- Business Essentials
- Leadership & Management
- Credential of Leadership, Impact, and Management in Business (CLIMB)
- Entrepreneurship & Innovation
- Digital Transformation
- Finance & Accounting
- Business in Society
- For Organizations
- Support Portal
- Media Coverage
- Founding Donors
- Leadership Team
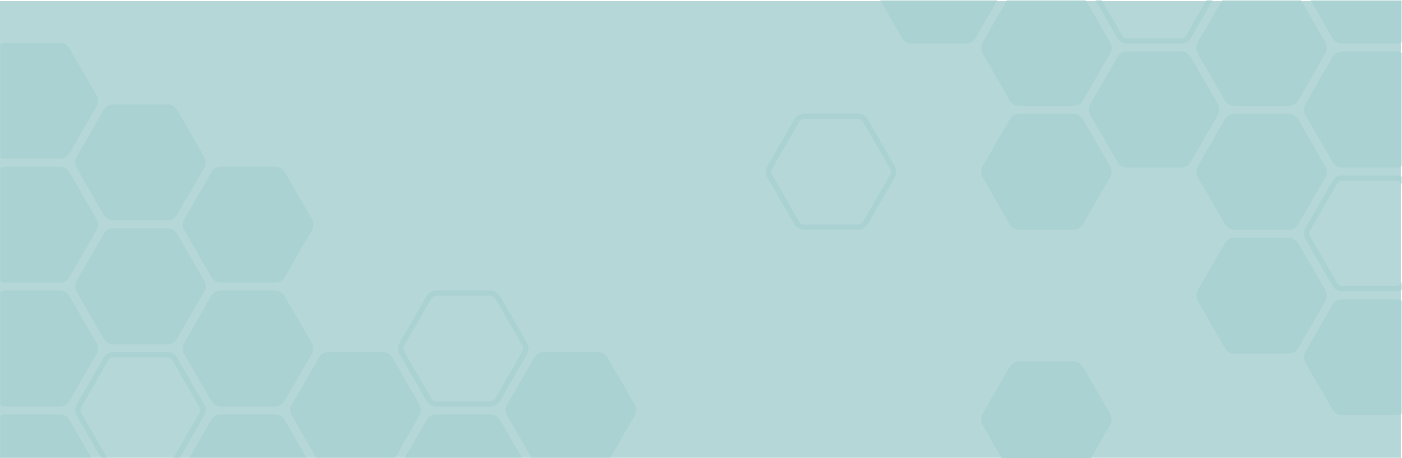
- Harvard Business School →
- HBS Online →
- Business Insights →

Business Insights
Harvard Business School Online's Business Insights Blog provides the career insights you need to achieve your goals and gain confidence in your business skills.
- Career Development
- Communication
- Decision-Making
- Earning Your MBA
- Negotiation
- News & Events
- Productivity
- Staff Spotlight
- Student Profiles
- Work-Life Balance
- AI Essentials for Business
- Alternative Investments
- Business Analytics
- Business Strategy
- Business and Climate Change
- Design Thinking and Innovation
- Digital Marketing Strategy
- Disruptive Strategy
- Economics for Managers
- Entrepreneurship Essentials
- Financial Accounting
- Global Business
- Launching Tech Ventures
- Leadership Principles
- Leadership, Ethics, and Corporate Accountability
- Leading with Finance
- Management Essentials
- Negotiation Mastery
- Organizational Leadership
- Power and Influence for Positive Impact
- Strategy Execution
- Sustainable Business Strategy
- Sustainable Investing
- Winning with Digital Platforms
A Beginner’s Guide to Hypothesis Testing in Business
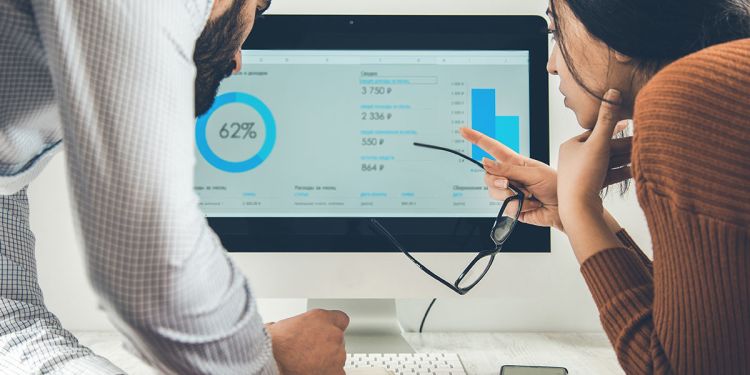
- 30 Mar 2021
Becoming a more data-driven decision-maker can bring several benefits to your organization, enabling you to identify new opportunities to pursue and threats to abate. Rather than allowing subjective thinking to guide your business strategy, backing your decisions with data can empower your company to become more innovative and, ultimately, profitable.
If you’re new to data-driven decision-making, you might be wondering how data translates into business strategy. The answer lies in generating a hypothesis and verifying or rejecting it based on what various forms of data tell you.
Below is a look at hypothesis testing and the role it plays in helping businesses become more data-driven.
Access your free e-book today.
What Is Hypothesis Testing?
To understand what hypothesis testing is, it’s important first to understand what a hypothesis is.
A hypothesis or hypothesis statement seeks to explain why something has happened, or what might happen, under certain conditions. It can also be used to understand how different variables relate to each other. Hypotheses are often written as if-then statements; for example, “If this happens, then this will happen.”
Hypothesis testing , then, is a statistical means of testing an assumption stated in a hypothesis. While the specific methodology leveraged depends on the nature of the hypothesis and data available, hypothesis testing typically uses sample data to extrapolate insights about a larger population.
Hypothesis Testing in Business
When it comes to data-driven decision-making, there’s a certain amount of risk that can mislead a professional. This could be due to flawed thinking or observations, incomplete or inaccurate data , or the presence of unknown variables. The danger in this is that, if major strategic decisions are made based on flawed insights, it can lead to wasted resources, missed opportunities, and catastrophic outcomes.
The real value of hypothesis testing in business is that it allows professionals to test their theories and assumptions before putting them into action. This essentially allows an organization to verify its analysis is correct before committing resources to implement a broader strategy.
As one example, consider a company that wishes to launch a new marketing campaign to revitalize sales during a slow period. Doing so could be an incredibly expensive endeavor, depending on the campaign’s size and complexity. The company, therefore, may wish to test the campaign on a smaller scale to understand how it will perform.
In this example, the hypothesis that’s being tested would fall along the lines of: “If the company launches a new marketing campaign, then it will translate into an increase in sales.” It may even be possible to quantify how much of a lift in sales the company expects to see from the effort. Pending the results of the pilot campaign, the business would then know whether it makes sense to roll it out more broadly.
Related: 9 Fundamental Data Science Skills for Business Professionals
Key Considerations for Hypothesis Testing
1. alternative hypothesis and null hypothesis.
In hypothesis testing, the hypothesis that’s being tested is known as the alternative hypothesis . Often, it’s expressed as a correlation or statistical relationship between variables. The null hypothesis , on the other hand, is a statement that’s meant to show there’s no statistical relationship between the variables being tested. It’s typically the exact opposite of whatever is stated in the alternative hypothesis.
For example, consider a company’s leadership team that historically and reliably sees $12 million in monthly revenue. They want to understand if reducing the price of their services will attract more customers and, in turn, increase revenue.
In this case, the alternative hypothesis may take the form of a statement such as: “If we reduce the price of our flagship service by five percent, then we’ll see an increase in sales and realize revenues greater than $12 million in the next month.”
The null hypothesis, on the other hand, would indicate that revenues wouldn’t increase from the base of $12 million, or might even decrease.
Check out the video below about the difference between an alternative and a null hypothesis, and subscribe to our YouTube channel for more explainer content.
2. Significance Level and P-Value
Statistically speaking, if you were to run the same scenario 100 times, you’d likely receive somewhat different results each time. If you were to plot these results in a distribution plot, you’d see the most likely outcome is at the tallest point in the graph, with less likely outcomes falling to the right and left of that point.

With this in mind, imagine you’ve completed your hypothesis test and have your results, which indicate there may be a correlation between the variables you were testing. To understand your results' significance, you’ll need to identify a p-value for the test, which helps note how confident you are in the test results.
In statistics, the p-value depicts the probability that, assuming the null hypothesis is correct, you might still observe results that are at least as extreme as the results of your hypothesis test. The smaller the p-value, the more likely the alternative hypothesis is correct, and the greater the significance of your results.
3. One-Sided vs. Two-Sided Testing
When it’s time to test your hypothesis, it’s important to leverage the correct testing method. The two most common hypothesis testing methods are one-sided and two-sided tests , or one-tailed and two-tailed tests, respectively.
Typically, you’d leverage a one-sided test when you have a strong conviction about the direction of change you expect to see due to your hypothesis test. You’d leverage a two-sided test when you’re less confident in the direction of change.

4. Sampling
To perform hypothesis testing in the first place, you need to collect a sample of data to be analyzed. Depending on the question you’re seeking to answer or investigate, you might collect samples through surveys, observational studies, or experiments.
A survey involves asking a series of questions to a random population sample and recording self-reported responses.
Observational studies involve a researcher observing a sample population and collecting data as it occurs naturally, without intervention.
Finally, an experiment involves dividing a sample into multiple groups, one of which acts as the control group. For each non-control group, the variable being studied is manipulated to determine how the data collected differs from that of the control group.

Learn How to Perform Hypothesis Testing
Hypothesis testing is a complex process involving different moving pieces that can allow an organization to effectively leverage its data and inform strategic decisions.
If you’re interested in better understanding hypothesis testing and the role it can play within your organization, one option is to complete a course that focuses on the process. Doing so can lay the statistical and analytical foundation you need to succeed.
Do you want to learn more about hypothesis testing? Explore Business Analytics —one of our online business essentials courses —and download our Beginner’s Guide to Data & Analytics .

About the Author
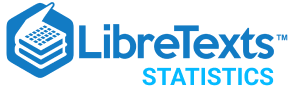
- school Campus Bookshelves
- menu_book Bookshelves
- perm_media Learning Objects
- login Login
- how_to_reg Request Instructor Account
- hub Instructor Commons
- Download Page (PDF)
- Download Full Book (PDF)
- Periodic Table
- Physics Constants
- Scientific Calculator
- Reference & Cite
- Tools expand_more
- Readability
selected template will load here
This action is not available.
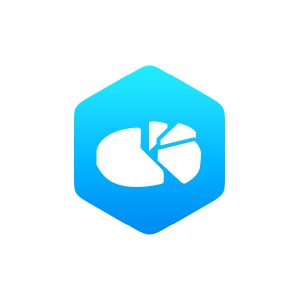
9.1: Introduction to Hypothesis Testing
- Last updated
- Save as PDF
- Page ID 10211
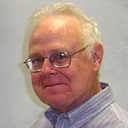
- Kyle Siegrist
- University of Alabama in Huntsville via Random Services
Basic Theory
Preliminaries.
As usual, our starting point is a random experiment with an underlying sample space and a probability measure \(\P\). In the basic statistical model, we have an observable random variable \(\bs{X}\) taking values in a set \(S\). In general, \(\bs{X}\) can have quite a complicated structure. For example, if the experiment is to sample \(n\) objects from a population and record various measurements of interest, then \[ \bs{X} = (X_1, X_2, \ldots, X_n) \] where \(X_i\) is the vector of measurements for the \(i\)th object. The most important special case occurs when \((X_1, X_2, \ldots, X_n)\) are independent and identically distributed. In this case, we have a random sample of size \(n\) from the common distribution.
The purpose of this section is to define and discuss the basic concepts of statistical hypothesis testing . Collectively, these concepts are sometimes referred to as the Neyman-Pearson framework, in honor of Jerzy Neyman and Egon Pearson, who first formalized them.
A statistical hypothesis is a statement about the distribution of \(\bs{X}\). Equivalently, a statistical hypothesis specifies a set of possible distributions of \(\bs{X}\): the set of distributions for which the statement is true. A hypothesis that specifies a single distribution for \(\bs{X}\) is called simple ; a hypothesis that specifies more than one distribution for \(\bs{X}\) is called composite .
In hypothesis testing , the goal is to see if there is sufficient statistical evidence to reject a presumed null hypothesis in favor of a conjectured alternative hypothesis . The null hypothesis is usually denoted \(H_0\) while the alternative hypothesis is usually denoted \(H_1\).
An hypothesis test is a statistical decision ; the conclusion will either be to reject the null hypothesis in favor of the alternative, or to fail to reject the null hypothesis. The decision that we make must, of course, be based on the observed value \(\bs{x}\) of the data vector \(\bs{X}\). Thus, we will find an appropriate subset \(R\) of the sample space \(S\) and reject \(H_0\) if and only if \(\bs{x} \in R\). The set \(R\) is known as the rejection region or the critical region . Note the asymmetry between the null and alternative hypotheses. This asymmetry is due to the fact that we assume the null hypothesis, in a sense, and then see if there is sufficient evidence in \(\bs{x}\) to overturn this assumption in favor of the alternative.
An hypothesis test is a statistical analogy to proof by contradiction, in a sense. Suppose for a moment that \(H_1\) is a statement in a mathematical theory and that \(H_0\) is its negation. One way that we can prove \(H_1\) is to assume \(H_0\) and work our way logically to a contradiction. In an hypothesis test, we don't prove anything of course, but there are similarities. We assume \(H_0\) and then see if the data \(\bs{x}\) are sufficiently at odds with that assumption that we feel justified in rejecting \(H_0\) in favor of \(H_1\).
Often, the critical region is defined in terms of a statistic \(w(\bs{X})\), known as a test statistic , where \(w\) is a function from \(S\) into another set \(T\). We find an appropriate rejection region \(R_T \subseteq T\) and reject \(H_0\) when the observed value \(w(\bs{x}) \in R_T\). Thus, the rejection region in \(S\) is then \(R = w^{-1}(R_T) = \left\{\bs{x} \in S: w(\bs{x}) \in R_T\right\}\). As usual, the use of a statistic often allows significant data reduction when the dimension of the test statistic is much smaller than the dimension of the data vector.
The ultimate decision may be correct or may be in error. There are two types of errors, depending on which of the hypotheses is actually true.
Types of errors:
- A type 1 error is rejecting the null hypothesis \(H_0\) when \(H_0\) is true.
- A type 2 error is failing to reject the null hypothesis \(H_0\) when the alternative hypothesis \(H_1\) is true.
Similarly, there are two ways to make a correct decision: we could reject \(H_0\) when \(H_1\) is true or we could fail to reject \(H_0\) when \(H_0\) is true. The possibilities are summarized in the following table:
Of course, when we observe \(\bs{X} = \bs{x}\) and make our decision, either we will have made the correct decision or we will have committed an error, and usually we will never know which of these events has occurred. Prior to gathering the data, however, we can consider the probabilities of the various errors.
If \(H_0\) is true (that is, the distribution of \(\bs{X}\) is specified by \(H_0\)), then \(\P(\bs{X} \in R)\) is the probability of a type 1 error for this distribution. If \(H_0\) is composite, then \(H_0\) specifies a variety of different distributions for \(\bs{X}\) and thus there is a set of type 1 error probabilities.
The maximum probability of a type 1 error, over the set of distributions specified by \( H_0 \), is the significance level of the test or the size of the critical region.
The significance level is often denoted by \(\alpha\). Usually, the rejection region is constructed so that the significance level is a prescribed, small value (typically 0.1, 0.05, 0.01).
If \(H_1\) is true (that is, the distribution of \(\bs{X}\) is specified by \(H_1\)), then \(\P(\bs{X} \notin R)\) is the probability of a type 2 error for this distribution. Again, if \(H_1\) is composite then \(H_1\) specifies a variety of different distributions for \(\bs{X}\), and thus there will be a set of type 2 error probabilities. Generally, there is a tradeoff between the type 1 and type 2 error probabilities. If we reduce the probability of a type 1 error, by making the rejection region \(R\) smaller, we necessarily increase the probability of a type 2 error because the complementary region \(S \setminus R\) is larger.
The extreme cases can give us some insight. First consider the decision rule in which we never reject \(H_0\), regardless of the evidence \(\bs{x}\). This corresponds to the rejection region \(R = \emptyset\). A type 1 error is impossible, so the significance level is 0. On the other hand, the probability of a type 2 error is 1 for any distribution defined by \(H_1\). At the other extreme, consider the decision rule in which we always rejects \(H_0\) regardless of the evidence \(\bs{x}\). This corresponds to the rejection region \(R = S\). A type 2 error is impossible, but now the probability of a type 1 error is 1 for any distribution defined by \(H_0\). In between these two worthless tests are meaningful tests that take the evidence \(\bs{x}\) into account.
If \(H_1\) is true, so that the distribution of \(\bs{X}\) is specified by \(H_1\), then \(\P(\bs{X} \in R)\), the probability of rejecting \(H_0\) is the power of the test for that distribution.
Thus the power of the test for a distribution specified by \( H_1 \) is the probability of making the correct decision.
Suppose that we have two tests, corresponding to rejection regions \(R_1\) and \(R_2\), respectively, each having significance level \(\alpha\). The test with region \(R_1\) is uniformly more powerful than the test with region \(R_2\) if \[ \P(\bs{X} \in R_1) \ge \P(\bs{X} \in R_2) \text{ for every distribution of } \bs{X} \text{ specified by } H_1 \]
Naturally, in this case, we would prefer the first test. Often, however, two tests will not be uniformly ordered; one test will be more powerful for some distributions specified by \(H_1\) while the other test will be more powerful for other distributions specified by \(H_1\).
If a test has significance level \(\alpha\) and is uniformly more powerful than any other test with significance level \(\alpha\), then the test is said to be a uniformly most powerful test at level \(\alpha\).
Clearly a uniformly most powerful test is the best we can do.
\(P\)-value
In most cases, we have a general procedure that allows us to construct a test (that is, a rejection region \(R_\alpha\)) for any given significance level \(\alpha \in (0, 1)\). Typically, \(R_\alpha\) decreases (in the subset sense) as \(\alpha\) decreases.
The \(P\)-value of the observed value \(\bs{x}\) of \(\bs{X}\), denoted \(P(\bs{x})\), is defined to be the smallest \(\alpha\) for which \(\bs{x} \in R_\alpha\); that is, the smallest significance level for which \(H_0\) is rejected, given \(\bs{X} = \bs{x}\).
Knowing \(P(\bs{x})\) allows us to test \(H_0\) at any significance level for the given data \(\bs{x}\): If \(P(\bs{x}) \le \alpha\) then we would reject \(H_0\) at significance level \(\alpha\); if \(P(\bs{x}) \gt \alpha\) then we fail to reject \(H_0\) at significance level \(\alpha\). Note that \(P(\bs{X})\) is a statistic . Informally, \(P(\bs{x})\) can often be thought of as the probability of an outcome as or more extreme than the observed value \(\bs{x}\), where extreme is interpreted relative to the null hypothesis \(H_0\).
Analogy with Justice Systems
There is a helpful analogy between statistical hypothesis testing and the criminal justice system in the US and various other countries. Consider a person charged with a crime. The presumed null hypothesis is that the person is innocent of the crime; the conjectured alternative hypothesis is that the person is guilty of the crime. The test of the hypotheses is a trial with evidence presented by both sides playing the role of the data. After considering the evidence, the jury delivers the decision as either not guilty or guilty . Note that innocent is not a possible verdict of the jury, because it is not the point of the trial to prove the person innocent. Rather, the point of the trial is to see whether there is sufficient evidence to overturn the null hypothesis that the person is innocent in favor of the alternative hypothesis of that the person is guilty. A type 1 error is convicting a person who is innocent; a type 2 error is acquitting a person who is guilty. Generally, a type 1 error is considered the more serious of the two possible errors, so in an attempt to hold the chance of a type 1 error to a very low level, the standard for conviction in serious criminal cases is beyond a reasonable doubt .
Tests of an Unknown Parameter
Hypothesis testing is a very general concept, but an important special class occurs when the distribution of the data variable \(\bs{X}\) depends on a parameter \(\theta\) taking values in a parameter space \(\Theta\). The parameter may be vector-valued, so that \(\bs{\theta} = (\theta_1, \theta_2, \ldots, \theta_n)\) and \(\Theta \subseteq \R^k\) for some \(k \in \N_+\). The hypotheses generally take the form \[ H_0: \theta \in \Theta_0 \text{ versus } H_1: \theta \notin \Theta_0 \] where \(\Theta_0\) is a prescribed subset of the parameter space \(\Theta\). In this setting, the probabilities of making an error or a correct decision depend on the true value of \(\theta\). If \(R\) is the rejection region, then the power function \( Q \) is given by \[ Q(\theta) = \P_\theta(\bs{X} \in R), \quad \theta \in \Theta \] The power function gives a lot of information about the test.
The power function satisfies the following properties:
- \(Q(\theta)\) is the probability of a type 1 error when \(\theta \in \Theta_0\).
- \(\max\left\{Q(\theta): \theta \in \Theta_0\right\}\) is the significance level of the test.
- \(1 - Q(\theta)\) is the probability of a type 2 error when \(\theta \notin \Theta_0\).
- \(Q(\theta)\) is the power of the test when \(\theta \notin \Theta_0\).
If we have two tests, we can compare them by means of their power functions.
Suppose that we have two tests, corresponding to rejection regions \(R_1\) and \(R_2\), respectively, each having significance level \(\alpha\). The test with rejection region \(R_1\) is uniformly more powerful than the test with rejection region \(R_2\) if \( Q_1(\theta) \ge Q_2(\theta)\) for all \( \theta \notin \Theta_0 \).
Most hypothesis tests of an unknown real parameter \(\theta\) fall into three special cases:
Suppose that \( \theta \) is a real parameter and \( \theta_0 \in \Theta \) a specified value. The tests below are respectively the two-sided test , the left-tailed test , and the right-tailed test .
- \(H_0: \theta = \theta_0\) versus \(H_1: \theta \ne \theta_0\)
- \(H_0: \theta \ge \theta_0\) versus \(H_1: \theta \lt \theta_0\)
- \(H_0: \theta \le \theta_0\) versus \(H_1: \theta \gt \theta_0\)
Thus the tests are named after the conjectured alternative. Of course, there may be other unknown parameters besides \(\theta\) (known as nuisance parameters ).
Equivalence Between Hypothesis Test and Confidence Sets
There is an equivalence between hypothesis tests and confidence sets for a parameter \(\theta\).
Suppose that \(C(\bs{x})\) is a \(1 - \alpha\) level confidence set for \(\theta\). The following test has significance level \(\alpha\) for the hypothesis \( H_0: \theta = \theta_0 \) versus \( H_1: \theta \ne \theta_0 \): Reject \(H_0\) if and only if \(\theta_0 \notin C(\bs{x})\)
By definition, \(\P[\theta \in C(\bs{X})] = 1 - \alpha\). Hence if \(H_0\) is true so that \(\theta = \theta_0\), then the probability of a type 1 error is \(P[\theta \notin C(\bs{X})] = \alpha\).
Equivalently, we fail to reject \(H_0\) at significance level \(\alpha\) if and only if \(\theta_0\) is in the corresponding \(1 - \alpha\) level confidence set. In particular, this equivalence applies to interval estimates of a real parameter \(\theta\) and the common tests for \(\theta\) given above .
In each case below, the confidence interval has confidence level \(1 - \alpha\) and the test has significance level \(\alpha\).
- Suppose that \(\left[L(\bs{X}, U(\bs{X})\right]\) is a two-sided confidence interval for \(\theta\). Reject \(H_0: \theta = \theta_0\) versus \(H_1: \theta \ne \theta_0\) if and only if \(\theta_0 \lt L(\bs{X})\) or \(\theta_0 \gt U(\bs{X})\).
- Suppose that \(L(\bs{X})\) is a confidence lower bound for \(\theta\). Reject \(H_0: \theta \le \theta_0\) versus \(H_1: \theta \gt \theta_0\) if and only if \(\theta_0 \lt L(\bs{X})\).
- Suppose that \(U(\bs{X})\) is a confidence upper bound for \(\theta\). Reject \(H_0: \theta \ge \theta_0\) versus \(H_1: \theta \lt \theta_0\) if and only if \(\theta_0 \gt U(\bs{X})\).
Pivot Variables and Test Statistics
Recall that confidence sets of an unknown parameter \(\theta\) are often constructed through a pivot variable , that is, a random variable \(W(\bs{X}, \theta)\) that depends on the data vector \(\bs{X}\) and the parameter \(\theta\), but whose distribution does not depend on \(\theta\) and is known. In this case, a natural test statistic for the basic tests given above is \(W(\bs{X}, \theta_0)\).
- Hypothesis Testing: Definition, Uses, Limitations + Examples

