

Research Variables 101
Independent variables, dependent variables, control variables and more
By: Derek Jansen (MBA) | Expert Reviewed By: Kerryn Warren (PhD) | January 2023
If you’re new to the world of research, especially scientific research, you’re bound to run into the concept of variables , sooner or later. If you’re feeling a little confused, don’t worry – you’re not the only one! Independent variables, dependent variables, confounding variables – it’s a lot of jargon. In this post, we’ll unpack the terminology surrounding research variables using straightforward language and loads of examples .
Overview: Variables In Research
What (exactly) is a variable.
The simplest way to understand a variable is as any characteristic or attribute that can experience change or vary over time or context – hence the name “variable”. For example, the dosage of a particular medicine could be classified as a variable, as the amount can vary (i.e., a higher dose or a lower dose). Similarly, gender, age or ethnicity could be considered demographic variables, because each person varies in these respects.
Within research, especially scientific research, variables form the foundation of studies, as researchers are often interested in how one variable impacts another, and the relationships between different variables. For example:
- How someone’s age impacts their sleep quality
- How different teaching methods impact learning outcomes
- How diet impacts weight (gain or loss)
As you can see, variables are often used to explain relationships between different elements and phenomena. In scientific studies, especially experimental studies, the objective is often to understand the causal relationships between variables. In other words, the role of cause and effect between variables. This is achieved by manipulating certain variables while controlling others – and then observing the outcome. But, we’ll get into that a little later…
The “Big 3” Variables
Variables can be a little intimidating for new researchers because there are a wide variety of variables, and oftentimes, there are multiple labels for the same thing. To lay a firm foundation, we’ll first look at the three main types of variables, namely:
- Independent variables (IV)
- Dependant variables (DV)
- Control variables
What is an independent variable?
Simply put, the independent variable is the “ cause ” in the relationship between two (or more) variables. In other words, when the independent variable changes, it has an impact on another variable.
For example:
- Increasing the dosage of a medication (Variable A) could result in better (or worse) health outcomes for a patient (Variable B)
- Changing a teaching method (Variable A) could impact the test scores that students earn in a standardised test (Variable B)
- Varying one’s diet (Variable A) could result in weight loss or gain (Variable B).
It’s useful to know that independent variables can go by a few different names, including, explanatory variables (because they explain an event or outcome) and predictor variables (because they predict the value of another variable). Terminology aside though, the most important takeaway is that independent variables are assumed to be the “cause” in any cause-effect relationship. As you can imagine, these types of variables are of major interest to researchers, as many studies seek to understand the causal factors behind a phenomenon.
Need a helping hand?
What is a dependent variable?
While the independent variable is the “ cause ”, the dependent variable is the “ effect ” – or rather, the affected variable . In other words, the dependent variable is the variable that is assumed to change as a result of a change in the independent variable.
Keeping with the previous example, let’s look at some dependent variables in action:
- Health outcomes (DV) could be impacted by dosage changes of a medication (IV)
- Students’ scores (DV) could be impacted by teaching methods (IV)
- Weight gain or loss (DV) could be impacted by diet (IV)
In scientific studies, researchers will typically pay very close attention to the dependent variable (or variables), carefully measuring any changes in response to hypothesised independent variables. This can be tricky in practice, as it’s not always easy to reliably measure specific phenomena or outcomes – or to be certain that the actual cause of the change is in fact the independent variable.
As the adage goes, correlation is not causation . In other words, just because two variables have a relationship doesn’t mean that it’s a causal relationship – they may just happen to vary together. For example, you could find a correlation between the number of people who own a certain brand of car and the number of people who have a certain type of job. Just because the number of people who own that brand of car and the number of people who have that type of job is correlated, it doesn’t mean that owning that brand of car causes someone to have that type of job or vice versa. The correlation could, for example, be caused by another factor such as income level or age group, which would affect both car ownership and job type.
To confidently establish a causal relationship between an independent variable and a dependent variable (i.e., X causes Y), you’ll typically need an experimental design , where you have complete control over the environmen t and the variables of interest. But even so, this doesn’t always translate into the “real world”. Simply put, what happens in the lab sometimes stays in the lab!
As an alternative to pure experimental research, correlational or “ quasi-experimental ” research (where the researcher cannot manipulate or change variables) can be done on a much larger scale more easily, allowing one to understand specific relationships in the real world. These types of studies also assume some causality between independent and dependent variables, but it’s not always clear. So, if you go this route, you need to be cautious in terms of how you describe the impact and causality between variables and be sure to acknowledge any limitations in your own research.
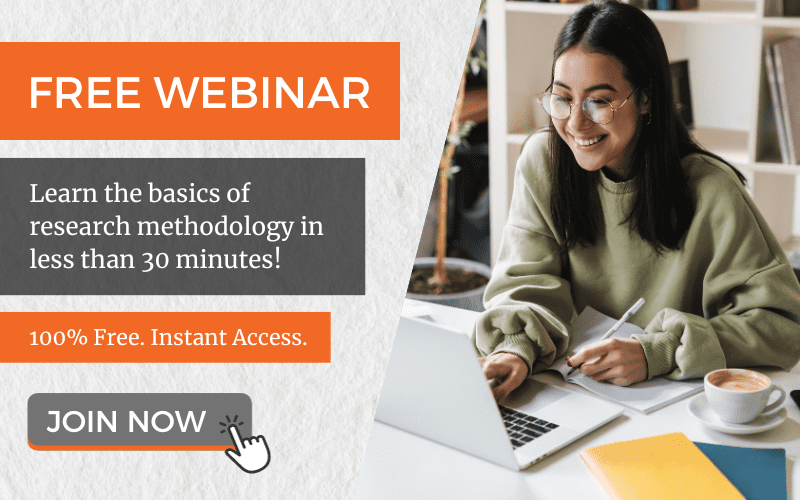
What is a control variable?
In an experimental design, a control variable (or controlled variable) is a variable that is intentionally held constant to ensure it doesn’t have an influence on any other variables. As a result, this variable remains unchanged throughout the course of the study. In other words, it’s a variable that’s not allowed to vary – tough life 🙂
As we mentioned earlier, one of the major challenges in identifying and measuring causal relationships is that it’s difficult to isolate the impact of variables other than the independent variable. Simply put, there’s always a risk that there are factors beyond the ones you’re specifically looking at that might be impacting the results of your study. So, to minimise the risk of this, researchers will attempt (as best possible) to hold other variables constant . These factors are then considered control variables.
Some examples of variables that you may need to control include:
- Temperature
- Time of day
- Noise or distractions
Which specific variables need to be controlled for will vary tremendously depending on the research project at hand, so there’s no generic list of control variables to consult. As a researcher, you’ll need to think carefully about all the factors that could vary within your research context and then consider how you’ll go about controlling them. A good starting point is to look at previous studies similar to yours and pay close attention to which variables they controlled for.
Of course, you won’t always be able to control every possible variable, and so, in many cases, you’ll just have to acknowledge their potential impact and account for them in the conclusions you draw. Every study has its limitations, so don’t get fixated or discouraged by troublesome variables. Nevertheless, always think carefully about the factors beyond what you’re focusing on – don’t make assumptions!
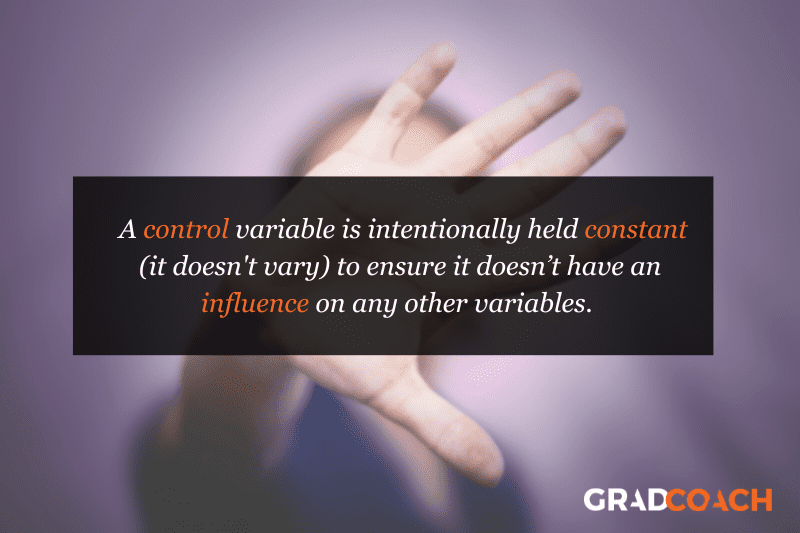
Other types of variables
As we mentioned, independent, dependent and control variables are the most common variables you’ll come across in your research, but they’re certainly not the only ones you need to be aware of. Next, we’ll look at a few “secondary” variables that you need to keep in mind as you design your research.
- Moderating variables
- Mediating variables
- Confounding variables
- Latent variables
Let’s jump into it…
What is a moderating variable?
A moderating variable is a variable that influences the strength or direction of the relationship between an independent variable and a dependent variable. In other words, moderating variables affect how much (or how little) the IV affects the DV, or whether the IV has a positive or negative relationship with the DV (i.e., moves in the same or opposite direction).
For example, in a study about the effects of sleep deprivation on academic performance, gender could be used as a moderating variable to see if there are any differences in how men and women respond to a lack of sleep. In such a case, one may find that gender has an influence on how much students’ scores suffer when they’re deprived of sleep.
It’s important to note that while moderators can have an influence on outcomes , they don’t necessarily cause them ; rather they modify or “moderate” existing relationships between other variables. This means that it’s possible for two different groups with similar characteristics, but different levels of moderation, to experience very different results from the same experiment or study design.
What is a mediating variable?
Mediating variables are often used to explain the relationship between the independent and dependent variable (s). For example, if you were researching the effects of age on job satisfaction, then education level could be considered a mediating variable, as it may explain why older people have higher job satisfaction than younger people – they may have more experience or better qualifications, which lead to greater job satisfaction.
Mediating variables also help researchers understand how different factors interact with each other to influence outcomes. For instance, if you wanted to study the effect of stress on academic performance, then coping strategies might act as a mediating factor by influencing both stress levels and academic performance simultaneously. For example, students who use effective coping strategies might be less stressed but also perform better academically due to their improved mental state.
In addition, mediating variables can provide insight into causal relationships between two variables by helping researchers determine whether changes in one factor directly cause changes in another – or whether there is an indirect relationship between them mediated by some third factor(s). For instance, if you wanted to investigate the impact of parental involvement on student achievement, you would need to consider family dynamics as a potential mediator, since it could influence both parental involvement and student achievement simultaneously.
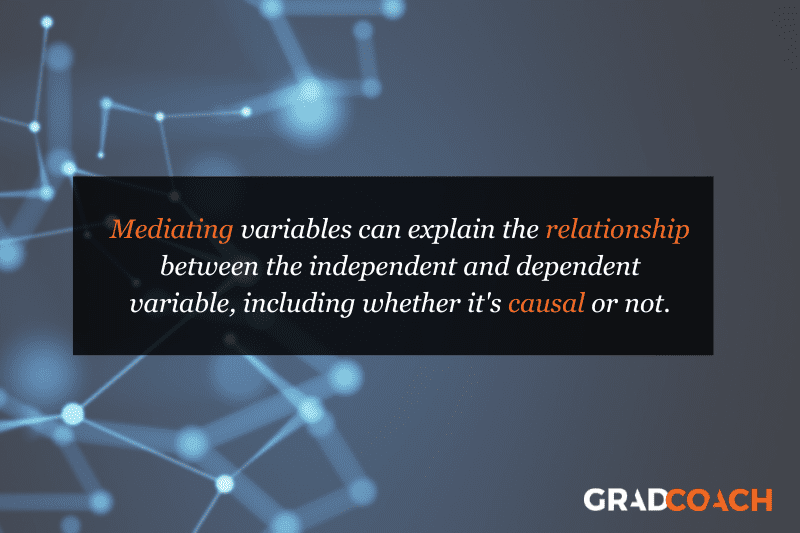
What is a confounding variable?
A confounding variable (also known as a third variable or lurking variable ) is an extraneous factor that can influence the relationship between two variables being studied. Specifically, for a variable to be considered a confounding variable, it needs to meet two criteria:
- It must be correlated with the independent variable (this can be causal or not)
- It must have a causal impact on the dependent variable (i.e., influence the DV)
Some common examples of confounding variables include demographic factors such as gender, ethnicity, socioeconomic status, age, education level, and health status. In addition to these, there are also environmental factors to consider. For example, air pollution could confound the impact of the variables of interest in a study investigating health outcomes.
Naturally, it’s important to identify as many confounding variables as possible when conducting your research, as they can heavily distort the results and lead you to draw incorrect conclusions . So, always think carefully about what factors may have a confounding effect on your variables of interest and try to manage these as best you can.
What is a latent variable?
Latent variables are unobservable factors that can influence the behaviour of individuals and explain certain outcomes within a study. They’re also known as hidden or underlying variables , and what makes them rather tricky is that they can’t be directly observed or measured . Instead, latent variables must be inferred from other observable data points such as responses to surveys or experiments.
For example, in a study of mental health, the variable “resilience” could be considered a latent variable. It can’t be directly measured , but it can be inferred from measures of mental health symptoms, stress, and coping mechanisms. The same applies to a lot of concepts we encounter every day – for example:
- Emotional intelligence
- Quality of life
- Business confidence
- Ease of use
One way in which we overcome the challenge of measuring the immeasurable is latent variable models (LVMs). An LVM is a type of statistical model that describes a relationship between observed variables and one or more unobserved (latent) variables. These models allow researchers to uncover patterns in their data which may not have been visible before, thanks to their complexity and interrelatedness with other variables. Those patterns can then inform hypotheses about cause-and-effect relationships among those same variables which were previously unknown prior to running the LVM. Powerful stuff, we say!
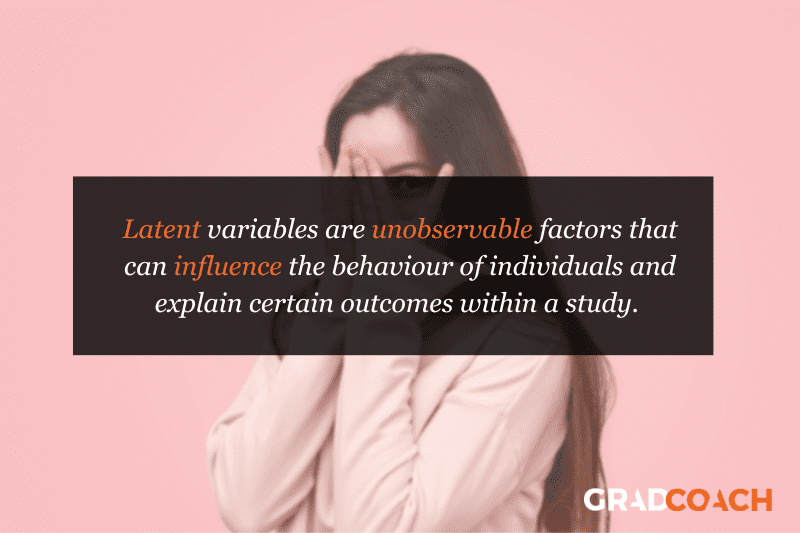
Let’s recap
In the world of scientific research, there’s no shortage of variable types, some of which have multiple names and some of which overlap with each other. In this post, we’ve covered some of the popular ones, but remember that this is not an exhaustive list .
To recap, we’ve explored:
- Independent variables (the “cause”)
- Dependent variables (the “effect”)
- Control variables (the variable that’s not allowed to vary)
If you’re still feeling a bit lost and need a helping hand with your research project, check out our 1-on-1 coaching service , where we guide you through each step of the research journey. Also, be sure to check out our free dissertation writing course and our collection of free, fully-editable chapter templates .
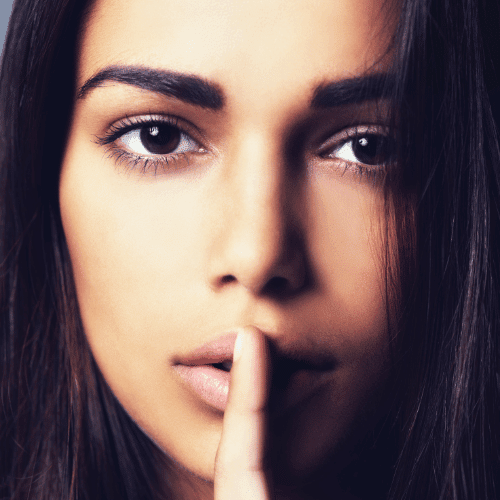
Psst... there’s more!
This post was based on one of our popular Research Bootcamps . If you're working on a research project, you'll definitely want to check this out ...
You Might Also Like:
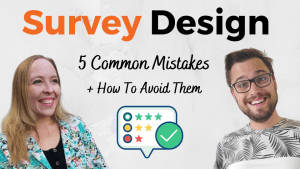
Very informative, concise and helpful. Thank you
Helping information.Thanks
practical and well-demonstrated
Very helpful and insightful
Submit a Comment Cancel reply
Your email address will not be published. Required fields are marked *
Save my name, email, and website in this browser for the next time I comment.
- Print Friendly
- USC Libraries
- Research Guides
Organizing Your Social Sciences Research Paper
- Independent and Dependent Variables
- Purpose of Guide
- Design Flaws to Avoid
- Glossary of Research Terms
- Reading Research Effectively
- Narrowing a Topic Idea
- Broadening a Topic Idea
- Extending the Timeliness of a Topic Idea
- Academic Writing Style
- Applying Critical Thinking
- Choosing a Title
- Making an Outline
- Paragraph Development
- Research Process Video Series
- Executive Summary
- The C.A.R.S. Model
- Background Information
- The Research Problem/Question
- Theoretical Framework
- Citation Tracking
- Content Alert Services
- Evaluating Sources
- Primary Sources
- Secondary Sources
- Tiertiary Sources
- Scholarly vs. Popular Publications
- Qualitative Methods
- Quantitative Methods
- Insiderness
- Using Non-Textual Elements
- Limitations of the Study
- Common Grammar Mistakes
- Writing Concisely
- Avoiding Plagiarism
- Footnotes or Endnotes?
- Further Readings
- Generative AI and Writing
- USC Libraries Tutorials and Other Guides
- Bibliography
Definitions
Dependent Variable The variable that depends on other factors that are measured. These variables are expected to change as a result of an experimental manipulation of the independent variable or variables. It is the presumed effect.
Independent Variable The variable that is stable and unaffected by the other variables you are trying to measure. It refers to the condition of an experiment that is systematically manipulated by the investigator. It is the presumed cause.
Cramer, Duncan and Dennis Howitt. The SAGE Dictionary of Statistics . London: SAGE, 2004; Penslar, Robin Levin and Joan P. Porter. Institutional Review Board Guidebook: Introduction . Washington, DC: United States Department of Health and Human Services, 2010; "What are Dependent and Independent Variables?" Graphic Tutorial.
Identifying Dependent and Independent Variables
Don't feel bad if you are confused about what is the dependent variable and what is the independent variable in social and behavioral sciences research . However, it's important that you learn the difference because framing a study using these variables is a common approach to organizing the elements of a social sciences research study in order to discover relevant and meaningful results. Specifically, it is important for these two reasons:
- You need to understand and be able to evaluate their application in other people's research.
- You need to apply them correctly in your own research.
A variable in research simply refers to a person, place, thing, or phenomenon that you are trying to measure in some way. The best way to understand the difference between a dependent and independent variable is that the meaning of each is implied by what the words tell us about the variable you are using. You can do this with a simple exercise from the website, Graphic Tutorial. Take the sentence, "The [independent variable] causes a change in [dependent variable] and it is not possible that [dependent variable] could cause a change in [independent variable]." Insert the names of variables you are using in the sentence in the way that makes the most sense. This will help you identify each type of variable. If you're still not sure, consult with your professor before you begin to write.
Fan, Shihe. "Independent Variable." In Encyclopedia of Research Design. Neil J. Salkind, editor. (Thousand Oaks, CA: SAGE, 2010), pp. 592-594; "What are Dependent and Independent Variables?" Graphic Tutorial; Salkind, Neil J. "Dependent Variable." In Encyclopedia of Research Design , Neil J. Salkind, editor. (Thousand Oaks, CA: SAGE, 2010), pp. 348-349;
Structure and Writing Style
The process of examining a research problem in the social and behavioral sciences is often framed around methods of analysis that compare, contrast, correlate, average, or integrate relationships between or among variables . Techniques include associations, sampling, random selection, and blind selection. Designation of the dependent and independent variable involves unpacking the research problem in a way that identifies a general cause and effect and classifying these variables as either independent or dependent.
The variables should be outlined in the introduction of your paper and explained in more detail in the methods section . There are no rules about the structure and style for writing about independent or dependent variables but, as with any academic writing, clarity and being succinct is most important.
After you have described the research problem and its significance in relation to prior research, explain why you have chosen to examine the problem using a method of analysis that investigates the relationships between or among independent and dependent variables . State what it is about the research problem that lends itself to this type of analysis. For example, if you are investigating the relationship between corporate environmental sustainability efforts [the independent variable] and dependent variables associated with measuring employee satisfaction at work using a survey instrument, you would first identify each variable and then provide background information about the variables. What is meant by "environmental sustainability"? Are you looking at a particular company [e.g., General Motors] or are you investigating an industry [e.g., the meat packing industry]? Why is employee satisfaction in the workplace important? How does a company make their employees aware of sustainability efforts and why would a company even care that its employees know about these efforts?
Identify each variable for the reader and define each . In the introduction, this information can be presented in a paragraph or two when you describe how you are going to study the research problem. In the methods section, you build on the literature review of prior studies about the research problem to describe in detail background about each variable, breaking each down for measurement and analysis. For example, what activities do you examine that reflect a company's commitment to environmental sustainability? Levels of employee satisfaction can be measured by a survey that asks about things like volunteerism or a desire to stay at the company for a long time.
The structure and writing style of describing the variables and their application to analyzing the research problem should be stated and unpacked in such a way that the reader obtains a clear understanding of the relationships between the variables and why they are important. This is also important so that the study can be replicated in the future using the same variables but applied in a different way.
Fan, Shihe. "Independent Variable." In Encyclopedia of Research Design. Neil J. Salkind, editor. (Thousand Oaks, CA: SAGE, 2010), pp. 592-594; "What are Dependent and Independent Variables?" Graphic Tutorial; “Case Example for Independent and Dependent Variables.” ORI Curriculum Examples. U.S. Department of Health and Human Services, Office of Research Integrity; Salkind, Neil J. "Dependent Variable." In Encyclopedia of Research Design , Neil J. Salkind, editor. (Thousand Oaks, CA: SAGE, 2010), pp. 348-349; “Independent Variables and Dependent Variables.” Karl L. Wuensch, Department of Psychology, East Carolina University [posted email exchange]; “Variables.” Elements of Research. Dr. Camille Nebeker, San Diego State University.
- << Previous: Design Flaws to Avoid
- Next: Glossary of Research Terms >>
- Last Updated: Apr 24, 2024 10:51 AM
- URL: https://libguides.usc.edu/writingguide
Independent Variable Definition and Examples
Understand the Independent Variable in an Experiment
- Chemical Laws
- Periodic Table
- Projects & Experiments
- Scientific Method
- Biochemistry
- Physical Chemistry
- Medical Chemistry
- Chemistry In Everyday Life
- Famous Chemists
- Activities for Kids
- Abbreviations & Acronyms
- Weather & Climate
- Ph.D., Biomedical Sciences, University of Tennessee at Knoxville
- B.A., Physics and Mathematics, Hastings College
The two main variables in a science experiment are the independent variable and the dependent variable . Here's the definition on independent variable and a look at how it's used:
Key Takeaways: Independent Variable
- The independent variable is the factor that you purposely change or control in order to see what effect it has.
- The variable that responds to the change in the independent variable is called the dependent variable. It depends on the independent variable.
- The independent variable is graphed on the x-axis.
Independent Variable Definition
An independent variable is defines as the variable that is changed or controlled in a scientific experiment. It represents the cause or reason for an outcome. Independent variables are the variables that the experimenter changes to test their dependent variable . A change in the independent variable directly causes a change in the dependent variable. The effect on the dependent variable is measured and recorded.
Common Misspellings: independant variable
Independent Variable Examples
- A scientist is testing the effect of light and dark on the behavior of moths by turning a light on and off. The independent variable is the amount of light and the moth's reaction is the dependent variable .
- In a study to determine the effect of temperature on plant pigmentation, the independent variable (cause) is the temperature, while the amount of pigment or color is the dependent variable (the effect).
Graphing the Independent Variable
When graphing data for an experiment, the independent variable is plotted on the x-axis, while the dependent variable is recorded on the y-axis. An easy way to keep the two variables straight is to use the acronym DRY MIX , which stands for:
- Dependent variable that Responds to change goes on the Y axis
- Manipulated or Independent variable goes on the X axis
Practice Identifying the Independent Variable
Students are often asked to identify the independent and dependent variable in an experiment. The difficulty is that the value of both of these variables can change. It's even possible for the dependent variable to remain unchanged in response to controlling the independent variable.
Example : You're asked to identify the independent and dependent variable in an experiment looking to see if there is a relationship between hours of sleep and student test scores.
There are two ways to identify the independent variable. The first is to write the hypothesis and see if it makes sense:
- Student test scores have no effect on the number of hours the students sleeps.
- The number of hours students sleep have no effect on their test scores.
Only one of these statements makes sense. This type of hypothesis is constructed to state the independent variable followed by the predicted impact on the dependent variable. So, the number of hours of sleep is the independent variable.
The other way to identify the independent variable is more intuitive. Remember, the independent variable is the one the experimenter controls to measures its effect on the dependent variable. A researcher can control the number of hours a student sleeps. On the other hand, the scientist has no control on the students' test scores.
The independent variable always changes in an experiment, even if there is just a control and an experimental group. The dependent variable may or may not change in response to the independent variable. In the example regarding sleep and student test scores, it's possible the data might show no change in test scores, no matter how much sleep students get (although this outcome seems unlikely). The point is that a researcher knows the values of the independent variable. The value of the dependent variable is measured .
- Babbie, Earl R. (2009). The Practice of Social Research (12th ed.). Wadsworth Publishing. ISBN 0-495-59841-0.
- Dodge, Y. (2003). The Oxford Dictionary of Statistical Terms . OUP. ISBN 0-19-920613-9.
- Everitt, B. S. (2002). The Cambridge Dictionary of Statistics (2nd ed.). Cambridge UP. ISBN 0-521-81099-X.
- Gujarati, Damodar N.; Porter, Dawn C. (2009). "Terminology and Notation". Basic Econometrics (5th international ed.). New York: McGraw-Hill. p. 21. ISBN 978-007-127625-2.
- Shadish, William R.; Cook, Thomas D.; Campbell, Donald T. (2002). Experimental and quasi-experimental designs for generalized causal inference . (Nachdr. ed.). Boston: Houghton Mifflin. ISBN 0-395-61556-9.
- Difference Between Independent and Dependent Variables
- Dependent Variable Definition and Examples
- What Is a Dependent Variable?
- What Are Independent and Dependent Variables?
- Scientific Variable
- What Is a Hypothesis? (Science)
- What Is an Experiment? Definition and Design
- Scientific Method Vocabulary Terms
- The Differences Between Explanatory and Response Variables
- What Are the Elements of a Good Hypothesis?
- DRY MIX Experiment Variables Acronym
- Scientific Method Flow Chart
- The Significance of Negative Slope
- Understanding Experimental Groups
- What Is a Variable in Science?
- Six Steps of the Scientific Method
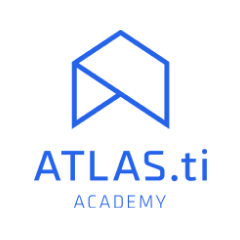
Variables in Research | Types, Definiton & Examples
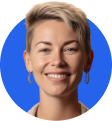
Introduction
What is a variable, what are the 5 types of variables in research, other variables in research.
Variables are fundamental components of research that allow for the measurement and analysis of data. They can be defined as characteristics or properties that can take on different values. In research design , understanding the types of variables and their roles is crucial for developing hypotheses , designing methods , and interpreting results .
This article outlines the the types of variables in research, including their definitions and examples, to provide a clear understanding of their use and significance in research studies. By categorizing variables into distinct groups based on their roles in research, their types of data, and their relationships with other variables, researchers can more effectively structure their studies and achieve more accurate conclusions.
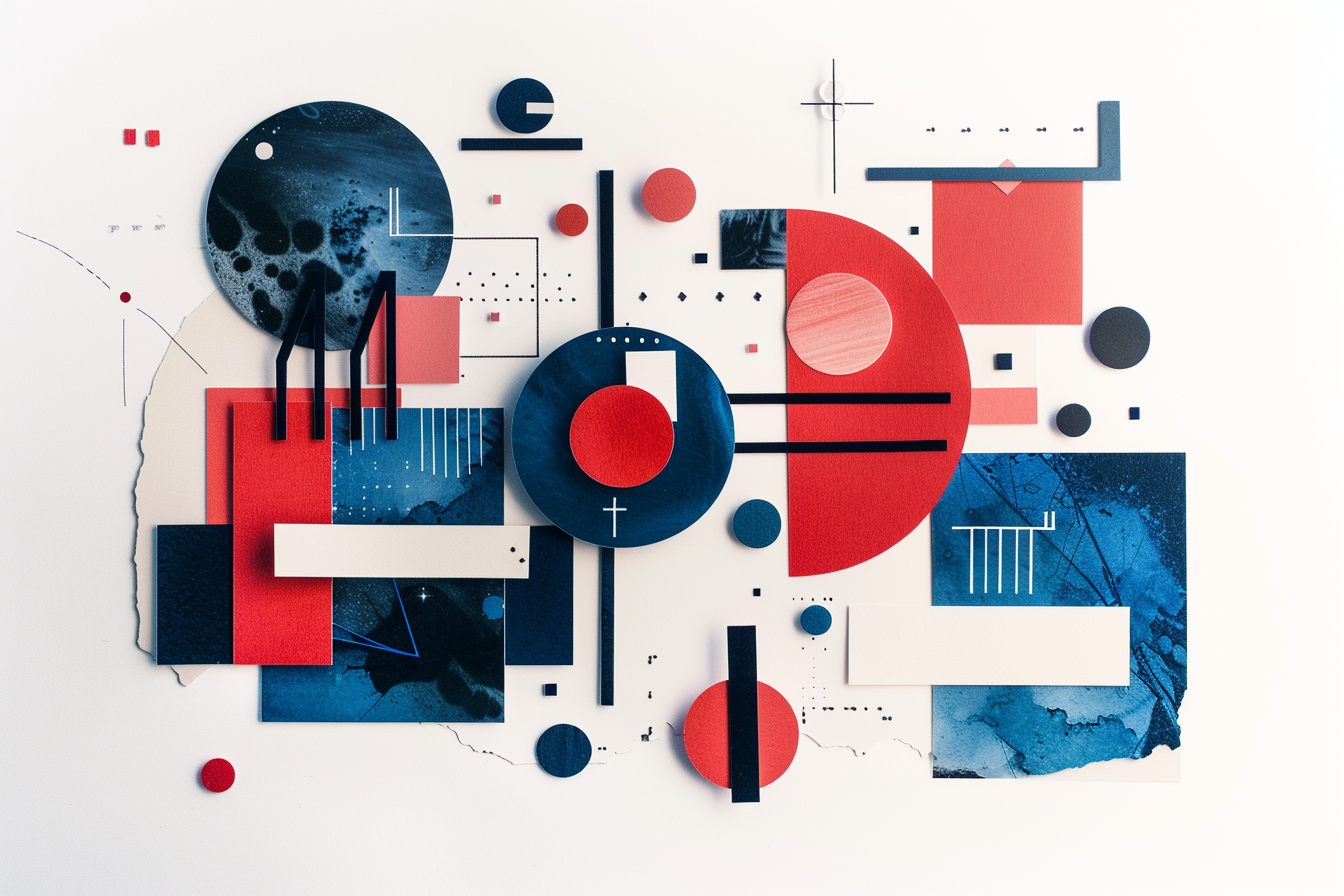
A variable represents any characteristic, number, or quantity that can be measured or quantified. The term encompasses anything that can vary or change, ranging from simple concepts like age and height to more complex ones like satisfaction levels or economic status. Variables are essential in research as they are the foundational elements that researchers manipulate, measure, or control to gain insights into relationships, causes, and effects within their studies. They enable the framing of research questions, the formulation of hypotheses, and the interpretation of results.
