

Research Topics & Ideas
Artifical Intelligence (AI) and Machine Learning (ML)
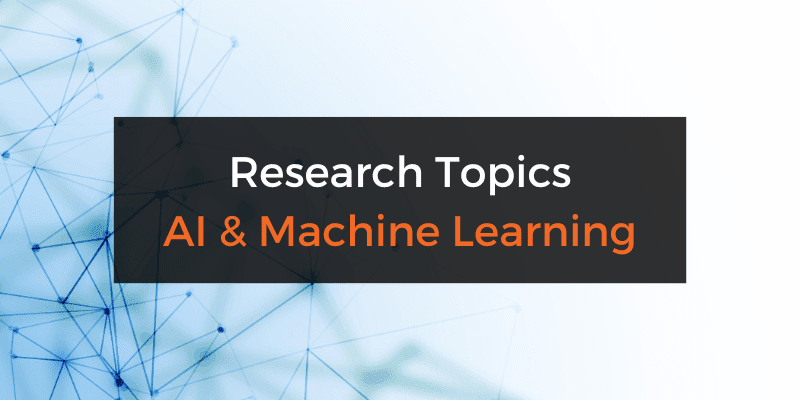
If you’re just starting out exploring AI-related research topics for your dissertation, thesis or research project, you’ve come to the right place. In this post, we’ll help kickstart your research topic ideation process by providing a hearty list of research topics and ideas , including examples from past studies.
PS – This is just the start…
We know it’s exciting to run through a list of research topics, but please keep in mind that this list is just a starting point . To develop a suitable research topic, you’ll need to identify a clear and convincing research gap , and a viable plan to fill that gap.
If this sounds foreign to you, check out our free research topic webinar that explores how to find and refine a high-quality research topic, from scratch. Alternatively, if you’d like hands-on help, consider our 1-on-1 coaching service .
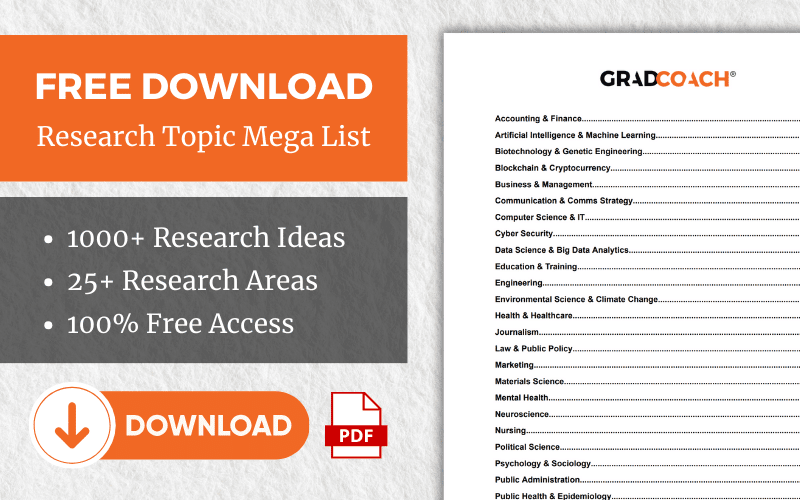
AI-Related Research Topics & Ideas
Below you’ll find a list of AI and machine learning-related research topics ideas. These are intentionally broad and generic , so keep in mind that you will need to refine them a little. Nevertheless, they should inspire some ideas for your project.
- Developing AI algorithms for early detection of chronic diseases using patient data.
- The use of deep learning in enhancing the accuracy of weather prediction models.
- Machine learning techniques for real-time language translation in social media platforms.
- AI-driven approaches to improve cybersecurity in financial transactions.
- The role of AI in optimizing supply chain logistics for e-commerce.
- Investigating the impact of machine learning in personalized education systems.
- The use of AI in predictive maintenance for industrial machinery.
- Developing ethical frameworks for AI decision-making in healthcare.
- The application of ML algorithms in autonomous vehicle navigation systems.
- AI in agricultural technology: Optimizing crop yield predictions.
- Machine learning techniques for enhancing image recognition in security systems.
- AI-powered chatbots: Improving customer service efficiency in retail.
- The impact of AI on enhancing energy efficiency in smart buildings.
- Deep learning in drug discovery and pharmaceutical research.
- The use of AI in detecting and combating online misinformation.
- Machine learning models for real-time traffic prediction and management.
- AI applications in facial recognition: Privacy and ethical considerations.
- The effectiveness of ML in financial market prediction and analysis.
- Developing AI tools for real-time monitoring of environmental pollution.
- Machine learning for automated content moderation on social platforms.
- The role of AI in enhancing the accuracy of medical diagnostics.
- AI in space exploration: Automated data analysis and interpretation.
- Machine learning techniques in identifying genetic markers for diseases.
- AI-driven personal finance management tools.
- The use of AI in developing adaptive learning technologies for disabled students.
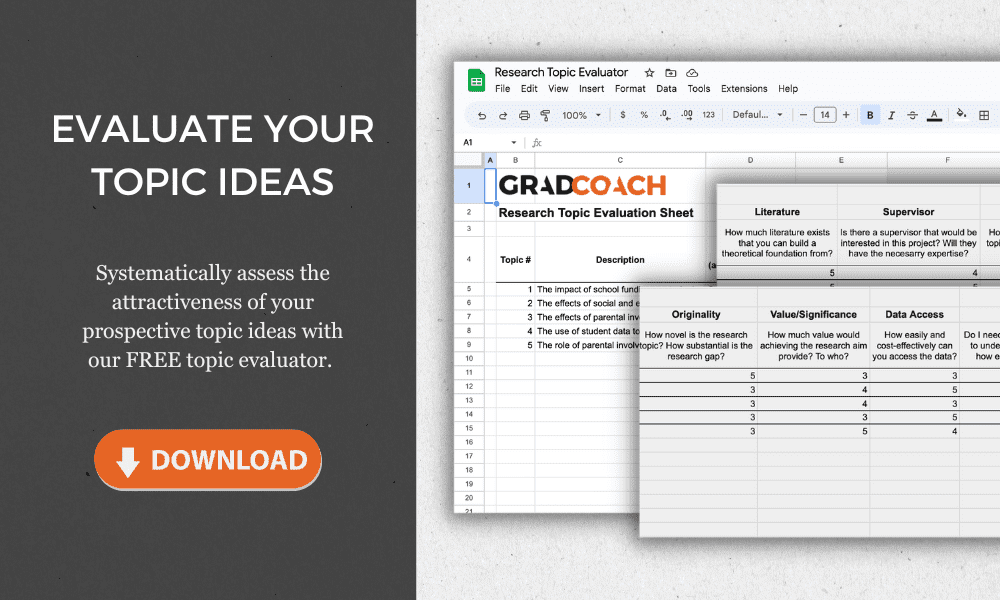
AI & ML Research Topic Ideas (Continued)
- Machine learning in cybersecurity threat detection and response.
- AI applications in virtual reality and augmented reality experiences.
- Developing ethical AI systems for recruitment and hiring processes.
- Machine learning for sentiment analysis in customer feedback.
- AI in sports analytics for performance enhancement and injury prevention.
- The role of AI in improving urban planning and smart city initiatives.
- Machine learning models for predicting consumer behaviour trends.
- AI and ML in artistic creation: Music, visual arts, and literature.
- The use of AI in automated drone navigation for delivery services.
- Developing AI algorithms for effective waste management and recycling.
- Machine learning in seismology for earthquake prediction.
- AI-powered tools for enhancing online privacy and data protection.
- The application of ML in enhancing speech recognition technologies.
- Investigating the role of AI in mental health assessment and therapy.
- Machine learning for optimization of renewable energy systems.
- AI in fashion: Predicting trends and personalizing customer experiences.
- The impact of AI on legal research and case analysis.
- Developing AI systems for real-time language interpretation for the deaf and hard of hearing.
- Machine learning in genomic data analysis for personalized medicine.
- AI-driven algorithms for credit scoring in microfinance.
- The use of AI in enhancing public safety and emergency response systems.
- Machine learning for improving water quality monitoring and management.
- AI applications in wildlife conservation and habitat monitoring.
- The role of AI in streamlining manufacturing processes.
- Investigating the use of AI in enhancing the accessibility of digital content for visually impaired users.
Recent AI & ML-Related Studies
While the ideas we’ve presented above are a decent starting point for finding a research topic in AI, they are fairly generic and non-specific. So, it helps to look at actual studies in the AI and machine learning space to see how this all comes together in practice.
Below, we’ve included a selection of AI-related studies to help refine your thinking. These are actual studies, so they can provide some useful insight as to what a research topic looks like in practice.
- An overview of artificial intelligence in diabetic retinopathy and other ocular diseases (Sheng et al., 2022)
- HOW DOES ARTIFICIAL INTELLIGENCE HELP ASTRONOMY? A REVIEW (Patel, 2022)
- Editorial: Artificial Intelligence in Bioinformatics and Drug Repurposing: Methods and Applications (Zheng et al., 2022)
- Review of Artificial Intelligence and Machine Learning Technologies: Classification, Restrictions, Opportunities, and Challenges (Mukhamediev et al., 2022)
- Will digitization, big data, and artificial intelligence – and deep learning–based algorithm govern the practice of medicine? (Goh, 2022)
- Flower Classifier Web App Using Ml & Flask Web Framework (Singh et al., 2022)
- Object-based Classification of Natural Scenes Using Machine Learning Methods (Jasim & Younis, 2023)
- Automated Training Data Construction using Measurements for High-Level Learning-Based FPGA Power Modeling (Richa et al., 2022)
- Artificial Intelligence (AI) and Internet of Medical Things (IoMT) Assisted Biomedical Systems for Intelligent Healthcare (Manickam et al., 2022)
- Critical Review of Air Quality Prediction using Machine Learning Techniques (Sharma et al., 2022)
- Artificial Intelligence: New Frontiers in Real–Time Inverse Scattering and Electromagnetic Imaging (Salucci et al., 2022)
- Machine learning alternative to systems biology should not solely depend on data (Yeo & Selvarajoo, 2022)
- Measurement-While-Drilling Based Estimation of Dynamic Penetrometer Values Using Decision Trees and Random Forests (García et al., 2022).
- Artificial Intelligence in the Diagnosis of Oral Diseases: Applications and Pitfalls (Patil et al., 2022).
- Automated Machine Learning on High Dimensional Big Data for Prediction Tasks (Jayanthi & Devi, 2022)
- Breakdown of Machine Learning Algorithms (Meena & Sehrawat, 2022)
- Technology-Enabled, Evidence-Driven, and Patient-Centered: The Way Forward for Regulating Software as a Medical Device (Carolan et al., 2021)
- Machine Learning in Tourism (Rugge, 2022)
- Towards a training data model for artificial intelligence in earth observation (Yue et al., 2022)
- Classification of Music Generality using ANN, CNN and RNN-LSTM (Tripathy & Patel, 2022)
As you can see, these research topics are a lot more focused than the generic topic ideas we presented earlier. So, in order for you to develop a high-quality research topic, you’ll need to get specific and laser-focused on a specific context with specific variables of interest. In the video below, we explore some other important things you’ll need to consider when crafting your research topic.
Get 1-On-1 Help
If you’re still unsure about how to find a quality research topic, check out our Research Topic Kickstarter service, which is the perfect starting point for developing a unique, well-justified research topic.
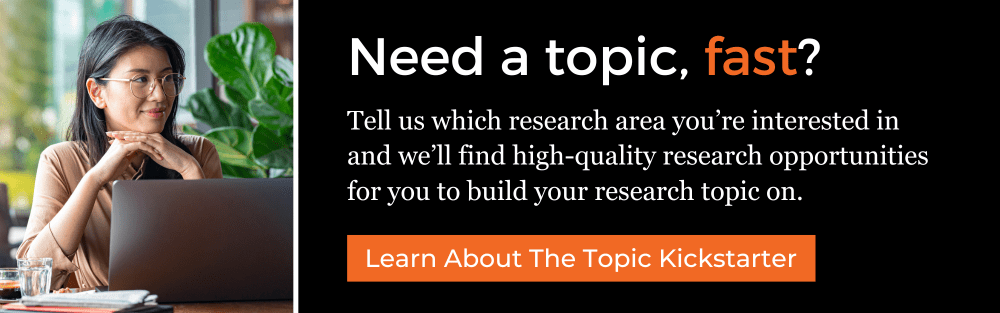
You Might Also Like:
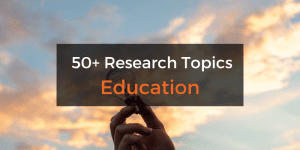
can one come up with their own tppic and get a search
can one come up with their own title and get a search
Submit a Comment Cancel reply
Your email address will not be published. Required fields are marked *
Save my name, email, and website in this browser for the next time I comment.
- Print Friendly

Exploring 250+ Machine Learning Research Topics

In recent years, machine learning has become super popular and grown very quickly. This happened because technology got better, and there’s a lot more data available. Because of this, we’ve seen lots of new and amazing things happen in different areas. Machine learning research is what makes all these cool things possible. In this blog, we’ll talk about machine learning research topics, why they’re important, how you can pick one, what areas are popular to study, what’s new and exciting, the tough problems, and where you can find help if you want to be a researcher.
Why Does Machine Learning Research Matter?
Table of Contents
Machine learning research is at the heart of the AI revolution. It underpins the development of intelligent systems capable of making predictions, automating tasks, and improving decision-making across industries. The importance of this research can be summarized as follows:
Advancements in Technology
The growth of machine learning research has led to the development of powerful algorithms, tools, and frameworks. Numerous industries, including healthcare, banking, autonomous cars, and natural language processing, have found use for these technology.
As researchers continue to push the boundaries of what’s possible, we can expect even more transformative technologies to emerge.
Real-world Applications
Machine learning research has brought about tangible changes in our daily lives. Voice assistants like Siri and Alexa, recommendation systems on streaming platforms, and personalized healthcare diagnostics are just a few examples of how this research impacts our world.
By working on new research topics, scientists can further refine these applications and create new ones.
Economic and Industrial Impacts
The economic implications of machine learning research are substantial. Companies that harness the power of machine learning gain a competitive edge in the market.
This creates a demand for skilled machine learning researchers, driving job opportunities and contributing to economic growth.
How to Choose the Machine Learning Research Topics?
Selecting the right machine learning research topics is crucial for your success as a machine learning researcher. Here’s a guide to help you make an informed decision:
- Understanding Your Interests
Start by considering your personal interests. Machine learning is a broad field with applications in virtually every sector. By choosing a topic that aligns with your passions, you’ll stay motivated and engaged throughout your research journey.
- Reviewing Current Trends
Stay updated on the latest trends in machine learning. Attend conferences, read research papers, and engage with the community to identify emerging research topics. Current trends often lead to exciting breakthroughs.
- Identifying Gaps in Existing Research
Sometimes, the most promising research topics involve addressing gaps in existing knowledge. These gaps may become evident through your own experiences, discussions with peers, or in the course of your studies.
- Collaborating with Experts
Collaboration is key in research. Working with experts in the field can help you refine your research topic and gain valuable insights. Seek mentors and collaborators who can guide you.
250+ Machine Learning Research Topics: Category-wise
Supervised learning.
- Explainable AI for Decision Support
- Few-shot Learning Methods
- Time Series Forecasting with Deep Learning
- Handling Imbalanced Datasets in Classification
- Regression Techniques for Non-linear Data
- Transfer Learning in Supervised Settings
- Multi-label Classification Strategies
- Semi-Supervised Learning Approaches
- Novel Feature Selection Methods
- Anomaly Detection in Supervised Scenarios
- Federated Learning for Distributed Supervised Models
- Ensemble Learning for Improved Accuracy
- Automated Hyperparameter Tuning
- Ethical Implications in Supervised Models
- Interpretability of Deep Neural Networks.
Unsupervised Learning
- Unsupervised Clustering of High-dimensional Data
- Semi-Supervised Clustering Approaches
- Density Estimation in Unsupervised Learning
- Anomaly Detection in Unsupervised Settings
- Transfer Learning for Unsupervised Tasks
- Representation Learning in Unsupervised Learning
- Outlier Detection Techniques
- Generative Models for Data Synthesis
- Manifold Learning in High-dimensional Spaces
- Unsupervised Feature Selection
- Privacy-Preserving Unsupervised Learning
- Community Detection in Complex Networks
- Clustering Interpretability and Visualization
- Unsupervised Learning for Image Segmentation
- Autoencoders for Dimensionality Reduction.
Reinforcement Learning
- Deep Reinforcement Learning in Real-world Applications
- Safe Reinforcement Learning for Autonomous Systems
- Transfer Learning in Reinforcement Learning
- Imitation Learning and Apprenticeship Learning
- Multi-agent Reinforcement Learning
- Explainable Reinforcement Learning Policies
- Hierarchical Reinforcement Learning
- Model-based Reinforcement Learning
- Curriculum Learning in Reinforcement Learning
- Reinforcement Learning in Robotics
- Exploration vs. Exploitation Strategies
- Reward Function Design and Ethical Considerations
- Reinforcement Learning in Healthcare
- Continuous Action Spaces in RL
- Reinforcement Learning for Resource Management.
Natural Language Processing (NLP)
- Multilingual and Cross-lingual NLP
- Contextualized Word Embeddings
- Bias Detection and Mitigation in NLP
- Named Entity Recognition for Low-resource Languages
- Sentiment Analysis in Social Media Text
- Dialogue Systems for Improved Customer Service
- Text Summarization for News Articles
- Low-resource Machine Translation
- Explainable NLP Models
- Coreference Resolution in NLP
- Question Answering in Specific Domains
- Detecting Fake News and Misinformation
- NLP for Healthcare: Clinical Document Understanding
- Emotion Analysis in Text
- Text Generation with Controlled Attributes.
Computer Vision
- Video Action Recognition and Event Detection
- Object Detection in Challenging Conditions (e.g., low light)
- Explainable Computer Vision Models
- Image Captioning for Accessibility
- Large-scale Image Retrieval
- Domain Adaptation in Computer Vision
- Fine-grained Image Classification
- Facial Expression Recognition
- Visual Question Answering
- Self-supervised Learning for Visual Representations
- Weakly Supervised Object Localization
- Human Pose Estimation in 3D
- Scene Understanding in Autonomous Vehicles
- Image Super-resolution
- Gaze Estimation for Human-Computer Interaction.
Deep Learning
- Neural Architecture Search for Efficient Models
- Self-attention Mechanisms and Transformers
- Interpretability in Deep Learning Models
- Robustness of Deep Neural Networks
- Generative Adversarial Networks (GANs) for Data Augmentation
- Neural Style Transfer in Art and Design
- Adversarial Attacks and Defenses
- Neural Networks for Audio and Speech Processing
- Explainable AI for Healthcare Diagnosis
- Automated Machine Learning (AutoML)
- Reinforcement Learning with Deep Neural Networks
- Model Compression and Quantization
- Lifelong Learning with Deep Learning Models
- Multimodal Learning with Vision and Language
- Federated Learning for Privacy-preserving Deep Learning.
Explainable AI
- Visualizing Model Decision Boundaries
- Saliency Maps and Feature Attribution
- Rule-based Explanations for Black-box Models
- Contrastive Explanations for Model Interpretability
- Counterfactual Explanations and What-if Analysis
- Human-centered AI for Explainable Healthcare
- Ethics and Fairness in Explainable AI
- Explanation Generation for Natural Language Processing
- Explainable AI in Financial Risk Assessment
- User-friendly Interfaces for Model Interpretability
- Scalability and Efficiency in Explainable Models
- Hybrid Models for Combined Accuracy and Explainability
- Post-hoc vs. Intrinsic Explanations
- Evaluation Metrics for Explanation Quality
- Explainable AI for Autonomous Vehicles.
Transfer Learning
- Zero-shot Learning and Few-shot Learning
- Cross-domain Transfer Learning
- Domain Adaptation for Improved Generalization
- Multilingual Transfer Learning in NLP
- Pretraining and Fine-tuning Techniques
- Lifelong Learning and Continual Learning
- Domain-specific Transfer Learning Applications
- Model Distillation for Knowledge Transfer
- Contrastive Learning for Transfer Learning
- Self-training and Pseudo-labeling
- Dynamic Adaption of Pretrained Models
- Privacy-Preserving Transfer Learning
- Unsupervised Domain Adaptation
- Negative Transfer Avoidance in Transfer Learning.
Federated Learning
- Secure Aggregation in Federated Learning
- Communication-efficient Federated Learning
- Privacy-preserving Techniques in Federated Learning
- Federated Transfer Learning
- Heterogeneous Federated Learning
- Real-world Applications of Federated Learning
- Federated Learning for Edge Devices
- Federated Learning for Healthcare Data
- Differential Privacy in Federated Learning
- Byzantine-robust Federated Learning
- Federated Learning with Non-IID Data
- Model Selection in Federated Learning
- Scalable Federated Learning for Large Datasets
- Client Selection and Sampling Strategies
- Global Model Update Synchronization in Federated Learning.
Quantum Machine Learning
- Quantum Neural Networks and Quantum Circuit Learning
- Quantum-enhanced Optimization for Machine Learning
- Quantum Data Compression and Quantum Principal Component Analysis
- Quantum Kernels and Quantum Feature Maps
- Quantum Variational Autoencoders
- Quantum Transfer Learning
- Quantum-inspired Classical Algorithms for ML
- Hybrid Quantum-Classical Models
- Quantum Machine Learning on Near-term Quantum Devices
- Quantum-inspired Reinforcement Learning
- Quantum Computing for Quantum Chemistry and Drug Discovery
- Quantum Machine Learning for Finance
- Quantum Data Structures and Quantum Databases
- Quantum-enhanced Cryptography in Machine Learning
- Quantum Generative Models and Quantum GANs.
Ethical AI and Bias Mitigation
- Fairness-aware Machine Learning Algorithms
- Bias Detection and Mitigation in Real-world Data
- Explainable AI for Ethical Decision Support
- Algorithmic Accountability and Transparency
- Privacy-preserving AI and Data Governance
- Ethical Considerations in AI for Healthcare
- Fairness in Recommender Systems
- Bias and Fairness in NLP Models
- Auditing AI Systems for Bias
- Societal Implications of AI in Criminal Justice
- Ethical AI Education and Training
- Bias Mitigation in Autonomous Vehicles
- Fair AI in Financial and Hiring Decisions
- Case Studies in Ethical AI Failures
- Legal and Policy Frameworks for Ethical AI.
Meta-Learning and AutoML
- Neural Architecture Search (NAS) for Efficient Models
- Transfer Learning in NAS
- Reinforcement Learning for NAS
- Multi-objective NAS
- Automated Data Augmentation
- Neural Architecture Optimization for Edge Devices
- Bayesian Optimization for AutoML
- Model Compression and Quantization in AutoML
- AutoML for Federated Learning
- AutoML in Healthcare Diagnostics
- Explainable AutoML
- Cost-sensitive Learning in AutoML
- AutoML for Small Data
- Human-in-the-Loop AutoML.
AI for Healthcare and Medicine
- Disease Prediction and Early Diagnosis
- Medical Image Analysis with Deep Learning
- Drug Discovery and Molecular Modeling
- Electronic Health Record Analysis
- Predictive Analytics in Healthcare
- Personalized Treatment Planning
- Healthcare Fraud Detection
- Telemedicine and Remote Patient Monitoring
- AI in Radiology and Pathology
- AI in Drug Repurposing
- AI for Medical Robotics and Surgery
- Genomic Data Analysis
- AI-powered Mental Health Assessment
- Explainable AI in Healthcare Decision Support
- AI in Epidemiology and Outbreak Prediction.
AI in Finance and Investment
- Algorithmic Trading and High-frequency Trading
- Credit Scoring and Risk Assessment
- Fraud Detection and Anti-money Laundering
- Portfolio Optimization with AI
- Financial Market Prediction
- Sentiment Analysis in Financial News
- Explainable AI in Financial Decision-making
- Algorithmic Pricing and Dynamic Pricing Strategies
- AI in Cryptocurrency and Blockchain
- Customer Behavior Analysis in Banking
- Explainable AI in Credit Decisioning
- AI in Regulatory Compliance
- Ethical AI in Financial Services
- AI for Real Estate Investment
- Automated Financial Reporting.
AI in Climate Change and Sustainability
- Climate Modeling and Prediction
- Renewable Energy Forecasting
- Smart Grid Optimization
- Energy Consumption Forecasting
- Carbon Emission Reduction with AI
- Ecosystem Monitoring and Preservation
- Precision Agriculture with AI
- AI for Wildlife Conservation
- Natural Disaster Prediction and Management
- Water Resource Management with AI
- Sustainable Transportation and Urban Planning
- Climate Change Mitigation Strategies with AI
- Environmental Impact Assessment with Machine Learning
- Eco-friendly Supply Chain Optimization
- Ethical AI in Climate-related Decision Support.
Data Privacy and Security
- Differential Privacy Mechanisms
- Federated Learning for Privacy-preserving AI
- Secure Multi-Party Computation
- Privacy-enhancing Technologies in Machine Learning
- Homomorphic Encryption for Machine Learning
- Ethical Considerations in Data Privacy
- Privacy-preserving AI in Healthcare
- AI for Secure Authentication and Access Control
- Blockchain and AI for Data Security
- Explainable Privacy in Machine Learning
- Privacy-preserving AI in Government and Public Services
- Privacy-compliant AI for IoT and Edge Devices
- Secure AI Models Sharing and Deployment
- Privacy-preserving AI in Financial Transactions
- AI in the Legal Frameworks of Data Privacy.
Global Collaboration in Research
- International Research Partnerships and Collaboration Models
- Multilingual and Cross-cultural AI Research
- Addressing Global Healthcare Challenges with AI
- Ethical Considerations in International AI Collaborations
- Interdisciplinary AI Research in Global Challenges
- AI Ethics and Human Rights in Global Research
- Data Sharing and Data Access in Global AI Research
- Cross-border Research Regulations and Compliance
- AI Innovation Hubs and International Research Centers
- AI Education and Training for Global Communities
- Humanitarian AI and AI for Sustainable Development Goals
- AI for Cultural Preservation and Heritage Protection
- Collaboration in AI-related Global Crises
- AI in Cross-cultural Communication and Understanding
- Global AI for Environmental Sustainability and Conservation.
Emerging Trends and Hot Topics in Machine Learning Research
The landscape of machine learning research topics is constantly evolving. Here are some of the emerging trends and hot topics that are shaping the field:
As AI systems become more prevalent, addressing ethical concerns and mitigating bias in algorithms are critical research areas.
Interpretable and Explainable Models
Understanding why machine learning models make specific decisions is crucial for their adoption in sensitive areas, such as healthcare and finance.
Meta-learning algorithms are designed to enable machines to learn how to learn, while AutoML aims to automate the machine learning process itself.
Machine learning is revolutionizing the healthcare sector, from diagnostic tools to drug discovery and patient care.
Algorithmic trading, risk assessment, and fraud detection are just a few applications of AI in finance, creating a wealth of research opportunities.
Machine learning research is crucial in analyzing and mitigating the impacts of climate change and promoting sustainable practices.
Challenges and Future Directions
While machine learning research has made tremendous strides, it also faces several challenges:
- Data Privacy and Security: As machine learning models require vast amounts of data, protecting individual privacy and data security are paramount concerns.
- Scalability and Efficiency: Developing efficient algorithms that can handle increasingly large datasets and complex computations remains a challenge.
- Ensuring Fairness and Transparency: Addressing bias in machine learning models and making their decisions transparent is essential for equitable AI systems.
- Quantum Computing and Machine Learning: The integration of quantum computing and machine learning has the potential to revolutionize the field, but it also presents unique challenges.
- Global Collaboration in Research: Machine learning research benefits from collaboration on a global scale. Ensuring that researchers from diverse backgrounds work together is vital for progress.
Resources for Machine Learning Researchers
If you’re looking to embark on a journey in machine learning research topics, there are various resources at your disposal:
- Journals and Conferences
Journals such as the “Journal of Machine Learning Research” and conferences like NeurIPS and ICML provide a platform for publishing and discussing research findings.
- Online Communities and Forums
Platforms like Stack Overflow, GitHub, and dedicated forums for machine learning provide spaces for collaboration and problem-solving.
- Datasets and Tools
Open-source datasets and tools like TensorFlow and PyTorch simplify the research process by providing access to data and pre-built models.
- Research Grants and Funding Opportunities
Many organizations and government agencies offer research grants and funding for machine learning projects. Seek out these opportunities to support your research.
Machine learning research is like a superhero in the world of technology. To be a part of this exciting journey, it’s important to choose the right machine learning research topics and keep up with the latest trends.
Machine learning research makes our lives better. It powers things like smart assistants and life-saving medical tools. It’s like the force driving the future of technology and society.
But, there are challenges too. We need to work together and be ethical in our research. Everyone should benefit from this technology. The future of machine learning research is incredibly bright. If you want to be a part of it, get ready for an exciting adventure. You can help create new solutions and make a big impact on the world.
Related Posts

Tips on How To Tackle A Machine Learning Project As A Beginner
Here in this blog, CodeAvail experts will explain to you tips on how to tackle a machine learning project as a beginner step by step…

Artificial Intelligence and Machine Learning Basics for Beginners
Here in this blog, CodeAvail experts will explain to you Artificial Intelligence and Machine Learning basics for beginners in detail step by step. What is…
Subscribe to the PwC Newsletter
Join the community, trending research, mambaout: do we really need mamba for vision.

