Change Password
Your password must have 6 characters or more:.
- a lower case character,
- an upper case character,
- a special character

Password Changed Successfully
Your password has been changed
Create your account
Forget yout password.
Enter your email address below and we will send you the reset instructions
If the address matches an existing account you will receive an email with instructions to reset your password
Forgot your Username?
Enter your email address below and we will send you your username
If the address matches an existing account you will receive an email with instructions to retrieve your username

- April 01, 2024 | VOL. 181, NO. 4 CURRENT ISSUE pp.255-346
- March 01, 2024 | VOL. 181, NO. 3 pp.171-254
- February 01, 2024 | VOL. 181, NO. 2 pp.83-170
- January 01, 2024 | VOL. 181, NO. 1 pp.1-82
The American Psychiatric Association (APA) has updated its Privacy Policy and Terms of Use , including with new information specifically addressed to individuals in the European Economic Area. As described in the Privacy Policy and Terms of Use, this website utilizes cookies, including for the purpose of offering an optimal online experience and services tailored to your preferences.
Please read the entire Privacy Policy and Terms of Use. By closing this message, browsing this website, continuing the navigation, or otherwise continuing to use the APA's websites, you confirm that you understand and accept the terms of the Privacy Policy and Terms of Use, including the utilization of cookies.
The Critical Relationship Between Anxiety and Depression
- Ned H. Kalin , M.D.
Search for more papers by this author
Anxiety and depressive disorders are among the most common psychiatric illnesses; they are highly comorbid with each other, and together they are considered to belong to the broader category of internalizing disorders. Based on statistics from the Substance Abuse and Mental Health Services Administration, the 12-month prevalence of major depressive disorder in 2017 was estimated to be 7.1% for adults and 13.3% for adolescents ( 1 ). Data for anxiety disorders are less current, but in 2001–2003, their 12-month prevalence was estimated to be 19.1% in adults, and 2001–2004 data estimated that the lifetime prevalence in adolescents was 31.9% ( 2 , 3 ). Both anxiety and depressive disorders are more prevalent in women, with an approximate 2:1 ratio in women compared with men during women’s reproductive years ( 1 , 2 ).
Across all psychiatric disorders, comorbidity is the rule ( 4 ), which is definitely the case for anxiety and depressive disorders, as well as their symptoms. With respect to major depression, a worldwide survey reported that 45.7% of individuals with lifetime major depressive disorder had a lifetime history of one or more anxiety disorder ( 5 ). These disorders also commonly coexist during the same time frame, as 41.6% of individuals with 12-month major depression also had one or more anxiety disorder over the same 12-month period. From the perspective of anxiety disorders, the lifetime comorbidity with depression is estimated to range from 20% to 70% for patients with social anxiety disorder ( 6 ), 50% for patients with panic disorder ( 6 ), 48% for patients with posttraumatic stress disorder (PTSD) ( 7 ), and 43% for patients with generalized anxiety disorder ( 8 ). Data from the well-known Sequenced Treatment Alternatives to Relieve Depression (STAR*D) study demonstrate comorbidity at the symptom level, as 53% of the patients with major depression had significant anxiety and were considered to have an anxious depression ( 9 ).
Anxiety and depressive disorders are moderately heritable (approximately 40%), and evidence suggests shared genetic risk across the internalizing disorders ( 10 ). Among internalizing disorders, the highest level of shared genetic risk appears to be between major depressive disorder and generalized anxiety disorder. Neuroticism is a personality trait or temperamental characteristic that is associated with the development of both anxiety and depression, and the genetic risk for developing neuroticism also appears to be shared with that of the internalizing disorders ( 11 ). Common nongenetic risk factors associated with the development of anxiety and depression include earlier life adversity, such as trauma or neglect, as well as parenting style and current stress exposure. At the level of neural circuits, alterations in prefrontal-limbic pathways that mediate emotion regulatory processes are common to anxiety and depressive disorders ( 12 , 13 ). These findings are consistent with meta-analyses that reveal shared structural and functional brain alterations across various psychiatric illnesses, including anxiety and major depression, in circuits involving emotion regulation ( 13 ), executive function ( 14 ), and cognitive control ( 15 ).
Anxiety disorders and major depression occur during development, with anxiety disorders commonly beginning during preadolescence and early adolescence and major depression tending to emerge during adolescence and early to mid-adulthood ( 16 – 18 ). In relation to the evolution of their comorbidity, studies demonstrate that anxiety disorders generally precede the presentation of major depressive disorder ( 17 ). A European community-based study revealed, beginning at age 15, the developmental relation between comorbid anxiety and major depression by specifically focusing on social phobia (based on DSM-IV criteria) and then asking the question regarding concurrent major depressive disorder ( 18 ). The findings revealed a 19% concurrent comorbidity between these disorders, and in 65% of the cases, social phobia preceded major depressive disorder by at least 2 years. In addition, initial presentation with social phobia was associated with a 5.7-fold increased risk of developing major depressive disorder. These associations between anxiety and depression can be traced back even earlier in life. For example, childhood behavioral inhibition in response to novelty or strangers, or an extreme anxious temperament, is associated with a three- to fourfold increase in the likelihood of developing social anxiety disorder, which in turn is associated with an increased risk to develop major depressive disorder and substance abuse ( 19 ).
It is important to emphasize that the presence of comor‐bid anxiety symptoms and disorders matters in relation to treatment. Across psychiatric disorders, the presence of significant anxiety symptoms generally predicts worse outcomes, and this has been well demonstrated for depression. In the STAR*D study, patients with anxious major depressive disorder were more likely to be severely depressed and to have more suicidal ideation ( 9 ). This is consistent with the study by Kessler and colleagues ( 5 ), in which patients with anxious major depressive disorder, compared with patients with nonanxious major depressive disorder, were found to have more severe role impairment and more suicidal ideation. Data from level 1 of the STAR*D study (citalopram treatment) nicely illustrate the impact of comorbid anxiety symptoms on treatment. Compared with patients with nonanxious major depressive disorder, those 53% of patients with an anxious depression were less likely to remit and also had a greater side effect burden ( 20 ). Other data examining patients with major depressive disorder and comorbid anxiety disorders support the greater difficulty and challenge in treating patients with these comorbidities ( 21 ).
This issue of the Journal presents new findings relevant to the issues discussed above in relation to understanding and treating anxiety and depressive disorders. Drs. Conor Liston and Timothy Spellman, from Weill Cornell Medicine, provide an overview for this issue ( 22 ) that is focused on understanding mechanisms at the neural circuit level that underlie the pathophysiology of depression. Their piece nicely integrates human neuroimaging studies with complementary data from animal models that allow for the manipulation of selective circuits to test hypotheses generated from the human data. Also included in this issue is a review of the data addressing the reemergence of the use of psychedelic drugs in psychiatry, particularly for the treatment of depression, anxiety, and PTSD ( 23 ). This timely piece, authored by Dr. Collin Reiff along with a subgroup from the APA Council of Research, provides the current state of evidence supporting the further exploration of these interventions. Dr. Alan Schatzberg, from Stanford University, contributes an editorial in which he comments on where the field is in relation to clinical trials with psychedelics and to some of the difficulties, such as adequate blinding, in reliably studying the efficacy of these drugs ( 24 ).
In an article by McTeague et al. ( 25 ), the authors use meta-analytic strategies to understand the neural alterations that are related to aberrant emotion processing that are shared across psychiatric disorders. Findings support alterations in the salience, reward, and lateral orbital nonreward networks as common across disorders, including anxiety and depressive disorders. These findings add to the growing body of work that supports the concept that there are common underlying factors across all types of psychopathology that include internalizing, externalizing, and thought disorder dimensions ( 26 ). Dr. Deanna Barch, from Washington University in St. Louis, writes an editorial commenting on these findings and, importantly, discusses criteria that should be met when we consider whether the findings are actually transdiagnostic ( 27 ).
Another article, from Gray and colleagues ( 28 ), addresses whether there is a convergence of findings, specifically in major depression, when examining data from different structural and functional neuroimaging modalities. The authors report that, consistent with what we know about regions involved in emotion processing, the subgenual anterior cingulate cortex, hippocampus, and amygdala were among the regions that showed convergence across multimodal imaging modalities.
In relation to treatment and building on our understanding of neural circuit alterations, Siddiqi et al. ( 29 ) present data suggesting that transcranial magnetic stimulation (TMS) targeting can be linked to symptom-specific treatments. Their findings identify different TMS targets in the left dorsolateral prefrontal cortex that modulate different downstream networks. The modulation of these different networks appears to be associated with a reduction in different types of symptoms. In an editorial, Drs. Sean Nestor and Daniel Blumberger, from the University of Toronto ( 30 ), comment on the novel approach used in this study to link the TMS-related engagement of circuits with symptom improvement. They also provide a perspective on how we can view these and other circuit-based findings in relation to conceptualizing personalized treatment approaches.
Kendler et al. ( 31 ), in this issue, contribute an article that demonstrates the important role of the rearing environment in the risk to develop major depression. Using a unique design from a Swedish sample, the analytic strategy involves comparing outcomes from high-risk full sibships and high-risk half sibships where at least one of the siblings was home reared and one was adopted out of the home. The findings support the importance of the quality of the rearing environment as well as the presence of parental depression in mitigating or enhancing the likelihood of developing major depression. In an accompanying editorial ( 32 ), Dr. Myrna Weissman, from Columbia University, reviews the methods and findings of the Kendler et al. article and also emphasizes the critical significance of the early nurturing environment in relation to general health.
This issue concludes with an intriguing article on anxiety disorders, by Gold and colleagues ( 33 ), that demonstrates neural alterations during extinction recall that differ in children relative to adults. With increasing age, and in relation to fear and safety cues, nonanxious adults demonstrated greater connectivity between the amygdala and the ventromedial prefrontal cortex compared with anxious adults, as the cues were being perceived as safer. In contrast, neural differences between anxious and nonanxious youths were more robust when rating the memory of faces that were associated with threat. Specifically, these differences were observed in the activation of the inferior temporal cortex. In their editorial ( 34 ), Dr. Dylan Gee and Sahana Kribakaran, from Yale University, emphasize the importance of developmental work in relation to understanding anxiety disorders, place these findings into the context of other work, and suggest the possibility that these and other data point to neuroscientifically informed age-specific interventions.
Taken together, the papers in this issue of the Journal present new findings that shed light onto alterations in neural function that underlie major depressive disorder and anxiety disorders. It is important to remember that these disorders are highly comorbid and that their symptoms are frequently not separable. The papers in this issue also provide a developmental perspective emphasizing the importance of early rearing in the risk to develop depression and age-related findings important for understanding threat processing in patients with anxiety disorders. From a treatment perspective, the papers introduce data supporting more selective prefrontal cortical TMS targeting in relation to different symptoms, address the potential and drawbacks for considering the future use of psychedelics in our treatments, and present new ideas supporting age-specific interventions for youths and adults with anxiety disorders.
Disclosures of Editors’ financial relationships appear in the April 2020 issue of the Journal .
