To read this content please select one of the options below:
Please note you do not have access to teaching notes, automobile insurance fraud detection in the age of big data – a systematic and comprehensive literature review.
Journal of Financial Regulation and Compliance
ISSN : 1358-1988
Article publication date: 8 April 2022
Issue publication date: 2 August 2022
The purpose of this paper is to survey the automobile insurance fraud detection literature in the past 31 years (1990–2021) and present a research agenda that addresses the challenges and opportunities artificial intelligence and machine learning bring to car insurance fraud detection.

Design/methodology/approach
Content analysis methodology is used to analyze 46 peer-reviewed academic papers from 31 journals plus eight conference proceedings to identify their research themes and detect trends and changes in the automobile insurance fraud detection literature according to content characteristics.
This study found that automobile insurance fraud detection is going through a transformation, where traditional statistics-based detection methods are replaced by data mining- and artificial intelligence-based approaches. In this study, it was also noticed that cost-sensitive and hybrid approaches are the up-and-coming avenues for further research.
Practical implications
This paper’s findings not only highlight the rise and benefits of data mining- and artificial intelligence-based automobile insurance fraud detection but also highlight the deficiencies observable in this field such as the lack of cost-sensitive approaches or the absence of reliable data sets.
Originality/value
This paper offers greater insight into how artificial intelligence and data mining challenges traditional automobile insurance fraud detection models and addresses the need to develop new cost-sensitive fraud detection methods that identify new real-world data sets.
- Literature review
- Data mining
- Automobile insurance fraud detection
Benedek, B. , Ciumas, C. and Nagy, B.Z. (2022), "Automobile insurance fraud detection in the age of big data – a systematic and comprehensive literature review", Journal of Financial Regulation and Compliance , Vol. 30 No. 4, pp. 503-523. https://doi.org/10.1108/JFRC-11-2021-0102
Emerald Publishing Limited
Copyright © 2022, Emerald Publishing Limited
Related articles
All feedback is valuable.
Please share your general feedback
Report an issue or find answers to frequently asked questions
Contact Customer Support
Information
- Author Services
Initiatives
You are accessing a machine-readable page. In order to be human-readable, please install an RSS reader.
All articles published by MDPI are made immediately available worldwide under an open access license. No special permission is required to reuse all or part of the article published by MDPI, including figures and tables. For articles published under an open access Creative Common CC BY license, any part of the article may be reused without permission provided that the original article is clearly cited. For more information, please refer to https://www.mdpi.com/openaccess .
Feature papers represent the most advanced research with significant potential for high impact in the field. A Feature Paper should be a substantial original Article that involves several techniques or approaches, provides an outlook for future research directions and describes possible research applications.
Feature papers are submitted upon individual invitation or recommendation by the scientific editors and must receive positive feedback from the reviewers.
Editor’s Choice articles are based on recommendations by the scientific editors of MDPI journals from around the world. Editors select a small number of articles recently published in the journal that they believe will be particularly interesting to readers, or important in the respective research area. The aim is to provide a snapshot of some of the most exciting work published in the various research areas of the journal.
Original Submission Date Received: .
- Active Journals
- Find a Journal
- Proceedings Series
- For Authors
- For Reviewers
- For Editors
- For Librarians
- For Publishers
- For Societies
- For Conference Organizers
- Open Access Policy
- Institutional Open Access Program
- Special Issues Guidelines
- Editorial Process
- Research and Publication Ethics
- Article Processing Charges
- Testimonials
- Preprints.org
- SciProfiles
- Encyclopedia
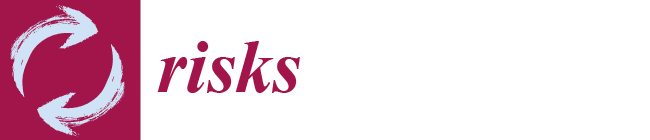
Article Menu
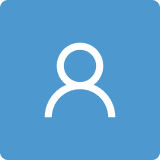
- Subscribe SciFeed
- Recommended Articles
- Google Scholar
- on Google Scholar
- Table of Contents
Find support for a specific problem in the support section of our website.
Please let us know what you think of our products and services.
Visit our dedicated information section to learn more about MDPI.
JSmol Viewer
Machine learning approaches for auto insurance big data.

1. Introduction
2. related work, 3. background, 3.1. machine learning, 3.2. machine learning approach to predict a driver’s risk, 3.3. classifiers, 3.3.1. regression analysis, 3.3.2. decision tree, 3.3.3. xgboost, 3.3.4. random forest, 3.3.5. k-nearest neighbor, 3.3.6. naïve bayes, 4. evaluation models (prediction performance), 4.1. confusion matrix, 4.2. kappa statistics, 4.3. sensitivity and specificity, 4.4. precision and recall, 4.5. the f-measure.
- Values of −1 indicate that the feature was missing from the observation.
- Feature names include the bin for binary features and cat for categorical features. ○ Binary data has two possible values, 0 or 1. ○ Categorical data (one of many possible values) have been processed into a value range for its lowest and highest value, respectively.
- Features are either continuous or ordinal. ○ The value range appears as a range that has used feature scaling; therefore, feature scaling is not required.
- Features belonging to similar groupings are tagged as ind, reg, car, and calc. ○ ind refers to a customer’s personal information, such as their name. ○ reg refers to a customer’s region or location information. ○ calc is Porto Seguro’s calculated features.
6. Proposed Model
6.1. data preprocessing, 6.1.1. claims occurrence variable, 6.1.2. details on missing values.
- The features of ps_car_03_cat and ps_car_05_cat have the largest number of missing values. They also share numerous instances where missing values occur in both for the same row.
- Some features share many missing value rows with other features, for instance, ps_reg_03. Other features have few missing values, like ps_car_12, ps_car_11, and ps_car_02.cat.
- We find that about 2.4% of the values are missing in total in each of the train and test datasets.
- From this figure, the features have a large proportion of missing values, being roughly 70% for ps_car_03_cat and 45% for ps_car_05_cat; therefore, these features are not that reliable, as there are too few values to represent the feature’s true meaning. Assigning new values that are missing to each customer record for these features may also not convey the feature’s purpose and negatively impact the learning algorithm’s performance. Due to these reasons, the features have been dropped and removed from the datasets.
- After we drop ps_car_03_cat and ps_car_05_cat, the features missing values in datasets become 0.18 instead of 2.4. The missing values for the rest of the variables are replaced such that missing values in every categorical and binary variable are replaced by the mode of the column values. In contrast, missing values in every continuous variable are replaced by the mean of the column values. This is because categorical data works well using the mode, and continuous data works well using the mean. Both methods are also quick and straightforward for inputting values ( Badr 2019 ).
6.1.3. Correlation Overview
6.1.4. hyper-parameter optimization, 6.1.5. features selection and implementation, variable importance, 8. conclusions, future research, author contributions, institutional review board statement, informed consent statement, data availability statement, conflicts of interest.
- Abdelhadi, Shady, Khaled Elbahnasy, and Mohamed Abdelsalam. 2020. A proposed model to predict auto insurance claims using machine learning techniques. Journal of Theoretical and Applied Information Technology 98: 3428–3437. [ Google Scholar ]
- Ariana, Diwan, Daniel E. Guyer, and Bim Shrestha. 2006. Integrating multispectral reflectance and fluorescence imaging for defect detection on apples. Computers and Electronics in Agriculture 50: 148–61. [ Google Scholar ] [ CrossRef ]
- Badr, W. 2019. Different Ways to Compensate for Missing Values in a Dataset (Data Imputation with Examples). Available online: https://towardsdatascience.com/6-different-ways-to-compensate-formissing-values-data-imputation-with-examples-6022d9ca0779 (accessed on 17 October 2019).
- Bradley, Andrew P. 1997. The use of the area under the ROC curve in the evaluation of machine learning algorithms. Pattern Recognition 30: 1145–59. [ Google Scholar ] [ CrossRef ] [ Green Version ]
- Breiman, Leo. 2001. Random forests. Machine Learning 45: 5–32. [ Google Scholar ] [ CrossRef ] [ Green Version ]
- Chen, Tianqi, and Carlos Guestrin. 2016. XGBoost: A scalable tree boosting system. Paper presented at the 22nd ACM SIGKDD International Conference on Knowledge Discovery and Data Mining, San Francisco, CA, USA, August 13–17. [ Google Scholar ]
- Columbus, Louis. 2017. McKinsey’s State of Machine Learning and AI, 2017. Forbes . Available online: https://www.forbes.com/sites/louiscolumbus/2017/07/09/mckinseys-state-of-machine-learning-and-ai-2017 (accessed on 17 December 2020).
- Columbus, Louis. 2018. Roundup of Machine Learning Forecasts and Market Estimates, 2018. Forbes Contrib . Available online: https://www.forbes.com/sites/louiscolumbus/2018/02/18/roundup-of-machine-learning-forecasts-and-marketestimates-2018 (accessed on 17 December 2020).
- Cunningham, Padraig, and Sarah Jane Delany. 2020. k-Nearest Neighbour Classifiers–. arXiv arXiv:2004.04523. [ Google Scholar ]
- D’Angelo, Gianni, Massimo Tipaldi, Luigi Glielmo, and Salvatore Rampone. 2017. Spacecraft Autonomy Modeled via Markov Decision Process and Associative Rule-Based Machine Learning. Paper presented at 2017 IEEE International Workshop on Metrology for Aerospace (MetroAeroSpace), Padua, Italy, June 21–23; pp. 324–29. [ Google Scholar ]
- D’Angelo, Gianni, Massimo Ficco, and Francesco Palmieri. 2020. Malware detection in mobile environments based on Autoencoders and API-images. Journal of Parallel and Distributed Computing 137: 26–33. [ Google Scholar ] [ CrossRef ]
- Dewi, Kartika Chandra, Hendri Murfi, and Sarini Abdullah. 2019. Analysis Accuracy of Random forest Model for Big Data—A Case Study of Claim Severity Prediction in Car Insurance. Paper presented at 2019 5th International Conference on Science in Information Technology (ICSITech), Yogyakarta, Indonesia, October 23–24; pp. 60–65. [ Google Scholar ]
- Fang, Kuangnan, Yefei Jiang, and Malin Song. 2016. Customer profitability forecasting using Big Data analytics: A case study of the insurance industry. Computers & Industrial Engineering 101: 554–64. [ Google Scholar ]
- Friedman, Nir, Dan Geiger, and Moises Goldszmidt. 1997. Bayesian network classifiers. Machine Learning 29: 131–63. [ Google Scholar ] [ CrossRef ] [ Green Version ]
- Ganganwar, Vaishali. 2012. An overview of classification algorithms for imbalanced datasets. International Journal of Emerging Technology and Advanced Engineering 2: 42–47. [ Google Scholar ]
- Gao, Guangyuan, and Mario V. Wüthrich. 2018. Feature extraction from telematics car driving heatmaps. European Actuarial Journal 8: 383–406. [ Google Scholar ] [ CrossRef ]
- Gao, Guangyuan, Shengwang Meng, and Mario V. Wüthrich. 2019. Claims frequency modeling using telematics car driving data. Scandinavian Actuarial Journal 2019: 143–62. [ Google Scholar ] [ CrossRef ]
- Géron, Aurélien. 2019. Hands-on Machine Learning with Scikit-Learn, Keras, and TensorFlow: Concepts, Tools, and Techniques to Build Intelligent Systems . Newton: O’Reilly Media. [ Google Scholar ]
- Gonçalves, Ivo, Sara Silva, Joana B. Melo, and João MB Carreiras. 2012. Random sampling technique for overfitting control in genetic programming. In European Conference on Genetic Programming . Berlin and Heidelberg: Springer, pp. 218–29. [ Google Scholar ]
- Goodfellow, Ian, Yoshua Bengio, and Aaron Courville. 2016. Machine learning basics. Deep Learning 1: 98–164. [ Google Scholar ]
- Grosan, C., and A. Abraham. 2011. Intelligent Systems . Berlin: Springer. [ Google Scholar ]
- Guillen, Montserrat, Jens Perch Nielsen, Mercedes Ayuso, and Ana M. Pérez-Marín. 2019. The use of telematics devices to improve automobile insurance rates. Risk Analysis 39: 662–72. [ Google Scholar ] [ CrossRef ]
- Günther, Clara-Cecilie, Ingunn Fride Tvete, Kjersti Aas, Geir Inge Sandnes, and Ørnulf Borgan. 2014. Modelling and predicting customer churn from an insurance company. Scandinavian Actuarial Journal 2014: 58–71. [ Google Scholar ] [ CrossRef ]
- Hossin, Mohammad, and M. N. Sulaiman. 2015. A review on evaluation metrics for data classification evaluations. International Journal of Data Mining & Knowledge Management Process 5: 1. [ Google Scholar ]
- Hultkrantz, Lars, Jan-Eric Nilsson, and Sara Arvidsson. 2012. Voluntary internalization of speeding externalities with vehicle insurance. Transportation Research Part A: Policy and Practice 46: 926–37. [ Google Scholar ] [ CrossRef ]
- Jiang, Shengyi, Guansong Pang, Meiling Wu, and Limin Kuang. 2012. An improved K-nearest-neighbor algorithm for text categorization. Expert Systems with Applications 39: 1503–9. [ Google Scholar ] [ CrossRef ]
- Jing, Longhao, Wenjing Zhao, Karthik Sharma, and Runhua Feng. 2018. Research on Probability-based Learning Application on Car Insurance Data. In 2017 4th International Conference on Machinery, Materials and Computer (MACMC 2017) . Amsterdam: Atlantis Press. [ Google Scholar ]
- Kansara, Dhvani, Rashika Singh, Deep Sanghvi, and Pratik Kanani. 2018. Improving Accuracy of Real Estate Valuation Using Stacked Regression. Int. J. Eng. Dev. Res. (IJEDR) 6: 571–77. [ Google Scholar ]
- Kayri, Murat, Ismail Kayri, and Muhsin Tunay Gencoglu. 2017. The performance comparison of multiple linear regression, random forest and artificial neural network by using photovoltaic and atmospheric data. Paper presented at 2017 14th International Conference on Engineering of Modern Electric Systems (EMES), Oradea, Romania, June 1–2; pp. 1–4. [ Google Scholar ]
- Kenett, Ron S., and Silvia Salini. 2011. Modern analysis of customer satisfaction surveys: Comparison of models and integrated analysis. Applied Stochastic Models in Business and Industry 27: 465–75. [ Google Scholar ] [ CrossRef ]
- Kotsiantis, Sotiris B., Ioannis D. Zaharakis, and Panayiotis E. Pintelas. 2006. Machine learning: A review of classification and combining techniques. Artificial Intelligence Review 26: 159–90. [ Google Scholar ] [ CrossRef ]
- Kotsiantis, Sotiris B., I. Zaharakis, and P. Pintelas. 2007. Supervised machine learning: A review of classification techniques. Emerging Artificial Intelligence Applications in Computer Engineering 160: 3–24. [ Google Scholar ]
- Kowshalya, G., and M. Nandhini. 2018. Predicting fraudulent claims in automobile insurance. In Proceedings of the 2018 Second International Conference on Inventive Communication and Computational Technologies (ICICCT), Coimbatore, India, April 20–21; pp. 1338–43. [ Google Scholar ]
- Kuhn, Max, and Kjell Johnson. 2013. Applied Predictive Modeling . New York: Springer, vol. 26. [ Google Scholar ]
- Lunardon, Nicola, Giovanna Menardi, and Nicola Torelli. 2014. ROSE: A Package for Binary Imbalanced Learning. R Journal 6: 79–89. [ Google Scholar ] [ CrossRef ] [ Green Version ]
- Mau, Stefan, Irena Pletikosa, and Joël Wagner. 2018. Forecasting the next likely purchase events of insurance customers: A case study on the value of data-rich multichannel environments. International Journal of Bank Marketing 36: 6. [ Google Scholar ] [ CrossRef ]
