Case Study Observational Research: A Framework for Conducting Case Study Research Where Observation Data Are the Focus
Affiliation.
- 1 1 University of Otago, Wellington, New Zealand.
- PMID: 27217290
- DOI: 10.1177/1049732316649160
Case study research is a comprehensive method that incorporates multiple sources of data to provide detailed accounts of complex research phenomena in real-life contexts. However, current models of case study research do not particularly distinguish the unique contribution observation data can make. Observation methods have the potential to reach beyond other methods that rely largely or solely on self-report. This article describes the distinctive characteristics of case study observational research, a modified form of Yin's 2014 model of case study research the authors used in a study exploring interprofessional collaboration in primary care. In this approach, observation data are positioned as the central component of the research design. Case study observational research offers a promising approach for researchers in a wide range of health care settings seeking more complete understandings of complex topics, where contextual influences are of primary concern. Future research is needed to refine and evaluate the approach.
Keywords: New Zealand; appreciative inquiry; case studies; case study observational research; health care; interprofessional collaboration; naturalistic inquiry; observation; primary health care; qualitative; research design.
- Observational Studies as Topic / methods*
- Observational Studies as Topic / standards
- Primary Health Care / organization & administration
- Research Design*
- Self Report / standards
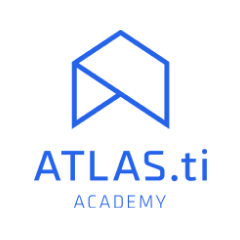

The Ultimate Guide to Qualitative Research - Part 1: The Basics
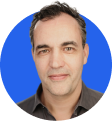
- Introduction and overview
- What is qualitative research?
- What is qualitative data?
- Examples of qualitative data
- Qualitative vs. quantitative research
- Mixed methods
- Qualitative research preparation
- Theoretical perspective
- Theoretical framework
- Literature reviews
Research question
- Conceptual framework
- Conceptual vs. theoretical framework
Data collection
- Qualitative research methods
- Focus groups
- Observational research
What is a case study?
Applications for case study research, what is a good case study, process of case study design, benefits and limitations of case studies.
- Ethnographical research
- Ethical considerations
- Confidentiality and privacy
- Power dynamics
- Reflexivity
Case studies
Case studies are essential to qualitative research , offering a lens through which researchers can investigate complex phenomena within their real-life contexts. This chapter explores the concept, purpose, applications, examples, and types of case studies and provides guidance on how to conduct case study research effectively.
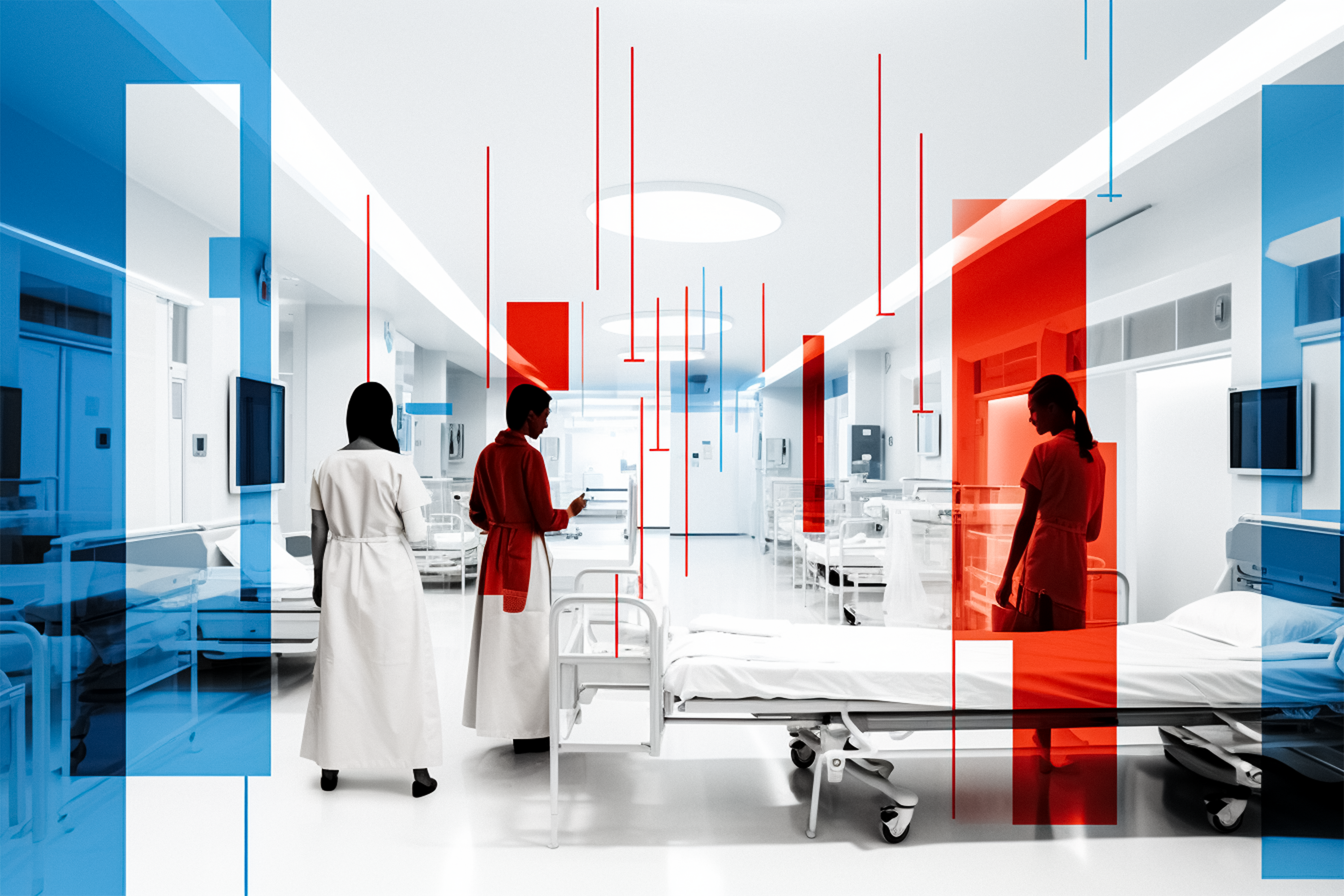
Whereas quantitative methods look at phenomena at scale, case study research looks at a concept or phenomenon in considerable detail. While analyzing a single case can help understand one perspective regarding the object of research inquiry, analyzing multiple cases can help obtain a more holistic sense of the topic or issue. Let's provide a basic definition of a case study, then explore its characteristics and role in the qualitative research process.
Definition of a case study
A case study in qualitative research is a strategy of inquiry that involves an in-depth investigation of a phenomenon within its real-world context. It provides researchers with the opportunity to acquire an in-depth understanding of intricate details that might not be as apparent or accessible through other methods of research. The specific case or cases being studied can be a single person, group, or organization – demarcating what constitutes a relevant case worth studying depends on the researcher and their research question .
Among qualitative research methods , a case study relies on multiple sources of evidence, such as documents, artifacts, interviews , or observations , to present a complete and nuanced understanding of the phenomenon under investigation. The objective is to illuminate the readers' understanding of the phenomenon beyond its abstract statistical or theoretical explanations.
Characteristics of case studies
Case studies typically possess a number of distinct characteristics that set them apart from other research methods. These characteristics include a focus on holistic description and explanation, flexibility in the design and data collection methods, reliance on multiple sources of evidence, and emphasis on the context in which the phenomenon occurs.
Furthermore, case studies can often involve a longitudinal examination of the case, meaning they study the case over a period of time. These characteristics allow case studies to yield comprehensive, in-depth, and richly contextualized insights about the phenomenon of interest.
The role of case studies in research
Case studies hold a unique position in the broader landscape of research methods aimed at theory development. They are instrumental when the primary research interest is to gain an intensive, detailed understanding of a phenomenon in its real-life context.
In addition, case studies can serve different purposes within research - they can be used for exploratory, descriptive, or explanatory purposes, depending on the research question and objectives. This flexibility and depth make case studies a valuable tool in the toolkit of qualitative researchers.
Remember, a well-conducted case study can offer a rich, insightful contribution to both academic and practical knowledge through theory development or theory verification, thus enhancing our understanding of complex phenomena in their real-world contexts.
What is the purpose of a case study?
Case study research aims for a more comprehensive understanding of phenomena, requiring various research methods to gather information for qualitative analysis . Ultimately, a case study can allow the researcher to gain insight into a particular object of inquiry and develop a theoretical framework relevant to the research inquiry.
Why use case studies in qualitative research?
Using case studies as a research strategy depends mainly on the nature of the research question and the researcher's access to the data.
Conducting case study research provides a level of detail and contextual richness that other research methods might not offer. They are beneficial when there's a need to understand complex social phenomena within their natural contexts.
The explanatory, exploratory, and descriptive roles of case studies
Case studies can take on various roles depending on the research objectives. They can be exploratory when the research aims to discover new phenomena or define new research questions; they are descriptive when the objective is to depict a phenomenon within its context in a detailed manner; and they can be explanatory if the goal is to understand specific relationships within the studied context. Thus, the versatility of case studies allows researchers to approach their topic from different angles, offering multiple ways to uncover and interpret the data .
The impact of case studies on knowledge development
Case studies play a significant role in knowledge development across various disciplines. Analysis of cases provides an avenue for researchers to explore phenomena within their context based on the collected data.
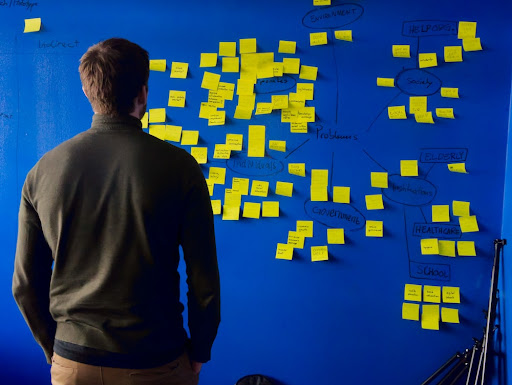
This can result in the production of rich, practical insights that can be instrumental in both theory-building and practice. Case studies allow researchers to delve into the intricacies and complexities of real-life situations, uncovering insights that might otherwise remain hidden.
Types of case studies
In qualitative research , a case study is not a one-size-fits-all approach. Depending on the nature of the research question and the specific objectives of the study, researchers might choose to use different types of case studies. These types differ in their focus, methodology, and the level of detail they provide about the phenomenon under investigation.
Understanding these types is crucial for selecting the most appropriate approach for your research project and effectively achieving your research goals. Let's briefly look at the main types of case studies.
Exploratory case studies
Exploratory case studies are typically conducted to develop a theory or framework around an understudied phenomenon. They can also serve as a precursor to a larger-scale research project. Exploratory case studies are useful when a researcher wants to identify the key issues or questions which can spur more extensive study or be used to develop propositions for further research. These case studies are characterized by flexibility, allowing researchers to explore various aspects of a phenomenon as they emerge, which can also form the foundation for subsequent studies.
Descriptive case studies
Descriptive case studies aim to provide a complete and accurate representation of a phenomenon or event within its context. These case studies are often based on an established theoretical framework, which guides how data is collected and analyzed. The researcher is concerned with describing the phenomenon in detail, as it occurs naturally, without trying to influence or manipulate it.
Explanatory case studies
Explanatory case studies are focused on explanation - they seek to clarify how or why certain phenomena occur. Often used in complex, real-life situations, they can be particularly valuable in clarifying causal relationships among concepts and understanding the interplay between different factors within a specific context.
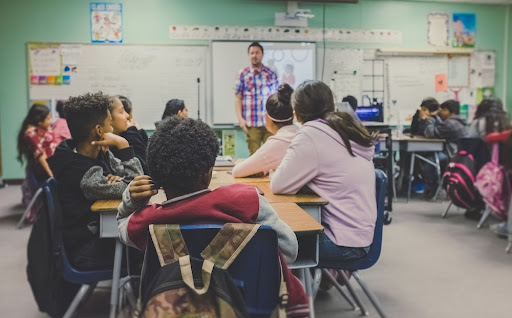
Intrinsic, instrumental, and collective case studies
These three categories of case studies focus on the nature and purpose of the study. An intrinsic case study is conducted when a researcher has an inherent interest in the case itself. Instrumental case studies are employed when the case is used to provide insight into a particular issue or phenomenon. A collective case study, on the other hand, involves studying multiple cases simultaneously to investigate some general phenomena.
Each type of case study serves a different purpose and has its own strengths and challenges. The selection of the type should be guided by the research question and objectives, as well as the context and constraints of the research.
The flexibility, depth, and contextual richness offered by case studies make this approach an excellent research method for various fields of study. They enable researchers to investigate real-world phenomena within their specific contexts, capturing nuances that other research methods might miss. Across numerous fields, case studies provide valuable insights into complex issues.
Critical information systems research
Case studies provide a detailed understanding of the role and impact of information systems in different contexts. They offer a platform to explore how information systems are designed, implemented, and used and how they interact with various social, economic, and political factors. Case studies in this field often focus on examining the intricate relationship between technology, organizational processes, and user behavior, helping to uncover insights that can inform better system design and implementation.
Health research
Health research is another field where case studies are highly valuable. They offer a way to explore patient experiences, healthcare delivery processes, and the impact of various interventions in a real-world context.
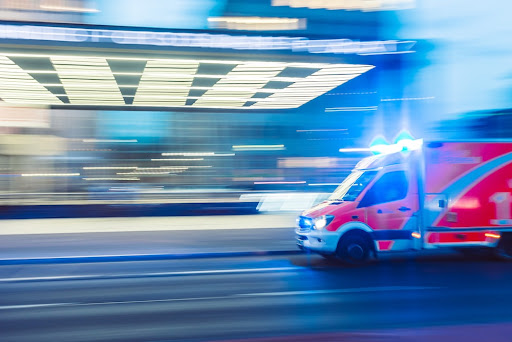
Case studies can provide a deep understanding of a patient's journey, giving insights into the intricacies of disease progression, treatment effects, and the psychosocial aspects of health and illness.
Asthma research studies
Specifically within medical research, studies on asthma often employ case studies to explore the individual and environmental factors that influence asthma development, management, and outcomes. A case study can provide rich, detailed data about individual patients' experiences, from the triggers and symptoms they experience to the effectiveness of various management strategies. This can be crucial for developing patient-centered asthma care approaches.
Other fields
Apart from the fields mentioned, case studies are also extensively used in business and management research, education research, and political sciences, among many others. They provide an opportunity to delve into the intricacies of real-world situations, allowing for a comprehensive understanding of various phenomena.
Case studies, with their depth and contextual focus, offer unique insights across these varied fields. They allow researchers to illuminate the complexities of real-life situations, contributing to both theory and practice.
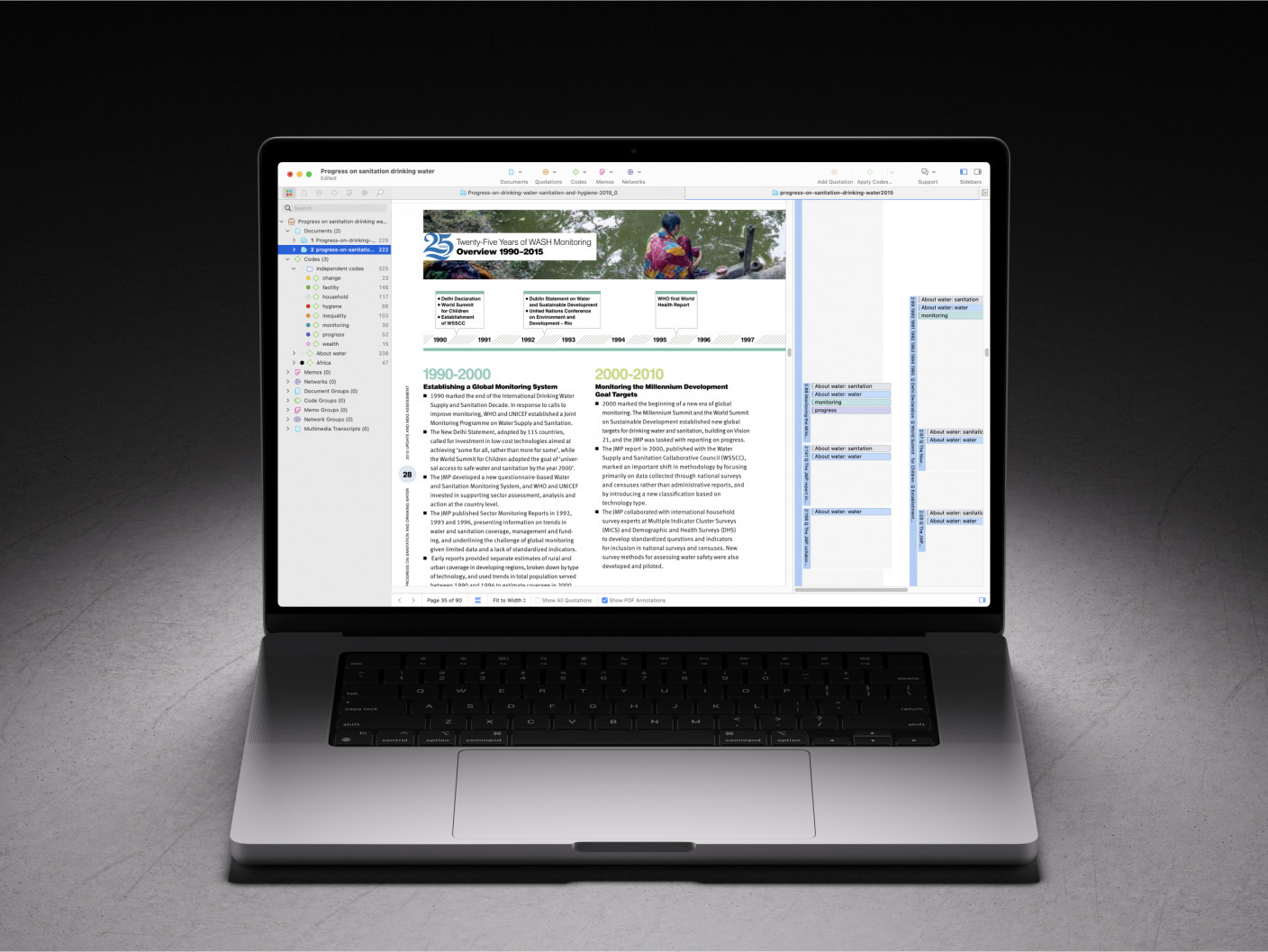
Whatever field you're in, ATLAS.ti puts your data to work for you
Download a free trial of ATLAS.ti to turn your data into insights.
Understanding the key elements of case study design is crucial for conducting rigorous and impactful case study research. A well-structured design guides the researcher through the process, ensuring that the study is methodologically sound and its findings are reliable and valid. The main elements of case study design include the research question , propositions, units of analysis, and the logic linking the data to the propositions.
The research question is the foundation of any research study. A good research question guides the direction of the study and informs the selection of the case, the methods of collecting data, and the analysis techniques. A well-formulated research question in case study research is typically clear, focused, and complex enough to merit further detailed examination of the relevant case(s).
Propositions
Propositions, though not necessary in every case study, provide a direction by stating what we might expect to find in the data collected. They guide how data is collected and analyzed by helping researchers focus on specific aspects of the case. They are particularly important in explanatory case studies, which seek to understand the relationships among concepts within the studied phenomenon.
Units of analysis
The unit of analysis refers to the case, or the main entity or entities that are being analyzed in the study. In case study research, the unit of analysis can be an individual, a group, an organization, a decision, an event, or even a time period. It's crucial to clearly define the unit of analysis, as it shapes the qualitative data analysis process by allowing the researcher to analyze a particular case and synthesize analysis across multiple case studies to draw conclusions.
Argumentation
This refers to the inferential model that allows researchers to draw conclusions from the data. The researcher needs to ensure that there is a clear link between the data, the propositions (if any), and the conclusions drawn. This argumentation is what enables the researcher to make valid and credible inferences about the phenomenon under study.
Understanding and carefully considering these elements in the design phase of a case study can significantly enhance the quality of the research. It can help ensure that the study is methodologically sound and its findings contribute meaningful insights about the case.
Ready to jumpstart your research with ATLAS.ti?
Conceptualize your research project with our intuitive data analysis interface. Download a free trial today.
Conducting a case study involves several steps, from defining the research question and selecting the case to collecting and analyzing data . This section outlines these key stages, providing a practical guide on how to conduct case study research.
Defining the research question
The first step in case study research is defining a clear, focused research question. This question should guide the entire research process, from case selection to analysis. It's crucial to ensure that the research question is suitable for a case study approach. Typically, such questions are exploratory or descriptive in nature and focus on understanding a phenomenon within its real-life context.
Selecting and defining the case
The selection of the case should be based on the research question and the objectives of the study. It involves choosing a unique example or a set of examples that provide rich, in-depth data about the phenomenon under investigation. After selecting the case, it's crucial to define it clearly, setting the boundaries of the case, including the time period and the specific context.
Previous research can help guide the case study design. When considering a case study, an example of a case could be taken from previous case study research and used to define cases in a new research inquiry. Considering recently published examples can help understand how to select and define cases effectively.
Developing a detailed case study protocol
A case study protocol outlines the procedures and general rules to be followed during the case study. This includes the data collection methods to be used, the sources of data, and the procedures for analysis. Having a detailed case study protocol ensures consistency and reliability in the study.
The protocol should also consider how to work with the people involved in the research context to grant the research team access to collecting data. As mentioned in previous sections of this guide, establishing rapport is an essential component of qualitative research as it shapes the overall potential for collecting and analyzing data.
Collecting data
Gathering data in case study research often involves multiple sources of evidence, including documents, archival records, interviews, observations, and physical artifacts. This allows for a comprehensive understanding of the case. The process for gathering data should be systematic and carefully documented to ensure the reliability and validity of the study.
Analyzing and interpreting data
The next step is analyzing the data. This involves organizing the data , categorizing it into themes or patterns , and interpreting these patterns to answer the research question. The analysis might also involve comparing the findings with prior research or theoretical propositions.
Writing the case study report
The final step is writing the case study report . This should provide a detailed description of the case, the data, the analysis process, and the findings. The report should be clear, organized, and carefully written to ensure that the reader can understand the case and the conclusions drawn from it.
Each of these steps is crucial in ensuring that the case study research is rigorous, reliable, and provides valuable insights about the case.
The type, depth, and quality of data in your study can significantly influence the validity and utility of the study. In case study research, data is usually collected from multiple sources to provide a comprehensive and nuanced understanding of the case. This section will outline the various methods of collecting data used in case study research and discuss considerations for ensuring the quality of the data.
Interviews are a common method of gathering data in case study research. They can provide rich, in-depth data about the perspectives, experiences, and interpretations of the individuals involved in the case. Interviews can be structured , semi-structured , or unstructured , depending on the research question and the degree of flexibility needed.
Observations
Observations involve the researcher observing the case in its natural setting, providing first-hand information about the case and its context. Observations can provide data that might not be revealed in interviews or documents, such as non-verbal cues or contextual information.
Documents and artifacts
Documents and archival records provide a valuable source of data in case study research. They can include reports, letters, memos, meeting minutes, email correspondence, and various public and private documents related to the case.
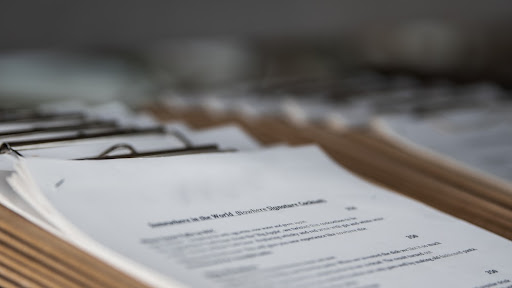
These records can provide historical context, corroborate evidence from other sources, and offer insights into the case that might not be apparent from interviews or observations.
Physical artifacts refer to any physical evidence related to the case, such as tools, products, or physical environments. These artifacts can provide tangible insights into the case, complementing the data gathered from other sources.
Ensuring the quality of data collection
Determining the quality of data in case study research requires careful planning and execution. It's crucial to ensure that the data is reliable, accurate, and relevant to the research question. This involves selecting appropriate methods of collecting data, properly training interviewers or observers, and systematically recording and storing the data. It also includes considering ethical issues related to collecting and handling data, such as obtaining informed consent and ensuring the privacy and confidentiality of the participants.
Data analysis
Analyzing case study research involves making sense of the rich, detailed data to answer the research question. This process can be challenging due to the volume and complexity of case study data. However, a systematic and rigorous approach to analysis can ensure that the findings are credible and meaningful. This section outlines the main steps and considerations in analyzing data in case study research.
Organizing the data
The first step in the analysis is organizing the data. This involves sorting the data into manageable sections, often according to the data source or the theme. This step can also involve transcribing interviews, digitizing physical artifacts, or organizing observational data.
Categorizing and coding the data
Once the data is organized, the next step is to categorize or code the data. This involves identifying common themes, patterns, or concepts in the data and assigning codes to relevant data segments. Coding can be done manually or with the help of software tools, and in either case, qualitative analysis software can greatly facilitate the entire coding process. Coding helps to reduce the data to a set of themes or categories that can be more easily analyzed.
Identifying patterns and themes
After coding the data, the researcher looks for patterns or themes in the coded data. This involves comparing and contrasting the codes and looking for relationships or patterns among them. The identified patterns and themes should help answer the research question.
Interpreting the data
Once patterns and themes have been identified, the next step is to interpret these findings. This involves explaining what the patterns or themes mean in the context of the research question and the case. This interpretation should be grounded in the data, but it can also involve drawing on theoretical concepts or prior research.
Verification of the data
The last step in the analysis is verification. This involves checking the accuracy and consistency of the analysis process and confirming that the findings are supported by the data. This can involve re-checking the original data, checking the consistency of codes, or seeking feedback from research participants or peers.
Like any research method , case study research has its strengths and limitations. Researchers must be aware of these, as they can influence the design, conduct, and interpretation of the study.
Understanding the strengths and limitations of case study research can also guide researchers in deciding whether this approach is suitable for their research question . This section outlines some of the key strengths and limitations of case study research.
Benefits include the following:
- Rich, detailed data: One of the main strengths of case study research is that it can generate rich, detailed data about the case. This can provide a deep understanding of the case and its context, which can be valuable in exploring complex phenomena.
- Flexibility: Case study research is flexible in terms of design , data collection , and analysis . A sufficient degree of flexibility allows the researcher to adapt the study according to the case and the emerging findings.
- Real-world context: Case study research involves studying the case in its real-world context, which can provide valuable insights into the interplay between the case and its context.
- Multiple sources of evidence: Case study research often involves collecting data from multiple sources , which can enhance the robustness and validity of the findings.
On the other hand, researchers should consider the following limitations:
- Generalizability: A common criticism of case study research is that its findings might not be generalizable to other cases due to the specificity and uniqueness of each case.
- Time and resource intensive: Case study research can be time and resource intensive due to the depth of the investigation and the amount of collected data.
- Complexity of analysis: The rich, detailed data generated in case study research can make analyzing the data challenging.
- Subjectivity: Given the nature of case study research, there may be a higher degree of subjectivity in interpreting the data , so researchers need to reflect on this and transparently convey to audiences how the research was conducted.
Being aware of these strengths and limitations can help researchers design and conduct case study research effectively and interpret and report the findings appropriately.
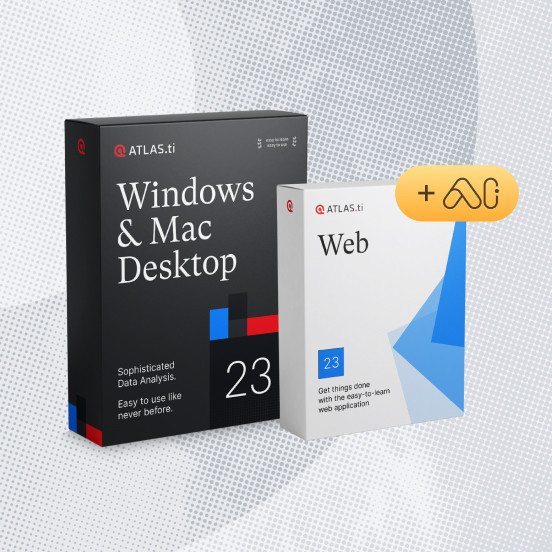
Ready to analyze your data with ATLAS.ti?
See how our intuitive software can draw key insights from your data with a free trial today.

An official website of the United States government
The .gov means it’s official. Federal government websites often end in .gov or .mil. Before sharing sensitive information, make sure you’re on a federal government site.
The site is secure. The https:// ensures that you are connecting to the official website and that any information you provide is encrypted and transmitted securely.
- Publications
- Account settings
Preview improvements coming to the PMC website in October 2024. Learn More or Try it out now .
- Advanced Search
- Journal List
- v.12(1); 2020 Jan

Observational Study Designs: Synopsis for Selecting an Appropriate Study Design
Assad a rezigalla.
1 Department of Basic Medical Sciences, College of Medicine, University of Bisha, Bisha, SAU
The selection of a study design is the most critical step in the research methodology. Crucial factors should be considered during the selection of the study design, which is the formulated research question, as well as the method of participant selection. Different study designs can be applied to the same research question(s). Research designs are classified as qualitative, quantitative, and mixed design. Observational design occupies the middle and lower parts of the hierarchy of evidence-based pyramid. The observational design is subdivided into descriptive, including cross-sectional, case report or case series, and correlational, and analytic which includes cross-section, case-control, and cohort studies. Each research design has its uses and points of strength and limitations. The aim of this article to provide a simplified approach for the selection of descriptive study design.
Introduction and background
A research design is defined as the “set up to decide on, among other issues, how to collect further data, analyze and interpret them, and finally, to provide an answer to the question” [ 1 ]. The primary objective of a research design is to guarantee that the collected evidence allows the answering of the initial question(s) as clearly as possible [ 2 ]. Various study designs have been described in the literature [ 1 - 3 ]. Each of them deals with the specific type of research or research questions and has points of strength and weakness. Broadly, research designs are classified into qualitative and quantitative research and mixed methods [ 3 ]. The quantitative study design is subdivided into descriptive versus analytical study designs or as observational versus interventional (Figure (Figure1). 1 ). Descriptive designs occupy the middle and lower parts of the hierarchy of evidence-based medicine pyramid. Study designs are organized in a hierarchy beginning from the basic "case report" to the highly valued "randomised clinical trial" [ 4 - 5 ].

Case report
The case report describes an individual case or cases in their natural settings. Also, it describes unrecognized syndromes or variants, abnormal findings or outcomes, or association between risk factors and disease. It is the lowest level and the first line of evidence and usually deals with the newly emerging issues and ideas (Table (Table1) 1 ) [ 4 , 6 - 10 ].
Case series
A case series is a report on data from a subject group (multiple patients) without control [ 6 , 11 - 12 ]. Commonly, this design is used for the illustration of novel, unusual, or atypical features identified in medical practice [ 6 ]. The investigator is governed by the availability and accuracy of the records, which can cause biases [ 13 - 14 ]. Bias in a case series can be decreased through consecutive patient enrollment and predefined inclusion and exclusion criteria, explicit specification of study duration, and enrollment of participants (Table 2 ) [ 11 - 12 ].
Correlational study design
Correlational studies (ecologic studies) explore the statistical relationships between the outcome of interest in population and estimate the exposures. It deals with the community rather than in individual cases. The correlational study design can compare two or more relevant variables and reports the association between them without controlling the variables. The aim of correlational study design or research is to uncover any types of systematic relationships between the studied variables. Ecological studies are often used to measure the prevalence and incidence of disease, mainly when the disease is rare. The populations compared can be defined in several ways, such as geographical, time trends, migrants, longitudinal, occupation, and social class. It should be considered that in ecological studies, the results are presented at the population (group) level rather than individuals. Ecological studies do not provide information about the degree or extent of exposure or outcome of interest for particular individuals within the study group (Table 3 ) [ 7 , 15 - 16 ]. For example, we do not know whether those individuals who died in the study group under observation had higher exposure than those remained alive.
Cross-sectional study design
The cross-sectional study examines the association between exposures and outcomes on a snap of time. The assessed associations are guided by sound hypotheses and seen as hypothesis-generating [ 17 ]. This design can be descriptive (when dealing with prevalence or survey) or analytic (when comparing groups) [ 17 - 18 ]. The selection of participants in a cross-sectional study design depends on the predefined inclusion and exclusion criteria [ 18 - 19 ]. This method of selection limits randomization (Table 4 ).
Case-control study
A case-control study is an observational analytic retrospective study design [ 12 ]. It starts with the outcome of interest (referred to as cases) and looks back in time for exposures that likely caused the outcome of interest [ 13 , 20 ]. This design compares two groups of participants - those with the outcome of interest and the matched control [ 12 ]. The controls should match the group of interest in most of the aspects, except for the outcome of interest [ 18 ]. The controls should be selected from the same localization or setting of the cases [ 13 , 21 - 22 ]. Case-control studies can determine the relative importance of a predictor variable about the presence or absence of the disease (Table (Table5 5 ).
Cohort study design
The cohort study design is classified as an observational analytic study design. This design compares two groups, with exposure of interest and control one [ 12 , 18 , 22 - 24 ].