Variables can be categorized based on their role in the study (such as independent and dependent variables ), the type of data they represent (quantitative or categorical), and their relationship to other variables (like confounding or control variables). Understanding what constitutes a variable and the various variable types available is a critical step in designing robust and meaningful research.
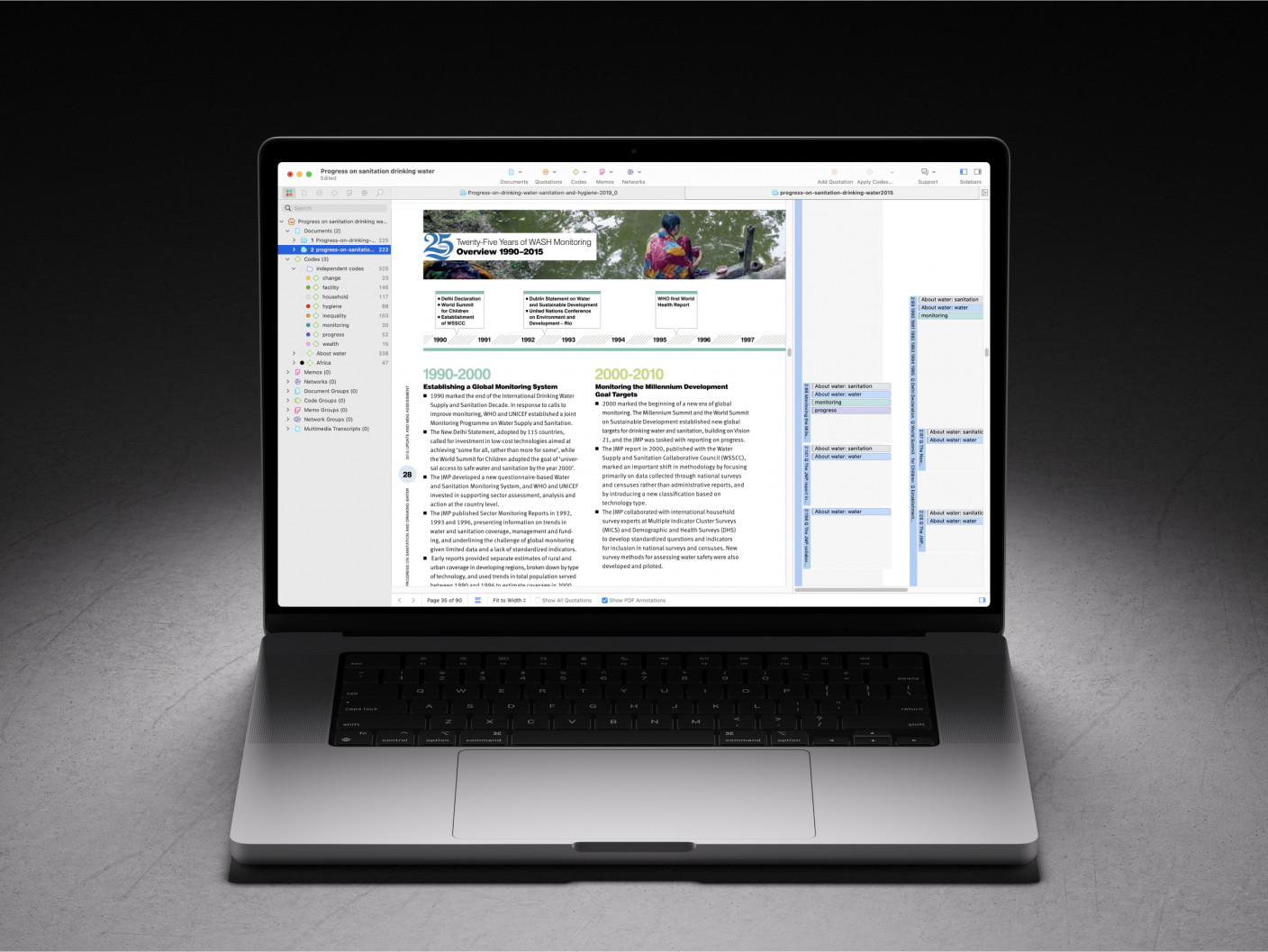
ATLAS.ti makes complex data easy to understand
Turn to our powerful data analysis tools to make the most of your research. Get started with a free trial.
Variables are crucial components in research, serving as the foundation for data collection , analysis , and interpretation . They are attributes or characteristics that can vary among subjects or over time, and understanding their types is essential for any study. Variables can be broadly classified into five main types, each with its distinct characteristics and roles within research.
This classification helps researchers in designing their studies, choosing appropriate measurement techniques, and analyzing their results accurately. The five types of variables include independent variables, dependent variables, categorical variables, continuous variables, and confounding variables. These categories not only facilitate a clearer understanding of the data but also guide the formulation of hypotheses and research methodologies.
Independent variables
Independent variables are foundational to the structure of research, serving as the factors or conditions that researchers manipulate or vary to observe their effects on dependent variables. These variables are considered "independent" because their variation does not depend on other variables within the study. Instead, they are the cause or stimulus that directly influences the outcomes being measured. For example, in an experiment to assess the effectiveness of a new teaching method on student performance, the teaching method applied (traditional vs. innovative) would be the independent variable.
The selection of an independent variable is a critical step in research design, as it directly correlates with the study's objective to determine causality or association. Researchers must clearly define and control these variables to ensure that observed changes in the dependent variable can be attributed to variations in the independent variable, thereby affirming the reliability of the results. In experimental research, the independent variable is what differentiates the control group from the experimental group, thereby setting the stage for meaningful comparison and analysis.
Dependent variables
Dependent variables are the outcomes or effects that researchers aim to explore and understand in their studies. These variables are called "dependent" because their values depend on the changes or variations of the independent variables.
Essentially, they are the responses or results that are measured to assess the impact of the independent variable's manipulation. For instance, in a study investigating the effect of exercise on weight loss, the amount of weight lost would be considered the dependent variable, as it depends on the exercise regimen (the independent variable).
The identification and measurement of the dependent variable are crucial for testing the hypothesis and drawing conclusions from the research. It allows researchers to quantify the effect of the independent variable , providing evidence for causal relationships or associations. In experimental settings, the dependent variable is what is being tested and measured across different groups or conditions, enabling researchers to assess the efficacy or impact of the independent variable's variation.
To ensure accuracy and reliability, the dependent variable must be defined clearly and measured consistently across all participants or observations. This consistency helps in reducing measurement errors and increases the validity of the research findings. By carefully analyzing the dependent variables, researchers can derive meaningful insights from their studies, contributing to the broader knowledge in their field.
Categorical variables
Categorical variables, also known as qualitative variables, represent types or categories that are used to group observations. These variables divide data into distinct groups or categories that lack a numerical value but hold significant meaning in research. Examples of categorical variables include gender (male, female, other), type of vehicle (car, truck, motorcycle), or marital status (single, married, divorced). These categories help researchers organize data into groups for comparison and analysis.
Categorical variables can be further classified into two subtypes: nominal and ordinal. Nominal variables are categories without any inherent order or ranking among them, such as blood type or ethnicity. Ordinal variables, on the other hand, imply a sort of ranking or order among the categories, like levels of satisfaction (high, medium, low) or education level (high school, bachelor's, master's, doctorate).
Understanding and identifying categorical variables is crucial in research as it influences the choice of statistical analysis methods. Since these variables represent categories without numerical significance, researchers employ specific statistical tests designed for a nominal or ordinal variable to draw meaningful conclusions. Properly classifying and analyzing categorical variables allow for the exploration of relationships between different groups within the study, shedding light on patterns and trends that might not be evident with numerical data alone.
Continuous variables
Continuous variables are quantitative variables that can take an infinite number of values within a given range. These variables are measured along a continuum and can represent very precise measurements. Examples of continuous variables include height, weight, temperature, and time. Because they can assume any value within a range, continuous variables allow for detailed analysis and a high degree of accuracy in research findings.
The ability to measure continuous variables at very fine scales makes them invaluable for many types of research, particularly in the natural and social sciences. For instance, in a study examining the effect of temperature on plant growth, temperature would be considered a continuous variable since it can vary across a wide spectrum and be measured to several decimal places.
When dealing with continuous variables, researchers often use methods incorporating a particular statistical test to accommodate a wide range of data points and the potential for infinite divisibility. This includes various forms of regression analysis, correlation, and other techniques suited for modeling and analyzing nuanced relationships between variables. The precision of continuous variables enhances the researcher's ability to detect patterns, trends, and causal relationships within the data, contributing to more robust and detailed conclusions.
Confounding variables
Confounding variables are those that can cause a false association between the independent and dependent variables, potentially leading to incorrect conclusions about the relationship being studied. These are extraneous variables that were not considered in the study design but can influence both the supposed cause and effect, creating a misleading correlation.
Identifying and controlling for a confounding variable is crucial in research to ensure the validity of the findings. This can be achieved through various methods, including randomization, stratification, and statistical control. Randomization helps to evenly distribute confounding variables across study groups, reducing their potential impact. Stratification involves analyzing the data within strata or layers that share common characteristics of the confounder. Statistical control allows researchers to adjust for the effects of confounders in the analysis phase.
Properly addressing confounding variables strengthens the credibility of research outcomes by clarifying the direct relationship between the dependent and independent variables, thus providing more accurate and reliable results.
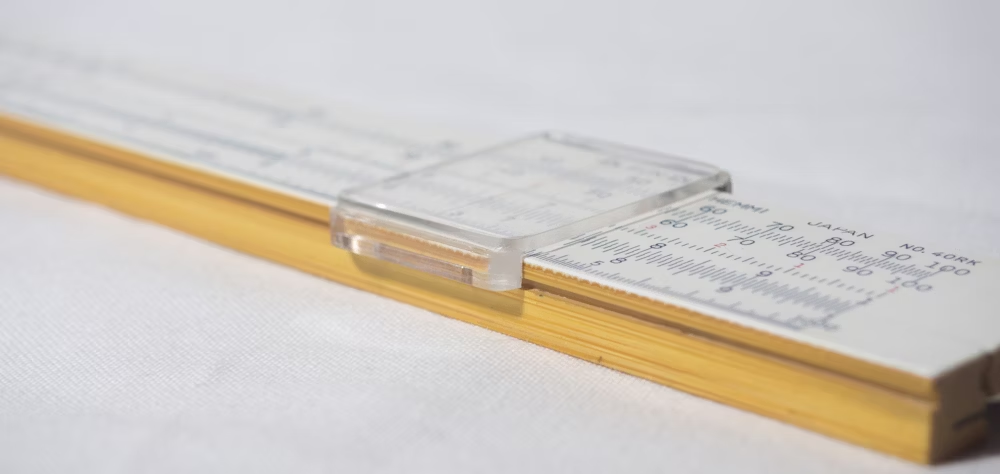
Beyond the primary categories of variables commonly discussed in research methodology , there exists a diverse range of other variables that play significant roles in the design and analysis of studies. Below is an overview of some of these variables, highlighting their definitions and roles within research studies:
- Discrete variables : A discrete variable is a quantitative variable that represents quantitative data , such as the number of children in a family or the number of cars in a parking lot. Discrete variables can only take on specific values.
- Categorical variables : A categorical variable categorizes subjects or items into groups that do not have a natural numerical order. Categorical data includes nominal variables, like country of origin, and ordinal variables, such as education level.
- Predictor variables : Often used in statistical models, a predictor variable is used to forecast or predict the outcomes of other variables, not necessarily with a causal implication.
- Outcome variables : These variables represent the results or outcomes that researchers aim to explain or predict through their studies. An outcome variable is central to understanding the effects of predictor variables.
- Latent variables : Not directly observable, latent variables are inferred from other, directly measured variables. Examples include psychological constructs like intelligence or socioeconomic status.
- Composite variables : Created by combining multiple variables, composite variables can measure a concept more reliably or simplify the analysis. An example would be a composite happiness index derived from several survey questions .
- Preceding variables : These variables come before other variables in time or sequence, potentially influencing subsequent outcomes. A preceding variable is crucial in longitudinal studies to determine causality or sequences of events.
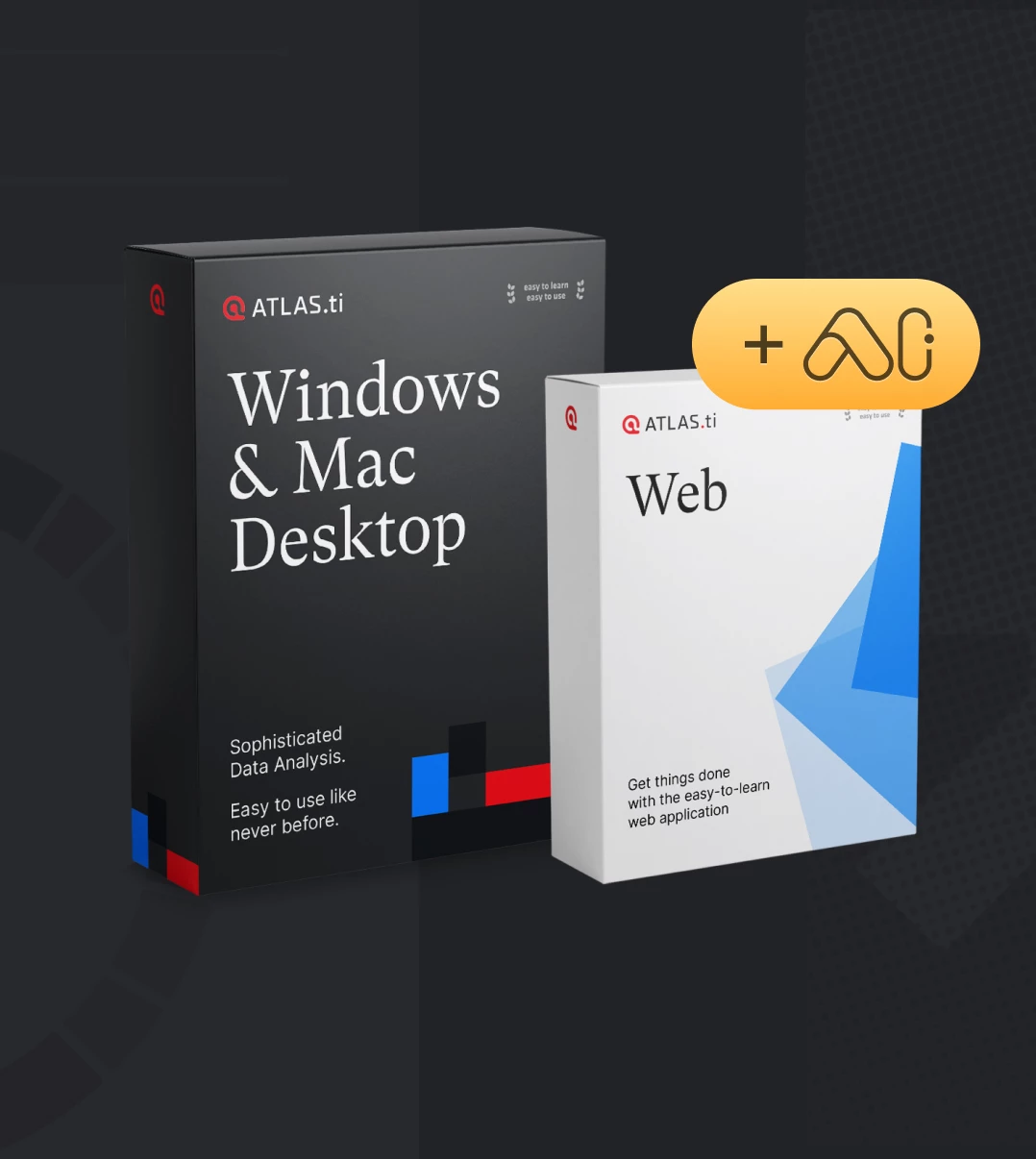
Master qualitative research with ATLAS.ti
Turn data into critical insights with our data analysis platform. Try out a free trial today.
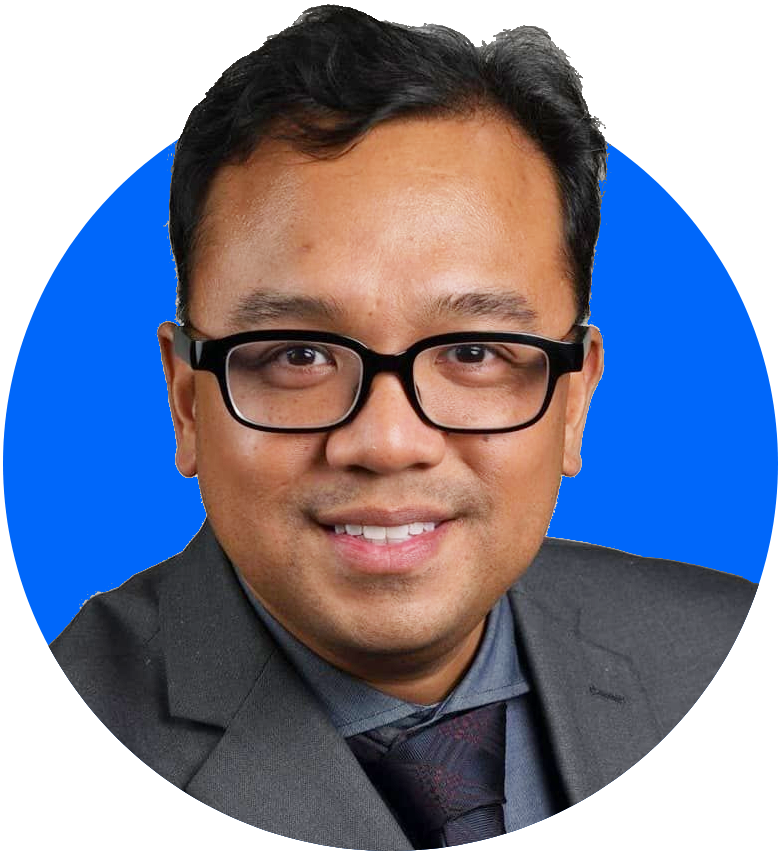
Independent Variables (Definition + 43 Examples)

Have you ever wondered how scientists make discoveries and how researchers come to understand the world around us? A crucial tool in their kit is the concept of the independent variable, which helps them delve into the mysteries of science and everyday life.
An independent variable is a condition or factor that researchers manipulate to observe its effect on another variable, known as the dependent variable. In simpler terms, it’s like adjusting the dials and watching what happens! By changing the independent variable, scientists can see if and how it causes changes in what they are measuring or observing, helping them make connections and draw conclusions.
In this article, we’ll explore the fascinating world of independent variables, journey through their history, examine theories, and look at a variety of examples from different fields.
History of the Independent Variable
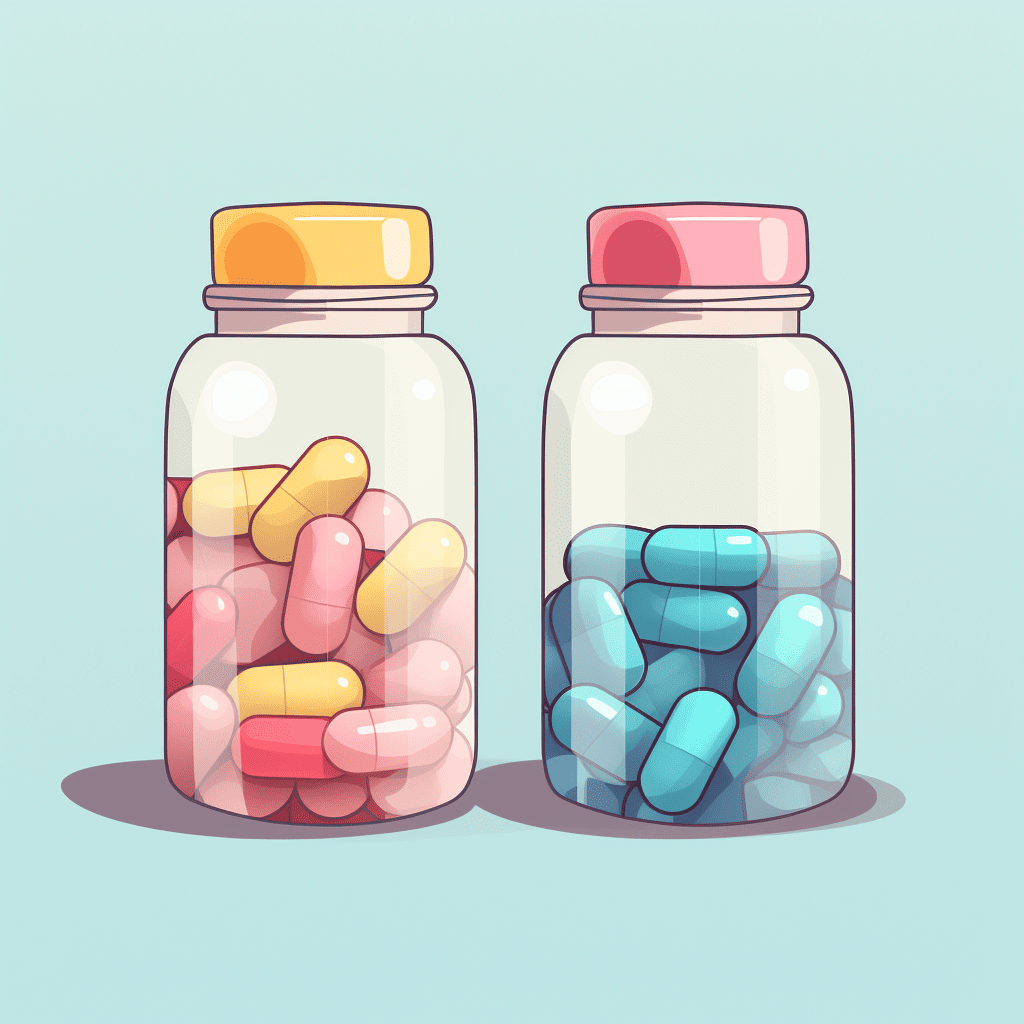
Once upon a time, in a world thirsty for understanding, people observed the stars, the seas, and everything in between, seeking to unlock the mysteries of the universe.
The story of the independent variable begins with a quest for knowledge, a journey taken by thinkers and tinkerers who wanted to explain the wonders and strangeness of the world.
Origins of the Concept
The seeds of the idea of independent variables were sown by Sir Francis Galton , an English polymath, in the 19th century. Galton wore many hats—he was a psychologist, anthropologist, meteorologist, and a statistician!
It was his diverse interests that led him to explore the relationships between different factors and their effects. Galton was curious—how did one thing lead to another, and what could be learned from these connections?
As Galton delved into the world of statistical theories , the concept of independent variables started taking shape.
He was interested in understanding how characteristics, like height and intelligence, were passed down through generations.
Galton’s work laid the foundation for later thinkers to refine and expand the concept, turning it into an invaluable tool for scientific research.
Evolution over Time
After Galton’s pioneering work, the concept of the independent variable continued to evolve and grow. Scientists and researchers from various fields adopted and adapted it, finding new ways to use it to make sense of the world.
They discovered that by manipulating one factor (the independent variable), they could observe changes in another (the dependent variable), leading to groundbreaking insights and discoveries.
Through the years, the independent variable became a cornerstone in experimental design . Researchers in fields like physics, biology, psychology, and sociology used it to test hypotheses, develop theories, and uncover the laws that govern our universe.
The idea that originated from Galton’s curiosity had bloomed into a universal key, unlocking doors to knowledge across disciplines.
Importance in Scientific Research
Today, the independent variable stands tall as a pillar of scientific research. It helps scientists and researchers ask critical questions, test their ideas, and find answers. Without independent variables, we wouldn’t have many of the advancements and understandings that we take for granted today.
The independent variable plays a starring role in experiments, helping us learn about everything from the smallest particles to the vastness of space. It helps researchers create vaccines, understand social behaviors, explore ecological systems, and even develop new technologies.
In the upcoming sections, we’ll dive deeper into what independent variables are, how they work, and how they’re used in various fields.
Together, we’ll uncover the magic of this scientific concept and see how it continues to shape our understanding of the world around us.
What is an Independent Variable?
Embarking on the captivating journey of scientific exploration requires us to grasp the essential terms and ideas. It's akin to a treasure hunter mastering the use of a map and compass.
In our adventure through the realm of independent variables, we’ll delve deeper into some fundamental concepts and definitions to help us navigate this exciting world.
Variables in Research
In the grand tapestry of research, variables are the gems that researchers seek. They’re elements, characteristics, or behaviors that can shift or vary in different circumstances.
Picture them as the myriad of ingredients in a chef’s kitchen—each variable can be adjusted or modified to create a myriad of dishes, each with a unique flavor!
Understanding variables is essential as they form the core of every scientific experiment and observational study.
Types of Variables
Independent Variable The star of our story, the independent variable, is the one that researchers change or control to study its effects. It’s like a chef experimenting with different spices to see how each one alters the taste of the soup. The independent variable is the catalyst, the initial spark that sets the wheels of research in motion.
Dependent Variable The dependent variable is the outcome we observe and measure . It’s the altered flavor of the soup that results from the chef’s culinary experiments. This variable depends on the changes made to the independent variable, hence the name!
Observing how the dependent variable reacts to changes helps scientists draw conclusions and make discoveries.
Control Variable Control variables are the unsung heroes of scientific research. They’re the constants, the elements that researchers keep the same to ensure the integrity of the experiment.
Imagine if our chef used a different type of broth each time he experimented with spices—the results would be all over the place! Control variables keep the experiment grounded and help researchers be confident in their findings.
Confounding Variables Imagine a hidden rock in a stream, changing the water’s flow in unexpected ways. Confounding variables are similar—they are external factors that can sneak into experiments and influence the outcome , adding twists to our scientific story.
These variables can blur the relationship between the independent and dependent variables, making the results of the study a bit puzzly. Detecting and controlling these hidden elements helps researchers ensure the accuracy of their findings and reach true conclusions.
There are of course other types of variables, and different ways to manipulate them called " schedules of reinforcement ," but we won't get into that too much here.
Role of the Independent Variable
Manipulation When researchers manipulate the independent variable, they are orchestrating a symphony of cause and effect. They’re adjusting the strings, the brass, the percussion, observing how each change influences the melody—the dependent variable.
This manipulation is at the heart of experimental research. It allows scientists to explore relationships, unravel patterns, and unearth the secrets hidden within the fabric of our universe.
Observation With every tweak and adjustment made to the independent variable, researchers are like seasoned detectives, observing the dependent variable for changes, collecting clues, and piecing together the puzzle.
Observing the effects and changes that occur helps them deduce relationships, formulate theories, and expand our understanding of the world. Every observation is a step towards solving the mysteries of nature and human behavior.
Identifying Independent Variables
Characteristics Identifying an independent variable in the vast landscape of research can seem daunting, but fear not! Independent variables have distinctive characteristics that make them stand out.
They’re the elements that are deliberately changed or controlled in an experiment to study their effects on the dependent variable. Recognizing these characteristics is like learning to spot footprints in the sand—it leads us to the heart of the discovery!
In Different Types of Research The world of research is diverse and varied, and the independent variable dons many guises! In the field of medicine, it might manifest as the dosage of a drug administered to patients.
In psychology, it could take the form of different learning methods applied to study memory retention. In each field, identifying the independent variable correctly is the golden key that unlocks the treasure trove of knowledge and insights.
As we forge ahead on our enlightening journey, equipped with a deeper understanding of independent variables and their roles, we’re ready to delve into the intricate theories and diverse examples that underscore their significance.
Independent Variables in Research
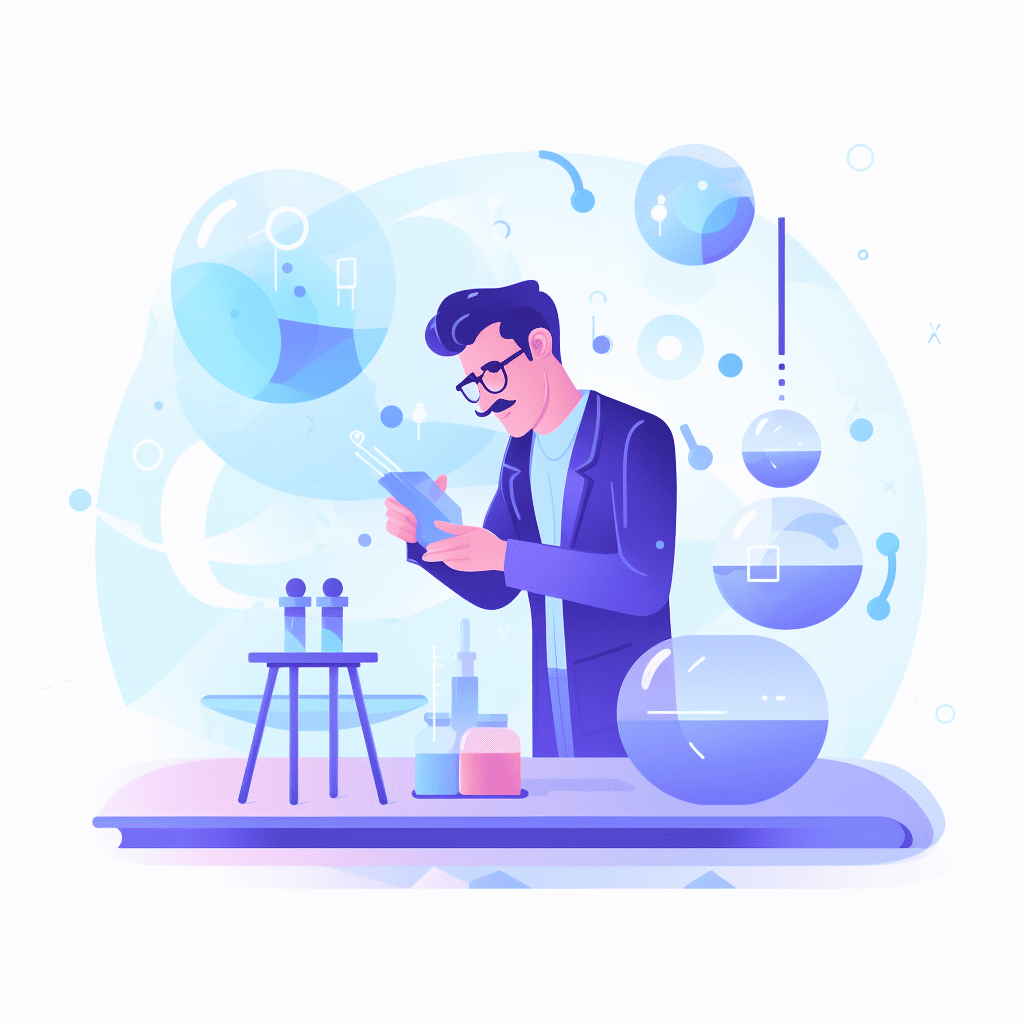
Now that we’re acquainted with the basic concepts and have the tools to identify independent variables, let’s dive into the fascinating ocean of theories and frameworks.
These theories are like ancient scrolls, providing guidelines and blueprints that help scientists use independent variables to uncover the secrets of the universe.
Scientific Method
What is it and How Does it Work? The scientific method is like a super-helpful treasure map that scientists use to make discoveries. It has steps we follow: asking a question, researching, guessing what will happen (that's a hypothesis!), experimenting, checking the results, figuring out what they mean, and telling everyone about it.
Our hero, the independent variable, is the compass that helps this adventure go the right way!
How Independent Variables Lead the Way In the scientific method, the independent variable is like the captain of a ship, leading everyone through unknown waters.
