Thank you for visiting nature.com. You are using a browser version with limited support for CSS. To obtain the best experience, we recommend you use a more up to date browser (or turn off compatibility mode in Internet Explorer). In the meantime, to ensure continued support, we are displaying the site without styles and JavaScript.
- View all journals
- Explore content
- About the journal
- Publish with us
- Sign up for alerts
- Open access
- Published: 01 July 2020

The effect of social media on well-being differs from adolescent to adolescent
- Ine Beyens ORCID: orcid.org/0000-0001-7023-867X 1 ,
- J. Loes Pouwels ORCID: orcid.org/0000-0002-9586-392X 1 ,
- Irene I. van Driel ORCID: orcid.org/0000-0002-7810-9677 1 ,
- Loes Keijsers ORCID: orcid.org/0000-0001-8580-6000 2 &
- Patti M. Valkenburg ORCID: orcid.org/0000-0003-0477-8429 1
Scientific Reports volume 10 , Article number: 10763 ( 2020 ) Cite this article
129k Accesses
197 Citations
127 Altmetric
Metrics details
- Human behaviour
The question whether social media use benefits or undermines adolescents’ well-being is an important societal concern. Previous empirical studies have mostly established across-the-board effects among (sub)populations of adolescents. As a result, it is still an open question whether the effects are unique for each individual adolescent. We sampled adolescents’ experiences six times per day for one week to quantify differences in their susceptibility to the effects of social media on their momentary affective well-being. Rigorous analyses of 2,155 real-time assessments showed that the association between social media use and affective well-being differs strongly across adolescents: While 44% did not feel better or worse after passive social media use, 46% felt better, and 10% felt worse. Our results imply that person-specific effects can no longer be ignored in research, as well as in prevention and intervention programs.
Similar content being viewed by others
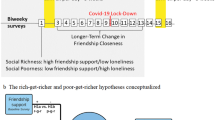
Some socially poor but also some socially rich adolescents feel closer to their friends after using social media
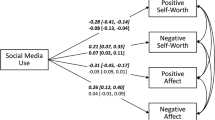
Associations between youth’s daily social media use and well-being are mediated by upward comparisons
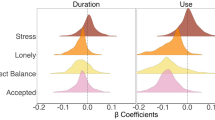
Variation in social media sensitivity across people and contexts
Introduction.
Ever since the introduction of social media, such as Facebook and Instagram, researchers have been studying whether the use of such media may affect adolescents’ well-being. These studies have typically reported mixed findings, yielding either small negative, small positive, or no effects of the time spent using social media on different indicators of well-being, such as life satisfaction and depressive symptoms (for recent reviews, see for example 1 , 2 , 3 , 4 , 5 ). Most of these studies have focused on between-person associations, examining whether adolescents who use social media more (or less) often than their peers experience lower (or higher) levels of well-being than these peers. While such between-person studies are valuable in their own right, several scholars 6 , 7 have recently called for studies that investigate within-person associations to understand whether an increase in an adolescent’s social media use is associated with an increase or decrease in that adolescent’s well-being. The current study aims to respond to this call by investigating associations between social media use and well-being within single adolescents across multiple points in time 8 , 9 , 10 .
Person-specific effects
To our knowledge, four recent studies have investigated within-person associations of social media use with different indicators of adolescent well-being (i.e., life satisfaction, depression), again with mixed results 6 , 11 , 12 , 13 . Orben and colleagues 6 found a small negative reciprocal within-person association between the time spent using social media and life satisfaction. Likewise, Boers and colleagues 12 found a small within-person association between social media use and increased depressive symptoms. Finally, Coyne and colleagues 11 and Jensen and colleagues 13 did not find any evidence for within-person associations between social media use and depression.
Earlier studies that investigated within-person associations of social media use with indicators of well-being have all only reported average effect sizes. However, it is possible, or even plausible, that these average within-person effects may have been small and nonsignificant because they result from sizeable heterogeneity in adolescents’ susceptibility to the effects of social media use on well-being (see 14 , 15 ). After all, an average within-person effect size can be considered an aggregate of numerous individual within-person effect sizes that range from highly positive to highly negative.
Some within-person studies have sought to understand adolescents’ differential susceptibility to the effects of social media by investigating differences between subgroups. For instance, they have investigated the moderating role of sex to compare the effects of social media on boys versus girls 6 , 11 . However, such a group-differential approach, in which potential differences in susceptibility are conceptualized by group-level moderators (e.g., gender, age) does not provide insights into more fine-grained differences at the level of the single individual 16 . After all, while girls and boys each represent a homogenous group in terms of sex, they may each differ on a wide array of other factors.
As such, although worthwhile, the average within-person effects of social media on well-being obtained in previous studies may have been small or non-significant because they are diluted across a highly heterogeneous population (or sub-population) of adolescents 14 , 15 . In line with the proposition of media effects theories that each adolescent may have a unique susceptibility to the effects of social media 17 , a viable explanation for the small and inconsistent findings in earlier studies may be that the effect of social media differs from adolescent to adolescent. The aim of the current study is to investigate this hypothesis and to obtain a better understanding of adolescents’ unique susceptibility to the effects of social media on their affective well-being.
Social media and affective well-being
Within-person studies have provided important insights into the associations of social media use with cognitive well-being (e.g., life satisfaction 6 ), which refers to adolescents’ cognitive judgment of how satisfied they are with their life 18 . However, the associations of social media use with adolescents’ affective well-being (i.e., adolescents’ affective evaluations of their moods and emotions 18 ) are still unknown. In addition, while earlier within-person studies have focused on associations with trait-like conceptualizations of well-being 11 , 12 , 13 , that is, adolescents’ average well-being across specific time periods 18 , there is a lack of studies that focus on well-being as a momentary affective state. Therefore, we extend previous research by examining the association between adolescents’ social media use and their momentary affective well-being. Like earlier experience sampling (ESM) studies among adults 19 , 20 , we measured adolescents’ momentary affective well-being with a single item. Adolescents’ momentary affective well-being was defined as their current feelings of happiness, a commonly used question to measure well-being 21 , 22 , which has high convergent validity, as evidenced by the strong correlations with the presence of positive affect and absence of negative affect.
To assess adolescents’ momentary affective well-being (henceforth referred to as well-being), we conducted a week-long ESM study among 63 middle adolescents ages 14 and 15. Six times a day, adolescents were asked to complete a survey using their own mobile phone, covering 42 assessments per adolescent, assessing their affective well-being and social media use. In total, adolescents completed 2,155 assessments (83.2% average compliance).
We focused on middle adolescence, since this is the period in life characterized by most significant fluctuations in well-being 23 , 24 . Also, in comparison to early and late adolescents, middle adolescents are more sensitive to reactions from peers and have a strong tendency to compare themselves with others on social media and beyond. Because middle adolescents typically use different social media platforms, in a complementary way 25 , 26 , 27 , each adolescent reported on his/her use of the three social media platforms that s/he used most frequently out of the five most popular social media platforms among adolescents: WhatsApp, followed by Instagram, Snapchat, YouTube, and, finally, the chat function of games 28 . In addition to investigating the association between overall social media use and well-being (i.e., the summed use of adolescents’ three most frequently used platforms), we examined the unique associations of the two most popular platforms, WhatsApp and Instagram 28 .
Like previous studies on social media use and well-being, we distinguished between active social media use (i.e., “activities that facilitate direct exchanges with others” 29 ) and passive social media use (i.e., “consuming information without direct exchanges” 29 ). Within-person studies among young adults have shown that passive but not active social media use predicts decreases in well-being 29 . Therefore, we examined the unique associations of adolescents’ overall active and passive social media use with their well-being, as well as active and passive use of Instagram and WhatsApp, specifically. We investigated categorical associations, that is, whether adolescents would feel better or worse if they had actively or passively used social media. And we investigated dose–response associations to understand whether adolescents’ well-being would change as a function of the time they had spent actively or passively using social media.
The hypotheses and the design, sampling and analysis plan were preregistered prior to data collection and are available on the Open Science Framework, along with the code used in the analyses ( https://osf.io/nhks2 ). For details about the design of the study and analysis approach, see Methods.
In more than half of all assessments (68.17%), adolescents had used social media (i.e., one or more of their three favorite social media platforms), either in an active or passive way. Instagram (50.90%) and WhatsApp (53.52%) were used in half of all assessments. Passive use of social media (66.21% of all assessments) was more common than active use (50.86%), both on Instagram (48.48% vs. 20.79%) and WhatsApp (51.25% vs. 40.07%).
Strong positive between-person correlations were found between the duration of active and passive social media use (overall: r = 0.69, p < 0.001; Instagram: r = 0.38, p < 0.01; WhatsApp: r = 0.85, p < 0.001): Adolescents who had spent more time actively using social media than their peers, had also spent more time passively using social media than their peers. Likewise, strong positive within-person correlations were found between the duration of active and passive social media use (overall: r = 0.63, p < 0.001; Instagram: r = 0.37, p < 0.001; WhatsApp: r = 0.57, p < 0.001): The more time an adolescent had spent actively using social media at a certain moment, the more time s/he had also spent passively using social media at that moment.
Table 1 displays the average number of minutes that adolescents had spent using social media in the past hour at each assessment, and the zero-order between- and within-person correlations between the duration of social media use and well-being. At the between-person level, the duration of active and passive social media use was not associated with well-being: Adolescents who had spent more time actively or passively using social media than their peers did not report significantly higher or lower levels of well-being than their peers. At the within-person level, significant but weak positive correlations were found between the duration of active and passive overall social media use and well-being. This indicates that adolescents felt somewhat better at moments when they had spent more time actively or passively using social media (overall), compared to moments when they had spent less time actively or passively using social media. When looking at specific platforms, a positive correlation was only found for passive WhatsApp use, but not for active WhatsApp use, and not for active and passive Instagram use.
Average and person-specific effects
The within-person associations of social media use with well-being and differences in these associations were tested in a series of multilevel models. We ran separate models for overall social media use (i.e., active use and passive use of adolescents’ three favorite social media platforms, see Table 2 ), Instagram use (see Table 3 ), and WhatsApp use (see Table 4 ). In a first step we examined the average categorical associations for each of these three social media uses using fixed effects models (Models 1A, 3A, and 5A) to investigate whether, on average, adolescents would feel better or worse at moments when they had used social media compared to moments when they had not (i.e., categorical predictors: active use versus no active use, and passive use versus no passive use). In a second step, we examined heterogeneity in the within-person categorical associations by adding random slopes to the fixed effects models (Models 1B, 3B, and 5B). Next, we examined the average dose–response associations using fixed effects models (Models 2A, 4A, and 6A), to investigate whether, on average, adolescents would feel better or worse when they had spent more time using social media (i.e., continuous predictors: duration of active use and duration of passive use). Finally, we examined heterogeneity in the within-person dose–response associations by adding random slopes to the fixed effects models (Models 2B, 4B, and 6B).
Overall social media use.
The model with the categorical predictors (see Table 2 ; Model 1A) showed that, on average, there was no association between overall use and well-being: Adolescents’ well-being did not increase or decrease at moments when they had used social media, either in a passive or active way. However, evidence was found that the association of passive (but not active) social media use with well-being differed from adolescent to adolescent (Model 1B), with effect sizes ranging from − 0.24 to 0.68. For 44.26% of the adolescents the association was non-existent to small (− 0.10 < r < 0.10). However, for 45.90% of the adolescents there was a weak (0.10 < r < 0.20; 8.20%), moderate (0.20 < r < 0.30; 22.95%) or even strong positive ( r ≥ 0.30; 14.75%) association between overall passive social media use and well-being, and for almost one in ten (9.84%) adolescents there was a weak (− 0.20 < r < − 0.10; 6.56%) or moderate negative (− 0.30 < r < − 0.20; 3.28%) association.
The model with continuous predictors (Model 2A) showed that, on average, there was a significant dose–response association for active use. At moments when adolescents had used social media, the time they spent actively (but not passively) using social media was positively associated with well-being: Adolescents felt better at moments when they had spent more time sending messages, posting, or sharing something on social media. The associations of the time spent actively and passively using social media with well-being did not differ across adolescents (Model 2B).
Instagram use
As shown in Model 3A in Table 3 , on average, there was a significant categorical association between passive (but not active) Instagram use and well-being: Adolescents experienced an increase in well-being at moments when they had passively used Instagram (i.e., viewing posts/stories of others). Adolescents did not experience an increase or decrease in well-being when they had actively used Instagram. The associations of passive and active Instagram use with well-being did not differ across adolescents (Model 3B).
On average, no significant dose–response association was found for Instagram use (Model 4A): At moments when adolescents had used Instagram, the time adolescents spent using Instagram (either actively or passively) was not associated with their well-being. However, evidence was found that the association of the time spent passively using Instagram differed from adolescent to adolescent (Model 4B), with effect sizes ranging from − 0.48 to 0.27. For most adolescents (73.91%) the association was non-existent to small (− 0.10 < r < 0.10), but for almost one in five adolescents (17.39%) there was a weak (0.10 < r < 0.20; 10.87%) or moderate (0.20 < r < 0.30; 6.52%) positive association, and for almost one in ten adolescents (8.70%) there was a weak (− 0.20 < r < − 0.10; 2.17%), moderate (− 0.30 < r < − 0.20; 4.35%), or strong ( r ≤ − 0.30; 2.17%) negative association. Figure 1 illustrates these differences in the dose–response associations.
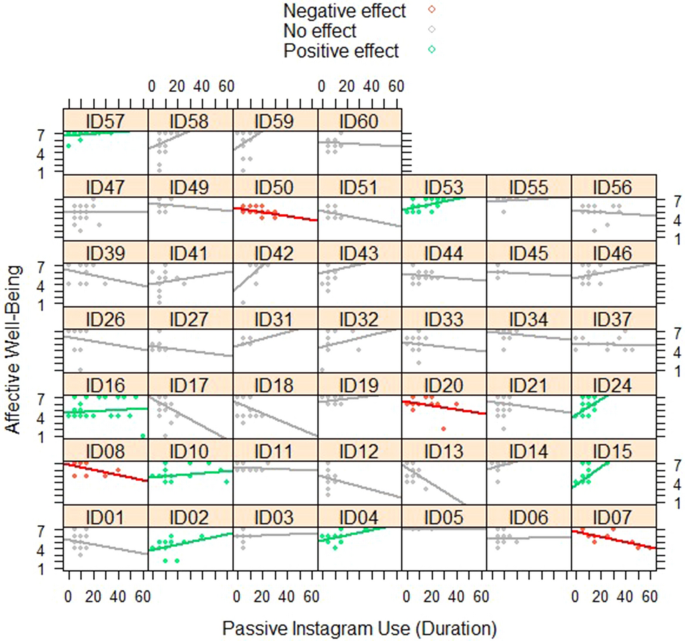
The dose–response association between passive Instagram use (in minutes per hour) and affective well-being for each individual adolescent (n = 46). Red lines represent significant negative within-person associations, green lines represent significant positive within-person associations, and gray lines represent non-significant within-person associations. A graph was created for each participant who had completed at least 10 assessments. A total of 13 participants were excluded because they had completed less than 10 assessments of passive Instagram use. In addition, one participant was excluded because no graph could be computed, since this participant's passive Instagram use was constant across assessments.
WhatsApp use
As shown in Model 5A in Table 4 , just as for Instagram, we found that, on average, there was a significant categorical association between passive (but not active) WhatsApp use and well-being: Adolescents reported that they felt better at moments when they had passively used WhatsApp (i.e., read WhatsApp messages). For active WhatsApp use, no significant association was found. Also, in line with the results for Instagram use, no differences were found regarding the associations of active and passive WhatsApp use (Model 5B).
In addition, a significant dose–response association was found for passive (but not active) use (Model 6A). At moments when adolescents had used WhatsApp, we found that, on average, the time adolescents spent passively using WhatsApp was positively associated with well-being: Adolescents felt better at moments when they had spent more time reading WhatsApp messages. The time spent actively using WhatsApp was not associated with well-being. No differences were found in the dose–response associations of active and passive WhatsApp use (Model 6B).
This preregistered study investigated adolescents’ unique susceptibility to the effects of social media. We found that the associations of passive (but not active) social media use with well-being differed substantially from adolescent to adolescent, with effect sizes ranging from moderately negative (− 0.24) to strongly positive (0.68). While 44.26% of adolescents did not feel better or worse if they had passively used social media, 45.90% felt better, and a small group felt worse (9.84%). In addition, for Instagram the majority of adolescents (73.91%) did not feel better or worse when they had spent more time viewing post or stories of others, whereas some felt better (17.39%), and others (8.70%) felt worse.
These findings have important implications for social media effects research, and media effects research more generally. For decades, researchers have argued that people differ in their susceptibility to the effects of media 17 , leading to numerous investigations of such differential susceptibility. These investigations have typically focused on moderators, based on variables such as sex, age, or personality. Yet, over the years, studies have shown that such moderators appear to have little power to explain how individuals differ in their susceptibility to media effects, probably because a group-differential approach does not account for the possibility that media users may differ across a range of factors, that are not captured by only one (or a few) investigated moderator variables.
By providing insights into each individual’s unique susceptibility, the findings of this study provide an explanation as to why, up until now, most media effects research has only found small effects. We found that the majority of adolescents do not experience any short-term changes in well-being related to their social media use. And if they do experience any changes, these are more often positive than negative. Because only small subsets of adolescents experience small to moderate changes in well-being, the true effects of social media reported in previous studies have probably been diluted across heterogeneous samples of individuals that differ in their susceptibility to media effects (also see 30 ). Several scholars have noted that overall effect sizes may mask more subtle individual differences 14 , 15 , which may explain why previous studies have typically reported small or no effects of social media on well-being or indicators of well-being 6 , 11 , 12 , 13 . The current study seems to confirm this assumption, by showing that while the overall effect sizes are small at best, the person-specific effect sizes vary considerably, from tiny and small to moderate and strong.
As called upon by other scholars 5 , 31 , we disentangled the associations of active and passive use of social media. Research among young adults found that passive (but not active) social media use is associated with lower levels of affective well-being 29 . In line with these findings, the current study shows that active and passive use yielded different associations with adolescents’ affective well-being. Interestingly though, in contrast to previous findings among adults, our study showed that, on average, passive use of Instagram and WhatsApp seemed to enhance rather than decrease adolescents’ well-being. This discrepancy in findings may be attributed to the fact that different mechanisms might be involved. Verduyn and colleagues 29 found that passive use of Facebook undermines adults’ well-being by enhancing envy, which may also explain the decreases in well-being found in our study among a small group of adolescents. Yet, adolescents who felt better by passively using Instagram and WhatsApp, might have felt so because they experienced enjoyment. After all, adolescents often seek positive content on social media, such as humorous posts or memes 32 . Also, research has shown that adolescents mainly receive positive feedback on social media 33 . Hence, their passive Instagram and WhatsApp use may involve the reading of positive feedback, which may explain the increases in well-being.
Overall, the time spent passively using WhatsApp improved adolescents’ well-being. This did not differ from adolescent to adolescent. However, the associations of the time spent passively using Instagram with well-being did differ from adolescent to adolescent. This discrepancy suggests that not all social media uses yield person-specific effects on well-being. A possible explanation may be that adolescents’ responses to WhatsApp are more homogenous than those to Instagram. WhatsApp is a more private platform, which is mostly used for one-to-one communication with friends and acquaintances 26 . Instagram, in contrast, is a more public platform, which allows its users to follow a diverse set of people, ranging from best friends to singers, actors, and influencers 28 , and to engage in intimate communication as well as self-presentation and social comparison. Such diverse uses could lead to more varied, or even opposing responses, such as envy versus inspiration.
Limitations and directions for future research
The current study extends our understanding of differential susceptibility to media effects, by revealing that the effect of social media use on well-being differs from adolescent to adolescent. The findings confirm our assumption that among the great majority of adolescents, social media use is unrelated to well-being, but that among a small subset, social media use is either related to decreases or increases in well-being. It must be noted, however, that participants in this study felt relatively happy, overall. Studies with more vulnerable samples, consisting of clinical samples or youth with lower social-emotional well-being may elicit different patterns of effects 27 . Also, the current study focused on affective well-being, operationalized as happiness. It is plausible that social media use relates differently with other types of well-being, such as cognitive well-being. An important next step is to identify which adolescents are particularly susceptible to experience declines in well-being. It is conceivable, for instance, that the few adolescents who feel worse when they use social media are the ones who receive negative feedback on social media 33 .
In addition, future ESM studies into the effects of social media should attempt to include one or more follow-up measures to improve our knowledge of the longer-term influence of social media use on affective well-being. While a week-long ESM is very common and applied in most earlier ESM studies 34 , a week is only a snapshot of adolescent development. Research is needed that investigates whether the associations of social media use with adolescents’ momentary affective well-being may cumulate into long-lasting consequences. Such investigations could help clarify whether adolescents who feel bad in the short term would experience more negative consequences in the long term, and whether adolescents who feel better would be more resistant to developing long-term negative consequences. And while most adolescents do not seem to experience any short-term increases or decreases in well-being, more research is needed to investigate whether these adolescents may experience a longer-term impact of social media.
While the use of different platforms may be differently associated with well-being, different types of use may also yield different effects. Although the current study distinguished between active and passive use of social media, future research should further differentiate between different activities. For instance, because passive use entails many different activities, from reading private messages (e.g., WhatsApp messages, direct messages on Instagram) to browsing a public feed (e.g., scrolling through posts on Instagram), research is needed that explores the unique effects of passive public use and passive private use. Research that seeks to explore the nuances in adolescents’ susceptibility as well as the nuances in their social media use may truly improve our understanding of the effects of social media use.
Participants
Participants were recruited via a secondary school in the south of the Netherlands. Our preregistered sampling plan set a target sample size of 100 adolescents. We invited adolescents from six classrooms to participate in the study. The final sample consisted of 63 adolescents (i.e., 42% consent rate, which is comparable to other ESM studies among adolescents; see, for instance 35 , 36 ). Informed consent was obtained from all participants and their parents. On average, participants were 15 years old ( M = 15.12 years, SD = 0.51) and 54% were girls. All participants self-identified as Dutch, and 41.3% were enrolled in the prevocational secondary education track, 25.4% in the intermediate general secondary education track, and 33.3% in the academic preparatory education track.
The study was approved by the Ethics Review Board of the Faculty of Social and Behavioral Sciences at the University of Amsterdam and was performed in accordance with the guidelines formulated by the Ethics Review Board. The study consisted of two phases: A baseline survey and a personalized week-long experience sampling (ESM) study. In phase 1, researchers visited the school during school hours. Researchers informed the participants of the objective and procedure of the study and assured them that their responses would be treated confidentially. Participants were asked to sign the consent form. Next, participants completed a 15-min baseline survey. The baseline survey included questions about demographics and assessed which social media each adolescent used most frequently, allowing to personalize the social media questions presented during the ESM study in phase 2. After completing the baseline survey, participants were provided detailed instructions about phase 2.
In phase 2, which took place two and a half weeks after the baseline survey, a 7-day ESM study was conducted, following the guidelines for ESM studies provided by van Roekel and colleagues 34 . Aiming for at least 30 assessments per participant and based on an average compliance rate of 70 to 80% reported in earlier ESM studies among adolescents 34 , we asked each participant to complete a total of 42 ESM surveys (i.e., six 2-min surveys per day). Participants completed the surveys using their own mobile phone, on which the ESM software application Ethica Data was installed during the instruction session with the researchers (phase 1). Each 2-min survey consisted of 22 questions, which assessed adolescents’ well-being and social media use. Two open-ended questions were added to the final survey of the day, which asked about adolescents’ most pleasant and most unpleasant events of the day.
The ESM sampling scheme was semi-random, to allow for randomization and avoid structural patterns in well-being, while taking into account that adolescents were not allowed to use their phone during school time. The Ethica Data app was programmed to generate six beep notifications per day at random time points within a fixed time interval that was tailored to the school’s schedule: before school time (1 beep), during school breaks (2 beeps), and after school time (3 beeps). During the weekend, the beeps were generated during the morning (1 beep), afternoon (3 beeps), and evening (2 beeps). To maximize compliance, a 30-min time window was provided to complete each survey. This time window was extended to one hour for the first survey (morning) and two hours for the final survey (evening) to account for travel time to school and time spent on evening activities. The average compliance rate was 83.2%. A total of 2,155 ESM assessments were collected: Participants completed an average of 34.83 surveys ( SD = 4.91) on a total of 42 surveys, which is high compared to previous ESM studies among adolescents 34 .
The questions of the ESM study were personalized based on the responses to the baseline survey. During the ESM study, each participant reported on his/her use of three different social media platforms: WhatsApp and either Instagram, Snapchat, YouTube, and/or the chat function of games (i.e., the most popular social media platforms among adolescents 28 ). Questions about Instagram and WhatsApp use were only included if the participant had indicated in the baseline survey that s/he used these platforms at least once a week. If a participant had indicated that s/he used Instagram or WhatsApp (or both) less than once a week, s/he was asked to report on the use of Snapchat, YouTube, or the chat function of games, depending on what platform s/he used at least once a week. In addition to Instagram and WhatsApp, questions were asked about a third platform, that was selected based on how frequently the participant used Snapchat, YouTube, or the chat function of games (i.e., at least once a week). This resulted in five different combinations of three platforms: Instagram, WhatsApp, and Snapchat (47 participants); Instagram, WhatsApp, and YouTube (11 participants); Instagram, WhatsApp, and chatting via games (2 participants); WhatsApp, Snapchat, and YouTube (1 participant); and WhatsApp, YouTube, and chatting via games (2 participants).
Frequency of social media use
In the baseline survey, participants were asked to indicate how often they used and checked Instagram, WhatsApp, Snapchat, YouTube, and the chat function of games, using response options ranging from 1 ( never ) to 7 ( more than 12 times per day ). These platforms are the five most popular platforms among Dutch 14- and 15-year-olds 28 . Participants’ responses were used to select the three social media platforms that were assessed in the personalized ESM study.
Duration of social media use
In the ESM study, duration of active and passive social media use was measured by asking participants how much time in the past hour they had spent actively and passively using each of the three platforms that were included in the personalized ESM surveys. Response options ranged from 0 to 60 min , with 5-min intervals. To measure active Instagram use, participants indicated how much time in the past hour they had spent (a) “posting on your feed or sharing something in your story on Instagram” and (b) “sending direct messages/chatting on Instagram.” These two items were summed to create the variable duration of active Instagram use. Sum scores exceeding 60 min (only 0.52% of all assessments) were recoded to 60 min. To measure duration of passive Instagram use, participants indicated how much time in the past hour they had spent “viewing posts/stories of others on Instagram.” To measure the use of WhatsApp, Snapchat, YouTube and game-based chatting, we asked participants how much time they had spent “sending WhatsApp messages” (active use) and “reading WhatsApp messages” (passive use); “sending snaps/messages or sharing something in your story on Snapchat” (active use) and “viewing snaps/stories/messages from others on Snapchat” (passive use); “posting YouTube clips” (active use) and “watching YouTube clips” (passive use); “sending messages via the chat function of a game/games” (active use) and “reading messages via the chat function of a game/games” (passive use). Duration of active and passive overall social media use were created by summing the responses across the three social media platforms for active and passive use, respectively. Sum scores exceeding 60 min (2.13% of all assessments for active overall use; 2.90% for passive overall use) were recoded to 60 min. The duration variables were used to investigate whether the time spent actively or passively using social media was associated with well-being (dose–response associations).
Use/no use of social media
Based on the duration variables, we created six dummy variables, one for active and one for passive overall social media use, one for active and one for passive Instagram use, and one for active and one for passive WhatsApp use (0 = no active use and 1 = active use , and 0 = no passive use and 1 = passive use , respectively). These dummy variables were used to investigate whether the use of social media, irrespective of the duration of use, was associated with well-being (categorical associations).
Consistent with previous ESM studies 19 , 20 , we measured affective well-being using one item, asking “How happy do you feel right now?” at each assessment. Adolescents indicated their response to the question using a 7-point scale ranging from 1 ( not at all ) to 7 ( completely ), with 4 ( a little ) as the midpoint. Convergent validity of this item was established in a separate pilot ESM study among 30 adolescents conducted by the research team of the fourth author: The affective well-being item was strongly correlated with the presence of positive affect and absence of negative affect (assessed by a 10-item positive and negative affect schedule for children; PANAS-C) at both the between-person (positive affect: r = 0.88, p < 0.001; negative affect: r = − 0.62, p < 0.001) and within-person level (positive affect: r = 0.74, p < 0.001; negative affect: r = − 0.58, p < 0.001).
Statistical analyses
Before conducting the analyses, several validation checks were performed (see 34 ). First, we aimed to only include participants in the analyses who had completed more than 33% of all ESM assessments (i.e., at least 14 assessments). Next, we screened participants’ responses to the open questions for unserious responses (e.g., gross comments, jokes). And finally, we inspected time series plots for patterns in answering tendencies. Since all participants completed more than 33% of all ESM assessments, and no inappropriate responses or low-quality data patterns were detected, all participants were included in the analyses.
Following our preregistered analysis plan, we tested the proposed associations in a series of multilevel models. Before doing so, we tested the homoscedasticity and linearity assumptions for multilevel analyses 37 . Inspection of standardized residual plots indicated that the data met these assumptions (plots are available on OSF at https://osf.io/nhks2 ). We specified separate models for overall social media use, use of Instagram, and use of WhatsApp. To investigate to what extent adolescents’ well-being would vary depending on whether they had actively or passively used social media/Instagram/WhatsApp or not during the past hour (categorical associations), we tested models including the dummy variables as predictors (active use versus no active use, and passive use versus no passive use; models 1, 3, and 5). To investigate whether, at moments when adolescents had used social media/Instagram/WhatsApp during the past hour, their well-being would vary depending on the duration of social media/Instagram/WhatsApp use (dose–response associations), we tested models including the duration variables as predictors (duration of active use and duration of passive use; models 2, 4, and 6). In order to avoid negative skew in the duration variables, we only included assessments during which adolescents had used social media in the past hour (overall, Instagram, or WhatsApp, respectively), either actively or passively. All models included well-being as outcome variable. Since multilevel analyses allow to include all available data for each individual, no missing data were imputed and no data points were excluded.
We used a model building approach that involved three steps. In the first step, we estimated an intercept-only model to assess the relative amount of between- and within-person variance in affective well-being. We estimated a three-level model in which repeated momentary assessments (level 1) were nested within adolescents (level 2), who, in turn, were nested within classrooms (level 3). However, because the between-classroom variance in affective well-being was small (i.e., 0.4% of the variance was explained by differences between classes), we proceeded with estimating two-level (instead of three-level) models, with repeated momentary assessments (level 1) nested within adolescents (level 2).
In the second step, we assessed the within-person associations of well-being with (a) overall active and passive social media use (i.e., the total of the three platforms), (b) active and passive use of Instagram, and (c) active and passive use of WhatsApp, by adding fixed effects to the model (Models 1A-6A). To facilitate the interpretation of the associations and control for the effects of time, a covariate was added that controlled for the n th assessment of the study week (instead of the n th assessment of the day, as preregistered). This so-called detrending is helpful to interpret within-person associations as correlated fluctuations beyond other changes in social media use and well-being 38 . In order to obtain within-person estimates, we person-mean centered all predictors 38 . Significance of the fixed effects was determined using the Wald test.
In the third and final step, we assessed heterogeneity in the within-person associations by adding random slopes to the models (Models 1B-6B). Significance of the random slopes was determined by comparing the fit of the fixed effects model with the fit of the random effects model, by performing the Satorra-Bentler scaled chi-square test 39 and by comparing the Bayesian information criterion (BIC 40 ) and Akaike information criterion (AIC 41 ) of the models. When the random effects model had a significantly better fit than the fixed effects model (i.e., pointing at significant heterogeneity), variance components were inspected to investigate whether heterogeneity existed in the association of either active or passive use. Next, when evidence was found for significant heterogeneity, we computed person-specific effect sizes, based on the random effect models, to investigate what percentages of adolescents experienced better well-being, worse well-being, and no changes in well-being. In line with Keijsers and colleagues 42 we only included participants who had completed at least 10 assessments. In addition, for the dose–response associations, we constructed graphical representations of the person-specific slopes, based on the person-specific effect sizes, using the xyplot function from the lattice package in R 43 .
Three improvements were made to our original preregistered plan. First, rather than estimating the models with multilevel modelling in R 43 , we ran the preregistered models in Mplus 44 . Mplus provides standardized estimates for the fixed effects models, which offers insight into the effect sizes. This allowed us to compare the relative strength of the associations of passive versus active use with well-being. Second, instead of using the maximum likelihood estimator, we used the maximum likelihood estimator with robust standard errors (MLR), which are robust to non-normality. Sensitivity tests, uploaded on OSF ( https://osf.io/nhks2 ), indicated that the results were almost identical across the two software packages and estimation approaches. Third, to improve the interpretation of the results and make the scales of the duration measures of social media use and well-being more comparable, we transformed the social media duration scores (0 to 60 min) into scales running from 0 to 6, so that an increase of 1 unit reflects 10 min of social media use. The model estimates were unaffected by this transformation.
Reporting summary
Further information on the research design is available in the Nature Research Reporting Summary linked to this article.
Data availability
The dataset generated and analysed during the current study is available in Figshare 45 . The preregistration of the design, sampling and analysis plan, and the analysis scripts used to analyse the data for this paper are available online on the Open Science Framework website ( https://osf.io/nhks2 ).
Best, P., Manktelow, R. & Taylor, B. Online communication, social media and adolescent wellbeing: A systematic narrative review. Child Youth Serv. Rev. 41 , 27–36. https://doi.org/10.1016/j.childyouth.2014.03.001 (2014).
Article Google Scholar
James, C. et al. Digital life and youth well-being, social connectedness, empathy, and narcissism. Pediatrics 140 , S71–S75. https://doi.org/10.1542/peds.2016-1758F (2017).
Article PubMed Google Scholar
McCrae, N., Gettings, S. & Purssell, E. Social media and depressive symptoms in childhood and adolescence: A systematic review. Adolesc. Res. Rev. 2 , 315–330. https://doi.org/10.1007/s40894-017-0053-4 (2017).
Sarmiento, I. G. et al. How does social media use relate to adolescents’ internalizing symptoms? Conclusions from a systematic narrative review. Adolesc Res Rev , 1–24, doi:10.1007/s40894-018-0095-2 (2018).
Orben, A. Teenagers, screens and social media: A narrative review of reviews and key studies. Soc. Psychiatry Psychiatr. Epidemiol. https://doi.org/10.1007/s00127-019-01825-4 (2020).
Orben, A., Dienlin, T. & Przybylski, A. K. Social media’s enduring effect on adolescent life satisfaction. Proc. Natl. Acad. Sci. USA 116 , 10226–10228. https://doi.org/10.1073/pnas.1902058116 (2019).
Article CAS PubMed Google Scholar
Whitlock, J. & Masur, P. K. Disentangling the association of screen time with developmental outcomes and well-being: Problems, challenges, and opportunities. JAMA https://doi.org/10.1001/jamapediatrics.2019.3191 (2019).
Hamaker, E. L. In Handbook of Research Methods for Studying Daily Life (eds Mehl, M. R. & Conner, T. S.) 43–61 (Guilford Press, New York, 2012).
Schmiedek, F. & Dirk, J. In The Encyclopedia of Adulthood and Aging (ed. Krauss Whitbourne, S.) 1–6 (Wiley, 2015).
Keijsers, L. & van Roekel, E. In Reframing Adolescent Research (eds Hendry, L. B. & Kloep, M.) (Routledge, 2018).
Coyne, S. M., Rogers, A. A., Zurcher, J. D., Stockdale, L. & Booth, M. Does time spent using social media impact mental health? An eight year longitudinal study. Comput. Hum. Behav. 104 , 106160. https://doi.org/10.1016/j.chb.2019.106160 (2020).
Boers, E., Afzali, M. H., Newton, N. & Conrod, P. Association of screen time and depression in adolescence. JAMA 173 , 853–859. https://doi.org/10.1001/jamapediatrics.2019.1759 (2019).
Jensen, M., George, M. J., Russell, M. R. & Odgers, C. L. Young adolescents’ digital technology use and mental health symptoms: Little evidence of longitudinal or daily linkages. Clin. Psychol. Sci. https://doi.org/10.1177/2167702619859336 (2019).
Valkenburg, P. M. The limited informativeness of meta-analyses of media effects. Perspect. Psychol. Sci. 10 , 680–682. https://doi.org/10.1177/1745691615592237 (2015).
Pearce, L. J. & Field, A. P. The impact of “scary” TV and film on children’s internalizing emotions: A meta-analysis. Hum. Commun.. Res. 42 , 98–121. https://doi.org/10.1111/hcre.12069 (2016).
Howard, M. C. & Hoffman, M. E. Variable-centered, person-centered, and person-specific approaches. Organ. Res. Methods 21 , 846–876. https://doi.org/10.1177/1094428117744021 (2017).
Valkenburg, P. M. & Peter, J. The differential susceptibility to media effects model. J. Commun. 63 , 221–243. https://doi.org/10.1111/jcom.12024 (2013).
Eid, M. & Diener, E. Global judgments of subjective well-being: Situational variability and long-term stability. Soc. Indic. Res. 65 , 245–277. https://doi.org/10.1023/B:SOCI.0000003801.89195.bc (2004).
Kross, E. et al. Facebook use predicts declines in subjective well-being in young adults. PLoS ONE 8 , e69841. https://doi.org/10.1371/journal.pone.0069841 (2013).
Article ADS CAS PubMed PubMed Central Google Scholar
Reissmann, A., Hauser, J., Stollberg, E., Kaunzinger, I. & Lange, K. W. The role of loneliness in emerging adults’ everyday use of facebook—An experience sampling approach. Comput. Hum. Behav. 88 , 47–60. https://doi.org/10.1016/j.chb.2018.06.011 (2018).
Rutledge, R. B., Skandali, N., Dayan, P. & Dolan, R. J. A computational and neural model of momentary subjective well-being. Proc. Natl. Acad. Sci. USA 111 , 12252–12257. https://doi.org/10.1073/pnas.1407535111 (2014).
Article ADS CAS PubMed Google Scholar
Tov, W. In Handbook of Well-being (eds Diener, E.D. et al. ) (DEF Publishers, 2018).
Harter, S. The Construction of the Self: Developmental and Sociocultural Foundations (Guilford Press, New York, 2012).
Steinberg, L. Adolescence . Vol. 9 (McGraw-Hill, 2011).
Rideout, V. & Fox, S. Digital Health Practices, Social Media Use, and Mental Well-being Among Teens and Young Adults in the US (HopeLab, San Francisco, 2018).
Google Scholar
Waterloo, S. F., Baumgartner, S. E., Peter, J. & Valkenburg, P. M. Norms of online expressions of emotion: Comparing Facebook, Twitter, Instagram, and WhatsApp. New Media Soc. 20 , 1813–1831. https://doi.org/10.1177/1461444817707349 (2017).
Article PubMed PubMed Central Google Scholar
Rideout, V. & Robb, M. B. Social Media, Social Life: Teens Reveal their Experiences (Common Sense Media, San Fransico, 2018).
van Driel, I. I., Pouwels, J. L., Beyens, I., Keijsers, L. & Valkenburg, P. M. 'Posting, Scrolling, Chatting & Snapping': Youth (14–15) and Social Media in 2019 (Center for Research on Children, Adolescents, and the Media (CcaM), Universiteit van Amsterdam, 2019).
Verduyn, P. et al. Passive Facebook usage undermines affective well-being: Experimental and longitudinal evidence. J. Exp. Psychol. 144 , 480–488. https://doi.org/10.1037/xge0000057 (2015).
Valkenburg, P. M. & Peter, J. Five challenges for the future of media-effects research. Int. J. Commun. 7 , 197–215 (2013).
Verduyn, P., Ybarra, O., Résibois, M., Jonides, J. & Kross, E. Do social network sites enhance or undermine subjective well-being? A critical review. Soc. Issues Policy Rev. 11 , 274–302. https://doi.org/10.1111/sipr.12033 (2017).
Radovic, A., Gmelin, T., Stein, B. D. & Miller, E. Depressed adolescents’ positive and negative use of social media. J. Adolesc. 55 , 5–15. https://doi.org/10.1016/j.adolescence.2016.12.002 (2017).
Valkenburg, P. M., Peter, J. & Schouten, A. P. Friend networking sites and their relationship to adolescents’ well-being and social self-esteem. Cyberpsychol. Behav. 9 , 584–590. https://doi.org/10.1089/cpb.2006.9.584 (2006).
van Roekel, E., Keijsers, L. & Chung, J. M. A review of current ambulatory assessment studies in adolescent samples and practical recommendations. J. Res. Adolesc. 29 , 560–577. https://doi.org/10.1111/jora.12471 (2019).
van Roekel, E., Scholte, R. H. J., Engels, R. C. M. E., Goossens, L. & Verhagen, M. Loneliness in the daily lives of adolescents: An experience sampling study examining the effects of social contexts. J. Early Adolesc. 35 , 905–930. https://doi.org/10.1177/0272431614547049 (2015).
Neumann, A., van Lier, P. A. C., Frijns, T., Meeus, W. & Koot, H. M. Emotional dynamics in the development of early adolescent psychopathology: A one-year longitudinal Study. J. Abnorm. Child Psychol. 39 , 657–669. https://doi.org/10.1007/s10802-011-9509-3 (2011).
Hox, J., Moerbeek, M. & van de Schoot, R. Multilevel Analysis: Techniques and Applications 3rd edn. (Routledge, London, 2018).
Wang, L. P. & Maxwell, S. E. On disaggregating between-person and within-person effects with longitudinal data using multilevel models. Psychol. Methods 20 , 63–83. https://doi.org/10.1037/met0000030 (2015).
Satorra, A. & Bentler, P. M. Ensuring positiveness of the scaled difference chi-square test statistic. Psychometrika 75 , 243–248. https://doi.org/10.1007/s11336-009-9135-y (2010).
Article MathSciNet PubMed PubMed Central MATH Google Scholar
Schwarz, G. Estimating the dimension of a model. Ann. Stat. 6 , 461–464. https://doi.org/10.1214/aos/1176344136 (1978).
Article MathSciNet MATH Google Scholar
Akaike, H. A new look at the statistical model identification. IEEE Trans. Autom. Control 19 , 716–723. https://doi.org/10.1109/TAC.1974.1100705 (1974).
Article ADS MathSciNet MATH Google Scholar
Keijsers, L. et al. What drives developmental change in adolescent disclosure and maternal knowledge? Heterogeneity in within-family processes. Dev. Psychol. 52 , 2057–2070. https://doi.org/10.1037/dev0000220 (2016).
R Core Team R: A Language and Environment for Statistical Computing. (R Foundation for Statistical Computing, Vienna, 2017).
Muthén, L. K. & Muthén, B. O. Mplus User’s Guide 8th edn. (Muthén & Muthén, Los Angeles, 2017).
Beyens, I., Pouwels, J. L., van Driel, I. I., Keijsers, L. & Valkenburg, P. M. Dataset belonging to Beyens et al. (2020). The effect of social media on well-being differs from adolescent to adolescent. https://doi.org/10.21942/uva.12497990 (2020).
Download references
Acknowledgements
This study was funded by the NWO Spinoza Prize and the Gravitation grant (NWO Grant 024.001.003; Consortium on Individual Development) awarded to P.M.V. by the Dutch Research Council (NWO). Additional funding was received from the VIDI grant (NWO VIDI Grant 452.17.011) awarded to L.K. by the Dutch Research Council (NWO). The authors would like to thank Savannah Boele (Tilburg University) for providing her pilot ESM results.
Author information
Authors and affiliations.
Amsterdam School of Communication Research, University of Amsterdam, 1001 NG, Amsterdam, The Netherlands
Ine Beyens, J. Loes Pouwels, Irene I. van Driel & Patti M. Valkenburg
Department of Developmental Psychology, Tilburg University, 5000 LE, Tilburg, The Netherlands
Loes Keijsers
You can also search for this author in PubMed Google Scholar
Contributions
I.B., J.L.P., I.I.v.D., L.K., and P.M.V. designed the study; I.B., J.L.P., and I.I.v.D. collected the data; I.B., J.L.P., and L.K. analyzed the data; and I.B., J.L.P., I.I.v.D., L.K., and P.M.V. contributed to writing and reviewing the manuscript.
Corresponding author
Correspondence to Ine Beyens .
Ethics declarations
Competing interests.
The authors declare no competing interests.
Additional information
Publisher's note.
Springer Nature remains neutral with regard to jurisdictional claims in published maps and institutional affiliations.
Rights and permissions
Open Access This article is licensed under a Creative Commons Attribution 4.0 International License, which permits use, sharing, adaptation, distribution and reproduction in any medium or format, as long as you give appropriate credit to the original author(s) and the source, provide a link to the Creative Commons license, and indicate if changes were made. The images or other third party material in this article are included in the article’s Creative Commons license, unless indicated otherwise in a credit line to the material. If material is not included in the article’s Creative Commons license and your intended use is not permitted by statutory regulation or exceeds the permitted use, you will need to obtain permission directly from the copyright holder. To view a copy of this license, visit http://creativecommons.org/licenses/by/4.0/ .
Reprints and permissions
About this article
Cite this article.
Beyens, I., Pouwels, J.L., van Driel, I.I. et al. The effect of social media on well-being differs from adolescent to adolescent. Sci Rep 10 , 10763 (2020). https://doi.org/10.1038/s41598-020-67727-7
Download citation
Received : 24 January 2020
Accepted : 11 June 2020
Published : 01 July 2020
DOI : https://doi.org/10.1038/s41598-020-67727-7
Share this article
Anyone you share the following link with will be able to read this content:
Sorry, a shareable link is not currently available for this article.
Provided by the Springer Nature SharedIt content-sharing initiative
This article is cited by
Problematic social media use and psychological symptoms in adolescents.
- Ramin Mojtabai
Social Psychiatry and Psychiatric Epidemiology (2024)
Mental health profiles of Finnish adolescents before and after the peak of the COVID-19 pandemic
- Jasmine Gustafsson
- Nelli Lyyra
- Leena Paakkari
Child and Adolescent Psychiatry and Mental Health (2023)
Heterogeneity in some relationships between social media use and emerging adults’ affective wellbeing
- Nastasia Griffioen
- Hanneke Scholten
- Isabela Granic
Current Psychology (2023)
Negative experiences, social exclusion and unwanted attention on social media: exploring the association with adolescent alcohol use
- Priya Ranganath
- Gunnhild Johnsen Hjetland
- Jens Christoffer Skogen
BMC Public Health (2022)
Dynamics of adolescents’ smartphone use and well-being are positive but ephemeral
- Laura Marciano
- Charles C. Driver
- Anne-Linda Camerini
Scientific Reports (2022)
By submitting a comment you agree to abide by our Terms and Community Guidelines . If you find something abusive or that does not comply with our terms or guidelines please flag it as inappropriate.
Quick links
- Explore articles by subject
- Guide to authors
- Editorial policies
Sign up for the Nature Briefing newsletter — what matters in science, free to your inbox daily.

