c = 2.5, 7.5 | H is rejected when c = 2.5, 7.5 (*) |
Sweden | 0.201 | 0.044 | H is rejected when c = 2.5, 7.5, 12.5 | H is rejected for all c values |
Switzerland | 0.118 | 0.120 | H is rejected for all c values | H is rejected when c = 2.5, 7.5 (*) |
UK | 0.154 | 0.084 | H is rejected when c = 2.5, 7.5, 12.5 | H is rejected for all c values |
- For the ADF test, most of real exchange rates are found to be stationary under a wide range of c values, showing little sensitivity to the X 0 * value. For eight time series, the null hypothesis is rejected for all c values or c ∊ {2.5, 7.5, 12.5}.
- For the DF–GLS test, most of real exchange rates are found to be stationary under a wide range of c values. For ten time series, the null hypothesis is rejected for all c values considered.
- When the DF–GLS test is used, there are cases where the results are sensitive to the choice of X 0 * value, which might be expected from the nature of the DF–GLS test.
P-Value | Sensitivity Analysis | |||
---|---|---|---|---|
ADF | DF–GLS | ADF | DF–GLS | |
Belgium | 0.200 | 0.045 | H is rejected when c = 2.5, 7.5, 12.5 | H is rejected for all c values |
Canada | 0.237 | 0.071 | H is rejected when c = 2.5, 7.5 | H is rejected when c = 2.5, 7.5, 12.5 (*) |
Denmark | 0.044 | 0.169 | H is rejected for all c values | H is rejected only when c = 2.5, 7.5 |
France | 0.253 | 0.025 | H is rejected when c = 2.5, 7.5 | H is rejected for all c values |
Ireland | 0.158 | 0.245 | H is rejected when c = 2.5, 7.5, 12.5 | H is rejected only when c = 2.5 (*) |
Italy | 0.138 | 0.139 | H is rejected when c = 2.5, 7.5, 12.5 | H is rejected only when c = 2.5, 7.5 (*) |
Japan | 0.120 | 0.038 | H is rejected for all c values | H is rejected for all c values |
Netherlands | 0.562 | 0.287 | H is rejected only when c = 2.5 | H is rejected only when c = 2.5, 7.5 |
New Zealand | 0.606 | 0.241 | H is accepted for all c values | H is rejected when c = 2.5, 7.5 (*) |
UK | 0.039 | 0.009 | H is rejected for all c values | H is rejected for all c values |
- For the ADF test most of real interest rates are found to be stationary under a wide range of c values, showing little sensitivity to the X 0 * value. For six time series, the null hypothesis is rejected for all c values or c ∊ {2.5, 7.5, 12.5}. Two additional time series are found to be stationary when c ∊ {2.5, 7.5}.
- For the DF–GLS test, most of the real exchange rates are found to be stationary under a wide range of c values. For five time series, the null hypothesis is rejected for all c values considered or c ∊ {2.5, 7.5, 12.5}. Three additional time series are found to be stationary when c ∊ {2.5, 7.5}.
- Andrews, Donald W. K., and Patrik Guggenberger. 2014. A Conditional-Heteroskedasticity-Robust Confidence Interval for the Autoregressive Parameter. Review of Economics and Statistics 96: 376–81. [ Google Scholar ] [ CrossRef ]
- Arrow, Kenneth. 1960. Decision theory and the choice of a level of significance for the t -test. In Contributions to Probability and Statistics: Essays in Honor of Harold Hotelling . Edited by Ingram Olkin. Palo Alto: Stanford University Press, pp. 70–8. [ Google Scholar ]
- Campbell, John Y., and N. Gregory Mankiw. 1987. Are Output Fluctuations Transitory? Quarterly Journal of Economics 102: 857–80. [ Google Scholar ] [ CrossRef ] [ Green Version ]
- Cheung, Yin-Wong, and Kon S. Lai. 1995. Lag order and critical values of a modified Dickey–Fuller test. Oxford Bulletin of Economics and Statistics 57: 411–19. [ Google Scholar ] [ CrossRef ]
- Choi, In. 2015. Almost All about Unit Roots: Foundations, Developments, and Applications . New York: Cambridge University Press. [ Google Scholar ]
- Cochrane, John H. 1991. A critique of application of unit root tests. Journal of Economic Dynamics and Control 15: 275–84. [ Google Scholar ] [ CrossRef ]
- Darné, Olivier. 2009. The uncertain unit root in real GNP: A re-examination. Journal of Macroeconomics 31: 153–66. [ Google Scholar ] [ CrossRef ]
- Das, C. 1994. Decision making by classical test procedures using an optimal level of significance. European Journal of Operational Research 73: 76–84. [ Google Scholar ] [ CrossRef ]
- Davidson, Russell, and James G. MacKinnon. 1993. Estimation and Inference in Econometrics . Oxford: Oxford University Press. [ Google Scholar ]
- DeJong, David N., John C. Nankervis, N. E. Savin, and Charles H. Whiteman. 1992. Integration versus trend stationary in time series. Econometrica 60: 423–33. [ Google Scholar ] [ CrossRef ]
- DeGroot, Morris. 1975. Probability and Statistics , 2nd ed. Reading: Addison-Wesley. [ Google Scholar ]
- Dickey, David A., and Wayne A. Fuller. 1979. Distribution of the estimators for autoregressive time series with a unit root. Journal of the American Statistical Association 74: 427–31. [ Google Scholar ]
- Diebold, Francis X., and Lutz Kilian. 2000. Unit-Root Tests Are Useful for Selecting Forecasting Models. Journal of Business and Economic Statistics 18: 265–73. [ Google Scholar ]
- Diebold, Francis X., and Abdelhak S. Senhadji. 1996. The uncertain root in real GNP: Comment. American Economic Review 86: 1291–98. [ Google Scholar ]
- Elliott, Graham, and Elena Pesavento. 2006. On the Failure of Purchasing Power Parity for Bilateral Exchange Rates after 1973. Journal of Money, Credit, and Banking 38: 1405–29. [ Google Scholar ] [ CrossRef ]
- Elliott, Graham, Thomas J. Rothenberg, and James H. Stock. 1996. Efficient tests for an autoregressive unit root. Econometrica 64: 813–36. [ Google Scholar ] [ CrossRef ]
- Engsted, Tom. 2009. Statistical vs. economic significance in economics and econometrics: Further comments on McCloskey and Ziliak. Journal of Economic Methodology 16: 393–408. [ Google Scholar ] [ CrossRef ]
- Fomby, Thomas B., and David K. Guilkey. 1978. On Choosing the Optimal Level of Significance for the Durbin–Watson test and the Bayesian alternative. Journal of Econometrics 8: 203–13. [ Google Scholar ] [ CrossRef ]
- Hausman, Jerry A. 1978. Specification Tests in Econometrics. Econometrica 46: 1251–71. [ Google Scholar ] [ CrossRef ]
- Keuzenkamp, Hugo A., and Jan R. Magnus. 1995. On tests and significance in econometrics. Journal of Econometrics 67: 103–28. [ Google Scholar ] [ CrossRef ]
- Kilian, Lutz. 1998. Small sample confidence intervals for impulse response functions. The Review of Economics and Statistics 80: 218–30. [ Google Scholar ] [ CrossRef ]
- Kim, Jae H. 2004. Bootstrap Prediction Intervals for Autoregression using Asymptotically Mean-Unbiased Parameter Estimators. International Journal of Forecasting 20: 85–97. [ Google Scholar ] [ CrossRef ]
- Kim, Jae H. 2015. BootPR: Bootstrap Prediction Intervals and Bias-Corrected Forecasting. R package version 0.60. Available online: http://CRAN.R-project.org/package=BootPR (accessed on 9 February 2016).
- Kim, Jae H., and Philip Inyeob Ji. 2015. Significance Testing in Empirical Finance: A Critical Review and Assessment. Journal of Empirical Finance 34: 1–14. [ Google Scholar ] [ CrossRef ]
- Kish, Leslie. 1959. Some statistical problems in research design. American Sociological Review 24: 328–38. [ Google Scholar ] [ CrossRef ]
- Koop, Gary, and Mark F. J. Steel. 1994. A Decision-Theoretic Analysis of the Unit-Root Hypothesis Using Mixtures of Elliptical Models. Journal of Business and Economic Statistics 12: 95–107. [ Google Scholar ]
- Leamer, Edward E. 1978. Specification Searches: Ad Hoc Inference with Nonexperimental Data . New York: Wiley. [ Google Scholar ]
- Lehmann, Erich L., and Joseph P. Romano. 2005. Testing Statistical Hypothesis , 3rd ed. New York: Springer. [ Google Scholar ]
- Lothian, James R., and Mark P. Taylor. 1996. Real exchange rate behavior: The recent float from the perspective of the past two centuries. Journal of Political Economy 104: 488–510. [ Google Scholar ] [ CrossRef ]
- Luo, Sui, and Richard Startz. 2014. Is it one break or ongoing permanent shocks that explains U.S. real GDP? Journal of Monetary Economics 66: 155–63. [ Google Scholar ] [ CrossRef ]
- MacKinnon, James G. 1996. Numerical distribution functions for unit root and cointegration tests. Journal of Applied Econometrics 11: 601–18. [ Google Scholar ] [ CrossRef ]
- MacKinnon, James G. 2002. Bootstrap inference in Econometrics. Canadian Journal of Economics 35: 615–44. [ Google Scholar ] [ CrossRef ]
- Maddala, G. S., and In-Moo Kim. 1998. Unit Roots, Cointegration and Structural Changes . Cambridge: Cambridge University Press. [ Google Scholar ]
- Manderscheid, Lester V. 1965. Significance Levels-0.05, 0.01, or? Journal of Farm Economics 47: 1381–85. [ Google Scholar ] [ CrossRef ]
- McCallum, Bennett T. 1986. On "Real" and "Sticky-Price" Theories of the Business Cycle. Journal of Money, Credit and Banking 18: 397–414. [ Google Scholar ] [ CrossRef ]
- Morrison, Denton E., and Ramon E. Henkel, eds. 1970. The Significance Test Controversy: A Reader . New Brunswick: Aldine Transactions. [ Google Scholar ]
- Müller, Ulrich K., and Graham Elliott. 2003. Testing for unit roots and the initial condition. Econometrica 71: 1269–86. [ Google Scholar ] [ CrossRef ]
- Murray, Christian J., and Charles R. Nelson. 2000. The uncertain trend in U.S. GDP. Journal of Monetary Economics 46: 79–95. [ Google Scholar ] [ CrossRef ]
- Neely, Christopher, and David E. Rapach. 2008. Real Interest Rate Persistence: Evidence and Implications. Federal Reserve Bank of St. Louis Review 90: 609–42. [ Google Scholar ]
- Nelson, Charles R., and Charles R. Plosser. 1982. Trends and random walks in macroeconomic time series. Journal of Monetary Economics 10: 139–62. [ Google Scholar ] [ CrossRef ]
- Orcutt, Guy H., and Herbert S. Winokur. 1969. First order autoregression: Inference, estimation and prediction. Econometrica 37: 1–14. [ Google Scholar ] [ CrossRef ]
- Papell, David H. 1997. Searching for stationarity: Purchasing power parity under the current float. Journal of International Economics 43: 313–32. [ Google Scholar ] [ CrossRef ]
- Papell, David H., and Ruxandra Prodan. 2004. The uncertain unit root in US real GDP: Evidence with restricted and unrestricted structural change. Journal of Money, Credit and Banking 36: 423–27. [ Google Scholar ] [ CrossRef ]
- Perez, María-Eglée, and Luis Raúl Pericchi. 2014. Changing statistical significance with the amount of information: The adaptive α significance level. Statistics and Probability Letters 85: 20–24. [ Google Scholar ] [ CrossRef ] [ PubMed ]
- Pericchi, Luis Raúl, and Carlos Pereira. 2016. Adaptive significance levels using optimal decision rules: Balancing by weighting the error probabilities. Brazilian Journal of Probability and Statistics 30: 70–90. [ Google Scholar ] [ CrossRef ]
- Pfaff, Bernhard. 2008. Analysis of Integrated and Cointegrated Time Series with R , 2nd ed. New York: Springer. [ Google Scholar ]
- Phillips, Peter, and Pierre Perron. 1988. Testing for a Unit Root in Time Series Regression. Biometrika 75: 335–46. [ Google Scholar ] [ CrossRef ]
- Poirier, Dale J. 1995. Intermediate Statistics and Econometrics: A Comparative Approach . Cambridge: MIT Press. [ Google Scholar ]
- R Core Team. 2014. R: A language and environment for statistical computing. Vienna, Austria: R Foundation for Statistical Computing. Available online: http://www.R-project.org/ (accessed on 9 February 2016).