Cohort design starts with exposure of interest comparing them to non-exposed participants at the time of study initiation [ 18 , 22 , 24 ]. The non-exposed serve as external control. A cohort design can be either prospective [ 18 ] or retrospective [ 12 , 20 , 24 - 25 ]. In prospective cohort studies, the investigator measures a variety of variables that might be a risk factor or relevant to the development of the outcome of interest. Over time, the participants are observed to detect whether they develop the outcome of interest or not. In this case, the participants who do not develop the outcome of interest can act as internal controls. Retrospective cohort studies use data records that were documented for other purposes. The study duration may vary according to the commencement of data recording. Completion of the study is limited to the analysis of the data [ 18 , 22 , 24 ]. In 2016, Setia reported that, in some instances, cohort design could not be well-defined as prospective or retrospective; this happened when retrospective and prospective data were collected from the same participants (Table (Table6) 6 ) [ 24 ].
The selection of the study design is the most critical step in research methodology [ 4 , 26 ]. An appropriate study design guarantees the achievement of the research objectives. The crucial factors that should be considered in the selection of the study design are the formulated research question, as well as the method of sampling [ 4 , 27 ]. The study design determines the way of sampling and data analysis [ 4 ]. The selection of a research study design depends on many factors. Two crucial points that should be noted during the process selection include different study designs that may be applicable for the same research question(s) and researches may have grey areas in which they have different views about the type of study design [ 4 ].
Conclusions
The selection of appropriate study designs for research is critical. Many research designs can apply to the same research. Appropriate selection guarantees that the author will achieve the research objectives and address the research questions.
Acknowledgments
The author would like to acknowledge Dr. M. Abass, Dr. I. Eljack, Dr. K. Salih, Dr. I. Jack, and my colleagues. Special thanks and appreciation to the college dean and administration of the College of Medicine, University of Bisha (Bisha, Saudi Arabia) for help and allowing the use of facilities.
The content published in Cureus is the result of clinical experience and/or research by independent individuals or organizations. Cureus is not responsible for the scientific accuracy or reliability of data or conclusions published herein. All content published within Cureus is intended only for educational, research and reference purposes. Additionally, articles published within Cureus should not be deemed a suitable substitute for the advice of a qualified health care professional. Do not disregard or avoid professional medical advice due to content published within Cureus.
The authors have declared that no competing interests exist.
6.5 Observational Research
Learning objectives.
- List the various types of observational research methods and distinguish between each
- Describe the strengths and weakness of each observational research method.
What Is Observational Research?
The term observational research is used to refer to several different types of non-experimental studies in which behavior is systematically observed and recorded. The goal of observational research is to describe a variable or set of variables. More generally, the goal is to obtain a snapshot of specific characteristics of an individual, group, or setting. As described previously, observational research is non-experimental because nothing is manipulated or controlled, and as such we cannot arrive at causal conclusions using this approach. The data that are collected in observational research studies are often qualitative in nature but they may also be quantitative or both (mixed-methods). There are several different types of observational research designs that will be described below.
Naturalistic Observation
Naturalistic observation is an observational method that involves observing people’s behavior in the environment in which it typically occurs. Thus naturalistic observation is a type of field research (as opposed to a type of laboratory research). Jane Goodall’s famous research on chimpanzees is a classic example of naturalistic observation. Dr. Goodall spent three decades observing chimpanzees in their natural environment in East Africa. She examined such things as chimpanzee’s social structure, mating patterns, gender roles, family structure, and care of offspring by observing them in the wild. However, naturalistic observation could more simply involve observing shoppers in a grocery store, children on a school playground, or psychiatric inpatients in their wards. Researchers engaged in naturalistic observation usually make their observations as unobtrusively as possible so that participants are not aware that they are being studied. Such an approach is called disguised naturalistic observation. Ethically, this method is considered to be acceptable if the participants remain anonymous and the behavior occurs in a public setting where people would not normally have an expectation of privacy. Grocery shoppers putting items into their shopping carts, for example, are engaged in public behavior that is easily observable by store employees and other shoppers. For this reason, most researchers would consider it ethically acceptable to observe them for a study. On the other hand, one of the arguments against the ethicality of the naturalistic observation of “bathroom behavior” discussed earlier in the book is that people have a reasonable expectation of privacy even in a public restroom and that this expectation was violated.
In cases where it is not ethical or practical to conduct disguised naturalistic observation, researchers can conduct undisguised naturalistic observation where the participants are made aware of the researcher presence and monitoring of their behavior. However, one concern with undisguised naturalistic observation is reactivity. Reactivity refers to when a measure changes participants’ behavior. In the case of undisguised naturalistic observation, the concern with reactivity is that when people know they are being observed and studied, they may act differently than they normally would. For instance, you may act much differently in a bar if you know that someone is observing you and recording your behaviors and this would invalidate the study. So disguised observation is less reactive and therefore can have higher validity because people are not aware that their behaviors are being observed and recorded. However, we now know that people often become used to being observed and with time they begin to behave naturally in the researcher’s presence. In other words, over time people habituate to being observed. Think about reality shows like Big Brother or Survivor where people are constantly being observed and recorded. While they may be on their best behavior at first, in a fairly short amount of time they are, flirting, having sex, wearing next to nothing, screaming at each other, and at times acting like complete fools in front of the entire nation.
Participant Observation
Another approach to data collection in observational research is participant observation. In participant observation , researchers become active participants in the group or situation they are studying. Participant observation is very similar to naturalistic observation in that it involves observing people’s behavior in the environment in which it typically occurs. As with naturalistic observation, the data that is collected can include interviews (usually unstructured), notes based on their observations and interactions, documents, photographs, and other artifacts. The only difference between naturalistic observation and participant observation is that researchers engaged in participant observation become active members of the group or situations they are studying. The basic rationale for participant observation is that there may be important information that is only accessible to, or can be interpreted only by, someone who is an active participant in the group or situation. Like naturalistic observation, participant observation can be either disguised or undisguised. In disguised participant observation, the researchers pretend to be members of the social group they are observing and conceal their true identity as researchers. In contrast with undisguised participant observation, the researchers become a part of the group they are studying and they disclose their true identity as researchers to the group under investigation. Once again there are important ethical issues to consider with disguised participant observation. First no informed consent can be obtained and second passive deception is being used. The researcher is passively deceiving the participants by intentionally withholding information about their motivations for being a part of the social group they are studying. But sometimes disguised participation is the only way to access a protective group (like a cult). Further, disguised participant observation is less prone to reactivity than undisguised participant observation.
Rosenhan’s study (1973) [1] of the experience of people in a psychiatric ward would be considered disguised participant observation because Rosenhan and his pseudopatients were admitted into psychiatric hospitals on the pretense of being patients so that they could observe the way that psychiatric patients are treated by staff. The staff and other patients were unaware of their true identities as researchers.
Another example of participant observation comes from a study by sociologist Amy Wilkins (published in Social Psychology Quarterly ) on a university-based religious organization that emphasized how happy its members were (Wilkins, 2008) [2] . Wilkins spent 12 months attending and participating in the group’s meetings and social events, and she interviewed several group members. In her study, Wilkins identified several ways in which the group “enforced” happiness—for example, by continually talking about happiness, discouraging the expression of negative emotions, and using happiness as a way to distinguish themselves from other groups.
One of the primary benefits of participant observation is that the researcher is in a much better position to understand the viewpoint and experiences of the people they are studying when they are apart of the social group. The primary limitation with this approach is that the mere presence of the observer could affect the behavior of the people being observed. While this is also a concern with naturalistic observation when researchers because active members of the social group they are studying, additional concerns arise that they may change the social dynamics and/or influence the behavior of the people they are studying. Similarly, if the researcher acts as a participant observer there can be concerns with biases resulting from developing relationships with the participants. Concretely, the researcher may become less objective resulting in more experimenter bias.
Structured Observation
Another observational method is structured observation. Here the investigator makes careful observations of one or more specific behaviors in a particular setting that is more structured than the settings used in naturalistic and participant observation. Often the setting in which the observations are made is not the natural setting, rather the researcher may observe people in the laboratory environment. Alternatively, the researcher may observe people in a natural setting (like a classroom setting) that they have structured some way, for instance by introducing some specific task participants are to engage in or by introducing a specific social situation or manipulation. Structured observation is very similar to naturalistic observation and participant observation in that in all cases researchers are observing naturally occurring behavior, however, the emphasis in structured observation is on gathering quantitative rather than qualitative data. Researchers using this approach are interested in a limited set of behaviors. This allows them to quantify the behaviors they are observing. In other words, structured observation is less global than naturalistic and participant observation because the researcher engaged in structured observations is interested in a small number of specific behaviors. Therefore, rather than recording everything that happens, the researcher only focuses on very specific behaviors of interest.
Structured observation is very similar to naturalistic observation and participant observation in that in all cases researchers are observing naturally occurring behavior, however, the emphasis in structured observation is on gathering quantitative rather than qualitative data. Researchers using this approach are interested in a limited set of behaviors. This allows them to quantify the behaviors they are observing. In other words, structured observation is less global than naturalistic and participant observation because the researcher engaged in structured observations is interested in a small number of specific behaviors. Therefore, rather than recording everything that happens, the researcher only focuses on very specific behaviors of interest.
Researchers Robert Levine and Ara Norenzayan used structured observation to study differences in the “pace of life” across countries (Levine & Norenzayan, 1999) [3] . One of their measures involved observing pedestrians in a large city to see how long it took them to walk 60 feet. They found that people in some countries walked reliably faster than people in other countries. For example, people in Canada and Sweden covered 60 feet in just under 13 seconds on average, while people in Brazil and Romania took close to 17 seconds. When structured observation takes place in the complex and even chaotic “real world,” the questions of when, where, and under what conditions the observations will be made, and who exactly will be observed are important to consider. Levine and Norenzayan described their sampling process as follows:
“Male and female walking speed over a distance of 60 feet was measured in at least two locations in main downtown areas in each city. Measurements were taken during main business hours on clear summer days. All locations were flat, unobstructed, had broad sidewalks, and were sufficiently uncrowded to allow pedestrians to move at potentially maximum speeds. To control for the effects of socializing, only pedestrians walking alone were used. Children, individuals with obvious physical handicaps, and window-shoppers were not timed. Thirty-five men and 35 women were timed in most cities.” (p. 186). Precise specification of the sampling process in this way makes data collection manageable for the observers, and it also provides some control over important extraneous variables. For example, by making their observations on clear summer days in all countries, Levine and Norenzayan controlled for effects of the weather on people’s walking speeds. In Levine and Norenzayan’s study, measurement was relatively straightforward. They simply measured out a 60-foot distance along a city sidewalk and then used a stopwatch to time participants as they walked over that distance.
As another example, researchers Robert Kraut and Robert Johnston wanted to study bowlers’ reactions to their shots, both when they were facing the pins and then when they turned toward their companions (Kraut & Johnston, 1979) [4] . But what “reactions” should they observe? Based on previous research and their own pilot testing, Kraut and Johnston created a list of reactions that included “closed smile,” “open smile,” “laugh,” “neutral face,” “look down,” “look away,” and “face cover” (covering one’s face with one’s hands). The observers committed this list to memory and then practiced by coding the reactions of bowlers who had been videotaped. During the actual study, the observers spoke into an audio recorder, describing the reactions they observed. Among the most interesting results of this study was that bowlers rarely smiled while they still faced the pins. They were much more likely to smile after they turned toward their companions, suggesting that smiling is not purely an expression of happiness but also a form of social communication.
When the observations require a judgment on the part of the observers—as in Kraut and Johnston’s study—this process is often described as coding . Coding generally requires clearly defining a set of target behaviors. The observers then categorize participants individually in terms of which behavior they have engaged in and the number of times they engaged in each behavior. The observers might even record the duration of each behavior. The target behaviors must be defined in such a way that different observers code them in the same way. This difficulty with coding is the issue of interrater reliability, as mentioned in Chapter 4. Researchers are expected to demonstrate the interrater reliability of their coding procedure by having multiple raters code the same behaviors independently and then showing that the different observers are in close agreement. Kraut and Johnston, for example, video recorded a subset of their participants’ reactions and had two observers independently code them. The two observers showed that they agreed on the reactions that were exhibited 97% of the time, indicating good interrater reliability.
One of the primary benefits of structured observation is that it is far more efficient than naturalistic and participant observation. Since the researchers are focused on specific behaviors this reduces time and expense. Also, often times the environment is structured to encourage the behaviors of interested which again means that researchers do not have to invest as much time in waiting for the behaviors of interest to naturally occur. Finally, researchers using this approach can clearly exert greater control over the environment. However, when researchers exert more control over the environment it may make the environment less natural which decreases external validity. It is less clear for instance whether structured observations made in a laboratory environment will generalize to a real world environment. Furthermore, since researchers engaged in structured observation are often not disguised there may be more concerns with reactivity.
Case Studies
A case study is an in-depth examination of an individual. Sometimes case studies are also completed on social units (e.g., a cult) and events (e.g., a natural disaster). Most commonly in psychology, however, case studies provide a detailed description and analysis of an individual. Often the individual has a rare or unusual condition or disorder or has damage to a specific region of the brain.
Like many observational research methods, case studies tend to be more qualitative in nature. Case study methods involve an in-depth, and often a longitudinal examination of an individual. Depending on the focus of the case study, individuals may or may not be observed in their natural setting. If the natural setting is not what is of interest, then the individual may be brought into a therapist’s office or a researcher’s lab for study. Also, the bulk of the case study report will focus on in-depth descriptions of the person rather than on statistical analyses. With that said some quantitative data may also be included in the write-up of a case study. For instance, an individuals’ depression score may be compared to normative scores or their score before and after treatment may be compared. As with other qualitative methods, a variety of different methods and tools can be used to collect information on the case. For instance, interviews, naturalistic observation, structured observation, psychological testing (e.g., IQ test), and/or physiological measurements (e.g., brain scans) may be used to collect information on the individual.
HM is one of the most notorious case studies in psychology. HM suffered from intractable and very severe epilepsy. A surgeon localized HM’s epilepsy to his medial temporal lobe and in 1953 he removed large sections of his hippocampus in an attempt to stop the seizures. The treatment was a success, in that it resolved his epilepsy and his IQ and personality were unaffected. However, the doctors soon realized that HM exhibited a strange form of amnesia, called anterograde amnesia. HM was able to carry out a conversation and he could remember short strings of letters, digits, and words. Basically, his short term memory was preserved. However, HM could not commit new events to memory. He lost the ability to transfer information from his short-term memory to his long term memory, something memory researchers call consolidation. So while he could carry on a conversation with someone, he would completely forget the conversation after it ended. This was an extremely important case study for memory researchers because it suggested that there’s a dissociation between short-term memory and long-term memory, it suggested that these were two different abilities sub-served by different areas of the brain. It also suggested that the temporal lobes are particularly important for consolidating new information (i.e., for transferring information from short-term memory to long-term memory).
www.youtube.com/watch?v=KkaXNvzE4pk
The history of psychology is filled with influential cases studies, such as Sigmund Freud’s description of “Anna O.” (see Note 6.1 “The Case of “Anna O.””) and John Watson and Rosalie Rayner’s description of Little Albert (Watson & Rayner, 1920) [5] , who learned to fear a white rat—along with other furry objects—when the researchers made a loud noise while he was playing with the rat.
The Case of “Anna O.”
Sigmund Freud used the case of a young woman he called “Anna O.” to illustrate many principles of his theory of psychoanalysis (Freud, 1961) [6] . (Her real name was Bertha Pappenheim, and she was an early feminist who went on to make important contributions to the field of social work.) Anna had come to Freud’s colleague Josef Breuer around 1880 with a variety of odd physical and psychological symptoms. One of them was that for several weeks she was unable to drink any fluids. According to Freud,
She would take up the glass of water that she longed for, but as soon as it touched her lips she would push it away like someone suffering from hydrophobia.…She lived only on fruit, such as melons, etc., so as to lessen her tormenting thirst. (p. 9)
But according to Freud, a breakthrough came one day while Anna was under hypnosis.
[S]he grumbled about her English “lady-companion,” whom she did not care for, and went on to describe, with every sign of disgust, how she had once gone into this lady’s room and how her little dog—horrid creature!—had drunk out of a glass there. The patient had said nothing, as she had wanted to be polite. After giving further energetic expression to the anger she had held back, she asked for something to drink, drank a large quantity of water without any difficulty, and awoke from her hypnosis with the glass at her lips; and thereupon the disturbance vanished, never to return. (p.9)
Freud’s interpretation was that Anna had repressed the memory of this incident along with the emotion that it triggered and that this was what had caused her inability to drink. Furthermore, her recollection of the incident, along with her expression of the emotion she had repressed, caused the symptom to go away.
As an illustration of Freud’s theory, the case study of Anna O. is quite effective. As evidence for the theory, however, it is essentially worthless. The description provides no way of knowing whether Anna had really repressed the memory of the dog drinking from the glass, whether this repression had caused her inability to drink, or whether recalling this “trauma” relieved the symptom. It is also unclear from this case study how typical or atypical Anna’s experience was.

Figure 10.1 Anna O. “Anna O.” was the subject of a famous case study used by Freud to illustrate the principles of psychoanalysis. Source: http://en.wikipedia.org/wiki/File:Pappenheim_1882.jpg
Case studies are useful because they provide a level of detailed analysis not found in many other research methods and greater insights may be gained from this more detailed analysis. As a result of the case study, the researcher may gain a sharpened understanding of what might become important to look at more extensively in future more controlled research. Case studies are also often the only way to study rare conditions because it may be impossible to find a large enough sample to individuals with the condition to use quantitative methods. Although at first glance a case study of a rare individual might seem to tell us little about ourselves, they often do provide insights into normal behavior. The case of HM provided important insights into the role of the hippocampus in memory consolidation. However, it is important to note that while case studies can provide insights into certain areas and variables to study, and can be useful in helping develop theories, they should never be used as evidence for theories. In other words, case studies can be used as inspiration to formulate theories and hypotheses, but those hypotheses and theories then need to be formally tested using more rigorous quantitative methods.
The reason case studies shouldn’t be used to provide support for theories is that they suffer from problems with internal and external validity. Case studies lack the proper controls that true experiments contain. As such they suffer from problems with internal validity, so they cannot be used to determine causation. For instance, during HM’s surgery, the surgeon may have accidentally lesioned another area of HM’s brain (indeed questioning into the possibility of a separate brain lesion began after HM’s death and dissection of his brain) and that lesion may have contributed to his inability to consolidate new information. The fact is, with case studies we cannot rule out these sorts of alternative explanations. So as with all observational methods case studies do not permit determination of causation. In addition, because case studies are often of a single individual, and typically a very abnormal individual, researchers cannot generalize their conclusions to other individuals. Recall that with most research designs there is a trade-off between internal and external validity, with case studies, however, there are problems with both internal validity and external validity. So there are limits both to the ability to determine causation and to generalize the results. A final limitation of case studies is that ample opportunity exists for the theoretical biases of the researcher to color or bias the case description. Indeed, there have been accusations that the woman who studied HM destroyed a lot of her data that were not published and she has been called into question for destroying contradictory data that didn’t support her theory about how memories are consolidated. There is a fascinating New York Times article that describes some of the controversies that ensued after HM’s death and analysis of his brain that can be found at: https://www.nytimes.com/2016/08/07/magazine/the-brain-that-couldnt-remember.html?_r=0
Archival Research
Another approach that is often considered observational research is the use of archival research which involves analyzing data that have already been collected for some other purpose. An example is a study by Brett Pelham and his colleagues on “implicit egotism”—the tendency for people to prefer people, places, and things that are similar to themselves (Pelham, Carvallo, & Jones, 2005) [7] . In one study, they examined Social Security records to show that women with the names Virginia, Georgia, Louise, and Florence were especially likely to have moved to the states of Virginia, Georgia, Louisiana, and Florida, respectively.
As with naturalistic observation, measurement can be more or less straightforward when working with archival data. For example, counting the number of people named Virginia who live in various states based on Social Security records is relatively straightforward. But consider a study by Christopher Peterson and his colleagues on the relationship between optimism and health using data that had been collected many years before for a study on adult development (Peterson, Seligman, & Vaillant, 1988) [8] . In the 1940s, healthy male college students had completed an open-ended questionnaire about difficult wartime experiences. In the late 1980s, Peterson and his colleagues reviewed the men’s questionnaire responses to obtain a measure of explanatory style—their habitual ways of explaining bad events that happen to them. More pessimistic people tend to blame themselves and expect long-term negative consequences that affect many aspects of their lives, while more optimistic people tend to blame outside forces and expect limited negative consequences. To obtain a measure of explanatory style for each participant, the researchers used a procedure in which all negative events mentioned in the questionnaire responses, and any causal explanations for them were identified and written on index cards. These were given to a separate group of raters who rated each explanation in terms of three separate dimensions of optimism-pessimism. These ratings were then averaged to produce an explanatory style score for each participant. The researchers then assessed the statistical relationship between the men’s explanatory style as undergraduate students and archival measures of their health at approximately 60 years of age. The primary result was that the more optimistic the men were as undergraduate students, the healthier they were as older men. Pearson’s r was +.25.
This method is an example of content analysis —a family of systematic approaches to measurement using complex archival data. Just as structured observation requires specifying the behaviors of interest and then noting them as they occur, content analysis requires specifying keywords, phrases, or ideas and then finding all occurrences of them in the data. These occurrences can then be counted, timed (e.g., the amount of time devoted to entertainment topics on the nightly news show), or analyzed in a variety of other ways.
Key Takeaways
- There are several different approaches to observational research including naturalistic observation, participant observation, structured observation, case studies, and archival research.
- Naturalistic observation is used to observe people in their natural setting, participant observation involves becoming an active member of the group being observed, structured observation involves coding a small number of behaviors in a quantitative manner, case studies are typically used to collect in-depth information on a single individual, and archival research involves analysing existing data.
- Describe one problem related to internal validity.
- Describe one problem related to external validity.
- Generate one hypothesis suggested by the case study that might be interesting to test in a systematic single-subject or group study.
- Rosenhan, D. L. (1973). On being sane in insane places. Science, 179 , 250–258. ↵
- Wilkins, A. (2008). “Happier than Non-Christians”: Collective emotions and symbolic boundaries among evangelical Christians. Social Psychology Quarterly, 71 , 281–301. ↵
- Levine, R. V., & Norenzayan, A. (1999). The pace of life in 31 countries. Journal of Cross-Cultural Psychology, 30 , 178–205. ↵
- Kraut, R. E., & Johnston, R. E. (1979). Social and emotional messages of smiling: An ethological approach. Journal of Personality and Social Psychology, 37 , 1539–1553. ↵
- Watson, J. B., & Rayner, R. (1920). Conditioned emotional reactions. Journal of Experimental Psychology, 3 , 1–14. ↵
- Freud, S. (1961). Five lectures on psycho-analysis . New York, NY: Norton. ↵
- Pelham, B. W., Carvallo, M., & Jones, J. T. (2005). Implicit egotism. Current Directions in Psychological Science, 14 , 106–110. ↵
- Peterson, C., Seligman, M. E. P., & Vaillant, G. E. (1988). Pessimistic explanatory style is a risk factor for physical illness: A thirty-five year longitudinal study. Journal of Personality and Social Psychology, 55 , 23–27. ↵

Share This Book
- Increase Font Size
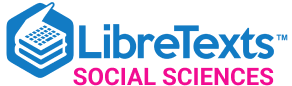
- school Campus Bookshelves
- menu_book Bookshelves
- perm_media Learning Objects
- login Login
- how_to_reg Request Instructor Account
- hub Instructor Commons
Margin Size
- Download Page (PDF)
- Download Full Book (PDF)
- Periodic Table
- Physics Constants
- Scientific Calculator
- Reference & Cite
- Tools expand_more
- Readability
selected template will load here
This action is not available.
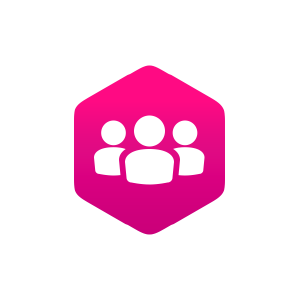
6.6: Observational Research
- Last updated
- Save as PDF
- Page ID 19655
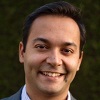
- Rajiv S. Jhangiani, I-Chant A. Chiang, Carrie Cuttler, & Dana C. Leighton
- Kwantlen Polytechnic U., Washington State U., & Texas A&M U.—Texarkana
\( \newcommand{\vecs}[1]{\overset { \scriptstyle \rightharpoonup} {\mathbf{#1}} } \)
\( \newcommand{\vecd}[1]{\overset{-\!-\!\rightharpoonup}{\vphantom{a}\smash {#1}}} \)
\( \newcommand{\id}{\mathrm{id}}\) \( \newcommand{\Span}{\mathrm{span}}\)
( \newcommand{\kernel}{\mathrm{null}\,}\) \( \newcommand{\range}{\mathrm{range}\,}\)
\( \newcommand{\RealPart}{\mathrm{Re}}\) \( \newcommand{\ImaginaryPart}{\mathrm{Im}}\)
\( \newcommand{\Argument}{\mathrm{Arg}}\) \( \newcommand{\norm}[1]{\| #1 \|}\)
\( \newcommand{\inner}[2]{\langle #1, #2 \rangle}\)
\( \newcommand{\Span}{\mathrm{span}}\)
\( \newcommand{\id}{\mathrm{id}}\)
\( \newcommand{\kernel}{\mathrm{null}\,}\)
\( \newcommand{\range}{\mathrm{range}\,}\)
\( \newcommand{\RealPart}{\mathrm{Re}}\)
\( \newcommand{\ImaginaryPart}{\mathrm{Im}}\)
\( \newcommand{\Argument}{\mathrm{Arg}}\)
\( \newcommand{\norm}[1]{\| #1 \|}\)
\( \newcommand{\Span}{\mathrm{span}}\) \( \newcommand{\AA}{\unicode[.8,0]{x212B}}\)
\( \newcommand{\vectorA}[1]{\vec{#1}} % arrow\)
\( \newcommand{\vectorAt}[1]{\vec{\text{#1}}} % arrow\)
\( \newcommand{\vectorB}[1]{\overset { \scriptstyle \rightharpoonup} {\mathbf{#1}} } \)
\( \newcommand{\vectorC}[1]{\textbf{#1}} \)
\( \newcommand{\vectorD}[1]{\overrightarrow{#1}} \)
\( \newcommand{\vectorDt}[1]{\overrightarrow{\text{#1}}} \)
\( \newcommand{\vectE}[1]{\overset{-\!-\!\rightharpoonup}{\vphantom{a}\smash{\mathbf {#1}}}} \)
Learning Objectives
- List the various types of observational research methods and distinguish between each.
- Describe the strengths and weakness of each observational research method.
What Is Observational Research?
The term observational research is used to refer to several different types of non-experimental studies in which behavior is systematically observed and recorded. The goal of observational research is to describe a variable or set of variables. More generally, the goal is to obtain a snapshot of specific characteristics of an individual, group, or setting. As described previously, observational research is non-experimental because nothing is manipulated or controlled, and as such we cannot arrive at causal conclusions using this approach. The data that are collected in observational research studies are often qualitative in nature but they may also be quantitative or both (mixed-methods). There are several different types of observational methods that will be described below.
Naturalistic Observation
Naturalistic observation is an observational method that involves observing people’s behavior in the environment in which it typically occurs. Thus naturalistic observation is a type of field research (as opposed to a type of laboratory research). Jane Goodall’s famous research on chimpanzees is a classic example of naturalistic observation. Dr. Goodall spent three decades observing chimpanzees in their natural environment in East Africa. She examined such things as chimpanzee’s social structure, mating patterns, gender roles, family structure, and care of offspring by observing them in the wild. However, naturalistic observation could more simply involve observing shoppers in a grocery store, children on a school playground, or psychiatric inpatients in their wards. Researchers engaged in naturalistic observation usually make their observations as unobtrusively as possible so that participants are not aware that they are being studied. Such an approach is called disguised naturalistic observation. Ethically, this method is considered to be acceptable if the participants remain anonymous and the behavior occurs in a public setting where people would not normally have an expectation of privacy. Grocery shoppers putting items into their shopping carts, for example, are engaged in public behavior that is easily observable by store employees and other shoppers. For this reason, most researchers would consider it ethically acceptable to observe them for a study. On the other hand, one of the arguments against the ethicality of the naturalistic observation of “bathroom behavior” discussed earlier in the book is that people have a reasonable expectation of privacy even in a public restroom and that this expectation was violated.
In cases where it is not ethical or practical to conduct disguised naturalistic observation, researchers can conduct undisguised naturalistic observation where the participants are made aware of the researcher presence and monitoring of their behavior. However, one concern with undisguised naturalistic observation is reactivity. Reactivity refers to when a measure changes participants’ behavior. In the case of undisguised naturalistic observation, the concern with reactivity is that when people know they are being observed and studied, they may act differently than they normally would. This type of reactivity is known as the Hawthorne effect . For instance, you may act much differently in a bar if you know that someone is observing you and recording your behaviors and this would invalidate the study. So disguised observation is less reactive and therefore can have higher validity because people are not aware that their behaviors are being observed and recorded. However, we now know that people often become used to being observed and with time they begin to behave naturally in the researcher’s presence. In other words, over time people habituate to being observed. Think about reality shows like Big Brother or Survivor where people are constantly being observed and recorded. While they may be on their best behavior at first, in a fairly short amount of time they are flirting, having sex, wearing next to nothing, screaming at each other, and occasionally behaving in ways that are embarrassing.
Participant Observation
Another approach to data collection in observational research is participant observation. In participant observation , researchers become active participants in the group or situation they are studying. Participant observation is very similar to naturalistic observation in that it involves observing people’s behavior in the environment in which it typically occurs. As with naturalistic observation, the data that are collected can include interviews (usually unstructured), notes based on their observations and interactions, documents, photographs, and other artifacts. The only difference between naturalistic observation and participant observation is that researchers engaged in participant observation become active members of the group or situations they are studying. The basic rationale for participant observation is that there may be important information that is only accessible to, or can be interpreted only by, someone who is an active participant in the group or situation. Like naturalistic observation, participant observation can be either disguised or undisguised. In disguised participant observation, the researchers pretend to be members of the social group they are observing and conceal their true identity as researchers.
In a famous example of disguised participant observation, Leon Festinger and his colleagues infiltrated a doomsday cult known as the Seekers, whose members believed that the apocalypse would occur on December 21, 1954. Interested in studying how members of the group would cope psychologically when the prophecy inevitably failed, they carefully recorded the events and reactions of the cult members in the days before and after the supposed end of the world. Unsurprisingly, the cult members did not give up their belief but instead convinced themselves that it was their faith and efforts that saved the world from destruction. Festinger and his colleagues later published a book about this experience, which they used to illustrate the theory of cognitive dissonance (Festinger, Riecken, & Schachter, 1956) [1] .
In contrast with undisguised participant observation, the researchers become a part of the group they are studying and they disclose their true identity as researchers to the group under investigation. Once again there are important ethical issues to consider with disguised participant observation. First no informed consent can be obtained and second deception is being used. The researcher is deceiving the participants by intentionally withholding information about their motivations for being a part of the social group they are studying. But sometimes disguised participation is the only way to access a protective group (like a cult). Further, disguised participant observation is less prone to reactivity than undisguised participant observation.
Rosenhan’s study (1973) [2] of the experience of people in a psychiatric ward would be considered disguised participant observation because Rosenhan and his pseudopatients were admitted into psychiatric hospitals on the pretense of being patients so that they could observe the way that psychiatric patients are treated by staff. The staff and other patients were unaware of their true identities as researchers.
Another example of participant observation comes from a study by sociologist Amy Wilkins on a university-based religious organization that emphasized how happy its members were (Wilkins, 2008) [3] . Wilkins spent 12 months attending and participating in the group’s meetings and social events, and she interviewed several group members. In her study, Wilkins identified several ways in which the group “enforced” happiness—for example, by continually talking about happiness, discouraging the expression of negative emotions, and using happiness as a way to distinguish themselves from other groups.
One of the primary benefits of participant observation is that the researchers are in a much better position to understand the viewpoint and experiences of the people they are studying when they are a part of the social group. The primary limitation with this approach is that the mere presence of the observer could affect the behavior of the people being observed. While this is also a concern with naturalistic observation, additional concerns arise when researchers become active members of the social group they are studying because that they may change the social dynamics and/or influence the behavior of the people they are studying. Similarly, if the researcher acts as a participant observer there can be concerns with biases resulting from developing relationships with the participants. Concretely, the researcher may become less objective resulting in more experimenter bias.
Structured Observation
Another observational method is structured observation . Here the investigator makes careful observations of one or more specific behaviors in a particular setting that is more structured than the settings used in naturalistic or participant observation. Often the setting in which the observations are made is not the natural setting. Instead, the researcher may observe people in the laboratory environment. Alternatively, the researcher may observe people in a natural setting (like a classroom setting) that they have structured some way, for instance by introducing some specific task participants are to engage in or by introducing a specific social situation or manipulation.
Structured observation is very similar to naturalistic observation and participant observation in that in all three cases researchers are observing naturally occurring behavior; however, the emphasis in structured observation is on gathering quantitative rather than qualitative data. Researchers using this approach are interested in a limited set of behaviors. This allows them to quantify the behaviors they are observing. In other words, structured observation is less global than naturalistic or participant observation because the researcher engaged in structured observations is interested in a small number of specific behaviors. Therefore, rather than recording everything that happens, the researcher only focuses on very specific behaviors of interest.
