Etiology and Treatment of Major Depressive Disorder: Where Research Stands and Suggestions for the Future
Journal title, journal issn, volume title, yu faculty profile.
A deeper understanding of Major Depressive Disorder (MDD) is crucial to identify and monitor those at risk for depression and more easily diagnose and effectively treat patients. This paper provides an in-depth look at the etiology of MDD, covering the biological and, briefly, the psychological theories of depression. Also included are environmental risk factors, genetic links to the disorder, biomarker measurements, and an overview of psychotherapy and pharmacological treatment options. Throughout, there is discussion of current obstacles in research and suggestions for future projects. Studies often focus on one or two aspects of depression or depression treatment, but combining the information allows for a much more complete understanding of the disease and for connections between topics to emerge. The author demonstrates how the hypotheses of the pathophysiology of depression complement one another, and explains why future research must focus on finding trends and subgroups within MDD, understanding the effect psychosocial risk factors and biomarker levels have on one another, and optimizing the synergistic relationship of psychotherapy and pharmacological treatment.

Description
Collections.

An official website of the United States government
The .gov means it’s official. Federal government websites often end in .gov or .mil. Before sharing sensitive information, make sure you’re on a federal government site.
The site is secure. The https:// ensures that you are connecting to the official website and that any information you provide is encrypted and transmitted securely.
- Publications
- Account settings
Preview improvements coming to the PMC website in October 2024. Learn More or Try it out now .
- Advanced Search
- Journal List
- Singapore Med J
- v.57(11); 2016 Nov

Major depression in primary care: making the diagnosis
Chung wai mark ng.
1 SingHealth Polyclinics, Changi General Hospital, Singapore
Choon How How
2 Care and Health Integration, Changi General Hospital, Singapore
Yin Ping Ng
3 Penang Medical College, Penang, Malaysia
4 Penang Adventist Hospital, Penang, Malaysia
Associated Data
Major depression is a common condition seen in the primary care setting, often presenting with somatic symptoms. It is potentially a chronic illness with considerable morbidity, and a high rate of relapse and recurrence. Major depression has a bidirectional relationship with chronic diseases, and a strong association with increased age and coexisting mental illnesses (e.g. anxiety disorders). Screening can be performed using clinical tools for major depression, such as the Patient Health Questionaire-2, Patient Health Questionaire-9 and Beck Depression Inventory, so that timely treatment can be initiated. An accurate diagnosis of major depression and its severity is essential for prompt treatment to reduce morbidity and mortality. This is the first of a series of articles that illustrates the approach to the management of major depression in primary care. Our next articles will cover suicide risk assessment in a depressed patient and outline the basic principles of management and treatment modalities.
Susan came to your clinic with her grandson Sam for an unscheduled consultation two months ahead of her next chronic diseases review. Susan kept looking down and heaving sighs now and then. Sam reported that his grandmother had not been herself since her close cousin passed away two months ago, and that she had since stopped knitting and following Korean drama serials. Her sleep and appetite had also been affected in the last month. His family had asked Sam to take his grandmother for an assessment .
WHAT IS MAJOR DEPRESSION?
Major depression is a mood disorder that presents with either a persistent feeling of sadness or loss of pleasure, or both.( 1 )
HOW COMMON IS THIS IN MY PRACTICE?
The Singapore Mental Health Study (SMHS) 2010 reported that major depression was the most common mental illness with a lifetime prevalence of 5.8%, followed by alcohol abuse and obsessive compulsive disorder.( 2 ) However, only 18% of patients with mental disorders sought help from primary care practitioners.( 2 ) The risk of major depression is significantly higher in the older population.( 3 ) As such, primary care doctors need to be familiar with the diagnosis and management of major depression.
WHAT CAN I DO IN MY PRACTICE?
Screening – particularly in patients at risk.
Major depression is a chronic illness of considerable morbidity, with high rates of relapse and recurrence;( 4 , 5 ) however, many patients suffering from major depression do not seek help early.( 2 , 6 ) This could be due to various factors: lack of insight into their medical condition; the stigma associated with the label of mental illness; and financial factors.( 6 ) The SMHS found that the median time between the onset of illness and help-seeking was five years.( 2 ) Hence, viewing screening as the first step, followed by diagnosis, early treatment and follow-up, was shown to result in better outcomes.( 7 , 8 )
There are many good recommendations that advocate screening for major depression. The clinical practice guidelines on major depression published by the Ministry of Health, Singapore, in 2012 recommend screening for major depression in high-risk persons where the benefits outweigh the risks.( 9 ) The United States Preventive Services Task Force recommends screening for major depression in the general adult population and having adequate systems in place to ensure proper diagnosis, treatment and follow-up.( 10 ) While the Canadian Task Force on Preventive Healthcare does not recommend routine screening of adults in primary care, it advocates vigilance for major depression in patients with risk factors and symptoms of insomnia, low mood, anhedonia and suicidal thoughts.( 11 ) The 2016 updated guidelines from the National Institute for Health and Care Excellence, United Kingdom, recommend that clinicians screen for major depression in persons who have chronic medical conditions with impaired function, as well as persons with a past history of major depression, by asking if low mood, hopelessness and anhedonia are also present.( 12 )
Which patients are at greater risk of major depression?
Anyone with a past depressive episode is at risk of further episodes, as the natural course of major depression involves frequent relapses.( 5 ) There is a bidirectional relationship between major depression and chronic disease. The SMHS showed that almost half of the persons with major depression had at least one chronic physical condition.( 2 ) Similarly, individuals with chronic physical conditions are known to be at greater risk of major depression.( 13 - 15 ) For example, the prevalence of major depression is higher in persons with chronic medical conditions such as heart disease, stroke and diabetes mellitus;( 16 , 17 ) coexisting major depression is associated with poorer prognosis and an increased rate of complications that are related to these conditions.( 18 ) A significant association was found between major depression and a number of diabetic complications. Those who were depressed were also more likely to die after a heart attack compared to non-depressed patients.( 19 )
Apart from the usual symptoms of major depression such as insomnia and low energy level, patients often present to primary care doctors with somatic symptoms.( 20 , 21 ) Physical symptoms associated with major depression include backaches, nonspecific musculoskeletal complaints, having multiple (three or more) somatic complaints, and having vague complaints.( 22 ) Patients may experience deteriorating memory as well. A review has shown that major depression is associated with attention deficit and poor cognitive functioning, particularly when the patient is acutely depressed.( 23 ) The elderly, in particular, are less likely to report low mood, instead presenting with physical complaints and deterioration in cognitive ability.( 24 )
Clinicians should also pay attention to life event stressors, as these are associated with the onset of major depression, particularly in persons with a genetic predisposition.( 25 , 26 ) Such stressors include recent loss or bereavement, physical or emotional abuse, incidents involving humiliation, and difficult relationships.( 27 ) Since major depression often coexists with other psychiatric disorders (e.g. anxiety disorders, substance abuse and somatoform disorders),( 28 , 29 ) patients who present with these diagnoses should be screened for major depression, and vice versa. Box 1 shows a summary of risk factors for major depression.

Persons at risk of major depression include those with
How do I screen for major depression in primary care?
The Patient Health Questionaire-2 (PHQ-2; Table I ) is a two-item tool that can be used by primary care practitioners in the busy outpatient setting with a high patient load.( 34 ) Both items in PHQ-2 are listed as key criteria for the diagnosis of major depression in the fifth edition of the Diagnostic and Statistical Manual of Mental Disorders (DSM-5).( 1 ) The specificity for major depression in primary care was as high as 90%, using a threshold score ≥ 3,( 34 ) but sensitivity findings were variable, ranging from 74% to 83%.( 34 , 35 ) Due to its brevity, the recommendation is to use the PHQ-2 simply as a screening tool for major depression and not for assessing disease severity. Among primary care doctors,( 35 ) some recommend using a threshold score ≥ 2 (instead of ≥ 3) to improve sensitivity. Patients who obtain a score ≥ 2 should proceed to the nine-item Patient Health Questionnaire-9 (PHQ-9).
Patient Health Questionnaire-2.( 34 )

The PHQ-9( 34 ) ( Table II ) exclusively focuses on the nine diagnostic criteria for major depression listed in the DSM-5.( 1 ) Major depression is diagnosed if a score ≥ 2 is obtained for five or more of the nine symptom criteria, with low mood or anhedonia being one of the criteria. For the criterion on suicide ideation (‘thoughts that you would be better off dead or of hurting yourself in some way’), a score ≥ 1 is counted, i.e. regardless of duration. The advantages of PHQ-9 are its brevity, sensitivity and specificity, and its utility as both a diagnostic and severity measure for major depression.
Patient Health Questionnaire-9.( 34 )

The Beck Depression Inventory (BDI)( 36 ) is a 21-item questionnaire that was first developed in 1961. The items cover affective, cognitive and somatic aspects of major depression. There were concerns that the somatic aspects of the inventory could lead to spuriously high estimates of major depression in patients with chronic medical conditions. A shorter version, the BDI-Fast Screen or BDI-FS (previously called BDI-Primary Care), was developed by removing the somatic components of the BDI. Each of the seven items (sadness, pessimism, past failure, anhedonia, self-dislike, self-criticalness and suicidal ideation) is rated on a four-point scale ranging from 0 to 3.( 37 ) A cutoff score ≥ 4 was found to be sensitive and specific for identifying major depression among outpatients.( 38 , 39 )
The Geriatric Depression Scale (GDS)( 40 ) is a 30-item depression questionnaire specifically designed for use in older adults. The GDS assesses the affective and cognitive aspects of major depression, but intentionally omits assessment for somatic symptoms. The rationale is that such an assessment may be non-discriminatory in the elderly due to the physiological effects of age and presence of chronic medical conditions. A score ≥ 11 on the GDS has a 84% sensitivity and 95% specificity for major depression in elderly patients.( 40 ) The questionnaire is easy to use, as the items require a yes-no response. However, the sheer number of items may be cumbersome in the busy outpatient setting. Shorter versions of the GDS, including 15-item, ten-item, four-item and one-item versions, have been found to be helpful in identifying depressive symptoms in elderly outpatients.( 41 )
Criteria and differentials for major depression
The primary care practitioner should be familiar with the criteria for the diagnosis of major depression, while being mindful of conditions that can mimic or coexist with major depression. An essential first step to management is making an accurate diagnosis.( 42 ) A meta-analysis has shown that, while primary care doctors are able to rule out major depression in persons who are not depressed, false positives are common in persons who are labelled as depressed.( 43 ) There is also reluctance to label patients as depressed even though they fulfil the diagnostic criteria. Underdiagnosing major depression leads to delay or non-treatment, while overdiagnosing it leads to antidepressant overuse, inappropriate referrals to psychiatric services and missing organic diseases that are mistaken as major depression.( 44 , 45 )
Major depression
The DSM-5 provides a set of criteria that should be fulfilled in order to diagnose major depression ( Box 2 ).( 1 ) The patient is said to have major depression if low mood or anhedonia (defined as loss of interest or pleasure) is present nearly every day for two or more weeks, together with other symptoms. However, it is important to note that the DSM-5, like any other diagnostic tool, serves as a guideline and should not replace clinical judgement. For example, a patient who presents with persistent low mood and anhedonia associated with hopelessness and suicidal ideation for ten days (hence not fulfilling the criteria of at least two weeks) should be managed in the same way as a patient with major depression. In the DSM-5 (which replaces the DSM-IV) criteria, recent bereavement is now recognised as one of the stressors that can precipitate major depression; it is thus not an exclusion criterion for the diagnosis of major depression.

DSM-5 criteria for major depressive episode( 1 )
The physician should also ensure that the symptoms of major depression are not attributable to another organic or psychiatric medical condition. Therefore, evaluation should take into consideration a number of organic illnesses that can mimic or coexist with major depression.( 46 ) The following conditions should be considered, as they determine both management and prognosis.
Persistent depressive disorder
Persistent depressive disorder (PDD) is characterised by milder depressive symptoms that persist for at least two years, or at least one year in children or adolescents.( 1 ) Patients should not be asymptomatic for more than two months. PDD can be underreported, as its symptoms are chronic and less severe, and form part of the patient’s regular day-to-day experience.( 1 ) Its symptoms are less likely to resolve compared to major depression,( 1 ) and may require a longer treatment period, more psychotherapy sessions, and/or higher doses of antidepressant medication. Psychotherapy may also be less effective for the treatment of PDD symptoms.( 47 )
Adjustment disorder with depressed mood
An adjustment disorder is an emotional response to a stressful event such as marital or relationship problems, loss of employment or acute illness.( 48 ) Patients can present with low mood, but this diagnosis is made only if the full criteria for a major depressive episode are not met.( 1 ) It can cause significant morbidity and increase suicide risk.
