

Research Topics & Ideas
Artifical Intelligence (AI) and Machine Learning (ML)
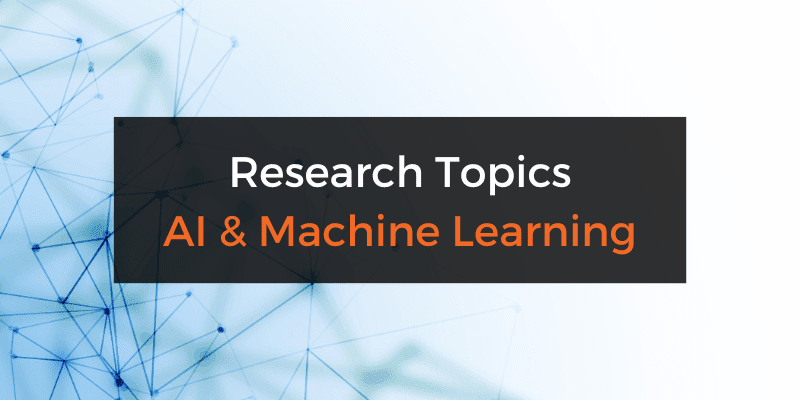
If you’re just starting out exploring AI-related research topics for your dissertation, thesis or research project, you’ve come to the right place. In this post, we’ll help kickstart your research topic ideation process by providing a hearty list of research topics and ideas , including examples from past studies.
PS – This is just the start…
We know it’s exciting to run through a list of research topics, but please keep in mind that this list is just a starting point . To develop a suitable research topic, you’ll need to identify a clear and convincing research gap , and a viable plan to fill that gap.
If this sounds foreign to you, check out our free research topic webinar that explores how to find and refine a high-quality research topic, from scratch. Alternatively, if you’d like hands-on help, consider our 1-on-1 coaching service .
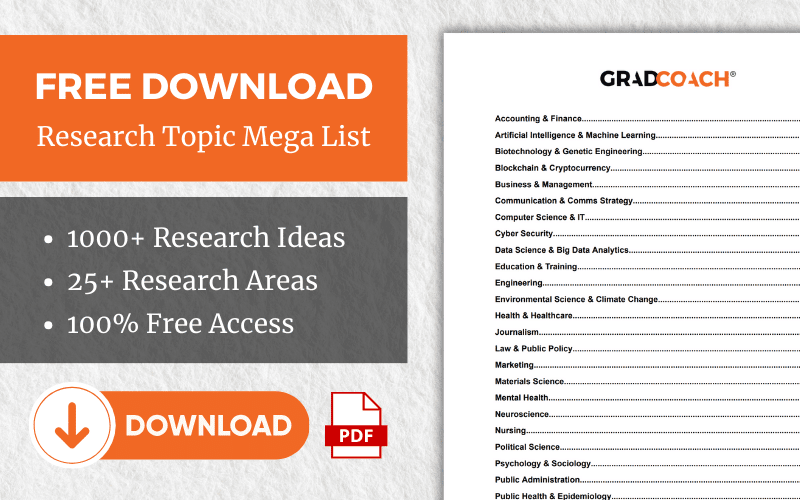
AI-Related Research Topics & Ideas
Below you’ll find a list of AI and machine learning-related research topics ideas. These are intentionally broad and generic , so keep in mind that you will need to refine them a little. Nevertheless, they should inspire some ideas for your project.
- Developing AI algorithms for early detection of chronic diseases using patient data.
- The use of deep learning in enhancing the accuracy of weather prediction models.
- Machine learning techniques for real-time language translation in social media platforms.
- AI-driven approaches to improve cybersecurity in financial transactions.
- The role of AI in optimizing supply chain logistics for e-commerce.
- Investigating the impact of machine learning in personalized education systems.
- The use of AI in predictive maintenance for industrial machinery.
- Developing ethical frameworks for AI decision-making in healthcare.
- The application of ML algorithms in autonomous vehicle navigation systems.
- AI in agricultural technology: Optimizing crop yield predictions.
- Machine learning techniques for enhancing image recognition in security systems.
- AI-powered chatbots: Improving customer service efficiency in retail.
- The impact of AI on enhancing energy efficiency in smart buildings.
- Deep learning in drug discovery and pharmaceutical research.
- The use of AI in detecting and combating online misinformation.
- Machine learning models for real-time traffic prediction and management.
- AI applications in facial recognition: Privacy and ethical considerations.
- The effectiveness of ML in financial market prediction and analysis.
- Developing AI tools for real-time monitoring of environmental pollution.
- Machine learning for automated content moderation on social platforms.
- The role of AI in enhancing the accuracy of medical diagnostics.
- AI in space exploration: Automated data analysis and interpretation.
- Machine learning techniques in identifying genetic markers for diseases.
- AI-driven personal finance management tools.
- The use of AI in developing adaptive learning technologies for disabled students.
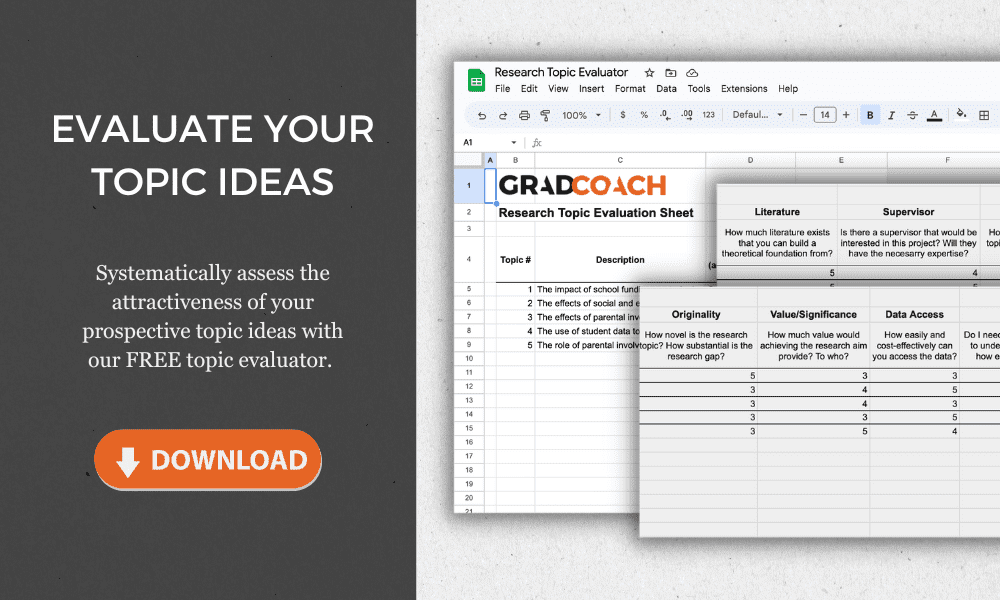
AI & ML Research Topic Ideas (Continued)
- Machine learning in cybersecurity threat detection and response.
- AI applications in virtual reality and augmented reality experiences.
- Developing ethical AI systems for recruitment and hiring processes.
- Machine learning for sentiment analysis in customer feedback.
- AI in sports analytics for performance enhancement and injury prevention.
- The role of AI in improving urban planning and smart city initiatives.
- Machine learning models for predicting consumer behaviour trends.
- AI and ML in artistic creation: Music, visual arts, and literature.
- The use of AI in automated drone navigation for delivery services.
- Developing AI algorithms for effective waste management and recycling.
- Machine learning in seismology for earthquake prediction.
- AI-powered tools for enhancing online privacy and data protection.
- The application of ML in enhancing speech recognition technologies.
- Investigating the role of AI in mental health assessment and therapy.
- Machine learning for optimization of renewable energy systems.
- AI in fashion: Predicting trends and personalizing customer experiences.
- The impact of AI on legal research and case analysis.
- Developing AI systems for real-time language interpretation for the deaf and hard of hearing.
- Machine learning in genomic data analysis for personalized medicine.
- AI-driven algorithms for credit scoring in microfinance.
- The use of AI in enhancing public safety and emergency response systems.
- Machine learning for improving water quality monitoring and management.
- AI applications in wildlife conservation and habitat monitoring.
- The role of AI in streamlining manufacturing processes.
- Investigating the use of AI in enhancing the accessibility of digital content for visually impaired users.
Recent AI & ML-Related Studies
While the ideas we’ve presented above are a decent starting point for finding a research topic in AI, they are fairly generic and non-specific. So, it helps to look at actual studies in the AI and machine learning space to see how this all comes together in practice.
Below, we’ve included a selection of AI-related studies to help refine your thinking. These are actual studies, so they can provide some useful insight as to what a research topic looks like in practice.
- An overview of artificial intelligence in diabetic retinopathy and other ocular diseases (Sheng et al., 2022)
- HOW DOES ARTIFICIAL INTELLIGENCE HELP ASTRONOMY? A REVIEW (Patel, 2022)
- Editorial: Artificial Intelligence in Bioinformatics and Drug Repurposing: Methods and Applications (Zheng et al., 2022)
- Review of Artificial Intelligence and Machine Learning Technologies: Classification, Restrictions, Opportunities, and Challenges (Mukhamediev et al., 2022)
- Will digitization, big data, and artificial intelligence – and deep learning–based algorithm govern the practice of medicine? (Goh, 2022)
- Flower Classifier Web App Using Ml & Flask Web Framework (Singh et al., 2022)
- Object-based Classification of Natural Scenes Using Machine Learning Methods (Jasim & Younis, 2023)
- Automated Training Data Construction using Measurements for High-Level Learning-Based FPGA Power Modeling (Richa et al., 2022)
- Artificial Intelligence (AI) and Internet of Medical Things (IoMT) Assisted Biomedical Systems for Intelligent Healthcare (Manickam et al., 2022)
- Critical Review of Air Quality Prediction using Machine Learning Techniques (Sharma et al., 2022)
- Artificial Intelligence: New Frontiers in Real–Time Inverse Scattering and Electromagnetic Imaging (Salucci et al., 2022)
- Machine learning alternative to systems biology should not solely depend on data (Yeo & Selvarajoo, 2022)
- Measurement-While-Drilling Based Estimation of Dynamic Penetrometer Values Using Decision Trees and Random Forests (García et al., 2022).
- Artificial Intelligence in the Diagnosis of Oral Diseases: Applications and Pitfalls (Patil et al., 2022).
- Automated Machine Learning on High Dimensional Big Data for Prediction Tasks (Jayanthi & Devi, 2022)
- Breakdown of Machine Learning Algorithms (Meena & Sehrawat, 2022)
- Technology-Enabled, Evidence-Driven, and Patient-Centered: The Way Forward for Regulating Software as a Medical Device (Carolan et al., 2021)
- Machine Learning in Tourism (Rugge, 2022)
- Towards a training data model for artificial intelligence in earth observation (Yue et al., 2022)
- Classification of Music Generality using ANN, CNN and RNN-LSTM (Tripathy & Patel, 2022)
As you can see, these research topics are a lot more focused than the generic topic ideas we presented earlier. So, in order for you to develop a high-quality research topic, you’ll need to get specific and laser-focused on a specific context with specific variables of interest. In the video below, we explore some other important things you’ll need to consider when crafting your research topic.
Get 1-On-1 Help
If you’re still unsure about how to find a quality research topic, check out our Research Topic Kickstarter service, which is the perfect starting point for developing a unique, well-justified research topic.
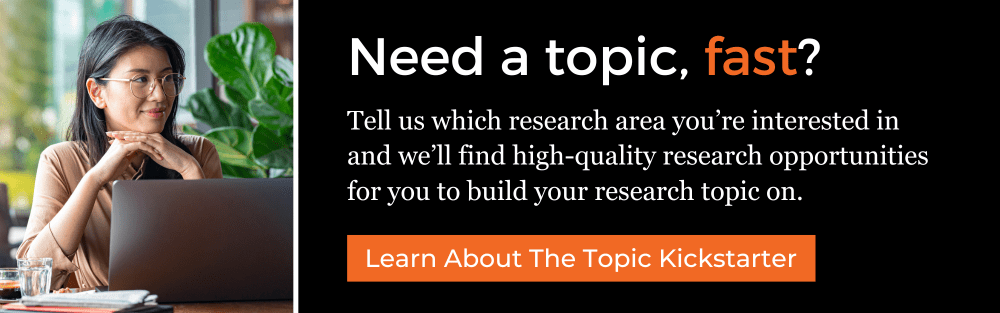
You Might Also Like:
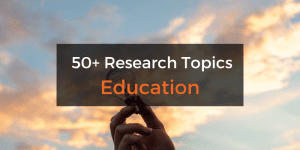
can one come up with their own tppic and get a search
can one come up with their own title and get a search
Submit a Comment Cancel reply
Your email address will not be published. Required fields are marked *
Save my name, email, and website in this browser for the next time I comment.
- Print Friendly
- Internal wiki
PhD Programme in Advanced Machine Learning
The Cambridge Machine Learning Group (MLG) runs a PhD programme in Advanced Machine Learning. The supervisors are Jose Miguel Hernandez-Lobato , Carl Rasmussen , Richard E. Turner , Adrian Weller , Hong Ge and David Krueger . Zoubin Ghahramani is currently on academic leave and not accepting new students at this time.
We encourage applications from outstanding candidates with academic backgrounds in Mathematics, Physics, Computer Science, Engineering and related fields, and a keen interest in doing basic research in machine learning and its scientific applications. There are no additional restrictions on the topic of the PhD, but for further information on our current research areas, please consult our webpages at http://mlg.eng.cam.ac.uk .
The typical duration of the PhD will be four years.
Applicants must formally apply through the Applicant Portal at the University of Cambridge by the deadline, indicating “PhD in Engineering” as the course (supervisor Hernandez-Lobato, Rasmussen, Turner, Weller, Ge and/or Krueger). Applicants who want to apply for University funding need to reply ‘Yes’ to the question ‘Apply for Cambridge Scholarships’. See http://www.admin.cam.ac.uk/students/gradadmissions/prospec/apply/deadlines.html for details. Note that applications will not be complete until all the required material has been uploaded (including reference letters), and we will not be able to see any applications until that happens.
Gates funding applicants (US or other overseas) need to fill out the dedicated Gates Cambridge Scholarships section later on the form which is sent on to the administrators of Gates funding.
Deadline for PhD Application: noon 5 December, 2023
Applications from outstanding individuals may be considered after this time, but applying later may adversely impact your chances for both admission and funding.
FURTHER INFORMATION ABOUT COMPLETING THE ADMISSIONS FORMS:
The Machine Learning Group is based in the Department of Engineering, not Computer Science.
We will assess your application on three criteria:
1 Academic performance (ensure evidence for strong academic achievement, e.g. position in year, awards, etc.) 2 references (clearly your references will need to be strong; they should also mention evidence of excellence as quotes will be drawn from them) 3 research (detail your research experience, especially that which relates to machine learning)
You will also need to put together a research proposal. We do not offer individual support for this. It is part of the application assessment, i.e. ascertaining whether you can write about a research area in a sensible way and pose interesting questions. It is not a commitment to what you will work on during your PhD. Most often PhD topics crystallise over the first year. The research proposal should be about 2 pages long and can be attached to your application (you can indicate that your proposal is attached in the 1500 character count Research Summary box). This aspect of the application does not carry a huge amount of weight so do not spend a large amount of time on it. Please also attach a recent CV to your application too.
INFORMATION ABOUT THE CAMBRIDGE-TUEBINGEN PROGRAMME:
We also offer a small number of PhDs on the Cambridge-Tuebingen programme. This stream is for specific candidates whose research interests are well-matched to both the machine learning group in Cambridge and the MPI for Intelligent Systems in Tuebingen. For more information about the Cambridge-Tuebingen programme and how to apply see here . IMPORTANT: remember to download your application form before you submit so that you can send a copy to the administrators in Tuebingen directly . Note that the application deadline for the Cambridge-Tuebingen programme is noon, 5th December, 2023, CET.
What background do I need?
An ideal background is a top undergraduate or Masters degree in Mathematics, Physics, Computer Science, or Electrical Engineering. You should be both very strong mathematically and have an intuitive and practical grasp of computation. Successful applicants often have research experience in statistical machine learning. Shortlisted applicants are interviewed.
Do you have funding?
There are a number of funding sources at Cambridge University for PhD students, including for international students. All our students receive partial or full funding for the full three years of the PhD. We do not give preference to “self-funded” students. To be eligible for funding it is important to apply early (see https://www.graduate.study.cam.ac.uk/finance/funding – current deadlines are 10 October for US students, and 1 December for others). Also make sure you tick the box on the application saying you wish to be considered for funding!
If you are applying to the Cambridge-Tuebingen programme, note that this source of funding will not be listed as one of the official funding sources, but if you apply to this programme, please tick the other possible sources of funding if you want to maximise your chances of getting funding from Cambridge.
What is my likelihood of being admitted?
Because we receive so many applications, unfortunately we can’t admit many excellent candidates, even some who have funding. Successful applicants tend to be among the very top students at their institution, have very strong mathematics backgrounds, and references, and have some research experience in statistical machine learning.
Do I have to contact one of the faculty members first or can I apply formally directly?
It is not necessary, but if you have doubts about whether your background is suitable for the programme, or if you have questions about the group, you are welcome to contact one of the faculty members directly. Due to their high email volume you may not receive an immediate response but they will endeavour to get back to you as quickly as possible. It is important to make your official application to Graduate Admissions at Cambridge before the funding deadlines, even if you don’t hear back from us; otherwise we may not be able to consider you.
Do you take Masters students, or part-time PhD students?
We generally don’t admit students for a part-time PhD. We also don’t usually admit students just for a pure-research Masters in machine learning , except for specific programs such as the Churchill and Marshall scholarships. However, please do note that we run a one-year taught Master’s Programme: The MPhil in Machine Learning, and Machine Intelligence . You are welcome to apply directly to this.
What Department / course should I indicate on my application form?
This machine learning group is in the Department of Engineering. The degree you would be applying for is a PhD in Engineering (not Computer Science or Statistics).
How long does a PhD take?
A typical PhD from our group takes 3-4 years. The first year requires students to pass some courses and submit a first-year research report. Students must submit their PhD before the 4th year.
What research topics do you have projects on?
We don’t generally pre-specify projects for students. We prefer to find a research area that suits the student. For a sample of our research, you can check group members’ personal pages or our research publications page.
What are the career prospects for PhD students from your group?
Students and postdocs from the group have moved on to excellent positions both in academia and industry. Have a look at our list of recent alumni on the Machine Learning group webpage . Research expertise in machine learning is in very high demand these days.
Machine Learning - CMU
Phd in machine learning.
Core Requirements The curriculum for the Machine Learning Ph.D. is built on a foundation of six core courses and one elective . A typical full-time, PhD student course load during the first two years consists each term of two classes (at 12 graduate units per class) plus 24 units of research. It is expected that all Ph.D. students engage in active research from their first semester. Roughly half of a student's time should be allocated to research and half to courses until the courses are completed.
Required Core courses:
- 10-715 Advanced Introduction to Machine Learning
- 10-716 Advanced Machine Learning: Theory & Methods
- 36-705 Intermediate Statistics
- 10-718* Machine Learning in Practice *Students who are in the joint PhD program in ML & Statistics may satisfy this requirement through the ADA project in Statistics. Students in the joint ML-CNBC program may satisfy it by completing a data intensive project for their second year milestone. Students in the joint ML-Heinz joint PhD also complete it in their program.