Researchers Robert Levine and Ara Norenzayan used structured observation to study differences in the “pace of life” across countries (Levine & Norenzayan, 1999) [4] . One of their measures involved observing pedestrians in a large city to see how long it took them to walk 60 feet. They found that people in some countries walked reliably faster than people in other countries. For example, people in Canada and Sweden covered 60 feet in just under 13 seconds on average, while people in Brazil and Romania took close to 17 seconds. When structured observation takes place in the complex and even chaotic “real world,” the questions of when, where, and under what conditions the observations will be made, and who exactly will be observed are important to consider. Levine and Norenzayan described their sampling process as follows:
“Male and female walking speed over a distance of 60 feet was measured in at least two locations in main downtown areas in each city. Measurements were taken during main business hours on clear summer days. All locations were flat, unobstructed, had broad sidewalks, and were sufficiently uncrowded to allow pedestrians to move at potentially maximum speeds. To control for the effects of socializing, only pedestrians walking alone were used. Children, individuals with obvious physical handicaps, and window-shoppers were not timed. Thirty-five men and 35 women were timed in most cities.” (p. 186).
Precise specification of the sampling process in this way makes data collection manageable for the observers, and it also provides some control over important extraneous variables. For example, by making their observations on clear summer days in all countries, Levine and Norenzayan controlled for effects of the weather on people’s walking speeds. In Levine and Norenzayan’s study, measurement was relatively straightforward. They simply measured out a 60-foot distance along a city sidewalk and then used a stopwatch to time participants as they walked over that distance.
As another example, researchers Robert Kraut and Robert Johnston wanted to study bowlers’ reactions to their shots, both when they were facing the pins and then when they turned toward their companions (Kraut & Johnston, 1979) [5] . But what “reactions” should they observe? Based on previous research and their own pilot testing, Kraut and Johnston created a list of reactions that included “closed smile,” “open smile,” “laugh,” “neutral face,” “look down,” “look away,” and “face cover” (covering one’s face with one’s hands). The observers committed this list to memory and then practiced by coding the reactions of bowlers who had been videotaped. During the actual study, the observers spoke into an audio recorder, describing the reactions they observed. Among the most interesting results of this study was that bowlers rarely smiled while they still faced the pins. They were much more likely to smile after they turned toward their companions, suggesting that smiling is not purely an expression of happiness but also a form of social communication.
In yet another example (this one in a laboratory environment), Dov Cohen and his colleagues had observers rate the emotional reactions of participants who had just been deliberately bumped and insulted by a confederate after they dropped off a completed questionnaire at the end of a hallway. The confederate was posing as someone who worked in the same building and who was frustrated by having to close a file drawer twice in order to permit the participants to walk past them (first to drop off the questionnaire at the end of the hallway and once again on their way back to the room where they believed the study they signed up for was taking place). The two observers were positioned at different ends of the hallway so that they could read the participants’ body language and hear anything they might say. Interestingly, the researchers hypothesized that participants from the southern United States, which is one of several places in the world that has a “culture of honor,” would react with more aggression than participants from the northern United States, a prediction that was in fact supported by the observational data (Cohen, Nisbett, Bowdle, & Schwarz, 1996) [6] .
When the observations require a judgment on the part of the observers—as in the studies by Kraut and Johnston and Cohen and his colleagues—a process referred to as coding is typically required . Coding generally requires clearly defining a set of target behaviors. The observers then categorize participants individually in terms of which behavior they have engaged in and the number of times they engaged in each behavior. The observers might even record the duration of each behavior. The target behaviors must be defined in such a way that guides different observers to code them in the same way. This difficulty with coding illustrates the issue of interrater reliability, as mentioned in Chapter 4. Researchers are expected to demonstrate the interrater reliability of their coding procedure by having multiple raters code the same behaviors independently and then showing that the different observers are in close agreement. Kraut and Johnston, for example, video recorded a subset of their participants’ reactions and had two observers independently code them. The two observers showed that they agreed on the reactions that were exhibited 97% of the time, indicating good interrater reliability.
One of the primary benefits of structured observation is that it is far more efficient than naturalistic and participant observation. Since the researchers are focused on specific behaviors this reduces time and expense. Also, often times the environment is structured to encourage the behaviors of interest which again means that researchers do not have to invest as much time in waiting for the behaviors of interest to naturally occur. Finally, researchers using this approach can clearly exert greater control over the environment. However, when researchers exert more control over the environment it may make the environment less natural which decreases external validity. It is less clear for instance whether structured observations made in a laboratory environment will generalize to a real world environment. Furthermore, since researchers engaged in structured observation are often not disguised there may be more concerns with reactivity.
Case Studies
A case study is an in-depth examination of an individual. Sometimes case studies are also completed on social units (e.g., a cult) and events (e.g., a natural disaster). Most commonly in psychology, however, case studies provide a detailed description and analysis of an individual. Often the individual has a rare or unusual condition or disorder or has damage to a specific region of the brain.
Like many observational research methods, case studies tend to be more qualitative in nature. Case study methods involve an in-depth, and often a longitudinal examination of an individual. Depending on the focus of the case study, individuals may or may not be observed in their natural setting. If the natural setting is not what is of interest, then the individual may be brought into a therapist’s office or a researcher’s lab for study. Also, the bulk of the case study report will focus on in-depth descriptions of the person rather than on statistical analyses. With that said some quantitative data may also be included in the write-up of a case study. For instance, an individual’s depression score may be compared to normative scores or their score before and after treatment may be compared. As with other qualitative methods, a variety of different methods and tools can be used to collect information on the case. For instance, interviews, naturalistic observation, structured observation, psychological testing (e.g., IQ test), and/or physiological measurements (e.g., brain scans) may be used to collect information on the individual.
HM is one of the most notorious case studies in psychology. HM suffered from intractable and very severe epilepsy. A surgeon localized HM’s epilepsy to his medial temporal lobe and in 1953 he removed large sections of his hippocampus in an attempt to stop the seizures. The treatment was a success, in that it resolved his epilepsy and his IQ and personality were unaffected. However, the doctors soon realized that HM exhibited a strange form of amnesia, called anterograde amnesia. HM was able to carry out a conversation and he could remember short strings of letters, digits, and words. Basically, his short term memory was preserved. However, HM could not commit new events to memory. He lost the ability to transfer information from his short-term memory to his long term memory, something memory researchers call consolidation. So while he could carry on a conversation with someone, he would completely forget the conversation after it ended. This was an extremely important case study for memory researchers because it suggested that there’s a dissociation between short-term memory and long-term memory, it suggested that these were two different abilities sub-served by different areas of the brain. It also suggested that the temporal lobes are particularly important for consolidating new information (i.e., for transferring information from short-term memory to long-term memory),
The history of psychology is filled with influential cases studies, such as Sigmund Freud’s description of “Anna O.” (see Note 6.1 “The Case of “Anna O.””) and John Watson and Rosalie Rayner’s description of Little Albert (Watson & Rayner, 1920) [7] , who allegedly learned to fear a white rat—along with other furry objects—when the researchers repeatedly made a loud noise every time the rat approached him.
The Case of “Anna O.”
Sigmund Freud used the case of a young woman he called “Anna O.” to illustrate many principles of his theory of psychoanalysis (Freud, 1961) [8] . (Her real name was Bertha Pappenheim, and she was an early feminist who went on to make important contributions to the field of social work.) Anna had come to Freud’s colleague Josef Breuer around 1880 with a variety of odd physical and psychological symptoms. One of them was that for several weeks she was unable to drink any fluids. According to Freud,
She would take up the glass of water that she longed for, but as soon as it touched her lips she would push it away like someone suffering from hydrophobia.…She lived only on fruit, such as melons, etc., so as to lessen her tormenting thirst. (p. 9)
But according to Freud, a breakthrough came one day while Anna was under hypnosis.
[S]he grumbled about her English “lady-companion,” whom she did not care for, and went on to describe, with every sign of disgust, how she had once gone into this lady’s room and how her little dog—horrid creature!—had drunk out of a glass there. The patient had said nothing, as she had wanted to be polite. After giving further energetic expression to the anger she had held back, she asked for something to drink, drank a large quantity of water without any difficulty, and awoke from her hypnosis with the glass at her lips; and thereupon the disturbance vanished, never to return. (p.9)
Freud’s interpretation was that Anna had repressed the memory of this incident along with the emotion that it triggered and that this was what had caused her inability to drink. Furthermore, he believed that her recollection of the incident, along with her expression of the emotion she had repressed, caused the symptom to go away.
As an illustration of Freud’s theory, the case study of Anna O. is quite effective. As evidence for the theory, however, it is essentially worthless. The description provides no way of knowing whether Anna had really repressed the memory of the dog drinking from the glass, whether this repression had caused her inability to drink, or whether recalling this “trauma” relieved the symptom. It is also unclear from this case study how typical or atypical Anna’s experience was.
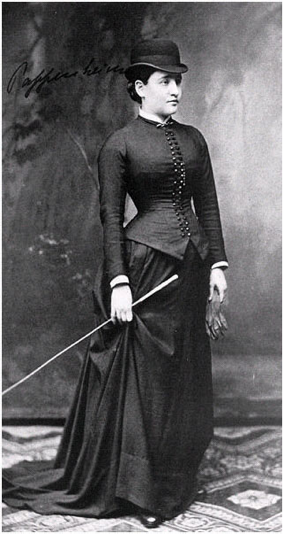
Case studies are useful because they provide a level of detailed analysis not found in many other research methods and greater insights may be gained from this more detailed analysis. As a result of the case study, the researcher may gain a sharpened understanding of what might become important to look at more extensively in future more controlled research. Case studies are also often the only way to study rare conditions because it may be impossible to find a large enough sample of individuals with the condition to use quantitative methods. Although at first glance a case study of a rare individual might seem to tell us little about ourselves, they often do provide insights into normal behavior. The case of HM provided important insights into the role of the hippocampus in memory consolidation.
However, it is important to note that while case studies can provide insights into certain areas and variables to study, and can be useful in helping develop theories, they should never be used as evidence for theories. In other words, case studies can be used as inspiration to formulate theories and hypotheses, but those hypotheses and theories then need to be formally tested using more rigorous quantitative methods. The reason case studies shouldn’t be used to provide support for theories is that they suffer from problems with both internal and external validity. Case studies lack the proper controls that true experiments contain. As such, they suffer from problems with internal validity, so they cannot be used to determine causation. For instance, during HM’s surgery, the surgeon may have accidentally lesioned another area of HM’s brain (a possibility suggested by the dissection of HM’s brain following his death) and that lesion may have contributed to his inability to consolidate new information. The fact is, with case studies we cannot rule out these sorts of alternative explanations. So, as with all observational methods, case studies do not permit determination of causation. In addition, because case studies are often of a single individual, and typically an abnormal individual, researchers cannot generalize their conclusions to other individuals. Recall that with most research designs there is a trade-off between internal and external validity. With case studies, however, there are problems with both internal validity and external validity. So there are limits both to the ability to determine causation and to generalize the results. A final limitation of case studies is that ample opportunity exists for the theoretical biases of the researcher to color or bias the case description. Indeed, there have been accusations that the woman who studied HM destroyed a lot of her data that were not published and she has been called into question for destroying contradictory data that didn’t support her theory about how memories are consolidated. There is a fascinating New York Times article that describes some of the controversies that ensued after HM’s death and analysis of his brain that can be found at: https://www.nytimes.com/2016/08/07/magazine/the-brain-that-couldnt-remember.html?_r=0
Archival Research
Another approach that is often considered observational research involves analyzing archival data that have already been collected for some other purpose. An example is a study by Brett Pelham and his colleagues on “implicit egotism”—the tendency for people to prefer people, places, and things that are similar to themselves (Pelham, Carvallo, & Jones, 2005) [9] . In one study, they examined Social Security records to show that women with the names Virginia, Georgia, Louise, and Florence were especially likely to have moved to the states of Virginia, Georgia, Louisiana, and Florida, respectively.
As with naturalistic observation, measurement can be more or less straightforward when working with archival data. For example, counting the number of people named Virginia who live in various states based on Social Security records is relatively straightforward. But consider a study by Christopher Peterson and his colleagues on the relationship between optimism and health using data that had been collected many years before for a study on adult development (Peterson, Seligman, & Vaillant, 1988) [10] . In the 1940s, healthy male college students had completed an open-ended questionnaire about difficult wartime experiences. In the late 1980s, Peterson and his colleagues reviewed the men’s questionnaire responses to obtain a measure of explanatory style—their habitual ways of explaining bad events that happen to them. More pessimistic people tend to blame themselves and expect long-term negative consequences that affect many aspects of their lives, while more optimistic people tend to blame outside forces and expect limited negative consequences. To obtain a measure of explanatory style for each participant, the researchers used a procedure in which all negative events mentioned in the questionnaire responses, and any causal explanations for them were identified and written on index cards. These were given to a separate group of raters who rated each explanation in terms of three separate dimensions of optimism-pessimism. These ratings were then averaged to produce an explanatory style score for each participant. The researchers then assessed the statistical relationship between the men’s explanatory style as undergraduate students and archival measures of their health at approximately 60 years of age. The primary result was that the more optimistic the men were as undergraduate students, the healthier they were as older men. Pearson’s r was +.25.
This method is an example of content analysis —a family of systematic approaches to measurement using complex archival data. Just as structured observation requires specifying the behaviors of interest and then noting them as they occur, content analysis requires specifying keywords, phrases, or ideas and then finding all occurrences of them in the data. These occurrences can then be counted, timed (e.g., the amount of time devoted to entertainment topics on the nightly news show), or analyzed in a variety of other ways.
- Festinger, L., Riecken, H., & Schachter, S. (1956). When prophecy fails: A social and psychological study of a modern group that predicted the destruction of the world. University of Minnesota Press. ↵
- Rosenhan, D. L. (1973). On being sane in insane places. Science, 179 , 250–258. ↵
- Wilkins, A. (2008). “Happier than Non-Christians”: Collective emotions and symbolic boundaries among evangelical Christians. Social Psychology Quarterly, 71 , 281–301. ↵
- Levine, R. V., & Norenzayan, A. (1999). The pace of life in 31 countries. Journal of Cross-Cultural Psychology, 30 , 178–205. ↵
- Kraut, R. E., & Johnston, R. E. (1979). Social and emotional messages of smiling: An ethological approach. Journal of Personality and Social Psychology, 37 , 1539–1553. ↵
- Cohen, D., Nisbett, R. E., Bowdle, B. F., & Schwarz, N. (1996). Insult, aggression, and the southern culture of honor: An "experimental ethnography." Journal of Personality and Social Psychology, 70 (5), 945-960. ↵
- Watson, J. B., & Rayner, R. (1920). Conditioned emotional reactions. Journal of Experimental Psychology, 3 , 1–14. ↵
- Freud, S. (1961). Five lectures on psycho-analysis . New York, NY: Norton. ↵
- Pelham, B. W., Carvallo, M., & Jones, J. T. (2005). Implicit egotism. Current Directions in Psychological Science, 14 , 106–110. ↵
- Peterson, C., Seligman, M. E. P., & Vaillant, G. E. (1988). Pessimistic explanatory style is a risk factor for physical illness: A thirty-five year longitudinal study. Journal of Personality and Social Psychology, 55 , 23–27. ↵
Observational Case Studies
- First Online: 01 January 2014
Cite this chapter
- Roel J. Wieringa 2
9774 Accesses
1 Citations
An observational case study is a study of a real-world case without performing an intervention. Measurement may influence the measured phenomena, but as in all forms of research, the researcher tries to restrict this to a minimum.
This is a preview of subscription content, log in via an institution to check access.
Access this chapter
- Available as EPUB and PDF
- Read on any device
- Instant download
- Own it forever
- Compact, lightweight edition
- Dispatched in 3 to 5 business days
- Free shipping worldwide - see info
- Durable hardcover edition
Tax calculation will be finalised at checkout
Purchases are for personal use only
Institutional subscriptions
D. Damian, J. Chisan, An empirical study of the complex relationships between requirements engineering processes and other processes that lead to payoffs in productivity, quality and risk management. IEEE Trans. Softw. Eng. 32 (7), 433–453 (2006)
Article Google Scholar
M. Denscombe, The Good Research Guide For Small-Scale Social Research Projects , 4th edn. (Open University Press, Maidenhead, 2010)
Google Scholar
K.M. Eisenhardt, Building theories from case study research. Acad. Manag. Rev. 14 (4), 532–550 (1989)
B. Flyvberg, Five misunderstandings about case-study research. Qual. Inq. 12 (2), 219–245 (2006)
R.L. Glass, Pilot studies: What, why, and how. J. Syst. Softw. 36 , 85–97 (1997)
M.M. Kennedy, Generalizing from single case studies. Eval. Q. 3 (4), 661–678 (1979)
B. Kitchenham, L. Pickard, S.L. Pfleeger, Case studies for method and tool evaluation. IEEE Softw. 12 (4), 52–62 (1995)
C. Robson, Real World Research , 2nd edn. (Blackwell, Oxford, 2002)
P. Runeson, M. Höst, A. Rainer, B. Regnell, Case Study Research in Software Engineering: Guidelines and Examples (Wiley, Hoboken, 2012)
Book Google Scholar
J.M. Verner, J. Sampson, V. Tosic, N.A.A. Bakar, B.A. Kitchenham, Guidelines for industrially-based multiple case studies in software engineering, in Research Challenges in Information Science, 2009. RCIS 2009. Third International Conference on , 2009, pp. 313–324
L. Warne, D. Hart, The impact of organizational politics on information systems project failure-a case study, in Proceedings of the Twenty-Ninth Hawaii International Conference on System Sciences , vol. 4, 1996, pp. 191–201
R.J. Wieringa, Towards a unified checklist for empirical research in software engineering: first proposal, in 16th International Conference on Evaluation and Assessment in Software Engineering (EASE 2012) , ed. by T. Baldaresse, M. Genero, E. Mendes, M. Piattini (IET, Ciudad Real, 2012), pp. 161–165
R.J. Wieringa, A unified checklist for observational and experimental research in software engineering (version 1). Technical Report TR-CTIT-12-07, Centre for Telematics and Information Technology University of Twente (2012)
R.K. Yin, Case Study research: Design and Methods (Sage, Thousand Oaks, 1984)
R.K. Yin, Case Study research: Design and Methods , 3rd edn. (Sage, Thousand Oaks, 2003)
Download references
Author information
Authors and affiliations.
University of Twente, Enschede, The Netherlands
Roel J. Wieringa
You can also search for this author in PubMed Google Scholar
Rights and permissions
Reprints and permissions
Copyright information
© 2014 Springer-Verlag Berlin Heidelberg
About this chapter
Wieringa, R.J. (2014). Observational Case Studies. In: Design Science Methodology for Information Systems and Software Engineering. Springer, Berlin, Heidelberg. https://doi.org/10.1007/978-3-662-43839-8_17
Download citation
DOI : https://doi.org/10.1007/978-3-662-43839-8_17
Published : 20 August 2014
Publisher Name : Springer, Berlin, Heidelberg
Print ISBN : 978-3-662-43838-1
Online ISBN : 978-3-662-43839-8
eBook Packages : Computer Science Computer Science (R0)
Share this chapter
Anyone you share the following link with will be able to read this content:
Sorry, a shareable link is not currently available for this article.
Provided by the Springer Nature SharedIt content-sharing initiative
- Publish with us
Policies and ethics
- Find a journal
- Track your research
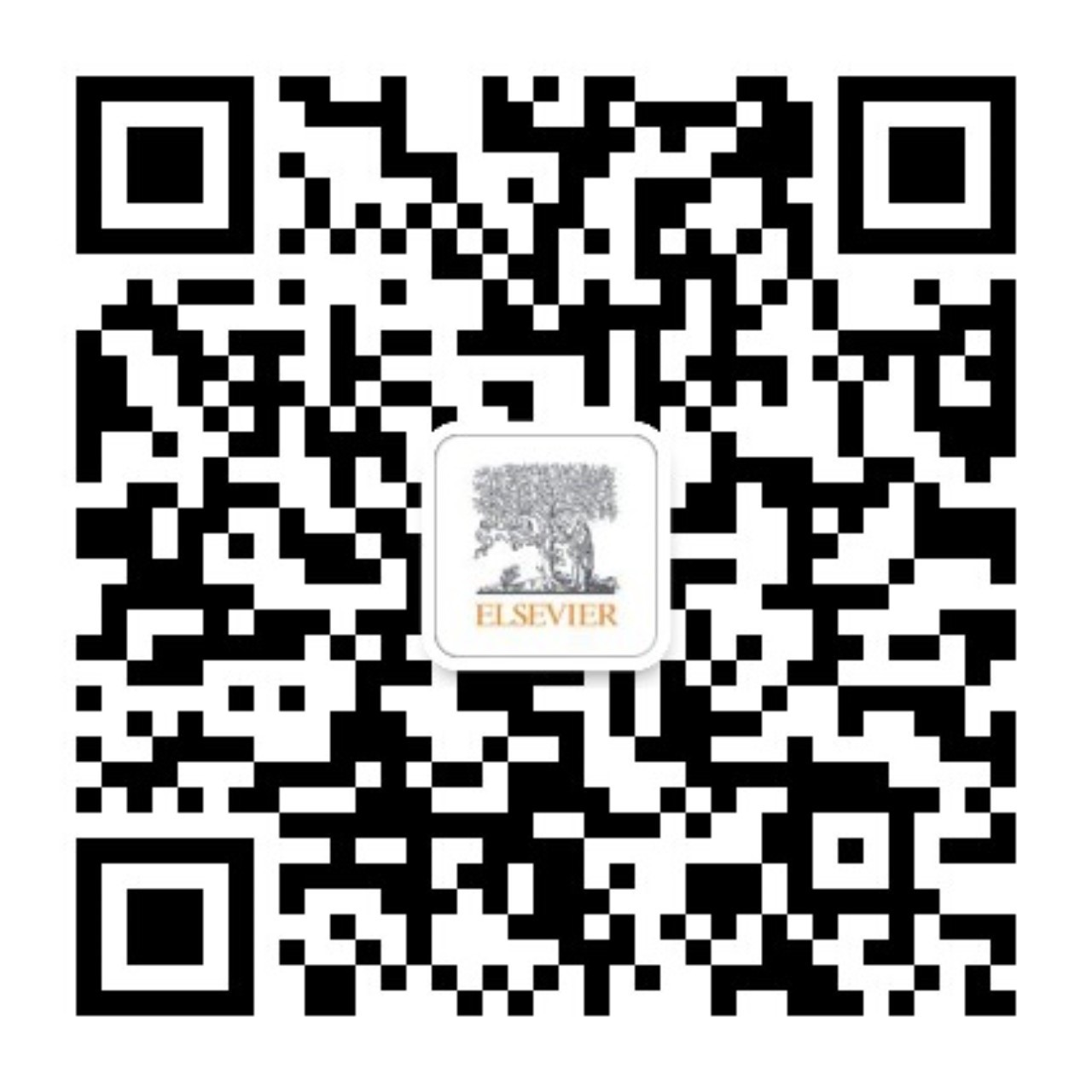
- Research Process
What is Observational Study Design and Types
- 4 minute read
- 117.2K views
Table of Contents
Most people think of a traditional experimental design when they consider research and published research papers. There is, however, a type of research that is more observational in nature, and it is appropriately referred to as “observational studies.”
There are many valuable reasons to utilize an observational study design. But, just as in research experimental design, different methods can be used when you’re considering this type of study. In this article, we’ll look at the advantages and disadvantages of an observational study design, as well as the 3 types of observational studies.
What is Observational Study Design?
An observational study is when researchers are looking at the effect of some type of intervention, risk, a diagnostic test or treatment, without trying to manipulate who is, or who isn’t, exposed to it.
This differs from an experimental study, where the scientists are manipulating who is exposed to the treatment, intervention, etc., by having a control group, or those who are not exposed, and an experimental group, or those who are exposed to the intervention, treatment, etc. In the best studies, the groups are randomized, or chosen by chance.
Any evidence derived from systematic reviews is considered the best in the hierarchy of evidence, which considers which studies are deemed the most reliable. Next would be any evidence that comes from randomized controlled trials. Cohort studies and case studies follow, in that order.
Cohort studies and case studies are considered observational in design, whereas the randomized controlled trial would be an experimental study.
Let’s take a closer look at the different types of observational study design.
The 3 types of Observational Studies
The different types of observational studies are used for different reasons. Selecting the best type for your research is critical to a successful outcome. One of the main reasons observational studies are used is when a randomized experiment would be considered unethical. For example, a life-saving medication used in a public health emergency. They are also used when looking at aetiology, or the cause of a condition or disease, as well as the treatment of rare conditions.
Case Control Observational Study
Researchers in case control studies identify individuals with an existing health issue or condition, or “cases,” along with a similar group without the condition, or “controls.” These two groups are then compared to identify predictors and outcomes. This type of study is helpful to generate a hypothesis that can then be researched.
Cohort Observational Study
This type of observational study is often used to help understand cause and effect. A cohort observational study looks at causes, incidence and prognosis, for example. A cohort is a group of people who are linked in a particular way, for example, a birth cohort would include people who were born within a specific period of time. Scientists might compare what happens to the members of the cohort who have been exposed to some variable to what occurs with members of the cohort who haven’t been exposed.
Cross Sectional Observational Study
Unlike a cohort observational study, a cross sectional observational study does not explore cause and effect, but instead looks at prevalence. Here you would look at data from a particular group at one very specific period of time. Researchers would simply observe and record information about something present in the population, without manipulating any variables or interventions. These types of studies are commonly used in psychology, education and social science.
Advantages and Disadvantages of Observational Study Design
Observational study designs have the distinct advantage of allowing researchers to explore answers to questions where a randomized controlled trial, or RCT, would be unethical. Additionally, if the study is focused on a rare condition, studying existing cases as compared to non-affected individuals might be the most effective way to identify possible causes of the condition. Likewise, if very little is known about a condition or circumstance, a cohort study would be a good study design choice.
A primary advantage to the observational study design is that they can generally be completed quickly and inexpensively. A RCT can take years before the data is compiled and available. RCTs are more complex and involved, requiring many more logistics and details to iron out, whereas an observational study can be more easily designed and completed.
The main disadvantage of observational study designs is that they’re more open to dispute than an RCT. Of particular concern would be confounding biases. This is when a cohort might share other characteristics that affect the outcome versus the outcome stated in the study. An example would be that people who practice good sleeping habits have less heart disease. But, maybe those who practice effective sleeping habits also, in general, eat better and exercise more.
Language Editing Plus Service
Need help with your research writing? With our Language Editing Plus service , we’ll help you improve the flow and writing of your paper, including UNLIMITED editing support. Use the simulator below to check the price for your manuscript, using the total number of words of the document.
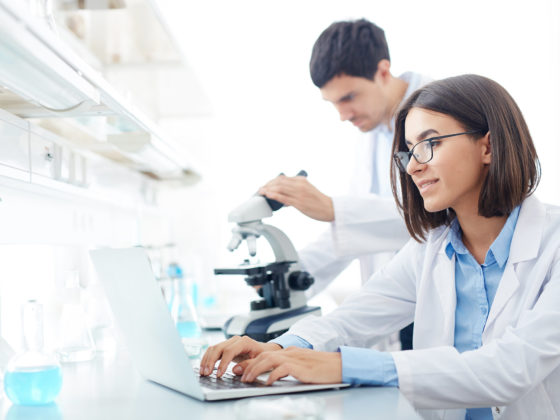
Clinical Questions: PICO and PEO Research
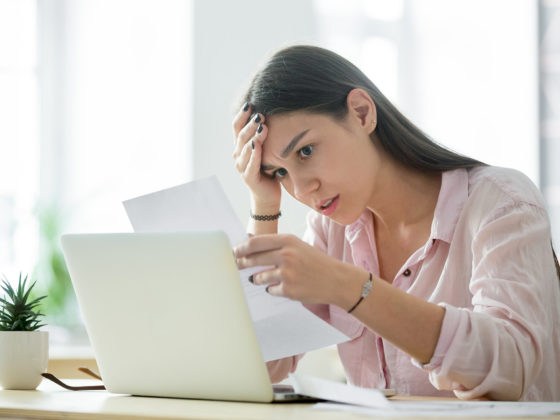
Paper Retraction: Meaning and Main Reasons
You may also like.
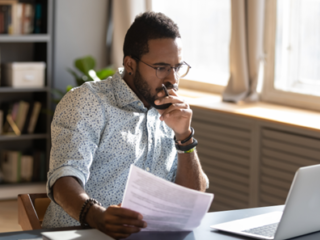
Descriptive Research Design and Its Myriad Uses
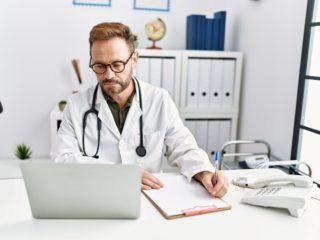
Five Common Mistakes to Avoid When Writing a Biomedical Research Paper
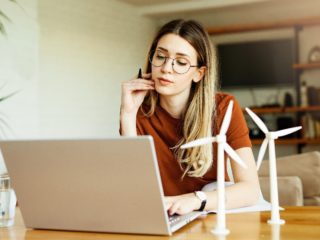
Making Technical Writing in Environmental Engineering Accessible
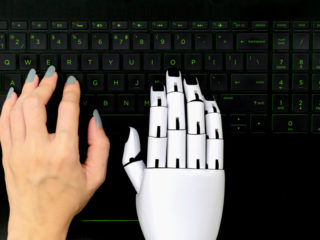
To Err is Not Human: The Dangers of AI-assisted Academic Writing
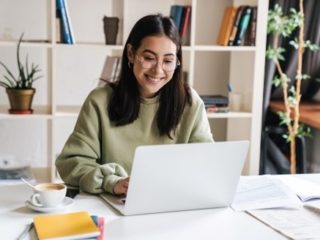
When Data Speak, Listen: Importance of Data Collection and Analysis Methods
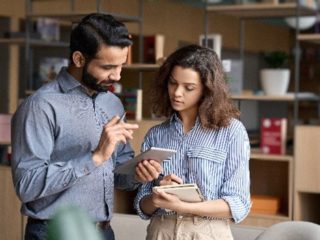
Choosing the Right Research Methodology: A Guide for Researchers
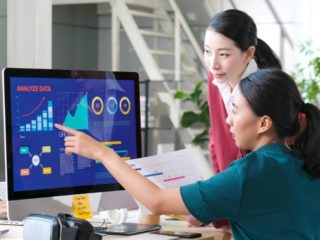
Why is data validation important in research?
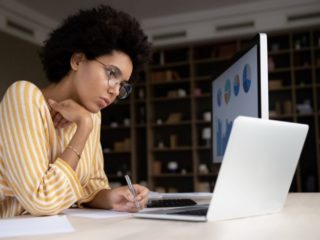
Writing a good review article
Input your search keywords and press Enter.
- Bipolar Disorder
- Therapy Center
- When To See a Therapist
- Types of Therapy
- Best Online Therapy
- Best Couples Therapy
- Best Family Therapy
- Managing Stress
- Sleep and Dreaming
- Understanding Emotions
- Self-Improvement
- Healthy Relationships
- Student Resources
- Personality Types
- Guided Meditations
- Verywell Mind Insights
- 2024 Verywell Mind 25
- Mental Health in the Classroom
- Editorial Process
- Meet Our Review Board
- Crisis Support
What Is a Case Study?
Weighing the pros and cons of this method of research
Kendra Cherry, MS, is a psychosocial rehabilitation specialist, psychology educator, and author of the "Everything Psychology Book."
:max_bytes(150000):strip_icc():format(webp)/IMG_9791-89504ab694d54b66bbd72cb84ffb860e.jpg)
Cara Lustik is a fact-checker and copywriter.
:max_bytes(150000):strip_icc():format(webp)/Cara-Lustik-1000-77abe13cf6c14a34a58c2a0ffb7297da.jpg)
Verywell / Colleen Tighe
- Pros and Cons
What Types of Case Studies Are Out There?
Where do you find data for a case study, how do i write a psychology case study.
A case study is an in-depth study of one person, group, or event. In a case study, nearly every aspect of the subject's life and history is analyzed to seek patterns and causes of behavior. Case studies can be used in many different fields, including psychology, medicine, education, anthropology, political science, and social work.
The point of a case study is to learn as much as possible about an individual or group so that the information can be generalized to many others. Unfortunately, case studies tend to be highly subjective, and it is sometimes difficult to generalize results to a larger population.
While case studies focus on a single individual or group, they follow a format similar to other types of psychology writing. If you are writing a case study, we got you—here are some rules of APA format to reference.
At a Glance
A case study, or an in-depth study of a person, group, or event, can be a useful research tool when used wisely. In many cases, case studies are best used in situations where it would be difficult or impossible for you to conduct an experiment. They are helpful for looking at unique situations and allow researchers to gather a lot of˜ information about a specific individual or group of people. However, it's important to be cautious of any bias we draw from them as they are highly subjective.
What Are the Benefits and Limitations of Case Studies?
A case study can have its strengths and weaknesses. Researchers must consider these pros and cons before deciding if this type of study is appropriate for their needs.
One of the greatest advantages of a case study is that it allows researchers to investigate things that are often difficult or impossible to replicate in a lab. Some other benefits of a case study:
- Allows researchers to capture information on the 'how,' 'what,' and 'why,' of something that's implemented
- Gives researchers the chance to collect information on why one strategy might be chosen over another
- Permits researchers to develop hypotheses that can be explored in experimental research
On the other hand, a case study can have some drawbacks:
- It cannot necessarily be generalized to the larger population
- Cannot demonstrate cause and effect
- It may not be scientifically rigorous
- It can lead to bias
Researchers may choose to perform a case study if they want to explore a unique or recently discovered phenomenon. Through their insights, researchers develop additional ideas and study questions that might be explored in future studies.