- Open access
- Published: 06 July 2023
Pros & cons: impacts of social media on mental health
- Ágnes Zsila 1 , 2 &
- Marc Eric S. Reyes ORCID: orcid.org/0000-0002-5280-1315 3
BMC Psychology volume 11 , Article number: 201 ( 2023 ) Cite this article
492k Accesses
21 Citations
119 Altmetric
Metrics details
The use of social media significantly impacts mental health. It can enhance connection, increase self-esteem, and improve a sense of belonging. But it can also lead to tremendous stress, pressure to compare oneself to others, and increased sadness and isolation. Mindful use is essential to social media consumption.
Social media has become integral to our daily routines: we interact with family members and friends, accept invitations to public events, and join online communities to meet people who share similar preferences using these platforms. Social media has opened a new avenue for social experiences since the early 2000s, extending the possibilities for communication. According to recent research [ 1 ], people spend 2.3 h daily on social media. YouTube, TikTok, Instagram, and Snapchat have become increasingly popular among youth in 2022, and one-third think they spend too much time on these platforms [ 2 ]. The considerable time people spend on social media worldwide has directed researchers’ attention toward the potential benefits and risks. Research shows excessive use is mainly associated with lower psychological well-being [ 3 ]. However, findings also suggest that the quality rather than the quantity of social media use can determine whether the experience will enhance or deteriorate the user’s mental health [ 4 ]. In this collection, we will explore the impact of social media use on mental health by providing comprehensive research perspectives on positive and negative effects.
Social media can provide opportunities to enhance the mental health of users by facilitating social connections and peer support [ 5 ]. Indeed, online communities can provide a space for discussions regarding health conditions, adverse life events, or everyday challenges, which may decrease the sense of stigmatization and increase belongingness and perceived emotional support. Mutual friendships, rewarding social interactions, and humor on social media also reduced stress during the COVID-19 pandemic [ 4 ].
On the other hand, several studies have pointed out the potentially detrimental effects of social media use on mental health. Concerns have been raised that social media may lead to body image dissatisfaction [ 6 ], increase the risk of addiction and cyberbullying involvement [ 5 ], contribute to phubbing behaviors [ 7 ], and negatively affects mood [ 8 ]. Excessive use has increased loneliness, fear of missing out, and decreased subjective well-being and life satisfaction [ 8 ]. Users at risk of social media addiction often report depressive symptoms and lower self-esteem [ 9 ].
Overall, findings regarding the impact of social media on mental health pointed out some essential resources for psychological well-being through rewarding online social interactions. However, there is a need to raise awareness about the possible risks associated with excessive use, which can negatively affect mental health and everyday functioning [ 9 ]. There is neither a negative nor positive consensus regarding the effects of social media on people. However, by teaching people social media literacy, we can maximize their chances of having balanced, safe, and meaningful experiences on these platforms [ 10 ].
We encourage researchers to submit their research articles and contribute to a more differentiated overview of the impact of social media on mental health. BMC Psychology welcomes submissions to its new collection, which promises to present the latest findings in the emerging field of social media research. We seek research papers using qualitative and quantitative methods, focusing on social media users’ positive and negative aspects. We believe this collection will provide a more comprehensive picture of social media’s positive and negative effects on users’ mental health.
Data Availability
Not applicable.
Statista. (2022). Time spent on social media [Chart]. Accessed June 14, 2023, from https://www.statista.com/chart/18983/time-spent-on-social-media/ .
Pew Research Center. (2023). Teens and social media: Key findings from Pew Research Center surveys. Retrieved June 14, 2023, from https://www.pewresearch.org/short-reads/2023/04/24/teens-and-social-media-key-findings-from-pew-research-center-surveys/ .
Boer, M., Van Den Eijnden, R. J., Boniel-Nissim, M., Wong, S. L., Inchley, J. C.,Badura, P.,… Stevens, G. W. (2020). Adolescents’ intense and problematic social media use and their well-being in 29 countries. Journal of Adolescent Health , 66(6), S89-S99. https://doi.org/10.1016/j.jadohealth.2020.02.011.
Marciano L, Ostroumova M, Schulz PJ, Camerini AL. Digital media use and adolescents’ mental health during the COVID-19 pandemic: a systematic review and meta-analysis. Front Public Health. 2022;9:2208. https://doi.org/10.3389/fpubh.2021.641831 .
Article Google Scholar
Naslund JA, Bondre A, Torous J, Aschbrenner KA. Social media and mental health: benefits, risks, and opportunities for research and practice. J Technol Behav Sci. 2020;5:245–57. https://doi.org/10.1007/s41347-020-00094-8 .
Article PubMed PubMed Central Google Scholar
Harriger JA, Thompson JK, Tiggemann M. TikTok, TikTok, the time is now: future directions in social media and body image. Body Image. 2023;44:222–6. https://doi.org/10.1016/j.bodyim.2021.12.005 .
Article PubMed Google Scholar
Chi LC, Tang TC, Tang E. The phubbing phenomenon: a cross-sectional study on the relationships among social media addiction, fear of missing out, personality traits, and phubbing behavior. Curr Psychol. 2022;41(2):1112–23. https://doi.org/10.1007/s12144-022-0135-4 .
Valkenburg PM. Social media use and well-being: what we know and what we need to know. Curr Opin Psychol. 2022;45:101294. https://doi.org/10.1016/j.copsyc.2020.101294 .
Bányai F, Zsila Á, Király O, Maraz A, Elekes Z, Griffiths MD, Urbán R, Farkas J, Rigó P Jr, Demetrovics Z. Problematic social media use: results from a large-scale nationally representative adolescent sample. PLoS ONE. 2017;12(1):e0169839. https://doi.org/10.1371/journal.pone.0169839 .
American Psychological Association. (2023). APA panel issues recommendations for adolescent social media use. Retrieved from https://apa-panel-issues-recommendations-for-adolescent-social-media-use-774560.html .
Download references
Acknowledgements
Ágnes Zsila was supported by the ÚNKP-22-4 New National Excellence Program of the Ministry for Culture and Innovation from the source of the National Research, Development and Innovation Fund.
Author information
Authors and affiliations.
Institute of Psychology, Pázmány Péter Catholic University, Budapest, Hungary
Ágnes Zsila
Institute of Psychology, ELTE Eötvös Loránd University, Budapest, Hungary
Department of Psychology, College of Science, University of Santo Tomas, Manila, 1008, Philippines
Marc Eric S. Reyes
You can also search for this author in PubMed Google Scholar
Contributions
AZ conceived and drafted the Editorial. MESR wrote the abstract and revised the Editorial. All authors read and approved the final manuscript.
Corresponding author
Correspondence to Marc Eric S. Reyes .
Ethics declarations
Ethics approval and consent to participate, consent for publication, competing interests.
The authors have no competing interests to declare relevant to the content of this article.
Additional information
Publisher’s note.
Springer Nature remains neutral with regard to jurisdictional claims in published maps and institutional affiliations.
Rights and permissions
Open Access This article is licensed under a Creative Commons Attribution 4.0 International License, which permits use, sharing, adaptation, distribution and reproduction in any medium or format, as long as you give appropriate credit to the original author(s) and the source, provide a link to the Creative Commons licence, and indicate if changes were made. The images or other third party material in this article are included in the article’s Creative Commons licence, unless indicated otherwise in a credit line to the material. If material is not included in the article’s Creative Commons licence and your intended use is not permitted by statutory regulation or exceeds the permitted use, you will need to obtain permission directly from the copyright holder. To view a copy of this licence, visit http://creativecommons.org/licenses/by/4.0/ . The Creative Commons Public Domain Dedication waiver ( http://creativecommons.org/publicdomain/zero/1.0/ ) applies to the data made available in this article, unless otherwise stated in a credit line to the data.
Reprints and permissions
About this article
Cite this article.
Zsila, Á., Reyes, M.E.S. Pros & cons: impacts of social media on mental health. BMC Psychol 11 , 201 (2023). https://doi.org/10.1186/s40359-023-01243-x
Download citation
Received : 15 June 2023
Accepted : 03 July 2023
Published : 06 July 2023
DOI : https://doi.org/10.1186/s40359-023-01243-x
Share this article
Anyone you share the following link with will be able to read this content:
Sorry, a shareable link is not currently available for this article.
Provided by the Springer Nature SharedIt content-sharing initiative
- Social media
- Mental health
BMC Psychology
ISSN: 2050-7283
- General enquiries: [email protected]
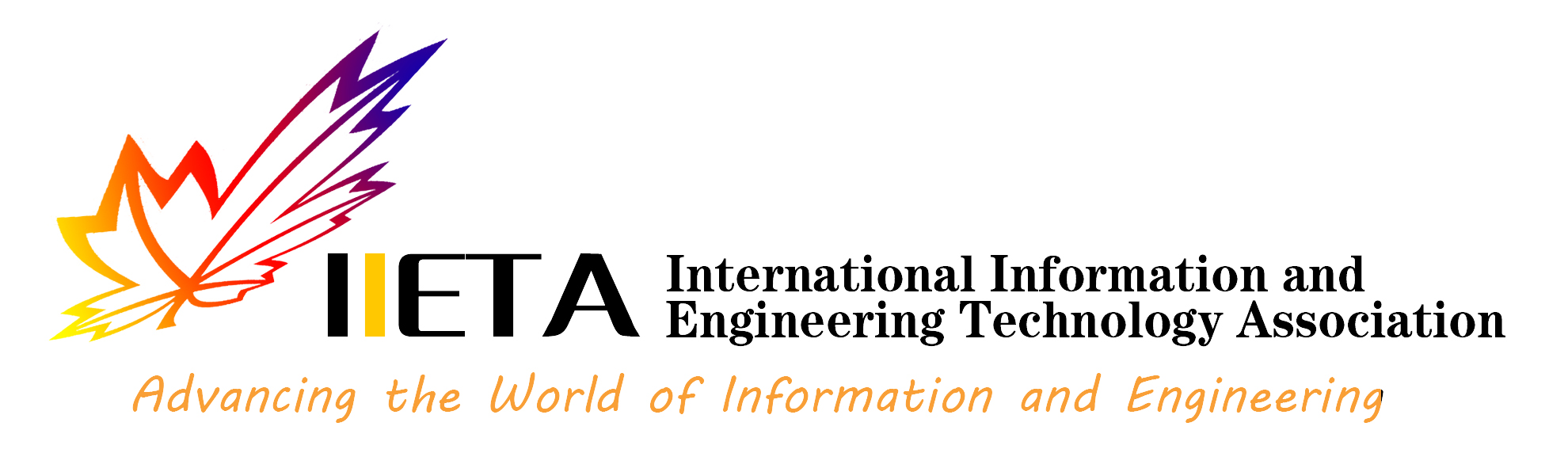
Search form
How does social media effect on students' health and academic performance using principal component analysis (pca): case study of simad university.
© 2024 The authors. This article is published by IIETA and is licensed under the CC BY 4.0 license ( http://creativecommons.org/licenses/by/4.0/ ).
OPEN ACCESS
In today's digital age, the pervasive influence of social media is undeniable, with over 5 billion people globally connected to various platforms. Against this backdrop, this study delves into the causal relationship between social media usage, student health, and academic performance, focusing specifically on SIMAD University students in Somalia. Its primary objective is to comprehensively examine how social media impacts students' physical and mental well-being and their educational achievements. Employing a quantitative research approach and the data analysis was conducted using the Statistical Package for the Social Sciences (SPSS) software, version 27. The significance level was set at α=0.05. the study utilizes Rifhd regression analysis and principal component analysis (PCA) to analyze primary data collected through a meticulously designed questionnaire administered to a randomly selected sample of stakeholders. The findings significantly highlight the connection between social media usage patterns and both student health and academic outcomes. Notably, the computed model demonstrates that even minor adjustments in the distribution of social media usage, student performance, and student health yield marginal increases, albeit with positive yet negligible values of 0.002 and 0.098, respectively. In conclusion, the study underscores the importance of self-regulation in social media usage among students, as higher levels of self-regulation are associated with improved mental well-being and enhanced academic performance.
social media, student’s health, academic performance, Rifhdreg model
Social media (SM) has become an indispensable component of daily existence for numerous individuals, especially the youth. Social media websites are digital platforms that facilitate the connection of individuals both locally and globally, serving as a means to foster interpersonal interactions. The global number of social media users reached 3.2 billion in 2018, with an annual growth rate of up to 13%. It is projected that by 2020, around 5 billion individuals will be linked to social media platforms (Social Media Week). Adolescents and young adults employ technology in novel and inventive ways. Although they are at the developmental stage of their lives, they exhibit distinct patterns of thinking, working, and communication compared to previous generations. Nevertheless, the utilization of social media platforms has resulted in a significant number of contemporary young individuals developing a dependency on technology and experiencing social isolation [1, 2]. Without a doubt, technical and mobile devices possess favorable attributes and greatly facilitate several parts of individuals' lives [3]. The utilization of social media by students can facilitate expedient access to essential information for their academic pursuits. Excessive utilization of social media can lead to issues such as ocular strain, exhaustion, insufficient physical exercise, diminished focus, and sleep disturbances [4, 5]. Moreover, there exists a positive correlation between the amount of time individuals allocate to social media usage and their susceptibility to mental health disorders. These diseases encompass a range of significant concerns, including diminished self-esteem, eating disorders, anxiety, feelings of inferiority, and impaired concentration [6]. Adolescents who are raised in an era of technology rely on social media platforms to engage with others, resulting in a decrease in in-person interpersonal communication. Nevertheless, virtual contacts fail to impart effective social and communication skills, and the absence of in-person interactions can result in issues such as social isolation, depressive symptoms, and various mental diseases.
Social media is a useful method of communication but may be harmful to health. Social media has ‘pros and cons,’ but the effect depends on the individual’s use of these platforms [7]. A recent study found that although students felt competent using social media platforms for academic purposes, they did not have the desire or willingness to do so; however, students with more self-regulation were better able to control social media use [6]. Another study reported that academic performance was a function of attention span, time management skills, student characteristics, academic competence, and time spent on online social networking [8]. Research suggests that college students who socialize rather than for academic pursuits mainly use social media, and the time spent on social media takes time from studying [9]. Yahaya [10] reported that addicted users of social media platforms (e.g., Facebook, WhatsApp) often devoted less time to their studies compared with non-users and subsequently had lower grade point averages [11]. That study also reported that social media use was negatively associated with students’ academic performance, with this association being much more reliable than the advantages derived from using social media platforms. Another study argued that social networks divert students’ attention and concentration from learning and redirect them towards non-educational activities (e.g., unnecessary chatting) [11, 12]. Previous studies have also found that social media may affect students’ attention, span, memory, sleep, vision, and overall physical, mental, and social health [13]. A recent study confirmed that unnecessary use of social media platforms affected students’ psychological and physical health and found that students often did not have their meals on time or get proper rest [14].
Social networking sites (SNS) experienced swift and widespread appeal in reaction to the rise and growth of the Internet. Currently, these sites are predominantly used for communication and the dissemination of information. The user population on these social networking sites (SNS) has undergone a quick and substantial expansion. Social networking sites (SNS) are frequently known as social media [15]. Social media (SM) is widely employed for the distribution of content, fostering discussions, promoting businesses, and reaping benefits over traditional media. Technology is crucial for augmenting the security and dependability of social media platforms [16]. As of January 2022, the worldwide Internet user population surpassed 4.95 billion, with over 4.62 billion actively participating in social media platforms [17]. As of January 2022, the number of internet users in India has reached 680 million, out of whom 487 million are actively engaged in using social media platforms [18].
As per the Statista Research Department [19], YouTube and Facebook are the dominant social media platforms in India. YouTube boasts a user population of 467 million, while Facebook has a somewhat smaller user count of 329 million. Research indicates that while individuals of various age groups utilize social media platforms for connecting and communicating with friends, young people, specifically students, exhibit a pronounced inclination towards these websites. Laura et al. [20] extensively employ social media (SM) for both personal and academic reasons. Furthermore, social media (SM) has played a role in the increased popularity of other online platforms like Microsoft Teams, Zoom, and Google Meet. Over the past two years, these platforms have gained popularity as preferred options for hosting virtual meetings, webinars, and online lectures. Amidst the outbreak, these platforms were widely employed worldwide to disseminate and promote knowledge among the target user population. Facebook, YouTube, Instagram, WhatsApp, and blogs are easily accessible social media platforms that function as communication channels for both public and private groups. Originally, social media platforms were developed to facilitate communication between individuals and their acquaintances and relatives. Gradually, these platforms have transformed into essential teaching tools for pupils [21]. Dzogbenuku et al. [21] assert that various educational communities employ social media platforms to enhance the process of teaching and learning. The integration of social media (SM) in educational environments has both advantages and disadvantages. Social media possesses the capacity to augment academic performance, although it can also divert students from their educational pursuits and engross them in non-academic endeavors [22].
Rahman et al. [6] investigate the influence of social media utilization on the welfare and academic accomplishments of students enrolled at the University of Sharjah. The study concluded that the utilization of social media has a noticeable effect on the academic achievement and well-being of students enrolled at the University of Sharjah. Considering the detrimental consequences of excessive social media consumption, it is crucial for universities to implement awareness campaigns and incorporate this topic into health education and awareness curricula. The objective of this study is to incorporate a causal effect model like RIFs (recentered influence functions) to examine the social media effect on the health and academic performance of students, which is the novelty of this research, and this model was not applied in the previous related studies to the best of my knowledge.
However, due to contextual factors, the direct relevance of these worldwide and Indian data to the situation at SIMAD University in Somalia may be restricted [23]. Similar to many other developing nations, social media usage and internet penetration have increased recently in Somalia. Although there may not be much precise data on social media usage in Somalia [24], anecdotal evidence points to a rising reliance on Facebook, Twitter, and Instagram among young Somalis for social contact, communication, and information sharing [25]. In addition, students face both opportunities and challenges as a result of social media's inclusion in academic environments, including institutions like SIMAD. The goal is to offer insights that are unique to the Somali setting on how social media affects academic performance and health by concentrating on students at SIMAD University. Comprehending the impact of social media on Somalian pupils is vital in order to devise focused treatments and tactics that foster favorable results in both academic and health-related areas.
The emergence of social media platforms in the last decade has resulted in a substantial revolution in the online domain. People of all age groups are using social media platforms more and more to share their own stories, feelings, multimedia content, and different types of public information at a rapidly expanding rate [1, 6, 26]. Adolescents, specifically individuals aged 16 to 24, eagerly embraced social media platforms as a way to interact with their peers and family members, exchange information, and showcase their social status [15]. Using social media platforms in academia provides a multitude of advantages. Social networking platforms offer a fun method for students to maintain connections with their peers and professors, which helps in the acquisition of knowledge [27]. Social media enhances communication between professors and students by minimizing ambiguity and misinterpretation, eventually improving students' academic accomplishments [28].
Massive open online courses (MOOCs) have gained popularity since 2020 as a result of the COVID-19 pandemic [29]. MOOCs are typically offered at no cost and are accessible to anybody who wishes to enroll online. Several prestigious colleges offer their courses on the MOOC platform, allowing students to engage in flexible learning. Students find them valuable for augmenting their knowledge base and facilitating job advancement. Several independent universities have formed partnerships with MOOC platforms and integrated these courses into their academic programs [30]. Besides, Rahman et al. [6] conducted a study to investigate the impact of social media usage on the well-being and scholastic achievements of students enrolled at the University of Sharjah. The study determined that social media usage had a discernible impact on the academic performance and health of University of Sharjah students. Given the adverse effects of excessive social media usage, it is imperative for colleges to establish awareness initiatives and include this subject in health education and awareness curricula.
Using social media for educational purposes has the capacity to improve academic performance, but it is crucial to recognize the associated challenges [31]. Integrating social media into academic environments may lead to students engaging in non-academic conversations as well [32]. The main reason for this diversion is its intentional design as a social networking tool [33]. Englander et al. [34] argue that the utilization of social media in academia yields a greater number of detrimental consequences compared to favorable outcomes. Social media has a significant negative impact on a student's academic achievement. Research conducted by Nalwa and Anand [35] indicates that students who are engaged in higher education are more likely to experience a greater occurrence of social media addiction, which in turn has a detrimental effect on their academic achievements. Facebook users exhibited inferior academic achievement compared to nonusers or users of other social media platforms within the social media user population. As per the findings of Kirschner and Karpinski [36], Facebook is the primary source of diversion for pupils. However, alternative research contradicts these findings and argues that students really benefit from participating in chat chats [37], as it improves their vocabulary and writing skills [38]. Social media possesses the capacity to either augment academic performance or significantly impede it. The result depends on how the students utilize it. Hence, the goal of this study is to examine the impact of social media on the well-being and academic achievement of students, utilizing a causal effect model such as Recentered Influence Functions (RIFs), which was not applied in the previous study.
Al-Rahmi et al. [39] contribute to the discourse on social media's role in contemporary higher education by emphasizing the importance of constructivist learning and task-technology fit in enhancing student satisfaction and performance. Their study underscores the need for collaborative learning opportunities, easy access to social media, and aligned performance expectations to maximize educational quality. Notably, while actual social media use predicts student performance, it surprisingly doesn't directly influence student satisfaction.
Safeer and Awan [40] underscore the importance of addressing the adverse effects of excessive social media use on students' academic success. By identifying usage patterns influenced by geography and gender, the study emphasizes the need for targeted interventions to counteract social media's negative impact on learning outcomes.
Adhikari [26] explores the widespread use of social media, particularly among youth, and its swift adoption by institutions for communication. While acknowledging its benefits in fostering global connections and cost-effective communication, the paper raises concerns about its negative effects on students, including time and energy waste. The study assesses the impact of social media specifically on Navodit College students, employing both qualitative and quantitative research methods.
Sharma and Behl [41] explore the transformative impact of technology in education, particularly focusing on how social media affects students' academic performance based on their extraversion and introversion personalities. Their study also examines the differences between postgraduate and undergraduate students, as well as gender disparities. They begin by identifying factors influencing academic performance through social media and developing a scale specifically validated for the Indian context. Data from 408 students, including 202 males and 206 females, is analyzed, with 234 in postgraduate and 174 in undergraduate programs. Using one-way ANOVA, the research uncovers significant variations between extraversion and introversion students in terms of social media's impact on academic performance, offering insights into the complex interplay between personality traits and technology usage in educational settings.
We can therefore develop a hypothesis in this regard as follows:
H1: Social media is significantly connected with a student’s health and academic performance.
3.1 Research design
The study employs a causal research methodology to examine the impact of social media on the health and academic performance of students. In order to elucidate the relationships between the factors, this study also concentrates on analysing a specific situation. Alternatively, while causality may be deduced, it cannot be definitively demonstrated with a high level of assurance. In addition, the causal research study is considered ideal for the objectives of this investigation since it allows for an investigation of the impact of social media on students' health and academic performance.
3.2 Sample size determination and sampling technique
The sample size will be calculated using Fisher’s formula for calculating minimum sample size for this study as follows:
$N=\frac{Z^2 P(1-P)}{d^2}$
where, N=Minimum sample size; Z=Standard normal deviation at 95% level of confidence=1.96; P=Assumed population proportion=0.5; d=Tolerance margin of error=0.05.
$N=\frac{(1.96)^2 (0.5)(0.5)}{(0.05)^2}$
N=384.16 approximately=384
In order to enhance the accuracy and precision of the study, the sample size of the participants will be expanded to 385. This will be accomplished by distributing 385 questionnaires to the target stakeholders, specifically, the students of SIMAD University in Somalia, using purposive sampling.
3.3 Questionnaire design
The questionnaire covers various aspects related to social media usage, including the frequency and duration of usage, preferred platforms, reasons for usage, perceived impact on academic performance and health, and behaviors related to social media use. Demographic information such as gender, age, academic year, and CGPA (Cumulative Grade Point Average) is also collected to understand the characteristics of the respondents. Likert scale items are used to measure participants' agreement or disagreement with statements related to the impact of social media on academic performance, health, and other aspects. Before distributing the questionnaire to the target sample, a pre-test was conducted with a small group of individuals, representing a diverse range of characteristics similar to the target population. The purpose of the pre-test was to assess the clarity, comprehensibility, and relevance of the questionnaire items. Feedback from pre-test participants was used to refine and finalize the questionnaire, ensuring that it effectively captured the intended information and aligned with the research questions. Content validity of the questionnaire was assessed by subject matter experts, including researchers familiar with social media usage and its impact on academic performance and health. Face validity was also evaluated to ensure that the questionnaire appeared relevant and appropriate to the target respondents. The questionnaire was reviewed to ensure that it covered all relevant dimensions of social media usage and its potential effects on students' academic performance and health.
3.4 Method of data analysis
The acquired primary data was analysed using quantitative research methods, specifically employing a regression model. The primary data for this study was collected by administering a questionnaire to randomly selected stakeholders, ensuring that there is no compulsion involved, in accordance with ethical research considerations. Meanwhile, the demographic profile of the respondents was examined using the techniques of frequency and percentage analysis. The study utilised frequencies and percentages to depict the characteristics of the respondents as derived from the survey questions. The principal component analysis was also applied as a variable reduction technique to identify the construct items that are very crucial and the ones that are less important. The data analysis was conducted using the Statistical Package for the Social Sciences (SPSS) software, version 27. The significance level was set at α = 0.05.
3.5 Principal component analysis (PCA)
PCA is a dimensionality reduction technique used to identify patterns in multivariate data by transforming the original variables into a new set of uncorrelated variables called principal components. The mathematical model for PCA involves the following steps:
Standardization: Standardize the data to have a mean of 0 and a standard deviation of 1 to ensure that all variables contribute equally to the analysis.
Compute the Covariance Matrix: Calculate the covariance matrix of the standardized data to understand the relationships between variables.
Eigenvalue Decomposition: Perform eigenvalue decomposition on the covariance matrix to obtain the eigenvectors and eigenvalues.
Eigenvectors: These represent the directions or principal components of the data.
Eigenvalues: These indicate the variance explained by each principal component.
Select Principal Components: Sort the eigenvalues in descending order and select the top k eigenvectors corresponding to the largest eigenvalues to retain the most important components.
Transform Data: Project the original data onto the new principal component space defined by the selected eigenvectors.
PCA can be represented mathematically as follows:
Given a dataset X consisting of n observations and p variables, where X is an n × p matrix, PCA aims to find a transformation matrix W such that the transformed data Z is given by:
where, Z is the n × k matrix of principal components; W is the p × k matrix of eigenvectors; k is the number of principal components selected.
The eigenvalue decomposition of the covariance matrix Σ of X is given by:
where, V is the matrix of eigenvectors; W is the diagonal matrix of eigenvalues.
The principal components are then obtained by multiplying the original data X by the matrix of eigenvectors V:
PCA helps in reducing the dimensionality of the data while retaining most of its variance, making it easier to interpret and visualize complex datasets.
3.5.1 RIF regression: Rifhdreg
According to Rios-Avila [42], Rifhdreg is an extension of RIFs (Recentered influence functions) with robustness against outliers and provides a simple framework for analyzing the impact of changes in the distribution of X’s on distribution statistics at margin which can be used to fit a linear model to capture how small changes in the distribution of the independent variables X affect v(Fy) and therefore has slight changes in the interpretation of the coefficient which is different from OLS regression.
The model can be specified mathematically with an equation as follows:
$R I F=\{y, v(F y)\}=x \beta+\varepsilon_i, E\left(\varepsilon_i\right)=0$
where, v(Fy) is the response variables which is the students’ academic performance while X’s is the small changes in the distribution of the independent variables identified as the social media, student health and socio-demographic factors and the β is the coefficient estimate of X’s while the εi is the stochastic error term. Similarly, the same model estimation is estimated with the response variable as student’s health and the independent variables as social media, academic performance and socio-demographic variables.
3.5.2 Principles of Rifhdreg model
Recentered Influence Functions (RIFs): RIFs are statistical metrics employed to evaluate the impact of independent variable modifications on distribution statistics. They offer a versatile methodology for examining causal connections and exhibit resilience in the face of outliers.
Causal inference: Causal inference is facilitated through the utilisation of the Rifhdreg model [42], which computes the effect of alterations in student health and social media utilisation on academic achievement. The ability of researchers to isolate the causal effect of social media on student outcomes is achieved through the control of confounding variables.
Robustness: The Rifhdreg model is appropriate for analysing complex relationships in real-world data due to its resistance to outliers and deviations from normality.
3.5.3 Justification for selecting the Rifhdreg model
The Rifhdreg model was selected due to its capacity to offer valuable insights regarding the causal connection that exists among student health, academic performance, and social media usage. Rifhdreg, in contrast to conventional regression models, provides robustness against outliers and deviations from normality, rendering it well-suited for examining the intricate and multifaceted effects of social media on student outcomes. Furthermore, causal inference is facilitated by the model, which empowers researchers to deduce the reasons behind the correlation between social media usage and health outcomes and academic achievement [5].
Table 1 shows that gender of the students under study consist of 233 males representing 60.5% and 152 females representing 39.5%, the students age group categories that is within 17-20 years are 181 representing 47%, those within 21-24 years are also 181 representing 47%, those within 25-28 years are 20 representing 5.2%, the student within 29-32 years is just 1 representing 0.3%, and the students above 32 years are 2 representing 0.5%, the students in the 1st year are 103 representing 26.8%, students in the 2nd year are 74 representing 19.2%, the students in the 3rd year are 117 representing 30.4% and those in the 4th year are 91 representing 23.6%, the students with semester CGPA less than 1.66 are 39 representing 10.1%, those with CGPA within 1.67-2.66 are 72 representing 18.7%, those with CGPA within 2.67-3.66 are 168 representing 43.6% and those with CGPA within 3.67-4.00 are 106 representing 27.5%, the students that spend less than 1hour on social media networking sites are 52 representing 13.5%, those that spend above 4hours on social networking sites are 81 representing 21%, those that spend within 1-2hours on social networking sites are 134 representing 34.8%, and the students that spend within 3-4hours on social networking sites are 118 representing 30.6%, the students investigated under study who feel that social media network sites had negative effect on academic performance are 51 representing 13.2%, those who believe that social had no effect on students’ performance are 95 representing 24.7%, those who feel social media had positive effect on students’ academic performance are 138 representing 35.8% (majority), those students who feel social media had a very negative effect on academic performance are 23 representing 6% and those students who feel that social media had a very positive effect on academic performance are 78 representing 20.3%. Meanwhile, gender on average is 1.39 with a variability of 0.49, age categories on average are 1.60 with a variability of 0.65, the average of the student's year of study is 2.51 with a variability of 1.12, the student’s CGPA on the average is 2.89 with variability of about 0.93, the average hours students spend on social media sites are about 3 hours with a variability of about 1.01 hours, and the average number of students who feel social media network sites have an effect on their grade or performance is 2.95 with a variability of about 1.28.
Note: Only items that are greater or equal to 0.5 are left in the communalities and they represent construct that are valid.
Table 2 shows that all the items have communalities with extraction above 0.5, indicating that the construct items are valid and therefore measure what they are designed to measure.
Table 3 shows that the first four construct items have eigen value above 1 which indicate that they are the most crucial or important items while the other three with eigen value less than 1 are the least important items. Meanwhile, the % of variance of the item with the highest eigen value is 22.305% indicating that the construct items do not occur the problem of common method bias.
Figure 1 is the scree plot of the construct items that demonstrated the first four items with eigen values above 1 indicating the most important items and those other three items with a decline pattern had eigen value below 1, indicating that they are the least important items.
Table 1 . Socio-demographic characteristics
|
|
|
| ||
Gender | Male | 233 | 60.5 | 1.39 | 0.49 |
Female | 152 | 39.5 |
|
| |
Total | 385 | 100 |
|
| |
Age | 17-20 | 181 | 47 | 1.60 | 0.65 |
21-24 | 181 | 47 |
|
| |
25-28 | 20 | 5.2 |
|
| |
29-32 | 1 | 0.3 |
|
| |
>32 | 2 | 0.5 |
|
| |
Total | 385 | 100 |
|
| |
Which year are you | Freshman (1st year) | 103 | 26.8 | 2.51 | 1.12 |
Sophomore (2nd year) | 74 | 19.2 |
|
| |
Junior (3rd year) | 117 | 30.4 |
|
| |
Senior (4th year) | 91 | 23.6 |
|
| |
Total | 385 | 100 |
|
| |
What is your last semester CGPA | <1.66 | 39 | 10.1 | 2.89 | 0.93 |
1.67-2.66 | 72 | 18.7 |
|
| |
2.67-3.66 | 168 | 43.6 |
|
| |
3.67-4.00 | 106 | 27.5 |
|
| |
Total | 385 | 100 |
|
| |
How many hours do you spend on these sites | <1 hour | 52 | 13.5 | 2.83 | 1.01 |
>4 hours | 81 | 21 |
|
| |
1 - 2 hours | 134 | 34.8 |
|
| |
3 - 4 hours | 118 | 30.6 |
|
| |
Total | 385 | 100 |
|
| |
Do you feel social media network sites had an effect on your grade or performance | Negative effect | 51 | 13.2 | 2.95 | 1.28 |
No effect | 95 | 24.7 |
|
| |
positive effect | 138 | 35.8 |
|
| |
Very negative effect | 23 | 6 |
|
| |
Very positive effect | 78 | 20.3 |
|
| |
Total | 385 | 100 |
|
|
Table 2. Communalities
|
|
|
Do you believe that social media is essential for young people today | 1.000 | .681 |
Do you think that social media is helpful to create awareness among youth | 1.000 | .648 |
What kind of effect do you think social network sites have on teaching and learning | 1.000 | .712 |
Do you feel social media network sites had an effect on your grade or performance | 1.000 | .595 |
Do you post or respond while completing homework | 1.000 | .522 |
Do you use social network sites to discuss educational work | 1.000 | .833 |
Do you face any health problem | 1.000 | .798 |
Source: Author’s computation using SPSS Software
Table 3. PCA
|
|
| ||||
|
|
|
|
|
| |
1. Do you believe that social media is essential for young people today | 1.561 | 22.305 | 22.305 | 1.561 | 22.305 | 22.305 |
2. Do you think that social media is helpful to create awareness among youth | 1.176 | 16.795 | 39.100 | 1.176 | 16.795 | 39.100 |
3. What kind of effect do you think social network sites have on teaching and learning | 1.041 | 14.871 | 53.971 | 1.041 | 14.871 | 53.971 |
4. Do you feel social media network sites had an effect on your grade or performance | 1.010 | 14.430 | 68.401 | 1.010 | 14.430 | 68.401 |
5. Do you post or respond while completing homework | .860 | 12.288 | 80.689 |
|
|
|
6. Do you use social network sites to discuss educational work | .699 | 9.986 | 90.675 |
|
|
|
7. Do you face any health problem | .653 | 9.325 | 100.000 |
|
|
|
Extraction Method: Principal Component Analysis. |

