

10 Case Study Advantages and Disadvantages
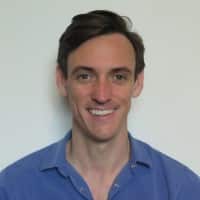
Chris Drew (PhD)
Dr. Chris Drew is the founder of the Helpful Professor. He holds a PhD in education and has published over 20 articles in scholarly journals. He is the former editor of the Journal of Learning Development in Higher Education. [Image Descriptor: Photo of Chris]
Learn about our Editorial Process
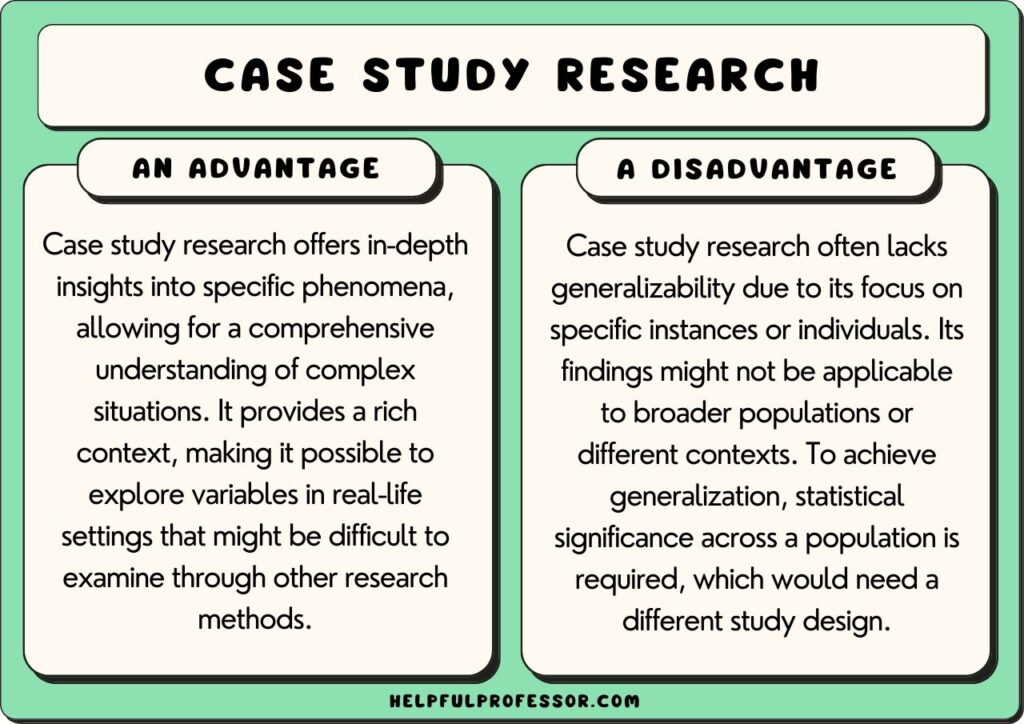
A case study in academic research is a detailed and in-depth examination of a specific instance or event, generally conducted through a qualitative approach to data.
The most common case study definition that I come across is is Robert K. Yin’s (2003, p. 13) quote provided below:
“An empirical inquiry that investigates a contemporary phenomenon within its real-life context, especially when the boundaries between phenomenon and context are not clearly evident.”
Researchers conduct case studies for a number of reasons, such as to explore complex phenomena within their real-life context, to look at a particularly interesting instance of a situation, or to dig deeper into something of interest identified in a wider-scale project.
While case studies render extremely interesting data, they have many limitations and are not suitable for all studies. One key limitation is that a case study’s findings are not usually generalizable to broader populations because one instance cannot be used to infer trends across populations.
Case Study Advantages and Disadvantages
1. in-depth analysis of complex phenomena.
Case study design allows researchers to delve deeply into intricate issues and situations.
By focusing on a specific instance or event, researchers can uncover nuanced details and layers of understanding that might be missed with other research methods, especially large-scale survey studies.
As Lee and Saunders (2017) argue,
“It allows that particular event to be studies in detail so that its unique qualities may be identified.”
This depth of analysis can provide rich insights into the underlying factors and dynamics of the studied phenomenon.
2. Holistic Understanding
Building on the above point, case studies can help us to understand a topic holistically and from multiple angles.
This means the researcher isn’t restricted to just examining a topic by using a pre-determined set of questions, as with questionnaires. Instead, researchers can use qualitative methods to delve into the many different angles, perspectives, and contextual factors related to the case study.
We can turn to Lee and Saunders (2017) again, who notes that case study researchers “develop a deep, holistic understanding of a particular phenomenon” with the intent of deeply understanding the phenomenon.
3. Examination of rare and Unusual Phenomena
We need to use case study methods when we stumble upon “rare and unusual” (Lee & Saunders, 2017) phenomena that would tend to be seen as mere outliers in population studies.
Take, for example, a child genius. A population study of all children of that child’s age would merely see this child as an outlier in the dataset, and this child may even be removed in order to predict overall trends.
So, to truly come to an understanding of this child and get insights into the environmental conditions that led to this child’s remarkable cognitive development, we need to do an in-depth study of this child specifically – so, we’d use a case study.
4. Helps Reveal the Experiences of Marginalzied Groups
Just as rare and unsual cases can be overlooked in population studies, so too can the experiences, beliefs, and perspectives of marginalized groups.
As Lee and Saunders (2017) argue, “case studies are also extremely useful in helping the expression of the voices of people whose interests are often ignored.”
Take, for example, the experiences of minority populations as they navigate healthcare systems. This was for many years a “hidden” phenomenon, not examined by researchers. It took case study designs to truly reveal this phenomenon, which helped to raise practitioners’ awareness of the importance of cultural sensitivity in medicine.
5. Ideal in Situations where Researchers cannot Control the Variables
Experimental designs – where a study takes place in a lab or controlled environment – are excellent for determining cause and effect . But not all studies can take place in controlled environments (Tetnowski, 2015).
When we’re out in the field doing observational studies or similar fieldwork, we don’t have the freedom to isolate dependent and independent variables. We need to use alternate methods.
Case studies are ideal in such situations.
A case study design will allow researchers to deeply immerse themselves in a setting (potentially combining it with methods such as ethnography or researcher observation) in order to see how phenomena take place in real-life settings.
6. Supports the generation of new theories or hypotheses
While large-scale quantitative studies such as cross-sectional designs and population surveys are excellent at testing theories and hypotheses on a large scale, they need a hypothesis to start off with!
This is where case studies – in the form of grounded research – come in. Often, a case study doesn’t start with a hypothesis. Instead, it ends with a hypothesis based upon the findings within a singular setting.
The deep analysis allows for hypotheses to emerge, which can then be taken to larger-scale studies in order to conduct further, more generalizable, testing of the hypothesis or theory.
7. Reveals the Unexpected
When a largescale quantitative research project has a clear hypothesis that it will test, it often becomes very rigid and has tunnel-vision on just exploring the hypothesis.
Of course, a structured scientific examination of the effects of specific interventions targeted at specific variables is extermely valuable.
But narrowly-focused studies often fail to shine a spotlight on unexpected and emergent data. Here, case studies come in very useful. Oftentimes, researchers set their eyes on a phenomenon and, when examining it closely with case studies, identify data and come to conclusions that are unprecedented, unforeseen, and outright surprising.
As Lars Meier (2009, p. 975) marvels, “where else can we become a part of foreign social worlds and have the chance to become aware of the unexpected?”
Disadvantages
1. not usually generalizable.
Case studies are not generalizable because they tend not to look at a broad enough corpus of data to be able to infer that there is a trend across a population.
As Yang (2022) argues, “by definition, case studies can make no claims to be typical.”
Case studies focus on one specific instance of a phenomenon. They explore the context, nuances, and situational factors that have come to bear on the case study. This is really useful for bringing to light important, new, and surprising information, as I’ve already covered.
But , it’s not often useful for generating data that has validity beyond the specific case study being examined.
2. Subjectivity in interpretation
Case studies usually (but not always) use qualitative data which helps to get deep into a topic and explain it in human terms, finding insights unattainable by quantitative data.
But qualitative data in case studies relies heavily on researcher interpretation. While researchers can be trained and work hard to focus on minimizing subjectivity (through methods like triangulation), it often emerges – some might argue it’s innevitable in qualitative studies.
So, a criticism of case studies could be that they’re more prone to subjectivity – and researchers need to take strides to address this in their studies.
3. Difficulty in replicating results
Case study research is often non-replicable because the study takes place in complex real-world settings where variables are not controlled.
So, when returning to a setting to re-do or attempt to replicate a study, we often find that the variables have changed to such an extent that replication is difficult. Furthermore, new researchers (with new subjective eyes) may catch things that the other readers overlooked.
Replication is even harder when researchers attempt to replicate a case study design in a new setting or with different participants.
Comprehension Quiz for Students
Question 1: What benefit do case studies offer when exploring the experiences of marginalized groups?
a) They provide generalizable data. b) They help express the voices of often-ignored individuals. c) They control all variables for the study. d) They always start with a clear hypothesis.
Question 2: Why might case studies be considered ideal for situations where researchers cannot control all variables?
a) They provide a structured scientific examination. b) They allow for generalizability across populations. c) They focus on one specific instance of a phenomenon. d) They allow for deep immersion in real-life settings.
Question 3: What is a primary disadvantage of case studies in terms of data applicability?
a) They always focus on the unexpected. b) They are not usually generalizable. c) They support the generation of new theories. d) They provide a holistic understanding.
Question 4: Why might case studies be considered more prone to subjectivity?
a) They always use quantitative data. b) They heavily rely on researcher interpretation, especially with qualitative data. c) They are always replicable. d) They look at a broad corpus of data.
Question 5: In what situations are experimental designs, such as those conducted in labs, most valuable?
a) When there’s a need to study rare and unusual phenomena. b) When a holistic understanding is required. c) When determining cause-and-effect relationships. d) When the study focuses on marginalized groups.
Question 6: Why is replication challenging in case study research?
a) Because they always use qualitative data. b) Because they tend to focus on a broad corpus of data. c) Due to the changing variables in complex real-world settings. d) Because they always start with a hypothesis.
Lee, B., & Saunders, M. N. K. (2017). Conducting Case Study Research for Business and Management Students. SAGE Publications.
Meir, L. (2009). Feasting on the Benefits of Case Study Research. In Mills, A. J., Wiebe, E., & Durepos, G. (Eds.). Encyclopedia of Case Study Research (Vol. 2). London: SAGE Publications.
Tetnowski, J. (2015). Qualitative case study research design. Perspectives on fluency and fluency disorders , 25 (1), 39-45. ( Source )
Yang, S. L. (2022). The War on Corruption in China: Local Reform and Innovation . Taylor & Francis.
Yin, R. (2003). Case Study research. Thousand Oaks, CA: Sage.
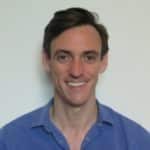
- Chris Drew (PhD) https://helpfulprofessor.com/author/chris-drew-phd/ 25 No-Prep Games for the Classroom (Emergency Games!)
- Chris Drew (PhD) https://helpfulprofessor.com/author/chris-drew-phd/ 101 Class Group Name Ideas (for School Students)
- Chris Drew (PhD) https://helpfulprofessor.com/author/chris-drew-phd/ 19 Top Cognitive Psychology Theories (Explained)
- Chris Drew (PhD) https://helpfulprofessor.com/author/chris-drew-phd/ 119 Bloom’s Taxonomy Examples
Leave a Comment Cancel Reply
Your email address will not be published. Required fields are marked *

Case Study Method – 18 Advantages and Disadvantages
The case study method uses investigatory research as a way to collect data about specific demographics. This approach can apply to individuals, businesses, groups, or events. Each participant receives an equal amount of participation, offering information for collection that can then find new insights into specific trends, ideas, of hypotheses.
Interviews and research observation are the two standard methods of data collection used when following the case study method.
Researchers initially developed the case study method to develop and support hypotheses in clinical medicine. The benefits found in these efforts led the approach to transition to other industries, allowing for the examination of results through proposed decisions, processes, or outcomes. Its unique approach to information makes it possible for others to glean specific points of wisdom that encourage growth.
Several case study method advantages and disadvantages can appear when researchers take this approach.
List of the Advantages of the Case Study Method
1. It requires an intensive study of a specific unit. Researchers must document verifiable data from direct observations when using the case study method. This work offers information about the input processes that go into the hypothesis under consideration. A casual approach to data-gathering work is not effective if a definitive outcome is desired. Each behavior, choice, or comment is a critical component that can verify or dispute the ideas being considered.
Intensive programs can require a significant amount of work for researchers, but it can also promote an improvement in the data collected. That means a hypothesis can receive immediate verification in some situations.
2. No sampling is required when following the case study method. This research method studies social units in their entire perspective instead of pulling individual data points out to analyze them. That means there is no sampling work required when using the case study method. The hypothesis under consideration receives support because it works to turn opinions into facts, verifying or denying the proposals that outside observers can use in the future.
Although researchers might pay attention to specific incidents or outcomes based on generalized behaviors or ideas, the study itself won’t sample those situations. It takes a look at the “bigger vision” instead.
3. This method offers a continuous analysis of the facts. The case study method will look at the facts continuously for the social group being studied by researchers. That means there aren’t interruptions in the process that could limit the validity of the data being collected through this work. This advantage reduces the need to use assumptions when drawing conclusions from the information, adding validity to the outcome of the study over time. That means the outcome becomes relevant to both sides of the equation as it can prove specific suppositions or invalidate a hypothesis under consideration.
This advantage can lead to inefficiencies because of the amount of data being studied by researchers. It is up to the individuals involved in the process to sort out what is useful and meaningful and what is not.
4. It is a useful approach to take when formulating a hypothesis. Researchers will use the case study method advantages to verify a hypothesis under consideration. It is not unusual for the collected data to lead people toward the formulation of new ideas after completing this work. This process encourages further study because it allows concepts to evolve as people do in social or physical environments. That means a complete data set can be gathered based on the skills of the researcher and the honesty of the individuals involved in the study itself.
Although this approach won’t develop a societal-level evaluation of a hypothesis, it can look at how specific groups will react in various circumstances. That information can lead to a better decision-making process in the future for everyone involved.
5. It provides an increase in knowledge. The case study method provides everyone with analytical power to increase knowledge. This advantage is possible because it uses a variety of methodologies to collect information while evaluating a hypothesis. Researchers prefer to use direct observation and interviews to complete their work, but it can also advantage through the use of questionnaires. Participants might need to fill out a journal or diary about their experiences that can be used to study behaviors or choices.
Some researchers incorporate memory tests and experimental tasks to determine how social groups will interact or respond in specific situations. All of this data then works to verify the possibilities that a hypothesis proposes.
6. The case study method allows for comparisons. The human experience is one that is built on individual observations from group situations. Specific demographics might think, act, or respond in particular ways to stimuli, but each person in that group will also contribute a small part to the whole. You could say that people are sponges that collect data from one another every day to create individual outcomes.
The case study method allows researchers to take the information from each demographic for comparison purposes. This information can then lead to proposals that support a hypothesis or lead to its disruption.
7. Data generalization is possible using the case study method. The case study method provides a foundation for data generalization, allowing researches to illustrate their statistical findings in meaningful ways. It puts the information into a usable format that almost anyone can use if they have the need to evaluate the hypothesis under consideration. This process makes it easier to discover unusual features, unique outcomes, or find conclusions that wouldn’t be available without this method. It does an excellent job of identifying specific concepts that relate to the proposed ideas that researchers were verifying through their work.
Generalization does not apply to a larger population group with the case study method. What researchers can do with this information is to suggest a predictable outcome when similar groups are placed in an equal situation.
8. It offers a comprehensive approach to research. Nothing gets ignored when using the case study method to collect information. Every person, place, or thing involved in the research receives the complete attention of those seeking data. The interactions are equal, which means the data is comprehensive and directly reflective of the group being observed.
This advantage means that there are fewer outliers to worry about when researching an idea, leading to a higher level of accuracy in the conclusions drawn by the researchers.
9. The identification of deviant cases is possible with this method. The case study method of research makes it easier to identify deviant cases that occur in each social group. These incidents are units (people) that behave in ways that go against the hypothesis under consideration. Instead of ignoring them like other options do when collecting data, this approach incorporates the “rogue” behavior to understand why it exists in the first place.
This advantage makes the eventual data and conclusions gathered more reliable because it incorporates the “alternative opinion” that exists. One might say that the case study method places as much emphasis on the yin as it does the yang so that the whole picture becomes available to the outside observer.
10. Questionnaire development is possible with the case study method. Interviews and direct observation are the preferred methods of implementing the case study method because it is cheap and done remotely. The information gathered by researchers can also lead to farming questionnaires that can farm additional data from those being studied. When all of the data resources come together, it is easier to formulate a conclusion that accurately reflects the demographics.
Some people in the case study method may try to manipulate the results for personal reasons, but this advantage makes it possible to identify this information readily. Then researchers can look into the thinking that goes into the dishonest behaviors observed.
List of the Disadvantages of the Case Study Method
1. The case study method offers limited representation. The usefulness of the case study method is limited to a specific group of representatives. Researchers are looking at a specific demographic when using this option. That means it is impossible to create any generalization that applies to the rest of society, an organization, or a larger community with this work. The findings can only apply to other groups caught in similar circumstances with the same experiences.
It is useful to use the case study method when attempting to discover the specific reasons why some people behave in a specific way. If researchers need something more generalized, then a different method must be used.
2. No classification is possible with the case study method. This disadvantage is also due to the sample size in the case study method. No classification is possible because researchers are studying such a small unit, group, or demographic. It can be an inefficient process since the skills of the researcher help to determine the quality of the data being collected to verify the validity of a hypothesis. Some participants may be unwilling to answer or participate, while others might try to guess at the outcome to support it.
Researchers can get trapped in a place where they explore more tangents than the actual hypothesis with this option. Classification can occur within the units being studied, but this data cannot extrapolate to other demographics.
3. The case study method still offers the possibility of errors. Each person has an unconscious bias that influences their behaviors and choices. The case study method can find outliers that oppose a hypothesis fairly easily thanks to its emphasis on finding facts, but it is up to the researchers to determine what information qualifies for this designation. If the results from the case study method are surprising or go against the opinion of participating individuals, then there is still the possibility that the information will not be 100% accurate.
Researchers must have controls in place that dictate how data gathering work occurs. Without this limitation in place, the results of the study cannot be guaranteed because of the presence of bias.
4. It is a subjective method to use for research. Although the purpose of the case study method of research is to gather facts, the foundation of what gets gathered is still based on opinion. It uses the subjective method instead of the objective one when evaluating data, which means there can be another layer of errors in the information to consider.
Imagine that a researcher interprets someone’s response as “angry” when performing direct observation, but the individual was feeling “shame” because of a decision they made. The difference between those two emotions is profound, and it could lead to information disruptions that could be problematic to the eventual work of hypothesis verification.
5. The processes required by the case study method are not useful for everyone. The case study method uses a person’s memories, explanations, and records from photographs and diaries to identify interactions on influences on psychological processes. People are given the chance to describe what happens in the world around them as a way for researchers to gather data. This process can be an advantage in some industries, but it can also be a worthless approach to some groups.
If the social group under study doesn’t have the information, knowledge, or wisdom to provide meaningful data, then the processes are no longer useful. Researchers must weigh the advantages and disadvantages of the case study method before starting their work to determine if the possibility of value exists. If it does not, then a different method may be necessary.
6. It is possible for bias to form in the data. It’s not just an unconscious bias that can form in the data when using the case study method. The narrow study approach can lead to outright discrimination in the data. Researchers can decide to ignore outliers or any other information that doesn’t support their hypothesis when using this method. The subjective nature of this approach makes it difficult to challenge the conclusions that get drawn from this work, and the limited pool of units (people) means that duplication is almost impossible.
That means unethical people can manipulate the results gathered by the case study method to their own advantage without much accountability in the process.
7. This method has no fixed limits to it. This method of research is highly dependent on situational circumstances rather than overarching societal or corporate truths. That means the researcher has no fixed limits of investigation. Even when controls are in place to limit bias or recommend specific activities, the case study method has enough flexibility built into its structures to allow for additional exploration. That means it is possible for this work to continue indefinitely, gathering data that never becomes useful.
Scientists began to track the health of 268 sophomores at Harvard in 1938. The Great Depression was in its final years at that point, so the study hoped to reveal clues that lead to happy and healthy lives. It continues still today, now incorporating the children of the original participants, providing over 80 years of information to sort through for conclusions.
8. The case study method is time-consuming and expensive. The case study method can be affordable in some situations, but the lack of fixed limits and the ability to pursue tangents can make it a costly process in most situations. It takes time to gather the data in the first place, and then researchers must interpret the information received so that they can use it for hypothesis evaluation. There are other methods of data collection that can be less expensive and provide results faster.
That doesn’t mean the case study method is useless. The individualization of results can help the decision-making process advance in a variety of industries successfully. It just takes more time to reach the appropriate conclusion, and that might be a resource that isn’t available.
The advantages and disadvantages of the case study method suggest that the helpfulness of this research option depends on the specific hypothesis under consideration. When researchers have the correct skills and mindset to gather data accurately, then it can lead to supportive data that can verify ideas with tremendous accuracy.
This research method can also be used unethically to produce specific results that can be difficult to challenge.
When bias enters into the structure of the case study method, the processes become inefficient, inaccurate, and harmful to the hypothesis. That’s why great care must be taken when designing a study with this approach. It might be a labor-intensive way to develop conclusions, but the outcomes are often worth the investments needed.
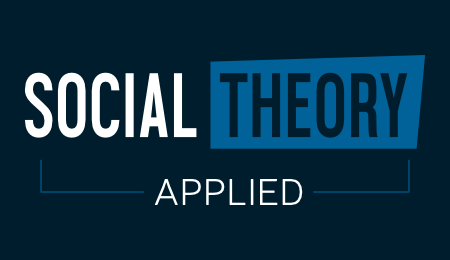
- About This Site
- What is social theory?
- Habermas/Parsons
- Frankfurt School
- Inequalities
- Research Students
- Dirty Looks
- Latest Posts
- Pedagogy & Curriculum
- Contributors
- Publications
Select Page
What are the benefits and drawbacks of case study research?
Posted by Mark Murphy | May 24, 2014 | Method , Research Students | 0
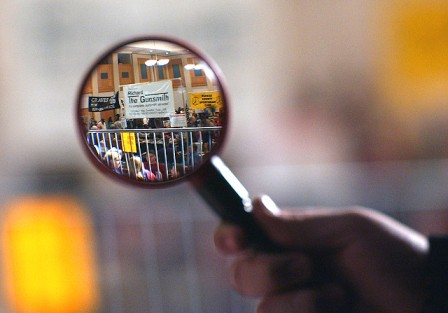
There should be no doubt that with case studies what you gain in depth you lose in breadth – this is the unavoidable compromise that needs to be understood from the beginning of the research process. So this is neither an advantage nor a disadvantage as one aspect cancels out the benefits/drawbacks of the other – there are other benefits and drawbacks that need attention however …
- Their flexibility: case studies are popular for a number of reasons, one being that they can be conducted at various points in the research process. Researchers are known to favour them as a way to develop ideas for more extensive research in the future – pilot studies often take the form of case studies. They are also effective conduits for a broad range of research methods; in that sense they are non-prejudicial against any particular type of research – focus groups are just as welcome in case study research as are questionnaires or participant observation.
- Capturing reality: One of their key benefits is their ability to capture what Hodkinson and Hodkinson call ‘lived reality’ (2001: 3). As they put it, case studies have the potential, when applied successfully, to ‘retain more of the “noise” of real life than many other types of research’ (Hodkinson and Hodkinson, 2001: 3). The importance of ‘noise’ and its place in research is especially important in contexts such as education, for example in schools where background noise is unavoidable. Educational contexts are always complex, and as a result it is difficult to exclude other unwanted variables, ‘some of which may only have real significance for one of their students’ (Hodkinson and Hodkinson, 2001, 4).
- The challenge of generality: At the same time, given their specificity, care needs to be taken when attempting to generalise from the findings. While there’s no inherent flaw in case study design that precludes its broader application, it is preferable that researchers choose their case study sites carefully, while also basing their analysis within existing research findings that have been generated via other research designs. No design is infallible but so often has the claim against case studies been made, that some of the criticism (unwarranted and unfair in many cases) has stuck.
- Suspicion of amateurism: Less partisan researchers might wonder whether the case study offers the time and finance-strapped researcher a convenient and pragmatic source of data, providing findings and recommendations that, given the nature of case studies, can neither be confirmed nor denied, in terms of utility or veracity. Who is to say that case studies offer anything more than a story to tell, and nothing more than that?
- But alongside this suspicion is another more insiduous one – a notion that ‘stories’ are not what social science research is about. This can be a concern for those who favour case study research, as the political consequences can be hard to ignore. That said, so much research is based either on peoples’ lives or the impact of other issues (poverty, institutional policy) on their lives, so the stories of what actually occurs in their lives or in professional environments tend to be an invaluable source of evidence. The fact is that stories (individual, collective, institutional) have a vital role to play in the world of research. And to play the specific v. general card against case study design suggests a tendency towards forms of research fundamentalism as opposed to any kind of rational and objective take on case study’s strengths and limitations.
- Preciousness: Having said that, researchers should not fall into the trap (surprising how often this happens) of assuming that case study data speaks for itself – rarely is this ever the case, an assumption that is as patronising to research subjects as it is false. The role of the researcher is both to describe social phenomena and also to explain – i.e., interpret. Without interpretation the research findings lack meaningful presentation – they present themselves as fact when of course the reality of ‘facts’ is one of the reasons why such research is carried out.
- Conflation of political/research objectives: Another trap that case study researchers sometimes fall into is presenting research findings as if they were self-evidently true, as if the stories were beyond criticism. This is often accompanied by a vague attachment to the notion that research is a political process – one that is performed as a form of liberation against for example policies that seek to ignore the stories of those who ‘suffer’ at the hands of overbearing political or economic imperatives. Case study design should not be viewed as a mechanism for providing a ‘local’ bulwark against the ‘global’ – bur rather as a mechanism for checking the veracity of universalist claims (at least one of its objectives). The valorisation of particularism can only get you so far in social research.
[This post is adapted from material in ‘Research and Education’ (Curtis, Murphy and Shields , Routledge 2014), pp. 80-82].
Reference: Hodkinson, P. and H. Hodkinson (2001). The strengths and limitations of case study research. Paper presented to the Learning and Skills Development Agency conference, Making an impact on policy and practice , Cambridge, 5-7 December 2001, downloaded from h ttp://education.exeter.ac.uk/tlc/docs/publications/LE_PH_PUB_05.12.01.rtf.26.01.2013
About The Author

Mark Murphy
Mark Murphy is a Reader in Education and Public Policy at the University of Glasgow. He previously worked as an academic at King’s College, London, University of Chester, University of Stirling, National University of Ireland, Maynooth, University College Dublin and Northern Illinois University. Mark is an active researcher in the fields of education and public policy. His research interests include educational sociology, critical theory, accountability in higher education, and public sector reform.
Related Posts

Theory shopping – a supervisor perspective
May 3, 2013

Call for book proposals: Theory as method in education research
January 2, 2016

Finding your writing flow
July 15, 2015

What is this thing called ‘Social Theory’?
April 27, 2015
Recent Posts
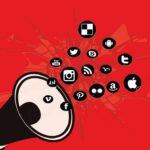
The Advantages and Limitations of Single Case Study Analysis
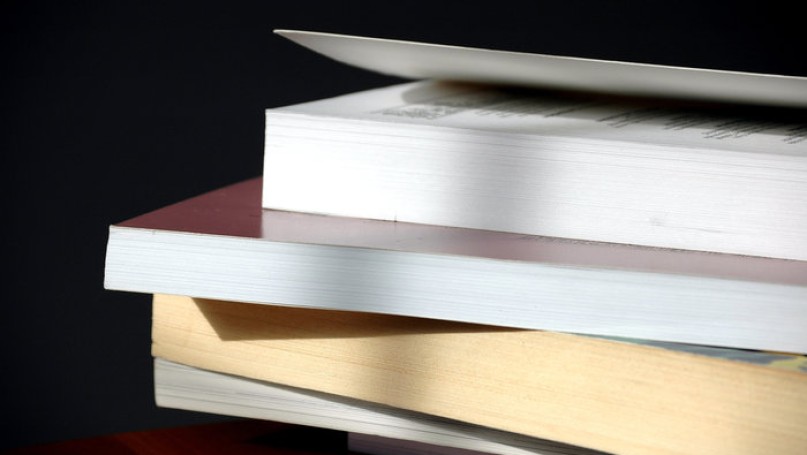
As Andrew Bennett and Colin Elman have recently noted, qualitative research methods presently enjoy “an almost unprecedented popularity and vitality… in the international relations sub-field”, such that they are now “indisputably prominent, if not pre-eminent” (2010: 499). This is, they suggest, due in no small part to the considerable advantages that case study methods in particular have to offer in studying the “complex and relatively unstructured and infrequent phenomena that lie at the heart of the subfield” (Bennett and Elman, 2007: 171). Using selected examples from within the International Relations literature[1], this paper aims to provide a brief overview of the main principles and distinctive advantages and limitations of single case study analysis. Divided into three inter-related sections, the paper therefore begins by first identifying the underlying principles that serve to constitute the case study as a particular research strategy, noting the somewhat contested nature of the approach in ontological, epistemological, and methodological terms. The second part then looks to the principal single case study types and their associated advantages, including those from within the recent ‘third generation’ of qualitative International Relations (IR) research. The final section of the paper then discusses the most commonly articulated limitations of single case studies; while accepting their susceptibility to criticism, it is however suggested that such weaknesses are somewhat exaggerated. The paper concludes that single case study analysis has a great deal to offer as a means of both understanding and explaining contemporary international relations.
The term ‘case study’, John Gerring has suggested, is “a definitional morass… Evidently, researchers have many different things in mind when they talk about case study research” (2006a: 17). It is possible, however, to distil some of the more commonly-agreed principles. One of the most prominent advocates of case study research, Robert Yin (2009: 14) defines it as “an empirical enquiry that investigates a contemporary phenomenon in depth and within its real-life context, especially when the boundaries between phenomenon and context are not clearly evident”. What this definition usefully captures is that case studies are intended – unlike more superficial and generalising methods – to provide a level of detail and understanding, similar to the ethnographer Clifford Geertz’s (1973) notion of ‘thick description’, that allows for the thorough analysis of the complex and particularistic nature of distinct phenomena. Another frequently cited proponent of the approach, Robert Stake, notes that as a form of research the case study “is defined by interest in an individual case, not by the methods of inquiry used”, and that “the object of study is a specific, unique, bounded system” (2008: 443, 445). As such, three key points can be derived from this – respectively concerning issues of ontology, epistemology, and methodology – that are central to the principles of single case study research.
First, the vital notion of ‘boundedness’ when it comes to the particular unit of analysis means that defining principles should incorporate both the synchronic (spatial) and diachronic (temporal) elements of any so-called ‘case’. As Gerring puts it, a case study should be “an intensive study of a single unit… a spatially bounded phenomenon – e.g. a nation-state, revolution, political party, election, or person – observed at a single point in time or over some delimited period of time” (2004: 342). It is important to note, however, that – whereas Gerring refers to a single unit of analysis – it may be that attention also necessarily be given to particular sub-units. This points to the important difference between what Yin refers to as an ‘holistic’ case design, with a single unit of analysis, and an ’embedded’ case design with multiple units of analysis (Yin, 2009: 50-52). The former, for example, would examine only the overall nature of an international organization, whereas the latter would also look to specific departments, programmes, or policies etc.
Secondly, as Tim May notes of the case study approach, “even the most fervent advocates acknowledge that the term has entered into understandings with little specification or discussion of purpose and process” (2011: 220). One of the principal reasons for this, he argues, is the relationship between the use of case studies in social research and the differing epistemological traditions – positivist, interpretivist, and others – within which it has been utilised. Philosophy of science concerns are obviously a complex issue, and beyond the scope of much of this paper. That said, the issue of how it is that we know what we know – of whether or not a single independent reality exists of which we as researchers can seek to provide explanation – does lead us to an important distinction to be made between so-called idiographic and nomothetic case studies (Gerring, 2006b). The former refers to those which purport to explain only a single case, are concerned with particularisation, and hence are typically (although not exclusively) associated with more interpretivist approaches. The latter are those focused studies that reflect upon a larger population and are more concerned with generalisation, as is often so with more positivist approaches[2]. The importance of this distinction, and its relation to the advantages and limitations of single case study analysis, is returned to below.
Thirdly, in methodological terms, given that the case study has often been seen as more of an interpretivist and idiographic tool, it has also been associated with a distinctly qualitative approach (Bryman, 2009: 67-68). However, as Yin notes, case studies can – like all forms of social science research – be exploratory, descriptive, and/or explanatory in nature. It is “a common misconception”, he notes, “that the various research methods should be arrayed hierarchically… many social scientists still deeply believe that case studies are only appropriate for the exploratory phase of an investigation” (Yin, 2009: 6). If case studies can reliably perform any or all three of these roles – and given that their in-depth approach may also require multiple sources of data and the within-case triangulation of methods – then it becomes readily apparent that they should not be limited to only one research paradigm. Exploratory and descriptive studies usually tend toward the qualitative and inductive, whereas explanatory studies are more often quantitative and deductive (David and Sutton, 2011: 165-166). As such, the association of case study analysis with a qualitative approach is a “methodological affinity, not a definitional requirement” (Gerring, 2006a: 36). It is perhaps better to think of case studies as transparadigmatic; it is mistaken to assume single case study analysis to adhere exclusively to a qualitative methodology (or an interpretivist epistemology) even if it – or rather, practitioners of it – may be so inclined. By extension, this also implies that single case study analysis therefore remains an option for a multitude of IR theories and issue areas; it is how this can be put to researchers’ advantage that is the subject of the next section.
Having elucidated the defining principles of the single case study approach, the paper now turns to an overview of its main benefits. As noted above, a lack of consensus still exists within the wider social science literature on the principles and purposes – and by extension the advantages and limitations – of case study research. Given that this paper is directed towards the particular sub-field of International Relations, it suggests Bennett and Elman’s (2010) more discipline-specific understanding of contemporary case study methods as an analytical framework. It begins however, by discussing Harry Eckstein’s seminal (1975) contribution to the potential advantages of the case study approach within the wider social sciences.
Eckstein proposed a taxonomy which usefully identified what he considered to be the five most relevant types of case study. Firstly were so-called configurative-idiographic studies, distinctly interpretivist in orientation and predicated on the assumption that “one cannot attain prediction and control in the natural science sense, but only understanding ( verstehen )… subjective values and modes of cognition are crucial” (1975: 132). Eckstein’s own sceptical view was that any interpreter ‘simply’ considers a body of observations that are not self-explanatory and “without hard rules of interpretation, may discern in them any number of patterns that are more or less equally plausible” (1975: 134). Those of a more post-modernist bent, of course – sharing an “incredulity towards meta-narratives”, in Lyotard’s (1994: xxiv) evocative phrase – would instead suggest that this more free-form approach actually be advantageous in delving into the subtleties and particularities of individual cases.
Eckstein’s four other types of case study, meanwhile, promote a more nomothetic (and positivist) usage. As described, disciplined-configurative studies were essentially about the use of pre-existing general theories, with a case acting “passively, in the main, as a receptacle for putting theories to work” (Eckstein, 1975: 136). As opposed to the opportunity this presented primarily for theory application, Eckstein identified heuristic case studies as explicit theoretical stimulants – thus having instead the intended advantage of theory-building. So-called p lausibility probes entailed preliminary attempts to determine whether initial hypotheses should be considered sound enough to warrant more rigorous and extensive testing. Finally, and perhaps most notably, Eckstein then outlined the idea of crucial case studies , within which he also included the idea of ‘most-likely’ and ‘least-likely’ cases; the essential characteristic of crucial cases being their specific theory-testing function.
Whilst Eckstein’s was an early contribution to refining the case study approach, Yin’s (2009: 47-52) more recent delineation of possible single case designs similarly assigns them roles in the applying, testing, or building of theory, as well as in the study of unique cases[3]. As a subset of the latter, however, Jack Levy (2008) notes that the advantages of idiographic cases are actually twofold. Firstly, as inductive/descriptive cases – akin to Eckstein’s configurative-idiographic cases – whereby they are highly descriptive, lacking in an explicit theoretical framework and therefore taking the form of “total history”. Secondly, they can operate as theory-guided case studies, but ones that seek only to explain or interpret a single historical episode rather than generalise beyond the case. Not only does this therefore incorporate ‘single-outcome’ studies concerned with establishing causal inference (Gerring, 2006b), it also provides room for the more postmodern approaches within IR theory, such as discourse analysis, that may have developed a distinct methodology but do not seek traditional social scientific forms of explanation.
Applying specifically to the state of the field in contemporary IR, Bennett and Elman identify a ‘third generation’ of mainstream qualitative scholars – rooted in a pragmatic scientific realist epistemology and advocating a pluralistic approach to methodology – that have, over the last fifteen years, “revised or added to essentially every aspect of traditional case study research methods” (2010: 502). They identify ‘process tracing’ as having emerged from this as a central method of within-case analysis. As Bennett and Checkel observe, this carries the advantage of offering a methodologically rigorous “analysis of evidence on processes, sequences, and conjunctures of events within a case, for the purposes of either developing or testing hypotheses about causal mechanisms that might causally explain the case” (2012: 10).
Harnessing various methods, process tracing may entail the inductive use of evidence from within a case to develop explanatory hypotheses, and deductive examination of the observable implications of hypothesised causal mechanisms to test their explanatory capability[4]. It involves providing not only a coherent explanation of the key sequential steps in a hypothesised process, but also sensitivity to alternative explanations as well as potential biases in the available evidence (Bennett and Elman 2010: 503-504). John Owen (1994), for example, demonstrates the advantages of process tracing in analysing whether the causal factors underpinning democratic peace theory are – as liberalism suggests – not epiphenomenal, but variously normative, institutional, or some given combination of the two or other unexplained mechanism inherent to liberal states. Within-case process tracing has also been identified as advantageous in addressing the complexity of path-dependent explanations and critical junctures – as for example with the development of political regime types – and their constituent elements of causal possibility, contingency, closure, and constraint (Bennett and Elman, 2006b).
Bennett and Elman (2010: 505-506) also identify the advantages of single case studies that are implicitly comparative: deviant, most-likely, least-likely, and crucial cases. Of these, so-called deviant cases are those whose outcome does not fit with prior theoretical expectations or wider empirical patterns – again, the use of inductive process tracing has the advantage of potentially generating new hypotheses from these, either particular to that individual case or potentially generalisable to a broader population. A classic example here is that of post-independence India as an outlier to the standard modernisation theory of democratisation, which holds that higher levels of socio-economic development are typically required for the transition to, and consolidation of, democratic rule (Lipset, 1959; Diamond, 1992). Absent these factors, MacMillan’s single case study analysis (2008) suggests the particularistic importance of the British colonial heritage, the ideology and leadership of the Indian National Congress, and the size and heterogeneity of the federal state.
Most-likely cases, as per Eckstein above, are those in which a theory is to be considered likely to provide a good explanation if it is to have any application at all, whereas least-likely cases are ‘tough test’ ones in which the posited theory is unlikely to provide good explanation (Bennett and Elman, 2010: 505). Levy (2008) neatly refers to the inferential logic of the least-likely case as the ‘Sinatra inference’ – if a theory can make it here, it can make it anywhere. Conversely, if a theory cannot pass a most-likely case, it is seriously impugned. Single case analysis can therefore be valuable for the testing of theoretical propositions, provided that predictions are relatively precise and measurement error is low (Levy, 2008: 12-13). As Gerring rightly observes of this potential for falsification:
“a positivist orientation toward the work of social science militates toward a greater appreciation of the case study format, not a denigration of that format, as is usually supposed” (Gerring, 2007: 247, emphasis added).
In summary, the various forms of single case study analysis can – through the application of multiple qualitative and/or quantitative research methods – provide a nuanced, empirically-rich, holistic account of specific phenomena. This may be particularly appropriate for those phenomena that are simply less amenable to more superficial measures and tests (or indeed any substantive form of quantification) as well as those for which our reasons for understanding and/or explaining them are irreducibly subjective – as, for example, with many of the normative and ethical issues associated with the practice of international relations. From various epistemological and analytical standpoints, single case study analysis can incorporate both idiographic sui generis cases and, where the potential for generalisation may exist, nomothetic case studies suitable for the testing and building of causal hypotheses. Finally, it should not be ignored that a signal advantage of the case study – with particular relevance to international relations – also exists at a more practical rather than theoretical level. This is, as Eckstein noted, “that it is economical for all resources: money, manpower, time, effort… especially important, of course, if studies are inherently costly, as they are if units are complex collective individuals ” (1975: 149-150, emphasis added).
Limitations
Single case study analysis has, however, been subject to a number of criticisms, the most common of which concern the inter-related issues of methodological rigour, researcher subjectivity, and external validity. With regard to the first point, the prototypical view here is that of Zeev Maoz (2002: 164-165), who suggests that “the use of the case study absolves the author from any kind of methodological considerations. Case studies have become in many cases a synonym for freeform research where anything goes”. The absence of systematic procedures for case study research is something that Yin (2009: 14-15) sees as traditionally the greatest concern due to a relative absence of methodological guidelines. As the previous section suggests, this critique seems somewhat unfair; many contemporary case study practitioners – and representing various strands of IR theory – have increasingly sought to clarify and develop their methodological techniques and epistemological grounding (Bennett and Elman, 2010: 499-500).
A second issue, again also incorporating issues of construct validity, concerns that of the reliability and replicability of various forms of single case study analysis. This is usually tied to a broader critique of qualitative research methods as a whole. However, whereas the latter obviously tend toward an explicitly-acknowledged interpretive basis for meanings, reasons, and understandings:
“quantitative measures appear objective, but only so long as we don’t ask questions about where and how the data were produced… pure objectivity is not a meaningful concept if the goal is to measure intangibles [as] these concepts only exist because we can interpret them” (Berg and Lune, 2010: 340).
The question of researcher subjectivity is a valid one, and it may be intended only as a methodological critique of what are obviously less formalised and researcher-independent methods (Verschuren, 2003). Owen (1994) and Layne’s (1994) contradictory process tracing results of interdemocratic war-avoidance during the Anglo-American crisis of 1861 to 1863 – from liberal and realist standpoints respectively – are a useful example. However, it does also rest on certain assumptions that can raise deeper and potentially irreconcilable ontological and epistemological issues. There are, regardless, plenty such as Bent Flyvbjerg (2006: 237) who suggest that the case study contains no greater bias toward verification than other methods of inquiry, and that “on the contrary, experience indicates that the case study contains a greater bias toward falsification of preconceived notions than toward verification”.
The third and arguably most prominent critique of single case study analysis is the issue of external validity or generalisability. How is it that one case can reliably offer anything beyond the particular? “We always do better (or, in the extreme, no worse) with more observation as the basis of our generalization”, as King et al write; “in all social science research and all prediction, it is important that we be as explicit as possible about the degree of uncertainty that accompanies out prediction” (1994: 212). This is an unavoidably valid criticism. It may be that theories which pass a single crucial case study test, for example, require rare antecedent conditions and therefore actually have little explanatory range. These conditions may emerge more clearly, as Van Evera (1997: 51-54) notes, from large-N studies in which cases that lack them present themselves as outliers exhibiting a theory’s cause but without its predicted outcome. As with the case of Indian democratisation above, it would logically be preferable to conduct large-N analysis beforehand to identify that state’s non-representative nature in relation to the broader population.
There are, however, three important qualifiers to the argument about generalisation that deserve particular mention here. The first is that with regard to an idiographic single-outcome case study, as Eckstein notes, the criticism is “mitigated by the fact that its capability to do so [is] never claimed by its exponents; in fact it is often explicitly repudiated” (1975: 134). Criticism of generalisability is of little relevance when the intention is one of particularisation. A second qualifier relates to the difference between statistical and analytical generalisation; single case studies are clearly less appropriate for the former but arguably retain significant utility for the latter – the difference also between explanatory and exploratory, or theory-testing and theory-building, as discussed above. As Gerring puts it, “theory confirmation/disconfirmation is not the case study’s strong suit” (2004: 350). A third qualification relates to the issue of case selection. As Seawright and Gerring (2008) note, the generalisability of case studies can be increased by the strategic selection of cases. Representative or random samples may not be the most appropriate, given that they may not provide the richest insight (or indeed, that a random and unknown deviant case may appear). Instead, and properly used , atypical or extreme cases “often reveal more information because they activate more actors… and more basic mechanisms in the situation studied” (Flyvbjerg, 2006). Of course, this also points to the very serious limitation, as hinted at with the case of India above, that poor case selection may alternatively lead to overgeneralisation and/or grievous misunderstandings of the relationship between variables or processes (Bennett and Elman, 2006a: 460-463).
As Tim May (2011: 226) notes, “the goal for many proponents of case studies […] is to overcome dichotomies between generalizing and particularizing, quantitative and qualitative, deductive and inductive techniques”. Research aims should drive methodological choices, rather than narrow and dogmatic preconceived approaches. As demonstrated above, there are various advantages to both idiographic and nomothetic single case study analyses – notably the empirically-rich, context-specific, holistic accounts that they have to offer, and their contribution to theory-building and, to a lesser extent, that of theory-testing. Furthermore, while they do possess clear limitations, any research method involves necessary trade-offs; the inherent weaknesses of any one method, however, can potentially be offset by situating them within a broader, pluralistic mixed-method research strategy. Whether or not single case studies are used in this fashion, they clearly have a great deal to offer.
References
Bennett, A. and Checkel, J. T. (2012) ‘Process Tracing: From Philosophical Roots to Best Practice’, Simons Papers in Security and Development, No. 21/2012, School for International Studies, Simon Fraser University: Vancouver.
Bennett, A. and Elman, C. (2006a) ‘Qualitative Research: Recent Developments in Case Study Methods’, Annual Review of Political Science , 9, 455-476.
Bennett, A. and Elman, C. (2006b) ‘Complex Causal Relations and Case Study Methods: The Example of Path Dependence’, Political Analysis , 14, 3, 250-267.
Bennett, A. and Elman, C. (2007) ‘Case Study Methods in the International Relations Subfield’, Comparative Political Studies , 40, 2, 170-195.
Bennett, A. and Elman, C. (2010) Case Study Methods. In C. Reus-Smit and D. Snidal (eds) The Oxford Handbook of International Relations . Oxford University Press: Oxford. Ch. 29.
Berg, B. and Lune, H. (2012) Qualitative Research Methods for the Social Sciences . Pearson: London.
Bryman, A. (2012) Social Research Methods . Oxford University Press: Oxford.
David, M. and Sutton, C. D. (2011) Social Research: An Introduction . SAGE Publications Ltd: London.
Diamond, J. (1992) ‘Economic development and democracy reconsidered’, American Behavioral Scientist , 35, 4/5, 450-499.
Eckstein, H. (1975) Case Study and Theory in Political Science. In R. Gomm, M. Hammersley, and P. Foster (eds) Case Study Method . SAGE Publications Ltd: London.
Flyvbjerg, B. (2006) ‘Five Misunderstandings About Case-Study Research’, Qualitative Inquiry , 12, 2, 219-245.
Geertz, C. (1973) The Interpretation of Cultures: Selected Essays by Clifford Geertz . Basic Books Inc: New York.
Gerring, J. (2004) ‘What is a Case Study and What Is It Good for?’, American Political Science Review , 98, 2, 341-354.
Gerring, J. (2006a) Case Study Research: Principles and Practices . Cambridge University Press: Cambridge.
Gerring, J. (2006b) ‘Single-Outcome Studies: A Methodological Primer’, International Sociology , 21, 5, 707-734.
Gerring, J. (2007) ‘Is There a (Viable) Crucial-Case Method?’, Comparative Political Studies , 40, 3, 231-253.
King, G., Keohane, R. O. and Verba, S. (1994) Designing Social Inquiry: Scientific Inference in Qualitative Research . Princeton University Press: Chichester.
Layne, C. (1994) ‘Kant or Cant: The Myth of the Democratic Peace’, International Security , 19, 2, 5-49.
Levy, J. S. (2008) ‘Case Studies: Types, Designs, and Logics of Inference’, Conflict Management and Peace Science , 25, 1-18.
Lipset, S. M. (1959) ‘Some Social Requisites of Democracy: Economic Development and Political Legitimacy’, The American Political Science Review , 53, 1, 69-105.
Lyotard, J-F. (1984) The Postmodern Condition: A Report on Knowledge . University of Minnesota Press: Minneapolis.
MacMillan, A. (2008) ‘Deviant Democratization in India’, Democratization , 15, 4, 733-749.
Maoz, Z. (2002) Case study methodology in international studies: from storytelling to hypothesis testing. In F. P. Harvey and M. Brecher (eds) Evaluating Methodology in International Studies . University of Michigan Press: Ann Arbor.
May, T. (2011) Social Research: Issues, Methods and Process . Open University Press: Maidenhead.
Owen, J. M. (1994) ‘How Liberalism Produces Democratic Peace’, International Security , 19, 2, 87-125.
Seawright, J. and Gerring, J. (2008) ‘Case Selection Techniques in Case Study Research: A Menu of Qualitative and Quantitative Options’, Political Research Quarterly , 61, 2, 294-308.
Stake, R. E. (2008) Qualitative Case Studies. In N. K. Denzin and Y. S. Lincoln (eds) Strategies of Qualitative Inquiry . Sage Publications: Los Angeles. Ch. 17.
Van Evera, S. (1997) Guide to Methods for Students of Political Science . Cornell University Press: Ithaca.
Verschuren, P. J. M. (2003) ‘Case study as a research strategy: some ambiguities and opportunities’, International Journal of Social Research Methodology , 6, 2, 121-139.
Yin, R. K. (2009) Case Study Research: Design and Methods . SAGE Publications Ltd: London.
[1] The paper follows convention by differentiating between ‘International Relations’ as the academic discipline and ‘international relations’ as the subject of study.
[2] There is some similarity here with Stake’s (2008: 445-447) notion of intrinsic cases, those undertaken for a better understanding of the particular case, and instrumental ones that provide insight for the purposes of a wider external interest.
[3] These may be unique in the idiographic sense, or in nomothetic terms as an exception to the generalising suppositions of either probabilistic or deterministic theories (as per deviant cases, below).
[4] Although there are “philosophical hurdles to mount”, according to Bennett and Checkel, there exists no a priori reason as to why process tracing (as typically grounded in scientific realism) is fundamentally incompatible with various strands of positivism or interpretivism (2012: 18-19). By extension, it can therefore be incorporated by a range of contemporary mainstream IR theories.
— Written by: Ben Willis Written at: University of Plymouth Written for: David Brockington Date written: January 2013
Further Reading on E-International Relations
- Identity in International Conflicts: A Case Study of the Cuban Missile Crisis
- Imperialism’s Legacy in the Study of Contemporary Politics: The Case of Hegemonic Stability Theory
- Recreating a Nation’s Identity Through Symbolism: A Chinese Case Study
- Ontological Insecurity: A Case Study on Israeli-Palestinian Conflict in Jerusalem
- Terrorists or Freedom Fighters: A Case Study of ETA
- A Critical Assessment of Eco-Marxism: A Ghanaian Case Study
Please Consider Donating
Before you download your free e-book, please consider donating to support open access publishing.
E-IR is an independent non-profit publisher run by an all volunteer team. Your donations allow us to invest in new open access titles and pay our bandwidth bills to ensure we keep our existing titles free to view. Any amount, in any currency, is appreciated. Many thanks!
Donations are voluntary and not required to download the e-book - your link to download is below.
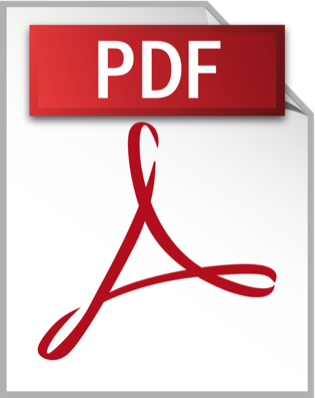
- Privacy Policy

