When you choose to publish with PLOS, your research makes an impact. Make your work accessible to all, without restrictions, and accelerate scientific discovery with options like preprints and published peer review that make your work more Open.
- PLOS Biology
- PLOS Climate
- PLOS Complex Systems
- PLOS Computational Biology
- PLOS Digital Health
- PLOS Genetics
- PLOS Global Public Health
- PLOS Medicine
- PLOS Mental Health
- PLOS Neglected Tropical Diseases
- PLOS Pathogens
PLOS Sustainability and Transformation
- PLOS Collections

Precision agriculture
PLOS publishes a broad range of Open interdisciplinary precision agriculture research that is essential to the wider understanding, advancement and adoption of precision agriculture practices and technology.
Discover precision agriculture research from PLOS
PLOS’ precision agriculture research explores and assesses the very latest agricultural technologies. Whether in controlled environments or directly in the field, our research highlights new methods and technologies for agricultural surveillance and intervention, such as sensors and chemical testing, or high-tech farm machinery and machine learning that measures, analyses, and improves crop yield, soil quality, and animal husbandry practices.
These efforts are fundamental to guaranteeing food security and sustainable crop production in the context of rising demand and increasingly challenging farming environments.
Stay up-to-date on precision agriculture research from PLOS
Research spotlights
As a leading publisher in the field, these articles showcase research that has influenced academia, industry and/or policy.

Role of biotechnology in creating sustainable agriculture

Maize yield in smallholder agriculture system—An approach integrating socio-economic and crop management factors

Forecasting severe grape downy mildew attacks using machine learning
Precision agriculture research topics.
PLOS publishes research across a broad range of topics. Take a look at the latest work in your field.
Data visualization
Digital soil mapping
Food security
Genetic engineering
Internet of Things (IoT)
Unmanned Aerial Vehicles (UAV)
Machine learning
Remote sensing
Robotic systems
Sustainable agriculture
Read the latest research developments in your field
Study the latest insights, technologies, and strategies that are shaping the global agricultural landscape and helping drive sustainable, efficient farming practices.
A meta-analysis of the adoption of agricultural technology in Sub-Saharan Africa
A multi-omics approach to solving problems in plant disease ecology
An embedded system for the automated generation of labeled plant images to enable machine learning applications in agriculture
A novel semi-supervised framework for UAV based crop/weed classification
Estimation of forage biomass and vegetation cover in grasslands using UAV imagery
Real-time recognition of spraying area for UAV sprayers using a deep learning approach
Fruit classification using attention-based MobileNetV2 for industrial applications
Harnessing digital technology to improve agricultural productivity?
Pheno4D: A spatio-temporal dataset of maize and tomato plant point clouds for phenotyping and advanced plant analysis
Browse the full PLOS portfolio of Open Access precision agriculture articles
14,898 authors from 131 countries chose PLOS to publish their precision agriculture research*
Reaching a global audience, this research has received over 4,000 news and blog mentions ^ , research in this field has been cited 80,259 times after authors published in a plos journal*, related plos research collections.
Covering a connected body of work and evaluated by leading experts in their respective fields, our Collections make it easier to delve deeper into specific research topics from across the breadth of the PLOS portfolio.
Check out our highlighted PLOS research Collections:

Future Crops

Plant Phenomics and Precision Agriculture

Sustainable Cropping
Stay up-to-date on the latest precision agriculture research from PLOS
Related journals in precision agriculture
We provide a platform for precision agriculture research across various PLOS journals, allowing interdisciplinary researchers to examine smart farming techniques through various academic lenses, enabling a more collaborative and holistic understanding of precision agriculture worldwide.
*Data source: Web of Science . © Copyright Clarivate 2023 | January 2004 – December 2023 ^Data source: Altmetric.com | January 2004 – December 2023
Breaking boundaries. Empowering researchers. Opening science.
PLOS is a nonprofit, Open Access publisher empowering researchers to accelerate progress in science and medicine by leading a transformation in research communication.
Open Access
All PLOS journals are fully Open Access, which means the latest published research is immediately available for all to learn from, share, and reuse with attribution. No subscription fees, no delays, no barriers.
Leading responsibly
PLOS is working to eliminate financial barriers to Open Access publishing, facilitate diversity and broad participation of voices in knowledge-sharing, and ensure inclusive policies shape our journals. We’re committed to openness and transparency, whether it’s peer review, our data policy, or sharing our annual financial statement with the community.
Breaking boundaries in Open Science
We push the boundaries of “Open” to create a more equitable system of scientific knowledge and understanding. All PLOS articles are backed by our Data Availability policy, and encourage the sharing of preprints, code, protocols, and peer review reports so that readers get more context.
Interdisciplinary
PLOS journals publish research from every discipline across science and medicine and related social sciences. Many of our journals are interdisciplinary in nature to facilitate an exchange of knowledge across disciplines, encourage global collaboration, and influence policy and decision-making at all levels
Community expertise
Our Editorial Boards represent the full diversity of the research and researchers in the field. They work in partnership with expert peer reviewers to evaluate each manuscript against the highest methodological and ethical standards in the field.
Rigorous peer review
Our rigorous editorial screening and assessment process is made up of several stages. All PLOS journals use anonymous peer review by default, but we also offer authors and reviewers options to make the peer review process more transparent.
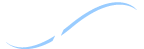
U.S. Government Accountability Office
Precision Agriculture: Benefits and Challenges for Technology Adoption and Use
Precision agriculture uses technologies such as GPS or automation to make farms more efficient. For example, farmers can use auto-steering equipment to precisely plant a field, and activity monitors can help dairy farmers collect data about their cows' health.
In this Technology Assessment , we review the benefits and challenges of precision agriculture technologies. They can make farms more profitable and have environmental benefits—like reducing fertilizer runoff in waterways. But they can also be complex and have high up-front costs, making it hard for some farmers to access them. We examine policy options to help address these challenges.
Artist’s concept of an autonomous tractor working in a corn field
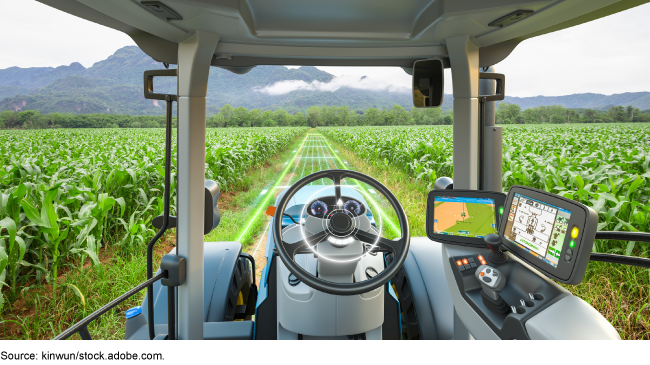
What GAO Found
Precision agriculture technologies can improve resource management through the precise application of inputs, such as water, fertilizer, and feed, leading to more efficient agricultural production. Precision agriculture can be implemented through a suite of technologies that can be used in isolation or in conjunction with other technologies. Examples of emerging precision agriculture technologies are in the table below.
Examples of emerging precision agriculture technologies
Source: GAO summary of literature, interviews, and agency documentation. | GAO-24-105962
While precision agriculture technologies, such as variable rate fertilizer applications and yield monitoring, have been available since the 1990s, only 27 percent of U.S. farms or ranches used precision agriculture practices to manage crops or livestock, based on 2023 U.S. Department of Agriculture (USDA) reporting.
Use of precision agriculture practices by U.S. farms, June 2022–June 2023
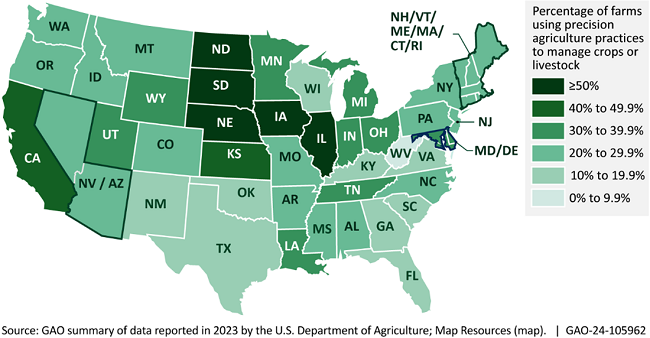
Federal agencies support precision agriculture adoption, research and development, education, and training. USDA supports precision agriculture technology adoption with financial assistance and loan programs, such as through payments for implementing practices that provide a conservation benefit. USDA and the National Science Foundation (NSF) have provided almost $200 million for precision agriculture research and development funding in fiscal years 2017—2021. This funding includes partnerships between the two agencies to support artificial intelligence (AI) research institutes.
Benefits to using precision agriculture technologies include:
- Increased profits. Farmers can increase yields and thus profits with the same amount of inputs or achieve an equivalent yield with fewer inputs.
- Reduced application of crop inputs. Technologies can reduce the application of crop inputs such as fertilizer, herbicide, fuel, and water. They can also address water scarcity by promoting the efficient use of water in agriculture.
- Environmental benefits. Technologies can prevent excessive use of chemicals and nutrients in a field, potentially reducing runoff into soil and waterways.
Challenges limiting the broader adoption and use of precision agriculture include:
- High up-front acquisition costs. Acquisition costs for the latest technologies can be prohibitive for farmers with limited resources or access to capital.
- Farm data sharing and ownership issues. Concerns regarding farm data sharing and ownership can pose obstacles to the widespread use of AI in agriculture.
- Lack of standards. An absence of uniform standards can hamper interoperability between different precision agriculture technologies.
GAO examined three policy goals and associated options that could help address adoption challenges or enhance the benefits of precision agriculture technologies. These policy options identify possible actions by policymakers, which include Congress, federal agencies, state and local governments, academic and research institutions, and industry. In addition, for each policy goal, policymakers may choose no additional policy interventions, maintaining the status quo by continuing existing activities.
Policy Goals and Options That Could Address Challenges or Help Enhance Benefits of Adoption and Use of Precision Agriculture Technologies
Source: GAO. | GAO-24-105962
Why GAO Did This Study
Precision agriculture involves collecting, analyzing, and taking actions based on data. It can help the agricultural sector meet increasing demand for food products, while also helping farmers improve efficiencies such as through reduced input costs.
