

How to Write the Methods Section of a Scientific Article
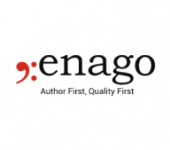
What Is the Methods Section of a Research Paper?
The Methods section of a research article includes an explanation of the procedures used to conduct the experiment. For authors of scientific research papers, the objective is to present their findings clearly and concisely and to provide enough information so that the experiment can be duplicated.
Research articles contain very specific sections, usually dictated by either the target journal or specific style guides. For example, in the social and behavioral sciences, the American Psychological Association (APA) style guide is used to gather information on how the manuscript should be arranged . As with most styles, APA’s objectives are to ensure that manuscripts are written with minimum distractions to the reader. Every research article should include a detailed Methods section after the Introduction.
Why is the Methods Section Important?
The Methods section (also referred to as “Materials and Methods”) is important because it provides the reader enough information to judge whether the study is valid and reproducible.
Structure of the Methods Section in a Research Paper
While designing a research study, authors typically decide on the key points that they’re trying to prove or the “ cause-and-effect relationship ” between objects of the study. Very simply, the study is designed to meet the objective. According to APA, a Methods section comprises of the following three subsections: participants, apparatus, and procedure.
How do You Write a Method Section in Biology?
In biological sciences, the Methods section might be more detailed, but the objectives are the same—to present the study clearly and concisely so that it is understandable and can be duplicated.
If animals (including human subjects) were used in the study, authors should ensure to include statements that they were treated according to the protocols outlined to ensure that treatment is as humane as possible.
- The Declaration of Helsinki is a set of ethical principles developed by The World Medical Association to provide guidance to scientists and physicians in medical research involving human subjects.
Research conducted at an institution using human participants is overseen by the Institutional Review Board (IRB) with which it is affiliated. IRB is an administrative body whose purpose is to protect the rights and welfare of human subjects during their participation in the study.
Literature Search
Literature searches are performed to gather as much information as relevant from previous studies. They are important for providing evidence on the topic and help validate the research. Most are accomplished using keywords or phrases to search relevant databases. For example, both MEDLINE and PubMed provide information on biomedical literature. Google Scholar, according to APA, is “one of the best sources available to an individual beginning a literature search.” APA also suggests using PsycINFO and refers to it as “the premier database for locating articles in psychological science and related literature.”
Authors must make sure to have a set of keywords (usually taken from the objective statement) to stay focused and to avoid having the search move far from the original objective. Authors will benefit by setting limiting parameters, such as date ranges, and avoiding getting pulled into the trap of using non-valid resources, such as social media, conversations with people in the same discipline, or similar non-valid sources, as references.
Related: Ready with your methods section and looking forward to manuscript submission ? Check these journal selection guidelines now!
What Should be Included in the Methods Section of a Research Paper?
One commonly misused term in research papers is “methodology.” Methodology refers to a branch of the Philosophy of Science which deals with scientific methods, not to the methods themselves, so authors should avoid using it. Here is the list of main subsections that should be included in the Methods section of a research paper ; authors might use subheadings more clearly to describe their research.
- Literature search : Authors should cite any sources that helped with their choice of methods. Authors should indicate timeframes of past studies and their particular parameters.
- Study participants : Authors should cite the source from where they received any non-human subjects. The number of animals used, the ages, sex, their initial conditions, and how they were housed and cared for, should be listed. In case of human subjects, authors should provide the characteristics, such as geographical location; their age ranges, sex, and medical history (if relevant); and the number of subjects. In case hospital records were used, authors should include the subjects’ basic health information and vital statistics at the beginning of the study. Authors should also state that written informed consent was provided by each subject.
- Inclusion/exclusion criteria : Authors should describe their inclusion and exclusion criteria, how they were determined, and how many subjects were eliminated.
- Group characteristics (could be combined with “Study participants”) : Authors should describe how the chosen group was divided into subgroups and their characteristics, including the control. Authors should also describe any specific equipment used, such as housing needs and feed (usually for animal studies). If patient records are reviewed and assessed, authors should mention whether the reviewers were blinded to them.
- Procedures : Authors should describe their study design. Any necessary preparations (e.g., tissue samples, drugs) and instruments must be explained. Authors should describe how the subjects were “ manipulated to answer the experimental question .” Timeframes should be included to ensure that the procedures are clear (e.g., “Rats were given XX drug for 14 d”). For animals sacrificed, the methods used and the protocols followed should be outlined.
- Statistical analyses: The type of data, how they were measured, and which statistical tests were performed, should be described. (Note: This is not the “results” section; any relevant tables and figures should be referenced later.) Specific software used must be cited.
What Should not be Included in Your Methods Section?
Common pitfalls can make the manuscript cumbersome to read or might make the readers question the validity of the research. The University of Southern California provides some guidelines .
- Background information that is not helpful must be avoided.
- Authors must avoid providing a lot of detail.
- Authors should focus more on how their method was used to meet their objective and less on mechanics .
- Any obstacles faced and how they were overcome should be described (often in your “Study Limitations”). This will help validate the results.
According to the University of Richmond , authors must avoid including extensive details or an exhaustive list of equipment that have been used as readers could quickly lose attention. These unnecessary details add nothing to validate the research and do not help the reader understand how the objective was satisfied. A well-thought-out Methods section is one of the most important parts of the manuscript. Authors must make a note to always prepare a draft that lists all parts, allow others to review it, and revise it to remove any superfluous information.

m so confused about ma research but now m okay so thank uh so mxh
Mil gracias por su ayuda.
Rate this article Cancel Reply
Your email address will not be published.
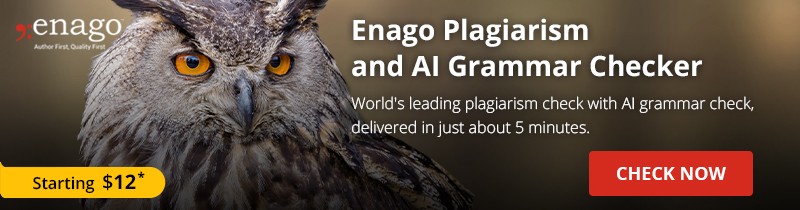
Enago Academy's Most Popular Articles
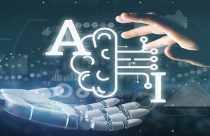
- AI in Academia
- Infographic
- Manuscripts & Grants
- Reporting Research
- Trending Now
Can AI Tools Prepare a Research Manuscript From Scratch? — A comprehensive guide
As technology continues to advance, the question of whether artificial intelligence (AI) tools can prepare…
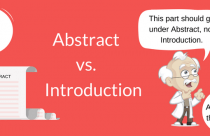
Abstract Vs. Introduction — Do you know the difference?
Ross wants to publish his research. Feeling positive about his research outcomes, he begins to…
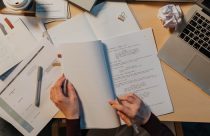
- Old Webinars
- Webinar Mobile App
Demystifying Research Methodology With Field Experts
Choosing research methodology Research design and methodology Evidence-based research approach How RAxter can assist researchers
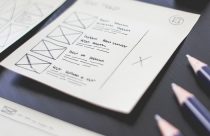
- Manuscript Preparation
- Publishing Research
How to Choose Best Research Methodology for Your Study
Successful research conduction requires proper planning and execution. While there are multiple reasons and aspects…
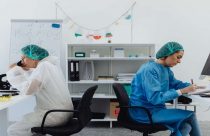
Top 5 Key Differences Between Methods and Methodology
While burning the midnight oil during literature review, most researchers do not realize that the…
How to Draft the Acknowledgment Section of a Manuscript
Discussion Vs. Conclusion: Know the Difference Before Drafting Manuscripts

Sign-up to read more
Subscribe for free to get unrestricted access to all our resources on research writing and academic publishing including:
- 2000+ blog articles
- 50+ Webinars
- 10+ Expert podcasts
- 50+ Infographics
- 10+ Checklists
- Research Guides
We hate spam too. We promise to protect your privacy and never spam you.
I am looking for Editing/ Proofreading services for my manuscript Tentative date of next journal submission:
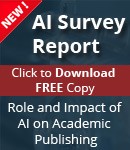
As a researcher, what do you consider most when choosing an image manipulation detector?

How To Write a Methodology for a Publishable Journal Article
How To Write a Methodology for a Publishable Journal Article Writing about the significant results and exciting implications of advanced research necessitates writing about the methodology employed to achieve those results. Although a well-written methodology for an academic or scientific journal article may come across as straightforward and even simple, the process of writing it is usually neither. Research procedures are often complicated, and decisions about the inclusion or exclusion of details in the methodology written for an article can be as challenging as initial decisions about the research design were. Unfortunately, there is no single set of rules or practices that apply to every project or article: journal requirements vary, and each research project as well as its methodology is unique. The following tips therefore do not address every situation and possibility, but they do outline a number of common requirements and concerns for the scholarly author who is working to write a suitable and informative methodology for an article that will prove worthy of publication in a reputable peer-reviewed journal. • The heading, subheadings, length, content and arrangement of material for an article methodology varies among journals, so always read the author instructions or guidelines and follow them precisely to ensure that you write an acceptable methodology. • A research methodology section in a journal article should inform readers about exactly what was done during the research, including the initial preparations and the way in which information or evidence (data) was gathered or generated, measured and analysed. • A methodology should also offer a rationale for the research methods, so be sure to explain why the research design and individual procedures are appropriate for examining the problem investigated, answering the research questions or testing the hypotheses. • A discussion of the broader contexts of the methodology may be desirable. This might involve introducing theoretical concepts and situating the methodology within the body of current knowledge or practice through descriptions and citations of published studies. • Established methods in a field can usually be simply named or identified rather than described in detail, but if those methods have been modified in any way, the modifications will require more extensive explanation as well as some justification. • Instruments commonly used in the field need only be named in most cases, but new instruments developed or adapted for the research should be carefully explained, perhaps with illustrations. Readers should be told exactly how all instruments were used. • The subject or subjects studied should always be described. Whether the article reports medical research on human subjects or palaeographical research on ancient books, give all the details about the objects or individuals studied and explain why they were chosen. • The variables considered and manipulated should be introduced and discussed. Independent variables should be distinguished from dependent ones, and the risks and precautions associated with confounding variables should be disclosed and explained. PhD Thesis Editing Services • Study and control groups and categories should be described in detail. The criteria for selection and division should be explained with precision, and any changes or problems associated with groups and categories as the research progressed should be itemised. • If ethical approval or informed consent was required for the research, the fact that it was obtained as appropriate should be stated. The same is the case with any kind of approval or permission required to make use of instruments, methods or data in the research. • Remember that a methodology should let readers know that the research procedures used are consistent with sound and accepted practice in the field. Any procedures that may seem questionable should therefore be justified in relation to the research objectives, • A methodology should provide the information necessary for experts in the field to judge whether the methods used are valid, reliable and replicable. Providing the details required for such judgements, such as statements about limitations, is therefore imperative. • The written style of a methodology should be clear, direct and concise. Errors and ambiguities must be avoided to prevent confusion, and unnecessary words should be removed. The procedures described have been done, so the past tense is appropriate. • Like all parts of an academic or scientific article, a methodology should be carefully proofread, edited and revised before submission. Ensure that all mistakes are corrected and that procedures and processes are presented in a chronological or logical order. Why Our Editing and Proofreading Services? At Proof-Reading-Service.com we offer the highest quality journal article editing , phd thesis editing and proofreading services via our large and extremely dedicated team of academic and scientific professionals. All of our proofreaders are native speakers of English who have earned their own postgraduate degrees, and their areas of specialisation cover such a wide range of disciplines that we are able to help our international clientele with research editing to improve and perfect all kinds of academic manuscripts for successful publication. Many of the carefully trained members of our expert editing and proofreading team work predominantly on articles intended for publication in scholarly journals, applying painstaking journal editing standards to ensure that the references and formatting used in each paper are in conformity with the journal’s instructions for authors and to correct any grammar, spelling, punctuation or simple typing errors. In this way, we enable our clients to report their research in the clear and accurate ways required to impress acquisitions proofreaders and achieve publication.
Our scientific proofreading services for the authors of a wide variety of scientific journal papers are especially popular, but we also offer manuscript proofreading services and have the experience and expertise to proofread and edit manuscripts in all scholarly disciplines, as well as beyond them. We have team members who specialise in medical proofreading services , and some of our experts dedicate their time exclusively to PhD proofreading and master’s proofreading , offering research students the opportunity to improve their use of formatting and language through the most exacting PhD thesis editing and dissertation proofreading practices. Whether you are preparing a conference paper for presentation, polishing a progress report to share with colleagues, or facing the daunting task of editing and perfecting any kind of scholarly document for publication, a qualified member of our professional team can provide invaluable assistance and give you greater confidence in your written work.
If you are in the process of preparing an article for an academic or scientific journal, or planning one for the near future, you may well be interested in a new book, Guide to Journal Publication , which is available on our Tips and Advice on Publishing Research in Journals website.
Guide to Academic and Scientific Publication
How to get your writing published in scholarly journals.
It provides practical advice on planning, preparing and submitting articles for publication in scholarly journals.
PhD Success
How to write a doctoral thesis.
If you are in the process of preparing a PhD thesis for submission, or planning one for the near future, you may well be interested in the book, How to Write a Doctoral Thesis , which is available on our thesis proofreading website.
PhD Success: How to Write a Doctoral Thesis provides guidance for students familiar with English and the procedures of English universities, but it also acknowledges that many theses in the English language are now written by candidates whose first language is not English, so it carefully explains the scholarly styles, conventions and standards expected of a successful doctoral thesis in the English language.
Why Is Proofreading Important?
To improve the quality of papers.
Effective proofreading is absolutely vital to the production of high-quality scholarly and professional documents. When done carefully, correctly and thoroughly, proofreading can make the difference between writing that communicates successfully with its intended readers and writing that does not. No author creates a perfect text without reviewing, reflecting on and revising what he or she has written, and proofreading is an extremely important part of this process.

- Interlibrary Loan and Scan & Deliver
- Course Reserves
- Purchase Request
- Collection Development & Maintenance
- Current Negotiations
- Ask a Librarian
- Instructor Support
- Library How-To
- Research Guides
- Research Support
- Study Rooms
- Research Rooms
- Partner Spaces
- Loanable Equipment
- Print, Scan, Copy
- 3D Printers
- Poster Printing
- OSULP Leadership
- Strategic Plan
Scholarly Articles: How can I tell?
- Journal Information
- Literature Review
- Author and affiliation
- Introduction
- Specialized Vocabulary
Methodology
- Research sponsors
- Peer-review
The methodology section or methods section tells you how the author(s) went about doing their research. It should let you know a) what method they used to gather data (survey, interviews, experiments, etc.), why they chose this method, and what the limitations are to this method.
The methodology section should be detailed enough that another researcher could replicate the study described. When you read the methodology or methods section:
- What kind of research method did the authors use? Is it an appropriate method for the type of study they are conducting?
- How did the authors get their tests subjects? What criteria did they use?
- What are the contexts of the study that may have affected the results (e.g. environmental conditions, lab conditions, timing questions, etc.)
- Is the sample size representative of the larger population (i.e., was it big enough?)
- Are the data collection instruments and procedures likely to have measured all the important characteristics with reasonable accuracy?
- Does the data analysis appear to have been done with care, and were appropriate analytical techniques used?
A good researcher will always let you know about the limitations of his or her research.
- << Previous: Specialized Vocabulary
- Next: Results >>
- Last Updated: Apr 15, 2024 3:26 PM
- URL: https://guides.library.oregonstate.edu/ScholarlyArticle
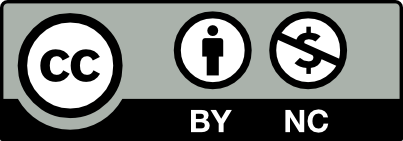
Contact Info
121 The Valley Library Corvallis OR 97331–4501
Phone: 541-737-3331
Services for Persons with Disabilities
In the Valley Library
- Oregon State University Press
- Special Collections and Archives Research Center
- Undergrad Research & Writing Studio
- Graduate Student Commons
- Tutoring Services
- Northwest Art Collection
Digital Projects
- Oregon Explorer
- Oregon Digital
- ScholarsArchive@OSU
- Digital Publishing Initiatives
- Atlas of the Pacific Northwest
- Marilyn Potts Guin Library  
- Cascades Campus Library
- McDowell Library of Vet Medicine


- Expert Journal of Finance
- Expert Journal of Economics
- Expert Journal of Marketing
- Expert Journal of Business and Management
- Send Your Article
- Google Plus
How to Write a Research Methodology for Your Academic Article
This article is part of an ongoing series on academic writing help of scholarly articles. Previous parts explored how to write an introduction for a research paper and a literature review outline and format .
The Methodology section portrays the reasoning for the application of certain techniques and methods in the context of the study.
For your academic article, when you describe and explain your chosen methods it is very important to correlate them to your research questions and/or hypotheses. The description of the methods used should include enough details so that the study can be replicated by other Researchers, or at least repeated in a similar situation or framework.
Every stage of your research needs to be explained and justified with clear information on why you chose those particular methods, and how they help you answer your research question or purpose.
As the Authors, in this section you get to explain the rationale of your article for other Researchers. You should focus on answering the following questions:
- How did you collect the data or how did you generate the data?
- Which research methods did you use?
- Why did you choose these methods and techniques?
- How did you use these methods for analyzing the research question or problem?
The responses to these questions should be clear and precise, and the answers should be written in past tense.
First off, let’s establish the differences between research methods and research methodology.
Research Methods and Research Methodology
As an Academic and Author of valuable research papers, it’s important not to confuse these two terms.
Research Methodology Definition
Research Methodology refers the discussion regarding the specific methods chosen and used in a research paper. This discussion also encompasses the theoretical concepts that further provide information about the methods selection and application.
In other words, you should highlight how these theoretical concepts are connected with these methods in a larger knowledge framework and explain their relevance in examining the purpose, problem and questions of your study. Thus, the discussion that forms your academic article’s research methodology also incorporates an extensive literature review about similar methods, used by other Authors to examine a certain research subject.
Research Method Definition
A Research Method represents the technical steps involved in conducting the research. Details about the methods focus on characterizing and defining them, but also explaining your chosen techniques, and providing a full account on the procedures used for selecting, collecting and analyzing the data.
Important Tips for a Good Methodology Section
The methodology section is very important for the credibility of your article and for a professional academic writing style.
Data Collection or Generation for Your Academic Article
Readers, academics and other researchers need to know how the information used in your academic article was collected. The research methods used for collecting or generating data will influence the discoveries and, by extension, how you will interpret them and explain their contribution to general knowledge.
The most basic methods for data collection are:
Secondary data
Secondary data are data that have been previously collected or gathered for other purposes than the aim of the academic article’s study. This type of data is already available, in different forms, from a variety of sources.
Secondary data collection could lead to Internal or External secondary data research.
Primary data
Primary data represent data originated for the specific purpose of the study, with its research questions. The methods vary on how Authors and Researchers conduct an experiment, survey or study, but, in general, it uses a particular scientific method.
Primary data collection could lead to Quantitative and Qualitative research.
Readers need to understand how the information was gathered or generated in a way that is consistent with research practices in a field of study . For instance, if you are using a multiple choice survey, the readers need to know which questionnaire items you have examined in your primary quantitative research. Similarly, if your academic article involves secondary data from FED or Eurostat it is important to mention the variables used in your study, their values, and their time-frame.
For primary research, that involve surveys, experiments or observations, for a valuable academic article, Authors should provide information about:
- Study participants or group participants,
- Inclusion or exclusion criteria
Selecting and Applying Research Methods
Establishing the main premises of methodology is pivotal for any research because a method or technique that is not reliable for a certain study context will lead to unreliable results, and the outcomes’ interpretation (and overall academic article) will not be valuable.
In most cases, there is a wide variety of methods and procedures that you can use to explore a research topic in your academic article. The methods section should fully explain the reasons for choosing a specific methodology or technique .
Also, it’s essential that you describe the specific research methods of data collection you are going to use , whether they are primary or secondary data collection.
For primary research methods, describe the surveys, interviews, observation methods, etc.
For secondary research methods, describe how the data was originally created, gathered and which institution created and published it.
Reasons for Choosing Specific Research Methods
For this aspect that characterizes a good research methodology, indicate how the research approach fits with the general study , considering the literature review outline and format , and the following sections.
The methods you choose should have a clear connection with the overall research approach and you need to explain the reasons for choosing the research techniques in your study, and how they help you towards understanding your study’s purpose.
Data Analysis Methods
This section should also focus on information on how you intend to analyze your results .
Describe how you plan and intend to achieve an accurate assessment of the hypotheses, relationships, patterns, trends, distributions associated with your data and research purpose.
The data type, how it was measured, and which statistical tests were conducted and performed, should be detailed and reported in an accurate manner.
For explaining the data analysis methods, you should aim to answer questions, such as:
- Will your research be based on statistical analysis?
- Will you use theoretical frameworks to help you (and your Readers) analyze a set of hypotheses or relationships?
- Which data analysis methods will you choose?
- Which other Authors or studies have used the same methods and should be cited in your academic article?
Issues to Avoid
There are certain aspects that you need to pay extra attention in relation to your research methodology section. The most common issues to avoid are:
- Irrelevant details and complicated background information that provides too information and does not provide accurate understanding for Readers
- Unnecessary description and explanations of basic or well-known procedures, for an academic audience who is already has a basin understanding of the study
- For unconventional research approaches, it is important to provide accurate details and explain why your innovative method contributes to general knowledge (save more details for your Discussion/ Conclusion section in which you can highlight your contributions)
- Research limitations and obstacles should be described in a separate section (Research Limitations)
- The methodology should include sources and references that support your choice of methods and procedures, compared to the literature review that provides a general outlook and framework for your study.
Which aspects are you generally focusing on when writing your academic article’s research methodology section?
You may also like, related policies and links, responsibilities of the publisher in the relationship with journal editors, general duties of publisher.
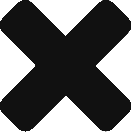
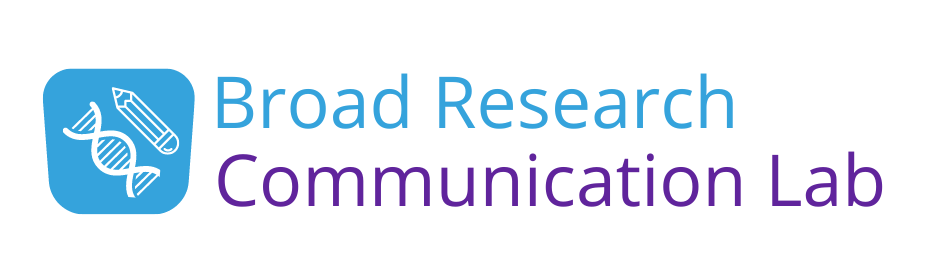
Journal Article: Methods
Criteria for success.
A successful Methods section:
- provides the reasons for choosing your methodology
- allows readers to confirm your findings through replication
Compare Authentic Annotated Examples for Methods and Results . Note the correspondence of subheadings between the two sections.
Identify Your Purpose
The purpose of a Methods section is to describe how the questions/knowledge gap posed in the Introduction were answered in the Results section. Not all readers will be interested in this information. For those who are, the Methods section has two purposes:
1. Allow readers to judge whether the results and conclusions of the study are valid.
The interpretation of your results depends on the methods you used to obtain them. A reader who is skeptical of your results will read your Methods section to see if they can be trusted. They’ll want to know that you chose the most appropriate methods and performed the necessary controls. Without this content, skeptical readers might think your data and any conclusions drawn from them are unreliable.
2. Allow readers to repeat the study.
For readers interested in replicating your study, the Methods section should provide enough information for them to obtain the same or similar results.
Analyze your audience
Typically, only readers in your field will want to replicate your study or have the knowledge to assess your methodology. More general audiences will read the Introduction and then proceed straight to the Results. You can therefore assume that people reading your Methods understand methodologies that are frequently used in your field. To gauge the level of detail necessary for a given method, you can look at articles previously published in your target journal.
If your paper is designed to appeal to experts in more than one field, you still need to write your Methods for a single set of experts. For example, say you applied a novel computational approach to gain new insight into a well-characterized biological system. Is your goal to get to show biologists the value of your computational tool or to show computational scientists how they can help study biology? In the former case, assume less computational expertise: provide more extensive explanations for how methods work and why they were chosen.
State the reasons for choosing your methodology
A reader looking to assess your methodology will read your Methods section to judge your experimental design. When describing your approach, place more emphasis on how you applied a method rather than on how you performed the method. For example, you don’t need to explain how to perform a western blot, but you might want to describe why a western blot is an appropriate approach for the task at hand (and, potentially, why you didn’t use another method).
Use subheadings to organize content
As recommended for your Results section , use subheadings within your Methods to group related experiments and establish a logical flow. Write your Results section first, and then follow the order of Results subheadings when writing your Methods. The parallel structure will make it easy for readers to locate corresponding information in the two sections.
Subheadings for Methods and Results may not exactly correspond. Sometimes you may need multiple Methods subheadings to explain one Results subheading. Other times, one Method subheading is enough to explain multiple Result subheadings.
Provide minimal essential detail
Provide only those details necessary for a reader to replicate the experiments presented in your study; anything more is extraneous. Remember that readers use Methods to help them assess the validity of your conclusions, so specify any methodological details that might cause someone to reach a different conclusion.
You can cite papers for standard methods, but any modifications or alterations should be clearly stated. When citing methods, cite the original paper in which a method was described instead of a paper that used the method. This helps avoid chains of citations that your reader must follow to find information about the method.
Avoid “we did…” or “the authors did…”
The Methods section should focus on the experiments, not the authors. Avoid phrasing your experiments as “We/The authors did ___”, even if it requires you to write in the passive voice.
“Samples were processed with standard DNA extraction protocols.”
“We processed the samples with standard DNA extraction protocols.”
This content was adapted from from an article originally created by the MIT Biological Engineering Communication Lab .
Resources and Annotated Examples
Annotated example 1.
Zetsche et al. , "Cpf1 is a single RNA-guided endonuclease...", Cell 2015. Compare to the annotated examples in the Results section. 2 MB
- Open access
- Published: 07 September 2020
A tutorial on methodological studies: the what, when, how and why
- Lawrence Mbuagbaw ORCID: orcid.org/0000-0001-5855-5461 1 , 2 , 3 ,
- Daeria O. Lawson 1 ,
- Livia Puljak 4 ,
- David B. Allison 5 &
- Lehana Thabane 1 , 2 , 6 , 7 , 8
BMC Medical Research Methodology volume 20 , Article number: 226 ( 2020 ) Cite this article
38k Accesses
53 Citations
58 Altmetric
Metrics details
Methodological studies – studies that evaluate the design, analysis or reporting of other research-related reports – play an important role in health research. They help to highlight issues in the conduct of research with the aim of improving health research methodology, and ultimately reducing research waste.
We provide an overview of some of the key aspects of methodological studies such as what they are, and when, how and why they are done. We adopt a “frequently asked questions” format to facilitate reading this paper and provide multiple examples to help guide researchers interested in conducting methodological studies. Some of the topics addressed include: is it necessary to publish a study protocol? How to select relevant research reports and databases for a methodological study? What approaches to data extraction and statistical analysis should be considered when conducting a methodological study? What are potential threats to validity and is there a way to appraise the quality of methodological studies?
Appropriate reflection and application of basic principles of epidemiology and biostatistics are required in the design and analysis of methodological studies. This paper provides an introduction for further discussion about the conduct of methodological studies.
Peer Review reports
The field of meta-research (or research-on-research) has proliferated in recent years in response to issues with research quality and conduct [ 1 , 2 , 3 ]. As the name suggests, this field targets issues with research design, conduct, analysis and reporting. Various types of research reports are often examined as the unit of analysis in these studies (e.g. abstracts, full manuscripts, trial registry entries). Like many other novel fields of research, meta-research has seen a proliferation of use before the development of reporting guidance. For example, this was the case with randomized trials for which risk of bias tools and reporting guidelines were only developed much later – after many trials had been published and noted to have limitations [ 4 , 5 ]; and for systematic reviews as well [ 6 , 7 , 8 ]. However, in the absence of formal guidance, studies that report on research differ substantially in how they are named, conducted and reported [ 9 , 10 ]. This creates challenges in identifying, summarizing and comparing them. In this tutorial paper, we will use the term methodological study to refer to any study that reports on the design, conduct, analysis or reporting of primary or secondary research-related reports (such as trial registry entries and conference abstracts).
In the past 10 years, there has been an increase in the use of terms related to methodological studies (based on records retrieved with a keyword search [in the title and abstract] for “methodological review” and “meta-epidemiological study” in PubMed up to December 2019), suggesting that these studies may be appearing more frequently in the literature. See Fig. 1 .
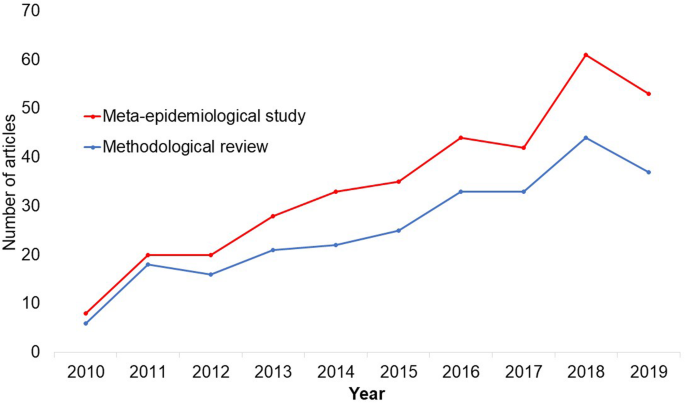
Trends in the number studies that mention “methodological review” or “meta-
epidemiological study” in PubMed.
The methods used in many methodological studies have been borrowed from systematic and scoping reviews. This practice has influenced the direction of the field, with many methodological studies including searches of electronic databases, screening of records, duplicate data extraction and assessments of risk of bias in the included studies. However, the research questions posed in methodological studies do not always require the approaches listed above, and guidance is needed on when and how to apply these methods to a methodological study. Even though methodological studies can be conducted on qualitative or mixed methods research, this paper focuses on and draws examples exclusively from quantitative research.
The objectives of this paper are to provide some insights on how to conduct methodological studies so that there is greater consistency between the research questions posed, and the design, analysis and reporting of findings. We provide multiple examples to illustrate concepts and a proposed framework for categorizing methodological studies in quantitative research.
What is a methodological study?
Any study that describes or analyzes methods (design, conduct, analysis or reporting) in published (or unpublished) literature is a methodological study. Consequently, the scope of methodological studies is quite extensive and includes, but is not limited to, topics as diverse as: research question formulation [ 11 ]; adherence to reporting guidelines [ 12 , 13 , 14 ] and consistency in reporting [ 15 ]; approaches to study analysis [ 16 ]; investigating the credibility of analyses [ 17 ]; and studies that synthesize these methodological studies [ 18 ]. While the nomenclature of methodological studies is not uniform, the intents and purposes of these studies remain fairly consistent – to describe or analyze methods in primary or secondary studies. As such, methodological studies may also be classified as a subtype of observational studies.
Parallel to this are experimental studies that compare different methods. Even though they play an important role in informing optimal research methods, experimental methodological studies are beyond the scope of this paper. Examples of such studies include the randomized trials by Buscemi et al., comparing single data extraction to double data extraction [ 19 ], and Carrasco-Labra et al., comparing approaches to presenting findings in Grading of Recommendations, Assessment, Development and Evaluations (GRADE) summary of findings tables [ 20 ]. In these studies, the unit of analysis is the person or groups of individuals applying the methods. We also direct readers to the Studies Within a Trial (SWAT) and Studies Within a Review (SWAR) programme operated through the Hub for Trials Methodology Research, for further reading as a potential useful resource for these types of experimental studies [ 21 ]. Lastly, this paper is not meant to inform the conduct of research using computational simulation and mathematical modeling for which some guidance already exists [ 22 ], or studies on the development of methods using consensus-based approaches.
When should we conduct a methodological study?
Methodological studies occupy a unique niche in health research that allows them to inform methodological advances. Methodological studies should also be conducted as pre-cursors to reporting guideline development, as they provide an opportunity to understand current practices, and help to identify the need for guidance and gaps in methodological or reporting quality. For example, the development of the popular Preferred Reporting Items of Systematic reviews and Meta-Analyses (PRISMA) guidelines were preceded by methodological studies identifying poor reporting practices [ 23 , 24 ]. In these instances, after the reporting guidelines are published, methodological studies can also be used to monitor uptake of the guidelines.
These studies can also be conducted to inform the state of the art for design, analysis and reporting practices across different types of health research fields, with the aim of improving research practices, and preventing or reducing research waste. For example, Samaan et al. conducted a scoping review of adherence to different reporting guidelines in health care literature [ 18 ]. Methodological studies can also be used to determine the factors associated with reporting practices. For example, Abbade et al. investigated journal characteristics associated with the use of the Participants, Intervention, Comparison, Outcome, Timeframe (PICOT) format in framing research questions in trials of venous ulcer disease [ 11 ].
How often are methodological studies conducted?
There is no clear answer to this question. Based on a search of PubMed, the use of related terms (“methodological review” and “meta-epidemiological study”) – and therefore, the number of methodological studies – is on the rise. However, many other terms are used to describe methodological studies. There are also many studies that explore design, conduct, analysis or reporting of research reports, but that do not use any specific terms to describe or label their study design in terms of “methodology”. This diversity in nomenclature makes a census of methodological studies elusive. Appropriate terminology and key words for methodological studies are needed to facilitate improved accessibility for end-users.
Why do we conduct methodological studies?
Methodological studies provide information on the design, conduct, analysis or reporting of primary and secondary research and can be used to appraise quality, quantity, completeness, accuracy and consistency of health research. These issues can be explored in specific fields, journals, databases, geographical regions and time periods. For example, Areia et al. explored the quality of reporting of endoscopic diagnostic studies in gastroenterology [ 25 ]; Knol et al. investigated the reporting of p -values in baseline tables in randomized trial published in high impact journals [ 26 ]; Chen et al. describe adherence to the Consolidated Standards of Reporting Trials (CONSORT) statement in Chinese Journals [ 27 ]; and Hopewell et al. describe the effect of editors’ implementation of CONSORT guidelines on reporting of abstracts over time [ 28 ]. Methodological studies provide useful information to researchers, clinicians, editors, publishers and users of health literature. As a result, these studies have been at the cornerstone of important methodological developments in the past two decades and have informed the development of many health research guidelines including the highly cited CONSORT statement [ 5 ].
Where can we find methodological studies?
Methodological studies can be found in most common biomedical bibliographic databases (e.g. Embase, MEDLINE, PubMed, Web of Science). However, the biggest caveat is that methodological studies are hard to identify in the literature due to the wide variety of names used and the lack of comprehensive databases dedicated to them. A handful can be found in the Cochrane Library as “Cochrane Methodology Reviews”, but these studies only cover methodological issues related to systematic reviews. Previous attempts to catalogue all empirical studies of methods used in reviews were abandoned 10 years ago [ 29 ]. In other databases, a variety of search terms may be applied with different levels of sensitivity and specificity.
Some frequently asked questions about methodological studies
In this section, we have outlined responses to questions that might help inform the conduct of methodological studies.
Q: How should I select research reports for my methodological study?
A: Selection of research reports for a methodological study depends on the research question and eligibility criteria. Once a clear research question is set and the nature of literature one desires to review is known, one can then begin the selection process. Selection may begin with a broad search, especially if the eligibility criteria are not apparent. For example, a methodological study of Cochrane Reviews of HIV would not require a complex search as all eligible studies can easily be retrieved from the Cochrane Library after checking a few boxes [ 30 ]. On the other hand, a methodological study of subgroup analyses in trials of gastrointestinal oncology would require a search to find such trials, and further screening to identify trials that conducted a subgroup analysis [ 31 ].
The strategies used for identifying participants in observational studies can apply here. One may use a systematic search to identify all eligible studies. If the number of eligible studies is unmanageable, a random sample of articles can be expected to provide comparable results if it is sufficiently large [ 32 ]. For example, Wilson et al. used a random sample of trials from the Cochrane Stroke Group’s Trial Register to investigate completeness of reporting [ 33 ]. It is possible that a simple random sample would lead to underrepresentation of units (i.e. research reports) that are smaller in number. This is relevant if the investigators wish to compare multiple groups but have too few units in one group. In this case a stratified sample would help to create equal groups. For example, in a methodological study comparing Cochrane and non-Cochrane reviews, Kahale et al. drew random samples from both groups [ 34 ]. Alternatively, systematic or purposeful sampling strategies can be used and we encourage researchers to justify their selected approaches based on the study objective.
Q: How many databases should I search?
A: The number of databases one should search would depend on the approach to sampling, which can include targeting the entire “population” of interest or a sample of that population. If you are interested in including the entire target population for your research question, or drawing a random or systematic sample from it, then a comprehensive and exhaustive search for relevant articles is required. In this case, we recommend using systematic approaches for searching electronic databases (i.e. at least 2 databases with a replicable and time stamped search strategy). The results of your search will constitute a sampling frame from which eligible studies can be drawn.
Alternatively, if your approach to sampling is purposeful, then we recommend targeting the database(s) or data sources (e.g. journals, registries) that include the information you need. For example, if you are conducting a methodological study of high impact journals in plastic surgery and they are all indexed in PubMed, you likely do not need to search any other databases. You may also have a comprehensive list of all journals of interest and can approach your search using the journal names in your database search (or by accessing the journal archives directly from the journal’s website). Even though one could also search journals’ web pages directly, using a database such as PubMed has multiple advantages, such as the use of filters, so the search can be narrowed down to a certain period, or study types of interest. Furthermore, individual journals’ web sites may have different search functionalities, which do not necessarily yield a consistent output.
Q: Should I publish a protocol for my methodological study?
A: A protocol is a description of intended research methods. Currently, only protocols for clinical trials require registration [ 35 ]. Protocols for systematic reviews are encouraged but no formal recommendation exists. The scientific community welcomes the publication of protocols because they help protect against selective outcome reporting, the use of post hoc methodologies to embellish results, and to help avoid duplication of efforts [ 36 ]. While the latter two risks exist in methodological research, the negative consequences may be substantially less than for clinical outcomes. In a sample of 31 methodological studies, 7 (22.6%) referenced a published protocol [ 9 ]. In the Cochrane Library, there are 15 protocols for methodological reviews (21 July 2020). This suggests that publishing protocols for methodological studies is not uncommon.
Authors can consider publishing their study protocol in a scholarly journal as a manuscript. Advantages of such publication include obtaining peer-review feedback about the planned study, and easy retrieval by searching databases such as PubMed. The disadvantages in trying to publish protocols includes delays associated with manuscript handling and peer review, as well as costs, as few journals publish study protocols, and those journals mostly charge article-processing fees [ 37 ]. Authors who would like to make their protocol publicly available without publishing it in scholarly journals, could deposit their study protocols in publicly available repositories, such as the Open Science Framework ( https://osf.io/ ).
Q: How to appraise the quality of a methodological study?
A: To date, there is no published tool for appraising the risk of bias in a methodological study, but in principle, a methodological study could be considered as a type of observational study. Therefore, during conduct or appraisal, care should be taken to avoid the biases common in observational studies [ 38 ]. These biases include selection bias, comparability of groups, and ascertainment of exposure or outcome. In other words, to generate a representative sample, a comprehensive reproducible search may be necessary to build a sampling frame. Additionally, random sampling may be necessary to ensure that all the included research reports have the same probability of being selected, and the screening and selection processes should be transparent and reproducible. To ensure that the groups compared are similar in all characteristics, matching, random sampling or stratified sampling can be used. Statistical adjustments for between-group differences can also be applied at the analysis stage. Finally, duplicate data extraction can reduce errors in assessment of exposures or outcomes.
Q: Should I justify a sample size?
A: In all instances where one is not using the target population (i.e. the group to which inferences from the research report are directed) [ 39 ], a sample size justification is good practice. The sample size justification may take the form of a description of what is expected to be achieved with the number of articles selected, or a formal sample size estimation that outlines the number of articles required to answer the research question with a certain precision and power. Sample size justifications in methodological studies are reasonable in the following instances:
Comparing two groups
Determining a proportion, mean or another quantifier
Determining factors associated with an outcome using regression-based analyses
For example, El Dib et al. computed a sample size requirement for a methodological study of diagnostic strategies in randomized trials, based on a confidence interval approach [ 40 ].
Q: What should I call my study?
A: Other terms which have been used to describe/label methodological studies include “ methodological review ”, “methodological survey” , “meta-epidemiological study” , “systematic review” , “systematic survey”, “meta-research”, “research-on-research” and many others. We recommend that the study nomenclature be clear, unambiguous, informative and allow for appropriate indexing. Methodological study nomenclature that should be avoided includes “ systematic review” – as this will likely be confused with a systematic review of a clinical question. “ Systematic survey” may also lead to confusion about whether the survey was systematic (i.e. using a preplanned methodology) or a survey using “ systematic” sampling (i.e. a sampling approach using specific intervals to determine who is selected) [ 32 ]. Any of the above meanings of the words “ systematic” may be true for methodological studies and could be potentially misleading. “ Meta-epidemiological study” is ideal for indexing, but not very informative as it describes an entire field. The term “ review ” may point towards an appraisal or “review” of the design, conduct, analysis or reporting (or methodological components) of the targeted research reports, yet it has also been used to describe narrative reviews [ 41 , 42 ]. The term “ survey ” is also in line with the approaches used in many methodological studies [ 9 ], and would be indicative of the sampling procedures of this study design. However, in the absence of guidelines on nomenclature, the term “ methodological study ” is broad enough to capture most of the scenarios of such studies.
Q: Should I account for clustering in my methodological study?
A: Data from methodological studies are often clustered. For example, articles coming from a specific source may have different reporting standards (e.g. the Cochrane Library). Articles within the same journal may be similar due to editorial practices and policies, reporting requirements and endorsement of guidelines. There is emerging evidence that these are real concerns that should be accounted for in analyses [ 43 ]. Some cluster variables are described in the section: “ What variables are relevant to methodological studies?”
A variety of modelling approaches can be used to account for correlated data, including the use of marginal, fixed or mixed effects regression models with appropriate computation of standard errors [ 44 ]. For example, Kosa et al. used generalized estimation equations to account for correlation of articles within journals [ 15 ]. Not accounting for clustering could lead to incorrect p -values, unduly narrow confidence intervals, and biased estimates [ 45 ].
Q: Should I extract data in duplicate?
A: Yes. Duplicate data extraction takes more time but results in less errors [ 19 ]. Data extraction errors in turn affect the effect estimate [ 46 ], and therefore should be mitigated. Duplicate data extraction should be considered in the absence of other approaches to minimize extraction errors. However, much like systematic reviews, this area will likely see rapid new advances with machine learning and natural language processing technologies to support researchers with screening and data extraction [ 47 , 48 ]. However, experience plays an important role in the quality of extracted data and inexperienced extractors should be paired with experienced extractors [ 46 , 49 ].
Q: Should I assess the risk of bias of research reports included in my methodological study?
A : Risk of bias is most useful in determining the certainty that can be placed in the effect measure from a study. In methodological studies, risk of bias may not serve the purpose of determining the trustworthiness of results, as effect measures are often not the primary goal of methodological studies. Determining risk of bias in methodological studies is likely a practice borrowed from systematic review methodology, but whose intrinsic value is not obvious in methodological studies. When it is part of the research question, investigators often focus on one aspect of risk of bias. For example, Speich investigated how blinding was reported in surgical trials [ 50 ], and Abraha et al., investigated the application of intention-to-treat analyses in systematic reviews and trials [ 51 ].
Q: What variables are relevant to methodological studies?
A: There is empirical evidence that certain variables may inform the findings in a methodological study. We outline some of these and provide a brief overview below:
Country: Countries and regions differ in their research cultures, and the resources available to conduct research. Therefore, it is reasonable to believe that there may be differences in methodological features across countries. Methodological studies have reported loco-regional differences in reporting quality [ 52 , 53 ]. This may also be related to challenges non-English speakers face in publishing papers in English.