Bipolar disorder
Patients with bipolar disorder are often misdiagnosed as having unipolar major depression, particularly at initial presentation and in the primary care setting. A large proportion may remain misdiagnosed for up to ten years.( 49 , 50 ) There are various reasons for this. Patients with bipolar disorder are much more likely to present with low mood rather than mania or hypomania,( 51 , 52 ) and thus are labelled as having major depression. Hypomania in bipolar II disorder is often associated with increased creativity and energy with no impairment in function,( 53 ) which patients may not see as negative and often do not seek medical attention for. Misdiagnosis of bipolar disorder as major depression leads to inappropriate treatment with antidepressants instead of a mood stabiliser. As this contributes to worsening outcomes and may induce mania or rapid cycling,( 54 - 56 ) any patient who presents with symptoms of major depression should be evaluated for possible bipolar disorder.
A history of mania or hypomania is the main feature that distinguishes bipolar disorder from major depression. Manic episodes are present in bipolar I disorder, whereas the patient with bipolar II disorder experiences only hypomania and major depression without any manic episodes.( 1 ) Both mania and hypomania are characterised by an abnormally elevated or irritable mood, with persistent increased energy and a noticeable change from baseline behaviour. Behavioural changes may include a decreased need for sleep, an inflated self-esteem or grandiosity, increased goal-directed activity, being more talkative, and an excessive involvement in activities that are likely to have ill consequences, such as uncontrolled spending sprees, bad investments and sexual promiscuity.( 1 ) In mania, unlike hypomania, the patient’s symptoms are of at least a week’s duration and result in impaired functioning or require hospitalisation. Psychosis may or may not be present. It is important to check that the symptoms during these episodes are different, more prominent and more persistent as compared to the baseline.( 1 ) When taking a history, useful questions include: Have you ever experienced a period of time when you felt happier or more energetic than usual, for no particular reason? And if so, did you notice during such times that your thoughts were more rapid, or you had more ideas, required less sleep or were more talkative than usual? Did others notice this too? What did they say? How long did these last? Did they have any impact or effect on your life, work or relationships?
Other features that may help distinguish bipolar disorder from major depression include younger age of onset, a family history of bipolar disorder, a higher number of previous depressive episodes (e.g. too numerous to recall), atypical depressive features (e.g. hypersomnia instead of insomnia or hyperphagia instead of poor appetite), fewer somatic symptoms and increased phobias (e.g. of the dark, strangers or crowds).( 57 - 59 ) In patients who are misdiagnosed with major depression and started on antidepressants, rapid ‘switching’ from low mood to a manic or hypomanic state may occur.( 54 , 55 , 58 ) Hence, responding to antidepressant therapy too rapidly should raise the suspicion of a misdiagnosis as well.
Neurological conditions
Neurological conditions such as dementia, Parkinson’s disease and multiple sclerosis have symptoms that overlap with those of major depression.( 60 ) In Parkinson’s disease, low mood and other affective symptoms may even precede motor symptoms. Persons with cognitive impairment may present with low mood; conversely, those who have major depression may have poor concentration with impaired executive functioning and perform poorly at cognitive tests.( 23 , 61 ) Major depression itself may be a risk factor for developing dementia.( 61 , 62 ) Assessment should be guided by clinical suspicion. Neurological examination and cognitive assessment using well-known tools such as the Mini-Mental State Examination and Abbreviated Mental Test are important aspects of evaluation, particularly for older patients presenting with low mood.
Other organic conditions
In a person who presents predominantly with somatic symptoms, the primary care physician needs to first exclude any organic disease.( 21 , 31 ) Depending on the symptomatology, the scope of organic conditions to consider can be wide, especially with elderly patients. Organic conditions can also coexist or masquerade as major depression (e.g. occult malignancy or even infections).( 63 , 64 ) Patients should have their thyroid function tested, as thyroid dysfunction may present with low mood and other nonspecific somatic symptoms. However, minor abnormalities in thyroid function should be interpreted with caution; major depression may be associated with subtle changes in thyroid function.( 65 , 66 ) A useful method of differentiating major depression in medical patients, who may present with somatic symptoms similar to those found in major depression, is to ask about cognitive symptoms such as negative thinking, inappropriate guilt and low self-esteem.( 67 )
Drugs and substance abuse
Major depression is a risk factor for and is often associated with substance abuse, including that of alcohol,( 33 ) which may be under-recognised in elderly patients.( 68 , 69 ) In terms of lifetime prevalence, it has been shown that alcohol abuse is the second most common mental disorder among adults in Singapore.( 2 ) Tactful enquiry using an open-ended question about alcohol intake has been shown to enhance the sensitivity of the CAGE questionnaire.( 70 , 71 ) The patient’s medication history is important, as even prescription drugs have been cited as potential causes of major depression.( 72 ) A review found strong association between major depression and finasteride, isotretinoin and a smoking cessation drug, varenicline.( 73 ) The authors recommended that physicians exercise caution when prescribing these medications and strongly weigh risk-benefit considerations, particularly in persons who are predisposed to major depression.( 73 ) Fortunately, evidence implicating medications commonly prescribed in primary care was shown to be inconclusive. These medications include beta-blockers, calcium channel blockers, angiotensin-converting enzyme inhibitors and angiotensin II receptor blockers.( 73 )
Assessing the severity of major depression
The DSM-5( 1 ) defines the severity of major depression based on the number of criterion symptoms, severity of those symptoms and degree of functional disability. Major depression is classified as mild if (a) the patient has very few, if any, symptoms in excess of the five required to fulfil the criteria for diagnosis; (b) the symptoms are manageable; and (c) functional impairment is minor (e.g. the patient is still able to work). At the opposite end of the spectrum, severe major depression has (a) a substantially greater number of symptoms than that required to make the diagnosis; (b) seriously distressing and unmanageable symptoms; and (c) extensive impairment of social and occupational functioning. For moderate major depression, the number of symptoms, the intensity of symptoms and/or functional impairment are in-between those specified for ‘mild’ and ‘severe’.
The severity of major depression has an important bearing on the urgency and mode of treatment, setting in which the patient is to be managed and frequency of follow-up visits. For example, psychotherapy may be used as the first-line treatment for mild to moderate major depression, whereas pharmacotherapy is recommended for moderate to severe major depression.( 74 ) In patients with previous episodes of moderate to severe depression, who present with mild symptoms, the first-line treatment should be drug therapy.( 12 ) Those who have psychotic features should be managed by psychiatric services in tertiary care, while patients who are acutely suicidal should be hospitalised for urgent psychiatric evaluation.
Major depression is the most prevalent mental disorder in Singapore. Patients often present with somatic nonspecific complaints apart from the usual symptoms. Major depression is also common among patients with chronic conditions; there is a bidirectional relationship between the two factors. As the first point of contact for patients, the primary care practitioner is in a unique position to diagnose and manage major depression. Another important aspect of evaluating a person with major depression is performing a suicide risk assessment, which is described in our next article in this three-part series.
You identified the death of Susan’s cousin as a life event that had a significant emotional impact on her. Using the PHQ-2 and PHQ-9, you diagnosed Susan with major depression. You started her on a serotonin-specific reuptake inhibitor and referred her to a clinical psychologist for cognitive behaviour therapy .
TAKE HOME MESSAGES
- Major depression is the most common mental disorder in the community and patients often present with somatic symptoms.
- Major depression is potentially a chronic illness that has considerable morbidity, and high relapse and recurrence rates.
- There is a bidirectional relationship between major depression and chronic diseases.
- Clinical tools available for screening for major depression include the common PHQ-2, PHQ-9 and BDI.
- The severity of major depression, according to the DSM-5, increases with the number of criterion symptoms present and degree of functional disability.
Advertisement
Deep learning for prediction of depressive symptoms in a large textual dataset
- Original Article
- Open access
- Published: 27 August 2021
- Volume 34 , pages 721–744, ( 2022 )
Cite this article
You have full access to this open access article
- Md Zia Uddin ORCID: orcid.org/0000-0002-5215-1834 1 ,
- Kim Kristoffer Dysthe 2 ,
- Asbjørn Følstad 1 &
- Petter Bae Brandtzaeg 1 , 2
21k Accesses
60 Citations
25 Altmetric
Explore all metrics
Depression is a common illness worldwide with potentially severe implications. Early identification of depressive symptoms is a crucial first step towards assessment, intervention, and relapse prevention. With an increase in data sets with relevance for depression, and the advancement of machine learning, there is a potential to develop intelligent systems to detect symptoms of depression in written material. This work proposes an efficient approach using Long Short-Term Memory (LSTM)-based Recurrent Neural Network (RNN) to identify texts describing self-perceived symptoms of depression. The approach is applied on a large dataset from a public online information channel for young people in Norway. The dataset consists of youth’s own text-based questions on this information channel. Features are then provided from a one-hot process on robust features extracted from the reflection of possible symptoms of depression pre-defined by medical and psychological experts. The features are better than conventional approaches, which are mostly based on the word frequencies (i.e., some topmost frequent words are chosen as features from the whole text dataset and applied to model the underlying events in any text message) rather than symptoms. Then, a deep learning approach is applied (i.e., RNN) to train the time-sequential features discriminating texts describing depression symptoms from posts with no such descriptions (non-depression posts). Finally, the trained RNN is used to automatically predict depression posts. The system is compared against conventional approaches where it achieved superior performance than others. The linear discriminant space clearly reveals the robustness of the features by generating better clustering than other traditional features. Besides, since the features are based on the possible symptoms of depression, the system may generate meaningful explanations of the decision from machine learning models using an explainable Artificial Intelligence (XAI) algorithm called Local Interpretable Model-Agnostic Explanations (LIME). The proposed depression symptom feature-based approach shows superior performance compared to the traditional general word frequency-based approaches where frequency of the features gets more importance than the specific symptoms of depression. Although the proposed approach is applied on a Norwegian dataset, a similar robust approach can be applied on other depression datasets developed in other languages with proper annotations and symptom-based feature extraction. Thus, the depression prediction approach can be adopted to contribute to develop better mental health care technologies such as intelligent chatbots.

Similar content being viewed by others
Depression Detection Using Hybrid Transformer Networks
1D CNN Model: BERT-Based Approach for Depression and Suicide Identification
Early Detection of Mental Disorder Via Social Media Posts Using Deep Learning Models
Explore related subjects.
- Artificial Intelligence
Avoid common mistakes on your manuscript.
1 Introduction
Depression, or depressive disorder, is a common disease. According to the World Health Organization (WHO), the number of people with depression was estimated at more than 300 million affected worldwide [ 1 ]. Depression may severely impact well-being and functioning at work, school, and family, and can even lead to self-harm. Adolescent depression is associated with mood disorders and severe mental illness in adult life [ 2 , 3 ]. Nearly 0.8 million people die from suicide each year and suicide is the fourth leading cause of death in 15–19-year-olds, according to WHO [ 1 ]. Amongst the top major diseases causing disability or incapability, five are mental illnesses—depression being the most prominent of these [ 4 ]. Hence, the disease burden due to depression is vast. The prevalence of depression in the adult population is approximately 5% across cultures, and 20% in its milder forms (i.e., partial symptoms, mild depression, and probable depression) [ 5 ]. Among adults, those most at risk are within the middle-aged population. Also, the world-wide occurrence of depression is increasing, with a rise of 18% between 2005 and 2015. However, early professional intervention can improve mental symptoms (e.g., absence of self-confidence and rumination) and resolve somatic problems (e.g., gastrointestinal problems and sleeping disorders) in most of the cases [ 6 , 7 ].
Early detection of depressive symptoms followed by assessment and treatment can considerably improve chances for curbing symptoms and the underlying disease; mitigate negative implications for well-being and health as well as personal, economic, and social life [ 7 , 8 , 9 , 10 ]. However, detection of depressive symptoms is challenging and resource demanding. Current approaches are mainly based on clinical interviews and questionnaire surveys by hospitals or agencies [ 11 ], where psychological evaluation tables are utilized to make predictions on mental disorder. This approach is mostly based on one-to-one questionnaires and can roughly diagnose the psychological disorder for depression.
An alternative approach to interview or questionnaire-based predictions of depression is the analysis of informal texts provided by users. Previous studies in clinical psychology have shown that the relationship between the user of a language (e.g., speaker or writer) and their text is meaningful and has potential for the future [ 12 ]. A recent study by Havigerová et al. indicate a potential for text-based detection of persons at risk for depression, using a sample of informal text written about a holiday [ 12 ]. Hence, online records and data are increasingly seen as a valuable data source in supporting health care with decision support. The approach to identify depression symptoms from informal texts is promising, as it allows for benefitting from recent advances in natural language processing and Artificial Intelligence (AI). AI applied for natural language processing employs linguistics and computing techniques to help machines to understand underlying phenomena such as sentiments or emotions from texts. In that case, the core intent is to analyse opinions, ideas, and thoughts via the assignment of polarities either negative or positive.
