Thank you for visiting nature.com. You are using a browser version with limited support for CSS. To obtain the best experience, we recommend you use a more up to date browser (or turn off compatibility mode in Internet Explorer). In the meantime, to ensure continued support, we are displaying the site without styles and JavaScript.
- View all journals
- Explore content
- About the journal
- Publish with us
- Sign up for alerts
- Open access
- Published: 08 June 2023

Alcohol consumption and risks of more than 200 diseases in Chinese men
- Pek Kei Im ORCID: orcid.org/0000-0002-2624-9766 1 ,
- Neil Wright ORCID: orcid.org/0000-0002-3946-1870 1 ,
- Ling Yang ORCID: orcid.org/0000-0001-5750-6588 1 , 2 ,
- Ka Hung Chan ORCID: orcid.org/0000-0002-3700-502X 1 , 3 ,
- Yiping Chen ORCID: orcid.org/0000-0002-4973-0296 1 , 2 ,
- Huaidong Du ORCID: orcid.org/0000-0002-9814-0049 1 , 2 ,
- Xiaoming Yang 1 ,
- Daniel Avery ORCID: orcid.org/0000-0002-9823-9575 1 ,
- Shaojie Wang 5 ,
- Canqing Yu ORCID: orcid.org/0000-0002-0019-0014 6 , 7 ,
- Jun Lv 6 , 7 ,
- Robert Clarke ORCID: orcid.org/0000-0002-9802-8241 1 ,
- Junshi Chen 8 ,
- Rory Collins 1 ,
- Robin G. Walters ORCID: orcid.org/0000-0002-9179-0321 1 , 2 ,
- Richard Peto 1 ,
- Liming Li ORCID: orcid.org/0000-0001-5873-7089 6 , 7 na1 ,
- Zhengming Chen ORCID: orcid.org/0000-0001-6423-105X 1 , 2 na1 ,
- Iona Y. Millwood ORCID: orcid.org/0000-0002-0807-0682 1 , 2 na1 &
China Kadoorie Biobank Collaborative Group
Nature Medicine volume 29 , pages 1476–1486 ( 2023 ) Cite this article
31k Accesses
25 Citations
824 Altmetric
Metrics details
- Epidemiology
- Genetics research
- Risk factors
Alcohol consumption accounts for ~3 million annual deaths worldwide, but uncertainty persists about its relationships with many diseases. We investigated the associations of alcohol consumption with 207 diseases in the 12-year China Kadoorie Biobank of >512,000 adults (41% men), including 168,050 genotyped for ALDH2 - rs671 and ADH1B - rs1229984 , with >1.1 million ICD-10 coded hospitalized events. At baseline, 33% of men drank alcohol regularly. Among men, alcohol intake was positively associated with 61 diseases, including 33 not defined by the World Health Organization as alcohol-related, such as cataract ( n = 2,028; hazard ratio 1.21; 95% confidence interval 1.09–1.33, per 280 g per week) and gout ( n = 402; 1.57, 1.33–1.86). Genotype-predicted mean alcohol intake was positively associated with established ( n = 28,564; 1.14, 1.09–1.20) and new alcohol-associated ( n = 16,138; 1.06, 1.01–1.12) diseases, and with specific diseases such as liver cirrhosis ( n = 499; 2.30, 1.58–3.35), stroke ( n = 12,176; 1.38, 1.27–1.49) and gout ( n = 338; 2.33, 1.49–3.62), but not ischemic heart disease ( n = 8,408; 1.04, 0.94–1.14). Among women, 2% drank alcohol resulting in low power to assess associations of self-reported alcohol intake with disease risks, but genetic findings in women suggested the excess male risks were not due to pleiotropic genotypic effects. Among Chinese men, alcohol consumption increased multiple disease risks, highlighting the need to strengthen preventive measures to reduce alcohol intake.
Similar content being viewed by others
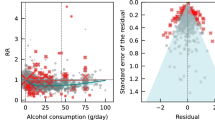
A burden of proof study on alcohol consumption and ischemic heart disease
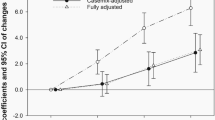
Effect of alcohol consumption on kidney function: population-based cohort study
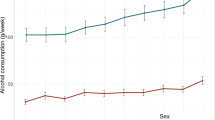
Genomic prediction of alcohol-related morbidity and mortality
Alcohol consumption is a major risk factor for poor physical and mental health, accounting for about 3 million deaths and over 130 million disability-adjusted life years worldwide in 2016 (ref. 1 ). Since the 1990s, alcohol consumption has increased in many low- and middle-income countries, including China, where it almost exclusively involves men 2 , 3 . Among Chinese men, those who reported alcohol consumption in the past 12 months increased from 59% to 85% and yearly per-capita alcohol consumption increased from 7.1 to 11.2 l between 1990 and 2017 and these have been predicted to increase in future years 2 .
Previous epidemiological studies conducted in mainly western populations have provided consistent evidence about the hazards of alcohol drinking for several major diseases, including several types of cancers and cardiovascular diseases (CVDs), liver cirrhosis, infectious diseases (for example tuberculosis and pneumonia) and injuries 4 , 5 , 6 , 7 , 8 , 9 . Large western cohort studies with linkage to hospital records have also investigated the associations of alcohol with risks of several less-common or non-fatal disease outcomes (for example certain site-specific cancers 10 , 11 , 12 , dementia 13 , falls 14 and cataract surgery 15 ). For some (for example stomach cancer), there was suggestive evidence of weak positive associations with heavy drinking 10 , 11 , whereas for others (for example cataract) the limited available evidence has been contradictory 10 , 12 , 13 , 15 ; however, the evidence from western populations, even for diseases known to be associated with alcohol, may not be generalizable to Chinese populations, where the prevalence and types of alcohol drinking (mainly spirits), patterns of diseases (for example high stroke rates) and differences in the ability to metabolize alcohol 8 , 9 , 16 differ markedly from those in western populations 4 , 17 .
For many diseases, including those considered by the World Health Organization (WHO) 4 to be alcohol-related (for example ischemic heart disease (IHD) and diabetes), uncertainty remains about the causal relevance of these associations, which can be assessed in genetic studies using a Mendelian randomization (MR) approach 18 . In such studies, genetic variants can be used as instruments for alcohol consumption to investigate the potential causal relevance of alcohol drinking for diseases, which can limit the biases of confounding and reverse causality common in conventional observational studies 18 . Such studies are particularly informative in East Asian populations where two common genetic variants ( ALDH2 - rs671 and ADH1B - rs1229984 ), which are both rare in western populations, greatly alter alcohol metabolism and strongly affect alcohol intake 19 . Several studies have explored the causal relevance of alcohol consumption with CVD risk factors and morbidity 19 , 20 , 21 , 22 and cancer 16 using these genetic variants, yet findings remain inconclusive for certain diseases (for example IHD) and evidence for other diseases is sparse.
To address these questions, we conducted analyses using observational and genetic approaches to evaluate the associations between alcohol consumption and the risks of a wide range of disease outcomes in the prospective China Kadoorie Biobank (CKB).
Among the 512,724 participants (Supplementary Fig. 1 ), the mean age at baseline was 52 (s.d. 10.7) years, 41% were men and 56% lived in rural areas. Among men, 33% reported drinking alcohol regularly (at least once a week) at baseline (current drinkers), consuming on average 286 g of alcohol per week, mainly from spirits (Supplementary Tables 1 and 2 ). Non-drinkers and ex-drinkers were older and more likely to report poor self-rated health or previous chronic diseases, compared to occasional or current drinkers (Table 1 ). Compared to moderate drinkers (<140 g per week), heavier drinkers were more likely to be rural residents, had received lower education and had more unhealthy lifestyle factors (for example smoking and infrequent fresh fruit intake), higher mean blood pressure and longer duration of drinking (Supplementary Table 3 ). Among male current drinkers, 62% reported drinking daily and 37% engaging in heavy episodic drinking (Supplementary Table 2 ). Among women, only 2% drank alcohol at least weekly (mean intake 116 g per week), but there were similar associations with other baseline characteristics (Table 1 and Supplementary Tables 3 and 4 ) compared to those in men.
During a median of 12.1 (interquartile range 11.1–13.1) years of follow-up, 134,641 men (44,027 drinkers) and 198,430 women (4,420 drinkers) experienced at least one reported hospitalization event or death at age-at-risk 35–84 years, involving a total of 1,111,495 hospitalization episodes. Among men, there were 333,541 (107,857 in current drinkers) recorded events from 207 diseases across 17 International Classification of Diseases Tenth Revision (ICD-10) chapters studied that had at least 80 cases each among current drinkers (Table 2 ), while among women there were 476,986 (11,773) events from 48 diseases across 18 ICD-10 chapters (Supplementary Table 5 ).
Observational associations of alcohol with disease risks
Among men, alcohol drinking was significantly associated with higher risks of 61 disease outcomes from 15 ICD-10 chapters based on two separate analyses, (1) comparing ever-regular versus occasional drinkers and (2) dose–response among current drinkers (Table 2 and Extended Data Fig. 1 ). In each of the analyses in men, there were significant associations of alcohol consumption with 42 diseases (or outcomes), of which 23 were significant in both analyses and the remainder were directionally consistent with one exception (transient cerebral ischemic attacks, ICD-10 code G45) (Fig. 1 ). In further analyses covering all alcohol consumption categories, there were typical U-shaped or J-shaped associations, with excess risks in male ex-drinkers and non-drinkers compared to occasional or moderate drinkers for most of these diseases (Supplementary Table 6 ). Among male ex-drinkers, the overall excess morbidity risks were more considerable for alcohol-associated diseases than for other diseases, but these excess risks were lower with increasing duration after stopping drinking (Extended Data Fig. 2 ).
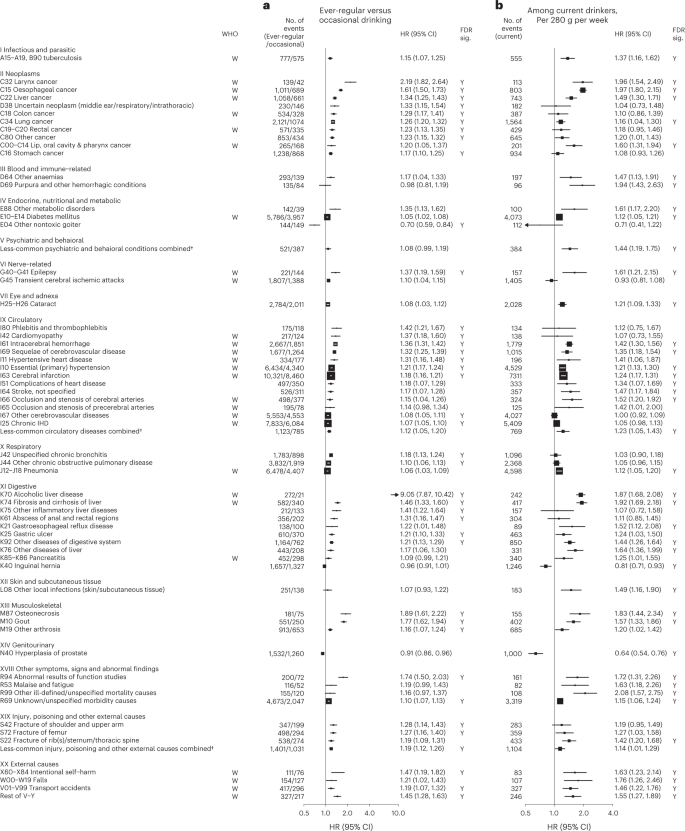
Cox models ( a ) comparing ever-regular drinkers with occasional drinkers or ( b ) assessing the dose–response per 280 g per week higher usual alcohol intake within current drinkers, were stratified by age at risk and study area and were adjusted for education and smoking. Each solid square represents HR with the area inversely proportional to the variance of the log HR. The horizontal lines indicate 95% CIs. Diseases considered to be alcohol-related by the WHO are indicated with ‘W’ under the ‘WHO’ column. The individual diseases listed included all that showed FDR-adjusted significant associations with alcohol (FDR-adjusted P < 0.05, indicated with ‘Y’ under the ‘FDR sig.’ column) and WHO alcohol-related diseases that showed nominally significant associations with alcohol ( P < 0.05). All P values are two-sided. † Included less-common ICD-10 codes within the corresponding ICD-10 chapter that were not individually investigated in the present study. ‘Less-common psychiatric and behavioral conditions’ consisted of ICD-10 codes F00–F99, excluding F32, F33 and F99. ‘Less-common circulatory diseases’ consisted of ICD-10 codes I00–I99, excluding I10, I11, I20, I21, I24, I25, I27, I42, I46, I48–I51, I60–I67, I69, I70, I80 and I83. ‘Less-common injury, poisoning and other external causes’ consisted of ICD-10 codes S00–T98, excluding S06, S09, S22, S32, S42, S52, S62, S72, S82, S92 and T14.
Of the 61 diseases positively associated with alcohol intake in male participants, 28 were considered by the WHO to be alcohol-related diseases, including tuberculosis (A15–A19 and B90), six site-specific cancers including cancers in the larynx (C32), esophagus (C15), liver (C22), colon (C18), rectum (C19 and C20) and lips, oral cavity and pharynx (C00–C14), diabetes (E10–E14), epilepsy (G40 and G41), several hypertensive diseases (I10 and I11) and cerebrovascular diseases (I61, I63, I65, I66, I67, I69 and G45), chronic IHD (I25), cardiomyopathy (I42), pneumonia (J12–J18), alcoholic liver disease (K70) and liver cirrhosis (K74), pancreatitis (K85 and K86) and external causes including self-harm (X60–X84), falls (W00–W19), transport accidents (V01–V99) and other external causes (rest of V–Y) (Fig. 1 and Extended Data Fig. 3 ). Of these 28 diseases, 22 showed significant dose–response associations with alcohol intake. The hazard ratios (HRs) per 280 g per week higher intake for the aggregated WHO alcohol-related diseases were 1.22 (95% confidence interval (CI) 1.19–1.25) (Supplementary Table 7 for detailed outcome classification), ranging from 1.12 (1.05–1.20) for pneumonia to 1.97 (1.80–2.15) for esophageal cancer.
The 33 other diseases showing false discovery rate (FDR)-adjusted significant positive associations with alcohol drinking in men included lung (C34) and stomach (C16) cancers, cataract (H25 and H26), six digestive diseases such as gastroesophageal reflux disease (K21) and gastric ulcer (K25), three musculoskeletal conditions, including gout (M10), three fracture types (S22, S42 and S72), and the aggregates of less-common psychiatric and behavioral conditions and circulatory diseases (Fig. 1 and Extended Data Fig. 4 ). Of these 33 diseases, 22 showed significant dose–response associations, with HRs per 280 g per week higher intake ranging from 1.16 (95% CI 1.04–1.30) for lung cancer to 1.94 (1.43–2.63) for purpura and other hemorrhagic conditions (D69) and 1.20 (1.16–1.24) for the aggregated CKB new alcohol-associated diseases. In contrast, three diseases showed FDR-adjusted significant inverse associations with alcohol drinking (other nontoxic goiter (E04), hyperplasia of prostate (N40) and inguinal hernia (K40)). Overall, for all-cause morbidity, the HR per 280 g per week higher intake was 1.12 (1.10–1.14) in male current drinkers.
Supplementary Figs. 2 – 4 show the dose–response associations for all disease outcomes investigated in male current drinkers. For alcohol-associated diseases and for total morbidity, the dose–response associations were unaltered after additional covariate adjustments or excluding participants with poor baseline health conditions (Supplementary Fig. 5 and Supplementary Table 8 ). Moreover, the associations were similar across various male population subgroups, but seemed to be stronger in younger men, urban residents and higher socioeconomic groups for new alcohol-associated diseases (Supplementary Fig. 6 ).