Scientists change this variable to see what happens and to learn new things. It’s like having a compass that points us towards uncharted lands full of knowledge!
Experimental Design
The Basics of Building Constructing an experiment is like building a castle, and the independent variable is the cornerstone. It’s carefully chosen and manipulated to see how it affects the dependent variable. Researchers also identify control and confounding variables, ensuring the castle stands strong, and the results are reliable.
Keeping Everything in Check In every experiment, maintaining control is key to finding the treasure. Scientists use control variables to keep the conditions consistent, ensuring that any changes observed are truly due to the independent variable. It’s like ensuring the castle’s foundation is solid, supporting the structure as it reaches for the sky.
Hypothesis Testing
Making Educated Guesses Before they start experimenting, scientists make educated guesses called hypotheses . It’s like predicting which X marks the spot of the treasure! It often includes the independent variable and the expected effect on the dependent variable, guiding researchers as they navigate through the experiment.
Independent Variables in the Spotlight When testing these guesses, the independent variable is the star of the show! Scientists change and watch this variable to see if their guesses were right. It helps them figure out new stuff and learn more about the world around us!
Statistical Analysis
Figuring Out Relationships After the experimenting is done, it’s time for scientists to crack the code! They use statistics to understand how the independent and dependent variables are related and to uncover the hidden stories in the data.
Experimenters have to be careful about how they determine the validity of their findings, which is why they use statistics. Something called "experimenter bias" can get in the way of having true (valid) results, because it's basically when the experimenter influences the outcome based on what they believe to be true (or what they want to be true!).
How Important are the Discoveries? Through statistical analysis, scientists determine the significance of their findings. It’s like discovering if the treasure found is made of gold or just shiny rocks. The analysis helps researchers know if the independent variable truly had an effect, contributing to the rich tapestry of scientific knowledge.
As we uncover more about how theories and frameworks use independent variables, we start to see how awesome they are in helping us learn more about the world. But we’re not done yet!
Up next, we’ll look at tons of examples to see how independent variables work their magic in different areas.
Examples of Independent Variables
Independent variables take on many forms, showcasing their versatility in a range of experiments and studies. Let’s uncover how they act as the protagonists in numerous investigations and learning quests!
Science Experiments
1) plant growth.
Consider an experiment aiming to observe the effect of varying water amounts on plant height. In this scenario, the amount of water given to the plants is the independent variable!
2) Freezing Water
Suppose we are curious about the time it takes for water to freeze at different temperatures. The temperature of the freezer becomes the independent variable as we adjust it to observe the results!
3) Light and Shadow
Have you ever observed how shadows change? In an experiment, adjusting the light angle to observe its effect on an object’s shadow makes the angle of light the independent variable!
4) Medicine Dosage
In medical studies, determining how varying medicine dosages influence a patient’s recovery is essential. Here, the dosage of the medicine administered is the independent variable!
5) Exercise and Health
Researchers might examine the impact of different exercise forms on individuals’ health. The various exercise forms constitute the independent variable in this study!
6) Sleep and Wellness
Have you pondered how the sleep duration affects your well-being the following day? In such research, the hours of sleep serve as the independent variable!
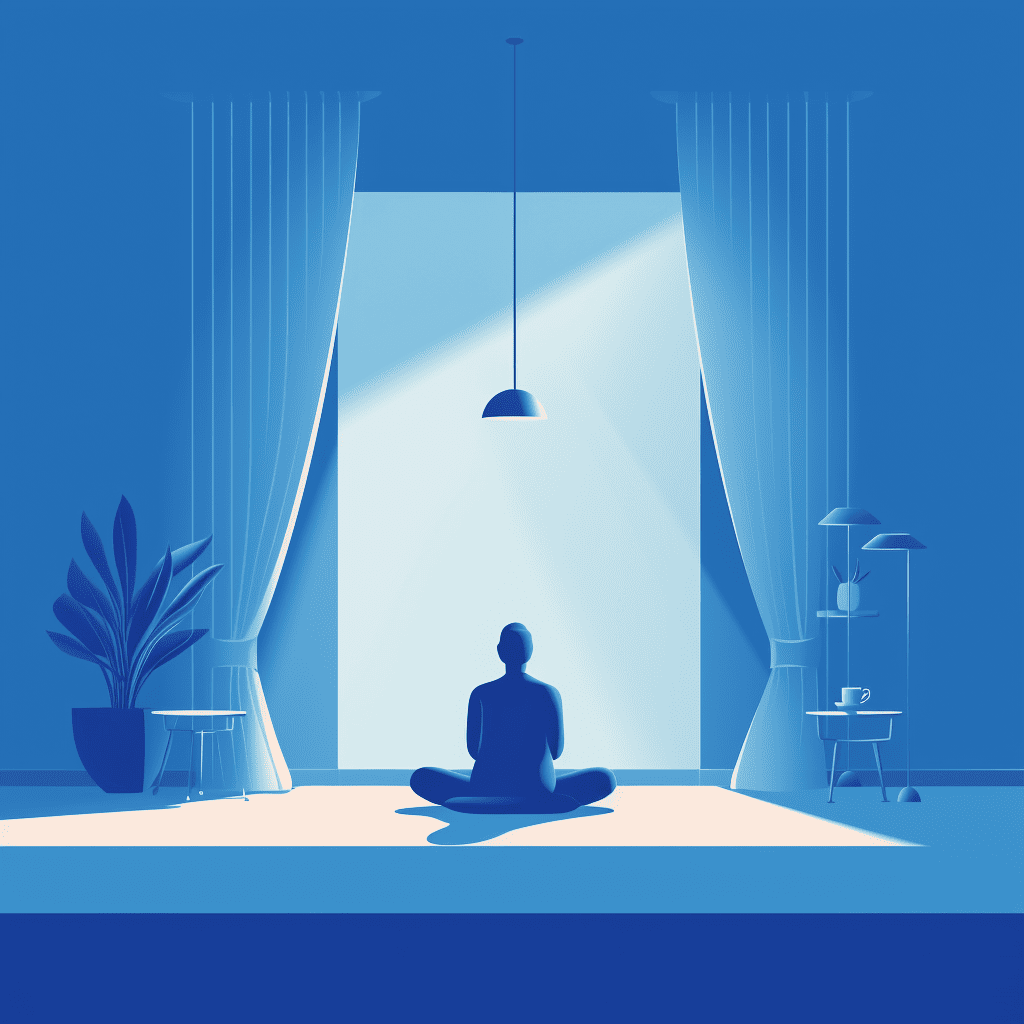
7) Learning Methods
Psychologists might investigate how diverse study methods influence test outcomes. Here, the different study methods adopted by students are the independent variable!
8) Mood and Music
Have you experienced varied emotions with different music genres? The genre of music played becomes the independent variable when researching its influence on emotions!
9) Color and Feelings
Suppose researchers are exploring how room colors affect individuals’ emotions. In this case, the room colors act as the independent variable!
Environment
10) rainfall and plant life.
Environmental scientists may study the influence of varying rainfall levels on vegetation. In this instance, the amount of rainfall is the independent variable!
11) Temperature and Animal Behavior
Examining how temperature variations affect animal behavior is fascinating. Here, the varying temperatures serve as the independent variable!
12) Pollution and Air Quality
Investigating the effects of different pollution levels on air quality is crucial. In such studies, the pollution level is the independent variable!
13) Internet Speed and Productivity
Researchers might explore how varying internet speeds impact work productivity. In this exploration, the internet speed is the independent variable!
14) Device Type and User Experience
Examining how different devices affect user experience is interesting. Here, the type of device used is the independent variable!
15) Software Version and Performance
Suppose a study aims to determine how different software versions influence system performance. The software version becomes the independent variable!
16) Teaching Style and Student Engagement
Educators might investigate the effect of varied teaching styles on student engagement. In such a study, the teaching style is the independent variable!
17) Class Size and Learning Outcome
Researchers could explore how different class sizes influence students’ learning. Here, the class size is the independent variable!
18) Homework Frequency and Academic Achievement
Examining the relationship between the frequency of homework assignments and academic success is essential. The frequency of homework becomes the independent variable!
19) Telescope Type and Celestial Observation
Astronomers might study how different telescopes affect celestial observation. In this scenario, the telescope type is the independent variable!
20) Light Pollution and Star Visibility
Investigating the influence of varying light pollution levels on star visibility is intriguing. Here, the level of light pollution is the independent variable!
21) Observation Time and Astronomical Detail
Suppose a study explores how observation duration affects the detail captured in astronomical images. The duration of observation serves as the independent variable!
22) Community Size and Social Interaction
Sociologists may examine how the size of a community influences social interactions. In this research, the community size is the independent variable!
23) Cultural Exposure and Social Tolerance
Investigating the effect of diverse cultural exposure on social tolerance is vital. Here, the level of cultural exposure is the independent variable!
24) Economic Status and Educational Attainment
Researchers could explore how different economic statuses impact educational achievements. In such studies, economic status is the independent variable!
25) Training Intensity and Athletic Performance
Sports scientists might study how varying training intensities affect athletes’ performance. In this case, the training intensity is the independent variable!
26) Equipment Type and Player Safety
Examining the relationship between different sports equipment and player safety is crucial. Here, the type of equipment used is the independent variable!
27) Team Size and Game Strategy
Suppose researchers are investigating how the size of a sports team influences game strategy. The team size becomes the independent variable!
28) Diet Type and Health Outcome
Nutritionists may explore the impact of various diets on individuals’ health. In this exploration, the type of diet followed is the independent variable!
29) Caloric Intake and Weight Change
Investigating how different caloric intakes influence weight change is essential. In such a study, the caloric intake is the independent variable!
30) Food Variety and Nutrient Absorption
Researchers could examine how consuming a variety of foods affects nutrient absorption. Here, the variety of foods consumed is the independent variable!
Real-World Examples of Independent Variables
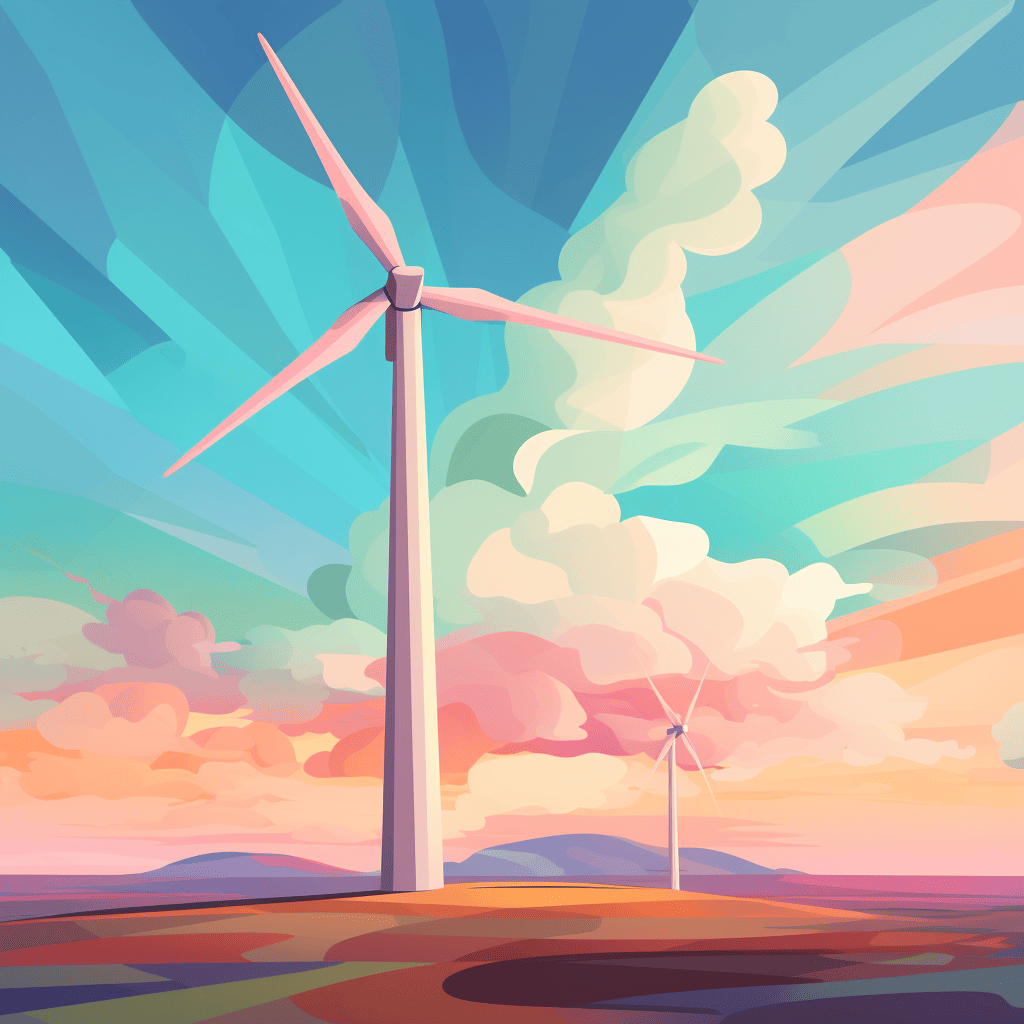
Isn't it fantastic how independent variables play such an essential part in so many studies? But the excitement doesn't stop there!
Now, let’s explore how findings from these studies, led by independent variables, make a big splash in the real world and improve our daily lives!
Healthcare Advancements
31) treatment optimization.
By studying different medicine dosages and treatment methods as independent variables, doctors can figure out the best ways to help patients recover quicker and feel better. This leads to more effective medicines and treatment plans!
32) Lifestyle Recommendations
Researching the effects of sleep, exercise, and diet helps health experts give us advice on living healthier lives. By changing these independent variables, scientists uncover the secrets to feeling good and staying well!
Technological Innovations
33) speeding up the internet.
When scientists explore how different internet speeds affect our online activities, they’re able to develop technologies to make the internet faster and more reliable. This means smoother video calls and quicker downloads!
34) Improving User Experience
By examining how we interact with various devices and software, researchers can design technology that’s easier and more enjoyable to use. This leads to cooler gadgets and more user-friendly apps!
Educational Strategies
35) enhancing learning.
Investigating different teaching styles, class sizes, and study methods helps educators discover what makes learning fun and effective. This research shapes classrooms, teaching methods, and even homework!
36) Tailoring Student Support
By studying how students with diverse needs respond to different support strategies, educators can create personalized learning experiences. This means every student gets the help they need to succeed!
Environmental Protection
37) conserving nature.
Researching how rainfall, temperature, and pollution affect the environment helps scientists suggest ways to protect our planet. By studying these independent variables, we learn how to keep nature healthy and thriving!
38) Combating Climate Change
Scientists studying the effects of pollution and human activities on climate change are leading the way in finding solutions. By exploring these independent variables, we can develop strategies to combat climate change and protect the Earth!
Social Development
39) building stronger communities.
Sociologists studying community size, cultural exposure, and economic status help us understand what makes communities happy and united. This knowledge guides the development of policies and programs for stronger societies!
40) Promoting Equality and Tolerance
By exploring how exposure to diverse cultures affects social tolerance, researchers contribute to fostering more inclusive and harmonious societies. This helps build a world where everyone is respected and valued!
Enhancing Sports Performance
41) optimizing athlete training.
Sports scientists studying training intensity, equipment type, and team size help athletes reach their full potential. This research leads to better training programs, safer equipment, and more exciting games!
42) Innovating Sports Strategies
By investigating how different game strategies are influenced by various team compositions, researchers contribute to the evolution of sports. This means more thrilling competitions and matches for us to enjoy!
Nutritional Well-Being
43) guiding healthy eating.
Nutritionists researching diet types, caloric intake, and food variety help us understand what foods are best for our bodies. This knowledge shapes dietary guidelines and helps us make tasty, yet nutritious, meal choices!
44) Promoting Nutritional Awareness
By studying the effects of different nutrients and diets, researchers educate us on maintaining a balanced diet. This fosters a greater awareness of nutritional well-being and encourages healthier eating habits!
As we journey through these real-world applications, we witness the incredible impact of studies featuring independent variables. The exploration doesn’t end here, though!
Let’s continue our adventure and see how we can identify independent variables in our own observations and inquiries! Keep your curiosity alive, and let’s delve deeper into the exciting realm of independent variables!
Identifying Independent Variables in Everyday Scenarios
So, we’ve seen how independent variables star in many studies, but how about spotting them in our everyday life?
Recognizing independent variables can be like a treasure hunt – you never know where you might find one! Let’s uncover some tips and tricks to identify these hidden gems in various situations.
1) Asking Questions
One of the best ways to spot an independent variable is by asking questions! If you’re curious about something, ask yourself, “What am I changing or manipulating in this situation?” The thing you’re changing is likely the independent variable!
For example, if you’re wondering whether the amount of sunlight affects how quickly your laundry dries, the sunlight amount is your independent variable!
2) Making Observations
Keep your eyes peeled and observe the world around you! By watching how changes in one thing (like the amount of rain) affect something else (like the height of grass), you can identify the independent variable.
In this case, the amount of rain is the independent variable because it’s what’s changing!
3) Conducting Experiments
Get hands-on and conduct your own experiments! By changing one thing and observing the results, you’re identifying the independent variable.
If you’re growing plants and decide to water each one differently to see the effects, the amount of water is your independent variable!
4) Everyday Scenarios
In everyday scenarios, independent variables are all around!
When you adjust the temperature of your oven to bake cookies, the oven temperature is the independent variable.
Or if you’re deciding how much time to spend studying for a test, the study time is your independent variable!
5) Being Curious
Keep being curious and asking “What if?” questions! By exploring different possibilities and wondering how changing one thing could affect another, you’re on your way to identifying independent variables.
If you’re curious about how the color of a room affects your mood, the room color is the independent variable!
6) Reviewing Past Studies
Don’t forget about the treasure trove of past studies and experiments! By reviewing what scientists and researchers have done before, you can learn how they identified independent variables in their work.
This can give you ideas and help you recognize independent variables in your own explorations!
Exercises for Identifying Independent Variables
Ready for some practice? Let’s put on our thinking caps and try to identify the independent variables in a few scenarios.
Remember, the independent variable is what’s being changed or manipulated to observe the effect on something else! (You can see the answers below)
Scenario One: Cooking Time
You’re cooking pasta for dinner and want to find out how the cooking time affects its texture. What is the independent variable?
Scenario Two: Exercise Routine
You decide to try different exercise routines each week to see which one makes you feel the most energetic. What is the independent variable?
Scenario Three: Plant Fertilizer
You’re growing tomatoes in your garden and decide to use different types of fertilizer to see which one helps them grow the best. What is the independent variable?
Scenario Four: Study Environment
You’re preparing for an important test and try studying in different environments (quiet room, coffee shop, library) to see where you concentrate best. What is the independent variable?
Scenario Five: Sleep Duration
You’re curious to see how the number of hours you sleep each night affects your mood the next day. What is the independent variable?
By practicing identifying independent variables in different scenarios, you’re becoming a true independent variable detective. Keep practicing, stay curious, and you’ll soon be spotting independent variables everywhere you go.
Independent Variable: The cooking time is the independent variable. You are changing the cooking time to observe its effect on the texture of the pasta.
Independent Variable: The type of exercise routine is the independent variable. You are trying out different exercise routines each week to see which one makes you feel the most energetic.
Independent Variable: The type of fertilizer is the independent variable. You are using different types of fertilizer to observe their effects on the growth of the tomatoes.
Independent Variable: The study environment is the independent variable. You are studying in different environments to see where you concentrate best.
Independent Variable: The number of hours you sleep is the independent variable. You are changing your sleep duration to see how it affects your mood the next day.
Whew, what a journey we’ve had exploring the world of independent variables! From understanding their definition and role to diving into a myriad of examples and real-world impacts, we’ve uncovered the treasures hidden in the realm of independent variables.
The beauty of independent variables lies in their ability to unlock new knowledge and insights, guiding us to discoveries that improve our lives and the world around us.
By identifying and studying these variables, we embark on exciting learning adventures, solving mysteries and answering questions about the universe we live in.
Remember, the joy of discovery doesn’t end here. The world is brimming with questions waiting to be answered and mysteries waiting to be solved.
Keep your curiosity alive, continue exploring, and who knows what incredible discoveries lie ahead.
Related posts:
- Confounding Variable in Psychology (Examples + Definition)
- 19+ Experimental Design Examples (Methods + Types)
- Variable Interval Reinforcement Schedule (Examples)
- Variable Ratio Reinforcement Schedule (Examples)
- State Dependent Memory + Learning (Definition and Examples)
Reference this article:
About The Author
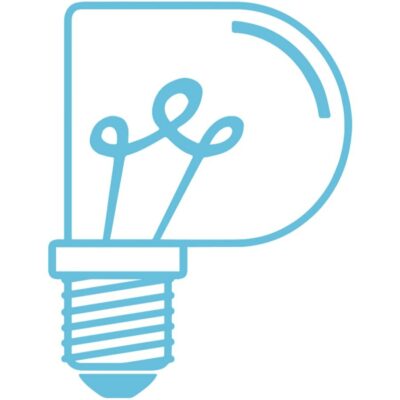
Free Personality Test
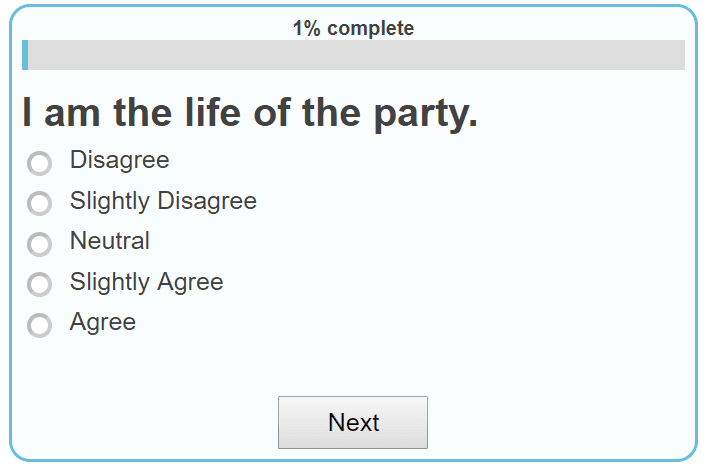
Free Memory Test
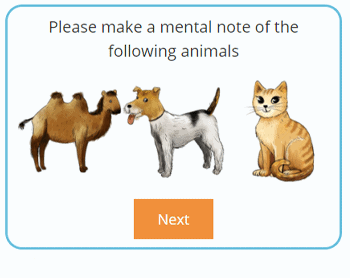
Free IQ Test
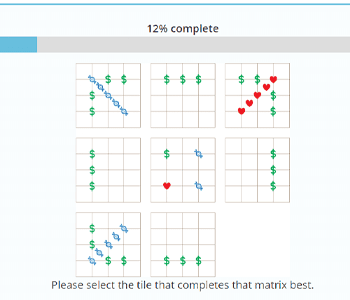
PracticalPie.com is a participant in the Amazon Associates Program. As an Amazon Associate we earn from qualifying purchases.
Follow Us On:
Youtube Facebook Instagram X/Twitter
Psychology Resources
Developmental
Personality
Relationships
Psychologists
Serial Killers
Psychology Tests
Personality Quiz
Memory Test
Depression test
Type A/B Personality Test
© PracticalPsychology. All rights reserved
Privacy Policy | Terms of Use
- Bipolar Disorder
- Therapy Center
- When To See a Therapist
- Types of Therapy
- Best Online Therapy
- Best Couples Therapy
- Best Family Therapy
- Managing Stress
- Sleep and Dreaming
- Understanding Emotions
- Self-Improvement
- Healthy Relationships
- Student Resources
- Personality Types
- Guided Meditations
- Verywell Mind Insights
- 2023 Verywell Mind 25
- Mental Health in the Classroom
- Editorial Process
- Meet Our Review Board
- Crisis Support
Independent Variables in Psychology
Kendra Cherry, MS, is a psychosocial rehabilitation specialist, psychology educator, and author of the "Everything Psychology Book."
:max_bytes(150000):strip_icc():format(webp)/IMG_9791-89504ab694d54b66bbd72cb84ffb860e.jpg)
Amanda Tust is a fact-checker, researcher, and writer with a Master of Science in Journalism from Northwestern University's Medill School of Journalism.
:max_bytes(150000):strip_icc():format(webp)/Amanda-Tust-1000-ffe096be0137462fbfba1f0759e07eb9.jpg)
Adam Berry / Getty Images
- Identifying
Potential Pitfalls
The independent variable (IV) in psychology is the characteristic of an experiment that is manipulated or changed by researchers, not by other variables in the experiment.
For example, in an experiment looking at the effects of studying on test scores, studying would be the independent variable. Researchers are trying to determine if changes to the independent variable (studying) result in significant changes to the dependent variable (the test results).
In general, experiments have these three types of variables: independent, dependent, and controlled.
Identifying the Independent Variable
If you are having trouble identifying the independent variables of an experiment, there are some questions that may help:
- Is the variable one that is being manipulated by the experimenters?
- Are researchers trying to identify how the variable influences another variable?
- Is the variable something that cannot be changed but that is not dependent on other variables in the experiment?
Researchers are interested in investigating the effects of the independent variable on other variables, which are known as dependent variables (DV). The independent variable is one that the researchers either manipulate (such as the amount of something) or that already exists but is not dependent upon other variables (such as the age of the participants).
Below are the key differences when looking at an independent variable vs. dependent variable.
Expected to influence the dependent variable
Doesn't change as a result of the experiment
Can be manipulated by researchers in order to study the dependent variable
Expected to be affected by the independent variable
Expected to change as a result of the experiment
Not manipulated by researchers; its changes occur as a result of the independent variable
There can be all different types of independent variables. The independent variables in a particular experiment all depend on the hypothesis and what the experimenters are investigating.
Independent variables also have different levels. In some experiments, there may only be one level of an IV. In other cases, multiple levels of the IV may be used to look at the range of effects that the variable may have.
In an experiment on the effects of the type of diet on weight loss, for example, researchers might look at several different types of diet. Each type of diet that the experimenters look at would be a different level of the independent variable while weight loss would always be the dependent variable.
To understand this concept, it's helpful to take a look at the independent variable in research examples.
In Organizations
A researcher wants to determine if the color of an office has any effect on worker productivity. In an experiment, one group of workers performs a task in a yellow room while another performs the same task in a blue room. In this example, the color of the office is the independent variable.
In the Workplace
A business wants to determine if giving employees more control over how to do their work leads to increased job satisfaction. In an experiment, one group of workers is given a great deal of input in how they perform their work, while the other group is not. The amount of input the workers have over their work is the independent variable in this example.
In Educational Research
Educators are interested in whether participating in after-school math tutoring can increase scores on standardized math exams. In an experiment, one group of students attends an after-school tutoring session twice a week while another group of students does not receive this additional assistance. In this case, participation in after-school math tutoring is the independent variable.
In Mental Health Research
Researchers want to determine if a new type of treatment will lead to a reduction in anxiety for patients living with social phobia. In an experiment, some volunteers receive the new treatment, another group receives a different treatment, and a third group receives no treatment. The independent variable in this example is the type of therapy .
Sometimes varying the independent variables will result in changes in the dependent variables. In other cases, researchers might find that changes in the independent variables have no effect on the variables that are being measured.
At the outset of an experiment, it is important for researchers to operationally define the independent variable. An operational definition describes exactly what the independent variable is and how it is measured. Doing this helps ensure that the experiments know exactly what they are looking at or manipulating, allowing them to measure it and determine if it is the IV that is causing changes in the DV.
Choosing an Independent Variable
If you are designing an experiment, here are a few tips for choosing an independent variable (or variables):
- Select independent variables that you think will cause changes in another variable. Come up with a hypothesis for what you expect to happen.
- Look at other experiments for examples and identify different types of independent variables.
- Keep your control group and experimental groups similar in other characteristics, but vary only the treatment they receive in terms of the independent variable. For example, your control group will receive either no treatment or no changes in the independent variable while your experimental group will receive the treatment or a different level of the independent variable.
It is also important to be aware that there may be other variables that might influence the results of an experiment. Two other kinds of variables that might influence the outcome include:
- Extraneous variables : These are variables that might affect the relationships between the independent variable and the dependent variable; experimenters usually try to identify and control for these variables.
- Confounding variables : When an extraneous variable cannot be controlled for in an experiment, it is known as a confounding variable.
Extraneous variables can also include demand characteristics (which are clues about how the participants should respond) and experimenter effects (which is when the researchers accidentally provide clues about how a participant will respond).
Kaliyadan F, Kulkarni V. Types of variables, descriptive statistics, and sample size . Indian Dermatol Online J . 2019;10(1):82-86. doi:10.4103/idoj.IDOJ_468_18
Weiten, W. Psychology: Themes and Variations, 10th ed . Boston, MA: Cengage Learning; 2017.
National Library of Medicine. Dependent and independent variables .
By Kendra Cherry, MSEd Kendra Cherry, MS, is a psychosocial rehabilitation specialist, psychology educator, and author of the "Everything Psychology Book."
What is an independent variable?
Last updated
14 February 2023
Reviewed by
Independent variables are features or values fixed within the population or study under investigation. An example might be a subject's age within a study - other variables, such as what they eat, how long they sleep, and how much TV they watch wouldn't change the subject's age.
On the other hand, a dependent variable can be influenced by other factors or variables. For example, how well you perform on a series of tests (a dependent variable) could be influenced by how long you study or how much sleep you get before the night of the exam.
A better understanding of independent variables, specifically the types, how they function in research contexts, and how to distinguish them from dependent variables, will assist you in determining how to identify them in your studies.
Make research less tedious
Dovetail streamlines research to help you uncover and share actionable insights
- Types of independent variables
Independent variables can be of several types, depending on the hypothesis and research. However, the most common types are experimental independent variables and subject variables.
Experimental independent variables
Experimental variables are those that can be directly manipulated in a study. In other words, these are independent variables that you can manipulate to discover how they influence your dependent variables.
For example, you may have two study groups split by independent variables: one receiving a new drug treatment and one receiving a placebo. These types of studies generally require the random assignment of research participants to different groups to observe how results vary based on the influence of different independent variables.
A proper experiment requires you to randomly assign different levels of an independent variable to your participants.
Random assignment helps you control participant characteristics, so they don't affect your experimental results. This helps you to have confidence that your dependent variable results come solely from the experimental independent variable manipulation.
Subject variables
Subject variables are independent variables that can't be changed in a study but can be used to categorize study participants. They are mostly features that differ between study subjects. For instance, as a social researcher, you can use gender identification, race, education level, or income as key independent variables to classify your research subjects.
Unlike experimental variables, subject variables necessitate a quasi-experimental approach because there is no random assignment. This type of independent variable comprises features and attributes inherent within study participants; therefore, they cannot be assigned randomly.
Instead, you can develop a research approach in which you evaluate the findings of different groups of participants based on their features. It is important to note that any research design that uses non-random assignment is vulnerable to study biases such as sampling and selection bias.
- What is the importance of independent variables?
As noted previously, independent variables are critical in developing a study design. This is because they assist researchers in determining cause-and-effect relationships. Controlled experiments require minimal to no outside influence to make conclusions.
Identifying independent variables is one way to eliminate external influences and achieve greater certainty that research results are representative. By controlling for outside influences as much as possible, you can make meaningful inferences about the link between independent and dependent variables.
In most cases, changes in the independent variables cause changes in the dependent variables. For example, if you change an independent variable such as age, you might expect a dependent variable such as cognitive function or running speed to change if the age difference is large. However, there are situations when variations in the independent variables do not influence the dependent variable.
- How can you choose an independent variable?
Choosing independent variables within your research will be driven by the objectives of your study. Start by formulating a hypothesis about the outcome you anticipate, and then choose independent variables that you believe will significantly influence the dependent variables.
Make sure you have experimental and control groups that have identical features. They should only differ based on the treatment they get for the independent variable. In this case, your control group will undergo no treatment or changes in the independent variable, versus the experimental group, which will receive the treatment or a wide variation of the independent variable.
- How to include an independent variable in an experiment
The type of study or experiment greatly impacts the nature of an independent variable. If you are doing an experiment involving a control condition or group, you will need to monitor and define the values of the independent variables you are using within test condition groups.
