- Search Menu
- Sign in through your institution
- Advance articles
- Editor's Choice
- Browse content in C - Mathematical and Quantitative Methods
- Browse content in C0 - General
- C01 - Econometrics
- Browse content in C1 - Econometric and Statistical Methods and Methodology: General
- C10 - General
- C11 - Bayesian Analysis: General
- C12 - Hypothesis Testing: General
- C13 - Estimation: General
- C14 - Semiparametric and Nonparametric Methods: General
- C15 - Statistical Simulation Methods: General
- C18 - Methodological Issues: General
- C19 - Other
- Browse content in C2 - Single Equation Models; Single Variables
- C20 - General
- C21 - Cross-Sectional Models; Spatial Models; Treatment Effect Models; Quantile Regressions
- C22 - Time-Series Models; Dynamic Quantile Regressions; Dynamic Treatment Effect Models; Diffusion Processes
- C23 - Panel Data Models; Spatio-temporal Models
- C24 - Truncated and Censored Models; Switching Regression Models; Threshold Regression Models
- C25 - Discrete Regression and Qualitative Choice Models; Discrete Regressors; Proportions; Probabilities
- C26 - Instrumental Variables (IV) Estimation
- Browse content in C3 - Multiple or Simultaneous Equation Models; Multiple Variables
- C30 - General
- C31 - Cross-Sectional Models; Spatial Models; Treatment Effect Models; Quantile Regressions; Social Interaction Models
- C32 - Time-Series Models; Dynamic Quantile Regressions; Dynamic Treatment Effect Models; Diffusion Processes; State Space Models
- C33 - Panel Data Models; Spatio-temporal Models
- C34 - Truncated and Censored Models; Switching Regression Models
- C35 - Discrete Regression and Qualitative Choice Models; Discrete Regressors; Proportions
- C36 - Instrumental Variables (IV) Estimation
- C38 - Classification Methods; Cluster Analysis; Principal Components; Factor Models
- Browse content in C4 - Econometric and Statistical Methods: Special Topics
- C40 - General
- C41 - Duration Analysis; Optimal Timing Strategies
- C44 - Operations Research; Statistical Decision Theory
- C45 - Neural Networks and Related Topics
- Browse content in C5 - Econometric Modeling
- C50 - General
- C51 - Model Construction and Estimation
- C52 - Model Evaluation, Validation, and Selection
- C53 - Forecasting and Prediction Methods; Simulation Methods
- C54 - Quantitative Policy Modeling
- C55 - Large Data Sets: Modeling and Analysis
- C57 - Econometrics of Games and Auctions
- C58 - Financial Econometrics
- Browse content in C6 - Mathematical Methods; Programming Models; Mathematical and Simulation Modeling
- C60 - General
- C61 - Optimization Techniques; Programming Models; Dynamic Analysis
- C63 - Computational Techniques; Simulation Modeling
- Browse content in C7 - Game Theory and Bargaining Theory
- C78 - Bargaining Theory; Matching Theory
- Browse content in C8 - Data Collection and Data Estimation Methodology; Computer Programs
- C81 - Methodology for Collecting, Estimating, and Organizing Microeconomic Data; Data Access
- Browse content in D - Microeconomics
- Browse content in D0 - General
- D04 - Microeconomic Policy: Formulation; Implementation, and Evaluation
- Browse content in D1 - Household Behavior and Family Economics
- D10 - General
- D12 - Consumer Economics: Empirical Analysis
- Browse content in D2 - Production and Organizations
- D22 - Firm Behavior: Empirical Analysis
- D24 - Production; Cost; Capital; Capital, Total Factor, and Multifactor Productivity; Capacity
- Browse content in D3 - Distribution
- D31 - Personal Income, Wealth, and Their Distributions
- Browse content in D4 - Market Structure, Pricing, and Design
- D41 - Perfect Competition
- D44 - Auctions
- Browse content in D6 - Welfare Economics
- D63 - Equity, Justice, Inequality, and Other Normative Criteria and Measurement
- Browse content in D9 - Micro-Based Behavioral Economics
- D91 - Role and Effects of Psychological, Emotional, Social, and Cognitive Factors on Decision Making
- Browse content in E - Macroeconomics and Monetary Economics
- Browse content in E2 - Consumption, Saving, Production, Investment, Labor Markets, and Informal Economy
- E24 - Employment; Unemployment; Wages; Intergenerational Income Distribution; Aggregate Human Capital; Aggregate Labor Productivity
- Browse content in E3 - Prices, Business Fluctuations, and Cycles
- E30 - General
- E31 - Price Level; Inflation; Deflation
- E32 - Business Fluctuations; Cycles
- E37 - Forecasting and Simulation: Models and Applications
- Browse content in E5 - Monetary Policy, Central Banking, and the Supply of Money and Credit
- E52 - Monetary Policy
- E58 - Central Banks and Their Policies
- Browse content in G - Financial Economics
- Browse content in G1 - General Financial Markets
- G10 - General
- G11 - Portfolio Choice; Investment Decisions
- G12 - Asset Pricing; Trading volume; Bond Interest Rates
- G17 - Financial Forecasting and Simulation
- Browse content in G2 - Financial Institutions and Services
- G21 - Banks; Depository Institutions; Micro Finance Institutions; Mortgages
- Browse content in H - Public Economics
- Browse content in H1 - Structure and Scope of Government
- H12 - Crisis Management
- Browse content in I - Health, Education, and Welfare
- Browse content in I1 - Health
- I12 - Health Behavior
- I13 - Health Insurance, Public and Private
- I14 - Health and Inequality
- I18 - Government Policy; Regulation; Public Health
- I19 - Other
- Browse content in I2 - Education and Research Institutions
- I21 - Analysis of Education
- I24 - Education and Inequality
- Browse content in J - Labor and Demographic Economics
- Browse content in J1 - Demographic Economics
- J16 - Economics of Gender; Non-labor Discrimination
- Browse content in J3 - Wages, Compensation, and Labor Costs
- J31 - Wage Level and Structure; Wage Differentials
- Browse content in J6 - Mobility, Unemployment, Vacancies, and Immigrant Workers
- J64 - Unemployment: Models, Duration, Incidence, and Job Search
- Browse content in J7 - Labor Discrimination
- J71 - Discrimination
- Browse content in L - Industrial Organization
- Browse content in L2 - Firm Objectives, Organization, and Behavior
- L24 - Contracting Out; Joint Ventures; Technology Licensing
- Browse content in M - Business Administration and Business Economics; Marketing; Accounting; Personnel Economics
- M3 - Marketing and Advertising
- Browse content in O - Economic Development, Innovation, Technological Change, and Growth
- Browse content in O1 - Economic Development
- O10 - General
- O18 - Urban, Rural, Regional, and Transportation Analysis; Housing; Infrastructure
- Browse content in O5 - Economywide Country Studies
- O57 - Comparative Studies of Countries
- Browse content in P - Economic Systems
- Browse content in P1 - Capitalist Systems
- P16 - Political Economy
- Browse content in Q - Agricultural and Natural Resource Economics; Environmental and Ecological Economics
- Browse content in Q0 - General
- Q02 - Commodity Markets
- Browse content in Q1 - Agriculture
- Q11 - Aggregate Supply and Demand Analysis; Prices
- Browse content in Q5 - Environmental Economics
- Q54 - Climate; Natural Disasters; Global Warming
- Browse content in R - Urban, Rural, Regional, Real Estate, and Transportation Economics
- Browse content in R0 - General
- R00 - General
- Browse content in R1 - General Regional Economics
- R15 - Econometric and Input-Output Models; Other Models
- Browse content in R2 - Household Analysis
- R23 - Regional Migration; Regional Labor Markets; Population; Neighborhood Characteristics
- Author Guidelines
- Submission Site
- Open Access
- Self-Archiving Policy
- About The Econometrics Journal
- About the Royal Economic Society
- Editorial Board
- Advertising and Corporate Services
- Journals on Oxford Academic
- Books on Oxford Academic

Article Contents
- 1. Introduction
- 2. Theoretical Background
- 3. Empirical Methodology
- 5. Empirical Results
- 6. Conclusions
- Acknowledgments
- < Previous
The impact of homework on student achievement
- Article contents
- Figures & tables
- Supplementary Data
Ozkan Eren, Daniel J. Henderson, The impact of homework on student achievement, The Econometrics Journal , Volume 11, Issue 2, 1 July 2008, Pages 326–348, https://doi.org/10.1111/j.1368-423X.2008.00244.x
- Permissions Icon Permissions
Utilizing parametric and nonparametric techniques, we assess the role of a heretofore relatively unexplored ‘input’ in the educational process, homework, on academic achievement. Our results indicate that homework is an important determinant of student test scores. Relative to more standard spending related measures, extra homework has a larger and more significant impact on test scores. However, the effects are not uniform across different subpopulations. Specifically, we find additional homework to be most effective for high and low achievers, which is further confirmed by stochastic dominance analysis. Moreover, the parametric estimates of the educational production function overstate the impact of schooling related inputs. In all estimates, the homework coefficient from the parametric model maps to the upper deciles of the nonparametric coefficient distribution and as a by‐product the parametric model understates the percentage of students with negative responses to additional homework.
Email alerts
Citing articles via.
- X (formerly Twitter)
- Recommend to your Librarian
Affiliations
- Online ISSN 1368-423X
- Print ISSN 1368-4221
- Copyright © 2024 Royal Economic Society
- About Oxford Academic
- Publish journals with us
- University press partners
- What we publish
- New features
- Open access
- Institutional account management
- Rights and permissions
- Get help with access
- Accessibility
- Advertising
- Media enquiries
- Oxford University Press
- Oxford Languages
- University of Oxford
Oxford University Press is a department of the University of Oxford. It furthers the University's objective of excellence in research, scholarship, and education by publishing worldwide
- Copyright © 2024 Oxford University Press
- Cookie settings
- Cookie policy
- Privacy policy
- Legal notice
This Feature Is Available To Subscribers Only
Sign In or Create an Account
This PDF is available to Subscribers Only
For full access to this pdf, sign in to an existing account, or purchase an annual subscription.
ORIGINAL RESEARCH article
Students' achievement and homework assignment strategies.
- 1 Department of Education Sciences, University of Oviedo, Oviedo, Spain
- 2 Department of Education, Principality of Asturias Government, Oviedo, Spain
- 3 Department of Psychology, University of Oviedo, Oviedo, Spain
The optimum time students should spend on homework has been widely researched although the results are far from unanimous. The main objective of this research is to analyze how homework assignment strategies in schools affect students' academic performance and the differences in students' time spent on homework. Participants were a representative sample of Spanish adolescents ( N = 26,543) with a mean age of 14.4 (±0.75), 49.7% girls. A test battery was used to measure academic performance in four subjects: Spanish, Mathematics, Science, and Citizenship. A questionnaire allowed the measurement of the indicators used for the description of homework and control variables. Two three-level hierarchical-linear models (student, school, autonomous community) were produced for each subject being evaluated. The relationship between academic results and homework time is negative at the individual level but positive at school level. An increase in the amount of homework a school assigns is associated with an increase in the differences in student time spent on homework. An optimum amount of homework is proposed which schools should assign to maximize gains in achievement for students overall.
The role of homework in academic achievement is an age-old debate ( Walberg et al., 1985 ) that has swung between times when it was thought to be a tool for improving a country's competitiveness and times when it was almost outlawed. So Cooper (2001) talks about the battle over homework and the debates and rows continue ( Walberg et al., 1985 , 1986 ; Barber, 1986 ). It is considered a complicated subject ( Corno, 1996 ), mysterious ( Trautwein and Köller, 2003 ), a chameleon ( Trautwein et al., 2009b ), or Janus-faced ( Flunger et al., 2015 ). One must agree with Cooper et al. (2006) that homework is a practice full of contradictions, where positive and negative effects coincide. As such, depending on our preferences, it is possible to find data which support the argument that homework benefits all students ( Cooper, 1989 ), or that it does not matter and should be abolished ( Barber, 1986 ). Equally, one might argue a compensatory effect as it favors students with more difficulties ( Epstein and Van Voorhis, 2001 ), or on the contrary, that it is a source of inequality as it specifically benefits those better placed on the social ladder ( Rømming, 2011 ). Furthermore, this issue has jumped over the school wall and entered the home, contributing to the polemic by becoming a common topic about which it is possible to have an opinion without being well informed, something that Goldstein (1960) warned of decades ago after reviewing almost 300 pieces of writing on the topic in Education Index and finding that only 6% were empirical studies.