Among male current drinkers, drinking daily, heavy episodic drinking and drinking spirits were each associated with higher risks for alcohol-related diseases, but most of these associations were attenuated to the null after adjusting for total alcohol intake (Extended Data Fig. 5 ); however, for a given total alcohol intake among male current drinkers, drinking daily was associated with 30–40% higher risks of alcohol-related cancers (1.30, 1.17–1.45) and liver cirrhosis (1.39, 1.13–1.72), compared to non-daily drinking. Similarly, heavy episodic drinking was associated with higher risks of diabetes (1.23, 1.12–1.34) and IHD (1.11, 1.03–1.19), whereas drinking outside of meals was associated with 49% (1.49, 1.19–1.86) higher risk of liver cirrhosis than drinking with meals. The risks of all major alcohol-associated diseases were higher with longer duration of alcohol consumption in men (Extended Data Fig. 6 ).
Among women, due to few reported current drinkers there was a lack of statistical power to detect any associations of self-reported alcohol intake with disease risks (Supplementary Table 5 , Extended Data Fig. 7 and Supplementary Fig. 7 ).
Genetic associations of alcohol with disease risks
A genetic instrument for alcohol intake was derived using ALDH2 - rs671 (G > A) and ADH1B - rs1229984 (G > A) genotypes. The overall A-allele frequency was 0.21 for ALDH2 - rs671 and 0.69 for ADH1B - rs1229984 , with both A-alleles being more common in southern than northern study areas (Supplementary Table 9 ). Both ALDH2 - rs671 and, to a lesser extent, ADH1B - rs1229984 were strongly associated with alcohol drinking in men, but much less so in women (Supplementary Table 10 ). In men, the derived genetic instrument predicted a >60-fold difference (range 4–255 g per week, C1 to C6) in mean alcohol intake, whereas in women mean alcohol intake remained low (<10 g per week) across genetic categories (Supplementary Table 11 ). Both variants and the derived instrument were not associated with smoking or other major self-reported baseline characteristics, except for a small difference in fresh fruit intake by ALDH2 - rs671 genotype in men.
Among men, genotype-predicted mean alcohol intake was positively associated with higher risks of CKB WHO alcohol-related (HR per 280 g per week higher genotype-predicted mean male alcohol intake: 1.14, 95% CI 1.09–1.20) and CKB new alcohol-associated (1.06, 1.01–1.12) diseases (Fig. 2 ), both of which were slightly weaker than the conventional associations. For certain diseases, however, the genetic associations were stronger, with HRs of 1.38 (1.27–1.49) for stroke, 2.30 (1.58–3.35) for liver cirrhosis and 2.33 (1.49–3.62) for gout, in men (Fig. 3 and Extended Data Fig. 8 ). For individual genetic variants, the associations were directionally consistent (Extended Data Figs. 9 and 10 ). Conversely, there were no significant dose–response genotypic associations with IHD, inguinal hernia or hyperplasia of prostate in men. For other alcohol-associated diseases, higher genotype-predicted mean male alcohol intake was significantly associated with higher risks of esophageal cancer, cataract, occlusion and stenosis of cerebral arteries, sequelae of cerebrovascular disease, essential primary hypertension and fractures of ribs, sternum or thoracic spine. There were also suggestive positive genotypic associations with several digestive tract cancer types (liver, colon and stomach) and circulatory and digestive diseases, and significant inverse associations with lung cancer and other chronic obstructive pulmonary disease (J44) in men (Extended Data Figs. 8 – 10 ). Sensitivity analyses using different analytical methods to adjust for confounding by study area, or a two-stage least-squares MR approach, did not alter the main genetic findings in men (Supplementary Table 12 ). In contrast, genotypes that increased alcohol intake in men were not adversely associated with most alcohol-related disease risks among women (for example HR 1.00 (0.97–1.04) for all morbidity among female non-drinkers; Supplementary Fig. 7 and Extended Data Figs. 8 – 10 ).
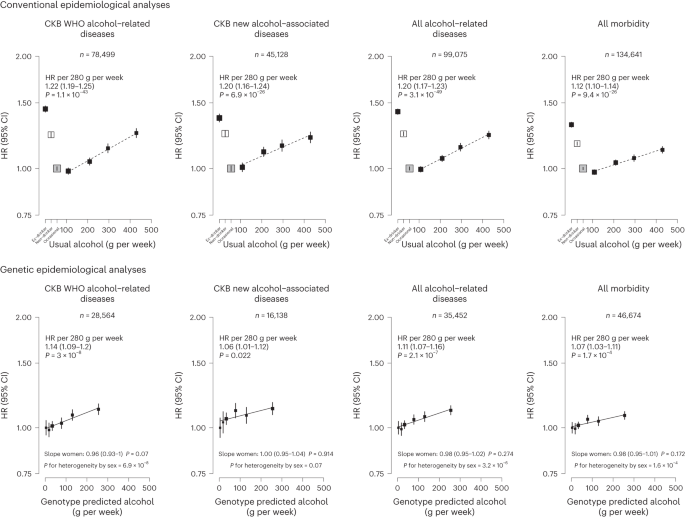
Each box represents HR with the area inversely proportional to the variance of the group-specific log hazard within subplot. The vertical lines indicate group-specific 95% CIs. Conventional epidemiological analyses relate self-reported drinking patterns to risks of diseases (reference group is occasional drinkers), using Cox models stratified by age at risk and study area and adjusted for education and smoking. Within current drinkers, HRs were plotted against usual alcohol intake and were calculated per 280 g per week higher usual alcohol intake. Genetic epidemiological analyses relate genetic categories to risks of diseases (reference group is the genotype group with lowest genotype-predicted mean male alcohol intake), using Cox models stratified by age at risk and study area and adjusted for genomic principal components. The HR per 280 g per week higher genotype-predicted mean male alcohol intake was calculated from the inverse-variance-weighted mean of the slopes of the fitted lines in each study area. The corresponding slopes in women were summarized in text and the slopes of the fitted line by sex were compared and assessed for heterogeneity using chi-squared tests (indicated by P for heterogeneity by sex). All P values are two-sided. Analyses of these aggregated outcomes were based on first recorded event of the aggregate during follow-up and participants may have had multiple events of different types of diseases. ‘All alcohol-related diseases’ includes the first recorded event from ‘CKB WHO alcohol-related diseases’ or ‘CKB new alcohol-associated diseases’ during follow-up.
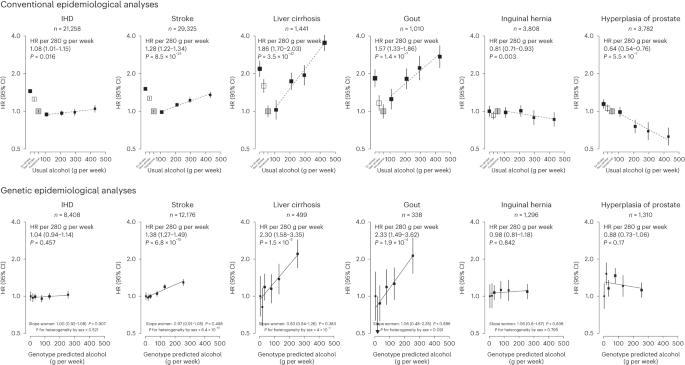
Each box represents HR with the area inversely proportional to the variance of the group-specific log hazard within subplot. The vertical lines indicate group-specific 95% CIs. Conventional epidemiological analyses relate self-reported drinking patterns to risks of diseases (reference group is occasional drinkers), using Cox models stratified by age at risk and study area and adjusted for education and smoking. Within current drinkers, HRs were plotted against usual alcohol intake and were calculated per 280 g per week higher usual alcohol intake. Genetic epidemiological analyses relate genetic categories to risks of diseases (reference group is the genotype group with lowest genotype-predicted mean male alcohol intake), using Cox models stratified by age at risk and study area and adjusted for genomic principal components. The HR per 280 g per week higher genotype-predicted mean male alcohol intake was calculated from the inverse-variance-weighted mean of the slopes of the fitted lines in each study area. The corresponding slopes in women were summarized in text and the slopes of the fitted line by sex were compared and assessed for heterogeneity using chi-squared tests (indicated by P for heterogeneity by sex). All P values are two-sided. Corresponding ICD-10 codes, IHD (I20–I25); stroke (I60, I61, I63 and I64); liver cirrhosis (K70 and K74); gout (M10); inguinal hernia (K40); hyperplasia of prostate (N40).
Hospitalizations associated with alcohol drinking
Among men, ever-regular drinkers had higher numbers of hospitalizations for any causes than occasional drinkers, particularly for cancer hospitalizations, and these differences increased with increasing age at risk, except for CVD hospitalizations (Supplementary Fig. 8 ).
This prospective study provides a comprehensive assessment of the impact of alcohol consumption on a very wide range of disease outcomes in Chinese adults. Among men, alcohol consumption was associated with significantly higher risks of 61 diseases, including 33 not previously reported as alcohol-related diseases by the WHO, and higher risks of hospitalizations for any causes. For a given total amount, drinking daily, heavy episodic drinking and drinking outside of meals exacerbated the risks of four major diseases in Chinese men. Moreover, most of these associations in Chinese men were confirmed in genetic analyses, at least when assessed collectively, and are likely to reflect the effects alcohol consumption itself rather than any pleiotropic effects of the genetic instruments.
Based primarily on observational findings in western populations, alcohol consumption has been considered by the WHO 4 and the Global Burden of Disease (GBD) study 23 to be related to about 20 distinct disease categories, involving chronic diseases and cancers largely in the gastrointestinal system, several CVD types, infectious diseases and injuries. The observational analyses largely confirmed these known associations (Supplementary Table 13 ), but also provided insights into additional hazards of certain drinking patterns suggested by previous studies 8 , 9 , 24 , 25 . Moreover, this study discovered 33 additional alcohol-associated diseases across various body systems in Chinese men that had not been previously reported by the WHO. For these 33 disease outcomes, their associations with alcohol intake were confirmed in genetic analyses, at least collectively as well as for certain specific diseases (for example gout), as was the case for a similar number of WHO alcohol-related diseases. The somewhat smaller relative (but not absolute) risks of alcohol drinking with major diseases at older than younger age in men from observational analyses were consistent with previous studies of other risk factors (for example blood pressure 26 and smoking 27 ), which could be driven by a number of factors such as selection bias 27 and comorbidities.
For certain major WHO alcohol-related diseases, particularly IHD and ischemic stroke, observational studies, including this study, have consistently reported J-shaped associations, with those who drank moderately (for example 1–2 units a day) having the lowest risks 6 , 28 ; however, these apparent protective effects of moderate drinking probably largely reflect residual confounding (for example non-drinkers having worse health and socioeconomic profiles than occasional drinkers) and uncontrolled reverse causation (for example sick-quitter effect where pre-existing poor health or changes in health conditions lead to alcohol cessation), including the difficulty in defining abstainers (for example ex-drinkers may be reported as non-drinkers) as the reference group in many previous studies 3 , 29 . In this study, we used occasional drinkers rather than non-drinkers as the reference group, which, together with separate dose–response analyses among current drinkers, helped to reduce but not eliminate any such biases, which could largely be mitigated in genetic analyses using an MR approach.
To date the existing MR studies for alcohol have focused mainly on CVD types 30 , 31 , 32 and cancers 33 , 34 , 35 , with limited data for other diseases. Moreover, previous studies mainly involved European-ancestry populations and hence were constrained by availability of relatively weak genetic instruments. Using genetic instruments specific to East Asian populations that predicted >60-fold difference in alcohol consumption, we previously reported evidence for the causal relevance and apparent dose–response effects of alcohol consumption on upper-aerodigestive tract cancers 16 and stroke 19 . These findings were further corroborated by subsequent European ancestry-based MR studies 30 , 32 , 36 and the analyses presented in this study with additional follow-up data. In contrast to stroke, we found no reliable genetic evidence for a cardioprotective, nor harmful, effect of moderate drinking on risk of IHD in men, consistent with findings in other MR studies 30 , 32 . The present study also demonstrated a log-linear genetic association of alcohol with liver cirrhosis and suggestive positive associations for several WHO alcohol-related digestive tract cancers in men. Moreover, separate genetic analyses among women suggests that the excess risks observed among men were due chiefly to alcohol per se rather than to potential pleiotropic effects of the alcohol-related genotypes. Further larger genetic studies are required to confirm and elucidate the potential causal relevance for each of the other WHO alcohol-related diseases individually.
For the new alcohol-associated diseases identified in this study, the available prospective epidemiological evidence has been sparse and mostly confined to western populations. For gout, previous western prospective studies have reported positive associations 37 , 38 and an MR study of 8,000 Korean men has also reported positive associations of alcohol consumption with hyperuricemia, a risk factor for gout 39 . The present study provides genetic evidence that alcohol drinking increases the risk of gout. Consistent with the present study, previous European-ancestry-based observational studies 40 , 41 and one MR study 42 also reported positive associations of alcohol intake with risks of several fracture types. The available prospective evidence on associations between alcohol drinking and risk of cataract has been conflicting 15 , 43 and one European-ancestry-based MR study reported no genetic associations 44 . We found a significant dose–response association between alcohol and risk of cataract among Chinese men in observational analyses, which was supported by the present genetic analyses.
For several other diseases (for example gastroesophageal reflux disease and gastric ulcer), the observational findings provide additional evidence to the existing literature 5 , 45 , 46 , 47 , but the supporting genetic evidence is still constrained by limited statistical power. Similarly, our observational findings for lung and stomach cancers were generally consistent with evidence provided by previous prospective studies 7 , 11 , 48 , 49 ; however, the causal relevance of these associations remains to be elucidated in future larger MR studies with appropriate consideration of the potential gene–environment interactions between ALDH2 - rs671 and alcohol intake (the effect of alcohol intake on cancer risks being modified by ALDH2 - rs671 genotype due to excessive acetaldehyde) 16 and other aldehyde exposures 50 in cancer risks, which might similarly affect the genetic associations for respiratory diseases and other potential acetaldehyde-related diseases. In observational analyses, we found significant inverse associations for inguinal hernia, prostate hyperplasia and other nontoxic goiter, but not for several other diseases previously inversely associated with alcohol drinking, including non-Hodgkin lymphoma 48 , kidney cancer 48 , thyroid cancer 48 and gallstones 51 . The genetic analyses, albeit with limited power, did not provide reliable evidence supporting the inverse associations with these outcomes. Future well-powered genetic investigations are warranted for less-common diseases in different populations.
The strengths of this study include the prospective design, large sample size, detailed information on alcohol consumption and drinking patterns, completeness of follow-up and a wide range of morbidity outcomes analyzed. We were also able to assess the potential causal relevance of the associations using two powerful East Asian genetic variants. Moreover, the extremely low drinking prevalence in women (regardless of their genotypes) enabled assessment for potential pleiotropy, further supporting the genetic findings among men.
Nevertheless, the study also has limitations. First, it is still possible that heavy drinking was under-reported, which could have underestimated the hazards of heavy episodic drinking. Second, as in many population-based cohort studies, extreme problematic drinkers and certain alcohol-related disease events may be under-represented, but this should not affect the assessment of the associations of alcohol with most disease outcomes. Third, while the repeated measures of alcohol consumption available in the re-survey subsets allowed us to estimate long-term usual mean alcohol intake at the group level to account for regression dilution bias, we were unable to study the effects of longitudinal alcohol drinking trajectories on health. Fourth, we were unable or underpowered to study diseases that do not normally require hospitalization (for example dementia and depression), nor alcohol-related diseases only affecting women, given the low proportion of female drinkers (for example <70 cases of breast cancer in female drinkers). While the low female drinking prevalence in CKB was consistent with findings in a nationwide survey 52 , it is possible that women may be more likely to under-report drinking than men for cultural and social reasons. Hence our null findings in women should be interpreted with caution and not be taken as a lack of alcohol-related harms in women in general, especially in the context of rising alcohol consumption among Asian women 2 . Fifth, as spirits were the main beverage type and our genetic instrument did not distinguish between beverage types, we were unable to assess beverage-specific effects on disease risks, including wine consumption, which is uncommon in China 17 and has been proposed as potentially cardioprotective due to other non-alcoholic components in red wine 53 . Sixth, although our genetic analyses allowed comparison of the overall genetic effects of negligible, moderate and high mean alcohol intake levels for major and overall morbidities, we had limited power to confidently clarify any small threshold effects in the low consumption end, especially for individual diseases. Finally, the genetic analyses lacked statistical power to assess the associations with several individual alcohol-associated diseases so these findings should still be viewed as hypothesis-generating.