Previous work has found that automatic analysis of depression symptoms from texts can be applied in, for example, sentiment retrieval from suicide notes and detecting insulting or depressive words or sentences in conversations or blog posts [ 13 , 14 , 15 , 16 , 7 , 18 ]. However, there is still substantial untapped potential in research on extracting depressive symptoms from texts. Key challenges include portraying significant cues of depression from texts. Also, there is a substantial hurdle in detecting depression symptoms from short texts.
To contribute towards solving these challenges, we aim to develop an automatic algorithm for detecting depression symptoms in texts, using a text-based sample of young people seeking advice about self-perceived depressive symptoms. We believe our automatic detection approach, describing the problems of the users in natural language, can be a substantial contribution to this research field. Hence, the current study focuses on how symptoms of depression are manifested through text in natural language using AI.
To visualize sample data of different groups in different applications, Linear Discriminant Analysis (LDA) is a good tool for data visualization based on discriminations [ 19 , 20 , 21 , 22 ]. It works on grouping of samples of similar classes. It tries to find the directions where the classes are best separated by considering minimizing the within-class scatter while maximizing the between-class scatter. LDA has already been used in various practical applications such as facial emotion recognition and human activity recognition. LDA projects the sample data of different classes onto a lower-dimensional vector space. Thus, the ratios of the between-class scatter and the within-class scatter is maximized to achieve highest discrimination.
Deep neural network has been contributing a lot recently in enormous fields of research, especially in pattern recognition and AI [ 23 , 24 , 25 , 26 , 27 , 28 , 29 , 30 , 31 , 32 , 33 , 34 ]. Though it is more robust than typical neural networks, it however consists of two major disadvantages. The first disadvantage is overfitting problem most of the time. The last one is taking much time for modelling the underlying data. The first successful deep learning algorithm was deep belief network that consisted of Restricted Boltzmann Machines (RBMs) that made the training quite faster than other previous learning approaches. Later, convolutional neural networks (CNN) was proposed and got popular especially in image processing fields. It showed better discriminative power compared to other approaches. CNN also extracts features alongside training the data. It has some convolutional stacks to generate a progressive hierarchy of abstract features via convolution, pooling, tangent squashing, rectifier, and normalization [ 24 ]. CNN is mostly applied for image and video pattern analysis rather than temporal information decoding. Hence, it has not been adopted for time-sequential data analysis. Recurrent Neural Networks (RNNs) is however a better choice than CNN since it consists of better discriminative power over others in case of sequential data and pattern analysis [ 30 ]. Since the basic RNNs usually consist of vanishing gradient problem due to long-term dependencies when it handles high-dimensional and time-sequential data, Long Short-Term Memory (LSTM) was introduced in RNN to overcome it. Hence, this work utilizes the advantage of LSTM-based RNN to model different emotional states in text data.
Among different approaches to analyse physical and mental states of human being from different data sources, machine learning has been very widely used [ 35 , 36 , 37 , 38 , 39 , 40 , 41 ]. Since machine learning models are progressively being employed to do significant predictions in crucial contexts day by day, the demand of transparency rises in such contexts from the various stakeholders in AI industry [ 42 ]. The high risk in this regard is making and applying the AI decisions that are unjustifiable and lacks explanations of the models' behaviour. Hence, explanations of the output of a model are vital. For example, specialists in precision medicine fields need further information from the machine learning models than simple prediction for supporting their diagnosis. Such necessities may also arise in other fields as well, such as medical emergencies. Hence, focusing merely on the performances of the AI models, gradually makes the systems towards unacceptance in some cases. Therefore, current research has highlighted the importance of explainable Artificial Intelligence (XAI) for establishing trust in machine learning-based decisions through the explanations of the black-box models. Popular state-of-the-art explanation algorithms include Local Interpretable Model-Agnostic Explanations (LIME), SHapley Additive exPlanations (SHAP), and layer-wise relevance propagation (LRP). From which, LIME is very light-weight and yet tries to generate quick and satisfactory post-hoc explanations. Hence, this work adopts LIME to see the explanations (i.e., importance of the features) once the decision is provided by the model.
1.1 Contribution
This work focuses on processing text data, features, and depression symptoms text recognition with the target of chatbot as a smart application. Figure 1 shows a schematic setup of a text-based depression symptoms text detection system in a smart application where a user provides a query in text format and a server processes the text to apply feature extraction and deep learning. Based on the results, the server can suggest further advices to the user. Figure 2 shows the basic architecture of the proposed system consisting of training and testing procedure for the classification of texts describing symptoms of depression. In the training part, text data from all the users is obtained and then the features are trained using RNN. In the testing part, features from a sample test are applied to the trained model to take the decision whether the user describes depression or not. LDA is applied to show the robustness of the proposed features compared to other traditional ones. Finally, we apply one of the most popular algorithms (i.e., LIME) for post-hoc, local, and meaningful explanations of the machine learning decision regarding the existence of a potential depression or not, in the text. The contribution of the paper can be summarized as bellow:
A large dataset of text is obtained from a public Norwegian online information channel: ung.no .
Novel features are extracted representing the possible symptoms of depression defined by the experts from medical and psychology domains.
RNN is applied based on LSTM, attention, and dense layers for modelling the emotional states.
The machine learning decisions are explained using a state-of-the-art XAI approach, LIME to see the importance of the features.
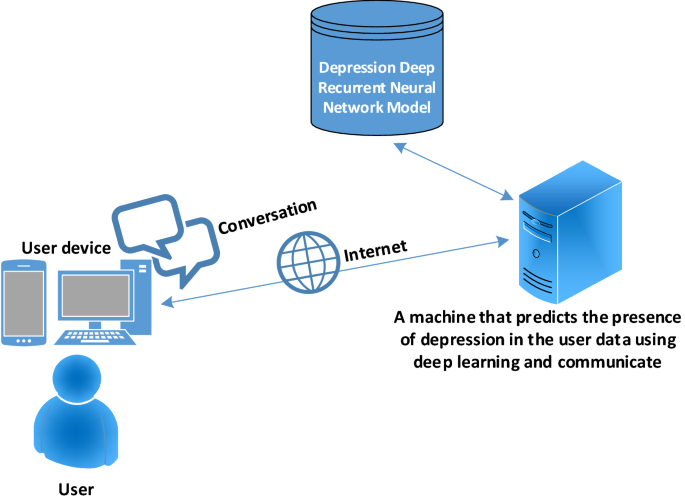
A schematic setup for classifying texts containing symptoms of depression
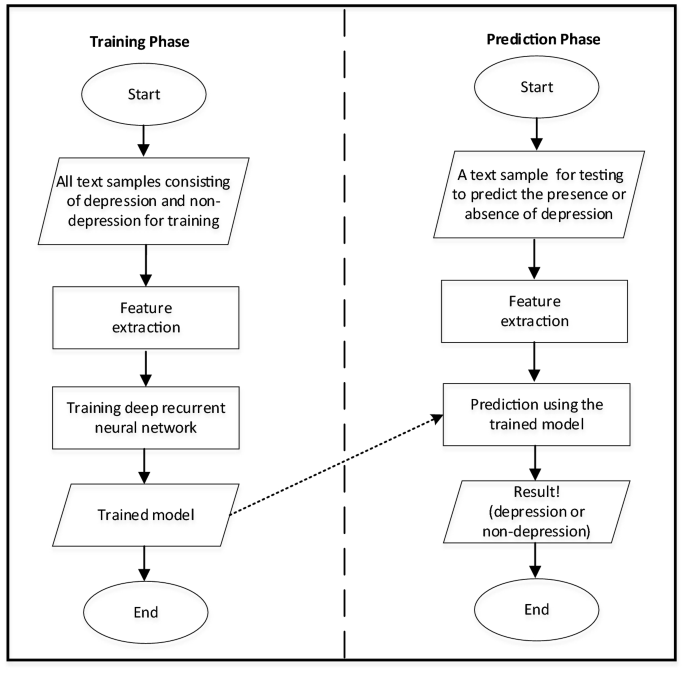
Flowcharts of the proposed depression prediction system from text
2 Data collection and processing
To reliably detect symptoms of mental health issues, the collection of data for the detection model is crucial. For instance, data from social media such as Facebook status updates does not seem to be sufficiently detailed to develop reliable models to decode emotional states from data [ 43 ]. For this work, we obtained a large text-based dataset from a public Norwegian information website: ung.no . At ung.no, youth have the opportunity to post questions anonymously in Norwegian about their various challenges and problems in their everyday life. In response, corresponding professional experts (e.g. doctors, psychologist, nurses etc.) provide answers and offer advise. These questions and answers are published online and publicly available for everyone. Prior to submitting a question on ung.no , young people pre-define and categorize the topic of their post. We focused herein on the category “Mental health and emotions”. Even if the texts are relatively short, they typically describe the activating factors leading to the mental state and the ensuing symptoms and behaviour. First, a proportion of the texts describe depressive conditions already diagnosed by a health professional. Second, many of the texts describe the narrative and the ensuing symptoms, either asking if it could represent depression or suggesting depression as a possible diagnosis. We believe these texts to present self-perceived depressive symptoms. Previous research suggest that self-perceived mental states correspond well with later clinical diagnoses [ 44 , 45 , 46 ]. Last, some of the texts describe the narratives and the succeeding mental states without mentioning a possible depression. The staff interpret the texts as describing symptoms of depression. Accordingly, the data is classified into categories, depression being one of them. Then, a trained GP went through the posts, confirming descriptions of depressive symptoms. A list of sentences and words are summarized analysing the messages in the database where they may indicate the person having depression. A medical practitioner validated the sentences and words. Table 1 shows some important features from “Appendix” representing the possible sentences and/or words may occur in the queries by the youth having depression.
The sentences and words are used to obtain features for each message of the dataset. Five translated and paraphrased examples of depression texts derived from a Norwegian text dataset at ung.no are shown in Fig. 3 . The Norwegian dataset consists of 277,552 free-text posts in different categories including depression texts. From that dataset, we utilized 11,807 and 21,470 posts of different length for our two different experiments in this work. For feature extraction process to model depression and non-depression machine learning model, we augment all the feature rows of “Appendix” first. Then, all rows in the “Appendix” are tokenized word by word and stemmed for feature extraction process. The stemmed words from the list of symptoms are represented as F = " all hat meg alt er jæv … noe mer å lev for" . To extract features from a text input, one-hot process is applied on the stemmed words of the input text based on each word of F (i.e., 1 if a word from F is present and 0 otherwise). Thus, the features for the texts represents binary patterns to be applied with machine learning model of depression prediction. The collection of 189 unique words extracted from the list of possible symptoms is shown in Fig. 4 where the words in Norwegian are in alphabetical order in Fig. 4 a and the corresponding translated words are in Fig. 4 b. Unique extractions of stemmed words are listed to illustrate the diversity of possible words associated with symptoms of depression.
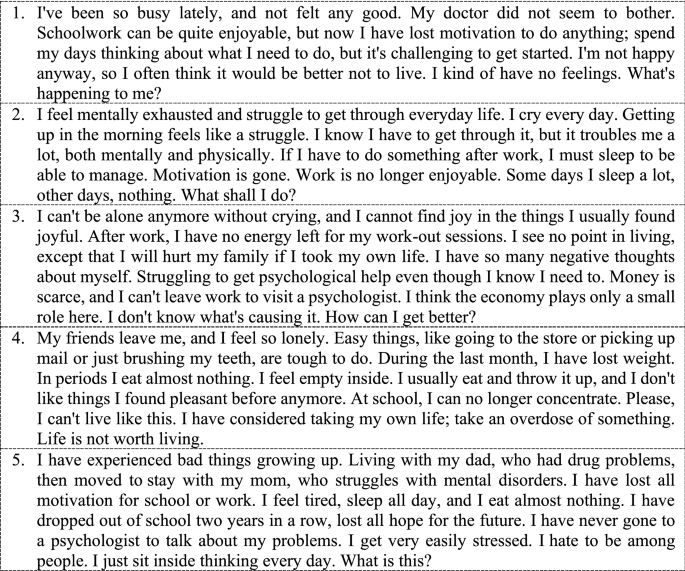
Five translated and paraphrased examples of depression posts derived from the Norwegian dataset used in the work
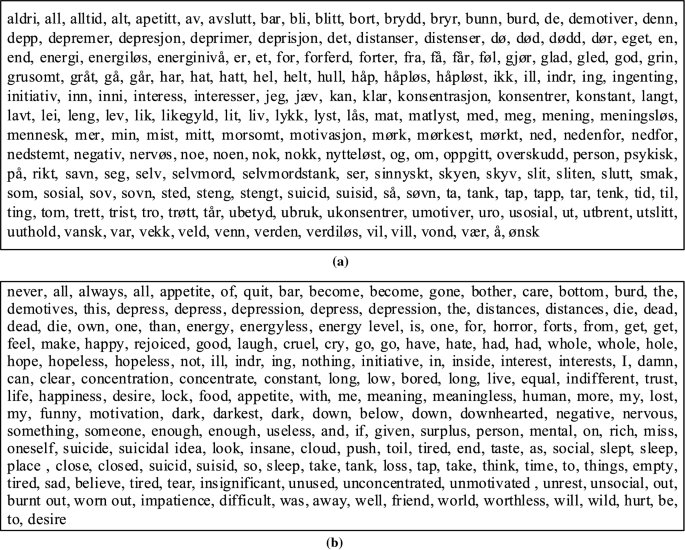
Unique words extracted from the stemmed words of possible symptoms reported in APENDIX A: a Norwegian words in alphabetical order and b translated in English
The symptoms presented in “Appendix” are obtained with the help of Norwegian professionals (e.g., medical doctors and psychologists). However, the way of expressing the emotions in Norwegian texts may be linguistically different from other languages. Therefore, professionals in those languages can contribute to building dataset and features in modelling depression and non-depression. To be noted, the English texts are shown in Table 1 , Figs. 3 , 4 , and “Appendix” only for the readability of international readers and researchers. Otherwise, whole approaches from input text to emotional state modelling via feature processing, is done based on the Norwegian language.