- Rapach, David E., and Christian E. Weber. 2004. Are real interest rates really nonstationary? New evidence from tests with good size and power. Journal of Macroeconomics 26: 409–30. [ Google Scholar ] [ CrossRef ]
- Rose, Andrew K. 1988. Is the real interest rate stable? Journal of Finance 43: 1095–112. [ Google Scholar ] [ CrossRef ]
- Rudebusch, Glenn D. 1993. The uncertain unit root in real GNP. American Economic Review 83: 264–72. [ Google Scholar ]
- Schotman, Peter C., and Herman K. van Dijk. 1991. On Bayesian Roots to Unit Roots. Journal of Applied Econometrics 6: 387–401. [ Google Scholar ] [ CrossRef ]
- Schwert, G. William. 1989. Testing for unit roots: A Monte Carlo investigation. Journal of Business and Economic Statistics 7: 147–59. [ Google Scholar ]
- Sims, Christopher. 1988. Bayesian Scepticism on Unit Root Econometrics. Journal of Economics Dynamics and Control 12: 463–74. [ Google Scholar ] [ CrossRef ]
- Sims, Christopher, and Harald Uhlig. 1991. Understanding Unit Rooters: A Helicopter Tour. Econometrica 59: 1591–99. [ Google Scholar ] [ CrossRef ]
- Skipper, James K., Anthony L. Guenther, and Gilbert Nass. 1967. The sacredness of 0.05: A note on concerning the use of statistical levels of significance in social science. The American Sociologist 2: 16–18. [ Google Scholar ]
- Startz, Richard. 2014. Choosing the More Likely Hypothesis. Foundations and Trends in Econometrics 7: 119–89. [ Google Scholar ] [ CrossRef ]
- Stine, Robert A., and Paul Shaman. 1989. A fixed point characterization for bias of autoregressive estimators. The Annals of Statistics 17: 1275–84. [ Google Scholar ] [ CrossRef ]
- Stock, James H. 1991. Confidence Intervals for the Largest Autoregressive Root in U.S. Macroeconomic Time Series. Journal of Monetary Economics 28: 435–59. [ Google Scholar ] [ CrossRef ]
- Wasserstein, Ronald L., and Nicole A. Lazar. 2016. The ASA’s statement on p -values: Context, process, and purpose. The American Statistician 70: 129–33. [ Google Scholar ] [ CrossRef ]
- Winer, Benjamin J. 1962. Statistical Principles in Experimental Design . New York: McGraw-Hill. [ Google Scholar ]
- Ziliak, Stephen Thomas, and Deirdre N. McCloskey. 2008. The Cult of Statistical Significance: How the Standard Error Costs Us Jobs, Justice, and Lives . Ann Arbor: The University of Michigan Press. [ Google Scholar ]
1 | and β in the context of unit root testing will be formally defined in . |
2 | ( ). |
3 | ( ). |
4 | ( ) and ( ) also propose the same method for choosing the decision-based significance level, without introducing the line of enlightened judgement. |
5 | ( ). |
6 | ( ) called the minimum oomph, which is the smallest value where the null hypothesis is violated economically. |
7 | where the calibration rules for the decision-based significance levels are constructed under a wide range of starting values. |
8 | ( ) provide response surface estimates for the critical values of the DF–GLS test for the model with a linear trend. However, they are only applicable for 5% and 10% levels of significance. |
9 | ) provides computational resources for this bias-corrected estimation. |
Click here to enlarge figure
α fixed at 0.05 | minimize α + β | |||||||
60 | 0.05 | 0.88 | 0.12 | −3.49 | 0.47 | 0.27 | 0.73 | −2.22 |
70 | 0.05 | 0.86 | 0.14 | −3.48 | 0.37 | 0.31 | 0.69 | −2.41 |
80 | 0.05 | 0.82 | 0.18 | −3.47 | 0.37 | 0.25 | 0.75 | −2.41 |
90 | 0.05 | 0.78 | 0.22 | −3.46 | 0.37 | 0.21 | 0.79 | −2.41 |
100 | 0.05 | 0.74 | 0.26 | −3.46 | 0.31 | 0.22 | 0.78 | −2.54 |
110 | 0.05 | 0.69 | 0.31 | −3.46 | 0.29 | 0.19 | 0.81 | −2.58 |
120 | 0.05 | 0.64 | 0.36 | −3.45 | 0.27 | 0.16 | 0.84 | −2.63 |
130 | 0.05 | 0.58 | 0.42 | −3.44 | 0.23 | 0.16 | 0.84 | −2.72 |
α fixed at 0.05 | minimize α + β | |||||||
60 | 0.05 | 0.72 | 0.28 | −2.89 | 0.29 | 0.21 | 0.79 | −2.05 |
70 | 0.05 | 0.68 | 0.32 | −2.89 | 0.29 | 0.17 | 0.83 | −2.05 |
80 | 0.05 | 0.62 | 0.38 | −2.89 | 0.25 | 0.15 | 0.85 | −2.13 |
90 | 0.05 | 0.56 | 0.44 | −2.89 | 0.25 | 0.12 | 0.88 | −2.13 |
100 | 0.05 | 0.51 | 0.49 | −2.89 | 0.21 | 0.12 | 0.88 | −2.24 |
110 | 0.05 | 0.44 | 0.56 | −2.89 | 0.19 | 0.10 | 0.90 | −2.29 |
120 | 0.05 | 0.38 | 0.62 | −2.89 | 0.15 | 0.11 | 0.89 | −2.40 |
130 | 0.05 | 0.31 | 0.69 | −2.89 | 0.15 | 0.08 | 0.92 | −2.40 |
α fixed at 0.05 | minimize α + β | |||||||
80 | 0.05 | 0.91 | 0.09 | −2.90 | 0.55 | 0.23 | 0.77 | −1.46 |
120 | 0.05 | 0.87 | 0.13 | −2.89 | 0.47 | 0.20 | 0.80 | −1.62 |
160 | 0.05 | 0.81 | 0.19 | −2.88 | 0.39 | 0.18 | 0.82 | −1.78 |
180 | 0.05 | 0.78 | 0.22 | −2.88 | 0.33 | 0.19 | 0.81 | −1.90 |
200 | 0.05 | 0.73 | 0.27 | −2.88 | 0.29 | 0.18 | 0.82 | −1.99 |
240 | 0.05 | 0.63 | 0.37 | −2.87 | 0.27 | 0.13 | 0.87 | −2.04 |
α fixed at 0.05 | minimize α + β | |||||||
80 | 0.05 | 0.68 | 0.32 | −1.94 | 0.25 | 0.15 | 0.85 | −1.08 |
120 | 0.05 | 0.56 | 0.44 | −1.94 | 0.23 | 0.08 | 0.92 | −1.14 |
160 | 0.05 | 0.41 | 0.59 | −1.94 | 0.17 | 0.08 | 0.92 | −1.33 |
180 | 0.05 | 0.34 | 0.66 | −1.94 | 0.15 | 0.07 | 0.93 | −1.40 |
200 | 0.05 | 0.27 | 0.73 | −1.94 | 0.13 | 0.06 | 0.94 | −1.48 |
240 | 0.05 | 0.16 | 0.84 | −1.94 | 0.11 | 0.04 | 0.96 | −1.57 |
ADF | DF–GLS | |||||||
---|---|---|---|---|---|---|---|---|
ρ | −0.5 | 0 | 0.5 | 0.9 | −0.5 | 0 | 0.5 | 0.9 |
X * | ||||||||
0 | 0.45 | 0.47 | 0.51 | 0.51 | 0.19 | 0.21 | 0.21 | 0.27 |
1 | 0.47 | 0.45 | 0.49 | 0.47 | 0.23 | 0.21 | 0.21 | 0.27 |
2 | 0.45 | 0.49 | 0.51 | 0.53 | 0.29 | 0.25 | 0.21 | 0.27 |
3 | 0.45 | 0.47 | 0.51 | 0.51 | 0.35 | 0.29 | 0.23 | 0.27 |
4 | 0.45 | 0.49 | 0.49 | 0.47 | 0.45 | 0.35 | 0.25 | 0.27 |
5 | 0.43 | 0.47 | 0.47 | 0.49 | 0.55 | 0.41 | 0.27 | 0.29 |
6 | 0.37 | 0.43 | 0.47 | 0.49 | 0.63 | 0.49 | 0.31 | 0.29 |
8 | 0.33 | 0.37 | 0.45 | 0.51 | 0.75 | 0.59 | 0.35 | 0.27 |
10 | 0.27 | 0.35 | 0.43 | 0.47 | 0.81 | 0.67 | 0.43 | 0.27 |
, k) | ||||
(0.25, 0.25) | 0.27 | 0.30 | 0.25 | 0.26 |
(0.25, 1) | 0.63 | 0.65 | 0.49 | 0.49 |
(0.25, 4) | 0.83 | 0.84 | 0.67 | 0.67 |
(0.5, 0.25) | 0.03 | 0.03 | 0.07 | 0.05 |
(0.5, 1) | 0.39 | 0.37 | 0.29 | 0.30 |
(0.5, 4) | 0.71 | 0.70 | 0.53 | 0.53 |
(0.75, 0.25) | 0.01 | 0.01 | 0.01 | 0.01 |
(0.75, 1) | 0.07 | 0.06 | 0.11 | 0.10 |
(0.75, 4) | 0.47 | 0.46 | 0.37 | 0.36 |
(0.25, 0.25) | 0.27 | 0.29 | 0.25 | 0.26 |
(0.25, 1) | 0.63 | 0.64 | 0.49 | 0.49 |
(0.25, 4) | 0.83 | 0.84 | 0.69 | 0.67 |
(0.5, 0.25) | 0.03 | 0.03 | 0.07 | 0.05 |
(0.5, 1) | 0.35 | 0.36 | 0.31 | 0.31 |
(0.5, 4) | 0.67 | 0.69 | 0.55 | 0.54 |
(0.75, 0.25) | 0.01 | 0.01 | 0.01 | 0.01 |
(0.75, 1) | 0.07 | 0.06 | 0.11 | 0.10 |
(0.75, 4) | 0.43 | 0.43 | 0.37 | 0.35 |
n | ADF | DF–GLS | |||||||
---|---|---|---|---|---|---|---|---|---|
p-Value | Decision (α = 0.01/0.05) | α* | Decision* | p-Value | Decision (α = 0.01/0.05) | α* | Decision* | ||
Real GNP | 80 | 0.05 | Accept | 0.37 | Reject | 0.05 | Accept | 0.25 | Reject |
Nominal GNP | 80 | 0.58 | Accept | 0.37 | Accept | 0.49 | Accept | 0.25 | Accept |
Real per capital GNP | 80 | 0.04 | Accept/Reject | 0.37 | Reject | 0.06 | Accept | 0.25 | Reject |
Industrial Production | 129 | 0.26 | Accept | 0.23 | Accept | 0.27 | Accept | 0.15 | Accept |
Employment | 99 | 0.18 | Accept | 0.31 | Reject | 0.04 | Accept/Reject | 0.21 | Reject |
Unemployment Rate | 99 | 0.01 | Reject | 0.31 | Reject | 0.01 | Reject | 0.21 | Reject |
GNP deflator | 100 | 0.70 | Accept | 0.31 | Accept | 0.73 | Accept | 0.21 | Accept |
Consumer Prices | 129 | 0.91 | Accept | 0.22 | Accept | 0.61 | Accept | 0.15 | Accept |
Wages | 89 | 0.53 | Accept | 0.37 | Accept | 0.37 | Accept | 0.25 | Accept |
Real Wages | 89 | 0.75 | Accept | 0.37 | Accept | 0.51 | Accept | 0.25 | Accept |
Money Stock | 100 | 0.18 | Accept | 0.31 | Reject | 0.10 | Accept | 0.21 | Reject |
Velocity | 120 | 0.78 | Accept | 0.27 | Accept | 0.87 | Accept | 0.15 | Accept |
Interest Rate | 89 | 0.98 | Accept | 0.37 | Accept | 0.33 | Accept | 0.25 | Accept |
Common Stock Prices | 118 | 0.64 | Accept | 0.27 | Accept | 0.63 | Accept | 0.15 | Accept |
ADF | DF–GLS | |||||||
---|---|---|---|---|---|---|---|---|
Statistic | p-Value | Decision (α = 0.01/0.05) | Decision* (α* = 0.47) | Statistic | p-Value | Decision (α = 0.01/0.05) | Decision* (α* = 0.23) | |
Austria | −1.729 | 0.414 | Accept | Reject | −1.155 | 0.225 | Accept | Reject |
Belgium | −2.319 | 0.168 | Accept | Reject | −2.133 | 0.032 | Accept/Reject | Reject |
Canada | −1.297 | 0.629 | Accept | Accept | −0.487 | 0.503 | Accept | Accept |
Denmark | −2.507 | 0.116 | Accept | Reject | −2.318 | 0.020 | Accept/Reject | Reject |
Finland | −2.377 | 0.150 | Accept | Reject | −1.847 | 0.061 | Accept | Reject |
France | −1.955 | 0.306 | Accept | Reject | −1.965 | 0.047 | Accept/Reject | Reject |