- Mccord, Michael, and M. Chuah. 2011. Spam detection on twitter using traditional classifiers. In International Conference on Autonomic and Trusted Computing . Berlin and Heidelberg: Springer, pp. 175–86. [ Google Scholar ]
- Musa, Abdallah Bashir. 2013. Comparative study on classification performance between support vector machine and logistic regression. International Journal of Machine Learning and Cybernetics 4: 13–24. [ Google Scholar ] [ CrossRef ]
- Pesantez-Narvaez, Jessica, Montserrat Guillen, and Manuela Alcañiz. 2019. Predicting motor insurance claims using telematics data—XGBoost versus logistic regression. Risks 7: 70. [ Google Scholar ] [ CrossRef ] [ Green Version ]
- Roel, Verbelen, Katrien Antonio, and Gerda Claeskens. 2017. Unraveling the predictive power of telematics data in car insurance pricing. Journal of the Royal Statistical Society SSRN. , 2872112. [ Google Scholar ] [ CrossRef ] [ Green Version ]
- Sabbeh, Sahar F. 2018. Machine-learning techniques for customer retention: A comparative study. International Journal of Advanced Computer Science and Applications 9: 273–81. [ Google Scholar ]
- Schmidt, Jonathan, Mário R. G. Marques, Silvana Botti, and Miguel A. L. Marques. 2019. Recent advances and applications of machine learning in solid-state materials science. npj Computational Materials 5: 1–36. [ Google Scholar ] [ CrossRef ]
- Singh, Ranjodh, Meghna P. Ayyar, Tata Venkata Sri Pavan, Sandeep Gosain, and Rajiv Ratn Shah. 2019. Automating Car Insurance Claims Using Deep Learning Techniques. Paper presented at 2019 IEEE Fifth International Conference on Multimedia Big Data (BigMM), Singapore, September 11–13; pp. 199–207. [ Google Scholar ]
- Smith, Kate A., Robert J. Willis, and Malcolm Brooks. 2000. An analysis of customer retention and insurance claim patterns using data mining: A case study. Journal of the Operational Research Society 51: 532–41. [ Google Scholar ] [ CrossRef ]
- Song, Yan Yan, and L. U. Ying. 2015. Decision tree methods: Applications for classification and prediction. Shanghai Archives of Psychiatry 27: 130. [ Google Scholar ] [ PubMed ]
- Stucki, Oskar. 2019. Predicting the Customer Churn with Machine Learning Methods: Case: Private Insurance Customer Data. Master’s dissertation, LUT University, Lappeenranta, Finland. [ Google Scholar ]
- Subudhi, Sharmila, and Suvasini Panigrahi. 2017. Use of optimized Fuzzy C-Means clustering and supervised classifiers for automobile insurance fraud detection. Journal of King Saud University-Computer and Information Sciences 32: 568–75. [ Google Scholar ] [ CrossRef ]
- Weerasinghe, K. P. M. L. P., and M. C. Wijegunasekara. 2016. A comparative study of data mining algorithms in the prediction of auto insurance claims. European International Journal of Science and Technology 5: 47–54. [ Google Scholar ]
- Wu, Shaomin, and Peter Flach. 2005. A scored AUC metric for classifier evaluation and selection. Paper presented at Second Workshop on ROC Analysis in ML, Bonn, Germany, August 11. [ Google Scholar ]
- Wüthrich, Mario V. 2017. Covariate selection from telematics car driving data. European Actuarial Journal 7: 89–108. [ Google Scholar ] [ CrossRef ]
- Yerpude, Prajakta. 2020. Predictive Modelling of Crime Dataset Using Data Mining. International Journal of Data Mining & Knowledge Management Process (IJDKP) 7: 4. [ Google Scholar ]
- Zhou, Zhi Hua. 2012. Ensemble Methods: Foundations and Algorithms . Boca Raton: CRC Press. [ Google Scholar ]
1 | (accessed on 21 December 2020). |
2 | (accessed on 19 December 2020). |
3 | (accessed on 15 December 2020). |
4 | (accessed on 15 December 2020). |
5 | (accessed on 15 December 2020). |
Click here to enlarge figure
ARTICLE & YEAR | PURPOSE | Algorithms | Performance Metrics | The Best Model |
---|---|---|---|---|
( ) | Classification to predict customer retention patterns | DT, NN | Accuracy ROC | NN |
( ) | Classification to predict the risk of leaving | LR and GAMS | ROC | LR |
( ) | Classification to predict the number of claims (low, fair, or high) | LR, DT, NN | Precision Recall Specificity | NN |
( ) | Regression to forecast insurance customer profitability | RF, LR, DT, SVM, GBM | R-squares RMSE | RF |
( ) | Classification to predict insurance fraud | DT, SVM, MLP | Sensitivity Specificity Accuracy | SVM |
( ) | Classification to predict churn, retention, and cross-selling | RF | Accuracy AUC ROC F-score | RF |
( ) | Classification to predict claims occurrence | Naïve Bayes, Bayesian, Network model | Accuracy | Both have the same accuracy. |
( ) | Classification to predict insurance fraud and percentage of premium amount | J48, RF, Naïve Bayes | Accuracy Precision Recall | RF |
( ) | Classification to predict churn problem | RF, AB, MLP, SGB, SVM, KNN, CART, Naïve Bayes, LR, LDA. | Accuracy | AB |
( ) | Classification to predict churn and retention | LR, RF, KNN, AB, and NN | Accuracy F-Score AUC | RF |
( ) | Regression to predict claims severity | Random forest | MSE | RF |
( ) | Classification to predict claims occurrence | XGBoost, LR | Sensitivity Specificity Accuracy RMSE ROC | XGBoost |
( ) | Classification to predict claims occurrence | J48, NN, XGBoost, naïve base | Accuracy ROC | XGBoost |
Actual Positive | Actual Negative | |
---|---|---|
Predicted positive | True positive (TP) | False negative(FN) |
Predicted negative | False positive (FP) | True negative (TN) |
Model | Parameters | Range | Optimal Value |
---|---|---|---|
1. mtry | [2,28,54] | 28 | |
1. Model 2. Winnow 3. Trials | [rules, tree] [FALSE, TRUE] [1,10,20] | Tree FALSE 20 | |
1. Eta 2. max_depth 3. colsample_bytree 4. Subsample 5. nrounds 6. Gamma | [3,4] [1,2,3] [0.6,0.8] [0.50,0.75,1] [50,100,150] [0 to 1] | 0.4 3 0.6 1 150 0 | |
1. C 2. M | [0.010, 0.255,0.500] [1,2,3] | 0.5 1 | |
1. K | [1 to 10] | 3 | |
1. cp | 0 to 0.1 | 0.00274052 | |
1. Laplace 2. Adjust 3. Usekernel | [0 to 1] [0 to 1] [FALSE, TRUE] | 0 1 FALSE |
Model | Accuracy | Error Rate | Kappa | AUC | Sensitivity | Specificity | Precision | Recall | F1 |
---|---|---|---|---|---|---|---|---|---|
RF | 0.8677 | 0.1323 | 0.7117 | 0.84 | 0.9717 | 0.71 | 0.9429 | 0.71 | 0.8101 |
C50 | 0.7913 | 0.2087 | 0.5546 | 0.769 | 0.8684 | 0.6743 | 0.7717 | 0.6743 | 0.7197 |
XGBoost | 0.7067 | 0.2933 | 0.3589 | 0.671 | 0.8434 | 0.4994 | 0.6777 | 0.4994 | 0.575 |
J48 | 0.6994 | 0.3006 | 0.3761 | 0.689 | 0.7385 | 0.6399 | 0.6174 | 0.6399 | 0.6284 |
knn | 0.6629 | 0.3371 | 0.2513 | 0.628 | 0.836 | 0.4003 | 0.6167 | 0.4003 | 0.4855 |
LR | 0.6192 | 0.3808 | 0.1173 | 0.615 | 0.8761 | 0.2296 | 0.55 | 0.2296 | 0.3239 |
caret | 0.6148 | 0.3852 | 0.0786 | 0.534 | 0.9264 | 0.1422 | 0.5601 | 0.1422 | 0.2268 |
Naïve Bayes | 0.6056 | 0.3944 | 0.1526 | 0.574 | 0.421 | 0.7273 | 0.6558 | 0.7273 | 0.6897 |
MDPI stays neutral with regard to jurisdictional claims in published maps and institutional affiliations. |
Share and Cite
Hanafy, M.; Ming, R. Machine Learning Approaches for Auto Insurance Big Data. Risks 2021 , 9 , 42. https://doi.org/10.3390/risks9020042
Hanafy M, Ming R. Machine Learning Approaches for Auto Insurance Big Data. Risks . 2021; 9(2):42. https://doi.org/10.3390/risks9020042
Hanafy, Mohamed, and Ruixing Ming. 2021. "Machine Learning Approaches for Auto Insurance Big Data" Risks 9, no. 2: 42. https://doi.org/10.3390/risks9020042
Article Metrics
Article access statistics, further information, mdpi initiatives, follow mdpi.
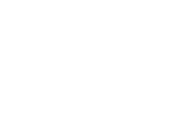
Subscribe to receive issue release notifications and newsletters from MDPI journals
Thank you for visiting nature.com. You are using a browser version with limited support for CSS. To obtain the best experience, we recommend you use a more up to date browser (or turn off compatibility mode in Internet Explorer). In the meantime, to ensure continued support, we are displaying the site without styles and JavaScript.
- View all journals
- Explore content
- About the journal
- Publish with us
- Sign up for alerts
- Open access
- Published: 08 February 2024
Women and insurance pricing policies: a gender-based analysis with GAMLSS on two actuarial datasets
- Giuseppe Pernagallo ORCID: orcid.org/0000-0002-6035-7728 1 ,
- Antonio Punzo ORCID: orcid.org/0000-0001-7742-1821 2 &
- Benedetto Torrisi ORCID: orcid.org/0000-0002-1829-5412 2
Scientific Reports volume 14 , Article number: 3239 ( 2024 ) Cite this article
926 Accesses
1 Citations
Metrics details
- Engineering
- Mathematics and computing
In most of the United States, insurance companies may use gender to determine car insurance rates. In addition, several studies have shown that women over the age of 25 generally pay more than men for car insurance. Then, we investigate whether the distributions of claims for women and men differ in location, scale and shape by means of the GAMLSS regression framework, using microdata provided by U.S. and Australian insurance companies, to use this evidence to support policy makers’ decisions. We also develop a parametric-bootstrap test to investigate the tail behavior of the distributions. When covariates are not considered, the distribution of claims does not appear to differ by gender. When covariates are included, the regressions provide mixed evidence for the location parameter. However, for female claimants, the spread of the distribution is lower. Our research suggests that, at least for the contexts analyzed, there is no clear statistical reason for charging higher rates to women. While providing evidence to support unisex insurance pricing policies, given the limitations represented by the use of country-specific data, this paper aims to promote further research on this topic with different datasets to corroborate our findings and draw more general conclusions.
Similar content being viewed by others
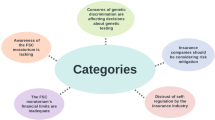
“Uninsurable because of a genetic test”: a qualitative study of consumer views about the use of genetic test results in Australian life insurance
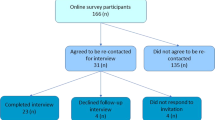
Health professionals’ views and experiences of the Australian moratorium on genetic testing and life insurance: A qualitative study
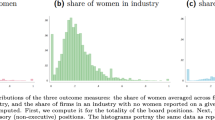
Gender board diversity across Europe throughout four decades
Introduction.
The research question of this paper stems from a popular belief, common in many countries. There are numerous quips regarding female drivers, who are often depicted as less skilled drivers than men. In Italy, for example, men usually say “donne al volante, pericolo costante”, which can be (approximately) translated as “women driving, peril thriving”. Albeit the issue may seem frivolous, it assumes great importance from the perspective of insurers, risk analysts and policy makers. If women were indeed worse customers for insurers, gender would represent an important variable to model insurance-related data. This study aims to provide evidence to determine whether insurers are statistically justified in treating women and men differently using claims data.
We have two main research objectives. Firstly, we look for the best model for the loss distribution (a largely debated issue in literature) and we investigate whether gender makes differences in some aspects of the distribution such as, for example, location or scale. Secondly, we evaluate whether gender affects the magnitude of losses, controlling for other available covariates. In particular, we give emphasis to the largest claims (the right tail of the loss distribution), which are of relevant importance for insurance companies.
In summary, our contribution is mainly empirical in nature, but also partly methodological. Empirically, we aim to provide evidence to answer the important policy question of whether gender is a relevant variable for insurers. These results are limited by the use of available data, but have important economic value for both insurers and policy makers (see “ Potential limitations ” for a discussion). On the other hand, we also contribute to the methodological literature by proposing the use of many statistical models neglected in similar works and introducing a bootstrap test to test for differences between groups in the tails of their distributions.
There are several studies related to the issue. Sivak and Schoettle 1 study the representativeness of gender in six different crash scenarios. Even though the results may be influenced by different factors, the authors find that, in some scenarios, male-to-male crashes tend to be underrepresented, whereas female-to-female crashes tend to be over-represented.
A study of prominent interest for insurers was carried out by Massie et al. 2 on passenger-vehicle travel data. The authors find that elevated crash involvement rates per vehicle-mile of travel are registered for young individuals (aged 16–19) and old drivers (75 and over). Men are more likely to experience a fatal crash whereas women are more frequently involved in injury crashes and in all police-reported crashes. Santamariña-Rubio et al. 3 provide contrasting evidences: first, the authors find the presence of an interaction effect between gender and age in road traffic injury risk; second, in some age groups men show excess risk compared to women, while in others they observe the opposite, with a dependence on the severity of the injury and the mode of transport.
Several studies have shown that, in general, women drive more cautiously than men 2 , 4 , 5 , 6 , 7 . Moreover, as documented in Regev et al. 8 , p. 131, “ driver’s age and gender have also been shown to affect the severity of crash outcomes (i.e. the risk of fatal injury given a crash) ”, with a higher likelihood to be exposed to fatal injuries in a crash for male and elderly drivers than female and young drivers 9 , 10 .
The theme of this paper is merely economic: if gender affects the likelihood of being involved in a crash or the severity of a car accident (and therefore economic losses for a company), then insurance companies may require different rates. The debate is still open. For example, a recent article of the HuffPost (Car Insurance Companies Charge Women Higher Rates Than Men Because They Can, by Elaine S. Povich, 2019, HuffPost) revealed that several studies in 2017 and 2018 showed that women over 25 generally pay more than men for auto insurance. As reported in the article, in many cases (and for the same policy) women paid $500 more than men for no reason other than their gender. The European Union, as reported by The Guardian (How an EU gender equality ruling widened inequality, by Patrick Collinson, 2019, The Guardian), introduced rules to avoid gender discrimination by car insurance companies, a practice detrimental for the principle of unisex pricing. One may argue that the variable “gender” is fully controlled by legislators, but this is not true for many relevant geographical contexts. As reported by the Business Insider (Car insurance rates are going up for women across the US—here’s where they pay more than men, by Shayanne Gal and Tanza Loudenback, 2019, Business Insider), in 44 US states insurance companies can use gender to determine a driver’s car insurance rate, whereas only the states of California, Hawaii, Massachusetts, Montana, North Carolina, and Pennsylvania have banned the practice. Therefore, the present study is of prominent interest for legislators of many states.
Risk classification is necessary in the insurance industry. Hence, some sort of differentiation is needed to operate optimally in the market, but such decisions require a “fair justification” 11 . As analysts, this means that gender-based price discrimination should be statistically motivated. Loss or claims data have been treated in literature generally without differentiating by gender (which is surprising given the huge quantity of studies in the field). These studies (see “ Literature review ”) consider many aspects of the data, from the distributional properties to predictive models. With this paper we want to check whether similar results hold when we separate data based on the claimant’s gender, using two important datasets provided in R packages.