Authors’ expertise: The inclusion of authors with expertise in research methodology, biostatistics, and scientific writing is likely to influence the end-product. Oltean et al. found that among randomized trials in orthopaedic surgery, the use of analyses that accounted for clustering was more likely when specialists (e.g. statistician, epidemiologist or clinical trials methodologist) were included on the study team [ 54 ]. Fleming et al. found that including methodologists in the review team was associated with appropriate use of reporting guidelines [ 55 ].
Source of funding and conflicts of interest: Some studies have found that funded studies report better [ 56 , 57 ], while others do not [ 53 , 58 ]. The presence of funding would indicate the availability of resources deployed to ensure optimal design, conduct, analysis and reporting. However, the source of funding may introduce conflicts of interest and warrant assessment. For example, Kaiser et al. investigated the effect of industry funding on obesity or nutrition randomized trials and found that reporting quality was similar [ 59 ]. Thomas et al. looked at reporting quality of long-term weight loss trials and found that industry funded studies were better [ 60 ]. Kan et al. examined the association between industry funding and “positive trials” (trials reporting a significant intervention effect) and found that industry funding was highly predictive of a positive trial [ 61 ]. This finding is similar to that of a recent Cochrane Methodology Review by Hansen et al. [ 62 ]
Journal characteristics: Certain journals’ characteristics may influence the study design, analysis or reporting. Characteristics such as journal endorsement of guidelines [ 63 , 64 ], and Journal Impact Factor (JIF) have been shown to be associated with reporting [ 63 , 65 , 66 , 67 ].
Study size (sample size/number of sites): Some studies have shown that reporting is better in larger studies [ 53 , 56 , 58 ].
Year of publication: It is reasonable to assume that design, conduct, analysis and reporting of research will change over time. Many studies have demonstrated improvements in reporting over time or after the publication of reporting guidelines [ 68 , 69 ].
Type of intervention: In a methodological study of reporting quality of weight loss intervention studies, Thabane et al. found that trials of pharmacologic interventions were reported better than trials of non-pharmacologic interventions [ 70 ].
Interactions between variables: Complex interactions between the previously listed variables are possible. High income countries with more resources may be more likely to conduct larger studies and incorporate a variety of experts. Authors in certain countries may prefer certain journals, and journal endorsement of guidelines and editorial policies may change over time.
Q: Should I focus only on high impact journals?
A: Investigators may choose to investigate only high impact journals because they are more likely to influence practice and policy, or because they assume that methodological standards would be higher. However, the JIF may severely limit the scope of articles included and may skew the sample towards articles with positive findings. The generalizability and applicability of findings from a handful of journals must be examined carefully, especially since the JIF varies over time. Even among journals that are all “high impact”, variations exist in methodological standards.
Q: Can I conduct a methodological study of qualitative research?
A: Yes. Even though a lot of methodological research has been conducted in the quantitative research field, methodological studies of qualitative studies are feasible. Certain databases that catalogue qualitative research including the Cumulative Index to Nursing & Allied Health Literature (CINAHL) have defined subject headings that are specific to methodological research (e.g. “research methodology”). Alternatively, one could also conduct a qualitative methodological review; that is, use qualitative approaches to synthesize methodological issues in qualitative studies.
Q: What reporting guidelines should I use for my methodological study?
A: There is no guideline that covers the entire scope of methodological studies. One adaptation of the PRISMA guidelines has been published, which works well for studies that aim to use the entire target population of research reports [ 71 ]. However, it is not widely used (40 citations in 2 years as of 09 December 2019), and methodological studies that are designed as cross-sectional or before-after studies require a more fit-for purpose guideline. A more encompassing reporting guideline for a broad range of methodological studies is currently under development [ 72 ]. However, in the absence of formal guidance, the requirements for scientific reporting should be respected, and authors of methodological studies should focus on transparency and reproducibility.
Q: What are the potential threats to validity and how can I avoid them?
A: Methodological studies may be compromised by a lack of internal or external validity. The main threats to internal validity in methodological studies are selection and confounding bias. Investigators must ensure that the methods used to select articles does not make them differ systematically from the set of articles to which they would like to make inferences. For example, attempting to make extrapolations to all journals after analyzing high-impact journals would be misleading.
Many factors (confounders) may distort the association between the exposure and outcome if the included research reports differ with respect to these factors [ 73 ]. For example, when examining the association between source of funding and completeness of reporting, it may be necessary to account for journals that endorse the guidelines. Confounding bias can be addressed by restriction, matching and statistical adjustment [ 73 ]. Restriction appears to be the method of choice for many investigators who choose to include only high impact journals or articles in a specific field. For example, Knol et al. examined the reporting of p -values in baseline tables of high impact journals [ 26 ]. Matching is also sometimes used. In the methodological study of non-randomized interventional studies of elective ventral hernia repair, Parker et al. matched prospective studies with retrospective studies and compared reporting standards [ 74 ]. Some other methodological studies use statistical adjustments. For example, Zhang et al. used regression techniques to determine the factors associated with missing participant data in trials [ 16 ].
With regard to external validity, researchers interested in conducting methodological studies must consider how generalizable or applicable their findings are. This should tie in closely with the research question and should be explicit. For example. Findings from methodological studies on trials published in high impact cardiology journals cannot be assumed to be applicable to trials in other fields. However, investigators must ensure that their sample truly represents the target sample either by a) conducting a comprehensive and exhaustive search, or b) using an appropriate and justified, randomly selected sample of research reports.
Even applicability to high impact journals may vary based on the investigators’ definition, and over time. For example, for high impact journals in the field of general medicine, Bouwmeester et al. included the Annals of Internal Medicine (AIM), BMJ, the Journal of the American Medical Association (JAMA), Lancet, the New England Journal of Medicine (NEJM), and PLoS Medicine ( n = 6) [ 75 ]. In contrast, the high impact journals selected in the methodological study by Schiller et al. were BMJ, JAMA, Lancet, and NEJM ( n = 4) [ 76 ]. Another methodological study by Kosa et al. included AIM, BMJ, JAMA, Lancet and NEJM ( n = 5). In the methodological study by Thabut et al., journals with a JIF greater than 5 were considered to be high impact. Riado Minguez et al. used first quartile journals in the Journal Citation Reports (JCR) for a specific year to determine “high impact” [ 77 ]. Ultimately, the definition of high impact will be based on the number of journals the investigators are willing to include, the year of impact and the JIF cut-off [ 78 ]. We acknowledge that the term “generalizability” may apply differently for methodological studies, especially when in many instances it is possible to include the entire target population in the sample studied.
Finally, methodological studies are not exempt from information bias which may stem from discrepancies in the included research reports [ 79 ], errors in data extraction, or inappropriate interpretation of the information extracted. Likewise, publication bias may also be a concern in methodological studies, but such concepts have not yet been explored.
A proposed framework
In order to inform discussions about methodological studies, the development of guidance for what should be reported, we have outlined some key features of methodological studies that can be used to classify them. For each of the categories outlined below, we provide an example. In our experience, the choice of approach to completing a methodological study can be informed by asking the following four questions:
What is the aim?
Methodological studies that investigate bias
A methodological study may be focused on exploring sources of bias in primary or secondary studies (meta-bias), or how bias is analyzed. We have taken care to distinguish bias (i.e. systematic deviations from the truth irrespective of the source) from reporting quality or completeness (i.e. not adhering to a specific reporting guideline or norm). An example of where this distinction would be important is in the case of a randomized trial with no blinding. This study (depending on the nature of the intervention) would be at risk of performance bias. However, if the authors report that their study was not blinded, they would have reported adequately. In fact, some methodological studies attempt to capture both “quality of conduct” and “quality of reporting”, such as Richie et al., who reported on the risk of bias in randomized trials of pharmacy practice interventions [ 80 ]. Babic et al. investigated how risk of bias was used to inform sensitivity analyses in Cochrane reviews [ 81 ]. Further, biases related to choice of outcomes can also be explored. For example, Tan et al investigated differences in treatment effect size based on the outcome reported [ 82 ].
Methodological studies that investigate quality (or completeness) of reporting
Methodological studies may report quality of reporting against a reporting checklist (i.e. adherence to guidelines) or against expected norms. For example, Croituro et al. report on the quality of reporting in systematic reviews published in dermatology journals based on their adherence to the PRISMA statement [ 83 ], and Khan et al. described the quality of reporting of harms in randomized controlled trials published in high impact cardiovascular journals based on the CONSORT extension for harms [ 84 ]. Other methodological studies investigate reporting of certain features of interest that may not be part of formally published checklists or guidelines. For example, Mbuagbaw et al. described how often the implications for research are elaborated using the Evidence, Participants, Intervention, Comparison, Outcome, Timeframe (EPICOT) format [ 30 ].
Methodological studies that investigate the consistency of reporting
Sometimes investigators may be interested in how consistent reports of the same research are, as it is expected that there should be consistency between: conference abstracts and published manuscripts; manuscript abstracts and manuscript main text; and trial registration and published manuscript. For example, Rosmarakis et al. investigated consistency between conference abstracts and full text manuscripts [ 85 ].
Methodological studies that investigate factors associated with reporting
In addition to identifying issues with reporting in primary and secondary studies, authors of methodological studies may be interested in determining the factors that are associated with certain reporting practices. Many methodological studies incorporate this, albeit as a secondary outcome. For example, Farrokhyar et al. investigated the factors associated with reporting quality in randomized trials of coronary artery bypass grafting surgery [ 53 ].
Methodological studies that investigate methods
Methodological studies may also be used to describe methods or compare methods, and the factors associated with methods. Muller et al. described the methods used for systematic reviews and meta-analyses of observational studies [ 86 ].
Methodological studies that summarize other methodological studies
Some methodological studies synthesize results from other methodological studies. For example, Li et al. conducted a scoping review of methodological reviews that investigated consistency between full text and abstracts in primary biomedical research [ 87 ].
Methodological studies that investigate nomenclature and terminology
Some methodological studies may investigate the use of names and terms in health research. For example, Martinic et al. investigated the definitions of systematic reviews used in overviews of systematic reviews (OSRs), meta-epidemiological studies and epidemiology textbooks [ 88 ].
Other types of methodological studies
In addition to the previously mentioned experimental methodological studies, there may exist other types of methodological studies not captured here.
What is the design?
Methodological studies that are descriptive
Most methodological studies are purely descriptive and report their findings as counts (percent) and means (standard deviation) or medians (interquartile range). For example, Mbuagbaw et al. described the reporting of research recommendations in Cochrane HIV systematic reviews [ 30 ]. Gohari et al. described the quality of reporting of randomized trials in diabetes in Iran [ 12 ].
Methodological studies that are analytical
Some methodological studies are analytical wherein “analytical studies identify and quantify associations, test hypotheses, identify causes and determine whether an association exists between variables, such as between an exposure and a disease.” [ 89 ] In the case of methodological studies all these investigations are possible. For example, Kosa et al. investigated the association between agreement in primary outcome from trial registry to published manuscript and study covariates. They found that larger and more recent studies were more likely to have agreement [ 15 ]. Tricco et al. compared the conclusion statements from Cochrane and non-Cochrane systematic reviews with a meta-analysis of the primary outcome and found that non-Cochrane reviews were more likely to report positive findings. These results are a test of the null hypothesis that the proportions of Cochrane and non-Cochrane reviews that report positive results are equal [ 90 ].
What is the sampling strategy?
Methodological studies that include the target population
Methodological reviews with narrow research questions may be able to include the entire target population. For example, in the methodological study of Cochrane HIV systematic reviews, Mbuagbaw et al. included all of the available studies ( n = 103) [ 30 ].
Methodological studies that include a sample of the target population
Many methodological studies use random samples of the target population [ 33 , 91 , 92 ]. Alternatively, purposeful sampling may be used, limiting the sample to a subset of research-related reports published within a certain time period, or in journals with a certain ranking or on a topic. Systematic sampling can also be used when random sampling may be challenging to implement.
What is the unit of analysis?
Methodological studies with a research report as the unit of analysis
Many methodological studies use a research report (e.g. full manuscript of study, abstract portion of the study) as the unit of analysis, and inferences can be made at the study-level. However, both published and unpublished research-related reports can be studied. These may include articles, conference abstracts, registry entries etc.
Methodological studies with a design, analysis or reporting item as the unit of analysis
Some methodological studies report on items which may occur more than once per article. For example, Paquette et al. report on subgroup analyses in Cochrane reviews of atrial fibrillation in which 17 systematic reviews planned 56 subgroup analyses [ 93 ].
This framework is outlined in Fig. 2 .
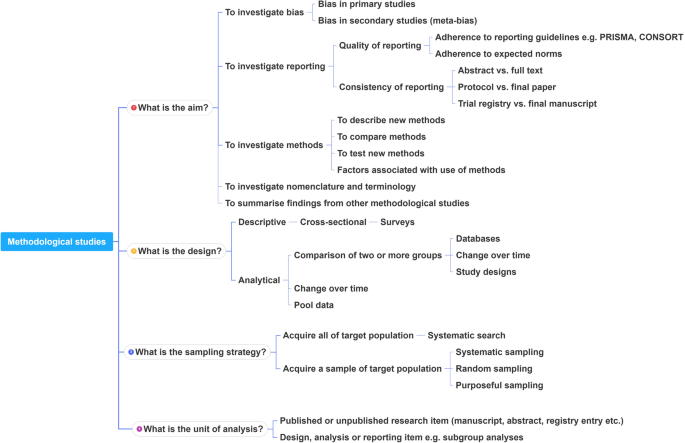
A proposed framework for methodological studies
Conclusions
Methodological studies have examined different aspects of reporting such as quality, completeness, consistency and adherence to reporting guidelines. As such, many of the methodological study examples cited in this tutorial are related to reporting. However, as an evolving field, the scope of research questions that can be addressed by methodological studies is expected to increase.
In this paper we have outlined the scope and purpose of methodological studies, along with examples of instances in which various approaches have been used. In the absence of formal guidance on the design, conduct, analysis and reporting of methodological studies, we have provided some advice to help make methodological studies consistent. This advice is grounded in good contemporary scientific practice. Generally, the research question should tie in with the sampling approach and planned analysis. We have also highlighted the variables that may inform findings from methodological studies. Lastly, we have provided suggestions for ways in which authors can categorize their methodological studies to inform their design and analysis.
Availability of data and materials
Data sharing is not applicable to this article as no new data were created or analyzed in this study.
Abbreviations
Consolidated Standards of Reporting Trials
Evidence, Participants, Intervention, Comparison, Outcome, Timeframe
Grading of Recommendations, Assessment, Development and Evaluations
Participants, Intervention, Comparison, Outcome, Timeframe
Preferred Reporting Items of Systematic reviews and Meta-Analyses
Studies Within a Review
Studies Within a Trial
Chalmers I, Glasziou P. Avoidable waste in the production and reporting of research evidence. Lancet. 2009;374(9683):86–9.
PubMed Google Scholar
Chan AW, Song F, Vickers A, Jefferson T, Dickersin K, Gotzsche PC, Krumholz HM, Ghersi D, van der Worp HB. Increasing value and reducing waste: addressing inaccessible research. Lancet. 2014;383(9913):257–66.
PubMed PubMed Central Google Scholar
Ioannidis JP, Greenland S, Hlatky MA, Khoury MJ, Macleod MR, Moher D, Schulz KF, Tibshirani R. Increasing value and reducing waste in research design, conduct, and analysis. Lancet. 2014;383(9912):166–75.
Higgins JP, Altman DG, Gotzsche PC, Juni P, Moher D, Oxman AD, Savovic J, Schulz KF, Weeks L, Sterne JA. The Cochrane Collaboration's tool for assessing risk of bias in randomised trials. BMJ. 2011;343:d5928.
Moher D, Schulz KF, Altman DG. The CONSORT statement: revised recommendations for improving the quality of reports of parallel-group randomised trials. Lancet. 2001;357.
Liberati A, Altman DG, Tetzlaff J, Mulrow C, Gotzsche PC, Ioannidis JP, Clarke M, Devereaux PJ, Kleijnen J, Moher D. The PRISMA statement for reporting systematic reviews and meta-analyses of studies that evaluate health care interventions: explanation and elaboration. PLoS Med. 2009;6(7):e1000100.
Shea BJ, Hamel C, Wells GA, Bouter LM, Kristjansson E, Grimshaw J, Henry DA, Boers M. AMSTAR is a reliable and valid measurement tool to assess the methodological quality of systematic reviews. J Clin Epidemiol. 2009;62(10):1013–20.
Shea BJ, Reeves BC, Wells G, Thuku M, Hamel C, Moran J, Moher D, Tugwell P, Welch V, Kristjansson E, et al. AMSTAR 2: a critical appraisal tool for systematic reviews that include randomised or non-randomised studies of healthcare interventions, or both. Bmj. 2017;358:j4008.
Lawson DO, Leenus A, Mbuagbaw L. Mapping the nomenclature, methodology, and reporting of studies that review methods: a pilot methodological review. Pilot Feasibility Studies. 2020;6(1):13.
Puljak L, Makaric ZL, Buljan I, Pieper D. What is a meta-epidemiological study? Analysis of published literature indicated heterogeneous study designs and definitions. J Comp Eff Res. 2020.
Abbade LPF, Wang M, Sriganesh K, Jin Y, Mbuagbaw L, Thabane L. The framing of research questions using the PICOT format in randomized controlled trials of venous ulcer disease is suboptimal: a systematic survey. Wound Repair Regen. 2017;25(5):892–900.
Gohari F, Baradaran HR, Tabatabaee M, Anijidani S, Mohammadpour Touserkani F, Atlasi R, Razmgir M. Quality of reporting randomized controlled trials (RCTs) in diabetes in Iran; a systematic review. J Diabetes Metab Disord. 2015;15(1):36.
Wang M, Jin Y, Hu ZJ, Thabane A, Dennis B, Gajic-Veljanoski O, Paul J, Thabane L. The reporting quality of abstracts of stepped wedge randomized trials is suboptimal: a systematic survey of the literature. Contemp Clin Trials Commun. 2017;8:1–10.
Shanthanna H, Kaushal A, Mbuagbaw L, Couban R, Busse J, Thabane L: A cross-sectional study of the reporting quality of pilot or feasibility trials in high-impact anesthesia journals Can J Anaesthesia 2018, 65(11):1180–1195.
Kosa SD, Mbuagbaw L, Borg Debono V, Bhandari M, Dennis BB, Ene G, Leenus A, Shi D, Thabane M, Valvasori S, et al. Agreement in reporting between trial publications and current clinical trial registry in high impact journals: a methodological review. Contemporary Clinical Trials. 2018;65:144–50.
Zhang Y, Florez ID, Colunga Lozano LE, Aloweni FAB, Kennedy SA, Li A, Craigie S, Zhang S, Agarwal A, Lopes LC, et al. A systematic survey on reporting and methods for handling missing participant data for continuous outcomes in randomized controlled trials. J Clin Epidemiol. 2017;88:57–66.
CAS PubMed Google Scholar
Hernández AV, Boersma E, Murray GD, Habbema JD, Steyerberg EW. Subgroup analyses in therapeutic cardiovascular clinical trials: are most of them misleading? Am Heart J. 2006;151(2):257–64.
Samaan Z, Mbuagbaw L, Kosa D, Borg Debono V, Dillenburg R, Zhang S, Fruci V, Dennis B, Bawor M, Thabane L. A systematic scoping review of adherence to reporting guidelines in health care literature. J Multidiscip Healthc. 2013;6:169–88.
Buscemi N, Hartling L, Vandermeer B, Tjosvold L, Klassen TP. Single data extraction generated more errors than double data extraction in systematic reviews. J Clin Epidemiol. 2006;59(7):697–703.
Carrasco-Labra A, Brignardello-Petersen R, Santesso N, Neumann I, Mustafa RA, Mbuagbaw L, Etxeandia Ikobaltzeta I, De Stio C, McCullagh LJ, Alonso-Coello P. Improving GRADE evidence tables part 1: a randomized trial shows improved understanding of content in summary-of-findings tables with a new format. J Clin Epidemiol. 2016;74:7–18.
The Northern Ireland Hub for Trials Methodology Research: SWAT/SWAR Information [ https://www.qub.ac.uk/sites/TheNorthernIrelandNetworkforTrialsMethodologyResearch/SWATSWARInformation/ ]. Accessed 31 Aug 2020.
Chick S, Sánchez P, Ferrin D, Morrice D. How to conduct a successful simulation study. In: Proceedings of the 2003 winter simulation conference: 2003; 2003. p. 66–70.
Google Scholar
Mulrow CD. The medical review article: state of the science. Ann Intern Med. 1987;106(3):485–8.
Sacks HS, Reitman D, Pagano D, Kupelnick B. Meta-analysis: an update. Mount Sinai J Med New York. 1996;63(3–4):216–24.
CAS Google Scholar
Areia M, Soares M, Dinis-Ribeiro M. Quality reporting of endoscopic diagnostic studies in gastrointestinal journals: where do we stand on the use of the STARD and CONSORT statements? Endoscopy. 2010;42(2):138–47.
Knol M, Groenwold R, Grobbee D. P-values in baseline tables of randomised controlled trials are inappropriate but still common in high impact journals. Eur J Prev Cardiol. 2012;19(2):231–2.
Chen M, Cui J, Zhang AL, Sze DM, Xue CC, May BH. Adherence to CONSORT items in randomized controlled trials of integrative medicine for colorectal Cancer published in Chinese journals. J Altern Complement Med. 2018;24(2):115–24.
Hopewell S, Ravaud P, Baron G, Boutron I. Effect of editors' implementation of CONSORT guidelines on the reporting of abstracts in high impact medical journals: interrupted time series analysis. BMJ. 2012;344:e4178.
The Cochrane Methodology Register Issue 2 2009 [ https://cmr.cochrane.org/help.htm ]. Accessed 31 Aug 2020.
Mbuagbaw L, Kredo T, Welch V, Mursleen S, Ross S, Zani B, Motaze NV, Quinlan L. Critical EPICOT items were absent in Cochrane human immunodeficiency virus systematic reviews: a bibliometric analysis. J Clin Epidemiol. 2016;74:66–72.
Barton S, Peckitt C, Sclafani F, Cunningham D, Chau I. The influence of industry sponsorship on the reporting of subgroup analyses within phase III randomised controlled trials in gastrointestinal oncology. Eur J Cancer. 2015;51(18):2732–9.
Setia MS. Methodology series module 5: sampling strategies. Indian J Dermatol. 2016;61(5):505–9.
Wilson B, Burnett P, Moher D, Altman DG, Al-Shahi Salman R. Completeness of reporting of randomised controlled trials including people with transient ischaemic attack or stroke: a systematic review. Eur Stroke J. 2018;3(4):337–46.
Kahale LA, Diab B, Brignardello-Petersen R, Agarwal A, Mustafa RA, Kwong J, Neumann I, Li L, Lopes LC, Briel M, et al. Systematic reviews do not adequately report or address missing outcome data in their analyses: a methodological survey. J Clin Epidemiol. 2018;99:14–23.
De Angelis CD, Drazen JM, Frizelle FA, Haug C, Hoey J, Horton R, Kotzin S, Laine C, Marusic A, Overbeke AJPM, et al. Is this clinical trial fully registered?: a statement from the International Committee of Medical Journal Editors*. Ann Intern Med. 2005;143(2):146–8.
Ohtake PJ, Childs JD. Why publish study protocols? Phys Ther. 2014;94(9):1208–9.
Rombey T, Allers K, Mathes T, Hoffmann F, Pieper D. A descriptive analysis of the characteristics and the peer review process of systematic review protocols published in an open peer review journal from 2012 to 2017. BMC Med Res Methodol. 2019;19(1):57.
Grimes DA, Schulz KF. Bias and causal associations in observational research. Lancet. 2002;359(9302):248–52.
Porta M (ed.): A dictionary of epidemiology, 5th edn. Oxford: Oxford University Press, Inc.; 2008.
El Dib R, Tikkinen KAO, Akl EA, Gomaa HA, Mustafa RA, Agarwal A, Carpenter CR, Zhang Y, Jorge EC, Almeida R, et al. Systematic survey of randomized trials evaluating the impact of alternative diagnostic strategies on patient-important outcomes. J Clin Epidemiol. 2017;84:61–9.
Helzer JE, Robins LN, Taibleson M, Woodruff RA Jr, Reich T, Wish ED. Reliability of psychiatric diagnosis. I. a methodological review. Arch Gen Psychiatry. 1977;34(2):129–33.
Chung ST, Chacko SK, Sunehag AL, Haymond MW. Measurements of gluconeogenesis and Glycogenolysis: a methodological review. Diabetes. 2015;64(12):3996–4010.
CAS PubMed PubMed Central Google Scholar
Sterne JA, Juni P, Schulz KF, Altman DG, Bartlett C, Egger M. Statistical methods for assessing the influence of study characteristics on treatment effects in 'meta-epidemiological' research. Stat Med. 2002;21(11):1513–24.
Moen EL, Fricano-Kugler CJ, Luikart BW, O’Malley AJ. Analyzing clustered data: why and how to account for multiple observations nested within a study participant? PLoS One. 2016;11(1):e0146721.
Zyzanski SJ, Flocke SA, Dickinson LM. On the nature and analysis of clustered data. Ann Fam Med. 2004;2(3):199–200.
Mathes T, Klassen P, Pieper D. Frequency of data extraction errors and methods to increase data extraction quality: a methodological review. BMC Med Res Methodol. 2017;17(1):152.
Bui DDA, Del Fiol G, Hurdle JF, Jonnalagadda S. Extractive text summarization system to aid data extraction from full text in systematic review development. J Biomed Inform. 2016;64:265–72.
Bui DD, Del Fiol G, Jonnalagadda S. PDF text classification to leverage information extraction from publication reports. J Biomed Inform. 2016;61:141–8.
Maticic K, Krnic Martinic M, Puljak L. Assessment of reporting quality of abstracts of systematic reviews with meta-analysis using PRISMA-A and discordance in assessments between raters without prior experience. BMC Med Res Methodol. 2019;19(1):32.
Speich B. Blinding in surgical randomized clinical trials in 2015. Ann Surg. 2017;266(1):21–2.
Abraha I, Cozzolino F, Orso M, Marchesi M, Germani A, Lombardo G, Eusebi P, De Florio R, Luchetta ML, Iorio A, et al. A systematic review found that deviations from intention-to-treat are common in randomized trials and systematic reviews. J Clin Epidemiol. 2017;84:37–46.
Zhong Y, Zhou W, Jiang H, Fan T, Diao X, Yang H, Min J, Wang G, Fu J, Mao B. Quality of reporting of two-group parallel randomized controlled clinical trials of multi-herb formulae: A survey of reports indexed in the Science Citation Index Expanded. Eur J Integrative Med. 2011;3(4):e309–16.
Farrokhyar F, Chu R, Whitlock R, Thabane L. A systematic review of the quality of publications reporting coronary artery bypass grafting trials. Can J Surg. 2007;50(4):266–77.
Oltean H, Gagnier JJ. Use of clustering analysis in randomized controlled trials in orthopaedic surgery. BMC Med Res Methodol. 2015;15:17.
Fleming PS, Koletsi D, Pandis N. Blinded by PRISMA: are systematic reviewers focusing on PRISMA and ignoring other guidelines? PLoS One. 2014;9(5):e96407.
Balasubramanian SP, Wiener M, Alshameeri Z, Tiruvoipati R, Elbourne D, Reed MW. Standards of reporting of randomized controlled trials in general surgery: can we do better? Ann Surg. 2006;244(5):663–7.
de Vries TW, van Roon EN. Low quality of reporting adverse drug reactions in paediatric randomised controlled trials. Arch Dis Child. 2010;95(12):1023–6.
Borg Debono V, Zhang S, Ye C, Paul J, Arya A, Hurlburt L, Murthy Y, Thabane L. The quality of reporting of RCTs used within a postoperative pain management meta-analysis, using the CONSORT statement. BMC Anesthesiol. 2012;12:13.
Kaiser KA, Cofield SS, Fontaine KR, Glasser SP, Thabane L, Chu R, Ambrale S, Dwary AD, Kumar A, Nayyar G, et al. Is funding source related to study reporting quality in obesity or nutrition randomized control trials in top-tier medical journals? Int J Obes. 2012;36(7):977–81.
Thomas O, Thabane L, Douketis J, Chu R, Westfall AO, Allison DB. Industry funding and the reporting quality of large long-term weight loss trials. Int J Obes. 2008;32(10):1531–6.
Khan NR, Saad H, Oravec CS, Rossi N, Nguyen V, Venable GT, Lillard JC, Patel P, Taylor DR, Vaughn BN, et al. A review of industry funding in randomized controlled trials published in the neurosurgical literature-the elephant in the room. Neurosurgery. 2018;83(5):890–7.
Hansen C, Lundh A, Rasmussen K, Hrobjartsson A. Financial conflicts of interest in systematic reviews: associations with results, conclusions, and methodological quality. Cochrane Database Syst Rev. 2019;8:Mr000047.
Kiehna EN, Starke RM, Pouratian N, Dumont AS. Standards for reporting randomized controlled trials in neurosurgery. J Neurosurg. 2011;114(2):280–5.
Liu LQ, Morris PJ, Pengel LH. Compliance to the CONSORT statement of randomized controlled trials in solid organ transplantation: a 3-year overview. Transpl Int. 2013;26(3):300–6.
Bala MM, Akl EA, Sun X, Bassler D, Mertz D, Mejza F, Vandvik PO, Malaga G, Johnston BC, Dahm P, et al. Randomized trials published in higher vs. lower impact journals differ in design, conduct, and analysis. J Clin Epidemiol. 2013;66(3):286–95.
Lee SY, Teoh PJ, Camm CF, Agha RA. Compliance of randomized controlled trials in trauma surgery with the CONSORT statement. J Trauma Acute Care Surg. 2013;75(4):562–72.
Ziogas DC, Zintzaras E. Analysis of the quality of reporting of randomized controlled trials in acute and chronic myeloid leukemia, and myelodysplastic syndromes as governed by the CONSORT statement. Ann Epidemiol. 2009;19(7):494–500.
Alvarez F, Meyer N, Gourraud PA, Paul C. CONSORT adoption and quality of reporting of randomized controlled trials: a systematic analysis in two dermatology journals. Br J Dermatol. 2009;161(5):1159–65.
Mbuagbaw L, Thabane M, Vanniyasingam T, Borg Debono V, Kosa S, Zhang S, Ye C, Parpia S, Dennis BB, Thabane L. Improvement in the quality of abstracts in major clinical journals since CONSORT extension for abstracts: a systematic review. Contemporary Clin trials. 2014;38(2):245–50.
Thabane L, Chu R, Cuddy K, Douketis J. What is the quality of reporting in weight loss intervention studies? A systematic review of randomized controlled trials. Int J Obes. 2007;31(10):1554–9.
Murad MH, Wang Z. Guidelines for reporting meta-epidemiological methodology research. Evidence Based Med. 2017;22(4):139.
METRIC - MEthodological sTudy ReportIng Checklist: guidelines for reporting methodological studies in health research [ http://www.equator-network.org/library/reporting-guidelines-under-development/reporting-guidelines-under-development-for-other-study-designs/#METRIC ]. Accessed 31 Aug 2020.
Jager KJ, Zoccali C, MacLeod A, Dekker FW. Confounding: what it is and how to deal with it. Kidney Int. 2008;73(3):256–60.
Parker SG, Halligan S, Erotocritou M, Wood CPJ, Boulton RW, Plumb AAO, Windsor ACJ, Mallett S. A systematic methodological review of non-randomised interventional studies of elective ventral hernia repair: clear definitions and a standardised minimum dataset are needed. Hernia. 2019.
Bouwmeester W, Zuithoff NPA, Mallett S, Geerlings MI, Vergouwe Y, Steyerberg EW, Altman DG, Moons KGM. Reporting and methods in clinical prediction research: a systematic review. PLoS Med. 2012;9(5):1–12.
Schiller P, Burchardi N, Niestroj M, Kieser M. Quality of reporting of clinical non-inferiority and equivalence randomised trials--update and extension. Trials. 2012;13:214.
Riado Minguez D, Kowalski M, Vallve Odena M, Longin Pontzen D, Jelicic Kadic A, Jeric M, Dosenovic S, Jakus D, Vrdoljak M, Poklepovic Pericic T, et al. Methodological and reporting quality of systematic reviews published in the highest ranking journals in the field of pain. Anesth Analg. 2017;125(4):1348–54.
Thabut G, Estellat C, Boutron I, Samama CM, Ravaud P. Methodological issues in trials assessing primary prophylaxis of venous thrombo-embolism. Eur Heart J. 2005;27(2):227–36.
Puljak L, Riva N, Parmelli E, González-Lorenzo M, Moja L, Pieper D. Data extraction methods: an analysis of internal reporting discrepancies in single manuscripts and practical advice. J Clin Epidemiol. 2020;117:158–64.
Ritchie A, Seubert L, Clifford R, Perry D, Bond C. Do randomised controlled trials relevant to pharmacy meet best practice standards for quality conduct and reporting? A systematic review. Int J Pharm Pract. 2019.
Babic A, Vuka I, Saric F, Proloscic I, Slapnicar E, Cavar J, Pericic TP, Pieper D, Puljak L. Overall bias methods and their use in sensitivity analysis of Cochrane reviews were not consistent. J Clin Epidemiol. 2019.
Tan A, Porcher R, Crequit P, Ravaud P, Dechartres A. Differences in treatment effect size between overall survival and progression-free survival in immunotherapy trials: a Meta-epidemiologic study of trials with results posted at ClinicalTrials.gov. J Clin Oncol. 2017;35(15):1686–94.
Croitoru D, Huang Y, Kurdina A, Chan AW, Drucker AM. Quality of reporting in systematic reviews published in dermatology journals. Br J Dermatol. 2020;182(6):1469–76.
Khan MS, Ochani RK, Shaikh A, Vaduganathan M, Khan SU, Fatima K, Yamani N, Mandrola J, Doukky R, Krasuski RA: Assessing the Quality of Reporting of Harms in Randomized Controlled Trials Published in High Impact Cardiovascular Journals. Eur Heart J Qual Care Clin Outcomes 2019.
Rosmarakis ES, Soteriades ES, Vergidis PI, Kasiakou SK, Falagas ME. From conference abstract to full paper: differences between data presented in conferences and journals. FASEB J. 2005;19(7):673–80.
Mueller M, D’Addario M, Egger M, Cevallos M, Dekkers O, Mugglin C, Scott P. Methods to systematically review and meta-analyse observational studies: a systematic scoping review of recommendations. BMC Med Res Methodol. 2018;18(1):44.
Li G, Abbade LPF, Nwosu I, Jin Y, Leenus A, Maaz M, Wang M, Bhatt M, Zielinski L, Sanger N, et al. A scoping review of comparisons between abstracts and full reports in primary biomedical research. BMC Med Res Methodol. 2017;17(1):181.
Krnic Martinic M, Pieper D, Glatt A, Puljak L. Definition of a systematic review used in overviews of systematic reviews, meta-epidemiological studies and textbooks. BMC Med Res Methodol. 2019;19(1):203.
Analytical study [ https://medical-dictionary.thefreedictionary.com/analytical+study ]. Accessed 31 Aug 2020.
Tricco AC, Tetzlaff J, Pham B, Brehaut J, Moher D. Non-Cochrane vs. Cochrane reviews were twice as likely to have positive conclusion statements: cross-sectional study. J Clin Epidemiol. 2009;62(4):380–6 e381.
Schalken N, Rietbergen C. The reporting quality of systematic reviews and Meta-analyses in industrial and organizational psychology: a systematic review. Front Psychol. 2017;8:1395.
Ranker LR, Petersen JM, Fox MP. Awareness of and potential for dependent error in the observational epidemiologic literature: A review. Ann Epidemiol. 2019;36:15–9 e12.
Paquette M, Alotaibi AM, Nieuwlaat R, Santesso N, Mbuagbaw L. A meta-epidemiological study of subgroup analyses in cochrane systematic reviews of atrial fibrillation. Syst Rev. 2019;8(1):241.
Download references
Acknowledgements
This work did not receive any dedicated funding.
Author information
Authors and affiliations.
Department of Health Research Methods, Evidence and Impact, McMaster University, Hamilton, ON, Canada
Lawrence Mbuagbaw, Daeria O. Lawson & Lehana Thabane
Biostatistics Unit/FSORC, 50 Charlton Avenue East, St Joseph’s Healthcare—Hamilton, 3rd Floor Martha Wing, Room H321, Hamilton, Ontario, L8N 4A6, Canada
Lawrence Mbuagbaw & Lehana Thabane
Centre for the Development of Best Practices in Health, Yaoundé, Cameroon
Lawrence Mbuagbaw
Center for Evidence-Based Medicine and Health Care, Catholic University of Croatia, Ilica 242, 10000, Zagreb, Croatia
Livia Puljak
Department of Epidemiology and Biostatistics, School of Public Health – Bloomington, Indiana University, Bloomington, IN, 47405, USA
David B. Allison
Departments of Paediatrics and Anaesthesia, McMaster University, Hamilton, ON, Canada
Lehana Thabane
Centre for Evaluation of Medicine, St. Joseph’s Healthcare-Hamilton, Hamilton, ON, Canada
Population Health Research Institute, Hamilton Health Sciences, Hamilton, ON, Canada
You can also search for this author in PubMed Google Scholar
Contributions
LM conceived the idea and drafted the outline and paper. DOL and LT commented on the idea and draft outline. LM, LP and DOL performed literature searches and data extraction. All authors (LM, DOL, LT, LP, DBA) reviewed several draft versions of the manuscript and approved the final manuscript.
Corresponding author
Correspondence to Lawrence Mbuagbaw .
Ethics declarations
Ethics approval and consent to participate.
Not applicable.
Consent for publication
Competing interests.
DOL, DBA, LM, LP and LT are involved in the development of a reporting guideline for methodological studies.
Additional information
Publisher’s note.
Springer Nature remains neutral with regard to jurisdictional claims in published maps and institutional affiliations.
Rights and permissions
Open Access This article is licensed under a Creative Commons Attribution 4.0 International License, which permits use, sharing, adaptation, distribution and reproduction in any medium or format, as long as you give appropriate credit to the original author(s) and the source, provide a link to the Creative Commons licence, and indicate if changes were made. The images or other third party material in this article are included in the article's Creative Commons licence, unless indicated otherwise in a credit line to the material. If material is not included in the article's Creative Commons licence and your intended use is not permitted by statutory regulation or exceeds the permitted use, you will need to obtain permission directly from the copyright holder. To view a copy of this licence, visit http://creativecommons.org/licenses/by/4.0/ . The Creative Commons Public Domain Dedication waiver ( http://creativecommons.org/publicdomain/zero/1.0/ ) applies to the data made available in this article, unless otherwise stated in a credit line to the data.
Reprints and permissions
About this article
Cite this article.
Mbuagbaw, L., Lawson, D.O., Puljak, L. et al. A tutorial on methodological studies: the what, when, how and why. BMC Med Res Methodol 20 , 226 (2020). https://doi.org/10.1186/s12874-020-01107-7
Download citation
Received : 27 May 2020
Accepted : 27 August 2020
Published : 07 September 2020
DOI : https://doi.org/10.1186/s12874-020-01107-7
Share this article
Anyone you share the following link with will be able to read this content:
Sorry, a shareable link is not currently available for this article.
Provided by the Springer Nature SharedIt content-sharing initiative
- Methodological study
- Meta-epidemiology
- Research methods
- Research-on-research
BMC Medical Research Methodology
ISSN: 1471-2288
- General enquiries: [email protected]
REVIEW article
A review of common statistical methods for dealing with multiple pollutant mixtures and multiple exposures.
- 1 Department of Health Statistics, School of Public Health, Shanxi Medical University, Taiyuan, China
- 2 Key Laboratory of Coal Environmental Pathogenicity and Prevention (Shanxi Medical University), Ministry of Education, Taiyuan, China
Traditional environmental epidemiology has consistently focused on studying the impact of single exposures on specific health outcomes, considering concurrent exposures as variables to be controlled. However, with the continuous changes in environment, humans are increasingly facing more complex exposures to multi-pollutant mixtures. In this context, accurately assessing the impact of multi-pollutant mixtures on health has become a central concern in current environmental research. Simultaneously, the continuous development and optimization of statistical methods offer robust support for handling large datasets, strengthening the capability to conduct in-depth research on the effects of multiple exposures on health. In order to examine complicated exposure mixtures, we introduce commonly used statistical methods and their developments, such as weighted quantile sum, bayesian kernel machine regression, toxic equivalency analysis, and others. Delineating their applications, advantages, weaknesses, and interpretability of results. It also provides guidance for researchers involved in studying multi-pollutant mixtures, aiding them in selecting appropriate statistical methods and utilizing R software for more accurate and comprehensive assessments of the impact of multi-pollutant mixtures on human health.
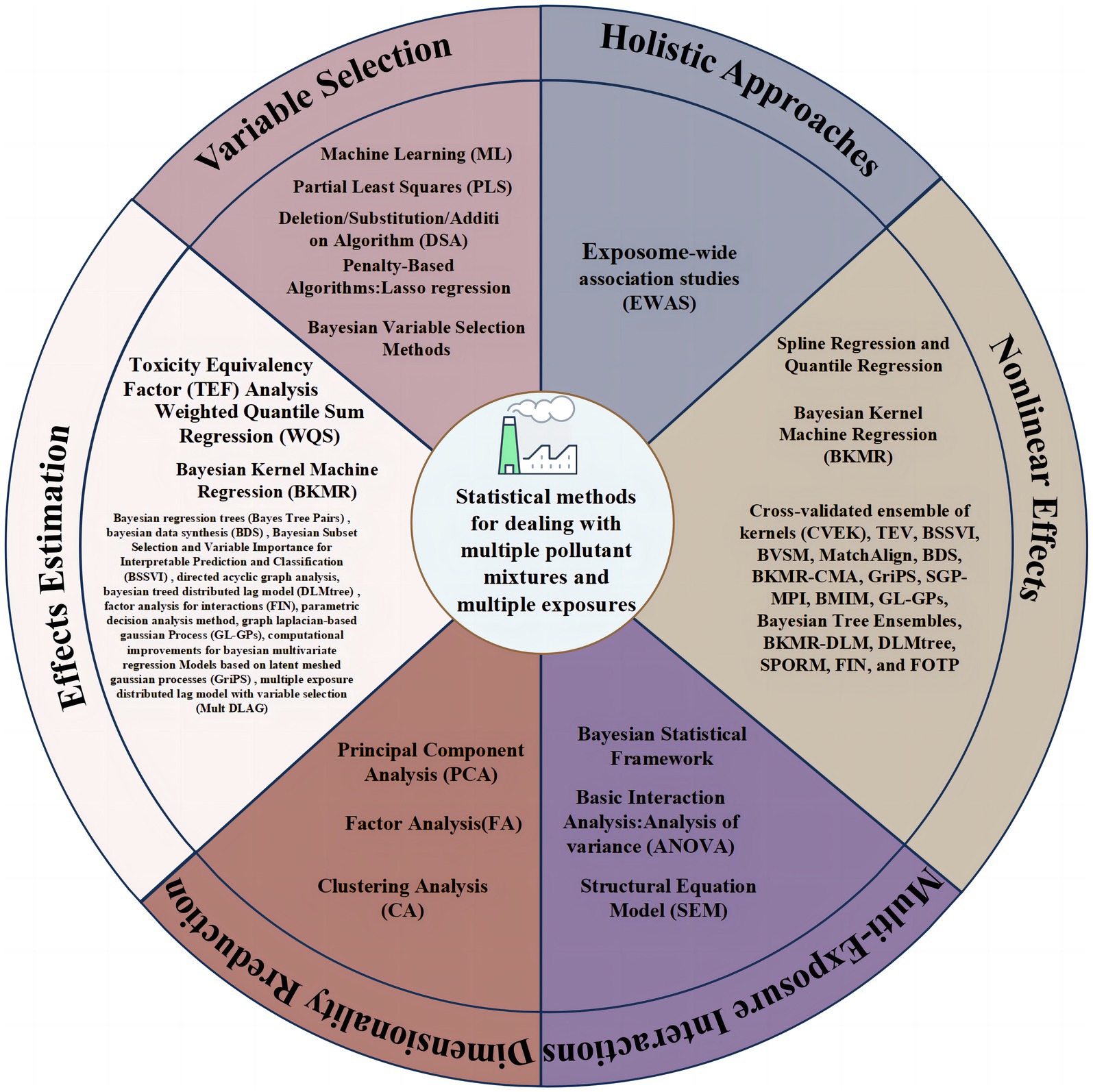
Graphical Abstract .