It's important to remember that the insights from case studies cannot be used to determine cause-and-effect relationships between variables. However, case studies may be used to develop hypotheses that can then be addressed in experimental research.
Case Study Examples
There have been a number of notable case studies in the history of psychology. Much of Freud's work and theories were developed through individual case studies. Some great examples of case studies in psychology include:
- Anna O : Anna O. was a pseudonym of a woman named Bertha Pappenheim, a patient of a physician named Josef Breuer. While she was never a patient of Freud's, Freud and Breuer discussed her case extensively. The woman was experiencing symptoms of a condition that was then known as hysteria and found that talking about her problems helped relieve her symptoms. Her case played an important part in the development of talk therapy as an approach to mental health treatment.
- Phineas Gage : Phineas Gage was a railroad employee who experienced a terrible accident in which an explosion sent a metal rod through his skull, damaging important portions of his brain. Gage recovered from his accident but was left with serious changes in both personality and behavior.
- Genie : Genie was a young girl subjected to horrific abuse and isolation. The case study of Genie allowed researchers to study whether language learning was possible, even after missing critical periods for language development. Her case also served as an example of how scientific research may interfere with treatment and lead to further abuse of vulnerable individuals.
Such cases demonstrate how case research can be used to study things that researchers could not replicate in experimental settings. In Genie's case, her horrific abuse denied her the opportunity to learn a language at critical points in her development.
This is clearly not something researchers could ethically replicate, but conducting a case study on Genie allowed researchers to study phenomena that are otherwise impossible to reproduce.
There are a few different types of case studies that psychologists and other researchers might use:
- Collective case studies : These involve studying a group of individuals. Researchers might study a group of people in a certain setting or look at an entire community. For example, psychologists might explore how access to resources in a community has affected the collective mental well-being of those who live there.
- Descriptive case studies : These involve starting with a descriptive theory. The subjects are then observed, and the information gathered is compared to the pre-existing theory.
- Explanatory case studies : These are often used to do causal investigations. In other words, researchers are interested in looking at factors that may have caused certain things to occur.
- Exploratory case studies : These are sometimes used as a prelude to further, more in-depth research. This allows researchers to gather more information before developing their research questions and hypotheses .
- Instrumental case studies : These occur when the individual or group allows researchers to understand more than what is initially obvious to observers.
- Intrinsic case studies : This type of case study is when the researcher has a personal interest in the case. Jean Piaget's observations of his own children are good examples of how an intrinsic case study can contribute to the development of a psychological theory.
The three main case study types often used are intrinsic, instrumental, and collective. Intrinsic case studies are useful for learning about unique cases. Instrumental case studies help look at an individual to learn more about a broader issue. A collective case study can be useful for looking at several cases simultaneously.
The type of case study that psychology researchers use depends on the unique characteristics of the situation and the case itself.
There are a number of different sources and methods that researchers can use to gather information about an individual or group. Six major sources that have been identified by researchers are:
- Archival records : Census records, survey records, and name lists are examples of archival records.
- Direct observation : This strategy involves observing the subject, often in a natural setting . While an individual observer is sometimes used, it is more common to utilize a group of observers.
- Documents : Letters, newspaper articles, administrative records, etc., are the types of documents often used as sources.
- Interviews : Interviews are one of the most important methods for gathering information in case studies. An interview can involve structured survey questions or more open-ended questions.
- Participant observation : When the researcher serves as a participant in events and observes the actions and outcomes, it is called participant observation.
- Physical artifacts : Tools, objects, instruments, and other artifacts are often observed during a direct observation of the subject.
If you have been directed to write a case study for a psychology course, be sure to check with your instructor for any specific guidelines you need to follow. If you are writing your case study for a professional publication, check with the publisher for their specific guidelines for submitting a case study.
Here is a general outline of what should be included in a case study.
Section 1: A Case History
This section will have the following structure and content:
Background information : The first section of your paper will present your client's background. Include factors such as age, gender, work, health status, family mental health history, family and social relationships, drug and alcohol history, life difficulties, goals, and coping skills and weaknesses.
Description of the presenting problem : In the next section of your case study, you will describe the problem or symptoms that the client presented with.
Describe any physical, emotional, or sensory symptoms reported by the client. Thoughts, feelings, and perceptions related to the symptoms should also be noted. Any screening or diagnostic assessments that are used should also be described in detail and all scores reported.
Your diagnosis : Provide your diagnosis and give the appropriate Diagnostic and Statistical Manual code. Explain how you reached your diagnosis, how the client's symptoms fit the diagnostic criteria for the disorder(s), or any possible difficulties in reaching a diagnosis.
Section 2: Treatment Plan
This portion of the paper will address the chosen treatment for the condition. This might also include the theoretical basis for the chosen treatment or any other evidence that might exist to support why this approach was chosen.
- Cognitive behavioral approach : Explain how a cognitive behavioral therapist would approach treatment. Offer background information on cognitive behavioral therapy and describe the treatment sessions, client response, and outcome of this type of treatment. Make note of any difficulties or successes encountered by your client during treatment.
- Humanistic approach : Describe a humanistic approach that could be used to treat your client, such as client-centered therapy . Provide information on the type of treatment you chose, the client's reaction to the treatment, and the end result of this approach. Explain why the treatment was successful or unsuccessful.
- Psychoanalytic approach : Describe how a psychoanalytic therapist would view the client's problem. Provide some background on the psychoanalytic approach and cite relevant references. Explain how psychoanalytic therapy would be used to treat the client, how the client would respond to therapy, and the effectiveness of this treatment approach.
- Pharmacological approach : If treatment primarily involves the use of medications, explain which medications were used and why. Provide background on the effectiveness of these medications and how monotherapy may compare with an approach that combines medications with therapy or other treatments.
This section of a case study should also include information about the treatment goals, process, and outcomes.
When you are writing a case study, you should also include a section where you discuss the case study itself, including the strengths and limitiations of the study. You should note how the findings of your case study might support previous research.
In your discussion section, you should also describe some of the implications of your case study. What ideas or findings might require further exploration? How might researchers go about exploring some of these questions in additional studies?
Need More Tips?
Here are a few additional pointers to keep in mind when formatting your case study:
- Never refer to the subject of your case study as "the client." Instead, use their name or a pseudonym.
- Read examples of case studies to gain an idea about the style and format.
- Remember to use APA format when citing references .
Crowe S, Cresswell K, Robertson A, Huby G, Avery A, Sheikh A. The case study approach . BMC Med Res Methodol . 2011;11:100.
Crowe S, Cresswell K, Robertson A, Huby G, Avery A, Sheikh A. The case study approach . BMC Med Res Methodol . 2011 Jun 27;11:100. doi:10.1186/1471-2288-11-100
Gagnon, Yves-Chantal. The Case Study as Research Method: A Practical Handbook . Canada, Chicago Review Press Incorporated DBA Independent Pub Group, 2010.
Yin, Robert K. Case Study Research and Applications: Design and Methods . United States, SAGE Publications, 2017.
By Kendra Cherry, MSEd Kendra Cherry, MS, is a psychosocial rehabilitation specialist, psychology educator, and author of the "Everything Psychology Book."
Want to create or adapt books like this? Learn more about how Pressbooks supports open publishing practices.
Ch 2: Psychological Research Methods
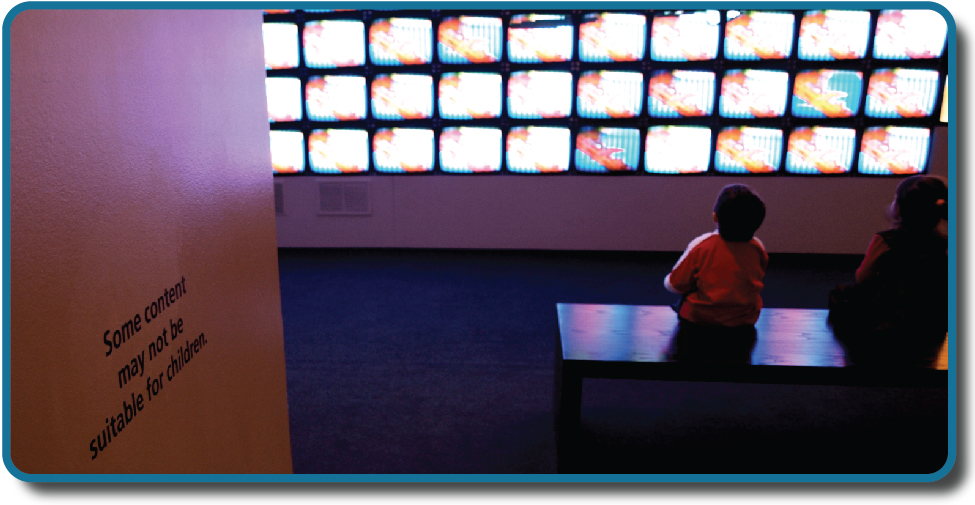
Have you ever wondered whether the violence you see on television affects your behavior? Are you more likely to behave aggressively in real life after watching people behave violently in dramatic situations on the screen? Or, could seeing fictional violence actually get aggression out of your system, causing you to be more peaceful? How are children influenced by the media they are exposed to? A psychologist interested in the relationship between behavior and exposure to violent images might ask these very questions.
The topic of violence in the media today is contentious. Since ancient times, humans have been concerned about the effects of new technologies on our behaviors and thinking processes. The Greek philosopher Socrates, for example, worried that writing—a new technology at that time—would diminish people’s ability to remember because they could rely on written records rather than committing information to memory. In our world of quickly changing technologies, questions about the effects of media continue to emerge. Is it okay to talk on a cell phone while driving? Are headphones good to use in a car? What impact does text messaging have on reaction time while driving? These are types of questions that psychologist David Strayer asks in his lab.
Watch this short video to see how Strayer utilizes the scientific method to reach important conclusions regarding technology and driving safety.
You can view the transcript for “Understanding driver distraction” here (opens in new window) .
How can we go about finding answers that are supported not by mere opinion, but by evidence that we can all agree on? The findings of psychological research can help us navigate issues like this.
Introduction to the Scientific Method
Learning objectives.
- Explain the steps of the scientific method
- Describe why the scientific method is important to psychology
- Summarize the processes of informed consent and debriefing
- Explain how research involving humans or animals is regulated
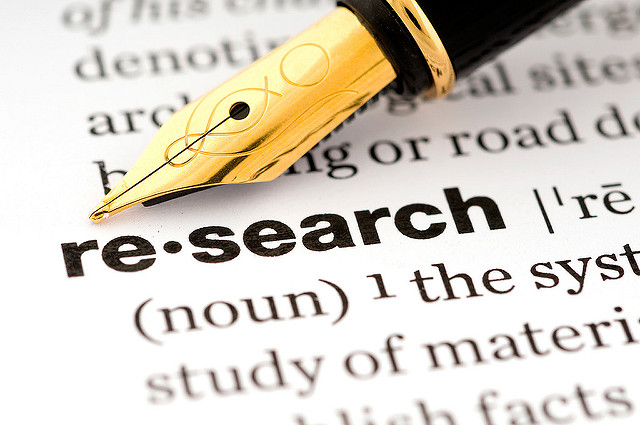
Scientists are engaged in explaining and understanding how the world around them works, and they are able to do so by coming up with theories that generate hypotheses that are testable and falsifiable. Theories that stand up to their tests are retained and refined, while those that do not are discarded or modified. In this way, research enables scientists to separate fact from simple opinion. Having good information generated from research aids in making wise decisions both in public policy and in our personal lives. In this section, you’ll see how psychologists use the scientific method to study and understand behavior.
The Scientific Process
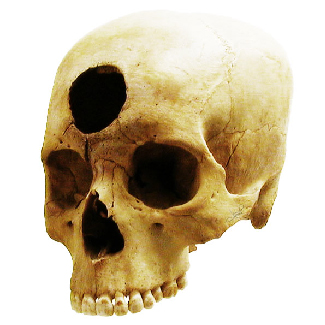
The goal of all scientists is to better understand the world around them. Psychologists focus their attention on understanding behavior, as well as the cognitive (mental) and physiological (body) processes that underlie behavior. In contrast to other methods that people use to understand the behavior of others, such as intuition and personal experience, the hallmark of scientific research is that there is evidence to support a claim. Scientific knowledge is empirical : It is grounded in objective, tangible evidence that can be observed time and time again, regardless of who is observing.
While behavior is observable, the mind is not. If someone is crying, we can see the behavior. However, the reason for the behavior is more difficult to determine. Is the person crying due to being sad, in pain, or happy? Sometimes we can learn the reason for someone’s behavior by simply asking a question, like “Why are you crying?” However, there are situations in which an individual is either uncomfortable or unwilling to answer the question honestly, or is incapable of answering. For example, infants would not be able to explain why they are crying. In such circumstances, the psychologist must be creative in finding ways to better understand behavior. This module explores how scientific knowledge is generated, and how important that knowledge is in forming decisions in our personal lives and in the public domain.
Process of Scientific Research
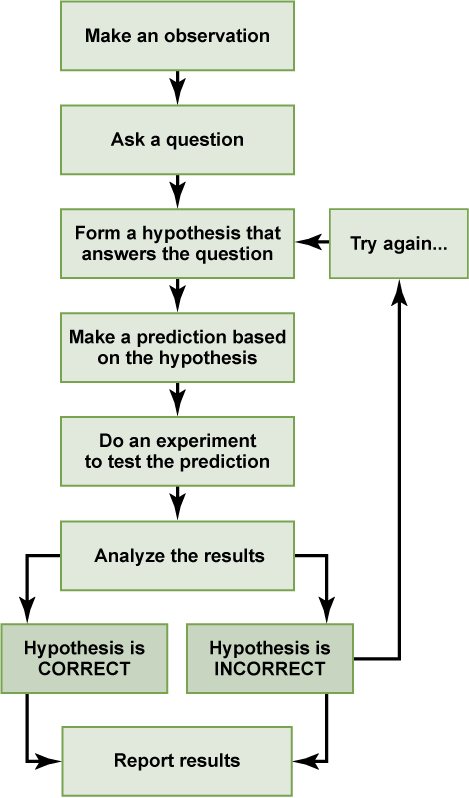
Scientific knowledge is advanced through a process known as the scientific method. Basically, ideas (in the form of theories and hypotheses) are tested against the real world (in the form of empirical observations), and those empirical observations lead to more ideas that are tested against the real world, and so on.
The basic steps in the scientific method are:
- Observe a natural phenomenon and define a question about it
- Make a hypothesis, or potential solution to the question
- Test the hypothesis
- If the hypothesis is true, find more evidence or find counter-evidence
- If the hypothesis is false, create a new hypothesis or try again
- Draw conclusions and repeat–the scientific method is never-ending, and no result is ever considered perfect
In order to ask an important question that may improve our understanding of the world, a researcher must first observe natural phenomena. By making observations, a researcher can define a useful question. After finding a question to answer, the researcher can then make a prediction (a hypothesis) about what he or she thinks the answer will be. This prediction is usually a statement about the relationship between two or more variables. After making a hypothesis, the researcher will then design an experiment to test his or her hypothesis and evaluate the data gathered. These data will either support or refute the hypothesis. Based on the conclusions drawn from the data, the researcher will then find more evidence to support the hypothesis, look for counter-evidence to further strengthen the hypothesis, revise the hypothesis and create a new experiment, or continue to incorporate the information gathered to answer the research question.
Basic Principles of the Scientific Method
Two key concepts in the scientific approach are theory and hypothesis. A theory is a well-developed set of ideas that propose an explanation for observed phenomena that can be used to make predictions about future observations. A hypothesis is a testable prediction that is arrived at logically from a theory. It is often worded as an if-then statement (e.g., if I study all night, I will get a passing grade on the test). The hypothesis is extremely important because it bridges the gap between the realm of ideas and the real world. As specific hypotheses are tested, theories are modified and refined to reflect and incorporate the result of these tests.
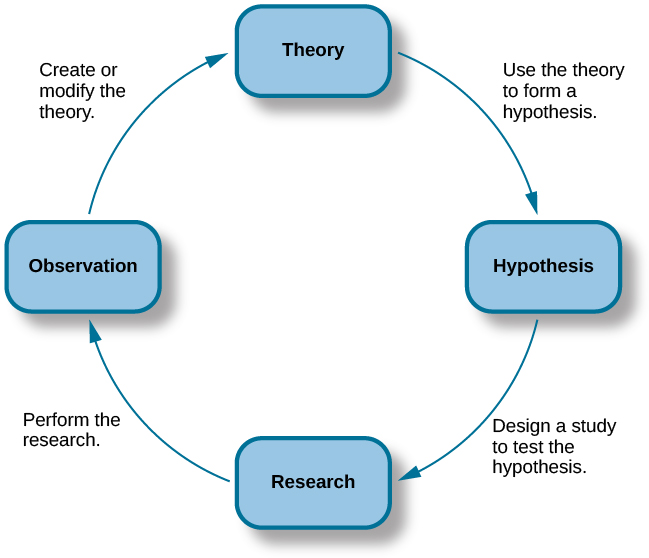
Other key components in following the scientific method include verifiability, predictability, falsifiability, and fairness. Verifiability means that an experiment must be replicable by another researcher. To achieve verifiability, researchers must make sure to document their methods and clearly explain how their experiment is structured and why it produces certain results.
Predictability in a scientific theory implies that the theory should enable us to make predictions about future events. The precision of these predictions is a measure of the strength of the theory.
Falsifiability refers to whether a hypothesis can be disproved. For a hypothesis to be falsifiable, it must be logically possible to make an observation or do a physical experiment that would show that there is no support for the hypothesis. Even when a hypothesis cannot be shown to be false, that does not necessarily mean it is not valid. Future testing may disprove the hypothesis. This does not mean that a hypothesis has to be shown to be false, just that it can be tested.
To determine whether a hypothesis is supported or not supported, psychological researchers must conduct hypothesis testing using statistics. Hypothesis testing is a type of statistics that determines the probability of a hypothesis being true or false. If hypothesis testing reveals that results were “statistically significant,” this means that there was support for the hypothesis and that the researchers can be reasonably confident that their result was not due to random chance. If the results are not statistically significant, this means that the researchers’ hypothesis was not supported.
Fairness implies that all data must be considered when evaluating a hypothesis. A researcher cannot pick and choose what data to keep and what to discard or focus specifically on data that support or do not support a particular hypothesis. All data must be accounted for, even if they invalidate the hypothesis.
Applying the Scientific Method
To see how this process works, let’s consider a specific theory and a hypothesis that might be generated from that theory. As you’ll learn in a later module, the James-Lange theory of emotion asserts that emotional experience relies on the physiological arousal associated with the emotional state. If you walked out of your home and discovered a very aggressive snake waiting on your doorstep, your heart would begin to race and your stomach churn. According to the James-Lange theory, these physiological changes would result in your feeling of fear. A hypothesis that could be derived from this theory might be that a person who is unaware of the physiological arousal that the sight of the snake elicits will not feel fear.
Remember that a good scientific hypothesis is falsifiable, or capable of being shown to be incorrect. Recall from the introductory module that Sigmund Freud had lots of interesting ideas to explain various human behaviors (Figure 5). However, a major criticism of Freud’s theories is that many of his ideas are not falsifiable; for example, it is impossible to imagine empirical observations that would disprove the existence of the id, the ego, and the superego—the three elements of personality described in Freud’s theories. Despite this, Freud’s theories are widely taught in introductory psychology texts because of their historical significance for personality psychology and psychotherapy, and these remain the root of all modern forms of therapy.
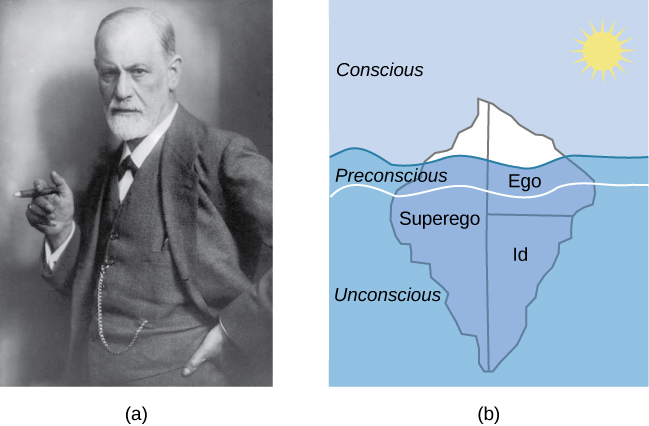
In contrast, the James-Lange theory does generate falsifiable hypotheses, such as the one described above. Some individuals who suffer significant injuries to their spinal columns are unable to feel the bodily changes that often accompany emotional experiences. Therefore, we could test the hypothesis by determining how emotional experiences differ between individuals who have the ability to detect these changes in their physiological arousal and those who do not. In fact, this research has been conducted and while the emotional experiences of people deprived of an awareness of their physiological arousal may be less intense, they still experience emotion (Chwalisz, Diener, & Gallagher, 1988).
Link to Learning
Why the scientific method is important for psychology.
The use of the scientific method is one of the main features that separates modern psychology from earlier philosophical inquiries about the mind. Compared to chemistry, physics, and other “natural sciences,” psychology has long been considered one of the “social sciences” because of the subjective nature of the things it seeks to study. Many of the concepts that psychologists are interested in—such as aspects of the human mind, behavior, and emotions—are subjective and cannot be directly measured. Psychologists often rely instead on behavioral observations and self-reported data, which are considered by some to be illegitimate or lacking in methodological rigor. Applying the scientific method to psychology, therefore, helps to standardize the approach to understanding its very different types of information.
The scientific method allows psychological data to be replicated and confirmed in many instances, under different circumstances, and by a variety of researchers. Through replication of experiments, new generations of psychologists can reduce errors and broaden the applicability of theories. It also allows theories to be tested and validated instead of simply being conjectures that could never be verified or falsified. All of this allows psychologists to gain a stronger understanding of how the human mind works.
Scientific articles published in journals and psychology papers written in the style of the American Psychological Association (i.e., in “APA style”) are structured around the scientific method. These papers include an Introduction, which introduces the background information and outlines the hypotheses; a Methods section, which outlines the specifics of how the experiment was conducted to test the hypothesis; a Results section, which includes the statistics that tested the hypothesis and state whether it was supported or not supported, and a Discussion and Conclusion, which state the implications of finding support for, or no support for, the hypothesis. Writing articles and papers that adhere to the scientific method makes it easy for future researchers to repeat the study and attempt to replicate the results.
Ethics in Research
Today, scientists agree that good research is ethical in nature and is guided by a basic respect for human dignity and safety. However, as you will read in the Tuskegee Syphilis Study, this has not always been the case. Modern researchers must demonstrate that the research they perform is ethically sound. This section presents how ethical considerations affect the design and implementation of research conducted today.
Research Involving Human Participants
Any experiment involving the participation of human subjects is governed by extensive, strict guidelines designed to ensure that the experiment does not result in harm. Any research institution that receives federal support for research involving human participants must have access to an institutional review board (IRB) . The IRB is a committee of individuals often made up of members of the institution’s administration, scientists, and community members (Figure 6). The purpose of the IRB is to review proposals for research that involves human participants. The IRB reviews these proposals with the principles mentioned above in mind, and generally, approval from the IRB is required in order for the experiment to proceed.
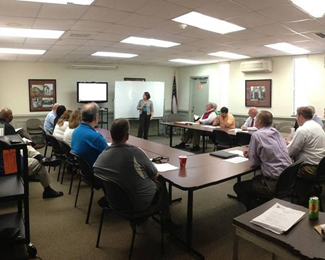
An institution’s IRB requires several components in any experiment it approves. For one, each participant must sign an informed consent form before they can participate in the experiment. An informed consent form provides a written description of what participants can expect during the experiment, including potential risks and implications of the research. It also lets participants know that their involvement is completely voluntary and can be discontinued without penalty at any time. Furthermore, the informed consent guarantees that any data collected in the experiment will remain completely confidential. In cases where research participants are under the age of 18, the parents or legal guardians are required to sign the informed consent form.
While the informed consent form should be as honest as possible in describing exactly what participants will be doing, sometimes deception is necessary to prevent participants’ knowledge of the exact research question from affecting the results of the study. Deception involves purposely misleading experiment participants in order to maintain the integrity of the experiment, but not to the point where the deception could be considered harmful. For example, if we are interested in how our opinion of someone is affected by their attire, we might use deception in describing the experiment to prevent that knowledge from affecting participants’ responses. In cases where deception is involved, participants must receive a full debriefing upon conclusion of the study—complete, honest information about the purpose of the experiment, how the data collected will be used, the reasons why deception was necessary, and information about how to obtain additional information about the study.
Dig Deeper: Ethics and the Tuskegee Syphilis Study
Unfortunately, the ethical guidelines that exist for research today were not always applied in the past. In 1932, poor, rural, black, male sharecroppers from Tuskegee, Alabama, were recruited to participate in an experiment conducted by the U.S. Public Health Service, with the aim of studying syphilis in black men (Figure 7). In exchange for free medical care, meals, and burial insurance, 600 men agreed to participate in the study. A little more than half of the men tested positive for syphilis, and they served as the experimental group (given that the researchers could not randomly assign participants to groups, this represents a quasi-experiment). The remaining syphilis-free individuals served as the control group. However, those individuals that tested positive for syphilis were never informed that they had the disease.
While there was no treatment for syphilis when the study began, by 1947 penicillin was recognized as an effective treatment for the disease. Despite this, no penicillin was administered to the participants in this study, and the participants were not allowed to seek treatment at any other facilities if they continued in the study. Over the course of 40 years, many of the participants unknowingly spread syphilis to their wives (and subsequently their children born from their wives) and eventually died because they never received treatment for the disease. This study was discontinued in 1972 when the experiment was discovered by the national press (Tuskegee University, n.d.). The resulting outrage over the experiment led directly to the National Research Act of 1974 and the strict ethical guidelines for research on humans described in this chapter. Why is this study unethical? How were the men who participated and their families harmed as a function of this research?
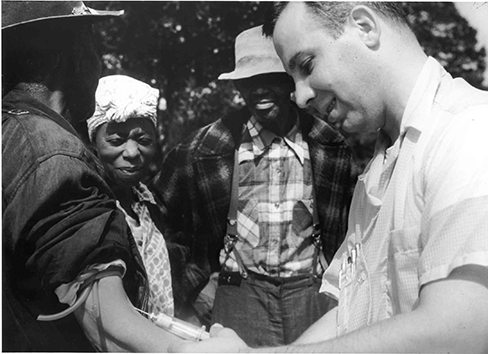
Learn more about the Tuskegee Syphilis Study on the CDC website .
Research Involving Animal Subjects
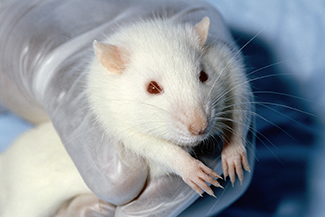
This does not mean that animal researchers are immune to ethical concerns. Indeed, the humane and ethical treatment of animal research subjects is a critical aspect of this type of research. Researchers must design their experiments to minimize any pain or distress experienced by animals serving as research subjects.
Whereas IRBs review research proposals that involve human participants, animal experimental proposals are reviewed by an Institutional Animal Care and Use Committee (IACUC) . An IACUC consists of institutional administrators, scientists, veterinarians, and community members. This committee is charged with ensuring that all experimental proposals require the humane treatment of animal research subjects. It also conducts semi-annual inspections of all animal facilities to ensure that the research protocols are being followed. No animal research project can proceed without the committee’s approval.
Introduction to Approaches to Research
- Differentiate between descriptive, correlational, and experimental research
- Explain the strengths and weaknesses of case studies, naturalistic observation, and surveys
- Describe the strength and weaknesses of archival research
- Compare longitudinal and cross-sectional approaches to research
- Explain what a correlation coefficient tells us about the relationship between variables
- Describe why correlation does not mean causation
- Describe the experimental process, including ways to control for bias
- Identify and differentiate between independent and dependent variables
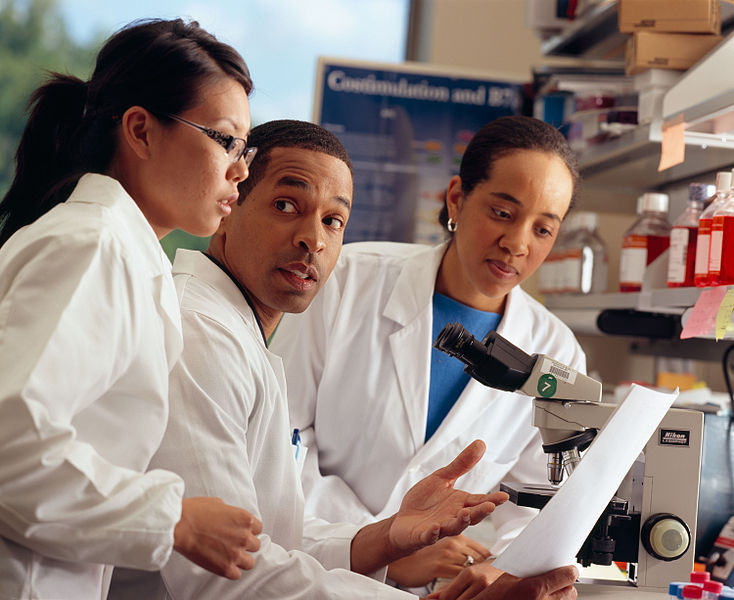
Psychologists use descriptive, experimental, and correlational methods to conduct research. Descriptive, or qualitative, methods include the case study, naturalistic observation, surveys, archival research, longitudinal research, and cross-sectional research.
Experiments are conducted in order to determine cause-and-effect relationships. In ideal experimental design, the only difference between the experimental and control groups is whether participants are exposed to the experimental manipulation. Each group goes through all phases of the experiment, but each group will experience a different level of the independent variable: the experimental group is exposed to the experimental manipulation, and the control group is not exposed to the experimental manipulation. The researcher then measures the changes that are produced in the dependent variable in each group. Once data is collected from both groups, it is analyzed statistically to determine if there are meaningful differences between the groups.
When scientists passively observe and measure phenomena it is called correlational research. Here, psychologists do not intervene and change behavior, as they do in experiments. In correlational research, they identify patterns of relationships, but usually cannot infer what causes what. Importantly, with correlational research, you can examine only two variables at a time, no more and no less.
Watch It: More on Research
If you enjoy learning through lectures and want an interesting and comprehensive summary of this section, then click on the Youtube link to watch a lecture given by MIT Professor John Gabrieli . Start at the 30:45 minute mark and watch through the end to hear examples of actual psychological studies and how they were analyzed. Listen for references to independent and dependent variables, experimenter bias, and double-blind studies. In the lecture, you’ll learn about breaking social norms, “WEIRD” research, why expectations matter, how a warm cup of coffee might make you nicer, why you should change your answer on a multiple choice test, and why praise for intelligence won’t make you any smarter.
You can view the transcript for “Lec 2 | MIT 9.00SC Introduction to Psychology, Spring 2011” here (opens in new window) .
Descriptive Research
There are many research methods available to psychologists in their efforts to understand, describe, and explain behavior and the cognitive and biological processes that underlie it. Some methods rely on observational techniques. Other approaches involve interactions between the researcher and the individuals who are being studied—ranging from a series of simple questions to extensive, in-depth interviews—to well-controlled experiments.
The three main categories of psychological research are descriptive, correlational, and experimental research. Research studies that do not test specific relationships between variables are called descriptive, or qualitative, studies . These studies are used to describe general or specific behaviors and attributes that are observed and measured. In the early stages of research it might be difficult to form a hypothesis, especially when there is not any existing literature in the area. In these situations designing an experiment would be premature, as the question of interest is not yet clearly defined as a hypothesis. Often a researcher will begin with a non-experimental approach, such as a descriptive study, to gather more information about the topic before designing an experiment or correlational study to address a specific hypothesis. Descriptive research is distinct from correlational research , in which psychologists formally test whether a relationship exists between two or more variables. Experimental research goes a step further beyond descriptive and correlational research and randomly assigns people to different conditions, using hypothesis testing to make inferences about how these conditions affect behavior. It aims to determine if one variable directly impacts and causes another. Correlational and experimental research both typically use hypothesis testing, whereas descriptive research does not.
Each of these research methods has unique strengths and weaknesses, and each method may only be appropriate for certain types of research questions. For example, studies that rely primarily on observation produce incredible amounts of information, but the ability to apply this information to the larger population is somewhat limited because of small sample sizes. Survey research, on the other hand, allows researchers to easily collect data from relatively large samples. While this allows for results to be generalized to the larger population more easily, the information that can be collected on any given survey is somewhat limited and subject to problems associated with any type of self-reported data. Some researchers conduct archival research by using existing records. While this can be a fairly inexpensive way to collect data that can provide insight into a number of research questions, researchers using this approach have no control on how or what kind of data was collected.
Correlational research can find a relationship between two variables, but the only way a researcher can claim that the relationship between the variables is cause and effect is to perform an experiment. In experimental research, which will be discussed later in the text, there is a tremendous amount of control over variables of interest. While this is a powerful approach, experiments are often conducted in very artificial settings. This calls into question the validity of experimental findings with regard to how they would apply in real-world settings. In addition, many of the questions that psychologists would like to answer cannot be pursued through experimental research because of ethical concerns.
The three main types of descriptive studies are, naturalistic observation, case studies, and surveys.