Germany | −1.996 | 0.288 | Accept | Reject | −2.006 | 0.043 | Accept/Reject | Reject |
Italy | −1.966 | 0.301 | Accept | Reject | −1.975 | 0.047 | Accept/Reject | Reject |
Japan | −2.265 | 0.185 | Accept | Reject | −1.208 | 0.207 | Accept | Reject |
Netherlands | −1.755 | 0.401 | Accept | Reject | −1.714 | 0.082 | Accept | Reject |
Norway | −2.178 | 0.215 | Accept | Reject | −2.135 | 0.032 | Accept/Reject | Reject |
Spain | −1.928 | 0.319 | Accept | Reject | −1.478 | 0.130 | Accept | Reject |
Sweden | −2.219 | 0.201 | Accept | Reject | −1.997 | 0.044 | Accept/Reject | Reject |
Switzerland | −2.499 | 0.118 | Accept | Reject | −1.521 | 0.120 | Accept | Reject |
UK | −2.363 | 0.154 | Accept | Reject | −1.703 | 0.084 | Accept | Reject |
ADF | DF–GLS | |||||||
---|---|---|---|---|---|---|---|---|
Statistic | p-Value | Decision (α = 0.01/0.05) | Decision* (α* = 0.33) | Statistic | p-Value | Decision (α = 0.01/0.05) | Decision* (α* = 0.15) | |
Belgium | −2.22 | 0.200 | Accept | Reject | −1.99 | 0.045 | Accept/Reject | Reject |
Canada | −2.12 | 0.237 | Accept | Reject | −1.78 | 0.071 | Accept | Reject |
Denmark | −2.93 | 0.044 | Accept/Reject | Reject | −1.33 | 0.169 | Accept | Accept |
France | −2.08 | 0.253 | Accept | Reject | −2.23 | 0.025 | Accept/Reject | Reject |
Ireland | −2.35 | 0.158 | Accept | Reject | −1.10 | 0.245 | Accept | Accept |
Italy | −2.42 | 0.138 | Accept | Reject | −1.44 | 0.139 | Accept | Reject |
Japan | −2.49 | 0.120 | Accept | Reject | −2.06 | 0.038 | Accept/Reject | Reject |
Netherlands | −1.44 | 0.562 | Accept | Accept | −0.99 | 0.287 | Accept | Accept |
New Zealand | −1.35 | 0.606 | Accept | Accept | −1.11 | 0.241 | Accept | Accept |
UK | −2.98 | 0.039 | Accept/Reject | Reject | −2.64 | 0.009 | Reject | Reject |
Phillips–Perron | ERS–P | |||
---|---|---|---|---|
X * | c = 5 | c = 15 | c = 5 | c = 15 |
0 | 0.52 | 0.25 | 0.49 | 0.17 |
5 | 0.50 | 0.24 | 0.49 | 0.27 |
ADF | DF–GLS | |||
---|---|---|---|---|
δ = 1 | δ = 5 | δ = 1 | δ = 5 | |
c = 9.37 | ||||
X * = 1 | ||||
p = P(H ) | ||||
0.1 | 0.64 | 0.45 | 0.24 | 0.17 |
0.5 | 0.32 | 0.05 | 0.13 | 0.07 |
0.9 | 0.01 | 0.01 | 0.03 | 0.01 |
X * = 3 | ||||
p = P(H ) | ||||
0.1 | 0.63 | 0.45 | 0.32 | 0.22 |
0.5 | 0.31 | 0.05 | 0.18 | 0.09 |
0.9 | 0.01 | 0.01 | 0.03 | 0.01 |
c = 7 | ||||
X * = 1 | ||||
p = P(H ) | ||||
0.1 | 0.89 | 0.70 | 0.51 | 0.33 |
0.5 | 0.48 | 0.01 | 0.25 | 0.07 |
0.9 | 0.01 | 0.01 | 0.01 | 0.01 |
X * = 3 | ||||
p = P(H ) | ||||
0.1 | 0.88 | 0.70 | 0.55 | 0.37 |
0.5 | 0.48 | 0.01 | 0.28 | 0.07 |
0.9 | 0.01 | 0.01 | 0.01 | 0.01 |
c = 13 | ||||
X * = 1 | ||||
p = P(H ) | ||||
0.1 | 0.45 | 0.30 | 0.14 | 0.11 |
0.5 | 0.21 | 0.07 | 0.09 | 0.05 |
0.9 | 0.02 | 0.01 | 0.03 | 0.01 |
X * = 3 | ||||
p = P(H ) | ||||
0.1 | 0.43 | 0.30 | 0.22 | 0.17 |
0.5 | 0.21 | 0.07 | 0.13 | 0.08 |
0.9 | 0.02 | 0.01 | 0.03 | 0.01 |
Share and Cite
Kim, J.H.; Choi, I. Unit Roots in Economic and Financial Time Series: A Re-Evaluation at the Decision-Based Significance Levels. Econometrics 2017 , 5 , 41. https://doi.org/10.3390/econometrics5030041
Kim JH, Choi I. Unit Roots in Economic and Financial Time Series: A Re-Evaluation at the Decision-Based Significance Levels. Econometrics . 2017; 5(3):41. https://doi.org/10.3390/econometrics5030041
Kim, Jae H., and In Choi. 2017. "Unit Roots in Economic and Financial Time Series: A Re-Evaluation at the Decision-Based Significance Levels" Econometrics 5, no. 3: 41. https://doi.org/10.3390/econometrics5030041
Article Metrics
Article access statistics, supplementary material.
Supplementary (ZIP, 741 KiB)
Further Information
Mdpi initiatives, follow mdpi.
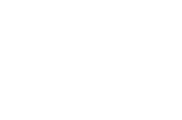
Subscribe to receive issue release notifications and newsletters from MDPI journals
- Search Menu
- Sign in through your institution
- Advance articles
- Author Guidelines
- Submission Site
- Open Access
- Reviewer Guidelines
- About Biometrika
- Editorial Board
- Advertising and Corporate Services
- Self-Archiving Policy
- Dispatch Dates
- Journals on Oxford Academic
- Books on Oxford Academic

Article Contents
1. introduction, 2. main results, 3. simulation study, acknowledgement, supplementary material.
- < Previous
Testing for unit roots based on sample autocovariances

- Article contents
- Figures & tables
- Supplementary Data
Jinyuan Chang, Guanghui Cheng, Qiwei Yao, Testing for unit roots based on sample autocovariances, Biometrika , Volume 109, Issue 2, June 2022, Pages 543–550, https://doi.org/10.1093/biomet/asab034
- Permissions Icon Permissions
We propose a new unit-root test for a stationary null hypothesis |$H_0$| against a unit-root alternative |$H_1$| . Our approach is nonparametric as |$H_0$| assumes only that the process concerned is |$I(0)$| , without specifying any parametric forms. The new test is based on the fact that the sample autocovariance function converges to the finite population autocovariance function for an |$I(0)$| process, but diverges to infinity for a process with unit roots. Therefore, the new test rejects |$H_0$| for large values of the sample autocovariance function. To address the technical question of how large is large, we split the sample and establish an appropriate normal approximation for the null distribution of the test statistic. The substantial discriminative power of the new test statistic is due to the fact that it takes finite values under |$H_0$| and diverges to infinity under |$H_1$| . This property allows one to truncate the critical values of the test so that it has asymptotic power 1; it also alleviates the loss of power due to the sample-splitting. The test is implemented in |$\texttt{R}$| .
Models with unit roots are frequently used for nonstationary time series. The importance of the unit-root concept stems from the fact that many economic, financial, business and social-domain data exhibit segmented trend-like or random wandering phenomena. While the random-walk-like behaviour of stock prices was noticed much earlier, for example by Jules Regnault, a French broker, in 1863 and by Louis Bachelier in his 1900 PhD thesis, the development of statistical inference for unit roots started only in the late 1970s. Nevertheless, the literature on unit-root tests is by now immense and diverse. We review only a selection of important developments below, leading naturally to the new test presented in this paper.
The Dickey–Fuller tests ( Dickey & Fuller, 1979 , 1981 ) deal with Gaussian random walks with independent errors. Efforts to relax the condition of independent Gaussian errors have led to, among others, the augmented Dickey–Fuller tests ( Said & Dickey, 1984 ; Elliott et al., 1996 ), which deal with auto-regressive errors, and the Phillips–Perron test ( Phillips, 1987 ; Phillips & Perron, 1988 ), which estimates the long-run variance of the error process nonparametrically. The augmented Dickey–Fuller tests have been further extended to deal with structural breaks in trend ( Zivot & Andrews, 1992 ), long memory processes ( Robinson, 1994 ), seasonal unit roots ( Chan & Wei, 1988 ; Hylleberg et al., 1990 ), bootstrap unit-root tests ( Paparoditis & Politis, 2005 ), nonstationary volatility ( Cavaliere & Taylor, 2007 ), panel data ( Pesaran, 2007 ) and local stationary processes ( Rho & Shao, 2019 ); see the survey papers by Stock (1994) and Phillips & Xiao (1998) , and the monographs by Hatanaka (1996) and Maddala & Kim (1998) for further references.
The Dickey–Fuller tests and their variants are based on regression of a time series on its first lag, in which the existence of a unit root is postulated as a null hypothesis in the form of the regression coefficient being equal to 1. This null hypothesis is tested against a stationary alternative hypothesis that the regression coefficient is smaller than 1. This setting leads to innate indecisive inference for ascertaining the existence of unit roots, as a statistical test is incapable of accepting a null hypothesis. To place the assertion of unit roots on firmer ground, Kwiatkowski et al. (1992) adopted a different approach: their proposed test considers a stationary null hypothesis against a unit-root alternative. It is based on a plausible representation of possible nonstationary time series in which a unit root is represented as an additive random-walk component. Then, under the null hypothesis, the variance of the random-walk component is zero. The test of Kwiatkowski et al. (1992) is the one-sided Lagrange multiplier test for testing the variance being zero against it being greater than zero.