1 Introduction
In the contemporary industrialized society, environmental concerns such as air pollution, water pollution, and soil contamination have gained significant attention ( 1 – 4 ). Some pollutants are metabolized because of their shorter half-lives, others, such as heavy metals, insecticides, flame retardants, persistent organic pollutants, and other endocrine-disrupting chemicals continue to accumulate in the human body and have significant and long-term effects on human health ( 5 – 8 ). For instance, the association between heavy metals toxicity and the development of neurodegenerative diseases and various ocular pathologies has been established, while concurrent exposure to heavy metals can elevate the risk of prostate cancer and thyroid enlargement ( 9 – 11 ). Polybrominated diphenyl ether is a persistent and pervasive environmental pollutant that disrupts the human endocrine system, leading to health implications such as developmental, thyroidal, and reproductive toxicity ( 12 , 13 ). Particulate matter along with nitrogen oxides in the atmospheric environment exhibit a close correlation with stroke incidence rate and mortality. The higher the concentration of particulate matter exposure, the greater risk of stroke ( 14 ). However, most studies are on single pollutants, they do not accurately reflect the real world since people are exposed to a combination of several dangerous substances at any given time, which might have antagonistic or synergistic effects. What’s more, single-pollutant analysis methods often fall short in capturing the complexity and interactive effects of multi-pollutant mixtures ( 15 ). Lastly, the potential for spurious associations has increased in single-pollutant models, contributing to disagreements between studies. Consequently, accurately assessing the health effects of exposure to mixtures of environmental pollutants has become a focal point in current environmental epidemiology. In recent years, the focus of health effect assessments of environmental risk factors has shifted from the traditional single-pollutant approach to the study of mixtures of multiple pollutants ( 16 – 18 ). This shift aims to more accurately reflect the impact of environmental risk factors on human health.
In order to handle the multi-pollutant mixtures exposure, researchers have raised a number of problems that need to be addressed, as shown in Table 1 .
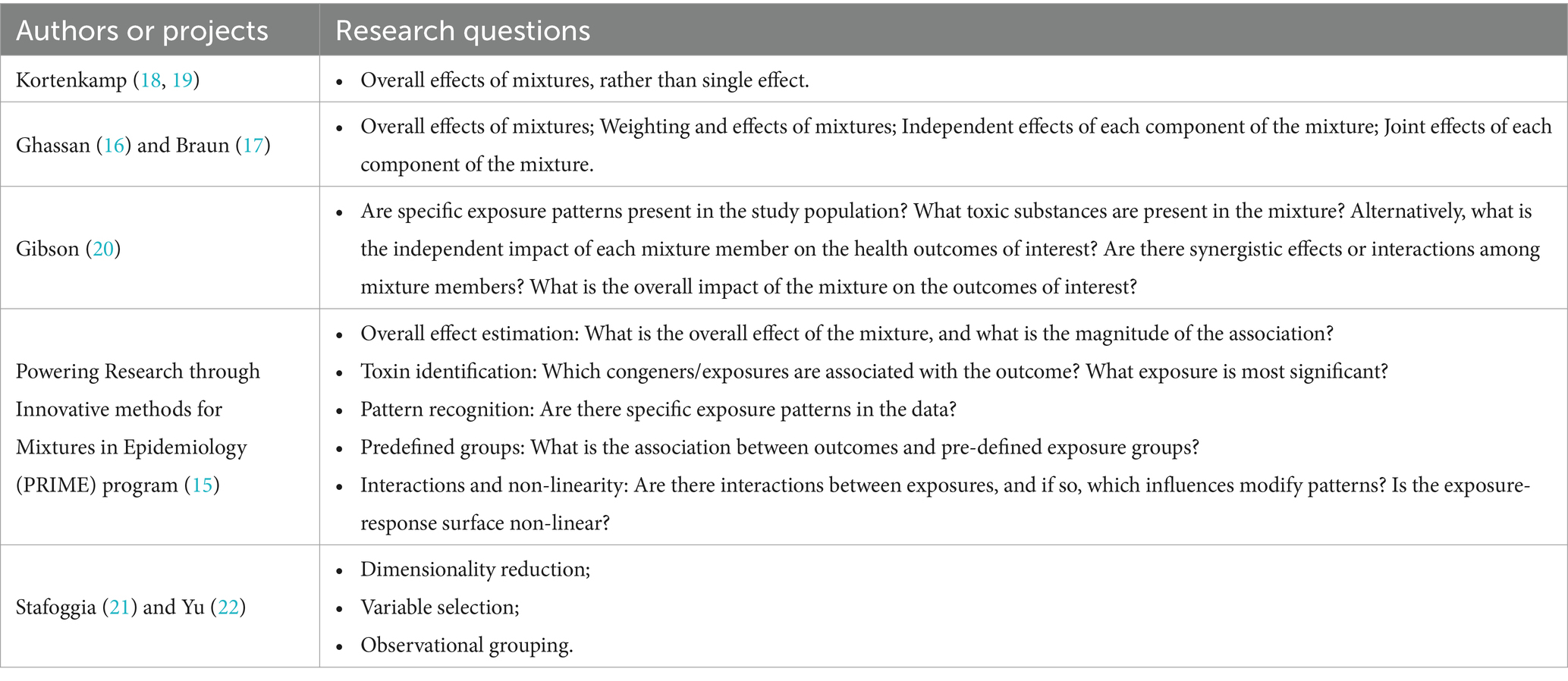
Table 1 . Key research questions on multi-pollutant mixtures exposure.
The study of multi-pollutant mixtures is characterized by two primary focuses: (A) Estimating the effects of pollutants and (B) addressing the complexity associated with multi-pollutant mixtures.
Regarding effects estimation, the focus is divided into three aspects: (1) Overall effects of multi-pollutant mixtures; (2) Independent effects of components within multi-pollutant mixtures; and (3) Joint effects of mixture components.
Addressing the complexity of multi-pollutant mixtures involves three main aspects: (1) Addressing the challenge of high-dimensional data when multiple chemical substances are present in the model; (2) Resolving the issue of high correlations among pollutants to assess synergistic or antagonistic effects; and (3) Addressing interplay and non-linear effects among pollutants.
Thus, diverse statistical methods are introduced in this paper to address specific issues, as shown in Graphical abstract.
2 Methods for effects estimation
In estimating overall effects of multi-pollutant mixtures, two main approaches are commonly used: treating the mixtures as a single exposure or analyzing the weighted sum of exposures ( 17 ). For example, particle matter concentration serves as a comprehensive measure of particulate matter components in ambient air, assuming equal impact on health for all components ( 23 ). Alternatively, overall effect estimation can involve the weighted sum of individual component effects, with weights based on toxicological potency or contribution percentage ( 17 ). For instance, modeling phthalate metabolites’ concentrations using molar sum or potency-weighted sum methods ( 24 , 25 ). Independent effects estimation considers diverse pollution components’ varied adverse effects, requiring the evaluation of overall mixture impact before assessing individual component effects ( 26 ). Joint effects estimation accounts for component interactions beyond additive impacts ( 18 ), considering mechanisms and pathways. For example, non-volatile carbonaceous particles like black carbon may require specific attention when studying joint health effects with volatile organic compounds. In this section we briefly introduce several methods of effects estimation. Figure 1 shows details of the effect estimation methods and R packages for their implementation.
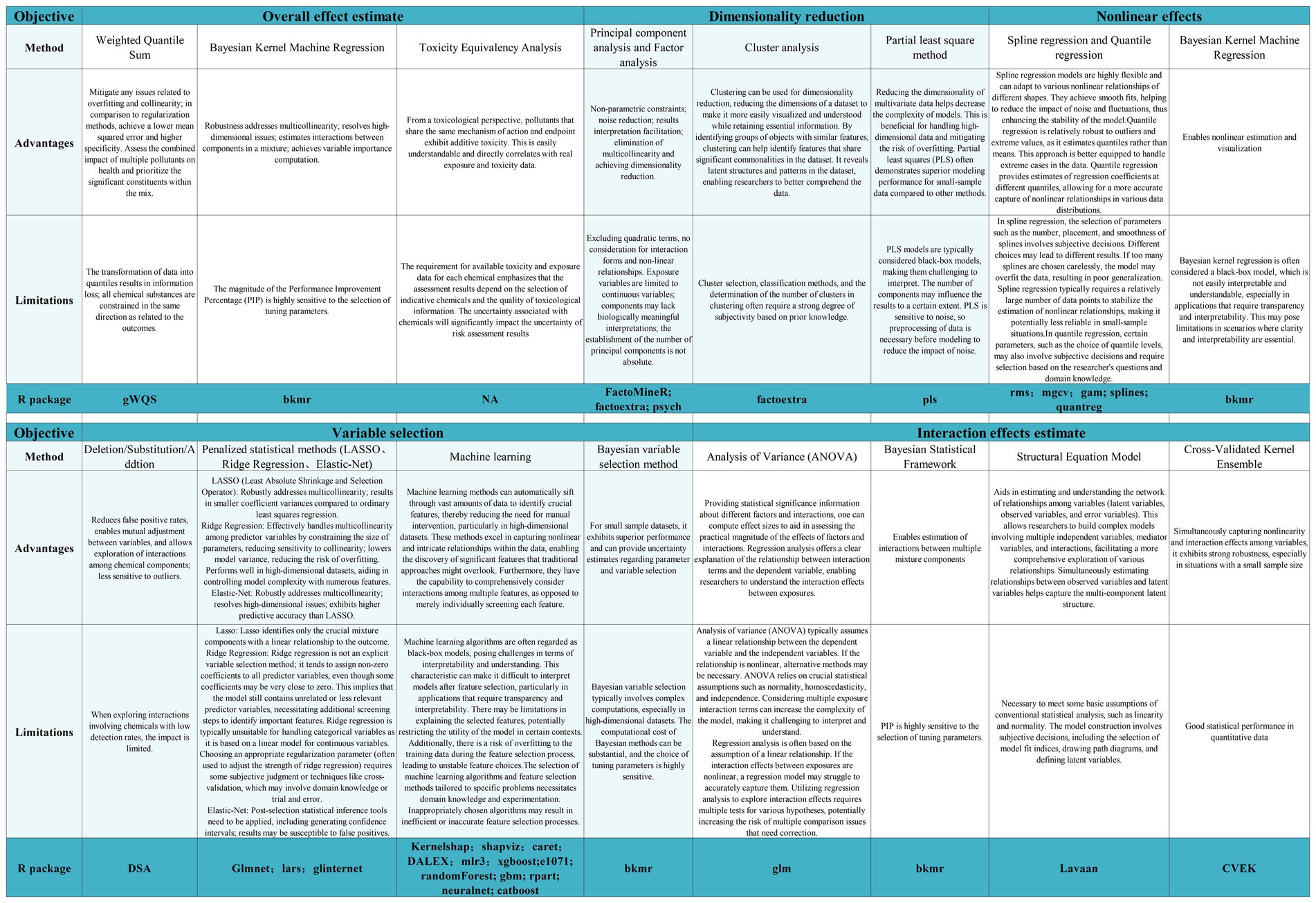
Figure 1 . Overview of the methods and R packages for implementation.
2.1 Weighted quantile sum regression
Weighted quantile sum (WQS) regression is a convenient tool for addressing effect estimation problem, the high-dimensional and highly correlated issues among multiple pollutants, particularly among homogenous pollutants ( 27 ). It is widely used in studies of environmental exposure to multi-pollutant mixtures and enables the identification of high-risk factors. This model allows the construction of a weighted index in a supervised manner to assess the overall effects of environmental exposure and the contribution of each component in the mixture to the overall effect. WQS calculates individual exposure characteristics by weighting based on the correlation between exposure and outcome, resulting in a composite index value for various exposure components. This index characterizes the levels of mixed exposure to a range of exposure components and evaluates the impact of each component on health outcomes. Following Tanner’s recommendation, introducing a bootstrap step in the WQS yields stable weights for exposure components and WQS index estimates ( 28 ). The core idea is to construct WQS to achieve dimensionality reduction, address multicollinearity issues, and filter high-risk factors through the weighting process. The most recent weight coefficient for each component in the exposure index represents its contribution to health outcomes.
WQS has advantages in analyzing multifactor exposure due to their simple model structure, small computational burden, and fast analysis speed. But the “directional consistency” precondition must be met, i.e., the effects of each component in the mixture are all in the same direction (all positive or all negative). Recent research has explored and developed new methods for WQS. For unidirectional hypotheses, methods such as quantile g-computation combined with the g-algorithm ( 29 ), grouped WQS ( 30 ), and Bayes group WQS model ( 31 ) have been developed. Focus has also been given to the lagged WQS to address time-varying exposure mixtures ( 32 , 33 ).
2.2 Bayesian kernel machine regression
Bayesian kernel machine regression (BKMR) also provides a new approach to analyze multi-pollutant mixtures ( 34 , 35 ). In contrast to WQS, BKMR provides probabilities included in the total effects of multi-pollutant mixtures, rather than estimate the percentage contribution of this effect but provides probabilities included in the total effects of multi-pollutant mixtures. It visualizes various exposure-response shapes. BKMR can also examine the independent impacts of mixture components by considering the effects of keeping other components constant at predetermined percentiles, such as the 50th percentile of the exposure distribution. BKMR does not require setting a parameter expression, allowing for the presence of nonlinear effects and interactions. It generates kernel functions based on the mixture variables included in the model, followed by Bayesian sampling and analysis methods to generate relationship curves between mixture components and disease variables included in the model.
In addition to analyzing the mixture’s overall impacts and each component’s effects separately, BKMR also estimates any possible interactions between the distinct components. Posterior inclusion probabilities (PIPs) generated by BKMR range from 0 (least important) to 1 (most important). Components with PIP ≥ 0.5 are identified as relatively important mixture components. BKMR can also be used to study possible three-way interactions. This is achieved by fixing one of the exposures at different quantile levels and visualizing the exposure-response functions for the remaining two exposures. Overall, BKMR has been widely used in environmental health research, including the analysis of continuous variables, binary variables, and repeated measurement data ( 36 , 37 ). The advantages of this method include the ability to simultaneously assess the importance of each variable, analyze data with uncertainty, and easily extend the obtained results to longitudinal data.
Although BKMR can effectively assess the health effects of multi-pollutant mixtures, it has certain limitations. Firstly, when using the BKMR, the studied exposure variables must be continuous, and the size of PIPs is easily influenced by adjustment parameters. So, caution is required when interpreting results, as this method may obscure the underlying complex features of the data. If some components in the mixture are positively correlated while others are equally negatively correlated, the final overall result will appear as if there is no correlation, and other methods are needed to verify the estimation of their interactions. In addition, considering causality, time-varying exposure, or computational efficiency in massive datasets, the traditional implementation of BKMR may be limited. Several new methods have extended the BKMR strategy to address these limitations, such as bayesian kernel machine regression – causal mediation analysis (BKMR-CMA) ( 38 ), bayesian kernel machine regression distributed lag model (BKMR-DLM) ( 39 ).
2.3 Toxicity equivalency analysis
In addition to the two commonly used estimation methods mentioned above, pollutants with similar mechanisms of action and the same endpoint from a toxicological perspective exhibit additive toxicity. However, individual pollutants contribute differently to the overall health risk. Therefore, a normalization method, known as toxicity equivalency factor (TEF) analysis, a normalizing technique, is required. TEF is generally obtained by comparing the “starting point” of health risk assessments for standard reference compounds with the respective compounds. The exposure dose of a mixture, commonly represented as toxicity equivalent quantity (TEQ), is calculated by multiplying the TEF for each compound by its exposure metric and summing them. By combining TEQ with reference metrics such as the reference dose (RfD) or carcinogenic slope factor, the health risk of the mixture can be assessed ( 40 – 43 ). TEF represents the relative toxicity of an isomer of a compound and is set to 1 for the most toxic 2,3,7,8-TCDD. Other pollutants’ toxicities are converted to their corresponding relative toxic intensities. Alternatively, TEF can be the toxicity equivalency factor for individual Polycyclic Aromatic Hydrocarbons, with a TEF of 0.001 for Pyrene. Daily total intake exposure metric from plasma polycyclic aromatic hydrocarbon levels based on pharmacokinetic models ( 40 – 42 ). The results can be compared against specified standards to determine the presence of carcinogenic risk ( 43 ). To address non-linear problems, the acceptable concentration range model has been developed based on the RfD concept ( 44 ).
TEF has the advantage of being easy to understand and directly associated with real exposure and toxicity data. However, it requires available toxicity and exposure data for each chemical, making the assessment results dependent on the selection of indicative chemicals and the quality of toxicological information. Uncertainty in the chemicals significantly affects the uncertainty of risk assessment results.
2.4 Other methods for effects estimation
In addition to the three statistical methods for estimating effects mentioned above, there are also novel and unique methods for effect estimation, although they may have a narrower audience. These include bayesian regression trees ( 45 ), bayesian data synthesis (BDS) ( 46 ), bayesian subset selection and variable importance for interpretable prediction and classification (BSSVI) ( 47 ), directed acyclic graph analysis ( 48 ), bayesian treed distributed lag model (DLMtree) ( 49 ), factor analysis for interactions (FIN) ( 50 ), parametric decision analysis method ( 51 ), graph laplacian-based gaussian Process (GL-GPs) ( 52 ), computational improvements for bayesian multivariate regression models based on latent meshed gaussian processes (GriPS) ( 53 ), and multiple exposure distributed lag model with variable selection ( 54 ).
3 Methods for dimensionality reduction
When analyzing multi-pollutant mixtures with fewer components, the process is relatively simple, but the dimensionality of the data increases dramatically when multi-pollutant mixtures contain several components. Many statistical methods lack the capability to address this issue, and even methods designed to handle the complexity of high-dimensional data incur exponential time costs as the data dimensionality grows. Furthermore, the high correlation among components may lead to multicollinearity. For instance, analyzing correlated components with similar sources, exposure pathways, or metabolic processes, regardless of which one is individually studied, may yield biased conclusions. Faced with the challenges of high dimensionality and multicollinearity, a crucial aspect of studying the health impacts of multi-pollutant mixtures involves learning low-dimensional structures in the data to enhance interpretability and statistical efficiency, employing methods of dimensionality reduction proves to be a favorable approach. In this section we briefly introduce several dimensionality reduction methods. Figure 1 shows details of the methods for dimensionality reduction and R packages for their implementation.
3.1 Principal component analysis and factor analysis
Principal component analysis (PCA), introduced by Pearson for non-random variables and later extended to random vectors by Hotelling, transforms a set of potentially correlated variables into a set of linearly uncorrelated variables, referred to as principal components, through orthogonal transformation ( 55 ). The primary objective of PCA is to explain the majority of variance in the original data using fewer variables, converting highly correlated variables into ones that are independent or uncorrelated. When analyzing the relationship between multiple pollutant indicators and health, PCA can reduce the number of indicators for analysis, minimizing information loss from the original indicators and facilitating comprehensive data analysis. It simplifies high-dimensional exposure data into several orthogonal components usable for regression models, thus mitigating multicollinearity issues. For example, Smit applied PCA to estimate the relationship between the risk of asthma and eczema in school-age children and 16 pollutants in their mothers’ serum. The study ultimately incorporated indicators from five principal components, explaining 70% of the variance in the outcome variable ( 56 ).
PCA’s main limitations include difficulty in interpreting results, as the components are not in the same units as the original exposure variables, and the derived components may lack a direct relationship with study outcomes as they are derived in an unsupervised manner. Subsequently, PCA has evolved into methods like supervised PCA, which overcomes these issues by excluding “pollutants” that do not provide information directly related to the outcomes ( 57 ). Roberts applied this method to air pollution analysis, proposing a recursive algorithm that identifies the optimal predictor for study outcomes and combines it into several relevant principal components ( 58 ). Other developments include principal component pursuit (PCP), an analysis method based on matrix factorization, extended to multi-pollutant mixtures by Gibson. Through cross-validation in simulations, PCP identified the true number of patterns in all simulations, while PCA achieved this in only 32% of simulations, demonstrating PCP’s superiority in most simulation scenarios ( 59 ). In addition to the above methods, Positive matrix factorization (PMF) is a variant of PCA applicable to multi-pollutant profiles, deriving air pollution sources from individual chemical components ( 60 ). Specifically, PMF decomposes the matrix of mixture data into two matrices—source contributions and source profiles. Source contributions represent the mass contribution of each source to the mixture measurements, while source profiles reflect the emission types from a given source. Source contributions are constrained to be non-negative, and the method can incorporate uncertainty measurements related to the data at each point ( 61 – 64 ).
Factor analysis (FA) is another commonly used dimensionality reduction method that groups variables based on the correlation matrix, creating common factors that represent the fundamental structure of the data. It decomposes multidimensional variables into a small number of common factors, where the fundamental idea is to break down original variables into two parts: one part is a linear combination of common factors that condenses a vast majority of information in the original variables, and the other part is special factors unrelated to common factors, reflecting the gap between the linear combination of common factors and the original variables. In other words, FA aggregates numerous variables into a few independent common factors with minimal loss or little loss of original data information. These common factors can reflect the essential information of numerous variables, reduce the number of variables, and reveal the inherent connections among variables. Perturbation FA is commonly used in multi-pollutant mixtures studies, focusing on exploring the similarities and differences in exposure conditions among different groups. For example, Roy used this method to assessed the differences in exposure characteristics in biological or social structures based on race/ethnicity ( 65 ).
Both PCA and FA seek a small number of variables to comprehensively reflect the majority of information in all variables. While the number of variables is fewer than the original variables, the information contained is substantial, and the reliability of using these new variables for analysis remains high. Moreover, these new variables are uncorrelated, eliminating multicollinearity and achieving dimensionality reduction. In PCA, the newly determined variables are linear combinations of the original variables, obtained through coordinate transformation. In contrast, FA aims to explain the complex relationships present in many observed variables using a small number of common factors. It does not recombine original variables but decomposes them.
3.2 Clustering analysis
Clustering analysis (CA) organizes all data into clusters, groups of similar elements, where instances within the same cluster are similar to each other, while those in different clusters are dissimilar. Similarity among data is determined by defining a distance or similarity coefficient ( 66 ). Once several clusters are identified, the next step is to select a representative prototype for each cluster. CA can be matched with exposure data to define groups, and indicators of group members can then be used as predictor variables in health outcome regression models.
Clustering can be categorized into different groups based on techniques, with partition-based clustering being the most widely used, where k-means is a common approach ( 67 ). One advantage of the k-means method is its linear complexity, making its execution time proportional to the number of individuals, making it suitable for large datasets. However, the choice of initial centers and the number of clusters is arbitrary and can influence the results. Nevertheless, hierarchical classification can be applied to the cluster centers obtained from the k-means method. Clustering has been used in several studies to assess the impact of various pollutants. For instance, in time series analysis of air pollution, one study used k-means to divide days into five groups representing days with low pollution levels, high concentrations of crustal particles, high particle content from traffic and combustion of oil, days influenced by regional pollution sources, and days with high concentrations of particles from wood or oil burning ( 68 ). Some clusters were associated with pulse amplitude. Similarly, based on pollutant characteristics and community background, an evaluation was conducted on the correlation between NO 2 , NO, and PM 2.5 concentrations and low birth weight ( 69 ).
The challenges of CA lie in the selection of clusters, classification methods, and the number of clusters. CA facilitates the distinct grouping of various entities, making it challenging to summarize them under a single label. The process typically necessitates the initial selection of appropriate distance metrics, clustering algorithms, and the number of clusters. These choices often based on users’ subjective judgments by the user, and different selections may yield disparate clustering outcomes, thereby rendering the results subjective.
4 Methods for variable selection
When analyzing multi-pollutant mixtures with many components, it is not necessary to estimate the impact of each component of mixture, rather, the focus is on investigating the effects of a few crucial components that exhibit the maximum toxicity to human health and/or have the highest predictive power for the outcomes of interest. Therefore, it is imperative to employ appropriate methods for identifying or selecting important variables that represent the exposure-response relationship between individual exposures in the mixture and the outcomes. Uch methods are frequently known as “variable selection.” In this section we briefly introduce several methods of variable selection. Figure 1 shows details of the methods for variable selection and R packages for their implementation.
4.1 Partial least squares
Partial least squares (PLS) regression combines principal component analysis and multivariate regression, taking into account the correlation between the outcomes and exposure variables ( 70 ). In essence, PLS regression searches for a linear decomposition of the exposure matrix that maximizes the covariance between exposure and outcomes. The exposed variable has a higher weight in the linear combination, the stronger the association between it and the outcome. PLS regression can also include multiple outcome variables. The optimal number of components can be selected based on cross-validated mean squared error ( 71 ). However, a drawback of PLS regression is that the interpretation of the linear combination can be challenging, especially in the presence of a large number of original exposure variables.
To address this limitation, Chun and Keles introduced a method called sparse PLS regression, which simultaneously combines variable selection and dimensionality reduction ( 72 ). This method results in a linear combination of exposure variables with reduced quantity. Sparsity is introduced into the loadings of exposure variables through penalty terms. The optimal number of components and sparsity parameters are selected based on cross-validated performance. This method has been applied in simulation studies related to exposure-health associations. In one simulation study involving 237 generated exposure covariates, 0 to 25 of which were related to the outcomes, sparse PLS regression demonstrated better sensitivity in distinguishing true predictive factors from correlated covariates ( 73 ).
4.2 Deletion/substitution/addition algorithm
The Deletion/Substitution/Addition (DSA) algorithm is a variable selection method ( 74 , 75 ). The main steps include: (1) removal of selected variables; (2) substitution of selected variables with unselected ones; and (3) addition of new variables. By using five-fold cross-validation to minimize the root mean square error (L2 loss function) of the prediction equation, the number and particular kinds of variables included in the model are ascertained. To ensure selection stability, DSA is run with different seed numbers for 50 iterations. Subsequently, a binomial generalized linear model evaluation is conducted for multi-exposure variables. Variables included in the final model are those selected in at least 6% ( n ≥ 3 times) or 10% ( n ≥ 5 times) of DSA iterations, and multicollinearity is validated in the final model.
Compared to traditional linear regression equations, this method reduces the false-positive rate, allows for mutual adjustments between variables, and explores interactions between chemicals. However, its effectiveness is limited when exploring interactions involving chemicals with low detection rates. The algorithm also provides the possibility of including interaction terms. In contrast to stepwise model selection procedures, DSA has the advantage of being less sensitive to outliers and permits movement between non-nested statistical models. In previous applications, the DSA algorithm has been utilized in multi-pollutant mixtures analysis, estimating the relationship between O 3 , CO, NO 2 , PM 10 and lung function ( 76 ). However, DSA has faced criticism, particularly when the ratio of sample size to the number of candidate predictors is small, leading to inconsistent estimates. Moreover, its statistical properties for confidence intervals are compromised, when there is substantial correlation between predictors ( 77 ).
4.3 Penalty-based algorithms
Least absolute shrinkage and selection operator (LASSO) regression is highly similar to ordinary least squares, with the key difference lying in the estimation of coefficients through the minimization of a slightly different quantity, resulting in a shrinkage penalty on the coefficients’ magnitudes ( 78 ). It penalizes the absolute size of regression coefficients based on the value of the tuning parameter λ. Consequently, LASSO can drive coefficients of irrelevant variables to zero, thereby performing automatic variable selection. When the tuning parameter λ is small, the results essentially converge to least squares estimation. Elastic net (ENET) combines the LASSO method with ridge regression (RR) ( 79 ),it includes first and second-order penalty terms on the regression coefficients. Thus, it not only selects the best subset of variables by precisely shrinking some effect estimates to zero through LASSO but also retains a set of highly correlated variables in a RR model with similar effect estimates. For instance, in the Veterans Affairs Normative Aging Study, the use of LASSO enables the selection of PM 2.5 components related to blood pressure ( 80 ). In a recent study, based on ENET penalized regression, two metabolites of phthalates were found to be consistently associated with impaired fetal growth ( 81 ). The group-lasso interaction-net method extends LASSO to select bidirectional interaction terms ( 82 ), allowing for the simultaneous use of LASSO while controlling the false discovery rate ( 83 ). A key characteristic of LASSO is the introduction of an L1 regularization term in estimation, leading to the precise compression of certain coefficients to zero, thereby achieving feature selection. Nevertheless, this excessive sparsity may render the model overly sensitive to noise, and the selected features may prove unstable across different datasets.
4.4 Machine learning approaches
Machine learning (ML) is a research methodology focused on discovering patterns within data and utilizing these patterns to make predictions. Variable selection is a crucial issue in the field of ML, as the predictive performance of models is influenced to some extent by the variables included in the model. The number of variables, variables’ correlations, and the inclusion of important variables significantly impact the accuracy and efficiency of predictive models. Therefore, variable selection plays an indispensable role in constructing predictive models. Numerous ML algorithms are currently available for variable selection based on variable importance. Common methods include classification and regression trees ( 84 ), random forest (RF) models ( 85 ), support vector regression (SVM) ( 86 ), K-nearest neighbors (KNN) ( 87 ), naive bayes ( 88 ), neural networks ( 89 ), adaptive boosting (AdaBoost) ( 90 ), gradient boosting (GBM) ( 91 ), eXtreme gradient boosting (XGBoost) ( 92 ), light gradient boosting machine (LightGBM) ( 93 ), CatBoost ( 94 ), and others are examples of common techniques.
While ML demonstrates effective results, they often face challenges related to interpretability. For instance, models like XGBoost or LightGBM, comprised of N trees, make it difficult to understand how the features of a specific sample influence the final result. To address this issue, SHAP (shapley additive explanations) provides a method for explaining ML, offering detailed and interpretable information about model predictions ( 95 ). As the demand for incorporating complex high-dimensional data in environmental health research continues to grow, researchers are increasingly turning to ML. Recent studies have employed various ML such as AdaBoost, SVM, RF, decision tree classifier (DT), and KNN to identify the relationship between heavy metal exposure and coronary heart disease. Integrated with SHAP, these studies explained ML, determining the contributions of heavy metals such as cesium, thallium, antimony, dimethyl arsenic acid, barium, and arsenic acid in urine to the risk of coronary heart disease. This increases the likelihood that coronary heart disease can be detected and treated early ( 96 ). Some studies have also used multilayer perceptron, RR, gradient boosting decision tree, voting classifier, and KNN algorithms for generating optimal predictive models for multiple heavy metals causing hypertension. These studies integrated permutation feature importance analysis, Partial Dependence Plots, and SHAP methods into a single process, embedded within ML for model interpretation ( 97 ). However, most of the mentioned ML models are used for prediction and require comparisons based on accuracy, sensitivity/recall, specificity, negative predictive value, false positive rate, false negative rate, and F1 score.
4.5 Bayesian variable selection methods
ML algorithms such as RF can provide measures of variable importance for mixed components, but these measures do not succinctly capture the overall magnitude or direction of their associations. Variable selection techniques within the regression framework, such as LASSO, shrink individual regression coefficients to zero. However, these techniques are typically based on relatively simple models of mixed components parameters. To systematically address highly correlated exposures, the BKMR employs a hierarchical variable selection approach. This method can incorporate prior knowledge about the exposure variable/mixed component correlation structure to provide PIPs, as detailed in Section 2.2.
5 Methods for identifying multi-exposure interactions
Although various components in multi-pollutant mixtures may have completely independent effects on health outcomes, in many cases, there may be interactions among components in the mixtures. Interactions represent the mutual dependence effects of two or more variables and can manifest as synergistic, additive, or antagonistic effects ( 98 ). A typical example of interaction is the additive synergistic effect of O 3 and particulate matter on the incidence of cardiovascular diseases ( 99 ). In the real world, interactions among various exposure pollutants may exist, and the analysis of these interactions aims to identify and explain their effects. Analyzing and interpreting interactions among multiple exposures can provide a more comprehensive understanding of exposure patterns and identify cooperative effects between specific exposures under certain conditions. In this section we briefly introduce several methods for identifying interaction effects. Figure 1 shows details of the methods for identifying multi-exposure interactions and R packages for their implementation.
5.1 Basic interaction analysis
In interaction factor analysis, analysis of variance (ANOVA) is commonly used to test whether interaction effects among multiple exposures are significant. By comparing the F -values or p -values of individual factors and interactions, it is possible to determine which factors exhibit interaction effects. Additionally, regression analysis can also be used to explore the interaction effects of exposures, perform significance tests, and create a relationship model between exposure interaction terms and outcomes.
5.2 Bayesian statistical framework
Apart from BKMR, Antonelli utilized a semi-parametric Bayesian sparse prior regression framework to generate variable importance scores for each exposure and each pairwise interaction in the mixture ( 100 ).
5.3 Structural equation model
A technique called the structural equation model (SEM) combines particular covariance and regression sets between certain variables into a single coherent model ( 101 ). It is used to test and estimate relationships between observed data and latent variables, as well as to assess the fit of theoretical models. SEM combines various techniques such as FA and path analysis, allowing researchers to simultaneously explore complex relationships between multiple variables. In SEM, a measurement model can be constructed to capture measurement errors and covariances among different exposure factors and health outcomes. This aids in accurately measuring these factors and accounting for measurement errors. It is also possible to determine the causal links between various exposure factors and health outcomes using structural models.
SEM is useful for estimating and understanding the network of relationships between variables (latent, observed, and error variables) and it is also employed to estimate the degree of model fit and allows for the presence of measurement errors in independent and dependent variables ( 102 ). As researchers turn to modeling multi-pollutant mixtures, SEM is increasingly used to estimate the impact of multi-pollutant mixtures on health ( 103 , 104 ). For instance, SEM assessed the relationship between respiratory function, tobacco smoke exposure, and volatile organic compound exposure in a nationally representative sample of adolescents, revealing associations between respiratory function and certain types of volatile organic compounds ( 104 ). It is noteworthy that a critical feature of SEM analysis is its requirement to meet some basic assumptions of traditional statistical analyses, such as linearity and normality; otherwise, the obtained statistical data may be unreliable.
6 Methods for nonlinear effects
Numerous epidemiological studies have identified nonlinear associations (U-shaped, inverted U-shaped, J-shaped, etc.) between mixed pollutant exposures and health outcomes. For example, the relationship between plasma heavy metals concentrations and type 2 diabetes ( 105 ), as well as the association between volatile organic compounds and heart rate variability index ( 106 ). Ignoring the potential nonlinearity may result in biased conclusions. Therefore, a better approach is to fit the nonlinear relationship between exposure and outcome. In this section we briefly introduce several methods for estimating nonlinear effects. Figure 1 shows details of the methods for nonlinear effects and R packages for their implementation.
6.1 Spline regression and quantile regression
To overcome the limitations of polynomials, spline methods are often used for curve fitting, employing a piecewise function strategy instead of complex polynomials. One commonly used method in pollution studies is restricted cubic spline (RCS), which fits the curve relationship between a variable and an outcome using restricted cubic spline terms. For instance, Zhou combined RCS with logistic regression to estimate the relationship between typical heavy metal contents (lead, cadmium, mercury, and manganese) in the blood of adults and the metabolic syndrome ( 107 ). Similarly, generalized additive models can fit spline line models without specifying nodes automatically, allowing the fitting of spline terms like B-splines, natural splines, thin plates, etc., to control the impact of nonlinear confounding factors. This is achieved by fitting curves of corresponding nonlinear terms of pollutants.
Quantile regression (QR) is a regression analysis method that allows modeling different quantiles of the dependent variable, it can handle issues like non-normal error distribution, heteroscedasticity, and outliers. QR can also be used to fit the nonlinear relationship between pollutants and outcomes. For example, a study used linear regression and QR to investigate the relationship between the increase in concentrations of pollutants (PM 10 , PM 2.5 , NO 2 , and O 3 ) and changes in birth weight by using linear regression and QR ( 108 ).
6.2 Bayesian kernel machine regression
BKMR can also handle nonlinear relationships between exposures. Exposure and result interactions are frequently nonlinear, and BKMR is an efficient way to capture these kinds of nonlinear relationships between contaminants. BKMR has been applied to a dataset on metal exposure and neurodevelopment in Bangladeshi children, indicating the presence of non-additive and nonlinear exposure-response functions between metals and a summary measure of psychomotor development ( 109 ).
6.3 Other methods for nonlinear effects
Methods highlighted in other categories can also be employed to address nonlinear problems, including cross-validated ensemble of kernels ( 110 ), TEV, BSSVI, BVSM, MatchAlign, BDS, BKMR-CMA, GriPS, SGP-MPI, BMIM, GL-GPs, Bayesian Tree Ensembles, BKMR-DLM, DLMtree, SPORM, FIN, and FOTP.
7 Holistic approaches to mixture studies
As understanding of environmental pollution deepens and technology advances, the study of a single pollutant can no longer match the analysis of the total health impact of pollutants on the human body. So, researchers are increasingly recognizing the need to analyze complex interactions leading to or exacerbating diseases in mixtures. It is imperative to evaluate the connections between various risk factors and modifying factors from multiple biological dimensions. Similar to exploring the impact of genetic factors on chronic diseases through genome-wide association studies, exposome-wide association studies (EWAS) facilitate the investigation of non-genetic risk factors.
Initially proposed by Wild ( 111 ), EWAS can be represented as P = G + E, where an individual’s phenotype, encompassing health and physical characteristics, is the sum of genetic factors (G) and environmental factors (E) ( 112 ). Rappaport also argue that exposure should not be limited to directly encountered chemicals but should consider a broader range of exposures, such as microbial exposure and life stress ( 113 ). EWAS provides a conceptual framework to understand the complex network of interactions between genes and the environment, as well as their causal relationships with diseases. It facilitates a holistic analysis of the impact of genetics and the environment on human diseases, including DNA sequences, epigenetic DNA modifications, gene expression, metabolite analysis, and the intricate and dynamic interactions among environmental factors, all of which can influence disease phenotypes.
EWAS research does not solely focus on a single exposure but systematically addresses multiple exposures and their mutual influences, thereby increasing the complexity of the study ( 114 , 115 ). For instance, a recent study utilized data from the National Health and Nutrition Examination Survey (NHANES) and retained exposure factors, including 75 laboratory variables (clinical and biological biomarkers of environmental chemical exposure) and 64 lifestyle variables (63 dietary variables and 1 physical exercise variable). This study described the associations between body mass index, nutrition, clinical factors, and environmental factors among adolescents ( 116 ).

8 Conclusion
In conclusion, the statistical analysis of health effects resulting from the multi-pollutant mixtures is a key challenge in current environmental epidemiological research. In this paper, we review multi-pollutant mixtures statistical methods. It is essential to note that while examining scientific ideas, complementary approaches should be taken into account and statistical methods should be selected with the particular scientific problems in mind. By selecting appropriate statistical methods, considering the combined effects of various pollutants, incorporating interdisciplinary collaboration and emerging technological tools, a more accurate and comprehensive assessment of the impact of mixed environmental pollutant exposure on human health can be achieved. This will contribute to the scientific basis for environmental protection and the formulation of public health policies, promoting sustainable development for human health.
To facilitate the application of the discussed statistical methods, we summarize the advantages and limitations of commonly used statistical methods, corresponding R packages, and the above basic statistical analyses were conducted using the NHANES dataset within gWQS package (Please refer to Figure 1 ; Supplementary material for statistical analysis code). This serves as a convenient resource for researchers to directly apply these methods.
Author contributions
GZ: Writing – review & editing, Visualization, Writing – original draft. YW: Visualization, Writing – original draft, Writing – review & editing. KC: Visualization, Writing – review & editing. SH: Visualization, Writing – review & editing. TW: Writing – review & editing, Funding acquisition, Supervision.
The author(s) declare that financial support was received for the research, authorship, and/or publication of this article. This study was supported by the National Natural Science Foundation of China (Grant numbers: 82073674 and 82373692).
Conflict of interest
The authors declare that the research was conducted in the absence of any commercial or financial relationships that could be construed as a potential conflict of interest.
Publisher’s note
All claims expressed in this article are solely those of the authors and do not necessarily represent those of their affiliated organizations, or those of the publisher, the editors and the reviewers. Any product that may be evaluated in this article, or claim that may be made by its manufacturer, is not guaranteed or endorsed by the publisher.