Figure 1. Scree plot
Table 4 shows that the overall Rifhdreg model has a p-value of 0.0019 which is below the 0.05 significant level, which means that we reject the null hypothesis at the 5% level, indicating that social media is significantly connected with a student’s health and academic performance while accounting for socio-demographic variables like age, semester CGPA, and hours spent on social media sites. This supported the research hypothesis, and the results also suggest that the fitted Rifhdreg model is a good fit for the dataset since the overall model is statistically significant.
More so, Table 4 shows that the coefficient estimate of the students' health has a positive and significant effect on their academic performance, which suggests that students’ academic performance improves greatly when they have good health, while the coefficient estimate of the social media is not statistically significant and therefore has no significant effect on their academic performance, which supports the coefficient estimate value of 0.002, indicating that with a small change in the distribution of the social media, student performance will also rise slightly, with small value changes of 0.002 considered positive but insignificant.
Table 5 shows that the overall Rifhdreg model has a p-value of 0.0292 which is below the 0.05 significant level, which means that we reject the null hypothesis at the 5% level, indicating that social media is significantly connected with a student’s health and academic performance while accounting for socio-demographic variables like age, semester CGPA, and hours spent on social media sites. Meanwhile, academic performance has a significant positive effect on the student’s health, indicating that students become happier with better health when their academic performance improves greatly. Besides, the coefficient of social media is positive but has no significant effect on the student’s health. Meanwhile, Figures 2 and 3 demonstrated the coefficient estimates plot of the student academic performance and student’s health respectively.
Table 4. Rifhdreg model for students’ academic performance, social media, student’s health and other socio-demographic variables
| |||
|
|
|
|
Students health | 0.067 | 3.03 | 0.003 |
Social media | 0.002 | 0.10 | 0.916 |
Age | -0.366 | -1.09 | 0.278 |
Semester CGPA | 0.754 | 2.98 | 0.003 |
Hours spent on social media sites | 0.216 | 0.97 | 0.330 |
Constant | 11.143 | 8.35 | 0.000 |
Table 5. Rifhdreg model for students’ health, social media, academic performance and other socio-demographic variables
| |||
|
|
|
|
Students’ academic performance | 0.581 | 2.85 | 0.005 |
Social media | 0.098 | 1.45 | 0.149 |
Age | -0.996 | -0.87 | 0.384 |
Semester CGPA | 0.556 | 0.76 | 0.446 |
Hours spent on social media sites | 0.540 | 0.90 | 0.370 |
Constant | -1.663 | -0.38 | 0.707 |

Figure 2. Coefficient estimates plot of students’ academic performance and the predictor variables