1 Substance Abuse and Mental Health Services Administration (SAMHSA): Key substance use and mental health indicators in the United States: results from the 2017 National Survey on Drug Use and Health (HHS Publication No. SMA 18-5068, NSDUH Series H-53). Rockville, Md, Center for Behavioral Health Statistics and Quality, SAMHSA, 2018. https://www.samhsa.gov/data/sites/default/files/cbhsq-reports/NSDUHFFR2017/NSDUHFFR2017.htm Google Scholar
2 Kessler RC, Chiu WT, Demler O, et al. : Prevalence, severity, and comorbidity of 12-month DSM-IV disorders in the National Comorbidity Survey Replication . Arch Gen Psychiatry 2005 ; 62:617–627, correction, 62:709 Crossref , Medline , Google Scholar
3 Merikangas KR, He JP, Burstein M, et al. : Lifetime prevalence of mental disorders in U.S. adolescents: results from the National Comorbidity Survey Replication–Adolescent Supplement (NCS-A) . J Am Acad Child Adolesc Psychiatry 2010 ; 49:980–989 Crossref , Medline , Google Scholar
4 Kessler RC, McGonagle KA, Zhao S, et al. : Lifetime and 12-month prevalence of DSM-III-R psychiatric disorders in the United States: results from the National Comorbidity Survey . Arch Gen Psychiatry 1994 ; 51:8–19 Crossref , Medline , Google Scholar
5 Kessler RC, Sampson NA, Berglund P, et al. : Anxious and non-anxious major depressive disorder in the World Health Organization World Mental Health Surveys . Epidemiol Psychiatr Sci 2015 ; 24:210–226 Crossref , Medline , Google Scholar
6 Dunner DL : Management of anxiety disorders: the added challenge of comorbidity . Depress Anxiety 2001 ; 13:57–71 Crossref , Medline , Google Scholar
7 Kessler RC, Sonnega A, Bromet E, et al. : Posttraumatic stress disorder in the National Comorbidity Survey . Arch Gen Psychiatry 1995 ; 52:1048–1060 Crossref , Medline , Google Scholar
8 Brawman-Mintzer O, Lydiard RB, Emmanuel N, et al. : Psychiatric comorbidity in patients with generalized anxiety disorder . Am J Psychiatry 1993 ; 150:1216–1218 Link , Google Scholar
9 Fava M, Alpert JE, Carmin CN, et al. : Clinical correlates and symptom patterns of anxious depression among patients with major depressive disorder in STAR*D . Psychol Med 2004 ; 34:1299–1308 Crossref , Medline , Google Scholar
10 Hettema JM : What is the genetic relationship between anxiety and depression? Am J Med Genet C Semin Med Genet 2008 ; 148C:140–146 Crossref , Medline , Google Scholar
11 Hettema JM, Neale MC, Myers JM, et al. : A population-based twin study of the relationship between neuroticism and internalizing disorders . Am J Psychiatry 2006 ; 163:857–864 Link , Google Scholar
12 Kovner R, Oler JA, Kalin NH : Cortico-limbic interactions mediate adaptive and maladaptive responses relevant to psychopathology . Am J Psychiatry 2019 ; 176:987–999 Link , Google Scholar
13 Etkin A, Schatzberg AF : Common abnormalities and disorder-specific compensation during implicit regulation of emotional processing in generalized anxiety and major depressive disorders . Am J Psychiatry 2011 ; 168:968–978 Link , Google Scholar
14 Goodkind M, Eickhoff SB, Oathes DJ, et al. : Identification of a common neurobiological substrate for mental illness . JAMA Psychiatry 2015 ; 72:305–315 Crossref , Medline , Google Scholar
15 McTeague LM, Huemer J, Carreon DM, et al. : Identification of common neural circuit disruptions in cognitive control across psychiatric disorders . Am J Psychiatry 2017 ; 174:676–685 Link , Google Scholar
16 Beesdo K, Knappe S, Pine DS : Anxiety and anxiety disorders in children and adolescents: developmental issues and implications for DSM-V . Psychiatr Clin North Am 2009 ; 32:483–524 Crossref , Medline , Google Scholar
17 Kessler RC, Wang PS : The descriptive epidemiology of commonly occurring mental disorders in the United States . Annu Rev Public Health 2008 ; 29:115–129 Crossref , Medline , Google Scholar
18 Ohayon MM, Schatzberg AF : Social phobia and depression: prevalence and comorbidity . J Psychosom Res 2010 ; 68:235–243 Crossref , Medline , Google Scholar
19 Clauss JA, Blackford JU : Behavioral inhibition and risk for developing social anxiety disorder: a meta-analytic study . J Am Acad Child Adolesc Psychiatry 2012 ; 51:1066–1075 Crossref , Medline , Google Scholar
20 Fava M, Rush AJ, Alpert JE, et al. : Difference in treatment outcome in outpatients with anxious versus nonanxious depression: a STAR*D report . Am J Psychiatry 2008 ; 165:342–351 Link , Google Scholar
21 Dold M, Bartova L, Souery D, et al. : Clinical characteristics and treatment outcomes of patients with major depressive disorder and comorbid anxiety disorders: results from a European multicenter study . J Psychiatr Res 2017 ; 91:1–13 Crossref , Medline , Google Scholar
22 Spellman T, Liston C : Toward circuit mechanisms of pathophysiology in depression . Am J Psychiatry 2020 ; 177:381–390 Link , Google Scholar
23 Reiff CM, Richman EE, Nemeroff CB, et al. : Psychedelics and psychedelic-assisted psychotherapy . Am J Psychiatry 2020 ; 177:391–410 Link , Google Scholar
24 Schatzberg AF : Some comments on psychedelic research (editorial). Am J Psychiatry 2020 ; 177:368–369 Link , Google Scholar
25 McTeague LM, Rosenberg BM, Lopez JW, et al. : Identification of common neural circuit disruptions in emotional processing across psychiatric disorders . Am J Psychiatry 2020 ; 177:411–421 Link , Google Scholar
26 Caspi A, Moffitt TE : All for one and one for all: mental disorders in one dimension . Am J Psychiatry 2018 ; 175:831–844 Link , Google Scholar
27 Barch DM : What does it mean to be transdiagnostic and how would we know? (editorial). Am J Psychiatry 2020 ; 177:370–372 Abstract , Google Scholar
28 Gray JP, Müller VI, Eickhoff SB, et al. : Multimodal abnormalities of brain structure and function in major depressive disorder: a meta-analysis of neuroimaging studies . Am J Psychiatry 2020 ; 177:422–434 Link , Google Scholar
29 Siddiqi SH, Taylor SF, Cooke D, et al. : Distinct symptom-specific treatment targets for circuit-based neuromodulation . Am J Psychiatry 2020 ; 177:435–446 Link , Google Scholar
30 Nestor SM, Blumberger DM : Mapping symptom clusters to circuits: toward personalizing TMS targets to improve treatment outcomes in depression (editorial). Am J Psychiatry 2020 ; 177:373–375 Abstract , Google Scholar
31 Kendler KS, Ohlsson H, Sundquist J, et al. : The rearing environment and risk for major depression: a Swedish national high-risk home-reared and adopted-away co-sibling control study . Am J Psychiatry 2020 ; 177:447–453 Abstract , Google Scholar
32 Weissman MM : Is depression nature or nurture? Yes (editorial). Am J Psychiatry 2020 ; 177:376–377 Abstract , Google Scholar
33 Gold AL, Abend R, Britton JC, et al. : Age differences in the neural correlates of anxiety disorders: an fMRI study of response to learned threat . Am J Psychiatry 2020 ; 177:454–463 Link , Google Scholar
34 Gee DG, Kribakaran S : Developmental differences in neural responding to threat and safety: implications for treating youths with anxiety (editorial). Am J Psychiatry 2020 ; 177:378–380 Abstract , Google Scholar
- Cited by None
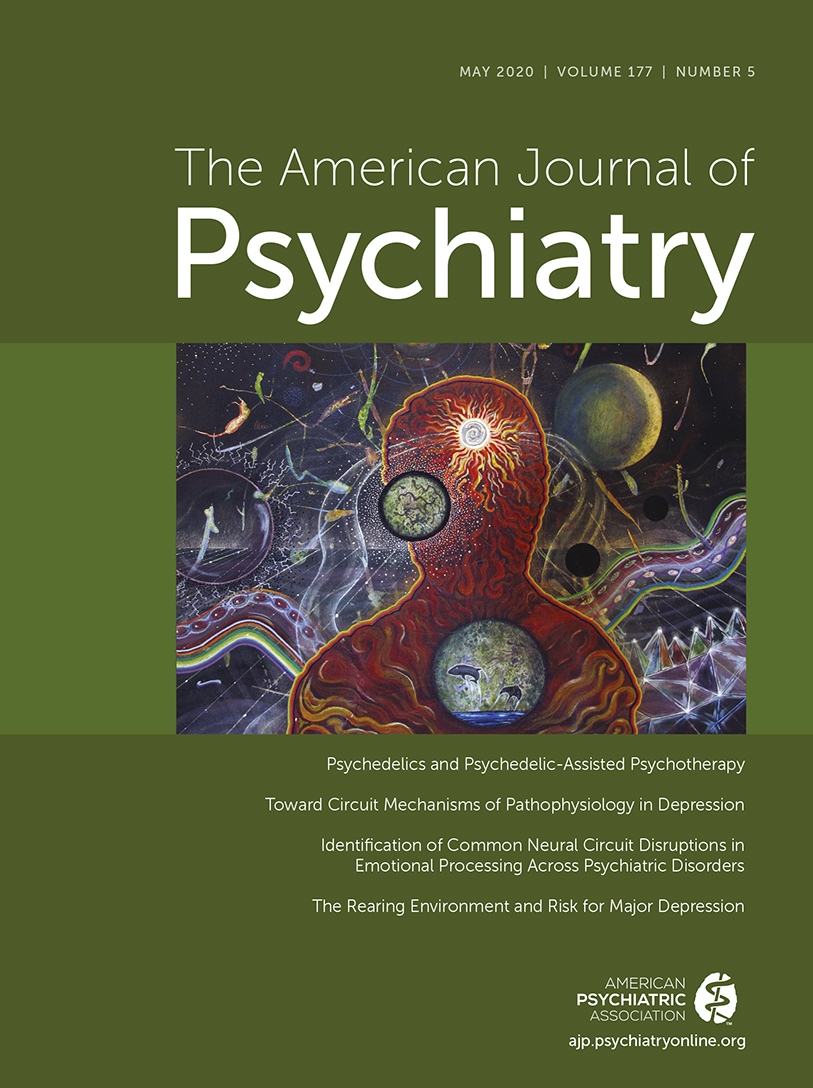
- Neuroanatomy
- Neurochemistry
- Neuroendocrinology
- Other Research Areas
Thank you for visiting nature.com. You are using a browser version with limited support for CSS. To obtain the best experience, we recommend you use a more up to date browser (or turn off compatibility mode in Internet Explorer). In the meantime, to ensure continued support, we are displaying the site without styles and JavaScript.
- View all journals
- My Account Login
- Explore content
- About the journal
- Publish with us
- Sign up for alerts
- Open access
- Published: 09 June 2022
Loneliness associates strongly with anxiety and depression during the COVID pandemic, especially in men and younger adults
- Olivier D. Steen 1 ,
- Anil P. S. Ori 1 , 2 ,
- Klaas J. Wardenaar 1 &
- Hanna M. van Loo 1
Scientific Reports volume 12 , Article number: 9517 ( 2022 ) Cite this article
5022 Accesses
13 Citations
41 Altmetric
Metrics details
- Human behaviour
Loneliness is associated with major depressive disorder (MDD), and likely also with generalized anxiety disorder (GAD). It is unclear if these associations are moderated by age, sex, or genetic susceptibility for MDD. We included 75,279 individuals from the Lifelines COVID-19 study, a longitudinal study of a Dutch population-based cohort. Participants completed up to sixteen digital questionnaires between March 2020 and January 2021, yielding a total of 616,129 observations. Loneliness was assessed with the Three-Item Loneliness Scale, and MDD and GAD with the Mini-International Neuropsychiatric Interview. We used generalized estimating equations to investigate the association between loneliness and MDD and GAD, and whether this association varied across time, age, sex and MDD polygenic risk. Loneliness was strongly associated with all MDD and GAD outcomes. Individuals with the highest loneliness scores were around 14 times more likely to have MDD, and 11 times more likely to have GAD, compared to individuals who reported the least loneliness. The association between loneliness and MDD symptoms was stronger in men, younger individuals, and increased across time. While MDD polygenic risk predicted MDD and GAD outcomes, we did not find an interaction effect with loneliness. Our study, which is the largest to date, confirms that loneliness is an important risk factor for MDD, GAD, depressive and anxiety symptoms, especially in men and younger individuals. Future studies should investigate the mechanisms of these associations and explore loneliness-based interventions to prevent and treat MDD and GAD.