In an observational experiment, the explanatory variables' values are not predetermined, but instead are observed in their natural surroundings.
Model specification is the process of deciding which independent variables to incorporate into a statistical model. It involves extensive study, numerous specific topics, and statistical aspects.
Including one independent variable in a regression model entails performing a simple regression, while for more than one independent variable, it is a multiple regression. The names might be different, but the analysis, interpretation, and assumptions are all the same.
- What are some examples of independent variables?
To better understand the concept of independent variables, have a look at these few examples used in different contexts:
Mental health context: As a medical researcher, you may be interested in finding out whether a new type of treatment can reduce anxiety in people suffering from a social anxiety disorder. Your study can include three groups of patients. One group receives the new treatment, another gets a different treatment, and the last gets no treatment. The type of treatment is the independent variable.
Workplace context: In this case, you may want to know if giving employees greater control over how they perform their duties results in increased job satisfaction. Your study will involve two groups of employees, one with a lot of say over how they do their jobs and the other without. In this scenario, the independent variable is the amount of control the employees have over their job.
Educational context: You can conduct a study to see if after-school math tutoring improves student performance on standardized math tests. In this example, one group of students will attend an after-school tutoring session three times a week, whereas another group will not receive this extra help. The independent variable is the involvement in after-school math tutoring sessions.
Organization context: You may want to know if the color of an office affects work efficiency. Your research will consider a group of employees working in white or yellow rooms. The independent variable is the color of the office.
- What is a dependent variable?
A dependent variable changes as a result of the manipulation of the independent variable. In a nutshell, it is what you test or measure in an experiment. It is also known as a response variable since it responds to changes in another variable, or known as an outcome variable because it represents the outcome you want to measure.
Statisticians also denote these as left-hand side variables because they are typically found on the left-hand side of a regression model. Typically, dependent variables are plotted on the y-axis of graphs.
For instance, in a study designed to evaluate how a certain treatment affects the symptoms of psychological disorders, the dependent variable might be identified as the severity of the symptoms a patient experiences. The treatment used would be the independent variable.
The results of an experiment are important because they can assist you in determining the extent to which changes in your independent variable cause variations in your dependent variable. They can also help forecast the degree to which your dependent variable will vary due to changes in the independent variable.
- Identifying independent vs. dependent variables
It can be challenging to differentiate between independent and dependent variables, especially when designing comprehensive research. In some circumstances, a dependent variable from one research study will be used as an independent variable in another. The key is to pay close attention to the study design.
Recognizing independent variables
To recognize independent variables in research, focus on determining whether the variable causes variation in another variable. Independent variables are also manipulated variables whose values are determined by the researchers. In certain experiments, notably in medicine, they are described as risk factors; whereas in others, they are referred to as experimental factors.
Keep in mind that control groups and treatments are often independent variables. And studies that use this approach tend to classify independent variables as categorical grouping variables that establish the experimental groups.
The approaches used to identify independent variables in observational research differ slightly. In these studies, independent variables explain, predict, or correlate with variation in the dependent variable. The study results are also changed or regulated by a variable. If you see an estimated impact size, it is an independent variable, irrespective of the type of study you are reading or designing.
Recognizing dependent variables
To identify dependent variables, you must first determine if the variable is measurable within the research. Also, determine whether the variable relies on another variable in the experiment. If you discover that a variable is only subject to change or variability after other variables have been changed, it may be a dependent variable.
- Independent and dependent variables in research
Both independent and dependent variables are mainly used in quasi-experimental and experimental studies. When conducting research, you can generate descriptive statistics to illustrate results. Following that, you would choose a suitable statistical test to validate your hypothesis.
The kind of variable, measurement level, and several independent variable levels will significantly influence your chosen test. Many studies use either the ANOVA or the t-test for data analysis and to obtain answers to research questions .
- Other key variables
Other variables, in addition to independent and dependent variables, may have a major impact on a research outcome. Thus, it is vital to identify and take control of extraneous variables since they can cause variation in the relationship between the independent and dependent variables.
Some examples of extraneous variables include demand characteristics and experimenter effects. When these variables cannot be controlled in an experiment, they are usually called confounding variables .
- Visualizing independent and dependent variables
You can use either a chart or a graph to visualize quantitative research results. Graphs have a typical display in which the independent variables lie on the horizontal x-axis and the dependent variables on the vertical y-axis. The presentation of data will depend on the nature of the variables in your research questions.
- The lowdown
Having a working knowledge of independent and dependent variables is key to understanding how research projects work. There are various ways to think of independent variables. However, the best approach is to picture the independent variable as what you change and the dependent variable as what is influenced due to the variation.
In other words, consider the independent variable the cause and the dependent variable the effect. When visualizing these variables in a graph, place the independent variable on the x-axis and the dependent variable on the y-axis.
It is also essential to remember that there are other variables aside from the independent and dependent variables that might impact the outcome of an experiment. As a result, you should identify and control extraneous variables as much as possible to make a valid conclusion about the study findings.

What are the dependent and independent variables in research?
An independent variable in research or an experiment is what the researcher manipulates or changes. The dependent variable, on the other hand, is what is measured. In general, the independent variable is in charge of influencing the dependent variable.
What are the variables in research examples?
In research or an experiment, a variable refers to something that can be tested. You can use independent and dependent variables to design research .
Can a variable be both independent and dependent at the same time?
No, because a dependent variable is reliant on the independent variable. Thus, a variable in a study can only be the cause (independent) or the effect (dependent). However, there are also cases in which a dependent variable from one study is used as an independent variable in another.
Can a study have more than one independent or dependent variable?
Yes, however, a study must include various research questions for multiple independent and dependent variables to be effective.
Get started today
Go from raw data to valuable insights with a flexible research platform
Editor’s picks
Last updated: 21 December 2023
Last updated: 16 December 2023
Last updated: 6 October 2023
Last updated: 25 November 2023
Last updated: 12 May 2023
Last updated: 15 February 2024
Last updated: 11 March 2024
Last updated: 12 December 2023
Last updated: 18 May 2023
Last updated: 6 March 2024
Last updated: 10 April 2023
Last updated: 20 December 2023
Latest articles
Related topics, log in or sign up.
Get started for free

An official website of the United States government
The .gov means it's official. Federal government websites often end in .gov or .mil. Before sharing sensitive information, make sure you're on a federal government site.
The site is secure. The https:// ensures that you are connecting to the official website and that any information you provide is encrypted and transmitted securely.
- Publications
- Account settings
- Browse Titles
NCBI Bookshelf. A service of the National Library of Medicine, National Institutes of Health.
StatPearls [Internet]. Treasure Island (FL): StatPearls Publishing; 2024 Jan-.

StatPearls [Internet].
Types of variables and commonly used statistical designs.
Jacob Shreffler ; Martin R. Huecker .
Affiliations
Last Update: March 6, 2023 .
- Definition/Introduction
Suitable statistical design represents a critical factor in permitting inferences from any research or scientific study. [1] Numerous statistical designs are implementable due to the advancement of software available for extensive data analysis. [1] Healthcare providers must possess some statistical knowledge to interpret new studies and provide up-to-date patient care. We present an overview of the types of variables and commonly used designs to facilitate this understanding. [2]
- Issues of Concern
Individuals who attempt to conduct research and choose an inappropriate design could select a faulty test and make flawed conclusions. This decision could lead to work being rejected for publication or (worse) lead to erroneous clinical decision-making, resulting in unsafe practice. [1] By understanding the types of variables and choosing tests that are appropriate to the data, individuals can draw appropriate conclusions and promote their work for an application. [3]
To determine which statistical design is appropriate for the data and research plan, one must first examine the scales of each measurement. [4] Multiple types of variables determine the appropriate design.
Ordinal data (also sometimes referred to as discrete) provide ranks and thus levels of degree between the measurement. [5] Likert items can serve as ordinal variables, but the Likert scale, the result of adding all the times, can be treated as a continuous variable. [6] For example, on a 20-item scale with each item ranging from 1 to 5, the item itself can be an ordinal variable, whereas if you add up all items, it could result in a range from 20 to 100. A general guideline for determining if a variable is ordinal vs. continuous: if the variable has more than ten options, it can be treated as a continuous variable. [7] The following examples are ordinal variables:
- Likert items
- Cancer stages
- Residency Year
Nominal, Categorical, Dichotomous, Binary
Other types of variables have interchangeable terms. Nominal and categorical variables describe samples in groups based on counts that fall within each category, have no quantitative relationships, and cannot be ranked. [8] Examples of these variables include:
- Service (i.e., emergency, internal medicine, psychiatry, etc.)
- Mode of Arrival (ambulance, helicopter, car)
A dichotomous or a binary variable is in the same family as nominal/categorical, but this type has only two options. Binary logistic regression, which will be discussed below, has two options for the outcome of interest/analysis. Often used as (yes/no), examples of dichotomous or binary variables would be:
- Alive (yes vs. no)
- Insurance (yes vs. no)
- Readmitted (yes vs. no)
With this overview of the types of variables provided, we will present commonly used statistical designs for different scales of measurement. Importantly, before deciding on a statistical test, individuals should perform exploratory data analysis to ensure there are no issues with the data and consider type I, type II errors, and power analysis. Furthermore, investigators should ensure appropriate statistical assumptions. [9] [10] For example, parametric tests, including some discussed below (t-tests, analysis of variance (ANOVA), correlation, and regression), require the data to have a normal distribution and that the variances within each group are similar. [6] [11] After eliminating any issues based on exploratory data analysis and reducing the likelihood of committing type I and type II errors, a statistical test can be chosen. Below is a brief introduction to each of the commonly used statistical designs with examples of each type. An example of one research focus, with each type of statistical design discussed, can be found in Table 1 to provide more examples of commonly used statistical designs.
Commonly Used Statistical Designs
Independent Samples T-test
An independent samples t-test allows a comparison of two groups of subjects on one (continuous) variable. Examples in biomedical research include comparing results of treatment vs. control group and comparing differences based on gender (male vs. female).
Example: Does adherence to the ketogenic diet (yes/no; two groups) have a differential effect on total sleep time (minutes; continuous)?
Paired T-test
A paired t-test analyzes one sample population, measuring the same variable on two different occasions; this is often useful for intervention and educational research.
Example : Does participating in a research curriculum (one group with intervention) improve resident performance on a test to measure research competence (continuous)?
One-Way Analysis of Variance (ANOVA)
Analysis of variance (ANOVA), as an extension of the t-test, determines differences amongst more than two groups, or independent variables based on a dependent variable. [11] ANOVA is preferable to conducting multiple t-tests as it reduces the likelihood of committing a type I error.
Example: Are there differences in length of stay in the hospital (continuous) based on the mode of arrival (car, ambulance, helicopter, three groups)?
Repeated Measures ANOVA
Another procedure commonly used if the data for individuals are recurrent (repeatedly measured) is a repeated-measures ANOVA. [1] In these studies, multiple measurements of the dependent variable are collected from the study participants. [11] A within-subjects repeated measures ANOVA determines effects based on the treatment variable alone, whereas mixed ANOVAs allow both between-group effects and within-subjects to be considered.
Within-Subjects Example: How does ketamine effect mean arterial pressure (continuous variable) over time (repeated measurement)?
Mixed Example: Does mean arterial pressure (continuous) differ between males and females (two groups; mixed) on ketamine throughout a surgical procedure (over time; repeated measurement)?
Nonparametric Tests
Nonparametric tests, such as the Mann-Whitney U test (two groups; nonparametric t-test), Kruskal Wallis test (multiple groups; nonparametric ANOVA), Spearman’s rho (nonparametric correlation coefficient) can be used when data are ordinal or lack normality. [3] [5] Not requiring normality means that these tests allow skewed data to be analyzed; they require the meeting of fewer assumptions. [11]
Example: Is there a relationship between insurance status (two groups) and cancer stage (ordinal)?
A Chi-square test determines the effect of relationships between categorical variables, which determines frequencies and proportions into which these variables fall. [11] Similar to other tests discussed, variants and extensions of the chi-square test (e.g., Fisher’s exact test, McNemar’s test) may be suitable depending on the variables. [8]
Example: Is there a relationship between individuals with methamphetamine in their system (yes vs. no; dichotomous) and gender (male or female; dichotomous)?
Correlation
Correlations (used interchangeably with ‘associations’) signal patterns in data between variables. [1] A positive association occurs if values in one variable increase as values in another also increase. A negative association occurs if variables in one decrease while others increase. A correlation coefficient, expressed as r, describes the strength of the relationship: a value of 0 means no relationship, and the relationship strengthens as r approaches 1 (positive relationship) or -1 (negative association). [5]
Example: Is there a relationship between age (continuous) and satisfaction with life survey scores (continuous)?
Linear Regression
Regression allows researchers to determine the degrees of relationships between a dependent variable and independent variables and results in an equation for prediction. [11] A large number of variables are usable in regression methods.
Example: Which admission to the hospital metrics (multiple continuous) best predict the total length of stay (minutes; continuous)?
Binary Logistic Regression
This type of regression, which aims to predict an outcome, is appropriate when the dependent variable or outcome of interest is binary or dichotomous (yes/no; cured/not cured). [12]
Example: Which panel results (multiple of continuous, ordinal, categorical, dichotomous) best predict whether or not an individual will have a positive blood culture (dichotomous/binary)?
An example of one research focus, with each type of statistical design discussed, can be found in Table 1 to provide more examples of commonly used statistical designs.
(See Types of Variables and Statistical Designs Table 1)
- Clinical Significance
Though numerous other statistical designs and extensions of methods covered in this article exist, the above information provides a starting point for healthcare providers to become acquainted with variables and commonly used designs. Researchers should study types of variables before determining statistical tests to obtain relevant measures and valid study results. [6] There is a recommendation to consult a statistician to ensure appropriate usage of the statistical design based on the variables and that the assumptions are upheld. [1] With the variety of statistical software available, investigators must a priori understand the type of statistical tests when designing a study. [13] All providers must interpret and scrutinize journal publications to make evidence-based clinical decisions, and this becomes enhanced by a limited but sound understanding of variables and commonly used study designs. [14]
- Nursing, Allied Health, and Interprofessional Team Interventions
All interprofessional healthcare team members need to be familiar with study design and the variables used in studies to accurately evaluate new data and studies as they are published and apply the latest data to patient care and drive optimal outcomes.
- Review Questions
- Access free multiple choice questions on this topic.
- Comment on this article.
Types of Variables and Statistical Designs Table 1 Contributed by Martin Huecker, MD and Jacob Shreffler, PhD
Disclosure: Jacob Shreffler declares no relevant financial relationships with ineligible companies.
Disclosure: Martin Huecker declares no relevant financial relationships with ineligible companies.
This book is distributed under the terms of the Creative Commons Attribution-NonCommercial-NoDerivatives 4.0 International (CC BY-NC-ND 4.0) ( http://creativecommons.org/licenses/by-nc-nd/4.0/ ), which permits others to distribute the work, provided that the article is not altered or used commercially. You are not required to obtain permission to distribute this article, provided that you credit the author and journal.
- Cite this Page Shreffler J, Huecker MR. Types of Variables and Commonly Used Statistical Designs. [Updated 2023 Mar 6]. In: StatPearls [Internet]. Treasure Island (FL): StatPearls Publishing; 2024 Jan-.
In this Page
Bulk download.
- Bulk download StatPearls data from FTP
Related information
- PMC PubMed Central citations
- PubMed Links to PubMed
Similar articles in PubMed
- Suicidal Ideation. [StatPearls. 2024] Suicidal Ideation. Harmer B, Lee S, Duong TVH, Saadabadi A. StatPearls. 2024 Jan
- Prescription of Controlled Substances: Benefits and Risks. [StatPearls. 2024] Prescription of Controlled Substances: Benefits and Risks. Preuss CV, Kalava A, King KC. StatPearls. 2024 Jan
- The future of Cochrane Neonatal. [Early Hum Dev. 2020] The future of Cochrane Neonatal. Soll RF, Ovelman C, McGuire W. Early Hum Dev. 2020 Nov; 150:105191. Epub 2020 Sep 12.
- Review How to study improvement interventions: a brief overview of possible study types. [BMJ Qual Saf. 2015] Review How to study improvement interventions: a brief overview of possible study types. Portela MC, Pronovost PJ, Woodcock T, Carter P, Dixon-Woods M. BMJ Qual Saf. 2015 May; 24(5):325-36. Epub 2015 Mar 25.
- Review How to study improvement interventions: a brief overview of possible study types. [Postgrad Med J. 2015] Review How to study improvement interventions: a brief overview of possible study types. Portela MC, Pronovost PJ, Woodcock T, Carter P, Dixon-Woods M. Postgrad Med J. 2015 Jun; 91(1076):343-54.
Recent Activity
- Types of Variables and Commonly Used Statistical Designs - StatPearls Types of Variables and Commonly Used Statistical Designs - StatPearls
Your browsing activity is empty.
Activity recording is turned off.
Turn recording back on
Connect with NLM
National Library of Medicine 8600 Rockville Pike Bethesda, MD 20894
Web Policies FOIA HHS Vulnerability Disclosure
Help Accessibility Careers
Types of Variable
All experiments examine some kind of variable(s). A variable is not only something that we measure, but also something that we can manipulate and something we can control for. To understand the characteristics of variables and how we use them in research, this guide is divided into three main sections. First, we illustrate the role of dependent and independent variables. Second, we discuss the difference between experimental and non-experimental research. Finally, we explain how variables can be characterised as either categorical or continuous.
Dependent and Independent Variables
An independent variable, sometimes called an experimental or predictor variable, is a variable that is being manipulated in an experiment in order to observe the effect on a dependent variable, sometimes called an outcome variable.
Imagine that a tutor asks 100 students to complete a maths test. The tutor wants to know why some students perform better than others. Whilst the tutor does not know the answer to this, she thinks that it might be because of two reasons: (1) some students spend more time revising for their test; and (2) some students are naturally more intelligent than others. As such, the tutor decides to investigate the effect of revision time and intelligence on the test performance of the 100 students. The dependent and independent variables for the study are:
Dependent Variable: Test Mark (measured from 0 to 100)
Independent Variables: Revision time (measured in hours) Intelligence (measured using IQ score)
The dependent variable is simply that, a variable that is dependent on an independent variable(s). For example, in our case the test mark that a student achieves is dependent on revision time and intelligence. Whilst revision time and intelligence (the independent variables) may (or may not) cause a change in the test mark (the dependent variable), the reverse is implausible; in other words, whilst the number of hours a student spends revising and the higher a student's IQ score may (or may not) change the test mark that a student achieves, a change in a student's test mark has no bearing on whether a student revises more or is more intelligent (this simply doesn't make sense).
Therefore, the aim of the tutor's investigation is to examine whether these independent variables - revision time and IQ - result in a change in the dependent variable, the students' test scores. However, it is also worth noting that whilst this is the main aim of the experiment, the tutor may also be interested to know if the independent variables - revision time and IQ - are also connected in some way.
In the section on experimental and non-experimental research that follows, we find out a little more about the nature of independent and dependent variables.
Experimental and Non-Experimental Research
- Experimental research : In experimental research, the aim is to manipulate an independent variable(s) and then examine the effect that this change has on a dependent variable(s). Since it is possible to manipulate the independent variable(s), experimental research has the advantage of enabling a researcher to identify a cause and effect between variables. For example, take our example of 100 students completing a maths exam where the dependent variable was the exam mark (measured from 0 to 100), and the independent variables were revision time (measured in hours) and intelligence (measured using IQ score). Here, it would be possible to use an experimental design and manipulate the revision time of the students. The tutor could divide the students into two groups, each made up of 50 students. In "group one", the tutor could ask the students not to do any revision. Alternately, "group two" could be asked to do 20 hours of revision in the two weeks prior to the test. The tutor could then compare the marks that the students achieved.
- Non-experimental research : In non-experimental research, the researcher does not manipulate the independent variable(s). This is not to say that it is impossible to do so, but it will either be impractical or unethical to do so. For example, a researcher may be interested in the effect of illegal, recreational drug use (the independent variable(s)) on certain types of behaviour (the dependent variable(s)). However, whilst possible, it would be unethical to ask individuals to take illegal drugs in order to study what effect this had on certain behaviours. As such, a researcher could ask both drug and non-drug users to complete a questionnaire that had been constructed to indicate the extent to which they exhibited certain behaviours. Whilst it is not possible to identify the cause and effect between the variables, we can still examine the association or relationship between them. In addition to understanding the difference between dependent and independent variables, and experimental and non-experimental research, it is also important to understand the different characteristics amongst variables. This is discussed next.
Categorical and Continuous Variables
Categorical variables are also known as discrete or qualitative variables. Categorical variables can be further categorized as either nominal , ordinal or dichotomous .
- Nominal variables are variables that have two or more categories, but which do not have an intrinsic order. For example, a real estate agent could classify their types of property into distinct categories such as houses, condos, co-ops or bungalows. So "type of property" is a nominal variable with 4 categories called houses, condos, co-ops and bungalows. Of note, the different categories of a nominal variable can also be referred to as groups or levels of the nominal variable. Another example of a nominal variable would be classifying where people live in the USA by state. In this case there will be many more levels of the nominal variable (50 in fact).
- Dichotomous variables are nominal variables which have only two categories or levels. For example, if we were looking at gender, we would most probably categorize somebody as either "male" or "female". This is an example of a dichotomous variable (and also a nominal variable). Another example might be if we asked a person if they owned a mobile phone. Here, we may categorise mobile phone ownership as either "Yes" or "No". In the real estate agent example, if type of property had been classified as either residential or commercial then "type of property" would be a dichotomous variable.
- Ordinal variables are variables that have two or more categories just like nominal variables only the categories can also be ordered or ranked. So if you asked someone if they liked the policies of the Democratic Party and they could answer either "Not very much", "They are OK" or "Yes, a lot" then you have an ordinal variable. Why? Because you have 3 categories, namely "Not very much", "They are OK" and "Yes, a lot" and you can rank them from the most positive (Yes, a lot), to the middle response (They are OK), to the least positive (Not very much). However, whilst we can rank the levels, we cannot place a "value" to them; we cannot say that "They are OK" is twice as positive as "Not very much" for example.
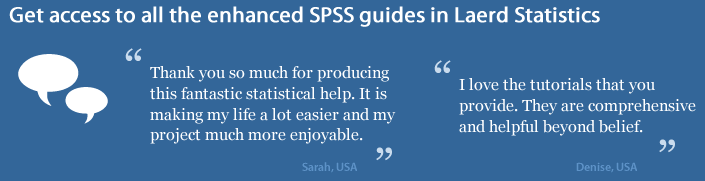
Continuous variables are also known as quantitative variables. Continuous variables can be further categorized as either interval or ratio variables.
- Interval variables are variables for which their central characteristic is that they can be measured along a continuum and they have a numerical value (for example, temperature measured in degrees Celsius or Fahrenheit). So the difference between 20°C and 30°C is the same as 30°C to 40°C. However, temperature measured in degrees Celsius or Fahrenheit is NOT a ratio variable.
- Ratio variables are interval variables, but with the added condition that 0 (zero) of the measurement indicates that there is none of that variable. So, temperature measured in degrees Celsius or Fahrenheit is not a ratio variable because 0°C does not mean there is no temperature. However, temperature measured in Kelvin is a ratio variable as 0 Kelvin (often called absolute zero) indicates that there is no temperature whatsoever. Other examples of ratio variables include height, mass, distance and many more. The name "ratio" reflects the fact that you can use the ratio of measurements. So, for example, a distance of ten metres is twice the distance of 5 metres.
Ambiguities in classifying a type of variable
In some cases, the measurement scale for data is ordinal, but the variable is treated as continuous. For example, a Likert scale that contains five values - strongly agree, agree, neither agree nor disagree, disagree, and strongly disagree - is ordinal. However, where a Likert scale contains seven or more value - strongly agree, moderately agree, agree, neither agree nor disagree, disagree, moderately disagree, and strongly disagree - the underlying scale is sometimes treated as continuous (although where you should do this is a cause of great dispute).
It is worth noting that how we categorise variables is somewhat of a choice. Whilst we categorised gender as a dichotomous variable (you are either male or female), social scientists may disagree with this, arguing that gender is a more complex variable involving more than two distinctions, but also including measurement levels like genderqueer, intersex and transgender. At the same time, some researchers would argue that a Likert scale, even with seven values, should never be treated as a continuous variable.
Have a language expert improve your writing
Run a free plagiarism check in 10 minutes, automatically generate references for free.
- Knowledge Base
- Methodology
- Independent vs Dependent Variables | Definition & Examples
Independent vs Dependent Variables | Definition & Examples
Published on 4 May 2022 by Pritha Bhandari . Revised on 17 October 2022.
In research, variables are any characteristics that can take on different values, such as height, age, temperature, or test scores.
Researchers often manipulate or measure independent and dependent variables in studies to test cause-and-effect relationships.
- The independent variable is the cause. Its value is independent of other variables in your study.
- The dependent variable is the effect. Its value depends on changes in the independent variable.
Your independent variable is the temperature of the room. You vary the room temperature by making it cooler for half the participants, and warmer for the other half.
Table of contents
What is an independent variable, types of independent variables, what is a dependent variable, identifying independent vs dependent variables, independent and dependent variables in research, visualising independent and dependent variables, frequently asked questions about independent and dependent variables.
An independent variable is the variable you manipulate or vary in an experimental study to explore its effects. It’s called ‘independent’ because it’s not influenced by any other variables in the study.
Independent variables are also called:
- Explanatory variables (they explain an event or outcome)
- Predictor variables (they can be used to predict the value of a dependent variable)
- Right-hand-side variables (they appear on the right-hand side of a regression equation).
These terms are especially used in statistics , where you estimate the extent to which an independent variable change can explain or predict changes in the dependent variable.
Prevent plagiarism, run a free check.
There are two main types of independent variables.
- Experimental independent variables can be directly manipulated by researchers.
- Subject variables cannot be manipulated by researchers, but they can be used to group research subjects categorically.
Experimental variables
In experiments, you manipulate independent variables directly to see how they affect your dependent variable. The independent variable is usually applied at different levels to see how the outcomes differ.
You can apply just two levels in order to find out if an independent variable has an effect at all.
You can also apply multiple levels to find out how the independent variable affects the dependent variable.
You have three independent variable levels, and each group gets a different level of treatment.
You randomly assign your patients to one of the three groups:
- A low-dose experimental group
- A high-dose experimental group
- A placebo group
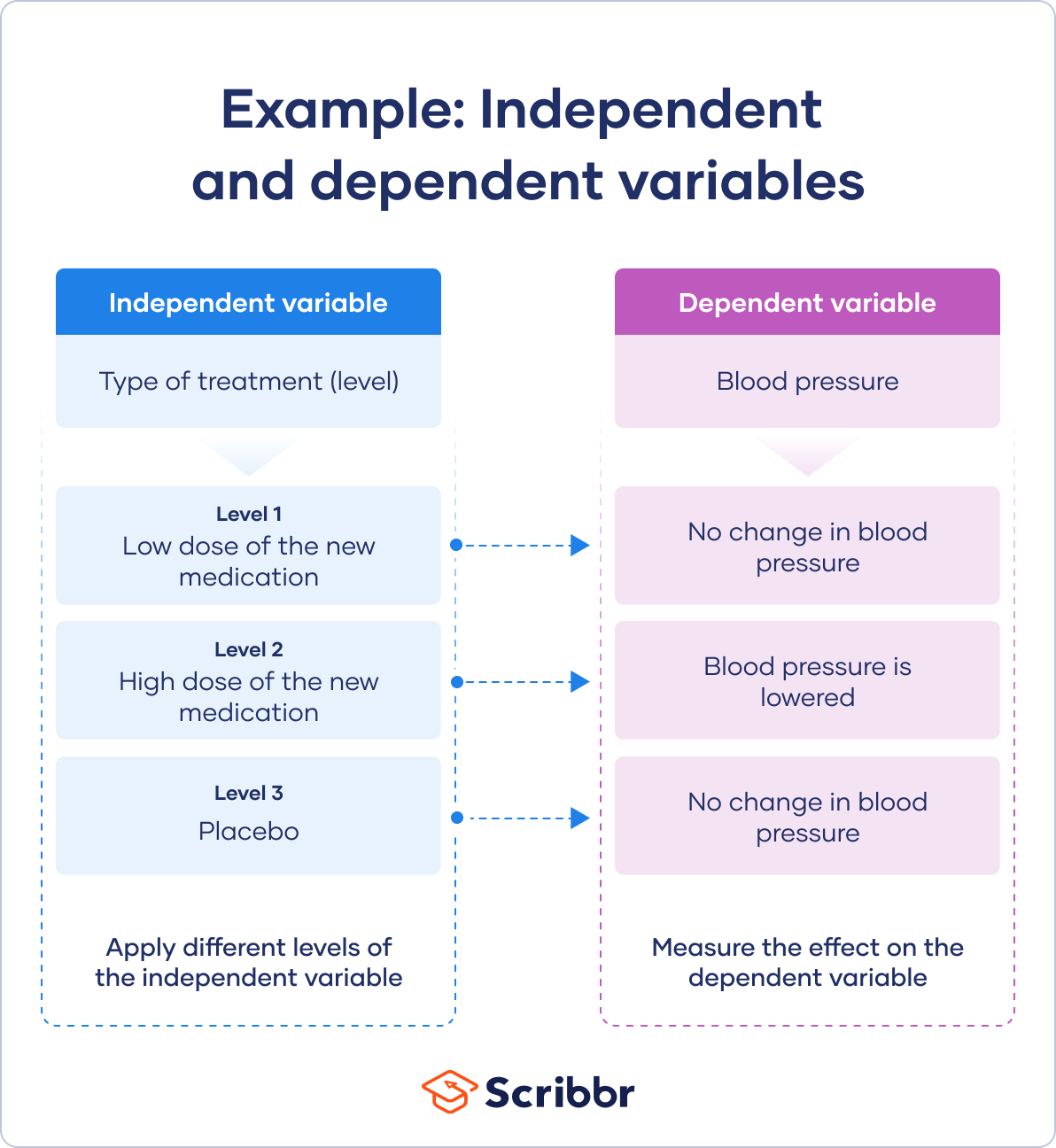
A true experiment requires you to randomly assign different levels of an independent variable to your participants.
Random assignment helps you control participant characteristics, so that they don’t affect your experimental results. This helps you to have confidence that your dependent variable results come solely from the independent variable manipulation.
Subject variables
Subject variables are characteristics that vary across participants, and they can’t be manipulated by researchers. For example, gender identity, ethnicity, race, income, and education are all important subject variables that social researchers treat as independent variables.
It’s not possible to randomly assign these to participants, since these are characteristics of already existing groups. Instead, you can create a research design where you compare the outcomes of groups of participants with characteristics. This is a quasi-experimental design because there’s no random assignment.
Your independent variable is a subject variable, namely the gender identity of the participants. You have three groups: men, women, and other.
Your dependent variable is the brain activity response to hearing infant cries. You record brain activity with fMRI scans when participants hear infant cries without their awareness.
A dependent variable is the variable that changes as a result of the independent variable manipulation. It’s the outcome you’re interested in measuring, and it ‘depends’ on your independent variable.
In statistics , dependent variables are also called:
- Response variables (they respond to a change in another variable)
- Outcome variables (they represent the outcome you want to measure)
- Left-hand-side variables (they appear on the left-hand side of a regression equation)
The dependent variable is what you record after you’ve manipulated the independent variable. You use this measurement data to check whether and to what extent your independent variable influences the dependent variable by conducting statistical analyses.
Based on your findings, you can estimate the degree to which your independent variable variation drives changes in your dependent variable. You can also predict how much your dependent variable will change as a result of variation in the independent variable.
Distinguishing between independent and dependent variables can be tricky when designing a complex study or reading an academic paper.
A dependent variable from one study can be the independent variable in another study, so it’s important to pay attention to research design.
Here are some tips for identifying each variable type.
Recognising independent variables
Use this list of questions to check whether you’re dealing with an independent variable:
- Is the variable manipulated, controlled, or used as a subject grouping method by the researcher?