The relationship between homework time and educational outcomes has traditionally been the most researched aspect ( Cooper, 1989 ; Cooper et al., 2006 ; Fan et al., 2017 ), although conclusions have evolved over time. The first experimental studies ( Paschal et al., 1984 ) worked from the hypothesis that time spent on homework was a reflection of an individual student's commitment and diligence and as such the relationship between time spent on homework and achievement should be positive. This was roughly the idea at the end of the twentieth century, when more positive effects had been found than negative ( Cooper, 1989 ), although it was also known that the relationship was not strictly linear ( Cooper and Valentine, 2001 ), and that its strength depended on the student's age- stronger in post-compulsory secondary education than in compulsory education and almost zero in primary education ( Cooper et al., 2012 ). With the turn of the century, hierarchical-linear models ran counter to this idea by showing that homework was a multilevel situation and the effect of homework on outcomes depended on classroom factors (e.g., frequency or amount of assigned homework) more than on an individual's attitude ( Trautwein and Köller, 2003 ). Research with a multilevel approach indicated that individual variations in time spent had little effect on academic results ( Farrow et al., 1999 ; De Jong et al., 2000 ; Dettmers et al., 2010 ; Murillo and Martínez-Garrido, 2013 ; Fernández-Alonso et al., 2014 ; Núñez et al., 2014 ; Servicio de Evaluación Educativa del Principado de Asturias, 2016 ) and that when statistically significant results were found, the effect was negative ( Trautwein, 2007 ; Trautwein et al., 2009b ; Lubbers et al., 2010 ; Chang et al., 2014 ). The reasons for this null or negative relationship lie in the fact that those variables which are positively associated with homework time are antagonistic when predicting academic performance. For example, some students may not need to spend much time on homework because they learn quickly and have good cognitive skills and previous knowledge ( Trautwein, 2007 ; Dettmers et al., 2010 ), or maybe because they are not very persistent in their work and do not finish homework tasks ( Flunger et al., 2015 ). Similarly, students may spend more time on homework because they have difficulties learning and concentrating, low expectations and motivation or because they need more direct help ( Trautwein et al., 2006 ), or maybe because they put in a lot of effort and take a lot of care with their work ( Flunger et al., 2015 ). Something similar happens with sociological variables such as gender: Girls spend more time on homework ( Gershenson and Holt, 2015 ) but, compared to boys, in standardized tests they have better results in reading and worse results in Science and Mathematics ( OECD, 2013a ).
On the other hand, thanks to multilevel studies, systematic effects on performance have been found when homework time is considered at the class or school level. De Jong et al. (2000) found that the number of assigned homework tasks in a year was positively and significantly related to results in mathematics. Equally, the volume or amount of homework (mean homework time for the group) and the frequency of homework assignment have positive effects on achievement. The data suggests that when frequency and volume are considered together, the former has more impact on results than the latter ( Trautwein et al., 2002 ; Trautwein, 2007 ). In fact, it has been estimated that in classrooms where homework is always assigned there are gains in mathematics and science of 20% of a standard deviation over those classrooms which sometimes assign homework ( Fernández-Alonso et al., 2015 ). Significant results have also been found in research which considered only homework volume at the classroom or school level. Dettmers et al. (2009) concluded that the school-level effect of homework is positive in the majority of participating countries in PISA 2003, and the OECD (2013b) , with data from PISA 2012, confirms that schools in which students have more weekly homework demonstrate better results once certain school and student-background variables are discounted. To put it briefly, homework has a multilevel nature ( Trautwein and Köller, 2003 ) in which the variables have different significance and effects according to the level of analysis, in this case a positive effect at class level, and a negative or null effect in most cases at the level of the individual. Furthermore, the fact that the clearest effects are seen at the classroom and school level highlights the role of homework policy in schools and teaching, over and above the time individual students spend on homework.
From this complex context, this current study aims to explore the relationships between the strategies schools use to assign homework and the consequences that has on students' academic performance and on the students' own homework strategies. There are two specific objectives, firstly, to systematically analyze the differential effect of time spent on homework on educational performance, both at school and individual level. We hypothesize a positive effect for homework time at school level, and a negative effect at the individual level. Secondly, the influence of homework quantity assigned by schools on the distribution of time spent by students on homework will be investigated. This will test the previously unexplored hypothesis that an increase in the amount of homework assigned by each school will create an increase in differences, both in time spent on homework by the students, and in academic results. Confirming this hypothesis would mean that an excessive amount of homework assigned by schools would penalize those students who for various reasons (pace of work, gaps in learning, difficulties concentrating, overexertion) need to spend more time completing their homework than their peers. In order to resolve this apparent paradox we will calculate the optimum volume of homework that schools should assign in order to benefit the largest number of students without contributing to an increase in differences, that is, without harming educational equity.
Participants
The population was defined as those students in year 8 of compulsory education in the academic year 2009/10 in Spain. In order to provide a representative sample, a stratified random sampling was carried out from the 19 autonomous regions in Spain. The sample was selected from each stratum according to a two-stage cluster design ( OECD, 2009 , 2011 , 2014a ; Ministerio de Educación, 2011 ). In the first stage, the primary units of the sample were the schools, which were selected with a probability proportional to the number of students in the 8th grade. The more 8th grade students in a given school, the higher the likelihood of the school being selected. In the second stage, 35 students were selected from each school through simple, systematic sampling. A detailed, step-by-step description of the sampling procedure may be found in OECD (2011) . The subsequent sample numbered 29,153 students from 933 schools. Some students were excluded due to lack of information (absences on the test day), or for having special educational needs. The baseline sample was finally made up of 26,543 students. The mean student age was 14.4 with a standard deviation of 0.75, rank of age from 13 to 16. Some 66.2% attended a state school; 49.7% were girls; 87.8% were Spanish nationals; 73.5% were in the school year appropriate to their age, the remaining 26.5% were at least 1 year behind in terms of their age.
Test application, marking, and data recording were contracted out via public tendering, and were carried out by qualified personnel unconnected to the schools. The evaluation, was performed on two consecutive days, each day having two 50 min sessions separated by a break. At the end of the second day the students completed a context questionnaire which included questions related to homework. The evaluation was carried out in compliance with current ethical standards in Spain. Families of the students selected to participate in the evaluation were informed about the study by the school administrations, and were able to choose whether those students would participate in the study or not.
Instruments
Tests of academic performance.
The performance test battery consisted of 342 items evaluating four subjects: Spanish (106 items), mathematics (73 items), science (78), and citizenship (85). The items, completed on paper, were in various formats and were subject to binary scoring, except 21 items which were coded on a polytomous scale, between 0 and 2 points ( Ministerio de Educación, 2011 ). As a single student is not capable of answering the complete item pool in the time given, the items were distributed across various booklets following a matrix design ( Fernández-Alonso and Muñiz, 2011 ). The mean Cronbach α for the booklets ranged from 0.72 (mathematics) to 0.89 (Spanish). Student scores were calculated adjusting the bank of items to Rasch's IRT model using the ConQuest 2.0 program ( Wu et al., 2007 ) and were expressed in a scale with mean and standard deviation of 500 and 100 points respectively. The student's scores were divided into five categories, estimated using the plausible values method. In large scale assessments this method is better at recovering the true population parameters (e.g., mean, standard deviation) than estimates of scores using methods of maximum likelihood or expected a-posteriori estimations ( Mislevy et al., 1992 ; OECD, 2009 ; von Davier et al., 2009 ).
Homework Variables
A questionnaire was made up of a mix of items which allowed the calculation of the indicators used for the description of homework variables. Daily minutes spent on homework was calculated from a multiple choice question with the following options: (a) Generally I don't have homework; (b) 1 h or less; (c) Between 1 and 2 h; (d) Between 2 and 3 h; (e) More than 3 h. The options were recoded as follows: (a) = 0 min.; (b) = 45 min.; (c) = 90 min.; (d) = 150 min.; (e) = 210 min. According to Trautwein and Köller (2003) the average homework time of the students in a school could be regarded as a good proxy for the amount of homework assigned by the teacher. So the mean of this variable for each school was used as an estimator of Amount or volume of homework assigned .
Control Variables
Four variables were included to describe sociological factors about the students, three were binary: Gender (1 = female ); Nationality (1 = Spanish; 0 = other ); School type (1 = state school; 0 = private ). The fourth variable was Socioeconomic and cultural index (SECI), which is constructed with information about family qualifications and professions, along with the availability of various material and cultural resources at home. It is expressed in standardized points, N(0,1) . Three variables were used to gather educational history: Appropriate School Year (1 = being in the school year appropriate to their age ; 0 = repeated a school year) . The other two adjustment variables were Academic Expectations and Motivation which were included for two reasons: they are both closely connected to academic achievement ( Suárez-Álvarez et al., 2014 ). Their position as adjustment factors is justified because, in an ex-post facto descriptive design such as this, both expectations and motivation may be thought of as background variables that the student brings with them on the day of the test. Academic expectations for finishing education was measured with a multiple-choice item where the score corresponds to the years spent in education in order to reach that level of qualification: compulsory secondary education (10 points); further secondary education (12 points); non-university higher education (14 points); University qualification (16 points). Motivation was constructed from the answers to six four-point Likert items, where 1 means strongly disagree with the sentence and 4 means strongly agree. Students scoring highly in this variable are agreeing with statements such as “at school I learn useful and interesting things.” A Confirmatory Factor Analysis was performed using a Maximum Likelihood robust estimation method (MLMV) and the items fit an essentially unidimensional scale: CFI = 0.954; TLI = 0.915; SRMR = 0.037; RMSEA = 0.087 (90% CI = 0.084–0.091).
As this was an official evaluation, the tests used were created by experts in the various fields, contracted by the Spanish Ministry of Education in collaboration with the regional education authorities.
Data Analyses
Firstly the descriptive statistics and Pearson correlations between the variables were calculated. Then, using the HLM 6.03 program ( Raudenbush et al., 2004 ), two three-level hierarchical-linear models (student, school, autonomous community) were produced for each subject being evaluated: a null model (without predictor variables) and a random intercept model in which adjustment variables and homework variables were introduced at the same time. Given that HLM does not return standardized coefficients, all of the variables were standardized around the general mean, which allows the interpretation of the results as classical standardized regression analysis coefficients. Levels 2 and 3 variables were constructed from means of standardized level 1 variables and were not re-standardized. Level 1 variables were introduced without centering except for four cases: study time, motivation, expectation, and socioeconomic and cultural level which were centered on the school mean to control composition effects ( Xu and Wu, 2013 ) and estimate the effect of differences in homework time among the students within the same school. The range of missing variable cases was very small, between 1 and 3%. Recovery was carried out using the procedure described in Fernández-Alonso et al. (2012) .
The results are presented in two ways: the tables show standardized coefficients while in the figures the data are presented in a real scale, taking advantage of the fact that a scale with a 100 point standard deviation allows the expression of the effect of the variables and the differences between groups as percentage increases in standardized points.
Table 1 shows the descriptive statistics and the matrix of correlations between the study variables. As can be seen in the table, the relationship between the variables turned out to be in the expected direction, with the closest correlations between the different academic performance scores and socioeconomic level, appropriate school year, and student expectations. The nationality variable gave the highest asymmetry and kurtosis, which was to be expected as the majority of the sample are Spanish.
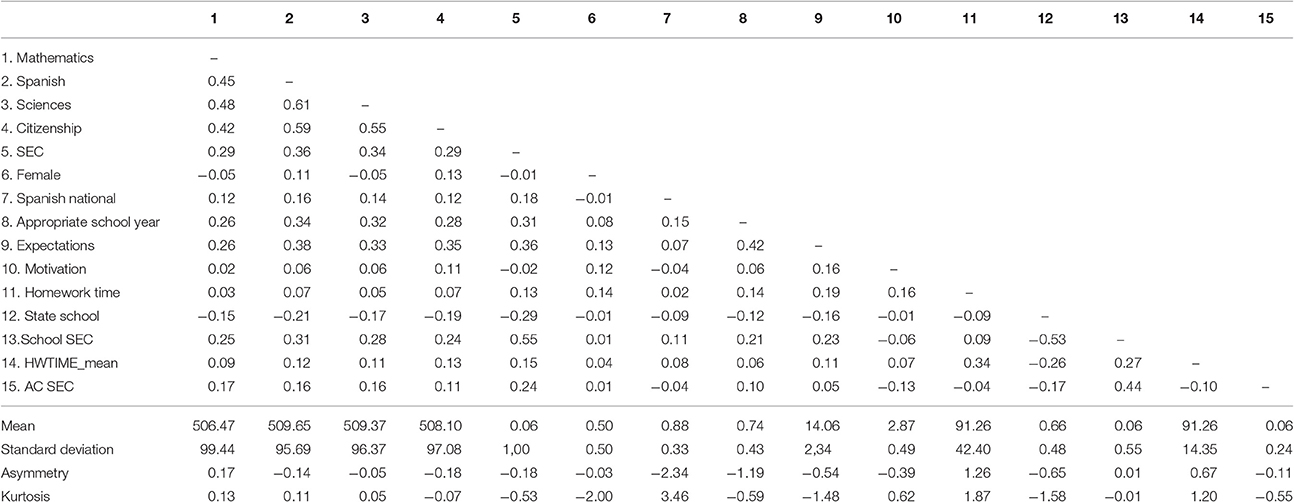
Table 1. Descriptive statistics and Pearson correlation matrix between the variables .