The main reason to go for using one-hot on the robust depression features rather than traditional ones such as typical one-hot and Term Frequency—Inverse Document Frequency (TF-IDF) [ 47 ] that are related to typical word frequencies rather than word importance is, the features describing depression symptoms are much more important than just word frequencies to predict depression in the text. Figure 5 shows the algorithm for one-hot feature extraction based on the unique feature words in the list of depression symptoms. Thus, the one-hot binary features based on the depression symptoms for the i th text in the dataset can be represented as L i .
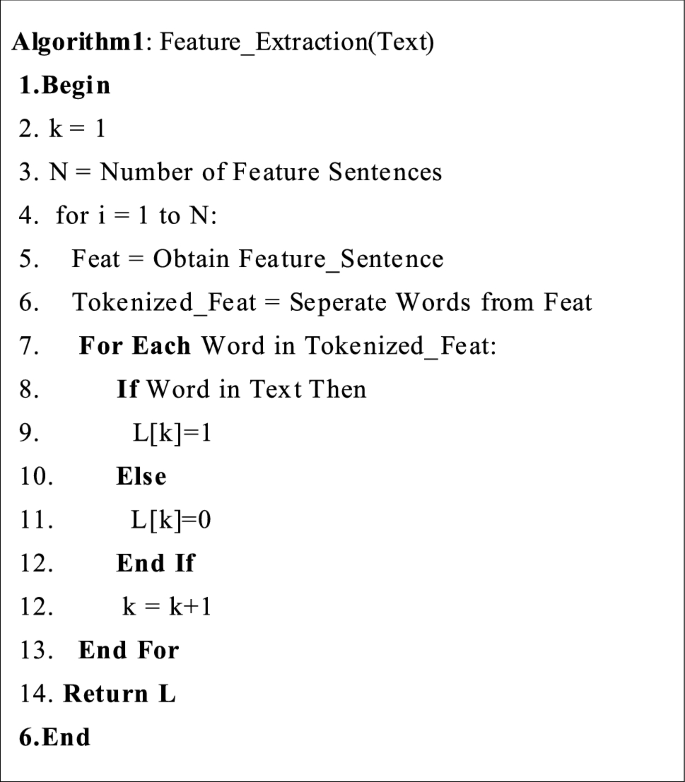
The algorithm of one-hot depression symptom feature extraction
3 Linear discriminant analysis (LDA) for visualization
To visualize different features, we adopt linear discriminant analysis (LDA) here. LDA is basically an eigenvalue decomposition problem trying to maximize the inter-class scatterings of the samples whereas minimizing the inner-class scatterings of them. The formulas for the inter-class scattering, \(M_{B}\) and inner-class scattering matrix, \(M_{W}\) are shown as follows:
where c is the total number of classes, \(N_{i}\) the number samples in class \(C_{i}\) , \(m_{k}\) the feature vectors from class C , \(m_{i}\) the mean of class i , and \(m_{j}\) the mean of all feature vectors. The LDA feature space representing the optimal discrimination matrix can be found by maximizing the ratio of the determinant of \(M_{B}\) and \(M_{W}\) as
where Q basically represents the set of discriminant vectors. Thus, the discriminant ratio of inner as well as inter-class samples of different classes can be found by solving an eigenvalue problem as
where \(\Lambda\) is the eigenvalue matrix in the singular value decomposition process. Figures 6 , 7 , 8 , and 9 show the feature visualizations using 3-D plots of typical one-hot in LDA, typical TF-IDF in LDA, and proposed features in PCA, and proposed features in LDA features spaces, respectively. In the figures, the proposed features (i.e., Fig. 9 ) shows superior clustering of the samples of same class and better separation among the samples of different classes compare to the two other approaches, indicating the robustness of the proposed features in this regard. However, the traditional PCA projection on the features Thus, the text feature matrix F is projected to the LDA feature space \(Q_{{{\text{opt}}}}\) as
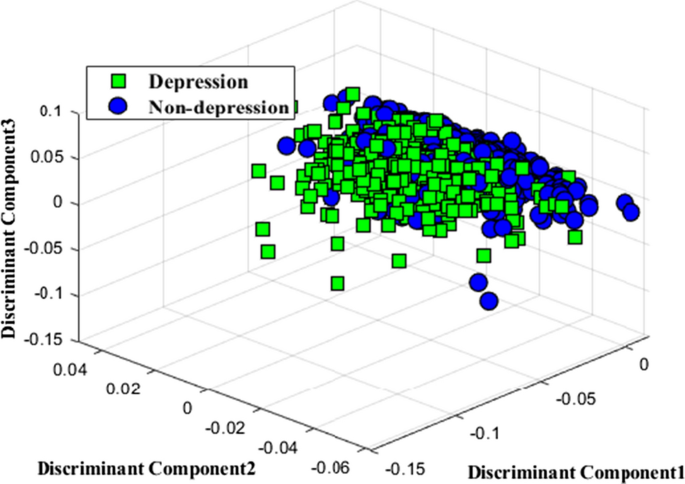
3-D plot after LDA on the traditional one-hot features of two emotional states
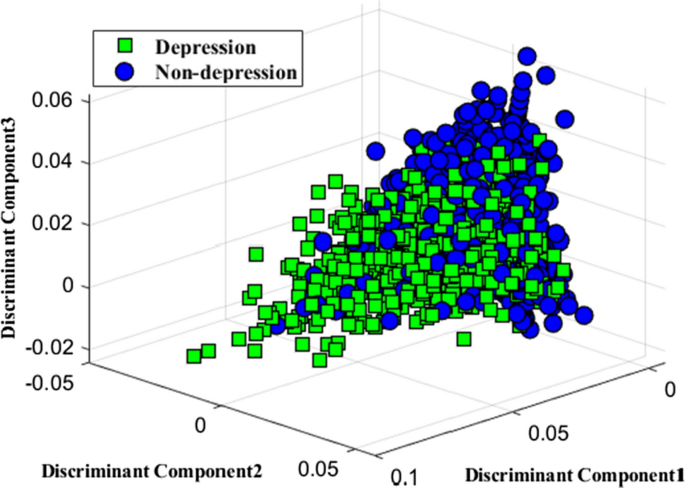
3-D plot after LDA on the traditional TF-IDF features of two emotional states
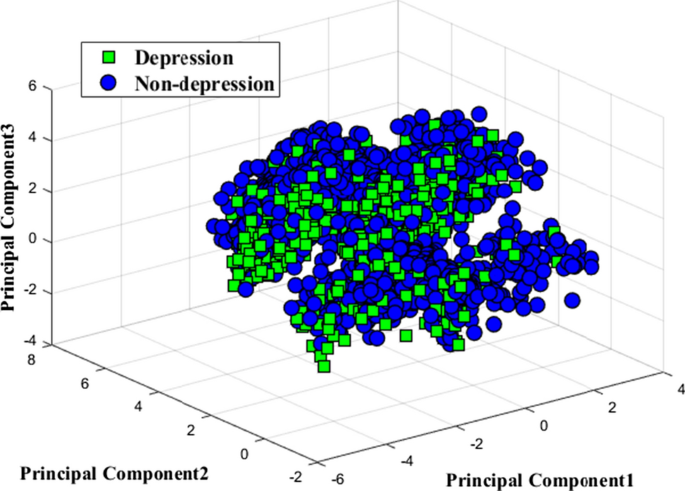
3-D plot after PCA on the proposed robust features of two emotional states
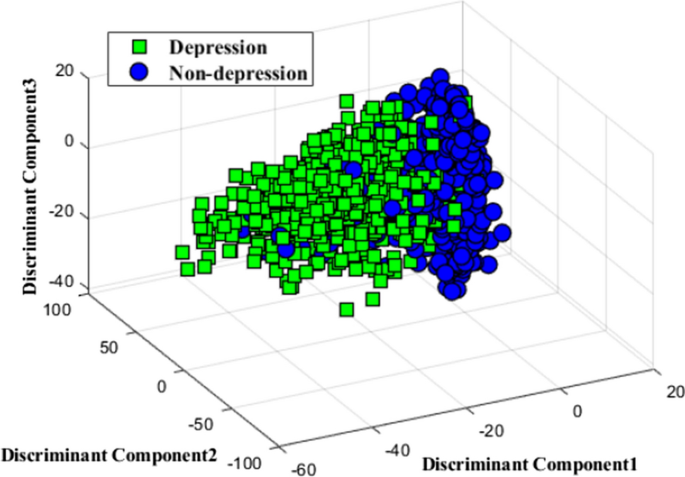
3-D plot after LDA on the proposed robust features of two emotional states
4 Deep recurrent neural network (RNN) for modelling emotional states
Emotional states can be represented as time-sequential words in text data while conversating with others. Hence, a machine learning model capable of encoding time-sequential data is quite suitable for such kind of work. Hence, Recurrent Neural Networks (RNNs) is adopted in this work. RNN can be considered as most popular deep learning approaches used to model time-sequential information [ 22 ]. RNNs basically consists of recurrent connections between history to present state and hidden states. That is a quite important role of the memory in neural networks. The usual RNN algorithms very often face a vanishing gradient problem, a limitation of processing long-term data which is mostly known as Long-Term Dependencies. To overcome the problem, Long Short-Term Memory (LSTM) was developed [ 23 ]. Figure 10 shows a sample deep neural network consists of 50 LSTM units.
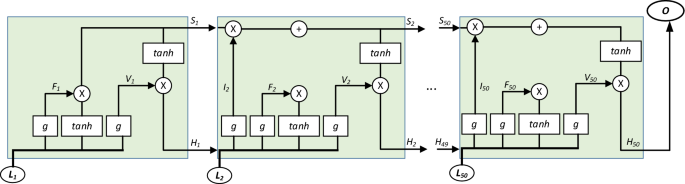
A basic structure of LSTM-based RNN
Each LSTM memory block has a cell state as well as three gates, which are input, forget, and the output gates. The input gate F t can be represented as
where W is weight matrix, b bias vectors, and β a logistic function. The forget gate F can be expressed as
The long-term memory is stored in a cell state vector S that is expressed as
The output gate V produces the output for the unit and can be expressed as
The hidden state H is expressed as
We adopt an attention layer over the LSTM units before applying dense layer [ 48 ] as
The attention technique is basically used for emphasising important information in the current task rather than other useless information. Hence, it can be applied on top of the LSTM layers to improve the model's accuracy. Finally, the output can be determined using a softmax function as
where W and b represent weights and bias, respectively. Figures 11 and 12 show the algorithms for training and prediction of depression or non-depression through RNN, respectively.