In recent decades, several studies have estimated the alcohol-attributable disease burden, involving predominantly WHO alcohol-related diseases. These estimates were based mainly on observational evidence and included the potentially biased U- or J-shaped associations with IHD and ischemic stroke 1 , 23 , 54 . We have demonstrated in both conventional and genetic analyses that alcohol drinking is associated with hazards in a dose–response manner with a much wider range of disease outcomes than previously considered by the WHO 4 and the GBD study 23 and do not find any evidence for protective effects for IHD or stroke, suggesting that the actual alcohol-attributable disease burden is likely to be much greater than widely believed.
Overall, the present study demonstrated substantial hazards of alcohol consumption with a wide range of disease outcomes among Chinese men. The findings reinforce the need to lower population mean levels of alcohol consumption as a public health priority in China. Future estimation of the alcohol-attributable disease burden worldwide and in specific regions should incorporate new genetic evidence from the present and any future studies about the likely causal relevance of alcohol consumption for a broad range of disease outcomes.
Study population
Details of the CKB study design and methods have been previously reported 55 . Briefly, 512,724 adults aged 30–79 years were recruited from ten geographically diverse (five rural and five urban) areas across China during 2004–2008. At local study assessment clinics, trained health workers administered a laptop-based questionnaire recording sociodemographic factors, lifestyle (for example alcohol drinking, smoking, diet and physical activity) and medical history; undertook physical measurements (for example blood pressure and anthropometry); and collected a blood sample for long-term storage. Two resurveys of ~5% randomly selected surviving participants were subsequently conducted in 2008 and 2013–2014 using similar procedures.
Ethics approval
Ethical approval was obtained from the Ethical Review Committee of the Chinese Centre for Disease Control and Prevention (Beijing, China, 005/2004) and the Oxford Tropical Research Ethics Committee, University of Oxford (UK, 025-04). All participants provided written informed consent.
Assessment of alcohol consumption
Detailed questionnaire assessment of alcohol consumption has been described previously 3 , 17 , 56 . In the baseline questionnaire, participants were asked how often they had drunk alcohol during the past 12 months (never or almost never, occasionally, only at certain seasons, every month but less than weekly or usually at least once a week). Those who had not drunk alcohol at least weekly in the past 12 months were asked whether there was a period of at least a year before that when they had drunk some alcohol at least once a week. Based on their past and current drinking history, participants were classified into: non-drinkers (had never drunk alcohol in the past year and had not drunk in most weeks in the past); ex-drinkers (had not drunk alcohol in most weeks in the past year but had done so in the past); occasional drinkers (had drunk alcohol but less than weekly in the past year and had not drunk alcohol in most weeks in the past); and current drinkers (had drunk alcohol on a weekly basis (regularly) in the past year).
Current drinkers were asked further questions about their drinking patterns, including frequency, beverage type (beer, grape wine, rice wine, weak spirits with <40% alcohol content and strong spirits with ≥40% alcohol content) and amount consumed on a typical drinking day, mealtime drinking habits, age started drinking in most week and their experience of flushing or dizziness after drinking.
Alcohol intake level was estimated based on the reported frequency (taken as the median of the reported frequency intervals; 1.5 for 1–2 d per week, 4 for 3–5 d per week, 6.5 for 6–7 d per week), beverage type and amount consumed, assuming the following alcohol content by volume (v/v) typically seen in China: beer 4%, grape wine 12%, rice wine 15%, weak spirits 38% and strong spirits 53% 57 . Among current drinkers, men were grouped into four consumption categories (<140, 140–279, 280–419 and 420+ g per week) and women into three categories (<70, 70–139 and 140+ g per week), broadly based on the recommended cutoffs for alcohol categories by the WHO 58 and national drinking guidelines. Heavy episodic drinking was defined as consuming >60 g of alcohol on a typical drinking occasion for men and >40 g per occasion for women 58 . Drinking outside of meals was defined as usually drinking between or after meals or having no regular patterns (versus usually drinking with meals). Duration of drinking was derived by the difference in years between age at baseline and age started drinking.
Ex-drinkers were asked how long (in years) ago they had stopped drinking in most weeks. Ex-drinkers were grouped with current drinkers as ‘ever-regular drinkers’.
Follow-up for mortality and morbidity
The vital status of participants was obtained periodically from local death registries, supplemented by annual active confirmation through local residential, health insurance and administrative records. Additional information on morbidity was collected through linkage with disease registries (for cancer, stroke, IHD and diabetes) and the national health insurance system, which record any episodes of hospitalization and almost has universal coverage. All events were coded with ICD-10 codes, blinded to the baseline information. By 1 January 2019, 56,550 (11%) participants had died, 311,338 (61%) were ever hospitalized, but only 4,028 (<1%) were lost to follow-up.
Outcome measures
To enable a ‘phenome-wide’ investigation, all recorded diseases and injuries (referred to as ‘diseases’ for simplicity) coded by three-character ICD-10 codes were reviewed. ICD-10 codes were combined (where appropriate) based on disease characteristics and their potential relationships with alcohol consumption 4 , 8 , 10 , 59 . Disease end points were curated based on diseases considered to be causally impacted by alcohol by the WHO 4 , 59 and major diseases previously shown to be related to alcohol in CKB and other large prospective cohort studies 8 , 10 , while retaining maximal granularity. Diseases with at least 80 cases recorded during follow-up among current drinkers, separately by sex, were analyzed individually to capture a wide range of specific conditions while ensuring reasonable statistical power (around 60–80% power to detect a HR of 2.00 per 280 g per week higher usual alcohol intake at P < 0.01 and P < 0.05, respectively). Within each ICD-10 chapter, diseases with <80 events were grouped into a ‘less-common’ category. Several ICD-10 chapters considered not directly relevant in this population (for example perinatal-origin diseases (chapter XVI) and congenital conditions (XVII); pregnancy-related diseases (XV) in men) were excluded.
Major diseases defined by the WHO as likely to be causally related with alcohol consumption 4 , including several cancers (mouth and throat, esophagus, colon-rectum, liver and female breast), diabetes mellitus, IHD, stroke, liver cirrhosis and external causes, were also selected a priori for detailed analyses of associations with drinking patterns (daily drinking, heavy episodic drinking, mealtime habit, spirit drinking and drinking duration). Similarly, diseases that were significantly and adversely associated with alcohol in the ‘phenome-wide’ investigations (either with ever-regular versus occasional drinking or in dose–response associations with amounts consumed) were further categorized as ‘CKB WHO alcohol-related diseases’ and ‘CKB new alcohol-associated diseases’ respectively for genetic investigation of causality. Detailed outcome classifications are reported in Supplementary Table 7 .
Genotyping and alcohol genetic instruments
The two East Asian genetic variants ( ALDH2 - rs671 and ADH1B - rs1229984 ) were genotyped in 168,050 participants (151,347 randomly selected, 16,703 selected as part of nested case–control studies of CVD and chronic obstructive pulmonary disease, which were only included in analyses of relevant outcomes; Supplementary Fig. 1 ) using Affymetrix Axiom ( n = 100,396) or custom Illumina GoldenGate ( n = 93,125) arrays at BGI (Shenzhen, China), with some overlap between them. Among 25,471 participants genotyped with both arrays, the concordance was >99.9% for both variants. Where discordant, genotypes obtained from the Affymetrix Axiom array were used.
The genetic instrument for alcohol was derived from ALDH2 - rs671 and ADH1B - rs1229984 and ten study areas from the random genotyped subset of male participants to avoid potential selection bias, using a previously developed method in CKB 19 . Briefly, nine genotype combinations were defined based on the genotypes for each of the two variants (each AA, AG or GG). As alcohol use varies greatly by study area, among men, mean alcohol intake was calculated for each of these nine genotype across ten study areas (that is a total of 90 genotype-area combinations) to reflect a wide range of alcohol consumption, assigning an intake of 5 g per week to occasional drinkers and excluding ex-drinkers from the calculation. Ex-drinkers were excluded from the calculation of mean alcohol intake as their baseline intake did not reflect their long-term intake; nevertheless, they were included in subsequent genetic analyses once they had been assigned a genetic group. These 90 combinations were then grouped into six categories (C1–C6) according to their corresponding mean intake values, at cutoff points of 10, 25, 50, 100 and 150 g per week, selected to facilitate investigation of the causal effects of alcohol across a wide range of mean alcohol intakes while allowing adequate sample size in each category for reliable comparisons. In this way participants (including ex-drinkers) were classified only based on their genotypes and study area, but not on individual self-reported drinking patterns. Comparisons of these six genetic categories can, where analyses are stratified by area, be used to estimate the genotypic effects on disease risks.
To facilitate the comparison of genotypic effects between sexes (pleiotropic effects), women were classified into the same six categories as men based on their genotypes and study area, regardless of female alcohol intake. This allowed comparison of genotypic effects between men (where genotype were strongly associated with alcohol intake) and women (where alcohol intake was low in all genotypic categories) (Supplementary Tables 10 and 11 ).
Statistical analysis
Given the extremely low alcohol use among women 3 , 17 , the analyses were conducted separately by sex but focused chiefly on men. All CKB participants and the genotyped subset with genomic principal components (PCs; derived from genome-wide genotyping array data and were informative for CKB population structure) 60 were included in conventional and genetic analyses, respectively (Supplementary Fig. 1 ). Means and percentages of baseline characteristics were calculated by self-reported alcohol consumption patterns and by genotype categories, adjusted for age (in 10-year intervals), ten study areas and (for genetic analysis) genomic PCs 60 to control for differences in genetic distribution due to population stratification, as appropriate.
For conventional observational analyses, Cox proportional hazard models were used to estimate HRs for individual diseases associated with different alcohol consumption categories (in three broad categories: occasional drinkers, ever-regular drinkers, non-drinkers; and in 6–7 detailed categories: occasional drinkers, ex-drinkers, non-drinkers, 3–4 further current drinker groups defined by alcohol intake level) and among current drinkers with continuous levels of alcohol intake (per 280 g per week in men, per 100 g per week in women) or with categories of alcohol intake (<140, 140–279, 280–419 and 420+ g per week in men; <70, 70–139 and 140+ g per week in women). The Cox models were stratified by age at risk (5-year groups between 35–84 years) and ten areas and adjusted for education (four groups: no formal school, primary school, middle or high school and technical school/college or above) and smoking status (six groups in men: never, occasional, ex-regular, current <15, current 15–24, current ≥25 cigarettes equivalent per day; four groups in women: never, occasional, ex-regular and current). Smoking data have been previously validated against exhaled carbon monoxide 61 . Competing risks from all-cause mortality for disease events were handled by censoring participants at death from any cause to estimate cause-specific HRs comparing event rates in participants who were alive and free of the disease of interest 62 . To reduce biases from residual confounding and uncontrolled reverse causation related to the choice of using non-drinkers (for example sick-quitter effect, pre-existing poor health or social disadvantages leading to alcohol cessation or abstinence) as the reference group 3 , 29 , we used occasional drinkers as the reference group, together with separate dose–response analyses among current drinkers. To account for within-person variation of alcohol intake over the follow-up period, repeat alcohol measures for participants who attended the two resurveys were used to estimate usual alcohol intake (Supplementary Table 1 ) and correct for regression dilution bias 9 , 63 . The shapes of dose–response associations between alcohol and disease risks were assessed among current drinkers by plotting the HRs of predefined baseline consumption categories against the corresponding mean usual alcohol intake. Log HR estimates and the corresponding standard errors for baseline alcohol intake, modeled as a continuous variable, were divided by the regression dilution ratio (0.53 for both men and women; calculated using the McMahon–Peto method 64 ) to obtain estimated HRs per 280 g per week higher usual alcohol intake among male current drinkers and HRs per 100 g per week among female current drinkers. For analyses involving drinking patterns, additional adjustments were conducted for total alcohol intake (continuous) and baseline age (continuous; for drinking duration analysis) where appropriate.
Sensitivity analyses were performed by (1) additional adjustments for further covariates (household income (<10,000, 10,000–19,999, 20,000–34,999 and ≥35,000 yuan per year), fresh fruit intake (4–7 d per week and ≤3 d per week), physical activity (continuous, in metabolic equivalent of task per hour per day), body mass index (<22, 22–24.9, 25–26.9 and ≥27 kg m 2 ); and (2) excluding individuals with poor self-reported health or previous major chronic diseases (including self-reported coronary heart diseases, stroke, transient ischemic attack, tuberculosis, emphysema or bronchitis, liver cirrhosis or chronic hepatitis, peptic ulcer, gallstone or gallbladder disease, kidney disease, rheumatoid arthritis, cancer and diabetes) at baseline. For all aggregated end points (for example CKB WHO alcohol-related, CKB new alcohol-associated and all morbidity), subgroup analyses were conducted by baseline age (<55, 55–64 and ≥65 years), area (urban and rural), education (primary school or below, middle school, high school or above), household income (<10,000, 10,000–19,999 and 20,000+ yuan per year) and smoking status (ever-regular and never-regular), with heterogeneity or trend assessed by chi-squared tests 65 . HRs for diseases associated with years of stopping among ex-drinkers compared to occasional drinkers were also estimated.
In genetic analyses, Cox regression, stratified by age at risk and study area and adjusted for 11 genomic PCs 60 , were used to estimate HRs for major alcohol-related diseases associated with the six genetic categories (C1–C6). Log HRs were plotted against the genotype-predicted mean male alcohol intake in the six categories. To control for potential confounding by population structure, similar analyses were repeated within each study area using age-at-risk-stratified and genomic PC-adjusted Cox models. A line of best fit was fitted through the log HRs against genotype-predicted mean male alcohol intake in the genetic categories present in the corresponding study area, using meta-regression. These within-area slopes (each reflecting purely genotypic effects) were combined by inverse-variance-weighted meta-analysis to yield the overall area-stratified genotypic associations, which controlled for any potential bias resulted from variations due to population structure, summarized as HR per 280 g per week higher genotype-predicted mean male alcohol intake. For total morbidity and aggregated alcohol-associated outcomes, sensitivity analyses were performed by (1) using age-at-risk- and area-stratified and genomic PC-adjusted Cox models to estimate HR per 280 g per week (area-adjusted genotypic associations); and (2) using a two-stage least-squares approach 66 .
Genotypic analyses in women were conducted not to assess the health effects of alcohol in women, but to investigate the extent to which the genotypes studied in men had pleiotropic effects (genotypic effects not mediated by drinking patterns). As few women consumed alcohol, any genotypic effects of the six genetic categories that are mediated by drinking alcohol should be much smaller in women than in men, but any other pleiotropic genotypic effects should be similar in both sexes. Hence, among women, we used the same genetic categories as in men and related the genotypic effects in women to the mean male alcohol intake in these six categories, which allows comparisons of genetic findings by sex and assessment of potential pleiotropy. To further remove the small genotypic effects on alcohol use in women (Supplementary Tables 10 and 11 ), we restricted the genetic analyses to female non-drinkers in sensitivity analyses.
The genotypic associations of individual genetic variants ( rs671 , rs1229984 ; GG versus AG genotype) with alcohol-related disease risks were also assessed using a similar area-stratified approach.
The proportional hazards assumption was tested using scaled Schoenfeld residuals for the pre-specified major diseases (no clear evidence of violation was found). For analyses involving more than two exposure categories, the floating absolute risks were used to estimate group-specific 95% CIs for all categories including the reference group 9 , 19 , 67 . All P values were two-sided. Statistical significance (at the 5% level) was evaluated using both FDR-adjusted P values applied within ICD-10 chapters to correct for multiple testing in the ‘phenome-wide’ investigation 68 , 69 , 70 and conventional P values for hypothesis testing for observational analyses of WHO alcohol-related diseases, analyses of drinking patterns and genetic analyses.
To assess the cumulative burden of alcohol consumption, the total number of hospitalizations were estimated for ever-regular versus occasional drinkers using the mean cumulative count, which does not assume independence between hospitalizations and all-cause mortality 71 , 72 , 73 . All analyses used R software (v.4.0.5).