The Advancing IoT for Precision Agriculture Act of 2021, contained in what is commonly referred to as the CHIPS and Science Act of 2022, included provisions for GAO to conduct a technology assessment and review federal programs.
This report examines (1) emerging precision agriculture technologies and precision agriculture technology adoption; (2) federal programs providing support for precision agriculture; (3) benefits and challenges of adopting and using precision agriculture technologies; and (4) policy options that could address challenges or help enhance benefits of adopting and using precision agriculture technologies.
To conduct this technology assessment and review, GAO reviewed scientific literature and other key reports; interviewed officials and representatives from government, industry, academia, and associations; conducted two site visits to observe technology operations and obtain stakeholder perspectives; and convened a 3-day virtual expert meeting in collaboration with the National Academies of Sciences, Engineering, and Medicine. GAO is identifying policy options in this report.
For more information, contact Brian Bothwell at (202) 512-6888 or [email protected] , or Steve D. Morris at (202) 512-3841, [email protected] .
Full Report
Gao contacts.
Steve Morris Director [email protected] (202) 512-3841
Brian Bothwell Director [email protected] (202) 512-6888
Office of Public Affairs
Chuck Young Managing Director [email protected] (202) 512-4800

An official website of the United States government
Here's how you know
Official websites use .gov A .gov website belongs to an official government organization in the United States.
Secure .gov websites use HTTPS A lock ( Lock Locked padlock ) or https:// means you’ve safely connected to the .gov website. Share sensitive information only on official, secure websites.
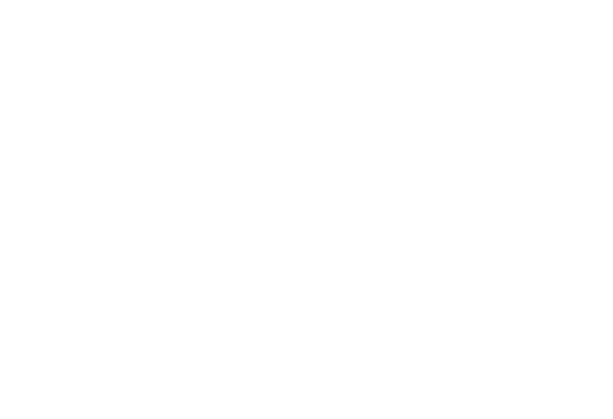
Precision Agriculture
Applying the right resources in the right place at the right time can be a win-win for farmers and the environment. This approach, known as precision agriculture , lets farmers use cutting-edge tools to get specific, detailed information on individual bits of land or even plants in their fields. This knowledge enables them to apply only as much fertilizer, water, or other inputs as necessary – producing better results for them and their crops. Overall, researchers have found that using these methods costs no more than traditional approaches, but produces more consistent crop yields, and improves soil and water quality by reducing excess nutrient run-off from fertilizer. ARS conducts research on precision agriculture as part of its long-term agroecological research project, which has locations throughout the country.
The secret to precision agriculture is understanding that plants, and land, are not all the same; some parts of a field may require more or less fertilizer, for instance. By figuring out what is, or isn’t, needed, and responding appropriately, farmers use scarce resources like water most effectively. Watch our video to learn more about this innovative new approach that’s helping farmers grow their crops more efficiently – and sustainably.

An official website of the United States government
The .gov means it’s official. Federal government websites often end in .gov or .mil. Before sharing sensitive information, make sure you’re on a federal government site.
The site is secure. The https:// ensures that you are connecting to the official website and that any information you provide is encrypted and transmitted securely.
- Publications
- Account settings
Preview improvements coming to the PMC website in October 2024. Learn More or Try it out now .
- Advanced Search
- Journal List
- Animals (Basel)

Precision Agriculture for Crop and Livestock Farming—Brief Review
António monteiro.
1 Agrarian Superior School, Polytechnic Institute of Viseu, Quinta da Alagoa, 3500-606 Viseu, Portugal; moc.liamg@sotssevlaoigres
2 CERNAS, Research Centre for Natural Resources, Environment and Society, Polytechnic Institute of Viseu, Polytechnic Campus, 3500-606 Viseu, Portugal
Sérgio Santos
Pedro gonçalves.
3 Escola Superior de Tecnologia e Gestão de Águeda and Instituto de Telecomunicações, Campus Universitário de Santiago, Universidade de Aveiro, 3810-193 Aveiro, Portugal; tp.au@gsap
Associated Data
Not applicable.
Simple Summary
Precision agriculture has the potential to contribute to the broader objective of meeting the growing demand for food, ensuring the sustainability of primary production, based on a more accurate and resource-efficient approach to crop and livestock management. The aim of this paper consists of a brief review of the recent scientific and technological tools and sensors in precision agriculture and their application in crop and livestock farming. This literature review allowed us to realize that precision agriculture has been proven to be a highly researched and constantly evolving area due to the needs of farmers to use resources in a more optimized way.
In the last few decades, agriculture has played an important role in the worldwide economy. The need to produce more food for a rapidly growing population is creating pressure on crop and animal production and a negative impact to the environment. On the other hand, smart farming technologies are becoming increasingly common in modern agriculture to assist in optimizing agricultural and livestock production and minimizing the wastes and costs. Precision agriculture (PA) is a technology-enabled, data-driven approach to farming management that observes, measures, and analyzes the needs of individual fields and crops. Precision livestock farming (PLF), relying on the automatic monitoring of individual animals, is used for animal growth, milk production, and the detection of diseases as well as to monitor animal behavior and their physical environment, among others. This study aims to briefly review recent scientific and technological trends in PA and their application in crop and livestock farming, serving as a simple research guide for the researcher and farmer in the application of technology to agriculture. The development and operation of PA applications involve several steps and techniques that need to be investigated further to make the developed systems accurate and implementable in commercial environments.
1. Introduction
Agriculture has played a key role in the global economy in recent years [ 1 ]. Estimates show that current agricultural production must increase 60–100 percent with everything else unchanged to meet the nutritional needs of a future human population of 9–10 billion. In addition, agricultural intensification over the last few decades has had negative environmental impacts [ 2 ]. As a result, the pressure on the agricultural system is greater than ever before [ 1 ]. In order to minimize these issues, traditional agricultural management methods have been complemented by new sensing and driving technologies and improved information and communication technologies (ICT) [ 3 ]. Based on the concept of “produce more with less” [ 4 ], precision agriculture, also known as precision farming or smart farming, has the potential to contribute to the wider goal of meeting the increasing demand for food whilst ensuring the sustainability of primary production, based on a more precise and resource-efficient approach to production management [ 5 ].
PA technologies are used in the important stages of the crop growth cycle (soil preparation, seeding, crop management, and harvesting). However, it is not just crop and fruit farming that has benefited from precision farming technologies—farmers engaged in livestock rearing are also experiencing the positive benefits derived from precision farming technologies [ 5 ]. PA could be divided into two categories: precision crop farming, which consists of the application of precision farming technologies to manage spatial and temporal variability for improving crop performance and environmental quality, and PLF, which is based on the use of advanced technologies to optimize the contribution of each animal. Through this “per animal” approach, the farmer aims to achieve better results in livestock farming [ 4 ]. Precision crop farming and PLF are currently being shaped by two major technological trends: big-data and advanced-analytics capabilities on the one hand, and aerial imagery, feeding and milking robots, and intelligent sensors, on the other [ 6 ].
The current paper aims to briefly review the recent scientific and technological trends of precision farming and its application in crop and livestock farming. This study can serve as a research guide for both the researcher and the farmer in applying technology to agriculture. The remainder of this paper is organized as follows. Section 2 presents precision crop farming including different farming activities such as soil monitoring, precision seeding, smart irrigation and fertilization, and grass yield monitoring. It is also an approach to farm machinery and its important role in precision farming. Section 3 presents the PLF and scientific and technological developments concerning animal behavior, welfare, feed and live weight measurement, and automatic milking systems. In Section 4 , slight considerations are made based on the risks and concerns inherent to precision farming. Finally, in Section 4 , we present the conclusions.
2. Precision Crop Farming
Precision crop farming or site-specific crop management is a concept based on sensing or observing and responding with management actions to spatial and temporal variability in crops. The “sensing” component of the concept is a fundamental element of precision crop farming [ 7 ]. Sensors in fields and crops are starting to provide granular data points on soil conditions as well as detailed information on climate, fertilizer requirements, water availability, and pest infestations. In addition, aerial images captured by non-aircraft vehicles, crews, or drones can patrol fields, alert farmers to crop maturation or potential problems, and provide early warning of deviations from expected growth rates or quality. Satellites can also be at the service of precision crop farming, facilitating the detection of relevant changes in the field by using satellite imagery [ 8 ]. In this section, we introduce some of the scientific research and technological developments involved with smart crop farming ( Table 1 ) as well as the important role of machinery in precision farming.
Overview of precision crop farming technology and applications.
2.1. Evaluation of Soil Properties by Sensor Measurements
There are a range of sensors available used to measure and calculate the parameters of an agricultural field, namely the soil sensors [ 9 ], which can save labor and be a useful management tool, often providing more timely results, if they are accurate and the data are correctly interpreted [ 10 ].