We believe that our study is of interest for five main reasons. First, to our knowledge this is the first study that implements distribution fitting to claims data separating by gender. Studies in this field are generally concerned only with finding the best model for the whole distribution. Second, our empirical analysis shows the good performance of many statistical models neglected in the field. Third, we introduce a new parametric test to check whether VaR computed for females data differs from VaR computed for males data. The test has been conceived for our case study but can be used also in different contexts. Fourth, we show the power of GAMLSS modelling when dealing with asymmetric and/or non-mesokurtic data, or when a researcher aims to modify existing distributions, for example, via truncation or adjusting for zeros. Indeed, this approach can yield enormous benefits in modelling economic or financial data. Last but not least, we provide guidance for policy makers, encouraging the application of a fair pricing.
The paper is structured as follows. “ Literature review ” presents a review of the existing literature. “ Data ” describes the data used in the empirical analysis. “ Methodology ” illustrates the adopted statistical methodology. “ Distribution fitting results ” describes the results of the regression analysis when the available covariates are not included (hereafter often referred to as “distribution modelling/fitting”) where we also test for differences in the two distributions. “ Regression results ” shows the regression results when the available covariates are included in the analysis: we check whether gender is related to insurers’ claims, considering the whole distribution and the tail of the data. In “ Potential limitations ”, we discuss a series of shortcomings that could undermine the validity of our results. “ Conclusions and policy implications ” concludes the paper. Appendices (A, B, and C) are distributed as online supplementary material .
Literature review
Distribution modelling.
Regarding the first research question of this paper, we need to understand whether the claims of females and males behave differently in distribution. It has been shown in many works that the distribution of insurance losses is generally heavy-tailed 12 , 13 , unimodal hump-shaped or multimodal 14 , 15 , 16 and skewed 13 , 17 , 18 . Moreover, it is important to account for the positive support of the distribution 16 , 19 , 20 , 21 , 22 .
Among the many parametric models proposed in literature for the loss distribution 19 , Eling 18 assesses the performance of the following classical distributions: Normal, Student’s t , hyperbolic, generalized hyperbolic, normal inverse Gaussian, variance gamma, gamma, Weibull, Cauchy, skew-normal, skew- t , logistic, log-normal, exponential, Pareto, chi-square and geometric. As pointed out by Eling 18 , the Pareto distribution is a relevant statistical model in catastrophe insurance to describe, especially, large losses, and many authors have used it as a starting framework for modelling losses and lifetime data, or in any context characterised by heavy-tailed distributions 23 , 24 , 25 . The more flexible family of the generalized Pareto distributions, albeit promising to fit insurance data, has not found the same favour by researchers, probably because estimation methods like the maximum likelihood and method-of-moments are undefined in some regions of the parameter space, making the fitting procedure a difficult routine 26 .
Recently, some authors have focused their attention on more sophisticated, but also more flexible, composite 14 , 24 , compound 16 , 22 and mixture 20 , 27 , 28 models. All these approaches share the common principle to combine the characteristics of two or more distributions, so modelling many aspects that a single distribution cannot represent.
We provide novelty to this already large stream of papers in different ways. Firstly, we fit renowned, but also less used, parametric models to important car insurance datasets. Secondly, we avoid the boundary bias issue 29 , 30 , that in our case means allocation of probability mass to negative values, by considering distributions with a positive support or by applying convenient transformations to distributions defined on the whole real line. We accomplish the latter task by truncation or using a log-transformation. Thirdly, while the aforementioned works are concerned with the whole amount of claims, we fit the competing models splitting the data by gender to see whether relevant differences exist. Finally, we test whether gender makes differences in all (or some of) the parameters of the model used to describe the distribution of claims, and we introduce a bootstrap-based parametric test to see whether significant statistical differences exist between the value at risk (VaR) predicted by the various fitted models for females and males.
Regression modelling
With the second research question we want to assess whether gender has an effect on the magnitude of the claims, controlling for other available covariates. However, traditional regression techniques are problematic when dealing with actuarial data. Rousseeuw et al. 31 point out that in many applications (such as insurance data), outliers have relevant effects on the estimates. Traditional ordinary least squares (OLS) regression does not satisfy the requisite of robustness, because it is sensitive to outliers. Indeed, in the OLS method the underlying distribution is Gaussian 32 whereas insurance data, as discussed in “ Distribution modelling ”, depart severely from a Gaussian distribution. For these reasons, traditional OLS cannot be used for our purpose. Among the many alternative models that can solve these problems, quantile regression gained the favour of many analysts thanks to the fact that quantiles, such as the median, are less sensitive to outliers; moreover, quantile regression models are distribution-free 33 . However, Rigby et al. 34 note that “ quantile (and expectile) regressions are less reliable in the extreme tails of the distribution because of sparsity of data points ”. For this reason, the authors consider an alternative procedure for modelling the tail of a distribution under a regression perspective, which is used in the present work (see “ Regression results ”). From the point of view of an insurer, knowing the behaviour of the data in the tail of the distribution is fundamental to prevent and assess adequately the largest losses. Then, we also explore the relationship between extreme losses and gender.
We worked with two important insurance datasets. The choice of these datasets descends from the need of having enough covariates and a variable for gender. It is important to note that while these are large and reliable datasets, they are country-specific and therefore our results are difficult to generalize. An in-depth discussion of this issue is provided in “ Potential limitations ”.
The automobile bodily injury claims ( AutoBi ) dataset
The first dataset is freely available in the R package insuranceData and is called “Automobile Bodily Injury Claims” ( AutoBi ). This dataset derives from a 2002 survey conducted by the Insurance Research Council (IRC), a division of the American Institute for Chartered Property Casualty Underwriters and the Insurance Institute of America. The survey asked participating companies to report claims closed with payment during a designated 2-week period. The sample available in the package is made by 1340 bodily injury liability claims.
The variable of our interest is the claimant’s total economic loss (abbreviated as Loss ) in thousands of dollars from a single state. Furthermore, thanks to the variable Clmsex , i.e. the claimant’s gender, we were able to subset the original data dividing the losses for men and women. The split of the data causes the loss of some observations since the claimant’s sex is not available for all the reported losses. The variable Loss is also used in the regression model as dependent variable; however, for the description of the model and the included covariates we invite the reader to look at “ AutoBi ”. This dataset is also used, among the others, by Frees 35 in his book.
The automobile claim datasets in Australia ( ausprivauto0405 )
The second dataset is freely available in the R package CASdatasets and is named “Automobile claim datasets in Australia”. Specifically, we use the dataset ausprivauto0405 , made of 67,856 observations, which represent 1-year vehicle insurance policies taken out in 2004 or 2005 in Australia. Among the available policies, 4624 have at least one claim, the rest of the data are all zeros. All the losses are expressed in Australian dollars, but for scaling purposes, we rescaled the data to work with hundreds of dollars. In this case there are no missing observations. The rescaled variable ClaimAmount is also the dependent variable for the regression model, but all the information regarding the model are provided in “ ausprivauto0405 ”. This dataset is also used, among the others, by De Jong and Heller 36 in their book. It is important to note that given the presence of many zeros, all the models considered for this dataset have been zero adjusted, which means including a probability mass at 0 37 . In this way we have two different views for the phenomenon: the first dataset is focused only on losses, whereas the second one considers also policy holders without reported losses, in this way accounting for the possibility that car accidents can be more frequent depending on driver’s gender.
Methodology
As already detailed in “ Literature review ”, we evaluate the variable of interest, namely the Loss variable (denoted by Y ), from the point of view of its distribution (“ Distribution modelling ”) and as a function of some covariates of interest \({\varvec{Z}}\) (“ Regression modelling ”), giving particular attention to the Gender variable. For uniformity sake, we handle both these research objectives under a model-based paradigm which uses the very flexible family of generalized additive models for location, scale and shape (GAMLSS), proposed by Rigby and Stasinopoulos 38 to overcome some of the limitations associated with the generalized linear models (GLMs)—such as, for example, the exponential family distribution assumption for the response variable—and generalized additive models (GAMs). In the GAMLSS methodology, the systematic part of the model is expanded to allow equations not only for the mean, but also for the other parameters (scale and shape) of the distribution of the response variable.
The GAMLSS regression framework
A GAMLSS model can be expressed as
where \({\mathcal {D}}(\mu ,\sigma ,\nu ,\tau )\) is a four-parameter distribution (but it can have less or more parameters), with \(\mu\) and \(\sigma\) usually characterizing location and scale, respectively, and with \(\nu\) and \(\tau\) known as shape parameters (i.e., skewness and kurtosis). We denote with \(i=1,\ldots ,4\) the generic i th equation in the system, \(\eta _i\) is a predictor of the parameter (one for each of the four parameters), \(g_i(\cdot )\) is a function to model the parameter of the distribution (in the empirical part of the paper we use the default functions of the commands gamlss , gamlssML , and gamlssZadj ), \(\varvec{Z}_i\) is a vector of covariates, \(\varvec{\beta }_i\) is the coefficient vector, and \(s_{ij}(\cdot )\) is a nonparametric smoothing function applied to the covariate \(\varvec{z}_{ij}\) , \(j=1,\ldots ,J\) , with J denoting the number of covariates. The smoothing terms \(s_{ij}(\cdot )\) introduce nonlinearities in the model, and are unspecified functions estimated using a scatterplot smoother, in an iterative procedure called the local scoring algorithm 39 .
The form of \({\mathcal {D}}(\mu ,\sigma ,\nu ,\tau )\) is general and only implies that the distribution should be in parametric form; it can be any distribution (including highly skew and kurtotic continuous and discrete distributions) and it can model heterogeneity (e.g., cases where the scale or shape of the distribution of the response variable changes with explanatory variables). All the distributions defined on \((0,\infty )\) can be zero adjusted to \([0,\infty )\) by including a probability mass at zero using the gamlss.inf package 40 . The resulting new distribution can then have up to five parameters, the four parameters of the original distribution defined on \((0,\infty )\) plus a parameter \(\xi _0=p_0=P(Y=0)\in \left( 0,1\right)\) that represents the probability mass at 0. Computationally, the function gen.Zadj() creates a mixed (continuous-discrete) probability density function (pdf) given by
where \(f(y|\mu ,\sigma ,\nu ,\tau )\) denotes the pdf on \((0,\infty )\) .
How the research objectives of the paper are handled
Firstly, we look for the best model for the loss distribution (see “ Distribution modelling ” for related literature) and we investigate whether Gender makes differences in some aspects of the distribution such as, for example, location or scale. We handle this first objective by regressing all the parameters \(\mu\) , \(\sigma\) , \(\nu\) and \(\tau\) of \({\mathcal {D}}(\mu ,\sigma ,\nu ,\tau )\) on Gender , i.e. on only one covariate ( \(Z_1=Z_2=Z_3=Z_4=Z\) ) in ( 1 ). Thus, in case of differences due to gender in the loss distribution, that we can identify by looking at the significance of the coefficients \(\beta _1\) , \(\beta _2\) , \(\beta _3\) and \(\beta _4\) in ( 1 ), we have the advantage to detect the aspect(s) (location, scale and/or shape) affected by this variable.
We try several models for the loss distribution not only to have a large set of models within which to look for the best one, but also to make the evaluation of gender differences more robust with respect to a wrong model specification. Thanks to the package gamlss and its extensions 41 , 42 , we consider both classical distributions already defined on \((0,\infty )\) and new distributions on \((0,\infty )\) . These new distributions are created from those with support \((-\infty ,\infty )\) , using the inverse log (i.e. the exponential) transformation through the function gen.Family() with argument type=“log” , and by truncation using the function gen.trun() 42 . In detail, we consider the following 30 parametric models: Box-Cox Cole and Green, Box-Cox Power Exponential, Box-Cox t , Burr, Dagum (Burr III), Exponential, Gamma, Generalized Beta type 2, Generalized Gamma, Generalized Inverse Gaussian, Generalized Pareto, Inverse Gamma, Inverse Gaussian, Log-Gumbel, Log-Johnson’s SU, Log-Logistic, Log-Normal, Log-Power Exponential, Log-Skew Normal Type 2, Log-Skew t Type 5 43 , Log- t Family, Pareto Type 2, Truncated Exponential Gaussian, Truncated Johnson’s SU, Truncated Logistic, Truncated Normal, Truncated Power Exponential, Truncated Skew t Type 5 43 , Truncated t Family, Weibull.
The distributions were fitted via the maximum likelihood (ML) approach. It must be noted that, for the ausprivauto0405 dataset, we did not implement all the distributions because of computational problems related with the zero adjusted routine 44 . However, considering that we use a large number of distributions, it should not be a great loss to exclude these models from the analysis. Once the regression models are fitted, we rank them via the Akaike information criterion (AIC 45 ) and by the Bayesian information criterion (BIC 46 ), which represent the most popular criteria in the actuarial literature 16 , 18 , 27 , 28 .
Secondly, as concerns the objective of assessing the impact of Gender on Loss , controlling for other covariates, we always use the GAMLSS regression framework to model the whole distribution and its tail. The research question in this case pertains to whether female claimants generate higher losses for insurers such that the application of higher rates can be supported by a “fair justification” 11 . The use of heavy-tailed distributions overcomes the problem of extreme values in actuarial datasets. Nonetheless, knowing how gender impacts the mean or one of the other parameters of the losses distribution is less interesting for insurers than knowing the impact of gender on the tail of the distribution, where the highest losses are placed. To study this portion of the data, without recurring to nonparametric methods like the less reliable quantile regression 34 or more complex approaches like entropic/symbolic methods 47 , we use a procedure that can be found in “ Regression results ” of the present paper 34 , 48 .
Comparing the tail behaviour
Comparing the female and male distributions in their tails is important information for insurers because of its relation to VaR. In detail, we define a parametric (model-based) bootstrap test that can be schematized as follows.
Compute the sample values at risk, \(\text {VaR}^F_\alpha\) and \(\text {VaR}^M_\alpha\) , separately for females and males, but at the same probability level \(\alpha\) , and compute the test statistic \(\text {AD}_{\text {obs}}=\left| \text {VaR}^F_\alpha -\text {VaR}^M_\alpha \right|\) .
Fit the GAMLSS model of interest— \({\mathcal {D}}(\mu ,\sigma ,\nu ,\tau )\) or \({\mathcal {D}}(\mu ,\sigma ,\nu ,\tau ,{\xi }_0)\) , depending on the available data—to the whole data of size \(n=n_{\text {F}}+n_{\text {M}}\) , where \(n_{\text {F}}\) and \(n_{\text {M}}\) are the sample sizes for females and males, respectively.
For \(r=1,\ldots ,B\) :
generate two samples of sizes \(n_{\text {F}}\) and \(n_{\text {M}}\) from the model fitted at step 2;
compute the AD statistic, say \(\text {AD}_r\) , on the generated samples.
Under \(H_0\) (VaRs for males and females are statistically non-different), \(\text {AD}_1,\ldots ,\text {AD}_B\) are equally likely and the p value of the testing procedure can be computed as
where \(F_{\text {Boot}}\left( \cdot \right)\) is the (stepwise) cumulative distribution function of \(\text {AD}_1,\ldots ,\text {AD}_B\) 49 .
In real data analyses, whose results are described in “ Distribution fitting results ”–“ Regression results ”, we consider a sufficiently large number of bootstrap replicates ( \(B=1000\) ); moreover, as usual in the insurance practice/literature, we consider the probability levels 0.95 and 0.99.
Distribution fitting results
Autobi data.
We start with the AutoBi data described in “ The automobile bodily injury claims ( AutoBi ) dataset ”. Supplementary figures C.1 – C.3 in Appendix C (online) show histograms and normal Q–Q plots for the total amount of losses (Supplementary figure C.1 ), for the losses reported by female claimants (Supplementary figure C.2 ), and for the losses reported by male claimants (Supplementary figure C.3 ). On the histograms we superimpose also a kernel density estimate (the red line) to give an idea on how the density of the observed data should look like. The horizontal axis of the histograms in Supplementary figures C.1 – C.2 is restricted to 250 for the sake of readability.