Ethics and inclusion statement
In accordance with the Nature Portfolio journals’ editorial policies, the research has included local researchers from China throughout the research process, including study design, study implementation, data ownership and authorship. The roles and responsibilities were agreed among collaborators ahead of the research and capacity-building plans, including data collection and study implementation skills for local researchers, were discussed and delivered. This research is locally relevant to the studied country and included local collaborative partners in all aspects of the study, thus, will provide local and regional organizations with epidemiological evidence on the health impacts of alcohol consumption to inform public health policies.
This research was not restricted nor prohibited in the setting of the researchers. The study was approved by local ethics review committee. The research raised no risks related to stigmatization, incrimination, discrimination, animal welfare, the environment, health, safety, security or other personal or biorisks. No biological materials, cultural artifacts or associated traditional knowledge has been transferred out of the country. In preparing the manuscript, the authors have reviewed and cited local and regional relevant studies.
Reporting summary
Further information on research design is available in the Nature Portfolio Reporting Summary linked to this article.
Data availability
The CKB is a global resource for the investigation of lifestyle, environmental, blood biochemical and genetic factors as determinants of common diseases. The CKB study group is committed to making the cohort data available to the scientific community in China, the United Kingdom and worldwide to advance knowledge about the causes, prevention and treatment of disease. For detailed information on what data are currently available to open access users, how to apply for them and the timeline for data access (12–16 weeks), please visit the CKB website: https://www.ckbiobank.org/data-access . Researchers who are interested in obtaining the raw data from the CKB study that underlines this paper should contact [email protected]. A research proposal will be requested to ensure that any analysis is performed by bona fide researchers and, where data are not currently available to open access researchers, is restricted to the topic covered in this paper. Further information is available from the corresponding authors upon request.
Code availability
The codes used for the data analyses in this study can be made available by contacting the corresponding authors. Access to codes will be granted for requests for academic use within 4 weeks of application.
Shield, K. et al. National, regional, and global burdens of disease from 2000 to 2016 attributable to alcohol use: a comparative risk assessment study. Lancet Public Health 5 , e51–e61 (2020).
PubMed Google Scholar
Manthey, J., Shield, K. D., Rylett, M., Hasan, O. S. M., Probst, C. & Rehm, J. Global alcohol exposure between 1990 and 2017 and forecasts until 2030: a modelling study. Lancet 393 , 2493–2502 (2019).
Im, P. K. et al. Patterns and trends of alcohol consumption in rural and urban areas of China: findings from the China Kadoorie Biobank. BMC Public Health 19 , 217 (2019).
PubMed PubMed Central Google Scholar
World Health Organization. Global Status Report on Alcohol and Health 2018 (World Health Organization, 2018).
Corrao, G., Bagnardi, V., Zambon, A., La & Vecchia, C. A meta-analysis of alcohol consumption and the risk of 15 diseases. Prev. Med. 38 , 613–619 (2004).
Ferrari, P. et al. Lifetime alcohol use and overall and cause-specific mortality in the European Prospective Investigation into Cancer and nutrition (EPIC) study. BMJ Open 4 , e005245 (2014).
Yang, L. et al. Alcohol drinking and overall and cause-specific mortality in China: nationally representative prospective study of 220,000 men with 15 years of follow-up. Int. J. Epidemiol. 41 , 1101–1113 (2012).
Im, P. K. et al. Alcohol drinking and risks of total and site-specific cancers in China: a 10-year prospective study of 0.5 million adults. Int J. Cancer 149 , 522–534 (2021).
CAS PubMed PubMed Central Google Scholar
Im, P. K. et al. Alcohol drinking and risks of liver cancer and non-neoplastic chronic liver diseases in China: a 10-year prospective study of 0.5 million adults. BMC Med. 19 , 216 (2021).
Allen, N. E. et al. Moderate alcohol intake and cancer incidence in women. J. Natl Cancer Inst. 101 , 296–305 (2009).
Jayasekara, H. et al. Lifetime alcohol intake, drinking patterns over time and risk of stomach cancer: a pooled analysis of data from two prospective cohort studies. Int J. Cancer 148 , 2759–2773 (2021).
Botteri, E. et al. Alcohol consumption and risk of urothelial cell bladder cancer in the European prospective investigation into cancer and nutrition cohort. Int J. Cancer 141 , 1963–1970 (2017).
CAS PubMed Google Scholar
Sabia, S. et al. Alcohol consumption and risk of dementia: 23 year follow-up of Whitehall II cohort study. BMJ 362 , k2927 (2018).
Tan, G. J. al. The relationship between alcohol intake and falls hospitalization: results from the EPIC-Norfolk. Geriatr. Gerontol. Int. https://doi.org/10.1111/ggi.14219 (2021).
Chua, S. Y. L. et al. Alcohol consumption and incident cataract surgery in two large UK cohorts. Ophthalmology https://doi.org/10.1016/j.ophtha.2021.02.007 (2021).
Im, P. K. et al. Alcohol metabolism genes and risks of site-specific cancers in Chinese adults: an 11-year prospective study. Int. J. Cancer 150 , 1627–1639 (2022).
Millwood, I. Y. et al. Alcohol consumption in 0.5 million people from 10 diverse regions of China: prevalence, patterns and socio-demographic and health-related correlates. Int. J. Epidemiol. 42 , 816–827 (2013).
Davey Smith, G. & Hemani, G. Mendelian randomization: genetic anchors for causal inference in epidemiological studies. Hum. Mol. Genet. 23 , R89–R98 (2014).
Millwood, I. Y. et al. Conventional and genetic evidence on alcohol and vascular disease aetiology: a prospective study of 500 000 men and women in China. Lancet 393 , 1831–1842 (2019).
Au Yeung, S. L. et al. Moderate alcohol use and cardiovascular disease from Mendelian randomization. PLoS ONE 8 , e68054 (2013).
Shin, M. J., Cho, Y., Davey & Smith, G. Alcohol consumption, aldehyde dehydrogenase 2 gene polymorphisms, and cardiovascular health in Korea. Yonsei Med J. 58 , 689–696 (2017).
Taylor, A. E. et al. Exploring causal associations of alcohol with cardiovascular and metabolic risk factors in a Chinese population using Mendelian randomization analysis. Sci. Rep. 5 , 14005 (2015).
Griswold, M. G. et al. Alcohol use and burden for 195 countries and territories, 1990-2016: a systematic analysis for the Global Burden of Disease Study 2016. Lancet 392 , 1015–1035 (2018).
Google Scholar
Simpson, R. F. et al. Alcohol drinking patterns and liver cirrhosis risk: analysis of the prospective UK Million Women Study. Lancet Public Health 4 , e41–e48 (2019).
Roerecke, M. & Rehm, J. Alcohol consumption, drinking patterns, and ischemic heart disease: a narrative review of meta-analyses and a systematic review and meta-analysis of the impact of heavy drinking occasions on risk for moderate drinkers. BMC Med. 12 , 182 (2014).
Lacey, B. et al. Age-specific association between blood pressure and vascular and non-vascular chronic diseases in 0.5 million adults in China: a prospective cohort study. Lancet Glob. Health 6 , e641–e649 (2018).
Hernán, M. A., Alonso, A. & Logroscino, G. Cigarette smoking and dementia: potential selection bias in the elderly. Epidemiology 19 , 448–450 (2008).
Rehm, J., Rovira, P., Llamosas-Falcón, L. & Shield, K. D. Dose-response relationships between levels of alcohol use and risks of mortality or disease, for all people, by age, sex, and specific risk factors. Nutrients 13 , 2652 (2021).
Rehm, J., Irving, H., Ye, Y., Kerr, W. C., Bond, J. & Greenfield, T. K. Are lifetime abstainers the best control group in alcohol epidemiology? On the stability and validity of reported lifetime abstention. Am. J. Epidemiol. 168 , 866–871 (2008).
Lankester, J., Zanetti, D., Ingelsson, E. & Assimes, T. L. Alcohol use and cardiometabolic risk in the UK Biobank: a Mendelian randomization study. PLoS ONE 16 , e0255801 (2021).
Rosoff, D. B., Davey Smith, G., Mehta, N., Clarke, T.-K. & Lohoff, F. W. Evaluating the relationship between alcohol consumption, tobacco use, and cardiovascular disease: a multivariable Mendelian randomization study. PLoS Med. 17 , e1003410 (2020).
Larsson, S. C., Burgess, S., Mason, A. M. & Michaelsson, K. Alcohol consumption and cardiovascular disease: a Mendelian randomization study. Circ. Genom. Precis. Med. 13 , e002814 (2020).
Larsson, S. C. et al. Smoking, alcohol consumption, and cancer: a mendelian randomisation study in UK Biobank and international genetic consortia participants. PLoS Med. 17 , e1003178 (2020).
Zhou, X. et al. Alcohol consumption, DNA methylation and colorectal cancer risk: results from pooled cohort studies and Mendelian randomization analysis. Int. J. Cancer https://doi.org/10.1002/ijc.33945 (2022).
Zhou, X. et al. Alcohol consumption, blood DNA methylation and breast cancer: a Mendelian randomisation study. Eur. J. Epidemiol. 37 , 701–712 (2022).
Biddinger, K. J. et al. Association of habitual alcohol intake with risk of cardiovascular disease. JAMA Netw. Open 5 , e223849 (2022).
Wang, M., Jiang, X., Wu, W. & Zhang, D. A meta-analysis of alcohol consumption and the risk of gout. Clin. Rheumatol. 32 , 1641–1648 (2013).
Neogi, T., Chen, C., Niu, J., Chaisson, C., Hunter, D. J. & Zhang, Y. Alcohol quantity and type on risk of recurrent gout attacks: an internet-based case-crossover study. Am. J. Med 127 , 311–318 (2014).
Jee, Y. H., Jung, K. J., Park, Y. B., Spiller, W. & Jee, S. H. Causal effect of alcohol consumption on hyperuricemia using a Mendelian randomization design. Int J. Rheum. Dis. 22 , 1912–1919 (2019).
Berg, K. M. et al. Association between alcohol consumption and both osteoporotic fracture and bone density. Am. J. Med. 121 , 406–418 (2008).
Søgaard, A. J. et al. The association between alcohol consumption and risk of hip fracture differs by age and gender in Cohort of Norway: a NOREPOS study. Osteoporos. Int. 29 , 2457–2467 (2018).
Yuan, S., Michaëlsson, K., Wan, Z. & Larsson, S. C. Associations of smoking and alcohol and coffee intake with fracture and bone mineral density: a Mendelian randomization study. Calcif. Tissue Int. 105 , 582–588 (2019).
Gong, Y., Feng, K., Yan, N., Xu, Y. & Pan, C.-W. Different amounts of alcohol consumption and cataract: a meta-analysis. Optom. Vis. Sci. 92 , 471–479 (2015).
Yuan, S., Wolk, A. & Larsson, S. C. Metabolic and lifestyle factors in relation to senile cataract: a Mendelian randomization study. Sci. Rep. 12 , 409 (2022).
Pan, J., Cen, L., Chen, W., Yu, C., Li, Y. & Shen, Z. Alcohol consumption and the risk of gastroesophageal reflux disease: a systematic review and meta-analysis. Alcohol Alcohol. 54 , 62–69 (2019).
Strate, L. L., Singh, P., Boylan, M. R., Piawah, S., Cao, Y. & Chan, A. T. A prospective study of alcohol consumption and smoking and the risk of major gastrointestinal bleeding in men. PLoS ONE 11 , e0165278 (2016).
Yuan, S. & Larsson, S. C. Adiposity, diabetes, lifestyle factors and risk of gastroesophageal reflux disease: a Mendelian randomization study. Eur. J. Epidemiol. 37 , 747–754 (2022).
Bagnardi, V. et al. Alcohol consumption and site-specific cancer risk: a comprehensive dose–response meta-analysis. Br. J. Cancer 112 , 580–593 (2015).
Shen, C., Schooling, C. M., Chan, W. M., Xu, L., Lee, S. Y. & Lam, T. H. Alcohol intake and death from cancer in a prospective Chinese elderly cohort study in Hong Kong. J. Epidemiol. Community Health 67 , 813–820 (2013).
Kuroda, A. et al. Effects of the common polymorphism in the human aldehyde dehydrogenase 2 (ALDH2) gene on the lung. Respir. Res. 18 , 69 (2017).
Cha, B. H., Jang, M. J. & Lee, S. H. Alcohol consumption can reduce the risk of gallstone disease: a systematic review with a dose–response meta-analysis of case–control and cohort studies. Gut Liver 13 , 114–131 (2019).
Ma, G. S., Xhu, D. H., Hu, X. Q., Luan, D. C., Kong, L. Z. & Yang, X. Q. The drinking practice of people in China. Acta Nutrimenta Sin. 27 , 362–365 (2005).
Arranz, S., Chiva-Blanch, G., Valderas-Martínez, P., Medina-Remón, A., Lamuela-Raventós, R. M. & Estruch, R. Wine, beer, alcohol and polyphenols on cardiovascular disease and cancer. Nutrients 4 , 759–781 (2012).
Bryazka, D. et al. Population-level risks of alcohol consumption by amount, geography, age, sex, and year: a systematic analysis for the Global Burden of Disease Study 2020. Lancet 400 , 185–235 (2022).
Chen, Z. et al. China Kadoorie Biobank of 0.5 million people: survey methods, baseline characteristics and long-term follow-up. Int. J. Epidemiol. 40 , 1652–1666 (2011).
Im, P. K. et al. Problem drinking, wellbeing and mortality risk in Chinese men: findings from the China Kadoorie Biobank. Addiction 115 , 850–862 (2020).
Cochrane, J., Chen, H., Conigrave, K. M. & Hao, W. Alcohol use in China. Alcohol Alcohol. 38 , 537–542 (2003).
World Health Organization. International Guide for Monitoring Alcohol Consumption and Related Harm (World Health Organization, 2000).
Rehm, J. et al. The relationship between different dimensions of alcohol use and the burden of disease-an update. Addiction 112 , 968–1001 (2017).
Walters, R. G. et al. Genotyping and population structure of the China Kadoorie Biobank. Preprint at medRxiv https://doi.org/10.1101/2022.05.02.22274487 (2022).
Zhang, Q. et al. Exhaled carbon monoxide and its associations with smoking, indoor household air pollution and chronic respiratory diseases among 512,000 Chinese adults. Int. J. Epidemiol. 42 , 1464–1475 (2013).
Lau, B., Cole, S. R. & Gange, S. J. Competing risk regression models for epidemiologic data. Am. J. Epidemiol. 170 , 244–256 (2009).
Clarke, R., Shipley, M. & Lewington, S. et al. Underestimation of risk associations due to regression dilution in long-term follow-up of prospective studies. Am. J. Epidemiol. 150 , 341–353 (1999).
MacMahon, S., Peto, R. & Cutler, J. et al. Blood pressure, stroke, and coronary heart disease. Part 1, prolonged differences in blood pressure: prospective observational studies corrected for the regression dilution bias. Lancet 335 , 765–774 (1990).
Early Breast Cancer Trialists’ Collaborative Group. Section 5: Statistical Methods. Treatment of Early Breast Cancer. Worldwide Evidence, 1985–1990 (Oxford University Press, 1990).
Burgess, S. et al. Guidelines for performing Mendelian randomization investigations. Wellcome Open Res. 4 , 186 (2019).
Easton, D. F., Peto, J. & Babiker, A. G. Floating absolute risk: an alternative to relative risk in survival and case-control analysis avoiding an arbitrary reference group. Stat. Med. 10 , 1025–1035 (1991).
Benjamini, Y. & Hochberg, Y. Controlling the false discovery rate: a practical and powerful approach to multiple testing. J. R. Stat. Soc. B 57 , 289–300 (1995).
Braun, J. M., Kalloo, G., Kingsley, S. L. & Li, N. Using phenome-wide association studies to examine the effect of environmental exposures on human health. Environ. Int. 130 , 104877 (2019).
Glickman, M. E., Rao, S. R. & Schultz, M. R. False discovery rate control is a recommended alternative to Bonferroni-type adjustments in health studies. J. Clin. Epidemiol. 67 , 850–857 (2014).
Cook, R. J. & Lawless, J. F. Marginal analysis of recurrent events and a terminating event. Stat. Med. 16 , 911–924 (1997).