Supplementary material
The Supplementary material for this article can be found online at: https://www.frontiersin.org/articles/10.3389/fpubh.2024.1377685/full#supplementary-material
1. Holgate, S . Air pollution is a public health emergency. BMJ . (2022) 378:o1664. doi: 10.1136/bmj.o1664
Crossref Full Text | Google Scholar
2. Münzel, T, Hahad, O, Daiber, A, and Landrigan, PJ. Soil and water pollution and human health: what should cardiologists worry about? Cardiovasc Res . (2023) 119:440–9. doi: 10.1093/cvr/cvac082
PubMed Abstract | Crossref Full Text | Google Scholar
3. Boelee, E, Geerling, G, van der Zaan, B, Blauw, A, and Vethaak, AD. Water and health: from environmental pressures to integrated responses. Acta Trop . (2019) 193:217–26. doi: 10.1016/j.actatropica.2019.03.011
4. Tariq, M, Iqbal, B, Khan, I, Khan, AR, Jho, EH, Salam, A, et al. Microplastic contamination in the agricultural soil-mitigation strategies, heavy metals contamination, and impact on human health: a review. Plant Cell Rep . (2024) 43:65. doi: 10.1007/s00299-024-03162-6
5. Fu, Z, and Xi, S. The effects of heavy metals on human metabolism. Toxicol Mech Methods . (2020) 30:167–76. doi: 10.1080/15376516.2019.1701594
6. Zhang, D, and Lu, S. Human exposure to neonicotinoids and the associated health risks: a review. Environ Int . (2022) 163:107201. doi: 10.1016/j.envint.2022.107201
7. Feiteiro, J, Mariana, M, and Cairrão, E. Health toxicity effects of brominated flame retardants: from environmental to human exposure. Environ Pollut . (2021) 285:117475. doi: 10.1016/j.envpol.2021.117475
8. Yu, Y, Quan, X, Wang, H, Zhang, B, Hou, Y, and Su, C. Assessing the health risk of hyperuricemia in participants with persistent organic pollutants exposure – a systematic review and meta-analysis. Ecotoxicol Environ Saf . (2023) 251:114525. doi: 10.1016/j.ecoenv.2023.114525
9. He, JL, Li, GA, Zhu, ZY, Hu, MJ, Wu, HB, Zhu, JL, et al. Associations of exposure to multiple trace elements with the risk of goiter: a case-control study. Environ Pollut . (2021) 288:117739. doi: 10.1016/j.envpol.2021.117739
10. Vennam, S, Georgoulas, S, Khawaja, A, Chua, S, Strouthidis, NG, and Foster, PJ. Heavy metal toxicity and the aetiology of glaucoma. Eye (Lond) . (2020) 34:129–37. doi: 10.1038/s41433-019-0672-z
11. Lim, JT, Tan, YQ, Valeri, L, Lee, J, Geok, PP, Chia, SE, et al. Association between serum heavy metals and prostate cancer risk – a multiple metal analysis. Environ Int . (2019) 132:105109. doi: 10.1016/j.envint.2019.105109
12. Gomes, J, Begum, M, and Kumarathasan, P. Polybrominated diphenyl ether (PBDE) exposure and adverse maternal and infant health outcomes: systematic review. Chemosphere . (2024) 347:140367. doi: 10.1016/j.chemosphere.2023.140367
13. Linares, V, Bellés, M, and Domingo, JL. Human exposure to PBDE and critical evaluation of health hazards. Arch Toxicol . (2015) 89:335–56. doi: 10.1007/s00204-015-1457-1
14. Tian, F, Cai, M, Li, H, Qian, Z(M), Chen, L, Zou, H, et al. Air pollution associated with incident stroke, Poststroke cardiovascular events, and death: a trajectory analysis of a prospective cohort. Neurology . (2022) 99:e2474–84. doi: 10.1212/WNL.0000000000201316
15. Joubert, BR, Kioumourtzoglou, MA, Chamberlain, T, Chen, HY, Gennings, C, Turyk, ME, et al. Powering research through innovative methods for mixtures in epidemiology (PRIME) program: novel and expanded statistical methods. Int J Environ Res Public Health . (2022) 19:1378. doi: 10.3390/ijerph19031378
16. Hamra, GB, and Buckley, JP. Environmental exposure mixtures: questions and methods to address them. Curr Epidemiol Rep . (2018) 5:160–5. doi: 10.1007/s40471-018-0145-0
17. Braun, JM, Gennings, C, Hauser, R, and Webster, TF. What can epidemiological studies tell us about the impact of chemical mixtures on human health? Environ Health Perspect . (2016) 124:A6–9. doi: 10.1289/ehp.1510569
18. Kortenkamp, A . Ten years of mixing cocktails: a review of combination effects of endocrine-disrupting chemicals. Environ Health Perspect . (2007) 115:98–105. doi: 10.1289/ehp.9357
19. Kortenkamp, A . Low dose mixture effects of endocrine disrupters: implications for risk assessment and epidemiology. Int J Androl . (2008) 31:233–40. doi: 10.1111/j.1365-2605.2007.00862.x
20. Gibson, EA, Goldsmith, J, and Kioumourtzoglou, MA. Complex mixtures, complex analyses: an emphasis on interpretable results. Curr Environ Health Rep . (2019) 6:53–61. doi: 10.1007/s40572-019-00229-5
21. Stafoggia, M, Breitner, S, Hampel, R, and Basagaña, X. Statistical approaches to address multi-pollutant mixtures and multiple exposures: the state of the science. Curr Environ Health Rep . (2017) 4:481–90. doi: 10.1007/s40572-017-0162-z
22. Yu, L, Liu, W, Wang, X, Ye, Z, Tan, Q, Qiu, W, et al. A review of practical statistical methods used in epidemiological studies to estimate the health effects of multi-pollutant mixture. Environ Pollut . (2022) 306:119356. doi: 10.1016/j.envpol.2022.119356
23. Hamra, GB, Guha, N, Cohen, A, Laden, F, Raaschou-Nielsen, O, Samet, JM, et al. Outdoor particulate matter exposure and lung cancer: a systematic review and meta-analysis. Environ Health Perspect . (2014) 122:906–11. doi: 10.1289/ehp/1408092
24. Wolff, MS, Engel, SM, Berkowitz, GS, Ye, X, Silva, MJ, Zhu, C, et al. Prenatal phenol and phthalate exposures and birth outcomes. Environ Health Perspect . (2008) 116:1092–7. doi: 10.1289/ehp.11007
25. Varshavsky, JR, Zota, AR, and Woodruff, TJ. A novel method for calculating potency-weighted cumulative phthalates exposure with implications for identifying racial/ethnic disparities among U.S. reproductive-aged women in NHANES 2001–2012. Environ Sci Technol . (2016) 50:10616–24. doi: 10.1021/acs.est.6b00522
26. Zhang, B, Weuve, J, Langa, KM, D’Souza, J, Szpiro, A, Faul, J, et al. Comparison of particulate air pollution from different emission sources and incident dementia in the US. JAMA Intern Med . (2023) 183:1080–9. doi: 10.1001/jamainternmed.2023.3300
27. Carrico, C, Gennings, C, Wheeler, DC, and Factor-Litvak, P. Characterization of weighted quantile sum regression for highly correlated data in a risk analysis setting. J Agric Biol Environ Stat . (2015) 20:100–20. doi: 10.1007/s13253-014-0180-3
28. Tanner, EM, Bornehag, CG, and Gennings, C. Repeated holdout validation for weighted quantile sum regression. MethodsX . (2019) 6:2855–60. doi: 10.1016/j.mex.2019.11.008
29. Zhang, Y, Dong, T, Hu, W, Wang, X, Xu, B, Lin, Z, et al. Association between exposure to a mixture of phenols, pesticides, and phthalates and obesity: comparison of three statistical models. Environ Int . (2019) 123:325–36. doi: 10.1016/j.envint.2018.11.076
30. Wheeler, DC, Rustom, S, Carli, M, Whitehead, TP, Ward, MH, and Metayer, C. Assessment of grouped weighted quantile sum regression for modeling chemical mixtures and Cancer risk. Int J Environ Res Public Health . (2021) 18:504. doi: 10.3390/ijerph18020504
31. Wheeler, DC, Rustom, S, Carli, M, Whitehead, TP, Ward, MH, and Metayer, C. Bayesian group index regression for modeling chemical mixtures and Cancer risk. Int J Environ Res Public Health . (2021) 18:3486. doi: 10.3390/ijerph18073486
32. Gennings, C, Curtin, P, Bello, G, Wright, R, Arora, M, and Austin, C. Lagged WQS regression for mixtures with many components. Environ Res . (2020) 186:109529. doi: 10.1016/j.envres.2020.109529
33. Bello, GA, Arora, M, Austin, C, Horton, MK, Wright, RO, and Gennings, C. Extending the distributed lag model framework to handle chemical mixtures. Environ Res . (2017) 156:253–64. doi: 10.1016/j.envres.2017.03.031
34. Bobb, JF, Valeri, L, Claus Henn, B, Christiani, DC, Wright, RO, Mazumdar, M, et al. Bayesian kernel machine regression for estimating the health effects of multi-pollutant mixtures. Biostatistics . (2015) 16:493–508. doi: 10.1093/biostatistics/kxu058
35. Bobb, JF, Claus Henn, B, Valeri, L, and Coull, BA. Statistical software for analyzing the health effects of multiple concurrent exposures via Bayesian kernel machine regression. Environ Health . (2018) 17:67. doi: 10.1186/s12940-018-0413-y
36. Chen, L, Sun, Q, Peng, S, Tan, T, Mei, G, Chen, H, et al. Associations of blood and urinary heavy metals with rheumatoid arthritis risk among adults in NHANES, 1999–2018. Chemosphere . (2022) 289:133147. doi: 10.1016/j.chemosphere.2021.133147
37. Tan, Y, Fu, Y, Yao, H, Wu, X, Yang, Z, Zeng, H, et al. Relationship between phthalates exposures and hyperuricemia in U.S. general population, a multi-cycle study of NHANES 2007–2016. Sci Total Environ . (2023) 859:160208. doi: 10.1016/j.scitotenv.2022.160208
38. Devick, KL, Bobb, JF, Mazumdar, M, Claus Henn, B, Bellinger, DC, Christiani, DC, et al. Bayesian kernel machine regression-causal mediation analysis. Stat Med . (2022) 41:860–76. doi: 10.1002/sim.9255
39. Wilson, A, Hsu, HL, Chiu, YM, Wright, RO, Wright, RJ, and Coull, BA. Kernel machine and distributed lag models for assessing windows of susceptibility to environmental mixtures in children's health studies. Ann Appl Stat . (2022) 16:1090–110. doi: 10.1214/21-aoas1533
40. Yang, Z, Guo, C, Li, Q, Zhong, Y, Ma, S, Zhou, J, et al. Human health risks estimations from polycyclic aromatic hydrocarbons in serum and their hydroxylated metabolites in paired urine samples. Environ Pollut . (2021) 290:117975. doi: 10.1016/j.envpol.2021.117975
41. Haddad, S, Withey, J, Laparé, S, Law, F, and Krishnan, K. Physiologically-based pharmacokinetic modeling of pyrene in the rat. Environ Toxicol Pharmacol . (1998) 5:245–55. doi: 10.1016/S1382-6689(98)00008-8
42. Viau, C, Diakité, AS, Ruzgyté, A, Tuchweber, B, Blais, C, Bouchard, M, et al. Is 1-hydroxypyrene a reliable bioindicator of measured dietary polycyclic aromatic hydrocarbon under normal conditions? J Chromatogr B . (2002) 778:165–77. doi: 10.1016/S0378-4347(01)00465-0
43. Lei, B, Zhang, K, An, J, Zhang, X, and Yu, Y. Human health risk assessment of multiple contaminants due to consumption of animal-based foods available in the markets of Shanghai, China. Environ Sci Pollut Res . (2015) 22:4434–46. doi: 10.1007/s11356-014-3683-0
44. Gennings, C, Shu, H, Rudén, C, Öberg, M, Lindh, C, Kiviranta, H, et al. Incorporating regulatory guideline values in analysis of epidemiology data. Environ Int . (2018) 120:535–43. doi: 10.1016/j.envint.2018.08.039
45. Mork, D, and Wilson, A. Estimating perinatal critical windows of susceptibility to environmental mixtures via structured Bayesian regression tree pairs. Biometrics . (2023) 79:449–61. doi: 10.1111/biom.13568
46. Feldman, J, and Kowal, DR. A Bayesian framework for generation of fully synthetic mixed datasets. arXiv: Methodology . (2021). doi: 10.48550/arXiv.2102.08255
47. Kowal, DR . Bayesian subset selection and variable importance for interpretable prediction and classification. J Mach Learn Res . (2021) 23:108. doi: 10.48550/arXiv.2104.10150
48. Jin, B, Peruzzi, M, and Dunson, D B. Bag of DAGs: flexible & scalable modeling of Spatiotem-poral dependence , (2021).
Google Scholar
49. Mork, D, and Wilson, A. Treed distributed lag nonlinear models. Biostatistics . (2020) 23:754–71. doi: 10.1093/biostatistics/kxaa051
50. Ferrari, F, and Dunson, DB. Bayesian Factor analysis for inference on interactions. J Am Stat Assoc . (2021) 116:1521–32. doi: 10.1080/01621459.2020.1745813
51. Kowal, DR . Fast, optimal, and targeted predictions using parameterized decision analysis. J Am Stat Assoc . (2020) 117:1875–86. doi: 10.1080/01621459.2021.1891926
52. Dunson, DB, Wu, HT, and Wu, N. Diffusion based Gaussian processes on restricted domains. arXiv: Methodology . (2020). doi: 10.48550/arXiv.2010.07242
53. Peruzzi, M, Banerjee, S, Dunson, D B, and Finley, AO. Grid-parametrize-Split (GriPS) for improved scalable inference in spatial big data analysis , (2021).
54. Antonelli, J, Wilson, A, and Coull, B. Multiple exposure distributed lag models with variable selection. Biostatistics . (2021) 2021:1. doi: 10.1289/isee.2021.O-SY-069
55. Ben Salem, K, and Ben, AA. Principal component analysis (PCA). Tunis Med . (2021) 99:383–9. doi: 10.1201/b10345-2
56. Smit, LA, Lenters, V, Høyer, BB, Lindh, CH, Pedersen, HS, Liermontova, I, et al. Prenatal exposure to environmental chemical contaminants and asthma and eczema in school-age children. Allergy . (2015) 70:653–60. doi: 10.1111/all.12605
57. Bair, E, Hastie, T, Paul, D, and Tibshirani, R. Prediction by supervised principal components. J Am Stat Assoc . (2006) 101:119–37. doi: 10.1198/016214505000000628
58. Roberts, S, and Martin, MA. Using supervised principal components analysis to assess multiple pollutant effects. Environ Health Perspect . (2006) 114:1877–82. doi: 10.1289/ehp.9226
59. Gibson, EA, Zhang, J, Yan, J, Chillrud, L, Benavides, J, Nunez, Y, et al. Principal component pursuit for pattern identification in environmental mixtures. Environ Health Perspect . (2022) 130:117008. doi: 10.1289/EHP10479
60. Paatero, P, and Tapper, U. Positive matrix factorization: a non-negative factor model with optimal utilization of error estimates of data values†. Environmetrics . (1994) 5:111–26. doi: 10.1002/env.3170050203
61. Krall, JR, and Strickland, MJ. Recent approaches to estimate associations between source-specific air pollution and health. Curr Environ Health Rep . (2017) 4:68–78. doi: 10.1007/s40572-017-0124-5
62. Krall, JR, Mulholland, JA, Russell, AG, Balachandran, S, Winquist, A, Tolbert, PE, et al. Associations between source-specific fine particulate matter and emergency department visits for respiratory disease in four U.S. cities. Environ Health Perspect . (2016) 125:97–103. doi: 10.1289/EHP271
63. Dai, L, Bind, MA, Koutrakis, P, Coull, BA, Sparrow, D, Vokonas, PS, et al. Fine particles, genetic pathways, and markers of inflammation and endothelial dysfunction: analysis on particulate species and sources. J Expo Sci Environ Epidemiol . (2016) 26:415–21. doi: 10.1038/jes.2015.83
64. Siponen, T, Yli-Tuomi, T, Aurela, M, Dufva, H, Hillamo, R, Hirvonen, MR, et al. Source-specific fine particulate air pollution and systemic inflammation in ischaemic heart disease patients. Occup Environ Med . (2014) 72:277–83. doi: 10.1136/oemed-2014-102240
65. Roy, A, Lavine, I, Herring, AH, and Dunson, DB. Perturbed factor analysis: accounting for group differences in exposure profiles. Ann Appl Stat . (2021) 15:1386. doi: 10.1214/20-AOAS1435
66. Reid, S, and Tibshirani, R. Sparse regression and marginal testing using cluster prototypes. Biostatistics . (2016) 17:364–76. doi: 10.1093/biostatistics/kxv049
67. Steinley, D . K-means clustering: a half-century synthesis. Br J Math Stat Psychol . (2006) 59:1–34. doi: 10.1348/000711005X48266
68. Ljungman, PL, Wilker, EH, Rice, MB, Austin, E, Schwartz, J, Gold, DR, et al. The impact of multipollutant clusters on the association between fine particulate air pollution and microvascular function. Epidemiology . (2016) 27:194–201. doi: 10.1097/EDE.0000000000000415
69. Coker, E, Liverani, S, Ghosh, JK, Jerrett, M, Beckerman, B, Li, A, et al. Multi-pollutant exposure profiles associated with term low birth weight in Los Angeles County. Environ Int . (2016) 91:1–13. doi: 10.1016/j.envint.2016.02.011
70. Wold, H . Estimation of principal components and related models by iterative least squares. Multivar Anal . (1966):1.
71. Mevik, B-H, and Wehrens, R. The pls package: principal component and partial least squares regression in R. J Stat Softw . (2007) 18:1–23. doi: 10.18637/jss.v018.i02
72. Chun, H, and Keleş, S. Sparse partial least squares regression for simultaneous dimension reduction and variable selection. J R Stat Soc Series B Stat Methodol . (2010) 72:3–25. doi: 10.1111/j.1467-9868.2009.00723.x
73. Agier, L, Portengen, L, Chadeau-Hyam, M, Basagaña, X, Giorgis-Allemand, L, Siroux, V, et al. A systematic comparison of linear regression–based statistical methods to assess Exposome-health associations. Environ Health Perspect . (2016) 124:1848–56. doi: 10.1289/EHP172
74. Sinisi, SE, and Van Der Laan, MJ. Deletion/substitution/addition algorithm in learning with applications in genomics. Stat Appl Genet Mol Biol . (2004) 3:1–38. doi: 10.2202/1544-6115.1069
75. Sun, Z, Tao, Y, Li, S, Ferguson, KK, Meeker, JD, Park, SK, et al. Statistical strategies for constructing health risk models with multiple pollutants and their interactions: possible choices and comparisons. Environ Health . (2013) 12:85. doi: 10.1186/1476-069X-12-85
76. Beckerman, BS, Jerrett, M, Martin, RV, van Donkelaar, A, Ross, Z, and Burnett, RT. Application of the deletion/substitution/addition algorithm to selecting land use regression models for interpolating air pollution measurements in California. Atmos Environ . (2013) 77:172–7. doi: 10.1016/j.atmosenv.2013.04.024
77. Dominici, F, Wang, C, Crainiceanu, C, and Parmigiani, G. Model selection and health effect estimation in environmental epidemiology. Epidemiology . (2008) 19:558–60. doi: 10.1097/EDE.0b013e31817307dc
78. Tibshirani, R . Regression shrinkage and selection via the lasso. J R Stat Soc Series B Stat Methodol . (1996) 58:267–88. doi: 10.1111/j.2517-6161.1996.tb02080.x
79. Zou, H, and Hastie, TJ. Regularization and variable selection via the elastic net. J R Stat Soc Series B Stat Methodol . (2005) 67:301–20. doi: 10.1111/j.1467-9868.2005.00503.x
80. Dai, L, Koutrakis, P, Coull, BA, Sparrow, D, Vokonas, PS, and Schwartz, JD. Use of the adaptive LASSO method to identify PM2.5 components associated with blood pressure in elderly men: the veterans affairs normative aging study. Environ Health Perspect . (2016) 124:120–5. doi: 10.1289/ehp.1409021
81. Lenters, V, Portengen, L, Rignell-Hydbom, A, Jönsson, BAG, Lindh, CH, Piersma, AH, et al. Prenatal phthalate, Perfluoroalkyl acid, and organochlorine exposures and term birth weight in three birth cohorts: multi-pollutant models based on elastic net regression. Environ Health Perspect . (2016) 124:365–72. doi: 10.1289/ehp.1408933
82. Lim, M, and Hastie, T. Learning interactions via hierarchical group-lasso regularization. J Comput Graph Stat . (2015) 24:627–54. doi: 10.1080/10618600.2014.938812
83. Huang, H . Controlling the false discoveries in LASSO. Biometrics . (2017) 73:1102–10. doi: 10.1111/biom.12665
84. Loh, WY . Classification and regression trees. Wiley Interdiscip Rev Data Min Knowl Discov . (2011) 1:14–23. doi: 10.1002/widm.8
85. Biau, G . Analysis of a random forests model. J Mach Learn Res . (2012) 13:1063–95.
86. Smola, AJ, and Schölkopf, B. A tutorial on support vector regression. Stat Comput . (2004) 14:199–222. doi: 10.1023/B:STCO.0000035301.49549.88
87. Peterson, LE . K-nearest neighbor. Scholarpedia . (2009) 4:1883. doi: 10.4249/scholarpedia.1883
88. Webb, GI, Keogh, E, and Miikkulainen, R. Naïve Bayes. Encycl Mach Learn . (2010) 15:713–4. doi: 10.1007/978-0-387-30164-8_576
89. Bishop, CM . Neural networks and their applications. Rev Sci Instrum . (1994) 65:1803–32. doi: 10.1063/1.1144830
90. Margineantu, D D, and Dietterich, T G. Pruning adaptive boosting. ICML , (1997): 211–218.
91. Friedman, JH . Stochastic gradient boosting. Comput Stat Data Anal . (2002) 38:367–78. doi: 10.1016/S0167-9473(01)00065-2
92. Chen, T, and Guestrin, C. Xgboost: a scalable tree boosting system. Proceedings of the 22nd acm sigkdd international conference on knowledge discovery and data mining, (2016): 785–794.
93. Ke, G, Meng, Q, Finley, T, Wang, T, Chen, W, Ma, W, et al. Lightgbm: a highly efficient gradient boosting decision tree. Adv Neural Inf Proces Syst . (2017) 30:3146–3154. doi: 10.5555/3294996.3295074
94. Prokhorenkova, L, Gusev, G, Vorobev, A, Dorogush, AV, and Gulin, A. CatBoost: unbiased boosting with categorical features. Adv Neural Inf Proces Syst . (2018) 31. doi: 10.48550/arXiv.1706.09516
95. Lundberg, SM, and Lee, S-I. A unified approach to interpreting model predictions. Adv Neural Inf Proces Syst . (2017) 30:4768–4777. doi: 10.48550/arXiv.1705.07874
96. Li, X, Zhao, Y, Zhang, D, Kuang, L, Huang, H, Chen, W, et al. Development of an interpretable machine learning model associated with heavy metals' exposure to identify coronary heart disease among US adults via SHAP: findings of the US NHANES from 2003 to 2018. Chemosphere . (2023) 311:137039. doi: 10.1016/j.chemosphere.2022.137039
97. Li, W, Huang, G, Tang, N, Lu, P, Jiang, L, Lv, J, et al. Effects of heavy metal exposure on hypertension: a machine learning modeling approach. Chemosphere . (2023) 337:139435. doi: 10.1016/j.chemosphere.2023.139435
98. Mauderly, JL, and Samet, JM. Is there evidence for synergy among air pollutants in causing health effects? Environ Health Perspect . (2009) 117:1–6. doi: 10.1289/ehp.11654
99. Liu, C, Chen, R, Sera, F, Vicedo-Cabrera, AM, Guo, Y, Tong, S, et al. Interactive effects of ambient fine particulate matter and ozone on daily mortality in 372 cities: two stage time series analysis. BMJ . (2023) 383:e075203. doi: 10.1136/bmj-2023-075203
100. Antonelli, J, Mazumdar, M, Bellinger, DC, Christiani, D, Wright, R, and Coull, B. Estimating the health effects of environmental mixtures using Bayesian semiparametric regression and sparsity inducing priors. Ann Appl Stat . (2017) 14:275–75. doi: 10.48550/arXiv.1711.11239
101. Davalos, AD, Luben, TJ, Herring, AH, and Sacks, JD. Current approaches used in epidemiologic studies to examine short-term multipollutant air pollution exposures. Ann Epidemiol . (2017) 27:145–153.e1. doi: 10.1016/j.annepidem.2016.11.016
102. Tomarken, AJ, and Waller, NG. Structural equation modeling: strengths, limitations, and misconceptions. Annu Rev Clin Psychol . (2005) 1:31–65. doi: 10.1146/annurev.clinpsy.1.102803.144239
103. Stein, CM, Morris, NJ, and Nock, NL. Structural equation modeling. Methods Mol Biol . (2012) 850:495–512. doi: 10.1007/978-1-61779-555-8_27
104. Shook-Sa, BE, Chen, DG, and Zhou, H. Using structural equation modeling to assess the links between tobacco smoke exposure, volatile organic compounds, and respiratory function for adolescents aged 6 to 18 in the United States. Int J Environ Res Public Health . (2017) 14:1112. doi: 10.3390/ijerph14101112
105. Shan, Z, Chen, S, Sun, T, Luo, C, Guo, Y, Yu, X, et al. U-shaped association between plasma manganese levels and type 2 diabetes. Environ Health Perspect . (2016) 124:1876–81. doi: 10.1289/EHP176
106. Wang, B, Cheng, M, Yang, S, Qiu, W, Li, W, Zhou, Y, et al. Exposure to acrylamide and reduced heart rate variability: the mediating role of transforming growth factor-β. J Hazard Mater . (2020) 395:122677. doi: 10.1016/j.jhazmat.2020.122677
107. Zhou, J, Meng, X, Deng, L, and Liu, N. Non-linear associations between metabolic syndrome and four typical heavy metals: data from NHANES 2011–2018. Chemosphere . (2022) 291:132953. doi: 10.1016/j.chemosphere.2021.132953
108. Lamichhane, DK, Lee, S-Y, Ahn, K, Kim, KW, Shin, YH, Suh, DI, et al. Quantile regression analysis of the socioeconomic inequalities in air pollution and birth weight. Environ Int . (2020) 142:105875. doi: 10.1016/j.envint.2020.105875
109. Valeri, L, Mazumdar, MM, Bobb, JF, Claus Henn, B, Rodrigues, E, Sharif, OIA, et al. The joint effect of prenatal exposure to metal mixtures on neurodevelopmental outcomes at 20–40 months of age: evidence from rural Bangladesh. Environ Health Perspect . (2017) 125:067015. doi: 10.1289/EHP614
110. Liu, JZ, Deng, W, Lee, J, Lin, PD, Valeri, L, Christiani, DC, et al. A cross-validated ensemble approach to robust hypothesis testing of continuous nonlinear interactions: application to nutrition-environment studies. J Am Stat Assoc . (2022) 117:561–73. doi: 10.1080/01621459.2021.1962889
111. Wild, CP . Complementing the genome with an "exposome": the outstanding challenge of environmental exposure measurement in molecular epidemiology. Cancer Epidemiol Biomarkers Prev . (2005) 14:1847–50. doi: 10.1158/1055-9965.EPI-05-0456
112. Wild, CP . The exposome: from concept to utility. Int J Epidemiol . (2012) 41:24–32. doi: 10.1093/ije/dyr236
113. Rappaport, SM, and Smith, MT. Epidemiology. Environment and disease risks. Science . (2010) 330:460–1. doi: 10.1126/science.1192603
114. Khoury, MJ, and Wacholder, S. Invited commentary: from genome-wide association studies to gene-environment-wide interaction studies--challenges and opportunities. Am J Epidemiol . (2009) 169:227–30. doi: 10.1093/aje/kwn351
115. Thomas, D . Gene--environment-wide association studies: emerging approaches. Nat Rev Genet . (2010) 11:259–72. doi: 10.1038/nrg2764
116. Haddad, N, Andrianou, X, Parrish, C, Oikonomou, S, and Makris, KC. An exposome-wide association study on body mass index in adolescents using the National Health and nutrition examination survey (NHANES) 2003–2004 and 2013–2014 data. Sci Rep . (2022) 12:8856. doi: 10.1038/s41598-022-12459-z
Keywords: health effects, epidemiology, statistical methods, multi-pollutant mixtures, environment
Citation: Zhu G, Wen Y, Cao K, He S and Wang T (2024) A review of common statistical methods for dealing with multiple pollutant mixtures and multiple exposures. Front. Public Health . 12:1377685. doi: 10.3389/fpubh.2024.1377685
Received: 28 January 2024; Accepted: 15 April 2024; Published: 09 May 2024.
Reviewed by:
Copyright © 2024 Zhu, Wen, Cao, He and Wang. This is an open-access article distributed under the terms of the Creative Commons Attribution License (CC BY) . The use, distribution or reproduction in other forums is permitted, provided the original author(s) and the copyright owner(s) are credited and that the original publication in this journal is cited, in accordance with accepted academic practice. No use, distribution or reproduction is permitted which does not comply with these terms.
*Correspondence: Tong Wang, [email protected]
Disclaimer: All claims expressed in this article are solely those of the authors and do not necessarily represent those of their affiliated organizations, or those of the publisher, the editors and the reviewers. Any product that may be evaluated in this article or claim that may be made by its manufacturer is not guaranteed or endorsed by the publisher.
Thank you for visiting nature.com. You are using a browser version with limited support for CSS. To obtain the best experience, we recommend you use a more up to date browser (or turn off compatibility mode in Internet Explorer). In the meantime, to ensure continued support, we are displaying the site without styles and JavaScript.
- View all journals
- My Account Login
- Explore content
- About the journal
- Publish with us
- Sign up for alerts
- Open access
- Published: 10 May 2024
Metabolic network analysis of pre-ASD newborns and 5-year-old children with autism spectrum disorder
- Sai Sachin Lingampelly 1 , 2 ,
- Jane C. Naviaux 1 , 3 ,
- Luke S. Heuer 4 ,
- Jonathan M. Monk 1 , 2 ,
- Kefeng Li ORCID: orcid.org/0000-0002-7233-4347 1 , 2 nAff8 ,
- Lin Wang 1 , 2 ,
- Lori Haapanen 4 ,
- Chelsea A. Kelland 4 ,
- Judy Van de Water ORCID: orcid.org/0000-0003-1193-5875 4 , 5 &
- Robert K. Naviaux ORCID: orcid.org/0000-0001-5832-2297 1 , 2 , 6 , 7
Communications Biology volume 7 , Article number: 536 ( 2024 ) Cite this article
598 Accesses
353 Altmetric
Metrics details
Metabolomics
- Pathogenesis
Classical metabolomic and new metabolic network methods were used to study the developmental features of autism spectrum disorder (ASD) in newborns ( n = 205) and 5-year-old children ( n = 53). Eighty percent of the metabolic impact in ASD was caused by 14 shared biochemical pathways that led to decreased anti-inflammatory and antioxidant defenses, and to increased physiologic stress molecules like lactate, glycerol, cholesterol, and ceramides. CIRCOS plots and a new metabolic network parameter, \(\dot{{{\boldsymbol{V}}}}\!\) net , revealed differences in both the kind and degree of network connectivity. Of 50 biochemical pathways and 450 polar and lipid metabolites examined, the developmental regulation of the purine network was most changed. Purine network hub analysis revealed a 17-fold reversal in typically developing children. This purine network reversal did not occur in ASD. These results revealed previously unknown metabolic phenotypes, identified new developmental states of the metabolic correlation network, and underscored the role of mitochondrial functional changes, purine metabolism, and purinergic signaling in autism spectrum disorder.
Similar content being viewed by others
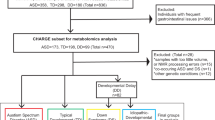
Metabolomics analysis of children with autism, idiopathic-developmental delays, and Down syndrome
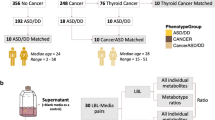
Distinct metabolic profiles associated with autism spectrum disorder versus cancer in individuals with germline PTEN mutations
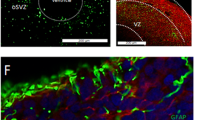
Proteomic phenotype of cerebral organoids derived from autism spectrum disorder patients reveal disrupted energy metabolism, cellular components, and biological processes
Introduction.
Autism spectrum disorder (ASD) now affects 1 in 36 children born in the United States 1 . Despite clinical heterogeneity, all children with ASD share the three core features of difficulty with language, social communication, and restricted or repetitive behaviors or interests. With current therapy, only about 20% of children diagnosed with ASD in childhood gain independence as adults 2 . Advanced methods in biochemical genetics and metabolomics over the past 65 years have shown that many metabolic changes can be found in children and adults with ASD, but the specific differences change by age, sex, and severity of symptoms 3 , 4 , 5 , 6 , 7 , 8 , 9 . The developmental nature of metabolic changes in ASD has been attributed in part to a delayed maturational program 10 , 11 , 12 , which in turn, is coupled to corresponding developmental changes in brain structure and function, immunity, the microbiome, and the autonomic nervous system 4 , 13 , 14 , 15 . A root regulator of these multisystem changes in ASD has not yet been discovered.
Whole genome sequencing efforts have identified 134 genes and structural DNA variants in 5100 children and adults with ASD 16 . However, no single genetic change accounted for more than 0.5% of the children. Diverse environmental factors are also known to increase the risk of ASD. These range from gestational fever 17 to early exposure to environmental pollutants 18 , but no single environmental risk factor is found in every child with ASD. Exhaustive DNA sequencing and epidemiologic studies in ASD over the past 10 years have reinforced the findings that genes do not work in isolation, and that polygenic and gene-environment interactions are the dominant contributors to the development of ASD 19 .
The conceptual framework of the cell danger response (CDR) was developed to explain how genes and environment are connected through metabolism 20 , 21 . The CDR is a universal response to genetic or environmental stress 21 and describes how different genetic and environmental stressors alter development and increase the risk of ASD 22 . Starting with mitochondria and the cell, the CDR propagates from local to remote systems to coordinate the metabolic, inflammatory, autonomic, neuroendocrine, and other responses needed to heal and recover from any stress or injury, and to adapt to future exposures 23 . When triggered by chronic or repeated exposure to certain environmental pollutants or other early life stresses 24 , elements of the CDR that were acutely adaptive, become chronic and pathologic. When the triggering factors occur during gestation or early in childhood, ASD and several other neurodevelopmental disorders can result. In the case of ASD, four features of systemic stress are universally present. These include changes in mitochondrial function, oxidative stress, innate immune activation, and changes in the microbiome 25 . These are each established features of the CDR 20 .
The root regulator of the CDR is extracellular adenosine triphosphate (eATP). eATP release is triggered by both genetic and environmental factors 26 . eATP is released in a graded fashion in vesicles and through pannexin 1 and other membrane channels in proportion to the magnitude of stress 27 , 28 . ATP is metabolically expensive to make, and proper compartmentalization is essential for normal cell function. The intracellular functions of ATP (iATP) in metabolism are well known 29 . More universal than any gene, iATP is used as an energy carrier by all forms of life on Earth 30 . However, when released to the outside of the cell, the function of ATP shifts seamlessly from matter to information. Outside the cell, eATP becomes a signaling molecule that binds purinergic receptors 31 , and signals danger. eATP signaling changes metabolism and initiates a cascade of events that starts with innate immunity, and spreads from acute, short-distance responses at the site of infection or injury, to chronic, long-distance responses that affect organ systems and regulate child development 32 . eATP is one of the most powerful signaling molecules known, capable of binding to receptors found on every cell in the body and regulating their function. Acute injection of eATP profoundly regulates mitochondria and over 200 molecules in the metabolome 33 . When given during pregnancy in a mouse model, a single injection of eATP causes life-long neurodevelopmental changes and ASD-like behaviors in the offspring 34 . Specific aspects of the CDR are triggered by specific threats like a viral infection, toxic metals, drugs, or environmental pollutants. The generalized functions of the CDR are triggered directly by eATP and its metabolites.
Differences in purine metabolism and ATP-related purinergic signaling have now been reported in every experimental model and every human study of ASD in which purines have been interrogated 5 , 8 , 34 , 35 , 36 , 37 . Unbiased multiomics analysis has independently underscored this discovery 38 . eATP fundamentally regulates mast cells 39 , microglia 40 , neuronal sensitization 41 , vagal function 42 , and synaptic plasticity 43 . Each of these is a known issue in ASD 44 , 45 . Mitochondria make over 90% of ATP used for energy and purinergic signaling. Mitochondria also serve as the information processing, early warning, and early response system that allows cells to adapt to changing environmental conditions 46 , 47 . The mitochondrial proteome contains the enzymes for up to 789 metabolic reactions 48 . These enzymes drive the functional changes in cells needed for growth, differentiation, healing, adaptation to stress, child development, and healthy aging. Over half of these enzymes are regulated by ATP and related nucleotides 48 , and are thus responsive to changes in the CDR that regulate ATP production and release. Chronic changes in mitochondrial functions are an established feature of ASD that are capable of remodeling the metabolic network 8 , regulating gene expression 49 , 50 , and shifting the trajectory of neurodevelopment 51 . In the following study, we tested the hypothesis that developmental patterns in the metabolic network of infants at risk and children with ASD can provide mechanistic insight into the developmental neurobiology of ASD.
The aims of this study were: 1) to identify the developmental patterns in metabolism that distinguish children with ASD, and 2) to identify the changes in metabolic networks that can be used as early biomarkers of risk and to gain new insights into the pathophysiologic mechanisms of ASD between birth and 5-years of age.
Participant characteristics
Cohort 1—the newborn study.
Figure 1 illustrates the biological systems interrogated and the analytical workflow of this study. Participant characteristics are shown in Supplementary Table 1 . There were no differences between the pre-ASD and typically developing (TD) groups by birth weight, or the frequency of births by in vitro fertilization, gestational diabetes, or the occurrence of a gestational fever. There was also no difference in the number of medications prescribed during pregnancy, C-section, infant age at sample collection, child immunization history, maternal or paternal age, or ethnicity. We found that 48% of the children in the pre-ASD group had at least one episode of developmental regression. The frequency of regression in the TD group was 2% ( p < 0.0001). The mean age of the first spoken word was 14.7 months in the ASD group and 11.4 months in the TD group ( p < 0.0001). The mean age of diagnosis of ASD was 3.3 ± 1.1 years (Supplementary Table 1 ).
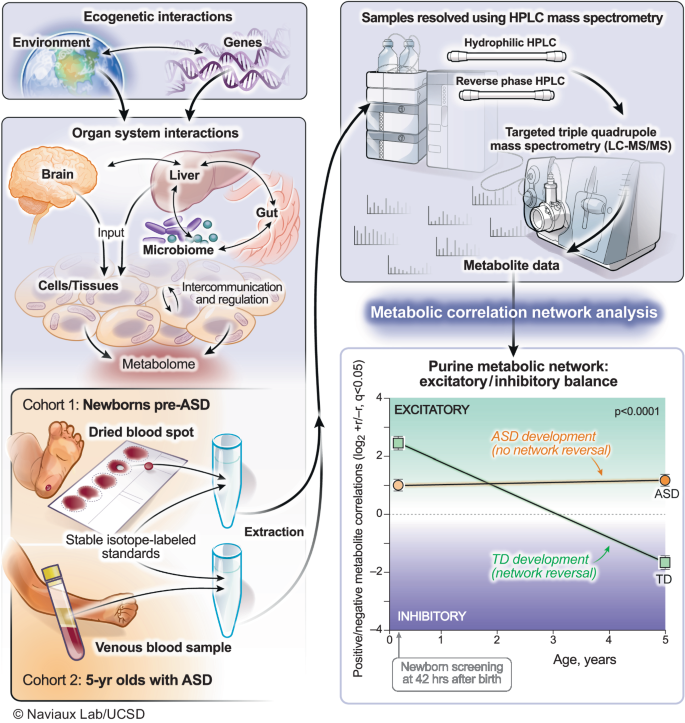
Metabolomics was used to interrogate real-time gene-environment interactions. The purine metabolic network was the most changed of 50 biochemical pathways analyzed in autism spectrum disorder. Developmental reversal of the excitatory (+r) to inhibitory (−r) purine network was a feature of neurotypical development. This reversal did not occur in children with ASD. ASD autism spectrum disorder, TD typically developing.
Cohort 2—The 5-year-old child study
Participant characteristics are shown in Supplementary Table 2 . Fifty-three (53) children were enrolled in this study. All children had been diagnosed with ASD by a healthcare professional or were typically developing children enrolled as controls. This cohort consisted of children recruited prior to planned enrollment in kindergarten and before routine pre-kindergarten immunizations. The mean age was 5.3 ± 0.8 years. There were 23 males and 8 females with ASD, and 16 TD control males and 6 TD females (Supplementary Table 2 ).
Metabolomics overview
Developmental progression of asd-associated metabolic changes.
Multivariate partial least squares discriminant analysis (PLS-DA) showed that newborns who later developed ASD could not be completely separated from TD newborns using classical metabolomics (Fig. 2a ). The metabolic differences between 5-year-olds with ASD and TD controls allowed for greater separation, but still showed significant overlap (Fig. 2b ). The top 60 most discriminating metabolites were aggregated into pathways by variable importance in projection (VIP) scores ≥ 1.5 for comparison of the metabolic impacts in newborn and 5-year-old groups. Fourteen of 24 dysregulated metabolic pathways (58%) were shared by pre-ASD newborns and 5-year-old children with ASD (Supplementary Fig. 1a, b , shaded pathways). Raw metabolomics data for newborns and 5-year-olds are reported in Supplementary Data 1 and 2 , respectively. Detailed statistical analysis is reported in Supplementary Data 3 and 4 . The pathways shared between the two cohorts accounted for 79% of the metabolic impact in newborns and 80% of the impact in 5-year-olds. Altered lipid metabolism accounted for 63% and 71% of the fractional impact in the newborn pre-ASD and 5-year-old ASD cohorts, respectively. About 25% of the impact was produced by changes in sphingolipids like sphingomyelins, ceramides, and glycosphingolipids, and another 20–26% was produced by changes in phospholipids (Supplementary Fig. 1a, b ). See Supplementary Results and Discussion for additional details.
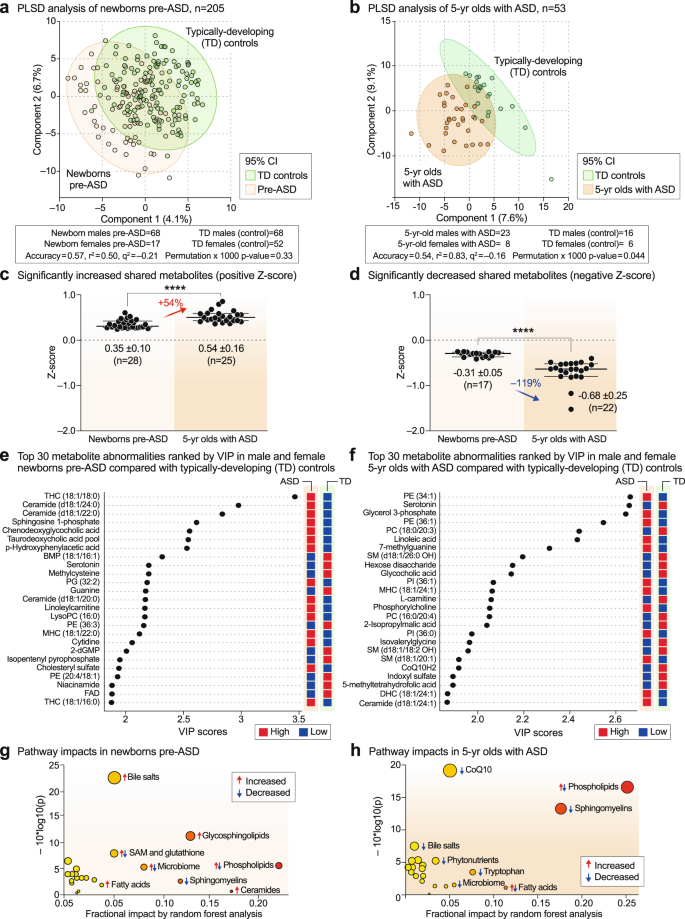
a , b Multivariate partial least squares discriminant analysis (PLS-DA). Cross-validation accuracy, r 2 , q 2 , and permutation p values are reported for a multivariate model with three components. c , d The magnitude of metabolic differences between ASD and TD increased with age. **** p < 0.0001. e , f Metabolite changes and pre-ASD newborns and 5-year-olds with ASD ranked by variable importance in projection (VIP) scores . g , h Bubble impact plot summary of biochemical pathway changes ranked by random forest analysis and mean decrease in accuracy (MDA). n = 205 newborns (68 males and 17 females in the pre-ASD group. 68 males and 52 females in the TD group), n = 53 5-year-olds (23 males and 8 females with ASD. 16 males and 6 females in the TD group).
Quantitatively, the magnitude of metabolic disturbances in 5-year-olds with ASD was greater than in the pre-ASD newborns (Fig. 2c, d ). The mean z-scores for metabolites that were significantly increased (+z-score and VIP ≥ 1.5) or decreased (-z-score and VIP score ≥ 1.5) were compared in the pre-ASD newborns and 5-year-olds. We found that the metabolites that were increased in newborns were increased by 54% more in the 5-year-old cohort ( p < 0.0001; Fig. 2c , Supplementary Data 3 and 4 ). Molecules that were increased in ASD included several stress response metabolites like lactate, alanine, glycerol, glycerol-3-phosphate, threonine, linoleic acid, linoleylcarnitine, cholesterol, ceramides, and the mRNA capping purine 7-methylguanine. Metabolites that were decreased at birth were decreased by 119% more in 5-year-olds ( p < 0.0001; Fig. 2d ). Molecules that were decreased in ASD were anti-inflammatory, 1-carbon, and antioxidant molecules like glutathione, carnosine, carnitine, betaine, 5’-methyltetrahydrofolic acid, and CoQ10, and neurotransmitters like dopamine and serotonin.
Metabolite changes in pre-ASD newborns
The top 4 most dysregulated metabolites in the pre-ASD newborns were sphingolipids (Fig. 2e ). Sphingosine-1-phosphate (S1P), ceramides and glycosphingolipids, like the mono and trihexosylceramides (MHCs and THCs), were increased, and their parent molecules, the SM lipids (sphingomyelins), were decreased. Two conjugated bile acids, chenodeoxyglycocholic acid and taurodeoxcycholic acid, were increased. Four of 27 purines were dysregulated in newborns who later developed ASD. The mRNA capping purine, 7-methylguanosine was increased, and guanine (Gua), dGMP, and AICAR (5-aminoimidazole-4-carboxamide ribonucleotide) were each decreased compared to TD controls (Fig. 2e , Supplementary Data 3 ). Serotonin, dopamine, and the levels of two B vitamins, niacin (niacinamide, vitamin B3) and FAD (from riboflavin, vitamin B2), were decreased in pre-ASD newborns (Fig. 2e and Supplementary Data 3 ).
Metabolite changes in 5-year-olds with ASD
The metabolomes of 5-year-olds with ASD contained increased levels of several phosphatidylethanolamine (PE) and phosphatidylinositol (PI) lipids. Phosphorylcholine, the head group of phosphatidylcholine (PC) and sphingomyelin (SM) lipids, which is produced by the action of phospholipase Cs (PLCs) and sphingomyelinases (SMSs), was increased. Several cardiolipins needed for mitochondrial biogenesis and oxidative phosphorylation were decreased in ASD (Supplementary Data 4 ). Several PC and sphingomyelin lipids that are substrates for PLCs and SMSs, were also decreased. A decrease in 2-hydroxy sphingomyelins like SM(d18:1/26:0 OH) and SM(d18:1/18:2 OH) needed for myelin stabilization 52 was observed (Fig. 2f , Supplementary Data 4 ). The mRNA capping purine 7-methylguanine was increased in the ASD group. Serotonin, and 3 vitamins and cofactors, L-carnitine, CoQ10, and 5’-methyltetrahydrofolic acid, were decreased. In contrast to increased levels in pre-ASD newborns, the bile acids glycocholic and chenodeoxyglycocholic acid, and the microbiome product indoxyl-3-sulfate were decreased (Fig. 2f , Supplementary Data 4 ). The most dysregulated metabolic pathways found in newborns who later developed ASD and 5-year-olds with ASD are summarized in Fig. 2g, h . Using metabolic classifiers of 6-7 biomarkers, the use of metabolomics was able to discriminate pre-ASD from TD newborns with an accuracy of 75%, and with an accuracy of 88% in 5-year-olds (Fig. 3a, b ). A summary of the metabolic changes that were unique and shared between the two cohorts is summarized in the Venn diagram in Fig. 3c . Additional metabolomic results from classical methods of area under the curve (AUC) and z-score analysis are reported in the Supplementary Results .