Similar content being viewed by others
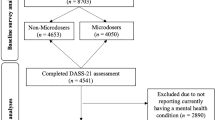
Adults who microdose psychedelics report health related motivations and lower levels of anxiety and depression compared to non-microdosers
Joseph M. Rootman, Pamela Kryskow, … Zach Walsh
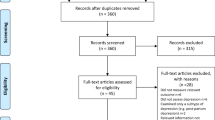
The serotonin theory of depression: a systematic umbrella review of the evidence
Joanna Moncrieff, Ruth E. Cooper, … Mark A. Horowitz
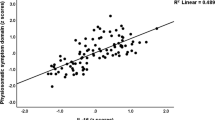
In major dysmood disorder, physiosomatic, chronic fatigue and fibromyalgia symptoms are driven by immune activation and increased immune-associated neurotoxicity
Michael Maes, Abbas F. Almulla, … Pimpayao Sodsai
Introduction
Major depressive disorder (MDD) and generalized anxiety disorder (GAD) are two of the most common mental illnesses worldwide, with substantial morbidity and mortality 1 , 2 . Gaining a better understanding of the mechanisms that underlie their development is important to reduce the burden of these illnesses. The identification of modifiable risk factors is of particular interest because they provide targets for intervention.
The current COVID-19 pandemic has highlighted the importance of loneliness as a modifiable risk factor for MD 3 . Loneliness is the subjective negative experience of deficient social relationships and is distinct from objective social isolation 4 . Research on loneliness and depression indicates a bidirectional relationship between both constructs, with greater experienced loneliness predicting future depressive symptoms, and vice versa 5 . Furthermore, loneliness influences the course of MDD, impeding the chances of recovery 6 . Recent findings furthermore suggest an association between loneliness and GAD as well, but fewer studies have been performed 7 , 8 .
However, it is not clear which groups are most vulnerable to the effect of loneliness on depression and anxiety. Different factors may moderate this association, including sex, age and genetic susceptibility. Findings regarding age are mixed. Some studies suggest an increased vulnerability in younger individuals, but few studies included sufficient numbers of adolescents and children to reliably demonstrate this 9 , 10 . Whether sex moderates the effect of loneliness is also unclear. One study found the effect of loneliness on depression to be more pronounced in men 5 , while others did not observe a moderating effect 9 . Thus, it remains inconclusive whether age or sex moderate the association between loneliness and depression and anxiety outcomes.
Furthermore, the association between loneliness and depressive and anxiety outcomes may be influenced by genetic factors. Loneliness, MDD, and GAD have moderate heritability, which means that genetic factors contribute to their aetiology 11 , 12 , 13 . Genome-wide association studies (GWAS) have identified multiple overlapping regions in the genome which are associated with both experiencing loneliness 14 and developing MDD 15 , 16 . This genetic overlap suggests that loneliness and depression could have a shared genetic aetiology, or that individuals with a higher genetic risk for MDD could be more sensitive to developing depression after exposure to loneliness.
The COVID-19 pandemic offers a unique situation to study the relationship of loneliness with depression and anxiety. The COVID-19 pandemic, and subsequent lockdowns, have had a profound impact on social relationships and increased the prevalence of loneliness 3 , 17 , 18 . This is akin to a natural experiment: a large share of the population is subjected to sustained loneliness, and not only subjects with a psychiatric history or other specific groups. Some studies have investigated the impact of loneliness on anxiety and depression amidst the COVID pandemic, and report an association between loneliness and anxiety and depression 19 , with young women most at risk 20 , but their findings are challenging to interpret due to the use of unrepresentative and smaller samples. The present study makes use of the Lifelines Cohort Study, which represents a large multi-generational population-based study in the North of the Netherlands with repeated and structured assessments of loneliness, MDD and GAD during the COVID-19 pandemic.
Here, we investigate the association of loneliness with depression and anxiety, as well as moderators of this association, in the largest study to date on this relationship.
Lifelines COVID-19 cohort
Data were derived from the Lifelines COVID-19 study, a longitudinal extension of the Lifelines cohort 21 . Lifelines is a large multidisciplinary prospective population-based cohort that monitors the health and health-related behaviours in a detailed manner of 167,729 persons living in the North of the Netherlands. The cohort consists primarily (98%) of white Dutch native people 22 , 23 . All subjects provided written informed consent for participation in Lifelines, and the Medical Ethical Committee of the University Medical Center Groningen approved the study protocol.
Starting on March 30th 2020, Lifelines invited all adult participants of whom an email address was known (n = 139,679) to participate in the first COVID-19 digital questionnaire, which assessed somatic and mental health, COVID-19 infection status, and loneliness, among other domains. The first questionnaire was distributed on March 30, 2020. The first six questionnaires (Q1–Q6) were sent out weekly with items assessing participants' experiences in the 7 days prior to filling out the questionnaire. Starting from Q7, questionnaires were sent biweekly or monthly, with items assessing participants’ experiences in the 14 days prior to assessment (Supplementary Table A ). A further description of the Lifelines COVID-19 cohort can be found elsewhere 24 .
Data from the first nineteen consecutive assessments (March 30th, 2020, to January 29th, 2021) were available. Questionnaires eleven, twelve and thirteen did not include measures of loneliness, so data from these measurements were not included in our analyses. A total of n = 75,279 individuals participated in at least one of the remaining sixteen questionnaires and are included in this study. In some instances (n = 14,763), participants completed multiple different assessments on the same date. In this case, we included only the questionnaire completed first.
Measurements
Depression and anxiety outcomes.
Symptoms of GAD and MDD were assessed with a self-report digital questionnaire based on the Mini-International Neuropsychiatric Interview (MINI) 25 , which assesses all symptoms that are part of the DSM-IV diagnostic criteria. Symptoms that are part of both MDD and GAD (being easily fatigued, difficulty concentrating and sleep disturbance) were assessed once in every questionnaire to avoid repetition.
The presence of current MDD and GAD were established according to the diagnostic criteria of the DSM-IV. For every questionnaire, we also calculated a sum score of MDD (range 0–9) and GAD (range 0–7) criterion symptoms, resulting in two outcomes (symptoms and diagnoses) for both MDD and GAD.
The experience of loneliness was assessed through the previously validated three-item UCLA scale 26 . This scale consists of three items (‘How often do you feel that you lack companionship?’, ‘How often do you feel left out?’ and ‘How often do you feel isolated from others?’), with a three-point (0–2) Likert scale. We calculated a loneliness sum score ranging from 0 to 6, with 6 being the maximum score.
Demographic variables
Age was defined as subjects’ age when completing the first questionnaire. The sex variable refers to biological sex assigned at birth, which was determined by linking the Lifelines data to information stored in personal records of the municipalities in the Netherlands.
Polygenic risk score
We calculated MDD polygenic risk scores (PRS) in 19,128 subjects who had genotype data available. MDD PRS was constructed as a weighted sum of risk alleles for MDD with weights defined as single nucleotide polymorphism (SNP) effect sizes derived from the meta-analysis of the Psychiatric Genomics Consortium (PGC) and UKBiobank GWASs 15 . The Lifelines sample was not included in the base GWAS, but was used as a target sample to calculate PRS for each participant. Details of PRS calculation are discussed in Supplementary Methods .
Missing data
While all subjects were invited to participate in all assessments, few completed all 16 assessments. Out of 75,279 subjects, 11,528 (15.3%) completed one assessment, 54,409 (72.3%) completed > 1 and < 16 assessments, and 9342 (12.4%) completed all assessments. As expected, there were thus a large number of non-responses for different time points.
Some data were missing due to design choices of the questionnaire (e.g., suicidal ideation was not assessed in every instance). As the questionnaire was web-based, some data were missing due to technical glitches (e.g., a failing internet connection on the participants’ end). Further details on missing data are provided in the Supplementary Methods , while rates of missing data are reported in Supplementary Table B .
As missing data were limited, we implemented multivariate imputation by chained equation (MICE) to impute missing data in one dataset 27 . For imputation, all available data, including data on loneliness, lifetime history of MDD and GAD and accompanying age of onset and number of experienced episodes, stressful life events, and the NEO personality inventory neuroticism score that were collected in previous Lifelines assessments, were used as predictors in MICE. Items were only imputed if a participant filled out other items on that specific questionnaire. Questionnaires with a non-response (i.e. complete missing data) were not imputed. Detailed imputation parameters are presented in Supplementary Methods .
Statistical methods
Model specification.
We used generalized estimating equations (GEE) to investigate the association between loneliness and the four depression and anxiety outcomes. GEE is a technique for estimating parameters of a generalized linear model with correlated measurements, yielding population-averaged coefficients. Because our data included repeated measurements within subjects, we used an exchangeable working correlation structure to account for the dependence of measurements within individuals. A further description of GEE can be found elsewhere 28 .
We performed GEE Poisson regression for the sum scores of MDD and GAD symptoms as both of these outcomes were count-variables. We used GEE logistic regression for the dichotomous outcomes of MDD and GAD. The fitted models included a linear and squared effect of time to account for seasonality. Furthermore, we included loneliness, age and sex as main effects. We added an interaction term between loneliness and time to investigate a possible change in the impact of loneliness on MDD and GAD outcomes across the study duration. We furthermore added interaction terms between loneliness and age, and loneliness and sex (main model) to identify possible moderating effects of age and/or sex. For the model including PRS in participants with genetic data available, we also added a main effect for MDD polygenic risk to the model, as well as an interaction term of PRS with loneliness. Furthermore, for the model including PRS, we included ten principal components as model terms to account for population structure confounding.
Multiple testing correction was implemented by Bonferroni correction. We conducted ten hypothesis tests across four outcomes, yielding a total of 40 hypothesis tests and a Bonferroni-corrected alpha of 0.00125. We performed GEE using the ‘geepack’ R package version 1.3.2 29 , and all analyses were performed in R version 4.0.3 30 .
Sensitivity analyses
Attrition bias.
Individuals experiencing much loneliness, or who suffer from mental illnesses, may more likely be lost to attrition in longitudinal studies 31 . To determine if results were sensitive to attrition, we conducted a sensitivity analysis with individuals who participated at least three times across three intervals covering the entire study duration. As assessment dates were variable, we constructed these intervals in such a way that the number of assessments was equal across intervals. The first time interval covered the period March 30, 2020 until 29 April 2020. The second interval covered the interval of 30 April 2020 until 10 July 2020. The third interval covered 10 July 2020 until 31 January 2021. 42,001 (55.8%) participants had completed at least one assessment in each of the three intervals and were included in this sensitivity analysis. Participants in this subsample completed a median of 13 [IQR 10–15] responses.
Family structure
Lifelines is a multi-generational sample and includes family members, which means that our longitudinal data were not only nested within individuals, but also within families. GEE cannot appropriately account for correlated measurements across multiple levels of clustering 28 . Therefore, to test whether our results were influenced by pedigree structures, we conducted a sensitivity analysis in a subsample consisting of one randomly selected subject for every family. As there were 42,089 pedigrees part of the present study, this analysis included 42,089 (55.9%) subjects.
For both sensitivity analyses, we estimated models in the full sample, and in the sample with PRS data available. Because only a limited number of participants had PRS data available, subsamples in the sensitivity analyses are comparatively small (n = 10,955 and n = 7036), and possibly underpowered to detect interaction effects. The latter was therefore only used to test the robustness of a possible interaction term between loneliness and PRS.
Ethics approval and consent to participate
All subjects provided written informed consent for participation in Lifelines, and the Medical Ethical Committee of the University Medical Center Groningen approved the study protocol. All methods were carried out in accordance with relevant guidelines and regulations.