For vision tasks, as image classification does not align with either characteristic, we hypothesize that Mamba is not necessary for this task; Detection and segmentation tasks are also not autoregressive, yet they adhere to the long-sequence characteristic, so we believe it is still worthwhile to explore Mamba's potential for these tasks.

Hunyuan-DiT: A Powerful Multi-Resolution Diffusion Transformer with Fine-Grained Chinese Understanding
For fine-grained language understanding, we train a Multimodal Large Language Model to refine the captions of the images.

Grounding DINO 1.5: Advance the "Edge" of Open-Set Object Detection
idea-research/grounding-dino-1.5-api • 16 May 2024
Empirical results demonstrate the effectiveness of Grounding DINO 1. 5, with the Grounding DINO 1. 5 Pro model attaining a 54. 3 AP on the COCO detection benchmark and a 55. 7 AP on the LVIS-minival zero-shot transfer benchmark, setting new records for open-set object detection.
How Far Are We to GPT-4V? Closing the Gap to Commercial Multimodal Models with Open-Source Suites
Compared to both open-source and proprietary models, InternVL 1. 5 shows competitive performance, achieving state-of-the-art results in 8 of 18 benchmarks.

A decoder-only foundation model for time-series forecasting

Motivated by recent advances in large language models for Natural Language Processing (NLP), we design a time-series foundation model for forecasting whose out-of-the-box zero-shot performance on a variety of public datasets comes close to the accuracy of state-of-the-art supervised forecasting models for each individual dataset.
How Far Are We From AGI
ulab-uiuc/agi-survey • 16 May 2024
The evolution of artificial intelligence (AI) has profoundly impacted human society, driving significant advancements in multiple sectors.
AniTalker: Animate Vivid and Diverse Talking Faces through Identity-Decoupled Facial Motion Encoding
The paper introduces AniTalker, an innovative framework designed to generate lifelike talking faces from a single portrait.

Sakuga-42M Dataset: Scaling Up Cartoon Research
zhenglinpan/SakugaDataset • 13 May 2024
Can we harness the success of the scaling paradigm to benefit cartoon research?

Lumina-T2X: Transforming Text into Any Modality, Resolution, and Duration via Flow-based Large Diffusion Transformers
Sora unveils the potential of scaling Diffusion Transformer for generating photorealistic images and videos at arbitrary resolutions, aspect ratios, and durations, yet it still lacks sufficient implementation details.
KAN: Kolmogorov-Arnold Networks
Inspired by the Kolmogorov-Arnold representation theorem, we propose Kolmogorov-Arnold Networks (KANs) as promising alternatives to Multi-Layer Perceptrons (MLPs).
Thank you for visiting nature.com. You are using a browser version with limited support for CSS. To obtain the best experience, we recommend you use a more up to date browser (or turn off compatibility mode in Internet Explorer). In the meantime, to ensure continued support, we are displaying the site without styles and JavaScript.
- View all journals
Machine learning articles from across Nature Portfolio
Machine learning is the ability of a machine to improve its performance based on previous results. Machine learning methods enable computers to learn without being explicitly programmed and have multiple applications, for example, in the improvement of data mining algorithms.
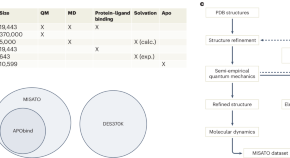
A multidimensional dataset for structure-based machine learning
MISATO, a dataset for structure-based drug discovery combines quantum mechanics property data and molecular dynamics simulations on ~20,000 protein–ligand structures, substantially extends the amount of data available to the community and holds potential for advancing work in drug discovery.
- Matthew Holcomb
- Stefano Forli
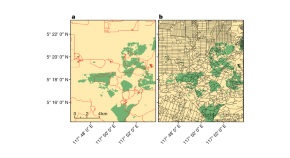
‘Ghost roads’ could be the biggest direct threat to tropical forests
By using volunteers to map roads in forests across Borneo, Sumatra and New Guinea, an innovative study shows that existing maps of the Asia-Pacific region are rife with errors. It also reveals that unmapped roads are extremely common — up to seven times more abundant than mapped ones. Such ‘ghost roads’ are promoting illegal logging, mining, wildlife poaching and deforestation in some of the world’s biologically richest ecosystems.
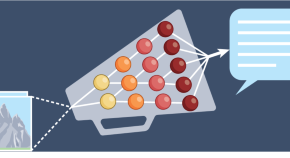
Adapting vision–language AI models to cardiology tasks
Vision–language models can be trained to read cardiac ultrasound images with implications for improving clinical workflows, but additional development and validation will be required before such models can replace humans.
- Rima Arnaout
Latest Research and Reviews
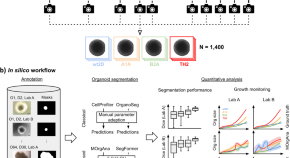
A large and diverse brain organoid dataset of 1,400 cross-laboratory images of 64 trackable brain organoids
- Julian Schröter
- Luca Deininger
- Sabine Jung-Klawitter
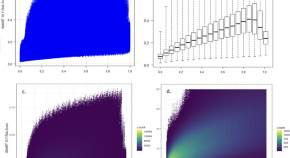
Medical calculators derived synthetic cohorts: a novel method for generating synthetic patient data
- Francis Jeanson
- Michael E. Farkouh
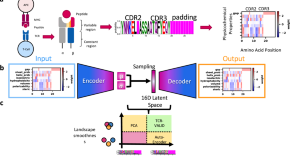
Designing meaningful continuous representations of T cell receptor sequences with deep generative models
Relating T cell receptor (TCR) sequencing to antigen specificity is a challenge especially when TCR specificity is unclear. Here the authors use a low dimensional generative approach to model TCR sequence similarity and to associate TCR sequences with the same specificity.
- Allen Y. Leary
- Darius Scott
- Peter G. Hawkins
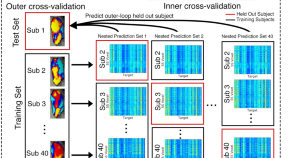
Predicting high-level visual areas in the absence of task fMRI
- M. Fiona Molloy
- Zeynep M. Saygin
- David E. Osher
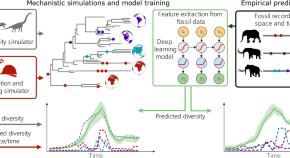
DeepDive: estimating global biodiversity patterns through time using deep learning
Estimates of palaeodiversity are biased by the incompleteness of the fossil record. Here, the authors develop DeepDive, a deep learning approach that infers richness while accounting for record heterogeneity, and test it with two empirical datasets.
- Rebecca B. Cooper
- Joseph T. Flannery-Sutherland
- Daniele Silvestro
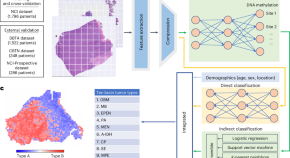
Prediction of DNA methylation-based tumor types from histopathology in central nervous system tumors with deep learning
A deep learning model is used to classify central nervous system tumors based on their DNA methylation profile directly from histopathology, and showed high accuracy in a large set of external validation cohorts, potentially informing downstream treatment.
- Danh-Tai Hoang
- Eldad D. Shulman
- Kenneth Aldape
News and Comment
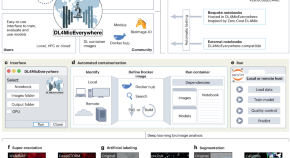
DL4MicEverywhere: deep learning for microscopy made flexible, shareable and reproducible
- Iván Hidalgo-Cenalmor
- Joanna W. Pylvänäinen
- Estibaliz Gómez-de-Mariscal
The potential and perils of generative artificial intelligence in psychiatry and psychology
Generative artificial intelligence (AI), exemplified by large language models such as ChatGPT, shows promise in mental health practice, aiding research, training and therapy. However, bias, inaccuracy and trust issues necessitate careful integration with human expertise.
- Arun J. Thirunavukarasu
- Jessica O’Logbon
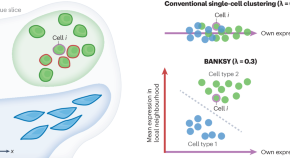
BANKSY: scalable cell typing and domain segmentation for spatial omics
In this Tools of the Trade article, Vipul Singhal and Nigel Chou describe BANKSY, a machine learning tool that harnesses gene expression gradients from the neighbourhood of a cell for cell typing and domain segmentation.
- Vipul Singhal
Investigating immunity
Recent methods development in immunology has galvanized our understanding of immune responses.
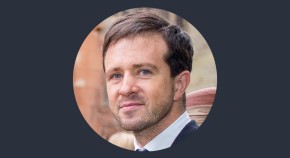
Why mathematics is set to be revolutionized by AI
Cheap data and the absence of coincidences make maths an ideal testing ground for AI-assisted discovery — but only humans will be able to tell good conjectures from bad ones.
- Thomas Fink
Teaching artificial intelligence in medicine
Artificial intelligence (AI) is finding its way into healthcare. Therefore, medical students need to be trained to be ‘bilingual’ in both medical and computational terminology and concepts to allow them to understand, implement and evaluate AI-related research.
- Yosra Magdi Mekki
- Susu M. Zughaier
Quick links
- Explore articles by subject
- Guide to authors
- Editorial policies


- Conferences
- Last updated October 25, 2022
- In AI Origins & Evolution
Top 10 Machine Learning Papers of 2022

- Published on October 25, 2022
- by Tasmia Ansari
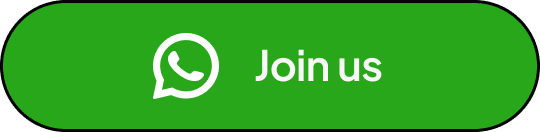
The relevance of any field depends on the ongoing research and studies around it. This especially holds for advancing fields like machine learning.
To bring you up to speed on the critical ideas driving machine learning in 2022, we handpicked the top 10 research papers for all AI/ML enthusiasts out there!
Let’s dive in!
- Artificial Replay: A Meta-Algorithm for Harnessing Historical Data in Bandits
Author(s) – Sean R. Sinclair et al .
Ways to incorporate historical data are still unclear: initialising reward estimates with historical samples can suffer from bogus and imbalanced data coverage, leading to computational and storage issues—particularly in continuous action spaces. The paper addresses the obstacles by proposing ‘Artificial Replay’, an algorithm to incorporate historical data into any arbitrary base bandit algorithm.
Read the full paper here .
- Bootstrapped Meta-Learning
Author(s) – Sebastian Flennerhag et al.
The paper proposes an algorithm in which the meta-learner teaches itself to overcome the meta-optimisation challenge. The algorithm focuses on meta-learning with gradients, which guarantees performance improvements. Furthermore, the paper also looks at how bootstrapping opens up possibilities.
Read the full paper here .
- LaMDA: Language Models for Dialog Applications
Author(s) – Romal Thoppilan et al.
The research describes the LaMDA system which caused chaos in AI this summer when a former Google engineer claimed that it had shown signs of sentience. LaMDA is a family of large language models for dialogue applications based on Transformer architecture. The interesting feature of the model is its fine-tuning with human-annotated data and the possibility of consulting external sources. This is a very interesting model family, which we might encounter in many applications we use daily.
- Competition-Level Code Generation with AlphaCode
Author(s) – Yujia Li et al.
Systems can help programmers become more productive. The following research addresses the problems with incorporating innovations in AI into these systems. AlphaCode is a system that creates solutions for problems that require deeper reasoning.
- Privacy for Free: How does Dataset Condensation Help Privacy?
Author(s) – Tian Dong et al.
The paper focuses on Privacy Preserving Machine Learning, specifically deducting the leakage of sensitive data in machine learning. It puts forth one of the first propositions of using dataset condensation techniques to preserve the data efficiency during model training and furnish membership privacy.
- Why do tree-based models still outperform deep learning on tabular data?
Author(s) – Léo Grinsztajn, Edouard Oyallon and Gaël Varoquaux
The research answers why deep learning models still find it hard to compete on tabular data compared to tree-based models. It is shown that MLP-like architectures are more sensitive to uninformative features in data compared to their tree-based counterparts.
- Multi-Objective Bayesian Optimisation over High-Dimensional Search Spaces
Author(s) – Samuel Daulton et al.
The paper proposes ‘MORBO’, a scalable method for multiple-objective BO as it performs better than that of high-dimensional search spaces. MORBO significantly improves the sample efficiency and, where existing BO algorithms fail, MORBO provides improved sample efficiencies over the current approach.
- A Path Towards Autonomous Machine Intelligence Version 0.9.2
Author(s) – Yann LeCun
The research offers a vision about how to progress towards general AI. The study combines several concepts: a configurable predictive world model, behaviour driven through intrinsic motivation, and hierarchical joint embedding architectures trained with self-supervised
learning.
- TranAD: Deep Transformer Networks for Anomaly Detection in Multivariate Time Series Data
Author(s) – Shreshth Tuli, Giuliano Casale and Nicholas R. Jennings
This is a specialised paper applying transformer architecture to the problem of unsupervised anomaly detection in multivariate time series. Many architectures which were successful in other fields are, at some point, also being applied to time series. The research shows improved performance on some known data sets.
- Differentially Private Bias-Term only Fine-tuning of Foundation Models
Author(s) – Zhiqi Bu et al.
In the paper, researchers study the problem of differentially private (DP) fine-tuning of large pre-trained models—a recent privacy-preserving approach suitable for solving downstream tasks with sensitive data. Existing work has demonstrated that high accuracy is possible under strong privacy constraints yet requires significant computational overhead or modifications to the network architecture.
Read the full paper here .
Access all our open Survey & Awards Nomination forms in one place

Tasmia Ansari
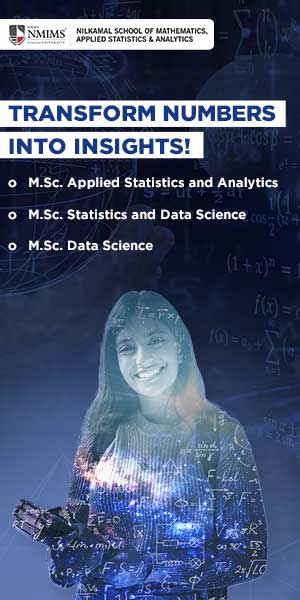
CORPORATE TRAINING PROGRAMS ON GENERATIVE AI
Generative ai skilling for enterprises, our customized corporate training program on generative ai provides a unique opportunity to empower, retain, and advance your talent., upcoming large format conference, data engineering summit 2024, may 30 and 31, 2024 | 📍 bangalore, india, download the easiest way to stay informed.
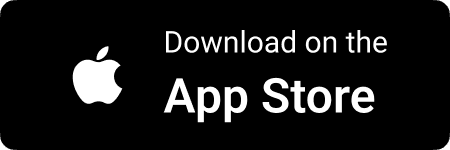
Why India Needs More AI4Bharats
In a podcast with AIM, Indic AI developers said that what India needs is more initiatives like AI4Bharat, with industry-academia collaboration.
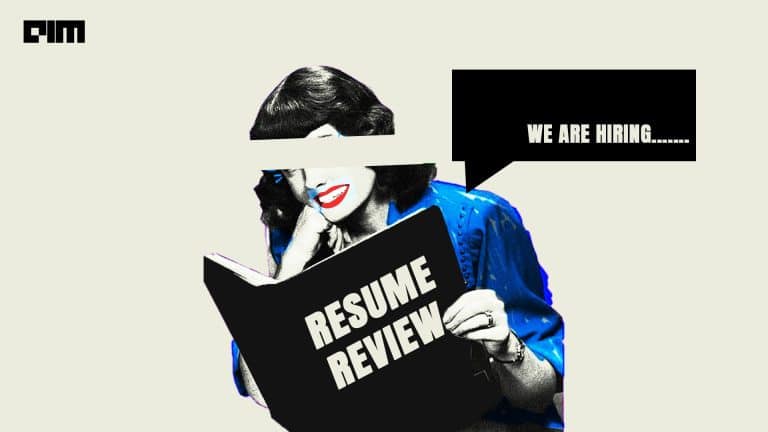
6 Indian Generative AI Platforms for Recruiters and HR Professionals
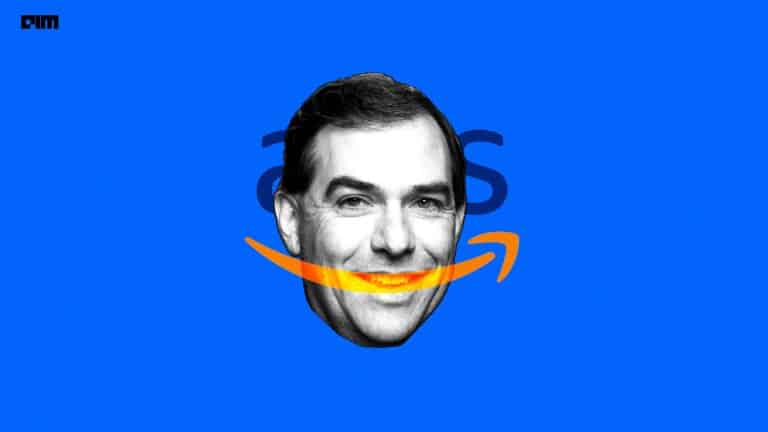
Meet AWS’ New CEO, Matt Garman, Filling Adam Selipsky’s Shoes
Top editorial picks, ai to help you understand this indian classical dance, indian it is training a genai workforce to eventually replace them with ai, yotta appoints anil pawar as chief ai officer, marks next phase in ai strategy, openai brings generative ai search experience to chatgpt, subscribe to the belamy: our weekly newsletter, biggest ai stories, delivered to your inbox every week., also in news.