Plus any 2 of the following Menu* of Core courses:
- 10-703 Deep Reinforcement Learning or 10-707 Topics in Deep Learning
- 10-708 Probabilistic Graphical Models
- 10-725 Convex Optimization
- 10-805 Machine Learning with Large Datasets
- 15-750 Algorithms in the Real World or 15-850 Advanced Algorithms
- 15-780 Graduate Artificial Intelligence
- 36-707 Regression Analysis
- 36-709 Advanced Statistical Theory I
- 36-710 Advanced Statistical Theory II
* Students in the Statistics & ML joint program must choose two of the Menu of Core courses with a prefix in a department that is not their home department. Thus, Statistics joint students should choose two 10- and 15- prefix courses, and Machine Learning joint students should choose two 36- and 15- courses. Students accepted to the Statistics & ML joint program before Spring 2021 are grandfathered and follow the previous rules.
Plus 1 elective:
- An additional course from the Menu Core list above
- Any course at the 700 or higher level in SCS or Statistics (36-xxx)
- Other 700 or higher level courses by approval
Note: Some students will have taken some of the above courses before entering the MLD PhD program: for example, as MS students at CMU. If students have previously taken the above-named courses at Carnegie Mellon before joining the MLD PhD, those may be used to satisfy the requirements and do not need to be repeated. (Note that courses can only be used for a single Master's degree.)
Some students will have taken similar courses at other universities before entering the MLD PhD program. Based on such equivalent coursework, any student can apply to replace (not reduce) up to two courses with either menu cores or electives. All requests must be supported by the advisor, and will be evaluated by the PhD Director.
Typical schedule of Courses & Milestones
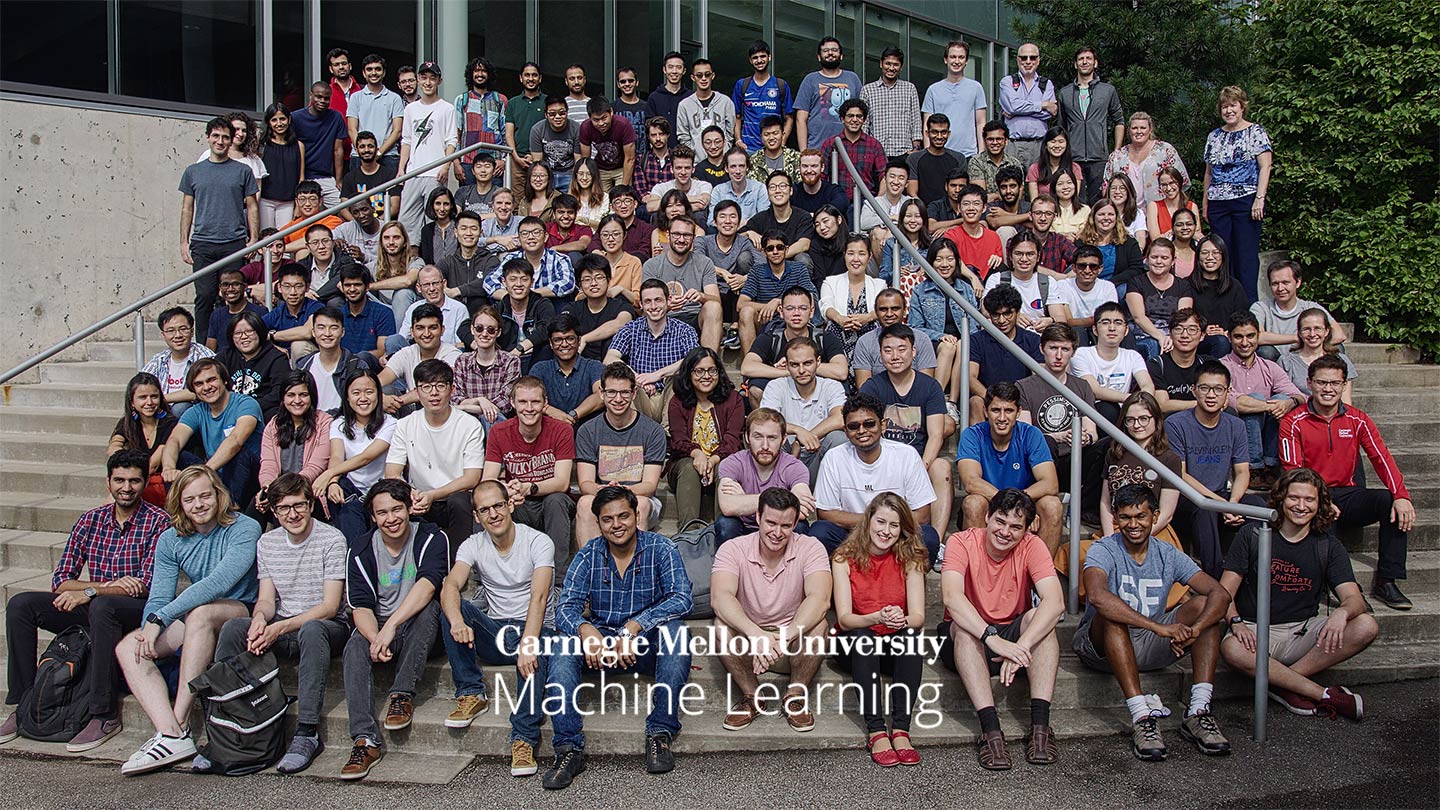
- Who’s Teaching What
- Subject Updates
- MEng program
- Opportunities
- Minor in Computer Science
- Resources for Current Students
- Program objectives and accreditation
- Graduate program requirements
- Admission process
- Degree programs
- Graduate research
- EECS Graduate Funding
- Resources for current students
- Student profiles
- Instructors
- DEI data and documents
- Recruitment and outreach
- Community and resources
- Get involved / self-education
- Rising Stars in EECS
- Graduate Application Assistance Program (GAAP)
- MIT Summer Research Program (MSRP)
- Sloan-MIT University Center for Exemplary Mentoring (UCEM)
- Electrical Engineering
- Computer Science
- Artificial Intelligence + Decision-making
- AI and Society
- AI for Healthcare and Life Sciences
- Artificial Intelligence and Machine Learning
- Biological and Medical Devices and Systems
- Communications Systems
- Computational Biology
- Computational Fabrication and Manufacturing
- Computer Architecture
- Educational Technology
- Electronic, Magnetic, Optical and Quantum Materials and Devices
- Graphics and Vision
- Human-Computer Interaction
- Information Science and Systems
- Integrated Circuits and Systems
- Nanoscale Materials, Devices, and Systems
- Natural Language and Speech Processing
- Optics + Photonics
- Optimization and Game Theory
- Programming Languages and Software Engineering
- Quantum Computing, Communication, and Sensing
- Security and Cryptography
- Signal Processing
- Systems and Networking
- Systems Theory, Control, and Autonomy
- Theory of Computation
- Departmental History
- Departmental Organization
- Visiting Committee
- Explore all research areas
Our research covers a wide range of topics of this fast-evolving field, advancing how machines learn, predict, and control, while also making them secure, robust and trustworthy. Research covers both the theory and applications of ML. This broad area studies ML theory (algorithms, optimization, etc.); statistical learning (inference, graphical models, causal analysis, etc.); deep learning; reinforcement learning; symbolic reasoning ML systems; as well as diverse hardware implementations of ML.
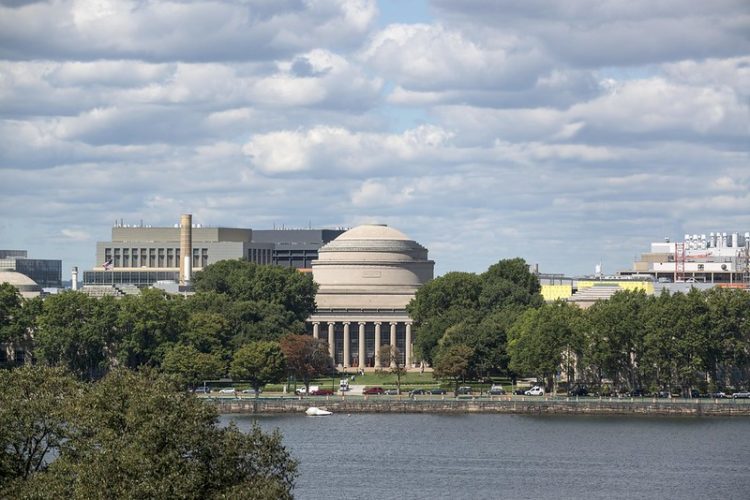
Latest news in artificial intelligence and machine learning
Creating bespoke programming languages for efficient visual ai systems.
Associate Professor Jonathan Ragan-Kelley optimizes how computer graphics and images are processed for the hardware of today and tomorrow.
QS World University Rankings rates MIT No. 1 in 11 subjects for 2024
The Institute also ranks second in five subject areas.
Three from MIT awarded 2024 Guggenheim Fellowships
MIT professors Roger Levy, Tracy Slatyer, and Martin Wainwright appointed to the 2024 class of “trail-blazing fellows.”
To build a better AI helper, start by modeling the irrational behavior of humans
A new technique can be used to predict the actions of human or AI agents who behave suboptimally while working toward unknown goals.
Priya Donti named AI2050 Early Career Fellow
Assistant Professor Priya Donti has been named an AI2050 Early Career Fellow by Schmidt Sciences, a philanthropic initiative from Eric and Wendy Schmidt aimed at helping to solve hard problems in AI.
Upcoming events
Doctoral thesis: guiding deep probabilistic models.
Doctor of Philosophy with a major in Machine Learning
The Doctor of Philosophy with a major in Machine Learning program has the following principal objectives, each of which supports an aspect of the Institute’s mission:
- Create students that are able to advance the state of knowledge and practice in machine learning through innovative research contributions.
- Create students who are able to integrate and apply principles from computing, statistics, optimization, engineering, mathematics and science to innovate, and create machine learning models and apply them to solve important real-world data intensive problems.
- Create students who are able to participate in multidisciplinary teams that include individuals whose primary background is in statistics, optimization, engineering, mathematics and science.
- Provide a high quality education that prepares individuals for careers in industry, government (e.g., national laboratories), and academia, both in terms of knowledge, computational (e.g., software development) skills, and mathematical modeling skills.
- Foster multidisciplinary collaboration among researchers and educators in areas such as computer science, statistics, optimization, engineering, social science, and computational biology.
- Foster economic development in the state of Georgia.
- Advance Georgia Tech’s position of academic leadership by attracting high quality students who would not otherwise apply to Tech for graduate study.
All PhD programs must incorporate a standard set of Requirements for the Doctoral Degree .
The central goal of the PhD program is to train students to perform original, independent research. The most important part of the curriculum is the successful defense of a PhD Dissertation, which demonstrates this research ability. The academic requirements are designed in service of this goal.
The curriculum for the PhD in Machine Learning is truly multidisciplinary, containing courses taught in nine schools across three colleges at Georgia Tech: the Schools of Computational Science and Engineering, Computer Science, and Interactive Computing in the College of Computing; the Schools of Aerospace Engineering, Chemical and Biomolecular Engineering, Industrial and Systems Engineering, Electrical and Computer Engineering, and Biomedical Engineering in the College of Engineering; and the School of Mathematics in the College of Science.
Summary of General Requirements for a PhD in Machine Learning
- Core curriculum (4 courses, 12 hours). Machine Learning PhD students will be required to complete courses in four different areas: Mathematical Foundations, Probabilistic and Statistical Methods in Machine Learning, ML Theory and Methods, and Optimization.
- Area electives (5 courses, 15 hours).
- Responsible Conduct of Research (RCR) (1 course, 1 hour, pass/fail). Georgia Tech requires that all PhD students complete an RCR requirement that consists of an online component and in-person training. The online component is completed during the student’s first semester enrolled at Georgia Tech. The in-person training is satisfied by taking PHIL 6000 or their associated academic program’s in-house RCR course.
- Qualifying examination (1 course, 3 hours). This consists of a one-semester independent literature review followed by an oral examination.
- Doctoral minor (2 courses, 6 hours).
- Research Proposal. The purpose of the proposal is to give the faculty an opportunity to give feedback on the student’s research direction, and to make sure they are developing into able communicators.
- PhD Dissertation.
Almost all of the courses in both the core and elective categories are already taught regularly at Georgia Tech. However, two core courses (designated in the next section) are being developed specifically for this program. The proposed outlines for these courses can be found in the Appendix. Students who complete these required courses as part of a master’s program will not need to repeat the courses if they are admitted to the ML PhD program.
Core Courses
Machine Learning PhD students will be required to complete courses in four different areas. With the exception of the Foundations course, each of these area requirements can be satisfied using existing courses from the College of Computing or Schools of ECE, ISyE, and Mathematics.
Machine Learning core:
Mathematical Foundations of Machine Learning. This required course is the gateway into the program, and covers the key subjects from applied mathematics needed for a rigorous graduate program in ML. Particular emphasis will be put on advanced concepts in linear algebra and probabilistic modeling. This course is cross-listed between CS, CSE, ECE, and ISyE.
ECE 7750 / ISYE 7750 / CS 7750 / CSE 7750 Mathematical Foundations of Machine Learning
Probabilistic and Statistical Methods in Machine Learning
- ISYE 6412 , Theoretical Statistics
- ECE 7751 / ISYE 7751 / CS 7751 / CSE 7751 Probabilistic Graphical Models
- MATH 7251 High Dimension Probability
- MATH 7252 High Dimension Statistics
Machine Learning: Theory and Methods. This course serves as an introduction to the foundational problems, algorithms, and modeling techniques in machine learning. Each of the courses listed below treats roughly the same material using a mix of applied mathematics and computer science, and each has a different balance between the two.
- CS 7545 Machine Learning Theory and Methods
- CS 7616 , Pattern Recognition
- CSE 6740 / ISYE 6740 , Computational Data Analysis
- ECE 6254 , Statistical Machine Learning
- ECE 6273 , Methods of Pattern Recognition with Applications to Voice
Optimization. Optimization plays a crucial role in both developing new machine learning algorithms and analyzing their performance. The three courses below all provide a rigorous introduction to this topic; each emphasizes different material and provides a unique balance of mathematics and algorithms.
- ECE 8823 , Convex Optimization: Theory, Algorithms, and Applications
- ISYE 6661 , Linear Optimization
- ISYE 6663 , Nonlinear Optimization
- ISYE 7683 , Advanced Nonlinear Programming
After core requirements are satisfied, all courses listed in the core not already taken can be used as (appropriately classified) electives.
In addition to meeting the core area requirements, each student is required to complete five elective courses. These courses are required for getting a complete breadth in ML. These courses must be chosen from at least two of the five subject areas listed below. In addition, students can use up to six special problems research hours to satisfy this requirement.
i. Statistics and Applied Probability : To build breadth and depth in the areas of statistics and probability as applied to ML.
- AE 6505 , Kalman Filtering
- AE 8803 Gaussian Processes
- BMED 6700 , Biostatistics
- ECE 6558 , Stochastic Systems
- ECE 6601 , Random Processes
- ECE 6605 , Information Theory
- ISYE 6402 , Time Series Analysis
- ISYE 6404 , Nonparametric Data Analysis
- ISYE 6413 , Design and Analysis of Experiments
- ISYE 6414 , Regression Analysis
- ISYE 6416 , Computational Statistics
- ISYE 6420 , Bayesian Statistics
- ISYE 6761 , Stochastic Processes I
- ISYE 6762 , Stochastic Processes II
- ISYE 7400 , Adv Design-Experiments
- ISYE 7401 , Adv Statistical Modeling
- ISYE 7405 , Multivariate Data Analysis
- ISYE 8803 , Statistical and Probabilistic Methods for Data Science
- ISYE 8813 , Special Topics in Data Science
- MATH 6221 , Probability Theory for Scientists and Engineers
- MATH 6266 , Statistical Linear Modeling
- MATH 6267 , Multivariate Statistical Analysis
- MATH 7244 , Stochastic Processes and Stochastic Calculus I
- MATH 7245 , Stochastic Processes and Stochastic Calculus II
ii. Advanced Theory: To build a deeper understanding of foundations of ML.
- AE 8803 , Optimal Transport Theory and Applications
- CS 7280 , Network Science
- CS 7510 , Graph Algorithms
- CS 7520 , Approximation Algorithms
- CS 7530 , Randomized Algorithms
- CS 7535 , Markov Chain Monte Carlo Algorithms
- CS 7540 , Spectral Algorithms
- CS 8803 , Continuous Algorithms
- ECE 6283 , Harmonic Analysis and Signal Processing
- ECE 6555 , Linear Estimation
- ISYE 7682 , Convexity
- MATH 6112 , Advanced Linear Algebra
- MATH 6241 , Probability I
- MATH 6262 , Advanced Statistical Inference
- MATH 6263 , Testing Statistical Hypotheses
- MATH 6580 , Introduction to Hilbert Space
- MATH 7338 , Functional Analysis
- MATH 7586 , Tensor Analysis
- MATH 88XX, Special Topics: High Dimensional Probability and Statistics
iii. Applications: To develop a breadth and depth in variety of applications domains impacted by/with ML.
- AE 6373 , Advanced Design Methods
- AE 8803 , Machine Learning for Control Systems
- AE 8803 , Nonlinear Stochastic Optimal Control
- BMED 6780 , Medical Image Processing
- BMED 6790 / ECE 6790 , Information Processing Models in Neural Systems
- BMED 7610 , Quantitative Neuroscience
- BMED 8813 BHI, Biomedical and Health Informatics
- BMED 8813 MHI, mHealth Informatics
- BMED 8813 MLB, Machine Learning in Biomedicine
- BMED 8823 ALG, OMICS Data and Bioinformatics Algorithms
- CHBE 6745 , Data Analytics for Chemical Engineers
- CHBE 6746 , Data-Driven Process Engineering
- CS 6440 , Introduction to Health Informatics
- CS 6465 , Computational Journalism
- CS 6471 , Computational Social Science
- CS 6474 , Social Computing
- CS 6475 , Computational Photography
- CS 6476 , Computer Vision
- CS 6601 , Artificial Intelligence
- CS 7450 , Information Visualization
- CS 7476 , Advanced Computer Vision
- CS 7630 , Autonomous Robots
- CS 7632 , Game AI
- CS 7636 , Computational Perception
- CS 7643 , Deep Learning
- CS 7646 , Machine Learning for Trading
- CS 7647 , Machine Learning with Limited Supervision
- CS 7650 , Natural Language Processing
- CSE 6141 , Massive Graph Analysis
- CSE 6240 , Web Search and Text Mining
- CSE 6242 , Data and Visual Analytics
- CSE 6301 , Algorithms in Bioinformatics and Computational Biology
- ECE 4580 , Computational Computer Vision
- ECE 6255 , Digital Processing of Speech Signals
- ECE 6258 , Digital Image Processing
- ECE 6260 , Data Compression and Modeling
- ECE 6273 , Methods of Pattern Recognition with Application to Voice
- ECE 6550 , Linear Systems and Controls
- ECE 8813 , Network Security
- ISYE 6421 , Biostatistics
- ISYE 6810 , Systems Monitoring and Prognosis
- ISYE 7201 , Production Systems
- ISYE 7204 , Info Prod & Ser Sys
- ISYE 7203 , Logistics Systems
- ISYE 8813 , Supply Chain Inventory Theory
- HS 6000 , Healthcare Delivery
- MATH 6759 , Stochastic Processes in Finance
- MATH 6783 , Financial Data Analysis
iv. Computing and Optimization: To provide more breadth and foundation in areas of math, optimization and computation for ML.