Characteristics of the study population
The 75,279 individuals included in this study completed a total of 616,129 assessments across 16 time points. Out of these individuals, 19,128 (25.4%) had genotype data available. The median number of collected questionnaires per individual was 8 (IQR 3–14) (Table 1 ). The mean age at first assessment was 53.7 (standard deviation 12.9). The majority of participants in the sample were female (60.8%), which is similar to the full Lifelines cohort 23 . MDD was present in 5442 (7.2%) participants and GAD in 6733 (8.9%) participants in the 1/2 weeks preceding at least one questionnaire assessment. Loneliness scores were highest during the start and end of data collection, corresponding to periods of nationwide lockdown as a result of government action in response to the COVID-19 pandemic (Fig. 1 A).
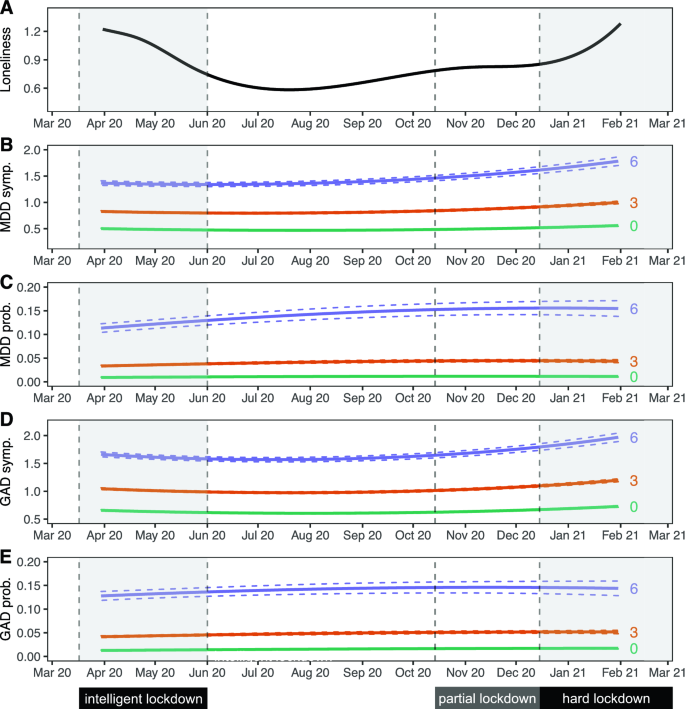
Trajectories of loneliness and MDD and GAD outcomes over time. Shaded areas represent periods during which lockdown measures were enforced in the Netherlands. The first 'intelligent' lockdown consisted of restrictions such as social distancing and bans on public gatherings. During the partial lockdown, some restrictions were reintroduced. The 'hard' lockdown introduced more restrictions and a curfew. ( A ) Marginal GEE effect plot of a GEE model of loneliness scores predicted by a polynomial spline time term. The 95% confidence interval of model-predicted values is not visible because the interval is very small. ( B – E ) Marginal GEE effect plot of predicted counts of MDD symptoms ( B ), odds of MDD diagnoses ( C ), counts of GAD symptoms ( D ) and odds of GAD diagnoses ( E ) across different levels (0/green; lowest, 3/orange; moderate, 6/purple; highest) of loneliness scores. Predicted values represent symptoms counts and diagnosis probabilities, and are based on main model fits (Tables 2 and 3 ). Dashed lines represent a 95% confidence interval of model-predicted values.
Loneliness is associated with MDD and GAD symptoms and diagnoses
We observed an association between loneliness and MDD and GAD. We found that a one-point increase in loneliness was associated with a 55.9% increased odds of MDD, and a 48.9% increased odds of GAD. This means that the group scoring highest on loneliness had a 14.4 times increased odds of MDD and a 10.9 times increased odds of GAD compared to those with the lowest loneliness scores (Tables 2 and 3 , Fig. 1 C, E ). Sensitivity analyses showed that these effects were robust for attrition and family structures (Supplementary Tables D 1-3 and E 1-3).
We found a similar association between loneliness and symptom severity of both MDD and GAD, which was robust across both sensitivity analyses (Tables 2 and 3 ; Fig. 1 B, D ; Supplementary Tables D 1-3 and E 1-3). A one-point increase in loneliness was associated with a 27.5% increase in the number of MDD symptoms, and a 19.9% increase in the number of GAD symptoms. This means that the group scoring highest on loneliness had on average 4.3 times as many MDD symptoms, and 3.0 times as many GAD symptoms, compared to the group with the lowest loneliness score.
We observed a significant interaction between loneliness and time, meaning that the magnitude of the association between loneliness and MDD symptoms increased slightly across time (Tables 2 and 3 , Fig. 1 B,D). A 1 week increment in time was associated with a 0.06% increase in effect size, which corresponds to a 2.4% stronger association between loneliness and MDD symptoms after 10 months (roughly the duration covered by the repeated questionnaires). This interaction effect remained significant in both sensitivity analyses. Taken together, these analyses demonstrate a strong association between loneliness and MDD and GAD outcomes.
Moderators of the association between loneliness and depression
We found a significant interaction effect of loneliness and sex, indicating that the association between loneliness and MDD symptoms was stronger in men than in women (Table 2 , Fig. 2 ). This effect was consistent across both sensitivity analyses. We observed a similar result for GAD symptoms, but this was not robust in the sensitivity analysis for attrition. We performed a sex-stratified analysis for MDD symptoms to estimate effect sizes for men and women separately (Supplementary Table C 5). Results were in agreement with the main analysis, with a point increase in loneliness score being associated with a 32.1% increase in MDD symptoms in men, compared to 22.5% in women. These analyses suggest that the association between loneliness and symptoms of MDD is stronger in men.
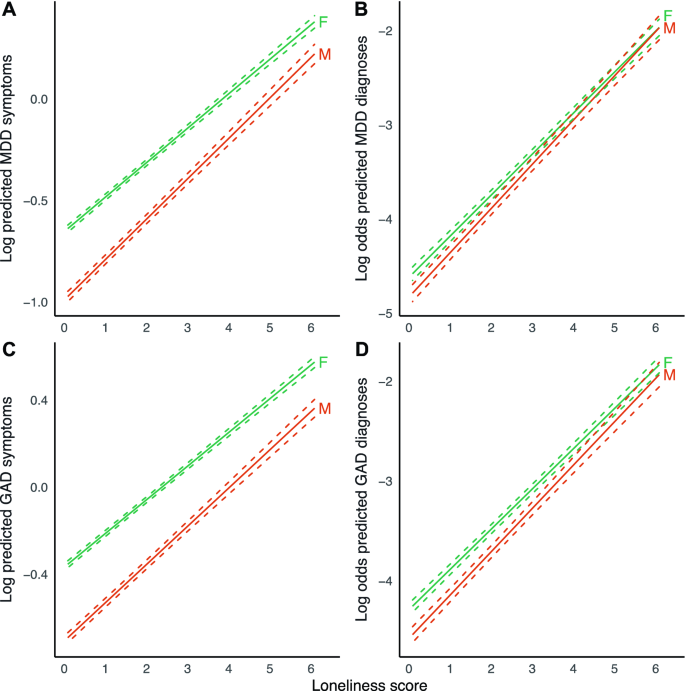
Marginal effect plots of associations between loneliness and MDD and GAD outcomes across sex. Displayed are log predicted counts of MDD symptoms ( A ) and log odds of MDD diagnosis ( B ), as well as log predicted counts of GAD symptoms ( C ) and log odds of GAD ( D ), across different loneliness scores, for men (M) and women (F) (plotted estimates are derived from Tables 2 and 3 , main models). Dashed lines represent 95% confidence intervals of model-predicted values.
We found a significant interaction effect between loneliness and age for MDD symptoms (Table 2 , Fig. 3 ), indicating that the magnitude of the association between loneliness and MDD symptoms increased slightly across age. This finding was robust across both sensitivity analyses. A 1 year increment in age was associated with a 0.08% reduction in effect size, which corresponds to a 4.7% weaker association between loneliness and MDD symptoms with a 60-year age difference. A point increase in loneliness is then associated with an increase in the number of MDD symptoms of 25.5% in 20-year-olds, compared to 19.6% in 80-year-olds. We observed no moderating effect of age for the other outcomes.
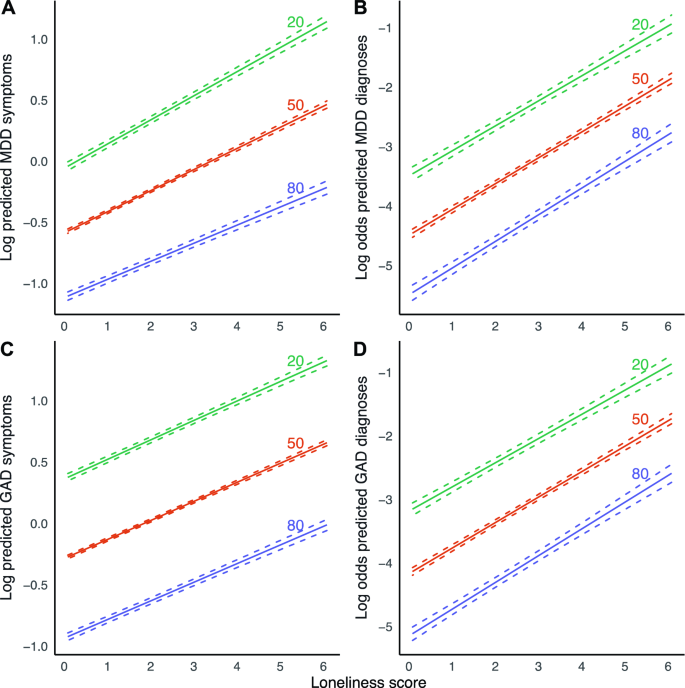
Marginal effect plots of associations between loneliness and MDD and GAD outcomes across age. Displayed are log predicted counts of MDD symptoms ( A ) and log odds of MDD diagnosis ( B ), as well as log predicted counts of GAD symptoms ( C ) and log odds of GAD ( D ), across different loneliness scores, across three levels of age in years (plotted estimates are derived from Tables 2 and 3 , main models). Dashed lines represent 95% confidence intervals of model-predicted values.
We found a significant main effect of PRS on symptoms of both MDD and GAD, as well MDD and GAD diagnoses, with a one standard deviation increase in PRS Z-score being associated with 26.5% increased odds of MDD, and 20.0% increased odds of GAD. We however did not observe a modifying effect of PRS on the association between loneliness and the four outcomes in any of the models.
Summary of main findings
This study investigated the association between loneliness and (symptoms of) MDD and GAD, and whether this association differs across age, sex and MDD PRS. We found significant associations between loneliness and MDD, GAD, MDD symptoms and GAD symptoms. On average, individuals who experienced the most loneliness were about 14 times more likely to report MDD, and around 11 times more likely to report GAD compared to individuals experiencing the least loneliness. For MDD symptoms, the strength of this association increased across time, becoming ~ 2.4% stronger between April 2020 and February 2021.
The association between loneliness and symptoms of MDD was significantly stronger in men than in women. The association of loneliness and MDD symptoms was also stronger in younger individuals. MDD polygenic risk did not modify the association between loneliness and the different outcomes.
Interpretation of findings and comparison with previous studies
We found a robust association between loneliness and MDD symptom severity. We found a similar association between loneliness and MDD. These findings are in line with multiple previous population-based studies 5 , 9 , 32 , which we now replicate in, to our knowledge, the largest study conducted to date. The strength of the association between loneliness and symptoms of MDD, but not MDD diagnoses, increased across the study duration. This could be due to several reasons. It may, for example, suggest that, as the COVID-19 pandemic lingered on, its influence magnified the effect of loneliness on specific depressive symptoms, but that this increase in symptoms did not necessarily cause a participant to develop MDD. Future studies could test this hypothesis, and assess if loneliness associates with only specific depressive symptoms. Alternatively, although the depressive symptom score and MDD are positively associated, they are distinct phenotypic constructs. MDD will likely display less variation across the period of data collection compared to MDD symptoms. Furthermore, the association of the two constructs with loneliness may also be different. Lastly, the present study may be underpowered to detect a significant interaction term for dichotomous outcomes (MDD), compared to continuous outcomes (MDD symptoms). However, we think that our sample of > 75,000 individuals and > 600,000 measurements should be large enough to trace any interaction effect with a relevant effect size.