From the Q–Q plots we see that the distribution of losses for both females and males cannot be approximated by a Gaussian distribution (which is quite obvious); furthermore, the underlying distributions appear to be right skewed and heavy-tailed, as we expected. From all the histograms we confirm another recurrent feature of insurance loss data: the presence of a large amount of small losses and a lower number of high losses 16 , 18 . However, it should be noted that the maximum loss is registered for female claimants (1067.70), whereas the maximum for male claimants is much smaller (222.41). The kernel density estimate in the three cases seems to suggest a similar distribution, highly right-skewed and highly peaked. Further detailed information on the differences among the data can be obtained looking at the descriptive statistics in Table 1 . The mean loss is higher for females than males; however, looking at the median (less sensitive to extreme values) we see that there are no remarkable differences. Nonetheless, the variability (and then the risk) is much higher for females, as evidenced by the range and by the standard deviation. The females data are also more skewed and exhibit a more pronounced leptokurtosis. The VaR shows that an insurer should expect (with confidence at 99%) higher losses for male policy holders.
Supplementary Tables A.1 – A.3 in Appendix A (online) show the results of the distribution fitting. The results can be summarized as follows. First, we see that among the best models we have the Box-Cox t (selected by both the AIC and BIC as the best model for the total losses and females’ losses), the Truncated t and the Truncated Skew- t . Similar results are obtained for the female and male claimants, with a good performance of the Log-Johnson’s SU model, whereas also the Generalized Pareto and the Log-Power Exponential are competitive models. Second, we do not observe drastic differences in the selection of models for females and males. Finally, we see that distributions often neglected in applied works, such as the generalized Pareto or the log-Johnson’s SU, represent good alternatives to traditional models, whereas the variants of the normal distribution perform poorly for these data.
In order to check whether gender may explain differences in the loss distribution, we ran a GAMLSS regression for each model as described in the first part of “ How the research objectives of the paper are handled ”. The results are reported in Table 2 . The coefficient of gender was significant only for few distributions parameters and for an exiguous number of distributions. This is a strong evidence against the fact that the loss distribution is affected by gender, regardless of the considered parametric model.
Supplemntary tables A.4 – A.6 in Appendix A (online) show the VaR at 95% and 99% (computed numerically) for the three typologies of data for each of the selected models. We compared these results with the observed VaRs. In this case the ranking is very different because is based on the fact that the best distribution is the one that minimises the absolute distance from the empirical VaR. Summarily, we note that the results are very different if we consider a different confidence level. Furthermore, the results for the males in this case seem to differ from the results for the females. This is reasonable since extreme values are placed in the tail of the distribution. To test if these tail differences are statistically significant, we performed the parametric bootstrap test illustrated in “ Comparing the tail behaviour ”; the results are reported in the left part of Table 3 . For many models the differences resulted statistically significant; therefore, we should conclude that for these models the tail behaviour differs by gender. This does not necessarily imply that female claimants are riskier than male claimants, it simply means that VaRs are different.
ausprivauto0405 data
We now analyse the distribution fitting results for the ausprivauto0405 data. Supplementary figures C.4 – C.6 in Appendix C (online) show histograms and normal Q–Q plots for the total amount of losses (Supplementary figure C.4 ), for the losses reported by female claimants (Supplementary figure C.5 ) and for the losses reported by male claimants (Supplementary figure C.6 ). We remember that for scaling purposes the variable ClaimAmount is expressed in hundreds of dollars; furthermore, since we are considering only reported losses, we have excluded for the moment the zeros. In this case there was no need to restrict the horizontal axis of the histograms. The analysis of the histograms and of the normal Q–Q plots confirm the findings observed in the first dataset and characterising the majority of claims data: non-normality deriving from severe right skewness and heavy-tailed distributions, and the fact that the majority of the observations are concentrated in the first bins of the histograms. The analysis of the plots including also the zeros is redundant.
Table 4 shows the descriptive statistics for the ausprivauto0405 data (zeros excluded), whereas Table 5 shows the same statistics including also the zeros. We note that with respect to the other dataset, the losses for males are higher, on average and median, and more variable than the females. The females’ loss distribution is slightly more peaked but less skewed, whereas the males’ distribution including also the zeros shows higher kurtosis and skeweness. The VaR shows that an insurer should expect (with confidence at 99%) higher losses for male policy holders.
Supplementary Tables B.1 – B.3 in Appendix B (online) show the results of the distribution fitting. The ZA Generalized Gamma was selected as the best model by both the AIC and BIC for the total claims, and both the females and males claims. The ZA Log-Skew Normal, the ZA Log-Johnson’s SU and the ZA Generalized Inverse Gaussian were competitive models for all the three groups of data. Table 6 shows that, for this dataset, gender seems to play a role in explaining differences in the location parameter, and for some distributions also the dispersion parameter. As for the AutoBi data there is weak evidence that gender could explain the shape of the distribution.
Supplementary Tables B.4 – B.6 in Appendix B (online) show the estimated VaR values at 95% and 99% using the ZA parametric models. We can say that ZA Truncated Power Exponential, ZA Generalized Pareto and ZA Log-Skew Normal are good models to describe the tail behaviour of these data. As in the previous dataset there are differences between the ranks obtained using the two different levels. However, in this case the VaR bootstrap tests highlight that there are no significant differences in the tail of the distribution of male and female claimants when we consider a level of 95%, whereas significant differences emerge for a level of 99% (see Table 3 ).
Regression results
In this section we tackle the second research question of the paper, i.e. whether gender affects the claims distribution controlling for other available covariates. We fit regression models on the whole dataset and on the right tail of the data. The former approach is useful to quantify the effect of gender on the conditional location, scale and shape of the losses, the latter to quantify the effect of gender on the largest claims. For insurance companies this information is of relevant importance because it influences the solvency of the company and its policies. The GAMLSS framework consents to exploit the results of the distribution fitting in order to use the best model as underlying distribution.
The choice of functions \(g_i(\cdot )\) , \(i=1,\ldots ,4\) , to model the parameters of the considered models (refer to “ The GAMLSS regression framework ”) is limited to those available in the gamlss package. To model the tail of the data we used a different approach 34 , 48 . These are synthetically the steps followed.
We fitted a \(\alpha\) (95% and 99%) smooth quantile curve for LOSS (or ClaimAmount) against the explanatory variables using the R package cobs with automatic smoothing parameter selection.
We selected the cases above the \(\alpha\) quantile curve to work only with the tail of data.
We fitted a suitable GAMLSS truncated distribution to the tail data with the fitted \(\alpha\) quantile as truncation parameter. Since fitting via regression all the distributions is computationally prohibitive, the choice of an adequate distribution is determined using the best models obtained in “ Distribution fitting results ”. For the whole dataset we used the best model on the total claims distribution, while for the tail of data we used the best model as suggested by the VaR difference between the empirical VaR and the theoretical VaR. For the asprivauto0405 dataset we used GAMLSS zero-adjusted distributions.
We fitted regression models to assess the magnitude of the gender coefficient on the distribution of claims using, for the tail of data, the truncated distribution as determined in step 3.
The AutoBi dataset contains the following explanatory variables:
Attorney : whether the claimant is represented by an attorney.
Clmsex : claimant’s gender.
Marital : claimant’s marital status (= 1 if married, =2 if single, = 3 if widowed, and = 4 if divorced/separated).
Clminsur : whether or not the driver of the claimant’s vehicle was uninsured.
Seabelt : whether or not the claimant was wearing a seatbelt.
Clmage : claimant’s age.
As before, the dependent variable of the regression model is Loss , the claimant’s total economic loss (in thousands of dollars). In order to perform the regression model, we create dummy variables for Attorney (1 if yes), for Clmsex (1 if female), for each marital status, for Clminsur (1 if yes) and for Seatbelt (1 if yes). To avoid the dummy variables trap we exclude from the regression the dummy relative to divorced/separated, which becomes the benchmark category. Due to the presence of missing observations we use listwise deletion to eliminate the rows with missing information, therefore, the final dimension of the dataset in terms of rows is 1091.
Tables 8 and 9 show the result of the GAMLSS regressions. We could not fit the best model for the 99% quantile because the cases above it are too few to fit a suitable regression model. Figure 1 shows the wormplots for the AutoBi data. We used also other graphical tools for diagnostics and we estimated many models but we omit them from this paper for the sake of synthesis. The interested reader can contact the corresponding author for further elaborations.
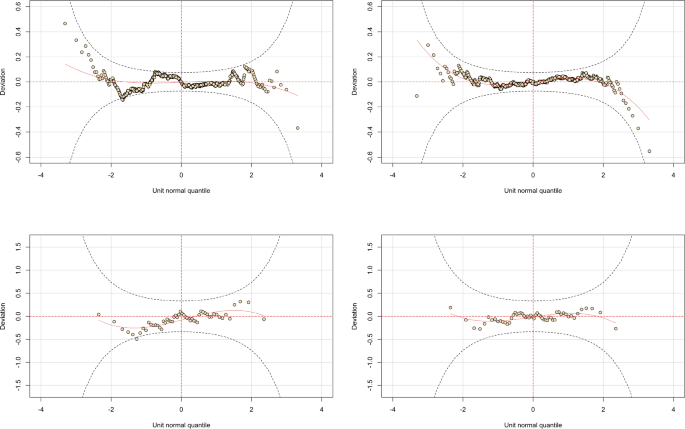
Wormplots of models I–IV (Tables 7 , 8 ) for the AutoBi data. Upper panels: model I on the left, model II on the right. Lower panels: model III on the left, model IV on the right.
AutoBi : regression model on total claims
In Table 7 , we report the results of two regression models. In model I we model only the equation of the parameter \(\mu\) using all the data and all the explanatory variables. The best model, as suggested by the analysis performed in “ AutoBi data ”, is the Box-Cox t distribution. The coefficient of our interest is the coefficient of Clmsex . Female claimants are associated with a positive and significant (at 5%) increase in the insurer losses (in thousands of dollars). The fit of the model is good enough as evidenced by the wormplot of the model in Fig. 1 (upper-left panel). However, we can obtain better estimates if we model also the other parameters, i.e. the scale parameter \(\sigma\) and the skeweness and kurtosis parameters \(\nu\) and \(\tau\) . To achieve this purpose, we gone through several models estimation. These models do not exhaust all the possible cases: given the fact that we can model four equations using several explanatory variables, the number of cases is high. This happens because not only can we create many models by simply changing the set of explanatory variables among those available (all models with one variable, with two variables, with three variables, and so on) but we can test these different combinations in four different equations (one for the mean, one for the dispersion parameter, and so on). However, we tried to cover all the relevant cases for the research question of this paper. These relevant cases are all those in which it was possible to retain the gender variable (given the research question of this paper), and were considered the best (using information criteria and graphical tools such as wormplots) among those with the gender variable for which the algorithm was able to converge.
Model II represents the best model, with respect to the many models that we estimated, in terms of computational feasibility(with this term we refer to the fact that some models were not computationally feasible and/or showed excessive time complexity), AIC and BIC, and goodness of fit as exhibited by the worm plot. The wormplot (Fig. 1 , upper-right panel) shows a better fit since all the points lie within the 95% confidence intervals given by the two elliptic curves. The coefficient of Clmsex preserved the same sign and approximately the same magnitude. On the other hand, Clmsex does not affect significantly the other parameters of the distribution. Finally, the significant coefficients of the other explanatory variables are economically reasonable. For example, considering the \(\mu\) equation, if the claimant is represented by an attorney, the insurance company tends to pay bigger amounts; if the age of the claimant increases, also the loss for the company increases, probably because elder people suffer more physical damages in car accidents.
AutoBi : regression model on the tail of data
The analysis for the tail of the data is reported in Table 8 . In this case the best distribution is selected according the result for the VaR estimation reported in online Supplementary table A.4 . Once again, we first estimate a model (III) only for the \(\mu\) equation and with all the explanatory variables ( Widowed is dropped because on 54 cases there were not sufficient observations for this variable). The other model (IV) is again the best one in the sense specified in “ AutoBi ”. In model IV we include a smoother for Clmsex ( pb is a smoothing additive term based on P-splines) for both the \(\mu\) and \(\sigma\) equations. Modeling also the other equations is not possible due to the low number of cases available in the tail of data.
These results are probably more interesting for an insurer. The coefficient of Clmsex is strongly significant and negative in both models. This means that female claimants entail lower losses for insurers, which means that the biggest losses are made for male claimants as confirmed by other works 9 , 10 . In model IV we also learn that the variable Clmsex has a negative effect also on the scale parameter, which means that female claimants decrease the spread in the tail of the distribution. Both the wormplots of model III and IV show a satisfactory fit (Fig. 1 , respectively, lower-left and lower-right panels). Once again, the presence of an attorney is associated with the biggest losses for the company.
ausprivauto0405
The dataset asprivauto0405 contains 9 variables. The dependent variable in our study is ClaimAmount , which is the sum of claim payments. In this case we do not use the term loss because the variable ClaimAmount contains also zeros. The explanatory variables available in the dataset are:
Exposure : the number of policy years.
VehValue : the vehicle value in thousand of Australian dollars.
VehAge : The vehicle age group divided into 4 classes: old cars, oldest cars, young cars and youngest cars. We created a dummy variable for each category.
VehBody : the vehicle body group divided into 13 classes: Bus, Convertible, Coupe, Hardtop, Hatchback, Minibus, Motorized caravan, Panel van, Roadster, Sedan, Station wagon, Truck and Utility. We created a dummy variable for each category.
Gender : the gender of the policyholder. We created a dummy variable for female claimants ( Female ).
DrivAge : the age of the policyholder divided into 6 classes: old people, older working people, oldest people, working people, young people and youngest people.
ClaimOcc : a dummy variable that indicates occurence of a claim.
ClaimNb : the number of claims.
We proceed as for the AutoBi dataset with the only difference that for this dataset we use the zero-adjusted GAMLSS framework. Also in this case we estimated several models but we report only the relevant cases for the sake of synthesis, which are, as mentioned earlier, those for which the gender variable could be retained and were selected as the best model among those for which the algorithm was able to converge.
ausprivauto0405 : regression model on total claims
We started with the ZA Generalized Gamma (GG) as underlying distribution since it was the best one to model the total amount of claims (online Supplementary table B.1 , Appendix B). Unfortunately, for this model the regression algorithm cannot reach convergence and this affects the reliability of the estimates. Given the problem of convergence, we tried the second and third best models as suggested by the analysis of Supplementary table B.1 (Appendix B, online), but for the ZA Log-Skew Normal Type 2 and the ZA Truncated Power Exponential we had also the same problem. Consequently, in order to improve the reliability of the regression model we discarded them. For the fourth best model, the ZA Log-Johnson’s SU, the algorithm converged.
Model V in Table 9 is the best in terms of computational feasibility, AIC, BIC, and wormplot. Nonetheless, we should warn the reader that better models could be obtained removing the variable Female , but this is not the purpose of this paper. Even though the coefficient of the variable ClaimOcc in the \(\xi _0\) equation is not significant, we include it to obtain a satisfactory wormplot (Fig. 2 , upper-left panel). We did not model also the equation for the \(\tau\) parameter because this would have increased enormously the time complexity. Just to give an idea, Model V in Table 9 converged after 220 iterations, a model with all variables in the four parameters did not converge even after 1500 iterations (a routine of about 24 h on a computer Intel Core i7-6500U CPU with 16 GB of RAM).
The variable Female affects significantly both the \(\mu\) and \(\sigma\) parameters and the sign is negative, which means that for female claimants the location and spread of claims is lower respect to male claimants. No significant effect resulted for the coefficient of Female on the parameter \(\nu\) . We also tried a model where the variable Female appeared also in the \(\xi _0\) equation, but the coefficient was highly non-significant. As in the AutoBi dataset we find the same effect of gender on the spread, but in this dataset, where we consider also the case of no-claims, we find that female claimants seem to be better clients for insurers also in terms of the location parameter.
ausprivauto0405 : regression model on the tail of data
We shift now our attention to the tail of the distribution. Since now we deal with data above the 95% and 99% quantiles, we are eliminating from the analysis all the zeros and dealing only with losses. In this case the regression framework becomes again the traditional GAMLSS without any need for zero-adjustment. Moreover, including the variable ClaimOcc becomes redundant because in the tail there are only realised claims.