Hypothesis testing is as old as the scientific method and is at the heart of the research process.
Research exists to validate or disprove assumptions about various phenomena. The process of validation involves testing and it is in this context that we will explore hypothesis testing.
What is a Hypothesis?
A hypothesis is a calculated prediction or assumption about a population parameter based on limited evidence. The whole idea behind hypothesis formulation is testing—this means the researcher subjects his or her calculated assumption to a series of evaluations to know whether they are true or false.
Typically, every research starts with a hypothesis—the investigator makes a claim and experiments to prove that this claim is true or false . For instance, if you predict that students who drink milk before class perform better than those who don’t, then this becomes a hypothesis that can be confirmed or refuted using an experiment.
Read: What is Empirical Research Study? [Examples & Method]
What are the Types of Hypotheses?
1. simple hypothesis.
Also known as a basic hypothesis, a simple hypothesis suggests that an independent variable is responsible for a corresponding dependent variable. In other words, an occurrence of the independent variable inevitably leads to an occurrence of the dependent variable.
Typically, simple hypotheses are considered as generally true, and they establish a causal relationship between two variables.
Examples of Simple Hypothesis
- Drinking soda and other sugary drinks can cause obesity.
- Smoking cigarettes daily leads to lung cancer.
2. Complex Hypothesis
A complex hypothesis is also known as a modal. It accounts for the causal relationship between two independent variables and the resulting dependent variables. This means that the combination of the independent variables leads to the occurrence of the dependent variables .
Examples of Complex Hypotheses
- Adults who do not smoke and drink are less likely to develop liver-related conditions.
- Global warming causes icebergs to melt which in turn causes major changes in weather patterns.
3. Null Hypothesis
As the name suggests, a null hypothesis is formed when a researcher suspects that there’s no relationship between the variables in an observation. In this case, the purpose of the research is to approve or disapprove this assumption.
Examples of Null Hypothesis
- This is no significant change in a student’s performance if they drink coffee or tea before classes.
- There’s no significant change in the growth of a plant if one uses distilled water only or vitamin-rich water.
Read: Research Report: Definition, Types + [Writing Guide]
4. Alternative Hypothesis
To disapprove a null hypothesis, the researcher has to come up with an opposite assumption—this assumption is known as the alternative hypothesis. This means if the null hypothesis says that A is false, the alternative hypothesis assumes that A is true.
An alternative hypothesis can be directional or non-directional depending on the direction of the difference. A directional alternative hypothesis specifies the direction of the tested relationship, stating that one variable is predicted to be larger or smaller than the null value while a non-directional hypothesis only validates the existence of a difference without stating its direction.
Examples of Alternative Hypotheses
- Starting your day with a cup of tea instead of a cup of coffee can make you more alert in the morning.
- The growth of a plant improves significantly when it receives distilled water instead of vitamin-rich water.
5. Logical Hypothesis
Logical hypotheses are some of the most common types of calculated assumptions in systematic investigations. It is an attempt to use your reasoning to connect different pieces in research and build a theory using little evidence. In this case, the researcher uses any data available to him, to form a plausible assumption that can be tested.
Examples of Logical Hypothesis
- Waking up early helps you to have a more productive day.
- Beings from Mars would not be able to breathe the air in the atmosphere of the Earth.
6. Empirical Hypothesis
After forming a logical hypothesis, the next step is to create an empirical or working hypothesis. At this stage, your logical hypothesis undergoes systematic testing to prove or disprove the assumption. An empirical hypothesis is subject to several variables that can trigger changes and lead to specific outcomes.
Examples of Empirical Testing
- People who eat more fish run faster than people who eat meat.
- Women taking vitamin E grow hair faster than those taking vitamin K.
7. Statistical Hypothesis
When forming a statistical hypothesis, the researcher examines the portion of a population of interest and makes a calculated assumption based on the data from this sample. A statistical hypothesis is most common with systematic investigations involving a large target audience. Here, it’s impossible to collect responses from every member of the population so you have to depend on data from your sample and extrapolate the results to the wider population.
Examples of Statistical Hypothesis
- 45% of students in Louisiana have middle-income parents.
- 80% of the UK’s population gets a divorce because of irreconcilable differences.
What is Hypothesis Testing?
Hypothesis testing is an assessment method that allows researchers to determine the plausibility of a hypothesis. It involves testing an assumption about a specific population parameter to know whether it’s true or false. These population parameters include variance, standard deviation, and median.
Typically, hypothesis testing starts with developing a null hypothesis and then performing several tests that support or reject the null hypothesis. The researcher uses test statistics to compare the association or relationship between two or more variables.
Explore: Research Bias: Definition, Types + Examples
Researchers also use hypothesis testing to calculate the coefficient of variation and determine if the regression relationship and the correlation coefficient are statistically significant.
How Hypothesis Testing Works
The basis of hypothesis testing is to examine and analyze the null hypothesis and alternative hypothesis to know which one is the most plausible assumption. Since both assumptions are mutually exclusive, only one can be true. In other words, the occurrence of a null hypothesis destroys the chances of the alternative coming to life, and vice-versa.
Interesting: 21 Chrome Extensions for Academic Researchers in 2021
What Are The Stages of Hypothesis Testing?
To successfully confirm or refute an assumption, the researcher goes through five (5) stages of hypothesis testing;
- Determine the null hypothesis
- Specify the alternative hypothesis
- Set the significance level
- Calculate the test statistics and corresponding P-value
- Draw your conclusion
- Determine the Null Hypothesis
Like we mentioned earlier, hypothesis testing starts with creating a null hypothesis which stands as an assumption that a certain statement is false or implausible. For example, the null hypothesis (H0) could suggest that different subgroups in the research population react to a variable in the same way.
- Specify the Alternative Hypothesis
Once you know the variables for the null hypothesis, the next step is to determine the alternative hypothesis. The alternative hypothesis counters the null assumption by suggesting the statement or assertion is true. Depending on the purpose of your research, the alternative hypothesis can be one-sided or two-sided.
Using the example we established earlier, the alternative hypothesis may argue that the different sub-groups react differently to the same variable based on several internal and external factors.
- Set the Significance Level
Many researchers create a 5% allowance for accepting the value of an alternative hypothesis, even if the value is untrue. This means that there is a 0.05 chance that one would go with the value of the alternative hypothesis, despite the truth of the null hypothesis.
Something to note here is that the smaller the significance level, the greater the burden of proof needed to reject the null hypothesis and support the alternative hypothesis.
Explore: What is Data Interpretation? + [Types, Method & Tools]
- Calculate the Test Statistics and Corresponding P-Value
Test statistics in hypothesis testing allow you to compare different groups between variables while the p-value accounts for the probability of obtaining sample statistics if your null hypothesis is true. In this case, your test statistics can be the mean, median and similar parameters.
If your p-value is 0.65, for example, then it means that the variable in your hypothesis will happen 65 in100 times by pure chance. Use this formula to determine the p-value for your data:
- Draw Your Conclusions
After conducting a series of tests, you should be able to agree or refute the hypothesis based on feedback and insights from your sample data.
Applications of Hypothesis Testing in Research
Hypothesis testing isn’t only confined to numbers and calculations; it also has several real-life applications in business, manufacturing, advertising, and medicine.
In a factory or other manufacturing plants, hypothesis testing is an important part of quality and production control before the final products are approved and sent out to the consumer.
During ideation and strategy development, C-level executives use hypothesis testing to evaluate their theories and assumptions before any form of implementation. For example, they could leverage hypothesis testing to determine whether or not some new advertising campaign, marketing technique, etc. causes increased sales.
In addition, hypothesis testing is used during clinical trials to prove the efficacy of a drug or new medical method before its approval for widespread human usage.
What is an Example of Hypothesis Testing?
An employer claims that her workers are of above-average intelligence. She takes a random sample of 20 of them and gets the following results:
Mean IQ Scores: 110
Standard Deviation: 15
Mean Population IQ: 100
Step 1: Using the value of the mean population IQ, we establish the null hypothesis as 100.
Step 2: State that the alternative hypothesis is greater than 100.
Step 3: State the alpha level as 0.05 or 5%
Step 4: Find the rejection region area (given by your alpha level above) from the z-table. An area of .05 is equal to a z-score of 1.645.
Step 5: Calculate the test statistics using this formula
Z = (110–100) ÷ (15÷√20)
10 ÷ 3.35 = 2.99
If the value of the test statistics is higher than the value of the rejection region, then you should reject the null hypothesis. If it is less, then you cannot reject the null.
In this case, 2.99 > 1.645 so we reject the null.
Importance/Benefits of Hypothesis Testing
The most significant benefit of hypothesis testing is it allows you to evaluate the strength of your claim or assumption before implementing it in your data set. Also, hypothesis testing is the only valid method to prove that something “is or is not”. Other benefits include:
- Hypothesis testing provides a reliable framework for making any data decisions for your population of interest.
- It helps the researcher to successfully extrapolate data from the sample to the larger population.
- Hypothesis testing allows the researcher to determine whether the data from the sample is statistically significant.
- Hypothesis testing is one of the most important processes for measuring the validity and reliability of outcomes in any systematic investigation.
- It helps to provide links to the underlying theory and specific research questions.
Criticism and Limitations of Hypothesis Testing
Several limitations of hypothesis testing can affect the quality of data you get from this process. Some of these limitations include:
- The interpretation of a p-value for observation depends on the stopping rule and definition of multiple comparisons. This makes it difficult to calculate since the stopping rule is subject to numerous interpretations, plus “multiple comparisons” are unavoidably ambiguous.
- Conceptual issues often arise in hypothesis testing, especially if the researcher merges Fisher and Neyman-Pearson’s methods which are conceptually distinct.
- In an attempt to focus on the statistical significance of the data, the researcher might ignore the estimation and confirmation by repeated experiments.
- Hypothesis testing can trigger publication bias, especially when it requires statistical significance as a criterion for publication.
- When used to detect whether a difference exists between groups, hypothesis testing can trigger absurd assumptions that affect the reliability of your observation.
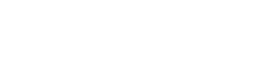
Connect to Formplus, Get Started Now - It's Free!
- alternative hypothesis
- alternative vs null hypothesis
- complex hypothesis
- empirical hypothesis
- hypothesis testing
- logical hypothesis
- simple hypothesis
- statistical hypothesis
- busayo.longe
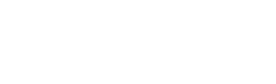
You may also like:
Type I vs Type II Errors: Causes, Examples & Prevention
This article will discuss the two different types of errors in hypothesis testing and how you can prevent them from occurring in your research

Alternative vs Null Hypothesis: Pros, Cons, Uses & Examples
We are going to discuss alternative hypotheses and null hypotheses in this post and how they work in research.
What is Pure or Basic Research? + [Examples & Method]
Simple guide on pure or basic research, its methods, characteristics, advantages, and examples in science, medicine, education and psychology
Internal Validity in Research: Definition, Threats, Examples
In this article, we will discuss the concept of internal validity, some clear examples, its importance, and how to test it.
Formplus - For Seamless Data Collection
Collect data the right way with a versatile data collection tool. try formplus and transform your work productivity today..
- Prompt Library
- DS/AI Trends
- Stats Tools
- Interview Questions
- Generative AI
- Machine Learning
- Deep Learning
Hypothesis Testing Steps & Examples
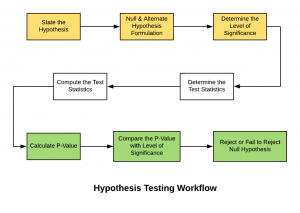
Table of Contents
What is a Hypothesis testing?
As per the definition from Oxford languages, a hypothesis is a supposition or proposed explanation made on the basis of limited evidence as a starting point for further investigation. As per the Dictionary page on Hypothesis , Hypothesis means a proposition or set of propositions, set forth as an explanation for the occurrence of some specified group of phenomena, either asserted merely as a provisional conjecture to guide investigation (working hypothesis) or accepted as highly probable in the light of established facts.
The hypothesis can be defined as the claim that can either be related to the truth about something that exists in the world, or, truth about something that’s needs to be established a fresh . In simple words, another word for the hypothesis is the “claim” . Until the claim is proven to be true, it is called the hypothesis. Once the claim is proved, it becomes the new truth or new knowledge about the thing. For example , let’s say that a claim is made that students studying for more than 6 hours a day gets more than 90% of marks in their examination. Now, this is just a claim or a hypothesis and not the truth in the real world. However, in order for the claim to become the truth for widespread adoption, it needs to be proved using pieces of evidence, e.g., data. In order to reject this claim or otherwise, one needs to do some empirical analysis by gathering data samples and evaluating the claim. The process of gathering data and evaluating the claims or hypotheses with the goal to reject or otherwise (failing to reject) can be called as hypothesis testing . Note the wordings – “failing to reject”. It means that we don’t have enough evidence to reject the claim. Thus, until the time that new evidence comes up, the claim can be considered the truth. There are different techniques to test the hypothesis in order to reach the conclusion of whether the hypothesis can be used to represent the truth of the world.
One must note that the hypothesis testing never constitutes a proof that the hypothesis is absolute truth based on the observations. It only provides added support to consider the hypothesis as truth until the time that new evidences can against the hypotheses can be gathered. We can never be 100% sure about truth related to those hypotheses based on the hypothesis testing.
Simply speaking, hypothesis testing is a framework that can be used to assert whether the claim or the hypothesis made about a real-world/real-life event can be seen as the truth or otherwise based on the given data (evidences).
Hypothesis Testing Examples
Before we get ahead and start understanding more details about hypothesis and hypothesis testing steps, lets take a look at some real-world examples of how to think about hypothesis and hypothesis testing when dealing with real-world problems :
- Customers are churning because they ain’t getting response to their complaints or issues
- Customers are churning because there are other competitive services in the market which are providing these services at lower cost.
- Customers are churning because there are other competitive services which are providing more services at the same cost.
- It is claimed that a 500 gm sugar packet for a particular brand, say XYZA, contains sugar of less than 500 gm, say around 480gm. Can this claim be taken as truth? How do we know that this claim is true? This is a hypothesis until proved.
- A group of doctors claims that quitting smoking increases lifespan. Can this claim be taken as new truth? The hypothesis is that quitting smoking results in an increase in lifespan.
- It is claimed that brisk walking for half an hour every day reverses diabetes. In order to accept this in your lifestyle, you may need evidence that supports this claim or hypothesis.
- It is claimed that doing Pranayama yoga for 30 minutes a day can help in easing stress by 50%. This can be termed as hypothesis and would require testing / validation for it to be established as a truth and recommended for widespread adoption.
- One common real-life example of hypothesis testing is election polling. In order to predict the outcome of an election, pollsters take a sample of the population and ask them who they plan to vote for. They then use hypothesis testing to assess whether their sample is representative of the population as a whole. If the results of the hypothesis test are significant, it means that the sample is representative and that the poll can be used to predict the outcome of the election. However, if the results are not significant, it means that the sample is not representative and that the poll should not be used to make predictions.
- Machine learning models make predictions based on the input data. Each of the machine learning model representing a function approximation can be taken as a hypothesis. All different models constitute what is called as hypothesis space .
- As part of a linear regression machine learning model , it is claimed that there is a relationship between the response variables and predictor variables? Can this hypothesis or claim be taken as truth? Let’s say, the hypothesis is that the housing price depends upon the average income of people already staying in the locality. How true is this hypothesis or claim? The relationship between response variable and each of the predictor variables can be evaluated using T-test and T-statistics .
- For linear regression model , one of the hypothesis is that there is no relationship between the response variable and any of the predictor variables. Thus, if b1, b2, b3 are three parameters, all of them is equal to 0. b1 = b2 = b3 = 0. This is where one performs F-test and use F-statistics to test this hypothesis.
You may note different hypotheses which are listed above. The next step would be validate some of these hypotheses. This is where data scientists will come into picture. One or more data scientists may be asked to work on different hypotheses. This would result in these data scientists looking for appropriate data related to the hypothesis they are working. This section will be detailed out in near future.
State the Hypothesis to begin Hypothesis Testing
The first step to hypothesis testing is defining or stating a hypothesis. Before the hypothesis can be tested, we need to formulate the hypothesis in terms of mathematical expressions. There are two important aspects to pay attention to, prior to the formulation of the hypothesis. The following represents different types of hypothesis that could be put to hypothesis testing:
- Claim made against the well-established fact : The case in which a fact is well-established, or accepted as truth or “knowledge” and a new claim is made about this well-established fact. For example , when you buy a packet of 500 gm of sugar, you assume that the packet does contain at the minimum 500 gm of sugar and not any less, based on the label of 500 gm on the packet. In this case, the fact is given or assumed to be the truth. A new claim can be made that the 500 gm sugar contains sugar weighing less than 500 gm. This claim needs to be tested before it is accepted as truth. Such cases could be considered for hypothesis testing if this is claimed that the assumption or the default state of being is not true. The claim to be established as new truth can be stated as “alternate hypothesis”. The opposite state can be stated as “null hypothesis”. Here the claim that the 500 gm packet consists of sugar less than 500 grams would be stated as alternate hypothesis. The opposite state which is the sugar packet consists 500 gm is null hypothesis.
- Claim to establish the new truth : The case in which there is some claim made about the reality that exists in the world (fact). For example , the fact that the housing price depends upon the average income of people already staying in the locality can be considered as a claim and not assumed to be true. Another example could be the claim that running 5 miles a day would result in a reduction of 10 kg of weight within a month. There could be varied such claims which when required to be proved as true have to go through hypothesis testing. The claim to be established as new truth can be stated as “alternate hypothesis”. The opposite state can be stated as “null hypothesis”. Running 5 miles a day would result in reduction of 10 kg within a month would be stated as alternate hypothesis.
Based on the above considerations, the following hypothesis can be stated for doing hypothesis testing.
- The packet of 500 gm of sugar contains sugar of weight less than 500 gm. (Claim made against the established fact). This is a new knowledge which requires hypothesis testing to get established and acted upon.
- The housing price depends upon the average income of the people staying in the locality. This is a new knowledge which requires hypothesis testing to get established and acted upon.
- Running 5 miles a day results in a reduction of 10 kg of weight within a month. This is a new knowledge which requires hypothesis testing to get established for widespread adoption.
Formulate Null & Alternate Hypothesis as Next Step
Once the hypothesis is defined or stated, the next step is to formulate the null and alternate hypothesis in order to begin hypothesis testing as described above.
What is a null hypothesis?
In the case where the given statement is a well-established fact or default state of being in the real world, one can call it a null hypothesis (in the simpler word, nothing new). Well-established facts don’t need any hypothesis testing and hence can be called the null hypothesis. In cases, when there are any new claims made which is not well established in the real world, the null hypothesis can be thought of as the default state or opposite state of that claim. For example , in the previous section, the claim or hypothesis is made that the students studying for more than 6 hours a day gets more than 90% of marks in their examination. The null hypothesis, in this case, will be that the claim is not true or real. The null hypothesis can be stated that there is no relationship or association between the students reading more than 6 hours a day and they getting 90% of the marks. Any occurrence is only a chance occurrence. Another example of hypothesis is when somebody is alleged that they have performed a crime.
Null hypothesis is denoted by letter H with 0, e.g., [latex]H_0[/latex]
What is an alternate hypothesis?
When the given statement is a claim (unexpected event in the real world) and not yet proven, one can call/formulate it as an alternate hypothesis and accordingly define a null hypothesis which is the opposite state of the hypothesis. The alternate hypothesis is a new knowledge or truth that needs to be established. In simple words, the hypothesis or claim that needs to be tested against reality in the real world can be termed the alternate hypothesis. In order to reach a conclusion that the claim (alternate hypothesis) can be considered the new knowledge or truth (based on the available evidence), it would be important to reject the null hypothesis. It should be noted that null and alternate hypotheses are mutually exclusive and at the same time asymmetric. In the example given in the previous section, the claim that the students studying for more than 6 hours get more than 90% of marks can be termed as the alternate hypothesis.
Alternate hypothesis is denoted with H subscript a, e.g., [latex]H_a[/latex]
Once the hypothesis is formulated as null([latex]H_0[/latex]) and alternate hypothesis ([latex]H_a[/latex]), there are two possible outcomes that can happen from hypothesis testing. These outcomes are the following:
- Reject the null hypothesis : There is enough evidence based on which one can reject the null hypothesis. Let’s understand this with the help of an example provided earlier in this section. The null hypothesis is that there is no relationship between the students studying more than 6 hours a day and getting more than 90% marks. In a sample of 30 students studying more than 6 hours a day, it was found that they scored 91% marks. Given that the null hypothesis is true, this kind of hypothesis testing result will be highly unlikely. This kind of result can’t happen by chance. That would mean that the claim can be taken as the new truth or new knowledge in the real world. One can go and take further samples of 30 students to perform some more testing to validate the hypothesis. If similar results show up with other tests, it can be said with very high confidence that there is enough evidence to reject the null hypothesis that there is no relationship between the students studying more than 6 hours a day and getting more than 90% marks. In such cases, one can go to accept the claim as new truth that the students studying more than 6 hours a day get more than 90% marks. The hypothesis can be considered the new truth until the time that new tests provide evidence against this claim.
- Fail to reject the null hypothesis : There is not enough evidence-based on which one can reject the null hypothesis (well-established fact or reality). Thus, one would fail to reject the null hypothesis. In a sample of 30 students studying more than 6 hours a day, the students were found to score 75%. Given that the null hypothesis is true, this kind of result is fairly likely or expected. With the given sample, one can’t reject the null hypothesis that there is no relationship between the students studying more than 6 hours a day and getting more than 90% marks.
Examples of formulating the null and alternate hypothesis
The following are some examples of the null and alternate hypothesis.
Hypothesis Testing Steps
Here is the diagram which represents the workflow of Hypothesis Testing.
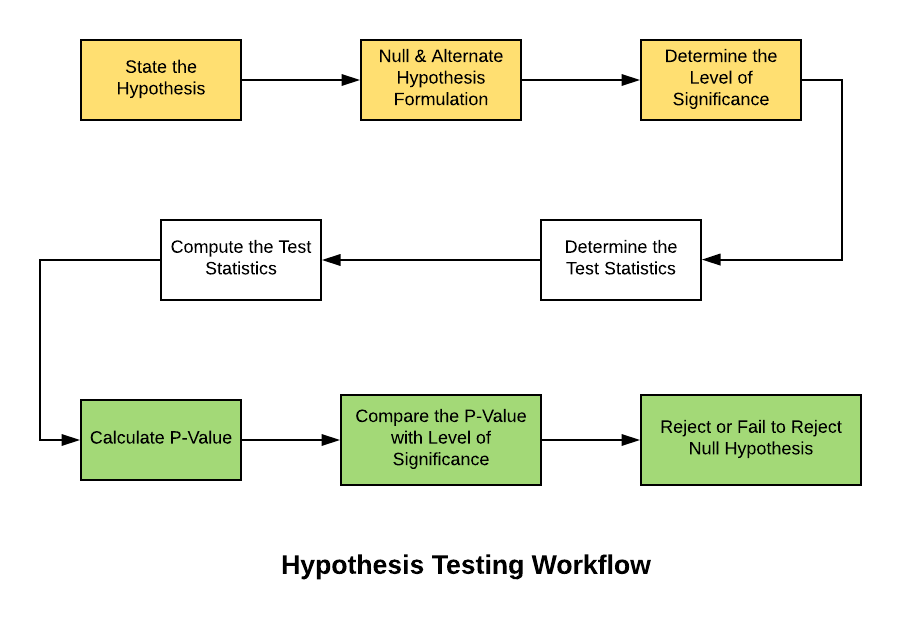
Figure 1. Hypothesis Testing Steps
Based on the above, the following are some of the steps to be taken when doing hypothesis testing:
- State the hypothesis : First and foremost, the hypothesis needs to be stated. The hypothesis could either be the statement that is assumed to be true or the claim which is made to be true.
- Formulate the hypothesis : This step requires one to identify the Null and Alternate hypotheses or in simple words, formulate the hypothesis. Take an example of the canned sauce weighing 500 gm as the Null Hypothesis.
- Set the criteria for a decision : Identify test statistics that could be used to assess the Null Hypothesis. The test statistics with the above example would be the average weight of the sugar packet, and t-statistics would be used to determine the P-value. For different kinds of problems, different kinds of statistics including Z-statistics, T-statistics, F-statistics, etc can be used.
- Identify the level of significance (alpha) : Before starting the hypothesis testing, one would be required to set the significance level (also called as alpha ) which represents the value for which a P-value less than or equal to alpha is considered statistically significant. Typical values of alpha are 0.1, 0.05, and 0.01. In case the P-value is evaluated as statistically significant, the null hypothesis is rejected. In case, the P-value is more than the alpha value, the null hypothesis is failed to be rejected.
- Compute the test statistics : Next step is to calculate the test statistics (z-test, t-test, f-test, etc) to determine the P-value. If the sample size is more than 30, it is recommended to use z-statistics. Otherwise, t-statistics could be used. In the current example where 20 packets of canned sauce is selected for hypothesis testing, t-statistics will be calculated for the mean value of 505 gm (sample mean). The t-statistics would then be calculated as the difference of 505 gm (sample mean) and the population means (500 gm) divided by the sample standard deviation divided by the square root of sample size (20).
- Calculate the P-value of the test statistics : Once the test statistics have been calculated, find the P-value using either of t-table or a z-table. P-value is the probability of obtaining a test statistic (t-score or z-score) equal to or more extreme than the result obtained from the sample data, given that the null hypothesis H0 is true.
- Compare P-value with the level of significance : The significance level is set as the allowable range within which if the value appears, one will be failed to reject the Null Hypothesis. This region is also called as Non-rejection region . The value of alpha is compared with the p-value. If the p-value is less than the significance level, the test is statistically significant and hence, the null hypothesis will be rejected.
P-Value: Key to Statistical Hypothesis Testing
Once you formulate the hypotheses, there is the need to test those hypotheses. Meaning, say that the null hypothesis is stated as the statement that housing price does not depend upon the average income of people staying in the locality, it would be required to be tested by taking samples of housing prices and, based on the test results, this Null hypothesis could either be rejected or failed to be rejected . In hypothesis testing, the following two are the outcomes:
- Reject the Null hypothesis
- Fail to Reject the Null hypothesis
Take the above example of the sugar packet weighing 500 gm. The Null hypothesis is set as the statement that the sugar packet weighs 500 gm. After taking a sample of 20 sugar packets and testing/taking its weight, it was found that the average weight of the sugar packets came to 495 gm. The test statistics (t-statistics) were calculated for this sample and the P-value was determined. Let’s say the P-value was found to be 15%. Assuming that the level of significance is selected to be 5%, the test statistic is not statistically significant (P-value > 5%) and thus, the null hypothesis fails to get rejected. Thus, one could safely conclude that the sugar packet does weigh 500 gm. However, if the average weight of canned sauce would have found to be 465 gm, this is way beyond/away from the mean value of 500 gm and one could have ended up rejecting the Null Hypothesis based on the P-value .
Hypothesis Testing for Problem Analysis & Solution Implementation
Hypothesis testing can be applied in both problem analysis and solution implementation. The following represents method on how you can apply hypothesis testing technique for both problem and solution space:
- Problem Analysis : Hypothesis testing is a systematic way to validate assumptions or educated guesses during problem analysis. It allows for a structured investigation into the nature of a problem and its potential root causes. In this process, a null hypothesis and an alternative hypothesis are usually defined. The null hypothesis generally asserts that no significant change or effect exists, while the alternative hypothesis posits the opposite. Through controlled experiments, data collection, or statistical analysis, these hypotheses are then tested to determine their validity. For example, if a software company notices a sudden increase in user churn rate, they might hypothesize that the recent update to their application is the root cause. The null hypothesis could be that the update has no effect on churn rate, while the alternative hypothesis would assert that the update significantly impacts the churn rate. By analyzing user behavior and feedback before and after the update, and perhaps running A/B tests where one user group has the update and another doesn’t, the company can test these hypotheses. If the alternative hypothesis is confirmed, the company can then focus on identifying specific issues in the update that may be causing the increased churn, thereby moving closer to a solution.
- Solution Implementation : Hypothesis testing can also be a valuable tool during the solution implementation phase, serving as a method to evaluate the effectiveness of proposed remedies. By setting up a specific hypothesis about the expected outcome of a solution, organizations can create targeted metrics and KPIs to measure success. For example, if a retail business is facing low customer retention rates, they might implement a loyalty program as a solution. The hypothesis could be that introducing a loyalty program will increase customer retention by at least 15% within six months. The null hypothesis would state that the loyalty program has no significant effect on retention rates. To test this, the company can compare retention metrics from before and after the program’s implementation, possibly even setting up control groups for more robust analysis. By applying statistical tests to this data, the company can determine whether their hypothesis is confirmed or refuted, thereby gauging the effectiveness of their solution and making data-driven decisions for future actions.
- Tests of Significance
- Hypothesis testing for the Mean
- z-statistics vs t-statistics (Khan Academy)
Hypothesis testing quiz
The claim that needs to be established is set as ____________, the outcome of hypothesis testing is _________.
Please select 2 correct answers
P-value is defined as the probability of obtaining the result as extreme given the null hypothesis is true
There is a claim that doing pranayama yoga results in reversing diabetes. which of the following is true about null hypothesis.
In this post, you learned about hypothesis testing and related nuances such as the null and alternate hypothesis formulation techniques, ways to go about doing hypothesis testing etc. In data science, one of the reasons why one needs to understand the concepts of hypothesis testing is the need to verify the relationship between the dependent (response) and independent (predictor) variables. One would, thus, need to understand the related concepts such as hypothesis formulation into null and alternate hypothesis, level of significance, test statistics calculation, P-value, etc. Given that the relationship between dependent and independent variables is a sort of hypothesis or claim , the null hypothesis could be set as the scenario where there is no relationship between dependent and independent variables.
Recent Posts
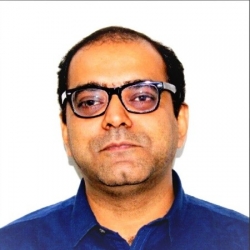
- Mean Squared Error vs Cross Entropy Loss Function - April 28, 2024
- Cross Entropy Loss Explained with Python Examples - April 28, 2024
- Logistic Regression in Machine Learning: Python Example - April 26, 2024
Ajitesh Kumar
Leave a reply cancel reply.
Your email address will not be published. Required fields are marked *
- Search for:
- Excellence Awaits: IITs, NITs & IIITs Journey
ChatGPT Prompts (250+)
- Generate Design Ideas for App
- Expand Feature Set of App
- Create a User Journey Map for App
- Generate Visual Design Ideas for App
- Generate a List of Competitors for App
- Mean Squared Error vs Cross Entropy Loss Function
- Cross Entropy Loss Explained with Python Examples
- Logistic Regression in Machine Learning: Python Example
- MSE vs RMSE vs MAE vs MAPE vs R-Squared: When to Use?
- Gradient Descent in Machine Learning: Python Examples
Data Science / AI Trends
- • Prepend any arxiv.org link with talk2 to load the paper into a responsive chat application
- • Custom LLM and AI Agents (RAG) On Structured + Unstructured Data - AI Brain For Your Organization
- • Guides, papers, lecture, notebooks and resources for prompt engineering
- • Common tricks to make LLMs efficient and stable
- • Machine learning in finance
Free Online Tools
- Create Scatter Plots Online for your Excel Data
- Histogram / Frequency Distribution Creation Tool
- Online Pie Chart Maker Tool
- Z-test vs T-test Decision Tool
- Independent samples t-test calculator
Recent Comments
I found it very helpful. However the differences are not too understandable for me
Very Nice Explaination. Thankyiu very much,
in your case E respresent Member or Oraganization which include on e or more peers?
Such a informative post. Keep it up
Thank you....for your support. you given a good solution for me.
How to Generate and Validate Product Hypotheses