Naturalistic Observation
If you want to understand how behavior occurs, one of the best ways to gain information is to simply observe the behavior in its natural context. However, people might change their behavior in unexpected ways if they know they are being observed. How do researchers obtain accurate information when people tend to hide their natural behavior? As an example, imagine that your professor asks everyone in your class to raise their hand if they always wash their hands after using the restroom. Chances are that almost everyone in the classroom will raise their hand, but do you think hand washing after every trip to the restroom is really that universal?
This is very similar to the phenomenon mentioned earlier in this module: many individuals do not feel comfortable answering a question honestly. But if we are committed to finding out the facts about hand washing, we have other options available to us.
Suppose we send a classmate into the restroom to actually watch whether everyone washes their hands after using the restroom. Will our observer blend into the restroom environment by wearing a white lab coat, sitting with a clipboard, and staring at the sinks? We want our researcher to be inconspicuous—perhaps standing at one of the sinks pretending to put in contact lenses while secretly recording the relevant information. This type of observational study is called naturalistic observation : observing behavior in its natural setting. To better understand peer exclusion, Suzanne Fanger collaborated with colleagues at the University of Texas to observe the behavior of preschool children on a playground. How did the observers remain inconspicuous over the duration of the study? They equipped a few of the children with wireless microphones (which the children quickly forgot about) and observed while taking notes from a distance. Also, the children in that particular preschool (a “laboratory preschool”) were accustomed to having observers on the playground (Fanger, Frankel, & Hazen, 2012).
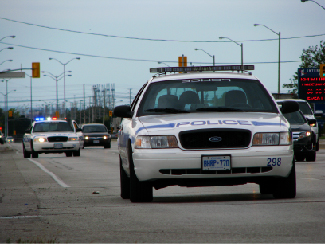
It is critical that the observer be as unobtrusive and as inconspicuous as possible: when people know they are being watched, they are less likely to behave naturally. If you have any doubt about this, ask yourself how your driving behavior might differ in two situations: In the first situation, you are driving down a deserted highway during the middle of the day; in the second situation, you are being followed by a police car down the same deserted highway (Figure 9).
It should be pointed out that naturalistic observation is not limited to research involving humans. Indeed, some of the best-known examples of naturalistic observation involve researchers going into the field to observe various kinds of animals in their own environments. As with human studies, the researchers maintain their distance and avoid interfering with the animal subjects so as not to influence their natural behaviors. Scientists have used this technique to study social hierarchies and interactions among animals ranging from ground squirrels to gorillas. The information provided by these studies is invaluable in understanding how those animals organize socially and communicate with one another. The anthropologist Jane Goodall, for example, spent nearly five decades observing the behavior of chimpanzees in Africa (Figure 10). As an illustration of the types of concerns that a researcher might encounter in naturalistic observation, some scientists criticized Goodall for giving the chimps names instead of referring to them by numbers—using names was thought to undermine the emotional detachment required for the objectivity of the study (McKie, 2010).
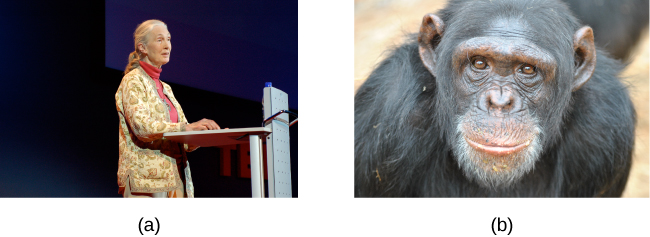
The greatest benefit of naturalistic observation is the validity, or accuracy, of information collected unobtrusively in a natural setting. Having individuals behave as they normally would in a given situation means that we have a higher degree of ecological validity, or realism, than we might achieve with other research approaches. Therefore, our ability to generalize the findings of the research to real-world situations is enhanced. If done correctly, we need not worry about people or animals modifying their behavior simply because they are being observed. Sometimes, people may assume that reality programs give us a glimpse into authentic human behavior. However, the principle of inconspicuous observation is violated as reality stars are followed by camera crews and are interviewed on camera for personal confessionals. Given that environment, we must doubt how natural and realistic their behaviors are.
The major downside of naturalistic observation is that they are often difficult to set up and control. In our restroom study, what if you stood in the restroom all day prepared to record people’s hand washing behavior and no one came in? Or, what if you have been closely observing a troop of gorillas for weeks only to find that they migrated to a new place while you were sleeping in your tent? The benefit of realistic data comes at a cost. As a researcher you have no control of when (or if) you have behavior to observe. In addition, this type of observational research often requires significant investments of time, money, and a good dose of luck.
Sometimes studies involve structured observation. In these cases, people are observed while engaging in set, specific tasks. An excellent example of structured observation comes from Strange Situation by Mary Ainsworth (you will read more about this in the module on lifespan development). The Strange Situation is a procedure used to evaluate attachment styles that exist between an infant and caregiver. In this scenario, caregivers bring their infants into a room filled with toys. The Strange Situation involves a number of phases, including a stranger coming into the room, the caregiver leaving the room, and the caregiver’s return to the room. The infant’s behavior is closely monitored at each phase, but it is the behavior of the infant upon being reunited with the caregiver that is most telling in terms of characterizing the infant’s attachment style with the caregiver.
Another potential problem in observational research is observer bias . Generally, people who act as observers are closely involved in the research project and may unconsciously skew their observations to fit their research goals or expectations. To protect against this type of bias, researchers should have clear criteria established for the types of behaviors recorded and how those behaviors should be classified. In addition, researchers often compare observations of the same event by multiple observers, in order to test inter-rater reliability : a measure of reliability that assesses the consistency of observations by different observers.

Case Studies
In 2011, the New York Times published a feature story on Krista and Tatiana Hogan, Canadian twin girls. These particular twins are unique because Krista and Tatiana are conjoined twins, connected at the head. There is evidence that the two girls are connected in a part of the brain called the thalamus, which is a major sensory relay center. Most incoming sensory information is sent through the thalamus before reaching higher regions of the cerebral cortex for processing.
The implications of this potential connection mean that it might be possible for one twin to experience the sensations of the other twin. For instance, if Krista is watching a particularly funny television program, Tatiana might smile or laugh even if she is not watching the program. This particular possibility has piqued the interest of many neuroscientists who seek to understand how the brain uses sensory information.
These twins represent an enormous resource in the study of the brain, and since their condition is very rare, it is likely that as long as their family agrees, scientists will follow these girls very closely throughout their lives to gain as much information as possible (Dominus, 2011).
In observational research, scientists are conducting a clinical or case study when they focus on one person or just a few individuals. Indeed, some scientists spend their entire careers studying just 10–20 individuals. Why would they do this? Obviously, when they focus their attention on a very small number of people, they can gain a tremendous amount of insight into those cases. The richness of information that is collected in clinical or case studies is unmatched by any other single research method. This allows the researcher to have a very deep understanding of the individuals and the particular phenomenon being studied.
If clinical or case studies provide so much information, why are they not more frequent among researchers? As it turns out, the major benefit of this particular approach is also a weakness. As mentioned earlier, this approach is often used when studying individuals who are interesting to researchers because they have a rare characteristic. Therefore, the individuals who serve as the focus of case studies are not like most other people. If scientists ultimately want to explain all behavior, focusing attention on such a special group of people can make it difficult to generalize any observations to the larger population as a whole. Generalizing refers to the ability to apply the findings of a particular research project to larger segments of society. Again, case studies provide enormous amounts of information, but since the cases are so specific, the potential to apply what’s learned to the average person may be very limited.
Often, psychologists develop surveys as a means of gathering data. Surveys are lists of questions to be answered by research participants, and can be delivered as paper-and-pencil questionnaires, administered electronically, or conducted verbally (Figure 11). Generally, the survey itself can be completed in a short time, and the ease of administering a survey makes it easy to collect data from a large number of people.
Surveys allow researchers to gather data from larger samples than may be afforded by other research methods . A sample is a subset of individuals selected from a population , which is the overall group of individuals that the researchers are interested in. Researchers study the sample and seek to generalize their findings to the population.
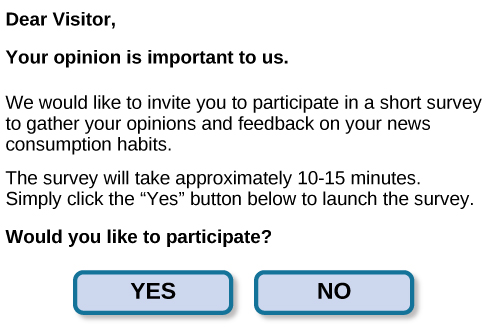
There is both strength and weakness of the survey in comparison to case studies. By using surveys, we can collect information from a larger sample of people. A larger sample is better able to reflect the actual diversity of the population, thus allowing better generalizability. Therefore, if our sample is sufficiently large and diverse, we can assume that the data we collect from the survey can be generalized to the larger population with more certainty than the information collected through a case study. However, given the greater number of people involved, we are not able to collect the same depth of information on each person that would be collected in a case study.
Another potential weakness of surveys is something we touched on earlier in this chapter: people don’t always give accurate responses. They may lie, misremember, or answer questions in a way that they think makes them look good. For example, people may report drinking less alcohol than is actually the case.
Any number of research questions can be answered through the use of surveys. One real-world example is the research conducted by Jenkins, Ruppel, Kizer, Yehl, and Griffin (2012) about the backlash against the US Arab-American community following the terrorist attacks of September 11, 2001. Jenkins and colleagues wanted to determine to what extent these negative attitudes toward Arab-Americans still existed nearly a decade after the attacks occurred. In one study, 140 research participants filled out a survey with 10 questions, including questions asking directly about the participant’s overt prejudicial attitudes toward people of various ethnicities. The survey also asked indirect questions about how likely the participant would be to interact with a person of a given ethnicity in a variety of settings (such as, “How likely do you think it is that you would introduce yourself to a person of Arab-American descent?”). The results of the research suggested that participants were unwilling to report prejudicial attitudes toward any ethnic group. However, there were significant differences between their pattern of responses to questions about social interaction with Arab-Americans compared to other ethnic groups: they indicated less willingness for social interaction with Arab-Americans compared to the other ethnic groups. This suggested that the participants harbored subtle forms of prejudice against Arab-Americans, despite their assertions that this was not the case (Jenkins et al., 2012).
Think It Over
Archival research.
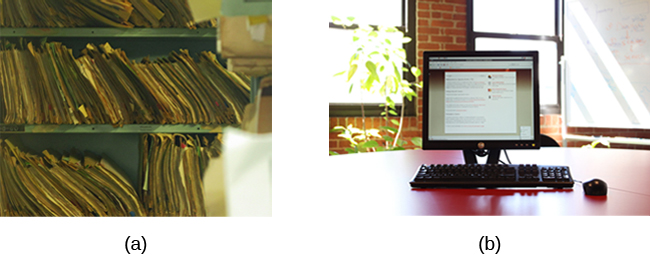
In comparing archival research to other research methods, there are several important distinctions. For one, the researcher employing archival research never directly interacts with research participants. Therefore, the investment of time and money to collect data is considerably less with archival research. Additionally, researchers have no control over what information was originally collected. Therefore, research questions have to be tailored so they can be answered within the structure of the existing data sets. There is also no guarantee of consistency between the records from one source to another, which might make comparing and contrasting different data sets problematic.
Longitudinal and Cross-Sectional Research
Sometimes we want to see how people change over time, as in studies of human development and lifespan. When we test the same group of individuals repeatedly over an extended period of time, we are conducting longitudinal research. Longitudinal research is a research design in which data-gathering is administered repeatedly over an extended period of time. For example, we may survey a group of individuals about their dietary habits at age 20, retest them a decade later at age 30, and then again at age 40.
Another approach is cross-sectional research . In cross-sectional research, a researcher compares multiple segments of the population at the same time. Using the dietary habits example above, the researcher might directly compare different groups of people by age. Instead of observing a group of people for 20 years to see how their dietary habits changed from decade to decade, the researcher would study a group of 20-year-old individuals and compare them to a group of 30-year-old individuals and a group of 40-year-old individuals. While cross-sectional research requires a shorter-term investment, it is also limited by differences that exist between the different generations (or cohorts) that have nothing to do with age per se, but rather reflect the social and cultural experiences of different generations of individuals make them different from one another.
To illustrate this concept, consider the following survey findings. In recent years there has been significant growth in the popular support of same-sex marriage. Many studies on this topic break down survey participants into different age groups. In general, younger people are more supportive of same-sex marriage than are those who are older (Jones, 2013). Does this mean that as we age we become less open to the idea of same-sex marriage, or does this mean that older individuals have different perspectives because of the social climates in which they grew up? Longitudinal research is a powerful approach because the same individuals are involved in the research project over time, which means that the researchers need to be less concerned with differences among cohorts affecting the results of their study.
Often longitudinal studies are employed when researching various diseases in an effort to understand particular risk factors. Such studies often involve tens of thousands of individuals who are followed for several decades. Given the enormous number of people involved in these studies, researchers can feel confident that their findings can be generalized to the larger population. The Cancer Prevention Study-3 (CPS-3) is one of a series of longitudinal studies sponsored by the American Cancer Society aimed at determining predictive risk factors associated with cancer. When participants enter the study, they complete a survey about their lives and family histories, providing information on factors that might cause or prevent the development of cancer. Then every few years the participants receive additional surveys to complete. In the end, hundreds of thousands of participants will be tracked over 20 years to determine which of them develop cancer and which do not.
Clearly, this type of research is important and potentially very informative. For instance, earlier longitudinal studies sponsored by the American Cancer Society provided some of the first scientific demonstrations of the now well-established links between increased rates of cancer and smoking (American Cancer Society, n.d.) (Figure 13).
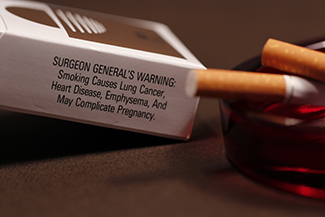
As with any research strategy, longitudinal research is not without limitations. For one, these studies require an incredible time investment by the researcher and research participants. Given that some longitudinal studies take years, if not decades, to complete, the results will not be known for a considerable period of time. In addition to the time demands, these studies also require a substantial financial investment. Many researchers are unable to commit the resources necessary to see a longitudinal project through to the end.
Research participants must also be willing to continue their participation for an extended period of time, and this can be problematic. People move, get married and take new names, get ill, and eventually die. Even without significant life changes, some people may simply choose to discontinue their participation in the project. As a result, the attrition rates, or reduction in the number of research participants due to dropouts, in longitudinal studies are quite high and increases over the course of a project. For this reason, researchers using this approach typically recruit many participants fully expecting that a substantial number will drop out before the end. As the study progresses, they continually check whether the sample still represents the larger population, and make adjustments as necessary.
Correlational Research
Did you know that as sales in ice cream increase, so does the overall rate of crime? Is it possible that indulging in your favorite flavor of ice cream could send you on a crime spree? Or, after committing crime do you think you might decide to treat yourself to a cone? There is no question that a relationship exists between ice cream and crime (e.g., Harper, 2013), but it would be pretty foolish to decide that one thing actually caused the other to occur.
It is much more likely that both ice cream sales and crime rates are related to the temperature outside. When the temperature is warm, there are lots of people out of their houses, interacting with each other, getting annoyed with one another, and sometimes committing crimes. Also, when it is warm outside, we are more likely to seek a cool treat like ice cream. How do we determine if there is indeed a relationship between two things? And when there is a relationship, how can we discern whether it is attributable to coincidence or causation?
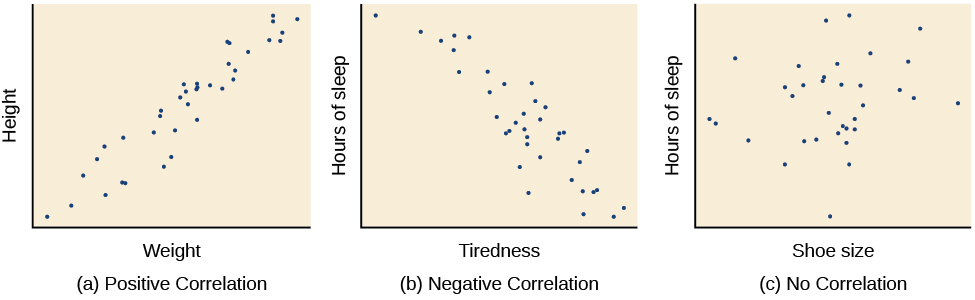
Correlation Does Not Indicate Causation
Correlational research is useful because it allows us to discover the strength and direction of relationships that exist between two variables. However, correlation is limited because establishing the existence of a relationship tells us little about cause and effect . While variables are sometimes correlated because one does cause the other, it could also be that some other factor, a confounding variable , is actually causing the systematic movement in our variables of interest. In the ice cream/crime rate example mentioned earlier, temperature is a confounding variable that could account for the relationship between the two variables.
Even when we cannot point to clear confounding variables, we should not assume that a correlation between two variables implies that one variable causes changes in another. This can be frustrating when a cause-and-effect relationship seems clear and intuitive. Think back to our discussion of the research done by the American Cancer Society and how their research projects were some of the first demonstrations of the link between smoking and cancer. It seems reasonable to assume that smoking causes cancer, but if we were limited to correlational research , we would be overstepping our bounds by making this assumption.
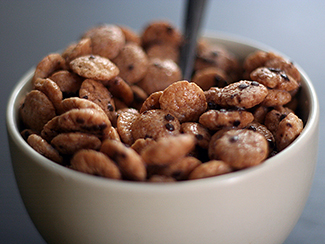
Unfortunately, people mistakenly make claims of causation as a function of correlations all the time. Such claims are especially common in advertisements and news stories. For example, recent research found that people who eat cereal on a regular basis achieve healthier weights than those who rarely eat cereal (Frantzen, Treviño, Echon, Garcia-Dominic, & DiMarco, 2013; Barton et al., 2005). Guess how the cereal companies report this finding. Does eating cereal really cause an individual to maintain a healthy weight, or are there other possible explanations, such as, someone at a healthy weight is more likely to regularly eat a healthy breakfast than someone who is obese or someone who avoids meals in an attempt to diet (Figure 15)? While correlational research is invaluable in identifying relationships among variables, a major limitation is the inability to establish causality. Psychologists want to make statements about cause and effect, but the only way to do that is to conduct an experiment to answer a research question. The next section describes how scientific experiments incorporate methods that eliminate, or control for, alternative explanations, which allow researchers to explore how changes in one variable cause changes in another variable.
Watch this clip from Freakonomics for an example of how correlation does not indicate causation.
You can view the transcript for “Correlation vs. Causality: Freakonomics Movie” here (opens in new window) .
Illusory Correlations
The temptation to make erroneous cause-and-effect statements based on correlational research is not the only way we tend to misinterpret data. We also tend to make the mistake of illusory correlations, especially with unsystematic observations. Illusory correlations , or false correlations, occur when people believe that relationships exist between two things when no such relationship exists. One well-known illusory correlation is the supposed effect that the moon’s phases have on human behavior. Many people passionately assert that human behavior is affected by the phase of the moon, and specifically, that people act strangely when the moon is full (Figure 16).
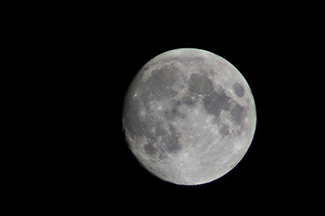
There is no denying that the moon exerts a powerful influence on our planet. The ebb and flow of the ocean’s tides are tightly tied to the gravitational forces of the moon. Many people believe, therefore, that it is logical that we are affected by the moon as well. After all, our bodies are largely made up of water. A meta-analysis of nearly 40 studies consistently demonstrated, however, that the relationship between the moon and our behavior does not exist (Rotton & Kelly, 1985). While we may pay more attention to odd behavior during the full phase of the moon, the rates of odd behavior remain constant throughout the lunar cycle.
Why are we so apt to believe in illusory correlations like this? Often we read or hear about them and simply accept the information as valid. Or, we have a hunch about how something works and then look for evidence to support that hunch, ignoring evidence that would tell us our hunch is false; this is known as confirmation bias . Other times, we find illusory correlations based on the information that comes most easily to mind, even if that information is severely limited. And while we may feel confident that we can use these relationships to better understand and predict the world around us, illusory correlations can have significant drawbacks. For example, research suggests that illusory correlations—in which certain behaviors are inaccurately attributed to certain groups—are involved in the formation of prejudicial attitudes that can ultimately lead to discriminatory behavior (Fiedler, 2004).
We all have a tendency to make illusory correlations from time to time. Try to think of an illusory correlation that is held by you, a family member, or a close friend. How do you think this illusory correlation came about and what can be done in the future to combat them?
Experiments
Causality: conducting experiments and using the data, experimental hypothesis.
In order to conduct an experiment, a researcher must have a specific hypothesis to be tested. As you’ve learned, hypotheses can be formulated either through direct observation of the real world or after careful review of previous research. For example, if you think that children should not be allowed to watch violent programming on television because doing so would cause them to behave more violently, then you have basically formulated a hypothesis—namely, that watching violent television programs causes children to behave more violently. How might you have arrived at this particular hypothesis? You may have younger relatives who watch cartoons featuring characters using martial arts to save the world from evildoers, with an impressive array of punching, kicking, and defensive postures. You notice that after watching these programs for a while, your young relatives mimic the fighting behavior of the characters portrayed in the cartoon (Figure 17).
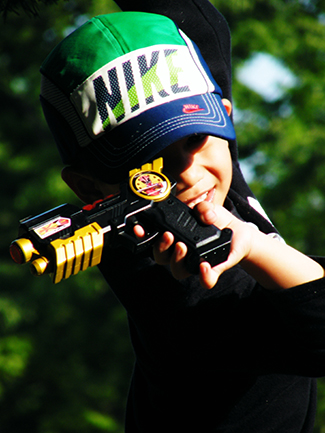
These sorts of personal observations are what often lead us to formulate a specific hypothesis, but we cannot use limited personal observations and anecdotal evidence to rigorously test our hypothesis. Instead, to find out if real-world data supports our hypothesis, we have to conduct an experiment.
Designing an Experiment
The most basic experimental design involves two groups: the experimental group and the control group. The two groups are designed to be the same except for one difference— experimental manipulation. The experimental group gets the experimental manipulation—that is, the treatment or variable being tested (in this case, violent TV images)—and the control group does not. Since experimental manipulation is the only difference between the experimental and control groups, we can be sure that any differences between the two are due to experimental manipulation rather than chance.
In our example of how violent television programming might affect violent behavior in children, we have the experimental group view violent television programming for a specified time and then measure their violent behavior. We measure the violent behavior in our control group after they watch nonviolent television programming for the same amount of time. It is important for the control group to be treated similarly to the experimental group, with the exception that the control group does not receive the experimental manipulation. Therefore, we have the control group watch non-violent television programming for the same amount of time as the experimental group.
We also need to precisely define, or operationalize, what is considered violent and nonviolent. An operational definition is a description of how we will measure our variables, and it is important in allowing others understand exactly how and what a researcher measures in a particular experiment. In operationalizing violent behavior, we might choose to count only physical acts like kicking or punching as instances of this behavior, or we also may choose to include angry verbal exchanges. Whatever we determine, it is important that we operationalize violent behavior in such a way that anyone who hears about our study for the first time knows exactly what we mean by violence. This aids peoples’ ability to interpret our data as well as their capacity to repeat our experiment should they choose to do so.
Once we have operationalized what is considered violent television programming and what is considered violent behavior from our experiment participants, we need to establish how we will run our experiment. In this case, we might have participants watch a 30-minute television program (either violent or nonviolent, depending on their group membership) before sending them out to a playground for an hour where their behavior is observed and the number and type of violent acts is recorded.
Ideally, the people who observe and record the children’s behavior are unaware of who was assigned to the experimental or control group, in order to control for experimenter bias. Experimenter bias refers to the possibility that a researcher’s expectations might skew the results of the study. Remember, conducting an experiment requires a lot of planning, and the people involved in the research project have a vested interest in supporting their hypotheses. If the observers knew which child was in which group, it might influence how much attention they paid to each child’s behavior as well as how they interpreted that behavior. By being blind to which child is in which group, we protect against those biases. This situation is a single-blind study , meaning that one of the groups (participants) are unaware as to which group they are in (experiment or control group) while the researcher who developed the experiment knows which participants are in each group.
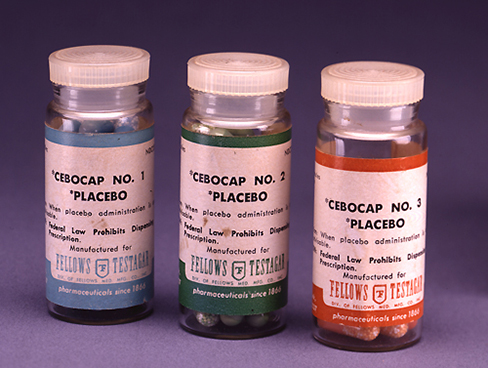
In a double-blind study , both the researchers and the participants are blind to group assignments. Why would a researcher want to run a study where no one knows who is in which group? Because by doing so, we can control for both experimenter and participant expectations. If you are familiar with the phrase placebo effect, you already have some idea as to why this is an important consideration. The placebo effect occurs when people’s expectations or beliefs influence or determine their experience in a given situation. In other words, simply expecting something to happen can actually make it happen.
The placebo effect is commonly described in terms of testing the effectiveness of a new medication. Imagine that you work in a pharmaceutical company, and you think you have a new drug that is effective in treating depression. To demonstrate that your medication is effective, you run an experiment with two groups: The experimental group receives the medication, and the control group does not. But you don’t want participants to know whether they received the drug or not.
Why is that? Imagine that you are a participant in this study, and you have just taken a pill that you think will improve your mood. Because you expect the pill to have an effect, you might feel better simply because you took the pill and not because of any drug actually contained in the pill—this is the placebo effect.
To make sure that any effects on mood are due to the drug and not due to expectations, the control group receives a placebo (in this case a sugar pill). Now everyone gets a pill, and once again neither the researcher nor the experimental participants know who got the drug and who got the sugar pill. Any differences in mood between the experimental and control groups can now be attributed to the drug itself rather than to experimenter bias or participant expectations (Figure 18).
Independent and Dependent Variables
In a research experiment, we strive to study whether changes in one thing cause changes in another. To achieve this, we must pay attention to two important variables, or things that can be changed, in any experimental study: the independent variable and the dependent variable. An independent variable is manipulated or controlled by the experimenter. In a well-designed experimental study, the independent variable is the only important difference between the experimental and control groups. In our example of how violent television programs affect children’s display of violent behavior, the independent variable is the type of program—violent or nonviolent—viewed by participants in the study (Figure 19). A dependent variable is what the researcher measures to see how much effect the independent variable had. In our example, the dependent variable is the number of violent acts displayed by the experimental participants.
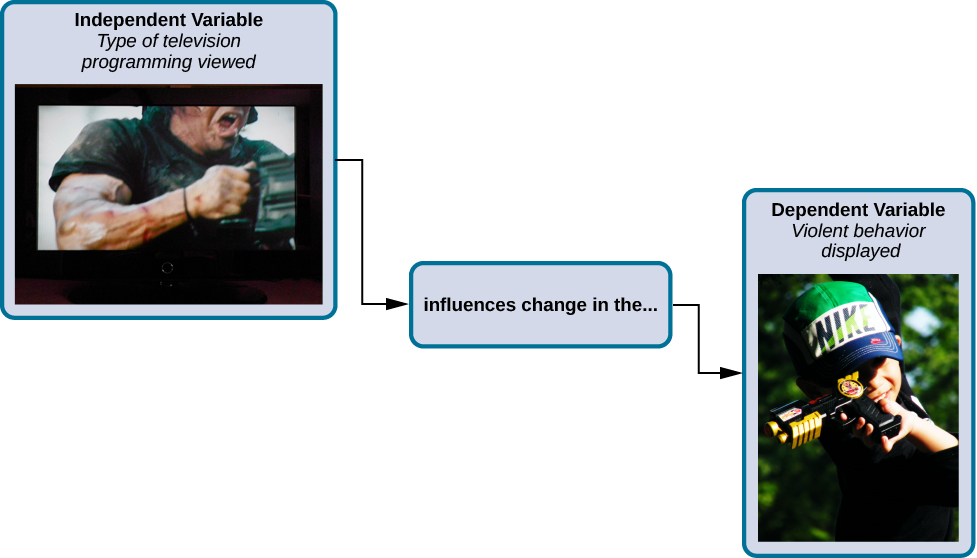
We expect that the dependent variable will change as a function of the independent variable. In other words, the dependent variable depends on the independent variable. A good way to think about the relationship between the independent and dependent variables is with this question: What effect does the independent variable have on the dependent variable? Returning to our example, what effect does watching a half hour of violent television programming or nonviolent television programming have on the number of incidents of physical aggression displayed on the playground?
Selecting and Assigning Experimental Participants
Now that our study is designed, we need to obtain a sample of individuals to include in our experiment. Our study involves human participants so we need to determine who to include. Participants are the subjects of psychological research, and as the name implies, individuals who are involved in psychological research actively participate in the process. Often, psychological research projects rely on college students to serve as participants. In fact, the vast majority of research in psychology subfields has historically involved students as research participants (Sears, 1986; Arnett, 2008). But are college students truly representative of the general population? College students tend to be younger, more educated, more liberal, and less diverse than the general population. Although using students as test subjects is an accepted practice, relying on such a limited pool of research participants can be problematic because it is difficult to generalize findings to the larger population.
Our hypothetical experiment involves children, and we must first generate a sample of child participants. Samples are used because populations are usually too large to reasonably involve every member in our particular experiment (Figure 20). If possible, we should use a random sample (there are other types of samples, but for the purposes of this section, we will focus on random samples). A random sample is a subset of a larger population in which every member of the population has an equal chance of being selected. Random samples are preferred because if the sample is large enough we can be reasonably sure that the participating individuals are representative of the larger population. This means that the percentages of characteristics in the sample—sex, ethnicity, socioeconomic level, and any other characteristics that might affect the results—are close to those percentages in the larger population.
In our example, let’s say we decide our population of interest is fourth graders. But all fourth graders is a very large population, so we need to be more specific; instead we might say our population of interest is all fourth graders in a particular city. We should include students from various income brackets, family situations, races, ethnicities, religions, and geographic areas of town. With this more manageable population, we can work with the local schools in selecting a random sample of around 200 fourth graders who we want to participate in our experiment.
In summary, because we cannot test all of the fourth graders in a city, we want to find a group of about 200 that reflects the composition of that city. With a representative group, we can generalize our findings to the larger population without fear of our sample being biased in some way.
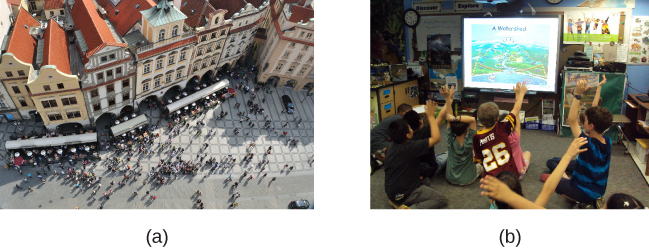
Now that we have a sample, the next step of the experimental process is to split the participants into experimental and control groups through random assignment. With random assignment , all participants have an equal chance of being assigned to either group. There is statistical software that will randomly assign each of the fourth graders in the sample to either the experimental or the control group.
Random assignment is critical for sound experimental design. With sufficiently large samples, random assignment makes it unlikely that there are systematic differences between the groups. So, for instance, it would be very unlikely that we would get one group composed entirely of males, a given ethnic identity, or a given religious ideology. This is important because if the groups were systematically different before the experiment began, we would not know the origin of any differences we find between the groups: Were the differences preexisting, or were they caused by manipulation of the independent variable? Random assignment allows us to assume that any differences observed between experimental and control groups result from the manipulation of the independent variable.
Issues to Consider
While experiments allow scientists to make cause-and-effect claims, they are not without problems. True experiments require the experimenter to manipulate an independent variable, and that can complicate many questions that psychologists might want to address. For instance, imagine that you want to know what effect sex (the independent variable) has on spatial memory (the dependent variable). Although you can certainly look for differences between males and females on a task that taps into spatial memory, you cannot directly control a person’s sex. We categorize this type of research approach as quasi-experimental and recognize that we cannot make cause-and-effect claims in these circumstances.
Experimenters are also limited by ethical constraints. For instance, you would not be able to conduct an experiment designed to determine if experiencing abuse as a child leads to lower levels of self-esteem among adults. To conduct such an experiment, you would need to randomly assign some experimental participants to a group that receives abuse, and that experiment would be unethical.
Introduction to Statistical Thinking
Psychologists use statistics to assist them in analyzing data, and also to give more precise measurements to describe whether something is statistically significant. Analyzing data using statistics enables researchers to find patterns, make claims, and share their results with others. In this section, you’ll learn about some of the tools that psychologists use in statistical analysis.
- Define reliability and validity
- Describe the importance of distributional thinking and the role of p-values in statistical inference
- Describe the role of random sampling and random assignment in drawing cause-and-effect conclusions
- Describe the basic structure of a psychological research article
Interpreting Experimental Findings
Once data is collected from both the experimental and the control groups, a statistical analysis is conducted to find out if there are meaningful differences between the two groups. A statistical analysis determines how likely any difference found is due to chance (and thus not meaningful). In psychology, group differences are considered meaningful, or significant, if the odds that these differences occurred by chance alone are 5 percent or less. Stated another way, if we repeated this experiment 100 times, we would expect to find the same results at least 95 times out of 100.