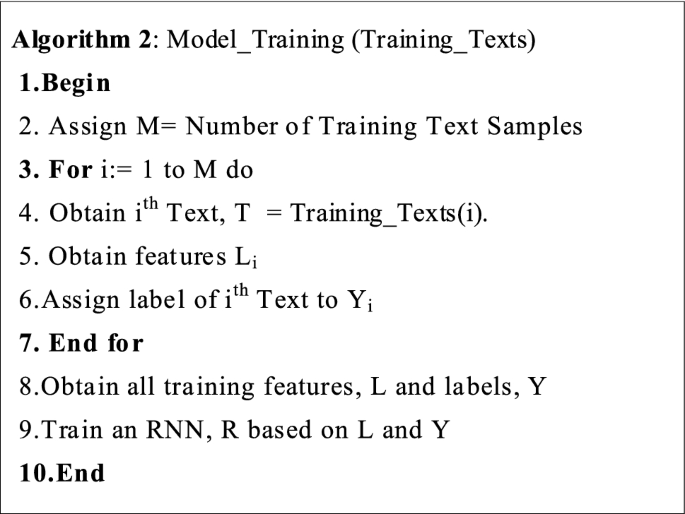
The algorithm of training features from all texts with RNN
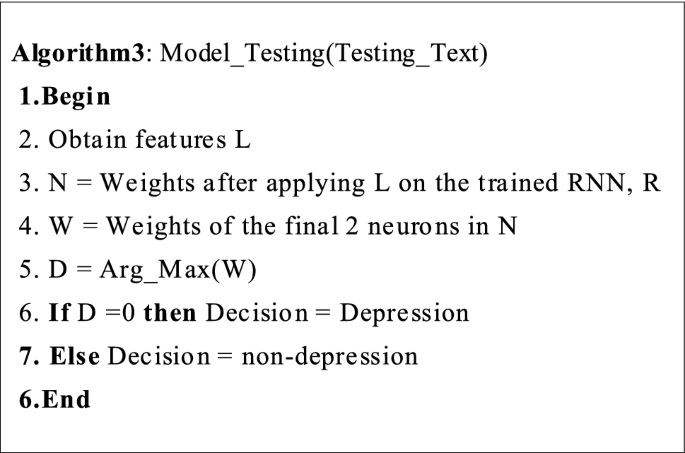
The algorithm of testing of a test text message with the trained RNN
5 Experimental results and discussion
For experiments, two text datasets were obtained from the queries and answers from ung.no website. The dataset comprises of several categories including depression texts. The annotations of the messages were done with the help of professionals such as medical doctors and psychologists. All the experiments are done on a computer that has Intel(R) Core(TM) i7-7700HQ CPU with the speed of 2.80 GHz and 2.81 GHz, memory of 32 GB, Windows® 10 operating system, and TensorFlow deep learning tool version 2.4.1.
5.1 First dataset and experiments
From the whole collection of texts of different categories, 11,807 of them were extracted for the first dataset and experiments that consisted of 1820 texts categorized as depression texts (describing symptoms of depression) and the other 9987 as non-depression texts (not describing symptoms of depression). Tables 2 , 3 , 4 , 5 , 6 , 7 , 8 , 9 , 10 , 11 represent the classification reports of tenfold used in the experiments where each fold consist of 90% data as training and rest as testing. Figures 13 , 14 , 15 , 16 , 17 , 18 , 19 , 20 , 21 , 22 show the confusion matrices of each fold. Figure 23 depicts the accuracy and loss for 100 epochs during the training of the ten different folds. The overall training of the folds looks good except a little negligible fluctuation. Figure 24 shows the attention-based LSTM model used in this work where there are 53,358 parameters represented by an LSTM layer with 50 memory units, an attention layer, and a dense layer for 2 different emotional states (i.e., depression and non-depression).
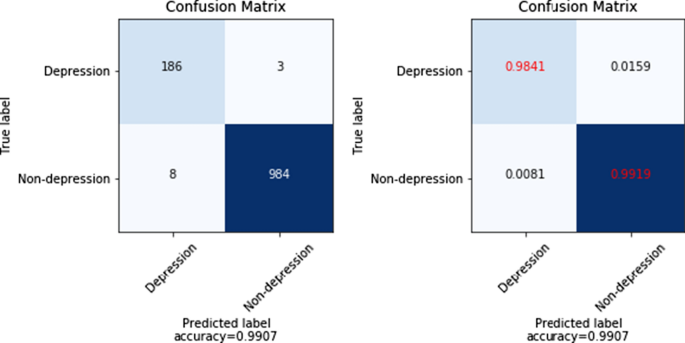
Confusion matrix of fold-1 in the first dataset using proposed approach
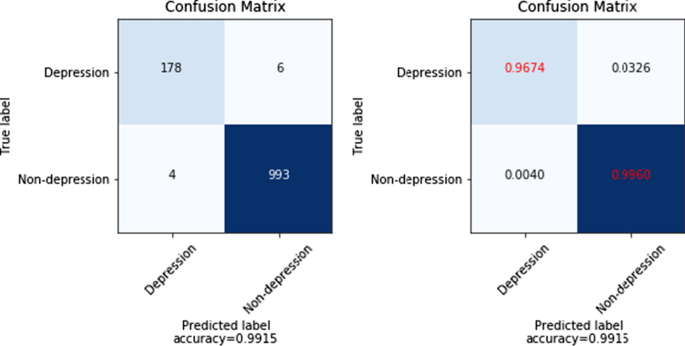
Confusion matrix of fold-2 in the first dataset using proposed approach
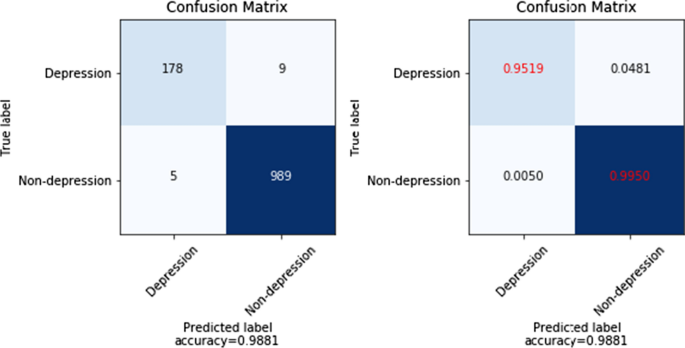
Confusion matrix of fold-3 in the first dataset using proposed approach
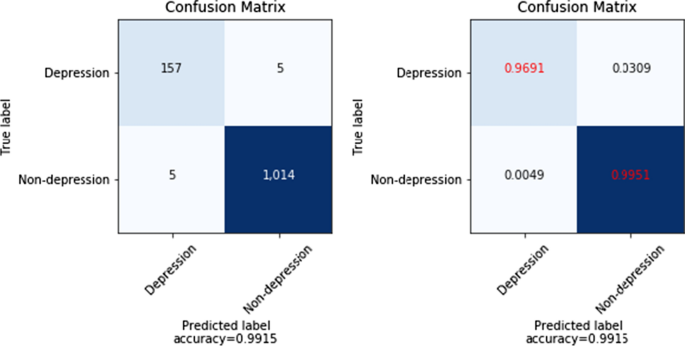
Confusion matrix of fold-4 in the first dataset using proposed approach
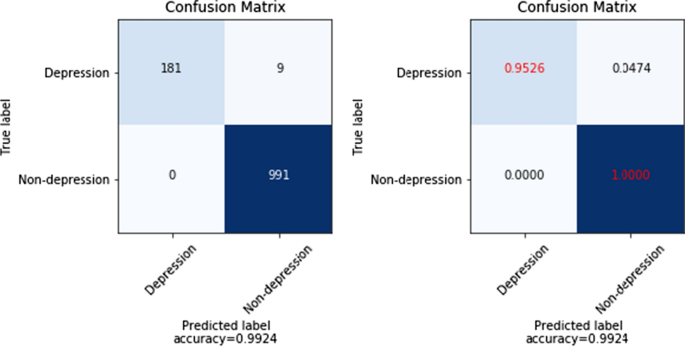
Confusion matrix of fold-5 in the first dataset using proposed approach
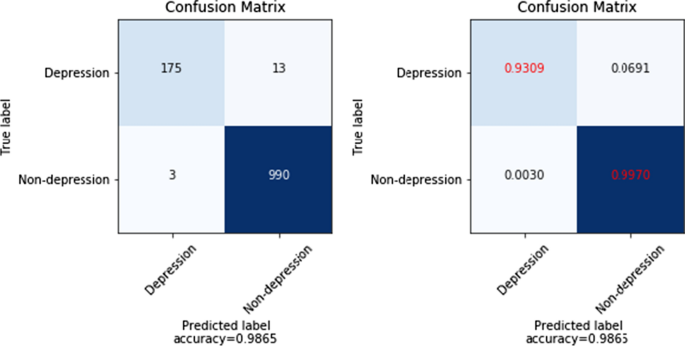
Confusion matrix of fold-6 in the first dataset using proposed approach
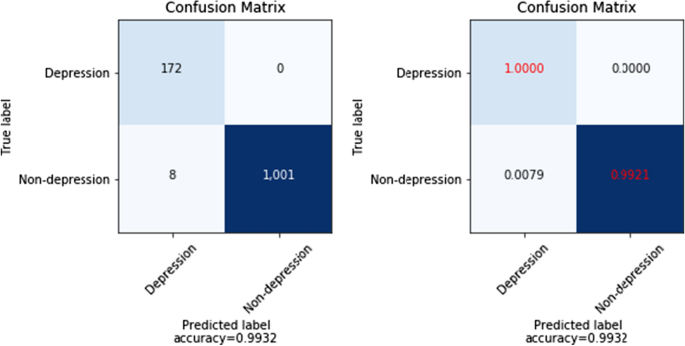
Confusion matrix of fold-7 in the first dataset using proposed approach
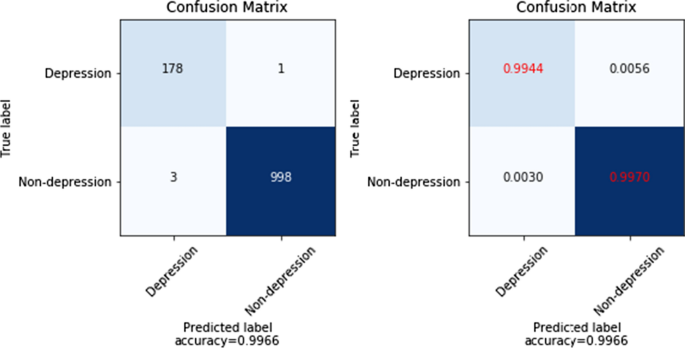
Confusion matrix of fold-8 in the first dataset using proposed approach
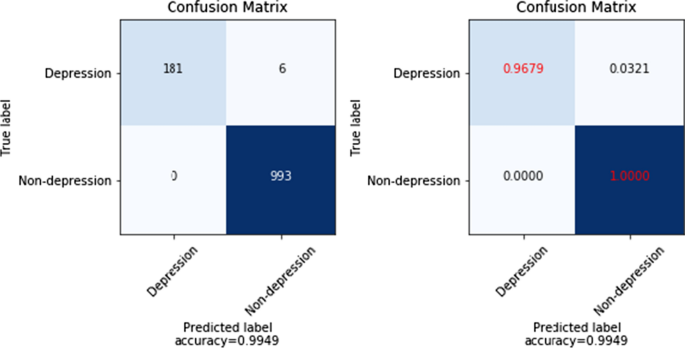
Confusion matrix of fold-9in the first dataset using proposed approach
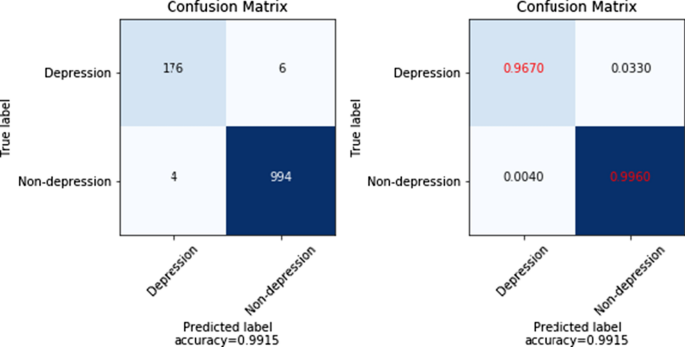
Confusion matrix of fold-10 in the first dataset using proposed approach
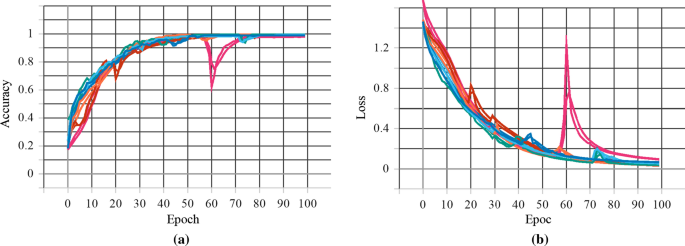
a Accuracy and b loss of 10-folds during experiments on the first dataset using the proposed approach
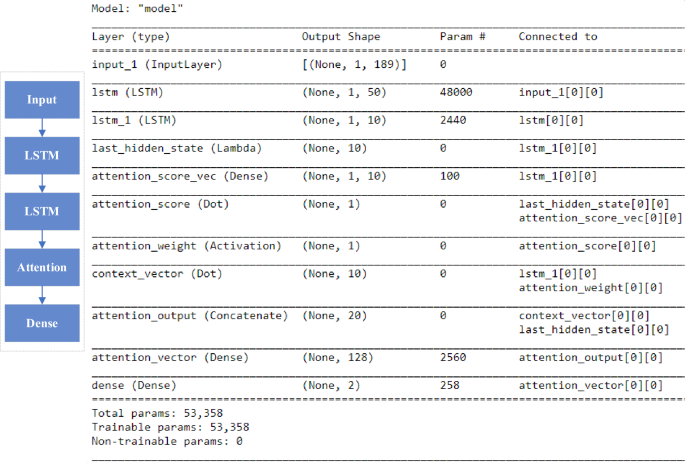
The emotional state model structure and parameters based on attention over LSTM units
5.2 Comparison with traditional approaches
We compared the proposed approach with traditional approaches where the proposed one showed superior results than others. We first applied traditional machine learning approaches using different features (i.e., typical one-hot, TF-IDF, and proposed features) with other conventional machine learning algorithms (i.e., logistic regression, decision trees, support vector machines (SVM), typical large artificial neural network (ANN), DBN, and CNN) but could not achieve more than 91% of mean accuracy as shown in Table 12 . Furthermore, we tried LSTM with the traditional as well as proposed features to decode and model the time-sequential information to determine the emotional states. Table 13 and chart in Fig. 25 show the performance of three different approaches to the first dataset where the proposed approach shows the superiority by achieving 98% of mean accuracy over two other approaches.