Despite the many exciting developments mentioned above, testing for the existence of unit roots remains a challenge in time series analysis, as most available methods suffer from lack of accurate size control and low power. In this paper we propose a new test that is based on a radically different idea from existing approaches. Our setting is similar in spirit to that of Kwiatkowski et al. (1992) , in that we test a stationary null hypothesis |$H_0$| against a unit-root alternative |$H_1$| . However, our approach is nonparametric as |$H_0$| assumes only that the process concerned is |$I(0)$| , without specifying any parametric forms. The new test is based on the simple fact that under |$H_0$| the sample autocovariance function converges to the finite population autocovariance function, while under |$H_1$| it diverges to infinity. Therefore, we can reject |$H_0$| for large absolute values of the sample autocovariance function. To address the technical question of how large is large, we split the sample and establish an appropriate normal approximation for the null distribution of the test statistic. Our sample autocovariance function-based test statistic offers substantial discriminative power as it takes finite values under |$H_0$| and diverges to infinity under |$H_1$| . This property allows us to truncate the critical values determined by the normal approximation to ensure that the test has asymptotic power 1; furthermore, it alleviates the loss of power due to the sample-splitting, so that our test outperforms the test of Kwiatkowski et al. (1992) in a power comparison simulation. Another advantage of the new method is that it has remarkable discriminative power, being able to tell the difference between, for example, a random walk and an ar |$(1)$| with autoregressive coefficient close to but still smaller than 1, a case in which most available unit-root tests, including the method of Kwiatkowski et al. (1992) , suffer from weak discriminative power. Admittedly, the new test is technically sophisticated, which we argue is inevitable in order to gain improvement over existing methods. Nevertheless, we have developed an |$\texttt{R}$| ( R Development Core Team, 2022 ) function |$\texttt{ur.test}$| in the package |$\texttt{HDTSA}$| that implements the test in an automatic manner.
2.1. A power-one test
Let |$Y_t$| satisfy ( 2 ) with independent |$\epsilon_t\sim(0,\sigma_{\epsilon}^2)$| and |$\sum_{j=1}^\infty j|\psi_j|<\infty$| . Write |$a=\sum_{j=0}^\infty\psi_j$| and |$V_{d-1}(t)=F_{d-1}(t)-\int_0^1F_{d-1}(t)\,{\rm d}t$| where |$F_{d-1}(t)$| is the scalar multi-fold integrated Brownian motion defined recursively by |$F_j(t)=\int_0^tF_{j-1}(x)\,{\rm d}x$| for any |$j\geqslant1$| , with |$F_0(t)$| the standard Brownian motion. For any given integer |$k\geqslant 0$| , as |$n\rightarrow\infty$| we have that (i) |$n^{-(2d-1)}\hat{\gamma}(k)\rightarrow a^2\sigma_{\epsilon}^2\int_0^1V_{d-1}^2(t)\,{\rm d}t$| in distribution if |$\mu_d=0$| , and (ii) |$n^{-2d}\hat{\gamma}(k)\rightarrow\phi_{d,k}\mu_d^2$| in probability if |$\mu_d\neq 0$| , where |$\phi_{d,k}>0$| is a bounded constant depending only on |$d$| and |$k$| .
Formally, we reject |$H_0$| at the significance level |$\phi \in (0, 1)$| if |$T_n>{\small{\text{cv}}}_\phi$| , where |${\small{\text{cv}}}_\phi$| is the critical value satisfying |${\mathrm{pr}}_{H_0}(T_n>{\small{\text{cv}}}_\phi)\rightarrow\phi$| . As we will see in ( 3 ), |$\{\hat{\gamma}_1(k)\}_{k=0}^{K_0}$| are used to determine the critical value |${\small{\text{cv}}}_\phi$| . One obvious concern with splitting the sample into two halves is loss of testing power. However, the fact that |$T_n$| takes finite values under |$H_0$| and diverges to infinity under |$H_1$| implies that |$T_n$| has strong discriminative power to tell |$H_1$| apart from |$H_0$| , which is enough to provide more power than, for example, the test of Kwiatkowski et al. (1992) . Our simulation results indicate that the sample-splitting works well even for sample size |$n=80$| . Under |$H_0$| , write |$y_{t,k}=2\{(Y_t-\mu)(Y_{t+k}-\mu)-\gamma(k)\}{\rm sgn}(k+t-N-1/2)$| . For |$\ell\geqslant1$| define |$B_\ell^2=E\{(\sum_{t=1}^\ell Q_t)^2\}$| where |$Q_t=\sum_{k=0}^{K_0}\xi_{t,k}$| with |$\xi_{t,k}=2y_{t,k}\gamma(k)$| . The following regularity conditions are needed; see the Supplementary Material for a discussion of their validity.
Under |$H_0$| , |$\max_{1\leqslant t \leqslant n}E(|Y_t|^{2s_1})\leqslant c_1$| for two constants |$s_1\in(2,3]$| and |$c_1>0$| .
Under |$H_0$| , |$\{Y_t\}$| is |$\alpha$| -mixing with |$\alpha(\tau)=\sup_{t}\,\sup_{A \in {\mathcal F}_{-\infty}^t, B \in {\mathcal F}_{t+\tau}^{\infty}}|{\mathrm{pr}}(AB)-{\mathrm{pr}}(A){\mathrm{pr}}(B)|\leqslant c_2\tau^{-\beta_1}$| for any |$\tau\geqslant1$| , where |${\mathcal F}_{-\infty}^t$| and |$\mathcal{F}_{t+\tau}^\infty$| denote the |$\sigma$| -fields generated by |$\{Y_u\}_{u\leqslant t}$| and |$\{Y_u\}_{u\geqslant t+\tau}$| , respectively, and |$c_2>0$| and |$\beta_1>2(s_1-1)s_1/(s_1-2)^2$| are two constants, with |$s_1$| as specified in Condition 1.
Under |$H_0$| , there is a constant |$c_3>0$| such that |$B_\ell^2\geqslant c_3\ell$| for any |$\ell\geqslant1$| .
Let |${\small{\text{cv}}}_\phi$| be defined by ( 3 ) with |${\mathcal T}$| satisfying |${\mathrm{pr}}_{H_0}(\mathcal{T} )\rightarrow1$| and |${\mathrm{pr}}_{H_1}(\mathcal{T}^{\rm c} )\rightarrow1$| , and suppose that |$\hat{B}_{2N-K_0}/B_{2N-K_0} \rightarrow1$| in probability under |$H_0$| as |$n\rightarrow\infty$| . Then (i) |${\mathrm{pr}}_{H_0}(T_n>{\small{\text{cv}}}_\phi)\rightarrow\phi$| if the conditions of Theorem 1 hold, and (ii) |${\mathrm{pr}}_{H_1}(T_n>{\small{\text{cv}}}_\phi)\rightarrow1$| if |$Y_t$| satisfies ( 2 ) with independent |$\epsilon_t\sim (0,\sigma_{\epsilon}^2)$| and |$\sum_{j=1}^\infty j|\psi_j|<\infty$| .
2.2. Determining the event |$\mathcal{T}$| in ( 3 )
To use |$\mathcal{T}$| with finite samples, |$C_*$| must be specified according to the underlying process.
Let |$Y_t\sim I(1)$| satisfy ( 2 ) with independent |$\epsilon_t\sim(0,\sigma_{\epsilon}^2)$| and |$\sum_{j=1}^\infty j|\psi_j|<\infty$| . Write |$\eta=\sum_{j=0}^{\infty}\psi_j^2+\sum_{j=0}^{\infty}\psi_j\psi_{j+1}$| . As |$n\rightarrow\infty$| , we have that (i) |${n}^{-1}R \rightarrow 2a^2\eta^{-1}\int_0^1V_{0}^2(t)\,{\rm d}t$| in distribution if |$\mu_1=0$| , where |$a$| and |$V_0(t)$| are defined in Proposition 1, and (ii) |$n^{-2}R\rightarrow 6^{-1}\sigma_{\epsilon}^{-2}\eta^{-1}\mu_1^2$| in probability if |$\mu_1\neq 0$| .
Although the above specification was derived for |$Y_t \sim I(1)$| , our simulation results indicate that it also works well for |$I(2)$| processes. Testing |$I(0)$| against |$I(d)$| with |$d>1$| is easier than doing so with |$d=1$| , as the autocovariances are of order at least |$n^{2d-1}$| for |$I(d)$| processes; hence the difference between the values of |$T_n$| under |$H_1$| and those under |$H_0$| increases as |$d$| increases.
2.3. Estimation of |$B_{2N-K_0}^2$|
The kernel function |$\mathcal{K}(\cdot):\mathbb{R}\rightarrow[-1,1]$| is continuously differentiable on |$\mathbb{R}$| and is such that (i) |$\mathcal{K}(0)=1$| , (ii) |$\mathcal{K}(x)=\mathcal{K}(-x)$| for any |$x\in\mathbb{R}$| , and (iii) |$\int_{-\infty}^{\infty}|\mathcal{K}(x)|\,{\rm d}x<\infty$| . Let |$K_*=K_0+2$| satisfy |$K_*^{13}\log K_*=o(n^{1-2/s_2})$| with |$s_2$| as specified in Condition 5. The bandwidth |$b_m\rightarrow\infty$| as |$n\rightarrow\infty$| satisfies |$b_m=o\{n^{1/2-1/s_2}(K_*^5\log K_*)^{-1/2}\}$| and |$K_*^4=o(b_m)$| .
Under |$H_0$| , |$\max_{1\leqslant t \leqslant n}E(|Y_t|^{2s_2})\leqslant c_4$| for two constants |$s_2>4$| and |$c_4>0$| , and the |$\alpha$| -mixing coefficients |$\{\alpha(\tau)\}_{\tau\geqslant1}$| satisfy |$\alpha(\tau)\leqslant c_5\tau^{-\beta_2}$| for two constants |$c_5\,{>}\,0$| and |$\beta_2\,{>}\,\max\{2s_2/(s_2-2), s_2/(s_2-4)\}$| , where |$\alpha(\tau)$| is as defined in Condition 2.
Suppose that Conditions 4 and 5 hold. Then, as |$n\rightarrow\infty$| , |$\hat{B}_{2N-K_0}/B_{2N-K_0}\rightarrow1$| in probability under |$H_0$| .
2.4. Implementation of the test
Based on § 2.2 and § 2.3 , Algorithm 1 outlines the steps of performing our test, which includes two tuning parameters. The algorithm is implemented in an |$\texttt{R}$| function |$\texttt{ur.test}$| in the package |$\texttt{HDTSA}$| ( Lin et al., 2021 ). To perform the test using function |$\texttt{ur.test}$| , one merely needs to input the time series |$\{Y_t\}_{t=1}^n$| and the nominal level |$\phi$| . The package sets the default value |$c_\kappa=0.55$| and returns the five testing results for |$K_0=0, 1, \ldots, 4$| . One can also set |$(c_\kappa,K_0)$| subjectively. We recommend using |$c_\kappa \in [0.45, 0.65]$| and |$K_0\in\{0,1,2,3,4\}$| .
To illustrate robustness with respect to the choice of |$(c_\kappa,K_0)$| , we apply our test to 14 U.S. annual economic time series ( Nelson & Plosser, 1982 ) that are often used for testing unit roots in the literature. The results with |$c_\kappa \in\{0.45, 0.55,0.65\}$| and |$K_0\in\{0,1,2, 3,4\}$| are exactly the same for each of the 14 time series; see the Supplementary Material for details.
Input : Time series |$\{Y_t\}_{t=1}^n$| , nominal level |$\phi$| , and two (optional) tuning parameters |$(c_\kappa,K_0)$| .
Step 1. Compute |$\hat{\gamma}(k)$| , |$\hat{\gamma}_1(k)$| , |$\hat{\gamma}_2(k)$| and |$\hat{\gamma}_x(k)$| . Put |$\hat{\rho}=\hat{\gamma}_x(1)/\hat{\gamma}_x(0)$| .
Step 2. Call function |$\texttt{lrvar}$| from the |$\texttt{R}$| package |$\texttt{sandwich}$| , with the default bandwidth of the function, to compute the long-run variances of |$\{\tilde{Q}_t\}$| and |$\{X_t\}$| , denoted by |$\tilde{V}_{2N-K_0}$| and |$\hat{\sigma}_{\rm L}^2$| , respectively, where |$\tilde{Q}_t$| is defined in § 2.3 . Put |$\hat{\lambda}=\hat{\gamma}_x(0)/\hat{\sigma}_{\rm L}^2$| .