Table 2 shows the distribution of variance in the null model. In the four subjects taken together, 85% of the variance was found at the student level, 10% was variance between schools, and 5% variance between regions. Although the 10% of variance between schools could seem modest, underlying that there were large differences. For example, in Spanish the 95% plausible value range for the school means ranged between 577 and 439 points, practically 1.5 standard deviations, which shows that schools have a significant impact on student results.
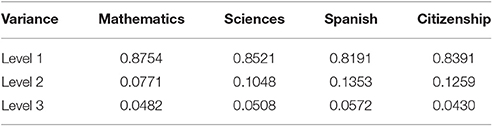
Table 2. Distribution of the variance in the null model .
Table 3 gives the standardized coefficients of the independent variables of the four multilevel models, as well as the percentage of variance explained by each level.
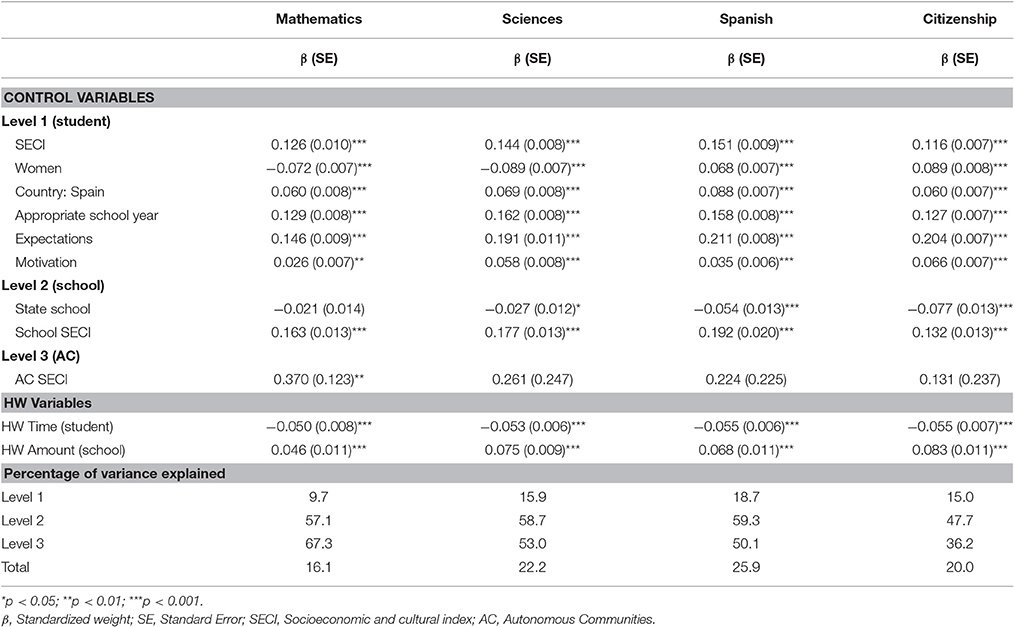
Table 3. Multilevel models for prediction of achievement in four subjects .
The results indicated that the adjustment variables behaved satisfactorily, with enough control to analyze the net effects of the homework variables. This was backed up by two results, firstly, the two variables with highest standardized coefficients were those related to educational history: academic expectations at the time of the test, and being in the school year corresponding to age. Motivation demonstrated a smaller effect but one which was significant in all cases. Secondly, the adjustment variables explained the majority of the variance in the results. The percentages of total explained variance in Table 2 were calculated with all variables. However, if the strategy had been to introduce the adjustment variables first and then add in the homework variables, the explanatory gain in the second model would have been about 2% in each subject.
The amount of homework turned out to be positively and significantly associated with the results in the four subjects. In a 100 point scale of standard deviation, controlling for other variables, it was estimated that for each 10 min added to the daily volume of homework, schools would achieve between 4.1 and 4.8 points more in each subject, with the exception of mathematics where the increase would be around 2.5 points. In other words, an increase of between 15 and 29 points in the school mean is predicted for each additional hour of homework volume of the school as a whole. This school level gain, however, would only occur if the students spent exactly the same time on homework as their school mean. As the regression coefficient of student homework time is negative and the variable is centered on the level of the school, the model predicts deterioration in results for those students who spend more time than their class mean on homework, and an improvement for those who finish their homework more quickly than the mean of their classmates.
Furthermore, the results demonstrated a positive association between the amount of homework assigned in a school and the differences in time needed by the students to complete their homework. Figure 1 shows the relationship between volume of homework (expressed as mean daily minutes of homework by school) and the differences in time spent by students (expressed as the standard deviation from the mean school daily minutes). The correlation between the variables was 0.69 and the regression gradient indicates that schools which assigned 60 min of homework per day had a standard deviation in time spent by students on homework of approximately 25 min, whereas in those schools assigning 120 min of homework, the standard deviation was twice as long, and was over 50 min. So schools which assigned more homework also tended to demonstrate greater differences in the time students need to spend on that homework.
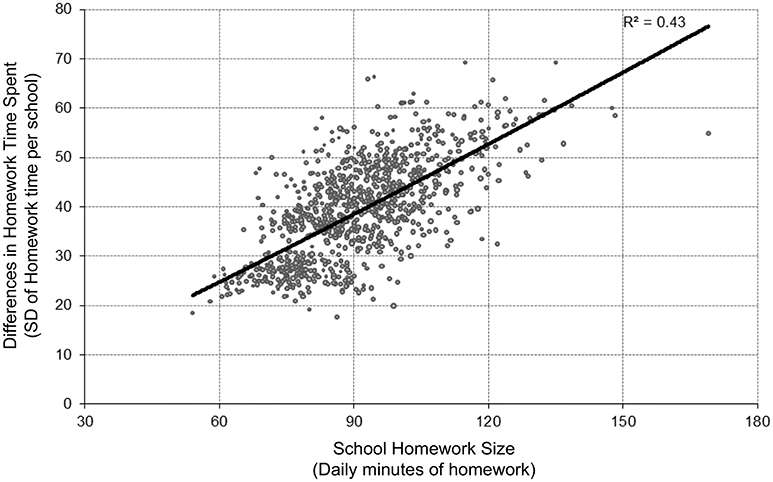
Figure 1. Relationship between school homework volume and differences in time needed by students to complete homework .
Figure 2 shows the effect on results in mathematics of the combination of homework time, homework amount, and the variance of homework time associated with the amount of homework assigned in two types of schools: in type 1 schools the amount of homework assigned is 1 h, and in type 2 schools the amount of homework 2 h. The result in mathematics was used as a dependent variable because, as previously noted, it was the subject where the effect was smallest and as such is the most conservative prediction. With other subjects the results might be even clearer.
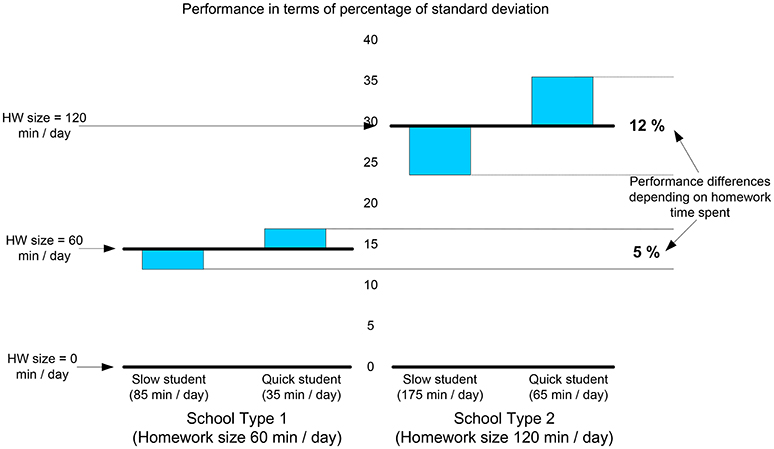
Figure 2. Prediction of results for quick and slow students according to school homework size .
Looking at the first standard deviation of student homework time shown in the first graph, it was estimated that in type 1 schools, which assign 1 h of daily homework, a quick student (one who finishes their homework before 85% of their classmates) would spend a little over half an hour (35 min), whereas the slower student, who spends more time than 85% of classmates, would need almost an hour and a half of work each day (85 min). In type 2 schools, where the homework amount is 2 h a day, the differences increase from just over an hour (65 min for a quick student) to almost 3 h (175 min for a slow student). Figure 2 shows how the differences in performance would vary within a school between the more and lesser able students according to amount of homework assigned. In type 1 schools, with 1 h of homework per day, the difference in achievement between quick and slow students would be around 5% of a standard deviation, while in schools assigning 2 h per day the difference would be 12%. On the other hand, the slow student in a type 2 school would score 6 points more than the quick student in a type 1 school. However, to achieve this, the slow student in a type 2 school would need to spend five times as much time on homework in a week (20.4 weekly hours rather than 4.1). It seems like a lot of work for such a small gain.
Discussion and Conclusions
The data in this study reaffirm the multilevel nature of homework ( Trautwein and Köller, 2003 ) and support this study's first hypothesis: the amount of homework (mean daily minutes the student spends on homework) is positively associated with academic results, whereas the time students spent on homework considered individually is negatively associated with academic results. These findings are in line with previous research, which indicate that school-level variables, such as amount of homework assigned, have more explanatory power than individual variables such as time spent ( De Jong et al., 2000 ; Dettmers et al., 2010 ; Scheerens et al., 2013 ; Fernández-Alonso et al., 2015 ). In this case it was found that for each additional hour of homework assigned by a school, a gain of 25% of a standard deviation is expected in all subjects except mathematics, where the gain is around 15%. On the basis of this evidence, common sense would dictate the conclusion that frequent and abundant homework assignment may be one way to improve school efficiency.
However, as noted previously, the relationship between homework and achievement is paradoxical- appearances are deceptive and first conclusions are not always confirmed. Analysis demonstrates another two complementary pieces of data which, read together, raise questions about the previous conclusion. In the first place, time spent on homework at the individual level was found to have a negative effect on achievement, which confirms the findings of other multilevel-approach research ( Trautwein, 2007 ; Trautwein et al., 2009b ; Chang et al., 2014 ; Fernández-Alonso et al., 2016 ). Furthermore, it was found that an increase in assigned homework volume is associated with an increase in the differences in time students need to complete it. Taken together, the conclusion is that, schools with more homework tend to exhibit more variation in student achievement. These results seem to confirm our second hypothesis, as a positive covariation was found between the amount of homework in a school (the mean homework time by school) and the increase in differences within the school, both in student homework time and in the academic results themselves. The data seem to be in line with those who argue that homework is a source of inequity because it affects those less academically-advantaged students and students with greater limitations in their home environments ( Kohn, 2006 ; Rømming, 2011 ; OECD, 2013b ).
This new data has clear implications for educational action and school homework policies, especially in compulsory education. If quality compulsory education is that which offers the best results for the largest number ( Barber and Mourshed, 2007 ; Mourshed et al., 2010 ), then assigning an excessive volume of homework at those school levels could accentuate differences, affecting students who are slower, have more gaps in their knowledge, or are less privileged, and can make them feel overwhelmed by the amount of homework assigned to them ( Martinez, 2011 ; OECD, 2014b ; Suárez et al., 2016 ). The data show that in a school with 60 min of assigned homework, a quick student will need just 4 h a week to finish their homework, whereas a slow student will spend 10 h a week, 2.5 times longer, with the additional aggravation of scoring one twentieth of a standard deviation below their quicker classmates. And in a school assigning 120 min of homework per day, a quick student will need 7.5 h per week whereas a slow student will have to triple this time (20 h per week) to achieve a result one eighth worse, that is, more time for a relatively worse result.
It might be argued that the differences are not very large, as between 1 and 2 h of assigned homework, the level of inequality increases 7% on a standardized scale. But this percentage increase has been estimated after statistically, or artificially, accounting for sociological and psychological student factors and other variables at school and region level. The adjustment variables influence both achievement and time spent on homework, so it is likely that in a real classroom situation the differences estimated here might be even larger. This is especially important in comprehensive education systems, like the Spanish ( Eurydice, 2015 ), in which the classroom groups are extremely heterogeneous, with a variety of students in the same class in terms of ability, interest, and motivation, in which the aforementioned variables may operate more strongly.