Figure 3. Coefficient estimates plot of students’ health and the predictor variable
Based on global statistics, over 5 billion people are currently connected to social media platforms. Research has revealed the adverse consequences of excessive use of social media (SM) on students' well-being, encompassing impaired concentration, memory, sleep patterns, eyesight, and overall physical, mental, and social health. Conversely, moderate and positive use of SM has been found to have a beneficial impact on academic performance. The aim of this study is to examine the impact of social media on the physical and mental well-being, as well as the educational achievements, of students.
According to data from Table 1, 138 students, accounting for 35.8% of the total, believe that social media has a positive impact on academic performance. This result is in line with the findings of Oueder and Abousaber's (2018) study, which also found that social media enhances academic performance. Table 1 indicates that the gender distribution of the students in the study consists of 233 males, accounting for 60.5% of the total, and 152 females, representing 39.5%. In terms of semester CGPA, 39 students (10.1%) have a CGPA below 1.66. 72 students (18.7%) have a CGPA between 1.67 and 2.66, 168 students (43.6%) have a CGPA between 2.67 and 3.66, and 106 students (27.5%) have a CGPA between 3.67 and 4.00. Additionally, 52 students (13.5%) spend less than 1 hour on social media networking sites, 81 students (21%) spend more than 4 hours, 134 students (34.8%) spend between 1 and 2 hours, and 118 students (30.6%) spend between 3 and 4 hours.
Table 3 indicates that the first four construct elements have eigenvalues greater than 1, suggesting that they are the most significant or essential items. Conversely, the last three items with eigenvalues less than 1 are considered less important. The item with the highest eigenvalue is responsible for explaining 22.305% of the variance. This suggests that the problem of common method bias is not affecting the construct items.
Table 4 demonstrates a substantial correlation between social media usage and a student's health and academic performance. This correlation takes into account socio-demographic factors such as age, semester CGPA, and the amount of time spent on social media sites. Conversely, there is a notable correlation between academic achievement and student health, suggesting that students experience more happiness and improved health when their academic performance significantly improves. In addition, the coefficient of social media is positively correlated with student health, although the impact is not statistically significant. This contradicts the findings of Englander et al. (2010), who contend that the use of social media in academia is more harmful than good. According to Amin et al. (2016), who found a significant correlation between social media usage and students' academic achievement, social media has a significant negative impact on students' academic performance. The computed model indicates that tiny changes in the distribution of social media, student performance, and students' health will result in a slight increase, with positive but negligible values of 0.002 and 0.098, respectively. Hence, individuals who possess higher levels of self-regulation exhibit greater mastery over their utilization of social media, thereby leading to enhanced mental well-being and improved academic achievements. In light of this, schools and families need to work together to enhance the student’s self-regulation ability, which will guide students to use social media in a moral and standardized way.
Funding by SIMAD University.
Stata do-file
Rifhdreg StudentsHealth StudentsAcademicPerformance SocialMedia Age SemesterCGPA Hoursspentonsocialmediasite, rif(q(10)) robust
Rifhdreg StudentsAcademicPerformance StudentsHealth SocialMedia Age SemesterCGPA Hoursspentonsocialmediasite, rif(q(25)) robust
[1] Social Media Week. https://socialmediaweek.org/london/, accessed on Nov. 4, 2021. [2] Tateno, M., Teo, A.R., Ukai, W., Kanazawa, J., Katsuki, R., Kubo, H., Kato, T.A. (2019). Internet addiction, smartphone addiction, and Hikikomori trait in Japanese young adult: Social isolation and social network. Frontiers in Psychiatry, 10: 455. https://doi.org/10.3389/fpsyt.2019.00455 [3] Qiu, L., Ono, L.K., Qi, Y. (2018). Advances and challenges to the commercialization of organic-inorganic halide perovskite solar cell technology. Materials Today Energy, 7: 169-189. https://doi.org/10.1016/j.mtener.2017.09.008 [4] Nakshine, V.S., Thute, P., Khatib, M.N., Sarkar, B. (2022). Increased screen time as a cause of declining physical, psychological health, and sleep patterns: A literary review. Cureus, 14(10): e30051. https://doi.org/10.7759/cureus.30051 [5] Ramzan, M., Javaid, Z.K., Fatima, M. (2023). Empowering ESL students: Harnessing the potential of social media to enhance academic motivation in higher education. Global Digital & Print Media Review, VI(2): 224-237. https://doi.org/10.31703/gdpmr.2023(VI-II).15 [6] Rahman, S.A., Al Marzouqi, A., Variyath, S., Rahman, S., Rabbani, M., Ahamed, S.I. (2020). Effects of social media use on health and academic performance among students at the University of Sharjah. In 2020 IEEE 44th Annual Computers, Software, and Applications Conference (COMPSAC), Madrid, Spain, pp. 711-716. https://doi.org/10.1109/COMPSAC48688.2020.0-176 [7] Kolan, B.J., Dzandza, P.E. (2018). Effect of social media on academic performance of students in Ghanaian Universities: A case study of University of Ghana, Legon. Library Philosophy and Practice, 1-24. https://core.ac.uk/download/pdf/189476898.pdf. [8] Murad, A., Gul, A., Changezi, R., Naz, A., Khan, N. (2019). Effects of Facebook usage on the academic performance on the undergraduate students of Quetta City. Clinical Social Work. https://clinicalsocialwork.eu/wp-content/uploads/2019/06/CSW-2-2019-cel%C3%BD.pdf#page=72. [9] AlFaris, E., Irfan, F., Ponnamperuma, G., Jamal, A., Van der Vleuten, C., Al Maflehi, N., Al-Qeas, S., Alenezi, A., Alrowaished, M., Alsalman, R., Ahmed, A.M.A. (2018). The pattern of social media use and its association with academic performance among medical students. Medical Teacher, 40(1): S77-S82. https://doi.org/10.1080/0142159X.2018.1465536 [10] Yahaya, I.N. (2019). The effects of WhatsApp and Facebook on students’ academic performance in the University for Development Studies (UDS), Wa Campus. https://www.academia.edu/download/63733350/The_Effects_of_WhatsApp_and_Facebook_on_students_academic_performance_Nuhu_Yahaya_UDS20200624-61615-ygdds4.pdf. [11] Rather, A.A. (2013). Overuse of Facebook and academic grades: An inverse correlation. IOSR Journal of Humanities and Social Science (IOSR-JHSS), 12(6): 68-72. [12] Gutmo, E. (2020). Assessment of the effect of social media on secondary school students’ academic performances in Kinondoni Municipality. Doctoral dissertation, The Open University of Tanzania. http://ir.cuea.edu/jspui/bitstream/1/6824/1/Ferister%20Final%20Thesis.pdf. [13] Shanmugasundaram, M., Tamilarasu, A. (2023). The impact of digital technology, social media, and artificial intelligence on cognitive functions: A review. Frontiers in Cognition, 2: 1203077. https://doi.org/10.3389/fcogn.2023.1203077 [14] Mukhtar, A., Mukhtar, S., Mukhtar, A., Shahid, C., Raza, H., Razzaq, S.U.R. (2023). The use of social media and its impact on the learning behavior of ESL University students for sustainable education in Pakistan. https://journals.kozminski.cems-j.com/index.php/pl_cemj/pdf/2023/02_398.pdf. [15] Boyd, D.M., Ellison, N.B. (2008). Social network sites: Definition, history and scholarship. Journal of Computer-Mediatied Communication, 13: 210-230. https://doi.org/10.1111/j.1083-6101.2007.00393.x [16] Stergiou, C., Psannis, K.E, Kim, B.G, Gupta, B. (2018) Secure integration of IoT and cloud computing. Future Generation Computer System, 78: 964-975. https://doi.org/10.1016/j.future.2016.11.031 [17] Johnson, J. (2022). Worldwide digital population as of January 2022. https://www.statista.com/statistics/617136/digital-population-worldwide/, accessed on Jan. 23, 2022. [18] Basuray, T. (2022). Social media usage in India: Statistics & facts. https://www.statista.com/topics/5113/social-media-usage-in-india/#topicHeader__wrapper, accessed on Mar. 25, 2024. [19] Statista Research Department. (2022). Countries with the most Facebook users 2022. https://www.statista.com/statistics/268136/top-15-countries-based-on-number-of-facebook-users/, accessed on Mar. 25, 2024. [20] Laura, R., Jose, B., Braojos, J. (2017). From traditional education technologies to student satisfaction in Management education: A theory of the role of social media applications. Information & Management, 54(8): 1059-1071. https://doi.org/10.1016/j.im.2017.06.002 [21] Dzogbenuku, R.K., George, A.K., Kumi, D.K. (2019). Social media and student performance: The moderating role of ICT knowledge. Journal of Information, Communication and Ethics in Society, 18(2): 197-219. https://doi.org/10.1108/JICES-08-2019-0092 [22] Alshuaibi, M., Ibrahim, S., Ibrahim, A.A.S., Mohd, F., Shamsudin, D.A., Arshad. (2018). Use of social media, student engagement, and academic performance of business students in Malaysia. International Journal of Educational Management, 32(4): 625-640. https://doi.org/10.1108/IJEM-08-2016-0182 [23] Pellini, A., Abdi, D.I., Salah, G., Ali, H.Y., Quintin, K.L., Hassan, M.A., Said, S., Khan, A., Laws, E. (2020). Research in Somalia: Opportunities for cooperation. ODI Report. https://hdl.handle.net/10419/216987. [24] Molony, T. (2019). Social media warfare and Kenya’s conflict with Al Shabaab in Somalia: A right to know? African Affairs, 118(471): 328-351. https://doi.org/10.1093/afraf/ady035 [25] Brudvig, I. (2019). (Im) mobility, digital technologies and transnational spaces of belonging: An ethnographic study of Somali migrants in Cape Town. Doctoral dissertation, Faculty of Humanities, University of Cape Town. [26] Adhikari, A. (2020). The impact of social media on Navodit college students. Nepal Journal of Multidisciplinary Research, 3(2): 97-108. https://doi.org/10.3126/njmr.v3i2.33040 [27] Amin, Z., Mansoor, A., Hussain, S.R., Hashmat, F. (2016). Impact of social media of student’s academic performance. International Journal of Business and Management Invention, 5(4): 22-29. [28] Oueder, M., Abousaber, I. (2018). A study on the impact of social media usage on student academic performance: University of Tabuk an example. American Academic Scientific Research Journal for Engineering, Technology, and Sciences, 40(1): 77-88. [29] Raja, M.A.S., Kallarakal, T.K. (2020). “COVID-19 and students’ perception about MOOCs” a case of Indian higher educational institutions. Interactive Technology and Smart Education, 18(3): 450-474. https://doi.org/10.1108/ITSE-07-2020-0106 [30] Chen, J. (2013). Opportunities and challenges of MOOCs: Perspectives from Asia. In International Federation of Library Associations and Institutions, The Hague, Netherlands. [31] Rithika, M., Selvaraj, S. (2013). Impact of social media on students’ academic performance. International Journal of Logistics & Supply Chain Management Perspectives, 2(4): 636-640. [32] Arnold, N., Paulus, T. (2010). Using a social networking site for experiential learning: Appropriating, lurking, modeling and community building. The Internet and Higher Education, 13(4): 188-196. https://doi.org/10.1016/j.iheduc.2010.04.002 [33] Qiu, L., Lin, H., Leung, A.K.Y. (2013). Cultural differences and switching of in-group sharing behavior between an American (Facebook) and a Chinese (Renren) social networking site. Journal of Cross-Cultural Psychology, 44(1): 106-121. https://doi.org/10.1177/0022022111434597 [34] Englander, F., Terregrossa, R.A., Wang, Z. (2010). Internet use among college students: Tool or toy? Educational Review, 62(1): 85-96. https://doi.org/10.1080/00131910903519793 [35] Nalwa, K., Anand, A.P. (2003). Internet addiction in students: A cause of concern. Cyberpsychology & Behavior, 6(6): 653-656. https://doi.org/10.1089/109493103322725441 [36] Kirschner, P.A., Karpinski, A.C. (2010). Facebook® and academic performance. Computers in Human Behavior, 26(6): 1237-1245. https://doi.org/10.1016/j.chb.2010.03.024 [37] Jain, N., Verma, A., Verma, R., Tiwari, P. (2012). Going social: The impact of social networking in promoting education. International Journal of Computer Science, 9(1): 483-485. [38] Yunus, M., Salehi, H. (2012). The effectiveness of Facebook groups on teaching and improving writing: Students’ perceptions. International Journal of Education and Information Technologies, 6: 87-96. [39] Al-Rahmi, A.M., Shamsuddin, A., Wahab, E., Al-Rahmi, W.M., Alyoussef, I.Y., Crawford, J. (2022). Social media use in higher education: Building a structural equation model for student satisfaction and performance. Frontiers in Public Health, 10: 1003007. https://doi.org/10.3389/fpubh.2022.1003007 [40] Safeer, R., Awan, A.G. (2021). Effects of social media on student’s performance in examination. Global Journal of Management, Social Sciences and Humanities, 7(1): 182-204. https://orcid.org/0000-0001-5767-6229 [41] Sharma, S., Behl, R. (2022). Analysing the impact of social media on students’ academic performance: A comparative study of extraversion and introversion personality. Psychological Studies, 67(4): 549-559. https://doi.org/10.1007/s12646-022-00675-6 [42] Rios-Avila, F. (2020). Recentered influence functions (RIFs) in Stata: RIF regression and RIF decomposition. The Stata Journal, 20(1): 51-94. https://doi.org/10.1177/1536867X20909690
Phone: + 1 825 436 9306
Email: [email protected]
Subscription
Language support
Please sign up to receive notifications on new issues and newsletters from IIETA
Select Journal/Journals:
Copyright © 2024 IIETA. All Rights Reserved.

Potential risks of content, features, and functions: The science of how social media affects youth

Almost a year after APA issued its health advisory on social media use in adolescence , society continues to wrestle with ways to maximize the benefits of these platforms while protecting youth from the potential harms associated with them. 1
By early 2024, few meaningful changes to social media platforms had been enacted by industry, and no federal policies had been adopted. There remains a need for social media companies to make fundamental changes to their platforms.
Psychological science continues to reveal benefits from social media use , as well as risks and opportunities that certain content, features, and functions present to young social media users. The science discussed below highlights the need to enact new, responsible safety standards to mitigate harm. 2
Download in PDF format (267KB)
Related content
- APA report calls on social media companies to take responsibility to protect youth
- How much is too much social media use?
Elaboration of science on social media content, features, and functions
Platforms built for adults are not inherently suitable for youth. i Youth require special protection due to areas of competence or vulnerability as they progress through the childhood, teenage, and late adolescent years. ii This is especially true for youth experiencing psychological, physical, intellectual, mental health, or other developmental challenges; chronological age is not directly associated with social media readiness . iii
Hypersensitivity to social feedback
Brain development starting at ages 10–13 (i.e., the outset of puberty) until approximately the mid-twenties is linked with hypersensitivity to social feedback/stimuli. iv In other words, youth become especially invested in behaviors that will help them get personalized feedback, praise, or attention from peers.
- AI-recommended content has the potential to be especially influential and hard to resist within this age range. v It is critical that AI-recommended content be designed to prioritize youth safety and welfare over engagement. This suggests potentially restricting the use of personalized recommendations using youth data, design features that may prioritize content evoking extreme emotions, or content that may depict illegal or harmful behavior.
- Likes and follower counts activate neural regions that trigger repetitive behavior, and thus may exert greater influence on youths’ attitudes and behavior than among adults. vi Youth are especially sensitive to both positive social feedback and rejection from others. Using these metrics to maintain platform engagement capitalizes on youths’ vulnerabilities and likely leads to problematic use.
- The use of youth data for tailored ad content similarly is influential for youth who are biologically predisposed toward peer influence at this stage and sensitive to personalized content. vii

Need for relationship skill building
Adolescence is a critical period for the development of more complex relationship skills, characterized by the ability to form emotionally intimate relationships. viii The adolescent years should provide opportunities to practice these skills through one-on-one or small group interactions.
- The focus on metrics of followers, likes, and views focuses adolescents’ attention on unilateral, depersonalized interactions and may discourage them from building healthier and psychologically beneficial relationship skills. ix
Susceptibility to harmful content
Adolescence is a period of heightened susceptibility to peer influence, impressionability, and sensitivity to social rejection. x Harmful content, including cyberhate, the depiction of illegal behavior, and encouragement to engage in self-harm (e.g., cutting or eating-disordered behavior) is associated with increased mental health difficulties among both the targets and witnesses of such content. xi
- The absence of clear and transparent processes for addressing reports of harmful content makes it harder for youth to feel protected or able to get help in the face of harmful content.
Underdeveloped impulse control
Youths’ developing cortical system (particularly in the brain’s inhibitory control network) makes them less capable of resisting impulses or stopping themselves from behavior that may lead to temporary benefit despite negative longer-term consequences. xii This can lead to adolescents making decisions based on short-term gain, lower appreciation of long-term risks, and interference with focus on tasks that require concentration.
- Infinite scroll is particularly risky for youth since their ability to monitor and stop engagement on social media is more limited than among adults. xiii This contributes to youths’ difficulty disengaging from social media and may contribute to high rates of youth reporting symptoms of clinical dependency on social media. xiv
- The lack of time limits on social media use similarly is challenging for youth, particularly during the school day or at times when they should be doing homework. xv
- Push notifications capitalize on youths’ sensitivity to distraction. Task-shifting is a higher order cognitive ability not fully developed until early adulthood and may interfere with youths’ focus during class time and when they should be doing homework. xvi
- The use and retention of youths’ data without appropriate parental consent, and/or child assent in developmentally appropriate language, capitalizes on youths’ relatively poor appreciation for long-term consequences of their actions, permanence of online content, or their ability to weigh the risks of their engagement on social media. xvii
Reliance on sleep for healthy brain development
Other than the first year of life, puberty is the most important period of brain growth and reorganization in our lifetimes. xviii Sleep is essential for healthy brain development and mental health in adolescence. xix Sleep delay or disruptions have significant negative effects on youths’ attention, behavior, mood, safety, and academic performance.
- A lack of limits on the time of day when youth can use social media has been cited as the predominant reason why adolescents are getting less than the recommended amount of sleep, with significant implications for brain and mental health. xx

Vulnerability to malicious actors
Youth are easily deceived by predators and other malicious actors who may attempt to interact with them on social media channels. xxi
- Connection and direct messaging with adult strangers places youth at risk of identity theft and potentially dangerous interactions, including sexploitation.

Need for parental/caregiver partnership
Research indicates that youth benefit from parental support to guide them toward safe decisions and to help them understand and appropriately respond to complex social interactions. xxii Granting parents oversight of youths’ accounts should be offered in balance with adolescents’ needs for autonomy, privacy, and independence. However, it should be easier for parents to partner with youth online in a manner that fits their family’s needs.
- The absence of transparent and easy-to-use parental/caregiver tools increases parents’ or guardians’ difficulty in supporting youths’ experience on social media. xxiii
Health advisory on social media use in adolescence
Related topics
- Social media and the internet
- Mental health
Recommended Reading

Science Spotlight
Get the most relevant news and information for psychological scientists delivered to your inbox every two weeks
Welcome! Thank you for subscribing.
Six Things Psychologists are Talking About
The APA Monitor on Psychology ® sister e-newsletter offers fresh articles on psychology trends, new research, and more.
A path forward based on science
Change is needed soon. Solutions should reflect a greater understanding of the science in at least three ways.
First, youth vary considerably in how they use social media. Some uses may promote healthy development and others may create harm. As noted in the APA health advisory , using social media is not inherently beneficial or harmful to young people. The effects of social media depend not only on what teens can do and see online, but teens’ pre-existing strengths or vulnerabilities, and the contexts in which they grow up.
Second, science has highlighted biological and psychological abilities/vulnerabilities that interact with the content, functions, and features built into social media platforms, and it is these aspects of youths’ social media experience that must be addressed to attenuate risks. xxiv Social media use, functionality, and permissions/consenting should be tailored to youths’ developmental capabilities. Design features created for adults may not be appropriate for children.
Third, youth are adept at working around age restrictions. Substantial data reveal a remarkable number of children aged 12 years and younger routinely using social media, indicating that current policies and practices to restrict use to older youth are not working. xxv
Policies will not protect youth unless technology companies are required to reduce the risks embedded within the platforms themselves.
As policymakers at every level assess their approach to this complex issue, it is important to note the limitations of frequently proposed policies, which are often misreported and fall far short of comprehensive safety solutions that will achieve meaningful change.
Restricting downloads
Restricting application downloads at the device level does not fully restrict youths’ access and will not meaningfully improve the safety of social media platforms. Allowing platforms to delegate responsibility to app stores does not address the vulnerabilities and harms built into the platforms.

Requiring age restrictions
Focusing only on age restrictions does not improve the platforms or address the biological and psychological vulnerabilities that persist past age 18. While age restriction proposals could offer some benefits if effectively and equitably implemented, they do not represent comprehensive improvements to social media platforms, for at least four reasons:
- Creating a bright line age limit ignores individual differences in adolescents’ maturity and competency
- These proposals fail to mitigate the harms for those above the age limit and can lead to a perception that social media is safe for adolescents above the threshold age, though neurological changes continue until age 25
- Completely limiting access to social media may disadvantage those who are experiencing psychological benefits from social media platforms, such as community support and access to science-based resources, which particularly impact those in marginalized populations
- The process of age-verification requires more thoughtful consideration to ensure that the storage of official identification documents does not systematically exclude subsets of youth, create risks for leaks, or circumvent the ability of young people to maintain anonymity on social platforms.
Use of parental controls
Granting parents and caregivers greater access to their children’s social media accounts will not address risks embedded within platforms themselves. More robust and easy-to-use parental controls would help some younger age groups, but as a sole strategy, this approach ignores the complexities of adolescent development, the importance of childhood autonomy and privacy, and disparities in time or resources available for monitoring across communities. xxvi
[Related: Keeping teens safe on social media: What parents should know to protect their kids ]
Some parents might be technologically ill-equipped, lack the time or documentation to complete requirements, or simply be unavailable to complete these requirements. Disenfranchising some young people from these platforms creates inequities. xxvii