Table 10 shows the results of the best model for cases above the 95% quantile among many competing models. The choice of the Truncated Power Exponential was determined by the results obtained comparing the empirical VaR with the VaR predicted by the models (online Supplementary table B.4 , Appendix B). One may notice that the analysis of VaR was conducted using ZA distributions, but this is a minor concern since the wormplot shows that the model offers a good fit for the data (Fig. 2 , upper-right panel). The coefficient of Female is significant and positive in the \(\mu\) equation, which means that claims in the tail increase for female claimants, whereas the coefficient of Female for the scale parameter is non-significant. We excluded the variable from the \(\nu\) equation because it was non-significant and it affected severely the goodness of fit of the model.
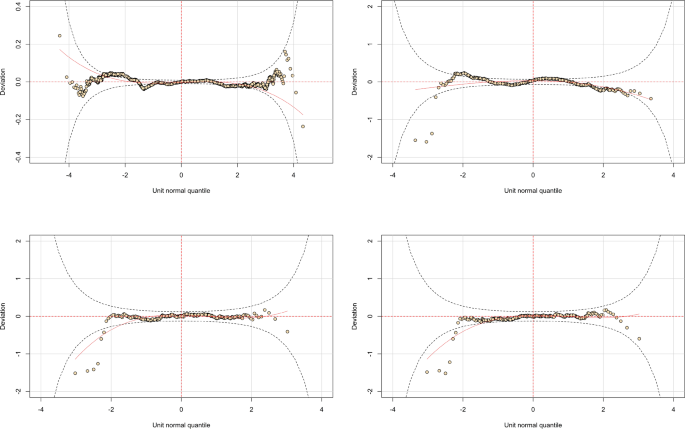
Wormplots of model V–VIII (Tables 9 , 10 , 11 ) for the ausprivauto0405 dataset. Upper panels: model V on the left, model VI on the right. Lower panels: model VII on the left, model VIII on the right.
Table 11 shows two possible models to describe the behaviour of extreme losses. Both models are good in terms of fit as highlighted by the wormplots in Fig. 2 . However, model VII should be preferred in terms of AIC and BIC. In model VIII the variable Female was removed from the equation for the location parameter because it was non-significant. The choice of the underlying distributions is again determined by computational feasibility and the results of Supplementary table B.4 (Appendix B, online). The coefficient of the variable Female is negative and significant at 10% for the location parameter in model VII and for the dispersion parameter in model VIII. These results are in line with the observed tail behaviour in the AutoBi dataset (Table 8 ).
Potential limitations
In this section, we address a series of shortcomings that could undermine the validity of our results.
Finding adequate data when dealing with actuarial studies is a relevant problem. Since in most cases researchers need micro-data, these data should contain enough information, especially when one aims to run regressions. In our case a suitable dataset must report the claimant’s gender and a sufficient number of other variables to avoid endogeneity problems. Furthermore, the ideal dataset should include an high number of observations and should contain data on a relevant geographical context to draw useful policy proposals. Nonetheless, the search of these data was not painless. We think that the data used in our study are a good compromise. The AutoBi dataset allows us to study the American context, where the problem of pricing based on gender is currently relevant. Moreover, the ausprivauto0405 dataset allows us to extend the analysis to a different geographical context, including also policy holders with no claims.
One may argue that the data used are old. We think that this is not a serious problem for many reasons. It is customary in actuarial studies to work with important and established datasets. Working with reliable and significant data is more important than working with new data. Furthermore, as already mentioned, finding data is very difficult. The literature is plenty of works dealing with older but established datasets. Just to mention: the Danish Fire losses dataset contains data gathered over the period 1980–1990, yet it is still one of the most used in contemporary studies 18 , 27 ; Fuzi et al. 33 used private car policies in year 2001; Blostein and Miljkovic 28 used data for the time period 1988–2001. Another relevant aspect to consider is that the distribution of claims generally presents the same statistical features over time and across countries.
We are aware of the fact that many other variables should have been added in the model, such as locations of accident, time of the accident, reason of the accident (drug, traffic rule disregard, etc.) and so on. Nonetheless, a dataset with such a detailed information, to our knowledge, is not freely accessible. The data used in this paper are among the most complete we could have found. Nevertheless, we must stress that the use of country-specific data limits the conclusions drawn from these datasets to the cases analyzed; therefore, further research using the same methodology but different data would help corroborate the results of this work. In this regard, the hope is that more insurers will make the data freely available to advance actuarial research.
The regression models used in our analysis served to study the relationship between gender and claims; however, no causal effect can be drawn from this setup. The point is that even conceiving a study capable of assessing the existence of a causal effect is troublesome because car accidents, and hence the amount of claims, are too complex to ideate any experiment. The lack of data makes this problem even worse. Nonetheless, the study of correlations is important to investigate whether a fair justification supporting a pricing practice exists.
External validity
One major drawback from using data of US and Australian companies is the impossibility of drawing general conclusions also for other countries. In general, a representative sample is needed to generalize the results to different countries. As one of the referees pointed out, it is reasonable to assume that our data are not representative of the many policy holders who have contracts with insurance companies. This obviously limits the application of our results to the scenarios analyzed, and their application to broader contexts depends strictly on how close one thinks our data are to a representative sample.
Despite this, our results are useful for different reasons. First, as we point out in “ Introduction ”, the problem of price discrimination based on gender is particularly relevant in the US. This work therefore can be used to provide statistical substance to the debate. Second, Australia and USA are two prominent markets for insurers worldwide. Third, even though driving habits are very different from country to country, countries with similar backgrounds can still use the results of our analysis. Fourth, the loss distribution is characterized by stylized facts that make the present study useful also for different data. Finally, our work can serve as a stimulus to produce further empirical evidence on this topic, providing new insights into the external validity of our results.
Conclusions and policy implications
This paper provides several results that extend and enrich the existing literature. These results can be split into two parts. In the first part of the paper, we focus our attention on finding the best statistical model to describe the distribution of claims. The variables investigated are taken from two important R packages. The Autobi dataset allows us to work on losses, as is commonly done in the literature 16 , 18 , 27 , whereas the ausprivauto0405 includes also zeros, allowing us to adopt the zero-adjusted distribution framework. Moreover, we conduct the analysis not only on total claims but also distinguishing by gender and analysing the tail behaviour of the data.
In the first part of the paper, we learn that male and female claims can be approximated by similar distributions, for example the Truncated Skew t Type 5 or the Truncated t Family for the AutoBi dataset. Secondly, regarding the effect of gender on the parameters of the distribution, we find a significant difference for the location parameter of many distributions for the second dataset (Table 3 ). Finally, thanks to a parametric bootstrap test based on the difference between VaRs, we can conclude that for many distributions a significant difference exists between the tail distribution of male and female claimants. Based on this evidence, few statistical differences seem to exist between male and females. However, this just evidences that the best model to describe the data may differ by gender. Unfortunately, these results are limited by the use of the only available data we could find. Therefore, this evidence, although based on sound statistical methodology, should be supported by the analysis on additional data to be generalized.
The second part of the paper is devoted to build a GAMLSS regression model to capture the “effect” of gender on the claims reported by the insurer. In this case we conduct the analysis using all the data and the tail (cases above the 95% and 99% quantiles). It seems that for female claimants the spread of losses is lower than for male claimants. For the \(\mu\) parameter the results are contrasting. For the AutoBi dataset we find evidence of a positive effect of female claimants on the location parameter when we consider all the data, whereas the effect is negative when we consider only the cases above the 95% quantile. For the ausprivauto0405 dataset we find evidence of a negative effect on location when considering all the data and on extreme losses (cases above 99%), and a positive effect when considering cases above 95%. The negative effect on the location parameter on the whole dataset is, in our opinion, a more reliable result than the positive effect for the AutoBi dataset because the inclusion of zeros accounts for the fact that females can be safer policy holders.
Nonetheless, the regression framework presents some limits. The principal limits are related to the high complexity of the computational routines and to the lack of data. We must rely on the adequacy of the control variables provided in the R packages. The strength of the empirical analysis is that the GAMLSS framework allowed us to study the phenomenon thoroughly, including also equations for the other parameters of the distribution (quite often neglected in empirical works) and weighting also the information carried by the zeros. The main limitation is the use of old, country-specific data, which reduces the scope of these results, although the analysis is robust and allows useful policy implications to be drawn for many countries.
In conclusion, our research enlightened that finding a “fair justification” 11 for applying different rates to male and female claimants is difficult. However, female claimants seem in most of the investigated cases to decrease the location parameter for extreme losses and when zeros are included. Furthermore, in our data female claimants have a beneficial effect on the scale parameter of claims, since for females the spread of losses decreases. We do not think that these results represent incontestable statistical reasons to differentiate policy rates by gender. Indeed, if we read our results together with other works that show that female policy holders are safer than men, we do not see any clear reason to charge women with higher rates. The same argument can be made for male policy holders. The evidence collected suggests in part that men may be riskier for insurance companies in some cases, but the evidence is not strong enough to justify charging higher rates. Future research can make use of the methodology presented in this paper to see if similar results are obtained for different data. In any case, this paper offers guidance to policy makers in the countries considered on whether unisex pricing policies should be promoted.
Data availability
Data can be accessed downloading the R packages reported in the paper.
Sivak, M. & Schoettle, B. Toward understanding on-road interactions of male and female drivers. Traffic Inj. Prev. 12 (3), 235–238 (2011).
Article PubMed Google Scholar
Massie, D. L., Campbell, K. L. & Williams, A. F. Traffic accident involvement rates by driver age and gender. Accid. Analy. Prev. 27 (1), 73–87 (1995).
Article CAS Google Scholar
Santamariña-Rubio, E., Pérez, K., Olabarria, M. & Novoa, A. M. Gender differences in road traffic injury rate using time travelled as a measure of exposure. Accid. Anal. Prev. 65 , 1–7 (2014).
Åkerstedt, T. & Kecklund, G. Age, gender and early morning highway accidents. J. Sleep Res. 10 (2), 105–110 (2001).
Kim, K., Brunner, I. M. & Yamashita, E. Modeling fault among accident—involved pedestrians and motorists in Hawaii. Accid. Anal. Prev. 40 (6), 2043–2049 (2008).
Ma, L. & Yan, X. Examining the nonparametric effect of drivers’ age in rear-end accidents through an additive logistic regression model. Accid. Anal. Prev. 67 , 129–136 (2014).
Zhou, H., Zhao, J., Pour-Rouholamin, M. & Tobias, P. A. Statistical characteristics of wrong-way driving crashes on Illinois freeways. Traffic Inj. Prev. 16 (8), 760–767 (2015).
Regev, S., Rolison, J. J. & Moutari, S. Crash risk by driver age, gender, and time of day using a new exposure methodology. J. Saf. Res. 66 , 131–140 (2018).
Article Google Scholar
Vorko-Jović, A., Kern, J. & Biloglav, Z. Risk factors in urban road traffic accidents. J. Saf. Res. 37 (1), 93–98 (2006).
Kim, J.-K., Ulfarsson, G. F., Kim, S. & Shankar, V. N. Driver-injury severity in single-vehicle crashes in California: A mixed logit analysis of heterogeneity due to age and gender. Accid. Anal. Prev. 50 , 1073–1081 (2013).
Thiery, Y. & Van Schoubroeck, C. Fairness and equality in insurance classification. Geneva Pap. Risk Insur. Issues Pract. 31 (2), 190–211 (2006).
Embrechts, P., McNeil, A. & Straumann, D. Correlation and dependence in risk management: Properties and pitfalls. Risk Manage. Value Risk Beyond 1 , 176–223 (2002).
Article MathSciNet Google Scholar
Bernardi, M. & Maruotti, A. Skew mixture models for loss distributions: A Bayesian approach. Insur. Math. Econom. 51 , 617–623 (2012).
Cooray, K. & Ananda, M. M. A. Modeling actuarial data with a composite lognormal-pareto model. Scand. Actuar. J. 2005 (5), 321–334 (2005).
Jeon, Y. & Kim, J. H. T. A gamma kernel density estimation for insurance loss data. Insur. Math. Econom. 53 (3), 569–579 (2013).
Punzo, A., Bagnato, L. & Maruotti, A. Compound unimodal distributions for insurance losses. Insur. Math. Econom. 81 , 95–107 (2018a).
Lane, M. N. Pricing risk transfer transactions. ASTIN Bull. J. IAA 30 (2), 259–293 (2000).
Eling, M. Fitting insurance claims to skewed distributions: Are the skew-normal and skew-student good models?. Insur. Math. Econom. 51 , 239–248. https://doi.org/10.1016/j.insmatheco.2012.04.001 (2012).
Klugman, S. A., Panjer, H. H. & Willmot, G. E. Loss Models: From Data to Decisions Vol. 715 (Wiley, 2012).
Punzo, A., Mazza, A. & Maruotti, A. Fitting insurance and economic data with outliers: A flexible approach based on finite mixtures of contaminated gamma distributions. J. Appl. Stat. 45 (14), 2563–2584 (2018).
Punzo, A. A new look at the inverse Gaussian distribution with applications to insurance and economic data. J. Appl. Stat. 46 (7), 1260–1287 (2019).
Tomarchio, S. D. & Punzo, A. Dichotomous unimodal compound models: Application to the distribution of insurance losses. J. Appl. Stat. 47 (13–15), 2328–2353. https://doi.org/10.1080/02664763.2020.1789076 (2020).
Article MathSciNet PubMed PubMed Central Google Scholar
Guillen, M., Prieto, F. & Sarabia, J. M. Modelling losses and locating the tail with the Pareto positive stable distribution. Insur. Math. Econom. 49 (3), 454–461 (2011).
Scollnik, D. P. M. & Sun, C. Modeling with Weibull–Pareto models. N. Am. Actuar. J. 16 (2), 260–272 (2012).
Pernagallo, G. & Torrisi, B. An empirical analysis on the degree of gaussianity and long memory of financial returns in emerging economies. Phys. A Stat. Mech. Appl. 527 , 121296. https://doi.org/10.1016/j.physa.2019.121296 (2019).
Brazauskas, V. & Kleefeld, A. Robust and efficient fitting of the generalized pareto distribution with actuarial applications in view. Insur. Math. Econom. 45 (3)), 424–435 (2009).
Miljkovic, T. & Grün, B. Modeling loss data using mixtures of distributions. Insur. Math. Econom. 70 , 387–396 (2016).
Blostein, M. & Miljkovic, T. On modeling left-truncated loss data using mixtures of distributions. Insur. Math. Econom. 85 , 35–46 (2019).
Mazza, A. & Punzo, A. DBKGrad: An R package for mortality rates graduation by discrete beta kernel techniques. J. Stat. Softw. 57 (Code Snippet 2), 1–18 (2014).
Mazza, A. & Punzo, A. Bivariate discrete beta kernel graduation of mortality data. Lifetime Data Anal. 21 (3), 419–433 (2015).
Article MathSciNet PubMed Google Scholar
Rousseeuw, P., Daniels, B. & Leroy, A. Applying robust regression to insurance. Insur. Math. Econom. 3 (1), 67–72 (1984).
Hill, R. C., Griffiths, W. E. & Lim, G. C. Principles of Econometrics (Wiley, 2018) ( ISBN 9781119342854 ).
Google Scholar
Fuzi, M. F., Jemain, A. A. & Ismail, N. Bayesian quantile regression model for claim count data. Insur. Math. Econ. 66 , 124–137 (2016).
Rigby, R. A., Stasinopoulos, M. D. & Voudouris, V. Discussion: A comparison of GAMLSS with quantile regression. Stat. Model. 13 (4), 335–348 (2013).
Frees, E. W. Regression Modeling with Actuarial and Financial Applications. International Series on Actuarial Science (Cambridge University Press, 2010).