The results of this research must be interpreted bearing in mind a number of limitations. The most significant limitation in the research design is the lack of a measure of previous achievement, whether an ad hoc test ( Murillo and Martínez-Garrido, 2013 ) or school grades ( Núñez et al., 2014 ), which would allow adjustment of the data. In an attempt to alleviate this, our research has placed special emphasis on the construction of variables which would work to exclude academic history from the model. The use of the repetition of school year variable was unavoidable because Spain has one of the highest levels of repetition in the European Union ( Eurydice, 2011 ) and repeating students achieve worse academic results ( Ministerio de Educación, 2011 ). Similarly, the expectation and motivation variables were included in the group of adjustment factors assuming that in this research they could be considered background variables. In this way, once the background factors are discounted, the homework variables explain 2% of the total variance, which is similar to estimations from other multilevel studies ( De Jong et al., 2000 ; Trautwein, 2007 ; Dettmers et al., 2009 ; Fernández-Alonso et al., 2016 ). On the other hand, the statistical models used to analyze the data are correlational, and as such, one can only speak of an association between variables and not of directionality or causality in the analysis. As Trautwein and Lüdtke (2009) noted, the word “effect” must be understood as “predictive effect.” In other words, it is possible to say that the amount of homework is connected to performance; however, it is not possible to say in which direction the association runs. Another aspect to be borne in mind is that the homework time measures are generic -not segregated by subject- when it its understood that time spent and homework behavior are not consistent across all subjects ( Trautwein et al., 2006 ; Trautwein and Lüdtke, 2007 ). Nonetheless, when the dependent variable is academic results it has been found that the relationship between homework time and achievement is relatively stable across all subjects ( Lubbers et al., 2010 ; Chang et al., 2014 ) which leads us to believe that the results given here would have changed very little even if the homework-related variables had been separated by subject.
Future lines of research should be aimed toward the creation of comprehensive models which incorporate a holistic vision of homework. It must be recognized that not all of the time spent on homework by a student is time well spent ( Valle et al., 2015 ). In addition, research has demonstrated the importance of other variables related to student behavior such as rate of completion, the homework environment, organization, and task management, autonomy, parenting styles, effort, and the use of study techniques ( Zimmerman and Kitsantas, 2005 ; Xu, 2008 , 2013 ; Kitsantas and Zimmerman, 2009 ; Kitsantas et al., 2011 ; Ramdass and Zimmerman, 2011 ; Bembenutty and White, 2013 ; Xu and Wu, 2013 ; Xu et al., 2014 ; Rosário et al., 2015a ; Osorio and González-Cámara, 2016 ; Valle et al., 2016 ), as well as the role of expectation, value given to the task, and personality traits ( Lubbers et al., 2010 ; Goetz et al., 2012 ; Pedrosa et al., 2016 ). Along the same lines, research has also indicated other important variables related to teacher homework policies, such as reasons for assignment, control and feedback, assignment characteristics, and the adaptation of tasks to the students' level of learning ( Trautwein et al., 2009a ; Dettmers et al., 2010 ; Patall et al., 2010 ; Buijs and Admiraal, 2013 ; Murillo and Martínez-Garrido, 2013 ; Rosário et al., 2015b ). All of these should be considered in a comprehensive model of homework.
In short, the data seem to indicate that in year 8 of compulsory education, 60–70 min of homework a day is a recommendation that, slightly more optimistically than Cooper's (2001) “10 min rule,” gives a reasonable gain for the whole school, without exaggerating differences or harming students with greater learning difficulties or who work more slowly, and is in line with other available evidence ( Fernández-Alonso et al., 2015 ). These results have significant implications when it comes to setting educational policy in schools, sending a clear message to head teachers, teachers and those responsible for education. The results of this research show that assigning large volumes of homework increases inequality between students in pursuit of minimal gains in achievement for those who least need it. Therefore, in terms of school efficiency, and with the aim of improving equity in schools it is recommended that educational policies be established which optimize all students' achievement.
Ethics Statement
This study was carried out in accordance with the recommendations of the University of Oviedo with written informed consent from all subjects. All subjects gave written informed consent in accordance with the Declaration of Helsinki. The protocol was approved by the University of Oviedo.
Author Contributions
RF and JM have designed the research; RF and JS have analyzed the data; MA and JM have interpreted the data; RF, MA, and JS have drafted the paper; JM has revised it critically; all authors have provided final approval of the version to be published and have ensured the accuracy and integrity of the work.
This research was funded by the Ministerio de Economía y Competitividad del Gobierno de España. References: PSI2014-56114-P, BES2012-053488. We would like to express our utmost gratitude to the Ministerio de Educación Cultura y Deporte del Gobierno de España and to the Consejería de Educación y Cultura del Gobierno del Principado de Asturias, without whose collaboration this research would not have been possible.
Conflict of Interest Statement
The authors declare that the research was conducted in the absence of any commercial or financial relationships that could be construed as a potential conflict of interest.
Barber, B. (1986). Homework does not belong on the agenda for educational reform. Educ. Leadersh. 43, 55–57.
Google Scholar
Barber, M., and Mourshed, M. (2007). How the World's Best-Performing School Systems Come Out on Top. McKinsey and Company . Available online at: http://mckinseyonsociety.com/downloads/reports/Education/Worlds_School_Systems_Final.pdf (Accessed January 25, 2016).
Bembenutty, H., and White, M. C. (2013). Academic performance and satisfaction with homework completion among college students. Learn. Individ. Differ. 24, 83–88. doi: 10.1016/j.lindif.2012.10.013
CrossRef Full Text | Google Scholar
Buijs, M., and Admiraal, W. (2013). Homework assignments to enhance student engagement in secondary education. Eur. J. Psychol. Educ. 28, 767–779. doi: 10.1007/s10212-012-0139-0
Chang, C. B., Wall, D., Tare, M., Golonka, E., and Vatz, K. (2014). Relations of attitudes toward homework and time spent on homework to course outcomes: the case of foreign language learning. J. Educ. Psychol. 106, 1049–1065. doi: 10.1037/a0036497
Cooper, H. (1989). Synthesis of research on homework. Educ. Leadersh. 47, 85–91.
Cooper, H. (2001). The Battle Over Homework: Common Ground for Administrators, Teachers, and Parents . Thousand Oaks, CA: Sage.
Cooper, H., Robinson, J. C., and Patall, E. A. (2006). Does homework improve academic achievement? A synthesis of research, 1987-2003. Rev. Educ. Res. 76, 1–62. doi: 10.3102/00346543076001001
Cooper, H., Steenbergen-Hu, S., and Dent, A. L. (2012). “Homework,” in APA Educational Psychology Handbook , Vol. 3: Application to Learning and Teaching , eds K. R. Harris, S. Graham, and T. Urdan (Washington, DC: American Psychological Association), 475–495.
Cooper, H., and Valentine, J. C. (2001). Using research to answer practical questions about homework. Educ. Psychol. 36, 143–153. doi: 10.1207/S15326985EP3603_1
Corno, L. (1996). Homework is a complicated thing. Educ. Res. 25, 27–30. doi: 10.3102/0013189X025008027
De Jong, R., Westerhof, K. J., and Creemers, B. P. M. (2000). Homework and student math achievement in junior high schools. Educ. Res. Eval. 6, 130–157. doi: 10.1076/1380-3611(200006)6:2;1-E;F130
Dettmers, S., Trautwein, U., Lüdtke, M., Kunter, M., and Baumert, J. (2010). Homework works if homework quality is high: using multilevel modeling to predict the development of achievement in mathematics. J. Educ. Psychol. 102, 467–482. doi: 10.1037/a0018453
Dettmers, S., Trautwein, U., and Lüdtke, O. (2009). The relationship between homework time and achievement is not universal: evidence from multilevel analyses in 40 countries. Sch. Eff. Sch. Improv. 20, 375–405. doi: 10.1080/09243450902904601
Epstein, J. L., and Van Voorhis, F. L. (2001). More than minutes: teachers' roles in designing homework. Educ. Psychol. 36, 181–193. doi: 10.1207/S15326985EP3603_4
Eurydice (2015). The Structure of the European Education Systems 2015/16: Schematic Diagrams. Luxembourg: Publications Office of the European Union . Available online at: https://webgate.ec.europa.eu/fpfis/mwikis/eurydice/index.php/Publications:The_Structure_of_the_European_Education_Systems_2015/16:_Schematic_Diagrams (Accessed January 25, 2016).
Eurydice (2011). Grade Retention during Compulsory Education in Europe: Regulations and Statistics . Luxembourg: Publications Office of the European Union.
Fan, H., Xu, J., Cai, Z., He, J., and Fan, X. (2017). Homework and students' achievement in math and science: a 30-year meta-analysis, 1986-2015. Educ. Res. Rev. 20, 35–54. doi: 10.1016/j.edurev.2016.11.003
Farrow, S., Tymms, P., and Henderson, B. (1999). Homework and attainment in primary schools. Br. Educ. Res. J. 25, 323–341. doi: 10.1080/0141192990250304
Fernández-Alonso, R., and Muñiz, J. (2011). Diseños de cuadernillos para la evaluación de competencias b1sicas. Aula Abierta 39, 3–34.
Fernández-Alonso, R., Suárez-Álvarez, J., and Muñiz, J. (2012). Imputación de datos perdidos en las evaluaciones diagnósticas educativas. [Imputation methods for missing data in educational diagnostic evaluation]. Psicothema 24, 167–175.
Fernández-Alonso, R., Suárez-Álvarez, J., and Muñiz, J. (2014). Tareas escolares en el hogar y rendimiento en matemáticas: una aproximación multinivel con estudiantes de enseñanza primaria. [Homework and academic performance in mathematics: A multilevel approach with primary school student]. Rev. Psicol. Educ. 9, 15–30.
Fernández-Alonso, R., Suárez-Álvarez, J., and Muñiz, J. (2015). Adolescents' homework performance in mathematics and science: personal factors and teaching practices. J. Educ. Psychol. 107, 1075–1085. doi: 10.1037/edu0000032
Fernández-Alonso, R., Suárez-Álvarez, J., and Muñiz, J. (2016). Homework and performance in mathematics: the role of the teacher, the family and the student's background. Rev. Psicod. 21, 5–23. doi: 10.1387/RevPsicodidact.13939
CrossRef Full Text
Flunger, B., Trautwein, U., Nagengast, B., Lüdtke, O., Niggli, A., and Schnyder, I. (2015). The Janus-faced nature of time spent on homework: using latent profile analyses to predict academic achievement over a school year. Lear. Instr. 39, 97–106. doi: 10.1016/j.learninstruc.2015.05.008
Gershenson, S., and Holt, S. B. (2015). Gender gaps in high school students' homework time. Educ. Res. 44, 432–441. doi: 10.3102/0013189X15616123
Goetz, T., Nett, U. E., Martiny, S. E., Hall, N. C., Pekrun, R., Dettmers, S., et al. (2012). Students' emotions during homework: structures, self-concept antecedents, and achievement outcomes. Learn. Individ. Differ. 22, 225–234. doi: 10.1016/j.lindif.2011.04.006
Goldstein, A. (1960). Does homework help? A review of research. Elementary Sch. J. 60, 212–224. doi: 10.1086/459804
Kitsantas, A., Cheema, J., and Ware, H. (2011). The role of homework support resources, time spent on homework, and self-efficacy beliefs in mathematics achievement. J. Adv. Acad. 22, 312–341. doi: 10.1177/1932202X1102200206
Kitsantas, A., and Zimmerman, B. J. (2009). College students homework and academic achievement: the mediating role of self-regulatory beliefs. Metacognition Learn. 4, 1556–1623. doi: 10.1007/s11409-008-9028-y
Kohn, A. (2006). Abusing research: the study of homework and other examples. Phi Delta Kappan 88, 9–22. doi: 10.1177/003172170608800105
Lubbers, M. J., Van Der Werf, M. P. C., Kuyper, H., and Hendriks, A. A. J. (2010). Does homework behavior mediate the relation between personality and academic performance? Learn. Individ. Differ. 20, 203–208. doi: 10.1016/j.lindif.2010.01.005
Martinez, S. (2011). An examination of Latino students' homework routines. J. Latinos Educ. 10, 354–368. doi: 10.1080/15348431.2011.605688
Mislevy, R. J., Beaton, A. E., Kaplan, B., and Sheehan, K. M. (1992). Estimating population characteristics from sparse matrix samples of item responses. J. Educ. Meas. 29, 133–161. doi: 10.1111/j.1745-3984.1992.tb00371.x
Ministerio de Educación (2011). Evaluación General de Diagnóstico 2010. Educación Secundaria Obligatoria. Informe de Resultados . Madrid: Instituto de Evaluación. Available online at: http://www.mecd.gob.es/dctm/ievaluacion/informe-egd-2010.pdf?documentId=0901e72b80d5ad3e (Accessed January 25, 2016).
Mourshed, M., Chijioke, C., and Barber, M. (2010). How the World's Most Improved School Systems Keep Getting Better. McKinsey and Company . Available online at: http://mckinseyonsociety.com/downloads/reports/Education/How-the-Worlds-Most-Improved-School-Systems-Keep-Getting-Better_Download-version_Final.pdf (Accessed January 25, 2016).
Murillo, F. J., and Martínez-Garrido, C. (2013). Homework influence on academic performance. A study of iberoamerican students of primary education. J. Psychodidactics 18, 157–171. doi: 10.1387/RevPsicodidact.6156
Núñez, J. C., Vallejo, G., Rosário, P., Tuero, E., and Valle, A. (2014). Student, teacher, and school context variables predicting academic achievement in biology: analysis from a multilevel perspective. J. Psychodidactics 19, 145–171. doi: 10.1387/RevPsicodidact.7127
OECD (2009). PISA Data Analysis Manual: SPSS, 2nd Edn . Paris: OECD Publishing.