Step 3. Calculate the test statistic |$T_n=\sum_{k=0}^{K_0}|\hat{\gamma}_2(k)|^2$| and the critical value |${\small{\text{cv}}}_\phi$| as in ( 3 ) with |$\hat{B}_{2N-K_0}=(2N-K_0)^{1/2}\tilde{V}_{2N-K_0}^{1/2}$| and |$\mathcal{T}$| given in ( 4 ) for |$C_*$| specified in ( 5 ).
Step 4. Reject |$H_0$| if |$T_n>{\small{\text{cv}}}_\phi$| .
We investigate the finite-sample properties of our test |$T_n$| by simulation with |$K_0\in\{0,1,2,3,4\}$| and |$c_\kappa\in\{0.45, 0.55, 0.65\}$| . We also consider |$T_n$| with the untruncated critical value |${\small{\text{cv}}}_{\phi,{\rm naive}}$| , i.e., |$c_\kappa=\infty$| in ( 5 ). Hualde & Robinson (2011) proposed the pseudo maximum likelihood estimator |$\hat{d}$| for the integration order |$d$| in the autoregressive fractionally integrated moving average models that can be used to construct a |$t$| -statistic |$\hat{d}/{\rm sd}(\hat{d})$| for |$H_0: d=0$| versus |$H_1:d\geqslant1$| . We call the test that rejects |$H_0$| if |$\hat{d}/{\rm sd}(\hat{d})>z_{1-\phi}$| the hr test, where |$z_{1-\phi}$| is the |$(1-\phi)$| -quantile of |$\mathcal{N}(0,1)$| . For comparison, we include the test of Kwiatkowski et al. (1992) and the hr test in our experiments. We set |$N=40, 70, 100$| and repeat each setting 2000 times. To examine the rejection probability of the tests under |$H_0$| , we consider the following three models.
Model 1: |$Y_t=\rho Y_{t-1}+\epsilon_t$| .
Model 2: |$Y_t=\epsilon_t+\phi_1\epsilon_{t-1}+\phi_2\epsilon_{t-2}$| .
Model 3: |$Y_t-\rho_1Y_{t-1}-\rho_2 Y_{t-2}=\epsilon_t+ 0.5 \epsilon_{t-1}+ 0.3 \epsilon_{t-2}$| .
To examine the rejection probability of the tests under |$H_1$| , we consider the following four models.
Model 4: |$\nabla Y_t=Z_t$| , |$Z_t=\rho Z_{t-1}+\epsilon_t$| .
Model 5: |$\nabla Y_t=Z_t$| , |$Z_t=\epsilon_t+\phi_1\epsilon_{t}+\phi_2\epsilon_{t-1}$| .
Model 6: |$\nabla Y_t=Z_t$| , |$Z_t-\rho_1Z_{t-1}-\rho_2 Z_{t-2}=\epsilon_t+0.5\epsilon_{t}+0.3\epsilon_{t-1}$| .
Model 7: |$\nabla^2 Y_t=Z_t$| , |$Z_t=\epsilon_t+\phi_1\epsilon_{t}+\phi_2\epsilon_{t-1}$| .
Unless specified otherwise, we always assume that |$\epsilon_t\sim\mathcal{N}(0, \sigma_{\epsilon}^2)$| independently with |$\sigma_\epsilon^2=1$| or 2 and set the nominal level |$\phi$| to |$5\%$| . The results with different |$(c_\kappa,K_0)$| are similar, indicating once again that our test is robust with respect to the choice of |$(c_\kappa,K_0)$| . We list the results with |$K_0=0$| and |$\sigma_{\epsilon}^2=1$| in Table 1 , and report other results and the |$\epsilon_t\sim t(2)$| and |$\epsilon_t\sim t(5)$| cases in the Supplementary Material .
Rejection probabilities |$(\%)$| of the proposed test |$T_n$| with |$K_0\,{=}\,0$| and |$c_\kappa\,{=}\,0.45, 0.55, 0.65, \infty$| , the test of Kwiatkowski et al. (1992) , and the hr test; the nominal level is |$5\%$|
Model 1 . | Model 4 . | ||||||||||||||
---|---|---|---|---|---|---|---|---|---|---|---|---|---|---|---|
|$\rho$| . | |$N$| . | |$\infty$| . | |$0.45$| . | |$0.55$| . | |$0.65$| . | KPSS . | HR . | |$\rho$| . | |$N$| . | |$\infty$| . | |$0.45$| . | |$0.55$| . | |$0.65$| . | KPSS . | HR . |
0.5 | 40 | 6.0 | 6.0 | 6.0 | 6.0 | 10.4 | 5.7 | 0.5 | 40 | 11.7 | 94.2 | 88.4 | 84.0 | 84.2 | 96.4 |
70 | 6.9 | 6.9 | 6.9 | 6.9 | 10.1 | 7.0 | 70 | 11.7 | 96.5 | 92.9 | 88.4 | 90.9 | 99.8 | ||
100 | 6.1 | 6.1 | 6.1 | 6.1 | 10.2 | 8.4 | 100 | 11.3 | 98.0 | 95.5 | 92.2 | 95.5 | 100.0 | ||
0.9 | 40 | 7.2 | 41.9 | 30.0 | 20.3 | 51.2 | 46.8 | 0.9 | 40 | 13.1 | 99.2 | 97.3 | 94.6 | 91.1 | 98.9 |
70 | 7.8 | 23.7 | 14.6 | 10.4 | 46.7 | 58.8 | 70 | 14.8 | 99.8 | 99.1 | 97.9 | 95.3 | 100.0 | ||
100 | 8.5 | 12.7 | 9.4 | 8.6 | 49.2 | 61.1 | 100 | 16.4 | 99.9 | 99.5 | 99.1 | 97.2 | 100.0 | ||
|$-$|0.5 | 40 | 7.4 | 7.4 | 7.4 | 7.4 | 1.8 | 0.1 | |$-$|0.5 | 40 | 5.6 | 82.2 | 75.1 | 67.6 | 81.5 | 99.7 |
70 | 6.9 | 6.9 | 6.9 | 6.9 | 2.5 | 0.2 | 70 | 6.3 | 92.1 | 86.1 | 80.0 | 90.1 | 100.0 | ||
100 | 6.4 | 6.4 | 6.4 | 6.4 | 1.8 | 0.3 | 100 | 5.8 | 94.2 | 89.5 | 85.2 | 94.5 | 100.0 | ||
Model 2 | Model 5 | ||||||||||||||
|$(\phi_1,\phi_2)$| | |$N$| | |$\infty$| | |$0.45$| | |$0.55$| | |$0.65$| | KPSS | HR | |$(\phi_1,\phi_2)$| | |$N$| | |$\infty$| | |$0.45$| | |$0.55$| | |$0.65$| | KPSS | HR |
(0.8, 0.3) | 40 | 6.2 | 6.2 | 6.2 | 6.2 | 7.6 | 0.9 | (0.8, 0.3) | 40 | 11.8 | 94.3 | 88.8 | 82.3 | 82.0 | 99.4 |
70 | 6.4 | 6.4 | 6.4 | 6.4 | 6.2 | 0.4 | 70 | 11.8 | 96.6 | 92.7 | 88.3 | 90.1 | 100.0 | ||
100 | 7.2 | 7.2 | 7.2 | 7.2 | 7.0 | 0.4 | 100 | 12.1 | 98.4 | 95.4 | 91.8 | 95.3 | 100.0 | ||
(0.9, 0.5) | 40 | 6.7 | 6.7 | 6.7 | 6.7 | 8.5 | 0.4 | (0.9, 0.5) | 40 | 11.8 | 95.3 | 90.0 | 84.2 | 83.5 | 99.8 |
70 | 6.5 | 6.5 | 6.5 | 6.5 | 8.1 | 0.0 | 70 | 12.2 | 97.2 | 93.8 | 89.8 | 89.2 | 100.0 | ||
100 | 5.6 | 5.6 | 5.6 | 5.6 | 7.4 | 0.0 | 100 | 11.6 | 98.6 | 96.4 | 92.7 | 94.8 | 100.0 | ||
|$(0.95, 0.9)$| | 40 | 7.2 | 7.2 | 7.2 | 7.2 | 9.0 | 0.0 | |$(0.95, 0.9)$| | 40 | 13.1 | 95.0 | 90.0 | 83.9 | 83.0 | 99.6 |
70 | 7.1 | 7.1 | 7.1 | 7.1 | 7.3 | 0.2 | 70 | 11.6 | 97.3 | 93.8 | 89.7 | 90.2 | 100.0 | ||
100 | 5.5 | 5.5 | 5.5 | 5.5 | 8.1 | 0.0 | 100 | 13.7 | 99.0 | 96.4 | 92.3 | 95.2 | 100.0 | ||
Model 3 | Model 6 | ||||||||||||||
|$(\rho_1,\rho_2)$| | |$N$| | |$\infty$| | |$0.45$| | |$0.55$| | |$0.65$| | KPSS | HR | |$(\rho_1,\rho_2)$| | |$N$| | |$\infty$| | |$0.45$| | |$0.55$| | |$0.65$| | KPSS | HR |
(0.4, 0.2) | 40 | 7.2 | 8.2 | 7.4 | 7.3 | 22.5 | 4.8 | (0.4, 0.2) | 40 | 14.8 | 98.0 | 95.2 | 90.6 | 85.9 | 29.2 |
70 | 7.7 | 7.7 | 7.7 | 7.7 | 17.3 | 5.0 | 70 | 15.4 | 99.1 | 97.0 | 93.8 | 92.0 | 43.4 | ||
100 | 7.2 | 7.2 | 7.2 | 7.2 | 18.0 | 5.1 | 100 | 16.6 | 99.6 | 98.8 | 96.5 | 96.5 | 54.5 | ||
(0.5, 0.1) | 40 | 8.5 | 8.9 | 8.5 | 8.5 | 19.6 | 5.4 | (0.5, 0.1) | 40 | 14.2 | 99.1 | 95.9 | 91.3 | 84.7 | 30.2 |
70 | 8.0 | 8.0 | 8.0 | 8.0 | 16.6 | 6.2 | 70 | 14.8 | 99.4 | 97.2 | 94.0 | 91.2 | 47.8 | ||
100 | 6.3 | 6.3 | 6.3 | 6.3 | 17.4 | 5.9 | 100 | 15.0 | 99.6 | 98.5 | 96.2 | 95.5 | 60.9 | ||
(0.6, 0.1) | 40 | 8.5 | 12.7 | 9.6 | 8.7 | 26.2 | 6.0 | (0.6, 0.1) | 40 | 14.5 | 99.2 | 97.1 | 93.3 | 87.2 | 27.6 |
70 | 7.3 | 7.3 | 7.3 | 7.3 | 22.4 | 6.8 | 70 | 15.7 | 99.7 | 98.5 | 96.2 | 93.5 | 37.3 | ||
100 | 7.6 | 7.6 | 7.6 | 7.6 | 20.3 | 7.0 | 100 | 16.4 | 99.8 | 99.1 | 97.7 | 95.7 | 44.0 | ||
Model 7 | Model 7 | ||||||||||||||
|$(\phi_1,\phi_2)$| | |$N$| | |$\infty$| | |$0.45$| | |$0.55$| | |$0.65$| | KPSS | HR | |$(\phi_1,\phi_2)$| | |$N$| | |$\infty$| | |$0.45$| | |$0.55$| | |$0.65$| | KPSS | HR |
(0.8, 0.3) | 40 | 6.7 | 100.0 | 100.0 | 99.9 | 98.5 | 100.0 | (0.9, 0.5) | 40 | 7.0 | 100.0 | 100.0 | 100.0 | 98.4 | 100.0 |
70 | 6.3 | 100.0 | 100.0 | 100.0 | 99.7 | 100.0 | 70 | 5.5 | 100.0 | 100.0 | 100.0 | 99.5 | 100.0 | ||
100 | 7.0 | 100.0 | 100.0 | 100.0 | 99.8 | 100.0 | 100 | 5.9 | 100.0 | 100.0 | 100.0 | 99.9 | 100.0 | ||
(0.95, 0.9) | 40 | 8.0 | 100.0 | 100.0 | 100.0 | 98.5 | 100.0 | ||||||||
70 | 7.3 | 100.0 | 100.0 | 100.0 | 99.2 | 100.0 | |||||||||
100 | 6.1 | 100.0 | 100.0 | 100.0 | 99.9 | 100.0 |
Model 1 . | Model 4 . | ||||||||||||||
---|---|---|---|---|---|---|---|---|---|---|---|---|---|---|---|
|$\rho$| . | |$N$| . | |$\infty$| . | |$0.45$| . | |$0.55$| . | |$0.65$| . | KPSS . | HR . | |$\rho$| . | |$N$| . | |$\infty$| . | |$0.45$| . | |$0.55$| . | |$0.65$| . | KPSS . | HR . |
0.5 | 40 | 6.0 | 6.0 | 6.0 | 6.0 | 10.4 | 5.7 | 0.5 | 40 | 11.7 | 94.2 | 88.4 | 84.0 | 84.2 | 96.4 |