- AE 6513 , Mathematical Planning and Decision-Making for Autonomy
- AE 8803 , Optimization-Based Learning Control and Games
- CS 6515 , Introduction to Graduate Algorithms
- CS 6550 , Design and Analysis of Algorithms
- CSE 6140 , Computational Science and Engineering Algorithms
- CSE 6643 , Numerical Linear Algebra
- CSE 6644 , Iterative Methods for Systems of Equations
- CSE 6710 , Numerical Methods I
- CSE 6711 , Numerical Methods II
- ECE 6553 , Optimal Control and Optimization
- ISYE 6644 , Simulation
- ISYE 6645 , Monte Carlo Methods
- ISYE 6662 , Discrete Optimization
- ISYE 6664 , Stochastic Optimization
- ISYE 6679 , Computational methods for optimization
- ISYE 7686 , Advanced Combinatorial Optimization
- ISYE 7687 , Advanced Integer Programming
v. Platforms : To provide breadth and depth in computing platforms that support ML and Computation.
- CS 6421 , Temporal, Spatial, and Active Databases
- CS 6430 , Parallel and Distributed Databases
- CS 6290 , High-Performance Computer Architecture
- CSE 6220 , High Performance Computing
- CSE 6230 , High Performance Parallel Computing
Qualifying Examination
The purpose of the Qualifying Examination is to judge the candidate’s potential as an independent researcher.
The Ph.D. qualifying exam consists of a focused literature review that will take place over the course of one semester. At the beginning of the second semester of their second year, a qualifying committee consisting of three members of the ML faculty will assign, in consultation with the student and the student’s advisor, a course of study consisting of influential papers, books, or other intellectual artifacts relevant to the student’s research interests. The student’s focus area and current research efforts (and related portfolio) will be considered in defining the course of study.
At the end of the semester, the student will submit a written summary of each artifact which highlights their understanding of the importance (and weaknesses) of the work in question and the relationship of this work to their current research. Subsequently, the student will have a closed oral exam with the three members of the committee. The exam will be interactive, with the student and the committee discussing and criticizing each work and posing questions related the students current research to determine the breadth of student’s knowledge in that specific area.
The success of the examination will be determined by the committee’s qualitative assessment of the student’s understanding of the theory, methods, and ultimate impact of the assigned syllabus.
The student will be given a passing grade for meeting the requirements of the committee in both the written and the oral part. Unsatisfactory performance on either part will require the student to redo the entire qualifying exam in the following semester year. Each student will be allowed only two attempts at the exam.
Students are expected to perform the review by the end of their second year in the program.
Doctoral Dissertation
The primary requirement of the PhD student is to do original and substantial research. This research is reported for review in the PhD dissertation, and presented at the final defense. As the first step towards completing a dissertation, the student must prepare and defend a Research Proposal. The proposal is a document of no more than 20 pages in length that carefully describes the topic of the dissertation, including references to prior work, and any preliminary results to date. The written proposal is submitted to a committee of three faculty members from the ML PhD program, and is presented in a public seminar shortly thereafter. The committee members provide feedback on the proposed research directions, comments on the strength of writing and oral presentation skills, and might suggest further courses to solidify the student’s background. Approval of the Research Proposal by the committee is required at least six months prior to the scheduling of the PhD defense. It is expected that the student complete this proposal requirement no later than their fourth year in the program. The PhD thesis committee consists of five faculty members: the student’s advisor, three additional members from the ML PhD program, and one faculty member external to the ML program. The committee is charged with approving the written dissertation and administering the final defense. The defense consists of a public seminar followed by oral examination from the thesis committee.
Doctoral minor (2 courses, 6 hours):
The minor follows the standard Georgia Tech requirement: 6 hours, preferably outside the student’s home unit, with a GPA in those graduate-level courses of at least 3.0. The courses for the minor should form a cohesive program of study outside the area of Machine Learning; no ML core or elective courses may be used to fulfill this requirement and must be approved by your thesis advisor and ML Academic Advisor. Typical programs will consist of three courses two courses from the same school (any school at the Institute) or two courses from the same area of study.
This site uses cookies. Review the Privacy & Legal Notice . Email questions to [email protected]
Print Options
Send Page to Printer
Print this page.
Download Page (PDF)
The PDF will include all information unique to this page.
- Interesting
- Scholarships
- UGC-CARE Journals
100 Machine Learning PhD viva Questions
100 machine learning phd viva questions: a comprehensive guide for defending your thesis.
Are you preparing for your Machine Learning PhD viva and feeling overwhelmed by the thought of the questions you might be asked? You’re not alone. The viva, or oral examination, is a crucial part of the PhD process, and it can be challenging to know how to prepare for it.
Fortunately, we’ve got you covered. In this article, we’ve compiled a list of 100 Machine Learning PhD viva questions that are likely to come up during your examination. By reviewing these questions and preparing thoughtful answers, you can boost your confidence and improve your chances of success.
- What is your research topic, and what motivated you to pursue it?
- What are the key research questions you aimed to answer in your PhD project?
- What is the main contribution of your research to the field of Machine Learning?
- Can you explain the technical approach you used to tackle your research problem?
- What are the limitations of your research, and how did you address them?
- What are the potential applications of your research in industry or academia?
- How does your work fit into the broader context of the Machine Learning field?
- What were the key challenges you faced during your research, and how did you overcome them?
- Can you explain the methodology you used to collect and analyze your data?
- What are the key insights you gained from your research , and how do they contribute to the field?
- What are the main strengths and weaknesses of the Machine Learning methods you used in your research?
- Can you explain the differences between supervised and unsupervised learning, and when to use each method?
- What are the key steps in building a successful Machine Learning model?
- What are the advantages and disadvantages of using deep learning models?
- How do you handle overfitting in your models, and what techniques do you use to prevent it?
- What is regularization, and how does it help prevent overfitting?
- How do you handle missing data in your Machine Learning models?
- What is cross-validation, and how does it help evaluate the performance of your models?
- Can you explain the difference between precision and recall, and why they are important metrics in Machine Learning?
- What is the curse of dimensionality, and how does it affect the performance of Machine Learning models?
- Can you explain the difference between a generative and a discriminative model?
- What are the advantages and disadvantages of using decision trees for Machine Learning?
- Can you explain the difference between gradient descent and stochastic gradient descent?
- What is backpropagation, and how does it work in training neural networks?
- What are convolutional neural networks, and how are they used in computer vision?
- Can you explain the difference between a convolutional layer and a pooling layer in a CNN?
- What are recurrent neural networks, and how are they used in natural language processing?
- Can you explain the difference between a LSTM and a GRU?
- What are autoencoders, and how are they used in unsupervised learning?
- What is transfer learning, and how can it be used to improve the performance of Machine Learning models?
- What are adversarial attacks, and how do they affect the robustness of Machine Learning models?
- What is reinforcement learning, and how does it differ from supervised and unsupervised learning?
- Can you explain the difference between on-policy and off-policy learning in reinforcement learning?
- What is Q-learning, and how does it work in reinforcement learning?
- What are the challenges in scaling Machine Learning models, and how can they be addressed?
- How do you evaluate the performance of a Machine Learning model, and what metrics do you use?
- Can you explain the difference between accuracy and F1 score, and when to use each metric?
- How do you interpret the results of a confusion matrix, and what insights can you gain from it?
- What are the different techniques for feature selection, and how do you decide which one to use?
- Can you explain the difference between feature extraction and feature selection, and when to use each technique?
- What are the different types of clustering algorithms, and how do they differ from each other?
- Can you explain the difference between K-means clustering and hierarchical clustering?
- What are the challenges in clustering high-dimensional data, and how can they be addressed?
- What are the different types of classification algorithms, and how do they differ from each other?
- Can you explain the difference between logistic regression and support vector machines?
- What are the different types of ensemble learning methods, and how do they work?
- Can you explain the difference between bagging and boosting?
- What are the advantages and disadvantages of using random forests for classification tasks?
- What are the different types of time-series forecasting models, and how do they differ from each other?
- Can you explain the difference between ARIMA and exponential smoothing models?
- What are the different types of anomaly detection algorithms, and how do they work?
- Can you explain the difference between unsupervised and semi-supervised anomaly detection?
- What are the different types of deep learning architectures, and how do they differ from each other?
- Can you explain the difference between a feedforward neural network and a convolutional neural network?
- What are the different types of optimization algorithms, and how do they differ from each other?
- Can you explain the difference between batch gradient descent and mini-batch gradient descent?
- What are the different regularization techniques, and how do they prevent overfitting?
- Can you explain the difference between L1 and L2 regularization?
- What are the different types of data augmentation techniques, and how do they improve the performance of Machine Learning models?
- Can you explain the difference between data augmentation and transfer learning?
- What are the different types of hyperparameter tuning techniques, and how do they help optimize Machine Learning models?
- Can you explain the difference between grid search and random search?
- What are the different types of model interpretability techniques, and how do they help understand the inner workings of Machine Learning models?
- Can you explain the difference between LIME and SHAP?
- What are the ethical considerations in Machine Learning, and how do you ensure that your research is ethically sound?
- Can you explain the difference between bias and variance in Machine Learning, and how do you balance them?
- What are the challenges in building Machine Learning models for real-world applications, and how do you address them?
- Can you explain the difference between batch learning and online learning, and when to use each method?
- What are the different types of data preprocessing techniques, and how do they prepare data for Machine Learning?
- Can you explain the difference between normalization and standardization?
- What are the different types of distance metrics, and how do they measure similarity between data points?
- What are the different types of optimization problems in Machine Learning, and how do you solve them?
- Can you explain the difference between convex and non-convex optimization problems?
- What are the different types of regularization techniques, and how do they prevent overfitting?
- Can you explain the difference between dropout and weight decay regularization?
- What are the different types of learning rate schedules, and how do they impact the training of Machine Learning models?
- Can you explain the difference between a fixed learning rate and an adaptive learning rate?
- What are the different types of data imbalance, and how do you handle them in Machine Learning?
- Can you explain the difference between oversampling and undersampling?
- What are the different types of neural network architectures for sequence modeling, and how do they differ from each other?
- Can you explain the difference between a Recurrent Neural Network and a Transformer?
- What are the different types of attention mechanisms, and how do they improve the performance of Machine Learning models?
- What are the challenges in building Machine Learning models for natural language processing, and how do you address them?
- Can you explain the difference between word embeddings and character embeddings?
- What are the different types of text classification algorithms, and how do they differ from each other?
- What are the different types of named entity recognition algorithms, and how do they work?
- What are the different types of sentiment analysis algorithms, and how do they determine the sentiment of a piece of text?
- What are the different types of topic modeling algorithms, and how do they discover topics in a corpus of text?
- What are the different types of Machine Learning models for image classification, and how do they differ from each other?
- Can you explain the difference between a Convolutional Neural Network and a Residual Neural Network?
- What are the different types of object detection algorithms, and how do they detect objects in an image?
- What are the different types of image segmentation algorithms, and how do they segment an image into regions?
- What are the different types of generative models, and how do they generate new data samples?
- Can you explain the difference between a Generative Adversarial Network and a Variational Autoencoder?
- What are the different types of Machine Learning models for speech recognition, and how do they differ from each other?
- Can you explain the difference between a Hidden Markov Model and a Deep Neural Network for speech recognition?
- What are the different types of Machine Learning models for time-series forecasting, and how do they differ from each other?
- Can you explain the difference between a recurrent neural network and a convolutional neural network for time-series forecasting?
- What are the different types of Machine Learning models for recommender systems, and how do they recommend items to users?
- Can you explain the difference between collaborative filtering and content-based filtering for recommender systems?
These 100 Machine Learning PhD viva questions cover a wide range of topics and concepts in Machine Learning, from basic concepts such as linear regression and decision trees, to more advanced topics such as deep learning, natural language processing, and image recognition.
By preparing for these questions, you will be well-equipped to defend your thesis and demonstrate your knowledge and expertise in the field of Machine Learning. Remember to keep practicing and honing your skills, as the field of Machine Learning is constantly evolving and there is always something new to learn. Good luck with your viva!
Also Read: Top 50 Possible PhD Viva Questions
- artificial intelligence
- Data Science
- decision trees
- Deep Learning
- graduate school
- image recognition
- linear regression
- Machine Learning
- natural language processing
- neural networks
- PhD Viva Questions
- thesis defense
Six Effective Tips to Identify Research Gap
Types of research variable in research with example, what is hypothesis in research types, examples, & importance, most popular, iitm & birmingham – joint master program, anna’s archive – download research papers for free, fulbright-kalam climate fellowship: fostering us-india collaboration, fulbright specialist program 2024-25, 24 best free plagiarism checkers in 2024, how to check scopus indexed journals 2024, how to write a research paper a complete guide, best for you, what is phd, popular posts, 480 ugc-care list of journals – science – 2024, popular category.
- POSTDOC 317
- Interesting 259
- Journals 234
- Fellowship 128
- Research Methodology 102
- All Scopus Indexed Journals 92
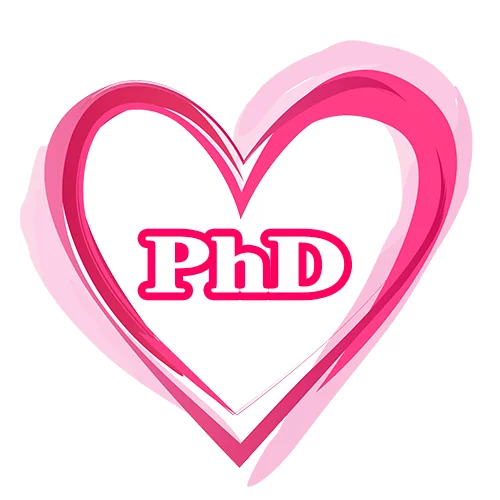
iLovePhD is a research education website to know updated research-related information. It helps researchers to find top journals for publishing research articles and get an easy manual for research tools. The main aim of this website is to help Ph.D. scholars who are working in various domains to get more valuable ideas to carry out their research. Learn the current groundbreaking research activities around the world, love the process of getting a Ph.D.
Contact us: [email protected]
Google News
Copyright © 2024 iLovePhD. All rights reserved
- Artificial intelligence


Exploring 250+ Machine Learning Research Topics

In recent years, machine learning has become super popular and grown very quickly. This happened because technology got better, and there’s a lot more data available. Because of this, we’ve seen lots of new and amazing things happen in different areas. Machine learning research is what makes all these cool things possible. In this blog, we’ll talk about machine learning research topics, why they’re important, how you can pick one, what areas are popular to study, what’s new and exciting, the tough problems, and where you can find help if you want to be a researcher.
Why Does Machine Learning Research Matter?
Table of Contents
Machine learning research is at the heart of the AI revolution. It underpins the development of intelligent systems capable of making predictions, automating tasks, and improving decision-making across industries. The importance of this research can be summarized as follows:
Advancements in Technology
The growth of machine learning research has led to the development of powerful algorithms, tools, and frameworks. Numerous industries, including healthcare, banking, autonomous cars, and natural language processing, have found use for these technology.
As researchers continue to push the boundaries of what’s possible, we can expect even more transformative technologies to emerge.
Real-world Applications
Machine learning research has brought about tangible changes in our daily lives. Voice assistants like Siri and Alexa, recommendation systems on streaming platforms, and personalized healthcare diagnostics are just a few examples of how this research impacts our world.
By working on new research topics, scientists can further refine these applications and create new ones.
Economic and Industrial Impacts
The economic implications of machine learning research are substantial. Companies that harness the power of machine learning gain a competitive edge in the market.
This creates a demand for skilled machine learning researchers, driving job opportunities and contributing to economic growth.
How to Choose the Machine Learning Research Topics?
Selecting the right machine learning research topics is crucial for your success as a machine learning researcher. Here’s a guide to help you make an informed decision:
- Understanding Your Interests
Start by considering your personal interests. Machine learning is a broad field with applications in virtually every sector. By choosing a topic that aligns with your passions, you’ll stay motivated and engaged throughout your research journey.
- Reviewing Current Trends
Stay updated on the latest trends in machine learning. Attend conferences, read research papers, and engage with the community to identify emerging research topics. Current trends often lead to exciting breakthroughs.
- Identifying Gaps in Existing Research
Sometimes, the most promising research topics involve addressing gaps in existing knowledge. These gaps may become evident through your own experiences, discussions with peers, or in the course of your studies.
- Collaborating with Experts
Collaboration is key in research. Working with experts in the field can help you refine your research topic and gain valuable insights. Seek mentors and collaborators who can guide you.
250+ Machine Learning Research Topics: Category-wise
Supervised learning.
- Explainable AI for Decision Support
- Few-shot Learning Methods
- Time Series Forecasting with Deep Learning
- Handling Imbalanced Datasets in Classification
- Regression Techniques for Non-linear Data
- Transfer Learning in Supervised Settings
- Multi-label Classification Strategies
- Semi-Supervised Learning Approaches
- Novel Feature Selection Methods
- Anomaly Detection in Supervised Scenarios
- Federated Learning for Distributed Supervised Models
- Ensemble Learning for Improved Accuracy
- Automated Hyperparameter Tuning
- Ethical Implications in Supervised Models
- Interpretability of Deep Neural Networks.
Unsupervised Learning
- Unsupervised Clustering of High-dimensional Data
- Semi-Supervised Clustering Approaches
- Density Estimation in Unsupervised Learning
- Anomaly Detection in Unsupervised Settings
- Transfer Learning for Unsupervised Tasks
- Representation Learning in Unsupervised Learning
- Outlier Detection Techniques
- Generative Models for Data Synthesis
- Manifold Learning in High-dimensional Spaces
- Unsupervised Feature Selection
- Privacy-Preserving Unsupervised Learning
- Community Detection in Complex Networks
- Clustering Interpretability and Visualization
- Unsupervised Learning for Image Segmentation
- Autoencoders for Dimensionality Reduction.
Reinforcement Learning
- Deep Reinforcement Learning in Real-world Applications
- Safe Reinforcement Learning for Autonomous Systems
- Transfer Learning in Reinforcement Learning
- Imitation Learning and Apprenticeship Learning
- Multi-agent Reinforcement Learning
- Explainable Reinforcement Learning Policies
- Hierarchical Reinforcement Learning
- Model-based Reinforcement Learning
- Curriculum Learning in Reinforcement Learning
- Reinforcement Learning in Robotics
- Exploration vs. Exploitation Strategies
- Reward Function Design and Ethical Considerations
- Reinforcement Learning in Healthcare
- Continuous Action Spaces in RL
- Reinforcement Learning for Resource Management.
Natural Language Processing (NLP)
- Multilingual and Cross-lingual NLP
- Contextualized Word Embeddings
- Bias Detection and Mitigation in NLP
- Named Entity Recognition for Low-resource Languages
- Sentiment Analysis in Social Media Text
- Dialogue Systems for Improved Customer Service
- Text Summarization for News Articles
- Low-resource Machine Translation
- Explainable NLP Models
- Coreference Resolution in NLP
- Question Answering in Specific Domains
- Detecting Fake News and Misinformation
- NLP for Healthcare: Clinical Document Understanding
- Emotion Analysis in Text
- Text Generation with Controlled Attributes.
Computer Vision
- Video Action Recognition and Event Detection
- Object Detection in Challenging Conditions (e.g., low light)
- Explainable Computer Vision Models
- Image Captioning for Accessibility
- Large-scale Image Retrieval
- Domain Adaptation in Computer Vision
- Fine-grained Image Classification
- Facial Expression Recognition
- Visual Question Answering
- Self-supervised Learning for Visual Representations
- Weakly Supervised Object Localization
- Human Pose Estimation in 3D
- Scene Understanding in Autonomous Vehicles
- Image Super-resolution
- Gaze Estimation for Human-Computer Interaction.