- Does this variable come before the other variable in time?
- Is the researcher trying to understand whether or how this variable affects another variable?
Recognising dependent variables
Check whether you’re dealing with a dependent variable:
- Is this variable measured as an outcome of the study?
- Is this variable dependent on another variable in the study?
- Does this variable get measured only after other variables are altered?
Independent and dependent variables are generally used in experimental and quasi-experimental research.
Here are some examples of research questions and corresponding independent and dependent variables.
For experimental data, you analyse your results by generating descriptive statistics and visualising your findings. Then, you select an appropriate statistical test to test your hypothesis .
The type of test is determined by:
- Your variable types
- Level of measurement
- Number of independent variable levels
You’ll often use t tests or ANOVAs to analyse your data and answer your research questions.
In quantitative research , it’s good practice to use charts or graphs to visualise the results of studies. Generally, the independent variable goes on the x -axis (horizontal) and the dependent variable on the y -axis (vertical).
The type of visualisation you use depends on the variable types in your research questions:
- A bar chart is ideal when you have a categorical independent variable.
- A scatterplot or line graph is best when your independent and dependent variables are both quantitative.
To inspect your data, you place your independent variable of treatment level on the x -axis and the dependent variable of blood pressure on the y -axis.
You plot bars for each treatment group before and after the treatment to show the difference in blood pressure.
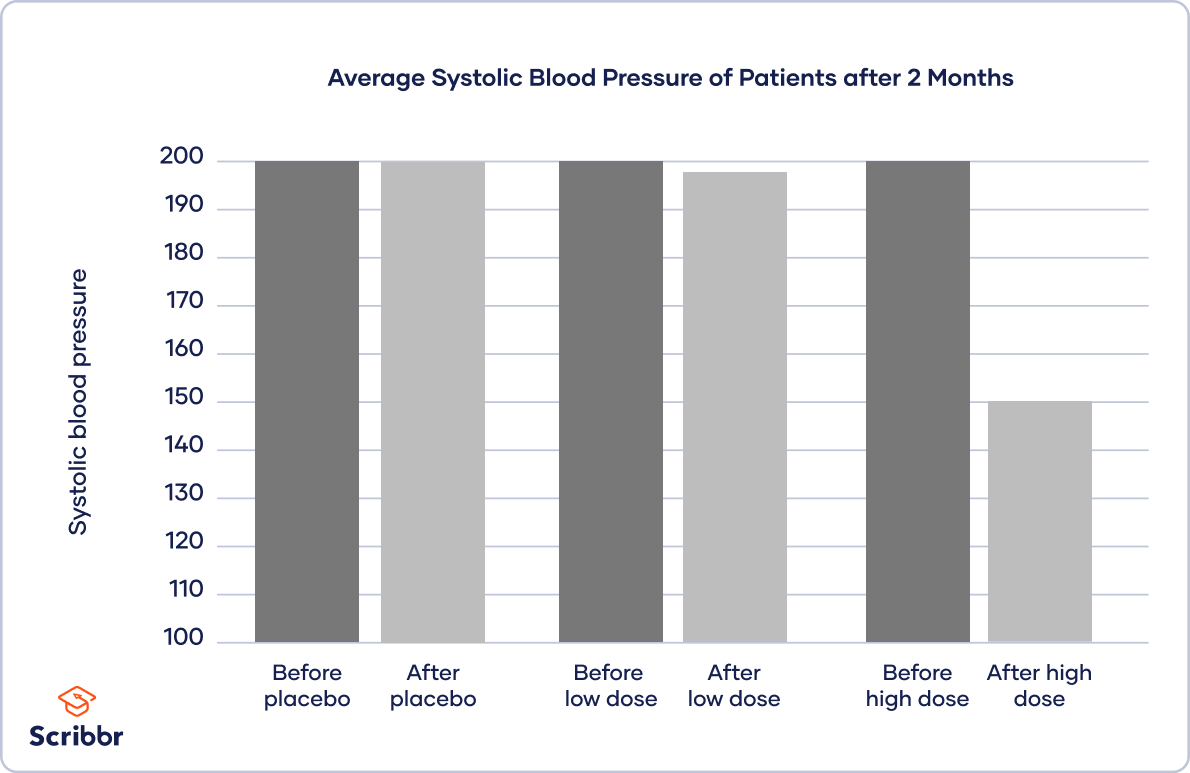
An independent variable is the variable you manipulate, control, or vary in an experimental study to explore its effects. It’s called ‘independent’ because it’s not influenced by any other variables in the study.
- Right-hand-side variables (they appear on the right-hand side of a regression equation)
A dependent variable is what changes as a result of the independent variable manipulation in experiments . It’s what you’re interested in measuring, and it ‘depends’ on your independent variable.
In statistics, dependent variables are also called:
Determining cause and effect is one of the most important parts of scientific research. It’s essential to know which is the cause – the independent variable – and which is the effect – the dependent variable.
You want to find out how blood sugar levels are affected by drinking diet cola and regular cola, so you conduct an experiment .
- The type of cola – diet or regular – is the independent variable .
- The level of blood sugar that you measure is the dependent variable – it changes depending on the type of cola.
Yes, but including more than one of either type requires multiple research questions .
For example, if you are interested in the effect of a diet on health, you can use multiple measures of health: blood sugar, blood pressure, weight, pulse, and many more. Each of these is its own dependent variable with its own research question.
You could also choose to look at the effect of exercise levels as well as diet, or even the additional effect of the two combined. Each of these is a separate independent variable .
To ensure the internal validity of an experiment , you should only change one independent variable at a time.
No. The value of a dependent variable depends on an independent variable, so a variable cannot be both independent and dependent at the same time. It must be either the cause or the effect, not both.
Cite this Scribbr article
If you want to cite this source, you can copy and paste the citation or click the ‘Cite this Scribbr article’ button to automatically add the citation to our free Reference Generator.
Bhandari, P. (2022, October 17). Independent vs Dependent Variables | Definition & Examples. Scribbr. Retrieved 22 April 2024, from https://www.scribbr.co.uk/research-methods/independent-vs-dependent-variables/
Is this article helpful?
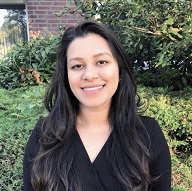
Pritha Bhandari
Other students also liked, a quick guide to experimental design | 5 steps & examples, quasi-experimental design | definition, types & examples, types of variables in research | definitions & examples.
- What is New
- Download Your Software
- Behavioral Research
- Software for Consumer Research
- Software for Human Factors R&D
- Request Live Demo
- Contact Sales
Sensor Hardware
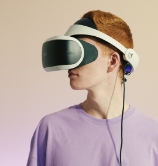
We carry a range of biosensors from the top hardware producers. All compatible with iMotions
iMotions for Higher Education
Imotions for business.
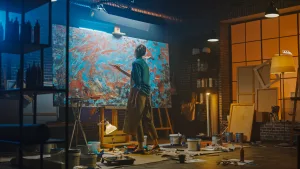
How to Measure the 4 Types of Attention – with Biosensors
Work and Safety
Morten Pedersen
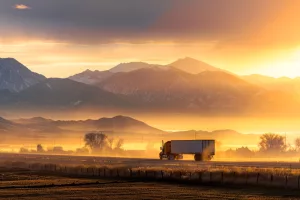
Enhancing Safety in Road-Based Transportation through Human Factors R&D
News & events.
- iMotions Lab
- iMotions Online
- Eye Tracking
- Eye Tracking Screen Based
- Eye Tracking VR
- Eye Tracking Glasses
- Eye Tracking Webcam
- FEA (Facial Expression Analysis)
- Voice Analysis
- EDA/GSR (Electrodermal Activity)
- EEG (Electroencephalography)
- ECG (Electrocardiography)
- EMG (Electromyography)
- Respiration
- iMotions Lab: New features
- iMotions Lab: Developers
- EEG sensors
- Sensory and Perceptual
- Consumer Inights
- Human Factors R&D
- Work Environments, Training and Safety
- Customer Stories
- Published Research Papers
- Document Library
- Customer Support Program
- Help Center
- Release Notes
- Contact Support
- Partnerships
- Mission Statement
- Ownership and Structure
- Executive Management
- Job Opportunities
Publications
- Newsletter Sign Up
Roles of Independent and Dependent Variables in Research
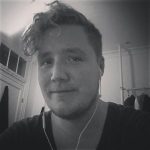
Explore the essential roles of independent and dependent variables in research. This guide delves into their definitions, significance in experiments, and their critical relationship. Learn how these variables are the foundation of research design, influencing hypothesis testing, theory development, and statistical analysis, empowering researchers to understand and predict outcomes of research studies.
Table of Contents
Introduction.
At the very base of scientific inquiry and research design , variables act as the fundamental steps, guiding the rhythm and direction of research. This is particularly true in human behavior research, where the quest to understand the complexities of human actions and reactions hinges on the meticulous manipulation and observation of these variables. At the heart of this endeavor lie two different types of variables, namely: independent and dependent variables, whose roles and interplay are critical in scientific discovery.
Understanding the distinction between independent and dependent variables is not merely an academic exercise; it is essential for anyone venturing into the field of research. This article aims to demystify these concepts, offering clarity on their definitions, roles, and the nuances of their relationship in the study of human behavior, and in science generally. We will cover hypothesis testing and theory development, illuminating how these variables serve as the cornerstone of experimental design and statistical analysis.
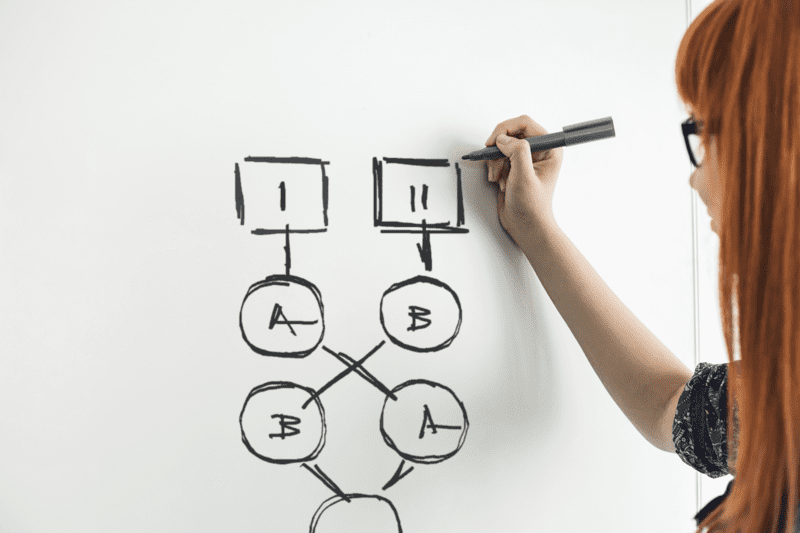
The significance of grasping the difference between independent and dependent variables extends beyond the confines of academia. It empowers researchers to design robust studies, enables critical evaluation of research findings, and fosters an appreciation for the complexity of human behavior research. As we delve into this exploration, our objective is clear: to equip readers with a deep understanding of these fundamental concepts, enhancing their ability to contribute to the ever-evolving field of human behavior research.
Chapter 1: The Role of Independent Variables in Human Behavior Research
In the realm of human behavior research, independent variables are the keystones around which studies are designed and hypotheses are tested. Independent variables are the factors or conditions that researchers manipulate or observe to examine their effects on dependent variables, which typically reflect aspects of human behavior or psychological phenomena. Understanding the role of independent variables is crucial for designing robust research methodologies, ensuring the reliability and validity of findings.
Defining Independent Variables
Independent variables are those variables that are changed or controlled in a scientific experiment to test the effects on dependent variables. In studies focusing on human behavior, these can range from psychological interventions (e.g., cognitive-behavioral therapy), environmental adjustments (e.g., noise levels, lighting, smells, etc), to societal factors (e.g., social media use). For example, in an experiment investigating the impact of sleep on cognitive performance, the amount of sleep participants receive is the independent variable.
Selection and Manipulation
Selecting an independent variable requires careful consideration of the research question and the theoretical framework guiding the study. Researchers must ensure that their chosen variable can be effectively, and consistently manipulated or measured and is ethically and practically feasible, particularly when dealing with human subjects.
Manipulating an independent variable involves creating different conditions (e.g., treatment vs. control groups) to observe how changes in the variable affect outcomes. For instance, researchers studying the effect of educational interventions on learning outcomes might vary the type of instructional material (digital vs. traditional) to assess differences in student performance.
Challenges in Human Behavior Research
Manipulating independent variables in human behavior research presents unique challenges. Ethical considerations are paramount, as interventions must not harm participants. For example, studies involving vulnerable populations or sensitive topics require rigorous ethical oversight to ensure that the manipulation of independent variables does not result in adverse effects.
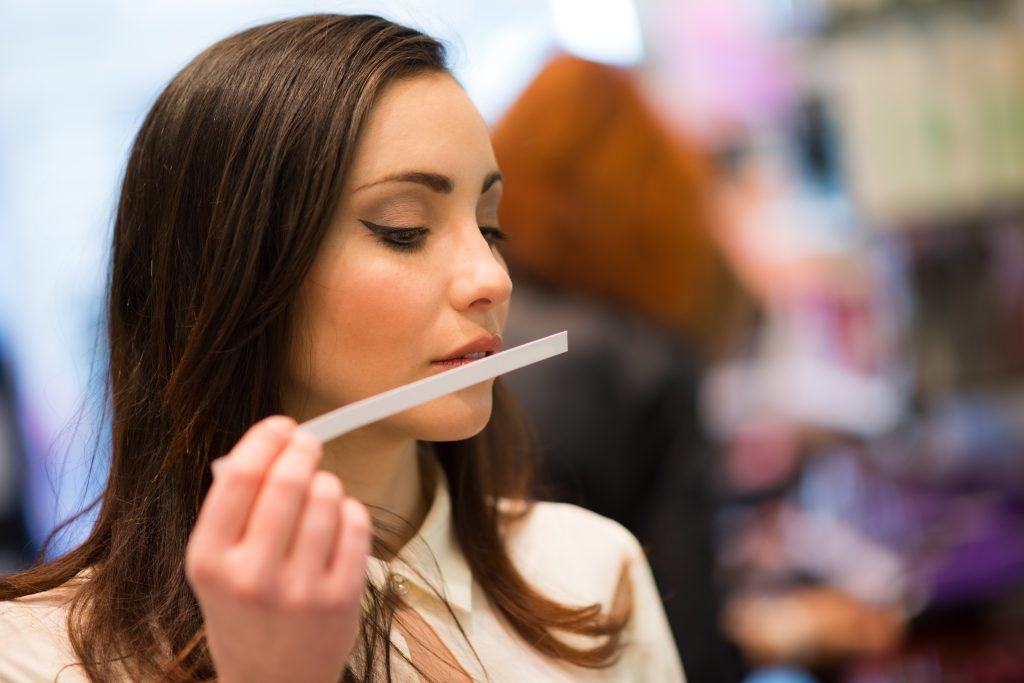
Practical limitations also come into play, such as controlling for extraneous variables that could influence the outcomes. In the aforementioned example of sleep and cognitive performance, factors like caffeine consumption or stress levels could confound the results. Researchers employ various methodological strategies, such as random assignment and controlled environments, to mitigate these influences.
Chapter 2: Dependent Variables: Measuring Human Behavior
The dependent variable in human behavior research acts as a mirror, reflecting the outcomes or effects resulting from variations in the independent variable. It is the aspect of human experience or behavior that researchers aim to understand, predict, or change through their studies. This section explores how dependent variables are measured, the significance of their accurate measurement, and the inherent challenges in capturing the complexities of human behavior.
Defining Dependent Variables
Dependent variables are the responses or outcomes that researchers measure in an experiment, expecting them to vary as a direct result of changes in the independent variable. In the context of human behavior research, dependent variables could include measures of emotional well-being, cognitive performance, social interactions, or any other aspect of human behavior influenced by the experimental manipulation. For instance, in a study examining the effect of exercise on stress levels, stress level would be the dependent variable, measured through various psychological assessments or physiological markers.
Measurement Methods and Tools
Measuring dependent variables in human behavior research involves a diverse array of methodologies, ranging from self-reported questionnaires and interviews to physiological measurements and behavioral observations. The choice of measurement tool depends on the nature of the dependent variable and the objectives of the study.
- Self-reported Measures: Often used for assessing psychological states or subjective experiences, such as anxiety, satisfaction, or mood. These measures rely on participants’ introspection and honesty, posing challenges in terms of accuracy and bias.
- Behavioral Observations: Involve the direct observation and recording of participants’ behavior in natural or controlled settings. This method is used for behaviors that can be externally observed and quantified, such as social interactions or task performance.
- Physiological Measurements: Include the use of technology to measure physical responses that indicate psychological states, such as heart rate, cortisol levels, or brain activity. These measures can provide objective data about the physiological aspects of human behavior.
Reliability and Validity
The reliability and validity of the measurement of dependent variables are critical to the integrity of human behavior research.
- Reliability refers to the consistency of a measure; a reliable tool yields similar results under consistent conditions.
- Validity pertains to the accuracy of the measure; a valid tool accurately reflects the concept it aims to measure.
Ensuring reliability and validity often involves the use of established measurement instruments with proven track records, pilot testing new instruments, and applying rigorous statistical analyses to evaluate measurement properties.
Challenges in Measuring Human Behavior
Measuring human behavior presents challenges due to its complexity and the influence of multiple, often interrelated, variables. Researchers must contend with issues such as participant bias, environmental influences, and the subjective nature of many psychological constructs. Additionally, the dynamic nature of human behavior means that it can change over time, necessitating careful consideration of when and how measurements are taken.
Section 3: Relationship between Independent and Dependent Variables
Understanding the relationship between independent and dependent variables is at the core of research in human behavior. This relationship is what researchers aim to elucidate, whether they seek to explain, predict, or influence human actions and psychological states. This section explores the nature of this relationship, the means by which it is analyzed, and common misconceptions that may arise.
The Nature of the Relationship
The relationship between independent and dependent variables can manifest in various forms—direct, indirect, linear, nonlinear, and may be moderated or mediated by other variables. At its most basic, this relationship is often conceptualized as cause and effect: the independent variable (the cause) influences the dependent variable (the effect). For instance, increased physical activity (independent variable) may lead to decreased stress levels (dependent variable).
Analyzing the Relationship
Statistical analyses play a pivotal role in examining the relationship between independent and dependent variables. Techniques vary depending on the nature of the variables and the research design, ranging from simple correlation and regression analyses for quantifying the strength and form of relationships, to complex multivariate analyses for exploring relationships among multiple variables simultaneously.
- Correlation Analysis : Used to determine the degree to which two variables are related. However, it’s crucial to note that correlation does not imply causation.
- Regression Analysis : Goes a step further by not only assessing the strength of the relationship but also predicting the value of the dependent variable based on the independent variable.
- Experimental Design : Provides a more robust framework for inferring causality, where manipulation of the independent variable and control of confounding factors allow researchers to directly observe the impact on the dependent variable.
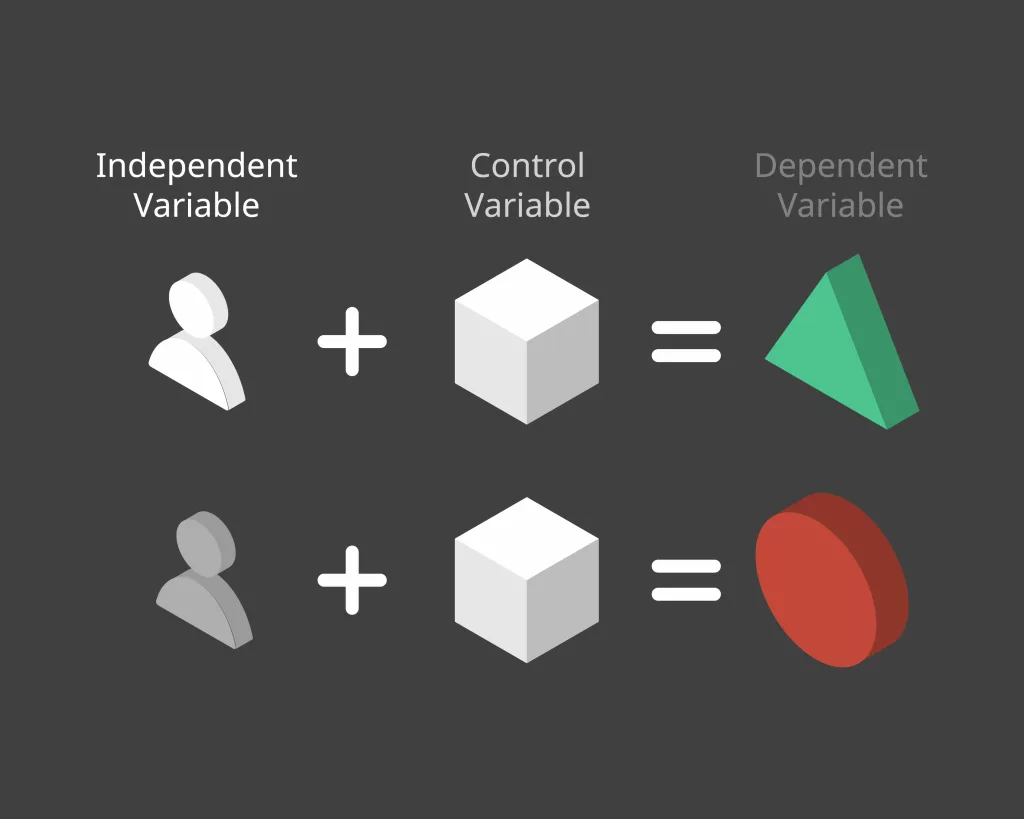
Causality vs. Correlation
A fundamental consideration in human behavior research is the distinction between causality and correlation. Causality implies that changes in the independent variable cause changes in the dependent variable. Correlation, on the other hand, indicates that two variables are related but does not establish a cause-effect relationship. Confounding variables may influence both, creating the appearance of a direct relationship where none exists. Understanding this distinction is crucial for accurate interpretation of research findings.
Common Misinterpretations
The complexity of human behavior and the myriad factors that influence it often lead to challenges in interpreting the relationship between independent and dependent variables. Researchers must be wary of:
- Overestimating the strength of causal relationships based on correlational data.
- Ignoring potential confounding variables that may influence the observed relationship.
- Assuming the directionality of the relationship without adequate evidence.
This exploration highlights the importance of understanding independent and dependent variables in human behavior research. Independent variables act as the initiating factors in experiments, influencing the observed behaviors, while dependent variables reflect the results of these influences, providing insights into human emotions and actions.
Ethical and practical challenges arise, especially in experiments involving human participants, necessitating careful consideration to respect participants’ well-being. The measurement of these variables is critical for testing theories and validating hypotheses, with their relationship offering potential insights into causality and correlation within human behavior.
Rigorous statistical analysis and cautious interpretation of findings are essential to avoid misconceptions. Overall, the study of these variables is fundamental to advancing human behavior research, guiding researchers towards deeper understanding and potential interventions to improve the human condition.
Free 44-page Experimental Design Guide
For Beginners and Intermediates
- Introduction to experimental methods
- Respondent management with groups and populations
- How to set up stimulus selection and arrangement
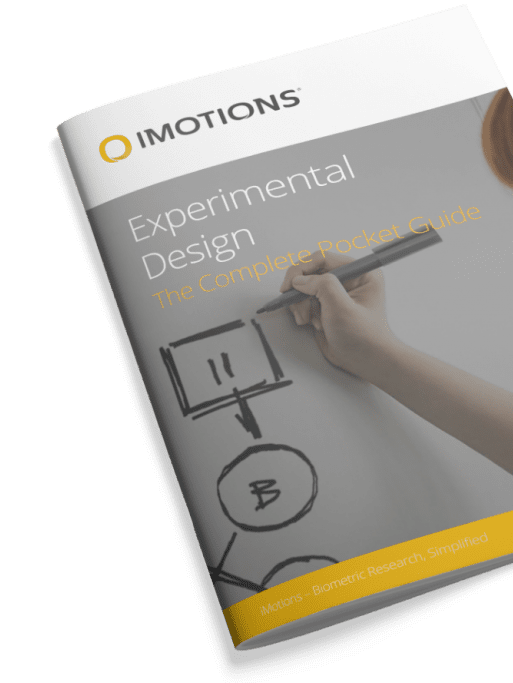
Last edited
About the author
See what is next in human behavior research
Follow our newsletter to get the latest insights and events send to your inbox.
Related Posts
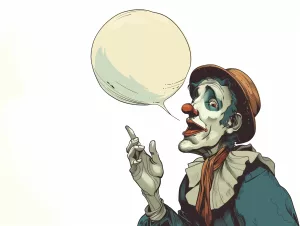
Beyond Words: Behavioral Expressions in Communication
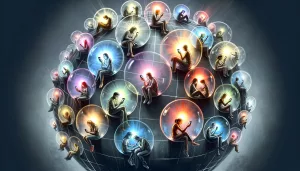
Technological Influences on Behavior: Insights and Implications
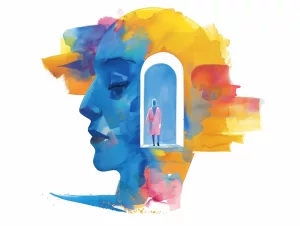
Understanding Human Behavior: An Essential Guide
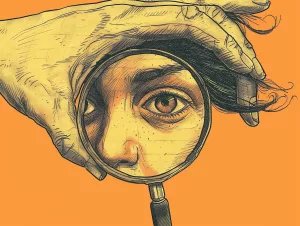
Studying Human Behavior: Methods and Insights
You might also like these.
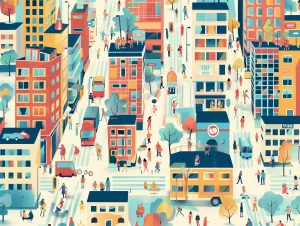
Human Behavior in Practice: Applications Across Fields
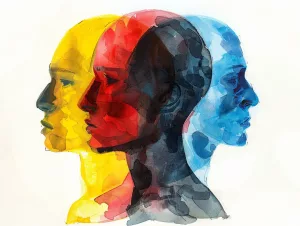
Theoretical Frameworks in Understanding Human Behavior
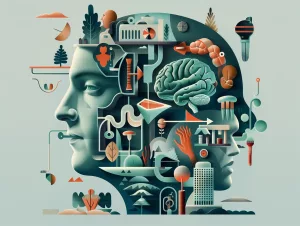
Foundations of Human Behavior
Case Stories
Explore Blog Categories
Best Practice
Collaboration, product guides, product news, research fundamentals, research insights, 🍪 use of cookies.
We are committed to protecting your privacy and only use cookies to improve the user experience.
Chose which third-party services that you will allow to drop cookies. You can always change your cookie settings via the Cookie Settings link in the footer of the website. For more information read our Privacy Policy.
- gtag This tag is from Google and is used to associate user actions with Google Ad campaigns to measure their effectiveness. Enabling this will load the gtag and allow for the website to share information with Google.
- Livechat Livechat provides you with direct access to the experts in our office. The service tracks visitors to the website but does not store any information unless consent is given. This service is essential and can not be disabled.
- Pardot Collects information such as the IP address, browser type, and referring URL. This information is used to create reports on website traffic and track the effectiveness of marketing campaigns.
- Third-party iFrames Allows you to see thirdparty iFrames.
Our websites may use cookies to personalize and enhance your experience. By continuing without changing your cookie settings, you agree to this collection. For more information, please see our University Websites Privacy Notice .
Neag School of Education
Educational Research Basics by Del Siegle
Each person/thing we collect data on is called an OBSERVATION (in our work these are usually people/subjects. Currently, the term participant rather than subject is used when describing the people from whom we collect data).
OBSERVATIONS (participants) possess a variety of CHARACTERISTICS .
If a CHARACTERISTIC of an OBSERVATION (participant) is the same for every member of the group (doesn’t vary) it is called a CONSTANT .
If a CHARACTERISTIC of an OBSERVATION (participant) differs for group members it is called a VARIABLE . In research we don’t get excited about CONSTANTS (since everyone is the same on that characteristic); we’re more interested in VARIABLES. Variables can be classified as QUANTITATIVE or QUALITATIVE (also known as CATEGORICAL).
QUANTITATIVE variables are ones that exist along a continuum that runs from low to high. Ordinal, interval, and ratio variables are quantitative. QUANTITATIVE variables are sometimes called CONTINUOUS VARIABLES because they have a variety (continuum) of characteristics. Height in inches and scores on a test would be examples of quantitative variables.
QUALITATIVE variables do not express differences in amount, only differences. They are sometimes referred to as CATEGORICAL variables because they classify by categories. Nominal variables such as gender, religion, or eye color are CATEGORICAL variables. Generally speaking, categorical variables
A special case of a CATEGORICAL variable is a DICHOTOMOUS VARIABLE. DICHOTOMOUS variables have only two CHARACTERISTICS (male or female). When naming QUALITATIVE variables, it is important to name the category rather than the levels (i.e., gender is the variable name, not male and female).
Variables have different purposes or roles…
Independent (Experimental, Manipulated, Treatment, Grouping) Variable- That factor which is measured, manipulated, or selected by the experimenter to determine its relationship to an observed phenomenon. “In a research study, independent variables are antecedent conditions that are presumed to affect a dependent variable. They are either manipulated by the researcher or are observed by the researcher so that their values can be related to that of the dependent variable. For example, in a research study on the relationship between mosquitoes and mosquito bites, the number of mosquitoes per acre of ground would be an independent variable” (Jaeger, 1990, p. 373)
While the independent variable is often manipulated by the researcher, it can also be a classification where subjects are assigned to groups. In a study where one variable causes the other, the independent variable is the cause. In a study where groups are being compared, the independent variable is the group classification.
Dependent (Outcome) Variable- That factor which is observed and measured to determine the effect of the independent variable, i.e., that factor that appears, disappears, or varies as the experimenter introduces, removes, or varies the independent variable. “In a research study, the independent variable defines a principal focus of research interest. It is the consequent variable that is presumably affected by one or more independent variables that are either manipulated by the researcher or observed by the researcher and regarded as antecedent conditions that determine the value of the dependent variable. For example, in a study of the relationship between mosquitoes and mosquito bites, the number of mosquito bites per hour would be the dependent variable” (Jaeger, 1990, p. 370). The dependent variable is the participant’s response.
The dependent variable is the outcome. In an experiment, it may be what was caused or what changed as a result of the study. In a comparison of groups, it is what they differ on.
Moderator Variable- That factor which is measured, manipulated, or selected by the experimenter to discover whether it modifies the relationship of the independent variable to an observed phenomenon. It is a special type of independent variable.
The independent variable’s relationship with the dependent variable may change under different conditions. That condition is the moderator variable. In a study of two methods of teaching reading, one of the methods of teaching reading may work better with boys than girls. Method of teaching reading is the independent variable and reading achievement is the dependent variable. Gender is the moderator variable because it moderates or changes the relationship between the independent variable (teaching method) and the dependent variable (reading achievement).
Suppose we do a study of reading achievement where we compare whole language with phonics, and we also include students’ social economic status (SES) as a variable. The students are randomly assigned to either whole language instruction or phonics instruction. There are students of high and low SES in each group.
Let’s assume that we found that whole language instruction worked better than phonics instruction with the high SES students, but phonics instruction worked better than whole language instruction with the low SES students. Later you will learn in statistics that this is an interaction effect. In this study, language instruction was the independent variable (with two levels: phonics and whole language). SES was the moderator variable (with two levels: high and low). Reading achievement was the dependent variable (measured on a continuous scale so there aren’t levels).
With a moderator variable, we find the type of instruction did make a difference, but it worked differently for the two groups on the moderator variable. We select this moderator variable because we think it is a variable that will moderate the effect of the independent on the dependent. We make this decision before we start the study.