70 | 6.9 | 6.9 | 6.9 | 6.9 | 10.1 | 7.0 | 70 | 11.7 | 96.5 | 92.9 | 88.4 | 90.9 | 99.8 | ||
100 | 6.1 | 6.1 | 6.1 | 6.1 | 10.2 | 8.4 | 100 | 11.3 | 98.0 | 95.5 | 92.2 | 95.5 | 100.0 | ||
0.9 | 40 | 7.2 | 41.9 | 30.0 | 20.3 | 51.2 | 46.8 | 0.9 | 40 | 13.1 | 99.2 | 97.3 | 94.6 | 91.1 | 98.9 |
70 | 7.8 | 23.7 | 14.6 | 10.4 | 46.7 | 58.8 | 70 | 14.8 | 99.8 | 99.1 | 97.9 | 95.3 | 100.0 | ||
100 | 8.5 | 12.7 | 9.4 | 8.6 | 49.2 | 61.1 | 100 | 16.4 | 99.9 | 99.5 | 99.1 | 97.2 | 100.0 | ||
|$-$|0.5 | 40 | 7.4 | 7.4 | 7.4 | 7.4 | 1.8 | 0.1 | |$-$|0.5 | 40 | 5.6 | 82.2 | 75.1 | 67.6 | 81.5 | 99.7 |
70 | 6.9 | 6.9 | 6.9 | 6.9 | 2.5 | 0.2 | 70 | 6.3 | 92.1 | 86.1 | 80.0 | 90.1 | 100.0 | ||
100 | 6.4 | 6.4 | 6.4 | 6.4 | 1.8 | 0.3 | 100 | 5.8 | 94.2 | 89.5 | 85.2 | 94.5 | 100.0 | ||
Model 2 | Model 5 | ||||||||||||||
|$(\phi_1,\phi_2)$| | |$N$| | |$\infty$| | |$0.45$| | |$0.55$| | |$0.65$| | KPSS | HR | |$(\phi_1,\phi_2)$| | |$N$| | |$\infty$| | |$0.45$| | |$0.55$| | |$0.65$| | KPSS | HR |
(0.8, 0.3) | 40 | 6.2 | 6.2 | 6.2 | 6.2 | 7.6 | 0.9 | (0.8, 0.3) | 40 | 11.8 | 94.3 | 88.8 | 82.3 | 82.0 | 99.4 |
70 | 6.4 | 6.4 | 6.4 | 6.4 | 6.2 | 0.4 | 70 | 11.8 | 96.6 | 92.7 | 88.3 | 90.1 | 100.0 | ||
100 | 7.2 | 7.2 | 7.2 | 7.2 | 7.0 | 0.4 | 100 | 12.1 | 98.4 | 95.4 | 91.8 | 95.3 | 100.0 | ||
(0.9, 0.5) | 40 | 6.7 | 6.7 | 6.7 | 6.7 | 8.5 | 0.4 | (0.9, 0.5) | 40 | 11.8 | 95.3 | 90.0 | 84.2 | 83.5 | 99.8 |
70 | 6.5 | 6.5 | 6.5 | 6.5 | 8.1 | 0.0 | 70 | 12.2 | 97.2 | 93.8 | 89.8 | 89.2 | 100.0 | ||
100 | 5.6 | 5.6 | 5.6 | 5.6 | 7.4 | 0.0 | 100 | 11.6 | 98.6 | 96.4 | 92.7 | 94.8 | 100.0 | ||
|$(0.95, 0.9)$| | 40 | 7.2 | 7.2 | 7.2 | 7.2 | 9.0 | 0.0 | |$(0.95, 0.9)$| | 40 | 13.1 | 95.0 | 90.0 | 83.9 | 83.0 | 99.6 |
70 | 7.1 | 7.1 | 7.1 | 7.1 | 7.3 | 0.2 | 70 | 11.6 | 97.3 | 93.8 | 89.7 | 90.2 | 100.0 | ||
100 | 5.5 | 5.5 | 5.5 | 5.5 | 8.1 | 0.0 | 100 | 13.7 | 99.0 | 96.4 | 92.3 | 95.2 | 100.0 | ||
Model 3 | Model 6 | ||||||||||||||
|$(\rho_1,\rho_2)$| | |$N$| | |$\infty$| | |$0.45$| | |$0.55$| | |$0.65$| | KPSS | HR | |$(\rho_1,\rho_2)$| | |$N$| | |$\infty$| | |$0.45$| | |$0.55$| | |$0.65$| | KPSS | HR |
(0.4, 0.2) | 40 | 7.2 | 8.2 | 7.4 | 7.3 | 22.5 | 4.8 | (0.4, 0.2) | 40 | 14.8 | 98.0 | 95.2 | 90.6 | 85.9 | 29.2 |
70 | 7.7 | 7.7 | 7.7 | 7.7 | 17.3 | 5.0 | 70 | 15.4 | 99.1 | 97.0 | 93.8 | 92.0 | 43.4 | ||
100 | 7.2 | 7.2 | 7.2 | 7.2 | 18.0 | 5.1 | 100 | 16.6 | 99.6 | 98.8 | 96.5 | 96.5 | 54.5 | ||
(0.5, 0.1) | 40 | 8.5 | 8.9 | 8.5 | 8.5 | 19.6 | 5.4 | (0.5, 0.1) | 40 | 14.2 | 99.1 | 95.9 | 91.3 | 84.7 | 30.2 |
70 | 8.0 | 8.0 | 8.0 | 8.0 | 16.6 | 6.2 | 70 | 14.8 | 99.4 | 97.2 | 94.0 | 91.2 | 47.8 | ||
100 | 6.3 | 6.3 | 6.3 | 6.3 | 17.4 | 5.9 | 100 | 15.0 | 99.6 | 98.5 | 96.2 | 95.5 | 60.9 | ||
(0.6, 0.1) | 40 | 8.5 | 12.7 | 9.6 | 8.7 | 26.2 | 6.0 | (0.6, 0.1) | 40 | 14.5 | 99.2 | 97.1 | 93.3 | 87.2 | 27.6 |
70 | 7.3 | 7.3 | 7.3 | 7.3 | 22.4 | 6.8 | 70 | 15.7 | 99.7 | 98.5 | 96.2 | 93.5 | 37.3 | ||
100 | 7.6 | 7.6 | 7.6 | 7.6 | 20.3 | 7.0 | 100 | 16.4 | 99.8 | 99.1 | 97.7 | 95.7 | 44.0 | ||
Model 7 | Model 7 | ||||||||||||||
|$(\phi_1,\phi_2)$| | |$N$| | |$\infty$| | |$0.45$| | |$0.55$| | |$0.65$| | KPSS | HR | |$(\phi_1,\phi_2)$| | |$N$| | |$\infty$| | |$0.45$| | |$0.55$| | |$0.65$| | KPSS | HR |
(0.8, 0.3) | 40 | 6.7 | 100.0 | 100.0 | 99.9 | 98.5 | 100.0 | (0.9, 0.5) | 40 | 7.0 | 100.0 | 100.0 | 100.0 | 98.4 | 100.0 |
70 | 6.3 | 100.0 | 100.0 | 100.0 | 99.7 | 100.0 | 70 | 5.5 | 100.0 | 100.0 | 100.0 | 99.5 | 100.0 | ||
100 | 7.0 | 100.0 | 100.0 | 100.0 | 99.8 | 100.0 | 100 | 5.9 | 100.0 | 100.0 | 100.0 | 99.9 | 100.0 | ||
(0.95, 0.9) | 40 | 8.0 | 100.0 | 100.0 | 100.0 | 98.5 | 100.0 | ||||||||
70 | 7.3 | 100.0 | 100.0 | 100.0 | 99.2 | 100.0 | |||||||||
100 | 6.1 | 100.0 | 100.0 | 100.0 | 99.9 | 100.0 |
KPSS, the test of Kwiatkowski et al. (1992) ; HR, the test that rejects |$H_0$| if |$\hat{d}/{\rm sd}(\hat{d})>z_{1-\phi}$| where |$z_{1-\phi}$| is the |$(1-\phi)$| -quantile of |$\mathcal{N}(0,1)$| .
Overall the rejection probabilities of our test under |$H_0$| are close to the nominal level |$\phi=5\%$| , especially when |$n$| is large, such as |$N=100$| . The performance of our test is stable across different models with different parameters, different |$K_0$| and different innovation distributions, whereas that of Kwiatkowski et al. ’s test and of the hr test vary and are adequate only in some settings. Table 1 indicates that our test works well for Model 1 with both positive and negative |$\rho$| , while Kwiatkowski et al. ’s test and the hr test perform poorly when |$\rho<0$| and even worse when |$\rho>0$| . Kwiatkowski et al. ’s test and the hr test completely fail when |$\rho=0.9$| , as the rejection probabilities are at least 46.7%. This is due to the fact that when |$\rho$| is close to 1, Kwiatkowski et al. ’s test and the hr test have difficulties distinguishing |$\rho$| from 1, which is a unit root; see also Table 3 of Kwiatkowski et al. (1992) . Our test does not suffer from this closeness to 1, as the order of the magnitude of the autocovariance function matters. Our test and that of Kwiatkowski et al. (1992) work well for Model 2, while the hr test is too conservative. For Model 3, the rejection probabilities of our test and the hr test are close to |$5\%$| , while Kwiatkowski et al. ’s test does not work as its rejection probabilities range from 16.6% to 26.2%. Our test with |$c_\kappa=\infty$| has no power, which shows that the truncation step for the critical value in ( 3 ) is necessary. The test of Kwiatkowski et al. (1992) has impressive power owing to the fact that it has a tendency to overestimate the rejection probability under |$H_0$| , leading to inflated power. Nevertheless, our test exhibits greater power in most cases. The hr test has good power for Models 4 and 5, but performs poorly for Model 6. The power-one property of our test is observable in the simulation since the rejection probability tends to 1 as |$N$| increases. Comparing the results of Models 5 and 7, we find that our test displays the power-one property more distinctly as our test statistic has more discriminative power between |$I(2)$| and |$I(0)$| than between |$I(1)$| and |$I(0)$| .
All authors contributed equally to the paper. We thank the editor, associate editor and referees for their constructive comments. Chang and Cheng were supported by the National Natural Science Foundation of China. Chang was also supported by the Center of Statistical Research and the Joint Lab of Data Science and Business Intelligence at Southwestern University of Finance and Economics. Yao was supported in part by the U.K. Engineering and Physical Sciences Research Council.
Supplementary Material available at Biometrika online includes all the technical proofs and some additional numerical results.
Andrews, D. W. K. ( 1991 ). Heteroskedasticity and autocorrelation consistent covariance matrix estimation . Econometrica 59 , 817 – 58 .
Google Scholar
Cavaliere, G. & Taylor, A. M. R. ( 2007 ). Testing for unit roots in time series models with non-stationary volatility . J. Economet. 140 , 919 – 47 .
Chan, N. H. & Wei, C. Z. ( 1988 ). Limiting distributions of least squares estimates of unstable autoregressive processes . Ann. Statist. 16 , 367 – 401 .
Chang, J. , Zheng, C. , Zhou, W.-X. & Zhou, W. ( 2017 ). Simulation-based hypothesis testing of high dimensional means under covariance heterogeneity . Biometrics 73 , 1300 – 10 .
