
- Table of Contents
- Random Entry
- Chronological
- Editorial Information
- About the SEP
- Editorial Board
- How to Cite the SEP
- Special Characters
- Advanced Tools
- Support the SEP
- PDFs for SEP Friends
- Make a Donation
- SEPIA for Libraries
- Entry Contents

Bibliography
Academic tools.
- Friends PDF Preview
- Author and Citation Info
- Back to Top
Ethics of Artificial Intelligence and Robotics
Artificial intelligence (AI) and robotics are digital technologies that will have significant impact on the development of humanity in the near future. They have raised fundamental questions about what we should do with these systems, what the systems themselves should do, what risks they involve, and how we can control these.
After the Introduction to the field (§1), the main themes (§2) of this article are: Ethical issues that arise with AI systems as objects , i.e., tools made and used by humans. This includes issues of privacy (§2.1) and manipulation (§2.2), opacity (§2.3) and bias (§2.4), human-robot interaction (§2.5), employment (§2.6), and the effects of autonomy (§2.7). Then AI systems as subjects , i.e., ethics for the AI systems themselves in machine ethics (§2.8) and artificial moral agency (§2.9). Finally, the problem of a possible future AI superintelligence leading to a “singularity” (§2.10). We close with a remark on the vision of AI (§3).
For each section within these themes, we provide a general explanation of the ethical issues , outline existing positions and arguments , then analyse how these play out with current technologies and finally, what policy consequences may be drawn.
1.1 Background of the Field
1.2 ai & robotics, 1.3 a note on policy, 2.1 privacy & surveillance, 2.2 manipulation of behaviour, 2.3 opacity of ai systems, 2.4 bias in decision systems, 2.5 human-robot interaction, 2.6 automation and employment, 2.7 autonomous systems, 2.8 machine ethics, 2.9 artificial moral agents, 2.10 singularity, research organizations, conferences, policy documents, other relevant pages, related entries, 1. introduction.
The ethics of AI and robotics is often focused on “concerns” of various sorts, which is a typical response to new technologies. Many such concerns turn out to be rather quaint (trains are too fast for souls); some are predictably wrong when they suggest that the technology will fundamentally change humans (telephones will destroy personal communication, writing will destroy memory, video cassettes will make going out redundant); some are broadly correct but moderately relevant (digital technology will destroy industries that make photographic film, cassette tapes, or vinyl records); but some are broadly correct and deeply relevant (cars will kill children and fundamentally change the landscape). The task of an article such as this is to analyse the issues and to deflate the non-issues.
Some technologies, like nuclear power, cars, or plastics, have caused ethical and political discussion and significant policy efforts to control the trajectory these technologies, usually only once some damage is done. In addition to such “ethical concerns”, new technologies challenge current norms and conceptual systems, which is of particular interest to philosophy. Finally, once we have understood a technology in its context, we need to shape our societal response, including regulation and law. All these features also exist in the case of new AI and Robotics technologies—plus the more fundamental fear that they may end the era of human control on Earth.
The ethics of AI and robotics has seen significant press coverage in recent years, which supports related research, but also may end up undermining it: the press often talks as if the issues under discussion were just predictions of what future technology will bring, and as though we already know what would be most ethical and how to achieve that. Press coverage thus focuses on risk, security (Brundage et al. 2018, in the Other Internet Resources section below, hereafter [OIR]), and prediction of impact (e.g., on the job market). The result is a discussion of essentially technical problems that focus on how to achieve a desired outcome. Current discussions in policy and industry are also motivated by image and public relations, where the label “ethical” is really not much more than the new “green”, perhaps used for “ethics washing”. For a problem to qualify as a problem for AI ethics would require that we do not readily know what the right thing to do is. In this sense, job loss, theft, or killing with AI is not a problem in ethics, but whether these are permissible under certain circumstances is a problem. This article focuses on the genuine problems of ethics where we do not readily know what the answers are.
A last caveat: The ethics of AI and robotics is a very young field within applied ethics, with significant dynamics, but few well-established issues and no authoritative overviews—though there is a promising outline (European Group on Ethics in Science and New Technologies 2018) and there are beginnings on societal impact (Floridi et al. 2018; Taddeo and Floridi 2018; S. Taylor et al. 2018; Walsh 2018; Bryson 2019; Gibert 2019; Whittlestone et al. 2019), and policy recommendations (AI HLEG 2019 [OIR]; IEEE 2019). So this article cannot merely reproduce what the community has achieved thus far, but must propose an ordering where little order exists.
The notion of “artificial intelligence” (AI) is understood broadly as any kind of artificial computational system that shows intelligent behaviour, i.e., complex behaviour that is conducive to reaching goals. In particular, we do not wish to restrict “intelligence” to what would require intelligence if done by humans , as Minsky had suggested (1985). This means we incorporate a range of machines, including those in “technical AI”, that show only limited abilities in learning or reasoning but excel at the automation of particular tasks, as well as machines in “general AI” that aim to create a generally intelligent agent.
AI somehow gets closer to our skin than other technologies—thus the field of “philosophy of AI”. Perhaps this is because the project of AI is to create machines that have a feature central to how we humans see ourselves, namely as feeling, thinking, intelligent beings. The main purposes of an artificially intelligent agent probably involve sensing, modelling, planning and action, but current AI applications also include perception, text analysis, natural language processing (NLP), logical reasoning, game-playing, decision support systems, data analytics, predictive analytics, as well as autonomous vehicles and other forms of robotics (P. Stone et al. 2016). AI may involve any number of computational techniques to achieve these aims, be that classical symbol-manipulating AI, inspired by natural cognition, or machine learning via neural networks (Goodfellow, Bengio, and Courville 2016; Silver et al. 2018).
Historically, it is worth noting that the term “AI” was used as above ca. 1950–1975, then came into disrepute during the “AI winter”, ca. 1975–1995, and narrowed. As a result, areas such as “machine learning”, “natural language processing” and “data science” were often not labelled as “AI”. Since ca. 2010, the use has broadened again, and at times almost all of computer science and even high-tech is lumped under “AI”. Now it is a name to be proud of, a booming industry with massive capital investment (Shoham et al. 2018), and on the edge of hype again. As Erik Brynjolfsson noted, it may allow us to
virtually eliminate global poverty, massively reduce disease and provide better education to almost everyone on the planet. (quoted in Anderson, Rainie, and Luchsinger 2018)
While AI can be entirely software, robots are physical machines that move. Robots are subject to physical impact, typically through “sensors”, and they exert physical force onto the world, typically through “actuators”, like a gripper or a turning wheel. Accordingly, autonomous cars or planes are robots, and only a minuscule portion of robots is “humanoid” (human-shaped), like in the movies. Some robots use AI, and some do not: Typical industrial robots blindly follow completely defined scripts with minimal sensory input and no learning or reasoning (around 500,000 such new industrial robots are installed each year (IFR 2019 [OIR])). It is probably fair to say that while robotics systems cause more concerns in the general public, AI systems are more likely to have a greater impact on humanity. Also, AI or robotics systems for a narrow set of tasks are less likely to cause new issues than systems that are more flexible and autonomous.
Robotics and AI can thus be seen as covering two overlapping sets of systems: systems that are only AI, systems that are only robotics, and systems that are both. We are interested in all three; the scope of this article is thus not only the intersection, but the union, of both sets.
Policy is only one of the concerns of this article. There is significant public discussion about AI ethics, and there are frequent pronouncements from politicians that the matter requires new policy, which is easier said than done: Actual technology policy is difficult to plan and enforce. It can take many forms, from incentives and funding, infrastructure, taxation, or good-will statements, to regulation by various actors, and the law. Policy for AI will possibly come into conflict with other aims of technology policy or general policy. Governments, parliaments, associations, and industry circles in industrialised countries have produced reports and white papers in recent years, and some have generated good-will slogans (“trusted/responsible/humane/human-centred/good/beneficial AI”), but is that what is needed? For a survey, see Jobin, Ienca, and Vayena (2019) and V. Müller’s list of PT-AI Policy Documents and Institutions .
For people who work in ethics and policy, there might be a tendency to overestimate the impact and threats from a new technology, and to underestimate how far current regulation can reach (e.g., for product liability). On the other hand, there is a tendency for businesses, the military, and some public administrations to “just talk” and do some “ethics washing” in order to preserve a good public image and continue as before. Actually implementing legally binding regulation would challenge existing business models and practices. Actual policy is not just an implementation of ethical theory, but subject to societal power structures—and the agents that do have the power will push against anything that restricts them. There is thus a significant risk that regulation will remain toothless in the face of economical and political power.
Though very little actual policy has been produced, there are some notable beginnings: The latest EU policy document suggests “trustworthy AI” should be lawful, ethical, and technically robust, and then spells this out as seven requirements: human oversight, technical robustness, privacy and data governance, transparency, fairness, well-being, and accountability (AI HLEG 2019 [OIR]). Much European research now runs under the slogan of “responsible research and innovation” (RRI), and “technology assessment” has been a standard field since the advent of nuclear power. Professional ethics is also a standard field in information technology, and this includes issues that are relevant in this article. Perhaps a “code of ethics” for AI engineers, analogous to the codes of ethics for medical doctors, is an option here (Véliz 2019). What data science itself should do is addressed in (L. Taylor and Purtova 2019). We also expect that much policy will eventually cover specific uses or technologies of AI and robotics, rather than the field as a whole. A useful summary of an ethical framework for AI is given in (European Group on Ethics in Science and New Technologies 2018: 13ff). On general AI policy, see Calo (2018) as well as Crawford and Calo (2016); Stahl, Timmermans, and Mittelstadt (2016); Johnson and Verdicchio (2017); and Giubilini and Savulescu (2018). A more political angle of technology is often discussed in the field of “Science and Technology Studies” (STS). As books like The Ethics of Invention (Jasanoff 2016) show, concerns in STS are often quite similar to those in ethics (Jacobs et al. 2019 [OIR]). In this article, we discuss the policy for each type of issue separately rather than for AI or robotics in general.
2. Main Debates
In this section we outline the ethical issues of human use of AI and robotics systems that can be more or less autonomous—which means we look at issues that arise with certain uses of the technologies which would not arise with others. It must be kept in mind, however, that technologies will always cause some uses to be easier, and thus more frequent, and hinder other uses. The design of technical artefacts thus has ethical relevance for their use (Houkes and Vermaas 2010; Verbeek 2011), so beyond “responsible use”, we also need “responsible design” in this field. The focus on use does not presuppose which ethical approaches are best suited for tackling these issues; they might well be virtue ethics (Vallor 2017) rather than consequentialist or value-based (Floridi et al. 2018). This section is also neutral with respect to the question whether AI systems truly have “intelligence” or other mental properties: It would apply equally well if AI and robotics are merely seen as the current face of automation (cf. Müller forthcoming-b).
There is a general discussion about privacy and surveillance in information technology (e.g., Macnish 2017; Roessler 2017), which mainly concerns the access to private data and data that is personally identifiable. Privacy has several well recognised aspects, e.g., “the right to be let alone”, information privacy, privacy as an aspect of personhood, control over information about oneself, and the right to secrecy (Bennett and Raab 2006). Privacy studies have historically focused on state surveillance by secret services but now include surveillance by other state agents, businesses, and even individuals. The technology has changed significantly in the last decades while regulation has been slow to respond (though there is the Regulation (EU) 2016/679)—the result is a certain anarchy that is exploited by the most powerful players, sometimes in plain sight, sometimes in hiding.
The digital sphere has widened greatly: All data collection and storage is now digital, our lives are increasingly digital, most digital data is connected to a single Internet, and there is more and more sensor technology in use that generates data about non-digital aspects of our lives. AI increases both the possibilities of intelligent data collection and the possibilities for data analysis. This applies to blanket surveillance of whole populations as well as to classic targeted surveillance. In addition, much of the data is traded between agents, usually for a fee.
At the same time, controlling who collects which data, and who has access, is much harder in the digital world than it was in the analogue world of paper and telephone calls. Many new AI technologies amplify the known issues. For example, face recognition in photos and videos allows identification and thus profiling and searching for individuals (Whittaker et al. 2018: 15ff). This continues using other techniques for identification, e.g., “device fingerprinting”, which are commonplace on the Internet (sometimes revealed in the “privacy policy”). The result is that “In this vast ocean of data, there is a frighteningly complete picture of us” (Smolan 2016: 1:01). The result is arguably a scandal that still has not received due public attention.
The data trail we leave behind is how our “free” services are paid for—but we are not told about that data collection and the value of this new raw material, and we are manipulated into leaving ever more such data. For the “big 5” companies (Amazon, Google/Alphabet, Microsoft, Apple, Facebook), the main data-collection part of their business appears to be based on deception, exploiting human weaknesses, furthering procrastination, generating addiction, and manipulation (Harris 2016 [OIR]). The primary focus of social media, gaming, and most of the Internet in this “surveillance economy” is to gain, maintain, and direct attention—and thus data supply. “Surveillance is the business model of the Internet” (Schneier 2015). This surveillance and attention economy is sometimes called “surveillance capitalism” (Zuboff 2019). It has caused many attempts to escape from the grasp of these corporations, e.g., in exercises of “minimalism” (Newport 2019), sometimes through the open source movement, but it appears that present-day citizens have lost the degree of autonomy needed to escape while fully continuing with their life and work. We have lost ownership of our data, if “ownership” is the right relation here. Arguably, we have lost control of our data.
These systems will often reveal facts about us that we ourselves wish to suppress or are not aware of: they know more about us than we know ourselves. Even just observing online behaviour allows insights into our mental states (Burr and Christianini 2019) and manipulation (see below section 2.2 ). This has led to calls for the protection of “derived data” (Wachter and Mittelstadt 2019). With the last sentence of his bestselling book, Homo Deus , Harari asks about the long-term consequences of AI:
What will happen to society, politics and daily life when non-conscious but highly intelligent algorithms know us better than we know ourselves? (2016: 462)
Robotic devices have not yet played a major role in this area, except for security patrolling, but this will change once they are more common outside of industry environments. Together with the “Internet of things”, the so-called “smart” systems (phone, TV, oven, lamp, virtual assistant, home,…), “smart city” (Sennett 2018), and “smart governance”, they are set to become part of the data-gathering machinery that offers more detailed data, of different types, in real time, with ever more information.
Privacy-preserving techniques that can largely conceal the identity of persons or groups are now a standard staple in data science; they include (relative) anonymisation , access control (plus encryption), and other models where computation is carried out with fully or partially encrypted input data (Stahl and Wright 2018); in the case of “differential privacy”, this is done by adding calibrated noise to encrypt the output of queries (Dwork et al. 2006; Abowd 2017). While requiring more effort and cost, such techniques can avoid many of the privacy issues. Some companies have also seen better privacy as a competitive advantage that can be leveraged and sold at a price.
One of the major practical difficulties is to actually enforce regulation, both on the level of the state and on the level of the individual who has a claim. They must identify the responsible legal entity, prove the action, perhaps prove intent, find a court that declares itself competent … and eventually get the court to actually enforce its decision. Well-established legal protection of rights such as consumer rights, product liability, and other civil liability or protection of intellectual property rights is often missing in digital products, or hard to enforce. This means that companies with a “digital” background are used to testing their products on the consumers without fear of liability while heavily defending their intellectual property rights. This “Internet Libertarianism” is sometimes taken to assume that technical solutions will take care of societal problems by themselves (Mozorov 2013).
The ethical issues of AI in surveillance go beyond the mere accumulation of data and direction of attention: They include the use of information to manipulate behaviour, online and offline, in a way that undermines autonomous rational choice. Of course, efforts to manipulate behaviour are ancient, but they may gain a new quality when they use AI systems. Given users’ intense interaction with data systems and the deep knowledge about individuals this provides, they are vulnerable to “nudges”, manipulation, and deception. With sufficient prior data, algorithms can be used to target individuals or small groups with just the kind of input that is likely to influence these particular individuals. A ’nudge‘ changes the environment such that it influences behaviour in a predictable way that is positive for the individual, but easy and cheap to avoid (Thaler & Sunstein 2008). There is a slippery slope from here to paternalism and manipulation.
Many advertisers, marketers, and online sellers will use any legal means at their disposal to maximise profit, including exploitation of behavioural biases, deception, and addiction generation (Costa and Halpern 2019 [OIR]). Such manipulation is the business model in much of the gambling and gaming industries, but it is spreading, e.g., to low-cost airlines. In interface design on web pages or in games, this manipulation uses what is called “dark patterns” (Mathur et al. 2019). At this moment, gambling and the sale of addictive substances are highly regulated, but online manipulation and addiction are not—even though manipulation of online behaviour is becoming a core business model of the Internet.
Furthermore, social media is now the prime location for political propaganda. This influence can be used to steer voting behaviour, as in the Facebook-Cambridge Analytica “scandal” (Woolley and Howard 2017; Bradshaw, Neudert, and Howard 2019) and—if successful—it may harm the autonomy of individuals (Susser, Roessler, and Nissenbaum 2019).
Improved AI “faking” technologies make what once was reliable evidence into unreliable evidence—this has already happened to digital photos, sound recordings, and video. It will soon be quite easy to create (rather than alter) “deep fake” text, photos, and video material with any desired content. Soon, sophisticated real-time interaction with persons over text, phone, or video will be faked, too. So we cannot trust digital interactions while we are at the same time increasingly dependent on such interactions.
One more specific issue is that machine learning techniques in AI rely on training with vast amounts of data. This means there will often be a trade-off between privacy and rights to data vs. technical quality of the product. This influences the consequentialist evaluation of privacy-violating practices.
The policy in this field has its ups and downs: Civil liberties and the protection of individual rights are under intense pressure from businesses’ lobbying, secret services, and other state agencies that depend on surveillance. Privacy protection has diminished massively compared to the pre-digital age when communication was based on letters, analogue telephone communications, and personal conversation and when surveillance operated under significant legal constraints.
While the EU General Data Protection Regulation (Regulation (EU) 2016/679) has strengthened privacy protection, the US and China prefer growth with less regulation (Thompson and Bremmer 2018), likely in the hope that this provides a competitive advantage. It is clear that state and business actors have increased their ability to invade privacy and manipulate people with the help of AI technology and will continue to do so to further their particular interests—unless reined in by policy in the interest of general society.
Opacity and bias are central issues in what is now sometimes called “data ethics” or “big data ethics” (Floridi and Taddeo 2016; Mittelstadt and Floridi 2016). AI systems for automated decision support and “predictive analytics” raise “significant concerns about lack of due process, accountability, community engagement, and auditing” (Whittaker et al. 2018: 18ff). They are part of a power structure in which “we are creating decision-making processes that constrain and limit opportunities for human participation” (Danaher 2016b: 245). At the same time, it will often be impossible for the affected person to know how the system came to this output, i.e., the system is “opaque” to that person. If the system involves machine learning, it will typically be opaque even to the expert, who will not know how a particular pattern was identified, or even what the pattern is. Bias in decision systems and data sets is exacerbated by this opacity. So, at least in cases where there is a desire to remove bias, the analysis of opacity and bias go hand in hand, and political response has to tackle both issues together.
Many AI systems rely on machine learning techniques in (simulated) neural networks that will extract patterns from a given dataset, with or without “correct” solutions provided; i.e., supervised, semi-supervised or unsupervised. With these techniques, the “learning” captures patterns in the data and these are labelled in a way that appears useful to the decision the system makes, while the programmer does not really know which patterns in the data the system has used. In fact, the programs are evolving, so when new data comes in, or new feedback is given (“this was correct”, “this was incorrect”), the patterns used by the learning system change. What this means is that the outcome is not transparent to the user or programmers: it is opaque. Furthermore, the quality of the program depends heavily on the quality of the data provided, following the old slogan “garbage in, garbage out”. So, if the data already involved a bias (e.g., police data about the skin colour of suspects), then the program will reproduce that bias. There are proposals for a standard description of datasets in a “datasheet” that would make the identification of such bias more feasible (Gebru et al. 2018 [OIR]). There is also significant recent literature about the limitations of machine learning systems that are essentially sophisticated data filters (Marcus 2018 [OIR]). Some have argued that the ethical problems of today are the result of technical “shortcuts” AI has taken (Cristianini forthcoming).
There are several technical activities that aim at “explainable AI”, starting with (Van Lent, Fisher, and Mancuso 1999; Lomas et al. 2012) and, more recently, a DARPA programme (Gunning 2017 [OIR]). More broadly, the demand for
a mechanism for elucidating and articulating the power structures, biases, and influences that computational artefacts exercise in society (Diakopoulos 2015: 398)
is sometimes called “algorithmic accountability reporting”. This does not mean that we expect an AI to “explain its reasoning”—doing so would require far more serious moral autonomy than we currently attribute to AI systems (see below §2.10 ).
The politician Henry Kissinger pointed out that there is a fundamental problem for democratic decision-making if we rely on a system that is supposedly superior to humans, but cannot explain its decisions. He says we may have “generated a potentially dominating technology in search of a guiding philosophy” (Kissinger 2018). Danaher (2016b) calls this problem “the threat of algocracy” (adopting the previous use of ‘algocracy’ from Aneesh 2002 [OIR], 2006). In a similar vein, Cave (2019) stresses that we need a broader societal move towards more “democratic” decision-making to avoid AI being a force that leads to a Kafka-style impenetrable suppression system in public administration and elsewhere. The political angle of this discussion has been stressed by O’Neil in her influential book Weapons of Math Destruction (2016), and by Yeung and Lodge (2019).
In the EU, some of these issues have been taken into account with the (Regulation (EU) 2016/679), which foresees that consumers, when faced with a decision based on data processing, will have a legal “right to explanation”—how far this goes and to what extent it can be enforced is disputed (Goodman and Flaxman 2017; Wachter, Mittelstadt, and Floridi 2016; Wachter, Mittelstadt, and Russell 2017). Zerilli et al. (2019) argue that there may be a double standard here, where we demand a high level of explanation for machine-based decisions despite humans sometimes not reaching that standard themselves.
Automated AI decision support systems and “predictive analytics” operate on data and produce a decision as “output”. This output may range from the relatively trivial to the highly significant: “this restaurant matches your preferences”, “the patient in this X-ray has completed bone growth”, “application to credit card declined”, “donor organ will be given to another patient”, “bail is denied”, or “target identified and engaged”. Data analysis is often used in “predictive analytics” in business, healthcare, and other fields, to foresee future developments—since prediction is easier, it will also become a cheaper commodity. One use of prediction is in “predictive policing” (NIJ 2014 [OIR]), which many fear might lead to an erosion of public liberties (Ferguson 2017) because it can take away power from the people whose behaviour is predicted. It appears, however, that many of the worries about policing depend on futuristic scenarios where law enforcement foresees and punishes planned actions, rather than waiting until a crime has been committed (like in the 2002 film “Minority Report”). One concern is that these systems might perpetuate bias that was already in the data used to set up the system, e.g., by increasing police patrols in an area and discovering more crime in that area. Actual “predictive policing” or “intelligence led policing” techniques mainly concern the question of where and when police forces will be needed most. Also, police officers can be provided with more data, offering them more control and facilitating better decisions, in workflow support software (e.g., “ArcGIS”). Whether this is problematic depends on the appropriate level of trust in the technical quality of these systems, and on the evaluation of aims of the police work itself. Perhaps a recent paper title points in the right direction here: “AI ethics in predictive policing: From models of threat to an ethics of care” (Asaro 2019).
Bias typically surfaces when unfair judgments are made because the individual making the judgment is influenced by a characteristic that is actually irrelevant to the matter at hand, typically a discriminatory preconception about members of a group. So, one form of bias is a learned cognitive feature of a person, often not made explicit. The person concerned may not be aware of having that bias—they may even be honestly and explicitly opposed to a bias they are found to have (e.g., through priming, cf. Graham and Lowery 2004). On fairness vs. bias in machine learning, see Binns (2018).
Apart from the social phenomenon of learned bias, the human cognitive system is generally prone to have various kinds of “cognitive biases”, e.g., the “confirmation bias”: humans tend to interpret information as confirming what they already believe. This second form of bias is often said to impede performance in rational judgment (Kahnemann 2011)—though at least some cognitive biases generate an evolutionary advantage, e.g., economical use of resources for intuitive judgment. There is a question whether AI systems could or should have such cognitive bias.
A third form of bias is present in data when it exhibits systematic error, e.g., “statistical bias”. Strictly, any given dataset will only be unbiased for a single kind of issue, so the mere creation of a dataset involves the danger that it may be used for a different kind of issue, and then turn out to be biased for that kind. Machine learning on the basis of such data would then not only fail to recognise the bias, but codify and automate the “historical bias”. Such historical bias was discovered in an automated recruitment screening system at Amazon (discontinued early 2017) that discriminated against women—presumably because the company had a history of discriminating against women in the hiring process. The “Correctional Offender Management Profiling for Alternative Sanctions” (COMPAS), a system to predict whether a defendant would re-offend, was found to be as successful (65.2% accuracy) as a group of random humans (Dressel and Farid 2018) and to produce more false positives and less false negatives for black defendants. The problem with such systems is thus bias plus humans placing excessive trust in the systems. The political dimensions of such automated systems in the USA are investigated in Eubanks (2018).
There are significant technical efforts to detect and remove bias from AI systems, but it is fair to say that these are in early stages: see UK Institute for Ethical AI & Machine Learning (Brownsword, Scotford, and Yeung 2017; Yeung and Lodge 2019). It appears that technological fixes have their limits in that they need a mathematical notion of fairness, which is hard to come by (Whittaker et al. 2018: 24ff; Selbst et al. 2019), as is a formal notion of “race” (see Benthall and Haynes 2019). An institutional proposal is in (Veale and Binns 2017).
Human-robot interaction (HRI) is an academic fields in its own right, which now pays significant attention to ethical matters, the dynamics of perception from both sides, and both the different interests present in and the intricacy of the social context, including co-working (e.g., Arnold and Scheutz 2017). Useful surveys for the ethics of robotics include Calo, Froomkin, and Kerr (2016); Royakkers and van Est (2016); Tzafestas (2016); a standard collection of papers is Lin, Abney, and Jenkins (2017).
While AI can be used to manipulate humans into believing and doing things (see section 2.2 ), it can also be used to drive robots that are problematic if their processes or appearance involve deception, threaten human dignity, or violate the Kantian requirement of “respect for humanity”. Humans very easily attribute mental properties to objects, and empathise with them, especially when the outer appearance of these objects is similar to that of living beings. This can be used to deceive humans (or animals) into attributing more intellectual or even emotional significance to robots or AI systems than they deserve. Some parts of humanoid robotics are problematic in this regard (e.g., Hiroshi Ishiguro’s remote-controlled Geminoids), and there are cases that have been clearly deceptive for public-relations purposes (e.g. on the abilities of Hanson Robotics’ “Sophia”). Of course, some fairly basic constraints of business ethics and law apply to robots, too: product safety and liability, or non-deception in advertisement. It appears that these existing constraints take care of many concerns that are raised. There are cases, however, where human-human interaction has aspects that appear specifically human in ways that can perhaps not be replaced by robots: care, love, and sex.
2.5.1 Example (a) Care Robots
The use of robots in health care for humans is currently at the level of concept studies in real environments, but it may become a usable technology in a few years, and has raised a number of concerns for a dystopian future of de-humanised care (A. Sharkey and N. Sharkey 2011; Robert Sparrow 2016). Current systems include robots that support human carers/caregivers (e.g., in lifting patients, or transporting material), robots that enable patients to do certain things by themselves (e.g., eat with a robotic arm), but also robots that are given to patients as company and comfort (e.g., the “Paro” robot seal). For an overview, see van Wynsberghe (2016); Nørskov (2017); Fosch-Villaronga and Albo-Canals (2019), for a survey of users Draper et al. (2014).
One reason why the issue of care has come to the fore is that people have argued that we will need robots in ageing societies. This argument makes problematic assumptions, namely that with longer lifespan people will need more care, and that it will not be possible to attract more humans to caring professions. It may also show a bias about age (Jecker forthcoming). Most importantly, it ignores the nature of automation, which is not simply about replacing humans, but about allowing humans to work more efficiently. It is not very clear that there really is an issue here since the discussion mostly focuses on the fear of robots de-humanising care, but the actual and foreseeable robots in care are assistive robots for classic automation of technical tasks. They are thus “care robots” only in a behavioural sense of performing tasks in care environments, not in the sense that a human “cares” for the patients. It appears that the success of “being cared for” relies on this intentional sense of “care”, which foreseeable robots cannot provide. If anything, the risk of robots in care is the absence of such intentional care—because less human carers may be needed. Interestingly, caring for something, even a virtual agent, can be good for the carer themselves (Lee et al. 2019). A system that pretends to care would be deceptive and thus problematic—unless the deception is countered by sufficiently large utility gain (Coeckelbergh 2016). Some robots that pretend to “care” on a basic level are available (Paro seal) and others are in the making. Perhaps feeling cared for by a machine, to some extent, is progress for come patients.
2.5.2 Example (b) Sex Robots
It has been argued by several tech optimists that humans will likely be interested in sex and companionship with robots and be comfortable with the idea (Levy 2007). Given the variation of human sexual preferences, including sex toys and sex dolls, this seems very likely: The question is whether such devices should be manufactured and promoted, and whether there should be limits in this touchy area. It seems to have moved into the mainstream of “robot philosophy” in recent times (Sullins 2012; Danaher and McArthur 2017; N. Sharkey et al. 2017 [OIR]; Bendel 2018; Devlin 2018).
Humans have long had deep emotional attachments to objects, so perhaps companionship or even love with a predictable android is attractive, especially to people who struggle with actual humans, and already prefer dogs, cats, birds, a computer or a tamagotchi . Danaher (2019b) argues against (Nyholm and Frank 2017) that these can be true friendships, and is thus a valuable goal. It certainly looks like such friendship might increase overall utility, even if lacking in depth. In these discussions there is an issue of deception, since a robot cannot (at present) mean what it says, or have feelings for a human. It is well known that humans are prone to attribute feelings and thoughts to entities that behave as if they had sentience,even to clearly inanimate objects that show no behaviour at all. Also, paying for deception seems to be an elementary part of the traditional sex industry.
Finally, there are concerns that have often accompanied matters of sex, namely consent (Frank and Nyholm 2017), aesthetic concerns, and the worry that humans may be “corrupted” by certain experiences. Old fashioned though this may seem, human behaviour is influenced by experience, and it is likely that pornography or sex robots support the perception of other humans as mere objects of desire, or even recipients of abuse, and thus ruin a deeper sexual and erotic experience. In this vein, the “Campaign Against Sex Robots” argues that these devices are a continuation of slavery and prostitution (Richardson 2016).
It seems clear that AI and robotics will lead to significant gains in productivity and thus overall wealth. The attempt to increase productivity has often been a feature of the economy, though the emphasis on “growth” is a modern phenomenon (Harari 2016: 240). However, productivity gains through automation typically mean that fewer humans are required for the same output. This does not necessarily imply a loss of overall employment, however, because available wealth increases and that can increase demand sufficiently to counteract the productivity gain. In the long run, higher productivity in industrial societies has led to more wealth overall. Major labour market disruptions have occurred in the past, e.g., farming employed over 60% of the workforce in Europe and North-America in 1800, while by 2010 it employed ca. 5% in the EU, and even less in the wealthiest countries (European Commission 2013). In the 20 years between 1950 and 1970 the number of hired agricultural workers in the UK was reduced by 50% (Zayed and Loft 2019). Some of these disruptions lead to more labour-intensive industries moving to places with lower labour cost. This is an ongoing process.
Classic automation replaced human muscle, whereas digital automation replaces human thought or information-processing—and unlike physical machines, digital automation is very cheap to duplicate (Bostrom and Yudkowsky 2014). It may thus mean a more radical change on the labour market. So, the main question is: will the effects be different this time? Will the creation of new jobs and wealth keep up with the destruction of jobs? And even if it is not different, what are the transition costs, and who bears them? Do we need to make societal adjustments for a fair distribution of costs and benefits of digital automation?
Responses to the issue of unemployment from AI have ranged from the alarmed (Frey and Osborne 2013; Westlake 2014) to the neutral (Metcalf, Keller, and Boyd 2016 [OIR]; Calo 2018; Frey 2019) to the optimistic (Brynjolfsson and McAfee 2016; Harari 2016; Danaher 2019a). In principle, the labour market effect of automation seems to be fairly well understood as involving two channels:
(i) the nature of interactions between differently skilled workers and new technologies affecting labour demand and (ii) the equilibrium effects of technological progress through consequent changes in labour supply and product markets. (Goos 2018: 362)
What currently seems to happen in the labour market as a result of AI and robotics automation is “job polarisation” or the “dumbbell” shape (Goos, Manning, and Salomons 2009): The highly skilled technical jobs are in demand and highly paid, the low skilled service jobs are in demand and badly paid, but the mid-qualification jobs in factories and offices, i.e., the majority of jobs, are under pressure and reduced because they are relatively predictable, and most likely to be automated (Baldwin 2019).
Perhaps enormous productivity gains will allow the “age of leisure” to be realised, something (Keynes 1930) had predicted to occur around 2030, assuming a growth rate of 1% per annum. Actually, we have already reached the level he anticipated for 2030, but we are still working—consuming more and inventing ever more levels of organisation. Harari explains how this economic development allowed humanity to overcome hunger, disease, and war—and now we aim for immortality and eternal bliss through AI, thus his title Homo Deus (Harari 2016: 75).
In general terms, the issue of unemployment is an issue of how goods in a society should be justly distributed. A standard view is that distributive justice should be rationally decided from behind a “veil of ignorance” (Rawls 1971), i.e., as if one does not know what position in a society one would actually be taking (labourer or industrialist, etc.). Rawls thought the chosen principles would then support basic liberties and a distribution that is of greatest benefit to the least-advantaged members of society. It would appear that the AI economy has three features that make such justice unlikely: First, it operates in a largely unregulated environment where responsibility is often hard to allocate. Second, it operates in markets that have a “winner takes all” feature where monopolies develop quickly. Third, the “new economy” of the digital service industries is based on intangible assets, also called “capitalism without capital” (Haskel and Westlake 2017). This means that it is difficult to control multinational digital corporations that do not rely on a physical plant in a particular location. These three features seem to suggest that if we leave the distribution of wealth to free market forces, the result would be a heavily unjust distribution: And this is indeed a development that we can already see.
One interesting question that has not received too much attention is whether the development of AI is environmentally sustainable: Like all computing systems, AI systems produce waste that is very hard to recycle and they consume vast amounts of energy, especially for the training of machine learning systems (and even for the “mining” of cryptocurrency). Again, it appears that some actors in this space offload such costs to the general society.
There are several notions of autonomy in the discussion of autonomous systems. A stronger notion is involved in philosophical debates where autonomy is the basis for responsibility and personhood (Christman 2003 [2018]). In this context, responsibility implies autonomy, but not inversely, so there can be systems that have degrees of technical autonomy without raising issues of responsibility. The weaker, more technical, notion of autonomy in robotics is relative and gradual: A system is said to be autonomous with respect to human control to a certain degree (Müller 2012). There is a parallel here to the issues of bias and opacity in AI since autonomy also concerns a power-relation: who is in control, and who is responsible?
Generally speaking, one question is the degree to which autonomous robots raise issues our present conceptual schemes must adapt to, or whether they just require technical adjustments. In most jurisdictions, there is a sophisticated system of civil and criminal liability to resolve such issues. Technical standards, e.g., for the safe use of machinery in medical environments, will likely need to be adjusted. There is already a field of “verifiable AI” for such safety-critical systems and for “security applications”. Bodies like the IEEE (The Institute of Electrical and Electronics Engineers) and the BSI (British Standards Institution) have produced “standards”, particularly on more technical sub-problems, such as data security and transparency. Among the many autonomous systems on land, on water, under water, in air or space, we discuss two samples: autonomous vehicles and autonomous weapons.
2.7.1 Example (a) Autonomous Vehicles
Autonomous vehicles hold the promise to reduce the very significant damage that human driving currently causes—approximately 1 million humans being killed per year, many more injured, the environment polluted, earth sealed with concrete and tarmac, cities full of parked cars, etc. However, there seem to be questions on how autonomous vehicles should behave, and how responsibility and risk should be distributed in the complicated system the vehicles operates in. (There is also significant disagreement over how long the development of fully autonomous, or “level 5” cars (SAE International 2018) will actually take.)
There is some discussion of “trolley problems” in this context. In the classic “trolley problems” (Thomson 1976; Woollard and Howard-Snyder 2016: section 2) various dilemmas are presented. The simplest version is that of a trolley train on a track that is heading towards five people and will kill them, unless the train is diverted onto a side track, but on that track there is one person, who will be killed if the train takes that side track. The example goes back to a remark in (Foot 1967: 6), who discusses a number of dilemma cases where tolerated and intended consequences of an action differ. “Trolley problems” are not supposed to describe actual ethical problems or to be solved with a “right” choice. Rather, they are thought-experiments where choice is artificially constrained to a small finite number of distinct one-off options and where the agent has perfect knowledge. These problems are used as a theoretical tool to investigate ethical intuitions and theories—especially the difference between actively doing vs. allowing something to happen, intended vs. tolerated consequences, and consequentialist vs. other normative approaches (Kamm 2016). This type of problem has reminded many of the problems encountered in actual driving and in autonomous driving (Lin 2016). It is doubtful, however, that an actual driver or autonomous car will ever have to solve trolley problems (but see Keeling 2020). While autonomous car trolley problems have received a lot of media attention (Awad et al. 2018), they do not seem to offer anything new to either ethical theory or to the programming of autonomous vehicles.
The more common ethical problems in driving, such as speeding, risky overtaking, not keeping a safe distance, etc. are classic problems of pursuing personal interest vs. the common good. The vast majority of these are covered by legal regulations on driving. Programming the car to drive “by the rules” rather than “by the interest of the passengers” or “to achieve maximum utility” is thus deflated to a standard problem of programming ethical machines (see section 2.9 ). There are probably additional discretionary rules of politeness and interesting questions on when to break the rules (Lin 2016), but again this seems to be more a case of applying standard considerations (rules vs. utility) to the case of autonomous vehicles.
Notable policy efforts in this field include the report (German Federal Ministry of Transport and Digital Infrastructure 2017), which stresses that safety is the primary objective. Rule 10 states
In the case of automated and connected driving systems, the accountability that was previously the sole preserve of the individual shifts from the motorist to the manufacturers and operators of the technological systems and to the bodies responsible for taking infrastructure, policy and legal decisions.
(See section 2.10.1 below). The resulting German and EU laws on licensing automated driving are much more restrictive than their US counterparts where “testing on consumers” is a strategy used by some companies—without informed consent of the consumers or their possible victims.
2.7.2 Example (b) Autonomous Weapons
The notion of automated weapons is fairly old:
For example, instead of fielding simple guided missiles or remotely piloted vehicles, we might launch completely autonomous land, sea, and air vehicles capable of complex, far-ranging reconnaissance and attack missions. (DARPA 1983: 1)
This proposal was ridiculed as “fantasy” at the time (Dreyfus, Dreyfus, and Athanasiou 1986: ix), but it is now a reality, at least for more easily identifiable targets (missiles, planes, ships, tanks, etc.), but not for human combatants. The main arguments against (lethal) autonomous weapon systems (AWS or LAWS), are that they support extrajudicial killings, take responsibility away from humans, and make wars or killings more likely—for a detailed list of issues see Lin, Bekey, and Abney (2008: 73–86).
It appears that lowering the hurdle to use such systems (autonomous vehicles, “fire-and-forget” missiles, or drones loaded with explosives) and reducing the probability of being held accountable would increase the probability of their use. The crucial asymmetry where one side can kill with impunity, and thus has few reasons not to do so, already exists in conventional drone wars with remote controlled weapons (e.g., US in Pakistan). It is easy to imagine a small drone that searches, identifies, and kills an individual human—or perhaps a type of human. These are the kinds of cases brought forward by the Campaign to Stop Killer Robots and other activist groups. Some seem to be equivalent to saying that autonomous weapons are indeed weapons …, and weapons kill, but we still make them in gigantic numbers. On the matter of accountability, autonomous weapons might make identification and prosecution of the responsible agents more difficult—but this is not clear, given the digital records that one can keep, at least in a conventional war. The difficulty of allocating punishment is sometimes called the “retribution gap” (Danaher 2016a).
Another question is whether using autonomous weapons in war would make wars worse, or make wars less bad. If robots reduce war crimes and crimes in war, the answer may well be positive and has been used as an argument in favour of these weapons (Arkin 2009; Müller 2016a) but also as an argument against them (Amoroso and Tamburrini 2018). Arguably the main threat is not the use of such weapons in conventional warfare, but in asymmetric conflicts or by non-state agents, including criminals.
It has also been said that autonomous weapons cannot conform to International Humanitarian Law, which requires observance of the principles of distinction (between combatants and civilians), proportionality (of force), and military necessity (of force) in military conflict (A. Sharkey 2019). It is true that the distinction between combatants and non-combatants is hard, but the distinction between civilian and military ships is easy—so all this says is that we should not construct and use such weapons if they do violate Humanitarian Law. Additional concerns have been raised that being killed by an autonomous weapon threatens human dignity, but even the defenders of a ban on these weapons seem to say that these are not good arguments:
There are other weapons, and other technologies, that also compromise human dignity. Given this, and the ambiguities inherent in the concept, it is wiser to draw on several types of objections in arguments against AWS, and not to rely exclusively on human dignity. (A. Sharkey 2019)
A lot has been made of keeping humans “in the loop” or “on the loop” in the military guidance on weapons—these ways of spelling out “meaningful control” are discussed in (Santoni de Sio and van den Hoven 2018). There have been discussions about the difficulties of allocating responsibility for the killings of an autonomous weapon, and a “responsibility gap” has been suggested (esp. Rob Sparrow 2007), meaning that neither the human nor the machine may be responsible. On the other hand, we do not assume that for every event there is someone responsible for that event, and the real issue may well be the distribution of risk (Simpson and Müller 2016). Risk analysis (Hansson 2013) indicates it is crucial to identify who is exposed to risk, who is a potential beneficiary , and who makes the decisions (Hansson 2018: 1822–1824).
Machine ethics is ethics for machines, for “ethical machines”, for machines as subjects , rather than for the human use of machines as objects. It is often not very clear whether this is supposed to cover all of AI ethics or to be a part of it (Floridi and Saunders 2004; Moor 2006; Anderson and Anderson 2011; Wallach and Asaro 2017). Sometimes it looks as though there is the (dubious) inference at play here that if machines act in ethically relevant ways, then we need a machine ethics. Accordingly, some use a broader notion:
machine ethics is concerned with ensuring that the behavior of machines toward human users, and perhaps other machines as well, is ethically acceptable. (Anderson and Anderson 2007: 15)
This might include mere matters of product safety, for example. Other authors sound rather ambitious but use a narrower notion:
AI reasoning should be able to take into account societal values, moral and ethical considerations; weigh the respective priorities of values held by different stakeholders in various multicultural contexts; explain its reasoning; and guarantee transparency. (Dignum 2018: 1, 2)
Some of the discussion in machine ethics makes the very substantial assumption that machines can, in some sense, be ethical agents responsible for their actions, or “autonomous moral agents” (see van Wynsberghe and Robbins 2019). The basic idea of machine ethics is now finding its way into actual robotics where the assumption that these machines are artificial moral agents in any substantial sense is usually not made (Winfield et al. 2019). It is sometimes observed that a robot that is programmed to follow ethical rules can very easily be modified to follow unethical rules (Vanderelst and Winfield 2018).
The idea that machine ethics might take the form of “laws” has famously been investigated by Isaac Asimov, who proposed “three laws of robotics” (Asimov 1942):
First Law—A robot may not injure a human being or, through inaction, allow a human being to come to harm. Second Law—A robot must obey the orders given it by human beings except where such orders would conflict with the First Law. Third Law—A robot must protect its own existence as long as such protection does not conflict with the First or Second Laws.
Asimov then showed in a number of stories how conflicts between these three laws will make it problematic to use them despite their hierarchical organisation.
It is not clear that there is a consistent notion of “machine ethics” since weaker versions are in danger of reducing “having an ethics” to notions that would not normally be considered sufficient (e.g., without “reflection” or even without “action”); stronger notions that move towards artificial moral agents may describe a—currently—empty set.
If one takes machine ethics to concern moral agents, in some substantial sense, then these agents can be called “artificial moral agents”, having rights and responsibilities. However, the discussion about artificial entities challenges a number of common notions in ethics and it can be very useful to understand these in abstraction from the human case (cf. Misselhorn 2020; Powers and Ganascia forthcoming).
Several authors use “artificial moral agent” in a less demanding sense, borrowing from the use of “agent” in software engineering in which case matters of responsibility and rights will not arise (Allen, Varner, and Zinser 2000). James Moor (2006) distinguishes four types of machine agents: ethical impact agents (e.g., robot jockeys), implicit ethical agents (e.g., safe autopilot), explicit ethical agents (e.g., using formal methods to estimate utility), and full ethical agents (who “can make explicit ethical judgments and generally is competent to reasonably justify them. An average adult human is a full ethical agent”.) Several ways to achieve “explicit” or “full” ethical agents have been proposed, via programming it in (operational morality), via “developing” the ethics itself (functional morality), and finally full-blown morality with full intelligence and sentience (Allen, Smit, and Wallach 2005; Moor 2006). Programmed agents are sometimes not considered “full” agents because they are “competent without comprehension”, just like the neurons in a brain (Dennett 2017; Hakli and Mäkelä 2019).
In some discussions, the notion of “moral patient” plays a role: Ethical agents have responsibilities while ethical patients have rights because harm to them matters. It seems clear that some entities are patients without being agents, e.g., simple animals that can feel pain but cannot make justified choices. On the other hand, it is normally understood that all agents will also be patients (e.g., in a Kantian framework). Usually, being a person is supposed to be what makes an entity a responsible agent, someone who can have duties and be the object of ethical concerns. Such personhood is typically a deep notion associated with phenomenal consciousness, intention and free will (Frankfurt 1971; Strawson 1998). Torrance (2011) suggests “artificial (or machine) ethics could be defined as designing machines that do things that, when done by humans, are indicative of the possession of ‘ethical status’ in those humans” (2011: 116)—which he takes to be “ethical productivity and ethical receptivity ” (2011: 117)—his expressions for moral agents and patients.
2.9.1 Responsibility for Robots
There is broad consensus that accountability, liability, and the rule of law are basic requirements that must be upheld in the face of new technologies (European Group on Ethics in Science and New Technologies 2018, 18), but the issue in the case of robots is how this can be done and how responsibility can be allocated. If the robots act, will they themselves be responsible, liable, or accountable for their actions? Or should the distribution of risk perhaps take precedence over discussions of responsibility?
Traditional distribution of responsibility already occurs: A car maker is responsible for the technical safety of the car, a driver is responsible for driving, a mechanic is responsible for proper maintenance, the public authorities are responsible for the technical conditions of the roads, etc. In general
The effects of decisions or actions based on AI are often the result of countless interactions among many actors, including designers, developers, users, software, and hardware.… With distributed agency comes distributed responsibility. (Taddeo and Floridi 2018: 751).
How this distribution might occur is not a problem that is specific to AI, but it gains particular urgency in this context (Nyholm 2018a, 2018b). In classical control engineering, distributed control is often achieved through a control hierarchy plus control loops across these hierarchies.
2.9.2 Rights for Robots
Some authors have indicated that it should be seriously considered whether current robots must be allocated rights (Gunkel 2018a, 2018b; Danaher forthcoming; Turner 2019). This position seems to rely largely on criticism of the opponents and on the empirical observation that robots and other non-persons are sometimes treated as having rights. In this vein, a “relational turn” has been proposed: If we relate to robots as though they had rights, then we might be well-advised not to search whether they “really” do have such rights (Coeckelbergh 2010, 2012, 2018). This raises the question how far such anti-realism or quasi-realism can go, and what it means then to say that “robots have rights” in a human-centred approach (Gerdes 2016). On the other side of the debate, Bryson has insisted that robots should not enjoy rights (Bryson 2010), though she considers it a possibility (Gunkel and Bryson 2014).
There is a wholly separate issue whether robots (or other AI systems) should be given the status of “legal entities” or “legal persons” in a sense natural persons, but also states, businesses, or organisations are “entities”, namely they can have legal rights and duties. The European Parliament has considered allocating such status to robots in order to deal with civil liability (EU Parliament 2016; Bertolini and Aiello 2018), but not criminal liability—which is reserved for natural persons. It would also be possible to assign only a certain subset of rights and duties to robots. It has been said that “such legislative action would be morally unnecessary and legally troublesome” because it would not serve the interest of humans (Bryson, Diamantis, and Grant 2017: 273). In environmental ethics there is a long-standing discussion about the legal rights for natural objects like trees (C. D. Stone 1972).
It has also been said that the reasons for developing robots with rights, or artificial moral patients, in the future are ethically doubtful (van Wynsberghe and Robbins 2019). In the community of “artificial consciousness” researchers there is a significant concern whether it would be ethical to create such consciousness since creating it would presumably imply ethical obligations to a sentient being, e.g., not to harm it and not to end its existence by switching it off—some authors have called for a “moratorium on synthetic phenomenology” (Bentley et al. 2018: 28f).
2.10.1 Singularity and Superintelligence
In some quarters, the aim of current AI is thought to be an “artificial general intelligence” (AGI), contrasted to a technical or “narrow” AI. AGI is usually distinguished from traditional notions of AI as a general purpose system, and from Searle’s notion of “strong AI”:
computers given the right programs can be literally said to understand and have other cognitive states. (Searle 1980: 417)
The idea of singularity is that if the trajectory of artificial intelligence reaches up to systems that have a human level of intelligence, then these systems would themselves have the ability to develop AI systems that surpass the human level of intelligence, i.e., they are “superintelligent” (see below). Such superintelligent AI systems would quickly self-improve or develop even more intelligent systems. This sharp turn of events after reaching superintelligent AI is the “singularity” from which the development of AI is out of human control and hard to predict (Kurzweil 2005: 487).
The fear that “the robots we created will take over the world” had captured human imagination even before there were computers (e.g., Butler 1863) and is the central theme in Čapek’s famous play that introduced the word “robot” (Čapek 1920). This fear was first formulated as a possible trajectory of existing AI into an “intelligence explosion” by Irvin Good:
Let an ultraintelligent machine be defined as a machine that can far surpass all the intellectual activities of any man however clever. Since the design of machines is one of these intellectual activities, an ultraintelligent machine could design even better machines; there would then unquestionably be an “intelligence explosion”, and the intelligence of man would be left far behind. Thus the first ultraintelligent machine is the last invention that man need ever make, provided that the machine is docile enough to tell us how to keep it under control. (Good 1965: 33)
The optimistic argument from acceleration to singularity is spelled out by Kurzweil (1999, 2005, 2012) who essentially points out that computing power has been increasing exponentially, i.e., doubling ca. every 2 years since 1970 in accordance with “Moore’s Law” on the number of transistors, and will continue to do so for some time in the future. He predicted in (Kurzweil 1999) that by 2010 supercomputers will reach human computation capacity, by 2030 “mind uploading” will be possible, and by 2045 the “singularity” will occur. Kurzweil talks about an increase in computing power that can be purchased at a given cost—but of course in recent years the funds available to AI companies have also increased enormously: Amodei and Hernandez (2018 [OIR]) thus estimate that in the years 2012–2018 the actual computing power available to train a particular AI system doubled every 3.4 months, resulting in an 300,000x increase—not the 7x increase that doubling every two years would have created.
A common version of this argument (Chalmers 2010) talks about an increase in “intelligence” of the AI system (rather than raw computing power), but the crucial point of “singularity” remains the one where further development of AI is taken over by AI systems and accelerates beyond human level. Bostrom (2014) explains in some detail what would happen at that point and what the risks for humanity are. The discussion is summarised in Eden et al. (2012); Armstrong (2014); Shanahan (2015). There are possible paths to superintelligence other than computing power increase, e.g., the complete emulation of the human brain on a computer (Kurzweil 2012; Sandberg 2013), biological paths, or networks and organisations (Bostrom 2014: 22–51).
Despite obvious weaknesses in the identification of “intelligence” with processing power, Kurzweil seems right that humans tend to underestimate the power of exponential growth. Mini-test: If you walked in steps in such a way that each step is double the previous, starting with a step of one metre, how far would you get with 30 steps? (answer: almost 3 times further than the Earth’s only permanent natural satellite.) Indeed, most progress in AI is readily attributable to the availability of processors that are faster by degrees of magnitude, larger storage, and higher investment (Müller 2018). The actual acceleration and its speeds are discussed in (Müller and Bostrom 2016; Bostrom, Dafoe, and Flynn forthcoming); Sandberg (2019) argues that progress will continue for some time.
The participants in this debate are united by being technophiles in the sense that they expect technology to develop rapidly and bring broadly welcome changes—but beyond that, they divide into those who focus on benefits (e.g., Kurzweil) and those who focus on risks (e.g., Bostrom). Both camps sympathise with “transhuman” views of survival for humankind in a different physical form, e.g., uploaded on a computer (Moravec 1990, 1998; Bostrom 2003a, 2003c). They also consider the prospects of “human enhancement” in various respects, including intelligence—often called “IA” (intelligence augmentation). It may be that future AI will be used for human enhancement, or will contribute further to the dissolution of the neatly defined human single person. Robin Hanson provides detailed speculation about what will happen economically in case human “brain emulation” enables truly intelligent robots or “ems” (Hanson 2016).
The argument from superintelligence to risk requires the assumption that superintelligence does not imply benevolence—contrary to Kantian traditions in ethics that have argued higher levels of rationality or intelligence would go along with a better understanding of what is moral and better ability to act morally (Gewirth 1978; Chalmers 2010: 36f). Arguments for risk from superintelligence say that rationality and morality are entirely independent dimensions—this is sometimes explicitly argued for as an “orthogonality thesis” (Bostrom 2012; Armstrong 2013; Bostrom 2014: 105–109).
Criticism of the singularity narrative has been raised from various angles. Kurzweil and Bostrom seem to assume that intelligence is a one-dimensional property and that the set of intelligent agents is totally-ordered in the mathematical sense—but neither discusses intelligence at any length in their books. Generally, it is fair to say that despite some efforts, the assumptions made in the powerful narrative of superintelligence and singularity have not been investigated in detail. One question is whether such a singularity will ever occur—it may be conceptually impossible, practically impossible or may just not happen because of contingent events, including people actively preventing it. Philosophically, the interesting question is whether singularity is just a “myth” (Floridi 2016; Ganascia 2017), and not on the trajectory of actual AI research. This is something that practitioners often assume (e.g., Brooks 2017 [OIR]). They may do so because they fear the public relations backlash, because they overestimate the practical problems, or because they have good reasons to think that superintelligence is an unlikely outcome of current AI research (Müller forthcoming-a). This discussion raises the question whether the concern about “singularity” is just a narrative about fictional AI based on human fears. But even if one does find negative reasons compelling and the singularity not likely to occur, there is still a significant possibility that one may turn out to be wrong. Philosophy is not on the “secure path of a science” (Kant 1791: B15), and maybe AI and robotics aren’t either (Müller 2020). So, it appears that discussing the very high-impact risk of singularity has justification even if one thinks the probability of such singularity ever occurring is very low.
2.10.2 Existential Risk from Superintelligence
Thinking about superintelligence in the long term raises the question whether superintelligence may lead to the extinction of the human species, which is called an “existential risk” (or XRisk): The superintelligent systems may well have preferences that conflict with the existence of humans on Earth, and may thus decide to end that existence—and given their superior intelligence, they will have the power to do so (or they may happen to end it because they do not really care).
Thinking in the long term is the crucial feature of this literature. Whether the singularity (or another catastrophic event) occurs in 30 or 300 or 3000 years does not really matter (Baum et al. 2019). Perhaps there is even an astronomical pattern such that an intelligent species is bound to discover AI at some point, and thus bring about its own demise. Such a “great filter” would contribute to the explanation of the “Fermi paradox” why there is no sign of life in the known universe despite the high probability of it emerging. It would be bad news if we found out that the “great filter” is ahead of us, rather than an obstacle that Earth has already passed. These issues are sometimes taken more narrowly to be about human extinction (Bostrom 2013), or more broadly as concerning any large risk for the species (Rees 2018)—of which AI is only one (Häggström 2016; Ord 2020). Bostrom also uses the category of “global catastrophic risk” for risks that are sufficiently high up the two dimensions of “scope” and “severity” (Bostrom and Ćirković 2011; Bostrom 2013).
These discussions of risk are usually not connected to the general problem of ethics under risk (e.g., Hansson 2013, 2018). The long-term view has its own methodological challenges but has produced a wide discussion: (Tegmark 2017) focuses on AI and human life “3.0” after singularity while Russell, Dewey, and Tegmark (2015) and Bostrom, Dafoe, and Flynn (forthcoming) survey longer-term policy issues in ethical AI. Several collections of papers have investigated the risks of artificial general intelligence (AGI) and the factors that might make this development more or less risk-laden (Müller 2016b; Callaghan et al. 2017; Yampolskiy 2018), including the development of non-agent AI (Drexler 2019).
2.10.3 Controlling Superintelligence?
In a narrow sense, the “control problem” is how we humans can remain in control of an AI system once it is superintelligent (Bostrom 2014: 127ff). In a wider sense, it is the problem of how we can make sure an AI system will turn out to be positive according to human perception (Russell 2019); this is sometimes called “value alignment”. How easy or hard it is to control a superintelligence depends significantly on the speed of “take-off” to a superintelligent system. This has led to particular attention to systems with self-improvement, such as AlphaZero (Silver et al. 2018).
One aspect of this problem is that we might decide a certain feature is desirable, but then find out that it has unforeseen consequences that are so negative that we would not desire that feature after all. This is the ancient problem of King Midas who wished that all he touched would turn into gold. This problem has been discussed on the occasion of various examples, such as the “paperclip maximiser” (Bostrom 2003b), or the program to optimise chess performance (Omohundro 2014).
Discussions about superintelligence include speculation about omniscient beings, the radical changes on a “latter day”, and the promise of immortality through transcendence of our current bodily form—so sometimes they have clear religious undertones (Capurro 1993; Geraci 2008, 2010; O’Connell 2017: 160ff). These issues also pose a well-known problem of epistemology: Can we know the ways of the omniscient (Danaher 2015)? The usual opponents have already shown up: A characteristic response of an atheist is
People worry that computers will get too smart and take over the world, but the real problem is that they’re too stupid and they’ve already taken over the world (Domingos 2015)
The new nihilists explain that a “techno-hypnosis” through information technologies has now become our main method of distraction from the loss of meaning (Gertz 2018). Both opponents would thus say we need an ethics for the “small” problems that occur with actual AI and robotics ( sections 2.1 through 2.9 above), and that there is less need for the “big ethics” of existential risk from AI ( section 2.10 ).
The singularity thus raises the problem of the concept of AI again. It is remarkable how imagination or “vision” has played a central role since the very beginning of the discipline at the “Dartmouth Summer Research Project” (McCarthy et al. 1955 [OIR]; Simon and Newell 1958). And the evaluation of this vision is subject to dramatic change: In a few decades, we went from the slogans “AI is impossible” (Dreyfus 1972) and “AI is just automation” (Lighthill 1973) to “AI will solve all problems” (Kurzweil 1999) and “AI may kill us all” (Bostrom 2014). This created media attention and public relations efforts, but it also raises the problem of how much of this “philosophy and ethics of AI” is really about AI rather than about an imagined technology. As we said at the outset, AI and robotics have raised fundamental questions about what we should do with these systems, what the systems themselves should do, and what risks they have in the long term. They also challenge the human view of humanity as the intelligent and dominant species on Earth. We have seen issues that have been raised and will have to watch technological and social developments closely to catch the new issues early on, develop a philosophical analysis, and learn for traditional problems of philosophy.
NOTE: Citations in the main text annotated “[OIR]” may be found in the Other Internet Resources section below, not in the Bibliography.
- Abowd, John M, 2017, “How Will Statistical Agencies Operate When All Data Are Private?”, Journal of Privacy and Confidentiality , 7(3): 1–15. doi:10.29012/jpc.v7i3.404
- AI4EU, 2019, “Outcomes from the Strategic Orientation Workshop (Deliverable 7.1)”, (June 28, 2019). https://www.ai4eu.eu/ai4eu-project-deliverables
- Allen, Colin, Iva Smit, and Wendell Wallach, 2005, “Artificial Morality: Top-down, Bottom-up, and Hybrid Approaches”, Ethics and Information Technology , 7(3): 149–155. doi:10.1007/s10676-006-0004-4
- Allen, Colin, Gary Varner, and Jason Zinser, 2000, “Prolegomena to Any Future Artificial Moral Agent”, Journal of Experimental & Theoretical Artificial Intelligence , 12(3): 251–261. doi:10.1080/09528130050111428
- Amoroso, Daniele and Guglielmo Tamburrini, 2018, “The Ethical and Legal Case Against Autonomy in Weapons Systems”, Global Jurist , 18(1): art. 20170012. doi:10.1515/gj-2017-0012
- Anderson, Janna, Lee Rainie, and Alex Luchsinger, 2018, Artificial Intelligence and the Future of Humans , Washington, DC: Pew Research Center.
- Anderson, Michael and Susan Leigh Anderson, 2007, “Machine Ethics: Creating an Ethical Intelligent Agent”, AI Magazine , 28(4): 15–26.
- ––– (eds.), 2011, Machine Ethics , Cambridge: Cambridge University Press. doi:10.1017/CBO9780511978036
- Aneesh, A., 2006, Virtual Migration: The Programming of Globalization , Durham, NC and London: Duke University Press.
- Arkin, Ronald C., 2009, Governing Lethal Behavior in Autonomous Robots , Boca Raton, FL: CRC Press.
- Armstrong, Stuart, 2013, “General Purpose Intelligence: Arguing the Orthogonality Thesis”, Analysis and Metaphysics , 12: 68–84.
- –––, 2014, Smarter Than Us , Berkeley, CA: MIRI.
- Arnold, Thomas and Matthias Scheutz, 2017, “Beyond Moral Dilemmas: Exploring the Ethical Landscape in HRI”, in Proceedings of the 2017 ACM/IEEE International Conference on Human-Robot Interaction—HRI ’17 , Vienna, Austria: ACM Press, 445–452. doi:10.1145/2909824.3020255
- Asaro, Peter M., 2019, “AI Ethics in Predictive Policing: From Models of Threat to an Ethics of Care”, IEEE Technology and Society Magazine , 38(2): 40–53. doi:10.1109/MTS.2019.2915154
- Asimov, Isaac, 1942, “Runaround: A Short Story”, Astounding Science Fiction , March 1942. Reprinted in “I, Robot”, New York: Gnome Press 1950, 1940ff.
- Awad, Edmond, Sohan Dsouza, Richard Kim, Jonathan Schulz, Joseph Henrich, Azim Shariff, Jean-François Bonnefon, and Iyad Rahwan, 2018, “The Moral Machine Experiment”, Nature , 563(7729): 59–64. doi:10.1038/s41586-018-0637-6
- Baldwin, Richard, 2019, The Globotics Upheaval: Globalisation, Robotics and the Future of Work , New York: Oxford University Press.
- Baum, Seth D., Stuart Armstrong, Timoteus Ekenstedt, Olle Häggström, Robin Hanson, Karin Kuhlemann, Matthijs M. Maas, James D. Miller, Markus Salmela, Anders Sandberg, Kaj Sotala, Phil Torres, Alexey Turchin, and Roman V. Yampolskiy, 2019, “Long-Term Trajectories of Human Civilization”, Foresight , 21(1): 53–83. doi:10.1108/FS-04-2018-0037
- Bendel, Oliver, 2018, “Sexroboter aus Sicht der Maschinenethik”, in Handbuch Filmtheorie , Bernhard Groß and Thomas Morsch (eds.), (Springer Reference Geisteswissenschaften), Wiesbaden: Springer Fachmedien Wiesbaden, 1–19. doi:10.1007/978-3-658-17484-2_22-1
- Bennett, Colin J. and Charles Raab, 2006, The Governance of Privacy: Policy Instruments in Global Perspective , second edition, Cambridge, MA: MIT Press.
- Benthall, Sebastian and Bruce D. Haynes, 2019, “Racial Categories in Machine Learning”, in Proceedings of the Conference on Fairness, Accountability, and Transparency - FAT* ’19 , Atlanta, GA, USA: ACM Press, 289–298. doi:10.1145/3287560.3287575
- Bentley, Peter J., Miles Brundage, Olle Häggström, and Thomas Metzinger, 2018, “Should We Fear Artificial Intelligence? In-Depth Analysis”, European Parliamentary Research Service, Scientific Foresight Unit (STOA), March 2018, PE 614.547, 1–40. [ Bentley et al. 2018 available online ]
- Bertolini, Andrea and Giuseppe Aiello, 2018, “Robot Companions: A Legal and Ethical Analysis”, The Information Society , 34(3): 130–140. doi:10.1080/01972243.2018.1444249
- Binns, Reuben, 2018, “Fairness in Machine Learning: Lessons from Political Philosophy”, Proceedings of the 1st Conference on Fairness, Accountability and Transparency , in Proceedings of Machine Learning Research , 81: 149–159.
- Bostrom, Nick, 2003a, “Are We Living in a Computer Simulation?”, The Philosophical Quarterly , 53(211): 243–255. doi:10.1111/1467-9213.00309
- –––, 2003b, “Ethical Issues in Advanced Artificial Intelligence”, in Cognitive, Emotive and Ethical Aspects of Decision Making in Humans and in Artificial Intelligence, Volume 2 , Iva Smit, Wendell Wallach, and G.E. Lasker (eds), (IIAS-147-2003), Tecumseh, ON: International Institute of Advanced Studies in Systems Research and Cybernetics, 12–17. [ Botstrom 2003b revised available online ]
- –––, 2003c, “Transhumanist Values”, in Ethical Issues for the Twenty-First Century , Frederick Adams (ed.), Bowling Green, OH: Philosophical Documentation Center Press.
- –––, 2012, “The Superintelligent Will: Motivation and Instrumental Rationality in Advanced Artificial Agents”, Minds and Machines , 22(2): 71–85. doi:10.1007/s11023-012-9281-3
- –––, 2013, “Existential Risk Prevention as Global Priority”, Global Policy , 4(1): 15–31. doi:10.1111/1758-5899.12002
- –––, 2014, Superintelligence: Paths, Dangers, Strategies , Oxford: Oxford University Press.
- Bostrom, Nick and Milan M. Ćirković (eds.), 2011, Global Catastrophic Risks , New York: Oxford University Press.
- Bostrom, Nick, Allan Dafoe, and Carrick Flynn, forthcoming, “Policy Desiderata for Superintelligent AI: A Vector Field Approach (V. 4.3)”, in Ethics of Artificial Intelligence , S Matthew Liao (ed.), New York: Oxford University Press. [ Bostrom, Dafoe, and Flynn forthcoming – preprint available online ]
- Bostrom, Nick and Eliezer Yudkowsky, 2014, “The Ethics of Artificial Intelligence”, in The Cambridge Handbook of Artificial Intelligence , Keith Frankish and William M. Ramsey (eds.), Cambridge: Cambridge University Press, 316–334. doi:10.1017/CBO9781139046855.020 [ Bostrom and Yudkowsky 2014 available online ]
- Bradshaw, Samantha, Lisa-Maria Neudert, and Phil Howard, 2019, “Government Responses to Malicious Use of Social Media”, Working Paper 2019.2, Oxford: Project on Computational Propaganda. [ Bradshaw, Neudert, and Howard 2019 available online/ ]
- Brownsword, Roger, Eloise Scotford, and Karen Yeung (eds.), 2017, The Oxford Handbook of Law, Regulation and Technology , Oxford: Oxford University Press. doi:10.1093/oxfordhb/9780199680832.001.0001
- Brynjolfsson, Erik and Andrew McAfee, 2016, The Second Machine Age: Work, Progress, and Prosperity in a Time of Brilliant Technologies , New York: W. W. Norton.
- Bryson, Joanna J., 2010, “Robots Should Be Slaves”, in Close Engagements with Artificial Companions: Key Social, Psychological, Ethical and Design Issues , Yorick Wilks (ed.), (Natural Language Processing 8), Amsterdam: John Benjamins Publishing Company, 63–74. doi:10.1075/nlp.8.11bry
- –––, 2019, “The Past Decade and Future of Ai’s Impact on Society”, in Towards a New Enlightenment: A Transcendent Decade , Madrid: Turner - BVVA. [ Bryson 2019 available online ]
- Bryson, Joanna J., Mihailis E. Diamantis, and Thomas D. Grant, 2017, “Of, for, and by the People: The Legal Lacuna of Synthetic Persons”, Artificial Intelligence and Law , 25(3): 273–291. doi:10.1007/s10506-017-9214-9
- Burr, Christopher and Nello Cristianini, 2019, “Can Machines Read Our Minds?”, Minds and Machines , 29(3): 461–494. doi:10.1007/s11023-019-09497-4
- Butler, Samuel, 1863, “Darwin among the Machines: Letter to the Editor”, Letter in The Press (Christchurch) , 13 June 1863. [ Butler 1863 available online ]
- Callaghan, Victor, James Miller, Roman Yampolskiy, and Stuart Armstrong (eds.), 2017, The Technological Singularity: Managing the Journey , (The Frontiers Collection), Berlin, Heidelberg: Springer Berlin Heidelberg. doi:10.1007/978-3-662-54033-6
- Calo, Ryan, 2018, “Artificial Intelligence Policy: A Primer and Roadmap”, University of Bologna Law Review , 3(2): 180-218. doi:10.6092/ISSN.2531-6133/8670
- Calo, Ryan, A. Michael Froomkin, and Ian Kerr (eds.), 2016, Robot Law , Cheltenham: Edward Elgar.
- Čapek, Karel, 1920, R.U.R. , Prague: Aventium. Translated by Peter Majer and Cathy Porter, London: Methuen, 1999.
- Capurro, Raphael, 1993, “Ein Grinsen Ohne Katze: Von der Vergleichbarkeit Zwischen ‘Künstlicher Intelligenz’ und ‘Getrennten Intelligenzen’”, Zeitschrift für philosophische Forschung , 47: 93–102.
- Cave, Stephen, 2019, “To Save Us from a Kafkaesque Future, We Must Democratise AI”, The Guardian , 04 January 2019. [ Cave 2019 available online ]
- Chalmers, David J., 2010, “The Singularity: A Philosophical Analysis”, Journal of Consciousness Studies , 17(9–10): 7–65. [ Chalmers 2010 available online ]
- Christman, John, 2003 [2018], “Autonomy in Moral and Political Philosophy”, (Spring 2018) Stanford Encyclopedia of Philosophy (EDITION NEEDED), URL = < https://plato.stanford.edu/archives/spr2018/entries/autonomy-moral/ >
- Coeckelbergh, Mark, 2010, “Robot Rights? Towards a Social-Relational Justification of Moral Consideration”, Ethics and Information Technology , 12(3): 209–221. doi:10.1007/s10676-010-9235-5