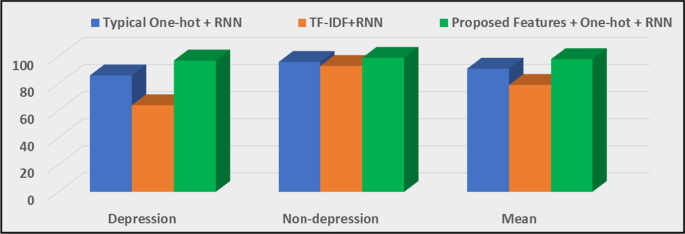
Performance of three different approaches to the first dataset
Besides, another straight-forward approach was applied where the direct presence of the symptoms from “Appendix” was checked to take the binary decision of depression or non-depression. This approach was applied on the whole dataset rather than splitting into training and testing since it was a simple rule-based classification. The direct presence of one or more symptoms-based approach achieved the accuracy of 84.20% where 1684 depression texts were correctly classified among a total of 1807 depression texts and 1730 non-depression texts correctly classified among 10,000 non-depression text. Since there are different ways to express self-depression in texts of different length, it is hard to apply just a binary rule to determine the depression in the text. Hence, it is better to combine the base words from all the symptoms to define collection of features for depression to apply some complicated algorithms such as sequence-based machine learning algorithm using LSTM-based RNN that has been applied in this work.
5.3 Second dataset and experiments
For the second dataset, a total of 21,470 text samples were obtained consisting of depression—and non-depressions texts. From which, 1470 were depression texts and rest of the 20,000 were non-depression texts. We applied fivefold cross validation for the second phase experiments with the proposed approach, i.e. using RNN on the robust features. Only the results using the proposed approach is reported here since it showed the best results than the other approaches as shown in the experiments of the first phase, i.e. first dataset. Figures 26 , 27 , 28 , 29 , 30 represent the confusion matrices of fivefold used in the second experiments where each fold consist of 80% data as training and rest 20% as testing. The experimental results show a remarkable performance of the proposed features followed by one-hot and LSTM where the mean recall rate of depression and non-depression is 0.98 and 0.99, respectively. The mean accuracy is 0.99 that shows the robustness of the proposed approach.
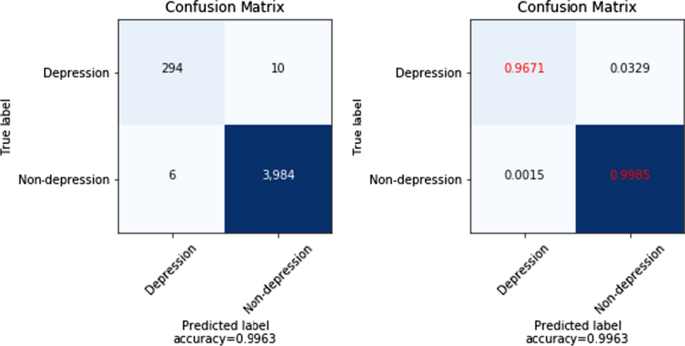
Confusion matrix of fold-1 in the second dataset using proposed approach
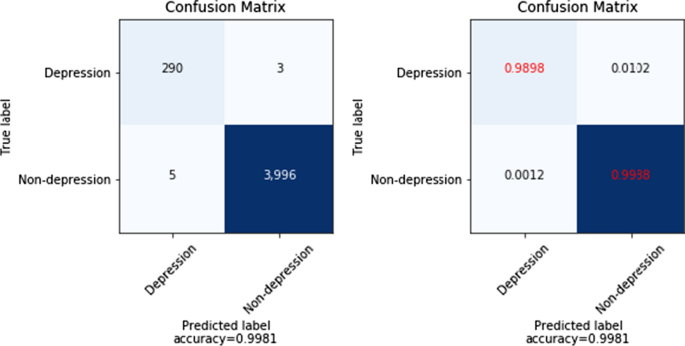
Confusion matrix of fold-2 in the second dataset using proposed approach
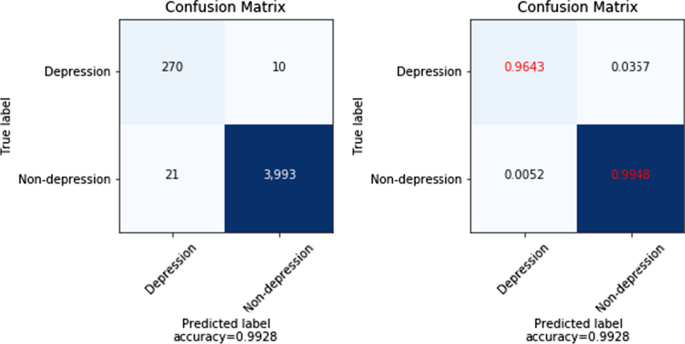
Confusion matrix of fold-3 in the second dataset using proposed approach
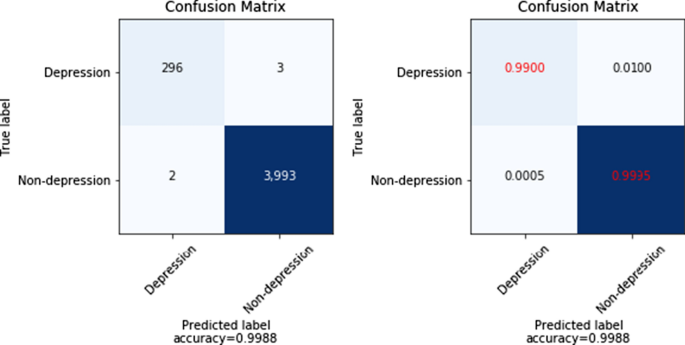
Confusion matrix of fold-4 in the second dataset using proposed approach
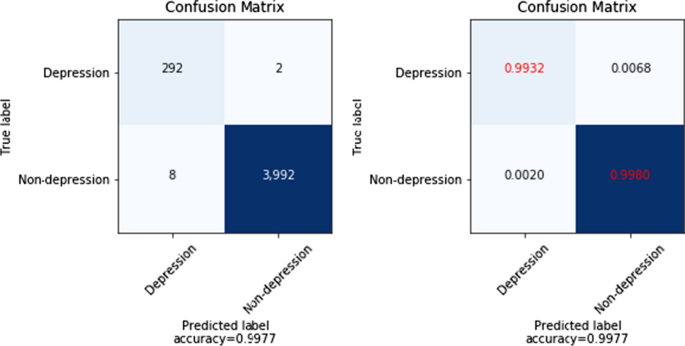
Confusion matrix of fold-5 in the second dataset using proposed approach
In summary, the above experimental results show the overall efficiency of the proposed depression prediction system using depression symptom-based features and time-sequential LSTM-based machine learning model. The proposed system shows better results than existing latest approaches for depression prediction. For instance, in [ 12 ], the work is basically based on a measuring scale considering depression, anxiety and stress, which is a point-based measuring scale obtained by writing four different kind of letters by the candidates. The candidates collected by formal advertisements were asked to write these letters whereas in our database, the participants wrote the text spontaneously expressing their necessity to seek assistance over a national portal. The model used [ 12 ] is logistic regression, a simple and basic machine learning model which is usually simple linear model and hence, should not generally fit well where the sample data is distributed non-linearly. On the contrary, our work adopted time-sequential LSTM-based machine learning model that can separate both linearly and nonlinearly distributed samples from different classes. The proposed approach also overpowers other popular deep learning models such as DBN and CNN which are usually used for non-sequential event modelling.
5.4 XAI to explain the ML decisions
Humans are basically restrained to accept approaches that are not interpretable or trustworthy, pushes the demand for transparent AI to increase. Hence, focusing only on performance of the AI models, gradually makes the systems towards unacceptance. Though there is a trade-off between the performance and transparency of machine learning models, improvements in the understanding of the models via explainability can however lead to the correction of the model's deficiencies as well. Therefore, with the target of overcoming the limitations of accepting the current generation AI models, XAI should focus on machine learning techniques to produce more and more explainable models while upholding a high level of accuracy. Besides, they can also make it happen for humans to appropriately understand, trust, and manage the emerging AI phenomena as much as possible. Explainability is a main factor to gain confidence of whether a model would act as intended for a given problem. Most certainly, it is a property of any explainable model. Local explanations in AI models handle explainability by dividing the model's complex solutions space into several less complex solution subspaces which are relevant for the whole model. These explanations can utilize some approaches with the differentiating property to explain the model to some basic extent.
Most of the techniques of model simplification are based on rule extraction techniques. The most popular contributions for local post-hoc explanation is based on the approach called Local Interpretable Model-Agnostic Explanations (LIME) [ 35 ]. LIME basically generates locally linear models for the predictions of a machine learning model to explain it. It falls under category of the rule-based local explanations by simplification. Explanations by simplification builds a whole new system based on the trained model to be explained. Then, the new simplified model usually tries to optimize its resemblance to its predecessor model functions while reducing the complexity and at the same time, keeping a similar performance. Therefore, once the machine learning decision is obtained, XAI algorithm LIME is applied to see the importance of the features and probabilities towards the decision. Hence, we can understand the presence of the feature importance in the input for the decision, that helps understanding the outcomes of the system. Figure 31 shows the total class probabilities, top 10 features, their probabilities, and automatically highlighted features in a sample input text using LIME. As can be seen in right side of the figure, features towards depression get higher weights altogether than non-depression class, indicates the person to be in depression mode. The input text, features, and highlights were originally in Norwegian language since the database is from a Norwegian national portal to interact with youth, but the figure shows the corresponding translated text in English for better readability and understanding of the approach. According to the decision from machine learning model and explanations from LIME, the sample text consists of depression. To be noted, the ground truth for the sample text in the figure was the same as the model's prediction (i.e., depression), indicating the robustness of the model's decision and explanation.
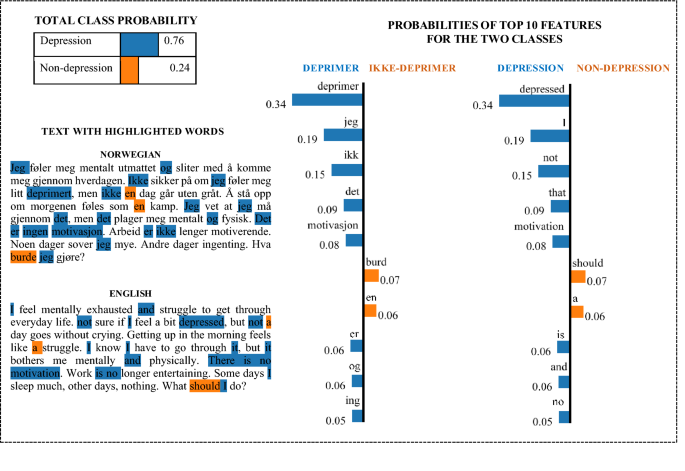
Total class probabilities, top 10 features, their probabilities, and automatically highlighted features in a sample input text using LIME
Furthermore, Fig. 32 shows summarized probabilities of top 10 features for a paraphrased non-depression example text using LIME. In the figure, left side represents the original part after applying the algorithm and right side the corresponding representation in English for better readability as well as understandability. The overall probabilities of the non-depression text from the machine learning model for depression and non-depression classes were 0.001 and 0.999, respectively.