Most Software Engineers Know Nothing About Hardware
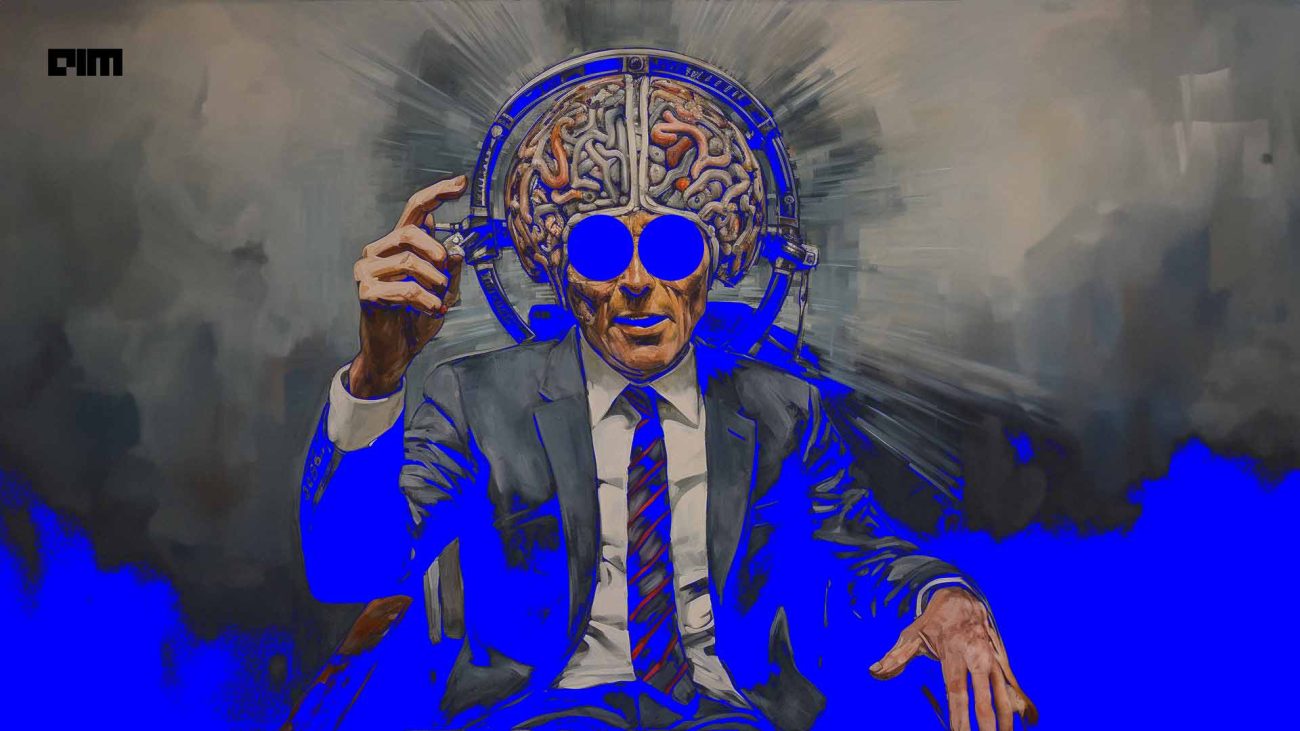
6 Techniques to Reduce Hallucinations in LLMs
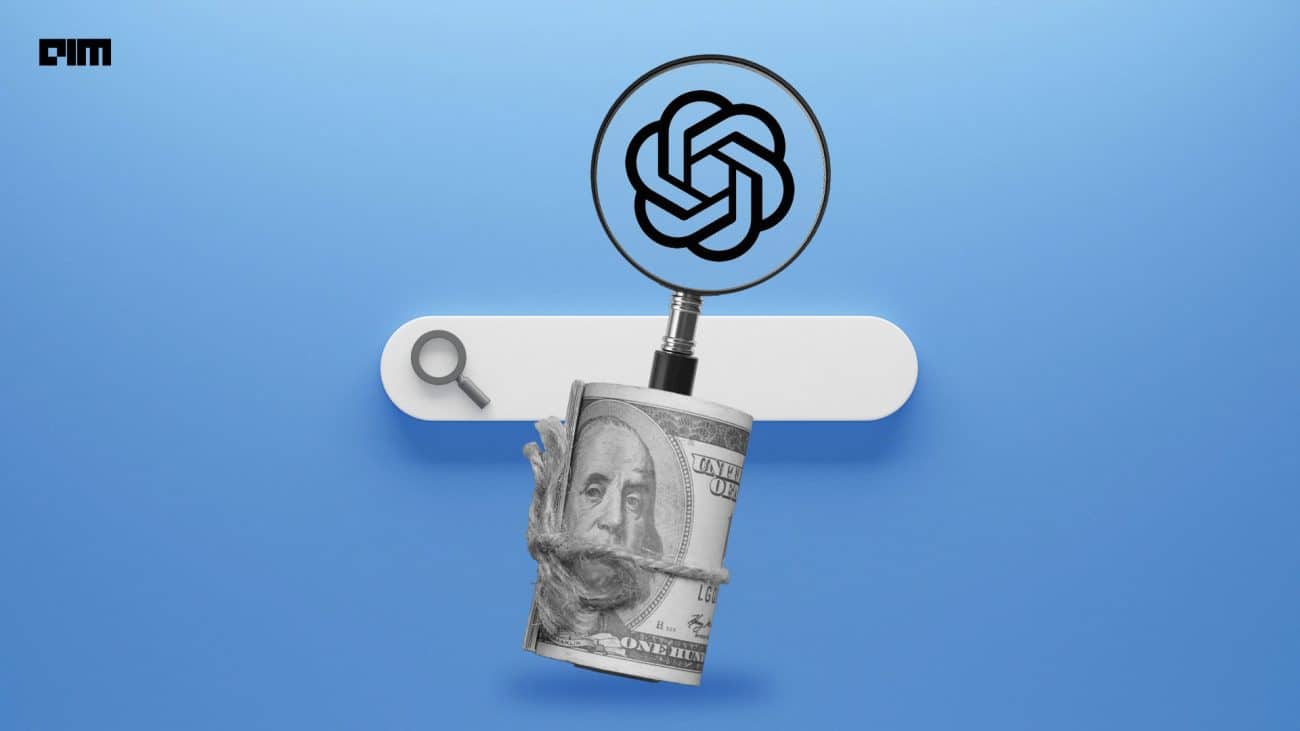
Bad Times for Perplexity AI Begins
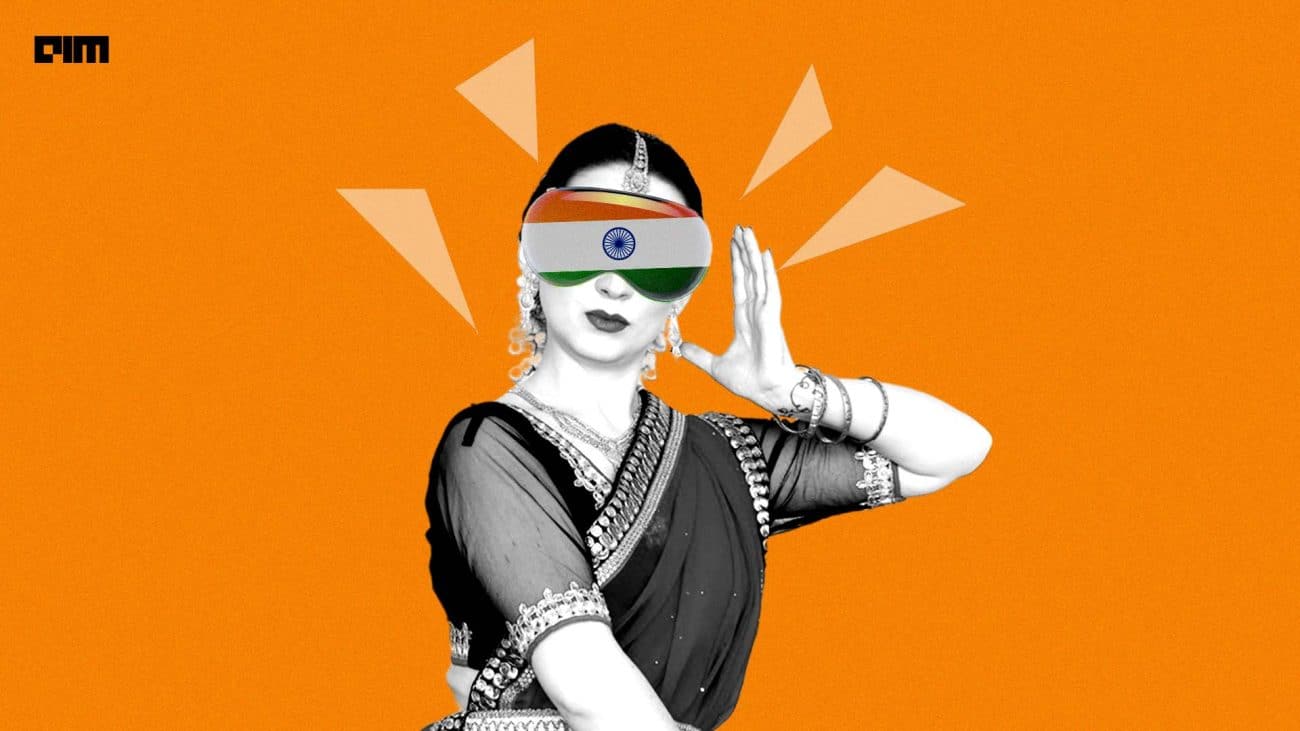
Top 9 Apple Vision Pro Use Cases in India
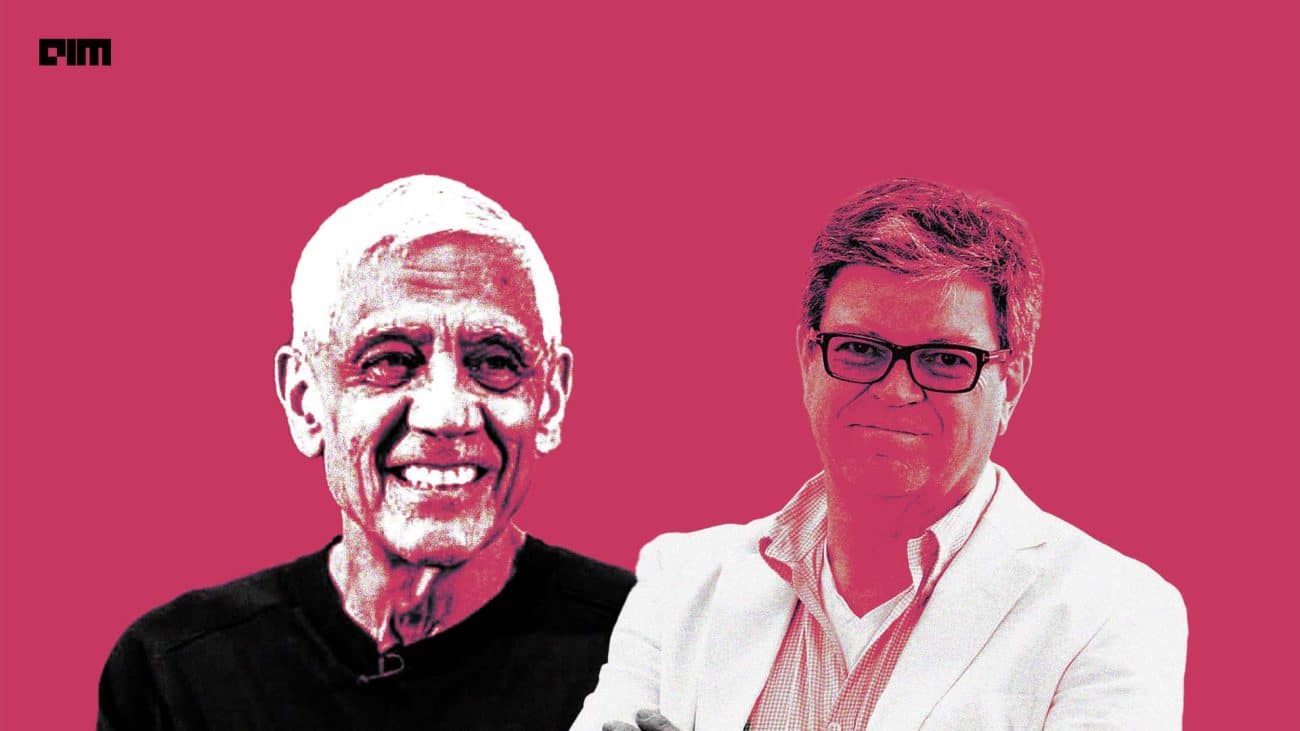
US Fears China’s Rise in AI Could Dominate Global Economy and Politics
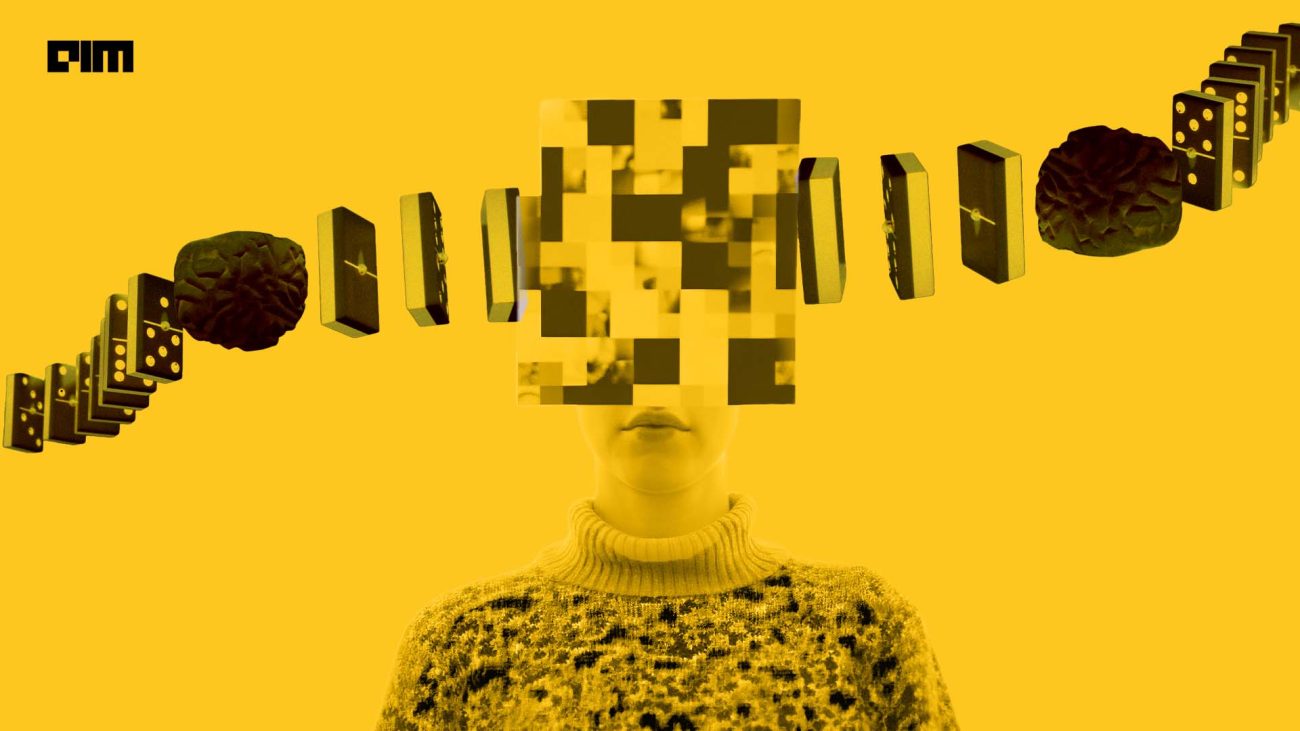
We Live in an Era Where it’s Easy to Build but Difficult to Figure Out What to Build

Indian Companies are Good at Copying Ideas Generated Elsewhere

Why Ollama is Good for Running LLMs on Computer

AI Forum for India
Our discord community for ai ecosystem, in collaboration with nvidia. , "> "> flagship events, rising 2024 | de&i in tech summit, april 4 and 5, 2024 | 📍 hilton convention center, manyata tech park, bangalore, machinecon gcc summit 2024, june 28 2024 | 📍bangalore, india, machinecon usa 2024, 26 july 2024 | 583 park avenue, new york, cypher india 2024, september 25-27, 2024 | 📍bangalore, india, cypher usa 2024, nov 21-22 2024 | 📍santa clara convention center, california, usa, genai corner.

Kunal Shah Says ‘GPT Makes Him 10x More Efficient in Sharing Ideas with the Team’

10 Free Courses to Build AI Agents in 2024

ChatGPT Brings Data to Life With Interactive Charts and Tables Directly from Google Drive and Microsoft OneDrive

After Stack Overflow, Reddit Succumbs to OpenAI

VMware Makes Workstation Pro and Fusion Pro Free for Personal Use
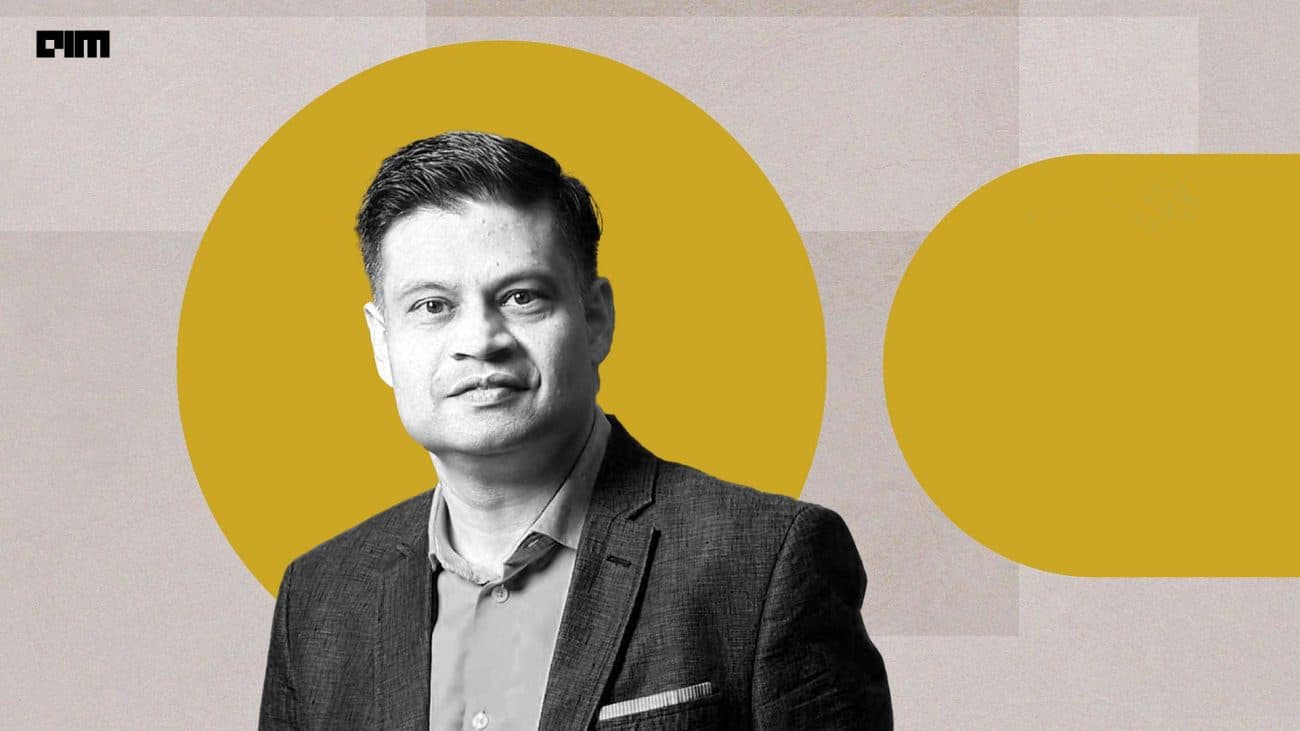
L&T Technology Services Trains 3,000 Engineers in GenAI

Zoho Ventures into Chipmaking, Plans $700M Investment
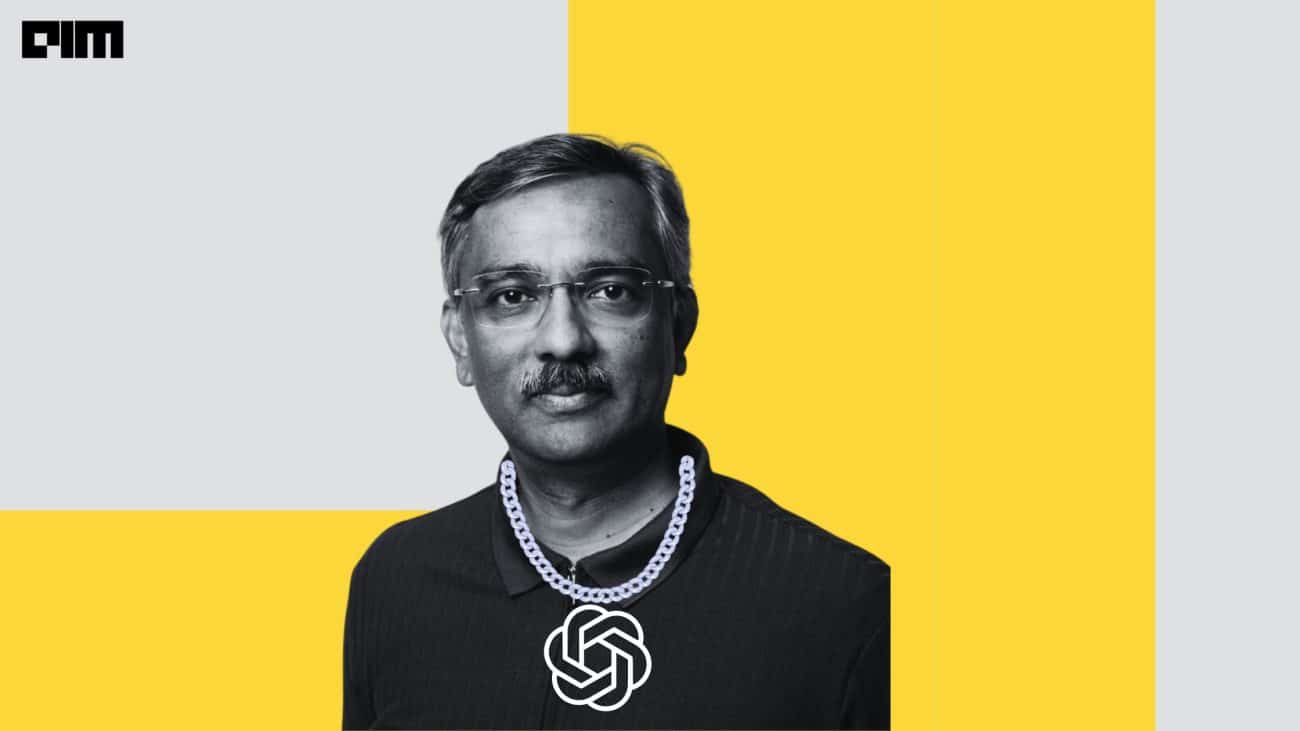
OpenAI Hires Google Veteran to Build ‘Google Search Alternative’
World's biggest media & analyst firm specializing in ai, advertise with us, aim publishes every day, and we believe in quality over quantity, honesty over spin. we offer a wide variety of branding and targeting options to make it easy for you to propagate your brand., branded content, aim brand solutions, a marketing division within aim, specializes in creating diverse content such as documentaries, public artworks, podcasts, videos, articles, and more to effectively tell compelling stories., corporate upskilling, adasci corporate training program on generative ai provides a unique opportunity to empower, retain and advance your talent, with machinehack you can not only find qualified developers with hiring challenges but can also engage the developer community and your internal workforce by hosting hackathons., talent assessment, conduct customized online assessments on our powerful cloud-based platform, secured with best-in-class proctoring, research & advisory, aim research produces a series of annual reports on ai & data science covering every aspect of the industry. request customised reports & aim surveys for a study on topics of your interest., conferences & events, immerse yourself in ai and business conferences tailored to your role, designed to elevate your performance and empower you to accomplish your organization’s vital objectives., aim launches the 3rd edition of data engineering summit. may 30-31, bengaluru.
Join the forefront of data innovation at the Data Engineering Summit 2024, where industry leaders redefine technology’s future.
© Analytics India Magazine Pvt Ltd & AIM Media House LLC 2024
- Terms of use
- Privacy Policy
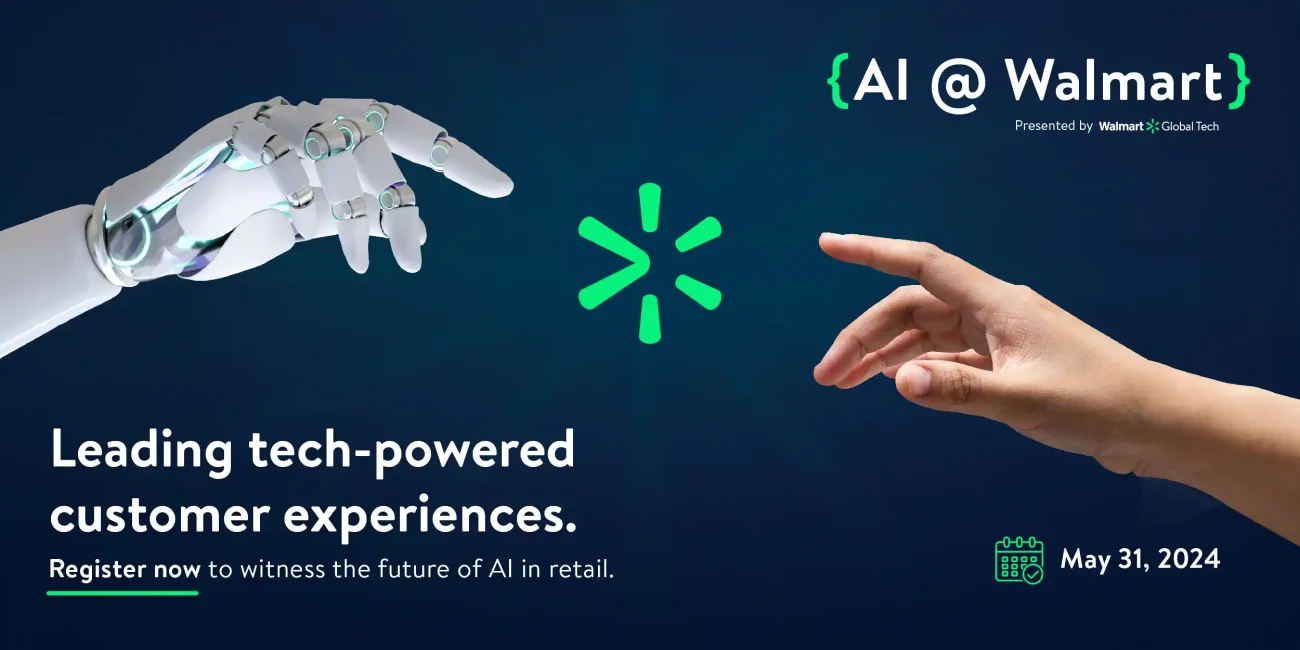
Machine Learning
- Reports substantive results on a wide range of learning methods applied to various learning problems.
- Provides robust support through empirical studies, theoretical analysis, or comparison to psychological phenomena.
- Demonstrates how to apply learning methods to solve significant application problems.
- Improves how machine learning research is conducted.
- Prioritizes verifiable and replicable supporting evidence in all published papers.
- Hendrik Blockeel
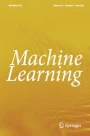
Latest issue
Volume 113, Issue 6
Latest articles
Comadout—a robust outlier detection algorithm based on comad.
- Andreas Lohrer
- Daniyal Kazempour
- Peer Kröger
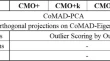
Finite-time error bounds for Greedy-GQ
- Shaofeng Zou
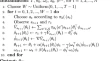
SWoTTeD : an extension of tensor decomposition to temporal phenotyping
- Thomas Guyet
- Etienne Audureau
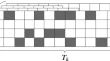
Semantic-enhanced graph neural networks with global context representation
- Youcheng Qian
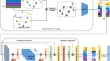
Explaining Siamese networks in few-shot learning
- Andrea Fedele
- Riccardo Guidotti
- Dino Pedreschi
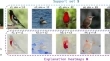
Journal updates
Cfp: discovery science 2023.
Submission Deadline: March 4, 2024
Guest Editors: Rita P. Ribeiro, Albert Bifet, Ana Carolina Lorena
CfP: IJCLR Learning and reasoning
Call for papers: conformal prediction and distribution-free uncertainty quantification.
Submission Deadline: January 7th, 2024
Guest Editors: Henrik Boström, Eyke Hüllermeier, Ulf Johansson, Khuong An Nguyen, Aaditya Ramdas
Call for Papers: DSAA 2024 Journal Track with Machine Learning Journal
Guest Editors: Longbing Cao, David C. Anastasiu, Qi Zhang, Xiaolin Huang,
Journal information
- ACM Digital Library
- Current Contents/Engineering, Computing and Technology
- EI Compendex
- Google Scholar
- Japanese Science and Technology Agency (JST)
- Mathematical Reviews
- OCLC WorldCat Discovery Service
- Science Citation Index Expanded (SCIE)
- TD Net Discovery Service
- UGC-CARE List (India)
Rights and permissions
Editorial policies
© Springer Science+Business Media LLC, part of Springer Nature
- Find a journal
- Publish with us
- Track your research
- Who’s Teaching What
- Subject Updates
- MEng program
- Opportunities
- Minor in Computer Science
- Resources for Current Students
- Program objectives and accreditation
- Graduate program requirements
- Admission process
- Degree programs
- Graduate research
- EECS Graduate Funding
- Resources for current students
- Student profiles
- Instructors
- DEI data and documents
- Recruitment and outreach
- Community and resources
- Get involved / self-education
- Rising Stars in EECS
- Graduate Application Assistance Program (GAAP)
- MIT Summer Research Program (MSRP)
- Sloan-MIT University Center for Exemplary Mentoring (UCEM)
- Electrical Engineering
- Computer Science
- Artificial Intelligence + Decision-making
- AI and Society
- AI for Healthcare and Life Sciences
- Artificial Intelligence and Machine Learning
- Biological and Medical Devices and Systems
- Communications Systems
- Computational Biology
- Computational Fabrication and Manufacturing
- Computer Architecture
- Educational Technology
- Electronic, Magnetic, Optical and Quantum Materials and Devices
- Graphics and Vision
- Human-Computer Interaction
- Information Science and Systems
- Integrated Circuits and Systems
- Nanoscale Materials, Devices, and Systems
- Natural Language and Speech Processing
- Optics + Photonics
- Optimization and Game Theory
- Programming Languages and Software Engineering
- Quantum Computing, Communication, and Sensing
- Security and Cryptography
- Signal Processing
- Systems and Networking
- Systems Theory, Control, and Autonomy
- Theory of Computation
- Departmental History
- Departmental Organization
- Visiting Committee
- Explore all research areas
Our research covers a wide range of topics of this fast-evolving field, advancing how machines learn, predict, and control, while also making them secure, robust and trustworthy. Research covers both the theory and applications of ML. This broad area studies ML theory (algorithms, optimization, etc.); statistical learning (inference, graphical models, causal analysis, etc.); deep learning; reinforcement learning; symbolic reasoning ML systems; as well as diverse hardware implementations of ML.
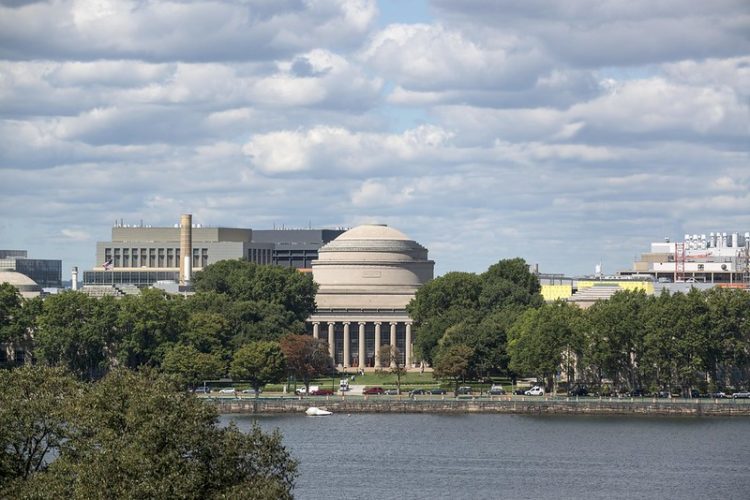
Latest news in artificial intelligence and machine learning
Creating bespoke programming languages for efficient visual ai systems.
Associate Professor Jonathan Ragan-Kelley optimizes how computer graphics and images are processed for the hardware of today and tomorrow.
QS World University Rankings rates MIT No. 1 in 11 subjects for 2024
The Institute also ranks second in five subject areas.
Three from MIT awarded 2024 Guggenheim Fellowships
MIT professors Roger Levy, Tracy Slatyer, and Martin Wainwright appointed to the 2024 class of “trail-blazing fellows.”
To build a better AI helper, start by modeling the irrational behavior of humans
A new technique can be used to predict the actions of human or AI agents who behave suboptimally while working toward unknown goals.
Priya Donti named AI2050 Early Career Fellow
Assistant Professor Priya Donti has been named an AI2050 Early Career Fellow by Schmidt Sciences, a philanthropic initiative from Eric and Wendy Schmidt aimed at helping to solve hard problems in AI.