Soil electrical conductivity (ECa) sensors measure the soil solute concentration while assessing the soil salinity hazard [ 11 ]. Mobile measurements of ECa have become widely used to map soil variability. The greatest potential use of ECa scanning is in the survey of spatial soil variability and delineating potential site-specific management zones. This, in turn, would allow for better resource allocation and long-term management planning [ 10 ]. Soil water content sensors such as frequency domain reflectometry (FDR) or time domain reflectometry (TDR) sensors measure the amount of water (volume or mass) contained in a unit volume or mass of soil by using electrodes. It is expressed by the change in capacitance value, which depends on the dielectric constant of the soil. It can range from 0 (completely dry) to the value of the materials’ porosity at saturation [ 12 ]. The sensor must be calibrated for each location because the measurements depend on the type of soil [ 9 ]. Soil moisture content sensors (or “volumetric water content sensors”) such as tensiometers evaluate the soil water tension or suction, which is a denotation of the plant root system effort while extracting water from the soil. It can be used to estimate the amount of stored water in the soil or how much irrigation is required to reach a desired amount of water in the soil [ 13 ]. Soil moisture content can also be determined by Photodiode, an optical sensor that uses the light to measure soil properties, namely clay, organic matter, and moisture content of the soil [ 9 ]. Smolka et al. [ 14 ] recently presented a mobile sensor aimed for on-site analysis of soil sample extracts used to detect the primary plant nutrients in their available form, at a fraction of the time and cost associated with traditional laboratory soil analysis. The sensor was particularly appropriate for the analysis of NO 3 , NH 4 , K, and PO 4 and followed on from previous studies exploring the potential for on the-go soil sampling for nitrate using electrochemical sensor platforms and ion-selective electrodes [ 10 ]. There are also other types of sensors such as ground penetrating radar (GPR) and gamma ray spectrometry (GRS), which can be used through the ground cover vegetation. GPR data were correlated with soil hydrology parameters, and GRS data were related to some soil nutrients and other soil texture characteristics. Sensors based on optical reflectance as well as multi-spectral and hyperspectral sensors also have good correlation with soil properties [ 15 , 16 ]. Additionally, researchers from the EU-funded MISTRALE project have designed a system that can measure soil moisture from a drone flying at low altitude using Global Navigation Satellite Systems (GNSS) reflectometry. The system, still a prototype, can produce high-resolution maps of soil moisture by harnessing signals from either the Galileo or GPS global satellite systems. This could help farmers make better decisions about when and where to irrigate, and to help water managers understand weather events such as flooding and water logging [ 5 ].
2.2. Precision Seeding
Precision seeding has become the main feature and developing direction of modern seeding technologies in recent years. Precision seeding can save seeds and effectively control the sowing depth, densities, or distance. According to statistics, the output of precision seeding increases by 10–30% compared with that of the conventional drill [ 17 ]. Recent research has been carried out in order to make the sowing process more precise and efficient.
One of the most important aspects to achieve the consistent emergence of the crop desired is the use of a uniform seeding depth. Consistent crop emergence influences final crop yields, as the seedling growth process affects the variability of crop biomass. In this sense, Nielsen et al. [ 18 ] developed and studied a proof-of-concept dynamic coulter depth control system for a low-cost seed drill in a field experiment. The performance of the active control system was evaluated based on coulter depth measurements, obtained by coulter position sensors combined with ultrasonic soil surface sensors. The system was found to be capable of maintaining a more accurate and stable coulter depth across the field [ 18 ].
In light of the problem that small seeds are not easy for mechanized seeding at present, Jin et al. [ 19 ] developed and tested an electric seeder for small-size vegetable seeds based on the power drive and optical fiber detection technology. The system can perform precision seeding and real-time monitoring of the quality of seeding, and can complete the functions of furrow, seeding, and repression at a time, improving the quality of field operation and the accuracy of monitoring the seeding effect.
Due to the particular characteristics of the wheat seed grain geometry, Haibo et al. [ 20 ] designed and developed a wheel mobile robot for wheat precision seeding. A kinematic model was built for the four-wheel drive robot, and some experiments were taken using this machine. It provided a reference for the design and product of the wheat precision seeding robot. The experiment results showed that the qualified rates of seeding exceeded 93% at different sowing speeds.
Jianbo et al. [ 17 ] designed a control system based on a single chip microcomputer that could make the seed-metering device maintain synchronization with the working speed of the seeder. The experimental results proved that the control system was reliable and also showed that uniformity could be maintained between the speed of the seed metering-device and the seeder concerning the amount of seeding.
A high-speed precision seeding device enables one to obtain better seeding quality. This system consists of a seed supply device (including a seed box and a wheel) and a venture tube. In this sense, Gao et al. [ 21 ] designed a new air-assisted high speed precision seed metering device that could solve the problems of short filling time during high-speed operation and reduce the accumulation of seeds in the venturi tube. The results proved that for better design of the quantitative seed feeding system, the proper nozzle convergence angle is 70°, and the feeding angle around 45°.
2.3. Smart Irrigation Systems
The efficient and effective management of water in irrigation is one of the main benefits that comes from precision agriculture technologies [ 4 ], being of critical importance for sustainable agricultural development, food security, and overall economic growth. This is particularly true considering climate changes and the competing demand for water from other economic sectors. Precision irrigation allows farmers to apply a precise amount of water to crops at precise times [ 5 ]. In fact, instead of traditional irrigation, increasing attention has been paid to knowledge-and technology-driven smart irrigation due to advantages such as automatic controllability and feasibility in optimizing crop yield and irrigation water use efficiency (IWUE) [ 22 ].
In recent years, the literature has provided several studies on the optimization of irrigation water management. Ortega et al. [ 23 ] evaluated the effects of different temperatures on greenhouse tomato growth using an automatic irrigation system to suggest an optimal irrigation strategy for improving the IWUE. Goap et al. [ 24 ] proposed an IoT based smart irrigation system along with a hybrid machine learning based approach to predict the soil moisture with very encouraging results. The proposed algorithm uses the sensors’ data of the recent past and the weather forecasted data for the prediction of soil moisture for upcoming days. Furthermore, the prediction approach is integrated into a cost-effective standalone system prototype as it is based on open-source technologies. The auto mode makes it a smart system and it can be further customized for application specific scenarios.
In the south of Italy, Corbari et al. [ 25 ] developed an operational irrigation water management system using satellite LANDSAT data and meteo-hydrological modeling, which is based on the coupling of remote sensing data, a hydrological model of distributed water-energy, and meteorological forecasts. As a result, the authors concluded that operative applications of parsimonious irrigation are feasible by integrating data from satellites for updating and parameterizing the state of the hydrological model and meteorological forecasts, thus improving the management of irrigation scheduling.
Krishnan et al. [ 26 ] proposed a smart irrigation system to help farmers water their agricultural fields using Global System for Mobile Communication (GSM). According to the authors, this system provides a long-term sustainable solution for automatic irrigation control. Based on the data received from the soil moisture sensor, temperature sensor, and rain sensor, water is supplied to the agricultural field, helping in the consumption of water. By using GSM technology, the full system is automated to drastically reduce manual work [ 26 ].
Al-Ali et al. [ 27 ] developed an IoT-based renewable solar energy system important for regions that face water scarcity and power shortages around the world. This system uses a single board system-on-a-chip controller, which has built-in WiFi connectivity, and connections to a solar cell to provide the required operating power. The controller reads the field’s soil moisture, humidity, and temperature sensors, and emits actuation command signals to operate irrigation pumps.
Benyezza et al. [ 28 ] designed an intelligent and low-cost irrigation system based on zoning to make water use and energy consumption more efficient. This system consists of the collection and transmission of data on the soil and environmental conditions to a base station (server) using a wireless sensor network based on radio frequency communication. Since these data were collected and stored on a server, the time needed for irrigation in each zone was calculated using a fuzzy logic controller (FLC). When combining FLC with a zoning strategy, the system showed better results in terms of minimizing water and energy consumption when compared to other methods.
Recently, Liao et al. [ 29 ] developed a smart irrigation system based on real-time soil moisture data to estimate the dynamic crop water uptake depth (WUD) using the spatiotemporal characteristics of soil moisture distributions. According to the authors, this study estimated the tomato WUD from the distribution characteristics of soil moisture in the profile, providing a real-time and effortless method to determine the dynamic designed irrigation depth for guiding irrigation events.
2.4. Smart Fertilization Systems
Precise fertilization techniques are the future of agriculture, in which nutrients are supplied in a controlled way with minimized losses to the environment, caused by over-fertilization and leaching of microelements to deeper soil layers, ground water, and surface water [ 30 ].
As an important part of precision agriculture, variable-rate technology (VRT) offers an effective way to protect the environment and increase economic benefit while farming with site-specific fertilizer inputs. Song et al. [ 31 ] developed a variable-rate fertilizer control system applied to a UAV-based granular fertilizer spreader for automatically adjusting the fertilizer application rate based on a prescription map. The optimized metering apparatus showed high reliability in adjusting the rotational speed of a fluted roller motor to control the application rate as the specific relationship between them. The control system showed good stability with small error and rapid response whenever in a fixed-rate pattern application, where the target discharge rate changed unregularly or in a variable-rate pattern where the prescription map was given [ 31 ].
To reduce the labor workload and costs, thus increasing the efficiency of the organic fertilizer mixing process, Ishak et al. [ 32 ] developed an improved organic fertilizer mixer based on the Internet of Things technology, which can monitor the status of fertilizer production remotely, thus providing updates and alerts to the farmers. According to the authors, the automated organic fertilizer prototype provides operational cost savings of over five times when compared to current automated systems.
From another aspect, robots are perfectly suited to be used in agricultural operations, particularly in the spraying of fertilizer, which can carry large storage reservoirs, be operated safely and autonomously, and be deployed at a fraction of the costs compared to the traditional methods. In this sense, Ghafar et al. [ 33 ] developed a low-cost agricultural robot to spray fertilizers and pesticides in agriculture fields as well as for general crop monitoring. The prototype system is a two-wheeled robot that consists of a mobile base, a spraying mechanism, a wireless controller to control the movement of the robot, and a camera for crop health and growth monitoring. According to the authors, tests conducted on the agricultural robot prototype showed that it could perform as required under real-world usage scenarios.
Additionally, fertigation technology is recognized as a new agricultural technology with high-efficiency water and fertilizer control. Lin et al. [ 34 ] developed a framework for the IoT-based fertigation system in which both long-term and short-term planning were considered. Based on the framework, an integer linear programming model was developed to allocate limited resources among multiple crops with the goal of maximizing the economic profits and environmental benefits. The results confirmed that the optimization model can promote sustainable irrigation and fertilization management in precision agriculture by offering more economic and environmental benefits than empirical models. In addition, Bai and Gao [ 35 ] proposed an optimization method for the maize nitrogen (N) fertilizer schedule by combining a decision support system for agrotechnology transfer (DSSAT) model with a genetic algorithm, after calibration and validation of the DSSAT model. According to the authors, GA and the DSSAT model can promote the optimized fertilizer schedule.
2.5. Grass Yield Monitoring
Monitoring and predicting above ground biomass yield are of key importance for crop management. According to Beukes et al. [ 36 ], a 15% increase in farm profitability could be achieved by carrying out regular herbage measurements [ 37 ].