Deep Learning
- Neural Architecture Search for Efficient Models
- Self-attention Mechanisms and Transformers
- Interpretability in Deep Learning Models
- Robustness of Deep Neural Networks
- Generative Adversarial Networks (GANs) for Data Augmentation
- Neural Style Transfer in Art and Design
- Adversarial Attacks and Defenses
- Neural Networks for Audio and Speech Processing
- Explainable AI for Healthcare Diagnosis
- Automated Machine Learning (AutoML)
- Reinforcement Learning with Deep Neural Networks
- Model Compression and Quantization
- Lifelong Learning with Deep Learning Models
- Multimodal Learning with Vision and Language
- Federated Learning for Privacy-preserving Deep Learning.
Explainable AI
- Visualizing Model Decision Boundaries
- Saliency Maps and Feature Attribution
- Rule-based Explanations for Black-box Models
- Contrastive Explanations for Model Interpretability
- Counterfactual Explanations and What-if Analysis
- Human-centered AI for Explainable Healthcare
- Ethics and Fairness in Explainable AI
- Explanation Generation for Natural Language Processing
- Explainable AI in Financial Risk Assessment
- User-friendly Interfaces for Model Interpretability
- Scalability and Efficiency in Explainable Models
- Hybrid Models for Combined Accuracy and Explainability
- Post-hoc vs. Intrinsic Explanations
- Evaluation Metrics for Explanation Quality
- Explainable AI for Autonomous Vehicles.
Transfer Learning
- Zero-shot Learning and Few-shot Learning
- Cross-domain Transfer Learning
- Domain Adaptation for Improved Generalization
- Multilingual Transfer Learning in NLP
- Pretraining and Fine-tuning Techniques
- Lifelong Learning and Continual Learning
- Domain-specific Transfer Learning Applications
- Model Distillation for Knowledge Transfer
- Contrastive Learning for Transfer Learning
- Self-training and Pseudo-labeling
- Dynamic Adaption of Pretrained Models
- Privacy-Preserving Transfer Learning
- Unsupervised Domain Adaptation
- Negative Transfer Avoidance in Transfer Learning.
Federated Learning
- Secure Aggregation in Federated Learning
- Communication-efficient Federated Learning
- Privacy-preserving Techniques in Federated Learning
- Federated Transfer Learning
- Heterogeneous Federated Learning
- Real-world Applications of Federated Learning
- Federated Learning for Edge Devices
- Federated Learning for Healthcare Data
- Differential Privacy in Federated Learning
- Byzantine-robust Federated Learning
- Federated Learning with Non-IID Data
- Model Selection in Federated Learning
- Scalable Federated Learning for Large Datasets
- Client Selection and Sampling Strategies
- Global Model Update Synchronization in Federated Learning.
Quantum Machine Learning
- Quantum Neural Networks and Quantum Circuit Learning
- Quantum-enhanced Optimization for Machine Learning
- Quantum Data Compression and Quantum Principal Component Analysis
- Quantum Kernels and Quantum Feature Maps
- Quantum Variational Autoencoders
- Quantum Transfer Learning
- Quantum-inspired Classical Algorithms for ML
- Hybrid Quantum-Classical Models
- Quantum Machine Learning on Near-term Quantum Devices
- Quantum-inspired Reinforcement Learning
- Quantum Computing for Quantum Chemistry and Drug Discovery
- Quantum Machine Learning for Finance
- Quantum Data Structures and Quantum Databases
- Quantum-enhanced Cryptography in Machine Learning
- Quantum Generative Models and Quantum GANs.
Ethical AI and Bias Mitigation
- Fairness-aware Machine Learning Algorithms
- Bias Detection and Mitigation in Real-world Data
- Explainable AI for Ethical Decision Support
- Algorithmic Accountability and Transparency
- Privacy-preserving AI and Data Governance
- Ethical Considerations in AI for Healthcare
- Fairness in Recommender Systems
- Bias and Fairness in NLP Models
- Auditing AI Systems for Bias
- Societal Implications of AI in Criminal Justice
- Ethical AI Education and Training
- Bias Mitigation in Autonomous Vehicles
- Fair AI in Financial and Hiring Decisions
- Case Studies in Ethical AI Failures
- Legal and Policy Frameworks for Ethical AI.
Meta-Learning and AutoML
- Neural Architecture Search (NAS) for Efficient Models
- Transfer Learning in NAS
- Reinforcement Learning for NAS
- Multi-objective NAS
- Automated Data Augmentation
- Neural Architecture Optimization for Edge Devices
- Bayesian Optimization for AutoML
- Model Compression and Quantization in AutoML
- AutoML for Federated Learning
- AutoML in Healthcare Diagnostics
- Explainable AutoML
- Cost-sensitive Learning in AutoML
- AutoML for Small Data
- Human-in-the-Loop AutoML.
AI for Healthcare and Medicine
- Disease Prediction and Early Diagnosis
- Medical Image Analysis with Deep Learning
- Drug Discovery and Molecular Modeling
- Electronic Health Record Analysis
- Predictive Analytics in Healthcare
- Personalized Treatment Planning
- Healthcare Fraud Detection
- Telemedicine and Remote Patient Monitoring
- AI in Radiology and Pathology
- AI in Drug Repurposing
- AI for Medical Robotics and Surgery
- Genomic Data Analysis
- AI-powered Mental Health Assessment
- Explainable AI in Healthcare Decision Support
- AI in Epidemiology and Outbreak Prediction.
AI in Finance and Investment
- Algorithmic Trading and High-frequency Trading
- Credit Scoring and Risk Assessment
- Fraud Detection and Anti-money Laundering
- Portfolio Optimization with AI
- Financial Market Prediction
- Sentiment Analysis in Financial News
- Explainable AI in Financial Decision-making
- Algorithmic Pricing and Dynamic Pricing Strategies
- AI in Cryptocurrency and Blockchain
- Customer Behavior Analysis in Banking
- Explainable AI in Credit Decisioning
- AI in Regulatory Compliance
- Ethical AI in Financial Services
- AI for Real Estate Investment
- Automated Financial Reporting.
AI in Climate Change and Sustainability
- Climate Modeling and Prediction
- Renewable Energy Forecasting
- Smart Grid Optimization
- Energy Consumption Forecasting
- Carbon Emission Reduction with AI
- Ecosystem Monitoring and Preservation
- Precision Agriculture with AI
- AI for Wildlife Conservation
- Natural Disaster Prediction and Management
- Water Resource Management with AI
- Sustainable Transportation and Urban Planning
- Climate Change Mitigation Strategies with AI
- Environmental Impact Assessment with Machine Learning
- Eco-friendly Supply Chain Optimization
- Ethical AI in Climate-related Decision Support.
Data Privacy and Security
- Differential Privacy Mechanisms
- Federated Learning for Privacy-preserving AI
- Secure Multi-Party Computation
- Privacy-enhancing Technologies in Machine Learning
- Homomorphic Encryption for Machine Learning
- Ethical Considerations in Data Privacy
- Privacy-preserving AI in Healthcare
- AI for Secure Authentication and Access Control
- Blockchain and AI for Data Security
- Explainable Privacy in Machine Learning
- Privacy-preserving AI in Government and Public Services
- Privacy-compliant AI for IoT and Edge Devices
- Secure AI Models Sharing and Deployment
- Privacy-preserving AI in Financial Transactions
- AI in the Legal Frameworks of Data Privacy.
Global Collaboration in Research
- International Research Partnerships and Collaboration Models
- Multilingual and Cross-cultural AI Research
- Addressing Global Healthcare Challenges with AI
- Ethical Considerations in International AI Collaborations
- Interdisciplinary AI Research in Global Challenges
- AI Ethics and Human Rights in Global Research
- Data Sharing and Data Access in Global AI Research
- Cross-border Research Regulations and Compliance
- AI Innovation Hubs and International Research Centers
- AI Education and Training for Global Communities
- Humanitarian AI and AI for Sustainable Development Goals
- AI for Cultural Preservation and Heritage Protection
- Collaboration in AI-related Global Crises
- AI in Cross-cultural Communication and Understanding
- Global AI for Environmental Sustainability and Conservation.
Emerging Trends and Hot Topics in Machine Learning Research
The landscape of machine learning research topics is constantly evolving. Here are some of the emerging trends and hot topics that are shaping the field:
As AI systems become more prevalent, addressing ethical concerns and mitigating bias in algorithms are critical research areas.
Interpretable and Explainable Models
Understanding why machine learning models make specific decisions is crucial for their adoption in sensitive areas, such as healthcare and finance.
Meta-learning algorithms are designed to enable machines to learn how to learn, while AutoML aims to automate the machine learning process itself.
Machine learning is revolutionizing the healthcare sector, from diagnostic tools to drug discovery and patient care.
Algorithmic trading, risk assessment, and fraud detection are just a few applications of AI in finance, creating a wealth of research opportunities.
Machine learning research is crucial in analyzing and mitigating the impacts of climate change and promoting sustainable practices.
Challenges and Future Directions
While machine learning research has made tremendous strides, it also faces several challenges:
- Data Privacy and Security: As machine learning models require vast amounts of data, protecting individual privacy and data security are paramount concerns.
- Scalability and Efficiency: Developing efficient algorithms that can handle increasingly large datasets and complex computations remains a challenge.
- Ensuring Fairness and Transparency: Addressing bias in machine learning models and making their decisions transparent is essential for equitable AI systems.
- Quantum Computing and Machine Learning: The integration of quantum computing and machine learning has the potential to revolutionize the field, but it also presents unique challenges.
- Global Collaboration in Research: Machine learning research benefits from collaboration on a global scale. Ensuring that researchers from diverse backgrounds work together is vital for progress.
Resources for Machine Learning Researchers
If you’re looking to embark on a journey in machine learning research topics, there are various resources at your disposal:
- Journals and Conferences
Journals such as the “Journal of Machine Learning Research” and conferences like NeurIPS and ICML provide a platform for publishing and discussing research findings.
- Online Communities and Forums
Platforms like Stack Overflow, GitHub, and dedicated forums for machine learning provide spaces for collaboration and problem-solving.
- Datasets and Tools
Open-source datasets and tools like TensorFlow and PyTorch simplify the research process by providing access to data and pre-built models.
- Research Grants and Funding Opportunities
Many organizations and government agencies offer research grants and funding for machine learning projects. Seek out these opportunities to support your research.
Machine learning research is like a superhero in the world of technology. To be a part of this exciting journey, it’s important to choose the right machine learning research topics and keep up with the latest trends.
Machine learning research makes our lives better. It powers things like smart assistants and life-saving medical tools. It’s like the force driving the future of technology and society.
But, there are challenges too. We need to work together and be ethical in our research. Everyone should benefit from this technology. The future of machine learning research is incredibly bright. If you want to be a part of it, get ready for an exciting adventure. You can help create new solutions and make a big impact on the world.
Related Posts

Tips on How To Tackle A Machine Learning Project As A Beginner
Here in this blog, CodeAvail experts will explain to you tips on how to tackle a machine learning project as a beginner step by step…


Artificial Intelligence and Machine Learning Basics for Beginners
Here in this blog, CodeAvail experts will explain to you Artificial Intelligence and Machine Learning basics for beginners in detail step by step. What is…
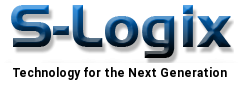
Office Address
- #5, First Floor, 4th Street Dr. Subbarayan Nagar Kodambakkam, Chennai-600 024 Landmark : Samiyar Madam
- [email protected]
- +91- 81240 01111
Social List
Latest phd thesis topics in machine learning.

- With progressive technological development, the exploration of machine learning has increased a huge number of applications. Consequentially. Machine learning instigates an essential part of implementing smart and automated applications by intelligent data analysis.
- The applicability of machine learning is abundant in many real-world application fields, such as predictive analytics and intelligent decision-making, cyber-security systems, smart cities, healthcare, e-commerce, agriculture, finance, retail, social media, traffic prediction and transportation, computer vision applications, user behavior analytics and context-aware smartphone applications, bioinformatics, cheminformatics, computer networks, DNA sequence classification, economics and banking, robotics, advanced engineering, and many more.
- Recently described branches of machine learning are computational learning theory, adversarial machine learning, quantum machine learning, robot learning, and meta-learning. Efficient data processing and handling the diverse learning algorithms are the constraints that are needed to be the focus in machine learning. PHD thesis on machine learning contributes to the challenges, promising research opportunities, and effective solutions in various application areas. Below is the list of PHD thesis topics on machine learning to effectively explore, scrutinize and discover the new findings of machine learning systems.
List of Sample PHD Thesis in Machine Learning
- Incremental Learning for Large-Scale Data Stream Analytics in a Complex Environment
- Neural Sequential Transfer Learning for Relation Extraction
- Predicting Depression and Suicide Ideation in the Canadian Population Using Social Media Data
- Neural Aspect-based Text Generation
- Leveraging Social Media and Machine Learning for enhanced Communication and Understanding between Organizations and Stakeholders
- Deep Learning Methods for Short,Informal, and Multilingual Text Analytics
- Deep Learning Based Cursive Text Detection and Recognition in Natural Scene Images
- Deep Learning-Based Text Detection and Recognition
- Explaining Deep Neural Networks
- Machine Learning Techniques in Spam Filtering
- Anomaly-Based Network Intrusion Detection Using Machine Learning
- Machine Learning for Financial Products Recommendation
- Sentiment Analysis of Textual Content in Social Networks
- Deep Learning For Time Series Classification
- Deep Learning for Traffic Time Series Data Analysis
- Novel applications of Machine Learning to Network Traffic Analysis and Prediction
- Deep Learning for Animal Recognition
- Neural Transfer Learning for Natural Language Processing
- Scalable and Ensemble Learning for Big Data
- Ensembles for Time Series Forecasting
- Sample-Efficient Deep Reinforcement Learning for Continuous Control
- Towards Generalization and Efficiency in Reinforcement Learning
- Transfer Learning with Deep Neural Networks for Computer Vision
- Deep Learning for Recommender Systems
- CHAMELEON: A Deep Learning Meta-Architecture For News Recommender Systems
- Learning in Dynamic Data-Streams with a Scarcity of Labels
- Learning Meaning Representations For Text Generation With Deep Generative Models
- Social Media Sentiment Analysis with a Deep Neural Network: An Enhanced Approach Using User Behavioral Information
- Global-Local Word Embedding for Text Classification
- Measuring Generalization and Overfitting in Machine Learning
- Handling Class Imbalance Using Swarm Intelligence Techniques, Hybrid Data and Algorithmic Level Solutions
- Using Data Science and Predictive Analytics to Understand 4-Year University Student Churn
- Deep Learning Based Imbalanced Data Classification and Information Retrieval for Multimedia Big Data
- Improving Geospatial Data Search Ranking Using Deep Learning and User Behaviour Data
- An Investigation Into Machine Learning Solutions Involving Time Series Across Different Problem Domains
- Deep Learning Applications for Biomedical Data and Natural Language Processing
- Deep Neural Network Models for Image Classification and Regression
- Deep learning for medical report texts
- Deep multi-agent reinforcement learning
- Artificial intelligence methods to support people management in organisations
- An Intelligent Recommender System Based on Short-term Disease Risk Prediction for Patients with Chronic Diseases in a Telehealth Environment
- Bringing Interpretability and Visualization with Artificial Neural Networks
- Investigating machine learning methods in Recommender systems
- Adaptive Machine Learning Algorithms For Data Streams Subject To Concept Drifts
- Active Learning for Data Streams
- Heart Diseases Diagnosis Using Artificial Neural Networks
- Advanced Natural Language Processing and Temporal Mining for Clinical Discovery
- Uncertainty in Deep Learning
- Parallel Transfer Learning: Accelerating Reinforcement Learning in Multi-Agent Systems
- Sentiment analysis on students Real-time Feedback
- Aspect-Based Opinion Mining From Customer Reviews
- Word Embeddings for Natural Language Processing
- On Effectively Creating Ensembles of Classifiers
- Design of Intelligent Ensembled Classifiers Combination Methods
- ELSE: Ensemble Learning System with Evolution for Content Based Image Retrieval
- Using Hybrid Algorithm to Improve Intrusion Detection in Multi Layer Feed Forward Neural Networks
- Achieving Consistent Near-Optimal Pattern Recognition Accuracy Using Particle Swarm Optimization to Pre-Train Artificial Neural Networks
- Using Assessments of Contextual Learning to Identify Characteristics of Adaptive Transfer in Medical Students
- Recursive Deep Learning for Natural Language Processing and Computer Vision
- Machine learning strategies for multi-step-ahead time series forecasting
- General Attention Mechanism for Artificial Intelligence Systems
- Defense Acquisition University: A Study of Employee Perceptions on Web Based Learning Transfer
- Incremental Learning with Large Datasets
- Machine Learning and Data Mining Methods for Recommender Systems and Chemical Informatics
- Transfer of Learning in Leadership Development: Lived Experiences of HPI Practitioners
- Online Ensemble Learning in the Presence of Concept Drift
- Learning From Data Streams With Concept Drift
- PhD Guidance and Support Enquiry
- Masters and PhD Project Enquiry
- Research Topics in Machine Learning
- PhD Research Guidance in Machine Learning
- PhD Research Proposal in Machine Learning
- Latest Research Papers in Machine Learning
- Literature Survey in Machine Learning
- PhD Projects in Machine Learning
- Python Project Titles in Machine Learning
- Python Sample Source Code
- Python Projects in Machine Learning
- Leading Journals in Machine Learning
- Leading Research Books in Machine Learning
- Research Topics in Depression Detection based on Deep Learning
- Research Topics in Deep Learning for Intelligent Vehicular Networks
- Research Topics in Recent Advances in Deep Recurrent Neural Networks
- Research Proposal on Hyperparameter Optimization and Fine-Tuning in Deep Neural Network
- Research Proposal Topics on Convolutional Neural Networks Research Challenges and Future Impacts
- Research Proposal Topics on Deep Learning Models for Epilepsy Detection
- Research Topics in Computer Science
- PhD Thesis Writing Services in Computer Science
- PhD Paper Writing Services in Computer Science
- How to Write a PhD Research Proposal in Computer Science
- Ph.D Support Enquiry
- Project Enquiry
- Research Guidance in Machine Learning
- Research Proposal in Machine Learning
- Research Papers in Machine Learning
- Ph.D Thesis in Machine Learning
- Research Projects in Machine Learning
- Project Titles in Machine Learning
- Project Source Code in Machine Learning
Reflections on my (Machine Learning) PhD Journey
December 31, 2020
2020 has been an incredibly challenging year, and on a personal note, has marked an important milestone — graduating with my PhD in computer science from Cornell University. This has been a six year journey, where my personal growth as a machine learning researcher (from thrills of first discoveries to the laborious grind through publication rejections to identifying a broader research vision) also took place against the backdrop of the rapid growth and change of the entire field ( 2014 NeurIPS : ~2k attendees, 2020 NeurIPS : ~20k attendees).
With this year coming to an end, I’ve put together some of my reflections and lessons learned from my (Machine Learning) PhD experiences. I discuss topics including expectations going in, common challenges during the PhD (and some strategies for helping with them), keeping up with papers, the community nature of research and developing a research vision. I hope that these topics are helpful in navigating the PhD and research in Machine Learning!
Expectations going into the PhD
Feeling completely stuck, feeling overwhelmed with keeping up with ml progress, feeling isolated, three useful personal skills, keeping notes on papers and ideas, the importance of community, developing a research vision.
In the post title, I’ve referred to the PhD as a “journey”, an aspect often underappreciated, particularly if one is coming straight out of undergrad (which was my experience). A typical Machine Learning PhD is going to be ~5-6 years of relatively unstructured time, and during this, not only will you learn research skills and knowledge about the field, but you’ll also develop personal preferences on how interesting specific problems are, the aesthetics of different subfields, and even perspectives on the type of work being done across academia/industry/policy/nonprofits.