Dong, H., Robison, L. L., Leisenring, W. M., Martin, L. J., Armstrong, G. T. & Yasui, Y. Estimating the burden of recurrent events in the presence of competing risks: the method of mean cumulative count. Am. J. Epidemiol. 181 , 532–540 (2015).
Ghosh, D. & Lin, D. Y. Nonparametric analysis of recurrent events and death. Biometrics 56 , 554–562 (2000).
Download references
Acknowledgements
The chief acknowledgment is to the participants, the project staff and the China National Centre for Disease Control and Prevention (CDC) and its regional offices for assisting with the fieldwork. We thank J. Mackay in Hong Kong; Y. Wang, G. Yang, Z. Qiang, L. Feng, M. Zhou, W. Zhao. and Y. Zhang in China CDC; L. Kong, X. Yu and K. Li in the Chinese Ministry of Health; and S. Clark, M. Radley and M. Hill in the CTSU, Oxford, for assisting with the design, planning, organization and conduct of the study. A complete list of members of the China Kadoorie Collaborative Group is provided in the Supplementary Information. The CKB baseline survey and the first re-survey were supported by the Kadoorie Charitable Foundation in Hong Kong. The long-term follow-up of the CKB study has been supported by Wellcome grants to Z.C. at Oxford University (212946/Z/18/Z, 202922/Z/16/Z, 104085/Z/14/Z, 088158/Z/09/Z) and grants to L.L. from the National Natural Science Foundation of China (82192901, 82192904 and 82192900) and from the National Key Research and Development Program of China (2016YFC0900500). DNA extraction and genotyping was supported by grants to Z.C. from GlaxoSmithKline and the UK Medical Research Council (MC-PC-13049, MC-PC-14135). The UK Medical Research Council (MC_UU_00017/1, MC_UU_12026/2, MC_U137686851), Cancer Research UK (C16077/A29186; C500/A16896) and the British Heart Foundation (CH/1996001/9454) provide core funding to the CTSU and Epidemiological Studies Unit at Oxford University for the project. P.K.I. is supported by an Early Career Research Fellowship from the Nuffield Department of Population Health, University of Oxford. K.H.C. acknowledges support from the British Heart Foundation Centre of Research Excellence, University of Oxford (RE/18/3/34214). The funders had no role in study design, data collection and analysis, decision to publish or preparation of the manuscript. For the purpose of Open Access, the author has applied a CC-BY public copyright license to any author accepted manuscript version arising from this submission.
Author information
These authors jointly supervised this work: Liming Li, Zhengming Chen, Iona Y. Millwood.
Authors and Affiliations
Clinical Trial Service Unit and Epidemiological Studies Unit (CTSU), Nuffield Department of Population Health, University of Oxford, Oxford, UK
Pek Kei Im, Neil Wright, Ling Yang, Ka Hung Chan, Yiping Chen, Huaidong Du, Xiaoming Yang, Daniel Avery, Robert Clarke, Rory Collins, Robin G. Walters, Richard Peto, Zhengming Chen, Iona Y. Millwood, Maxim Barnard, Derrick Bennett, Ruth Boxall, Johnathan Clarke, Ahmed Edris Mohamed, Hannah Fry, Simon Gilbert, Andri Iona, Maria Kakkoura, Christiana Kartsonaki, Hubert Lam, Kuang Lin, James Liu, Mohsen Mazidi, Sam Morris, Qunhua Nie, Alfred Pozarickij, Paul Ryder, Saredo Said, Dan Schmidt, Becky Stevens, Iain Turnbull, Baihan Wang, Lin Wang & Pang Yao
Medical Research Council Population Health Research Unit (MRC PHRU), Nuffield Department of Population Health, University of Oxford, Oxford, UK
Ling Yang, Yiping Chen, Huaidong Du, Robin G. Walters, Zhengming Chen, Iona Y. Millwood, Derrick Bennett, Ruth Boxall & Christiana Kartsonaki
Oxford British Heart Foundation Centre of Research Excellence, University of Oxford, Oxford, UK
Ka Hung Chan
Fuwai Hospital, Chinese Academy of Medical Sciences, Beijing, China
NCD Prevention and Control Department, Qingdao CDC, Qingdao, China
Shaojie Wang, Liang Cheng, Ranran Du, Ruqin Gao, Feifei Li, Shanpeng Li, Yongmei Liu, Feng Ning, Zengchang Pang, Xiaohui Sun, Xiaocao Tian, Yaoming Zhai & Hua Zhang
Department of Epidemiology and Biostatistics, School of Public Health, Peking University, Beijing, China
Canqing Yu, Jun Lv, Liming Li & Dianjianyi Sun
Peking University Center for Public Health and Epidemic Preparedness & Response, Beijing, China
Canqing Yu, Jun Lv, Liming Li, Xiao Han, Can Hou, Qingmei Xia, Chao Liu, Pei Pei & Dianjianyi Sun
China National Center for Food Safety Risk Assessment, Beijing, China
- Junshi Chen
WHO Collaborating Center for Tobacco Cessation and Respiratory Diseases Prevention, China-Japan Friendship Hospital, Beijing, China
Institute of Respiratory Medicine, Chinese Academy of Medical Sciences, Beijing, China
NCD Prevention and Control Department, Guangxi Provincial CDC, Nanning, China
Naying Chen, Duo Liu & Zhenzhu Tang
NCD Prevention and Control Department, Liuzhou CDC, Liubei, Liuzhou, China
Ningyu Chen, Qilian Jiang, Jian Lan, Mingqiang Li, Yun Liu, Fanwen Meng, Jinhuai Meng, Rong Pan, Yulu Qin, Ping Wang, Sisi Wang, Liuping Wei & Liyuan Zhou
NCD Prevention and Control Department, Gansu Provincial CDC, Lanzhou, China
Caixia Dong, Pengfei Ge & Xiaolan Ren
NCD Prevention and Control Department, Maijixiang CDC, Maijixiang, Tianshui, China
Zhongxiao Li, Enke Mao, Tao Wang, Hui Zhang & Xi Zhang
NCD Prevention and Control Department, Hainan Provincial CDC, Haikou, China
Jinyan Chen, Ximin Hu & Xiaohuan Wang
NCD Prevention and Control Department, Meilan CDC, Meilan, Haikou, China
Zhendong Guo, Huimei Li, Yilei Li, Min Weng & Shukuan Wu
NCD Prevention and Control Department, Heilongjiang CDC, Harbin, China
Shichun Yan, Mingyuan Zou & Xue Zhou
NCD Prevention and Control Department, Nangang CDC, Harbin, China
Ziyan Guo, Quan Kang, Yanjie Li, Bo Yu & Qinai Xu
NCD Prevention and Control Department, Henan Provincial CDC, Zhengzhou, China
Liang Chang, Lei Fan, Shixian Feng, Ding Zhang & Gang Zhou
NCD Prevention and Control Department, Huixian CDC, Huixian, China
Yulian Gao, Tianyou He, Pan He, Chen Hu, Huarong Sun & Xukui Zhang
NCD Prevention and Control Department, Hunan Provincial CDC, Changsha, China
Biyun Chen, Zhongxi Fu, Yuelong Huang, Huilin Liu, Qiaohua Xu & Li Yin
NCD Prevention and Control Department, Liuyang CDC, Liuyang, China
Huajun Long, Xin Xu, Hao Zhang & Libo Zhang
NCD Prevention and Control Department, Jiangsu Provincial CDC, Nanjing, China
Jian Su, Ran Tao, Ming Wu, Jie Yang, Jinyi Zhou & Yonglin Zhou
NCD Prevention and Control Department, Wuzhong CDC, Wuzhong, Suzhou, China
Yihe Hu, Yujie Hua, Jianrong Jin, Fang Liu, Jingchao Liu, Yan Lu, Liangcai Ma, Aiyu Tang & Jun Zhang
NCD Prevention and Control Department, Licang CDC, Qingdao, China
Wei Hou, Silu Lv & Junzheng Wang
NCD Prevention and Control Department, Sichuan Provincial CDC, Chengdu, China
Xiaofang Chen, Xianping Wu, Ningmei Zhang & Xiaoyu Chang
NCD Prevention and Control Department, Pengzhou CDC, Pengzhou, Chengdu, China
Xiaofang Chen, Jianguo Li, Jiaqiu Liu, Guojin Luo, Qiang Sun & Xunfu Zhong
NCD Prevention and Control Department, Zhejiang Provincial CDC, Hangzhou, China
Weiwei Gong, Ruying Hu, Hao Wang, Meng Wang & Min Yu
NCD Prevention and Control Department, Tongxiang CDC, Tongxiang, China
Lingli Chen, Qijun Gu, Dongxia Pan, Chunmei Wang, Kaixu Xie & Xiaoyi Zhang
You can also search for this author in PubMed Google Scholar
- , Zhengming Chen
- , Robert Clarke
- , Rory Collins
- , Liming Li
- , Chen Wang
- , Richard Peto
- , Robin G. Walters
- , Daniel Avery
- , Maxim Barnard
- , Derrick Bennett
- , Ruth Boxall
- , Ka Hung Chan
- , Yiping Chen
- , Johnathan Clarke
- , Huaidong Du
- , Ahmed Edris Mohamed
- , Hannah Fry
- , Simon Gilbert
- , Pek Kei Im
- , Andri Iona
- , Maria Kakkoura
- , Christiana Kartsonaki
- , Hubert Lam
- , Kuang Lin
- , James Liu
- , Mohsen Mazidi
- , Iona Y. Millwood
- , Sam Morris
- , Qunhua Nie
- , Alfred Pozarickij
- , Paul Ryder
- , Saredo Said
- , Dan Schmidt
- , Becky Stevens
- , Iain Turnbull
- , Baihan Wang
- , Neil Wright
- , Ling Yang
- , Xiaoming Yang
- , Qingmei Xia
- , Dianjianyi Sun
- , Canqing Yu
- , Naying Chen
- , Zhenzhu Tang
- , Ningyu Chen
- , Qilian Jiang
- , Mingqiang Li
- , Fanwen Meng
- , Jinhuai Meng
- , Ping Wang
- , Sisi Wang
- , Liuping Wei
- , Liyuan Zhou
- , Caixia Dong
- , Pengfei Ge
- , Xiaolan Ren
- , Zhongxiao Li
- , Hui Zhang
- , Jinyan Chen
- , Xiaohuan Wang
- , Zhendong Guo
- , Huimei Li
- , Shukuan Wu
- , Shichun Yan
- , Mingyuan Zou
- , Ziyan Guo
- , Quan Kang
- , Yanjie Li
- , Liang Chang
- , Shixian Feng
- , Ding Zhang
- , Gang Zhou
- , Yulian Gao
- , Tianyou He
- , Huarong Sun
- , Xukui Zhang
- , Biyun Chen
- , Zhongxi Fu
- , Yuelong Huang
- , Huilin Liu
- , Qiaohua Xu
- , Huajun Long
- , Hao Zhang
- , Libo Zhang
- , Jinyi Zhou
- , Yonglin Zhou
- , Yujie Hua
- , Jianrong Jin
- , Jingchao Liu
- , Liangcai Ma
- , Aiyu Tang
- , Jun Zhang
- , Liang Cheng
- , Ranran Du
- , Ruqin Gao
- , Feifei Li
- , Shanpeng Li
- , Yongmei Liu
- , Feng Ning
- , Zengchang Pang
- , Xiaohui Sun
- , Xiaocao Tian
- , Shaojie Wang
- , Yaoming Zhai
- , Hua Zhang
- , Junzheng Wang
- , Xiaofang Chen
- , Xianping Wu
- , Ningmei Zhang
- , Xiaoyu Chang
- , Jianguo Li
- , Jiaqiu Liu
- , Guojin Luo
- , Qiang Sun
- , Xunfu Zhong
- , Weiwei Gong
- , Ruying Hu
- , Meng Wang
- , Lingli Chen
- , Dongxia Pan
- , Chunmei Wang
- , Kaixu Xie
- & Xiaoyi Zhang
Contributions
P.K.I., I.Y.M., L.Y. and Z.C. contributed to the conception of this paper. P.K.I., N.W., K.H.C., I.Y.M. and Z.C. planned the statistical analysis. P.K.I. analyzed the data and drafted the manuscript. P.K.I., I.Y.M. and Z.C. contributed to the interpretation of the results and the revision of manuscript. R. Collins, R.P., J.C., L.L. and Z.C. designed the study. L.L., Z.C., I.Y.M., L.Y., Y.C., Y.G., H.D., S.W., C.Y., J.L., J.C., R. Collins, R. Clarke and R.G.W. contributed to data acquisition and general study management. X.Y. and D.A. provided administrative and technical support. All authors critically reviewed the manuscript and approved the final submission.
Corresponding authors
Correspondence to Zhengming Chen or Iona Y. Millwood .
Ethics declarations
Competing interests.
The authors declare no competing interests.
Peer review
Peer review information.
Nature Medicine thanks Shiu Lun Au Yeung, Yan-Bo Zhang and the other, anonymous, reviewer(s) for their contribution to the peer review of this work. Primary Handling Editor: Ming Yang, in collaboration with the Nature Medicine team.

Additional information
Publisher’s note Springer Nature remains neutral with regard to jurisdictional claims in published maps and institutional affiliations.
Extended data
Extended data fig. 1 adjusted hrs for icd−10 chapter−specific morbidities associated with ever-regular drinking and with usual alcohol intake, in men..
Cox models comparing ever-regular drinkers with occasional drinkers, or assessing the dose–response per 280 g/week higher usual alcohol intake within current drinkers, were stratified by age-at-risk and study area and adjusted for education and smoking. Each solid square or diamond represents HR with the area inversely proportional to the variance of the log HR. The horizontal lines indicate 95% CIs. CI, confidence interval; HR hazard ratio; ICD-10, International Classification of Diseases, 10th Revision.
Extended Data Fig. 2 Adjusted HRs for different aggregated and all-cause morbidities associated with years after stopping drinking, in men.
Cox models comparing ex-drinker groups with occasional drinkers were stratified by age-at-risk and study area and were adjusted for education and smoking. Each box represents HR with the area inversely proportional to the variance of the group-specific log hazard within subplot. The vertical lines indicate group-specific 95% CIs for various ex-drinker groups. The shaded strip indicate the group-specific 95% CIs for occasional drinkers. The numbers above the error bars are point estimates for HRs. CI, confidence interval; HR hazard ratio; CKB, China Kadoorie Biobank; WHO, World Health Organization.
Extended Data Fig. 3 Associations of alcohol consumption with risks of 28 diseases previously defined as alcohol-related by the WHO, in male current drinkers.
Cox models were stratified by age-at-risk and study area and were adjusted for education and smoking. HRs were plotted against usual alcohol intake and were calculated per 280 g/week higher usual alcohol intake. All specific diseases displayed were significantly associated with alcohol intake (ever-regular drinking or per 280 g/week higher usual alcohol intake) after multiple testing correction (FDR-adjusted p<0.05), except transient cerebral ischemic attacks and related syndromes (ICD-10 code: G45), occlusion and stenosis of precerebral arteries (I65) and pancreatitis (K85-K86) which showed statistical significance at nominal level (p<0.05). Each box represents HR with the area inversely proportional to the variance of the group-specific log hazard within subplot. The vertical lines indicate group-specific 95% CIs. The numbers above the error bars are point estimates for HRs and the numbers below are number of events. All P values are two-sided. CI, confidence interval; HR hazard ratio; CKB, China Kadoorie Biobank; FDR, false discovery rate; ICD-10, International Classification of Diseases, 10th Revision; WHO, World Health Organization.
Extended Data Fig. 4 Associations of alcohol consumption with risks of 36 diseases not previously defined as alcohol-related, in male current drinkers.
Cox models were stratified by age-at-risk and study area and were adjusted for education and smoking. HRs were plotted against usual alcohol intake and were calculated per 280 g/week higher usual alcohol intake. All specific diseases displayed were significantly associated with alcohol intake (ever-regular drinking or per 280 g/week higher usual alcohol intake) after multiple testing correction (FDR-adjusted p<0.05). Each box represents HR with the area inversely proportional to the variance of the group-specific log hazard within subplot. The vertical lines indicate group-specific 95% CIs. The numbers above the error bars are point estimates for HRs and the numbers below are number of events. All P values are two-sided. CI, confidence interval; HR hazard ratio; CKB, China Kadoorie Biobank; FDR, false discovery rate.