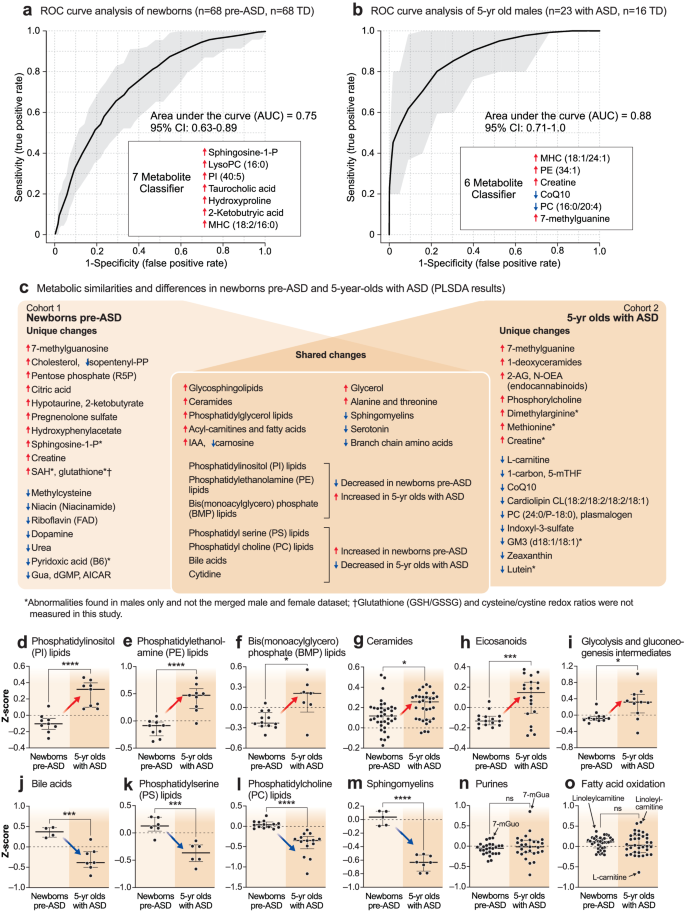
a Receiver operator characteristic (ROC) curve analysis by random forest analysis. Newborn males. n = 136 newborn males (n = 68 ASD and 68 TD). b 5-year-old males. n = 39 5-year-old males ( n = 23 ASD and 16 TD). c Blood metabolome changes found in pre-ASD newborns are on the left. Changes found in 5-year-olds with ASD are on the right. Shared changes are shown at the intersection. *Changes found in males only. †Glutathione (GSH/GSSG) and Cysteine/Cystine redox ratios were not measured in this study. Developmental regulation of discriminating metabolites, d Phosphatidylinositol (PI) lipids, e Phosphatidyl ethanolamine (PE) lipids, f Bis(monoacylglycero)phosphate (BMP) lipids, g Ceramides, h Eicosanoids, i Glycolysis, j Bile acids. k Phosphatidylserine (PS) lipids, l Phosphatidylcholine (PC) lipids, m Sphingomyelins, n Purines, o Fatty acid oxidation and synthesis intermediates. Error bars indicate the z-score medians and interquartile ranges. Red arrows indicate metabolites that were low in pre-ASD newborns and increased in 5-year-olds with ASD. Blue arrows indicate metabolites that were increased in pre-ASD newborns and low in 5-year-olds with ASD. 5-mTHF 5’-methyltetrahydrofolate, 7-mGuo 7-methylguanosine, 7-mGua 7-methylguanine, 2-KB 2-ketobutyric acid, Gua guanine, dGMP deoxyguanosine monophosphate, AICAR 5-aminoimidazole-4-carboxamide ribonucleotide, PI phosphatidylinositol lipids, PS phosphatidyl serine lipids, PE phosphatidyl ethanolamine lipids, PC phosphatidyl choline lipids, BMP bis(monoacylglycero)phosphate lipids, IAA imidazole acetic acid, FAs fatty acids, R5P ribose-5-phosphate, SAM S-adenosyl methionine, SAH S-adenosylhomocysteine, 2-AG 2-arachidonylglycerol, N-OEA N-oleoylethanolamine, GM3(d18:1/18:1) is a monosialic, trihexosyl ganglioside, PG phosphatidylglyerol lipids. * p < 0.05, ** p < 0.01, *** p < 0.001, **** p < 0.0001.
Developmentally regulated metabolic changes
The z-scores of several important classes of metabolites that were significantly changed by VIP scores ≥1.5, were found to be changed in opposite directions in the newborn pre-ASD and 5-year-old ASD groups. The top 6 classes of metabolites that were changed in newborns were either increased or underwent a developmental reversal in 5-year-olds. These were PI, PE, and BMP (bis(monoacylglycero)phosphate) lipids, ceramides, eicosanoids, and products of glycolysis like lactate and glycerol-3-phosphate (Fig. 3d–i ). The top 4 classes of molecules that were decreased with age in ASD compared to TD controls were bile acids, PS and PC lipids, and sphingomyelins (Fig. 3j–m ). Notably, purines and fatty acid oxidation intermediates were not dysregulated as a group. However, individual purines like the mRNA capping purines 7-methylguanosine (7-mGuo) and 7-methylguanine (7-mGua, Fig. 3n ), and the polyunsaturated long-chain acyl-carnitine linoleylcarnitine, were elevated in both groups, and L-carnitine was decreased in the 5-year-olds with ASD (Fig. 3o ).
Metabolic network studies
We next explored how changes in the network of pair-wise correlations between metabolites might predict the chance of future ASD in newborns or provide new insights into the biology of ASD in 5-year-old children.
CIRCOS analysis
Newborn correlation networks.
CIRCOS plots were constructed to visualize the global metabolic networks of cases and controls. Positive (+r) and negative (− r ) correlations were displayed separately. The global metabolic network in pre-ASD newborn males had 102% fewer positive correlations than typically developing newborns (515 vs 1038; p < 1 × 10 −6 ; Fig. 4a, b ). The sequence of metabolites around the rim of the CIRCOS plots is shown in Supplementary Data 5 and 6 . Major positive correlation trunks connected ceramides and phosphatidylinositol (PI) phospholipids (segment \({ab}^{\atop\longleftrightarrow}\) ), and sphingomyelins and phosphatidylcholine (PC) phospholipids (segment \(\overline{{cd}}\) ). In contrast to the loss of positive correlations in the pre-ASD network, there was a 97% gain of negative correlations in the pre-ASD group (449 vs 228; p < 1 × 10 −6 ; Fig. 4c, d , Supplementary Data 7 and 8 ). Prominent among the increased negative correlations was the appearance of two new major trunks from sphingomyelins to eicosanoids (segment \(\overline{{ab}}\) ), and from purines to the eicosanoids (segment \(\overline{{bc}}\) ).
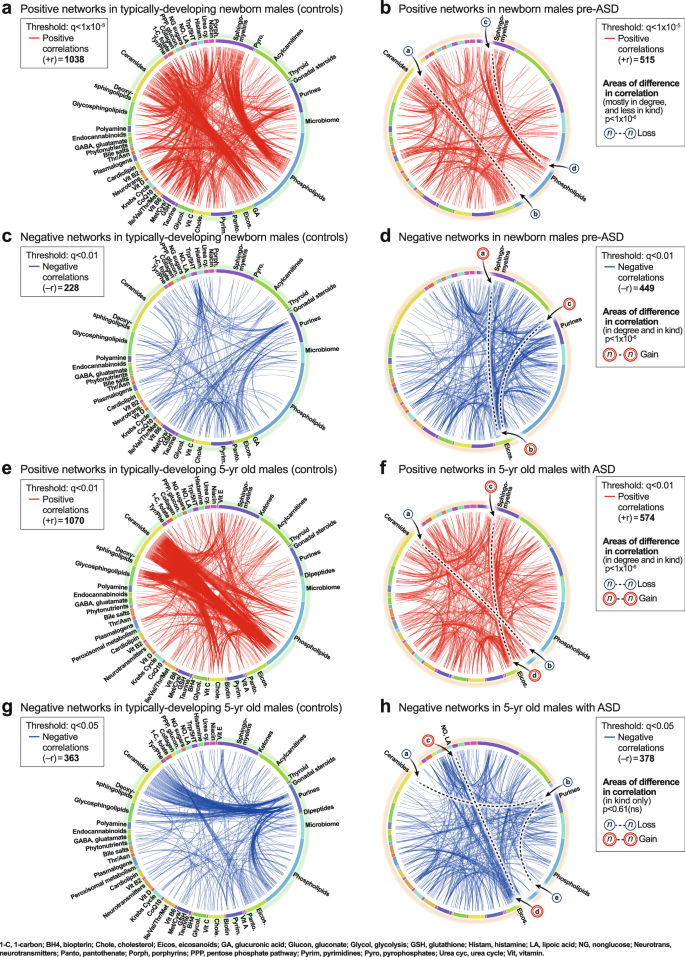
a , b Positive correlation networks (+r), TD and pre-ASD in newborn males. q < 1 × 10 -5 . c , d Negative correlation networks (-r), TD and ASD newborn males. q < 0.01. e , f Positive correlation networks (+r), TD, and ASD in 5-year-old males. q < 0.01. g , h Negative correlation networks (−r), TD and ASD in 5-year-old males. q < 0.05. n = 68 pre-ASD newborn males and 68 newborn TD male controls. n = 23 5-year-old ASD males and 16 TD male controls. See Results for description of the specific metabolite-pair correlations that were gained or lost.
5-year-old correlation networks
The ASD network in 5-year-olds had 184% fewer positive correlations than the TD network (574 vs 1070; 1 × 10 −6 ; Fig. 4e, f ). The sequence of metabolites around the rim of the CIRCOS plots is shown in Supplementary Data 6 . Normal positive connections between ceramides and phosphatidylinositol (PI) phospholipids were lost in the ASD group (segment \(\overline{{ab}}\) ). New positive connections between sphingomyelins and eicosanoids (segment \(\overline{{cd}}\) ) occurred (Fig. 4f ). There was no significant difference in the number of negative edges in the ASD and TD networks (378 vs 362 at q < 0.05; p = 0.61 (ns); Fig. 4g, h ; Supplementary Data 9 and 10 ). However, there were several differences in the major trunks that connected metabolites. For example, normal negative correlations between ceramides and purines (segment \(\overline{{ab}}\) , Fig. 4g ), and purines to PI lipids (segment \(\overline{{be}}\) ) were lost in the ASD group (Fig. 4h ). New negative correlations between lipoic acid in the nitric oxide/lipoic acid/ROS pathway and several eicosanoids were gained (segment \(\overline{{cd}}\) , Fig. 4h ).
Complementarity of network and classical metabolomic methods
We next calculated statistical parameters for all possible pair-wise correlations between metabolites in the metabolome and ranked each metabolite by its ability to discriminate between the ASD and TD networks (Supplementary Data 7 – 10 ). The network rank of a metabolite was poorly correlated with its rank determined by classical tools in metabolomics like multivariate VIP score, Random Forest mean decrease in accuracy (MDA), Welch’s adjusted p values, or Mann-Whitney U test. Classical metabolomics methods explained less than 3% of the variance in the network rank ( r 2 ≤ 0.03; Supplementary Fig. 2a–d ). In contrast, the ranking of metabolites by classical AUC-based metabolomic methods correlated with each other (Supplementary Fig. 2e–h ). Since 97% of the information obtained by network analysis could not be predicted by standard univariate or multivariate analysis of the metabolomics data, these results showed that network-based and concentration-based analyses were independent and complementary.
Metabolic network hub-and-spoke analysis
Based on network changes that were most significant in the 5-year-olds with established ASD, we selected the purine, ceramide, PI lipid, and eicosanoid pathways for further analysis in both cohorts.
The purine hub
Unbiased network correlation analysis showed that the purine hub was the most dysregulated of all 50 metabolic pathways interrogated in the 5-year-olds with established ASD ( p < 1.0 × 10 −22 ; Supplementary Data 11 and 13 ). The purine network was also highly dysregulated in the pre-ASD newborns ( p < 6.3 × 10 −22 ; Supplementary Data 12 and 14 ). The purine hub in newborns consisted of 26 measured nucleobases, nucleosides, nucleotides, and associated metabolites. Comparing the purine hubs in TD and pre-ASD newborns, the first impression obtained from looking at the networks from a distance is that the purine hub in the TD group is relatively polymorphic, undifferentiated, and diffusely connected (Fig. 5a, e ). The negative edges (−r) were few and sparsely connected (Fig. 5 c ). The purine hub in the pre-ASD group on the other hand, had more structured features and appeared prematurely differentiated. New positive edges (+r) were found between xanthosine and the eicosanoids in the pre-ASD group, labeled [1] (Fig. 5b ). Several purines lost positive correlations with the riboflavin/FAD pathway associated with mitochondrial fatty acid oxidation [2] (Fig. 5b ). New negative correlations connected purines with several other important metabolic pathways like the sphingomyelins [3] and the eicosanoids [4] (Fig. 5d ). The negative correlations between XMP and GMP and the eicosanoids were found in both the TD and pre-ASD groups (Fig. 5c, d ). However, new negative correlations between AMP and the eicosanoids were found in the pre-ASD group [4] (Fig. 5d ). Overall, the newborn pre-ASD purine network had fewer positive and more negative correlations than the TD network ( p < 0.0001; Fig. 5a–d ). The rise and fall of purines in pre-ASD newborn males was correlated with changes in over 400 metabolites from 31 different metabolic pathways (Fig. 5a–f ).
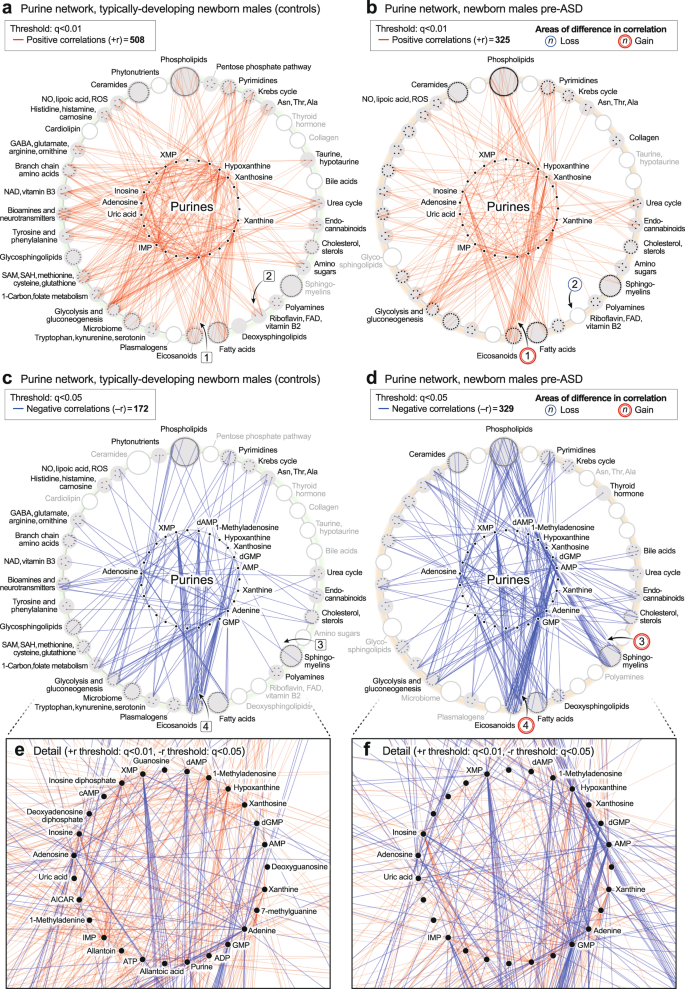
a , b Positively correlated (+r, red) network. c , d Negatively correlated (−r, blue) network. e , f Magnified purine hubs in newborn males. n = 68 pre-ASD newborn males and 68 newborn TD male controls. TD vs pre-ASD +r/−r edge ratio p < 0.0001; total purine network correlations p < 0.0003.
The purine hub in 5-year-olds consisted of 27 measured purines and associated metabolites. Comparing the purine hubs in TD and ASD groups, the first impression obtained from looking at the networks from a distance is that the purine hub in the TD group is highly structured and well-differentiated by 5 years of age (Fig. 6a, c, e ). In contrast, the purine hub in the ASD group was polymorphic, diffusely connected, and developmentally underdifferentiated (Fig. 6b, d, f ). The early structuring of the purine network at birth (Fig. 5b, d ) led to an underdifferentiated purine network in children with ASD at age 5 (Fig. 6b, d ). The ASD group lost positive correlations between 7-methylguanine and the ceramides [1] (Fig. 6b ). Positive correlations were also lost between xanthine and several neurotransmitters like N-acetylaspartate and dopamine [2] (Fig. 6b ). New positive correlations occurred between xanthine and xanthosine and several phospholipids [3] (Fig. 6b ), and from deoxyguanosine to glycine and serine in the 1-carbon pathway [4] (Fig. 6b ). The negative correlations between XMP, dGMP, and AMP, and the eicosanoids that occurred in the pre-ASD group of newborns (Fig. 5d, f ), were lost by 5-years of age (Fig. 6d, f ). Negative correlations between AMP, GMP, ADP, IMP, and the ceramides [5] (Fig. 6d, f ), phospholipids [6], glycosphingolipids [7], and cholesterol [8] did not develop in 5-year-olds with ASD (Fig. 6c–f ). The rise and fall of purines was correlated with changes in over 150 metabolites from 33 different metabolic pathways. Overall, the purine nucleobase xanthine gained the most positive correlations (+r, Fig. 6b ), and GMP lost the most negative correlations (−r, Fig. 6d ) of all the purines in the 5-year-old ASD metabolic network (Supplementary Data 11 ). New xanthine stimulatory (+r) correlations in ASD included biomarkers of mitochondrial dysfunction like myristoylcarnitine and lactic acid, and several phosphatidylserine (PS) lipids like PS(18:0/20:4) that are markers of apoptosis (Supplementary Data 9 ).
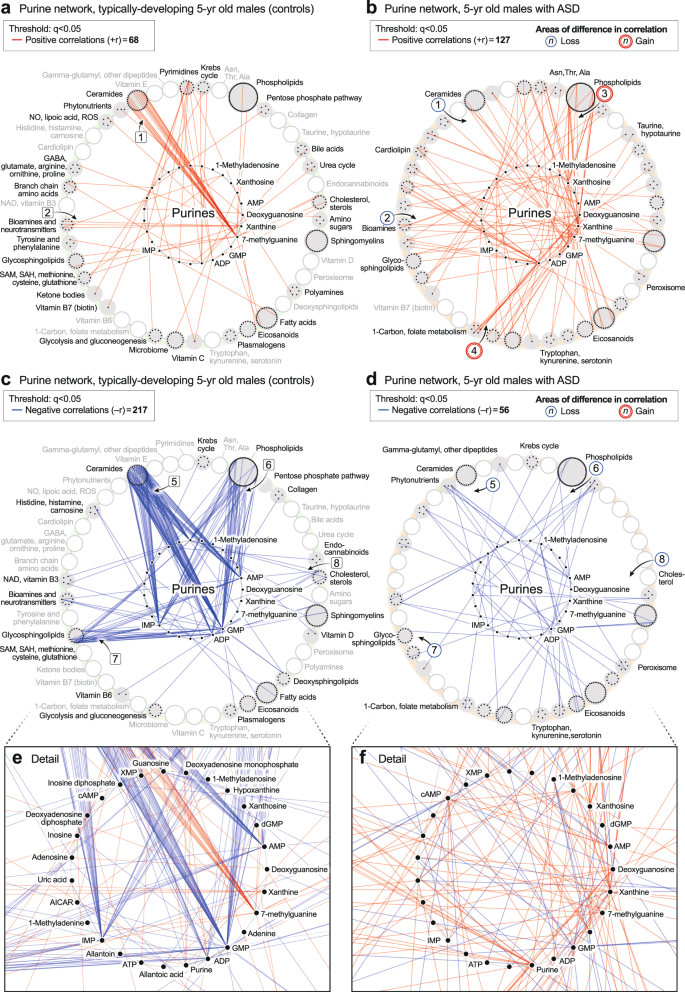
a , b Positively correlated (+r, red) network. c , d . Negatively correlated (−r, blue) network. e , f Magnified purine hubs in 5-year-old males. TD vs ASD +r/−r edge ratio p < 0.0001; total purine network correlations p < 0.0001. n = 23 5-year-old ASD males and 16 TD male controls.
Purine network developmental arrest and failed reversal in ASD
The ratio of positive to negative correlations—the +r/-r ratio—of purines in TD children was higher in newborns and underwent a reversal during normal development so that by 5-years of age, the +r/−r ratio was low (+r/−r = 5.5 ± 0.27 in TD newborns to 0.31 ± 0.02 in TD 5-year-olds; p < 0.0001; Fig. 7a, b ). The magnitude of this change was 17.7-fold (5.5/0.31 = 17.7 ± 1.1). The pattern in the pre-ASD newborns was much different. The +r/−r ratio in pre-ASD newborns was just 36% of the TD group (+r/−r = 2.0 ± 0.11 in pre-ASD newborns vs 5.5 ± 0.27 in the TD newborns; p < 0.0001; Fig. 7a, b ). In addition, the normal developmental reversal of the +r/−r ratio in the purine network did not occur in 5-year-olds with ASD. Instead, the +r/−r ratio of purine correlations in the metabolome remained statistically unchanged between birth and 5-year-old children with ASD (+r/−r = 2.0 ± 0.11 in the pre-ASD newborns vs 2.3 ± 0.12 in 5-year-olds with ASD; p = ns; Fig. 7a, b ). To place this in physiologic context, the relationship of five correlated developmental systems is shown in Fig. 7c . Mitochondrial DNA (mtDNA) copy number increases rapidly from the 2nd trimester, continues postnatally, and nears a plateau at about age 3 years. The increase in mtDNA is associated with increased expression of the highly hydrophobic core subunits of the mitochondrial respiratory chain. These mtDNA-encoded subunits are used to nucleate assembly of the multi-subunit complexes and supercomplexes of the electron transport chain. Increased oxidative phosphorylation and increased bioenergetic capacity—the capacity for ATP synthesis—accompany the increase in mtDNA. Increasing metabolic and bioenergetic capacity permits increasing cellular metabolic specialization and differentiation 53 , 54 , and myelination 55 , 56 , 57 (Fig. 7c ). Epidemiologic studies have shown that the sensitivity to ASD risk factors like maternal infection, gestational maternal autoantibodies, pesticides, perfluoroalkyl substances (PFAS), phthalates, brominated diphenyl ether (BDE) flame retardants, and mixtures of endocrine disruptors and other potentially hazardous prenatal and postnatal environmental exposures, extends from near the beginning of the 2nd trimester, to 3 years of age 18 , 58 , 59 . The sensitivity to environmental factors and the risk of ASD decline together as the GABA signaling network reverses from net excitatory signaling at birth, to net inhibitory signaling by 2–3 years of age 60 (Fig. 7c ).
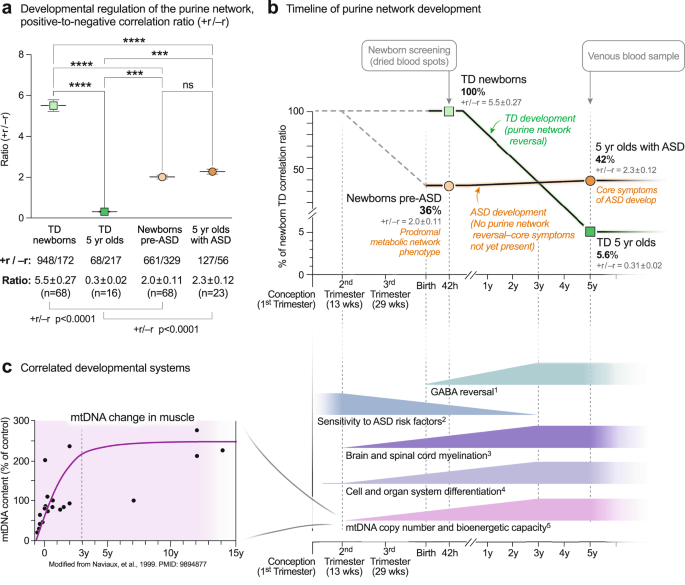
a 2-way ANOVA of the +r/−r ratio. +r and −r correlations at q < 0.05. * p < 0.05, ** p < 0.01, *** p < 0.001, **** p < 0.0001. b Timeline of purine metabolic network reversal. +r/−r ratios were expressed as the percent of the TD ratio in newborn males. n = 68 pre-ASD newborn males and 68 newborn TD male controls. n = 23 5-year-old ASD males and 16 TD male controls. Means are indicated in the figure. SEMs are reported numerically in gray text and were smaller than the outline of the points. c Correlated developmental systems. 1 GABA (γ-aminobutyric acid) network reversal 60 . 2 Sensitivity to ASD risk factors 18 , 59 . 3 Brain and spinal cord myelination 55 , 56 , 57 . 4 Cell and organ system differentiation 54 . 5 Mitochondrial DNA (mtDNA) copy number and cell bioenergetic capacity 53 .
Lipid network dysregulation in ASD
Lipids represented 58% (270 of 465) of the metabolites measured in this study. Yet we found that 80% of the TD metabolic network in 5-year-old males (4395 of 5510 edges with q < 0.05) was formed from nodes created by lipids ( p < 0.0001; Supplementary Data 11 ). In comparison, 60% of the ASD metabolic network (2256 of 3782 edges at a threshold of q < 0.05) was formed from lipids (TD vs ASD p < 0.0001). The fraction of the metabolic network formed by polar non-lipid metabolites like purines, amino acids, and neurotransmitters doubled from 20% in TD to 40% in ASD. After the purine hub, some of the largest changes in the ASD network were hubs formed by ceramides, phosphatidylinositol (PI) lipids, and eicosanoids. These are described below.
The ceramide hub
Ceramide correlations were a dominant feature in typically developing 5-year-olds (Fig. 5e, g ). These correlations are shown in more detail in Fig. 8a, b . The ceramide hub consisted of 33 ceramides connected by a total of 1455 edges in the TD network, but by only 322 edges in the ASD network ( p < 0.006; Fig. 8a,b ). Over 90% of the ceramide correlations (1350 of 1455 edges) in the TD network were positive (red) correlations. 100% (105 of 105) negative (blue) correlations in the TD network from the ceramide hub were to purines, labeled [1] (Fig. 8a ). These negative correlations between ceramides and purines were lost in the ASD network [1] (Fig. 8b ). Normal positive correlations between bile acids [2], pantothenic acid [CoA, 3], cholesterol [4], plasmalogens [5], glutamate in the neurotransmitter pathway [6], NAD+ metabolism [7], histidine, histamine, and carnosine [8], and dimethylarginine in the nitric oxide/lipoic acid pathway [9], were lost in the ceramide network of ASD (Fig. 8a, b ). Strong positive correlations were maintained between ceramides, sphingomyelins, and glycosphingolipids in both TD and ASD groups (Fig. 8a, b ).
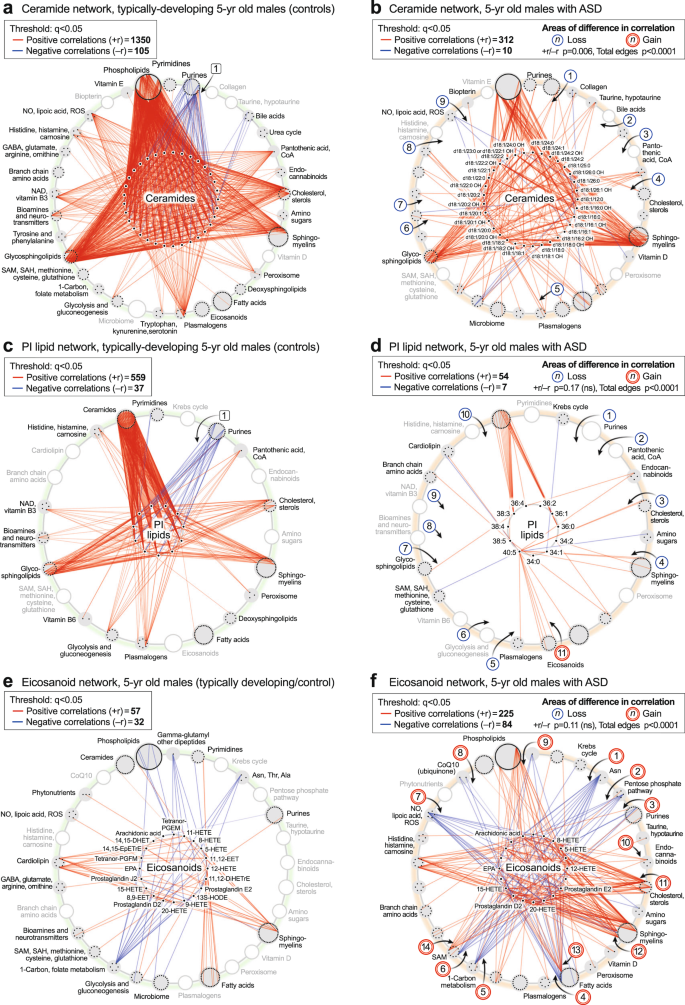
a , b Ceramide hub analysis. c , d Phosphatidylinositol (PI) lipid hub analysis. e , f Eicosanoid hub analysis. n = 23 5-year-old ASD males and 16 TD male controls. All edges with q < 0.05. EPA eicosapentaenoic acid, HETE hydroxyeicosatetraenoic acid.
The PI lipid hub
Phospholipids were another dominant pathway revealed by the CIRCOS analysis. The PI lipid hub consisted of 11 phosphatidylinositol lipids connected by a total of 596 edges in the TD network, but by only 61 edges in the ASD network (total edge p value < 0.0001; Fig. 8c, d ). The PI lipid network in TD was comprised of 93% positive correlations. Most of the negative correlations (blue edges) in the TD network were to purines, labeled [1] (Fig. 8c ). All the normal negative correlations between PI lipids and purines were lost in the ASD network [1] (Fig. 8d ). In addition, normal positive correlations were lost in the PI network of ASD to pantothenic acid (CoA) [2], cholesterol [3] sphingomyelins [4], plasmalogens [5], glycolysis [6], glycosphingolipids [7], glutamate in the neurotransmitter pathway [8], NAD+ metabolism [9] and histidine and carnosine in the histamine pathway [10]. New positive correlations were found between the eicosapentaenoic acid (EPA; 20:5)-containing PI lipid PI(40:5) and several eicosanoids in the ASD network [11] (Fig. 8c, d ).
The eicosanoid hub
The eicosanoid hub in ASD had nearly 4-times more positive, and nearly 3-times more negative correlations than in the TD control group (total edge p value < 0.0001; Fig. 8e, f ). Several new negative correlations were found in the ASD network between the eicosanoids and L-asparagine [1], the pentose phosphate pathway for NADPH synthesis [2], the purine 1-methyladenosine [3], the C6-saturated medium chain acyl-carnitine hexanoyl-carnitine in the fatty acid oxidation pathway [4], 5’-methyltetrahydrofolic acid in the 1-Carbon pathway [5], cysteine-S-sulfate in the SAM/glutathione pathway [6], lipoic acid [7], and CoQ10 [8]. New positive correlations occurred between the eicosanoids and BMP(18:1/16:1) in the phospholipid pathway [9], 2-arachidonylglycerol in the endocannabinoid pathway [10], desmosterol and 7-dehydrocholesterol [11], 2-hydroxy sphingomyelins [12], docosahexaenoic acid (DHA) in the fatty acid pathway [13], and 3-methylthiopropionate from the polyamine, methionine salvage, and the SAMe pathway [14].
The ASD hypercorrelator hub
We next studied the top 15 metabolites that were increased in the correlation network of ASD compared to TD (Fig. 9a, b ; Supplementary Data 13 ). The ratio of positive to negative edges (the +r/−r ratio) was not different between ASD and TD ( p = 0.14). However, the kind and degree (number) of the correlations were distinct (total edges p < 0.0001). A striking set of negative (blue) correlations were found between L-asparagine (Asn) and several eicosanoids like 18-, 15-, 12-, 9-, and 8-HETE, in the ASD network [1] (Fig. 8f , and the hub of 9b). Asparagine is known to be a mediator of mitochondrial signaling pathways needed for cell growth 61 and mitochondrial ROS-mediated activation of hypoxia-inducible factor 1α (HIF1α) 62 . However, the negative correlation of asparagine with eicosanoids was a novel finding of our study. New correlations were also found between the hypercorrelators hub and pyrimidines, the mitochondrial Krebs cycle, pentose phosphate pathway, and purines [2]. Hub hypercorrelators gained new correlations with cholesterol [3], glucosamine in the amino sugar pathway [4], sphingomyelins [5], and between 2-hydroxy sphingomyelins like SM(d18:1/20:0 OH) and several eicosanoids [6], ceramides [7], and phospholipids [8]. Thirty-five (35) of 423 correlations in the ASD hypercorrelator hub (8.3%) occurred with other hypercorrelators within the hub (Fig. 9b ). Similar ASD hypercorrelator analysis in the pre-ASD newborn males showed new correlations with eicosanoids, plasmalogens, androsterone-sulfate, and hypoxanthine (Supplementary Data 14 ).
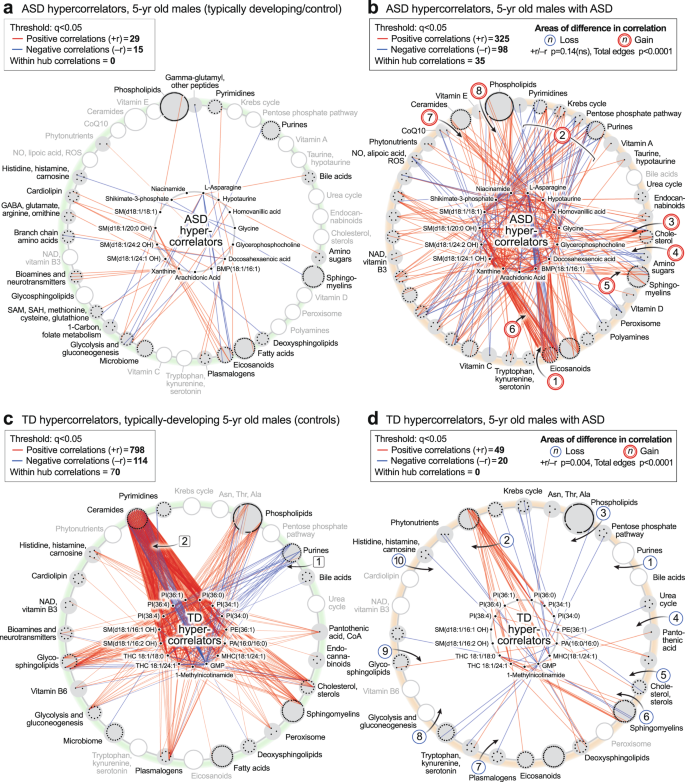
a , b ASD hypercorrelators, 5-year-old males. c , d TD hypercorrelators, 5-year-old males. The top 15 metabolite hypercorrelators shown in each network. 467 metabolites measured. n = 23 ASD males and 16 TD males. All edges with q < 0.05.
The TD Hypercorrelator hub
The top 15 metabolites with the most correlations in the TD correlation network of 5-year-old males are shown in Fig. 9c, d and Supplementary Data 13 . The ratio of positive to negative edges (the +r/-r ratio) was significantly different between the TD and ASD hypercorrelator networks ( p < 0.0004). Lipids, which made up 13 of the top 15 (87%) hypercorrelated nodes in TD metabolome, lost over 90% of their correlations in the ASD metabolome (912 edges in TD vs 69 edges in ASD (92% loss; p < 0.0001). Phosphatidylinositol (PI) lipids make up 6 of the top 15 metabolites. Several negative correlations between lipids in the TD hypercorrelator network to purines [1] (Fig. 9c ) were lost in the ASD network [1] (Fig. 9d ). The purine GMP had multiple negative (blue) correlations with ceramides in the TD network that were lost in the ASD network [2] (Fig. 9c, d ). Positive correlations that were lost in ASD occurred with phospholipids [3], pantothenic acid [4], 7-dehydrocholesterol [5], sphingomyelins [6], plasmalogens [7], glycolysis [8], and glycosphingolipids [9], and carnosine in the histidine/histamine pathway [10]. Seventy of 912 total correlations (7.7%) occurred within the hub of the TD hypercorrelator network. Similar TD hypercorrelator analysis in the pre-ASD newborn males showed new correlations with neurotransmitters like GABA and N-acetylaspartate, the Krebs cycle intermediate malate, the inhibitor of nitric oxide synthase (NOS) dimethylarginine, IMP, the gluconeogenesis metabolites phosphoenolpyruvate, dihydroxyacetone phosphate, and glycerol-3-phosphate, and the gluconeogenic amino acids proline and hydroxyproline (Supplementary Data 14 ).
Metabolic network growth ( \(\dot{{{\boldsymbol{V}}}}\!_{{{\boldsymbol{net}}}}\) ) analysis
Using ramped random resampling, we developed a new parameter, \(\dot{{{\boldsymbol{V}}}}\!\) net , to quantify the rate at which the metabolic network grows as clinical samples from the group were added (see Supplementary Fig. 3 , Supplementary Table 3 , and Supplementary Data 15 and 16 ). This method balanced statistical power by using equally sized subsamples and allowed for a comparison of the correlation network between differently sized case and control groups. Linear regression analysis showed that rates of metabolic network growth were different in ASD and TD. We found that the \(\dot{{{\boldsymbol{V}}}}\!\) net in pre-ASD newborns was significantly lower than \(\dot{{{\boldsymbol{V}}}}\!\) net for the TD network (+r \(\,\dot{{{\boldsymbol{V}}}}\!\) net = 121 ± 2.2 in pre-ASD vs 159 ± 1.1 in TD; p < 0.0001; ASD/TD = 0.76 ± 0.02 at birth; Fig. 10a–c ; Supplementary Data 15 ). The \(\dot{{{\boldsymbol{V}}}}\!\) net for ASD was only 41% of the \(\dot{{{\boldsymbol{V}}}}\!\) net for TD in 5-year-old children (+r \(\dot{{{\boldsymbol{V}}}}\!\) net = 112 ± 6.4 in ASD vs 275 ± 12 in TD; p < 0.0001; ASD/TD = 0.41 ± 0.04; Fig. 10d–f ; Supplementary Data 16 ).
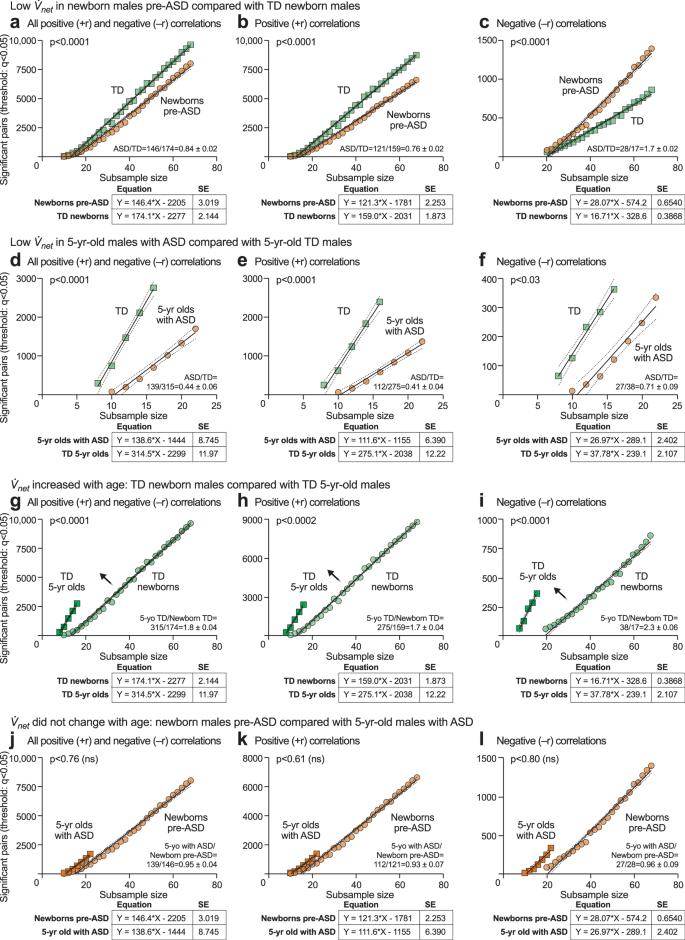
Newborn males, a–c \(\dot{{{{{{\boldsymbol{V}}}}}}}\!\) net for the positive (+r) network was decreased in newborns who later developed ASD compared to age-matched typically developing controls . a All +r and -r out-of-pathway correlations. b Positive (+r) out-of-pathway correlations. c \(\dot{{{{{{\boldsymbol{V}}}}}}}\!\) net for the negative (−r) network was increased in pre-ASD newborns. n = 68 pre-ASD males and 68 TD control males. \(\dot{{{{{{\boldsymbol{V}}}}}}}\) net for both the positive (+r) and negative (−r) networks was decreased in 5-year-olds with ASD compared to age-matched typically developing controls, d All +r and -r out-of-pathway correlations e Positive (+r) out-of-pathway correlations. f Negative (−r) out-of-pathway correlations. Green squares: TD. Tan circles: ASD. Numbers indicated are for edges with q < 0.05 at each indicated subsample size. n = 23 ASD males and 16 TD males. \(\dot{{{{{{\boldsymbol{V}}}}}}}\!\) net in typically developing 5-year-old males was increased compared to typically developing newborn males, g All +r and -r out-of-pathway correlations h Positive (+r) out-of-pathway correlations . i Negative (-r) out-of-pathway correlations. Dark green squares: 5-year-old TD. Light green circles: newborn TD. N = 68 newborn males TD and 16 5-year-old males TD. \(\dot{{{{{{\boldsymbol{V}}}}}}}\) net in 5-year-old males with ASD was unchanged compared to pre-ASD newborns, j All +r and −r out-of-pathway correlations k Positive (+r) out-of-pathway correlations . l Negative (−r) out-of-pathway correlations. Dark tan squares: n = 23 5-year-old males with ASD. Light tan circles: n = 68 pre-ASD newborn males.
Developmental arrest of \(\dot{{{\boldsymbol{V}}}}\!_{{{\boldsymbol{net}}}}\) in ASD
A comparison of the ASD to TD networks revealed that the metabolic network growth rate ( \(\dot{{{\boldsymbol{V}}}}\!\) net ) in typically developing children increased by 173% between birth and 5-years of age (+r \(\dot{{{\boldsymbol{V}}}}\!\) net = 159 ± 1.9 for TD newborns vs 275 ± 12 for TD 5-year-olds; p < 0.0001; 5-years/newborn TD = 275/159 = 1.73 ± 0.04 = 173%; Fig. 10g–i , Supplementary Table 3 ; Supplementary Data 15 and 16 ). In contrast, \(\dot{{{\boldsymbol{V}}}}\!\) net in pre-ASD newborns was unchanged in 5-year-olds diagnosed with ASD, and failed to show the typical developmental increase ( \(\dot{{{\boldsymbol{V}}}}\!\) net = 121 ± 2.2 in pre-ASD newborns vs 112 ± 6.4 in 5-year-olds with ASD; p = ns; 5-years-old ASD/pre-ASD newborns = 121/112 = 1.1 ± 0.04; Fig. 10j–l , Supplementary Table 3 ; Supplementary Data 15 and 16 ).