Upcoming events
Doctoral thesis: guiding deep probabilistic models.
How to Read Research Papers: A Pragmatic Approach for ML Practitioners
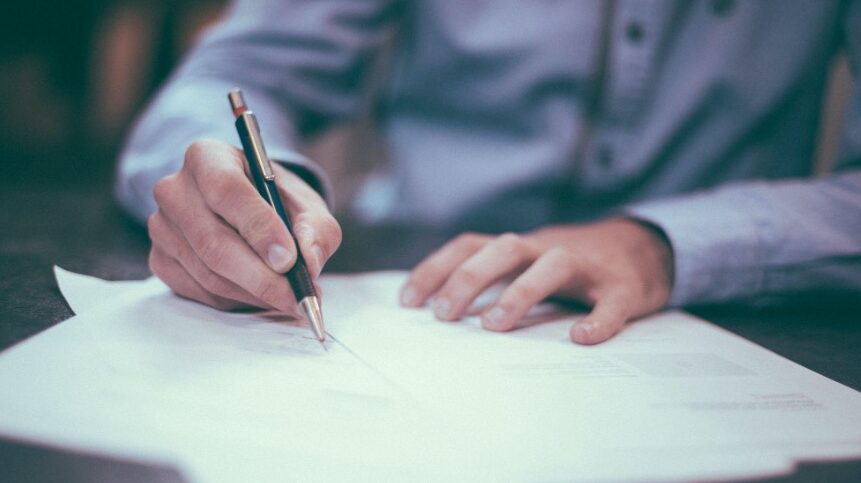
Is it necessary for data scientists or machine-learning experts to read research papers?
The short answer is yes. And don’t worry if you lack a formal academic background or have only obtained an undergraduate degree in the field of machine learning.
Reading academic research papers may be intimidating for individuals without an extensive educational background. However, a lack of academic reading experience should not prevent Data scientists from taking advantage of a valuable source of information and knowledge for machine learning and AI development .
This article provides a hands-on tutorial for data scientists of any skill level to read research papers published in academic journals such as NeurIPS , JMLR , ICML, and so on.
Before diving wholeheartedly into how to read research papers, the first phases of learning how to read research papers cover selecting relevant topics and research papers.
Step 1: Identify a topic
The domain of machine learning and data science is home to a plethora of subject areas that may be studied. But this does not necessarily imply that tackling each topic within machine learning is the best option.
Although generalization for entry-level practitioners is advised, I’m guessing that when it comes to long-term machine learning, career prospects, practitioners, and industry interest often shifts to specialization.
Identifying a niche topic to work on may be difficult, but good. Still, a rule of thumb is to select an ML field in which you are either interested in obtaining a professional position or already have experience.
Deep Learning is one of my interests, and I’m a Computer Vision Engineer that uses deep learning models in apps to solve computer vision problems professionally. As a result, I’m interested in topics like pose estimation, action classification, and gesture identification.
Based on roles, the following are examples of ML/DS occupations and related themes to consider.
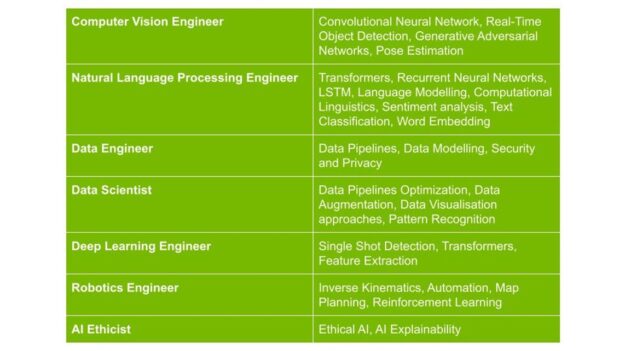
For this article, I’ll select the topic Pose Estimation to explore and choose associated research papers to study.
Step 2: Finding research papers
One of the most excellent tools to use while looking at machine learning-related research papers, datasets, code, and other related materials is PapersWithCode .
We use the search engine on the PapersWithCode website to get relevant research papers and content for our chosen topic, “Pose Estimation.” The following image shows you how it’s done.
The search results page contains a short explanation of the searched topic, followed by a table of associated datasets, models, papers, and code. Without going into too much detail, the area of interest for this use case is the “Greatest papers with code”. This section contains the relevant papers related to the task or topic. For the purpose of this article, I’ll select the DensePose: Dense Human Pose Estimation In The Wild .
Step 3: First pass (gaining context and understanding)
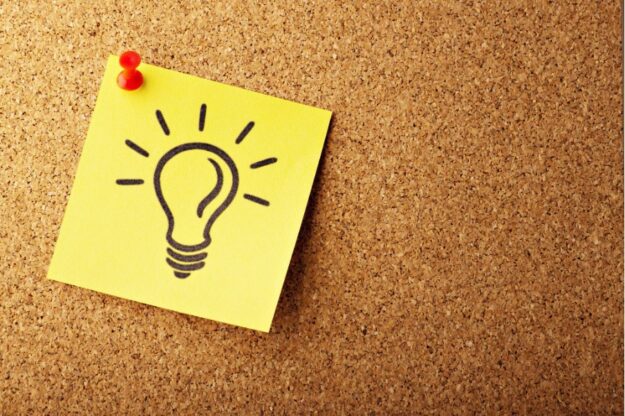
At this point, we’ve selected a research paper to study and are prepared to extract any valuable learnings and findings from its content.
It’s only natural that your first impulse is to start writing notes and reading the document from beginning to end, perhaps taking some rest in between. However, having a context for the content of a study paper is a more practical way to read it. The title, abstract, and conclusion are three key parts of any research paper to gain an understanding.
The goal of the first pass of your chosen paper is to achieve the following:
- Assure that the paper is relevant.
- Obtain a sense of the paper’s context by learning about its contents, methods, and findings.
- Recognize the author’s goals, methodology, and accomplishments.
The title is the first point of information sharing between the authors and the reader. Therefore, research papers titles are direct and composed in a manner that leaves no ambiguity.
The research paper title is the most telling aspect since it indicates the study’s relevance to your work. The importance of the title is to give a brief perception of the paper’s content.
In this situation, the title is “DensePose: Dense Human Pose Estimation in the Wild.” This gives a broad overview of the work and implies that it will look at how to provide pose estimations in environments with high levels of activity and realistic situations properly.
The abstract portion gives a summarized version of the paper. It’s a short section that contains 300-500 words and tells you what the paper is about in a nutshell. The abstract is a brief text that provides an overview of the article’s content, researchers’ objectives, methods, and techniques.
When reading an abstract of a machine-learning research paper, you’ll typically come across mentions of datasets, methods, algorithms, and other terms. Keywords relevant to the article’s content provide context. It may be helpful to take notes and keep track of all keywords at this point.
For the paper: “ DensePose: Dense Human Pose Estimation In The Wild “, I identified in the abstract the following keywords: pose estimation, COCO dataset, CNN, region-based models, real-time.
It’s not uncommon to experience fatigue when reading the paper from top to bottom at your first initial pass, especially for Data Scientists and practitioners with no prior advanced academic experience. Although extracting information from the later sections of a paper might seem tedious after a long study session, the conclusion sections are often short. Hence reading the conclusion section in the first pass is recommended.
The conclusion section is a brief compendium of the work’s author or authors and/or contributions and accomplishments and promises for future developments and limitations.
Before reading the main content of a research paper, read the conclusion section to see if the researcher’s contributions, problem domain, and outcomes match your needs.
Following this particular brief first pass step enables a sufficient understanding and overview of the research paper’s scope and objectives, as well as a context for its content. You’ll be able to get more detailed information out of its content by going through it again with laser attention.
Step 4: Second pass (content familiarization)
Content familiarization is a process that’s relevant to the initial steps. The systematic approach to reading the research paper presented in this article. The familiarity process is a step that involves the introduction section and figures within the research paper.
As previously mentioned, the urge to plunge straight into the core of the research paper is not required because knowledge acclimatization provides an easier and more comprehensive examination of the study in later passes.
Introduction
Introductory sections of research papers are written to provide an overview of the objective of the research efforts. This objective mentions and explains problem domains, research scope, prior research efforts, and methodologies.
It’s normal to find parallels to past research work in this area, using similar or distinct methods. Other papers’ citations provide the scope and breadth of the problem domain, which broadens the exploratory zone for the reader. Perhaps incorporating the procedure outlined in Step 3 is sufficient at this point.
Another aspect of the benefit provided by the introduction section is the presentation of requisite knowledge required to approach and understand the content of the research paper.
Graph, diagrams, figures
Illustrative materials within the research paper ensure that readers can comprehend factors that support problem definition or explanations of methods presented. Commonly, tables are used within research papers to provide information on the quantitative performances of novel techniques in comparison to similar approaches.
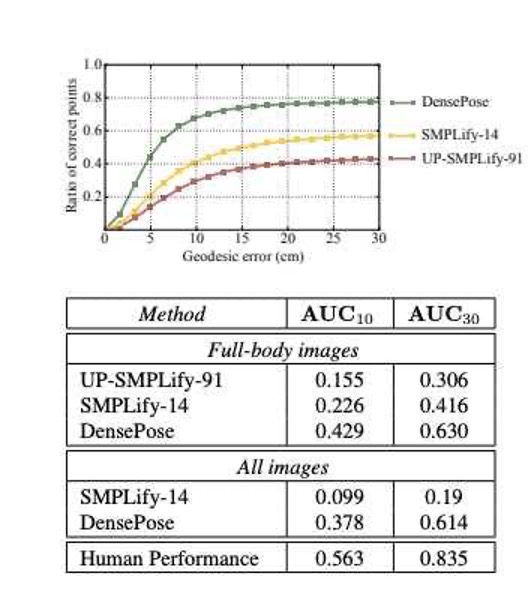
Generally, the visual representation of data and performance enables the development of an intuitive understanding of the paper’s context. In the Dense Pose paper mentioned earlier, illustrations are used to depict the performance of the author’s approach to pose estimation and create. An overall understanding of the steps involved in generating and annotating data samples.
In the realm of deep learning, it’s common to find topological illustrations depicting the structure of artificial neural networks. Again this adds to the creation of intuitive understanding for any reader. Through illustrations and figures, readers may interpret the information themselves and gain a fuller perspective of it without having any preconceived notions about what outcomes should be.
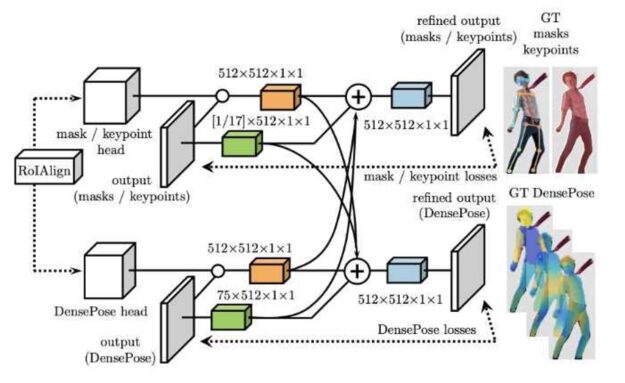
Step 5: Third pass (deep reading)
The third pass of the paper is similar to the second, though it covers a greater portion of the text. The most important thing about this pass is that you avoid any complex arithmetic or technique formulations that may be difficult for you. During this pass, you can also skip over any words and definitions that you don’t understand or aren’t familiar with. These unfamiliar terms, algorithms, or techniques should be noted to return to later.
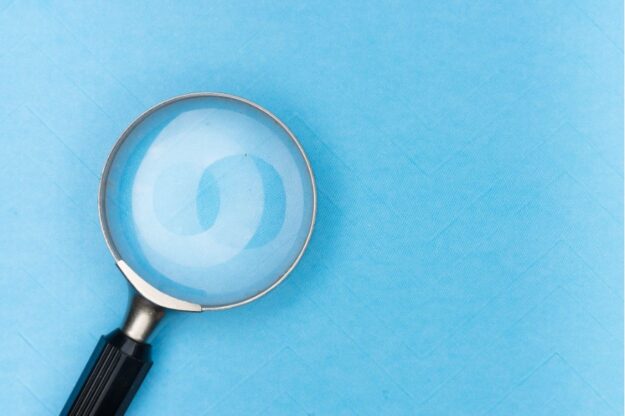
During this pass, your primary objective is to gain a broad understanding of what’s covered in the paper. Approach the paper, starting again from the abstract to the conclusion, but be sure to take intermediary breaks in between sections. Moreover, it’s recommended to have a notepad, where all key insights and takeaways are noted, alongside the unfamiliar terms and concepts.
The Pomodoro Technique is an effective method of managing time allocated to deep reading or study. Explained simply, the Pomodoro Technique involves the segmentation of the day into blocks of work, followed by short breaks.
What works for me is the 50/15 split, that is, 50 minutes studying and 15 minutes allocated to breaks. I tend to execute this split twice consecutively before taking a more extended break of 30 minutes. If you are unfamiliar with this time management technique, adopt a relatively easy division such as 25/5 and adjust the time split according to your focus and time capacity.
Step 6: Forth pass (final pass)
The final pass is typically one that involves an exertion of your mental and learning abilities, as it involves going through the unfamiliar terms, terminologies, concepts, and algorithms noted in the previous pass. This pass focuses on using external material to understand the recorded unfamiliar aspects of the paper.
In-depth studies of unfamiliar subjects have no specified time length, and at times efforts span into the days and weeks. The critical factor to a successful final pass is locating the appropriate sources for further exploration.
Unfortunately, there isn’t one source on the Internet that provides the wealth of information you require. Still, there are multiple sources that, when used in unison and appropriately, fill knowledge gaps. Below are a few of these resources.
- The Machine Learning Subreddit
- The Deep Learning Subreddit
- PapersWithCode
- Top conferences such as NIPS , ICML , ICLR
- Research Gate
- Machine Learning Apple
The Reference sections of research papers mention techniques and algorithms. Consequently, the current paper either draws inspiration from or builds upon, which is why the reference section is a useful source to use in your deep reading sessions.
Step 7: Summary (optional)
In almost a decade of academic and professional undertakings of technology-associated subjects and roles, the most effective method of ensuring any new information learned is retained in my long-term memory through the recapitulation of explored topics. By rewriting new information in my own words, either written or typed, I’m able to reinforce the presented ideas in an understandable and memorable manner.
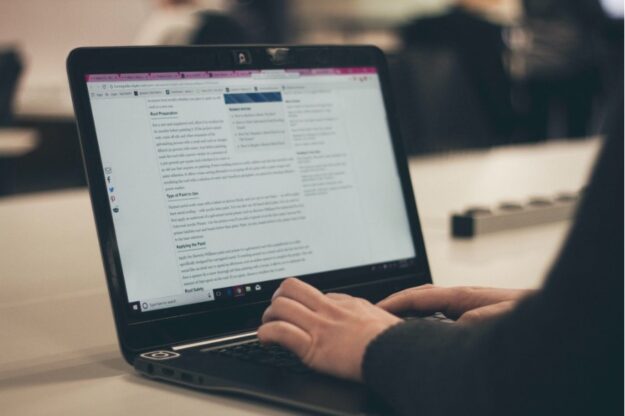
To take it one step further, it’s possible to publicize learning efforts and notes through the utilization of blogging platforms and social media. An attempt to explain the freshly explored concept to a broad audience, assuming a reader isn’t accustomed to the topic or subject, requires understanding topics in intrinsic details.
Undoubtedly, reading research papers for novice Data Scientists and ML practitioners can be daunting and challenging; even seasoned practitioners find it difficult to digest the content of research papers in a single pass successfully.
The nature of the Data Science profession is very practical and involved. Meaning, there’s a requirement for its practitioners to employ an academic mindset, more so as the Data Science domain is closely associated with AI, which is still a developing field.
To summarize, here are all of the steps you should follow to read a research paper:
- Identify A Topic.
- Finding associated Research Papers
- Read title, abstract, and conclusion to gain a vague understanding of the research effort aims and achievements.
- Familiarize yourself with the content by diving deeper into the introduction; including the exploration of figures and graphs presented in the paper.
- Use a deep reading session to digest the main content of the paper as you go through the paper from top to bottom.
- Explore unfamiliar terms, terminologies, concepts, and methods using external resources.
- Summarize in your own words essential takeaways, definitions, and algorithms.
Thanks for reading!
Related resources
- DLI course: Building Transformer-Based Natural Language Processing
- GTC session: Enterprise MLOps 101
- GTC session: Intro to Large Language Models: LLM Tutorial and Disease Diagnosis LLM Lab
- GTC session: Build AI Applications with GPU Vector Databases
- NGC Containers: MATLAB
- Webinar: Empowering Future Engineers and Scientists With AI and NVIDIA Modulus
About the Authors
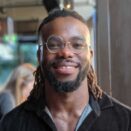
Related posts
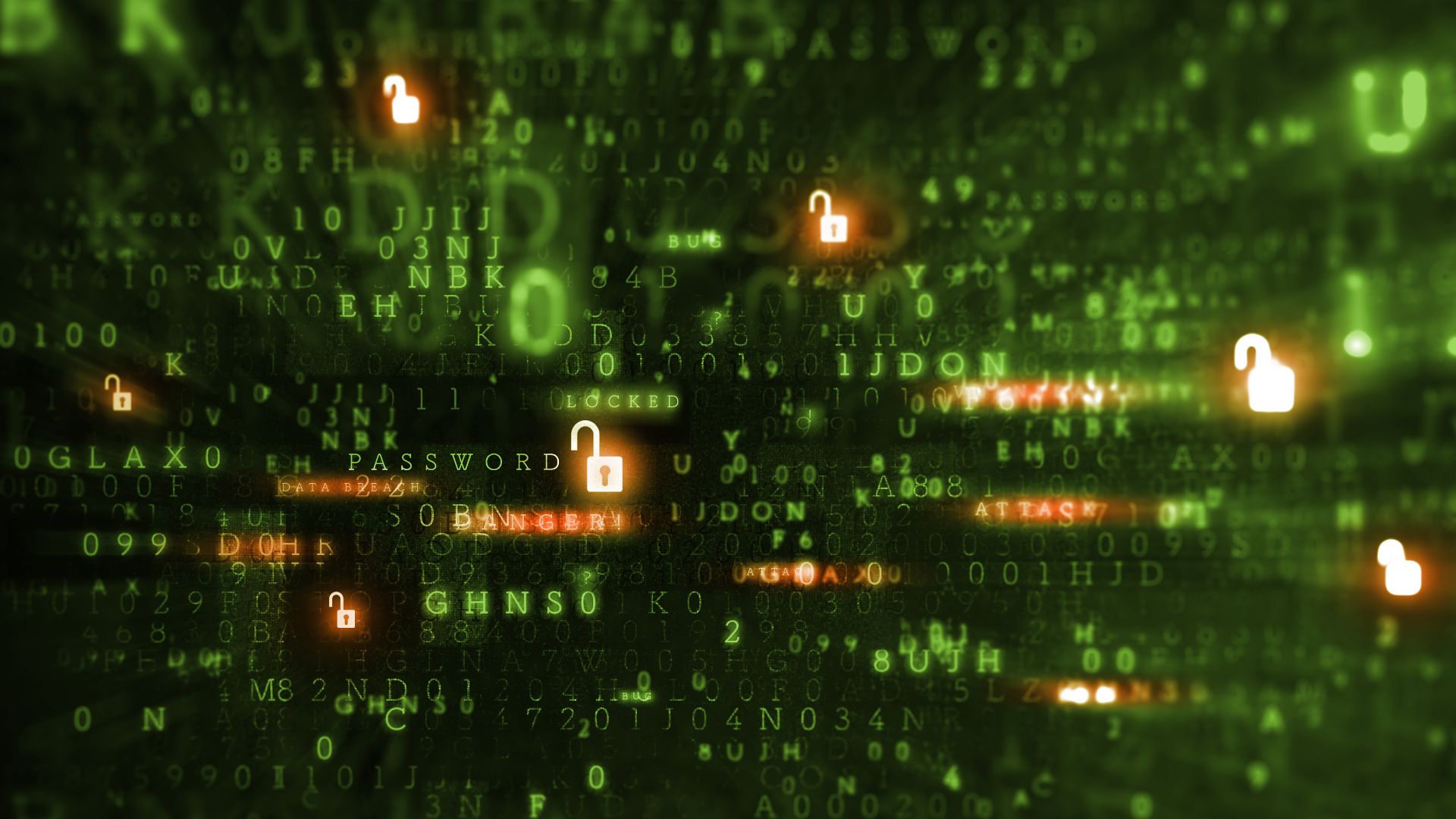
Improving Machine Learning Security Skills at a DEF CON Competition