OECD (2011). School Sampling Preparation Manual. PISA 2012 Main Survey. Paris: OECD Publishing. Available online at: https://www.oecd.org/pisa/pisaproducts/PISA2012MS-SamplingGuidelines-.pdf (Accessed January 6, 2017).
OECD (2013a). PISA 2012 Results: What Students Know and Can Do. Student Performance in Mathematics, Reading and Science (Volume I) . Paris: OECD Publishing.
OECD (2013b). PISA 2012 Results: What Makes Schools Successful? Resources, Policies and Practices (Volume IV). Paris: OECD Publishing.
OECD (2014a). PISA 2012 Technical Report. Paris: OECD Publishing. Available online at: http://www.oecd.org/pisa/pisaproducts/PISA-2012-technical-report-final.pdf (Accessed January 25, 2016).
OECD (2014b). Does Homework Perpetuate Inequities in Education? PISA in Focus . Paris: OECD Publishing.
Osorio, A., and González-Cámara, M. (2016). Testing the alleged superiority of the indulgent parenting style among Spanish adolescents. Psicothema 28, 414–420. doi: 10.7334/psicothema2015.314
PubMed Abstract | CrossRef Full Text | Google Scholar
Paschal, R. A., Weinstein, T., and Walberg, H. J. (1984). The effects of homework on learning: a quantitative synthesis. J. Educ. Res. 78, 97–104. doi: 10.1080/00220671.1984.10885581
Patall, E. A., Cooper, H., and Wynn, S. R. (2010). The effectiveness and relative importance of providing choices in the classroom. J. Educ. Psychol. 102, 896–915. doi: 10.1037/a0019545
Pedrosa, I., Suárez-Álvarez, J., García-Cueto, E., and Muñiz, J. (2016). A computerized adaptive test for enterprising personality assessment in youth. Psicothema 28, 471–478. doi: 10.7334/psicothema2016.68
Ramdass, D., and Zimmerman, B. J. (2011). Developing self-regulation skills: the important role of homework. J. Adv. Acad. 22, 194–218. doi: 10.1177/1932202X1102200202
Raudenbush, S. W., Bryk, A. S., Cheong, Y. F., and Congdon, R. T. (2004). HLM6: Hierarchical Linear and Nonlinear Modeling . Chicago: Scientific Software International.
Rømming, M. (2011). Who benefits from homework assignments? Econ. Educ. Rev. 30, 55–64. doi: 10.1016/j.econedurev.2010.07.001
Rosário, P., Núñez, J. C., Vallejo, G., Cunha, J., Nunes, T., Mourão, R., et al. (2015a). Does homework design matter? The role of homework's purpose in student mathematics achievement. Contemp. Educ. Psychol. 43, 10–24. doi: 10.1016/j.cedpsych.2015.08.001
Rosário, P., Núñez, J. C., Vallejo, G., Cunha, J., Nunes, T., Suárez, N., et al. (2015b). The effects of teachers' homework follow-up practices on students' EFL performance: a randomized-group design. Front. Psychol. 6:1528. doi: 10.3389/fpsyg.2015.01528
Servicio de Evaluación Educativa del Principado de Asturias (2016). La relación entre el tiempo de deberes y los resultados académicos [The Relationship between Homework Time and Academic Performance]. Informes de Evaluación, 1 . Oviedo: Consejería de Educación y Cultura del Gobierno del Principado de Asturias.
Scheerens, J., Hendriks, M., Luyten, H., Sleegers, P., and Cees, G. (2013). Productive Time in Education. A Review of the Effectiveness of Teaching Time at School, Homework and Extended Time Outside School Hours. Enschede: University of Twente . Available online at: http://doc.utwente.nl/86371/ (Accessed January 25, 2016).
Suárez-Álvarez, J., Fernández-Alonso, R., and Muñiz, J. (2014). Self-concept, motivation, expectations and socioeconomic level as predictors of academic performance in mathematics. Learn. Indiv. Diff. 30, 118–123. doi: 10.1016/j.lindif.2013.10.019
Suárez, N., Regueiro, B., Epstein, J. L., Piñeiro, I., Díaz, S. M., and Valle, A. (2016). Homework involvement and academic achievement of native and immigrant students. Front. Psychol. 7:1517. doi: 10.3389/fpsyg.2016.01517
Trautwein, U. (2007). The homework–achievement relation reconsidered: differentiating homework time, homework frequency, and homework effort. Learn. Instr. 17, 372–388. doi: 10.1016/j.learninstruc.2007.02.009
Trautwein, U., and Köller, O. (2003). The relationship between homework and achievement: still much of a mystery. Educ. Psychol. Rev. 15, 115–145. doi: 10.1023/A:1023460414243
Trautwein, U., Köller, O., Schmitz, B., and Baumert, J. (2002). Do homework assignments enhance achievement? A multilevel analysis in 7th grade mathematics. Contemp. Educ. Psychol. 27, 26–50. doi: 10.1006/ceps.2001.1084
Trautwein, U., Lüdtke, O., Schnyder, I., and Niggli, A. (2006). Predicting homework effort: support for a domain-specific, multilevel homework model. J. Educ. Psychol. 98, 438–456. doi: 10.1037/0022-0663.98.2.438
Trautwein, U., and Lüdtke, O. (2007). Students' self-reported effort and time on homework in six school subjects: between-student differences and within-student variation. J. Educ. Psychol. 99, 432–444. doi: 10.1037/0022-0663.99.2.432
Trautwein, U., and Lüdtke, O. (2009). Predicting homework motivation and homework effort in six school subjects: the role of person and family characteristics, classroom factors, and school track. Learn. Instr. 19, 243–258. doi: 10.1016/j.learninstruc.2008.05.001
Trautwein, U., Niggli, A., Schnyder, I., and Lüdtke, O. (2009a). Between-teacher differences in homework assignments and the development of students' homework effort, homework emotions, and achievement. J. Educ. Psychol. 101, 176–189. doi: 10.1037/0022-0663.101.1.176
Trautwein, U., Schnyder, I., Niggli, A., Neumann, M., and Lüdtke, O. (2009b). Chameleon effects in homework research: the homework–achievement association depends on the measures used and the level of analysis chosen. Contemp. Educ. Psychol. 34, 77–88. doi: 10.1016/j.cedpsych.2008.09.001
Valle, A., Pan, I., Regueiro, B., Suárez, N., Tuero, E., and Nunes, A. R. (2015). Predicting approach to homework in primary school students. Psicothema 27, 334–340. doi: 10.7334/psicothema2015.118
Valle, A., Regueiro, B., Núñez, J. C., Rodríguez, S., Piñero, I., and Rosário, P. (2016). Academic goals, student homework engagement, and academic achievement in elementary school. Front. Psychol. 7:463. doi: 10.3389/fpsyg.2016.00463
von Davier, M., Gonzalez, E., and Mislevy, R. J. (2009). What are Plausible Values and Why are They Useful?. IERI Monograph Series. Issues and Methodologies in Large-Scale Assessments. Available online at: http://www.ierinstitute.org/fileadmin/Documents/IERI_Monograph/IERI_Monograph_Volume_02.pdf (Accessed January 15, 2017).
Walberg, H. J., Paschal, R. A., and Weinstein, T. (1985). Homework's powerful effects on learning. Educ. Leadersh. 42, 76–79.
Walberg, H. J., Paschal, R. A., and Weinstein, T. (1986). Walberg and colleagues reply: effective schools use homework effectively. Educ. Leadersh. 43, 58.
Wu, M. L., Adams, R. J., Wilson, M. R., and Haldane, S. A. (2007). ACER ConQuest 2.0: Generalised Item Response Modelling Software . Camberwell, VIC: Australian Council for Educational Research.
Xu, J. (2008). Models of secondary school students' interest in homework: a multilevel analysis. Am. Educ. Res. J. 45, 1180–1205. doi: 10.3102/0002831208323276
Xu, J. (2013). Why do students have difficulties completing homework? The need for homework management. J. Educ. Train. Stud. 1, 98–105. doi: 10.11114/jets.v1i1.78
Xu, J., and Wu, H. (2013). Self-regulation of homework behavior: homework management at the secondary school level. J. Educ. Res. 106, 1–13. doi: 10.1080/00220671.2012.658457
Xu, J., Yuan, R., Xu, B., and Xu, M. (2014). Modeling students' time management in math homework. Learn. Individ. Differ. 34, 33–42. doi: 10.1016/j.lindif.2014.05.011
Zimmerman, B. J., and Kitsantas, A. (2005). Homework practices and academic achievement: the mediating role of self-efficacy and perceived responsibility beliefs. Contemp. Educ. Psychol. 30, 397–417. doi: 10.1016/j.cedpsych.2005.05.003
Keywords: homework time, equity, compulsory secondary education, hierarchical modeling, adolescents
Citation: Fernández-Alonso R, Álvarez-Díaz M, Suárez-Álvarez J and Muñiz J (2017) Students' Achievement and Homework Assignment Strategies. Front. Psychol . 8:286. doi: 10.3389/fpsyg.2017.00286
Received: 16 November 2016; Accepted: 14 February 2017; Published: 07 March 2017.
Reviewed by:
Copyright © 2017 Fernández-Alonso, Álvarez-Díaz, Suárez-Álvarez and Muñiz. This is an open-access article distributed under the terms of the Creative Commons Attribution License (CC BY) . The use, distribution or reproduction in other forums is permitted, provided the original author(s) or licensor are credited and that the original publication in this journal is cited, in accordance with accepted academic practice. No use, distribution or reproduction is permitted which does not comply with these terms.
*Correspondence: Javier Suárez-Álvarez, [email protected]
Disclaimer: All claims expressed in this article are solely those of the authors and do not necessarily represent those of their affiliated organizations, or those of the publisher, the editors and the reviewers. Any product that may be evaluated in this article or claim that may be made by its manufacturer is not guaranteed or endorsed by the publisher.
An official website of the United States government
Official websites use .gov A .gov website belongs to an official government organization in the United States.
Secure .gov websites use HTTPS A lock ( Lock Locked padlock icon ) or https:// means you've safely connected to the .gov website. Share sensitive information only on official, secure websites.
- Publications
- Account settings
- Advanced Search
- Journal List

PROTOCOL: The relationship between homework time and academic performance among K‐12 students: A systematic review
Xiaoling hu, chunyan liu, howard white.
- Author information
- Article notes
- Copyright and License information
Correspondence Kehu Yang, Evidence‐Based Medicine Center, School of Basic Medical Sciences, and Evidence‐based Social Sciences Research Center, School of Public Health, Lanzhou University, Lanzhou 730000, China. Email: [email protected]
Corresponding author.
Collection date 2021 Dec.
This is an open access article under the terms of the http://creativecommons.org/licenses/by/4.0/ License, which permits use, distribution and reproduction in any medium, provided the original work is properly cited.
This review will synthesize the results from publications focused on homework time and academic performance, and estimate the relationship between the two. Our objectives are: (1) To identify the extent of the relationship between homework time and students' academic performance; (2) To analyze the differences in the effectiveness of homework time across genders, grades, subject and regions; and (3) To identify the potential factors that affect homework time, such as academic subject, task difficulty, type of homework, mode of homework, parental involvement, and feedback on homework.
1. BACKGROUND
1.1. description of the condition.
Homework is defined as “any task assigned by schoolteachers intended for students to carry out during non‐school hours” (Cooper, 1989 ). This definition explicitly excludes (a) in‐school guided study; (b) home study courses delivered through the mail, television, audio or videocassette, or the internet; and (c) extracurricular activities such as sports and participation in clubs (Cooper et al., 2006 ). With the development of technology, web‐based homework has become more popular among teachers, with online platforms such as Blackboard, WebCT ( www.webct.com ), Homework Service ( https://hw.utexas.edu/bur/overview.html ), WebWorK, Study Island, and PowerSchool (Mendicino et al., 2009 ), as these allow students to do their homework online, and teachers to give feedback to students immediately (Callahan, 2016 ; Lucas, 2012 ; Mendicino et al., 2009 ). Therefore, in this systematic review, homework also includes online tasks performed outside the school.
The purpose of homework can be divided into instructional and noninstructional objectives (Lee & Pruitt, 1979 ). The most common instructional purpose of homework includes review, preview, and extension (Becker & Epstein, 1982 ; Lee & Pruitt, 1979 ; Mulhenbruck et al., 1999 ). The review assignments mainly offer the students an opportunity to practise newly acquired skills or review material learnt in class. The preview assignments introduce new skills or materials to help students prepare for unfamiliar knowledge before the class (Mulhenbruck et al., 1999 ), and the extension assignments involve the transfer of previously learned skills to new situations (Cooper et al., 2006 ; Lee & Pruitt, 1979 ). The noninstructional purpose of homework varies. It can be used to form better study habits, increase the students' sense of responsibility, enhance awareness of independent learning, and build communication between parents, children, and teachers (Becker & Epstein, 1982 ; González et al., 2001 ; Lee & Pruitt, 1979 ; Mulhenbruck et al., 1999 ; Van Voorhis, 2003 ). Homework can also be used to punish students (Epstein & Van Voorhis, 2001 ).