Dickey, D. A. & Fuller, W. A. ( 1979 ). Distribution of the estimators for autoregressive time series with a unit root . J. Am. Statist. Assoc. 74 , 427 – 31 .
Dickey, D. A. & Fuller, W. A. ( 1981 ). Likelihood ratio statistics for autoregressive time series with a unit root . Econometrica 49 , 1057 – 72 .
Elliott, G. , Rothenberg, T. J. & Stock, J. H. ( 1996 ). Efficient tests for an autoregressive unit root . Econometrica 64 , 813 – 36 .
Hatanaka, M. ( 1996 ). Time-Series-Based Econometrics: Unit Roots and Cointegration . Oxford : Oxford University Press .
Google Preview
Hualde, J. & Robinson, P. M. ( 2011 ). Gaussian pseudo-maximum likelihood estimation of fractional time series models . Ann. Statist. 39 , 3152 – 81 .
Hylleberg, S. , Engle, R. F. , Granger, C. F. J. & Yoo, S. ( 1990 ). Seasonal integration and cointegration . J. Economet. 44 , 215 – 38 .
Kwiatkowski, D. , Phillips, P. C. B. , Schmidt, P. & Shin, Y. ( 1992 ). Testing the null hypothesis of stationarity against the alternative of a unit root . J. Economet. 54 , 159 – 78 .
Lin, C. , Cheng, G. , Chang, J. & Yao, Q. ( 2021 ). HDTSA: High Dimensional Time Series Analysis Tools . R package version 1.0.0 , available at https://cran.r-project.org/web/packages/HDTSA/ .
Maddala, G. S. & Kim, I.-M. ( 1998 ). Unit roots, Cointegration and Structural Change . Cambridge : Cambridge University Press .
Nelson, C. R. & Plosser, C. I. ( 1982 ). Trends versus random walks in macroeconomic time series: Some evidence and implications . J. Monet. Econ. 10 , 139 – 62 .
Paparoditis, E. & Politis, D. N. ( 2005 ). Bootstrapping unit root tests for autoregressive time series . J. Am. Statist. Assoc. 100 , 545 – 53 .
Pesaran, M. H. ( 2007 ). A simple panel unit root test in the presence of cross-section dependence . Appl. Economet. 22 , 265 – 317 .
Phillips, P. C. B. ( 1987 ). Time series regression with a unit root . Econometrica 55 , 277 – 301 .
Phillips, P. C. B. & Perron, P. ( 1988 ). Testing for a unit root in time series regression . Biometrika , 75 , 335 – 46 .
Phillips, P. C. B. & Xiao, Z. ( 1998 ). A primer on unit root testing . J. Econ. Surv. 12 , 423 – 69 .
R Development Core Team ( 2022 ). R: A Language and Environment for Statistical Computing . Vienna, Austria: R Foundation for Statistical Computing. ISBN 3-900051-07-0, http://www.R-project.org .
Rho, R. & Shao, X. ( 2019 ). Bootstrap-assisted unit root testing with piecewise locally stationary errors . Economet. Theory 35 , 143 – 66 .
Robinson, P. M. ( 1994 ). Efficient tests of nonstationary hypothesis . J. Am. Statist. Assoc. 89 , 1420 – 37 .
Said, S. E. & Dickey, D. A. ( 1984 ). Testing for unit roots in autoregressive-moving average models of unknown order . Biometrika 71 , 599 – 608 .
Stock, J. H. ( 1994 ). Unit roots, structural breaks and trends . In Handbook of Econometrics , vol. 4 , Engle R. F. and McFadden D. L. (eds). vol. 4 . Amsterdam : Elsevier .
Zeileis, A. , Lumley, T. , Graham, N. & Koell, S. ( 2021 ). sandwich: Robust Covariance Matrix Estimators . R package version 3.0-1, available at https://sandwich.R-Forge.R-project.org/ .
Zivot, E. & Andrews, D. W. K. ( 1992 ). Further evidence on the great crash, the oil price shock, and the unit root hypothesis . J. Bus. Econ. Statist. 10 , 251 – 70 .
Supplementary data
Month: | Total Views: |
---|---|
June 2021 | 27 |
July 2021 | 41 |
August 2021 | 13 |
September 2021 | 32 |
October 2021 | 20 |
November 2021 | 28 |
December 2021 | 22 |
January 2022 | 6 |
February 2022 | 11 |
March 2022 | 9 |
April 2022 | 16 |
May 2022 | 45 |
June 2022 | 74 |
July 2022 | 161 |
August 2022 | 98 |
September 2022 | 72 |
October 2022 | 87 |
November 2022 | 42 |
December 2022 | 55 |
January 2023 | 53 |
February 2023 | 43 |
March 2023 | 59 |
April 2023 | 45 |
May 2023 | 41 |
June 2023 | 33 |
July 2023 | 38 |
August 2023 | 42 |
September 2023 | 59 |
October 2023 | 45 |
November 2023 | 43 |
December 2023 | 23 |
January 2024 | 47 |
February 2024 | 24 |
March 2024 | 30 |
April 2024 | 45 |
May 2024 | 51 |
June 2024 | 34 |
July 2024 | 22 |
August 2024 | 18 |
September 2024 | 15 |
Email alerts
Citing articles via.
- Recommend to your Library
Affiliations
- Online ISSN 1464-3510
- Copyright © 2024 Biometrika Trust
- About Oxford Academic
- Publish journals with us
- University press partners
- What we publish
- New features
- Open access
- Institutional account management
- Rights and permissions
- Get help with access
- Accessibility
- Advertising
- Media enquiries
- Oxford University Press
- Oxford Languages
- University of Oxford
Oxford University Press is a department of the University of Oxford. It furthers the University's objective of excellence in research, scholarship, and education by publishing worldwide
- Copyright © 2024 Oxford University Press
- Cookie settings
- Cookie policy
- Privacy policy
- Legal notice

This Feature Is Available To Subscribers Only
Sign In or Create an Account
This PDF is available to Subscribers Only
For full access to this pdf, sign in to an existing account, or purchase an annual subscription.
Unit Root Tests Are Useful for Selecting Forecasting Models
We study the usefulness of root tests as diagnostic tools for selecting forecasting models. Difference stationary and trend stationary models of economic and financial time series often imply very different predictions, so deciding which model to use is tremendously important for applied forecasters. Forecasters face three choices: always difference the data, never difference, or use a unit-root pretest. We characterize the predictive loss of these strategies for the canonical AR(1) process with trend, focusing on the effects of sample size, forecast horizon, and degree of persistence. We show that pretesting routinely improves forecast accuracy relative to forecasts from models in differences, and we give conditions under which pretesting is likely to improve forecast accuracy relative to forecasts from models in levels.
- Acknowledgements and Disclosures
MARC RIS BibTeΧ
Download Citation Data
Published Versions
More from nber.
In addition to working papers , the NBER disseminates affiliates’ latest findings through a range of free periodicals — the NBER Reporter , the NBER Digest , the Bulletin on Retirement and Disability , the Bulletin on Health , and the Bulletin on Entrepreneurship — as well as online conference reports , video lectures , and interviews .
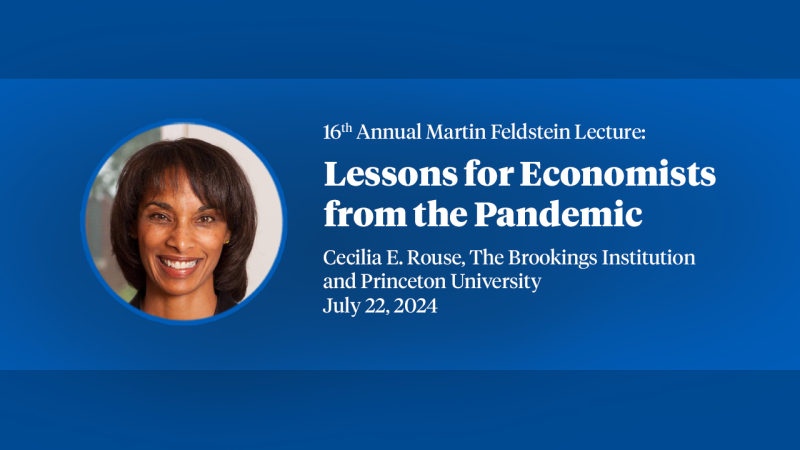
A Unit Root Test for an AR(1) Process with AR Errors by Using Random Weighted Bootstrap
- Published: 15 September 2023
- Volume 39 , pages 1834–1854, ( 2023 )
Cite this article
- Xiao Hui Liu 1 , 2 ,
- Ya Wen Fan 1 , 2 ,
- Yu Zi Liu 1 , 2 &
- Shi Hua Luo 1 , 2
64 Accesses
2 Citations
Explore all metrics
A great deal of economic problems are related to detecting the stability of time series data, where the main interest is in the unit root test. In this paper, we consider the unit root testing problem with errors being long-memory processes with the GARCH structure. A new test statistic is developed by using the random weighted bootstrap method. It turns out that the proposed statistic has a chi-squared distribution asymptotically regardless of the process being stationary or nonstationary, and with or without an intercept term. The simulation results show that the statistic has a desired finite sample performance in terms of both size and power. A real data application is also given relying on the inflation rate data of 17 countries.
This is a preview of subscription content, log in via an institution to check access.
Access this article
Subscribe and save.
- Get 10 units per month
- Download Article/Chapter or eBook
- 1 Unit = 1 Article or 1 Chapter
- Cancel anytime
Price includes VAT (Russian Federation)
Instant access to the full article PDF.