We also found a robust association between loneliness and symptoms of GAD as well as GAD diagnoses, which is in line with previous studies 7 , 8 . This could mean that loneliness is a risk factor for GAD, or it could mean that GAD is a risk factor for loneliness or both. We however did not find that this association changed over time, suggesting that the course of the COVID-19 pandemic did not impact the relationship between loneliness and (symptoms of) GAD.
We found that the association between loneliness and depressive symptoms was stronger in men than in women. Results in previous studies were inconsistent. Cacioppo et al. found a stronger association in men in a cross-sectional study of 1939 older adults 5 . However, Lee et al. did not find a moderation effect of sex in a longitudinal study with baseline loneliness as a predictor and depressive symptoms at follow-up as an outcome, in a sample of 4211 older adults 9 . The present study assessed loneliness and outcomes across multiple contemporaneous timepoints. It may be that the moderating effect of sex disappears in time-lagged scenarios—possibly, loneliness has a stronger transient impact in men, without having a stronger effect on sustained depressive symptoms or disorders. Another explanation is that previous studies may not have been sufficiently powered to consistently detect a moderating effect of sex. We stress the importance of conducting such analyses in large population-based samples. Furthermore, differences in study populations, such as demographic, social, and environmental factors could contribute to different study outcomes as well.
We found a modifying effect of younger age on the association between loneliness and MDD symptoms. One previous study conducted in 1006 adults observed a significant interaction effect between loneliness and age on both anxiety and depressive symptoms 10 , while this effect was not found in a sample of 4211 adults above 50 years of age 9 . A lack of young adults in these previous studies may have contributed to these mixed findings. As MDD and GAD often first develop in adolescents or young adults, it is warranted that this age group is included in study cohorts, as is the case for the Lifelines cohort.
The present study did not find a significant moderation effect of MDD PRS on the association between loneliness and anxiety or depression. As far as we know, no comparable studies exist. A previous study by Lee et al. did assess the impact of both loneliness and MDD PRS on the association between loneliness and depression, but only as a confounder, not as an interaction term with loneliness. The authors found that the effect of loneliness persisted also in a model adjusted for PRS 9 . PRS for MDD currently explains only a limited amount of variance (Nagelkerke’s R 2 1.5–3.2%) of MDD 15 , thereby capturing only a small proportion of the heritability. As PRS does not capture the complete genetic architecture of MDD, we cannot exclude that genetic factors may impact the relationship between loneliness and MDD and GAD outcomes. Furthermore, while loneliness and depression share genes and heritability, both constructs likely also have phenotype-specific heritability, while the present study only employed genetic susceptibility for MDD. Perhaps, a PRS for loneliness or a PRS calculated from gene variants shared between the two constructs might be a significant moderator. Future work should revisit such analyses as sample sizes of GWASs, continue to increase. Finally, genetic analyses are worthwhile to explore for GAD and loneliness PRS as well, especially now that larger GWASs have been conducted 14 , 33 .
While loneliness is strongly associated with MDD and GAD outcomes, many individuals experiencing significant loneliness do not develop MDD or GAD. While age and sex explain some of these differences, future research should include other variables as well to further understand how loneliness impacts MDD and GAD. These might include socioeconomic variables, specific environmental variables, or psychological traits related to (tolerance of) loneliness and isolation.
Strengths and limitations
A strength of the present study is its large sample size and longitudinal design, with repeated measures of loneliness and depression and anxiety outcomes that are measured using multi-item and well-validated instruments.
As our study was conducted amidst the COVID-19 pandemic in the North of the Netherlands, it raises the question of whether our results are generalizable to populations not or no longer affected by the pandemic or to other regions or countries. We think results may be robust, given that the effect sizes of the association between loneliness and MDD and GAD outcomes are comparable to those found in studies conducted before the pandemic and in other populations 5 , 9 .
Because of the initial weekly and later biweekly/monthly assessments of symptoms, we only assessed MDD and GAD symptoms during the past week or 2 weeks. The DSM requires GAD symptoms to be present for 6 months, which means that our GAD diagnoses likely do not correspond to those of the cases seen in clinical practice. A similar point can be made for MDD diagnoses, which require 14 days of symptoms according to the DSM.
The present study focused on contemporaneous associations but did not investigate temporal relations between loneliness and MDD or GAD. While previous longitudinal studies showed that loneliness predicts (symptoms of) MDD 9 , and vice versa 5 , such studies have not yet been performed for GAD. In addition, studies involving loneliness-targeted interventions can further elucidate the causal relevance of loneliness towards depression and anxiety outcomes, besides evaluating its value as a target for treatment.
As we imputed one single dataset, we have sub-optimally accounted for the uncertainty introduced by our missing data handling approach 34 . However, as we had limited missing data and most data were missing by design (i.e., missing completely at random), we deem it unlikely to have significantly influenced our results.
The present study used a GEE marginal model as opposed to a conditional approach such as generalized linear mixed-effects models. The latter approach also allows the estimation of population-averaged estimates and can adjust for multiple correlation structures (such as for correlation within both individuals and families). This approach however was not feasible as it required excessive computational resources given our large sample size. However, in this study, the estimates from GEE models are likely to be in line with results that would have been derived from conditional models. First, estimates obtained from a GEE model agree closely with those from a conditional model if the right assumptions are being met, as is the case for the present study 35 . Second, our sample size was large, which means that we had sufficient power to trace small effects, even with GEE.
Implications
The current study replicates that loneliness is strongly associated with MDD and demonstrates a significant association in a large population-based sample for GAD. We furthermore observed that the association between loneliness and MDD symptoms became stronger over time. As COVID-19-related restrictions have led to more loneliness in the population, this might precipitate a subsequent increase in diagnoses, possibly even as government restrictions are being loosened. Our findings warrant extra vigilance in groups such as younger individuals, who experienced more loneliness.
Besides its association with MDD and GAD, loneliness is strongly predictive of a myriad of adverse health outcomes, such as cardiovascular disease and mortality 36 . Currently, few effective interventions are used routinely in clinical practice or the community, while effective interventions do exist 37 , 38 . Furthermore, there is a stigma surrounding loneliness 39 , and it has not received significant attention in clinical practice or policy. This is unfortunate, as its amelioration could entail a large health gain across somatic and mental domains. If nationwide restrictions on social relationships can lead to more loneliness and a higher prevalence of depression and anxiety in the population, public health policies aimed at nurturing social interactions may achieve the reverse. Some interventions already exist 37 , 38 , but have not yet been widely employed in clinical or public health settings.

Conclusions
In the largest study on loneliness and mental health to date, we found that loneliness is strongly associated with MDD, GAD and the symptoms thereof during the COVID-19 pandemic in the Netherlands. The association between loneliness and symptoms of MDD was stronger in men and in younger adults. Finally, we found the association between loneliness and symptoms of MDD to become stronger over time during the COVID-19 pandemic.
Data availability
All data is available through the Lifelines Cohort Study. Application for data access can be sent to the Lifelines Research Office: https://www.lifelines.nl/researcher/how-to-apply .
Meier, S. M. et al. Increased mortality among people with anxiety disorders: Total population study. Br. J. Psychiatry 209 , 216–221 (2016).
Article Google Scholar
Cuijpers, P. & Schoevers, R. A. Increased mortality in depressive disorders: A review. Curr. Psychiatry Rep. 6 , 430–437 (2004).
Killgore, W. D. S., Cloonan, S. A., Taylor, E. C. & Dailey, N. S. Loneliness: A signature mental health concern in the era of COVID-19. Psychiatry Res. 290 , 113117 (2020).
Article CAS Google Scholar
Cacioppo, J. T. & Hawkley, L. C. Loneliness. (2009).
Cacioppo, J. T., Hughes, M. E., Waite, L. J., Hawkley, L. C. & Thisted, R. A. Loneliness as a specific risk factor for depressive symptoms: Cross-sectional and longitudinal analyses. Psychol. Aging 21 , 140 (2006).
Van Den Brink, R. H. S. et al. Prognostic significance of social network, social support and loneliness for course of major depressive disorder in adulthood and old age. Epidemiol. Psychiatr. Sci. 27 , 266 (2018).
Beutel, M. E. et al. Loneliness in the general population: Prevalence, determinants and relations to mental health. BMC Psychiatry 17 , 97 (2017).
Domènech-Abella, J., Mundó, J., Haro, J. M. & Rubio-Valera, M. Anxiety, depression, loneliness and social network in the elderly: Longitudinal associations from The Irish Longitudinal Study on Ageing (TILDA). J. Affect. Disord. 246 , 82–88 (2019).
Lee, S. L. et al. The association between loneliness and depressive symptoms among adults aged 50 years and older: A 12-year population-based cohort study. Lancet Psychiatry 8 , 48–57 (2021).
Hubbard, G., den Daas, C., Johnston, M. & Dixon, D. Sociodemographic and psychological risk factors for anxiety and depression: Findings from the Covid-19 health and adherence research in Scotland on mental health (CHARIS-MH) cross-sectional survey. Int. J. Behav. Med. 28 , 788–800 (2021).
Distel, M. A. et al. Familial resemblance for loneliness. Behav. Genet. 40 , 480–494 (2010).
Sullivan, P. F., Neale, M. C. & Kendler, K. S. Genetic epidemiology of major depression: Review and meta-analysis. Am. J. Psychiatry 157 , 1552–1562 (2000).
Hettema, J. M., Neale, M. C. & Kendler, K. S. A review and meta-analysis of the genetic epidemiology of anxiety disorders. Am. J. Psychiatry 158 , 1568–1578 (2001).
Day, F. R., Ong, K. K. & Perry, J. R. B. Elucidating the genetic basis of social interaction and isolation. Nat. Commun. 9 , 2457 (2018).
Article ADS Google Scholar
Howard, D. M. et al. Genome-wide meta-analysis of depression identifies 102 independent variants and highlights the importance of the prefrontal brain regions. Nat. Neurosci. 22 , 343–352 (2019).
Wray, N. R. et al. Genome-wide association analyses identify 44 risk variants and refine the genetic architecture of major depression. Nat. Genet. 50 , 668–681 (2018).
McGinty, E. E., Presskreischer, R., Han, H. & Barry, C. L. Psychological distress and loneliness reported by US adults in 2018 and April 2020. JAMA 324 , 93–94 (2020).
Bu, F., Steptoe, A. & Fancourt, D. Loneliness during a strict lockdown: Trajectories and predictors during the COVID-19 pandemic in 38,217 United Kingdom adults. Soc. Sci. Med. 265 , 113521 (2020).
Robb, C. E. et al. Associations of social isolation with anxiety and depression during the early COVID-19 pandemic: A survey of older adults in London. UK. Front. Psychiatry 11 , 991 (2020).
Google Scholar
McQuaid, R. J., Cox, S. M. L., Ogunlana, A. & Jaworska, N. The burden of loneliness: Implications of the social determinants of health during COVID-19. Psychiatry Res. 296 , 113648 (2021).
McIntyre, K. et al. Lifelines COVID-19 cohort: Investigating COVID-19 infection and its health and societal impacts in a Dutch population-based cohort. BMJ Open 11 , e044474 (2021).
Scholtens, S. et al. Cohort Profile: LifeLines, a three-generation cohort study and biobank. Int. J. Epidemiol. 44 , 1172–1180 (2015).
Klijs, B. et al. Representativeness of the LifeLines cohort study. PLoS ONE 10 , e0137203 (2015).
Mc Intyre, K. et al. Lifelines COVID-19 cohort: Investigating COVID-19 infection and its health and societal impacts in a Dutch population-based cohort. BMJ Open 11 , e044474 (2021).
Sheehan, D. V. et al. The Mini-International Neuropsychiatric Interview (MINI): The development and validation of a structured diagnostic psychiatric interview for DSM-IV and ICD-10. J. Clin. Psychiatry 59 , 22–33 (1998).