- –––, 2012, Growing Moral Relations: Critique of Moral Status Ascription , London: Palgrave. doi:10.1057/9781137025968
- –––, 2016, “Care Robots and the Future of ICT-Mediated Elderly Care: A Response to Doom Scenarios”, AI & Society , 31(4): 455–462. doi:10.1007/s00146-015-0626-3
- –––, 2018, “What Do We Mean by a Relational Ethics? Growing a Relational Approach to the Moral Standing of Plants, Robots and Other Non-Humans”, in Plant Ethics: Concepts and Applications , Angela Kallhoff, Marcello Di Paola, and Maria Schörgenhumer (eds.), London: Routledge, 110–121.
- Crawford, Kate and Ryan Calo, 2016, “There Is a Blind Spot in AI Research”, Nature , 538(7625): 311–313. doi:10.1038/538311a
- Cristianini, Nello, forthcoming, “Shortcuts to Artificial Intelligence”, in Machines We Trust , Marcello Pelillo and Teresa Scantamburlo (eds.), Cambridge, MA: MIT Press. [ Cristianini forthcoming – preprint available online ]
- Danaher, John, 2015, “Why AI Doomsayers Are Like Sceptical Theists and Why It Matters”, Minds and Machines , 25(3): 231–246. doi:10.1007/s11023-015-9365-y
- –––, 2016a, “Robots, Law and the Retribution Gap”, Ethics and Information Technology , 18(4): 299–309. doi:10.1007/s10676-016-9403-3
- –––, 2016b, “The Threat of Algocracy: Reality, Resistance and Accommodation”, Philosophy & Technology , 29(3): 245–268. doi:10.1007/s13347-015-0211-1
- –––, 2019a, Automation and Utopia: Human Flourishing in a World without Work , Cambridge, MA: Harvard University Press.
- –––, 2019b, “The Philosophical Case for Robot Friendship”, Journal of Posthuman Studies , 3(1): 5–24. doi:10.5325/jpoststud.3.1.0005
- –––, forthcoming, “Welcoming Robots into the Moral Circle: A Defence of Ethical Behaviourism”, Science and Engineering Ethics , first online: 20 June 2019. doi:10.1007/s11948-019-00119-x
- Danaher, John and Neil McArthur (eds.), 2017, Robot Sex: Social and Ethical Implications , Boston, MA: MIT Press.
- DARPA, 1983, “Strategic Computing. New-Generation Computing Technology: A Strategic Plan for Its Development an Application to Critical Problems in Defense”, ADA141982, 28 October 1983. [ DARPA 1983 available online ]
- Dennett, Daniel C, 2017, From Bacteria to Bach and Back: The Evolution of Minds , New York: W.W. Norton.
- Devlin, Kate, 2018, Turned On: Science, Sex and Robots , London: Bloomsbury.
- Diakopoulos, Nicholas, 2015, “Algorithmic Accountability: Journalistic Investigation of Computational Power Structures”, Digital Journalism , 3(3): 398–415. doi:10.1080/21670811.2014.976411
- Dignum, Virginia, 2018, “Ethics in Artificial Intelligence: Introduction to the Special Issue”, Ethics and Information Technology , 20(1): 1–3. doi:10.1007/s10676-018-9450-z
- Domingos, Pedro, 2015, The Master Algorithm: How the Quest for the Ultimate Learning Machine Will Remake Our World , London: Allen Lane.
- Draper, Heather, Tom Sorell, Sandra Bedaf, Dag Sverre Syrdal, Carolina Gutierrez-Ruiz, Alexandre Duclos, and Farshid Amirabdollahian, 2014, “Ethical Dimensions of Human-Robot Interactions in the Care of Older People: Insights from 21 Focus Groups Convened in the UK, France and the Netherlands”, in International Conference on Social Robotics 2014 , Michael Beetz, Benjamin Johnston, and Mary-Anne Williams (eds.), (Lecture Notes in Artificial Intelligence 8755), Cham: Springer International Publishing, 135–145. doi:10.1007/978-3-319-11973-1_14
- Dressel, Julia and Hany Farid, 2018, “The Accuracy, Fairness, and Limits of Predicting Recidivism”, Science Advances , 4(1): eaao5580. doi:10.1126/sciadv.aao5580
- Drexler, K. Eric, 2019, “Reframing Superintelligence: Comprehensive AI Services as General Intelligence”, FHI Technical Report, 2019-1, 1-210. [ Drexler 2019 available online ]
- Dreyfus, Hubert L., 1972, What Computers Still Can’t Do: A Critique of Artificial Reason , second edition, Cambridge, MA: MIT Press 1992.
- Dreyfus, Hubert L., Stuart E. Dreyfus, and Tom Athanasiou, 1986, Mind over Machine: The Power of Human Intuition and Expertise in the Era of the Computer , New York: Free Press.
- Dwork, Cynthia, Frank McSherry, Kobbi Nissim, and Adam Smith, 2006, Calibrating Noise to Sensitivity in Private Data Analysis , Berlin, Heidelberg.
- Eden, Amnon H., James H. Moor, Johnny H. Søraker, and Eric Steinhart (eds.), 2012, Singularity Hypotheses: A Scientific and Philosophical Assessment , (The Frontiers Collection), Berlin, Heidelberg: Springer Berlin Heidelberg. doi:10.1007/978-3-642-32560-1
- Eubanks, Virginia, 2018, Automating Inequality: How High-Tech Tools Profile, Police, and Punish the Poor , London: St. Martin’s Press.
- European Commission, 2013, “How Many People Work in Agriculture in the European Union? An Answer Based on Eurostat Data Sources”, EU Agricultural Economics Briefs , 8 (July 2013). [ Anonymous 2013 available online ]
- European Group on Ethics in Science and New Technologies, 2018, “Statement on Artificial Intelligence, Robotics and ‘Autonomous’ Systems”, 9 March 2018, European Commission, Directorate-General for Research and Innovation, Unit RTD.01. [ European Group 2018 available online ]
- Ferguson, Andrew Guthrie, 2017, The Rise of Big Data Policing: Surveillance, Race, and the Future of Law Enforcement , New York: NYU Press.
- Floridi, Luciano, 2016, “Should We Be Afraid of AI? Machines Seem to Be Getting Smarter and Smarter and Much Better at Human Jobs, yet True AI Is Utterly Implausible. Why?”, Aeon , 9 May 2016. URL = < Floridi 2016 available online >
- Floridi, Luciano, Josh Cowls, Monica Beltrametti, Raja Chatila, Patrice Chazerand, Virginia Dignum, Christoph Luetge, Robert Madelin, Ugo Pagallo, Francesca Rossi, Burkhard Schafer, Peggy Valcke, and Effy Vayena, 2018, “AI4People—An Ethical Framework for a Good AI Society: Opportunities, Risks, Principles, and Recommendations”, Minds and Machines , 28(4): 689–707. doi:10.1007/s11023-018-9482-5
- Floridi, Luciano and Jeff W. Sanders, 2004, “On the Morality of Artificial Agents”, Minds and Machines , 14(3): 349–379. doi:10.1023/B:MIND.0000035461.63578.9d
- Floridi, Luciano and Mariarosaria Taddeo, 2016, “What Is Data Ethics?”, Philosophical Transactions of the Royal Society A: Mathematical, Physical and Engineering Sciences , 374(2083): 20160360. doi:10.1098/rsta.2016.0360
- Foot, Philippa, 1967, “The Problem of Abortion and the Doctrine of the Double Effect”, Oxford Review , 5: 5–15.
- Fosch-Villaronga, Eduard and Jordi Albo-Canals, 2019, “‘I’ll Take Care of You,’ Said the Robot”, Paladyn, Journal of Behavioral Robotics , 10(1): 77–93. doi:10.1515/pjbr-2019-0006
- Frank, Lily and Sven Nyholm, 2017, “Robot Sex and Consent: Is Consent to Sex between a Robot and a Human Conceivable, Possible, and Desirable?”, Artificial Intelligence and Law , 25(3): 305–323. doi:10.1007/s10506-017-9212-y
- Frankfurt, Harry G., 1971, “Freedom of the Will and the Concept of a Person”, The Journal of Philosophy , 68(1): 5–20.
- Frey, Carl Benedict, 2019, The Technology Trap: Capital, Labour, and Power in the Age of Automation , Princeton, NJ: Princeton University Press.
- Frey, Carl Benedikt and Michael A. Osborne, 2013, “The Future of Employment: How Susceptible Are Jobs to Computerisation?”, Oxford Martin School Working Papers, 17 September 2013. [ Frey and Osborne 2013 available online ]
- Ganascia, Jean-Gabriel, 2017, Le Mythe De La Singularité , Paris: Éditions du Seuil.
- EU Parliament, 2016, “Draft Report with Recommendations to the Commission on Civil Law Rules on Robotics (2015/2103(Inl))”, Committee on Legal Affairs , 10.11.2016. https://www.europarl.europa.eu/doceo/document/A-8-2017-0005_EN.html
- EU Regulation, 2016/679, “General Data Protection Regulation: Regulation (EU) 2016/679 of the European Parliament and of the Council of 27 April 2016 on the Protection of Natural Persons with Regard to the Processing of Personal Data and on the Free Movement of Such Data, and Repealing Directive 95/46/Ec”, Official Journal of the European Union , 119 (4 May 2016), 1–88. [ Regulation (EU) 2016/679 available online ]
- Geraci, Robert M., 2008, “Apocalyptic AI: Religion and the Promise of Artificial Intelligence”, Journal of the American Academy of Religion , 76(1): 138–166. doi:10.1093/jaarel/lfm101
- –––, 2010, Apocalyptic AI: Visions of Heaven in Robotics, Artificial Intelligence, and Virtual Reality , Oxford: Oxford University Press. doi:10.1093/acprof:oso/9780195393026.001.0001
- Gerdes, Anne, 2016, “The Issue of Moral Consideration in Robot Ethics”, ACM SIGCAS Computers and Society , 45(3): 274–279. doi:10.1145/2874239.2874278
- German Federal Ministry of Transport and Digital Infrastructure, 2017, “Report of the Ethics Commission: Automated and Connected Driving”, June 2017, 1–36. [ GFMTDI 2017 available online ]
- Gertz, Nolen, 2018, Nihilism and Technology , London: Rowman & Littlefield.
- Gewirth, Alan, 1978, “The Golden Rule Rationalized”, Midwest Studies in Philosophy , 3(1): 133–147. doi:10.1111/j.1475-4975.1978.tb00353.x
- Gibert, Martin, 2019, “Éthique Artificielle (Version Grand Public)”, in L’Encyclopédie Philosophique , Maxime Kristanek (ed.), accessed: 16 April 2020, URL = < Gibert 2019 available online >
- Giubilini, Alberto and Julian Savulescu, 2018, “The Artificial Moral Advisor. The ‘Ideal Observer’ Meets Artificial Intelligence”, Philosophy & Technology , 31(2): 169–188. doi:10.1007/s13347-017-0285-z
- Good, Irving John, 1965, “Speculations Concerning the First Ultraintelligent Machine”, in Advances in Computers 6 , Franz L. Alt and Morris Rubinoff (eds.), New York & London: Academic Press, 31–88. doi:10.1016/S0065-2458(08)60418-0
- Goodfellow, Ian, Yoshua Bengio, and Aaron Courville, 2016, Deep Learning , Cambridge, MA: MIT Press.
- Goodman, Bryce and Seth Flaxman, 2017, “European Union Regulations on Algorithmic Decision-Making and a ‘Right to Explanation’”, AI Magazine , 38(3): 50–57. doi:10.1609/aimag.v38i3.2741
- Goos, Maarten, 2018, “The Impact of Technological Progress on Labour Markets: Policy Challenges”, Oxford Review of Economic Policy , 34(3): 362–375. doi:10.1093/oxrep/gry002
- Goos, Maarten, Alan Manning, and Anna Salomons, 2009, “Job Polarization in Europe”, American Economic Review , 99(2): 58–63. doi:10.1257/aer.99.2.58
- Graham, Sandra and Brian S. Lowery, 2004, “Priming Unconscious Racial Stereotypes about Adolescent Offenders”, Law and Human Behavior , 28(5): 483–504. doi:10.1023/B:LAHU.0000046430.65485.1f
- Gunkel, David J., 2018a, “The Other Question: Can and Should Robots Have Rights?”, Ethics and Information Technology , 20(2): 87–99. doi:10.1007/s10676-017-9442-4
- –––, 2018b, Robot Rights , Boston, MA: MIT Press.
- Gunkel, David J. and Joanna J. Bryson (eds.), 2014, Machine Morality: The Machine as Moral Agent and Patient special issue of Philosophy & Technology , 27(1): 1–142.
- Häggström, Olle, 2016, Here Be Dragons: Science, Technology and the Future of Humanity , Oxford: Oxford University Press. doi:10.1093/acprof:oso/9780198723547.001.0001
- Hakli, Raul and Pekka Mäkelä, 2019, “Moral Responsibility of Robots and Hybrid Agents”, The Monist , 102(2): 259–275. doi:10.1093/monist/onz009
- Hanson, Robin, 2016, The Age of Em: Work, Love and Life When Robots Rule the Earth , Oxford: Oxford University Press.
- Hansson, Sven Ove, 2013, The Ethics of Risk: Ethical Analysis in an Uncertain World , New York: Palgrave Macmillan.
- –––, 2018, “How to Perform an Ethical Risk Analysis (eRA)”, Risk Analysis , 38(9): 1820–1829. doi:10.1111/risa.12978
- Harari, Yuval Noah, 2016, Homo Deus: A Brief History of Tomorrow , New York: Harper.
- Haskel, Jonathan and Stian Westlake, 2017, Capitalism without Capital: The Rise of the Intangible Economy , Princeton, NJ: Princeton University Press.
- Houkes, Wybo and Pieter E. Vermaas, 2010, Technical Functions: On the Use and Design of Artefacts , (Philosophy of Engineering and Technology 1), Dordrecht: Springer Netherlands. doi:10.1007/978-90-481-3900-2
- IEEE, 2019, Ethically Aligned Design: A Vision for Prioritizing Human Well-Being with Autonomous and Intelligent Systems (First Version), < IEEE 2019 available online >.
- Jasanoff, Sheila, 2016, The Ethics of Invention: Technology and the Human Future , New York: Norton.
- Jecker, Nancy S., forthcoming, Ending Midlife Bias: New Values for Old Age , New York: Oxford University Press.
- Jobin, Anna, Marcello Ienca, and Effy Vayena, 2019, “The Global Landscape of AI Ethics Guidelines”, Nature Machine Intelligence , 1(9): 389–399. doi:10.1038/s42256-019-0088-2
- Johnson, Deborah G. and Mario Verdicchio, 2017, “Reframing AI Discourse”, Minds and Machines , 27(4): 575–590. doi:10.1007/s11023-017-9417-6
- Kahnemann, Daniel, 2011, Thinking Fast and Slow , London: Macmillan.
- Kamm, Frances Myrna, 2016, The Trolley Problem Mysteries , Eric Rakowski (ed.), Oxford: Oxford University Press. doi:10.1093/acprof:oso/9780190247157.001.0001
- Kant, Immanuel, 1781/1787, Kritik der reinen Vernunft . Translated as Critique of Pure Reason , Norman Kemp Smith (trans.), London: Palgrave Macmillan, 1929.
- Keeling, Geoff, 2020, “Why Trolley Problems Matter for the Ethics of Automated Vehicles”, Science and Engineering Ethics , 26(1): 293–307. doi:10.1007/s11948-019-00096-1
- Keynes, John Maynard, 1930, “Economic Possibilities for Our Grandchildren”. Reprinted in his Essays in Persuasion , New York: Harcourt Brace, 1932, 358–373.
- Kissinger, Henry A., 2018, “How the Enlightenment Ends: Philosophically, Intellectually—in Every Way—Human Society Is Unprepared for the Rise of Artificial Intelligence”, The Atlantic , June 2018. [ Kissinger 2018 available online ]
- Kurzweil, Ray, 1999, The Age of Spiritual Machines: When Computers Exceed Human Intelligence , London: Penguin.
- –––, 2005, The Singularity Is Near: When Humans Transcend Biology , London: Viking.
- –––, 2012, How to Create a Mind: The Secret of Human Thought Revealed , New York: Viking.
- Lee, Minha, Sander Ackermans, Nena van As, Hanwen Chang, Enzo Lucas, and Wijnand IJsselsteijn, 2019, “Caring for Vincent: A Chatbot for Self-Compassion”, in Proceedings of the 2019 CHI Conference on Human Factors in Computing Systems—CHI ’19 , Glasgow, Scotland: ACM Press, 1–13. doi:10.1145/3290605.3300932
- Levy, David, 2007, Love and Sex with Robots: The Evolution of Human-Robot Relationships , New York: Harper & Co.
- Lighthill, James, 1973, “Artificial Intelligence: A General Survey”, Artificial intelligence: A Paper Symposion , London: Science Research Council. [ Lighthill 1973 available online ]
- Lin, Patrick, 2016, “Why Ethics Matters for Autonomous Cars”, in Autonomous Driving , Markus Maurer, J. Christian Gerdes, Barbara Lenz, and Hermann Winner (eds.), Berlin, Heidelberg: Springer Berlin Heidelberg, 69–85. doi:10.1007/978-3-662-48847-8_4
- Lin, Patrick, Keith Abney, and Ryan Jenkins (eds.), 2017, Robot Ethics 2.0: From Autonomous Cars to Artificial Intelligence , New York: Oxford University Press. doi:10.1093/oso/9780190652951.001.0001
- Lin, Patrick, George Bekey, and Keith Abney, 2008, “Autonomous Military Robotics: Risk, Ethics, and Design”, ONR report, California Polytechnic State University, San Luis Obispo, 20 December 2008), 112 pp. [ Lin, Bekey, and Abney 2008 available online ]
- Lomas, Meghann, Robert Chevalier, Ernest Vincent Cross, Robert Christopher Garrett, John Hoare, and Michael Kopack, 2012, “Explaining Robot Actions”, in Proceedings of the Seventh Annual ACM/IEEE International Conference on Human-Robot Interaction—HRI ’12 , Boston, MA: ACM Press, 187–188. doi:10.1145/2157689.2157748
- Macnish, Kevin, 2017, The Ethics of Surveillance: An Introduction , London: Routledge.
- Mathur, Arunesh, Gunes Acar, Michael J. Friedman, Elena Lucherini, Jonathan Mayer, Marshini Chetty, and Arvind Narayanan, 2019, “Dark Patterns at Scale: Findings from a Crawl of 11K Shopping Websites”, Proceedings of the ACM on Human-Computer Interaction , 3(CSCW): art. 81. doi:10.1145/3359183
- Minsky, Marvin, 1985, The Society of Mind , New York: Simon & Schuster.
- Misselhorn, Catrin, 2020, “Artificial Systems with Moral Capacities? A Research Design and Its Implementation in a Geriatric Care System”, Artificial Intelligence , 278: art. 103179. doi:10.1016/j.artint.2019.103179
- Mittelstadt, Brent Daniel and Luciano Floridi, 2016, “The Ethics of Big Data: Current and Foreseeable Issues in Biomedical Contexts”, Science and Engineering Ethics , 22(2): 303–341. doi:10.1007/s11948-015-9652-2
- Moor, James H., 2006, “The Nature, Importance, and Difficulty of Machine Ethics”, IEEE Intelligent Systems , 21(4): 18–21. doi:10.1109/MIS.2006.80
- Moravec, Hans, 1990, Mind Children , Cambridge, MA: Harvard University Press.
- –––, 1998, Robot: Mere Machine to Transcendent Mind , New York: Oxford University Press.
- Mozorov, Eygeny, 2013, To Save Everything, Click Here: The Folly of Technological Solutionism , New York: Public Affairs.
- Müller, Vincent C., 2012, “Autonomous Cognitive Systems in Real-World Environments: Less Control, More Flexibility and Better Interaction”, Cognitive Computation , 4(3): 212–215. doi:10.1007/s12559-012-9129-4
- –––, 2016a, “Autonomous Killer Robots Are Probably Good News”, In Drones and Responsibility: Legal, Philosophical and Socio-Technical Perspectives on the Use of Remotely Controlled Weapons , Ezio Di Nucci and Filippo Santoni de Sio (eds.), London: Ashgate, 67–81.
- ––– (ed.), 2016b, Risks of Artificial Intelligence , London: Chapman & Hall - CRC Press. doi:10.1201/b19187
- –––, 2018, “In 30 Schritten zum Mond? Zukünftiger Fortschritt in der KI”, Medienkorrespondenz , 20: 5–15. [ Müller 2018 available online ]
- –––, 2020, “Measuring Progress in Robotics: Benchmarking and the ‘Measure-Target Confusion’”, in Metrics of Sensory Motor Coordination and Integration in Robots and Animals , Fabio Bonsignorio, Elena Messina, Angel P. del Pobil, and John Hallam (eds.), (Cognitive Systems Monographs 36), Cham: Springer International Publishing, 169–179. doi:10.1007/978-3-030-14126-4_9
- –––, forthcoming-a, Can Machines Think? Fundamental Problems of Artificial Intelligence , New York: Oxford University Press.
- ––– (ed.), forthcoming-b, Oxford Handbook of the Philosophy of Artificial Intelligence , New York: Oxford University Press.
- Müller, Vincent C. and Nick Bostrom, 2016, “Future Progress in Artificial Intelligence: A Survey of Expert Opinion”, in Fundamental Issues of Artificial Intelligence , Vincent C. Müller (ed.), Cham: Springer International Publishing, 555–572. doi:10.1007/978-3-319-26485-1_33
- Newport, Cal, 2019, Digital Minimalism: On Living Better with Less Technology , London: Penguin.
- Nørskov, Marco (ed.), 2017, Social Robots , London: Routledge.
- Nyholm, Sven, 2018a, “Attributing Agency to Automated Systems: Reflections on Human–Robot Collaborations and Responsibility-Loci”, Science and Engineering Ethics , 24(4): 1201–1219. doi:10.1007/s11948-017-9943-x
- –––, 2018b, “The Ethics of Crashes with Self-Driving Cars: A Roadmap, II”, Philosophy Compass , 13(7): e12506. doi:10.1111/phc3.12506
- Nyholm, Sven, and Lily Frank, 2017, “From Sex Robots to Love Robots: Is Mutual Love with a Robot Possible?”, in Danaher and McArthur 2017: 219–243.
- O’Connell, Mark, 2017, To Be a Machine: Adventures among Cyborgs, Utopians, Hackers, and the Futurists Solving the Modest Problem of Death , London: Granta.
- O’Neil, Cathy, 2016, Weapons of Math Destruction: How Big Data Increases Inequality and Threatens Democracy , Largo, ML: Crown.
- Omohundro, Steve, 2014, “Autonomous Technology and the Greater Human Good”, Journal of Experimental & Theoretical Artificial Intelligence , 26(3): 303–315. doi:10.1080/0952813X.2014.895111
- Ord, Toby, 2020, The Precipice: Existential Risk and the Future of Humanity , London: Bloomsbury.
- Powers, Thomas M. and Jean-Gabriel Ganascia, forthcoming, “The Ethics of the Ethics of AI”, in Oxford Handbook of Ethics of Artificial Intelligence , Markus D. Dubber, Frank Pasquale, and Sunnit Das (eds.), New York: Oxford.
- Rawls, John, 1971, A Theory of Justice , Cambridge, MA: Belknap Press.
- Rees, Martin, 2018, On the Future: Prospects for Humanity , Princeton: Princeton University Press.
- Richardson, Kathleen, 2016, “Sex Robot Matters: Slavery, the Prostituted, and the Rights of Machines”, IEEE Technology and Society Magazine , 35(2): 46–53. doi:10.1109/MTS.2016.2554421
- Roessler, Beate, 2017, “Privacy as a Human Right”, Proceedings of the Aristotelian Society , 117(2): 187–206. doi:10.1093/arisoc/aox008
- Royakkers, Lambèr and Rinie van Est, 2016, Just Ordinary Robots: Automation from Love to War , Boca Raton, LA: CRC Press, Taylor & Francis. doi:10.1201/b18899
- Russell, Stuart, 2019, Human Compatible: Artificial Intelligence and the Problem of Control , New York: Viking.
- Russell, Stuart, Daniel Dewey, and Max Tegmark, 2015, “Research Priorities for Robust and Beneficial Artificial Intelligence”, AI Magazine , 36(4): 105–114. doi:10.1609/aimag.v36i4.2577
- SAE International, 2018, “Taxonomy and Definitions for Terms Related to Driving Automation Systems for on-Road Motor Vehicles”, J3016_201806, 15 June 2018. [ SAE International 2015 available online ]
- Sandberg, Anders, 2013, “Feasibility of Whole Brain Emulation”, in Philosophy and Theory of Artificial Intelligence , Vincent C. Müller (ed.), (Studies in Applied Philosophy, Epistemology and Rational Ethics, 5), Berlin, Heidelberg: Springer Berlin Heidelberg, 251–264. doi:10.1007/978-3-642-31674-6_19
- –––, 2019, “There Is Plenty of Time at the Bottom: The Economics, Risk and Ethics of Time Compression”, Foresight , 21(1): 84–99. doi:10.1108/FS-04-2018-0044
- Santoni de Sio, Filippo and Jeroen van den Hoven, 2018, “Meaningful Human Control over Autonomous Systems: A Philosophical Account”, Frontiers in Robotics and AI , 5(February): 15. doi:10.3389/frobt.2018.00015
- Schneier, Bruce, 2015, Data and Goliath: The Hidden Battles to Collect Your Data and Control Your World , New York: W. W. Norton.
- Searle, John R., 1980, “Minds, Brains, and Programs”, Behavioral and Brain Sciences , 3(3): 417–424. doi:10.1017/S0140525X00005756
- Selbst, Andrew D., Danah Boyd, Sorelle A. Friedler, Suresh Venkatasubramanian, and Janet Vertesi, 2019, “Fairness and Abstraction in Sociotechnical Systems”, in Proceedings of the Conference on Fairness, Accountability, and Transparency—FAT* ’19 , Atlanta, GA: ACM Press, 59–68. doi:10.1145/3287560.3287598
- Sennett, Richard, 2018, Building and Dwelling: Ethics for the City , London: Allen Lane.
- Shanahan, Murray, 2015, The Technological Singularity , Cambridge, MA: MIT Press.
- Sharkey, Amanda, 2019, “Autonomous Weapons Systems, Killer Robots and Human Dignity”, Ethics and Information Technology , 21(2): 75–87. doi:10.1007/s10676-018-9494-0
- Sharkey, Amanda and Noel Sharkey, 2011, “The Rights and Wrongs of Robot Care”, in Robot Ethics: The Ethical and Social Implications of Robotics , Patrick Lin, Keith Abney and George Bekey (eds.), Cambridge, MA: MIT Press, 267–282.
- Shoham, Yoav, Perrault Raymond, Brynjolfsson Erik, Jack Clark, James Manyika, Juan Carlos Niebles, … Zoe Bauer, 2018, “The AI Index 2018 Annual Report”, 17 December 2018, Stanford, CA: AI Index Steering Committee, Human-Centered AI Initiative, Stanford University. [ Shoam et al. 2018 available online ]
- SIENNA, 2019, “Deliverable Report D4.4: Ethical Issues in Artificial Intelligence and Robotics”, June 2019, published by the SIENNA project (Stakeholder-informed ethics for new technologies with high socio-economic and human rights impact), University of Twente, pp. 1–103. [ SIENNA 2019 available online ]
- Silver, David, Thomas Hubert, Julian Schrittwieser, Ioannis Antonoglou, Matthew Lai, Arthur Guez, Marc Lanctot, Laurent Sifre, Dharshan Kumaran, Thore Graepel, Timothy Lillicrap, Karen Simonyan, and Demis Hassabis, 2018, “A General Reinforcement Learning Algorithm That Masters Chess, Shogi, and Go through Self-Play”, Science , 362(6419): 1140–1144. doi:10.1126/science.aar6404
- Simon, Herbert A. and Allen Newell, 1958, “Heuristic Problem Solving: The Next Advance in Operations Research”, Operations Research , 6(1): 1–10. doi:10.1287/opre.6.1.1
- Simpson, Thomas W. and Vincent C. Müller, 2016, “Just War and Robots’ Killings”, The Philosophical Quarterly , 66(263): 302–322. doi:10.1093/pq/pqv075
- Smolan, Sandy (director), 2016, “The Human Face of Big Data”, PBS Documentary, 24 February 2016, 56 mins.
- Sparrow, Robert, 2007, “Killer Robots”, Journal of Applied Philosophy , 24(1): 62–77. doi:10.1111/j.1468-5930.2007.00346.x
- –––, 2016, “Robots in Aged Care: A Dystopian Future?”, AI & Society , 31(4): 445–454. doi:10.1007/s00146-015-0625-4
- Stahl, Bernd Carsten, Job Timmermans, and Brent Daniel Mittelstadt, 2016, “The Ethics of Computing: A Survey of the Computing-Oriented Literature”, ACM Computing Surveys , 48(4): art. 55. doi:10.1145/2871196
- Stahl, Bernd Carsten and David Wright, 2018, “Ethics and Privacy in AI and Big Data: Implementing Responsible Research and Innovation”, IEEE Security Privacy , 16(3): 26–33.
- Stone, Christopher D., 1972, “Should Trees Have Standing - toward Legal Rights for Natural Objects”, Southern California Law Review , 45: 450–501.
- Stone, Peter, Rodney Brooks, Erik Brynjolfsson, Ryan Calo, Oren Etzioni, Greg Hager, Julia Hirschberg, Shivaram Kalyanakrishnan, Ece Kamar, Sarit Kraus, Kevin Leyton-Brown, David Parkes, William Press, AnnaLee Saxenian, Julie Shah, Milind Tambe, and Astro Teller, 2016, “Artificial Intelligence and Life in 2030”, One Hundred Year Study on Artificial Intelligence: Report of the 2015–2016 Study Panel, Stanford University, Stanford, CA, September 2016. [ Stone et al. 2016 available online ]
- Strawson, Galen, 1998, “Free Will”, in Routledge Encyclopedia of Philosophy , Taylor & Francis. doi:10.4324/9780415249126-V014-1
- Sullins, John P., 2012, “Robots, Love, and Sex: The Ethics of Building a Love Machine”, IEEE Transactions on Affective Computing , 3(4): 398–409. doi:10.1109/T-AFFC.2012.31
- Susser, Daniel, Beate Roessler, and Helen Nissenbaum, 2019, “Technology, Autonomy, and Manipulation”, Internet Policy Review , 8(2): 30 June 2019. [ Susser, Roessler, and Nissenbaum 2019 available online ]
- Taddeo, Mariarosaria and Luciano Floridi, 2018, “How AI Can Be a Force for Good”, Science , 361(6404): 751–752. doi:10.1126/science.aat5991
- Taylor, Linnet and Nadezhda Purtova, 2019, “What Is Responsible and Sustainable Data Science?”, Big Data & Society, 6(2): art. 205395171985811. doi:10.1177/2053951719858114
- Taylor, Steve, et al., 2018, “Responsible AI – Key Themes, Concerns & Recommendations for European Research and Innovation: Summary of Consultation with Multidisciplinary Experts”, June. doi:10.5281/zenodo.1303252 [ Taylor, et al. 2018 available online ]
- Tegmark, Max, 2017, Life 3.0: Being Human in the Age of Artificial Intelligence , New York: Knopf.
- Thaler, Richard H and Sunstein, Cass, 2008, Nudge: Improving decisions about health, wealth and happiness , New York: Penguin.
- Thompson, Nicholas and Ian Bremmer, 2018, “The AI Cold War That Threatens Us All”, Wired , 23 November 2018. [ Thompson and Bremmer 2018 available online ]
- Thomson, Judith Jarvis, 1976, “Killing, Letting Die, and the Trolley Problem”, Monist , 59(2): 204–217. doi:10.5840/monist197659224
- Torrance, Steve, 2011, “Machine Ethics and the Idea of a More-Than-Human Moral World”, in Anderson and Anderson 2011: 115–137. doi:10.1017/CBO9780511978036.011
- Trump, Donald J, 2019, “Executive Order on Maintaining American Leadership in Artificial Intelligence”, 11 February 2019. [ Trump 2019 available online ]
- Turner, Jacob, 2019, Robot Rules: Regulating Artificial Intelligence , Berlin: Springer. doi:10.1007/978-3-319-96235-1
- Tzafestas, Spyros G., 2016, Roboethics: A Navigating Overview , (Intelligent Systems, Control and Automation: Science and Engineering 79), Cham: Springer International Publishing. doi:10.1007/978-3-319-21714-7
- Vallor, Shannon, 2017, Technology and the Virtues: A Philosophical Guide to a Future Worth Wanting , Oxford: Oxford University Press. doi:10.1093/acprof:oso/9780190498511.001.0001
- Van Lent, Michael, William Fisher, and Michael Mancuso, 2004, “An Explainable Artificial Intelligence System for Small-Unit Tactical Behavior”, in Proceedings of the 16th Conference on Innovative Applications of Artifical Intelligence, (IAAI’04) , San Jose, CA: AAAI Press, 900–907.
- van Wynsberghe, Aimee, 2016, Healthcare Robots: Ethics, Design and Implementation , London: Routledge. doi:10.4324/9781315586397
- van Wynsberghe, Aimee and Scott Robbins, 2019, “Critiquing the Reasons for Making Artificial Moral Agents”, Science and Engineering Ethics , 25(3): 719–735. doi:10.1007/s11948-018-0030-8
- Vanderelst, Dieter and Alan Winfield, 2018, “The Dark Side of Ethical Robots”, in Proceedings of the 2018 AAAI/ACM Conference on AI, Ethics, and Society , New Orleans, LA: ACM, 317–322. doi:10.1145/3278721.3278726
- Veale, Michael and Reuben Binns, 2017, “Fairer Machine Learning in the Real World: Mitigating Discrimination without Collecting Sensitive Data”, Big Data & Society , 4(2): art. 205395171774353. doi:10.1177/2053951717743530
- Véliz, Carissa, 2019, “Three Things Digital Ethics Can Learn from Medical Ethics”, Nature Electronics , 2(8): 316–318. doi:10.1038/s41928-019-0294-2
- Verbeek, Peter-Paul, 2011, Moralizing Technology: Understanding and Designing the Morality of Things , Chicago: University of Chicago Press.
- Wachter, Sandra and Brent Daniel Mittelstadt, 2019, “A Right to Reasonable Inferences: Re-Thinking Data Protection Law in the Age of Big Data and AI”, Columbia Business Law Review , 2019(2): 494–620.
- Wachter, Sandra, Brent Mittelstadt, and Luciano Floridi, 2017, “Why a Right to Explanation of Automated Decision-Making Does Not Exist in the General Data Protection Regulation”, International Data Privacy Law , 7(2): 76–99. doi:10.1093/idpl/ipx005
- Wachter, Sandra, Brent Mittelstadt, and Chris Russell, 2018, “Counterfactual Explanations Without Opening the Black Box: Automated Decisions and the GDPR”, Harvard Journal of Law & Technology , 31(2): 842–887. doi:10.2139/ssrn.3063289
- Wallach, Wendell and Peter M. Asaro (eds.), 2017, Machine Ethics and Robot Ethics , London: Routledge.
- Walsh, Toby, 2018, Machines That Think: The Future of Artificial Intelligence , Amherst, MA: Prometheus Books.
- Westlake, Stian (ed.), 2014, Our Work Here Is Done: Visions of a Robot Economy , London: Nesta. [ Westlake 2014 available online ]
- Whittaker, Meredith, Kate Crawford, Roel Dobbe, Genevieve Fried, Elizabeth Kaziunas, Varoon Mathur, … Jason Schultz, 2018, “AI Now Report 2018”, New York: AI Now Institute, New York University. [ Whittaker et al. 2018 available online ]
- Whittlestone, Jess, Rune Nyrup, Anna Alexandrova, Kanta Dihal, and Stephen Cave, 2019, “Ethical and Societal Implications of Algorithms, Data, and Artificial Intelligence: A Roadmap for Research”, Cambridge: Nuffield Foundation, University of Cambridge. [ Whittlestone 2019 available online ]
- Winfield, Alan, Katina Michael, Jeremy Pitt, and Vanessa Evers (eds.), 2019, Machine Ethics: The Design and Governance of Ethical AI and Autonomous Systems , special issue of Proceedings of the IEEE , 107(3): 501–632.
- Woollard, Fiona and Frances Howard-Snyder, 2016, “Doing vs. Allowing Harm”, Stanford Encyclopedia of Philosophy (Winter 2016 edition), Edward N. Zalta (ed.), URL = < https://plato.stanford.edu/archives/win2016/entries/doing-allowing/ >
- Woolley, Samuel C. and Philip N. Howard (eds.), 2017, Computational Propaganda: Political Parties, Politicians, and Political Manipulation on Social Media , Oxford: Oxford University Press. doi:10.1093/oso/9780190931407.001.0001
- Yampolskiy, Roman V. (ed.), 2018, Artificial Intelligence Safety and Security , Boca Raton, FL: Chapman and Hall/CRC. doi:10.1201/9781351251389
- Yeung, Karen and Martin Lodge (eds.), 2019, Algorithmic Regulation , Oxford: Oxford University Press. doi:10.1093/oso/9780198838494.001.0001
- Zayed, Yago and Philip Loft, 2019, “Agriculture: Historical Statistics”, House of Commons Briefing Paper , 3339(25 June 2019): 1-19. [ Zayed and Loft 2019 available online ]
- Zerilli, John, Alistair Knott, James Maclaurin, and Colin Gavaghan, 2019, “Transparency in Algorithmic and Human Decision-Making: Is There a Double Standard?”, Philosophy & Technology , 32(4): 661–683. doi:10.1007/s13347-018-0330-6
- Zuboff, Shoshana, 2019, The Age of Surveillance Capitalism: The Fight for a Human Future at the New Frontier of Power , New York: Public Affairs.
How to cite this entry . Preview the PDF version of this entry at the Friends of the SEP Society . Look up topics and thinkers related to this entry at the Internet Philosophy Ontology Project (InPhO). Enhanced bibliography for this entry at PhilPapers , with links to its database.
Other Internet Resources
- AI HLEG, 2019, “ High-Level Expert Group on Artificial Intelligence: Ethics Guidelines for Trustworthy AI ”, European Commission , accessed: 9 April 2019.
- Amodei, Dario and Danny Hernandez, 2018, “ AI and Compute ”, OpenAI Blog , 16 July 2018.
- Aneesh, A., 2002, Technological Modes of Governance: Beyond Private and Public Realms , paper in the Proceedings of the 4th International Summer Academy on Technology Studies, available at archive.org.
- Brooks, Rodney, 2017, “ The Seven Deadly Sins of Predicting the Future of AI ”, on Rodney Brooks: Robots, AI, and Other Stuff , 7 September 2017.
- Brundage, Miles, Shahar Avin, Jack Clark, Helen Toner, Peter Eckersley, Ben Garfinkel, Allan Dafoe, Paul Scharre, Thomas Zeitzoff, Bobby Filar, Hyrum Anderson, Heather Roff, Gregory C. Allen, Jacob Steinhardt, Carrick Flynn, Seán Ó hÉigeartaigh, Simon Beard, Haydn Belfield, Sebastian Farquhar, Clare Lyle, et al., 2018, “ The Malicious Use of Artificial Intelligence: Forecasting, Prevention, and Mitigation ”, unpublished manuscript, ArXiv:1802.07228 [Cs].
- Costa, Elisabeth and David Halpern, 2019, “ The Behavioural Science of Online Harm and Manipulation, and What to Do About It: An Exploratory Paper to Spark Ideas and Debate ”, The Behavioural Insights Team Report, 1-82.
- Gebru, Timnit, Jamie Morgenstern, Briana Vecchione, Jennifer Wortman Vaughan, Hanna Wallach, Hal Daumeé III, and Kate Crawford, 2018, “ Datasheets for Datasets ”, unpublished manuscript, arxiv:1803.09010, 23 March 2018.
- Gunning, David, 2017, “ Explainable Artificial Intelligence (XAI) ”, Defense Advanced Research Projects Agency (DARPA) Program.
- Harris, Tristan, 2016, “ How Technology Is Hijacking Your Mind—from a Magician and Google Design Ethicist ”, Thrive Global , 18 May 2016.
- International Federation of Robotics (IFR), 2019, World Robotics 2019 Edition .
- Jacobs, An, Lynn Tytgat, Michel Maus, Romain Meeusen, and Bram Vanderborght (eds.), Homo Roboticus: 30 Questions and Answers on Man, Technology, Science & Art, 2019, Brussels: ASP .
- Marcus, Gary, 2018, “ Deep Learning: A Critical Appraisal ”, unpublished manuscript, 2 January 2018, arxiv:1801.00631.
- McCarthy, John, Marvin Minsky, Nathaniel Rochester, and Claude E. Shannon, 1955, “ A Proposal for the Dartmouth Summer Research Project on Artificial Intelligence ”, 31 August 1955.
- Metcalf, Jacob, Emily F. Keller, and Danah Boyd, 2016, “ Perspectives on Big Data, Ethics, and Society ”, 23 May 2016, Council for Big Data, Ethics, and Society.
- National Institute of Justice (NIJ), 2014, “ Overview of Predictive Policing ”, 9 June 2014.
- Searle, John R., 2015, “ Consciousness in Artificial Intelligence ”, Google’s Singularity Network, Talks at Google (YouTube video).
- Sharkey, Noel, Aimee van Wynsberghe, Scott Robbins, and Eleanor Hancock, 2017, “ Report: Our Sexual Future with Robots ”, Responsible Robotics , 1–44.
- Turing Institute (UK): Data Ethics Group
- Leverhulme Centre for the Future of Intelligence
- Future of Humanity Institute
- Future of Life Institute
- Stanford Center for Internet and Society
- Berkman Klein Center
- Digital Ethics Lab
- Open Roboethics Institute
- Philosophy & Theory of AI
- Ethics and AI 2017
- We Robot 2018
- Robophilosophy
- EUrobotics TG ‘robot ethics’ collection of policy documents
- PhilPapers section on Ethics of Artificial Intelligence
- PhilPapers section on Robot Ethics
computing: and moral responsibility | ethics: internet research | ethics: search engines and | information technology: and moral values | information technology: and privacy | manipulation, ethics of | social networking and ethics
Acknowledgments
Early drafts of this article were discussed with colleagues at the IDEA Centre of the University of Leeds, some friends, and my PhD students Michael Cannon, Zach Gudmunsen, Gabriela Arriagada-Bruneau and Charlotte Stix. Later drafts were made publicly available on the Internet and publicised via Twitter and e-mail to all (then) cited authors that I could locate. These later drafts were presented to audiences at the INBOTS Project Meeting (Reykjavik 2019), the Computer Science Department Colloquium (Leeds 2019), the European Robotics Forum (Bucharest 2019), the AI Lunch and the Philosophy & Ethics group (Eindhoven 2019)—many thanks for their comments.
I am grateful for detailed written comments by John Danaher, Martin Gibert, Elizabeth O’Neill, Sven Nyholm, Etienne B. Roesch, Emma Ruttkamp-Bloem, Tom Powers, Steve Taylor, and Alan Winfield. I am grateful for further useful comments by Colin Allen, Susan Anderson, Christof Wolf-Brenner, Rafael Capurro, Mark Coeckelbergh, Yazmin Morlet Corti, Erez Firt, Vasilis Galanos, Anne Gerdes, Olle Häggström, Geoff Keeling, Karabo Maiyane, Brent Mittelstadt, Britt Östlund, Steve Petersen, Brian Pickering, Zoë Porter, Amanda Sharkey, Melissa Terras, Stuart Russell, Jan F Veneman, Jeffrey White, and Xinyi Wu.
Parts of the work on this article have been supported by the European Commission under the INBOTS project (H2020 grant no. 780073).
Copyright © 2020 by Vincent C. Müller < vincent . c . mueller @ fau . de >
- Accessibility
Support SEP
Mirror sites.
View this site from another server:
- Info about mirror sites
The Stanford Encyclopedia of Philosophy is copyright © 2023 by The Metaphysics Research Lab , Department of Philosophy, Stanford University
Library of Congress Catalog Data: ISSN 1095-5054
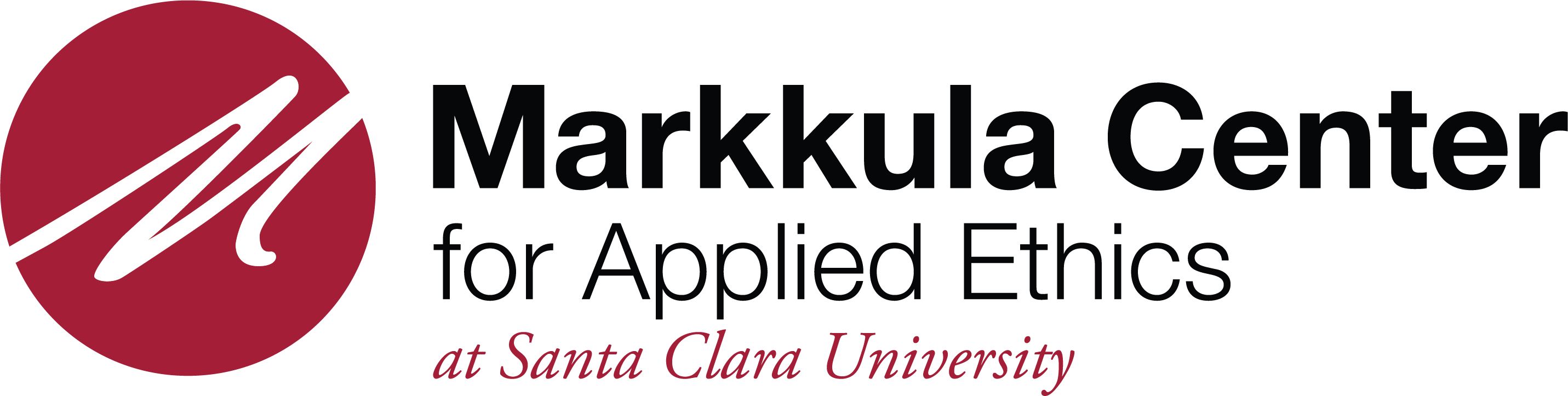
Artificial Intelligence and Ethics: Sixteen Challenges and Opportunities
- Markkula Center for Applied Ethics
- Ethics Resources
- Ethics Blogs
- All About Ethics
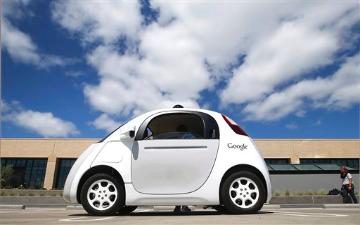
Artificial intelligence offers great opportunity, but it also brings potential hazards—this article presents 16 of them.
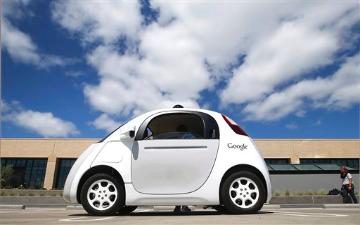
a white Google AI car parked against a backdrop of blue sky with white clouds
Tony Avelar/Associated Press
Brian Patrick Green is the director of Technology Ethics at the Markkula Center for Applied Ethics. This article is an update of an earlier article [1]. Views are his own.
Artificial intelligence and machine learning technologies are rapidly transforming society and will continue to do so in the coming decades. This social transformation will have deep ethical impact, with these powerful new technologies both improving and disrupting human lives. AI, as the externalization of human intelligence, offers us in amplified form everything that humanity already is, both good and evil. Much is at stake. At this crossroads in history we should think very carefully about how to make this transition, or we risk empowering the grimmer side of our nature, rather than the brighter.
Why is AI ethics becoming a problem now? Machine learning (ML) through neural networks is advancing rapidly for three reasons: 1) Huge increase in the size of data sets; 2) Huge increase in computing power; 3) Huge improvement in ML algorithms and more human talent to write them. All three of these trends are centralizing of power, and “With great power comes great responsibility” [2].
As an institution, the Markkula Center for Applied Ethics has been thinking deeply about the ethics of AI for several years. This article began as presentations delivered at academic conferences and has since expanded to an academic paper (links below) and most recently to a presentation of “Artificial Intelligence and Ethics: Sixteen Issues” I have given in the U.S. and internationally [3]. In that spirit, I offer this current list:
1. Technical Safety
The first question for any technology is whether it works as intended. Will AI systems work as they are promised or will they fail? If and when they fail, what will be the results of those failures? And if we are dependent upon them, will we be able to survive without them?
For example, several people have died in a semi-autonomous car accident because vehicles encountered situations in which they failed to make safe decisions. While writing very detailed contracts that limit liability might legally reduce a manufacturer’s responsibility, from a moral perspective, not only is responsibility still with the company, but the contract itself can be seen as an unethical scheme to avoid legitimate responsibility.
The question of technical safety and failure is separate from the question of how a properly-functioning technology might be used for good or for evil (questions 3 and 4, below). This question is merely one of function, yet it is the foundation upon which all the rest of the analysis must build.
2. Transparency and Privacy
Once we have determined that the technology functions adequately, can we actually understand how it works and properly gather data on its functioning? Ethical analysis always depends on getting the facts first—only then can evaluation begin.
It turns out that with some machine learning techniques such as deep learning in neural networks it can be difficult or impossible to really understand why the machine is making the choices that it makes. In other cases, it might be that the machine can explain something, but the explanation is too complex for humans to understand.
For example, in 2014 a computer proved a mathematical theorem, using a proof that was, at the time at least, longer than the entire Wikipedia encyclopedia [4]. Explanations of this sort might be true explanations, but humans will never know for sure.
As an additional point, in general, the more powerful someone or something is, the more transparent it ought to be, while the weaker someone is, the more right to privacy he or she should have. Therefore the idea that powerful AIs might be intrinsically opaque is disconcerting.
3. Beneficial Use & Capacity for Good
The main purpose of AI is, like every other technology, to help people lead longer, more flourishing, more fulfilling lives. This is good, and therefore insofar as AI helps people in these ways, we can be glad and appreciate the benefits it gives to us.
Additional intelligence will likely provide improvements in nearly every field of human endeavor, including, for example, archaeology, biomedical research, communication, data analytics, education, energy efficiency, environmental protection, farming, finance, legal services, medical diagnostics, resource management, space exploration, transportation, waste management, and so on.
As just one concrete example of a benefit from AI, some farm equipment now has computer systems capable of visually identifying weeds and spraying them with tiny targeted doses of herbicide. This not only protects the environment by reducing the use of chemicals on crops, but it also protects human health by reducing exposure to these chemicals.
4. Malicious Use & Capacity for Evil
A perfectly well functioning technology, such as a nuclear weapon, can, when put to its intended use, cause immense evil. Artificial intelligence, like human intelligence, will be used maliciously, there is no doubt.
For example, AI-powered surveillance is already widespread, in both appropriate contexts (e.g., airport-security cameras), perhaps inappropriate ones (e.g., products with always-on microphones in our homes), and conclusively inappropriate ones (e.g., products which help authoritarian regimes identify and oppress their citizens). Other nefarious examples can include AI-assisted computer-hacking and lethal autonomous weapons systems (LAWS), a.k.a. “killer robots.” Additional fears, of varying degrees of plausibility, include scenarios like those in the movies “2001: A Space Odyssey,” “Wargames,” and “Terminator.”
While movies and weapons technologies might seem to be extreme examples of how AI might empower evil, we should remember that competition and war are always primary drivers of technological advance, and that militaries and corporations are working on these technologies right now. History also shows that great evils are not always completely intended (e.g., stumbling into World War I and various nuclear close-calls in the Cold War), and so having destructive power, even if not intending to use it, still risks catastrophe. Because of this, forbidding, banning, and relinquishing certain types of technology would be the most prudent solution.
5. Bias in Data, Training Sets, etc.
One of the interesting things about neural networks, the current workhorses of artificial intelligence, is that they effectively merge a computer program with the data that is given to it. This has many benefits, but it also risks biasing the entire system in unexpected and potentially detrimental ways.
Already algorithmic bias has been discovered, for example, in areas ranging from criminal punishment to photograph captioning. These biases are more than just embarrassing to the corporations which produce these defective products; they have concrete negative and harmful effects on the people who are the victims of these biases, as well as reducing trust in corporations, government, and other institutions which might be using these biased products. Algorithmic bias is one of the major concerns in AI right now and will remain so in the future unless we endeavor to make our technological products better than we are. As one person said at the first meeting of the Partnership on AI, “We will reproduce all of our human faults in artificial form unless we strive right now to make sure that we don’t” [5].
6. Unemployment / Lack of Purpose & Meaning
Many people have already perceived that AI will be a threat to certain categories of jobs. Indeed, automation of industry has been a major contributing factor in job losses since the beginning of the industrial revolution. AI will simply extend this trend to more fields, including fields that have been traditionally thought of as being safer from automation, for example law, medicine, and education. It is not clear what new careers unemployed people ultimately will be able to transition into, although the more that labor has to do with caring for others, the more likely people will want to be dealing with other humans and not AIs.
Attached to the concern for employment is the concern for how humanity spends its time and what makes a life well-spent. What will millions of unemployed people do? What good purposes can they have? What can they contribute to the well-being of society? How will society prevent them from becoming disillusioned, bitter, and swept up in evil movements such as white supremacy and terrorism?
7. Growing Socio-Economic Inequality
Related to the unemployment problem is the question of how people will survive if unemployment rises to very high levels. Where will they get money to maintain themselves and their families? While prices may decrease due to lowered cost of production, those who control AI will also likely rake in much of the money that would have otherwise gone into the wages of the now-unemployed, and therefore economic inequality will increase. This will also affect international economic disparity, and therefore is likely a major threat to less-developed nations.
Some have suggested a universal basic income (UBI) to address the problem, but this will require a major restructuring of national economies. Various other solutions to this problem may be possible, but they all involve potentially major changes to human society and government. Ultimately this is a political problem, not a technical one, so this solution, like those to many of the problems described here, needs to be addressed at the political level.
8. Environmental Effects
Machine learning models require enormous amounts of energy to train, so much energy that the costs can run into the tens of millions of dollars or more. Needless to say, if this energy is coming from fossil fuels, this is a large negative impact on climate change, not to mention being harmful at other points in the hydrocarbon supply chain.
Machine learning can also make electrical distribution and use much more efficient, as well as working on solving problems in biodiversity, environmental research, resource management, etc. AI is in some very basic ways a technology focused on efficiency, and energy efficiency is one way that its capabilities can be directed.
On balance, it looks like AI could be a net positive for the environment [6]—but only if it is actually directed towards that positive end, and not just towards consuming energy for other uses.
9. Automating Ethics
One strength of AI is that it can automate decision-making, thus lowering the burden on humans and speeding up – potentially greatly speeding up—some kinds of decision-making processes. However, this automation of decision making will presents huge problems for society, because if these automated decisions are good, society will benefit, but if they are bad, society will be harmed.
As AI agents are given more powers to make decisions, they will need to have ethical standards of some sort encoded into them. There is simply no way around it: the ethical decision-making process might be as simple as following a program to fairly distribute a benefit, wherein the decision is made by humans and executed by algorithms, but it also might entail much more detailed ethical analysis, even if we humans would prefer that it did not—this is because Ai will operate so much faster than humans can, that under some circumstances humans will be left “out of the loop” of control due to human slowness. This already occurs with cyberattacks, and high-frequency trading (both of which are filled with ethical questions which are typically ignored) and it will only get worse as AI expands its role in society.
Since AI can be so powerful, the ethical standards we give to it had better be good.
10. Moral Deskilling & Debility
If we turn over our decision-making capacities to machines, we will become less experienced at making decisions. For example, this is a well-known phenomenon among airline pilots: the autopilot can do everything about flying an airplane, from take-off to landing, but pilots intentionally choose to manually control the aircraft at crucial times (e.g., take-off and landing) in order to maintain their piloting skills.
Because one of the uses of AI will be to either assist or replace humans at making certain types of decisions (e.g. spelling, driving, stock-trading, etc.), we should be aware that humans may become worse at these skills. In its most extreme form, if AI starts to make ethical and political decisions for us, we will become worse at ethics and politics. We may reduce or stunt our moral development precisely at the time when our power has become greatest and our decisions the most important.
This means that the study of ethics and ethics training are now more important than ever. We should determine ways in which AI can actually enhance our ethical learning and training. We should never allow ourselves to become deskilled and debilitated at ethics, or when our technology finally does present us with hard choices to make and problems we must solve—choices and problems that, perhaps, our ancestors would have been capable of solving—future humans might not be able to do it.
For more on deskilling, see this article [7] and Shannon Vallor’s original article on the topic [8].
11. AI Consciousness, Personhood, and “Robot Rights”
Some thinkers have wondered whether AIs might eventually become self-conscious, attain their own volition, or otherwise deserve recognition as persons like ourselves. Legally speaking, personhood has been given to corporations and (in other countries) rivers, so there is certainly no need for consciousness even before legal questions may arise.
Morally speaking, we can anticipate that technologists will attempt to make the most human-like AIs and robots possible, and perhaps someday they will be such good imitations that we will wonder if they might be conscious and deserve rights—and we might not be able to determine this conclusively. If future humans do conclude AIs and robots might be worthy of moral status, then we ought to err on the side of caution and give it.
In the midst of this uncertainty about the status of our creations, what we will know is that we humans have moral characters and that, to follow an inexact quote of Aristotle, “we become what we repeatedly do” [9]. So we ought not to treat AIs and robots badly, or we might be habituating ourselves towards having flawed characters, regardless of the moral status of the artificial beings we are interacting with. In other words, no matter the status of AIs and robots, for the sake of our own moral characters we ought to treat them well, or at least not abuse them.
12. AGI and Superintelligence
If or when AI reaches human levels of intelligence, doing everything that humans can do as well the average human can, then it will be an Artificial General Intelligence—an AGI—and it will be the only other such intelligence to exist on Earth at the human level.
If or when AGI exceeds human intelligence, it will become a superintelligence, an entity potentially vastly more clever and capable than we are: something humans have only ever related to in religions, myths, and stories.
Importantly here, AI technology is improving exceedingly fast. Global corporations and governments are in a race to claim the powers of AI as their own. Equally importantly, there is no reason why the improvement of AI would stop at AGI. AI is scalable and fast. Unlike a human brain, if we give AI more hardware it will do more and more, faster and faster.
The advent of AGI or superintelligence will mark the dethroning of humanity as the most intelligent thing on Earth. We have never faced (in the material world) anything smarter than us before. Every time Homo sapiens encountered other intelligent human species in the history of life on Earth, the other species either genetically merged with us (as Neanderthals did) or was driven extinct. As we encounter AGI and superintelligence, we ought to keep this in mind; though, because AI is a tool, there may be ways yet to maintain an ethical balance between human and machine.
13. Dependency on AI
Humans depend on technology. We always have, ever since we have been “human;” our technological dependency is almost what defines us as a species. What used to be just rocks, sticks, and fur clothes has now become much more complex and fragile, however. Losing electricity or cell connectivity can be a serious problem, psychologically or even medically (if there is an emergency). And there is no dependence like intelligence dependence.
Intelligence dependence is a form of dependence like that of a child to an adult. Much of the time, children rely on adults to think for them, and in our older years, as some people experience cognitive decline, the elderly rely on younger adults too. Now imagine that middle-aged adults who are looking after children and the elderly are themselves dependent upon AI to guide them. There would be no human “adults” left—only “AI adults.” Humankind would have become a race of children to our AI caregivers.
This, of course, raises the question of what an infantilized human race would do if our AI parents ever malfunctioned. Without that AI, if dependent on it, we could become like lost children not knowing how to take care of ourselves or our technological society. This “lostness” already happens when smartphone navigation apps malfunction (or the battery just runs out), for example.
We are already well down the path to technological dependency. How can we prepare now so that we can avoid the dangers of specifically intelligence dependency on AI?
14. AI-powered Addiction
Smartphone app makers have turned addiction into a science, and AI-powered video games and apps can be addictive like drugs. AI can exploit numerous human desires and weaknesses including purpose-seeking, gambling, greed, libido, violence, and so on.
Addiction not only manipulates and controls us; it also prevents us from doing other more important things—educational, economic, and social. It enslaves us and wastes our time when we could be doing something worthwhile. With AI constantly learning more about us and working harder to keep us clicking and scrolling, what hope is there for us to escape its clutches? Or, rather, the clutches of the app makers who create these AIs to trap us—because it is not the AIs that choose to treat people this way, it is other people.
When I talk about this topic with any group of students, I discover that all of them are “addicted” to one app or another. It may not be a clinical addiction, but that is the way that the students define it, and they know they are being exploited and harmed. This is something that app makers need to stop doing: AI should not be designed to intentionally exploit vulnerabilities in human psychology.
15. Isolation and Loneliness
Society is in a crisis of loneliness. For example, recently a study found that “200,000 older people in the UK have not had a conversation with a friend or relative in more than a month” [10]. This is a sad state of affairs because loneliness can literally kill [11]. It is a public health nightmare, not to mention destructive of the very fabric of society: our human relationships. Technology has been implicated in so many negative social and psychological trends, including loneliness, isolation, depression, stress, and anxiety, that it is easy to forget that things could be different, and in fact were quite different only a few decades ago.
One might think that “social” media, smartphones, and AI could help, but in fact they are major causes of loneliness since people are facing screens instead of each other. What does help are strong in-person relationships, precisely the relationships that are being pushed out by addictive (often AI-powered) technology.
Loneliness can be helped by dropping devices and building quality in-person relationships. In other words: caring.
This may not be easy work and certainly at the societal level it may be very difficult to resist the trends we have already followed so far. But resist we should, because a better, more humane world is possible. Technology does not have to make the world a less personal and caring place—it could do the opposite, if we wanted it to.
16. Effects on the Human Spirit
All of the above areas of interest will have effects on how humans perceive themselves, relate to each other, and live their lives. But there is a more existential question too. If the purpose and identity of humanity has something to do with our intelligence (as several prominent Greek philosophers believed, for example), then by externalizing our intelligence and improving it beyond human intelligence, are we making ourselves second-class beings to our own creations?
This is a deeper question with artificial intelligence which cuts to the core of our humanity, into areas traditionally reserved for philosophy, spirituality, and religion. What will happen to the human spirit if or when we are bested by our own creations in everything that we do? Will human life lose meaning? Will we come to a new discovery of our identity beyond our intelligence?
Perhaps intelligence is not really as important to our identity as we might think it is, and perhaps turning over intelligence to machines will help us to realize that. If we instead find our humanity not in our brains, but in our hearts, perhaps we will come to recognize that caring, compassion, kindness, and love are ultimately what make us human and what make life worth living. Perhaps by taking away some of the tedium of life, AI can help us to fulfill this vision of a more humane world.
There are more issues in the ethics of AI; here I have just attempted to point out some major ones. Much more time could be spent on topics like AI-powered surveillance, the role of AI in promoting misinformation and disinformation, the role of AI in politics and international relations, the governance of AI, and so on.
New technologies are always created for the sake of something good—and AI offers us amazing new abilities to help people and make the world a better place. But in order to make the world a better place we need to choose to do that, in accord with ethics.
Through the concerted effort of many individuals and organizations, we can hope that AI technology will help us to make a better world.
This article builds upon the following previous works: “AI: Ethical Challenges and a Fast Approaching Future” (Oct. 2017) [12], “Some Ethical and Theological Reflections on Artificial Intelligence,” (Nov. 2017) [13], Artificial Intelligence and Ethics: Ten areas of interest (Nov. 2017) [1], “AI and Ethics” (Mar. 2018) [14], “Ethical Reflections on Artificial Intelligence”(Aug. 2018) [15], and several presentations of “Artificial Intelligence and Ethics: Sixteen Issues” (2019-20) [3].
[1] Brian Patrick Green, “Artificial Intelligence and Ethics: Ten areas of interest,” Markkula Center for Applied Ethics website , Nov 21, 2017.
[2] Originally paraphrased in Stan Lee and Steve Ditko, “Spider-Man,” Amazing Fantasy vol. 1, #15 (August 1962), exact phrase from Uncle Ben in J. Michael Straczynski, Amazing Spider-Man vol. 2, #38 (February 2002). For more information: https://en.wikipedia.org/wiki/With_great_power_comes_great_responsibility
[3] Brian Patrick Green, “Artificial Intelligence and Ethics: Sixteen Issues,” various locations and dates: Los Angeles, Mexico City, San Francisco, Santa Clara University (2019-2020).
[4] Bob Yirka, “Computer generated math proof is too large for humans to check,” Phys.org , February 19, 2014, available at: https://phys.org/news/2014-02-math-proof-large-humans.html
[5] The Partnership on AI to Benefit People and Society, Inaugural Meeting, Berlin, Germany, October 23-24, 2017.
[6] Leila Scola, “AI and the Ethics of Energy Efficiency,” Markkula Center for Applied Ethics website , May 26, 2020, available at: https://www.scu.edu/environmental-ethics/resources/ai-and-the-ethics-of-energy-efficiency/
[7] Brian Patrick Green, “Artificial Intelligence, Decision-Making, and Moral Deskilling,” Markkula Center for Applied Ethics website , Mar 15, 2019, available at: https://www.scu.edu/ethics/focus-areas/technology-ethics/resources/artificial-intelligence-decision-making-and-moral-deskilling/
[8] Shannon Vallor, “Moral Deskilling and Upskilling in a New Machine Age: Reflections on the Ambiguous Future of Character.” Philosophy of Technology 28 (2015):107–124., available at: https://link.springer.com/article/10.1007/s13347-014-0156-9
[9] Brad Sylvester, “Fact Check: Did Aristotle Say, ‘We Are What We Repeatedly Do’?” Check Your Fact website , June 26, 2019, available at: https://checkyourfact.com/2019/06/26/fact-check-aristotle-excellence-habit-repeatedly-do/
[10] Lee Mannion, “Britain appoints minister for loneliness amid growing isolation,” Reuters , January 17, 2018, available at: https://www.reuters.com/article/us-britain-politics-health/britain-appoints-minister-for-loneliness-amid-growing-isolation-idUSKBN1F61I6
[11] Julianne Holt-Lunstad, Timothy B. Smith, Mark Baker,Tyler Harris, and David Stephenson, “Loneliness and Social Isolation as Risk Factors for Mortality: A Meta-Analytic Review,” Perspectives on Psychological Science 10(2) (2015): 227–237, available at: https://journals.sagepub.com/doi/full/10.1177/1745691614568352
[12] Markkula Center for Applied Ethics Staff, “AI: Ethical Challenges and a Fast Approaching Future: A panel discussion on artificial intelligence,” with Maya Ackerman, Sanjiv Das, Brian Green, and Irina Raicu, Santa Clara University, California, October 24, 2017, posted to the All About Ethics Blog , Oct 31, 2017, video available at: https://www.scu.edu/ethics/all-about-ethics/ai-ethical-challenges-and-a-fast-approaching-future/
[13] Brian Patrick Green, “Some Ethical and Theological Reflections on Artificial Intelligence,” Pacific Coast Theological Society (PCTS) meeting, Graduate Theological Union, Berkeley, 3-4 November, 2017, available at: http://dx.doi.org/10.12775/SetF.2018.015
[14] Brian Patrick Green, “AI and Ethics,” guest lecture in PACS003: What is an Ethical Life? , University of the Pacific, Stockton, March 21, 2018.
[15] Brian Patrick Green, “Ethical Reflections on Artificial Intelligence,” Scientia et Fides 6(2), 24 August 2018. Available at: https://apcz.umk.pl/SetF/article/view/SetF.2018.015/15729
Thank you to many people for all the helpful feedback which has helped me develop this list, including Maya Ackermann, Kirk Bresniker, Sanjiv Das, Kirk Hanson, Brian Klunk, Thane Kreiner, Angelus McNally, Irina Raicu, Leila Scola, Lili Tavlan, Shannon Vallor, the employees of several tech companies, the attendees of the PCTS Fall 2017 meeting, the attendees of the needed.education meetings, several anonymous reviewers, the professors and students of PACS003 at the University of the Pacific, the students of my ENGR 344: AI and Ethics course, as well as many more.
Subscribe to Our Blogs
- Benison: The Practice of Ethical Leadership
- Center News
- Ethical Dilemmas in the Social Sector
- Internet Ethics: Views from Silicon Valley
- Internet Ethics: Views from Silicon Valley
IEEE Account
- Change Username/Password
- Update Address
Purchase Details
- Payment Options
- Order History
- View Purchased Documents
Profile Information
- Communications Preferences
- Profession and Education
- Technical Interests
- US & Canada: +1 800 678 4333
- Worldwide: +1 732 981 0060
- Contact & Support
- About IEEE Xplore
- Accessibility
- Terms of Use
- Nondiscrimination Policy
- Privacy & Opting Out of Cookies
A not-for-profit organization, IEEE is the world's largest technical professional organization dedicated to advancing technology for the benefit of humanity. © Copyright 2024 IEEE - All rights reserved. Use of this web site signifies your agreement to the terms and conditions.
- Search Menu
Sign in through your institution
- Browse content in Arts and Humanities
- Browse content in Archaeology
- Anglo-Saxon and Medieval Archaeology
- Archaeological Methodology and Techniques
- Archaeology by Region
- Archaeology of Religion
- Archaeology of Trade and Exchange
- Biblical Archaeology
- Contemporary and Public Archaeology
- Environmental Archaeology
- Historical Archaeology
- History and Theory of Archaeology
- Industrial Archaeology
- Landscape Archaeology
- Mortuary Archaeology
- Prehistoric Archaeology
- Underwater Archaeology
- Zooarchaeology
- Browse content in Architecture
- Architectural Structure and Design
- History of Architecture
- Residential and Domestic Buildings
- Theory of Architecture
- Browse content in Art
- Art Subjects and Themes
- History of Art
- Industrial and Commercial Art
- Theory of Art
- Biographical Studies
- Byzantine Studies
- Browse content in Classical Studies
- Classical History
- Classical Philosophy
- Classical Mythology
- Classical Numismatics
- Classical Literature
- Classical Reception
- Classical Art and Architecture
- Classical Oratory and Rhetoric
- Greek and Roman Epigraphy
- Greek and Roman Law
- Greek and Roman Papyrology
- Greek and Roman Archaeology
- Late Antiquity
- Religion in the Ancient World
- Social History
- Digital Humanities
- Browse content in History
- Colonialism and Imperialism
- Diplomatic History
- Environmental History
- Genealogy, Heraldry, Names, and Honours
- Genocide and Ethnic Cleansing
- Historical Geography
- History by Period
- History of Emotions
- History of Agriculture
- History of Education
- History of Gender and Sexuality
- Industrial History
- Intellectual History
- International History
- Labour History
- Legal and Constitutional History
- Local and Family History
- Maritime History
- Military History
- National Liberation and Post-Colonialism
- Oral History
- Political History
- Public History
- Regional and National History
- Revolutions and Rebellions
- Slavery and Abolition of Slavery
- Social and Cultural History
- Theory, Methods, and Historiography
- Urban History
- World History
- Browse content in Language Teaching and Learning
- Language Learning (Specific Skills)
- Language Teaching Theory and Methods
- Browse content in Linguistics
- Applied Linguistics
- Cognitive Linguistics
- Computational Linguistics
- Forensic Linguistics
- Grammar, Syntax and Morphology
- Historical and Diachronic Linguistics
- History of English
- Language Acquisition
- Language Evolution
- Language Reference
- Language Variation
- Language Families
- Lexicography
- Linguistic Anthropology
- Linguistic Theories
- Linguistic Typology
- Phonetics and Phonology
- Psycholinguistics
- Sociolinguistics
- Translation and Interpretation
- Writing Systems
- Browse content in Literature
- Bibliography
- Children's Literature Studies
- Literary Studies (Asian)
- Literary Studies (European)
- Literary Studies (Eco-criticism)
- Literary Studies (Romanticism)
- Literary Studies (American)
- Literary Studies (Modernism)
- Literary Studies - World
- Literary Studies (1500 to 1800)
- Literary Studies (19th Century)
- Literary Studies (20th Century onwards)
- Literary Studies (African American Literature)
- Literary Studies (British and Irish)
- Literary Studies (Early and Medieval)
- Literary Studies (Fiction, Novelists, and Prose Writers)
- Literary Studies (Gender Studies)
- Literary Studies (Graphic Novels)
- Literary Studies (History of the Book)
- Literary Studies (Plays and Playwrights)
- Literary Studies (Poetry and Poets)
- Literary Studies (Postcolonial Literature)
- Literary Studies (Queer Studies)
- Literary Studies (Science Fiction)
- Literary Studies (Travel Literature)
- Literary Studies (War Literature)
- Literary Studies (Women's Writing)
- Literary Theory and Cultural Studies
- Mythology and Folklore
- Shakespeare Studies and Criticism
- Browse content in Media Studies
- Browse content in Music
- Applied Music
- Dance and Music
- Ethics in Music
- Ethnomusicology
- Gender and Sexuality in Music
- Medicine and Music
- Music Cultures
- Music and Religion
- Music and Media
- Music and Culture
- Music Education and Pedagogy
- Music Theory and Analysis
- Musical Scores, Lyrics, and Libretti
- Musical Structures, Styles, and Techniques
- Musicology and Music History
- Performance Practice and Studies
- Race and Ethnicity in Music
- Sound Studies
- Browse content in Performing Arts
- Browse content in Philosophy
- Aesthetics and Philosophy of Art
- Epistemology
- Feminist Philosophy
- History of Western Philosophy
- Meta-Philosophy
- Metaphysics
- Moral Philosophy
- Non-Western Philosophy
- Philosophy of Science
- Philosophy of Language
- Philosophy of Mind
- Philosophy of Perception
- Philosophy of Action
- Philosophy of Law
- Philosophy of Religion
- Philosophy of Mathematics and Logic
- Practical Ethics
- Social and Political Philosophy
- Browse content in Religion
- Biblical Studies
- Christianity
- East Asian Religions
- History of Religion
- Judaism and Jewish Studies
- Qumran Studies
- Religion and Education
- Religion and Health
- Religion and Politics
- Religion and Science
- Religion and Law
- Religion and Art, Literature, and Music
- Religious Studies
- Browse content in Society and Culture
- Cookery, Food, and Drink
- Cultural Studies
- Customs and Traditions
- Ethical Issues and Debates
- Hobbies, Games, Arts and Crafts
- Natural world, Country Life, and Pets
- Popular Beliefs and Controversial Knowledge
- Sports and Outdoor Recreation
- Technology and Society
- Travel and Holiday
- Visual Culture
- Browse content in Law
- Arbitration
- Browse content in Company and Commercial Law
- Commercial Law
- Company Law
- Browse content in Comparative Law
- Systems of Law
- Competition Law
- Browse content in Constitutional and Administrative Law
- Government Powers
- Judicial Review
- Local Government Law
- Military and Defence Law
- Parliamentary and Legislative Practice
- Construction Law
- Contract Law
- Browse content in Criminal Law
- Criminal Procedure
- Criminal Evidence Law
- Sentencing and Punishment
- Employment and Labour Law
- Environment and Energy Law
- Browse content in Financial Law
- Banking Law
- Insolvency Law
- History of Law
- Human Rights and Immigration
- Intellectual Property Law
- Browse content in International Law
- Private International Law and Conflict of Laws
- Public International Law
- IT and Communications Law
- Jurisprudence and Philosophy of Law
- Law and Politics
- Law and Society
- Browse content in Legal System and Practice
- Courts and Procedure
- Legal Skills and Practice
- Legal System - Costs and Funding
- Primary Sources of Law
- Regulation of Legal Profession
- Medical and Healthcare Law
- Browse content in Policing
- Criminal Investigation and Detection
- Police and Security Services
- Police Procedure and Law
- Police Regional Planning
- Browse content in Property Law
- Personal Property Law
- Restitution
- Study and Revision
- Terrorism and National Security Law
- Browse content in Trusts Law
- Wills and Probate or Succession
- Browse content in Medicine and Health
- Browse content in Allied Health Professions
- Arts Therapies
- Clinical Science
- Dietetics and Nutrition
- Occupational Therapy
- Operating Department Practice
- Physiotherapy
- Radiography
- Speech and Language Therapy
- Browse content in Anaesthetics
- General Anaesthesia
- Browse content in Clinical Medicine
- Acute Medicine
- Cardiovascular Medicine
- Clinical Genetics
- Clinical Pharmacology and Therapeutics
- Dermatology
- Endocrinology and Diabetes
- Gastroenterology
- Genito-urinary Medicine
- Geriatric Medicine
- Infectious Diseases
- Medical Toxicology
- Medical Oncology
- Pain Medicine
- Palliative Medicine
- Rehabilitation Medicine
- Respiratory Medicine and Pulmonology
- Rheumatology
- Sleep Medicine
- Sports and Exercise Medicine
- Clinical Neuroscience
- Community Medical Services
- Critical Care
- Emergency Medicine
- Forensic Medicine
- Haematology
- History of Medicine
- Browse content in Medical Dentistry
- Oral and Maxillofacial Surgery
- Paediatric Dentistry
- Restorative Dentistry and Orthodontics
- Surgical Dentistry
- Browse content in Medical Skills
- Clinical Skills
- Communication Skills
- Nursing Skills
- Surgical Skills
- Medical Ethics
- Medical Statistics and Methodology
- Browse content in Neurology
- Clinical Neurophysiology
- Neuropathology
- Nursing Studies
- Browse content in Obstetrics and Gynaecology
- Gynaecology
- Occupational Medicine
- Ophthalmology
- Otolaryngology (ENT)
- Browse content in Paediatrics
- Neonatology
- Browse content in Pathology
- Chemical Pathology
- Clinical Cytogenetics and Molecular Genetics
- Histopathology
- Medical Microbiology and Virology
- Patient Education and Information
- Browse content in Pharmacology
- Psychopharmacology
- Browse content in Popular Health
- Caring for Others
- Complementary and Alternative Medicine
- Self-help and Personal Development
- Browse content in Preclinical Medicine
- Cell Biology
- Molecular Biology and Genetics
- Reproduction, Growth and Development
- Primary Care
- Professional Development in Medicine
- Browse content in Psychiatry
- Addiction Medicine
- Child and Adolescent Psychiatry
- Forensic Psychiatry
- Learning Disabilities
- Old Age Psychiatry
- Psychotherapy
- Browse content in Public Health and Epidemiology
- Epidemiology
- Public Health
- Browse content in Radiology
- Clinical Radiology
- Interventional Radiology
- Nuclear Medicine
- Radiation Oncology
- Reproductive Medicine
- Browse content in Surgery
- Cardiothoracic Surgery
- Gastro-intestinal and Colorectal Surgery
- General Surgery
- Neurosurgery
- Paediatric Surgery
- Peri-operative Care
- Plastic and Reconstructive Surgery
- Surgical Oncology
- Transplant Surgery
- Trauma and Orthopaedic Surgery
- Vascular Surgery
- Browse content in Science and Mathematics
- Browse content in Biological Sciences
- Aquatic Biology
- Biochemistry
- Bioinformatics and Computational Biology
- Developmental Biology
- Ecology and Conservation
- Evolutionary Biology
- Genetics and Genomics
- Microbiology
- Molecular and Cell Biology
- Natural History
- Plant Sciences and Forestry
- Research Methods in Life Sciences
- Structural Biology
- Systems Biology
- Zoology and Animal Sciences
- Browse content in Chemistry
- Analytical Chemistry
- Computational Chemistry
- Crystallography
- Environmental Chemistry
- Industrial Chemistry
- Inorganic Chemistry
- Materials Chemistry
- Medicinal Chemistry
- Mineralogy and Gems
- Organic Chemistry
- Physical Chemistry
- Polymer Chemistry
- Study and Communication Skills in Chemistry
- Theoretical Chemistry
- Browse content in Computer Science
- Artificial Intelligence
- Computer Architecture and Logic Design
- Game Studies
- Human-Computer Interaction
- Mathematical Theory of Computation
- Programming Languages
- Software Engineering
- Systems Analysis and Design
- Virtual Reality
- Browse content in Computing
- Business Applications
- Computer Security
- Computer Games
- Computer Networking and Communications
- Digital Lifestyle
- Graphical and Digital Media Applications
- Operating Systems
- Browse content in Earth Sciences and Geography
- Atmospheric Sciences
- Environmental Geography
- Geology and the Lithosphere
- Maps and Map-making
- Meteorology and Climatology
- Oceanography and Hydrology
- Palaeontology
- Physical Geography and Topography
- Regional Geography
- Soil Science
- Urban Geography
- Browse content in Engineering and Technology
- Agriculture and Farming
- Biological Engineering
- Civil Engineering, Surveying, and Building
- Electronics and Communications Engineering
- Energy Technology
- Engineering (General)
- Environmental Science, Engineering, and Technology
- History of Engineering and Technology
- Mechanical Engineering and Materials
- Technology of Industrial Chemistry
- Transport Technology and Trades
- Browse content in Environmental Science
- Applied Ecology (Environmental Science)
- Conservation of the Environment (Environmental Science)
- Environmental Sustainability
- Environmentalist Thought and Ideology (Environmental Science)
- Management of Land and Natural Resources (Environmental Science)
- Natural Disasters (Environmental Science)
- Nuclear Issues (Environmental Science)
- Pollution and Threats to the Environment (Environmental Science)
- Social Impact of Environmental Issues (Environmental Science)
- History of Science and Technology
- Browse content in Materials Science
- Ceramics and Glasses
- Composite Materials
- Metals, Alloying, and Corrosion
- Nanotechnology
- Browse content in Mathematics
- Applied Mathematics
- Biomathematics and Statistics
- History of Mathematics
- Mathematical Education
- Mathematical Finance
- Mathematical Analysis
- Numerical and Computational Mathematics
- Probability and Statistics
- Pure Mathematics
- Browse content in Neuroscience
- Cognition and Behavioural Neuroscience
- Development of the Nervous System
- Disorders of the Nervous System
- History of Neuroscience
- Invertebrate Neurobiology
- Molecular and Cellular Systems
- Neuroendocrinology and Autonomic Nervous System
- Neuroscientific Techniques
- Sensory and Motor Systems
- Browse content in Physics
- Astronomy and Astrophysics
- Atomic, Molecular, and Optical Physics
- Biological and Medical Physics
- Classical Mechanics
- Computational Physics
- Condensed Matter Physics
- Electromagnetism, Optics, and Acoustics
- History of Physics
- Mathematical and Statistical Physics
- Measurement Science
- Nuclear Physics
- Particles and Fields
- Plasma Physics
- Quantum Physics
- Relativity and Gravitation
- Semiconductor and Mesoscopic Physics
- Browse content in Psychology
- Affective Sciences
- Clinical Psychology
- Cognitive Psychology
- Cognitive Neuroscience
- Criminal and Forensic Psychology
- Developmental Psychology
- Educational Psychology
- Evolutionary Psychology
- Health Psychology
- History and Systems in Psychology
- Music Psychology
- Neuropsychology
- Organizational Psychology
- Psychological Assessment and Testing
- Psychology of Human-Technology Interaction
- Psychology Professional Development and Training
- Research Methods in Psychology
- Social Psychology
- Browse content in Social Sciences
- Browse content in Anthropology
- Anthropology of Religion
- Human Evolution
- Medical Anthropology
- Physical Anthropology
- Regional Anthropology
- Social and Cultural Anthropology
- Theory and Practice of Anthropology
- Browse content in Business and Management
- Business Strategy
- Business Ethics
- Business History
- Business and Government
- Business and Technology
- Business and the Environment
- Comparative Management
- Corporate Governance
- Corporate Social Responsibility
- Entrepreneurship
- Health Management
- Human Resource Management
- Industrial and Employment Relations
- Industry Studies
- Information and Communication Technologies
- International Business
- Knowledge Management
- Management and Management Techniques
- Operations Management
- Organizational Theory and Behaviour
- Pensions and Pension Management
- Public and Nonprofit Management
- Social Issues in Business and Management
- Strategic Management
- Supply Chain Management
- Browse content in Criminology and Criminal Justice
- Criminal Justice
- Criminology
- Forms of Crime
- International and Comparative Criminology
- Youth Violence and Juvenile Justice
- Development Studies
- Browse content in Economics
- Agricultural, Environmental, and Natural Resource Economics
- Asian Economics
- Behavioural Finance
- Behavioural Economics and Neuroeconomics
- Econometrics and Mathematical Economics
- Economic Systems
- Economic History
- Economic Methodology
- Economic Development and Growth
- Financial Markets
- Financial Institutions and Services
- General Economics and Teaching
- Health, Education, and Welfare
- History of Economic Thought
- International Economics
- Labour and Demographic Economics
- Law and Economics
- Macroeconomics and Monetary Economics
- Microeconomics
- Public Economics
- Urban, Rural, and Regional Economics
- Welfare Economics
- Browse content in Education
- Adult Education and Continuous Learning
- Care and Counselling of Students
- Early Childhood and Elementary Education
- Educational Equipment and Technology
- Educational Strategies and Policy
- Higher and Further Education
- Organization and Management of Education
- Philosophy and Theory of Education
- Schools Studies
- Secondary Education
- Teaching of a Specific Subject
- Teaching of Specific Groups and Special Educational Needs
- Teaching Skills and Techniques
- Browse content in Environment
- Applied Ecology (Social Science)
- Climate Change
- Conservation of the Environment (Social Science)
- Environmentalist Thought and Ideology (Social Science)
- Management of Land and Natural Resources (Social Science)
- Natural Disasters (Environment)
- Pollution and Threats to the Environment (Social Science)
- Social Impact of Environmental Issues (Social Science)
- Sustainability
- Browse content in Human Geography
- Cultural Geography
- Economic Geography
- Political Geography
- Browse content in Interdisciplinary Studies
- Communication Studies
- Museums, Libraries, and Information Sciences
- Browse content in Politics
- African Politics
- Asian Politics
- Chinese Politics
- Comparative Politics
- Conflict Politics
- Elections and Electoral Studies
- Environmental Politics
- Ethnic Politics
- European Union
- Foreign Policy
- Gender and Politics
- Human Rights and Politics
- Indian Politics
- International Relations
- International Organization (Politics)
- Irish Politics
- Latin American Politics
- Middle Eastern Politics
- Political Methodology
- Political Communication
- Political Philosophy
- Political Sociology
- Political Behaviour
- Political Economy
- Political Institutions
- Political Theory
- Politics and Law
- Politics of Development
- Public Administration
- Public Policy
- Qualitative Political Methodology
- Quantitative Political Methodology
- Regional Political Studies
- Russian Politics
- Security Studies
- State and Local Government
- UK Politics
- US Politics
- Browse content in Regional and Area Studies
- African Studies
- Asian Studies
- East Asian Studies
- Japanese Studies
- Latin American Studies
- Middle Eastern Studies
- Native American Studies
- Scottish Studies
- Browse content in Research and Information
- Research Methods
- Browse content in Social Work
- Addictions and Substance Misuse
- Adoption and Fostering
- Care of the Elderly
- Child and Adolescent Social Work
- Couple and Family Social Work
- Direct Practice and Clinical Social Work
- Emergency Services
- Human Behaviour and the Social Environment
- International and Global Issues in Social Work
- Mental and Behavioural Health
- Social Justice and Human Rights
- Social Policy and Advocacy
- Social Work and Crime and Justice
- Social Work Macro Practice
- Social Work Practice Settings
- Social Work Research and Evidence-based Practice
- Welfare and Benefit Systems
- Browse content in Sociology
- Childhood Studies
- Community Development
- Comparative and Historical Sociology
- Disability Studies
- Economic Sociology
- Gender and Sexuality
- Gerontology and Ageing
- Health, Illness, and Medicine
- Marriage and the Family
- Migration Studies
- Occupations, Professions, and Work
- Organizations
- Population and Demography
- Race and Ethnicity
- Social Theory
- Social Movements and Social Change
- Social Research and Statistics
- Social Stratification, Inequality, and Mobility
- Sociology of Religion
- Sociology of Education
- Sport and Leisure
- Urban and Rural Studies
- Browse content in Warfare and Defence
- Defence Strategy, Planning, and Research
- Land Forces and Warfare
- Military Administration
- Military Life and Institutions
- Naval Forces and Warfare
- Other Warfare and Defence Issues
- Peace Studies and Conflict Resolution
- Weapons and Equipment