Speaking of Psychology
Subscribe to APA’s audio podcast series highlighting some of the most important and relevant psychological research being conducted today.
Subscribe to Speaking of Psychology and download via:
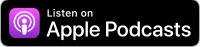
1 These recommendations enact policies and resolutions approved by the APA Council of Representatives including the APA Resolution on Child and Adolescent Mental and Behavioral Health and the APA Resolution on Dismantling Systemic Racism in contexts including social media. These are not professional practice guidelines but are intended to provide information based on psychological science.
2 This report seeks to elaborate on extant psychological science findings, which may be particularly relevant in the creation of policy solutions that protect young people, and to inform the development of social media safety standards.
Recommendations from APA’s health advisory on social media use in adolescence
- Youth using social media should be encouraged to use functions that create opportunities for social support, online companionship, and emotional intimacy that can promote healthy socialization.
- Social media use, functionality, and permissions/consenting should be tailored to youths’ developmental capabilities; designs created for adults may not be appropriate for children.
- In early adolescence (i.e., typically 10–14 years), adult monitoring (i.e., ongoing review, discussion, and coaching around social media content) is advised for most youths’ social media use; autonomy may increase gradually as kids age and if they gain digital literacy skills. However, monitoring should be balanced with youths’ appropriate needs for privacy.
- To reduce the risks of psychological harm, adolescents’ exposure to content on social media that depicts illegal or psychologically maladaptive behavior, including content that instructs or encourages youth to engage in health-risk behaviors, such as self-harm (e.g., cutting, suicide), harm to others, or those that encourage eating-disordered behavior (e.g., restrictive eating, purging, excessive exercise) should be minimized, reported, and removed; moreover, technology should not drive users to this content.
- To minimize psychological harm, adolescents’ exposure to “cyberhate” including online discrimination, prejudice, hate, or cyberbullying especially directed toward a marginalized group (e.g., racial, ethnic, gender, sexual, religious, ability status), or toward an individual because of their identity or allyship with a marginalized group should be minimized.
- Adolescents should be routinely screened for signs of “problematic social media use” that can impair their ability to engage in daily roles and routines, and may present risk for more serious psychological harms over time.
- The use of social media should be limited so as to not interfere with adolescents’ sleep and physical activity.
- Adolescents should limit use of social media for social comparison, particularly around beauty- or appearance-related content.
- Adolescents’ social media use should be preceded by training in social media literacy to ensure that users have developed psychologically-informed competencies and skills that will maximize the chances for balanced, safe, and meaningful social media use.
- Substantial resources should be provided for continued scientific examination of the positive and negative effects of social media on adolescent development.
Acknowledgments
We wish to acknowledge the outstanding contributions to this report made by the following individuals:
Expert advisory panel
Mary Ann McCabe, PhD, ABPP, member-at-large, Board of Directors, American Psychological Association; associate clinical professor of pediatrics, The George Washington University School of Medicine and Health Sciences
Mitchell J. Prinstein, PhD, ABPP, chief science officer, American Psychological Association; John Van Seters Distinguished Professor of Psychology and Neuroscience, University of North Carolina at Chapel Hill
Mary K. Alvord, PhD, founder, Alvord, Baker & Associates; board president, Resilience Across Borders; adjunct associate professor of psychiatry and behavioral sciences, The George Washington University School of Medicine and Health Sciences
Dawn T. Bounds, PhD, PMHNP-BC, FAAN, assistant professor, Sue & Bill Gross School of Nursing, University of California, Irvine
Linda Charmaraman, PhD, senior research scientist, Wellesley Centers for Women, Wellesley College
Sophia Choukas-Bradley, PhD, assistant professor, Department of Psychology, University of Pittsburgh
Dorothy L. Espelage, PhD, William C. Friday Distinguished Professor of Education, University of North Carolina at Chapel Hill
Joshua A. Goodman, PhD, assistant professor, Department of Psychology, Southern Oregon University
Jessica L. Hamilton, PhD, assistant professor, Department of Psychology, Rutgers University
Brendesha M. Tynes, PhD, Dean’s Professor of Educational Equity, University of Southern California
L. Monique Ward, PhD, professor, Department of Psychology (Developmental), University of Michigan
Lucía Magis-Weinberg, MD, PhD, assistant professor, Department of Psychology, University of Washington
We also wish to acknowledge the contributions to this report made by Katherine B. McGuire, chief advocacy officer, and Corbin Evans, JD, senior director of congressional and federal relations, American Psychological Association.
Selected references
i Maza, M. T., Fox, K. A., Kwon, S. J., Flannery, J. E., Lindquist, K. A., Prinstein, M. J., & Telzer, E. H. (2023). Association of habitual checking behaviors on social media with longitudinal functional brain development. JAMA Pediatrics , 177 (2), 160–167; Prinstein, M. J., Nesi, J., & Telzer, E. H. (2020). Commentary: An updated agenda for the study of digital media use and adolescent development—Future directions following Odgers & Jensen (2020). Journal of Child Psychology and Psychiatry , 61 (3), 349–352. https://doi.org/10.1111/jcpp.13219
ii Nesi, J., Choukas-Bradley, S., & Prinstein, M. J. (2018). Transformation of adolescent peer relations in the social media context: Part 1—A theoretical framework and application to dyadic peer relationships. Clinical Child and Family Psychology Review , 21 (3), 267–294. https://doi.org/10.1007/s10567-018-0261-x
iii Valkenburg, P. M., & Peter, J. (2013). The differential susceptibility to media effects model. Journal of Communication , 63 (2), 221–243. https://doi.org/10.1111/jcom.12024
iv Fareri, D. S., Martin, L. N., & Delgado, M. R. (2008). Reward-related processing in the human brain: Developmental considerations. Development and Psychopathology , 20 (4), 1191–1211; Somerville, L. H., & Casey, B. J. (2010). Developmental neurobiology of cognitive control and motivational systems. Current Opinion in Neurobiology , 20 (2), 236–241. https://doi.org/10.1016/j.conb.2010.01.006
v Shin, D. (2020). How do users interact with algorithm recommender systems? The interaction of users, algorithms, and performance. Computers in Human Behavior , 109 , 106344. https://doi.org/10.1016/j.chb.2020.106344
vi Sherman, L. E., Payton, A. A., Hernandez, L. M., Greenfield, P. M., & Dapretto, M. (2016). The power of the Like in adolescence: Effects of peer influence on neural and behavioral responses to social media. Psychological Science , 27 (7), 1027–1035. https://doi.org/10.1177/0956797616645673
vii Albert, D., Chein, J., & Steinberg, L. (2013). The teenage brain: Peer influences on adolescent decision making. Current Directions in Psychological Science , 22 (2), 114–120. https://doi.org/10.1177/0963721412471347
viii Armstrong-Carter, E., & Telzer, E. H. (2021). Advancing measurement and research on youths’ prosocial behavior in the digital age. Child Development Perspectives , 15 (1), 31–36. https://doi.org/10.1111/cdep.12396 ; Newcomb, A. F., & Bagwell, C. L. (1995). Children’s friendship relations: A meta-analytic review. Psychological Bulletin , 117 (2), 306.
ix Nesi, J., & Prinstein, M. J. (2019). In search of likes: Longitudinal associations between adolescents’ digital status seeking and health-risk behaviors. Journal of Clinical Child & Adolescent Psychology , 48 (5), 740–748. https://doi.org/10.1080/15374416.2018.1437733 ; Rotondi, V., Stanca, L., & Tomasuolo, M. (2017). Connecting alone: Smartphone use, quality of social interactions and well-being. Journal of Economic Psychology , 63 , 17–26. https://doi.org/10.1016/j.joep.2017.09.001
x Sherman, L. E., Payton, A. A., Hernandez, L. M., Greenfield, P. M., & Dapretto, M. (2016). The power of the Like in adolescence: Effects of peer influence on neural and behavioral responses to social media. Psychological Science , 27 (7), 1027–1035. https://doi.org/10.1177/0956797616645673
xi Susi, K., Glover-Ford, F., Stewart, A., Knowles Bevis, R., & Hawton, K. (2023). Research review: Viewing self-harm images on the internet and social media platforms: Systematic review of the impact and associated psychological mechanisms. Journal of Child Psychology and Psychiatry , 64 (8), 1115–1139.
xii Hartley, C. A., & Somerville, L. H. (2015). The neuroscience of adolescent decision-making. Current Opinion in Behavioral Sciences , 5 , 108–115. https://doi.org/10.1016/j.cobeha.2015.09.004
xiii Atherton, O. E., Lawson, K. M., & Robins, R. W. (2020). The development of effortful control from late childhood to young adulthood. Journal of Personality and Social Psychology , 119 (2), 417–456. https://doi.org/10.1037/pspp0000283
xiv Boer, M., Stevens, G. W., Finkenauer, C., & Van den Eijnden, R. J. (2022). The course of problematic social media use in young adolescents: A latent class growth analysis. Child Development , 93 (2), e168–e187.
xv Hall, A. C. G., Lineweaver, T. T., Hogan, E. E., & O’Brien, S. W. (2020). On or off task: The negative influence of laptops on neighboring students’ learning depends on how they are used. Computers & Education , 153 , 103901. https://doi.org/10.1016/j.compedu.2020.103901 ; Sana, F., Weston, T., & Cepeda, N. J. (2013). Laptop multitasking hinders classroom learning for both users and nearby peers. Computers & Education , 62 , 24–31. https://doi.org/10.1016/j.compedu.2012.10.003
xvi von Bastian, C. C., & Druey, M. D. (2017). Shifting between mental sets: An individual differences approach to commonalities and differences of task switching components. Journal of Experimental Psychology: General , 146 (9), 1266–1285. https://doi.org/10.1037/xge0000333
xvii Andrews, J. C., Walker, K. L., & Kees, J. (2020). Children and online privacy protection: Empowerment from cognitive defense strategies. Journal of Public Policy & Marketing , 39 (2), 205–219. https://doi.org/10.1177/0743915619883638 ; Romer D. (2010). Adolescent risk taking, impulsivity, and brain development: Implications for prevention. Developmental Psychobiology , 52 (3), 263–276. https://doi.org/10.1002/dev.20442
xviii Orben, A., Przybylski, A. K., Blakemore, S.-J., Kievit, R. A. (2022). Windows of developmental sensitivity to social media. Nature Communications , 13 (1649). https://doi.org/10.1038/s41467-022-29296-3
xix Paruthi, S., Brooks, L. J., D’Ambrosio, C., Hall, W. A., Kotagal, S., Lloyd, R. M., Malow, B. A., Maski, K., Nichols, C., Quan, S. F., Rosen, C. L., Troester, M. M., & Wise, M. S. (2016). Recommended amount of sleep for pediatric populations: A consensus statement of the American Academy of Sleep Medicine. Journal of Clinical Sleep Medicine , 12 (6), 785–786. https://doi.org/10.5664/jcsm.5866
xx Perrault, A. A., Bayer, L., Peuvrier, M., Afyouni, A., Ghisletta, P., Brockmann, C., Spiridon, M., Hulo Vesely, S., Haller, D. M., Pichon, S., Perrig, S., Schwartz, S., & Sterpenich, V. (2019). Reducing the use of screen electronic devices in the evening is associated with improved sleep and daytime vigilance in adolescents. Sleep , 42 (9), zsz125. https://doi.org/10.1093/sleep/zsz125 ; Telzer, E. H., Goldenberg, D., Fuligni, A. J., Lieberman, M. D., & Gálvan, A. (2015). Sleep variability in adolescence is associated with altered brain development. Developmental Cognitive Neuroscience , 14, 16–22. https://doi.org/10.1016/j.dcn.2015.05.007
xxi Livingstone, S., & Smith, P. K. (2014). Annual research review: Harms experienced by child users of online and mobile technologies: The nature, prevalence and management of sexual and aggressive risks in the digital age. Journal of Child Psychology and Psychiatry , 55 (6), 635–654. https://doi.org/10.1111/jcpp.12197 ; Wolak, J., Finkelhor, D., Mitchell, K. J., & Ybarra, M. L. (2008). Online “predators” and their victims: Myths, realities, and implications for prevention and treatment. American Psychologist , 63 (2), 111–128. https://doi.org/10.1037/0003-066X.63.2.111
xxii Wachs, S., Costello, M., Wright, M. F., Flora, K., Daskalou, V., Maziridou, E., Kwon, Y., Na, E.-Y., Sittichai, R., Biswal, R., Singh, R., Almendros, C., Gámez-Guadix, M., Görzig, A., & Hong, J. S. (2021). “DNT LET ’EM H8 U!”: Applying the routine activity framework to understand cyberhate victimization among adolescents across eight countries. Computers & Education , 160 , Article 104026. https://doi.org/10.1016/j.compedu.2020.104026 ; Padilla-Walker, L. M., Stockdale, L. A., & McLean, R. D. (2020). Associations between parental media monitoring, media use, and internalizing symptoms during adolescence. Psychology of Popular Media , 9 (4), 481. https://doi.org/10.1037/ppm0000256
xxiii Dietvorst, E., Hiemstra, M., Hillegers, M. H. J., & Keijsers, L. (2018). Adolescent perceptions of parental privacy invasion and adolescent secrecy: An illustration of Simpson’s paradox. Child Development , 89 (6), 2081–2090. https://doi.org/10.1111/cdev.13002 ; Auxier, B. (2020, July 28). Parenting Children in the Age of Screens. Pew Research Center: Internet, Science & Tech; Pew Research Center. https://www.pewresearch.org/internet/2020/07/28/parenting-children-in-the-age-of-screens/
xxiv National Academies of Sciences, Engineering, and Medicine. (2024). Social media and adolescent health . The National Academies Press. https://doi.org/10.17226/27396
xxv Charmaraman, L., Lynch, A. D., Richer, A. M., & Zhai, E. (2022). Examining early adolescent positive and negative social technology behaviors and well-being during the Covid -19 pandemic. Technology, Mind, and Behavior , 3 (1), Feb 17 2022. https://doi.org/10.1037/tmb0000062
xxvi Dietvorst, E., Hiemstra, M., Hillegers, M.H.J., & Keijsers, L. (2018). Adolescent perceptions of parental privacy invasion and adolescent secrecy: An illustration of Simpson’s paradox. Child Development , 89 (6), 2081–2090. https://doi.org/10.1111/cdev.13002
xxvii Charmaraman, L., Lynch, A. D., Richer, A. M., & Zhai, E. (2022). Examining early adolescent positive and negative social technology behaviors and well-being during the Covid -19 pandemic. Technology, Mind, and Behavior , 3 (1), Feb 17 2022. https://doi.org/10.1037/tmb0000062
Adolescent Social Media Use and Well-Being: A Systematic Review and Thematic Meta-synthesis
- Systematic Review
- Published: 17 April 2021
- Volume 6 , pages 471–492, ( 2021 )
Cite this article
- Michael Shankleman ORCID: orcid.org/0000-0002-7150-8827 1 ,
- Linda Hammond 1 &
- Fergal W. Jones ORCID: orcid.org/0000-0001-9459-6631 1
4939 Accesses
24 Citations
25 Altmetric
Explore all metrics
Qualitative research into adolescents’ experiences of social media use and well-being has the potential to offer rich, nuanced insights, but has yet to be systematically reviewed. The current systematic review identified 19 qualitative studies in which adolescents shared their views and experiences of social media and well-being. A critical appraisal showed that overall study quality was considered relatively high and represented geographically diverse voices across a broad adolescent age range. A thematic meta-synthesis revealed four themes relating to well-being: connections, identity, learning, and emotions. These findings demonstrated the numerous sources of pressures and concerns that adolescents experience, providing important contextual information. The themes appeared related to key developmental processes, namely attachment, identity, attention, and emotional regulation, that provided theoretical links between social media use and well-being. Taken together, the findings suggest that well-being and social media are related by a multifaceted interplay of factors. Suggestions are made that may enhance future research and inform developmentally appropriate social media guidance.
This is a preview of subscription content, log in via an institution to check access.
Access this article
Subscribe and save.
- Get 10 units per month
- Download Article/Chapter or eBook
- 1 Unit = 1 Article or 1 Chapter
- Cancel anytime
Price includes VAT (Russian Federation)
Instant access to the full article PDF.
Rent this article via DeepDyve
Institutional subscriptions
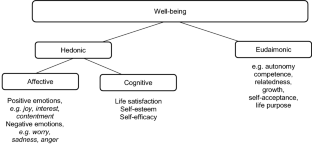
Similar content being viewed by others
Social media and depressive symptoms in childhood and adolescence: a systematic review.
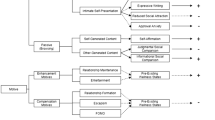
Social Media and Psychological Well-Being Among Youth: The Multidimensional Model of Social Media Use
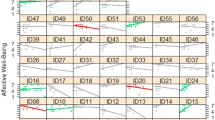
The effect of social media on well-being differs from adolescent to adolescent
Aboujaoude, E., Savage, M. W., Starcevic, V., & Salame, W. O. (2015). Cyberbullying: Review of an old problem gone viral. Journal of Adolescent Health, 57 (1), 10–18. https://doi.org/10.1016/j.jadohealth.2015.04.011 .
Article Google Scholar
Allen, J. P., & Tan J. S (2018). The multiple facets of attachment in adolescence. In J. Cassidy & P. R. Shaver (Eds) Handbook of attachment (pp. 399–415). Guilford Press.
Atkins, S., Lewin, S., Smith, H., Engel, M., Fretheim, A., & Volmink, J. (2008). Conducting a meta-ethnography of qualitative literature: Lessons learnt. BMC Medical Research Methodology, 8 , 21. https://doi.org/10.1186/1471-2288-8-21 .
Article PubMed PubMed Central Google Scholar
Bartsch, A., & Oliver, M. B. (2017). Appreciation of meaningful entertainment experiences and eudaimonic well-being. In L. Reinecke & M. B. Oliver (Eds.), The Routledge handbook of media use and well-being: International perspectives on theory and research on positive media effects (pp. 80–92). Routledge.
Bazarova, N. N., & Choi, Y. H. (2014). Self-disclosure in social media: Extending the functional approach to disclosure motivations and characteristics on social network sites. Journal of Communication, 64 (4), 635–657. https://doi.org/10.1111/jcom.12106 .
Braun, V., & Clarke, V. (2006). Using thematic analysis in psychology. Qualitative Research in Psychology, 3 (2), 77–101. https://doi.org/10.1191/1478088706qp063oa .
Bell, B. T. (2019). “You take fifty photos, delete forty-nine and use one”: A qualitative study of adolescent image-sharing practices on social media. International Journal of Child–Computer Interaction, 20 , 64–71. https://doi.org/10.1016/j.ijcci.2019.03.002 .
Best, P., Manktelow, R., & Taylor, B. (2014). Online communication, social media and adolescent wellbeing: A systematic narrative review. Children and Youth Services Review, 41 , 27–36. https://doi.org/10.1016/j.childyouth.2014.03.001 .
Best, P., Taylor, B., & Manktelow, R. (2015). I’ve 500 friends, but who are my mates? Investigating the influence of online friend networks on adolescent wellbeing. Journal of Public Mental Health, 14 (3), 135–148. https://doi.org/10.1108/JPMH-05-2014-0022 .
Bharucha, J. (2018). Social network use and youth well-being: A study in India. Safer Communities, 17 (2), 119–131. https://doi.org/10.1108/SC-07-2017-0029 .
Blumberg, F. C., Rice, J. L., & Dickmeis, A. (2016). Social media as a venue for emotion regulation among adolescents. In S. Y. Tettegah, Emotions and technology: Communication of feelings for, with, and through digital media. Emotions, technology, and social media (pp. 105–116). Academic.
Bondas, T., & Hall, E. O. (2007). Challenges in approaching metasynthesis research. Qualitative Health Research, 17 (1), 113–121. https://doi.org/10.1177/1049732306295879 .
Article PubMed Google Scholar
Burnette, C. B., Kwitowski, M. A., & Mazzeo, S. E. (2017). “I don’t need people to tell me I’m pretty on social media:” A qualitative study of social media and body image in early adolescent girls. Body Image, 23 , 114–125. https://doi.org/10.1016/j.bodyim.2017.09.001 .
Calancie, O., Ewing, L., Narducci, L. D., Horgan, S., & Khalid-Khan, S. (2017). Exploring how social networking sites impact youth with anxiety: A qualitative study of Facebook stressors among adolescents with an anxiety disorder diagnosis. Cyberpsychology . https://doi.org/10.5817/CP2017-4-2 .
Chua, T. H. H., & Chang, L. (2016). Follow me and like my beautiful selfies: Singapore teenage girls’ engagement in self-presentation and peer comparison on social media. Computers in Human Behavior, 55 , 190–197. https://doi.org/10.1016/j.chb.2015.09.011 .
Coyne, S. M., Rogers, A. A., Zurcher, J. D., Stockdale, L., & Booth, M. (2020). Does time spent using social media impact mental health?: An eight year longitudinal study. Computers in Human Behavior , 104 , 106160. https://doi.org/10.1016/j.chb.2019.106160
Critical Appraisal Skills Programme (CASP). (2018). CASP Qualitative Checklist . https://casp-uk.net/casp-tools-checklists/ .
Crogan, P., & Kinsley, S. (2012). Paying attention: Towards a critique of the attention economy. Culture Machine . https://tinyurl.com/u28jo47 .
Davis, K. (2012). Friendship 2.0: Adolescents’ experiences of belonging and self-disclosure online. Journal of Adolescence, 35 (6), 1527–1536. https://doi.org/10.1016/j.adolescence.2012.02.013 .
Dickson, K., Richardson, M., Kwan, I., MacDowall, W., Burchett, H., Stansfield, C., Brunton, G., Sutcliffe, K., & Thomas, J. (2018). Screen-based activities and children and young people’s mental health: A Systematic Map of Reviews . EPPI-Centre, Social Science Research Unit, UCL Institute of Education, University College London. http://eppi.ioe.ac.uk/ .
Diener, E. (2000). Subjective well-being: The science of happiness and a proposal for a national index. American Psychologist, 55 (1), 34. https://doi.org/10.1037/0003-066X.55.1.34 .
Dowell, E. (2009). Clustering of Internet risk behaviours in a middle school student population. Journal of School Health, 79 , 547–553. https://doi.org/10.1111/j.1746-1561.2009.00447.x .
Duvenage, M., Correia, H., Uink, B., Barber, B. L., Donovan, C. L., & Modecki, K. L. (2020). Technology can sting when reality bites: Adolescents’ frequent online coping is ineffective with momentary stress. Computers in Human Behavior, 102 , 248–259. https://doi.org/10.1016/j.chb.2019.08.024 .
Erfani, S. S., & Abedin, B. (2018). Impacts of the use of social network sites on users’ psychological well-being: A systematic review. Journal of the Association for Information Science and Technology, 69 (7), 900–912. https://doi.org/10.1002/asi.24015 .
Erikson, E. H. (1968). Identity: Youth and crisis . Norton.
Finfgeld-Connett, D. (2010). Generalizability and transferability of meta-synthesis research findings. Journal of Advanced Nursing, 66 , 246–254. https://doi.org/10.1111/j.1365-2648.2009.05250.x .
Gardner, H., & Davis, K. (2013). The app generation: How today’s youth navigate identity, intimacy, and imagination in a digital world . Yale University Press.
Gikas, J., & Grant, M. M. (2013). Mobile computing devices in higher education: Student perspectives on learning with cellphones, smartphones and social media. The Internet and Higher Education, 19 , 18–26. https://doi.org/10.1016/j.iheduc.2013.06.002 .
Griffin, A. (2017). Adolescent neurological development and implications for health and well-being. Healthcare, 5 , 62–76. https://doi.org/10.3390/healthcare5040062 .
Article PubMed Central Google Scholar
Goossens, L. (2001). Global versus domain-specific statuses in identity research: A comparison of two self-report measures. Journal of Adolescence, 24 , 681–699. https://doi.org/10.1006/jado.2001.0438 .
Guyer, A. E., Silk, J. S., & Nelson, E. E. (2016). The neurobiology of the emotional adolescent: From the inside out. Neuroscience and Biobehavioral Reviews, 70 , 74–85. https://doi.org/10.1016/j.neubiorev.2016.07.037 .
Haidt, J., & Allen, N. (2020). Scrutinizing the effects of digital technology on mental health. Nature, 578 , 226–227. https://doi.org/10.1038/d41586-020-00296-x .
Harter, S. (1990). Causes, correlates, and the functional role of global self-worth: A life-span perspective. In R. J. Sternberg & J. Kolligan Jr. (Eds.), Competence considered (pp. 67–98). Yale University Press.
Hayes, A. F. (2017). Introduction to mediation, moderation, and conditional process analysis: A regression-based approach . Guilford Publications.
Heffer, T., Good, M., Daly, O., MacDonell, E., & Willoughby, T. (2019). The longitudinal association between social-media use and depressive symptoms among adolescents and young adults: An empirical reply to Twenge et al. (2018). Clinical Psychological Science, 7 (3), 462–470. https://doi.org/10.1177/2167702618812727 .
Jong, S. T., & Drummond, M. J. N. (2016). Hurry up and ‘like’ me: Immediate feedback on social networking sites and the impact on adolescent girls. Asia–Pacific Journal of Health, Sport and Physical Education, 7 (3), 251–267. https://doi.org/10.1080/18377122.2016.1222647 .
Kelly, Y., Zilanawala, A., Booker, C., & Sacker, A. (2018). Social media use and adolescent mental health: Findings from the UK Millennium Cohort Study. EClinicalMedicine, 6 , 59–68. https://doi.org/10.1016/j.eclinm.2018.12.005 .
Keyes, K. M., Gary, D., O’Malley, P. M., Hamilton, A., & Schulenberg, J. (2019). Recent increases in depressive symptoms among US adolescents: Trends from 1991 to 2018. Social Psychiatry and Psychiatric Epidemiology, 54 (8), 987–996. https://doi.org/10.1007/s00127-019-01697-8 .
Lachal, J., Revah-Levy, A., Orri, M., & Moro, M. R. (2017). Metasynthesis: An original method to synthesize qualitative literature in psychiatry. Frontiers in Psychiatry, 8 , 269. https://doi.org/10.3389/fpsyt.2017.00269 .
Landau, A., Eisikovits, Z., & Rafaeli, S. (2019). Coping strategies for youth suffering from online interpersonal rejection. In Proceedings of the 52nd Hawaii international conference on system sciences. http://hdl.handle.net/10125/59656 .
Leary, M. R. (2015). Emotional responses to interpersonal rejection. Dialogues in Clinical Neuroscience, 17 (4), 435. https://doi.org/10.31887/DCNS.2015.17.4/mleary .
Long, H. A., French, D. P., & Brooks, J. M. (2020). Optimising the value of the critical appraisal skills programme (CASP) tool for quality appraisal in qualitative evidence synthesis. Research Methods in Medicine and Health Sciences, 1 (1), 31–42. https://doi.org/10.1177/2632084320947559 .
Liu, M., Wu, L., & Yao, S. (2016). Dose–response association of screen time-based sedentary behavior in children and adolescents and depression: A meta-analysis of observational studies. British Journal of Sports Medicine, 50 (20), 1252–1258. https://doi.org/10.1136/bjsports-2015-095084 .
Lyons-Ruth, K. (1991). Rapprochement or approachment: Mahler’s theory reconsidered from the vantage point of recent research on early attachment relationships. Psychoanalytic Psychology, 8 , 1–23. https://doi.org/10.1037/h0079237 .
MacIsaac, S., Kelly, J., & Gray, S. (2018). ‘She has like 4000 followers!’: The celebrification of self within school social networks. Journal of Youth Studies, 21 (6), 816–835. https://doi.org/10.1080/13676261.2017.1420764 .
Manago, A. M., Taylor, T., & Greenfield, P. M. (2012). Me and my 400 friends: The anatomy of college students’ Facebook networks, their communication patterns, and well-being. Developmental Psychology, 48 (2), 369. https://doi.org/10.1037/a0026338 .
Mihálik, J., Garaj, M., Sakellariou, A., Koronaiou, A., Alexias, G., Nico, M., Nico, M., de Almeida Alves, N., Unt, M., & Taru, M. (2018). Similarity and difference in conceptions of well-being among children and young people in four contrasting European countries. In G. Pollock, J. Ozan, H. Goswami, G. Rees & A. Stasulane (Eds.), Measuring youth well-being (pp. 55–69). Springer.
McQuail, D. (2010). Mass communication theory: An introduction (6 th Ed., pp. 420–430). Sage.
Nabi, R. L., Prestin, A., & So, J. (2013). Facebook friends with (health) benefits? Exploring social network site use and perceptions of social support, stress, and well-being. Cyberpsychology, Behavior, and Social Networking, 16 (10), 721–727. https://doi.org/10.1089/cyber.2012.0521 .
Odgers, C. L., Schueller, S. M., & Ito, M. (2020). Screen time, social media use, and adolescent development. Annual Review of Developmental Psychology, 2 (1), 485–502. https://doi.org/10.1146/annurev-devpsych-121318-084815 .
Oh, H. J., Ozkaya, E., & LaRose, R. (2014). How does online social networking enhance life satisfaction? The relationships among online supportive interaction, affect, perceived social support, sense of community, and life satisfaction. Computers in Human Behavior, 30 , 69–78. https://doi.org/10.1016/j.chb.2013.07.053 .
ONS. (2017). Social networking by age group, 2011 to 2017 . https://tinyurl.com/yc9lhjdk .
Opitz, P. C., Gross, J. J., & Urry, H. L. (2012). Selection, optimization, and compensation in the domain of emotion regulation: Applications to adolescence, older age, and major depressive disorder. Social and Personality Psychology Compass, 6 , 142–155. https://doi.org/10.1111/j.1751-9004.2011.00413.x .
Orben, A., & Przybylski, A. K. (2019). The association between adolescent well-being and digital technology use. Nature Human Behaviour , 3 (2), 173–182. https://doi.org/10.1038/s41562-018-0506-1
O’Reilly, M. (2020). Social media and adolescent mental health: The good, the bad and the ugly. Journal of Mental Health . https://doi.org/10.1080/09638237.2020.1714007 Advanced online publication.
O’Reilly, M., Dogra, N., Whiteman, N., Hughes, J., Eruyar, S., & Reilly, P. (2018). Is social media bad for mental health and wellbeing? Exploring the perspectives of adolescents. Clinical Child Psychology and Psychiatry, 23 (4), 601–613. https://doi.org/10.1177/1359104518775154 .
Ozan, J., Mierina, I., & Koroleva, I. (2018). A comparative expert survey on measuring and enhancing children and young people’s well-being in Europe. In G. Pollock, J. Ozan, H. Goswami, G. Rees & A. Stasulane (Eds.), Measuring youth well-being. Children’s well-being: Indicators and research (Vol. 19). Springer.
Pajares, F. (2006). Self-efficacy during childhood and adolescence. In T. Urdan & F. Pajares (Eds.), Self-efficacy beliefs of adolescents (pp. 339–367). IAP-Information Age Publishing.
Quoidbach, J., Mikolajczak, M., & Gross, J. J. (2015). Positive interventions: An emotion regulation perspective. Psychology Bulletin, 141 , 655–693. https://doi.org/10.1037/a0038648 .
QSR International Pty Ltd. (2015). NVivo (released in March 2015). https://www.qsrinternational.com/nvivo-qualitative-data-analysis-software/home .
Radovic, A., Gmelin, T., Stein, B. D., & Miller, E. (2017). Depressed adolescents’ positive and negative use of social media. Journal of Adolescence, 55 , 5–15. https://doi.org/10.1016/j.adolescence.2016.12.002 .
Rideout, V. J., & Robb, M. B. (2019). The common sense census: Media use by tweens and teens . Common Sense Media. https://www.commonsensemedia.org/sites/default/files/uploads/research/2019-census-8-to-18-key-findings-updated.pdf .
Riediger, M., & Klipker, K. (2014). Emotion regulation in adolescence. In J. J. Gross (Ed.), Handbook of emotion regulation (pp. 187–202). The Guilford Press.
Rigby, E., Hagell, A., & Starbuck, L. (2018). What do children and young people tell us about what supports their wellbeing? Evidence from existing research. Health and Wellbeing Alliance. http://www.youngpeopleshealth.org.uk/wp-content/uploads/2019/10/Scoping-paper-CYP-views-on-wellbeing-FINAL.pdf .
Rogers, C. R. (1961). On becoming a person: A therapist’s view of psychotherapy . Houghton Mifflin
Ryff, C. D. (1989). Happiness is everything, or is it? Explorations on the meaning of psychological well-being. Journal of Personality and Social Psychology, 57 (6), 1069–1081. https://doi.org/10.1037/0022-3514.57.6.1069 .
Scott, H., Biello, S. M., & Woods, H. C. (2019). Identifying drivers for bedtime social media use despite sleep costs: The adolescent perspective. Sleep Health, 6 , 539–545. https://doi.org/10.1016/j.sleh.2019.07.006 .
Singleton, A., Abeles, P., & Smith, I. C. (2016). Online social networking and psychological experiences: The perceptions of young people with mental health difficulties. Computers in Human Behavior, 61 , 394–403. https://doi.org/10.1016/j.chb.2016.03.011 .
Suchert, V., Hanewinkel, R., & Isensee, B. (2015). Sedentary behavior and indicators of mental health in school-aged children and adolescents: A systematic review. Preventive Medicine, 76 , 48–57. https://doi.org/10.1016/j.ypmed.2015.03.026 .
Thomas, J., & Harden, A. (2008). Methods for the thematic synthesis of qualitative research in systematic reviews. BMC Medical Research Methodology, 8 , 45. https://doi.org/10.1186/1471-2288-8-45 .
Throuvala, M. A., Griffiths, M. D., Rennoldson, M., & Kuss, D. J. (2019). Motivational processes and dysfunctional mechanisms of social media use among adolescents: A qualitative focus group study. Computers in Human Behavior, 93 , 164–175. https://doi.org/10.1016/j.chb.2018.12.012 .
Tong, A., Flemming, K., McInnes, E., Oliver, S., & Craig, J. (2012). Enhancing transparency in reporting the synthesis of qualitative research: ENTREQ. BMC Medical Research Methodology, 12 , 181. https://doi.org/10.1186/1471-2288-12-181 .
Twenge, J. M., Joiner, T. E., Rogers, M. L., & Martin, G. N. (2018). Increases in depressive symptoms, suicide-related outcomes, and suicide rates among US adolescents after 2010 and links to increased new media screen time. Clinical Psychological Science, 6 , 3–17. https://doi.org/10.1177/2167702617723376 .
Twenge, J. M. (2020). Why increases in adolescent depression may be linked to the technological environment. Current Opinion in Psychology, 32 , 89–94. https://doi.org/10.1016/j.copsyc.2019.06.036 .
Twomey, C., & O’Reilly, G. (2017). Associations of self-presentation on Facebook with mental health and personality variables: A systematic review. Cyberpsychology, Behavior, and Social Networking, 20 , 587–595. https://doi.org/10.1089/cyber.2017.0247 .
Vermeulen, A., Vandebosch, H., & Heirman, W. (2018). Shall I call, text, post it online or just tell it face-to-face? How and why Flemish adolescents choose to share their emotions on- or offline. Journal of Children and Media, 12 (1), 81–97. https://doi.org/10.1080/17482798.2017.1386580 .
Vignoles, V. L., Regalia, C., Manzi, C., Golledge, J., & Scabini, E. (2006). Beyond self-esteem: Influence of multiple motives on identity construction. Journal of Personality and Social Psychology, 17 , 251–268. https://doi.org/10.1037/0022-3514.90.2.308 .
Vignoles, V. L. (2011). Identity motives. In S. J. Schwartz, K. Luyckx & V. L. Vignoles (Eds.), Handbook of identity theory and research (pp. 403–432). Springer.
Vorderer, P., & Reinecke, L. (2015). From mood to meaning: The changing model of the user in entertainment research. Communication Theory, 25 (4), 447–453. https://doi.org/10.1111/comt.12082 .
Weinstein, E. (2018). The social media see-saw: Positive and negative influences on adolescents’ affective well-being. New Media and Society, 20 (10), 3597–3623. https://doi.org/10.1177/1461444818755634 .
White, M. (2000). Reflecting Team work as definitional ceremony revisited. In Reflections on narrative practice: essays and interviews. Dulwich Centre Publications.
Download references
Acknowlegement
We extend our gratitude to the authors of the original studies for bringing forth the perspectives of young people.
Preregistration
The review protocol including review question, search strategy, inclusion criteria data extraction, quality assessment, data synthesis was preregistered and is accessible at: https://www.crd.york.ac.uk/prospero/display_record.php?RecordID=156922 .
Author information
Authors and affiliations.
Salomons Institute for Applied Psychology, Canterbury Christ Church University, Lucy Fildes Building, 1 Meadow Rd, Royal Tunbridge Wells, TN1 2YG, UK
Michael Shankleman, Linda Hammond & Fergal W. Jones
You can also search for this author in PubMed Google Scholar
Contributions
MS conceived of the study, participated in its design, coordination, interpretation of the data and drafted the manuscript; LH participated in the design and interpretation of the data; FWJ participated in the design and interpretation of the data. All authors read, helped to draft, and approved the final manuscript.
Corresponding author
Correspondence to Michael Shankleman .
Ethics declarations
Conflict of interest.
The authors report no conflict of interests.
Additional information
Publisher's note.
Springer Nature remains neutral with regard to jurisdictional claims in published maps and institutional affiliations.
Rights and permissions
Reprints and permissions
About this article
Shankleman, M., Hammond, L. & Jones, F.W. Adolescent Social Media Use and Well-Being: A Systematic Review and Thematic Meta-synthesis. Adolescent Res Rev 6 , 471–492 (2021). https://doi.org/10.1007/s40894-021-00154-5
Download citation
Received : 14 December 2020
Accepted : 03 April 2021
Published : 17 April 2021
Issue Date : December 2021
DOI : https://doi.org/10.1007/s40894-021-00154-5
Share this article
Anyone you share the following link with will be able to read this content:
Sorry, a shareable link is not currently available for this article.
Provided by the Springer Nature SharedIt content-sharing initiative
- Adolescents
- Qualitative
- Social media
- Meta-synthesis
- Find a journal
- Publish with us
- Track your research

An official website of the United States government
The .gov means it’s official. Federal government websites often end in .gov or .mil. Before sharing sensitive information, make sure you’re on a federal government site.
The site is secure. The https:// ensures that you are connecting to the official website and that any information you provide is encrypted and transmitted securely.
- Publications
- Account settings
Preview improvements coming to the PMC website in October 2024. Learn More or Try it out now .
- Advanced Search
- Journal List
- Front Psychol
Social Media Use and Mental Health and Well-Being Among Adolescents – A Scoping Review
Viktor schønning.
1 Department of Health Promotion, Norwegian Institute of Public Health, Bergen, Norway
Gunnhild Johnsen Hjetland
Leif edvard aarø, jens christoffer skogen.
2 Alcohol and Drug Research Western Norway, Stavanger University Hospital, Stavanger, Norway
3 Faculty of Health Sciences, University of Stavanger, Stavanger, Norway
Associated Data
Introduction: Social media has become an integrated part of daily life, with an estimated 3 billion social media users worldwide. Adolescents and young adults are the most active users of social media. Research on social media has grown rapidly, with the potential association of social media use and mental health and well-being becoming a polarized and much-studied subject. The current body of knowledge on this theme is complex and difficult-to-follow. The current paper presents a scoping review of the published literature in the research field of social media use and its association with mental health and well-being among adolescents.
Methods and Analysis: First, relevant databases were searched for eligible studies with a vast range of relevant search terms for social media use and mental health and well-being over the past five years. Identified studies were screened thoroughly and included or excluded based on prior established criteria. Data from the included studies were extracted and summarized according to the previously published study protocol.
Results: Among the 79 studies that met our inclusion criteria, the vast majority (94%) were quantitative, with a cross-sectional design (57%) being the most common study design. Several studies focused on different aspects of mental health, with depression (29%) being the most studied aspect. Almost half of the included studies focused on use of non-specified social network sites (43%). Of specified social media, Facebook (39%) was the most studied social network site. The most used approach to measuring social media use was frequency and duration (56%). Participants of both genders were included in most studies (92%) but seldom examined as an explanatory variable. 77% of the included studies had social media use as the independent variable.
Conclusion: The findings from the current scoping review revealed that about 3/4 of the included studies focused on social media and some aspect of pathology. Focus on the potential association between social media use and positive outcomes seems to be rarer in the current literature. Amongst the included studies, few separated between different forms of (inter)actions on social media, which are likely to be differentially associated with mental health and well-being outcomes.
In just a few decades, the use of social media have permeated most areas of our society. For adolescents, social media play a particularly large part in their lives as indicated by their extensive use of several different social media platforms ( Ofcom, 2018 ). Furthermore, the use of social media and types of platforms offered have increased at such a speed that there is reason to believe that scientific knowledge about social media in relation to adolescents’ health and well-being is scattered and incomplete ( Orben, 2020 ). Nevertheless, research findings indicating the potential negative effects of social media on mental health and well-being are frequently reported in traditional media (newspapers, radio, TV) ( Bell et al., 2015 ). Within the scientific community, however, there are ongoing debates regarding the impact and relevance of social media in relation to mental health and well-being. For instance, Twenge and Campbell (2019) stated that use of digital technology and social media have a negative impact on well-being, while Orben and Przybylski (2019) argued that the association between digital technology use and adolescent well-being is so small that it is more or less inconsequential. Research on social media use is a new focus area, and it is therefore important to get an overview of the studies performed to date, and describe the subject matter studies have investigated in relation to the effect of social media use on adolescents mental health and well-being. Also, research gaps in this emerging research field is important to highlight as it may guide future research in new and meritorious directions. A scoping review is therefore deemed necessary to provide a foundation for further research, which in time will provide a knowledge base for policymaking and service delivery.
This scoping review will help provide an overall understanding of the main foci of research within the field of social media and mental health and well-being among adolescents, as well as the type of data sources and research instruments used so far. Furthermore, we aim to highlight potential gaps in the research literature ( Arksey and O’Malley, 2005 ). Even though a large number of studies on social media use and mental health with different vantage points has been conducted over the last decade, we are not aware of any broad-sweeping scoping review covering this area.
This scoping review aims to give an overview of the main research questions that have been focused on with regard to use of social media among adolescents in relation to mental health and well-being. Both quantitative and qualitative studies are of interest. Three specific secondary research questions will be addressed and together with the main research question serve as a template for organizing the results:
- • Which aspects of mental health and well-being have been the focus or foci of research so far?
- • Has the research focused on different research aims across gender, ethnicity, socio-economic status, geographic location? What kind of findings are reported across these groups?
- • Organize and describe the main sources of evidence related to social media that have been used in the studies identified.
Defining Adolescence and Social Media
In the present review, adolescents are defined as those between 13 and 19 years of age. We chose the mean age of 13 as our lower limit as nearly all social media services require users to be at least 13 years of age to access and use their services ( Childnet International, 2018 ). All pertinent studies which present results relevant for this age range is within the scope of this review. For social media we used the following definition by Kietzmann et al. (2011 , p. 1): “Social media employ mobile and web-based technologies to create highly interactive platforms via which individuals and communities share, co-create, discuss, and modify user-generated content.” We also employed the typology described by Kaplan and Haenlein’s classification scheme across two axes: level of self-presentation and social presence/media richness ( Kaplan and Haenlein, 2010 ). The current scoping review adheres to guidelines and recommendations stated by Tricco et al. (2018) .
See protocol for further details about the definitions used ( Schønning et al., 2020 ).
Data Sources and Search Strategy
A literature search was performed in OVID Medline, OVID Embase, OVID PsycINFO, Sociological Abstracts (proquest), Social Services Abstracts (proquest), ERIC (proquest), and CINAHL. The search strategy combined search terms for adolescents, social media and mental health or wellbeing. The database-controlled vocabulary was used for searching subject headings, and a large spectrum of synonyms with appropriate truncations was used for searching title, abstract, and author keywords. A filter for observational studies was applied to limit the results. The search was also limited to publications from 2014 to current. The search strategy was translated between each database. An example of full strategy for Embase is attached as Supplementary Material .
Study Selection: Exclusion and Inclusion Criteria
The exclusion and inclusion criteria are detailed in the protocol ( Schønning et al., 2020 ). Briefly, we included English language peer-reviewed quantitative- or qualitative papers or systematic reviews published within the last 5 years with an explicit focus on mental health/well-being and social media. Non-empirical studies, intervention studies, clinical studies and publications not peer-reviewed were excluded. Intervention studies and clinical studies were excluded as we sought to not introduce too much heterogeneity in design and our focus was on observational studies. The criteria used for study selection was part of an iterative process which was described in detail in the protocol ( Schønning et al., 2020 ). As per the study protocol ( Schønning et al., 2020 ), and in line with scoping review guidelines ( Peters et al., 2015 , 2017 ; Tricco et al., 2018 ), we did not assess methodological quality or risk of bias of the included studies.
The selection process is illustrated by a flow-chart indicating the stages from unsorted search results to the number of included studies (see Figure 1 ). Study selection was accomplished and organized using the Rayyan QCRI software 1 . The inclusion and exclusion process was performed independently by VS and JCS. The interrater agreement was κ = 0.87, indicating satisfactory agreement.