PubMed Google Scholar
Hughes, M. E., Waite, L. J., Hawkley, L. C. & Cacioppo, J. T. A short scale for measuring loneliness in large surveys: Results from two population-based studies. Res. Aging 26 , 655–672 (2004).
Azur, M. J., Stuart, E. A., Frangakis, C. & Leaf, P. J. Multiple imputation by chained equations: What is it and how does it work?. Int. J. Methods Psychiatr. Res. 20 , 40–49 (2011).
Pekár, S. & Brabec, M. Generalized estimating equations: A pragmatic and flexible approach to the marginal GLM modelling of correlated data in the behavioural sciences. Ethology 124 , 86–93 (2018).
Højsgaard, S., Halekoh, U. & Yan, J. The R package geepack for generalized estimating equations. J. Stat. Softw. 15 , 1–11 (2005).
R Core Team. R: A Language and Environment for Statistical Computing. (2019).
Nunan, D., Aronson, J. & Bankhead, C. Catalogue of bias: Attrition bias. BMJ Evid.-Based Med. 23 , 21–22 (2018).
Wang, J., Mann, F., Lloyd-Evans, B., Ma, R. & Johnson, S. Associations between loneliness and perceived social support and outcomes of mental health problems: A systematic review. BMC Psychiatry 18 , 1–16 (2018).
Levey, D. F. et al. Reproducible genetic risk loci for anxiety: Results from ∼ 200,000 participants in the Million Veteran Program. Am. J. Psychiatry 177 , 223–232 (2020).
Pedersen, A. B. et al. Missing data and multiple imputation in clinical epidemiological research. Clin. Epidemiol. 9 , 157 (2017).
Hedeker, D., du Toit, S. H. C., Demirtas, H. & Gibbons, R. D. A note on marginalization of regression parameters from mixed models of binary outcomes. Biometrics 74 , 354–361 (2018).
Article MathSciNet Google Scholar
Holt-Lunstad, J., Smith, T. B., Baker, M., Harris, T. & Stephenson, D. Loneliness and social isolation as risk factors for mortality: A meta-analytic review. Perspect. Psychol. Sci. 10 , 227–237 (2015).
Masi, C. M., Chen, H.-Y., Hawkley, L. C. & Cacioppo, J. T. A meta-analysis of interventions to reduce loneliness. Pers. Soc. Psychol. Rev. 15 , 219–266 (2011).
Kahlon, M. K. et al. Effect of layperson-delivered, empathy-focused program of telephone calls on loneliness, depression, and anxiety among adults during the COVID-19 pandemic: A randomized clinical trial. JAMA Psychiat. 78 , 616–622 (2021).
Rotenberg, K. J. & MacKie, J. Stigmatization of social and intimacy loneliness. Psychol. Rep. 84 , 147–148 (1999).
Download references
Acknowledgements
We acknowledge funding for the Lifelines Corona Research project from the University of Groningen and the University Medical Centre Groningen. The authors wish to acknowledge the efforts of the Lifelines Corona Research Initiative and the following initiative participants:
H. M. Boezen 1 , Jochen O. Mierau 2,3 , Lude H. Franke 4 , Jackie Dekens 4,6 , Patrick Deelen 4 , Pauline Lanting 4 , Judith M. Vonk 1 , Ilja Nolte 1 , Anil P.S. Ori 4,5 , Annique Claringbould 4 , Floranne Boulogne 4 , Marjolein X.L. Dijkema 4 , Henry H. Wiersma 4 , Robert Warmerdam 4 , Soesma A. Jankipersadsing 4 , Irene van Blokland 4,7 .
1 Department of Epidemiology, University of Groningen, University Medical Center Groningen, Groningen, The Netherlands. 2 Faculty of Economics and Business, University of Groningen, Groningen, The Netherlands. 3 Aletta Jacobs School of Public Health, Groningen, The Netherlands. 4 Department of Genetics, University of Groningen, University Medical Center Groningen, Groningen, The Netherlands. 5 Department of Psychiatry, University of Groningen, University Medical Center Groningen, Groningen, The Netherlands. 6 Center of Development and Innovation, University of Groningen, University Medical Center Groningen, Groningen, The Netherlands. 7 Department of Cardiology, University of Groningen, University Medical Center Groningen, Groningen, The Netherlands.
The generation and management of GWAS genotype data for the Lifelines Cohort Study is supported by the UMCG Genetics Lifelines Initiative (UGLI). The authors wish to acknowledge the services of the Lifelines Cohort Study, the contributing research centres delivering data to Lifelines:
Raul Aguirre-Gamboa 1 , Patrick Deelen 1 , Lude Franke 1 , Jan A. Kuivenhoven 2 , Esteban A. Lopera Maya 1 , Ilja M Nolte 3 , Serena Sanna 1 , Harold Snieder 3 , Morris A. Swertz 1 , Judith M. Vonk 3 , Cisca Wijmenga 1 .
1 Department of Genetics, University of Groningen, University Medical Center Groningen, The Netherlands. 2 Department of Paediatrics, University of Groningen, University Medical Center Groningen, The Netherlands. 3 Department of Epidemiology, University of Groningen, University Medical Center Groningen, The Netherlands
As well as all the study participants.
We thank the UMCG Genomics Coordination Center, the UG Center for Information Technology and their sponsors BBMRI-NL & TarGet for storage and computing infrastructure.
We thank Lian Beijers, Aranka Ballering, Judith Rosmalen and Nord van den Bos for their collaboration and feedback as part of our COVID Psychiatry working group.
The Lifelines Biobank initiative has been made possible by funding from the Dutch Ministry of Health, Welfare and Sport, the Dutch Ministry of Economic Affairs, the University Medical Center Groningen (UMCG the Netherlands), the University of Groningen, the Northern Provinces of the Netherlands, FES (Fonds Economische Structuurversterking), SNN (Samenwerkingsverband Noord Nederland) and REP (Ruimtelijk Economisch Programma). Hanna M. van Loo was supported by a NARSAD Young Investigator Grant from the Brain & Behavior Research Foundation and a VENI grant from the Talent Programme of the Netherlands Organization of Scientific Research (NWO-ZonMW 09150161810021).
Author information
Authors and affiliations.
Department of Psychiatry, University Medical Center Groningen, University of Groningen, Hanzeplein 1, PO Box 30.001, 9700 RB, Groningen, The Netherlands
Olivier D. Steen, Anil P. S. Ori, Klaas J. Wardenaar & Hanna M. van Loo
Department of Genetics, University Medical Center Groningen, University of Groningen, Groningen, The Netherlands
Anil P. S. Ori
You can also search for this author in PubMed Google Scholar
Contributions
O.D.S., A.P.S.O., H.M.v.L. designed the study. A.P.S.O. and H.M.v.L. were involved in data collection. O.D.S. performed the statistical analyses and primary drafting of the manuscript with critical input from A.P.S.O., K.J.W., and H.M.v.L. A.P.S.O. and H.M.v.L. oversaw the study. All authors contributed to and approved the final manuscript.
Corresponding author
Correspondence to Hanna M. van Loo .
Ethics declarations
Competing interests.
The authors declare no competing interests.
Additional information
Publisher's note.
Springer Nature remains neutral with regard to jurisdictional claims in published maps and institutional affiliations.
Supplementary Information
Supplementary information., rights and permissions.
Open Access This article is licensed under a Creative Commons Attribution 4.0 International License, which permits use, sharing, adaptation, distribution and reproduction in any medium or format, as long as you give appropriate credit to the original author(s) and the source, provide a link to the Creative Commons licence, and indicate if changes were made. The images or other third party material in this article are included in the article's Creative Commons licence, unless indicated otherwise in a credit line to the material. If material is not included in the article's Creative Commons licence and your intended use is not permitted by statutory regulation or exceeds the permitted use, you will need to obtain permission directly from the copyright holder. To view a copy of this licence, visit http://creativecommons.org/licenses/by/4.0/ .
Reprints and permissions
About this article
Cite this article.
Steen, O.D., Ori, A.P.S., Wardenaar, K.J. et al. Loneliness associates strongly with anxiety and depression during the COVID pandemic, especially in men and younger adults. Sci Rep 12 , 9517 (2022). https://doi.org/10.1038/s41598-022-13049-9
Download citation
Received : 09 November 2021
Accepted : 09 May 2022
Published : 09 June 2022
DOI : https://doi.org/10.1038/s41598-022-13049-9
Share this article
Anyone you share the following link with will be able to read this content:
Sorry, a shareable link is not currently available for this article.
Provided by the Springer Nature SharedIt content-sharing initiative
By submitting a comment you agree to abide by our Terms and Community Guidelines . If you find something abusive or that does not comply with our terms or guidelines please flag it as inappropriate.
Quick links
- Explore articles by subject
- Guide to authors
- Editorial policies
Sign up for the Nature Briefing newsletter — what matters in science, free to your inbox daily.


An official website of the United States government
The .gov means it’s official. Federal government websites often end in .gov or .mil. Before sharing sensitive information, make sure you’re on a federal government site.
The site is secure. The https:// ensures that you are connecting to the official website and that any information you provide is encrypted and transmitted securely.
- Publications
- Account settings
Preview improvements coming to the PMC website in October 2024. Learn More or Try it out now .
- Advanced Search
- Journal List
- Front Neurol
The Effect of Anxiety and Depression on Sleep Quality of Individuals With High Risk for Insomnia: A Population-Based Study
Chang-myung oh.
1 Division of Endocrinology and Metabolism, CHA Bundang Medical Center, School of Medicine CHA University, Seongnam-si, South Korea
2 Biostatistics Collaboration Unit, Yonsei University College of Medicine, Seoul, South Korea
3 Department of Neurology, Yonsei University College of Medicine, Seoul, South Korea
Kyoo Ho Cho
Min kyung chu, associated data.
The datasets analyzed in this manuscript are not publicly available. Requests to access the datasets should be directed to ca.shuy@kmuhc .
Introduction: One of the most common sleep disorders, insomnia is a significant public health concern. Several psychiatric disorders, such as anxiety disorders and depression, have shown strong relationships with insomnia. However, the clinical impact of the combination of these two conditions on insomnia severity and sleep quality remains unknown. We investigated the relationship between sleep disturbance and psychiatric comorbidities in subjects with high risk for insomnia.
Methods: We analyzed data from a nation-wide cross-sectional survey of Korean adults aged 19 ~ 69 years conducted from November 2011 to January 2012. The survey was performed via face-to-face interviews using a structured questionnaire. We used the insomnia severity index (ISI) to evaluate insomnia and defined respondents with ISI scores of ≥10 were considered to be at high risk for insomnia. To diagnose anxiety and depression, we used the Goldberg anxiety scale (GAS) and Patient Health Questionnaire-9 (PHQ-9), respectively.
Results: Of the 2,762 respondents, 290 (10.5%) were classified as subjects with high risk for insomnia; anxiety [odds ratio (OR), 9.8; 95% confidence interval (CI), 7.3–13.1] and depression (OR, 19.7; 95% CI, 13.1–29.6) were more common in this population than in participants without insomnia. Of the participants with insomnia, 152 (52.4%) had neither anxiety nor depression, 63 (21.7%) only had anxiety, 21 (7.2%) only had depression, and 54 (18.6%) had both anxiety and depression. The group with both anxiety and depression was associated with worse scores on sleep-related scales than the other groups [high ISI, Pittsburgh Sleep Quality Index (PSQI), and Epworth Sleepiness Scale]. The relationship between outcome measures (ISI and PSQI) and psychiatric problems was significant only when anxiety and depression were present. The PSQI has a significant mediation effect on the relationship between psychiatric comorbidities and insomnia severity.
Conclusion: Among the respondents with insomnia, psychiatric comorbidities may have a negative impact on daytime alertness, general sleep quality, and insomnia severity, especially when the two conditions are present at the same time. Clinicians should, therefore, consider psychiatric comorbidities when treating insomnia.