Ethics of Artificial Intelligence
- Cite Icon Cite
- Permissions Icon Permissions
Featuring seventeen original essays on the ethics of artificial intelligence (AI) by today’s most prominent AI scientists and academic philosophers, this volume represents state-of-the-art thinking in this fast-growing field. It highlights central themes in AI and morality such as how to build ethics into AI, how to address mass unemployment caused by automation, how to avoid designing AI systems that perpetuate existing biases, and how to determine whether an AI is conscious. As AI technologies progress, questions about the ethics of AI, in both the near future and the long term, become more pressing than ever. Should a self-driving car prioritize the lives of the passengers over those of pedestrians? Should we as a society develop autonomous weapon systems capable of identifying and attacking a target without human intervention? What happens when AIs become smarter and more capable than us? Could they have greater than human-level moral status? Can we prevent superintelligent AIs from harming us or causing our extinction? At a critical time in this fast-moving debate, thirty leading academics and researchers at the forefront of AI technology development have come together to explore these existential questions.
Personal account
- Sign in with email/username & password
- Get email alerts
- Save searches
- Purchase content
- Activate your purchase/trial code
- Add your ORCID iD
Institutional access
Sign in with a library card.
- Sign in with username/password
- Recommend to your librarian
- Institutional account management
- Get help with access
Access to content on Oxford Academic is often provided through institutional subscriptions and purchases. If you are a member of an institution with an active account, you may be able to access content in one of the following ways:
IP based access
Typically, access is provided across an institutional network to a range of IP addresses. This authentication occurs automatically, and it is not possible to sign out of an IP authenticated account.
Choose this option to get remote access when outside your institution. Shibboleth/Open Athens technology is used to provide single sign-on between your institution’s website and Oxford Academic.
- Click Sign in through your institution.
- Select your institution from the list provided, which will take you to your institution's website to sign in.
- When on the institution site, please use the credentials provided by your institution. Do not use an Oxford Academic personal account.
- Following successful sign in, you will be returned to Oxford Academic.
If your institution is not listed or you cannot sign in to your institution’s website, please contact your librarian or administrator.
Enter your library card number to sign in. If you cannot sign in, please contact your librarian.
Society Members
Society member access to a journal is achieved in one of the following ways:
Sign in through society site
Many societies offer single sign-on between the society website and Oxford Academic. If you see ‘Sign in through society site’ in the sign in pane within a journal:
- Click Sign in through society site.
- When on the society site, please use the credentials provided by that society. Do not use an Oxford Academic personal account.
If you do not have a society account or have forgotten your username or password, please contact your society.
Sign in using a personal account
Some societies use Oxford Academic personal accounts to provide access to their members. See below.
A personal account can be used to get email alerts, save searches, purchase content, and activate subscriptions.
Some societies use Oxford Academic personal accounts to provide access to their members.
Viewing your signed in accounts
Click the account icon in the top right to:
- View your signed in personal account and access account management features.
- View the institutional accounts that are providing access.
Signed in but can't access content
Oxford Academic is home to a wide variety of products. The institutional subscription may not cover the content that you are trying to access. If you believe you should have access to that content, please contact your librarian.
For librarians and administrators, your personal account also provides access to institutional account management. Here you will find options to view and activate subscriptions, manage institutional settings and access options, access usage statistics, and more.
Our books are available by subscription or purchase to libraries and institutions.
Month: | Total Views: |
---|---|
October 2022 | 15 |
October 2022 | 13 |
October 2022 | 13 |
October 2022 | 25 |
October 2022 | 9 |
October 2022 | 12 |
October 2022 | 51 |
October 2022 | 46 |
October 2022 | 14 |
October 2022 | 82 |
October 2022 | 38 |
October 2022 | 18 |
October 2022 | 45 |
October 2022 | 35 |
October 2022 | 45 |
October 2022 | 23 |
October 2022 | 26 |
October 2022 | 7 |
October 2022 | 44 |
October 2022 | 17 |
October 2022 | 16 |
October 2022 | 2 |
October 2022 | 7 |
November 2022 | 28 |
November 2022 | 12 |
November 2022 | 26 |
November 2022 | 34 |
November 2022 | 16 |
November 2022 | 12 |
November 2022 | 40 |
November 2022 | 35 |
November 2022 | 4 |
November 2022 | 11 |
November 2022 | 35 |
November 2022 | 37 |
November 2022 | 30 |
November 2022 | 6 |
November 2022 | 39 |
November 2022 | 16 |
November 2022 | 12 |
November 2022 | 6 |
November 2022 | 83 |
November 2022 | 3 |
November 2022 | 24 |
November 2022 | 7 |
November 2022 | 15 |
December 2022 | 5 |
December 2022 | 1 |
December 2022 | 29 |
December 2022 | 18 |
December 2022 | 33 |
December 2022 | 39 |
December 2022 | 19 |
December 2022 | 32 |
December 2022 | 12 |
December 2022 | 32 |
December 2022 | 35 |
December 2022 | 2 |
December 2022 | 29 |
December 2022 | 2 |
December 2022 | 61 |
December 2022 | 79 |
December 2022 | 10 |
December 2022 | 17 |
December 2022 | 33 |
December 2022 | 19 |
December 2022 | 15 |
December 2022 | 14 |
January 2023 | 4 |
January 2023 | 27 |
January 2023 | 3 |
January 2023 | 12 |
January 2023 | 19 |
January 2023 | 16 |
January 2023 | 44 |
January 2023 | 23 |
January 2023 | 14 |
January 2023 | 8 |
January 2023 | 14 |
January 2023 | 46 |
January 2023 | 6 |
January 2023 | 5 |
January 2023 | 28 |
January 2023 | 3 |
January 2023 | 131 |
January 2023 | 9 |
January 2023 | 45 |
January 2023 | 8 |
January 2023 | 12 |
January 2023 | 15 |
February 2023 | 3 |
February 2023 | 12 |
February 2023 | 38 |
February 2023 | 8 |
February 2023 | 16 |
February 2023 | 34 |
February 2023 | 43 |
February 2023 | 6 |
February 2023 | 23 |
February 2023 | 20 |
February 2023 | 20 |
February 2023 | 3 |
February 2023 | 1 |
February 2023 | 3 |
February 2023 | 41 |
February 2023 | 4 |
February 2023 | 38 |
February 2023 | 13 |
February 2023 | 12 |
February 2023 | 10 |
February 2023 | 93 |
March 2023 | 26 |
March 2023 | 2 |
March 2023 | 26 |
March 2023 | 14 |
March 2023 | 34 |
March 2023 | 41 |
March 2023 | 24 |
March 2023 | 22 |
March 2023 | 12 |
March 2023 | 34 |
March 2023 | 44 |
March 2023 | 54 |
March 2023 | 2 |
March 2023 | 15 |
March 2023 | 56 |
March 2023 | 70 |
March 2023 | 19 |
March 2023 | 13 |
March 2023 | 26 |
March 2023 | 19 |
April 2023 | 135 |
April 2023 | 5 |
April 2023 | 3 |
April 2023 | 47 |
April 2023 | 26 |
April 2023 | 38 |
April 2023 | 18 |
April 2023 | 30 |
April 2023 | 15 |
April 2023 | 12 |
April 2023 | 75 |
April 2023 | 44 |
April 2023 | 4 |
April 2023 | 11 |
April 2023 | 1 |
April 2023 | 2 |
April 2023 | 57 |
April 2023 | 70 |
April 2023 | 15 |
April 2023 | 74 |
April 2023 | 18 |
April 2023 | 14 |
April 2023 | 27 |
May 2023 | 1 |
May 2023 | 23 |
May 2023 | 13 |
May 2023 | 23 |
May 2023 | 18 |
May 2023 | 51 |
May 2023 | 14 |
May 2023 | 25 |
May 2023 | 8 |
May 2023 | 13 |
May 2023 | 44 |
May 2023 | 2 |
May 2023 | 40 |
May 2023 | 8 |
May 2023 | 38 |
May 2023 | 2 |
May 2023 | 44 |
May 2023 | 17 |
May 2023 | 83 |
May 2023 | 17 |
May 2023 | 9 |
June 2023 | 2 |
June 2023 | 59 |
June 2023 | 1 |
June 2023 | 21 |
June 2023 | 16 |
June 2023 | 28 |
June 2023 | 18 |
June 2023 | 23 |
June 2023 | 17 |
June 2023 | 13 |
June 2023 | 48 |
June 2023 | 23 |
June 2023 | 3 |
June 2023 | 1 |
June 2023 | 12 |
June 2023 | 28 |
June 2023 | 26 |
June 2023 | 59 |
June 2023 | 20 |
June 2023 | 12 |
June 2023 | 18 |
June 2023 | 21 |
July 2023 | 1 |
July 2023 | 36 |
July 2023 | 4 |
July 2023 | 34 |
July 2023 | 17 |
July 2023 | 27 |
July 2023 | 33 |
July 2023 | 25 |
July 2023 | 25 |
July 2023 | 18 |
July 2023 | 27 |
July 2023 | 35 |
July 2023 | 34 |
July 2023 | 23 |
July 2023 | 11 |
July 2023 | 16 |
July 2023 | 43 |
July 2023 | 1 |
July 2023 | 43 |
July 2023 | 14 |
July 2023 | 14 |
July 2023 | 23 |
August 2023 | 27 |
August 2023 | 7 |
August 2023 | 8 |
August 2023 | 22 |
August 2023 | 16 |
August 2023 | 18 |
August 2023 | 21 |
August 2023 | 21 |
August 2023 | 34 |
August 2023 | 16 |
August 2023 | 26 |
August 2023 | 39 |
August 2023 | 23 |
August 2023 | 26 |
August 2023 | 9 |
August 2023 | 3 |
August 2023 | 16 |
August 2023 | 6 |
August 2023 | 31 |
August 2023 | 18 |
August 2023 | 21 |
August 2023 | 52 |
August 2023 | 18 |
September 2023 | 3 |
September 2023 | 2 |
September 2023 | 12 |
September 2023 | 23 |
September 2023 | 73 |
September 2023 | 29 |
September 2023 | 18 |
September 2023 | 27 |
September 2023 | 18 |
September 2023 | 36 |
September 2023 | 41 |
September 2023 | 35 |
September 2023 | 6 |
September 2023 | 35 |
September 2023 | 2 |
September 2023 | 18 |
September 2023 | 62 |
September 2023 | 16 |
September 2023 | 20 |
September 2023 | 17 |
September 2023 | 16 |
September 2023 | 9 |
October 2023 | 6 |
October 2023 | 41 |
October 2023 | 8 |
October 2023 | 26 |
October 2023 | 60 |
October 2023 | 28 |
October 2023 | 24 |
October 2023 | 54 |
October 2023 | 96 |
October 2023 | 34 |
October 2023 | 82 |
October 2023 | 60 |
October 2023 | 2 |
October 2023 | 22 |
October 2023 | 10 |
October 2023 | 113 |
October 2023 | 20 |
October 2023 | 26 |
October 2023 | 25 |
October 2023 | 58 |
October 2023 | 105 |
October 2023 | 76 |
October 2023 | 25 |
November 2023 | 54 |
November 2023 | 56 |
November 2023 | 5 |
November 2023 | 5 |
November 2023 | 18 |
November 2023 | 34 |
November 2023 | 37 |
November 2023 | 17 |
November 2023 | 38 |
November 2023 | 56 |
November 2023 | 17 |
November 2023 | 90 |
November 2023 | 10 |
November 2023 | 16 |
November 2023 | 68 |
November 2023 | 2 |
November 2023 | 121 |
November 2023 | 5 |
November 2023 | 66 |
November 2023 | 11 |
November 2023 | 17 |
November 2023 | 17 |
November 2023 | 29 |
December 2023 | 2 |
December 2023 | 20 |
December 2023 | 43 |
December 2023 | 2 |
December 2023 | 35 |
December 2023 | 27 |
December 2023 | 26 |
December 2023 | 24 |
December 2023 | 36 |
December 2023 | 55 |
December 2023 | 2 |
December 2023 | 3 |
December 2023 | 36 |
December 2023 | 38 |
December 2023 | 18 |
December 2023 | 52 |
December 2023 | 24 |
December 2023 | 15 |
December 2023 | 33 |
December 2023 | 10 |
December 2023 | 13 |
December 2023 | 35 |
December 2023 | 22 |
January 2024 | 2 |
January 2024 | 14 |
January 2024 | 4 |
January 2024 | 105 |
January 2024 | 59 |
January 2024 | 23 |
January 2024 | 27 |
January 2024 | 6 |
January 2024 | 13 |
January 2024 | 5 |
January 2024 | 12 |
January 2024 | 205 |
January 2024 | 5 |
January 2024 | 23 |
January 2024 | 3 |
January 2024 | 16 |
January 2024 | 61 |
January 2024 | 38 |
January 2024 | 35 |
January 2024 | 11 |
January 2024 | 20 |
January 2024 | 63 |
January 2024 | 31 |
February 2024 | 90 |
February 2024 | 9 |
February 2024 | 5 |
February 2024 | 23 |
February 2024 | 95 |
February 2024 | 47 |
February 2024 | 161 |
February 2024 | 96 |
February 2024 | 19 |
February 2024 | 51 |
February 2024 | 31 |
February 2024 | 14 |
February 2024 | 8 |
February 2024 | 18 |
February 2024 | 131 |
February 2024 | 5 |
February 2024 | 4 |
February 2024 | 182 |
February 2024 | 28 |
February 2024 | 14 |
February 2024 | 23 |
February 2024 | 99 |
February 2024 | 37 |
March 2024 | 18 |
March 2024 | 33 |
March 2024 | 52 |
March 2024 | 11 |
March 2024 | 46 |
March 2024 | 21 |
March 2024 | 24 |
March 2024 | 72 |
March 2024 | 36 |
March 2024 | 15 |
March 2024 | 5 |
March 2024 | 9 |
March 2024 | 6 |
March 2024 | 99 |
March 2024 | 55 |
March 2024 | 5 |
March 2024 | 83 |
March 2024 | 48 |
March 2024 | 18 |
April 2024 | 4 |
April 2024 | 41 |
April 2024 | 5 |
April 2024 | 48 |
April 2024 | 32 |
April 2024 | 10 |
April 2024 | 71 |
April 2024 | 69 |
April 2024 | 43 |
April 2024 | 12 |
April 2024 | 63 |
April 2024 | 64 |
April 2024 | 6 |
April 2024 | 35 |
April 2024 | 1 |
April 2024 | 157 |
April 2024 | 3 |
April 2024 | 8 |
April 2024 | 114 |
April 2024 | 12 |
April 2024 | 132 |
April 2024 | 17 |
April 2024 | 28 |
May 2024 | 12 |
May 2024 | 2 |
May 2024 | 2 |
May 2024 | 15 |
May 2024 | 17 |
May 2024 | 33 |
May 2024 | 19 |
May 2024 | 25 |
May 2024 | 23 |
May 2024 | 8 |
May 2024 | 23 |
May 2024 | 47 |
May 2024 | 59 |
May 2024 | 10 |
May 2024 | 8 |
May 2024 | 4 |
May 2024 | 65 |
May 2024 | 34 |
May 2024 | 2 |
May 2024 | 46 |
May 2024 | 14 |
May 2024 | 13 |
May 2024 | 13 |
June 2024 | 9 |
June 2024 | 24 |
June 2024 | 14 |
June 2024 | 25 |
June 2024 | 17 |
June 2024 | 17 |
June 2024 | 26 |
June 2024 | 28 |
June 2024 | 21 |
June 2024 | 14 |
June 2024 | 12 |
June 2024 | 16 |
June 2024 | 25 |
June 2024 | 37 |
June 2024 | 14 |
June 2024 | 11 |
June 2024 | 4 |
June 2024 | 5 |
June 2024 | 54 |
June 2024 | 32 |
June 2024 | 28 |
June 2024 | 16 |
June 2024 | 20 |
July 2024 | 11 |
July 2024 | 8 |
July 2024 | 6 |
July 2024 | 10 |
July 2024 | 18 |
July 2024 | 14 |
July 2024 | 35 |
July 2024 | 12 |
July 2024 | 8 |
July 2024 | 9 |
July 2024 | 45 |
July 2024 | 22 |
July 2024 | 45 |
July 2024 | 8 |
July 2024 | 8 |
July 2024 | 6 |
July 2024 | 2 |
July 2024 | 27 |
July 2024 | 39 |
July 2024 | 13 |
July 2024 | 10 |
July 2024 | 11 |
July 2024 | 43 |
August 2024 | 12 |
August 2024 | 1 |
August 2024 | 21 |
August 2024 | 8 |
August 2024 | 17 |
August 2024 | 15 |
August 2024 | 15 |
August 2024 | 23 |
August 2024 | 7 |
August 2024 | 14 |
August 2024 | 24 |
August 2024 | 18 |
August 2024 | 4 |
August 2024 | 34 |
August 2024 | 9 |
August 2024 | 2 |
August 2024 | 1 |
August 2024 | 33 |
August 2024 | 8 |
August 2024 | 38 |
August 2024 | 18 |
August 2024 | 4 |
August 2024 | 3 |
September 2024 | 2 |
September 2024 | 1 |
September 2024 | 3 |
September 2024 | 3 |
September 2024 | 7 |
September 2024 | 2 |
September 2024 | 3 |
September 2024 | 5 |
September 2024 | 7 |
September 2024 | 1 |
September 2024 | 19 |
September 2024 | 20 |
September 2024 | 16 |
September 2024 | 4 |
September 2024 | 13 |
September 2024 | 3 |
September 2024 | 4 |
September 2024 | 4 |
- About Oxford Academic
- Publish journals with us
- University press partners
- What we publish
- New features
- Open access
- Rights and permissions
- Accessibility
- Advertising
- Media enquiries
- Oxford University Press
- Oxford Languages
- University of Oxford
Oxford University Press is a department of the University of Oxford. It furthers the University's objective of excellence in research, scholarship, and education by publishing worldwide
- Copyright © 2024 Oxford University Press
- Cookie settings
- Cookie policy
- Privacy policy
- Legal notice
This Feature Is Available To Subscribers Only
Sign In or Create an Account
This PDF is available to Subscribers Only
For full access to this pdf, sign in to an existing account, or purchase an annual subscription.