Homework is a common and widespread educational activity for many students across the world. As an achievement of the educational excellence movement, the level of homework was generalized, which was supported by the parents at the beginning. However, as homework increased more and more, parents and scholars realized the burden of homework on students. They complained that the students lost their childhood and called for less homework (Gill & Schlossman, 2003 ). Similarly, in the United Kingdom homework became common in the mid‐19th century, and was a matter of much debate in the 1880s as levels of homework increased in response to the introduction of payment by results for teachers and other factors (Hallam, 2004 ).
Recently, the World Health Organization (WHO) found that students feel the most pressured by the amount of homework (WHO, 2016 ). Meanwhile, parents continued to complain about excessive homework assigned to their children (Gill & Schlossman, 2003 , 2004 ; Jerrima et al., 2019 ; Xue & Zhang, 2019 ).
Homework is often argued to improve academic performance. However, the relationship between homework and academic performance has been debated for more than one hundred years (Cheema & Sheridan, 2015 ; Cooper, 1989 ; Cooper et al., 2006 ; Kitsantas et al., 2011 ; Kralovec & Buell, 2000 ; Trautwein, 2007 ). Although several meta‐analyses of the relationship between homework and performance have found a positive correlation between homework time and academic performance (Baş et al., 2017 ; Cooper, 1989 ; Cooper et al., 2006 ; Fan et al., 2017 ), it is difficult to establish causality. More academically inclined students, who get better grades regardless, may complete their homework more conscientiously. Conversely, students who are doing badly may study harder at home to catch up.
Still, it may also be the case that the effect is not linear. Some evidence has shown that academic performance increases with the increase in homework time, but begins to decline when homework time exceeds the optimal amount of time (Ackerman et al., 2011 ; Krzysztof et al., 2018 ; Reteig et al., 2019 ). Based on data from the National Center for Education Statistics (NCES) survey of 58,000 high school students in grades one and two, Keith ( 1982 ) found that for anyone with any level of ability, increasing the amount of homework will improve their performance. Homework plays a compensatory role; however, the amount of homework cannot be increased indefinitely, only moderately. If it exceeds a certain limit, it will lead to a decline in performance (Keith, 1982 ).
1.2. Description of the intervention
The intervention is the homework assigned by schoolteachers for nonschool hours, and completed independently by students without additional teaching, such as online tasks and activities in study club. The comparison condition is different time spent on the homework, and we plan to divide the comparisons into several groups, such as 0–15, 16–30, 31–45, 46–60, 61–90, 90–120 min, and more than 120 min. Any type of homework will be included, such as written, oral, or practical homework. We excluded homework allocated by other people such as parents or teachers from extracurricular schools, and in‐school guided study, home study courses, and extracurricular activities such as sports and participation in clubs were excluded. Homework related to psychotherapy was also outside our definition of homework.
1.3. Conceptual framework
The conceptual framework for this review is the theory of change that describes how homework may affect academic performance. Figure 1 below demonstrates the conceptual framework through which the interventions are hypothesized to lead to the intended outcomes.
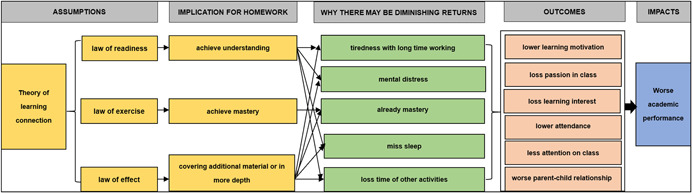
Conceptual framework for intervention and outcomes of homework
As in Figure 1 , the law of readiness reveals that before commencing a certain learning activity, if the learners do well in the preparatory stages (including physiological and psychological) related to the corresponding learning activities, they can grasp the learning content more rapidly (Muhammad, 2015 ). Second, the law of exercise suggests that for a certain kind of connection formed by learners, the correct repetition of this action in practice will effectively enhance this connection. And, third, the law of effect indicates that all kinds of positive or negative feedback that learners get in the process of learning will strengthen or weaken the connection that learners have formed in their mind.
However, there are limits to our ability to stay focused, and when students spend too much time on homework, their cognitive load and mental fatigue increase, and they will feel tired and even lead to mental distress, such as anxiety, which may reduce rather than improve readiness.
Furthermore, if the purpose of homework is to achieve mastery or covering additional material, then when the content of homework has been fully understood by a student, doing more homework won't help. That is there are diminishing returns to the time spent on homework, which can reach zero.
Finally, if the students miss sleep because of long homework hours, they may be tired in school and so do worse in class or tests. Hence readiness is reduced, and the law of effect undermined as the student is not in a good condition to receive feedback or perform well.
In addition, a student's development should be multifaceted, while if they spent too much time on homework, they will lose time to take part in other activities which can contribute to their overall development.
1.4. Why it is important to do this review
Several systematic reviews have explored the effectiveness of homework in improving students' performance, but all the conclusions were based on the assumption of a linear correlation between homework time and performance, and none of them considered the impact of homework time on students' autonomous motivation. A summary of the evidence by Hallam ( 2004 ) makes no recommendation on the time spent on the homework. The UK Education Endowment Foundation's toolkit entry for homework for secondary school students notes quality is more important than quantity but gives no explicit recommendation on the amount of time that should be spent on homework.
Existing reviews leave the important practical question of homework time unanswered.
In 1989, Cooper conducted a review related to the relationship between homework and performance. The results showed that the average correlation for students in primary school, middle school, and high school between the amount of homework and performance was nearly r = 0; for students in middle school, it was r = .07; and for high school students, it was r = .25 (Cooper, 1989 ). In 2006, Cooper et al. ( 2006 ) conducted another systematic review to explore the effectiveness of homework to improve academic performance. The results showed that the correlation between homework time and performance for high school students was still 0.25, but for middle school students, it was nearly 0. However, they did not look explicitly at homework time.
All the above studies assumed that the correlation between homework and performance was linear, that is, that either more or less homework was better. Indeed, their reported effect size is the correlation coefficient, which is a measure of the linearity of a relationship. While homework can be a dull task that requires full mental effort, and there are limits to our ability to stay focused. Therefore, it is important for teachers, school managers, parents, and children themselves to establish the optimum duration of homework to improve its effectiveness.
1.5. The contribution of this review
Regardless of its aims of preparation, practice, extension or application, homework can be an effective means to improve student's academic achievement. Previous reviews indeed testify to the effectiveness of homework in relation to academic performance. More is not always better, and is restricted by students' ability to maintain their attention for a long time. The present systematic review plans to divide the participants into several groups according to the amount of time spent on homework, such as 0–15, 16–30, 31–45, 46–60, 61–90, 90–120 min, and more than 120 min to compare the test scores of different groups to identify the extent of the relationship between homework time and students' academic performance. We aim to investigate the role of homework in academic achievement, and to determine the optimum homework time by comparing the differences in outcomes between different groupings of homework time. This will be helpful for teachers and parents to better understand the role and utility of homework, and provide theoretical support for teachers to arrange homework.
2. OBJECTIVES
This review will synthesize the results from publications focused on homework time and academic performance, and estimate the relationship between the two. Our objectives are:
To identify the extent of the relationship between homework time and students' academic performance;
To analyze the differences in the effectiveness of homework time across genders, grades, subject and regions; and
To identify the potential factors that affect homework time, such as academic subject, task difficulty, type of homework, mode of homework, parental involvement, and feedback on homework.
3.1. Criteria for considering studies for this review
3.1.1. types of studies.
We will include treatment‐control group design or a comparison group design studies, to adequately address the effect of differing homework time on the academic performance of K‐12 students.
Randomized controlled trials (RCTs) that aim to explore the effect of homework time on academic performance by comparing the test score differences between different groups before and after the intervention will be included. In addition, non‐RCTs such as cohort studies (NRCTs), controlled before and after studies, and interrupted time series studies also will be included if they explicitly take homework as intervention, and report the time spent on homework and the mean and standard deviation of academic achievement.
Relevant correlational studies without mean and standard deviation of academic achievement will be excluded. Other study designs such as case studies, narrative reviews, and nonprimary studies such as editorials, book reviews, commentaries, and letters to the editor, will also be excluded. Qualitative evidence is also beyond the inclusion criteria in the present systematic review.
3.1.2. Types of participants
This review will include studies of K‐12 school students. We excluded children with disabilities, since the context and effects may be different for that population group. Students from special education schools are excluded. If the primary study includes mixed samples (e.g., special education and nonspecial education students), we will use the sub sample excluding special needs students if reported.

3.1.3. Types of interventions
In this review, we will explore the relationship between homework time and academic performance by comparing the academic scores with different amounts of time spent on homework. The eligible intervention studies must be clear that the intervention is homework assigned to students to complete during nonschool hours regularly by schoolteachers which aims to improve academic achievement. This does not mean that the intervention must consist of academic activities, but rather that the explicit expectation must be that the homework, regardless of the nature of the homework content, will result in improved academic performance. Furthermore, we will only include school‐based interventions, that is, homework allocated by other people such as parents or teachers from extracurricular schools, study clubs, and extracurricular activities such as sports and participation in clubs are excluded. Homework related to psychotherapy will also be excluded.
The comparison condition is different time spend on the homework, and we plan to divide the comparisons into several groups, such as 0–15, 16–30, 31–45, 46–60, 61–90, 90–120 min, and more than 120 min.
3.1.4. Types of outcome measures
The objective of the review is to explore the impact of homework on students' academic outcomes. We will extract the homework time and academic performance provided in the primary study. The homework time is the exact time or a time frame reported by students or parents. Academic performance will be measured by the teacher, exam results and/or by the research team using any valid standardized test and reported as test scores.
As valid standardized tests, we will consider norm‐referenced tests (e.g., Gates‐MacGinitie Reading Tests and Star Math), state‐wide tests (e.g., Iowa Test of Basic Skills), national tests (e.g., National Assessment of Educational Progress). If it is not clear from the description of outcome measures in the studies, we will use electronic sources to determine whether a test is standardized or not.
3.1.5. Primary outcomes
The primary outcome is academic performance (test score and standard deviation), and studies that have measured academic performance (and homework time) will be included.
3.1.6. Secondary outcomes
Academic motivation and quality of homework will be included as secondary outcomes.
3.2. Search methods for identification of studies
3.2.1. electronic searches.
The following databases will be searched from inception to present:
Social Sciences Citation Index (Web of Science)
ScienceDirect ( https://www.sciencedirect.com/ )
Taylor & Francis Online Database ( https://www.tandfonline.com/ )
The Campbell Library ( https://www.campbellcollaboration.org/better-evidence.html )
ERCI (EBSCOhost)
EBSCO ( http://search.ebscohost.com/ )
JSTOR ( https://www.jstor.org/ )
PsychArticles (ProQuest)
PsychInfo (EBSCOhost)
ProQuest Dissertations ( https://www.proquest.com/index )
OCLC FirstSearch ( https://firstsearch.oclc.org/ )
Below, the search strategy for Web of Science is provided:
#1 TI = homework OR AB = homework
#2 TI = home‐work OR AB = home‐work
#3 #1 OR #2
#4 TS = K‐12 OR TS = preschool student* OR TS = pre‐school student* OR TS = Kindergarten
student* OR TS = middle school student* OR TS = high school student* OR TS = senior school
student* OR TS = primary school student* OR TS = pupil OR TS = schoolchild OR TS = junior
high school student* OR TS = school‐age
#5 TS = achievement OR TS = performance OR TS = grade OR TS = score OR TS = academic
achievement* OR TS = GPA OR TS = academic performance
#6 #3 AND #4 AND #5
3.2.2. Searching other resources
We will consult the following sources of gray literature, and search the websites of organizations devoted to the education research, to identify relevant unpublished studies and reports. The following gray literature resources will be searched with the keyword “homework”:
What Works Clearinghouse ( https://ies.ed.gov/ncee/wwc/ )
Education Endowment Foundation ( https://educationendowmentfoundation.org.uk/ )
European Educational Research Association ( http://www.eera-ecer.de/ )
American Educational Research Association ( http://www.aera.net/ )
Best Evidence Encyclopedia ( http://www.bestevidence.org/ )
Open Grey ( http://www.opengrey.eu/ )
We will also search the Google Scholar with the keyword “homework,” and we will stop scan if there are 5 consecutive pages with no relevant studies.
The following international journals will be hand searched for relevant studies with the keyword “homework”:
American Educational Research Journal
Educational Psychologist
Learning and Instruction
Journal of Educational Research
Journal of Educational Psychology
Journal of Research on Educational Effectiveness
Journal of Experimental Education.