Home » Case Study – Methods, Examples and Guide
Case Study – Methods, Examples and Guide
Table of Contents

A case study is a research method that involves an in-depth examination and analysis of a particular phenomenon or case, such as an individual, organization, community, event, or situation.
It is a qualitative research approach that aims to provide a detailed and comprehensive understanding of the case being studied. Case studies typically involve multiple sources of data, including interviews, observations, documents, and artifacts, which are analyzed using various techniques, such as content analysis, thematic analysis, and grounded theory. The findings of a case study are often used to develop theories, inform policy or practice, or generate new research questions.
Types of Case Study
Types and Methods of Case Study are as follows:
Single-Case Study
A single-case study is an in-depth analysis of a single case. This type of case study is useful when the researcher wants to understand a specific phenomenon in detail.
For Example , A researcher might conduct a single-case study on a particular individual to understand their experiences with a particular health condition or a specific organization to explore their management practices. The researcher collects data from multiple sources, such as interviews, observations, and documents, and uses various techniques to analyze the data, such as content analysis or thematic analysis. The findings of a single-case study are often used to generate new research questions, develop theories, or inform policy or practice.
Multiple-Case Study
A multiple-case study involves the analysis of several cases that are similar in nature. This type of case study is useful when the researcher wants to identify similarities and differences between the cases.
For Example, a researcher might conduct a multiple-case study on several companies to explore the factors that contribute to their success or failure. The researcher collects data from each case, compares and contrasts the findings, and uses various techniques to analyze the data, such as comparative analysis or pattern-matching. The findings of a multiple-case study can be used to develop theories, inform policy or practice, or generate new research questions.
Exploratory Case Study
An exploratory case study is used to explore a new or understudied phenomenon. This type of case study is useful when the researcher wants to generate hypotheses or theories about the phenomenon.
For Example, a researcher might conduct an exploratory case study on a new technology to understand its potential impact on society. The researcher collects data from multiple sources, such as interviews, observations, and documents, and uses various techniques to analyze the data, such as grounded theory or content analysis. The findings of an exploratory case study can be used to generate new research questions, develop theories, or inform policy or practice.
Descriptive Case Study
A descriptive case study is used to describe a particular phenomenon in detail. This type of case study is useful when the researcher wants to provide a comprehensive account of the phenomenon.
For Example, a researcher might conduct a descriptive case study on a particular community to understand its social and economic characteristics. The researcher collects data from multiple sources, such as interviews, observations, and documents, and uses various techniques to analyze the data, such as content analysis or thematic analysis. The findings of a descriptive case study can be used to inform policy or practice or generate new research questions.
Instrumental Case Study
An instrumental case study is used to understand a particular phenomenon that is instrumental in achieving a particular goal. This type of case study is useful when the researcher wants to understand the role of the phenomenon in achieving the goal.
For Example, a researcher might conduct an instrumental case study on a particular policy to understand its impact on achieving a particular goal, such as reducing poverty. The researcher collects data from multiple sources, such as interviews, observations, and documents, and uses various techniques to analyze the data, such as content analysis or thematic analysis. The findings of an instrumental case study can be used to inform policy or practice or generate new research questions.
Case Study Data Collection Methods
Here are some common data collection methods for case studies:
Interviews involve asking questions to individuals who have knowledge or experience relevant to the case study. Interviews can be structured (where the same questions are asked to all participants) or unstructured (where the interviewer follows up on the responses with further questions). Interviews can be conducted in person, over the phone, or through video conferencing.
Observations
Observations involve watching and recording the behavior and activities of individuals or groups relevant to the case study. Observations can be participant (where the researcher actively participates in the activities) or non-participant (where the researcher observes from a distance). Observations can be recorded using notes, audio or video recordings, or photographs.
Documents can be used as a source of information for case studies. Documents can include reports, memos, emails, letters, and other written materials related to the case study. Documents can be collected from the case study participants or from public sources.
Surveys involve asking a set of questions to a sample of individuals relevant to the case study. Surveys can be administered in person, over the phone, through mail or email, or online. Surveys can be used to gather information on attitudes, opinions, or behaviors related to the case study.
Artifacts are physical objects relevant to the case study. Artifacts can include tools, equipment, products, or other objects that provide insights into the case study phenomenon.
How to conduct Case Study Research
Conducting a case study research involves several steps that need to be followed to ensure the quality and rigor of the study. Here are the steps to conduct case study research:
- Define the research questions: The first step in conducting a case study research is to define the research questions. The research questions should be specific, measurable, and relevant to the case study phenomenon under investigation.
- Select the case: The next step is to select the case or cases to be studied. The case should be relevant to the research questions and should provide rich and diverse data that can be used to answer the research questions.
- Collect data: Data can be collected using various methods, such as interviews, observations, documents, surveys, and artifacts. The data collection method should be selected based on the research questions and the nature of the case study phenomenon.
- Analyze the data: The data collected from the case study should be analyzed using various techniques, such as content analysis, thematic analysis, or grounded theory. The analysis should be guided by the research questions and should aim to provide insights and conclusions relevant to the research questions.
- Draw conclusions: The conclusions drawn from the case study should be based on the data analysis and should be relevant to the research questions. The conclusions should be supported by evidence and should be clearly stated.
- Validate the findings: The findings of the case study should be validated by reviewing the data and the analysis with participants or other experts in the field. This helps to ensure the validity and reliability of the findings.
- Write the report: The final step is to write the report of the case study research. The report should provide a clear description of the case study phenomenon, the research questions, the data collection methods, the data analysis, the findings, and the conclusions. The report should be written in a clear and concise manner and should follow the guidelines for academic writing.
Examples of Case Study
Here are some examples of case study research:
- The Hawthorne Studies : Conducted between 1924 and 1932, the Hawthorne Studies were a series of case studies conducted by Elton Mayo and his colleagues to examine the impact of work environment on employee productivity. The studies were conducted at the Hawthorne Works plant of the Western Electric Company in Chicago and included interviews, observations, and experiments.
- The Stanford Prison Experiment: Conducted in 1971, the Stanford Prison Experiment was a case study conducted by Philip Zimbardo to examine the psychological effects of power and authority. The study involved simulating a prison environment and assigning participants to the role of guards or prisoners. The study was controversial due to the ethical issues it raised.
- The Challenger Disaster: The Challenger Disaster was a case study conducted to examine the causes of the Space Shuttle Challenger explosion in 1986. The study included interviews, observations, and analysis of data to identify the technical, organizational, and cultural factors that contributed to the disaster.
- The Enron Scandal: The Enron Scandal was a case study conducted to examine the causes of the Enron Corporation’s bankruptcy in 2001. The study included interviews, analysis of financial data, and review of documents to identify the accounting practices, corporate culture, and ethical issues that led to the company’s downfall.
- The Fukushima Nuclear Disaster : The Fukushima Nuclear Disaster was a case study conducted to examine the causes of the nuclear accident that occurred at the Fukushima Daiichi Nuclear Power Plant in Japan in 2011. The study included interviews, analysis of data, and review of documents to identify the technical, organizational, and cultural factors that contributed to the disaster.
Application of Case Study
Case studies have a wide range of applications across various fields and industries. Here are some examples:
Business and Management
Case studies are widely used in business and management to examine real-life situations and develop problem-solving skills. Case studies can help students and professionals to develop a deep understanding of business concepts, theories, and best practices.
Case studies are used in healthcare to examine patient care, treatment options, and outcomes. Case studies can help healthcare professionals to develop critical thinking skills, diagnose complex medical conditions, and develop effective treatment plans.
Case studies are used in education to examine teaching and learning practices. Case studies can help educators to develop effective teaching strategies, evaluate student progress, and identify areas for improvement.
Social Sciences
Case studies are widely used in social sciences to examine human behavior, social phenomena, and cultural practices. Case studies can help researchers to develop theories, test hypotheses, and gain insights into complex social issues.
Law and Ethics
Case studies are used in law and ethics to examine legal and ethical dilemmas. Case studies can help lawyers, policymakers, and ethical professionals to develop critical thinking skills, analyze complex cases, and make informed decisions.
Purpose of Case Study
The purpose of a case study is to provide a detailed analysis of a specific phenomenon, issue, or problem in its real-life context. A case study is a qualitative research method that involves the in-depth exploration and analysis of a particular case, which can be an individual, group, organization, event, or community.
The primary purpose of a case study is to generate a comprehensive and nuanced understanding of the case, including its history, context, and dynamics. Case studies can help researchers to identify and examine the underlying factors, processes, and mechanisms that contribute to the case and its outcomes. This can help to develop a more accurate and detailed understanding of the case, which can inform future research, practice, or policy.
Case studies can also serve other purposes, including:
- Illustrating a theory or concept: Case studies can be used to illustrate and explain theoretical concepts and frameworks, providing concrete examples of how they can be applied in real-life situations.
- Developing hypotheses: Case studies can help to generate hypotheses about the causal relationships between different factors and outcomes, which can be tested through further research.
- Providing insight into complex issues: Case studies can provide insights into complex and multifaceted issues, which may be difficult to understand through other research methods.
- Informing practice or policy: Case studies can be used to inform practice or policy by identifying best practices, lessons learned, or areas for improvement.
Advantages of Case Study Research
There are several advantages of case study research, including:
- In-depth exploration: Case study research allows for a detailed exploration and analysis of a specific phenomenon, issue, or problem in its real-life context. This can provide a comprehensive understanding of the case and its dynamics, which may not be possible through other research methods.
- Rich data: Case study research can generate rich and detailed data, including qualitative data such as interviews, observations, and documents. This can provide a nuanced understanding of the case and its complexity.
- Holistic perspective: Case study research allows for a holistic perspective of the case, taking into account the various factors, processes, and mechanisms that contribute to the case and its outcomes. This can help to develop a more accurate and comprehensive understanding of the case.
- Theory development: Case study research can help to develop and refine theories and concepts by providing empirical evidence and concrete examples of how they can be applied in real-life situations.
- Practical application: Case study research can inform practice or policy by identifying best practices, lessons learned, or areas for improvement.
- Contextualization: Case study research takes into account the specific context in which the case is situated, which can help to understand how the case is influenced by the social, cultural, and historical factors of its environment.
Limitations of Case Study Research
There are several limitations of case study research, including:
- Limited generalizability : Case studies are typically focused on a single case or a small number of cases, which limits the generalizability of the findings. The unique characteristics of the case may not be applicable to other contexts or populations, which may limit the external validity of the research.
- Biased sampling: Case studies may rely on purposive or convenience sampling, which can introduce bias into the sample selection process. This may limit the representativeness of the sample and the generalizability of the findings.
- Subjectivity: Case studies rely on the interpretation of the researcher, which can introduce subjectivity into the analysis. The researcher’s own biases, assumptions, and perspectives may influence the findings, which may limit the objectivity of the research.
- Limited control: Case studies are typically conducted in naturalistic settings, which limits the control that the researcher has over the environment and the variables being studied. This may limit the ability to establish causal relationships between variables.
- Time-consuming: Case studies can be time-consuming to conduct, as they typically involve a detailed exploration and analysis of a specific case. This may limit the feasibility of conducting multiple case studies or conducting case studies in a timely manner.
- Resource-intensive: Case studies may require significant resources, including time, funding, and expertise. This may limit the ability of researchers to conduct case studies in resource-constrained settings.
About the author
Muhammad Hassan
Researcher, Academic Writer, Web developer
You may also like

Focus Groups – Steps, Examples and Guide

Descriptive Research Design – Types, Methods and...

Triangulation in Research – Types, Methods and...

Qualitative Research – Methods, Analysis Types...

Textual Analysis – Types, Examples and Guide

Exploratory Research – Types, Methods and...
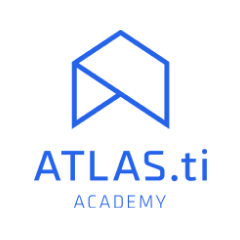
The Ultimate Guide to Qualitative Research - Part 1: The Basics
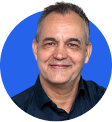
- Introduction and overview
- What is qualitative research?
- What is qualitative data?
- Examples of qualitative data
- Qualitative vs. quantitative research
- Mixed methods
- Qualitative research preparation
- Theoretical perspective
- Theoretical framework
- Literature reviews
Research question
- Conceptual framework
- Conceptual vs. theoretical framework
Data collection
- Qualitative research methods
- Focus groups
- Observational research
What is a case study?
Applications for case study research, what is a good case study, process of case study design, benefits and limitations of case studies.
- Ethnographical research
- Ethical considerations
- Confidentiality and privacy
- Power dynamics
- Reflexivity
Case studies
Case studies are essential to qualitative research , offering a lens through which researchers can investigate complex phenomena within their real-life contexts. This chapter explores the concept, purpose, applications, examples, and types of case studies and provides guidance on how to conduct case study research effectively.
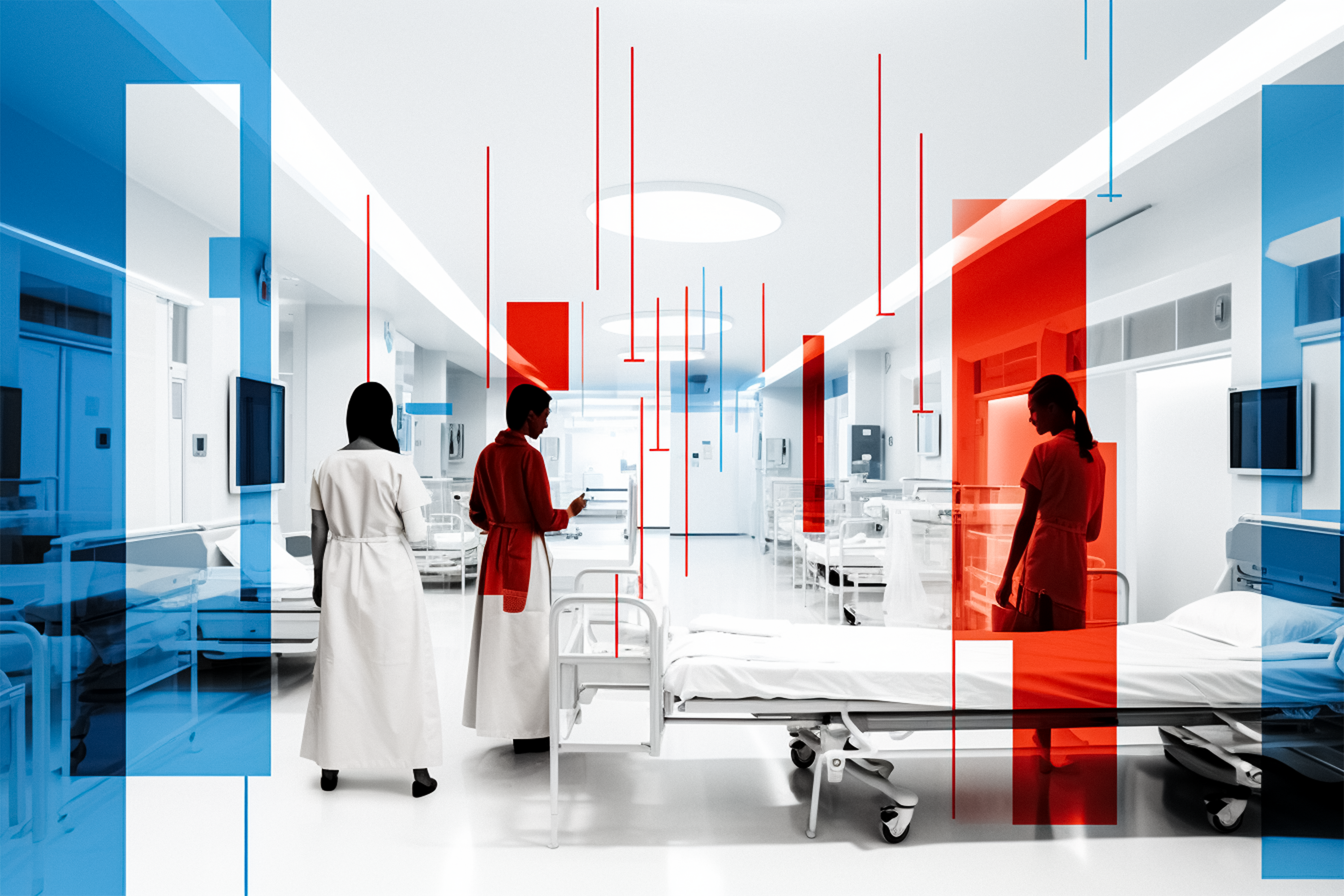
Whereas quantitative methods look at phenomena at scale, case study research looks at a concept or phenomenon in considerable detail. While analyzing a single case can help understand one perspective regarding the object of research inquiry, analyzing multiple cases can help obtain a more holistic sense of the topic or issue. Let's provide a basic definition of a case study, then explore its characteristics and role in the qualitative research process.
Definition of a case study
A case study in qualitative research is a strategy of inquiry that involves an in-depth investigation of a phenomenon within its real-world context. It provides researchers with the opportunity to acquire an in-depth understanding of intricate details that might not be as apparent or accessible through other methods of research. The specific case or cases being studied can be a single person, group, or organization – demarcating what constitutes a relevant case worth studying depends on the researcher and their research question .
Among qualitative research methods , a case study relies on multiple sources of evidence, such as documents, artifacts, interviews , or observations , to present a complete and nuanced understanding of the phenomenon under investigation. The objective is to illuminate the readers' understanding of the phenomenon beyond its abstract statistical or theoretical explanations.
Characteristics of case studies
Case studies typically possess a number of distinct characteristics that set them apart from other research methods. These characteristics include a focus on holistic description and explanation, flexibility in the design and data collection methods, reliance on multiple sources of evidence, and emphasis on the context in which the phenomenon occurs.
Furthermore, case studies can often involve a longitudinal examination of the case, meaning they study the case over a period of time. These characteristics allow case studies to yield comprehensive, in-depth, and richly contextualized insights about the phenomenon of interest.
The role of case studies in research
Case studies hold a unique position in the broader landscape of research methods aimed at theory development. They are instrumental when the primary research interest is to gain an intensive, detailed understanding of a phenomenon in its real-life context.
In addition, case studies can serve different purposes within research - they can be used for exploratory, descriptive, or explanatory purposes, depending on the research question and objectives. This flexibility and depth make case studies a valuable tool in the toolkit of qualitative researchers.
Remember, a well-conducted case study can offer a rich, insightful contribution to both academic and practical knowledge through theory development or theory verification, thus enhancing our understanding of complex phenomena in their real-world contexts.
What is the purpose of a case study?
Case study research aims for a more comprehensive understanding of phenomena, requiring various research methods to gather information for qualitative analysis . Ultimately, a case study can allow the researcher to gain insight into a particular object of inquiry and develop a theoretical framework relevant to the research inquiry.
Why use case studies in qualitative research?
Using case studies as a research strategy depends mainly on the nature of the research question and the researcher's access to the data.
Conducting case study research provides a level of detail and contextual richness that other research methods might not offer. They are beneficial when there's a need to understand complex social phenomena within their natural contexts.
The explanatory, exploratory, and descriptive roles of case studies
Case studies can take on various roles depending on the research objectives. They can be exploratory when the research aims to discover new phenomena or define new research questions; they are descriptive when the objective is to depict a phenomenon within its context in a detailed manner; and they can be explanatory if the goal is to understand specific relationships within the studied context. Thus, the versatility of case studies allows researchers to approach their topic from different angles, offering multiple ways to uncover and interpret the data .
The impact of case studies on knowledge development
Case studies play a significant role in knowledge development across various disciplines. Analysis of cases provides an avenue for researchers to explore phenomena within their context based on the collected data.
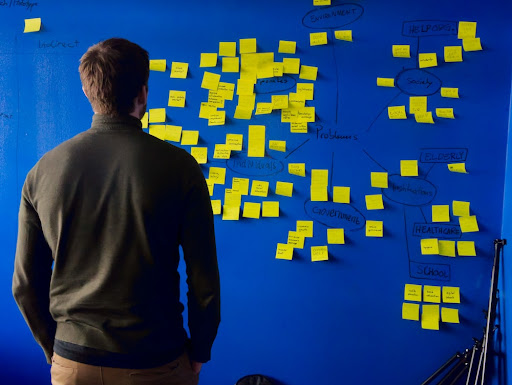
This can result in the production of rich, practical insights that can be instrumental in both theory-building and practice. Case studies allow researchers to delve into the intricacies and complexities of real-life situations, uncovering insights that might otherwise remain hidden.
Types of case studies
In qualitative research , a case study is not a one-size-fits-all approach. Depending on the nature of the research question and the specific objectives of the study, researchers might choose to use different types of case studies. These types differ in their focus, methodology, and the level of detail they provide about the phenomenon under investigation.
Understanding these types is crucial for selecting the most appropriate approach for your research project and effectively achieving your research goals. Let's briefly look at the main types of case studies.
Exploratory case studies
Exploratory case studies are typically conducted to develop a theory or framework around an understudied phenomenon. They can also serve as a precursor to a larger-scale research project. Exploratory case studies are useful when a researcher wants to identify the key issues or questions which can spur more extensive study or be used to develop propositions for further research. These case studies are characterized by flexibility, allowing researchers to explore various aspects of a phenomenon as they emerge, which can also form the foundation for subsequent studies.
Descriptive case studies
Descriptive case studies aim to provide a complete and accurate representation of a phenomenon or event within its context. These case studies are often based on an established theoretical framework, which guides how data is collected and analyzed. The researcher is concerned with describing the phenomenon in detail, as it occurs naturally, without trying to influence or manipulate it.
Explanatory case studies
Explanatory case studies are focused on explanation - they seek to clarify how or why certain phenomena occur. Often used in complex, real-life situations, they can be particularly valuable in clarifying causal relationships among concepts and understanding the interplay between different factors within a specific context.
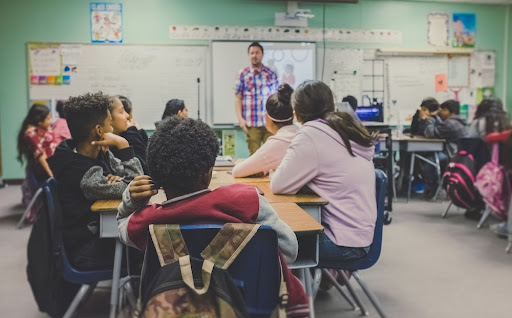
Intrinsic, instrumental, and collective case studies
These three categories of case studies focus on the nature and purpose of the study. An intrinsic case study is conducted when a researcher has an inherent interest in the case itself. Instrumental case studies are employed when the case is used to provide insight into a particular issue or phenomenon. A collective case study, on the other hand, involves studying multiple cases simultaneously to investigate some general phenomena.
Each type of case study serves a different purpose and has its own strengths and challenges. The selection of the type should be guided by the research question and objectives, as well as the context and constraints of the research.
The flexibility, depth, and contextual richness offered by case studies make this approach an excellent research method for various fields of study. They enable researchers to investigate real-world phenomena within their specific contexts, capturing nuances that other research methods might miss. Across numerous fields, case studies provide valuable insights into complex issues.
Critical information systems research
Case studies provide a detailed understanding of the role and impact of information systems in different contexts. They offer a platform to explore how information systems are designed, implemented, and used and how they interact with various social, economic, and political factors. Case studies in this field often focus on examining the intricate relationship between technology, organizational processes, and user behavior, helping to uncover insights that can inform better system design and implementation.
Health research
Health research is another field where case studies are highly valuable. They offer a way to explore patient experiences, healthcare delivery processes, and the impact of various interventions in a real-world context.
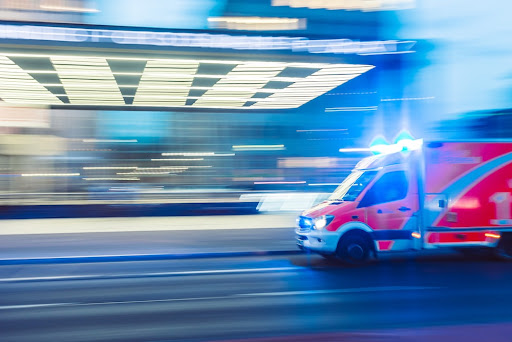
Case studies can provide a deep understanding of a patient's journey, giving insights into the intricacies of disease progression, treatment effects, and the psychosocial aspects of health and illness.
Asthma research studies
Specifically within medical research, studies on asthma often employ case studies to explore the individual and environmental factors that influence asthma development, management, and outcomes. A case study can provide rich, detailed data about individual patients' experiences, from the triggers and symptoms they experience to the effectiveness of various management strategies. This can be crucial for developing patient-centered asthma care approaches.
Other fields
Apart from the fields mentioned, case studies are also extensively used in business and management research, education research, and political sciences, among many others. They provide an opportunity to delve into the intricacies of real-world situations, allowing for a comprehensive understanding of various phenomena.
Case studies, with their depth and contextual focus, offer unique insights across these varied fields. They allow researchers to illuminate the complexities of real-life situations, contributing to both theory and practice.
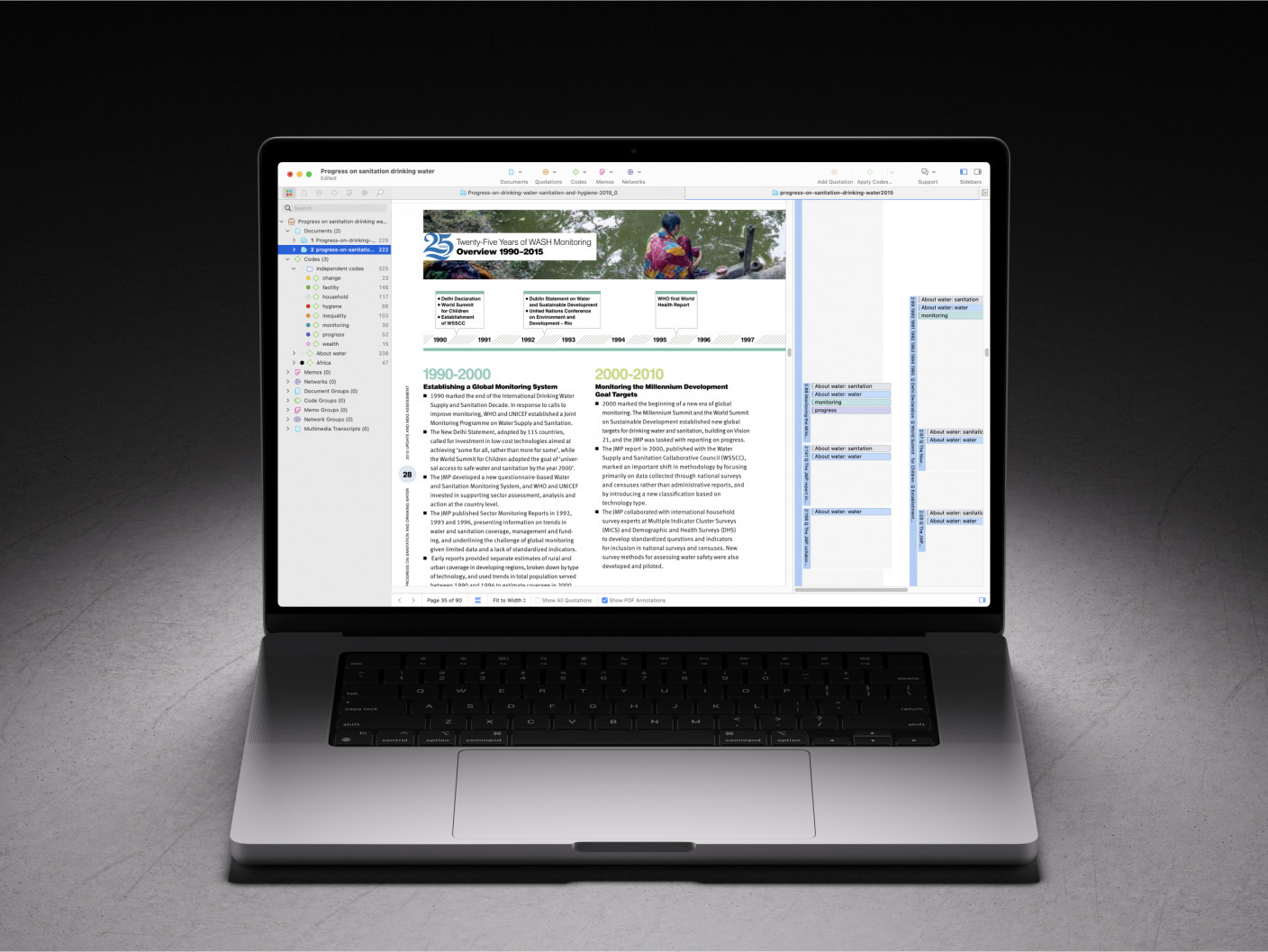
Whatever field you're in, ATLAS.ti puts your data to work for you
Download a free trial of ATLAS.ti to turn your data into insights.
Understanding the key elements of case study design is crucial for conducting rigorous and impactful case study research. A well-structured design guides the researcher through the process, ensuring that the study is methodologically sound and its findings are reliable and valid. The main elements of case study design include the research question , propositions, units of analysis, and the logic linking the data to the propositions.
The research question is the foundation of any research study. A good research question guides the direction of the study and informs the selection of the case, the methods of collecting data, and the analysis techniques. A well-formulated research question in case study research is typically clear, focused, and complex enough to merit further detailed examination of the relevant case(s).
Propositions
Propositions, though not necessary in every case study, provide a direction by stating what we might expect to find in the data collected. They guide how data is collected and analyzed by helping researchers focus on specific aspects of the case. They are particularly important in explanatory case studies, which seek to understand the relationships among concepts within the studied phenomenon.
Units of analysis
The unit of analysis refers to the case, or the main entity or entities that are being analyzed in the study. In case study research, the unit of analysis can be an individual, a group, an organization, a decision, an event, or even a time period. It's crucial to clearly define the unit of analysis, as it shapes the qualitative data analysis process by allowing the researcher to analyze a particular case and synthesize analysis across multiple case studies to draw conclusions.
Argumentation
This refers to the inferential model that allows researchers to draw conclusions from the data. The researcher needs to ensure that there is a clear link between the data, the propositions (if any), and the conclusions drawn. This argumentation is what enables the researcher to make valid and credible inferences about the phenomenon under study.
Understanding and carefully considering these elements in the design phase of a case study can significantly enhance the quality of the research. It can help ensure that the study is methodologically sound and its findings contribute meaningful insights about the case.
Ready to jumpstart your research with ATLAS.ti?
Conceptualize your research project with our intuitive data analysis interface. Download a free trial today.
Conducting a case study involves several steps, from defining the research question and selecting the case to collecting and analyzing data . This section outlines these key stages, providing a practical guide on how to conduct case study research.
Defining the research question
The first step in case study research is defining a clear, focused research question. This question should guide the entire research process, from case selection to analysis. It's crucial to ensure that the research question is suitable for a case study approach. Typically, such questions are exploratory or descriptive in nature and focus on understanding a phenomenon within its real-life context.
Selecting and defining the case
The selection of the case should be based on the research question and the objectives of the study. It involves choosing a unique example or a set of examples that provide rich, in-depth data about the phenomenon under investigation. After selecting the case, it's crucial to define it clearly, setting the boundaries of the case, including the time period and the specific context.
Previous research can help guide the case study design. When considering a case study, an example of a case could be taken from previous case study research and used to define cases in a new research inquiry. Considering recently published examples can help understand how to select and define cases effectively.
Developing a detailed case study protocol
A case study protocol outlines the procedures and general rules to be followed during the case study. This includes the data collection methods to be used, the sources of data, and the procedures for analysis. Having a detailed case study protocol ensures consistency and reliability in the study.
The protocol should also consider how to work with the people involved in the research context to grant the research team access to collecting data. As mentioned in previous sections of this guide, establishing rapport is an essential component of qualitative research as it shapes the overall potential for collecting and analyzing data.
Collecting data
Gathering data in case study research often involves multiple sources of evidence, including documents, archival records, interviews, observations, and physical artifacts. This allows for a comprehensive understanding of the case. The process for gathering data should be systematic and carefully documented to ensure the reliability and validity of the study.
Analyzing and interpreting data
The next step is analyzing the data. This involves organizing the data , categorizing it into themes or patterns , and interpreting these patterns to answer the research question. The analysis might also involve comparing the findings with prior research or theoretical propositions.
Writing the case study report
The final step is writing the case study report . This should provide a detailed description of the case, the data, the analysis process, and the findings. The report should be clear, organized, and carefully written to ensure that the reader can understand the case and the conclusions drawn from it.
Each of these steps is crucial in ensuring that the case study research is rigorous, reliable, and provides valuable insights about the case.
The type, depth, and quality of data in your study can significantly influence the validity and utility of the study. In case study research, data is usually collected from multiple sources to provide a comprehensive and nuanced understanding of the case. This section will outline the various methods of collecting data used in case study research and discuss considerations for ensuring the quality of the data.
Interviews are a common method of gathering data in case study research. They can provide rich, in-depth data about the perspectives, experiences, and interpretations of the individuals involved in the case. Interviews can be structured , semi-structured , or unstructured , depending on the research question and the degree of flexibility needed.
Observations
Observations involve the researcher observing the case in its natural setting, providing first-hand information about the case and its context. Observations can provide data that might not be revealed in interviews or documents, such as non-verbal cues or contextual information.
Documents and artifacts
Documents and archival records provide a valuable source of data in case study research. They can include reports, letters, memos, meeting minutes, email correspondence, and various public and private documents related to the case.
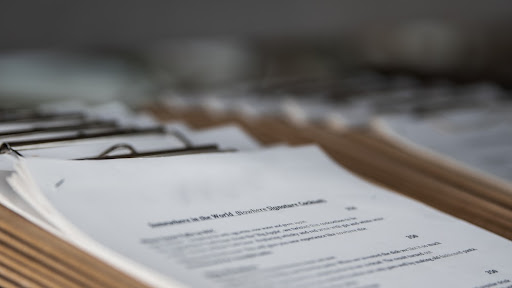
These records can provide historical context, corroborate evidence from other sources, and offer insights into the case that might not be apparent from interviews or observations.
Physical artifacts refer to any physical evidence related to the case, such as tools, products, or physical environments. These artifacts can provide tangible insights into the case, complementing the data gathered from other sources.
Ensuring the quality of data collection
Determining the quality of data in case study research requires careful planning and execution. It's crucial to ensure that the data is reliable, accurate, and relevant to the research question. This involves selecting appropriate methods of collecting data, properly training interviewers or observers, and systematically recording and storing the data. It also includes considering ethical issues related to collecting and handling data, such as obtaining informed consent and ensuring the privacy and confidentiality of the participants.
Data analysis
Analyzing case study research involves making sense of the rich, detailed data to answer the research question. This process can be challenging due to the volume and complexity of case study data. However, a systematic and rigorous approach to analysis can ensure that the findings are credible and meaningful. This section outlines the main steps and considerations in analyzing data in case study research.
Organizing the data
The first step in the analysis is organizing the data. This involves sorting the data into manageable sections, often according to the data source or the theme. This step can also involve transcribing interviews, digitizing physical artifacts, or organizing observational data.
Categorizing and coding the data
Once the data is organized, the next step is to categorize or code the data. This involves identifying common themes, patterns, or concepts in the data and assigning codes to relevant data segments. Coding can be done manually or with the help of software tools, and in either case, qualitative analysis software can greatly facilitate the entire coding process. Coding helps to reduce the data to a set of themes or categories that can be more easily analyzed.
Identifying patterns and themes
After coding the data, the researcher looks for patterns or themes in the coded data. This involves comparing and contrasting the codes and looking for relationships or patterns among them. The identified patterns and themes should help answer the research question.
Interpreting the data
Once patterns and themes have been identified, the next step is to interpret these findings. This involves explaining what the patterns or themes mean in the context of the research question and the case. This interpretation should be grounded in the data, but it can also involve drawing on theoretical concepts or prior research.
Verification of the data
The last step in the analysis is verification. This involves checking the accuracy and consistency of the analysis process and confirming that the findings are supported by the data. This can involve re-checking the original data, checking the consistency of codes, or seeking feedback from research participants or peers.
Like any research method , case study research has its strengths and limitations. Researchers must be aware of these, as they can influence the design, conduct, and interpretation of the study.
Understanding the strengths and limitations of case study research can also guide researchers in deciding whether this approach is suitable for their research question . This section outlines some of the key strengths and limitations of case study research.
Benefits include the following:
- Rich, detailed data: One of the main strengths of case study research is that it can generate rich, detailed data about the case. This can provide a deep understanding of the case and its context, which can be valuable in exploring complex phenomena.
- Flexibility: Case study research is flexible in terms of design , data collection , and analysis . A sufficient degree of flexibility allows the researcher to adapt the study according to the case and the emerging findings.
- Real-world context: Case study research involves studying the case in its real-world context, which can provide valuable insights into the interplay between the case and its context.
- Multiple sources of evidence: Case study research often involves collecting data from multiple sources , which can enhance the robustness and validity of the findings.
On the other hand, researchers should consider the following limitations:
- Generalizability: A common criticism of case study research is that its findings might not be generalizable to other cases due to the specificity and uniqueness of each case.
- Time and resource intensive: Case study research can be time and resource intensive due to the depth of the investigation and the amount of collected data.
- Complexity of analysis: The rich, detailed data generated in case study research can make analyzing the data challenging.
- Subjectivity: Given the nature of case study research, there may be a higher degree of subjectivity in interpreting the data , so researchers need to reflect on this and transparently convey to audiences how the research was conducted.
Being aware of these strengths and limitations can help researchers design and conduct case study research effectively and interpret and report the findings appropriately.
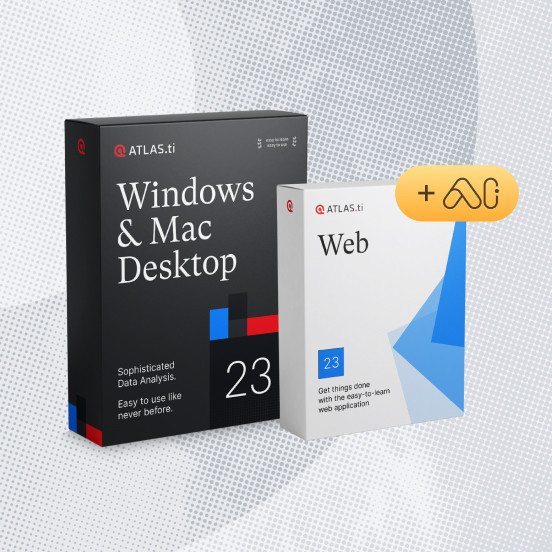
Ready to analyze your data with ATLAS.ti?
See how our intuitive software can draw key insights from your data with a free trial today.
Case Study Research Method in Psychology
Saul Mcleod, PhD
Editor-in-Chief for Simply Psychology
BSc (Hons) Psychology, MRes, PhD, University of Manchester
Saul Mcleod, PhD., is a qualified psychology teacher with over 18 years of experience in further and higher education. He has been published in peer-reviewed journals, including the Journal of Clinical Psychology.
Learn about our Editorial Process
Olivia Guy-Evans, MSc
Associate Editor for Simply Psychology
BSc (Hons) Psychology, MSc Psychology of Education
Olivia Guy-Evans is a writer and associate editor for Simply Psychology. She has previously worked in healthcare and educational sectors.
On This Page:
Case studies are in-depth investigations of a person, group, event, or community. Typically, data is gathered from various sources using several methods (e.g., observations & interviews).
The case study research method originated in clinical medicine (the case history, i.e., the patient’s personal history). In psychology, case studies are often confined to the study of a particular individual.
The information is mainly biographical and relates to events in the individual’s past (i.e., retrospective), as well as to significant events that are currently occurring in his or her everyday life.
The case study is not a research method, but researchers select methods of data collection and analysis that will generate material suitable for case studies.
Freud (1909a, 1909b) conducted very detailed investigations into the private lives of his patients in an attempt to both understand and help them overcome their illnesses.
This makes it clear that the case study is a method that should only be used by a psychologist, therapist, or psychiatrist, i.e., someone with a professional qualification.
There is an ethical issue of competence. Only someone qualified to diagnose and treat a person can conduct a formal case study relating to atypical (i.e., abnormal) behavior or atypical development.
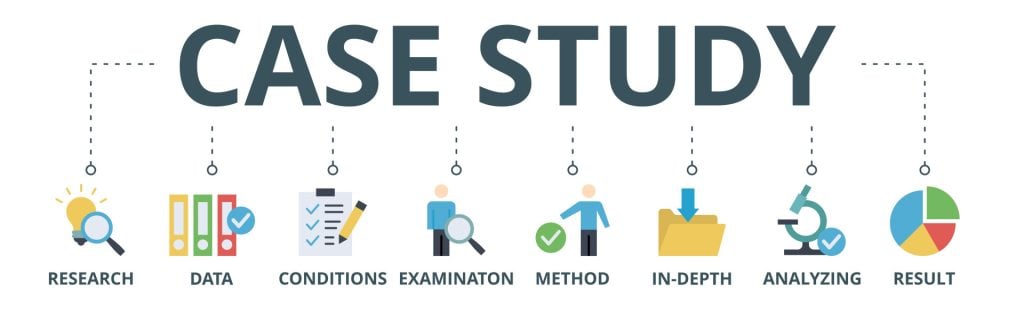
Famous Case Studies
- Anna O – One of the most famous case studies, documenting psychoanalyst Josef Breuer’s treatment of “Anna O” (real name Bertha Pappenheim) for hysteria in the late 1800s using early psychoanalytic theory.
- Little Hans – A child psychoanalysis case study published by Sigmund Freud in 1909 analyzing his five-year-old patient Herbert Graf’s house phobia as related to the Oedipus complex.
- Bruce/Brenda – Gender identity case of the boy (Bruce) whose botched circumcision led psychologist John Money to advise gender reassignment and raise him as a girl (Brenda) in the 1960s.
- Genie Wiley – Linguistics/psychological development case of the victim of extreme isolation abuse who was studied in 1970s California for effects of early language deprivation on acquiring speech later in life.
- Phineas Gage – One of the most famous neuropsychology case studies analyzes personality changes in railroad worker Phineas Gage after an 1848 brain injury involving a tamping iron piercing his skull.
Clinical Case Studies
- Studying the effectiveness of psychotherapy approaches with an individual patient
- Assessing and treating mental illnesses like depression, anxiety disorders, PTSD
- Neuropsychological cases investigating brain injuries or disorders
Child Psychology Case Studies
- Studying psychological development from birth through adolescence
- Cases of learning disabilities, autism spectrum disorders, ADHD
- Effects of trauma, abuse, deprivation on development
Types of Case Studies
- Explanatory case studies : Used to explore causation in order to find underlying principles. Helpful for doing qualitative analysis to explain presumed causal links.
- Exploratory case studies : Used to explore situations where an intervention being evaluated has no clear set of outcomes. It helps define questions and hypotheses for future research.
- Descriptive case studies : Describe an intervention or phenomenon and the real-life context in which it occurred. It is helpful for illustrating certain topics within an evaluation.
- Multiple-case studies : Used to explore differences between cases and replicate findings across cases. Helpful for comparing and contrasting specific cases.
- Intrinsic : Used to gain a better understanding of a particular case. Helpful for capturing the complexity of a single case.
- Collective : Used to explore a general phenomenon using multiple case studies. Helpful for jointly studying a group of cases in order to inquire into the phenomenon.
Where Do You Find Data for a Case Study?
There are several places to find data for a case study. The key is to gather data from multiple sources to get a complete picture of the case and corroborate facts or findings through triangulation of evidence. Most of this information is likely qualitative (i.e., verbal description rather than measurement), but the psychologist might also collect numerical data.
1. Primary sources
- Interviews – Interviewing key people related to the case to get their perspectives and insights. The interview is an extremely effective procedure for obtaining information about an individual, and it may be used to collect comments from the person’s friends, parents, employer, workmates, and others who have a good knowledge of the person, as well as to obtain facts from the person him or herself.
- Observations – Observing behaviors, interactions, processes, etc., related to the case as they unfold in real-time.
- Documents & Records – Reviewing private documents, diaries, public records, correspondence, meeting minutes, etc., relevant to the case.
2. Secondary sources
- News/Media – News coverage of events related to the case study.
- Academic articles – Journal articles, dissertations etc. that discuss the case.
- Government reports – Official data and records related to the case context.
- Books/films – Books, documentaries or films discussing the case.
3. Archival records
Searching historical archives, museum collections and databases to find relevant documents, visual/audio records related to the case history and context.
Public archives like newspapers, organizational records, photographic collections could all include potentially relevant pieces of information to shed light on attitudes, cultural perspectives, common practices and historical contexts related to psychology.
4. Organizational records
Organizational records offer the advantage of often having large datasets collected over time that can reveal or confirm psychological insights.
Of course, privacy and ethical concerns regarding confidential data must be navigated carefully.
However, with proper protocols, organizational records can provide invaluable context and empirical depth to qualitative case studies exploring the intersection of psychology and organizations.
- Organizational/industrial psychology research : Organizational records like employee surveys, turnover/retention data, policies, incident reports etc. may provide insight into topics like job satisfaction, workplace culture and dynamics, leadership issues, employee behaviors etc.
- Clinical psychology : Therapists/hospitals may grant access to anonymized medical records to study aspects like assessments, diagnoses, treatment plans etc. This could shed light on clinical practices.
- School psychology : Studies could utilize anonymized student records like test scores, grades, disciplinary issues, and counseling referrals to study child development, learning barriers, effectiveness of support programs, and more.
How do I Write a Case Study in Psychology?
Follow specified case study guidelines provided by a journal or your psychology tutor. General components of clinical case studies include: background, symptoms, assessments, diagnosis, treatment, and outcomes. Interpreting the information means the researcher decides what to include or leave out. A good case study should always clarify which information is the factual description and which is an inference or the researcher’s opinion.
1. Introduction
- Provide background on the case context and why it is of interest, presenting background information like demographics, relevant history, and presenting problem.
- Compare briefly to similar published cases if applicable. Clearly state the focus/importance of the case.
2. Case Presentation
- Describe the presenting problem in detail, including symptoms, duration,and impact on daily life.
- Include client demographics like age and gender, information about social relationships, and mental health history.
- Describe all physical, emotional, and/or sensory symptoms reported by the client.
- Use patient quotes to describe the initial complaint verbatim. Follow with full-sentence summaries of relevant history details gathered, including key components that led to a working diagnosis.
- Summarize clinical exam results, namely orthopedic/neurological tests, imaging, lab tests, etc. Note actual results rather than subjective conclusions. Provide images if clearly reproducible/anonymized.
- Clearly state the working diagnosis or clinical impression before transitioning to management.
3. Management and Outcome
- Indicate the total duration of care and number of treatments given over what timeframe. Use specific names/descriptions for any therapies/interventions applied.
- Present the results of the intervention,including any quantitative or qualitative data collected.
- For outcomes, utilize visual analog scales for pain, medication usage logs, etc., if possible. Include patient self-reports of improvement/worsening of symptoms. Note the reason for discharge/end of care.
4. Discussion
- Analyze the case, exploring contributing factors, limitations of the study, and connections to existing research.
- Analyze the effectiveness of the intervention,considering factors like participant adherence, limitations of the study, and potential alternative explanations for the results.
- Identify any questions raised in the case analysis and relate insights to established theories and current research if applicable. Avoid definitive claims about physiological explanations.
- Offer clinical implications, and suggest future research directions.
5. Additional Items
- Thank specific assistants for writing support only. No patient acknowledgments.
- References should directly support any key claims or quotes included.
- Use tables/figures/images only if substantially informative. Include permissions and legends/explanatory notes.
- Provides detailed (rich qualitative) information.
- Provides insight for further research.
- Permitting investigation of otherwise impractical (or unethical) situations.
Case studies allow a researcher to investigate a topic in far more detail than might be possible if they were trying to deal with a large number of research participants (nomothetic approach) with the aim of ‘averaging’.
Because of their in-depth, multi-sided approach, case studies often shed light on aspects of human thinking and behavior that would be unethical or impractical to study in other ways.
Research that only looks into the measurable aspects of human behavior is not likely to give us insights into the subjective dimension of experience, which is important to psychoanalytic and humanistic psychologists.
Case studies are often used in exploratory research. They can help us generate new ideas (that might be tested by other methods). They are an important way of illustrating theories and can help show how different aspects of a person’s life are related to each other.
The method is, therefore, important for psychologists who adopt a holistic point of view (i.e., humanistic psychologists ).
Limitations
- Lacking scientific rigor and providing little basis for generalization of results to the wider population.
- Researchers’ own subjective feelings may influence the case study (researcher bias).
- Difficult to replicate.
- Time-consuming and expensive.
- The volume of data, together with the time restrictions in place, impacted the depth of analysis that was possible within the available resources.
Because a case study deals with only one person/event/group, we can never be sure if the case study investigated is representative of the wider body of “similar” instances. This means the conclusions drawn from a particular case may not be transferable to other settings.
Because case studies are based on the analysis of qualitative (i.e., descriptive) data , a lot depends on the psychologist’s interpretation of the information she has acquired.
This means that there is a lot of scope for Anna O , and it could be that the subjective opinions of the psychologist intrude in the assessment of what the data means.
For example, Freud has been criticized for producing case studies in which the information was sometimes distorted to fit particular behavioral theories (e.g., Little Hans ).
This is also true of Money’s interpretation of the Bruce/Brenda case study (Diamond, 1997) when he ignored evidence that went against his theory.
Breuer, J., & Freud, S. (1895). Studies on hysteria . Standard Edition 2: London.
Curtiss, S. (1981). Genie: The case of a modern wild child .
Diamond, M., & Sigmundson, K. (1997). Sex Reassignment at Birth: Long-term Review and Clinical Implications. Archives of Pediatrics & Adolescent Medicine , 151(3), 298-304
Freud, S. (1909a). Analysis of a phobia of a five year old boy. In The Pelican Freud Library (1977), Vol 8, Case Histories 1, pages 169-306
Freud, S. (1909b). Bemerkungen über einen Fall von Zwangsneurose (Der “Rattenmann”). Jb. psychoanal. psychopathol. Forsch ., I, p. 357-421; GW, VII, p. 379-463; Notes upon a case of obsessional neurosis, SE , 10: 151-318.
Harlow J. M. (1848). Passage of an iron rod through the head. Boston Medical and Surgical Journal, 39 , 389–393.
Harlow, J. M. (1868). Recovery from the Passage of an Iron Bar through the Head . Publications of the Massachusetts Medical Society. 2 (3), 327-347.
Money, J., & Ehrhardt, A. A. (1972). Man & Woman, Boy & Girl : The Differentiation and Dimorphism of Gender Identity from Conception to Maturity. Baltimore, Maryland: Johns Hopkins University Press.
Money, J., & Tucker, P. (1975). Sexual signatures: On being a man or a woman.
Further Information
- Case Study Approach
- Case Study Method
- Enhancing the Quality of Case Studies in Health Services Research
- “We do things together” A case study of “couplehood” in dementia
- Using mixed methods for evaluating an integrative approach to cancer care: a case study