These evolving personal preferences will influence the type of research you decide to work on, and even the post-PhD career path you pick. But particularly at the start of the PhD, it’s hard to predict how these personal perspectives will evolve. In my case, I started my PhD fully assuming I’d stay in industry, part way through began seriously considering academia, and at the end made the very difficult decision to turn down academic offers and stay on in industry. So going into the PhD program, it’s often helpful to take it step by step, and focus on getting the most out of the experience (learning/research/community participation), instead of a very specific desired outcome (which is prone to change, and may also add unnecessary pressure.)
Common Challenges through the Journey
Doing a PhD can be an immensely rewarding experience, and, particularly in Machine Learning, offers the chance to contribute to fundamental scientific understanding as well as impactful technology deployment. I’ve been grateful to my PhD for providing many opportunities to experience both of these! However, the duration and unstructured nature of the PhD can also make it challenging. My journey definitely consisted of ups and downs, and at various times I’ve struggled with feeling isolated, completely stuck, and even overwhelmed by trying to keep up with the rapid pace of progress. Looking back, and through discussion with peers, I now know these low points can unfortunately be quite common. But because these experiences are shared across many people, there can also be strategies for working through them. Below I discuss some of these experiences and strategies.
One very common challenge is feeling completely stuck, either on a specific project or with regards to the research process on the whole.
If the challenge is a specific project, where you’ve pushed hard and it’s still not quite working, then some strategies that might help are
- Making a write up : Collect any partial experimental results, mathematical insights, jotted notes on motivation, etc and take time to put together a write up. This can help with providing a picture of where things stand and where the important gaps are.
- Pivot : if there’s a specific part of the project that’s not working, is there the possibility to reframe the question (possibly taking inspiration from related work) to make it more tractable?
- Forming connections : are there links between what the current project focuses on and other areas of study? Can that connection be explored in this project? This can both help progress and in making the project relevant to a broader community.
- Feedback on writeup : It might also be helpful to get feedback on the project write up from peers, collaborators and friends in the research community. They may be able to offer new perspectives or suggest improvements.
- Workshop submission : it can also be useful to make a workshop submission. This also provides a chance to help collect together all the research results and get useful feedback. (For some time now, I’ve gained the most out of the workshops at machine learning conferences, due to being able to discuss/get feedback on ongoing directions and meeting other researchers working on the same area.)
- Wrap up and move on : Occasionally, there may be a project which sounded promising in the beginning, but has been difficult to make work, and is also inherently challenging to reframe or form connections to other areas. In this (difficult) situation, it may make most sense to wrap up the project quickly and move on. If you have partial results, it’s likely worthwhile to create a final writeup of those and share, so one option is to do this, get confirmation from collaborators and final feedback, and keep it as an arXiv preprint or workshop paper.
If the feeling of being stuck originates from the research process more broadly, one important point I’ve realised is that gaining research maturity can often be very hard to measure, especially when evaluating yourself! Midway through my PhD, I started working on some healthcare applications, and was only making slow headway on learning about the area/writing papers. This had me feeling stuck and somewhat frustrated at my slowdown in research progress. But when I re-read some papers that I’d first come across at the start of my PhD, the depth and context with which I could understand their results was strikingly different from earlier on.
Critical aspects of research maturity — understanding the broader context of results, being able to form connections between different areas, quickly narrowing in on novel key contributions in your subfield — don’t immediately translate to tangible outputs (more papers). But they’re central for becoming an independent researcher with a rich research vision — arguably the main research goal of the PhD. And if you’re reading papers, learning about the field, and working on research directions yourself, (and maybe even teaching/mentoring) most likely you’re making progress on all of these important aspects!
Machine Learning is a vibrant, fast-paced field. But the flipside of this is drowning in the flood of new papers, new preprints, new blogposts, new implementations, new frameworks, etc, etc. (Fun statistic: NeurIPS this past year had ~10k submissions and ~2k accepted papers — no wonder we’re feeling overwhelmed!)
My strategy in dealing with this has been
- Have a number of go-to links to find references to related papers. For me, this has been a combination of (i) subscribing to the arxiv stat.ML cs.LG mailing lists, arXiv-sanity , Twitter, (occasionally) reddit/MachineLearning , paperswithcode and perusing Semantic Scholar / Google Scholar .
- Keep a reading list of papers If I come across an interesting paper but don’t have time to read it then (often the case), I make a note of it and try to return to it later.
- Have a paper reading strategy If a paper is very close to research directions I’m actively working on, I’ll read it in detail, otherwise I’ll skim the abstract to get a high level picture.
- Occasionally read up on different areas Occasionally (maybe once per year), I’ll look into a few interesting areas I’m not working on, and read several papers in each to get a sense of what is being worked on.
It’s also helpful to remember that (i) everyone feels overwhelmed with the rate of publishing and (ii) many papers may rely on the same underlying idea, and often being familiar with the idea is enough for keeping up with the field.
Another common challenge in the PhD is struggling with feelings of isolation. In the first couple of years of my PhD, some projects required that I kept laser focus on very narrow, specific questions, which were also highly laborious and (felt) never-ending. During those times, it was hard not to feel completely cut-off from other researchers and the broader field, and I’m very grateful for all the support and guidance from my PhD advisor in pushing through that situation.
More broadly, this scenario can be common especially earlier on during the PhD, where you might simultaneously be learning how to see through a research project from start to finish, and at the same time have less context and connections to the broader research field/community. Maintaining connections to the field/community can be very helpful in making sure you don’t feel isolated. Some ways to do this could be: (i) setting up collaborations with (senior) students/postdocs (ii) getting feedback on your work in progress – this might be your advisor/lab, but could also be other peers/mentors working in the field (iii) actively participating in the broader community, whether that’s through simply attending conferences, mentoring or organizing workshops.
Having discussed some of the common challenges faced in the PhD and ways to help address them, the rest of this post will overview some useful considerations for research progress.
In particular, I’ll begin by discussing three personal skills I found to be very helpful throughout the PhD: initiative, focus and perseverance. This is of course drawing on my personal experiences, and there are varying opinions on useful personal skills! But for me, coming out of undergrad, a key difference I noticed in the PhD program was the need to take initiative — whether that meant reading important relevant papers, doing rapid preliminary studies of the feasibility of different approaches, talking to peers doing related research, or even attending and being an active participant in conferences. Because the PhD broadly consists of unstructured time, being productive largely relies on your initiative for learning and conducting research.
Two other skills that I’ve found very helpful are focus and perseverance. When getting started with a new research direction, focus is very helpful to peruse related work, distill the key points, quickly learn from initial exploration and determine the main project directions. Perseverance on the other hand is very useful (especially) for wrapping up the research project: there’s often a long tail of edits/additions for the paper to be submission ready, and post-submission, further edits to respond to peer-review and paper rejections. It can be hard to muster the motivation to make all of these edits (especially when preparing the paper for yet another resubmission, and having newer, more interesting projects also going on), but the variability of the peer-review process often means it’s worth persevering through.
Through my PhD, there are two documents, one started in my first year, and the other in my third year, that I’ve continuously kept updated. The first keeps track of papers that I’ve read – every time I read a new paper, I add it into the doc, along with a short summary of my takeaways. The document is now over fifty pages (which maybe means I should switch to Mendeley or Paperpile), and has been a very useful way to quickly flick back to papers I’ve read years earlier and get key points. The other document keeps track of research ideas. Everytime I have a promising new idea, I make a note of it. Over time, this has helped inform my research directions and highlight key themes.
One important property of (Machine Learning) research that took me time to realise is that research is fundamentally a community endeavor. The problems that we aim to tackle are incredibly difficult, and progress relies on the cycle of you building off of others’ ideas and others building off of your ideas. This is a crucial factor to keep in mind when exploring research directions. What is the community excited about, and why? Are there shortcomings or gaps? Are there natural next steps to study? Taking the time to discuss these questions and others with peers in the community is vital to developing well-informed, germane research questions. And if you identify an exciting, new research direction of interest to the field, it’s often useful to build a community around that direction — this can happen through initiating collaborations, disseminating key open questions and organizing workshops. From very early on in my PhD, I was interested in understanding the key empirical phenomena exhibited by our modern deep learning systems. But working on this topic then was very challenging. The field was evolving rapidly, making the focus of any kind of analysis a moving target, and significantly adding to the challenge of building a new community around this topic. So publishing my first deep learning analysis papers was pretty difficult, and definitely an act of perseverance! But since then, it’s been wonderful to witness and contribute to the growth of this exciting research area!
While I described earlier that when getting started with the PhD, it may be better to take things one step at a time and focus on the experience instead of a specific goal, from the research maturity perspective, the PhD does have a specific goal: to make you an independent researcher, with a rich (articulable) research vision.
In current Machine Learning research, with the deluge of papers, it’s easy to feel stressed about the need to continuously churn out publications. But while paper writing is an important skill, I think the crucial test of research maturity is being able to have knowledgeable perspectives on your field which help identify key research questions, connected by overarching themes — a research vision.
Having a well developed research vision is enormously motivating. As an analogy, it’s a little like completing a “paint by numbers” kit: instead of just seeing the color of each individual square, you suddenly appreciate the full picture.
So how does one develop a research vision?
As a first note, from my PhD journey, I think it’s hard to develop a full-fledged research vision without some years of research experience. In my first couple of years of PhD, I remember reading papers and watching talks of senior researchers, and being frustrated that I couldn’t identify/articulate interesting research questions nearly as well. In the years since then, the compounding effects of all the papers I’ve read, projects worked on, seminars attended, have significantly improved my ability to do this. (There is of course room to improve! Going forwards, this ability will continue to develop, as I gain more context and understanding of larger subfields.)
Being more specific about the stages that led to a (better developed) research vision: it started off with exploration, with my first few projects giving me diverse exposures and helping me understand what I found intrinsically interesting. From there, there were natural followup projects to study, which finally also led to some related questions on applications/deployment. All of this started coming together under the broad theme of Machine Learning Design and Human-AI interaction at Deployment, and, as research visions are good at doing, also inspired new questions. (I am deeply grateful to my PhD advisor for his insights, guidance and encouragement through all of this!)
As a final point, I want to emphasise that the years of experience really do have a compounding effect. As you work on research projects, it becomes easier to identify the salient ideas in research papers, this informs your personal perspectives and promising questions for next projects, working on these next projects makes it easier to absorb/give talks, which then circles back to help with identifying new interesting research directions, which eventually coalesce to form a broader vision.
In summary, doing a PhD can be very fulfilling. It is however a journey, and has its ups and downs, personal discoveries, and evolution of (research) perspectives. I’m very grateful for the many rich experiences during my PhD, and hope this post might be helpful for others on the journey!
Explore your training options in 10 minutes Get Started
- Graduate Stories
- Partner Spotlights
- Bootcamp Prep
- Bootcamp Admissions
- University Bootcamps
- Coding Tools
- Software Engineering
- Web Development
- Data Science
- Tech Guides
- Tech Resources
- Career Advice
- Online Learning
- Internships
- Apprenticeships
- Tech Salaries
- Associate Degree
- Bachelor's Degree
- Master's Degree
- University Admissions
- Best Schools
- Certifications
- Bootcamp Financing
- Higher Ed Financing
- Scholarships
- Financial Aid
- Best Coding Bootcamps
- Best Online Bootcamps
- Best Web Design Bootcamps
- Best Data Science Bootcamps
- Best Technology Sales Bootcamps
- Best Data Analytics Bootcamps
- Best Cybersecurity Bootcamps
- Best Digital Marketing Bootcamps
- Los Angeles
- San Francisco
- Browse All Locations
- Digital Marketing
- Machine Learning
- See All Subjects
- Bootcamps 101
- Full-Stack Development
- Career Changes
- View all Career Discussions
- Mobile App Development
- Cybersecurity
- Product Management
- UX/UI Design
- What is a Coding Bootcamp?
- Are Coding Bootcamps Worth It?
- How to Choose a Coding Bootcamp
- Best Online Coding Bootcamps and Courses
- Best Free Bootcamps and Coding Training
- Coding Bootcamp vs. Community College
- Coding Bootcamp vs. Self-Learning
- Bootcamps vs. Certifications: Compared
- What Is a Coding Bootcamp Job Guarantee?
- How to Pay for Coding Bootcamp
- Ultimate Guide to Coding Bootcamp Loans
- Best Coding Bootcamp Scholarships and Grants
- Education Stipends for Coding Bootcamps
- Get Your Coding Bootcamp Sponsored by Your Employer
- GI Bill and Coding Bootcamps
- Tech Intevriews
- Our Enterprise Solution
- Connect With Us
- Publication
- Reskill America
- Partner With Us
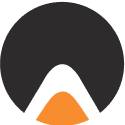
- Resource Center
- Bachelor’s Degree
- Master’s Degree
Best Online Doctorates in Machine Learning: Top PhD Programs, Career Paths, and Salary
Machine learning is a rapidly growing, fascinating field dealing with algorithm development that can be used to make predictions from data. The best online PhD in Machine Learning prepares students for a career in this promising field.
The best online doctorates in machine learning offer students a comprehensive education in all aspects of the field. Students are also provided with the opportunity to choose a specialization such as deep learning, natural language processing , or computer vision. Find out in this article what machine learning PhD online degree program best fits you and the machine learning jobs for graduates.
Find your bootcamp match
Can you get a phd in machine learning online.
Yes, you can get a PhD in Machine Learning online. The online learning system has seen rapid growth in many academic fields and has given students the opportunity to virtually access the academic curriculum remotely.
Many online PhD programs in the United States are accredited and designed with working professionals in mind. Online learning is a great way to earn a doctorate without sacrificing your day job, and in most cases, online students can complete their entire academic journey without stepping foot on campus.
Is an Online PhD Respected?
Yes, an online PhD is respected when it is obtained from an accredited institution in the US. A PhD from an unaccredited school is regarded as just an expensive piece of paper by many other academic institutions.
In regard to employment, many companies and organizations respect an online PhD, holding it to the same standard as an in-person PhD. However, some employers prefer in-person degrees and will disregard online degrees. Ensure your potential future employer accepts online degrees as credible education.
What Is the Best Online PhD Program in Machine Learning?
The best online PhD program in machine learning is at Clarkson University in Potsdam, New York. It is regionally accredited by the Middle States Commission on Higher Education and has an excellent reputation within the academic community, a student-to-faculty ratio of 12 to one, and one in five of its 44,000 alumni is a CEO or executive.
Why Clarkson University Has the Best Online PhD Program in Machine Learning
Clarkson University has the best machine learning PhD program not only because it is accredited by the Middle States Commission on Higher Education (MSCHE) but also because of its US News & World Report ranking. MSCHE is a regionally recognized accreditation association that uses a rigorous and comprehensive system for the purpose of accreditation.
Referring to US News & World Report, Clarkson University is ranked 127 for best national universities out of 4000 degree-granting academic institutions in the United States and 49 for best value schools.
Best Online Master’s Degrees
[query_class_embed] online-*subject-masters-degrees
Online PhD in Machine Learning Admission Requirements
The admission requirements for an online PhD in Machine Learning typically include a master’s degree or Bachelor’s in Machine Learning or a related subject like the field of engineering. Moreover, prepare to submit official transcripts from previously attended postsecondary institutions and GRE test scores.
Additionally, you may be asked to submit three letters of recommendation, a statement of purpose, a CV or resume, and prove your knowledge of calculus and your fluency in computer programming languages like Python and Java. Below is a list of the typical admission requirements needed by distinct schools that offer a machine learning PhD program.
- Master’s or bachelor’s degree in a relevant field
- Official transcripts and GRE test scores
- Letters of recommendation
- Statement of purpose
- CV or resume
- Knowledge of programming and calculus
Best Online PhDs in Machine Learning: Top Degree Program Details
Best online phds in machine learning: top university programs to get a phd in machine learning online.
The top university programs to get a PhD in Machine Learning are at Clarkson University, Aspen University, Capitol Technology University, The University of Rhode Island, and The University of the Cumberlands, among other distinct schools.
This section discusses the properties, requirements, and descriptions of the best universities offering online PhD in Machine Learning programs. We have created this list below to narrow down your school search for these graduate-level in-depth study programs.
Aspen University is a Distance Education Accrediting Commission accredited university. It was established in 1987 as a private for-profit online university offering undergraduate and graduate degrees in computer science, business information systems, and project management.
Aspen University in Phoenix, Arizona is a known member of the Council for Adult and Experiential Learning and is dedicated to supporting adult learners in achieving a professional career in whatever field they desire.
DSc in Computer Science
This doctoral degree teaches students the theory and practical application of computer science in data science, application design, and computer architecture. It contains 20 courses, including artificial intelligence, risk analysis, and system metrics.
These courses are offered online and aim to impart students with the necessary skills for improving existing technology, as well as evaluating and applying them. It also contains courses that aid doctoral students in carrying out their research dissertations.
DSc in Computer Science Overview
- Accreditation: Distance Education Accrediting Commission
- Program Length: 5 years and 7 months
- Acceptance Rate: N/A
- Tuition and Fees: $375/month
DSc in Computer Science Admission Requirements
- Master’s degree
- Statement of goals
- Minimum of 3.0 GPA
- Must know about object-oriented development
Capitol Technology University was founded in 1927 and offers online programs at the undergraduate, graduate, and doctoral levels. The areas of study in which these online programs are offered include business, technology, and the field of engineering.
PhD in Artificial Intelligence
This is a research-based PhD program that offers students the opportunity to conduct research in any field of their choice. Throughout the program, student work must be approved by the academic supervisor. Students are to submit a thesis and give an oral presentation which will be supervised by an expert in the field.
PhD in Artificial Intelligence Overview
- Accreditation: Middle States Commission on Higher Education
- Program Length: 2 to 3 years
- Tuition and Fees: $933/credit
PhD in Artificial Intelligence Admission Requirements
- Application fee of $100
- Master’s degree in a relevant field
- Minimum of five years of related work experience
- Two recommendation letters
Founded in 1973, City University of Seattle is recognized as a top 10 educator of adults nationwide, as ranked by the US News & World Report for school rankings. It offers online undergraduate, graduate, and doctoral programs designed for working professionals
PhD in Information Technology
The program’s curriculum consists of courses in machine and deep learning. Candidates are given the option to propose their depth of study, which requires approval from the academic director. The program consists of core courses, concentration courses, a comprehensive examination, a research core, and a dissertation.
PhD in Information Technology Overview
- Accreditation: Northwest Commission on Colleges and Universities
- Program Length: Flexible
- Acceptance Rate: 100% due to open admission policy
- Tuition and Fees: $765/credit
PhD in Information Technology Admission Requirements
- A master’s degree from an accredited or recognized institution
- CV and resume, and three references letters
- Proof of English proficiency
- Interview with admissions advisor
- State goals related to your academic work
Founded in 1896 to honor Thomas S. Clarkson, Clarkson University offers flexible online degree programs at the undergraduate and graduate levels. It is a research university that leads in technology education.
PhD in Computer Science
This doctoral program places emphasis on areas such as artificial intelligence , software, security, and networking. Current students are required to complete 36 credits of computer science foundation and research-oriented courses, elective courses, achieve candidacy within the first two years of the program, and propose and defend a thesis.
PhD in Computer Science Overview
- Program Length: 3 years
- Tuition and Fees: $1,533/credit
PhD in Computer Science Admission Requirements
- Complete the online application form
- Resume, statement of purpose, and three letters of recommendation
- English proficiency test for international applicants (TOEFL, IELTS, PTE, and Duolingo English Test)
Northcentral University is a private university established in 1996 and is designed for flexibility by offering programs of distance learning for working professionals. It practices a distinctive one-to-one learning system and has a dedicated doctoral faculty.