Traditional methods of measuring grass yield include destructive grass sampling coupled with weighing systems on mowers, trailers, or balers [ 38 ]. Optical sensing techniques have recently evolved and, according to Schaefer and Lamb [ 39 ], it is now possible to estimate herbage biomass levels by combining a measure of pasture canopy height using light detection and ranging (LiDAR) and pasture canopy reflectance measurements using active optical sensors. Additionally, the prediction accuracy of herbage mass from ultrasonic height measurements was promising and could be improved further by using spectral reflectance signatures in combination with the ultrasonic sensor [ 10 ].
Current developments in unmanned aerial vehicle-based (UAV) sensing systems allow for the acquisition of image data at a ultrahigh spatial resolution for important stages of plant phenological growth [ 11 , 38 ]. Furthermore, new photogrammetric software products support the analysis of such image data to produce 3D point clouds and digital surface models (DSMs). The analysis of multi-temporal DSMs supports the monitoring of sward or crop height development in high spatial resolution [ 39 ]. A research by Lussem et al. [ 40 ] evaluated the potential of sward height metrics derived from low-cost UAV image data to predict forage yield. According to the authors, dry biomass yield can be predicted using sward height derived from multi-temporal DSMs derived from low-cost UAV-based imaging with consistent results over three years. The on-going miniaturization and cost efficiency of sensors and platforms as well as powerful algorithms and computer hardware can open new paths to sustainable grassland management [ 38 ]. In addition, research by Oliveira et al. [ 41 ] showed that technology drone-based spectral remote sensing and photogrammetry could be used to predict the yield with an adequate degree of accuracy and to study the spatial differences within a field and between different fields, thus indicating great potential for remote sensing and drones to support the management of silage production for animal feeding [ 41 ].
The capacity to yield-map crops is relevant and provides a decision support tool for improved crop management. An on-the-go pasture meter was developed in New Zealand to meet this need. The equipment uses optical sensors to determine sward height and needs to be calibrated for herbage density. It can be used as a stand-alone sward meter or with GPS for sward-yield mapping [ 42 ].
Finally, we should also refer to the grass measurement optimization tool (GMOT), which is designed to utilize basic pasture management and geo-spatial information to develop a spatially balanced and non-biased grass measurement protocol. Calibrations to predict herbage mass that were built into the GMOT could utilize herbage management information such as fertilization rates and the number of previously performed paddock grazing or cutting events to increase herbage mass prediction precision [ 37 ].

2.6. Linking Technology to Farm Machinery
Mechanization is one of the main drivers of efficient farming systems, involving numerous operations in the crop’s production cycle and throughout the value chain [ 43 ]. Agricultural mechanization started with steam powered reapers and traction engines, and then advanced with the invention of mobile hydraulics and the electronic control systems that are currently used in modern machinery [ 44 ]. Indeed, mechanized farming has been adopting increased levels of automation and intelligence to improve management and increase productivity in field operations. Nowadays, farmers can use, for example, auto-steered agricultural vehicles for many different field operations including tilling, planting, chemical applications, and harvesting. Intelligent machinery for automated thinning or precise weeding in vegetable and other crops has recently been introduced to farmers [ 45 ].
Recently, the accomplishment of agricultural operations carried-out with tractors has been heading toward the integration of traditional mechanics with geolocation technologies to bring farming procedures closer to the logic of precision agriculture. The possibility of controlling the tractor steering by using GNSS is an important contribution to improving the efficiency of agricultural practices and allows savings of time, fuel, labor, and production factors contributing to the economic and environmental sustainability of the agricultural process [ 46 ]. The automatic steering system or autopilot system tractor combines digital analysis and the image processing method, cameras, and GPS. In general, the system is comprised of two major elements that are hardware (various sensor and actuator such as GPS, a steering angle sensor) and software (path planner, a navigation control and steering controller). With high accuracy in the automated steering system, the farmer is able to automatically run the tractor throughout the desired path without causing any damage to the vegetation while maintaining a wide range of measurement [ 47 ].
The latest developments in ICT and the prevailing lack of interoperability between agricultural tractors, implements, and on-board computers has led to the development of the ISO 11783 (ISOBUS) international standard for securing a more effective communication between these entities. Precision agriculture requires an increasing amount of information in order to be sufficiently managed and the abilities of the ISOBUS protocol is a significant step toward this goal as it will provide a wealth of automated data acquisition for improving the management of crop production [ 48 ].
In addition, mechatronic systems can also play an essential role in modern agricultural machinery, especially on intelligent and robotic vehicles [ 45 ]. The term “agricultural robot” or “agrobot” applies to autonomous machines that are capable of performing different repetitive agricultural tasks on the farm without direct human intervention [ 49 ]. Agricultural robots allow the farmer to reduce inputs—pesticides, herbicides, and fertilizers—with positive implications for the environment. Mechanical weed control is already a reality; other functions under development include the microapplication of inputs and early detection of pests, which will considerably decrease, even eliminate, the need for inputs. Agrobots are also lighter than tractors with implements or specific equipment for spraying or harvesting and can thus alleviate problems associated with soil compaction and are able to access fields not suitable for heavy machinery (e.g., vineyards on slopes or land affected by wet conditions) [ 43 ].
Mechatronics enables the practical implementation of variable rate application (VRA) equipment for precision agriculture. As VRA technology is progressing quickly, intelligent applicators are becoming available commercially. A smart system can automatically adjust the number of inputs dispersed in response to needs, even allowing for the simultaneous use of different types of treatments, resulting in new ways of managing agricultural production. Thus, an intelligent VRA seeder can, for instance, change the number of seeds laid out in the soil according to its potential, either provided by prescription maps or detected using on-board sensors. Control of the seeding rate is achieved by actuating the opening of the distributing device to allow the desired number of seeds to go through. In many cases, a feedback control system is required to achieve accurate control of the application rate. For example, in applying liquid chemicals, the application rate may be affected by changes in the moving speed of the vehicle as well as the environmental conditions. Some smart sprayers are programmed to accurately control the amount of liquid chemical by adjusting the nozzles in response to changes of sprayer forward speed. This is normally accomplished using electronically controlled nozzle valves that are commanded from the on-board processor [ 45 ].
Today’s commercial farmer, who has a full command of the existing farming skills and knowledge, will need to become a sort of information technology (IT) manager operating from an office or in front of a screen (computer, mobile phone, tablet etc.), rather than a machine operator working in the field, handling machine steering, and adjusting equipment manually. For livestock management, skilled operators will still be needed, but with new sets of skills related to ICT and automatization [ 43 ].
3. Precision Livestock Farming
As part of precision farming, managing livestock is one of the current challenges for agriculture [ 50 ]. The term ‘precision livestock farming’ (PLF) appeared in the early 21st century, with the first PLF conference held in 2003 [ 51 ] as an innovative production system approach [ 52 ], playing a key role in the fourth industrial revolution, also known as Industry 4.0 [ 53 ]. PLF is potentially one of the most powerful developments amongst a few interesting new and upcoming technologies that have the potential to revolutionize the livestock farming industries [ 54 ].
PLF uses a combination of tools and methods to measure different variables from each animal with high precision, supporting farmers to make decisions concerning the livestock production systems [ 55 ]. Decisions are often based on the acquisition, collection, and analysis of quantitative data obtained by continuous real-time from animals and the environment [ 56 , 57 ]. These tools include sensor technology cameras [ 58 ], microphones, wireless communication tools, Internet connections, and cloud storage [ 59 ], among others. However, the application of the existing tools for PLF can be challenging under extensive livestock management because this occurs on natural pastures that are large, heterogeneous, and highly dynamic environments [ 55 ]. Therefore, the main purpose of PLF is to enhance farm profitability, efficiency, and sustainability [ 58 , 59 ] by improving on-farm acquisition, management, and utilization of data management and the utilization of data, in order to enhance the nutritional and other management aspects from distinct species of animals [ 59 ]. PLF could also deliver additional food safety, traceability, welfare, and environmental benefits [ 52 , 54 , 59 , 60 ]. In addition, PLF aims the management of crop processes to create perfect synergy with livestock feeding [ 3 ]. If properly implemented, PLF could (a) promote product segmentation and better marketing of livestock products; (b) reduce illegal trading of livestock products; and (c) improve the economic stability of rural areas [ 54 ]. In this section, we present some of the technological advancements and scientific research involved with PLF ( Table 2 ).
Overview of PLF technology and applications.
3.1. Animal Monitoring
Successful grazing and pasture management require an understanding of the adjustment mechanisms behind the grazing behavior [ 61 ] that enables adaptation to grazing conditions [ 62 ]. As well as facilitating the precise management of grazing, the monitoring of animal position, foraging, and other behaviors can bring considerable benefits for animal health and welfare by continuously monitoring each animal in the flock, any small deviation from ‘normal’ behavior (for that individual animal) can be quickly identified and flagged to the farmer [ 63 ].
The use of GNSS technology allows for the characterization of grazing behavior including grazing patterns, paths, and favored areas. Grazing activities can also be differentiated based on the speed of movements. The increased knowledge conveyed using GNSS receptors in grazing sheep can become a valuable tool to support the decisions that are essential to a more precise pasture management [ 62 ].
Tracking location on pasture, through the large dissemination of global positioning system (GPS) sensors have been successfully used to detect static or dynamic unitary behaviors differentiated through changes in path speeds: foraging or grazing, resting, and walking [ 64 ]. Likewise, the use of GPS “collars” for livestock has opened the possibility of recording detailed position data for extended periods of time, thus allowing for a more complete understanding of the habits and causes of the spatial distribution of ruminants. Current GPS technology can determine the position of individual animals with a precision of 10 m or better. The position information can be stored on small flash cards together with substantial amounts of behavior and physiological data and can be transmitted to a management center in real time or in periodical sessions [ 65 ].
Behavior of cattle can also be monitored by means of a collar characterized by a behavior-monitoring series of sensors. Through analysis of rumination rate and the feeding and resting behavior, estrus events can be detected, according to a study by [ 58 ]. Similar collars were used, showing that the highest accuracy was achieved with these instruments (>90%) whereas visual human observation was far less accurate [ 66 ].
Posture analysis was developed using accelerometers and based on the position of the head: up or down. This information, in combination with GPS-based data, allowed for discrimination between several kinds of feeding related behaviors for grazing animals with high accuracies (>90%). These accuracies were obtained with a brief time window of 5 to 10 s while the data acquisition from the GPS and the accelerometer ran between 4 Hz and 10 Hz [ 67 ].