Additionally, the primary studies included in the previous systematic reviews on the relationship between homework and academic performance will be scanned, and the reference lists will also be searched. Furthermore, the studies of experts in the research of homework (such as Cooper Harris, Trautwein Ulric, and Xu jianzhong) will be searched systematically to check our search strategy, and they will be contacted to help identify other relevant studies if possible.
3.3. Data collection and analysis
3.3.1. selection of studies.
The selection of studies will be performed independently by the first two reviewers (Guo LP and Jieyun Li) in Rayyan ( https://rayyan.qcri.org/ ). All titles and abstracts of the records identified after retrieval will be screened, the potentially relevant references will be located with full‐text, and the primary studies that meet our criteria will be included for further analysis. Studies that meet the selection criteria and have the outcomes of interest measured, but do not report these outcome data, will be included and described in the results section of the review. Any discrepancies between the two reviewers will be resolved by consensus with another reviewer involved (Kehu Yang). The whole process of study screening will be based on the PRISMA guidelines (Moher et al., 2009 ).
3.3.2. Data extraction and management
Information extraction and coding will consist of two parts. The first is general information, including the information of primary study (publication, the year of publication, and the year of data collection), sample characteristics (e.g., sample size, gender, grade level, region, family economic status, parental education level), methodological characteristics (e.g., sampling method, measures of homework time, and the measure of academic performance), and the intervention characteristics (e.g., subject, mode of homework and the type of homework). The other is the effect size, including the homework time and the test score (The details are shown in Supporting Information Annex 1 ). This process will be conducted independently by two authors (Zheng Xu and Xing Xin), and disagreements between coders will be resolved by discussions with another author (Xiuxia Li).
If a study contains multiple interventions (e.g., different homework modalities such as online vs. book‐based), the reviewers will only extract data that are eligible for this review.
For academic performance, means, standard deviations (or information to estimate standard deviations), and the number of participants in each group will be extracted. If more than one measure is reported, we will extract all of them and analyze the measurement method as a moderator.
For the homework time, we will extract the homework time interval reported in the primary studies and code the data as presented, either categorical or continuous. We will then create a continuous variable measure data set (using the mid‐point for data reported in categorical form) and at least two categorical data sets. The multiple categorical data sets will be used to test for sensitivity to the chosen thresholds, and then calculate the mean and standard deviations in each group. If the weekly homework time is reported instead of the daily homework time, we divide the total homework time by 5, and if the homework time is in hours, we convert it to minutes.
In case of controlled before and after studies, mean or median change from baseline scores will be extracted or computed by the reviewers if all necessary data are available. If change scores are not available or cannot be computed, post‐intervention values will be extracted by the reviewers.
3.3.3. Assessment of risk of bias in included studies
For RCTs, the Cochrane bias risk tool will be used to assess the quality of the method and potential defects (Higgins & Green, 2011 ). For nonrandomized studies (including cohort studies, controlled before and after studies, and interrupted time series studies), the risk of bias in nonrandomized studies of interventions (ROBINS‐I) will be used to check the quality of the individual study (Sterne et al., 2016 ). In addition, the grade will be used to rate the overall quality of the evidence included in this review, ranging from high, moderate, low, and very low, based on the assessment to study design, imprecision, inconsistency, indirectness, and publication bias (Atkins et al., 2004 ; Schünemann et al., 2013 ). The risk of bias assessment will be conducted by the two authors (Zheng Xu and Xing Xin), and any disaccord will be solved by discussion with another author (Xiuxia Li).
3.3.4. Dealing with missing data
If there are any missing data, we will contact the author at least twice to obtain more information if the correspondence address is available. If these data are unavailable, we will only analyze the available data, and the studies with missing data will be described in the Results section. Besides, the potential impact of missing data on comprehensive estimates will be considered in the Discussion section.
3.3.5. Assessment of heterogeneity
Forest plots will be inspected to visually investigate overlaps in the confidence intervals (CIs) of the results of the individual studies. The χ 2 test will be performed, and the Q statistics, I 2 and τ 2 index will be adopted to evaluate heterogeneity across studies. For Q statistics, a p value of .05 will be used as a threshold for statistical significance. The I 2 index refers to the truly observed variation ratio (Borenstein et al., 2009 ), and 25%, 50%, and 75% of the I 2 indicate low, medium, and high heterogeneity (Higgins & Thompson, 2002 ). And the parameter τ 2 is the between‐studies variance (the variance of the effect size parameters across the population of studies), that is, the variance of true effect sizes (across an infinite number of studies).
3.3.6. Assessment of reporting biases
Reporting bias, also called publication bias, refers to the potential of the studies with statistically significant findings to be accepted for publication, whilst those with statistically nonsignificant findings would hardly be accepted for publication. These studies would have a higher probability of being left in the “file drawer,” according to the so‐called “file drawer problem.” If 10 or more studies were identified, visual funnel plots and Egger's test of funnel plot symmetry were performed to evaluate potential publication bias (Rosenthal, 1979 ). If there is evidence of funnel plot asymmetry from a test, we will attempt to conduct the comparisons between the effect with that after trim and fill. The possible reasons for this (e.g., nonreporting biases, poor methodological quality leading to spuriously inflated effects in smaller studies, true heterogeneity, artefactual, and chance) will also be considered (Page et al., 2019 ).
3.3.7. Data synthesis
We will include all intervention studies that meet our inclusion criteria and extract the mean (M) and SD of test scores. We will take the standard mean difference (SMD) and CI as our effect size index. For subgroups, we plan to divide the outcomes into several groups by time spent on the homework, such as 0–15, 16–30, 31–45, 46–60, 61–90, 90–120 min, and more than 120 min, and then compare the difference of SMD between groups to explore the role of homework time on academic achievement.
If two or more studies are identified that have investigated the effect of homework time on academic performance with sufficiently available data, a random‐effects meta‐analysis will be performed to estimate the comprehensive effect due to the expected heterogeneity between groups using the Review Manager 5 software. The pooled estimates will be presented in forest plots. If quantitative synthesis is not suitable, narrative synthesis will be adopted.
3.3.8. Planned moderators
If statistically significant heterogeneity is detected, subgroup analyses with stratification analysis will be conducted to explore the source of heterogeneity based on the available data.
Gender. Previous studies showed that girls more frequently reported managing their homework than boys (Mau & Lynn, 2000 ; Xu, 2007 ), and zero‐time homework students are most often male (Hagborg, 1991 ). Therefore, it is worth identifying the influence of gender on homework time and academic performance.
Grade level. Existing reviews on homework suggest that the relationship between homework and performance is mediated by grade level (Baş et al., 2017 ; Cooper, 1989 ; Cooper et al., 2006 ; Fan et al., 2017 ). Thus, we include the grade level as a potential factor that moderates the linkage of homework time and academic performance.
Region. Numerous studies indicated that there are regional differences in homework policies and practices (Chen & Stevenson, 1989 ; Tam & Chan, 2009 ; Zhu, 2015 ). The samples involved in the primary study on homework are from different regions (e.g., the United States and Asia); thus, we included the sampling region as a potential moderator in the present review.
Publication year: Education systems are susceptible to influence of societal changes; thus, publication year is likely to be a moderator, as the homework time may change systematically over time (Cooper et al., 2006 ; Gill & Schlossman, 2004 ; Twenge et al., 2004 ). Thus, publication year may also potentially affect the effect sizes of homework time on performance.
Mode of homework. With web‐learning popularized in education, online homework is being adopted by more teachers, and several researchers have argued about the effects of online homework compared to traditional homework (Callahan, 2016 ; Elias et al., 2017 ; Jonsdottir et al., 2017 ; Mendicino et al., 2009 ). In the present review, we will explore whether the relationship between homework time and academic performance is affected by the mode of homework.
Type of homework. Teachers typically assign different kinds of homework according to their purpose. Such as reading story to parents, writing math exercises, and trying scientific experiments. In this review, we will divide the academic achievement into three groups: oral homework, paper homework and practical homework and explore whether the relationship between homework time and academic performance is different depending on the type of homework.
The measure of academic performance: The methods used in previous homework studies included standardized tests and unstandardized assessments (Fan et al., 2017 ). Several studies suggested that the influence of homework on performance is larger with unstandardized assessments than with standardized tests (e.g., Cooper et al., 1998 ); therefore, there is a need to consider the measure of performance as a potential moderator.
Subject. Several studies showed that time and effort input in homework varies depending on the subject (e.g., Trautwein, 2007 ; Trautwein & Lüdtke, 2009 ), and it is reasonable to suspect that homework may play a different role in different subjects (e.g., Cooper et al., 2006 ; Fan et al., 2017 ; Paschal et al., 1984 ).
3.3.9. Sensitivity analysis
In addition to the implicit sensitivity analysis in both the analysis of heterogeneity and subgroup analysis, the “One‐leave‐out” method is adopted for sensitivity analysis to check for outliers that potentially influence the overall results, and test the robustness of the meta‐analysis.
CONTRIBUTIONS OF AUTHORS
Liping Guo drafted the protocol, and all authors reviewed the draft and approved the final version.
DECLARATIONS OF INTEREST
All authors declare no potential interest.
INTERNAL SOURCES
This review is supported by funding of the Major Project of the National Social Science Fund of China: Research on the Theoretical System, International Experience, and Chinese Path of Evidence‐based Social Science.
Supporting information
Supplementary Information
ACKNOWLEDGEMENTS
This review is supported by funding of the Major Project of the National Social Science Fund of China: Research on the Theoretical System, International Experience, and Chinese Path of Evidence‐based Social Science (No.: 19ZDA142).