Extended Data Fig. 5 Adjusted HRs for major diseases associated with drinking patterns, in male current drinkers.
Cox models were stratified by age-at-risk and study area and were adjusted for education and smoking and for total alcohol intake where indicated. Each solid square or diamond represents HR with the area inversely proportional to the variance of the log HR. The horizontal lines indicate 95% CIs. CI, confidence interval; HR hazard ratio; HED, heavy episodic drinking; CKB, China Kadoorie Biobank; WHO, World Health Organization.
Extended Data Fig. 6 Adjusted HRs for major diseases associated with duration of drinking, in male current drinkers.
Cox models were stratified by age-at-risk and study area and were adjusted for education, smoking, total alcohol intake and baseline age in (A). (B) had the same model specifications as (A) plus further adjustments for income, physical activity, fruit intake and body mass index. (C) had the same model specifications as (A) and excluded participants with poor self-reported health or prior chronic disease at baseline. Each box represents HR with the area inversely proportional to the variance of the group-specific log hazard. The horizontal lines indicate group-specific 95% CIs. All P values are two-sided. CI, confidence interval; HR hazard ratio; CKB, China Kadoorie Biobank; WHO, World Health Organization.
Extended Data Fig. 7 Adjusted HRs for ICD−10 chapter−specific morbidities associated with ever-regular drinking and with usual alcohol intake, in women.
Cox models comparing ever-regular drinkers with occasional drinkers, or assessing the dose–response per 100 g/week higher usual alcohol intake within current drinkers, were stratified by age-at-risk and study area and adjusted for education and smoking. Each solid square or diamond represents HR with the area inversely proportional to the variance of the log HR. The horizontal lines indicate 95% CIs. CI, confidence interval; HR hazard ratio; ICD-10, International Classification of Diseases, 10th Revision.
Extended Data Fig. 8 Adjusted HRs per 280 g/week higher genotype-predicted mean male alcohol intake for specific alcohol-associated diseases by ICD-10 chapters, in men and women.
Cox modes, stratified by age-at-risk and adjusted for genomic principal components, were used to relate genetic categories to risks of diseases within each study area. The HR per 280 g/week higher genotype-predicted mean male alcohol intake was calculated from the inverse-variance-weighted mean of the slopes of the fitted lines in each study area. Each solid square or diamond represents HR per 280 g/week higher genetically-predicted mean male alcohol intake, with the area inversely proportional to the variance of the log HR. The horizontal lines indicate 95% CIs. Diseases considered to be alcohol-related by the WHO are indicated with ‘Y’ under the ‘WHO’ column. The ‘RC’ column indicates the number of study areas that contributed to the overall area-stratified genotypic associations, as for certain less common diseases some study areas may not have enough number of cases to contribute to the inverse-variance-weighted meta-analysis. The ‘P het’ column indicates the p-value from a \(\chi\) 2 test for heterogeneity between sexes. All P values are two-sided. † Included less common ICD-10 codes within the corresponding ICD-10 chapter which were not individually investigated in the present study. CI, confidence interval; HR hazard ratio; ICD-10, International Classification of Diseases, 10th Revision; WHO, World Health Organization.
Extended Data Fig. 9 Adjusted HRs associated with GG versus AG genotype of ALDH2 - rs671 for specific alcohol-associated diseases by ICD-10 chapters, in men and women.
Area-specific genotypic effects (GG vs. AG genotype) were estimated within each study area (thus each reflecting the purely genotypic effects) using age-at-risk-stratified and genomic principal components-adjusted Cox models and were combined by inverse-variance-weighted meta-analysis to yield the overall area-stratified genotypic associations. Each solid square represents HR for GG vs. AG genotype, with the area inversely proportional to the variance of the log HR. The horizontal lines indicate 95% CIs. Diseases considered to be alcohol-related by the WHO are indicated with ‘Y’ under the ‘WHO’ column. The ‘RC’ column indicates the number of study areas that contributed to the overall area-stratified genotypic associations, as for certain less common diseases some study areas may not have enough number of cases to contribute to the inverse-variance-weighted meta-analysis. The ‘P het’ column indicates the P value from a \(\chi\) 2 test for heterogeneity between sexes. All P values are two-sided. † Included less common ICD-10 codes within the corresponding ICD-10 chapter which were not individually investigated in the present study. CI, confidence interval; HR hazard ratio; ICD-10, International Classification of Diseases, 10th Revision; WHO, World Health Organization.
Extended Data Fig. 10 Adjusted HRs associated with GG versus AG genotype of ADH1B - rs1229984 for specific alcohol-associated diseases by ICD-10 chapters, in men and women.
Supplementary information, supplementary information.
Supplementary Figs. 1–8 and Supplementary Tables 1–13.
Reporting Summary
Rights and permissions.
Open Access This article is licensed under a Creative Commons Attribution 4.0 International License, which permits use, sharing, adaptation, distribution and reproduction in any medium or format, as long as you give appropriate credit to the original author(s) and the source, provide a link to the Creative Commons license, and indicate if changes were made. The images or other third party material in this article are included in the article’s Creative Commons license, unless indicated otherwise in a credit line to the material. If material is not included in the article’s Creative Commons license and your intended use is not permitted by statutory regulation or exceeds the permitted use, you will need to obtain permission directly from the copyright holder. To view a copy of this license, visit http://creativecommons.org/licenses/by/4.0/ .
Reprints and permissions
About this article
Cite this article.
Im, P.K., Wright, N., Yang, L. et al. Alcohol consumption and risks of more than 200 diseases in Chinese men. Nat Med 29 , 1476–1486 (2023). https://doi.org/10.1038/s41591-023-02383-8
Download citation
Received : 16 December 2022
Accepted : 02 May 2023
Published : 08 June 2023
Issue Date : June 2023
DOI : https://doi.org/10.1038/s41591-023-02383-8
Share this article
Anyone you share the following link with will be able to read this content:
Sorry, a shareable link is not currently available for this article.
Provided by the Springer Nature SharedIt content-sharing initiative
This article is cited by
Uncovering newly identified aldehyde dehydrogenase 2 genetic variants that lead to acetaldehyde accumulation after an alcohol challenge.
- Freeborn Rwere
- Joseph R. White
- Eric R. Gross
Journal of Translational Medicine (2024)
Alcohol consumption may be a risk factor for cerebrovascular stenosis in acute ischemic stroke and transient ischemic attack
- Xiaoyan Guo
BMC Neurology (2024)
Western diets and chronic diseases
- Timon E. Adolph
- Herbert Tilg
Nature Medicine (2024)
Mapping multimorbidity progression among 190 diseases
Communications Medicine (2024)
The aldehyde dehydrogenase 2 rs671 variant enhances amyloid β pathology
Nature Communications (2024)
Quick links
- Explore articles by subject
- Guide to authors
- Editorial policies
Sign up for the Nature Briefing newsletter — what matters in science, free to your inbox daily.


An official website of the United States government
The .gov means it’s official. Federal government websites often end in .gov or .mil. Before sharing sensitive information, make sure you’re on a federal government site.
The site is secure. The https:// ensures that you are connecting to the official website and that any information you provide is encrypted and transmitted securely.
- Publications
- Account settings
Preview improvements coming to the PMC website in October 2024. Learn More or Try it out now .
- Advanced Search
- Journal List
- Alcohol Res Health
- v.33(1-2); 2010

The Past and Future of Research on Treatment of Alcohol Dependence
Research on the treatment of alcoholism has gained significant ground over the past 40 years. Studies such as the National Institute on Alcohol Abuse and Alcoholism’s Project MATCH, which examined the prospect of tailoring treatments for particular people to better suit their needs, and Project COMBINE, which examined in-depth, cognitive–behavioral therapy and medical management, helped pave the way for a new way of approaching alcoholism treatment. New findings garnered through the National Epidemiologic Survey on Alcohol and Related Conditions further defined the problem. At the heart of this research has been the development of procedures to characterize, measure, and monitor the fidelity to a particular conceptual psychotherapeutic approach so that clear comparisons can be made between conceptually and technically distinct approaches. Advances in scientific methodology and statistics have provided tools to analyze complex datasets. The resulting findings mark an improvement over the first models of treatment developed decades ago, which tended to focus on anecdotal findings and assumptions. This hard-earned progress has enabled scientists today to move ahead and address the next set of challenges. Future research, coupled with a restructured treatment system capable of making new scientific findings rapidly available to the community, hold the key to significantly improving treatment outcomes and reducing suffering from alcohol-related disorders.
Remarkable progress has been made in the treatment of alcohol use disorders (AUDs) over the past 40 years. We have a better understanding of the natural history of heavy drinking and the development of dependence. We understand better the course of recovery and the risk factors and prognostic indicators for AUDs. Most importantly, we have made significant strides in the behavioral and pharmacological treatments available to people, and their families, who suffer from alcoholism. Research supported by the National Institute on Alcohol Abuse and Alcoholism (NIAAA) has been instrumental in advancing treatment, moving our understanding from anecdotal approaches to those that are based firmly on evidence.
Of course, new scientific findings almost always generate more questions than they resolve, and alcohol treatment research is no different. Research conducted over the past four decades has created a number of new scientific challenges. The most central of these challenges is to truly understand the scientific basis underlying health behavior, such as alcohol consumption. This calls for careful understanding of behavior and the steps involved in decisionmaking, as well as the social determinants that influence those decisions; in short, we need to know who we are and why we do what we do. It is especially important to identify potentially modifiable operators within the systems that determine these behaviors in order to develop new and more powerful ways to help people overcome addiction to alcohol. Medications offer one method to do so, which will require identifying neurophysiological and genomic targets for development of new medications with novel mechanisms ( Koob 2006 ). Better-targeted behavioral approaches that address these habits (such as addiction) also are needed. Ultimately, our goals are to ensure that more people respond to treatment and that they are able to experience long-lasting effects from that treatment.
In addition to addressing these scientific challenges, there is a pressing need to create a new system of providing risk reduction and treatment for heavy drinkers and people with alcohol dependence. The current treatment system model, the Minnesota Model, was developed by professionals at a State hospital in Minnesota and promulgated most famously by the Johnson Institute and the Hazelden Foundation. Based on what was known at the time (primarily through the folk wisdom of Alcoholics Anonymous [AA]), the Minnesota Model combined the first five steps of AA with lectures on the disease concept of alcoholism and some practical supportive psychotherapy. Central to its concept was the use of staff members who themselves were in recovery from alcohol dependence, along with others ( Anderson et al. 1999 ).
Unfortunately, because this model of care was developed without the benefit of a scientific basis, it was not configured in such a way as to rapidly change in response to scientific advances. Currently, more than 90 percent of community treatment programs in the United States offer lectures, group counseling, and referral to AA, and nothing else ( McLellan and Meyers 2004 ). It is common that treatment staff members are poorly trained and supervised, are paid little, and frequently do not stay long ( McLellan et al. 2004 ). In fact, the “counseling” that is provided often consists of casual talk rather than sophisticated psychotherapy ( Carroll et al. 2008 ). To assert this is not to disparage the dedicated professionals who devote their lives to helping others with addiction. However, most addiction counselors have relatively little education and almost no supervision, which is in stark contrast to the counselors who provide behavioral treatment in efficacy trials ( Anton et al. 2006 ; McLellan and Meyers 2004 ). In addition, very few treatment programs use integrated teams with other professionals such as physicians, psychologists, nurses, and social workers ( McLellan and Meyers 2004 ). As a result, there is a disconnect between what has been discovered through research and what is actually implemented in everyday practice, or, for that matter, what can be implemented given the state of the treatment system.
Challenging Current Treatment Models
The first decades of research on treatment of alcohol dependence were characterized by several assumptions: (1) that change occurred because of, or was substantially influenced by, interaction between a client or patient and a professional—in a word, psychotherapy; (2) that the technical differences between different psychotherapeutic approaches would result in different outcomes, or at least different outcomes for different patients; (3) that most people with alcohol dependence had severe, recurrent, or chronic dependence; (4) that change depended on the development of insight and the conscious application of techniques or methods taught by an expert (who in this case would include an experienced AA member, such as a sponsor); and (5) perhaps most importantly, that the problem to be addressed was alcoholism, not heavy drinking, which were considered to be quite different entities.
For the most part, these assumptions have been proven wrong, or at best incomplete. About three-quarters of people with alcohol dependence reduce or stop drinking without any kind of professional treatment or even interaction with a community support group such as AA ( Dawson et al. 2005 ). Psychotherapies that are conceptually and technically distinct have very similar results ( Project MATCH Research Group 1998 ). Almost 70 percent of people who develop alcohol dependence have mild-to-moderate forms that are self-limiting ( Hasin and Stinson 2007 ; Moss et al. 2007 ). Although some differences in therapist technique, such as use of an empathic, engaging approach, are associated with marginally better outcomes ( Miller and Rollnick 2002 ), it still is unclear what drives change. Finally, there is no clear distinction between heavy drinking, per se, and “addiction.” In fact, non-symptomatic heavy drinking blends imperceptibly into mild then moderate dependence and, in a minority of those affected, severe and recurrent dependence. Alcohol dependence is not inevitably progressive but may have long periods of stability or alternate back and forth between heavy and lighter drinking and abstinence ( Dawson and Grant 2006 ; Dawson et al. 2005 ; Vaillant 2003 ).
Many of these conclusions, however counterintuitive they may seem, are the result of rigorous research by a dedicated scientific community. At the heart of this research was the development of procedures to characterize, measure, and monitor the fidelity to a particular conceptual psychotherapeutic approach so that clear comparisons could be made between conceptually and technically distinct approaches. Advances in scientific methodology and statistics also have provided tools to analyze complex datasets. This hard-earned progress has enabled scientists today to move ahead and address the next set of challenges.
For example, NIAAA’s Project MATCH compared three different approaches: cognitive–behavioral, which focuses on teaching skills such as drink refusal and relapse prevention; motivational enhancement, which focuses more on addressing ambivalence about and motivation to change; and 12-step facilitation, which focuses on teaching that alcoholism is a disease that requires abstinence and affiliation with AA ( Project MATCH Research Group 1998 ). In addition, 20 different hypotheses were proffered concerning interactions between study participant characteristics and the specific therapy approach—that is, that participants with certain characteristics, such as antisocial traits or anger, would respond differentially to different therapies.
The result, for the most part, showed that all three groups had highly similar (and positive) results, and most matching hypotheses were not supported ( Project MATCH Research Group 1998 ). Far from a failure, however, this outcome showed that treatment works. Unfortunately, no attribution could be made as to what caused these positive outcomes, because all three therapeutic approaches had similar outcomes. Perhaps the most significant outcome was that Project MATCH provided a formidable challenge to existing thinking. Technical differences between therapies did not seem to result in different outcomes, even for extremely well-characterized participants across multiple domains. In addition, although it was not recognized at the time, it also provided a clue as to where scientists might look next. The concept emerging from the Project MATCH study (and many other studies with similar outcomes) was this: If technical differences among therapies (such as cognitive–behavioral therapy, motivational enhancement therapy, and 12-step facilitation therapy) do not account for differences in change, then it is unclear what indeed is driving the large change that occurs in most people who seek treatment.
Understanding the Mechanisms of Change
Looking carefully at treatment studies for alcohol dependence, some interesting features emerge. First, the results of these studies are remarkably similar. Studies comparing different behavioral approaches, placebo-controlled medication trials, and studies combining the two have essentially identical average baseline and 12-month outcomes ( Anton et al. 2006 ; Project MATCH Research Group 1998 ; Miller et al. 2001 ).
Overall, treatment outcomes are much better than might be expected given the widespread pessimism about outcomes. For example, about one-third of people who enter treatment trials are in full remission from alcohol dependence for the following year, and the other two-thirds show substantial improvement, from an average of more than 70 drinks per week to less than 10 drinks per week ( Miller et al. 2001 ). Nevertheless, the specific treatment received (as long as it is high quality, which is the case in research studies comparing treatments) does not seem to account for differences in outcomes.