Finally, we monitored cattle movements using accelerometers. Through diverse analysis methods, accelerometers recording data at 10 Hz could be used to classify behaviors using a basic statistical method to classify lame and non-lame cows, reaching an average accuracy of 91% [ 64 ]. Similarly, in [ 67 ], they were classified as multiple behaviors using a machine learning method with accuracies ranging from 29% to 86% with samples windowed for 10 s for all behavior classifications.
Among the solutions to detect the animal behavior and collect data with a reduced uncertainty [ 68 ], image and sound analyses are also promising. However, video recordings require a large amount of time to be analyzed and manually checked, involving potential mismatches in the interpretation of observers. According to Meen et al. [ 69 ], there is a correlation between sounds and behavior, as a significant difference emerged between the average maximum frequency of murmurings during the lying and ruminating phase and that of calls during the other phases [ 66 ].
In addition, a review carried out by Meunier et al. [ 70 ] described machine learning algorithms such as pig-face recognition. Recent advancements in facial recognition have been extended to identify and recognize the patterns of several animal behaviors. Different facial detection and recognition methods such as the VGG-face model, Fisherfaces, and convolutional neural networks can now discern individual animal faces in complex real-time scenarios, in the presence of some shape deformation and even in instances where there is insufficient data. This non-invasive imaging system recognizes the faces of individual pigs in a real farm setting with 96.7% accuracy [ 71 ].
3.2. Animal Health and Welfare
Animal health is of key importance in the livestock industry as it impairs production efficiency through growth retardation or even mortality, animal welfare through pain and discomfort, and it can even impair human health through the misuse of antibiotics or zoonosis [ 72 ]. In fact, the large density of animals living so close to humans in some countries can transfer a high number of zoonosis diseases to humans [ 73 ]. The monitoring of health problems in the early detection of clinical signs of diseases on the farm is one of the key issues from which PLF has arisen [ 66 ]. Most diseases are easily treated when detected in an early phase, although prevention is always the priority [ 72 ]. Modern technologies such as sensors, big data, artificial intelligence (AI), and machine learning (ML) algorithms enable farmers to react to diseases after they become evident, or pro-actively using vet services, and also provide an opportunity to constantly monitor key animal health parameters such as movement, air quality, or consumption of feed and water. By constantly collecting these data and using advanced technology to predict deviations or abnormalities, farmers can identify, predict, and prevent disease outbreaks. Therefore, this technology has a significant cost advantage over older detection methods [ 71 ].
Animals can be monitored by methods based on the sound, with the potential to be automated for large-scale farming [ 70 ]. A sound-based tool (Pig Cough Monitor™ (PCM), Soundtalks ® , and Fancom B.V.) has been developed for automated pig cough detection that is based on a mathematical algorithm that processes all incoming sound and identifies the number of coughs automatically [ 69 ]. In addition, Van Hertem et al. [ 74 ] evaluated the effect of using a microphone and subsequent advanced methods for labelling in the early detection of cough in calves and highlighted how the adoption of an algorithm with >90% precision allowed reducing the emergence of bovine respiratory disease (BRD). In addition, distress can be vocalized by animals or shown though unusual activity. Vocalization could be measured via microphones, whereas activity could be observed and recorded using staff observations or surveillance cameras, with the interpretation of sounds and images to produce meaningful information [ 75 ].
Nowadays, automated sensors and algorithms can reliably predict and reduce the risk of mastitis in cows. Air sensors in the poultry industry can predict the onset of Coccidiosis by constantly monitoring the concentration of volatile organic compounds in the air increase, as the number of infected birds increases. Air sensors could detect this change much earlier than a farmer or a vet could [ 71 ]. In other cases, by carrying out image analysis and calculating model parameters from the image information, it was possible to develop an algorithm for automatic detection of lameness based on animal locomotion [ 71 , 76 ]. In the case of cattle health, a few common diseases can be identified using non-invasive, cheap sensor technologies. More complex sensor platforms exist, for instance, camera systems to detect back posture, and ingestible pills for heart rate determination [ 77 ]. Furthermore, the continuous feed and water registration in the farm makes it possible to assess the first freedom from hunger and thirst. Climate control sensors such as temperature sensors, relative humidity probes, and CO 2 sensors will allow the automatic evaluation of thermal discomfort in the house [ 72 ].
3.3. Feed and Live Weight Measurement
Precision feeding is a key component of PLF [ 60 ]. Based on real-time feedback from sensors [ 78 ], precision livestock feeding aims to provide to individuals or a group of animals with the amount of nutrients that maximizes its utilization without loss of performance [ 60 ]. Therefore, accurately and automatically measuring the amount of feed used per day per animal or distinct group of animals is extremely important [ 59 ]. The use of precision livestock feeding can decrease protein intake by 25%, and nitrogen excretion into the environment by 40%, while increasing profitability by nearly 10% [ 60 ].
The implementation of automated feeding systems (AFS) can provide a cost-effective alternative to manual regimes. Feeding units have been developed for a variety of animal systems including cattle, sheep, and pigs. These systems can be advantageous by providing an interface that monitors time and date of feeding, the electronic identification of each animal, the weight of the feed consumed, and the duration of feeding [ 79 ].
Demmers et al. [ 80 ] used an automated feeding system to control the amount of feed delivered to pens and the ambient temperature to optimize growth and reduce ammonia emissions [ 60 ]. In Australia, a feed sensor was developed to measure and control the amount of feed delivered to individual feeders quite precisely [ 59 ]. In Canada, a next generation feeding system was recently developed that will provide the additional capability to provide feed with a variety of nutrient specifications to tailor both the amount and composition of the feed. In the next few years, we might be able to adjust the nutrient intake to match the requirements of individual animals in real-time, based on their state-specific needs, as estimated from the sensor data [ 77 ]. We must also emphasize a study by Evangelista et al. [ 81 ] that highlights the use of portable near infrared spectroscopy (NIRS) to evaluate the physio-chemical composition of total mixed ration (TMR) and manure in dairy farms. According to the authors, the use on barn NIRS, through appropriate calibrations, is a rapid and accurate analytical technique with high potential benefits.
RGB-D cameras can also help farmers to measure feed intake for individual cows [ 82 ]. In addition, several advanced algorithms can help farmers calibrate and optimize feed expenses according to their animals’ needs [ 72 ]. Finally, mathematical nutrition models can be useful components to correctly estimate the contribution of ruminants to greenhouse gas emissions (GHG) [ 77 ].
The measurement of average live weight gain (speed of growth) of a distinct group of animals is one of the most important measurements to be undertaken on livestock farm as the speed of growth will affect both the financial performance of the farming enterprise as well as the final body composition of the animals [ 59 ]. Recent systems have appeared on the market (such as the Osborne Weight-Watcher™). Weighing systems based on image analysis techniques have been designed to determine the weight of individual or groups of animals (specifically pigs) with acceptable precision by correlating dimensional measurements of the animals to weight. Recent studies carried out by Banhazi et al. [ 82 ] have demonstrated that those systems can reliably provide a performance record of successive batches of animals and in a timely manner.
3.4. Automatic Milking Systems
The milking robot is a classical PLF application [ 50 ]. The growing popularity of this technology is evident in its rapid rate of adoption. Automatic milking systems (AMS) have gained widespread acceptance, particularly in western Europe to reduce labor on dairy farms, increase production per cow, and improve the lifestyle of dairy farm families [ 83 ]. The first installations were typically associated with ‘indoor’ systems and nearby grazing fields. Nowadays, there is an increasing interest regarding the integration of robots into larger scale pasture-based dairy systems. The milking process can now be spread over a 24-hour period, allowing the animal to choose when to be milked. The AMS can be applied both to indoor and pasture-based feeding systems [ 84 ].
There are numerous offers for automatic milking systems (AMSs) in the market. Recent technological advances have included the integration of more modern sensors/vision systems, the addition of animal monitoring features, and the integration of robots in rotary milking parlors. Time of flight (ToF) depth sensing cameras have been used in many recent developments in AMSs. Acceptable accuracy (teat location detection within 5 mm) has been achieved when the search space is limited to a region of interest 150 mm wide by 80 mm deep [ 85 ]. Teat detection and tracking using algorithmic solutions from depth images and point-cloud data were also achieved according a study by Rodenburg et al. [ 86 ]. Other vision technologies have also been investigated using a Kinect structured light camera and a Haar Cascade classifier [ 87 ], or using a combination of thermal imaging and stereovision techniques [ 88 ]. The task of attaching the milking clusters to the cows’ teats is a challenging one as the shape of the udder is variable between cows and between distinct stages of lactation [ 85 ].
Cycle time of the milking operation must also be minimal so that both the cow and farmer can be more productive with their time. Therefore, an intelligent control of the robot is required using visual feedback to navigate the cluster onto the cow accurately, safely, and quickly. A system that can milk cows with unusually shaped udders or that do not take to a robot milker, which would otherwise have to be culled as is the case with some existing AMSs, would also be a considerable advantage [ 85 ].
4. Risks and Concerns about Precision Farming
It is important to understand how farmers interpret the value of technology in the context of their farms. On one hand, farmers look at value to their farming business in the adoption of the usage of new technologies to solve future problems [ 73 ]. On the other hand, many producers perceive that adopting high productive management systems involves increased risk [ 54 ]. The perceived risks involve the risk of financial failure because of unforeseen environmental or market circumstances, damage to the farm infrastructure such as soils and pasture, compromises to animal health and welfare, and the risk of increased stress on them from managing an intensified system [ 54 , 56 ]. Another risk that precision farming shares with other technologies is the further consolidation of farms as far as wealthier participants in a sector can benefit the most from recent technologies [ 91 ]. There is also the concern about some instances where technology cannot be used effectively. In some cases, farmers are either reluctant or may not be able to use the latest technology on their farms. The selling of pre-mature technology to farmers by companies without sufficient trials or evidence could result in costly losses for the farmers, namely, when it comes to predicting epidemic diseases in large scale animal farms. Furthermore, use of the data is itself a problem. Vast amounts of data from the technology products and services get stored in remote cloud servers. This is often monetized for commercial benefits. Big corporations can now collect, use, and even sell data from farmers. The rising tension between corporations and farmers over data misuse is a considerable threat [ 71 ].