- Ethics of AI
- AI in Education
- Digital Inclusion
- Digital Policy, Capacities and Inclusion
- Women’s access to and participation in technological developments
- Internet Universality Indicators
- All publications
- Recommendation on the Ethics of AI
- Report on ethics in robotics
- Map of emerging AI areas in the Global South
- 7 minutes to understand AI
- On the ethics of AI
- On a possible normative instrument for the ethics of AI
- On technical and legal aspects of the desirability of a standard-setting instrument for AI ethics
Ethics of Artificial Intelligence

Global AI Ethics and Governance Observatory
Getting AI governance right is one of the most consequential challenges of our time, calling for mutual learning based on the lessons and good practices emerging from the different jurisdictions around the world.
The aim of the Global AI Ethics and Governance Observatory is to provide a global resource for policymakers, regulators, academics, the private sector and civil society to find solutions to the most pressing challenges posed by Artificial Intelligence.
The Observatory showcases information about the readiness of countries to adopt AI ethically and responsibly.
It also hosts the AI Ethics and Governance Lab, which gathers contributions, impactful research, toolkits and good practices.
With its unique mandate, UNESCO has led the international effort to ensure that science and technology develop with strong ethical guardrails for decades.
Be it on genetic research, climate change, or scientific research, UNESCO has delivered global standards to maximize the benefits of the scientific discoveries, while minimizing the downside risks, ensuring they contribute to a more inclusive, sustainable, and peaceful world. It has also identified frontier challenges in areas such as the ethics of neurotechnology, on climate engineering, and the internet of things.
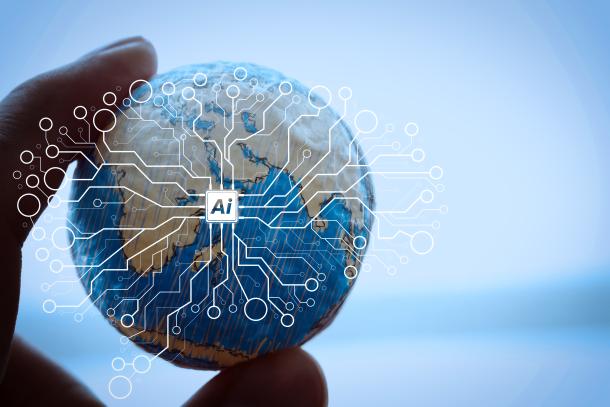
The rapid rise in artificial intelligence (AI) has created many opportunities globally , from facilitating healthcare diagnoses to enabling human connections through social media and creating labour efficiencies through automated tasks.
However, these rapid changes also raise profound ethical concerns . These arise from the potential AI systems have to embed biases, contribute to climate degradation, threaten human rights and more. Such risks associated with AI have already begun to compound on top of existing inequalities, resulting in further harm to already marginalised groups.

In no other field is the ethical compass more relevant than in artificial intelligence. These general-purpose technologies are re-shaping the way we work, interact, and live. The world is set to change at a pace not seen since the deployment of the printing press six centuries ago. AI technology brings major benefits in many areas, but without the ethical guardrails , it risks reproducing real world biases and discrimination, fueling divisions and threatening fundamental human rights and freedoms.

Recommendation on the Ethics of Artificial Intelligence
UNESCO produced the first-ever global standard on AI ethics – the ‘Recommendation on the Ethics of Artificial Intelligence ’ in November 2021. This framework was adopted by all 193 Member States. The protection of human rights and dignity is the cornerstone of the Recommendation, based on the advancement of fundamental principles such as transparency and fairness, always remembering the importance of human oversight of AI systems. However, what makes the Recommendation exceptionally applicable are its extensive Policy Action Areas , which allow policymakers to translate the core values and principles into action with respect to data governance, environment and ecosystems, gender, education and research, and health and social wellbeing, among many other spheres.
Four core values
Respect, protection and promotion of human rights and fundamental freedoms and human dignity
just, and interconnected societies
A dynamic understanding of AI
The Recommendation interprets AI broadly as systems with the ability to process data in a way which resembles intelligent behaviour.
This is crucial as the rapid pace of technological change would quickly render any fixed, narrow definition outdated, and make future-proof policies infeasible.
A human rights approach to AI
The use of AI systems must not go beyond what is necessary to achieve a legitimate aim. Risk assessment should be used to prevent harms which may result from such uses.
Unwanted harms (safety risks) as well as vulnerabilities to attack (security risks) should be avoided and addressed by AI actors.
Privacy must be protected and promoted throughout the AI lifecycle. Adequate data protection frameworks should also be established.
International law & national sovereignty must be respected in the use of data. Additionally, participation of diverse stakeholders is necessary for inclusive approaches to AI governance.
AI systems should be auditable and traceable. There should be oversight, impact assessment, audit and due diligence mechanisms in place to avoid conflicts with human rights norms and threats to environmental wellbeing.
The ethical deployment of AI systems depends on their transparency & explainability (T&E). The level of T&E should be appropriate to the context, as there may be tensions between T&E and other principles such as privacy, safety and security.
Member States should ensure that AI systems do not displace ultimate human responsibility and accountability.
AI technologies should be assessed against their impacts on ‘sustainability’, understood as a set of constantly evolving goals including those set out in the UN’s Sustainable Development Goals.
Public understanding of AI and data should be promoted through open & accessible education, civic engagement, digital skills & AI ethics training, media & information literacy.
AI actors should promote social justice, fairness, and non-discrimination while taking an inclusive approach to ensure AI’s benefits are accessible to all.
Actionable policies
Key policy areas make clear arenas where Member States can make strides towards responsible developments in AI
While values and principles are crucial to establishing a basis for any ethical AI framework, recent movements in AI ethics have emphasised the need to move beyond high-level principles and toward practical strategies.
The Recommendation does just this by setting out eleven key areas for policy actions .

Implementing the Recommendation
The RAM is designed to help assess whether Member States are prepared to effectively implement the Recommendation. It will help them identify their status of preparedness & provide a basis for UNESCO to custom-tailor its capacity-building support.
EIA is a structured process which helps AI project teams, in collaboration with the affected communities, to identify & assess the impacts an AI system may have. It allows to reflect on its potential impact & to identify needed harm prevention actions.
Women4Ethical AI expert platform to advance gender equality
UNESCO's Women4Ethical AI is a new collaborative platform to support governments and companies’ efforts to ensure that women are represented equally in both the design and deployment of AI . The platform’s members will also contribute to the advancement of all the ethical provisions in the Recommendation on the Ethics of AI.
The platform unites 17 leading female experts from academia, civil society, the private sector and regulatory bodies, from around the world. They will share research and contribute to a repository of good practices. The platform will drive progress on non-discriminatory algorithms and data sources, and incentivize girls, women and under-represented groups to participate in AI.

Business Council for Ethics of AI
The Business Council for Ethics of AI is a collaborative initiative between UNESCO and companies operating in Latin America that are involved in the development or use of artificial intelligence (AI) in various sectors.
The Council serves as a platform for companies to come together, exchange experiences, and promote ethical practices within the AI industry. By working closely with UNESCO, it aims to ensure that AI is developed and utilized in a manner that respects human rights and upholds ethical standards.
Currently co-chaired by Microsoft and Telefonica, the Council is committed to strengthening technical capacities in ethics and AI, designing and implementing the Ethical Impact Assessment tool mandated by the Recommendation on the Ethics of AI, and contributing to the development of intelligent regional regulations. Through these efforts, it strives to create a competitive environment that benefits all stakeholders and promotes the responsible and ethical use of AI.

Ideas, news & stories
Examples of ethical dilemmas
Examples of gender bias in artificial intelligence, originating from stereotypical representations deeply rooted in our societies.
The use of AI in judicial systems around the world is increasing, creating more ethical questions to explore.
The use of AI in culture raises interesting ethical reflections. For instance, what happens when AI has the capacity to create works of art itself?
An autonomous car is a vehicle that is capable of sensing its environment and moving with little or no human involvement.
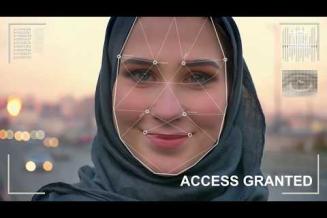
Discover our resources

Related items
- Social and human sciences
- Natural sciences
- Artificial intelligence
- Norms & Standards
- Policy Advice
- UN & International cooperation
- High technology
- Information technology
- Information technology (hardware)
- Information technology (software)
- Computer science
- Ethics of science
- Science and development
- Science and society
- Science policy
- Social science policy
- Social sciences
- Ethics of artificial intelligence
- Ethics of technology
- See more add
Thank you for visiting nature.com. You are using a browser version with limited support for CSS. To obtain the best experience, we recommend you use a more up to date browser (or turn off compatibility mode in Internet Explorer). In the meantime, to ensure continued support, we are displaying the site without styles and JavaScript.
- View all journals
- Explore content
- About the journal
- Publish with us
- Sign up for alerts
- Open access
- Published: 17 June 2020
Ethical principles in machine learning and artificial intelligence: cases from the field and possible ways forward
- Samuele Lo Piano ORCID: orcid.org/0000-0002-2625-483X 1 , 2
Humanities and Social Sciences Communications volume 7 , Article number: 9 ( 2020 ) Cite this article
99k Accesses
104 Citations
175 Altmetric
Metrics details
- Science, technology and society
Decision-making on numerous aspects of our daily lives is being outsourced to machine-learning (ML) algorithms and artificial intelligence (AI), motivated by speed and efficiency in the decision process. ML approaches—one of the typologies of algorithms underpinning artificial intelligence—are typically developed as black boxes. The implication is that ML code scripts are rarely scrutinised; interpretability is usually sacrificed in favour of usability and effectiveness. Room for improvement in practices associated with programme development have also been flagged along other dimensions, including inter alia fairness, accuracy, accountability, and transparency. In this contribution, the production of guidelines and dedicated documents around these themes is discussed. The following applications of AI-driven decision-making are outlined: (a) risk assessment in the criminal justice system, and (b) autonomous vehicles, highlighting points of friction across ethical principles. Possible ways forward towards the implementation of governance on AI are finally examined.
Similar content being viewed by others
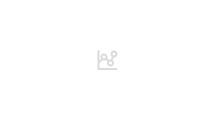
Principles alone cannot guarantee ethical AI
How ai can learn from the law: putting humans in the loop only on appeal.

Algorithmic fairness in artificial intelligence for medicine and healthcare
Introduction.
Artificial intelligence (AI) is the branch of computer science that deals with the simulation of intelligent behaviour in computers as regards their capacity to mimic, and ideally improve , human behaviour. To achieve this, the simulation of human cognition and functions, including learning and problem-solving, is required (Russell, 2010 ). This simulation may limit itself to some simple predictable features, thus limiting human complexity (Cowls, 2019 ).
AI became a self-standing discipline in the year 1955 (McCarthy et al., 2006 ) with significant development over the last decades. AI resorts to ML to implement a predictive functioning based on data acquired from a given context. The strength of ML resides in its capacity to learn from data without need to be explicitly programmed (Samuel, 1959 ); ML algorithms are autonomous and self-sufficient when performing their learning function. This is the reason why they are ubiquitous in AI developments. Further to this, ML implementations in data science and other applied fields are conceptualised in the context of a final decision-making application, hence their prominence.
Applications in our daily lives encompass fields, such as (precision) agriculture (Sennaar, 2019 ), air combat and military training (Gallagher, 2016 ; Wong, 2020 ), education (Sears, 2018 ), finance (Bahrammirzaee, 2010 ), health care (Beam and Kohane, 2018 ), human resources and recruiting (Hmoud and Laszlo, 2019 ), music composition (Cheng, 2009/09 ), customer service (Kongthon et al., 2009 ), reliable engineering and maintenance (Dragicevic et al., 2019 ), autonomous vehicles and traffic management (Ye, 2018 ), social-media news-feed (Rader et al., 2018 ), work scheduling and optimisation (O’Neil, 2016 ), and several others.
In all these fields, an increasing amount of functions are being ceded to algorithms to the detriment of human control, raising concern for loss of fairness and equitability (Sareen et al., 2020 ). Furthermore, issues of garbage-in-garbage-out (Saltelli and Funtowicz, 2014 ) may be prone to emerge in contexts when external control is entirely removed. This issue may be further exacerbated by the offer of new services of auto-ML (Chin, 2019 ), where the entire algorithm development workflow is automatised and the residual human control practically removed.
In the following sections, we will (i) detail a series of research questions around the ethical principles in AI; (ii) take stock of the production of guidelines elaborated in the field; (iii) showcase their prominence in practical examples; and (iv) discuss actions towards the inclusion of these dimensions in the future of AI ethics.
Research questions on the ethical dimensions of artificial intelligence
Critical aspects in AI deployment have already gained traction in mainstreaming literature and media. For instance, according to O’Neil ( 2016 ), a main shortcoming of ML approaches is the fact these resort to proxies for driving trends, such as person’s ZIP code or language in relation with the capacity of an individual to pay back a loan or handle a job, respectively. However, these correlations may be discriminatory, if not illegal.
Potential black swans (Taleb, 2007 ) in the code should also be considered. These have been documented, for instance, in the case of the Amazon website, for which errors, such as the quotation of plain items (often books) up to 10,000 dollars (Smith, 2018 ) have been reported. While mistakes about monetary values may be easy to spot, the situation may become more complex and less intelligible when incommensurable dimensions come to play. That is the reason why a number of guidelines on the topic of ethics in AI have been proliferating over the last few years.
While reflections around the ethical implications of machines and automation deployment were already put forth in the ’50s and ’60s (Samuel, 1959 ; Wiener, 1988 ), the increasing use of AI in many fields raises new important questions about its suitability (Yu et al., 2018 ). This stems from the complexity of the aspects undertaken and the plurality of views, stakes, and values at play. A fundamental aspect is how and to what extent the values and the perspectives of the involved stakeholders have been taken care of in the design of the decision-making algorithm (Saltelli, 2020 ). In addition to this ex-ante evaluation, an ex-post evaluation would need to be put in place so as to monitor the consequences of AI-driven decisions in making winners and losers.
To wrap up, it is fundamental to assess how and if ethical aspects have been included in the AI-driven decision-making implemented by asking questions such as:
What are the most prominent ethical concerns raised by large-scale deployment of AI applications?
How are these multiple dimensions interwoven?
What are the actions the involved stakeholders are carrying out to address these concerns?
What are possible ways forward to improve ML and AI development and use over their full life-cycle?
We will firstly examine the production of relevant guidelines in the fields along with academic secondary literature. These aspects will then be discussed in the context of two applied cases: (i) recidivism-risk assessment in the criminal justice system, and (ii) autonomous vehicles.
Guidelines and secondary literature on AI ethics, its dimensions and stakes
The production of dedicated documents has been skyrocketing from 2016 (Jobin et al., 2019 ). We here report on the most prominent international initiatives. A suggested reading on national and international AI strategies providing a comprehensive list of documents (Future of Earth Institute, 2020 ).
The France’s Digital Republic Act gives the right to an explanation as regards decisions on an individual made through the use of administrative algorithms (Edwards and Veale, 2018 ). This law touches upon several aspects including:
how and to what extent the algorithmic processing contributed to the decision-making;
which data was processed and its source;
how parameters were treated and weighted;
which operations were carried out in the treatment.
Sensitive governmental areas, such as national security and defence, and the private sector (the largest user and producer of ML algorithms by far) are excluded from this document.
An international European initiative is the multi-stakeholder European Union High-Level Expert Group on Artificial Intelligence , which is composed by 52 experts from academia, civil society, and industry. The group produced a deliverable on the required criteria for AI trustworthiness (Daly, 2019 ). Even articles 21 and 22 of the recent European Union General Data Protection Regulation include passages functional to AI governance, although further action has been recently demanded from the European Parliament (De Sutter, 2019 ). In this context, China has also been allocating efforts on privacy and data protection (Roberts, 2019 ).
As regards secondary literature, Floridi and Cowls ( 2019 ) examined a list of statements/declarations elaborated since 2016 from multi-stakeholder organisations. A set of 47 principles has been identified, which mapped onto five overarching dimensions (Floridi and Cowls, 2019 ): beneficence, non-maleficence, autonomy, justice and, explicability . The latter is a new dimension specifically acknowledged in the case of AI, while the others were already identified in the controversial domain of bioethics .
Jobin et al. ( 2019 ) reviewed 84 documents, which were produced by several actors of the field, almost half of which from private companies or governmental agencies. The classification proposed by Jobin et al. ( 2019 ) is around a slightly different set of values: transparency, justice and fairness, non-maleficience, responsibility and privacy . Other potentially relevant dimensions, such as accountability and responsibility, were rarely defined in the studies reviewed by these authors.
Seven of the most prominent value statements from the AI/ML fields were examined in Greene et al. ( 2019 ): The Partnership on AI to Benefit People and Society ; The Montreal Declaration for a Responsible Development of Artificial Intelligence ; The Toronto Declaration Protecting the rights to equality and non-discrimination in machine-learning systems ; OpenAI ; The Centre for Humane Technology ; Fairness, Accountability and Transparency in Machine Learning ; Axon’s AI Ethics Board for Public Safety . Greene et al. ( 2019 ) found seven common core elements across these documents: (i) design’s moral background (universal concerns, objectively measured); (ii) expert oversight; (iii) values-driven determinism; (iv) design as locus of ethical scrutiny; (v) better building; (vi) stakeholder-driven legitimacy; and, (vii) machine translation.
Mittelstadt ( 2019 ) critically analysed the current debate and actions in the field of AI ethics and noted that the dimensions addressed in AI ethics are converging towards those of medical ethics. However, this process appears problematic due to four main differences between medicine and the medical professionals on one side, and AI and its developers on the other. Firstly, the medical professional rests on common aims and fiduciary duties, which AI developers lack. Secondly, a formal profession with a set of clearly defined and governed good-behaviour practices exists in medicine. This is not the case for AI, which also lacks a full understanding of the consequences of the actions enacted by algorithms (Wallach and Allen, 2008 ). Thirdly, AI faces the difficulty of translating overarching principle into practices. Even its current setting of seeking maximum speed, efficiency and profit clashes with the resource and time requirements of an ethical assessment and/or counselling. Finally, the accountability of professionals or institutions is at this stage mainly theoretical, having the vast majority of these guidelines been defined on a merely voluntary basis and hence with the total lack of a sanctionary scheme for non-compliance.
Points of friction between ethical dimensions
Higher transparency is a common refrain when discussing ethics of algorithms, in relation to dimensions such as how an algorithmic decision is arrived at, based on what assumptions, and how this could be corrected to incorporate feedback from the involved parties. Rudin ( 2019 ) argued that the community of algorithm developers should go beyond explaining black-box models by developing interpretable models in the first place.
On a larger scale, the use of open-source software in the context of ML applications has already been advocated for over a decade (Thimbleby, 2003 ) with an indirect call for tools to execute more interpretable and reproducible programming such as Jupyter Notebooks , available from 2015 onwards. However, publishing scripts expose their developers to the public scrutiny of professional programmers, who may find shortcomings in the development of the code (Sonnenburg, 2007 ).
Ananny and Crawford ( 2018 ) comment that resorting to full algorithmic transparency may not be an adequate means to address their ethical dimensions; opening up the black-box would not suffice to disclose their modus operandi . Moreover, developers of algorithm may not be capable of explaining in plain language how a given tool works and what functional elements it is based on. A more social relevant understanding would encompass the human/non-human interface (i.e., looking across the system rather than merely inside ). Algorithmic complexity and all its implications unravel at this level, in terms of relationships rather than as mere self-standing properties.
Other authors pointed to possible points of friction between transparency and other relevant ethical dimensions. de Laat ( 2018 ) argues that transparency and accountability may even be at odds in the case of algorithms. Hence, he argues against full transparency along four main lines of reasoning: (i) leaking of privacy sensitive data into the open; (ii) backfiring into an implicit invitation to game the system; (iii) harming of the company property rights with negative consequences on their competitiveness (and on the developers reputation as discussed above); (iv) inherent opacity of algorithms, whose interpretability may be even hard for experts (see the example below about the code adopted in some models of autonomous vehicles). All these arguments suggest limitations to full disclosure of algorithms, be it that the normative implications behind these objections should be carefully scrutinised.
Raji et al. ( 2020 ) suggest that a process of algorithmic auditing within the software-development company could help in tackling some of the ethical issues raised. Larger interpretability could be in principle achieved by using simpler algorithms, although this may come at the expenses of accuracy. To this end, Watson and Floridi ( 2019 ) defined a formal framework for interpretable ML, where explanatory accuracy can be assessed against algorithmic simplicity and relevance.
Loss in accuracy may be produced by the exclusion of politically critical features (such as gender, race, age, etc.) from the pool of training predictive variables. For instance, Amazon scrapped a gender-biased recruitment algorithm once it realised that despite excluding gender, the algorithm was resorting to surrogate gender variables to implement its decisions (Dastin, 2018 ). This aspect points again to possible political issues of a trade-off between fairness, demanded by society, and algorithmic accuracy, demanded by, e.g., a private actor.
Fairness may be further hampered by reinforcement effects. This is the case of algorithms attributing credit scores, that have a reinforcement effect proportional to people wealth that de facto rules out credit access for people in a more socially difficult condition (O’Neil, 2016 ).
According to Floridi and Cowls ( 2019 ) a prominent role is also played by the autonomy dimension; the possibility of refraining from ceding decision power to AI for overriding reasons (e.g., the gain of efficacy is not deemed fit to justify the loss of control over decision-making). In other words, machines autonomy could be reduced in favour of human autonomy according to this meta-autonomy dimension.
Contrasting dimensions in terms of the theoretical framing of the issue also emerged from the review of Jobin et al. ( 2019 ), as regards interpretation of ethical principles, reasons for their importance, ownership and responsibility of their implementation. This also applies to different ethical principles, resulting in the trade-offs previously discussed, difficulties in setting prioritisation strategies, operationalisation and actual compliance with the guidelines. For instance, while private actors demand and try to cultivate trust from their users, this runs counter to the need for society to scrutinise the operation of algorithms in order to maintain developer accountability (Cowls, 2019 ). Attributing responsibilities in complicated projects where many parties and developers may be involved, an issue known as the problem of many hands (Nissenbaum, 1996 ), may indeed be very difficult.
Conflicts may also emerge between the requirements to overcome potential algorithm deficits in accuracy associated with large data bases and the individual rights to privacy and autonomy of decision. Such conflicts may exacerbate tensions, further complicating agreeing on standards and practices.
In the following two sections, the issues and points of friction raised are examined in two practical case studies, criminal justice and autonomous vehicles. These examples have been selected due to their prominence in the public debate on the ethical aspects of AI and ML algorithms.
Machine-learning algorithms in the field of criminal justice
ML algorithms have been largely used to assist juridical deliberation in many states of the USA (Angwin and Larson, 2016 ). This country faces the issue of the world’s highest incarcerated population, both in absolute and per-capita terms (Brief, 2020 ). The COMPAS algorithm, developed by the private company Northpointe , attributes a 2-year recidivism-risk score to arrested people. It also evaluates the risk of violent recidivism as a score.
The fairness of the algorithm has been questioned in an investigative report, that examined a pool of cases where a recidivism score was attributed to >18,000 criminal defendants in Broward County, Florida and flagged up a potential racial bias in the application of the algorithm (Angwin and Larson, 2016 ). According to the authors of the report, the recidivism-risk was systematically overestimated for black people: the decile distribution of white defendants was skewed towards the lower end. Conversely, the decile distribution of black defendants was only slightly decreasing towards the higher end. The risk of violent recidivism within 2 years followed a similar trend. This analysis was debunked by the company, which, however, refused to disclose the full details of its proprietary code. While the total number of variables amounts to about 140, only the core variables were disclosed (Northpointe, 2012 ). The race of the subject was not one of those.
Here, a crucial point is how this fairness is to be attained: whether it is more important a fair treatment across groups of individuals or within the same group. For instance, let us take the case of gender, where men are overrepresented in prison in comparison with women. As to account for this aspect, the algorithm may discount violent priors for men in order to reduce their recidivism-risk score. However, attaining this sort of algorithmic fairness would imply inequality of treatment across genders (Berk et al., 2018 ).
Fairness could be further hampered by the combined use of this algorithm with others driving decisions on neighbourhood police patrolling. The fact these algorithms may be prone to drive further patrolling in poor neighbourhoods may result from a training bias as crimes occurring in public tend to be more frequently reported (Karppi, 2018 ). One can easily understand how these algorithms may jointly produce a vicious cycle—more patrolling would lead to more arrests that would worsen the neighbourhood average recidivism-risk score , which would in turn trigger more patrolling. All this would result in exacerbated inequalities, likewise the case of credit scores previously discussed (O’Neil, 2016 ).
A potential point of friction may also emerge between the algorithm dimensions of fairness and accuracy. The latter may be theoretically defined as the classification error in terms of rate of false positive (individuals labelled at risk of recidivism, that did not re-offend within 2 years) and false negative (individuals labelled at low risk of recidivism, that did re-offend within the same timeframe) (Loi and Christen, 2019 ). Different classification accuracy (the fraction of observed outcomes in disagreement with the predictions) and forecasting accuracy (the fraction of predictions in disagreement with the observed outcomes) may exist across different classes of individuals (e.g., black or white defendants). Seeking equal rates of false positive and false negative across these two pools would imply a different forecasting error (and accuracy) given the different characteristics of the two different training pools available for the algorithm. Conversely, having the same forecasting accuracy would come at the expense of different classification errors between these two pools (Corbett-Davies et al., 2016 ). Hence, a trade-off exists between these two different shades of fairness, which derives from the very statistical properties of the data population distributions the algorithm has been trained on. However, the decision-making rests again on the assumptions the algorithm developers have adopted, e.g., on the relative importance of false positive and false negative (i.e., the weights attributed to the different typologies of errors, and the accuracy sought (Berk, 2019 )). When it comes to this point, an algorithm developer may decide (or be instructed) to train his/her algorithm to attribute, e.g., a five/ten/twenty times higher weight for a false negative (re-offender, low recidivism-risk score) in comparison with a false positive (non re-offender, high recidivism-risk score).
As with all ML, an issue of transparency exists as no one knows what type of inference is drawn on the variables out of which the recidivism-risk score is estimated. Reverse-engineering exercises have been run so as to understand what are the key drivers on the observed scores. Rudin ( 2019 ) found that the algorithm seemed to behave differently from the intentions of their creators (Northpointe, 2012 ) with a non-linear dependence on age and a weak correlation with one’s criminal history. These exercises (Rudin, 2019 ; Angelino et al., 2018 ) showed that it is possible to implement interpretable classification algorithms that lead to a similar accuracy as COMPAS. Dressel and Farid ( 2018 ) achieved this result by using a linear predictor-logistic regressor that made use of only two variables (age and total number of previous convictions of the subject).
Machine-learning algorithms in the field of autonomous vehicles
The case of autonomous vehicles, also known as self-driving vehicles, poses different challenges as a continuity of decisions is to be enacted while the vehicle is moving. It is not a one-off decision as in the case of the assessment of recidivism risk.
An exercise to appreciate the value-ladenness of these decisions is the moral-machine experiment (Massachussets Institute of Technology 2019 )—a serious game where users are requested to fulfil the function of an autonomous-vehicle decision-making algorithm in a situation of danger. This experiment entails performing choices that would prioritise the safety of some categories of users over others. For instance, choosing over the death of car occupants, pedestrians, or occupants of other vehicles, et cetera. While such extreme situations may be a simplification of reality, one cannot exclude that the algorithms driving an autonomous-vehicle may find themselves in circumstances where their decisions may result in harming some of the involved parties (Bonnefon et al., 2019 ).
In practice, the issue would be framed by the algorithm in terms of a statistical trolley dilemma in the words of Bonnefon et al. ( 2019 ), whereby the risk of harm for some road users will be increased. This corresponds to a risk management situation by all means, with a number of nuances and inherent complexity (Goodall, 2016 ).
Hence, autonomous vehicles are not bound to play the role of silver bullets, solving once and forever the vexing issue of traffic fatalities (Smith, 2018 ). Furthermore, the way decisions enacted could backfire in complex contexts to which the algorithms had no extrapolative power, is an unpredictable issue one has to deal with (Wallach and Allen, 2008 ; Yurtsever et al., 2020 ).
Coding algorithms that assure fairness in autonomous vehicles can be a very challenging issue. Contrasting and incommensurable dimensions are likely to emerge (Goodall, 2014 ) when designing an algorithm to reduce the harm of a given crash. For instance, in terms of material damage against human harm. Odds may emerge between the interest of the vehicle owner and passengers, on one side, and the collective interest of minimising the overall harm, on the other. Minimising the overall physical harm may be achieved by implementing an algorithm that, in the circumstance of an unavoidable collision, would target the vehicles with the highest safety standards. However, one may want to question the fairness of targeting those who have invested more in their own and others’ safety. The algorithm may also face a dilemma between low probability of a serious harm and higher probability of a mild harm. Unavoidable normative rules will need to be included in the decision-making algorithms to tackle these types of situations.
Accuracy in the context of self-autonomous vehicles rests on their capacity to correctly simulate the course of the events. While this is based on physics and can be informed by the numerous sensors these vehicles are equipped with, unforeseen events can still play a prominent role, and profoundly affect the vehicles behaviour and reactions (Yurtsever et al., 2020 ). For instance, fatalities due to autonomous-vehicle malfunctioning were reported as caused by the following failures: (i) the incapability of perceiving a pedestrian as such (National Transport Safety Board 2018 ); (ii) the acceleration of the vehicle in a situation when braking was required due to contrasting instructions from different algorithms the vehicle was hinged upon (Smith, 2018 ). In this latter case, the complexity of autonomous-vehicle algorithms was witnessed by the millions lines of code composing their scripts, a universe no one fully understands in the words of The Guardian (Smith, 2018 ), so that the causality of the decisions made was practically impossible to scrutinise. Hence, no corrective action in the algorithm code may be possible at this stage, with no room for improvement in accuracy.
One should also not forget that these algorithms are learning by direct experience and they may still end up conflicting with the initial set of ethical rules around which they have been conceived. Learning may occur through algorithms interaction taking place at a higher hierarchical level than the one imagined in the first place (Smith, 2018 ). This aspect would represent a further open issue to be taken into account in their development (Markham et al., 2018 ). It also poses further tension between the accuracy a vehicle manufacturer seeks and the capability to keep up the agreed fairness standards upstream from the algorithm development process.
Discussion and conclusions
In this contribution, we have examined the ethical dimensions affected by the application of algorithm-driven decision-making. These are entailed both ex-ante, in terms of the assumptions underpinning the algorithm development, and ex-post as regards the consequences upon society and social actors on whom the elaborated decisions are to be enforced.
Decision-making-based algorithms rest inevitably on assumptions, even silent ones, such as the quality of data the algorithm is trained on (Saltelli and Funtowicz, 2014 ), or the actual modelling relations adopted (Hoerl, 2019 ), with all the implied consequences (Saltelli, 2019 ).
A decision-making algorithm will always be based on a formal system, which is a representation of a real system (Rosen, 2005 ). As such, it will always be based on a restricted set of relevant relations, causes, and effects. It does not matter how complicated the algorithm may be (how many relations may be factored in), it will always represent one-specific vision of the system being modelled (Laplace, 1902 ).
Eventually, the set of decision rules underpinning the AI algorithm derives from human-made assumptions, such as, where to define the boundary between action and no action, between different possible choices. This can only take place at the human/non-human interface: the response of the algorithm is driven by these human-made assumptions and selection rules. Even the data on which an algorithm is trained on are not an objective truth, they are dependent upon the context in which they have been produced (Neff et al., 2017 ).
Tools for technically scrutinising the potential behaviour of an algorithm and its uncertainty already exist and could be included in the workflow of algorithm development. For instance, global sensitivity analysis (Saltelli, 2008 ) may help in exploring how the uncertainty in the input parameters and modelling assumptions would affect the output. Additionally, a modelling of the modelling process would assist in the model transparency and in addressing questions such as: Are the results from a particular model more sensitive to changes in the model and the methods used to estimate its parameters, or to changes in the data? (Majone, 1989 ).
Tools of post-normal-science inspiration for knowledge and modelling quality assessment could be adapted to the analysis of algorithms, such as the NUSAP (Numeral Unit Spread Assessment Pedigree) notation system for the management and communication of uncertainty (Funtowicz and Ravetz, 1990 ; Van Der Sluijs, 2005 ) and sensitivity auditing (Saltelli and Funtowicz, 2014 ), respectively. Ultimately, developers should acknowledge the limits of AI, and what its ultimate function should be in the equivalent of an Hippocratic Oath for ML developers (O’Neil, 2016 ). An example comes from the field of financial modelling, with a manifesto elaborated in the aftermath of the 2008 financial crisis (Derman and Wilmott, 2009 ).
As to address these dimensions, value statements and guidelines have been elaborated by political and multi-stakeholder organisations. For instance, The Alan Turing Institute released a guide for responsible design and implementation of AI (Leslie, 2019 ) that covers the whole life-cycle of design, use, and monitoring. However, the field of AI ethics is just at its infancy and it is still to be conceptualised how AI developments that encompass ethical dimensions could be attained. Some authors are pessimistic, such as Supiot ( 2017 ) who speaks of governance by numbers , where quantification is replacing the traditional decision-making system and profoundly affecting the pillar of equality of judgement. Trying to revert the current state of affairs may expose the first movers in the AI field to a competitive disadvantage (Morley et al., 2019 ). One should also not forget that points of friction across ethical dimensions may emerge, e.g., between transparency and accountability, or accuracy and fairness as highlighted in the case studies. Hence, the development process of the algorithm cannot be perfect in this setting, one has to be open to negotiation and unavoidably work with imperfections and clumsiness (Ravetz, 1987 ).
The development of decision-making algorithms remains quite obscure in spite of the concerns raised and the intentions manifested to address them. Attempts to expose to public scrutiny the algorithms developed are yet scant. As are the attempt to make the process more inclusive, with a higher participation from all the stakeholders. Identifying a relevant pool of social actors may require an important effort in terms of stakeholders’ mapping so as to assure a complete, but also effective, governance in terms of number of participants and simplicity of working procedures. The post-normal-science concept of extended peer communities could assist also in this endeavour (Funtowicz and Ravetz, 1997 ). Example-based explanations (Molnar, 2020 ) may also contribute to an effective engagement of all the parties by helping in bridging technical divides across developers, experts in other fields, and lay-people.
An overarching meta-framework for the governance of AI in experimental technologies (i.e., robot use) has also been proposed (Rego de Almeida et al., 2020 ). This initiative stems from the attempt to include all the forms of governance put forth and would rest on an integrated set of feedback and interactions across dimensions and actors. An interesting proposal comes from Berk ( 2019 ), who asked for the intervention of super partes authorities to define standards of transparency, accuracy and fairness for algorithm developers in line with the role of the Food and Drug administration in the US and other regulation bodies. A shared regulation could help in tackling the potential competitive disadvantage a first mover may suffer. The development pace of new algorithms would be necessarily reduced so as to comply with the standards defined and the required clearance processes. In this setting, seeking algorithm transparency would not be harmful for their developers as scrutiny would be delegated to entrusted intermediate parties, to take place behind closed doors (de Laat, 2018 ).
As noted by a perceptive reviewer, ML systems that keep learning are dangerous and hard to understand because they can quickly change. Thus, could a ML system with real world consequences be “locked down” to increase transparency? If yes, the algorithm could become defective. If not, transparency today may not be helpful in understanding what the system does tomorrow. This issue could be tackled by hard-coding the set of rules on the behaviour of the algorithm, once these are agreed upon among the involved stakeholders. This would prevent the algorithm-learning process from conflicting with the standards agreed. Making mandatory to deposit these algorithms in a database owned and operated by this entrusted super-partes body could ease the development of this overall process.
Ananny M, Crawford K (2018) Seeing without knowing: Limitations of the transparency ideal and its application to algorithmic accountability. New Media & Society 20:973–989
Article Google Scholar
Angelino E, Larus-Stone N, Alabi D, Seltzer M, Rudin C (2018) Learning certifiably optimal rule lists for categorical data. http://arxiv.org/abs/1704.01701
Angwin J, Larson J (2016) Machine bias. https://www.propublica.org/article/machine-bias-risk-assessments-in-criminal-sentencing
Bahrammirzaee A (2010) A comparative survey of artificial intelligence applications in finance: artificial neural networks, expert system and hybrid intelligent systems. Neural Comput Appl 19:1165–1195
Beam AL, Kohane IS (2018) Big data and machine learning in health care. JAMA 319:1317
Berk R (2019) Machine learning risk assessments in criminal justice settings. Springer International Publishing, Cham
Berk R, Heidari H, Jabbari S, Kearns M, Roth A (2018) Fairness in criminal justice risk assessments: the state of the art. Soc Methods Res 004912411878253
Board NTS (2018) Vehicle automation report. Tech. Rep. HWY18MH010, Office of Highway Safety, Washington, D.C.
Bonnefon J-F, Shariff A, Rahwan I (2019) The trolley, the bull bar, and why engineers should care about the ethics of autonomous cars [point of view]. Proc IEEE 107:502–504
Brief WP (2020) World prison brief- an online database comprising information on prisons and the use of imprisonment around the world. https://www.prisonstudies.org/
Cheng J (2009) Virtual composer makes beautiful music and stirs controversy. https://arstechnica.com/science/news/2009/09/virtual-composer-makes-beautiful-musicand-stirs-controversy.ars
Chin J (2019) The death of data scientists. https://towardsdatascience.com/the-death-of-data-scientists-c243ae167701
Corbett-Davies S, Pierson E, Feller A, Goel S (2016) A computer program used for bail and sentencing decisions was labeled biased against blacks. It’s actually not that clear. Washington Post. https://www.washingtonpost.com/news/monkey-cage/wp/2016/10/17/can-an-algorithm-be-racist-our-analysis-is-more-cautious-than-propublicas/
Cowls J (2020) Deciding how to decide: six key questions for reducing AI’s democratic deficit. In: Burr C, Milano S (eds) The 2019 Yearbook of the Digital Ethics Lab, Digital ethics lab yearbook. Springer International Publishing, Cham. pp. 101–116. https://doi.org/10.1007/978-3-030-29145-7_7
Daly A et al. (2019) Artificial intelligence, governance and ethics: global perspectives. SSRN Electron J. https://www.ssrn.com/abstract=3414805
Dastin J (2018) Amazon scraps secret AI recruiting tool that showed bias against women. Reuters. https://www.reuters.com/article/us-amazon-com-jobs-automation-insight-idUSKCN1MK08G
De Sutter P (2020) Automated decision-making processes: ensuring consumer protection, and free movement of goods and services. https://www.europarl.europa.eu/meetdocs/2014_2019/plmrep/COMMITTEES/IMCO/DV/2020/01-22/Draft_OQ_Automated_decision-making_EN.pdf
Derman E, Wilmott P (2009) The financial modelers’ manifesto. SSRN Electron J. http://www.ssrn.com/abstract=1324878 .
Dragičević T, Wheeler P, Blaabjerg F (2019) Artificial intelligence aided automated design for reliability of power electronic systems. IEEE Trans Power Electron 34:7161–7171
Article ADS Google Scholar
Dressel J, Farid H (2018) The accuracy, fairness, and limits of predicting recidivism. Sci Adv 4:eaao5580
Edwards L, Veale M (2018) Enslaving the algorithm: from A -right to an explanation- to A -right to better decisions-? IEEE Security, Priv 16:46–54
Floridi L, Cowls J (2019) A unified framework of five principles for AI in society. Harvard Data Science Review. https://hdsr.mitpress.mit.edu/pub/l0jsh9d1
Funtowicz SO, Ravetz JR (1990) Uncertainty and quality in science for policy. Springer Science, Business Media, Berlin, Heidelberg
Book Google Scholar
Funtowicz S, Ravetz J (1997) Environmental problems, post-normal science, and extended peer communities. Études et Recherches sur les Systémes Agraires et le Développement. INRA Editions. pp. 169–175
Future of Earth Institute (2020) National and International AI Strategies. https://futureoflife.org/national-international-ai-strategies/
Gallagher S (2016) AI bests Air Force combat tactics experts in simulated dogfights. https://arstechnica.com/information-technology/2016/06/ai-bests-air-force-combat-tactics-experts-in-simulated-dogfights/
Goodall NJ (2014) Ethical decision making during automated vehicle crashes. Transportation Res Rec: J Transportation Res Board 2424:58–65
Goodall NJ (2016) Away from trolley problems and toward risk management. Appl Artif Intell 30:810–821
Greene D, Hoffmann AL, Stark L (2019) Better, nicer, clearer, fairer: a critical assessment of the movement for ethical artificial intelligence and machine learning. In Proceedings of the 52nd Hawaii International Conference on System Sciences
Hmoud B, Laszlo V (2019) Will artificial intelligence take over human-resources recruitment and selection? Netw Intell Stud VII:21–30
Hoerl RW (2019) The integration of big data analytics into a more holistic approach-JMP. Tech. Rep., SAS Institute. https://www.jmp.com/en_us/whitepapers/jmp/integration-of-big-data-analytics-holistic-approach.html
Jobi A, Ienca M, Vayena E (2019) Artificial intelligence: the global landscape of ethics guidelines. Nat Mach Intell 1:389–399
Karppi T (2018) The computer said so-: on the ethics, effectiveness, and cultural techniques of predictive policing. Soc Media + Soc 4:205630511876829
Kongthon A, Sangkeettrakarn C, Kongyoung S, Haruechaiyasak C (2009) Implementing an online help desk system based on conversational agent. In: Proceedings of the International Conference on Management of Emergent Digital EcoSystems, MEDES ’09, vol. 69. ACM, New York, NY, USA. pp. 450–69:451. Event-place: France. https://doi.org/10.1145/1643823.1643908
de Laat PB (2018) Algorithmic decision-making based on machine learning from big data: can transparency restore accountability? Philos Technol 31:525–541
Laplace PS (1902) A philosophical essay on probabilities. J. Wiley, New York; Chapman, Hall, London. http://archive.org/details/philosophicaless00lapliala
Leslie D (2019) Understanding artificial intelligence ethics and safety. http://arxiv.org/abs/1906.05684
Loi M, Christen M (2019) How to include ethics in machine learning research. https://ercim-news.ercim.eu/en116/r-s/how-to-include-ethics-in-machine-learning-research
Majone G (1989) Evidence, argument, and persuasion in the policy process. Yale University Press, Yale
Google Scholar
Markham AN, Tiidenberg K, Herman A (2018) Ethics as methods: doing ethics in the era of big data research-introduction. Soc Media + Soc 4:205630511878450
Massachussets Institute of Technology (2019) Moral machine. Massachussets Institute of Technology. http://moralmachine.mit.edu
McCarthy J, Minsky ML, Rochester N, Shannon CE (2006) A proposal for the dartmouth summer research project on artificial intelligence, August 31, 1955. AI Mag 27:12–12
Mittelstadt B (2019) Principles alone cannot guarantee ethical AI. Nat Mach Intell 1:501–507
Molnar C (2020) Interpretable machine learning (2020). https://christophm.github.io/interpretable-ml-book/
Morley J, Floridi L, Kinsey K, Elhalal A (2019) From what to how: an initial review of publicly available AI ethics tools, methods and research to translate principles into practices. Tech Rep. https://arxiv.org/abs/1905.06876
Neff G, Tanweer A, Fiore-Gartland B, Osburn L (2017) Critique and contribute: a practice-based framework for improving critical data studies and data science. Big Data 5:85–97
Nissenbaum H (1996) Accountability in a computerized society. Sci Eng Ethics 2:25–42
Northpointe (2012) Practitioner’s guide to COMPAS. northpointeinc.com/files/technical_documents/FieldGuide2_081412.pdf
O’Neil C (2016) Weapons of math destruction: how big data increases inequality and threatens democracy, 1st edn. Crown, New York
MATH Google Scholar
Rader E, Cotter K, Cho J (2018) Explanations as mechanisms for supporting algorithmic transparency. In: Proceedings of the 2018 CHI Conference on Human Factors in Computing Systems - CHI ’1 8 . ACM Press, Montreal QC, Canada. pp. 1–13. http://dl.acm.org/citation.cfm?doid=3173574.3173677
Raji ID et al. Closing the AI accountability gap: defining an end-to-end framework for internal algorithmic auditing. In Proceedings of the 2020 Conference on Fairness, Accountability, and Transparency pp 33–44 (Association for Computing Machinery, 2020). https://doi.org/10.1145/3351095.3372873
Ravetz JR (1987) Usable knowledge, usable ignorance: incomplete science with policy implications. Knowledge 9:87–116
Rêgo de Almeida PG, Denner dos Santos C, Silva Farias J (2020) Artificial intelligence regulation: a meta-framework for formulation and governance. In: Proceedings of the 53rd Hawaii International Conference on System Sciences (2020). http://hdl.handle.net/10125/64389
Roberts H et al. (2019) The Chinese approach to artificial intelligence: an analysis of policy and regulation. SSRN Electron J. https://www.ssrn.com/abstract=3469784
Rosen R (2005) Life itself: a comprehensive inquiry into the nature, origin, and fabrication of life. Columbia University Press, New York
Rudin C (2019) Stop explaining black box machine learning models for high stakes decisions and use interpretable models instead. http://arxiv.org/abs/1811.10154
Russell SJ (2010) Artificial intelligence : a modern approach. Prentice Hall, Upper Saddle River, NJ
Saltelli A et al. (2008) Global sensitivity analysis: the primer. Wiley, Hoboken, NJ
Saltelli A (2019) A short comment on statistical versus mathematical modelling. Nat Commun 10:3870
Saltelli A (2020) Ethics of quantification or quantification of ethics? Futures 116:102509
Saltelli A, Funtowicz S (2014) When all models are wrong. Issues Sci Technol 30:79–85
Samuel AL (1959) Some studies in machine learning using the game of checkers. IBM J Res Dev 3:210–229
Article MathSciNet Google Scholar
Sareen S, Saltelli A, Rommetveit K (2020) Ethics of quantification: illumination, obfuscation and performative legitimation. Palgrave Commun 6:1–5
Sears (2018) The role of artificial intelligence in the classroom. https://elearningindustry.com/artificial-intelligence-in-the-classroom-role
Sennaar K (2019) AI in agriculture-present applications and impact. https://emerj.com/ai-sector-overviews/ai-agriculture-present-applications-impact/
Van Der Sluijs JP et al. (2005) Combining quantitative and qualitative measures of uncertainty in model-based environmental assessment: The NUSAP system. Risk Anal 25:481–492
Smith A (2018) Franken-algorithms: the deadly consequences of unpredictable code. The Guardian. https://www.theguardian.com/technology/2018/aug/29/coding-algorithms-frankenalgos-program-danger
Sonnenburg S et al. (2007) The need for open source software in machine learning. J Mach Learn Res 8:2443–2466
Supiot A (2017) Governance by numbers: the making of a legal model of allegiance. Hart Publishing, Oxford; Portland, Oregon
Taleb NN (2007) The Black Swan: the impact of the highly improbable. Random House Publishing Group, New York, NY
Thimbleby H (2003) Explaining code for publication. Softw: Pract Experience 33:975–1001
Wallach W, Allen C (2008) Moral machines: teaching robots right from wrong. Oxford University Press, Oxford, USA
Watson D, Floridi L (2019) The explanation game: A formal framework for interpretable machine learning. https://papers.ssrn.com/abstract=3509737
Wiener N (1988) The human use of human beings: cybernetics and society. Da Capo Press, New York, N.Y, new edition
Wong YH et al. (2020). Deterrence in the age of thinking machines: product page. RAND Corporation. https://www.rand.org/pubs/research_reports/RR2797.html
Ye H et al. (2018) Machine learning for vehicular networks: recent advances and application examples. IEEE Vehicular Technol Mag 13:94–101
Yu H et al. (2018) Building ethics into artificial intelligence. http://arxiv.org/abs/1812.02953
Yurtsever E, Capito L, Redmill K, Ozguner U (2020) Integrating deep reinforcement learning with model-based path planners for automated driving. http://arxiv.org/abs/2002.00434
Download references
Acknowledgements
I would like to thank Kjetil Rommetveit, Andrea Saltelli and Siddarth Sareen for the organisation of the Workshop Ethics of Quantification , and the Centre for the Study of Sciences and the Humanities of the University of Bergen for the travel grant, at which a previous version of this paper was presented. Thomas Hodgson, Jill Walter Rettberg, Elizabeth Chatterjee, Ragnar Fjelland and Marta Kuc-Czarnecka for their useful comments in this venue. Finally, Stefn Thor Smith and Andrea Saltelli for their suggestions and constructive criticism on a draft version of the present manuscript.
Author information
Authors and affiliations.
School of the Built Environment, University of Reading, Reading, UK
Samuele Lo Piano
Open Evidence, Universitat Oberta de Catalunya, Barcelona, Catalonia, Spain
You can also search for this author in PubMed Google Scholar
Corresponding author
Correspondence to Samuele Lo Piano .
Ethics declarations
Competing interests.
The author declares no competing interests.
Additional information
Publisher’s note Springer Nature remains neutral with regard to jurisdictional claims in published maps and institutional affiliations.
Rights and permissions
Open Access This article is licensed under a Creative Commons Attribution 4.0 International License, which permits use, sharing, adaptation, distribution and reproduction in any medium or format, as long as you give appropriate credit to the original author(s) and the source, provide a link to the Creative Commons license, and indicate if changes were made. The images or other third party material in this article are included in the article’s Creative Commons license, unless indicated otherwise in a credit line to the material. If material is not included in the article’s Creative Commons license and your intended use is not permitted by statutory regulation or exceeds the permitted use, you will need to obtain permission directly from the copyright holder. To view a copy of this license, visit http://creativecommons.org/licenses/by/4.0/ .
Reprints and permissions
About this article
Cite this article.
Lo Piano, S. Ethical principles in machine learning and artificial intelligence: cases from the field and possible ways forward. Humanit Soc Sci Commun 7 , 9 (2020). https://doi.org/10.1057/s41599-020-0501-9
Download citation
Received : 29 January 2020
Accepted : 12 May 2020
Published : 17 June 2020
DOI : https://doi.org/10.1057/s41599-020-0501-9
Share this article
Anyone you share the following link with will be able to read this content:
Sorry, a shareable link is not currently available for this article.
Provided by the Springer Nature SharedIt content-sharing initiative
This article is cited by
A survey on interpretable reinforcement learning.
- Claire Glanois
Machine Learning (2024)
Reframing data ethics in research methods education: a pathway to critical data literacy
- Javiera Atenas
- Leo Havemann
- Cristian Timmermann
International Journal of Educational Technology in Higher Education (2023)
AI ethics: from principles to practice
- Jianlong Zhou
AI & SOCIETY (2023)
The Challenge of Quantification: An Interdisciplinary Reading
- Monica Di Fiore
- Marta Kuc-Czarnecka
- Andrea Saltelli
Minerva (2023)
Quick links
- Explore articles by subject
- Guide to authors
- Editorial policies