De Jong, P. & Heller, G. Z. Generalized Linear Models for Insurance Data (Cambridge Books, 2008).
Book Google Scholar
Stasinopoulos, M., Enea, M., & Rigby, R. A. Zero adjusted distributions on the positive real line. (2017a). http://www.gamlss.com/wp-content/uploads/2018/01/ZeroAdjustedDistributions.pdf .
Rigby, R. A. & Stasinopoulos, M. D. Generalized additive models for location, scale and shape. J. R. Stat. Soc. Ser. C (Appl. Stat.) 54 (3), 507–554 (2005).
Hastie, T. J. & Tibshirani, R. J. Generalized Additive Models (CRC Press, 2017) ( ISBN 9781351445962 ).
Enea, M., Stasinopoulos, M., Rigby, B., & Hossain, A. gamlss.inf : Fitting Mixed (Inflated and Adjusted) Distributions (2019). https://CRAN.R-project.org/package=gamlss.inf.Version1.0-1 . Accessed 12 Mar 2019.
Stasinopoulos, M. D. & Rigby, R. A. Generalized additive models for location scale and shape (gamlss) in R . J. Stat. Softw. 23 (7), 1–46. https://doi.org/10.18637/jss.v023.i07 (2007).
Stasinopoulos, M. D., Rigby, R. A., Heller, G. Z., Voudouris, V. & De Bastiani, F. Flexible Regression and Smoothing: Using GAMLSS in R (CRC Press, 2017).
Chris Jones, M. & Faddy, M. J. A skew extension of the \(t\) -distribution, with applications. J. R. Stat. Soc. Ser. B (Stat. Methodol.) 65 (1), 159–174 (2003).
Tomarchio, S. D. & Punzo, A. Modelling the loss given default distribution via a family of zero-and-one inflated mixture models. J. R. Stat. Soc. A. Stat. Soc. 182 (4), 1247–1266 (2019).
Akaike, H. A new look at the statistical model identification. IEEE Trans. Autom. Control 19 (6), 716–723 (1974).
Article MathSciNet ADS Google Scholar
Schwarz, G. Estimating the dimension of a model. Ann. Stat. 6 (2), 461–464 (1978).
Pernagallo, G. An entropy-based measure of correlation for time series. Inf. Sci. 643 , 119272. https://doi.org/10.1016/j.ins.2023.119272 (2023).
Rigby, R. A., Stasinopoulos, M. D., Heller, G. Z. & De Bastiani, F. Distributions for Modeling Location, Scale, and Shape: Using GAMLSS in R . Chapman & Hall/CRC The R Series (CRC Press, 2019) ( ISBN 9781000699968 ).
Bagnato, L., De Capitani, L. & Punzo, A. Testing serial independence via density-based measures of divergence. Methodol. Comput. Appl. Probab. 16 (3), 627–641 (2014).
Download references
Acknowledgements
The authors are grateful for the comments made by the three anonymous Reviewers and the Editor.
This research did not receive any specific grant from funding agencies in the public, commercial, or not-for-profit sectors.
Author information
Authors and affiliations.
Department of Economics and Statistics “Cognetti de Martiis”, University of Turin, Lungo Dora Siena, 100A, 10153, Turin, Italy
Giuseppe Pernagallo
Department of Economics and Business, University of Catania, Corso Italia 55, 95129, Catania, Italy
Antonio Punzo & Benedetto Torrisi
You can also search for this author in PubMed Google Scholar
Contributions
All the authors contributed to all sections. The programming codes were written in R by G.P. and A.P.
Corresponding author
Correspondence to Antonio Punzo .
Ethics declarations
Competing interests.
The authors declare no competing interests.
Additional information
Publisher's note.
Springer Nature remains neutral with regard to jurisdictional claims in published maps and institutional affiliations.
Supplementary Information
Supplementary information., rights and permissions.
Open Access This article is licensed under a Creative Commons Attribution 4.0 International License, which permits use, sharing, adaptation, distribution and reproduction in any medium or format, as long as you give appropriate credit to the original author(s) and the source, provide a link to the Creative Commons licence, and indicate if changes were made. The images or other third party material in this article are included in the article's Creative Commons licence, unless indicated otherwise in a credit line to the material. If material is not included in the article's Creative Commons licence and your intended use is not permitted by statutory regulation or exceeds the permitted use, you will need to obtain permission directly from the copyright holder. To view a copy of this licence, visit http://creativecommons.org/licenses/by/4.0/ .
Reprints and permissions
About this article
Cite this article.
Pernagallo, G., Punzo, A. & Torrisi, B. Women and insurance pricing policies: a gender-based analysis with GAMLSS on two actuarial datasets. Sci Rep 14 , 3239 (2024). https://doi.org/10.1038/s41598-024-52959-8
Download citation
Received : 24 May 2023
Accepted : 25 January 2024
Published : 08 February 2024
DOI : https://doi.org/10.1038/s41598-024-52959-8
Share this article
Anyone you share the following link with will be able to read this content:
Sorry, a shareable link is not currently available for this article.
Provided by the Springer Nature SharedIt content-sharing initiative
By submitting a comment you agree to abide by our Terms and Community Guidelines . If you find something abusive or that does not comply with our terms or guidelines please flag it as inappropriate.
Quick links
- Explore articles by subject
- Guide to authors
- Editorial policies
Sign up for the Nature Briefing: AI and Robotics newsletter — what matters in AI and robotics research, free to your inbox weekly.

Advertisement

A data science approach to risk assessment for automobile insurance policies
- Regular Paper
- Published: 22 March 2023
- Volume 17 , pages 127–138, ( 2024 )
Cite this article
- Patrick Hosein 1
549 Accesses
7 Citations
1 Altmetric
Explore all metrics
In order to determine a suitable automobile insurance policy premium, one needs to take into account three factors: the risk associated with the drivers and cars on the policy, the operational costs associated with management of the policy and the desired profit margin. The premium should then be some function of these three values. We focus on risk assessment using a data science approach. Instead of using the traditional frequency and severity metrics, we instead predict the total claims that will be made by a new customer using historical data of current and past policies. Given multiple features of the policy (age and gender of drivers, value of car, previous accidents, etc.), one can potentially try to provide personalized insurance policies based specifically on these features as follows. We can compute the average claims made per year of all past and current policies with identical features and then take an average over these claim rates. Unfortunately there may not be sufficient samples to obtain a robust average. We can instead try to include policies that are “similar” to obtain sufficient samples for a robust average. We therefore face a trade-off between personalization (only using closely similar policies) and robustness (extending the domain far enough to capture sufficient samples). This is known as the bias–variance trade-off. We model this problem and determine the optimal trade-off between the two (i.e., the balance that provides the highest prediction accuracy) and apply it to the claim rate prediction problem. We demonstrate our approach using real data.
This is a preview of subscription content, log in via an institution to check access.
Access this article
Subscribe and save.
- Get 10 units per month
- Download Article/Chapter or eBook
- 1 Unit = 1 Article or 1 Chapter
- Cancel anytime
Price includes VAT (Russian Federation)
Instant access to the full article PDF.
Rent this article via DeepDyve
Institutional subscriptions
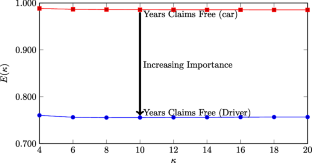
Similar content being viewed by others
Risk Assessment for Personalized Health Insurance Products
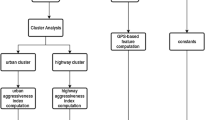
A “pay-how-you-drive” car insurance approach through cluster analysis
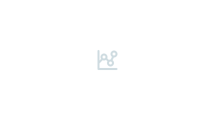
Unpriced and unseen: private information and taxi insurance purchases in Taiwan
Explore related subjects.
- Artificial Intelligence
Availability of data and materials
The data used for this publication are confidential, and hence, we are only permitted to provide results but cannot share the data.
Code Availability
The code used to generate results is also proprietary to the company, but we hope that our pseudo-code can be used if one wishes to apply the model to their datasets.
Albrecher, H., Bommier, A., Filipović, D., et al.: Insurance: models, digitalization, and data science. Eur. Actuar. J. 9 , 349–360 (2019)
Article MathSciNet Google Scholar
Bian, Y., Yang, C., Zhao, J.L., et al.: Good drivers pay less: a study of usage-based vehicle insurance models. Transp. Res. A: Policy Pract. 107 , 20–34 (2018). https://doi.org/10.1016/j.tra.2017.10.018
Article Google Scholar
David, M., Jemna, D.V.: Modeling the frequency of auto insurance claims by means of poisson and negative binomial models. Analele stiintifice ale Universitatii “Al I Cuza” din Iasi Stiinte economice/Scientific Annals of the“ Al I Cuza” (2015)
Denuit, M., Trufin, J.: Effective Statistical Learning Methods for Actuaries. Springer Actuarial Lecture Notes (2019)
Errais, E.: Pricing insurance premia: a top down approach. Annals of Operations Research, pp. 1–16 (2019)
Esfandabadi, Z.S., Ranjbari, M., Scagnelli, S.D.: (0) Prioritizing risk-level factors in comprehensive automobile insurance management: A hybrid multi-criteria decision-making model. Glob. Bus. Rev. https://doi.org/10.1177/0972150920932287 ,
Guelman, L.: Gradient boosting trees for auto insurance loss cost modeling and prediction. Expert Syst. Appl. 39 (3), 3659–3667 (2012)
Hanafy, M., Ming, R.: Machine learning approaches for auto insurance big data. Risks 9 (2), 42 (2021)
Hassani, H., Unger, S., Beneki, C.: Big data and actuarial science. Big Data Cogn. Comput. 4 , 40 (2020)
He, B., Zhang, D., Liu, S., et al.: Profiling driver behavior for personalized insurance pricing and maximal profit. In: 2018 IEEE International Conference on Big Data (Big Data), pp. 1387–1396. https://doi.org/10.1109/BigData.2018.8622491 (2018)
Hosein, P.: On the prediction of automobile insurance claims: the personalization versus confidence trade-off. In: 2021 IEEE International Conference on Technology Management, pp. 1–6. Operations and Decisions (ICTMOD), IEEE (2021)
Hosein, P., Rahaman, I., Nichols, K., et al.: Recommendations for long-term profit optimization. In: ImpactRS@ RecSys (2019)
Jeong, H., Valdez, E.A.: Predictive compound risk models with dependence. Insurance Math. Econom. 94 , 182–195 (2020)
Kanchinadam, T., Qazi, M., Bockhorst, J., et al.: Using discriminative graphical models for insurance recommender systems. In: 2018 17th IEEE International Conference on Machine Learning and Applications (ICMLA), pp. 421–428 (2018). https://doi.org/10.1109/ICMLA.2018.00069
Liu, Y., Wang, B.J., Lv, S.G.: Using multi-class adaboost tree for prediction frequency of auto insurance. J. Appl. Finance Bank. 4 (5), 45 (2014)
Google Scholar
Lundberg, S.M., Lee, S.I.: A unified approach to interpreting model predictions. In: Guyon, I., Luxburg, U.V., Bengio, S., et al. (Eds.) Advances in Neural Information Processing Systems, vol 30. Curran Associates, Inc (2017). https://proceedings.neurips.cc/paper/2017/file/8a20a8621978632d76c43dfd28b67767-Paper.pdf
Qazi, M., Fung, G.M., Meissner, K.J., et al.: An insurance recommendation system using bayesian networks. In: Proceedings of the Eleventh ACM Conference on Recommender Systems. Association for Computing Machinery, New York, NY, USA, RecSys ’17, pp. 274–278 (2017). https://doi.org/10.1145/3109859.3109907
Qazi, M., Tollas, K., Kanchinadam, T., et al.: Designing and deploying insurance recommender systems using machine learning. WIREs Data Min. Knowl. Discovery 10 (4), e1363 (2020). https://doi.org/10.1002/widm.1363
Su, X., Bai, M.: Stochastic gradient boosting frequency-severity model of insurance claims. PLoS ONE 15 (8), e0238000 (2020)
Zhang, Y., Dukic, V.: Predicting multivariate insurance loss payments under the bayesian copula framework. J. Risk Insurance 80 (4), 891–919 (2013)
Download references
The authors did not receive support from any organization for the submitted work.
Author information
Authors and affiliations.
Department of Computer Science, The University of the West Indies, St. Augustine, Trinidad and Tobago
Patrick Hosein
You can also search for this author in PubMed Google Scholar
Contributions
The sole author performed the research, wrote the code for evaluating the solution and wrote the entire paper
Corresponding author
Correspondence to Patrick Hosein .
Ethics declarations
Conflict of interest.
The authors have no conflicts of interest to declare that are relevant to the content of this article.
Ethics approval
Not applicable.
Consent to participate
Consent for publication, additional information, publisher's note.
Springer Nature remains neutral with regard to jurisdictional claims in published maps and institutional affiliations.
Rights and permissions
Springer Nature or its licensor (e.g. a society or other partner) holds exclusive rights to this article under a publishing agreement with the author(s) or other rightsholder(s); author self-archiving of the accepted manuscript version of this article is solely governed by the terms of such publishing agreement and applicable law.
Reprints and permissions
About this article
Hosein, P. A data science approach to risk assessment for automobile insurance policies. Int J Data Sci Anal 17 , 127–138 (2024). https://doi.org/10.1007/s41060-023-00392-x
Download citation
Received : 13 September 2022
Accepted : 05 March 2023
Published : 22 March 2023
Issue Date : January 2024
DOI : https://doi.org/10.1007/s41060-023-00392-x
Share this article
Anyone you share the following link with will be able to read this content:
Sorry, a shareable link is not currently available for this article.
Provided by the Springer Nature SharedIt content-sharing initiative
- Motor insurance
- Machine learning
- Premium pricing
- Claims prediction
Mathematics subject classification
- Find a journal
- Publish with us
- Track your research
- Insights and Resources
- White Paper
- Auto Insurance Trends Report
LexisNexis® U.S. Auto Insurance Trends Report
Top 5 auto insurance trends to watch.
The annual LexisNexis® Risk Solutions U.S. Auto Insurance Trends Report explores key trends from the previous year and offers insights to help insurers make more informed business decisions. This year, we identified five trends impacting U.S. consumer auto insurance shopping, claims, driving violations and more.
As U.S. auto insurers steer through the challenges of the current market, these trends offer insights to help navigate the road ahead. Insurers can leverage proprietary and industry consortium data to help gain a clearer view of performance benchmarks and plan for the road ahead.
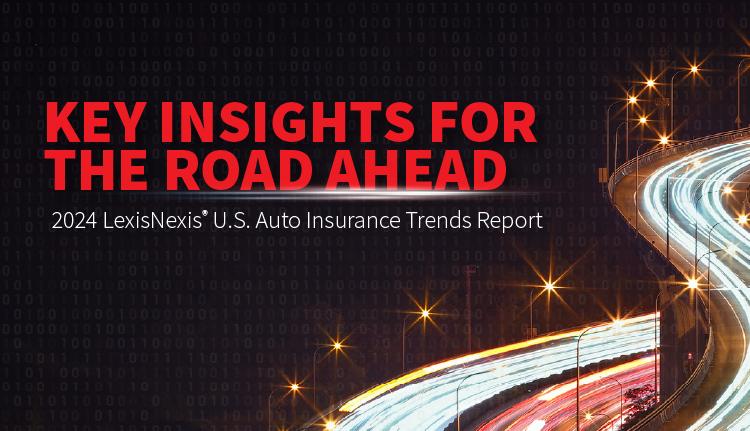
Access the report to explore :
- High claim severities show little signs of slowing down
- Insurers take aggressive steps to address profitability challenges
- Consumers respond to market conditions by shopping and switching auto policies
- Risky driving behavior persists
- As electric vehicle sales grow, so do insurance risks
Access the report now
We appreciate your interest.
Explore each section to gain additional insights
High claim severities show little signs of slowing down.