5. Conclusions
With increasing population pressure around the world and the need to increase agricultural production, there is a concern for improved management of the world’s agricultural resources while minimizing the negative impact on the environment. Information on crops, rangeland, livestock, and other agricultural resources is critical for effective management of depleting and scarce resources. The implementation of the PA involves the integration of smart technologies in both farming and livestock, allowing the farmer to manage field variability to maximize the cost–benefit ratio and also to continuously and/or automatically monitor the main animal performance indicators.
This literature review allowed us to realize that PA has been proven to be a highly researched and constantly evolving area due to the needs of farmers to use resources in a more optimized way. Many techniques presented in this study were applied under strict controlled conditions for research and their implementation on the farms would also require some ability for auto-calibration of the device or tools. The development and operation of PA applications involve several steps and techniques that need to be investigated further to make the developed systems accurate and implementable in commercial environments and diverse farming systems. In addition, future research should also focus on the current need for farmers to acquire more knowledge regarding PA, as this may be one of the main factors dissuading them from implementing smart technologies in their fields.
Author Contributions
Conceptualization, A.M. and S.S.; methodology, A.M.; software, S.S. and P.G.; validation, A.M., S.S. and P.G.; formal analysis, A.M.; investigation, A.M. and S.S.; resources, A.M., S.S. and P.G.; data curation, S.S.; writing—original draft preparation, A.M. and S.S.; writing—review and editing, A.M.; visualization, S.S. and P.G.; supervision, A.M.; project administration, A.M.; funding acquisition, A.M. and P.G. All authors have read and agreed to the published version of the manuscript.
This research received no external funding.
Institutional Review Board Statement
Data availability statement, conflicts of interest.
The authors declare no conflict of interest.
Publisher’s Note: MDPI stays neutral with regard to jurisdictional claims in published maps and institutional affiliations.
Department of Plant and Soil Science
- Davis College
- Plant and Soil Science
Guo Precision Ag Lab
Crop ecophysiology & precision agriculture.
Conventional farming practices treat an agricultural field uniformly despite the inherent variability in soil properties and crop growth conditions. The challenge and necessity of feeding 9 billion people on the earth by 2050 urges us to use our land and water resources more wisely.
At the Crop Ecophysiology & Precision Agriculture Laboratory, we strive to improve the bottom line of production agriculture through optimization of crop inputs, management, while protecting the environment.
Creating a More Efficient Future
The challenge and necessity of feeding 9 billion people on the earth by 2050 stimulates us to use our land and water resources more wisely. Conventional farming practices treat an agricultural field uniformly despite the inherent variability in soil properties and crop growth conditions. Uniform management may result in over- or under-application of resources in specific locations within a field, which may have a negative impact on the environment, profitability, and sustainability of agricultural production.
One solution is to manage our soil and water more efficiently and effectively by applying crop inputs at the right location with the right amount and at the right time. Precision agriculture offers a suite of technologies to implement such intensive management. These technologies include global navigation satellite systems, geographic information systems, remote and proximal sensing, yield monitoring, variable rate technology, and data science. This list is expanding. The goal of precision agriculture is to optimize crop input and management to enhance productivity, profitability, sustainability, while minimizing the impact of agriculture on the environment.

At the Crop Ecophysiology & Precision Agriculture Laboratory, we strive to improve the bottom line of production agriculture through optimization of crop inputs, management, while protecting the environment. Our mission is to build on partnerships with the industry and research institutions to develop and expand innovative research and teaching programs in precision agriculture and environmental modeling that align well with the department and university plans, address important local and national agricultural needs, and support the economic development of Texas and the nation through education and research.
Our research interests include studies at a range of scales, from individual plant, experimental plot, commercial field, to regional, national, or even international levels. Specific research projects include irrigation scheduling based on weather and satellite imagery, variable rate water and nutrient application, remote sensing for plant phenotyping and growth monitoring, crop growth modeling and simulation, environmental modeling and hazard prediction, etc. The lab maintains state-of-the-art facilities to support our research, including sophisticated UAS platforms and sensors, high performance computation power and software programs, as well as various radiometers, field spectrometers, calibration equipment, and ecosystem measurement instrumentation.
Publications
Google Scholar
ResearchGate
- Karn, R. Hillin, D., Helwi, P., Scheiner, J., Guo, W. 2024. Assessing grapevine vigor as affected by soil physicochemical properties and topographic attributes for precision vineyard management. Scientia Horticulturae, 328: 112857.
- Abdalla, A., Wheeler, T.W., Dever, J. Lin, J., Arce, J., and Guo, W. 2024. Assessing fusarium oxysporum disease severity in cotton using unmanned aerial system images and a hybrid domain adaptation deep learning time series model. Biosystems Engineering 237, 220-231.
- Singh, A., Deb, S.K., Slaughter, L.C., Singh, S., Ritchie, G.L., Guo, W., and Saini, R. 2024. Simulation of root zone soil water dynamics under cotton-silverleaf nightshade interactions in drip-irrigated cotton. Agricultural Water Management, 288, 108479.
- Rabia, A., J. Neupane, Z. Lin, K. Lewis, G. Cao, and W. Guo. 2022. Principles and Applications of Topography in Precision Agriculture. Advances in Agronomy, 171: 143-189. https://doi.org/10.1016/bs.agron.2021.08.005.
- Neupane, J., W. Guo, G. Cao, F. Zhang, L. Slaughter, and S. Deb. 2022. Spatial patterns of soil microbial communities and implications for precision soil management at the field scale. Precision Agriculture. 23: 1008–1026. DOI: 10.1007/s11119-021-09872-1.
- Neupane, J., W. Guo, C. West, F. Zhang, and Z. Lin. 2021. Effects of Irrigation Rates on Cotton Yield as Affected by Soil Physical Properties and Topography in the Southern High Plains. Plos One 16(10): e0258496. https://doi.org/10.1371/journal.pone.0258496.
- Gikunda, R. M., D. Lawver, M. Baker, A. Boren, and W. Guo. 2021. Extension education needs for improved adoption of sustainable organic agriculture in Central Kenya. American Journal of Geographic Information System, 10(2): 61-71.
- Lin, Z., and W. Guo. 2021. Cotton Stand Counting from Unmanned Aerial System Imagery using MobileNet and CenterNet Deep Learning Models. Remote Sensing, 13(14), 2822.
- Sun, Y., W. Guo, D. Weindorf, F. Sun, S. Deb, G. Cao, J. Neupane, Z. Lin, A. Raihan. 2021. Field-scale Calcium Spatial Variability: Implications for Soil Erosion and Site-specific Management. Pedosphere. 31(5): 705-714.
- Wen M., W. Zhao, W. Guo, X. Wang, P. Li, J. Cui, Y. Liu, and F. Ma. 2021. Coupling effects of reduced nitrogen, phosphorus and potassium on drip-irrigated cotton growth and yield formation in northern XinJiang. Archives of Agronomy and Soil Science. 68(9): 1239-1250. https://doi.org/10.1080/03650340.2021.1881776.
- Gu, H., Z. Lin, W. Guo, and S. Deb. 2021. Retrieving Surface Soil Water Content Using a Soil Texture Adjusted Vegetation Index and Unmanned Aerial System Images. Remote Sensing 2021, 13(1), 145; https://doi.org/10.3390/rs13010145.
- Lin, Z., and W. Guo. 2020. Sorghum Panicle Detection and Counting using Unmanned Aerial System Images and Deep Learning. Frontiers in Plant Science, 11:534853. doi: 10.3389/fpls.2020.534853.
- Lin, Y., Z. Zhu, W. Guo, Y. Sun, X. Yang, and X. Kovalskyy. 2020. Continuous Monitoring of Cotton Stem Water Potential using Sentinel-2 Imagery. Remote Sens. 12, 1176.
- Pabuayon, I. L., Y. Sun, W. Guo, and G. Ritchie. 2019. High-Throughput Phenotyping in Cotton: A review. Journal of Cotton Research, 2(1), 2-18.
- Gusso, A., W. Guo, and S. Rolim. 2019. Reflectance-based model for soybean mapping in United States at common land unit scale with Landsat 8. European Journal of Remote Sensing, 52(1): 522-531.
- Thompson, C., W. Guo, B. Sharma, and G. Ritchie. 2019. Using Normalized Difference Red Edge index to assess maturity in cotton. Crop Science, 59(5): 2167-2177.
- Neupane, J., and W. Guo. 2019. Agronomic basis, technology, and benefits of precision water management: a review. Agronomy 9(2): 87. doi:10.3390/agronomy9020087.
- Guo, W. 2018. Spatial and temporal trends of irrigated cotton yield in the Southern High Plains. Agronomy 8(12): 298. doi:10.3390/agronomy8120298.
- Guo, W. 2018. Application of geographic information system and automated guidance system in optimizing contour and terrace farming. Agriculture 8(9): 142. doi: 10.3390/agriculture8090142.
- Chen, T., R. Zeng, W. Guo, X. Hou, Y. Lan, and L. Zhang. 2018. Detection of stress in cotton (Gossypium hirsutum L.) caused by aphids using leaf level hyperspectral measurements. Sensors 18(9), 2798. doi:10.3390/s18092798.
- Guo, W., S. Cui, J. Torrion, and N. Rajan. 2015. Data-Driven Precision Agriculture: Opportunities and Challenges. In Soil-Specific Farming: Precision Agriculture. L. Rattan and B. A. Stewart (eds.). CRC Press, Boca Raton, FL. P.353–372. doi: 10.1201/b18759-15.
- Torrion, J. A., S. J. Maas, W. Guo, J. P. Bordovsky, and A.M. Cranmer. 2014. A three-dimensional index for characterizing crop water stress. Remote Sensing 6: 4025-4042 .
- Guo, W., S.J. Maas, and K.F. Bronson. 2012. Relationship between cotton yield and soil electrical conductivity, topography, and Landsat imagery. Precision Agriculture 13: 678-692.
- Guo, W., and S.J. Maas. 2012. Terrace layout design utilizing geographic information system and automated guidance system. Applied Engineering in Agriculture 28:31-38.
- Ko, J., G. Piccinni, W. Guo, and E. Steglich. 2009. Parameterization of EPIC crop model for simulation of cotton growth in South Texas. Journal of Agricultural Science 147: 169-178.
- Todd, R.W., N.A. Cole, R.N. Clark, W.C. Rice, and W. Guo. 2008. Soil nitrogen distribution and deposition on shortgrass prairie adjacent to a beef cattle feedyard. Biology and Fertility of Soils. 44(8): 1099-1102. doi: 10.1007/s00374-008-0286-2.