In this doctorate program, besides writing papers about past research, students are allowed to propose their research. Its curriculum consists of subjects such as software engineering , artificial intelligence, data mining, and cyber security. Through the course, students conduct research and examine real-world issues in the field of computer science.
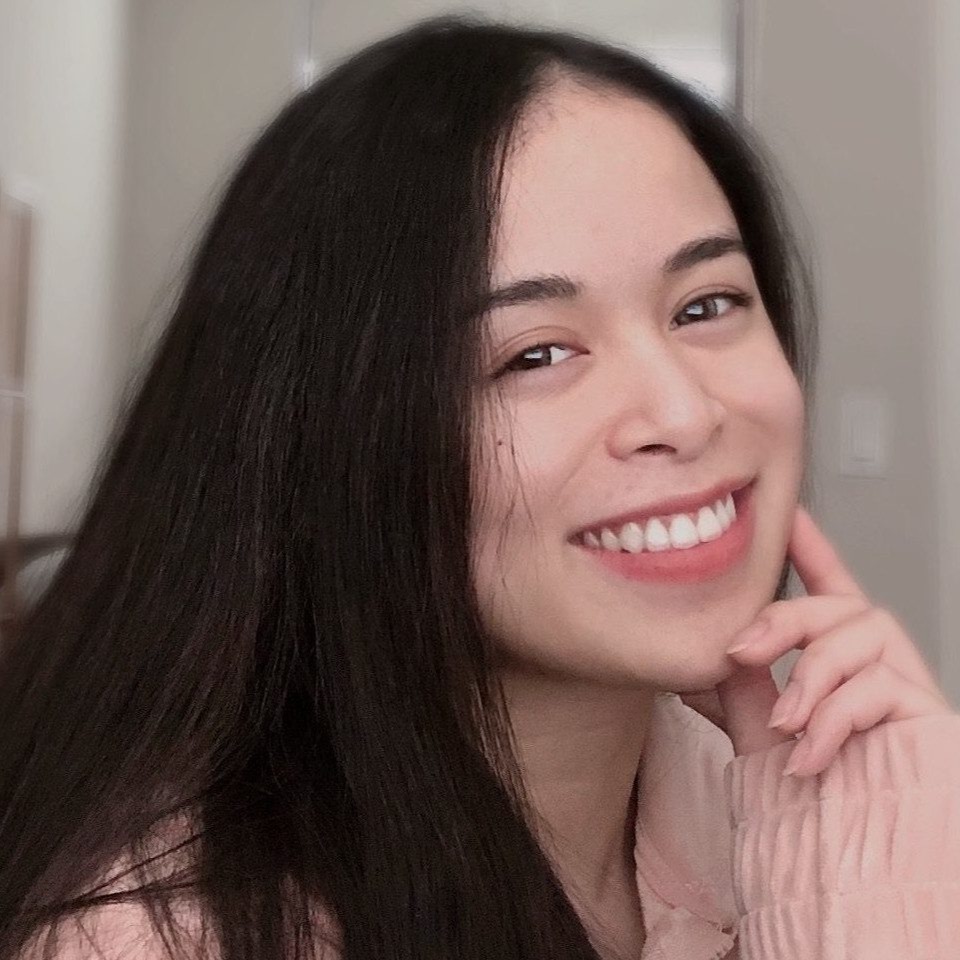
"Career Karma entered my life when I needed it most and quickly helped me match with a bootcamp. Two months after graduating, I found my dream job that aligned with my values and goals in life!"
Venus, Software Engineer at Rockbot
- Accreditation: WASC Senior College and University Commission
- Program Length: 3 years and 4 months
- Tuition and Fees: $1,094/credit
- Master’s degree from an accredited institution
- Official transcripts
- English proficiency exam score for international students
Nova Southeastern University was founded in 1964 in Fort Lauderdale, Florida. It offers online graduate and undergraduate courses and conducts a wide variety of interdisciplinary healthcare research. It is home to national athletics champions and Olympians.
This program provides research in computer science. Its format of learning combines both traditional and online instruction designed with consideration for working professionals . Its coursework consists of research in computer science areas, including cyber security, software engineering, and artificial intelligence.
- Accreditation: Southern Association of Colleges and Schools, Commission on Colleges
- Program Length: Not specified
- Tuition and Fees: $1,282/credit
- Online application and $50 application fee
- A bachelor’s or master’s degree in a relevant field from a regionally accredited institution
- GPA of at least 3.20
- Official transcripts from all institutions attended
- A resume
- Essay, and three letters of recommendation
The University of North Dakota was founded in 1883, six years before North Dakota was made a state. Today, it offers several academic programs in undergraduate, graduate, and doctoral fields and is known for conducting research in areas that include medicine, aerospace, and engineering.
This PhD in Computer Science curriculum consists of courses in machine learning, software engineering, applications of AI, computer forensics, and computer networks which benefit students by granting them proficiencies in areas such as artificial intelligence, compiler design, operating systems, simulation, databases, and networks.
- Accreditation: Higher Learning Commission
- Program Length: 4 to 5 years
- Tuition and Fees: $545.16/credit (in state); $817.73/ credit (out of state)
- Application fee of $35
- Master’s or bachelor’s degree in engineering or a related science field
- GPA of 3.0 on a 4.0 scale and GRE test score
- Official copy of all college and university academic transcripts
- Statement of academic goals and three letters of recommendation
- Expertise in a high-level programming language and basic knowledge of data structures, formal languages, computer architecture and OS, calculus, statistics, and linear algebra
- English language proficiency
The University of Rhode Island is a public research institution founded in 1892. It conducts extensive research in the field of science. It offers online, on-site, and hybrid programs at the graduate and undergraduate levels, as well as certificate programs.
In this PhD in Computer Science program, students are involved in research geared toward producing new intellectual and innovative contributions to the field of computer science. It offers a combination of on-campus, online, and day and evening classes. It consists of courses in machine learning, artificial intelligence, software engineering, and systems simulation.
- Accreditation: New England Commission of Higher Education
- Program Length: 4 years
- Tuition and Fees: $14,454/year (in-state); $27,906/ year (out of state)
- An undergraduate degree from a regionally accredited institution in the US
- A minimum GPA of 3.0
- All official college transcripts
- Personal statement
- An application fee of $65
Founded in 1888 by Baptist ministers in Williamsburg KY, today the University of the Cumberlands offers online master's and doctoral degree programs in the fields of education, information technology, and business.
The program requires 18 credit hours of core courses which include information technology geared toward creating machine learning engineers . Its curriculum focuses on predictive analytics and other skills students need to become experts in cyber crime security, big data, and smart technologies.
Students have the option to specialize in information systems security, information technology, digital forensics, or blockchain technologies. Students will complete 21 credit hours of professional research while working toward a dissertation.
- Tuition and Fees: $500/credit
- A master’s degree from a regionally accredited institution
- TOEFL for non-native English speakers
- Application fee of $30
Wright State University was first seen in 1964 as a branch campus for Ohio State University and Miami University. It is a Carnegie classified research university and offers research at the undergraduate, graduate, and doctoral levels.
PhD in Computer Science and Engineering
This degree is awarded to students who show excellence in study and research that significantly contributes to the field of computer science and engineering. The degree requirements include an A grade completion of the core coursework in two areas and at least a B in the third.
Students are to complete a minimum of 18 hours of residency research before taking the candidacy exam, which must be completed with a satisfactory grade. Also, a minimum of 12 hours of dissertation research is needed before the dissertation defense, which has to be approved.
PhD in Computer Science and Engineering Overview
- Program Length: 10 years time limit
- Tuition and Fees: $660/credit (in state); $1,125/ credit (out of state)
- Bachelor’s or master’s degree in a related discipline (computer science or engineering)
- Minimum GPA of 3.0 if admitted with a bachelor’s degree or 3.3 with a master’s degree
- GRE general test portion
- TOEFL score for non-native English speakers
- Knowledge of high-level programming languages, computer organization, operating systems, data structures, and computer systems design
- A record that indicates potential for a career in research
Online Machine Learning PhD Graduation Rates: How Hard Is It to Complete an Online PhD Program in Machine Learning?
It is very hard to complete an online PhD in Machine Learning. According to a paper published in the International Journal of Doctoral Studies, there is a PhD attrition rate of 50 percent in the US within the past 50 years. Therefore, the graduation rate for doctorate students is approximately 50 percent.
How Long Does It Take to Get a PhD in Machine Learning Online?
It takes about four years to get a PhD in Machine Learning online, which is fast when compared to a traditional in-person PhD program which may take over seven years to complete. Online PhD programs are accelerated by default, so the curriculum focuses on the major needs of a PhD graduate in the areas of research, thesis, and dissertation.
Students may be able to reduce the time spent pursuing a PhD in Machine Learning by first acquiring a master’s degree in the field. If you choose to pursue a PhD on a part-time schedule as opposed to full-time study, it will significantly increase the time it takes to acquire the degree.
How Hard Is an Online Doctorate in Machine Learning?
Getting an online doctorate in machine learning is very hard, as are most graduate programs. Besides the rigorous research, strict requirements, deadlines, qualification examinations, and dissertations, other challenges may exist, such as limited student connection with the faculty members, isolation, financial issues, and lack of an adequate work-life balance .
Getting a doctorate in any field is not easy. In fact, there is research to suggest that online doctorate students face challenges regarding culture and academia. As a result of these challenges, many students drop out from their PhD programs.
Best PhD Programs
[query_class_embed] phd-in-*subject
What Courses Are in an Online Machine Learning PhD Program?
The courses in an online machine learning PhD program include an introduction to machine learning and deep learning, artificial intelligence, statistical theories, data mining , system simulation, computer programming, and software development.
Main Areas of Study in a Machine Learning PhD Program
- Machine learning
- Deep learning
- Artificial intelligence
- Databases and data mining
- Statistical theory
- Software engineering
- Systems simulation
How Much Does Getting an Online Machine Learning PhD Cost?
On average, it costs $19,314 per year to get a PhD in Machine Learning, according to the National Center of Education Statistics (NCES). However, this figure is not fixed, as the total tuition for a PhD program varies from school to school.
Private institutions generally cost more than public institutions, but there are funding opportunities for PhD students. Some PhD programs may guarantee financial aid for all their students regardless of merit.
How to Pay for an Online PhD Program in Machine Learning
You can pay for an online PhD in Machine Learning by taking advantage of student loans, scholarships, grants, teaching and research assistantships, graduate assistantships, and fellowship assistantships. As a result, most PhD students spend less than the tuition fee displayed on a school’s website.
How to Get an Online PhD for Free
You cannot get an online PhD in Machine Learning for free. However, there are ways to reduce the cost, or get partial tuition discounts and stipends through graduate assistantships, fellowships, scholarships, or grants.
What Is the Most Affordable Online PhD in Machine Learning Degree Program?
The most affordable online PhD in Machine Learning based on cost per credit is at Aspen University in Phoenix, Arizona. It charges $375 per month, which, when multiplied by the 67 months it takes to complete the program, results in a total of $25,125 for the entire program. This is more affordable compared to a school like Clarkson University, which charges $1,533 per credit hour.
Most Affordable Online PhD Programs in Machine Learning: In Brief
Why you should get an online phd in machine learning.
You should get an online PhD in Machine Learning because having a PhD offers you a stronger advantage in terms of employability, salary, and in your career in general that would otherwise be unavailable with just a bachelor’s and master’s degree.
Top Reasons for Getting a PhD in Machine Learning
- Research opportunities. PhD students get the opportunity to be involved in rigorous and innovative research that may positively impact humanity, add to the world’s knowledge, and improve the lives of others.
- Expertise development. A PhD is the highest level of academic degree, and as a result, PhD holders have expert-level knowledge in whichever field they acquire a PhD in. However, it is advised to only get a PhD if you are very interested in the field and willing to explore your interest and expand your understanding through cutting-edge research.
- Access to better jobs. There are lots of bachelor’s and master’s degree graduates in the job market, and earning a PhD will help you stick out from the crowd. A PhD reveals career opportunities that may not be available to bachelor’s and master’s degree grads.
- Networking opportunities . During a PhD program, students are in contact with top lecturers and academic experts by attending guest lectures, conferences, seminars, and workshops. Students can network with colleagues and classmates, which helps put them in a good position after their academic journey.
Best Master’s Degree Programs
[query_class_embed] *subject-masters-degrees
What Is the Difference Between an On-Campus Machine Learning PhD and an Online PhD in Machine Learning?
The difference between an on-campus machine learning PhD and an online PhD in Machine Learning is primarily the mode of learning. Online PhDs are as rigorous and effective as their on-campus counterparts.
However, there may be some slight differences between the two in terms of cost, schedule, quality, and funding. Some of the differences that may exist are discussed below.
Online PhD vs On-Campus PhD: Key Differences
- Affordability. An online PhD is more affordable compared to the traditional on-campus alternative. An on-campus PhD can cost as much as $30,000 per year, while an online PhD may be as low as $20,000 per year.
- Flexibility. Online PhD students have the liberty to conduct in-depth study and research at their own time as opposed to the schedule of an in-person PhD program. Moreover, most online PhD programs don’t have an enrollment date, and some online PhD work is asynchronous, meaning students can take classes from anywhere at their convenience.
- Quality. Traditionally acquired PhDs are thought to be superior to their online counterparts by some employers and academics, probably due to sentiment. However, the quality of an online PhD is dependent on the research subject, the school’s reputation, and accreditation.
- Availability of funding. Funding available for online PhD programs may be limited due to some geographical constraints. For example, online PhD students cannot take up teaching assistantship positions unless they are willing to be physically present.
How to Get a PhD in Machine Learning Online: A Step-by-Step Guide
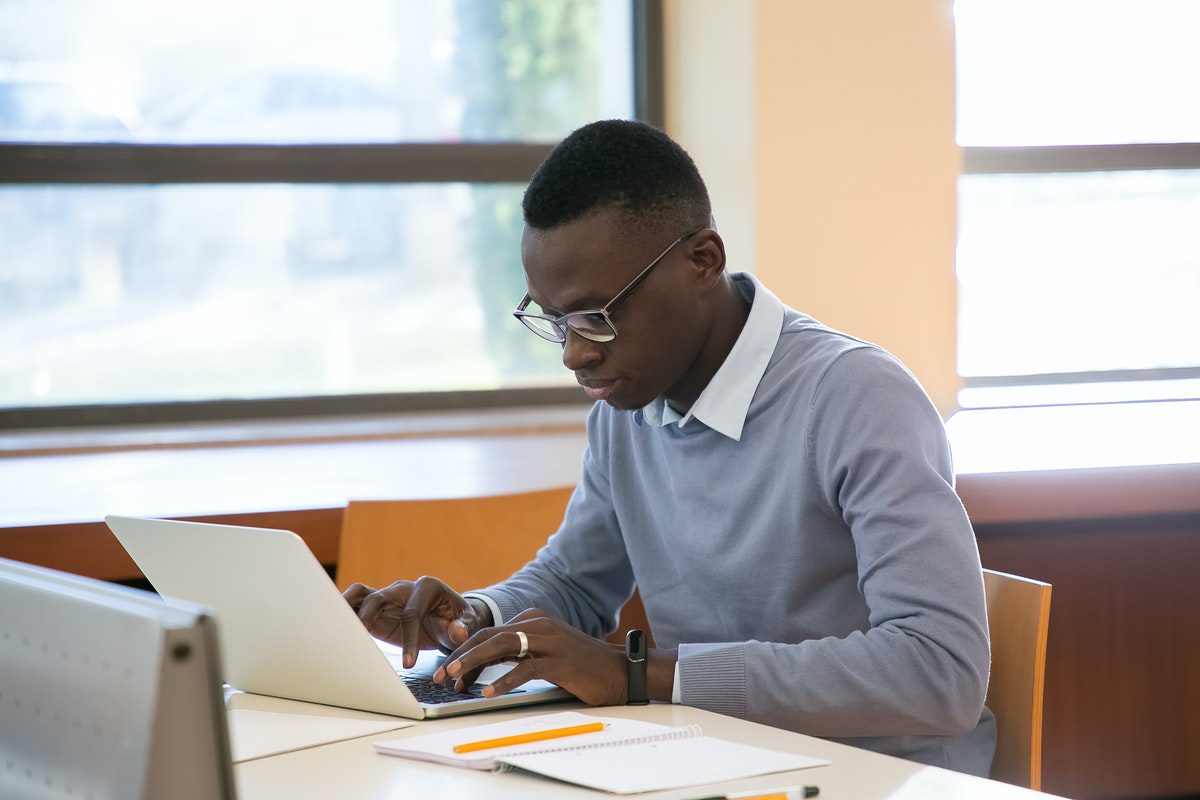
To get a PhD in Machine Learning, you need to first apply online to a PhD program. If accepted, you must enroll in the required classes and complete the academic coursework, research, and a series of academic milestones, which include attaining candidacy, passing the qualification examinations, proposing, writing, and defending your dissertation.
To begin your journey to acquiring a PhD in Machine Learning, you first need to apply online to the school of your choice. You also need to fulfill the admission requirements, including possessing a master's or bachelor's degree–depending on the school–in a relevant field, a minimum grade point average, letters of recommendation, and GRE test scores .
Many online PhD programs require students to take and pass a minimum number of credit hours in core and elective courses. A typical online PhD in Machine Learning program consists of about 70 to 90 credit hours that involve intensive research in a provided or chosen area of concentration.
Obtaining a PhD in Machine Learning allows an individual to become a world-renowned expert in the field. After completing a rigorous course of study and passing a series of exams, the doctoral candidate would then undertake an original research project that contributes new knowledge to the field. Upon successful completion of the degree, the graduate would be able to pursue a career in academia or industry.
Examinations are an essential part of any education. They test a student's understanding of the material and help them to learn and remember the information. If you want to earn a machine learning PhD, you must pass the examinations for various core and required courses. Then, you will need to complete and defend your dissertation.
A dissertation is a research paper that is submitted to and defended by a graduate student to earn a graduate degree. To graduate with a PhD in Machine Learning, you are required to write a dissertation on a topic related to machine learning. Your doctoral dissertation must demonstrate your knowledge and understanding of the field of machine learning, as well as your ability to conduct original research in the field.
Online PhD in Machine Learning Salary and Job Outlook
The job outlook for machine learning jobs is 22 percent between 2020 and 2030 , with the number of new jobs expected in this time frame being 7,200, according to the US Bureau of Labor Statistics. The average salary for computer and information research scientists, which is a category that machine learning professionals belong to, is $131,490 per year .
What Can You Do With an Online Doctorate in Machine Learning?
With an online doctorate in machine learning, you can qualify for specialization roles and lead machine learning positions, including senior machine learning engineer and computer research scientist.
Depending on your preferences, you may also opt for a research and academic career path to become a university professor. The list below is a list of the best jobs for PhD in Machine Learning graduates.
Best Jobs with a PhD in Machine Learning
- Senior Machine Learning Engineer
- Computer and Information Research Scientist
- Data Scientist
- Software Engineer
- Postsecondary Teacher
Potential Careers With a Machine Learning Degree
[query_class_embed] how-to-become-a-*profession
What Is the Average Salary for an Online PhD Holder in Machine Learning?
The average salary for a PhD in Machine Learning holder is $108,000 per year , according to PayScale’s salary for skills in machine learning. The average salary a PhD holder receives depends on the location and position you apply for.
Highest-Paying Machine Learning Jobs for PhD Grads
Best machine learning jobs for online phd holders.