Featured Topics
Featured series.
A series of random questions answered by Harvard experts.
Explore the Gazette
Read the latest.
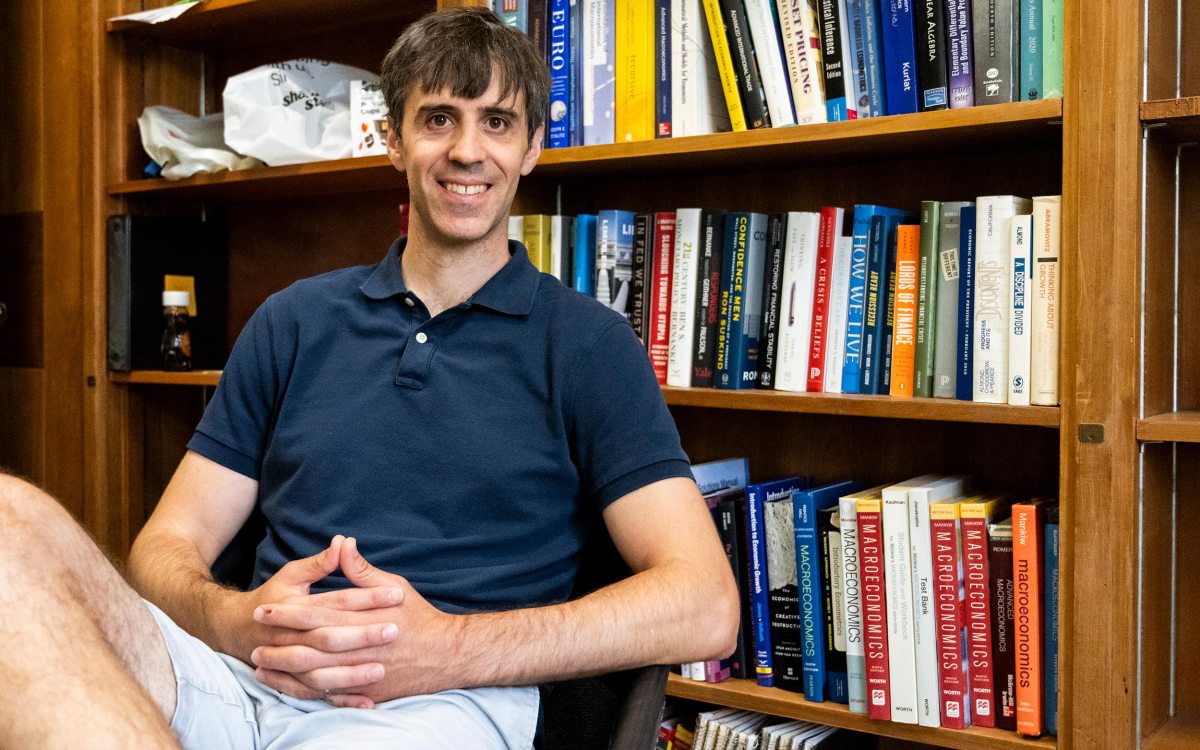
Raise corporate tax rates! No, cut them! Maybe take a look first?
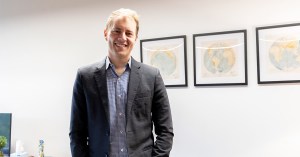
You want to be boss. You probably won’t be good at it.

Revising the cost of climate change
Illustration by Ben Boothman
Great promise but potential for peril
Christina Pazzanese
Harvard Staff Writer
Ethical concerns mount as AI takes bigger decision-making role in more industries
Second in a four-part series that taps the expertise of the Harvard community to examine the promise and potential pitfalls of the rising age of artificial intelligence and machine learning , and how to humanize them .
For decades, artificial intelligence, or AI, was the engine of high-level STEM research. Most consumers became aware of the technology’s power and potential through internet platforms like Google and Facebook, and retailer Amazon. Today, AI is essential across a vast array of industries, including health care, banking, retail, and manufacturing.
Also in the series
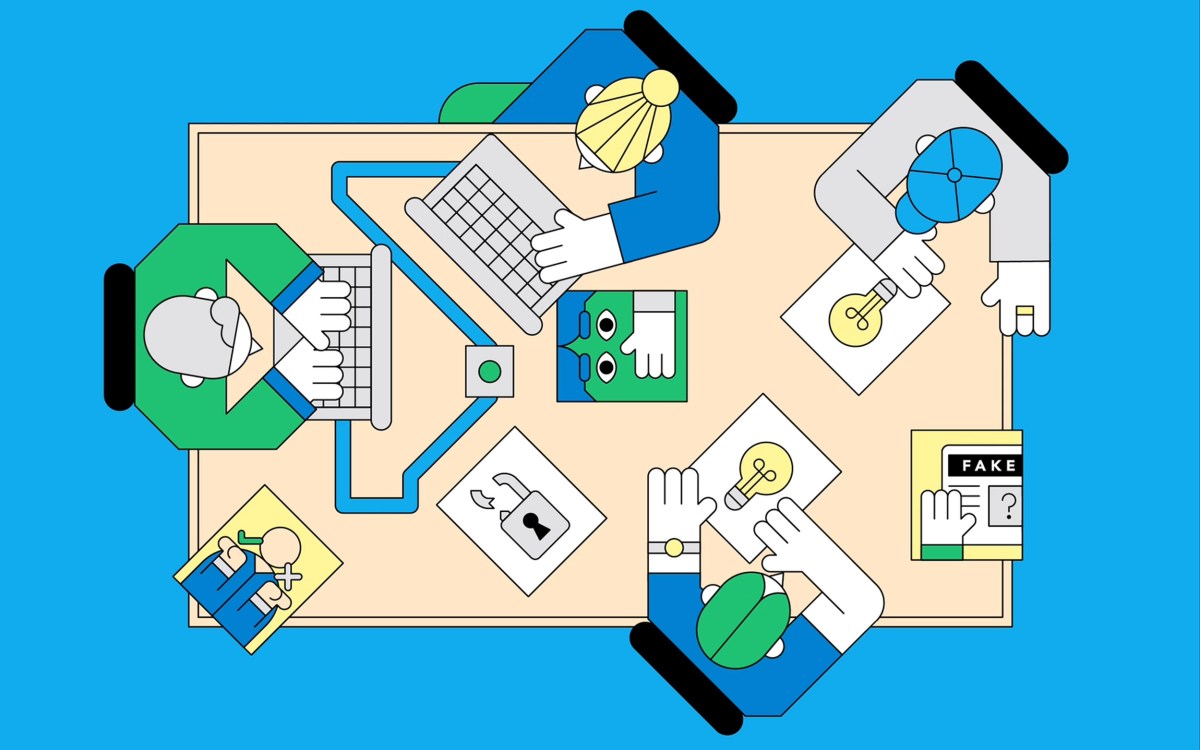
Trailblazing initiative marries ethics, tech
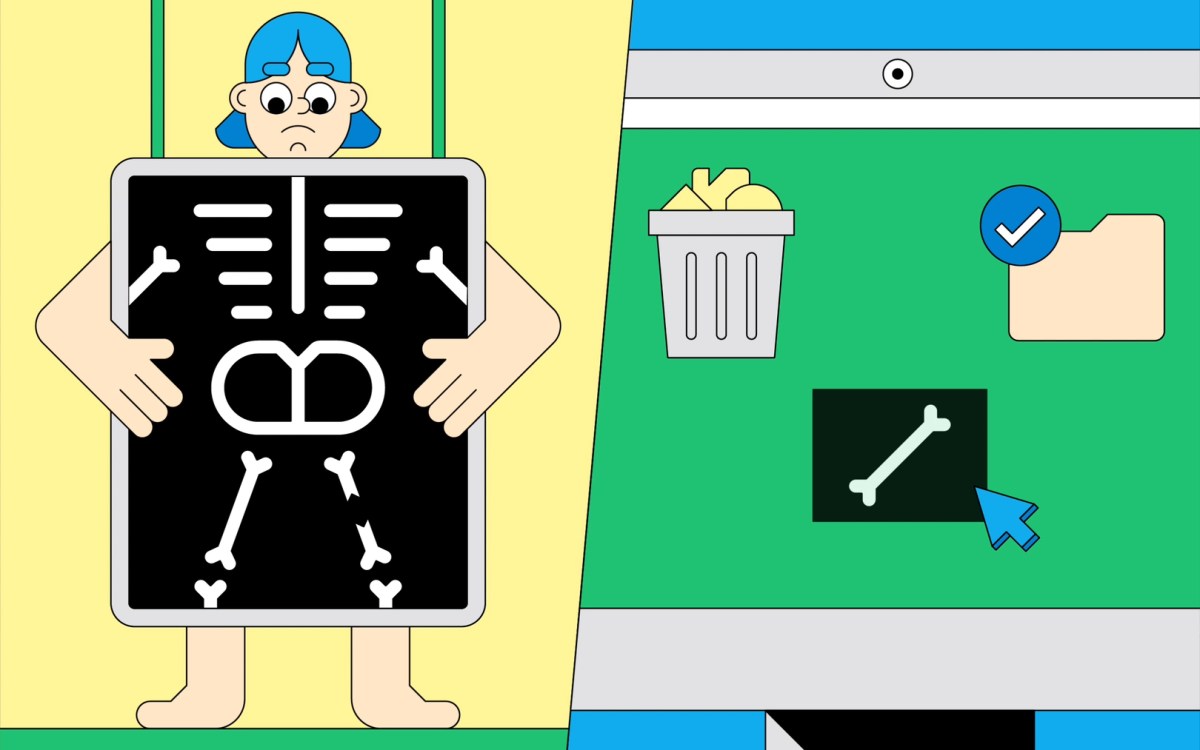
AI revolution in medicine
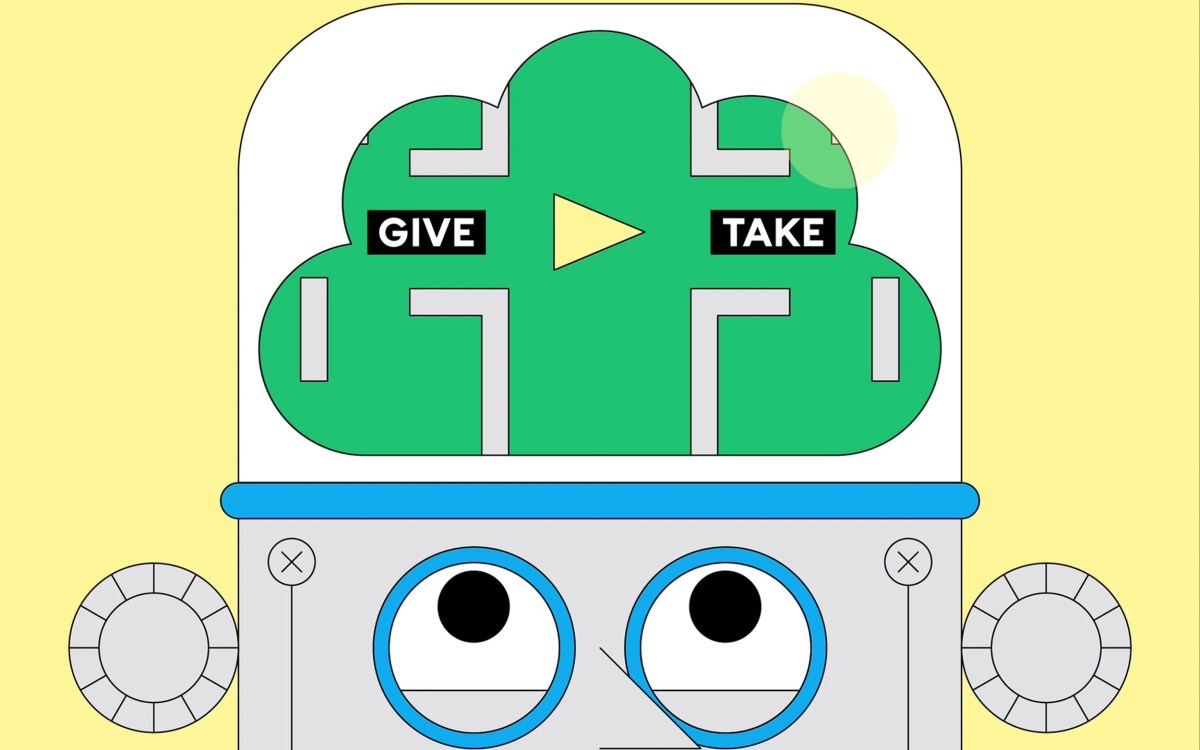
Imagine a world in which AI is in your home, at work, everywhere
But its game-changing promise to do things like improve efficiency, bring down costs, and accelerate research and development has been tempered of late with worries that these complex, opaque systems may do more societal harm than economic good. With virtually no U.S. government oversight, private companies use AI software to make determinations about health and medicine, employment, creditworthiness, and even criminal justice without having to answer for how they’re ensuring that programs aren’t encoded, consciously or unconsciously, with structural biases.
Its growing appeal and utility are undeniable. Worldwide business spending on AI is expected to hit $50 billion this year and $110 billion annually by 2024, even after the global economic slump caused by the COVID-19 pandemic, according to a forecast released in August by technology research firm IDC. Retail and banking industries spent the most this year, at more than $5 billion each. The company expects the media industry and federal and central governments will invest most heavily between 2018 and 2023 and predicts that AI will be “the disrupting influence changing entire industries over the next decade.”
“Virtually every big company now has multiple AI systems and counts the deployment of AI as integral to their strategy,” said Joseph Fuller , professor of management practice at Harvard Business School, who co-leads Managing the Future of Work , a research project that studies, in part, the development and implementation of AI, including machine learning, robotics, sensors, and industrial automation, in business and the work world.
Early on, it was popularly assumed that the future of AI would involve the automation of simple repetitive tasks requiring low-level decision-making. But AI has rapidly grown in sophistication, owing to more powerful computers and the compilation of huge data sets. One branch, machine learning, notable for its ability to sort and analyze massive amounts of data and to learn over time, has transformed countless fields, including education.
Firms now use AI to manage sourcing of materials and products from suppliers and to integrate vast troves of information to aid in strategic decision-making, and because of its capacity to process data so quickly, AI tools are helping to minimize time in the pricey trial-and-error of product development — a critical advance for an industry like pharmaceuticals, where it costs $1 billion to bring a new pill to market, Fuller said.
Health care experts see many possible uses for AI, including with billing and processing necessary paperwork. And medical professionals expect that the biggest, most immediate impact will be in analysis of data, imaging, and diagnosis. Imagine, they say, having the ability to bring all of the medical knowledge available on a disease to any given treatment decision.
In employment, AI software culls and processes resumes and analyzes job interviewees’ voice and facial expressions in hiring and driving the growth of what’s known as “hybrid” jobs. Rather than replacing employees, AI takes on important technical tasks of their work, like routing for package delivery trucks, which potentially frees workers to focus on other responsibilities, making them more productive and therefore more valuable to employers.
“It’s allowing them to do more stuff better, or to make fewer errors, or to capture their expertise and disseminate it more effectively in the organization,” said Fuller, who has studied the effects and attitudes of workers who have lost or are likeliest to lose their jobs to AI.
“Can smart machines outthink us, or are certain elements of human judgment indispensable in deciding some of the most important things in life?”
— Michael Sandel, political philosopher and Anne T. and Robert M. Bass Professor of Government
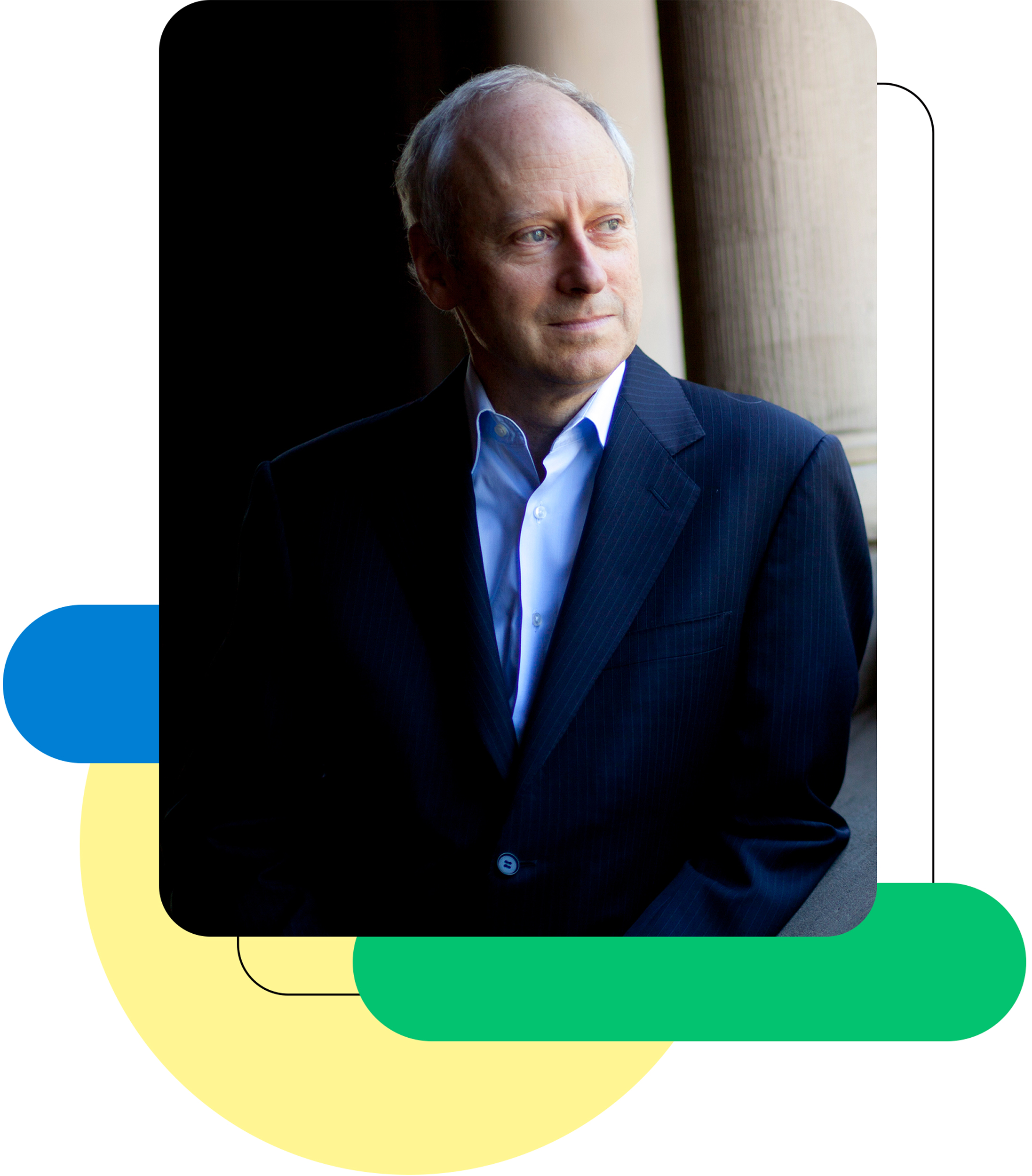
Though automation is here to stay, the elimination of entire job categories, like highway toll-takers who were replaced by sensors because of AI’s proliferation, is not likely, according to Fuller.
“What we’re going to see is jobs that require human interaction, empathy, that require applying judgment to what the machine is creating [will] have robustness,” he said.
While big business already has a huge head start, small businesses could also potentially be transformed by AI, says Karen Mills ’75, M.B.A. ’77, who ran the U.S. Small Business Administration from 2009 to 2013. With half the country employed by small businesses before the COVID-19 pandemic, that could have major implications for the national economy over the long haul.
Rather than hamper small businesses, the technology could give their owners detailed new insights into sales trends, cash flow, ordering, and other important financial information in real time so they can better understand how the business is doing and where problem areas might loom without having to hire anyone, become a financial expert, or spend hours laboring over the books every week, Mills said.
One area where AI could “completely change the game” is lending, where access to capital is difficult in part because banks often struggle to get an accurate picture of a small business’s viability and creditworthiness.
“It’s much harder to look inside a business operation and know what’s going on” than it is to assess an individual, she said.
Information opacity makes the lending process laborious and expensive for both would-be borrowers and lenders, and applications are designed to analyze larger companies or those who’ve already borrowed, a built-in disadvantage for certain types of businesses and for historically underserved borrowers, like women and minority business owners, said Mills, a senior fellow at HBS.
But with AI-powered software pulling information from a business’s bank account, taxes, and online bookkeeping records and comparing it with data from thousands of similar businesses, even small community banks will be able to make informed assessments in minutes, without the agony of paperwork and delays, and, like blind auditions for musicians, without fear that any inequity crept into the decision-making.
“All of that goes away,” she said.
A veneer of objectivity
Not everyone sees blue skies on the horizon, however. Many worry whether the coming age of AI will bring new, faster, and frictionless ways to discriminate and divide at scale.
“Part of the appeal of algorithmic decision-making is that it seems to offer an objective way of overcoming human subjectivity, bias, and prejudice,” said political philosopher Michael Sandel , Anne T. and Robert M. Bass Professor of Government. “But we are discovering that many of the algorithms that decide who should get parole, for example, or who should be presented with employment opportunities or housing … replicate and embed the biases that already exist in our society.”
“If we’re not thoughtful and careful, we’re going to end up with redlining again.”
— Karen Mills, senior fellow at the Business School and head of the U.S. Small Business Administration from 2009 to 2013
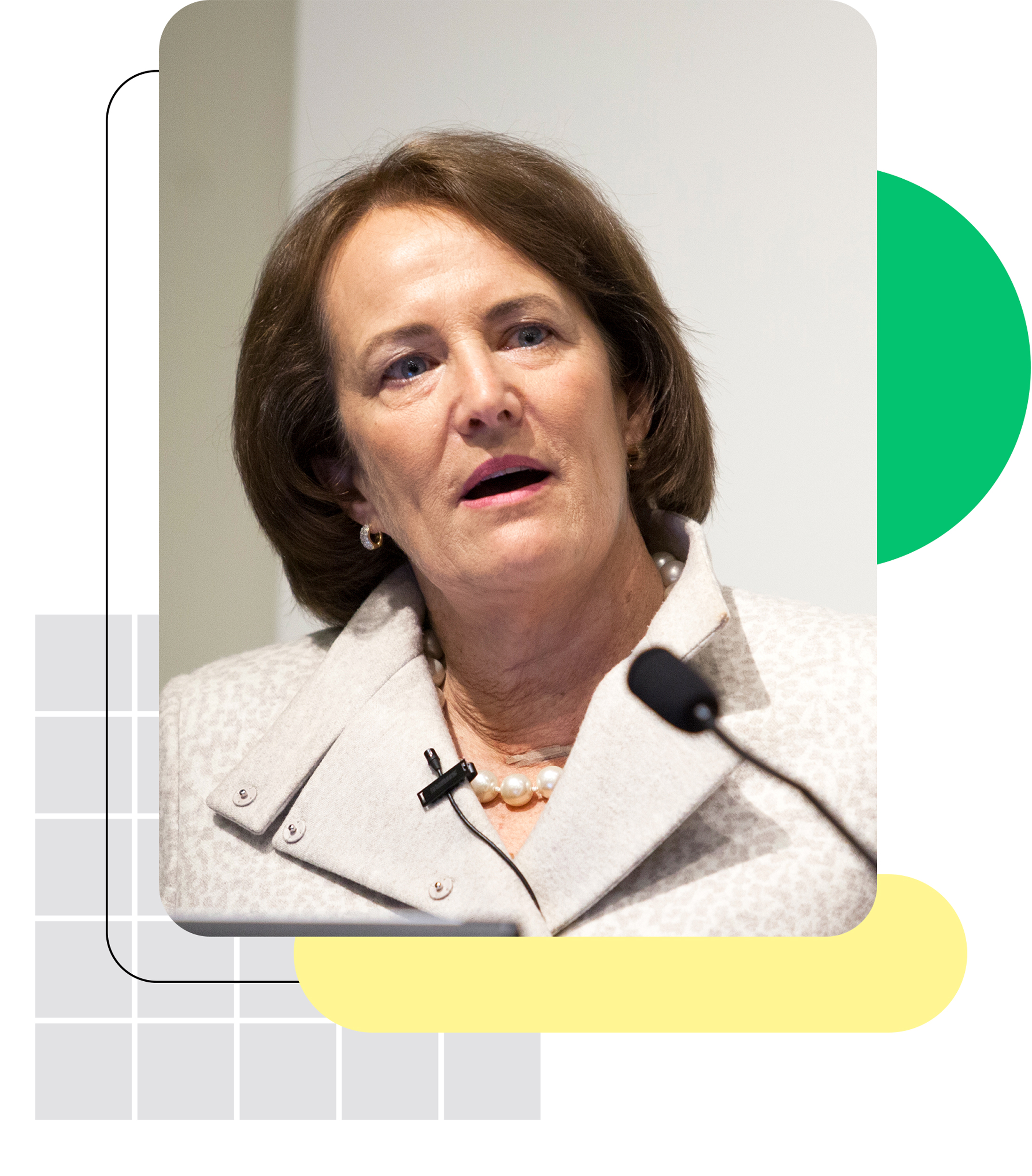
AI presents three major areas of ethical concern for society: privacy and surveillance, bias and discrimination, and perhaps the deepest, most difficult philosophical question of the era, the role of human judgment, said Sandel, who teaches a course in the moral, social, and political implications of new technologies.
“Debates about privacy safeguards and about how to overcome bias in algorithmic decision-making in sentencing, parole, and employment practices are by now familiar,” said Sandel, referring to conscious and unconscious prejudices of program developers and those built into datasets used to train the software. “But we’ve not yet wrapped our minds around the hardest question: Can smart machines outthink us, or are certain elements of human judgment indispensable in deciding some of the most important things in life?”
Panic over AI suddenly injecting bias into everyday life en masse is overstated, says Fuller. First, the business world and the workplace, rife with human decision-making, have always been riddled with “all sorts” of biases that prevent people from making deals or landing contracts and jobs.
When calibrated carefully and deployed thoughtfully, resume-screening software allows a wider pool of applicants to be considered than could be done otherwise, and should minimize the potential for favoritism that comes with human gatekeepers, Fuller said.
Sandel disagrees. “AI not only replicates human biases, it confers on these biases a kind of scientific credibility. It makes it seem that these predictions and judgments have an objective status,” he said.
In the world of lending, algorithm-driven decisions do have a potential “dark side,” Mills said. As machines learn from data sets they’re fed, chances are “pretty high” they may replicate many of the banking industry’s past failings that resulted in systematic disparate treatment of African Americans and other marginalized consumers.
“If we’re not thoughtful and careful, we’re going to end up with redlining again,” she said.
A highly regulated industry, banks are legally on the hook if the algorithms they use to evaluate loan applications end up inappropriately discriminating against classes of consumers, so those “at the top levels” in the field are “very focused” right now on this issue, said Mills, who closely studies the rapid changes in financial technology, or “fintech.”
“They really don’t want to discriminate. They want to get access to capital to the most creditworthy borrowers,” she said. “That’s good business for them, too.”
Oversight overwhelmed
Given its power and expected ubiquity, some argue that the use of AI should be tightly regulated. But there’s little consensus on how that should be done and who should make the rules.
Thus far, companies that develop or use AI systems largely self-police, relying on existing laws and market forces, like negative reactions from consumers and shareholders or the demands of highly-prized AI technical talent to keep them in line.
“There’s no businessperson on the planet at an enterprise of any size that isn’t concerned about this and trying to reflect on what’s going to be politically, legally, regulatorily, [or] ethically acceptable,” said Fuller.
Firms already consider their own potential liability from misuse before a product launch, but it’s not realistic to expect companies to anticipate and prevent every possible unintended consequence of their product, he said.
Few think the federal government is up to the job, or will ever be.
“The regulatory bodies are not equipped with the expertise in artificial intelligence to engage in [oversight] without some real focus and investment,” said Fuller, noting the rapid rate of technological change means even the most informed legislators can’t keep pace. Requiring every new product using AI to be prescreened for potential social harms is not only impractical, but would create a huge drag on innovation.
“I wouldn’t have a central AI group that has a division that does cars, I would have the car people have a division of people who are really good at AI.”
— Jason Furman, a professor of the practice of economic policy at the Kennedy School and a former top economic adviser to President Barack Obama
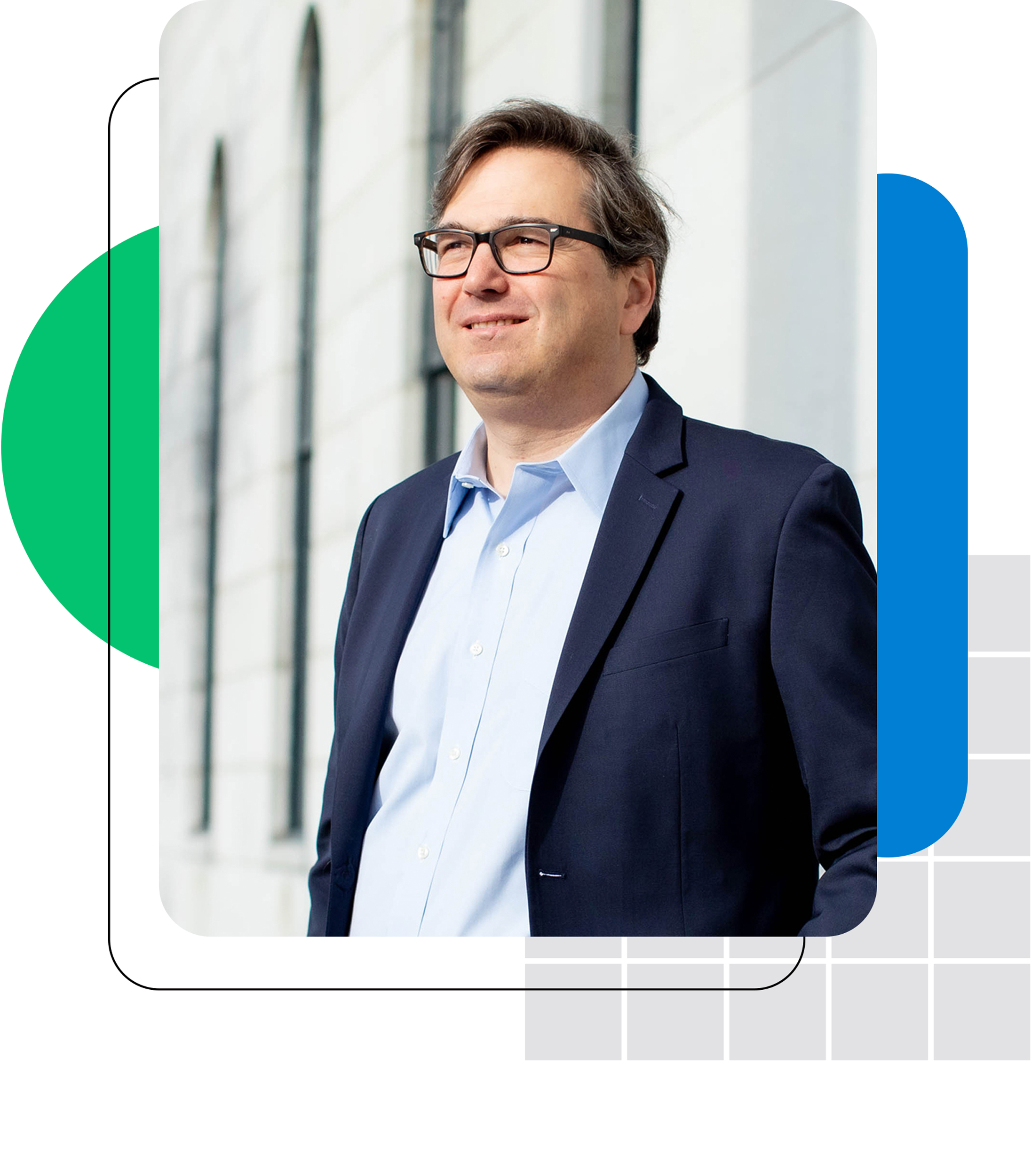
Jason Furman , a professor of the practice of economic policy at Harvard Kennedy School, agrees that government regulators need “a much better technical understanding of artificial intelligence to do that job well,” but says they could do it.
Existing bodies like the National Highway Transportation Safety Association, which oversees vehicle safety, for example, could handle potential AI issues in autonomous vehicles rather than a single watchdog agency, he said.
“I wouldn’t have a central AI group that has a division that does cars, I would have the car people have a division of people who are really good at AI,” said Furman, a former top economic adviser to President Barack Obama.
Though keeping AI regulation within industries does leave open the possibility of co-opted enforcement, Furman said industry-specific panels would be far more knowledgeable about the overarching technology of which AI is simply one piece, making for more thorough oversight.
While the European Union already has rigorous data-privacy laws and the European Commission is considering a formal regulatory framework for ethical use of AI, the U.S. government has historically been late when it comes to tech regulation.
“I think we should’ve started three decades ago, but better late than never,” said Furman, who thinks there needs to be a “greater sense of urgency” to make lawmakers act.
Business leaders “can’t have it both ways,” refusing responsibility for AI’s harmful consequences while also fighting government oversight, Sandel maintains.
More like this

The robots are coming, but relax
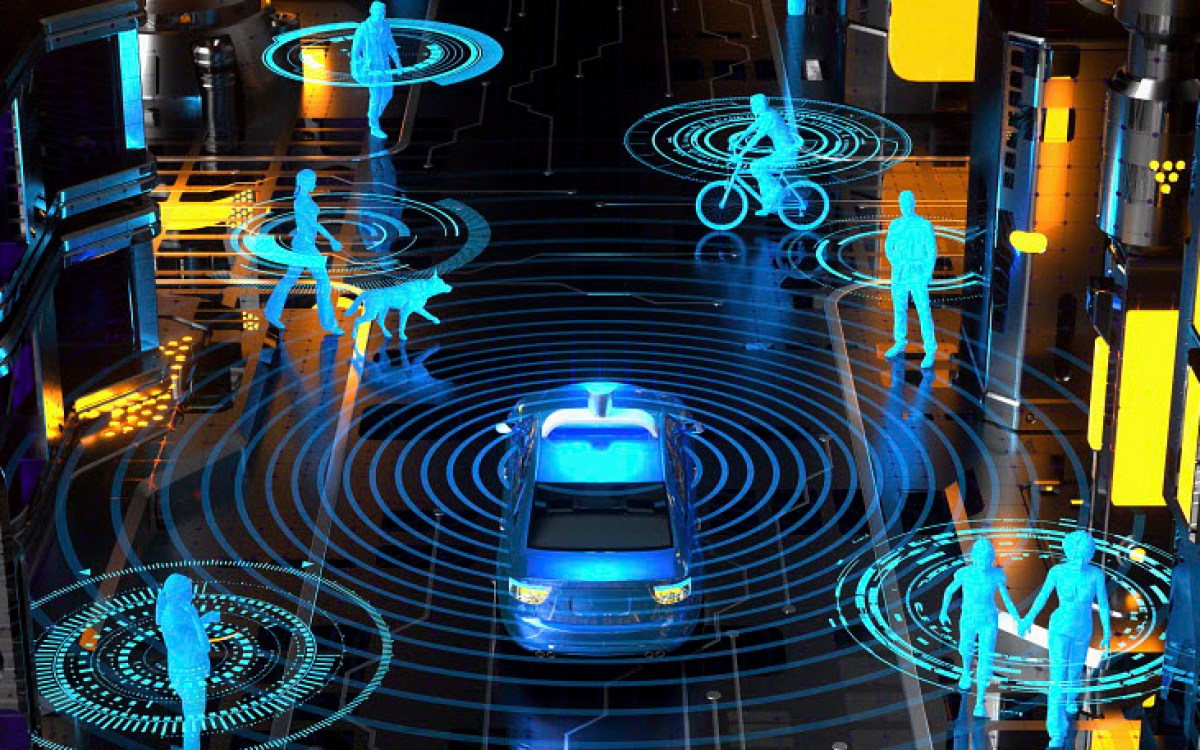
The good, bad, and scary of the Internet of Things
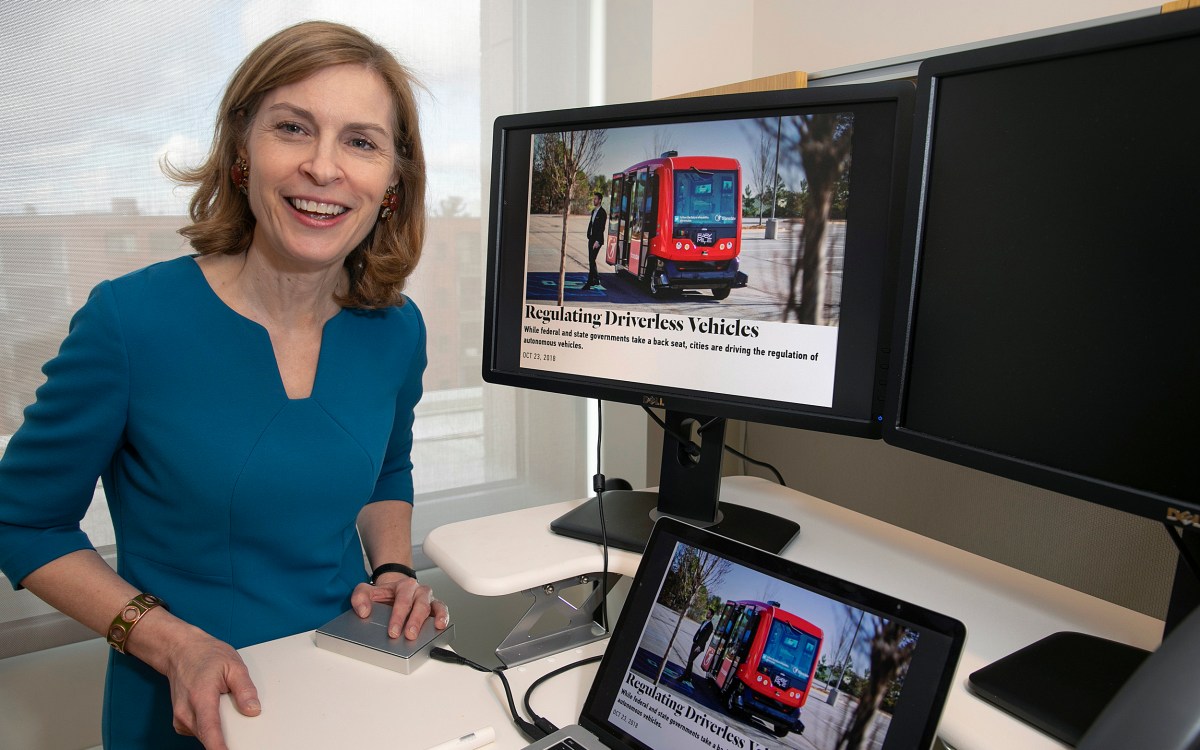
Paving the way for self-driving cars
“The problem is these big tech companies are neither self-regulating, nor subject to adequate government regulation. I think there needs to be more of both,” he said, later adding: “We can’t assume that market forces by themselves will sort it out. That’s a mistake, as we’ve seen with Facebook and other tech giants.”
Last fall, Sandel taught “ Tech Ethics ,” a popular new Gen Ed course with Doug Melton, co-director of Harvard’s Stem Cell Institute. As in his legendary “Justice” course, students consider and debate the big questions about new technologies, everything from gene editing and robots to privacy and surveillance.
“Companies have to think seriously about the ethical dimensions of what they’re doing and we, as democratic citizens, have to educate ourselves about tech and its social and ethical implications — not only to decide what the regulations should be, but also to decide what role we want big tech and social media to play in our lives,” said Sandel.
Doing that will require a major educational intervention, both at Harvard and in higher education more broadly, he said.
“We have to enable all students to learn enough about tech and about the ethical implications of new technologies so that when they are running companies or when they are acting as democratic citizens, they will be able to ensure that technology serves human purposes rather than undermines a decent civic life.”
Next: The AI revolution in medicine may lift personalized treatment, fill gaps in access to care, and cut red tape. Yet risks abound.
Share this article
You might like.
New study scrutinizes what did, did not work as disputed 2017 law becomes partisan football in election year
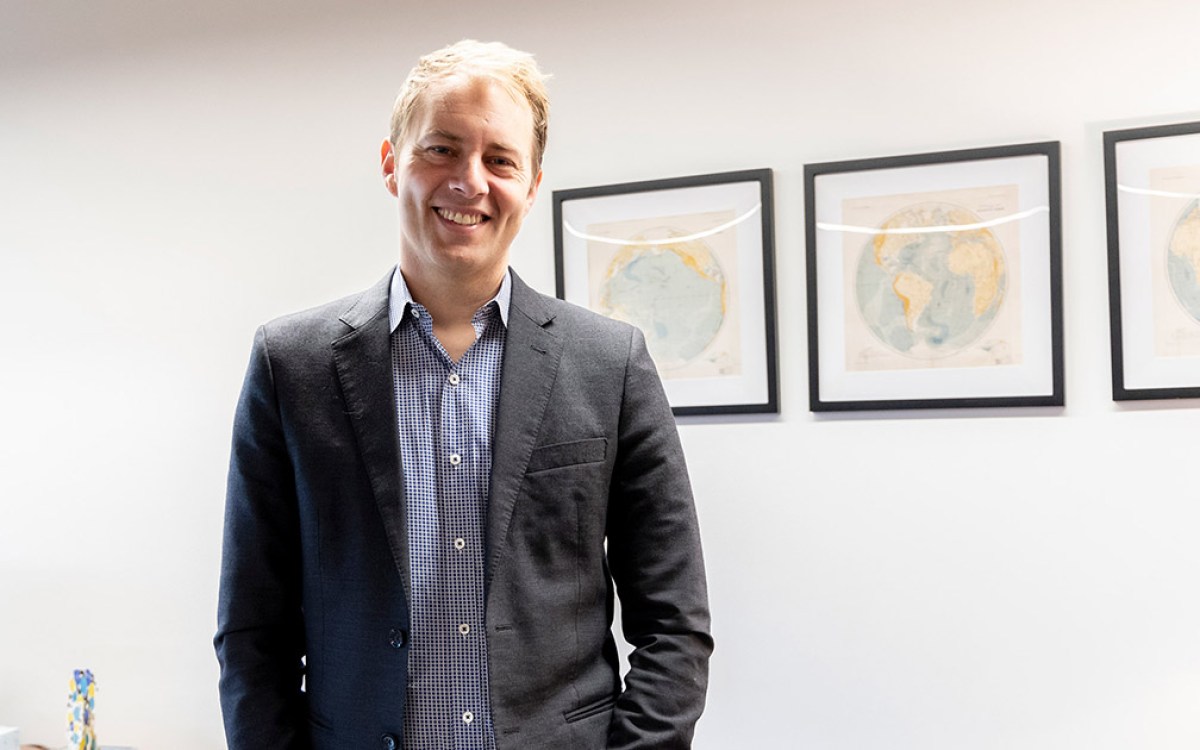
Study pinpoints two measures that predict effective managers

New study of economic toll yields projections ‘six times larger than previous estimates’
Harvard releases race data for Class of 2028
Cohort is first to be impacted by Supreme Court’s admissions ruling
Parkinson’s may take a ‘gut-first’ path
Damage to upper GI lining linked to future risk of Parkinson’s disease, says new study
Professor tailored AI tutor to physics course. Engagement doubled.
Preliminary findings inspire other large Harvard classes to test approach this fall
Help | Advanced Search
Computer Science > Computers and Society
Title: ethics of ai: a systematic literature review of principles and challenges.
Abstract: Ethics in AI becomes a global topic of interest for both policymakers and academic researchers. In the last few years, various research organizations, lawyers, think tankers and regulatory bodies get involved in developing AI ethics guidelines and principles. However, there is still debate about the implications of these principles. We conducted a systematic literature review (SLR) study to investigate the agreement on the significance of AI principles and identify the challenging factors that could negatively impact the adoption of AI ethics principles. The results reveal that the global convergence set consists of 22 ethical principles and 15 challenges. Transparency, privacy, accountability and fairness are identified as the most common AI ethics principles. Similarly, lack of ethical knowledge and vague principles are reported as the significant challenges for considering ethics in AI. The findings of this study are the preliminary inputs for proposing a maturity model that assess the ethical capabilities of AI systems and provide best practices for further improvements.
Comments: | 21 pages, 8 figures |
Subjects: | Computers and Society (cs.CY); Artificial Intelligence (cs.AI) |
Cite as: | [cs.CY] |
(or [cs.CY] for this version) | |
Focus to learn more arXiv-issued DOI via DataCite |
Submission history
Access paper:.
- Other Formats

References & Citations
- Google Scholar
- Semantic Scholar

DBLP - CS Bibliography
Bibtex formatted citation.

Bibliographic and Citation Tools
Code, data and media associated with this article, recommenders and search tools.
- Institution
arXivLabs: experimental projects with community collaborators
arXivLabs is a framework that allows collaborators to develop and share new arXiv features directly on our website.
Both individuals and organizations that work with arXivLabs have embraced and accepted our values of openness, community, excellence, and user data privacy. arXiv is committed to these values and only works with partners that adhere to them.
Have an idea for a project that will add value for arXiv's community? Learn more about arXivLabs .