- Todd, R., W. Guo, B.A. Stewart, and C. Robinson. 2004. Vegetation, phosphorus, and dust gradients downwind from a cattle feedyard. Journal of Range Management 57: 291-299.
- Meng, Q., A. Meng, J. Wang, Z. Liu, W. Guo, and M. Cui. 1998. The path analysis of main quantity characters and dry leaf yield of Stevia Rebaudiano Bertoni. Journal of Jilin Agricultural University 20:17-19.
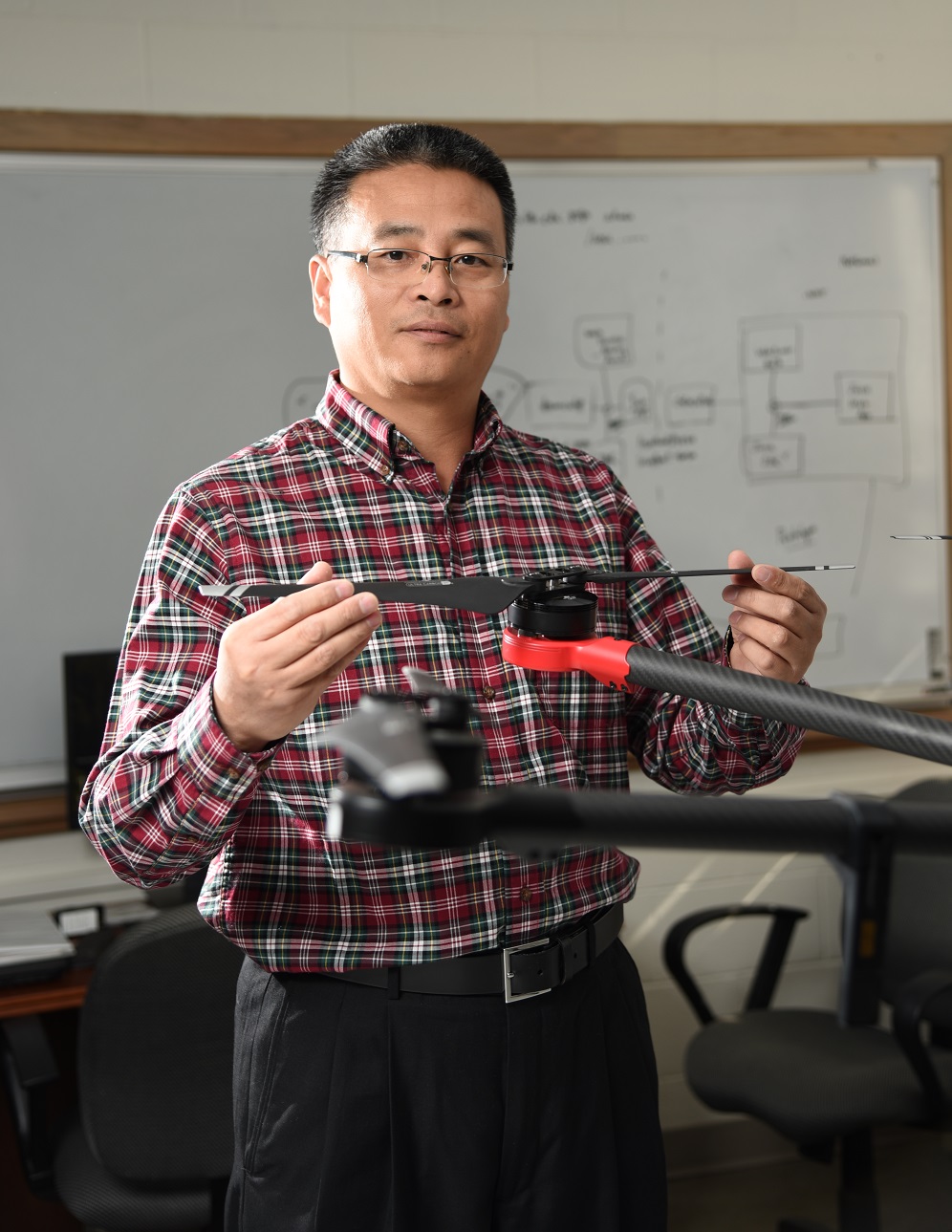
Wenxuan Guo, Ph.D. Associate Professor of Crop Ecophysiology & Precision Agriculture
Dr. Guo's research efforts are centered in precision agriculture, environmental sciences, and remote sensing in agriculture, particularly UAV application in precision plant phenotyping. One of his primary goals is to lead interdisciplinary research and teaching programs that leverage state-of-the-art technologies to improve agricultural production with limited resources, especially water. He teaches PSS5329 - Precision Agriculture, PSS6301 - Quantitative Agricultural Remote Sensing, and PSS6302 - Plant Growth Modeling.
Learn more »
Current Lab Members
Past lab members, in the news.
Texas Tech-led study aims to transform precision ag with AI, UAV technologies (Davis College NewsCenter, October 2023)
Texas Tech researchers looking for sustainable solutions to water shortages (Texas Tech Today, May 2022)
Sensor-equipped drones help detect crop stress, aid decision making (Cotton Farming, July 1, 2020)
Jasmine Neupane wins thesis award (CASNR NewsCenter, June 2019)
Texas Tech on the cutting edge for agriculture research (KLBK, Dec 11, 2018)
Drones, new technology could be the future for West Texas farming (Doublet973.com, December 2018)
Smart Drones Take Flight for Precision Agriculture Use in Plant and Soil Science (Texas Tech Today, Dec 7, 2018)
Texas Tech earns 'Top 25' ranking for its efforts in precision agriculture (CASNR News, April 2018)
25 Best Colleges for Precision Agriculture (Precisionag.com, March 2018)
Texas Tech earns Top 25 ranking in Precision Agriculture (http://ramar.worldnow.com, Apr 2018)
Where the Grass Grows Greener (the Agriculturist, Fall 2018)
Join the Lab
We are currently seeking to fill several positions in the Precision Ag Lab:
Part-time Student Worker — Laboratory and field assistance
Post-Doctoral Research Associate — Remote Sensing and Precision Agriculture
PhD Research Assistantship — Water Stress Assessment and Precision Water Management
PhD Research Assistantship — Precision Agriculture and Remote Sensing
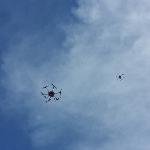
Dr. Wenxuan Guo
Associate Professor of Crop Ecophysiology & Precision Agriculture Texas Tech University Dept. of Plant and Soil Science
(806) 834-2266
Bayer Plant Science, Box 42122 2911 15th Street Lubbock, TX 79409
- Like Department of Plant and Soil Science on Facebook Like Department of Plant and Soil Science on Facebook
- Follow Department of Plant and Soil Science on Instagram Follow Department of Plant and Soil Science on Instagram
- Connect with Department of Plant and Soil Science on LinkedIn Connect with Department of Plant and Soil Science on LinkedIn
- Skip to primary navigation
- Skip to main content
Precision Agriculture Programs
Precision agriculture research is at the forefront of revolutionizing modern farming practices. Integrating advanced technologies like satellite imagery, drones, and sensor networks, researchers aim to create a data-driven paradigm for agriculture. This approach enables farmers to make informed decisions based on real-time information about soil conditions, crop health, and weather patterns. By optimizing the use of resources, such as water and fertilizers, precision agriculture not only enhances crop yields but also contributes to sustainability by minimizing environmental impact. This innovative field holds the promise of transforming traditional farming into a more efficient, precise, and environmentally friendly endeavor.
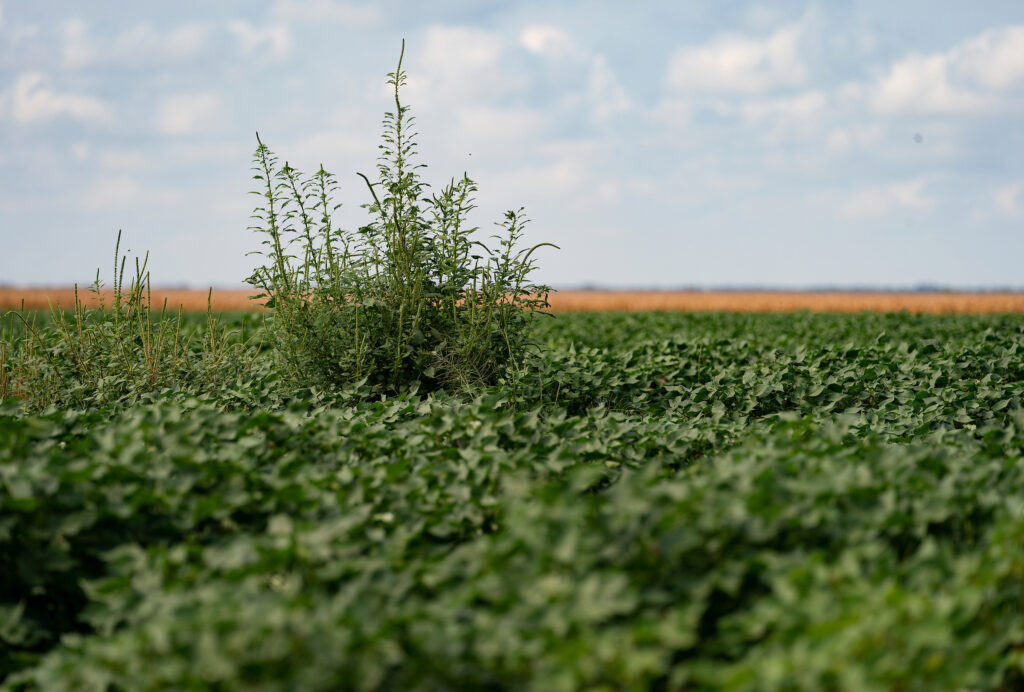
Research Interests
Faculty and staff.