Introduction
As one of the most common sleep disorders, insomnia has become a significant public health problem. While the prevalence of insomnia varies considerably across countries, its global prevalence of insomnia as defined by the Diagnostic and Statistical Manual of Mental Disorders, Fourth Edition (DSM-IV) criteria is estimated to be ~6–10% ( 1 – 3 ).
Insomnia reportedly increases the rate of car accidents ( 4 ), decreases job performance, results in self-medication with alcohol as well as socio-economic problems ( 5 – 7 ), and has been associated with the onset of cardiovascular diseases ( 8 ).
Although a previous (second) edition of the International Classification of Sleep Disorders (ICSD-2) distinguished psychophysiological insomnia from mental-illness induced insomnia ( 9 ), there are substantial overlap features between the two: e.g., conditioned arousal, poor sleep hygiene, and excessive worry about sleep ( 10 ). Also, many subjects with insomnia have psychiatric comorbidities, rendering the discrimination of insomnia subtypes difficult. Because of these issues (overlapping primary and secondary insomnia), in the next version, ICSD-3, insomnia was recategorized according to time course ( 10 ). Nevertheless, it is still of great value to recognize the most common psychiatric comorbidities such as anxiety disorders and depression in patients with insomnia ( 11 ). Among patients with insomnia, the prevalence of anxiety disorder, including generalized anxiety disorder, panic disorder, post-traumatic stress disorder, and phobia, is 24–36% ( 11 , 12 ), while that of major depression is 14–31% ( 11 , 12 ). Conversely, about 90% of patients with depression complain of sleep disturbance ( 13 , 14 ). Similarly, sleep problems are much more common among individuals with anxiety disorders ( 15 , 16 ).
Multiple studies have investigated the relationship between sleep disorders and psychiatric comorbidity. A study that used data collected by the national survey in the United States revealed that individuals with any comorbid sleep problem (especially non-restorative sleep) are more prone to impairments of daytime activities ( 17 ). Using data obtained by the same survey, another investigation reported that the rate of insomnia complaints was highest among individuals with anxiety and mood disorders (42–63%) ( 18 ). However, to date, it remains unclear whether the combination of anxiety and mood (depression) problems aggravate the severity of insomnia itself and the sleep quality of people at high risk for insomnia.
This study explored the relationships among insomnia severity, sleep quality and daytime sleepiness, and comorbidities with anxiety and depression among individuals at high risk for insomnia. In addition, we used mediation modeling to evaluate whether insomnia severity is mediated by changes in general sleep quality.
Survey Procedure
We used data from a nationwide, cross-sectional survey of headache and anxiety in the general Korean population conducted from November 2011 to January 2012 ( 19 ). Trained interviewers performed structured, face-to-face interviews that included questionnaires regarding sleep and headache disorders and mood problems (anxiety and depression). Adults between the ages of 19 and 69 years were included. Besides, we collected the respondents' demographic and geographic information. The target area, sampling method, and detailed survey procedures were same as the previously documented process ( 19 ). The distribution in this study and the total population were not significantly different in sex, age groups, size of a residential area, and educational level.
Diagnosis of Insomnia, Anxiety, and Depression
We used the insomnia severity index (ISI) to define high risk for insomnia. Subjects who received an ISI score of ≥10 were classified as high risk for insomnia according to a previous community-based study ( 20 ). Using an ISI score of 10 as the cutoff, insomnia was detected with 86.1% sensitivity and 87.7% specificity ( 20 ).
For the diagnosis of anxiety and depression, we used the Goldberg anxiety scale (GAS) and Patient Health Questionnaire-9 (PHQ-9), respectively. The GAS consists of four screening and five supplementary questions. The validated Korean version of the GAS features a sensitivity of 82.0% and a specificity of 94.4% ( 21 ). At least two positive answers to screening and five or more positive answers to complementary questions in GAS questions indicated anxiety. The PHQ-9 was used to diagnose depression ( 22 ). The Korean version of the PHQ-9 features 81% sensitivity and 89.9% specificity ( 23 ). Participants with a PHQ-9 score of ≥10 were considered to have depression. We classified the respondents into four groups: individuals (1) without anxiety or depression, (2) with anxiety but without depression, (3) without anxiety but with depression, and (4) with both anxiety and depression. The demographic data of the participants are shown in Table 1 .
Sociodemographic distribution of all survey participants, the total Korean population, and of cases identified as insomnia, anxiety, and depression.
Total subjects: 2,695; P, p-value;
Measures Related to Sleep Quality
Each individual was asked to complete questionnaires, including the Pittsburgh Sleep Quality Index (PSQI), which accesses the multifactorial construct of sleep dysfunction with strong reliability and validity ( 24 ), and the Epworth Sleepiness Scale (ESS). which assesses each participant's daytime sleepiness ( 25 ). The subjective and objective sleep qualities measured with ISI feature a high concordance with daytime disability ( 26 ). Even young adults with insomnia frequently complain of daytime sleepiness ( 27 ). Additionally, each subject's ISI score was categorized into one of three groups (mild insomnia, 10 ≤ ISI < 15; moderate, 15 ≤ ISI < 20; severe, ISI > 20).
This study was approved by the institutional review board/ethics committee of the Severance Hospital, and written informed consent was obtained from each participant.
Statistical Analysis
We used the chi-square test to examine whether the number of diagnoses differed according to sex, age, size of a residential area, educational level, and types of work shift. We compared group differences in sleep time and ESS and PSQI scores using the Kruskal-Wallis test followed by Bonferroni's multiple comparison correction. Group differences in ISI severity and insomnia characteristics were compared with Jonckheere's trend test. Univariate and multivariate logistic regression analysis was performed to evaluate the odds ratio (OR) of anxiety and depression in individuals with high risk for insomnia compared to those without high risk for insomnia. The same procedure was used to compare the PSQI and ISI scores stratified by the presence of depression or anxiety between groups; sex, age group, size of residential area, and education level were included in the multivariate analysis. To examine whether the association between anxiety and depression comorbidities and insomnia risk is mediated by sleep quality, we performed a mediation analysis based on a previously developed method ( 28 ). In all the statistical analyses, two-tailed p <0.05 were considered statistically significant.
Demographic Data of the Subjects
Of the total 7,430 interviewees, 3,114 completed the survey (acceptance rate of 41.9%), and 352 subjects suspended the interview. The final sample that completed the survey and the interview included 2,762 individuals ( 19 ). The enrollment flow chart of this study is presented in Figure 1 . The prevalence of insomnia, anxiety, and depression according to sex, age distribution, size of the residential area, and education level is depicted in Table 1 . Insomnia, depression, and anxiety were significantly more prevalent among women. Participants with the lowest educational levels were associated with a higher prevalence of insomnia and anxiety than were those who had completed high school, college, or graduate school.

Flow chart of participants in the Korean Headache-Sleep study. Whether individuals had anxiety (A) or depression (D) are denoted by positive (+) and negative (–). A–/D–, without anxiety or depression; A+/D–, with anxiety only; A–/D+, with depression only; A+/D+, with both anxiety and depression.
Prevalence of Insomnia, Anxiety, and Depression and Mean Scores on Sleep-Related Scales
Of the 2,762 participants, 290 (10.5%) were classified as having a high risk for insomnia. The frequencies of insomnia, anxiety, and depression are presented in Table 1 according to sex, age group, size of the residential area, and education level. The mean ISI, ESS, and PSQI scores of individuals with high risk for insomnia were 14.37 ± 4.39, 7.73 ± 4.87, and 7.42 ± 2.59, respectively. Of the 290 subjects with high risk for insomnia, 152 (52.4%) had neither anxiety nor depression (Group 1), 63 (21.7 %) had anxiety only (Group 2), 21 (7.2%) had depression only (Group 3), and 54 (18.6%) had both anxiety and depression (Group 4). Anxiety was more common among individuals who had a high risk for insomnia than among those who did not [40.3% vs. 6.1%; OR, 10.1; 95% confidence interval (CI), 7.6–13.4]; this finding remained consistent after adjustment for demographic variables (OR, 9.8; 95% CI, 7.3–13.1). Depression was also more common among individuals who had a high risk for insomnia than those who did not (25.9% vs. 1.7%; OR, 20.1; 95% CI, 13.4–30.2); this finding also remained consistent after adjustment for demographic variables (OR, 19.7l 95% CI, 13.1–29.6) ( Table 2 ).
Logistic regression analysis.
Insomnia vs. non-insomnia population. OR, odds ratio; CI, 95% confidence interval;
Difference in Sleep-Related Scales Among the Four Groups
Participants at high risk for insomnia with both anxiety and depression were associated with significantly higher ESS and PSQI scores relative to the anxiety-only group ( Figure 2 ). Meanwhile, individuals with no psychiatric comorbidities were associated with lower PSQI scores relative to the three other groups. ISI scores were significantly higher among participants with both anxiety and depression than those with any psychiatric comorbidities. When stratified by the presence of anxiety and depression, severe ISI tended to increase from Groups 1–4 ( Figure 3 ).

Differences in scores on sleep-related scale across four groups. (A) Epworth sleepiness scale and (B) Pittsburg sleep quality index is depicted in box plots. (C) Proportion of each insomnia severity index category is shown in a stacked bar plot. A–/D–, without anxiety or depression; A+/D–, with anxiety only; A–/D+, with depression only; A+/D+, with both anxiety and depression; * p < 0.05, ** p < 0.01, and *** p < 0.001.

Stacked bar plot showing the proportion of each insomnia characteristic scale 1–5 across groups. A–/D–, without anxiety or depression; A+/D–, with anxiety only; A–/D+, with depression only; A+/D+, with both anxiety and depression. Insomnia characteristic scale (ICS) was graded on a scale of 1–5.
Insomnia Characteristics According to the Presence of Anxiety or Depression
No statistical differences were observed among the 4 groups in average sleep time during workdays, weekends, and overall sleep. The three insomnia-related symptom scales of each group are presented in Figure 3 . Regarding the difficulty in initiating sleep and feelings of impaired quality of life, only Groups 1 and 4 featured a statistically significant difference. Group 4 was more likely to have difficulties in maintaining sleep than were Groups 1 and 2. The groups did not differ in their scores on the frequent awakening scale.
Mediation Model Analysis
When insomnia was comorbid with both anxiety and depression, PSQI and ISI were significantly correlated with each other ( Tables 3 , ,4). 4 ). The relationship between anxiety and ISI (beta = 4.21) was attenuated when PSQI was used as a mediator ( Figure 4 ). The Sobel test revealed the significance of the indirect effect of PSQI in mediating both anxiety and depression and ISI ( Table 5 ).
Mediation analysis.
ISI, insomnia severity index; PSQI, Pittsburgh Sleep Quality Index; SE, standard error; P, p-value .
Linear regression analysis, endpoint: ISI.
ISI, insomnia severity index;

Mediation model: the indirect effect of combined anxiety and depression on ISI scores through PSQI. Unstandardized regression coefficients are presented for each path *** p < 0.001.
Linear regression analysis, endpoint: PSQI.
PSQI, Pittsburgh Sleep Quality Index;
We found the prevalence of high risk for insomnia in the Korean adult population to be 10.5%, which is significantly different from previously reported rates in the Korean population (about 20%). Differences between the methodology of our study and those of previous investigations, which used telephone-based interviews without validated questionnaires, may account for the divergent findings ( 29 , 30 ). Measuring residential noise levels in addition to employing a structured questionnaire, a population-based study conducted in Japan, reported a crude prevalence of insomnia of 8.8% ( 31 ), which better accords with our results. The prevalence of overall anxiety and depression observed in our study was also similar to or slightly higher than the rates reported by prior Korean epidemiologic studies ( 32 , 33 ). Similar to a previous study ( 34 ), we found that the prevalence of insomnia to be higher among women and individuals with low levels of education. We also found no association between age and the prevalence of insomnia. This discrepancy may be due to the changes in the lifestyles of young Korean adults, which might cause higher rates of insomnia among adults below the age of 30 ( 35 ).