Flowchart of exclusion process from unsorted results to included studies.
Data Extraction and Organization
Details of the data extracted is described in the protocol. Three types of information were extracted, bibliographic information, information about study design and subject matter information. Subject matter information included aim of study, how social media and mental health/well-being was measured, and main findings of the study.
Visualization of Words From the Titles of the Included Studies
The most frequently occurring words and bigrams in the titles of the included studies are presented in Figures 2 , ,3. 3 . The following procedure was used to generate Figure 1 : First, a text file containing all titles were imported into R as a data frame ( R Core Team, 2014 ). The data frame was processed using the “tidy text”-package with required additional packages ( Silge and Robinson, 2016 ). Second, numbers and commonly used words with little inherent meaning (so called “stop words,” such as “and,” “of,” and “in”), were removed from the data frame using the three available lexicons in the “tidy-text”-package ( Silge and Robinson, 2016 ). Furthermore, variations of “adolescents” (e.g., “adolescent,” “adolescence,” and “adolescents”) and “social media” (e.g., “social media,” “social networking,” “online social networks”) were removed from the data frame. Third, the resulting data frame was sorted based on frequency of unique words, and words occurring only once were removed. The final data frame is presented as a word cloud in Figure 1 ( N = 113). The same procedure as described above was employed to generate commonly occurring bigrams (two words occurring adjacent to each other), but without removing bigrams occurring only once ( N = 231). The word clouds were generated using the “wordcloud2”-package in R ( Lang and Chien, 2018 ). For Figure 1 , shades of blue indicate word frequencies >2 and green a frequency of 2. For Figure 2 , shades of blue indicate bigram frequencies of >1 and green a frequency of 1.

Word cloud from the titles of the included studies. Most frequent words, excluding variations of “adolescence” and “social media.” N = 113. Shades of blue indicate word frequencies >2 and green a frequency of 2. The size of each word is indicative of its relative frequency of occurrence.