Metabolism and child development are inextricably connected. Classical metabolomic analysis by laboratories around the world has shown that children with ASD have distinct metabolic profiles that vary by age, sex, and severity of symptoms 3 , 4 , 5 , 6 , 7 , 8 . The developmental neurobiology of ASD is driven in part by the patterned changes in metabolism that occur during child development. Using classical methods of concentration-based mass spectrometry and new methods in network metabolomic analysis, we found that the top discriminating changes in 5-year-olds with ASD occurred in phospholipid, fatty acid oxidation and acyl-carnitines, cardiolipins, ceramides, sphingomyelin, and glycosphingolipid metabolism. Fourteen pathways shared between pre-ASD newborns and 5-year-olds with ASD accounted for 80% of the metabolic impact. The metabolic phenotype of ASD was characterized by a decrease in anti-inflammatory and antioxidant molecules like glutathione, carnosine, 5’-methyltetrahydrofolic acid, and CoQ10, and a coordinated increase in stress response metabolites like lactate, glycerol, alanine, threonine, cholesterol, and ceramides. The magnitude of these metabolic changes increased with child age.
A major result of this research was that the developmental differences observed in ASD were not the result of an increase or decrease of one causal metabolite, or an isolated change in the gut-brain axis, or neuroendocrine, autonomic, cytokine, or immunologic circuits. Instead, it was the interconnectedness and developmental state of the metabolic network that underlies all these systems that was fundamentally changed. The implication of this finding is that the metabolic changes found in children with ASD were not the result of cell dysfunction or damage. Instead, the measured changes were the result of normal physiologic and neurodevelopmental responses to metabolic signals that cells received in ASD that were not being sent in typically developing children.
New methods for metabolic correlation network analysis were used to reveal previously hidden phenotypes in ASD. CIRCOS and hub-and-spoke analysis provided global, and pathway-specific views, respectively. In newborns, pre-ASD males had twice as many negative correlations and half as many positive network correlations compared to the TD group. A major trunk of positive correlations between ceramides and phosphatidylinositol (PI) lipids in the TD network was diminished in the pre-ASD network. New negative correlations between sphingomyelin and eicosanoids, and purines to eicosanoids were formed in the pre-ASD group. New positive correlations developed between the eicosanoids, cholesterol, and 2-hydroxy sphingomyelins used for myelin stabilization in 5-year-olds with ASD. Eicosanoid abnormalities have been previously reported in ASD 63 .
Dysregulated calcium homeostasis is an established feature of ASD 64 , 65 . In the current study, there was a loss of negative correlations between ceramides and purines, and between purines and PI lipids that regulate calcium homeostasis in 5-year-olds with ASD. Ceramides and PI lipids are functionally related. These lipids co-aggregate in microdomains of membrane lipid rafts (MLRs) and mitochondria associated membranes (MAMs) in stressed cells. Cooperativity between ceramides and PI lipids is used for activation of the phosphatidylinositol-3-dependent kinase 1 (PI3K)/protein kinase B (AKT)/mammalian target of rapamycin (mTOR) pathway that is used during conditions of cell stress 66 . IP3-activated release of intracellular stores of calcium in the ER is used for stress signaling 67 , and the activation of the AKT/mTOR pathway, play key roles in the neurobiology and immunobiology of ASD 68 . The TD network was comprised of dozens of strong inhibitory connections between purines like AMP, ADP, and ATP and the PI lipids, creating a natural dampening effect that prevents runaway cell activation by IP3-mediated calcium signaling. The ASD network lacked these natural dampeners to cell activation. The loss of inhibitory correlations between purines and PI lipids has implications for poorly regulated IP3-stimulated calcium release, persistent cell activation, and hypersensitive sensory responses in ASD. Ceramides are inhibitors of mitochondrial complex III that can trigger apoptosis and inhibit PTEN-induced kinase 1 (PINK1)-dependent mitochondrial quality control by mitophagy 69 . The loss of negative correlations between purines and ceramides in ASD can result in accumulation of dysfunctional mitochondria and excessive apoptotic cell death in response to non-injurious environmental stimuli.
Of all 50 metabolic pathways interrogated, the purine correlation network was the most changed in ASD. Purine network hub analyses revealed a 17-fold reversal from excess positive (+r) correlations in typically developing newborns, to excess negative (−r) correlations in TD 5-year-olds. This reversal failed in newborns who later developed ASD. The developmental reversal of positive (+r, stimulatory) and negative (−r, inhibitory) correlations in the purine network was similar to the reversal of the effects of GABA (γ-aminobutyric acid) signaling in normal child development 70 . The GABA-A receptor is a chloride channel 71 . GABA is an excitatory and stimulatory (depolarizing) neurotransmitter at birth and early development, then becomes inhibitory (relaxing, hyperpolarizing) during postnatal development as nicotinic cholinergic safety signaling drives mitochondrial biogenesis 72 , and facilitates the shift to the mature pattern of inhibitory GABA signaling 73 . This change is mediated in part by decreased intracellular chloride (Cl - ) concentrations that occur with normal brain development 74 . Early excitatory GABAergic activity in striatal cholinergic-GABA-ergic interneurons is required to facilitate later developmental reversal to inhibitory GABA function. If early excitation is blocked prematurely with bumetanide to inhibit the chloride importer NKCC1, then the later reversal to inhibitory GABA signaling is incomplete 75 . Interestingly, our data showed a similar pattern during maturation of the ATP-related purine network. In contrast to the TD network, infants at risk for future ASD had a decreased ratio of excitatory to inhibitory (+r/−r) correlations in the ATP network at birth, and this ratio did not decrease during ASD development.
ATP, chloride, GABA signaling, and mitochondria are fundamentally interrelated. Increasing mitochondrial bioenergetic (ATP-producing) capacity is made possible by a phase of rapid increase in mitochondrial DNA (mtDNA) copy number that occurs in cells as they develop from the 2nd trimester to 3 years of age 53 , 54 . As mitochondrial biogenesis and oxidative phosphorylation increase, cellular ATP reserves increase. The increased ATP reserve creates increased capacity of the sodium-potassium ATPase (Na + /K + ATPase) pump and permits more rapid reestablishment of ion gradients and membrane potential after depolarization. Intracellular chloride concentration is decreased as mitochondrial biogenesis continues and neurons mature. This occurs in part because of decreased expression of the bumetanide-sensitive, sodium, potassium, and chloride co-importer NKCC1 and increased expression of the chloride-extruding potassium-chloride cotransporter KCC2 76 . Combined with higher intracellular potassium produced by a more active Na + /K + ATPase, the increase in KCC2 expression enables the developmental reduction in intracellular chloride. These data support the hypothesis that failure of the normal reversal of the purine network underlies the failed reversal of the GABA signaling network, and underlies the imbalance between excitatory-inhibitory circuits in ASD that was first described by Rubenstein and Merzenich 77 . The discovery of developmental arrest of the purine network creates a strong biological foundation for the increasing number of pre-clinical studies 34 , 36 , 78 , 79 , 80 and randomized clinical trials 81 , 82 that have shown safety and efficacy of low-dose suramin, a non-selective purinergic antagonist that treats the core symptoms and metabolic abnormalities in ASD. If confirmed in larger clinical trials, entirely new antipurinergic drugs that have a range of receptor subtype selectivities, and pannexin channel blockers that decrease the loss of eATP during chronic stress 27 , might create new options for treatment that have not existed before.
Metabolome correlation analysis led to the characterization of a new parameter, \(\dot{{{\boldsymbol{V}}}}\!\) net , to quantify the rate of metabolic network growth. We found that \(\dot{{{\boldsymbol{V}}}}\!\) net in pre-ASD newborns was low compared to age-matched controls. \(\dot{{{\boldsymbol{V}}}}\!\) net remained low in 5-year-olds with ASD. In contrast, typically developing newborns had a higher \(\dot{{{\boldsymbol{V}}}}\!\) net than pre-ASD infants. By 5-years of age, the \(\dot{{{\boldsymbol{V}}}}\!\) net increased by 173% in the TD group. Although the mathematical meaning of metabolic network growth rate \(\dot{{{\boldsymbol{V}}}}\!\) net is clear—fewer connections were created in ASD as each new sample was added to the network—the deeper biological meaning in other disorders and across other states of health and disease, and the broader implications for child development in ASD, will require further study. One hypothesis that fits the facts is that metabolic network hypoconnectivity in children with ASD is produced by persistence of local cell danger signaling that blocks or inhibits receptivity to long-distance coordinating signals from the brain, vagal autonomic, neuroendocrine, endocrine, and immune systems. Receptivity to long-distance, coordinating signals from the brain is needed to produce phase synchronization of chemical changes across organs and subsystems. The loss of long-distance phase synchronization will have the effect of decreasing the total number of edges and the rate at which new correlations are added to the network in ASD.
The cell danger response model provides a conceptual framework for understanding the genetics, epidemiology, neurobiology, and complex multisystem developmental biology of ASD. Acute activation of the CDR triggers a cascade of responses that starts with mitochondria and the cell, then expands to coordinate every system needed to respond to the triggering stress. Acute activation of the CDR is universal and required to heal from any injury 23 . The root regulator of the CDR is eATP signaling. Once an infection is cleared, or other triggering stress has passed, normal mitochondrial oxygen consumption is restored, reactive oxygen species (ROS) and reactive nitrogen species are reduced, intracellular redox is restored, eATP release is decreased, and the CDR becomes self-limited. However, when exposure to a stress or stressors is severe or chronic, excessive eATP release can persist. The associated drain on energy and metabolic resources places the eATP-releasing cell in peril. Only cells that can decrease their metabolic losses during chronic stress can survive. We hypothesize that the observed changes in purinergic receptor expression in the brains of children with ASD 35 and in animal models of ASD-like behavior 80 sensitize the cell to purinergic signaling. This adaptation permits cells to survive chronic stress by lowering the amount of eATP release needed to manage the stress. The increased bioenergetic efficiency conserves precious intracellular resources and protects the cell from death. However, the cost of sensitized purinergic signaling is a decreased threshold for activating of the CDR. When the threshold for the CDR is set too low, cells become hypersensitive and react inappropriately to non-injurious stimuli. Repeated or chronic activation of the CDR by eATP suppresses mitochondrial oxygen consumption 33 . The resulting excess in dissolved oxygen concentration within the cell causes mitochondrial and cellular redox changes that remodel the lipid composition of cellular membranes. Cellular cholesterol is biophysically concentrated in phospholipid membranes that are chronically exposed to high concentrations of dissolved oxygen. This enables mitochondrial and cell membranes to buffer excess intracellular oxygen by absorbing it from the aqueous environment of the cytosol and sequestering it in the cholesterol-enriched lipid environment of cellular and organellar phospholipid membranes 83 . The degree of cholesterol and ceramide accumulation in cell surface membranes and in MAMs, acts as a rheostat that regulates future eATP release and the production of ROS in response to stress during development 84 , 85 . Cholesterol and ceramide accumulation also leads to stiffened cell membranes, impaired mitochondrial fusion-fission dynamics, oxidative stress 86 , altered synaptogenesis 87 , 88 , and delayed and amplified responses to environmental stimuli in ASD 89 , 90 .
During neurotypical development, inhibitory correlations between purines and lipids develop that prevent excessive calcium activation and prevent overexcitation in response to environmental change. These self-calming connections in metabolism failed to develop in ASD. The natural consequence of the loss of these metabolic safeguards to overexcitation is for children with ASD to seek sameness to avoid the anxiety produced by change 91 , and to be more sensitive to environmental changes across many sensory domains. These include increased sensitivity to both exteroceptive and interoceptive stimuli. From an exteroceptive perspective, children with ASD have heightened sensitivity to subtle somatosensory changes to touch, new tastes, textures, colors, and new patterns in a changing environment 92 . From an interoceptive perspective, children with ASD have heightened sensitivity to adverse childhood experiences (ACEs) 93 , environmental pollutants 94 , triggers of innate immunity 95 , and to gastrointestinal 96 , microbiome 4 , and vagal autonomic 14 changes.
In the current study, xanthine was the purine that gained the most stimulatory (+r) correlations in 5-year-olds with ASD. Xanthine is one of the end-products of eATP metabolism 97 . Xanthine is known to trigger a cascade of events that leads to mitochondrial network fragmentation, reactive oxygen species and reactive nitrogen species (ROS and RNS), eicosanoid (e.g., leukotriene, HETE, and prostaglandin) signaling, immune activation, anxiety-associated behaviors, and consolidates long-term aversive memories that make the animal hypersensitive to future environmental changes that warn of environmental danger, cause fear, and trigger anxiety in mice, and is elevated in the blood of adults with anxiety disorders 98 . Anxiety is a common but under-recognized problem in autism 99 . Purine nucleobases like xanthine and its derivatives are metabokines and ancient CDR signaling molecules that are conserved across species and were called Schreckstoff —alarm substances—by the Nobel Prize winning ethologist Karl von Frisch 100 . Our results support the finding from other studies that sensory over-responsivity (SOR) and anxiety in ASD are related but separable biological phenomena 101 . Our results suggest: 1) that SOR is the result of chronic hypersensitivity of the eATP signaling network that leads to brittle calcium release in response to sensory stimuli, and 2) anxiety is the result of acute effects of the metabolic byproduct of eATP, xanthine, acting as an interoceptive mediator, producing ROS 102 , fragmenting mitochondria 98 , and acutely perturbing the metabolic network in ASD.
New methods of pre-symptomatic risk stratification for ASD during newborn screening and early well-baby checks create the opportunity to identify infants at increased risk even before the first behavioral symptoms occur. Several new methods might be combined to increase the accuracy of screening for the risk of developmental delays. For example, maternal blood metabolomics 103 , maternal chemokines and cytokines 104 , 105 , maternal autoantibodies 106 , newborn dried blood spot metabolomics, cytokine analysis 13 , infant hair analysis 107 , and others 108 , have each shown efficacy. And because the complex chemistry of mother’s milk for nursing infants in the first few months of life integrates a number of environmental stresses, physiologic factors, and biological needs of the child, metabolomic and exposomic analysis of milk has been shown to have about an 80% accuracy in identifying children at risk for neurodevelopmental delay, including children who later develop the ASD phenotype 109 . If these combined methods are successful, then early ASD diagnosis and intervention can be used not only to improve outcomes 110 , but also to document the decrease in the incidence of ASD made possible by multimodal infant screening and intervention programs.
This study has some limitations. In the newborn cohort, we were unable to recruit sufficient females with ASD to perform an analysis of the metabolic network in females at birth. The number of females with ASD and TD controls in the 5-year-old cohort was also small and insufficient for analysis. Cross validation of the partial least squares discriminant analysis results showed that the separation between ASD and TD groups was incomplete. The negative q 2 values showed that the global metabolome was not the only predictor of the developmental outcome. Random forest methods were used to select the most discriminating metabolites, minimize the risk of overfitting, and to aggregate metabolites into pathways to gain biological insight. Classical z-score analysis was used for metabolite quantitation. Z-score results are quantitative but relative and based on a comparison of mass spectral areas under the curve (AUCs) in case and control groups. The absolute concentrations of metabolites in micromoles per liter in the two cohorts and two sample types—dried blood spots and plasma—were not measured. Another limitation is that in contrast to concentration-based metabolic phenotypes, which can be used to identify individuals who later develop ASD, metabolic network parameters calculated from a group of samples measured a single time point, identify a group phenotype. The group correlation phenotype illuminates the shared biology but does not yet allow the identification of individuals at risk. Longitudinal data from samples collected over time from the same subject are needed to calculate the metabolic network parameters for an individual. This was a single-sample-per-patient, non-prospective study, so time-series data were not available. This was also a non-interventional study, so data in response to treatment were not available. Future prospective studies will allow the developmental transitions in metabolism and the metabolic network to be studied in real time. Interventional studies, with accompanying metabolomic, neurophysiologic, electrophysiologic, behavioral, and pharmacologic analyses will help to confirm the practical importance of purinergic signaling, and its normalization, in ASD. The proposed mechanistic studies have been hampered by the lack of FDA-approved, antipurinergic drugs available for human use.
By studying both newborns and 5-year-olds, the developmental patterns of metabolism and metabolic network formation were highlighted in this report. Classical concentration-based metabolomics and new metabolic correlation network analyses provided complementary insights into the biology of autism spectrum disorder. The classical methods showed that metabolites and pathways associated with decreased anti-inflammatory and antioxidant defenses, and increased bioenergetic stress response molecules were markers of the pre-ASD phenotype at birth and of ASD in 5-year-old children. The new correlation network methods showed that of the 50 biochemical pathways interrogated, the purine network was the most changed. In typically developing children, the purine network underwent a 17-fold reversal between birth and 5-years of age. This reversal did not occur in ASD. In typically developing children, negative correlations between purines and phosphatidylinositol (PI) lipids developed that were hypothesized to prevent or dampen spikes in intracellular calcium release in response to commonly encountered, non-injurious environmental stimuli. In addition, negative correlations between purines and ceramides developed in typically developing children that were hypothesized to prevent excessive apoptotic cell death in response to non-injurious environmental stimuli, and to support mitochondrial quality control through PINK1-dependent mitophagy. These metabolic safeguards to overexcitation did not develop in children with ASD. The failed reversal of purine correlation network was reminiscent of, and may underlie, the failed excitatory-to-inhibitory GABAergic signaling reversal and the excitatory-to-inhibitory imbalance model of ASD by Rubenstein and Merzenich 77 .
These findings support the hypothesis that the absence of the developmental reversal of the eATP signaling and purine networks in ASD leads to dysregulated calcium homeostasis and brittle regulation of the cell danger response. If these metabolic changes drive differences in child development, then further research and development of new antipurinergic medications and devices designed to normalize the hypersensitive cell danger response may one day help to improve developmental outcomes and create new options for treatment and services for children and adults with ASD.
Study design
Children in the newborn cohort were enrolled when they were between the ages of 3 and 10 years and their newborn and demographic data studied retrospectively. After determining if the child had ASD or was typically developing at the time of enrollment, we received permission from the parents or guardians to go back in time to analyze their dried blood spots that were collected at birth and archived as part of the California newborn screening program 111 . Both conventional metabolomics and new methods for metabolic network analysis were used. The cohorts were analyzed first by combining data that included samples from both males and females. Next, males were analyzed separately. Fewer females with ASD were enrolled (17 females in the newborn cohort, and 8 in the 5-year-old cohort). Detailed analysis of females with ASD, separate from males, was underpowered statistically, and was not performed. All network studies were performed using data from males only. Future studies will be needed to enroll a larger number of females with ASD for sex-specific metabolomic and network analysis.
Participant inclusion and exclusion criteria
Cohort 1 consisted of participants in a newborn screening study designed to test if a latent signature of the pre-ASD phenotype can be detected at birth. This study utilized dried blood spots collected at birth as part of the universal newborn screening program in California 111 . Secure responses to the study questionnaire were collected online using REDCap ( https://projectredcap.org/about/ ). Signed informed consent was obtained from the parents or guardians of all participants. Inclusion criteria were a DSM-IV diagnosis of autism spectrum disorder in children between the ages of 3 and 10 years, or typically developing controls 3-10 years old. Exclusion criteria were: 1) not born in California, 2) sibling already enrolled in the study, 3) non-term pregnancy duration of either <37 weeks or >42 weeks, 4) complicated labor and delivery requiring perinatal resuscitation or hospital stay over 7 days, 5) readmission to the hospital for any reason in the first month of life, 6) any chronic disease diagnosis in a typically developing control subject. Dried blood spot samples were collected at birth from 11/23/2007 to 1/29/2018. There were 68 males with ASD and 68 TD male controls (n = 136 males), 17 females with ASD, and 52 TD female controls (n = 69 females). The male to female ratio in the ASD group was 3.9 to 1. The total number of samples analyzed in this study was n = 205.
Cohort 2 consisted of a study of 5-year-old children with ASD or TD controls. The children were originally recruited as part of the CHARGE (CHildhood Autism Risks from Genetics and Environment) Study. The CHARGE Study is an ongoing population-based case-control study that evaluates a broad range of risk factors, including environmental chemicals, in relation to neurodevelopmental outcomes. Consenting families were enrolled in CHARGE, which is associated with the Center for Children’s Environmental Health (CCEH) and the MIND (Medical Investigation of Neurodevelopmental Disorders) Institute at the University of California at Davis as described previously 112 . The CHARGE Study protocol was approved by the institutional review board of the University of California, Davis, and the State of California Committee for the Protection of Human Subjects. Families provided written informed consent before participating. Inclusion criteria included children between 24 to 60 months old upon initial enrollment, born in California, living with at least one biological parent who spoke English or Spanish, and residing in one of the selected regional center catchment areas. No exclusions were made on the basis of genetics. An autism diagnosis was identified from records provided by the California Department of Developmental Services (DDS), which is an agency responsible for providing services to individuals with developmental disabilities via a network of regional centers throughout California. TD controls were randomly selected from the general population using birth records obtained from the California Department of Public Health Vital Statistics 112 . Children from the CHARGE study were enrolled prior to receiving their planned pre-kindergarten immunizations as part of a study of the immunologic features of ASD and TD controls. Samples were collected 8/25/2010 to 1/9/2013. In this study, the results of the baseline blood samples were analyzed. There were 23 males with ASD and 16 typically developing male controls ( n = 39 males). There were eight females with ASD and six typically developing female controls ( n = 14 females). The male to female ratio in this cohort was 2.9 to 1. The total number of samples analyzed in this study was n = 53. All ethical regulations relevant to human research participants were followed.
Dried blood spots were obtained from the California State Universal Newborn Screening and biobank program 111 and analyzed as previously described 113 with minor modifications. Briefly, Dried blood spots were received on Whatman 903 protein saver cards. A single 3.0 mm (~1/8th in.) punch was made using a Harris Uni-core device and transferred to a 1.7 ml Eppendorf tube. 36 µl of water and 4 µl of internal standard mix were added, mixed, and the samples stored overnight at 4 °C. Then 160 µl chilled methanol/acetonitrile (50:50) was added to bring the total volume to 200 µl and vortexed. Samples were incubated on ice for 15 min, then centrifuged at 16,000 g for 10 min. at 4 °C. The supernatant was transferred to a fresh vial and stored at −80 °C for future analysis. For the 5-year-old cohort, venous blood was collected in acid citrate dextrose solution A vacutainer tubes (BD# 364606), mixed by gentle inversion, then centrifuged at 2100 rpm (810 × g ) for 10 min at room temperature. Citrate-anticoagulated plasma was then removed from the top layer, aliquoted into cryotubes and stored at −80 °C until analysis. Targeted, broad-spectrum, metabolomic analysis of 690 metabolites was performed by high performance liquid chromatography using a Shimadzu LC-20AD UHPLC system coupled by electrospray ionization to a SCIEX Qtrap 5500 triple quadrupole mass spectrometer (LC-ESI-MS/MS) as described 114 with minor modifications to accommodate an expanded list of targeted chemicals. MS/MS detection was performed using scheduled multiple reaction monitoring (MRM) of precursor and product ions. Compound-specific source and fragmentation parameters for 2–6 MRMs for each targeted compound, along with MS/MS spectral data, and retention times by hydrophilic interaction liquid chromatography (HILIC) and reverse phase (RP) chromatography, were optimized using purified standards 114 . A total of 431 (dried blood spot study) or 467 (plasma study) of the 687 targeted metabolites were measurable in both males and females. These metabolites, their associated retention times, and MRM data are reported in Supplementary Data 19 . 100% of samples provided AUC data on these chemicals. There were no missing values, and no data were imputed. This targeted metabolomics platform interrogated 46 (newborn screening) and 53 (5-year-olds) different biochemical pathways and permitted analysis of many of the metabolites known to be core features of the CDR and integrated stress response (ISR) 21 , 115 , 116 .
Metabolic network and hub-and-spoke analysis
Metabolic network results were for the males only. Insufficient females were enrolled for correlation analysis. Metabolite AUCs were log-transformed and converted to z-scores. Pair-wise Pearson correlations between the z-scores were then calculated. The sign (+ or −) and strength (r value) of the correlation coefficient, q-values, false discovery rates (FDR), and p values were calculated for all the possible pair-wise metabolite correlations. To minimize overfitting, only correlations with q values < 0.05 were tallied. Correlations among the metabolites belonging to the same pathway were designated as in-pathway, or “in” for short. Correlations between metabolites belonging the different pathway were designated out-of-pathway, or “out” for short. About 5% of the correlations were in-pathway, and the other 95% were out-of-pathway. Metabolites were the nodes, and the pair-wise correlation was displayed as an edge in the network. Each metabolite was assigned to one of 50 biochemical pathways. The number of positive correlations, negative correlations, in- and out-of-pathway correlations, mean and median correlation strengths, and corresponding statistical parameters were calculated. CIRCOS 117 was used to visualize the global metabolic connectome. MetScape 3.0, a plug-in for Cytoscape 3.8.2 was used to visualize the metabolic hub and metabolite connections for the most dysregulated pathways 118 .
Network growth ( \(\dot{{{\boldsymbol{V}}}}\!_{{{\boldsymbol{net}}}}\) ) analysis
A ramped random resampling method was developed to study the properties of the metabolic network. This method permitted control for changes in statistical power produced by unequal case and control sample sizes. Pearson’s r , p , FDR, and q values were calculated for all possible pair-wise correlations between measured metabolites using the python package scipy v1.9.1. All correlations with q value ≤ 0.05 were considered significant and counted. A subset size starting at four samples, ramping to n −1 samples, was incremented by 2 (e.g., 4, 6, 8… to 21 for 22 controls and 4, 6, 8… to 30 for 31 cases). Subsamples from cases and controls were randomly selected using the random.sample function in python. Results obtained by ramped random resampling with and without replacement were compared and reported in the Supplementary Figs. 4 – 6 and Supplementary Data 15 – 18 . Fifty (50) random samples at each subsample size were taken to estimate the population statistics based on the central limit theorem. In some cohorts, fewer than 51 samples were available and the maximum subsample size was limited to n −1 samples as described above. Mean, median, standard deviation, standard error of the mean (SEM), and standard error coefficients of variation defined as the SEM/mean for the significant correlations were calculated at each subsample size across all iterations. The number of significant metabolite pairs (edges) was plotted on the y-axis as a function of the subsample size on the x-axis. Least mean squares linear regression was used to identify the best fit equation for the line, y = mx + b , and to test the differences between the slopes for cases and controls. The slope ( m ) of the metabolic network growth curve was used as a new parameter, \(\dot{{{\boldsymbol{V}}}}\!\) net . The computer code for executing this analysis is available on the GitHub site for this paper. See Supplementary Methods for additional details.
Hypercorrelator analysis
The top 15 ASD hypercorrelators were identified by ranking all 467 measured metabolites by the total difference in out-of-pathway correlations (edges) meeting a q value of <0.05 and calculating the difference of ASD-TD. The top 15 had the most positive (+) differences between ASD and TD. Reciprocally, the top 15 TD hypercorrelators had the most negative (−) differences between ASD and TD. This ranking is reported in Supplementary Data 13 and 14 .
Statistics and reproducibility
Demographic data were analyzed by t-tests or non-parametric Mann-Whitney U tests in GraphPad Prism ( https://www.graphpad.com ). Categorical data and 2 × 2 tables were analyzed by Fisher’s exact test. AUC data from metabolomics were log 2 transformed, scaled by control standard deviations, and the resulting z scores were analyzed by VIP scores calculated by multivariate PLS-DA in MetaboAnalyst 5.0 119 . Cross-validation was used to calculate accuracy, r 2 , and q 2 statistics 120 . Permutation analysis (×1000) was used to calculate the p value for the model. Mean decrease in accuracy (MDA) scores were calculated by random forest analysis from 5000 trees in R 121 . FDRs were calculated by the method of Benjamini and Hochberg 122 and Bayesian false discovery rates by Storey q value 123 . Metabolites with VIP ≥ 1.5 and MDA > 0 were considered significant and were used for pathway enrichment analysis 121 . Bubble impact plots were visualized in Python. Significance was quantified as the hypergeometric p value and plotted on the y-axis. The k-nearest neighbor algorithm 124 , a non-parametric classification method used to cluster metabolites and pathways that behave in similar ways, was used to identify the metabolite clusters. Classifiers of 4–7 metabolites were selected and tested for diagnostic accuracy using area under the receiver operator characteristic curve and random forest analysis. Five hundred bootstrappings were used to calculate the 95% confidence intervals of the ROC curve. The 2 × 2 confusion matrix generated in MetaboAnalyst was used to calculate the sensitivity, specificity, and the accuracy of the diagnostic classifier. Diagnostic accuracy was calculated using the 1000 permutations 120 . Classifiers were validated within sample using repeated double cross-validation with bootstrapping 100 times to test random subsamples of 2/3 in and 1/3 out, and by permutation analysis 125 . See Supplementary Information for additional methods.
Reporting summary
Further information on research design is available in the Nature Portfolio Reporting Summary linked to this article.
A special note from the authors
Although the words “normal” and “abnormal”, “regulated” and “dysregulated”, and “functional” and “dysfunctional” are used in this paper, a major result of this research was the finding that the ASD phenotype is the result of developmental changes caused by a normal physiologic response to the signals that cells are receiving in autistic children. The cells of neurotypical children do not receive the same cell danger signals, and therefore do not show the same set of mitochondrial, metabolic, immunologic, microbiome, autonomic, neuroendocrine, neurodevelopmental, and behavioral changes. We are sensitive to the negative impact that poorly chosen words can have 126 , 127 , 128 , 129 and have endeavored to use neutral, specific, and descriptive language whenever possible.
Study approvals
This study was approved by the University of California, San Diego (#140072, #171940, #190972), California state (#1162, #2018-020), and University of California, Davis (#226004-4) institutional review boards (IRBs). Informed signed consent was obtained prior to participation.
Data availability
Metabolomics data are available in Supplementary Data 1 and 2 . All numerical source data for the figures are available as a single Excel file containing tabs labeled Supplementary Data 1 – 19 .
Code availability
Software for metabolic network growth ( \(\dot{{{\boldsymbol{V}}}}\!_{{net}}\) ) analysis is publicly available at: https://github.com/BDNav/metabotools
Maenner, M. J. et al. Prevalence and characteristics of autism spectrum disorder among children aged 8 years - autism and developmental disabilities monitoring network, 11 sites, United States, 2020. MMWR Surveill. Summ. 72 , 1–14 (2023).
Article PubMed PubMed Central Google Scholar
Pickles, A., McCauley, J. B., Pepa, L. A., Huerta, M. & Lord, C. The adult outcome of children referred for autism: typology and prediction from childhood. J. Child Psychol. Psychiatry, Allied Discip. 61 , 760–767 (2020).
Article Google Scholar
Wang L., et al. Altered metabolic characteristics in plasma of young boys with autism spectrum disorder. J. Autism Dev. Disord. 52 , 4897–4907 (2022).
Needham, B. D. et al. Plasma and fecal metabolite profiles in autism spectrum disorder. Biol. Psychiatry 89 , 451–462 (2021).
Article CAS PubMed Google Scholar
Gevi, F., Zolla, L., Gabriele, S. & Persico, A. M. Urinary metabolomics of young Italian autistic children supports abnormal tryptophan and purine metabolism. Mol. Autism 7 , 47 (2016).
Smith, A. M. et al. A metabolomics approach to screening for autism risk in the children’s autism metabolome project. Autism Res. 13 , 1270–1285 (2020).
Likhitweerawong, N. et al. Profiles of urine and blood metabolomics in autism spectrum disorders. Metab. Brain Dis. 36 , 1641–1671 (2021).
Brister, D. et al. Metabolomic signatures of autism spectrum disorder. J. Pers. Med 12 , 1727 (2022).
Courraud, J., Ernst, M., Svane Laursen, S., Hougaard, D. M. & Cohen, A. S. Studying autism using untargeted metabolomics in newborn screening samples. J. Mol. Neurosci. 71 , 1378–1393 (2021).
Article CAS PubMed PubMed Central Google Scholar
Hoxha, E. et al. Maturation, refinement, and serotonergic modulation of cerebellar cortical circuits in normal development and in murine models of autism. Neural Plast. 2017 , 6595740 (2017).
Anderson, G. M. et al. Whole blood serotonin in autistic and normal subjects. J. Child Psychol. Psychiatry Allied Discip. 28 , 885–900 (1987).
Article CAS Google Scholar
Eagleson, K. L., Xie, Z. & Levitt, P. The pleiotropic MET receptor network: circuit development and the neural-medical interface of autism. Biol. Psychiatry 81 , 424–433 (2017).
Kim, D. H., Krakowiak, P., Meltzer, A., Hertz-Picciotto, I. & Van de Water, J. Neonatal chemokine markers predict subsequent diagnosis of autism spectrum disorder and delayed development. Brain Behav. Immun. 100 , 121–133 (2022).
Article PubMed Google Scholar
Patriquin, M. A., Hartwig, E. M., Friedman, B. H., Porges, S. W. & Scarpa, A. Autonomic response in autism spectrum disorder: relationship to social and cognitive functioning. Biol. Psychol. 145 , 185–197 (2019).
Raznahan, A. et al. Compared to what? Early brain overgrowth in autism and the perils of population norms. Biol. Psychiatry 74 , 563–575 (2013).
Trost, B. et al. Genomic architecture of autism from comprehensive whole-genome sequence annotation. Cell 185 , 4409–4427.e4418 (2022).
Zerbo, O. et al. Is maternal influenza or fever during pregnancy associated with autism or developmental delays? Results from the CHARGE (CHildhood Autism Risks from Genetics and Environment) Study. J. Autism Dev. Disord. 43 , 25–33 (2013).
Hertz-Picciotto, I., Schmidt, R. J. & Krakowiak, P. Understanding environmental contributions to autism: causal concepts and the state of science. Autism Res. 11 , 554–586 (2018).
Cirnigliaro, M. et al. The contributions of rare inherited and polygenic risk to ASD in multiplex families. Proc. Natl Acad. Sci. USA 120 , e2215632120 (2023).
Naviaux, R. K. Perspective: Cell danger response biology-The new science that connects environmental health with mitochondria and the rising tide of chronic illness. Mitochondrion 51 , 40–45 (2020).
Naviaux, R. K. Metabolic features of the cell danger response. Mitochondrion 16 , 7–17 (2014).
Naviaux, R. K. Metabolic features and regulation of the healing cycle—a new model for chronic disease pathogenesis and treatment. Mitochondrion 46 , 278–297 (2019).
Naviaux, R. K. Mitochondrial and metabolic features of salugenesis and the healing cycle. Mitochondrion 70 , 131–163 (2023).
Cameron, J. L., Eagleson, K. L., Fox, N. A., Hensch, T. K. & Levitt, P. Social origins of developmental risk for mental and physical illness. J. Neurosci. 37 , 10783–10791 (2017).
Panisi, C. et al. Autism spectrum disorder from the womb to adulthood: suggestions for a paradigm shift. J. Pers. Med. 11 , 70 (2021).
Burnstock, G. An introduction to the roles of purinergic signalling in neurodegeneration, neuroprotection and neuroregeneration. Neuropharmacology 104 , 4–17 (2016).
Seo, J. H., Dalal, M. S. & Contreras, J. E. Pannexin-1 channels as mediators of neuroinflammation. Int. J. Mol. Sci. 22 , 5189 (2021).
Linden, J., Koch-Nolte, F. & Dahl, G. Purine release, metabolism, and signaling in the inflammatory response. Annu. Rev. Immunol. 37 , 325–347 (2019).
Judge, A. & Dodd, M. S. Metabolism. Essays Biochem. 64 , 607–647 (2020).
Pinna, S. et al. A prebiotic basis for ATP as the universal energy currency. PLoS Biol. 20 , e3001437 (2022).
Burnstock, G. Introduction to purinergic signaling. Methods Mol. Biol. 2041 , 1–15 (2020).
Fumagalli, M., Lecca, D., Abbracchio, M. P. & Ceruti, S. Pathophysiological role of purines and pyrimidines in neurodevelopment: unveiling new pharmacological approaches to congenital brain diseases. Front. Pharm. 8 , 941 (2017).
Zolkipli-Cunningham, Z. et al. Metabolic and behavioral features of acute hyperpurinergia and the maternal immune activation mouse model of autism spectrum disorder. PloS One 16 , e0248771 (2021).
Horvath, G. et al. P2X7 receptors drive poly(I:C) induced autism-like behavior in mice. J. Neurosci. 39 , 2542–2561 (2019).
CAS PubMed PubMed Central Google Scholar
Ginsberg, M. R., Rubin, R. A., Falcone, T., Ting, A. H. & Natowicz, M. R. Brain transcriptional and epigenetic associations with autism. PloS One 7 , e44736 (2012).
Hirsch, M. M. et al. Effects of single-dose antipurinergic therapy on behavioral and molecular alterations in the valproic acid-induced animal model of autism. Neuropharmacology 167 , 107930 (2020).
Babiec, L. et al. Alterations of purinergic receptors levels and their involvement in the glial cell morphology in a pre-clinical model of autism spectrum disorders. Brain Sci. 13 , 1088 (2023).
Dai, S. et al. Purine signaling pathway dysfunction in autism spectrum disorders: Evidence from multiple omics data. Front. Mol. Neurosci. 16 , 1089871 (2023).
Schulman, E. S., Nishi, H. & Pelleg, A. Degranulation of human mast cells: modulation by P2 receptors’ agonists. Front. Immunol. 14 , 1216580 (2023).
Calovi, S., Mut-Arbona, P. & Sperlagh, B. Microglia and the purinergic signaling system. Neuroscience 405 , 137–147 (2019).
Fabbretti, E. ATP P2X3 receptors and neuronal sensitization. Front. Cell. Neurosci. 7 , 236 (2013).
Gourine, A. V., Wood, J. D. & Burnstock, G. Purinergic signalling in autonomic control. Trends Neurosci. 32 , 241–248 (2009).
Lalo, U. & Pankratov, Y. ATP-mediated signalling in the central synapses. Neuropharmacology 229 , 109477 (2023).
Garcia-Gutierrez, E., Narbad, A. & Rodriguez, J. M. Autism spectrum disorder associated with gut microbiota at immune, metabolomic, and neuroactive level. Front Neurosci. 14 , 578666 (2020).
Xiong, Y., Chen, J. & Li, Y. Microglia and astrocytes underlie neuroinflammation and synaptic susceptibility in autism spectrum disorder. Front Neurosci. 17 , 1125428 (2023).
Wu, Z. et al. Mitochondrial DNA stress signalling protects the nuclear genome. Nat. Metab. 1 , 1209–1218 (2019).
Picard, M. & Shirihai, O. S. Mitochondrial signal transduction. Cell Metab. 34 , 1620–1653 (2022).
Naviaux, R. K. Incomplete healing as a cause of aging: the role of mitochondria and the cell danger response. Biology 8 , 27 (2019).
Matilainen, O., Quiros, P. M. & Auwerx, J. Mitochondria and epigenetics—crosstalk in homeostasis and stress. Trends Cell Biol. 27 , 453–463 (2017).
Hunt, R. J. & Bateman, J. M. Mitochondrial retrograde signaling in the nervous system. FEBS Lett. 592 , 663–678 (2018).
Rose, S. et al. Mitochondrial and redox abnormalities in autism lymphoblastoid cells: a sibling control study. FASEB J. 31 , 904–909 (2017).
Barnes-Velez, J. A., Aksoy Yasar, F. B. & Hu, J. Myelin lipid metabolism and its role in myelination and myelin maintenance. Innovation 4 , 100360 (2023).
CAS PubMed Google Scholar
Naviaux, R. K. et al. Mitochondrial DNA polymerase gamma deficiency and mtDNA depletion in a child with Alpers’ syndrome. Ann. Neurol. 45 , 54–58 (1999).
Lee, W. T. & St John, J. The control of mitochondrial DNA replication during development and tumorigenesis. Ann. N.Y. Acad. Sci. 1350 , 95–106 (2015).
Aggarwal, S., Yurlova, L. & Simons, M. Central nervous system myelin: structure, synthesis and assembly. Trends Cell Biol. 21 , 585–593 (2011).
Kinney, H. C., Karthigasan, J., Borenshteyn, N. I., Flax, J. D. & Kirschner, D. A. Myelination in the developing human brain: biochemical correlates. Neurochem. Res. 19 , 983–996 (1994).
Gil, M. & Gama, V. Emerging mitochondrial-mediated mechanisms involved in oligodendrocyte development. J. Neurosci. Res. 101 , 354–366 (2023).
Braun, J. M. et al. Gestational exposure to endocrine-disrupting chemicals and reciprocal social, repetitive, and stereotypic behaviors in 4- and 5-year-old children: the HOME study. Environ. Health Perspect. 122 , 513–520 (2014).
Bolte, S., Girdler, S. & Marschik, P. B. The contribution of environmental exposure to the etiology of autism spectrum disorder. Cell Mol. Life Sci. 76 , 1275–1297 (2019).
Hui, K. K., Chater, T. E., Goda, Y. & Tanaka, M. How staying negative is good for the (adult) brain: maintaining chloride homeostasis and the GABA-shift in neurological disorders. Front. Mol. Neurosci. 15 , 893111 (2022).
Krall, A. S. et al. Asparagine couples mitochondrial respiration to ATF4 activity and tumor growth. Cell Metab. 33 , 1013–1026.e1016 (2021).
Emerling, B. M. et al. Mitochondrial reactive oxygen species activation of p38 mitogen-activated protein kinase is required for hypoxia signaling. Mol. Cell. Biol. 25 , 4853–4862 (2005).
El-Ansary, A. & Al-Ayadhi, L. Lipid mediators in plasma of autism spectrum disorders. Lipids Health Dis. 11 , 160 (2012).
Nguyen, R. L., Medvedeva, Y. V., Ayyagari, T. E., Schmunk, G. & Gargus, J. J. Intracellular calcium dysregulation in autism spectrum disorder: an analysis of converging organelle signaling pathways. Biochim. Biophys. Acta Mol. Cell Res. 1865 , 1718–1732 (2018).
Smith, M. et al. Mitochondrial and ion channel gene alterations in autism. Biochim. Biophys. Acta 1817 , 1796–1802 (2012).
Gao, X. et al. PI3K/Akt signaling requires spatial compartmentalization in plasma membrane microdomains. Proc. Natl Acad. Sci. USA 108 , 14509–14514 (2011).
Schmunk, G. et al. High-throughput screen detects calcium signaling dysfunction in typical sporadic autism spectrum disorder. Sci. Rep. 7 , 40740 (2017).
Onore, C., Yang, H., Van de Water, J. & Ashwood, P. Dynamic Akt/mTOR signaling in children with autism spectrum disorder. Front. Pediatr. 5 , 43 (2017).
Wang, X. et al. CERS6-derived ceramides aggravate kidney fibrosis by inhibiting PINK1-mediated mitophagy in diabetic kidney disease. Am. J. Physiol. Cell Physiol. 325 , C538–C549 (2023).
Kilb, W. Development of the GABAergic system from birth to adolescence. Neuroscientist Neurol. Psychiatry 18 , 613–630 (2012).
Google Scholar
Ghit, A., Assal, D., Al-Shami, A. S. & Hussein, D. E. E. GABA(A) receptors: structure, function, pharmacology, and related disorders. J. Genet. Eng. Biotechnol. 19 , 123 (2021).
Godoy, J. A., Valdivieso, A. G. & Inestrosa, N. C. Nicotine modulates mitochondrial dynamics in hippocampal neurons. Mol. Neurobiol. 55 , 8965–8977 (2018).
Liu, Z., Neff, R. A. & Berg, D. K. Sequential interplay of nicotinic and GABAergic signaling guides neuronal development. Science 314 , 1610–1613 (2006).
Kahle, K. T. & Staley, K. J. The bumetanide-sensitive Na-K-2Cl cotransporter NKCC1 as a potential target of a novel mechanism-based treatment strategy for neonatal seizures. Neurosurg. Focus 25 , E22 (2008).
Lozovaya, N., Eftekhari, S. & Hammond, C. The early excitatory action of striatal cholinergic-GABAergic microcircuits conditions the subsequent GABA inhibitory shift. Commun. Biol. 6 , 723 (2023).
Liu, R., Wang, J., Liang, S., Zhang, G. & Yang, X. Role of NKCC1 and KCC2 in epilepsy: from expression to function. Front. Neurol. 10 , 1407 (2019).
Rubenstein, J. L. & Merzenich, M. M. Model of autism: increased ratio of excitation/inhibition in key neural systems. Genes Brain Behav. 2 , 255–267 (2003).