Every product owner knows that it takes effort to build something that'll cater to user needs. You'll have to make many tough calls if you wish to grow the company and evolve the product so it delivers more value. But how do you decide what to change in the product, your marketing strategy, or the overall direction to succeed? And how do you make a product that truly resonates with your target audience?
There are many unknowns in business, so many fundamental decisions start from a simple "what if?". But they can't be based on guesses, as you need some proof to fill in the blanks reasonably.
Because there's no universal recipe for successfully building a product, teams collect data, do research, study the dynamics, and generate hypotheses according to the given facts. They then take corresponding actions to find out whether they were right or wrong, make conclusions, and most likely restart the process again.
On this page, we thoroughly inspect product hypotheses. We'll go over what they are, how to create hypothesis statements and validate them, and what goes after this step.
What Is a Hypothesis in Product Management?
A hypothesis in product development and product management is a statement or assumption about the product, planned feature, market, or customer (e.g., their needs, behavior, or expectations) that you can put to the test, evaluate, and base your further decisions on . This may, for instance, regard the upcoming product changes as well as the impact they can result in.
A hypothesis implies that there is limited knowledge. Hence, the teams need to undergo testing activities to validate their ideas and confirm whether they are true or false.
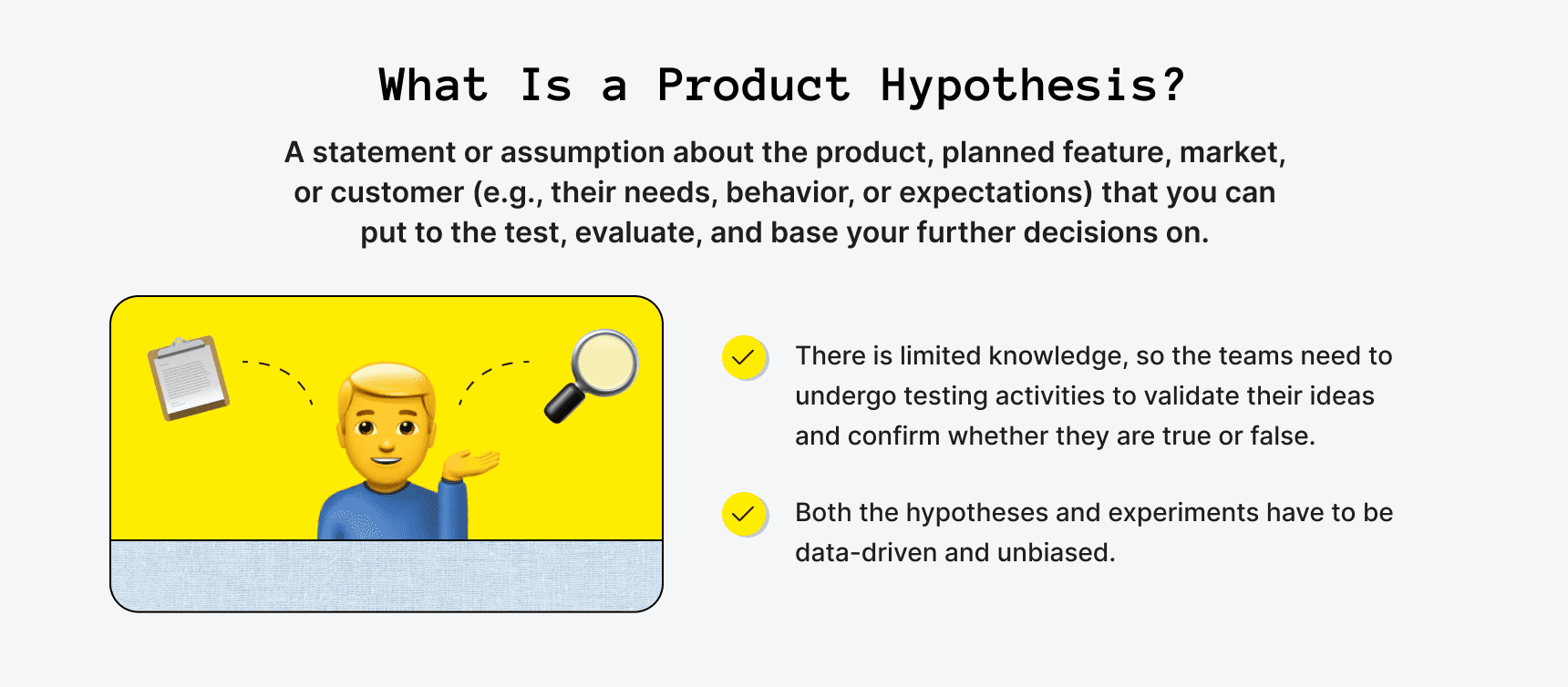
Hypotheses guide the product development process and may point at important findings to help build a better product that'll serve user needs. In essence, teams create hypothesis statements in an attempt to improve the offering, boost engagement, increase revenue, find product-market fit quicker, or for other business-related reasons.
It's sort of like an experiment with trial and error, yet, it is data-driven and should be unbiased . This means that teams don't make assumptions out of the blue. Instead, they turn to the collected data, conducted market research , and factual information, which helps avoid completely missing the mark. The obtained results are then carefully analyzed and may influence decision-making.
Such experiments backed by data and analysis are an integral aspect of successful product development and allow startups or businesses to dodge costly startup mistakes .
When do teams create hypothesis statements and validate them? To some extent, hypothesis testing is an ongoing process to work on constantly. It may occur during various product development life cycle stages, from early phases like initiation to late ones like scaling.
In any event, the key here is learning how to generate hypothesis statements and validate them effectively. We'll go over this in more detail later on.
Idea vs. Hypothesis Compared
You might be wondering whether ideas and hypotheses are the same thing. Well, there are a few distinctions.
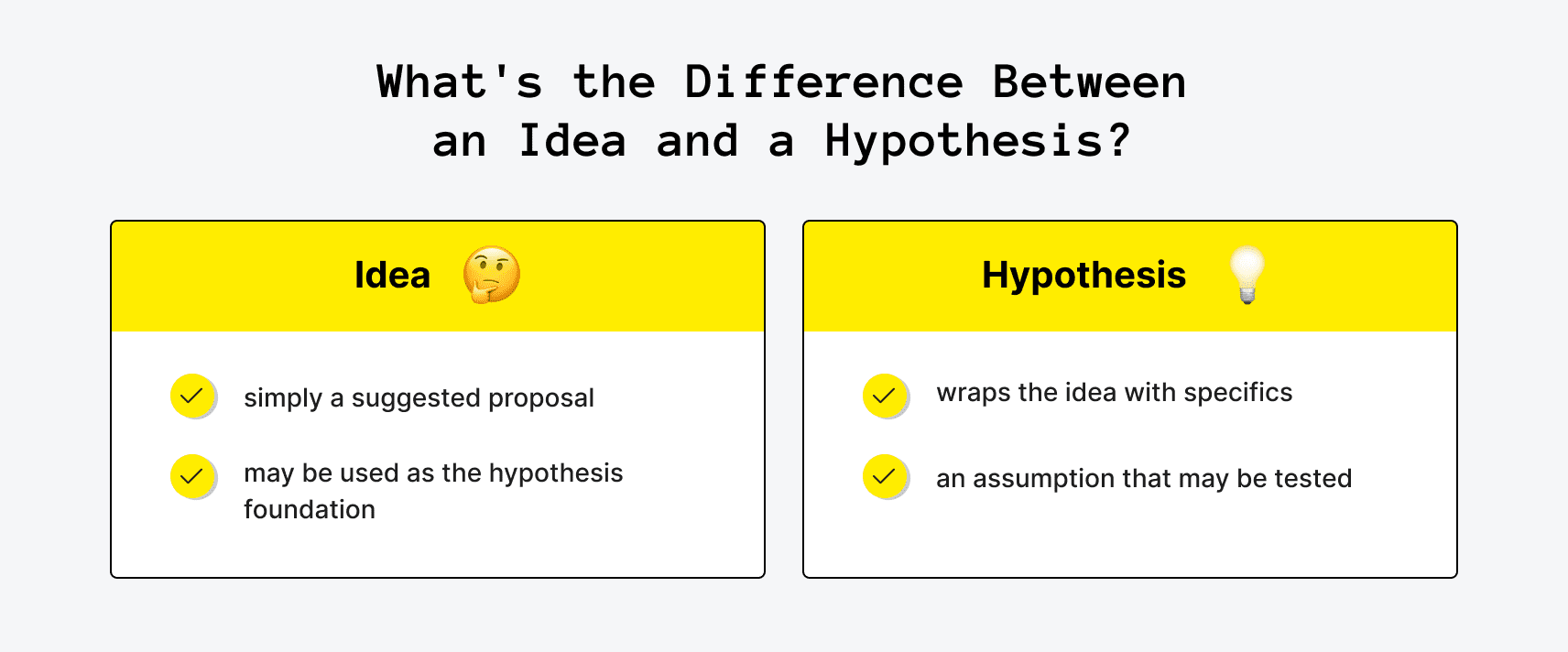
An idea is simply a suggested proposal. Say, a teammate comes up with something you can bring to life during a brainstorming session or pitches in a suggestion like "How about we shorten the checkout process?". You can jot down such ideas and then consider working on them if they'll truly make a difference and improve the product, strategy, or result in other business benefits. Ideas may thus be used as the hypothesis foundation when you decide to prove a concept.
A hypothesis is the next step, when an idea gets wrapped with specifics to become an assumption that may be tested. As such, you can refine the idea by adding details to it. The previously mentioned idea can be worded into a product hypothesis statement like: "The cart abandonment rate is high, and many users flee at checkout. But if we shorten the checkout process by cutting down the number of steps to only two and get rid of four excessive fields, we'll simplify the user journey, boost satisfaction, and may get up to 15% more completed orders".
A hypothesis is something you can test in an attempt to reach a certain goal. Testing isn't obligatory in this scenario, of course, but the idea may be tested if you weigh the pros and cons and decide that the required effort is worth a try. We'll explain how to create hypothesis statements next.
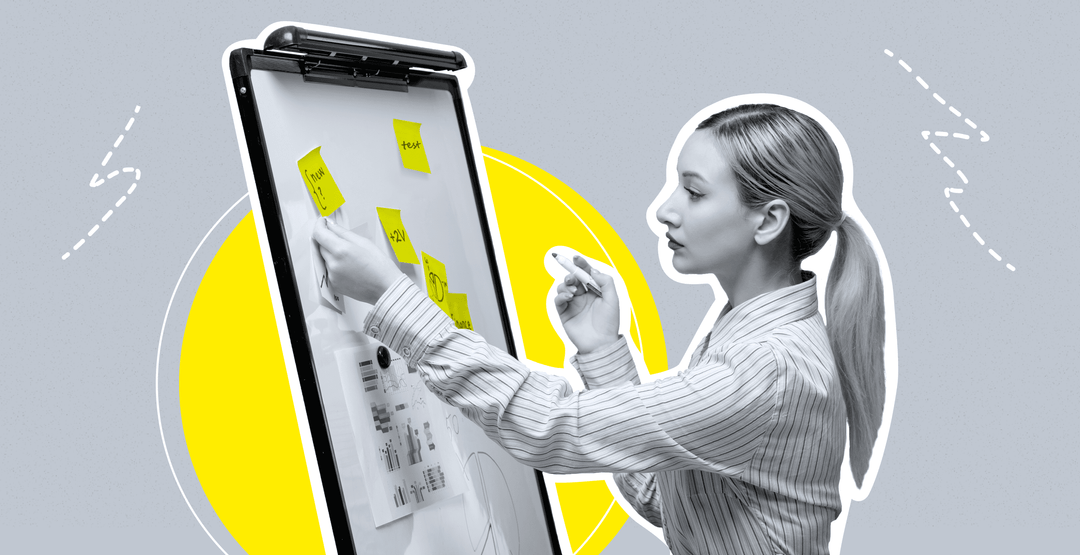
How to Generate a Hypothesis for a Product
The last thing those developing a product want is to invest time and effort into something that won't bring any visible results, fall short of customer expectations, or won't live up to their needs. Therefore, to increase the chances of achieving a successful outcome and product-led growth , teams may need to revisit their product development approach by optimizing one of the starting points of the process: learning to make reasonable product hypotheses.
If the entire procedure is structured, this may assist you during such stages as the discovery phase and raise the odds of reaching your product goals and setting your business up for success. Yet, what's the entire process like?
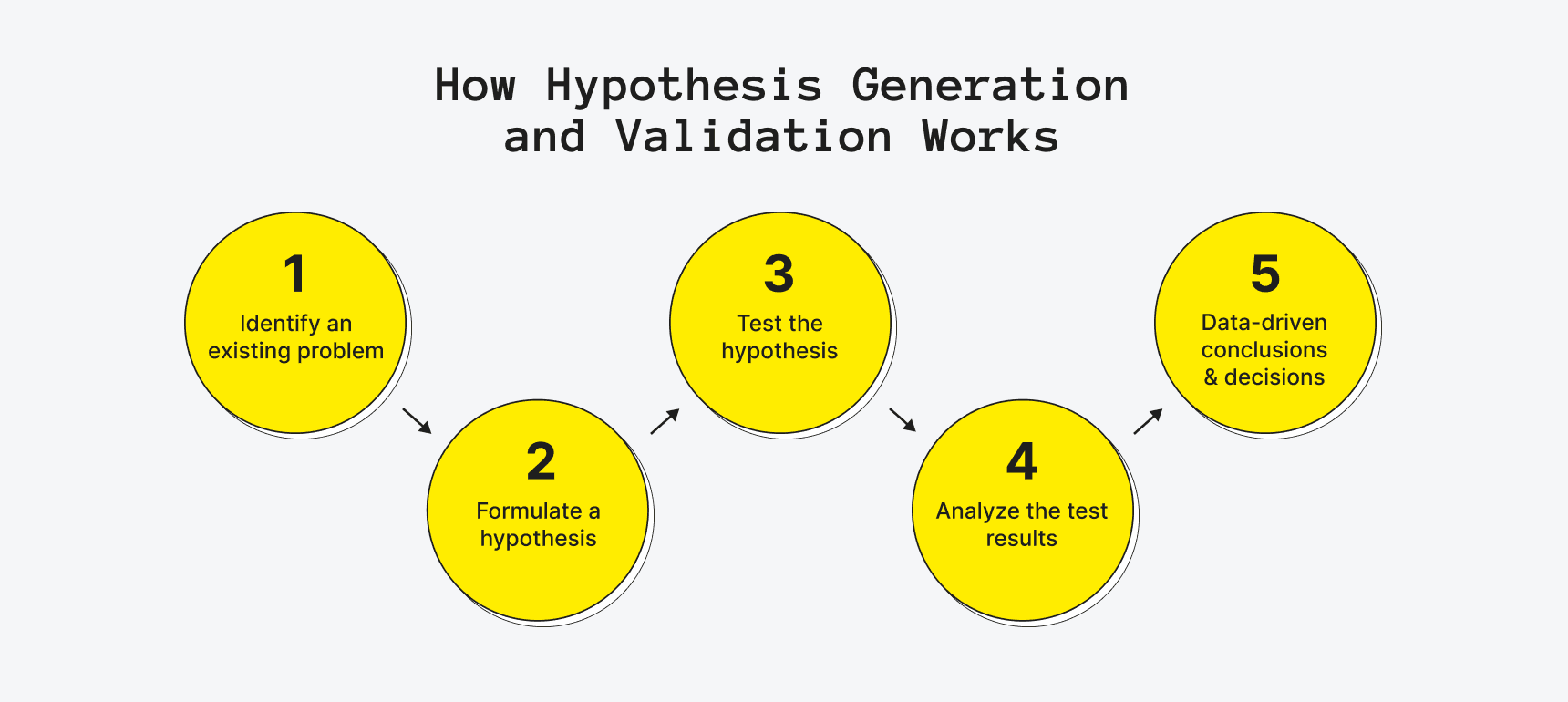
- It all starts with identifying an existing problem . Is there a product area that's experiencing a downfall, a visible trend, or a market gap? Are users often complaining about something in their feedback? Or is there something you're willing to change (say, if you aim to get more profit, increase engagement, optimize a process, expand to a new market, or reach your OKRs and KPIs faster)?
- Teams then need to work on formulating a hypothesis . They put the statement into concise and short wording that describes what is expected to achieve. Importantly, it has to be relevant, actionable, backed by data, and without generalizations.
- Next, they have to test the hypothesis by running experiments to validate it (for instance, via A/B or multivariate testing, prototyping, feedback collection, or other ways).
- Then, the obtained results of the test must be analyzed . Did one element or page version outperform the other? Depending on what you're testing, you can look into various merits or product performance metrics (such as the click rate, bounce rate, or the number of sign-ups) to assess whether your prediction was correct.
- Finally, the teams can make conclusions that could lead to data-driven decisions. For example, they can make corresponding changes or roll back a step.
How Else Can You Generate Product Hypotheses?
Such processes imply sharing ideas when a problem is spotted by digging deep into facts and studying the possible risks, goals, benefits, and outcomes. You may apply various MVP tools like (FigJam, Notion, or Miro) that were designed to simplify brainstorming sessions, systemize pitched suggestions, and keep everyone organized without losing any ideas.
Besides, you can settle on one of the many frameworks that facilitate decision-making processes , ideation phases, or feature prioritization . Such frameworks are best applicable if you need to test your assumptions and structure the validation process. These are a few common ones if you're looking toward a systematic approach:
- Business Model Canvas (used to establish the foundation of the business model and helps find answers to vitals like your value proposition, finding the right customer segment, or the ways to make revenue);
- Lean Startup framework (the lean startup framework uses a diagram-like format for capturing major processes and can be handy for testing various hypotheses like how much value a product brings or assumptions on personas, the problem, growth, etc.);
- Design Thinking Process (is all about interactive learning and involves getting an in-depth understanding of the customer needs and pain points, which can be formulated into hypotheses followed by simple prototypes and tests).
Need a hand with product development?
Upsilon's team of pros is ready to share our expertise in building tech products.
How to Make a Hypothesis Statement for a Product
Once you've indicated the addressable problem or opportunity and broken down the issue in focus, you need to work on formulating the hypotheses and associated tasks. By the way, it works the same way if you want to prove that something will be false (a.k.a null hypothesis).
If you're unsure how to write a hypothesis statement, let's explore the essential steps that'll set you on the right track.
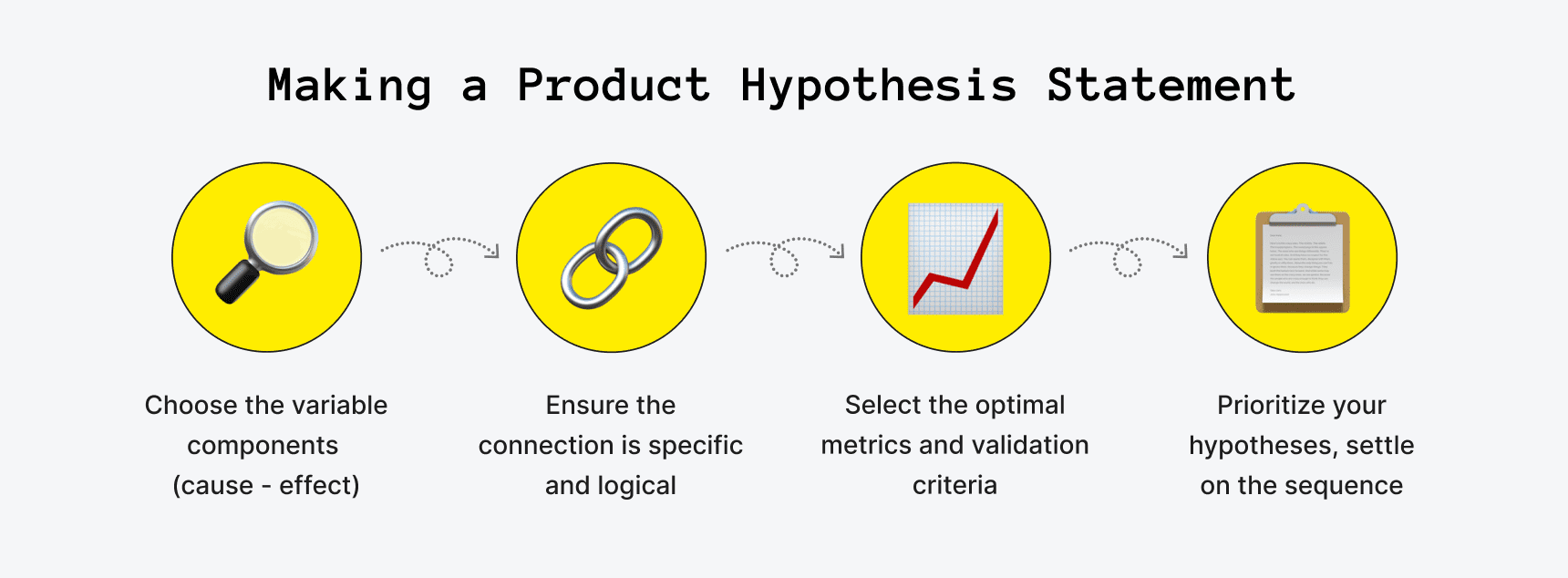
Step 1: Allocate the Variable Components
Product hypotheses are generally different for each case, so begin by pinpointing the major variables, i.e., the cause and effect . You'll need to outline what you think is supposed to happen if a change or action gets implemented.
Put simply, the "cause" is what you're planning to change, and the "effect" is what will indicate whether the change is bringing in the expected results. Falling back on the example we brought up earlier, the ineffective checkout process can be the cause, while the increased percentage of completed orders is the metric that'll show the effect.
Make sure to also note such vital points as:
- what the problem and solution are;
- what are the benefits or the expected impact/successful outcome;
- which user group is affected;
- what are the risks;
- what kind of experiments can help test the hypothesis;
- what can measure whether you were right or wrong.
Step 2: Ensure the Connection Is Specific and Logical
Mind that generic connections that lack specifics will get you nowhere. So if you're thinking about how to word a hypothesis statement, make sure that the cause and effect include clear reasons and a logical dependency .
Think about what can be the precise and link showing why A affects B. In our checkout example, it could be: fewer steps in the checkout and the removed excessive fields will speed up the process, help avoid confusion, irritate users less, and lead to more completed orders. That's much more explicit than just stating the fact that the checkout needs to be changed to get more completed orders.
Step 3: Decide on the Data You'll Collect
Certainly, multiple things can be used to measure the effect. Therefore, you need to choose the optimal metrics and validation criteria that'll best envision if you're moving in the right direction.
If you need a tip on how to create hypothesis statements that won't result in a waste of time, try to avoid vagueness and be as specific as you can when selecting what can best measure and assess the results of your hypothesis test. The criteria must be measurable and tied to the hypotheses . This can be a realistic percentage or number (say, you expect a 15% increase in completed orders or 2x fewer cart abandonment cases during the checkout phase).
Once again, if you're not realistic, then you might end up misinterpreting the results. Remember that sometimes an increase that's even as little as 2% can make a huge difference, so why make 50% the merit if it's not achievable in the first place?
Step 4: Settle on the Sequence
It's quite common that you'll end up with multiple product hypotheses. Some are more important than others, of course, and some will require more effort and input.
Therefore, just as with the features on your product development roadmap , prioritize your hypotheses according to their impact and importance. Then, group and order them, especially if the results of some hypotheses influence others on your list.
Product Hypothesis Examples
To demonstrate how to formulate your assumptions clearly, here are several more apart from the example of a hypothesis statement given above:
- Adding a wishlist feature to the cart with the possibility to send a gift hint to friends via email will increase the likelihood of making a sale and bring in additional sign-ups.
- Placing a limited-time promo code banner stripe on the home page will increase the number of sales in March.
- Moving up the call to action element on the landing page and changing the button text will increase the click-through rate twice.
- By highlighting a new way to use the product, we'll target a niche customer segment (i.e., single parents under 30) and acquire 5% more leads.
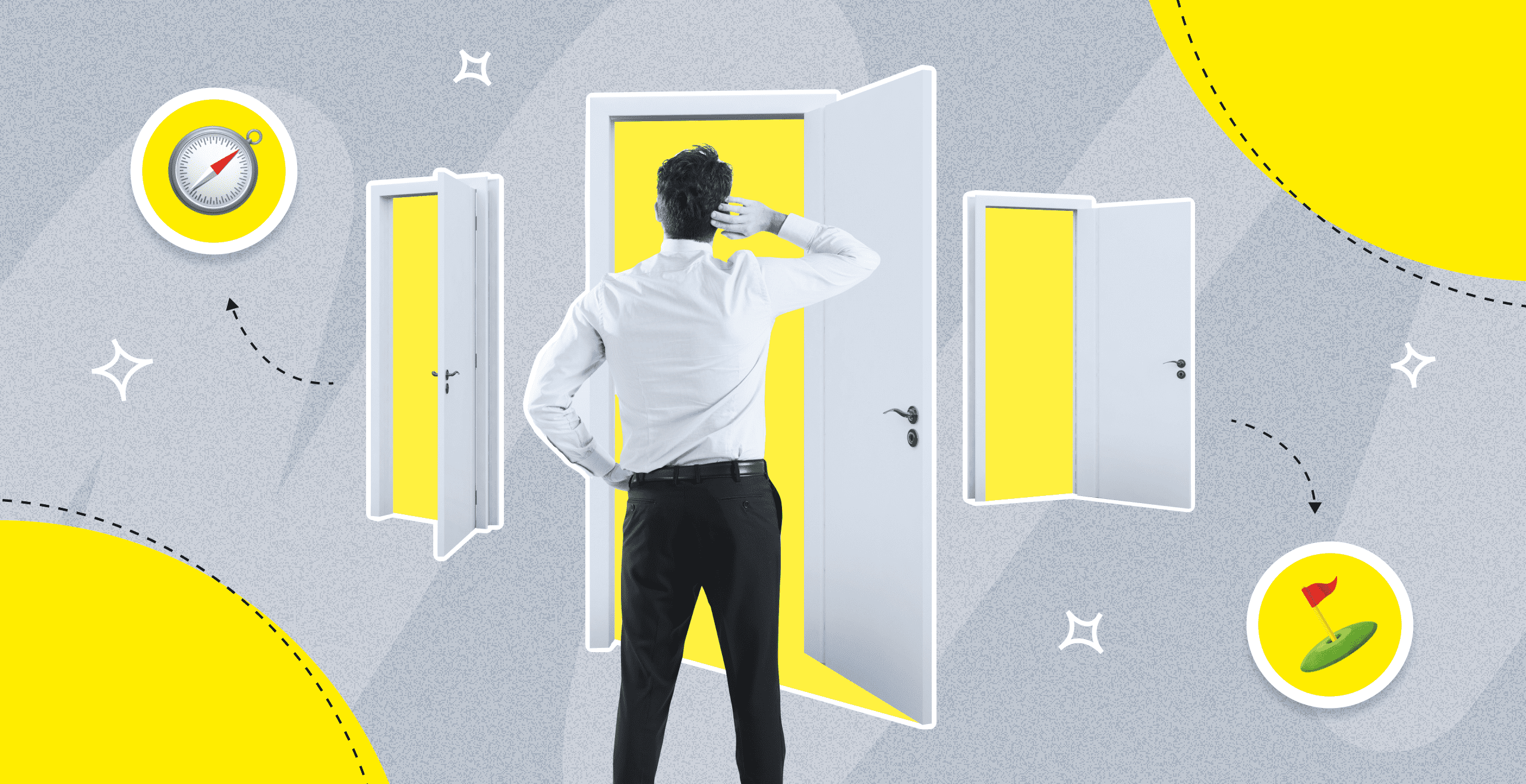
How to Validate Hypothesis Statements: The Process Explained
There are multiple options when it comes to validating hypothesis statements. To get appropriate results, you have to come up with the right experiment that'll help you test the hypothesis. You'll need a control group or people who represent your target audience segments or groups to participate (otherwise, your results might not be accurate).
What can serve as the experiment you may run? Experiments may take tons of different forms, and you'll need to choose the one that clicks best with your hypothesis goals (and your available resources, of course). The same goes for how long you'll have to carry out the test (say, a time period of two months or as little as two weeks). Here are several to get you started.
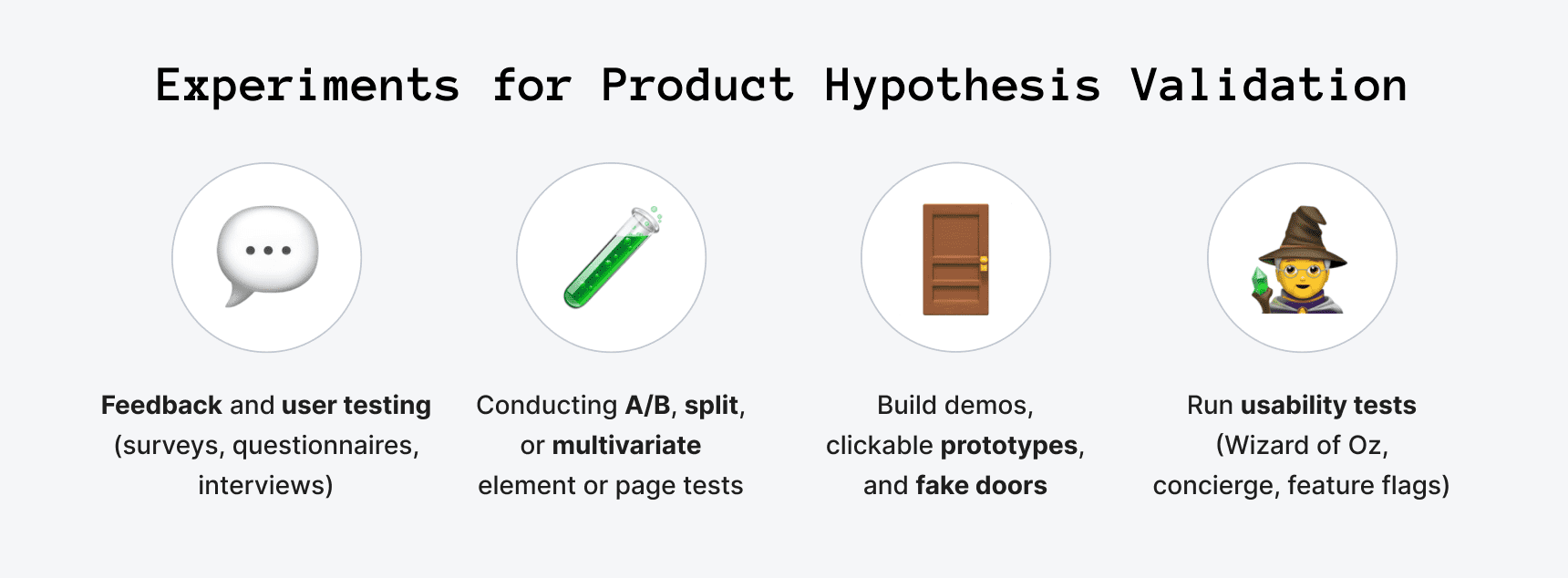
Feedback and User Testing
Talking to users, potential customers, or members of your own online startup community can be another way to test your hypotheses. You may use surveys, questionnaires, or opt for more extensive interviews to validate hypothesis statements and find out what people think. This assumption validation approach involves your existing or potential users and might require some additional time, but can bring you many insights.
Conduct A/B or Multivariate Tests
One of the experiments you may develop involves making more than one version of an element or page to see which option resonates with the users more. As such, you can have a call to action block with different wording or play around with the colors, imagery, visuals, and other things.
To run such split experiments, you can apply tools like VWO that allows to easily construct alternative designs and split what your users see (e.g., one half of the users will see version one, while the other half will see version two). You can track various metrics and apply heatmaps, click maps, and screen recordings to learn more about user response and behavior. Mind, though, that the key to such tests is to get as many users as you can give the tests time. Don't jump to conclusions too soon or if very few people participated in your experiment.
Build Prototypes and Fake Doors
Demos and clickable prototypes can be a great way to save time and money on costly feature or product development. A prototype also allows you to refine the design. However, they can also serve as experiments for validating hypotheses, collecting data, and getting feedback.
For instance, if you have a new feature in mind and want to ensure there is interest, you can utilize such MVP types as fake doors . Make a short demo recording of the feature and place it on your landing page to track interest or test how many people sign up.
Usability Testing
Similarly, you can run experiments to observe how users interact with the feature, page, product, etc. Usually, such experiments are held on prototype testing platforms with a focus group representing your target visitors. By showing a prototype or early version of the design to users, you can view how people use the solution, where they face problems, or what they don't understand. This may be very helpful if you have hypotheses regarding redesigns and user experience improvements before you move on from prototype to MVP development.
You can even take it a few steps further and build a barebone feature version that people can really interact with, yet you'll be the one behind the curtain to make it happen. There were many MVP examples when companies applied Wizard of Oz or concierge MVPs to validate their hypotheses.
Or you can actually develop some functionality but release it for only a limited number of people to see. This is referred to as a feature flag , which can show really specific results but is effort-intensive.
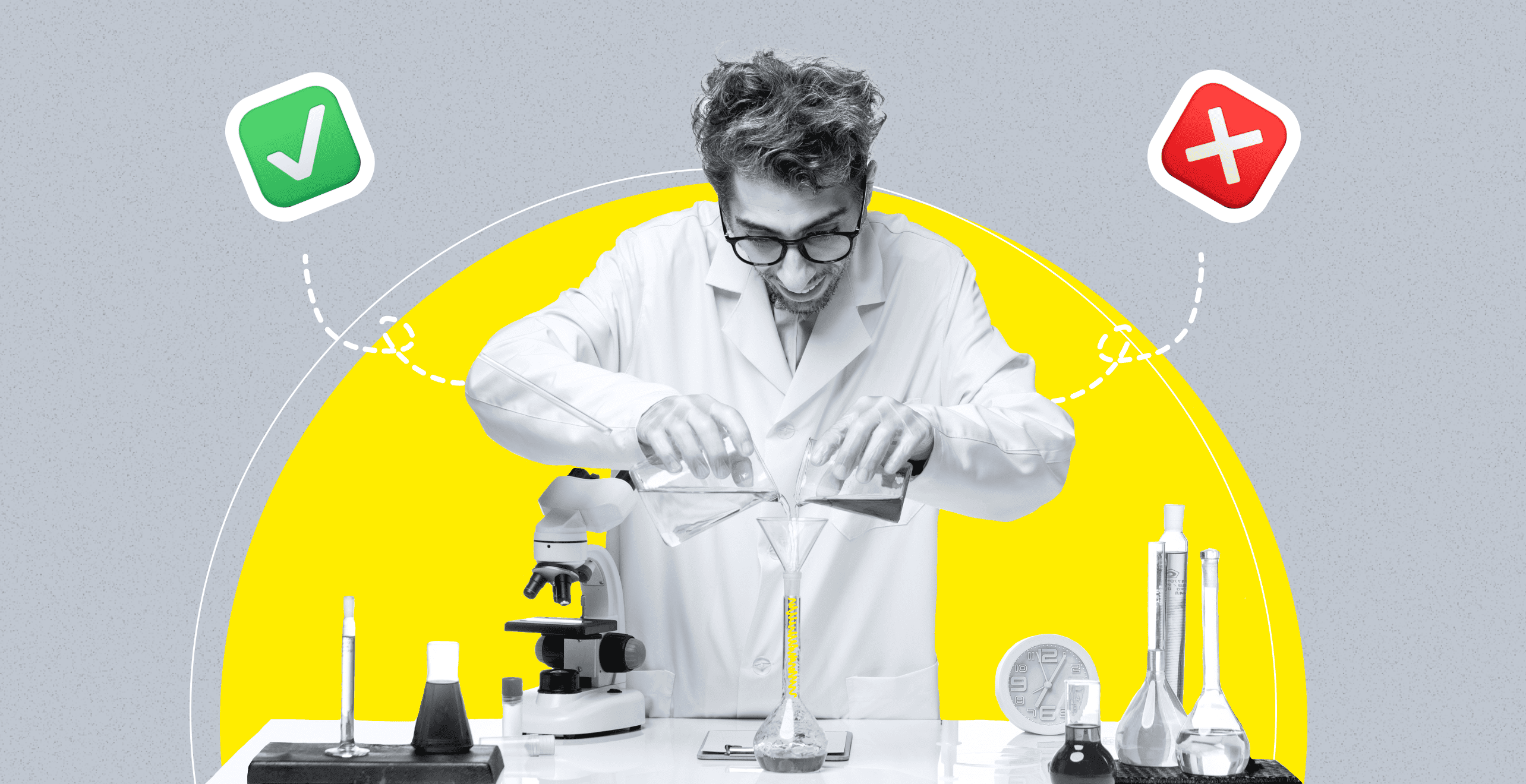
What Comes After Hypothesis Validation?
Analysis is what you move on to once you've run the experiment. This is the time to review the collected data, metrics, and feedback to validate (or invalidate) the hypothesis.
You have to evaluate the experiment's results to determine whether your product hypotheses were valid or not. For example, if you were testing two versions of an element design, color scheme, or copy, look into which one performed best.
It is crucial to be certain that you have enough data to draw conclusions, though, and that it's accurate and unbiased . Because if you don't, this may be a sign that your experiment needs to be run for some additional time, be altered, or held once again. You won't want to make a solid decision based on uncertain or misleading results, right?
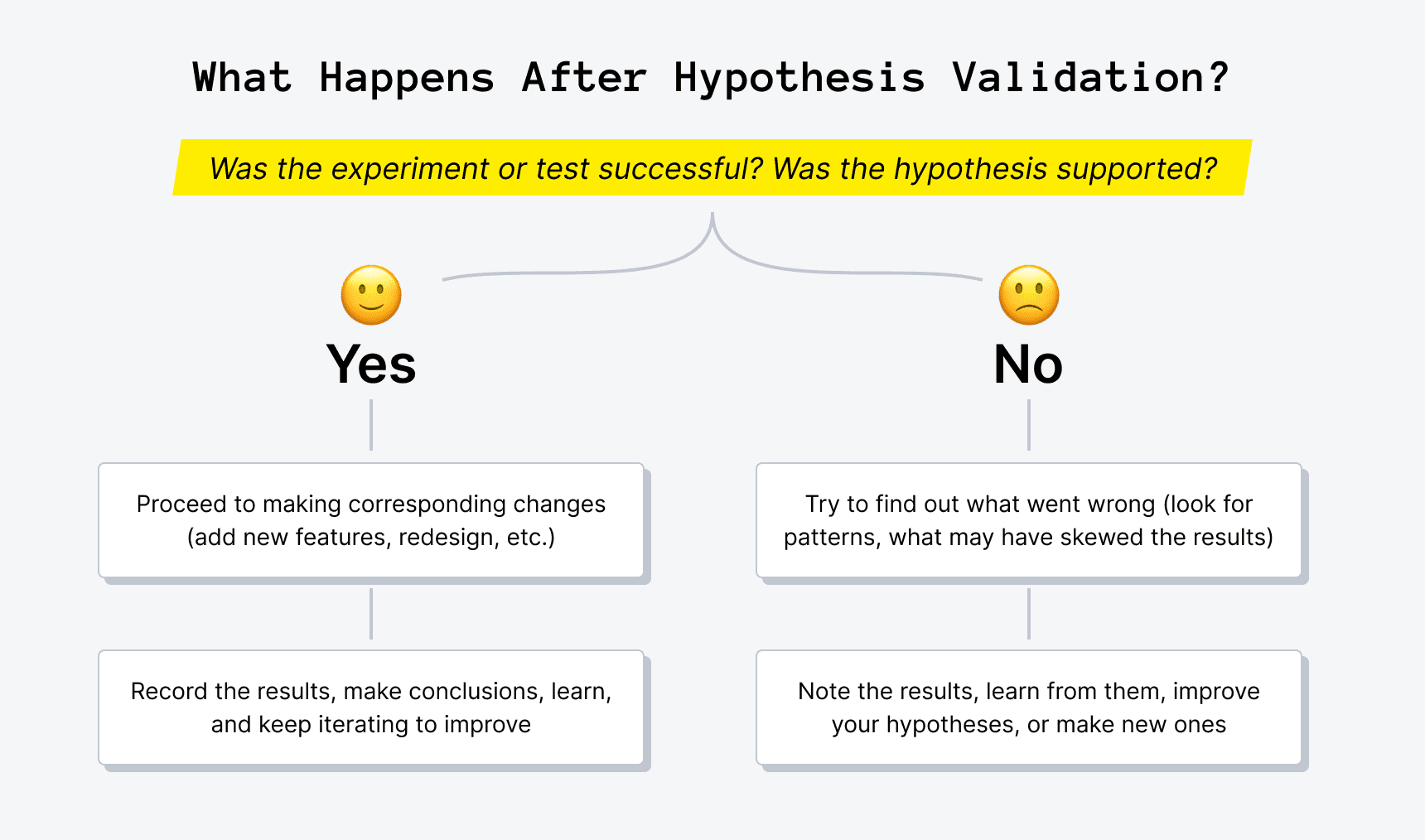
- If the hypothesis was supported , proceed to making corresponding changes (such as implementing a new feature, changing the design, rephrasing your copy, etc.). Remember that your aim was to learn and iterate to improve.
- If your hypothesis was proven false , think of it as a valuable learning experience. The main goal is to learn from the results and be able to adjust your processes accordingly. Dig deep to find out what went wrong, look for patterns and things that may have skewed the results. But if all signs show that you were wrong with your hypothesis, accept this outcome as a fact, and move on. This can help you make conclusions on how to better formulate your product hypotheses next time. Don't be too judgemental, though, as a failed experiment might only mean that you need to improve the current hypothesis, revise it, or create a new one based on the results of this experiment, and run the process once more.
On another note, make sure to record your hypotheses and experiment results . Some companies use CRMs to jot down the key findings, while others use something as simple as Google Docs. Either way, this can be your single source of truth that can help you avoid running the same experiments or allow you to compare results over time.
Have doubts about how to bring your product to life?
Upsilon's team of pros can help you build a product most optimally.
Final Thoughts on Product Hypotheses
The hypothesis-driven approach in product development is a great way to avoid uncalled-for risks and pricey mistakes. You can back up your assumptions with facts, observe your target audience's reactions, and be more certain that this move will deliver value.
However, this only makes sense if the validation of hypothesis statements is backed by relevant data that'll allow you to determine whether the hypothesis is valid or not. By doing so, you can be certain that you're developing and testing hypotheses to accelerate your product management and avoiding decisions based on guesswork.
Certainly, a failed experiment may bring you just as much knowledge and findings as one that succeeds. Teams have to learn from their mistakes, boost their hypothesis generation and testing knowledge, and make improvements according to the results of their experiments. This is an ongoing process, of course, as no product can grow if it isn't iterated and improved.
If you're only planning to or are currently building a product, Upsilon can lend you a helping hand. Our team has years of experience providing product development services for growth-stage startups and building MVPs for early-stage businesses , so you can use our expertise and knowledge to dodge many mistakes. Don't be shy to contact us to discuss your needs!
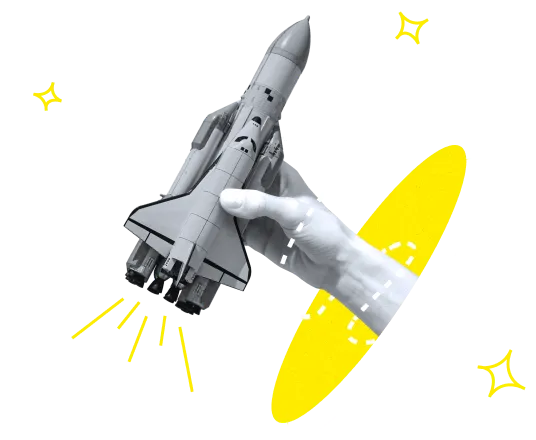
How to Prototype a Product: Steps, Tips, and Best Practices
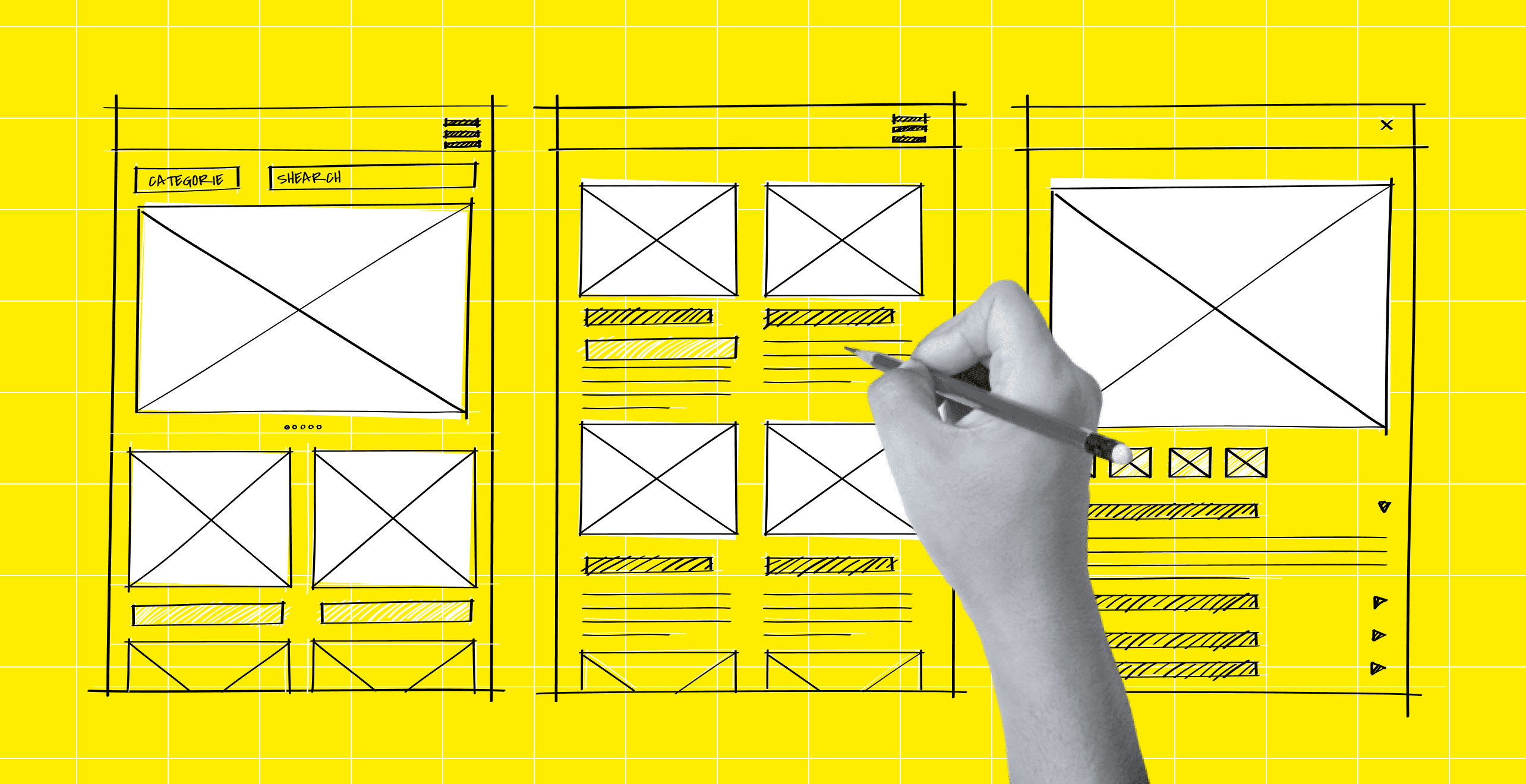
What Is Wireframing and Its Role in Product Development
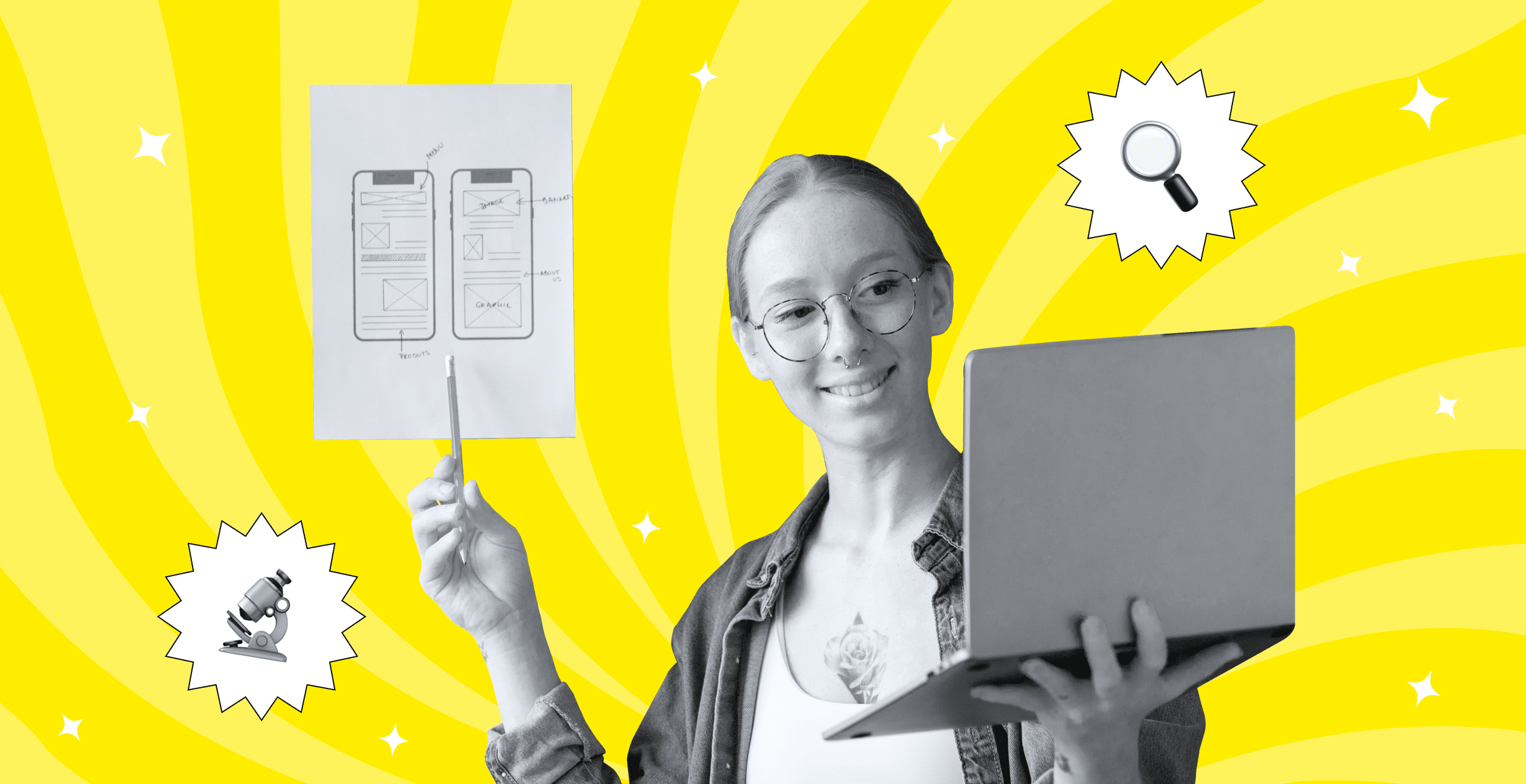
UX Discovery: Deliverables, Process, and Importance
Never miss an update.