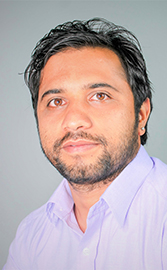
Mahendra Bhandari
Assistant Professor Digital Agriculture
361-265-9201
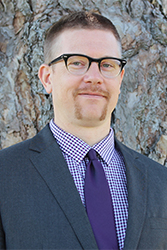
Seth C. Murray
Professor Butler Chair Corn Breeding and Genetics
979-321-5933
Agronomy Field Lab 110/111
Related Sites of Interest
- Texas AgriLife Research
Learn More About Research Areas of Focus in Soil and Crop Sciences
In the realm of soil and crop sciences, research focuses on a myriad of critical areas that drive sustainable agricultural practices and global food security. Soil health and management take center stage, exploring ways to optimize soil fertility, structure, and nutrient cycling to enhance crop productivity. Genetic advancements in crop breeding and biotechnology are pursued to develop resilient varieties that withstand environmental stressors and ensure high yields. Precision agriculture, integrating technology and data analytics, aims to fine-tune farming practices, reducing resource waste and maximizing efficiency. The exploration of sustainable agroecosystems delves into the intricate relationships between crops, soil, and the environment, fostering environmentally friendly approaches. Such research not only informs farming practices but also contributes to vital policy discussions, shaping the future of agriculture in a rapidly changing world.
Reimagining Design with Nature: ecological urbanism in Moscow
- Reflective Essay
- Published: 10 September 2019
- Volume 1 , pages 233–247, ( 2019 )
Cite this article
- Brian Mark Evans ORCID: orcid.org/0000-0003-1420-1682 1
977 Accesses
2 Citations
Explore all metrics
The twenty-first century is the era when populations of cities will exceed rural communities for the first time in human history. The population growth of cities in many countries, including those in transition from planned to market economies, is putting considerable strain on ecological and natural resources. This paper examines four central issues: (a) the challenges and opportunities presented through working in jurisdictions where there are no official or established methods in place to guide regional, ecological and landscape planning and design; (b) the experience of the author’s practice—Gillespies LLP—in addressing these challenges using techniques and methods inspired by McHarg in Design with Nature in the Russian Federation in the first decade of the twenty-first century; (c) the augmentation of methods derived from Design with Nature in reference to innovations in technology since its publication and the contribution that the art of landscape painters can make to landscape analysis and interpretation; and (d) the application of this experience to the international competition and colloquium for the expansion of Moscow. The text concludes with a comment on how the application of this learning and methodological development to landscape and ecological planning and design was judged to be a central tenant of the winning design. Finally, a concluding section reflects on lessons learned and conclusions drawn.
This is a preview of subscription content, log in via an institution to check access.
Access this article
Price includes VAT (Russian Federation)
Instant access to the full article PDF.
Rent this article via DeepDyve
Institutional subscriptions
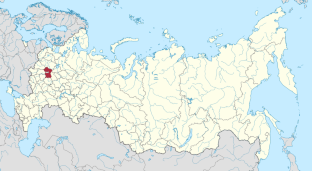
Similar content being viewed by others
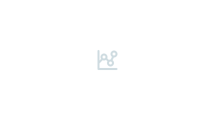
The politics of designing with nature: reflections from New Orleans and Dhaka
Zachary Lamb
Acknowledgements
The landscape team from Gillespies Glasgow Studio (Steve Nelson, Graeme Pert, Joanne Walker, Rory Wilson and Chris Swan) led by the author and all our collaborators in the Capital Cities Planning Group.
Author information
Authors and affiliations.
Mackintosh School of Architecture, The Glasgow School of Art, 167 Renfrew Street, Glasgow, G3 6BY, UK
Brian Mark Evans
You can also search for this author in PubMed Google Scholar
Corresponding author
Correspondence to Brian Mark Evans .
Rights and permissions
Reprints and permissions
About this article
Evans, B.M. Reimagining Design with Nature: ecological urbanism in Moscow. Socio Ecol Pract Res 1 , 233–247 (2019). https://doi.org/10.1007/s42532-019-00031-5
Download citation
Received : 17 March 2019
Accepted : 13 August 2019
Published : 10 September 2019
Issue Date : October 2019
DOI : https://doi.org/10.1007/s42532-019-00031-5
Share this article
Anyone you share the following link with will be able to read this content:
Sorry, a shareable link is not currently available for this article.
Provided by the Springer Nature SharedIt content-sharing initiative
- Design With Nature
- Find a journal
- Publish with us
- Track your research

IMAGES
VIDEO
COMMENTS
Precision Agriculture is an internationl journal that publishes innovative research on resource use efficiency, productivity, quality, profitability, and sustainability of agricultural production. Addresses within-field natural resources variability, including soil and crop variability and characteristics.
Precision agriculture is a management concept, which relies on intensive data collection and data processing for guiding targeted actions that improve the efficiency, productivity, and sustainability of agricultural operations. Several studies have assessed the adoption rate of precision agriculture technologies at regional or national scale, but the literature lacks global evaluations of the ...
The focus of the research is to help small farmers apply fertilizer, seed, and herbicide with more precision and less waste. The image shows the difference in fertilizer overlap (red) when the tractor-guidance system is off (left) versus when it is on (right). UM - Do the benefits of precision agriculture reach consumers?
This report documents trends in the U.S. farm sector's adoption of digital agriculture technologies between 1996 and 2019, with emphasis on changes since 2016, using data from USDA's Agricultural Resource Management Survey (ARMS). The adoption of yield maps, soil maps, and variable rate technologies (VRT), in addition to other technologies ...
PLOS' precision agriculture research explores and assesses the very latest agricultural technologies. Whether in controlled environments or directly in the field, our research highlights new methods and technologies for agricultural surveillance and intervention, such as sensors and chemical testing, or high-tech farm machinery and machine learning that measures, analyses, and improves crop ...
Precision agriculture is the collection of hardware and software technologies that allow a farmer to make informed, differentiated decisions regarding agricultural operations such as planting ...
Precision agriculture (PA) is a management strategy for addressing geographical and temporal variabilities in agricultural fields [1,2,3] that involves data and contemporary technologies.With a forecasted human population of between 9 and 10 billion by 2050 [3,4,5], precision agriculture is becoming more and more important to contemporary agricultural research.
Mapping hailstorm damage on winter wheat ( Triticum aestivum L.) using a microscale UAV hyperspectral approach. Precision Agriculture is an internationl journal that publishes innovative research on resource use efficiency, productivity, quality, profitability, and ...
Precision agriculture, driven by the convergence of smart sensors and advanced technologies, has emerged as a transformative force in modern farming practices. The present review synthesizes insights from a multitude of research papers, exploring the dynamic landscape of precision agriculture. The main focus is on the integration of smart sensors, coupled with technologies such as the Internet ...
Federal agencies support precision agriculture adoption, research and development, education, and training. USDA supports precision agriculture technology adoption with financial assistance and loan programs, such as through payments for implementing practices that provide a conservation benefit. USDA and the National Science Foundation (NSF ...
Precision Agriculture. Applying the right resources in the right place at the right time can be a win-win for farmers and the environment. This approach, known as precision agriculture, lets farmers use cutting-edge tools to get specific, detailed information on individual bits of land or even plants in their fields.This knowledge enables them to apply only as much fertilizer, water, or other ...
Precision agriculture is a key component of the third wave of modern agricultural revolutions.The first agricultural revolution was the increase of mechanized agriculture, from 1900 to 1930.Each farmer produced enough food to feed about 26 people during this time. The 1960s prompted the Green Revolution with new methods of genetic modification, which led to each farmer feeding about 156 people.
The current paper aims to briefly review the recent scientific and technological trends of precision farming and its application in crop and livestock farming. This study can serve as a research guide for both the researcher and the farmer in applying technology to agriculture.
Research. The Precision Agriculture Center is a hub for conducting multi- and inter-disciplinary research in Minnesota, the United States, and in countries around the world. Center affiliates develop and implement innovative techniques to help understand the spatial and temporal variability that exists in agriculture. Our researchers are driven ...
Wenxuan Guo, Ph.D. Associate Professor of Crop Ecophysiology & Precision Agriculture. Dr. Guo's research efforts are centered in precision agriculture, environmental sciences, and remote sensing in agriculture, particularly UAV application in precision plant phenotyping. One of his primary goals is to lead interdisciplinary research and ...
Aims and scope. Precision Agriculture promotes the most innovative results coming from the research in the field of precision agriculture. It provides an effective forum for disseminating original and fundamental research and experience in the rapidly advancing area of precision farming. There are many topics in the field of precision ...
Precision agriculture research is at the forefront of revolutionizing modern farming practices. Integrating advanced technologies like satellite imagery, drones, and sensor networks, researchers aim to create a data-driven paradigm for agriculture. This approach enables farmers to make informed decisions based on real-time information about soil conditions, crop health, and weather patterns ...
Precision Farming or Precision Agr iculture is generally defined as informat ion and technology based farm. management system to identify, analyse an d manage spatial and temporal variability ...
Precision Agriculture is a leading international journal on advances in precision farming. Established in 1999, it focuses on natural resource variability, engineering technology, profitability, environment, and technology transfer. It serves as an effective platform for disseminating original and fundamental research and understanding in the continuously evolving field of precision farming ...
Above the Field with UAVs in Precision Agriculture: Discover How and Why UAVs are Set to Impact and Change the Way Precision Agriculture Professionals Operate . Las Vegas: Commercial U AV Expo, pp. 6.
Significant challenges of the COVID-19 pandemic highlighted that features of a modern, sustainable and resilient city should not only relate to fulfilling economic and social urban strategies, but also to functional urban design, in particular, related to urban blue and green infrastructure (BGI). Using results from a web-based questionnaire survey conducted May-July 2020 in Moscow (Russia ...
Precision agriculture (PA) has a huge potential for growth in sub-Saharan Africa (SSA), but it faces a number of social-economic and technological challenges. This study sought to map existing PA research and application in SSA countries following the methodology for systematic mapping in environmental sciences. After screening for relevance, the initial about 7715 articles was reduced to 128 ...
The main driver for the precision farming market worldwide is cost optimization and increased yields. It is these two factors that basically induce farmers to introduce precision farming into their farms and rebuild their work for new technologies. ... Research Institute VNII of Labor, Zemlyanoy Val, 34, 105064, Moscow, Russia. Marina Danilina ...
The twenty-first century is the era when populations of cities will exceed rural communities for the first time in human history. The population growth of cities in many countries, including those in transition from planned to market economies, is putting considerable strain on ecological and natural resources. This paper examines four central issues: (a) the challenges and opportunities ...