Naviaux, J. C. et al. Antipurinergic therapy corrects the autism-like features in the Fragile X (Fmr1 knockout) mouse model. Mol. Autism 6 , 1 (2015).
Naviaux, J. C. et al. Reversal of autism-like behaviors and metabolism in adult mice with single-dose antipurinergic therapy. Transl. Psychiatry 4 , e400 (2014).
Naviaux, R. K. et al. Antipurinergic therapy corrects the autism-like features in the poly(IC) mouse model. PloS One 8 , e57380 (2013).
Naviaux, R. K. et al. Low-dose suramin in autism spectrum disorder: a small, phase I/II, randomized clinical trial. Ann. Clin. Transl. Neurol. 4 , 491–505 (2017).
Hough, D. et al. Randomized clinical trial of low dose suramin intravenous infusions for treatment of autism spectrum disorder. Ann. Gen. Psychiatry 22 , 45 (2023).
Al-Samir, S. et al. O(2) permeability of lipid bilayers is low, but increases with membrane cholesterol. Cell Mol. Life Sci. 78 , 7649–7662 (2021).
Roy A., Patra S. K. Lipid raft facilitated receptor organization and signaling: a functional rheostat in embryonic development, stem cell biology and cancer. Stem Cell Rev. Rep . 19 , 2–25 (2023).
Fujimoto, M., Hayashi, T. & Su, T. P. The role of cholesterol in the association of endoplasmic reticulum membranes with mitochondria. Biochem. Biophys. Res. Commun. 417 , 635–639 (2012).
Goicoechea, L., Conde de la Rosa, L., Torres, S., Garcia-Ruiz, C. & Fernandez-Checa, J. C. Mitochondrial cholesterol: Metabolism and impact on redox biology and disease. Redox Biol. 61 , 102643 (2023).
Lauwers, E., Goodchild, R. & Verstreken, P. Membrane lipids in presynaptic function and disease. Neuron 90 , 11–25 (2016).
Tulodziecka, K. et al. Remodeling of the postsynaptic plasma membrane during neural development. Mol. Biol. Cell 27 , 3480–3489 (2016).
Matsuzaki, J. et al. Delayed auditory evoked responses in autism spectrum disorder across the life span. Dev. Neurosci. 41 , 223–233 (2019).
Ebishima, K. et al. Relationship of the acoustic startle response and its modulation to adaptive and maladaptive behaviors in typically developing children and those with autism spectrum disorders: a pilot study. Front. Hum. Neurosci. 13 , 5 (2019).
Baribeau, D. A. et al. Co-occurring trajectories of anxiety and insistence on sameness behaviour in autism spectrum disorder. Br. J. Psychiatry J. Ment. Sci. 218 , 20–27 (2021).
Baron-Cohen S. The Pattern Seekers: How Autism Drives Human Invention (Basic Books, 2020).
Hartley, G., Sirois, F., Purrington, J. & Rabey, Y. Adverse childhood experiences and autism: a meta-analysis. Trauma Violence Abus. 1 , 15248380231213314 (2023).
Song, A. Y. et al. Associations of prenatal exposure to a mixture of persistent organic pollutants with social traits and cognitive and adaptive function in early childhood: findings from the EARLI study. Environ. Res. 229 , 115978 (2023).
Hughes, H. K., Moreno, R. J. & Ashwood, P. Innate immune dysfunction and neuroinflammation in autism spectrum disorder (ASD). Brain Behav. Immun. 108 , 245–254 (2023).
Gorrindo, P. et al. Gastrointestinal dysfunction in autism: parental report, clinical evaluation, and associated factors. Autism Res. 5 , 101–108 (2012).
Moriwaki, Y., Yamamoto, T. & Higashino, K. Enzymes involved in purine metabolism—a review of histochemical localization and functional implications. Histol. Histopathol. 14 , 1321–1340 (1999).
Fan, K. Q. et al. Stress-induced metabolic disorder in peripheral CD4(+) T cells leads to anxiety-like behavior. Cell 179 , 864–879.e819 (2019).
Winder-Patel, B. et al. Often undiagnosed but treatable: case vignettes and clinical considerations for assessing anxiety disorders in youth with autism spectrum disorder and intellectual disability. Evid. Based Pract. Child Adolesc. Ment. Health 7 , 24–40 (2022).
Frisch Kv Über einen Schreckstoff der Fischhaut und seine biologische Bedeutung. Z. Vgl. Physiol. 29 , 46–145 (1942).
Cummings K. K., et al. Shared and distinct biological mechanisms for anxiety and sensory over-responsivity in youth with autism versus anxiety disorders. J. Neurosci. Res . 102 , e25250 (2024).
Furuhashi, M. New insights into purine metabolism in metabolic diseases: role of xanthine oxidoreductase activity. Am. J. Physiol. Endocrinol. Metab. 319 , E827–E834 (2020).
Hollowood-Jones, K. et al. Altered metabolism of mothers of young children with autism spectrum disorder: a case control study. BMC Pediatr. 20 , 557 (2020).
Jones, K. L. et al. Autism with intellectual disability is associated with increased levels of maternal cytokines and chemokines during gestation. Mol. Psychiatry 22 , 273–279 (2017).
Heuer, L. S. et al. An exploratory examination of neonatal cytokines and chemokines as predictors of autism risk: the early markers for autism study. Biol. Psychiatry 86 , 255–264 (2019).
McLellan, J., Kim, D. H. J., Bruce, M., Ramirez-Celis, A. & Van de Water, J. Maternal immune dysregulation and autism-understanding the role of cytokines, chemokines and autoantibodies. Front Psychiatry 13 , 834910 (2022).
Austin, C. et al. Elemental dynamics in hair accurately predict future autism spectrum disorder diagnosis: an international multi-center study. J. Clin. Med. 11 , 7154 (2022).
Lyall, K. et al. A profile and review of findings from the Early Markers for Autism study: unique contributions from a population-based case-control study in California. Mol. Autism 12 , 24 (2021).
Li K., et al. Metabolomic and exposomic biomarkers of risk of future neurodevelopmental delay in human milk. Pediatric Res. 93 , 1710–1720 (2023).
Pierce, K. et al. Get SET early to identify and treatment refer autism spectrum disorder at 1 year and discover factors that influence early diagnosis. J. Pediatr. 236 , 179–188 (2021).
California Department of Public Health. The California newborn screening program. (California Department of Public Health, 2022).
Hertz-Picciotto, I. et al. The CHARGE study: an epidemiologic investigation of genetic and environmental factors contributing to autism. Environ. Health Perspect. 114 , 1119–1125 (2006).
Li K., Naviaux J. C., Monk J. M., Wang L., Naviaux R. K. Improved dried blood spot-based metabolomics: a targeted, broad-spectrum, single-injection method. Metabolites 10 , 82–96 (2020).
Li, K., Naviaux, J. C., Bright, A. T., Wang, L. & Naviaux, R. K. A robust, single-injection method for targeted, broad-spectrum plasma metabolomics. Metabolomics 13 , 122 (2017).
Buzkova, J. et al. Metabolomes of mitochondrial diseases and inclusion body myositis patients: treatment targets and biomarkers. EMBO Mol. Med. 10 , e9091 (2018).
Pakos-Zebrucka, K. et al. The integrated stress response. EMBO Rep. 17 , 1374–1395 (2016).
Krzywinski, M. et al. Circos: an information aesthetic for comparative genomics. Genome Res. 19 , 1639–1645 (2009).
Basu, S. et al. Sparse network modeling and metscape-based visualization methods for the analysis of large-scale metabolomics data. Bioinformatics 33 , 1545–1553 (2017).
Pang, Z. et al. Using MetaboAnalyst 5.0 for LC-HRMS spectra processing, multi-omics integration and covariate adjustment of global metabolomics data. Nat. Protoc. 17 , 1735–1761 (2022).
Szymanska, E., Saccenti, E., Smilde, A. K. & Westerhuis, J. A. Double-check: validation of diagnostic statistics for PLS-DA models in metabolomics studies. Metabolomics 8 , 3–16 (2012).
Breiman, L. Random forests. Mach. Learn. 45 , 5–32 (2001).
Benjamini, Y. & Hochberg, Y. Controlling the false discovery rate—a practical and powerful approach to multiple testing. J. R. Stat. Soc. Ser. B Methodol. 57 , 289–300 (1995).
Storey, J. D. The positive false discovery rate: a Bayesian interpretation and the q-value. Ann. Stat. 31 , 2013–2035 (2003).
Abu Alfeilat, H. A. et al. Effects of distance measure choice on K-nearest neighbor classifier performance: a review. Big Data 7 , 221–248 (2019).
Filzmoser, P., Liebmann, B. & Varmuza, K. Repeated double cross validation. J. Chemom. 23 , 160–171 (2009).
Keating, C. T. et al. Autism-related language preferences of English-speaking individuals across the globe: a mixed methods investigation. Autism Res. 16 , 406–428 (2023).
Zamzow, R. Autism researchers face off over language. Science 379 , 523–524 (2023).
Botha, M. & Cage, E. “Autism research is in crisis”: a mixed method study of researcher’s constructions of autistic people and autism research. Front. Psychol. 13 , 1050897 (2022).
Bottema-Beutel, K., Kapp, S. K., Lester, J. N., Sasson, N. J. & Hand, B. N. Avoiding Ableist language: suggestions for autism researchers. Autism Adulth. 3 , 18–29 (2021).
Download references
Acknowledgements
R.K.N. and J.V. thank the participants and their families who donated their time and effort in helping to make this study possible. R.K.N. thanks Rachel Riggs for being the community liaison for the newborn screening project, and Cassio Lynm/Amino Creative, LLC, for help in creating the medical illustrations and for harmonizing the figure style across all elements of this study. This work was funded in part by a grant from Autism Speaks (grant number 7274) to J.V.W. and by philanthropic gifts to the Naviaux Lab from the UCSD Christini Fund, the Lennox Foundation, the William Wright Family Foundation, Malone Family Foundation, the Brain Foundation, the Westreich Foundation, the Aloe family, the Harb family, Marc Spilo and all the others who contributed to the Aloe family autism research fund, the N of One Autism Research Foundation, the UCSD Mitochondrial Disease Research Fund, the JMS Fund, Linda Clark, Jeanne Conrad, David Cannistraro, the Kirby and Katie Mano Family, Simon and Evelyn Foo, Wing-kun Tam, Gita and Anurag Gupta, the Brent Kaufman Family, and the Daniel and Kelly White Family, and grassroots support from over 2000 individuals from around the world who have each provided gifts in the past year to support Naviaux Lab research. The REDCap software system used in this study was provided by the UCSD Clinical and Translational Research Center and supported by Award Number UL1TR001442 from the National Center for Research Resources. Financial supporters for this study had no role in study design, data collection, analysis, interpretation, writing, or publication of this work.
Author information
Present address: Macao Polytechnic University, Macau, China
Authors and Affiliations
The Mitochondrial and Metabolic Disease Center, University of California, San Diego School of Medicine, San Diego, CA, 92103-8467, USA
Sai Sachin Lingampelly, Jane C. Naviaux, Jonathan M. Monk, Kefeng Li, Lin Wang & Robert K. Naviaux
Department of Medicine, University of California, San Diego School of Medicine, San Diego, CA, 92103-8467, USA
Sai Sachin Lingampelly, Jonathan M. Monk, Kefeng Li, Lin Wang & Robert K. Naviaux
Department of Neuroscience, University of California, San Diego School of Medicine, San Diego, CA, 92103-8467, USA
Jane C. Naviaux
The UC Davis MIND Institute, University of California, Davis, Davis, CA, 95616, USA
Luke S. Heuer, Lori Haapanen, Chelsea A. Kelland & Judy Van de Water
Department of Rheumatology and Allergy, School of Veterinary Medicine, University of California, Davis, Davis, CA, 95616, USA
Judy Van de Water
Department of Pediatrics, University of California, San Diego School of Medicine, San Diego, CA, 92103-8467, USA
Robert K. Naviaux
Department of Pathology, University of California, San Diego School of Medicine, San Diego, CA, 92103-8467, USA
You can also search for this author in PubMed Google Scholar
Contributions
R.K.N. designed the newborn screening study and received UC San Diego IRB approval for both the newborn and 5-year-old child studies. R.K.N. and J.V. designed and directed the 5-year-old child metabolomics study. J.V. received UC Davis IRB approval for the 5-year-old child study. S.S.L., J.C.N. and K.L. did the metabolomics. J.M.M. and R.K.N. developed the ramped random resampling for \(\dot{{{\boldsymbol{V}}}}\!\) net analysis and other correlation network tools used to analyze and visualize the metabolomic data. L.W. coordinated the California IRB application and renewal for the newborn study. L.W. and J.C.N. developed the REDCap questionnaires and coordinated the enrollment for the newborn screening study. C.A.K. assisted with the demographic analyses and edited the manuscript. J.V., L.He and L.Ha recruited the participants in the 5-year-old study and processed the blood samples. S.S.L., R.K.N. and J.C.N. wrote the paper. All authors read and approved the final manuscript.
Corresponding author
Correspondence to Robert K. Naviaux .
Ethics declarations
Competing interests.
The authors declare the following competing interests: R.K.N. is a scientific advisory board member for The Autism Community in Action (TACA), the Open Medicine Foundation (OMF), Pannex Therapeutics, Yuva Biosciences, Kuzani, and Paxmedica. J.V. is a founder and advisor for MaraBio Systems, Inc. All other authors declare no competing interests.
Peer review
Peer review information.
Communications Biology thanks Douglas Wallace and the other, anonymous, reviewer(s) for their contribution to the peer review of this work. Primary Handling Editor: Joao Valente.
Additional information
Publisher’s note Springer Nature remains neutral with regard to jurisdictional claims in published maps and institutional affiliations.
Supplementary information
Supplementary information, description of additional supplementary files, supplementary data 1-19, reporting summary, rights and permissions.
Open Access This article is licensed under a Creative Commons Attribution 4.0 International License, which permits use, sharing, adaptation, distribution and reproduction in any medium or format, as long as you give appropriate credit to the original author(s) and the source, provide a link to the Creative Commons licence, and indicate if changes were made. The images or other third party material in this article are included in the article’s Creative Commons licence, unless indicated otherwise in a credit line to the material. If material is not included in the article’s Creative Commons licence and your intended use is not permitted by statutory regulation or exceeds the permitted use, you will need to obtain permission directly from the copyright holder. To view a copy of this licence, visit http://creativecommons.org/licenses/by/4.0/ .
Reprints and permissions
About this article
Cite this article.
Lingampelly, S.S., Naviaux, J.C., Heuer, L.S. et al. Metabolic network analysis of pre-ASD newborns and 5-year-old children with autism spectrum disorder. Commun Biol 7 , 536 (2024). https://doi.org/10.1038/s42003-024-06102-y
Download citation
Received : 19 October 2023
Accepted : 22 March 2024
Published : 10 May 2024
DOI : https://doi.org/10.1038/s42003-024-06102-y
Share this article
Anyone you share the following link with will be able to read this content:
Sorry, a shareable link is not currently available for this article.
Provided by the Springer Nature SharedIt content-sharing initiative
By submitting a comment you agree to abide by our Terms and Community Guidelines . If you find something abusive or that does not comply with our terms or guidelines please flag it as inappropriate.
Quick links
- Explore articles by subject
- Guide to authors
- Editorial policies
Sign up for the Nature Briefing: Translational Research newsletter — top stories in biotechnology, drug discovery and pharma.


Analytical Methods
A one-pot crispr-rca strategy for ultrasensitive and specific detection of circrna †.

* Corresponding authors
a Children's Hospital, Zhejiang University School of Medicine, National Clinical Research Center for Child Health, Hangzhou, China E-mail: [email protected]
b School of Basic Medical Sciences & Forensic Medicine, Hangzhou Medical College, Hangzhou 310000, China
c School of Materials Science and Engineering, University of Jinan, Jinan 250022, China
d Department of Obstetrics, Shanghai First Maternity and Infant Hospital, Obstetrics and Gynecology Hospital, School of Medicine, Tongji University, Shanghai 201204, China
Accurate and precise detection of circular RNA (circRNA) is imperative for its clinical use. However, the inherent challenges in circRNA detection, arising from its low abundance and potential interference from linear isomers, necessitate innovative solutions. In this study, we introduce, for the first time, the application of the CRISPR/Cas12a system to establish a one-pot, rapid (30 minutes to 2 hours), specific and ultrasensitive circRNA detection strategy, termed RETA-CRISPR (reverse transcription-rolling circle amplification (RT-RCA) with the CRISPR/Cas12a). This method comprises two steps: (1) the RT-RCA process of circRNA amplification, generating repeat units containing the back-splicing junction (BSJ) sequences; and (2) leveraging the protospacer adjacent motif (PAM)-independent Cas12a/crRNA complex to precisely recognize target sequences with BSJ, thereby initiating the collateral cleavage activity of Cas12a to generate a robust fluorescence signal. Remarkably, this approach exhibits the capability to detect circRNAs at a concentration as low as 300 aM. The sensor has been successfully employed for accurate detection of a potential hepatocellular carcinoma biomarker hsa_circ_0001445 (circRNA1445) in various cell lines. In conclusion, RETA-CRISPR seamlessly integrates the advantages of exponential amplification reaction and the robust collateral cleavage activity of Cas12a, positioning it as a compelling tool for practical CRISPR-based diagnostics.
Supplementary files
- Supplementary information PDF (518K)
Article information
Download citation, permissions.

A one-pot CRISPR-RCA strategy for ultrasensitive and specific detection of circRNA
X. Ke, A. Liang, C. Chen and T. Hu, Anal. Methods , 2024, Advance Article , DOI: 10.1039/D4AY00693C
To request permission to reproduce material from this article, please go to the Copyright Clearance Center request page .
If you are an author contributing to an RSC publication, you do not need to request permission provided correct acknowledgement is given.
If you are the author of this article, you do not need to request permission to reproduce figures and diagrams provided correct acknowledgement is given. If you want to reproduce the whole article in a third-party publication (excluding your thesis/dissertation for which permission is not required) please go to the Copyright Clearance Center request page .
Read more about how to correctly acknowledge RSC content .
Social activity
Search articles by author.
This article has not yet been cited.
Advertisements
A Generative AI approach to improve in-situ vision tool wear monitoring with scarce data
- Published: 10 May 2024
Cite this article
- Alberto Garcia-Perez ORCID: orcid.org/0000-0002-3755-1661 1 ,
- Maria Jose Gomez-Silva 2 &
- Arturo de la Escalera-Hueso 3
15 Accesses
Explore all metrics
Most aerospace turbine casings are mechanised using a vertical lathe. This paper presents a tool wear monitoring system using computer vision that analyses tool inserts once that the machining process has been completed. By installing a camera in the robot magazine room and a tool cleaning device to remove chips and cooling residuals, a neat tool image can be acquired. A subsequent Deep Learning (DL) model classifies the tool as acceptable or not, avoiding the drawbacks of alternative computer vision algorithms based on edges, dedicated features etc. Such model was trained with a significantly reduced number of images, in order to minimise the costly process to acquire and classify images during production. This could be achieved by introducing a special lens and some generative Artificial Intelligence (AI) models. This paper proposes two novel architectures: SCWGAN-GP, Scalable Condition Wasserstein Generative Adversarial Network (WGAN) with Gradient Penalty, and Focal Stable Diffusion (FSD) model, with class injection and dedicated loss function, to artificially increase the number of images to train the DL model. In addition, a K|Lens special optics was used to get multiple views of the vertical lathe inserts as a means of further increase data augmentation by hardware with a reduced number of production samples. Given an initial dataset, the classification accuracy was increased from 80.0 % up to 96.0 % using the FSD model. We also found that using as low as 100 real images, our methodology can achieve up to 93.3 % accuracy. Using only 100 original images for each insert type and wear condition results in 93.3 % accuracy and up to 94.6 % if 200 images are used. This accuracy is considered to be within human inspector uncertainty for this use-case. Fine-tuning the FSD model, with nearly 1 billion training parameters, showed superior performance compared to the SCWGAN-GP model, with only 80 million parameters, besides of requiring less training samples to produced higher quality output images. Furthermore, the visualization of the output activation mapping confirms that the model takes a decision on the right image features. Time to create the dataset was reduced from 3 months to 2 days using generative AI. So our approach enables to create industrial dataset with minimum effort and significant time speed-up compared with the conventional approach of acquiring a large number of images that DL models usually requires to avoid over-fitting. Despite the good results, this methodology is only applicable to relatively simple cases, such as our inserts where the images are not complex.
This is a preview of subscription content, log in via an institution to check access.
Access this article
Price includes VAT (Russian Federation)
Instant access to the full article PDF.
Rent this article via DeepDyve
Institutional subscriptions
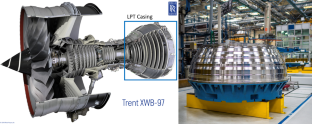
Abbreviations
Automated Defect Recognition
Artificial Intelligence
Conditional GAN
Contrastive Language-Image Pre-training
Convolutional Neural Network
- Computer Vision
Conditional Variational Auto-Encoder
Deep Learning
Floating Point Operations per Second
Focal Stable Diffusion
Generative Adversarial Network
Generative Artificial Intelligence
Graphics Processing Unit
Gradient-weighted Class Activation Mapping
Low Pressure Turbine
Mathews Correlation Coefficient
Neural Network
Random Access Memory
Root Mean Squared Error
Scalable Conditional Wasserstein GAN with Gradient Penalty
- Stable Diffusion
Super Resolution GAN
Structural Similarity Index
Support Vector Machine
Tool Condition Monitoring
Variational Auto Encoders
Vision Transformer
Wasserstein Generative Adversarial Network
Wasserstein GAN with Gradient Penalty
Arjovsky, M., Chintala, S., & Bottou, L. (2017). Wasserstein GAN. arXiv:1701.07875 [cs, stat].
Bagga, P. J., Makhesana, M. A., & Patel, K. M. (2021). A novel approach of combined edge detection and segmentation for tool wear measurement in machining. Production Engineering?: Research & Development, 15 (3), 519–533. https://doi.org/10.1007/s11740-021-01035-5
Bagga, P. J., Makhesana, M. A., Patel, K., & Patel, K. M. (2021). Tool wear monitoring in turning using image processing techniques. Materials Today: Proceedings, 44 , 771–775. https://doi.org/10.1016/j.matpr.2020.10.680
Article Google Scholar
Banda, T., Farid, A. A., Li, C., Jauw, V. L., & Lim, C. S. (2022). Application of machine vision for tool condition monitoring and tool performance optimization-a review. International Journal of Advanced Manufacturing Technology, 121 (11), 7057–7086. https://doi.org/10.1007/s00170-022-09696-x
Barreiro, J., Fernández-Abia, A. I., González-Laguna, A., & Pereira, O. (2017). TCM system in contour milling of very thick-very large steel plates based on vibration and AE signals. Journal of Materials Processing Technology, 246 , 144–157. https://doi.org/10.1016/j.jmatprotec.2017.03.016
Bergs, T., Holst, C., Gupta, P., & Augspurger, T. (2020). Digital image processing with deep learning for automated cutting tool wear detection. Procedia Manufacturing, 48 , 947–958. https://doi.org/10.1016/j.promfg.2020.05.134
Castejón, M., Alegre, E., Barreiro, J., & Hernández, L. K. (2007). On-line tool wear monitoring using geometric descriptors from digital images. International Journal of Machine Tools and Manufacture, 47 (12), 1847–1853. https://doi.org/10.1016/j.ijmachtools.2007.04.001
Chen, N., Hao, B., Guo, Y., Li, L., Khan, M. A., & He, N. (2020). Research on tool wear monitoring in drilling process based on APSO-LS-SVM approach. International Journal of Advanced Manufacturing Technology, 108 (7), 2091–2101. https://doi.org/10.1007/s00170-020-05549-7
Chicco, D., & Jurman, G. (2020). The advantages of the Matthews correlation coefficient (MCC) over F1 score and accuracy in binary classification evaluation. BMC Genomics, 21 (1), 6. https://doi.org/10.1186/s12864-019-6413-7
Dai, Y., & Zhu, K. (2018). A machine vision system for micro-milling tool condition monitoring. Precision Engineering, 52 , 183–191. https://doi.org/10.1016/j.precisioneng.2017.12.006
Domínguez-Monferrer, C., Fernández-Pérez, J., De Santos, R., Miguélez, M. H., & Cantero, J. L. (2022). Machine learning approach in non-intrusive monitoring of tool wear evolution in massive CFRP automatic drilling processes in the aircraft industry. Journal of Manufacturing Systems, 65 , 622–639. https://doi.org/10.1016/j.jmsy.2022.10.018
Dosovitskiy, A., Beyer, L., Kolesnikov, A., Weissenborn, D., Zhai, X., Unterthiner, T., Dehghani, M., Minderer, M., Heigold, G., Gelly, S., Uszkoreit, J., & Houlsby, N. (2021). An Image Is Worth 16x16 Words: Transformers for Image Recognition at Scale. arXiv:2010.11929
Dou, J., Dong, H., Zhang, J., Meng, J., Tian, Y., Pang, M., Luo, W., & Xu, C. (2023). An adaptive method of measuring the rake face wear of end mills based on image feature point set registration. Journal of Manufacturing Processes, 98 , 149–158. https://doi.org/10.1016/j.jmapro.2023.05.027
Drury, C.G., & Fox, J.G. (1976). Human reliability in quality control. Taylor & Francis Ltd. 10–14 Macklin St, London WC2B 5NF, pp 328, £7.00. Applied Ergonomics 7 (1), 46 (1976) https://doi.org/10.1016/0003-6870(76)90012-0
Feng, Y. (2022). Improving Tool Wear Prediction with Synthetic Features from Conditional Generative Adversarial Networks. TechRxiv. https://doi.org/10.36227/techrxiv.21253308.v1
Fernández-Robles, L., Azzopardi, G., Alegre, E., & Petkov, N. (2017). Machine-vision-based identification of broken inserts in edge profile milling heads. Robotics and Computer-Integrated Manufacturing, 44 , 276–283. https://doi.org/10.1016/j.rcim.2016.10.004
Fernández-Robles, L., Sánchez-González, L., Díez-González, J., Castejón-Limas, M., & Pérez, H. (2021). Use of image processing to monitor tool wear in micro milling. Neurocomputing, 452 , 333–340. https://doi.org/10.1016/j.neucom.2019.12.146
Fong, K. M., Wang, X., Kamaruddin, S., & Ismadi, M.-Z. (2021). Investigation on universal tool wear measurement technique using image-based cross-correlation analysis. Measurement, 169 , 108489. https://doi.org/10.1016/j.measurement.2020.108489
Friedrich, M., Gerber, T., Dumler, J., & Döpper, F. (2023). A system for automated tool wear monitoring and classification using computer vision. Procedia CIRP, 118 , 425–430. https://doi.org/10.1016/j.procir.2023.06.073
Gantala, T., & Balasubramaniam, K. (2021). Automated defect recognition for welds using simulation assisted TFM imaging with artificial intelligence. Journal of Nondestructive Evaluation, 40 (1), 28. https://doi.org/10.1007/s10921-021-00761-1
García Pérez, A., Gómez Silva, M. J., & de La Escalera Hueso, A. (2021). Automated defect recognition of castings defects using neural networks. Journal of Nondestructive Evaluation, 41 (1), 11. https://doi.org/10.1007/s10921-021-00842-1
García-Ordás, M. T., Alegre, E., González-Castro, V., & Alaiz-Rodríguez, R. (2017). A computer vision approach to analyze and classify tool wear level in milling processes using shape descriptors and machine learning techniques. International Journal of Advanced Manufacturing Technology, 90 (5), 1947–1961. https://doi.org/10.1007/s00170-016-9541-0
García-Pérez, A., Ziegenbein, A., Schmidt, E., Shamsafar, F., Fernández-Valdivielso, A., Llorente-Rodríguez, R., & Weigold, M. (2023). CNN-based in situ tool wear detection: A study on model training and data augmentation in turning inserts. Journal of Manufacturing Systems, 68 , 85–98. https://doi.org/10.1016/j.jmsy.2023.03.005
Gomes, M. C., Brito, L. C., da Silva, M. B., & Viana Duarte, M. A. (2021). Tool wear monitoring in micromilling using Support Vector Machine with vibration and sound sensors. Precision Engineering, 67 , 137–151. https://doi.org/10.1016/j.precisioneng.2020.09.025
Goodfellow, I.J. (2015). On distinguishability criteria for estimating generative models. arXiv:1412.6515 [stat]
Goodfellow, I.J., Pouget-Abadie, J., Mirza, M., Xu, B., Warde-Farley, D., Ozair, S., Courville, A., & Bengio, Y. (2014). Generative Adversarial Networks. arXiv:1406.2661 [cs, stat]
Han, J., Tan, X., Li, T., Tang, Z., Liao, G., & Shi, T. (2021). In-situ measurement of cutting edge temperature and its effect on tool wear in turning by a near-infrared fiber-optic two-color pyrometer. Procedia CIRP, 101 , 89–92. https://doi.org/10.1016/j.procir.2021.02.012
Han, G., Ye, Z., Xu, J., Ma, Y., Xu, C., Zhao, X., Yu, L., Feng, P., & Feng, F. (2023). Investigation on cutting forces and tool wear in high-speed milling of Ti-6Al-4V assisted by longitudinal torsional ultrasonic vibrations. International Journal of Advanced Manufacturing Technology . https://doi.org/10.1007/s00170-023-12306-z
He, K., Zhang, X., Ren, S., & Sun, J. (2015). Deep residual learning for image recognition. arXiv:1512.03385 [cs].
Ho, J., Jain, A., & Abbeel, P. (2020). Denoising Diffusion Probabilistic Models. arXiv:2006.11239
Hüter, C., Yin, X., Vo, T., & Braun, S. (2020). A pragmatic dataset augmentation approach for transformation temperature prediction in steels. Computational Materials Science, 176 , 109488. https://doi.org/10.1016/j.commatsci.2019.109488
Iglesias, G., Talavera, E., & Díaz-Álvarez, A. (2023). A survey on GANs for computer vision: Recent research, analysis and taxonomy. Computer Science Review, 48 , 100553. https://doi.org/10.1016/j.cosrev.2023.100553
Illgner, K., Restrepo, J., Jaiswal, S.P., & Ihrke, I. (2020). Lightfield imaging for industrial applications. In: SPIE Future Sensing Technologies, vol. 11525, pp. 493–504. SPIE. https://doi.org/10.1117/12.2580328
Jang, Woong-Ki., Kim, Dong-Wook., Seo, Young-Ho., & Kim, Byeong-Hee. (2023). Tool-wear-estimation system in milling using multi-view CNN based on reflected infrared images. Sensors, 23 (3), 1208. https://doi.org/10.3390/s23031208
Kingma, D.P., & Welling, M. (2014). Auto-encoding variational bayes. arXiv:1312.6114 [cs, stat]
Kurada, S., & Bradley, C. (1997). A machine vision system for tool wear assessment. Tribology International, 30 (4), 295–304. https://doi.org/10.1016/S0301-679X(96)00058-8
Laion/Laion-High-Resolution \(\cdot \) Datasets at Hugging Face. https://huggingface.co/datasets/laion/laion-high-resolution
Ledig, C., Theis, L., Huszar, F., Caballero, J., Cunningham, A., Acosta, A., Aitken, A., Tejani, A., Totz, J., Wang, Z., & Shi, W. (2017). Photo-realistic single image super-resolution using a generative adversarial network. arXiv:1609.04802 [cs, stat]
Lee, J., Lee, Y. C., & Kim, J. T. (2020). Fault detection based on one-class deep learning for manufacturing applications limited to an imbalanced database. Journal of Manufacturing Systems, 57 , 357–366. https://doi.org/10.1016/j.jmsy.2020.10.013
Leng, S., Wang, Z., Min, T., Dai, Z., & Chen, G. (2020). Detection of tool wear in drilling CFRP/TC4 stacks by acoustic emission. Journal of Vibration Engineering & Technologies, 8 (3), 463–470. https://doi.org/10.1007/s42417-019-00190-5
Li, L., & An, Q. (2016). An in-depth study of tool wear monitoring technique based on image segmentation and texture analysis. Measurement, 79 , 44–52. https://doi.org/10.1016/j.measurement.2015.10.029
Li, Z., Liu, X., Incecik, A., Gupta, M. K., Królczyk, G. M., & Gardoni, P. (2022). A novel ensemble deep learning model for cutting tool wear monitoring using audio sensors. Journal of Manufacturing Processes, 79 , 233–249. https://doi.org/10.1016/j.jmapro.2022.04.066
Lim, M. L., Derani, M. N., Ratnam, M. M., & Yusoff, A. R. (2022). Tool wear prediction in turning using workpiece surface profile images and deep learning neural networks. International Journal of Advanced Manufacturing Technology, 120 (11), 8045–8062. https://doi.org/10.1007/s00170-022-09257-2
Lins, R. G., Guerreiro, B., Marques de Araujo, P. R., & Schmitt, R. (2020). In-Process Tool Wear Measurement System Based on Image Analysis for CNC Drilling Machines. IEEE Transactions on Instrumentation and Measurement, 69 (8), 5579–5588. https://doi.org/10.1109/TIM.2019.2961572
Li, T., Shi, T., Tang, Z., Liao, G., Duan, J., Han, J., & He, Z. (2021). Real-time tool wear monitoring using thin-film thermocouple. Journal of Materials Processing Technology, 288 , 116901. https://doi.org/10.1016/j.jmatprotec.2020.116901
Liu, R. (2023). An edge-based algorithm for tool wear monitoring in repetitive milling processes. Journal of Intelligent Manufacturing, 34 (5), 2333–2343. https://doi.org/10.1007/s10845-022-01925-0
Liu, L., Cao, D., Wu, Y., & Wei, T. (2019). Defective samples simulation through adversarial training for automatic surface inspection. Neurocomputing, 360 , 230–245. https://doi.org/10.1016/j.neucom.2019.05.080
Liu, Y., Guo, L., Gao, H., You, Z., Ye, Y., & Zhang, B. (2022). Machine vision based condition monitoring and fault diagnosis of machine tools using information from machined surface texture: A review. Mechanical Systems and Signal Processing, 164 , 108068. https://doi.org/10.1016/j.ymssp.2021.108068
Liu, R., Kothuru, A., & Zhang, S. (2020). Calibration-based tool condition monitoring for repetitive machining operations. Journal of Manufacturing Systems, 54 , 285–293. https://doi.org/10.1016/j.jmsy.2020.01.005
Liu, H., Liu, Z., Jia, W., Lin, X., & Zhang, S. (2020). A novel transformer-based neural network model for tool wear estimation. Measurement Science and Technology, 31 (6), 065106. https://doi.org/10.1088/1361-6501/ab7282
Liu, C., & Zhu, L. (2020). A two-stage approach for predicting the remaining useful life of tools using bidirectional long short-term memory. Measurement, 164 , 108029. https://doi.org/10.1016/j.measurement.2020.108029
Li, X., Yue, C., Liu, X., Zhou, J., & Wang, L. (2024). ACWGAN-GP for milling tool breakage monitoring with imbalanced data. Robotics and Computer-Integrated Manufacturing, 85 , 102624. https://doi.org/10.1016/j.rcim.2023.102624
Madhusudana, C. K., Kumar, H., & Narendranath, S. (2016). Condition monitoring of face milling tool using K-star algorithm and histogram features of vibration signal. Engineering Science and Technology, an International Journal, 19 (3), 1543–1551. https://doi.org/10.1016/j.jestch.2016.05.009
Ma, J., Luo, D., Liao, X., Zhang, Z., Huang, Y., & Lu, J. (2021). Tool wear mechanism and prediction in milling TC18 titanium alloy using deep learning. Measurement, 173 , 108554. https://doi.org/10.1016/j.measurement.2020.108554
Meister, S., Möller, N., Stüve, J., & Groves, R. M. (2021). Synthetic image data augmentation for fibre layup inspection processes: Techniques to enhance the data set. Journal of Intelligent Manufacturing, 32 (6), 1767–1789. https://doi.org/10.1007/s10845-021-01738-7
Metz, L., Poole, B., Pfau, D., & Sohl-Dickstein, J. (2016). Unrolled generative adversarial networks.
Milling Wear | NASA Open Data Portal. https://data.nasa.gov/Raw-Data/Milling-Wear/vjv9-9f3x
Molitor, D. A., Kubik, C., Becker, M., Hetfleisch, R. H., Lyu, F., & Groche, P. (2022). Towards high-performance deep learning models in tool wear classification with generative adversarial networks. Journal of Materials Processing Technology, 302 , 117484. https://doi.org/10.1016/j.jmatprotec.2021.117484
Nair, V., & Hinton, G.E. (2010). Rectified linear units improve restricted boltzmann machines. In: Proceedings of the 27th International Conference on International Conference on Machine Learning. ICML’10, pp. 807–814. Omnipress, Madison, WI, USA.
Niranjan Prasad, K., & Ramamoorthy, B. (2001). Tool wear evaluation by stereo vision and prediction by artificial neural network. Journal of Materials Processing Technology, 112 (1), 43–52. https://doi.org/10.1016/S0924-0136(00)00896-7
Ong, P., Lee, W. K., & Lau, R. J. H. (2019). Tool condition monitoring in CNC end milling using wavelet neural network based on machine vision. International Journal of Advanced Manufacturing Technology, 104 (1), 1369–1379. https://doi.org/10.1007/s00170-019-04020-6
Papenberg, B., Hogreve, S., & Tracht, K. (2023). Visualization of relevant areas of milling tools for the classification of tool wear by machine learning methods. Procedia CIRP, 118 , 525–530. https://doi.org/10.1016/j.procir.2023.06.090
Parvaiz, A., Khalid, M. A., Zafar, R., Ameer, H., Ali, M., & Fraz, M. M. (2023). Vision Transformers in medical computer vision-A contemplative retrospection. Engineering Applications of Artificial Intelligence, 122 , 106126. https://doi.org/10.1016/j.engappai.2023.106126
Peng, R., Liu, J., Fu, X., Liu, C., & Zhao, L. (2021). Application of machine vision method in tool wear monitoring. International Journal of Advanced Manufacturing Technology, 116 (3), 1357–1372. https://doi.org/10.1007/s00170-021-07522-4
Peng, Ruitao, Pang, H., Jiang, H., & Hu, Y. (2020). Study of Tool Wear Monitoring Using Machine Vision. Automatic Control and Computer Sciences, 54 (3), 259–270. https://doi.org/10.3103/S0146411620030062
Pérez, E., & Ventura, S. (2023). Progressive growing of Generative Adversarial Networks for improving data augmentation and skin cancer diagnosis. Artificial Intelligence in Medicine, 141 , 102556. https://doi.org/10.1016/j.artmed.2023.102556
Pimenov, D. Y., Bustillo, A., Wojciechowski, S., Sharma, V. S., Gupta, M. K., & Kuntoğlu, M. (2023). Artificial intelligence systems for tool condition monitoring in machining: Analysis and critical review. Journal of Intelligent Manufacturing, 34 (5), 2079–2121. https://doi.org/10.1007/s10845-022-01923-2
Radford, A., Kim, J.W., Hallacy, C., Ramesh, A., Goh, G., Agarwal, S., Sastry, G., Askell, A., Mishkin, P., Clark, J., Krueger, G., & Sutskever, I. (2021). Learning Transferable Visual Models From Natural Language Supervision. arXiv:2103.00020
Ramesh, A., Dhariwal, P., Nichol, A., Chu, C., & Chen, M. (2022). Hierarchical text-conditional image generation with CLIP Latents. arXiv.
Riego, V., Castejón-Limas, M., Sánchez-González, L., Fernández-Robles, L., Perez, H., Diez-Gonzalez, J., & Guerrero-Higueras, Á. -M. (2021). Strong classification system for wear identification on milling processes using computer vision and ensemble learning. Neurocomputing, 456 , 678–684. https://doi.org/10.1016/j.neucom.2020.07.131
Rmili, W., Ouahabi, A., Serra, R., & Leroy, R. (2016). An automatic system based on vibratory analysis for cutting tool wear monitoring. Measurement, 77 , 117–123. https://doi.org/10.1016/j.measurement.2015.09.010
Rombach, R., Blattmann, A., Lorenz, D., Esser, P., & Ommer, B. (2022). High-resolution image synthesis with latent diffusion models. arXiv:2112.10752
Ronneberger, O., Fischer, P., & Brox, T. (2015). U-Net: convolutional networks for biomedical image segmentation. In: Navab, N., Hornegger, J., Wells, W.M., Frangi, A.F. (eds.) Medical image computing and computer-assisted intervention – MICCAI 2015. Lecture Notes in Computer Science, pp. 234–241. Springer, Cham. https://doi.org/10.1007/978-3-319-24574-4_28
Ruiz, N., Li, Y., Jampani, V., Pritch, Y., Rubinstein, M., & Aberman, K. (2022). DreamBooth: Fine tuning text-to-image diffusion models for subject-driven generation. arxiv:2208.12242 .
Saharia, C., Chan, W., Saxena, S., Li, L., Whang, J., Denton, E., Ghasemipour, S.K.S., Ayan, B.K., Mahdavi, S.S., Lopes, R.G., Salimans, T., Ho, J., Fleet, D.J., & Norouzi, M. (2022). Photorealistic text-to-image diffusion models with deep language understanding. arXiv:2205.11487
Scharf, H. P., Cambraia, H. N., & da Costa, D. D. (2023). A new embedded vision system for monitoring tool conditions in production lines using a combination of direct and indirect methods. Journal of Manufacturing Processes, 102 , 143–153. https://doi.org/10.1016/j.jmapro.2023.07.028
Selvaraj, B., Vignesh, H., & Prabakaran (2013). Tool wear monitoring by image processing. International Journal of Engineering Research & Technology (IJERT) 2 Issue B .
Selvaraju, R.R., Cogswell, M., Das, A., Vedantam, R., Parikh, D., & Batra, D. (2020). Grad-CAM: visual explanations from deep networks via gradient-based localization. International Journal of Computer Vision, 128 (2), 336–359. https://doi.org/10.1007/s11263-019-01228-7 arxiv:1610.02391
Sensors | Free Full-Text | Tool-Wear-Estimation System in Milling Using Multi-View CNN Based on Reflected Infrared Images. https://www.mdpi.com/1424-8220/23/3/1208
Shin, H.-C., Tenenholtz, N.A., Rogers, J.K., Schwarz, C.G., Senjem, M.L., Gunter, J.L., Andriole, K., & Michalski, M. (2018). Medical image synthesis for data augmentation and anonymization using generative adversarial networks. arXiv:1807.10225 [cs, stat].
Singh, S. A., & Desai, K. A. (2023). Automated surface defect detection framework using machine vision and convolutional neural networks. Journal of Intelligent Manufacturing, 34 (4), 1995–2011. https://doi.org/10.1007/s10845-021-01878-w
Stavropoulos, P., Souflas, T., Papaioannou, C., Bikas, H., & Mourtzis, D. (2023). An adaptive, artificial intelligence-based chatter detection method for milling operations. The International Journal of Advanced Manufacturing Technology, 124 (7), 2037–2058. https://doi.org/10.1007/s00170-022-09920-8
Tan, M., & Le, Q.V. (2020). EfficientNet: rethinking model scaling for convolutional neural networks. arXiv:1905.11946 [cs, stat].