Shipping Your Product in Iterations: A Guide to Hypothesis Testing
Glancing at the App Store on any phone will reveal that most installed apps have had updates released within the last week. Software products today are shipped in iterations to validate assumptions and hypotheses about what makes the product experience better for users.
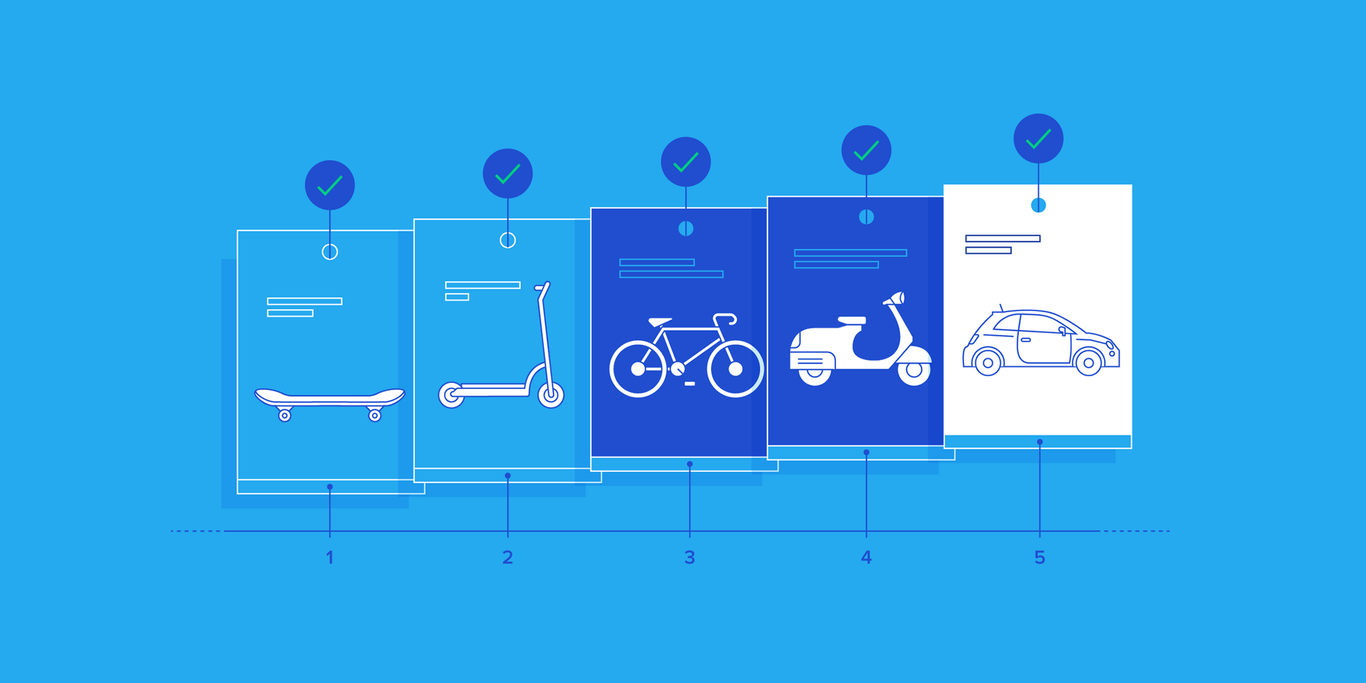
By Kumara Raghavendra
Kumara has successfully delivered high-impact products in various industries ranging from eCommerce, healthcare, travel, and ride-hailing.
PREVIOUSLY AT
A look at the App Store on any phone will reveal that most installed apps have had updates released within the last week. A website visit after a few weeks might show some changes in the layout, user experience, or copy.
Today, software is shipped in iterations to validate assumptions and the product hypothesis about what makes a better user experience. At any given time, companies like booking.com (where I worked before) run hundreds of A/B tests on their sites for this very purpose.
For applications delivered over the internet, there is no need to decide on the look of a product 12-18 months in advance, and then build and eventually ship it. Instead, it is perfectly practical to release small changes that deliver value to users as they are being implemented, removing the need to make assumptions about user preferences and ideal solutions—for every assumption and hypothesis can be validated by designing a test to isolate the effect of each change.
In addition to delivering continuous value through improvements, this approach allows a product team to gather continuous feedback from users and then course-correct as needed. Creating and testing hypotheses every couple of weeks is a cheaper and easier way to build a course-correcting and iterative approach to creating product value .
What Is Hypothesis Testing in Product Management?
While shipping a feature to users, it is imperative to validate assumptions about design and features in order to understand their impact in the real world.
This validation is traditionally done through product hypothesis testing , during which the experimenter outlines a hypothesis for a change and then defines success. For instance, if a data product manager at Amazon has a hypothesis that showing bigger product images will raise conversion rates, then success is defined by higher conversion rates.
One of the key aspects of hypothesis testing is the isolation of different variables in the product experience in order to be able to attribute success (or failure) to the changes made. So, if our Amazon product manager had a further hypothesis that showing customer reviews right next to product images would improve conversion, it would not be possible to test both hypotheses at the same time. Doing so would result in failure to properly attribute causes and effects; therefore, the two changes must be isolated and tested individually.
Thus, product decisions on features should be backed by hypothesis testing to validate the performance of features.
Different Types of Hypothesis Testing
A/b testing.
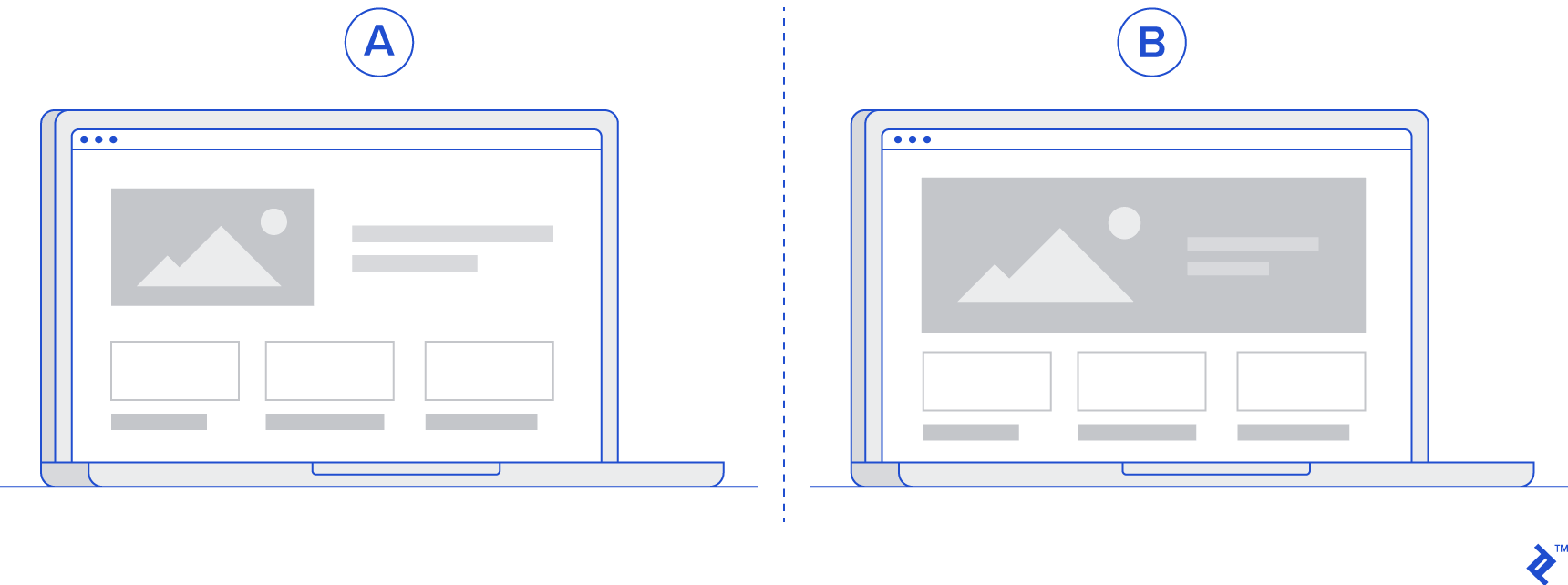
One of the most common use cases to achieve hypothesis validation is randomized A/B testing, in which a change or feature is released at random to one-half of users (A) and withheld from the other half (B). Returning to the hypothesis of bigger product images improving conversion on Amazon, one-half of users will be shown the change, while the other half will see the website as it was before. The conversion will then be measured for each group (A and B) and compared. In case of a significant uplift in conversion for the group shown bigger product images, the conclusion would be that the original hypothesis was correct, and the change can be rolled out to all users.
Multivariate Testing
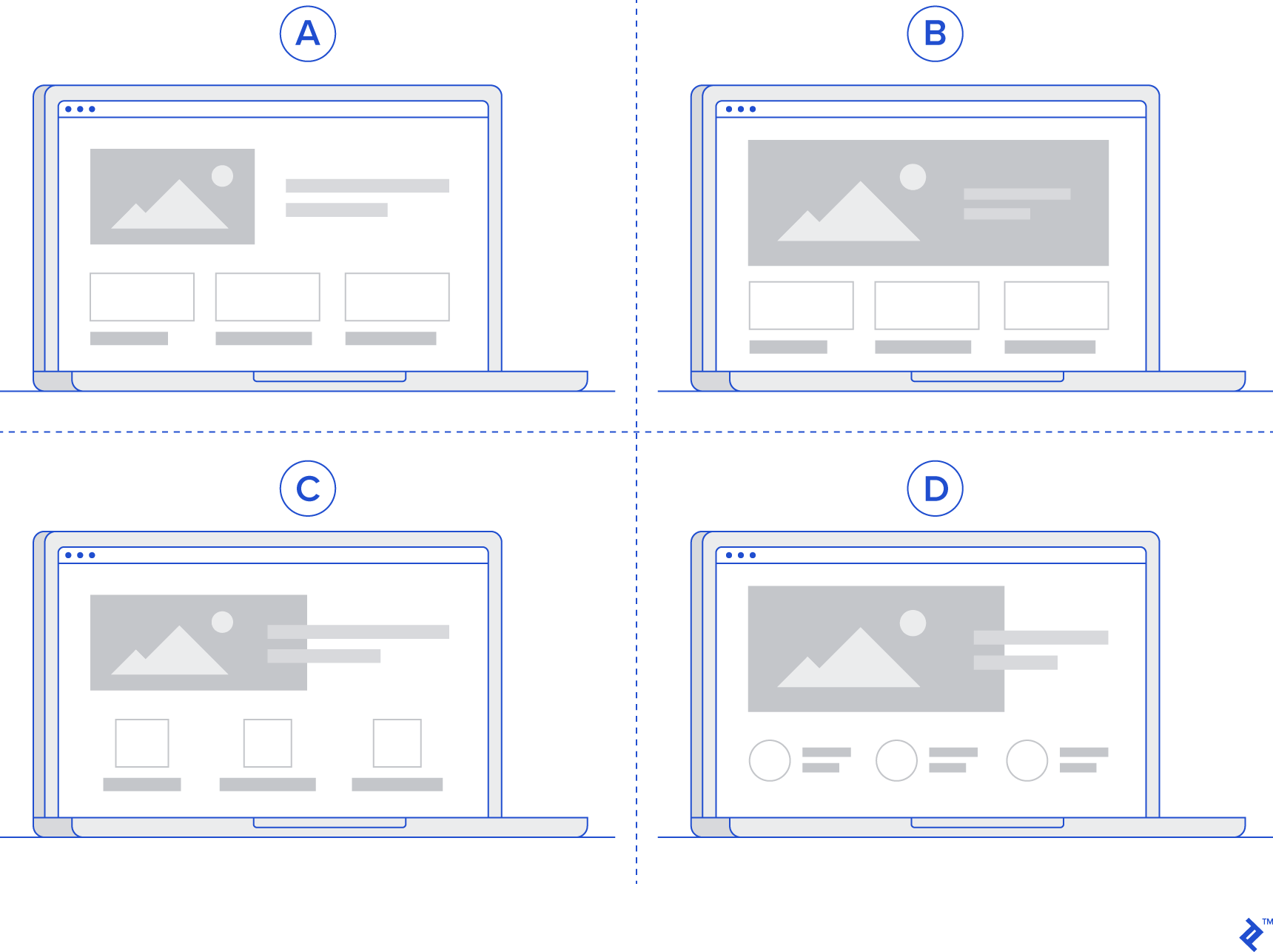
Ideally, each variable should be isolated and tested separately so as to conclusively attribute changes. However, such a sequential approach to testing can be very slow, especially when there are several versions to test. To continue with the example, in the hypothesis that bigger product images lead to higher conversion rates on Amazon, “bigger” is subjective, and several versions of “bigger” (e.g., 1.1x, 1.3x, and 1.5x) might need to be tested.
Instead of testing such cases sequentially, a multivariate test can be adopted, in which users are not split in half but into multiple variants. For instance, four groups (A, B, C, D) are made up of 25% of users each, where A-group users will not see any change, whereas those in variants B, C, and D will see images bigger by 1.1x, 1.3x, and 1.5x, respectively. In this test, multiple variants are simultaneously tested against the current version of the product in order to identify the best variant.
Before/After Testing
Sometimes, it is not possible to split the users in half (or into multiple variants) as there might be network effects in place. For example, if the test involves determining whether one logic for formulating surge prices on Uber is better than another, the drivers cannot be divided into different variants, as the logic takes into account the demand and supply mismatch of the entire city. In such cases, a test will have to compare the effects before the change and after the change in order to arrive at a conclusion.