The greatest strength of experiments is the ability to assert that any significant differences in the findings are caused by the independent variable. This occurs because random selection, random assignment, and a design that limits the effects of both experimenter bias and participant expectancy should create groups that are similar in composition and treatment. Therefore, any difference between the groups is attributable to the independent variable, and now we can finally make a causal statement. If we find that watching a violent television program results in more violent behavior than watching a nonviolent program, we can safely say that watching violent television programs causes an increase in the display of violent behavior.
Reporting Research
When psychologists complete a research project, they generally want to share their findings with other scientists. The American Psychological Association (APA) publishes a manual detailing how to write a paper for submission to scientific journals. Unlike an article that might be published in a magazine like Psychology Today, which targets a general audience with an interest in psychology, scientific journals generally publish peer-reviewed journal articles aimed at an audience of professionals and scholars who are actively involved in research themselves.
A peer-reviewed journal article is read by several other scientists (generally anonymously) with expertise in the subject matter. These peer reviewers provide feedback—to both the author and the journal editor—regarding the quality of the draft. Peer reviewers look for a strong rationale for the research being described, a clear description of how the research was conducted, and evidence that the research was conducted in an ethical manner. They also look for flaws in the study’s design, methods, and statistical analyses. They check that the conclusions drawn by the authors seem reasonable given the observations made during the research. Peer reviewers also comment on how valuable the research is in advancing the discipline’s knowledge. This helps prevent unnecessary duplication of research findings in the scientific literature and, to some extent, ensures that each research article provides new information. Ultimately, the journal editor will compile all of the peer reviewer feedback and determine whether the article will be published in its current state (a rare occurrence), published with revisions, or not accepted for publication.
Peer review provides some degree of quality control for psychological research. Poorly conceived or executed studies can be weeded out, and even well-designed research can be improved by the revisions suggested. Peer review also ensures that the research is described clearly enough to allow other scientists to replicate it, meaning they can repeat the experiment using different samples to determine reliability. Sometimes replications involve additional measures that expand on the original finding. In any case, each replication serves to provide more evidence to support the original research findings. Successful replications of published research make scientists more apt to adopt those findings, while repeated failures tend to cast doubt on the legitimacy of the original article and lead scientists to look elsewhere. For example, it would be a major advancement in the medical field if a published study indicated that taking a new drug helped individuals achieve a healthy weight without changing their diet. But if other scientists could not replicate the results, the original study’s claims would be questioned.
Dig Deeper: The Vaccine-Autism Myth and the Retraction of Published Studies
Some scientists have claimed that routine childhood vaccines cause some children to develop autism, and, in fact, several peer-reviewed publications published research making these claims. Since the initial reports, large-scale epidemiological research has suggested that vaccinations are not responsible for causing autism and that it is much safer to have your child vaccinated than not. Furthermore, several of the original studies making this claim have since been retracted.
A published piece of work can be rescinded when data is called into question because of falsification, fabrication, or serious research design problems. Once rescinded, the scientific community is informed that there are serious problems with the original publication. Retractions can be initiated by the researcher who led the study, by research collaborators, by the institution that employed the researcher, or by the editorial board of the journal in which the article was originally published. In the vaccine-autism case, the retraction was made because of a significant conflict of interest in which the leading researcher had a financial interest in establishing a link between childhood vaccines and autism (Offit, 2008). Unfortunately, the initial studies received so much media attention that many parents around the world became hesitant to have their children vaccinated (Figure 21). For more information about how the vaccine/autism story unfolded, as well as the repercussions of this story, take a look at Paul Offit’s book, Autism’s False Prophets: Bad Science, Risky Medicine, and the Search for a Cure.
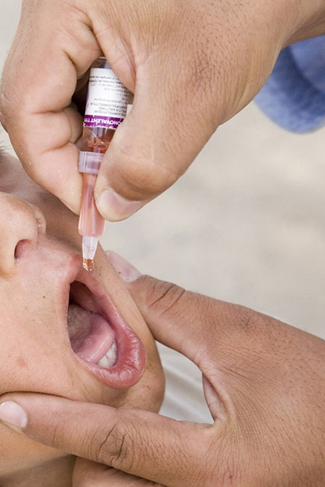
Reliability and Validity
Dig deeper: everyday connection: how valid is the sat.
Standardized tests like the SAT are supposed to measure an individual’s aptitude for a college education, but how reliable and valid are such tests? Research conducted by the College Board suggests that scores on the SAT have high predictive validity for first-year college students’ GPA (Kobrin, Patterson, Shaw, Mattern, & Barbuti, 2008). In this context, predictive validity refers to the test’s ability to effectively predict the GPA of college freshmen. Given that many institutions of higher education require the SAT for admission, this high degree of predictive validity might be comforting.
However, the emphasis placed on SAT scores in college admissions has generated some controversy on a number of fronts. For one, some researchers assert that the SAT is a biased test that places minority students at a disadvantage and unfairly reduces the likelihood of being admitted into a college (Santelices & Wilson, 2010). Additionally, some research has suggested that the predictive validity of the SAT is grossly exaggerated in how well it is able to predict the GPA of first-year college students. In fact, it has been suggested that the SAT’s predictive validity may be overestimated by as much as 150% (Rothstein, 2004). Many institutions of higher education are beginning to consider de-emphasizing the significance of SAT scores in making admission decisions (Rimer, 2008).
In 2014, College Board president David Coleman expressed his awareness of these problems, recognizing that college success is more accurately predicted by high school grades than by SAT scores. To address these concerns, he has called for significant changes to the SAT exam (Lewin, 2014).
Statistical Significance
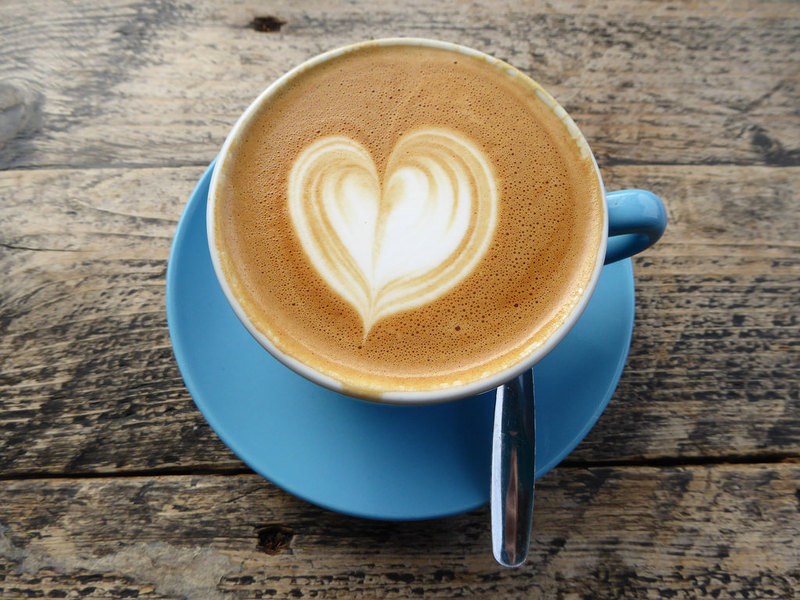
Does drinking coffee actually increase your life expectancy? A recent study (Freedman, Park, Abnet, Hollenbeck, & Sinha, 2012) found that men who drank at least six cups of coffee a day also had a 10% lower chance of dying (women’s chances were 15% lower) than those who drank none. Does this mean you should pick up or increase your own coffee habit? We will explore these results in more depth in the next section about drawing conclusions from statistics. Modern society has become awash in studies such as this; you can read about several such studies in the news every day.
Conducting such a study well, and interpreting the results of such studies requires understanding basic ideas of statistics , the science of gaining insight from data. Key components to a statistical investigation are:
- Planning the study: Start by asking a testable research question and deciding how to collect data. For example, how long was the study period of the coffee study? How many people were recruited for the study, how were they recruited, and from where? How old were they? What other variables were recorded about the individuals? Were changes made to the participants’ coffee habits during the course of the study?
- Examining the data: What are appropriate ways to examine the data? What graphs are relevant, and what do they reveal? What descriptive statistics can be calculated to summarize relevant aspects of the data, and what do they reveal? What patterns do you see in the data? Are there any individual observations that deviate from the overall pattern, and what do they reveal? For example, in the coffee study, did the proportions differ when we compared the smokers to the non-smokers?
- Inferring from the data: What are valid statistical methods for drawing inferences “beyond” the data you collected? In the coffee study, is the 10%–15% reduction in risk of death something that could have happened just by chance?
- Drawing conclusions: Based on what you learned from your data, what conclusions can you draw? Who do you think these conclusions apply to? (Were the people in the coffee study older? Healthy? Living in cities?) Can you draw a cause-and-effect conclusion about your treatments? (Are scientists now saying that the coffee drinking is the cause of the decreased risk of death?)
Notice that the numerical analysis (“crunching numbers” on the computer) comprises only a small part of overall statistical investigation. In this section, you will see how we can answer some of these questions and what questions you should be asking about any statistical investigation you read about.
Distributional Thinking
When data are collected to address a particular question, an important first step is to think of meaningful ways to organize and examine the data. Let’s take a look at an example.
Example 1 : Researchers investigated whether cancer pamphlets are written at an appropriate level to be read and understood by cancer patients (Short, Moriarty, & Cooley, 1995). Tests of reading ability were given to 63 patients. In addition, readability level was determined for a sample of 30 pamphlets, based on characteristics such as the lengths of words and sentences in the pamphlet. The results, reported in terms of grade levels, are displayed in Figure 23.

- Data vary . More specifically, values of a variable (such as reading level of a cancer patient or readability level of a cancer pamphlet) vary.
- Analyzing the pattern of variation, called the distribution of the variable, often reveals insights.
Addressing the research question of whether the cancer pamphlets are written at appropriate levels for the cancer patients requires comparing the two distributions. A naïve comparison might focus only on the centers of the distributions. Both medians turn out to be ninth grade, but considering only medians ignores the variability and the overall distributions of these data. A more illuminating approach is to compare the entire distributions, for example with a graph, as in Figure 24.
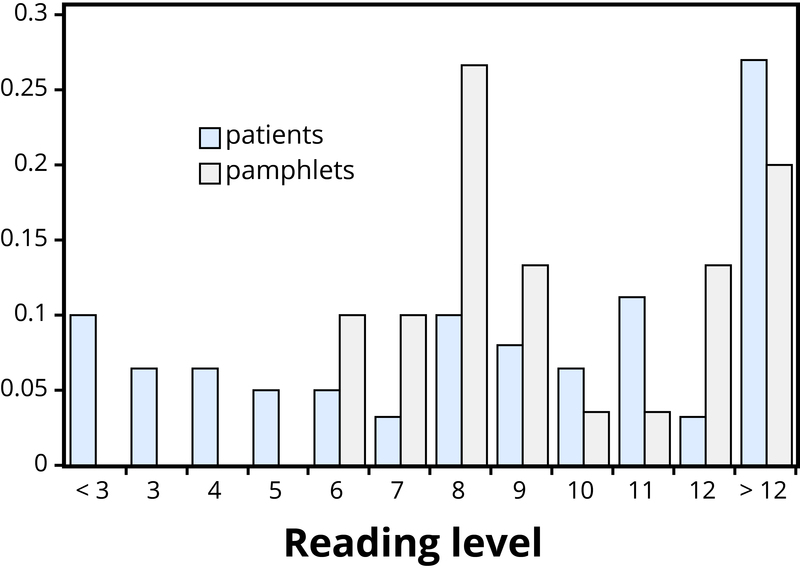
Figure 24 makes clear that the two distributions are not well aligned at all. The most glaring discrepancy is that many patients (17/63, or 27%, to be precise) have a reading level below that of the most readable pamphlet. These patients will need help to understand the information provided in the cancer pamphlets. Notice that this conclusion follows from considering the distributions as a whole, not simply measures of center or variability, and that the graph contrasts those distributions more immediately than the frequency tables.
Finding Significance in Data
Even when we find patterns in data, often there is still uncertainty in various aspects of the data. For example, there may be potential for measurement errors (even your own body temperature can fluctuate by almost 1°F over the course of the day). Or we may only have a “snapshot” of observations from a more long-term process or only a small subset of individuals from the population of interest. In such cases, how can we determine whether patterns we see in our small set of data is convincing evidence of a systematic phenomenon in the larger process or population? Let’s take a look at another example.
Example 2 : In a study reported in the November 2007 issue of Nature , researchers investigated whether pre-verbal infants take into account an individual’s actions toward others in evaluating that individual as appealing or aversive (Hamlin, Wynn, & Bloom, 2007). In one component of the study, 10-month-old infants were shown a “climber” character (a piece of wood with “googly” eyes glued onto it) that could not make it up a hill in two tries. Then the infants were shown two scenarios for the climber’s next try, one where the climber was pushed to the top of the hill by another character (“helper”), and one where the climber was pushed back down the hill by another character (“hinderer”). The infant was alternately shown these two scenarios several times. Then the infant was presented with two pieces of wood (representing the helper and the hinderer characters) and asked to pick one to play with.
The researchers found that of the 16 infants who made a clear choice, 14 chose to play with the helper toy. One possible explanation for this clear majority result is that the helping behavior of the one toy increases the infants’ likelihood of choosing that toy. But are there other possible explanations? What about the color of the toy? Well, prior to collecting the data, the researchers arranged so that each color and shape (red square and blue circle) would be seen by the same number of infants. Or maybe the infants had right-handed tendencies and so picked whichever toy was closer to their right hand?
Well, prior to collecting the data, the researchers arranged it so half the infants saw the helper toy on the right and half on the left. Or, maybe the shapes of these wooden characters (square, triangle, circle) had an effect? Perhaps, but again, the researchers controlled for this by rotating which shape was the helper toy, the hinderer toy, and the climber. When designing experiments, it is important to control for as many variables as might affect the responses as possible. It is beginning to appear that the researchers accounted for all the other plausible explanations. But there is one more important consideration that cannot be controlled—if we did the study again with these 16 infants, they might not make the same choices. In other words, there is some randomness inherent in their selection process.
Maybe each infant had no genuine preference at all, and it was simply “random luck” that led to 14 infants picking the helper toy. Although this random component cannot be controlled, we can apply a probability model to investigate the pattern of results that would occur in the long run if random chance were the only factor.
If the infants were equally likely to pick between the two toys, then each infant had a 50% chance of picking the helper toy. It’s like each infant tossed a coin, and if it landed heads, the infant picked the helper toy. So if we tossed a coin 16 times, could it land heads 14 times? Sure, it’s possible, but it turns out to be very unlikely. Getting 14 (or more) heads in 16 tosses is about as likely as tossing a coin and getting 9 heads in a row. This probability is referred to as a p-value . The p-value represents the likelihood that experimental results happened by chance. Within psychology, the most common standard for p-values is “p < .05”. What this means is that there is less than a 5% probability that the results happened just by random chance, and therefore a 95% probability that the results reflect a meaningful pattern in human psychology. We call this statistical significance .
So, in the study above, if we assume that each infant was choosing equally, then the probability that 14 or more out of 16 infants would choose the helper toy is found to be 0.0021. We have only two logical possibilities: either the infants have a genuine preference for the helper toy, or the infants have no preference (50/50) and an outcome that would occur only 2 times in 1,000 iterations happened in this study. Because this p-value of 0.0021 is quite small, we conclude that the study provides very strong evidence that these infants have a genuine preference for the helper toy.
If we compare the p-value to some cut-off value, like 0.05, we see that the p=value is smaller. Because the p-value is smaller than that cut-off value, then we reject the hypothesis that only random chance was at play here. In this case, these researchers would conclude that significantly more than half of the infants in the study chose the helper toy, giving strong evidence of a genuine preference for the toy with the helping behavior.
Drawing Conclusions from Statistics
Generalizability.
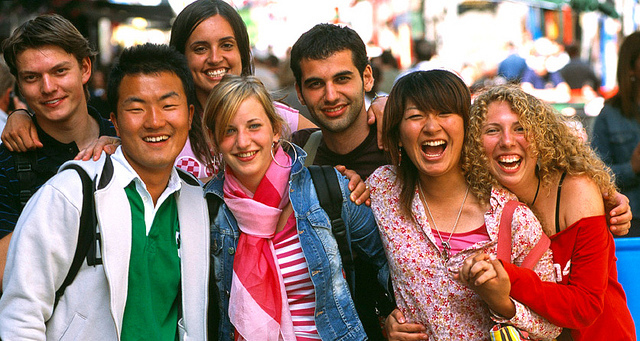
One limitation to the study mentioned previously about the babies choosing the “helper” toy is that the conclusion only applies to the 16 infants in the study. We don’t know much about how those 16 infants were selected. Suppose we want to select a subset of individuals (a sample ) from a much larger group of individuals (the population ) in such a way that conclusions from the sample can be generalized to the larger population. This is the question faced by pollsters every day.
Example 3 : The General Social Survey (GSS) is a survey on societal trends conducted every other year in the United States. Based on a sample of about 2,000 adult Americans, researchers make claims about what percentage of the U.S. population consider themselves to be “liberal,” what percentage consider themselves “happy,” what percentage feel “rushed” in their daily lives, and many other issues. The key to making these claims about the larger population of all American adults lies in how the sample is selected. The goal is to select a sample that is representative of the population, and a common way to achieve this goal is to select a r andom sample that gives every member of the population an equal chance of being selected for the sample. In its simplest form, random sampling involves numbering every member of the population and then using a computer to randomly select the subset to be surveyed. Most polls don’t operate exactly like this, but they do use probability-based sampling methods to select individuals from nationally representative panels.
In 2004, the GSS reported that 817 of 977 respondents (or 83.6%) indicated that they always or sometimes feel rushed. This is a clear majority, but we again need to consider variation due to random sampling . Fortunately, we can use the same probability model we did in the previous example to investigate the probable size of this error. (Note, we can use the coin-tossing model when the actual population size is much, much larger than the sample size, as then we can still consider the probability to be the same for every individual in the sample.) This probability model predicts that the sample result will be within 3 percentage points of the population value (roughly 1 over the square root of the sample size, the margin of error. A statistician would conclude, with 95% confidence, that between 80.6% and 86.6% of all adult Americans in 2004 would have responded that they sometimes or always feel rushed.
The key to the margin of error is that when we use a probability sampling method, we can make claims about how often (in the long run, with repeated random sampling) the sample result would fall within a certain distance from the unknown population value by chance (meaning by random sampling variation) alone. Conversely, non-random samples are often suspect to bias, meaning the sampling method systematically over-represents some segments of the population and under-represents others. We also still need to consider other sources of bias, such as individuals not responding honestly. These sources of error are not measured by the margin of error.
Cause and Effect
In many research studies, the primary question of interest concerns differences between groups. Then the question becomes how were the groups formed (e.g., selecting people who already drink coffee vs. those who don’t). In some studies, the researchers actively form the groups themselves. But then we have a similar question—could any differences we observe in the groups be an artifact of that group-formation process? Or maybe the difference we observe in the groups is so large that we can discount a “fluke” in the group-formation process as a reasonable explanation for what we find?
Example 4 : A psychology study investigated whether people tend to display more creativity when they are thinking about intrinsic (internal) or extrinsic (external) motivations (Ramsey & Schafer, 2002, based on a study by Amabile, 1985). The subjects were 47 people with extensive experience with creative writing. Subjects began by answering survey questions about either intrinsic motivations for writing (such as the pleasure of self-expression) or extrinsic motivations (such as public recognition). Then all subjects were instructed to write a haiku, and those poems were evaluated for creativity by a panel of judges. The researchers conjectured beforehand that subjects who were thinking about intrinsic motivations would display more creativity than subjects who were thinking about extrinsic motivations. The creativity scores from the 47 subjects in this study are displayed in Figure 26, where higher scores indicate more creativity.
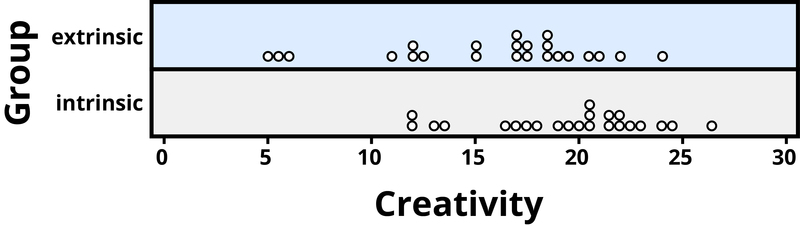
In this example, the key question is whether the type of motivation affects creativity scores. In particular, do subjects who were asked about intrinsic motivations tend to have higher creativity scores than subjects who were asked about extrinsic motivations?
Figure 26 reveals that both motivation groups saw considerable variability in creativity scores, and these scores have considerable overlap between the groups. In other words, it’s certainly not always the case that those with extrinsic motivations have higher creativity than those with intrinsic motivations, but there may still be a statistical tendency in this direction. (Psychologist Keith Stanovich (2013) refers to people’s difficulties with thinking about such probabilistic tendencies as “the Achilles heel of human cognition.”)
The mean creativity score is 19.88 for the intrinsic group, compared to 15.74 for the extrinsic group, which supports the researchers’ conjecture. Yet comparing only the means of the two groups fails to consider the variability of creativity scores in the groups. We can measure variability with statistics using, for instance, the standard deviation: 5.25 for the extrinsic group and 4.40 for the intrinsic group. The standard deviations tell us that most of the creativity scores are within about 5 points of the mean score in each group. We see that the mean score for the intrinsic group lies within one standard deviation of the mean score for extrinsic group. So, although there is a tendency for the creativity scores to be higher in the intrinsic group, on average, the difference is not extremely large.
We again want to consider possible explanations for this difference. The study only involved individuals with extensive creative writing experience. Although this limits the population to which we can generalize, it does not explain why the mean creativity score was a bit larger for the intrinsic group than for the extrinsic group. Maybe women tend to receive higher creativity scores? Here is where we need to focus on how the individuals were assigned to the motivation groups. If only women were in the intrinsic motivation group and only men in the extrinsic group, then this would present a problem because we wouldn’t know if the intrinsic group did better because of the different type of motivation or because they were women. However, the researchers guarded against such a problem by randomly assigning the individuals to the motivation groups. Like flipping a coin, each individual was just as likely to be assigned to either type of motivation. Why is this helpful? Because this random assignment tends to balance out all the variables related to creativity we can think of, and even those we don’t think of in advance, between the two groups. So we should have a similar male/female split between the two groups; we should have a similar age distribution between the two groups; we should have a similar distribution of educational background between the two groups; and so on. Random assignment should produce groups that are as similar as possible except for the type of motivation, which presumably eliminates all those other variables as possible explanations for the observed tendency for higher scores in the intrinsic group.
But does this always work? No, so by “luck of the draw” the groups may be a little different prior to answering the motivation survey. So then the question is, is it possible that an unlucky random assignment is responsible for the observed difference in creativity scores between the groups? In other words, suppose each individual’s poem was going to get the same creativity score no matter which group they were assigned to, that the type of motivation in no way impacted their score. Then how often would the random-assignment process alone lead to a difference in mean creativity scores as large (or larger) than 19.88 – 15.74 = 4.14 points?
We again want to apply to a probability model to approximate a p-value , but this time the model will be a bit different. Think of writing everyone’s creativity scores on an index card, shuffling up the index cards, and then dealing out 23 to the extrinsic motivation group and 24 to the intrinsic motivation group, and finding the difference in the group means. We (better yet, the computer) can repeat this process over and over to see how often, when the scores don’t change, random assignment leads to a difference in means at least as large as 4.41. Figure 27 shows the results from 1,000 such hypothetical random assignments for these scores.
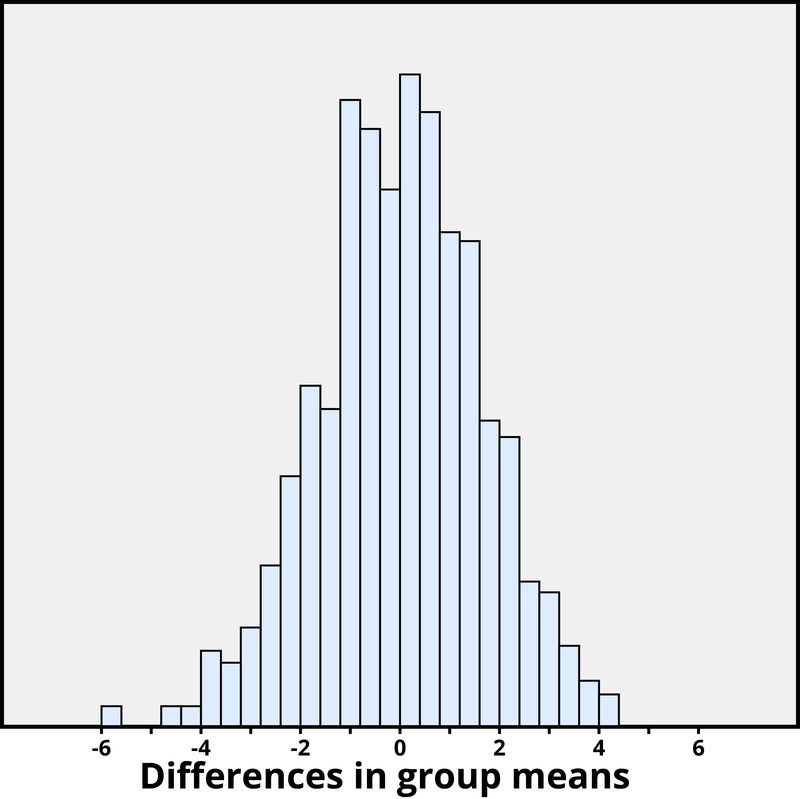
Only 2 of the 1,000 simulated random assignments produced a difference in group means of 4.41 or larger. In other words, the approximate p-value is 2/1000 = 0.002. This small p-value indicates that it would be very surprising for the random assignment process alone to produce such a large difference in group means. Therefore, as with Example 2, we have strong evidence that focusing on intrinsic motivations tends to increase creativity scores, as compared to thinking about extrinsic motivations.
Notice that the previous statement implies a cause-and-effect relationship between motivation and creativity score; is such a strong conclusion justified? Yes, because of the random assignment used in the study. That should have balanced out any other variables between the two groups, so now that the small p-value convinces us that the higher mean in the intrinsic group wasn’t just a coincidence, the only reasonable explanation left is the difference in the type of motivation. Can we generalize this conclusion to everyone? Not necessarily—we could cautiously generalize this conclusion to individuals with extensive experience in creative writing similar the individuals in this study, but we would still want to know more about how these individuals were selected to participate.
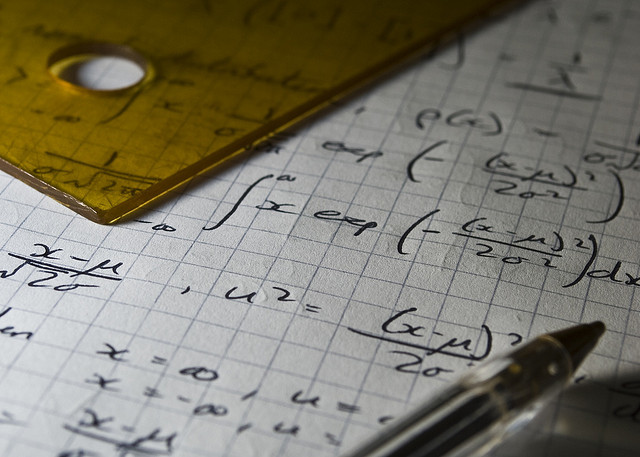
Statistical thinking involves the careful design of a study to collect meaningful data to answer a focused research question, detailed analysis of patterns in the data, and drawing conclusions that go beyond the observed data. Random sampling is paramount to generalizing results from our sample to a larger population, and random assignment is key to drawing cause-and-effect conclusions. With both kinds of randomness, probability models help us assess how much random variation we can expect in our results, in order to determine whether our results could happen by chance alone and to estimate a margin of error.
So where does this leave us with regard to the coffee study mentioned previously (the Freedman, Park, Abnet, Hollenbeck, & Sinha, 2012 found that men who drank at least six cups of coffee a day had a 10% lower chance of dying (women 15% lower) than those who drank none)? We can answer many of the questions:
- This was a 14-year study conducted by researchers at the National Cancer Institute.
- The results were published in the June issue of the New England Journal of Medicine , a respected, peer-reviewed journal.
- The study reviewed coffee habits of more than 402,000 people ages 50 to 71 from six states and two metropolitan areas. Those with cancer, heart disease, and stroke were excluded at the start of the study. Coffee consumption was assessed once at the start of the study.
- About 52,000 people died during the course of the study.
- People who drank between two and five cups of coffee daily showed a lower risk as well, but the amount of reduction increased for those drinking six or more cups.
- The sample sizes were fairly large and so the p-values are quite small, even though percent reduction in risk was not extremely large (dropping from a 12% chance to about 10%–11%).
- Whether coffee was caffeinated or decaffeinated did not appear to affect the results.
- This was an observational study, so no cause-and-effect conclusions can be drawn between coffee drinking and increased longevity, contrary to the impression conveyed by many news headlines about this study. In particular, it’s possible that those with chronic diseases don’t tend to drink coffee.
This study needs to be reviewed in the larger context of similar studies and consistency of results across studies, with the constant caution that this was not a randomized experiment. Whereas a statistical analysis can still “adjust” for other potential confounding variables, we are not yet convinced that researchers have identified them all or completely isolated why this decrease in death risk is evident. Researchers can now take the findings of this study and develop more focused studies that address new questions.
Explore these outside resources to learn more about applied statistics:
- Video about p-values: P-Value Extravaganza
- Interactive web applets for teaching and learning statistics
- Inter-university Consortium for Political and Social Research where you can find and analyze data.
- The Consortium for the Advancement of Undergraduate Statistics
- Find a recent research article in your field and answer the following: What was the primary research question? How were individuals selected to participate in the study? Were summary results provided? How strong is the evidence presented in favor or against the research question? Was random assignment used? Summarize the main conclusions from the study, addressing the issues of statistical significance, statistical confidence, generalizability, and cause and effect. Do you agree with the conclusions drawn from this study, based on the study design and the results presented?
- Is it reasonable to use a random sample of 1,000 individuals to draw conclusions about all U.S. adults? Explain why or why not.
How to Read Research
In this course and throughout your academic career, you’ll be reading journal articles (meaning they were published by experts in a peer-reviewed journal) and reports that explain psychological research. It’s important to understand the format of these articles so that you can read them strategically and understand the information presented. Scientific articles vary in content or structure, depending on the type of journal to which they will be submitted. Psychological articles and many papers in the social sciences follow the writing guidelines and format dictated by the American Psychological Association (APA). In general, the structure follows: abstract, introduction, methods, results, discussion, and references.
- Abstract : the abstract is the concise summary of the article. It summarizes the most important features of the manuscript, providing the reader with a global first impression on the article. It is generally just one paragraph that explains the experiment as well as a short synopsis of the results.
- Introduction : this section provides background information about the origin and purpose of performing the experiment or study. It reviews previous research and presents existing theories on the topic.
- Method : this section covers the methodologies used to investigate the research question, including the identification of participants , procedures , and materials as well as a description of the actual procedure . It should be sufficiently detailed to allow for replication.
- Results : the results section presents key findings of the research, including reference to indicators of statistical significance.
- Discussion : this section provides an interpretation of the findings, states their significance for current research, and derives implications for theory and practice. Alternative interpretations for findings are also provided, particularly when it is not possible to conclude for the directionality of the effects. In the discussion, authors also acknowledge the strengths and limitations/weaknesses of the study and offer concrete directions about for future research.
Watch this 3-minute video for an explanation on how to read scholarly articles. Look closely at the example article shared just before the two minute mark.
https://digitalcommons.coastal.edu/kimbel-library-instructional-videos/9/
Practice identifying these key components in the following experiment: Food-Induced Emotional Resonance Improves Emotion Recognition.
In this chapter, you learned to
- define and apply the scientific method to psychology
- describe the strengths and weaknesses of descriptive, experimental, and correlational research
- define the basic elements of a statistical investigation
Putting It Together: Psychological Research
Psychologists use the scientific method to examine human behavior and mental processes. Some of the methods you learned about include descriptive, experimental, and correlational research designs.
Watch the CrashCourse video to review the material you learned, then read through the following examples and see if you can come up with your own design for each type of study.
You can view the transcript for “Psychological Research: Crash Course Psychology #2” here (opens in new window).
Case Study: a detailed analysis of a particular person, group, business, event, etc. This approach is commonly used to to learn more about rare examples with the goal of describing that particular thing.
- Ted Bundy was one of America’s most notorious serial killers who murdered at least 30 women and was executed in 1989. Dr. Al Carlisle evaluated Bundy when he was first arrested and conducted a psychological analysis of Bundy’s development of his sexual fantasies merging into reality (Ramsland, 2012). Carlisle believes that there was a gradual evolution of three processes that guided his actions: fantasy, dissociation, and compartmentalization (Ramsland, 2012). Read Imagining Ted Bundy (http://goo.gl/rGqcUv) for more information on this case study.
Naturalistic Observation : a researcher unobtrusively collects information without the participant’s awareness.
- Drain and Engelhardt (2013) observed six nonverbal children with autism’s evoked and spontaneous communicative acts. Each of the children attended a school for children with autism and were in different classes. They were observed for 30 minutes of each school day. By observing these children without them knowing, they were able to see true communicative acts without any external influences.
Survey : participants are asked to provide information or responses to questions on a survey or structure assessment.
- Educational psychologists can ask students to report their grade point average and what, if anything, they eat for breakfast on an average day. A healthy breakfast has been associated with better academic performance (Digangi’s 1999).