Community Spotlight: Democratizing Computer Vision and Conversational AI in Kenya
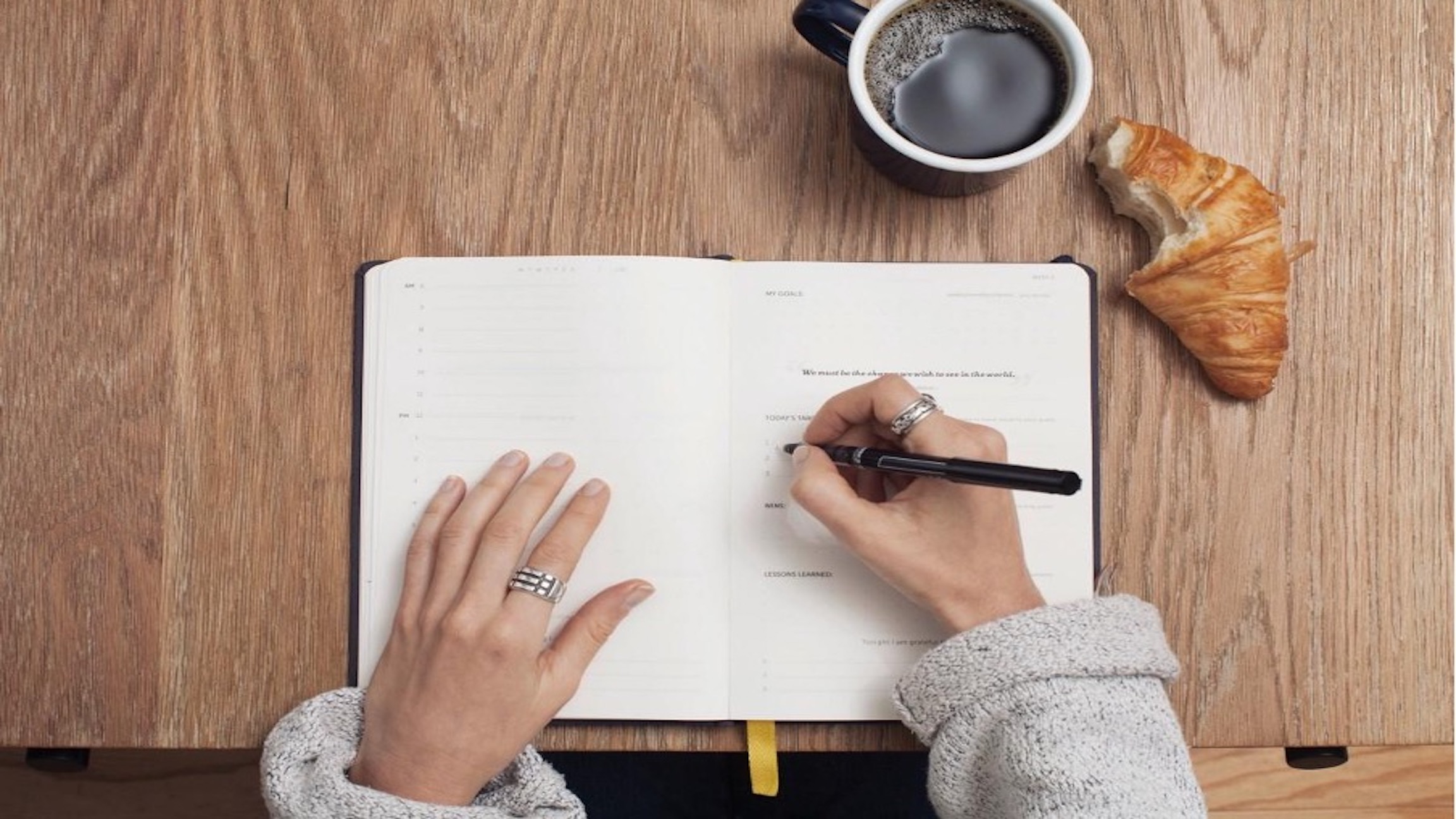
An Important Skill for Data Scientists and Machine Learning Practitioners
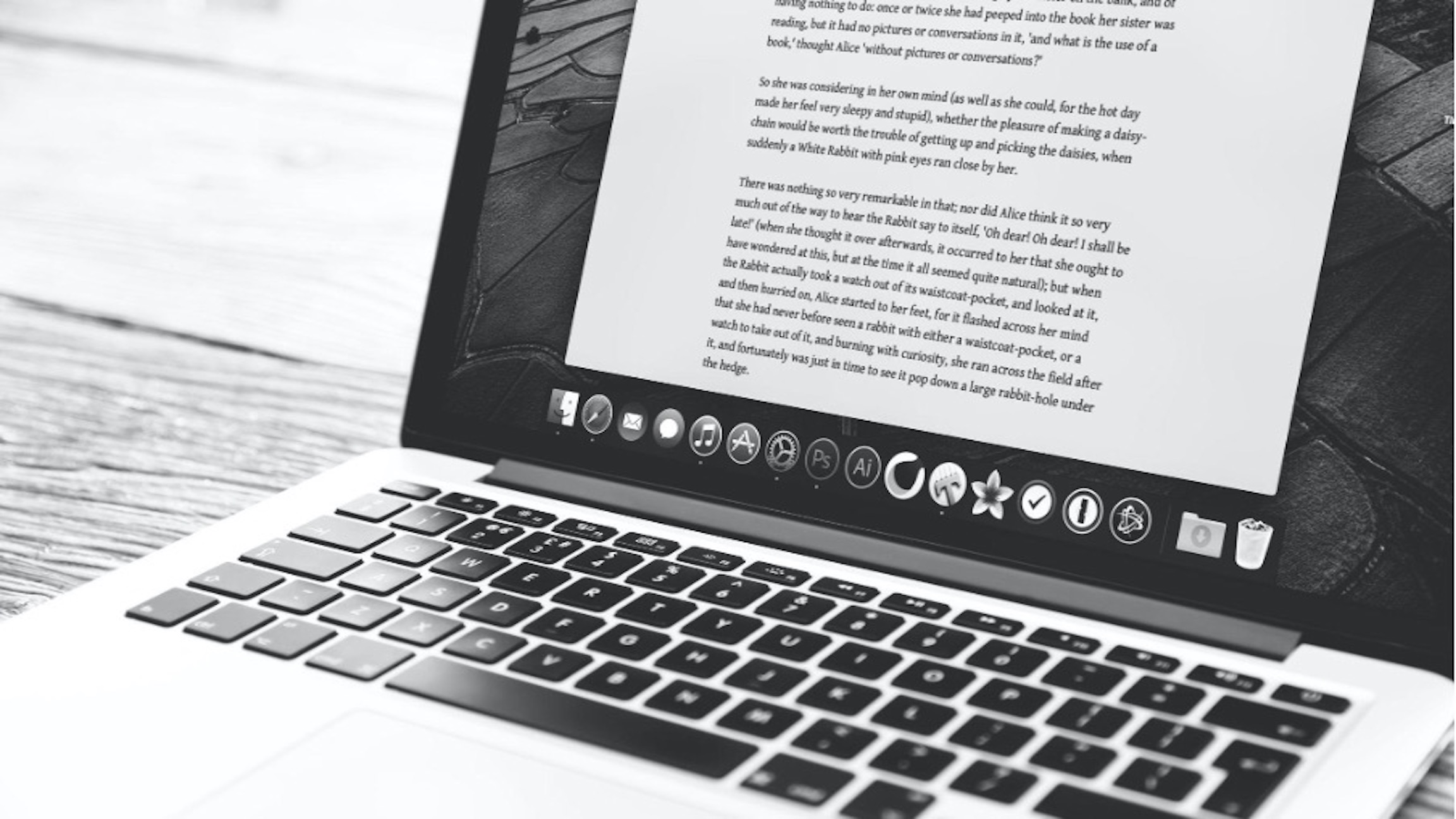
AI Pioneers Write So Should Data Scientists
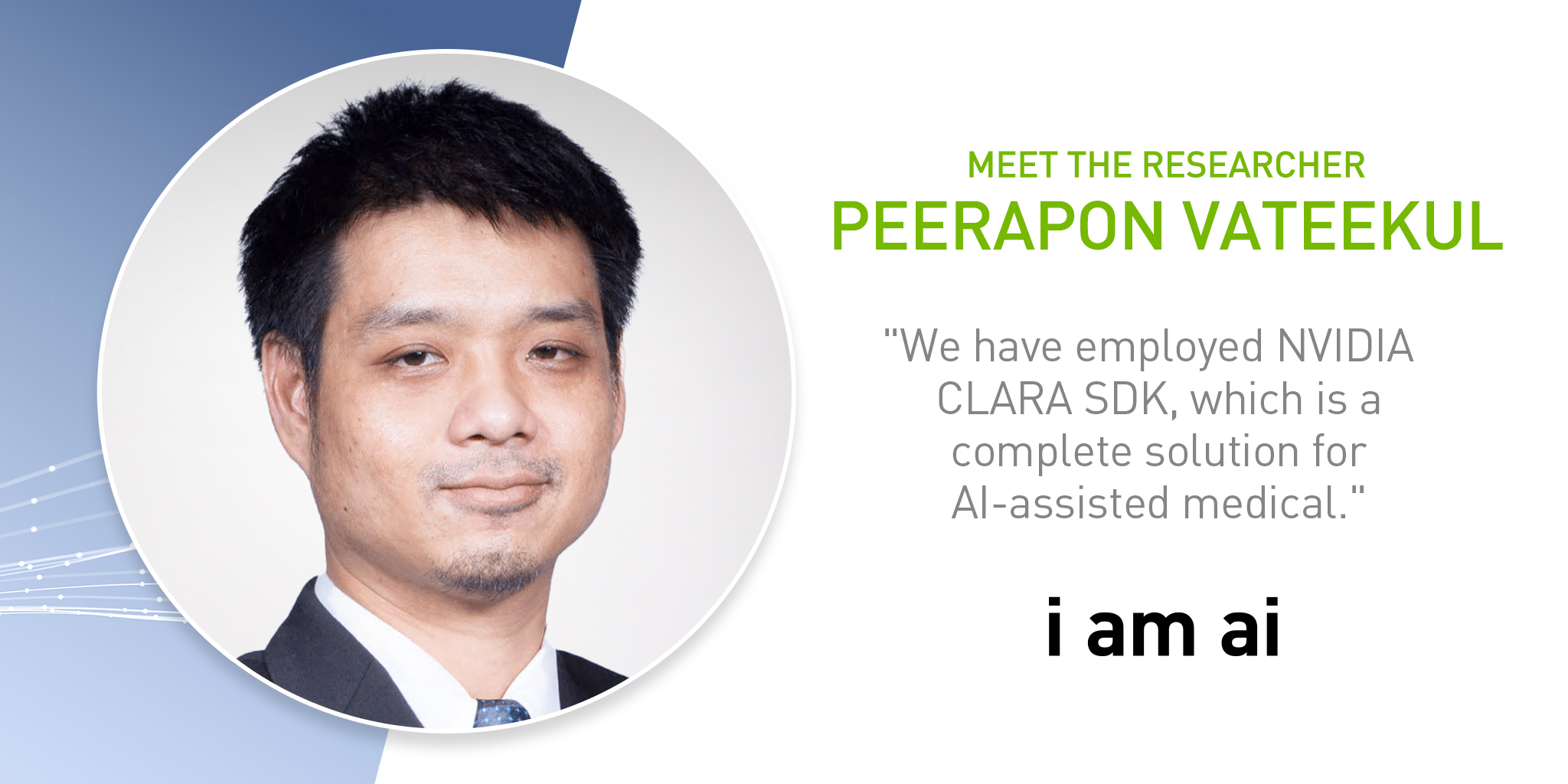
Meet the Researcher: Peerapon Vateekul, Deep Learning Solutions for Medical Diagnosis and NLP
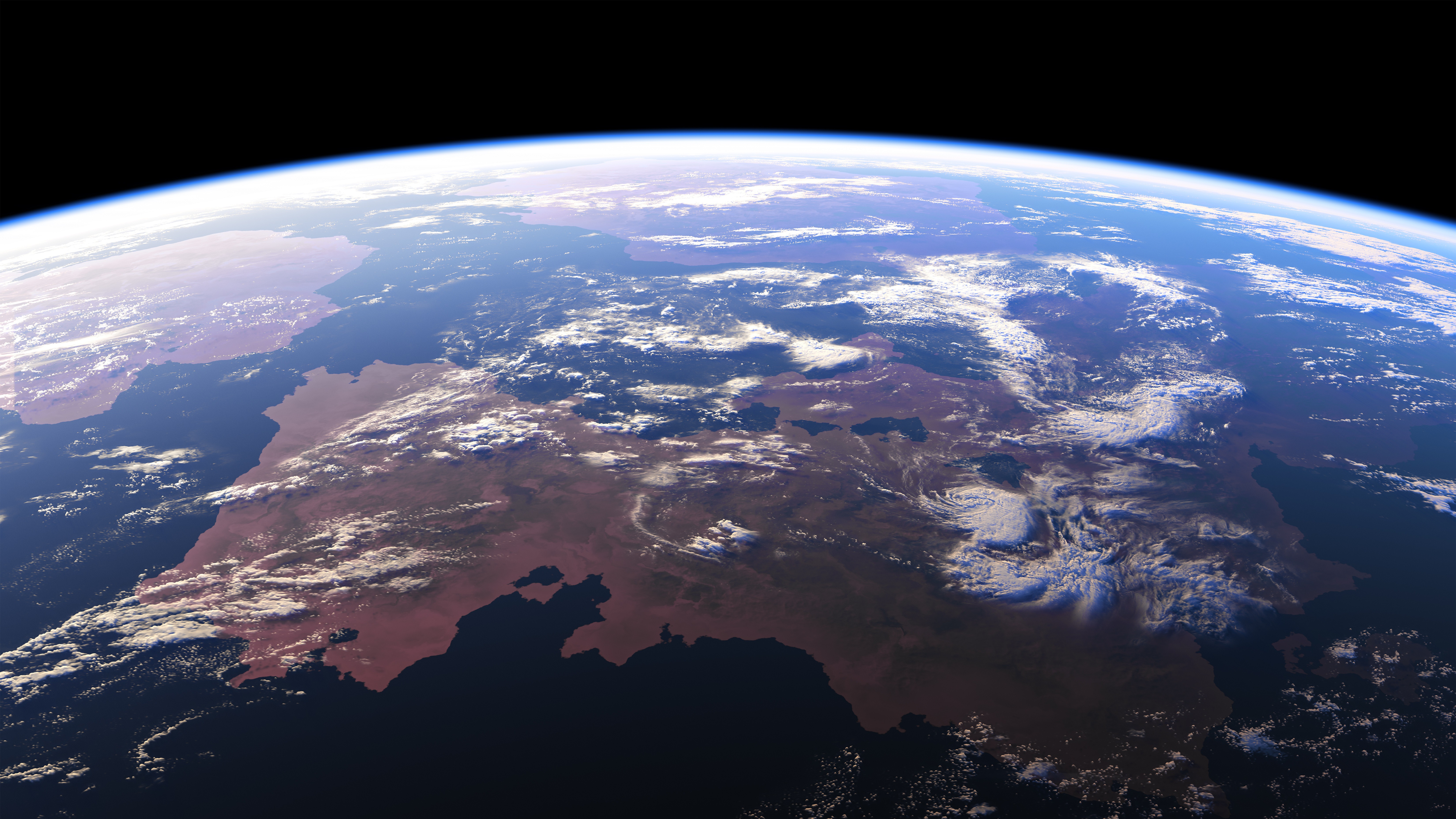
Next-Generation Seismic Monitoring with Neural Operators
Simulating realistic traffic behavior with a bi-level imitation learning ai model.
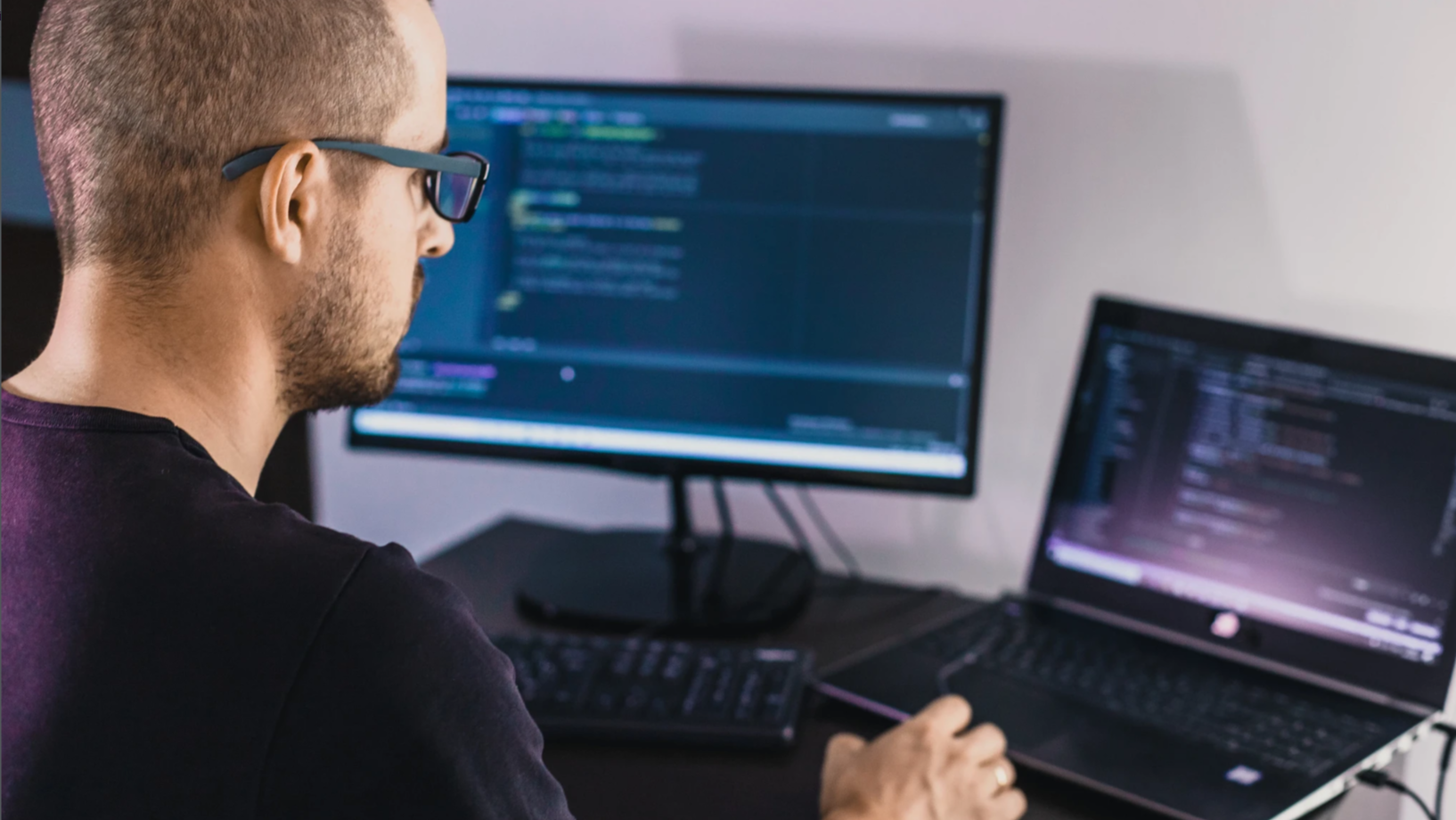
Analyzing the Security of Machine Learning Research Code
Research unveils breakthrough deep learning tool for understanding neural activity and movement control, generative ai research empowers creators with guided image structure control.
FOR DEVELOPERS
How to write a good research paper in the machine learning area.
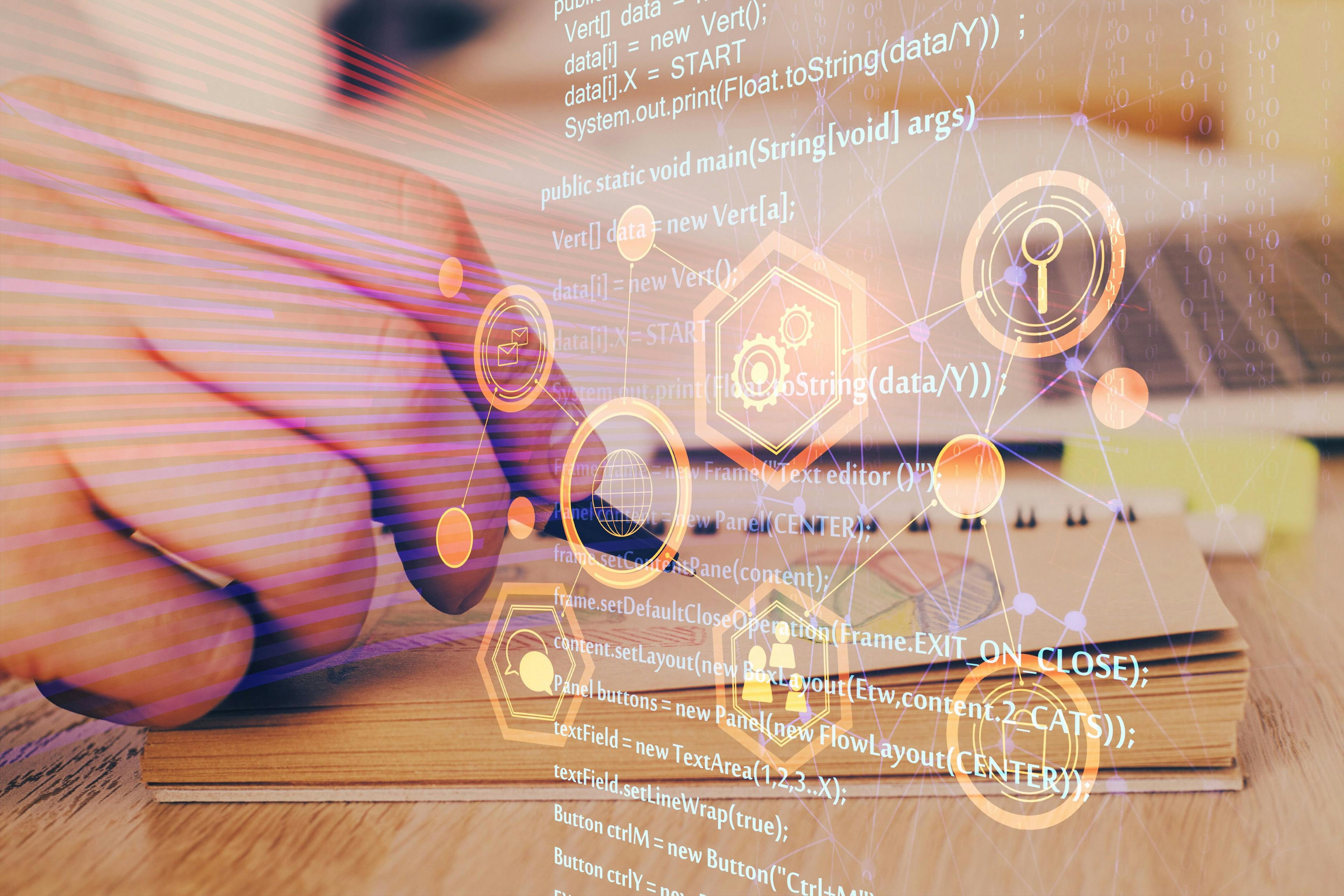
Author is a seasoned writer with a reputation for crafting highly engaging, well-researched, and useful content that is widely read by many of today's skilled programmers and developers.
Frequently Asked Questions
Yes, AI can write a research paper for you in less time than you would take to write it manually.
We have listed down some of the top journals where you can publish machine learning papers below.
- Elsevier Pattern Recognition
- Journal of Machine Learning Research
- IEEE Transactions on Pattern Analysis and Machine Intelligence
- Wiley International Journal of Intelligent Systems
- IEEE Transactions on Neural Networks and Learning Systems
Here is a list of some of the best research papers for machine learning.
- Unbiased Gradient Estimation in Unrolled Computation Graphs with Persistent Evolution By Paul Vicol, Luke Metz, and Jascha Sohl-Dickstein
- Scalable nearest neighbor algorithms for high dimensional data By Lowe, D.G., & Muja, M.
- Trends in extreme learning machines By Huang, G., Huang, G., Song, S., & You, K.
- Solving high-dimensional parabolic PDEs using the tensor train format By Lorenz Richter, Leon Sallandt, and Nikolas Nüsken
- Optimal complexity in decentralized training By researchers at Cornell University, Yucheng Lu and Christopher De Sa
Follow the procedure given below to write a dataset in your research paper.
Step 1: Navigate to your study folder and then “Manage” tab.
Step 2: Select “Manage datasets.”
Step 3: Select “Create new dataset.”
Check out some free platforms which will publish your machine learning papers for free.
- ScienceOpen
- Social Science Research Network
- Directory of Open Access Journals
- Education Resources Information Center
- arXiv e-Print Archive
An abstract is something that summarises your paper in a small paragraph. So, when you write it for your research paper, ensure that:
- Its word count is 300 or less.
- It includes the purpose of your paper.
- Your discovery or findings as an outcome of your research paper
Hire remote developers
Tell us the skills you need and we'll find the best developer for you in days, not weeks.
machine learning Recently Published Documents
Total documents.
- Latest Documents
- Most Cited Documents
- Contributed Authors
- Related Sources
- Related Keywords
An explainable machine learning model for identifying geographical origins of sea cucumber Apostichopus japonicus based on multi-element profile
A comparison of machine learning- and regression-based models for predicting ductility ratio of rc beam-column joints, alexa, is this a historical record.
Digital transformation in government has brought an increase in the scale, variety, and complexity of records and greater levels of disorganised data. Current practices for selecting records for transfer to The National Archives (TNA) were developed to deal with paper records and are struggling to deal with this shift. This article examines the background to the problem and outlines a project that TNA undertook to research the feasibility of using commercially available artificial intelligence tools to aid selection. The project AI for Selection evaluated a range of commercial solutions varying from off-the-shelf products to cloud-hosted machine learning platforms, as well as a benchmarking tool developed in-house. Suitability of tools depended on several factors, including requirements and skills of transferring bodies as well as the tools’ usability and configurability. This article also explores questions around trust and explainability of decisions made when using AI for sensitive tasks such as selection.
Automated Text Classification of Maintenance Data of Higher Education Buildings Using Text Mining and Machine Learning Techniques
Data-driven analysis and machine learning for energy prediction in distributed photovoltaic generation plants: a case study in queensland, australia, modeling nutrient removal by membrane bioreactor at a sewage treatment plant using machine learning models, big five personality prediction based in indonesian tweets using machine learning methods.
<span lang="EN-US">The popularity of social media has drawn the attention of researchers who have conducted cross-disciplinary studies examining the relationship between personality traits and behavior on social media. Most current work focuses on personality prediction analysis of English texts, but Indonesian has received scant attention. Therefore, this research aims to predict user’s personalities based on Indonesian text from social media using machine learning techniques. This paper evaluates several machine learning techniques, including <a name="_Hlk87278444"></a>naive Bayes (NB), K-nearest neighbors (KNN), and support vector machine (SVM), based on semantic features including emotion, sentiment, and publicly available Twitter profile. We predict the personality based on the big five personality model, the most appropriate model for predicting user personality in social media. We examine the relationships between the semantic features and the Big Five personality dimensions. The experimental results indicate that the Big Five personality exhibit distinct emotional, sentimental, and social characteristics and that SVM outperformed NB and KNN for Indonesian. In addition, we observe several terms in Indonesian that specifically refer to each personality type, each of which has distinct emotional, sentimental, and social features.</span>
Compressive strength of concrete with recycled aggregate; a machine learning-based evaluation
Temperature prediction of flat steel box girders of long-span bridges utilizing in situ environmental parameters and machine learning, computer-assisted cohort identification in practice.
The standard approach to expert-in-the-loop machine learning is active learning, where, repeatedly, an expert is asked to annotate one or more records and the machine finds a classifier that respects all annotations made until that point. We propose an alternative approach, IQRef , in which the expert iteratively designs a classifier and the machine helps him or her to determine how well it is performing and, importantly, when to stop, by reporting statistics on a fixed, hold-out sample of annotated records. We justify our approach based on prior work giving a theoretical model of how to re-use hold-out data. We compare the two approaches in the context of identifying a cohort of EHRs and examine their strengths and weaknesses through a case study arising from an optometric research problem. We conclude that both approaches are complementary, and we recommend that they both be employed in conjunction to address the problem of cohort identification in health research.
Export Citation Format
Share document.
Machine Intelligence
Google is at the forefront of innovation in Machine Intelligence, with active research exploring virtually all aspects of machine learning, including deep learning and more classical algorithms. Exploring theory as well as application, much of our work on language, speech, translation, visual processing, ranking and prediction relies on Machine Intelligence. In all of those tasks and many others, we gather large volumes of direct or indirect evidence of relationships of interest, applying learning algorithms to understand and generalize.
Machine Intelligence at Google raises deep scientific and engineering challenges, allowing us to contribute to the broader academic research community through technical talks and publications in major conferences and journals. Contrary to much of current theory and practice, the statistics of the data we observe shifts rapidly, the features of interest change as well, and the volume of data often requires enormous computation capacity. When learning systems are placed at the core of interactive services in a fast changing and sometimes adversarial environment, combinations of techniques including deep learning and statistical models need to be combined with ideas from control and game theory.
Recent Publications
Some of our teams.
Algorithms & optimization
Applied science
Climate and sustainability
Graph mining
Impact-Driven Research, Innovation and Moonshots
Learning theory
Market algorithms
Operations research
Security, privacy and abuse
System performance
We're always looking for more talented, passionate people.
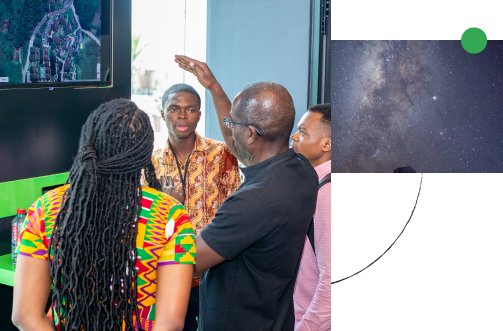
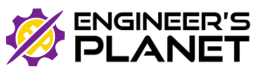
Machine Learning Project Topics With Abstracts and Base Papers 2024
Embark on a journey into the realm of machine learning with our curated list of M.Tech project topics for 2024, complemented by trending IEEE base papers. These projects cover a spectrum of innovative applications and advancements in the field, offering an invaluable resource for M.Tech students seeking to push the boundaries of knowledge and skill. Our comprehensive collection encompasses diverse Machine Learning project topics, each accompanied by a meticulously selected base paper and a concise abstract. From natural language processing and computer vision to reinforcement learning and predictive analytics, these projects reflect the latest trends in the ever-evolving landscape of artificial intelligence. Stay ahead of the curve by exploring projects that align with the current demands and challenges faced by industries worldwide. Whether you are a student, researcher, or industry professional, our compilation serves as a gateway to the forefront of machine learning innovation. The project titles are strategically chosen to include relevant keywords, ensuring alignment with the latest IEEE standards and technological advancements. Dive into the abstracts to gain a quick insight into the scope, methodology, and potential impact of each project.
M.Tech Projects Topics List In Machine Learning
Shivam Kashyap
Cloud computing project topics with abstracts and base papers 2024, computer network project topics with abstracts and base papers 2024, you may also like, major projects on cyber security final year, tensorflow based projects for final year, machine learning final year projects 2024 | ai, cse,..., intensity analysis (build your own model using nlp..., faultfindy (build intelligence using machine learning to predict..., recommender (build intelligence to help customers discover products..., propensity model to identify how likely certain target..., nutrigro (building a recommender system), moodformusic (an intelligent mood detection and music recommendation..., fire detection model using deep learning, leave a reply cancel reply.
This site uses Akismet to reduce spam. Learn how your comment data is processed .
- Mentorship Sessions
- M.Tech Projects R&D
- Ph.D Projects R&D
- B.Tech Projects R&D
- Plagiarism Report
- Bootcamp Hot
IMPORTANT LINKS
- News & Updates
- Project Downloads
- Career / Internship
- Privacy Policy
- Terms & Conditions
- MTech Projects Hot
- PhD Projects New
- BTech Projects
- AMIE Projects
- DBMS Projects Hot
- ECE & EEE Projects
- Research Paper
- Thesis Report
Fabrum Planet Solutions Pvt. Ltd.
- [email protected]
- +91 8882479344
- Delhi | Mumbai | Kolkata | Chennai
© 2024 All Rights Reserved Engineer’s Planet
Digital Media Partner #magdigit
- Terms & Conditions
This website uses cookies to improve your experience. We'll assume you're ok with this, but you can opt-out if you wish. OK Read More
- Write my thesis
- Thesis writers
- Buy thesis papers
- Bachelor thesis
- Master's thesis
- Thesis editing services
- Thesis proofreading services
- Buy a thesis online
- Write my dissertation
- Dissertation proposal help
- Pay for dissertation
- Custom dissertation
- Dissertation help online
- Buy dissertation online
- Cheap dissertation
- Dissertation editing services
- Write my research paper
- Buy research paper online
- Pay for research paper
- Research paper help
- Order research paper
- Custom research paper
- Cheap research paper
- Research papers for sale
- Thesis subjects
- How It Works
177 Great Artificial Intelligence Research Paper Topics to Use
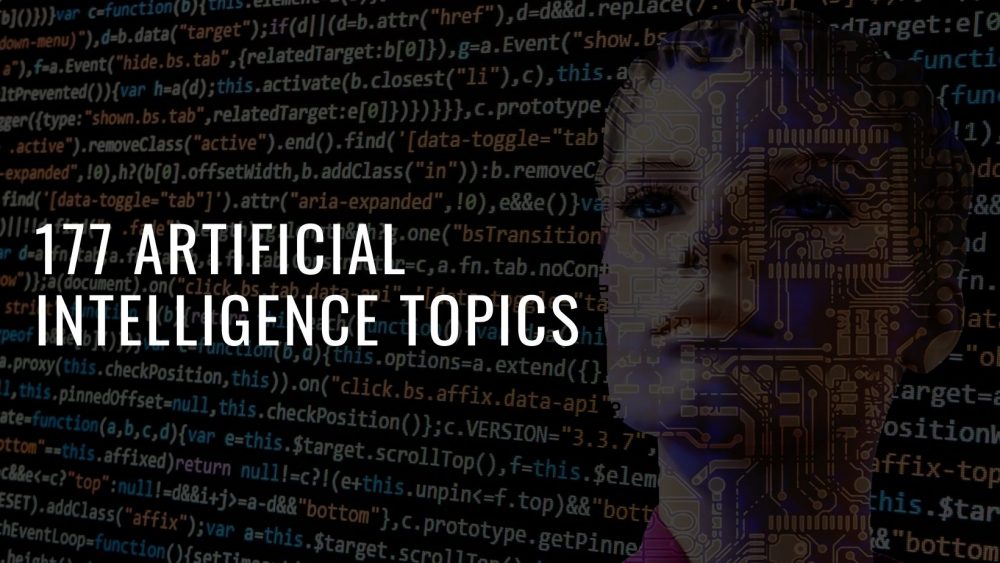
In this top-notch post, we will look at the definition of artificial intelligence, its applications, and writing tips on how to come up with AI topics. Finally, we shall lock at top artificial intelligence research topics for your inspiration.
What Is Artificial Intelligence?
It refers to intelligence as demonstrated by machines, unlike that which animals and humans display. The latter involves emotionality and consciousness. The field of AI has gained proliferation in recent days, with many scientists investing their time and effort in research.
How To Develop Topics in Artificial Intelligence
Developing AI topics is a critical thinking process that also incorporates a lot of creativity. Due to the ever-dynamic nature of the discipline, most students find it hard to develop impressive topics in artificial intelligence. However, here are some general rules to get you started:
Read widely on the subject of artificial intelligence Have an interest in news and other current updates about AI Consult your supervisor
Once you are ready with these steps, nothing is holding you from developing top-rated topics in artificial intelligence. Now let’s look at what the pros have in store for you.
Artificial Intelligence Research Paper Topics
- The role of artificial intelligence in evolving the workforce
- Are there tasks that require unique human abilities apart from machines?
- The transformative economic impact of artificial intelligence
- Managing a global autonomous arms race in the face of AI
- The legal and ethical boundaries of artificial intelligence
- Is the destructive role of AI more than its constructive role in society?
- How to build AI algorithms to achieve the far-reaching goals of humans
- How privacy gets compromised with the everyday collection of data
- How businesses and governments can suffer at the hands of AI
- Is it possible for AI to devolve into social oppression?
- Augmentation of the work humans do through artificial intelligence
- The role of AI in monitoring and diagnosing capabilities
Artificial Intelligence Topics For Presentation
- How AI helps to uncover criminal activity and solve serial crimes
- The place of facial recognition technologies in security systems
- How to use AI without crossing an individual’s privacy
- What are the disadvantages of using a computer-controlled robot in performing tasks?
- How to develop systems endowed with intellectual processes
- The challenge of programming computers to perform complex tasks
- Discuss some of the mathematical theorems for artificial intelligence systems
- The role of computer processing speed and memory capacity in AI
- Can computer machines achieve the performance levels of human experts?
- Discuss the application of artificial intelligence in handwriting recognition
- A case study of the key people involved in developing AI systems
- Computational aesthetics when developing artificial intelligence systems
Topics in AI For Tip-Top Grades
- Describe the necessities for artificial programming language
- The impact of American companies possessing about 2/3 of investments in AI
- The relationship between human neural networks and A.I
- The role of psychologists in developing human intelligence
- How to apply past experiences to analogous new situations
- How machine learning helps in achieving artificial intelligence
- The role of discernment and human intelligence in developing AI systems
- Discuss the various methods and goals in artificial intelligence
- What is the relationship between applied AI, strong AI, and cognitive simulation
- Discuss the implications of the first AI programs
- Logical reasoning and problem-solving in artificial intelligence
- Challenges involved in controlled learning environments
AI Research Topics For High School Students
- How quantum computing is affecting artificial intelligence
- The role of the Internet of Things in advancing artificial intelligence
- Using Artificial intelligence to enable machines to perform programming tasks
- Why do machines learn automatically without human hand holding
- Implementing decisions based on data processing in the human mind
- Describe the web-like structure of artificial neural networks
- Machine learning algorithms for optimal functions through trial and error
- A case study of Google’s AlphaGo computer program
- How robots solve problems in an intelligent manner
- Evaluate the significant role of M.I.T.’s artificial intelligence lab