If the moderator had not been in the study above, we would have said that there was no difference in reading achievement between the two types of reading instruction. This would have happened because the average of the high and low scores of each SES group within a reading instruction group would cancel each other an produce what appears to be average reading achievement in each instruction group (i.e., Phonics: Low—6 and High—2; Whole Language: Low—2 and High—6; Phonics has an average of 4 and Whole Language has an average of 4. If we just look at the averages (without regard to the moderator), it appears that the instruction types produced similar results).
Extraneous Variable- Those factors which cannot be controlled. Extraneous variables are independent variables that have not been controlled. They may or may not influence the results. One way to control an extraneous variable which might influence the results is to make it a constant (keep everyone in the study alike on that characteristic). If SES were thought to influence achievement, then restricting the study to one SES level would eliminate SES as an extraneous variable.
Here are some examples similar to your homework:
Null Hypothesis: Students who receive pizza coupons as a reward do not read more books than students who do not receive pizza coupon rewards. Independent Variable: Reward Status Dependent Variable: Number of Books Read
High achieving students do not perform better than low achieving student when writing stories regardless of whether they use paper and pencil or a word processor. Independent Variable: Instrument Used for Writing Moderator Variable: Ability Level of the Students Dependent Variable: Quality of Stories Written When we are comparing two groups, the groups are the independent variable. When we are testing whether something influences something else, the influence (cause) is the independent variable. The independent variable is also the one we manipulate. For example, consider the hypothesis “Teachers given higher pay will have more positive attitudes toward children than teachers given lower pay.” One approach is to ask ourselves “Are there two or more groups being compared?” The answer is “Yes.” “What are the groups?” Teachers who are given higher pay and teachers who are given lower pay. Therefore, the independent variable is teacher pay (it has two levels– high pay and low pay). The dependent variable (what the groups differ on) is attitude towards school.
We could also approach this another way. “Is something causing something else?” The answer is “Yes.” “What is causing what?” Teacher pay is causing attitude towards school. Therefore, teacher pay is the independent variable (cause) and attitude towards school is the dependent variable (outcome).
Research Questions and Hypotheses
The research question drives the study. It should specifically state what is being investigated. Statisticians often convert their research questions to null and alternative hypotheses. The null hypothesis states that no relationship (correlation study) or difference (experimental study) exists. Converting research questions to hypotheses is a simple task. Take the questions and make it a positive statement that says a relationship exists (correlation studies) or a difference exists (experiment study) between the groups and we have the alternative hypothesis. Write a statement that a relationship does not exist or a difference does not exist and we have the null hypothesis.
Format for sample research questions and accompanying hypotheses:
Research Question for Relationships: Is there a relationship between height and weight? Null Hypothesis: There is no relationship between height and weight. Alternative Hypothesis: There is a relationship between height and weight.
When a researcher states a nondirectional hypothesis in a study that compares the performance of two groups, she doesn’t state which group she believes will perform better. If the word “more” or “less” appears in the hypothesis, there is a good chance that we are reading a directional hypothesis. A directional hypothesis is one where the researcher states which group she believes will perform better. Most researchers use nondirectional hypotheses.
We usually write the alternative hypothesis (what we believe might happen) before we write the null hypothesis (saying it won’t happen).
Directional Research Question for Differences: Do boys like reading more than girls? Null Hypothesis: Boys do not like reading more than girls. Alternative Hypothesis: Boys do like reading more than girls.
Nondirectional Research Question for Differences: Is there a difference between boys’ and girls’ attitude towards reading? –or– Do boys’ and girls’ attitude towards reading differ? Null Hypothesis: There is no difference between boys’ and girls’ attitude towards reading. –or– Boys’ and girls’ attitude towards reading do not differ. Alternative Hypothesis: There is a difference between boys’ and girls’ attitude towards reading. –or– Boys’ and girls’ attitude towards reading differ.
Del Siegle, Ph.D. Neag School of Education – University of Connecticut [email protected] www.delsiegle.com
Sciencing_Icons_Science SCIENCE
Sciencing_icons_biology biology, sciencing_icons_cells cells, sciencing_icons_molecular molecular, sciencing_icons_microorganisms microorganisms, sciencing_icons_genetics genetics, sciencing_icons_human body human body, sciencing_icons_ecology ecology, sciencing_icons_chemistry chemistry, sciencing_icons_atomic & molecular structure atomic & molecular structure, sciencing_icons_bonds bonds, sciencing_icons_reactions reactions, sciencing_icons_stoichiometry stoichiometry, sciencing_icons_solutions solutions, sciencing_icons_acids & bases acids & bases, sciencing_icons_thermodynamics thermodynamics, sciencing_icons_organic chemistry organic chemistry, sciencing_icons_physics physics, sciencing_icons_fundamentals-physics fundamentals, sciencing_icons_electronics electronics, sciencing_icons_waves waves, sciencing_icons_energy energy, sciencing_icons_fluid fluid, sciencing_icons_astronomy astronomy, sciencing_icons_geology geology, sciencing_icons_fundamentals-geology fundamentals, sciencing_icons_minerals & rocks minerals & rocks, sciencing_icons_earth scructure earth structure, sciencing_icons_fossils fossils, sciencing_icons_natural disasters natural disasters, sciencing_icons_nature nature, sciencing_icons_ecosystems ecosystems, sciencing_icons_environment environment, sciencing_icons_insects insects, sciencing_icons_plants & mushrooms plants & mushrooms, sciencing_icons_animals animals, sciencing_icons_math math, sciencing_icons_arithmetic arithmetic, sciencing_icons_addition & subtraction addition & subtraction, sciencing_icons_multiplication & division multiplication & division, sciencing_icons_decimals decimals, sciencing_icons_fractions fractions, sciencing_icons_conversions conversions, sciencing_icons_algebra algebra, sciencing_icons_working with units working with units, sciencing_icons_equations & expressions equations & expressions, sciencing_icons_ratios & proportions ratios & proportions, sciencing_icons_inequalities inequalities, sciencing_icons_exponents & logarithms exponents & logarithms, sciencing_icons_factorization factorization, sciencing_icons_functions functions, sciencing_icons_linear equations linear equations, sciencing_icons_graphs graphs, sciencing_icons_quadratics quadratics, sciencing_icons_polynomials polynomials, sciencing_icons_geometry geometry, sciencing_icons_fundamentals-geometry fundamentals, sciencing_icons_cartesian cartesian, sciencing_icons_circles circles, sciencing_icons_solids solids, sciencing_icons_trigonometry trigonometry, sciencing_icons_probability-statistics probability & statistics, sciencing_icons_mean-median-mode mean/median/mode, sciencing_icons_independent-dependent variables independent/dependent variables, sciencing_icons_deviation deviation, sciencing_icons_correlation correlation, sciencing_icons_sampling sampling, sciencing_icons_distributions distributions, sciencing_icons_probability probability, sciencing_icons_calculus calculus, sciencing_icons_differentiation-integration differentiation/integration, sciencing_icons_application application, sciencing_icons_projects projects, sciencing_icons_news news.
- Share Tweet Email Print
- Home ⋅
- Math ⋅
- Algebra ⋅
- Factorization
What Is an Independent Variable in Quantitative Research?
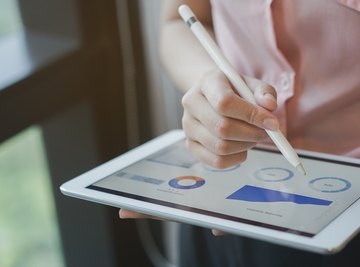
What Are the Independent Variables for a Moldy Bread Experiment?
The foundations of quantitative research are variables and there are three main types: dependent, independent and controlled. The researcher will manipulate an independent variable in an effort to understand its effect on the dependent or controlled variable. In other cases when manipulation is not an option, the independent variable is presumed to have an effect on the dependent variable and is called a “status variable” but often treated as an independent variable. However, to draw precise conclusions about the effects of an independent variable, the scientist must use a controlled variable for consistency.
An independent variable is a variable in research that causes a change -- or is presumed will cause a change -- to other variables in the research conducted. Scientists can control the independent variable to monitor those changes or he can presume a change and look for evidence of those changes to the other variables.
How it Works
Let’s say a researcher wants to study the growth of coffee beans. The dependent variables of such study include the number of coffee beans used, the weight of the plants, height of the plant, the size of the leaves and time it takes for the plant to mature.
The independent variables will impact the results of the dependent variable. Those variables may include the amount of water present, the use of fertilizer, the amount of fertilizer used, and temperature; the amount of exposure to sunlight will also affect the dependent variables.
Controlled Variable Importance
If a scientist wants to monitor how two different types of fertilizer (independent variables) effect the growth of the coffee beans, he will need to control all other variables. First he must use the same kind of coffee beans and the same amount of fertilizer to grow both sets of plants. He will need to make to make sure both sets are exposed to the exactly the same amount of water, sunlight and temperatures. These are all controlled variables for the research.
Status Variable
In some situations a researcher cannot manipulate an independent variable, although it may have an effect on the dependent variable. As a technical term scientists may refer to this independent variable as a status variable, but still treat it as an independent variable to further research and record results.
For example, if a social scientist is attempting a quantitative study on cigarette smoking and lung cancer, he cannot manipulate ethnicity of gender of individual subjects; although he suspects both independent variables may affect the body’s reaction to cigarette smoking. These are labeled as status variables and the scientist may look for consistent effects in both gender and ethnicity, while comparing those results to other ethnicities and the opposite gender, to ascertain the impact of the independent variable.
Related Articles
What are the independent variables for a moldy bread..., definitions of control, constant, independent and dependent..., difference between manipulative & responding variable, what is a responding variable in science projects, what are constants & controls of a science project..., distinguishing between descriptive & causal studies, can a science experiment have two manipulated variables, does music affect plant growth, what are comparative experiments, what is a constant in a science fair project, what is a standardized variable in biology, difference between correlation and causality, how to calculate an adjusted odds ratio, what is a constant in the scientific method, ideas for controlled variable science projects, how to get rid of a variable that is cubed, essential tenets of the scientific method, why is constant temperature important in an experiment, why should you only test for one variable at a time..., the effect of alcohol on plants.
- Penn State University: Define the Variables
About the Author
Kenneth W. Michael Wills is a writer on culture, society and business. With more than 15 years of experience in sales, public relations and written communications, Wills' passion is delighting audiences with invigorating perspectives and refreshing ideas. He has ghostwritten articles on a diverse range of topics for corporate websites and composed proposals for organizations seeking growth opportunities.
Find Your Next Great Science Fair Project! GO
This paper is in the following e-collection/theme issue:
Published on 25.4.2024 in Vol 26 (2024)
Effect of Prosocial Behaviors on e-Consultations in a Web-Based Health Care Community: Panel Data Analysis
Authors of this article:

Original Paper
- Xiaoxiao Liu 1, 2 , PhD ;
- Huijing Guo 3 , PhD ;
- Le Wang 4 , PhD ;
- Mingye Hu 5 , PhD ;
- Yichan Wei 1 , BBM ;
- Fei Liu 6 , PhD ;
- Xifu Wang 7 , MCM
1 School of Management, Xi’an Jiaotong University, Xi'an, China
2 China Institute of Hospital Development and Reform, Xi'an Jiaotong University, Xi'an, China
3 School of Economics and Management, China University of Mining and Technology, Xuzhou, China
4 College of Business, City University of Hong Kong, Hong Kong, China (Hong Kong)
5 School of Economics and Management, Xi’an University of Technology, Xi'an, China
6 School of Management, Harbin Engineering University, Harbin, China
7 Healthcare Simulation Center, Guangzhou First People’s Hospital, Guangzhou, China
Corresponding Author:
Xifu Wang, MCM
Healthcare Simulation Center
Guangzhou First People’s Hospital
1 Pan Fu Road
Yuexiu District
Guangzhou, 510180
Phone: 86 13560055951
Email: [email protected]
Background: Patients using web-based health care communities for e-consultation services have the option to choose their service providers from an extensive digital market. To stand out in this crowded field, doctors in web-based health care communities often engage in prosocial behaviors, such as proactive and reactive actions, to attract more users. However, the effect of these behaviors on the volume of e-consultations remains unclear and warrants further exploration.
Objective: This study investigates the impact of various prosocial behaviors on doctors’ e-consultation volume in web-based health care communities and the moderating effects of doctors’ digital and offline reputations.
Methods: A panel data set containing information on 2880 doctors over a 22-month period was obtained from one of the largest web-based health care communities in China. Data analysis was conducted using a 2-way fixed effects model with robust clustered SEs. A series of robustness checks were also performed, including alternative measurements of independent variables and estimation methods.
Results: Results indicated that both types of doctors’ prosocial behaviors, namely, proactive and reactive actions, positively impacted their e-consultation volume. In terms of the moderating effects of external reputation, doctors’ offline professional titles were found to negatively moderate the relationship between their proactive behaviors and their e-consultation volume. However, these titles did not significantly affect the relationship between doctors’ reactive behaviors and their e-consultation volume ( P =.45). Additionally, doctors’ digital recommendations from patients negatively moderated both the relationship between doctors’ proactive behaviors and e-consultation volume and the relationship between doctors’ reactive behaviors and e-consultation volume.
Conclusions: Drawing upon functional motives theory and social exchange theory, this study categorizes doctors’ prosocial behaviors into proactive and reactive actions. It provides empirical evidence that prosocial behaviors can lead to an increase in e-consultation volume. This study also illuminates the moderating roles doctors’ digital and offline reputations play in the relationships between prosocial behaviors and e-consultation volume.
Introduction
e-Consultations, offered through web-based health care communities [ 1 ], are increasingly becoming vital complements to traditional hospital services [ 2 - 4 ]. In hospital consultations, patients can only passively accept treatment [ 5 ] from a limited pool of medical resources within a geographical radius. However, when engaging with web-based health care communities, patients can search for primary care solutions [ 6 ] from an extensive digital market in a relatively short time [ 7 ]. Given that the diagnostic accuracy of e-consultations matches that of hospital consultations [ 8 - 10 ], e-consultations are becoming increasingly attractive to patients [ 3 , 11 ].
Doctors are also showing a growing interest in e-consultations, motivated by economic and social benefits. First, doctors can achieve economic gains by participating in e-consultations [ 7 , 12 ]. Web-based consultation platforms facilitate an efficient reputation system, enabling patients to easily provide feedback about doctors. Consequently, doctors can use e-consultation to strengthen their relationship with patients [ 13 , 14 ] and foster positive word-of-mouth [ 15 ]. More e-consultations can benefit doctors by retaining current patients, attracting new ones, and boosting in-person hospital visits [ 16 , 17 ]. Second, doctors could also receive social returns from engaging in e-consultation [ 7 ]. Active participation in e-consultations allows doctors to demonstrate their skills, attitude, and experience, aiding in accumulating professional capital [ 7 ], building their reputation [ 18 ], and increasing their social influence [ 19 ]. Given these tangible and intangible benefits, it is essential for doctors to diligently provide the desired e-consultations and make additional efforts to highlight their service attributes to stand out [ 6 , 20 , 21 ]. This involves engaging in prosocial behaviors in web-based health care communities, which is the primary research focus of this study.
Prior studies have examined the effects of prosocial behaviors on financial outcomes, such as actions reflecting social responsibility in the workplace [ 22 ]. In the health care sector, previous research has explored doctors’ prosocial behaviors within traditional, offline medical services. Doctors, working in established medical institutes and serving patients with limited choices of clinical service providers, often aim for self-satisfaction and patient satisfaction with their offline prosocial behaviors. For example, research indicates that doctors may act prosocially to regulate their self-oriented feelings [ 23 ] and foster a caring and understanding attitude toward patients [ 24 , 25 ]. Additionally, doctors who demonstrate more empathy and care can elicit positive emotions in patients and improve the doctor-patient relationship [ 26 , 27 ].
Compared to the offline context, doctors’ prosocial behaviors in a digital context may differ in 2 aspects. First, the internet allows patients to choose from a broader, more diverse range of doctors without the constraints of time and space [ 7 ]. However, the uncertainty inherent in the digital environment creates a more pronounced information asymmetry between patients and doctors [ 28 ], consequently making it more challenging for patients to establish trust. Therefore, doctors’ prosocial behaviors are crucial in building their self-image, establishing patients’ trust, and assisting patients in identifying suitable doctors [ 29 , 30 ]. Second, unlike offline environments, web-based medical platforms offer a range of functions, including asynchronous activities such as publishing articles, as well as real-time interactional actions such as answering questions during live streams. This array of functions facilitates the adoption of more diverse prosocial behaviors by doctors.
Although these differences underscore the importance of studying doctors’ prosocial behavior, there has been limited research focusing on the impact of such behaviors in the digital context. One previous study has scrutinized the impact of prosocial behaviors, such as answering patients’ questions freely, on patient engagement within web-based health care communities [ 31 ]. An aspect that requires further exploration is how doctors’ motivations and patients’ involvement vary in doctors’ helping behaviors. Consequently, studies on web-based health care communities should differentiate between diverse prosocial actions to understand their effects on doctors’ web-based service outcomes. This study aims to contribute new knowledge regarding the full breadth of doctors’ prosocial behaviors.
Unlike the previous study that exclusively investigated doctors’ asynchronous behaviors in web-based health care communities [ 31 ], this study also explores the role of synchronous reactive actions in achieving optimal doctors’ e-consultation volume. Recently, web-based health care communities have developed and released live-streaming functions to assist doctors in providing voluntary interactions with patients. The effect of doctors’ engagement in medical live streaming on e-consultation services remains unexplored. While these behaviors could demonstrate doctors’ ethical traits and ability to fulfill an e-consultation workflow, a potential trade-off with e-consultations may exist when doctors engage in prosocial behaviors.
In summary, this study examines the effects of doctors’ proactive and reactive prosocial behaviors, considering their digital and offline reputations as potential moderating factors. First, drawing from functional motives theory (FMT), we explore the impact of doctors’ web-based proactive actions on their e-consultation volume. Proactive behaviors are actions in which individuals exceed their assigned work, focusing on long-term goals to prevent future problems [ 32 , 33 ]. According to FMT, these behaviors reflect helping actions that satisfy personal needs [ 34 ], driven by self-focused motivations [ 35 ], such as impression management and the realization of self-worth goals. For example, knowledge-based proactive behaviors, such as disseminating expertise to preempt future issues, are self-initiated and not reactions to immediate requests [ 36 ]. This study categorizes doctors’ sharing of professional articles as a form of proactive behavior that creates a professional image for their patient audience. This is because these actions aim to assist patients with future health concerns rather than directly responding to patients’ immediate needs.
Second, this study explores the role of doctors’ reactive prosocial behaviors in increasing e-consultations, guided by social exchange theory (SET). Unlike proactive behaviors, reactive behaviors are characterized by instances of individuals engaging in helping activities [ 35 ], typically in response to others’ needs [ 34 ]. SET posits that individuals incurring additional social costs in relationships may anticipate reciprocal value [ 37 , 38 ]. Reactive prosocial behaviors, per SET, are initiated by the motivation to satisfy others’ desires, leading to the development of cooperative social values. In our context, medical live streams facilitate real-time, synchronized interactions, enabling patients to ask questions and doctors to provide immediate responses. Patients’ health questions during these streams indicate their immediate needs. Thus, a higher frequency of live streams within a certain period suggests doctors are increasingly responding to patients’ needs during that time. Therefore, this study uses the number of medical live-streaming sessions conducted by doctors as a measure for their synchronous reactive behaviors.
Finally, considering that doctors’ reputations play a crucial role in their workflow on web-based health care communities [ 39 , 40 ], we test the moderating roles of digital and offline reputation—measured by doctors’ offline professional titles and patients’ recommendations in the digital context, respectively—on the main effects.
Based on previous studies and practices within web-based health care communities, we aim to extend the literature by testing the impact of 2 types of web-based prosocial behaviors by doctors: proactive and synchronous reactive actions on e-consultation volume. We then explore the moderating roles of doctors’ offline and digital reputations on these main effects.
Research Framework and Hypothesis Development
We have developed a research framework, shown in Figure 1 , to identify effective prosocial strategies used by doctors within web-based health care communities to achieve a preferred e-consultation volume from the supply side.

Primarily, we explore the relationships between doctors’ prosocial behaviors and e-consultation volume, drawing on FMT and SET. These theories are widely adopted for measuring and classifying the outcomes of prosocial behaviors from 2 fundamental perspectives based on human nature [ 34 ]. While doctors’ offline prosocial behaviors may help satisfy patients [ 24 , 25 ], who are already service acceptors, the outcomes of doctors’ web-based prosocial behaviors still need careful distinction. It is essential to clearly differentiate between various types of doctors’ prosocial behaviors to identify their nature. In this study, following the leads of FMT and SET, we test 2 kinds of prosocial behavior: proactive (posting professional articles to achieve self-worth) and reactive (conducting medical live streaming to create cooperative social values).
Subsequently, we examine how doctors’ external reputation moderates the impacts of doctors’ proactive and reactive prosocial behaviors. This examination is conducted from the perspectives of reducing uncertainty and building trust, respectively.
Doctors’ Proactive Behaviors and e-Consultation Volume
FMT places emphasis on the primary motivations behind individuals’ behaviors, adopting an atheoretical stance [ 41 ]. Through the exploratory process, previous studies have provided examples to identify the functional motivations behind prosocial behaviors [ 42 ], such as expressing important personal values. In web-based health care communities, doctors have the opportunity to demonstrate personal traits through proactive behaviors. According to FMT, these proactive behaviors stem from the actors’ active efforts to satisfy their own needs and achieve self-worth [ 34 , 35 ].
Doctors might post professional articles, such as clinical notes and scientific papers, on web-based health care communities to help patient readers handle future health problems. These proactive prosocial behaviors are primarily driven by a desire to showcase personal medical competence, a crucial characteristic of a professional image [ 43 ], in medical consultations. By posting professional articles, doctors can display their medical knowledge, care delivery capability, and service quality, thereby enhancing their professional image. We hypothesize that this effort will lead to an increase in the e-consultation volume. Therefore, we propose the following hypothesis:
- Hypothesis 1: The posting of professional articles by doctors positively impacts their e-consultation volume on web-based health care communities.
Doctors’ Reactive Behaviors and e-Consultation Volume
Considering the social environment in the working context, SET suggests that reactive prosocial behaviors stem from responding to others’ needs [ 34 ]. Engaging in such behaviors can foster positive perceptions among the audience and build cooperative social values [ 44 ] through reactive social exchange. People with a high orientation toward cooperative social values act to maximize mutual interests [ 45 ], a trait highly valued in the medical field.
We use medical live streaming as a measure of doctors’ reactive behaviors on web-based health care communities. Volunteering to provide interactional live streaming, a typical reactive behavior that may generate cooperative social value, gives the patient audience the impression that the doctors will prioritize demand-side interests during e-consultation services. Additionally, engaging in medical live streaming allows doctors to present themselves as authentic and recognized experts. This enhances their social presence [ 46 ], potentially leading to increased service use [ 47 ] and greater popularity [ 48 ]. Consequently, patients are more likely to perceive doctors who participate in medical live streaming as trustworthy for consultations. Given that e-consultations are closely related to the health conditions of the demand side, a credible doctor is likely to attract more e-consultations. Therefore, we propose the following hypothesis:
- Hypothesis 2: The conduct of medical live streaming by doctors positively impacts their e-consultation volume on web-based health care communities.
Moderating Roles of Offline and Digital Reputation
As doctors’ proactive and reactive behaviors potentially affect their consultation performance, based on 2 distinct theoretical foundations of human nature, there exists a discrepancy in how doctors’ reputations influence the relationship between various prosocial behaviors and e-consultation.
We formulate hypotheses regarding the moderating effects within the context of digital health care, by taking into account the inherent information asymmetry and the significance of establishing patient trust. Specifically, our hypotheses explore the influence of reputation on the relationship between doctors’ proactive behaviors and e-consultation volume, with a focus on reducing uncertainty. Additionally, we examine how reputation moderates the impact of doctors’ reactive behaviors, emphasizing the perspective of trust building.
First, in the marketing literature, service providers’ reputations, which can reduce information asymmetry and purchase uncertainty [ 49 ], are key factors influencing purchasing behavior and sales performance in the digital context [ 50 - 52 ]. Similarly, for doctors, reputations are related to the experiences and beliefs of other stakeholders [ 53 ]. As health care services are credence goods [ 54 ]—whose quality patients cannot discern even after experiencing the services—and given the nature of web-based platforms (eg, the absence of face-to-face meetings), there is a significant information asymmetry [ 51 ]. This increases patients’ uncertainty regarding the quality of doctors. Consequently, doctors’ reputations play crucial roles in patients’ decision-making processes [ 18 , 39 ]. We use doctors’ professional titles and patients’ recommendations on web-based health care communities to measure doctors’ offline and digital reputations.
Proactive behaviors by low-reputation doctors can create deeper professional impressions [ 34 , 35 ] to reduce uncertainty in e-consultations than high-reputation doctors, who are less uncertain in medical services. Then, doctors’ reputations—measured by offline professional titles and digital patients’ recommendations on web-based health care communities—will negatively moderate the relationship between proactive behavior and e-consultation volume. Thus, we propose the following hypotheses:
- Hypothesis 3a: Doctors’ offline professional titles negatively moderate the relationship between the posting of professional articles and e-consultation volume on web-based health care communities.
- Hypothesis 3b: Doctors’ digital recommendations from patients negatively moderate the relationship between the posting of professional articles and e-consultation volume on web-based health care communities.
Second, one of the central elements of SET is the concept of trust between actors in the exchange process [ 55 - 58 ]. In the context of digital health, patient’s trust in doctors is important to establish in order to refine the doctor-patient relationship. Doctors’ reputations can reflect their personality traits [ 39 ] and promote trust from patients [ 53 ]. Conducting medical live streaming, a form of reactive prosocial behavior, includes doctors’ cooperative social value orientations that are preferred in e-consultations. For low-reputation doctors, such as those with relatively junior professional titles and few digital patient recommendations, conducting medical live streaming will build patients’ confidence in e-consultations to a greater extent than doctors with high reputations, who are usually already highly trusted. Then, offline and digital reputation may negatively moderate the relationship between engaging in medical live streaming and e-consultation volume. Thus, we propose the final hypotheses:
- Hypothesis 4a: Doctors’ offline professional titles will negatively moderate the relationship between conducting medical live streaming and e-consultation volume on web-based health care communities.
- Hypothesis 4b: Doctors’ digital recommendations from patients will negatively moderate the relationship between conducting medical live streaming and e-consultation volume on web-based health care communities.
Research Context and Data Collection
Our research context is one of the largest web-based health care communities in China. This platform, established in 2006, offers e-consultation services to patients. As of July 2023, it boasts over 260,000 active doctors from 10,000 hospitals nationwide and has provided web-based medical services to 79 million patients.
The platform allows doctors to create home pages where they can display relevant information such as offline professional titles, experiences shared by other patients, and personal introductions. Patients can select doctors for e-consultation by browsing this information. Besides e-consultation, doctors can engage in prosocial behavior primarily focused on knowledge sharing. This includes posting professional articles in various formats (text, voice, and short videos) and conducting medical live streams for real-time interaction with patients.
We collected data over a 22-month period, from January 2021 to October 2022, focusing on common diseases such as diabetes, depression, infertility, skin diseases, and gynecological diseases. To ensure that our findings are generalizable to a typical and active doctor on the platform, we included doctors who had posted at least 1 article and conducted at least 1 live stream before the end of the study period in our analysis [ 59 - 61 ]. Our sample consists of 2880 doctors and includes the following information for each doctor: professional title, patient recommendations, records of experiences shared by the doctor’s patients, records of professional articles posted, records of live streams conducted, and records of the doctor’s e-consultations.
Variable Operationalization
Our unit of analysis is each doctor. We investigate how doctors’ prosocial behaviors, including proactive behaviors (posting professional articles) and reactive behaviors (conducting medical live streams), influence their e-consultation volume.
Dependent Variable
Our dependent variable is the doctors’ e-consultation volume, denoted as Consultation it , which is measured by the number of e-consultations of doctor i in month t .
Independent Variables
Our independent variables are doctors’ proactive behaviors and reactive behaviors. Doctors’ proactive behavior is operationalized as the posting of professional articles. Specifically, we denote proactive behavior as Articles it , which is measured by the number of professional articles posted by doctor i in month t . Doctors’ reactive behavior is operationalized as medical live streaming. This variable is denoted as LiveStreaming it , which is calculated as the number of medical live streams conducted by doctor i in month t .
Moderating Variables
We are also interested in how doctors’ external reputation, including their offline professional titles and digital recommendations from patients, influences the relationship between prosocial behaviors and e-consultation volume. A doctor’s offline professional title is denoted as Title i , which is a dummy variable indicating whether doctor i is a chief doctor ( Title i =1 indicates the doctor is a chief doctor, and Title i =0 indicates the doctor has a lower-ranked title). Digital recommendations are captured by Recommendations i , which is the digital recommendation level of doctor i as calculated by the platform based on the recommendations provided by their past patients.
Control Variables
We incorporated several control variables to account for factors that may influence patient’s choices of doctors in the digital context. The shared experiences of patients regarding a doctor’s treatment [ 39 ], as well as the number of patients who have previously consulted with the doctors [ 17 , 62 ], can indicate the doctor’s overall popularity. This, in turn, may affect patient choice. Therefore, we controlled for (1) the total number of patients who consulted with doctor i in the digital context before month t ( TotalPatients it ) and (2) the total number of patient-shared experiences about offline treatment by doctor i before month t ( TotalExperiences it ). Furthermore, doctors’ past behaviors, including article publishing and live streaming, can influence their current practices in posting articles and conducting live streams. Simultaneously, these factors may also act as signals affecting patients’ judgments and selection of doctors [ 12 ]. To account for these influences, we also controlled for (1) the total number of articles posted by doctor i before month t ( TotalArticles it ) and (2) the total number of medical live streams conducted by doctor i before month t ( TotalLiveStreaming it ).
To control for both observed and unobserved doctor-specific factors that do not change over time, individual-fixed effects were added. Additionally, time-fixed effects were introduced into our analysis to account for both observed and unobserved factors that vary over time but remain constant across doctors. Table 1 shows the variables and their definitions.
Estimation Model
To estimate the direct impact of doctors’ proactive behaviors and reactive behaviors on their e-consultation volume, the following 2-way fixed effects regression model was used:
Consultation it = β 0 + β 1 Articles it + β 2 LiveStreaming it + β 3 TotalPatients it + β 4 TotalExperiences it + β 5 TotalArticles it + β 6 TotalLiveStreaming it + α i + δ t + μ it (1)
where i denotes doctor, t denotes month, α i is doctor-fixed effects, δ t is month-fixed effects, Consultation it is the number of e-consultations of doctor i in month t , Articles it is the number of professional articles posted by doctor i in month t , LiveStreaming it is the number of medical live streams conducted by doctor i in month t , TotalPatients it is the total number of patients who consulted doctor i in the digital context before month t , TotalExperiences it is the total number of patient-shared experiences about offline treatment by doctor i before month t , TotalArticles it is the total number of articles posted by doctor i before month t , TotalLiveStreaming it is the total number of medical live streams doctor i conducted before month t , β is the coefficient, and μ it is the error term. We took the log transformation for our continuous variables in the model to reduce the skewness of the variables [ 63 ].
Next, the moderating effects of doctors’ offline professional titles and digital recommendations by patients were investigated based on the following specification:
Consultation it = β 0 + β 1 Articles it + β 2 LiveStreaming it + β 3 Articles it × Title i + β 4 LiveStreaming it × Title i + β 5 Articles it × Recommendation i + β 6 LiveStreaming it × Recommendation i + β 7 TotalPatients it + β 8 TotalExperiences it + β 9 TotalArticles it + β 10 TotalLiveStreaming it + α i + δ t + μ it (2)
where Title i indicates whether doctor i is a chief doctor ( Title i =1 indicates the doctor is a chief doctor, and Title i =0 indicates the doctor has a lower-ranked title). Recommendations i is the digital recommendation level of doctor i by other patients.
Ethical Considerations
This study used secondary publicly available data obtained from a website and did not involve the collection of original data pertaining to human participants. As such, there is no evidence of unethical behavior in the study. Consequently, ethics approval by an ethics committee or institutional review board was not deemed necessary.