However, the constraint here is the inability to isolate the effects of seasonality and externality that can differently affect the test and control periods. Suppose a change to the logic that determines surge pricing on Uber is made at time t , such that logic A is used before and logic B is used after. While the effects before and after time t can be compared, there is no guarantee that the effects are solely due to the change in logic. There could have been a difference in demand or other factors between the two time periods that resulted in a difference between the two.
Time-based On/Off Testing

The downsides of before/after testing can be overcome to a large extent by deploying time-based on/off testing, in which the change is introduced to all users for a certain period of time, turned off for an equal period of time, and then repeated for a longer duration.
For example, in the Uber use case, the change can be shown to drivers on Monday, withdrawn on Tuesday, shown again on Wednesday, and so on.
While this method doesn’t fully remove the effects of seasonality and externality, it does reduce them significantly, making such tests more robust.
Test Design
Choosing the right test for the use case at hand is an essential step in validating a hypothesis in the quickest and most robust way. Once the choice is made, the details of the test design can be outlined.
The test design is simply a coherent outline of:
- The hypothesis to be tested: Showing users bigger product images will lead them to purchase more products.
- Success metrics for the test: Customer conversion
- Decision-making criteria for the test: The test validates the hypothesis that users in the variant show a higher conversion rate than those in the control group.
- Metrics that need to be instrumented to learn from the test: Customer conversion, clicks on product images
In the case of the product hypothesis example that bigger product images will lead to improved conversion on Amazon, the success metric is conversion and the decision criteria is an improvement in conversion.
After the right test is chosen and designed, and the success criteria and metrics are identified, the results must be analyzed. To do that, some statistical concepts are necessary.
When running tests, it is important to ensure that the two variants picked for the test (A and B) do not have a bias with respect to the success metric. For instance, if the variant that sees the bigger images already has a higher conversion than the variant that doesn’t see the change, then the test is biased and can lead to wrong conclusions.
In order to ensure no bias in sampling, one can observe the mean and variance for the success metric before the change is introduced.
Significance and Power
Once a difference between the two variants is observed, it is important to conclude that the change observed is an actual effect and not a random one. This can be done by computing the significance of the change in the success metric.
In layman’s terms, significance measures the frequency with which the test shows that bigger images lead to higher conversion when they actually don’t. Power measures the frequency with which the test tells us that bigger images lead to higher conversion when they actually do.
So, tests need to have a high value of power and a low value of significance for more accurate results.
While an in-depth exploration of the statistical concepts involved in product management hypothesis testing is out of scope here, the following actions are recommended to enhance knowledge on this front:
- Data analysts and data engineers are usually adept at identifying the right test designs and can guide product managers, so make sure to utilize their expertise early in the process.
- There are numerous online courses on hypothesis testing, A/B testing, and related statistical concepts, such as Udemy , Udacity , and Coursera .
- Using tools such as Google’s Firebase and Optimizely can make the process easier thanks to a large amount of out-of-the-box capabilities for running the right tests.
Using Hypothesis Testing for Successful Product Management
In order to continuously deliver value to users, it is imperative to test various hypotheses, for the purpose of which several types of product hypothesis testing can be employed. Each hypothesis needs to have an accompanying test design, as described above, in order to conclusively validate or invalidate it.
This approach helps to quantify the value delivered by new changes and features, bring focus to the most valuable features, and deliver incremental iterations.
- How to Conduct Remote User Interviews [Infographic]
- A/B Testing UX for Component-based Frameworks
- Building an AI Product? Maximize Value With an Implementation Framework