- A case study of Robonaut developed by NASA to work with astronauts in space
- Discuss natural language processing where machines analyze language and speech
Argument Debate Topics on AI
- How chatbots use ML and N.L.P. to interact with the users
- How do computers use and understand images?
- The impact of genetic engineering on the life of man
- Why are micro-chips not recommended in human body systems?
- Can humans work alongside robots in a workplace system?
- Have computers contributed to the intrusion of privacy for many?
- Why artificial intelligence systems should not be made accessible to children
- How artificial intelligence systems are contributing to healthcare problems
- Does artificial intelligence alleviate human problems or add to them?
- Why governments should put more stringent measures for AI inventions
- How artificial intelligence is affecting the character traits of children born
- Is virtual reality taking people out of the real-world situation?
Quality AI Topics For Research Paper
- The use of recommender systems in choosing movies and series
- Collaborative filtering in designing systems
- How do developers arrive at a content-based recommendation
- Creation of systems that can emulate human tasks
- How IoT devices generate a lot of data
- Artificial intelligence algorithms convert data to useful, actionable results.
- How AI is progressing rapidly with the 5G technology
- How to develop robots with human-like characteristics
- Developing Google search algorithms
- The role of artificial intelligence in developing autonomous weapons
- Discuss the long-term goal of artificial intelligence
- Will artificial intelligence outperform humans at every cognitive task?
Computer Science AI Topics
- Computational intelligence magazine in computer science
- Swarm and evolutionary computation procedures for college students
- Discuss computational transactions on intelligent transportation systems
- The structure and function of knowledge-based systems
- A review of the artificial intelligence systems in developing systems
- Conduct a review of the expert systems with applications
- Critique the various foundations and trends in information retrieval
- The role of specialized systems in transactions on knowledge and data engineering
- An analysis of a journal on ambient intelligence and humanized computing
- Discuss the various computer transactions on cognitive communications and networking
- What is the role of artificial intelligence in medicine?
- Computer engineering applications of artificial intelligence
AI Ethics Topics
- How the automation of jobs is going to make many jobless
- Discuss inequality challenges in distributing wealth created by machines
- The impact of machines on human behavior and interactions
- How artificial intelligence is going to affect how we act accordingly
- The process of eliminating bias in Artificial intelligence: A case of racist robots
- Measures that can keep artificial intelligence safe from adversaries
- Protecting artificial intelligence discoveries from unintended consequences
- How a man can stay in control despite the complex, intelligent systems
- Robot rights: A case of how man is mistreating and misusing robots
- The balance between mitigating suffering and interfering with set ethics
- The role of artificial intelligence in negative outcomes: Is it worth it?
- How to ethically use artificial intelligence for bettering lives
Advanced AI Topics
- Discuss how long it will take until machines greatly supersede human intelligence
- Is it possible to achieve superhuman artificial intelligence in this century?
- The impact of techno-skeptic prediction on the performance of A.I
- The role of quarks and electrons in the human brain
- The impact of artificial intelligence safety research institutes
- Will robots be disastrous for humanity shortly?
- Robots: A concern about consciousness and evil
- Discuss whether a self-driving car has a subjective experience or not
- Should humans worry about machines turning evil in the end?
- Discuss how machines exhibit goal-oriented behavior in their functions
- Should man continue to develop lethal autonomous weapons?
- What is the implication of machine-produced wealth?
AI Essay Topics Technology
- Discuss the implication of the fourth technological revelation in cloud computing
- Big database technologies used in sensors
- The combination of technologies typical of the technological revolution
- Key determinants of the civilization process of industry 4.0
- Discuss some of the concepts of technological management
- Evaluate the creation of internet-based companies in the U.S.
- The most dominant scientific research in the field of artificial intelligence
- Discuss the application of artificial intelligence in the literature
- How enterprises use artificial intelligence in blockchain business operations
- Discuss the various immersive experiences as a result of digital AI
- Elaborate on various enterprise architects and technology innovations
- Mega-trends that are future impacts on business operations
Interesting Topics in AI
- The role of the industrial revolution of the 18 th century in A.I
- The electricity era of the late 19 th century and its contribution to the development of robots
- How the widespread use of the internet contributes to the AI revolution
- The short-term economic crisis as a result of artificial intelligence business technologies
- Designing and creating artificial intelligence production processes
- Analyzing large collections of information for technological solutions
- How biotechnology is transforming the field of agriculture
- Innovative business projects that work using artificial intelligence systems
- Process and marketing innovations in the 21 st century
- Medical intelligence in the era of smart cities
- Advanced data processing technologies in developed nations
- Discuss the development of stelliform technologies
Good Research Topics For AI
- Development of new technological solutions in I.T
- Innovative organizational solutions that develop machine learning
- How to develop branches of a knowledge-based economy
- Discuss the implications of advanced computerized neural network systems
- How to solve complex problems with the help of algorithms
- Why artificial intelligence systems are predominating over their creator
- How to determine artificial emotional intelligence
- Discuss the negative and positive aspects of technological advancement
- How internet technology companies like Facebook are managing large social media portals
- The application of analytical business intelligence systems
- How artificial intelligence improves business management systems
- Strategic and ongoing management of artificial intelligence systems
Graduate AI NLP Research Topics
- Morphological segmentation in artificial intelligence
- Sentiment analysis and breaking machine language
- Discuss input utterance for language interpretation
- Festival speech synthesis system for natural language processing
- Discuss the role of the Google language translator
- Evaluate the various analysis methodologies in N.L.P.
- Native language identification procedure for deep analytics
- Modular audio recognition framework
- Deep linguistic processing techniques
- Fact recognition and extraction techniques
- Dialogue and text-based applications
- Speaker verification and identification systems
Controversial Topics in AI
- Ethical implication of AI in movies: A case study of The Terminator
- Will machines take over the world and enslave humanity?
- Does human intelligence paint a dark future for humanity?
- Ethical and practical issues of artificial intelligence
- The impact of mimicking human cognitive functions
- Why the integration of AI technologies into society should be limited
- Should robots get paid hourly?
- What if AI is a mistake?
- Why did Microsoft shut down chatbots immediately?
- Should there be AI systems for killing?
- Should machines be created to do what they want?
- Is the computerized gun ethical?
Hot AI Topics
- Why predator drones should not exist
- Do the U.S. laws restrict meaningful innovations in AI
- Why did the campaign to stop killer robots fail in the end?
- Fully autonomous weapons and human safety
- How to deal with rogues artificial intelligence systems in the United States
- Is it okay to have a monopoly and control over artificial intelligence innovations?
- Should robots have human rights or citizenship?
- Biases when detecting people’s gender using Artificial intelligence
- Considerations for the adoption of a particular artificial intelligence technology
Are you a university student seeking research paper writing services or dissertation proposal help ? We offer custom help for college students in any field of artificial intelligence.
Leave a Reply Cancel reply
Collaborative ML research projects within a single cloud environment
Andika rachman.
AVP, Head of AI, Bank Rakyat Indonesia
Yoga Yustiawan
AI Research Lead, Bank Rakyat Indonesia
Try Gemini 1.5 models
Google's most advanced multimodal models in Vertex AI
As one of the largest banks in Indonesia and Southeast Asia, Bank Rakyat Indonesia (BRI) focuses on small-to-medium businesses and microfinance. At BRI, we established a Digital Banking Development and Operation Division to implement digital banking and digitalization. Within this division, a department we call Digital BRIBRAIN develops a range of AI solutions that span customer engagement, credit underwriting, anti-fraud and risk analytics, and smart services and operations for our business and operational teams.
Within Digital BRIBRAIN, our AI research team works on projects like the BRIBRAIN Academy — a collaborative initiative with higher education institutions that aims to nurture AI and ML in banking and finance, expand BRI’s AI capabilities, and contribute to the academic community. The program enables students from partner universities to study the application of AI in the financial sector, selecting from topics such as unfair bias and fairness, explainable AI, Graph ML, federated learning, unified product recommendations, natural language processing and computer vision.
Based on our long history and work with Google Cloud, with some Vertex AI technology implemented in other use cases, we selected its products and services to provide a sandbox environment for this research effort with partner universities. This research covers a range of use cases and concepts, including the following:
1. Fairness analysis on credit scoring research in banking
Industry-wide, banks and other financial institutions use credit scoring to determine an individual’s or organization’s credit risk when applying for a loan. Historically, this is a manual and paper-driven process that uses statistical techniques and historical data. There is considerable potential benefit to apply automation to the credit scoring process, but only if it can be done responsibly.
The use of AI in credit scoring is a noted and well-documented area of concern for algorithmic unfairness. Providers should know which variables are used in credit scoring AI models and take steps to reduce the risk of disparate model outputs across marginalized groups. To help bring the industry closer to a solution where unfair bias is appropriately mitigated, we decided to work on fairness analysis in credit scoring as one of our BRIBRAIN Academy research projects.
Fairness has different meanings in different situations. To help minimize poor outcomes for lenders and applicants, we measured bias in our models with two fairness constraints, demographic parity difference and equalized odds difference, and reduced unfair bias with post-processing and reduction algorithms. As a result, we found that the fairness of demographic parity improved from 0.127 to 0.0004, and equalized odds from 0.09 to 0.01. All of the work we have done thus far is still in the research and exploration stage, as we continue to discover the limitations that need to be navigated to improve fairness.
2. Interpreting ML model decisions for credit scoring using explainable AI
Historical data is used to train a model to evaluate the creditworthiness of an application. However, the lack of transparency in these data can make it challenging to understand, and the ability to help others interpret results and predictions from AI models is becoming more important.
An explanation that truly represents a model’s behavior and earns the trust of concerned stakeholders is critical. With explainable AI, we can get a deeper level of understanding of how a credit score is created. We can also use the features we built in the model as filters for different credit scoring decisions. To conduct this research collaboration, we needed to leverage a secure platform with strict access controls for data storage and maintenance.
3. Sentiment analysis of financial chatbots using graph ML
Chatbots are computer programs that simulate human conversations, with users communicating via a chat interface. Some chatbots can interpret and process users' words or phrases and provide instant preset answers without sentiment knowledge.
Unfortunately, responses are sometimes taken out of context because they do not recognize the relationship between words. This means we had to represent chatbot data that can learn relationships between words through preprocessing using graph representation learning. These methods help to account for linguistic, semantic, and grammatical features that other natural language processing techniques like bag-of-words (BOW) models and Term Frequency-Inverse Document Frequency (TF-IDF) representation cannot catch.
We built a sentiment analysis model for financial chatbot responses using graph ML, allowing us to identify which conversations are positive, neutral, or negative. This helps the chatbot avoid mistakes in categorizing user responses.
Deploying data warehousing, ML, and access management tools
Google Cloud met our needs for these projects with infrastructure and services, such as its cloud data warehouse BigQuery and its unified machine learning (ML) development platform Vertex AI , which offers a range of fully-managed tools that enabled us to undertake our ML builds.
We also used Vertex AI Workbench , a Jupyter notebook-based development environment, to create and manage virtual machine instances adjusted to researchers’ needs. This enabled us to perform data preparation, model training, and evaluation of our use case model.
Using the structured data stored in BigQuery, we were able to write our own training code and train custom models through our preferred ML framework. Furthermore, we employed Identity and Access Management (IAM) to deliver fine-grained control and management of access to resources.
The general architecture we used to support each research topic is below:
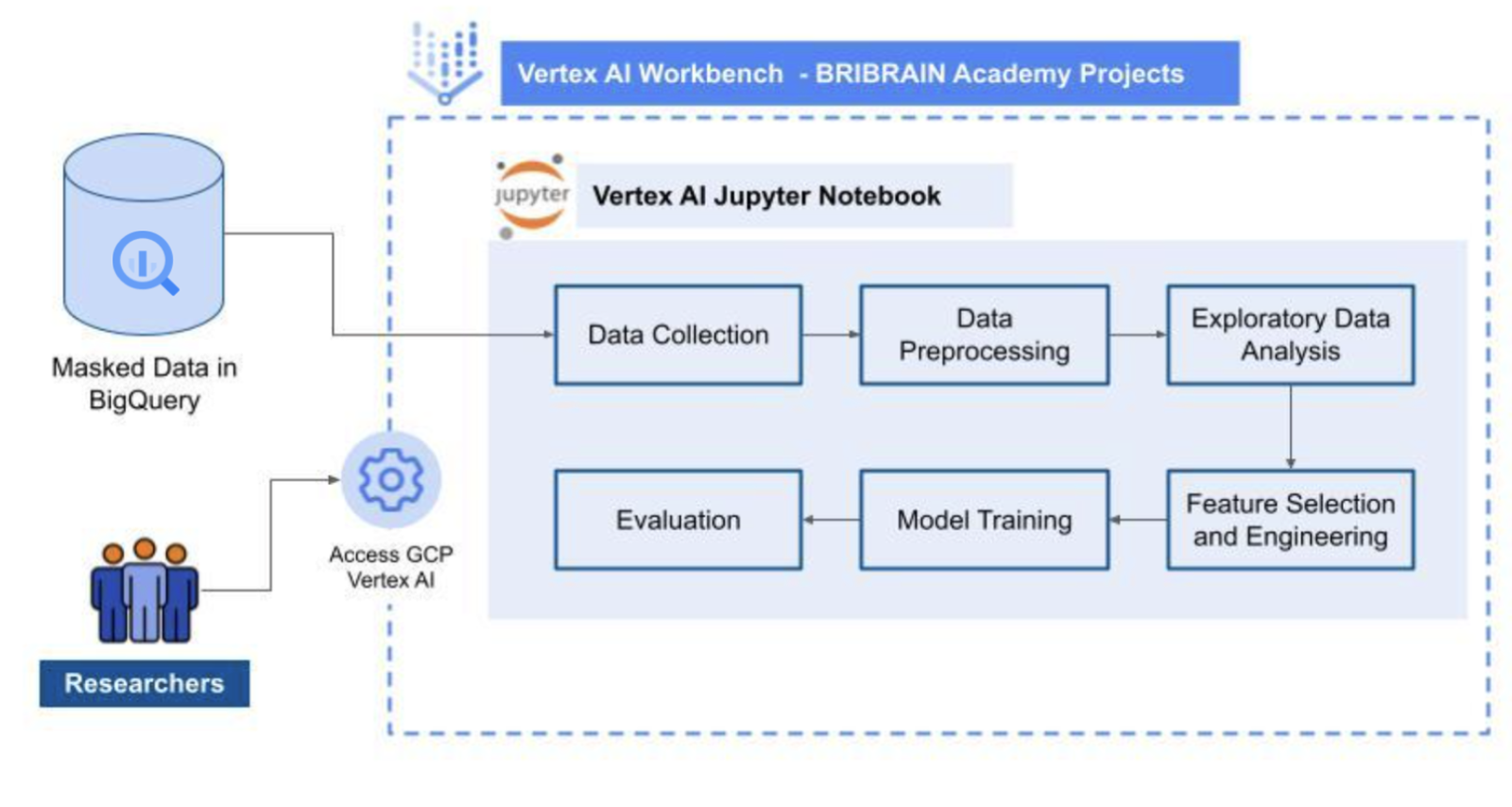
We loaded masked research into BigQuery and gave researchers access to Vertex AI for specific BRIBRAIN Academy projects, assigning a virtual machine on which to conduct research. They could then use Vertex AI Workbench to perform the pipeline steps illustrated above in Vertex AI Workbench and access required data in BRIBRAIN Academy projects via BigQuery.
To build and run our ML solution efficiently and cost-effectively, we limited the resources available to each user. However, Vertex AI enabled us to modify instance resources to accommodate cases where significant data volumes were needed to create a model.
At the same time, Google Cloud data security services allowed us to protect data at rest and in transit from unauthorized access while creating and managing specific access to project data and resources. We provided specific access to researchers through BigQuery and notebook custom roles, while developers received administration roles.
Undertaking research projects within a single platform
With Google Cloud, Digital BRIBRAIN now has the power to explore use cases from BRIBRAIN Academy and apply lessons learned in live business projects.
For example, we have already used research around AI explainability to help us develop end-to-end ML solutions for recommender systems in our branchless banking services, known as BRILink agents. We also built a mobile application containing recommendations with AI explanations. In an environment where many users are unfamiliar with ML and its complexities, AI explainability can help make ML solutions more transparent so they can understand the rationales behind recommendations and decisions.
With our success to date, we plan to evolve our ML and data management capabilities. At present, we use BigQuery to store mostly tabular data for training and building models. Now, we are expanding these capabilities to store, process, and manage unstructured data, such as text, files and images, with Cloud Storage . In addition, we plan to monitor app usage using reports generated through Google Analytics for Firebase with some of the ML solutions available in our web-based applications.
Google Cloud gives us the ability to store our data, build and train ML model workflows, monitor access control, and maintain data security — all within a single platform. With the promising results we’ve seen, we hope to be able to tap into more of Vertex AI capabilities to support ongoing developments at BRIBRAIN Academy.
- AI & Machine Learning
- Financial Services
Related articles