Guo, L. , Li, J. , Xu, Z. , Hu, X. , Liu, C. , Xing, X. , Li, X. , White, H. , & Yang, K. (2021). PROTOCOL: The relationship between homework time and academic performance among K‐12 students: A systematic review. Campbell Systematic Reviews, 17, e1199. 10.1002/cl2.1199
- Ackerman, P. L. , Chamorro‐Premuzic, T. , & Furnham, A. (2011). Trait complexes and academic achievement: Old and new ways of examining personality in educational contexts. British Journal of Educational Psychology, 81(1), 27–40. 10.1348/000709910X522564 [ DOI ] [ PubMed ] [ Google Scholar ]
- Atkins, D. , Eccles, M. , Flottorp, S. , Guyatt, G. H. , Henry, D. , Hill, S. , Liberati, A. , O'Connell, D. , Oxman, A. D. , Phillips, B. , Schünemann, H. , Edejer, T. T.‐T. , Vist, G. E. , Williams Jr, J. W. , & The GRADE Working Group . (2004). Systems for grading the quality of evidence and the strength of recommendations I: Critical appraisal of existing approaches The GRADE Working Group. BMC Health Services Research, 4(1), 38. 10.1186/1472-6963-4-38 [ DOI ] [ PMC free article ] [ PubMed ] [ Google Scholar ]
- Baş, G. , Şentürk, C. , & Ciğerci, F. M. (2017). Homework and academic achievement: A meta‐analytic review of research. Issues in Educational Research, 27(1), 30–50. https://eric.ed.gov/?id=EJ1130406 [ Google Scholar ]
- Becker, H. J. , & Epstein, J. L. (1982). Parent involvement: A survey of teacher practices. Elementary School Journal, 83, 85–102. 10.2307/1001098 [ DOI ] [ Google Scholar ]
- Borenstein, M. , Hedges, L. V. , Higgins, J. P. T. , & Rothstein, H. R. (2009). Introduction to meta‐analysis. John Wiley & Sons. [ Google Scholar ]
- Callahan, J. T. (2016). Assessing online homework in first‐semester calculus. PRIMUS, 26(6), 545–556. 10.1080/10511970.2015.1128501 [ DOI ] [ Google Scholar ]
- Cheema, J. R. , & Sheridan, K. (2015). Time spent on homework, mathematics anxiety and mathematics achievement: Evidence from a US sample. Issues in Educational Research, 25, 246–259. http://www.iier.org.au/iier25/cheema.pdf [ Google Scholar ]
- Chen, C. , & Stevenson, H. W. (1989). Homework: A cross‐cultural examination. Child Development, 60(3), 551–561. 10.2307/1130721 [ DOI ] [ PubMed ] [ Google Scholar ]
- Cooper, H. (1989). Homework. Longman. [ Google Scholar ]
- Cooper, H. , Lindsay, J. J. , Nye, B. , & Greathouse, S. (1998). Relationships among attitudes about homework, the amount of homework assigned and completed, and student achievement. Journal of Educational Psychology, 90(1), 70–83. 10.1037/0022-0663.90.1.70 [ DOI ] [ Google Scholar ]
- Cooper, H. , Robinson, J. C. , & Patall, E. A. (2006). Does homework improve academic achievement? A synthesis of research, 1987−2003. Review of educational research, 76, 1–62. 10.3102/00346543076001001 [ DOI ] [ Google Scholar ]
- Elias, A. L. , Elliott, D. G. , & Elliott, J. A. W. (2017). Student perceptions and instructor experiences in implementing an online homework system in a large second‐year engineering course. Education for Chemical Engineers, 21, 40–49. 10.1016/j.ece.2017.07.005 [ DOI ] [ Google Scholar ]
- Epstein, J. L. , & Van Voorhis, F. L. (2001). More than minutes: Teachers' roles in designing homework. Educational Psychologist, 36, 181–193. 10.1207/S15326985EP3603_4 [ DOI ] [ Google Scholar ]
- Fan, H. , Xu, J. , He, J. , Cai, Z. , & Fan, X. (2017). Homework and students' achievement in math and science: A 30‐year meta‐analysis, 1986‐2015. Educational Research Review, 20(1), 35–54. 10.1016/j.edurev.2016.11.003 [ DOI ] [ Google Scholar ]
- Gill, B. P. , & Schlossman, S. L. (2003). Homework and the elusive voice of parents: Some historical perspectives. Teachers College Record, 105(5), 846–871. 10.1111/1467-9620.00270 [ DOI ] [ Google Scholar ]
- Gill, B. P. , & Schlossman, S. L. (2004). Villain or savior? The American Discourse on homework, 1850‐2003. Theory Into Practice, 43(3), 174–181. 10.1207/s15430421tip4303_2 [ DOI ] [ Google Scholar ]
- González, N. , Andrade, R. , Civil, M. , & Moll, L. (2001). Bridging funds of distributed knowledge: Creating zones of practice in mathematics. Journal of Education of Students Placed at Risk, 6, 115–132. 10.1207/S15327671ESPR0601-2_7 [ DOI ] [ Google Scholar ]
- Hagborg, W. J. (1991). A study of homework time of a high school sample. Perceptual and Motor Skills, 73(1), 103–106. 10.2466/pms.1991.73.1.103 [ DOI ] [ Google Scholar ]
- Hallam, S. (2004). Homework: The evidence. University of London. [ Google Scholar ]
- Higgins, J. P. T. , & Green, S. (Eds). (2011). Cochrane handbook for systematic reviews of interventions version 5.1.0 [updated March 2011]. The Cochrane Collaboration. Available from www.handbook.cochrane.org [ Google Scholar ]
- Higgins, J. P. T. , & Thompson, S. G. (2002). Quantifying heterogeneity in a meta‐analysis. Statistics in Medicine, 21(11), 1539–1558. 10.1002/sim.1186 [ DOI ] [ PubMed ] [ Google Scholar ]
- Jerrima, J. , Lopez‐Agudob, J. A. , & Marcenaro‐Gutierrez, O. D. (2019). The relationship between homework and the academic progress of children in Spain during compulsory elementary education: A twin fixed‐effects approach. British Educational Research Journal, 45(5), 1021–1049. 10.1002/berj.3549 [ DOI ] [ Google Scholar ]
- Jonsdottir, A. H. , Bjornsdottir, A. , & Stefansson, G. (2017). Difference in learning among students doing pen‐and‐paper homework compared to web‐based homework in an introductory statistics course. Journal of Statistics Education, 25(1), 12–20. 10.1080/10691898.2017.1291289 [ DOI ] [ Google Scholar ]
- Keith, T. Z. (1982). Time spent on homework and high school grades: A large‐sample path analysis. Journal of Educational Psychology, 74(2), 248–253. 10.1037/0022-0663.74.2.248 [ DOI ] [ Google Scholar ]
- Kitsantas, A. , Cheema, J. , & Ware, H. W. (2011). Mathematics achievement: The role of homework and self‐efficacy beliefs. Journal of Advanced Academics, 22, 310–339. 10.1177/1932202x1102200206 [ DOI ] [ Google Scholar ]
- Kralovec, E. , & Buell, J. (2000). The end of homework: How homework disrupts families, overburdens children, and limits learning. Beacon Press. [ Google Scholar ]
- Krzysztof, K. , Duchowski, A. T. , Anna, N. , Cezary, B. , Izabela, K. , & Susana, M. C. (2018). Eye tracking cognitive load using pupil diameter and microsaccades with fixed gaze. PLOS One, 13(9), 1–23. 10.1371/journal.pone.0203629 [ DOI ] [ PMC free article ] [ PubMed ] [ Google Scholar ]
- Lee, J. F. , & Pruitt, K. W. (1979). Homework assignments: Classroom games or teaching tools? The Clearing House, 53(1), 31–35. 10.2307/30185241 [ DOI ] [ Google Scholar ]
- Lucas, A. R. (2012). Using webwork, a web‐based homework delivery and grading system, to help prepare students for active learning. Primus Problems Resources & Issues in Mathematics Undergraduate Studies, 22(2), 97–107. 10.1080/10511970.2010.497834 [ DOI ] [ Google Scholar ]
- Mau, W. C. , & Lynn, R. (2000). Gender differences in homework and test scores in mathematics, reading and science at tenth and twelfth grade. Psychology, Evolution & Gender, 2(2), 119–125. 10.1080/14616660050200904 [ DOI ] [ Google Scholar ]
- Mendicino, M. , Razzaq, L. , & Heffernan, N. T. (2009). A comparison of traditional homework to computer‐supported homework. Journal of Research on Technology in Education, 41(3), 331–359. 10.1080/15391523.2009.10782534 [ DOI ] [ Google Scholar ]
- Moher, D. , Liberati, A. , & Tetzlaff, J. , The PRISMA Group . (2009). Preferred reporting items for systematic reviews and meta‐analyses: The PRISMA statement. PLOS Medicine, 6(7), e1000097. 10.1016/j.jclinepi.2009.06.005 [ DOI ] [ PMC free article ] [ PubMed ] [ Google Scholar ]
- Muhammad, H. I. (2015). Thorndike theory and it's application in learning. At‐Ta'lim: Journal Pendidikan, 1(1), 37–47. https://ejournal.inzah.ac.id/index.php/attalim/article/view/166 [ Google Scholar ]
- Mulhenbruck, L. , Cooper, H. , Nye, B. , & Lindsay, J. J. (1999). Homework and achievement: Explaining the different strengths of relation at the elementary and secondary school levels. Social Psychology of Education, 3, 295–317. 10.1023/A:1009680513901 [ DOI ] [ Google Scholar ]
- Page, M. J. , Higgins, J. P. T. , & Sterne, J. A. C. (2019). Chapter 13: Assessing risk of bias due to missing results in a synthesis. In Higgins J. P. T., Thomas J., Chandler J., Cumpston M., Li T., Page M. J., & Welch V. A. (Eds.), Cochrane handbook for systematic reviews of interventions version 6.0 (updated July 2019). Cochrane. https://www.training.cochrane.org/handbook [ Google Scholar ]
- Paschal, R. A. , Weinstein, T. , & WAlberg, H. J. W. (1984). The effects of homework on learning: A quantitative synthesis. The Journal of Educational Research, 78(2), 97–104. 10.1080/00220671.1984.10885581 [ DOI ] [ Google Scholar ]
- Reteig, L. C. , Brinka, R. L. , Prinssena, S. , Cohena, M. X. , & Slagter, H. A. (2019). Sustaining attention for a prolonged period of time increases temporal variability in cortical responses. Cortex, 117, 16–32. 10.1016/j.cortex.2019.02.016 [ DOI ] [ PubMed ] [ Google Scholar ]
- Rosenthal, R. (1979). The file drawer problem and tolerance for null results. Psychological Bulletin, 86(3), 638–641. 10.1037/0033-2909.86.3.638 [ DOI ] [ Google Scholar ]
- Schünemann, H. J. , Tugwell, P. , Reeves, B. C. , Akl, E. A. , Santesso, N. , Spencer, F. A. , Shea, B. , Wells, G. , & Helfand, M. (2013). Non‐randomized studies as a source of complementary, sequential or replacement evidence for randomized controlled trials in systematic reviews on the effects of interventions. Research Synthesis Methods, 4(1), 49–62. 10.1002/jrsm.1078 [ DOI ] [ PubMed ] [ Google Scholar ]
- Sterne, J. A. , Hernán, M. A. , Reeves, B. C. , Savović, J. , Berkman, N. D. , Viswanathan, M. , Henry, D. , Altman, D. G. , Ansari, M. T. , Boutron, I. , Carpenter, J. R. , Chan, A. W. , Churchill, R. , Deeks, J. J. , Hróbjartsson, A. , Kirkham, J. , Jüni, P. , Loke, Y. K. , Pigott, T. D. , … Higgins, J. P. (2016). ROBINS‐I: A tool for assessing risk of bias in non‐randomized studies of interventions. BMJ, 355, i4919. 10.1136/bmj.i4919 [ DOI ] [ PMC free article ] [ PubMed ] [ Google Scholar ]
- Tam, V. C. , & Chan, R. M. (2009). Parental involvement in primary children's homework in Hong Kong. School Community Journal, 19(2), 81–100. http://www.adi.org/journal/fw09/TamChanFall2009.pdf [ Google Scholar ]
- Trautwein, U. (2007). The homework‐achievement relation reconsidered: Differentiating homework time, homework frequency, and homework effort. Learning and Instruction, 17, 372–388. 10.1016/j.learninstruc.2007.02.009 [ DOI ] [ Google Scholar ]
- Trautwein, U. , & Lüdtke, O. (2009). Predicting homework motivation and homework effort in six school subjects: The role of person and family characteristics, classroom factors, and school track. Learning and Instruction, 19(3), 243–258. 10.1016/j.learninstruc.2008.05.001 [ DOI ] [ Google Scholar ]
- Twenge, J. M. , Zhang, L. , & Im, C. (2004). It's beyond my control: A cross‐temporal meta‐analysis of increasing externality in locus of control, 1960−2002. Personality and Social Psychology Review, 8(3), 308–319. 10.1207/s15327957pspr0803_5 [ DOI ] [ PubMed ] [ Google Scholar ]
- Van Voorhis, F. L. (2003). Interactive homework in middle school: Effects on family involvement and science achievement. Journal of Educational Research, 96, 323–338. 10.1080/00220670309596616 [ DOI ] [ Google Scholar ]
- WHO . (2016). Growing up unequal: Gender and socioeconomic differences in young people's health and well‐being . Health Behaviours in School‐Aged Children (HBSC) Study: International Report from the 2013/14 Survey (Health Policy for Children and Adolescents, No. 7).
- Xu, J. (2007). Middle‐school homework management: More than just gender and family involvement. Educational Psychology, 27(2), 173–189. 10.1080/01443410601066669 [ DOI ] [ Google Scholar ]
- Xue, H. P. , & Zhang, Y. (2019). Analysis of the academic burden level and difference of junior high school students in China: An Empirical Study Based on CEPS2015 data. Journal of Capital Normal University (Social Sciences Edition), 1(5), 147–166. [ Google Scholar ]
- Zhu, Y. (2015). Homework and mathematics learning: What can we learn from the TIMSS series studies in the last two decades? In Middleton J. A., Cai J., & Hwang S. (Eds.), Large‐scale studies in mathematics education (pp. 209–234). Springer International Publishing. [ Google Scholar ]
Associated Data
This section collects any data citations, data availability statements, or supplementary materials included in this article.
Supplementary Materials
- View on publisher site
- PDF (1.0 MB)
- Collections
Similar articles
Cited by other articles, links to ncbi databases.
- Download .nbib .nbib
- Format: AMA APA MLA NLM
Add to Collections

IMAGES
VIDEO
COMMENTS
The results showed that the correlation between homework time and performance for high-school students was 0.25, but for middle-school students, it was nearly zero. However, they did not explicitly consider homework time (Cooper et al., 2006).
Summarizing, the current investigation examined whether the amount of time spent on homework affected students’ academic achievement and investigated some variables that may moderate the relationship between homework and achievement through the meta-analysis of TIMSS data.
The question of how homework effects student achievement is an important one considering the ultimate goal as a teacher is fo r students to be successful and make an impact in the world.
Utilizing parametric and nonparametric techniques, we assess the impact of a heretofore relatively unexplored ‘input’ in the educational process, homework, on tenth grade test performance. Our results indicate that homework is an important determinant of student achievement.
Two experiments explored the effectiveness of homework moderated by homework time. In Koch (1965), the effects of long daily homework (20–30 min) and short daily homework (10–15 min) were...
The reason most cited for giving homework to students is that the practice can improve students’ retention and understanding of the covered material (Collier, 2007). Homework has been researched many times to verify that students’ achievement is directly related to completing homework.
The optimum time students should spend on homework has been widely researched although the results are far from unanimous. The main objective of this research is to analyze how homework assignment strategies in schools affect students' academic performance and the differences in students' time spent on homework.
The amount of homework completed by students seems to be more positively associated with student achievement than the amount of homework assigned by teachers. Some research has shown that students who spend more time on homework score higher on measures of achievement and attitude.
The objective of the review is to explore the impact of homework on students' academic outcomes. We will extract the homework time and academic performance provided in the primary study. The homework time is the exact time or a time frame reported by students or parents.
An increase in the amount of homework a school assigns is associated with an increase in the differences in student time spent on homework. An optimum amount of homework is proposed which schools should assign to maximize gains in achievement for students overall.