In this section, we present our empirical results. The descriptive statistics are shown in Table 2 , and the correlation matrix is shown in Table 3 .
Empirical Results
Results for direct effects.
The analysis was conducted progressively. We first estimated the equation without control variables (model 1) and then added control variables in model 2. The estimated results are shown in Table 4 . From the results, we can see that the coefficient of Articles is significant and positive in model 2 (β=.093; P <.001), indicating that doctors’ proactive behaviors (ie, posting professional articles) can help them obtain more e-consultations. Thus, hypothesis 1 is supported. Regarding doctors’ engagement in medical live streaming, the results show that the coefficient of LiveStreaming is significantly positive (β=.214; P <.001), which suggests that doctors’ reactive behaviors (ie, conducting medical live streaming) can increase their e-consultation volume. This supports hypothesis 2.
a All models include doctor-fixed effects and month-fixed effects; robust SEs clustered by doctors are reported; the number of doctors is 2880, and the number of observations is 63,360.
b R 2 =0.843; F 2,2879 =175.98; P <.001.
c R 2 =0.851; F 6,2879 =119.72; P <.001.
d N/A: not applicable.
Results for Moderating Effects
The results for moderating effects are shown in Table 5 . In model 1, interaction terms were initially introduced between Title and Articles , as well as between Title and LiveStreaming , to estimate the moderating effect of doctors’ offline professional titles. The interaction terms were then added between Recommendations and Articles , as well as between Recommendations and LiveStreaming , to estimate the moderating effect of doctors’ digital recommendations in model 2. Finally, a full model was estimated by incorporating all interaction terms. We find that the results are consistent across all models. Wald tests and likelihood ratio were used to compare the fit among nested models [ 64 , 65 ], and the results show that the inclusion of moderating variables significantly enhances the model’s fit.
Regarding the moderating effect of doctors’ offline professional titles, we find that the coefficient of Articles × Title in model 1 of Table 5 is significantly negative (β=–.058; P <.001), which supports hypothesis 3a that doctors’ offline professional titles have a negative moderating effect on the relationship between doctors’ proactive behaviors and e-consultation volume. However, the coefficient of LiveStreaming × Title is insignificant (β=–.024; P =.45), which suggests that doctors’ offline professional titles have no moderating effect on the relationship between doctors’ reactive behaviors and e-consultation volume. Thus, hypothesis 4a is not supported.
b R 2 =0.851; F 8,2879 =89.98; P <.001; Wald test: P <.001; likelihood ratio: P <.001.
c R 2 =0.851; F 8,2879 =89.13; P <.001; Wald test: P <.001; likelihood ratio: P <.001.
d R 2 =0.852; F 10,2879 =71.44; P <.001; Wald test: P <.001; likelihood ratio: P <.001.
e N/A: not applicable.
For the moderating effect of digital patient recommendations, we find that both of the coefficients of Articles × Recommendations and LiveStreaming × Recommendations are negative and significant (β=–.055; P <.001 and β=–.100; P <.001, respectively, in model 2 of Table 5 ). This indicates that digital recommendations from patients have negative moderating effects on the relationship between doctors’ proactive behaviors and e-consultation volume as well as on the relationship between doctors’ reactive behaviors and e-consultation volume; this finding supports hypotheses 3b and 4b.
Robustness Check
First, additional analysis was performed to check whether our findings are robust to different measures of doctors’ reactive behaviors. In the main analysis, we used the number of medical live streams to construct doctors’ reactive behaviors. In the robustness check, doctors’ reactive behaviors were measured using the following measures: (1) the length of time spent in medical live streaming ( LSDuration it ), which is calculated as the total duration of all medical live streams conducted by doctor i in month t ; and (2) the number of doctor-patient interactions in the medical live streams ( LSInteractions it ), which is calculated as the total number of interactions between doctor i and patients in medical live streams in month t . This measure is likely to more effectively capture the reactive element of the behavior. The estimated results are shown in Table 6 , and we can see that the results are consistent with the main results.
Second, in the above analysis, the total number of articles posted by the doctors was used to measure doctors’ proactive behaviors. As doctors can post articles that are either their own original work or reposts from others, we further used the number of original articles ( OriArticles it ) to measure doctors’ proactive prosocial behaviors. Specifically, the number of articles was replaced with the number of original articles posted by doctor i in month t ( OriArticles it ). Models 1 and 2 in Table 7 show the results. We can see that using this alternative measure of proactive behavior does not materially change the results.
Third, as our dependent variable takes nonnegative values, negative binomial regression was further used to re-estimate our models. We find that the results (models 3 and 4 in Table 7 ) are similar to the main results.
Fourth, to further enhance the robustness and validity of our findings, article quality was used as a measure of doctors’ proactive behaviors. This approach is based on the premise that article quality more accurately reflects the effort and time invested by doctors in content creation. Specifically, we assessed article quality based on either the length of each article or the number of likes it received and then re-estimated our model. As indicated in Table 8 , the results remain consistent with our main findings, thereby further reinforcing the validity of our conclusions.
b R 2 =0.851; F 6,2879 =131.71; P <.001.
c R 2 =0.851; F 10,2879 =79.29; P <.001; Wald test: P <.001; likelihood ratio: P <.001.
d R 2 =0.850; F 6,2879 =112.52; P <.001.
e R 2 =0.851; F 10,2879 =68.43; P <.001; Wald test: P <.001; likelihood ratio: P <.001.
f N/A: not applicable.
a All models include doctor-fixed effects and month-fixed effects; robust SEs clustered by doctors are reported in models 1 and 2; bootstrap SEs in models 3 and 4.
b R 2 =0.851; F 6,2879 =118.99; P <.001.
c R 2 =0.852; F 10,2879 =71.04; P <.001; Wald test: P <.001; likelihood ratio: P <.001.
d Log likelihood=–150,015.36.
e Log likelihood=–149,888.24.
b R 2 =0.851; F 6,2879 =127.75; P <.001.
c R 2 =0.851; F 10,2879 =89.97; P <.001; Wald test: P <.001; likelihood ratio: P <.001.
d R 2 =0.851; F 6 , 2879 =133.39; P <.001.
e R 2 =0.852; F 10 , 2879 =84.94; P <.001; Wald test: P <.001; likelihood ratio: P <.001.
Analysis of Results
Web-based medical platforms offer a variety of functions to support doctors’ engagement in different types of prosocial behaviors. However, few studies have investigated the effects of these behaviors. Drawing on FMT and SET, this study categorized doctors’ prosocial practices in web-based health care communities into proactive and reactive actions and examined their effects on e-consultation volume. Briefly, prosocial behaviors positively impact on e-consultation, and a doctor’s digital and offline reputation moderates the relationship between prosocial behavior and e-consultation, albeit with some nuances.
First, we expanded upon existing literature on proactive prosocial behaviors, concluding that these actions can help doctors create professional images [ 43 ] in the medical consultation context. Our panel data analysis reveals that doctors’ posting of professional articles, which contribute to their professional image in the digital context, attracts more e-consultations. This finding aligns with the prior study [ 31 ], which observed that a health professional’s previous asynchronous prosocial behavior positively influences their future economic performance.
Second, drawing from SET, we analyzed the impact of synchronous reactive prosocial behaviors, a less explored area in prior literature. Our findings confirm that engaging in medical live streaming, a form of reactive prosocial behavior, leads to higher e-consultation volumes. Interestingly, we found that the positive impact of conducting a live stream exceeds that of posting an article.
Third, we expanded our research by testing the moderating roles of digital and offline reputations, measured by doctors’ offline professional titles and patients’ recommendations on web-based health care communities. We found that digital reputations significantly moderate the relationships between both types of prosocial behaviors and e-consultation volume. Specifically, doctors who post professional articles or conduct medical live streams attract more e-consultations when they have fewer patient recommendations compared to those with higher recommendations. Regarding offline professional titles, our results indicate a significant moderating effect on the relationship between proactive prosocial behaviors and e-consultation volume. Notably, junior doctors should focus more on posting articles in web-based health care communities to compensate for limitations associated with their titles [ 66 ]. However, the moderating effect of offline titles on the impact of reactive prosocial behaviors was found to be insignificant. We attribute this to the unique dynamics of trust conversion in Chinese health care settings. As doctors’ offline titles are granted by medical institutions, these titles could enhance patients’ trust in doctors only if there is a conversion of trust from the organization to the individual doctor, which represents different types of trust [ 67 ]. Consequently, doctors with the same offline titles from different hospitals may be perceived differently. For example, a senior doctor from a 3-A hospital is usually seen as highly professional in their clinical field, while a doctor with the same title in a 1-A hospital might typically handle primary diseases. Due to this trust conversion phenomenon, patients may not uniformly trust doctors from different hospitals with the same offline titles, leading to the insignificant moderating effect of offline titles on the impact of reactive prosocial behaviors.
In summary, this study underscores the importance of prosocial behaviors and reputation in shaping doctors’ e-consultation volumes on web-based health care communities, offering valuable insights for health care professionals aiming to increase their consultation outreach.
Implications
This study makes several theoretical implications. First, this study contributes to web-based health care community literature by offering a nuanced understanding of how doctors’ prosocial behaviors enhance e-consultation volume. While a limited number of studies have examined the effects of doctors’ freely provided behaviors in the digital context [ 31 ], the specific impact of different types of prosocial behaviors on e-consultation volume remains largely unexplored. This study addresses this knowledge gap by theoretically categorizing doctors’ prosocial behaviors in web-based health care communities into proactive and reactive types and exploring their impacts on e-consultations.
Second, this study enriches web-based health care communities and live streaming literature by validating the role of medical live streaming in web-based health services. Prior research on live streaming has mainly concentrated on e-commerce [ 68 ], web-based gaming [ 69 ], and web-based learning [ 70 ]. Our study extends this research to the health care context, highlighting the importance of live streaming on web-based health care platforms. Specifically, this study delves into how doctors’ synchronous, reactive volunteer interactions via live streaming influence patient decision-making.
Finally, this study advances FMT and SET by highlighting the importance of context in theory development and providing guidance for context-specific theorizing on web-based health platforms. It also sheds light on how the impact of different prosocial behaviors on e-consultation volume varies depending on a doctor’s offline and digital reputations. Notably, this study validates that proactive behaviors work more effectively in promoting e-consultations for doctors with lower titles or fewer digital recommendations, while reactive behaviors are more effective for doctors with fewer digital recommendations.
This study offers several practical implications for doctors and platform managers. First, the beneficial effects of prosocial behaviors suggest that doctors should adapt their engagement activities when participating in web-based health care platforms. Nowadays, an increasing number of doctors are joining web-based health care communities and focusing on e-consultations, attracted by the economic and social benefits. Based on our results, posting professional articles can help doctors establish a professional image, potentially leading to more e-consultations. Additionally, conducting medical live streams can bolster e-consultations by fostering cooperative social value for doctors and enhancing their credibility among patient audiences. Therefore, doctors may prefer engaging in both proactive and reactive prosocial activities in web-based health care communities to attract more patients to their e-consultation services.
Second, the boundary conditions of the effects of prosocial behaviors imply that doctors should strategically leverage the beneficial effect of proactive and reactive behaviors according to their offline and digital reputations. Doctors with fewer digital recommendations should focus more on prosocial behavior to attract patients to e-consultations. Meanwhile, doctors with lower titles should devote their efforts to proactive behaviors to demonstrate their capability in fulfilling the e-consultations, thereby reducing information asymmetry between patients and themselves.
Third, our findings offer implications for web-based health care platform managers in designing effective functions. An increasing number of platforms are launching various features to better serve doctors and patients, meeting the needs of both groups more effectively. Our empirical findings suggest that doctors’ proactive and reactive prosocial behaviors, such as posting professional articles and conducting medical live streams, can help them establish professional image and enhance patient trust, leading to improved performance. Importantly, these behaviors also benefit patients by enhancing their health knowledge and literacy. Thus, platform managers could introduce functions (eg, article posting, live streaming, and doctor-driven communities) to encourage more prosocial behaviors by doctors. Additionally, platform managers might consider incorporating guidelines or incentive mechanisms for prosocial behaviors into their platforms. For example, it is recommended that platforms collect and analyze doctors’ proactive and reactive prosocial behaviors and guide them on how to effectively use these functions and engage in different types of activities.
Limitations
Despite its contributions, this study also presents several limitations that future research should consider. First, various classifications of prosocial behavior are available; for instance, Richaud et al [ 71 ] classified such behavior as altruistic, compliant, emotional, public, anonymous, or dire actions. Given the intricacy of web-based medical services, future studies would benefit from further exploring the roles of these other types of prosocial behavior exhibited by doctors on web-based health care communities. Second, our research model was constructed primarily from the doctor’s perspective and thus did not investigate the influence of doctors’ prosocial behaviors on patients’ satisfaction and well-being. Future research should delve into these relationships to obtain a more comprehensive understanding of the impacts of doctors’ prosocial behaviors. Finally, this study focused only on the quantity of medical live-streaming sessions, overlooking the quality aspect, which could be a crucial factor influencing e-consultation volume. Future research will concentrate on exploring this aspect.
Conclusions
Building upon prior studies on doctors’ prosocial behaviors on web-based health care communities, this study further delineates doctors’ beneficial actions into proactive and synchronous reactive behaviors. This distinction is based on the divergence in doctors’ motives for engaging and patients’ levels of involvement. Drawing from FMT and SET, this study offers insights that could aid doctors in increasing their e-consultation volume by adopting these beneficial behaviors. Concurrently, this research augments our understanding of the roles a doctor’s reputation plays in the relationships between various prosocial behaviors—specifically, proactive and reactive actions—and their e-consultation volume. This study may inspire doctors with comparatively lower offline professional titles and digital popularity to achieve their desired e-consultation volume.
Acknowledgments
This work was supported by the National Natural Science Foundation of China (72001170 and 72102179), the Fundamental Research Funds for the Central Universities (SK2024028), the Ministry of Education in China Project of Humanities and Social Sciences (21XJC630003), the China Postdoctoral Science Foundation (2022T150515, 2023M742818, and 2020M673432), the National Natural Science Foundation of China (72004042), and the Heilongjiang Natural Science Foundation (YQ2023G003), and the grants from City University of Hong Kong (projects 7005959, 7006152, and 7200725).
Conflicts of Interest
None declared.
- Qi M, Cui J, Li X, Han Y. Influence of e-consultation on the intention of first-visit patients to select medical services: results of a scenario survey. J Med Internet Res. 2023;25:e40993. [ FREE Full text ] [ CrossRef ] [ Medline ]
- Cao B, Huang W, Chao N, Yang G, Luo N. Patient activeness during online medical consultation in China: multilevel analysis. J Med Internet Res. 2022;24(5):e35557. [ FREE Full text ] [ CrossRef ] [ Medline ]
- Jiang S. Talk to your doctors online: an internet-based intervention in China. Health Commun. 2021;36(4):405-411. [ FREE Full text ] [ CrossRef ] [ Medline ]
- Jiang J, Cameron AF, Yang M. Analysis of massive online medical consultation service data to understand physicians' economic return: observational data mining study. JMIR Med Inform. 2020;8(2):e16765. [ FREE Full text ] [ CrossRef ] [ Medline ]
- Street RL, Millay B. Analyzing patient participation in medical encounters. Health Commun. 2001;13(1):61-73. [ FREE Full text ] [ CrossRef ] [ Medline ]
- Ren D, Ma B. Effectiveness of interactive tools in online health care communities: social exchange theory perspective. J Med Internet Res. 2021;23(3):e21892. [ FREE Full text ] [ CrossRef ] [ Medline ]
- Guo S, Guo X, Fang Y, Vogel D. How doctors gain social and economic returns in online health-care communities: a professional capital perspective. J Manag Inf Syst. 2017;34(2):487-519. [ FREE Full text ] [ CrossRef ]
- Tande AJ, Berbari EF, Ramar P, Ponamgi SP, Sharma U, Philpot L, et al. Association of a remotely offered infectious diseases eConsult service with improved clinical outcomes. Open Forum Infect Dis. 2020;7(1):ofaa003. [ FREE Full text ] [ CrossRef ] [ Medline ]
- Richardson BR, Truter P, Blumke R, Russell TG. Physiotherapy assessment and diagnosis of musculoskeletal disorders of the knee via telerehabilitation. J Telemed Telecare. 2017;23(1):88-95. [ CrossRef ] [ Medline ]
- Castaneda PR, Duffy B, Andraska EA, Stevens J, Reschke K, Osborne N, et al. Outcomes and safety of electronic consult use in vascular surgery. J Vasc Surg. 2020;71(5):1726-1732. [ FREE Full text ] [ CrossRef ] [ Medline ]
- Jiang S. The relationship between face-to-face and online patient-provider communication: examining the moderating roles of patient trust and patient satisfaction. Health Commun. 2020;35(3):341-349. [ FREE Full text ] [ CrossRef ] [ Medline ]
- Li J, Tang J, Jiang L, Yen DC, Liu X. Economic success of physicians in the online consultation market: a signaling theory perspective. Int J Electron Commer. 2019;23(2):244-271. [ FREE Full text ] [ CrossRef ]
- Guo S, Guo X, Zhang X, Vogel D. Doctor–patient relationship strength’s impact in an online healthcare community. Inf Technol Dev. 2017;24(2):279-300. [ FREE Full text ] [ CrossRef ]
- Audrain-Pontevia AF, Menvielle L. Do online health communities enhance patient-physician relationship? an assessment of the impact of social support and patient empowerment. Health Serv Manage Res. 2018;31(3):154-162. [ FREE Full text ] [ CrossRef ] [ Medline ]
- Yang Y, Zhang X, Lee PK. Improving the effectiveness of online healthcare platforms: an empirical study with multi-period patient-doctor consultation data. Int J Prod Econ. 2019;207:70-80. [ FREE Full text ] [ CrossRef ]
- Fan W, Zhou Q, Qiu L, Kumar S. Should doctors open online consultation services? An empirical investigation of their impact on offline appointments. Inf Syst Res. 2023;34(2):629-651. [ FREE Full text ] [ CrossRef ]
- Liu QB, Liu X, Guo X. The effects of participating in a physician-driven online health community in managing chronic disease: evidence from two natural experiments. MIS Q. 2020;44(1):391-419. [ FREE Full text ] [ CrossRef ]
- Yan Z, Wang T, Chen Y, Zhang H. Knowledge sharing in online health communities: a social exchange theory perspective. Inf Manag. 2016;53(5):643-653. [ FREE Full text ] [ CrossRef ]
- Luo P, Chen K, Wu C, Li Y. Exploring the social influence of multichannel access in an online health community. J Assoc Inf Sci Technol. 2018;69(1):98-109. [ FREE Full text ] [ CrossRef ]
- Chai S, Bagchi-Sen S, Morrell C, Rao HR, Upadhyaya SJ. Internet and online information privacy: an exploratory study of preteens and early teens. IEEE Trans Profess Commun. 2009;52(2):167-182. [ FREE Full text ] [ CrossRef ]
- Ahearne M, Rapp A, Hughes DE, Jindal R. Managing sales force product perceptions and control systems in the success of new product introductions. J Mark Res. 2010;47(4):764-776. [ FREE Full text ] [ CrossRef ]
- Awaysheh A, Heron RA, Perry T, Wilson JI. On the relation between corporate social responsibility and financial performance. Strateg Manag J. 2020;41(6):965-987. [ FREE Full text ] [ CrossRef ]
- Coll MP, Grégoire M, Eugène F, Jackson PL. Neural correlates of prosocial behavior towards persons in pain in healthcare providers. Biol Psychol. 2017;128:1-10. [ FREE Full text ] [ CrossRef ] [ Medline ]
- Kapıkıran NA. Sources of ethnocultural empathy: personality, intergroup relations, affects. Curr Psychol. 2021;42(14):11510-11528. [ FREE Full text ] [ CrossRef ]
- Yin Y, Wang Y. Is empathy associated with more prosocial behaviour? a meta‐analysis. Asian J Soc Psychol. 2023;26(1):3-22. [ FREE Full text ] [ CrossRef ]
- Finset A. "I am worried, Doctor!" emotions in the doctor-patient relationship. Patient Educ Couns. 2012;88(3):359-363. [ FREE Full text ] [ CrossRef ] [ Medline ]
- Larson EB, Yao X. Clinical empathy as emotional labor in the patient-physician relationship. JAMA. 2005;293(9):1100-1106. [ FREE Full text ] [ CrossRef ] [ Medline ]
- Bloom G, Standing H, Lloyd R. Markets, information asymmetry and health care: towards new social contracts. Soc Sci Med. 2008;66(10):2076-2087. [ FREE Full text ] [ CrossRef ] [ Medline ]
- Guo F, Zou B, Guo J, Shi Y, Bo Q, Shi L. What determines academic entrepreneurship success? A social identity perspective. Int Entrep Manag J. 2019;15(3):929-952. [ FREE Full text ] [ CrossRef ]
- Yang H, Zhang X. Investigating the effect of paid and free feedback about physicians' telemedicine services on patients' and physicians' behaviors: panel data analysis. J Med Internet Res. 2019;21(3):e12156. [ FREE Full text ] [ CrossRef ] [ Medline ]
- Yan Z, Kuang L, Qiu L. Prosocial behaviors and economic performance: evidence from an online mental healthcare platform. Prod Oper Manag. 2022;31(10):3859-3876. [ FREE Full text ] [ CrossRef ]
- Frese M, Fay D. 4. Personal initiative: an active performance concept for work in the 21st century. Res Organ Behav. 2001;23:133-187. [ FREE Full text ] [ CrossRef ]
- Frese M, Kring W, Soose A, Zempel J. Personal initiative at work: differences between East and West Germany. Acad Manage J. 1996;39(1):37-63. [ FREE Full text ] [ CrossRef ]
- Spitzmuller M, Van Dyne L. Proactive and reactive helping: contrasting the positive consequences of different forms of helping. J Organ Behavior. 2013;34(4):560-580. [ FREE Full text ] [ CrossRef ]
- Bandura A. Social cognitive theory: an agentic perspective. Annu Rev Psychol. 2001;52(1):1-26. [ FREE Full text ] [ CrossRef ] [ Medline ]
- Mittal S, Sengupta A, Agrawal NM, Gupta S. How prosocial is proactive: developing and validating a scale and process model of knowledge-based proactive helping. J Manag Organ. 2018;26(4):625-650. [ FREE Full text ] [ CrossRef ]
- Walter A, Ritter T, Gemünden HG. Value creation in buyer–seller relationships: theoretical considerations and empirical results from a supplier's perspective. Ind Mark Manag. 2001;30(4):365-377. [ FREE Full text ] [ CrossRef ]
- Eggert A, Ulaga W, Schultz F. Value creation in the relationship life cycle: a quasi-longitudinal analysis. Ind Mark Manag. 2006;35(1):20-27. [ FREE Full text ] [ CrossRef ]
- Deng Z, Hong Z, Zhang W, Evans R, Chen Y. The effect of online effort and reputation of physicians on patients' choice: 3-wave data analysis of China's good doctor website. J Med Internet Res. 2019;21(3):e10170. [ FREE Full text ] [ CrossRef ] [ Medline ]
- Kurihara H, Maeno T, Maeno T. Importance of physicians' attire: factors influencing the impression it makes on patients, a cross-sectional study. Asia Pac Fam Med. 2014;13(1):2. [ FREE Full text ] [ CrossRef ] [ Medline ]
- Cooper ML, Shapiro CM, Powers AM. Motivations for sex and risky sexual behavior among adolescents and young adults: a functional perspective. J Pers Soc Psychol. 1998;75(6):1528-1558. [ CrossRef ] [ Medline ]
- Clary EG, Snyder M, Ridge RD, Copeland J, Stukas AA, Haugen J, et al. Understanding and assessing the motivations of volunteers: a functional approach. J Pers Soc Psychol. 1998;74(6):1516-1530. [ CrossRef ] [ Medline ]
- Epstein RM, Hundert EM. Defining and assessing professional competence. JAMA. 2002;287(2):226-235. [ FREE Full text ] [ CrossRef ] [ Medline ]
- Zhang L, Qiu Y, Zhang N, Li S. How difficult doctor‒patient relationships impair physicians' work engagement: the roles of prosocial motivation and problem-solving pondering. Psychol Rep. 2020;123(3):885-902. [ FREE Full text ] [ CrossRef ] [ Medline ]
- Dabholkar PA, Johnston WJ, Cathey AS. The dynamics of long-term business-to-business exchange relationships. J Acad Mark Sci. 1994;22(2):130-145. [ FREE Full text ] [ CrossRef ]
- Ochs M, Mestre D, de Montcheuil G, Pergandi JM, Saubesty J, Lombardo E, et al. Training doctors’ social skills to break bad news: evaluation of the impact of virtual environment displays on the sense of presence. J Multimodal User Interfaces. 2019;13(1):41-51. [ FREE Full text ] [ CrossRef ]
- Schroeder T, Seaman K, Nguyen A, Gewald H, Georgiou A. Enablers and inhibitors to the adoption of mHealth apps by patients—a qualitative analysis of German doctors' perspectives. Patient Educ Couns. 2023;114:107865. [ FREE Full text ] [ CrossRef ] [ Medline ]
- Liu X, Hu M, Xiao BS, Shao J. Is my doctor around me? investigating the impact of doctors’ presence on patients’ review behaviors on an online health platform. J Assoc Inf Sci Technol. 2022;73(9):1279-1296. [ FREE Full text ] [ CrossRef ]
- Chen Y, Xie J. Online consumer review: word-of-mouth as a new element of marketing communication mix. Manage Sci. 2008;54(3):477-491. [ FREE Full text ] [ CrossRef ]
- Ye Q, Li Y, Kiang M, Wu W. The impact of seller reputation on the performance of online sales: evidence from TaoBao Buy-It-Now (BIN) data. SIGMIS Database. 2009;40(1):12-19. [ FREE Full text ] [ CrossRef ]
- Liu X, Guo X, Wu H, Wu T. The impact of individual and organizational reputation on physicians’ appointments online. Int J Electron Commer. 2016;20(4):551-577. [ FREE Full text ] [ CrossRef ]
- Dewan S, Hsu V. Adverse selection in electronic markets: evidence from online stamp auctions. J Ind Econ. 2004;52(4):497-516. [ CrossRef ]
- Torres E, Vasquez-Parraga AZ, Barra C. The path of patient loyalty and the role of doctor reputation. Health Mark Q. 2009;26(3):183-197. [ FREE Full text ] [ CrossRef ] [ Medline ]
- Gottschalk F, Mimra W, Waibel C. Health services as credence goods: a field experiment. Econ J. 2020;130(629):1346-1383. [ FREE Full text ] [ CrossRef ]
- Nunkoo R, Ramkissoon H. Power, trust, social exchange and community support. Ann Tour Res. 2012;39(2):997-1023. [ FREE Full text ] [ CrossRef ]
- Blau PM. Exchange and Power in Social Life. New Brunswick. Transaction Publishers; 1964.
- Molm LD, Takahashi N, Peterson G. Risk and trust in social exchange: an experimental test of a classical proposition. Am J Sociol. 2000;105(5):1396-1427. [ FREE Full text ] [ CrossRef ]
- Lioukas CS, Reuer JJ. Isolating trust outcomes from exchange relationships: social exchange and learning benefits of prior ties in alliances. Acad Manage J. 2015;58(6):1826-1847. [ FREE Full text ] [ CrossRef ]
- Malgonde OS, Saldanha TJ, Mithas S. Resilience in the open source software community: how pandemic and unemployment shocks influence contributions to others’ and one’s own projects. MIS Q. 2023;47(1):361-390. [ FREE Full text ] [ CrossRef ]
- Mayya R, Ye S, Viswanathan S, Agarwal R. Who forgoes screening in online markets and why? Evidence from Airbnb. MIS Q. 2021;45(4):1745-1776. [ FREE Full text ] [ CrossRef ]
- Moqri M, Mei X, Qiu L, Bandyopadhyay S. Effect of “following” on contributions to open source communities. J Manag Inf Syst. 2018;35(4):1188-1217. [ FREE Full text ] [ CrossRef ]
- Chen Q, Jin J, Zhang T, Yan X. The effects of log-in behaviors and web reviews on patient consultation in online health communities: longitudinal study. J Med Internet Res. 2021;23(6):e25367. [ FREE Full text ] [ CrossRef ] [ Medline ]
- Mo J, Sarkar S, Menon S. Competing tasks and task quality: an empirical study of crowdsourcing contests. MIS Q. 2021;45(4):1921-1948. [ FREE Full text ] [ CrossRef ]
- Gourieroux C, Holly A, Monfort A. Likelihood ratio test, Wald test, and Kuhn-Tucker test in linear models with inequality constraints on the regression parameters. Econometrica. 1982;50(1):63. [ CrossRef ]
- Peng CH, Yin D, Zhang H. More than words in medical question-and-answer sites: a content-context congruence perspective. Inf Syst Res. 2020;31(3):913-928. [ FREE Full text ] [ CrossRef ]
- Guo L, Jin B, Yao C, Yang H, Huang D, Wang F. Which doctor to trust: a recommender system for identifying the right doctors. J Med Internet Res. 2016;18(7):e186. [ FREE Full text ] [ CrossRef ] [ Medline ]
- Zheng S, Hui SF, Yang Z. Hospital trust or doctor trust? A fuzzy analysis of trust in the health care setting. J Bus Res. 2017;78:217-225. [ FREE Full text ] [ CrossRef ]
- Mao Z, Du Z, Yuan R, Miao Q. Short-term or long-term cooperation between retailer and MCN? new launched products sales strategies in live streaming e-commerce. J Retail Consum Serv. 2022;67:102996. [ FREE Full text ] [ CrossRef ]
- Hilvert-Bruce Z, Neill JT, Sjöblom M, Hamari J. Social motivations of live-streaming viewer engagement on Twitch. Comput Hum Behav. 2018;84:58-67. [ FREE Full text ] [ CrossRef ]
- Tang YM, Chen PC, Law KMY, Wu CH, Lau YY, Guan J, et al. Comparative analysis of student's live online learning readiness during the coronavirus (COVID-19) pandemic in the higher education sector. Comput Educ. 2021;168:104211. [ FREE Full text ] [ CrossRef ] [ Medline ]
- Richaud MC, Mesurado B, Cortada AK. Analysis of dimensions of prosocial behavior in an Argentinean sample of children. Psychol Rep. 2012;111(3):687-696. [ FREE Full text ] [ CrossRef ] [ Medline ]
Abbreviations
Edited by G Eysenbach; submitted 11.09.23; peer-reviewed by P Luo, Y Zhu, C Fu; comments to author 05.10.23; revised version received 30.12.23; accepted 09.03.24; published 25.04.24.
©Xiaoxiao Liu, Huijing Guo, Le Wang, Mingye Hu, Yichan Wei, Fei Liu, Xifu Wang. Originally published in the Journal of Medical Internet Research (https://www.jmir.org), 25.04.2024.
This is an open-access article distributed under the terms of the Creative Commons Attribution License (https://creativecommons.org/licenses/by/4.0/), which permits unrestricted use, distribution, and reproduction in any medium, provided the original work, first published in the Journal of Medical Internet Research, is properly cited. The complete bibliographic information, a link to the original publication on https://www.jmir.org/, as well as this copyright and license information must be included.
Tactical Menu
Strip-grazing milo as a low-cost winter forage.
Rusty Lee Field Specialist in Agronomy
Drew Kientzy Research Analyst, Agricultural Business and Policy Extension
Eric Bailey Assistant Professor and State Beef Extension Specialist
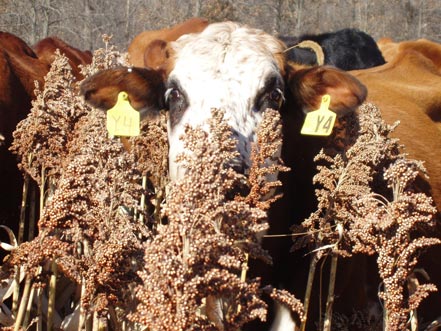
Minimizing the cost of winter feed is a key element of profitability on most pasture-based livestock operations. Baled dry hay is the industry standard for feeding cattle and small ruminants throughout the winter. Challenging weather often complicates the hay-making process. Some producers around the state have reduced their hay crop in exchange for stockpiled milo for their winter forage needs. Strip-grazing standing milo eliminates the costs associated with harvesting and transporting hay and forgoes milo harvesting costs; resulting in much lower daily feed costs compared to traditional baled hay.