Unlocking enhanced LLM capabilities with RAG in BigQuery
By Luis Velasco • 10-minute read
Creating marketing campaigns using BigQuery and Gemini models
By Adam Paternostro • 5-minute read
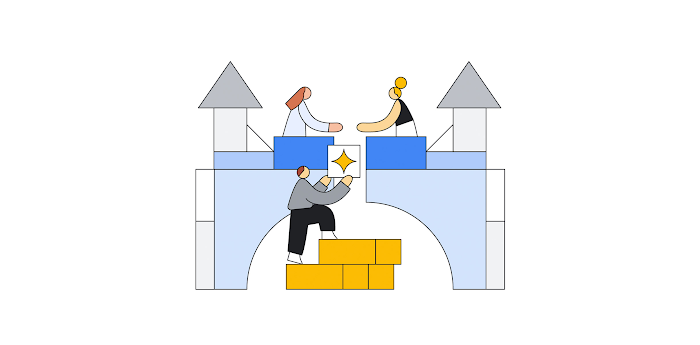
How Mantle uses Gemini to simplify equity management
By Merlin Yamssi • 4-minute read

To tune or not to tune? A guide to leveraging your data with LLMs
By Kamilla Kurta • 5-minute read
Suggestions or feedback?
MIT News | Massachusetts Institute of Technology
- Machine learning
- Social justice
- Black holes
- Classes and programs
Departments
- Aeronautics and Astronautics
- Brain and Cognitive Sciences
- Architecture
- Political Science
- Mechanical Engineering
Centers, Labs, & Programs
- Abdul Latif Jameel Poverty Action Lab (J-PAL)
- Picower Institute for Learning and Memory
- Lincoln Laboratory
- School of Architecture + Planning
- School of Engineering
- School of Humanities, Arts, and Social Sciences
- Sloan School of Management
- School of Science
- MIT Schwarzman College of Computing
Using ideas from game theory to improve the reliability of language models
Press contact :.
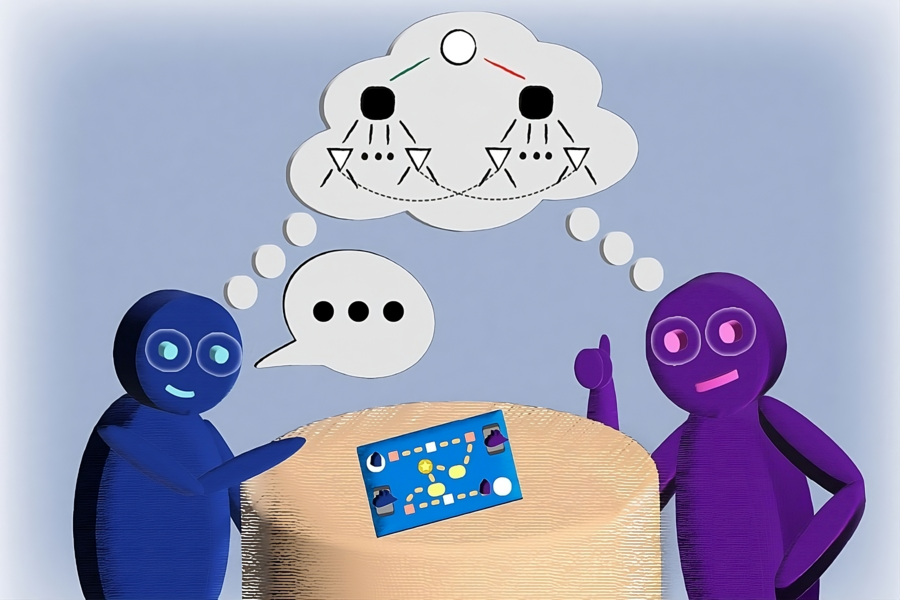
Previous image Next image
Imagine you and a friend are playing a game where your goal is to communicate secret messages to each other using only cryptic sentences. Your friend's job is to guess the secret message behind your sentences. Sometimes, you give clues directly, and other times, your friend has to guess the message by asking yes-or-no questions about the clues you've given. The challenge is that both of you want to make sure you're understanding each other correctly and agreeing on the secret message.
MIT Computer Science and Artificial Intelligence Laboratory (CSAIL) researchers have created a similar "game" to help improve how AI understands and generates text. It is known as a “consensus game” and it involves two parts of an AI system — one part tries to generate sentences (like giving clues), and the other part tries to understand and evaluate those sentences (like guessing the secret message).
The researchers discovered that by treating this interaction as a game, where both parts of the AI work together under specific rules to agree on the right message, they could significantly improve the AI's ability to give correct and coherent answers to questions. They tested this new game-like approach on a variety of tasks, such as reading comprehension, solving math problems, and carrying on conversations, and found that it helped the AI perform better across the board.
Traditionally, large language models answer one of two ways: generating answers directly from the model (generative querying) or using the model to score a set of predefined answers (discriminative querying), which can lead to differing and sometimes incompatible results. With the generative approach, "Who is the president of the United States?" might yield a straightforward answer like "Joe Biden." However, a discriminative query could incorrectly dispute this fact when evaluating the same answer, such as "Barack Obama."
So, how do we reconcile mutually incompatible scoring procedures to achieve coherent, efficient predictions?
"Imagine a new way to help language models understand and generate text, like a game. We've developed a training-free, game-theoretic method that treats the whole process as a complex game of clues and signals, where a generator tries to send the right message to a discriminator using natural language. Instead of chess pieces, they're using words and sentences," says Athul Jacob, an MIT PhD student in electrical engineering and computer science and CSAIL affiliate. "Our way to navigate this game is finding the 'approximate equilibria,' leading to a new decoding algorithm called 'equilibrium ranking.' It's a pretty exciting demonstration of how bringing game-theoretic strategies into the mix can tackle some big challenges in making language models more reliable and consistent."
When tested across many tasks, like reading comprehension, commonsense reasoning, math problem-solving, and dialogue, the team's algorithm consistently improved how well these models performed. Using the ER algorithm with the LLaMA-7B model even outshone the results from much larger models. "Given that they are already competitive, that people have been working on it for a while, but the level of improvements we saw being able to outperform a model that's 10 times the size was a pleasant surprise," says Jacob.
"Diplomacy," a strategic board game set in pre-World War I Europe, where players negotiate alliances, betray friends, and conquer territories without the use of dice — relying purely on skill, strategy, and interpersonal manipulation — recently had a second coming. In November 2022, computer scientists, including Jacob, developed “Cicero,” an AI agent that achieves human-level capabilities in the mixed-motive seven-player game, which requires the same aforementioned skills, but with natural language. The math behind this partially inspired the Consensus Game.
While the history of AI agents long predates when OpenAI's software entered the chat in November 2022, it's well documented that they can still cosplay as your well-meaning, yet pathological friend.
The consensus game system reaches equilibrium as an agreement, ensuring accuracy and fidelity to the model's original insights. To achieve this, the method iteratively adjusts the interactions between the generative and discriminative components until they reach a consensus on an answer that accurately reflects reality and aligns with their initial beliefs. This approach effectively bridges the gap between the two querying methods.
In practice, implementing the consensus game approach to language model querying, especially for question-answering tasks, does involve significant computational challenges. For example, when using datasets like MMLU, which have thousands of questions and multiple-choice answers, the model must apply the mechanism to each query. Then, it must reach a consensus between the generative and discriminative components for every question and its possible answers.
The system did struggle with a grade school right of passage: math word problems. It couldn't generate wrong answers, which is a critical component of understanding the process of coming up with the right one.
“The last few years have seen really impressive progress in both strategic decision-making and language generation from AI systems, but we’re just starting to figure out how to put the two together. Equilibrium ranking is a first step in this direction, but I think there’s a lot we’ll be able to do to scale this up to more complex problems,” says Jacob.
An avenue of future work involves enhancing the base model by integrating the outputs of the current method. This is particularly promising since it can yield more factual and consistent answers across various tasks, including factuality and open-ended generation. The potential for such a method to significantly improve the base model's performance is high, which could result in more reliable and factual outputs from ChatGPT and similar language models that people use daily.
"Even though modern language models, such as ChatGPT and Gemini, have led to solving various tasks through chat interfaces, the statistical decoding process that generates a response from such models has remained unchanged for decades," says Google Research Scientist Ahmad Beirami, who was not involved in the work. "The proposal by the MIT researchers is an innovative game-theoretic framework for decoding from language models through solving the equilibrium of a consensus game. The significant performance gains reported in the research paper are promising, opening the door to a potential paradigm shift in language model decoding that may fuel a flurry of new applications."
Jacob wrote the paper with MIT-IBM Watson Lab researcher Yikang Shen and MIT Department of Electrical Engineering and Computer Science assistant professors Gabriele Farina and Jacob Andreas, who is also a CSAIL member. They presented their work at the International Conference on Learning Representations (ICLR) earlier this month, where it was highlighted as a "spotlight paper." The research also received a “best paper award” at the NeurIPS R0-FoMo Workshop in December 2023.
Share this news article on:
Press mentions, quanta magazine.
MIT researchers have developed a new procedure that uses game theory to improve the accuracy and consistency of large language models (LLMs), reports Steve Nadis for Quanta Magazine . “The new work, which uses games to improve AI, stands in contrast to past approaches, which measured an AI program’s success via its mastery of games,” explains Nadis.
Previous item Next item
Related Links
- Article: "Game Theory Can Make AI More Correct and Efficient"
- Jacob Andreas
- Athul Paul Jacob
- Language & Intelligence @ MIT
- Computer Science and Artificial Intelligence Laboratory (CSAIL)
- Department of Electrical Engineering and Computer Science
- MIT-IBM Watson AI Lab
Related Topics
- Computer science and technology
- Artificial intelligence
- Human-computer interaction
- Natural language processing
- Game theory
- Electrical Engineering & Computer Science (eecs)
Related Articles
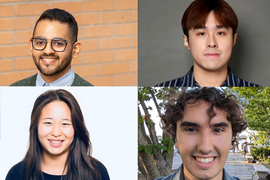
Reasoning and reliability in AI
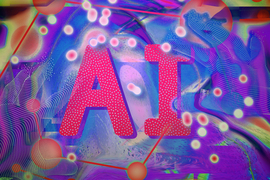
Explained: Generative AI
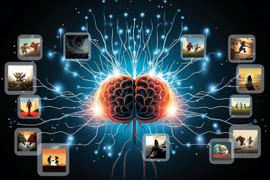
Synthetic imagery sets new bar in AI training efficiency
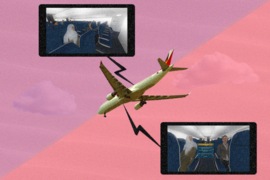
Simulating discrimination in virtual reality
More mit news.
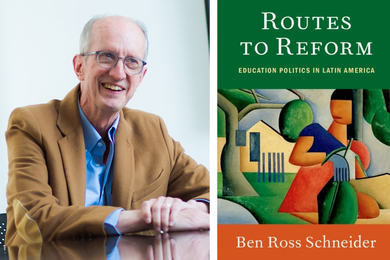
Trying to make the grade
Read full story →
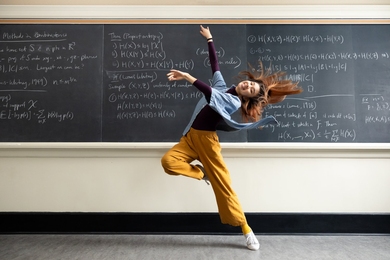
Janabel Xia: Algorithms, dance rhythms, and the drive to succeed
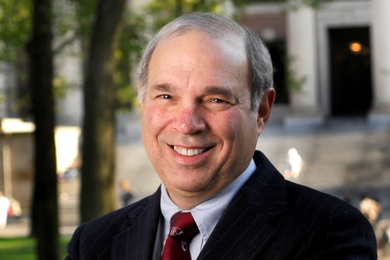
Jonathan Byrnes, MIT Center for Transportation and Logistics senior lecturer and visionary in supply chain management, dies at 75
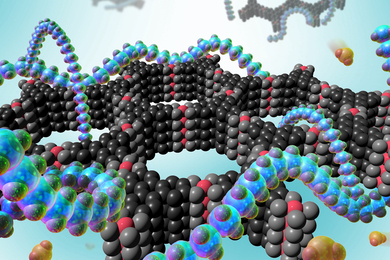
Researchers develop a detector for continuously monitoring toxic gases
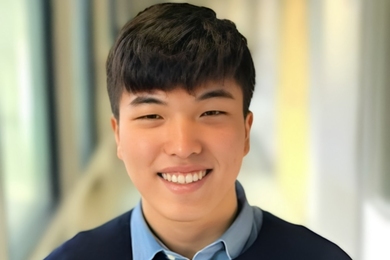
The beauty of biology
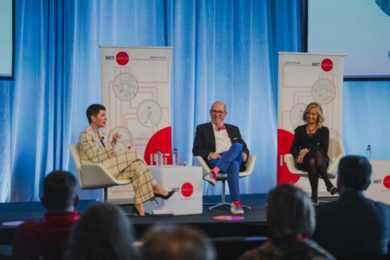
Navigating longevity with industry leaders at MIT AgeLab PLAN Forum
- More news on MIT News homepage →
Massachusetts Institute of Technology 77 Massachusetts Avenue, Cambridge, MA, USA
- Map (opens in new window)
- Events (opens in new window)
- People (opens in new window)
- Careers (opens in new window)
- Accessibility
- Social Media Hub
- MIT on Facebook
- MIT on YouTube
- MIT on Instagram
How Much Research Is Being Written by Large Language Models?
New studies show a marked spike in LLM usage in academia, especially in computer science. What does this mean for researchers and reviewers?
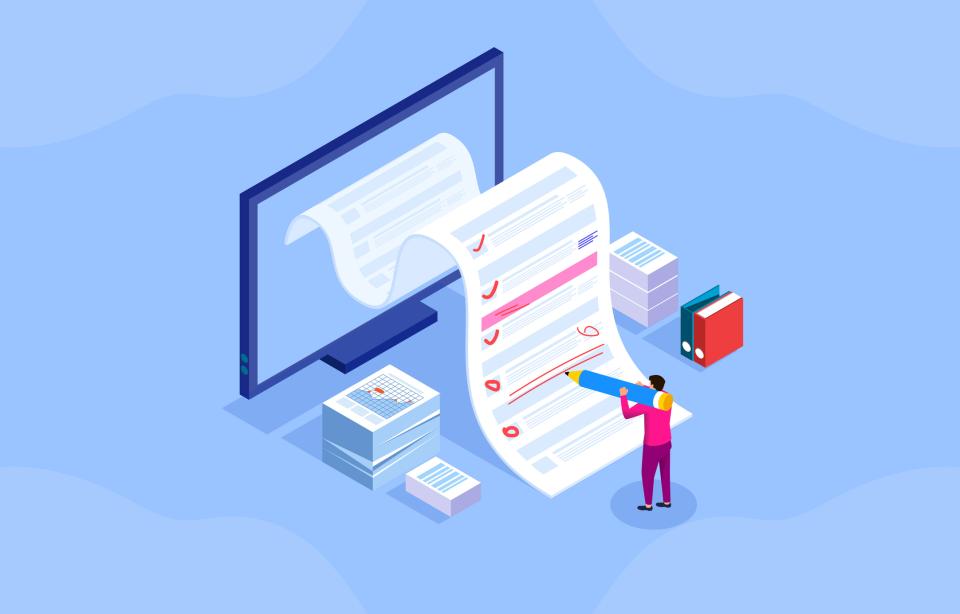
In March of this year, a tweet about an academic paper went viral for all the wrong reasons. The introduction section of the paper, published in Elsevier’s Surfaces and Interfaces , began with this line: Certainly, here is a possible introduction for your topic.
Look familiar?
It should, if you are a user of ChatGPT and have applied its talents for the purpose of content generation. LLMs are being increasingly used to assist with writing tasks, but examples like this in academia are largely anecdotal and had not been quantified before now.
“While this is an egregious example,” says James Zou , associate professor of biomedical data science and, by courtesy, of computer science and of electrical engineering at Stanford, “in many cases, it’s less obvious, and that’s why we need to develop more granular and robust statistical methods to estimate the frequency and magnitude of LLM usage. At this particular moment, people want to know what content around us is written by AI. This is especially important in the context of research, for the papers we author and read and the reviews we get on our papers. That’s why we wanted to study how much of those have been written with the help of AI.”
In two papers looking at LLM use in scientific publishings, Zou and his team* found that 17.5% of computer science papers and 16.9% of peer review text had at least some content drafted by AI. The paper on LLM usage in peer reviews will be presented at the International Conference on Machine Learning.
Read Mapping the Increasing Use of LLMs in Scientific Papers and Monitoring AI-Modified Content at Scale: A Case Study on the Impact of ChatGPT on AI Conference Peer Reviews
Here Zou discusses the findings and implications of this work, which was supported through a Stanford HAI Hoffman Yee Research Grant .
How did you determine whether AI wrote sections of a paper or a review?
We first saw that there are these specific worlds – like commendable, innovative, meticulous, pivotal, intricate, realm, and showcasing – whose frequency in reviews sharply spiked, coinciding with the release of ChatGPT. Additionally, we know that these words are much more likely to be used by LLMs than by humans. The reason we know this is that we actually did an experiment where we took many papers, used LLMs to write reviews of them, and compared those reviews to reviews written by human reviewers on the same papers. Then we quantified which words are more likely to be used by LLMs vs. humans, and those are exactly the words listed. The fact that they are more likely to be used by an LLM and that they have also seen a sharp spike coinciding with the release of LLMs is strong evidence.
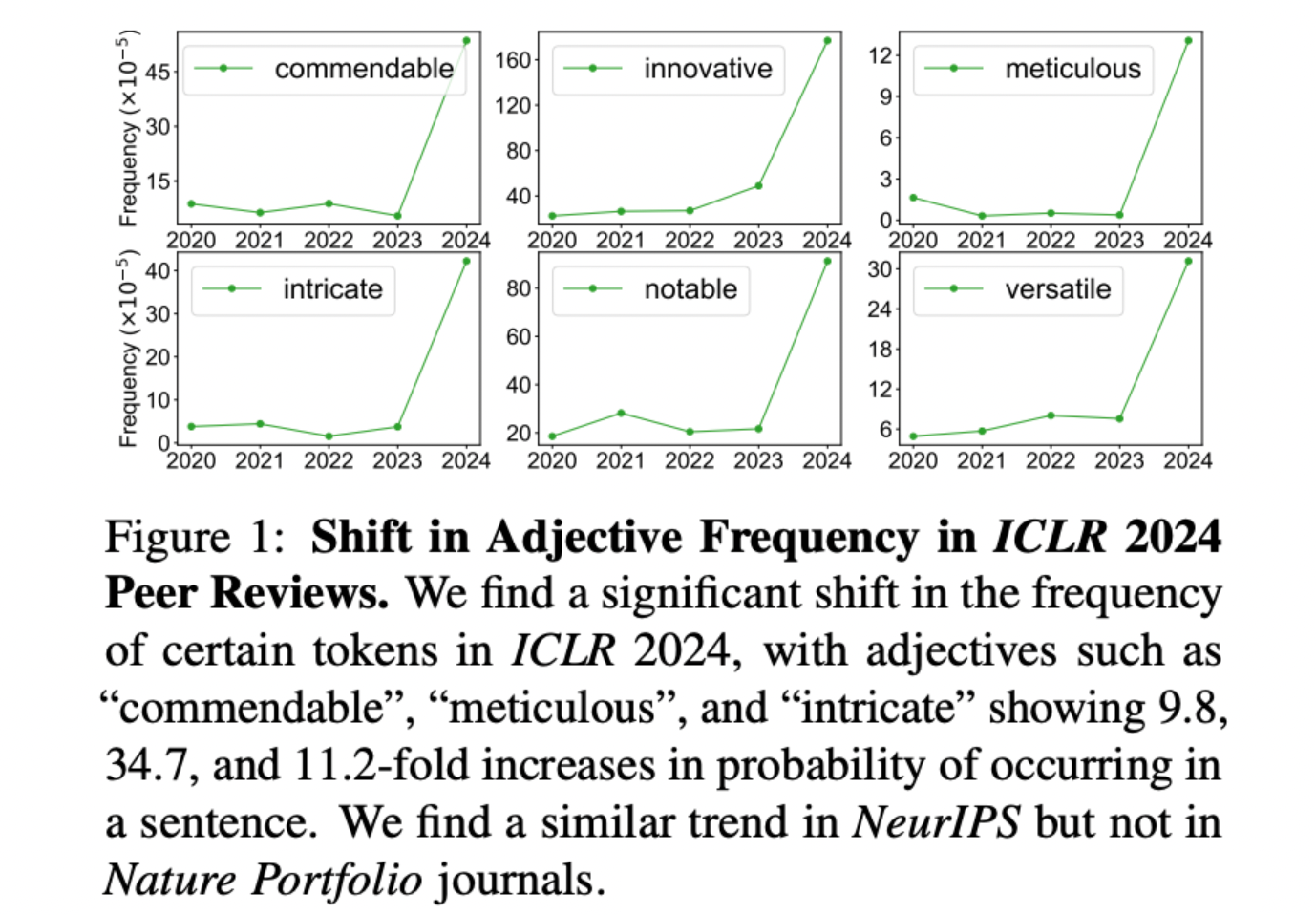
Some journals permit the use of LLMs in academic writing, as long as it’s noted, while others, including Science and the ICML conference, prohibit it. How are the ethics perceived in academia?
This is an important and timely topic because the policies of various journals are changing very quickly. For example, Science said in the beginning that they would not allow authors to use language models in their submissions, but they later changed their policy and said that people could use language models, but authors have to explicitly note where the language model is being used. All the journals are struggling with how to define this and what’s the right way going forward.
You observed an increase in usage of LLMs in academic writing, particularly in computer science papers (up to 17.5%). Math and Nature family papers, meanwhile, used AI text about 6.3% of the time. What do you think accounts for the discrepancy between these disciplines?
Artificial intelligence and computer science disciplines have seen an explosion in the number of papers submitted to conferences like ICLR and NeurIPS. And I think that’s really caused a strong burden, in many ways, to reviewers and to authors. So now it’s increasingly difficult to find qualified reviewers who have time to review all these papers. And some authors may feel more competition that they need to keep up and keep writing more and faster.
You analyzed close to a million papers on arXiv, bioRxiv, and Nature from January 2020 to February 2024. Do any of these journals include humanities papers or anything in the social sciences?
We mostly wanted to focus more on CS and engineering and biomedical areas and interdisciplinary areas, like Nature family journals, which also publish some social science papers. Availability mattered in this case. So, it’s relatively easy for us to get data from arXiv, bioRxiv, and Nature . A lot of AI conferences also make reviews publicly available. That’s not the case for humanities journals.
Did any results surprise you?
A few months after ChatGPT’s launch, we started to see a rapid, linear increase in the usage pattern in academic writing. This tells us how quickly these LLM technologies diffuse into the community and become adopted by researchers. The most surprising finding is the magnitude and speed of the increase in language model usage. Nearly a fifth of papers and peer review text use LLM modification. We also found that peer reviews submitted closer to the deadline and those less likely to engage with author rebuttal were more likely to use LLMs.
This suggests a couple of things. Perhaps some of these reviewers are not as engaged with reviewing these papers, and that’s why they are offloading some of the work to AI to help. This could be problematic if reviewers are not fully involved. As one of the pillars of the scientific process, it is still necessary to have human experts providing objective and rigorous evaluations. If this is being diluted, that’s not great for the scientific community.
What do your findings mean for the broader research community?
LLMs are transforming how we do research. It’s clear from our work that many papers we read are written with the help of LLMs. There needs to be more transparency, and people should state explicitly how LLMs are used and if they are used substantially. I don’t think it’s always a bad thing for people to use LLMs. In many areas, this can be very useful. For someone who is not a native English speaker, having the model polish their writing can be helpful. There are constructive ways for people to use LLMs in the research process; for example, in earlier stages of their draft. You could get useful feedback from a LLM in real time instead of waiting weeks or months to get external feedback.
But I think it’s still very important for the human researchers to be accountable for everything that is submitted and presented. They should be able to say, “Yes, I will stand behind the statements that are written in this paper.”
*Collaborators include: Weixin Liang , Yaohui Zhang , Zhengxuan Wu , Haley Lepp , Wenlong Ji , Xuandong Zhao , Hancheng Cao , Sheng Liu , Siyu He , Zhi Huang , Diyi Yang , Christopher Potts , Christopher D. Manning , Zachary Izzo , Yaohui Zhang , Lingjiao Chen , Haotian Ye , and Daniel A. McFarland .
Stanford HAI’s mission is to advance AI research, education, policy and practice to improve the human condition. Learn more .
More News Topics
Estimating the Effects of Political Pressure on the Fed: A Narrative Approach with New Data
This paper combines new data and a narrative approach to identify shocks to political pressure on the Federal Reserve. From archival records, I build a data set of personal interactions between U.S. Presidents and Fed officials between 1933 and 2016. Since personal interactions do not necessarily reflect political pressure, I develop a narrative identification strategy based on President Nixon's pressure on Fed Chair Burns. I exploit this narrative through restrictions on a structural vector autoregression that includes the personal interaction data. I find that political pressure shocks (i) increase inflation strongly and persistently, (ii) lead to statistically weak negative effects on activity, (iii) contributed to inflationary episodes outside of the Nixon era, and (iv) transmit differently from standard expansionary monetary policy shocks, by having a stronger effect on inflation expectations. Quantitatively, increasing political pressure by half as much as Nixon, for six months, raises the price level more than 8%.
I thank Juan Antolin-Diaz, Jonas Arias, Boragan Aruoba, Miguel Bandeira, Francesco Bianchi, Allan Drazen, Leland Farmer, Yuriy Gorodnichenko, Amy Handlan, Fatima Hussein, Hanno Lustig, Fernando Martin, Emi Nakamura, Evgenia Passari, Jon Steinsson and Sarah Zubairy for detailed discussions. Seminar and conference participants at UC Berkeley, Stanford SITE, the NBER Monetary Economics Spring Meeting 2024, the Federal Reserve Board, the Boston Fed, Philadelphia Fed, Minneapolis Fed, Richmond Fed, St. Louis Fed, Texas A&M University, and the University of Maryland provided very useful suggestions. I am grateful to Seho Kim, Ko Miura and Daniel Schwindt for excellent research assistance. The views expressed herein are those of the author and do not necessarily reflect the views of the National Bureau of Economic Research.
MARC RIS BibTeΧ
Download Citation Data
- President-Fed personal interaction time series data
Conferences
More from nber.
In addition to working papers , the NBER disseminates affiliates’ latest findings through a range of free periodicals — the NBER Reporter , the NBER Digest , the Bulletin on Retirement and Disability , the Bulletin on Health , and the Bulletin on Entrepreneurship — as well as online conference reports , video lectures , and interviews .
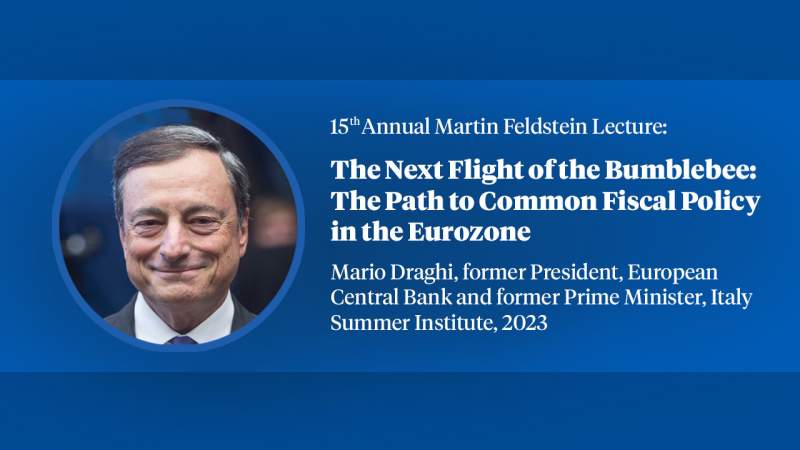
Better Siri is coming: what Apple’s research says about its AI plans
Apple hasn’t talked too much about ai so far — but it’s been working on stuff. a lot of stuff..
By David Pierce , editor-at-large and Vergecast co-host with over a decade of experience covering consumer tech. Previously, at Protocol, The Wall Street Journal, and Wired.
Share this story
:format(webp)/cdn.vox-cdn.com/uploads/chorus_asset/file/25430195/247097_Apple__AI__CVirginia_B2B.jpg)
It would be easy to think that Apple is late to the game on AI. Since late 2022, when ChatGPT took the world by storm, most of Apple’s competitors have fallen over themselves to catch up. While Apple has certainly talked about AI and even released some products with AI in mind, it seemed to be dipping a toe in rather than diving in headfirst.
But over the last few months, rumors and reports have suggested that Apple has, in fact, just been biding its time, waiting to make its move. There have been reports in recent weeks that Apple is talking to both OpenAI and Google about powering some of its AI features, and the company has also been working on its own model, called Ajax .
If you look through Apple’s published AI research, a picture starts to develop of how Apple’s approach to AI might come to life. Now, obviously, making product assumptions based on research papers is a deeply inexact science — the line from research to store shelves is windy and full of potholes. But you can at least get a sense of what the company is thinking about — and how its AI features might work when Apple starts to talk about them at its annual developer conference, WWDC, in June.
Smaller, more efficient models
I suspect you and I are hoping for the same thing here: Better Siri. And it looks very much like Better Siri is coming! There’s an assumption in a lot of Apple’s research (and in a lot of the tech industry, the world, and everywhere) that large language models will immediately make virtual assistants better and smarter. For Apple, getting to Better Siri means making those models as fast as possible — and making sure they’re everywhere.
In iOS 18, Apple plans to have all its AI features running on an on-device, fully offline model, Bloomberg recently reported . It’s tough to build a good multipurpose model even when you have a network of data centers and thousands of state-of-the-art GPUs — it’s drastically harder to do it with only the guts inside your smartphone. So Apple’s having to get creative.
In a paper called “ LLM in a flash: Efficient Large Language Model Inference with Limited Memory ” (all these papers have really boring titles but are really interesting, I promise!), researchers devised a system for storing a model’s data, which is usually stored on your device’s RAM, on the SSD instead. “We have demonstrated the ability to run LLMs up to twice the size of available DRAM [on the SSD],” the researchers wrote, “achieving an acceleration in inference speed by 4-5x compared to traditional loading methods in CPU, and 20-25x in GPU.” By taking advantage of the most inexpensive and available storage on your device, they found, the models can run faster and more efficiently.
Apple’s researchers also created a system called EELBERT that can essentially compress an LLM into a much smaller size without making it meaningfully worse. Their compressed take on Google’s Bert model was 15 times smaller — only 1.2 megabytes — and saw only a 4 percent reduction in quality. It did come with some latency tradeoffs, though.
In general, Apple is pushing to solve a core tension in the model world: the bigger a model gets, the better and more useful it can be, but also the more unwieldy, power-hungry, and slow it can become. Like so many others, the company is trying to find the right balance between all those things while also looking for a way to have it all.
Siri, but good
A lot of what we talk about when we talk about AI products is virtual assistants — assistants that know things, that can remind us of things, that can answer questions, and get stuff done on our behalf. So it’s not exactly shocking that a lot of Apple’s AI research boils down to a single question: what if Siri was really, really, really good?
A group of Apple researchers has been working on a way to use Siri without needing to use a wake word at all; instead of listening for “Hey Siri” or “Siri,” the device might be able to simply intuit whether you’re talking to it. “This problem is significantly more challenging than voice trigger detection,” the researchers did acknowledge, “since there might not be a leading trigger phrase that marks the beginning of a voice command.” That might be why another group of researchers developed a system to more accurately detect wake words . Another paper trained a model to better understand rare words, which are often not well understood by assistants.
In both cases, the appeal of an LLM is that it can, in theory, process much more information much more quickly. In the wake-word paper, for instance, the researchers found that by not trying to discard all unnecessary sound but, instead, feeding it all to the model and letting it process what does and doesn’t matter, the wake word worked far more reliably.
Once Siri hears you, Apple’s doing a bunch of work to make sure it understands and communicates better. In one paper, it developed a system called STEER (which stands for Semantic Turn Extension-Expansion Recognition, so we’ll go with STEER) that aims to improve your back-and-forth communication with an assistant by trying to figure out when you’re asking a follow-up question and when you’re asking a new one. In another, it uses LLMs to better understand “ambiguous queries” to figure out what you mean no matter how you say it. “In uncertain circumstances,” they wrote, “intelligent conversational agents may need to take the initiative to reduce their uncertainty by asking good questions proactively, thereby solving problems more effectively.” Another paper aims to help with that, too: researchers used LLMs to make assistants less verbose and more understandable when they’re generating answers.
:format(webp)/cdn.vox-cdn.com/uploads/chorus_asset/file/25431587/CleanShot_2024_05_03_at_09.50.52.png)
AI in health, image editors, in your Memojis
Whenever Apple does talk publicly about AI, it tends to focus less on raw technological might and more on the day-to-day stuff AI can actually do for you. So, while there’s a lot of focus on Siri — especially as Apple looks to compete with devices like the Humane AI Pin, the Rabbit R1, and Google’s ongoing smashing of Gemini into all of Android — there are plenty of other ways Apple seems to see AI being useful.
One obvious place for Apple to focus is on health: LLMs could, in theory, help wade through the oceans of biometric data collected by your various devices and help you make sense of it all. So, Apple has been researching how to collect and collate all of your motion data, how to use gait recognition and your headphones to identify you, and how to track and understand your heart rate data. Apple also created and released “the largest multi-device multi-location sensor-based human activity dataset” available after collecting data from 50 participants with multiple on-body sensors.
Apple also seems to imagine AI as a creative tool. For one paper, researchers interviewed a bunch of animators, designers, and engineers and built a system called Keyframer that “enable[s] users to iteratively construct and refine generated designs.” Instead of typing in a prompt and getting an image, then typing another prompt to get another image, you start with a prompt but then get a toolkit to tweak and refine parts of the image to your liking. You could imagine this kind of back-and-forth artistic process showing up anywhere from the Memoji creator to some of Apple’s more professional artistic tools.
In another paper , Apple describes a tool called MGIE that lets you edit an image just by describing the edits you want to make. (“Make the sky more blue,” “make my face less weird,” “add some rocks,” that sort of thing.) “Instead of brief but ambiguous guidance, MGIE derives explicit visual-aware intention and leads to reasonable image editing,” the researchers wrote. Its initial experiments weren’t perfect, but they were impressive.
We might even get some AI in Apple Music: for a paper called “ Resource-constrained Stereo Singing Voice Cancellation ,” researchers explored ways to separate voices from instruments in songs — which could come in handy if Apple wants to give people tools to, say, remix songs the way you can on TikTok or Instagram.
:format(webp)/cdn.vox-cdn.com/uploads/chorus_asset/file/25431565/CleanShot_2024_05_03_at_09.36.28.png)
Over time, I’d bet this is the kind of stuff you’ll see Apple lean into, especially on iOS. Some of it Apple will build into its own apps; some it will offer to third-party developers as APIs. (The recent Journaling Suggestions feature is probably a good guide to how that might work.) Apple has always trumpeted its hardware capabilities, particularly compared to your average Android device; pairing all that horsepower with on-device, privacy-focused AI could be a big differentiator.
But if you want to see the biggest, most ambitious AI thing going at Apple, you need to know about Ferret . Ferret is a multi-modal large language model that can take instructions, focus on something specific you’ve circled or otherwise selected, and understand the world around it. It’s designed for the now-normal AI use case of asking a device about the world around you, but it might also be able to understand what’s on your screen. In the Ferret paper, researchers show that it could help you navigate apps, answer questions about App Store ratings, describe what you’re looking at, and more. This has really exciting implications for accessibility but could also completely change the way you use your phone — and your Vision Pro and / or smart glasses someday.
We’re getting way ahead of ourselves here, but you can imagine how this would work with some of the other stuff Apple is working on. A Siri that can understand what you want, paired with a device that can see and understand everything that’s happening on your display, is a phone that can literally use itself. Apple wouldn’t need deep integrations with everything; it could simply run the apps and tap the right buttons automatically.
Again, all this is just research, and for all of it to work well starting this spring would be a legitimately unheard-of technical achievement. (I mean, you’ve tried chatbots — you know they’re not great.) But I’d bet you anything we’re going to get some big AI announcements at WWDC. Apple CEO Tim Cook even teased as much in February, and basically promised it on this week’s earnings call. And two things are very clear: Apple is very much in the AI race, and it might amount to a total overhaul of the iPhone. Heck, you might even start willingly using Siri! And that would be quite the accomplishment.
Sonos is teasing its ‘most requested product ever’ on Tuesday
Two students find security bug that could let millions do laundry for free, microsoft’s surface and windows ai event live blog: it’s arm time, the ai assistants are getting better fast, the five-year journey to make an adventure game out of ink and paper.