Word cloud from the titles of the included studies. Bigrams from the titles of the included studies, excluding variations of “adolescence” and “social media.” N = 231. Shades of blue indicate bigram frequencies of >1 and green a frequency of 1. The size of each bigram is indicative of its relative frequency of occurrence.
Characteristics of the Included Studies
Of 7927 unique studies, 79 (1%) met our inclusion criteria ( Aboujaoude et al., 2015 ; Banjanin et al., 2015 ; Banyai et al., 2017 ; Barry et al., 2017 ; Best et al., 2014 , 2015 ; Booker et al., 2018 ; Bourgeois et al., 2014 ; Boyle et al., 2016 ; Brunborg et al., 2017 ; Burnette et al., 2017 ; Colder Carras et al., 2017 ; Critchlow et al., 2019 ; Cross et al., 2015 ; Curtis et al., 2018 ; de Lenne et al., 2018 ; de Vries et al., 2016 ; Erfani and Abedin, 2018 ; Erreygers et al., 2018 ; Fahy et al., 2016 ; Ferguson et al., 2014 ; Fisher et al., 2016 ; Foerster and Roosli, 2017 ; Foody et al., 2017 ; Fredrick and Demaray, 2018 ; Frison and Eggermont, 2016 , 2017 ; Geusens and Beullens, 2017 , 2018 ; Hamm et al., 2015 ; Hanprathet et al., 2015 ; Harbard et al., 2016 ; Hase et al., 2015 ; Holfeld and Mishna, 2019 ; Houghton et al., 2018 ; Jafarpour et al., 2017 ; John et al., 2018 ; Kim et al., 2019 ; Kim, 2017 ; Koo et al., 2015 ; Lai et al., 2018 ; Larm et al., 2017 , 2019 ; Marchant et al., 2017 ; Marengo et al., 2018 ; Marques et al., 2018 ; Meier and Gray, 2014 ; Memon et al., 2018 ; Merelle et al., 2017 ; Neira and Barber, 2014 ; Nesi et al., 2017a , b ; Niu et al., 2018 ; Nursalam et al., 2018 ; Oberst et al., 2017 ; O’Connor et al., 2014 ; O’Reilly et al., 2018 ; Przybylski and Bowes, 2017 ; Przybylski and Weinstein, 2017 ; Richards et al., 2015 ; Rousseau et al., 2017 ; Salmela-Aro et al., 2017 ; Sampasa-Kanyinga and Chaput, 2016 ; Sampasa-Kanyinga and Lewis, 2015 ; Sampasa-Kanyinga et al., 2018 ; Scott and Woods, 2018 ; Settanni et al., 2018 ; Spears et al., 2015 ; Throuvala et al., 2019 ; Tiggemann and Slater, 2017 ; Tseng and Yang, 2015 ; Twenge and Campbell, 2019 ; Twenge et al., 2018 ; van den Eijnden et al., 2018 ; Wang et al., 2018 ; Wartberg et al., 2018 ; Wolke et al., 2017 ; Woods and Scott, 2016 ; Yan et al., 2017 ). Among the included studies, 74 (94%) are quantitative ( Aboujaoude et al., 2015 ; Banjanin et al., 2015 ; Banyai et al., 2017 ; Barry et al., 2017 ; Best et al., 2014 ; Booker et al., 2018 ; Bourgeois et al., 2014 ; Boyle et al., 2016 ; Brunborg et al., 2017 ; Colder Carras et al., 2017 ; Critchlow et al., 2019 ; Cross et al., 2015 ; Curtis et al., 2018 ; de Lenne et al., 2018 ; de Vries et al., 2016 ; Erfani and Abedin, 2018 ; Erreygers et al., 2018 ; Fahy et al., 2016 ; Ferguson et al., 2014 ; Fisher et al., 2016 ; Foerster and Roosli, 2017 ; Foody et al., 2017 ; Fredrick and Demaray, 2018 ; Frison and Eggermont, 2016 , 2017 ; Geusens and Beullens, 2017 , 2018 ; Hamm et al., 2015 ; Hanprathet et al., 2015 ; Harbard et al., 2016 ; Hase et al., 2015 ; Houghton et al., 2018 ; Jafarpour et al., 2017 ; John et al., 2018 ; Kim et al., 2019 ; Kim, 2017 ; Koo et al., 2015 ; Lai et al., 2018 ; Larm et al., 2017 , 2019 ; Marchant et al., 2017 ; Marengo et al., 2018 ; Marques et al., 2018 ; Meier and Gray, 2014 ; Memon et al., 2018 ; Merelle et al., 2017 ; Neira and Barber, 2014 ; Nesi et al., 2017a , b ; Niu et al., 2018 ; Nursalam et al., 2018 ; Oberst et al., 2017 ; O’Connor et al., 2014 ; Przybylski and Bowes, 2017 ; Przybylski and Weinstein, 2017 ; Richards et al., 2015 ; Rousseau et al., 2017 ; Salmela-Aro et al., 2017 ; Sampasa-Kanyinga and Chaput, 2016 ; Sampasa-Kanyinga and Lewis, 2015 ; Sampasa-Kanyinga et al., 2018 ; Scott and Woods, 2018 ; Settanni et al., 2018 ; Spears et al., 2015 ; Tiggemann and Slater, 2017 ; Tseng and Yang, 2015 ; Twenge and Campbell, 2019 ; Twenge et al., 2018 ; van den Eijnden et al., 2018 ; Wang et al., 2018 ; Wartberg et al., 2018 ; Wolke et al., 2017 ; Woods and Scott, 2016 ; Yan et al., 2017 ), three are qualitative ( O’Reilly et al., 2018 ; Burnette et al., 2017 ; Throuvala et al., 2019 ), and two use mixed methods ( Best et al., 2015 ; Holfeld and Mishna, 2019 ) (see Supplementary Tables 1 , 2 in the Supplementary Material for additional details extracted from all included studies). In relation to study design, 45 (57%) used a cross-sectional design ( Bourgeois et al., 2014 ; Ferguson et al., 2014 ; Meier and Gray, 2014 ; Neira and Barber, 2014 ; O’Connor et al., 2014 ; Banjanin et al., 2015 ; Hanprathet et al., 2015 ; Hase et al., 2015 ; Koo et al., 2015 ; Sampasa-Kanyinga and Lewis, 2015 ; Spears et al., 2015 ; Tseng and Yang, 2015 ; Frison and Eggermont, 2016 ; Sampasa-Kanyinga and Chaput, 2016 ; Woods and Scott, 2016 ; Banyai et al., 2017 ; Barry et al., 2017 ; Brunborg et al., 2017 ; Colder Carras et al., 2017 ; Larm et al., 2017 , 2019 ; Merelle et al., 2017 ; Oberst et al., 2017 ; Przybylski and Bowes, 2017 ; Przybylski and Weinstein, 2017 ; Tiggemann and Slater, 2017 ; Wolke et al., 2017 ; Yan et al., 2017 ; de Lenne et al., 2018 ; Erreygers et al., 2018 ; Fredrick and Demaray, 2018 ; Geusens and Beullens, 2018 ; Lai et al., 2018 ; Marengo et al., 2018 ; Marques et al., 2018 ; Niu et al., 2018 ; Nursalam et al., 2018 ; Sampasa-Kanyinga et al., 2018 ; Scott and Woods, 2018 ; Settanni et al., 2018 ; Wang et al., 2018 ; Wartberg et al., 2018 ; Critchlow et al., 2019 ; Kim et al., 2019 ; Twenge and Campbell, 2019 ), 17 used a longitudinal design ( Cross et al., 2015 ; Boyle et al., 2016 ; de Vries et al., 2016 ; Fahy et al., 2016 ; Frison and Eggermont, 2016 ; Harbard et al., 2016 ; Foerster and Roosli, 2017 ; Geusens and Beullens, 2017 ; Kim, 2017 ; Nesi et al., 2017a , b ; Rousseau et al., 2017 ; Salmela-Aro et al., 2017 ; Booker et al., 2018 ; Houghton et al., 2018 ; van den Eijnden et al., 2018 ; Holfeld and Mishna, 2019 ), seven were systematic reviews ( Aboujaoude et al., 2015 ; Best et al., 2015 ; Fisher et al., 2016 ; Marchant et al., 2017 ; Erfani and Abedin, 2018 ; John et al., 2018 ; Memon et al., 2018 ), two were meta-analyses ( Foody et al., 2017 : Curtis et al., 2018 ), one was a causal-comparative study ( Jafarpour et al., 2017 ), one was a review article ( Richards et al., 2015 ), one used a time-lag design ( Twenge et al., 2018 ), one was a scoping review ( Hamm et al., 2015 ), three used a focus-group interview design ( Burnette et al., 2017 ; O’Reilly et al., 2018 ; Throuvala et al., 2019 ), and one study used a combined survey and focus-group design ( Best et al., 2014 ).
The most common study settings were schools [ N = 42 (54%)] ( Best et al., 2014 ; Bourgeois et al., 2014 ; Meier and Gray, 2014 ; Neira and Barber, 2014 ; O’Connor et al., 2014 ; Banjanin et al., 2015 ; Hanprathet et al., 2015 ; Hase et al., 2015 ; Sampasa-Kanyinga and Lewis, 2015 ; Frison and Eggermont, 2016 ; Sampasa-Kanyinga and Chaput, 2016 ; Woods and Scott, 2016 ; Banyai et al., 2017 ; Brunborg et al., 2017 ; Colder Carras et al., 2017 ; Foerster and Roosli, 2017 ; Geusens and Beullens, 2017 , 2018 ; Kim, 2017 ; Larm et al., 2017 , 2019 ; Merelle et al., 2017 ; Nesi et al., 2017a , b ; Przybylski and Bowes, 2017 ; Rousseau et al., 2017 ; Salmela-Aro et al., 2017 ; Tiggemann and Slater, 2017 ; de Lenne et al., 2018 ; Fredrick and Demaray, 2018 ; Houghton et al., 2018 ; Lai et al., 2018 ; Marengo et al., 2018 ; Niu et al., 2018 ; Nursalam et al., 2018 ; Sampasa-Kanyinga et al., 2018 ; Scott and Woods, 2018 ; Settanni et al., 2018 ; van den Eijnden et al., 2018 ; Wang et al., 2018 ; Holfeld and Mishna, 2019 ; Kim et al., 2019 ). Fourteen of the included studies were based on data from a home setting ( Cross et al., 2015 ; Koo et al., 2015 ; Spears et al., 2015 ; Boyle et al., 2016 ; de Vries et al., 2016 ; Harbard et al., 2016 ; Barry et al., 2017 ; Frison and Eggermont, 2017 ; Oberst et al., 2017 ; Yan et al., 2017 ; Booker et al., 2018 ; Marques et al., 2018 ; Wartberg et al., 2018 ; Critchlow et al., 2019 ). Eleven publications were reviews or meta-analyses and included primary studies from different settings ( Aboujaoude et al., 2015 ; Best et al., 2015 ; Hamm et al., 2015 ; Richards et al., 2015 ; Fisher et al., 2016 ; Foody et al., 2017 ; Marchant et al., 2017 ; Curtis et al., 2018 ; Erfani and Abedin, 2018 ; John et al., 2018 ; Memon et al., 2018 ). One study used both a home and school setting ( Erreygers et al., 2018 ), and 11 (14%) of the included studies did not mention the study setting for data collection ( Ferguson et al., 2014 ; Tseng and Yang, 2015 ; Fahy et al., 2016 ; Burnette et al., 2017 ; Jafarpour et al., 2017 ; Przybylski and Weinstein, 2017 ; Wolke et al., 2017 ; O’Reilly et al., 2018 ; Twenge et al., 2018 ; Throuvala et al., 2019 ; Twenge and Campbell, 2019 ).
Mental Health Foci of Included Studies
For a visual overview of the mental health foci of the included studies see Figures 2 , ,3. 3 . Most studies had a focus on different negative aspects of mental health, as evident from the frequently used terms in Figures 2 , ,3. 3 . The most studied aspect was depression, with 23 (29%) studies examining the relationship between social media use and depressive symptoms ( Ferguson et al., 2014 ; Neira and Barber, 2014 ; O’Connor et al., 2014 ; Banjanin et al., 2015 ; Richards et al., 2015 ; Spears et al., 2015 ; Tseng and Yang, 2015 ; Fahy et al., 2016 ; Frison and Eggermont, 2016 , 2017 ; Woods and Scott, 2016 ; Banyai et al., 2017 ; Brunborg et al., 2017 ; Colder Carras et al., 2017 ; Larm et al., 2017 ; Nesi et al., 2017a ; Salmela-Aro et al., 2017 ; Fredrick and Demaray, 2018 ; Houghton et al., 2018 ; Niu et al., 2018 ; Twenge et al., 2018 ; Wang et al., 2018 ; Wartberg et al., 2018 ). Twenty of the included studies focused on different aspects of good mental health, such as well-being, happiness, or quality of life ( Best et al., 2014 , 2015 ; Bourgeois et al., 2014 ; Ferguson et al., 2014 ; Cross et al., 2015 ; Koo et al., 2015 ; Richards et al., 2015 ; Spears et al., 2015 ; Fahy et al., 2016 ; Foerster and Roosli, 2017 ; Przybylski and Bowes, 2017 ; Przybylski and Weinstein, 2017 ; Yan et al., 2017 ; Booker et al., 2018 ; de Lenne et al., 2018 ; Erfani and Abedin, 2018 ; Erreygers et al., 2018 ; Lai et al., 2018 ; van den Eijnden et al., 2018 ; Twenge and Campbell, 2019 ). Nineteen studies had a more broad-stroke approach, and covered general mental health or psychiatric problems ( Aboujaoude et al., 2015 ; Hanprathet et al., 2015 ; Hase et al., 2015 ; Sampasa-Kanyinga and Lewis, 2015 ; Spears et al., 2015 ; Fisher et al., 2016 ; Barry et al., 2017 ; Jafarpour et al., 2017 ; Kim, 2017 ; Merelle et al., 2017 ; Oberst et al., 2017 ; Wolke et al., 2017 ; Marengo et al., 2018 ; Marques et al., 2018 ; Sampasa-Kanyinga et al., 2018 ; Holfeld and Mishna, 2019 ; Kim et al., 2019 ; Larm et al., 2019 ). Eight studies examined the link between social media use and body dissatisfaction and eating disorder symptoms ( Ferguson et al., 2014 ; Meier and Gray, 2014 ; de Vries et al., 2016 ; Burnette et al., 2017 ; Rousseau et al., 2017 ; Tiggemann and Slater, 2017 ; Marengo et al., 2018 ; Wartberg et al., 2018 ). Anxiety was the focus of seven studies ( O’Connor et al., 2014 ; Koo et al., 2015 ; Spears et al., 2015 ; Fahy et al., 2016 ; Woods and Scott, 2016 ; Colder Carras et al., 2017 ; Yan et al., 2017 ), and 13 studies included a focus on the relationship between alcohol use and social media use ( O’Connor et al., 2014 ; Boyle et al., 2016 ; Sampasa-Kanyinga and Chaput, 2016 ; Brunborg et al., 2017 ; Geusens and Beullens, 2017 , 2018 ; Larm et al., 2017 ; Merelle et al., 2017 ; Nesi et al., 2017b ; Curtis et al., 2018 ; Sampasa-Kanyinga et al., 2018 ; Critchlow et al., 2019 ; Kim et al., 2019 ). Seven studies examined the effect of social media use on sleep ( Harbard et al., 2016 ; Woods and Scott, 2016 ; Yan et al., 2017 ; Nursalam et al., 2018 ; Sampasa-Kanyinga et al., 2018 ; Scott and Woods, 2018 ; Larm et al., 2019 ). Five studies saw how drug use and social media use affected each other ( O’Connor et al., 2014 ; Merelle et al., 2017 ; Sampasa-Kanyinga et al., 2018 ; Kim et al., 2019 ; Larm et al., 2019 ). Self-harm and suicidal behavior was the focus of eleven studies ( O’Connor et al., 2014 ; Sampasa-Kanyinga and Lewis, 2015 ; Tseng and Yang, 2015 ; Kim, 2017 ; Marchant et al., 2017 ; Merelle et al., 2017 ; Fredrick and Demaray, 2018 ; John et al., 2018 ; Memon et al., 2018 ; Twenge et al., 2018 ; Kim et al., 2019 ). Other areas of focus other than the aforementioned are loneliness, self-esteem, fear of missing out and other non-pathological measures ( Neira and Barber, 2014 ; Banyai et al., 2017 ; Barry et al., 2017 ; Colder Carras et al., 2017 ).
Social Media Metrics of Included Studies
The studies included in the current scoping review often focus on specific, widely used, social media and social networking services, such as 31 (39%) studies focusing on Facebook ( Bourgeois et al., 2014 ; Meier and Gray, 2014 ; Banjanin et al., 2015 ; Cross et al., 2015 ; Hanprathet et al., 2015 ; Richards et al., 2015 ; Sampasa-Kanyinga and Lewis, 2015 ; Spears et al., 2015 ; Boyle et al., 2016 ; de Vries et al., 2016 ; Frison and Eggermont, 2016 ; Harbard et al., 2016 ; Sampasa-Kanyinga and Chaput, 2016 ; Banyai et al., 2017 ; Barry et al., 2017 ; Brunborg et al., 2017 ; Larm et al., 2017 ; Merelle et al., 2017 ; Nesi et al., 2017a , b ; Rousseau et al., 2017 ; Tiggemann and Slater, 2017 ; Booker et al., 2018 ; de Lenne et al., 2018 ; Lai et al., 2018 ; Marengo et al., 2018 ; Marques et al., 2018 ; Memon et al., 2018 ; Sampasa-Kanyinga et al., 2018 ; Settanni et al., 2018 ; Twenge et al., 2018 ), 11 on Instagram ( Sampasa-Kanyinga and Lewis, 2015 ; Boyle et al., 2016 ; Sampasa-Kanyinga and Chaput, 2016 ; Barry et al., 2017 ; Brunborg et al., 2017 ; Frison and Eggermont, 2017 ; Nesi et al., 2017a ; Marengo et al., 2018 ; Memon et al., 2018 ; Sampasa-Kanyinga et al., 2018 ), 11 including Twitter ( Richards et al., 2015 ; Sampasa-Kanyinga and Lewis, 2015 ; Spears et al., 2015 ; Harbard et al., 2016 ; Sampasa-Kanyinga and Chaput, 2016 ; Barry et al., 2017 ; Brunborg et al., 2017 ; Merelle et al., 2017 ; Nesi et al., 2017a ; Memon et al., 2018 ; Sampasa-Kanyinga et al., 2018 ), and five studies asking about Snapchat ( Boyle et al., 2016 ; Barry et al., 2017 ; Brunborg et al., 2017 ; Nesi et al., 2017a ; Marengo et al., 2018 ). Eight studies mentioned Myspace ( Richards et al., 2015 ; Sampasa-Kanyinga and Lewis, 2015 ; de Vries et al., 2016 ; Harbard et al., 2016 ; Sampasa-Kanyinga and Chaput, 2016 ; Larm et al., 2017 ; Booker et al., 2018 ; Sampasa-Kanyinga et al., 2018 ) and two asked about Tumblr ( Barry et al., 2017 ; Nesi et al., 2017a ). Other media such as Skype ( Merelle et al., 2017 ), Youtube ( Richards et al., 2015 ), WhatsApp ( Brunborg et al., 2017 ), Ping ( Merelle et al., 2017 ), Bebo ( Booker et al., 2018 ), Hyves ( de Vries et al., 2016 ), Kik ( Brunborg et al., 2017 ), Ask ( Brunborg et al., 2017 ), and Qzone ( Niu et al., 2018 ) were only included in one study each.
Almost half ( n = 34, 43%) of the included studies focus on use of social network sites or online communication in general, without specifying particular social media sites, leaving this up to the study participants to decide ( Best et al., 2014 , 2015 ; Ferguson et al., 2014 ; Neira and Barber, 2014 ; O’Connor et al., 2014 ; Koo et al., 2015 ; Tseng and Yang, 2015 ; Fahy et al., 2016 ; Woods and Scott, 2016 ; Burnette et al., 2017 ; Colder Carras et al., 2017 ; Foerster and Roosli, 2017 ; Foody et al., 2017 ; Geusens and Beullens, 2017 , 2018 ; Jafarpour et al., 2017 ; Kim, 2017 ; Marchant et al., 2017 ; Oberst et al., 2017 ; Przybylski and Weinstein, 2017 ; Salmela-Aro et al., 2017 ; Yan et al., 2017 ; Curtis et al., 2018 ; Erfani and Abedin, 2018 ; Erreygers et al., 2018 ; Nursalam et al., 2018 ; Scott and Woods, 2018 ; van den Eijnden et al., 2018 ; Wartberg et al., 2018 ; Critchlow et al., 2019 ; Holfeld and Mishna, 2019 ; Larm et al., 2019 ; Throuvala et al., 2019 ; Twenge and Campbell, 2019 ). Seven of the included studies examined the relationship between virtual game worlds or socially oriented video games and mental health ( Ferguson et al., 2014 ; Best et al., 2015 ; Spears et al., 2015 ; Yan et al., 2017 ; van den Eijnden et al., 2018 ; Larm et al., 2019 ; Twenge and Campbell, 2019 ).
In the 79 studies included in this scoping review, several approaches to measuring social media use are utilized. The combination of frequency and duration of social media use is by far the most used measurement of social media use, and 44 (56%) of the included studies collected data on these parameters ( Ferguson et al., 2014 ; Meier and Gray, 2014 ; Neira and Barber, 2014 ; Banjanin et al., 2015 ; Best et al., 2015 ; Hanprathet et al., 2015 ; Sampasa-Kanyinga and Lewis, 2015 ; Tseng and Yang, 2015 ; Boyle et al., 2016 ; de Vries et al., 2016 ; Frison and Eggermont, 2016 , 2017 ; Harbard et al., 2016 ; Sampasa-Kanyinga and Chaput, 2016 ; Woods and Scott, 2016 ; Banyai et al., 2017 ; Brunborg et al., 2017 ; Colder Carras et al., 2017 ; Foerster and Roosli, 2017 ; Jafarpour et al., 2017 ; Kim, 2017 ; Larm et al., 2017 , 2019 ; Merelle et al., 2017 ; Nesi et al., 2017b ; Oberst et al., 2017 ; Rousseau et al., 2017 ; Tiggemann and Slater, 2017 ; Yan et al., 2017 ; Booker et al., 2018 ; de Lenne et al., 2018 ; Erreygers et al., 2018 ; Houghton et al., 2018 ; Lai et al., 2018 ; Marengo et al., 2018 ; Marques et al., 2018 ; Niu et al., 2018 ; Nursalam et al., 2018 ; Sampasa-Kanyinga et al., 2018 ; Scott and Woods, 2018 ; Settanni et al., 2018 ; Twenge et al., 2018 ; van den Eijnden et al., 2018 ; Twenge and Campbell, 2019 ). Eight studies focused on the relationship between social media addiction or excessive use and mental health ( Banjanin et al., 2015 ; Tseng and Yang, 2015 ; Banyai et al., 2017 ; Merelle et al., 2017 ; Nursalam et al., 2018 ; Settanni et al., 2018 ; Wang et al., 2018 ). Bergen Social Media Addiction Scale is a commonly used questionnaire amongst the included studies ( Hanprathet et al., 2015 ; Banyai et al., 2017 ; Settanni et al., 2018 ). Seven studies asked about various specific actions on social media, such as liking or commenting on photos, posting something or participating in a discussion ( Meier and Gray, 2014 ; Koo et al., 2015 ; Nesi et al., 2017b ; Geusens and Beullens, 2018 ; Marques et al., 2018 ; van den Eijnden et al., 2018 ; Critchlow et al., 2019 ).
Five studies had a specific and sole focus on the link between social media use and alcohol, and examined how various alcohol-related social media use affected alcohol intake ( Boyle et al., 2016 ; Geusens and Beullens, 2017 , 2018 ; Nesi et al., 2017b ; Critchlow et al., 2019 ). Some studies had a more theory-based focus and investigated themes such as peer comparison, social media intrusion or pro-social behavior on social media and its effect on mental health ( Bourgeois et al., 2014 ; Rousseau et al., 2017 ; de Lenne et al., 2018 ). One of the included studies looked into night-time specific social media use ( Scott and Woods, 2018 ) and one looked into pre-bedtime social media behavior ( Harbard et al., 2016 ) to study the link between this use and sleep.
Amongst the 79 included studies, only six (8%) studies had participants of one gender ( Ferguson et al., 2014 ; Meier and Gray, 2014 ; Best et al., 2015 ; Burnette et al., 2017 ; Jafarpour et al., 2017 ; Tiggemann and Slater, 2017 ). Sixteen studies (20%) did not mention the gender distribution of the participants ( Aboujaoude et al., 2015 ; Best et al., 2015 ; Hamm et al., 2015 ; Richards et al., 2015 ; Fisher et al., 2016 ; Woods and Scott, 2016 ; Foody et al., 2017 ; Marchant et al., 2017 ; Przybylski and Weinstein, 2017 ; Curtis et al., 2018 ; Erfani and Abedin, 2018 ; John et al., 2018 ; Memon et al., 2018 ; O’Reilly et al., 2018 ; Twenge et al., 2018 ; Twenge and Campbell, 2019 ). Several of these were meta-analyses or reviews ( Aboujaoude et al., 2015 ; Best et al., 2014 ; Curtis et al., 2018 ; Foody et al., 2017 ; John et al., 2018 ; Erfani and Abedin, 2018 ; Wallaroo, 2020 ). The studies that included both genders as participants generally had a well-balanced gender distribution with no gender below 40% of the participants. Eight of the studies did not report gender-specific results ( Harbard et al., 2016 ; Nesi et al., 2017b ; Curtis et al., 2018 ; de Lenne et al., 2018 ; Niu et al., 2018 ; Nursalam et al., 2018 ; Wang et al., 2018 ; Twenge and Campbell, 2019 ). Of the included studies, gender was seldom examined as an explanatory variable, and other sociodemographic variables (e.g., ethnicity, socioeconomic status) were not included at all.
Implicit Causation Based on Direction of Association
Sixty-one (77%) of the included studies has social media use as the independent variable and some of the mentioned measurements of mental health as the dependent variable ( Aboujaoude et al., 2015 ; Banjanin et al., 2015 ; Banyai et al., 2017 ; Barry et al., 2017 ; Best et al., 2014 ; Booker et al., 2018 ; Bourgeois et al., 2014 ; Boyle et al., 2016 ; Brunborg et al., 2017 ; Colder Carras et al., 2017 ; Critchlow et al., 2019 ; Cross et al., 2015 ; Curtis et al., 2018 ; de Lenne et al., 2018 ; de Vries et al., 2016 ; Erfani and Abedin, 2018 ; Fahy et al., 2016 ; Fisher et al., 2016 ; Foerster and Roosli, 2017 ; Fredrick and Demaray, 2018 ; Frison and Eggermont, 2016 ; Geusens and Beullens, 2018 ; Hamm et al., 2015 ; Hanprathet et al., 2015 ; Harbard et al., 2016 ; Hase et al., 2015 ; Holfeld and Mishna, 2019 ; Jafarpour et al., 2017 ; John et al., 2018 ; Kim et al., 2019 ; Kim, 2017 ; Lai et al., 2018 ; Larm et al., 2017 , 2019 ; Marengo et al., 2018 ; Marques et al., 2018 ; Meier and Gray, 2014 ; Memon et al., 2018 ; Neira and Barber, 2014 ; Nesi et al., 2017b ; Niu et al., 2018 ; Nursalam et al., 2018 ; O’Connor et al., 2014 ; O’Reilly et al., 2018 ; Przybylski and Bowes, 2017 ; Przybylski and Weinstein, 2017 ; Richards et al., 2015 ; Sampasa-Kanyinga and Chaput, 2016 ; Sampasa-Kanyinga and Lewis, 2015 ; Sampasa-Kanyinga et al., 2018 ; Scott and Woods, 2018 ; Spears et al., 2015 ; Tseng and Yang, 2015 ; Twenge and Campbell, 2019 ; Twenge et al., 2018 ; van den Eijnden et al., 2018 ; Wang et al., 2018 ; Wartberg et al., 2018 ; Wolke et al., 2017 ; Woods and Scott, 2016 ; Yan et al., 2017 ). Most of the included studies hypothesize social media use pattern will affect youth mental health in certain ways. The majority of the included studies tend to find a correlation between more frequent social media use and poor well-being and/or mental health (see Supplementary Table 2 ). The strength of this correlation is however heterogeneous as social media use is measured substantially different across studies. Four (5%) of the included studies focus explicitly on how mental health can affect social media use ( Merelle et al., 2017 ; Nesi et al., 2017a ; Erreygers et al., 2018 ; Settanni et al., 2018 ). Fourteen studies included a mediating factor or focus on reciprocal relationships between social media use and mental health ( Ferguson et al., 2014 ; Koo et al., 2015 ; Tseng and Yang, 2015 ; Frison and Eggermont, 2017 ; Geusens and Beullens, 2017 ; Marchant et al., 2017 ; Oberst et al., 2017 ; Rousseau et al., 2017 ; Salmela-Aro et al., 2017 ; Tiggemann and Slater, 2017 ; Houghton et al., 2018 ; Marques et al., 2018 ; Niu et al., 2018 ; Wang et al., 2018 ). An example is a cross-sectional study by Ferguson et al. (2014) suggesting that exposure to social media contribute to later peer competition which was found to be a predictor of negative mental health outcomes such as eating disorder symptoms.
Cyberbullying as a Nexus
Thirteen of the 79 (17%) included studies investigated cyberbullying as the measurement of social media use ( Aboujaoude et al., 2015 ; Cross et al., 2015 ; Hamm et al., 2015 ; Hase et al., 2015 ; Spears et al., 2015 ; Fahy et al., 2016 ; Fisher et al., 2016 ; Foody et al., 2017 ; Przybylski and Bowes, 2017 ; Wolke et al., 2017 ; Fredrick and Demaray, 2018 ; John et al., 2018 ; Holfeld and Mishna, 2019 ). Most of the systematic reviews and meta-analyses included focused on cyberbullying. A cross-sectional study from 2017 suggests that cyberbullying has similar negative effects as direct or relational bullying, and that cyberbullying is “mainly a new tool to harm victims already bullied by traditional means” ( Wolke et al., 2017 ). A meta-analysis from 2016 concludes that “peer cybervictimization is indeed associated with a variety of internalizing and externalizing problems among adolescents” ( Fisher et al., 2016 ). A systematic review from 2018 concludes that both victims and perpetrators of cyberbullying are at greater risk of suicidal behavior compared with non-victims and non-perpetrators ( John et al., 2018 ).
Strengths and Limitations of Present Study
The main strength of this scoping review lies in the effort to give a broad overview of published research related to use of social media, and mental health and well-being among adolescents. Although a range of reviews on screen-based activities in general and mental health and well-being exist ( Dickson et al., 2018 ; Orben, 2020 ), they do not necessarily discern between social media use and other types of technology-based media. Also, some previous reviews tend to be more particular regarding mental health outcome ( Best et al., 2014 ; Seabrook et al., 2016 ; Orben, 2020 ), or do not focus on adolescents per se ( Seabrook et al., 2016 ). The main limitation is that, despite efforts to make the search strategy as comprehensive and inclusive as possible, we probably have not been able to identify all relevant studies – this is perhaps especially true when studies do include relevant information about social media and mental health/well-being, but this information is part of sub-group analyses or otherwise not the main aim of the studies. In a similar manner, related to qualitative studies, we do not know if our search strategy were as efficient in identifying studies of relevance if this was not the main theme or focus of the study. Despite this, we believe that we were able to strike a balance between specificity and sensitivity in our search strategy.
Description of Central Themes and Core Concepts
The findings from the present scoping review on social media use and mental health and well-being among adolescents revealed that the majority (about 3/4) of the included studies focused on social media and pathology. The core concepts identified are social media use and its statistical association with symptoms of depression, general psychiatric symptoms and other symptoms of psychopathology. Similar findings were made by Keles et al. (2020) in a systematic review from 2019. Focus on the potential association between social media use and positive outcomes seems to be rarer in the current literature, even though some studies focused on well-being which also includes positive aspects of mental health. Studies focusing on screen-based media in general and well-being is more prevalent than studies linking social media specifically with well-being ( Orben, 2020 ). The notion that excessive social media use is associated with poor mental health is well established within mainstream media. Our observation that this preconception seems to be the starting point for much research is not conducive to increased knowledge, but also alluded to elsewhere ( Coyne et al., 2020 ).
Why the Focus on Poor Mental Health/Pathology?
The relationship between social media and mental health is likely to be complex, and social media use can be beneficial for maintaining friendships and enriching social life ( Seabrook et al., 2016 ; Birkjær and Kaats, 2019 ; Coyne et al., 2020 ; Orben, 2020 ). This scoping review reveals that the majority of studies focusing on effects of social media use has a clearly stated focus on pathology and detrimental results of social media use. Mainstream media and the public discourse has contributed in creating a culture of fear around social media, with a focus on its negative elements ( Ahn, 2012 ; O’Reilly et al., 2018 ). It is difficult to pin-point why the one-sided focus on the negative effects of social media has been established within the research literature. But likely reasons are elements of “moral panic,” and reports of increases in mental health problems among adolescents in the same period that social media were introduced and became wide-spread ( Birkjær and Kaats, 2019 ). The phenomenon of moral panic typically resurges with the introduction and increasing use of new technologies, as happened with video games, TV, and radio ( Mueller, 2019 ).
The Metrics of Social Media
Social media trends change rapidly, and it is challenging for the research field to keep up. The included studies covered some of the most frequently used social media, but the amount of studies focusing on each social media did not accurately reflect the contemporary distribution of users. Even though sites such as Instagram and Snapchat were covered in some studies, the coverage did not do justice to the amount of users these sites had. Newer social media sites such as TikTok were not mentioned in the included studies even though it has several hundred million daily users ( Mediakix, 2019 ; Wallaroo, 2020 ).
Across the included studies there was some variation in how social media were gauged, but the majority of studies focused on the mere frequency and duration of use. There were little focus on separating between different forms of (inter)actions on social media, as these can vary between being a victim of cyberbullying to participating in healthy community work. Also, few studies differentiated between types of actions (i.e., posting, scrolling, reading), active and passive modes of social media use (i.e., production versus consumption, and level of interactivity), a finding similar to other reports ( Seabrook et al., 2016 ; Verduyn et al., 2017 ; Orben, 2020 ). There is reason to believe that different modes of use on social media platforms are differentially associated with mental health, and a recent narrative review highlight the need to address this in future research ( Orben, 2020 ). One of the included studies found for instance that it is not the total time spent on Facebook or the internet, but the specific amount of time allocated to photo-related activities that is associated with greater symptoms of eating disorders such as thin ideal internalization, self-objectification, weight dissatisfaction, and drive for thinness ( Meier and Gray, 2014 ). This observation can possibly be explained by social comparison mechanisms ( Appel et al., 2016 ) and passive use of social media ( Verduyn et al., 2017 ). The lack of research differentiating social media use and its association with mental health is an important finding of this scoping review and will hopefully contribute to this being included in future studies.
Few studies examined the motivation behind choosing to use social media, or the mental health status of the users when beginning a social media session. It has been reported that young people sometimes choose to enter sites such as Facebook and Twitter as an escape from threats to their mental health such as experiencing overwhelming pressure in daily life ( Boyd, 2014 ). This kind of escapism can be explained through uses and gratifications theory [see for instance ( Coyne et al., 2020 )]. On the other hand, more recent research suggest that additional motivational factors may include the need to control relationships, content, presentation, and impressions ( Throuvala et al., 2019 ), and it is possible that social media use can act as an reinforcement of adolescents’ current moods and motivations ( Birkjær and Kaats, 2019 ). Regardless, it seems obvious that the interplay between online and offline use and underlying motivational mechanisms needs to be better understood.
There has also been some questions about the accuracy when it comes to deciding the amount and frequency of one’s personal social media use. Without measuring duration and frequency of use directly and objectively it is unlikely that subjective self-report of general use is reliable ( Kobayashi and Boase, 2012 ; Scharkow, 2016 , 2019 ; Naab et al., 2019 ). Especially since the potential for social media use is almost omnipresent and the use itself is diverse in nature. Also, due to processes such as social desirability, it is likely that some participants report lower amounts of social media use as excessive use is seen largely undesirable ( Krumpal, 2013 ). Inaccurate reporting of prior social media use could also be a threat to the validity of the reported numbers and thus bias the results reported. Real-time tracking of actual use and modes of use is therefore recommended in future studies to ensure higher accuracy of these aspects of social media use ( Coyne et al., 2020 ; Orben, 2020 ), despite obvious legal and ethical challenges. Another aspect of social media use which does not seem to be addressed is potential spill-over effects, where use of social media leads to potential interest in or thinking about use of – and events or contents on – social media when the individual is offline. When this aspect has been addressed, it seems to be in relation to preoccupations and with a focus on excessive use or addictive behaviors ( Griffiths et al., 2014 ). Conversely, given the ubiquitous and important role of social media, experiences on social media – for better or for worse – are likely to be interconnected with the rest of an individual’s lived experience ( Birkjær and Kaats, 2019 ).
The Studies Seem to Implicitly Think That the Use of Social Media “Causes”/“Affects” Mental Health (Problems)
Most of the included studies establish an implicit causation between social media and mental health. It is assumed that social media use has an impact on mental health. The majority of studies included establish some correlation between more frequent use of social media and poor well-being/mental health, as evident from Supplementary Table 2 . As formerly mentioned, most of the included studies are cross-sectional and cannot shed light into temporality or cause-and-effect. In total, only 16 studies had a longitudinal design, using different types of regression models, latent growth curve models and cross-lagged models. Yet there seems to be an unspoken expectation that the direction of the association is social media use affecting mental health. The reason for this supposition is unclear, but again it is likely that the mainstream media discourse dominated by mostly negative stories and reports of social media use has some impact together with the observed moral panic.
With the increased popularity of social media and internet arrived a reduction of face-to-face contact and supposed increased social isolation ( Kraut et al., 1998 ; Espinoza and Juvonen, 2011 ). This view is described as the displacement hypothesis [see for instance ( Coyne et al., 2020 )]. Having a thriving social life and community with meaningful relations are for many considered vital for well-being and good mental health, and the supposed reduction of sociality were undoubtedly met with skepticism by some. Social media use has increased rapidly among young people over the last two decades along with reports that mental health problems are increasing. Several studies report that there is a rising prevalence of symptom of anxiety and depression among our adolescents ( Bor et al., 2014 ; Olfson et al., 2015 ). The observation that increases in social media use and mental health issues happened in more or less the same time period can have contributed to focus on how use of social media affects mental health problems.
The existence of an implicit causation is supported by the study variables chosen and the lack of positively worded outcomes. Depression, anxiety, alcohol use, psychiatric problems, suicidal behavior and eating disorders are amongst the most studied outcome-variables. On the other side of the spectrum we have well-being, which can oscillate from positive to negative, whilst the measures of pathology only vary from “ill” to “not ill” with positive outcomes not possible.
What Is the Gap in the Literature?
The current literature on social media and mental health among youth is still developing and has several gaps and shortcomings, as evident from this scoping review and other publications ( Seabrook et al., 2016 ; Coyne et al., 2020 ; Keles et al., 2020 ; Orben, 2020 ). Some of the gaps and shortcomings in the field we propose solutions for has been identified in a systematic review from 2019 by Keles et al. (2020) . The majority of the included studies in the current scoping review were cross-sectional, were limited in their inclusion of potential confounders and 3rd variables such as sociodemographics and personality, preventing knowledge about possible cause-and-effect between social media and mental health. There is a lack of longitudinal studies examining the effects of social media over extended periods of time, as well as investigations longitudinally of how mental health impacts social media use. However, since the formal search was ended for this scoping review, some innovative studies have emerged using longitudinal data ( Brunborg and Andreas, 2019 ; Orben et al., 2019 ; Coyne et al., 2020 ). More high quality longitudinal studies of social media use and mental health could help us identify the patterns over time and help us learn about possible cause-and-effect relationships, as well as disentangling between- and within-person associations ( Coyne et al., 2020 ; Orben, 2020 ). Furthermore, both social media use and mental health are complex phenomena in themselves, and future studies need to consider which aspects they want to investigate when trying to understand their relationship. Mechanisms linking social media use and eating disorders are for instance likely to be different than mechanisms linking social media use and symptoms of ADHD.
Our literature search also revealed a paucity of qualitative studies exploring the why’s and how’s of social media use in relation to mental health among adolescents. Few studies examine how youth themselves experience and perceive the relationship between social media and mental health, and the reasons for their continued and frequent use. Qualitatively oriented studies would contribute to a deeper understanding of adolescent’s social media sphere, and their thoughts about the relationship between social media use and mental health [see for instance ( Burnette et al., 2017 )]. For instance, O’Reilly et al. (2018) found that adolescents viewed social media as a threat to mental well-being, and concluded that they buy into the idea that “inherently social media has negative effects on mental wellbeing” and seem to “reify the moral panic that has become endemic to contemporary discourses.” On the other hand, Weinstein found using both quantitative and qualitative data that adolescents’ perceptions of the relationship between social media use and well-being probably is more nuanced, and mostly positive. Another clear gap in the research literature is the lack of focus on potentially positive aspects of social media use. It is obvious that there are some positive sides of the use of social media, and these also need to be investigated further ( Weinstein, 2018 ; Birkjær and Kaats, 2019 ). Gender-specific analyses are also lacking in the research literature, and there is reason to believe that social media use have different characteristics between the genders with different relationships to mental health. In fact, recent findings indicate that not only gender should be considered an important factor when investigating the role of social media in adolescents’ lives, but individual characteristics in general ( Orben et al., 2019 ; Orben, 2020 ). Analyses of socioeconomic status and geographic location are also lacking and it is likely that these factors might play a role the potential association between social media use and mental health. And finally, several studies point to the fact that social media potentially could be a fruitful arena for promoting mental well-being among youth, and developing mental health literacy to better equip our adolescents for the challenges that will surely arise ( O’Reilly et al., 2018 ; Teesson et al., 2020 ).
Research into the association between social media use and mental health and well-being among adolescents is rapidly emerging. The field is characterized by a focus on the association between social media use and negative aspects of mental health and well-being, and where studies focusing on the potentially positive aspects of social media use are lacking. Presently, the majority of studies in the field are quantitatively oriented, with most utilizing a cross-sectional design. An increase in qualitatively oriented studies would add to the field of research by increasing the understanding of adolescents’ social-media life and their own experiences of its association with mental health and well-being. More studies using a longitudinal design would contribute to examining the effects of social media over extended periods of time and help us learn about possible cause-and-effect relationships. Few studies look into individual factors, which may be important for our understanding of the association. Social media use and mental health and well-being are complex phenomena, and future studies could benefit from specifying the type of social media use they focus on when trying to understand its link to mental health. In conclusion, studies including more specific aspects of social media, individual differences and potential intermediate variables, and more studies using a longitudinal design are needed as the research field matures.
Author Contributions
JS conceptualized the review approach and provided general guidance to the research team. VS and JS drafted the first version of this manuscript. JS, GH, and LA developed the draft further based on feedback from the author group. All authors reviewed and approved the final version of the manuscript and have made substantive intellectual contributions to the development of this manuscript.
Conflict of Interest
The authors declare that the research was conducted in the absence of any commercial or financial relationships that could be construed as a potential conflict of interest.
Acknowledgments
We would like to thank Bergen municipality, Hordaland County Council and Western Norway University of Applied Sciences for their collaboration and help with the review. We would also like to thank Senior Librarian Marita Heinz at the Norwegian Institute for Public Health for vital help conducting the literature search.
Funding. This review was partly funded by Regional Research Funds in Norway, funding #RFF297031. No other specific funding was received for the present project. The present project is associated with a larger innovation-project lead by Bergen municipality in Western Norway related to the use of social media and mental health and well-being. The innovation-project is funded by a program initiated by the Norwegian Directorate of Health, and in Vestland county coordinated by the County Council (County Authority). The project aims to explore social media as platform for health-promotion among adolescents.
1 https://rayyan.qcri.org/welcome
Supplementary Material
The Supplementary Material for this article can be found online at: https://www.frontiersin.org/articles/10.3389/fpsyg.2020.01949/full#supplementary-material
- Aboujaoude E., Savage M. W., Starcevic V., Salame W. O. (2015). Cyberbullying: review of an old problem gone viral. J. Adolesc. Health 57 10–18. 10.1016/j.jadohealth.2015.04.011 [ PubMed ] [ CrossRef ] [ Google Scholar ]
- Ahn J. (2012). Teenagers’ experiences with social network sites: relationships to bridging and bonding social capital. Inform. Soc. 28 99–109. 10.1080/01972243.2011.649394 [ CrossRef ] [ Google Scholar ]
- Appel H., Gerlach A. L., Crusius J. (2016). The interplay between Facebook use, social comparison, envy, and depression. Curr. Opin. Psychol. 9 44–49. 10.1016/j.copsyc.2015.10.006 [ CrossRef ] [ Google Scholar ]
- Arksey H., O’Malley L. (2005). Scoping studies: towards a methodological framework. Int. J. Soc. Res. Methodol. 8 19–32. 10.1080/1364557032000119616 [ CrossRef ] [ Google Scholar ]
- Banjanin N., Banjanin N., Dimitrijevic I., Pantic I. (2015). Relationship between internet use and depression: focus on physiological mood oscillations, social networking and online addictive behavior. Comput. Hum. Behav. 43 308–312. 10.1016/j.chb.2014.11.013 [ CrossRef ] [ Google Scholar ]
- Banyai F., Zsila A., Kiraly O., Maraz A., Elekes Z., Griffiths M. D., et al. (2017). Problematic social media use: results from a large-scale nationally representative adolescent sample. PLoS One 12 : e0169839 . 10.1371/journal.pone.0169839 [ PMC free article ] [ PubMed ] [ CrossRef ] [ Google Scholar ]
- Barry C. T., Sidoti C. L., Briggs S. M., Reiter S. R., Lindsey R. A. (2017). Adolescent social media use and mental health from adolescent and parent perspectives. J. Adolesc. 61 1–11. 10.1016/j.adolescence.2017.08.005 [ PubMed ] [ CrossRef ] [ Google Scholar ]
- Bell V., Bishop D. V., Przybylski A. K. (2015). The debate over digital technology and young people. BMJ 351 : h3064 . 10.1136/bmj.h3064 [ PubMed ] [ CrossRef ] [ Google Scholar ]
- Best P., Manktelow R., Taylor B. (2014). Online communication, social media and adolescent wellbeing: a systematic narrative review. Child. Youth Serv. Rev. 41 27–36. 10.1016/j.childyouth.2014.03.001 [ CrossRef ] [ Google Scholar ]
- Best P., Taylor B., Manktelow R. (2015). I’ve 500 friends, but who are my mates? Investigating the influence of online friend networks on adolescent wellbeing. J. Public Ment. Health 14 135–148. 10.1108/jpmh-05-2014-0022 [ CrossRef ] [ Google Scholar ]
- Birkjær M., Kaats M. (2019). in Er sociale Medier Faktisk en Truss for Unges Trivsel? [Does Social Media Really Pose a Threat to Young People’s Well-Being?] , ed. N.M.H.R. Institute (København: Nordic Co-operation; ). [ Google Scholar ]
- Booker C. L., Kelly Y. J., Sacker A. (2018). Gender differences in the associations between age trends of social media interaction and well-being among 10-15 year olds in the UK. BMC Public Health 18 : 321 . 10.1186/s12889-018-5220-4 [ PMC free article ] [ PubMed ] [ CrossRef ] [ Google Scholar ]
- Bor W., Dean A. J., Najman J., Hayatbakhsh R. (2014). Are child and adolescent mental health problems increasing in the 21st century? A systematic review. Austr. N. Z. J. Psychiatry 48 606–616. 10.1177/0004867414533834 [ PubMed ] [ CrossRef ] [ Google Scholar ]
- Bourgeois A., Bower J., Carroll A. (2014). Social networking and the social and emotional wellbeing of adolescents in Australia. J. Psychol. Counsell. Sch. 24 167–182. 10.1017/jgc.2014.14 [ CrossRef ] [ Google Scholar ]
- Boyd D. (2014). It’s Complicated: The Social Lives of Networked Teens. New Haven, CT: Yale University Press. [ Google Scholar ]
- Boyle S. C., LaBrie J. W., Froidevaux N. M., Witkovic Y. D. (2016). Different digital paths to the keg? How exposure to peers’ alcohol-related social media content influences drinking among male and female first-year college students. Addict. Behav. 57 21–29. 10.1016/j.addbeh.2016.01.011 [ PMC free article ] [ PubMed ] [ CrossRef ] [ Google Scholar ]
- Brunborg G. S., Andreas J. B. (2019). Increase in time spent on social media is associated with modest increase in depression, conduct problems, and episodic heavy drinking. J. Adolesc. 74 201–209. 10.1016/j.adolescence.2019.06.013 [ PubMed ] [ CrossRef ] [ Google Scholar ]
- Brunborg G. S., Andreas J. B., Kvaavik E. (2017). Social media use and episodic heavy drinking among adolescents. Psychol. Rep. 120 475–490. 10.1177/0033294117697090 [ PubMed ] [ CrossRef ] [ Google Scholar ]
- Burnette C. B., Kwitowski M. A., Mazzeo S. E. (2017). “I don’t need people to tell me I’m pretty on social media:” A qualitative study of social media and body image in early adolescent girls. Body Image 23 114–125. 10.1016/j.bodyim.2017.09.001 [ PubMed ] [ CrossRef ] [ Google Scholar ]
- Childnet International (2018). Age Restrictions on Social Media Services. Available online at: https://www.childnet.com/blog/age-restrictions-on-social-media-services (accessed September 30, 2019). [ Google Scholar ]
- Colder Carras M., Van Rooij A. J., Van de Mheen D., Musci R., Xue Q., Mendelson T. (2017). Video gaming in a hyperconnected world: a cross-sectional study of heavy gaming, problematic gaming symptoms, and online socializing in adolescents. Comput. Hum. Bahav. 68 472–479. 10.1016/j.chb.2016.11.060 [ PMC free article ] [ PubMed ] [ CrossRef ] [ Google Scholar ]
- Coyne S. M., Rogers A. A., Zurcher J. D., Stockdale L., Booth M. (2020). Does time spent using social media impact mental health?: an eight year longitudinal study. Comput. Hum. Behav. 104 : 106160 10.1016/j.chb.2019.106160 [ CrossRef ] [ Google Scholar ]
- Critchlow N., MacKintosh A. M., Hooper L., Thomas C., Vohra J. (2019). Participation with alcohol marketing and user-created promotion on social media, and the association with higher-risk alcohol consumption and brand identification among adolescents in the UK. Addict. Res. Theory 27 515–526. 10.1080/16066359.2019.1567715 [ PMC free article ] [ PubMed ] [ CrossRef ] [ Google Scholar ]
- Cross D., Lester L., Barnes A. (2015). A longitudinal study of the social and emotional predictors and consequences of cyber and traditional bullying victimisation. Int. J. Public Health 60 207–217. 10.1007/s00038-015-0655-1 [ PubMed ] [ CrossRef ] [ Google Scholar ]
- Curtis B. L., Lookatch S. J., Ramo D. E., McKay J. R., Feinn R. S., Kranzler H. R. (2018). Meta-analysis of the association of alcohol-related social media use with alcohol consumption and alcohol-related problems in adolescents and young adults. Alcohol. Clin. Exp. Res. 42 978–986. 10.1111/acer.13642 [ PMC free article ] [ PubMed ] [ CrossRef ] [ Google Scholar ]
- de Lenne O., Vandenbosch L., Eggermont S., Karsay K., Trekels J. (2018). Picture-perfect lives on social media: a cross-national study on the role of media ideals in adolescent well-being. Med. Psychol. 23 52–78. 10.1080/15213269.2018.1554494 [ CrossRef ] [ Google Scholar ]
- de Vries D. A., Peter J., de Graaf H., Nikken P. (2016). Adolescents’ social network site use, peer appearance-related feedback, and body dissatisfaction: testing a mediation model. J. Youth Adolesc. 45 211–224. 10.1007/s10964-015-0266-4 [ PMC free article ] [ PubMed ] [ CrossRef ] [ Google Scholar ]
- Dickson K., Richardson M., Kwan I., MacDowall W., Burchett H., Stansfield C., et al. (2018). Screen-Based Activities and Children and Young People’s Mental Health: A Systematic Map of Reviews. London: University College London. [ PMC free article ] [ PubMed ] [ Google Scholar ]
- Erfani S. S., Abedin B. (2018). Impacts of the use of social network sites on users’ psychological well-being: a systematic review. J. Assoc. Inform. Sci. Technol. 69 900–912. 10.1002/asi.24015 [ CrossRef ] [ Google Scholar ]
- Erreygers S., Vandebosch H., Vranjes I., Baillien E., De Witte H. (2018). Feel good, do good online? Spillover and crossover effects of happiness on adolescents’ online prosocial behavior. Happiness Stud. 20 1241–1258. 10.1007/s10902-018-0003-2 [ CrossRef ] [ Google Scholar ]
- Espinoza G., Juvonen J. (2011). The pervasiveness, connectedness, and intrusiveness of social network site use among young adolescents. Cyberpsychol. Behav. Soc. Netw. 14 705–709. 10.1089/cyber.2010.0492 [ PubMed ] [ CrossRef ] [ Google Scholar ]
- Fahy A. E., Stansfield S. A., Smuk M., Smith N. R., Cummins S., Clark C. (2016). Longitudinal associations between cyberbullying involvement and adolescent mental health. J. Adolesc. Health 59 502–509. 10.1016/j.jadohealth.2016.06.006 [ PubMed ] [ CrossRef ] [ Google Scholar ]
- Ferguson C. J., Munoz M. E., Garza A., Galindo M. (2014). Concurrent and prospective analyses of peer, television and social media influences on body dissatisfaction, eating disorder symptoms and life satisfaction in adolescent girls. J. Youth Adolesc. 43 1–14. 10.1007/s10964-012-9898-9 [ PubMed ] [ CrossRef ] [ Google Scholar ]
- Fisher B. W., Gardella J. H., Teurbe-Tolon A. R. (2016). Peer Cybervictimization among adolescents and the associated internalizing and externalizing problems: a meta-analysis. J. Youth Adolesc. 45 1727–1743. 10.1007/s10964-016-0541-z [ PubMed ] [ CrossRef ] [ Google Scholar ]
- Foerster M., Roosli M. (2017). A latent class analysis on adolescents media use and associations with health related quality of life. Comput. Huma. Bahav. 71 266–274. 10.1016/j.chb.2017.02.015 [ CrossRef ] [ Google Scholar ]
- Foody M., Samara M., O’Higgins Norman J. (2017). Bullying and cyberbullying studies in the school-aged population on the island of Ireland: a meta-analysis. Br. J. Educ. Psychol. 87 535–557. 10.1111/bjep.12163 [ PubMed ] [ CrossRef ] [ Google Scholar ]
- Fredrick S. S., Demaray M. K. (2018). Peer victimization and suicidal ideation: the role of gender and depression in a school. Based sample. J. Sch. Psychol. 67 1–15. 10.1016/j.jsp.2018.02.001 [ PubMed ] [ CrossRef ] [ Google Scholar ]
- Frison E., Eggermont S. (2016). Exploring the relationships between different types of Facebook use, perceived online social support, and adolescents’ depressed mood. Soc. Sci. Comput. Rev. 34 153–171. 10.1177/0894439314567449 [ CrossRef ] [ Google Scholar ]
- Frison E., Eggermont S. (2017). Browsing, posting, and liking on instagram: the reciprocal relationships between different types of instagram use and adolescents’. Depressed Mood. 20 603–609. 10.1089/cyber.2017.0156 [ PubMed ] [ CrossRef ] [ Google Scholar ]
- Geusens F., Beullens K. (2017). The reciprocal associations between sharing alcohol references on social networking sites and binge drinking: a longitudinal study among late adolescents. Comput. Hum. Behav. 73 499–506. 10.1016/j.chb.2017.03.062 [ CrossRef ] [ Google Scholar ]
- Geusens F., Beullens K. (2018). The association between social networking sites and alcohol abuse among Belgian adolescents: the role of attitudes and social norms. J. Media Psychol. 30 207–216. 10.1027/1864-1105/a000196 [ CrossRef ] [ Google Scholar ]
- Griffiths M. D., Kuss D. J., Demetrovics Z. (2014). “ Chapter 6 - social networking addiction: an overview of preliminary findings ,” in Behavioral Addictions , eds Rosenberg K. P., Feder L. C. (San Diego: Academic Press), 119–141. [ Google Scholar ]
- Hamm M. P., Newton A. S., Chisholm A., Shulhan J., Milne A., Sundar P., et al. (2015). Prevalence and effect of cyberbullying on children and young people: a scoping review of social media studies. JAMA Pediatr. 169 770–777. [ PubMed ] [ Google Scholar ]
- Hanprathet N., Manwong M., Khumsri J., Yingyeun R., Phanasathit M. (2015). Facebook addiction and its relationship with mental health among thai high school students. J. Med. Assoc. Thailand 98(Suppl. 3) S81–S90. [ PubMed ] [ Google Scholar ]
- Harbard E., Allen N. B., Trinder J., Bei B. (2016). What’s keeping teenagers up? prebedtime behaviors and actigraphy-assessed sleep over school and vacation. J. Adolesc. Health 58 426–432. 10.1016/j.jadohealth.2015.12.011 [ PubMed ] [ CrossRef ] [ Google Scholar ]
- Hase C. N., Goldberg S. B., Smith D., Stuck A., Campain J. (2015). Impacts of traditional bullying and cyberbullying on the mental health of middle school and high school students. Psychol. Sch. 52 607–617. 10.1002/pits.21841 [ CrossRef ] [ Google Scholar ]
- Holfeld B., Mishna F. (2019). , Internalizing symptoms and externalizing problems: risk factors for or consequences of cyber victimization? J. Youth Adolesc. 48 567–580. 10.1007/s10964-018-0974-7 [ PubMed ] [ CrossRef ] [ Google Scholar ]
- Houghton S., Lawrence D., Hunter S. C., Rosenberg M., Zadow C., Wood L., et al. (2018). Reciprocal relationships between trajectories of depressive symptoms and screen media use during adolescence. Youth Adolesc. 47 2453–2467. 10.1007/s10964-018-0901-y [ PMC free article ] [ PubMed ] [ CrossRef ] [ Google Scholar ]
- Jafarpour S., Jadidi H., Almadani S. A. H. (2017). Comparing personality traits, mental health and self-esteem in users and non-users of social networks. Razavi Int. J. Med. 5 :e61401. 10.5812/rijm.61401 [ CrossRef ] [ Google Scholar ]
- John A., Glendenning A. C., Marchant A., Montgomery P., Stewart A., Wood S., et al. (2018). Self-harm, suicidal behaviours, and cyberbullying in children and young people: systematic review. J. Med. Int. Res. 20 : e129 . 10.2196/jmir.9044 [ PMC free article ] [ PubMed ] [ CrossRef ] [ Google Scholar ]
- Kaplan A. M., Haenlein M. (2010). Users of the world, unite! The challenges and opportunities of social media. Bus. Horiz. 53 59–68. 10.1016/j.bushor.2009.09.003 [ CrossRef ] [ Google Scholar ]
- Keles B., McCrae N., Grealish A. (2020). A systematic review: the influence of social media on depression, anxiety and psychological distress in adolescents. Int. J. Adolesc. Youth 25 79–93. 10.1080/02673843.2019.1590851 [ CrossRef ] [ Google Scholar ]
- Kietzmann J. H., Hermkens K., McCarthy I. P., Silvestre B. S. (2011). Social media? Get serious! Understanding the functional building blocks of social media. Bus. Horiz. 54 241–251. 10.1016/j.bushor.2011.01.005 [ CrossRef ] [ Google Scholar ]
- Kim H. H.-S. (2017). The impact of online social networking on adolescent psychological well-being (WB): a population-level analysis of Korean school-aged children. Int. J. Adolesc. Youth 22 364–376. 10.1080/02673843.2016.1197135 [ CrossRef ] [ Google Scholar ]
- Kim S., Kimber M., Boyle M. H., Georgiades K. (2019). Sex differences in the association between cyberbullying victimization and mental health. Subst. Suicid. Ideation Adolesc. 64 126–135. 10.1177/0706743718777397 [ PMC free article ] [ PubMed ] [ CrossRef ] [ Google Scholar ]
- Kobayashi T., Boase J. (2012). No Such Effect? The implications of measurement error in self-report measures of mobile communication use. Commun. Methods Meas. 6 126–143. 10.1080/19312458.2012.679243 [ CrossRef ] [ Google Scholar ]
- Koo H. J., Woo S., Yang E., Kwon J. H. (2015). The double meaning of online social space: three-way interactions among social anxiety, online social behavior, and offline social behavior. Cyberpsychol. Behav. Soc. Netw. 18 514–520. 10.1089/cyber.2014.0396 [ PubMed ] [ CrossRef ] [ Google Scholar ]
- Kraut R., Patterson M., Lundmark V., Kiesler S., Mukophadhyay T., Scherlis W. (1998). Internet paradox: a social technology that reduces social involvement and psychological well-being? Am. Psychol. 53 1017 . 10.1037/0003-066x.53.9.1017 [ PubMed ] [ CrossRef ] [ Google Scholar ]
- Krumpal I. (2013). Determinants of social desirability bias in sensitive surveys: a literature review. Qua. Quant. 47 2025–2047. 10.1007/s11135-011-9640-9 [ CrossRef ] [ Google Scholar ]
- Lai H.-M., Hsieh P.-J., Zhang R.-C. (2018). Understanding adolescent students’ use of facebook and their subjective wellbeing: a gender-based comparison. Behav. Inform. Technol. 38 533–548. 10.1080/0144929x.2018.1543452 [ CrossRef ] [ Google Scholar ]
- Lang D., Chien G. (2018). “wordcloud2”: a fast visualization tool for creating wordclouds by using “wordcloud2.js”. R Package Version 0.2.1. Available online at: https://cran.r-project.org/web/packages/wordcloud2/index.html [ Google Scholar ]
- Larm P., Aslund C., Nilsson K. W. (2017). The role of online social network chatting for alcohol use in adolescence: testing three peer-related pathways in a Swedish population-based sample. Comput. Hum. Behav. 71 284–290. 10.1016/j.chb.2017.02.012 [ CrossRef ] [ Google Scholar ]
- Larm P., Raninen J., Åslund C., Svensson J., Nilsson K. W. (2019). The increased trend of non-drinking alcohol among adolescents: what role do internet activities have? Eur. J. Public Health 29 27–32. 10.1093/eurpub/cky168 [ PMC free article ] [ PubMed ] [ CrossRef ] [ Google Scholar ]
- Marchant A., Hawton K., Stewart A., Montgomery P., Singaravelu V., Lloyd K., et al. (2017). A systematic review of the relationship between internet use, self-harm and suicidal behaviour in young people: the good, the bad and the unknown. PLoS One 12 : e0181722 . 10.1371/journal.pone.0181722 [ PMC free article ] [ PubMed ] [ CrossRef ] [ Google Scholar ]
- Marengo D., Longobardi C., Fabris M. A., Settanni M. (2018). Highly-visual social media and internalizing symptoms in adolescence: the mediating role of body image concerns. Comput. Hum. Behav. 82 63–69. 10.1016/j.chb.2018.01.003 [ CrossRef ] [ Google Scholar ]
- Marques T. P., Marques-Pinto A., Alvarez M. J., Pereira C. R. (2018). Facebook: risks and opportunities in brazilian and portuguese youths with different levels of psychosocial adjustment. Spanish J. Psychol. 21 : E31 . [ PubMed ] [ Google Scholar ]
- Mediakix (2019). 20 Tiktok Statistics Marketers Need To Know: Tiktok Demographics & Key Data. 2019. Available online at: https://mediakix.com/blog/top-tik-tok-statistics-demographics/ (accessed February 20, 2020). [ Google Scholar ]
- Meier E. P., Gray J. (2014). Facebook photo activity associated with body image disturbance in adolescent girls. Cyberpsychol. Behav. Soc. Netw. 17 199–206. 10.1089/cyber.2013.0305 [ PubMed ] [ CrossRef ] [ Google Scholar ]
- Memon A. M., Sharma S. G., Mohite S. S., Jain S. (2018). The role of online social networking on deliberate self-harm and suicidality in adolescents: a systematized review of literature. Indian J. Psychiatry 60 384–392. [ PMC free article ] [ PubMed ] [ Google Scholar ]
- Merelle S. Y. M., Kleiboer A., Schotanus M., Cluitmans T. L. M., Waardenburg C. M., Kramer D., et al. (2017). Which health-related problems are associated with problematic video-gaming or social media use in adolescents? A large-scale cross-sectional study. Clin. Neuropsych. 14 11–19. [ Google Scholar ]
- Mueller M. (2019). Challenging the Social Media Moral Panic: Preserving Free Expression under Hypertransparency. Washington, DC: Cato Institute Policy Analysis. [ Google Scholar ]
- Naab T. K., Karnowski V., Schlütz D. (2019). Reporting mobile social media use: how survey and experience sampling measures differ. Commun. Methods Meas. 13 126–147. 10.1080/19312458.2018.1555799 [ CrossRef ] [ Google Scholar ]
- Neira C. J., Barber B. L. (2014). Social networking site use: linked to adolescents’ social self-concept, self-esteem, and depressed mood. Austr. J. Psychol. 66 56–64. 10.1111/ajpy.12034 [ CrossRef ] [ Google Scholar ]
- Nesi J., Miller A. B., Prinstein M. J. (2017a). Adolescents’ depressive symptoms and subsequent technology-based interpersonal behaviors: a multi-wave study. J. Appl. Dev. Psychol. 51 12–19. 10.1016/j.appdev.2017.02.002 [ PMC free article ] [ PubMed ] [ CrossRef ] [ Google Scholar ]
- Nesi J., Rothenberg W. A., Hussong A. M., Jackson K. M. (2017b). Friends’ alcohol-related social networking site activity predicts escalations in adolescent drinking: mediation by peer norms. J. Adolesc. Health 60 641–647. 10.1016/j.jadohealth.2017.01.009 [ PMC free article ] [ PubMed ] [ CrossRef ] [ Google Scholar ]
- Niu G. F., Luo Y. J., Sun X. J., Zhou Z. K., Yu F., Yang S. L., et al. (2018). Qzone use and depression among Chinese adolescents: a moderated mediation model. J. Affect. Disord. 231 58–62. 10.1016/j.jad.2018.01.013 [ PubMed ] [ CrossRef ] [ Google Scholar ]
- Nursalam N., Octavia M., Tristiana R. D., Efendi F. (2018). Association between insomnia and social network site use in Indonesian adolescents. Nurs. Forum 54 149–156. 10.1111/nuf.12308 [ PubMed ] [ CrossRef ] [ Google Scholar ]
- Oberst U., Wegmann E., Stodt B., Brand M., Chamarro A. (2017). Negative consequences from heavy social networking in adolescents: the mediating role of fear of missing out. J. Adolesc. 55 51–60. 10.1016/j.adolescence.2016.12.008 [ PubMed ] [ CrossRef ] [ Google Scholar ]
- O’Connor R. C., Rasmussen S., Hawton K. (2014). Adolescent self-harm: a school-based study in Northern Ireland. J. Affect. Disord. 159 46–52. 10.1016/j.jad.2014.02.015 [ PubMed ] [ CrossRef ] [ Google Scholar ]
- Ofcom (2018). Children and Parents: Media Use and Attitudes Report. Warrington: Ofcom. [ Google Scholar ]
- Olfson M., Druss B. G., Marcus S. C. (2015). Trends in mental health care among children and adolescents. N. Engl. J. Med. 372 2029–2038. 10.1056/nejmsa1413512 [ PubMed ] [ CrossRef ] [ Google Scholar ]
- Orben A. (2020). Teenagers, screens and social media: a narrative review of reviews and key studies. J. Soc. Psychiatry Psychiatr. Epidemiol. 55 407–414. 10.1007/s00127-019-01825-4 [ PubMed ] [ CrossRef ] [ Google Scholar ]
- Orben A., Dienlin T., Przybylski A. K. (2019). Social media’s enduring effect on adolescent life satisfaction. Pro. Natl. Acad. Sci. U.S.A. 116 10226–10228. 10.1073/pnas.1902058116 [ PMC free article ] [ PubMed ] [ CrossRef ] [ Google Scholar ]
- Orben A., Przybylski A. K. (2019). The association between adolescent well-being and digital technology use. Nat. Hum. Behav. 3 173–182. 10.1038/s41562-018-0506-1 [ PubMed ] [ CrossRef ] [ Google Scholar ]
- O’Reilly M., Dogra N., Whiteman N., Hughes J., Eruyar S., Reilly P. (2018). Is social media bad for mental health and wellbeing? Exploring the perspectives of adolescents. Clin. Chld Psychol. Psychiatry 23 601–613. 10.1177/1359104518775154 [ PubMed ] [ CrossRef ] [ Google Scholar ]
- Peters M., Godfrey C., McInerney P. (2017). “ Chapter 11: scoping reviews ,” in Joanna Briggs Institute Reviewer’s Manual , eds Aromataris E., Munn Z. (Adelaide: The Joanna Briggs Institute; ). [ Google Scholar ]
- Peters M. D., Godfrey C., Khalil H., McInerney P., Parker D., Soares C. B. (2015). Guidance for conducting systematic scoping reviews. Int. J. Evi. -Based Healthc. 13 141–146. 10.1097/xeb.0000000000000050 [ PubMed ] [ CrossRef ] [ Google Scholar ]
- Przybylski A. K., Bowes L. (2017). Cyberbullying and adolescent well-being in England: a population-based cross-sectional study. Lancet Child Adolesc. Health 1 19–26. 10.1016/s2352-4642(17)30011-1 [ PubMed ] [ CrossRef ] [ Google Scholar ]
- Przybylski A. K., Weinstein N. (2017). A large-scale test of the goldilocks hypothesis. Psychol. Sci. 28 204–215. 10.1177/0956797616678438 [ PubMed ] [ CrossRef ] [ Google Scholar ]
- R Core Team (2014). R: A Language and Environment for Statistical Computing. Vienna: R Foundation for Statistical Computing. [ Google Scholar ]
- Richards D., Caldwell P. H., Go H. (2015). Impact of social media on the health of children and young people. J. Paediatr. Child Health 51 1152–1157. 10.1111/jpc.13023 [ PubMed ] [ CrossRef ] [ Google Scholar ]
- Rousseau A., Eggermont S., Frison E. (2017). The reciprocal and indirect relationships between passive Facebook use, comparison on Facebook, and adolescents’ body dissatisfaction. Comput. Hum. Behav. 73 336–344. 10.1016/j.chb.2017.03.056 [ CrossRef ] [ Google Scholar ]
- Salmela-Aro K., Upadyaya K., Hakkarainen K., Lonka K., Alho K. (2017). The dark side of internet use: two longitudinal studies of excessive internet use. depressive symptoms, school burnout and engagement among finnish early and late adolescents. J. Youth Adolesc. 46 343–357. 10.1007/s10964-016-0494-2 [ PubMed ] [ CrossRef ] [ Google Scholar ]
- Sampasa-Kanyinga H., Chaput J. P. (2016). Use of social networking sites and alcohol consumption among adolescents. Public Health 139 88–95. 10.1016/j.puhe.2016.05.005 [ PubMed ] [ CrossRef ] [ Google Scholar ]
- Sampasa-Kanyinga H., Hamilton H. A., Chaput J. P. (2018). Use of social media is associated with short sleep duration in a dose-response manner in students aged 11 to 20 years. Acta Paediatr. 107 694–700. 10.1111/apa.14210 [ PubMed ] [ CrossRef ] [ Google Scholar ]
- Sampasa-Kanyinga H., Lewis R. F. (2015). Frequent use of social networking sites is associated with poor psychological functioning among children and adolescents. Cyberpsychol. Behav. Soc. Netw. 18 380–385. 10.1089/cyber.2015.0055 [ PubMed ] [ CrossRef ] [ Google Scholar ]
- Scharkow M. (2016). The accuracy of self-reported internet Use—A validation study using client log data. Commun. Methods Meas. 10 13–27. 10.1080/19312458.2015.1118446 [ CrossRef ] [ Google Scholar ]
- Scharkow M. (2019). The reliability and temporal stability of self-reported media exposure: a meta-analysis. Commun. Methods Meas. 13 198–211. 10.1080/19312458.2019.1594742 [ CrossRef ] [ Google Scholar ]
- Schønning V., Aarø L. E., Skogen J. C. (2020). Central themes, core concepts and knowledge gaps concerning social media use, and mental health and well-being among adolescents: a protocol of a scoping review of published literature. BMJ Open 10 : e031105 . 10.1136/bmjopen-2019-031105 [ PMC free article ] [ PubMed ] [ CrossRef ] [ Google Scholar ]
- Scott H., Woods H. C. (2018). Fear of missing out and sleep: cognitive behavioural factors in adolescents’ nighttime social media use. J. Adolesc. 68 61–65. 10.1016/j.adolescence.2018.07.009 [ PubMed ] [ CrossRef ] [ Google Scholar ]
- Seabrook E. M., Kern M. L., Rickard N. S. (2016). Social networking sites, depression, and anxiety: a systematic review. JMIR Ment. Health 3 : e50 . 10.2196/mental.5842 [ PMC free article ] [ PubMed ] [ CrossRef ] [ Google Scholar ]
- Settanni M., Marengo D., Fabris M. A., Longobardi C. (2018). The interplay between ADHD symptoms and time perspective in addictive social media use: a study on adolescent Facebook users. Child. Youth Serv. Rev. 89 165–170. 10.1016/j.childyouth.2018.04.031 [ CrossRef ] [ Google Scholar ]
- Silge J., Robinson D. (2016). tidytext: text mining and analysis using tidy data principles in RJ. Open Source Softw. 1 : 37 10.21105/joss.00037 [ CrossRef ] [ Google Scholar ]
- Spears B. A., Taddeo C. M., Daly A. L., Stretton A., Karklins L. T. (2015). Cyberbullying, help-seeking and mental health in young Australians: implications for public health. Int. J. Public Health 60 219–226. 10.1007/s00038-014-0642-y [ PubMed ] [ CrossRef ] [ Google Scholar ]
- Teesson M., Newton N. C., Slade T., Chapman C., Birrell L., Mewton L., et al. (2020). Combined prevention for substance use, depression, and anxiety in adolescence: a cluster-randomised controlled trial of a digital online intervention. Lancet Digital Health 2 e74–e84. 10.1016/s2589-7500(19)30213-4 [ PubMed ] [ CrossRef ] [ Google Scholar ]
- Throuvala M. A., Griffiths M. D., Rennoldson M., Kuss D. J. (2019). Motivational processes and dysfunctional mechanisms of social media use among adolescents: a qualitative focus group study. Comput. Hum. Behav. 93 164–175. 10.1016/j.chb.2018.12.012 [ CrossRef ] [ Google Scholar ]
- Tiggemann M., Slater A. (2017). Facebook and body image concern in adolescent girls: a prospective study. Int. J. Eat. Disord. 50 80–83. 10.1002/eat.22640 [ PubMed ] [ CrossRef ] [ Google Scholar ]
- Tricco A. C., Lillie E., Zarin W., O’Brien K. K., Colquhoun H., Levac D., et al. (2018). PRISMA extension for scoping reviews (PRISMA-ScR): checklist and explanation. Ann. Int. Med. 169 467–473. [ PubMed ] [ Google Scholar ]
- Tseng F.-Y., Yang H.-J. (2015). Internet use and web communication networks, sources of social support, and forms of suicidal and nonsuicidal self-injury among adolescents: different patterns between genders. Suicide Life Threat. Behav. 45 178–191. 10.1111/sltb.12124 [ PubMed ] [ CrossRef ] [ Google Scholar ]
- Twenge J. M., Campbell W. K. (2019). Media use is linked to lower psychological well-being: evidence from three datasets. Psychiatr. Q. 11 311–331. 10.1007/s11126-019-09630-7 [ PubMed ] [ CrossRef ] [ Google Scholar ]
- Twenge J. M., Joiner T. E., Rogers M. L., Martin G. N. (2018). Increases in depressive symptoms, suicide-related outcomes, and suicide rates among U.S. adolescents after 2010 and links to increased new media screen time. Clin. Psychol. Sci. 6 3–17. 10.1177/2167702617723376 [ CrossRef ] [ Google Scholar ]
- van den Eijnden R., Koning I., Doornwaard S., van Gurp F., ter Bogt T. (2018). The impact of heavy and disordered use of games and social media on adolescents’ psychological, social, and school functioning. J. Behav. Addict. 7 697–706. 10.1556/2006.7.2018.65 [ PMC free article ] [ PubMed ] [ CrossRef ] [ Google Scholar ]
- Verduyn P., Ybarra O., Resibois M., Jonides J., Kross E. (2017). Do social network sites enhance or undermine subjective well-being? a critical review: do social network sites enhance or undermine subjective well-being?. Soc. Issues Policy Rev. 11 274–302. 10.1111/sipr.12033 [ CrossRef ] [ Google Scholar ]
- Wallaroo (2020). TikTok Statistics – Updated February 2020. Available online at: https://wallaroomedia.com/blog/social-media/tiktok-statistics/ (accessed February 20, 2020). [ Google Scholar ]
- Wang P., Wang X., Wu Y., Xie X., Wang X., Zhao F., et al. (2018). Social networking sites addiction and adolescent depression: A moderated mediation model of rumination and self-esteem. Personal. Individ. Differ. 127 162–167. 10.1016/j.paid.2018.02.008 [ CrossRef ] [ Google Scholar ]
- Wartberg L., Kriston L., Thomasius R. (2018). Depressive symptoms in adolescents. Dtsch. Arztebl. Int. 115 549–555. [ PMC free article ] [ PubMed ] [ Google Scholar ]
- Weinstein E. (2018). The social media see-saw: positive and negative influences on adolescents’ affective well-being. New Media Soc. 20 3597–3623. 10.1177/1461444818755634 [ CrossRef ] [ Google Scholar ]
- Wolke D., Lee K., Guy A. (2017). Cyberbullying: a storm in a teacup? Eur. Child Adolesc. Psychiatry 26 899–908. 10.1007/s00787-017-0954-6 [ PMC free article ] [ PubMed ] [ CrossRef ] [ Google Scholar ]
- Woods H. C., Scott H. (2016). #Sleepyteens: social media use in adolescence is associated with poor sleep quality, anxiety, depression and low self-esteem. J. Adolesc. 51 41–49. 10.1016/j.adolescence.2016.05.008 [ PubMed ] [ CrossRef ] [ Google Scholar ]
- Yan H., Zhang R., Oniffrey T. M., Chen G., Wang Y., Wu Y., et al. (2017). Associations among screen time and unhealthy behaviors. academic performance, and well-being in chinese adolescents. Int. J. Envion. Res. Public Heath. 14 : 596 . 10.3390/ijerph14060596 [ PMC free article ] [ PubMed ] [ CrossRef ] [ Google Scholar ]
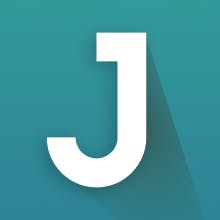
Research on the Impact of Social Media Among School-Age Children and Teenagers
While social media can enhance educational opportunities and social interaction, it also poses risks such as cyberbullying and mental health concerns..
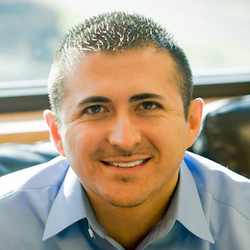
A recent review article in the Journal of Datta Meghe Institute of Medical Sciences University found that social media affects school-age children and teenagers in various ways. It has both benefits and drawbacks.
As for the benefits, social media can grant children easy access to educational resources. However, it poses risks such as cyberbullying, privacy issues, and negative impacts on their mental health. For instance, receiving negative comments or engaging in social comparison on platforms like Facebook can decrease their self-esteem.
Social media usage among children and teens has increased, with about 22% of teenagers interacting with social media more than ten times a day. Pew Research Center surveys show that only about one in five teens believe their parents are very concerned about their social media use.
Understanding the Research
The review conducted by Santosh Kumar Swain involved a comprehensive search of databases like Scopus, PubMed, and Google Scholar, which identified 65 relevant articles, including case reports, case series, and original studies.
These were the trends revealed by the research:
Benefits of Social Media:
- Social media allows students to quickly find resources for learning, and it makes learning more interactive and engaging. Platforms like blogs help students with their English language skills and ability to think creatively.
- Relationships are maintained or strengthened since students can stay connected with their close friends and family. The study also mentioned social media being a tool for getting involved in charitable causes.
Drawbacks of Social Media:
- Social media may be used for harassment or spreading embarrassing information, which can lead to anxiety, depression, and in severe cases, suicide.
- Depression is linked to excessive use of social media platforms like Facebook. There are also concerns regarding oversharing of personal information and an increased risk of scams and privacy violations.
- As for self-esteem, negative comments can lower self-esteem, encourage narcissistic tendencies, and result in jealousy (for users who frequently compare themselves to their friends).
- Spending a lot of time on screens is linked to poorer sleep quality as kids and teens are more likely to have trouble falling asleep at night.
Practical Strategies for Parents and Caregivers
As parents, we can help our children have a healthier relationship with social media and keep them safe by implementing these tips:
- Help your child balance screen time with other activities such as outdoor play and reading print books. These diverse activities prevent children from overly relying on screens for learning, entertainment, and connection.
- Teach them about screen time guidelines, such as limiting screen time to 2 hours per day and removing distracting apps (those that impact their productivity). Here’s a handout that discusses ways to regulate screen time .
- Educate your child about the effects of excessive screen time on their physical health, including their sleep and mental health . Moreover, explain how a balance of activities reduces stress and promotes personal development. Here are ideas to unplug for stress relief and healthy tech habits for stress relief .
- Set and discuss family values regarding age-appropriate social media use and content restrictions in your home. Consider the use of parental apps to set age-appropriate social media content and use restrictions on childrens’ devices. Be sure to sit with your child to explain the purpose and rationale behind the restrictions.
- Try to maintain a general awareness of your child’s social media use without being overbearing. This might include maintaining an open dialogue regarding their engagement on social media platforms. Have regular discussions with your children about their online social interactions, and provide education regarding healthy and unhealthy interactions.
Don’t forget to lead by example! Be conscious of how much time you spend on social media. Show your child that you can use social media purposefully and not excessively.
“Because social media can strongly affect how school-age children and teens behave, parents, teachers, and friends have a big impact on how they handle social media misuse,” the author wrote.
Along with healthy use of social media, encourage young people to adopt additional healthy habits that benefit them long-term with our Healthy Habits Posters .
No articles found...