Further Reading on the Toptal Blog:
- Evolving UX: Experimental Product Design with a CXO
- How to Conduct Usability Testing in Six Steps
- 3 Product-led Growth Frameworks to Build Your Business
- A Product Designer’s Guide to Competitive Analysis
Understanding the basics
What is a product hypothesis.
A product hypothesis is an assumption that some improvement in the product will bring an increase in important metrics like revenue or product usage statistics.
What are the three required parts of a hypothesis?
The three required parts of a hypothesis are the assumption, the condition, and the prediction.
Why do we do A/B testing?
We do A/B testing to make sure that any improvement in the product increases our tracked metrics.
What is A/B testing used for?
A/B testing is used to check if our product improvements create the desired change in metrics.
What is A/B testing and multivariate testing?
A/B testing and multivariate testing are types of hypothesis testing. A/B testing checks how important metrics change with and without a single change in the product. Multivariate testing can track multiple variations of the same product improvement.
Kumara Raghavendra
Dubai, United Arab Emirates
Member since August 6, 2019
About the author
World-class articles, delivered weekly.
By entering your email, you are agreeing to our privacy policy .
Toptal Product Managers
- Artificial Intelligence Product Managers
- Blockchain Product Managers
- Business Systems Analysts
- Cloud Product Managers
- Data Science Product Managers
- Digital Marketing Product Managers
- Digital Product Managers
- Directors of Product
- eCommerce Product Managers
- Enterprise Product Managers
- Enterprise Resource Planning Product Managers
- Freelance Product Managers
- Interim CPOs
- Jira Product Managers
- Kanban Product Managers
- Lean Product Managers
- Mobile Product Managers
- Product Consultants
- Product Development Managers
- Product Owners
- Product Portfolio Managers
- Product Strategy Consultants
- Product Tour Consultants
- Robotic Process Automation Product Managers
- Robotics Product Managers
- SaaS Product Managers
- Salesforce Product Managers
- Scrum Product Owner Contractors
- Web Product Managers
- View More Freelance Product Managers
Join the Toptal ® community.
- Product Management
How to Generate and Validate Product Hypotheses
What is a product hypothesis.
A hypothesis is a testable statement that predicts the relationship between two or more variables. In product development, we generate hypotheses to validate assumptions about customer behavior, market needs, or the potential impact of product changes. These experimental efforts help us refine the user experience and get closer to finding a product-market fit.
Product hypotheses are a key element of data-driven product development and decision-making. Testing them enables us to solve problems more efficiently and remove our own biases from the solutions we put forward.
Here’s an example: ‘If we improve the page load speed on our website (variable 1), then we will increase the number of signups by 15% (variable 2).’ So if we improve the page load speed, and the number of signups increases, then our hypothesis has been proven. If the number did not increase significantly (or not at all), then our hypothesis has been disproven.
In general, product managers are constantly creating and testing hypotheses. But in the context of new product development , hypothesis generation/testing occurs during the validation stage, right after idea screening .
Now before we go any further, let’s get one thing straight: What’s the difference between an idea and a hypothesis?
Idea vs hypothesis
Innovation expert Michael Schrage makes this distinction between hypotheses and ideas – unlike an idea, a hypothesis comes with built-in accountability. “But what’s the accountability for a good idea?” Schrage asks. “The fact that a lot of people think it’s a good idea? That’s a popularity contest.” So, not only should a hypothesis be tested, but by its very nature, it can be tested.
At Railsware, we’ve built our product development services on the careful selection, prioritization, and validation of ideas. Here’s how we distinguish between ideas and hypotheses:
Idea: A creative suggestion about how we might exploit a gap in the market, add value to an existing product, or bring attention to our product. Crucially, an idea is just a thought. It can form the basis of a hypothesis but it is not necessarily expected to be proven or disproven.
- We should get an interview with the CEO of our company published on TechCrunch.
- Why don’t we redesign our website?
- The Coupler.io team should create video tutorials on how to export data from different apps, and publish them on YouTube.
- Why not add a new ‘email templates’ feature to our Mailtrap product?
Hypothesis: A way of framing an idea or assumption so that it is testable, specific, and aligns with our wider product/team/organizational goals.
Examples:
- If we add a new ‘email templates’ feature to Mailtrap, we’ll see an increase in active usage of our email-sending API.
- Creating relevant video tutorials and uploading them to YouTube will lead to an increase in Coupler.io signups.
- If we publish an interview with our CEO on TechCrunch, 500 people will visit our website and 10 of them will install our product.
Now, it’s worth mentioning that not all hypotheses require testing . Sometimes, the process of creating hypotheses is just an exercise in critical thinking. And the simple act of analyzing your statement tells whether you should run an experiment or not. Remember: testing isn’t mandatory, but your hypotheses should always be inherently testable.
Let’s consider the TechCrunch article example again. In that hypothesis, we expect 500 readers to visit our product website, and a 2% conversion rate of those unique visitors to product users i.e. 10 people. But is that marginal increase worth all the effort? Conducting an interview with our CEO, creating the content, and collaborating with the TechCrunch content team – all of these tasks take time (and money) to execute. And by formulating that hypothesis, we can clearly see that in this case, the drawbacks (efforts) outweigh the benefits. So, no need to test it.
In a similar vein, a hypothesis statement can be a tool to prioritize your activities based on impact. We typically use the following criteria:
- The quality of impact
- The size of the impact
- The probability of impact
This lets us organize our efforts according to their potential outcomes – not the coolness of the idea, its popularity among the team, etc.
Now that we’ve established what a product hypothesis is, let’s discuss how to create one.
Start with a problem statement
Before you jump into product hypothesis generation, we highly recommend formulating a problem statement. This is a short, concise description of the issue you are trying to solve. It helps teams stay on track as they formalize the hypothesis and design the product experiments. It can also be shared with stakeholders to ensure that everyone is on the same page.
The statement can be worded however you like, as long as it’s actionable, specific, and based on data-driven insights or research. It should clearly outline the problem or opportunity you want to address.
Here’s an example: Our bounce rate is high (more than 90%) and we are struggling to convert website visitors into actual users. How might we improve site performance to boost our conversion rate?
How to generate product hypotheses
Now let’s explore some common, everyday scenarios that lead to product hypothesis generation. For our teams here at Railsware, it’s when:
- There’s a problem with an unclear root cause e.g. a sudden drop in one part of the onboarding funnel. We identify these issues by checking our product metrics or reviewing customer complaints.
- We are running ideation sessions on how to reach our goals (increase MRR, increase the number of users invited to an account, etc.)
- We are exploring growth opportunities e.g. changing a pricing plan, making product improvements , breaking into a new market.
- We receive customer feedback. For example, some users have complained about difficulties setting up a workspace within the product. So, we build a hypothesis on how to help them with the setup.
BRIDGES framework for ideation
When we are tackling a complex problem or looking for ways to grow the product, our teams use BRIDGeS – a robust decision-making and ideation framework. BRIDGeS makes our product discovery sessions more efficient. It lets us dive deep into the context of our problem so that we can develop targeted solutions worthy of testing.
Between 2-8 stakeholders take part in a BRIDGeS session. The ideation sessions are usually led by a product manager and can include other subject matter experts such as developers, designers, data analysts, or marketing specialists. You can use a virtual whiteboard such as Figjam or Miro (see our Figma template ) to record each colored note.
In the first half of a BRIDGeS session, participants examine the Benefits, Risks, Issues, and Goals of their subject in the ‘Problem Space.’ A subject is anything that is being described or dealt with; for instance, Coupler.io’s growth opportunities. Benefits are the value that a future solution can bring, Risks are potential issues they might face, Issues are their existing problems, and Goals are what the subject hopes to gain from the future solution. Each descriptor should have a designated color.
After we have broken down the problem using each of these descriptors, we move into the Solution Space. This is where we develop solution variations based on all of the benefits/risks/issues identified in the Problem Space (see the Uber case study for an in-depth example).
In the Solution Space, we start prioritizing those solutions and deciding which ones are worthy of further exploration outside of the framework – via product hypothesis formulation and testing, for example. At the very least, after the session, we will have a list of epics and nested tasks ready to add to our product roadmap.
How to write a product hypothesis statement
Across organizations, product hypothesis statements might vary in their subject, tone, and precise wording. But some elements never change. As we mentioned earlier, a hypothesis statement must always have two or more variables and a connecting factor.
1. Identify variables
Since these components form the bulk of a hypothesis statement, let’s start with a brief definition.
First of all, variables in a hypothesis statement can be split into two camps: dependent and independent. Without getting too theoretical, we can describe the independent variable as the cause, and the dependent variable as the effect . So in the Mailtrap example we mentioned earlier, the ‘add email templates feature’ is the cause i.e. the element we want to manipulate. Meanwhile, ‘increased usage of email sending API’ is the effect i.e the element we will observe.
Independent variables can be any change you plan to make to your product. For example, tweaking some landing page copy, adding a chatbot to the homepage, or enhancing the search bar filter functionality.
Dependent variables are usually metrics. Here are a few that we often test in product development:
- Number of sign-ups
- Number of purchases
- Activation rate (activation signals differ from product to product)
- Number of specific plans purchased
- Feature usage (API activation, for example)
- Number of active users
Bear in mind that your concept or desired change can be measured with different metrics. Make sure that your variables are well-defined, and be deliberate in how you measure your concepts so that there’s no room for misinterpretation or ambiguity.
For example, in the hypothesis ‘Users drop off because they find it hard to set up a project’ variables are poorly defined. Phrases like ‘drop off’ and ‘hard to set up’ are too vague. A much better way of saying it would be: If project automation rules are pre-defined (email sequence to responsible, scheduled tickets creation), we’ll see a decrease in churn. In this example, it’s clear which dependent variable has been chosen and why.
And remember, when product managers focus on delighting users and building something of value, it’s easier to market and monetize it. That’s why at Railsware, our product hypotheses often focus on how to increase the usage of a feature or product. If users love our product(s) and know how to leverage its benefits, we can spend less time worrying about how to improve conversion rates or actively grow our revenue, and more time enhancing the user experience and nurturing our audience.
2. Make the connection
The relationship between variables should be clear and logical. If it’s not, then it doesn’t matter how well-chosen your variables are – your test results won’t be reliable.
To demonstrate this point, let’s explore a previous example again: page load speed and signups.
Through prior research, you might already know that conversion rates are 3x higher for sites that load in 1 second compared to sites that take 5 seconds to load. Since there appears to be a strong connection between load speed and signups in general, you might want to see if this is also true for your product.
Here are some common pitfalls to avoid when defining the relationship between two or more variables:
Relationship is weak. Let’s say you hypothesize that an increase in website traffic will lead to an increase in sign-ups. This is a weak connection since website visitors aren’t necessarily motivated to use your product; there are more steps involved. A better example is ‘If we change the CTA on the pricing page, then the number of signups will increase.’ This connection is much stronger and more direct.
Relationship is far-fetched. This often happens when one of the variables is founded on a vanity metric. For example, increasing the number of social media subscribers will lead to an increase in sign-ups. However, there’s no particular reason why a social media follower would be interested in using your product. Oftentimes, it’s simply your social media content that appeals to them (and your audience isn’t interested in a product).
Variables are co-dependent. Variables should always be isolated from one another. Let’s say we removed the option “Register with Google” from our app. In this case, we can expect fewer users with Google workspace accounts to register. Obviously, it’s because there’s a direct dependency between variables (no registration with Google→no users with Google workspace accounts).
3. Set validation criteria
First, build some confirmation criteria into your statement . Think in terms of percentages (e.g. increase/decrease by 5%) and choose a relevant product metric to track e.g. activation rate if your hypothesis relates to onboarding. Consider that you don’t always have to hit the bullseye for your hypothesis to be considered valid. Perhaps a 3% increase is just as acceptable as a 5% one. And it still proves that a connection between your variables exists.
Secondly, you should also make sure that your hypothesis statement is realistic . Let’s say you have a hypothesis that ‘If we show users a banner with our new feature, then feature usage will increase by 10%.’ A few questions to ask yourself are: Is 10% a reasonable increase, based on your current feature usage data? Do you have the resources to create the tests (experimenting with multiple variations, distributing on different channels: in-app, emails, blog posts)?
Null hypothesis and alternative hypothesis
In statistical research, there are two ways of stating a hypothesis: null or alternative. But this scientific method has its place in hypothesis-driven development too…
Alternative hypothesis: A statement that you intend to prove as being true by running an experiment and analyzing the results. Hint: it’s the same as the other hypothesis examples we’ve described so far.
Example: If we change the landing page copy, then the number of signups will increase.
Null hypothesis: A statement you want to disprove by running an experiment and analyzing the results. It predicts that your new feature or change to the user experience will not have the desired effect.
Example: The number of signups will not increase if we make a change to the landing page copy.
What’s the point? Well, let’s consider the phrase ‘innocent until proven guilty’ as a version of a null hypothesis. We don’t assume that there is any relationship between the ‘defendant’ and the ‘crime’ until we have proof. So, we run a test, gather data, and analyze our findings — which gives us enough proof to reject the null hypothesis and validate the alternative. All of this helps us to have more confidence in our results.
Now that you have generated your hypotheses, and created statements, it’s time to prepare your list for testing.
Prioritizing hypotheses for testing
Not all hypotheses are created equal. Some will be essential to your immediate goal of growing the product e.g. adding a new data destination for Coupler.io. Others will be based on nice-to-haves or small fixes e.g. updating graphics on the website homepage.
Prioritization helps us focus on the most impactful solutions as we are building a product roadmap or narrowing down the backlog . To determine which hypotheses are the most critical, we use the MoSCoW framework. It allows us to assign a level of urgency and importance to each product hypothesis so we can filter the best 3-5 for testing.
MoSCoW is an acronym for Must-have, Should-have, Could-have, and Won’t-have. Here’s a breakdown:
- Must-have – hypotheses that must be tested, because they are strongly linked to our immediate project goals.
- Should-have – hypotheses that are closely related to our immediate project goals, but aren’t the top priority.
- Could-have – hypotheses of nice-to-haves that can wait until later for testing.
- Won’t-have – low-priority hypotheses that we may or may not test later on when we have more time.
How to test product hypotheses
Once you have selected a hypothesis, it’s time to test it. This will involve running one or more product experiments in order to check the validity of your claim.
The tricky part is deciding what type of experiment to run, and how many. Ultimately, this all depends on the subject of your hypothesis – whether it’s a simple copy change or a whole new feature. For instance, it’s not necessary to create a clickable prototype for a landing page redesign. In that case, a user-wide update would do.
On that note, here are some of the approaches we take to hypothesis testing at Railsware:
A/B testing
A/B or split testing involves creating two or more different versions of a webpage/feature/functionality and collecting information about how users respond to them.
Let’s say you wanted to validate a hypothesis about the placement of a search bar on your application homepage. You could design an A/B test that shows two different versions of that search bar’s placement to your users (who have been split equally into two camps: a control group and a variant group). Then, you would choose the best option based on user data. A/B tests are suitable for testing responses to user experience changes, especially if you have more than one solution to test.
Prototyping
When it comes to testing a new product design, prototyping is the method of choice for many Lean startups and organizations. It’s a cost-effective way of collecting feedback from users, fast, and it’s possible to create prototypes of individual features too. You may take this approach to hypothesis testing if you are working on rolling out a significant new change e.g adding a brand-new feature, redesigning some aspect of the user flow, etc. To control costs at this point in the new product development process , choose the right tools — think Figma for clickable walkthroughs or no-code platforms like Bubble.
Deliveroo feature prototype example
Let’s look at how feature prototyping worked for the food delivery app, Deliveroo, when their product team wanted to ‘explore personalized recommendations, better filtering and improved search’ in 2018. To begin, they created a prototype of the customer discovery feature using web design application, Framer.
One of the most important aspects of this feature prototype was that it contained live data — real restaurants, real locations. For test users, this made the hypothetical feature feel more authentic. They were seeing listings and recommendations for real restaurants in their area, which helped immerse them in the user experience, and generate more honest and specific feedback. Deliveroo was then able to implement this feedback in subsequent iterations.
Asking your users
Interviewing customers is an excellent way to validate product hypotheses. It’s a form of qualitative testing that, in our experience, produces better insights than user surveys or general user research. Sessions are typically run by product managers and involve asking in-depth interview questions to one customer at a time. They can be conducted in person or online (through a virtual call center , for instance) and last anywhere between 30 minutes to 1 hour.
Although CustDev interviews may require more effort to execute than other tests (the process of finding participants, devising questions, organizing interviews, and honing interview skills can be time-consuming), it’s still a highly rewarding approach. You can quickly validate assumptions by asking customers about their pain points, concerns, habits, processes they follow, and analyzing how your solution fits into all of that.
Wizard of Oz
The Wizard of Oz approach is suitable for gauging user interest in new features or functionalities. It’s done by creating a prototype of a fake or future feature and monitoring how your customers or test users interact with it.
For example, you might have a hypothesis that your number of active users will increase by 15% if you introduce a new feature. So, you design a new bare-bones page or simple button that invites users to access it. But when they click on the button, a pop-up appears with a message such as ‘coming soon.’
By measuring the frequency of those clicks, you could learn a lot about the demand for this new feature/functionality. However, while these tests can deliver fast results, they carry the risk of backfiring. Some customers may find fake features misleading, making them less likely to engage with your product in the future.
User-wide updates
One of the speediest ways to test your hypothesis is by rolling out an update for all users. It can take less time and effort to set up than other tests (depending on how big of an update it is). But due to the risk involved, you should stick to only performing these kinds of tests on small-scale hypotheses. Our teams only take this approach when we are almost certain that our hypothesis is valid.
For example, we once had an assumption that the name of one of Mailtrap ’s entities was the root cause of a low activation rate. Being an active Mailtrap customer meant that you were regularly sending test emails to a place called ‘Demo Inbox.’ We hypothesized that the name was confusing (the word ‘demo’ implied it was not the main inbox) and this was preventing new users from engaging with their accounts. So, we updated the page, changed the name to ‘My Inbox’ and added some ‘to-do’ steps for new users. We saw an increase in our activation rate almost immediately, validating our hypothesis.
Feature flags
Creating feature flags involves only releasing a new feature to a particular subset or small percentage of users. These features come with a built-in kill switch; a piece of code that can be executed or skipped, depending on who’s interacting with your product.
Since you are only showing this new feature to a selected group, feature flags are an especially low-risk method of testing your product hypothesis (compared to Wizard of Oz, for example, where you have much less control). However, they are also a little bit more complex to execute than the others — you will need to have an actual coded product for starters, as well as some technical knowledge, in order to add the modifiers ( only when… ) to your new coded feature.
Let’s revisit the landing page copy example again, this time in the context of testing.
So, for the hypothesis ‘If we change the landing page copy, then the number of signups will increase,’ there are several options for experimentation. We could share the copy with a small sample of our users, or even release a user-wide update. But A/B testing is probably the best fit for this task. Depending on our budget and goal, we could test several different pieces of copy, such as:
- The current landing page copy
- Copy that we paid a marketing agency 10 grand for
- Generic copy we wrote ourselves, or removing most of the original copy – just to see how making even a small change might affect our numbers.
Remember, every hypothesis test must have a reasonable endpoint. The exact length of the test will depend on the type of feature/functionality you are testing, the size of your user base, and how much data you need to gather. Just make sure that the experiment running time matches the hypothesis scope. For instance, there is no need to spend 8 weeks experimenting with a piece of landing page copy. That timeline is more appropriate for say, a Wizard of Oz feature.
Recording hypotheses statements and test results
Finally, it’s time to talk about where you will write down and keep track of your hypotheses. Creating a single source of truth will enable you to track all aspects of hypothesis generation and testing with ease.
At Railsware, our product managers create a document for each individual hypothesis, using tools such as Coda or Google Sheets. In that document, we record the hypothesis statement, as well as our plans, process, results, screenshots, product metrics, and assumptions.
We share this document with our team and stakeholders, to ensure transparency and invite feedback. It’s also a resource we can refer back to when we are discussing a new hypothesis — a place where we can quickly access information relating to a previous test.
Understanding test results and taking action
The other half of validating product hypotheses involves evaluating data and drawing reasonable conclusions based on what you find. We do so by analyzing our chosen product metric(s) and deciding whether there is enough data available to make a solid decision. If not, we may extend the test’s duration or run another one. Otherwise, we move forward. An experimental feature becomes a real feature, a chatbot gets implemented on the customer support page, and so on.
Something to keep in mind: the integrity of your data is tied to how well the test was executed, so here are a few points to consider when you are testing and analyzing results:
Gather and analyze data carefully. Ensure that your data is clean and up-to-date when running quantitative tests and tracking responses via analytics dashboards. If you are doing customer interviews, make sure to record the meetings (with consent) so that your notes will be as accurate as possible.
Conduct the right amount of product experiments. It can take more than one test to determine whether your hypothesis is valid or invalid. However, don’t waste too much time experimenting in the hopes of getting the result you want. Know when to accept the evidence and move on.
Choose the right audience segment. Don’t cast your net too wide. Be specific about who you want to collect data from prior to running the test. Otherwise, your test results will be misleading and you won’t learn anything new.
Watch out for bias. Avoid confirmation bias at all costs. Don’t make the mistake of including irrelevant data just because it bolsters your results. For example, if you are gathering data about how users are interacting with your product Monday-Friday, don’t include weekend data just because doing so would alter the data and ‘validate’ your hypothesis.
- Not all failed hypotheses should be treated as losses. Even if you didn’t get the outcome you were hoping for, you may still have improved your product. Let’s say you implemented SSO authentication for premium users, but unfortunately, your free users didn’t end up switching to premium plans. In this case, you still added value to the product by streamlining the login process for paying users.
- Yes, taking a hypothesis-driven approach to product development is important. But remember, you don’t have to test everything . Use common sense first. For example, if your website copy is confusing and doesn’t portray the value of the product, then you should still strive to replace it with better copy – regardless of how this affects your numbers in the short term.
Wrapping Up
The process of generating and validating product hypotheses is actually pretty straightforward once you’ve got the hang of it. All you need is a valid question or problem, a testable statement, and a method of validation. Sure, hypothesis-driven development requires more of a time commitment than just ‘giving it a go.’ But ultimately, it will help you tune the product to the wants and needs of your customers.
If you share our data-driven approach to product development and engineering, check out our services page to learn more about how we work with our clients!
12 min read
Value Hypothesis 101: A Product Manager's Guide
Talk to sales.
Humans make assumptions every day—it’s our brain’s way of making sense of the world around us, but assumptions are only valuable if they're verifiable . That’s where a value hypothesis comes in as your starting point.
A good hypothesis goes a step beyond an assumption. It’s a verifiable and validated guess based on the value your product brings to your real-life customers. When you verify your hypothesis, you confirm that the product has real-world value, thus you have a higher chance of product success.
What Is a Verifiable Value Hypothesis?
A value hypothesis is an educated guess about the value proposition of your product. When you verify your hypothesis , you're using evidence to prove that your assumption is correct. A hypothesis is verifiable if it does not prove false through experimentation or is shown to have rational justification through data, experiments, observation, or tests.
The most significant benefit of verifying a hypothesis is that it helps you avoid product failure and helps you build your product to your customers’ (and potential customers’) needs.
Verifying your assumptions is all about collecting data. Without data obtained through experiments, observations, or tests, your hypothesis is unverifiable, and you can’t be sure there will be a market need for your product.
A Verifiable Value Hypothesis Minimizes Risk and Saves Money
When you verify your hypothesis, you’re less likely to release a product that doesn’t meet customer expectations—a waste of your company’s resources. Harvard Business School explains that verifying a business hypothesis “...allows an organization to verify its analysis is correct before committing resources to implement a broader strategy.”
If you verify your hypothesis upfront, you’ll lower risk and have time to work out product issues.
UserVoice Validation makes product validation accessible to everyone. Consider using its research feature to speed up your hypothesis verification process.
Value Hypotheses vs. Growth Hypotheses
Your value hypothesis focuses on the value of your product to customers. This type of hypothesis can apply to a product or company and is a building block of product-market fit .
A growth hypothesis is a guess at how your business idea may develop in the long term based on how potential customers may find your product. It’s meant for estimating business model growth rather than individual products.
Because your value hypothesis is really the foundation for your growth hypothesis, you should focus on value hypothesis tests first and complete growth hypothesis tests to estimate business growth as a whole once you have a viable product.
4 Tips to Create and Test a Verifiable Value Hypothesis
A verifiable hypothesis needs to be based on a logical structure, customer feedback data , and objective safeguards like creating a minimum viable product. Validating your value significantly reduces risk . You can prevent wasting money, time, and resources by verifying your hypothesis in early-stage development.
A good value hypothesis utilizes a framework (like the template below), data, and checks/balances to avoid bias.
1. Use a Template to Structure Your Value Hypothesis
By using a template structure, you can create an educated guess that includes the most important elements of a hypothesis—the who, what, where, when, and why. If you don’t structure your hypothesis correctly, you may only end up with a flimsy or leap-of-faith assumption that you can’t verify.
A true hypothesis uses a few guesses about your product and organizes them so that you can verify or falsify your assumptions. Using a template to structure your hypothesis can ensure that you’re not missing the specifics.
You can’t just throw a hypothesis together and think it will answer the question of whether your product is valuable or not. If you do, you could end up with faulty data informed by bias , a skewed significance level from polling the wrong people, or only a vague idea of what your customer would actually pay for your product.
A template will help keep your hypothesis on track by standardizing the structure of the hypothesis so that each new hypothesis always includes the specifics of your client personas, the cost of your product, and client or customer pain points.
A value hypothesis template might look like:
[Client] will spend [cost] to purchase and use our [title of product/service] to solve their [specific problem] OR help them overcome [specific obstacle].
An example of your hypothesis might look like:
B2B startups will spend $500/mo to purchase our resource planning software to solve resource over-allocation and employee burnout.
By organizing your ideas and the important elements (who, what, where, when, and why), you can come up with a hypothesis that actually answers the question of whether your product is useful and valuable to your ideal customer.
2. Turn Customer Feedback into Data to Support Your Hypothesis
Once you have your hypothesis, it’s time to figure out whether it’s true—or, more accurately, prove that it’s valid. Since a hypothesis is never considered “100% proven,” it’s referred to as either valid or invalid based on the information you discover in your experiments or tests. Additionally, your results could lead to an alternative hypothesis, which is helpful in refining your core idea.
To support value hypothesis testing, you need data. To do that, you'll want to collect customer feedback . A customer feedback management tool can also make it easier for your team to access the feedback and create strategies to implement or improve customer concerns.
If you find that potential clients are not expressing pain points that could be solved with your product or you’re not seeing an interest in the features you hope to add, you can adjust your hypothesis and absorb a lower risk. Because you didn’t invest a lot of time and money into creating the product yet, you should have more resources to put toward the product once you work out the kinks.
On the other hand, if you find that customers are requesting features your product offers or pain points your product could solve, then you can move forward with product development, confident that your future customers will value (and spend money on) the product you’re creating.
A customer feedback management tool like UserVoice can empower you to challenge assumptions from your colleagues (often based on anecdotal information) which find their way into team decision making . Having data to reevaluate an assumption helps with prioritization, and it confirms that you’re focusing on the right things as an organization.
3. Validate Your Product
Since you have a clear idea of who your ideal customer is at this point and have verified their need for your product, it’s time to validate your product and decide if it’s better than your competitors’.
At this point, simply asking your customers if they would buy your product (or spend more on your product) instead of a competitor’s isn’t enough confirmation that you should move forward, and customers may be biased or reluctant to provide critical feedback.
Instead, create a minimum viable product (MVP). An MVP is a working, bare-bones version of the product that you can test out without risking your whole budget. Hypothesis testing with an MVP simulates the product experience for customers and, based on their actions and usage, validates that the full product will generate revenue and be successful.
If you take the steps to first verify and then validate your hypothesis using data, your product is more likely to do well. Your focus will be on the aspect that matters most—whether your customer actually wants and would invest money in purchasing the product.
4. Use Safeguards to Remain Objective
One of the pitfalls of believing in your product and attempting to validate it is that you’re subject to confirmation bias . Because you want your product to succeed, you may pay more attention to the answers in the collected data that affirm the value of your product and gloss over the information that may lead you to conclude that your hypothesis is actually false. Confirmation bias could easily cloud your vision or skew your metrics without you even realizing it.
Since it’s hard to know when you’re engaging in confirmation bias, it’s good to have safeguards in place to keep you in check and aligned with the purpose of objectively evaluating your value hypothesis.
Safeguards include sharing your findings with third-party experts or simply putting yourself in the customer’s shoes.
Third-party experts are the business version of seeking a peer review. External parties don’t stand to benefit from the outcome of your verification and validation process, so your work is verified and validated objectively. You gain the benefit of knowing whether your hypothesis is valid in the eyes of the people who aren’t stakeholders without the risk of confirmation bias.
In addition to seeking out objective minds, look into potential counter-arguments , such as customer objections (explicit or imagined). What might your customer think about investing the time to learn how to use your product? Will they think the value is commensurate with the monetary cost of the product?
When running an experiment on validating your hypothesis, it’s important not to elevate the importance of your beliefs over the objective data you collect. While it can be exciting to push for the validity of your idea, it can lead to false assumptions and the permission of weak evidence.
Validation Is the Key to Product Success
With your new value hypothesis in hand, you can confidently move forward, knowing that there’s a true need, desire, and market for your product.
Because you’ve verified and validated your guesses, there’s less of a chance that you’re wrong about the value of your product, and there are fewer financial and resource risks for your company. With this strong foundation and the new information you’ve uncovered about your customers, you can add even more value to your product or use it to make more products that fit the market and user needs.
If you think customer feedback management software would be useful in your hypothesis validation process, consider opting into our free trial to see how UserVoice can help.
Heather Tipton
Start your free trial.
Validating the Product Hypothesis
- First Online: 22 September 2022
Cite this chapter
- Mariya Breyter 2
1689 Accesses
In Chapter 3 , we discussed different techniques for identifying your customer. Whether you are delivering a fixed- scope project or iteratively building a new product, your business will not be possible without having customer needs at the center of your delivery model. Customers define which businesses succeed and which businesses fail. In addition, we discussed Lean UX techniques for identifying proto-personas and understanding their needs. We also covered multiple techniques for user segmentation and analysis, including empathy maps and customer journeys.
This is a preview of subscription content, log in via an institution to check access.
Access this chapter
- Available as PDF
- Read on any device
- Instant download
- Own it forever
- Available as EPUB and PDF
- Compact, lightweight edition
- Dispatched in 3 to 5 business days
- Free shipping worldwide - see info
Tax calculation will be finalised at checkout
Purchases are for personal use only
Institutional subscriptions
Author information
Authors and affiliations.
New York, NY, USA
Mariya Breyter
You can also search for this author in PubMed Google Scholar
Rights and permissions
Reprints and permissions
Copyright information
© 2022 The Author(s), under exclusive license to APress Media, LLC, part of Springer Nature
About this chapter
Breyter, M. (2022). Validating the Product Hypothesis. In: Agile Product and Project Management. Apress, Berkeley, CA. https://doi.org/10.1007/978-1-4842-8200-7_4
Download citation
DOI : https://doi.org/10.1007/978-1-4842-8200-7_4
Published : 22 September 2022
Publisher Name : Apress, Berkeley, CA
Print ISBN : 978-1-4842-8199-4
Online ISBN : 978-1-4842-8200-7
eBook Packages : Professional and Applied Computing Apress Access Books Professional and Applied Computing (R0)
Share this chapter
Anyone you share the following link with will be able to read this content:
Sorry, a shareable link is not currently available for this article.
Provided by the Springer Nature SharedIt content-sharing initiative
- Publish with us
Policies and ethics
- Find a journal
- Track your research
- Bipolar Disorder
- Therapy Center
- When To See a Therapist
- Types of Therapy
- Best Online Therapy
- Best Couples Therapy
- Best Family Therapy
- Managing Stress
- Sleep and Dreaming
- Understanding Emotions
- Self-Improvement
- Healthy Relationships
- Student Resources
- Personality Types
- Guided Meditations
- Verywell Mind Insights
- 2023 Verywell Mind 25
- Mental Health in the Classroom
- Editorial Process
- Meet Our Review Board
- Crisis Support
How to Write a Great Hypothesis
Hypothesis Definition, Format, Examples, and Tips
Kendra Cherry, MS, is a psychosocial rehabilitation specialist, psychology educator, and author of the "Everything Psychology Book."
:max_bytes(150000):strip_icc():format(webp)/IMG_9791-89504ab694d54b66bbd72cb84ffb860e.jpg)
Amy Morin, LCSW, is a psychotherapist and international bestselling author. Her books, including "13 Things Mentally Strong People Don't Do," have been translated into more than 40 languages. Her TEDx talk, "The Secret of Becoming Mentally Strong," is one of the most viewed talks of all time.
:max_bytes(150000):strip_icc():format(webp)/VW-MIND-Amy-2b338105f1ee493f94d7e333e410fa76.jpg)
Verywell / Alex Dos Diaz
- The Scientific Method
Hypothesis Format
Falsifiability of a hypothesis.
- Operationalization
Hypothesis Types
Hypotheses examples.
- Collecting Data
A hypothesis is a tentative statement about the relationship between two or more variables. It is a specific, testable prediction about what you expect to happen in a study. It is a preliminary answer to your question that helps guide the research process.
Consider a study designed to examine the relationship between sleep deprivation and test performance. The hypothesis might be: "This study is designed to assess the hypothesis that sleep-deprived people will perform worse on a test than individuals who are not sleep-deprived."
At a Glance
A hypothesis is crucial to scientific research because it offers a clear direction for what the researchers are looking to find. This allows them to design experiments to test their predictions and add to our scientific knowledge about the world. This article explores how a hypothesis is used in psychology research, how to write a good hypothesis, and the different types of hypotheses you might use.
The Hypothesis in the Scientific Method
In the scientific method , whether it involves research in psychology, biology, or some other area, a hypothesis represents what the researchers think will happen in an experiment. The scientific method involves the following steps:
- Forming a question
- Performing background research
- Creating a hypothesis
- Designing an experiment
- Collecting data
- Analyzing the results
- Drawing conclusions
- Communicating the results
The hypothesis is a prediction, but it involves more than a guess. Most of the time, the hypothesis begins with a question which is then explored through background research. At this point, researchers then begin to develop a testable hypothesis.
Unless you are creating an exploratory study, your hypothesis should always explain what you expect to happen.
In a study exploring the effects of a particular drug, the hypothesis might be that researchers expect the drug to have some type of effect on the symptoms of a specific illness. In psychology, the hypothesis might focus on how a certain aspect of the environment might influence a particular behavior.
Remember, a hypothesis does not have to be correct. While the hypothesis predicts what the researchers expect to see, the goal of the research is to determine whether this guess is right or wrong. When conducting an experiment, researchers might explore numerous factors to determine which ones might contribute to the ultimate outcome.
In many cases, researchers may find that the results of an experiment do not support the original hypothesis. When writing up these results, the researchers might suggest other options that should be explored in future studies.
In many cases, researchers might draw a hypothesis from a specific theory or build on previous research. For example, prior research has shown that stress can impact the immune system. So a researcher might hypothesize: "People with high-stress levels will be more likely to contract a common cold after being exposed to the virus than people who have low-stress levels."
In other instances, researchers might look at commonly held beliefs or folk wisdom. "Birds of a feather flock together" is one example of folk adage that a psychologist might try to investigate. The researcher might pose a specific hypothesis that "People tend to select romantic partners who are similar to them in interests and educational level."
Elements of a Good Hypothesis
So how do you write a good hypothesis? When trying to come up with a hypothesis for your research or experiments, ask yourself the following questions:
- Is your hypothesis based on your research on a topic?
- Can your hypothesis be tested?
- Does your hypothesis include independent and dependent variables?
Before you come up with a specific hypothesis, spend some time doing background research. Once you have completed a literature review, start thinking about potential questions you still have. Pay attention to the discussion section in the journal articles you read . Many authors will suggest questions that still need to be explored.
How to Formulate a Good Hypothesis
To form a hypothesis, you should take these steps:
- Collect as many observations about a topic or problem as you can.
- Evaluate these observations and look for possible causes of the problem.
- Create a list of possible explanations that you might want to explore.
- After you have developed some possible hypotheses, think of ways that you could confirm or disprove each hypothesis through experimentation. This is known as falsifiability.
In the scientific method , falsifiability is an important part of any valid hypothesis. In order to test a claim scientifically, it must be possible that the claim could be proven false.
Students sometimes confuse the idea of falsifiability with the idea that it means that something is false, which is not the case. What falsifiability means is that if something was false, then it is possible to demonstrate that it is false.
One of the hallmarks of pseudoscience is that it makes claims that cannot be refuted or proven false.
The Importance of Operational Definitions
A variable is a factor or element that can be changed and manipulated in ways that are observable and measurable. However, the researcher must also define how the variable will be manipulated and measured in the study.
Operational definitions are specific definitions for all relevant factors in a study. This process helps make vague or ambiguous concepts detailed and measurable.
For example, a researcher might operationally define the variable " test anxiety " as the results of a self-report measure of anxiety experienced during an exam. A "study habits" variable might be defined by the amount of studying that actually occurs as measured by time.
These precise descriptions are important because many things can be measured in various ways. Clearly defining these variables and how they are measured helps ensure that other researchers can replicate your results.
Replicability
One of the basic principles of any type of scientific research is that the results must be replicable.
Replication means repeating an experiment in the same way to produce the same results. By clearly detailing the specifics of how the variables were measured and manipulated, other researchers can better understand the results and repeat the study if needed.
Some variables are more difficult than others to define. For example, how would you operationally define a variable such as aggression ? For obvious ethical reasons, researchers cannot create a situation in which a person behaves aggressively toward others.
To measure this variable, the researcher must devise a measurement that assesses aggressive behavior without harming others. The researcher might utilize a simulated task to measure aggressiveness in this situation.
Hypothesis Checklist
- Does your hypothesis focus on something that you can actually test?
- Does your hypothesis include both an independent and dependent variable?
- Can you manipulate the variables?
- Can your hypothesis be tested without violating ethical standards?
The hypothesis you use will depend on what you are investigating and hoping to find. Some of the main types of hypotheses that you might use include:
- Simple hypothesis : This type of hypothesis suggests there is a relationship between one independent variable and one dependent variable.
- Complex hypothesis : This type suggests a relationship between three or more variables, such as two independent and dependent variables.
- Null hypothesis : This hypothesis suggests no relationship exists between two or more variables.
- Alternative hypothesis : This hypothesis states the opposite of the null hypothesis.
- Statistical hypothesis : This hypothesis uses statistical analysis to evaluate a representative population sample and then generalizes the findings to the larger group.
- Logical hypothesis : This hypothesis assumes a relationship between variables without collecting data or evidence.
A hypothesis often follows a basic format of "If {this happens} then {this will happen}." One way to structure your hypothesis is to describe what will happen to the dependent variable if you change the independent variable .
The basic format might be: "If {these changes are made to a certain independent variable}, then we will observe {a change in a specific dependent variable}."
A few examples of simple hypotheses:
- "Students who eat breakfast will perform better on a math exam than students who do not eat breakfast."
- "Students who experience test anxiety before an English exam will get lower scores than students who do not experience test anxiety."
- "Motorists who talk on the phone while driving will be more likely to make errors on a driving course than those who do not talk on the phone."
- "Children who receive a new reading intervention will have higher reading scores than students who do not receive the intervention."
Examples of a complex hypothesis include:
- "People with high-sugar diets and sedentary activity levels are more likely to develop depression."
- "Younger people who are regularly exposed to green, outdoor areas have better subjective well-being than older adults who have limited exposure to green spaces."
Examples of a null hypothesis include:
- "There is no difference in anxiety levels between people who take St. John's wort supplements and those who do not."
- "There is no difference in scores on a memory recall task between children and adults."
- "There is no difference in aggression levels between children who play first-person shooter games and those who do not."
Examples of an alternative hypothesis:
- "People who take St. John's wort supplements will have less anxiety than those who do not."
- "Adults will perform better on a memory task than children."
- "Children who play first-person shooter games will show higher levels of aggression than children who do not."
Collecting Data on Your Hypothesis
Once a researcher has formed a testable hypothesis, the next step is to select a research design and start collecting data. The research method depends largely on exactly what they are studying. There are two basic types of research methods: descriptive research and experimental research.
Descriptive Research Methods
Descriptive research such as case studies , naturalistic observations , and surveys are often used when conducting an experiment is difficult or impossible. These methods are best used to describe different aspects of a behavior or psychological phenomenon.
Once a researcher has collected data using descriptive methods, a correlational study can examine how the variables are related. This research method might be used to investigate a hypothesis that is difficult to test experimentally.
Experimental Research Methods
Experimental methods are used to demonstrate causal relationships between variables. In an experiment, the researcher systematically manipulates a variable of interest (known as the independent variable) and measures the effect on another variable (known as the dependent variable).
Unlike correlational studies, which can only be used to determine if there is a relationship between two variables, experimental methods can be used to determine the actual nature of the relationship—whether changes in one variable actually cause another to change.
The hypothesis is a critical part of any scientific exploration. It represents what researchers expect to find in a study or experiment. In situations where the hypothesis is unsupported by the research, the research still has value. Such research helps us better understand how different aspects of the natural world relate to one another. It also helps us develop new hypotheses that can then be tested in the future.
Thompson WH, Skau S. On the scope of scientific hypotheses . R Soc Open Sci . 2023;10(8):230607. doi:10.1098/rsos.230607
Taran S, Adhikari NKJ, Fan E. Falsifiability in medicine: what clinicians can learn from Karl Popper [published correction appears in Intensive Care Med. 2021 Jun 17;:]. Intensive Care Med . 2021;47(9):1054-1056. doi:10.1007/s00134-021-06432-z
Eyler AA. Research Methods for Public Health . 1st ed. Springer Publishing Company; 2020. doi:10.1891/9780826182067.0004
Nosek BA, Errington TM. What is replication ? PLoS Biol . 2020;18(3):e3000691. doi:10.1371/journal.pbio.3000691
Aggarwal R, Ranganathan P. Study designs: Part 2 - Descriptive studies . Perspect Clin Res . 2019;10(1):34-36. doi:10.4103/picr.PICR_154_18
Nevid J. Psychology: Concepts and Applications. Wadworth, 2013.
By Kendra Cherry, MSEd Kendra Cherry, MS, is a psychosocial rehabilitation specialist, psychology educator, and author of the "Everything Psychology Book."