- Sustained rise in claims severity
- Increase in uninsured motorist and attorney-represented claims
- Total loss claims
- Length of repair times
- Rising costs of medical bills, towing services and storage costs
Trend Details
Both the severity and frequency of claims, including severe auto physical damage and bodily injury, have increased since 2020. Bodily injury severity has increased 20% in the post-pandemic years.
More than a quarter of collision claims were deemed total losses in 2023. While that percentage is the same as the previous year, total loss claims have jumped 29% since 2020.
More claimants are seeking advice from attorneys before settling. In fact, according to our 2023 survey, a majority of claimants who hired an attorney for their last claim would most likely do so again.
Consumers respond sharply to market conditions by shopping and switching auto policies
As rate increases went into effect throughout 2023, many consumers reacted by shopping for lower policy prices. Many of those who shopped for lower rates ended up switching insurers, resulting in new policy growth of 6.2% last year.

Source: LexisNexis Risk Solutions Insurance Demand Meter
Get the latest auto insurance shopping trend data in our quarterly report.
Insurance Demand Meter
Driving behavior continues to change dramatically
As miles driven returned to 2019 levels in 2023, moving and non-moving violations returned as well. In fact, all driving violations have increased 4% from 2022 to 2023. Speeding, distracted driving and DUIs all increased year over year, resulting in escalating risk profiles. Get additional details in this blog post .
Both major and minor speeding violations continue to rise, consistent with the post-pandemic trend.
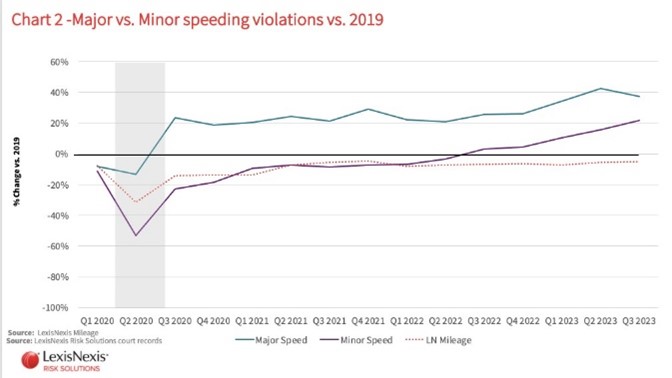
Unlike other violations, DUIs take longer to move through the court system, so they can be a lagging metric. Comparing the first six months of 2022 to the first six months of 2023, DUI violations increase 8%. Our latest driving violations blog dives into current unsafe driving trends.
Distracted Driving
Distracted driving violations continue to rise as people return to the roads. Younger drivers continue to be the more susceptible problematic age group when it comes to increases in distracted driving. This increase in violations, plus Gen Z’s inexperience compared to other generations, has implications for both personal lines and commercial lines insurers. Read more in our latest blog article .
Access the Report
Download previous lexisnexis® u.s. auto insurance trends reports.
2023 Auto Insurance Trends Report
2022 auto insurance trends report, 2021 auto insurance trends report .
- DOI: 10.1109/CCISP59915.2023.10355772
- Corpus ID: 266476702
Research on Vehicle Pricing Factors of Auto Insurance Based on Machine Leaning
- Xu Zhu , Yingnan Liu , Dongyu Li
- Published in 8th International Conference… 17 November 2023
- Business, Computer Science
- 2023 8th International Conference on Communication, Image and Signal Processing (CCISP)
10 References
Gradient boosting trees for auto insurance loss cost modeling and prediction, insurance premium prediction via gradient tree-boosted tweedie compound poisson models, delta boosting machine with application to general insurance, generalized additive models for location, scale and shape - discussion, related papers.
Showing 1 through 3 of 0 Related Papers
Academia.edu no longer supports Internet Explorer.
To browse Academia.edu and the wider internet faster and more securely, please take a few seconds to upgrade your browser .
Enter the email address you signed up with and we'll email you a reset link.
- We're Hiring!
- Help Center
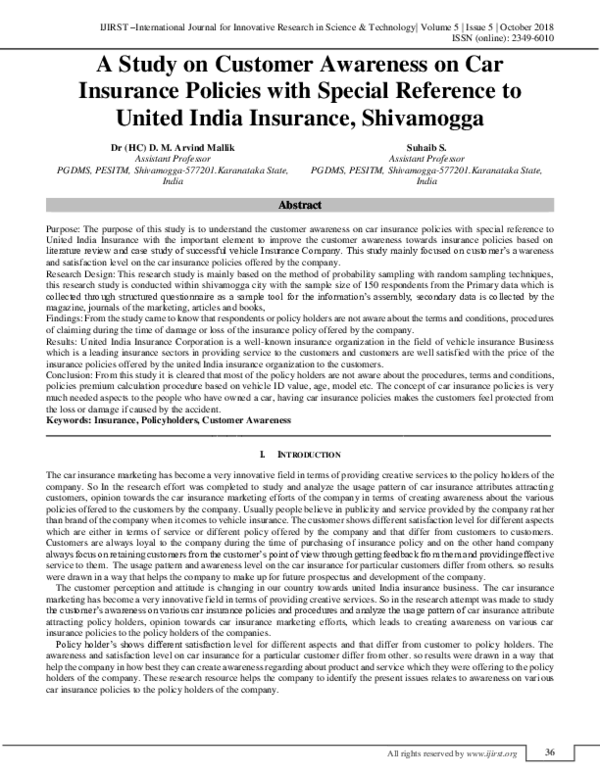
A Study on Customer Awareness on Car Insurance Policies with Special Reference to

Purpose: The purpose of this study is to understand the customer awareness on car insurance policies with special reference to United India Insurance with the important element to improve the customer awareness towards insurance policies based on literature review and case study of successful vehicle Insurance Company. This study mainly focused on customer's awareness and satisfaction level on the car insurance policies offered by the company. Research Design: This research study is mainly based on the method of probability sampling with random sampling techniques, this research study is conducted within shivamogga city with the sample size of 150 respondents from the Primary data which is collected through structured questionnaire as a sample tool for the information's assembly, secondary data is collected by the magazine, journals of the marketing, articles and books, Findings: From the study came to know that respondents or policy holders are not aware about the terms and conditions, procedures of claiming during the time of damage or loss of the insurance policy offered by the company. Results: United India Insurance Corporation is a well-known insurance organization in the field of vehicle insurance Business which is a leading insurance sectors in providing service to the customers and customers are well satisfied with the price of the insurance policies offered by the united India insurance organization to the customers. Conclusion: From this study it is cleared that most of the policy holders are not aware about the procedures, terms and conditions, policies premium calculation procedure based on vehicle ID value, age, model etc. The concept of car insurance policies is very much needed aspects to the people who have owned a car, having car insurance policies makes the customers feel protected from the loss or damage if caused by the accident.
Related Papers
Journal of emerging technologies and innovative research
Josephine Stella A.
Motor insurance contributes to one third of the premium income for the General Insurance industry in India. The growth of the economy and consequently, the standard of living of the people, further supported by the increased choice for the customer and entry of large number of automobile players led to a sharp increase in motor insurance. The main aim of the motor insurance is to protect the people from the loss arising out of accident. It covers loss made vehicle. The awareness of the people towards Insurance is low in India generally it is very difficult to create the buying attitude among the prospective buyers towards the different kinds of insurance. The General Insurance Corporation finds it difficult to identify and to make the clients believe the concepts of Insurance Policy. At the same time, the policy will be valued for only one year. The lack of insurance awareness is the main problem in general insurance particularly in motor insurance. Vehicle owners buy it only on the...
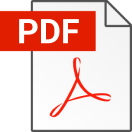
International Journal of Research in Commerce and Management
Dhiraj Jain
vikas kumar gautam
International Journal of Management, Technology, and Social Sciences (IJMTS)
Srinivas Publication , Swati Basu Ghose
The primary purpose of vehicle insurance is to cover the vehicle against damage, personal injury, and third-party liability. In addition to this, some insurance companies also provide value-added services such as roadside assistance and other services in return of the amount called as premium which attracts a large number of customers. However, our study shows that vehicle owners give maximum importance to the cost of insurance in terms of the annual premium. Primary data has been collected through questionnaire and analysed to ascertain about the factors responsible for taking out vehicle insurance, choice between private and public sector insurance companies, preferred insurance companies among the major players in the field, factors that play a role in the customers' choice of a particular insurance company, customers' opinion about the affordability of the premium to be paid, customers' satisfaction with their chosen company, whether customers consider fast and efficient service as a deciding factor, and whether the brand value of the company plays a role in the customers' choice.
Turkish Online Journal of Qualitative Inquiry (TOJQI) Volume 12, Issue 2, March 2021: 801-815
veera venkat satyanarayana penumarthi
An effort is being made in this study to show how Vijayawada customers see insurance services. Respondents to a five-point Likert scale questionnaire were used to compile the data for this research. More than 377 people were surveyed to determine their degree of knowledge and attitude about insurance services. According to a new study, Vijayawada customers' perceptions about insurance services are strongly influenced by socioeconomic and demographic factors. Insurance businesses in Vijayawada may use the results of this research as a basis for developing marketing plans that include socio-demographic and economic factors.
Dharmesh Motwani
IJIRIS:: AM Publications,India
IJIRIS International Journal of Innovative Research in Information Security
The journey of new India Insurances scheme has stated 17th century England. Insurances are the co– operative device who distribute the loss caused by a particular risk.When any insurances company face any types of mismanagement, they should look for a market for that policy instead of constantly lying to the public or with their clients. Market competition brings decrease in price of the insurances company and increase in the quality but customer play their part according to their views. In the unpredictable society insurances paly a secure part but customers face many other problems. In this paper we will discussed about the problems faced by the customers and also their reasons why many people don’t have faith in the insurances company. The paper is focused on the problems faces by the customer in insurances sector.
Pranjal Bezborah
Sathishkumar Ramasamy
the present study analyzes the attitudes of policyholders of Life Insurance Corporation of India with special reference to Tiruchirappalli district, the data were collected and analysed as per the requirement of the study. The primary data were collected from the respondents through interview schedule in June 2011 to March 2012. The study has adopted proportionate stratified random sampling method for selecting 500 respondents. The results revealed the fact that the factors, age, education, marital status, family size, number of earning members, income and awareness have influenced the level of attitude of the policyholders. Whereas the factors like sex, occupation and patronage mentality did not influence the level of attitude.
IP innovative publication pvt ltd
IP Innovative Publication Pvt. Ltd.
With the increase in risk there is need of insurance to bear the losses. Insurance is the instrument used as the financial protection against various contingency. This paper examines the customer perception towards the General Insurance. A study had been conducted at Gwalior region with the sample of 200 respondents to find out the perception of the customer (policyholders). In this context, the respondents’ opinion on the various related statements were collected with a 5 point scaling. Reliability, Factor analysis, multivariate technique had been applied on the data. The result concluded that loyalty, transparency, proficiency, reliable and convenient services are the five factors from the 18 statements on the basis of the expectation of the customers. This study signifies that various customer had different expectation from the insurance company in the studied area.
Loading Preview
Sorry, preview is currently unavailable. You can download the paper by clicking the button above.
RELATED PAPERS
Dr.Dhiraj Jain
iaeme iaeme
Carmelo Panepinto
Murangirwa Festus
International Journal of Engineering and Advanced Technology
Srimannarayana Gajula
Ahmed Salman Syed
SHS Web of Conferences
Sesilya Kempa
Mohammad Jamal Hossain
IOSR Journal of Business and Management
Festus Epetimehin
International Journal of Law Management & Humanities (IJLMH) A peer-reviewed, HeinOnline, MANUPATRA, Google Scholar & 23 databases Indexed Int'l Journal with IF of 6.530.
Jayshree Singh
Velmurugan Ramasamy
As-Syirkah: Islamic Economic & Financial Journal
Nurul Jannah
Journal of Asian Finance, Economics and Business
adinoto nursiana
Arti Sharma
Sarang S Bhola
PARIPEX INDIAN JOURNAL OF RESEARCH
Chitralekha Dhadhal
Restaurant Business
Bhavna Pathak
International Journal of Engineering & Technology
Dr. Arun Vijay
Nepalese Journal of Insurance and Social Security
Aayush Poudel
Lakshmi Sivaramakrishnan
The Journal of Risk Management
Bonny Bagenda
Sunil Kumar
IAEME PUBLICATION
IAEME Publication
Publishing India Group
https://www.ijrrjournal.com/IJRR_Vol.6_Issue.8_Aug2019/Abstract_IJRR0041.html
International Journal of Research & Review (IJRR)
RELATED TOPICS
- We're Hiring!
- Help Center
- Find new research papers in:
- Health Sciences
- Earth Sciences
- Cognitive Science
- Mathematics
- Computer Science
- Academia ©2024
- MyNewMarkets.com
- Claims Journal
- Insurance Journal TV
- Academy of Insurance
- Carrier Management
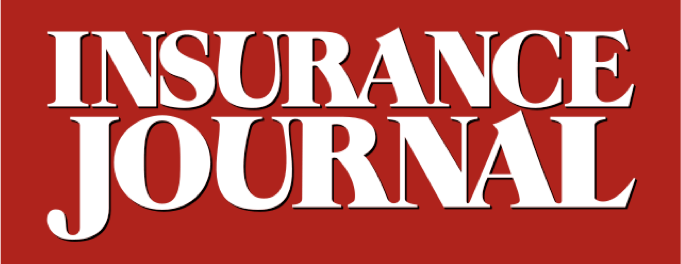
Featured Stories
- Liberty Mutual’s ‘Deliberate Actions’ to Profit
- Viewpoint: Generative AI in Insurance Isn’t Working
Current Magazine
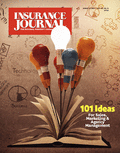
- Read Online
- Personal Auto Driving P/C Insurers to 2024 Underwriting Profit
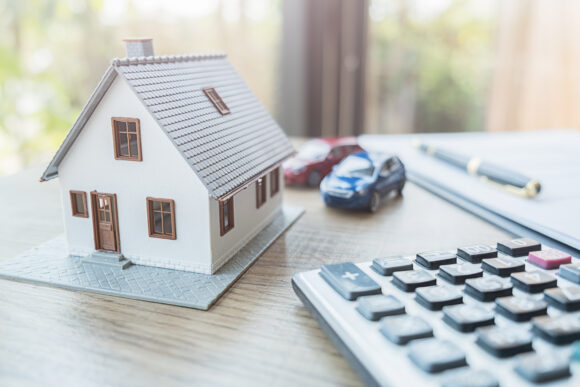
S&P Global Market Intelligence is forecasting a combined ratio of 99.2 for the U.S. P/C insurance industry overall in 2024, signifying a return to underwriting profitability for the first time since 2021.
According to the analysis, private passenger auto insurance is expected “to make a dramatic return to underwriting profitability,” with S&P GMI projecting a personal auto 2024 combined ratio of 98.4, down from 104.9 in 2023 and 112.2 in 2022. With personal auto representing almost 35% of overall P/C insurance industry premiums written, that turnaround is what will drive the overall underwriting profit for the industry, according to S&P GMI projections presented in the “S&P Global Market Intelligence 2024 U.S. P&C Insurance Market Report.”
The combined ratio for the industry overall will drop 2.5 points to 99.2 from a level of 101.7 in 2023, and improve a bit more to 99.0 in 2025, the report shows.
S&P GMI’s forecast that industrywide underwriting results will be back in the black this year contrasts projections from analysts at the Insurance Information Institute and Milliman. They indicate that the industry will record another overall underwriting loss — and an underwriting loss in the personal auto line — in 2024, with recovery not anticipated until 2025.
With homeowners results lagging behind personal auto, S&P GMI is not anticipating that the personal lines segment overall will record an underwriting profit this year or next year. The personal lines combined ratio will come close to breakeven in 2026, according to the five-year projections provided by line and segment in the report. The projected personal lines combined ratios are 101.2 for 2024 and 100.2 for 2025, compared to 106.7 in 2023.