Tušar, T., Gantar, K., Koblar, V., Ženko, B., & Filipič, B. (2017). A study of overfitting in optimization of a manufacturing quality control procedure. Applied Soft Computing, 59 , 77–87. https://doi.org/10.1016/j.asoc.2017.05.027
Vaswani, A., Shazeer, N., Parmar, N., Uszkoreit, J., Jones, L., Gomez, A.N., Kaiser, Ł., & Polosukhin, I. (2017). Attention is All you Need. In: Advances in neural information processing systems, vol. 30. Curran Associates, Inc.,
See, J. E. (2015). Visual inspection reliability for precision manufactured parts. Human Factors, 57 (8), 1427–1442. https://doi.org/10.1177/0018720815602389
Wager, S., Wang, S., & Liang, P. (2013). Dropout Training as Adaptive Regularization. arXiv:1307.1493
Wan, T., Chen, X., Li, C., & Tang, Y. (2018). An on-line tool wear monitoring method based on cutting power. In: 2018 IEEE 14th International Conference on Automation Science and Engineering (CASE), pp.205–210. https://doi.org/10.1109/COASE.2018.8560412
Wang, Z., Bovik, A. C., Sheikh, H. R., & Simoncelli, E. P. (2004). Image quality assessment: From error visibility to structural similarity. IEEE Transactions on Image Processing, 13 (4), 600–612. https://doi.org/10.1109/TIP.2003.819861
Wang, Y.-R., Sun, G.-D., & Jin, Q. (2020). Imbalanced sample fault diagnosis of rotating machinery using conditional variational auto-encoder generative adversarial network. Applied Soft Computing, 92 , 106333. https://doi.org/10.1016/j.asoc.2020.106333
Wang, Y., Sun, Z.-L., Zeng, Z., & Lam, K.-M. (2023). TRCT-GAN: CT reconstruction from biplane X-rays using transformer and generative adversarial networks. Digital Signal Processing . https://doi.org/10.1016/j.dsp.2023.104123
Wu, X., Liu, Y., Zhou, X., & Mou, A. (2019). Automatic Identification of Tool Wear Based on Convolutional Neural Network in Face Milling Process. Sensors (Basel), 19 (18), 3817. https://doi.org/10.3390/s19183817
Yang, M., Wang, Z., Chi, Z., & Du, W. (2023). ProtoGAN: Towards high diversity and fidelity image synthesis under limited data. Information Sciences, 632 , 698–714. https://doi.org/10.1016/j.ins.2023.03.042
You, Z., Gao, H., Guo, L., Liu, Y., & Li, J. (2020). On-line milling cutter wear monitoring in a wide field-of-view camera. Wear, 460–461 , 203479. https://doi.org/10.1016/j.wear.2020.203479
Yu, J., Cheng, X., Lu, L., & Wu, B. (2021). A machine vision method for measurement of machining tool wear. Measurement, 182 , 109683. https://doi.org/10.1016/j.measurement.2021.109683
Zhang, K., Chen, J., Zhang, T., He, S., Pan, T., & Zhou, Z. (2020). Intelligent fault diagnosis of mechanical equipment under varying working condition via iterative matching network augmented with selective Signal reuse strategy. Journal of Manufacturing Systems, 57 , 400–415. https://doi.org/10.1016/j.jmsy.2020.10.007
Zhang, X., Jiao, W., Wang, B., & Tian, X. (2023). CT-GAN: A conditional generative adversarial network of transformer architecture for text-to-image. Signal Processing: Image Communication, 115 , 116959. https://doi.org/10.1016/j.image.2023.116959
Zhang, C., & Zhang, J. (2013). On-line tool wear measurement for ball-end milling cutter based on machine vision. Computers in Industry, 64 (6), 708–719. https://doi.org/10.1016/j.compind.2013.03.010
Zhou, J., Yue, C., Liu, X., Xia, W., Wei, X., Qu, J., Liang, S. Y., & Wang, L. (2023). Classification of tool wear state based on dual attention mechanism network. Robotics and Computer-Integrated Manufacturing, 83 , 102575. https://doi.org/10.1016/j.rcim.2023.102575
Zhou, Y., Zhi, G., Chen, W., Qian, Q., He, D., Sun, B., & Sun, W. (2022). A new tool wear condition monitoring method based on deep learning under small samples. Measurement, 189 , 110622. https://doi.org/10.1016/j.measurement.2021.110622
Zhu, K., Guo, H., Li, S., & Lin, X. (2023). Online tool wear monitoring by super-resolution based machine vision. Computers in Industry, 144 , 103782. https://doi.org/10.1016/j.compind.2022.103782
Zou, Y., Ding, K., Shi, K., Lai, X., Zhang, K., Ding, G., & Qin, G. (2023). Wear identification of end mills based on a feature-weighted convolutional neural network under unbalanced samples. Journal of Manufacturing Processes, 89 , 64–76. https://doi.org/10.1016/j.jmapro.2023.01.054
Download references
Acknowledgements
The authors wish to thank ITP Aero for its support and permission to publish this paper. This work was partially conducted in the frame of the project AVISPA (Automation of Visual Inspection and Finishing Processes for Aero-engines), which has been co-funded by the European Union’s Horizon Europe Research and Innovation Programme under Grant Agreement 2021–2022 EIT Manufacturing.
Author information
Authors and affiliations.
Simulation Systems, ITP Aero, Francisca Delgado 9, Alcobendas, Madrid, Spain
Alberto Garcia-Perez
Computer Architecture and Automation Dept., Univ. Complutense de Madrid, Facultad de Ciencias Fisicas, Plaza de Ciencias, 1, Ciudad Universitaria, Madrid, Madrid, Spain
Maria Jose Gomez-Silva
Intelligent Systems Lab, Univ. Carlos III de Madrid, Avda de la Universidad 30, Leganes, Madrid, Spain
Arturo de la Escalera-Hueso
You can also search for this author in PubMed Google Scholar
Corresponding author
Correspondence to Alberto Garcia-Perez .
Ethics declarations
Conflicts of interest.
The authors declare that they have no Conflict of interest.
Additional information
Publisher's note.
Springer Nature remains neutral with regard to jurisdictional claims in published maps and institutional affiliations.
Rights and permissions
Springer Nature or its licensor (e.g. a society or other partner) holds exclusive rights to this article under a publishing agreement with the author(s) or other rightsholder(s); author self-archiving of the accepted manuscript version of this article is solely governed by the terms of such publishing agreement and applicable law.
Reprints and permissions
About this article
Garcia-Perez, A., Gomez-Silva, M.J. & de la Escalera-Hueso, A. A Generative AI approach to improve in-situ vision tool wear monitoring with scarce data. J Intell Manuf (2024). https://doi.org/10.1007/s10845-024-02379-2
Download citation
Received : 14 November 2023
Accepted : 17 March 2024
Published : 10 May 2024
DOI : https://doi.org/10.1007/s10845-024-02379-2
Share this article
Anyone you share the following link with will be able to read this content:
Sorry, a shareable link is not currently available for this article.
Provided by the Springer Nature SharedIt content-sharing initiative
- Tool Condition Monitoring (TCM)
- Vertical lathe
- Neural Networks
- Generative Artificial Intelligence (AI)
- Vision Transformers (ViT)
- Find a journal
- Publish with us
- Track your research
Click through the PLOS taxonomy to find articles in your field.
For more information about PLOS Subject Areas, click here .
Loading metrics
Open Access
Peer-reviewed
Research Article
Violence against women on Twitter in India: Testing a taxonomy for online misogyny and measuring its prevalence during COVID-19
Roles Conceptualization, Data curation, Formal analysis, Methodology, Writing – original draft, Writing – review & editing
* E-mail: [email protected]
Affiliation UNICEF, UNICEF HQ, New York, New York, United States of America

Roles Methodology, Supervision, Writing – review & editing
Affiliation Department of Computer Science, School of Engineering, University of California San Diego, San Diego, California, United States of America
Affiliation Center on Gender Equity and Health, Department of Medicine, University of California San Diego, San Diego, California, United States of America
Roles Supervision, Writing – review & editing
Affiliation Division of Health Promotion and Behavioral Science, School of Public Health, San Diego State University, San Diego, California, United States of America
Affiliation School of Social Work, San Diego State University, San Diego, California, United States of America
Roles Funding acquisition, Methodology, Supervision, Writing – review & editing
- Nabamallika Dehingia,
- Julian McAuley,
- Lotus McDougal,
- Elizabeth Reed,
- Jay G. Silverman,
- Lianne Urada,
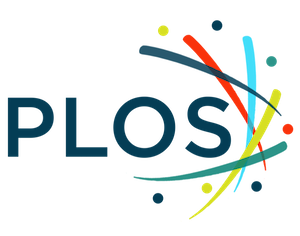
- Published: October 25, 2023
- https://doi.org/10.1371/journal.pone.0292121
- Peer Review
- Reader Comments
Online misogyny is a violation of women’s digital rights. Empirical studies on this topic are however lacking, particularly in low- and middle- income countries. The current study aimed to estimate whether prevalence of online misogyny on Twitter in India changed since the pandemic.
Based on prior theoretical work, we defined online misogyny as consisting of six overlapping forms: sexist abuses, sexual objectification, threatening to physically or sexually harm women, asserting women’s inferiority, justifying violence against women, and dismissing feminist efforts. Qualitative analysis of a small subset of tweets posted from India (40,672 tweets) substantiated this definition and taxonomy for online misogyny. Supervised machine learning models were used to predict the status of misogyny across a corpus of 30 million tweets posted from India between 2018 and 2021. Next, interrupted time series analysis examined changes in online misogyny prevalence, before and during COVID-19.
Qualitative assessment showed that online misogyny in India existed most in the form of sexual objectification and sexist abusive content, which demeans women and shames them for their presumed sexual activity. Around 2% of overall tweets posted from India between 2018 and 2021 included some form of misogynistic content. The absolute volume as well as proportion of misogynistic tweets showed significant increasing trends after the onset of COVID-19, relative to trends prior to the pandemic.
Findings highlight increasing gender inequalities on Twitter since the pandemic. Aggressive and hateful tweets that target women attempt to reinforce traditional gender norms, especially those relating to idealized sexual behavior and framing of women as sexual beings. There is an urgent need for future research and development of interventions to make digital spaces gender equitable and welcoming to women.
Citation: Dehingia N, McAuley J, McDougal L, Reed E, Silverman JG, Urada L, et al. (2023) Violence against women on Twitter in India: Testing a taxonomy for online misogyny and measuring its prevalence during COVID-19. PLoS ONE 18(10): e0292121. https://doi.org/10.1371/journal.pone.0292121
Editor: Mahdi Zareei, Technologico de Monterrey, MEXICO
Received: November 8, 2022; Accepted: September 13, 2023; Published: October 25, 2023
Copyright: © 2023 Dehingia et al. This is an open access article distributed under the terms of the Creative Commons Attribution License , which permits unrestricted use, distribution, and reproduction in any medium, provided the original author and source are credited.
Data Availability: All relevant data necessary for replicating the study findings can be found here: https://doi.org/10.7910/DVN/H3IXDN .
Funding: This study was funded by the Bill and Melinda Gates Foundation Grant No. OPP1163682. The funding body had no role in the design of the study and collection, analysis, and interpretation of data and in writing the manuscript.
Competing interests: The authors have declared that no competing interests exist.
Introduction
Violence against women (VAW) on online platforms is a violation of women’s digital rights. It can push women out of online spaces, and impact their social, economic, as well as health outcomes [ 1 ]. One of the most prevalent forms of online VAW, online misogyny or hate speech against women refers to any content that tries to threaten, intimidate, and shame women, or any rhetoric that emphasizes the authority of men over women [ 1 ]. According to a multi-country online survey in 2019, around two-thirds of women users of the internet reported having received some form of sexist or hateful language designed to attack or humiliate them [ 2 ]. Such experiences can often cause increased anxiety, depression, and lower self-esteem among women [ 3 , 4 ]. The extent and frequency of misogynistic attacks varies across different groups of women; younger women, and women belonging to marginalized racial or sexual identity groups are more at risk of experiencing online misogyny [ 2 , 5 – 9 ]. The past two years have thus noted an increasing number of calls, for hate speech regulation on social media platforms [ 10 , 11 ].
The majority of the existing research on online violence, including online misogyny, has focused on high-income countries, primarily due to greater digital access and use of social media platforms in these regions [ 12 ]. To our knowledge, no academic study has examined the characteristics of online misogyny in low- and middle-income countries (LMIC). This presents a key gap in literature, given that the use of social media, and consequently, the amount of online hateful content, has increased rapidly in LMICs in recent years [ 13 , 14 ]. India, in particular, is an important geography to examine online misogyny. Compared to many other LMICs, adverse gender norms and offline forms of gender-based violence are more prevalent in the country [ 15 , 16 ], putting women at a higher risk of experiencing violence in online spaces. Use of social media platforms is also on the rise in the country. As of January 2022, India had over 25 million active Twitter users, with a 30% increase in average daily users in 2020. Although Twitter has an urban bias; most Twitter users in the country are likely to be educated, living in cities, and of middle to high wealth status [ 17 ]. Nonetheless, Twitter is being frequently used as a key platform for feminist discussions and organizing in India [ 18 ], which can increase the likelihood of misogynist expression as a backlash [ 19 ]. The current study aims to contribute to the growing literature on online VAW on social media platforms, by measuring online misogyny on Twitter in India.
Limited global research suggests a potential increase in this form of violence since the pandemic, impacting millions of women daily [ 10 ]. An analysis of tweets and Facebook posts from South and South-east Asia by United Nations Women found 168 percent increase in misogynistic content during March-June 2020, when compared to the same period in 2019 [ 20 ]. However, despite many such analyses of the effect of COVID-19 on online content, little is known about the features and prevalence of online misogyny, and how it might have changed over the course of the pandemic. In this study, we take a first step to provide temporal estimates of online misogyny on Twitter in India from 2018 to 2021, and systematically examine any changes in its rates of increase/decrease before and during COVID-19.
As early as May 2020, the United Nations Secretary General made a global appeal to tackle COVID-19 influenced hate speech on digital platforms, with hateful content covering “ stereotyping , stigmatization , and the use of derogatory , misogynistic , racist , xenophobic , Islamophobic or antisemitic language ” [ 21 ]. Potential drivers of such hate speech during the pandemic include increased isolation due to stay-at-home orders, greater use of social media platforms, greater exposure to polarizing and differing views on the social media platforms, and fear, uncertainty, and anxiety of living through the pandemic [ 22 ]. Isolation is a strong motivational factor for hate speech on social media [ 23 ], and the confined living conditions, health and financial worries following the lockdown can create tension and stress, increasing the risk for online expression of hate speech. With regards to hate speech against women in particular, global evidence in support of increasing cases of gender-based violence during the pandemic further points to a potential increase in violence against women on online platforms [ 24 ]. An analysis of Twitter data before and after the onset of COVID-19 will allow us to test this hypothesis of increased levels of misogyny on Twitter since the pandemic.
Defining online misogyny
A key reason for the limited empirical evidence on online misogyny, is the lack of a standard taxonomy [ 25 ]. A majority of existing research on this topic has lacked theoretical considerations, classifying it as content that includes identifiable sexist slurs [ 26 – 28 ]. Earlier works on misogyny detection relied on lexicon-based methods, where misogynistic tweets were identified based on the presence of gendered abusive words [ 29 , 30 ]. Anzovino et. al (2018) introduced a benchmark dataset of tweets classified as misogynistic, with online misogyny defined as consisting of five forms: a) slurring, b) stereotypes, c) sexual harassment and threats, d) dominance to preserve men’s control, and e) derailing to justify VAW [ 31 , 32 ]. Multiple other studies have used this definition for misogyny detection [ 33 , 34 ]. We build on this taxonomy, by adapting it to the context of India, and locating it within a theoretical understanding of misogyny as a notion central to the feminist theory.
Feminist theory posits that misogyny, whether online or offline, stems from patriarchal values justifying men’s control over women via degradation and violence; misogyny actively seeks to silence women and maintain the status quo of patriarchal gender roles [ 35 ]. Sobieraj describes intimidating, shaming, and discrediting as the three key strategies often adopted by perpetrators of online misogyny, where attackers draw on women’s fear of sexual assault and physical violence to intimidate them, sexually objectify to publicly shame them, and discredit or dismiss their achievements as well as their fight to equality [ 1 ]. Asserting the inferiority of women, dismissing feminist movements, and objectifying women or viewing women primarily as an object of men’s desire, are thus at the heart of misogyny [ 36 ]. Our preliminary research on misogyny detection in South-Asian countries noted an emphasis on dismissal of feminist thought and discrediting of women’s claims of gender-based violence [ 37 ]. Guided by these theoretical and empirical works, we define online misogyny as any rhetoric or content that uses hostile and malicious language targeted at women, objectifies them, threatens them with physical or sexual harm, tries to assert their inferiority, justifies gender-based violence, or discredits feminist activists and their efforts. By qualitatively analyzing a large dataset of tweets (over 40,000 tweets), we first test whether this definition and taxonomy is valid for content posted on Twitter from India. This is followed by estimation of temporal online misogyny, using machine learning techniques that rely on the validated taxonomy. Our study objectives are two-fold: a) testing a taxonomy for online misogyny in India that is rooted in feminist theory, and b) using this taxonomy, estimate whether prevalence of online misogyny on Indian Twitter changed since the pandemic, across a sample of 30 million tweets posted between 2018 and 2021. Given the current lack of research on the extent and forms of misogyny on Twitter in LMICs, our study hopes to generate evidence that can be used to advocate for policies and strategies to address misogyny on Twitter.
Online misogyny taxonomy
We define online misogyny as consisting of six broad and overlapping forms: a) sexist abusive content, b) sexual objectification, c) threatening to physically or sexually harm women (presence of sexist language that indicates the threat being directed at women), d) asserting inferiority, e) justifying VAW, and f) dismissing feminist efforts. We provide definitions and example tweets for each form of online misogyny as Supporting Information. This taxonomy is guided by feminist theoretical work on misogyny [ 36 ], prior research on online misogyny detection, particularly the studies by Anzovino et. al (2018) , and our preliminary research on measuring online misogyny in South Asian countries.
Extracting geolocated tweets
We used geotagged English language tweets posted from India between January 2018 and December 2021 (30 million tweets). All tweets were extracted using the official Twitter API for academic research, which allows extraction of historical tweets. The most recent 800–1000 tweets published every hour from January 1 st 00:00 2018 to December 31 st 23:00 2021 were collected.
We used supervised machine learning models to classify tweets as misogynist/non-misogynist, as well as the six different forms of misogyny. Fig 1 depicts the different steps involved in our analysis. We describe each step in detail in the following sections.
- PPT PowerPoint slide
- PNG larger image
- TIFF original image
https://doi.org/10.1371/journal.pone.0292121.g001
Qualitative analysis of tweets for misogyny
Supervised machine learning models require a ground truth dataset. To that end, two trained undergraduate students first qualitatively coded a small subset of tweets, as misogynist/non-misogynist, and the six forms of misogyny. The selection of the subset of tweets was done using two approaches. First, from the large corpus of geotagged tweets between 2018 and 2020, we extracted posts containing representative keywords frequently used to harass and abuse women. To identify keywords, we contacted authors of an academic study on hate speech detection on Twitter. They used a list of keywords for different types of hate speech on Twitter, including misogyny [ 38 ]. We added a few words relevant to the Indian context to this list. Identified words included abusive terms, as well as generic words related to gender such as " woman ", " feminism " etc. It is key to note here that there may be certain tweets that contain abusive words, but might be non-offensive in their use and overall meaning. The inclusion of all such tweets that contained abusive words allowed us to build a ground truth dataset that identified the nuances in the use of abusive words. With this process, we selected a subset of 35,672 tweets.
Next, we chose a random subset of 5,000 tweets from the large corpus of geotagged tweets from 2018–2020. This dataset was included to ensure that tweets unrelated to women or gender were also represented in our ground truth sample. The main goal of using the two separate approaches to build the ground truth dataset was to collect a set of tweets that would have an adequate representation of both misogynist and non-misogynist tweets. A total of 40,672 tweets were thus selected for qualitative coding, with these two processes.
The qualitative coding of the tweets was carried out in two phases. First, the subset of tweets was coded as misogynist or non-misogynist by five trained undergraduate students. Every tweet was classified by at least two coders. Inter-rater kappa scores were calculated to examine reliability of the coding [ 39 ]. Any disagreement between the coders was resolved by the lead author of the study. Next, all tweets classified as misogynist were coded by two graduate students experienced in gender research, as the six forms of online misogyny. Inter-rater kappa scores were calculated for this step as well. The qualitative coding of misogynist tweets provided us with the opportunity to validate our taxonomy of misogyny. The two researchers did not identify any content that was outside the purview of the six predefined categories.
Selection of features or independent variables
Tweets were cleaned before running any machine learning model. This included deleting urls and ignoring punctuation and case. We also removed stop words, which are commonly used words such as “is”, “the”, “a” etc., from our text data. The decision to drop stop words was made after running multiple models with and without stop words. Next, the data was processed with text vectorization , in order for the machine learning regression models to mathematically interpret it. Text vectorization refers to the process of creating features or input variables in a model (independent variables in public health terminology). There are multiple methods for generating features or independent variables from text data. We used three types of feature selection methods: a) term frequency-inverse document frequency (TF-IDF) unigrams, b) TF-IDF unigrams and bigrams, and c) TF-IDF unigrams, bigrams, and linguistic features (number of words and number of adjectives). These methods were chosen based on guidelines suggested by a previous study on abuse detection [ 32 ]. TF-IDF is weighted word frequency, and reflects how important a word is to a document in a collection or corpus [ 40 ]. While TF-IDF with unigrams concerns with single words only, TF-IDF with unigrams and bigrams includes sequences of two words along with singular words. In addition to weighted word frequencies, linguistic features such as length of the tweet or number of adjectives in a tweet can also provide meaningful information about the content of the tweet. It is possible that tweets that are misogynist are shorter in length and have a relatively higher number of adjectives (abusive words or sentiments).
Running supervised machine learning models and predicting misogyny: Train and test models for best fit
We randomly split our data into a train and test dataset in a 70:30 ratio. The training dataset is used to train the machine learning model, while the test dataset is used to assess the performance of the trained model. To estimate the hyperparameters to be specified in the training model, we used a k-fold validation process. In this method, the training data are partitioned into k subsets of approximately equal size and one of the subsets becomes the validation set where the hyperparameters are validated. The remaining k-1 subsets are used as training data and this process is repeated.
We ran five different types of supervised machine learning models to classify tweets as misogynist/non-misogynist: a) Naïve Bayes (NB), b) Support Vector Machine (SVM), c) Ridge logistic regression, d) Multi-layer Perceptron Neural Network (MPNN), and e) Bidirectional Encoder Representations from Transformers (BERT). Naïve Bayes is a probabilistic model based on Bayes theorem and considers a strong independence assumption [ 41 ]. SVMs are based on a structural risk minimization principle, which aims to test a hypothesis for which we can guarantee the lowest true error. SVMs aim to create a hyperplane ( maximum-marginal hyperplane ), which separates the categories of the outcome label [ 42 ]. MPNN are complex non-linear models with a hierarchical or multi-layered structure. These models have been commonly used in text classification tasks [ 32 ]. BERT is a pre-trained language model (BERT is pretrained on a large corpus of English texts from Wikipedia and BookCorpus), with transformer architecture [ 43 ]. Transformers learn contextual relations between words; they are deep learning models where each output element is connected to every input element and the weightings between them are dynamically calculated. While previous models examine text sequences in a single direction (left-to-right or right-to-left training), BERT’s key difference and innovation is the application of bidirectional training. The BERT Transformer encoder reads the entire sequence of words at once. Specifically, we used the bert-large-uncased version, which consists of 24 layers (1024 hidden dimensions), 16 attention heads, and a total of 340M parameters. The Transformers library in Python was used to implement this approach.
The performance of each model was evaluated on the test set, by comparing actual labels or outcome variable values with the values predicted by the trained model. We used two evaluation metrics: (a) F1 score and (b) receiver operating characteristic—area under the curve (AUC). The receiver operating characteristic captures precision and recall at all potential decision thresholds, so the area under it is an appropriate metric to measure overall model performance. F1 score is the harmonic mean of precision and recall. The models with the best F1 and AUC estimates were chosen to predict the labels/outcome variable values for each tweet in our original dataset of over 30 million geotagged tweets posted between January 2018 to December 2021.
The analysis was repeated with each of the six forms of online misogyny as the outcome variable or label, with the sub-sample of tweets that was classified misogynist (N = 3894).
Analysis of misogyny prevalence pre and during COVID-19
Y t is the proportion of online misogyny related tweets, T is the time since COVID-19 related lockdown has been implemented in India (in days), X t is the dummy variable representing the imposition of lockdown, and X t T is an interaction term. β 1 represents the underlying pre-COVID-19 trend in misogyny prevalence, or the change in misogynistic tweets for every one unit time increase. β 2 captures the immediate effect of the onset of COVID-19, and β 3 represents the slope change in the proportion of misogynistic tweets following COVID-19, in comparison to trends prior to COVID-19. We ran interrupted time series regression models with two separate outcomes: a) total number of misogynistic tweets and b) proportion of misogynistic tweets per 100000 tweets.
Qualitative assessment of misogyny across tweets
Around 8% of the tweets that were qualitatively analyzed were classified as misogynist (N = 40,672). Among those identified as misogynist, 57% were related to sexual objectification, 34% covered sexist abusive content, 6% justified violence against women, and 5% were content that dismissed feminism or feminist efforts. A small proportion of misogynist tweets included threats of harm (1%) and assertion of authority (1%). The two researchers who conducted the qualitative coding did not identify any content that was outside the purview of the six predefined categories of online misogyny. The coding had good reliability, with an inter-rater kappa score of 0.87.
The tweets classified as sexual objectification (57%) included text that sexualized women’s body, shamed women for presumed sexual activity ( slut shaming ), and text that included lewd remarks and sexual solicitation. These tweets emphasized the treatment of women as sexual objects of men’s sexual desire. The category “justify violence against women” (6%) included tweets that highlighted the prevalence of rape myth acceptance and victim blaming among Twitter users. Tweets aggressively asserted that women needed to act a certain way to reduce the risk of rape and violence (e.g., not wear short clothes, not go out at night). This category also included tweets that aimed to derail the conversation from VAW, by focusing on “fake rape cases” in India. Around 10% of these tweets included the hashtag “#mentoo”, with tweets noting men’s victimization with fake rape allegations. Additionally, there were a small number of tweets (n = 3) that dismissed marital rape. For example, a tweet stated that all married women were free to be raped by their husbands.
The category on dismissal of feminist efforts included a number of tweets declaring feminism as a “virus”, with 13% of the tweets including the word “feminazis”. Around 16% of the tweets classified as dismissal of feminist efforts were also categorized as justifying VAW.
Machine learning models to predict misogyny
We ran the different types of machine learning models, separately for each outcome variable: misogyny, sexual objectification, sexist abusive content, justifying VAW, dismissing feminist efforts, threatening to harm, and asserting authority. For the analysis with misogyny as the outcome variable, BERT performed the best [ Table 1 ]. This was followed by the logistic regression models (with TF-IDF unigrams). The Naïve Bayes had the lowest values of AUC as well as F1 score, for misogyny prediction.
https://doi.org/10.1371/journal.pone.0292121.t001
Predicting online misogyny across tweets from 2018–2021 and examining prevalence
We used the specifications from our best performing model to predict misogyny across the large Twitter dataset of 30 million tweets. Each tweet in this dataset was classified as misogyny or non-misogyny by this model. Overall, 1.6% of the geotagged tweets collected from India included misogynist content.
We ran separate interrupted time series models with total number of misogynistic tweets, and proportion of misogynistic tweets per 100000 tweets as the outcomes ( Table 2 ). The volume of misogynistic tweets increased significantly by 30 times immediately after the onset of COVID-19, with increasing trends in the following months between April 2020-December 2021. The proportion of misogynistic tweets per 100000 tweets also showed significant and sustained increasing trends after the onset of COVID-19, relative to trends prior to the pandemic. However, there was a significant decrease immediately after the onset of COVID-19, followed by the increasing trends.
https://doi.org/10.1371/journal.pone.0292121.t002
Digital social media portals are often signaled to be democratic public spaces. However, we find that similar to the offline world, they are home to increasing gender inequalities. Our study observes online misogyny on Twitter in India to be prevalent, with increasing trends since COVID-19. Around 2% of overall daily tweets between 2018 and 2021 included some form of misogynistic content. This translates to millions of misogynistic tweets every day, given that around 20 billion tweets are posted daily on average. Our study draws attention to online misogyny as a topic for gender research in India, a country where internet use is on the rise and offline forms of gender-based violence are highly prevalent.
We find evidence in support of increasing trends in overall volume as well as proportion of misogynistic content on Twitter since the pandemic. However, our results show an immediate drop in the proportion of misogynistic tweets after the pandemic, followed by sustained and significant growth in the next two years. This could be because of an increase in tweets related to other relevant topics such as COVID-19, health services, and vaccines, right after the onset of the pandemic. Nonetheless, the sustained increasing trend after the initial drop is worrisome, and warrants further assessments of long-term changes. Our study contributes to the existing literature that shows significant increases in other offline forms of VAW since the pandemic.
We find that online misogyny exists in the form of sexual objectification, sexist abusive content, content that threatens to harm, asserts authority, justifies VAW, and dismisses feminism, with sexual objectification and sexual abuse of women being the most common forms. Multiple prior studies have shown online sexual harassment and victimization to be associated with adverse mental and psychological consequences, particularly for young girls [ 44 , 45 ]. Online sexual harassment is unique compared to offline experiences in that individuals who are not directly attacked by the abusers/social media users, are also exposed to the sexually charged posts. In addition to causing mental distress, for young users of the Internet, exposure to such content might cause endorsements of patriarchal beliefs that view women as sexual beings and normalize VAW, leading to a continuation of the cycle of violence [ 46 , 47 ]. While findings are related to the online world, this is indicative of an ongoing acceptability of sexual harassment in the country [ 48 ]. We find that sexual objectification related tweets also include content that shame women for their real or presumed sexual activity. Our findings show that online abusers often use women’s sexual autonomy as a weapon for demeaning and disrespecting them, further perpetuating adverse gender norms that deny women sexual freedom.
Another manifestation of online misogyny is the dismissal of feminist efforts, and justification of VAW. Resistance to advancement of gender equality is a common feature of the feminist struggle, and like the offline world, it is evidently present in the Indian Twitter space. This ‘backlash’ effect has been documented for offline movements, and it can take different forms such as denial of the issue, derailing of conversation, rejection of men’s responsibility, and discrediting of feminist activities [ 49 ]. Prior research has highlighted the disproportionate amount of online misogyny and violence directed at women politicians, journalists, and women engaging in feminist debate on Twitter. Our analysis did not classify tweets that were directed at specific women, but we found many posts that included words such as “ feminazis ”, and dismissed feminism as a “ problem ” and a “ virus ”. It is likely that such posts were directed at individuals who engage in conversations related to feminism or gender equality. Denial of the problem by focusing on fake rape allegations was another common theme across tweets categorized as justification of VAW. Such content, in addition to spreading disinformation, has the potential to widen digital gender inequalities by acting as triggering content for survivors of gender-based violence.
Currently, there are no dedicated legislations in India against gender-based cyber violence [ 50 ]. The laws protecting citizens from cyber hate do not specifically recognize sexist or misogynistic trolling. Our findings show that along with strengthening of laws and regulations to prevent online misogyny, shifting entrenched gender norms will be critical to achieving gender equality in digital spaces. Norms-based interventions addressing gender-based violence in general can consider inclusion of elements of digital violence in their programs.
In addition to providing insights on the prevalence and characteristics of online misogyny, our work is one of the first attempts to detect misogyny across a very large dataset of tweets from India. We tested multiple machine learning models, and found the pre-trained language model, BERT, to be the best performing models. However, our analysis is limited to English tweets only, and does not cover tweets in any Indian vernacular language. Future studies should consider building multilingual models to provide improved insights related to misogyny on Twitter in India.
Our study has a few additional limitations. First, our findings are likely to have an urban bias, representative of the English-speaking population, since our data was limited to English tweets only. Next, this study only includes geotagged tweets from India, which constitutes around 2% of the overall tweets. However, few prior studies have noted that the large number of tweets created daily ensure the adequateness of geotagged tweets in being a good representation of overall Twitter conversations [ 51 , 52 ]. Third, we examine the changes in prevalence of misogyny before and after the start of the pandemic. We do not take into account other relevant key events that might trigger changes in the amount of misogynistic expression. Finally, our study focusses solely on the misogyny directed against women in India, and does not cover transmisogyny or different intersectional categories as relates to race, ethnicity, age, and sexual orientation. Future research should consider including these aspects, given that younger individuals, and individuals belonging to minority groups are more likely to experience violence online [ 5 ].
Conclusions
The past couple of years have noted increasing activism against the growing levels of misogyny on Twitter as well as other social media platforms. Our findings emphasize the need for inclusion of digital violence in the broader policy discourse on gender-based violence; online misogyny is a continuum of violence experienced by women in their offline worlds in India. Aggressive and hateful tweets that target women in the country attempt to reinforce traditional gender norms, especially those relating to idealized sexual behavior and framing of women as sexual beings. Our study provides evidence on increasing trends of misogyny on Indian Twitter, and emphasizes the utility of machine learning methods in examination of aspects related to VAW, which can support future research and development of interventions to make these digital spaces equitable and welcome for women.
Supporting information
S1 file. annotation guidelines/manual for undergraduate student coders..
https://doi.org/10.1371/journal.pone.0292121.s001
S2 File. Definition and example tweet for each category of online misogyny.
https://doi.org/10.1371/journal.pone.0292121.s002
Acknowledgments
We are grateful to Dr. James Fowler who supported us in accessing the Twitter data. We thank Riley Saham, Mairen Oates, Aviram Raj-Silverman, Lucas Fowler, and Max Goldberg, for serving as qualitative coders of the Twitter dataset. We are grateful to Wendy Wei Cheung for carrying out the qualitative coding of misogynist tweets.
- 1. Sobieraj S. Credible threat: Attacks against women online and the future of democracy: Oxford University Press; 2020.
- 2. The Economist Intelligence Unit (EIU). Measuring the prevalence of online violence against women New York: The Economist Intelligence Unit; 2020 [ https://onlineviolencewomen.eiu.com/ .
- 3. Amnesty International. Amnesty reveals alarming impact of online abuse against women: Amnesty International; 2017 [ https://www.amnesty.org/en/latest/news/2017/11/amnesty-reveals-alarming-impact-of-online-abuse-against-women/ .
- 4. Amnesty TT. A Toxic Place for Women, 2018.
- 5. deSHAME P. Young people’s experiences of online sexual harassment. ChildNet; 2017.
- View Article
- Google Scholar
- 7. Amnesty I. Toxic Twitter: a Toxic Place for Women. Report. 2018.
- 9. Pew Research Center. Pew Report on Online Harassment 2017. 2017.
- 10. UN Women. Online and ICT facilitated violence against women and girls during COVID-19. 2020.
- 11. Wang S, Affoum N. Cyber harassment: A growing concern in the age of COVID. In: World Bank, editor. 2021.
- 13. Jeremy Liebowitz, Geoffrey Macdonald, Vivek Shivaram, Vignaraja S. The Digitalization of Hate Speech in South and Southeast Asia: Conflict-Mitigation Approaches. Georgetown University of International Affairs2021.
- 14. Minority Rights. South Asia State of Minorities Report 2021 –Hate Speech against Minorities. South Asia Collective; 2022.
- 15. National Crime Records B. Crime in India 2016: Statistics. Ministry of Home Affairs, Government of India New Delhi; 2016.
- 16. IIPS & ICF. National Family Health Survey (NFHS-4) 2015–16. Mumbai, India; 2017.
- 17. Ananya Bhattacharya. In India, the internet is a place for cityfolk and men. Quartz India. 2018.
- 20. UN Women. ELIMINATING ONLINE HATE SPEECH TO SECURE WOMEN’S POLITICAL PARTICIPATION. 2021.
- 21. United Nations. United Nations Guidance Note on Addressing and Countering COVID-19 related Hate Speech. United Nations; 2020.
- PubMed/NCBI
- 23. Von Behr I. Radicalisation in the digital era: The use of the internet in 15 cases of terrorism and extremism. 2013.
- 27. National Democratic Institute. Tweets That Chill: Analyzing Online Violence Against Women in Politics. 2019.
- 30. Hewitt S, Tiropanis T, Bokhove C, editors. The problem of identifying misogynist language on Twitter (and other online social spaces)2016.
- 32. Anzovino M, Fersini E, Rosso P, editors. Automatic identification and classification of misogynistic language on twitter2018: Springer.
- 35. Becker M. Patriarchy and inequality: Towards a substantive feminism. U Chi Legal F. 1999:21.
- 36. Manne K. Down girl: The logic of misogyny: Oxford University Press; 2017.
- 37. Dehingia N, Lundgren R, Dey AK, Raj A. Trends in online misogyny before and during the COVID-19 pandemic: Analysis of Twitter data from five South-Asian countries. Center on Gender Equity and Health2021. p. 1–4.
- 38. Siegel A, Sterling J, Pullen B, Bonneau R, Nagler J, Tucker J. Trumping Hate on Twitter? Online Hate Speech and White Nationalist Rhetoric in the 2016 US Election Campaign and its Aftermath. 2017.
- 41. Dey L, Chakraborty S, Biswas A, Bose B, Tiwari S. Sentiment analysis of review datasets using naive bayes and k-nn classifier. arXiv preprint arXiv:161009982. 2016.
- 42. Joachims T, editor Text categorization with support vector machines: Learning with many relevant features. European conference on machine learning; 1998: Springer.
- 43. Devlin J, Chang M-W, Lee K, Toutanova K. Bert: Pre-training of deep bidirectional transformers for language understanding. arXiv preprint arXiv:181004805. 2018.
- 50. Anita Gurumurthy, Chami N. Digital India as if women matter: A policy discussion paper. India Office of the Heinrich Böll Foundation; 2018.

IMAGES
VIDEO
COMMENTS
Your Methods Section contextualizes the results of your study, giving editors, reviewers and readers alike the information they need to understand and interpret your work. Your methods are key to establishing the credibility of your study, along with your data and the results themselves. A complete methods section should provide enough detail ...
The Methods section of a research article includes an explanation of the procedures used to conduct the experiment. For authors of scientific research papers, the objective is to present their findings clearly and concisely and to provide enough information so that the experiment can be duplicated. Research articles contain very specific ...
Methodological studies - studies that evaluate the design, analysis or reporting of other research-related reports - play an important role in health research. They help to highlight issues in the conduct of research with the aim of improving health research methodology, and ultimately reducing research waste.
Writing an excellent methodology is a challenging but essential aspect of reporting & publishing academic or scientific research in a journal article. This discussion lists the key ingredients common to most research methodologies, discusses journal guidelines & requirements, & offers helpful writing tips for authors.
Methodology. The methodology section or methods section tells you how the author (s) went about doing their research. It should let you know a) what method they used to gather data (survey, interviews, experiments, etc.), why they chose this method, and what the limitations are to this method. The methodology section should be detailed enough ...
Methodological Innovations is an international, open access journal and the principal venue for publishing peer-reviewed, social-research methods articles. Methodological Innovations is the forum for methodological advances and debates in social research … | View full journal description. This journal is a member of the Committee on ...
While many books and articles guide various qualitative research methods and analyses, there is currently no concise resource that explains and differentiates among the most common qualitative approaches. We believe novice qualitative researchers, students planning the design of a qualitative study or taking an introductory qualitative research course, and faculty teaching such courses can ...
There is a continuing need to support researchers in the creation of innovative research questions and hypotheses, as well as for journal articles that carefully review these elements.1 When research questions and hypotheses are not carefully thought of, unethical studies and poor outcomes usually ensue. Carefully formulated research questions ...
This article provides an overview of writing for publication in peer-reviewed journals. While the main focus is on writing a research article, it also provides guidance on factors influencing journal selection, including journal scope, intended audience for the findings, open access requirements, and journal citation metrics.
The bibliographies of the searched articles were specifically searched for missing manuscripts from the search engines and manually from the print journals in the library. The following text highlights/describes the basic essentials of methodology which needs to be adopted for conducting a good research.
The Methodology section portrays the reasoning for the application of certain techniques and methods in the context of the study. For your academic article, when you describe and explain your chosen methods it is very important to correlate them to your research questions and/or hypotheses. The description of the methods used should include ...
The purpose of a Methods section is to describe how the questions/knowledge gap posed in the Introduction were answered in the Results section. Not all readers will be interested in this information. For those who are, the Methods section has two purposes: 1. Allow readers to judge whether the results and conclusions of the study are valid.
This review aims to synthesize a published set of evaluative criteria for good qualitative research. The aim is to shed light on existing standards for assessing the rigor of qualitative research encompassing a range of epistemological and ontological standpoints. Using a systematic search strategy, published journal articles that deliberate criteria for rigorous research were identified. Then ...
many different venues (e.g., journal articles on empirical studies, review articles, conference symposium presentations, poster sessions). Communication also includes the media (dissemination of information to the public via TV, radio, and the web). Communication of findings has its own responsibilities and chal-lenges, as discussed later.
That is, to review every single article that could be relevant to the topic is simply not possible, so a different strategy must be developed. There are several examples of articles using this approach published in business journals (e.g., McColl-Kennedy et al., 2017). Besides the aim of overviewing a topic, a semi-systematic review often looks ...
Three high-impact qualitative methods journals were searched to locate case studies published in the past 5 years; 34 were selected for analysis. Articles were categorized as health and health services (n=12), social sciences and anthropology (n=7), or methods (n=15) case studies. The articles were reviewed using an adapted version of ...
Background Methodological studies - studies that evaluate the design, analysis or reporting of other research-related reports - play an important role in health research. They help to highlight issues in the conduct of research with the aim of improving health research methodology, and ultimately reducing research waste. Main body We provide an overview of some of the key aspects of ...
Advances in Social Sciences Research Journal, 7(3) 296-302. Practical Research Methods. Jan 2002; Catherine Dawson; ... The following research methods were used in writing the article: analysis of ...
About the journal. Methods publishes original review and research articles that cover emerging methodology in many areas of life and health sciences. The research areas covered by Methods include, but are not limited to, • Biochemistry and biophysics. • Cell, molecular, and developmental biology and genetics.
Mimansha Patel et.al. Exploring Research Methodology: Review Article International Journal of Researc h & Review (www.ijrrjournal.com) 51 Vol.6; Issue: 3; March 2019 with the problem. For this purpose, the abstracting and indexing journals and published or unpublished bibliographies are the first place to go to. Academic journals, conference ...
Although the existence of multiple approaches is a powerful source in the development of a research design, new public administration (PA) researchers and students may see it as a source of confusion because there is a lack of clarity in the literature about the approaches to research design, research methods, and research methodology in the ...
A research approach is a plan of action that gives direction to conduct research. systematically and efficientl y. There are three main research approaches as (Creswell 2009): i) quantitative ...
2.1 Weighted quantile sum regression. Weighted quantile sum (WQS) regression is a convenient tool for addressing effect estimation problem, the high-dimensional and highly correlated issues among multiple pollutants, particularly among homogenous pollutants ().It is widely used in studies of environmental exposure to multi-pollutant mixtures and enables the identification of high-risk factors.
Classical metabolomic and new metabolic network methods were used to study the developmental features of autism spectrum disorder (ASD) in newborns (n = 205) and 5-year-old children (n = 53).
Methods. A comprehensive literature search was conducted from September 14, 2022, to November 22, 2022, encompassing studies, reviews, and other evidence on human subjects that were published in English from PubMed, EMBASE, the Cochrane Library, the Agency for Healthcare Research and Quality, and other selected databases relevant to this guideline.
Soybeans are the number one source of plant proteins for food and feed, but the natural presence of protein protease inhibitors (PIs), namely, the Kunitz trypsin inhibitor (KTI) and the Bowman-Birk inhibitor (BBI), exerts antinutritional effects. This communication describes a new methodology for simultaneously quantitating all parameters of PIs in soybeans. It consists of seven steps and ...
Sage Research Methods & Evaluation is at the forefront of research and scholarship, providing classic and cutting-edge books, video collections, reference materials, cases built on real research, key journals, and our online platform for the community Methodspace.. Download special issues, collections, and a selection of most read articles.Browse our journal portfolio.
Accurate and precise detection of circular RNA (circRNA) is imperative for its clinical use. However, the inherent challenges in circRNA detection, arising from its low abundance and potential interference from linear isomers, necessitate innovative solutions. In this study, we introduce, for the first time, the ap
Most aerospace turbine casings are mechanised using a vertical lathe. This paper presents a tool wear monitoring system using computer vision that analyses tool inserts once that the machining process has been completed. By installing a camera in the robot magazine room and a tool cleaning device to remove chips and cooling residuals, a neat tool image can be acquired. A subsequent Deep ...
Background Online misogyny is a violation of women's digital rights. Empirical studies on this topic are however lacking, particularly in low- and middle- income countries. The current study aimed to estimate whether prevalence of online misogyny on Twitter in India changed since the pandemic. Methods Based on prior theoretical work, we defined online misogyny as consisting of six ...