The best machine learning jobs for online PhD holders are typically high-paying jobs that require advanced-level skills that coincide with the nature of the position they undertake. Below are some typical job titles that online machine learning PhD degree holders assume.
A senior machine learning engineer oversees a team of machine engineers charged with designing and developing effective machine learning and deep learning solutions implemented in machine learning systems.
- Salary with a Machine Learning PhD: $153,255
- Job Outlook: 22% job growth from 2020 to 2030
- Number of Jobs: 33,000
- Highest-Paying States: Oregon, Arizona, Texas
Computer and information research scientists research and develop new ways of solving complex computing problems and apply existing technology. They work to significantly increase the knowledge in the field of computer science, which will aid in the production of more efficient software and hardware technologies.
- Salary with a Machine Learning PhD: $131,490
A senior data scientist is responsible for developing data mining and machine learning techniques to solve complex business problems. They identify patterns and trends in large data sets, develop models to improve forecasting and decision making, and effectively communicate data-driven insights to non-technical stakeholders and lead a team of data analysts.
- Salary with a Machine Learning PhD: $127,455
A software engineer is a professional that develops and maintains software. They work on a variety of software, from operating systems to video games, and may be involved in the development of websites. They must also have an excellent understanding of computer programming languages and be able to solve complex problems.
- Salary with a Machine Learning PhD: $121,115
- Number of Jobs: 1,847,900
- Highest-Paying States: Washington, California, New York
Postsecondary teachers are in charge of lecturing students in colleges and universities. They are also responsible for instructing adults in several academic and non-academic subjects including career, work, and research.
- Salary with a Machine Learning PhD: $79,640
- Job Outlook: 12% job growth from 2020 to 2030
- Number of Jobs: 1,276,900
- Highest-Paying States: California, Oregon, District of Columbia
Is It Worth It to Do a PhD in Machine Learning Online?
Yes, it is worth it to do a PhD in Machine Learning online. Getting a PhD is not for everyone, as the process will require tremendous effort and discipline, but it can be rewarding. A PhD in Machine Learning online allows you to learn from some of the best minds in the field.
You can also specialize in an area of your choice, such as big data, natural language processing, or deep learning. Specializing in one area for your PhD in Machine Learning allows you to deep-dive into that subject and build doctorate-level expertise.
An online PhD in Machine Learning provides students with the same high-quality education as a traditional PhD but with more flexibility and affordability. You’ll have access to top-notch instructors, state-of-the-art technology, and a thriving online community of experts.
Additional Reading About Machine Learning
[query_class_embed] https://careerkarma.com/blog/machine-learning/ https://careerkarma.com/blog/best-machine-learning-bachelors-degrees/ https://careerkarma.com/blog/best-machine-learning-masters-degrees/
Online PhD in Machine Learning FAQ
Yes, you should get an online PhD in Machine Learning if it is critical for your career prospects. An online PhD in Machine Learning allows you to learn at your own pace and keep your day job while you pursue your degree. In the end, it sets you up for the highest-earning jobs in the machine learning industry , with better pay and a larger professional network.
The type of research you will carry out as a machine learning student includes research in deep learning, neural networks , machine learning algorithms, supervised and unsupervised machine learning, predictive learning, and computer vision. Students will make use of quantitative and experimental methods of research as well as the use of optimal feature selection.
You can choose a concentration for an online machine learning PhD by factoring in your interests, strengths, and career goals. You may also consider recent trends, the average salary of machine learning professionals , or the career options the machine learning industry has to offer when choosing a machine learning concentration.
Examples of online machine learning PhD dissertations include experimental quantum speed-up in reinforcement learning agents, improving automated medical diagnosis systems with machine learning technologies, regulating deep learning and robotics, and the use of machines and robotics in medical procedures.
About us: Career Karma is a platform designed to help job seekers find, research, and connect with job training programs to advance their careers. Learn about the CK publication .
What's Next?

Get matched with top bootcamps
Ask a question to our community, take our careers quiz.
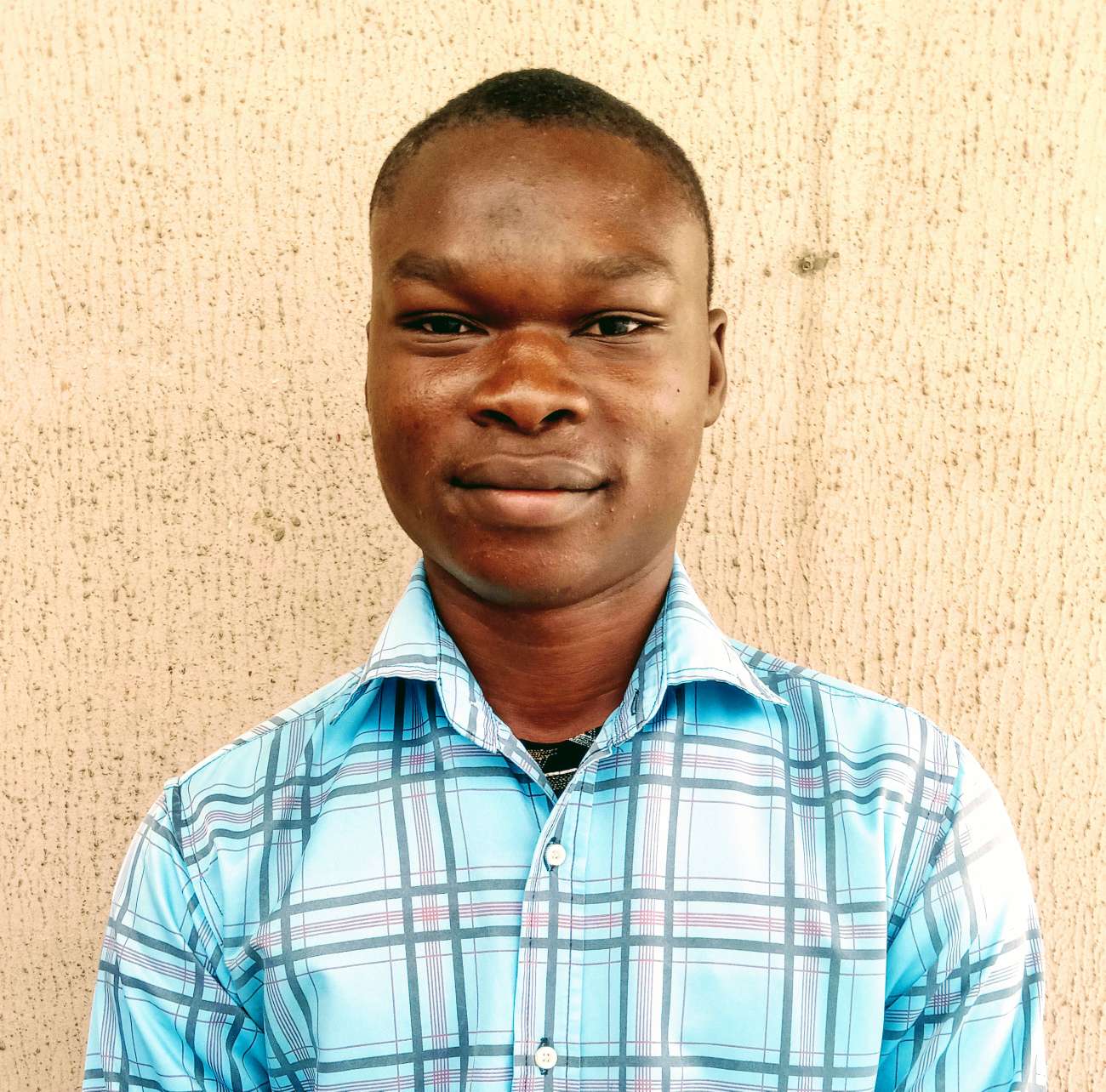
Leave a Reply Cancel reply
Your email address will not be published. Required fields are marked *
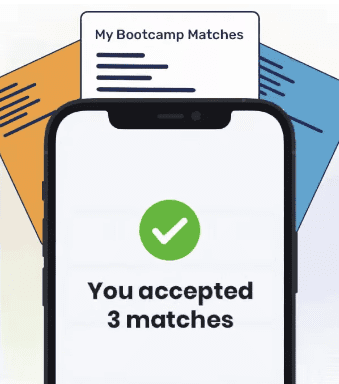
PhD Assistance
Recent phd topics in artificial intelligence 2023.
Artificial intelligence (AI) is expanding rapidly, and its applications are becoming more common in various sectors. As a result, researchers are always looking for new methods to improve AI algorithms and implementations. With the emergence of new technology and approaches, academics are researching novel artificial intelligence study subjects to progress the discipline even further.
This blog will look at the latest PhD research topics in artificial intelligence for 2023. These subjects include a combination of practical and theoretical challenges that might help influence the future of artificial intelligence. Let’s delve into AI research, from natural language processing to autonomous robots.
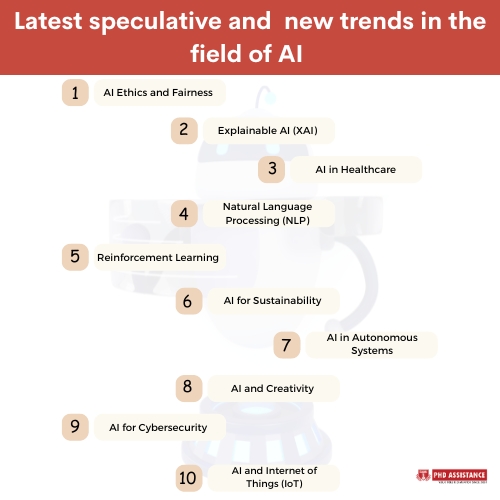
Introduction
What is PhD topic in Artificial Intelligence?
A PhD topic in Artificial Intelligence involves advanced research and exploration within the realm of AI. It encompasses a wide array of subjects, such as machine learning , natural language processing, computer vision, robotics, and neural networks selection of project topic introduction. Doctoral candidates delve into cutting-edge techniques, developing innovative algorithms and seeking novel applications to address complex challenges. These topics push the boundaries of AI, contributing to its growth and impact on various industries. From enhancing decision-making processes to enabling autonomous systems and tackling ethical considerations, AI PhD Topic selection paves the way for groundbreaking advancements shaping technology and society’s future.
However, some potential areas might be of interest and relevance in the field of AI in 2023. Keep in mind that these are speculative and that new trends may have emerged since my last update:
- AI Ethics and Fairness : With the increasing integration of AI in various domains, there’s a growing concern about ethical issues, bias, and fairness. Dissertation topics in English literature might focus on developing AI models that are more transparent, accountable, and unbiased.
- Explainable AI (XAI) : Explainability remains a crucial challenge in AI. Research in this area could explore methods and techniques to make AI models more interpretable and provide understandable explanations for their decisions.
- AI in Healthcare : AI has great potential to revolutionize healthcare . Research might delve into areas like medical image analysis, personalized treatment plans, drug discovery, and AI-assisted diagnostics.
- Natural Language Processing (NLP) : NLP continues to be a significant area of research. The focus could be improving language understanding, machine translation, sentiment analysis, and dialogue systems.
- Reinforcement Learning : Advancements in reinforcement learning have shown promise in various fields, such as robotics and gaming. Dissertation topics could explore more efficient algorithms and real-world applications.
- AI for Sustainability : AI can be critical in addressing environmental and sustainability challenges. PhD research might use interesting artificial intelligence (AI) topics to optimize resource management, climate modelling, and sustainability-driven decision-making.
- AI in Autonomous Systems : The development of autonomous vehicles and drones has accelerated, and research could focus on enhancing their safety, decision-making capabilities, and robustness.
- AI and Creativity : Exploring AI’s potential in creative domains like art, music, and storytelling could be a fascinating area of research.
- AI for Cybersecurity : As cyber threats evolve, AI can be leveraged to detect and mitigate attacks. Research might concentrate on building more robust and adaptive cybersecurity systems.
- AI and Internet of Things (IoT) : The integration of AI with IoT devices is becoming more prevalent. PhD research design might look into AI-enabled IoT applications, security concerns, and optimizing IoT systems using AI.
Remember that these are just general topics, and PhD research requires a more specific and well-defined research question within the chosen domain. To get the most recent and relevant information, I recommend checking the latest academic journals, conference proceedings, and university websites for updates on AI research topics in 2023.
- Check out our Sample Topic selection for the Project to see how the PhD topic selection is constructed.
Top 10 research topics for artificial intelligence in 2023
- Natural Language Processing (NLP)
- Computer Vision
- Deep Learning
- Reinforcement Learning
- Generative Adversarial Networks (GANs)
- Explainable AI (XAI)
- Autonomous Robotics
- Ethics in AI
- Quantum Computing for AI
- Edge Computing for AI
- Check out our study guide to learn more about PhD Topic selection. How do you choose a topic for your PhD research?
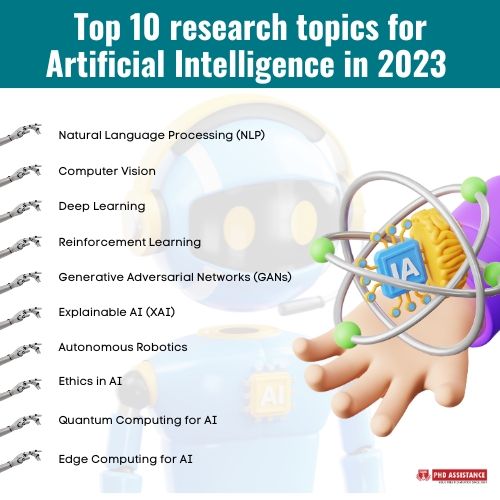
Important Points:
- Natural language processing (NLP) studies how computers perceive and interpret human language.
- Computer vision is the process of teaching machines how to recognize and understand pictures and movies.
- Deep learning is a subset of machine learning in which artificial neural networks are trained using massive volumes of data.
- Reinforcement learning is a sort of machine learning in which an agent is trained to make decisions based on incentives and penalties.
- GANs are a neural network that uses existing data to create new data.
- XAI aims to make AI more transparent and understandable to humans.
- Autonomous robotics is the development of robots that can function autonomously without human intervention.
- AI ethics is concerned with the proper development and application of AI technology.
- Quantum computing is an emerging field.
The 2023 PhD topics in Artificial Intelligence highlight the dynamic field’s growth and promise for revolutionizing industries and improving quality of life. The research emphasizes ethical AI, addressing bias, fairness, and transparency. Advancements in natural language processing make AI more accessible and intuitive. AI-driven approaches revolutionize decision-making, data analysis , and predictive modelling in healthcare, finance, and environmental sciences. Novel AI architectures, such as quantum-based and neuro-symbolic systems, demonstrate efficient algorithms and power.
Integrating AI in robotics and autonomous systems redefines machine interaction, with implications for automation, manufacturing, and transportation. Collaboration between academia, industry, and policymakers is crucial for responsible and ethical ai research topics for beginners in technology development.
About PhD Assistance
PhD Assistance , writers and researchers have extensive expertise in selecting the best topic and title for a PhD dissertation based on their specialization and personal interests. Furthermore, our specialists are drawn from international and top-ranked colleges in nations such as the United States, the United Kingdom, and India. Our authors have the expertise and understanding to choose a PhD research subject that is appropriate for your study and a catchy title that surely fits your research aim.
- Holmes, Wayne, Maya Bialik, and Charles Fadel. “Artificial intelligence in education.” Globethics Publications, 2023. 621-653. Doi: 58863/20.500.12424/4273108
- Bermejo, Belen, and Carlos Juiz. “Improving cloud/edge sustainability through artificial intelligence: A systematic review.” Journal of Parallel and Distributed Computing (2023). Doi: 1016/j.jpdc.2023.02.006
- Cerchia, Carmen, and Antonio Lavecchia. “New avenues in artificial-intelligence-assisted drug discovery.” Drug Discovery Today (2023): 103516. Doi: 1016/j.drudis.2023.103516
- AI research topics for beginners
- artificial intelligence topics
- artificial intelligence topics for Project
- artificial intelligence topics for research paper
- dissertation topics
- dissertation topics in education
- dissertation topics in English literature
- interesting topics in artificial intelligence
- PhD research topics in artificial intelligence
- PhD Topic Selection
- selection of project topic introduction
- thesis topics
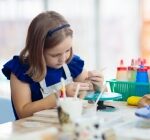
Quick Contact
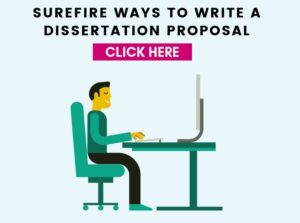
- Adversial Attacks
- Artificial Intelligence
- Artificial Intelligence (AI) and ML ( Machine Learning )
- Big Data Analysis
- Business and Management
- Categories of Research methodology – PhDAssistance
- Category of Research Proposal Services
- coding & algorithm
- Computer Data Science
- Category of Machine Learning – PhDassistance
- Computer Science/Research writing/Manuscript
- Course Work Service
- Data Analytics
- Data Processing
- Deep Networks
- Dissertation Statistics
- economics dissertation
- Editing Services
- Electrical Engineering Category
- Engineering & Technology
- finance dissertation writing
- Gap Identification
- Healthcare Dissertation Writing
- Intrusion-detection-system
- journals publishing
- Life Science Dissertation writing services
- literature review service
- Machine Learning
- medical thesis writing
- Peer review
- PhD Computer Programming
- PhD Dissertation
- Phd Journal Manuscript
- Annotated Bibliography
- PhD Publication Support
- Phd thesis writing services
- Phd Topic Selection
- Categories of PhdAssistance Dissertation
- Power Safety
- problem identification
- Quantitative Analysis
- quantitative research
- Recent Trends
- Research Gap
- research journals
- Research Methodology
- research paper
- Research Proposal Service
- secondary Data collection
- Statistical Consulting Services
- Uncategorized
PhD Assistance | Blog
Machine Learning Research Topics for PhD
Achieving a Ph.D. in machine learning provides a chance to gain skills in both basic ideas and new improvements. While choosing a project concept, it is very essential to think about the upcoming approach and possible societal effects in addition to the latest research techniques. More than 200+ Machine Learning experts are there in phddirection.com to guide in what encounters that you may face in your research process. If you are reading this it means that you are in need for topics help. Have one to one discussion with our experts to get innovative topic ideas as per your specifications.
Here we describe various Ph.D.-based machine learning project ideas:
- Foundational Theories in ML:
- Generalization & Overfitting: In this, we interpret the subject-based knowledge of why deep neural networks generalize efficiently even with a large number of parameters.
- Optimization in Deep Learning: Our work explores the factors of optimization methods such as SGD and aspects of loss functions in non-convex platforms.
- Explainability & Understandability:
- Transparent Techniques: We build efficiently understandable ML techniques, specifically deep learning.
- Post-hoc Explanation Methods: After the black-box frameworks carried out the forecasting process, we develop techniques to produce explanations for the framework.
- Fairness, Accountability & Transparency:
- Bias Reduction: To identify and rectify biases in ML frameworks to check the fairness among various groups, we create methods.
- Moral Considerations: Our work explores the wider moral considerations of automatic decision-making.
- Reinforcement Learning :
- Deep Meta-learning: In this, we utilize methods where the models learn the learning procedures and altering rapidly to new tasks.
- Multi-agent Systems: When there are various models communicating in a platform, our research intends to explore the aspects and learning techniques.
- Efficient ML:
- Model Compression & Distillation: To minimize the dimension of our framework while ensuring its efficiency, we utilize various approaches.
- On-device ML: For limited-resource devices such as IoT devices or smartphones, our research optimizes methods.