- Anderson (1987) tried to find the relationship between uncomfortably hot temperatures and aggressive behavior, which was then looked at with two studies done on violent and nonviolent crime. Based on previous research that had been done by Anderson and Anderson (1984), it was predicted that violent crimes would be more prevalent during the hotter time of year and the years in which it was hotter weather in general. The study confirmed this prediction.
Longitudinal Study: researchers recruit a sample of participants and track them for an extended period of time.
- In a study of a representative sample of 856 children Eron and his colleagues (1972) found that a boy’s exposure to media violence at age eight was significantly related to his aggressive behavior ten years later, after he graduated from high school.
Cross-Sectional Study: researchers gather participants from different groups (commonly different ages) and look for differences between the groups.
- In 1996, Russell surveyed people of varying age groups and found that people in their 20s tend to report being more lonely than people in their 70s.
Correlational Design: two different variables are measured to determine whether there is a relationship between them.
- Thornhill et al. (2003) had people rate how physically attractive they found other people to be. They then had them separately smell t-shirts those people had worn (without knowing which clothes belonged to whom) and rate how good or bad their body oder was. They found that the more attractive someone was the more pleasant their body order was rated to be.
- Clinical psychologists can test a new pharmaceutical treatment for depression by giving some patients the new pill and others an already-tested one to see which is the more effective treatment.
American Cancer Society. (n.d.). History of the cancer prevention studies. Retrieved from http://www.cancer.org/research/researchtopreventcancer/history-cancer-prevention-study
American Psychological Association. (2009). Publication Manual of the American Psychological Association (6th ed.). Washington, DC: Author.
American Psychological Association. (n.d.). Research with animals in psychology. Retrieved from https://www.apa.org/research/responsible/research-animals.pdf
Arnett, J. (2008). The neglected 95%: Why American psychology needs to become less American. American Psychologist, 63(7), 602–614.
Barton, B. A., Eldridge, A. L., Thompson, D., Affenito, S. G., Striegel-Moore, R. H., Franko, D. L., . . . Crockett, S. J. (2005). The relationship of breakfast and cereal consumption to nutrient intake and body mass index: The national heart, lung, and blood institute growth and health study. Journal of the American Dietetic Association, 105(9), 1383–1389. Retrieved from http://dx.doi.org/10.1016/j.jada.2005.06.003
Chwalisz, K., Diener, E., & Gallagher, D. (1988). Autonomic arousal feedback and emotional experience: Evidence from the spinal cord injured. Journal of Personality and Social Psychology, 54, 820–828.
Dominus, S. (2011, May 25). Could conjoined twins share a mind? New York Times Sunday Magazine. Retrieved from http://www.nytimes.com/2011/05/29/magazine/could-conjoined-twins-share-a-mind.html?_r=5&hp&
Fanger, S. M., Frankel, L. A., & Hazen, N. (2012). Peer exclusion in preschool children’s play: Naturalistic observations in a playground setting. Merrill-Palmer Quarterly, 58, 224–254.
Fiedler, K. (2004). Illusory correlation. In R. F. Pohl (Ed.), Cognitive illusions: A handbook on fallacies and biases in thinking, judgment and memory (pp. 97–114). New York, NY: Psychology Press.
Frantzen, L. B., Treviño, R. P., Echon, R. M., Garcia-Dominic, O., & DiMarco, N. (2013). Association between frequency of ready-to-eat cereal consumption, nutrient intakes, and body mass index in fourth- to sixth-grade low-income minority children. Journal of the Academy of Nutrition and Dietetics, 113(4), 511–519.
Harper, J. (2013, July 5). Ice cream and crime: Where cold cuisine and hot disputes intersect. The Times-Picaune. Retrieved from http://www.nola.com/crime/index.ssf/2013/07/ice_cream_and_crime_where_hot.html
Jenkins, W. J., Ruppel, S. E., Kizer, J. B., Yehl, J. L., & Griffin, J. L. (2012). An examination of post 9-11 attitudes towards Arab Americans. North American Journal of Psychology, 14, 77–84.
Jones, J. M. (2013, May 13). Same-sex marriage support solidifies above 50% in U.S. Gallup Politics. Retrieved from http://www.gallup.com/poll/162398/sex-marriage-support-solidifies-above.aspx
Kobrin, J. L., Patterson, B. F., Shaw, E. J., Mattern, K. D., & Barbuti, S. M. (2008). Validity of the SAT for predicting first-year college grade point average (Research Report No. 2008-5). Retrieved from https://research.collegeboard.org/sites/default/files/publications/2012/7/researchreport-2008-5-validity-sat-predicting-first-year-college-grade-point-average.pdf
Lewin, T. (2014, March 5). A new SAT aims to realign with schoolwork. New York Times. Retreived from http://www.nytimes.com/2014/03/06/education/major-changes-in-sat-announced-by-college-board.html.
Lowry, M., Dean, K., & Manders, K. (2010). The link between sleep quantity and academic performance for the college student. Sentience: The University of Minnesota Undergraduate Journal of Psychology, 3(Spring), 16–19. Retrieved from http://www.psych.umn.edu/sentience/files/SENTIENCE_Vol3.pdf
McKie, R. (2010, June 26). Chimps with everything: Jane Goodall’s 50 years in the jungle. The Guardian. Retrieved from http://www.theguardian.com/science/2010/jun/27/jane-goodall-chimps-africa-interview
Offit, P. (2008). Autism’s false prophets: Bad science, risky medicine, and the search for a cure. New York: Columbia University Press.
Perkins, H. W., Haines, M. P., & Rice, R. (2005). Misperceiving the college drinking norm and related problems: A nationwide study of exposure to prevention information, perceived norms and student alcohol misuse. J. Stud. Alcohol, 66(4), 470–478.
Rimer, S. (2008, September 21). College panel calls for less focus on SATs. The New York Times. Retrieved from http://www.nytimes.com/2008/09/22/education/22admissions.html?_r=0
Rothstein, J. M. (2004). College performance predictions and the SAT. Journal of Econometrics, 121, 297–317.
Rotton, J., & Kelly, I. W. (1985). Much ado about the full moon: A meta-analysis of lunar-lunacy research. Psychological Bulletin, 97(2), 286–306. doi:10.1037/0033-2909.97.2.286
Santelices, M. V., & Wilson, M. (2010). Unfair treatment? The case of Freedle, the SAT, and the standardization approach to differential item functioning. Harvard Education Review, 80, 106–134.
Sears, D. O. (1986). College sophomores in the laboratory: Influences of a narrow data base on social psychology’s view of human nature. Journal of Personality and Social Psychology, 51, 515–530.
Tuskegee University. (n.d.). About the USPHS Syphilis Study. Retrieved from http://www.tuskegee.edu/about_us/centers_of_excellence/bioethics_center/about_the_usphs_syphilis_study.aspx.
CC licensed content, Original
- Psychological Research Methods. Provided by : Karenna Malavanti. License : CC BY-SA: Attribution ShareAlike
CC licensed content, Shared previously
- Psychological Research. Provided by : OpenStax College. License : CC BY: Attribution . License Terms : Download for free at https://openstax.org/books/psychology-2e/pages/1-introduction. Located at : https://openstax.org/books/psychology-2e/pages/2-introduction .
- Why It Matters: Psychological Research. Provided by : Lumen Learning. License : CC BY: Attribution Located at: https://pressbooks.online.ucf.edu/lumenpsychology/chapter/introduction-15/
- Introduction to The Scientific Method. Provided by : Lumen Learning. License : CC BY: Attribution Located at: https://pressbooks.online.ucf.edu/lumenpsychology/chapter/outcome-the-scientific-method/
- Research picture. Authored by : Mediterranean Center of Medical Sciences. Provided by : Flickr. License : CC BY: Attribution Located at : https://www.flickr.com/photos/mcmscience/17664002728 .
- The Scientific Process. Provided by : Lumen Learning. License : CC BY-SA: Attribution ShareAlike Located at: https://pressbooks.online.ucf.edu/lumenpsychology/chapter/reading-the-scientific-process/
- Ethics in Research. Provided by : Lumen Learning. License : CC BY: Attribution Located at: https://pressbooks.online.ucf.edu/lumenpsychology/chapter/ethics/
- Ethics. Authored by : OpenStax College. Located at : https://openstax.org/books/psychology-2e/pages/2-4-ethics . License : CC BY: Attribution . License Terms : Download for free at https://openstax.org/books/psychology-2e/pages/1-introduction .
- Introduction to Approaches to Research. Provided by : Lumen Learning. License : CC BY-NC-SA: Attribution NonCommercial ShareAlike Located at: https://pressbooks.online.ucf.edu/lumenpsychology/chapter/outcome-approaches-to-research/
- Lec 2 | MIT 9.00SC Introduction to Psychology, Spring 2011. Authored by : John Gabrieli. Provided by : MIT OpenCourseWare. License : CC BY-NC-SA: Attribution-NonCommercial-ShareAlike Located at : https://www.youtube.com/watch?v=syXplPKQb_o .
- Paragraph on correlation. Authored by : Christie Napa Scollon. Provided by : Singapore Management University. License : CC BY-NC-SA: Attribution-NonCommercial-ShareAlike Located at : http://nobaproject.com/modules/research-designs?r=MTc0ODYsMjMzNjQ%3D . Project : The Noba Project.
- Descriptive Research. Provided by : Lumen Learning. License : CC BY-SA: Attribution ShareAlike Located at: https://pressbooks.online.ucf.edu/lumenpsychology/chapter/reading-clinical-or-case-studies/
- Approaches to Research. Authored by : OpenStax College. License : CC BY: Attribution . License Terms : Download for free at https://openstax.org/books/psychology-2e/pages/1-introduction. Located at : https://openstax.org/books/psychology-2e/pages/2-2-approaches-to-research
- Analyzing Findings. Authored by : OpenStax College. Located at : https://openstax.org/books/psychology-2e/pages/2-3-analyzing-findings . License : CC BY: Attribution . License Terms : Download for free at https://openstax.org/books/psychology-2e/pages/1-introduction.
- Experiments. Provided by : Lumen Learning. License : CC BY: Attribution Located at: https://pressbooks.online.ucf.edu/lumenpsychology/chapter/reading-conducting-experiments/
- Research Review. Authored by : Jessica Traylor for Lumen Learning. License : CC BY: Attribution Located at: https://pressbooks.online.ucf.edu/lumenpsychology/chapter/reading-conducting-experiments/
- Introduction to Statistics. Provided by : Lumen Learning. License : CC BY: Attribution Located at: https://pressbooks.online.ucf.edu/lumenpsychology/chapter/outcome-statistical-thinking/
- histogram. Authored by : Fisher’s Iris flower data set. Provided by : Wikipedia.
- License : CC BY-SA: Attribution-ShareAlike Located at : https://en.wikipedia.org/wiki/Wikipedia:Meetup/DC/Statistics_Edit-a-thon#/media/File:Fisher_iris_versicolor_sepalwidth.svg .
- Statistical Thinking. Authored by : Beth Chance and Allan Rossman . Provided by : California Polytechnic State University, San Luis Obispo.
- License : CC BY-NC-SA: Attribution-NonCommerci al-S hareAlike . License Terms : http://nobaproject.com/license-agreement Located at : http://nobaproject.com/modules/statistical-thinking . Project : The Noba Project.
- Drawing Conclusions from Statistics. Authored by: Pat Carroll and Lumen Learning. Provided by : Lumen Learning. License : CC BY: Attribution Located at: https://pressbooks.online.ucf.edu/lumenpsychology/chapter/reading-drawing-conclusions-from-statistics/
- Statistical Thinking. Authored by : Beth Chance and Allan Rossman, California Polytechnic State University, San Luis Obispo. Provided by : Noba. License: CC BY-NC-SA: Attribution-NonCommercial-ShareAlike Located at : http://nobaproject.com/modules/statistical-thinking .
- The Replication Crisis. Authored by : Colin Thomas William. Provided by : Ivy Tech Community College. License: CC BY: Attribution
- How to Read Research. Provided by : Lumen Learning. License : CC BY: Attribution Located at: https://pressbooks.online.ucf.edu/lumenpsychology/chapter/how-to-read-research/
- What is a Scholarly Article? Kimbel Library First Year Experience Instructional Videos. 9. Authored by: Joshua Vossler, John Watts, and Tim Hodge. Provided by : Coastal Carolina University License : CC BY NC ND: Attribution-NonCommercial-NoDerivatives Located at : https://digitalcommons.coastal.edu/kimbel-library-instructional-videos/9/
- Putting It Together: Psychological Research. Provided by : Lumen Learning. License : CC BY: Attribution Located at: https://pressbooks.online.ucf.edu/lumenpsychology/chapter/putting-it-together-psychological-research/
- Research. Provided by : Lumen Learning. License : CC BY: Attribution Located at:
All rights reserved content
- Understanding Driver Distraction. Provided by : American Psychological Association. License : Other. License Terms: Standard YouTube License Located at : https://www.youtube.com/watch?v=XToWVxS_9lA&list=PLxf85IzktYWJ9MrXwt5GGX3W-16XgrwPW&index=9 .
- Correlation vs. Causality: Freakonomics Movie. License : Other. License Terms : Standard YouTube License Located at : https://www.youtube.com/watch?v=lbODqslc4Tg.
- Psychological Research – Crash Course Psychology #2. Authored by : Hank Green. Provided by : Crash Course. License : Other. License Terms : Standard YouTube License Located at : https://www.youtube.com/watch?v=hFV71QPvX2I .
Public domain content
- Researchers review documents. Authored by : National Cancer Institute. Provided by : Wikimedia. Located at : https://commons.wikimedia.org/wiki/File:Researchers_review_documents.jpg . License : Public Domain: No Known Copyright
grounded in objective, tangible evidence that can be observed time and time again, regardless of who is observing
well-developed set of ideas that propose an explanation for observed phenomena
(plural: hypotheses) tentative and testable statement about the relationship between two or more variables
an experiment must be replicable by another researcher
implies that a theory should enable us to make predictions about future events
able to be disproven by experimental results
implies that all data must be considered when evaluating a hypothesis
committee of administrators, scientists, and community members that reviews proposals for research involving human participants
process of informing a research participant about what to expect during an experiment, any risks involved, and the implications of the research, and then obtaining the person’s consent to participate
purposely misleading experiment participants in order to maintain the integrity of the experiment
when an experiment involved deception, participants are told complete and truthful information about the experiment at its conclusion
committee of administrators, scientists, veterinarians, and community members that reviews proposals for research involving non-human animals
research studies that do not test specific relationships between variables
research investigating the relationship between two or more variables
research method that uses hypothesis testing to make inferences about how one variable impacts and causes another
observation of behavior in its natural setting
inferring that the results for a sample apply to the larger population
when observations may be skewed to align with observer expectations
measure of agreement among observers on how they record and classify a particular event
observational research study focusing on one or a few people
list of questions to be answered by research participants—given as paper-and-pencil questionnaires, administered electronically, or conducted verbally—allowing researchers to collect data from a large number of people
subset of individuals selected from the larger population
overall group of individuals that the researchers are interested in
method of research using past records or data sets to answer various research questions, or to search for interesting patterns or relationships
studies in which the same group of individuals is surveyed or measured repeatedly over an extended period of time
compares multiple segments of a population at a single time
reduction in number of research participants as some drop out of the study over time
relationship between two or more variables; when two variables are correlated, one variable changes as the other does
number from -1 to +1, indicating the strength and direction of the relationship between variables, and usually represented by r
two variables change in the same direction, both becoming either larger or smaller
two variables change in different directions, with one becoming larger as the other becomes smaller; a negative correlation is not the same thing as no correlation
changes in one variable cause the changes in the other variable; can be determined only through an experimental research design
unanticipated outside factor that affects both variables of interest, often giving the false impression that changes in one variable causes changes in the other variable, when, in actuality, the outside factor causes changes in both variables
seeing relationships between two things when in reality no such relationship exists
tendency to ignore evidence that disproves ideas or beliefs
group designed to answer the research question; experimental manipulation is the only difference between the experimental and control groups, so any differences between the two are due to experimental manipulation rather than chance
serves as a basis for comparison and controls for chance factors that might influence the results of the study—by holding such factors constant across groups so that the experimental manipulation is the only difference between groups
description of what actions and operations will be used to measure the dependent variables and manipulate the independent variables
researcher expectations skew the results of the study
experiment in which the researcher knows which participants are in the experimental group and which are in the control group
experiment in which both the researchers and the participants are blind to group assignments
people's expectations or beliefs influencing or determining their experience in a given situation
variable that is influenced or controlled by the experimenter; in a sound experimental study, the independent variable is the only important difference between the experimental and control group
variable that the researcher measures to see how much effect the independent variable had
subjects of psychological research
subset of a larger population in which every member of the population has an equal chance of being selected
method of experimental group assignment in which all participants have an equal chance of being assigned to either group
consistency and reproducibility of a given result
accuracy of a given result in measuring what it is designed to measure
determines how likely any difference between experimental groups is due to chance
statistical probability that represents the likelihood that experimental results happened by chance
Psychological Science is the scientific study of mind, brain, and behavior. We will explore what it means to be human in this class. It has never been more important for us to understand what makes people tick, how to evaluate information critically, and the importance of history. Psychology can also help you in your future career; indeed, there are very little jobs out there with no human interaction!
Because psychology is a science, we analyze human behavior through the scientific method. There are several ways to investigate human phenomena, such as observation, experiments, and more. We will discuss the basics, pros and cons of each! We will also dig deeper into the important ethical guidelines that psychologists must follow in order to do research. Lastly, we will briefly introduce ourselves to statistics, the language of scientific research. While reading the content in these chapters, try to find examples of material that can fit with the themes of the course.
To get us started:
- The study of the mind moved away Introspection to reaction time studies as we learned more about empiricism
- Psychologists work in careers outside of the typical "clinician" role. We advise in human factors, education, policy, and more!
- While completing an observation study, psychologists will work to aggregate common themes to explain the behavior of the group (sample) as a whole. In doing so, we still allow for normal variation from the group!
- The IRB and IACUC are important in ensuring ethics are maintained for both human and animal subjects
Psychological Science: Understanding Human Behavior Copyright © by Karenna Malavanti is licensed under a Creative Commons Attribution-NonCommercial-ShareAlike 4.0 International License , except where otherwise noted.
Share This Book
Observation Method in Psychology: Naturalistic, Participant and Controlled
Saul Mcleod, PhD
Editor-in-Chief for Simply Psychology
BSc (Hons) Psychology, MRes, PhD, University of Manchester
Saul Mcleod, PhD., is a qualified psychology teacher with over 18 years of experience in further and higher education. He has been published in peer-reviewed journals, including the Journal of Clinical Psychology.
Learn about our Editorial Process
Olivia Guy-Evans, MSc
Associate Editor for Simply Psychology
BSc (Hons) Psychology, MSc Psychology of Education
Olivia Guy-Evans is a writer and associate editor for Simply Psychology. She has previously worked in healthcare and educational sectors.
On This Page:
The observation method in psychology involves directly and systematically witnessing and recording measurable behaviors, actions, and responses in natural or contrived settings without attempting to intervene or manipulate what is being observed.
Used to describe phenomena, generate hypotheses, or validate self-reports, psychological observation can be either controlled or naturalistic with varying degrees of structure imposed by the researcher.
There are different types of observational methods, and distinctions need to be made between:
1. Controlled Observations 2. Naturalistic Observations 3. Participant Observations
In addition to the above categories, observations can also be either overt/disclosed (the participants know they are being studied) or covert/undisclosed (the researcher keeps their real identity a secret from the research subjects, acting as a genuine member of the group).
In general, conducting observational research is relatively inexpensive, but it remains highly time-consuming and resource-intensive in data processing and analysis.
The considerable investments needed in terms of coder time commitments for training, maintaining reliability, preventing drift, and coding complex dynamic interactions place practical barriers on observers with limited resources.
Controlled Observation
Controlled observation is a research method for studying behavior in a carefully controlled and structured environment.
The researcher sets specific conditions, variables, and procedures to systematically observe and measure behavior, allowing for greater control and comparison of different conditions or groups.
The researcher decides where the observation will occur, at what time, with which participants, and in what circumstances, and uses a standardized procedure. Participants are randomly allocated to each independent variable group.
Rather than writing a detailed description of all behavior observed, it is often easier to code behavior according to a previously agreed scale using a behavior schedule (i.e., conducting a structured observation).
The researcher systematically classifies the behavior they observe into distinct categories. Coding might involve numbers or letters to describe a characteristic or the use of a scale to measure behavior intensity.
The categories on the schedule are coded so that the data collected can be easily counted and turned into statistics.
For example, Mary Ainsworth used a behavior schedule to study how infants responded to brief periods of separation from their mothers. During the Strange Situation procedure, the infant’s interaction behaviors directed toward the mother were measured, e.g.,
- Proximity and contact-seeking
- Contact maintaining
- Avoidance of proximity and contact
- Resistance to contact and comforting
The observer noted down the behavior displayed during 15-second intervals and scored the behavior for intensity on a scale of 1 to 7.
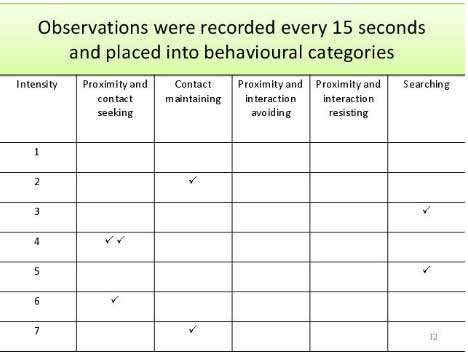
Sometimes participants’ behavior is observed through a two-way mirror, or they are secretly filmed. Albert Bandura used this method to study aggression in children (the Bobo doll studies ).
A lot of research has been carried out in sleep laboratories as well. Here, electrodes are attached to the scalp of participants. What is observed are the changes in electrical activity in the brain during sleep ( the machine is called an EEG ).
Controlled observations are usually overt as the researcher explains the research aim to the group so the participants know they are being observed.
Controlled observations are also usually non-participant as the researcher avoids direct contact with the group and keeps a distance (e.g., observing behind a two-way mirror).
- Controlled observations can be easily replicated by other researchers by using the same observation schedule. This means it is easy to test for reliability .
- The data obtained from structured observations is easier and quicker to analyze as it is quantitative (i.e., numerical) – making this a less time-consuming method compared to naturalistic observations.
- Controlled observations are fairly quick to conduct which means that many observations can take place within a short amount of time. This means a large sample can be obtained, resulting in the findings being representative and having the ability to be generalized to a large population.
Limitations
- Controlled observations can lack validity due to the Hawthorne effect /demand characteristics. When participants know they are being watched, they may act differently.
Naturalistic Observation
Naturalistic observation is a research method in which the researcher studies behavior in its natural setting without intervention or manipulation.
It involves observing and recording behavior as it naturally occurs, providing insights into real-life behaviors and interactions in their natural context.
Naturalistic observation is a research method commonly used by psychologists and other social scientists.
This technique involves observing and studying the spontaneous behavior of participants in natural surroundings. The researcher simply records what they see in whatever way they can.
In unstructured observations, the researcher records all relevant behavior with a coding system. There may be too much to record, and the behaviors recorded may not necessarily be the most important, so the approach is usually used as a pilot study to see what type of behaviors would be recorded.
Compared with controlled observations, it is like the difference between studying wild animals in a zoo and studying them in their natural habitat.
With regard to human subjects, Margaret Mead used this method to research the way of life of different tribes living on islands in the South Pacific. Kathy Sylva used it to study children at play by observing their behavior in a playgroup in Oxfordshire.
Collecting Naturalistic Behavioral Data
Technological advances are enabling new, unobtrusive ways of collecting naturalistic behavioral data.
The Electronically Activated Recorder (EAR) is a digital recording device participants can wear to periodically sample ambient sounds, allowing representative sampling of daily experiences (Mehl et al., 2012).
Studies program EARs to record 30-50 second sound snippets multiple times per hour. Although coding the recordings requires extensive resources, EARs can capture spontaneous behaviors like arguments or laughter.
EARs minimize participant reactivity since sampling occurs outside of awareness. This reduces the Hawthorne effect, where people change behavior when observed.
The SenseCam is another wearable device that passively captures images documenting daily activities. Though primarily used in memory research currently (Smith et al., 2014), systematic sampling of environments and behaviors via the SenseCam could enable innovative psychological studies in the future.
- By being able to observe the flow of behavior in its own setting, studies have greater ecological validity.
- Like case studies , naturalistic observation is often used to generate new ideas. Because it gives the researcher the opportunity to study the total situation, it often suggests avenues of inquiry not thought of before.
- The ability to capture actual behaviors as they unfold in real-time, analyze sequential patterns of interactions, measure base rates of behaviors, and examine socially undesirable or complex behaviors that people may not self-report accurately.
- These observations are often conducted on a micro (small) scale and may lack a representative sample (biased in relation to age, gender, social class, or ethnicity). This may result in the findings lacking the ability to generalize to wider society.
- Natural observations are less reliable as other variables cannot be controlled. This makes it difficult for another researcher to repeat the study in exactly the same way.
- Highly time-consuming and resource-intensive during the data coding phase (e.g., training coders, maintaining inter-rater reliability, preventing judgment drift).
- With observations, we do not have manipulations of variables (or control over extraneous variables), meaning cause-and-effect relationships cannot be established.
Participant Observation
Participant observation is a variant of the above (natural observations) but here, the researcher joins in and becomes part of the group they are studying to get a deeper insight into their lives.
If it were research on animals , we would now not only be studying them in their natural habitat but be living alongside them as well!
Leon Festinger used this approach in a famous study into a religious cult that believed that the end of the world was about to occur. He joined the cult and studied how they reacted when the prophecy did not come true.
Participant observations can be either covert or overt. Covert is where the study is carried out “undercover.” The researcher’s real identity and purpose are kept concealed from the group being studied.
The researcher takes a false identity and role, usually posing as a genuine member of the group.
On the other hand, overt is where the researcher reveals his or her true identity and purpose to the group and asks permission to observe.
- It can be difficult to get time/privacy for recording. For example, researchers can’t take notes openly with covert observations as this would blow their cover. This means they must wait until they are alone and rely on their memory. This is a problem as they may forget details and are unlikely to remember direct quotations.
- If the researcher becomes too involved, they may lose objectivity and become biased. There is always the danger that we will “see” what we expect (or want) to see. This problem is because they could selectively report information instead of noting everything they observe. Thus reducing the validity of their data.
Recording of Data
With controlled/structured observation studies, an important decision the researcher has to make is how to classify and record the data. Usually, this will involve a method of sampling.
In most coding systems, codes or ratings are made either per behavioral event or per specified time interval (Bakeman & Quera, 2011).
The three main sampling methods are:
Event-based coding involves identifying and segmenting interactions into meaningful events rather than timed units.
For example, parent-child interactions may be segmented into control or teaching events to code. Interval recording involves dividing interactions into fixed time intervals (e.g., 6-15 seconds) and coding behaviors within each interval (Bakeman & Quera, 2011).
Event recording allows counting event frequency and sequencing while also potentially capturing event duration through timed-event recording. This provides information on time spent on behaviors.
Coding Systems
The coding system should focus on behaviors, patterns, individual characteristics, or relationship qualities that are relevant to the theory guiding the study (Wampler & Harper, 2014).
Codes vary in how much inference is required, from concrete observable behaviors like frequency of eye contact to more abstract concepts like degree of rapport between a therapist and client (Hill & Lambert, 2004). More inference may reduce reliability.
Macroanalytic coding systems
Macroanalytic coding systems involve rating or summarizing behaviors using larger coding units and broader categories that reflect patterns across longer periods of interaction rather than coding small or discrete behavioral acts.
For example, a macroanalytic coding system may rate the overall degree of therapist warmth or level of client engagement globally for an entire therapy session, requiring the coders to summarize and infer these constructs across the interaction rather than coding smaller behavioral units.
These systems require observers to make more inferences (more time-consuming) but can better capture contextual factors, stability over time, and the interdependent nature of behaviors (Carlson & Grotevant, 1987).
Microanalytic coding systems
Microanalytic coding systems involve rating behaviors using smaller, more discrete coding units and categories.
For example, a microanalytic system may code each instance of eye contact or head nodding during a therapy session. These systems code specific, molecular behaviors as they occur moment-to-moment rather than summarizing actions over longer periods.
Microanalytic systems require less inference from coders and allow for analysis of behavioral contingencies and sequential interactions between therapist and client. However, they are more time-consuming and expensive to implement than macroanalytic approaches.
Mesoanalytic coding systems
Mesoanalytic coding systems attempt to balance macro- and micro-analytic approaches.
In contrast to macroanalytic systems that summarize behaviors in larger chunks, mesoanalytic systems use medium-sized coding units that target more specific behaviors or interaction sequences (Bakeman & Quera, 2017).
For example, a mesoanalytic system may code each instance of a particular type of therapist statement or client emotional expression. However, mesoanalytic systems still use larger units than microanalytic approaches coding every speech onset/offset.
The goal of balancing specificity and feasibility makes mesoanalytic systems well-suited for many research questions (Morris et al., 2014). Mesoanalytic codes can preserve some sequential information while remaining efficient enough for studies with adequate but limited resources.
For instance, a mesoanalytic couple interaction coding system could target key behavior patterns like validation sequences without coding turn-by-turn speech.
In this way, mesoanalytic coding allows reasonable reliability and specificity without requiring extensive training or observation. The mid-level focus offers a pragmatic compromise between depth and breadth in analyzing interactions.
Preventing Coder Drift
Coder drift results in a measurement error caused by gradual shifts in how observations get rated according to operational definitions, especially when behavioral codes are not clearly specified.
This type of error creeps in when coders fail to regularly review what precise observations constitute or do not constitute the behaviors being measured.
Preventing drift refers to taking active steps to maintain consistency and minimize changes or deviations in how coders rate or evaluate behaviors over time. Specifically, some key ways to prevent coder drift include:
- Operationalize codes : It is essential that code definitions unambiguously distinguish what interactions represent instances of each coded behavior.
- Ongoing training : Returning to those operational definitions through ongoing training serves to recalibrate coder interpretations and reinforce accurate recognition. Having regular “check-in” sessions where coders practice coding the same interactions allows monitoring that they continue applying codes reliably without gradual shifts in interpretation.
- Using reference videos : Coders periodically coding the same “gold standard” reference videos anchors their judgments and calibrate against original training. Without periodic anchoring to original specifications, coder decisions tend to drift from initial measurement reliability.
- Assessing inter-rater reliability : Statistical tracking that coders maintain high levels of agreement over the course of a study, not just at the start, flags any declines indicating drift. Sustaining inter-rater agreement requires mitigating this common tendency for observer judgment change during intensive, long-term coding tasks.
- Recalibrating through discussion : Having meetings for coders to discuss disagreements openly explores reasons judgment shifts may be occurring over time. Consensus on the application of codes is restored.
- Adjusting unclear codes : If reliability issues persist, revisiting and refining ambiguous code definitions or anchors can eliminate inconsistencies arising from coder confusion.
Essentially, the goal of preventing coder drift is maintaining standardization and minimizing unintentional biases that may slowly alter how observational data gets rated over periods of extensive coding.
Through the upkeep of skills, continuing calibration to benchmarks, and monitoring consistency, researchers can notice and correct for any creeping changes in coder decision-making over time.
Reducing Observer Bias
Observational research is prone to observer biases resulting from coders’ subjective perspectives shaping the interpretation of complex interactions (Burghardt et al., 2012). When coding, personal expectations may unconsciously influence judgments. However, rigorous methods exist to reduce such bias.
Coding Manual
A detailed coding manual minimizes subjectivity by clearly defining what behaviors and interaction dynamics observers should code (Bakeman & Quera, 2011).
High-quality manuals have strong theoretical and empirical grounding, laying out explicit coding procedures and providing rich behavioral examples to anchor code definitions (Lindahl, 2001).
Clear delineation of the frequency, intensity, duration, and type of behaviors constituting each code facilitates reliable judgments and reduces ambiguity for coders. Application risks inconsistency across raters without clarity on how codes translate to observable interaction.
Coder Training
Competent coders require both interpersonal perceptiveness and scientific rigor (Wampler & Harper, 2014). Training thoroughly reviews the theoretical basis for coded constructs and teaches the coding system itself.
Multiple “gold standard” criterion videos demonstrate code ranges that trainees independently apply. Coders then meet weekly to establish reliability of 80% or higher agreement both among themselves and with master criterion coding (Hill & Lambert, 2004).
Ongoing training manages coder drift over time. Revisions to unclear codes may also improve reliability. Both careful selection and investment in rigorous training increase quality control.
Blind Methods
To prevent bias, coders should remain unaware of specific study predictions or participant details (Burghardt et al., 2012). Separate data gathering versus coding teams helps maintain blinding.
Coders should be unaware of study details or participant identities that could bias coding (Burghardt et al., 2012).
Separate teams collecting data versus coding data can reduce bias.
In addition, scheduling procedures can prevent coders from rating data collected directly from participants with whom they have had personal contact. Maintaining coder independence and blinding enhances objectivity.