Addressing equity and ethics in artificial intelligence
Algorithms and humans both contribute to bias in AI, but AI may also hold the power to correct or reverse inequities among humans
Vol. 55 No. 3 Print version: page 24
- Artificial Intelligence
- Equity, Diversity, and Inclusion
- Technology and Design
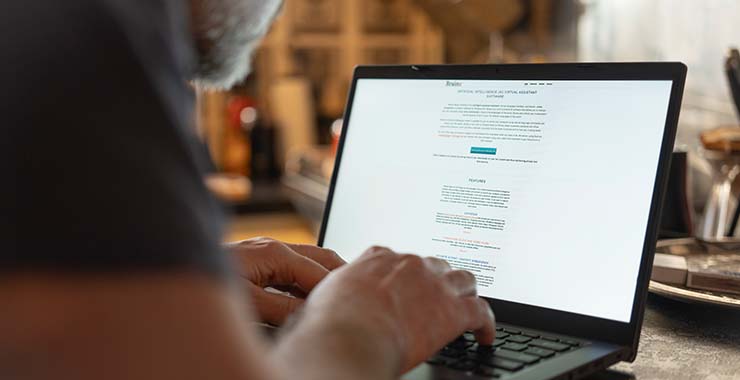
As artificial intelligence (AI) rapidly permeates our world, researchers and policymakers are scrambling to stay one step ahead. What are the potential harms of these new tools—and how can they be avoided?
“With any new technology, we always need to be thinking about what’s coming next. But AI is moving so fast that it’s difficult to grasp how significantly it’s going to change things,” said David Luxton, PhD, a clinical psychologist and an affiliate professor at the University of Washington’s School of Medicine who spoke at the 2024 Consumer Electronics Show (CES) on Harnessing the Power of AI Ethically .
Luxton and his colleagues dubbed recent AI advances “super-disruptive technology” because of their potential to profoundly alter society in unexpected ways. In addition to concerns about job displacement and manipulation, AI tools can cause unintended harm to individuals, relationships, and groups. Biased algorithms can promote discrimination or other forms of inaccurate decision-making that can cause systematic and potentially harmful errors; unequal access to AI can exacerbate inequality ( Proceedings of the Stanford Existential Risk Conference 2023, 60–74 ). On the flip side, AI may also hold the potential to reduce unfairness in today’s world—if people can agree on what “fairness” means.
“There’s a lot of pushback against AI because it can promote bias, but humans have been promoting biases for a really long time,” said psychologist Rhoda Au, PhD, a professor of anatomy and neurobiology at the Boston University Chobanian & Avedisian School of Medicine who also spoke at CES on harnessing AI ethically. “We can’t just be dismissive and say: ‘AI is good’ or ‘AI is bad.’ We need to embrace its complexity and understand that it’s going to be both.”
With that complexity in mind, world leaders are exploring how to maximize the benefits of AI and minimize its harms. In 2023, the Biden administration released an executive order on Safe, Secure, and Trustworthy AI and the European Union came close to passing its first comprehensive AI Act . Psychologists, with their expertise on cognitive biases and cultural inclusion, as well as in measuring the reliability and representativeness of datasets—have a growing role in those discussions.
“The conversation about AI bias is broadening,” said psychologist Tara Behrend, PhD, a professor at Michigan State University’s School of Human Resources and Labor Relations who studies human-technology interaction and spoke at CES about AI and privacy . “Agencies and various academic stakeholders are really taking the role of psychology seriously.”
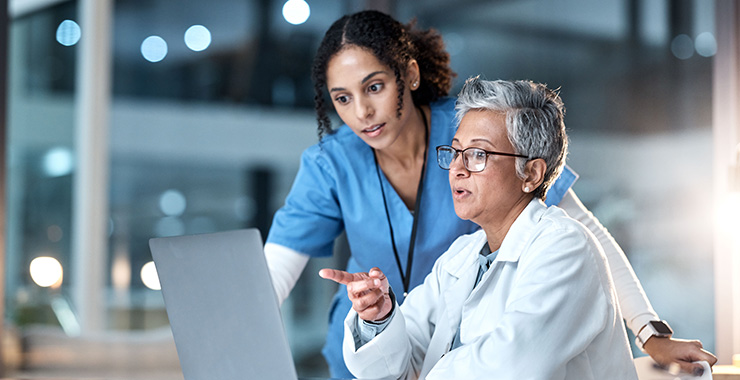
Bias in algorithms
Government officials and researchers are not the only ones worried that AI could perpetuate or worsen inequality. Research by Mindy Shoss, PhD, a professor of psychology at the University of Central Florida, shows that people in unequal societies are more likely to say AI adoption carries the threat of job loss ( Technology, Mind, and Behavior , Vol. 3, No. 2, 2022 ).
Those worries about job loss appear to be connected to overall mental well-being. For example, about half of employees who said they were worried that AI might make some or all of their job duties obsolete also said their work negatively impacted their mental health. Among those who did not report such worries about AI, only 29% said their work worsened their mental health, according to APA’s 2023 Work in America survey .
“In places where there’s a lot of inequality, those systems essentially create winners and losers,” Shoss said, so there is additional concern about how AI tools could be used irresponsibly—even maliciously—to make things worse.
Those fears are not unfounded. Biased algorithmic decision-making has been reported in health care, hiring, and other settings. It can happen when the data used to train a system is inaccurate or does not represent the population it intends to serve. With generative AI systems, such as ChatGPT, biased decision-making can also happen unexpectedly due to the “black box” issue , which refers to the fact that even an algorithm’s developers may not understand how it derives its answers.
“Even if we give a system the best available data, the AI may start doing things that are unpredictable,” Luxton said.
Examples include a recruiting tool at Amazon that preferred male candidates for technical jobs , and Replika, an AI companion that harassed some of its users . Avoiding such issues requires careful auditing of AI tools—including testing them in extreme scenarios before they are released—but it also requires significantly more transparency about how a given algorithm learns from data, Luxton said.
On top of technical audits, Behrend and Richard Landers, PhD, a professor of industrial-organizational psychology at the University of Minnesota Twin Cities, have published guidelines for conducting a “psychological audit” of an AI model, or evaluating how it might impact humans ( American Psychologist , Vol. 78, No. 1, 2023 ). That includes direct effects, such as who is recommended by a hiring algorithm, as well as broader ripple effects on organizations and communities.
The audit employs basic principles of psychological research to evaluate fairness and bias in AI systems. For example: Where is the data used to train an AI model coming from, and does it generalize to the population the tool intends to serve? Were the data collected using sound research methods, or were limitations introduced? Are developers making appropriate inferences from that data?
Conversations about algorithmic bias often center around high-stakes decision-making, such as educational and hiring selection, but Behrend said other applications of this technology are just as important to audit. For example, an AI-driven career guidance system could unintentionally steer a woman away from jobs in STEM (science, technology, engineering, and math), influencing her entire life trajectory.
“That can be potentially hugely consequential for a person’s future decisions and pathways,” she said. “It’s equally important to think about whether those tools are designed well.”
Even if an algorithm is well designed, it can be applied in an unfair way, Shoss said. For example, a system that determines salaries and bonuses could be implemented without transparency or human input—or it could be used as one of a series of factors to guide human decision-making. In that sense, using AI ethically requires asking the same questions that evaluate any other organizational change: Is it done with trust, transparency, and accountability?
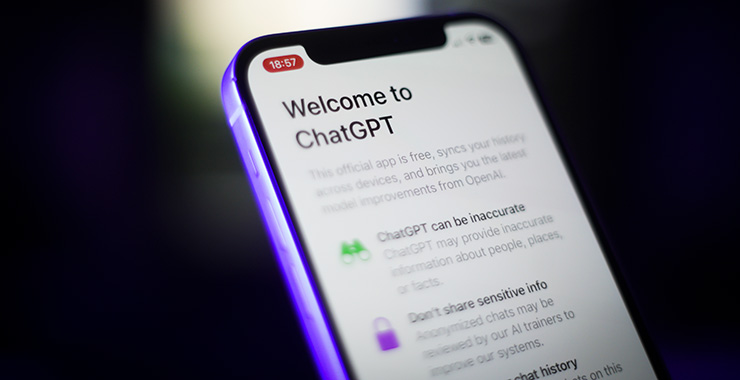
Human error
An algorithm itself may be biased, but humans can also introduce inaccuracies based on how they use AI tools.
“AI has many biases, but we’re often told not to worry, because there will always be a human in control,” said Helena Matute, PhD, a professor of experimental psychology at Universidad de Deusto in Bilbao, Spain. “But how do we know that AI is not influencing what a human believes and what a human can do?”
In a study she conducted with graduate student Lucía Vicente, participants classified images for a simulated medical diagnosis either with or without the help of AI. When the AI system made errors, humans inherited the same biased decision-making, even when they stopped using the AI ( Scientific Reports , Vol. 13, 2023 ).
“If you think of a doctor working with this type of assistance, will they be able to oppose the AI’s incorrect advice?” Matute said, adding that human users need the training to detect errors, the motivation to oppose them, and the job security to speak up about it.
Decades of psychological research clearly show that once humans inherit a bias or encounter misinformation, those beliefs are hard to revise. Celeste Kidd, PhD, an assistant professor of psychology at the University of California, Berkeley, argues that assumptions about AI’s capabilities, as well as the way many tools present information in a conversational, matter-of-fact way, make the risk of inheriting stubborn biases particularly high ( Science , Vol. 380, 2023 ).
“By the point [that] these systems have transmitted the information to the person…it may not be easy to correct,” Kidd said in a press release from the university ( Berkeley News , June 22, 2023 ).
Companies also can—and do—intentionally leverage AI to exploit human biases for gain, said Matute. In a study of simulated AI dating recommendations, she and graduate student Ujué Agudo found that participants were more likely to agree to date someone whose profile they viewed more than once, a choice she said is driven by the familiarity heuristic ( PLOS ONE , Vol. 16, No. 4, 2021 ). Guidelines for ethical AI should consider how it can be designed to intentionally play on cognitive biases and whether that constitutes safe use, she added.
“We all have cognitive biases, and AI can be used to exploit them in a very dangerous way,” Matute said.
Working toward “fairness”
While poorly designed algorithms can perpetuate real-world biases, AI may also hold the power to correct or reverse inequities among humans. For example, an algorithm could detect whether a company is less likely to hire or promote women, then nudge leaders to adjust job ads and decision-making criteria accordingly.
“There are risks here, too, and it’s equally important to have transparency about these types of systems—how they’re deriving answers and making decisions—so they don’t create distrust,” Luxton said.
Using AI to reverse bias also requires agreeing on what needs to change in society. The current approach to building AI tools involves collecting large quantities of data, looking for patterns, then applying them to the future. That strategy preserves the status quo, Behrend said—but it is not the only option.
“If you want to do something other than that, you have to know or agree what is best for people, which I don’t know that we do,” she said.
As a starting point, Behrend is working to help AI researchers, developers, and policymakers agree on how to conceptualize and discuss fairness. She and Landers distinguish between various uses of the term, including statistical bias versus equity-based differences in group outcomes, in their recent paper.
“These are noncomparable ways of using the word ‘fairness,’ and that was really shutting down a lot of conversations,” Behrend said.
Establishing a common language for discussing AI is an important step for regulating it effectively, which a growing contingent is seeking to do. In addition to Biden’s 2023 executive order, New York State passed a law requiring companies to tell employees if AI is used in hiring or promotion. At least 24 other states have either proposed or passed legislation aiming to curtail the use of AI, protect the privacy of users, or require various disclosures ( U.S. State-by-State AI Legislation Snapshot, BCLP Law, 2023 ).
“It’s pretty difficult to stay on top of what the best practice is at any given moment,” Behrend said. “That’s another reason why it’s important to emphasize the role of psychology, because basic psychological principles—reliability, validity, fairness—don’t change.”
Luxton argues that executive orders and piecemeal legislation can be politicized or inefficient, so policymakers should instead focus on establishing standard guidelines and best practices for AI. That includes requiring developers to show an audit trail, or a record of how an algorithm makes decisions. (Luxton is also writing a guidebook for behavioral health practitioners on integrating AI into practice.) When challenges arise, he suggests letting those play out through the judicial system.
“Government does need to play a role in AI regulation, but we also want to reduce the inefficiencies of government roadblocks in technological development,” Luxton said.
One thing is clear: AI is a moving target. Using it ethically will require continued dialogue as the technology grows ever more sophisticated.
“It’s not entirely clear what the shelf life of any of these conversations about bias will be,” said Shoss. “These discussions need to be ongoing, because the nature of generative AI is that it’s constantly changing.”
Further reading
How psychology is shaping the future of technology Straight, S., & Abrams, Z. APA, 2024
Speaking of Psychology: How to use AI ethically, with Nathanael Fast, PhD APA, 2024
Worried about AI in the workplace? You’re not alone Lerner, M. APA, 2024
The unstoppable momentum of generative AI Abrams, Z., APA 2024
Six Things Psychologists are Talking About
The APA Monitor on Psychology ® sister e-newsletter offers fresh articles on psychology trends, new research, and more.
Welcome! Thank you for subscribing.
Speaking of Psychology
Subscribe to APA’s audio podcast series highlighting some of the most important and relevant psychological research being conducted today.
Subscribe to Speaking of Psychology and download via:
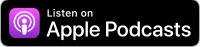
Contact APA
You may also like.
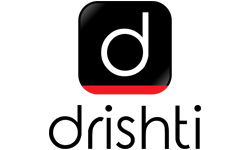
- Classroom Programme
- Interview Guidance
- Online Programme
- Drishti Store
- My Bookmarks
- My Progress
- Change Password
- From The Editor's Desk
- How To Use The New Website
- Help Centre
Achievers Corner
- Topper's Interview
- About Civil Services
- UPSC Prelims Syllabus
- GS Prelims Strategy
- Prelims Analysis
- GS Paper-I (Year Wise)
- GS Paper-I (Subject Wise)
- CSAT Strategy
- Previous Years Papers
- Practice Quiz
- Weekly Revision MCQs
- 60 Steps To Prelims
- Prelims Refresher Programme 2020
Mains & Interview
- Mains GS Syllabus
- Mains GS Strategy
- Mains Answer Writing Practice
- Essay Strategy
- Fodder For Essay
- Model Essays
- Drishti Essay Competition
- Ethics Strategy
- Ethics Case Studies
- Ethics Discussion
- Ethics Previous Years Q&As
- Papers By Years
- Papers By Subject
- Be MAINS Ready
- Awake Mains Examination 2020
- Interview Strategy
- Interview Guidance Programme
Current Affairs
- Daily News & Editorial
- Daily CA MCQs
- Sansad TV Discussions
- Monthly CA Consolidation
- Monthly Editorial Consolidation
- Monthly MCQ Consolidation
Drishti Specials
- To The Point
- Important Institutions
- Learning Through Maps
- PRS Capsule
- Summary Of Reports
- Gist Of Economic Survey
Study Material
- NCERT Books
- NIOS Study Material
- IGNOU Study Material
- Yojana & Kurukshetra
- Chhatisgarh
- Uttar Pradesh
- Madhya Pradesh
Test Series
- UPSC Prelims Test Series
- UPSC Mains Test Series
- UPPCS Prelims Test Series
- UPPCS Mains Test Series
- BPSC Prelims Test Series
- RAS/RTS Prelims Test Series
- Daily Editorial Analysis
- YouTube PDF Downloads
- Strategy By Toppers
- Ethics - Definition & Concepts
- Mastering Mains Answer Writing
- Places in News
- UPSC Mock Interview
- PCS Mock Interview
- Interview Insights
- Prelims 2019
- Product Promos
- Daily Updates
Make Your Note
Exploring the Ethical Implications of AI
- 25 Aug 2023
- 10 min read
- GS Paper - 3
- IT & Computers
- GS Paper - 4
This editorial is based on Can AI be Ethical and Moral? which was published in The Hindu on 24/08/2023. It talks about how programming ethics into machines is complex, and the world must proceed cautiously while using AI.
For Prelims: Artificial Intelligence (AI), Weak AI, Strong AI, Machine Learning (ML), Deep Learning (DL).
For Mains: Ethical Challenges of AI, Ethical Considerations of AI, Artificial Moral Agents (AMAs)
Increasingly, machines and Artificial Intelligence (AI) are assisting humans in decision-making, particularly in governance. Consequently, several countries are introducing AI regulations . Government agencies and policymakers are leveraging AI-powered tools to analyse complex patterns , forecast future scenarios, and provide more informed recommendations.
However, the use of AI in decision-making comes with challenges . AI can have built-in biases from the data it learns from or the viewpoints of its creators. This can result in unfair outcomes, posing a significant obstacle to effectively incorporating AI into governance.
What is Artificial Intelligence (AI)?
- Although there is no AI that can perform the wide variety of tasks an ordinary human can do, some AI can match humans in specific tasks.
- Deep Learning (DL) techniques enable this automatic learning through the absorption of huge amounts of unstructured data such as text, images, or video.
- Weak AI/ Narrow AI
How Does AI Relate to Certain Philosophical Ideas?
- autonomy (the ability to make one's own decisions),
- rationality (using logic and reason to make choices), and
- moral duty (following ethical obligations).
- Application to AI in Governance: The act of delegating decision-making processes to AI systems carries the risk of eroding the capacity for nuanced moral reasoning. Letting machines decide instead of humans might weaken the important ideas of Kantian ethics.
- This shows that the machine version of bounded ethicality is similar to how humans sometimes act against their own morals without feeling guilty, often using justifications.
Note : Bounded ethicality is people’s ability to make ethical choices is often limited or restricted because of internal and external pressures.
- Asimov’s laws were created to guide robots to behave ethically. However, in Asimov's fictional scenarios, these laws often resulted in unexpected and paradoxical outcomes, demonstrating the complexity of ethical decision-making even in machines designed to act ethically.
- Kant's emphasis on rational moral agency and Asimov's fictional exploration of ethical guidelines for robots are interconnected. This combination serves to illustrate the ethical difficulties and complexities that arise when human responsibilities and functions are delegated to artificial entities.
The Asimov’s Laws:
- A robot may not injure a human being or, through inaction, allow a human being to come to harm;
- A robot must obey the orders given to it by human beings except where such orders would conflict with the First Law;
- A robot must protect its own existence as long as such protection does not conflict with the First or Second Law.”
- Asimov later added another rule, known as the fourth or zeroth law , that superseded the others. It stated that “a robot may not harm humanity, or, by inaction, allow humanity to come to harm.”
What are the Ethical Challenges of AI?
- Job Displacement and Socioeconomic Impact: Automation powered by AI can lead to job displacement in certain industries. The resulting socioeconomic impact, including unemployment and income inequality , poses ethical questions about the responsibilities of governments and organisations in addressing these consequences.
- Threat to Moral Reasoning: When decisions that were traditionally made by humans are handed over to algorithms and AI, t here's a risk that the capacity for moral reasoning could be compromised . This implies that relying solely on AI might diminish the human ability to engage in thoughtful ethical thinking.
- Challenges of Codifying Ethics: Attempting to translate ethics into explicit rules for robots or AI-driven governmental decisions is highlighted as a challenging task. Human morals are very complex, and it's tough to make these complicated ideas fit into computer instructions.
- The inner workings of many AI systems are often opaque, making it difficult to understand how decisions are being made. This lack of transparency can lead to mistrust and skepticism among users.
- Informed Consent: AI systems can be used to collect and analyse personal data without the knowledge or consent of the individuals involved. This raises concerns about informed consent and the right to privacy.
Can Machines or AI be like Moral Decision-Makers/ Artificial Moral Agents (AMAs)?
- Ethical Impact Agents: These machines, like robot jockeys, don't make ethical choices themselves, but their actions have ethical effects. For example, they could change how a sport works.
- Implicit Ethical Agents: These machines have built-in safety or ethical rules, like the autopilot in planes. They follow set rules without actively deciding what's ethical.
- Explicit Ethical Agents: These go beyond fixed rules. They use specific methods to figure out the ethical value of choices. For instance, systems that balance money investments with social responsibility.
- Full Ethical Agents: These machines can make and explain ethical judgments. Adults and advanced AI with good ethical understanding fall into this category.
What are the Ethical Considerations of Responsible AI?
Currently, many machine predictions help with decisions, but humans still make the final call. In the future, governments might let machines make simple decisions. But what if a decision made by a machine is wrong or unethical? Who's responsible? Is it the AI system, the one who made the AI, or the person who used its data?
These are some of the tough questions that the world is going to face. Putting ethics into machines is tough, and everyone needs to be careful moving forward.
“Programming a computer to be ethical is much more difficult than programming a computer to play world-champion chess”. Discuss. |
UPSC Civil Services Examination, Previous Year Questions (PYQs)
Q. With the present state of development, Artificial Intelligence can effectively do which of the following? (2020)
- Bring down electricity consumption in industrial units
- Create meaningful short stories and songs
- Disease diagnosis
- Text-to-Speech Conversion
- Wireless transmission of electrical energy
Select the correct answer using the code given below:
(a) 1, 2, 3 and 5 only (b) 1, 3 and 4 only (c) 2, 4 and 5 only (d) 1, 2, 3, 4 and 5
Q. “The emergence of the Fourth Industrial Revolution (Digital Revolution) has initiated e-Governance as an integral part of government”. Discuss. (2020)
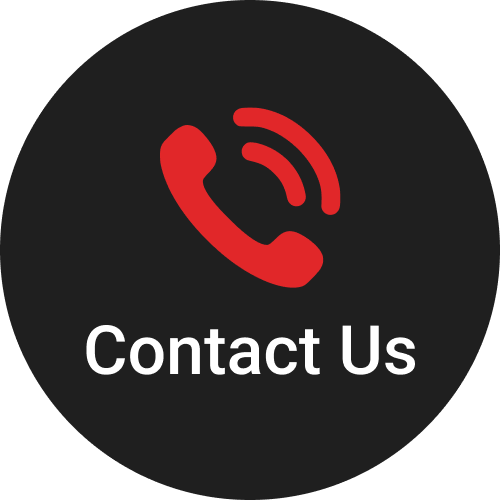
Ethics and Artificial Intelligence
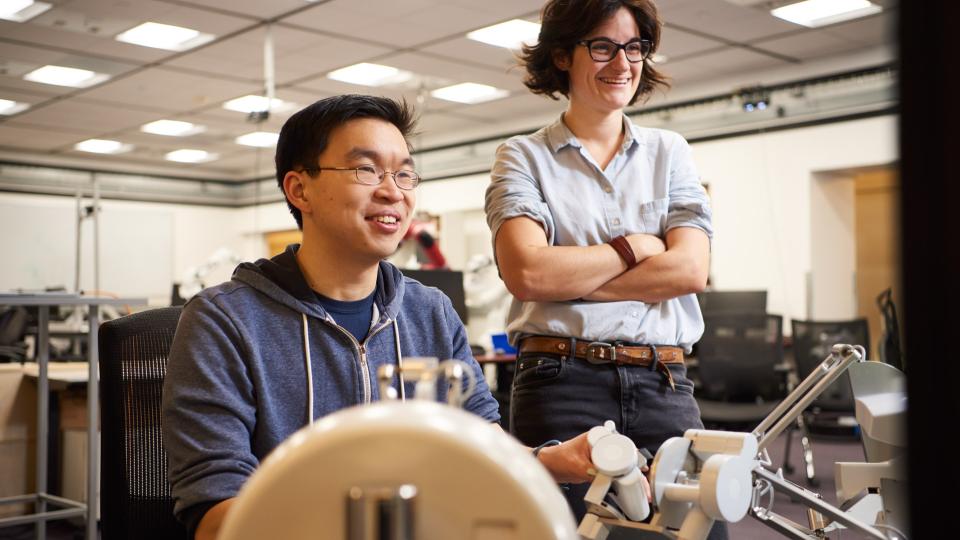
Guiding & Building the Future of AI
Stanford HAI's mission is to advance AI research, education, policy and practice to improve the human condition. One main focus area is to consider the societal implications of these technologies. Below find recent research and discussions on the impact and ethics of artificial intelligence.
Featured HAI Research
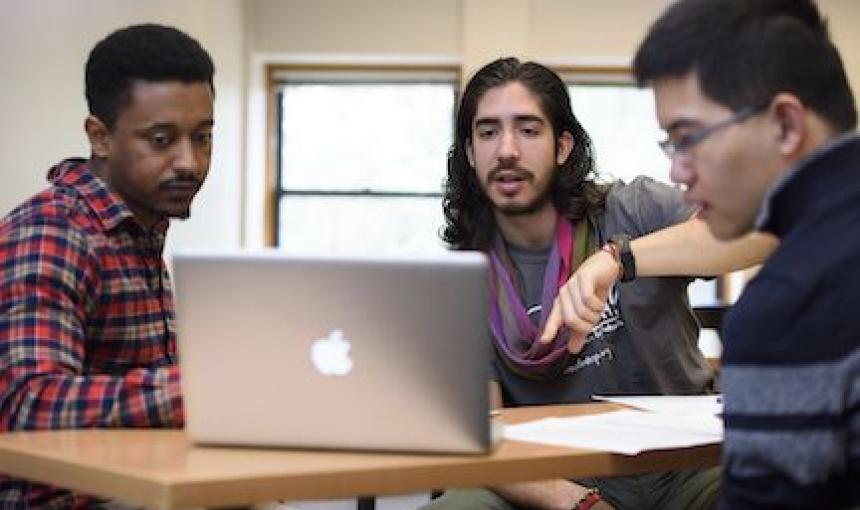
A New Approach To Mitigating AI’s Negative Impact
Stanford launches an Ethics and Society Review Board that asks researchers to take an early look at the impact of their...
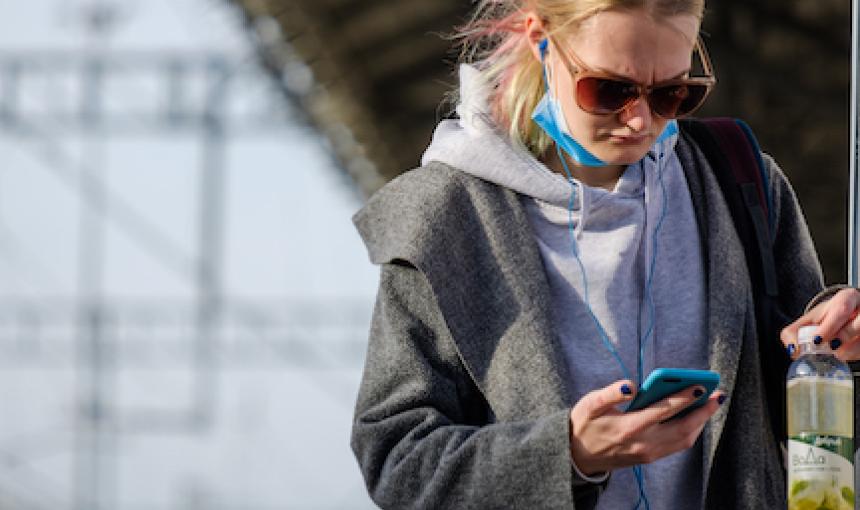
The Shibboleth Rule for Artificial Agents
Bots could one day dispense medical advice, teach our children, or call to collect debt. How can we avoid being deceived...
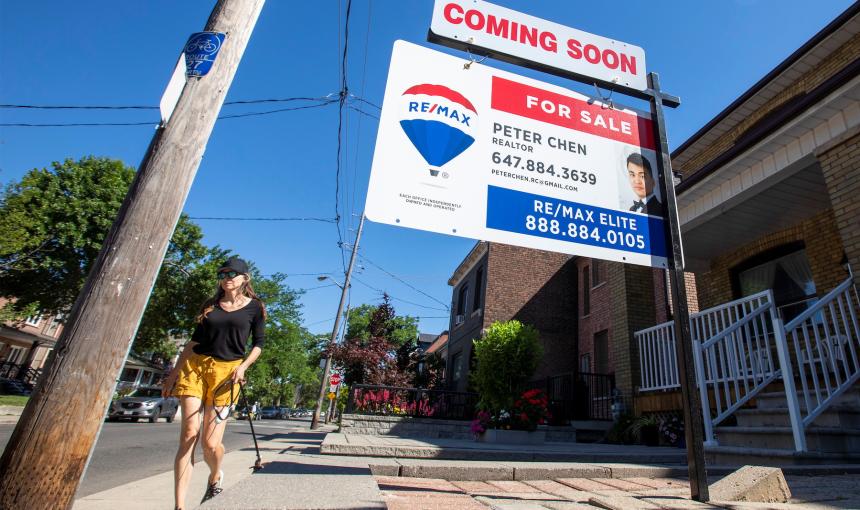
How Flawed Data Aggravates Inequality in Credit
AI offers new tools for calculating credit risk. But it can be tripped up by noisy data, leading to disadvantages for...
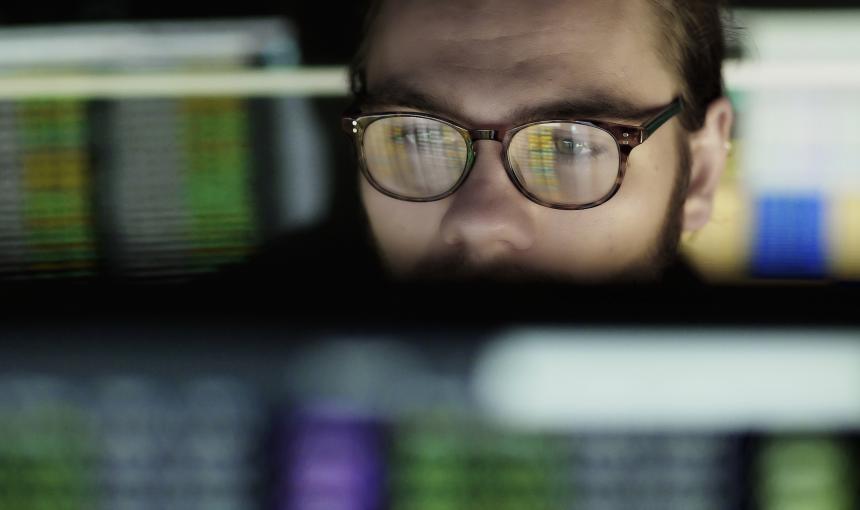
Rooting Out Anti-Muslim Bias in Popular Language Model GPT-3
This “severe” bias must be addressed before these language models become ingrained in real-world tasks.
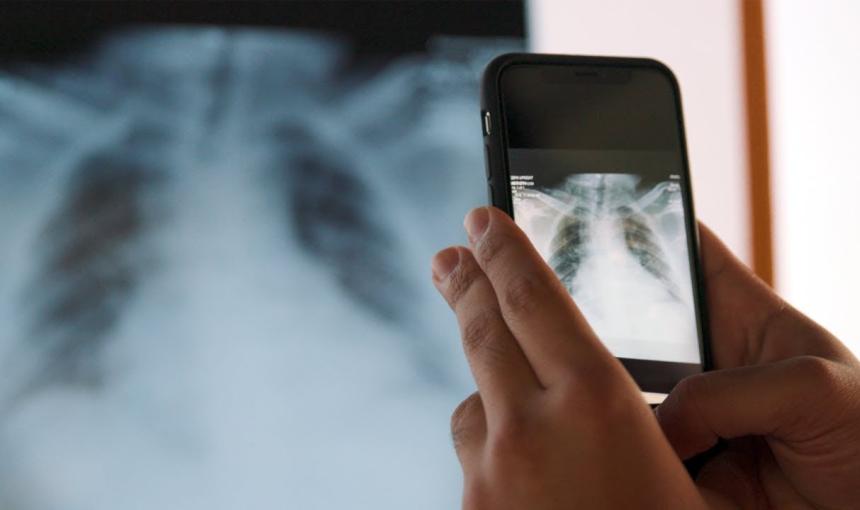
The Geographic Bias in Medical AI Tools
Patient data from just three states trains most AI diagnostic tools.
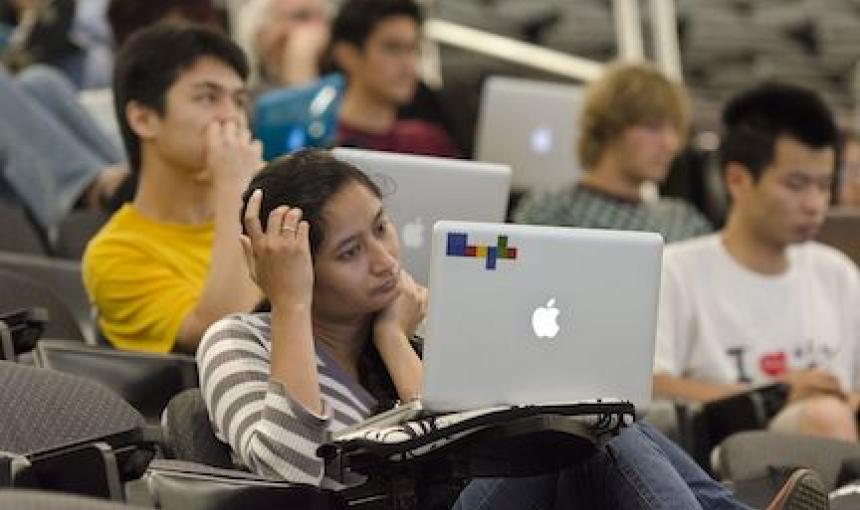
Building an Ethical Computational Mindset
Stanford launches an embedded EthiCS program to help students consistently think through the common issues that arise in...
Read more from the HAI blog
Featured HAI Videos
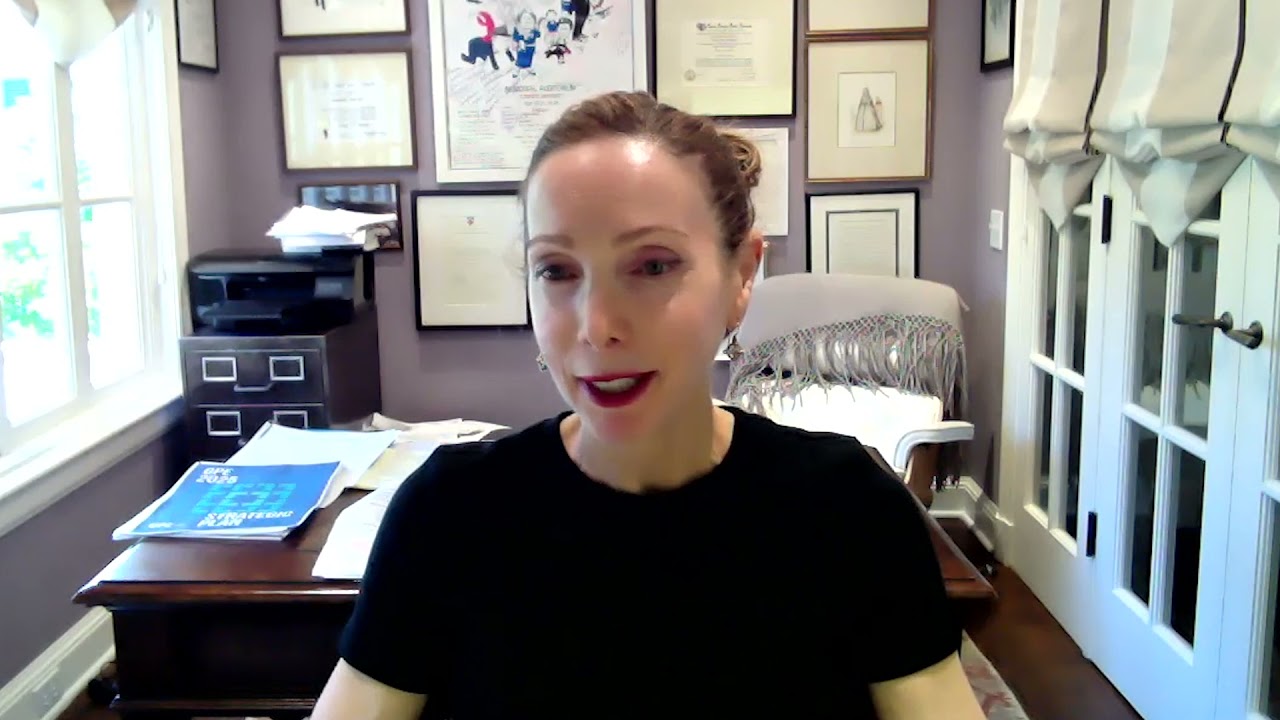
- Directors' Conversations: Susan Liautaud and Corporate Ethics
- Renata Avila: Prototyping Feminist AI
- Kathleen Creel: Arbitrariness, Fairness, and Opportunity in Algorithmic Decision-Making Systems
- Elizabeth Adams: The Path to Public Oversight of Surveillance Technology in Minneapolis
- Coded Bias: A Conversation with Director Shalini Kantayya
- Owning Ethics: Organizational Responsibility and Ethics in Silicon Valley
- Ethical Malice in Peer-Reviewed Machine Learning Literature
View More Stanford HAI Videos
HAI Policy Briefs
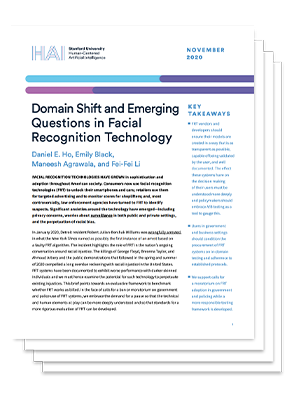
Domain Shift & Emerging Questions in Facial Recognition Technology
Facial recognition technologies have grown in sophistication and adoption throughout American society. Significant anxieties around the technology have emerged—including privacy concerns, worries about surveillance in both public and private settings, and the perpetuation of racial bias.
Read the full brief
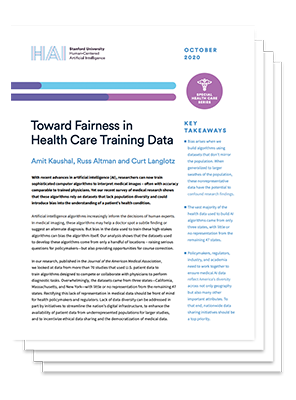
Toward Fairness in Health Care Training Data
With recent advances in artificial intelligence (AI), researchers can now train sophisticated computer algorithms to interpret medical images – often with accuracy comparable to trained physicians. Yet our recent survey of medical research shows that these algorithms rely on datasets that lack population diversity and could introduce bias into the understanding of a patient’s health condition.
Read more Policy Briefs
Newsletter Sign Up
Don’t miss out. Get Stanford HAI updates delivered directly to your inbox.

An official website of the United States government
The .gov means it’s official. Federal government websites often end in .gov or .mil. Before sharing sensitive information, make sure you’re on a federal government site.
The site is secure. The https:// ensures that you are connecting to the official website and that any information you provide is encrypted and transmitted securely.
- Publications
- Account settings
Preview improvements coming to the PMC website in October 2024. Learn More or Try it out now .
- Advanced Search
- Journal List
- J Korean Med Sci
- v.39(33); 2024 Aug 26
- PMC11347185