- Open access
- Published: 27 June 2011
The case study approach
- Sarah Crowe 1 ,
- Kathrin Cresswell 2 ,
- Ann Robertson 2 ,
- Guro Huby 3 ,
- Anthony Avery 1 &
- Aziz Sheikh 2
BMC Medical Research Methodology volume 11 , Article number: 100 ( 2011 ) Cite this article
788k Accesses
40 Altmetric
Metrics details
The case study approach allows in-depth, multi-faceted explorations of complex issues in their real-life settings. The value of the case study approach is well recognised in the fields of business, law and policy, but somewhat less so in health services research. Based on our experiences of conducting several health-related case studies, we reflect on the different types of case study design, the specific research questions this approach can help answer, the data sources that tend to be used, and the particular advantages and disadvantages of employing this methodological approach. The paper concludes with key pointers to aid those designing and appraising proposals for conducting case study research, and a checklist to help readers assess the quality of case study reports.
Peer Review reports
Introduction
The case study approach is particularly useful to employ when there is a need to obtain an in-depth appreciation of an issue, event or phenomenon of interest, in its natural real-life context. Our aim in writing this piece is to provide insights into when to consider employing this approach and an overview of key methodological considerations in relation to the design, planning, analysis, interpretation and reporting of case studies.
The illustrative 'grand round', 'case report' and 'case series' have a long tradition in clinical practice and research. Presenting detailed critiques, typically of one or more patients, aims to provide insights into aspects of the clinical case and, in doing so, illustrate broader lessons that may be learnt. In research, the conceptually-related case study approach can be used, for example, to describe in detail a patient's episode of care, explore professional attitudes to and experiences of a new policy initiative or service development or more generally to 'investigate contemporary phenomena within its real-life context' [ 1 ]. Based on our experiences of conducting a range of case studies, we reflect on when to consider using this approach, discuss the key steps involved and illustrate, with examples, some of the practical challenges of attaining an in-depth understanding of a 'case' as an integrated whole. In keeping with previously published work, we acknowledge the importance of theory to underpin the design, selection, conduct and interpretation of case studies[ 2 ]. In so doing, we make passing reference to the different epistemological approaches used in case study research by key theoreticians and methodologists in this field of enquiry.
This paper is structured around the following main questions: What is a case study? What are case studies used for? How are case studies conducted? What are the potential pitfalls and how can these be avoided? We draw in particular on four of our own recently published examples of case studies (see Tables 1 , 2 , 3 and 4 ) and those of others to illustrate our discussion[ 3 – 7 ].
What is a case study?
A case study is a research approach that is used to generate an in-depth, multi-faceted understanding of a complex issue in its real-life context. It is an established research design that is used extensively in a wide variety of disciplines, particularly in the social sciences. A case study can be defined in a variety of ways (Table 5 ), the central tenet being the need to explore an event or phenomenon in depth and in its natural context. It is for this reason sometimes referred to as a "naturalistic" design; this is in contrast to an "experimental" design (such as a randomised controlled trial) in which the investigator seeks to exert control over and manipulate the variable(s) of interest.
Stake's work has been particularly influential in defining the case study approach to scientific enquiry. He has helpfully characterised three main types of case study: intrinsic , instrumental and collective [ 8 ]. An intrinsic case study is typically undertaken to learn about a unique phenomenon. The researcher should define the uniqueness of the phenomenon, which distinguishes it from all others. In contrast, the instrumental case study uses a particular case (some of which may be better than others) to gain a broader appreciation of an issue or phenomenon. The collective case study involves studying multiple cases simultaneously or sequentially in an attempt to generate a still broader appreciation of a particular issue.
These are however not necessarily mutually exclusive categories. In the first of our examples (Table 1 ), we undertook an intrinsic case study to investigate the issue of recruitment of minority ethnic people into the specific context of asthma research studies, but it developed into a instrumental case study through seeking to understand the issue of recruitment of these marginalised populations more generally, generating a number of the findings that are potentially transferable to other disease contexts[ 3 ]. In contrast, the other three examples (see Tables 2 , 3 and 4 ) employed collective case study designs to study the introduction of workforce reconfiguration in primary care, the implementation of electronic health records into hospitals, and to understand the ways in which healthcare students learn about patient safety considerations[ 4 – 6 ]. Although our study focusing on the introduction of General Practitioners with Specialist Interests (Table 2 ) was explicitly collective in design (four contrasting primary care organisations were studied), is was also instrumental in that this particular professional group was studied as an exemplar of the more general phenomenon of workforce redesign[ 4 ].
What are case studies used for?
According to Yin, case studies can be used to explain, describe or explore events or phenomena in the everyday contexts in which they occur[ 1 ]. These can, for example, help to understand and explain causal links and pathways resulting from a new policy initiative or service development (see Tables 2 and 3 , for example)[ 1 ]. In contrast to experimental designs, which seek to test a specific hypothesis through deliberately manipulating the environment (like, for example, in a randomised controlled trial giving a new drug to randomly selected individuals and then comparing outcomes with controls),[ 9 ] the case study approach lends itself well to capturing information on more explanatory ' how ', 'what' and ' why ' questions, such as ' how is the intervention being implemented and received on the ground?'. The case study approach can offer additional insights into what gaps exist in its delivery or why one implementation strategy might be chosen over another. This in turn can help develop or refine theory, as shown in our study of the teaching of patient safety in undergraduate curricula (Table 4 )[ 6 , 10 ]. Key questions to consider when selecting the most appropriate study design are whether it is desirable or indeed possible to undertake a formal experimental investigation in which individuals and/or organisations are allocated to an intervention or control arm? Or whether the wish is to obtain a more naturalistic understanding of an issue? The former is ideally studied using a controlled experimental design, whereas the latter is more appropriately studied using a case study design.
Case studies may be approached in different ways depending on the epistemological standpoint of the researcher, that is, whether they take a critical (questioning one's own and others' assumptions), interpretivist (trying to understand individual and shared social meanings) or positivist approach (orientating towards the criteria of natural sciences, such as focusing on generalisability considerations) (Table 6 ). Whilst such a schema can be conceptually helpful, it may be appropriate to draw on more than one approach in any case study, particularly in the context of conducting health services research. Doolin has, for example, noted that in the context of undertaking interpretative case studies, researchers can usefully draw on a critical, reflective perspective which seeks to take into account the wider social and political environment that has shaped the case[ 11 ].
How are case studies conducted?
Here, we focus on the main stages of research activity when planning and undertaking a case study; the crucial stages are: defining the case; selecting the case(s); collecting and analysing the data; interpreting data; and reporting the findings.
Defining the case
Carefully formulated research question(s), informed by the existing literature and a prior appreciation of the theoretical issues and setting(s), are all important in appropriately and succinctly defining the case[ 8 , 12 ]. Crucially, each case should have a pre-defined boundary which clarifies the nature and time period covered by the case study (i.e. its scope, beginning and end), the relevant social group, organisation or geographical area of interest to the investigator, the types of evidence to be collected, and the priorities for data collection and analysis (see Table 7 )[ 1 ]. A theory driven approach to defining the case may help generate knowledge that is potentially transferable to a range of clinical contexts and behaviours; using theory is also likely to result in a more informed appreciation of, for example, how and why interventions have succeeded or failed[ 13 ].
For example, in our evaluation of the introduction of electronic health records in English hospitals (Table 3 ), we defined our cases as the NHS Trusts that were receiving the new technology[ 5 ]. Our focus was on how the technology was being implemented. However, if the primary research interest had been on the social and organisational dimensions of implementation, we might have defined our case differently as a grouping of healthcare professionals (e.g. doctors and/or nurses). The precise beginning and end of the case may however prove difficult to define. Pursuing this same example, when does the process of implementation and adoption of an electronic health record system really begin or end? Such judgements will inevitably be influenced by a range of factors, including the research question, theory of interest, the scope and richness of the gathered data and the resources available to the research team.
Selecting the case(s)
The decision on how to select the case(s) to study is a very important one that merits some reflection. In an intrinsic case study, the case is selected on its own merits[ 8 ]. The case is selected not because it is representative of other cases, but because of its uniqueness, which is of genuine interest to the researchers. This was, for example, the case in our study of the recruitment of minority ethnic participants into asthma research (Table 1 ) as our earlier work had demonstrated the marginalisation of minority ethnic people with asthma, despite evidence of disproportionate asthma morbidity[ 14 , 15 ]. In another example of an intrinsic case study, Hellstrom et al.[ 16 ] studied an elderly married couple living with dementia to explore how dementia had impacted on their understanding of home, their everyday life and their relationships.
For an instrumental case study, selecting a "typical" case can work well[ 8 ]. In contrast to the intrinsic case study, the particular case which is chosen is of less importance than selecting a case that allows the researcher to investigate an issue or phenomenon. For example, in order to gain an understanding of doctors' responses to health policy initiatives, Som undertook an instrumental case study interviewing clinicians who had a range of responsibilities for clinical governance in one NHS acute hospital trust[ 17 ]. Sampling a "deviant" or "atypical" case may however prove even more informative, potentially enabling the researcher to identify causal processes, generate hypotheses and develop theory.
In collective or multiple case studies, a number of cases are carefully selected. This offers the advantage of allowing comparisons to be made across several cases and/or replication. Choosing a "typical" case may enable the findings to be generalised to theory (i.e. analytical generalisation) or to test theory by replicating the findings in a second or even a third case (i.e. replication logic)[ 1 ]. Yin suggests two or three literal replications (i.e. predicting similar results) if the theory is straightforward and five or more if the theory is more subtle. However, critics might argue that selecting 'cases' in this way is insufficiently reflexive and ill-suited to the complexities of contemporary healthcare organisations.
The selected case study site(s) should allow the research team access to the group of individuals, the organisation, the processes or whatever else constitutes the chosen unit of analysis for the study. Access is therefore a central consideration; the researcher needs to come to know the case study site(s) well and to work cooperatively with them. Selected cases need to be not only interesting but also hospitable to the inquiry [ 8 ] if they are to be informative and answer the research question(s). Case study sites may also be pre-selected for the researcher, with decisions being influenced by key stakeholders. For example, our selection of case study sites in the evaluation of the implementation and adoption of electronic health record systems (see Table 3 ) was heavily influenced by NHS Connecting for Health, the government agency that was responsible for overseeing the National Programme for Information Technology (NPfIT)[ 5 ]. This prominent stakeholder had already selected the NHS sites (through a competitive bidding process) to be early adopters of the electronic health record systems and had negotiated contracts that detailed the deployment timelines.
It is also important to consider in advance the likely burden and risks associated with participation for those who (or the site(s) which) comprise the case study. Of particular importance is the obligation for the researcher to think through the ethical implications of the study (e.g. the risk of inadvertently breaching anonymity or confidentiality) and to ensure that potential participants/participating sites are provided with sufficient information to make an informed choice about joining the study. The outcome of providing this information might be that the emotive burden associated with participation, or the organisational disruption associated with supporting the fieldwork, is considered so high that the individuals or sites decide against participation.
In our example of evaluating implementations of electronic health record systems, given the restricted number of early adopter sites available to us, we sought purposively to select a diverse range of implementation cases among those that were available[ 5 ]. We chose a mixture of teaching, non-teaching and Foundation Trust hospitals, and examples of each of the three electronic health record systems procured centrally by the NPfIT. At one recruited site, it quickly became apparent that access was problematic because of competing demands on that organisation. Recognising the importance of full access and co-operative working for generating rich data, the research team decided not to pursue work at that site and instead to focus on other recruited sites.
Collecting the data
In order to develop a thorough understanding of the case, the case study approach usually involves the collection of multiple sources of evidence, using a range of quantitative (e.g. questionnaires, audits and analysis of routinely collected healthcare data) and more commonly qualitative techniques (e.g. interviews, focus groups and observations). The use of multiple sources of data (data triangulation) has been advocated as a way of increasing the internal validity of a study (i.e. the extent to which the method is appropriate to answer the research question)[ 8 , 18 – 21 ]. An underlying assumption is that data collected in different ways should lead to similar conclusions, and approaching the same issue from different angles can help develop a holistic picture of the phenomenon (Table 2 )[ 4 ].
Brazier and colleagues used a mixed-methods case study approach to investigate the impact of a cancer care programme[ 22 ]. Here, quantitative measures were collected with questionnaires before, and five months after, the start of the intervention which did not yield any statistically significant results. Qualitative interviews with patients however helped provide an insight into potentially beneficial process-related aspects of the programme, such as greater, perceived patient involvement in care. The authors reported how this case study approach provided a number of contextual factors likely to influence the effectiveness of the intervention and which were not likely to have been obtained from quantitative methods alone.
In collective or multiple case studies, data collection needs to be flexible enough to allow a detailed description of each individual case to be developed (e.g. the nature of different cancer care programmes), before considering the emerging similarities and differences in cross-case comparisons (e.g. to explore why one programme is more effective than another). It is important that data sources from different cases are, where possible, broadly comparable for this purpose even though they may vary in nature and depth.
Analysing, interpreting and reporting case studies
Making sense and offering a coherent interpretation of the typically disparate sources of data (whether qualitative alone or together with quantitative) is far from straightforward. Repeated reviewing and sorting of the voluminous and detail-rich data are integral to the process of analysis. In collective case studies, it is helpful to analyse data relating to the individual component cases first, before making comparisons across cases. Attention needs to be paid to variations within each case and, where relevant, the relationship between different causes, effects and outcomes[ 23 ]. Data will need to be organised and coded to allow the key issues, both derived from the literature and emerging from the dataset, to be easily retrieved at a later stage. An initial coding frame can help capture these issues and can be applied systematically to the whole dataset with the aid of a qualitative data analysis software package.
The Framework approach is a practical approach, comprising of five stages (familiarisation; identifying a thematic framework; indexing; charting; mapping and interpretation) , to managing and analysing large datasets particularly if time is limited, as was the case in our study of recruitment of South Asians into asthma research (Table 1 )[ 3 , 24 ]. Theoretical frameworks may also play an important role in integrating different sources of data and examining emerging themes. For example, we drew on a socio-technical framework to help explain the connections between different elements - technology; people; and the organisational settings within which they worked - in our study of the introduction of electronic health record systems (Table 3 )[ 5 ]. Our study of patient safety in undergraduate curricula drew on an evaluation-based approach to design and analysis, which emphasised the importance of the academic, organisational and practice contexts through which students learn (Table 4 )[ 6 ].
Case study findings can have implications both for theory development and theory testing. They may establish, strengthen or weaken historical explanations of a case and, in certain circumstances, allow theoretical (as opposed to statistical) generalisation beyond the particular cases studied[ 12 ]. These theoretical lenses should not, however, constitute a strait-jacket and the cases should not be "forced to fit" the particular theoretical framework that is being employed.
When reporting findings, it is important to provide the reader with enough contextual information to understand the processes that were followed and how the conclusions were reached. In a collective case study, researchers may choose to present the findings from individual cases separately before amalgamating across cases. Care must be taken to ensure the anonymity of both case sites and individual participants (if agreed in advance) by allocating appropriate codes or withholding descriptors. In the example given in Table 3 , we decided against providing detailed information on the NHS sites and individual participants in order to avoid the risk of inadvertent disclosure of identities[ 5 , 25 ].
What are the potential pitfalls and how can these be avoided?
The case study approach is, as with all research, not without its limitations. When investigating the formal and informal ways undergraduate students learn about patient safety (Table 4 ), for example, we rapidly accumulated a large quantity of data. The volume of data, together with the time restrictions in place, impacted on the depth of analysis that was possible within the available resources. This highlights a more general point of the importance of avoiding the temptation to collect as much data as possible; adequate time also needs to be set aside for data analysis and interpretation of what are often highly complex datasets.
Case study research has sometimes been criticised for lacking scientific rigour and providing little basis for generalisation (i.e. producing findings that may be transferable to other settings)[ 1 ]. There are several ways to address these concerns, including: the use of theoretical sampling (i.e. drawing on a particular conceptual framework); respondent validation (i.e. participants checking emerging findings and the researcher's interpretation, and providing an opinion as to whether they feel these are accurate); and transparency throughout the research process (see Table 8 )[ 8 , 18 – 21 , 23 , 26 ]. Transparency can be achieved by describing in detail the steps involved in case selection, data collection, the reasons for the particular methods chosen, and the researcher's background and level of involvement (i.e. being explicit about how the researcher has influenced data collection and interpretation). Seeking potential, alternative explanations, and being explicit about how interpretations and conclusions were reached, help readers to judge the trustworthiness of the case study report. Stake provides a critique checklist for a case study report (Table 9 )[ 8 ].
Conclusions
The case study approach allows, amongst other things, critical events, interventions, policy developments and programme-based service reforms to be studied in detail in a real-life context. It should therefore be considered when an experimental design is either inappropriate to answer the research questions posed or impossible to undertake. Considering the frequency with which implementations of innovations are now taking place in healthcare settings and how well the case study approach lends itself to in-depth, complex health service research, we believe this approach should be more widely considered by researchers. Though inherently challenging, the research case study can, if carefully conceptualised and thoughtfully undertaken and reported, yield powerful insights into many important aspects of health and healthcare delivery.
Yin RK: Case study research, design and method. 2009, London: Sage Publications Ltd., 4
Google Scholar
Keen J, Packwood T: Qualitative research; case study evaluation. BMJ. 1995, 311: 444-446.
Article CAS PubMed PubMed Central Google Scholar
Sheikh A, Halani L, Bhopal R, Netuveli G, Partridge M, Car J, et al: Facilitating the Recruitment of Minority Ethnic People into Research: Qualitative Case Study of South Asians and Asthma. PLoS Med. 2009, 6 (10): 1-11.
Article Google Scholar
Pinnock H, Huby G, Powell A, Kielmann T, Price D, Williams S, et al: The process of planning, development and implementation of a General Practitioner with a Special Interest service in Primary Care Organisations in England and Wales: a comparative prospective case study. Report for the National Co-ordinating Centre for NHS Service Delivery and Organisation R&D (NCCSDO). 2008, [ http://www.sdo.nihr.ac.uk/files/project/99-final-report.pdf ]
Robertson A, Cresswell K, Takian A, Petrakaki D, Crowe S, Cornford T, et al: Prospective evaluation of the implementation and adoption of NHS Connecting for Health's national electronic health record in secondary care in England: interim findings. BMJ. 2010, 41: c4564-
Pearson P, Steven A, Howe A, Sheikh A, Ashcroft D, Smith P, the Patient Safety Education Study Group: Learning about patient safety: organisational context and culture in the education of healthcare professionals. J Health Serv Res Policy. 2010, 15: 4-10. 10.1258/jhsrp.2009.009052.
Article PubMed Google Scholar
van Harten WH, Casparie TF, Fisscher OA: The evaluation of the introduction of a quality management system: a process-oriented case study in a large rehabilitation hospital. Health Policy. 2002, 60 (1): 17-37. 10.1016/S0168-8510(01)00187-7.
Stake RE: The art of case study research. 1995, London: Sage Publications Ltd.
Sheikh A, Smeeth L, Ashcroft R: Randomised controlled trials in primary care: scope and application. Br J Gen Pract. 2002, 52 (482): 746-51.
PubMed PubMed Central Google Scholar
King G, Keohane R, Verba S: Designing Social Inquiry. 1996, Princeton: Princeton University Press
Doolin B: Information technology as disciplinary technology: being critical in interpretative research on information systems. Journal of Information Technology. 1998, 13: 301-311. 10.1057/jit.1998.8.
George AL, Bennett A: Case studies and theory development in the social sciences. 2005, Cambridge, MA: MIT Press
Eccles M, the Improved Clinical Effectiveness through Behavioural Research Group (ICEBeRG): Designing theoretically-informed implementation interventions. Implementation Science. 2006, 1: 1-8. 10.1186/1748-5908-1-1.
Article PubMed Central Google Scholar
Netuveli G, Hurwitz B, Levy M, Fletcher M, Barnes G, Durham SR, Sheikh A: Ethnic variations in UK asthma frequency, morbidity, and health-service use: a systematic review and meta-analysis. Lancet. 2005, 365 (9456): 312-7.
Sheikh A, Panesar SS, Lasserson T, Netuveli G: Recruitment of ethnic minorities to asthma studies. Thorax. 2004, 59 (7): 634-
CAS PubMed PubMed Central Google Scholar
Hellström I, Nolan M, Lundh U: 'We do things together': A case study of 'couplehood' in dementia. Dementia. 2005, 4: 7-22. 10.1177/1471301205049188.
Som CV: Nothing seems to have changed, nothing seems to be changing and perhaps nothing will change in the NHS: doctors' response to clinical governance. International Journal of Public Sector Management. 2005, 18: 463-477. 10.1108/09513550510608903.
Lincoln Y, Guba E: Naturalistic inquiry. 1985, Newbury Park: Sage Publications
Barbour RS: Checklists for improving rigour in qualitative research: a case of the tail wagging the dog?. BMJ. 2001, 322: 1115-1117. 10.1136/bmj.322.7294.1115.
Mays N, Pope C: Qualitative research in health care: Assessing quality in qualitative research. BMJ. 2000, 320: 50-52. 10.1136/bmj.320.7226.50.
Mason J: Qualitative researching. 2002, London: Sage
Brazier A, Cooke K, Moravan V: Using Mixed Methods for Evaluating an Integrative Approach to Cancer Care: A Case Study. Integr Cancer Ther. 2008, 7: 5-17. 10.1177/1534735407313395.
Miles MB, Huberman M: Qualitative data analysis: an expanded sourcebook. 1994, CA: Sage Publications Inc., 2
Pope C, Ziebland S, Mays N: Analysing qualitative data. Qualitative research in health care. BMJ. 2000, 320: 114-116. 10.1136/bmj.320.7227.114.
Cresswell KM, Worth A, Sheikh A: Actor-Network Theory and its role in understanding the implementation of information technology developments in healthcare. BMC Med Inform Decis Mak. 2010, 10 (1): 67-10.1186/1472-6947-10-67.
Article PubMed PubMed Central Google Scholar
Malterud K: Qualitative research: standards, challenges, and guidelines. Lancet. 2001, 358: 483-488. 10.1016/S0140-6736(01)05627-6.
Article CAS PubMed Google Scholar
Yin R: Case study research: design and methods. 1994, Thousand Oaks, CA: Sage Publishing, 2
Yin R: Enhancing the quality of case studies in health services research. Health Serv Res. 1999, 34: 1209-1224.
Green J, Thorogood N: Qualitative methods for health research. 2009, Los Angeles: Sage, 2
Howcroft D, Trauth E: Handbook of Critical Information Systems Research, Theory and Application. 2005, Cheltenham, UK: Northampton, MA, USA: Edward Elgar
Book Google Scholar
Blakie N: Approaches to Social Enquiry. 1993, Cambridge: Polity Press
Doolin B: Power and resistance in the implementation of a medical management information system. Info Systems J. 2004, 14: 343-362. 10.1111/j.1365-2575.2004.00176.x.
Bloomfield BP, Best A: Management consultants: systems development, power and the translation of problems. Sociological Review. 1992, 40: 533-560.
Shanks G, Parr A: Positivist, single case study research in information systems: A critical analysis. Proceedings of the European Conference on Information Systems. 2003, Naples
Pre-publication history
The pre-publication history for this paper can be accessed here: http://www.biomedcentral.com/1471-2288/11/100/prepub
Download references
Acknowledgements
We are grateful to the participants and colleagues who contributed to the individual case studies that we have drawn on. This work received no direct funding, but it has been informed by projects funded by Asthma UK, the NHS Service Delivery Organisation, NHS Connecting for Health Evaluation Programme, and Patient Safety Research Portfolio. We would also like to thank the expert reviewers for their insightful and constructive feedback. Our thanks are also due to Dr. Allison Worth who commented on an earlier draft of this manuscript.
Author information
Authors and affiliations.
Division of Primary Care, The University of Nottingham, Nottingham, UK
Sarah Crowe & Anthony Avery
Centre for Population Health Sciences, The University of Edinburgh, Edinburgh, UK
Kathrin Cresswell, Ann Robertson & Aziz Sheikh
School of Health in Social Science, The University of Edinburgh, Edinburgh, UK
You can also search for this author in PubMed Google Scholar
Corresponding author
Correspondence to Sarah Crowe .
Additional information
Competing interests.
The authors declare that they have no competing interests.
Authors' contributions
AS conceived this article. SC, KC and AR wrote this paper with GH, AA and AS all commenting on various drafts. SC and AS are guarantors.
Rights and permissions
This article is published under license to BioMed Central Ltd. This is an Open Access article distributed under the terms of the Creative Commons Attribution License ( http://creativecommons.org/licenses/by/2.0 ), which permits unrestricted use, distribution, and reproduction in any medium, provided the original work is properly cited.
Reprints and permissions
About this article
Cite this article.
Crowe, S., Cresswell, K., Robertson, A. et al. The case study approach. BMC Med Res Methodol 11 , 100 (2011). https://doi.org/10.1186/1471-2288-11-100
Download citation
Received : 29 November 2010
Accepted : 27 June 2011
Published : 27 June 2011
DOI : https://doi.org/10.1186/1471-2288-11-100
Share this article
Anyone you share the following link with will be able to read this content:
Sorry, a shareable link is not currently available for this article.
Provided by the Springer Nature SharedIt content-sharing initiative
- Case Study Approach
- Electronic Health Record System
- Case Study Design
- Case Study Site
- Case Study Report
BMC Medical Research Methodology
ISSN: 1471-2288
- General enquiries: [email protected]

Strengths and Weaknesses of Case Studies
There is no doubt that case studies are a valuable and important form of research for all of the industries and fields that use them. However, along with all their advantages, they also have some disadvantages. In this article we are going to look at both.
Advantages of Case Studies
Intensive Study
Case study method is responsible for intensive study of a unit. It is the investigation and exploration of an event thoroughly and deeply. You get a very detailed and in-depth study of a person or event. This is especially the case with subjects that cannot be physically or ethically recreated.
This is one of the biggest advantages of the Genie case. You cannot lock up a child for 13 years and deprive them of everything. That would be morally and ethically wrong in every single way. So when the opportunity presented itself, researchers could not look away. It was a once in a lifetime opportunity to learn about feral children.
Genie was a feral child. She was raised in completed isolation, with little human contact. Because of the abuse she withstood, she was unable to develop cognitively. From infancy she was strapped to a potty chair, and therefore never acquired the physicality needed for walking, running and jumping.
If Genie made a noise, her father beat her. Therefore, she learned to not make a noise. Once she was found, researchers studied her language skills, and attempted to find ways to get her to communicate. They were successful. While she never gained the ability to speak, she did develop other ways to communicate. However, the public soon lost interest in her case, and with that, the funds to conduct the study.
However, her case was extremely important to child development psychology and linguistic theory. Because of her, we know that mental stimulation is needed for proper development. We also now know that there is a "critical period" for the learning of language.
Developing New Research
Case studies are one of the best ways to stimulate new research. A case study can be completed, and if the findings are valuable, they can lead to new and advanced research in the field. There has been a great deal of research done that wouldn't have been possible without case studies.
An example of this is the sociological study Nickel and Dimed. Nickel and Dimed is a book and study done by Barbara Ehrenreich. She wanted to study poverty in America, and did so by living and working as a person living on minimum wage.
Through her experiment, she discovered that poverty was almost inescapable. As soon as she saved a little money, she was hit with a crisis. She might get sick, or her car might break down, all occurrences that can be destructive when a person doesn't have a safety net to fall back on.
It didn't matter where she lived or what she did. Working a minimum wage job gave her no chances for advancement or improvement whatsoever. And she did the experiment as a woman with no children to support.
This study opened a lot of eyes to the problem of the working poor in America. By living and working as the experiment, Ehrenreich was able to show first-hand data regarding the issues surrounding poverty. The book didn't end with any solutions, just suggestions for the reader and points for them to think about.
Using this case study information, new studies could be organized to learn better ways to help people who are fighting poverty, or better ways to help the working poor.
Contradicting Established Ideas or Theories
Oftentimes there are theories that may be questioned with case studies. For example, in the John/John case study, it was believed that gender and sexual identity were a construct of nurture, not nature.
John-John focused on a set of twin boys, both of whom were circumcised at the age of 6 months. One of the twin's circumcisions failed, causing irreparable damage to the penis. His parents were concerned about the sexual health of their son, so they contacted Dr. John Money for a solution.
Dr. Money believed that sexuality came from nurture, not nature, and that the injured baby, Bruce, could be raised as a girl. His penis was removed and he was sexually reassigned to become a girl. Bruce's name was changed to Brenda, and his parents decided to raise him as a girl.
In this case, Dr. Money was dishonest. He believed that gender could be changed, which has since been proven false. Brenda's parents were also dishonest, stating that the surgery was a success, when in fact that wasn't the case.
As Brenda grew up, she always acted masculine and was teased for it at school. She did not socialize as a girl, and did not identify as a female. When Brenda was 13 she learned the truth, and was incredibly relieved. She changed her name to David, and lived the rest of her life as a male.
This case proved that the general theory was wrong, and is still valuable, even though the study author was dishonest.
Giving New Insight
Case studies have the ability to give insight into phenomena that cannot be learned in any other way. An example of this is the case study about Sidney Bradford. Bradford was blind from the age of 10 months old, and regained his sight at the age of 52 from a corneal transplant.
This unique situation allowed researchers to better learn how perception and motion changes when suddenly given sight. They were able to better understand how colors and dimensions affect the human process. For what it is worth, Bradford continued to live and work with his eyes closed, as he found sight too stimulating.
Another famous study was the sociological study of Milgram.
Stanley Milgram did a study from 1960 to 1974 in which he studied the effects of social pressure. The study was set up as an independent laboratory. A random person would walk in, and agree to be a part of the study. He was told to act as a teacher, and ask questions to another volunteer, who was the learner.
The teacher would ask the learner questions, and whenever he answered incorrectly, the teacher was instructed to give the learner an electric shock. Each time the learner was wrong, the shock would be increased by 15 volts. What the teacher didn't know was that the learner was a part of the experiment, and that no shocks were being given. However, the learner did act as if they were being shocked.
If the teachers tried to quit, they were strongly pushed to continue. The goal of the experiment was to see whether or not any of the teachers would go up to the highest voltage. As it turned out, 65% of the teachers did.
This study opened eyes when it comes to social pressure. If someone tells you it is okay to hurt someone, at what point will the person back off and say "this is not ok!" And in this study, the results were the same, regardless of income, race, gender or ethnicity.
This study opened up the sociological world of understanding the divide between social pressure and morality.
Disadvantages of Case Studies
Inability to Replicate
As demonstrated with the Genie case study, many studies cannot be replicated, and therefore, cannot be corroborated. Because the studies cannot be replicated, it means the data and results are only valid for that one person. Now, one could infer that that results of the Genie study would be the same with other feral children, without additional studies we can never be 100% certain.
Also, Genie was a white, American female. We do not know whether someone with a different gender, race or ethnicity would have a different result.
Key Term! Hawthorne Effect
The effect in which people change their behavior when they are aware they are being observed.
Researcher Bias
When conducting a case study, it is very possible for the author to form a bias. This bias can be for the subject; the form of data collection, or the way the data is interpreted. This is very common, since it is normal for humans to be subjective. It is well known that Sigmund Freud, the father of psychology, was often biased in his case histories and interpretations.
The researcher can become close to a study participant, or may learn to identify with the subject. When this happens the researcher loses their perspective as an outsider.
No Classification
Any classification is not possible due to studying a small unit. This generalization of results is limited, since the study is only focusing on one small group. However, this isn't always a problem, especially if generalization is not one of the study's goals.
Time Intensive
Case studies can be very time consuming. The data collection process can be very intensive and long, and this is something new researchers are not familiar with. It takes a long period of time to develop a case study, and develop a detailed analysis.
Many studies also require the authors to immerse themselves in the case. For example, in the Genie case, the lead researchers spent an abnormal amount of time with Genie, since so few people knew how to handle her. David Rigler, one of the lead researchers, actually had Genie live with him and his family for years. Because of this attachment, many questioned the veracity of the study data.
Possibility of Errors
Case study method may have errors of memory or judgment. Since reconstructing case history is based on memory, this can lead to errors. Also, how one person perceived the past could be different for another person, and this can and does lead to errors.
When considering various aspects of their lives, people tend to focus on issues that they find most important. This allows them to form a prejudice and can make them unaware of other possible options.
Ethical Issues
With small studies, there is always the question of ethics. At what point does a study become unethical? The Genie case was riddled with accusations of being unethical, and people still debate about it today.
Was it ethical to study Genie as deeply as she was studied?
Did Genie deserve to live out her life unbothered by researchers and academics trying to use her case to potentially further their careers?
At what point does the pursuit of scientific knowledge outweigh the right to a life free from research?
Also, because the researchers became so invested in the study, people questioned whether a researcher would report unethical behavior if they witnessed it.
Advantages and Disadvantages in Real-Life Studies
Two of these case studies are the Tylenol Scandal and the Genie language study.
Let's look at the advantages and disadvantages of these two studies.
Genie – Advantages
Uniqueness of study – Being able to study a feral child is a rare occurrence.
Genie – Disadvantages
Ethics - The lead researcher David Rigler provided a home for Genie, and was paid for being a foster parent. This is often seen as unethical, since Rigler had a financial interest in Genie and her case.
Tylenol – Advantages
Uniqueness of study – What happened to Tylenol was very unique and rare. While companies face crisis all the time, a public health crisis of this magnitude is very unique.
Tylenol – Disadvantages
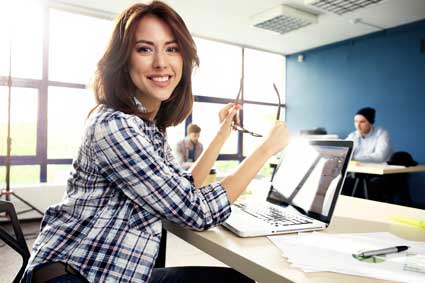
- Course Catalog
- Group Discounts
- Gift Certificates
- For Libraries
- CEU Verification
- Medical Terminology
- Accounting Course
- Writing Basics
- QuickBooks Training
- Proofreading Class
- Sensitivity Training
- Excel Certificate
- Teach Online
- Terms of Service
- Privacy Policy

- Search Menu
Sign in through your institution
- Browse content in Arts and Humanities
- Browse content in Archaeology
- Anglo-Saxon and Medieval Archaeology
- Archaeological Methodology and Techniques
- Archaeology by Region
- Archaeology of Religion
- Archaeology of Trade and Exchange
- Biblical Archaeology
- Contemporary and Public Archaeology
- Environmental Archaeology
- Historical Archaeology
- History and Theory of Archaeology
- Industrial Archaeology
- Landscape Archaeology
- Mortuary Archaeology
- Prehistoric Archaeology
- Underwater Archaeology
- Urban Archaeology
- Zooarchaeology
- Browse content in Architecture
- Architectural Structure and Design
- History of Architecture
- Residential and Domestic Buildings
- Theory of Architecture
- Browse content in Art
- Art Subjects and Themes
- History of Art
- Industrial and Commercial Art
- Theory of Art
- Biographical Studies
- Byzantine Studies
- Browse content in Classical Studies
- Classical History
- Classical Philosophy
- Classical Mythology
- Classical Literature
- Classical Reception
- Classical Art and Architecture
- Classical Oratory and Rhetoric
- Greek and Roman Epigraphy
- Greek and Roman Law
- Greek and Roman Papyrology
- Greek and Roman Archaeology
- Late Antiquity
- Religion in the Ancient World
- Social History
- Digital Humanities
- Browse content in History
- Colonialism and Imperialism
- Diplomatic History
- Environmental History
- Genealogy, Heraldry, Names, and Honours
- Genocide and Ethnic Cleansing
- Historical Geography
- History by Period
- History of Emotions
- History of Agriculture
- History of Education
- History of Gender and Sexuality
- Industrial History
- Intellectual History
- International History
- Labour History
- Legal and Constitutional History
- Local and Family History
- Maritime History
- Military History
- National Liberation and Post-Colonialism
- Oral History
- Political History
- Public History
- Regional and National History
- Revolutions and Rebellions
- Slavery and Abolition of Slavery
- Social and Cultural History
- Theory, Methods, and Historiography
- Urban History
- World History
- Browse content in Language Teaching and Learning
- Language Learning (Specific Skills)
- Language Teaching Theory and Methods
- Browse content in Linguistics
- Applied Linguistics
- Cognitive Linguistics
- Computational Linguistics
- Forensic Linguistics
- Grammar, Syntax and Morphology
- Historical and Diachronic Linguistics
- History of English
- Language Acquisition
- Language Evolution
- Language Reference
- Language Variation
- Language Families
- Lexicography
- Linguistic Anthropology
- Linguistic Theories
- Linguistic Typology
- Phonetics and Phonology
- Psycholinguistics
- Sociolinguistics
- Translation and Interpretation
- Writing Systems
- Browse content in Literature
- Bibliography
- Children's Literature Studies
- Literary Studies (Asian)
- Literary Studies (European)
- Literary Studies (Eco-criticism)
- Literary Studies (Romanticism)
- Literary Studies (American)
- Literary Studies (Modernism)
- Literary Studies - World
- Literary Studies (1500 to 1800)
- Literary Studies (19th Century)
- Literary Studies (20th Century onwards)
- Literary Studies (African American Literature)
- Literary Studies (British and Irish)
- Literary Studies (Early and Medieval)
- Literary Studies (Fiction, Novelists, and Prose Writers)
- Literary Studies (Gender Studies)
- Literary Studies (Graphic Novels)
- Literary Studies (History of the Book)
- Literary Studies (Plays and Playwrights)
- Literary Studies (Poetry and Poets)
- Literary Studies (Postcolonial Literature)
- Literary Studies (Queer Studies)
- Literary Studies (Science Fiction)
- Literary Studies (Travel Literature)
- Literary Studies (War Literature)
- Literary Studies (Women's Writing)
- Literary Theory and Cultural Studies
- Mythology and Folklore
- Shakespeare Studies and Criticism
- Browse content in Media Studies
- Browse content in Music
- Applied Music
- Dance and Music
- Ethics in Music
- Ethnomusicology
- Gender and Sexuality in Music
- Medicine and Music
- Music Cultures
- Music and Religion
- Music and Media
- Music and Culture
- Music Education and Pedagogy
- Music Theory and Analysis
- Musical Scores, Lyrics, and Libretti
- Musical Structures, Styles, and Techniques
- Musicology and Music History
- Performance Practice and Studies
- Race and Ethnicity in Music
- Sound Studies
- Browse content in Performing Arts
- Browse content in Philosophy
- Aesthetics and Philosophy of Art
- Epistemology
- Feminist Philosophy
- History of Western Philosophy
- Metaphysics
- Moral Philosophy
- Non-Western Philosophy
- Philosophy of Science
- Philosophy of Language
- Philosophy of Mind
- Philosophy of Perception
- Philosophy of Action
- Philosophy of Law
- Philosophy of Religion
- Philosophy of Mathematics and Logic
- Practical Ethics
- Social and Political Philosophy
- Browse content in Religion
- Biblical Studies
- Christianity
- East Asian Religions
- History of Religion
- Judaism and Jewish Studies
- Qumran Studies
- Religion and Education
- Religion and Health
- Religion and Politics
- Religion and Science
- Religion and Law
- Religion and Art, Literature, and Music
- Religious Studies
- Browse content in Society and Culture
- Cookery, Food, and Drink
- Cultural Studies
- Customs and Traditions
- Ethical Issues and Debates
- Hobbies, Games, Arts and Crafts
- Natural world, Country Life, and Pets
- Popular Beliefs and Controversial Knowledge
- Sports and Outdoor Recreation
- Technology and Society
- Travel and Holiday
- Visual Culture
- Browse content in Law
- Arbitration
- Browse content in Company and Commercial Law
- Commercial Law
- Company Law
- Browse content in Comparative Law
- Systems of Law
- Competition Law
- Browse content in Constitutional and Administrative Law
- Government Powers
- Judicial Review
- Local Government Law
- Military and Defence Law
- Parliamentary and Legislative Practice
- Construction Law
- Contract Law
- Browse content in Criminal Law
- Criminal Procedure
- Criminal Evidence Law
- Sentencing and Punishment
- Employment and Labour Law
- Environment and Energy Law
- Browse content in Financial Law
- Banking Law
- Insolvency Law
- History of Law
- Human Rights and Immigration
- Intellectual Property Law
- Browse content in International Law
- Private International Law and Conflict of Laws
- Public International Law
- IT and Communications Law
- Jurisprudence and Philosophy of Law
- Law and Politics
- Law and Society
- Browse content in Legal System and Practice
- Courts and Procedure
- Legal Skills and Practice
- Primary Sources of Law
- Regulation of Legal Profession
- Medical and Healthcare Law
- Browse content in Policing
- Criminal Investigation and Detection
- Police and Security Services
- Police Procedure and Law
- Police Regional Planning
- Browse content in Property Law
- Personal Property Law
- Study and Revision
- Terrorism and National Security Law
- Browse content in Trusts Law
- Wills and Probate or Succession
- Browse content in Medicine and Health
- Browse content in Allied Health Professions
- Arts Therapies
- Clinical Science
- Dietetics and Nutrition
- Occupational Therapy
- Operating Department Practice
- Physiotherapy
- Radiography
- Speech and Language Therapy
- Browse content in Anaesthetics
- General Anaesthesia
- Neuroanaesthesia
- Browse content in Clinical Medicine
- Acute Medicine
- Cardiovascular Medicine
- Clinical Genetics
- Clinical Pharmacology and Therapeutics
- Dermatology
- Endocrinology and Diabetes
- Gastroenterology
- Genito-urinary Medicine
- Geriatric Medicine
- Infectious Diseases
- Medical Toxicology
- Medical Oncology
- Pain Medicine
- Palliative Medicine
- Rehabilitation Medicine
- Respiratory Medicine and Pulmonology
- Rheumatology
- Sleep Medicine
- Sports and Exercise Medicine
- Clinical Neuroscience
- Community Medical Services
- Critical Care
- Emergency Medicine
- Forensic Medicine
- Haematology
- History of Medicine
- Browse content in Medical Dentistry
- Oral and Maxillofacial Surgery
- Paediatric Dentistry
- Restorative Dentistry and Orthodontics
- Surgical Dentistry
- Browse content in Medical Skills
- Clinical Skills
- Communication Skills
- Nursing Skills
- Surgical Skills
- Medical Ethics
- Medical Statistics and Methodology
- Browse content in Neurology
- Clinical Neurophysiology
- Neuropathology
- Nursing Studies
- Browse content in Obstetrics and Gynaecology
- Gynaecology
- Occupational Medicine
- Ophthalmology
- Otolaryngology (ENT)
- Browse content in Paediatrics
- Neonatology
- Browse content in Pathology
- Chemical Pathology
- Clinical Cytogenetics and Molecular Genetics
- Histopathology
- Medical Microbiology and Virology
- Patient Education and Information
- Browse content in Pharmacology
- Psychopharmacology
- Browse content in Popular Health
- Caring for Others
- Complementary and Alternative Medicine
- Self-help and Personal Development
- Browse content in Preclinical Medicine
- Cell Biology
- Molecular Biology and Genetics
- Reproduction, Growth and Development
- Primary Care
- Professional Development in Medicine
- Browse content in Psychiatry
- Addiction Medicine
- Child and Adolescent Psychiatry
- Forensic Psychiatry
- Learning Disabilities
- Old Age Psychiatry
- Psychotherapy
- Browse content in Public Health and Epidemiology
- Epidemiology
- Public Health
- Browse content in Radiology
- Clinical Radiology
- Interventional Radiology
- Nuclear Medicine
- Radiation Oncology
- Reproductive Medicine
- Browse content in Surgery
- Cardiothoracic Surgery
- Gastro-intestinal and Colorectal Surgery
- General Surgery
- Neurosurgery
- Paediatric Surgery
- Peri-operative Care
- Plastic and Reconstructive Surgery
- Surgical Oncology
- Transplant Surgery
- Trauma and Orthopaedic Surgery
- Vascular Surgery
- Browse content in Science and Mathematics
- Browse content in Biological Sciences
- Aquatic Biology
- Biochemistry
- Bioinformatics and Computational Biology
- Developmental Biology
- Ecology and Conservation
- Evolutionary Biology
- Genetics and Genomics
- Microbiology
- Molecular and Cell Biology
- Natural History
- Plant Sciences and Forestry
- Research Methods in Life Sciences
- Structural Biology
- Systems Biology
- Zoology and Animal Sciences
- Browse content in Chemistry
- Analytical Chemistry
- Computational Chemistry
- Crystallography
- Environmental Chemistry
- Industrial Chemistry
- Inorganic Chemistry
- Materials Chemistry
- Medicinal Chemistry
- Mineralogy and Gems
- Organic Chemistry
- Physical Chemistry
- Polymer Chemistry
- Study and Communication Skills in Chemistry
- Theoretical Chemistry
- Browse content in Computer Science
- Artificial Intelligence
- Computer Architecture and Logic Design
- Game Studies
- Human-Computer Interaction
- Mathematical Theory of Computation
- Programming Languages
- Software Engineering
- Systems Analysis and Design
- Virtual Reality
- Browse content in Computing
- Business Applications
- Computer Security
- Computer Games
- Computer Networking and Communications
- Digital Lifestyle
- Graphical and Digital Media Applications
- Operating Systems
- Browse content in Earth Sciences and Geography
- Atmospheric Sciences
- Environmental Geography
- Geology and the Lithosphere
- Maps and Map-making
- Meteorology and Climatology
- Oceanography and Hydrology
- Palaeontology
- Physical Geography and Topography
- Regional Geography
- Soil Science
- Urban Geography
- Browse content in Engineering and Technology
- Agriculture and Farming
- Biological Engineering
- Civil Engineering, Surveying, and Building
- Electronics and Communications Engineering
- Energy Technology
- Engineering (General)
- Environmental Science, Engineering, and Technology
- History of Engineering and Technology
- Mechanical Engineering and Materials
- Technology of Industrial Chemistry
- Transport Technology and Trades
- Browse content in Environmental Science
- Applied Ecology (Environmental Science)
- Conservation of the Environment (Environmental Science)
- Environmental Sustainability
- Environmentalist Thought and Ideology (Environmental Science)
- Management of Land and Natural Resources (Environmental Science)
- Natural Disasters (Environmental Science)
- Nuclear Issues (Environmental Science)
- Pollution and Threats to the Environment (Environmental Science)
- Social Impact of Environmental Issues (Environmental Science)
- History of Science and Technology
- Browse content in Materials Science
- Ceramics and Glasses
- Composite Materials
- Metals, Alloying, and Corrosion
- Nanotechnology
- Browse content in Mathematics
- Applied Mathematics
- Biomathematics and Statistics
- History of Mathematics
- Mathematical Education
- Mathematical Finance
- Mathematical Analysis
- Numerical and Computational Mathematics
- Probability and Statistics
- Pure Mathematics
- Browse content in Neuroscience
- Cognition and Behavioural Neuroscience
- Development of the Nervous System
- Disorders of the Nervous System
- History of Neuroscience
- Invertebrate Neurobiology
- Molecular and Cellular Systems
- Neuroendocrinology and Autonomic Nervous System
- Neuroscientific Techniques
- Sensory and Motor Systems
- Browse content in Physics
- Astronomy and Astrophysics
- Atomic, Molecular, and Optical Physics
- Biological and Medical Physics
- Classical Mechanics
- Computational Physics
- Condensed Matter Physics
- Electromagnetism, Optics, and Acoustics
- History of Physics
- Mathematical and Statistical Physics
- Measurement Science
- Nuclear Physics
- Particles and Fields
- Plasma Physics
- Quantum Physics
- Relativity and Gravitation
- Semiconductor and Mesoscopic Physics
- Browse content in Psychology
- Affective Sciences
- Clinical Psychology
- Cognitive Psychology
- Cognitive Neuroscience
- Criminal and Forensic Psychology
- Developmental Psychology
- Educational Psychology
- Evolutionary Psychology
- Health Psychology
- History and Systems in Psychology
- Music Psychology
- Neuropsychology
- Organizational Psychology
- Psychological Assessment and Testing
- Psychology of Human-Technology Interaction
- Psychology Professional Development and Training
- Research Methods in Psychology
- Social Psychology
- Browse content in Social Sciences
- Browse content in Anthropology
- Anthropology of Religion
- Human Evolution
- Medical Anthropology
- Physical Anthropology
- Regional Anthropology
- Social and Cultural Anthropology
- Theory and Practice of Anthropology
- Browse content in Business and Management
- Business Strategy
- Business Ethics
- Business History
- Business and Government
- Business and Technology
- Business and the Environment
- Comparative Management
- Corporate Governance
- Corporate Social Responsibility
- Entrepreneurship
- Health Management
- Human Resource Management
- Industrial and Employment Relations
- Industry Studies
- Information and Communication Technologies
- International Business
- Knowledge Management
- Management and Management Techniques
- Operations Management
- Organizational Theory and Behaviour
- Pensions and Pension Management
- Public and Nonprofit Management
- Strategic Management
- Supply Chain Management
- Browse content in Criminology and Criminal Justice
- Criminal Justice
- Criminology
- Forms of Crime
- International and Comparative Criminology
- Youth Violence and Juvenile Justice
- Development Studies
- Browse content in Economics
- Agricultural, Environmental, and Natural Resource Economics
- Asian Economics
- Behavioural Finance
- Behavioural Economics and Neuroeconomics
- Econometrics and Mathematical Economics
- Economic Systems
- Economic History
- Economic Methodology
- Economic Development and Growth
- Financial Markets
- Financial Institutions and Services
- General Economics and Teaching
- Health, Education, and Welfare
- History of Economic Thought
- International Economics
- Labour and Demographic Economics
- Law and Economics
- Macroeconomics and Monetary Economics
- Microeconomics
- Public Economics
- Urban, Rural, and Regional Economics
- Welfare Economics
- Browse content in Education
- Adult Education and Continuous Learning
- Care and Counselling of Students
- Early Childhood and Elementary Education
- Educational Equipment and Technology
- Educational Strategies and Policy
- Higher and Further Education
- Organization and Management of Education
- Philosophy and Theory of Education
- Schools Studies
- Secondary Education
- Teaching of a Specific Subject
- Teaching of Specific Groups and Special Educational Needs
- Teaching Skills and Techniques
- Browse content in Environment
- Applied Ecology (Social Science)
- Climate Change
- Conservation of the Environment (Social Science)
- Environmentalist Thought and Ideology (Social Science)
- Management of Land and Natural Resources (Social Science)
- Natural Disasters (Environment)
- Social Impact of Environmental Issues (Social Science)
- Sustainability
- Browse content in Human Geography
- Cultural Geography
- Economic Geography
- Political Geography
- Browse content in Interdisciplinary Studies
- Communication Studies
- Museums, Libraries, and Information Sciences
- Browse content in Politics
- African Politics
- Asian Politics
- Chinese Politics
- Comparative Politics
- Conflict Politics
- Elections and Electoral Studies
- Environmental Politics
- Ethnic Politics
- European Union
- Foreign Policy
- Gender and Politics
- Human Rights and Politics
- Indian Politics
- International Relations
- International Organization (Politics)
- International Political Economy
- Irish Politics
- Latin American Politics
- Middle Eastern Politics
- Political Methodology
- Political Communication
- Political Philosophy
- Political Sociology
- Political Behaviour
- Political Economy
- Political Institutions
- Political Theory
- Politics and Law
- Politics of Development
- Public Administration
- Public Policy
- Qualitative Political Methodology
- Quantitative Political Methodology
- Regional Political Studies
- Russian Politics
- Security Studies
- State and Local Government
- UK Politics
- US Politics
- Browse content in Regional and Area Studies
- African Studies
- Asian Studies
- East Asian Studies
- Japanese Studies
- Latin American Studies
- Middle Eastern Studies
- Native American Studies
- Scottish Studies
- Browse content in Research and Information
- Research Methods
- Browse content in Social Work
- Addictions and Substance Misuse
- Adoption and Fostering
- Care of the Elderly
- Child and Adolescent Social Work
- Couple and Family Social Work
- Direct Practice and Clinical Social Work
- Emergency Services
- Human Behaviour and the Social Environment
- International and Global Issues in Social Work
- Mental and Behavioural Health
- Social Justice and Human Rights
- Social Policy and Advocacy
- Social Work and Crime and Justice
- Social Work Macro Practice
- Social Work Practice Settings
- Social Work Research and Evidence-based Practice
- Welfare and Benefit Systems
- Browse content in Sociology
- Childhood Studies
- Community Development
- Comparative and Historical Sociology
- Economic Sociology
- Gender and Sexuality
- Gerontology and Ageing
- Health, Illness, and Medicine
- Marriage and the Family
- Migration Studies
- Occupations, Professions, and Work
- Organizations
- Population and Demography
- Race and Ethnicity
- Social Theory
- Social Movements and Social Change
- Social Research and Statistics
- Social Stratification, Inequality, and Mobility
- Sociology of Religion
- Sociology of Education
- Sport and Leisure
- Urban and Rural Studies
- Browse content in Warfare and Defence
- Defence Strategy, Planning, and Research
- Land Forces and Warfare
- Military Administration
- Military Life and Institutions
- Naval Forces and Warfare
- Other Warfare and Defence Issues
- Peace Studies and Conflict Resolution
- Weapons and Equipment