Blog » Value Hypothesis & Growth Hypothesis: lean startup validation
Value Hypothesis & Growth Hypothesis: lean startup validation
Posted on September 16, 2021 |
You’ve come up with a fantastic idea for a startup and you need to discuss the hypothesis and its value? But you’re not sure if it’s a viable one or not. What do you do next? It’s essential to get your ideas right before you start developing them. 95% of new products fail in their first year of launch. Or to put it another way, only one in twenty product ideas succeed. In this article, we’ll be taking a look at why it’s so important to validate your startup idea before you start spending a lot of time and money developing it. And that’s where the Lean Startup Validation process gets into, alongside the growth hypothesis and value hypothesis. We’ll also be looking at the questions that you need to ask.
Table of contents
The lean startup validation methodology, the benefits of validating your startup idea, the value hypothesis, the growth hypothesis, recommendations and questions for creating and running a good hypothesis, in conclusion – take the time to validate your product.

What does it mean to validate a lean startup?
Validating your lean startup idea may sound like a complicated process, but it’s a lot simpler than you may think. It may be the case that you were already planning on carrying out some of the work.
Essentially, validating your startup when you check your idea to see if it solves a problem that your prospective customers have. You can do this by creating hypotheses and then carrying out research to see if these hypotheses are true or false.
The best startups have always been about finding a gap in the market and offering a product or service that solves the problem. For example, take Airbnb . Before Airbnb launched, people only had the option of staying in hotels. Airbnb opened up the hospitality industry, offering cheaper accommodation to people who could not afford to stay inexpensive hotels.

“Don’t be in a rush to get big. Be in a rush to have a great product” – Eric Ries
Validation is a crucial part of the lean startup methodology, which was devised by entrepreneur Eric Ries. The lean startup methodology is all about optimizing the amount of time that is needed to ensure a product or service is viable.
Lean Startup Validation is a critical part of the lean startup process as it helps make sure that an idea will be successful before time is spent developing the final product.
As an example of a failed idea where more validation could have helped, take Google Glass . It sounded like a good idea on paper, but the technology failed spectacularly. Customer research would have shown that $1,500 was too much money, that people were worried about health and safety, and most importantly… there was no apparent benefit to the product.
Find out more about lean startup methodology on our blog
How to create a mobile app using lean startup methodology
The key benefit of validating your lean startup idea is to make sure that the idea you have is a viable one before you start using resources to build and promote it.
There are other less obvious benefits too:
- It can help you fine-tune your idea. So, it may be the case that you wanted your idea to go in a particular direction, but user research shows that pivoting may be the best thing to do
- It can help you get funding. Investors may be more likely to invest in your startup idea if you have evidence that your idea is a viable one
The value hypothesis and the growth hypothesis – are two ways to validate your idea
“To grow a successful business, validate your idea with customers” – Chad Boyda
In Eric Rie’s book ‘ The Lean Startup’ , he identifies two different types of hypotheses that entrepreneurs can use to validate their startup idea – the growth hypothesis and the value hypothesis.
Let’s look at the two different ideas, how they compare, and how you can use them to see if your startup idea could work.

The value hypothesis tests whether your product or service provides customers with enough value and most importantly, whether they are prepared to pay for this value.
For example, let’s say that you want to develop a mobile app to help dog owners find people to help walk their dogs while they are at work. Before you start spending serious time and money developing the app, you’ll want to see if it is something of interest to your target audience.
Your value hypothesis could say, “we believe that 60% of dog owners aged between 30 and 40 would be willing to pay upwards of €10 a month for this service.”
You then find dog owners in this age range and ask them the question. You’re pleased to see that 75% say that they would be willing to pay this amount! Your hypothesis has worked! This means that you should focus your app and your advertising on this target audience.
If the data comes back and says your prospective target audience isn’t willing to pay, then it means you have to rethink and reframe your app before running another hypothesis. For example, you may want to focus on another demographic, or look at reducing the price of the subscription.
Shoe retailer Zappos used a value hypothesis when starting out. Founder Nick Swinmurn went to local shoe stores, taking photos of the shoes and posting them on the Zappos website. Then, if customers bought the shoes, he’d buy them from the store and send them out to them. This allowed him to see if there was interest in his website, without having to spend lots of money on stock.

The growth hypothesis tests how your customers will find your product or service and shows how your potential product could grow over the years.
Let’s go back to the dog-walking app we talked about earlier. You think that 80% of app downloads will come from word-of-mouth recommendations.
You create a minimal viable product ( MVP for short ) – this is a basic version of your app that may not contain all of the features just yet. So, you then upload it to the app stores and wait for people to start downloading it. When you have a baseline of customers, you send them an email asking them how they heard of your app.
When the feedback comes back, it shows that only 30% of downloads have come from word-of-mouth recommendations. This means that your growth hypothesis has not been successful in this scenario.
Does this mean that your idea is a bad one? Not necessarily. It just means that you may have to look at other ways of promoting your app. If you are relying on word-of-mouth recommendations to advertise it, then it could potentially fail.
Dropbox used growth hypotheses to its advantage when creating its software. The file-storage company constantly tweaked its website, running A/B tests to see which features and changes were most popular with customers, using them in the final product.

Like any good science experiment, there are things that you need to bear in mind when running your hypotheses. Here are our recommendations:
- You may be wondering which type of hypothesis you should carry out first – a growth hypothesis or a value hypothesis. Eric Ries recommends carrying out a value hypothesis first, as it makes sense to see if there is interest before seeing how many people are interested. However, the precise order may depend on the type of product or service you want to sell;
- You will probably need to run multiple hypotheses to validate your product or service. If you do this, be sure to only test one hypothesis at a time. If you end up testing multiple ones in one go, you may not be sure which hypothesis has had which result;
- Test your most critical assumption first – this is one that you are most worried about, and could affect your idea the most. It may be that solving this issue makes your product or service a viable one;
- Specific – is your hypothesis simple? If it’s jumbled or confusing, you’re not going to get the best results from it. If you’re struggling to put together a clear hypothesis, it’s probably a sign to go back to the drawing board.
- Measurable – can your hypothesis be measured? You’ll want to get tangible results so you can check if the changes you have made have worked.
- Achievable – is your hypothesis attainable? If not, you may want to break it down into smaller goals.
- Relevant – will your hypothesis prove the validity of your product or service?
- Timely – can your hypothesis be measured in a set amount of time? You don’t want a goal that will take years to monitor and measure!
- Be as critical as possible. If you have created an idea, it is only natural that you want it to succeed. However, being objective rather than subjective will help your startup most in the long term;
- When you are carrying out customer research, use as vast a pool of people as time and money will allow. This will result in more accurate data. The great news is that you can use social media and other networking sites to reach out to potential customers and ask them their opinions;
- When carrying out customer research, be sure to ask the questions that matter. Bear in mind that liking your product or service isn’t the same as buying it. If a customer is enthusiastic about your idea, be sure to ask follow-on questions about why they like it, or if they would be willing to spend money on it. Otherwise, your data may end up being useless;
- While it is essential to have as many relevant hypotheses as possible, be careful not to have too many. While it may sound like a good idea to try out lots of different ideas, it can actually be counter-productive. As Eric Ries said:
“Don’t bog new teams down with too much information about falsifiable hypotheses. Because if we load our teams up with too much theory, they can easily get stuck in analysis paralysis. I’ve worked with teams that have come up with hundreds of leap-of-faith assumptions. They listed so many assumptions that were so detailed and complicated that they couldn’t decide what to do next. They were paralyzed by the just sheer quantity of the list.”

“We must learn what customers really want, not what they say they want or what we think they should want.” – Eric Ries
According to CB Insights , the number one reason why startups fail is that there is no demand for the product. Many entrepreneurs have gone ahead and launched a product that they think people want, only to find that there is no market at all.
Lean Startup Validation is essential in helping your business idea to succeed. While it may seem like extra work, the additional work you do in the beginning will be of a critical advantage later down the line.
Still not 100% convinced? Take HubSpot . Before HubSpot launched its sales and marketing services, it started off as a blog. Co-founders Dharmesh Shah and Brian Halligan used this blog to validate their ideas and see what their visitors wanted. This helped them confirm that their concept was on the right lines and meant they could launch a product that people actually wanted to use.
Validating a startup idea before development is crucial because it ensures that the idea is viable and addresses a real problem that customers have. With a high failure rate of new products, validation helps avoid wasting time and resources on ideas that might not succeed.
The value hypothesis tests whether customers find enough value in a product or service to pay for it. The growth hypothesis examines how customers will discover and adopt the product over time. Both hypotheses are essential for validating the viability of a startup idea.
Eric Ries recommends starting with a value hypothesis before a growth hypothesis. Validating whether the idea provides value is crucial before considering how to promote and grow it.
When creating and running a hypothesis, consider the following: 1. Focus on testing one hypothesis at a time. 2. Test your most critical assumptions first. 3. Ensure your hypothesis follows SMART goals (Specific, Measurable, Achievable, Relevant, Timely). 4. Use a wide pool of potential customers for accurate data. 5. Ask relevant and probing questions during customer research. 6. Avoid overwhelming your team with excessive hypotheses.
Validating your product idea before development helps you avoid the top reason for startup failure—lack of demand for the product. By confirming that there is a market need and interest in your idea, you increase the chances of building a successful product.
Lean Startup Validation helps entrepreneurs avoid the mistake of launching a product that doesn’t address a genuine need. By gathering evidence and feedback early, you can make informed decisions about pivoting or refining your idea before investing significant time and resources.
Certainly. Suppose you’re developing a mobile app for dog owners to find dog-walking services. Your value hypothesis could be: “We believe that 60% of dog owners aged between 30 and 40 would be willing to pay upwards of €10 a month for this service.” You then validate this hypothesis by surveying dog owners in that age range and analyzing their responses.
The growth hypothesis examines how customers will discover and adopt your product. If, for example, you expect 80% of app downloads to come from word-of-mouth recommendations, but feedback shows only 30% are from this source, you may need to reevaluate your promotion strategy.
Yes, Lean Startup Validation can be applied to startups across various industries. Whether you’re offering a product or service, the process of testing hypotheses and gathering evidence applies universally to ensure the viability of your idea.
To gather accurate data, focus on reaching a diverse pool of potential customers through various channels, including social media and networking sites. Ask relevant questions about their preferences, willingness to pay, and potential pain points related to your idea
Being critical and objective during validation helps you avoid confirmation bias and wishful thinking. Objectivity allows you to assess whether your idea truly addresses a problem and resonates with customers, ensuring that your startup’s foundation is built on solid evidence.
Launching Startups that get Success Stories
Contact us:
Quick links
© 2016 - 2024 URLAUNCHED LTD. All Rights Reserved
Hypothesis validation
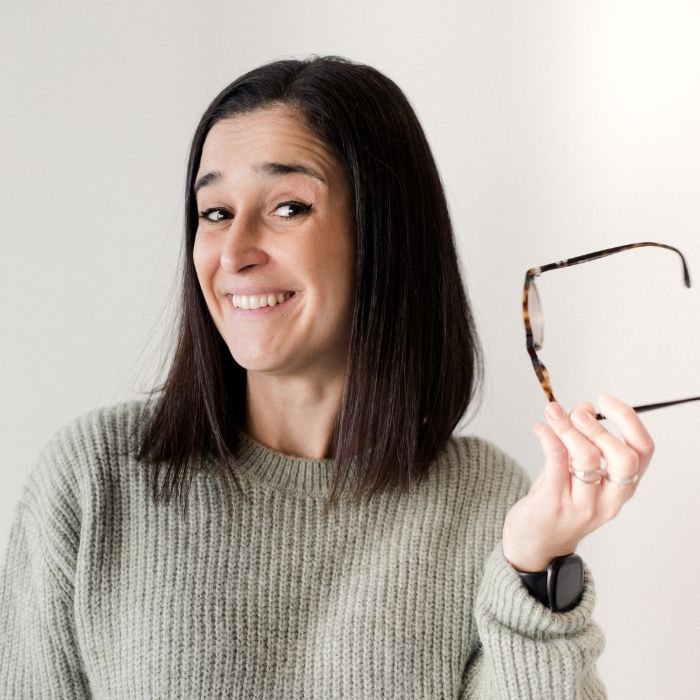
- May 24, 2022
- Product Ownership
- user stories
Share This Post
Table of Contents
Hi everyone!!
This article is based on the scenario presented in our article ‘ User Story Splitting ‘. If you didn’t have the chance yet to take a look at it, we encourage you to do it before continue with this article’s reading. We just want to be sure that you are not missing context but, just as a quick recap…
- …we are working in the product department of a company called ‘Frame Store’. We sell frames …
- … what we used to get from our business people is a big idea, inspirational ( sometimes not 100% realistic and rational! ) of a very nice and cool feature …
And… these are the assumptions we would like to validate:
- If the users are able to customize their menu, they will be able to find the options easily, improving performance and satisfaction.
- If the users are able to search menu options, they will be able to find the options easily, improving performance and satisfaction.
Validating these hypotheses we are seeking to reduce the risk of failure and to be sure we are giving real value to the user.
Let’s jump to the next sections where you will discover in more details how you can reduce the risks, this is ‘as simple as’ follow a hypothesis-driven validation or, what is the same, work on the hypothesis (with empiric data) before to take any decision and move forward.
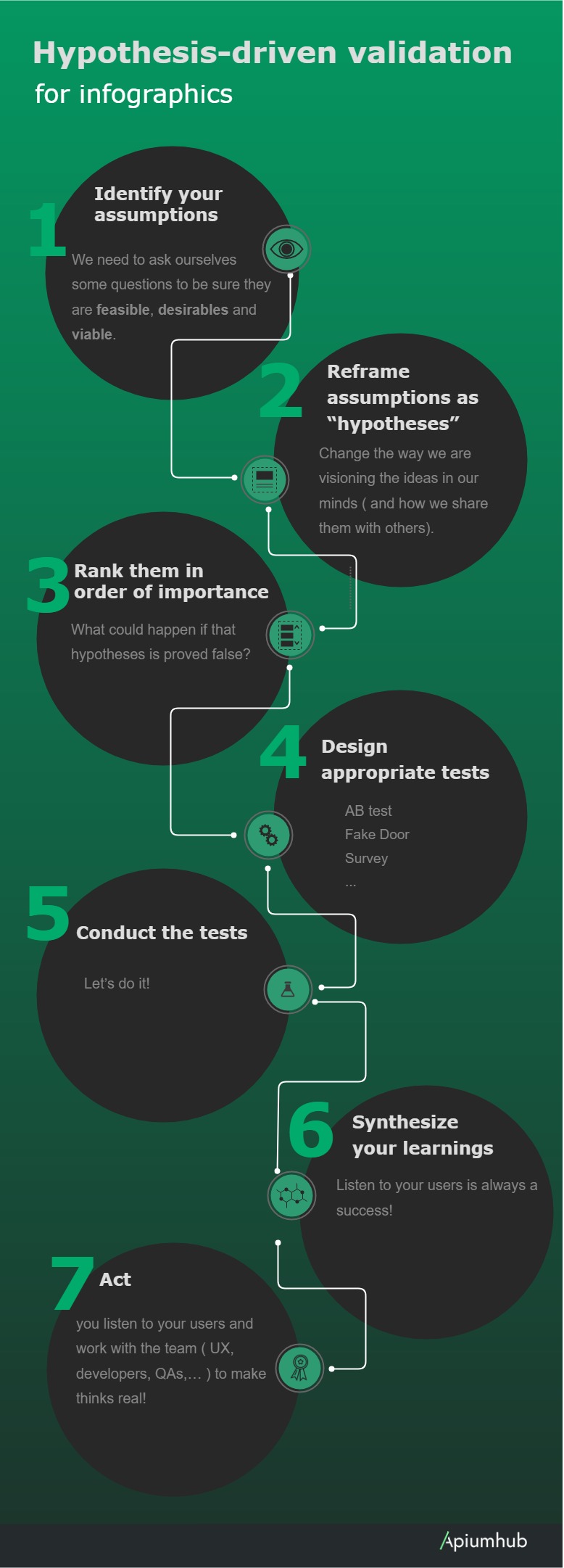
Identify your assumptions
For our example, we have the following assumptions:
We need to ask ourselves some questions to be sure they are feasible , desirables and viable .
Some examples of these questions could be…
- Are we facing some technological challenges that could make these hypotheses not realistic?
- Are we trying to solve a real pain point for our users?
- Does make sense for our company to improve that part of the process right now?
With this 3 questions we can have a very high level idea if our hypotheses is feasible, desirable and viable. We could iterate more in each of them, trying to go more deep on any of these characteristics. This is just a good point to start.
Reframe assumptions as “hypotheses”
This step could seems unnecessary but it’s really required to change the way we are visioning the ideas in our minds ( and how we share them with others).
Assumptions are something we take from granted, it is what it is. On the other hand, hypotheses are like a tentative assumption. We are implicitly saying that we have not proof of the certainty of the assumption.
- We believe that , if the users are able to customize their menu, they will be able to find them easily, improving performance and satisfaction.
- We believe that , if the users are able to search menu options, they will be able to find them easily, improving performance and satisfaction.
A good exercise in here is also the create ‘null hypotheses’, like:
- We believe that, if the users are able to customize their menu, they will be able to find the options in the same way than before, not improving performance and satisfaction.
- We believe that, if the users are able to search menu options, they will be able to find the options in the same way than before, not improving performance and satisfaction.
These are the ones we want to prove false.
Rank them in order of importance
One good advice in order to prioritize hypothesis is to evaluate what could happen if the hypothesis is proven false. In another words, what’s the Cost of Delay ( a concept some of you already know from Safe methodology).
What would be the impact for our app, or even higher level, for our company!
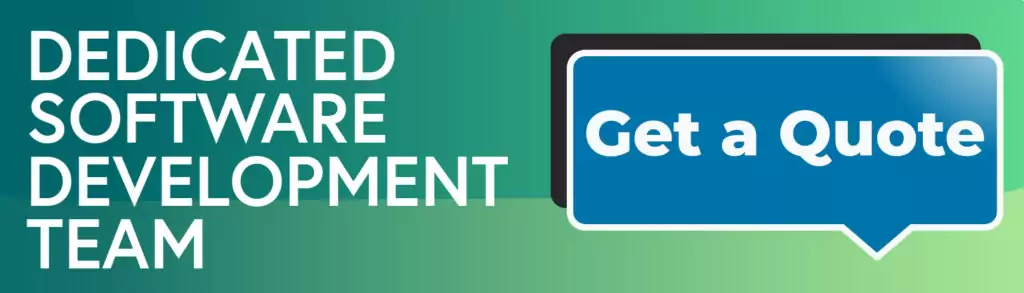
Design appropriate tests
As a good advice I would say ‘ test first what has more risk ‘. This could be apply to anything, do not start with the easy part just to realize that the more complex one is impossible to achieve. Let’s discard it ASAP.