- Neurosymbolic Computing :
- Integrated Frameworks: To overcome the issue among connectionist and symbolic AI approaches, we combine deep learning with symbolic reasoning.
- Out-of-distribution Generalization:
- We generalize the data that are not efficiently defined in the training set by exploring the capacity of our framework.
- Graph Neural Networks :
- Scalability: To effectively manage huge graphs, we aim to create GNNs.
- Dynamic Graph Learning: In this, our framework learns and alters to graphs that modify periodically.
- Transfer Learning & Domain Adaptation:
- To enhance the efficiency of one field or task, our project utilizes methods to alter the skills from another related field or task.
- Quantum Machine Learning :
- We investigate how quantum computing can improve machine learning methods and others.
- Multimodal Learning :
- Continuously, our techniques combine and reason beyond data from various sources such as images, text and audio.
- Neural Architecture Search:
- Efficient Search Plans: In this, we minimize the computational expense of examining for best network architectures.
- Privacy-preserving:
- Homomorphic Encryption: By using this, our work carries out the training and interpretation on encrypted data.
- Differential Privacy: By concatenating noise to computations, we make sure about data confidentiality.
- Self-supervised & Unsupervised Learning:
- Representation Learning: Without utilizing labeled data, we find out the potential data presentations.
- Contrastive Learning: By distinguishing positive and negative instances, our approaches learn embeddings.
- Bio-inspired:
- Neuromorphic Computing: We develop methods and hardware motivated by the design and task of the brain.
- Evolutionary Techniques: To optimize ML frameworks, our work utilizes algorithms motivated by natural development.
- Causal Inference:
- Causal Representation Learning : It is about learning of representations that not only capture correlations but also capture causal connections.
It is very significant to consider the following factors while choosing a concept for Ph.D. research:
- Find out domain experts or professionals with our selecting field-based knowledge.
- Check whether we have accessibility to required datasets and resources.
- Make sure that the selected concept relates with our passion and long-term professional objective.
We conclude that the Ph.D. research must intend to enhance the domain by solving important issues or problems in skills.
By following the above factors, we attain a 100% success in your work. Professional thesis support and help will be assisted in all your research needs. Thesis editing is also done by our editing department along with description. More than 120+ countries scholars we have provided best Machine Learning Research Topics for PhD and all have gained entire success. We are updated on trending technologies constantly and have huge resources to finish of the work.
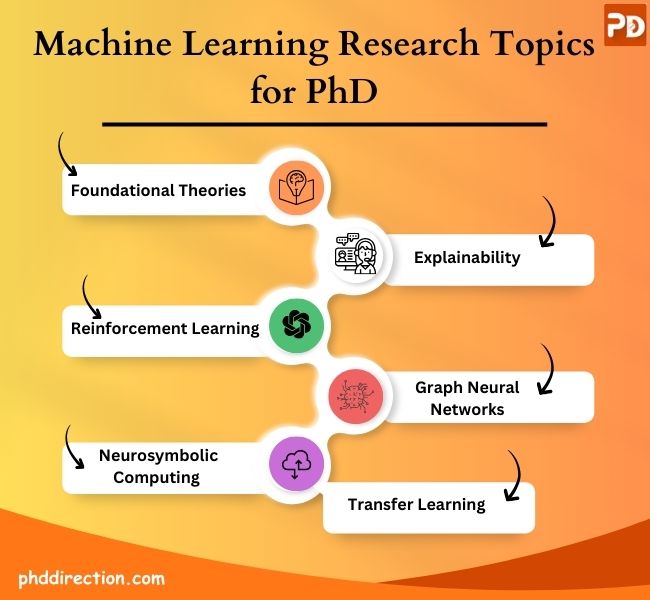
PhD Projects In Machine Learning
Wide varieties of PhD Projects in Machine Learning topics are covered by phddirection.com under machine learning. For both masters and doctorate degree students our team provide the best research service as per your needs. No matter where you are struck up with our lead technicians will follow the latest methodologies, correct algorithms and pattern and finish of your PhD Projects in Machine Learning with prospective research work.
- Machine Learning Algorithm in Network Traffic Classification
- On the Integration of Machine Learning and Array Databases
- Intrusion Detection of Imbalanced Network Traffic Based on Machine Learning and Deep Learning
- Sentiment Analysis on IMDB Movie Reviews using Machine Learning and Deep Learning Algorithms
- Horizon Detection Using Machine Learning Techniques
- Machine learning contributions on the field of security and privacy of android
- Performance Evaluation of Machine Learning Algorithms for Credit Card Fraud Detection
- Comparison of text sentiment analysis based on traditional machine learning and deep learning methods
- Advanced Machine Learning Scenarios for Real World Applications using Weka Platform
- A 4-way Matrix Multiply Unit for High Throughput Machine Learning Accelerator\
- A Intrusion Detection Algorithm Based on Improved Slime Mould Algorithm and Weighted Extreme Learning Machine
- IoT Botnet Malware Classification Using Weka Tool and Scikit-learn Machine Learning
- Understanding the learning of disabled students: An exploration of machine learning approaches
- Probabilistically shaped signaling and machine learning detection for optical interconnection
- Evaluation of Principal Component Analysis Algorithm for Locomotion Activities Detection in a Tiny Machine Learning Device
- Applications of Hybrid Machine Learning for Improved Content Based Image Classification
- Credit Card Fraud Detection Using Machine Learning Techniques
- Knowledge acquisition through machine learning: minimising expert’s effort
- Interpretable Machine Learning Techniques for Causal Inference Using Balancing Scores as Meta-features
- Research on Constant Power Loads Stability of DC Microgrid Based on Machine Learning
Why Work With Us ?
Senior research member, research experience, journal member, book publisher, research ethics, business ethics, valid references, explanations, paper publication, 9 big reasons to select us.
Our Editor-in-Chief has Website Ownership who control and deliver all aspects of PhD Direction to scholars and students and also keep the look to fully manage all our clients.
Our world-class certified experts have 18+years of experience in Research & Development programs (Industrial Research) who absolutely immersed as many scholars as possible in developing strong PhD research projects.
We associated with 200+reputed SCI and SCOPUS indexed journals (SJR ranking) for getting research work to be published in standard journals (Your first-choice journal).
PhDdirection.com is world’s largest book publishing platform that predominantly work subject-wise categories for scholars/students to assist their books writing and takes out into the University Library.
Our researchers provide required research ethics such as Confidentiality & Privacy, Novelty (valuable research), Plagiarism-Free, and Timely Delivery. Our customers have freedom to examine their current specific research activities.
Our organization take into consideration of customer satisfaction, online, offline support and professional works deliver since these are the actual inspiring business factors.
Solid works delivering by young qualified global research team. "References" is the key to evaluating works easier because we carefully assess scholars findings.
Detailed Videos, Readme files, Screenshots are provided for all research projects. We provide Teamviewer support and other online channels for project explanation.
Worthy journal publication is our main thing like IEEE, ACM, Springer, IET, Elsevier, etc. We substantially reduces scholars burden in publication side. We carry scholars from initial submission to final acceptance.
Related Pages
Our benefits, throughout reference, confidential agreement, research no way resale, plagiarism-free, publication guarantee, customize support, fair revisions, business professionalism, domains & tools, we generally use, wireless communication (4g lte, and 5g), ad hoc networks (vanet, manet, etc.), wireless sensor networks, software defined networks, network security, internet of things (mqtt, coap), internet of vehicles, cloud computing, fog computing, edge computing, mobile computing, mobile cloud computing, ubiquitous computing, digital image processing, medical image processing, pattern analysis and machine intelligence, geoscience and remote sensing, big data analytics, data mining, power electronics, web of things, digital forensics, natural language processing, automation systems, artificial intelligence, mininet 2.1.0, matlab (r2018b/r2019a), matlab and simulink, apache hadoop, apache spark mlib, apache mahout, apache flink, apache storm, apache cassandra, pig and hive, rapid miner, support 24/7, call us @ any time, +91 9444829042, [email protected].
Questions ?
Click here to chat with us

New machine learning algorithm promises advances in computing
Digital twin models may enhance future autonomous systems.
Systems controlled by next-generation computing algorithms could give rise to better and more efficient machine learning products, a new study suggests.
Using machine learning tools to create a digital twin, or a virtual copy, of an electronic circuit that exhibits chaotic behavior, researchers found that they were successful at predicting how it would behave and using that information to control it.
Many everyday devices, like thermostats and cruise control, utilize linear controllers -- which use simple rules to direct a system to a desired value. Thermostats, for example, employ such rules to determine how much to heat or cool a space based on the difference between the current and desired temperatures.
Yet because of how straightforward these algorithms are, they struggle to control systems that display complex behavior, like chaos.
As a result, advanced devices like self-driving cars and aircraft often rely on machine learning-based controllers, which use intricate networks to learn the optimal control algorithm needed to best operate. However, these algorithms have significant drawbacks, the most demanding of which is that they can be extremely challenging and computationally expensive to implement.
Now, having access to an efficient digital twin is likely to have a sweeping impact on how scientists develop future autonomous technologies, said Robert Kent, lead author of the study and a graduate student in physics at The Ohio State University.
"The problem with most machine learning-based controllers is that they use a lot of energy or power and they take a long time to evaluate," said Kent. "Developing traditional controllers for them has also been difficult because chaotic systems are extremely sensitive to small changes."
These issues, he said, are critical in situations where milliseconds can make a difference between life and death, such as when self-driving vehicles must decide to brake to prevent an accident.
The study was published recently in Nature Communications.
Compact enough to fit on an inexpensive computer chip capable of balancing on your fingertip and able to run without an internet connection, the team's digital twin was built to optimize a controller's efficiency and performance, which researchers found resulted in a reduction of power consumption. It achieves this quite easily, mainly because it was trained using a type of machine learning approach called reservoir computing.
"The great thing about the machine learning architecture we used is that it's very good at learning the behavior of systems that evolve in time," Kent said. "It's inspired by how connections spark in the human brain."
Although similarly sized computer chips have been used in devices like smart fridges, according to the study, this novel computing ability makes the new model especially well-equipped to handle dynamic systems such as self-driving vehicles as well as heart monitors, which must be able to quickly adapt to a patient's heartbeat.
"Big machine learning models have to consume lots of power to crunch data and come out with the right parameters, whereas our model and training is so extremely simple that you could have systems learning on the fly," he said.
To test this theory, researchers directed their model to complete complex control tasks and compared its results to those from previous control techniques. The study revealed that their approach achieved a higher accuracy at the tasks than its linear counterpart and is significantly less computationally complex than a previous machine learning-based controller.
"The increase in accuracy was pretty significant in some cases," said Kent. Though the outcome showed that their algorithm does require more energy than a linear controller to operate, this tradeoff means that when it is powered up, the team's model lasts longer and is considerably more efficient than current machine learning-based controllers on the market.
"People will find good use out of it just based on how efficient it is," Kent said. "You can implement it on pretty much any platform and it's very simple to understand." The algorithm was recently made available to scientists.
Outside of inspiring potential advances in engineering, there's also an equally important economic and environmental incentive for creating more power-friendly algorithms, said Kent.
As society becomes more dependent on computers and AI for nearly all aspects of daily life, demand for data centers is soaring, leading many experts to worry over digital systems' enormous power appetite and what future industries will need to do to keep up with it.
And because building these data centers as well as large-scale computing experiments can generate a large carbon footprint, scientists are looking for ways to curb carbon emissions from this technology.
To advance their results, future work will likely be steered toward training the model to explore other applications like quantum information processing, Kent said. In the meantime, he expects that these new elements will reach far into the scientific community.
"Not enough people know about these types of algorithms in the industry and engineering, and one of the big goals of this project is to get more people to learn about them," said Kent. "This work is a great first step toward reaching that potential."
This study was supported by the U.S. Air Force's Office of Scientific Research. Other Ohio State co-authors include Wendson A.S. Barbosa and Daniel J. Gauthier.
- Electronics
- Telecommunications
- Energy Technology
- Computers and Internet
- Neural Interfaces
- Information Technology
- Computer Science
- Artificial intelligence
- Alan Turing
- Computing power everywhere
- Grid computing
- Data mining
Story Source:
Materials provided by Ohio State University . Original written by Tatyana Woodall. Note: Content may be edited for style and length.
Journal Reference :
- Robert M. Kent, Wendson A. S. Barbosa, Daniel J. Gauthier. Controlling chaos using edge computing hardware . Nature Communications , 2024; 15 (1) DOI: 10.1038/s41467-024-48133-3
Cite This Page :
Explore More
- Autonomous Drones With Animal-Like 'Brains'
- How Practice Forms New Memory Pathways
- Reversing Brain Damage Caused by Ischemic Stroke
- Earth-Sized Planet Orbiting Ultra-Cool Dwarf
- Robots' Sense of Touch as Fast as Humans?
- Avian Flu Detected in NYC Wild Birds
- Metro-Area Quantum Computer Network Demo
- Iconic Baobab Tree's Origin Story
- 'Warm-Blooded' Dinos: 180 Million Years Ago
- Reaching 1,000 Degrees C With Solar Power
Trending Topics
Strange & offbeat.

IMAGES
VIDEO
COMMENTS
Get 1-On-1 Help. If you're still unsure about how to find a quality research topic, check out our Research Topic Kickstarter service, which is the perfect starting point for developing a unique, well-justified research topic. A comprehensive list of research topics ideas in the AI and machine learning area. Includes access to a free webinar ...
This dissertation revisits and makes progress on some old but challenging problems concerning least squares estimation, the work-horse of supervised machine learning. Two major problems are addressed: (i) least squares estimation with heavy-tailed errors, and (ii) least squares estimation in non-Donsker classes.
This dissertation explores three topics related to random forests: tree aggregation, variable importance, and robustness. 10. Climate Data Computing: Optimal Interpolation, Averaging, Visualization and Delivery. This dissertation solves two important problems in the modern analysis of big climate data.
PhD Dissertations [All are .pdf files] Probabilistic Reinforcement Learning: Using Data to Define Desired Outcomes, and Inferring How to Get There Benjamin Eysenbach, 2023. Data-driven Decisions - An Anomaly Detection Perspective Shubhranshu Shekhar, 2023. METHODS AND APPLICATIONS OF EXPLAINABLE MACHINE LEARNING Joon Sik Kim, 2023. Applied Mathematics of the Future Kin G. Olivares, 2023
The Machine Learning (ML) Ph.D. program is a fully-funded doctoral program in machine learning (ML), designed to train students to become tomorrow's leaders through a combination of interdisciplinary coursework, and cutting-edge research. Graduates of the Ph.D. program in machine learning are uniquely positioned to pioneer new developments in the field, and to be leaders in both industry and ...
The Cambridge Machine Learning Group (MLG) runs a PhD programme in Advanced Machine Learning. The supervisors are Jose Miguel Hernandez-Lobato, Carl Rasmussen, Richard E. Turner, Adrian Weller, Hong Ge and David Krueger. Zoubin Ghahramani is currently on academic leave and not accepting new students at this time.. We encourage applications from outstanding candidates with academic backgrounds ...
PhD in Machine Learning. Core Requirements The curriculum for the Machine Learning Ph.D. is built on a foundation of six core courses and one elective . A typical full-time, PhD student course load during the first two years consists each term of two classes (at 12 graduate units per class) plus 24 units of research.
Artificial Intelligence and Machine Learning. Our research covers a wide range of topics of this fast-evolving field, advancing how machines learn, predict, and control, while also making them secure, robust and trustworthy. Research covers both the theory and applications of ML. This broad area studies ML theory (algorithms, optimization, etc ...
Summary of General Requirements for a PhD in Machine Learning. Core curriculum (4 courses, 12 hours). Machine Learning PhD students will be required to complete courses in four different areas: Mathematical Foundations, Probabilistic and Statistical Methods in Machine Learning, ML Theory and Methods, and Optimization. Core Courses.
The Machine Learning (ML) Ph.D. program is a collaborative venture between Georgia Tech's colleges of Computing, Engineering, and Sciences. The central goal of the PhD program is to train students to perform original, independent research. The most important part of the curriculum is the successful defense of a PhD Dissertation, which
These 100 Machine Learning PhD viva questions cover a wide range of topics and concepts in Machine Learning, from basic concepts such as linear regression and decision trees, to more advanced topics such as deep learning, natural language processing, and image recognition. By preparing for these questions, you will be well-equipped to defend ...
Machine learning research is at the heart of the AI revolution. It underpins the development of intelligent systems capable of making predictions, automating tasks, and improving decision-making across industries. The importance of this research can be summarized as follows: Advancements in Technology.
We study a range of research areas related to machine learning and their applications for robotics, health care, language processing, information retrieval and more. Among these subjects include precision medicine, motion planning, computer vision, Bayesian inference, graphical models, statistical inference and estimation. Our work is ...
PHD thesis on machine learning contributes to the challenges, promising research opportunities, and effective solutions in various application areas. Below is the list of PHD thesis topics on machine learning to effectively explore, scrutinize and discover the new findings of machine learning systems.
I hope that these topics are helpful in navigating the PhD and research in Machine Learning! Expectations going into the PhD. Common Challenges through the Journey. Feeling completely stuck. Feeling overwhelmed with keeping up with ML progress. Feeling Isolated. Three Useful Personal Skills. Keeping Notes on Papers and Ideas.
#machinelearning #phd #howtoThis video is advice for new PhD students in the field of Machine Learning in 2021 and after. The field has shifted dramatically ...
In this tech-driven world, selecting research and thesis topics in machine learning projects is the first choice of masters and Doctorate scholars. Selecting and working on a thesis topic in machine learning is not an easy task as machine learning uses statistical algorithms to make computers work in a certain way without being explicitly ...
What is the cheapest PhD in machine learning? The cheapest PhD in machine learning is the PhD in Machine Learning and Data Science offered by University of California San Diego. The PhD in Machine Learning and Data Science tuition at University of California San Diego costs $11,442 per year for both residents and non-residents.
22,950 EUR / year. 3 years. This PhD project at Cranfield University will investigate the recent field of study of Causal Machine Learning in Aerospace, which aims to modify and augment Machine Learning by using Causal Analysis techniques as a way to solve its limitations. Ph.D. / Full-time / On Campus.
Photo by Sharon McCutcheon on Unsplash. For the role of a Machine Learning Researcher/Scientist, 90% of the ML Researcher roles within the United Kingdom require at least a PhD from applicants; some roles required applicants that had published papers in top conferences such as NAACL, or NeurIPS. Having a PhD certainly opens more doors to more positions. The average salary I found for the ...
The most affordable online PhD in Machine Learning based on cost per credit is at Aspen University in Phoenix, Arizona. It charges $375 per month, which, when multiplied by the 67 months it takes to complete the program, results in a total of $25,125 for the entire program.
A PhD topic in Artificial Intelligence involves advanced research and exploration within the realm of AI. It encompasses a wide array of subjects, such as machine learning, natural language processing, computer vision, robotics, and neural networks selection of project topic introduction. Doctoral candidates delve into cutting-edge techniques ...
Achieving a Ph.D. in machine learning provides a chance to gain skills in both basic ideas and new improvements. While choosing a project concept, it is very essential to think about the upcoming approach and possible societal effects in addition to the latest research techniques. More than 200+ Machine Learning experts are there in ...
2. Data Science: Machine Learning - Harvard. Data Science: Machine Learning is another course where you'll get to learn machine learning fundamentals by working on practical applications such as movie recommendation systems. The course goes over the following topics: Link: Data Science: Machine Learning. 3.
Ohio State University. "New machine learning algorithm promises advances in computing." ScienceDaily. ScienceDaily, 9 May 2024. <www.sciencedaily.com / releases / 2024 / 05 / 240509155536.htm ...