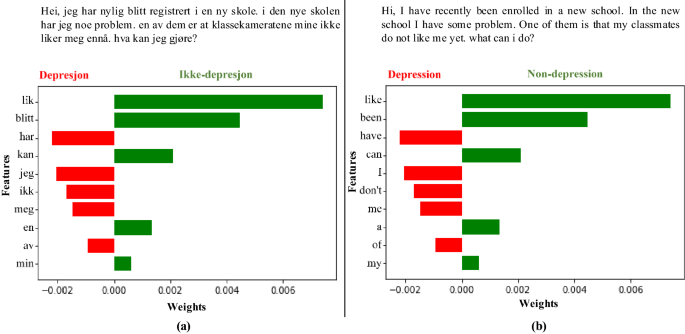
a Probabilities of top 10 features for a normal non-depression text (on the top) using LIME and b corresponding English on the right
6 Conclusion
To automatically detect depression symptoms in text for decision support in health care is important. In this work, a multimodal human depression prediction approach has been investigated based on one-hot approach on robust features based on describing depression symptoms and deep learning method, RNN. First, the young users' text data has been obtained from ung.no, a public information channel targeting young people in Norway. Then, one-hot method is applied after sequentially extracting the words from different sentences and words representing the symptoms of depression. Furthermore, the one-hot features have been applied to train a deep RNN based on LSTM method to model two different emotional states: depression and non-depression. Finally, the trained RNN has been used for predicting the underlying emotional state in unknown sensor text data. Using the proposed approach, 98% and 99% mean prediction performance has been achieved on first and second dataset consists of around 11,807 and 21,807 texts, respectively. Whereas, the traditional approaches could achieve maximum of 91% mean recognition performance, indicating the robustness of the proposed approach. The proposed approach outperforms the other traditional approaches such as using the proposed features with logistic regression, DBN, and CNN models as well as using typical one-hot and TF-IDF features with RNN. Besides, an XAI algorithm, LIME has been utilized to see whether the proposed system generates meaningful explanations to support its decision. Thus, the features used in this work can be used to support the machine learning decisions and to contribute to design effective user interface for better affective care. The deep learning-based efficient system can be explored in greater levels with comprehensive dataset. Detection of depression symptoms in texts can be applied in mental health care services for real-time analysing and predicting normal as well as severe states of mood disorders in smart environments combined with latest technologies. For instance, smart chatbot systems providing informational support about depression can be a feasible solution for both health professionals working with youth and youths struggling with mental health issues.
WHO (2021) https://www-who-int.ezproxy.uio.no/news-room/fact-sheets/detail/suicide .
Häfner H, Maurer K, Trendler G, an der Heiden W, Schmidt M (2005) The early course of schizophrenia and depression. Eur Arch Psychiatry Clin Neurosci 255(3):167–173
Article Google Scholar
Fusar-Poli P, Nelson B, Valmaggia L, Yung AR, McGuire PK (2014) Comorbid depressive and anxiety disorders in 509 individuals with an at-risk mental state: impact on psychopathology and transition to psychosis. Schizophr Bull 40(1):120–131
World Health Organization (2017) Depression and other common mental disorders: global health estimates (No. WHO/MSD/MER/2017.2)
Kessler RC, Bromet EJ (2013) The epidemiology of depression across cultures. Annu Rev Public Health 34:119–138. https://doi.org/10.1146/annurev-publhealth-031912-114409
Depression WHO (2017) Other common mental disorders: global health estimates. World Health Organization, Geneva, pp 1–24
Google Scholar
Weinberger AH, Gbedemah M, Martinez AM, Nash D, Galea S, Goodwin RD (2018) Trends in depression prevalence in the USA from 2005 to 2015: widening disparities in vulnerable groups. Psychol Med 48(8):1308–1315
Cambria E, White B (2014) Jumping NLP curves: a review of natural language processing research. IEEE Comput Intell Mag 9(2):48–57
Picard RW (2003) Affective computing: challenges. Int J Human Comput Stud 59(1–2):55–64
Cambria E (2016) Affective computing and sentiment analysis. IEEE Intell Syst 31(2):102–107
Shangfei W, Guozhu P, Zhuangqiang Z, Zhiwei Xu (2019) Capturing emotion distribution for multimedia emotion tagging. IEEE Trans Affect Comput. https://doi.org/10.1109/taffc.2019.2900240
Havigerová JM, Haviger J, Kučera D, Hoffmannová P (2019) Text-based detection of the risk of depression. Front Psychol 10:513
Tao J, Tan T (2005) Affective computing: a review. In: Proceedings of the international conference on affective computing and intelligent interaction, pp 981–995
Yang H, Willis A, De Roeck A, Nuseibeh B (2012) A hybrid model for automatic emotion recognition in suicide notes. Biomed Inform Insights 5(1):17–30
Desmet B, Hoste VR (2013) Emotion detection in suicide notes. Expert Syst Appl 40(16):6351–6358
Allouch M, Azaria A, Azoulay R, Ben‐Izchak E, Zwilling M, Zachor DA (2018) Automatic detection of insulting sentences in conversation. Paper presented at: Proceedings of the 2018 IEEE international conference on the science of electrical engineering in Israel (ICSEE); pp 1–4; IEEE.
John S, Ederyn W, Bruce C (1976) The social psychology of telecommunication. Wiley, London
Strapparava C, Mihalcea R (2008) Learning to identify emotions in text. Paper presented at: Proceedings of the 2008 ACM symposium on applied computing, pp 1556–1560; ACM
Ding Y, Chen X, Fu Q, Zhong S (2020) A depression recognition method for college students using deep integrated support vector algorithm. IEEE Access 8:75616–75629. https://doi.org/10.1109/ACCESS.2020.2987523
Yildirim E, Avci E (2018) Classificationof groutability of sands by linear discriminant analysis. ZBORNIK RADOVA GEO-EXPO 2018, Oct. 2018.
Vannatta RA, LaVenia KN (2020) Linear discriminant analysis. In Atkinson P, Delamont S, Cernat A, Sakshaug JW, Williams RA (eds) SAGE research methods foundations. doi: https://doi.org/10.4135/9781526421036889610
Gardner-Lubbe S (2020) Linear discriminant analysis for multiple functional data analysis. J Appl Stat pp 1–17
Uddin MZ, Torresen J (2019) Activity recognition using smartphone sensors, robust features, and recurrent neural network. In: 2019 13th international symposium on medical information and communication technology (ISMICT), May 2019.
Kiranyaz S, Ince T, Gabbouj M (2016) Real-time patient-specific ECG classification by 1-D convolutional neural networks. IEEE Trans Biomed Eng 63(3):664–675
Hinton GE, Osindero S, Teh Y-W (2006) A fast learning algorithm for deep belief nets. Neural Comput 18(7):1527–1554
Article MathSciNet Google Scholar
Deboeverie F, Roegiers S, Allebosch G, Veelaert P, Philips W (2016) Human gesture classification by brute-force machine learning for exergaming in physiotherapy. In: Proceedings of IEEE conference on computational intelligence and games (CIG), Santorini, pp 1–7
Graves A, Mohamed A, Hinton G (2013) Speech recognition with deep recurrent neural networks. In: 2013 IEEE international conference on acoustics, speech and signal processing (ICASSP). IEEE, pp 6645–6649
Hochreiter S, Schmidhuber J (1997) Long short-term memory. Neural Comput 9(8):1735–1780
Zaremba W, Sutskever I, Vinyals O (2014) Recurrent neural network regularization. arXiv preprint arXiv: 1409.2329
Gers FA, Schraudolph NN, Schmidhuber J (2003) Learning precise timing with LSTM recurrent networks. J Mach Learn Res 3:115–143
MathSciNet MATH Google Scholar
Sak H, Senior AW, Beaufays F (2014) Long short-term memory recurrent neural network architectures for large scale acoustic modeling. INTERSPEECH, pp 338–342
Kumari T, Mishra A (2020) Deep networks and deep learning algorithms. In: Artificial intelligence trends for data analytics using machine learning and deep learning approaches, pp 199–214
Liu P, Wang J, Guo Z (2020) Multiple and complete stability of recurrent neural networks with sinusoidal activation function. IEEE Trans Neural Netw Learn Syst, pp 1–12
Uddin MZ, Hassan M, Alsanad A, Savaglio C (2020) A body sensor data fusion and deep recurrent neural network-based behavior recognition approach for robust healthcare. Inform Fusion. https://doi.org/10.1016/j.inffus.2019.08.004
Govindasamy KA, Palanichamy N (2021) Depression detection using machine learning techniques on twitter data. In: 2021 5th international conference on intelligent computing and control systems (ICICCS), May 2021.
Dong Y, Yang X (2021) A hierarchical depression detection model based on vocal and emotional cues. Neurocomputing 441:279–290
Fortino G, Parisi D, Pirrone V, Di Fatta G (2014) BodyCloud: a SaaS approach for community Body Sensor Networks. Futur Gener Comput Syst 35:62–79
Fortino G, Galzarano S, Gravina R, Li W (2015) A framework for collaborative computing and multi-sensor data fusion in body sensor networks. Inform Fusion 22:50–70
Zhang Y, Gravina R, Lu H, Villari M, Fortino G (2018) PEA: Parallel electrocardiogram-based authentication for smart healthcare systems. J Netw Comput Appl 117:10–16
Wang R, Hao Y, Yu Q, Chen M, Humar I, Fortino G (2021) Depression Analysis and Recognition based on Functional Near-infrared Spectroscopy. IEEE J Biomed Health Inform. doi: https://doi.org/10.1109/JBHI.2021.3076762 . Epub ahead of print. PMID: 33929968.
Hassan MM, Alam MGR, Uddin MZ, Huda S, Almogren A, Fortino G (2019) Human emotion recognition using deep belief network architecture. Inform Fusion 51:10–18
Tjoa E, Guan C (2021) A survey on explainable artificial intelligence (XAI): toward medical XAI. IEEE Trans Neural Netw Learn Syst. https://doi.org/10.1109/TNNLS.2020.3027314
Wang N, Kosinski M, Stillwell DJ, Rust J (2014) Can well-being be measured using Facebook status updates? Validation of facebook’s gross national happiness index. Soc Indic Res 115(1):483–491
Baines T, Baines T, Wittkowski A, Wittkowski A (2013) A systematic review of the literature exploring illness perceptions in mental health utilising the self-regulation model. J Clin Psychol Med Settin 20(3):263–274
Cohen JR, So FK, Young JF, Hankin BL, Lee BA (2019) Youth depression screening with parent and self-reports: assessing current and prospective depression risk. Child Psychiatry Hum Dev 50(4):647–660
Coyne JC, Thompson R, Racioppo MW (2001) Validity and efficiency of screening for history of depression by self-report. Psychol Assess 13(2):163–170
Sim AYL, Borthwick A (2018) Record2Vec: unsupervised representation learning for structured records. IEEE Int Confer Data Min 2018:1236–1241. https://doi.org/10.1109/ICDM.2018.00165
Yuan X, Li L, Shardt YAW, Wang Y, Yang C (2021) Deep learning with spatiotemporal attention-based LSTM for industrial soft sensor model development. IEEE Trans Industr Electron 68(5):4404–4414. https://doi.org/10.1109/TIE.2020.2984443
Download references
Acknowledgements
This work was supported by the Research Council of Norway under Grant Number 262848.
Open access funding provided by SINTEF AS.
Author information
Authors and affiliations.
SINTEF Digital, Oslo, Norway
Md Zia Uddin, Asbjørn Følstad & Petter Bae Brandtzaeg
University of Oslo, Oslo, Norway
Kim Kristoffer Dysthe & Petter Bae Brandtzaeg
You can also search for this author in PubMed Google Scholar
Corresponding author
Correspondence to Md Zia Uddin .
Ethics declarations
Conflict of interest.
The authors declare that they have no conflict of interest.
Additional information
Publisher's note.
Springer Nature remains neutral with regard to jurisdictional claims in published maps and institutional affiliations.