These findings suggest that positive change is either a part of the natural history of the illness or the result of nonspecific factors, such as installation of hope, the decision to change, and encountering an empathic therapist who is willing to help and who has a way to do so. Conceptually and technically distinct forms of psychotherapy have roughly equivalent outcomes if they all are provided by trained professionals who are screened for the ability to form an empathic relationship with their clients ( Project MATCH Research Group 1998 ). Certain medications, such as naltrexone and topiramate, also provide meaningful improvements in outcomes, compared with placebo, but those differences pale in comparison to the overall improvement in both groups.
One interpretation of these findings is that the change process may be driven more by nontreatment factors than treatment-specific factors, or at least factors other than specific technical differences among therapies. Recent reanalyses of several clinical trials have examined the course of drinking among study participants before entering the trial, based on retrospective accounts obtained during the baseline evaluation. It appears that for most study participants the change process began before entering treatment, often by weeks ( Penberthy et al. 2007 ), and often includes stopping or nearly stopping drinking prior to study entry. Thus, treatment entry may be a result of change rather than an instigator of it.
Perhaps the decision to enter treatment is the crucial change point. A qualitative study of participants’ accounts of what occurred in their lives prior to study entry strongly suggests this is the case (Orford et al. 2005). Participants described a process of increasing distress and drinking and pressure from others to change. Then a trigger event, such as a drunk driving charge or domestic disturbance, led to a realization that “I can’t do this alone,” which, in turn, led to the decision to seek help. In addition, as the participants continued through the treatment process, many non-treatment factors were highly influential in what course they took. These nontreatment factors often are ignored in treatment research, when in fact they may be responsible for a much greater proportion of change processes than treatment. An additional concern is that most efficacy trials exclude many people who need treatment but do not meet rigorous inclusion and exclusion criteria, raising questions about how applicable the results of these studies are to community clinical populations ( Humphreys et al. 2005 ).
Taken together, these and other findings provide evidence that many of the assumptions underpinning the previous several decades of treatment research were wrong or at least incomplete. The mechanisms of change in drinking behavior among heavy drinkers are not well understood. In addition to these findings regarding treatment studies, it recently has become clear that most people with alcohol dependence change without exposure to treatment or AA ( Dawson et al. 2005 ; Fein and Landman 2005 ; Moss et al. 2007 ). Thus, the mechanisms of change for these individuals are not well characterized or understood and more attention needs to be paid to change outside the context of professional treatment or AA. Additionally, future research needs to focus on elucidating the actual mechanisms of change and how treatment professionals can best assist people in reducing or eliminating heavy drinking.
None of this is meant to suggest that providing treatment for people with alcohol dependence is either unnecessary or ineffective. Rather, progress in treatment research has raised important questions about how to improve already-decent outcomes for people seeking treatment. In addition, even though the majority of people with alcohol dependence have mild-to-moderate, rather than severe, dependence, they still require treatment to decrease the impact on those affected and to shorten the course. This is not significantly different from many other medical disorders. For example, most depressive episodes are ultimately self-limiting, but untreated they may result in substantial disability and misery ( O’Leary et al. 2010 ). The same could be said of asthma or arthritis. Another important goal is to provide treatments that are acceptable and accessible earlier in the course of illness rather than waiting until chronicity and severe disability already are present.
In 2005, NIAAA began to alter the direction of research funding concerning changes in drinking behavior. Staff of the Division of Treatment and Recovery Research branch first held a series of informal meetings among senior investigators representing a wide variety of scientific disciplines, including many with no prior experience in alcohol treatment research. The perspective outlined above was presented to them and they were asked to suggest possible new directions for behavior change research. Following those meetings, a strategic research plan was developed and presented to the Extramural Advisory Board, a subcommittee of the NIAAA National Advisory Council. After further discussion and refinement, the plan was approved by the Advisory Council and the NIAAA Director and was incorporated into the NIAAA Strategic Plan (see www.niaaa.nih.gov ).
What emerged was the NIAAA Mechanisms of Behavior Change (MOBC) Initiative. This initiative is an ambitious plan to fund interdisciplinary high-risk research projects and has the potential to transform our understanding of change in drinking behavior among heavy drinkers. One senior scientist, Dr. Jon Morgenstern, participating in the process described the goal as “developing the basic science of behavior change.” In this context, however, basic science refers not only to wet-lab basic research but also behavioral, psychological, and social components, which are essential to understanding the complexity of behavior. There is no “bottom-up” assumption that the “real” determinants are genomic or neurophysiological, because influence from one level to another is bidirectional: each level of analysis (genomic, cellular, physiological, individual, and social) influences all the others in a dynamic interplay. This entire system often is described as a complex dynamical system ( Barabasi 2009 ). In a complex dynamical system, the whole is more than the sum of its parts. One systems theorist ( Barabasi 2009 ) used the analogy that it is possible to know and be able to lay out every single part of an airplane, but that tells you nothing about how an airplane flies or how a particular airplane will respond to wind shear. With this in mind, NIAAA invited experts in the mathematical modeling of complex systems to participate in the initiative and they, in turn, have played a crucial role in shaping its direction and focus.
The NIAAA MOBC Initiative has stimulated several new lines of research. This research is in its earliest stages and it may take some time before results are obtained and even longer before clinically useful tools are developed as a result. Yet significant progress is being made. In the past 2 years, a cross–National Institutes of Health (NIH)-MOBC Initiative involving more than 15 NIH Institutes and Centers has developed and momentum continues to grow. In February 2010, NIH issued an NIH Roadmap request for applications on the science of behavior change, providing additional evidence of a sustained commitment to MOBC research.
Reconfiguring the Treatment System
No matter how elegant the research is and no matter how powerful the new treatments are that emerge from it, those findings must be accessible. For the most part, the scientific advances of the last 40 years have not been well embraced by treatment providers. Very few people with alcohol dependence seek or receive any kind of professional treatment ( Moss et al. 2007 ). Effective medications are prescribed very infrequently ( Mark et al. 2003 ). The model on which most currently available treatment relies was developed about 50 years ago, when scientific understanding of substance use disorders was rudimentary ( Anderson et al. 1999 ). More than 90 percent of U.S. treatment programs currently offer group counseling and referral to AA, without access to medications or evidence-based behavioral treatment ( McLellan and Meyers 2004 ). As a result, most consumers have little choice as to what treatment they wish to receive. Many counselors have minimal training, and the turnover among treatment program staff is more than 50 percent annually ( McLellan and Meyers 2004 ). There is a constant shortfall of funding so programs are unable to invest in infrastructure such as electronic medical records ( McLellan and Meyers 2004 ). Although program staff members often are dedicated and hard working, this environment makes providing modern treatment extremely challenging if not impossible.
Perhaps more importantly, most treatment programs are predicated on the idea that a relatively brief period of education and counseling will lead to a major shift in the trajectory of a serious chronic illness, an approach that has no scientific basis and which is not used for other chronic disorders. It is true that some types of psychotherapy for psychiatric disorders may be given in a time-limited way, but this generally occurs in the context of ongoing care management by a mental health or primary-care clinician. In addition, multiple courses of the same therapy usually are not prescribed when a disorder proves resistant to the first full course of well-administered therapy. For alcohol dependence, however, patients often undergo multiple courses of rehabilitation even when it is completely ineffective for a particular person simply because no alternatives are available.
A final important limitation of the current system is that it is focuses on the most severely affected people—those with severe and persistent alcohol dependence—most of whom have encountered serious life consequences. It is not configured to provide care to people with milder forms of dependence. That group, which comprises nearly three-fourths of all cases, typically remains functional and almost all eventually enter full remission ( Moss et al. 2007 ). Thus, the current treatment system reaches relatively few people with dependence, provides time-limited counseling for people with severe and persistent dependence, fails to offer consumers a choice of treatment approaches, and is not configured to deliver new approaches based on research. It is time to broadly reconsider what kind of services should be offered, where they should be offered, and who should provide them. Fortunately, 40 years of research provides a solid scientific basis to guide this process and suggests a framework for moving forward.
Building a Scientifically Based Continuum of Care
There is a broad spectrum of drinking behaviors and associated risk of alcohol-related problems spread across the entire adult population, as determined by the National Epidemiologic Survey on Alcohol and Related Conditions (NESARC) ( Grant et al. 2004 ; Hasin et al. 2007 ). NIAAA guidelines recommend that healthy adult men drink no more than 4 U.S. standard drinks in any day and no more than 14 drinks in any week and that healthy women drink no more than 3 drinks in any day and no more than 7 drinks in any week (NIAAA 2009). A standard drink in the United States contains about 14 g absolute ethyl alcohol by volume, the amount in 1.5 oz of 80-proof spirits, about 12 oz of beer, or about 5 oz of table wine. (Note that drink sizes vary widely by culture. For example in Australia and the United Kingdom, a standard drink “unit” contains about 10 g absolute alcohol by volume, the amount in 30 cc of 80-proof spirits.) Also, to be considered within the guidelines, both the daily and the weekly limits apply. Thus, someone drinking three drinks every day would not fall within these limits, nor would someone who drank eight drinks on 1 day in a week. Individuals who drink above the guidelines but who do not report having problems or symptoms related to drinking are considered to be at an elevated risk for developing consequences in the future. These “at-risk” drinkers are analogous to someone with high cholesterol but who has not yet developed coronary artery disease. Once several symptoms have developed (currently three for dependence and one for abuse), the individual is considered to have an AUD.
Thus, there are basically three groups of drinkers: people who never exceed the guidelines; at-risk drinkers who exceed the guidelines but have no current symptoms and who have never had alcohol dependence; and people with symptoms or consequences related to their drinking who thus can be diagnosed with an AUD. Currently, the diagnostic criteria for an AUD are based on the American Psychiatric Association’s (APA’s) Diagnostic and Statistical Manual, Fourth Edition, Text Revision ( DSM–IV–TR; APA 2000 ). The criteria have been undergoing review and revision for the fifth edition and are expected to be released in 2013. In the recently published draft guidelines, the categories of abuse and dependence are no longer separate disorders. Instead, the criteria for abuse and dependence have been combined in a single AUD. This revision is based on research demonstrating that the abuse and dependence diagnoses did not work as expected and that a single-dimensional construct offered a simpler solution that better fit the research findings. Thus, in the remainder of this paper, the term AUD will be used to describe what are now two different diagnoses.
In U.S. adults in any given year, 70 percent never exceed the NIAAA guidelines, either because they abstain or they drink within low-risk limits ( Hasin et al. 2007 ). Of the remaining 30 percent, most (21 percent) are at-risk drinkers and 4 percent have an AUD ( Hasin et al. 2007 ), currently diagnosed according to DSM–IV–TR as alcohol dependent. Among the 4 percent with dependence, 3 percent have functional dependence and 1 percent has severe recurrent or chronic dependence ( Hasin et al. 2007 ).
Functional alcohol dependence can be described in this way: Individuals repeatedly drink more or longer than they intend to, have a persistent desire to quit or cut down and have difficulty doing so, may drink and drive (without receiving a driving-while-intoxicated [DWI] citation), and often continue drinking in spite of physical or psychological symptoms such as hangover, headache, poor sleep, or nausea. Most people who develop an AUD have only three or four symptoms and do not develop severe life disruption as a result of their drinking. For example, they do not miss work, neglect their children or other responsibilities, have legal trouble, or lose their jobs. In many cases, only their closest friends and family members realize that their drinking is out of control. In addition, a striking and unexpected finding from NESARC is that 72 percent of people who develop alcohol dependence in their lives have a single episode lasting 3 to 4 years on average, after which it goes away and does not recur ( Hasin et al. 2007 ). The 28 percent who have recurrences have an average of five episodes ( Hasin et al. 2007 ). Thus, there appear to be two forms of this disorder, a milder self-limited form and a more severe recurrent form.
This new understanding differs drastically from what was traditionally described as alcoholism, a chronic, severe, progressive disease. This new insight was developed in part from NESARC findings. NESARC studied the general population and identified individuals who had gone through a period of years struggling with drinking too much but who eventually overcame it without seeking professional help or AA. This finding was in contrast to previous research efforts, which generalized to everyone findings from people in treatment programs or AA. As a result, researchers mistakenly assumed that unidentified “cases” in the community were similar to those in treatment, but that clearly is not the case. The same holds for every other medical disorder. For example, for people who have asthma, influenza, or depression, only a small proportion ever require hospital care, and it is because they have a milder, more treatment-responsive or less complex form of the illness. It turns out that the same is true for AUDs.
The result, then, is four distinct groups: abstainers and low-risk drinkers, at-risk or high-risk drinkers, people with functional alcohol dependence, and people with severe recurrent alcohol dependence. This new grouping, based on a large epidemiological research dataset, provides scientists with the information they need to devise a continuum of care for each group.
For abstainers and low-risk drinkers, the goal is to prevent a problem from developing, especially, but not exclusively, in young people. Universal prevention usually occurs in settings such as schools, workplaces, and health care settings. Such measures include public awareness campaigns establishing healthy limits and requirements for beverage labeling (Miller 2004). Such approaches provide consumers with the information they need to make informed decisions. A current example is the intense public health focus on overeating and obesity.
To support healthy choices in alcohol use, NIAAA recently published a consumer-oriented booklet and accompanying Web site (“Rethinking Drinking”) with information on defining a “standard” drink, recommended limits for alcohol use, and tips for cutting down on drinking. This material, though not expected to directly affect behavior change, may indirectly influence drinking behavior by supporting efforts to reduce drinking within the community at large. Studies to have not yet determined the efficacy of this approach.
For at-risk drinkers who do not have an AUD, the goal is to reduce the risk of later development of downstream consequences of heavy drinking. At-risk drinkers are similar to people with high blood pressure but who do not have symptoms or a cigarette smoker without lung cancer or heart disease. Fortunately, at-risk drinkers respond well to a variety of low-intensity interventions, including brief counseling by a physician ( Whitlock et al. 2004 ) and Internet-based approaches such as “Rethinking Drinking.” Although it has not been well studied, it is likely that work-place initiatives, toll-free telephone numbers, and other low-cost high-yield methods also will be successful in treating at-risk drinkers. There is some evidence that the medication, naltrexone, may be effective in this group as well, as most at-risk drinkers prefer to cut down rather than quit. Naltrexone reduces the “buzz” one gets from drinking and thus makes it easier to drink less ( O’Malley 1998 ; Volpicelli et al 1992 ).
Individuals with functional AUDs seldom, if ever, go to AA or an addiction treatment program, simply because they are able to manage their lives and not get into serious trouble even though they are drinking more than they wish ( Hasin et al. 2007 ). Onset varies from late teens to older age. Most seek no help at all and yet eventually are able to overcome their compulsive drinking either through abstaining or cutting back to low-risk drinking ( Moss et al. 2007 ). They typically suffer, however, for a period of years, and, although their lives do not fall apart, their excessive drinking may be a matter of significant concern for them and their loved ones. In this way, people with functional AUDs resemble others with major depression or anxiety disorders who are able to function but at a suboptimal level and with a significant level of distress. In both cases, effective medication treatment is available that can be prescribed by a physician. In the past, people with depression were rarely treated. Like AUDs now, only the most severely affected were identified and treated, typically with hospitalization. In 1987, when Prozac ® was introduced it changed the treatment landscape significantly. Now with many similar effective, safe, and easy-to-use medications available for treating depression, more than two-thirds of people receive treatment, usually from their primary-care doctor ( Ledoux et al. 2009 ). Only the most complex or treatment-resistant cases are referred to psychiatrists.
It is not widely known that medications currently are available to treat alcohol dependence and which have similar effectiveness to modern antidepressants (Bouza et al. 2005; Johnson 2007 ; Mann et al. 2004 ). Naltrexone and topiramate both reduce relapse in early abstinence patients by 20 to 30 percent, resulting in more people attaining sustained periods of abstinence compared with people taking placebo (Bouza et al. 2005; Johnson 2007 ; Johnson et al. 2003 ; Mann et al. 2004 ). A much older drug, disulfiram, is effective if used properly to ensure adherence ( O’Farrell et al. 1995 ), and it has the advantage of being very inexpensive. Several other medications have some evidence of effectiveness and many more are in various stages of development ( Johnson 2007 ; Srisurapanont and Jarusuraisin 2005 ). These medications are ideal for treating large numbers of people with functional AUDs in a discrete, familiar setting, such as the family doctor’s office. Coupled with brief support directed at encouraging medication adherence, attendance at community support groups, and abstinence, these medications are as effective as state-of-the-art behavioral treatment, at least among people seeking help for their drinking.