- Share on Facebook
- Share on Twitter
Leave a comment
Please note: comments must be approved before they are published.

IMAGES
VIDEO
COMMENTS
Given this research gap, this paper's main objective is to shed light on the effect of social media use on psychological well-being. As explained in detail in the next section, this paper explores the mediating effect of bonding and bridging social capital. ... The effects of social media usage on social capital have gained increasing scholarly ...
Abstract. Social media are responsible for aggravating mental health problems. This systematic study summarizes the effects of social network usage on mental health. Fifty papers were shortlisted from google scholar databases, and after the application of various inclusion and exclusion criteria, 16 papers were chosen and all papers were ...
Abstract. Social media has become an integral part of contemporary society, profoundly transforming communication, social behavior, political engagement, and cultural norms. This paper presents a ...
Recent studies have explored patterns of social media use, impact of social media use on mental health and wellbeing, and the potential to leverage the popularity and interactive features of social media to enhance the delivery of interventions. ... The ethical challenges of publishing Twitter data for research dissemination. Paper presented at ...
However, evidence was found that the association of passive (but not active) social media use with well-being differed from adolescent to adolescent (Model 1B), with effect sizes ranging from − ...
In this collection, we will explore the impact of social media use on mental health by providing comprehensive research perspectives on positive and negative effects. ... which promises to present the latest findings in the emerging field of social media research. We seek research papers using qualitative and quantitative methods, focusing on ...
Introduction. The past years have witnessed a staggering increase in empirical studies into the effects of social media use (SMU) on adolescents' mental health (e.g. [1∗∗, 2∗, 3]), defined as the absence of mental illness and the presence of well-being [4].This rapid increase may be due to at least two reasons.
Social media. The term 'social media' refers to the various internet-based networks that enable users to interact with others, verbally and visually (Carr & Hayes, Citation 2015).According to the Pew Research Centre (Citation 2015), at least 92% of teenagers are active on social media.Lenhart, Smith, Anderson, Duggan, and Perrin (Citation 2015) identified the 13-17 age group as ...
Social media use is an ever-increasing phenomenon of the 21st century. In the United States, about 7 of 10 individuals use social media to connect with others, receive news content, share information, and entertain themselves (Pew Research Center, 2018).According to a recent study, young individuals pervasively use social media for a variety of reasons including entertainment, identity ...
The potential impact of social media on psychosocial development is complex and is an emerging field of research. A systematic review was conducted to investigate existing research relating to social media's effects on psychosocial development. ... Deconstructing the quality appraisal tool in systematic review methodology [Conference paper ...
Depression, anxiety, catfishing, bullying, terro rism, and. criminal activities are some of the negative side s of social media on societies. Generall y, when peoples use social. media for ...
In a review of 43 studies in young people, many benefits of social media were cited, including increased self-esteem and opportunities for self-disclosure (Best et al. 2014). Yet, reported negative effects were an increased exposure to harm, social isolation, depres-sive symptoms, and bullying (Best et al. 2014).
Cyber bullying, which means a type of. harassment that is perpetrated using e lectronic technology, is one of the risks. I n this paper we cover every aspect of social. media with its positive and ...
The present paper reviews the findings of the exemplary published works of research to shed light on the positive and negative potential effects of the massive use of social media on students' emotional well-being. This review can be insightful for teachers who tend to take the potential psychological effects of social media for granted ...
Congruent with the growth of social media use, there are also increasing worries that social media might lead to social anxiety in users (Jelenchick et al., 2013).Social anxiety is one's state of avoiding social interactions and appearing inhibited in such interactions with other people (Schlenker & Leary, 1982).Scholars indicated that social anxiety could arise from managing a large network ...
Recent studies have explored patterns of social media use, impact of social media use on mental health and wellbeing, and the potential to leverage the popularity and interactive features of social media to enhance the delivery of interventions. ... The ethical challenges of publishing Twitter data for research dissemination. Paper presented at ...
negative effects of social media use on emotions were frustration, depression, and social ... Quinn, 2016). Research shows that 90% of adults own a smartphone (Pew Research Center, 2014). Additional research indicates that 72% of Americans and an ... primary purpose of this paper is to determine if a relationship exists between excessive social
Social media comprises communication websites that facilitate relationship forming between users from diverse backgrounds, resulting in a rich social structure. User generated content encourages inquiry and decision-making. Given the relevance of social media to various stakeholders, it has received significant attention from researchers of various fields, including information systems. There ...
Effect of social media use (duration of use) on relationships with family members and friends. ... competing financial interests or personal relationships that could have appeared to influence the work reported in this paper. Acknowledgments. We appreciate the Research Deanship of Prince Sattam Bin Abdulaziz University, Kingdom of Saudi Arabia ...
Bianca A Barton was a graduate student in the Department of Psychology, Counseling, and Family Therapy at Valdosta State University. Her research interests include differing variables that impact attention and self-regulating skills needed for academic achievement as well as social media usage on university students.
Research suggests that college students who socialize rather than for academic pursuits mainly use social media, and the time spent on social media takes time from studying [9]. Yahaya [10] reported that addicted users of social media platforms (e.g., Facebook, WhatsApp) often devoted less time to their studies compared with non-users and ...
Pediatricians should be aware of the risks associated to a problematic social media use for the young's health and identify sentinel signs in children as well as prevent negative outcomes in accordance with the family. Keywords: social media, adolescents, children, social network, health, COVID-19. 1.
Almost a year after APA issued its health advisory on social media use in adolescence, society continues to wrestle with ways to maximize the benefits of these platforms while protecting youth from the potential harms associated with them. 1. By early 2024, few meaningful changes to social media platforms had been enacted by industry, and no federal policies had been adopted.
Qualitative research into adolescents' experiences of social media use and well-being has the potential to offer rich, nuanced insights, but has yet to be systematically reviewed. The current systematic review identified 19 qualitative studies in which adolescents shared their views and experiences of social media and well-being. A critical appraisal showed that overall study quality was ...
Introduction: Social media has become an integrated part of daily life, with an estimated 3 billion social media users worldwide. Adolescents and young adults are the most active users of social media. Research on social media has grown rapidly, with the potential association of social media use and mental health and well-being becoming a polarized and much-studied subject.
A recent review article in the Journal of Datta Meghe Institute of Medical Sciences University found that social media affects school-age children and teenagers in various ways. It has both benefits and drawbacks. As for the benefits, social media can grant children easy access to educational resources.
This study explores the impact of wearable health technologies on individuals' confidence in managing their health, examining the mediating roles of social isolation, understanding of medical statistics, and confidence in finding online health resources via a national cross-sectional survey.