System basics
The basics of milo stockpile grazing are like other management-intensive grazing systems. Ideally, milo is planted into fields with existing perimeter fencing and a good water supply. When the milo is mature and feed supplies are transitioning to stored forages, it is time to begin allocating milo for daily strip-grazing.
The recommended daily allocation depends on cattle stocking rate and milo yield. Based on 15 years of research perfecting the milo grazing system, University of Missouri Extension agronomist Rusty Lee recommends feeding 10-13 pounds of grain per cow per day. A grain-only allocation is used as it is difficult to quantify the fodder in the field, but grain yield increases along with fodder so the two are closely connected. Forage utilization rates can be up to 75 percent of total milo biomass if the allotments of milo restrict loafing and encourage the animals to eat all the fodder.
Production costs
The first part of implementing an annual forage grazing system is growing the crop. Corn planting equipment can be used for milo. However, milo can be successfully planted with a grain drill if you do not have access to a row crop planter.
MU Extension publishes a Grain Sorghum Planning Budget , updated annually. Table 1 summarizes the operating costs of producing milo yielding 105 bushels per acre.
Annual operating costs per acre are lower for tall fescue-clover mixed hay, compared to milo. The full planning budget for mixed hay yielding three tons per acre in Missouri can be found in MU Extension’s Fescue-Clover Hay Planning Budget . Table 2 shows annual operating costs for mixed hay.
Table 1. Operating costs of producing milo in Missouri, less harvesting, in dollars per acre.
Table 2. Operating costs of growing and harvesting established fescue-clover hay, in dollars per acre.
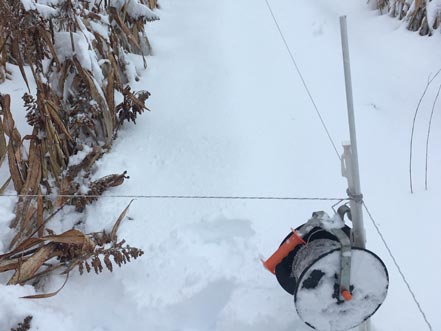
There are two types of fencing used in the milo grazing system: exterior and interior. Exterior fencing — high tensile wire, barb wire, woven wire or some combination of these three — may or may not be electrified. To maintain forage quality and keep utilization rate high, portable fencing must be used to separate the grazed area from the standing milo in the remainder of the field. Electric fence is an inexpensive and convenient way to keep cattle out of the milo not included in their daily allotment. Since milo is an annual crop, there is no need to install a back-fence to prevent the livestock from grazing previous allotments; only one stretch of temporary fencing is necessary.
Table 3 shows the costs per foot of constructing and maintaining both permanent perimeter and portable interior fences. The cost estimates below do not include labor to move the electric interior fence for each milo allotment. A general rule of one hour per one-quarter mile of fence assuming a single wire with step-in self-insulating posts. Driving down milo with an ATV or UTV creates the pathway for a single-strand fence utilizing step-in posts.
Table 3. Fence construction costs, per foot.
Daily allocations
There are four factors in estimating the daily allocation of milo for your herd: stocking rate, consumption rate, utilization rate and milo yield. Most mature cows will consume about three percent of their body mass daily. A 1,200-pound cow needs about 36 pounds of forage per day. Experienced milo grazers have found that 10-12 pounds of milo grain provides an appropriate intake, including the milo fodder. However, the grain-based allocation may change because varying growing conditions can change the ratio of grain to forage.
Milo yield is difficult to estimate prior to harvest. Components of milo yield include heads per acre, seeds per head and seed weight. Like corn, it is easy to calculate the number of heads in a stretch of row and extrapolate that area to find the yield per acre. However, milo heads are not uniform and can have over 4,000 seeds per head, making it nearly impossible to estimate yield. For a new grower of milo, it may be easiest and most accurate to consult your agronomist or an experienced milo producer to visually assess the yield. A visual assessment will likely not be accurate enough to determine the final daily allocation but can be a helpful starting point.
Ultimately, the allocation of milo comes down to cow-days per acre. These are the steps to determine cow days per acre in this scenario:
- 105 bushels/acre milo × 56 pounds/bushel = 5,880 pounds/acre
- 5,880 pounds/acre × 75% utilization = 4,410 usable pounds
- 4,410 usable pounds ÷ 11 pounds/cow/day = 400.9 cow days/acre.
Next, determine the daily allocation by:
- Dividing the number of cow-days/acre by the number of cows in the herd to effectively find herd-days/acre.
- Divide the area of an acre (43,560 sq. ft.) by the number of herd-days per acre to find the square footage needed for a daily allocation.
- Divide the daily allocation area by the width of your pasture where the temporary fence will run to find out how far you will move the wire every day.
Labor needs
Labor is required to manage daily allocations of milo. Giving an allocation larger than one day’s grazing results in over-feeding the first day and wasted grain from trampling and under-feeding on the additional days before moving to the next allocation. Cattle that respect an electric fence is a requirement as portable electric fencing keeps the labor manageable. Producers consistently report the daily time commitment being about 30 minutes.
Labor requirements are mostly independent of herd size. Producers report needing the same 30 minutes to feed 30 head or 300 head. However, wider paddocks (the distance spanned by the temporary fence moved daily) require more daily labor than narrower paddocks of the same area. The time required to feed hay increases as herd size increases. Moving feed bunks or supplement tubs are unnecessary in a milo grazing system. The ground near the prior day’s portable fence-line will be clear enough to feed due to driving down the milo before installing the fence and the natural inclination of the cattle to create a track near the boundary.
Nutritional value of feeding milo
The most common use of grazing standing milo is for beef cow diets. Given the mix of spring and fall calving herds across Missouri, distinguishing nutrient requirement differences between the groups is important. The spring-calving cow will be in the third trimester of gestation when grazing standing milo whereas a fall-calving cow will have calved and be near peak lactation, which coincides with a beef cow’s greatest nutrient requirements.
To avoid weight loss, beef cows in the last trimester of gestation require a diet that is greater than 55 percent total digestible nutrients (TDN), whereas an early lactation beef cow needs a diet of at least 60 percent TDN. Tall fescue hay harvested across Missouri rarely tests at 60 percent TDN and often near 50 percent TDN. When producers provide supplemental feed to beef in conjunction with hay, energy from the supplement is most needed by cows.
Analysis of milo heads regularly return TDN values above 70 percent and milo fodder (leaves and stems) routinely test approximately 55 percent TDN. Energy is not limiting when grazing standing milo, so long as cows are allocated 10-13 pounds of grain and 18-20 pounds of fodder. Table 4 contains a simple nutrient analysis of whole-plant milo forage. Note that weather, grain-to-fodder ratio, date of sampling and many other factors may affect the nutritional value of whole milo plants.
Table 4. Basic nutrient analysis for whole-plant milo forage.
Crude protein is often the first limiting nutrient when grazing standing milo. Use of a ration balancing program shows a 0.49 pounds of crude protein deficiency in a diet containing two parts milo stalks and one part milo grain. Producers grazing milo are encouraged to provide a minimum of 0.5 pounds of crude protein per cow per day to optimize feed digestion. For example, two pounds of dried distillers grains on an as-fed basis (88 percent dry matter; 30 percent crude protein) will provide 0.53 pounds of crude protein. Crude protein can be fed as little as twice a week, so long as the amount fed averages at least 0.5 pounds of crude protein per cow per day (6 pounds of dried distillers grains fed every third day). Self-fed protein supplements are also an option but producers should be aware of significant day-to-day variation in self-fed supplement consumption.
Adaptation from forage to grain-based diets is generally recommended when feeding beef cattle. Grazing standing milo may be an exception to this rule. The rate of rumen starch fermentation is slower for milo than corn. Milo grain has a hard outer shell that is resistant to digestion, and ruminants are not efficient at grinding whole milo due to their lacking of upper teeth. Do not give unrestricted access to a milo field due to the preference for grain over fodder, as well as the risk of rumen acidosis and other digestive disorders. However, the risk of acidosis is modest when allocating feed daily.
Winter weather can substantially increase nutrient requirements, with extreme cold and cool dampness increasing energy requirements. Do not hesitate to increase the daily allocation of grain by 20 to 30 percent during adverse weather. This provides extra energy to help offset the impacts of cold stress.
Feed value per acre
Acreage available for feed production is limited on many farms. The potential 401 cow-days per acre in a milo grazing system is higher than nearly all perennial forages, grazed or harvested. A Missouri hay crop may annually yield about 3 tons, or roughly 180 cow-days per acre. However, a fescue stockpile will often be no more than 60 cow-days per acre. This means that 6.5 acres of stockpiled fescue would be needed to provide the same winter grazing as an acre of milo. For fescue-clover hay, the land needed would cost $227.50, assuming a $35 per acre rental rate. If cropland for milo can be rented at $130, producers will experience $97.50 in land cost savings for each acre of milo grazed.
Milo grazing also offers a substantial advantage over feeding dry hay in the winter in terms of feed cost per cow per day. Assuming 105 bushel per acre milo yield with operating costs of $201.85, we find that the operating investment into the milo is $1.92 per bushel, or $0.03 per pound. If each cow is allotted 13 pounds of milo grain and the associated fodder daily, the cost of fed milo per cow/day is $0.45. This example reflects the cash-based costs of grazing milo. Alternative outcomes for the milo crop may value feed per cow per day differently.
Compared to grazing milo, feed costs are typically much higher in a hay-based winter feed program. If forage growers in Missouri can produce fescue-clover hay yielding 3 tons per acre with operating costs of $196.18, the variable cost of the hay is $65.39 per ton, or $0.03 per pound. A 1,200 pound cow consuming 3 percent of her body weight in dry matter daily would eat 36 pounds of hay daily at a cost of $1.18 per cow per day. In this scenario, the daily feed cost for a cow eating hay as its primary feed would be over 2.6 times that of one eating a milo-based diet.
Crop production implications
Grazing cattle in row crop fields can lead to excessive pocking from hoof traffic. Fields will likely be rough in spring if they were wet while grazing. For this reason, producers adopting this system will likely have to use conventional tillage practices on acres grazed. Additionally, significant investment must be made in perimeter fences and water access in each field where milo is grazed. These sunk costs incentivize continuously planting milo on the same fields. The early adopters of milo grazing systems have found that milo can be grown continuously for four to five years. Milo can also be planted into late June across Missouri with no yield loss, permitting a system using a cool season crop in the spring prior to seeding milo in early summer.
Field surface damage from hoof traffic can be managed by keeping cattle close to a separate area to be used as a sacrifice when there are excessively wet conditions. There, livestock can eat other stored forages to minimize the damage done to the milo field. The economic benefit of a sacrificial lot will typically not offset the higher feed costs of feeding stored forage. The benefit of pulling animals out of the field are mostly aesthetic. If animals damage wet fields, producers will bear those costs with added input costs or lost yield on the subsequent crop.
This guide is for informational purposes and the user assumes all risks associated with its use. If you have any questions about the information in this guide, please contact Rusty Lee at [email protected] for guidance.
Related programs
- Agricultural Business and Policy Extension
- Beef Extension
- Corn and Sorghum
- Drought Resources
- Forage-Livestock Group
- Pasture-Based Dairy
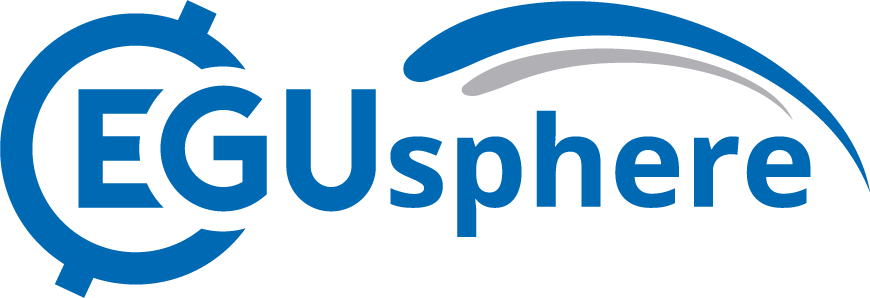
Preprint
- Preprint egusphere-2024-945
Evaluation of a reduced pressure chemical ion reactor utilizing adduct ionization for the detection of gaseous organic and inorganic species
Abstract. Volatile organic compounds (VOCs) and volatile inorganic compounds (VICs) provide critical information across many scientific fields including atmospheric chemistry, soil, and biological processes. Chemical ionization (CI) mass spectrometry has become a powerful tool for tracking these chemically complex and temporally variable compounds in a variety of laboratory and field environments. It is particularly powerful with time-of-flight mass spectrometers, which can measure hundreds of compounds in a fraction of a second and have enabled entirely new branches of VOC/VIC research in atmospheric and biological chemistry. To accurately describe each step of these chemical, physical, and biological processes, measurements across the entire range of gaseous products is crucial. Recently, chemically comprehensive gas-phase measurements have been performed using many CI mass spectrometers deployed in parallel, each utilizing a different ionization method to cover a broad range of compounds. Here we introduce the recently developed Vocus AIM (Adduct Ionization Mechanism) ion-molecule reactor (IMR), which samples trace vapors in air and ionizes them via chemical ionization at medium pressures. The Vocus AIM supports the use of many different reagent ions of positive and negative polarity and is largely independent of changes in the sample humidity. Within the present study, we present the performance and explore the capabilities of the Vocus AIM using various chemical ionization schemes, including Chloride (Cl – ), Bromide (Br – ), Iodide (I – ), Nitrate (NO 3 – ), Benzene cations (C 6 H 6 + ), Acetone dimers ((C 3 H 6 O) 2 H + ), and Ammonium (NH 4 + ) reagent ions primarily in laboratory and flow tube experiments. We report the technical characteristics, operational principles, and compare its performance in terms of time response, humidity dependence, and sensitivity to that of previous chemical ionization approaches. This work demonstrates the benefits of the Vocus AIM reactor which provides a versatile platform to characterize VOCs and VICs in real time at trace concentrations.
- Preprint (PDF, 2433 KB)
- Supplement (399 KB)
- Preprint (2433 KB)
- Metadata XML

Status : open (until 24 May 2024)
Report abuse
Please provide a reason why you see this comment as being abusive. You might include your name and email but you can also stay anonymous.
Please provide a reason why you see this comment as being abusive.
Please confirm reCaptcha.

https://doi.org/10.5194/egusphere-2024-945-supplement
- Supplement: 30
Viewed (geographical distribution)
Matthieu riva, veronika pospisilova, carla frege, sebastien perrier, priyanka bansal, spiro jorga, patrick sturm, joel thornton, felipe lopez-hilfiker.

An official website of the United States government
Here’s how you know
The .gov means it’s official. Federal government websites often end in .gov or .mil. Before sharing sensitive information, make sure you’re on a federal government site.
The site is secure. The https:// ensures that you are connecting to the official website and that any information you provide is encrypted and transmitted securely.
Take action
- Report an antitrust violation
- File adjudicative documents
- Find banned debt collectors
- View competition guidance
- Competition Matters Blog
New HSR thresholds and filing fees for 2024
View all Competition Matters Blog posts
We work to advance government policies that protect consumers and promote competition.
View Policy
Search or browse the Legal Library
Find legal resources and guidance to understand your business responsibilities and comply with the law.
Browse legal resources
- Find policy statements
- Submit a public comment
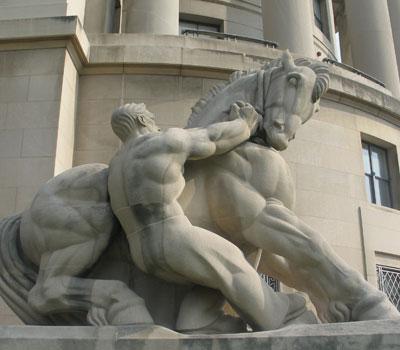
Vision and Priorities
Memo from Chair Lina M. Khan to commission staff and commissioners regarding the vision and priorities for the FTC.
Technology Blog
Consumer facing applications: a quote book from the tech summit on ai.
View all Technology Blog posts
Advice and Guidance
Learn more about your rights as a consumer and how to spot and avoid scams. Find the resources you need to understand how consumer protection law impacts your business.
- Report fraud
- Report identity theft
- Register for Do Not Call
- Sign up for consumer alerts
- Get Business Blog updates
- Get your free credit report
- Find refund cases
- Order bulk publications
- Consumer Advice
- Shopping and Donating
- Credit, Loans, and Debt
- Jobs and Making Money
- Unwanted Calls, Emails, and Texts
- Identity Theft and Online Security
- Business Guidance
- Advertising and Marketing
- Credit and Finance
- Privacy and Security
- By Industry
- For Small Businesses
- Browse Business Guidance Resources
- Business Blog
Servicemembers: Your tool for financial readiness
Visit militaryconsumer.gov
Get consumer protection basics, plain and simple
Visit consumer.gov
Learn how the FTC protects free enterprise and consumers
Visit Competition Counts
Looking for competition guidance?
- Competition Guidance
News and Events
Latest news, williams-sonoma will pay record $3.17 million civil penalty for violating ftc made in usa order.
View News and Events
Upcoming Event
Commissioner bedoya speaks at loyola university school of law’s 24th annual loyola antitrust colloquium.
View more Events
Sign up for the latest news
Follow us on social media
--> --> --> --> -->
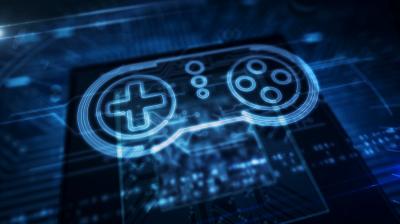
Playing it Safe: Explore the FTC's Top Video Game Cases
Learn about the FTC's notable video game cases and what our agency is doing to keep the public safe.
Latest Data Visualization
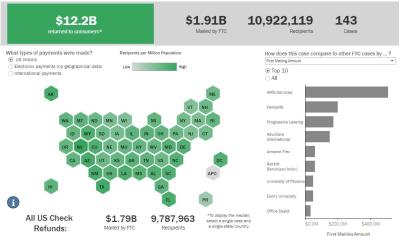
FTC Refunds to Consumers
Explore refund statistics including where refunds were sent and the dollar amounts refunded with this visualization.
About the FTC
Our mission is protecting the public from deceptive or unfair business practices and from unfair methods of competition through law enforcement, advocacy, research, and education.
Learn more about the FTC
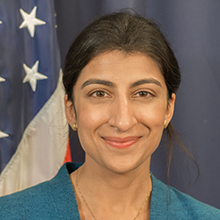
Meet the Chair
Lina M. Khan was sworn in as Chair of the Federal Trade Commission on June 15, 2021.
Chair Lina M. Khan
Looking for legal documents or records? Search the Legal Library instead.
- Cases and Proceedings
- Premerger Notification Program
- Merger Review
- Anticompetitive Practices
- Competition and Consumer Protection Guidance Documents
- Warning Letters
- Consumer Sentinel Network
- Criminal Liaison Unit
- FTC Refund Programs
- Notices of Penalty Offenses
- Advocacy and Research
- Advisory Opinions
- Cooperation Agreements
- Federal Register Notices
- Public Comments
- Policy Statements
- International
- Office of Technology Blog
- Military Consumer
- Consumer.gov
- Bulk Publications
- Data and Visualizations
- Stay Connected
- Commissioners and Staff
- Bureaus and Offices
- Budget and Strategy
- Office of Inspector General
- Careers at the FTC
FTC Announces Rule Banning Noncompetes
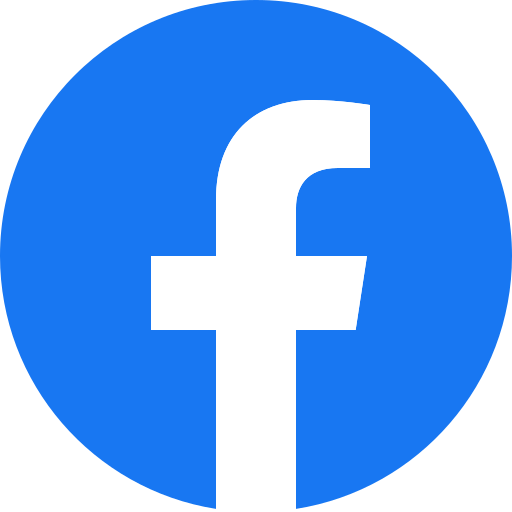
- Competition
- Office of Policy Planning
- Bureau of Competition
Today, the Federal Trade Commission issued a final rule to promote competition by banning noncompetes nationwide, protecting the fundamental freedom of workers to change jobs, increasing innovation, and fostering new business formation.
“Noncompete clauses keep wages low, suppress new ideas, and rob the American economy of dynamism, including from the more than 8,500 new startups that would be created a year once noncompetes are banned,” said FTC Chair Lina M. Khan. “The FTC’s final rule to ban noncompetes will ensure Americans have the freedom to pursue a new job, start a new business, or bring a new idea to market.”
The FTC estimates that the final rule banning noncompetes will lead to new business formation growing by 2.7% per year, resulting in more than 8,500 additional new businesses created each year. The final rule is expected to result in higher earnings for workers, with estimated earnings increasing for the average worker by an additional $524 per year, and it is expected to lower health care costs by up to $194 billion over the next decade. In addition, the final rule is expected to help drive innovation, leading to an estimated average increase of 17,000 to 29,000 more patents each year for the next 10 years under the final rule.
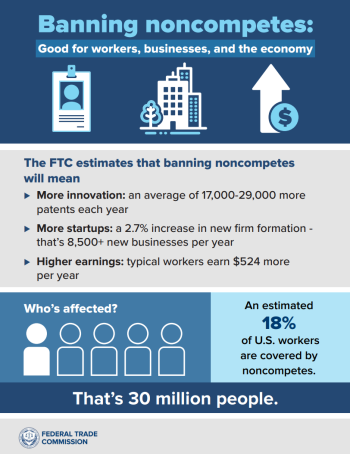
Noncompetes are a widespread and often exploitative practice imposing contractual conditions that prevent workers from taking a new job or starting a new business. Noncompetes often force workers to either stay in a job they want to leave or bear other significant harms and costs, such as being forced to switch to a lower-paying field, being forced to relocate, being forced to leave the workforce altogether, or being forced to defend against expensive litigation. An estimated 30 million workers—nearly one in five Americans—are subject to a noncompete.
Under the FTC’s new rule, existing noncompetes for the vast majority of workers will no longer be enforceable after the rule’s effective date. Existing noncompetes for senior executives - who represent less than 0.75% of workers - can remain in force under the FTC’s final rule, but employers are banned from entering into or attempting to enforce any new noncompetes, even if they involve senior executives. Employers will be required to provide notice to workers other than senior executives who are bound by an existing noncompete that they will not be enforcing any noncompetes against them.
In January 2023, the FTC issued a proposed rule which was subject to a 90-day public comment period. The FTC received more than 26,000 comments on the proposed rule, with over 25,000 comments in support of the FTC’s proposed ban on noncompetes. The comments informed the FTC’s final rulemaking process, with the FTC carefully reviewing each comment and making changes to the proposed rule in response to the public’s feedback.
In the final rule, the Commission has determined that it is an unfair method of competition, and therefore a violation of Section 5 of the FTC Act, for employers to enter into noncompetes with workers and to enforce certain noncompetes.
The Commission found that noncompetes tend to negatively affect competitive conditions in labor markets by inhibiting efficient matching between workers and employers. The Commission also found that noncompetes tend to negatively affect competitive conditions in product and service markets, inhibiting new business formation and innovation. There is also evidence that noncompetes lead to increased market concentration and higher prices for consumers.
Alternatives to Noncompetes
The Commission found that employers have several alternatives to noncompetes that still enable firms to protect their investments without having to enforce a noncompete.
Trade secret laws and non-disclosure agreements (NDAs) both provide employers with well-established means to protect proprietary and other sensitive information. Researchers estimate that over 95% of workers with a noncompete already have an NDA.
The Commission also finds that instead of using noncompetes to lock in workers, employers that wish to retain employees can compete on the merits for the worker’s labor services by improving wages and working conditions.
Changes from the NPRM
Under the final rule, existing noncompetes for senior executives can remain in force. Employers, however, are prohibited from entering into or enforcing new noncompetes with senior executives. The final rule defines senior executives as workers earning more than $151,164 annually and who are in policy-making positions.
Additionally, the Commission has eliminated a provision in the proposed rule that would have required employers to legally modify existing noncompetes by formally rescinding them. That change will help to streamline compliance.
Instead, under the final rule, employers will simply have to provide notice to workers bound to an existing noncompete that the noncompete agreement will not be enforced against them in the future. To aid employers’ compliance with this requirement, the Commission has included model language in the final rule that employers can use to communicate to workers.
The Commission vote to approve the issuance of the final rule was 3-2 with Commissioners Melissa Holyoak and Andrew N. Ferguson voting no. Commissioners Rebecca Kelly Slaughter , Alvaro Bedoya , Melissa Holyoak and Andrew N. Ferguson each issued separate statements. Chair Lina M. Khan will issue a separate statement.
The final rule will become effective 120 days after publication in the Federal Register.
Once the rule is effective, market participants can report information about a suspected violation of the rule to the Bureau of Competition by emailing [email protected] .
The Federal Trade Commission develops policy initiatives on issues that affect competition, consumers, and the U.S. economy. The FTC will never demand money, make threats, tell you to transfer money, or promise you a prize. Follow the FTC on social media , read consumer alerts and the business blog , and sign up to get the latest FTC news and alerts .
Press Release Reference
Contact information, media contact.
Victoria Graham Office of Public Affairs 415-848-5121

COMMENTS
Definition: Independent variable is a variable that is manipulated or changed by the researcher to observe its effect on the dependent variable. It is also known as the predictor variable or explanatory variable. The independent variable is the presumed cause in an experiment or study, while the dependent variable is the presumed effect or outcome.
A dependent variable from one study can be the independent variable in another study, so it's important to pay attention to research design. Here are some tips for identifying each variable type. Recognizing independent variables. Use this list of questions to check whether you're dealing with an independent variable:
While the independent variable is the " cause ", the dependent variable is the " effect " - or rather, the affected variable. In other words, the dependent variable is the variable that is assumed to change as a result of a change in the independent variable. Keeping with the previous example, let's look at some dependent variables ...
Parts of the experiment: Independent vs dependent variables. Experiments are usually designed to find out what effect one variable has on another - in our example, the effect of salt addition on plant growth.. You manipulate the independent variable (the one you think might be the cause) and then measure the dependent variable (the one you think might be the effect) to find out what this ...
Take the sentence, "The [independent variable] causes a change in [dependent variable] and it is not possible that [dependent variable] could cause a change in [independent variable]." Insert the names of variables you are using in the sentence in the way that makes the most sense. This will help you identify each type of variable.
Independent variables and dependent variables are the two fundamental types of variables in statistical modeling and experimental designs. Skip to secondary menu; ... This method is common in educational and social research, particularly when the goal is to understand or mitigate the impact of socioeconomic disparities on educational outcomes. ...
In research, the independent variable is manipulated to observe its effect, while the dependent variable is the measured outcome. Essentially, the independent variable is the presumed cause, and the dependent variable is the observed effect. Variables provide the foundation for examining relationships, drawing conclusions, and making ...
Independent variables (aka treatment variables) Variables you manipulate in order to affect the outcome of an experiment. The amount of salt added to each plant's water. Dependent variables (aka response variables) Variables that represent the outcome of the experiment.
Compare the independent variable and dependent variable in research. See other types of variables in research, including confounding and extraneous...
An independent variable is defines as the variable that is changed or controlled in a scientific experiment. It represents the cause or reason for an outcome. Independent variables are the variables that the experimenter changes to test their dependent variable. A change in the independent variable directly causes a change in the dependent ...
Examples of categorical variables include gender (male, female, other), type of vehicle (car, truck, motorcycle), or marital status (single, married, divorced). These categories help researchers organize data into groups for comparison and analysis. Categorical variables can be further classified into two subtypes: nominal and ordinal.
Independent Variables in Research. Now that we're acquainted with the basic concepts and have the tools to identify independent variables, let's dive into the fascinating ocean of theories and frameworks. ... Independent Variable: The type of fertilizer is the independent variable. You are using different types of fertilizer to observe ...
The independent variable (IV) in psychology is the characteristic of an experiment that is manipulated or changed by researchers, not by other variables in the experiment. For example, in an experiment looking at the effects of studying on test scores, studying would be the independent variable. Researchers are trying to determine if changes to ...
The independent variable is the involvement in after-school math tutoring sessions. Organization context: You may want to know if the color of an office affects work efficiency. Your research will consider a group of employees working in white or yellow rooms. The independent variable is the color of the office.
Suitable statistical design represents a critical factor in permitting inferences from any research or scientific study.[1] Numerous statistical designs are implementable due to the advancement of software available for extensive data analysis.[1] Healthcare providers must possess some statistical knowledge to interpret new studies and provide up-to-date patient care. We present an overview of ...
Experimental and Non-Experimental Research. Experimental research: In experimental research, the aim is to manipulate an independent variable(s) and then examine the effect that this change has on a dependent variable(s).Since it is possible to manipulate the independent variable(s), experimental research has the advantage of enabling a researcher to identify a cause and effect between variables.
Independent and dependent variables are crucial elements in research. The independent variable is the entity being tested and the dependent variable is the result. Check out this article to learn more about independent and dependent variable types and examples.
A dependent variable from one study can be the independent variable in another study, so it's important to pay attention to research design. Here are some tips for identifying each variable type. Recognising independent variables. Use this list of questions to check whether you're dealing with an independent variable:
The relationship between independent and dependent variables can manifest in various forms—direct, indirect, linear, nonlinear, and may be moderated or mediated by other variables. At its most basic, this relationship is often conceptualized as cause and effect: the independent variable (the cause) influences the dependent variable (the effect).
Here are several examples of independent and dependent variables in experiments: In a study to determine whether how long a student sleeps affects test scores, the independent variable is the length of time spent sleeping while the dependent variable is the test score. You want to know which brand of fertilizer is best for your plants.
Independent and Dependent Variables, Explained With Examples. Written by MasterClass. Last updated: Mar 21, 2022 • 4 min read. In experiments that test cause and effect, two types of variables come into play. One is an independent variable and the other is a dependent variable, and together they play an integral role in research design.
Categorical variables are groups…such as gender or type of degree sought. Quantitative variables are numbers that have a range…like weight in pounds or baskets made during a ball game. When we analyze data we do turn the categorical variables into numbers but only for identification purposes…e.g. 1 = male and 2 = female.
Definition. An independent variable is a variable in research that causes a change -- or is presumed will cause a change -- to other variables in the research conducted. Scientists can control the independent variable to monitor those changes or he can presume a change and look for evidence of those changes to the other variables.
A series of robustness checks were also performed, including alternative measurements of independent variables and estimation methods. Results: Results indicated that both types of doctors' prosocial behaviors, namely, proactive and reactive actions, positively impacted their e-consultation volume.
Compared to grazing milo, feed costs are typically much higher in a hay-based winter feed program. If forage growers in Missouri can produce fescue-clover hay yielding 3 tons per acre with operating costs of $196.18, the variable cost of the hay is $65.39 per ton, or $0.03 per pound.
Abstract. Volatile organic compounds (VOCs) and volatile inorganic compounds (VICs) provide critical information across many scientific fields including atmospheric chemistry, soil, and biological processes. Chemical ionization (CI) mass spectrometry has become a powerful tool for tracking these chemically complex and temporally variable compounds in a variety of laboratory and field environments.
Today, the Federal Trade Commission issued a final rule to promote competition by banning noncompetes nationwide, protecting the fundamental freedom of workers to change jobs, increasing innovation, and fostering new business formation. "Noncompete clauses keep wages low, suppress new ideas, and rob the American economy of dynamism, including from the more than 8,500 new startups that would ...