More from Apple
:format(webp)/cdn.vox-cdn.com/uploads/chorus_asset/file/25432052/installer.png)
The best new browser for Windows
:format(webp)/cdn.vox-cdn.com/uploads/chorus_asset/file/24205851/HT015_S_Haddad_ios_iphone_14_slideshow.jpg)
How to make the most of Apple Notes
:format(webp)/cdn.vox-cdn.com/uploads/chorus_asset/file/24401980/STK071_ACastro_apple_0003.jpg)
More details emerge about Apple’s plans for AI in iOS 18
:format(webp)/cdn.vox-cdn.com/uploads/chorus_asset/file/25431511/VST_0503_Site.jpg)
On The Vergecast: AI gadgets, iPads, and antitrust
How to Find Research Topics to Write About
So, you’ve got a research paper due, and the dread sets in – what to research about? We’ve all experienced that frustrating moment when finding a topic feels about as simple as finding a needle in a haystack.
But hold on! Before you check is essay pro legit enough to find someone to write your paper for you (tempting, I know!), take a deep breath. Finding a killer research topic doesn’t have to take weeks. It can actually be the spark that ignites your curiosity and leads you down a fascinating rabbit hole of discovery.
The key is knowing where to look for inspiration and how to narrow down those endless possibilities into a topic that’s manageable, meaningful, and maybe even a little bit exciting.
So, let’s come up with unconventional strategies, look for hidden sources of inspiration, and give you the tools to choose a topic that will give your research paper a head-start.
Source: https://www.pexels.com/photo/photo-of-a-woman-thinking-941555/
Look Beyond the Textbook
Sure, your course material is a good starting point for finding a research topic, but don’t let it be your only source of inspiration. Look around you – the world is full of fascinating questions just waiting to be explored.
What current events spark your interest? What social issues keep you up at night? Maybe there’s a scientific breakthrough that’s left you wanting to know more.
Don’t be afraid to let your curiosity guide you. Some of the most engaging research papers are born out of genuine interest and a desire to learn more about the world.
Another often-overlooked source of inspiration on what to research is your own life experiences. Have you ever faced a personal challenge or overcome an obstacle that could be relevant to others? Maybe you have a unique cultural background or a hobby that could be the basis for an intriguing research question.
Don’t undermine the potential of your own story to spark a meaningful topic.
Digg Deeper With Unconventional Sources
Now, let’s move beyond the obvious. While academic journals and textbooks are important resources, they’re not the only game in town.
Explore documentaries, podcasts, TED Talks, and even social media for potential research topic ideas. These sources often present complex issues in a more accessible way, making them a great way to spark your interest and notice new perspectives.
If you’re feeling stuck, try branching out into different fields of study. Maybe a sociology paper on the impact of social media on mental health or a history paper on the role of music in social movements could pique your interest.
Remember, research is about making connections, so don’t be afraid to get a little interdisciplinary with your topic choices.
Source: https://www.pexels.com/photo/box-with-brain-inscription-on-head-of-anonymous-woman-7203727/
Find Out What Makes a Good Research Paper Topic
So, you’ve got a whole bunch of potential research article topics swirling around in your head. Now what? It’s time to narrow it down and find the perfect fit.
A good topic should be several things:
- Interesting. You’re going to be spending a lot of time on this topic, so make sure it’s something you actually care about!
- Manageable. Choose a topic that’s narrow enough to be thoroughly researched within the scope of your assignment. Avoid topics that are too general or too niche.
- Relevant. Make sure your topic is in line with your course or field of study. If you’re unsure, talk to your professor for guidance.
- Original. While it’s okay to build on existing research, try to gauge a new angle or perspective on your topic.
If you still feel you can’t come up with the right topic, don’t hesitate to seek out help. Talk to your professor or librarian, or even consider consulting with one of the best coursework writing services to brainstorm ideas and get feedback on your choices.
Choosing a topic for a research paper is just the first step in the process. The real fun begins when you start diving into the research and getting new insights.
The Bottom Line
The journey of finding a research topic doesn’t have to be a dreaded chore. It can be an exciting opportunity to expand your knowledge and bring out new passions. Remember, there are no “easy research topics.” The best topics are the ones that ignite your curiosity and challenge you to think critically about the world around you.
So, feel free to get a little creative with your research topic choices. Whether you’re exploring a current event, a personal experience, or a complex social issue, the most important thing is to choose a topic that inspires you and makes you eager to dive into the research.
Learning how to find a research topic is an essential skill for any college student, and with the right approach, it can even be enjoyable! So, put on your explorer hat, embrace your curiosity, and let your research process begin.

IMAGES
VIDEO
COMMENTS
Get 1-On-1 Help. If you're still unsure about how to find a quality research topic, check out our Research Topic Kickstarter service, which is the perfect starting point for developing a unique, well-justified research topic. A comprehensive list of research topics ideas in the AI and machine learning area. Includes access to a free webinar ...
Collaboration is key in research. Working with experts in the field can help you refine your research topic and gain valuable insights. Seek mentors and collaborators who can guide you. 250+ Machine Learning Research Topics: Category-wise Supervised Learning. Explainable AI for Decision Support; Few-shot Learning Methods
OS-Copilot/FRIDAY • 12 Feb 2024. Autonomous interaction with the computer has been a longstanding challenge with great potential, and the recent proliferation of large language models (LLMs) has markedly accelerated progress in building digital agents. Papers With Code highlights trending Machine Learning research and the code to implement it.
Research Open Access 17 May 2024 Scientific Reports Volume: 14, P: 11263 Machine learning designs new GCGR/GLP-1R dual agonists with enhanced biological potency
This paper is ranked #1 on Image Classification on ImageNet (using extra training data). For the research paper, read here. For code, see here. SwinIR: Image Restoration Using Swin Transformer. The authors of this article suggest the SwinIR image restoration model, which is based on the Swin Transformer. The model comprises three modules ...
A variety of innovative topics are included in the agenda of the published papers in this special issue including topics such as: Stock market Prediction using Machine learning. Detection of Apple Diseases and Pests based on Multi-Model LSTM-based Convolutional Neural Networks. ML for Searching. Machine Learning for Learning Automata
In the current age of the Fourth Industrial Revolution (4IR or Industry 4.0), the digital world has a wealth of data, such as Internet of Things (IoT) data, cybersecurity data, mobile data, business data, social media data, health data, etc. To intelligently analyze these data and develop the corresponding smart and automated applications, the knowledge of artificial intelligence (AI ...
To bring you up to speed on the critical ideas driving machine learning in 2022, we handpicked the top 10 research papers for all AI/ML enthusiasts out there! Let's dive in! Artificial Replay: A Meta-Algorithm for Harnessing Historical Data in Bandits. Author (s) - Sean R. Sinclair et al. Ways to incorporate historical data are still ...
Register Now for ODSC West 2021. At our upcoming event this November 16th-18th in San Francisco, ODSC West 2021 will feature a plethora of talks, workshops, and training sessions on machine learning topics, deep learning, NLP, MLOps, and so on. You can register now for 20% off all ticket types, or register for a free AI Expo Pass to see what ...
Improves how machine learning research is conducted. Prioritizes verifiable and replicable supporting evidence in all published papers. Editor-in-Chief. Hendrik Blockeel; Impact factor 7.5 (2022) 5 year impact factor 6.3 (2022) Submission to first decision (median) 28 days. Downloads 1,349,126 (2023)
They mostly know all the related work and also all the relevant terms. But the closer these people are to your exact research topic, the fewer of them exist. So it's not very likely that they will be your peers. People in closely related research areas. These will not exactly know what your research is about and the specific problems.
Artificial Intelligence and Machine Learning. Our research covers a wide range of topics of this fast-evolving field, advancing how machines learn, predict, and control, while also making them secure, robust and trustworthy. Research covers both the theory and applications of ML. This broad area studies ML theory (algorithms, optimization, etc ...
Machine learning, especially its subfield of Deep Learning, had many amazing advances in the recent years, and important research papers may lead to breakthroughs in technology that get used by billio ns of people. The research in this field is developing very quickly and to help our readers monitor the progress we present the list of most important recent scientific papers published since 2014.
The abstract is a brief text that provides an overview of the article's content, researchers' objectives, methods, and techniques. When reading an abstract of a machine-learning research paper, you'll typically come across mentions of datasets, methods, algorithms, and other terms. Keywords relevant to the article's content provide context.
We recommend you get the results of your research first, run an analysis of them, and then move on to writing all about it in your research paper. 3. Review your paper like a critic. There are some things that, as a research paper writer, you should be accustomed to. We have listed them below for you.
Current practices for selecting records for transfer to The National Archives (TNA) were developed to deal with paper records and are struggling to deal with this shift. This article examines the background to the problem and outlines a project that TNA undertook to research the feasibility of using commercially available artificial ...
Every year, 1000s of research papers related to Machine Learning are published in popular publications like NeurIPS, ICML, ICLR, ACL, and MLDS. The criteria are using citation counts from three academic sources: scholar.google.com; academic.microsoft.com; and semanticscholar.org. "Key research papers in natural language processing ...
Google is at the forefront of innovation in Machine Intelligence, with active research exploring virtually all aspects of machine learning, including deep learning and more classical algorithms. Exploring theory as well as application, much of our work on language, speech, translation, visual processing, ranking and prediction relies on Machine ...
The convergence of. AI and ML has propelled innovation to unprecedented heights, revolutionizing the way we perceive and. interact with the world around us. This paper embarks on a comprehensive ...
Latest thesis topics in Machine Learning for research scholars: Choosing a research and thesis topics in Machine Learning is the first choice of masters and Doctorate scholars now a days. Though, choosing and working on a thesis topic in machine learning is not an easy task as Machine learning uses certain statistical algorithms to make computers work in a certain way without being explicitly ...
M.Tech Projects Topics List In Machine Learning. Project Topics. Base Paper. Abstract. 1.Analysis and Detection of Autism Spectrum Disorder Using Machine Learning Techniques. Get Help. Download. Abstract. 2.A Machine Learning-Based Recommender System for Improving Students Learning Experiences.
AI Ethics Topics. How the automation of jobs is going to make many jobless. Discuss inequality challenges in distributing wealth created by machines. The impact of machines on human behavior and interactions. How artificial intelligence is going to affect how we act accordingly.
Artificial Intelligence (AI) and Machine Learning (ML) are terms in computer science, but they have recently received tremendous attention from the entire scientific community. By mimicking human intelligence, AI and ML are becoming powerful tools in areas, including materials science, medicine, drug discovery, robotics, and sociology.
Within Digital BRIBRAIN, our AI research team works on projects like the BRIBRAIN Academy — a collaborative initiative with higher education institutions that aims to nurture AI and ML in banking and finance, expand BRI's AI capabilities, and contribute to the academic community. The program enables students from partner universities to ...
The analysis results will help researchers understand the trending and latest research topics in the related field, facilitate collaboration among researchers, and promote the exchange of innovative ideas and methods. ... As can be seen from Table 10 and Table 11, influential papers tend to use ML techniques to predict earthquakes occurring in ...
MIT researchers' "consensus game" is a game-theoretic approach for language model decoding. The equilibrium-ranking algorithm harmonizes generative and discriminative querying to enhance prediction accuracy across various tasks, outperforming larger models and demonstrating the potential of game theory in improving language model consistency and truthfulness.
That's why we wanted to study how much of those have been written with the help of AI.". In two papers looking at LLM use in scientific publishings, Zou and his team* found that 17.5% of computer science papers and 16.9% of peer review text had at least some content drafted by AI. The paper on LLM usage in peer reviews will be presented at ...
Estimating the Effects of Political Pressure on the Fed: A Narrative Approach with New Data. Thomas Drechsel. Working Paper 32461. DOI 10.3386/w32461. Issue Date May 2024. This paper combines new data and a narrative approach to identify shocks to political pressure on the Federal Reserve. From archival records, I build a data set of personal ...
Better Siri is coming: what Apple's research says about its AI plans. Apple hasn't talked too much about AI so far — but it's been working on stuff. A lot of stuff. By David Pierce, editor ...
Choosing a topic for a research paper is just the first step in the process. The real fun begins when you start diving into the research and getting new insights. The Bottom Line. The journey of finding a research topic doesn't have to be a dreaded chore. It can be an exciting opportunity to expand your knowledge and bring out new passions.