A newer edition of this book is available.
- < Previous chapter
- Next chapter >

22 Case Study Research: In-Depth Understanding in Context
Helen Simons, School of Education, University of Southampton
- Published: 01 July 2014
- Cite Icon Cite
- Permissions Icon Permissions
This chapter explores case study as a major approach to research and evaluation. After first noting various contexts in which case studies are commonly used, the chapter focuses on case study research directly Strengths and potential problematic issues are outlined and then key phases of the process. The chapter emphasizes how important it is to design the case, to collect and interpret data in ways that highlight the qualitative, to have an ethical practice that values multiple perspectives and political interests, and to report creatively to facilitate use in policy making and practice. Finally, it explores how to generalize from the single case. Concluding questions center on the need to think more imaginatively about design and the range of methods and forms of reporting requiredto persuade audiences to value qualitative ways of knowing in case study research.
Personal account
- Sign in with email/username & password
- Get email alerts
- Save searches
- Purchase content
- Activate your purchase/trial code
- Add your ORCID iD
Institutional access
Sign in with a library card.
- Sign in with username/password
- Recommend to your librarian
- Institutional account management
- Get help with access
Access to content on Oxford Academic is often provided through institutional subscriptions and purchases. If you are a member of an institution with an active account, you may be able to access content in one of the following ways:

IP based access
Typically, access is provided across an institutional network to a range of IP addresses. This authentication occurs automatically, and it is not possible to sign out of an IP authenticated account.
Choose this option to get remote access when outside your institution. Shibboleth/Open Athens technology is used to provide single sign-on between your institution’s website and Oxford Academic.
- Click Sign in through your institution.
- Select your institution from the list provided, which will take you to your institution's website to sign in.
- When on the institution site, please use the credentials provided by your institution. Do not use an Oxford Academic personal account.
- Following successful sign in, you will be returned to Oxford Academic.
If your institution is not listed or you cannot sign in to your institution’s website, please contact your librarian or administrator.
Enter your library card number to sign in. If you cannot sign in, please contact your librarian.
Society Members
Society member access to a journal is achieved in one of the following ways:
Sign in through society site
Many societies offer single sign-on between the society website and Oxford Academic. If you see ‘Sign in through society site’ in the sign in pane within a journal:
- Click Sign in through society site.
- When on the society site, please use the credentials provided by that society. Do not use an Oxford Academic personal account.
If you do not have a society account or have forgotten your username or password, please contact your society.
Sign in using a personal account
Some societies use Oxford Academic personal accounts to provide access to their members. See below.
A personal account can be used to get email alerts, save searches, purchase content, and activate subscriptions.
Some societies use Oxford Academic personal accounts to provide access to their members.
Viewing your signed in accounts
Click the account icon in the top right to:
- View your signed in personal account and access account management features.
- View the institutional accounts that are providing access.
Signed in but can't access content
Oxford Academic is home to a wide variety of products. The institutional subscription may not cover the content that you are trying to access. If you believe you should have access to that content, please contact your librarian.
For librarians and administrators, your personal account also provides access to institutional account management. Here you will find options to view and activate subscriptions, manage institutional settings and access options, access usage statistics, and more.
Our books are available by subscription or purchase to libraries and institutions.
Month: | Total Views: |
---|---|
October 2022 | 22 |
November 2022 | 17 |
December 2022 | 21 |
January 2023 | 10 |
February 2023 | 16 |
March 2023 | 42 |
April 2023 | 29 |
May 2023 | 19 |
June 2023 | 9 |
July 2023 | 7 |
August 2023 | 6 |
September 2023 | 12 |
October 2023 | 13 |
November 2023 | 14 |
December 2023 | 7 |
January 2024 | 7 |
February 2024 | 12 |
March 2024 | 44 |
April 2024 | 35 |
May 2024 | 25 |
June 2024 | 28 |
- About Oxford Academic
- Publish journals with us
- University press partners
- What we publish
- New features
- Open access
- Rights and permissions
- Accessibility
- Advertising
- Media enquiries
- Oxford University Press
- Oxford Languages
- University of Oxford
Oxford University Press is a department of the University of Oxford. It furthers the University's objective of excellence in research, scholarship, and education by publishing worldwide
- Copyright © 2024 Oxford University Press
- Cookie settings
- Cookie policy
- Privacy policy
- Legal notice
This Feature Is Available To Subscribers Only
Sign In or Create an Account
This PDF is available to Subscribers Only
For full access to this pdf, sign in to an existing account, or purchase an annual subscription.
Case Study Research Method | Benefits, Limitations
Case study research is a type of qualitative research that helps understand an individual case or a specific situation in detail.
A case study can be written as a final report or it can be presented as an article in a journal or conference proceedings. Before you begin your next case study, you must know about its scope and limitations.
Table of Contents
What is a Case Study?
Case studies concentrate on gathering data about a particular object, event, or activity, like a certain business unit or organization. The person, group, organization, event, or circumstance that the researcher is interested in is the case in a case study.
Most importantly, a case study helps you understand the reasons for the occurrence or failure of a specific event. Case studies are common in business and management research . They can be qualitative or quantitative in nature. Researchers explore a single case, typically a business-related event or experiment.
Researchers document findings as case studies and present them with the help of visuals such as
Case Study- Example
This might be stated as a case study of a nearby school that encourages active learning.
When to do a case study?
Step by step guide for conducting case study research, define the problem, create a research plan, conduct field research.
Once you have a research plan, start your field research . You can use a variety of methods to conduct field research in a case study. You can use observation, interviews, or document analysis to collect data related to your case study.
Analyze data
Organize data, present case findings.
Once you have organized data and summarized it, it’s time to present your case findings. You can write a case study report or present it in the form of an article in a journal or conference proceedings.
Benefits of Case Study Research
Multiple case studies are frequently more robust and trustworthy than single case studies. Studies with multiple cases enable the formation of theories and a more thorough investigation of research problems .
Limitations of Case Study Research
Bottom line, other articles.
Please read through some of our other articles with examples and explanations if you’d like to learn more about research methodology.
Related Posts
Google scholar: a complete guide for researchers, how to control extraneous variables, difference between conference papers and journal articles, academic curriculum vitae (cv) template and writing tips, research paper outline template: examples of structured research paper outlines, writing a research paper cover letter (example), get free access to 25 million peer-reviewed research papers, how to add social media citations in research article, clinical researcher: responsibilities, skills, and steps to pursue this career, alternative hypothesis: types and examples.
Human-generated content, empowered by AI.
FREE eBook: Establish Authorship & Build Authority Online
When and How to Use a Case Study for Research
May 17, 2021 (Updated: May 4, 2023)

Quick Navigation
What Is Case Study Research?
Types of case studies, when should you use a case study, case study benefits, case study limitations, how to write a case study.
Imagine your company receives a string of negative reviews online. You notice a few common themes among the complaints, but you still aren’t quite sure what went wrong. Or suppose an old blog post suddenly went viral, and you’d like to know why and how to do it again. In both of these situations, a case study could be the best way to find answers.
A case study is a process whereby researchers examine a specific subject in a thorough, detailed way. The subject of a case study could be an individual, a group, a community, a business, an organization, an event, or a phenomenon. Regardless of the type of subject, case studies are in-depth investigations designed to identify patterns and cause-and-effect relationships. Case studies are often used by researchers in the field of psychology , medicine, business, social work, anthropology, education, or political science.
Because they are singular in their focus and often rely on qualitative data, case studies tend to be highly subjective. The results of a single case study cannot always be generalized and applied to the larger population. However, case studies can be valuable tools for developing a thesis or illustrating a principle. They can help researchers understand, describe, compare, and evaluate different aspects of an issue or question.

Image via Flickr by plings
Case studies can be classified according to their purpose or their subject. For instance, a case study can focus on any of the following:
- A person: Some case studies focus on one particular person. Often, the subject will be an individual with some rare characteristic or experience.
- A group: Group case studies could look at a family, a group of coworkers, or a friend group. It could be people thrown together by circumstance or who share some bond or relationship. A group case study could even focus on an entire community of people.
- An organization: An organizational case study could focus on a business, a nonprofit, an institution, or any other formal entity. The study could look at the people in the organization, the processes they use, or an incident at the organization.
- A location: An event case study focuses on a specific area. It could be used to study environmental and population changes or to examine how people use the location.
- An event: Event case studies can be used to cover anything from a natural disaster to a political scandal. Often, these case studies are conducted retrospectively, as an investigation into a past event.
In addition to different types of subjects, case studies often have different designs or purposes. Here are a few of the most common types of case studies:
- Explanatory: An explanatory case study tries to explain the why or how behind something. This type of case study works well when studying an event or phenomenon, like an airplane crash or unexpected power outage.
- Descriptive: A descriptive, or illustrative, case study is designed to shed light on an unfamiliar subject. Case studies like this provide in-depth, real-world examples of whatever the researcher wants to help the audience understand. For instance, a descriptive case study could focus on the experience of a mother with postpartum depression or on a young adult who has aged out of the foster care system.
- Exploratory: An exploratory case study, or pilot case study, often serves as the first step in a larger research project. Researchers may use a case study to help them narrow their focus, draft a specific research question, and guide the parameters of a formal, large-scale study.
- Intrinsic: An intrinsic case study has no goal beyond a deeper understanding of its subject. In this type of study, researchers are not trying to make generalized conclusions, challenge existing assumptions, or make any compare-and-contrast connections. The most interesting thing about the study is the subject itself.
- Critical Instance: A critical instance case study is similar to an explanatory or intrinsic study. Like an intrinsic study, it may have no predetermined purpose beyond investigating the subject. Like an explanatory study, it may be used to explain a cause-and-effect relationship. A critical instance case study may also be used to call into question a commonly held assumption or popular theory.
- Instrumental: An instrumental case study is the opposite of an intrinsic study because it serves a purpose beyond understanding the immediate subject. In this type of study, researchers explore a larger question through an individual case or cases. For instance, researchers could use a handful of case studies to investigate the relationship between social media use and happiness.
- Cumulative: A cumulative, or collective, case study uses information from several past studies as the basis for a new study. Because it takes into account multiple case studies, a cumulative study allows for greater generalization than a single case study. It can also be a more time- and cost-effective option since it makes use of existing research.
Case studies are often used in the exploratory phase of research to gather qualitative data. They can also be used to create, support, or refute a hypothesis and guide future research. For instance, a marketing professional might conduct a case study to discover why a viral ad campaign was so successful . They can then take any lessons they glean from the case study and apply them to future marketing efforts. A psychologist could use a case study to form a theory about the best way to treat a specific disorder. That theory could then be tested later through a large-scale controlled study.
Case studies are a good way to explore a real-world topic in-depth, illustrate a point, discuss the implications or meaning of an event, or compare the experiences of different individuals. A trainer may use a case study to bring to life what would otherwise be an abstract series of recommended action steps or to spark a conversation about how to respond in a specific scenario. Similarly, professors can use case studies to highlight key concepts from a lecture and pose questions to test students’ understanding of the material.
In some situations, case studies are the only way to compile quantitative data in an ethical manner. For instance, many of the recommendations that doctors make regarding what is or is not safe during pregnancy are based on case studies. It wouldn’t be ethical to conduct a controlled study that exposes pregnant women to potentially harmful substances, so doctors rely on the anecdotal evidence provided by case studies to find correlations and draw their conclusions.
Case studies can also be used to gather data that would be otherwise impossible or impractical to obtain. Students often use case studies for their thesis or dissertation when they lack the time or resources to conduct large-scale research. Zoologists might use existing case studies to determine the success rate of reintroducing rehabilitated animals into the wild. A historian could use case studies to explore the strategies used by dictators to gain and maintain power.

Image via Flickr by calebmmartin
Case studies can be used on their own or as a complement to other research methods, depending on the situation. The examples above are just a few instances where case studies can be useful. Case studies also work well for the following:
Providing Insight Through Qualitative Data
Case studies generally provide more qualitative data as opposed to quantitative data , and that makes them an invaluable tool for gathering insight into complex topics. Psychologists, for instance, use case studies to better understand human behavior. Crafting theories on the motives behind human actions would be difficult with quantitative data alone. The information gleaned through case studies may be subjective, but so is much of what makes us human. As individuals, we each have a unique blend of emotions, attitudes, opinions, motivations, and behaviors. Objective quantitative data is rarely the best way to identify and explain these nuances.
By their very nature, case studies allow more more intensive, in-depth study than other research methods. Rather than aiming for a large sample size, case studies follow a single subject. Often case studies are conducted over a longer period of time, and the narrow focus allows researchers to gather more detail than would be possible in a study of thousands of people. The information gleaned may not be representative of the broader population, but it does provide richer insight into the subject than other research methods.
Identifying Avenues for Future Research
Case studies are often used as the first step in a larger research project. The results of a case study cannot necessarily be generalized, but they can help researchers narrow their focus. For instance, researchers in the medical field might conduct a case study on a patient who survived an injury that typically proves fatal.
Over the course of the study, researchers may identify two or three ways in which this patient’s situation differed from others they have seen. Perhaps they identify something unique in the patient’s DNA or lifestyle choices or in the steps doctors took to treat the injury. Letting that information guide them, researchers could use other methods to deepen their understanding of those factors and perhaps develop new treatments or preventative recommendations.
Case studies can also be used in the fields of social work, politics, and anthropology to draw attention to a widespread problem and spur more research. A detailed narrative about one person’s experience will inspire more compassion than an academic paper filled with quantitative data. Stories often have a greater impact than statistics.
Challenging, Testing, or Developing Theories
Case studies can be particularly useful in the process of forming and testing theories. A case study may lead researchers to form a new theory or call into a question an existing one. They are an invaluable tool for identifying exceptions to a rule or disproving conventional wisdom.
For instance, a medical professional may write a case study about a patient who exhibited atypical symptoms to assert that the list of symptoms for a condition should be expanded. A psychologist could use a case study to determine whether the new treatment they devised for depression is effective, or to demonstrate that existing treatment methods are flawed. As the result of a case study, a marketing professional could suggest that consumers values have changed and that marketing best practices should be updated accordingly.
Enabling the Study of Unique Subjects
Some subjects would be impossible, impractical, or unethical to study through other research methods. This is true in the case of extremely rare phenomenon, many aspects of human behavior, and even some medical conditions.
Suppose a medical professional would like to gather more information about multiple-birth pregnancies with four or more fetuses. More information would be helpful because we have less information about them, but the reason we have less information is because they are so rare. Conducting case studies of a few women who are currently pregnant with multiples or have given birth to multiples in the past may be the only practical way to research them.
Case studies can also be used to gain insight into historical events and natural phenomenon — things we are not able to repeat at will. Case studies have also been used to study subjects such as a feral child , child prodigies, rare psychological conditions, crisis response, and more.
Helping People Better Understand Nuanced Concepts
Educators incorporate case studies into their lectures for a reason. Walking students through a detailed case study can make the abstract seem more real and draw out the nuances of a concept. Case studies can facilitate engaging discussions, spark thoughtful questions, and give students a chance to apply what they have learned to real-world situations.
Outside the classroom, case studies can be used to illustrate complex ideas. For instance, a well-constructed case study can highlight the unintended consequences of a new piece of legislation or demonstrate that depression does not always manifest in an obvious way. Case studies can help readers and listeners understand and care about an issue that does not directly affect them.
Despite their benefits, case studies do come with a few limitations. Compared to other research methods, case studies are often at a disadvantage in terms of the following:
Replicability
In most cases, scientists strive to create experiments that can be repeated by others. That way, other scientists can perform their own research and compare their results to those of the initial study. Assuming these other scientists achieve similar results, the replicability of the experiment lends credibility to the findings and theories of the original researchers.
One limitation of case studies is that they are often difficult, if not impossible, to replicate. Although this fact does not diminish the value of case studies, it does demonstrate that case studies are not a good fit for every research problem — at least, not on their own. Additional research would have to be performed to corroborate the results and prove or disprove any generalized theories generated by a case study.
Generalization
Generalization is another area in which case studies cannot match other research methods. A case study can help us challenge existing theories and form new ones, but its results cannot necessarily be generalized. The data we gather from a case study is only valid for that specific subject, and we cannot assume that our conclusions apply to the broader population.
Researchers or readers can attempt to apply the principles from a particular case to similar situations or incorporate the results into a more comprehensive theory. However, a case study by itself can only prove the existence of certain possibilities and exceptions, not a general rule.
Reliability
The reliability of case studies may be called into question for two reasons. The first objection centers on the fallibility of human memory and the question of whether subjects are being honest. Many case studies rely on subjects to self-report biographical details, their state of mind, their thoughts and feelings, or their behaviors.
The second issue is the Hawthorne effect, which refers to the tendency of individuals to modify their behavior when they know they are being observed. This effect makes it nearly impossible for researchers to ensure that the observations and conclusions of their case study are reliable.
Researcher Bias
Researcher bias is another potential issue with case studies. The results of a case study are by nature subjective and qualitative rather than objective and qualitative, and any findings rely heavily on the observations and narrative provided by the researcher. Even the best researchers are still human, and no matter how hard they try to remain objective, they will not be able to keep their findings completely free of bias.
Researchers may have biases they are not even aware of. A researcher may over-identify with the subject and lose the benefit of a dispassionate outside perspective. If the researcher already has an opinion on the subject, they may subconsciously overlook or discount facts that contradict their pre-existing assumptions. Researcher bias can affect what the researcher observes and records, as well as how they interpret and apply their observations.
Case studies can be time-consuming and expensive to conduct. Crafting a thorough case study can be a lengthy project due to the intensive, detailed nature of this type of research. Plus, once the information has been gathered, it must be interpreted. Between the observation and analysis, a case study could take months or even years to complete. Researchers will need to be heavily involved in every step of the process, putting in a lot of time, energy, focus, and effort to ensure that the case study is as informative as possible.
Now that you understand the benefits, limitations, and types of case studies, you can follow these steps to write your own:
- Determine your objective. Write out your research problem, question, or goal. If you aren’t sure, ask yourself questions like, “What am I trying to accomplish? What do I need to know? What will success look like?” Be clear and specific. Your answers will help you choose the right type of case study for your needs.
- Review the research. Before delving into your case study, take some time to review the research that is already available. The information you gather during this preliminary research can help guide your efforts.
- Choose a subject. Decide what or who the subject of your case study will be. For instance, if you are conducting a case study to find out how businesses have been affected by new CDC guidelines, you will need to choose a specific restaurant or retailer. In some cases, you may need to draft a release form for the subject to sign so that you will be able to publish your study.
- Gather information. Case studies about a person, organization, or group may rely on questionnaires or interviews to gather information. If you are studying an event, you might use a combination of academic research and witness interviews. In some cases, you will record your own observations as part of the study.
- Write a report. Most case studies culminate in a written report, similar to a research paper. Most case studies include five sections : an introduction, a literature review, an explanation of your methods, a discussion of your findings and the implications, followed by a conclusion.
- Publish your findings. Once you’ve written your case study, consider the most engaging way to present your findings. A well-written research article is a good place to start, but going a step further will maximize the impact of your research. For instance, you could design an infographic to highlight key findings or commission an animated video to turn your case study into a visual narrative.
Whether research is your primary occupation or only an incidental part of your job, you can benefit from a solid understanding of what case studies are, how they work, and when to use them. Use the information and steps above to design and write a case study that will provide the answers you’re looking for.

CopyPress writer
More from the author:
Quick Navigation What Does a Content Manager Do? Who Does a Content Manager Report To? Are Content Managers Paid...
In our digital age, it’s important to know the best ways to reach your target audience and boost your...
The best content marketing conferences offer the chance to learn more about the industry and provide networking opportunities with...
RECENT ARTICLES
Read More About Measurement

27 Nov 2023

31 Oct 2023

23 May 2023

- SUGGESTED TOPICS
- The Magazine
- Newsletters
- Managing Yourself
- Managing Teams
- Work-life Balance
- The Big Idea
- Data & Visuals
- Reading Lists
- Case Selections
- HBR Learning
- Topic Feeds
- Account Settings
- Email Preferences
What the Case Study Method Really Teaches
- Nitin Nohria

Seven meta-skills that stick even if the cases fade from memory.
It’s been 100 years since Harvard Business School began using the case study method. Beyond teaching specific subject matter, the case study method excels in instilling meta-skills in students. This article explains the importance of seven such skills: preparation, discernment, bias recognition, judgement, collaboration, curiosity, and self-confidence.
During my decade as dean of Harvard Business School, I spent hundreds of hours talking with our alumni. To enliven these conversations, I relied on a favorite question: “What was the most important thing you learned from your time in our MBA program?”
- Nitin Nohria is the George F. Baker Jr. and Distinguished Service University Professor. He served as the 10th dean of Harvard Business School, from 2010 to 2020.
Partner Center

Home » Pros and Cons » 12 Case Study Method Advantages and Disadvantages
12 Case Study Method Advantages and Disadvantages
A case study is an investigation into an individual circumstance. The investigation may be of a single person, business, event, or group. The investigation involves collecting in-depth data about the individual entity through the use of several collection methods. Interviews and observation are two of the most common forms of data collection used.
The case study method was originally developed in the field of clinical medicine. It has expanded since to other industries to examine key results, either positive or negative, that were received through a specific set of decisions. This allows for the topic to be researched with great detail, allowing others to glean knowledge from the information presented.
Here are the advantages and disadvantages of using the case study method.
List of the Advantages of the Case Study Method
1. it turns client observations into useable data..
Case studies offer verifiable data from direct observations of the individual entity involved. These observations provide information about input processes. It can show the path taken which led to specific results being generated. Those observations make it possible for others, in similar circumstances, to potentially replicate the results discovered by the case study method.
2. It turns opinion into fact.
Case studies provide facts to study because you’re looking at data which was generated in real-time. It is a way for researchers to turn their opinions into information that can be verified as fact because there is a proven path of positive or negative development. Singling out a specific incident also provides in-depth details about the path of development, which gives it extra credibility to the outside observer.
3. It is relevant to all parties involved.
Case studies that are chosen well will be relevant to everyone who is participating in the process. Because there is such a high level of relevance involved, researchers are able to stay actively engaged in the data collection process. Participants are able to further their knowledge growth because there is interest in the outcome of the case study. Most importantly, the case study method essentially forces people to make a decision about the question being studied, then defend their position through the use of facts.
4. It uses a number of different research methodologies.
The case study method involves more than just interviews and direct observation. Case histories from a records database can be used with this method. Questionnaires can be distributed to participants in the entity being studies. Individuals who have kept diaries and journals about the entity being studied can be included. Even certain experimental tasks, such as a memory test, can be part of this research process.
5. It can be done remotely.
Researchers do not need to be present at a specific location or facility to utilize the case study method. Research can be obtained over the phone, through email, and other forms of remote communication. Even interviews can be conducted over the phone. That means this method is good for formative research that is exploratory in nature, even if it must be completed from a remote location.
6. It is inexpensive.
Compared to other methods of research, the case study method is rather inexpensive. The costs associated with this method involve accessing data, which can often be done for free. Even when there are in-person interviews or other on-site duties involved, the costs of reviewing the data are minimal.
7. It is very accessible to readers.
The case study method puts data into a usable format for those who read the data and note its outcome. Although there may be perspectives of the researcher included in the outcome, the goal of this method is to help the reader be able to identify specific concepts to which they also relate. That allows them to discover unusual features within the data, examine outliers that may be present, or draw conclusions from their own experiences.
List of the Disadvantages of the Case Study Method
1. it can have influence factors within the data..
Every person has their own unconscious bias. Although the case study method is designed to limit the influence of this bias by collecting fact-based data, it is the collector of the data who gets to define what is a “fact” and what is not. That means the real-time data being collected may be based on the results the researcher wants to see from the entity instead. By controlling how facts are collected, a research can control the results this method generates.
2. It takes longer to analyze the data.
The information collection process through the case study method takes much longer to collect than other research options. That is because there is an enormous amount of data which must be sifted through. It’s not just the researchers who can influence the outcome in this type of research method. Participants can also influence outcomes by given inaccurate or incomplete answers to questions they are asked. Researchers must verify the information presented to ensure its accuracy, and that takes time to complete.
3. It can be an inefficient process.
Case study methods require the participation of the individuals or entities involved for it to be a successful process. That means the skills of the researcher will help to determine the quality of information that is being received. Some participants may be quiet, unwilling to answer even basic questions about what is being studied. Others may be overly talkative, exploring tangents which have nothing to do with the case study at all. If researchers are unsure of how to manage this process, then incomplete data is often collected.
4. It requires a small sample size to be effective.
The case study method requires a small sample size for it to yield an effective amount of data to be analyzed. If there are different demographics involved with the entity, or there are different needs which must be examined, then the case study method becomes very inefficient.
5. It is a labor-intensive method of data collection.
The case study method requires researchers to have a high level of language skills to be successful with data collection. Researchers must be personally involved in every aspect of collecting the data as well. From reviewing files or entries personally to conducting personal interviews, the concepts and themes of this process are heavily reliant on the amount of work each researcher is willing to put into things.
These case study method advantages and disadvantages offer a look at the effectiveness of this research option. With the right skill set, it can be used as an effective tool to gather rich, detailed information about specific entities. Without the right skill set, the case study method becomes inefficient and inaccurate.
Related Posts:
- 25 Best Ways to Overcome the Fear of Failure
- Monroe's Motivated Sequence Explained [with Examples]
- 21 Most Effective Bundle Pricing Strategies with Examples
- Force Field Analysis Explained with Examples

Collaborations: A Journal of Community-Based Research and Practice

- Download XML (English) PDF (English)
- Alt. Display
Action-Research
Participatory research methods for examining lessons from covid-19 about local food systems vulnerabilities to exogenous shocks.
- Robert Newell
- Colin Dring
- Lenore Newman
Participatory processes are integral to sustainability and resilience planning; involving diverse stakeholders ensures planning practices and outcomes are grounded in local social, economic, environmental, and cultural contexts and realities. It follows that research and tools supporting planning processes should also be participatory, and such research approaches can lead to useful knowledge for developing appropriate, place-based approaches for addressing critical sustainability issues. Using the Fraser Valley region (British Columbia, Canada) as a case study, this research experiments with participatory research (PR) methods and tools for supporting long-term food systems planning by examining regional food vulnerabilities and opportunities/needs for building resilience to exogenous shocks. The research involved a survey and a series of workshops supported by an online collaboration platform, CoLabS, which engaged different food system stakeholders to first, reflect on what COVID-19 has revealed about regional food systems vulnerabilities, and second, discuss how these insights can be used for integrated long-term planning and increasing food resilience in the face of a variety of environmental and socioeconomic hazards. Strengths of this research include its place-based approach, relationship development and reciprocity aspects, multi-dimensional exploration of vulnerabilities and issues, and the use of dynamic digital tools. Limitations of the research include its lack of comprehensive participation and representation, capacity limitations of potential participants, influence of current real-world issues on research activities, and limited functionality of some online tools. Lessons and insights from this research demonstrate the importance of employing adaptable and flexible methods and tools when conducting PR on sustainability issues.
- community-engaged research
- food systems vulnerabilities
- integrated planning
- online engagement
Introduction
The COVID-19 pandemic has highlighted numerous vulnerabilities in local and regional food systems ( Clapp & Moseley, 2020 ). These vulnerabilities are not exclusive to the pandemic; they relate to other exogenous shocks such as climate change, which presents a need for comprehensive, integrated approaches to planning and resilience ( Newell & Dale, 2021 ). Accordingly, Carey et al. ( 2021 ) argues that communities should examine the lessons provided by the pandemic to identify and recognize food systems vulnerabilities and devise ways for increasing local resilience. Similarly, Hobbs ( 2020 ) notes that food systems stakeholders should reflect upon where food supply chains remained robust during the pandemic and where failures occurred. However, as there is no one-size-fits-all approach to food systems planning and development ( Knezevic et al., 2017 ), communities devise place-based solutions and ways of working appropriate to the local context.
The comments by Carey et al. ( 2021 ) and Hobbs ( 2020 ) about learning lessons from COVID-19 suggest that local governments and food systems stakeholders (i.e., the range of organizations and community actors involved in local food systems) should engage in exercises where they examine and reflect on what the pandemic has revealed about food systems vulnerabilities and ways toward resilience. Such reflective exercises are best facilitated through the use of participatory research (PR) approaches that center on real-world issues and aim to generate solutions to problems in collaboration with those affected by them ( Trimble & Berkes, 2013 ). PR has a long history in health and social sciences ( Israel et al., 1998 ); however, it also has been applied in broader and wider-ranging contexts, including food systems. PR is a valuable approach to researching complex sustainable issues as it draws from diverse knowledges and experiences ( Newell et al., 2020 ) and facilitates capacity-building for addressing a particular issue ( Greenhalgh et al., 2016 ), making it a useful research approach for examining the complexities around local and regional food systems challenges. Examples include Jacobi et al.’s ( 2019 ) work on developing and conducting a food systems mapping process with stakeholders in Kenya and Bolivia. Other examples include Domingo et al.’s ( 2021 ) research done in partnership with the Williams Treaties First Nations in Ontario, Canada, on the challenges and approaches for increasing local access to nutritious and culturally-relevant foods. Both examples demonstrate how PR can be used to comprehensively explore food systems considerations, including those related to health and nutrition, cultural values, agricultural production, environmental health, food economies, and others.
During the COVID-19 pandemic, using PR to engage in reflective exercises was challenging due to the nature of the health crisis and its public health measures. The need for physical distancing prevented in-person gathering, and as PR requires researcher-practitioner collaboration and stakeholder engagement ( Greenhalgh et al., 2016 ; Hacker et al., 2012 ; Moldenhauer & Sackey, 2016 ; Newell et al., 2020 ), these measures create complications for the development of relationships and for data collection. In response, online and remote engagement research innovations have been developed ( Newell & Dale, 2021 ), which can be applied in PR efforts where in-person engagement is not possible. Such online engagement continues to be relevant as researchers, practitioners, and stakeholders explore ways of collaborating on sustainability research while also attempting to (for instance) reduce transaction costs and travel-related greenhouse gas emissions ( Jost et al., 2021 ). It is thus important to experiment with innovative new ways of conducting PR on complex sustainability issues, such as food systems vulnerabilities, both through online and in-person methods to better understand the opportunities and challenges around such research exercises.
This paper documents a study that responds to both the opportunity (or need) for communities to examine lessons from COVID-19 regarding food systems vulnerabilities ( Carey et al., 2021 ) and the need for innovative approaches to PR that harness online technologies. The study was conducted in the Fraser Valley region, British Columbia (BC), Canada, and it engaged local and government, non-governmental organizations, food system stakeholders, and community members in an exploration of food systems vulnerabilities through a series of workshops and a survey. The research was conducted remotely, with the workshops being facilitated with the use of the CoLabS online engagement platform. This paper details the online methods used, successes and shortcomings of the study, and lessons for future PR work on complex sustainability issues.
The Participatory Research Approach
There are a variety of terms that describe participatory approaches to research, such as participatory action research, action research, collaborative inquiry, and community-based research. These terms commonly describe an approach to research inquiry that involves participants as co-researchers or collaborators ( Savan & Sider, 2003 ); however, the degree and nature of participation can vary depending on the approach used. For example, community-based participatory research is described as involving community members in all aspects of the research process, from design to dissemination, whereas community-engaged research is a broader term that refers to the use of academic-community partnerships in studies of issues which impact a community’s wellbeing ( Vaughn & Jacquez, 2020 ). PR involves community members in a range of research activities, such as the design of the research instruments (e.g., Moldenhauer & Sackey, 2016 ) and selection of stakeholder participants (e.g., Ciaccia et al., 2019 ). However, depending on the method used, PR can also involve researchers taking a more prominent role in certain activities such as workshop facilitation (e.g., Jacobi et al., 2019 ; Picketts et al., 2012 ) and data analysis (e.g., Domingo et al., 2021 ) in effort to support communities in their explorations of local challenges. In light of the wide range of PR methods, it is important to identify the degree and nature of participant involvement when reporting on PR studies.
This research best aligns with Vaughn and Jacquez’s ( 2020 ) description of community-engaged research, meaning it falls under a broad umbrella of research approaches that involve researchers, stakeholders, and community members working together to co-produce knowledge on issues affecting the community. In this work, researchers led activities, such as facilitation of workshops, initial drafts of reports, data analysis, and drafting survey; however, community input was involved throughout the process in multiple ways. The project was initiated through a collaboration with regional government collaborators, and discussions with the collaborators informed the research design. In addition, research instruments and analyses, such as survey and systems maps, were developed based on the discussions in and input from workshop participants. Furthermore, rather than predefining workshop designs and activities at the beginning of the project, the content and foci of workshops (following an initial workshop) were based on thoughts, interests, and food systems concerns expressed by participants in previous workshops. Workshop participants were also invited to bring forward ideas for what to explore in subsequent research activities. Finally, knowledge outputs (i.e., reports) were circulated to workshop participants to receive and incorporate their input before publishing, and participants also contributed to research dissemination by distributing the reports among their networks. Accordingly, the research aligns with PR approaches in that the relationships between researcher and participant differ from that of traditional science, with traditional science being more expert-driven and this project involving a more collaborative exploration of issues and co-production of knowledge ( Savan & Sider, 2003 ).
Regional Context
The Fraser Valley region is in the southwest of the Canadian province of BC ( Figure 1 ), and it has a population of over 340,000 people ( BC Stats, 2021 ). The region encompasses six municipalities, 30 First Nations bands, and eight electoral areas ( Fraser Valley Regional District [FVRD], n.d.-a. , n.d.-b ), with Abbotsford being the largest of the municipalities/communities. The region is located adjacent to Metro Vancouver, which is the largest urban centre in the province and supports a population of nearly 2.8 million people ( BC Stats, 2021 ).

Location of the Fraser Valley Regional District (yellow) within BC (red).
Note: Data sources: BC Data Catalogue, Esri, DigitalGlobe, GeoEye, Earthstar Geographies, CNES/Airbus DS, USDA, USGS, AeroGRID, and ISN.
The region makes for an interesting case study for research on food systems, as it is one of the most agriculturally productive areas in Canada. Over 71,000 hectares of FVRD’s land are within BC’s Agricultural Land Reserve ( BC Ministry of Agriculture, 2016 ), and the combination of nutrient-rich soils, agriculturally-favorable climate, and proximity to lucrative markets (e.g., Vancouver) have resulted in a thriving local agricultural industry ( FVRD, 2017 ). The Abbotsford Chamber of Commerce ( 2022 ) estimates that in Abbotsford alone, agriculture is responsible for $3.8 billion of economic activity. The significance of agriculture to the region has shaped the economic and sociocultural identities of the communities within the region, with food systems being central to said identities ( Newman et al., 2017 ).
The approach taken in this study involves aligning the research with previous local efforts and initiatives, as done in other PR work ( Newell et al., 2021 ). To this end, the study built upon previous and existing work toward enhancing local food systems resilience, such as the Fraser Valley agriculture and climate change strategy ( Climate Change Adaptation Program, 2015 ) and Clean Economy in the Fraser Valley report ( FVRD, 2019 ). The region has numerous food assets, relating to all aspects of food systems from production to consumption, including food banks, community meals, a food hub, food retailers, processors, and a variety of farms.
The research consisted of three virtual workshops and a survey delivered from July 2021 to March 2022. The main objectives of the research were (1) to explore local food systems vulnerabilities and impacts related to COVID-19, (2) to map and assess food systems vulnerabilities and potential impacts from multiple environmental, socioeconomic, and political hazards (e.g., flooding, fires, rapid population growth, economic recession, etc.), and (3) to enhance food systems resiliency through analysis of the region’s infrastructure, programs, and environmental features.
The workshops gathered food systems stakeholders and community members, and the numbers of participants for each workshop were: 25 in Workshop 1, 22 in Workshop 2, and 12 in Workshop 3. Note that the first workshop was conducted in two sessions, as there were community members that expressed interest in joining but were unable to attend the first date; however, for disambiguation, the two sessions will be collectively referred to as Workshop 1. Though groups were small, the participants included people with specific knowledge and involvement with different aspects of food systems, and in this way, the research follows a purposive sampling approach ( Etikan et al., 2016 ) and is similar to other work that employs small focus groups of people with specialized knowledge ( Onwuegbuzie, et al., 2009 ). Participants included representatives from regional and local government (elected officials and staff), the regional health authority, community development not-for-profit organisations, social services, community food programs (e.g., food banks), research, and local and regional food network organisations. With respect to the latter, researchers (i.e., first and second author) also joined two of these network organizations, namely the Abbotsford Fresh Network and the Mission Food Coalition. The researchers’ participation within these networks involved attending regular network meetings to share ideas on how research (including this project) and education at the local university, the University of the Fraser Valley, could better support local and regional food systems goals. At the time of the project, the authors were researchers based at this local university, and they contributed to network discussions by sharing their knowledge and expertise respectively on integrated community sustainability planning (i.e., first author) and food systems planning and food justice (i.e., second author).
As noted above, an aim of this research was to explore ways of engaging in PR, while following public health guidelines around physical distancing. To this end, the workshops were conducted online, using the Zoom virtual conferencing application for the workshop discussions and the CoLabS platform for workshop activities. CoLabS is a Drupal-based platform for supporting online collaboration on sustainable development issues and challenges ( www.changingtheconversation.ca/colabs ). It was designed as a flexible platform, with the ability to change its structure and add/embed different tools to meet needs of a particular project or group ( Jost et al., 2021 ). The CoLabS space for this project was customised with a series of virtual working tables, which used Padlet widgets that allowed for participant input in different ways, depending on the activity ( Figures 2 , 3 , & 4 ).

CoLabS Screenshot of the First Workshop’s Working Table.

CoLabS Screenshot of the Second Workshop’s Hazard-Impact Mapping Working Table.

CoLabS Screenshot of the Second Workshop’s Spatial Mapping Table.
Acknowledging the limited number of participants in the workshops, and the research needs of the FVRD, the project included a survey questionnaire. Survey questions were designed to align with activities and outcomes from Workshops 1 and 2. The survey was delivered to residents living both in the Fraser Valley and Metro Vancouver regions (i.e., the Lower Mainland). The case study region was broadened for this particular activity due to the Metro Vancouver and Fraser Valley regions being interconnected in terms of food flows and economies, as well as how workshop participants referred to both regions multiple times in the discussions of local food systems issues.
The timings of the research activities are noteworthy. In addition to the COVID-19 pandemic, a series of different food systems shocks coincided with the workshops. Workshop 1 was conducted in the summer of 2021, when the Pacific Northwest area was experiencing an extreme heat event. Workshop 2 occurred in the fall of 2021, during a severe flooding event in Abbotsford. Workshop 3 and the survey delivery occurred in early 2022, when the conflict in Ukraine began and supply chain disruptions were anticipated/emerging. Therefore, although the purpose of the exercise was to reflect on the lessons that emerged from COVID-19 in terms of vulnerabilities to broader range of shocks, the participants were able to draw from current experiences (rather than hypotheticals) when considering some of these other shocks.
For each workshop, participants were provided with a letter of consent. They were given the letter prior to the session and signed/returned electronically to indicate their consent to participate in the study, and at the beginning of the workshop, they were invited to ask questions about the consent letter. Survey participants were given a letter of informed consent, provided through a link to a PDF version found at the beginning of the survey. The research project and letters of consent were reviewed and approved by the Human Research Ethics Board at the University of the Fraser Valley in May 2021 (file number: 100755).
Following each workshop, a report was prepared for participants to summarize the main outcomes of the workshop and identify next steps. Each report was first sent to the workshop participants for review, and then after incorporating participant comments, the reports were made publicly available. A report was also prepared on the outcomes of the survey study.
The focus of this paper is the PR approach, and the following sections provide more details on the research activities and process. The findings reported in this paper relate to the strengths and shortcomings of the research approach designed and employed here. For more information on findings from the research itself (i.e., the study on food systems vulnerabilities and ways to increase resilience), refer to the reports prepared on the workshops ( Dring & Newell, 2021 , 2022a , 2022b ) and survey ( Newell & Dring, 2022 ).
Workshop 1 was held in two sessions in the summer of 2021 (July 29 and September 2), engaging a total of 25 participants and running for approximately two hours per session. Its purpose was to explore local food systems vulnerabilities and impacts related to COVID-19 in the Fraser Valley region to harness the opportunities for lessons learned. The food system framework of the Initiative for a Competitive Inner City (ICIC) was adapted and used in the workshop, which is a framework that categorizes food systems into four different areas: food processing, food distribution, food access, and government policies and practices ( Zeuli & Whalen, 2017 ). As ICIC’s framework focuses on urban environments, it excludes food production due to (generally speaking) the lack of farmland in these spaces. This study however added food production due to the importance of agriculture to the communities, economies, and cultural identities in FVRD. The modifications also included reframing the government policies and practices as “government response” to broaden and better capture the nature of government interventions toward COVID-19, that is, as a response to a public health crisis and its related impacts.
Researchers and scholars in the areas of food systems resilience (e.g., Carey et al., 2021 ; Savary et al., 2020 ) and sustainability planning (e.g., Ling et al., 2009 ) discuss the importance of examining issues through a temporal lens to be able to facilitate long-term planning. Accordingly, Workshop 1 explored how COVID-19 exerted impacts and revealed vulnerabilities across different timeframes, as well as how various issues, responses, and adaptations emerged in said timeframes. The timeframes examined in the workshop included short (0–3 months), medium (0–12 months), and long-term (>12 months) periods.
Workshop 1 examined impacts to and vulnerabilities of each component of the food system framework with respect to short-, medium-, and long-term timeframes. Virtual breakout rooms for each food system category were created, and workshop participants joined the room for whichever component they wished to examine. The activities used a Padlet-based working table for each food component where participants posted their thoughts/observations on food systems vulnerabilities and impacts for each of the three timeframes ( Figure 2 ). The activity was conducted in two 20-minute rounds, allowing participants to explore multiple food system categories.
The workshop concluded with a 30-minute plenary discussion, supported by questions and prompts) to expand on the outcomes of the breakout sessions and explore the implications of the impacts and vulnerabilities identified. In addition (and to facilitate a PR approach), participants were asked to identify further research and workshop directions and topics within the scope of the research. The workshop closed with a synthesis of the main outcomes of the workshop and an explanation of proposed next steps for the project.
Workshop 2 was held in November 2021, and it served to broaden the perspective of food systems vulnerabilities beyond the COVID-19 context by mapping said vulnerabilities from multiple hazards and exogenous shocks. The hazards were identified based on the discussions from the previous workshop and documents produced on food systems resilience in the FVRD (e.g., Climate Change Adaptation Program, 2015 ), and the final selection of hazards was supported by relevant literature ( Balfour & Keenan, 2007 ; Biehl et al. 2017 ; Intergovernmental Science-Policy Platform on Biodiversity and Ecosystem Services, 2019 ; Jahn et al. 2018 ). As shown in Table 1 , 10 hazards were explored in the workshop, with each falling under one of two categories: (1) environmental hazards, and (2) socioeconomic and political hazards.
Food System Hazards Explored in the Second Workshop.
TYPE OF HAZARD | HAZARDS |
---|---|
Environmental | Flooding; Wildfire; Extreme heat and drought; Air pollution; Water pollution; Loss of wildlife habitat and ecosystems |
Socioeconomic and political | Rapid population growth; Rapid population decline; Threat of war, conflict, or invasion; Economic recession and market failure |
A total of 22 participants joined Workshop 2, with 16 of the participants being people who attended the first workshop (i.e., about 73% were returning participants). Participants were provided with a copy of the Workshop 1 summary report prior to the second workshop, and Workshop 2 began with a presentation that referenced this previous work and its outcomes. Participants were then instructed on the activities to be performed in Workshop 2, which included systems mapping and spatial mapping exercises.
For the systems mapping exercise, a series of CoLabS pages were equipped with a Padlet-based concept mapping tool that allowed participants to create posts and links between the posts ( Figure 3 ). Workshop participants were randomly assigned to breakout rooms centered on different hazards, where they engaged in an activity that involved mapping hazard-impact relationships. Participants first identified direct impacts from the hazards by posting an impact and drawing a connecting line between the hazard and impact. Then, participants identified indirect impacts by posting a secondary impact occurring from the direct impact and drawing the appropriate connecting line. Two 20-minute rounds were held for the environmental hazards, allowing participants to explore multiple hazards. One 20-minute round was held for the socioeconomic and political hazards due to there being fewer hazards in this category (and because the workshop was running behind schedule).
For the spatial mapping exercise, participants located food system components/assets that they considered to be vulnerable to exogenous shocks (e.g., highways, food banks, the Fraser River, farms, etc.). The exercise was supported with a CoLabS page equipped with a Padlet-based mapping application tool, which allows users to place point data markers and captions on a Google base map ( Figure 4 ). In the captions, participants were asked to provide three pieces of information: (1) the name of the food system component/asset, (2) the hazard(s) threatening or impacting the component/assets, (3) reasons why the food system component is vulnerable to the hazard. Although the activity centred on the FVRD, participants were allowed to identify components located outside the region’s jurisdictional boundaries, if they felt the component was relevant to local food systems.
The workshop concluded with a 30-minute plenary discussion, where participants were asked to reflect on the exercises and explore how the systems and spatial mapping activities exhibit linkages among a variety of hazards and food system impacts. The group discussed how certain impacts emerged through various hazards, as well as how multiple food system shocks contribute to increased vulnerability. Similar to Workshop 1, the workshop closed with a summary of main outcomes from the workshop and a discussion on the next steps, including future activities and topics to be explored in the project.
As done following Workshop 1, a summary report was prepared on the Workshop 2 outcomes ( Dring & Newell, 2022a ). However, in addition to a synthesis of comments and ideas presented in the workshop, the Workshop 2 summary report included an analytical component. Data from the systems maps were tabulated to identify nodes (i.e., hazards, direct impacts, secondary impacts) and connections/edges between nodes. The data were coded to create consistent terminology for those identifying the same impact (for instance, “habitat degradation” could also be described as “reduced habitat quality”). Then, data were imported into the Gephi (v. 0.9.2) network visualisation and analysis software, and the full hazard-impact system was analysed to identify impacts with numerous connections to hazards and other impacts (see Dring & Newell, 2022a ).
Outputs from the spatial mapping exercise were analysed to see where concentrations of vulnerable food systems assets were located by participants by calculating the numbers of markers placed within certain jurisdictions (i.e., within municipal, electoral area, or community boundaries). Numbers and percentages of markers that referred to either environmental or socioeconomic/political hazards were also calculated. In some cases, marker captions referred to larger geographic areas, rather than specific sites, with respect to both the scales of impact and hazard activity; however, these vulnerabilities were still represented by point data markers, as this was the only data input type available, using the Padlet-based mapping application.
The survey was launched in February 2022, before Workshop 3, and its purpose was to broaden the engagement in the project and to confirm responses generated in Workshops 1 and 2. The survey was delivered through Decision Point Research (a Canada-based data collection company), and it specifically targeted people aged 18 or older, who live in the Lower Mainland in BC. The initial dataset consisted of 220 responses; however, six entries were removed due to the respondents noting their place-of-residence to be a community outside of the Lower Mainland or were unclear about its location, resulting in a final sample size of 214 in a total (i.e., the Lower Mainland’s) population of over 3 million people ( Newell & Dring, 2022 ). Although not a large enough sample to be considered representative of the Lower Mainland, the survey gathered useful demographic and place-of-residence information for gaining insights about people’s experiences and concerns about local food systems based on who they are and where they live.
The survey consisted of four sections: (a) demographic questions, (b) food systems concerns revealed by COVID-19, (c) food systems impacts experienced due to the pandemic, and (d) concerns and thoughts about impacts arising from a variety of environmental, socioeconomic, and political hazards. The survey questions were designed to align with the workshop series in that they explored the same issues (i.e., food system impacts and hazards) discussed in Workshops 1 and 2, and through systems-based and temporal lenses. For example, the survey posed questions about concerns regarding local and regional food systems vulnerabilities to the pandemic and same environmental, socioeconomic, and political hazards explored in Workshop 2, and using a similar approach to Workshop 1, respondents were asked to present the concerns in terms of different timeframes. In addition, the questions asking respondents about their concerns and experiences with food systems vulnerabilities and impacts during the pandemic were based on topics discussed and outcomes from the workshops. A full copy of the survey and more details on the methods and results can be found in Newell and Dring ( 2022 ).
Workshop 3 was held in March 2022, and it ran for three hours. It was attended by 15 participants, all of whom had attended previous workshops. Its goal was to stimulate thinking on how to build long-term food system resilience in the face of current and future shocks. The main objectives were to identify gaps in and ways to scale-up/enhance existing food system initiatives, programs, and assets. Building on previous workshops, Workshop 3 began with a presentation that summarized and reviewed the insights on local food systems vulnerabilities produced through Workshop 1 and 2 and the analyses of the survey and hazard-impact system data (see Dring & Newell, 2022a ; Newell & Dring, 2022 ).
The first activity of the workshop involved the facilitators introducing a food system future scenario ( Figure 5 ). The scenario was designed as a worst-case situation that could occur twenty years in the future (i.e., 2042), and it was developed from discussion topics and outcomes from the previous two workshops. This approach was taken instead of identifying an idyllic scenario, as it provided a method of building on the previous work done in the study by synthesizing and contextualizing the concerns and issues identified in the previous workshops. In this case, the scenario activity did not involve selecting an optimal or most desirable scenario, as typically done in scenario planning ( Amer et al., 2013 ); rather, it involved thinking about how to build resilience in ways that address and avoid the concerns and issues outlined in the worst-case scenario.