Thus, effective therapies exist for people with mild-to-moderate severity AUDs, who currently do not receive any treatment at all and for whom traditional rehabilitation programs, which better address a much smaller group of people with severe chronic AUDs, are not appropriate. For those who would prefer psychotherapy to medication, several behavioral approaches are effective in outpatient settings ( Miller et al. 2001 ), including cognitive–behavioral treatment, motivational enhancement therapy, community reinforcement, and 12-step facilitation ( Project MATCH Research Group 1998 ; Smith and Myers 2004 ). Emerging research also suggests that computer-based behavioral approaches (especially cognitive–behavioral treatment) may be effective either as a substitute for or augmentation of person-to-person treatment ( Carroll et al. 2008 ). It is likely that various combinations of computer-based and personal behavioral treatments will emerge, further increasing the options available to people who need help.
At the other end of the spectrum are those with more severe recurrent AUDs. These individuals are more likely to have a strong family history of AUDs, to have behavioral problems as children and antisocial behavior as adults, to come from chaotic families, and to experience the onset of AUDs in the early to mid-teens ( Moss et al. 2007 ). Many develop addiction to other substances such as cannabis, cocaine, or methamphetamine ( Grant 2004 ). They also may have other serious psychiatric disorders, such as major depression, serious anxiety disorders, bipolar disorder, or psychosis ( Grant et al. 2004 ; Hasin et al. 2007 ). This is the group who largely populates AA and treatment programs, especially as they age into mid-life ( Moss et al. 2007 ). What is striking is that in almost all treatment programs in the United States, the model for treating such a complicated and chronic illness consists of group counseling and AA, typically for only a few weeks or months ( McLellan and Meyers 2004 ). As noted earlier in this article, this model of treatment was developed when there were no formal treatments for AUDs and when no other methods of treatment were available. Recent findings on severe recurrent AUDs, however, suggest a different approach.
Based on the current understanding of the nature of the disorder, some principles seem relatively straightforward and resemble those for treating other severe chronic disorders. For example, treatment should continue as long as needed and not be stopped at some arbitrary point. The goal of treatment should always be full remission (for this group, this usually is abstinence), but it is not realistic to expect to reach that goal easily or quickly. For some affected individuals, it may not be possible to achieve long-term continuous abstinence at all. In that case, clinicians must be prepared to do everything possible to reduce the severity and impact of the disorder, to extend meaningful life, and to reduce suffering. Familiar examples exist in conditions such as diabetes, bipolar disorder, and cancer. In such cases, the aim is always for a cure, but we do not abandon the patient if cure is not forthcoming. Fortunately, many people with severe recurrent AUDs eventually do get well, which often is not the case with other chronic disorders. In addition to modifying the treatment approach and goals, it is important to attend to coexisting conditions such as psychiatric and physical disorders. Social and vocational functions also are commonly affected and should be addressed as well. Thus, long-term care coordination is essential to providing good care to this group over time.
Providing this level and type of care for severe recurrent AUDs requires new approaches to where services will be provided and by whom. Ideally, physicians (including psychiatrists), other mental health clinicians, nurses, and counselors will coordinate the provision of multiple services, individualized for each patient. Restructuring the continuum of care for at-risk drinking and AUDs is a formidable but not impossible task.
The Future of Treatment Research
The limited scope of this article cannot possibly do justice to the explosive growth in knowledge in neuroscience, genomics, pharmacology, psychology (including behavioral economics), social sciences, and mathematics and the implications for developing new approaches to help people change health behavior. Other articles in this issue summarize some of them. This explosion in knowledge holds great promise for developing new methods for helping people change their health behaviors. Medications aimed at novel targets and more direct behavioral approaches will become available that likely will be significantly more powerful than what are in use now. Research conducted during the past 40 years has enabled us to develop a continuum of care with a solid scientific base, an improvement over the first models of treatment developed decades ago. As the science develops, however, so will the complexity and difficulty of the questions to be answered. Research coupled with a restructured treatment system capable of making new scientific findings rapidly available to the community hold the key to significantly improving treatment outcomes and reducing suffering from alcohol-related disorders.
Behavioral Approaches for Treating Alcohol Dependence.
Focus on coping in the here and now; examining and changing irrational thoughts that lead to distress or increase risk for relapse; skill building | |
Focus on addressing ambivalence and barriers to change; enhancing motivation to change | |
Acceptance of addiction as a disease requiring abstinence to recover; willingness to engage fully in 12-step programs such as Alcoholics Anonymous | |
Eliminating positive reinforcement for drinking and increasing positive reinforcement for sobriety | |
Providing explicit incentives (e.g., money) for abstinence and for participating in treatment | |
Helping a significant other provide support for abstinence in the alcohol-dependent person, in particular by monitoring medication administration |
F inancial D isclosure
The author declares that he has no competing financial interests.
References:
- Anderson DJ, McGovern JP, DuPont RL. The origins of the Minnesota model of addictions treatment: A first person account. Journal of Addictive Diseases. 1999; 18 (1):107–114. [ PubMed ] [ Google Scholar ]
- Anton RF, O’Malley SS, Ciraulo DA, et al. Combined pharmacotherapies and behavioral interventions for alcohol dependence: The COMBINE study: A randomized controlled trial. JAMA: Journal of the American Medical Association. 2006; 295 (17):2003–2017. [ PubMed ] [ Google Scholar ]
- American Psychiatric Association . Diagnostic and Statistical Manual of Mental Disorders, Fourth Edition, Text Revision. Washington, DC: American Psychiatric Association; 2000. pp. 213–214. [ Google Scholar ]
- Barabási AL. Scale-free networks: A decade and beyond. Science. 2009; 325 (5939):412–413. [ PubMed ] [ Google Scholar ]
- Bouza C, Angeles M, Muñoz A, Amate JM. Efficacy and safety of naltrexone and acamprosate in the treatment of alcohol dependence: A systematic review. Addiction. 2004; 99 (7):811–828. Erratum in: Addiction 100(4):573, 2005. [ PubMed ] [ Google Scholar ]
- Carroll KM, Ball SA, Martino S, et al. Computer-assisted delivery of cognitive-behavioral therapy for addiction: A randomized trial of CBT4CB. American Journal of Psychiatry. 2008; 165 (7):881–888. [ PMC free article ] [ PubMed ] [ Google Scholar ]
- Dawson DA, Grant BF, Stinson FS, et al. Recovery from DSM-IV alcohol dependence: United States, 2001–2002. Addiction. 2005; 100 (3):281–292. [ PubMed ] [ Google Scholar ]
- Dawson DA, Grant BF, Stinson FS, Chou PS. Maturing out of alcohol dependence: The impact of transitional life events. Journal of Studies on Alcohol. 2006; 67 (2):195–203. [ PubMed ] [ Google Scholar ]
- Fein G, Landman B. Treated and treatment-naive alcoholics come from different populations. Alcohol. 2005; 35 (1):19–26. [published correction appears in Alcohol 36(2):137–138, 2005. [ PubMed ] [ Google Scholar ]
- Grant BF, Stinson FS, Dawson DA, et al. Prevalence and co-occurrence of substance use disorders and independent mood and anxiety disorders: Results from the National Epidemiologic Survey on Alcohol and Related Conditions. Archives of General Psychiatry. 2004; 61 (8):807–816. [ PubMed ] [ Google Scholar ]
- Hasin DS, Stinson FS, Ogburn E, Grant BF. Prevalence, correlates, disability, and comorbidity of DSM-IV alcohol abuse and dependence in the United States: Results from the National Epidemiologic Survey on Alcohol and Related Conditions. Archives of General Psychiatry. 2007; 64 (7):830–842. [ PubMed ] [ Google Scholar ]
- Humphreys K, Weingardt KR, Horst D, et al. Prevalence and predictors of research participant eligibility criteria in alcohol treatment outcome studies, 1970–98. Addiction. 2005; 100 (9):1249–1257. [ PubMed ] [ Google Scholar ]
- Johnson BA, Ait-Daoud N, Bowden CL, et al. Oral topiramate for treatment of alcohol dependence: A randomized controlled trial. Lancet. 2003; 361 (9370):1677–1685. [ PubMed ] [ Google Scholar ]
- Johnson BA, Rosenthal N, Capece JA, et al. Topiramate for treating alcohol dependence: A randomized controlled trial. JAMA: Journal of the American Medical Association. 2007; 298 (14):1641–1651. [ PubMed ] [ Google Scholar ]
- Johnson BA. Update on neuropharmacological treatments for alcoholism: Scientific basis and clinical findings. Biochemical Pharmacology. 2007; 75 (1):34–56. [ PMC free article ] [ PubMed ] [ Google Scholar ]
- Koob GF. The neurobiology of addiction: A neuroadaptational view relevant for diagnosis. Addiction. 2006; 101 (Suppl. 1):23–30. [ PubMed ] [ Google Scholar ]
- Kranzler HR, Van Kirk J. Efficacy of naltrexone and acamprosate for alcoholism treatment: A meta-analysis. Alcoholism: Clinical and Experimental Research. 2001; 25 (9):1335–1341. [ PubMed ] [ Google Scholar ]
- Ledoux T, Barnett MD, Garcini LM, Baker J. Predictors of recent mental health service use in a medical population: Implications for integrated care. Journal of Clinical Psychology in Medical Settings. 2009; 16 (4):304–310. [ PubMed ] [ Google Scholar ]
- Mann K, Lehert P, Morgan MY. The efficacy of acamprosate in the maintenance of abstinence in alcohol-dependent individuals: Results of a meta-analysis. Alcoholism: Clinical and Experimental Research. 2004; 28 (1):51–63. [ PubMed ] [ Google Scholar ]
- Mark TL, Kranzler HR, Song X, et al. Physicians’ opinions about medications to treat alcoholism. Addiction. 2003; 98 (5):617–626. [ PubMed ] [ Google Scholar ]
- McLellan AT, Meyers K. Contemporary addiction treatment: A review of systems problems for adults and adolescents. Biological Psychiatry. 2004; 56 :764–770. [ PubMed ] [ Google Scholar ]
- Miller W, editor. Combine Monograph Series, Vol. 1: Combined Behavioral Intervention Manual: A Clinical Research Guide for Therapists Treating People with Alcohol Abuse and Dependence. Bethesda, MD: NIAAA; (DHHS Publication no. [NIH] 04–5288). [ Google Scholar ]
- Miller WR, Rollnick S. Motivational Interviewing: Preparing People for Change. New York: Guilford Press; 2002. [ Google Scholar ]
- Miller WR, Walters ST, Bennett ME. How effective is alcoholism treatment in the United States? Journal of Studies on Alcohol. 2001; 62 (2):211–220. [ PubMed ] [ Google Scholar ]
- Moss HB, Chen CM, Yi HY. Subtypes of alcohol dependence in a nationally representative sample. Drug and Alcohol Dependence. 2007; 91 (2–3):149–158. [ PMC free article ] [ PubMed ] [ Google Scholar ]
- National Institute on Alcohol Abuse and Alcoholism . Helping Patients Who Drink too Much: A Clinician’s Guide. Rockville, MD: NIAAA; 2007. (NIH Publication no. 07–3769). Available at: http://pubs.niaaa.nih.gov/publications/Practitioner/CliniciansGuide2005/guide.pdf . [ Google Scholar ]
- O’Farrell TJ, Allen JP, Litten RZ. Disulfiram (Antabuse) Contracts in Treatment of Alcoholism. Rockville, MD: National Institute on Drug Abuse; 1995. (National Institute on Drug Abuse Research Monograph no. 150:65–91). [ PubMed ] [ Google Scholar ]
- O’Leary D, Hickey T, Lagendijk M, Webb M. Onset of remission and relapse in depression: Testing operational criteria through course description in a second Dublin cohort of first-admission participants. Journal of Affective Disorders. 2010 (Epub ahead of print). [ PubMed ] [ Google Scholar ]
- O’Malley SS. Naltrexone and Alcoholism Treatment: Treatment Improvement Protocol (TIP) Series 28. Rockville, MD: Substance Abuse and Mental Health Services Administration, Center for Substance Abuse Treatment; 1998. (DHHS Publication no. SMA–98–3206). [ PubMed ] [ Google Scholar ]
- Orford J, Hodgson R, Copello A, et al. The clients’ perspective on change during treatment for an alcohol problem: Qualitative analysis of follow-up interviews in the UK Alcohol Treatment Trial. Addiction. 2006; 101 (1):60–68. [ PubMed ] [ Google Scholar ]
- Penberthy JK, Ait-Daoud N, Breton M, et al. Evaluating readiness and treatment seeking effects in a pharmacotherapy trial for alcohol dependence. Alcoholism: Clinical and Experimental Research. 2007; 31 (9):1538–1544. [ PubMed ] [ Google Scholar ]
- Project MATCH Research Group Matching alcoholism treatments to client heterogeneity: Project MATCH three-year drinking outcomes. Alcoholism: Clinical and Experimental Research. 1998; 22 (6):1300–1311. [ PubMed ] [ Google Scholar ]
- Smith JE, Myers RJ. Motivating Substance Abusers to Enter Treatment. New York: Guilford Press; 2004. [ Google Scholar ]
- Srisurapanont M, Jarusuraisin N. Opioid antagonists for alcohol dependence. Cochrane Database System Reviews. 2005; 25 (1):CD001867. [ PubMed ] [ Google Scholar ]
- Vaillant GE. A 60-year follow-up of alcoholic men. Addiction. 2003; 98 (8):1043–1051. [ PubMed ] [ Google Scholar ]
- Volpicelli JR, Alterman AI, Hayashida M, O’Brien CP. Naltrexone in the treatment of alcohol dependence. Archives of General Psychiatry. 1992; 49 (11):876–880. [ PubMed ] [ Google Scholar ]
- Whitlock EP, Polen MR, Green CA, et al. Behavioral counseling interventions in primary care to reduce risky/harmful alcohol use by adults: A summary of the evidence for the U.S. Preventive Services Task Force. Annals of Internal Medicine. 2004; 140 (7):557–568. [ PubMed ] [ Google Scholar ]

IMAGES
COMMENTS
Alcohol use has negative effects on HIV disease progression through several mechanisms, including transmission, viral replication, host immunity, and treatment efficacy.
The findings of this paper recommend that daily consultation is suggested to reduce the addiction to alcohol drinking and enhance students' work rate in their academic success.
The results of several research studies conducted in various settings clearly indicate that increased intake of alcohol has increased adverse effects on our heart and its vasculature. Alcohol exerts its action on the cardiovascular system both directly and indirectly.
This paper examines the effects of alcohol use on high school students’ quality of learning. We estimate fixed-effects models using data from the National Longitudinal Study of Adolescent Health. Our primary measure of academic achievement is the student’s GPA abstracted from official school transcripts.
Jill Coyman. This study examines college students’ alcohol use and how it affects their academic performance, specifically looking at grade point average (GPA). An online survey was given out to male and female students at the University of New Hampshire.
Alcohol, Clinical and Experimental Research is a multi-disciplinary journal providing direct access to the most significant and current research findings on the nature and management of alcoholism and alcohol-related disorders.
Psychosocial theories of alcohol abuse can be utilized to identify and contextualize trends in major treatment approaches for the people of alcohol abuse and also provide possible future ...
1.1 Alcohol in the context of the United Nations 2030 Agenda for Sustainable Development..... 2 1.2 Alcohol and SDG 2030 health targets..... 3 1.2.1 Reproductive, maternal, newborn, child and adolescent health..... 5
We investigated the associations of alcohol consumption with 207 diseases in the 12-year China Kadoorie Biobank of >512,000 adults (41% men), including 168,050 genotyped for ALDH2 - rs671 and...
Future research, coupled with a restructured treatment system capable of making new scientific findings rapidly available to the community, hold the key to significantly improving treatment outcomes and reducing suffering from alcohol-related disorders.