Weather catastrophes continue to weigh on the homeowners business, and although the report notes (in a section outlining the methodology) that S&P GMI assumes a normal catastrophe load for exposed business lines, for homeowners, the combined ratio is still pegged at an unfavorable 107.3 for 2024. That’s down from 110.9 in 2023, which had been the worst result in a dozen years.
For personal lines insurers, results will also continue to be uneven as mutuals and reciprocals need more than rate hikes to recover from challenges to auto and homeowners lines in recent years, the report says. (Discussed further below.)
The commercial lines segment will remain profitable throughout the five-year projection period, although charts in the report reveal that the projected 2024 commercial lines combined ratio of 96.0 will creep up to 98.2 by 2028.
The highest combined ratio projection for the U.S. P/C insurance industry overall is also indicated for the last year of the forecast period — 99.9 for 2028 — with underwriting results remaining in the black throughout the five-year time frame.
The report calls out a “faster-than-expected erosion” in workers’ compensation results as the most significant risk to S&P GMI’s commercial lines outlook for continued underwriting profitability.
Commenting on drivers of earnings beyond underwriting profit, the S&P GMI report notes that “the industry stands to benefit materially from higher interest rates” after years and years of net yields on invested assets coming in at record low levels.
“The industry’s ongoing effort to rotate into high-quality, higher-yielding assets will serve as a catalyst for growth in net income even to the extent that underwriting margins remain relatively modest,” the report says.
“A key variable will be the industry’s continued adherence to disciplined underwriting in a more forgiving investment landscape,” the report says.
Top Line Growth Set to Drop
On the top line, S&P GMI projects a final year of double-digit industrywide growth in 2024, with premiums across all lines jumping 10 percent for full-year 2024 but then falling to 5.9% or lower in 2025-2028.
“A substantial decline in the pace of increases in private auto premium volumes serves as the primary contributor to our projection that the rate of growth in U.S. P/C industry direct premiums written will recede to the mid-single digits on an annual basis from 2025 through 2028,” said Tim Zawacki, insurance sector strategist at S&P Global Market Intelligence in a statement accompanying the report. Zawacki explained that the magnitude of the improvement in underwriting results is likely to prompt “fairly rapid retreats in the scope and scale” of the significant personal auto rate increases pursued by most market participants over the last three years.
According to the report, S&P GMI’s RateWatch application showed aggregate approved rate changes through the first half of 2024 dropping to 5.9%, down from an “outsized full-year 2023 tally” of 15.2%. Still, the rate hikes taking effect in the second half of 2023 have yet to fully impact income statements through 2024, the report notes, adding that rate momentum has persisted in the other major personal line: homeowners.
While the report shows direct premium growth for all personal lines hovering around 14% for 2023 and 2024, and dropping to the 4-7% range in the subsequent five years, commercial lines growth rates have already fallen below double-digit levels of 2021 and 2022. The projected commercial lines direct premium growth rate is 6.2% for 2024, with growth rates thereafter in the 5-6% range.
Overall, the 10% growth in direct premiums written across all lines marks the fourth straight year for growth of 9.5% or more.
“The duration of the expansion is without precedent in at least a generation as the previous comparable hard-market cycle in the early part of the 21st Century had only three years with annual growth rates of 9.5% or more,” the report notes.
Relative Performance
The report includes line-by-line details for historical combined ratio and growth rates going back to 2014 and projected forward to 2028, along with aggregations for the personal and commercial lines sector and the U.S. P/C insurance industry. For each line analyzed and for the segments, the report also presents charts displaying combined ratios and premiums for the top 15 players.
In private passenger auto, for example, the report shows that only Erie (ranked 12th by 2023 premium) and CSAA (ranked 14th) posted worse combined ratios than the biggest personal auto insurer, State Farm, in 2023. State Farm’s 115.3 combined ratio towered over the ratios recorded for the second- and third-largest writers — Progressive and GEICO, coming in at 94.2 and 92.1, respectively. Erie Insurance posted a 123.3 ratio and CSAA’s result was 117.1, according to S&P GMI.
The report includes a section devoted to S&P GMI’s performance rankings — determined by S&P GMI analysts using 13 financial metrics from 2023 statutory filings to measure rates of return, balance sheet expansion, investment performance and prior-accident-year reserve development, in addition to underwriting profitability and premium growth. Commercial lines players dominate those rankings, but balance could return, with tailwinds benefiting personal lines carriers while headwinds are already emerging in certain parts of the commercial lines, Zawacki observed in a recent article he wrote about the rankings for Carrier Management.
Analyzing relative premium growth rates for individual carriers, S&P GMI shows that Tesla Insurance was the biggest sprinter with a triple-digit growth rate of nearly 768 percent putting direct and net written premiums at roughly $110 million.
Personal v. Commercial; Stock vs. Mutual
The S&P GMI report reveals clear contrasts in underwriting results for commercial vs. personal lines insurers. Except for 2020, personal lines underwriting results haven’t bested commercial lines since 2012.
In 2023, the commercial segment combined ratio was more than 15 points better than personal lines, with the difference projected to narrow to about 11 points ’24.
Bifurcating the industry another way — by ownership structure — S&P GMI calculates a 107.9 cumulative combined ratio for the 2021-2023 period for mutuals, stock insurers that are part of mutual insurance holding company structures, and reciprocal exchanges (excluding policyholder dividends). That result was 11.3 percentage points higher than the rest of the industry.
“Mutuals tend to be more concentrated in the embattled home and auto business than stock insurers, conferring upon them the misfortune of experiencing the worst of the current cycle. But rate alone may be insufficient for them to overcome the depths of the challenges they face,” the report says.
Going forward, S&P GMI’s outlook anticipates greater market-share concentration among the largest private auto writers in the coming years, reasoning that “data, analytics and economies of scale remain critically important in a commoditized business.”
For homeowners, in contrast, S&P expects more market fragmentation as national carriers trim back exposure to loss-prone markets.
The report also includes analysis of growth in the E&S market, including premium rankings of E&S writers for homeowners and commercial property lines, drawing from a discussion of market trends included in an earlier report, The 2024 US Excess & Surplus Market.
This article first appeared in Carrier Management, a sister publication to Insurance Journal.
Topics Carriers Auto Profit Loss Personal Auto Underwriting Property Casualty
Was this article valuable?
Thank you! Please tell us what we can do to improve this article.
Thank you! % of people found this article valuable. Please tell us what you liked about it.
Here are more articles you may enjoy.
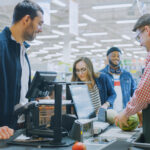
Written By Susanne Sclafane
Sclafane is Executive Editor of Carrier Management, a publication of Wells Media Group serving property/casualty insurance carrier executives. She is a media professional with deep background in the P/C insurance industry including 25 years as editor and reporter for trade magazines, online news services, digital journals. Her prior experience includes 14 years as a casualty actuary.
Latest Posts:
- Are Captives the Answer for Uninsured HOAs? Utah Opens Door
- How to Outperform: Don’t Outsource Underwriting to MGAs
From This Issue
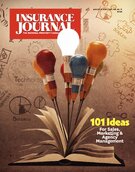
101 Sales, Marketing & Agency Management Ideas; Markets: Private Client, Non-Profits; Corporate Profiles, Summer Edition
Interested in auto .
Get automatic alerts for this topic.
- Categories: Features
- Have a hot lead? Email us at [email protected]
Insurance Jobs
- Property Adjuster – Field Estimating Kalamazoo, MI - Kalamazoo, MI
- AVP, Strategic Marketing, Personal Insurance - Hartford, CT
- Bilingual Entry Level Insurance Sales (2557) - Mobile, AL
- Enrollment Specialist – Health Insurance Carrier – REMOTE - Remote
- Senior Counsel - Las Vegas, NV

- Inflation, the Economy and Workers’ Comp: A Positive Outlook
- Umbrella Liability: The Triple Upsell Possibility for Personal Lines Clients
- Where the AI Risks Are: Swiss Re's Top 10 Ranking by Industry
- How to Succeed in the Entertainment Insurance Business: Get creative producing with a small cast of underwriters and limited capacity.
- How Do Insurers Define Systemic Cyber Risk?

- Why Onsite Inspections Are Essential for Accurate Large Loss Property Damage Claims
- Report: Auto Insurance Shopping Continued to Rise, but Rates Stabilized in Q2
- Beryl-Battered Grenada Becomes First to Utilize Government Bond Hurricane Clause
- U.S. State AGs Seek Triple Damages Against Live Nation for Concertgoers
- Texas Grid Faces Biggest Test of Summer With Extreme Heat

- August 22 HO-14: More Than a Mature HO-4
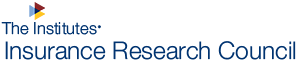
Search form
Research publications, personal auto insurance affordability in georgia.

- Purchase PDF ()
- Purchase Printed ()
- View News Release
Personal Auto Insurance Affordability in Michigan
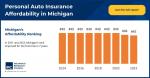
Homeowners Insurance Affordability: Trends and State Variations
- Purchase PDF (1000.00)
Underinsured Motorists, 2017-2022
- Purchase PDF (2500.00)
Personal Insurance Affordability in Louisiana
Homeowners insurance affordability: countrywide trends and state comparisons, uninsured motorists, 2017-2022.
Public Perceptions Regarding the Fairness of Insurance Rating Factors
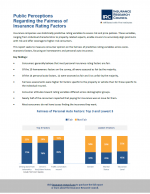
Trends in Personal Auto Insurance Claims: 2002–2022
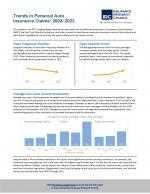
Trends in Homeowners Insurance Claims: 2001–2021
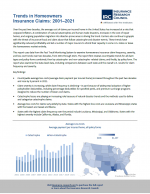

COMMENTS
Furthermore, as reported in this research, the analysis of keyword co-occurrence and its subsequent network visualisation contributed to highlighting the knowledge framework relevant to telematics-based automotive insurance. The knowledge structure of car insurance studies using telematics was mapped using keyword co-occurrence analysis, and ...
This study explored machine learning algorithms to det ect fraudulent. vehicle insurance claims. The r esearch evaluated AdaBoost, XGboostNB, SVM, LR, D T, ANN, and RF. AdaBoost and XGBoost classi ...
Denneberg first proposed the Poisson-gamma model to study the frequency of nonhomogeneous insurance policy claims and obtained good fitting results in empirical research on auto insurance . The generalized linear model (GLM) is a widely accepted model for premium ratemaking of automobile insurance in recent decades.
Abstract. With the continuous development of machine learning, enterprises using machine learning methods to mine potential data information has become a hot topic in the research of major insurance companies. In this paper, the features of auto insurance data are analyzed, and the most important features affecting auto renewal are mined.
Based on a data set of 91 papers and 22 industry studies, we analyse the impact of artificial intelligence on the insurance sector using Porter's (1985) value chain and Berliner's (1982) insurability criteria. Additionally, we present future research directions, from both the academic and practitioner points of view. The results illustrate that both cost efficiencies and new revenue ...
EC1 limits the scope of this study to the latest developments in automobile insurance fraud detection research. This allows the study to serve as an extension to Benedek et al. (2022), which includes papers from before 2022 only. Meanwhile, EC2 and EC4 shall aid in discovering peer-reviewed primary research, whereas EC3 and EC5 eliminate papers ...
The purpose of this paper is to survey the automobile insurance fraud detection literature in the past 31 years (1990-2021) and present a research agenda that addresses the challenges and opportunities artificial intelligence and machine learning bring to car insurance fraud detection.,Content analysis methodology is used to analyze 46 peer ...
The growing trend in the number and severity of auto insurance claims creates a need for new methods to efficiently handle these claims. Machine learning (ML) is one of the methods that solves this problem. As car insurers aim to improve their customer service, these companies have started adopting and applying ML to enhance the interpretation and comprehension of their data for efficiency ...
For example, a recent article of the HuffPost (Car Insurance Companies Charge Women Higher Rates Than Men Because They Can, by Elaine S. Povich, 2019, HuffPost) revealed that several studies in ...
Many past papers have focused on recommender systems for insurance companies where one of a small number of insurance products is offered. In [17, 18], they used historical data of existing and past customers to determine the most suitable policy for a new customer.In this case, a relatively small number of insurance products are available, and hence, the number of customers who have been ...
Low-Income Drivers Looking To Increase Auto Insurance Coverage Pay A Penalty Compared With Customers Who Already Had Higher Coverage Consumer Federation of America (2019) Auto insurance companies Allstate, Farmers, Geico, Liberty Mutual, Progressive, and State Farm, usually charge an average of $254 more annually for auto insurance to shoppers
It is found that UBI can benefit drivers, insurers and society, and recommends for insurers derived from users' views to provide to drivers more control over the user interface and over the way driving feedback is given to them. Usage-Based Insurance (UBI) is an application of Intelligent Transportation Systems (ITS) in the context of car insurance. UBI refers to insurance models in which ...
This study considers how automotive insurance pr oviders incorporate machinery learning in their. company, and explores how ML models can apply to insurance big data. We utilize various ML ...
Insurance Research Council (IRC). In addition to documenting that auto insurance has become more affordable for both the nation as a whole and across states, the study also showed that the ... Auto Insurance Expenditure to Income Ratio - Decade Average 1990s average 2000s average 2010s average. Author:
To investigate the impact that the widespread deployment of autonomous vehicles (AVs) could have on automobile insurance in the United States, RAND Corporation researchers interviewed 43 subject-matter experts from 35 stakeholder organizations and conducted an extensive literature review. A key finding from their research is that the existing ...
This paper examines loss development trends in personal auto liability insurance, as well as updating prior research on commercial auto liability insurance. It extends previous work in attempting to use Annual Statement data through year-end 2022 ... inflated claim costs are the cause of recent increases in auto insurance prices and attract the ...
Top 5 Auto Insurance Trends To Watch. The annual LexisNexis® Risk Solutions U.S. Auto Insurance Trends Report explores key trends from the previous year and offers insights to help insurers make more informed business decisions. This year, we identified five trends impacting U.S. consumer auto insurance shopping, claims, driving violations and ...
The research outcomes and model algorithm will provide an important methodological reference for the selection of auto insurance pricing elements in China, as well as a significant foundation for the personalization of auto insurance. In this paper, the claim data and vehicle configuration data of insurance companies are taken as the research object, and the vehicle pricing factors of auto ...
the insurance policy and the outcome of applying optimal premium rates in several customer segments are shown. I. Introduction The sensitivity of car insurance customers to price and how this affects their retention has been a subject of intense analysis in the insurance market research literature. Different
most insurance companies experience great loss as far as car insurance as shown in Figure 1, one of the main challenges face the insurance companies nowadays, is to define a proper premium for each risk represented by those customers [4], the majority of insurance companies keep the data on the history of its operations in a data warehouse
Motor insurance contributes to one third of the premium income for the General Insurance industry in India. The growth of the economy and consequently, the standard of living of the people, further supported by the increased choice for the customer and entry of large number of automobile players led to a sharp increase in motor insurance.
DOI: 10.4236/gep.2016.412002 December 1, 2016. Car Insurance Plans Could Make a Society Safer. Mohammad Zand, Amir Samimi, Khashayar Khavarian. Civil Engineering Department, Shar if University of ...
According to the analysis, private passenger auto insurance is expected "to make a dramatic return to underwriting profitability," with S&P GMI projecting a personal auto 2024 combined ratio ...
Research Report Summaries. Homeowners Insurance Affordability: Trends and State Variations. Underinsured Motorists, 2017-2022. Uninsured Motorists, 2017-2022. Trends in Personal Auto Insurance Claims: 2002-2022 . Public Opinions on Credit Scoring and the Use of Credit-Based Insurance Scores. State Variations in Auto Insurance Affordability
CHICAGO, Aug. 13, 2024 (GLOBE NEWSWIRE) -- Auto insurance shopping volume set a new record for the second consecutive quarter, according to new research from TransUnion (NYSE: TRU).
Car insurance rates are up 18.6% for the 12 months ended in July, according to Consumer Price Index data released Wednesday. That marked the third-largest jump in prices over the past year across ...