CoLabS Screenshot of the Future Scenario Narrative used in the Third Workshop.
Workshop 3 activities employed similar Padlet-based tools embedded in the CoLabS platform. The first activity involved participants posting comments and providing thoughts on the plausibility of the scenarios and additional elements and/or missing food system impacts that may occur in the scenarios. Participants were also asked to identify the kinds of strategies and interventions that might be employed by food system actors in the future scenario. The activity concluded with a plenary discussion, where participants examined, elaborated on, and further discussed the posted comments and topics.
The second activity conducted in Workshop 3 involved participants examining key assets, gaps, and needs for food systems ( Figure 6 ). The activity was structured into two parts: physical infrastructure and environmental features, and programs, initiatives, and policies. In both parts of the activity, participants were asked to identify the existing food systems assets (e.g., infrastructure, environmental features, policies, programs, or initiatives) that are critical for preventing the worst-case scenario, explaining how the asset needed to be protected and/or maintained to prevent the scenario. Participants were also asked to identify possible ways of changing or enhancing the assets to improve chances of preventing the scenario. Finally, participants identified gaps in infrastructure, environmental features, policies, programs, and initiatives to highlight areas of concern with respect to how future hazards may result in food systems impacts, if said gaps are not addressed.

CoLabS Screenshots of Third Workshop’s Working Tables.
Note: CoLabS Screenshots of Third Workshop’s Working Tables on (A) current and (B) missing/gaps in physical infrastructure and environmental features.
As done in the other workshops, Workshop 3 concluded with a final plenary session, where the group further discussed ideas and insights that emerged throughout the session. In addition, as all the Workshop 3 participants had previously engaged in the research, the plenary discussion also involved reflecting on the research process. The workshop concluded with a discussion on the next steps, including the preparation and release of the final workshop and survey reports (i.e., Dring & Newell, 2022b ; Newell & Dring, 2022 ) and plans for bringing the research knowledge into meetings and planning sessions hosted by participating local food network groups.
The findings in this paper shares lessons for future PR work that focuses on complex sustainability issues and that use online engagement methods, by presenting successes and shortcomings of the research approach. As discussed previously, the workshops included discussions with participants about the research process (including potential directions), and the findings presented in this research are based on both comments from the participants and participant observations. Accordingly, this section is structured following a thematic approach ( Clarke et al. 2015 ), the findings and insights are presented through a series of themes ( Table 2 ).
Thematic Findings on Strengths and Limitations of the Participatory Research Process.
CATEGORY | THEME | DESCRIPTION |
---|---|---|
Strengths | Place-based approach | The research focused on the Fraser Valley region for developing and advancing contextualized, local knowledge. Extending beyond the administrative boundary to include relationships and interactions between places. |
Relationship development & reciprocity | Relationship building was done by meeting community organizations and stakeholders on their terms and addressing their needs, and by participating in local networks. | |
Multi-dimensional exploration | The research involved participants/stakeholders in exercises that explored food systems vulnerabilities through temporal and spatial dimensions. | |
Dynamic digital tools | The variety of digital tools created an engaging workshop experience and opportunities for effective individual and group brainstorming. | |
Limitations | Participation | Comprehensive participation of food system actors (e.g., producers, labourers) and specific social groups (e.g. Indigenous, racialized, people experiencing poverty) was not fully achieved. |
Capacity limitations | Capacity limitations created challenges for certain stakeholders to participate, such as those working in food production and vulnerable groups with limited access to (or comfort with) online technologies. | |
Real-world issues | Real-world hazard events influenced topics and focus of workshop discussion and activities. | |
Online tool constraints | Some online tools were limiting in terms of the ideas and inputs participants wished to provide. | |
Place-Based Approach
The research used a place-based approach engaging participants living and working in the Fraser Valley region and centred the exploration specifically within the context of food systems issues and needs related to the region. Many of the issues and ideas presented through the workshops have broad applicability, such as, loss of livelihoods and overburdened social and health care systems (see Dring & Newell, 2021 ); however, the approach was still useful for identifying how issues commonly experienced in communities across the country and world occur in the local context. In this way, the research was effective in developing local, contextualized knowledge. For example, increases in food insecurity and (thus) usage of food banks were commonly observed issues in many communities during COVID-19 (e.g., Higgins et al., 2021 ), and such an issue was also observed in this work. However, the study also identified important nuances to the issue that were particular to the region, such as gaps in the types of food bank offerings to service to the local, significant South Asian population ( Dring & Newell, 2021 ). As indicated by one participant, the identification of both widely-experienced and locally-contextualised impacts can be useful for understanding local challenges while also gaining insights from other jurisdictions on how to address said challenges.
I think looking at some of the issues that have been identified in the discussion here today and just looking at how others are addressing those issues and just providing that information that can help those dealing with the challenges find the solutions. I think that would be really useful, just one place where people can look, this is the issue and this is how other jurisdictions have been successful at dealing with it, that would be just valuable. (Workshop 1 participant)
Relationship Development
The approach used in this research was effective for developing relationships among the researchers and participants. Relationships were developed and built by meeting community organizations and stakeholders on their terms and addressing their needs, as opposed to parachuting into a community with a specific research agenda and conducting the research in an extractive manner. In this study, the participants were provided space to explore topics of importance to them and their communities. Furthermore, as the work took a broader systems-based perspective, there were little constraints with respect to what was deemed relevant within this examination of local food systems vulnerabilities. One participant indicated feeling safe in the workshops with respect to being able to express the ideas they felt to be relevant and important.
I think the thing I was most impressed with is that I felt really safe and that I could say things that I think and I don’t often say some of the things that I said during our meetings in the general public because I think I’m thought of as being a weirdo. And I really appreciate the group and the expertise that was here, and I felt honored to participate. (Workshop 3 participant)
Researchers also built relationships and trust with community members and stakeholders by participating in local food systems networks. Such participation served the project in that it provided researchers with a greater understanding of the local food systems work and key challenges, while providing an avenue for putting research into practice. With respect to the latter, the researchers attended a strategic planning meeting held by one of the local food systems networks, where they brought forward ideas, analysis, and connections arising from the research to inform food system planning. In addition, researchers strengthened working relationships with participants by producing reports in-between research activities to communicate findings to participants, but also to offer further embellishment and directions for analysis of workshop/survey findings.
I really enjoyed the tools that you provided and the ability to actually see the reports in between the meetings so that we could kind of see refreshed on what’s been happening. And I hope this isn’t the end of this. I hope we get to continue with it at some point in time and in some capacity to continue working on solving these wicked problems. (Workshop 3 participant)
Multidimensional Exploration
The research explored temporal and spatial dimensions to stimulate thinking about present and future conditions in terms of food systems issues and initiatives for addressing these issues. Incorporating these dimensions added complexity to the workshop activities and discussions, which presented initial challenges but also resulted in expanded ways of thinking and approaching problem analysis and identification of root causes. This was particularly the case with the temporal component of the research. In the first workshop, participants struggled with the timelines, trying to identify whether impacts were short-, medium-, or long-term. However, in the second workshop, participants (many of whom participated in the first workshop) included temporal perspectives, without prompting, in their comments on impacts of various hazards to food systems. For example, a participant discussed the long-term, intergenerational consequences of an economic recession on local populations. Such findings suggest the research approach used here (i.e., one that directly involved local stakeholders in the examination of complex temporal issues) potentially increased capacity to do temporal analyses, which is important for long-term resilience planning.
With an economic downturn, some people lose their jobs and some kids would be living in poverty. But then I started thinking about the statistics I had heard about the impact it has on a child to grow up in poverty and to not have proper nutritional food. The impact on their education. Their ability as an adult to function their mental health. And, all of a sudden, they don’t do well in school because they don’t get good food at home. (Workshop 2 participant)
Dynamic Digital Tools and Activities
The use of online tools for facilitating the activities was advantageous in that it allowed for PR while also maintaining physical distancing during the on-going COVID-19 pandemic. Furthermore, workshop participants identified that the online approach held advantages even when physical distancing was not required. Some participants commented on the creative use of the CoLabS space in facilitating workshop activities using a variety of engagement tools. It was also noted that the use of said tools allowed for people to simultaneously engage in different aspects of a workshop, namely individual and group brainstorming, as participants could use the working tables while others were engaging in discussion. These comments suggest that there are particular benefits in running online PR workshops and focus groups.
I thought your CoLabS was very creative and just like, you know, usually as you’re brainstorming, which is sort of one person sort of thinking at a time kind of thing and people bouncing ideas off. But it was like, you know, one person can bounce ideas off while other people are writing on the Post-it board and all that kind of stuff. (Workshop 3 participant)
Limitations
Participation.
Although this project involved the participation of stakeholders with diverse involvement with food systems, comprehensive community and cultural representation was not achieved, nor possible. The topic of food system vulnerability is highly complex, and engaging every type of stakeholder and their specific relationships to food systems was not feasible in this project. Rather as researchers, we relied on our established relationships with organizations and individuals in the region. As such, the researchers operated under the assumption that participants exist in their own networks of relationships and engagement. Nonetheless, such challenges in participation resulted in gaps in food system actors, organizations, communities, and social groups that participated in the workshops.
Due to project resource and time constraints, the research did not effectively, and comprehensively, include relationship and trust building processes that are appropriate and sensitive to specific engagement considerations for food system actors (e.g., producers, labourers, processors) and certain impacted groups, such as people with lived experience of poverty or housing insecurity, racialized groups, and Indigenous communities. Such limitations ultimately resulted in conspicuous gaps in participation. The survey broadened participation; for example, unlike the workshops, the majority (77%) of survey respondents identified as being of Asian descent and multiple respondents indicating having lived experience with food insecurity issues due to the pandemic ( Newell & Dring, 2022 ). However, engagement through a survey is shallower than in the workshops, and it does not enable the bidirectional interaction needed for PR work ( Castleden et al., 2012 ); thus, lack of comprehensive representation was ultimately considered a major limitation of this work.
I just wanted to point out that I don’t think we really capture the Indigenous perspective here because many of our First Nations communities still rely on traditional ways and means of accessing food, and we haven’t really addressed what all of these environmental impacts will have on that. And I think at some point in time, it will be nice to connect with Elders and Knowledge Keepers from the various communities and see if they can add to this. (Workshop 2 participant)
Capacity Limitations
Capacity limitations of certain food systems stakeholders resulted in gaps in participation. Comprehensive sectoral representation was not achieved in this research, with notable gaps in those directly working in the areas of food production, processing, and retail. Unlike the challenges/issues related to engagement and recruitment noted above, these groups and communities were accessible to the researchers in that representatives were involved in the food networks previously discussed. However, barriers for these groups participating included challenges attending workshops while busy with their work. For example, Workshop 1 was in the summer, which is an extremely busy season for farmers, farm and food labourers, and food retailers. Therefore, although the workshops involved participants that work with various food system actors and organizations, these voices in many cases were not directly involved in the workshops.
The issue with capacity and participation in PR also relates to people’s ability to access research activities. As noted, online engagement was necessary for this project, and it carried benefits for the research process. However, it also created barriers to participation for certain vulnerable groups. This became apparent when a participant, who worked in the social services sector, discussed how reliable access to Internet was beneficial for their ability to access food during the pandemic; although this comment was directed toward food access, it also alluded to the ability (or lack thereof) of certain groups to participate in online workshops. Once again, the voices of these groups were represented by proxy (i.e., through social sector representatives), rather than directly.
I think in terms of at least impacts, I would say a massive amount has to do with socioeconomic status. I think about some of my privileges and what I’ve been able to do in the pandemic, especially as grocery stores became kind of like a place of threat and a place to avoid and the ability to have Internet access to be able to purchase things online. (Workshop 1 participant)
Real-World Issues
As noted above, the project activities occurred during times where a series of real-world hazard events were impacting food systems, perhaps the most salient example of this being the historic flooding event that impacted the FVRD in the fall of 2021. This synchronicity affected the research in multiple ways. One effect was to reduce workshop participation, as some were unable to attend workshops due to being too occupied with the emergency response to the flood event. Another way it affected the research was by elevating particular topics in the workshop discussions; for instance, although Workshop 2 involved exploring 10 different hazards and their potential impact on food systems, current and recent hazards (i.e., flooding, fires, and heat events) featured more prominently in the discussion, due to being top-of-mind.
We just acknowledged that these are not discrete hazards. They can happen at the same time, in fact, you could argue we’re doing that right now with the flood in the middle of the pandemic, for example, freshet season, which is a high-risk flooding season overlaps with the beginning of fire season. (Workshop 2 participant) Well, I started with flooding [in the spatial mapping activity] because that’s where my mind went. (Workshop 2 participant)
Online Tool Constraints
Participants for the most part expressed positive comments about the online activities; however, limitations in the tools were also clearly identified. In particular, a number of participants found the spatial mapping activity frustrating due to both technical and conceptual limitations. In terms of technical aspects, the tool embedded in the CoLabS platform only allowed for point data to be drawn on maps, whereas some participants indicated the issues and impacts that they identified relate to areas rather than specific locations and are better represented through polygon data. The trans-jurisdictional nature of certain vulnerabilities was noted during the workshop, and it was expressed by participants that the inability to identify areas (i.e., polygon data) was a limitation of the activity. In terms of conceptual issues, participants highlighted how certain hazards such as wildfire and conflict have difficult-to-pinpoint origins that exist outside of the provided map space. In both cases, these issues may not have been experienced in an in-person workshop, where freeform drawing on paper maps could be done. An in-person freeform drawing approach would allow for area/polygon mapping, and it would also enable creative methods for identifying difficult-to-pinpoint, outside-of-map issues such as drawing of arrows on the border of the map pointing inward. However, in the case of this research, the workshop activities were limited by the technical functionality of the tools and applications used.
I wanted to drop a pin and be able to show the effect across a larger area, such as, for example, there are a number of communities in North Mission and out in the electoral area to our east that are susceptible both to flight and to wildfire… a circle function for the map would be nice. (Workshop 2 participant) Difficult to address environmental hazards that come from outside places like how do I pinpoint an affected area when often, you know, if you’re dealing with wildfire in Chilliwack, you’re not actually dealing with a wildfire, you’re dealing with the smoke? (Workshop 2 participant)
The purpose of this project was to reflect upon the challenges and vulnerabilities that the COVID-19 pandemic has revealed about local food systems, ultimately to use this understanding to stimulate thinking about how to increase resilience to a variety of future shocks. Such work involves exploring linkages among different hazards, vulnerabilities, and impacts, which requires systems thinking and integrated planning perspectives. Researchers argue that integrated planning should involve participatory processes ( Ling et al., 2009 ), and by extension, applied research designed to support integrated planning should also be participatory in nature ( Newell et al., 2020 ). Illustrating these points is Jentoft and Chuenpagdee’s ( 2009 ) comment on governance, noting it to be “more effective if it occurs where the problem is felt (or where the opportunity actually appears), and in co-operation with those who are affected by it” (pg. 557). Instead of conducting vulnerability assessment based on historical environment and socioeconomic data such as done in other research (e.g., Bruno Soares et al., 2012 ), the PR approach employed in this work draws from both the professional and lived experiences of community members and their fellow community members during shock events. In this way, the study leveraged the expertise of community actors, while also engaging participants as humans and community members affected (or with friends and family affected) by hazards explored in the study. Such a PR approach enabled community reflections on the lessons COVID-19 provided on food systems vulnerabilities, using diverse expert and personal lenses.
Relationship and trust building are essential, and sometimes challenging activities, for conducting effective PR projects ( Hacker et al., 2012 ). One of the approaches used in this research for building and strengthening relationships involved the researchers participating in local food network organisations. Such an approach was effective in terms of developing interest and engaging participants in the project, as well as for enhancing the potential of the research being implemented into practice (e.g., researchers were subsequently invited to strategic planning meetings). However, the researchers’ participation in local food networks may have potentially affected recruitment and representation. Although the research primarily employed a purposive sampling method, inviting members of the food network organizations due to having access to this network could be considered akin to convenience sampling, which is a sampling approach with limitations to participant comprehensiveness ( Etikan et al., 2016 ).
Limitations around participation also occurred due to the nature of the engagement and relationship building activities. Jimmy et al. ( 2019 ) discuss how engaging with Indigenous and racialized communities in sustainability issues requires long-term engagement and relationship building, as well as recognition of differences in diverse ways of knowing. Project constraints did not enable sufficient time for long-term relationship building and additional workshops that are based on diverse ways of knowing, and as a result, the research experienced low representation from these communities. This being said, the survey broadened engagement in the project, with stronger representation from racialized groups ( Newell & Dring, 2022 ). However, surveys (particularly quantitative instruments) do not allow for transparent bidirectional engagement ( Andrews et al., 2011 ), which is needed for enabling the co-learning involved in PR work ( Castleden et al., 2012 ). To some degree, the research activities done in this project mirror different degrees of engagement outlined in Arnstein’s ( 1969 ) ladder of citizen participation, with the surveys fitting within lower “consultation” levels of the ladder and the workshops aligning with higher participation levels. Therefore, although the surveys were useful from broadening input and understanding of community experiences, it is fair to say that this research activity ultimately did little to increase inclusion in actual PR processes.
Another challenge experienced in this research included the real-time occurrence of hazards during the months that workshops were held. Such events created a context where the non-pandemic hazards were explored not as hypotheticals, but as real-world issues that are perhaps of more present concern than COVID-19. As a result, workshops with the initial aim to explore a variety of hazards tended toward particular issues and topics (e.g., the flooding in the Fraser Valley region) with respect to the workshop discussion and participant comments. The project ran over several seasons, and it explored a wide variety of locally-relevant environmental, socioeconomic, and political hazards; therefore, a distinct possibility existed that one of these hazard events would occur and be front-of-mind during the course of the research. A key learning here is that researchers who work with communities to examine real-world problems embedded in complex sustainability issues must be flexible in that they allow the focus of their research projects and activities to shift in accordance with current and most critical knowledge needs.
An interesting finding from this research was how the participants initially found the temporal aspects of the first workshop to be a challenge, but then employed temporal thinking in subsequent workshops. Although it adds complexity, exploring food systems issues through a temporal lens is valuable for long-term food systems resilience planning (e.g., Carey et al., 2021 ; Savary et al., 2020 ). Scholars that work in the areas of participatory planning and participatory research often discuss the importance of ensuring effective communication and engagement, which avoids overly complex presentation of topics/issues and jargon (e.g., De Bruin & Morgan, 2019 ; Portman et al., 2012 ). However, as evidenced by this research, this does not mean shying away from complexity altogether, as non-academic collaborators are able to expand their ability to engage in complex sustainability issues and topics. The challenge for PR work is to design activities and engage diverse team members in discussions in ways that do not overwhelm them with complexity, while also not over-simplifying the problems explored.
In addition to the application of a PR approach, this research experimented with the use of online tools for facilitating engagement and collaboration around a local sustainability issue. The CoLabS platform used in this work was designed to be flexible so that different tools can be embedded depending on the needs of the users ( Jost et al., 2021 ), and this degree of flexibility demonstrated to be useful as the workshops were developed in a manner that built on previous work, which required adding tools as new workshop activities were designed/planned. In addition, the digital platform offered new ways of engaging participants, allowing them to input ideas in the CoLabS space while also participating in online discussions, and this was noted to be advantageous for brainstorming.
The online approach also held shortcomings. This was in part due to limited functionality of the CoLabS tools; however, it is important to recognize that online engagement in PR will ultimately result in the participation of people who are comfortable with Internet technologies and have capacity to work in online spaces, potentially excluding some community members. Conversely, shifting completely to in-person engagement may discourage participation of those particularly vulnerable and concerned about contracting the COVID-19 virus. As the communities and societies continue to negotiate how to work and live within the new COVID-19 reality, it is important to consider and experiment diverse and hybrid options for engagement that enable higher degrees of inclusivity.
Participatory processes are integral to sustainability and resilience planning, as involving diverse stakeholders ensures planning practices and outcomes are grounded in local social, economic, environmental, and cultural contexts and realities ( Ling et al., 2009 ). It thus follows that research designed to support this type of planning should also be participatory in nature ( Newell et al., 2021 ). Accordingly, the project documented in this paper employed a PR approach to gain insights on food systems vulnerabilities and ways of building resilience in the Fraser Valley region. As seen with all research, this work had limitations and challenges. Food systems are complex, and due to time constraints, it was not feasible to engage every actor and stakeholder in local food systems. In addition, the researchers/participants contended with real-world hazard events during the project.
No research effort is perfect, and instead of dismissing the value of the work due to such limitations, researchers should be transparent about the shortcomings to provide appropriate context for knowledge outcomes. For example, the gaps in representation were identified by both researchers and participants in the workshops, as well as in knowledge products (e.g., Dring & Newell, 2021 ). Moreover, it is important for PR researchers to embrace the ‘messiness’ of this form of work, meaning their projects should be adaptable and responsive to emerging real-world issues. By taking such an approach, research can be nimble in that it can follow new pathways in pursuit of the production of useful knowledge for a community, as opposed to being rigid and mired in a set plan that does not necessarily respond to changing conditions and local needs.
Data Accessibility Statement
To comply with research ethics protocols, raw data are not made available; however, more details on the research activities, instruments, and outcomes can be found in reports available from the project’s webpage: www.ufv.ca/food-agriculture-institute/the-research/integrated-planning/food-systems-vulnerabilities .
Acknowledgements
We gratefully acknowledge the funding from the Social Sciences and Humanities Research Council (SSHRC) of Canada, provided through the Partnership Engage Grants (PEG) COVID-19 Special Initiative (File Number: 1008-2020-1011).
Funding Information
This research was supported by the Social Sciences and Humanities Research Council (SSHRC) of Canada, with funding provided through the Partnership Engage Grants (PEG) COVID-19 Special Initiative (File Number: 1008-2020-1011).
Competing Interests
The authors have no competing interests to declare.
Abbotsford Chamber of Commerce. (2022). Canada’s agricultural hub: An economic impact analysis of agriculture in Abbotsford . Retrieved from https://growthzonecmsprodeastus.azureedge.net/sites/92/2023/10/Canadas_Agricultural_Hub_-_An_Economic_Impact_Analysis_of_Agriculture_in_Abbotsford_May-25-2022-Report.pdf
Amer, M., Daim, T. U., & Jetter, A. (2013). A review of scenario planning. Futures , 46, 23–40. https://doi.org/10.1016/j.futures.2012.10.003
Andrews, J. O., Cox, M. J., Newman, S. D., & Meadows, O. (2011). Development and evaluation of a toolkit to assess partnership readiness for community-based participatory research. Progress in Community Health Partnerships , 5(2), 183–188. https://doi.org/10.1353/cpr.2011.0019
Arnstein, S. R. (1969). A ladder of citizen participation. Journal of the American Institute of planners , 35(4), 216–224. https://doi.org/10.1080/01944363.2018.1559388
Balfour, R. & Keenan, E.M. (2007). Strategic Sustainable Planning: A civil defense manual for cultural survival . Old City Foundation Press.
BC Ministry of Agriculture (2016). Fraser Valley Regional District – Agricultural Land Use Inventory. Retrieved from https://www2.gov.bc.ca/assets/gov/farming-natural-resources-and-industry/agriculture-and-seafood/agricultural-land-and-environment/strengthening-farming/land-use-inventories/fvrd_2011-13_aluireport.pdf
BC Stats (2021). Municipal and sub-provincial areas population, 2011 to 2021 . Government of British Columbia. Retrieved from https://www2.gov.bc.ca/gov/content/data/statistics/people-population-community/population/population-estimates
Biehl, E., Buzogany, S., Huang, A., Chodur, G., and Neff, R. (2017). Baltimore Food System Resilience Advisory Report . Johns Hopkins Center for a Livable Future. Retrieved from https://clf.jhsph.edu/sites/default/files/2019-01/baltimore-food-system-resilience-advisory-report.pdf
Bruno Soares, M., Gagnon, A. S., & Doherty, R. M. (2012). Conceptual elements of climate change vulnerability assessments: A review. International Journal of Climate Change Strategies and Management , 4(1), 6–35. https://doi.org/10.1108/17568691211200191
Carey, R., Murphy, M., & Alexandra, L. (2021). COVID-19 highlights the need to plan for healthy, equitable and resilient food systems. Cities & Health , 5(Suppl. 1), S123–S126. https://doi.org/10.1080/23748834.2020.1791442
Castleden, H., Morgan, V. S., & Lamb, C. (2012). “I spent the first year drinking tea”: Exploring Canadian university researchers’ perspectives on community-based participatory research involving Indigenous peoples. Canadian Geographer , 56(2), 160–179. https://doi.org/10.1111/j.1541-0064.2012.00432.x
Ciaccia, C., Pierro, M. di, Testani, E., Roccuzzo, G., Cutuli, M., & Ceccarelli, D. (2019). Participatory research towards food system redesign: Italian case study and perspectives. Sustainability , 11(24), 7138. https://doi.org/10.3390/su11247138
Clapp, J., & Moseley, W. G. (2020). This food crisis is different: COVID-19 and the fragility of the neoliberal food security order. Journal of Peasant Studies , 47(7), 1393–1417. https://doi.org/10.1080/03066150.2020.1823838
Clarke, V., Braun, V., & Hayfield, N. (2015). Thematic analysis. In J. Smith (Ed.), Qualitative Psychology: A Practical Guide to Research Methods . 3rd ed. (pp. 222–248). London, UK: Sage.
Climate Change Adaptation Program (2015). Fraser Valley: BC agriculture & climate change: Regional adaptation strategies series . British Columbia Agriculture & Food Climate Action Initiative. Retrieved from https://bcclimatechangeadaptation.ca/wp-content/uploads/2022/Resources/RegionalStrategies-FraserValley.pdf
De Bruin, W. B., & Morgan, M. G. (2019). Reflections on an interdisciplinary collaboration to inform public understanding of climate change, mitigation, and impacts. Proceedings of the National Academy of Sciences of the United States of America , 116(16), 7676–7683. https://doi.org/10.1073/pnas.1803726115
Domingo, A., Charles, K. A., Jacobs, M., Brooker, D., & Hanning, R. M. (2021). Indigenous community perspectives of food security, sustainable food systems and strategies to enhance access to local and traditional healthy food for partnering Williams Treaties First Nations (Ontario, Canada). International Journal of Environmental Research and Public Health , 18(9), 4404. https://doi.org/10.3390/ijerph18094404
Dring, C., & Newell, R. (2021). Fraser Valley food system vulnerability workshop: Summary report . Abbotsford, Canada: University of the Fraser Valley. https://doi.org/10.13140/RG.2.2.34634.08648
Dring, C., & Newell, R. (2022a). Mapping food system impacts, hazards, and vulnerabilities in the Fraser Valley region workshop: Summary report . Abbotsford, Canada: University of the Fraser Valley. https://doi.org/10.13140/RG.2.2.31197.69604
Dring, C., & Newell, R. (2022b). Building a foundation to resilient food systems in the Fraser Valley region: Summary report . Abbotsford, Canada: University of the Fraser Valley. https://doi.org/10.13140/RG.2.2.33906.79045
Etikan, I., Musa, S. A., & Alkassim, R. S. (2016) Comparison of convenience sampling and purposive sampling. American Journal of Theoretical and Applied Statistics , 5(1), 1–4. https://doi.org/10.11648/j.ajtas.20160501.11
Fraser Valley Regional District (2017). Regional Snapshot Series: Agriculture. Agricultural Economy in the Fraser Valley Regional District . Fraser Valley Regional District. Retrieved from https://www.fvrd.ca/assets/About~the~FVRD/Documents/RGS/AgricultureSnapshot.pdf
Fraser Valley Regional District (2019). Clean economy in the Fraser Valley: Resource guide . Fraser Valley Regional District. Retrieved from https://www.fvrd.ca/assets/About~the~FVRD/Documents/Clean~Economy/Clean%20Economy%20Resource%20Guide.pdf
Fraser Valley Regional District (n.d.-a.). First Nations . Fraser Valley Regional District. Retrieved from https://www.fvrd.ca/EN/main/about-the-fvrd/first-nations.html
Fraser Valley Regional District (n.d.-b.). What is the FVRD? Fraser Valley Regional District. Retrieved from https://www.fvrd.ca/EN/main/about-the-fvrd/what-is-the-fvrd.html
Greenhalgh, T., Jackson, C., Shaw, S., & Janamian, T. (2016). Achieving research impact through co-creation in community-based health services: Literature review and case study. The Milbank Quarterly , 94(2), 392–429. https://doi.org/10.1111/1468-0009.12197
Hacker, K., Tendulkar, S. A., Rideout, C., Bhuiya, N., Trinh-Shevrin, C., Savage, C. P., Grullon, M., Strelnick, H., Leung, C., & DiGirolamo, A. (2012). Community capacity building and sustainability: Outcomes of community-based participatory research. Progress in Community Health Partnerships: Research, Education, and Action , 6(3), 349–360. https://doi.org/10.1353/cpr.2012.0048
Higgins, C. D., Páez, A., Kim, G., & Wang, J. (2021). Changes in accessibility to emergency and community food services during COVID-19 and implications for low income populations in Hamilton, Ontario. Social Science & Medicine , 291, 114442. https://doi.org/10.1016/j.socscimed.2021.114442
Hobbs, J. (2020). Food supply chains during the COVID-19 pandemic. Canadian Journal of Agricultural Economics , 68, 171–176. https://doi.org/10.1111/cjag.12237
Intergovernmental Science-Policy Platform on Biodiversity and Ecosystem Services (2019). Global assessment report on biodiversity and ecosystem services of the Intergovernmental Science-Policy Platform on Biodiversity and Ecosystem Services. S. Díaz, J. Settele, E. S. Brondizio, H. T. Ngo, M. Guèze, J. Agard, A. Arneth, P. Balvanera, K. A. Brauman, S. H. M. Butchart, K. M. A. Chan, L. A. Garibaldi, K. Ichii, J. Liu, S. M. Subramanian, G. F. Midgley, P. Miloslavich, Z. Molnár, D. Obura, A. Pfaff, S. Polasky, A. Purvis, J. Razzaque, B. Reyers, R. Roy Chowdhury, Y. J. Shin, I. J. Visseren-Hamakers, K. J. Willis, and C. N. Zayas (eds.). Bonn, Germany: IPBES Secretariat.
Israel, B. A., Schulz, A. J., Parker, E. A., & Becker, A. N. (1998). Review of community-based research: assessing partnership approaches to improve public health. Annual Review of Public Health , 19, 173–202. https://doi.org/10.1146/annurev.publhealth.19.1.173
Jacobi, J., Wambugu, G., Ngutu, M., Augstburger, H., Mwangi, V., Zonta, A. L., Otieno, S., Kiteme, B. P., Burgoa, J. M. F. D., & Rist, S. (2019). Mapping food systems: A participatory research tool tested in Kenya and Bolivia. In Mountain Research and Development , 39(1), 1–11. https://doi.org/10.1659/MRD-JOURNAL-D-18-00024.1
Jahn, M., Jayamaha, J.B., Mulhern, W.S., Ross, D.E., Rose, M.A., and Treverton, G.F. (2018). Global food system stability and risk at the nexus of defense and development. Retrieved from https://www.thomsonreuters.com/content/dam/ewp-m/documents/thomsonreuters/en/pdf/reports/global-food-system-stability-and-risk-0718.pdf
Jentoft, S., & Chuenpagdee, R. (2009). Fisheries and coastal governance as a wicked problem. Marine Policy , 33(4), 553–560. https://doi.org/10.1016/j.marpol.2008.12.002
Jimmy, E., Andreotti, V., & Stein, S. (2019). Towards braiding . Guelph, Canada: Musagetes Foundation. Retrieved from https://musagetes.ca/wpcontent/uploads/2019/07/Braiding_ReaderWeb.pdf
Jost, F., Newell, R., & Dale, A. (2021). CoLabS: A collaborative space for transdisciplinary work in sustainable community development. Heliyon , 7(2), e05997. https://doi.org/10.1016/j.heliyon.2021.e05997
Knezevic, I., Blay-Palmer, A., Levkoe, C. Z., Mount, P., & Nelson, E. (2017). Nourishing communities: From fractured food systems to transformative pathways . Cham, Switzerland: Springer International Publishing.
Ling, C., Hanna, K., & Dale, A. (2009). A template for integrated community sustainability planning. Environmental Management , 44(2), 228–242. https://doi.org/10.1007/s00267-009-9315-7
Moldenhauer, J., & Sackey, D.J. (2016). Transdisciplinarity, community-based participatory research, and user-based information design research. In A. Marcus (Ed.), Design, User Experience, and Usability: Design Thinking and Methods. Lecture Notes in Computer Science (pp. 323–332). Springer: Cham, Switzerland.
Newell, R., & Dale, A. (2021). COVID-19 and climate change: An integrated perspective. Cities & Health , 5(Suppl. 1), S100–S104. https://doi.org/10.1080/23748834.2020.1778844
Newell, R., & Dring, C. (2022). Food systems hazards, vulnerabilities and impacts in the Lower Mainland, BC . Abbotsford, Canada: University of the Fraser Valley. https://doi.org/10.13140/RG.2.2.15365.42721
Newell, R., McCarthy, N., Picketts, I., Davis, F., Hovem, G., & Navarrete, S. (2021). Communicating complexity: Interactive model explorers and immersive visualizations as tools for local planning and community engagement. FACETS , 6, 287–316. https://doi.org/10.1139/facets-2020-0045
Newell, R., Picketts, I.M., & Dale, A. (2020). Community systems models and development scenarios for integrated planning: Lessons learned from a participatory approach. Community Development , 51(3), 261–282. https://doi.org/10.1080/15575330.2020.1772334
Newman, L., Powell, L. J., Nickel, J., Anderson, D., Jovanovic, L., Mendez, E., Mendez, E., & Kelly-Freiberg, K. (2017). Farm stores in agriburbia: The roles of agricultural retail on the rural-urban fringe. Canadian Food Studies/La Revue canadienne des études sur l’alimentation , 4(1), 4–23. https://doi.org/10.15353/cfs-rcea.v4i1.211
Onwuegbuzie, A. J., Dickinson, W. B., Leech, N. L., & Zoran, A. G. (2009). A qualitative framework for collecting and analyzing data in focus group research. International Journal of Qualitative Methods , 8(3), 1–21. https://doi.org/10.1177/160940690900800301
Portman, M. E., Esteves, L. S., Le, X. Q., & Khan, a Z. (2012). Improving integration for integrated coastal zone management: an eight country study. The Science of the Total Environment , 439, 194–201. https://doi.org/10.1016/j.scitotenv.2012.09.016
Picketts, I. M., Werner, A. T., Murdock, T. Q., Curry, J., Déry, S. J., & Dyer, D. (2012). Planning for climate change adaptation: Lessons learned from a community-based workshop. Environmental Science and Policy , 17, 82–93. https://doi.org/10.1016/j.envsci.2011.12.011
Savan, B., & Sider, D. (2003). Contrasting approaches to community-based research and a case study of community sustainability in Toronto, Canada. Local Environment , 8(3), 303–316. https://doi.org/10.1080/13549830306657
Savary, S., Akter, S., Almekinders, C., Harris, J., Korsten, L., Rötter, R., Waddington, S., & Watson, D. (2020). Mapping disruption and resilience mechanisms in food systems. Food Security , 12(4), 695–717. https://doi.org/10.1007/s12571-020-01093-0
Trimble, M., & Berkes, F. (2013). Participatory research towards co-management: Lessons from artisanal fisheries in coastal Uruguay. Journal of Environmental Management , 128, 768–778. https://doi.org/10.1016/j.jenvman.2013.06.032
Vaughn, L. M., & Jacquez, F. (2020). Participatory research methods – choice points in the research process. Journal of Participatory Research Methods , 1(1), 1–13. https://doi.org/10.35844/001c.13244
Zeuli, K., & Whalen, R. (2017). Resilient cities require resilient food systems. ICIC Blog . Retrieved from https://icic.org/blog/resilient-cities-require-resilient-food-systems
Thank you for visiting nature.com. You are using a browser version with limited support for CSS. To obtain the best experience, we recommend you use a more up to date browser (or turn off compatibility mode in Internet Explorer). In the meantime, to ensure continued support, we are displaying the site without styles and JavaScript.
- View all journals
- Explore content
- About the journal
- Publish with us
- Sign up for alerts
- Review Article
- Published: 04 July 2024
Harnessing EHR data for health research
- Alice S. Tang ORCID: orcid.org/0000-0003-4745-0714 1 ,
- Sarah R. Woldemariam 1 ,
- Silvia Miramontes 1 ,
- Beau Norgeot ORCID: orcid.org/0000-0003-2629-701X 2 ,
- Tomiko T. Oskotsky ORCID: orcid.org/0000-0001-7393-5120 1 &
- Marina Sirota ORCID: orcid.org/0000-0002-7246-6083 1 , 3
Nature Medicine ( 2024 ) Cite this article
4 Altmetric
Metrics details
- Computational biology and bioinformatics
- Machine learning
With the increasing availability of rich, longitudinal, real-world clinical data recorded in electronic health records (EHRs) for millions of patients, there is a growing interest in leveraging these records to improve the understanding of human health and disease and translate these insights into clinical applications. However, there is also a need to consider the limitations of these data due to various biases and to understand the impact of missing information. Recognizing and addressing these limitations can inform the design and interpretation of EHR-based informatics studies that avoid confusing or incorrect conclusions, particularly when applied to population or precision medicine. Here we discuss key considerations in the design, implementation and interpretation of EHR-based informatics studies, drawing from examples in the literature across hypothesis generation, hypothesis testing and machine learning applications. We outline the growing opportunities for EHR-based informatics studies, including association studies and predictive modeling, enabled by evolving AI capabilities—while addressing limitations and potential pitfalls to avoid.
This is a preview of subscription content, access via your institution
Access options
Access Nature and 54 other Nature Portfolio journals
Get Nature+, our best-value online-access subscription
24,99 € / 30 days
cancel any time
Subscribe to this journal
Receive 12 print issues and online access
195,33 € per year
only 16,28 € per issue
Buy this article
- Purchase on Springer Link
- Instant access to full article PDF
Prices may be subject to local taxes which are calculated during checkout
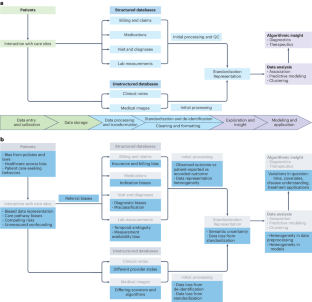
Gillum, R. F. From papyrus to the electronic tablet: a brief history of the clinical medical record with lessons for the digital age. Am. J. Med. 126 , 853–857 (2013).
Article PubMed Google Scholar
US Food and Drug Administration. Real-World Evidence. FDA https://www.fda.gov/science-research/science-and-research-special-topics/real-world-evidence/ (5 February 2023).
Office of the National Coordinator for Health Information Technology. National Trends in Hospital and Physician Adoption of Electronic Health Records. HealthIT.gov https://www.healthit.gov/data/quickstats/national-trends-hospital-and-physician-adoption-electronic-health-records/ (2021).
Liu, F. & Panagiotakos, D. Real-world data: a brief review of the methods, applications, challenges and opportunities. BMC Med. Res. Methodol. 22 , 287 (2022).
Article PubMed PubMed Central Google Scholar
Cowie, M. R. et al. Electronic health records to facilitate clinical research. Clin. Res. Cardiol. 106 , 1–9 (2017).
Kierkegaard, P. Electronic health record: wiring Europe’s healthcare. Comput. Law Secur. Rev. 27 , 503–515 (2011).
Article Google Scholar
Wen, H. -C., Chang, W. -P., Hsu, M. -H., Ho, C. -H. & Chu, C. -M. An assessment of the interoperability of electronic health record exchanges among hospitals and clinics in Taiwan. JMIR Med. Inform. 7 , e12630 (2019).
Johnson, A. E. W. et al. MIMIC-IV, a freely accessible electronic health record dataset. Sci. Data 10 , 1 (2023).
Article CAS PubMed PubMed Central Google Scholar
All of Us Research Program Investigators. The ‘All of Us’ Research Program. N. Engl. J. Med . 381 , 668–676 (2019).
Sudlow, C. et al. UK Biobank: an open access resource for identifying the causes of a wide range of complex diseases of middle and old age. PLoS Med. 12 , e1001779 (2015).
Sinha, P., Sunder, G., Bendale, P., Mantri, M. & Dande, A. Electronic Health Record: Standards, Coding Systems, Frameworks, and Infrastructures (Wiley, 2012); https://doi.org/10.1002/9781118479612
Overhage, J. M., Ryan, P. B., Reich, C. G., Hartzema, A. G. & Stang, P. E. Validation of a common data model for active safety surveillance research. J. Am. Med. Inform. Assoc. 19 , 54–60 (2012).
Murugadoss, K. et al. Building a best-in-class automated de-identification tool for electronic health records through ensemble learning. Patterns 2 , 100255 (2021).
Yogarajan, V., Pfahringer, B. & Mayo, M. A review of automatic end-to-end de-identification: is high accuracy the only metric? Appl. Artif. Intell. 34 , 251–269 (2020).
Mandl, K. D. & Perakslis, E. D. HIPAA and the leak of ‘deidentified’ EHR data. N. Engl. J. Med. 384 , 2171–2173 (2021).
Norgeot, B. et al. Protected Health Information filter (Philter): accurately and securely de-identifying free-text clinical notes. NPJ Digit. Med. 3 , 57 (2020).
Steurer, M. A. et al. Cohort study of respiratory hospital admissions, air quality and sociodemographic factors in preterm infants born in California. Paediatr. Perinat. Epidemiol. 34 , 130–138 (2020).
Costello, J. M., Steurer, M. A., Baer, R. J., Witte, J. S. & Jelliffe‐Pawlowski, L. L. Residential particulate matter, proximity to major roads, traffic density and traffic volume as risk factors for preterm birth in California. Paediatr. Perinat. Epidemiol. 36 , 70–79 (2022).
Yan, C. et al. Differences in health professionals’ engagement with electronic health records based on inpatient race and ethnicity. JAMA Netw. Open 6 , e2336383 (2023).
Lotfata, A., Moosazadeh, M., Helbich, M. & Hoseini, B. Socioeconomic and environmental determinants of asthma prevalence: a cross-sectional study at the U.S. county level using geographically weighted random forests. Int. J. Health Geogr. 22 , 18 (2023).
Li, L. et al. Identification of type 2 diabetes subgroups through topological analysis of patient similarity. Sci. Transl. Med. 7 , 311ra174 (2015).
De Freitas, J. K. et al. Phe2vec: automated disease phenotyping based on unsupervised embeddings from electronic health records. Patterns 2 , 100337 (2021).
Tang, A. S. et al. Deep phenotyping of Alzheimer’s disease leveraging electronic medical records identifies sex-specific clinical associations. Nat. Commun. 13 , 675 (2022).
Su, C. et al. Clinical subphenotypes in COVID-19: derivation, validation, prediction, temporal patterns, and interaction with social determinants of health. NPJ Digit. Med. 4 , 110 (2021).
Glicksberg, B. S. et al. PatientExploreR: an extensible application for dynamic visualization of patient clinical history from electronic health records in the OMOP common data model. Bioinformatics 35 , 4515–4518 (2019).
Huang, Z., Dong, W., Bath, P., Ji, L. & Duan, H. On mining latent treatment patterns from electronic medical records. Data Min. Knowl. Discov. 29 , 914–949 (2015).
Zaballa, O., Pérez, A., Gómez Inhiesto, E., Acaiturri Ayesta, T. & Lozano, J. A. Identifying common treatments from electronic health records with missing information. An application to breast cancer. PLoS ONE 15 , e0244004 (2020).
Lou, S. S., Liu, H., Harford, D., Lu, C. & Kannampallil, T. Characterizing the macrostructure of electronic health record work using raw audit logs: an unsupervised action embeddings approach. J. Am. Med. Inform. Assoc. 30 , 539–544 (2023).
Glicksberg, B. S. et al. Comparative analyses of population-scale phenomic data in electronic medical records reveal race-specific disease networks. Bioinformatics 32 , i101–i110 (2016).
Obermeyer, Z., Powers, B., Vogeli, C. & Mullainathan, S. Dissecting racial bias in an algorithm used to manage the health of populations. Science 366 , 447–453 (2019).
Article CAS PubMed Google Scholar
Smith, M. A. et al. Insights into measuring health disparities using electronic health records from a statewide network of health systems: a case study. J. Clin. Transl. Sci. 7 , e54 (2023).
Swerdel, J. N., Hripcsak, G. & Ryan, P. B. PheValuator: development and evaluation of a phenotype algorithm evaluator. J. Biomed. Inform. 97 , 103258 (2019).
Denny, J. C. et al. PheWAS: demonstrating the feasibility of a phenome-wide scan to discover gene-disease associations. Bioinformatics 26 , 1205–1210 (2010).
Chen, C., Ding, S. & Wang, J. Digital health for aging populations. Nat. Med. 29 , 1623–1630 (2023).
Woldemariam, S. R., Tang, A. S., Oskotsky, T. T., Yaffe, K. & Sirota, M. Similarities and differences in Alzheimer’s dementia comorbidities in racialized populations identified from electronic medical records. Commun. Med. 3 , 50 (2023).
Austin, P. C. An introduction to propensity score methods for reducing the effects of confounding in observational studies. Multivariate Behav. Res. 46 , 399–424 (2011).
Karlin, L. et al. Use of the propensity score matching method to reduce recruitment bias in observational studies: application to the estimation of survival benefit of non-myeloablative allogeneic transplantation in patients with multiple myeloma relapsing after a first autologous transplantation. Blood 112 , 1133 (2008).
Ho, D., Imai, K., King, G. & Stuart, E. A. MatchIt: nonparametric preprocessing for parametric causal inference. J. Stat. Softw. 42 , 8 (2011).
Zhang, Z., Kim, H. J., Lonjon, G. & Zhu, Y. Balance diagnostics after propensity score matching. Ann. Transl. Med. 7 , 16 (2019).
Landi, I. et al. Deep representation learning of electronic health records to unlock patient stratification at scale. NPJ Digit. Med. 3 , 96 (2020).
Bai, W. et al. A population-based phenome-wide association study of cardiac and aortic structure and function. Nat. Med . https://doi.org/10.1038/s41591-020-1009-y (2020).
Engels, E. A. et al. Comprehensive evaluation of medical conditions associated with risk of non-Hodgkin lymphoma using medicare claims (‘MedWAS’). Cancer Epidemiol. Biomark. Prev. 25 , 1105–1113 (2016).
Article CAS Google Scholar
Bastarache, L., Denny, J. C. & Roden, D. M. Phenome-wide association studies. J. Am. Med. Assoc. 327 , 75–76 (2022).
Yazdany, J. et al. Rheumatology informatics system for effectiveness: a national informatics‐enabled registry for quality improvement. Arthritis Care Res. 68 , 1866–1873 (2016).
Nelson, C. A., Bove, R., Butte, A. J. & Baranzini, S. E. Embedding electronic health records onto a knowledge network recognizes prodromal features of multiple sclerosis and predicts diagnosis. J. Am. Med. Inform. Assoc. 29 , 424–434 (2022).
Tang, A. S. et al. Leveraging electronic health records and knowledge networks for Alzheimer’s disease prediction and sex-specific biological insights. Nat. Aging 4 , 379–395 (2024).
Mullainathan, S. & Obermeyer, Z. Diagnosing physician error: a machine learning approach to low-value health care. Q. J. Econ. 137 , 679–727 (2022).
Makin, T. R. & Orban De Xivry, J. -J. Ten common statistical mistakes to watch out for when writing or reviewing a manuscript. eLife 8 , e48175 (2019).
Carrigan, G. et al. External comparator groups derived from real-world data used in support of regulatory decision making: use cases and challenges. Curr. Epidemiol. Rep. 9 , 326–337 (2022).
Hersh, W. R. et al. Caveats for the use of operational electronic health record data in comparative effectiveness research. Med. Care 51 , S30–S37 (2013).
Rudrapatna, V. A. & Butte, A. J. Opportunities and challenges in using real-world data for health care. J. Clin. Invest. 130 , 565–574 (2020).
Belthangady, C. et al. Causal deep learning reveals the comparative effectiveness of antihyperglycemic treatments in poorly controlled diabetes. Nat. Commun. 13 , 6921 (2022).
Roger, J. et al. Leveraging electronic health records to identify risk factors for recurrent pregnancy loss across two medical centers: a case–control study. Preprint at Res. Sq. https://doi.org/10.21203/rs.3.rs-2631220/v2 (2023).
Gervasi, S. S. et al. The potential for bias in machine learning and opportunities for health insurers to address it: article examines the potential for bias in machine learning and opportunities for health insurers to address it. Health Aff. 41 , 212–218 (2022).
Sai, S. et al. Generative AI for transformative healthcare: a comprehensive study of emerging models, applications, case studies, and limitations. IEEE Access 12 , 31078–31106 (2024).
Wang, M. et al. A systematic review of automatic text summarization for biomedical literature and EHRs. J. Am. Med. Inform. Assoc. 28 , 2287–2297 (2021).
Katsoulakis, E. et al. Digital twins for health: a scoping review. NPJ Digit. Med. 7 , 77 (2024).
Thirunavukarasu, A. J. et al. Large language models in medicine. Nat. Med. 29 , 1930–1940 (2023).
Meskó, B. & Topol, E. J. The imperative for regulatory oversight of large language models (or generative AI) in healthcare. NPJ Digit. Med. 6 , 120 (2023).
Hastings, J. Preventing harm from non-conscious bias in medical generative AI. Lancet Digit. Health 6 , e2–e3 (2024).
Lett, E., Asabor, E., Beltrán, S., Cannon, A. M. & Arah, O. A. Conceptualizing, contextualizing, and operationalizing race in quantitative health sciences research. Ann. Fam. Med. 20 , 157–163 (2022).
Belonwu, S. A. et al. Sex-stratified single-cell RNA-seq analysis identifies sex-specific and cell type-specific transcriptional responses in Alzheimer’s disease across two brain regions. Mol. Neurobiol. https://doi.org/10.1007/s12035-021-02591-8 (2021).
Krumholz, A. Driving and epilepsy: a review and reappraisal. J. Am. Med. Assoc. 265 , 622–626 (1991).
Xu, J. et al. Data-driven discovery of probable Alzheimer’s disease and related dementia subphenotypes using electronic health records. Learn. Health Syst. 4 , e10246 (2020).
Vyas, D. A., Eisenstein, L. G. & Jones, D. S. Hidden in plain sight—reconsidering the use of race correction in clinical algorithms. N. Engl. J. Med. 383 , 874–882 (2020).
Dagdelen, J. et al. Structured information extraction from scientific text with large language models. Nat. Commun. 15 , 1418 (2024).
Hu, Y. et al. Improving large language models for clinical named entity recognition via prompt engineering. J. Am. Med. Inform. Assoc. 27 , ocad259 (2024).
Microsoft. microsoft/FHIR-Converter (2024).
Torfi, A., Fox, E. A. & Reddy, C. K. Differentially private synthetic medical data generation using convolutional GANs. Inf. Sci. 586 , 485–500 (2022).
Yoon, J., Jordon, J. & van der Schaar, M. GAIN: missing data imputation using generative adversarial nets. Preprint at https://arxiv.org/abs/1806.02920v1 (2018).
Shi, J., Wang, D., Tesei, G. & Norgeot, B. Generating high-fidelity privacy-conscious synthetic patient data for causal effect estimation with multiple treatments. Front. Artif. Intell. 5 , 918813 (2022).
Stuart, E. A. Matching methods for causal inference: a review and a look forward. Stat. Sci. 25 , 1–21 (2010).
Murali, L., Gopakumar, G., Viswanathan, D. M. & Nedungadi, P. Towards electronic health record-based medical knowledge graph construction, completion, and applications: a literature study. J. Biomed. Inform. 143 , 104403 (2023).
Li, Y. et al. BEHRT: transformer for electronic health records. Sci. Rep. 10 , 7155 (2020).
Guo, L. L. et al. EHR foundation models improve robustness in the presence of temporal distribution shift. Sci. Rep. 13 , 3767 (2023).
Zhu, R. et al. Clinical pharmacology applications of real‐world data and real‐world evidence in drug development and approval—an industry perspective. Clin. Pharmacol. Ther. 114 , 751–767 (2023).
Voss, E. A. et al. Accuracy of an automated knowledge base for identifying drug adverse reactions. J. Biomed. Inform. 66 , 72–81 (2017).
Taubes, A. et al. Experimental and real-world evidence supporting the computational repurposing of bumetanide for APOE4-related Alzheimer’s disease. Nat. Aging 1 , 932–947 (2021).
Gold, R. et al. Using electronic health record-based clinical decision support to provide social risk-informed care in community health centers: protocol for the design and assessment of a clinical decision support tool. JMIR Res. Protoc. 10 , e31733 (2021).
Varga, A. N. et al. Dealing with confounding in observational studies: a scoping review of methods evaluated in simulation studies with single‐point exposure. Stat. Med. 42 , 487–516 (2023).
Carrigan, G. et al. Using electronic health records to derive control arms for early phase single‐arm lung cancer trials: proof‐of‐concept in randomized controlled trials. Clin. Pharmacol. Ther. 107 , 369–377 (2020).
Infante-Rivard, C. & Cusson, A. Reflection on modern methods: selection bias—a review of recent developments. Int. J. Epidemiol. 47 , 1714–1722 (2018).
Degtiar, I. & Rose, S. A review of generalizability and transportability. Annu. Rev. Stat. Appl. 10 , 501–524 (2023).
Badhwar, A. et al. A multiomics approach to heterogeneity in Alzheimer’s disease: focused review and roadmap. Brain 143 , 1315–1331 (2020).
Stuart, E. A. & Rubin, D. B. Matching with multiple control groups with adjustment for group differences. J. Educ. Behav. Stat. 33 , 279–306 (2008).
Hernan, M. A. & Robins, J. M. Causal Inference: What If (Taylor and Francis, 2024).
Hernan, M. A. Causal knowledge as a prerequisite for confounding evaluation: an application to birth defects epidemiology. Am. J. Epidemiol. 155 , 176–184 (2002).
Dang, L. E. et al. A causal roadmap for generating high-quality real-world evidence. J. Clin. Transl. Sci. 7 , e212 (2023).
Hernán, M. A. & Robins, J. M. Using big data to emulate a target trial when a randomized trial is not available. Am. J. Epidemiol. 183 , 758–764 (2016).
Oskotsky, T. et al. Mortality risk among patients with COVID-19 prescribed selective serotonin reuptake inhibitor antidepressants. JAMA Netw. Open 4 , e2133090 (2021).
Sperry, M. M. et al. Target-agnostic drug prediction integrated with medical record analysis uncovers differential associations of statins with increased survival in COVID-19 patients. PLoS Comput. Biol. 19 , e1011050 (2023).
Amit, G. et al. Antidepressant use during pregnancy and the risk of preterm birth – a cohort study. NPJ Womens Health 2 , 5 (2024); https://doi.org/10.1038/s44294-024-00008-0
Download references
Author information
Authors and affiliations.
Bakar Computational Health Sciences Institute, University of California, San Francisco, San Francisco, CA, USA
Alice S. Tang, Sarah R. Woldemariam, Silvia Miramontes, Tomiko T. Oskotsky & Marina Sirota
Qualified Health, Palo Alto, CA, USA
Beau Norgeot
Department of Pediatrics, University of California, San Francisco, San Francisco, CA, USA
Marina Sirota
You can also search for this author in PubMed Google Scholar
Corresponding author
Correspondence to Marina Sirota .
Ethics declarations
Competing interests.
B.N. is an employee at Qualified Health. The other authors declare no competing interests.
Peer review
Peer review information.
Nature Medicine thanks Wenbo Wu and the other, anonymous, reviewer(s) for their contribution to the peer review of this work. Primary Handling Editor: Karen O’Leary, in collaboration with the Nature Medicine team.
Additional information
Publisher’s note Springer Nature remains neutral with regard to jurisdictional claims in published maps and institutional affiliations.
Rights and permissions
Springer Nature or its licensor (e.g. a society or other partner) holds exclusive rights to this article under a publishing agreement with the author(s) or other rightsholder(s); author self-archiving of the accepted manuscript version of this article is solely governed by the terms of such publishing agreement and applicable law.
Reprints and permissions
About this article
Cite this article.
Tang, A.S., Woldemariam, S.R., Miramontes, S. et al. Harnessing EHR data for health research. Nat Med (2024). https://doi.org/10.1038/s41591-024-03074-8
Download citation
Received : 03 January 2024
Accepted : 17 May 2024
Published : 04 July 2024
DOI : https://doi.org/10.1038/s41591-024-03074-8
Share this article
Anyone you share the following link with will be able to read this content:
Sorry, a shareable link is not currently available for this article.
Provided by the Springer Nature SharedIt content-sharing initiative
Quick links
- Explore articles by subject
- Guide to authors
- Editorial policies
Sign up for the Nature Briefing newsletter — what matters in science, free to your inbox daily.