We observed that 47.6% of individuals with high risks for insomnia had comorbidities of anxiety or depression. This finding in the Korean population was similar to a previously reported rate: 40% of individuals with insomnia had comorbid psychiatric disorders ( 11 ), among which depression and anxiety disorders were reportedly the most common.
As expected, Group 1 had lower PSQI and ISI scores than those with anxiety-only, depression-only, or both (Groups 2, 3, and 4). The ESS scores of subjects with anxiety-only or depression-only (Groups 2 and 3), but not both (Group 4), did not differ significantly from those of individuals without any anxiety or depression (Group 1) as depicted in Figure 1 . This might be due to individuals with insomnia having excessive hyperarousal continuing throughout the day time rather than daytime sleepiness. Group 4 was consistently associated with poorer sleep-related indices than was Group 2. While Groups 3 and 4 differed significantly in the proportion of participants who had higher ISI scores ( Figure 3C ), they did not differ significantly in ESS and PSQI scores. This result might be due to a lack of statistical power associated with sample sizes (Group 3 was relatively small).
Individuals with high risk for insomnia are associated with an increased incidence of anxiety and depression relative to those without insomnia. After controlling for possible confounding variables, subjects with insomnia were found to be 9.8 times more likely to have anxiety than subjects without insomnia and 19.7 times more likely to have depression. In addition, we found Groups 2, 3, and 4, in order, had significantly higher rates of insomnia symptoms.
Numerous studies have reported an association between insomnia and depression and anxiety ( 36 – 40 ). Indeed, patients with persistent insomnia are reportedly predisposed to developing psychiatric illness and are more prone to the recurrence of depression ( 41 , 42 ). Among patients with partially treated depression, residual symptoms such as anxiety and insomnia are among the most powerful predictors for relapse ( 41 ). Additionally, insomnia and anxiety share a pathogenetic mechanism: hyperarousal caused by dysregulation of neurotransmitter systems including cholinergic and GABA (gamma-aminobutyric acid) eric mechanisms ( 43 ). Hyperarousal and insufficient sleep disrupt the function of corticolimbic circuitry, which leads to impaired affective reactivity and regulation ( 44 ). Genetic studies also showed a strong overlap between genetic influences on insomnia, depression, and anxiety ( 44 ). This is reflected in high comorbidity of the two conditions (70–90% of patients with anxiety report insomnia) ( 45 ).
The mediational model revealed that the effect of combined anxiety and depression on insomnia severity is mediated by poor sleep behaviors. Considering that the essential feature of insomnia is conditioned arousal affected by dysfunctional efforts to sleep and negative expectations, patients with insomnia become anxious and frustrated as insomnia symptoms persist. The mediator PSQI covers general sleep problems including sleep latency; sleep duration and efficiency; and sleep-disturbing factors, such as nocturia, nocturnal breathing problems, pain, feeling too hot or cold, use of sleeping pills, and daytime dysfunction. Even in the absence of direct insomnia-related factors, nearly all of these components are associated with somatic symptoms of depression ( 46 ), and more than half of patients with anxiety disorders report those somatic complaints ( 47 ). These observations are in agreement with the findings of our mediation analysis: depression and anxiety have indirect relationships with the degree of insomnia severity and poor sleep-related somatic symptoms, which in turn aggravates insomnia severity.
There was a systemic review on the relationships among anxiety, depression, and sleep disturbance. They showed that insomnia and sleep quality features have “bidirectional” relationships with anxiety and depression, respectively ( 48 ). Moreover, regarding treatment, cognitive behavioral therapy that focuses on attenuating anxiety and depression reduces insomnia severity and the symptoms of the two psychiatric conditions ( 49 ).
Our study is subject to several limitations. First, we did not consider comorbidities of anxiety and depression with other sleep disorders, including obstructive sleep apnea, narcolepsy, and restless legs syndrome. The presence of any pain or other significant medical illnesses was also not investigated. Second, even though we performed a population-based study with a low sampling error, the statistical power for the examination of subgroups might have been diminished due to the small samples (especially Group 3). Third, in the mediation analysis, we did not conduct a detailed investigation to elucidate the component of PSQI that features a close relationship with insomnia severity. Third, we did not analyze longitudinal data which causes a problem to establish causality, despite a quite large sample. Last, we didn't consider the impact of medication use when we analyzed our data. The medication status is an important factor that can change the subjects' mental status. Unfortunately, our research target was the general population. So, it was not easy to obtain optimal medical information from the survey method.
This report is, to the best of our knowledge, the first to evaluate how anxiety and depression affect sleep quality and the severity of insomnia. The prevalence of high risk for insomnia and the comorbidities with anxiety and depression is comparable to the findings of previous reports. Daytime sleepiness, general sleep quality, and insomnia severity were consistently poorer in subjects with both depression and anxiety. Also, we found that the effect of the combination of both psychiatric conditions was mediated by general sleep quality indices, which encompass insomnia and related somatic symptoms. We surmise that anxiety and depression affect insomnia in a supra-additive manner. When treating insomnia patients, clinicians should look for underlying comorbid psychiatric conditions to determine the appropriate therapy and enhance the therapeutic effect.
Data Availability
Author contributions.
C-MO, KC, and MC: study concept and design. C-MO, HK, KC, and MC: acquisition, analysis, and interpretation of data. C-MO and KC: drafting of the manuscript. HK, KC, and HN: statistical analysis. C-MO: obtained funding. KC and MC: study supervision. All authors read and approved the manuscript.
Conflict of Interest Statement
The authors declare that the research was conducted in the absence of any commercial or financial relationships that could be construed as a potential conflict of interest.
Acknowledgments
We would like to thank the Biostatistics Collaboration Unit members of Yonsei Medical University for their assistance in data analysis.
Funding. This research was supported by grant provided to C-MO by the Basic Science Research Program through the National Research Foundation of Korea (NRF), which was funded by the Ministry of Education (Grant No. 2016R1A6A3A04010466). KC was supported by a faculty research grant from Yonsei University College of Medicine 6-2018-0092.

COMMENTS
At the same time, studies of trait anxiety suggest that moderate (vs. low) ELS is associated with greater self-reported anxiety. This study tested the hypothesis that stress inoculation effects are evident for implicit (nonconscious) but not explicit (conscious) aspects of anxiety. Methods: Ninety-seven healthy women were assessed for ELS and ...
population aged 16-85 years, 14.4% have an anxiety. disorder. The pre valence of depression is 6.2%, with the. prevalence of unipolar depressive episodes being 4.1%, dysthymia, 1.3%, and bipolar ...
The findings revealed a 19% concurrent comorbidity between these disorders, and in 65% of the cases, social phobia preceded major depressive disorder by at least 2 years. In addition, initial presentation with social phobia was associated with a 5.7-fold increased risk of developing major depressive disorder. These associations between anxiety ...
This article is a systematic review of the literature on the impact of anxiety and depression on health-related quality of life (HRQoL) in patients with various medical conditions. It summarizes the evidence from 42 studies and discusses the possible mechanisms, implications and limitations of the findings. The article aims to provide a comprehensive overview of the topic and suggest ...
This review may be used as an evidence base by those in public health, clinical practice, and research. This paper discusses key areas in depression research; however, an exhaustive discussion of all the risk factors and determinants linked to depression and their mechanisms is not possible in one journal article—which, by its very nature, a ...
Meaning in life, anxiety and depression. Regarding the negative relationship between anxiety and meaning in life, there are at least two possible explanations. First, a high level of anxiety may make a person difficult to appreciate meaning in life because anxiety would make a person exhausted and confused.
level of anxiety, depression, and stress and second one to measure Emotional stability using a self-reported scale. The collected data was analyzed using SPSS version 22 to find result for this thesis. The results of the study outlined that there is a negative but significant correlation among depression, anxiety, and stress with emotional ...
Anxiety disorders refer to a group of mental disorders characterized by feelings of anxiety and fear, including generalised anxiety disorder (GAD), panic disorder, phobias, social anxiety disorder, obsessive-compulsive disorder (OCD) and post-traumatic stress disorder (PTSD). As with depression, symptoms can range from mild to severe.
Liu, C. et al. Prevalence and associated factors of depression and anxiety among doctoral students: The mediating effect of mentoring relationships on the association between research self ...
[31]. The levels of depression for the study were categorized as 'mild = 5-9', 'moderate = 10- 14,' 'moderately severe = 15-19,' 'severe = 20.' Anxiety. Anxiety was evaluated by using the Generalized Anxiety Disorder (GAD-7). In the questionnaire, the questions were used for screening anxiety state of an individual on a
Depression and Anxiety welcomes original research and synthetic review articles covering neurobiology (genetics and neuroimaging), epidemiology, experimental psychopathology, and treatment (psychotherapeutic and pharmacologic) aspects of mood and anxiety disorders and related phenomena in humans. Read the full Aims and Scope here.
Within-day bidirectional associations between physical activity and affect: A real-time ambulatory study in persons with and without depressive and anxiety disorders Sonia Difrancesco , Brenda W. J. H. Penninx , Kathleen R. Merikangas , Albert M. van Hemert , Harriëtte Riese , Femke Lamers ,
Main Findings. The results indicated that (i) anxiety partially mediated the effects of both stress and self-esteem upon depression, (ii) that stress partially mediated the effects of anxiety and positive affect upon depression, (iii) that stress completely mediated the effects of self-esteem on depression, and (iv) that there was a significant interaction between stress and negative affect ...
Even though there are few studies at the single level on depression, anxiety, and psychological distress, to the investigator's knowledge, this systematic review and meta-analysis study was the first of its kind that assessed the average prevalence of anxiety, depression, and psychological distress in the global community during the COVID-19 ...
In line with the Research Domain Criteria Project launched by the National Institute of Mental Health (Insel, 2014; Insel et al., 2010), a distinguished aim in developing an integrated neuroscientific model of depression therefore has to be the separation of distinct aetiological and pathophysiological trajectories which, although eventually ...
In this paper, we explored whether social psychological strains are related to depression, anxiety, and stress in non-clinical populations. Methods 6,305 college students (39.3% men; 60.7% women) from six Chinese provincial-level jurisdictions completed a paper-and-pencil survey with Psychological Strain Scales (PSS-40) and Depression, Anxiety ...
This paper concludes with various strategies to address stress, depression, and anxiety, in undergraduate STEM students including building resiliency, establishing peer support, and confiding in ...
Loneliness is associated with major depressive disorder (MDD), and likely also with generalized anxiety disorder (GAD). It is unclear if these associations are moderated by age, sex, or genetic ...
anxiety and depression on academic performance, as well as the role that social support has in relation to these variables. Research involving social support as it relates to academic achievement in college students is limited. Particularly, there is a gap in literature exploring this relationship with students that have anxiety and/or depression.
Introduction. Anxiety disorders are estimated to have the highest lifetime prevalence rates of all psychiatric disorders (18.0-3.7%; []) and result in considerable functional impairment and economic burden [2•, 3, 4].As a result, anxiety disorders have a significant and detrimental impact on QOL across the lifespan, particularly when left untreated [5, 6].
Box 1.1 Broad Street. The O'Sullivans live in a three-storey Victorian house in need of repair, in a northern English city. The extended family consists of Maria, 53, who is married to Ged; her parents, Bridie and Anthony; and Maria and Ged's sons, Patrick, 18, Francis, 20, and John-Paul, 23. Maria's brother, Frank, killed himself 10 ...
Abstract. Anxiety disorders are the most prevalent psychiatric disorders. There is a high comorbidity between anxiety (especially generalized anxiety disorders or panic disorders) and depressive disorders or between anxiety disorders, which renders treatment more complex. Current guidelines do not recommend benzodiazepines as first-line ...
Introduction: One of the most common sleep disorders, insomnia is a significant public health concern. Several psychiatric disorders, such as anxiety disorders and depression, have shown strong relationships with insomnia. However, the clinical impact of the combination of these two conditions on insomnia severity and sleep quality remains unknown.