Publication Ethics in the Era of Artificial Intelligence
Zafer kocak.
Department of Radiation Oncology, Trakya University School of Medicine, Edirne, Türkiye.
The application of new technologies, such as artificial intelligence (AI), to science affects the way and methodology in which research is conducted. While the responsible use of AI brings many innovations and benefits to science and humanity, its unethical use poses a serious threat to scientific integrity and literature. Even in the absence of malicious use, the Chatbot output itself, as a software application based on AI, carries the risk of containing biases, distortions, irrelevancies, misrepresentations and plagiarism. Therefore, the use of complex AI algorithms raises concerns about bias, transparency and accountability, requiring the development of new ethical rules to protect scientific integrity. Unfortunately, the development and writing of ethical codes cannot keep up with the pace of development and implementation of technology. The main purpose of this narrative review is to inform readers, authors, reviewers and editors about new approaches to publication ethics in the era of AI. It specifically focuses on tips on how to disclose the use of AI in your manuscript, how to avoid publishing entirely AI-generated text, and current standards for retraction.
INTRODUCTION
The emergence of ethical concerns regarding the use of artificial intelligence (AI) dates back to the early days of its development. Most sources claim that the modern development of AI began in the early 1950s with the work of Alan Mathison Turing. He performed the “Turing test,” which showed that computers could think like humans. His paper “Computing Machinery and Intelligence” brought about debates about machine intelligence that would eventually lead to ethical considerations. 1 The term “artificial intelligence” was first coined by John McCarthy at a conference in 1956. 2 , 3 He has done outstanding research in the field of AI. 2 With the advancement of computer technology, AI became more widely applied in the years of 1970 and 1980. This raised concerns about privacy and decision-making biases. In 1976, Joseph Weizenbaum’s book “Computer Power and Human Reason” addressed the moral responsibility of AI developers. 4 In the 1990s, Dr. Richard Wallace created ALICE (Artificial Linguistic Internet Computer Entity), the first chatbot to interact with humans. 5 Since the 1990s, ethical concerns in the use of AI have become more prominent and the need for ethical regulations and guidelines has been discussed by stakeholders.
What is the modern definition of AI? As pointed out by Chen J “The goal of AI is to build systems that can learn and adapt as they make well-informed decisions, that is, systems that have certain levels of autonomy (i.e., the capability of task and motion planning) as well as intelligence (i.e., the capability of decision-making and reasoning).” 6 The current definition in Britannica is as follows; AI is “the ability of a digital computer or computer-controlled robot to perform tasks commonly associated with intelligent beings.” 7 Many different AI applications are currently used in healthcare, such as robotics, image processing, big data analysis, machine learning, voice recognition, and predictive modeling. 8
One of the most widely used AI tools in scientific publishing is the Chatbots, which can generate text, code, video/images, and full research articles. From information retrieval to data analysis and plagiarism detection, AI tools facilitate the work of not only researchers but also editors and publishers in many areas ( Table 1 ). 9 , 10 , 11 However, we should be aware of not only the strengths but also the weaknesses or limitations of AI tools. 12 , 13 They lack consciousness. They lack creative or original ideas because they can only create content based on the libraries they were trained in. The information compiled by the chatbot may be inaccurate, out of date, and may contain subjective biases. They may even list references that do not exist. More worryingly, they can facilitate the production of fraudulent manuscripts such as paper mills. 14
• Literature search and information retrieval |
• Data analysis |
• Summarizing the content |
• Bibliography and citation management |
• Create abstracts, images, videos and manuscripts |
• Image quality control |
• Content formatting |
• Language translation and grammar check |
• Target journal selection |
• Peer review and statistical quality assessment |
• Similarity check to prevent plagiarism |
• Detection of data and image fabrication |
• Detection of paper mills |
AI = artificial intelligence.
While the responsible use of AI brings many innovations and benefits for science and humanity, its unethical use, such as fabricated articles, poses a serious threat to scientific integrity and literature. 15 , 16 , 17 Even without malicious use, the chatbot output itself is at risk of containing biases, distortions, irrelevancies, misrepresentations, and plagiarism. 18 , 19 Therefore, the use of complex AI algorithms raises concerns about bias, transparency, and accountability, which calls for the development of new ethical guidelines to protect scientific integrity.
We are now witnessing AI technologies reshaping the field of academic publishing. As researchers, authors, reviewers, and editors, we are in a period where we all have to renew and improve our knowledge on this subject. We need to recognize the rapidly changing dynamics in scholarly publishing and address some concerns and challenges to ensure that AI tools and chatbots are used ethically and responsibly in academia. Some of these issues include the debate on how to disclose the use of AI in a manuscript, how to prevent the publication of fabricated manuscripts and the changing standards of retraction.
The main purpose of this narrative review article is to inform readers, authors, reviewers, and editors about new approaches to publication ethics in the age of AI. It specifically focuses on how to disclose the use of AI in your writing, how to avoid publishing text entirely generated by AI, and tips on current standards for retraction.
SEARCH STRATEGY
I have prepared a list of keyword combinations such as ‘Artificial Intelligence,’ ‘Ethics in Publishing,’ ‘Scientific Fraud,’ ‘Scientific Integrity,’ ‘Scientific Misconduct,’ ‘Research Misconduct,’ and ‘Retraction of Publication.’ I took the presence of Medical Subject Headings terms into account when choosing my search queries. I searched MEDLINE/PubMed, Scopus, Web of Science, and the Directory of Open Access Journals. I also checked the “similar articles section” for additional PubMed citations closely related to the articles listed with the initial ‘MeSH’ terms. Google Scholar search was conducted for some terms that have no equivalent in MeSH (e.g. chatbot, paper mills, fabricated manuscripts). I did not set any time limits or intervals when creating our search strategy. All article types were included in the searches. Publications that are not suitable for my purpose, not in English and not full text are excluded. I focused specifically on articles related to standards for disclosure of AI, avoiding the publication of fabricated manuscripts, and new and changing standards for retracting articles.
AI AND DISCLOSURE
Journals vary in their policies on the use of generative AI for scientific writing. Some publishers prohibit the use of AI without explicit editorial authorization, 20 while others require detailed annotation in the manuscript. 21 , 22 In his article, Hosseini argues that banning these tools could encourage the undisclosed use of chatbots, which would undermine transparency and integrity in research. 23 He also emphasized that it would undermine the principle of equality and diversity in science for non-native speakers of English.
WAME revised its recommendations on “Chatbots and Generative AI in Relation to Scientific Publication” in May 2023. 18 These recommendations can be considered as general principles. The first version emphasizes transparency, honesty, and responsibility of authors. For the second version, the suggestion that editors and peer reviewers should inform authors and be transparent when using AI in the manuscript evaluation process was added ( Table 2 ).
• Chatbots cannot be authors |
• Authors should be transparent when chatbots are used and provide information about how they were used |
• Authors are responsible for material provided by a chatbot in their paper (including the accuracy of what is presented and the absence of plagiarism) and for appropriate attribution of all sources (including original sources for material generated by the chatbot) |
• Editors need appropriate tools to help them detect content generated or altered by AI. Such tools should be made available to editors regardless of ability to pay for them, for the good of science and the public, and to help ensure the integrity of healthcare information and reducing the risk of adverse health outcomes |
• |
a Written in bold italics is item added for version 2.
Where in your article and how do we disclose the use of AI in your manuscript? There is a bit more disagreement about where to disclose rather than how to disclose. Some journals require authors to detail the use of AI in the acknowledgments section, 24 , 25 , 26 while others prefer it to be described in the body of the text. 27 , 28 The logic behind the idea that it would not be appropriate to use it in the acknowledgments section is that they cannot be accepted as authors because they cannot take responsibility or accountability for the research. 23 APA recommends disclosure in the methods section in research articles and the introduction section in other types of articles. 28 If AI was used for data collection, analysis, or figure generation, ICMJE and COPE recommend describing its use in the methods section.
How do we disclose the use of AI? First of all, authors should read the journal AI policies before submission. Journals/Publishers expect you to be transparent and honest. You are asked to detail what you did and how you did it, and to indicate where the AI-generated content fits into your manuscript ( Table 3 ). You must keep all your prompts and answers and most journals require you to declare them.
Journals generally ask you to declare/indicate | |
• Which AI model was used, when and by whom? | |
• The rationale for the use of AI and how it is used | |
• All prompts and responses | |
• Where in your article the AI-generated content appears? | |
During the manuscript writing process | |
• Check the accuracy of all references | |
• Ensure that all concepts are properly attributed | |
• Ensure that the language used is neutral and inclusive | |
• Check the similarity of text for plagiarism | |
• Read the journal and/or publisher’s AI policy carefully |
Another important issue is how to cite AI. Of course, the journal instructions should be read, but it is necessary to include information such as the version used, the model used, and the date of use. For example, the following format suggested by Hosseini seems informative enough 23 :
“OpenAI (2023). ChatGPT (GPT-4, 12 May Release) [Large language model]. Response to query X.Y. Month/Day/Year. https://chat.openai.com/chat ”
AI AND AVOIDING FABRICATED MANUSCRIPTS
We all know that non-native English speakers face disadvantages in the primarily Anglophone publishing business and often have to send their work to expensive and time-consuming translation and editing agencies. AI tools like ChatGPT can play an important role in helping non-native English academics write and edit their papers. It also encourages researchers who shy away from peer review due to language difficulties to take on the role of reviewer. Therefore, AI tools, especially if freely available, can promote and improve scientific equity. 29 The important thing here is that the author informs the journal and the publisher transparently.
On the other hand, AI tools can easily be used in an unethical way, resulting in misconduct and even fraud. AI tools can now produce full papers, which threatens the integrity of science. Paper mills are one of the final points of scientific fraud. This business began to emerge as a response to the publish-or-perish policy. Paper mills are heavily dependent on AI-generated texts that often contain fake or low-quality data. 30 Authors pay to have their names appear in these papers. They try to bribe journal editors to get manuscripts accepted quickly. So, the need to distinguish human writing from AI is critical. AI tools can play an important role in detecting or suspecting this scientific misconduct/fraud.
Currently, AI tools such as PapermillAlarm, GPTZero, GPT-2 Output Detector, Profig, FigCheck, and ImaCheck are used by some publishers to detect fabricated papers and image manipulation. 31 , 32 , 33 However, journals and publishers should be aware of their limitations and that they are not infallible. They can produce false positive or false negative results, and many errors in images flagged by AI tools can result in false positive results. 32 Gao et al. 34 evaluated the abstracts generated by ChatGPT for 50 scientific medical articles. They collected titles and originals extracted abstracts from recent issues of five high-impact journals and compared them with the original abstracts. It was noted that only 68% of ChatGPT-generated abstracts and 86% of human-written abstracts were correctly identified. The effectiveness of AI text content detectors, such as OpenAI, Writer, Copyleaks, GPTZero, and CrossPlag, was the subject of another study. 35 The study’s findings show that there is a wide range in the tools’ capacity to distinguish between text that is correctly identified as AI-generated or human-written. Overall, the tools perform better at classifying text that is GPT 3.5-generated than content that is GPT 4-generated or written by humans. In the preliminary study by Habibzadeh, a total of 50 text fragments were used to determine the performance of Gptzero in distinguishing machine-generated texts from human-written texts. 36 It recorded an accuracy rate of 80% with positive and negative probabilities of 6.5 and 0.4, respectively. He concluded GPTZero had a low false-positive (classifying a human-written text as machine-generated) and a high false-negative rate (classifying a machine-generated text as human-written).
So, AI tools for discriminating from AI- from human-derived text is currently not good enough and need to enhance their accuracy and reliability. 35 , 37 They can also be easily bypassed by using an online rewording tool or by rewording it oneself. 38 Therefore, suspected misconduct identified by AI tools should be carefully scrutinized by humans to confirm accuracy as suggested by COPE. 39 As pointed out by Gasparyan, the advice of experienced editors on how the credibility of academic publications is threatened by blind reliance on online processing should not be ignored. 40 Sometimes the best way to ensure that this is a legitimate manuscript is to ask the author to provide the raw data of the study. 37
What makes the problem a little more complicated for us is that establishing strict rules on ethics in scientific publishing is not an easy task. In the age of AI, where do you draw the line between ethical and unethical behavior? More specifically, what should be the maximum percentage of AI content in an article? Is there a standard percentage set by journals for AI content? Unfortunately, editorial guidelines from global associations such as ICMJE, COPE and WAME generally outline the general framework for publishing ethics, but do not provide specific advice to authors and editors specifically on AI and ethics. 40
AI AND CURRENT STANDARDS FOR RETRACTION
In 2013, Steen et al. 41 conducted an important study showing that the reasons for retraction have expanded in recent years to include plagiarism and duplicate publication. One of the study’s conclusions was that lower barriers to retraction were apparent in an increase in retraction for “new” offenses such as plagiarism. Spanish group recently published two important trials. In the study by Candal-Pedreira et al., retracted articles originating from paper mills were evaluated. They reported that the ratio of paper mill retractions to all-cause retractions reached 21.8% (772/3,544) in 2021. 42 In their second trial, retracted papers from European institutions between 2000 and 2021 were analyzed. They noted retraction rates increased from 10.7 to 44.8 per 100,000 publications between 2000 and 2020. Research misconduct was the reason in 2/3 of retractions. From copyright and authorship issues in 2000 (2.5 per 100,000 publications) to duplications in 2020 (8.6 per 100,000 publications), they disclosed causes for retractions that changed over time. 43
In the recently published systematic review of studies of retraction notices, misconduct accounted for 60% of all retractions, confirming the results of the studies mentioned above. 44 According to the claim by “Retraction Watch,” hundreds of IEEE publications produced in the previous years contained plagiarized material, citation fraud, and distorted wording. 45 Voung et al. 46 reported that manipulated peer review was the most common reason for retraction in the 2010s. By analyzing 18,603 retractions compiled from the Retraction Watch database until 2019, they reported that manipulated peer review was responsible for 676 retractions in the period 2012–2019. Hard-to-believe findings were presented in Van Noorden’s research, which was just published in Nature. 47 A new annual record was set in 2023, with more than 10,000 retractions of research articles. The main reasons for this were the “paper mills” engaged in systematic manipulation of the peer review and publication processes. Even more worrying, integrity experts claimed this was just the “tip of the iceberg.” In 2015, WAME published an action plan to prevent “fake” reviewers from conducting reviews by searching for and verifying the ORCID IDs of potential reviewers in response to growing concerns about these activities worldwide. 40 However, the most decisive point here will be the approach and uncompromising attitude of journal editors towards publication ethics and scientific integrity.
In non-Anglophone countries, plagiarism in particular stands out as a reason for retraction. Koçyiğit et al. 48 drew attention to the increase in the number of retracted articles from Turkey in recent years and reported the most common reasons for retraction as plagiarism, duplication, and error. Gupta et al. 49 conducted to analyze plagiarism perceptions among researchers and journal editors, particularly from non-Anglophone countries. This survey confirmed again that despite increased global awareness of plagiarism, non-Anglophone medical researchers do not understand the issue sufficiently. While most agree that copying text and images is plagiarism, other behaviors, such as stealing ideas and paraphrasing previously published work, are considered outside the scope of plagiarism. They conclude that closing the knowledge gap by providing up-to-date training and widespread use of advanced anti-plagiarism software can address this unmet need.
These studies have shown us the changing concepts and practices for retractions over the last decade. What was the driver behind this? The use of advanced technology in publishing helps us to detect plagiarism and duplication. On the other hand, the misuse of technology raises some ethical issues such as paper mills, image manipulation, confidentiality issues, and non-disclosure of competing interests. Such an unethical act not only compromises the integrity of publishing and science but may also require the retraction of the article.
The first version of the COPE retraction guideline was published in 2009. A revised version was published in 2019 to set the current standards ( Table 4 ). 50 As can be seen in Table 4 , image manipulation, lack of authorization for material or data use, some legal issues, compromised peer review, and non-disclosure of conflict of interest were added as reasons for retraction. However, as Teixeria da Silva notes, the COPE, ICMJE, and CSE ethics guidelines are still incomplete in that they do not specifically address fake articles, authors, emails and affiliations associated with stings and hoaxes. 51
Editors should consider retracting a publication if: | |
• They have clear evidence that the findings are unreliable, either as a result of major error (eg, miscalculation or experimental error), or as a result of fabrication (eg, of data) or | |
• It constitutes plagiarism | |
• It reports unethical research | |
• The findings have previously been published elsewhere without proper attribution to previous sources or disclosure to the editor, permission to republish, or justification (ie, cases of redundant publication) | |
• | |
• | |
• | |
• |
a Written in bold italics are items added for version 2.
Another popular topic in recent years is self-retraction. As many experts have emphasized, self-retraction due to honest errors deserves more credit than it currently receives. Fanelli argues that such publications should be viewed as legitimate publications that scholars will treat as evidence of integrity. 52
FUTURE DIRECTIONS AND LIMITATIONS OF THE STUDY
Currently, international organizations such as COPE, ICMJE, and CSE share the same views on authorship, AI disclose, transparency and responsibility, and ethical use of AI in their recommendations on AI use in scholarly publishing ( Table 5 ). While acknowledging AI’s role in decision-making, COPE emphasizes the necessity of responsibility, transparency, and human oversight when incorporating AI tools into the peer review process. The ICMJE defines the use of AI in peer review but advocates restricting the use of AI by editors. The CSE recommendations are similar to those of ICJME and COPE but do not refer to reviewers and editors. As shown in the Table 2 , WAME details how to disclose the use of AI for your article and recommends that editors should have these AI tools.
Topic | COPE | ICMJE | CSE |
---|---|---|---|
AI and authorship | AI tools cannot be listed as authors | AI technologies cannot be listed as authors | AI tools should not be listed as authors |
AI use and transparency/responsibility | Authors are fully responsible for the content of their manuscript, even those parts produced by an AI tool, and are thus liable for any breach of publication ethics. Transparency of processes must ensure technical robustness and rigorous data governance. | Humans are responsible for any submitted material that included the use of AI-assisted technologies. Authors should carefully review and edit the result because AI can generate authoritative-sounding output that can be incorrect, incomplete, or biased. | Authors must be accountable for all aspects of a manuscript, including the accuracy of the content that was created with the assistance of AI, the absence of plagiarism, and for appropriate attributions of such sources. |
AI and disclose | Authors who use AI tools in the writing of a manuscript, production of images or graphical elements of the paper, or in the collection and analysis of data, must be transparent in disclosing in the Materials and Methods (or similar section) of the paper how the AI tool was used and which tool was used. | Authors who use such technology should describe, in both the cover letter and the submitted work in the appropriate section if applicable, how they used it. For example, if AI was used for writing assistance, describe this in the acknowledgment section. If AI was used for data collection, analysis, or figure generation, authors should describe this use in the methods. | Authors should disclose usage of AI tools and machine learning tools such as ChatGPT, Chatbots, and Large Language Models (LLM). CSE recommends that journals ask authors to attest at initial submission and revision to the usage of AI and describe its use in either a submission question or in the cover letter. Journals may want to ask for the technical specifications (name, version, model) of the LLM or AI and the method of the application (query structure, syntax). |
AI and editors/peer-reviewers | AI chatbots pose for journal editors, including issues with plagiarism detection. It suggests the application of human judgment and suitable software to overcome these challenges. | Reviewers must maintain the confidentiality of the manuscript as outlined above, which may prohibit the uploading of the manuscript to software or other AI technologies where confidentiality cannot be assured. Reviewers must request permission from the journal prior to using AI technology to facilitate their review. Reviewers should be aware that AI can generate authoritative-sounding output that can be incorrect, incomplete, or biased. Editors should be aware that using AI technology in the processing of manuscripts may violate confidentiality. |
AI = artificial intelligence, COPE = Committee on Publication Ethics, ICMJE = International Committee of Medical Journal Editors, CSE = Council of Science Editors.
AI technology will undoubtedly develop and play a bigger role in day-to-day living. Therefore, these developments in AI will reshape scientific publishing and ethics. More scientists, reviewers, and editors will endeavor to be more transparent about their work as well as be more aware of the ethical issues surrounding the use of AI. Education on ethics and AI will become more important and researchers will have to consider ethical issues in their projects. Shortly, equity and unrestricted access to these technologies may emerge as the most significant issues in AI ethics, particularly for non-native English speakers. So, it will be crucial to have common regulations and the same ethical standards for all countries. Governments and funding organizations will have to develop policies to further support ethical research on AI. Perhaps in the near future, a new concept, empathic AI, as proposed by Kasani et al. 53 could help protect research and publication ethics by overcoming the limitations of human empathy.
This narrative review has several restrictions. Excluding non-English articles could lead to bias. Another drawback was that some articles could not be accessed in full text, therefore they were excluded. It is also possible that publications in journals that are not listed in the indexes used for literature search may have been overlooked. These factors could affect the comprehensiveness and objectivity of the review.
The application of new technologies to science affects the way and methodology in which research is conducted. Unfortunately, the development and writing of ethical codes cannot keep up with the pace of development and application of technology. Moreover, preparing guidelines is not an easy task because codes of ethics are not completely black and white. Therefore, the fight against scientific misconduct is multi-faceted, continuous, and requires teamwork. 54 Table 3 is intended as a checklist for authors before writing and submitting an AI-assisted manuscript. I hope this review will guide authors, reviewers, and editors on the responsible use of AI and help raise awareness on this issue. Journals and publishers should have clear and transparent policies on the ethical use of AI for the drafting, editing, and reviewing of manuscripts. They should also avoid unfairly blaming authors when taking action against the unethical use of AI. Educating staff and editorial boards on this issue is not only a need but also an obligation.
Disclosure: The author has no potential conflicts of interest to disclose.
Advertisement
Why AI Ethics Is a Critical Theory
- Research Article
- Open access
- Published: 11 February 2022
- Volume 35 , article number 9 , ( 2022 )
Cite this article
You have full access to this open access article
- Rosalie Waelen ORCID: orcid.org/0000-0003-2812-8244 1
16k Accesses
19 Citations
24 Altmetric
Explore all metrics
The ethics of artificial intelligence (AI) is an upcoming field of research that deals with the ethical assessment of emerging AI applications and addresses the new kinds of moral questions that the advent of AI raises. The argument presented in this article is that, even though there exist different approaches and subfields within the ethics of AI, the field resembles a critical theory. Just like a critical theory, the ethics of AI aims to diagnose as well as change society and is fundamentally concerned with human emancipation and empowerment. This is shown through a power analysis that defines the most commonly addressed ethical principles and topics within the field of AI ethics as either to do with relational power or with dispositional power. Moreover, it is concluded that recognizing AI ethics as a critical theory and borrowing insights from the tradition of critical theory can help the field forward.
Similar content being viewed by others
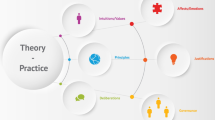
Reflections on Putting AI Ethics into Practice: How Three AI Ethics Approaches Conceptualize Theory and Practice
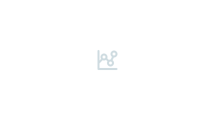
Towards Establishing Criteria for the Ethical Analysis of Artificial Intelligence
Decolonizing ai ethics: relational autonomy as a means to counter ai harms, explore related subjects.
- Artificial Intelligence
- Medical Ethics
Avoid common mistakes on your manuscript.
1 Introduction
The ethics of artificial intelligence (AI) is an emerging field within applied ethics and the philosophy of technology. It has gained attention and urgency due to the rapid development of AI technology during the past decade. Following Müller ( 2020 ), I understand the purpose of the field as twofold: AI ethics deals with the ethical issues that arise from AI systems as objects and with the moral questions raised by AI systems as subjects. Footnote 1 Various approaches for the ethical analysis of AI systems as objects have been proposed, but the most dominant one appears to be the principled approach . Over the past years, numerous initiatives have developed comparable sets of ethical principles and guidelines to ensure a desirable development and use of AI (Jobin et al., 2019 ; Ryan & Stahl, 2020 ). Common principles are for example transparency, justice and fairness, non-maleficence, responsibility, and privacy (Jobin et al., 2019 ). These principles function as a kind of soft law, they are not binding. The moral questions that AI systems raise as subjects are, among others, according to what norms AI should be programmed to act (also referred to as “machine ethics”), what the moral status of artificial moral agents would be, and whether AI systems can be held responsible or accountable for their actions and decisions.
The purpose of this article is to argue that AI ethics has the characteristics of a critical theory, by showing that the core concern of AI ethics is protecting and promoting human emancipation and empowerment. Furthermore, I propose that understanding the field as a critical theory can help to overcome some of the shortcomings of the currently popular principled approach to the ethical analysis of AI systems. In other words, I argue that we not only could, but should analyze the ethical implications of AI systems through the lens of critical theory. The focus of the article therefore lies on the ethical analysis of AI systems and the principled approach, but I also discuss what a critical understanding of AI ethics means for the other topics and questions that make up the field.
The structure of the article is as follows. In Sect. 2 , I start by briefly defining what it takes to be a critical theory. I conclude that a critical theory studies the power structures and relations in a society, with the goal of protecting and promoting human emancipation, and seeks not only to diagnose, but also to change society. In Sect. 3 , I take a more detailed look at the concept of power and explain how a pluralist understanding of the concept allows us to analyze power structures and relations, on the one hand, and (dis)empowerment, on the other hand. In Sect. 4 , I argue that the vast majority of the established AI ethics principles and topics in the field are fundamentally aimed at realizing human emancipation and empowerment, by defining these issues in terms of power. Next, in Sect. 5 , I propose that AI ethics should be seen as a critical theory, given that the discipline is fundamentally concerned with emancipation and empowerment, and meant not only to analyze the impact of emerging technologies on individuals and society, but also to change it. Moreover, I suggest that recognizing AI ethics as a critical theory can help the field forward—among other reasons because it promotes an interdisciplinary understanding of ethical issues, offers a target for change, helps to identify social and political implications of a technology, and helps to understand the social and political roots of ethical issues. I end the chapter with a brief conclusion in Sect. 6 .
2 What Constitutes a Critical Theory?
Marx’ famous thesis that philosophers should not only interpret the world but also change it, inspired a group of philosophers now known as the Frankfurt School. Footnote 2 The work of the Frankfurt School philosophers was given the name “critical theory.” Critical theory has two main, characteristic facets. First of all, critical theory has a practical goal; it is meant to diagnose as well as change society. Its unique approach to this is that of immanent transcendence, which implies that critical theorists argue how the world should be (transcendence) based on how it currently is (immanence), rather than always working towards a single predefined image of an ideal state of affairs (Delanty & Harris, 2021 ; Thompson, 2006 ). Horkheimer, who founded the Frankfurt School together with Adorno in the early 1930s, suggested that critical theory should be an interdisciplinary endeavor. History, economics, politics, psychology, and other social sciences can help to understand in what ways people’s freedom is limited, how the power relations causing this domination came about, and how to counter or resist them. A second important facet is critical theory’s emancipatory ambition. Horkheimer said critical theory is “an essential element in the historical effort to create a world which satisfies the needs and power of men” and defined its goal as “man’s emancipation from slavery” (Horkheimer, 1972 , 246). So critical theorists always seek to identify and overcome forms of domination or restraints that hinder human emancipation or empowerment. Emancipation can be defined as “overcoming social domination” (Forst, 2019 , 17) and gives people an equal opportunity for self-development (Allen & Mendieta, 2019 ). Empowerment implies “[i]ncreasing the scope of agency for individuals and collectives” (Forst, 2019 , 21).
There are distinct generations within critical theory. The first generation of critical theorists (among which were Theodor Adorno, Walter Benjamin, Max Horkheimer, and Herbert Marcuse) was preoccupied with criticizing modern capitalism and discussed typically Marxist subjects like alienation, exploitation, and reification. Later on, the focus of their critique became the enlightenment and the loss of individuality due to mass culture (Horkheimer & Adorno, 2002 ). Jürgen Habermas, a second-generation critical theorist, continued the tradition by studying the state of democracy and discussing power in relation to communication, which lead him to develop his discourse ethics (Habermas, 1984 , 1987 ). Axel Honneth, a student of Habermas, in turn focused his attention on the topic of recognition, which goes back to Hegel (Honneth, 1996 ). One of the contemporary, fourth-generation members of the school is Rainer Forst, who has continued the tradition by developing a critical theory of justice and redefining the notions of progress and power, among others.
Only the first generation of critical theorists explicitly concerned themselves with technology, mostly focusing on its relation to capitalism (Delanty & Harris, 2021 ). But, the types of technology that these early Frankfurt School members dealt with were nothing like AI and other digital technologies that exist today. Therefore, it is not easy and perhaps not very valuable either, to try to apply their theories of technology to today’s situation. However, that does not have to mean that the tradition of critical theory is not relevant to the philosophy and ethics of technology. Several contemporary thinkers have argued for the relevance of critical theory to understanding the societal role and impact of technology today. Most notably, Feenberg engaged with this tradition to develop his own critical theory of technology (a.o. Feenberg, 1991 ). Another example is Fuchs, who built on the work of Lukács, Adorno, Marcuse, Honneth, and Habermas to develop a critical theory of communication in the age of the internet (Fuchs, 2016 ). And in a recent article, Delanty and Harris argue that the general themes that are present in critical theory still offer a valuable framework for analyzing technology today (Delanty & Harris, 2021 ). So, the central idea of this paper, namely that the tradition of critical theory can support the analysis of modern technology, is not necessarily new. What is new, as will become clear in what follows, is my proposal to understand the emerging field of AI ethics as a critical theory and to conduct ethical analyses of AI systems through the lens of critical theory.
Finally, the understanding of critical theory as being the work of the Frankfurt School is a narrow understanding of the term. When understood in a broader sense, the term “critical theory” can refer to any theory or diagnosis of power structures and relations that ought to serve emancipatory ends. In this broad sense, the critical theory would also include other schools of thought, such as feminism or post-colonialism (Bohman, 2021 ). We could then say that specific critical approaches or theories focus on a particular oppressed societal group or a particular way in which people’s emancipation is hindered. Different critical theories deal with the struggle of a specific day and age (Bohman, 2021 ). AI ethics is a critical theory, as I further argue below, which deals with the ways in which AI—a radically new technology—(dis)empowers individuals and facilitates or exacerbates existing power structures in society.
3 Defining the Concept of Power
Given critical theory’s focus on emancipation and empowerment, and all the factors that enable or disable this, we can conclude that power is an important, central topic within the field of critical theory. However, power is also a contested concept—according to Steven Lukes it even is essentially contested (Lukes, 1974 , 2005 ). Therefore, it is difficult to understand power’s exact role in critical theory. When trying to define “power,” scholars disagree over a number of issues. For example, it is disputed whether power can be ascribed to structures or solely to agents; whether an exercise of power is necessarily intentional or whether someone or something can exercise power without intending to do so, and whether the exercise of power has to involve a conflict of interests or not (Brey, 2008 ). Furthermore, there is a divide between those who conceptualize power in dispositional terms, as “power-to,” and those who discuss power in relational terms, as “power-over.”
We could say that there are, broadly, four different views of power: the dispositional, episodic, systemic, and constitutive view (Allen, 2016 ; Haugaard, 2020 ; Sattarov, 2019 ). The first resembles “power-to,” the latter three are relational views, and therefore, they fall under the category of “power-over.” Those who defend the dispositional view of power argue that power is a capacity or ability—namely the capacity to bring about significant outcomes. Acquiring that capacity is also referred to as “empowerment,” losing it as “disempowerment.” One defender of the dispositional view is Morriss, who argued that two mistakes are commonly made in discussions of power: the vehicle fallacy and the exercise fallacy (Morriss, 2002 ). The vehicle fallacy is committed when the resources that give rise to power (e.g., AI technologies) are claimed to be power. The exercise fallacy occurs when one equates power with its exercise. Morriss argues that having power entails more than merely exercising it, it is a disposition that “can remain forever unmanifested” ( 2002 , 17). This is then a direct critique towards those who defend a relational view of power, in particular the episodic view.
The episodic view of power entails that power occurs when one party exercises power over another, for example, by means of force, coercion, manipulation, or through authority. Known for defending, this view of power is Weber, Dahl, and Lukes. Dahl famously formulated the intuitive notion of power as “A having power over B to the extent that A can get B to do something that B would not otherwise do” ( 1957 , 202). Lukes initially followed Dahl, by defining power as “A exercises power over B when A affects B in a manner contrary to B’s interests” ( 1974 , 30), but later in his career he accepted Morriss’ critique and acknowledged that power is “a capacity not the exercise of that capacity” and that “you can be powerful by satisfying and advancing others’ interests” (Lukes, 2005 , 12). But even though dispositional power appears to be more fundamental than episodic power, the episodic view of power is relevant because it highlights a specific aspect of power, namely the direct exercise thereof.
Other relational views of power are the systemic and constitutive view. While dispositional and episodic power focus on a single agent and specific instances of power, systemic and constitutive power are more structure-centric (Allen, 2016 ; Sattarov, 2019 ). Systemic power, to start with, refers to the ways in which societal institutions, social norms, values, laws, and group identities can have power over individuals. The systemic view complements the episodic and dispositional ones, because it enables us to look at the bigger picture and see what causes some to have dispositional power and exercise it, while others cannot or are constantly subjected to the power of others. Systemic power “highlights the ways in which broad historical, political, economic, cultural, and social forces enable some individuals to exercise power over others, or inculcate certain abilities and dispositions in some actors but not in others.” (Allen, 2016 ).
Constitutive power, finally, refers to the views or discussions of power that focus not on the oppressive character of power, but on the ways in which those subjected to power are also shaped by it. Systems of power not only determine one’s sphere of action or possibilities, as the systemic view of power highlights, they also constitute a person’s behavior, intentions, beliefs, and more. Foucault is probably most famous for developing this view of power in his work on discipline and biopolitics.
These four views of power are not necessarily incompatible, competing views. According to Mark Haugaard ( 2010 , 2020 ), Lukes is wrong in saying that power is essentially contested. Haugaard suggests that “power debates will advance more fruitfully if we treat power as a family resemblance concept, whereby their meaning varies depending upon language game ” (Haugaard, 2010 , p. 424). Footnote 3 A family-wide concept like power is so broad and vague that it explains little in itself; therefore, it is better understood through a cluster of concepts that refer to different aspects of the wider notion. Hence, we should reject the premise that there is a single best definition of power to be found and opt for a pluralist approach to power. The criterion for including a certain view of power in the pluralist approach should be “usefulness,” says Haugaard ( 2010 , 427). A definition or notion of power is useful, when it highlights a unique aspect of power.
Critical theorists are concerned with all four elements of power. They first and foremost study relational power, particularly systemic issues in society, in order to emancipate certain societal groups. But, critical theorists are also interested in increasing the scope of human agency, that is, empowering individuals and groups. This latter concern ties in with dispositional and constitutive power. Hence, all four notions of power are valuable in order to understand AI ethics as a critical theory and to conduct ethical analyses of AI systems through the lens of critical theory.
4 Analyzing AI Ethics Principles and Topics in Terms of Power
As explained in the introduction, the field of AI ethics is concerned with identifying and addressing the ethical implications of AI as well as the moral questions raised by this new technology. Müller ( 2020 ) discusses privacy, manipulation, opacity, bias, the future of work, and autonomy as main ethical issues that arise from AI systems as objects, and mentions machine ethics, artificial moral agency, and singularity as topics to do with AI systems as subjects. Gordon and Nyholm ( 2021 ) offer a similar list. As main debates in the ethics of AI they name machine ethics, autonomous systems, machine bias, opacity, machine consciousness, moral status, and singularity. To some extent, these lists overlap with sets of AI ethics principles or guidelines. It has namely become a trend, among academics as well as businesses and policymakers, to develop sets of ethical principles that should guide the development and use of AI in a desirable direction. This trend represents the aforementioned principled approach to AI ethics. Footnote 4 A comparative study of 84 sets of AI ethics principles showed that there is a lot of convergence between the principles that different parties have proposed (Jobin et al., 2019 ). More precisely, the study identified eleven clusters of values and principles that were brought forward in several documents: transparency (mentioned in 73 of the studied documents), justice and fairness (68), non-maleficence (60), responsibility (60), privacy (47), beneficence (41), freedom and autonomy (34), trust (28), sustainability (14), dignity (13), and solidarity (6). These principles touch upon the debates that are central in AI ethics according to Müller ( 2020 ) and Gordon and Nyholm ( 2021 ). The issue of opacity, for example, relates to the principle of transparency, and the issue of bias is addressed by the principle of justice.
In what follows I define the most-mentioned AI ethics principles (Sects. 4.1 , 4.2 , 4.3 , 4.4 , 4.5 , 4.6 , and 4.7 ) in terms of power. By doing so, I show that the fundamental concerns that underly these principles are emancipation, empowerment, or both. Furthermore, I also discuss how other topics in AI ethics, such as machine ethics or singularity, relate to the concept of power (Sect. 4.8 ). In the last section (Sect. 4.9 ), I briefly look at non-Western approaches to AI ethics and argue that the concern for emancipation and empowerment is present (although perhaps less dominant) in these approaches as well.
Finally, when I talk about AI as having or exercising power over humans, I do not mean to ascribe the technology as the kind of agency or intentionally required to purposefully seek power. Rather, I merely mean that technology can be used as an instrument or delegate by human beings to exercise power (Brey, 2008 ) or that AI can unintentionally create new power relations, exacerbate existing ones, or affect an individual’s autonomy by empowering or disempowering them. Footnote 5
4.1 Transparency
The most proposed principle for ethical AI is the principle of transparency. Transparency would be required, for example, with regard to data collection and processing (what is done with my personal information?), automated decision-making (how are the decisions that affect me made?), or personalized recommender systems (on the basis of what data am I shown a certain product or news article?). Transparency implies that the answers to these questions are both accessible and comprehensible. The idea behind this principle is that transparent, explainable, or interpretable AI would minimize harm by AI systems, improve human-AI interaction, advance trust in the technology, and potentially support democratic values (Jobin et al., 2019 ). A different way of explaining what makes transparency in AI so valuable is to say that it grants an individual epistemic agency, i.e., the ability to know what happens to their data and how AI affects them, as well as the ability to better control one’s own information and the ways in which they are affected by AI. This knowledge and control are dispositional powers—transparency implies having the power to know or understand what happens to one’s data and on what bases decisions are made. Therefore, we can say that what is really at stake when transparency is called for, is individual empowerment.
4.2 Justice, Fairness, and Solidarity
Justice is mentioned in AI ethics guidelines in relation to fairness, on the one hand, and bias and discrimination, on the other hand (Jobin et al., 2019 ). Fairness concerns have to do with equal access to AI and the equal share of the burdens and benefits of the technology. There is, for example, the concern about a digital divide between the countries that can afford to develop and use AI and those parts of the world that do not have access to the latest technology. The principle of non-discrimination has become pressing as many emerging technologies have been found to contain biases. Particularly algorithmic bias has received much attention in the field of AI ethics. Algorithms can contain biases, among other reasons, when they are built on non-inclusive training data. Biased algorithms or in other ways biased AI systems can lead to discriminatory outcomes (e.g., continuously misidentify certain demographics as a threat or potential criminal) and therefore violate the principle of just and fair AI. Mentioned less often in existing AI guidelines is the principle of solidarity. Solidarity has to do with the (fair) distribution of AI’s benefits and harms. Solidarity in AI would imply that the benefits of AI should be redistributed from those who are disproportionally benefitted by this new technology to those who turn out to be most vulnerable to it (e.g., those who are unemployed due to automation).
These justice-related concerns can be described in terms of relational power. Forst writes that “the question of power, qua social and political power that shapes collective processes, is central to justice” (Forst, 2015 , 8). When we speak of justice, we are referring to what we consider to be acceptable power relations or systems in society. The concept of justice can refer to the direct exercise of power by one actor over another (i.e., episodic power), but most often justice has to do with the systems of power that shape a society and, hence, determine the possibilities of certain individuals or groups in that society (systemic power). In other words, the principle of justice protects those subjected or vulnerable to systems of power or the exercise of power by others. In the context of AI, the principle of justice ought to see to it that the power relations that are created or reinforced by AI systems give people the space to develop themselves, and do so in an equal manner. By doing so, the principle of justice serves the goal of emancipation.
4.3 Non-maleficence and Beneficence
Although they are perhaps less strongly related to the concept of power than other principles, non-maleficence and beneficence too can be understood as principles meant to protect those who are vulnerable to the power of AI. As AI develops to be omnipresent in society, it becomes inescapable for people to use the technology, to be subject of data analysis, and to be affected by automated decision-making systems. Their lives are therefore increasingly controlled by this technology. Moreover, the fact that AI is inescapable to the modern citizen is in itself an instance of systemic power. Protecting that AI does not harm and potentially even benefits its users and subjects is a way of ensuring a desirable power relation and, hence, that AI does not stand in the way of people’s emancipation.
4.4 Responsibility and Accountability
Responsibility is frequently mentioned as a guiding principle for AI, because of the concern that automated decision-making will create responsibility gaps (Gordon & Nyholm, 2021 ). AI systems can take decisions that directly impact human beings, but cannot be held responsible or accountable for the consequences of their actions in the same ways humans can. This raises the question: who should (and can) be held responsible and accountable for the harm caused by AI systems? This question needs to be answered in order to assure that the power relations that AI creates, exacerbates or facilitates are not abused (Sattarov, 2019 ). Like justice, the principles of responsibility and accountability in AI ethics guidelines can be understood as a way of protecting those subjected to AI’s power. Therefore, responsibility too supports emancipation—it helps to overcome unjustifiable social domination.
4.5 Privacy
Privacy can be discussed as a value, moral right or legal right. Jobin et al. ( 2019 ) point out that AI ethics guidelines discuss privacy both as a value to uphold and a right that should be protected. Moreover, privacy is often discussed in relation to data protection, which is in line with the common definitions of privacy as “informational control” or “restricted access” (DeCew, 2018 ). Under both definitions, privacy is understood as a dispositional power, more precisely, as the capacity to control what happens to one’s information and to determine who has access to one’s information or other aspects of the self. AI, then, is perceived as a potential threat to this capacity because it entails the collection and analysis of large quantities and new types of personal data. Hence, AI could disempower individuals with respect to their privacy. Or put differently, privacy should be promoted because it empowers data subjects.
4.6 Freedom and Autonomy
Freedom and autonomy are related concepts and often mentioned together in AI ethics guidelines. Freedom can be defined in positive and negative terms; it can be understood as the lack of outside interference in one’s actions, or the possibility thereof, but it is also discussed as being free to act. The concept of autonomy relates to the positive definition of freedom, it means “self-rule” or “self-determination.” If empowerment entails increasing the scope of individual or collective agency, then autonomy and positive liberty clearly serve the goal of empowerment. Having the ability or power to act freely and rule oneself support one’s agency. This ability can be promoted, in the context of AI or other types of technology, by for example transparency or informed consent. Negative freedom can be defined in terms of episodic power and systemic power—it is the absence of power exercises by others or of systemic power relations. One is free, in this sense, when he or she is not subjected to the power of others or a system of power. Such freedom implies, for example, not being subject to technological experiments, manipulation, or surveillance (Jobin et al., 2019 ). In this sense, the principle of freedom serves the goal of emancipation. We want the values of freedom and autonomy to guide the development and use of AI, because we want to ensure that this new technology is emancipatory and empowering.
The call for trust or trustworthy AI can refer to the ways in which AI research and technology is done, to the organizations and persons that develop AI, to the underlying design principles, or to users’ relation to a technology (Jobin et al., 2019 ). Such trust can be fostered by transparency or by ensuring that AI meets the expectations of the public. People’s need to trust how AI is developed and functions, can be explained not in terms of dispositional power or empowerment, as is the case for transparency, but as a protection against the exercise of power by others. Trust is a desirable feature of the relation between technology and those using it or subjected to it. When trusting an AI system, one expects that the power that technology can exercise over the individual will not be misused. A trustworthy power relation is one where A holds power over B, without B needing to worry that A will take advantage of this situation. Footnote 6 Trust can therefore be understood as serving emancipation—by calling for trustworthy AI, we want to guarantee that AI cannot exercise power over us in arbitrary, harmful or excessive ways.
4.8 Other Topics in AI Ethics
Although there exists a significant amount of overlap between the main debates in the field of AI ethics and the principles that are most commonly mentioned in AI ethics guidelines, these guidelines do not cover topics like machine ethics, the moral status of AI systems, or technological singularity. Moreover, while there is wide agreement on what ethical principles should be reflected in the development and use of AI, the views on these other moral questions in AI are much more varied. But, there is nevertheless a shared concern present within all of these debates. All these topics address, each in their own way, the question of how we should relate to AI and exercise control over it. AI has the potential to become an unprecedentedly powerful technology, due to its intelligence, ability to function autonomously and also widespread reliance on technology. So debates regarding the norms according to which we want AI to act, whether we should grant AI rights, and whether the technology poses an existential risk or not, all express a concern for the human’s position in relation to the (potential) power of AI.
4.9 Non-Western Perspectives on AI Ethics
One of the criticisms raised against the principled approach to AI ethics is that the guidelines that have been established (including the ones discussed by Jobin et al., 2019 ) only represent Western views and values (Gordon & Nyholm, 2021 ). An AI ethics based on non-Western philosophy (e.g., Daoism, Confucianism, or Ubuntu) might not focus as much, or perhaps not at all, on the individual and their emancipation and empowerment. However, Gal ( 2020 ) shows that AI ethics guidelines developed in South Korea, China, and Japan show a lot of similarities with the guidelines studied by Jobin et al. ( 2019 ). The South Korean government presented an ethical framework in 2018, aimed at achieving a human-oriented intelligent information society. The framework consisted of four main principles: publicness, accountability, controllability, and transparency. Central to the Korean approach, Gal notes, is “a clear human-over-machine hierarchy” (Gal, 2020 , 609). Chinese approaches show the same emphasis on the idea that AI should first and foremost be a tool to benefit humans. Furthermore, China’s own big tech companies Baidu and Tencent developed AI ethics principles that show a lot of convergence with the principles mentioned before. Baidu lists safety and controllability, equal access, human development, and freedom. Tencent says AI should be available, reliable, comprehensive, and controllable. Japan deviates most from the trend, by envisioning a coexistence and coevolution between humans, on the one side, and AI and robots on the other side.
So although it is not unimaginable to have AI ethics approaches with an entirely different focus, the current state of AI ethics is that the field is predominantly concerned with human emancipation and empowerment. The goal of AI ethics is to ensure that the emerging technologies that promise to radically change life as we know it, do so for the better.
5 AI ethics as a Critical Theory
By defining the ethical principles and moral questions that are central in AI ethics in terms of power (under a pluralist understanding of power, that is), I have shown that the field is driven by a fundamental concern for human emancipation and empowerment. Transparency, privacy, freedom, and autonomy are valued because they are empowering—they grant individuals the ability to rule their own lives. Principles like trust, justice, responsibility, and non-maleficence are important because they protect individuals against the power that could be exercised by means of AI, or possibly even by AI itself. These central concerns are usually not made explicit. However, doing so helps us to see that AI ethics resembles a critical theory. Like any critical theory, the purpose of AI ethics is not merely to analyze or diagnose society, but also to change it. Both critical theory and AI ethics have a practical goal, namely that of empowering individuals and protecting them against systems of power. But while critical theory is concerned with society at large, AI ethics focuses on the part that a particular type of technology plays in society. Hence, we could say that AI ethics is a critical theory, which focuses on the ways in which human emancipation and empowerment are or could be hindered by AI technology.
Understanding AI ethics as a critical theory can help the field forward in a number of ways. First of all, defining ethical principles in terms of their relation to emancipation or empowerment (respectively, relational, or dispositional power) creates a common language to compare ethical issues and to discuss them in interdisciplinary contexts. Such a common language is welcome for two reasons: because the principles have been accused of being too abstract (Mittelstadt, 2019 ; Resseguier, 2021 ; Ryan and Stahl, 2020 ) and because improving AI is a necessarily interdisciplinary endeavor. Not just ethicists, but also tech developers, data scientists, policymakers, and legal experts need to be involved to realize the goal of ethical AI. The language of power could be more appropriate in interdisciplinary contexts than discussions about (sometimes highly contested) moral values and principles are. Furthermore, by defining how ethical issues relate to dispositional and relational power, we immediately have a clear target for change. While the principles of transparency, privacy, or justice might not be action guiding as such, empowering individuals with respect to their knowledge or informational control, or reducing the say AI systems have over their lives, are much more tangible goals.
A second benefit of the insight that AI ethics is a critical theory is that it offers us a new method for identifying and analyzing ethical issues: a power analysis. The principled approach to AI ethics functions as a kind of ethical checklist. However, the potential negative implications of a technology (and to some extent also the positive ones) could also be identified by analyzing in what ways the technology limits a person’s agency or freedom. The different aspects of power that I described in Sect. 3 could inform and guide such a power analysis. In addition to being more appropriate for interdisciplinary work, a power analysis also has the advantage that it could cover ethical issues that are left unaddressed by current AI principles. The principled approach has been accused of giving too little attention to the social and political context in which AI applications are developed and used, and because of which ethical issues arise. Using a power analysis to ethically assess emerging AI systems will improve our understanding of the ways in which ethical issues in AI tie into broader social, political, economic and historical matters, and understanding the broader context of an ethical issue will in turn make it easier to address the issue, not just on a technical level, but on a societal and political level.
Furthermore, a power analysis could be complemented by insights from the tradition of critical theory. Critical theory is not a full-fledged normative theory that explains what is right and what is wrong in the way that classic theories like consequentialism, deontology, or virtue ethics do, but it does take in a normative stance. Just like critical theory, AI ethics is not meant to be an ethical theory in the classic sense, but it should diagnose technological advancements in society and change them for the better. As others have argued before (Delanty & Harris, 2021 ; Feenberg, 1991 ; Fuchs, 2016 ), critical theory offers a valuable toolbox for analyzing the societal implications of modern technologies. I add to these arguments that many of these societal implications tie into ethical issues. Or in other words, critical theory can help to pinpoint ethically relevant issues that are not typically addressed by ethical principles or classic ethical theories. Critical theory could, for example, help to understand ethical issues that arise from AI’s relation to present-day capitalism (following first-generation critical theorists) or the potential ethical implications of misrecognition that is mediated by AI (following Honneth, 1996 ).
A final benefit of unmasking AI ethics as a critical theory is that we can now understand AI ethics principles as having a common aim. Mittelstadt ( 2019 ) criticizes the popular AI ethics principles for lacking a common aim. Without such a common aim, Mittelstadt argues, the principled approach to AI ethics could not have the same success as the principled approach in bioethics has. But by defining the AI ethics principles in terms of power, I showed that they do share a common aim: to protect human emancipation and empowerment in the face of this new, powerful technology. AI might imply technological progress, but that does not guarantee social progress. AI that decrease our freedom, our agency, and our ability to develop ourselves, would be a step back for the emancipation of individuals and societal groups. As Forst writes, “every progressive process must be constantly questioned as to whether it is in the social interest of those who are part of this process” (Forst, 2019 , 21).
6 Conclusion
The emerging field of AI ethics is unlike other fields in applied ethics. At the center of its attention is not human conduct, but the ways in which humans are affected by AI technology. It differs from the general ethics of technology too, in the sense that AI comes with radically new possibilities for action. This not only raises new moral questions, but also requires new approaches to conduct ethical analyses. But the most popular approach thus far—that is, the principled approach—has been met with criticism. Although there is a lot of convergence when it comes to determining which ethical issues or principles should shape the development, policy, and use of AI, AI ethics principles have been accused of being too abstract, little action guiding, and insufficiently attuned to the social and political context of ethical issues.
The aim of this paper was to show that AI ethics is just like a critical theory. I have explained that a critical theory is aimed at diagnosing and changing society for emancipatory purposes. I then showed that both the big debates in AI ethics and the most common AI ethics principles are fundamentally concerned with either individual empowerment (dispositional power) or the protection of those subjected to power relations (relational power). Approaching AI ethics as a critical theory, by diagnosing AI’s impact by means of a power analysis and the insights of critical theory, can help to overcome the shortcomings of the currently dominant principled approach to AI ethics. Further research could test the power analysis out in a concrete case study, further assess the extent to which the understanding of AI ethics as a critical theory resonates with non-western approaches to AI ethics, or investigate how related field (such as machine ethics) could benefit from the critical theory perspective.
Data availability
Not applicable.
For an overview of the field see Dubber et al., 2020 ; Gordon & Nyholm 2021 ; or Müller 2020 .
This is the 11th thesis on Feuerbach which Karl Marx wrote in 1845. It was later published as an appendix to Friedrich Engels’ Ludwig Feuerbach and the End of Classical German Philosophy in 1886.
Both the idea of family resemblance and language games are borrowed from Wittgenstein.
The principled approach to AI ethics resembles the dominant approach in the more established field of bioethics, where four ethical principles are widely recognized as the basis for policymaking and clinical decision-making in the medical field.
It should also be noted that there exist a number of examples of discussions of power within AI ethics or related fields. For instance, Cobbe ( 2020 ) argues that algorithmic censorship augments the societal power of social platforms; Danaher ( 2020 ) discusses the effects of “algocracy” on freedom; de Laat ( 2019 ) defends that predictive algorithms have disciplinary power; Mohamed et al. ( 2020 ) suggest the use of decolonial theory to understand how the values embedded in AI are shaped by existing power relations; Noble ( 2018 ) discusses the “oppressive algorithms” of search engines; and Zuboff ( 2019 ) critiques the dominance of “surveillance capitalism.” Also, Sattarov ( 2019 ) explores the relation between technology (e.g., algorithms), power and ethics. So there has certainly been recognition for the relevance of discussing power in relation to ethical issues in AI, but these issues are not covered or addressed by the dominant AI ethics guidelines. Moreover, each of the mentioned discussions of power focuses on a single ethical issue, a single technology, or a specific conception of power. The critical approach to AI ethics that will be put forward in this paper, which is based on a pluralist understanding of power, brings these different issues together.
For a more elaborate discussion of the relation between power and the moral concepts of trust, vulnerability, authenticity, and responsibility, see Sattarov 2019 .
Allen, A. (2016). Feminist perspectives on power. The Stanford Encyclopedia of Philosophy, edited by E.N. Zalta. Accessed October 15, 2021. https://plato.stanford.edu/archives/fall2016/entries/feminist-power/
Allen, A. & Mendieta, E. (2019). Introduction. In Justification and emancipation. The critical theory of Rainer Forst . Edited by Amy Allen and Eduardo Mendieta. The Pennsylvania State University Press.
Bohman, J. (2021). Critical Theory. The Stanford Encyclopedia of Philosophy, edited by E.N. Zalta. Accessed October 15, 2021. https://plato.stanford.edu/cgi-bin/encyclopedia/archinfo.cgi?entry=critical-theory
Brey, P. (2008). The technological construction of social power. Social Epistemology, 22 (1), 71–95. https://doi.org/10.1080/02691720701773551
Article Google Scholar
Cave, S., & Dihal, K. (2020). The Whiteness of AI. Philosophy & Technology, 33 (4), 685–703. https://doi.org/10.1007/s13347-020-00415-6
Cobbe, J. (2020). Algorithmic censorship by social platforms: Power and resistance. Philosophy & Technology . https://doi.org/10.1007/s13347-020-00429-0
Dahl, R. A. (1957). The concept of power. Behavioral Science , 201–215. https://doi.org/10.7312/popi17594-004
Danaher, J. (2020). Freedom in an age of algocracy. Oxford Handbook on the Philosophy of Technology , 1–32.
de Laat, P. B. (2019). The disciplinary power of predictive algorithms: A Foucauldian perspective. Ethics and Information Technology, 21 (4), 319–329. https://doi.org/10.1007/s10676-019-09509-y
DeCew, J. (2018). Privacy. Zalta, E.N. (Ed.). The Stanford Encyclopedia of Philosophy . First edition 2018. Accessed October 15, 2021. https://plato.stanford.edu/archives/spr2018/entries/privacy/
Delanty, G., & Harris, N. (2021). Critical theory and the question of technology: The Frankfurt School revisited. Thesis Eleven, 166 (1), 88–108.
Dubber, M.D., Pasquale, F. and Das, s. (eds) (2020). The Oxford handbook of ethics of AI . Oxford University Press. https://doi.org/10.1093/oxfordhb/9780190067397.001.0001
Engels, F. (1886). Ludwig Feuerbach und der Ausgang der klssischen deutschen Philosophie . Stuttgart: Neue Zeit.
Feenberg, A. (1991). Critical theory of technology . Oxford University Press.
Forst, R. (2015). Noumenal Power. Journal of Political Philosophy, 23 (2), 111–127. https://doi.org/10.1111/jopp.12046
Forst, R. (2019). The justification of progress and the progress of justification. In Justification and emancipation. The critical theory of Rainer Forst . Edited by Amy Allen and Eduardo Mendieta. The Pennsylvania State University Press.
Fuchs, C. (2016). Critical theory of communication: New readings of Lukács, Adorno, Marcuse, Honneth and Habermas in the age of the Internet. University of Westminster Press.
Gal, D. (2020). Perspectives and approaches in AI ethics. East Asia. In The Oxford Handbook of Ethics of AI . Edited by M. D. Dubber, F. Pasquale, and S. Das. Oxford University Press. DOI https://doi.org/10.1093/oxfordhb/9780190067397.001.0001
Gebru, T. (2020). Race and Gender. In The Oxford handbook of ethics of AI . Edited by M. D. Dubber, F. Pasquale, and S. Das. Oxford University Press. DOI https://doi.org/10.1093/oxfordhb/9780190067397.001.0001
Gordon, J. S. & Nyholm, S. (2021). Ethics of artificial intelligence. Internet Encyclopedia of Philosophy. Accessed January 21, 2022. https://iep.utm.edu/ethic-ai/
Habermas, J. (1984). The Theory of Communicative Action. Vol. I: Reason and the Rationalization of Society. Translated by T. McCarthy. Boston: Beacon Press. [Published in German in 1981]
Habermas, J. (1987). The theory of communicative action. Vol. II: Lifeworld and System. Translated by T. McCarthy. Boston: Beacon Press. [Published in German in 1981]
Haugaard, M. (2010). Power: A “family resemblance concept.” European Journal of Cultural Studies, 13 (4), 419–438.
Haugaard, M. (2020). The four dimensions of power: Understanding domination, empowerment and democracy . Manchester University Press.
Honneth, A. (1996). The struggle for recognition: The moral grammar of social conflicts . MIT Press.
Horkheimer, M. & Adorno, T.W. (2002). Dialectic of Enlightenment. Translated by Edmund Jephcott, edited by Gunzelin Schimdt Noeri. Stanford University Press.
Horkheimer, M. (1972). Critical theory selected essays . Translated by Matthew J. O'Connell. New York: The Continuum Publishing Company.
Jobin, A., Ienca, M. & Vayena, E. (2019). The global landscape of AI ethics guidelines. Nature Machine Intelligence . 10.1038/ s42256-019-0088-2
Lukes, S. (1974). Power: radical view . London: Macmillan.
Lukes, S. (2005). Power: A radical view. Second edition . London: Red Globe Press.
Mittelstadt, B. (2019). Principles alone cannot guarantee ethical AI. Nature Machine Intelligence . https://doi.org/10.1038/s42256-019-0114-4
Mohamed, S., Png, M. T., & Isaac, W. (2020). Decolonial AI: Decolonial theory as sociotechnical foresight in artificial intelligence. Philosophy & Technology, 33 , 659–684.
Morriss, P. (2002). Power: A philosophical analysis . Manchester, New York: Manchester University Press.
Müller, V. (2020). Ethics of artificial intelligence and robotics. Zalta, E.N. (Ed.). The Stanford Encyclopedia of Philosophy . First edition 2020. Accessed January 21, 2022. https://plato.stanford.edu/entries/ethics-ai/
Noble, S.U. (2018). Algorithms of oppression: How search engines reinforce racism . New York: New York University Press.
Resseguier (2021). Ethics as attention to context: Recommendations for AI ethics. In SIENNA D5.4: Multi-stakeholder strategy and practical tools for ethical AI and robotics . https://www.sienna-project.eu/publications/deliverable-reports/
Ryan, M., & Stahl, B. C. (2020). Artificial intelligence ethics guidelines for developers and users: Clarifying their content and normative implications. Journal of Information, Communication and Ethics in Society, 19 (1), 61–86. https://doi.org/10.1108/JICES-12-2019-0138
Sattarov, F. (2019). Power and technology: A philosophical and ethical analysis . London, New York: Rowman and Littlefield International.
Stahl, B. C., Doherty, N. F., Shaw, M., & Janicke, H. (2014). Critical theory as an approach to the ethics of information security. Science and Engineering Ethics, 20 , 675–699. https://doi.org/10.1007/s11948-013-9496-6
Thompson, S. (2006). The political theory of recognition. A critical introduction . Polity Press.
Zuboff, S. (2019). The age of surveillance capitalism: The fight for a human future at the new frontier of power. London: Profile Books.
Download references
The author is an early-stage researcher funded by MSCA ITN PROTECT project. This project has received funding from the European Union’s Horizon 2020 research and innovation program under the Marie Skłodowska-Curie grant agreement No 813497.
Author information
Authors and affiliations.
Section of Philosophy, University of Twente, Enschede, Netherlands
Rosalie Waelen
You can also search for this author in PubMed Google Scholar
Corresponding author
Correspondence to Rosalie Waelen .
Ethics declarations
Ethics approval and consent to participate, consent for publication, competing interests.
The author declares no competing interests.
Additional information
Publisher's note.
Springer Nature remains neutral with regard to jurisdictional claims in published maps and institutional affiliations.
Rights and permissions
Open Access This article is licensed under a Creative Commons Attribution 4.0 International License, which permits use, sharing, adaptation, distribution and reproduction in any medium or format, as long as you give appropriate credit to the original author(s) and the source, provide a link to the Creative Commons licence, and indicate if changes were made. The images or other third party material in this article are included in the article's Creative Commons licence, unless indicated otherwise in a credit line to the material. If material is not included in the article's Creative Commons licence and your intended use is not permitted by statutory regulation or exceeds the permitted use, you will need to obtain permission directly from the copyright holder. To view a copy of this licence, visit http://creativecommons.org/licenses/by/4.0/ .
Reprints and permissions
About this article
Waelen, R. Why AI Ethics Is a Critical Theory. Philos. Technol. 35 , 9 (2022). https://doi.org/10.1007/s13347-022-00507-5
Download citation
Received : 20 October 2021
Accepted : 01 February 2022
Published : 11 February 2022
DOI : https://doi.org/10.1007/s13347-022-00507-5
Share this article
Anyone you share the following link with will be able to read this content:
Sorry, a shareable link is not currently available for this article.
Provided by the Springer Nature SharedIt content-sharing initiative
- Artificial intelligence
- Critical theory
- Find a journal
- Publish with us
- Track your research
Peer Reviewed
GPT-fabricated scientific papers on Google Scholar: Key features, spread, and implications for preempting evidence manipulation
Article metrics.
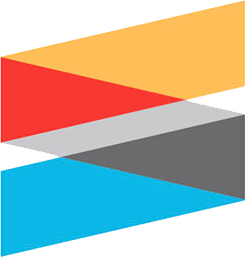
CrossRef Citations
Altmetric Score
PDF Downloads
Academic journals, archives, and repositories are seeing an increasing number of questionable research papers clearly produced using generative AI. They are often created with widely available, general-purpose AI applications, most likely ChatGPT, and mimic scientific writing. Google Scholar easily locates and lists these questionable papers alongside reputable, quality-controlled research. Our analysis of a selection of questionable GPT-fabricated scientific papers found in Google Scholar shows that many are about applied, often controversial topics susceptible to disinformation: the environment, health, and computing. The resulting enhanced potential for malicious manipulation of society’s evidence base, particularly in politically divisive domains, is a growing concern.
Swedish School of Library and Information Science, University of Borås, Sweden
Department of Arts and Cultural Sciences, Lund University, Sweden
Division of Environmental Communication, Swedish University of Agricultural Sciences, Sweden
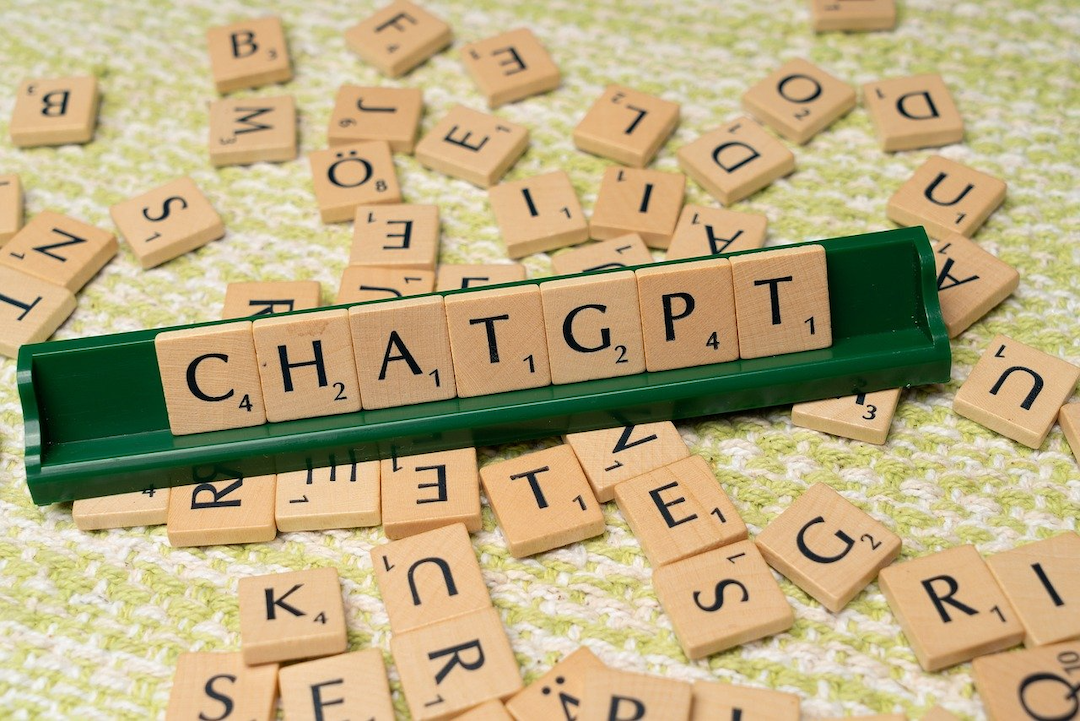
Research Questions
- Where are questionable publications produced with generative pre-trained transformers (GPTs) that can be found via Google Scholar published or deposited?
- What are the main characteristics of these publications in relation to predominant subject categories?
- How are these publications spread in the research infrastructure for scholarly communication?
- How is the role of the scholarly communication infrastructure challenged in maintaining public trust in science and evidence through inappropriate use of generative AI?
research note Summary
- A sample of scientific papers with signs of GPT-use found on Google Scholar was retrieved, downloaded, and analyzed using a combination of qualitative coding and descriptive statistics. All papers contained at least one of two common phrases returned by conversational agents that use large language models (LLM) like OpenAI’s ChatGPT. Google Search was then used to determine the extent to which copies of questionable, GPT-fabricated papers were available in various repositories, archives, citation databases, and social media platforms.
- Roughly two-thirds of the retrieved papers were found to have been produced, at least in part, through undisclosed, potentially deceptive use of GPT. The majority (57%) of these questionable papers dealt with policy-relevant subjects (i.e., environment, health, computing), susceptible to influence operations. Most were available in several copies on different domains (e.g., social media, archives, and repositories).
- Two main risks arise from the increasingly common use of GPT to (mass-)produce fake, scientific publications. First, the abundance of fabricated “studies” seeping into all areas of the research infrastructure threatens to overwhelm the scholarly communication system and jeopardize the integrity of the scientific record. A second risk lies in the increased possibility that convincingly scientific-looking content was in fact deceitfully created with AI tools and is also optimized to be retrieved by publicly available academic search engines, particularly Google Scholar. However small, this possibility and awareness of it risks undermining the basis for trust in scientific knowledge and poses serious societal risks.
Implications
The use of ChatGPT to generate text for academic papers has raised concerns about research integrity. Discussion of this phenomenon is ongoing in editorials, commentaries, opinion pieces, and on social media (Bom, 2023; Stokel-Walker, 2024; Thorp, 2023). There are now several lists of papers suspected of GPT misuse, and new papers are constantly being added. 1 See for example Academ-AI, https://www.academ-ai.info/ , and Retraction Watch, https://retractionwatch.com/papers-and-peer-reviews-with-evidence-of-chatgpt-writing/ . While many legitimate uses of GPT for research and academic writing exist (Huang & Tan, 2023; Kitamura, 2023; Lund et al., 2023), its undeclared use—beyond proofreading—has potentially far-reaching implications for both science and society, but especially for their relationship. It, therefore, seems important to extend the discussion to one of the most accessible and well-known intermediaries between science, but also certain types of misinformation, and the public, namely Google Scholar, also in response to the legitimate concerns that the discussion of generative AI and misinformation needs to be more nuanced and empirically substantiated (Simon et al., 2023).
Google Scholar, https://scholar.google.com , is an easy-to-use academic search engine. It is available for free, and its index is extensive (Gusenbauer & Haddaway, 2020). It is also often touted as a credible source for academic literature and even recommended in library guides, by media and information literacy initiatives, and fact checkers (Tripodi et al., 2023). However, Google Scholar lacks the transparency and adherence to standards that usually characterize citation databases. Instead, Google Scholar uses automated crawlers, like Google’s web search engine (Martín-Martín et al., 2021), and the inclusion criteria are based on primarily technical standards, allowing any individual author—with or without scientific affiliation—to upload papers to be indexed (Google Scholar Help, n.d.). It has been shown that Google Scholar is susceptible to manipulation through citation exploits (Antkare, 2020) and by providing access to fake scientific papers (Dadkhah et al., 2017). A large part of Google Scholar’s index consists of publications from established scientific journals or other forms of quality-controlled, scholarly literature. However, the index also contains a large amount of gray literature, including student papers, working papers, reports, preprint servers, and academic networking sites, as well as material from so-called “questionable” academic journals, including paper mills. The search interface does not offer the possibility to filter the results meaningfully by material type, publication status, or form of quality control, such as limiting the search to peer-reviewed material.
To understand the occurrence of ChatGPT (co-)authored work in Google Scholar’s index, we scraped it for publications, including one of two common ChatGPT responses (see Appendix A) that we encountered on social media and in media reports (DeGeurin, 2024). The results of our descriptive statistical analyses showed that around 62% did not declare the use of GPTs. Most of these GPT-fabricated papers were found in non-indexed journals and working papers, but some cases included research published in mainstream scientific journals and conference proceedings. 2 Indexed journals mean scholarly journals indexed by abstract and citation databases such as Scopus and Web of Science, where the indexation implies journals with high scientific quality. Non-indexed journals are journals that fall outside of this indexation. More than half (57%) of these GPT-fabricated papers concerned policy-relevant subject areas susceptible to influence operations. To avoid increasing the visibility of these publications, we abstained from referencing them in this research note. However, we have made the data available in the Harvard Dataverse repository.
The publications were related to three issue areas—health (14.5%), environment (19.5%) and computing (23%)—with key terms such “healthcare,” “COVID-19,” or “infection”for health-related papers, and “analysis,” “sustainable,” and “global” for environment-related papers. In several cases, the papers had titles that strung together general keywords and buzzwords, thus alluding to very broad and current research. These terms included “biology,” “telehealth,” “climate policy,” “diversity,” and “disrupting,” to name just a few. While the study’s scope and design did not include a detailed analysis of which parts of the articles included fabricated text, our dataset did contain the surrounding sentences for each occurrence of the suspicious phrases that formed the basis for our search and subsequent selection. Based on that, we can say that the phrases occurred in most sections typically found in scientific publications, including the literature review, methods, conceptual and theoretical frameworks, background, motivation or societal relevance, and even discussion. This was confirmed during the joint coding, where we read and discussed all articles. It became clear that not just the text related to the telltale phrases was created by GPT, but that almost all articles in our sample of questionable articles likely contained traces of GPT-fabricated text everywhere.
Evidence hacking and backfiring effects
Generative pre-trained transformers (GPTs) can be used to produce texts that mimic scientific writing. These texts, when made available online—as we demonstrate—leak into the databases of academic search engines and other parts of the research infrastructure for scholarly communication. This development exacerbates problems that were already present with less sophisticated text generators (Antkare, 2020; Cabanac & Labbé, 2021). Yet, the public release of ChatGPT in 2022, together with the way Google Scholar works, has increased the likelihood of lay people (e.g., media, politicians, patients, students) coming across questionable (or even entirely GPT-fabricated) papers and other problematic research findings. Previous research has emphasized that the ability to determine the value and status of scientific publications for lay people is at stake when misleading articles are passed off as reputable (Haider & Åström, 2017) and that systematic literature reviews risk being compromised (Dadkhah et al., 2017). It has also been highlighted that Google Scholar, in particular, can be and has been exploited for manipulating the evidence base for politically charged issues and to fuel conspiracy narratives (Tripodi et al., 2023). Both concerns are likely to be magnified in the future, increasing the risk of what we suggest calling evidence hacking —the strategic and coordinated malicious manipulation of society’s evidence base.
The authority of quality-controlled research as evidence to support legislation, policy, politics, and other forms of decision-making is undermined by the presence of undeclared GPT-fabricated content in publications professing to be scientific. Due to the large number of archives, repositories, mirror sites, and shadow libraries to which they spread, there is a clear risk that GPT-fabricated, questionable papers will reach audiences even after a possible retraction. There are considerable technical difficulties involved in identifying and tracing computer-fabricated papers (Cabanac & Labbé, 2021; Dadkhah et al., 2023; Jones, 2024), not to mention preventing and curbing their spread and uptake.
However, as the rise of the so-called anti-vaxx movement during the COVID-19 pandemic and the ongoing obstruction and denial of climate change show, retracting erroneous publications often fuels conspiracies and increases the following of these movements rather than stopping them. To illustrate this mechanism, climate deniers frequently question established scientific consensus by pointing to other, supposedly scientific, studies that support their claims. Usually, these are poorly executed, not peer-reviewed, based on obsolete data, or even fraudulent (Dunlap & Brulle, 2020). A similar strategy is successful in the alternative epistemic world of the global anti-vaccination movement (Carrion, 2018) and the persistence of flawed and questionable publications in the scientific record already poses significant problems for health research, policy, and lawmakers, and thus for society as a whole (Littell et al., 2024). Considering that a person’s support for “doing your own research” is associated with increased mistrust in scientific institutions (Chinn & Hasell, 2023), it will be of utmost importance to anticipate and consider such backfiring effects already when designing a technical solution, when suggesting industry or legal regulation, and in the planning of educational measures.
Recommendations
Solutions should be based on simultaneous considerations of technical, educational, and regulatory approaches, as well as incentives, including social ones, across the entire research infrastructure. Paying attention to how these approaches and incentives relate to each other can help identify points and mechanisms for disruption. Recognizing fraudulent academic papers must happen alongside understanding how they reach their audiences and what reasons there might be for some of these papers successfully “sticking around.” A possible way to mitigate some of the risks associated with GPT-fabricated scholarly texts finding their way into academic search engine results would be to provide filtering options for facets such as indexed journals, gray literature, peer-review, and similar on the interface of publicly available academic search engines. Furthermore, evaluation tools for indexed journals 3 Such as LiU Journal CheckUp, https://ep.liu.se/JournalCheckup/default.aspx?lang=eng . could be integrated into the graphical user interfaces and the crawlers of these academic search engines. To enable accountability, it is important that the index (database) of such a search engine is populated according to criteria that are transparent, open to scrutiny, and appropriate to the workings of science and other forms of academic research. Moreover, considering that Google Scholar has no real competitor, there is a strong case for establishing a freely accessible, non-specialized academic search engine that is not run for commercial reasons but for reasons of public interest. Such measures, together with educational initiatives aimed particularly at policymakers, science communicators, journalists, and other media workers, will be crucial to reducing the possibilities for and effects of malicious manipulation or evidence hacking. It is important not to present this as a technical problem that exists only because of AI text generators but to relate it to the wider concerns in which it is embedded. These range from a largely dysfunctional scholarly publishing system (Haider & Åström, 2017) and academia’s “publish or perish” paradigm to Google’s near-monopoly and ideological battles over the control of information and ultimately knowledge. Any intervention is likely to have systemic effects; these effects need to be considered and assessed in advance and, ideally, followed up on.
Our study focused on a selection of papers that were easily recognizable as fraudulent. We used this relatively small sample as a magnifying glass to examine, delineate, and understand a problem that goes beyond the scope of the sample itself, which however points towards larger concerns that require further investigation. The work of ongoing whistleblowing initiatives 4 Such as Academ-AI, https://www.academ-ai.info/ , and Retraction Watch, https://retractionwatch.com/papers-and-peer-reviews-with-evidence-of-chatgpt-writing/ . , recent media reports of journal closures (Subbaraman, 2024), or GPT-related changes in word use and writing style (Cabanac et al., 2021; Stokel-Walker, 2024) suggest that we only see the tip of the iceberg. There are already more sophisticated cases (Dadkhah et al., 2023) as well as cases involving fabricated images (Gu et al., 2022). Our analysis shows that questionable and potentially manipulative GPT-fabricated papers permeate the research infrastructure and are likely to become a widespread phenomenon. Our findings underline that the risk of fake scientific papers being used to maliciously manipulate evidence (see Dadkhah et al., 2017) must be taken seriously. Manipulation may involve undeclared automatic summaries of texts, inclusion in literature reviews, explicit scientific claims, or the concealment of errors in studies so that they are difficult to detect in peer review. However, the mere possibility of these things happening is a significant risk in its own right that can be strategically exploited and will have ramifications for trust in and perception of science. Society’s methods of evaluating sources and the foundations of media and information literacy are under threat and public trust in science is at risk of further erosion, with far-reaching consequences for society in dealing with information disorders. To address this multifaceted problem, we first need to understand why it exists and proliferates.
Finding 1: 139 GPT-fabricated, questionable papers were found and listed as regular results on the Google Scholar results page. Non-indexed journals dominate.
Most questionable papers we found were in non-indexed journals or were working papers, but we did also find some in established journals, publications, conferences, and repositories. We found a total of 139 papers with a suspected deceptive use of ChatGPT or similar LLM applications (see Table 1). Out of these, 19 were in indexed journals, 89 were in non-indexed journals, 19 were student papers found in university databases, and 12 were working papers (mostly in preprint databases). Table 1 divides these papers into categories. Health and environment papers made up around 34% (47) of the sample. Of these, 66% were present in non-indexed journals.
Indexed journals* | 5 | 3 | 4 | 7 | 19 |
Non-indexed journals | 18 | 18 | 13 | 40 | 89 |
Student papers | 4 | 3 | 1 | 11 | 19 |
Working papers | 5 | 3 | 2 | 2 | 12 |
Total | 32 | 27 | 20 | 60 | 139 |
Finding 2: GPT-fabricated, questionable papers are disseminated online, permeating the research infrastructure for scholarly communication, often in multiple copies. Applied topics with practical implications dominate.
The 20 papers concerning health-related issues are distributed across 20 unique domains, accounting for 46 URLs. The 27 papers dealing with environmental issues can be found across 26 unique domains, accounting for 56 URLs. Most of the identified papers exist in multiple copies and have already spread to several archives, repositories, and social media. It would be difficult, or impossible, to remove them from the scientific record.
As apparent from Table 2, GPT-fabricated, questionable papers are seeping into most parts of the online research infrastructure for scholarly communication. Platforms on which identified papers have appeared include ResearchGate, ORCiD, Journal of Population Therapeutics and Clinical Pharmacology (JPTCP), Easychair, Frontiers, the Institute of Electrical and Electronics Engineer (IEEE), and X/Twitter. Thus, even if they are retracted from their original source, it will prove very difficult to track, remove, or even just mark them up on other platforms. Moreover, unless regulated, Google Scholar will enable their continued and most likely unlabeled discoverability.
Environment | researchgate.net (13) | orcid.org (4) | easychair.org (3) | ijope.com* (3) | publikasiindonesia.id (3) |
Health | researchgate.net (15) | ieee.org (4) | twitter.com (3) | jptcp.com** (2) | frontiersin.org (2) |
A word rain visualization (Centre for Digital Humanities Uppsala, 2023), which combines word prominences through TF-IDF 5 Term frequency–inverse document frequency , a method for measuring the significance of a word in a document compared to its frequency across all documents in a collection. scores with semantic similarity of the full texts of our sample of GPT-generated articles that fall into the “Environment” and “Health” categories, reflects the two categories in question. However, as can be seen in Figure 1, it also reveals overlap and sub-areas. The y-axis shows word prominences through word positions and font sizes, while the x-axis indicates semantic similarity. In addition to a certain amount of overlap, this reveals sub-areas, which are best described as two distinct events within the word rain. The event on the left bundles terms related to the development and management of health and healthcare with “challenges,” “impact,” and “potential of artificial intelligence”emerging as semantically related terms. Terms related to research infrastructures, environmental, epistemic, and technological concepts are arranged further down in the same event (e.g., “system,” “climate,” “understanding,” “knowledge,” “learning,” “education,” “sustainable”). A second distinct event further to the right bundles terms associated with fish farming and aquatic medicinal plants, highlighting the presence of an aquaculture cluster. Here, the prominence of groups of terms such as “used,” “model,” “-based,” and “traditional” suggests the presence of applied research on these topics. The two events making up the word rain visualization, are linked by a less dominant but overlapping cluster of terms related to “energy” and “water.”
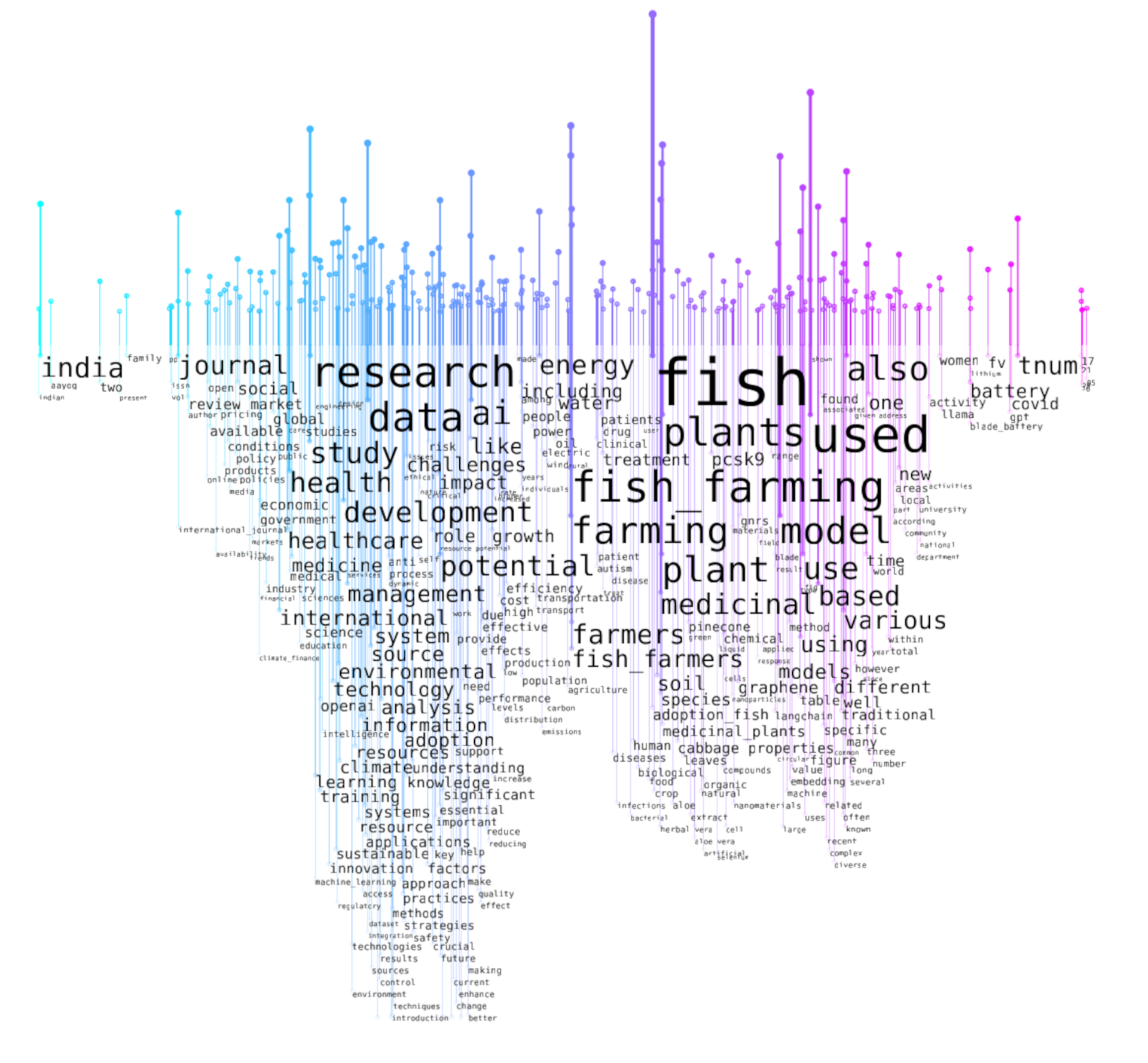
The bar chart of the terms in the paper subset (see Figure 2) complements the word rain visualization by depicting the most prominent terms in the full texts along the y-axis. Here, word prominences across health and environment papers are arranged descendingly, where values outside parentheses are TF-IDF values (relative frequencies) and values inside parentheses are raw term frequencies (absolute frequencies).
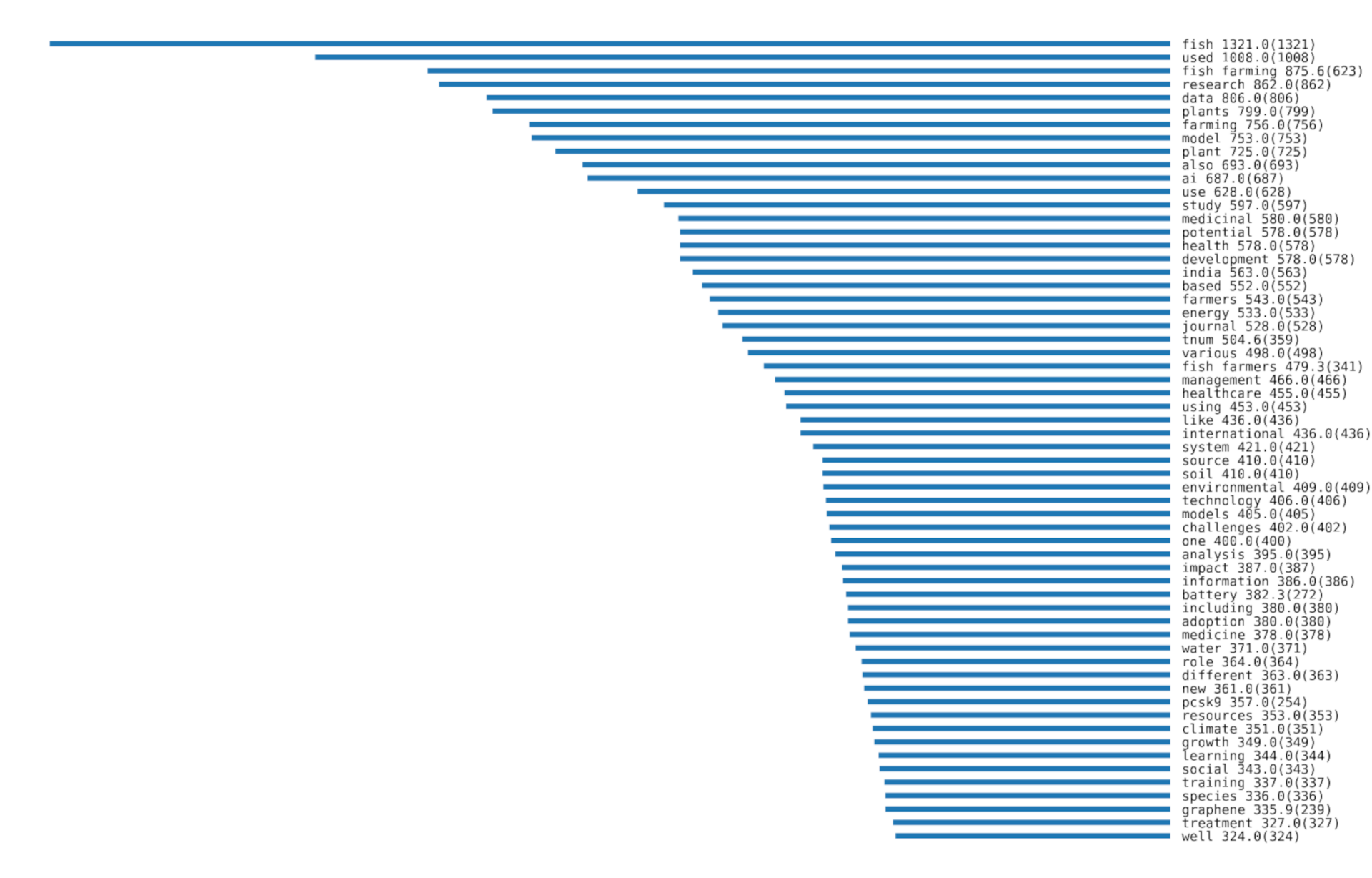
Finding 3: Google Scholar presents results from quality-controlled and non-controlled citation databases on the same interface, providing unfiltered access to GPT-fabricated questionable papers.
Google Scholar’s central position in the publicly accessible scholarly communication infrastructure, as well as its lack of standards, transparency, and accountability in terms of inclusion criteria, has potentially serious implications for public trust in science. This is likely to exacerbate the already-known potential to exploit Google Scholar for evidence hacking (Tripodi et al., 2023) and will have implications for any attempts to retract or remove fraudulent papers from their original publication venues. Any solution must consider the entirety of the research infrastructure for scholarly communication and the interplay of different actors, interests, and incentives.
We searched and scraped Google Scholar using the Python library Scholarly (Cholewiak et al., 2023) for papers that included specific phrases known to be common responses from ChatGPT and similar applications with the same underlying model (GPT3.5 or GPT4): “as of my last knowledge update” and/or “I don’t have access to real-time data” (see Appendix A). This facilitated the identification of papers that likely used generative AI to produce text, resulting in 227 retrieved papers. The papers’ bibliographic information was automatically added to a spreadsheet and downloaded into Zotero. 6 An open-source reference manager, https://zotero.org .
We employed multiple coding (Barbour, 2001) to classify the papers based on their content. First, we jointly assessed whether the paper was suspected of fraudulent use of ChatGPT (or similar) based on how the text was integrated into the papers and whether the paper was presented as original research output or the AI tool’s role was acknowledged. Second, in analyzing the content of the papers, we continued the multiple coding by classifying the fraudulent papers into four categories identified during an initial round of analysis—health, environment, computing, and others—and then determining which subjects were most affected by this issue (see Table 1). Out of the 227 retrieved papers, 88 papers were written with legitimate and/or declared use of GPTs (i.e., false positives, which were excluded from further analysis), and 139 papers were written with undeclared and/or fraudulent use (i.e., true positives, which were included in further analysis). The multiple coding was conducted jointly by all authors of the present article, who collaboratively coded and cross-checked each other’s interpretation of the data simultaneously in a shared spreadsheet file. This was done to single out coding discrepancies and settle coding disagreements, which in turn ensured methodological thoroughness and analytical consensus (see Barbour, 2001). Redoing the category coding later based on our established coding schedule, we achieved an intercoder reliability (Cohen’s kappa) of 0.806 after eradicating obvious differences.
The ranking algorithm of Google Scholar prioritizes highly cited and older publications (Martín-Martín et al., 2016). Therefore, the position of the articles on the search engine results pages was not particularly informative, considering the relatively small number of results in combination with the recency of the publications. Only the query “as of my last knowledge update” had more than two search engine result pages. On those, questionable articles with undeclared use of GPTs were evenly distributed across all result pages (min: 4, max: 9, mode: 8), with the proportion of undeclared use being slightly higher on average on later search result pages.
To understand how the papers making fraudulent use of generative AI were disseminated online, we programmatically searched for the paper titles (with exact string matching) in Google Search from our local IP address (see Appendix B) using the googlesearch – python library(Vikramaditya, 2020). We manually verified each search result to filter out false positives—results that were not related to the paper—and then compiled the most prominent URLs by field. This enabled the identification of other platforms through which the papers had been spread. We did not, however, investigate whether copies had spread into SciHub or other shadow libraries, or if they were referenced in Wikipedia.
We used descriptive statistics to count the prevalence of the number of GPT-fabricated papers across topics and venues and top domains by subject. The pandas software library for the Python programming language (The pandas development team, 2024) was used for this part of the analysis. Based on the multiple coding, paper occurrences were counted in relation to their categories, divided into indexed journals, non-indexed journals, student papers, and working papers. The schemes, subdomains, and subdirectories of the URL strings were filtered out while top-level domains and second-level domains were kept, which led to normalizing domain names. This, in turn, allowed the counting of domain frequencies in the environment and health categories. To distinguish word prominences and meanings in the environment and health-related GPT-fabricated questionable papers, a semantically-aware word cloud visualization was produced through the use of a word rain (Centre for Digital Humanities Uppsala, 2023) for full-text versions of the papers. Font size and y-axis positions indicate word prominences through TF-IDF scores for the environment and health papers (also visualized in a separate bar chart with raw term frequencies in parentheses), and words are positioned along the x-axis to reflect semantic similarity (Skeppstedt et al., 2024), with an English Word2vec skip gram model space (Fares et al., 2017). An English stop word list was used, along with a manually produced list including terms such as “https,” “volume,” or “years.”
- Artificial Intelligence
- / Search engines
Cite this Essay
Haider, J., Söderström, K. R., Ekström, B., & Rödl, M. (2024). GPT-fabricated scientific papers on Google Scholar: Key features, spread, and implications for preempting evidence manipulation. Harvard Kennedy School (HKS) Misinformation Review . https://doi.org/10.37016/mr-2020-156
- / Appendix B
Bibliography
Antkare, I. (2020). Ike Antkare, his publications, and those of his disciples. In M. Biagioli & A. Lippman (Eds.), Gaming the metrics (pp. 177–200). The MIT Press. https://doi.org/10.7551/mitpress/11087.003.0018
Barbour, R. S. (2001). Checklists for improving rigour in qualitative research: A case of the tail wagging the dog? BMJ , 322 (7294), 1115–1117. https://doi.org/10.1136/bmj.322.7294.1115
Bom, H.-S. H. (2023). Exploring the opportunities and challenges of ChatGPT in academic writing: A roundtable discussion. Nuclear Medicine and Molecular Imaging , 57 (4), 165–167. https://doi.org/10.1007/s13139-023-00809-2
Cabanac, G., & Labbé, C. (2021). Prevalence of nonsensical algorithmically generated papers in the scientific literature. Journal of the Association for Information Science and Technology , 72 (12), 1461–1476. https://doi.org/10.1002/asi.24495
Cabanac, G., Labbé, C., & Magazinov, A. (2021). Tortured phrases: A dubious writing style emerging in science. Evidence of critical issues affecting established journals . arXiv. https://doi.org/10.48550/arXiv.2107.06751
Carrion, M. L. (2018). “You need to do your research”: Vaccines, contestable science, and maternal epistemology. Public Understanding of Science , 27 (3), 310–324. https://doi.org/10.1177/0963662517728024
Centre for Digital Humanities Uppsala (2023). CDHUppsala/word-rain [Computer software]. https://github.com/CDHUppsala/word-rain
Chinn, S., & Hasell, A. (2023). Support for “doing your own research” is associated with COVID-19 misperceptions and scientific mistrust. Harvard Kennedy School (HSK) Misinformation Review, 4 (3). https://doi.org/10.37016/mr-2020-117
Cholewiak, S. A., Ipeirotis, P., Silva, V., & Kannawadi, A. (2023). SCHOLARLY: Simple access to Google Scholar authors and citation using Python (1.5.0) [Computer software]. https://doi.org/10.5281/zenodo.5764801
Dadkhah, M., Lagzian, M., & Borchardt, G. (2017). Questionable papers in citation databases as an issue for literature review. Journal of Cell Communication and Signaling , 11 (2), 181–185. https://doi.org/10.1007/s12079-016-0370-6
Dadkhah, M., Oermann, M. H., Hegedüs, M., Raman, R., & Dávid, L. D. (2023). Detection of fake papers in the era of artificial intelligence. Diagnosis , 10 (4), 390–397. https://doi.org/10.1515/dx-2023-0090
DeGeurin, M. (2024, March 19). AI-generated nonsense is leaking into scientific journals. Popular Science. https://www.popsci.com/technology/ai-generated-text-scientific-journals/
Dunlap, R. E., & Brulle, R. J. (2020). Sources and amplifiers of climate change denial. In D.C. Holmes & L. M. Richardson (Eds.), Research handbook on communicating climate change (pp. 49–61). Edward Elgar Publishing. https://doi.org/10.4337/9781789900408.00013
Fares, M., Kutuzov, A., Oepen, S., & Velldal, E. (2017). Word vectors, reuse, and replicability: Towards a community repository of large-text resources. In J. Tiedemann & N. Tahmasebi (Eds.), Proceedings of the 21st Nordic Conference on Computational Linguistics (pp. 271–276). Association for Computational Linguistics. https://aclanthology.org/W17-0237
Google Scholar Help. (n.d.). Inclusion guidelines for webmasters . https://scholar.google.com/intl/en/scholar/inclusion.html
Gu, J., Wang, X., Li, C., Zhao, J., Fu, W., Liang, G., & Qiu, J. (2022). AI-enabled image fraud in scientific publications. Patterns , 3 (7), 100511. https://doi.org/10.1016/j.patter.2022.100511
Gusenbauer, M., & Haddaway, N. R. (2020). Which academic search systems are suitable for systematic reviews or meta-analyses? Evaluating retrieval qualities of Google Scholar, PubMed, and 26 other resources. Research Synthesis Methods , 11 (2), 181–217. https://doi.org/10.1002/jrsm.1378
Haider, J., & Åström, F. (2017). Dimensions of trust in scholarly communication: Problematizing peer review in the aftermath of John Bohannon’s “Sting” in science. Journal of the Association for Information Science and Technology , 68 (2), 450–467. https://doi.org/10.1002/asi.23669
Huang, J., & Tan, M. (2023). The role of ChatGPT in scientific communication: Writing better scientific review articles. American Journal of Cancer Research , 13 (4), 1148–1154. https://www.ncbi.nlm.nih.gov/pmc/articles/PMC10164801/
Jones, N. (2024). How journals are fighting back against a wave of questionable images. Nature , 626 (8000), 697–698. https://doi.org/10.1038/d41586-024-00372-6
Kitamura, F. C. (2023). ChatGPT is shaping the future of medical writing but still requires human judgment. Radiology , 307 (2), e230171. https://doi.org/10.1148/radiol.230171
Littell, J. H., Abel, K. M., Biggs, M. A., Blum, R. W., Foster, D. G., Haddad, L. B., Major, B., Munk-Olsen, T., Polis, C. B., Robinson, G. E., Rocca, C. H., Russo, N. F., Steinberg, J. R., Stewart, D. E., Stotland, N. L., Upadhyay, U. D., & Ditzhuijzen, J. van. (2024). Correcting the scientific record on abortion and mental health outcomes. BMJ , 384 , e076518. https://doi.org/10.1136/bmj-2023-076518
Lund, B. D., Wang, T., Mannuru, N. R., Nie, B., Shimray, S., & Wang, Z. (2023). ChatGPT and a new academic reality: Artificial Intelligence-written research papers and the ethics of the large language models in scholarly publishing. Journal of the Association for Information Science and Technology, 74 (5), 570–581. https://doi.org/10.1002/asi.24750
Martín-Martín, A., Orduna-Malea, E., Ayllón, J. M., & Delgado López-Cózar, E. (2016). Back to the past: On the shoulders of an academic search engine giant. Scientometrics , 107 , 1477–1487. https://doi.org/10.1007/s11192-016-1917-2
Martín-Martín, A., Thelwall, M., Orduna-Malea, E., & Delgado López-Cózar, E. (2021). Google Scholar, Microsoft Academic, Scopus, Dimensions, Web of Science, and OpenCitations’ COCI: A multidisciplinary comparison of coverage via citations. Scientometrics , 126 (1), 871–906. https://doi.org/10.1007/s11192-020-03690-4
Simon, F. M., Altay, S., & Mercier, H. (2023). Misinformation reloaded? Fears about the impact of generative AI on misinformation are overblown. Harvard Kennedy School (HKS) Misinformation Review, 4 (5). https://doi.org/10.37016/mr-2020-127
Skeppstedt, M., Ahltorp, M., Kucher, K., & Lindström, M. (2024). From word clouds to Word Rain: Revisiting the classic word cloud to visualize climate change texts. Information Visualization , 23 (3), 217–238. https://doi.org/10.1177/14738716241236188
Swedish Research Council. (2017). Good research practice. Vetenskapsrådet.
Stokel-Walker, C. (2024, May 1.). AI Chatbots Have Thoroughly Infiltrated Scientific Publishing . Scientific American. https://www.scientificamerican.com/article/chatbots-have-thoroughly-infiltrated-scientific-publishing/
Subbaraman, N. (2024, May 14). Flood of fake science forces multiple journal closures: Wiley to shutter 19 more journals, some tainted by fraud. The Wall Street Journal . https://www.wsj.com/science/academic-studies-research-paper-mills-journals-publishing-f5a3d4bc
The pandas development team. (2024). pandas-dev/pandas: Pandas (v2.2.2) [Computer software]. Zenodo. https://doi.org/10.5281/zenodo.10957263
Thorp, H. H. (2023). ChatGPT is fun, but not an author. Science , 379 (6630), 313–313. https://doi.org/10.1126/science.adg7879
Tripodi, F. B., Garcia, L. C., & Marwick, A. E. (2023). ‘Do your own research’: Affordance activation and disinformation spread. Information, Communication & Society , 27 (6), 1212–1228. https://doi.org/10.1080/1369118X.2023.2245869
Vikramaditya, N. (2020). Nv7-GitHub/googlesearch [Computer software]. https://github.com/Nv7-GitHub/googlesearch
This research has been supported by Mistra, the Swedish Foundation for Strategic Environmental Research, through the research program Mistra Environmental Communication (Haider, Ekström, Rödl) and the Marcus and Amalia Wallenberg Foundation [2020.0004] (Söderström).
Competing Interests
The authors declare no competing interests.
The research described in this article was carried out under Swedish legislation. According to the relevant EU and Swedish legislation (2003:460) on the ethical review of research involving humans (“Ethical Review Act”), the research reported on here is not subject to authorization by the Swedish Ethical Review Authority (“etikprövningsmyndigheten”) (SRC, 2017).
This is an open access article distributed under the terms of the Creative Commons Attribution License , which permits unrestricted use, distribution, and reproduction in any medium, provided that the original author and source are properly credited.
Data Availability
All data needed to replicate this study are available at the Harvard Dataverse: https://doi.org/10.7910/DVN/WUVD8X
Acknowledgements
The authors wish to thank two anonymous reviewers for their valuable comments on the article manuscript as well as the editorial group of Harvard Kennedy School (HKS) Misinformation Review for their thoughtful feedback and input.
- Skip to Guides Search
- Skip to breadcrumb
- Skip to main content
- Skip to footer
- Skip to chat link
- Report accessibility issues and get help
- Go to Penn Libraries Home
- Go to Franklin catalog
Critical Writing Program Fall 2024 Critical Writing Seminar in PHIL: The Ethics of Artificial Intelligence: Researching the White Paper
- Getting started
- News and Opinion Sites
- Academic Sources
- Grey Literature
- Substantive News Sources
- What to Do When You Are Stuck
- Understanding a citation
- Examples of Quotation
- Examples of Paraphrase
- Chicago Manual of Style: Citing Images
- Researching the Op-Ed
- Researching Prospective Employers
- Resume Resources
- Cover Letter Resources
Research the White Paper
Researching the White Paper:
The process of researching and composing a white paper shares some similarities with the kind of research and writing one does for a high school or college research paper. What’s important for writers of white papers to grasp, however, is how much this genre differs from a research paper. First, the author of a white paper already recognizes that there is a problem to be solved, a decision to be made, and the job of the author is to provide readers with substantive information to help them make some kind of decision--which may include a decision to do more research because major gaps remain.
Thus, a white paper author would not “brainstorm” a topic. Instead, the white paper author would get busy figuring out how the problem is defined by those who are experiencing it as a problem. Typically that research begins in popular culture--social media, surveys, interviews, newspapers. Once the author has a handle on how the problem is being defined and experienced, its history and its impact, what people in the trenches believe might be the best or worst ways of addressing it, the author then will turn to academic scholarship as well as “grey” literature (more about that later). Unlike a school research paper, the author does not set out to argue for or against a particular position, and then devote the majority of effort to finding sources to support the selected position. Instead, the author sets out in good faith to do as much fact-finding as possible, and thus research is likely to present multiple, conflicting, and overlapping perspectives. When people research out of a genuine desire to understand and solve a problem, they listen to every source that may offer helpful information. They will thus have to do much more analysis, synthesis, and sorting of that information, which will often not fall neatly into a “pro” or “con” camp: Solution A may, for example, solve one part of the problem but exacerbate another part of the problem. Solution C may sound like what everyone wants, but what if it’s built on a set of data that have been criticized by another reliable source? And so it goes.
For example, if you are trying to write a white paper on the opioid crisis, you may focus on the value of providing free, sterilized needles--which do indeed reduce disease, and also provide an opportunity for the health care provider distributing them to offer addiction treatment to the user. However, the free needles are sometimes discarded on the ground, posing a danger to others; or they may be shared; or they may encourage more drug usage. All of those things can be true at once; a reader will want to know about all of these considerations in order to make an informed decision. That is the challenging job of the white paper author. The research you do for your white paper will require that you identify a specific problem, seek popular culture sources to help define the problem, its history, its significance and impact for people affected by it. You will then delve into academic and grey literature to learn about the way scholars and others with professional expertise answer these same questions. In this way, you will create creating a layered, complex portrait that provides readers with a substantive exploration useful for deliberating and decision-making. You will also likely need to find or create images, including tables, figures, illustrations or photographs, and you will document all of your sources.
Liaison Librarian
Connect to a Librarian Live Chat or "Ask a Question"
- Librarians staff live chat from 9-5 Monday through Friday . You can also text to chat: 215-543-7674
- You can submit a question 24 hours a day and we aim to respond within 24 hours
- You can click the "Schedule Appointment" button above in librarian's profile box (to the left), to schedule a consultation with her in person or by video conference.
- You can also make an appointment with a Librarian by subject specialization .
- Connect by email with a subject librarian
Find more easy contacts at our Quick Start Guide
- Next: Getting started >>
- Last Updated: Sep 13, 2024 8:59 AM
- URL: https://guides.library.upenn.edu/c.php?g=1423266
- Future Students
- Parents/Families
- Alumni/Friends
- Current Students
- Faculty/Staff
- MyOHIO Student Center
- Visit Athens Campus
- Regional Campuses
- OHIO Online
- Faculty/Staff Directory
- University Community
- Research & Impact
- Alumni & Friends
- Search All News
- OHIO Today Magazine
- Colleges & Campuses
- For the Media
Helpful Links
Navigate OHIO
Connect With Us
Call for papers for new publication focused on societal impact of AI