Wegovy, Ozempic linked with sight-threatening eye disorder in study
- Medium Text
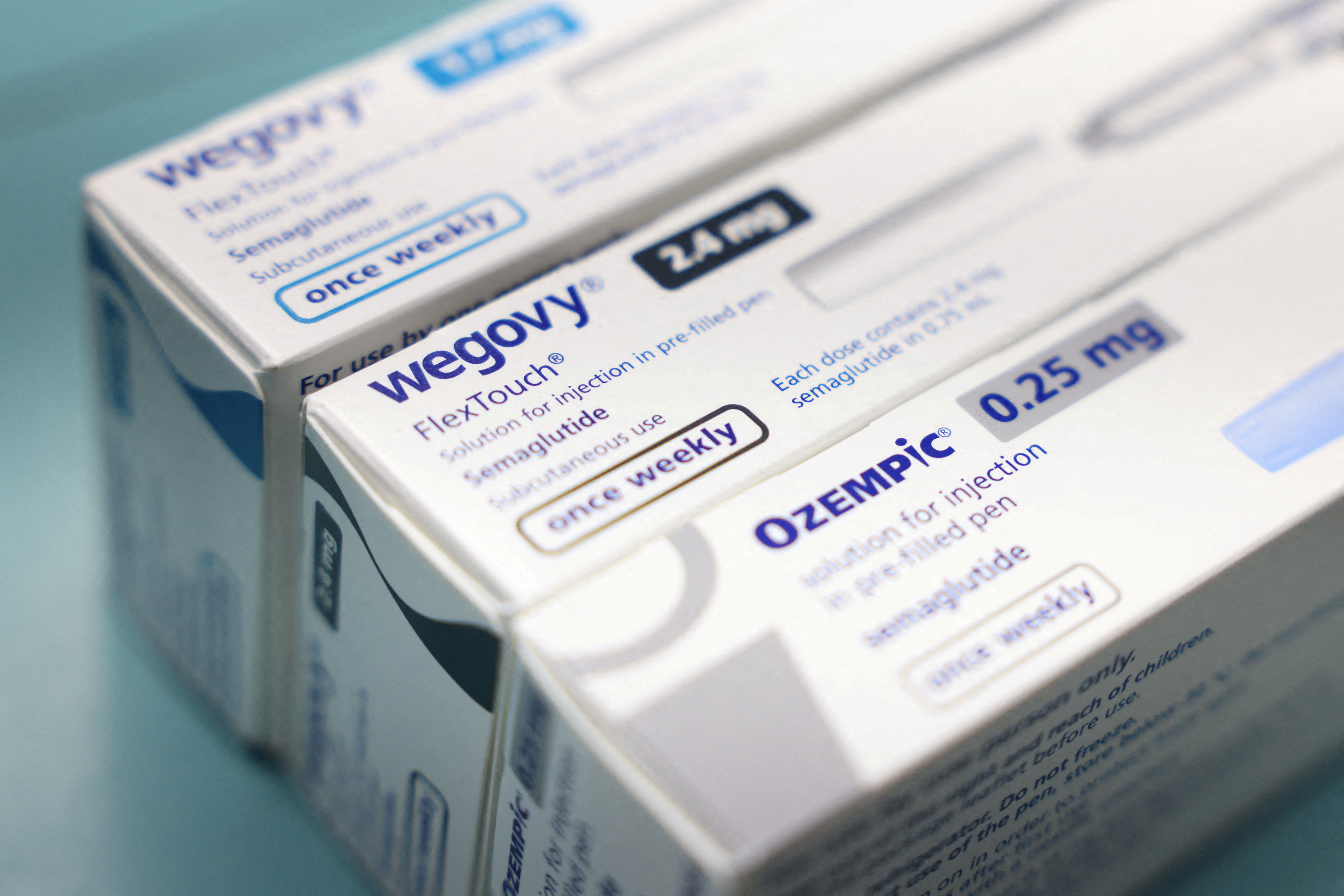
- Company Novo Nordisk A/S Follow
Sign up here.
Reporting by Nancy Lapid; Editing by Bill Berkrot
Our Standards: The Thomson Reuters Trust Principles. New Tab , opens new tab
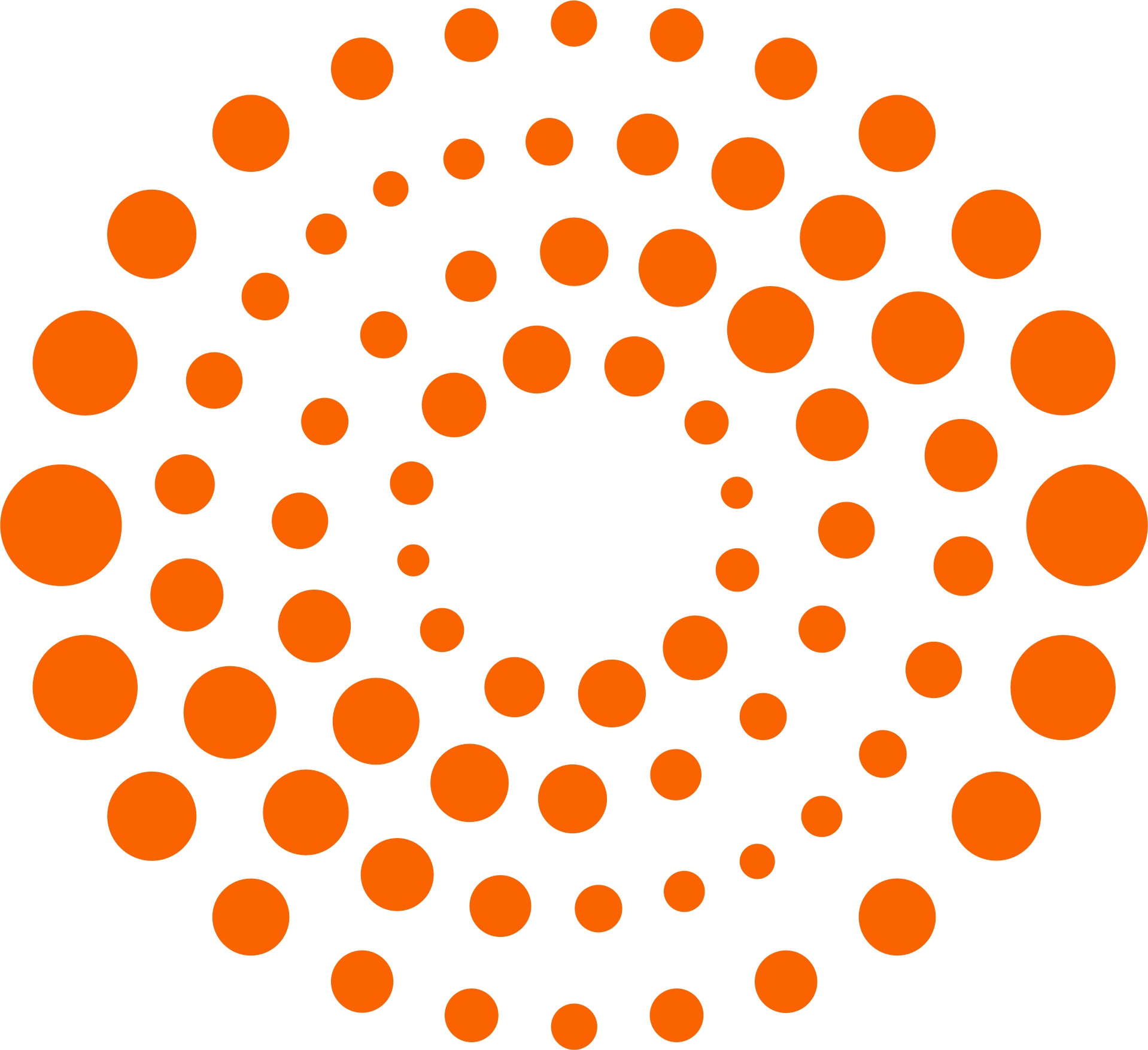
Thomson Reuters
Nancy has been a health news reporter and editor at Reuters for more than a decade, covering important medical research advances. She is the author of our twice-a-week Reuters Health Rounds newsletter.
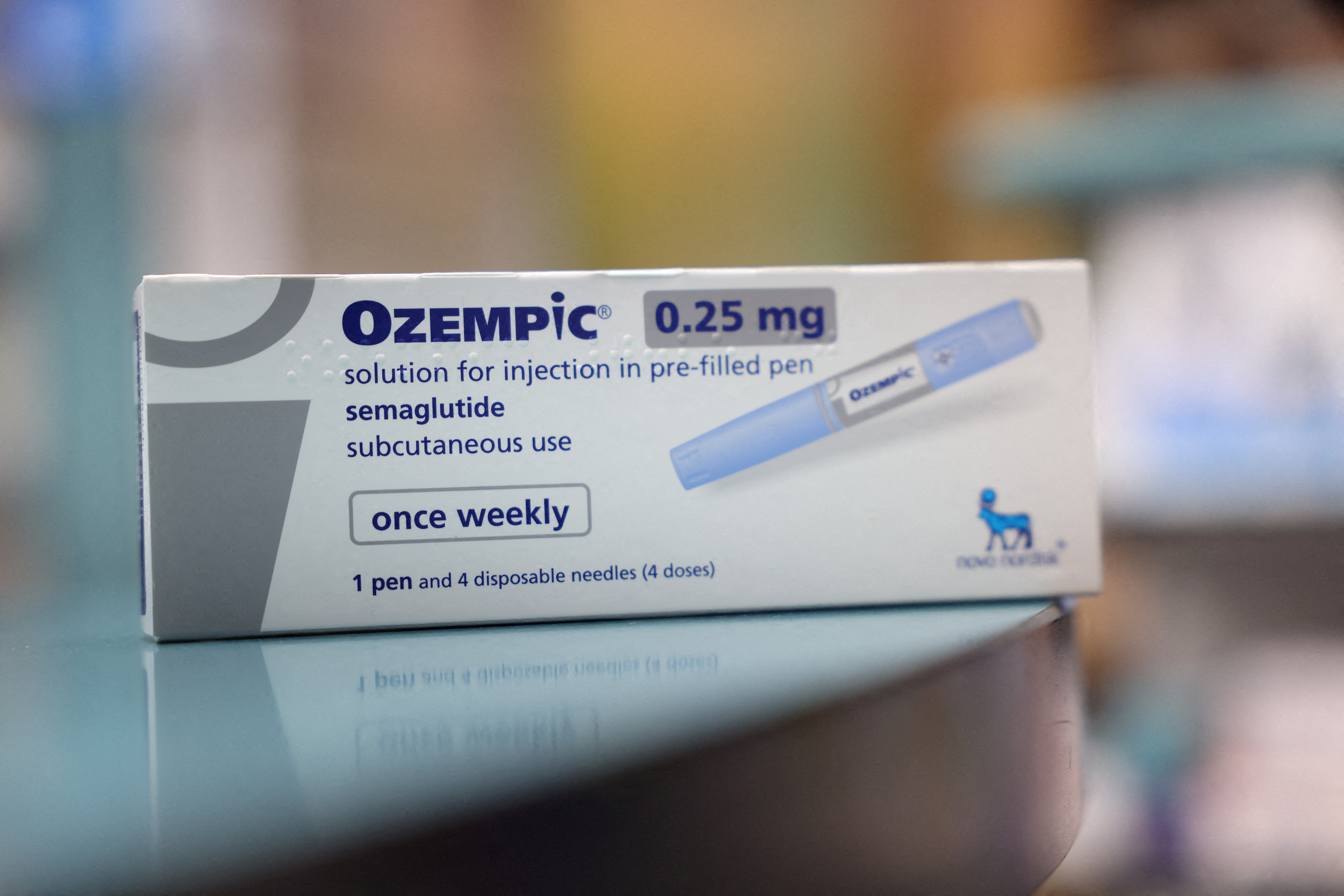
Business Chevron
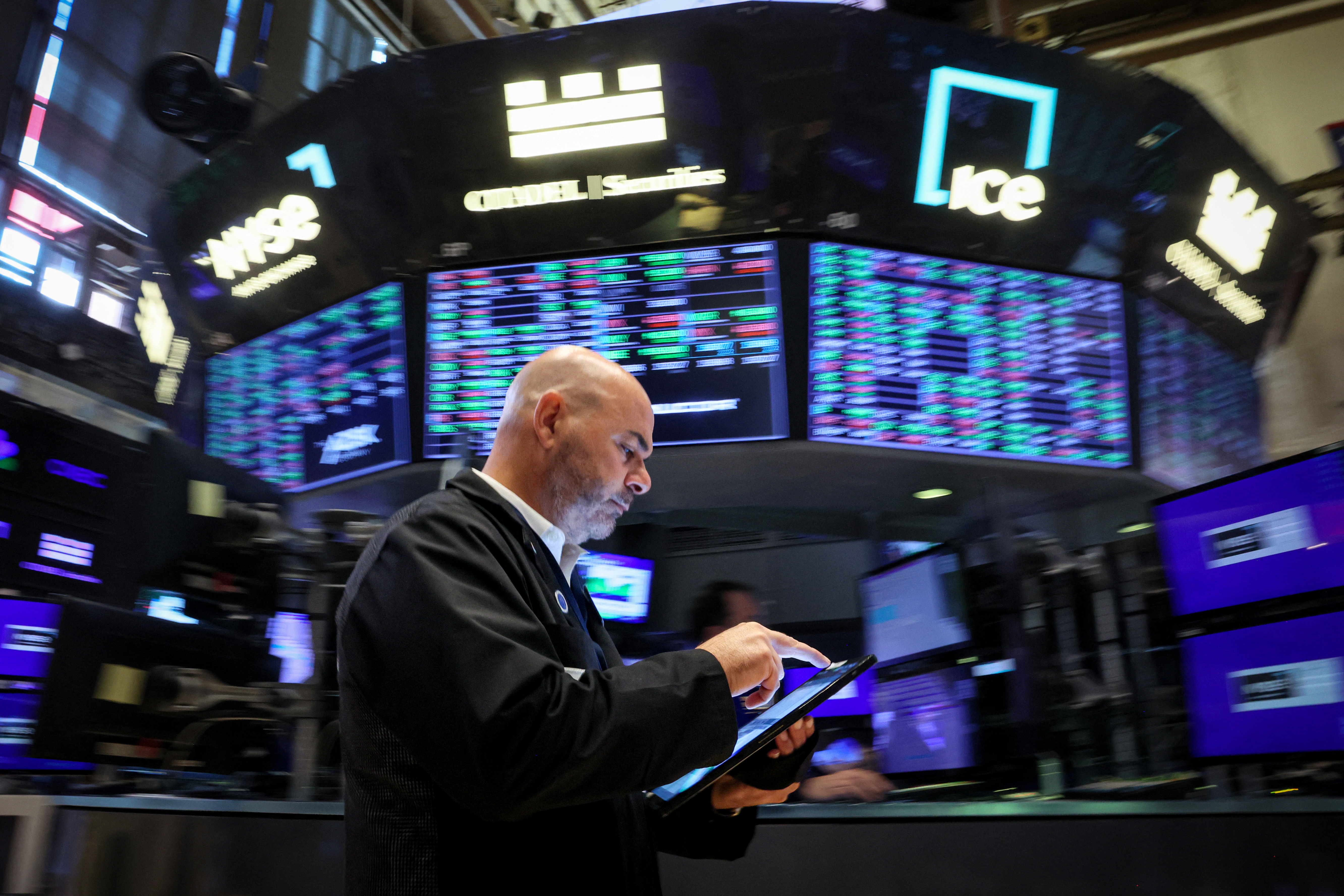
Nasdaq, S&P 500 hit record highs as payrolls data raises rate cut hopes
Wall Street stock indexes closed firmer on Friday, with the tech-heavy Nasdaq and benchmark S&P 500 hitting record highs, as new data showing U.S. labor market weakness boosted expectations for interest rate cuts as early as September.
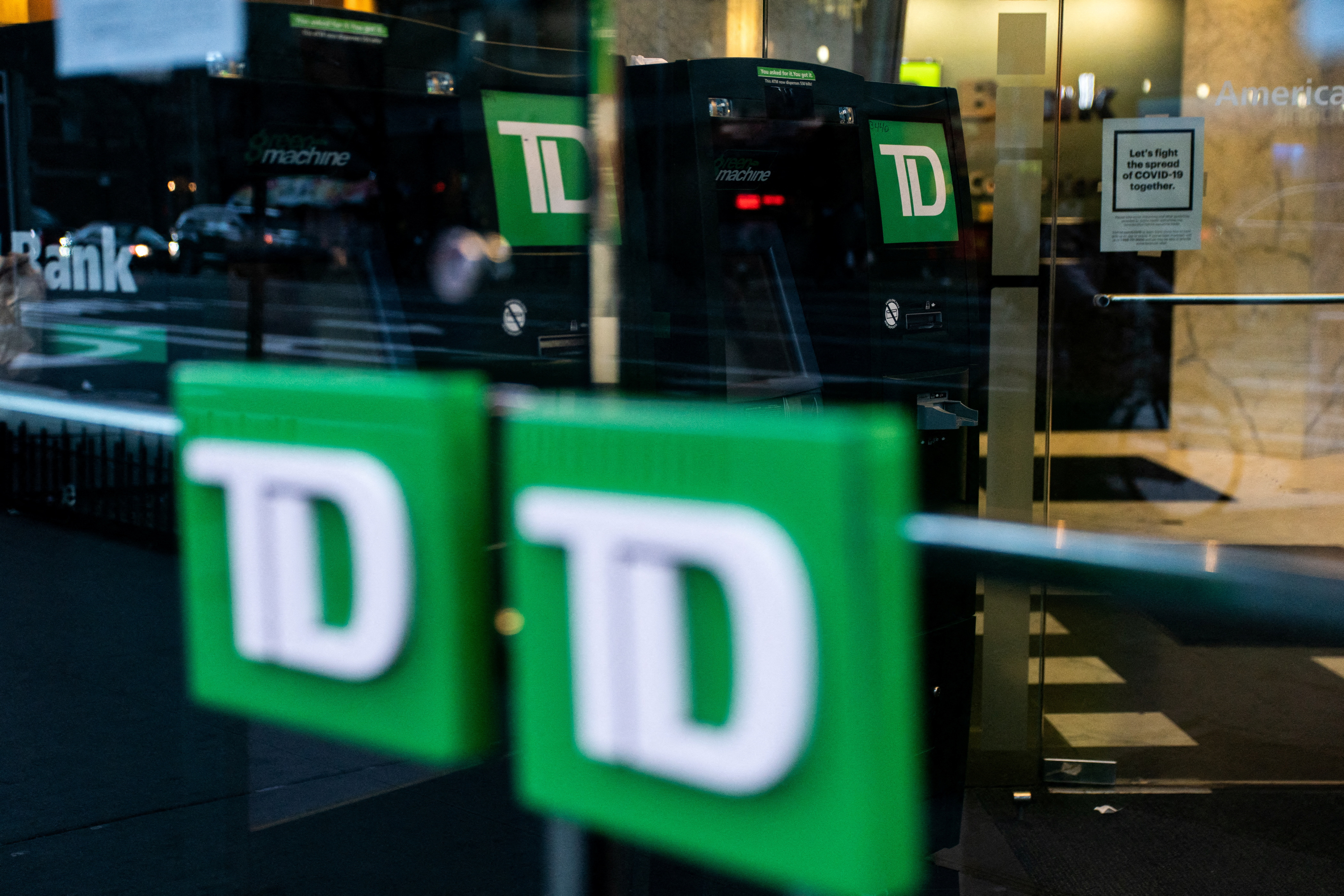

An official website of the United States government
The .gov means it’s official. Federal government websites often end in .gov or .mil. Before sharing sensitive information, make sure you’re on a federal government site.
The site is secure. The https:// ensures that you are connecting to the official website and that any information you provide is encrypted and transmitted securely.
- Publications
- Account settings
Preview improvements coming to the PMC website in October 2024. Learn More or Try it out now .
- Advanced Search
- Journal List
- BMC Med Res Methodol