Rent this article via DeepDyve
Institutional subscriptions
Amano, R.: Inflation persistence and monetary policy: A simple result. Economics Letters , 94 (1), 26–31 (2007)
Article Google Scholar
Baillie, R. T.: Long memory processes and fractional integration in econometrics. Journal of Econometrics , 73 (1), 5–59 (1996)
Article MathSciNet MATH Google Scholar
Ball, L., Cecchetti, S. G., Gordon, R. J.: Inflation and uncertainty at short and long horizons. Brookings Papers on Economic Activity , 1990 (1), 215–254 (1990)
Buchmann, B., Chan, N. H.: Asymptotic theory of least squares estimators for nearly unstable processes under strong dependence. Annals of Statistics , 35 (5), 2001–2017 (2007)
Chan, N. H., Zhang, R. M.: Inference for unit root models with infinite variance GARCH errors. Statistica Sinica , 20 , 1363–1393 (2010)
MathSciNet MATH Google Scholar
Cuestas, J. C., Harrison, B.: Inflation persistence and nonlinearities in Central and Eastern European countries. Economics Letters , 106 (2), 81–83 (2010)
Article MathSciNet Google Scholar
Culver, S. E., Papell, D. H.: Is there a unit root in the inflation rate? Evidence from sequential break and panel data models. Journal of Applied Econometrics , 12 (4), 435–444 (1997)
Doukhan, P., Oppenheim, G., Taqqu, M. (Eds.).: Theory and Applications of Long-range Dependence. Springer Science & Business Media, America, 2002
Google Scholar
Elliott, G., Rothenberg, T., Stock, J.: Efficient tests for an autoregressive unit root. Econometrica , 64 , 813–836 (1996)
Fuhrer, J. C.: The persistence of inflation and the cost of disinflation. New England Economic Review , 3–17 (1995)
Huang, H., Leng, X., Liu, X., et al.: Unified inference for an AR process with possible infinite variance GARCH errors. Journal of Financial Econometrics , 18 , 425–470 (2020)
Jain, M.: Perceived inflation persistence. Journal of Business & Economic Statistics , 37 (1), 110–120 (2019)
Jin, Z., Ying, Z., Wei, L. J.: A simple resampling method by perturbing the minimand. Biometrika , 88 , 381–390 (2001)
Kim, K., Schmidt, P.: Unit root tests with conditional heteroskedasticity. Journal of Econometrics , 59 (3), 287–300 (1993)
Kwiatkowski, D., Phillips, P. C. B., Schmidt, P., et al.: Testing the null hypothesis of stationarity against the alternative of a unit root. Journal of Econometrics , 54 , 159–178 (1992)
Article MATH Google Scholar
Kontonikas, A.: Inflation and inflation uncertainty in the United Kingdom, evidence from GARCH modelling. Economic Modelling , 21 (3), 525–543 (2004)
Ling, S., Li, W. K.: Asymptotic inference for unit root processes with GARCH (1, 1) errors. Econometric Theory , 19 , 541–564 (2003)
Ling, S., Li, W. K., McAleer, M.: Estimation and testing for unit root processes with GARCH (1, 1) errors: theory and Monte Carlo evidence. Econometric Reviews , 22 (2), 179–202 (2003)
Liu, X., Peng, L.: Asymptotic theory and unified confidence region for an autoregressive model. Journal of Time Series Analysis , 40 (1), 43–65 (2019)
Malliaropulos, D.: A note on nonstationarity, structural breaks, and the Fisher effect. Journal of Banking & Finance , 24 (5), 695–707 (2000)
Narayan, P. K., Popp, S.: An application of a new seasonal unit root test to inflation. International Review of Economics & Finance , 20 (4), 707–716 (2011)
Phillips, P. C. B.: Towards a unified asymptotic theory for autoregression. Biometrika , 74 , 535–547 (1987)
Phillips, P. C. B., Perron, P.: Testing for a unit root in time series regression. Biometrika , 75 , 335–346 (1988)
Robinson, P. M. (Ed.).: Time Series with Long Memory, Advanced Texts in Econometrics, New York, 2003
Rodrigues, P. M., Rubia, A.: The performance of unit root tests under level-dependent heteroskedasticity. Economics Letters , 89 (3), 262–268 (2005)
Said, S., Dickey, D.: Testing for unit roots in autoregressive-moving average models of unknown order. Biometrika , 71 , 599–607 (1984)
Seo, B.: Distribution theory for unit root tests with conditional heteroskedasticity. Journal of Econometrics , 91 (1), 113–144 (1999)
Taylor, J. B.: Aggregate dynamics and staggered contracts. Journal of Political Economy , 88 (1), 1–23 (1980)
Tsay, R. S.: Analysis of financial time series. John Wiley & Sons, America, 2005
Book MATH Google Scholar
Wang, G.: A note on unit root tests with heavy-tailed GARCH errors. Statistics & Probability Letters , 76 (10), 1075–1079 (2006)
Wu, W. B.: Unit root testing for functionals of linear processes. Econometric Theory , 22 (1), 1–14 (2006)
Zheng, Z. G.: Random weighting method. Acta Mathematicae Applicatae Sinica , 2 , 247–253 (1987)
Zhu, F., Cai, Z., Peng, L.: Predictive regressions for macroeconomic data. Annals of Applied Statistics , 8 , 577–594 (2014)
Zhu, K.: Bootstrapping the portmanteau tests in weak autoregressive moving average models. Journal of the Royal Statistical Society: Series B , 78 , 463–485 (2016)
Download references
Acknowledgements
We thank one referee and the Associate Editor for their insightful comments which led to many improvements to this paper.
Author information
Authors and affiliations.
School of Statistics and Data Science, Jiangxi University of Finance and Economics, Nanchang, 330013, P. R. China
Xiao Hui Liu, Ya Wen Fan, Yu Zi Liu & Shi Hua Luo
Key Laboratory of Data Science in Finance and Economics, Jiangxi University of Finance and Economics, Nanchang, 330013, P. R. China
You can also search for this author in PubMed Google Scholar
Corresponding author
Correspondence to Ya Wen Fan .
Ethics declarations
The authors declare no conflict of interest.
Additional information
Xiaohui Liu’s research is supported by the NNSF of China (Grant Nos. 11971208 and 11601197), the Outstanding Youth Fund Project of the Science and Technology Department of Jiangxi Province (Grant No. 20224ACB211003). Yawen Fan’s research is supported by the Science and Technology Research Project of Education Department of Jiangxi Province (Grant No. GJJ200545), the Postgraduate Innovation Project of Jiangxi Province (Grant No. YC2021-B124), and NSSF of China (Grant No. 21BTJ035). Shihua Luo’s research is supported by the National Major Social Science Project of China (Grant No. 21&ZD152), the NNSF of China (Grant No. 61973145) and Natural Science Project of Jiangxi Provincial Department of Science and Technology (Grant No. jxsq2023201048)
Rights and permissions
Reprints and permissions
About this article
Liu, X.H., Fan, Y.W., Liu, Y.Z. et al. A Unit Root Test for an AR(1) Process with AR Errors by Using Random Weighted Bootstrap. Acta. Math. Sin.-English Ser. 39 , 1834–1854 (2023). https://doi.org/10.1007/s10114-023-1535-x
Download citation
Received : 16 October 2021
Revised : 14 June 2022
Accepted : 19 September 2022
Published : 15 September 2023
Issue Date : September 2023
DOI : https://doi.org/10.1007/s10114-023-1535-x
Share this article
Anyone you share the following link with will be able to read this content:
Sorry, a shareable link is not currently available for this article.
Provided by the Springer Nature SharedIt content-sharing initiative
- Autoregressive model
- random weighted bootstrap
- autoregressive errors
- unit root test
MR(2010) Subject Classification
- Find a journal
- Publish with us
- Track your research
Academia.edu no longer supports Internet Explorer.
To browse Academia.edu and the wider internet faster and more securely, please take a few seconds to upgrade your browser .
- We're Hiring!
- Help Center
Unit Root Test
- Most Cited Papers
- Most Downloaded Papers
- Newest Papers
- Last »
- Energy Prices Follow Following
- Unit Root and Cointegration Analysis Follow Following
- Garch Models Follow Following
- Panel Data Follow Following
- Unit Root Follow Following
- Threshold Autoregressive Model Follow Following
- Descriptive Statistics Follow Following
- Early Warning System Follow Following
- Efficient Market Hypothesis Follow Following
- Investment Portfolio Management Follow Following
Enter the email address you signed up with and we'll email you a reset link.
- Academia.edu Journals
- We're Hiring!
- Help Center
- Find new research papers in:
- Health Sciences
- Earth Sciences
- Cognitive Science
- Mathematics
- Computer Science
- Academia ©2024
A New Unit Root Test Criterion
- December 2023
- 43(2):89-113

- Reserve Bank of India
Discover the world's research
- 25+ million members
- 160+ million publication pages
- 2.3+ billion citations
- Recruit researchers
- Join for free
- Login Email Tip: Most researchers use their institutional email address as their ResearchGate login Password Forgot password? Keep me logged in Log in or Continue with Google Welcome back! Please log in. Email · Hint Tip: Most researchers use their institutional email address as their ResearchGate login Password Forgot password? Keep me logged in Log in or Continue with Google No account? Sign up

IMAGES
VIDEO
COMMENTS
Unit root tests. Edward Herranz *. Unit roots are nonstationary autoregressive (AR) or autoregressive moving average. (ARMA) time series processes which may i nclude an intercept and/or a trend ...
E-mail address : [email protected]. Abstract: Over the last decade much research has been carried out on unit roots and. cointegration in panel-data with integrated time series, due to the ...
2.3 Harris-Tzavalis Test. The test for unit roots in panel data proposed by Harris and Tzavalis (1999) begins with. the observation that the "Nickell" bias in the estimated coefficient of the lagged endogenous. variable using LSDV (within) estimation is of known magnitude under some simple assumptions.
(a unit root), the signi… cance test will b e skewed from the normal and needs in principle to be simulated for each case when x t is an ARIMA( p; d; q ) process. This
Unit root tests have notoriously low power, especially if the AR coefficient is close to one. In this exercise you are asked to explore this for yourself. Generate 100 observations from a stationary zero-mean AR(1) process with β = 0.95. Draw the errors independently from a N(0,1) distribution.
This paper re-evaluates key past results of unit root tests, emphasizing that the use of a conventional level of significance is not in general optimal due to the test having low power. The decision-based significance levels for popular unit root tests, chosen using the line of enlightened judgement under a symmetric loss function, are found to be much higher than conventional ones. We also ...
Abstract. Using the augmented Dickey-Fuller test to verify the existence of a unit root in an autoregressive process often requires the correctly specified intercept, since the test statistics can be distinctive under different model specifications and lead to contradictory results at times. In this article, we develop a unified inference that ...
and test whether \(\gamma =0\) (i.e., that \(\beta =1\)).Equation emphasizes the fact that if the model were a random walk, then first differencing would render the model stationary.Unfortunately, under the null of a unit root, the sampling distribution of \(\beta _1\) does not follow a t-distribution, or any other standard distribution, neither in finite samples nor asymptotically.
Autoregressive unit root tests are based on testing the null hypothesis that 4> = 1 (difference stationary) against the alternative hypothesis that 4> < 1 (trend stationary). They are called unit root tests because under the null hypothesis the autoregressive polynomial of Zt, ¢(z) = (1 - ¢z) = 0, has a root equal to unity.
After a brief review of the -rst generation panel unit root tests, this paper focuses on the tests belonging to the second generation. The latter category of tests is characterized by the rejection of the cross-sectional independence hypothesis. Within this second generation of tests, two main approaches are distinguished.
The Dickey-Fuller test (DF test) and its various modified versions have been widely used for unit root or random walk testing, though advices are necessary regarding their proper use in hands-on statistical algorithm software. In this paper, we review the development of such tests over several decades.
Unit root tests for stationarity have relevancy in almost every practical time series analysis. Deciding on which unit root test to use is a topic of active interest. In this study, we compare the performance of the three commonly used unit root tests (i.e., Augmented Dickey-Fuller (ADF), Phillips-Perron (PP), and Kwiatkowski
The paper by Levin and Lin (1993) provides some new results on panel unit root tests. These tests are designed to take care of the problem of heteroscedasticity and autocorrelation. They involve the following steps. (i) Subtract cross-section averages from the data to eliminate the influ- ence of aggregate effects.
While the random-walk-like behaviour of stock prices was noticed much earlier, for example by Jules Regnault, a French broker, in 1863 and by Louis Bachelier in his 1900 PhD thesis, the development of statistical inference for unit roots started only in the late 1970s. Nevertheless, the literature on unit-root tests is by now immense and diverse.
Abstract. The occurrence of unit roots in economic time series has far reaching consequences for univariate as well as multivariate econometric modelling. Therefore, unit root tests are nowadays the starting point of most empirical time series studies. The oldest and most widely used test is due to Dickey and Fuller (1979).
This paper also reports the finite sample performance of our combination unit root tests and Im et al.'s [Mimeo (1995)] t-bar test. The results show that most of the combination tests are more ...
Over the last decade considerable work has been carried on unit root testing in panel data models. See, for example, Breitung and Pesaran (2008) for a recent survey of the literature. Most panel unit root tests are designed to test the null hypothesis of a unit root for each individual series in a panel. The formulation of
The Levin and Lin (1992, 1993) test ─ henceforth referred to as LL test─ treats panel data as being composed of homogeneous cross-sections, thus performing a test on a pooled data series. The LL test for unit roots in panel data is computed based on the following model: y y '. it i i , t 1 z.
Diebold, Francis X. and Lutz Kilian. "Unit-Root Tests Are Useful For Selecting Forecasting Models," Journal of Business and Economic Statistics, 2000, v18 (3,Jul), 265-273. citation courtesy of. Founded in 1920, the NBER is a private, non-profit, non-partisan organization dedicated to conducting economic research and to disseminating research ...
A great deal of economic problems are related to detecting the stability of time series data, where the main interest is in the unit root test. In this paper, we consider the unit root testing problem with errors being long-memory processes with the GARCH structure. A new test statistic is developed by using the random weighted bootstrap method. It turns out that the proposed statistic has a ...
The implications of unit root nonstationarity in series to be used in regression models have been studied by Granger and Newbold (1977, section 6.4), Plosser and Schwert (1978), and
Testing for unit roots in heterogeneous panels. This paper proposes unit root tests for dynamic heterogeneous panels based on the mean of individual unit root statistics. In particular it proposes a standardized t-bar test statistic based on the (augmented) Dickey-Fuller statistics... more. Download.
In this paper, a new criterion is proposed to test the presence of a unit root in any. zero-mean time series data with no deterministic trend and no structural break. The test is developed based ...