There are multiple ways to perform a test on the hypothesis (Qualitative and Quantitative) … we will go through few of them:
Implement a minimum valuable product with the hypotheses we need to validate. We don’t need to have the full functionality implemented, just the most important features.
If we want to validate our hypothesis ‘ We believe that, if the users are able to search menu options, they will be able to find them easily, improving performance and satisfaction. ‘, let’s implement a search field but that’s everything, nothing else, nothing fancy.
Let’s release it to half of our users and see how it goes. Do not spend a lot of time in something that maybe nobody want. Once we proof that our users likes it and is giving value, we will work to improve it!
This will require even less implementation than the AB testing of the feature BUT could generate more frustration to our users. To validate our hypothesis we will…
- Add the search field to our landing page
- Let the users write the information they need to search
- Do not perform any search, the portal could prompt a message like ‘Sorry, this feature is not still available, we are working on it!’.
- Send to our analytics system how many people is actually using the feature.
As I said before, I believe that this kind of communication need to be handle appropriately to reduce frustration from the users ( some companies uses messages like ‘sorry, this feature is not available in your country yet’… a kind of white lie but could be less harming ).
This validation process could be executed inside an AB test, just replacing the MVP implementation with this Fate Door.
Let’s ask to our users and see what’s the outcome. What is important in here is to not ask direct questions where we are driving the users to the answer we expect is the correct one.
During all this years, I heard like a hundred times ‘remember the yellow Walkman!’ … how can I forget about it!! That example teach us what we don’t have to ask but, the real value is on ‘what do we have to ask?’. It took me few days to come with just few ‘none interfering’ questions!
As advice, try to formulate questions following :
- Do you believe that …
- How do you feel when…
Try to avoid yes/no answers. I would always prefer to go with a scale from ‘less likely’ to ‘very likely’ or similar.
Take in consideration that our product needs to be designed and implemented considering hypotheses validation since day 1. We can not delay the integration of an AB test framework to the last one of our implementations, this needs to be prioritize high!
Conduct the tests
You can user tools like Firebase to perform your AB. Let’s place in there the segmentation of your users you want to move to the test and wait until the data you receive is significative enough ( some tools like Firebase tells it itself).
How long will take? Depends on the traffic you have on that part of the portal/app. Changes on the landing page will give you significative data sooner that changes on some detailed page, like users profiles or any other with less traffic.
About surveys, pick well your candidates to have a realistic representation of your users. Imagine that more than 75% of our users in the Frame Store Portal are people older than 50 years. Our candidates need to represents this distribution so, do not pick a lot of 20-30 years old candidates!
Synthesize your learnings
We need to be openminded at this stage of the hypothesis validation. Maybe, the hypothesis is proved false. This should never be consider a mistake! this is a success ! We where able to save time and money for the company stopping of pursuing something that has no value.
A lot of companies failed because they were unable to validate hypotheses but not just that. I saw cases where, after designed and conducted the appropriate test, they failed reading the results. How could that be possible?? that is just because people is too committed to the assumption that they do not accept that it could be proved false. People sold the idea to upper management before prove it right and now,… ‘ Wow… I can not go back and tell them that what I thought was not true, that I was wrong! ’. This is a big mistake, the biggest one we can commit at this point. We spend time and money to validate an hypothesis and now, we will spend time implementing it even if it not useful for anybody!. Crazy stuff…
Be openminded, change your thought to ‘ Wow… luckily we proved that our assumption where false before move forward with it. Now we know more about our users needs! we can formulate new assumptions! ’. Nobody failed!
Implementation with the certainty of giving value to your users is the best feeling ever! you listen to them and work with the team ( UX, developers, QAs,… ) to make thinks real!
Is there a small chance of failure ( will be almost impossible to reduce the risk to 0! ) but nothing if you compare it with the changes of failure when you put your team to work implementing an assumption that you didn’t validate!
Conclusions
Hope this article gives you some clues to improve your hypothesis validation process. Our advice…validate, validate and … iterate!
We wish you all the best on this long but very fulfilling process!
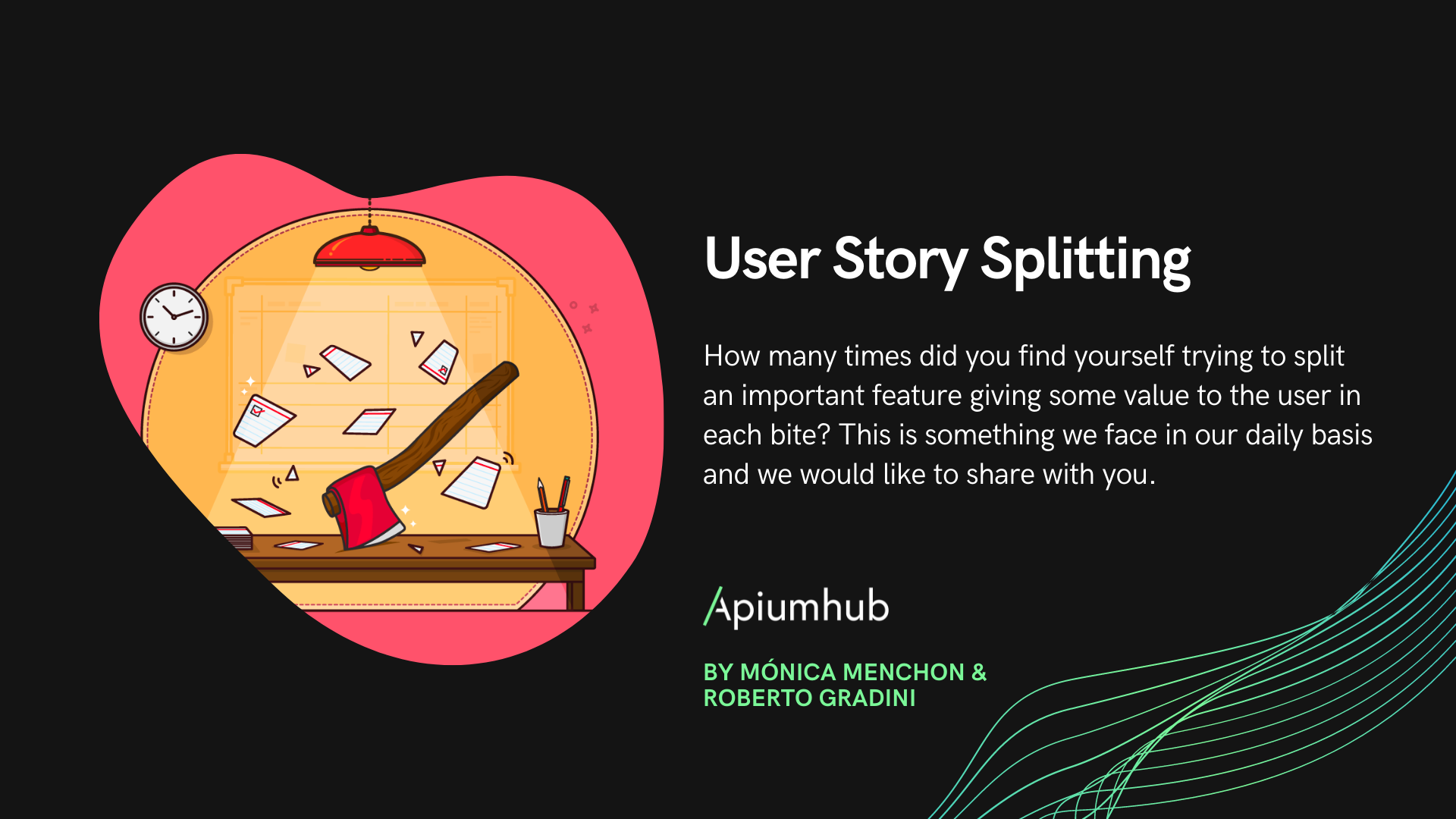
Leave a Reply Cancel Reply
Your email address will not be published. Required fields are marked *
You may use these HTML tags and attributes: <a href="" title=""> <abbr title=""> <acronym title=""> <b> <blockquote cite=""> <cite> <code> <del datetime=""> <em> <i> <q cite=""> <s> <strike> <strong>
Save my name, email, and website in this browser for the next time I comment.
Subscribe To Our Newsletter
Get updates from our latest tech findings
About Apiumhub
Apiumhub brings together a community of software developers & architects to help you transform your idea into a powerful and scalable product. Our Tech Hub specialises in Software Architecture , Web Development & Mobile App Development . Here we share with you industry tips & best practices, based on our experience.
Estimate Your Project
- Agile web and app development
- Data Science
- Offshoring and outsourcing
- Software architecture
- Software Architecture Sonar
- Software Quality Assurance Category
- Technology industry trends
- User Experience Design
Popular posts
- Custom Software Development Services & Modern Practices
- 5 Major Software Architecture Patterns
- Software Development Service Providers You Can Trust
- Software Outsourcing: Interesting Statistics, Predictions, Facts & Key Players
- Tech Of The Future: Technology Predictions For Our World In 2050
Get our Book : Software Architecture Metrics
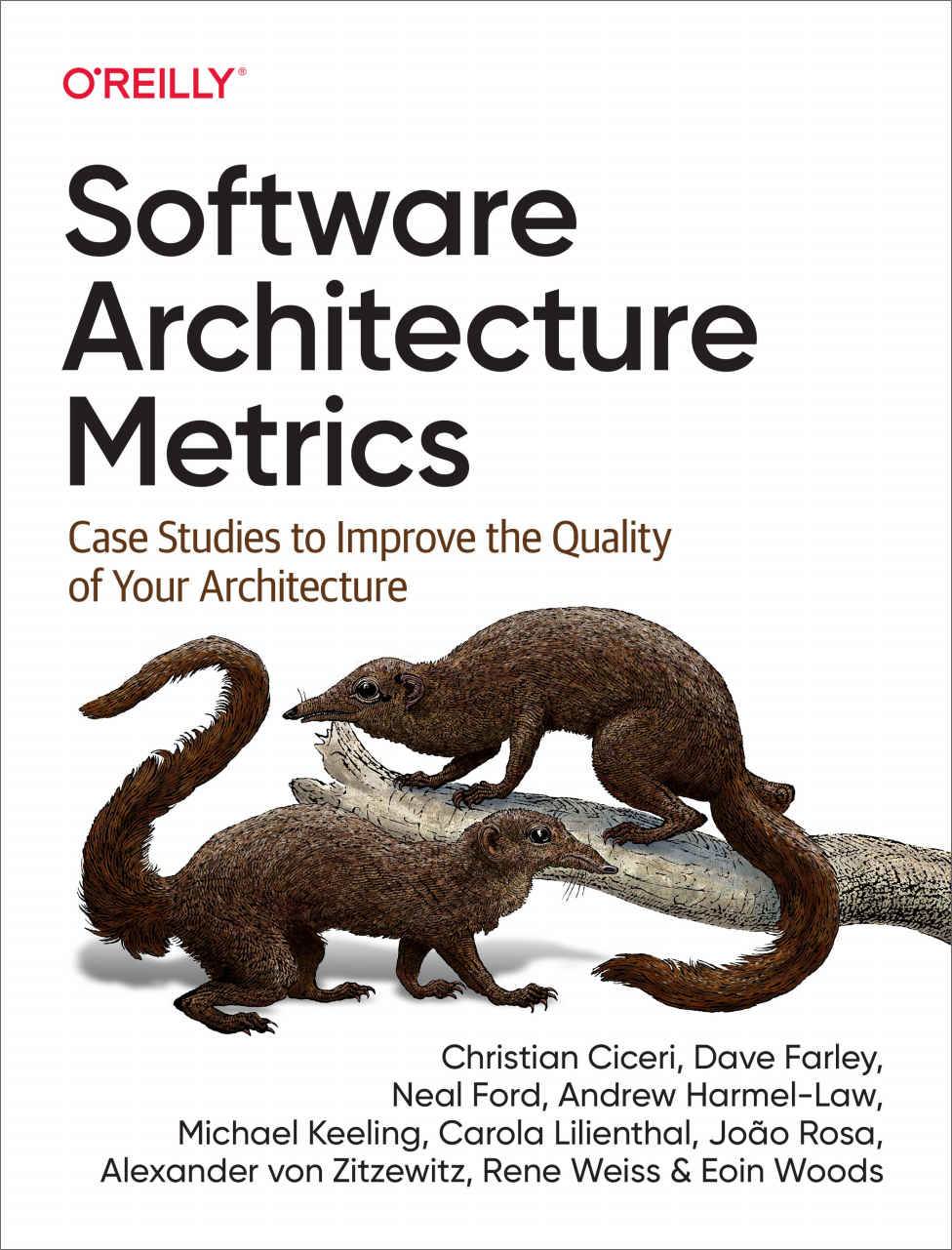
Have a challenging project?
We Can Work On It Together
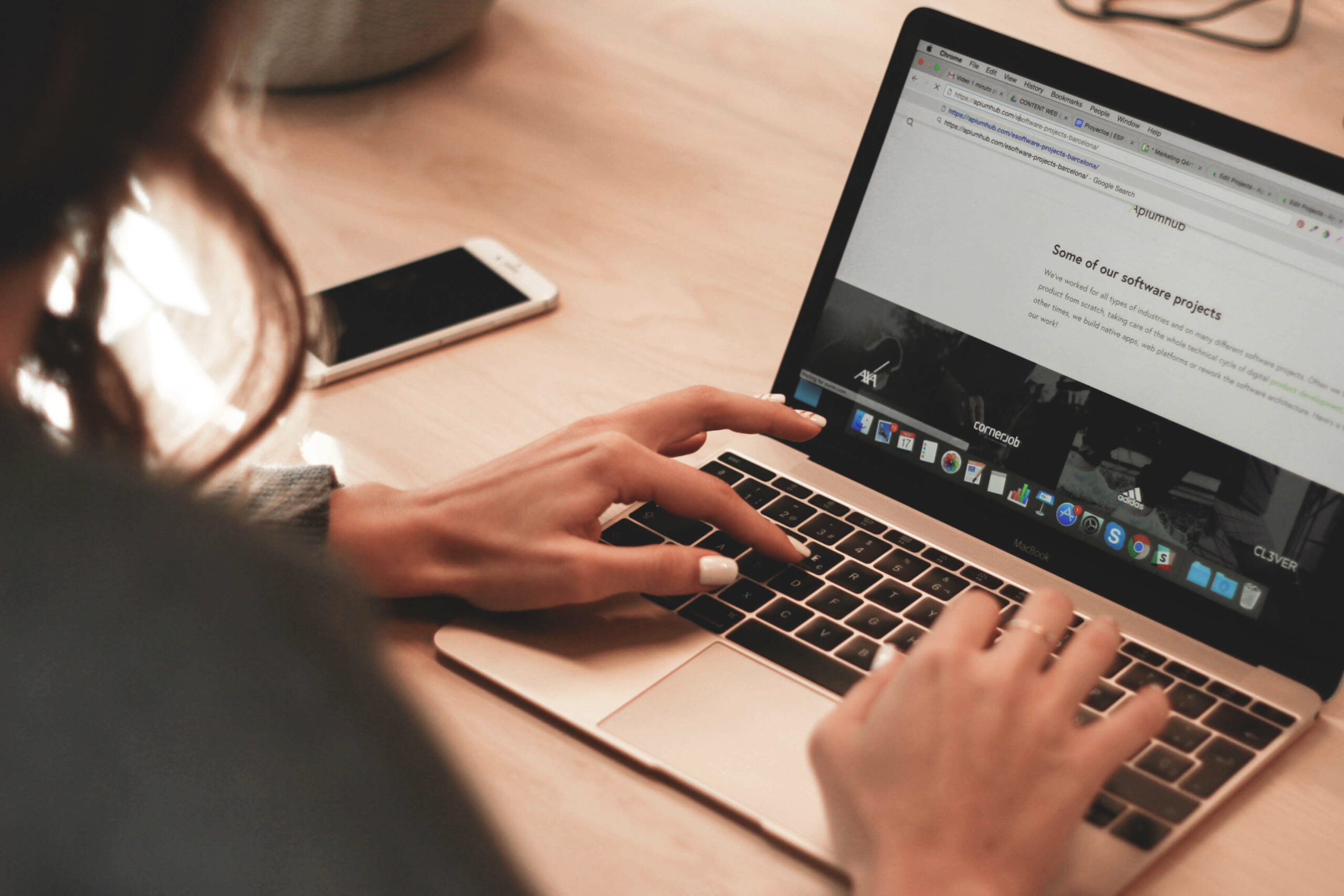
- (+34) 934 815 085
- [email protected]
- Consell de Cent, 333, 7o, 08007 Barcelona
OUR SERVICES
- Dedicated Team
- Team Extension
- Software Architecture
- Web Development
- Mobile App Development
- Agile Product Manager
- QA Automation
LATEST BLOG NEWS
7 Benefits of Using GitHub
HTMX vs React: Choosing the Right Frontend Approach for Your Project
5 Books for QA Engineers
Key Android and iOS Accessibility Features
USEFUL LINKS
- Free Discovery Session
- Green Software
- Become a Contributor
- Privacy Policy
- Whistleblower Channel
Receive the latest tech industry news and events


IMAGES
VIDEO
COMMENTS
There are 5 main steps in hypothesis testing: State your research hypothesis as a null hypothesis and alternate hypothesis (H o) and (H a or H 1 ). Collect data in a way designed to test the hypothesis. Perform an appropriate statistical test. Decide whether to reject or fail to reject your null hypothesis. Present the findings in your results ...
3. One-Sided vs. Two-Sided Testing. When it's time to test your hypothesis, it's important to leverage the correct testing method. The two most common hypothesis testing methods are one-sided and two-sided tests, or one-tailed and two-tailed tests, respectively. Typically, you'd leverage a one-sided test when you have a strong conviction ...
Hypothesis-driven validation sounds commonsensical enough. Yet most businesses tend to avoid these activities, preferring instead to minimize risk through planning, analysis, financial modelling ...
This page titled 9.1: Introduction to Hypothesis Testing is shared under a CC BY 2.0 license and was authored, remixed, and/or curated by Kyle Siegrist ( Random Services) via source content that was edited to the style and standards of the LibreTexts platform; a detailed edit history is available upon request. In hypothesis testing, the goal is ...
Hypothesis testing is as old as the scientific method and is at the heart of the research process. Research exists to validate or disprove assumptions about various phenomena. The process of validation involves testing and it is in this context that we will explore hypothesis testing. ...
Hypothesis testing is a technique that helps scientists, researchers, or for that matter, anyone test the validity of their claims or hypotheses about real-world or real-life events in order to establish new knowledge. Hypothesis testing techniques are often used in statistics and data science to analyze whether the claims about the occurrence of the events are true, whether the results ...
What Comes After Hypothesis Validation? Analysis is what you move on to once you've run the experiment. This is the time to review the collected data, metrics, and feedback to validate (or invalidate) the hypothesis. You have to evaluate the experiment's results to determine whether your product hypotheses were valid or not. For example, if you ...
A/B Testing. One of the most common use cases to achieve hypothesis validation is randomized A/B testing, in which a change or feature is released at random to one-half of users (A) and withheld from the other half (B). Returning to the hypothesis of bigger product images improving conversion on Amazon, one-half of users will be shown the ...
To produce valid results, the content of a test, survey or measurement method must cover all relevant parts of the subject it aims to measure. If some aspects are missing from the measurement (or if irrelevant aspects are included), the validity is threatened and the research is likely suffering from omitted variable bias.
Set validation criteria. First, build some confirmation criteria into your statement. Think in terms of percentages (e.g. increase/decrease by 5%) and choose a relevant product metric to track e.g. activation rate if your hypothesis relates to onboarding.
Validating your value significantly reduces risk. You can prevent wasting money, time, and resources by verifying your hypothesis in early-stage development. A good value hypothesis utilizes a framework (like the template below), data, and checks/balances to avoid bias. 1. Use a Template to Structure Your Value Hypothesis.
Photo by UX Indonesia on Unsplash 1. Card Sorting. Card Sorting is a user research method where participants are requested to group content and features into open or closed categories. The outcome of this exercise unveils valuable patterns that reflect users' expectations regarding the content organization, offering insights for refining navigation, menus, and categories.
Based on user research and related hypothesis validation, product teams define MVP (minimum viable product) based on customer needs and identify all the necessary details to build a prioritized list of features to start execution. This is followed by relentless prioritization based on customer needs once MVP is delivered to the customer. 7.
Hypothesis validation is really a puzzle that you need to solve, which each validation approach providing you with one more piece you can snap on. Always Remember Your Dependencies. It is important to remember that because you do not operate in a vacuum, many things, usually the result of poor prior decision making (which often leads to poor ...
A hypothesis is a tentative statement about the relationship between two or more variables. It is a specific, testable prediction about what you expect to happen in a study. It is a preliminary answer to your question that helps guide the research process. Consider a study designed to examine the relationship between sleep deprivation and test ...
In this tutorial, you will discover how to use statistical hypothesis tests for comparing machine learning algorithms. After completing this tutorial, you will know: Performing model selection based on the mean model performance can be misleading. The five repeats of two-fold cross-validation with a modified Student's t-Test is a good ...
What is Hypothesis Validation. Hypothesis validation is different. In this case, you already have an idea of what is wrong, and you have an idea of how you might possibly fix it. You now have to go out and do some research to figure out if your assumptions and decisions were correct. For our fictional shoe-buying product, hypothesis validation ...
The value hypothesis and the growth hypothesis - are two ways to validate your idea. "To grow a successful business, validate your idea with customers" - Chad Boyda. In Eric Rie's book ' The Lean Startup', he identifies two different types of hypotheses that entrepreneurs can use to validate their startup idea - the growth ...
How do you formulate a digital product hypothesis? How do you validate it? Can a negative hypothesis validation bring positive results? Find the answers in this article!
The Process. The hypothesis-driven validation process starts with extracting assumptions and writing hypotheses. Extracting assumptions helps to determine the amount of risk for each course of action. Next these hypotheses are ranked and tests are designed. These tests are then ranked followed by a period of building, measuring and learning.
Synthesize your learnings. We need to be openminded at this stage of the hypothesis validation. Maybe, the hypothesis is proved false. This should never be consider a mistake! this is a success! We where able to save time and money for the company stopping of pursuing something that has no value.