The case study approach
Sarah crowe.
1 Division of Primary Care, The University of Nottingham, Nottingham, UK
Kathrin Cresswell
2 Centre for Population Health Sciences, The University of Edinburgh, Edinburgh, UK
Ann Robertson
3 School of Health in Social Science, The University of Edinburgh, Edinburgh, UK
Anthony Avery
Aziz sheikh.
The case study approach allows in-depth, multi-faceted explorations of complex issues in their real-life settings. The value of the case study approach is well recognised in the fields of business, law and policy, but somewhat less so in health services research. Based on our experiences of conducting several health-related case studies, we reflect on the different types of case study design, the specific research questions this approach can help answer, the data sources that tend to be used, and the particular advantages and disadvantages of employing this methodological approach. The paper concludes with key pointers to aid those designing and appraising proposals for conducting case study research, and a checklist to help readers assess the quality of case study reports.
Introduction
The case study approach is particularly useful to employ when there is a need to obtain an in-depth appreciation of an issue, event or phenomenon of interest, in its natural real-life context. Our aim in writing this piece is to provide insights into when to consider employing this approach and an overview of key methodological considerations in relation to the design, planning, analysis, interpretation and reporting of case studies.
The illustrative 'grand round', 'case report' and 'case series' have a long tradition in clinical practice and research. Presenting detailed critiques, typically of one or more patients, aims to provide insights into aspects of the clinical case and, in doing so, illustrate broader lessons that may be learnt. In research, the conceptually-related case study approach can be used, for example, to describe in detail a patient's episode of care, explore professional attitudes to and experiences of a new policy initiative or service development or more generally to 'investigate contemporary phenomena within its real-life context' [ 1 ]. Based on our experiences of conducting a range of case studies, we reflect on when to consider using this approach, discuss the key steps involved and illustrate, with examples, some of the practical challenges of attaining an in-depth understanding of a 'case' as an integrated whole. In keeping with previously published work, we acknowledge the importance of theory to underpin the design, selection, conduct and interpretation of case studies[ 2 ]. In so doing, we make passing reference to the different epistemological approaches used in case study research by key theoreticians and methodologists in this field of enquiry.
This paper is structured around the following main questions: What is a case study? What are case studies used for? How are case studies conducted? What are the potential pitfalls and how can these be avoided? We draw in particular on four of our own recently published examples of case studies (see Tables Tables1, 1 , ,2, 2 , ,3 3 and and4) 4 ) and those of others to illustrate our discussion[ 3 - 7 ].
Example of a case study investigating the reasons for differences in recruitment rates of minority ethnic people in asthma research[ 3 ]
Minority ethnic people experience considerably greater morbidity from asthma than the White majority population. Research has shown however that these minority ethnic populations are likely to be under-represented in research undertaken in the UK; there is comparatively less marginalisation in the US. |
To investigate approaches to bolster recruitment of South Asians into UK asthma studies through qualitative research with US and UK researchers, and UK community leaders. |
Single intrinsic case study |
Centred on the issue of recruitment of South Asian people with asthma. |
In-depth interviews were conducted with asthma researchers from the UK and US. A supplementary questionnaire was also provided to researchers. |
Framework approach. |
Barriers to ethnic minority recruitment were found to centre around: |
1. The attitudes of the researchers' towards inclusion: The majority of UK researchers interviewed were generally supportive of the idea of recruiting ethnically diverse participants but expressed major concerns about the practicalities of achieving this; in contrast, the US researchers appeared much more committed to the policy of inclusion. |
2. Stereotypes and prejudices: We found that some of the UK researchers' perceptions of ethnic minorities may have influenced their decisions on whether to approach individuals from particular ethnic groups. These stereotypes centred on issues to do with, amongst others, language barriers and lack of altruism. |
3. Demographic, political and socioeconomic contexts of the two countries: Researchers suggested that the demographic profile of ethnic minorities, their political engagement and the different configuration of the health services in the UK and the US may have contributed to differential rates. |
4. Above all, however, it appeared that the overriding importance of the US National Institute of Health's policy to mandate the inclusion of minority ethnic people (and women) had a major impact on shaping the attitudes and in turn the experiences of US researchers'; the absence of any similar mandate in the UK meant that UK-based researchers had not been forced to challenge their existing practices and they were hence unable to overcome any stereotypical/prejudicial attitudes through experiential learning. |
Example of a case study investigating the process of planning and implementing a service in Primary Care Organisations[ 4 ]
Health work forces globally are needing to reorganise and reconfigure in order to meet the challenges posed by the increased numbers of people living with long-term conditions in an efficient and sustainable manner. Through studying the introduction of General Practitioners with a Special Interest in respiratory disorders, this study aimed to provide insights into this important issue by focusing on community respiratory service development. |
To understand and compare the process of workforce change in respiratory services and the impact on patient experience (specifically in relation to the role of general practitioners with special interests) in a theoretically selected sample of Primary Care Organisations (PCOs), in order to derive models of good practice in planning and the implementation of a broad range of workforce issues. |
Multiple-case design of respiratory services in health regions in England and Wales. |
Four PCOs. |
Face-to-face and telephone interviews, e-mail discussions, local documents, patient diaries, news items identified from local and national websites, national workshop. |
Reading, coding and comparison progressed iteratively. |
1. In the screening phase of this study (which involved semi-structured telephone interviews with the person responsible for driving the reconfiguration of respiratory services in 30 PCOs), the barriers of financial deficit, organisational uncertainty, disengaged clinicians and contradictory policies proved insurmountable for many PCOs to developing sustainable services. A key rationale for PCO re-organisation in 2006 was to strengthen their commissioning function and those of clinicians through Practice-Based Commissioning. However, the turbulence, which surrounded reorganisation was found to have the opposite desired effect. |
2. Implementing workforce reconfiguration was strongly influenced by the negotiation and contest among local clinicians and managers about "ownership" of work and income. |
3. Despite the intention to make the commissioning system more transparent, personal relationships based on common professional interests, past work history, friendships and collegiality, remained as key drivers for sustainable innovation in service development. |
It was only possible to undertake in-depth work in a selective number of PCOs and, even within these selected PCOs, it was not possible to interview all informants of potential interest and/or obtain all relevant documents. This work was conducted in the early stages of a major NHS reorganisation in England and Wales and thus, events are likely to have continued to evolve beyond the study period; we therefore cannot claim to have seen any of the stories through to their conclusion. |
Example of a case study investigating the introduction of the electronic health records[ 5 ]
Healthcare systems globally are moving from paper-based record systems to electronic health record systems. In 2002, the NHS in England embarked on the most ambitious and expensive IT-based transformation in healthcare in history seeking to introduce electronic health records into all hospitals in England by 2010. |
To describe and evaluate the implementation and adoption of detailed electronic health records in secondary care in England and thereby provide formative feedback for local and national rollout of the NHS Care Records Service. |
A mixed methods, longitudinal, multi-site, socio-technical collective case study. |
Five NHS acute hospital and mental health Trusts that have been the focus of early implementation efforts. |
Semi-structured interviews, documentary data and field notes, observations and quantitative data. |
Qualitative data were analysed thematically using a socio-technical coding matrix, combined with additional themes that emerged from the data. |
1. Hospital electronic health record systems have developed and been implemented far more slowly than was originally envisioned. |
2. The top-down, government-led standardised approach needed to evolve to admit more variation and greater local choice for hospitals in order to support local service delivery. |
3. A range of adverse consequences were associated with the centrally negotiated contracts, which excluded the hospitals in question. |
4. The unrealistic, politically driven, timeline (implementation over 10 years) was found to be a major source of frustration for developers, implementers and healthcare managers and professionals alike. |
We were unable to access details of the contracts between government departments and the Local Service Providers responsible for delivering and implementing the software systems. This, in turn, made it difficult to develop a holistic understanding of some key issues impacting on the overall slow roll-out of the NHS Care Record Service. Early adopters may also have differed in important ways from NHS hospitals that planned to join the National Programme for Information Technology and implement the NHS Care Records Service at a later point in time. |
Example of a case study investigating the formal and informal ways students learn about patient safety[ 6 ]
There is a need to reduce the disease burden associated with iatrogenic harm and considering that healthcare education represents perhaps the most sustained patient safety initiative ever undertaken, it is important to develop a better appreciation of the ways in which undergraduate and newly qualified professionals receive and make sense of the education they receive. | |
---|---|
To investigate the formal and informal ways pre-registration students from a range of healthcare professions (medicine, nursing, physiotherapy and pharmacy) learn about patient safety in order to become safe practitioners. | |
Multi-site, mixed method collective case study. | |
: Eight case studies (two for each professional group) were carried out in educational provider sites considering different programmes, practice environments and models of teaching and learning. | |
Structured in phases relevant to the three knowledge contexts: | |
Documentary evidence (including undergraduate curricula, handbooks and module outlines), complemented with a range of views (from course leads, tutors and students) and observations in a range of academic settings. | |
Policy and management views of patient safety and influences on patient safety education and practice. NHS policies included, for example, implementation of the National Patient Safety Agency's , which encourages organisations to develop an organisational safety culture in which staff members feel comfortable identifying dangers and reporting hazards. | |
The cultures to which students are exposed i.e. patient safety in relation to day-to-day working. NHS initiatives included, for example, a hand washing initiative or introduction of infection control measures. | |
1. Practical, informal, learning opportunities were valued by students. On the whole, however, students were not exposed to nor engaged with important NHS initiatives such as risk management activities and incident reporting schemes. | |
2. NHS policy appeared to have been taken seriously by course leaders. Patient safety materials were incorporated into both formal and informal curricula, albeit largely implicit rather than explicit. | |
3. Resource issues and peer pressure were found to influence safe practice. Variations were also found to exist in students' experiences and the quality of the supervision available. | |
The curriculum and organisational documents collected differed between sites, which possibly reflected gatekeeper influences at each site. The recruitment of participants for focus group discussions proved difficult, so interviews or paired discussions were used as a substitute. |
What is a case study?
A case study is a research approach that is used to generate an in-depth, multi-faceted understanding of a complex issue in its real-life context. It is an established research design that is used extensively in a wide variety of disciplines, particularly in the social sciences. A case study can be defined in a variety of ways (Table (Table5), 5 ), the central tenet being the need to explore an event or phenomenon in depth and in its natural context. It is for this reason sometimes referred to as a "naturalistic" design; this is in contrast to an "experimental" design (such as a randomised controlled trial) in which the investigator seeks to exert control over and manipulate the variable(s) of interest.
Definitions of a case study
Author | Definition |
---|---|
Stake[ ] | (p.237) |
Yin[ , , ] | (Yin 1999 p. 1211, Yin 1994 p. 13) |
• | |
• (Yin 2009 p18) | |
Miles and Huberman[ ] | (p. 25) |
Green and Thorogood[ ] | (p. 284) |
George and Bennett[ ] | (p. 17)" |
Stake's work has been particularly influential in defining the case study approach to scientific enquiry. He has helpfully characterised three main types of case study: intrinsic , instrumental and collective [ 8 ]. An intrinsic case study is typically undertaken to learn about a unique phenomenon. The researcher should define the uniqueness of the phenomenon, which distinguishes it from all others. In contrast, the instrumental case study uses a particular case (some of which may be better than others) to gain a broader appreciation of an issue or phenomenon. The collective case study involves studying multiple cases simultaneously or sequentially in an attempt to generate a still broader appreciation of a particular issue.
These are however not necessarily mutually exclusive categories. In the first of our examples (Table (Table1), 1 ), we undertook an intrinsic case study to investigate the issue of recruitment of minority ethnic people into the specific context of asthma research studies, but it developed into a instrumental case study through seeking to understand the issue of recruitment of these marginalised populations more generally, generating a number of the findings that are potentially transferable to other disease contexts[ 3 ]. In contrast, the other three examples (see Tables Tables2, 2 , ,3 3 and and4) 4 ) employed collective case study designs to study the introduction of workforce reconfiguration in primary care, the implementation of electronic health records into hospitals, and to understand the ways in which healthcare students learn about patient safety considerations[ 4 - 6 ]. Although our study focusing on the introduction of General Practitioners with Specialist Interests (Table (Table2) 2 ) was explicitly collective in design (four contrasting primary care organisations were studied), is was also instrumental in that this particular professional group was studied as an exemplar of the more general phenomenon of workforce redesign[ 4 ].
What are case studies used for?
According to Yin, case studies can be used to explain, describe or explore events or phenomena in the everyday contexts in which they occur[ 1 ]. These can, for example, help to understand and explain causal links and pathways resulting from a new policy initiative or service development (see Tables Tables2 2 and and3, 3 , for example)[ 1 ]. In contrast to experimental designs, which seek to test a specific hypothesis through deliberately manipulating the environment (like, for example, in a randomised controlled trial giving a new drug to randomly selected individuals and then comparing outcomes with controls),[ 9 ] the case study approach lends itself well to capturing information on more explanatory ' how ', 'what' and ' why ' questions, such as ' how is the intervention being implemented and received on the ground?'. The case study approach can offer additional insights into what gaps exist in its delivery or why one implementation strategy might be chosen over another. This in turn can help develop or refine theory, as shown in our study of the teaching of patient safety in undergraduate curricula (Table (Table4 4 )[ 6 , 10 ]. Key questions to consider when selecting the most appropriate study design are whether it is desirable or indeed possible to undertake a formal experimental investigation in which individuals and/or organisations are allocated to an intervention or control arm? Or whether the wish is to obtain a more naturalistic understanding of an issue? The former is ideally studied using a controlled experimental design, whereas the latter is more appropriately studied using a case study design.
Case studies may be approached in different ways depending on the epistemological standpoint of the researcher, that is, whether they take a critical (questioning one's own and others' assumptions), interpretivist (trying to understand individual and shared social meanings) or positivist approach (orientating towards the criteria of natural sciences, such as focusing on generalisability considerations) (Table (Table6). 6 ). Whilst such a schema can be conceptually helpful, it may be appropriate to draw on more than one approach in any case study, particularly in the context of conducting health services research. Doolin has, for example, noted that in the context of undertaking interpretative case studies, researchers can usefully draw on a critical, reflective perspective which seeks to take into account the wider social and political environment that has shaped the case[ 11 ].
Example of epistemological approaches that may be used in case study research
Approach | Characteristics | Criticisms | Key references |
---|---|---|---|
Involves questioning one's own assumptions taking into account the wider political and social environment. | It can possibly neglect other factors by focussing only on power relationships and may give the researcher a position that is too privileged. | Howcroft and Trauth[ ] Blakie[ ] Doolin[ , ] | |
Interprets the limiting conditions in relation to power and control that are thought to influence behaviour. | Bloomfield and Best[ ] | ||
Involves understanding meanings/contexts and processes as perceived from different perspectives, trying to understand individual and shared social meanings. Focus is on theory building. | Often difficult to explain unintended consequences and for neglecting surrounding historical contexts | Stake[ ] Doolin[ ] | |
Involves establishing which variables one wishes to study in advance and seeing whether they fit in with the findings. Focus is often on testing and refining theory on the basis of case study findings. | It does not take into account the role of the researcher in influencing findings. | Yin[ , , ] Shanks and Parr[ ] |
How are case studies conducted?
Here, we focus on the main stages of research activity when planning and undertaking a case study; the crucial stages are: defining the case; selecting the case(s); collecting and analysing the data; interpreting data; and reporting the findings.
Defining the case
Carefully formulated research question(s), informed by the existing literature and a prior appreciation of the theoretical issues and setting(s), are all important in appropriately and succinctly defining the case[ 8 , 12 ]. Crucially, each case should have a pre-defined boundary which clarifies the nature and time period covered by the case study (i.e. its scope, beginning and end), the relevant social group, organisation or geographical area of interest to the investigator, the types of evidence to be collected, and the priorities for data collection and analysis (see Table Table7 7 )[ 1 ]. A theory driven approach to defining the case may help generate knowledge that is potentially transferable to a range of clinical contexts and behaviours; using theory is also likely to result in a more informed appreciation of, for example, how and why interventions have succeeded or failed[ 13 ].
Example of a checklist for rating a case study proposal[ 8 ]
Clarity: Does the proposal read well? | |
Integrity: Do its pieces fit together? | |
Attractiveness: Does it pique the reader's interest? | |
The case: Is the case adequately defined? | |
The issues: Are major research questions identified? | |
Data Resource: Are sufficient data sources identified? | |
Case Selection: Is the selection plan reasonable? | |
Data Gathering: Are data-gathering activities outlined? | |
Validation: Is the need and opportunity for triangulation indicated? | |
Access: Are arrangements for start-up anticipated? | |
Confidentiality: Is there sensitivity to the protection of people? | |
Cost: Are time and resource estimates reasonable? |
For example, in our evaluation of the introduction of electronic health records in English hospitals (Table (Table3), 3 ), we defined our cases as the NHS Trusts that were receiving the new technology[ 5 ]. Our focus was on how the technology was being implemented. However, if the primary research interest had been on the social and organisational dimensions of implementation, we might have defined our case differently as a grouping of healthcare professionals (e.g. doctors and/or nurses). The precise beginning and end of the case may however prove difficult to define. Pursuing this same example, when does the process of implementation and adoption of an electronic health record system really begin or end? Such judgements will inevitably be influenced by a range of factors, including the research question, theory of interest, the scope and richness of the gathered data and the resources available to the research team.
Selecting the case(s)
The decision on how to select the case(s) to study is a very important one that merits some reflection. In an intrinsic case study, the case is selected on its own merits[ 8 ]. The case is selected not because it is representative of other cases, but because of its uniqueness, which is of genuine interest to the researchers. This was, for example, the case in our study of the recruitment of minority ethnic participants into asthma research (Table (Table1) 1 ) as our earlier work had demonstrated the marginalisation of minority ethnic people with asthma, despite evidence of disproportionate asthma morbidity[ 14 , 15 ]. In another example of an intrinsic case study, Hellstrom et al.[ 16 ] studied an elderly married couple living with dementia to explore how dementia had impacted on their understanding of home, their everyday life and their relationships.
For an instrumental case study, selecting a "typical" case can work well[ 8 ]. In contrast to the intrinsic case study, the particular case which is chosen is of less importance than selecting a case that allows the researcher to investigate an issue or phenomenon. For example, in order to gain an understanding of doctors' responses to health policy initiatives, Som undertook an instrumental case study interviewing clinicians who had a range of responsibilities for clinical governance in one NHS acute hospital trust[ 17 ]. Sampling a "deviant" or "atypical" case may however prove even more informative, potentially enabling the researcher to identify causal processes, generate hypotheses and develop theory.
In collective or multiple case studies, a number of cases are carefully selected. This offers the advantage of allowing comparisons to be made across several cases and/or replication. Choosing a "typical" case may enable the findings to be generalised to theory (i.e. analytical generalisation) or to test theory by replicating the findings in a second or even a third case (i.e. replication logic)[ 1 ]. Yin suggests two or three literal replications (i.e. predicting similar results) if the theory is straightforward and five or more if the theory is more subtle. However, critics might argue that selecting 'cases' in this way is insufficiently reflexive and ill-suited to the complexities of contemporary healthcare organisations.
The selected case study site(s) should allow the research team access to the group of individuals, the organisation, the processes or whatever else constitutes the chosen unit of analysis for the study. Access is therefore a central consideration; the researcher needs to come to know the case study site(s) well and to work cooperatively with them. Selected cases need to be not only interesting but also hospitable to the inquiry [ 8 ] if they are to be informative and answer the research question(s). Case study sites may also be pre-selected for the researcher, with decisions being influenced by key stakeholders. For example, our selection of case study sites in the evaluation of the implementation and adoption of electronic health record systems (see Table Table3) 3 ) was heavily influenced by NHS Connecting for Health, the government agency that was responsible for overseeing the National Programme for Information Technology (NPfIT)[ 5 ]. This prominent stakeholder had already selected the NHS sites (through a competitive bidding process) to be early adopters of the electronic health record systems and had negotiated contracts that detailed the deployment timelines.
It is also important to consider in advance the likely burden and risks associated with participation for those who (or the site(s) which) comprise the case study. Of particular importance is the obligation for the researcher to think through the ethical implications of the study (e.g. the risk of inadvertently breaching anonymity or confidentiality) and to ensure that potential participants/participating sites are provided with sufficient information to make an informed choice about joining the study. The outcome of providing this information might be that the emotive burden associated with participation, or the organisational disruption associated with supporting the fieldwork, is considered so high that the individuals or sites decide against participation.
In our example of evaluating implementations of electronic health record systems, given the restricted number of early adopter sites available to us, we sought purposively to select a diverse range of implementation cases among those that were available[ 5 ]. We chose a mixture of teaching, non-teaching and Foundation Trust hospitals, and examples of each of the three electronic health record systems procured centrally by the NPfIT. At one recruited site, it quickly became apparent that access was problematic because of competing demands on that organisation. Recognising the importance of full access and co-operative working for generating rich data, the research team decided not to pursue work at that site and instead to focus on other recruited sites.
Collecting the data
In order to develop a thorough understanding of the case, the case study approach usually involves the collection of multiple sources of evidence, using a range of quantitative (e.g. questionnaires, audits and analysis of routinely collected healthcare data) and more commonly qualitative techniques (e.g. interviews, focus groups and observations). The use of multiple sources of data (data triangulation) has been advocated as a way of increasing the internal validity of a study (i.e. the extent to which the method is appropriate to answer the research question)[ 8 , 18 - 21 ]. An underlying assumption is that data collected in different ways should lead to similar conclusions, and approaching the same issue from different angles can help develop a holistic picture of the phenomenon (Table (Table2 2 )[ 4 ].
Brazier and colleagues used a mixed-methods case study approach to investigate the impact of a cancer care programme[ 22 ]. Here, quantitative measures were collected with questionnaires before, and five months after, the start of the intervention which did not yield any statistically significant results. Qualitative interviews with patients however helped provide an insight into potentially beneficial process-related aspects of the programme, such as greater, perceived patient involvement in care. The authors reported how this case study approach provided a number of contextual factors likely to influence the effectiveness of the intervention and which were not likely to have been obtained from quantitative methods alone.
In collective or multiple case studies, data collection needs to be flexible enough to allow a detailed description of each individual case to be developed (e.g. the nature of different cancer care programmes), before considering the emerging similarities and differences in cross-case comparisons (e.g. to explore why one programme is more effective than another). It is important that data sources from different cases are, where possible, broadly comparable for this purpose even though they may vary in nature and depth.
Analysing, interpreting and reporting case studies
Making sense and offering a coherent interpretation of the typically disparate sources of data (whether qualitative alone or together with quantitative) is far from straightforward. Repeated reviewing and sorting of the voluminous and detail-rich data are integral to the process of analysis. In collective case studies, it is helpful to analyse data relating to the individual component cases first, before making comparisons across cases. Attention needs to be paid to variations within each case and, where relevant, the relationship between different causes, effects and outcomes[ 23 ]. Data will need to be organised and coded to allow the key issues, both derived from the literature and emerging from the dataset, to be easily retrieved at a later stage. An initial coding frame can help capture these issues and can be applied systematically to the whole dataset with the aid of a qualitative data analysis software package.
The Framework approach is a practical approach, comprising of five stages (familiarisation; identifying a thematic framework; indexing; charting; mapping and interpretation) , to managing and analysing large datasets particularly if time is limited, as was the case in our study of recruitment of South Asians into asthma research (Table (Table1 1 )[ 3 , 24 ]. Theoretical frameworks may also play an important role in integrating different sources of data and examining emerging themes. For example, we drew on a socio-technical framework to help explain the connections between different elements - technology; people; and the organisational settings within which they worked - in our study of the introduction of electronic health record systems (Table (Table3 3 )[ 5 ]. Our study of patient safety in undergraduate curricula drew on an evaluation-based approach to design and analysis, which emphasised the importance of the academic, organisational and practice contexts through which students learn (Table (Table4 4 )[ 6 ].
Case study findings can have implications both for theory development and theory testing. They may establish, strengthen or weaken historical explanations of a case and, in certain circumstances, allow theoretical (as opposed to statistical) generalisation beyond the particular cases studied[ 12 ]. These theoretical lenses should not, however, constitute a strait-jacket and the cases should not be "forced to fit" the particular theoretical framework that is being employed.
When reporting findings, it is important to provide the reader with enough contextual information to understand the processes that were followed and how the conclusions were reached. In a collective case study, researchers may choose to present the findings from individual cases separately before amalgamating across cases. Care must be taken to ensure the anonymity of both case sites and individual participants (if agreed in advance) by allocating appropriate codes or withholding descriptors. In the example given in Table Table3, 3 , we decided against providing detailed information on the NHS sites and individual participants in order to avoid the risk of inadvertent disclosure of identities[ 5 , 25 ].
What are the potential pitfalls and how can these be avoided?
The case study approach is, as with all research, not without its limitations. When investigating the formal and informal ways undergraduate students learn about patient safety (Table (Table4), 4 ), for example, we rapidly accumulated a large quantity of data. The volume of data, together with the time restrictions in place, impacted on the depth of analysis that was possible within the available resources. This highlights a more general point of the importance of avoiding the temptation to collect as much data as possible; adequate time also needs to be set aside for data analysis and interpretation of what are often highly complex datasets.
Case study research has sometimes been criticised for lacking scientific rigour and providing little basis for generalisation (i.e. producing findings that may be transferable to other settings)[ 1 ]. There are several ways to address these concerns, including: the use of theoretical sampling (i.e. drawing on a particular conceptual framework); respondent validation (i.e. participants checking emerging findings and the researcher's interpretation, and providing an opinion as to whether they feel these are accurate); and transparency throughout the research process (see Table Table8 8 )[ 8 , 18 - 21 , 23 , 26 ]. Transparency can be achieved by describing in detail the steps involved in case selection, data collection, the reasons for the particular methods chosen, and the researcher's background and level of involvement (i.e. being explicit about how the researcher has influenced data collection and interpretation). Seeking potential, alternative explanations, and being explicit about how interpretations and conclusions were reached, help readers to judge the trustworthiness of the case study report. Stake provides a critique checklist for a case study report (Table (Table9 9 )[ 8 ].
Potential pitfalls and mitigating actions when undertaking case study research
Potential pitfall | Mitigating action |
---|---|
Selecting/conceptualising the wrong case(s) resulting in lack of theoretical generalisations | Developing in-depth knowledge of theoretical and empirical literature, justifying choices made |
Collecting large volumes of data that are not relevant to the case or too little to be of any value | Focus data collection in line with research questions, whilst being flexible and allowing different paths to be explored |
Defining/bounding the case | Focus on related components (either by time and/or space), be clear what is outside the scope of the case |
Lack of rigour | Triangulation, respondent validation, the use of theoretical sampling, transparency throughout the research process |
Ethical issues | Anonymise appropriately as cases are often easily identifiable to insiders, informed consent of participants |
Integration with theoretical framework | Allow for unexpected issues to emerge and do not force fit, test out preliminary explanations, be clear about epistemological positions in advance |
Stake's checklist for assessing the quality of a case study report[ 8 ]
1. Is this report easy to read? |
2. Does it fit together, each sentence contributing to the whole? |
3. Does this report have a conceptual structure (i.e. themes or issues)? |
4. Are its issues developed in a series and scholarly way? |
5. Is the case adequately defined? |
6. Is there a sense of story to the presentation? |
7. Is the reader provided some vicarious experience? |
8. Have quotations been used effectively? |
9. Are headings, figures, artefacts, appendices, indexes effectively used? |
10. Was it edited well, then again with a last minute polish? |
11. Has the writer made sound assertions, neither over- or under-interpreting? |
12. Has adequate attention been paid to various contexts? |
13. Were sufficient raw data presented? |
14. Were data sources well chosen and in sufficient number? |
15. Do observations and interpretations appear to have been triangulated? |
16. Is the role and point of view of the researcher nicely apparent? |
17. Is the nature of the intended audience apparent? |
18. Is empathy shown for all sides? |
19. Are personal intentions examined? |
20. Does it appear individuals were put at risk? |
Conclusions
The case study approach allows, amongst other things, critical events, interventions, policy developments and programme-based service reforms to be studied in detail in a real-life context. It should therefore be considered when an experimental design is either inappropriate to answer the research questions posed or impossible to undertake. Considering the frequency with which implementations of innovations are now taking place in healthcare settings and how well the case study approach lends itself to in-depth, complex health service research, we believe this approach should be more widely considered by researchers. Though inherently challenging, the research case study can, if carefully conceptualised and thoughtfully undertaken and reported, yield powerful insights into many important aspects of health and healthcare delivery.
Competing interests
The authors declare that they have no competing interests.
Authors' contributions
AS conceived this article. SC, KC and AR wrote this paper with GH, AA and AS all commenting on various drafts. SC and AS are guarantors.
Pre-publication history
The pre-publication history for this paper can be accessed here:
http://www.biomedcentral.com/1471-2288/11/100/prepub
Acknowledgements
We are grateful to the participants and colleagues who contributed to the individual case studies that we have drawn on. This work received no direct funding, but it has been informed by projects funded by Asthma UK, the NHS Service Delivery Organisation, NHS Connecting for Health Evaluation Programme, and Patient Safety Research Portfolio. We would also like to thank the expert reviewers for their insightful and constructive feedback. Our thanks are also due to Dr. Allison Worth who commented on an earlier draft of this manuscript.
- Yin RK. Case study research, design and method. 4. London: Sage Publications Ltd.; 2009. [ Google Scholar ]
- Keen J, Packwood T. Qualitative research; case study evaluation. BMJ. 1995; 311 :444–446. [ PMC free article ] [ PubMed ] [ Google Scholar ]
- Sheikh A, Halani L, Bhopal R, Netuveli G, Partridge M, Car J. et al. Facilitating the Recruitment of Minority Ethnic People into Research: Qualitative Case Study of South Asians and Asthma. PLoS Med. 2009; 6 (10):1–11. [ PMC free article ] [ PubMed ] [ Google Scholar ]
- Pinnock H, Huby G, Powell A, Kielmann T, Price D, Williams S, The process of planning, development and implementation of a General Practitioner with a Special Interest service in Primary Care Organisations in England and Wales: a comparative prospective case study. Report for the National Co-ordinating Centre for NHS Service Delivery and Organisation R&D (NCCSDO) 2008. http://www.sdo.nihr.ac.uk/files/project/99-final-report.pdf
- Robertson A, Cresswell K, Takian A, Petrakaki D, Crowe S, Cornford T. et al. Prospective evaluation of the implementation and adoption of NHS Connecting for Health's national electronic health record in secondary care in England: interim findings. BMJ. 2010; 41 :c4564. [ PMC free article ] [ PubMed ] [ Google Scholar ]
- Pearson P, Steven A, Howe A, Sheikh A, Ashcroft D, Smith P. the Patient Safety Education Study Group. Learning about patient safety: organisational context and culture in the education of healthcare professionals. J Health Serv Res Policy. 2010; 15 :4–10. doi: 10.1258/jhsrp.2009.009052. [ PubMed ] [ CrossRef ] [ Google Scholar ]
- van Harten WH, Casparie TF, Fisscher OA. The evaluation of the introduction of a quality management system: a process-oriented case study in a large rehabilitation hospital. Health Policy. 2002; 60 (1):17–37. doi: 10.1016/S0168-8510(01)00187-7. [ PubMed ] [ CrossRef ] [ Google Scholar ]
- Stake RE. The art of case study research. London: Sage Publications Ltd.; 1995. [ Google Scholar ]
- Sheikh A, Smeeth L, Ashcroft R. Randomised controlled trials in primary care: scope and application. Br J Gen Pract. 2002; 52 (482):746–51. [ PMC free article ] [ PubMed ] [ Google Scholar ]
- King G, Keohane R, Verba S. Designing Social Inquiry. Princeton: Princeton University Press; 1996. [ Google Scholar ]
- Doolin B. Information technology as disciplinary technology: being critical in interpretative research on information systems. Journal of Information Technology. 1998; 13 :301–311. doi: 10.1057/jit.1998.8. [ CrossRef ] [ Google Scholar ]
- George AL, Bennett A. Case studies and theory development in the social sciences. Cambridge, MA: MIT Press; 2005. [ Google Scholar ]
- Eccles M. the Improved Clinical Effectiveness through Behavioural Research Group (ICEBeRG) Designing theoretically-informed implementation interventions. Implementation Science. 2006; 1 :1–8. doi: 10.1186/1748-5908-1-1. [ PMC free article ] [ PubMed ] [ CrossRef ] [ Google Scholar ]
- Netuveli G, Hurwitz B, Levy M, Fletcher M, Barnes G, Durham SR, Sheikh A. Ethnic variations in UK asthma frequency, morbidity, and health-service use: a systematic review and meta-analysis. Lancet. 2005; 365 (9456):312–7. [ PubMed ] [ Google Scholar ]
- Sheikh A, Panesar SS, Lasserson T, Netuveli G. Recruitment of ethnic minorities to asthma studies. Thorax. 2004; 59 (7):634. [ PMC free article ] [ PubMed ] [ Google Scholar ]
- Hellström I, Nolan M, Lundh U. 'We do things together': A case study of 'couplehood' in dementia. Dementia. 2005; 4 :7–22. doi: 10.1177/1471301205049188. [ CrossRef ] [ Google Scholar ]
- Som CV. Nothing seems to have changed, nothing seems to be changing and perhaps nothing will change in the NHS: doctors' response to clinical governance. International Journal of Public Sector Management. 2005; 18 :463–477. doi: 10.1108/09513550510608903. [ CrossRef ] [ Google Scholar ]
- Lincoln Y, Guba E. Naturalistic inquiry. Newbury Park: Sage Publications; 1985. [ Google Scholar ]
- Barbour RS. Checklists for improving rigour in qualitative research: a case of the tail wagging the dog? BMJ. 2001; 322 :1115–1117. doi: 10.1136/bmj.322.7294.1115. [ PMC free article ] [ PubMed ] [ CrossRef ] [ Google Scholar ]
- Mays N, Pope C. Qualitative research in health care: Assessing quality in qualitative research. BMJ. 2000; 320 :50–52. doi: 10.1136/bmj.320.7226.50. [ PMC free article ] [ PubMed ] [ CrossRef ] [ Google Scholar ]
- Mason J. Qualitative researching. London: Sage; 2002. [ Google Scholar ]
- Brazier A, Cooke K, Moravan V. Using Mixed Methods for Evaluating an Integrative Approach to Cancer Care: A Case Study. Integr Cancer Ther. 2008; 7 :5–17. doi: 10.1177/1534735407313395. [ PubMed ] [ CrossRef ] [ Google Scholar ]
- Miles MB, Huberman M. Qualitative data analysis: an expanded sourcebook. 2. CA: Sage Publications Inc.; 1994. [ Google Scholar ]
- Pope C, Ziebland S, Mays N. Analysing qualitative data. Qualitative research in health care. BMJ. 2000; 320 :114–116. doi: 10.1136/bmj.320.7227.114. [ PMC free article ] [ PubMed ] [ CrossRef ] [ Google Scholar ]
- Cresswell KM, Worth A, Sheikh A. Actor-Network Theory and its role in understanding the implementation of information technology developments in healthcare. BMC Med Inform Decis Mak. 2010; 10 (1):67. doi: 10.1186/1472-6947-10-67. [ PMC free article ] [ PubMed ] [ CrossRef ] [ Google Scholar ]
- Malterud K. Qualitative research: standards, challenges, and guidelines. Lancet. 2001; 358 :483–488. doi: 10.1016/S0140-6736(01)05627-6. [ PubMed ] [ CrossRef ] [ Google Scholar ]
- Yin R. Case study research: design and methods. 2. Thousand Oaks, CA: Sage Publishing; 1994. [ Google Scholar ]
- Yin R. Enhancing the quality of case studies in health services research. Health Serv Res. 1999; 34 :1209–1224. [ PMC free article ] [ PubMed ] [ Google Scholar ]
- Green J, Thorogood N. Qualitative methods for health research. 2. Los Angeles: Sage; 2009. [ Google Scholar ]
- Howcroft D, Trauth E. Handbook of Critical Information Systems Research, Theory and Application. Cheltenham, UK: Northampton, MA, USA: Edward Elgar; 2005. [ Google Scholar ]
- Blakie N. Approaches to Social Enquiry. Cambridge: Polity Press; 1993. [ Google Scholar ]
- Doolin B. Power and resistance in the implementation of a medical management information system. Info Systems J. 2004; 14 :343–362. doi: 10.1111/j.1365-2575.2004.00176.x. [ CrossRef ] [ Google Scholar ]
- Bloomfield BP, Best A. Management consultants: systems development, power and the translation of problems. Sociological Review. 1992; 40 :533–560. [ Google Scholar ]
- Shanks G, Parr A. Proceedings of the European Conference on Information Systems. Naples; 2003. Positivist, single case study research in information systems: A critical analysis. [ Google Scholar ]
Tracing sampling in applied linguistics: the case of quantitative research approach
- Published: 05 July 2024
Cite this article
- Mohammad Amini Farsani ORCID: orcid.org/0000-0002-0249-1996 1 &
- Reihaneh Barzegar 1
The popularity of research has opened some new doors to science and information. For this science to flourish, it requires sound and solid inference, which, in turn, is contingent upon proper sampling procedures. However, it is rare to find empirical studies that trace the use of sampling in research approaches. This review provides a hybrid analysis through quantitative and qualitative phases by examining the sampling implementation in 356 articles published in five top-tier journals of applied linguistics using a research synthesis approach. Our findings highlight the strengths and weaknesses of sampling conceptualization and implementation in the articles. The results show a high representation of convenience sampling in the dataset, with studies predominantly including adult participants from universities. Additionally, we found that most of the studies did not adhere to transparency, which is a crucial factor in shaping solid inference. Furthermore, an exploration of the limitation sections indicates that most of the studies did not report the reasons for or highlight challenges related to sampling drawbacks. We discuss methodological considerations and advocate for implementing justified and warranted sampling structures.
This is a preview of subscription content, log in via an institution to check access.
Access this article
Subscribe and save.
- Get 10 units per month
- Download Article/Chapter or Ebook
- 1 Unit = 1 Article or 1 Chapter
- Cancel anytime
Price includes VAT (Russian Federation)
Instant access to the full article PDF.
Rent this article via DeepDyve
Institutional subscriptions
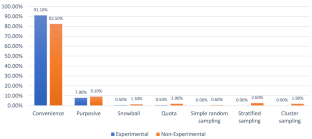
Amini Farsani, M., Asaie, M.: Exploring TEFL research methods courses in Iran: A path to research synthesis pedagogy. Qualitative Rep. 29 (1), 206–228 (2024). https://doi.org/10.46743/2160-3715/2024.5858
Article Google Scholar
Amini Farsani, M., Babaii, E.: Mapping past, current and future TEFL research trends in Iran: A synthetic review of topics and paradigms in three decades. J. Mod. Res. Engl. Lang. Stud. 5 (4), 81–108 (2019). https://doi.org/10.30479/jmrels.2019.10434.1299
Amini Farsani, M., Babaii, E.: Applied linguistics research in three decades: A methodological synthesis of graduate theses in an EFL context. Qual. Quantity. 54 (4), 1257–1283 (2020). https://doi.org/10.1007/s11135-020-00984-w
Amini Farsani, M., Jamali, H.R., Beikmohammadi, M., Ghorbani, B.D., Soleimani, L.: Methodological orientations, academic citations, and scientific collaboration in applied linguistics: What do research synthesis and bibliometrics indicate? System. 100 , 102547 (2021). https://doi.org/10.1016/j.system.2021.102547
Byrnes, H.: Notes from the editor. Mod. Lang. J. 97 (4), 825–827 (2013). https://doi.org/10.1111/j.1540-4781.2013.12051.x
Collins, K.M.T., Onwuegbuzie, A.J., Jiao, Q.G.: A mixed methods investigation of mixed methods sampling designs in social and health science research. J. Mixed Methods Res. 1 (3), 267–294 (2007). https://doi.org/10.1177/1558689807299526
Cooper, H.: Research synthesis and meta-analysis: A step-by-step Approach. Sage (2016). https://doi.org/10.4135/9781071878644
McKinley, J., Rose, H.: The Routledge Handbook of Research Methods in Applied Linguistics. Routledge (2020). https://doi.org/10.4324/9780367824471
Onwuegbuzie, A.J., Collins, K.M.T.: The role of sampling in mixed methods-research. Kölner Z. Für Soziologie Und Sozialpsychologie. 69 (S2), 133–156 (2017). https://doi.org/10.1007/s11577-017-0455-0
Plonsky, L.: Study quality in SLA: An assessment of designs, analyses, and reporting practices in quantitative L2 research. Stud. Second Lang. Acquisition. 35 (4), 655–687 (2013). https://doi.org/10.1017/S0272263113000399
Plonsky, L.: Study quality in quantitative L2 research (1990–2010): A methodological synthesis and call for reform. Mod. Lang. J. 98 (1), 450–470 (2014). https://doi.org/10.1111/j.1540-4781.2014.12058.x
Plonsky, L.: Quantitative research methods. In: Loewen, S., Sato, M. M (eds.) The Routledge Handbook of Instructed Second Language Acquisition, pp. 505–521. Taylor and Francis Inc (2017)
Plonsky, L., Gass, S.: Quantitative research methods, study quality, and outcomes: The case of interaction research. Lang. Learn. 61 (2), 325–366 (2011). https://doi.org/10.1111/j.1467-9922.2011.00640.x
Plonsky, L., Oswald, F.L.: How big is big? Interpreting effect sizes in L2 research. Lang. Learn. 64 (4), 878–912 (2014). https://doi.org/10.1111/lang.12079
Plonsky, L., Oswald, F. L.: Meta-analyzing second language research. In L. Plonsky (Ed.), Advancing quantitative methods in second language research, pp. 106–128. Routledge (2015)
Riazi, A.M., Farsani, M.A.: Mixed-methods research in applied linguistics: Charting the progress through the second decade of the twenty-first century. Lang. Teaching. 57 (2), 143–182 (2024). https://doi.org/10.1017/s0261444823000332
Sharma, G.: Pros and cons of different sampling techniques. Int. J. Appl. Res. 3 (7), 749–752 (2017). https://www.allresearchjournal.com/archives/2017/vol3issue7/PartK/3-7-69-542.pdf
Google Scholar
Download references
Author information
Authors and affiliations.
Department of Foreign Languages, Iran University of Science & Technology, Tehran, Iran
Mohammad Amini Farsani & Reihaneh Barzegar
You can also search for this author in PubMed Google Scholar
Corresponding author
Correspondence to Mohammad Amini Farsani .
Ethics declarations
Ethics approval.
All procedures performed in studies involving human participants were in accordance with the ethical standards of the Ethics Committee from the institutions involved, and with the 1964 Helsinki declaration and its later amendments or comparable ethical standards.
Informed consent
Informed consent was obtained from all individual participants included in the study.
Conflict of interest
The authors declare that there is no conflict of interest regarding the publication of this article.
Additional information
Publisher’s note.
Springer Nature remains neutral with regard to jurisdictional claims in published maps and institutional affiliations.
Rights and permissions
Springer Nature or its licensor (e.g. a society or other partner) holds exclusive rights to this article under a publishing agreement with the author(s) or other rightsholder(s); author self-archiving of the accepted manuscript version of this article is solely governed by the terms of such publishing agreement and applicable law.
Reprints and permissions
About this article
Amini Farsani, M., Barzegar, R. Tracing sampling in applied linguistics: the case of quantitative research approach. Qual Quant (2024). https://doi.org/10.1007/s11135-024-01929-3
Download citation
Accepted : 24 June 2024
Published : 05 July 2024
DOI : https://doi.org/10.1007/s11135-024-01929-3
Share this article
Anyone you share the following link with will be able to read this content:
Sorry, a shareable link is not currently available for this article.
Provided by the Springer Nature SharedIt content-sharing initiative
- Meta-research
- Research synthesis
- Sampling structures
- Sample size
- Find a journal
- Publish with us
- Track your research
To read this content please select one of the options below:
Please note you do not have access to teaching notes, mandatory csr regime strips the competitive advantage: a comparative study of pre-post csr mandate using the bandwagon-bias effect theory.
Society and Business Review
ISSN : 1746-5680
Article publication date: 8 July 2024
With corporate social responsibility (CSR) becoming mandatory, several firms in India have been compelled into spending resources on CSR while their business strategy and processes were unprepared to take up CSR activities, effectively. In this light, the CSR relationship with other business functions would be altered. Using Thomson Reuters data from 2010 to 2018 (pre-mandate to post-mandate) this study aims to re-examine the relationship between CSR and financial performance.
Design/methodology/approach
The current study is rooted in the bandwagon-bias effect theory and uses a long-term data (2010–2018) of Indian firms. It uses Refinitiv Thomson Reuters ESG rating to measure CSR and accounting measures for financial performance (FP) to make a pre-post analysis of the impact that mandatory CSR regime has had on firms performance. The study uses the weighted panel regression method.
The relationship between CSR and FP is different when CSR was voluntary than when it has been mandated by Law. CSR has a positive effect over the FP during the voluntary phase but this positive relationship weakens during the mandatory phase. The waning effect of CSR over FP substantiates the presence of bandwagon bias effect which can be explained by the crowding-in of several companies engaged in CSR activities because of the mandatory CSR law.
Research limitations/implicationsv
Few countries have made CSR mandatory therefore CSR literature is limited. But mandating CSR is a growing phenomenon so this study augments to the body of knowledge. Until now literature generally converged on a positive relationship between CSR performance and FP but the current study shows altering directions to this relationship in a changing CSR environment. The use of the bandwagon-bias theory contributes to the theoretical approaches. Theoretically, the findings add to the body CSR literature and offer impetus to the evolving domain of impact measurement and reporting.
Practical implications
Results of the study offer a clear indication to managers that they need to re-strategise their CSR activities during the mandatory CSR environment if they wish to draw instrumental benefits of a positive impact on the FP of their firms. CSR expenditure is now a leveller so managers may either exceed the mandatory 2% expenditure as some firms did during the voluntary CSR phase or else design their CSR implementation plans to bring about a more impactful positive change. Communicating the impact of CSR to influential and powerful stakeholders beyond the mandatory reporting to the government is yet another way through which managers can draw benefits of CSR activities. Additionally to draw positive results from CSR activities firms may consider adopting international reporting and benchmarking standards such as the GRI and ISO 26000. Finally, the results of the study can be used by policymakers to make a note that the CSR law is causing a weakening of the financial benefits and therefore.
Social implications
The results of the study can be used by policymakers also need to make a note that the CSR law is causing a weakening of the financial benefits and therefore firms are adopting shortcuts, by donating the required amount of funds. But donation of funds defeats the real purpose of mandatory CSR which is social impact, therefore the regulators may want to make the necessary changes unplug the gaps in the CSR law to ensure better adherence to the law in spirit and a real impact on the ground activities.
Originality/value
While CSR–FP relationship has been extensively explored but limited studies have explored this relationship in a mandatory CSR environment and no other work presents a comparative view of the CSR–FP relationship, namely, before and after the mandatory CSR policy. The current study is one of the limited few studying the impact of mandatory CSR policy on FP, and the only one that uses the bandwagon-bias effect to explain the phenomenon of weakening impact of CSR on FP of firms. Bandwagon-bias effect has been used in studying consumer behaviour, where group effect impacts behaviour of individuals and with mandatory CSR policy, firms following the other firms leading to crowding in. Using the bandwagon-bias effect has found limited attention from the CSR scholars, the current study uses this theoretical basis and therefore augments the CSR literature.
- Thomson Reuters
- Mandatory CSR
- Bandwagon-bias effect
- Financial performance instrumental CSR-normative CSR
Acknowledgements
Competing interest and funding: The authors declare none. The authors do not have any competing or conflict interests in this research work.
This research did not receive any funding from any agency. It was carried out independently by the researchers.
Consent: No human subjects were used to conduct this study.
Pathak, T. , Tewari, R. and Drempetic, S. (2024), "Mandatory CSR regime strips the competitive advantage: a comparative study of pre-post CSR mandate using the Bandwagon-bias effect theory", Society and Business Review , Vol. ahead-of-print No. ahead-of-print. https://doi.org/10.1108/SBR-01-2024-0002
Emerald Publishing Limited
Copyright © 2024, Emerald Publishing Limited
Related articles
All feedback is valuable.
Please share your general feedback
Report an issue or find answers to frequently asked questions
Contact Customer Support
This paper is in the following e-collection/theme issue:
Published on 3.7.2024 in Vol 8 (2024)
A Digital Sexual Health Education Web Application for Resource-Poor Regions in Kenya: Implementation-Oriented Case Study Using the Intercultural Research Model
Authors of this article:

Original Paper
- Clarissa Soehnchen 1 , MLB, BSciS ;
- Anja Burmann 2 , Dr rer med ;
- Maike Henningsen 3 , Dr med ;
- Sven Meister 1, 2 , Prof Dr
1 Health Informatics, School of Medicine, Faculty of Health, Witten/Herdecke University, Witten, Germany
2 Department Healthcare, Fraunhofer Institute for Software and Systems Engineering, Dortmund, Germany
3 School of Medicine, Faculty of Health, Witten/Herdecke University, Witten, Germany
Corresponding Author:
Clarissa Soehnchen, MLB, BSciS
Health Informatics
School of Medicine, Faculty of Health
Witten/Herdecke University
Alfred-Herrhausen-Straße 50
Witten, 58455
Phone: 49 2302 9260
Email: [email protected]
Background: Developing a digital educational application focused on sexual health education necessitates a framework that integrates cultural considerations effectively. Drawing from previous research, we identified the problem and essential requirements to incorporate cultural insights into the development of a solution.
Objective: This study aims to explore the Solution Room of the self-established Intercultural Research Model, with a focus on creating a reusable framework for developing and implementing a widely accessible digital educational tool for sexual health. The study centers on advancing from a low-fidelity prototype (She!Masomo) to a high-fidelity prototype (We!Masomo), while evaluating its system usability through differentiation. This research contributes to the pursuit of Sustainable Development Goals 3, 4, and 5.
Methods: The research methodology is anchored in the Solution Room of the self-expanded Intercultural Research Model, which integrates cultural considerations. It uses a multimethod, user-centered design thinking approach, focusing on extensive human involvement for the open web-based application. This includes gathering self-assessed textual user feedback, conducting a System Usability Scale (SUS) analysis, and conducting 4 face-to-face semistructured expert interviews, following COREQ (Consolidated Criteria for Reporting Qualitative Research) guidelines.
Results: Based on the identified limitations of the low-fidelity prototype, She!Masomo (SUS score 67), which were highlighted through textual user feedback (63/77) and prototype feature comparisons, iterative development and improvement were implemented. This process led to the creation of an enhanced high-fidelity prototype (We!Masomo). The improved effectiveness of the enhanced prototype was evaluated using the qualitative SUS analysis (82/90), resulting in a favorable score of 77.3, compared with the previous SUS score of 67 for the low-fidelity prototype. Highlighting the importance of accessible digital educational tools, this study conducted 4 expert interviews (4/4) and reported e-survey results following the CHERRIES (Checklist for Reporting Results of Internet E-Surveys) guideline. The digital educational platform, We!Masomo, is specifically designed to promote universal and inclusive free access to information. Therefore, the developed high-fidelity prototype was implemented in Kenya.
Conclusions: The primary outcome of this research provides a comprehensive exploration of utilizing a case study methodology to advance the development of digital educational web tools, particularly focusing on cultural sensitivity and sensitive educational subjects. It offers critical insights for effectively introducing such tools in regions with limited resources. Nonetheless, it is crucial to emphasize that the findings underscore the importance of integrating culture-specific components during the design phase. This highlights the necessity of conducting a thorough requirement engineering analysis and developing a low-fidelity prototype, followed by an SUS analysis. These measures are particularly critical when disseminating sensitive information, such as sexual health, through digital platforms.
International Registered Report Identifier (IRRID): RR2-10.1186/s12905-023-02839-6
Introduction
Sub-Saharan Africa has a population of approximately 1.1 billion people, representing roughly 14% of the global population. Despite a decrease in the global share of new HIV infections in the region, sub-Saharan Africa still accounted for 59% of all new infections worldwide in 2021 [ 1 ]. The region accounts for two-thirds of new sexually transmitted infection cases [ 2 ], and HIV continues to be the primary cause of death among adolescents in sub-Saharan Africa [ 3 ]. In 10 out of 11 countries in Western and Central Africa, less than half of the women surveyed reported being able to make their own decisions regarding sexual relations, contraceptive use, and their own health care [ 1 ]. Furthermore, over 80% of individuals report incidents of technology-facilitated sexual violence, gender-based violence, or other forms of violence [ 2 , 4 ]. Given these challenges in sub-Saharan Africa, it is imperative to reduce unplanned pregnancies and disease transmission. This entails providing adolescents with access to contraception information [ 4 ] and comprehensive sexual health education to help them make informed decisions. Access to knowledge empowers individuals to make sustainable choices regarding their bodies and health. Adolescents often lack access to crucial health care information [ 1 ]. One potential reason is the absence of comprehensive sex education in the national school curriculum, leaving teachers ill-equipped to address these topics in the classroom [ 5 ], particularly in Kenya. Kenya is selected as an example for this study due to its network of community centers, mobile infrastructure, and presence of low-income regions, which facilitate evaluation. The absence of structured, verified, valid, and reliable sexual information, particularly concerning contraception, menstruation, and female genital mutilation, leaves young women vulnerable to health risks [ 6 ]. Additionally, sexual health discussions are influenced by religious, tribal, and social affiliations, which can result in inconsistent or suppressed conversations [ 6 , 7 ]. The societal taboo surrounding contraceptive practices can drive some individuals to resort to fatal abortions to prevent unwanted pregnancies [ 8 ]. Given the challenges of limited infrastructure, financial resources, and access to education and sexual health information, the increased use of the internet and digital technologies presents an opportunity for promoting behavioral change [ 9 ]. The literature demonstrates a growing emphasis on digital tools to address these issues [ 6 , 8 , 10 - 15 ]. A study providing contraceptive information to young people [ 16 ] cites research indicating that mass media, particularly digital platforms, effectively reach and educate young adults about sexual health. Another study confirmed that adolescents in Kenya express a desire for easily accessible and trustworthy information about maintaining sexual health [ 17 ]. The use of digital tools and social media has bolstered confidence in addressing these topics, allowing for feedback and dispelling misconceptions [ 6 , 10 , 11 ]. Disseminating information can significantly reduce the risks of sexually transmitted infections such as HIV and unplanned pregnancies, with considerable potential for educating low-income and vulnerable communities [ 12 , 13 ]. However, ensuring lasting user engagement remains crucial [ 11 ].
Design thinking methodologies, as endorsed by Plattner et al [ 18 ], provide a creative framework for effectively addressing social and cultural challenges, specifically focusing on human needs. This involves collaborating with diverse groups, continually refining the process through interactions, and considering cultural-ergonomic factors to ensure the usability and accessibility of the final product [ 19 - 21 ]. The Double Diamond Model forms the foundation of the design thinking approach, providing a structured process; however, it typically lacks integration of intercultural principles. In the realm of design thinking processes, recognizing the significance of cultural consistency, as underscored by Kroeber and Kluckhohn [ 20 ], is essential for developing digital educational tools aimed at achieving cross-cultural usability.
The Intercultural Research Model
In a prior study by the author, the original Double Diamond Model (see Figure S1 in Multimedia Appendix 1 ) was adapted to the Intercultural Research Model, depicted in Figure 1 , to identify requirements and design principles, enhancing focus on user needs [ 22 ]. Hence, the research model was enriched with cultural considerations, drawing insights from Rau et al [ 23 ], Lachner et al [ 24 ], and Laws et al [ 25 ], culminating in the development of a solution as depicted in Figure 1 . The model empowers users from specific cultures to engage with products developed for foreign cultures, thereby enhancing accessibility and usability on a broader scale in the future. It establishes connections between diverse cultural spheres through varied perspectives. In the context of globalization, this process becomes imperative [ 26 ]. When addressing sexual health education in Kenya, it is essential to explore diverse perspectives beyond traditional literature to foster innovation. Culture plays a pivotal role in shaping both unconscious and conscious behaviors within social groups, influencing decision-making processes and various characteristics [ 20 ]. First, the Problem Room, divided into discover and define phases [ 24 ], conducts requirement engineering analysis. In phase 1, the discover phase identifies user requirements, defining primary, secondary, and anti-persona, alongside conducting empathy maps and storyboards [ 15 ]. Phase 2, the define phase, conceptualizes a cross-cultural design philosophy. Focusing on user, environment, and cultural components, based on Smith-Jackson et al’s [ 27 ] cultural ergonomics, results in a requirement engineering analysis (see Table S1 in Multimedia Appendix 1 ; also see [ 7 , 28 ]) [ 22 , 29 ]. In phase 3, the development phase meticulously outlines the product, integrating cultural and end user needs, while creating a low-fidelity prototype using a user interface/user experience design tool. The delivery phase, phase 4, encompasses high-fidelity prototype development, implementation, and delivery to the end user, building on the initial 3 phases [ 22 ]. Refer to Figure S2 in Multimedia Appendix 1 for the Intercultural Research Model, which is an adaptation based on the Nielsen Norman Group’s Double Diamond Model and insights from Soehnchen et al [ 22 , 29 ] and Rau et al [ 23 ].

From She!Masomo to We!Masomo
The initial phase of the Intercultural Research Model involves conducting a requirement engineering analysis, which provides the foundation for developing a low-fidelity prototype. In this development process, the low-fidelity prototype, named She!Masomo, is specifically designed to focus on women’s sexual health education [ 22 ]. The prototype, named She!Masomo, was developed using Balsamiq Software [ 30 ] to visualize requirements and demonstrate user interactions aligned with Kenyan culture and educational knowledge status. It underwent an end user Unified Theory of Acceptance and Use of Technology and System Usability Scale (SUS) evaluation, collecting feedback and improvement suggestions for the final high-fidelity prototype as part of the development phase in the Solution Room [ 29 ], depicted in Figure 1 . As a result, collected end user feedback was implemented to improve the development of the high-fidelity prototype, leading to the rebranding from She!Masomo to We!Masomo. We!Masomo is an all-gender inclusive web-based application for sexual health education, integrating She!Masomo, represented by its fictional character, Linda, and He!Masomo, featuring its fictional character Leo.
Study Objectives
This paper focuses on the Solution Room, encompassing the missing parts of the development and the not-yet-addressed delivery phases, culminating in the high-fidelity prototype—the digital educational tool named We!Masomo. The objective of We!Masomo is to provide free digital information on contraceptive methods and menstruation education to empower and educate users. Given that the targeted end users have diverse cultural backgrounds, their inclusion in the process is imperative. Societal stigmas, cultural and religious backgrounds, and misconceptions [ 7 , 8 , 28 ] are identified as barriers to sexual health knowledge. However, acceptance depends on various factors, and improving sexual health is deemed a major necessity [ 11 ], highlighting the need for tailored educational content to address specific circumstances. Recognizing the impact of cultural differences on product usability, Barber and Badre [ 31 ] encapsulate this concept as “culturability.” Consequently, this research aligns with cultural ergonomics, as outlined by Smith-Jackson et al [ 27 ], integrating culture into the development process of designing human-computer interaction systems. The purpose of this case study is to design and implement We!Masomo in a way that addresses the challenges of limited access to knowledge, especially in the field of sexual education. Therefore, our research question is “To what extent can the Intercultural Research Model support the development and delivery phases (Solution Room) with a focus on creating a high-fidelity prototype?” This question considers the following objectives:
- Objective 1: To evaluate system usability as a measure of the fit between the low-fidelity and high-fidelity prototypes during the transition from the Problem Room to the Solution Room.
- Objective 2: To differentiate between She!Masomo and the newly developed all-inclusive platform We!Masomo.
We hypothesize that not all established requirements can be fully met during the development of the high-fidelity prototype and may indicate levels of digital literacy. However, we posit that this shortfall will not negatively impact the measurement of system usability.
Case Study Approach Within the Development and Delivery Phases
Case study research is historically closely associated with the methodology outlined by Yin [ 32 ], encompassing preparation, data collection, analysis, and reporting. This approach is well-suited for investigating contemporary phenomena within the natural context of software engineering. Runeson and Höst [ 33 ] provide guidelines for conducting and reporting case study research in software engineering. They emphasize the importance of understanding what constitutes a case study and its real-life context, specifically in the case of We!Masomo. The authors compiled terminology and guidelines from various methodology handbooks in the social sciences and information systems fields, adapting them to meet the specific needs of software engineering. They stress the significance of checklists for researchers and readers, derived through a systematic analysis of existing literature [ 34 ]. The case study methodology is well suited for various types of software engineering research, as it focuses on contemporary phenomena that are difficult to study in isolation. This case study aligns with the process suggested by Runeson and Höst [ 33 ], which includes case study design, preparation for data collection, data collection, analysis of collected data, and reporting.
Case Study Design Within the Development and Delivery Phases: From the Low-Fidelity Prototype She!Masomo to Developing the High-Fidelity Prototype We!Masomo
The development phase of the Solution Room resulted in the creation of a low-fidelity prototype, She!Masomo, which analyzed the end user’s environment. This prototype visually represented user requirements and was demonstrated through abstract depictions in established storyboards [ 24 , 32 ]. In the subsequent delivery phase, the focus shifts to the high-fidelity prototype, now named We!Masomo, designed for sexual health education in sub-Saharan Africa. This evolution includes expanding content and broadening the target group to encompass both women and men. Our case study approach aims to be holistic, focusing on investigating and describing the transition from She!Masomo to We!Masomo, with attention to the objectives outlined in the introduction. The scope is confirmatory and explanatory, aiming to enhance the user experience of We!Masomo. Data and method triangulation are employed using a mixed methods approach, structured in the steps outlined in Textbox 1 .
- Conduct a descriptive analysis of textual user feedback by evaluating descriptive feedback collected from the low-fidelity prototype, She!Masomo.
- Identify improvement suggestions derived from end user evaluation and recommend enhancements.
- Conduct a prototype comparison by comparing the previously established low-fidelity click-dummy, She!Masomo, with the high-fidelity prototype, We!Masomo.
- Highlight eliminated or adjusted requirements during the development process.
- Conduct a System Usability Scale (SUS) analysis for the We!Masomo web application.
- Utilize the widely recognized qualitative SUS questionnaire to assess the usability and user perception of the high-fidelity prototype (We!Masomo).
- Apply descriptive statistics for a product-centered evaluation of SUS analysis to quantify the usability of the software product [ 35 ].
- Compare SUS analysis results with those of She!Masomo, analyzed in the development phase [ 29 ].
- Follow this with improvement suggestions derived from end user evaluation and enhancement recommendations for We!Masomo, highlighting the evolution of requirements during development.
- Conduct qualitative semistructured expert interviews with local supervisors of potential end users.
- Explore insights and gather long-term improvement suggestions using semistructured questions designed to facilitate open discussion and diverse feedback.
- Align the interview approach with the 32-item checklist known as the COREQ (Consolidated Criteria for Reporting Qualitative Research) guidelines [ 36 , 37 ].
Each step focuses on a specific aspect of the evaluation process, providing a clear and structured presentation of the analysis conducted throughout the development process leading to the final product, the high-fidelity prototype, We!Masomo. Figure 1 illustrates these essential steps.
Preparation for Data Collection and Recruiting
The preparation, recruitment, and collection of implementation user feedback data are divided into 3 distinct research phases to facilitate the subsequent 4-step analysis, in accordance with triangulation requirements.
The first research phase, which involved qualitative descriptive analysis of textual user feedback on She!Masomo, took place from December 1, 2022, to January 31, 2023. This phase was implemented through community centers, girls’ schools, and universities in rural regions of Kenya, as well as during a field trip. A convenience sampling technique was used to reach the predominantly female target group aged 18-35. To ensure participant support, the survey on the low-fidelity prototype was supplemented with in-person assistance from January 11 to January 23, 2023. Participants self-assessed through online questionnaires at various community centers in Eldoret (Making More Health, MOI University in Eldoret, and Learning Lions, each visited for 3 days). To ensure a comfortable environment for discussing the sensitive topic, the in-person support team, consisting of the main author and trusted local representatives, conducted a brief introduction to the tool and its mission. This was followed by a 20-minute testing session of the low-fidelity prototype using participants’ smartphones or provided smartphones. The survey was conducted using the online platform LimeSurvey (LimeSurvey Team), where all participants consented to participate, and no indication of unwillingness to participate in the study was detected [ 38 ]. The vulnerability of the target group is amplified by gendered social norms, practices, and inequalities, which are particularly pronounced in sub-Saharan Africa, where rates of child marriage are the highest globally [ 1 ].
The second research phase involved the SUS analysis of We!Masomo, conducted between November 15, 2023, and December 31, 2023. The online platform LimeSurvey was used, and the survey was distributed through community centers, high schools, and universities in both rural and urban areas of Kenya, using a convenience sampling technique. All participants provided consent, and no indication of unwillingness to participate in the study was detected [ 38 ]. The study targeted males and females aged 18-35 years. To ensure participant support and improve response rates, in-person survey facilitation took place from November 15 to December 15, 2023. This approach aimed to create a comfortable environment for discussing sensitive topics and addressing potential challenges related to comprehension of content and language. The web-based application enabled participants to access and review the provided content. After an exploration period, participants proceeded to complete the survey using their devices. For those without digital devices, smartphones or laptops were provided, allowing them a 20-minute window to explore the high-fidelity prototype through the web-based application. After exploration, participants completed the survey on the provided device.
The third data collection research phase involved qualitative expert interviews conducted by the author CS, who is female and the founder of We!Masomo. The interviews were recorded using Microsoft Teams for audio recording and coded by CS in alignment with COREQ guidelines [ 38 ]. As a result of data saturation, 4 expert interviews were deemed sufficient. All participants provided consent. No indication of unwillingness to participate during the interviews was detected [ 38 ]. Additionally, to ensure a supportive environment and address potential comprehension challenges, a local supervisor was present. The interviews had a duration ranging from 30 to 70 minutes [ 38 ].
Data Analysis and Reporting
Based on our triangulation approach, the data analysis was divided into 4 steps: step 1 involved a descriptive analysis of collected textual user feedback on the low-fidelity prototype; step 2 entailed prototype comparison; step 3 involved conducting an SUS analysis on We!Masomo and comparing the SUS score with that of the low-fidelity prototype; step 4 consisted of qualitative expert interviews using qualitative research methods on the high-fidelity prototype, as illustrated in Figure 2 .