A call for papers has been issued for a new publication that aims to provide a comprehensive overview of the societal impact of artificial intelligence (AI).
Ohio University Assistant Professor of Instruction Dr. Tamanna M. Shah has announced the call for chapters for the “Handbook on the Sociology of Artificial Intelligence,” with Emerald Publishers. This volume will explore the societal causes and consequences of AI, including its role in reshaping labor markets, privacy norms, political processes and social interactions.
Project overview
AI’s growing influence demands a rigorous sociological examination. This handbook will offer a theory-driven review of contemporary research, investigating AI's transformation of society, culture, and human relations. Contributions are requested on these key themes:
- Part I: Foundations of Artificial Intelligence and Society
- Part II: The Ontology and Epistemology of Human Data
- Part III: Uncovering and Debunking AI Myths
- Part IV: Imagining Equitable AI Futures
- Part V: AI, Ethics, and Policy
- Part VI: AI in Practice
- Part VII: Teaching a Sociology of AI
- Part VIII: Future Directions and Innovations
Submission guidelines
- Abstracts (300 words) should include three key references.
- A 50-word biography (including affiliation, if applicable) must accompany your submission.
- Deadline for abstracts: Oct. 10, 2024
- Deadline for full chapters (8000 words): March 30, 2025
Please submit abstracts and expressions of interest to [email protected] .
For more information, please visit the call for chapters website or email [email protected] .
- Fact sheets
- Facts in pictures
- Publications
- Questions and answers
- Tools and toolkits
- Endometriosis
- Excessive heat
- Mental disorders
- Polycystic ovary syndrome
- All countries
- Eastern Mediterranean
- South-East Asia
- Western Pacific
- Data by country
- Country presence
- Country strengthening
- Country cooperation strategies
- News releases
- Feature stories
- Press conferences
- Commentaries
- Photo library
- Afghanistan
- Cholera
- Coronavirus disease (COVID-19)
- Greater Horn of Africa
- Israel and occupied Palestinian territory
- Disease Outbreak News
- Situation reports
- Weekly Epidemiological Record
- Surveillance
- Health emergency appeal
- International Health Regulations
- Independent Oversight and Advisory Committee
- Classifications
- Data collections
- Global Health Observatory
- Global Health Estimates
- Mortality Database
- Sustainable Development Goals
- Health Inequality Monitor
- Global Progress
- World Health Statistics
- Partnerships
- Committees and advisory groups
- Collaborating centres
- Technical teams
- Organizational structure
- Initiatives
- General Programme of Work
- WHO Academy
- Investment in WHO
- WHO Foundation
- External audit
- Financial statements
- Internal audit and investigations
- Programme Budget
- Results reports
- Governing bodies
- World Health Assembly
- Executive Board
- Member States Portal
Kicking off the journey of artificial intelligence in traditional medicine
WHO Global Traditional Medicine Centre (GTMC) and Digital Health and Digital and Innovation (DHI) organized a global technical meeting on artificial intelligence (AI) applications in traditional medicine on 11–12 September at the All India Institute of Ayurveda (AIIA) in New Delhi, India. Sixty participants from 15 countries across all six WHO regions participated in the hybrid meeting to exchange experiences and enhance understanding of how AI can be used to advance traditional medicine.
Following scene-setting comments from AIIA, the Indian Ministry of Ayush and WHO’s Regional Office for South-East Asia, plenary sessions focused on equitable access and benefit-sharing through intellectual property (IP) agreements related to AI; strengthening capacity building for traditional medicine stakeholders across a range of AI applications; and how AI can accelerate knowledge-sharing across multiple stakeholder groups, from consumers to policymakers.
Consultations were also conducted among meeting participants on the development of a new WHO global technical brief on AI and traditional medicine, to launch in October 2024, as well as the development of a WHO global library on traditional medicine.
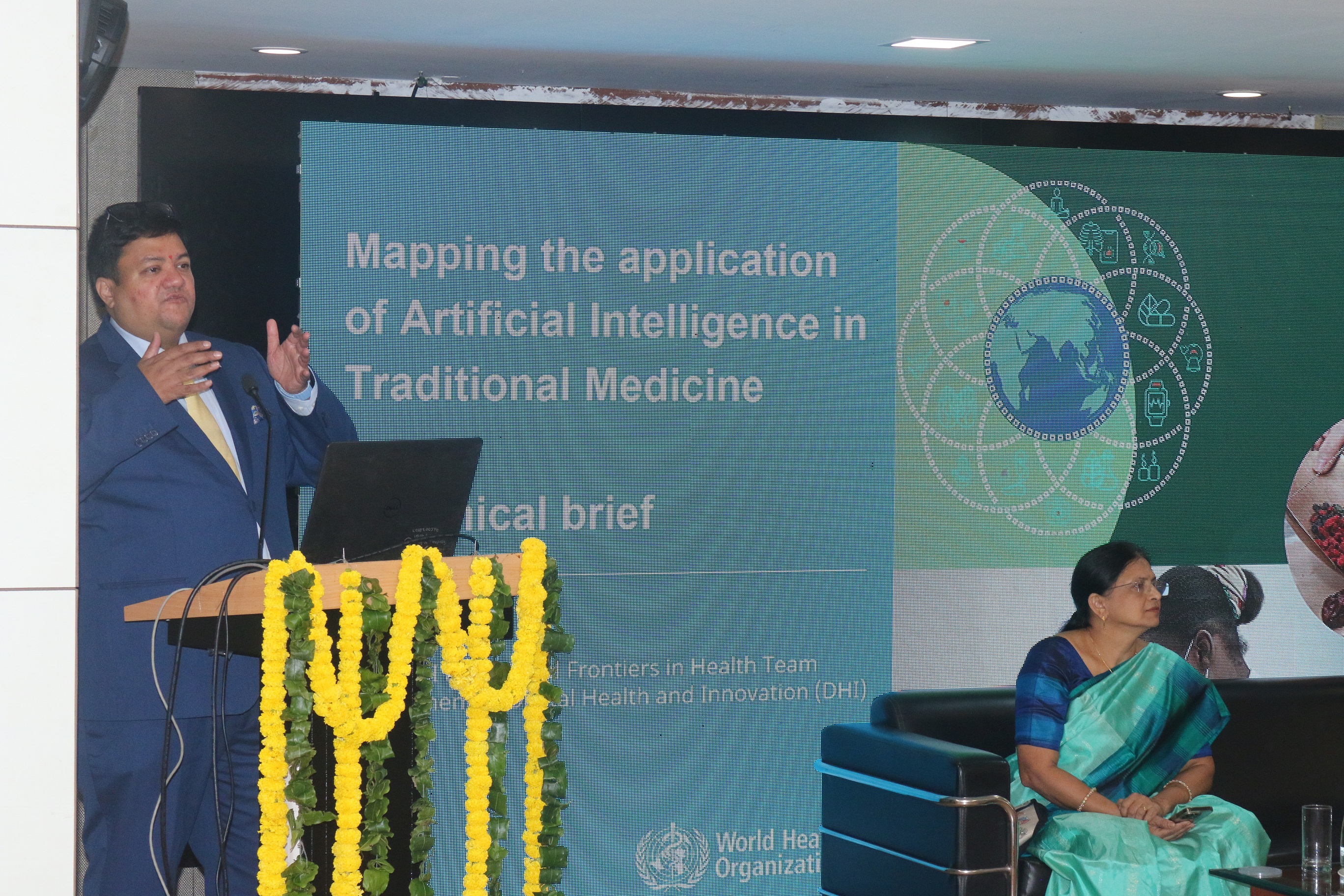
Sameer Pujari presenting AI technical brief in TM. Photo: AIIA
Capacity building in AI in relation to traditional medicine was also a core concern of the meeting, given the potential of AI to revolutionize healthcare outcomes. Since 2022, around 25 000 people from 178 countries have taken WHO’ online course on ethics and governance of AI for health . Meeting participants in Delhi discussed potential AI applications to support learning and understanding, as well as different types of AI learning opportunities required by different traditional medicine stakeholders. Meeting participants agreed on the need to develop a roadmap of learning requirements for AI in traditional medicine.
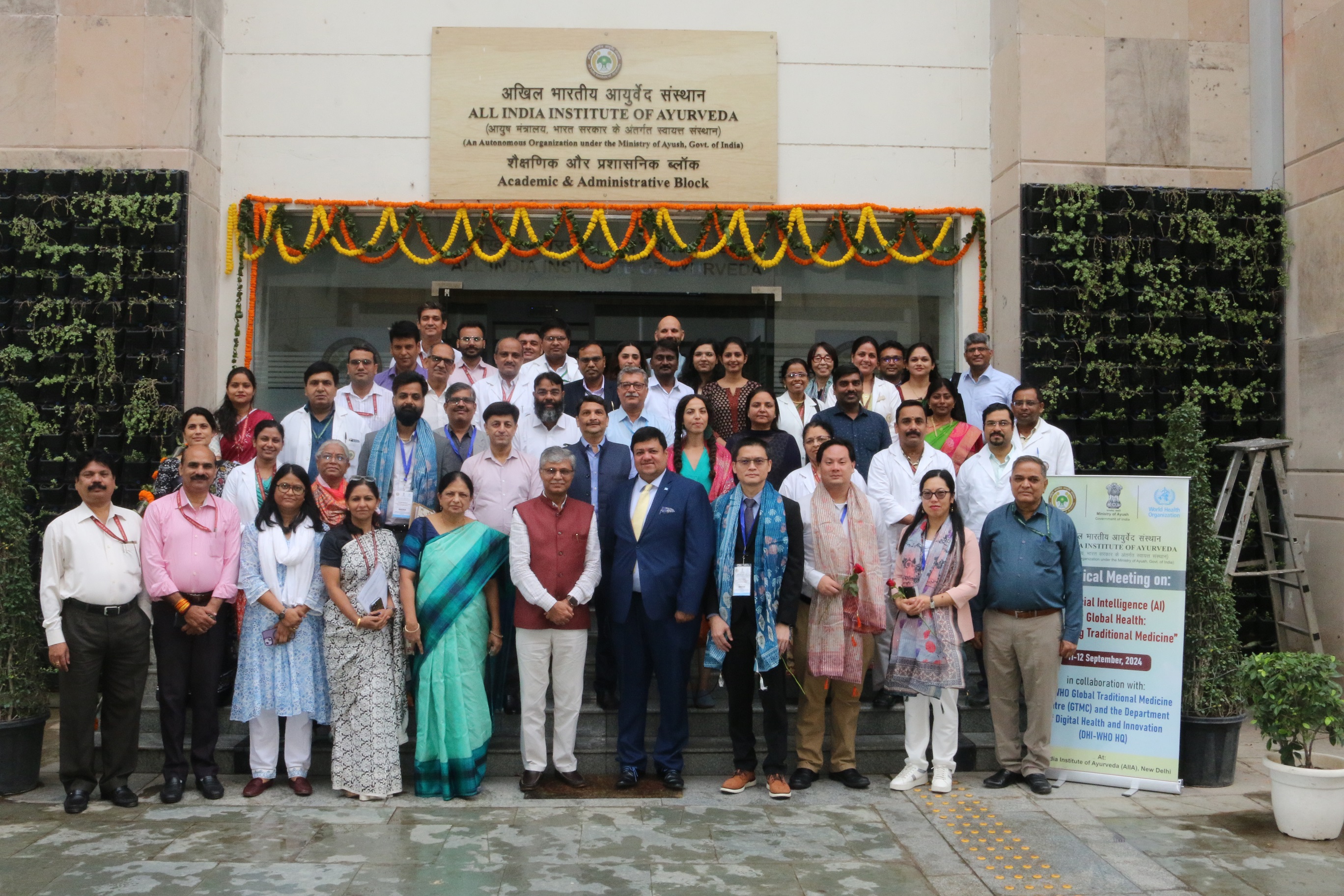
The participants with Secretary, Ministry of Ayush, India. Photo: AIIA
With respect to the development of the WHO Traditional Medicine Global Library, participants discussed how to synthesize evidence with AI, and what types of AI applications can support strengthening knowledge sharing through the library. The new WHO Traditional Medicine Global Library seeks to provide resources on traditional medicine, including Indigenous Knowledges, as widely as possible in a secure manner. Six regional portals and dedicated pages for 194 countries are planned as part of this global platform. The Library will undergo continuous testing during 2024–25 ahead of its public launch in conjunction with next WHO Global Summit on Traditional Medicine in November 2025.
The meeting concluded with agreement on developing specific workplans to take forward activities and initiatives discussed by participants.
“The global (AI) market size will be US$ 1.6 trillion by 2030,” said Karthik Adapa, Digital Health Advisor, WHO Regional Office for South-East Asia, pointing to the growing influence of AI on all aspects of health, sustainable development and equity goals, with respect for cultural and biodiversity heritage and rights.
WHO Global Traditional Medicine Centre
Traditional, Complementary, and Integrative Medicine Unit
Harnessing Artificial Intelligence for Health
Digital Health and Innovation
Development and Validation of Generative Artificial Intelligence Attitude Scale for Students
36 Pages Posted: 10 Sep 2024
Agostino Marengo
Università di Foggia
Fatma Gizem Karaoglan Yilmaz
Bartin University
Mehmet Ceylan
Kamal ahmed soomro.
Institute of Business Management (IoBM)

Generative artificial intelligence (AI) tools, notably exemplified by ChatGPT, have surged in prominence within educational landscapes, offering innovative avenues for learning. However, a crucial aspect that remains underexplored is the students’ attitude towards these technologies. This study aims to bridge this gap by creating a robust scale to assess university students' attitudes toward using generative AI tools like ChatGPT in educational settings. A three-stage process was employed to develop the scale, which included gathering data from 664 students across various faculties during the academic year 2022-2023. The scale underwent expert evaluations for face and content validity. Exploratory factor analysis (EFA) on 400 participants led to a two-factor, 14-item structure accounting for 78.440% of the variance in attitudes. Confirmatory factor analysis (CFA) on a separate sample of 264 students supported this structure but led to the elimination of one item, resulting in a 13-item scale. The scale exhibited high reliability with a Cronbach's alpha of .84 and test-retest reliability of .90. Discriminative power was assessed through corrected item-total correlations between lower and upper percentile groups, confirming the scale's efficacy in distinguishing attitudes toward generative AI in education. In sum, the Generative AI Attitude Scale is valid and reliable for measuring students' perspectives on the integration of generative AI tools in educational environments.
Keywords: generative artificial intelligence, ChatGPT, students' attitude, scale development, instrument development
Suggested Citation: Suggested Citation
Agostino Marengo (Contact Author)
Università di foggia ( email ).
FG 71121 Foggia Italy
Bartin University ( email )
Bartin Turkey
Institute of Business Management (IoBM) ( email )
Plot # 84 Korangi Creek Karachi, Sindh 75190 Pakistan 3332647994 (Phone) 75190 (Fax)
Do you have a job opening that you would like to promote on SSRN?
Paper statistics, related ejournals, human-computer interaction ejournal.
Subscribe to this fee journal for more curated articles on this topic

COMMENTS
Ethics of Artificial Intelligence and Robotics. First published Thu Apr 30, 2020. Artificial intelligence (AI) and robotics are digital technologies that will have significant impact on the development of humanity in the near future. They have raised fundamental questions about what we should do with these systems, what the systems themselves ...
Brian Patrick Green is the director of Technology Ethics at the Markkula Center for Applied Ethics. This article is an update of an earlier article [1]. Views are his own. Artificial intelligence and machine learning technologies are rapidly transforming society and will continue to do so in the coming decades.
An Overview of Artificial Intelligence Ethics. Impact Statement: AI ethics is an important emerging topic among academia, industry, government, society, and individuals. In the past decades, many efforts have been made to study the ethical issues in AI. This article offers a comprehensive overview of the AI ethics field, including a summary and ...
Ethics of Artificial Intelligence. This article provides a comprehensive overview of the main ethical issues related to the impact of Artificial Intelligence (AI) on human society. ... When Alan Turing introduced the so-called Turing test (which he called an 'imitation game') in his famous 1950 essay about whether machines can think, the ...
Abstract. This chapter introduces the themes covered by the book. It provides an overview of the concept of artificial intelligence (AI) and some of the technologies that have contributed to the current high level of visibility of AI. It explains why using case studies is a suitable approach to engage a broader audience with an interest in AI ...
Abstract. The book has two goals. The first goal is meta-theoretical and is fulfilled by Part One: an interpretation of the past (Chapter 1), the present (Chapter 2), and the future of AI (Chapter 3). Part One develops the thesis that AI is an unprecedented divorce between agency and intelligence.
In this context, the High-Level Expert Group on Artificial Intelligence Footnote 2 —which brings together AI experts, has been established to develop guidelines and recommendations on AI ethics ...
Featuring seventeen original essays on the ethics of artificial intelligence (AI) by today's most prominent AI scientists and academic philosophers, this volume represents state-of-the-art thinking in this fast-growing field. It highlights central themes in AI and morality such as how to build ethics into AI, how to address mass unemployment ...
The Business Council for Ethics of AI is a collaborative initiative between UNESCO and companies operating in Latin America that are involved in the development or use of artificial intelligence (AI) in various sectors. The Council serves as a platform for companies to come together, exchange experiences, and promote ethical practices within ...
Abstract. In the past five years, private companies, research institutions and public sector organizations have issued principles and guidelines for ethical artificial intelligence (AI). However ...
The ethics of artificial intelligence: Issues and initiatives . This study deals with the ethical implications and moral questions that arise from the development and implementation of artificial intelligence (AI) technologies. It also reviews the guidelines and frameworks which countries and regions around the world have created to address them.
2.1 Ethics in AI. This Special Issue addresses various ethical concerns related to the use of AI, such as psychological targeting, empathetic AI, cultural theories, fairness and discrimination, and accountability. Several papers face ethical concerns related to the development and deployment of artificial intelligence.
Artificial intelligence (AI) is the branch of computer science that deals with the simulation of intelligent behaviour in computers as regards their capacity to mimic, and ideally improve, human ...
Current advances in research, development and application of artificial intelligence (AI) systems have yielded a far-reaching discourse on AI ethics. In consequence, a number of ethics guidelines have been released in recent years. These guidelines comprise normative principles and recommendations aimed to harness the "disruptive" potentials of new AI technologies. Designed as a semi ...
Ethical concerns mount as AI takes bigger decision-making role in more industries. Second in a four-part series that taps the expertise of the Harvard community to examine the promise and potential pitfalls of the rising age of artificial intelligence and machine learning, and how to humanize them. For decades, artificial intelligence, or AI ...
Ethics in AI becomes a global topic of interest for both policymakers and academic researchers. In the last few years, various research organizations, lawyers, think tankers and regulatory bodies get involved in developing AI ethics guidelines and principles. However, there is still debate about the implications of these principles. We conducted a systematic literature review (SLR) study to ...
The essay concludes with thoughts on how the prospect of artificial general intelligence bears on this humanistic outlook. Ethics is, first and foremost, a domain of ordinary human thought, not a specialist academic discipline. It presupposes the existence of human choices that can be appraised by reference to a distinctive range of values.
Government officials and researchers are not the only ones worried that AI could perpetuate or worsen inequality. Research by Mindy Shoss, PhD, a professor of psychology at the University of Central Florida, shows that people in unequal societies are more likely to say AI adoption carries the threat of job loss (Technology, Mind, and Behavior, Vol. 3, No. 2, 2022).
Ethics of Artificial Intelligence." In Cambridge Handbook of Artificial Intelligence, edited. by Keith Frankish and William Ramsey. New York: Cambridge University Press. T. is version contains minor changes.1. Ethics in Machine Learning a. d Other Domain-Specific AI AlgorithmsImagine, in the near future, a bank using a machine learning ...
Immanuel Kant's ethical philosophy underscores three key principles: autonomy (the ability to make one's own decisions), rationality (using logic and reason to make choices), and. moral duty (following ethical obligations). Application to AI in Governance: The act of delegating decision-making processes to AI systems carries the risk of eroding ...
This study aimed to investigate artificial intelligence characteristics, clarify the legal responsibility resulting from the actions of artificial intelligence and to determine the penalty ...
Guiding & Building the Future of AI. Stanford HAI's mission is to advance AI research, education, policy and practice to improve the human condition. One main focus area is to consider the societal implications of these technologies. Below find recent research and discussions on the impact and ethics of artificial intelligence.
His paper "Computing Machinery and Intelligence" brought about debates about machine intelligence that would eventually lead to ethical considerations.1 The term "artificial intelligence" was first coined by John McCarthy at a conference in 1956.2,3 He has done outstanding research in the field of AI.2 With the advancement of computer ...
She has 12+ years of experience in IT leading various enterprise, agile, data, and cloud transformation initiatives. Her research interests include the business value of artificial intelligence (AI) systems, portfolio management and organizational agility, data analytics, and data-driven decision-making.
The ethics of artificial intelligence (AI) is an upcoming field of research that deals with the ethical assessment of emerging AI applications and addresses the new kinds of moral questions that the advent of AI raises. The argument presented in this article is that, even though there exist different approaches and subfields within the ethics of AI, the field resembles a critical theory. Just ...
ChatGPT and a new academic reality: Artificial Intelligence-written research papers and the ethics of the large language models in scholarly publishing. Journal of the Association for Information Science and Technology, 74 (5), 570-581.
Critical Writing Program Fall 2024 Critical Writing Seminar in PHIL: The Ethics of Artificial Intelligence: Researching the White Paper. Researching the White Paper Toggle Dropdown. Getting started ; ... What's important for writers of white papers to grasp, however, is how much this genre differs from a research paper.
A call for papers has been issued for a new publication that aims to provide a comprehensive overview of the societal impact of artificial intelligence (AI). Ohio University Assistant Professor of Instruction Dr. Tamanna M. Shah has announced the call for chapters for the "Handbook on the Sociology of Artificial Intelligence," with Emerald ...
WHO Global Traditional Medicine Centre (GTMC) and Digital Health and Digital Health and Innovation (DHI) (DHI) organized a global technical meeting on artificial intelligence (AI) applications in traditional medicine on 11-12 September at the All India Institute of Ayurveda (AIIA) in New Delhi, India. Sixty participants from 15 countries across all six WHO regions participated in the hybrid ...
Generative artificial intelligence (AI) tools, notably exemplified by ChatGPT, have surged in prominence within educational landscapes, offering innovative avenues for learning. However, a crucial aspect that remains underexplored is the students' attitude towards these technologies.