Symptoms (Norwegian) | Symptoms (Translated) |
---|---|
alle hater meg | everyone hates me |
alt er jævlig | everything is damn |
alt er så jævlig | everything is so damn |
alt var jævlig | everything was damn |
alt var så jævlig | everything was so damn |
ikke apetitt | no appetite |
lite apetitt | little appetite |
ingen apetitt | no appetite |
avslutte livet | end life |
bli borte fra alt | get away from it all |
bryr meg ikke om noe | I do not care about anything |
ikke bryr meg om noe | do not care about anything |
brydde meg ikke om noe | did not care about anything |
ikke brydde meg om noe | did not care about anything |
jeg burde bli glad | I should be happy |
depremert | depressed |
deprimert | depressed |
depresjon | depression |
deprisjon | depression |
Deppa | depressed |
distensert meg fra | distanced me from |
distansert meg fra | distanced me from |
Dø | die |
Død | death |
Dødd | died |
Dør | dying |
ikke mer energi | no more energy |
tom for energi | empty of energy |
lite energi | little energy |
ingen energi | no energy |
ikke har noen energi | have no energy |
Energiløs | energyless |
lavt energinivå | low energy level |
tappet for energi | drained of energy |
aldri nok energi | never enough energy |
som et forferdelig menneske | as a terrible human being |
vondt inni meg | hurt inside me |
meg god nok | me good enough |
meg god nokk | me good enough |
Gråt | crying |
Grine | grine |
går ikke ut mer | does not go out anymore |
ikke går ut mer | does not go out anymore |
ikke gå ut mer | do not go out anymore |
det grusomt | it cruel |
det ille | the bad |
har det vondt | is in pain |
hatt det vondt | had it hurt |
har det så vondt | is in so much pain |
hatt det så vondt | had it so painful |
har det så sinnyskt vondt | it hurts so insanely |
hatt det så sinnyskt vondt | had it so insanely painful |
hater å leve | hate living |
hater livet mitt | hate my life |
helt på bunnen | at the very bottom |
alt er håpløst | everything is hopeless |
alt føles helt håpløst | everything feels completely hopeless |
har mistet håp | have lost hope |
jeg mister håp | I lose hope |
Håpløshet | hopelessness |
jeg ikke får til noe | I do not get anything |
jeg ikke får til noen | I do not get to anyone |
jeg ikke kan gjøre noe riktig | I can not do anything right |
jeg ikke gjør noe riktig | I'm not doing anything right |
ikke klarer å tenke | unable to think |
ikke klarer og tenke | unable to think |
ikke leve lenger | no longer live |
ikke lyst til å gjøre noe | not wanting to do anything |
Meningsløs | meaningless |
ingenting har mening | nothing makes sense |
ingenting har noen mening | nothing has any meaning |
ser ikke noe mening | sees no meaning |
ikke morsomt lenger | no fun anymore |
ikke overskudd til noe | no profit to anything |
ikke tro på meg selv | do not believe in myself |
ikke være sosial lenger | not be social anymore |
blitt usosial | become antisocial |
indre uro | inner turmoil |
ingen bryr seg om meg | nobody cares about me |
ingen glede | no joy |
ingen liker meg | nobody likes me |
ingen som liker meg | no one like me |
ingen lykke | no happiness |
ingen liker meg | nobody likes me |
ingen som liker meg | no one like me |
ingen savner meg | no one misses me |
ingen vil savne meg | no one will miss me |
ingenting føles | nothing feels |
ingenting interesserer meg | nothing interests me |
mistet interesse | lost interest |
ingenting å leve for | nothing to live for |
jeg er en vanskelig person | I am a difficult person |
klarer ikke leve | unable to live |
Ukonsentrert | unconcentrated |
ikke konsentrere meg | do not concentrate |
ikke å konsentrere meg | not to concentrate |
ikke og konsentrere meg | not and concentrate |
til å konsentrere meg | to concentrate |
med å konsentrere meg | with concentrating |
ikke lenger konsentrasjon | no longer concentration |
mistet konsentrasjon | lost concentration |
mista konsentrasjon | lose concentration |
meg langt nede | me far down |
meg så langt nede | me so far down |
lei av livet | tired of life |
lei meg | sad |
leve med meg selv | live with myself |
meg likegyldig | me indifferent |
følelse av likegyldighet | feeling of indifference |
likegyldigheten | indifference |
jeg er likegyldig | I'm indifferent |
lite initiative | blu initiative |
låser meg inne | locks me inside |
mistet matlyst | lost appetite |
ikke matlyst | not appetite |
ingen matlyst | no appetite |
liten matlyst | small appetite |
har ikke matlyst | have no appetite |
meg ubetydelig | me insignificant |
mistet motivasjon | lost motivation |
ikke motivasjon | not motivation |
lite motivasjon | little motivation |
demotivert | demotivated |
motivasjonen er borte | the motivation is gone |
motivasjonen er vekk | the motivation is gone |
mørkeste tanker | darkest thoughts |
de mørke skyene | the dark clouds |
mørkt hull | dark hole |
mørkt sted | dark place |
mørke tanker | dark thoughts |
alt er mørkt | everything is dark |
nedfor | down |
nedenfor | below |
nedstemt | voted down |
tenke negativt | think negatively |
negative tanker | negative thoughts |
negativt inni meg | negative inside me |
nervøs følelse | nervous feeling |
nervøs hele tiden | nervous all the time |
nytteløst | useless |
oppgitt | tired |
selvmord | suicide |
selvmordstanker | suicidal thoughts |
skyver vennene mine vekk | pushes my friends away |
skyver venner vekk | pushes friends away |
sliten | tired |
jeg sliter | I'm struggling |
sliter med meg | struggling with me |
sluttet jeg å være med på | I stopped participating |
maten smaker ingenting | the food tastes nothing |
meg som en taper | me as a loser |
sove | sleep |
søvn | sleep |
sovne | to fall asleep |
sover bort | sleeping away |
stenger meg inne | shuts me in |
stengte meg inne | locked me inside |
suicid | suicide |
suisid | suicide |
ende livet mitt | end my life |
ta livet mitt | take my life |
ende mitt eget liv | end my own life |
ta livet av meg | take my life |
ta mitt eget liv | take my own life |
tar livet mitt | takes my life |
tar mitt eget liv | takes my own life |
tenke på døden | think of death |
jeg få ting til å gå fortere | I make things go faster |
helt tom | completely empty |
tomhet | emptiness |
er jeg tom | am I empty |
jeg er tom | I'm empty |
trist | sad |
konstant trøtt | constantly tired |
konstant trett | constantly tired |
alltid trøtt | always tired |
alltid trett | always tired |
tare | tear |
meg ubetydelig | me insignificant |
meg ubrukelig | me useless |
umotivert | unmotivated |
utbrent | burnt out |
jeg er utslitt | I'm exhausted |
jeg er så utslitt | I'm so exhausted |
føler meg så utslitt | feel so exhausted |
er bare helt utslitt | is just completely exhausted |
psykisk utslitt | mentally exhausted |
jeg er veldig utslitt | I'm very exhausted |
uutholdelig | unbearable |
vekk fra denne verdenen | away from this world |
vekker ikke følelser lenger | does not evoke emotions anymore |
ingenting vekker følelser | nothing evokes emotions |
meg verdiløs | me worthless |
verdiløs jeg er | worthless I am |
jeg er verdiløs | I'm worthless |
ønsker å være død | wants to be dead |
ønsket å være død | wanted to be dead |
vil ikke leve | will not live |
ikke vil leve | will not live |
ikke ville leve | would not live |
ville ikke leve | would not live |
noe mer å leve for | something more to live for |
Rights and permissions
Open Access This article is licensed under a Creative Commons Attribution 4.0 International License, which permits use, sharing, adaptation, distribution and reproduction in any medium or format, as long as you give appropriate credit to the original author(s) and the source, provide a link to the Creative Commons licence, and indicate if changes were made. The images or other third party material in this article are included in the article's Creative Commons licence, unless indicated otherwise in a credit line to the material. If material is not included in the article's Creative Commons licence and your intended use is not permitted by statutory regulation or exceeds the permitted use, you will need to obtain permission directly from the copyright holder. To view a copy of this licence, visit http://creativecommons.org/licenses/by/4.0/ .
Reprints and permissions
About this article
Uddin, M.Z., Dysthe, K.K., Følstad, A. et al. Deep learning for prediction of depressive symptoms in a large textual dataset. Neural Comput & Applic 34 , 721–744 (2022). https://doi.org/10.1007/s00521-021-06426-4
Download citation
Received : 18 March 2021
Accepted : 17 August 2021
Published : 27 August 2021
Issue Date : January 2022
DOI : https://doi.org/10.1007/s00521-021-06426-4
Share this article
Anyone you share the following link with will be able to read this content:
Sorry, a shareable link is not currently available for this article.
Provided by the Springer Nature SharedIt content-sharing initiative
- Find a journal
- Publish with us
- Track your research

IMAGES
COMMENTS
Depression is a prevalent psychiatric disorder that often leads to poor quality of life and impaired functioning. Treatment during the acute phase of a major depressive episode aims to help the patient reach a remission state and eventually return to their baseline level of functioning. Pharmacotherapy, especially selective serotonin reuptake ...
Major depressive disorder (MDD), also referred to as depression, is one of the most common psychiatric disorders with a high economic burden. The etiology of depression is still not clear, but it is generally believed that MDD is a multifactorial disease caused by the interaction of social, psychological, and biological aspects. Therefore, there is no exact pathological theory that can ...
Major depressive disorder (MDD) is one of the most prominent and debilitating. psychiatric disorders in the world. 17% of people in the world have a lifetime prevalence of. MDD while 5-9% experience a 12-month prevalence (Eaton et. al, 2008); meaning that 1 in.
Ph.D. Thesis - M. Maslej; McMaster University - Psychology iii Lay Abstract Depression is a mental health condition in part characterized by sadness and changes in thinking. One evolutionary perspective argues that depression is a response to complicated, personal problems, and that
major depressive disorder have increased at the end of the 20th century. Additionally, the research supports the notion that depression and melancholia should be considered as different disorders; therefore, the treatment strategies should vary (Healy, 2013). Depression was identified as one of the psychiatric symptoms of major depressive disorder.
Disorders. Major depressive disorder is a situation that feeling low energy and high sadness in. everyday life that lasts at least two weeks (NIMH, 2016). 1.1 Criteria for Major Depressive ...
depression scores (F (1,52) = 352.795, p<0.001) and a significant increase in perceived control scores (F (1,52) = 5.311, p=0.025) from T1 to T2. Depression, mindfulness, and perceived control were not significantly different between conditions at T2. The novelty-seeking only condition had the biggest drop in depression scores from T1 to T2 (10.75
depression in order to understand how my identity is socially constructed within that context. Through thematic analysis, I use Goffman's (1963) identity theory as a sensitizing concept. This thesis extends the literature on the issues of identity and major depressive disorder by suggesting my attempts at passing as normal essentially enable me
Introduction. Major depressive disorder (MDD) is a common mental disorder that affects ~185 million people globally1. Manifestations of MDD include depressed mood, reduced interest or pleasure in ...
demand for drug-free alternatives to treat depression. Group psychoeducation is a low threshold, drug-free intervention which has proven to be beneficial in the treatment of other mental disorders and which can be adapted to different populations. Use of group psychoeducation for major depressive disorder (MDD) will increase the availability of
MAJOR DEPRESSIVE DISORDER AND ACADEMIC ACHIEVEMENT 32 across all groups were absent for 1-10% of school days, however this percentage was highest for no disorder (82.6%), whereas for subthreshold and threshold level MDD, 79.1% and 56% adolescents were absent for 1-10% of school days respectively.
This thesis is submitted in partial fulfilment of the Honours degree of Bachelor of Psychological Science (Honours) Author Note ... 2020). Depressive disorders are now the single largest contributor to disability worldwide, and are likely to be the leading cause of disease by 2030 (WHO, 2012; 2017). In Australia, the current prevalence of ...
A deeper understanding of Major Depressive Disorder (MDD) is crucial to identify and monitor those at risk for depression and more easily diagnose and effectively treat patients. This paper provides an in-depth look at the etiology of MDD, covering the biological and, briefly, the psychological theories of depression. Also included are environmental risk factors, genetic links to the disorder ...
Amit Etkin, Maurizio Fava, David C. Mohr and Alan F. Schatzberg. Abstract Major depressive disorder (MDD) is a debilitating disease that is characterized by depressed. mood, diminished interests ...
Abstract. Major depression is a mood disorder characterized by a sense of inadequacy, despondency, decreased activity, pessimism, anhedonia and sadness where these symptoms severely disrupt and ...
Major depressive disorder (MDD) is a prevalent and highly disabling mental health disorder that has been identified as one of the leading causes of disease burden. 1 There are several preventative interventions and treatment options available for MDD (antidepressants and psychological interventions). 2 3 However, their effectiveness raises ...
Major depression is a risk factor for and is often associated with substance abuse, including that of alcohol, which may be under-recognised in elderly patients.(68,69) In terms of lifetime prevalence, it has been shown that alcohol abuse is the second most common mental disorder among adults in Singapore. Tactful enquiry using an open-ended ...
Depressive disorders are complex neurobiological conditions, and it is clear now that they are associated with a wide range of physiological and cognitive abnormalities. The large number of pathological features that have been identified in the last decades has stimulated the development of a whole array of (mutually not necessarily exclusive ...
Major depressive disorder (MDD) encompasses a large number of psychobiological syndromes with the core features of depressed mood and/or loss of interest associ-ated with cognitive and somatic disturbances, which causes significant functional impairment. Depressive disorders are classified as mood disorders and are distin-
Depression is a common illness worldwide with potentially severe implications. Early identification of depressive symptoms is a crucial first step towards assessment, intervention, and relapse prevention. With an increase in data sets with relevance for depression, and the advancement of machine learning, there is a potential to develop intelligent systems to detect symptoms of depression in ...
The serotonin deficit hypothesis explanation for major depressive disorder (MDD) has persisted among clinicians and the general public alike despite insufficient supporting evidence.
Abstract. Major Depressive Disorder is one of the most common and debilitating mental disorders. Cognitive behavioral therapy (CBT) for depression has received ample empirical support and is ...
This thesis is organized into ve main themes: (1) A depression classication model for understanding how the COVID-19 pandemic has affected people's depression; (2) Depression detection at the User level and its impact during the pandemic; (3) A new,