The first step involved descriptive analysis of textual user feedback to characterize the sample of 77 study respondents. Feedback collected during the initial fieldwork study from December 1, 2022, to January 31, 2023, was analyzed using grounded theory [ 33 , 39 ]. Grounded theory facilitates systematic collection and categorization of textual user feedback related to the research questions. Each idea was noted and organized into thematic areas, which facilitated a comprehensive understanding [ 33 ]. Ideas, content, and quotes from each participant were individually written on notes, using different colors to distinguish thematic areas. This approach allowed for a nuanced analysis of feedback, considering its origin and facilitating inductive categorization. The clustering process, guided by predefined dimensions, also incorporated additional categories and subcategories, resulting in the identification of 4 main clusters with subcategories. The textual feedback was systematically allocated to these categories and subcategories. In response to user feedback and improvement suggestions, the development of the high-fidelity prototype culminates in the rebranding to We!Masomo.
Step 2 involved a comparative study of the low- and high-fidelity prototypes to evaluate the usability and features of We!Masomo. Features and content were systematically analyzed to identify enhancements and improvements in the high-fidelity version.
Step 3 involved a qualitative SUS analysis of We!Masomo. The online SUS analysis, a quantitative method, assessed usability divided into 2 sections. Section A gathered demographic data and information on respondents’ patterns of electronic device usage for accessing health-related information. Section B incorporated the standardized SUS questionnaire. The SUS questionnaire consists of 10 items rated on a 5-point Likert scale, yielding scores between 0 and 100 [ 40 ]. Scores of 68 or above indicate good usability [ 40 , 41 ]. For reporting e-survey results, the recommended CHERRIES (Checklist for Reporting Results of Internet E-Surveys) checklist by Eysenbach [ 37 ] was followed (see Multimedia Appendix 2 and Table 1 ).
Feature | Low-fidelity prototype | High-fidelity prototype |
Explore page | Content: Information on menstruation, contraception methods, 15 female genital mutilation videos, potential list of health care centers, partnership organizations, about She!Masomo | Added content: Cervical cancer, breast cancer, mental health issues, parenting content, exchange platform, He!Masomo for male-focused content, period tracking option, Leo and Linda as comic figures, and extended user profile option |
Landing page | African Map, Linda is shown, She!Masomo logo | Changed color scheme, changed the logo to We!Masomo, directed to the “Explore” page, cookie request, and introduction by Leo and Linda |
Added content | Menstruation content, contraception methods, 15 female genital mutilation videos | Cervical cancer, breast cancer, parenting content, mental help support content, and Swahili audio files |
Hotline | — | Not yet—in discussion with a partner organization for emergency abuse cases |
Gender | Female (Linda) | Male and female (Leo and Linda comic figures) |
Free access to content | All content | Content of menstruation information, cervical and breast cancer, and contracepting methods |
Logo | She!Masomo | We!Masomo—as an all-inclusive platform for She!Masomo and He!Masomo |
User profiles | Not necessary | Added verified user profile via phone number or email address |
Exchange platform | — | Added a platform so users can ask questions only via verified user profiles |
Period tracker | — | Added a period tracking feature as a natural contraction option; can track mood and menstruation |
Contact formula | — | Contact option for specific questions and feedback |
Feedback option | — | Added a thumbs up/down option as a direct feedback option per provided content. |
Languages | Swahili and English | Swahili and English audio files for the read aloud function |
User profiles | — | General questions, family status, menstruation information, use of contraception method, female genital mutilation—besides the general information, filling out the rest is optional |
a Not applicable.
b When the user registers on the tool and creates a user profile, the user is not required to provide all information (ie, users are only required to provide certain information such as birth year, gender, and a creative username).
The fourth and final analysis step involved qualitative expert interviews using qualitative research methods. Expert interviews provide deep insights into user experiences, perceptions, and suggestions for improvement. This includes using one of Kuckartz’s [ 42 ] approaches for content-structuring qualitative analysis, utilizing inductive coding during the interviews.
The analysis of collected textual user feedback, prototype comparison, SUS score analysis and comparison, and qualitative expert interviews contribute to assessing the usability, effectiveness, and user satisfaction of We!Masomo, a web-based application aimed at promoting sexual health education in sub-Saharan Africa.
All findings from the Problem Room are consolidated in the subsequent study and available online through the web application We!Masomo [ 43 ].
Ethical Considerations
The research adheres to the principles outlined in the Declaration of Helsinki and has received approval from the Institutional Review Board (or Ethics Committee) at Witten/Herdecke University (protocol code S-119/2022; approval date August 6, 2022). All participants in the study provided informed consent for publication before participation. Participants in the study signed a consent form after receiving written information and a verbal explanation about the study’s context, procedures, and data usage. Each participant received a copy of the signed informed consent documentation. To protect confidentiality, all study data were anonymized to ensure participants could not be identified in any publications or research data. Importantly, participants did not receive any financial compensation for their involvement in the study.
Analyzing insights from various methodology handbooks in the social science and information systems fields, we refined the low-fidelity prototype She!Masomo by addressing cultural differences and overcoming limitations to enhance future usability. This evolution led to the delivery phase, where we analyzed the high-fidelity prototype We!Masomo through 4 distinct analysis steps tailored to the specific needs of the software engineering process. The case study methodology is well suited for this approach and study because contemporary phenomena are difficult to study in isolation.
Case Study Design Within the Development Phase: Using Textual User Feedback From the Low-Fidelity Prototype She!Masomo to Develop the High-Fidelity Prototype We!Masomo
The development phase resulted in the creation of a low-fidelity prototype named She!Masomo, along with an analysis of the end user’s environment. Moving into the delivery phase, we introduced the high-fidelity prototype, We!Masomo, specifically designed for sexual health education in sub-Saharan Africa. This version features expanded content and targets a broader audience, encompassing both women and men.
Implementing Textual User Feedback—Adjustment of the Low-Fidelity Prototype She!Masomo in the Development Phase
In the initial phase of analysis, adolescent women tested the low-fidelity prototype to evaluate its accessibility, voice control, and features aimed at establishing a secure familial environment, as highlighted by Rau et al [ 23 ]. User feedback was collected through self-assessment (63/77) and analyzed using the affinity mapping technique. This process revealed 4 distinct clusters through inductive coding.
In cluster 1, 36/63 (57%) participants provided nonconcrete feedback on the low-fidelity prototype. Responses varied; some suggested that no additional features or content are necessary, while others expressed positive sentiments about She!Masomo, stating, “I believe it's a valuable system as it enhances our understanding of health.” One participant notably underscored the significance of women’s health, asserting, “No changes are needed; it is crucial for women to maintain good health as men often neglect their well-being.” Participants also conveyed that the tool is easy to use, anticipating that most individuals would quickly grasp its functionality. A forward-looking perspective was evident as well, highlighting, “This tool is secure and beneficial for girls and women in the community.” However, several participants noted the tool’s ease of use and advocated for broader outreach, suggesting its introduction in schools and community centers to effectively reach a wider audience, including men. They emphasized the importance of informing both men and women about health topics. One participant indicated the need to include content relevant to males.
Cluster 2 consisted of technical improvement suggestions, with 7/63 (11%) focusing on enhancing the platform’s interface, user-friendliness, and visual appeal. Recommendations included the introduction of a log-in page for user profile verification, a search bar for specific content suggestions, integration with social media, and implementation of robust privacy regulations.
Cluster 3 encompassed content suggestions, with 17/63 (27%) participants defining 3 key areas of emphasis: gender equality and violence; the inclusion of men in conversations; and information on cervical and breast cancer, parenting styles, menstruation, and sexual reproductive health rights. There was a notable demand within the realm of gender equality and violence to expand the content to include the male perspective. A participant expressed, “Violence and abuse against women - what can we do? What do we have to do initially? Can we talk?” Another participant emphasized, “It is very attractive to use, but the men’s content is missing. Also, men need to know this information.” One participant conveyed, “This tool has helped me with family planning. It makes my marriage peaceful and nice, so my husband is nice to me. It also helps me understand why I need to go to the hospital regularly to check and when to go and to learn and understand more.” Participants expressed a need for content related to cervical and breast cancer, as well as information on sexually transmitted infections. They emphasized the importance of being able to identify and conduct home testing when access to hospital visits is limited. Additionally, the subcluster focusing on parenting styles and family planning received considerable attention. One participant inquired, “How to give birth to a child and how to raise it?” They highlighted the necessity for guidelines on parenting styles; developmental milestones for children; what activities are appropriate at various ages; and when children typically achieve milestones such as walking, talking, and engaging in tasks. Participants requested comprehensive and easily understandable content, preferably in video format, available in both English and Swahili. They also asked for information on nutritional intake. Additionally, participants expressed the need for a subsection on menstruation. They sought information on how the monthly cycle functions, the physiological changes in the female body, and details related to menopause. This prompted requests for more informative videos, as well as general information on the contextual framework of sexual and reproductive health (SRH) rights and services, which often differ in East Africa. They also wanted to understand the cultural differences and asked for guidance on whom to speak to regarding this topic.
In cluster 4, improvements focused on potential feature extensions. Only 3/63 (5%) participants expressed a desire for additional features, such as a period tracker and a 24-hour online service for inquiries related to sexual well-being and emergencies. Additionally, participants emphasized the importance of user engagement through features that facilitate questions and discussions on controversial topics. Furthermore, incorporating a period tracker could serve as an optional contraceptive method, aligning with the extensive list of contraceptive methods. Another participant expressed a need for a 24-hour online service, allowing users to inquire about any matters related to sexual well-being and emergencies at any time. This indicates a preference for an optional hotline feature to be included in the platform. Notably, a participant underscored the importance of user engagement, expressing the desire for an additional feature that allows for questions and discussions. This functionality could be facilitated through an exchange platform for user interaction. The evaluation marks the transition from She!Masomo to We!Masomo. We!Masomo, the developed high-fidelity prototype, is coded via GitHub [ 44 ], deployed on Vercel (Vercel Inc.), with Google Firebase (Google LLC/Alphabet Inc.) as the back end. This concludes the development phase of the Solution Room of the Intercultural Research Model, as shown in Figure 1 , and is followed by the delivery phase.
Comparing Low- and High-Fidelity Prototypes in the Delivery Phase
Step 2 of the analysis involved continuous engagement with potential users over an extended period. The Intercultural Research Model process concluded with the delivery phase, where the previous results were synthesized into a solution [ 38 ]. These findings guided the further development of a high-fidelity prototype to refine the detailed concept. This process included considerations of sizes, fonts, colors, and content. The high-fidelity prototype integrates advanced functionalities and serves as the final minimum viable product [ 38 ]. In terms of intercultural design, these mechanisms will be evaluated for their effectiveness within the target culture. The primary focus is on Kenyan users, ensuring that features are tailored to meet identified needs as comprehensively as possible.
Case Study Design Within the Delivery Phase: High-Fidelity Prototype We!Masomo—Introduction to the Web-Based Application
Based on the outcomes and feedback from the low-fidelity prototype, along with insights gathered from the SUS study [ 29 ] and textual user feedback, the development of the high-fidelity prototype as a web-based application with online user interactions has commenced. This advanced high-fidelity prototype, We!Masomo, serves as a visual representation of the user interface, integrating refined feedback. Figure 2 illustrates the freely accessible web-based application, We!Masomo [ 43 ] (screenshot taken on February 4, 2024), developed based on the structure of the low-fidelity prototype, She!Masomo. Table S1 in Multimedia Appendix 1 provides a full explanation of the requirements mentioned, including task appropriateness (TA), self-descriptiveness (SD), controllability (C), conformity to expectations (CE), learnability (L), and user retention (UR).
In accordance with requirement UR3, the tool is designed to be accessible without necessitating user log-in, outlining the application framework to ensure accessibility for all without requiring a user profile. The “Welcome” page introduces users to the tool, providing guidance on its optimal use and specifying the cookies that are tracked. Addressing user uncertainties regarding the sensitive content of the tool, we included an introductory text (L1) aimed at alleviating concerns ( Figure 2 ). It advises users to consider their environment, recommending a quiet, undisturbed moment for private engagement with the content. To create a welcoming atmosphere and provide guidance, a fictional character named Linda, portrayed as a supportive big sister figure, is introduced. Per requirement UR4, the placeholder is positioned in the upper-left corner of the introduction box, as outlined in the storyboard of the primary version (see Figure S3 in Multimedia Appendix 1 ). Users are offered the option to close the introduction using a cross located in the upper-right corner. If they wish to revisit the introduction, they can click on “Help.” The start screen is designed to be accessible without log-in credentials, intentionally crafted to encourage exploratory experiences. This approach eliminates the immediate requirement for users to disclose personal information and is provided free of charge (TA3). Subsequently, users are guided through the tool by the fictional characters Linda for She!Masomo and Leo for He!Masomo. After the initial presentation of the start screen, the next page is the “Explore” page. Beginning from the main page, various navigation options are provided to enhance the exploratory experience of the application. The core of the application is its “Content” page, which features menu points directing users to specific thematic areas covered by We!Masomo. These include details on contraceptive methods, expanded content on cervical and breast cancer, menstruation, parenting styles, mental health issues, and myths (TA8). One of these menu points is contraception methods (C2), displayed on the “Content” page. Upon selecting a specific topic, users are directed to an overview of categories within that topic (C2). From this point, users can delve into further subcategories. For instance, in the structure of “Contraception Methods,” if subdivisions exist, a tab bar will appear, featuring these subcategories (C2). These subcategories are visually presented in the bar to cater to users with varying levels of alphabetical knowledge. The selected option is highlighted in color while the unselected ones are grayed out. Additionally, auditory and textual versions are provided (TA12). To enhance accessibility, especially for users with limited literacy, each thematic area includes graphics and voice output. The interactive design integrates both written and auditory formats for all text passages, enabling a read aloud function supported in English and Swahili. This functionality is demonstrated on the “Content” page. Strategically placed speaker icons activate text-to-speech functionality for label reading, improving user comfort, particularly for those with limited reading proficiency. Structurally, the “Content” page is composed of modules, each organized consistently for ease of navigation and comprehension. Each module begins with a header stating the topic of discussion, similar to the header section, followed by textual, visual, and auditory representations. An explanatory text of varying length is placed below these representations. The speaker icon, distinct from the buttons, is positioned beneath the text. Furthermore, each module provides information on how users can access more detailed and personalized information. During the content-scrolling process, both the header section and tab bar remain visible, enabling users to seamlessly switch between subcategories or return to the previous page (C1). The content is designed to convey the informative and professionally validated nature of the application (TA1 and TA4). At the bottom of the page, an upward arrow appears to facilitate users’ return to the top of the page (SD1). Additionally, there is a feedback option available, represented by a thumbs up or down (dislike) option. Giving feedback is a valuable way for users to indicate if improvements to the content are needed.
In addition to the start screen, each page includes a header section at the top, which features the logo, language selection options, and a hamburger icon for navigation. The language selection feature (CE2) enables users to choose their preferred language and remains visible throughout their entire user journey. It is streamlined with globe icons representing the English and Kenyan flags. The currently selected language is highlighted, while the unused language appears in gray. Both the hamburger icon and the logo icon serve navigation purposes within the app. The hamburger icon opens a menu, as explained in the following section, while the logo icon links back to the main page, which aligns with requirement C1 and allows users to apply their knowledge (SD1). In future development stages, graphic placeholders will be replaced with sketches to provide a more abstract representation, which will help mitigate potential embarrassment (CE5). By contrast, navigating using the Hamburger icon introduces an additional step compared with navigating via the main page (C1). As the icon is accessible from any page, this approach involves an extra step by displaying all menu items. When the Hamburger icon is selected, it changes color to blue to indicate the current selection, aligning with the language selection feature. Clicking on another area closes the menu, and the icon returns to its original color (SD1). As shown in Figure 2 , a dedicated space for the We!Masomo logo (UR5) is included to inspire trust (CE6). In cases where user log-in is required, as depicted on the user log-in page, it is necessary to access newly developed features such as the exchange platform and period tracker, ensuring the privacy of users’ data.
Considering participants’ improvement suggestions from textual user feedback, the end user will be able to select their gender and indicate preferences for genders of interest. As a feature extension, in alignment with CE7, future development may include a health care center locator (TA15). This could potentially leverage tools such as Google Analytics (Google LLC/Alphabet Inc.) to enhance usability and accessibility. During the high-fidelity prototype development phase, the health care center locator feature was omitted. However, various other features have been successfully integrated into the prototype.
SUS Data Analysis of the High-Fidelity Prototype in the Delivery Phase
Assessing We!Masomo using the SUS methodology, as outlined by Brooke [ 40 ], represents step 3 of the multimethod approach to measure the improved usability of the web-based application. This evaluation compares with the prior SUS score of 67 obtained from the low-fidelity prototype (She!Masomo). The comprehensive SUS survey involved 90 individuals and was conducted as part of the reevaluation during phase 4 of the Intercultural Research Model. The repeat of the SUS analysis for We!Masomo was conducted in the fourth phase of the Intercultural Research Model. Out of the total responses received (N=90), 82 (91%) responses using We!Masomo were considered complete and included in the subsequent analysis; 8 observations were excluded as a result of missing values. Descriptive characteristics of the participants from the LimeSurvey are detailed in Table S2 in Multimedia Appendix 1 .
Conversely, for negatively worded questions, the score contribution is determined as 5 minus the scale position. The overall SUS score is calculated by multiplying the sum of the score contributions from each questionnaire by 2.5, following Brooke’s methodology [ 40 ]. An SUS score of 68 or higher is considered good [ 41 ]. The questionnaire details are illustrated in Table S3 in Multimedia Appendix 1 . The SUS questionnaire consists of 10 questions, each rated on a 5-point Likert scale, following Brooke’s guidelines [ 40 ]. For positively framed questions (1, 3, 5, 7, and 9), the score contribution is calculated as the scale position minus 1. For negatively framed questions (2, 4, 6, 8, and 10), the score contribution is determined using reversed scale positions (5 minus scale position). Based on these calculations, the computed SUS score, placed on a scale from 0 to 100, is determined to be 77.3. Interpreting this score, which falls within the “high marginal” to “acceptable” range, it is classified as “good” based on adjective ratings. Acceptability scores typically rank above 70 as acceptable, below 50 as unacceptable, and scores in between as marginal [ 34 , 38 ]. However, it is crucial to note that not all requirements from the initial requirement engineering analysis were fully met or significantly adjusted during the development phase, as originally suggested based on feedback from the low-fidelity prototype. This suggests areas for potential improvement or further refinement in subsequent iterations of the application. Despite this, the high-fidelity prototype remains acceptable, fostering a positive behavioral intention to use. However, continuous improvement and adjustment are essential to meet the evolving needs of its users.
Expert Interview—Review and Feedback on We!Masomo in the Delivery Phase
The 4 expert interviews, conducted as step 4 in the qualitative research methodologies, provide valuable insights into user feedback on the high-fidelity prototype, We!Masomo. These interviews highlight its superiority as a more user-friendly tool compared with She!Masomo. In the fourth and final analysis stage, these insights delve into user experiences, perceptions, and suggestions for further enhancements. Following Kuckartz’s [ 42 ] evaluation methodologies, qualitative structured content and inductive coding were applied during expert interviews, adhering to established qualitative research practices. This approach ensures systematic analysis and interpretation of the insights gathered from the interviews.
The main insights from the 4 expert interviews resulted in 105 Post-it notes categorized into 4 distinct clusters (see Figures S4 and S5 in Multimedia Appendix 1 ). These clusters offer a comprehensive understanding of various perspectives, including social belonging, education, technology aspects, and application insights. Notably, the experts emphasized the significance of We!Masomo as a digital educational application, particularly in addressing the challenges related to menstruation in sub-Saharan Africa and scaling knowledge and education effectively. They highlighted the complexities of societal norms, cultural beliefs, and educational barriers that impact sexual health awareness. Furthermore, the experts underscored the importance of technology accessibility, language inclusion, and creative engagement strategies in designing effective educational interventions. The experts provided perspectives from backgrounds in Kenyan social work and education.
In cluster 1, social belonging comprised participants’ characteristics from Kenyan nongovernmental organizations (NGOs), teachers, and social workers, offering diverse perspectives based on their backgrounds and experiences with end users. B1 (yellow) is a Kenyan male social worker and founder of a local information technology education campus in rural villages in northern Kenya. He expresses a supportive viewpoint, highlighting the significance of religion and culture, as well as the importance of empowering women and men through education. B2 (orange), from the slums around Nairobi, shared insights gained from working for a local NGO and founding a community center. His involvement in women’s empowerment groups and menstruation education adds depth to his perspectives. He has a daughter and oversees the technical aspects of the NGO. His perspective is shaped by interacting with users and his extensive experience in understanding the requirements needed to improve sexual health education. B3 (purple), a Kenyan start-up founder from Nairobi, previously worked for international development aid companies. She provides insights from the viewpoint of an educated urban dweller, where women have greater access to education. She established her business around a mentorship program, selling it to local partners and NGOs. Her mission is to mandate a mentorship program for all girls in secondary school to educate them about menstruation. B4 (green), originally from Nairobi, studied music at Nairobi University and founded her own NGO focusing on SRH and rights. Her diverse background, spanning Kenya and Denmark, equipped her to offer insights from an educated perspective, particularly regarding schooling systems and international education standards. This cluster highlighted the diverse social affiliations of the experts.
Cluster 2 focuses on education, addressing key aspects of the local schooling system, including reasons for school dropouts and the absence of sexual health education in the curriculum. In Kenya, most children are enrolled in school, benefiting from its free accessibility. However, secondary education typically requires fees, leading to a disparity where boys are more likely to attend secondary school compared with girls, as highlighted by B1, B2, and B3. The current government intends to increase school fees, which may lead to higher rates of school dropouts. Additionally, according to B2, most primary schools are not entirely free as school administrations still request money from parents. In certain regions, it remains uncommon for girls to pursue secondary education, as noted by B3. B3’s start-up focuses on menstruation education to encourage girls to stay in school. According to B3, only 30% of girls attend secondary school due to missing 1 week per month because of menstruation. Unfortunately, sexual education and contraceptive education are largely absent from secondary school curricula, as emphasized by B1, B2, and B3. B2 attributes the lack of comprehensive education to governmental policies and a conservative mindset. It is imperative to advocate for the inclusion of comprehensive sexual education in secondary school curricula, and initiatives such as We!Masomo could significantly contribute to this endeavor.
In cluster 3, focusing on technology aspects, B2 highlighted the divide between internet access in rural and urban areas. According to B1, smartphone and internet coverage reaches approximately 80% of the population in Kenya. Smartphone usage is increasing due to various initiatives, including government programs distributing smartphones in rural areas and support from Starlink (Starlink Services, LLC), which enhances accessibility. At B1’s NGO, every student receives a free computer to use and participate in the training program. B1 emphasizes that NGOs play a significant role in providing access to computers and internet facilities, especially in rural low-income areas. The widespread use of the Kenyan online payment system M-PESA ensures that most individuals already have a mobile phone, which facilitates digital transactions. M-PESA is a mobile money transfer and financial service for smartphones, owned by the local telecommunications provider Safaricom in Kenya. It allows users to deposit, withdraw, and transfer money, pay for goods and services, and access credit using their smartphones. M-PESA is widely used and deeply integrated into daily life, serving as a reference point for Kenya’s status as a digital pioneer. It has revolutionized financial inclusion in many parts of Africa by providing banking services to the unbanked population. B3 noted that at least one friend in every group in rural areas owns a smartphone. Additionally, schools, community centers, and NGOs provide computer rooms, which expand digital access. These factors underscore the potential reach and impact of a digital sexual health education application such as We!Masomo in urban areas across Kenya.
Cluster 4 highlights application insights, discussing improvement suggestions for We!Masomo. All 4 participants expressed strong support for We!Masomo and endorsed its introduction to their communities. They emphasized that while not everything needs to be included initially, the application serves as a foundational resource for learning. B1 emphasized how technology can motivate girls to pursue education, as he does at his NGO. B3 likened the application to a supportive elder sister, providing essential information about sexual health. Suggestions included incorporating more graphics and videos to enhance usability, as well as testimonials suggested by B2. Participants appreciated that everything was translated into Swahili and English, with audio files available. The expert interviews provided a nuanced understanding of user perceptions and preferences, affirming We!Masomo’s potential as a transformative tool.
The synthesized findings from expert interviews, along with other analytical components, inform continuous refinement and enhancement of We!Masomo to better serve its targeted audience and advance sexual health outcomes. The evaluation included textual user feedback, prototype comparisons, SUS analysis, and qualitative interviews, collectively contributing to assessing We!Masomo’s usability, effectiveness, and user satisfaction. Today, we have enrolled 250 user profiles in the educational digital tool.
Principal Findings
The analysis focuses on a practical triangulation case study approach, highlighting the development of a concept for educational digital tools aimed at achieving cross-cultural usability. This initiative aims to address culturally sensitive topics in resource-poor regions of sub-Saharan Africa, particularly in Kenya. The developed concept, tailored using this triangulated case study method approach, addresses taboos and the lack of sexual education and knowledge among adolescents [ 5 , 6 , 45 ]. It aims to tackle challenges associated with limited access to education and digital infrastructure, emphasizing the need for accessible content on intimate topics while respecting cultural and religious differences. By following the research objectives, we aim to evaluate system usability as a measure of the fit between the low- and high-fidelity prototypes, marking the transition from the Problem Room to the Solution Room. Additionally, research objective 2 involves assessing the differences between She!Masomo and the newly developed all-inclusive platform We!Masomo.
Therefore, the study utilizes the developed Intercultural Research Model, emphasizing cultural considerations throughout the tool development process and practical implementation in real-life usage. The case study accompanies the transition from the low-fidelity prototype, She!Masomo, to the high-fidelity prototype, We!Masomo, which has been successfully implemented. The results affirm the effectiveness of the Intercultural Research Model in enhancing usability and facilitating product development for culturally diverse settings.
The research objectives focus on evaluating how well the requirements of the Intercultural Research Model support the development and implementation of culturally sensitive SRH educational tools under challenging circumstances. The target group is specifically defined based on the social affiliations and circumstances of Kenyan men and women [ 7 , 8 ].
The objectives aim to ensure that all established requirements are met for the final tool implementation. However, despite the thorough requirement engineering analysis conducted, it is recognized that not all requirements need to be fulfilled to achieve high usability. This prompts a reassessment of the framework, anticipating that a shortfall in meeting certain requirements will not adversely impact the measurement of usability, as assessed by the SUS score. Answering objective 1, the study reveals a significant improvement in usability and acceptance of the high-fidelity prototype throughout its development process, as evidenced by its SUS score of 77. This is compared with the low-fidelity prototype, She!Masomo, which scored 67 [ 29 ]. For instance, the tool is designed to be accessible without requiring user log-in. However, to ensure data privacy, additional features that involve sensitive information require users to log-in. Furthermore, We!Masomo has been adjusted to include a feedback option, highlighting the iterative nature of the development process and the importance of user input in refining content. It is proven that integrating user- and human-centered evaluation, enriched by cultural ergonomics derived from the established Double Diamond Model, inspired by Rau et al [ 23 ], Lachner et al [ 24 ], and Laws et al [ 25 ], is essential but not sufficient for a successful development and implementation approach. Constant field testing and engagement with end users are essential requirements, emphasizing user- and youth-friendly services. School and health educators also play a significant role in the implementation and usage of such tools, as they provide positive feedback on their usage in communities. The study by Decker et al [ 46 ] demonstrated technology issues affecting the implementation of SRH technologies aimed at improving adolescent health, such as increasing condom usage and knowledge. Health educators are often not well-versed in technology or SRH education, leading to challenges in educating youth due to insufficient information provided. The study results are consistent with these difficulties, highlighting technological issues during data collection, problems with Wi-Fi hotspots, users forgetting log-in details, or malfunctioning technology on smartphones. Conversely, positive responses demonstrate acceptance of online resources and consent for using the tool as a local reference service in addition to on-the-ground services. The study conducted by Ouma et al [ 6 ] demonstrated that despite ongoing international commitments to increase accessibility and improve knowledge of contraceptive methods in Kenya, adolescents participating in the study still express safety concerns about the side effects of contraceptive methods.
Therefore, We!Masomo utilizes requirement CE6 by introducing the fictional characters Linda and Leo, designed to guide adolescents of both genders and create a trustworthy atmosphere, as specified in requirement L1. Enhancing usability is achieved through the implementation of additional content, such as male-specific content, information on HIV, child nutrition, and cervical and breast cancer. This expansion aims to support increased usage and address concerns highlighted in the study (O2), while also potentially increasing the frequency of visits, aligning with research objective 2. A study conducted by McMahon et al [ 47 ] demonstrated the feasibility and increased acceptability of eHealth interventions focusing on HIV content, while also offering insights into health-seeking behaviors [ 48 ]. It became evident that despite facing challenges in resource-poor regions, financial constraints, and cultural stigmatization, there is a pressing need for policy makers to adopt a variety of educational approaches to provide comprehensive SRH education to youth. Utilizing mass media for disseminating information has the potential to enhance awareness and utilization of modern contraceptives. Implementing similar initiatives focusing on segments of the population with low contraceptive uptake is advisable [ 49 ].
Looking ahead, future studies should involve a broader range of health and school educators in the evaluation process to help disseminate the tool and educate them about its usage. Local educators play a crucial role in determining accessibility and identifying potential limitations of the tool. Additionally, it is important to include the public sector and policy makers to ensure practical implementation of We!Masomo. Furthermore, exploring the transferability of the Intercultural Research Model to other cultural contexts and considering demographics such as men who have sex with men will be critical for comprehensive research efforts [ 50 ]. Moreover, beyond research studies, the tool is intended for long-term use, necessitating considerations for UR and engagement without requiring frequent relogging or intervention usage. Despite the study conducted by Norton and Tappis [ 51 ], there is an opportunity for a standardized research model to better inform the evidence base for improving the delivery of SRH interventions in humanitarian settings. Using this self-adjusted Intercultural Research Model, practical use cases should be implemented and analyzed more closely through case study examples in the future.
It is important to acknowledge potential limitations and biases related to the sensitive nature of the topic, as well as biases among participants when addressing intimate topics considered taboo in society. These factors may lead to feelings of shame and discomfort, compounded by language barriers that can make detailed statements challenging to analyze [ 52 ]. It is important to note that the applicability of the study’s findings on digital tools for sexual health information is restricted to the specifically developed web-based application prototype, We!Masomo, intended for practical and easy-to-use implementation. Furthermore, caution should be exercised when generalizing the results, as a convenience sample was used, which may not accurately represent the broader population. This limitation arises from restricted access to the internet and devices, as well as varying levels of digital literacy. To improve comparability with other studies, future research should include standardized measurements such as the widely accepted SUS measurement, alongside context-specific methods [ 38 ].
Conclusions
Individuals grappling with reproductive and sexual health challenges frequently confront educational disparities. Discussions on sexuality are often constrained by cultural stigmas and insufficient resources, yet sexual autonomy remains pivotal for well-being. Regrettably, data from 57 countries reveal that approximately 45% of women aged 15-49 in relationships or marriages lack this autonomy, particularly prevalent in sub-Saharan Africa [ 1 ]. This study aims to develop a reusable universal framework for creating a practical, scalable digital educational platform that addresses taboo and intimate topics. Specifically designed for resource-poor regions, this platform utilizes the concept of the Intercultural Research Model. This model, derived from the user-centered Double Diamond Model, serves as a guiding framework for the development process, emphasizing cultural considerations throughout. The study focuses on 14-35-year-old Kenyans as a case study, with the objective of providing accessible sexual health education that caters to the specific needs of both men and women in Kenya. The research methodology involves engaging with the targeted culture and reassessing the digital tools used to deliver educational sexual health information effectively. Requirement engineering analysis suggests enhancing text communication with visual and auditory channels. This includes incorporating fictional characters to guide users through the digital tool, aiming to reduce taboos and overcome language barriers. Additionally, features such as user log-in, an exchange platform between users, and feedback options are recommended. The study proposes conducting on-site evaluations of both low- and high-fidelity prototypes to explore interactive user feedback effectively. A triangulated multimethod approach, combining textual user feedback collection, prototype comparison, SUS analysis on We!Masomo, and comparing SUS scores with the low-fidelity prototype, alongside qualitative expert interviews, highlights the importance of accessible intimate content while respecting cultural and religious differences [ 31 ]. The findings emphasize the ongoing necessity to refine and explore methods to ensure inclusivity and effectiveness across diverse cultural contexts. The study’s objectives, which include evaluating system usability and discerning differences between prototypes, underscore the critical aspects of the Solution Room, spanning both the development and delivery phases. This process culminates in the creation of the high-fidelity prototype, We!Masomo. The primary goal of We!Masomo is to offer free digital information on contraceptive methods and menstruation education, with the aim of empowering and educating users, especially in regions where access to sexual health knowledge is limited. Social stigmas, cultural nuances, and religious beliefs have been identified as substantial barriers to sexual health knowledge, underscoring the need for tailored educational content that addresses specific circumstances. This discussion emphasizes the complex interplay between cultural considerations, usability evaluation, and existing research in the field. It stresses the significance of adaptive methodologies capable of accommodating diverse contexts while adhering to user-centered design principles. Looking ahead, there is consideration for expanding We!Masomo to other regions, despite not meeting all requirements of the self-established Intercultural Research Model. The digital tool, We!Masomo, shows a high behavioral intention to use, as indicated by its SUS score and successful implementation. In conclusion, this study underscores the substantial progress achieved in tackling reproductive and sexual health challenges using digital educational tools. The tailored survey methods highlight the potential effectiveness of adjusting the initially suggested requirements for successful implementation. This opens avenues for exploring the impact of cultural and religious factors on technology acceptance and paves the way for reaching a larger segment of the population in the future.
Acknowledgments
The authors express gratitude to the community workers in Eldoret, Lodwar, and Nairobi who assisted in recruiting participants. Special thanks are extended to all the participants, whose generosity in sharing information on this intimate topic was invaluable. Nongenerative artificial intelligence was utilized in a section of the manuscript.
Data Availability
The data sets generated and analyzed during this study are available from the corresponding author on reasonable request and under consideration of the ethics committee’s requirement to maintain anonymity.
Authors' Contributions
CS and SM conceptualized the study and developed the methodology. CS performed the validation and formal analysis, investigation, resource management, data curation, and writing—original draft preparation. CS, AB, and SM took care of writing—review and editing. MH and SM provided supervision.
Conflicts of Interest
CS is the product owner of the developed high-fidelity prototype, We!Masomo. Other authors have nothing to declare.
Details on the case study, approach, and results.
Survey questionnaire.
- Perehudoff K, Kibira D, Wuyts E, Pericas C, Omwoha J, van den Ham HA, et al. A comparative human rights analysis of laws and policies for adolescent contraception in Uganda and Kenya. Reprod Health. Feb 07, 2022;19(1):37. [ FREE Full text ] [ CrossRef ] [ Medline ]
- Kharsany ABM, Karim QA. HIV infection and AIDS in sub-Saharan Africa: current status, challenges and opportunities. Open AIDS J. Feb 07, 2016;10(1):34-48. [ FREE Full text ] [ CrossRef ] [ Medline ]
- Leddy AM, Weiss E, Yam E, Pulerwitz J. Gender-based violence and engagement in biomedical HIV prevention, care and treatment: a scoping review. BMC Public Health. Jul 08, 2019;19(1):897. [ FREE Full text ] [ CrossRef ] [ Medline ]
- Makinde OA, Olamijuwon E, Ichegbo NK, Onyemelukwe C, Ilesanmi MG. The nature of technology-facilitated violence and abuse among young adults in sub-Saharan Africa. In: Bailey J, Flynn A, Henry N, editors. The Emerald International Handbook of Technology-Facilitated Violence and Abuse. Bingley, UK. Emerald Publishing Limited; 2021:83-101.
- Ogolla M, Ondia M. Assessment of the implementation of comprehensive sexuality education in Kenya. Afr J Reprod Health. Jun 2019;23(2):110-120. [ CrossRef ] [ Medline ]
- Ouma L, Bozkurt B, Chanley J, Power C, Kakonge R, Adeyemi OC, et al. A cross-country qualitative study on contraceptive method mix: contraceptive decisionmaking among youth. Reprod Health. May 25, 2021;18(1):105. [ FREE Full text ] [ CrossRef ] [ Medline ]
- Ontiri S, Mutea L, Naanyu V, Kabue M, Biesma R, Stekelenburg J. A qualitative exploration of contraceptive use and discontinuation among women with an unmet need for modern contraception in Kenya. Reprod Health. Feb 09, 2021;18(1):33. [ FREE Full text ] [ CrossRef ] [ Medline ]
- Goodman ML, Gibson DC, Keiser PH, Gitari S, Raimer-Goodman L. Family, belonging and meaning in life among semi-rural Kenyans. J Happiness Stud. Aug 1, 2018;20(5):1627-1645. [ CrossRef ]
- Ferre C. Age at first child: does education delay fertility timing? The case of Kenya. World Bank. Mar 2009. URL: https://elibrary.worldbank.org/doi/abs/10.1596/1813-9450-4833 [accessed 2024-06-25]
- Branca Vergano L, Monesi M, Vicenti G, Bizzoca D, Solarino G, Moretti B. Posterior approaches in malleolar fracture: when, why and how. J Biol Regul Homeost Agents. 2020;34(3 Suppl. 2):89-95. Advances in Musculoskeletal Diseases and Infections. [ Medline ]
- Olamijuwon E, Odimegwu C. Sexuality education in the digital age: modelling the predictors of acceptance and behavioural intention to access and interact with sexuality information on social media. Sex Res Soc Policy. Jul 29, 2021;19(3):1241-1254. [ CrossRef ]
- Cilliers L, Viljoen KL, Chinyamurindi WT. A study on students' acceptance of mobile phone use to seek health information in South Africa. Health Inf Manag. May 2018;47(2):59-69. [ CrossRef ] [ Medline ]
- Obamiro K, West S, Lee S. Like, comment, tag, share: Facebook interactions in health research. Int J Med Inform. May 2020;137:104097. [ CrossRef ] [ Medline ]
- Gabarron E, Wynn R. Use of social media for sexual health promotion: a scoping review. Glob Health Action. Sep 19, 2016;9(1):32193. [ FREE Full text ] [ CrossRef ] [ Medline ]
- Jones K, Baldwin KA, Lewis PR. The potential influence of a social media intervention on risky sexual behavior and Chlamydia incidence. J Community Health Nurs. Apr 2012;29(2):106-120. [ CrossRef ] [ Medline ]
- Vahdat HL, L'Engle KL, Plourde KF, Magaria L, Olawo A. There are some questions you may not ask in a clinic: providing contraception information to young people in Kenya using SMS. Int J Gynaecol Obstet. Nov 2013;123 Suppl 1:e2-e6. [ FREE Full text ] [ CrossRef ] [ Medline ]
- Green EP, Whitcomb A, Kahumbura C, Rosen JG, Goyal S, Achieng D, et al. "What is the best method of family planning for me?": a text mining analysis of messages between users and agents of a digital health service in Kenya. Gates Open Res. May 29, 2019;3:1475. [ FREE Full text ] [ CrossRef ] [ Medline ]
- Plattner H, Meinel C, Weinberg U. Design Thinking. Berlin, Germany. Springer; Oct 01, 2009.
- Meinel C, von Thienen J. Design thinking. Informatik Spektrum. May 27, 2016;39(4):310-314. [ CrossRef ]
- Kroeber AL, Kluckhohn C. Culture: A Critical Review of Concepts and Definitions. Cambridge, MA. Peabody Museum Press; 1952:247.
- Köbler F, Eiting A. Design thinking als vorgehensmodell für die konzeption, gestaltung und evaluierung digitaler produkte und dienstleistungen. In: Wiesche M, Sauer P, Krimmling J, Krcmar H, editors. Management Digitaler Plattformen. Informationsmanagement und Digitale Transformation. Wiesbaden, Germany. Springer Gabler; Apr 20, 2018:323-330.
- Soehnchen C, Rietz A, Weirauch V, Meister S, Henningsen M. Creating an intercultural user-centric design for a digital sexual health education app for young women in resource-poor regions of Kenya: qualitative self-extended double diamond model for requirements engineering analysis. JMIR Form Res. Nov 03, 2023;7:e50304. [ FREE Full text ] [ CrossRef ] [ Medline ]
- Rau PL, Plocher T, Choong Y-Y. Cross-Cultural Design for IT Products and Services. Boca Raton, FL. CRC Press; Jan 01, 2012.
- Lachner F, Saucken C, Mueller F, Lindemann U. Cross-cultural user experience design helping product designers to consider cultural differences. In: Rau P, editor. Cross-Cultural Design Methods, Practice and Impact (CCD 2015). Cham, Switzerland. Springer; Jan 01, 2015:58-70.
- Laws S, Harper C, Jones N, Marcus R. Research for Development: A Practical Guide. Thousand Oaks, CA. Sage Publications; Jan 01, 2013.
- Heimgärtner R, Mandl T, Malaka R. Zur forschung im bereich der entwicklung interkultureller benutzungsschnittstellen. In: Boll-Westermann S, Maaß S, Malaka R, editors. Mensch & Computer 2013 – Workshopband: 13. Fachübergreifende Konferenz für Interaktive und Kooperative Medien. München, Germany. Oldenbourg Wissenschaftsverlag; 2013:441-450.
- Smith-Jackson T, Resnick M, Johnson K. Cultural Ergonomics: Theory, Methods, and Applications. Boca Raton, FL. CRC Press; 2014.
- Sedlander E, Bingenheimer JB, Lahiri S, Thiongo M, Gichangi P, Munar W, et al. Does the belief that contraceptive use causes infertility actually affect use? Findings from a social network study in Kenya. Stud Fam Plann. Sep 2021;52(3):343-359. [ FREE Full text ] [ CrossRef ] [ Medline ]
- Soehnchen C, Weirauch V, Schmook R, Henningsen M, Meister S. An acceptance analysis of a sexual health education digital tool in resource-poor regions of Kenya: an UTAUT based survey study. BMC Womens Health. Dec 19, 2023;23(1):676-623. [ FREE Full text ] [ CrossRef ] [ Medline ]
- Balsamiq SRL. Balsamiq. San Francisco, CA. Balsamiq Studios, LLC URL: https://balsamiq.com/ [accessed 2024-07-22]
- Barber W, Badre A. Culturability: the merging of culture and usability. 1998. Presented at: 4th Conference on Human Factors and the Web; June 1, 1998:1-10; Atlanta, GA.
- Yin R. Case Study Research and Applications: Design and Methods (6th Ed). Thousand Oaks, CA. SAGE Publications; Jan 01, 2018.
- Runeson P, Höst M. Guidelines for conducting and reporting case study research in software engineering. Empir Software Eng. Dec 19, 2008;14(2):131-164. [ CrossRef ]
- Wohlin C. Case study research in software engineering—it is a case, and it is a study, but is it a case study? Information and Software Technology. May 2021;133:106514. [ CrossRef ]
- Hyzy M, Bond R, Mulvenna M, Bai L, Dix A, Leigh S, et al. System Usability Scale benchmarking for digital health apps: meta-analysis. JMIR Mhealth Uhealth. Aug 18, 2022;10(8):e37290. [ FREE Full text ] [ CrossRef ] [ Medline ]
- Martins AI, Santinha G, Almeida AM, Ribeiro ?, Silva T, Rocha N, et al. Consensus on the terms and procedures for planning and reporting a usability evaluation of health-related digital solutions: delphi study and a resulting checklist. J Med Internet Res. Jun 06, 2023;25:e44326. [ FREE Full text ] [ CrossRef ] [ Medline ]
- Eysenbach G. Improving the quality of Web surveys: the Checklist for Reporting Results of Internet E-Surveys (CHERRIES). J Med Internet Res. Sep 29, 2004;6(3):e34. [ FREE Full text ] [ CrossRef ] [ Medline ]
- Tong A, Sainsbury P, Craig J. Consolidated Criteria for Reporting Qualitative Research (COREQ): a 32-item checklist for interviews and focus groups. Int J Qual Health Care. Dec 2007;19(6):349-357. [ CrossRef ] [ Medline ]
- Krause R, Pernice K. Affinity diagramming for collaboratively sorting UX findings and design ideas. Nielsen Norman Group. Apr 26, 2024. URL: https://www.nngroup.com/articles/affinity-diagram/ [accessed 2024-05-25]
- Brooke J. SUS: a retrospective. Journal of Usability Studies. Feb 2013;8(2):29-40. [ FREE Full text ]
- Bangor A, Kortum P, Miller J. Determining what individual SUS scores mean: adding an adjective rating scale. Journal of Usability Studies. May 01, 2009;4(3):114-123.
- Kuckartz U. Qualitative text analysis: a systematic approach. In: Kaiser G, Presmeg N, editors. Compendium for Early Career Researchers in Mathematics Education. Cham, Switzerland. Springer International Publishing; Jan 01, 2019:181-197.
- Clarissa S. We!Masomo: sexual health education for sub-Saharan Africa. We!Masomo. 2022. URL: https://www.wemasomo.com/ [accessed 2023-12-05]
- clarissasoehnchen. GitHub. URL: https://github.com/clarissasoehnchen [accessed 2024-06-25]
- Gahungu J, Vahdaninia M, Regmi PR. The unmet needs for modern family planning methods among postpartum women in sub-Saharan Africa: a systematic review of the literature. Reprod Health. Feb 10, 2021;18(1):35. [ FREE Full text ] [ CrossRef ] [ Medline ]
- Decker MJ, Harrison S, Price M, Gutmann-Gonzalez A, Yarger J, Tenney R. Educators' perspectives on integrating technology into sexual health education: implementation study. JMIR Hum Factors. Jan 12, 2022;9(1):e31381. [ FREE Full text ] [ CrossRef ] [ Medline ]
- McMahon SA, Musoke DK, Wachinger J, Nakitende A, Amongin J, Nanyiri E, et al. Unintended uses, meanings, and consequences: HIV self-testing among female sex workers in urban Uganda. AIDS Care. Oct 2021;33(10):1278-1285. [ FREE Full text ] [ CrossRef ] [ Medline ]
- Habyarimana F, Ramroop S. Spatial analysis of socio-economic and demographic factors associated with contraceptive use among women of childbearing age in Rwanda. Int J Environ Res Public Health. Oct 27, 2018;15(11):2383. [ FREE Full text ] [ CrossRef ] [ Medline ]
- Ochako R, Temmerman M, Mbondo M, Askew I. Determinants of modern contraceptive use among sexually active men in Kenya. Reprod Health. Apr 27, 2017;14(1):56. [ FREE Full text ] [ CrossRef ] [ Medline ]
- Hessou PHS, Glele-Ahanhanzo Y, Adekpedjou R, Ahouada C, Johnson RC, Boko M, et al. Comparison of the prevalence rates of HIV infection between men who have sex with men (MSM) and men in the general population in sub-Saharan Africa: a systematic review and meta-analysis. BMC Public Health. Dec 04, 2019;19(1):1634. [ FREE Full text ] [ CrossRef ] [ Medline ]
- Norton A, Tappis H. Sexual and reproductive health implementation research in humanitarian contexts: a scoping review. Reprod Health. May 13, 2024;21(1):64. [ FREE Full text ] [ CrossRef ] [ Medline ]
- Corrocher R, Tedesco F, Rabusin P, De Sandre G. Effect of human erythrocyte stromata on complement activation. Br J Haematol. Feb 12, 1975;29(2):235-241. [ CrossRef ]
Abbreviations
Checklist for Reporting Results of Internet E-Surveys |
Consolidated Criteria for Reporting Qualitative Research |
nongovernmental organization |
Sexual and reproductive health |
System Usability Scale |
Edited by A Mavragani; submitted 18.03.24; peer-reviewed by F Tian, P Bandiera-Paiva; comments to author 01.05.24; revised version received 19.05.24; accepted 22.05.24; published 03.07.24.
©Clarissa Soehnchen, Anja Burmann, Maike Henningsen, Sven Meister. Originally published in JMIR Formative Research (https://formative.jmir.org), 03.07.2024.
This is an open-access article distributed under the terms of the Creative Commons Attribution License (https://creativecommons.org/licenses/by/4.0/), which permits unrestricted use, distribution, and reproduction in any medium, provided the original work, first published in JMIR Formative Research, is properly cited. The complete bibliographic information, a link to the original publication on https://formative.jmir.org, as well as this copyright and license information must be included.

COMMENTS
Advantages. 1. In-depth analysis of complex phenomena. Case study design allows researchers to delve deeply into intricate issues and situations. By focusing on a specific instance or event, researchers can uncover nuanced details and layers of understanding that might be missed with other research methods, especially large-scale survey studies.
A case study is one of the most commonly used methodologies of social research. This article attempts to look into the various dimensions of a case study research strategy, the different epistemological strands which determine the particular case study type and approach adopted in the field, discusses the factors which can enhance the effectiveness of a case study research, and the debate ...
Case study research also has methodological limitations. Case study has been criticized for its perceived lack of rigor and/or quality, lack of consensus on design methods, as well as its ...
List of the Advantages of the Case Study Method. 1. It requires an intensive study of a specific unit. Researchers must document verifiable data from direct observations when using the case study method. This work offers information about the input processes that go into the hypothesis under consideration.
Case study method enables a researcher to closely examine the data within a specific context. In most cases, a case study method selects a small geograph ical area or a very li mited number. of ...
The fact is that stories (individual, collective, institutional) have a vital role to play in the world of research. And to play the specific v. general card against case study design suggests a tendency towards forms of research fundamentalism as opposed to any kind of rational and objective take on case study's strengths and limitations.
The term 'case study', John Gerring has suggested, is "a definitional morass… Evidently, researchers have many different things in mind when they talk about case study research" (2006a: 17). It is possible, however, to distil some of the more commonly-agreed principles.
A case study is a research method that involves an in-depth examination and analysis of a particular phenomenon or case, such as an individual, organization, community, event, or situation. It is a qualitative research approach that aims to provide a detailed and comprehensive understanding of the case being studied.
Using case studies as a research strategy depends mainly on the nature of the research question and the researcher's access to the data. ... Understanding the strengths and limitations of case study research can also guide researchers in deciding whether this approach is suitable for their research question. This section outlines some of the ...
The definitions of case study evolved over a period of time. Case study is defined as "a systematic inquiry into an event or a set of related events which aims to describe and explain the phenomenon of interest" (Bromley, 1990).Stoecker defined a case study as an "intensive research in which interpretations are given based on observable concrete interconnections between actual properties ...
Definitions of qualitative case study research. Case study research is an investigation and analysis of a single or collective case, intended to capture the complexity of the object of study (Stake, 1995).Qualitative case study research, as described by Stake (), draws together "naturalistic, holistic, ethnographic, phenomenological, and biographic research methods" in a bricoleur design ...
Case studies are in-depth investigations of a person, group, event, or community. Typically, data is gathered from various sources using several methods (e.g., observations & interviews). The case study research method originated in clinical medicine (the case history, i.e., the patient's personal history). In psychology, case studies are ...
Although case studies have been discussed extensively in the literature, little has been written about the specific steps one may use to conduct case study research effectively (Gagnon, 2010; Hancock & Algozzine, 2016).Baskarada (2014) also emphasized the need to have a succinct guideline that can be practically followed as it is actually tough to execute a case study well in practice.
A case study is a research approach that is used to generate an in-depth, multi-faceted understanding of a complex issue in its real-life context. It is an established research design that is used extensively in a wide variety of disciplines, particularly in the social sciences. A case study can be defined in a variety of ways (Table 5 ), the ...
Tylenol - Disadvantages. The main disadvantage is that the study cannot be recreated, and what happens in one industry, doesn't necessarily resonate in other industries. Case study method is responsible for intensive study of a unit. It is the investigation and exploration of an event thoroughly and deeply.
Abstract. This chapter explores case study as a major approach to research and evaluation. After first noting various contexts in which case studies are commonly used, the chapter focuses on case study research directly Strengths and potential problematic issues are outlined and then key phases of the process.
Definition of case study. Case study method enables a researcher to closely examine the data within a specific context. In most cases, a case study method selects a small geographical area or a very limited number of individuals as the subjects of study. Case studies, in their true essence, explore and investigate contemporary real-life ...
A case study is a type of qualitative research that examines one particular case (or several cases) in-depth. It is often used for exploring a single phenomenon or event, such as a successful marketing campaign, a product, or a service. Case study researchers collect data through a variety of methods, such as interviews, document analysis, and ...
Case Study Limitations. Despite their benefits, case studies do come with a few limitations. Compared to other research methods, case studies are often at a disadvantage in terms of the following: Replicability. In most cases, scientists strive to create experiments that can be repeated by others.
What the Case Study Method Really Teaches. Summary. It's been 100 years since Harvard Business School began using the case study method. Beyond teaching specific subject matter, the case study ...
Even interviews can be conducted over the phone. That means this method is good for formative research that is exploratory in nature, even if it must be completed from a remote location. 6. It is inexpensive. Compared to other methods of research, the case study method is rather inexpensive.
Using the Fraser Valley region (British Columbia, Canada) as a case study, this research experiments with participatory research (PR) methods and tools for supporting long-term food systems planning by examining regional food vulnerabilities and opportunities/needs for building resilience to exogenous shocks. ... Limitations of the research ...
Sai, S. et al. Generative AI for transformative healthcare: a comprehensive study of emerging models, applications, case studies, and limitations. IEEE Access 12 , 31078-31106 (2024). Article ...
In the next step of the study, the research team can use uncertainty analysis to understand the different historical construction methods and materials. The third limitation is related to the scope of the study. ... & Kraniotis, D. (2023). Adaptive reuse of industrial heritage building - comparative life cycle assessment using a case study in ...
July 3 (Reuters) - Patients using Novo Nordisk's (NOVOb.CO) , opens new tab wildly popular weigh-loss drug Wegovy and its similar medicines for type 2 diabetes may be at increased risk for a sight ...
A case study is a research approach that is used to generate an in-depth, multi-faceted understanding of a complex issue in its real-life context. It is an established research design that is used extensively in a wide variety of disciplines, particularly in the social sciences. A case study can be defined in a variety of ways (Table.
The popularity of research has opened some new doors to science and information. For this science to flourish, it requires sound and solid inference, which, in turn, is contingent upon proper sampling procedures. However, it is rare to find empirical studies that trace the use of sampling in research approaches. This review provides a hybrid analysis through quantitative and qualitative phases ...
Using Thomson Reuters data from 2010 to 2018 (pre-mandate to post-mandate) this study aims to re-examine the relationship between CSR and financial performance.,The current study is rooted in the bandwagon-bias effect theory and uses a long-term data (2010-2018) of Indian firms.
Background: Developing a digital educational application focused on sexual health education necessitates a framework that integrates cultural considerations effectively. Drawing from previous research, we identified the problem and essential requirements to incorporate cultural insights into the development of a solution. Objective: This study aims to explore the Solution Room of the self ...
The complication rate remained low, with 0.37% dissection and 1.56% SAH. LIMITATIONS: Limitations of the study include the following: 1) a large number of case reports or small series, 2) a meta-analysis of proportions with no statistical comparison with a control group, and 3) the lack of access to patient-level data.