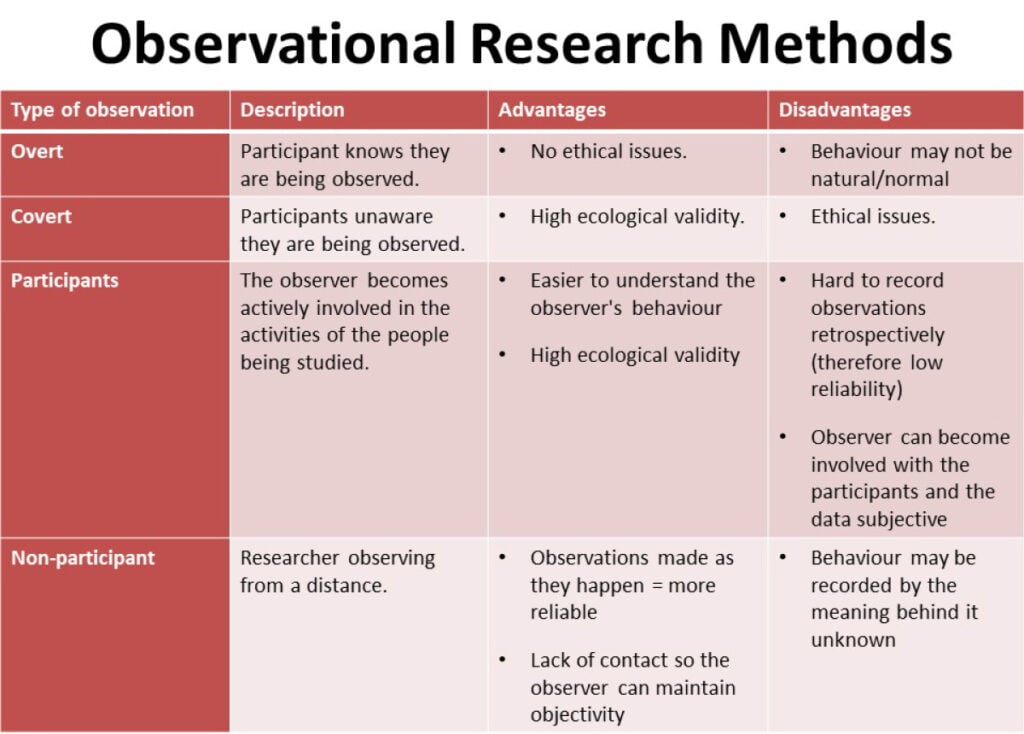
Bakeman, R., & Quera, V. (2017). Sequential analysis and observational methods for the behavioral sciences. Cambridge University Press.
Burghardt, G. M., Bartmess-LeVasseur, J. N., Browning, S. A., Morrison, K. E., Stec, C. L., Zachau, C. E., & Freeberg, T. M. (2012). Minimizing observer bias in behavioral studies: A review and recommendations. Ethology, 118 (6), 511-517.
Hill, C. E., & Lambert, M. J. (2004). Methodological issues in studying psychotherapy processes and outcomes. In M. J. Lambert (Ed.), Bergin and Garfield’s handbook of psychotherapy and behavior change (5th ed., pp. 84–135). Wiley.
Lindahl, K. M. (2001). Methodological issues in family observational research. In P. K. Kerig & K. M. Lindahl (Eds.), Family observational coding systems: Resources for systemic research (pp. 23–32). Lawrence Erlbaum Associates.
Mehl, M. R., Robbins, M. L., & Deters, F. G. (2012). Naturalistic observation of health-relevant social processes: The electronically activated recorder methodology in psychosomatics. Psychosomatic Medicine, 74 (4), 410–417.
Morris, A. S., Robinson, L. R., & Eisenberg, N. (2014). Applying a multimethod perspective to the study of developmental psychology. In H. T. Reis & C. M. Judd (Eds.), Handbook of research methods in social and personality psychology (2nd ed., pp. 103–123). Cambridge University Press.
Smith, J. A., Maxwell, S. D., & Johnson, G. (2014). The microstructure of everyday life: Analyzing the complex choreography of daily routines through the automatic capture and processing of wearable sensor data. In B. K. Wiederhold & G. Riva (Eds.), Annual Review of Cybertherapy and Telemedicine 2014: Positive Change with Technology (Vol. 199, pp. 62-64). IOS Press.
Traniello, J. F., & Bakker, T. C. (2015). The integrative study of behavioral interactions across the sciences. In T. K. Shackelford & R. D. Hansen (Eds.), The evolution of sexuality (pp. 119-147). Springer.
Wampler, K. S., & Harper, A. (2014). Observational methods in couple and family assessment. In H. T. Reis & C. M. Judd (Eds.), Handbook of research methods in social and personality psychology (2nd ed., pp. 490–502). Cambridge University Press.

Related Articles
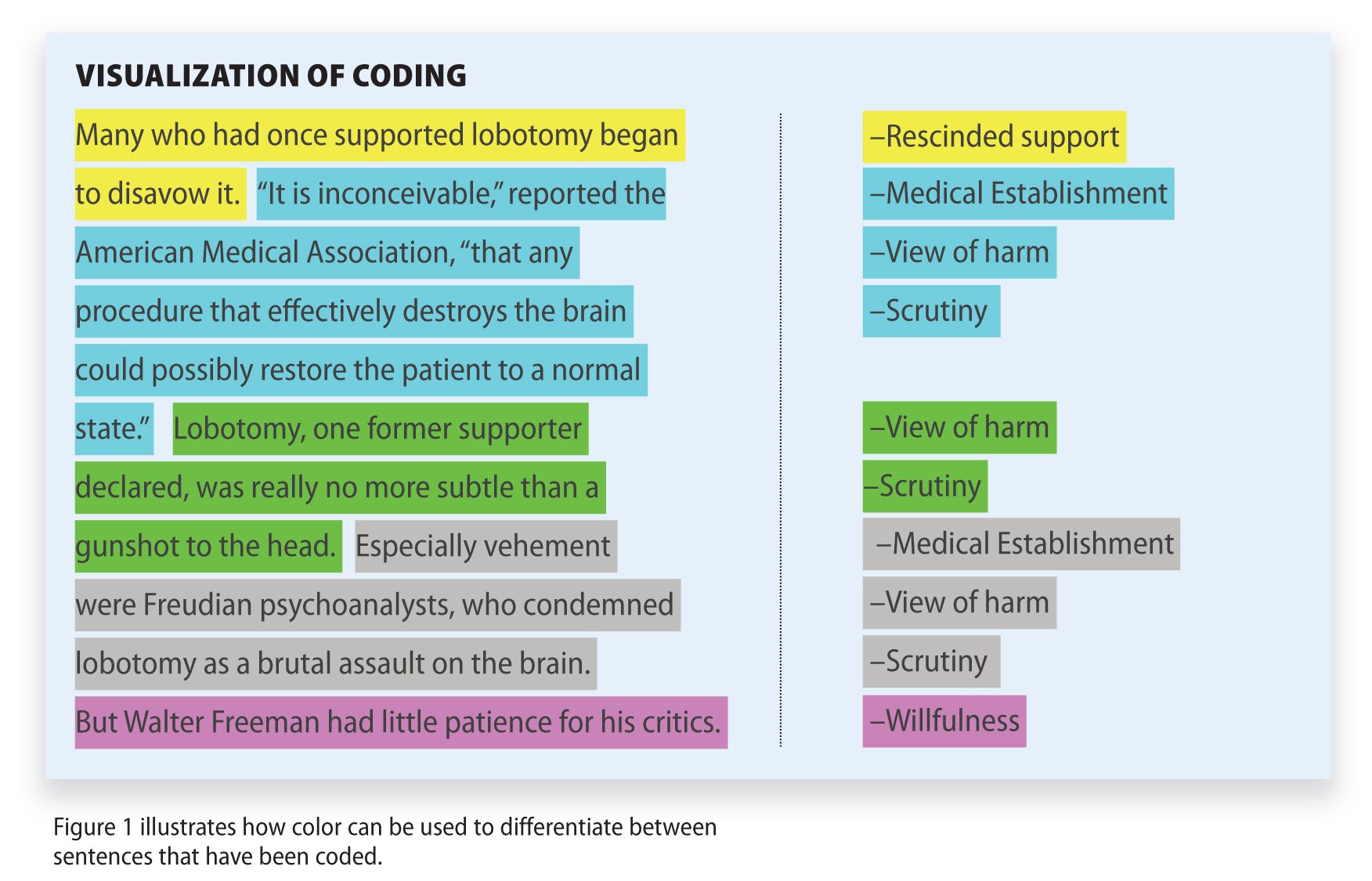
Research Methodology
Qualitative Data Coding
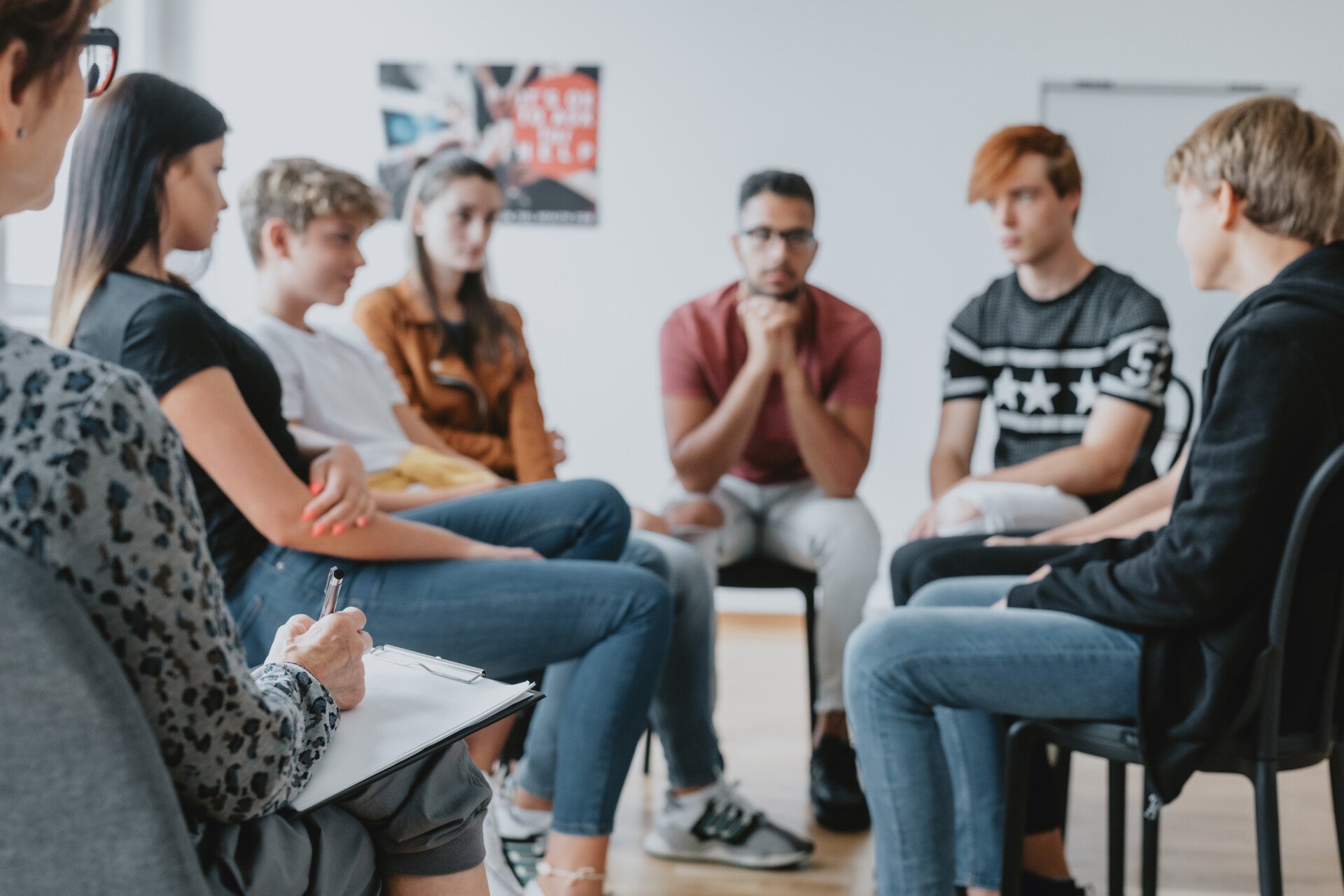
What Is a Focus Group?
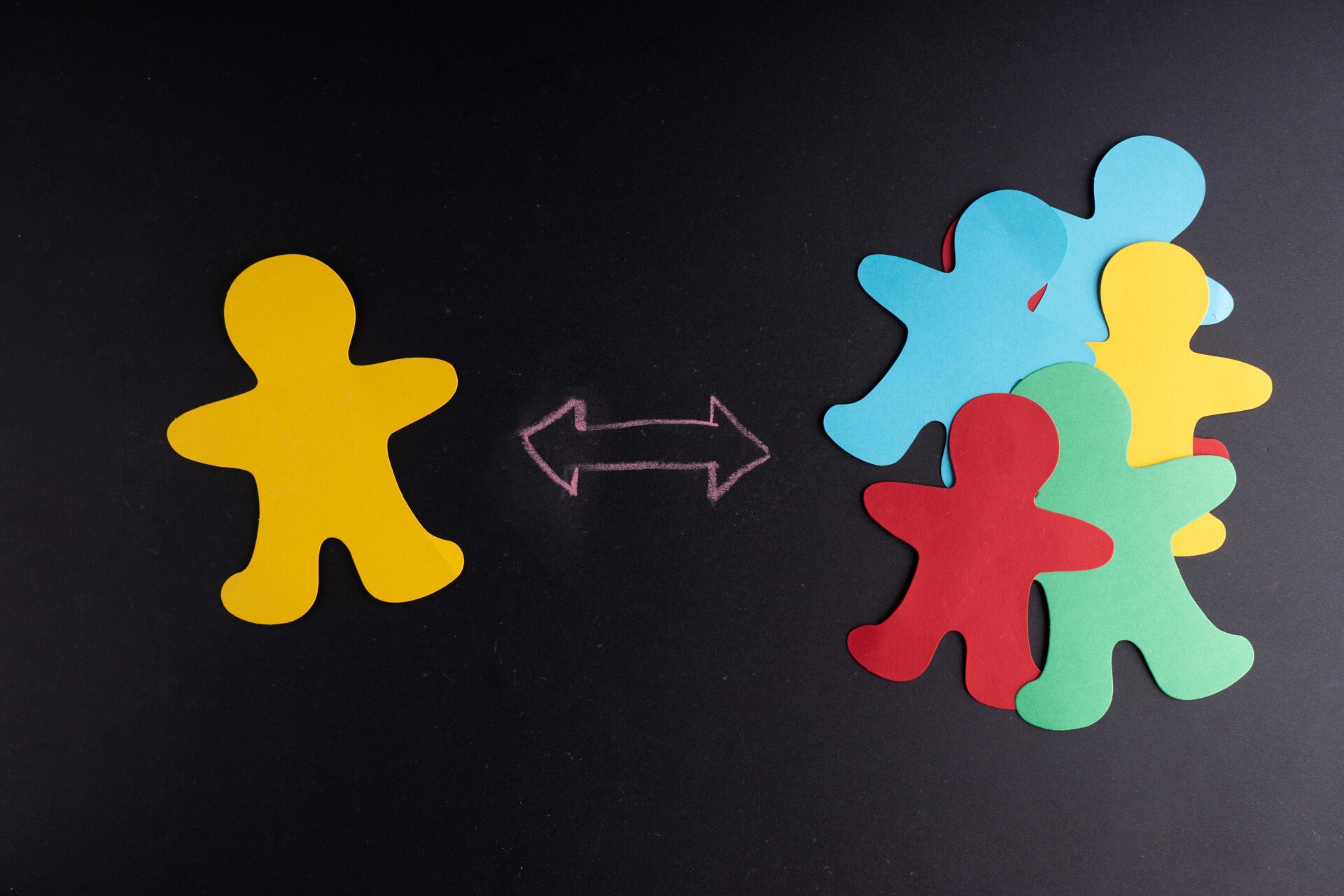
Cross-Cultural Research Methodology In Psychology
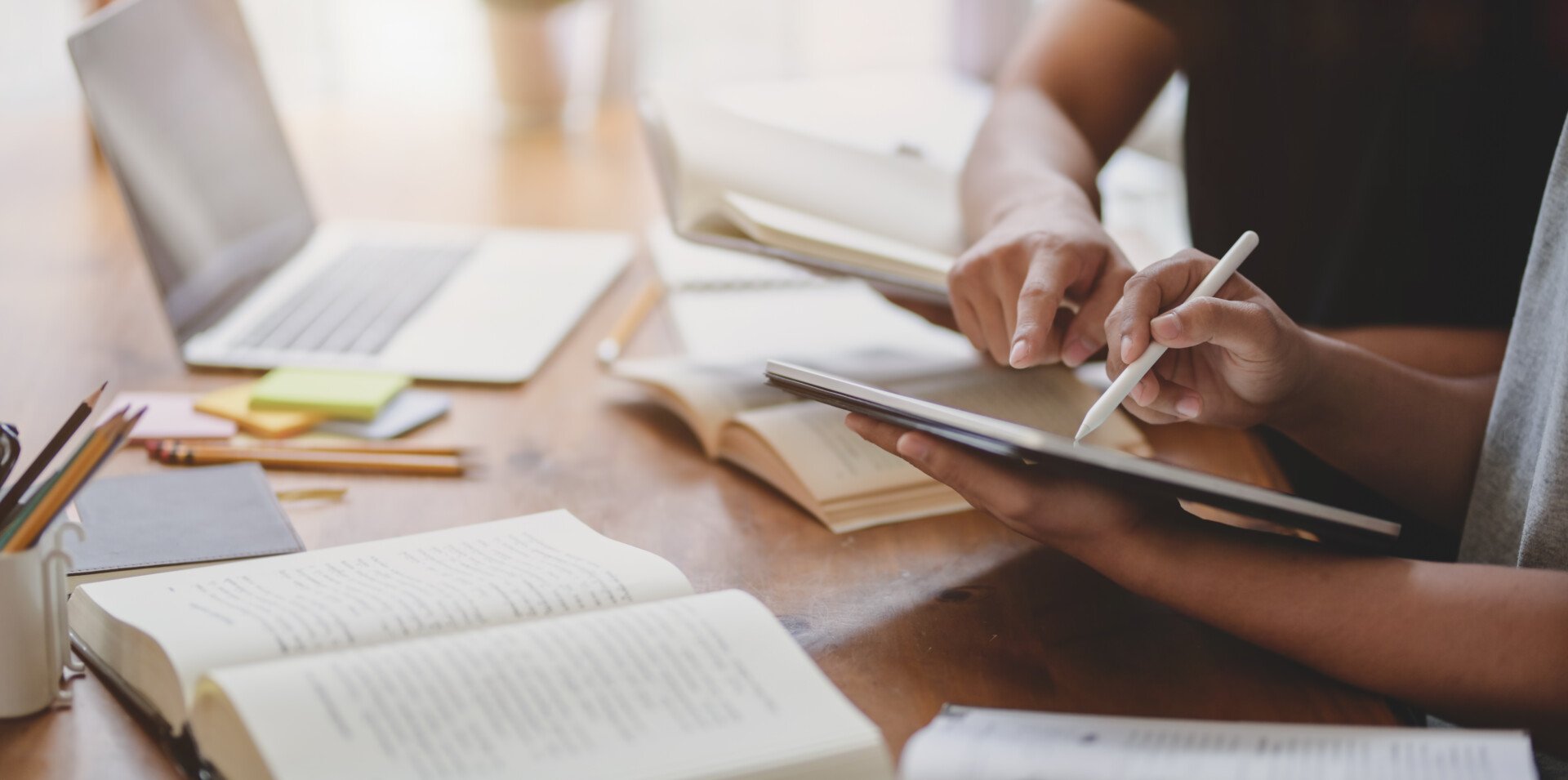
What Is Internal Validity In Research?
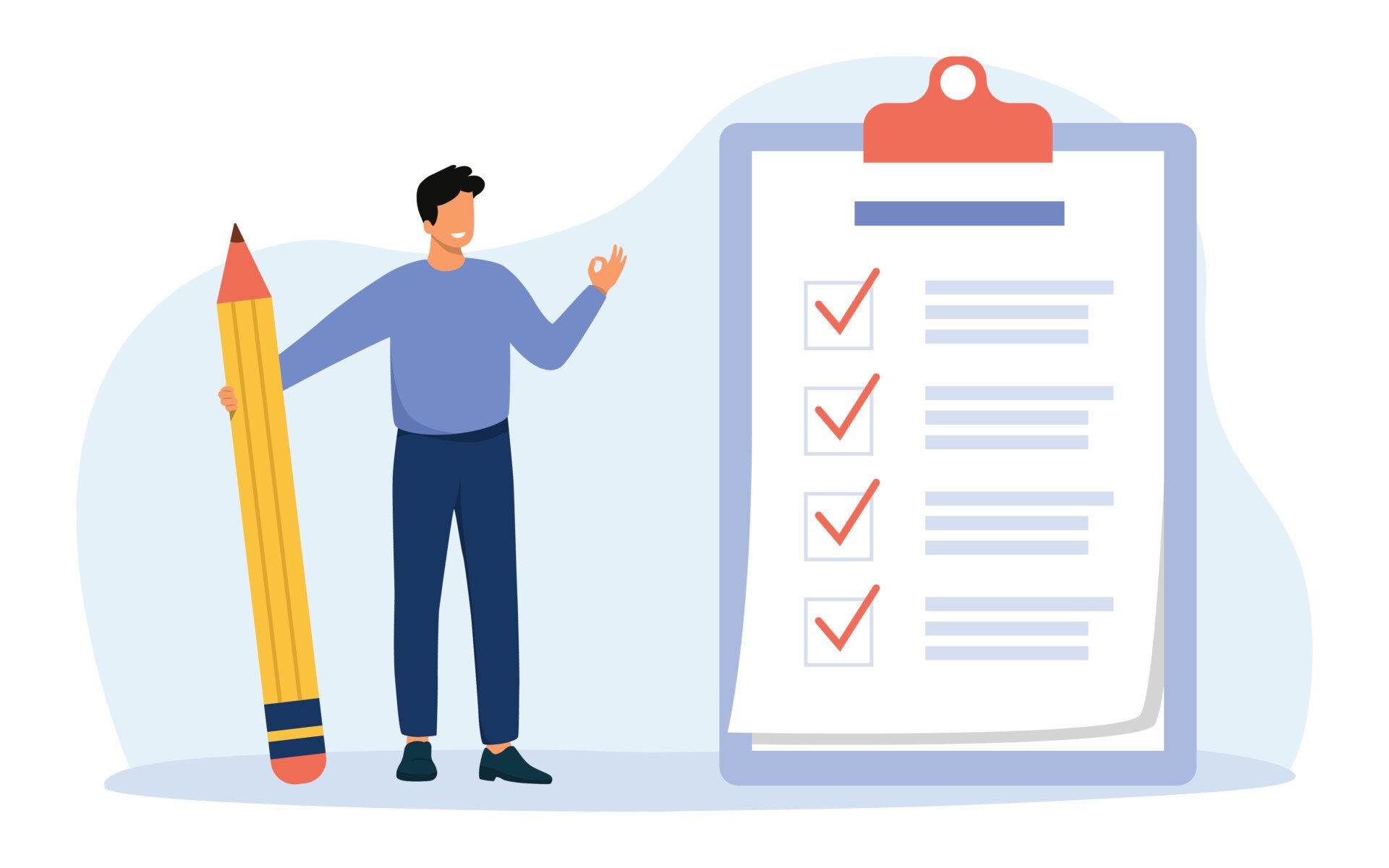
Research Methodology , Statistics
What Is Face Validity In Research? Importance & How To Measure
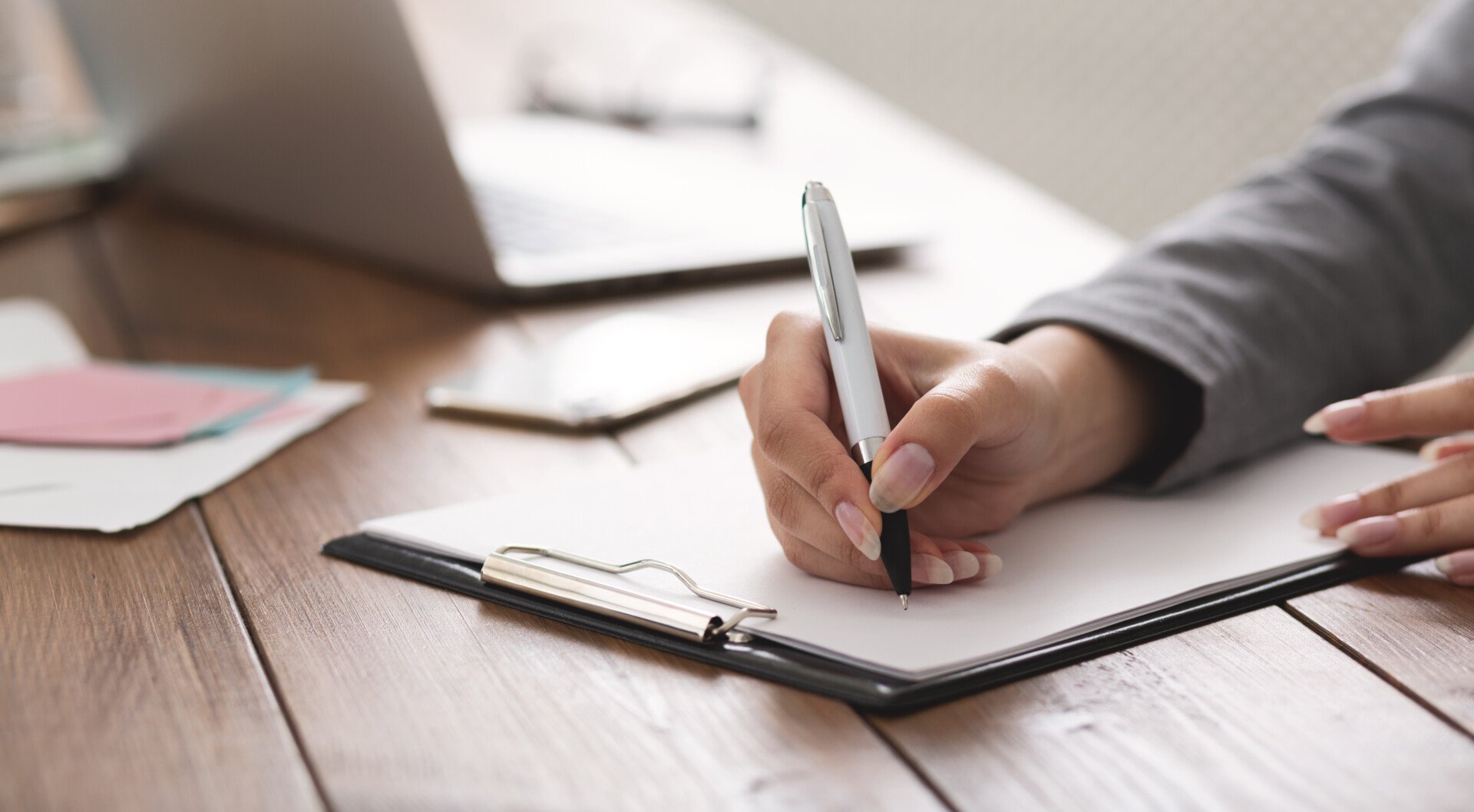
Criterion Validity: Definition & Examples
2.2 Approaches to Research
Learning objectives.
By the end of this section, you will be able to:
- Describe the different research methods used by psychologists
- Discuss the strengths and weaknesses of case studies, naturalistic observation, surveys, and archival research
- Compare longitudinal and cross-sectional approaches to research
- Compare and contrast correlation and causation
There are many research methods available to psychologists in their efforts to understand, describe, and explain behavior and the cognitive and biological processes that underlie it. Some methods rely on observational techniques. Other approaches involve interactions between the researcher and the individuals who are being studied—ranging from a series of simple questions to extensive, in-depth interviews—to well-controlled experiments.
Each of these research methods has unique strengths and weaknesses, and each method may only be appropriate for certain types of research questions. For example, studies that rely primarily on observation produce incredible amounts of information, but the ability to apply this information to the larger population is somewhat limited because of small sample sizes. Survey research, on the other hand, allows researchers to easily collect data from relatively large samples. While this allows for results to be generalized to the larger population more easily, the information that can be collected on any given survey is somewhat limited and subject to problems associated with any type of self-reported data. Some researchers conduct archival research by using existing records. While this can be a fairly inexpensive way to collect data that can provide insight into a number of research questions, researchers using this approach have no control on how or what kind of data was collected. All of the methods described thus far are correlational in nature. This means that researchers can speak to important relationships that might exist between two or more variables of interest. However, correlational data cannot be used to make claims about cause-and-effect relationships.
Correlational research can find a relationship between two variables, but the only way a researcher can claim that the relationship between the variables is cause and effect is to perform an experiment. In experimental research, which will be discussed later in this chapter, there is a tremendous amount of control over variables of interest. While this is a powerful approach, experiments are often conducted in artificial settings. This calls into question the validity of experimental findings with regard to how they would apply in real-world settings. In addition, many of the questions that psychologists would like to answer cannot be pursued through experimental research because of ethical concerns.
Clinical or Case Studies
In 2011, the New York Times published a feature story on Krista and Tatiana Hogan, Canadian twin girls. These particular twins are unique because Krista and Tatiana are conjoined twins, connected at the head. There is evidence that the two girls are connected in a part of the brain called the thalamus, which is a major sensory relay center. Most incoming sensory information is sent through the thalamus before reaching higher regions of the cerebral cortex for processing.
Link to Learning
Watch this CBC video about Krista's and Tatiana's lives to learn more.
The implications of this potential connection mean that it might be possible for one twin to experience the sensations of the other twin. For instance, if Krista is watching a particularly funny television program, Tatiana might smile or laugh even if she is not watching the program. This particular possibility has piqued the interest of many neuroscientists who seek to understand how the brain uses sensory information.
These twins represent an enormous resource in the study of the brain, and since their condition is very rare, it is likely that as long as their family agrees, scientists will follow these girls very closely throughout their lives to gain as much information as possible (Dominus, 2011).
Over time, it has become clear that while Krista and Tatiana share some sensory experiences and motor control, they remain two distinct individuals, which provides invaluable insight for researchers interested in the mind and the brain (Egnor, 2017).
In observational research, scientists are conducting a clinical or case study when they focus on one person or just a few individuals. Indeed, some scientists spend their entire careers studying just 10–20 individuals. Why would they do this? Obviously, when they focus their attention on a very small number of people, they can gain a precious amount of insight into those cases. The richness of information that is collected in clinical or case studies is unmatched by any other single research method. This allows the researcher to have a very deep understanding of the individuals and the particular phenomenon being studied.
If clinical or case studies provide so much information, why are they not more frequent among researchers? As it turns out, the major benefit of this particular approach is also a weakness. As mentioned earlier, this approach is often used when studying individuals who are interesting to researchers because they have a rare characteristic. Therefore, the individuals who serve as the focus of case studies are not like most other people. If scientists ultimately want to explain all behavior, focusing attention on such a special group of people can make it difficult to generalize any observations to the larger population as a whole. Generalizing refers to the ability to apply the findings of a particular research project to larger segments of society. Again, case studies provide enormous amounts of information, but since the cases are so specific, the potential to apply what’s learned to the average person may be very limited.
Naturalistic Observation
If you want to understand how behavior occurs, one of the best ways to gain information is to simply observe the behavior in its natural context. However, people might change their behavior in unexpected ways if they know they are being observed. How do researchers obtain accurate information when people tend to hide their natural behavior? As an example, imagine that your professor asks everyone in your class to raise their hand if they always wash their hands after using the restroom. Chances are that almost everyone in the classroom will raise their hand, but do you think hand washing after every trip to the restroom is really that universal?
This is very similar to the phenomenon mentioned earlier in this chapter: many individuals do not feel comfortable answering a question honestly. But if we are committed to finding out the facts about hand washing, we have other options available to us.
Suppose we send a classmate into the restroom to actually watch whether everyone washes their hands after using the restroom. Will our observer blend into the restroom environment by wearing a white lab coat, sitting with a clipboard, and staring at the sinks? We want our researcher to be inconspicuous—perhaps standing at one of the sinks pretending to put in contact lenses while secretly recording the relevant information. This type of observational study is called naturalistic observation : observing behavior in its natural setting. To better understand peer exclusion, Suzanne Fanger collaborated with colleagues at the University of Texas to observe the behavior of preschool children on a playground. How did the observers remain inconspicuous over the duration of the study? They equipped a few of the children with wireless microphones (which the children quickly forgot about) and observed while taking notes from a distance. Also, the children in that particular preschool (a “laboratory preschool”) were accustomed to having observers on the playground (Fanger, Frankel, & Hazen, 2012).
It is critical that the observer be as unobtrusive and as inconspicuous as possible: when people know they are being watched, they are less likely to behave naturally. If you have any doubt about this, ask yourself how your driving behavior might differ in two situations: In the first situation, you are driving down a deserted highway during the middle of the day; in the second situation, you are being followed by a police car down the same deserted highway ( Figure 2.7 ).
It should be pointed out that naturalistic observation is not limited to research involving humans. Indeed, some of the best-known examples of naturalistic observation involve researchers going into the field to observe various kinds of animals in their own environments. As with human studies, the researchers maintain their distance and avoid interfering with the animal subjects so as not to influence their natural behaviors. Scientists have used this technique to study social hierarchies and interactions among animals ranging from ground squirrels to gorillas. The information provided by these studies is invaluable in understanding how those animals organize socially and communicate with one another. The anthropologist Jane Goodall , for example, spent nearly five decades observing the behavior of chimpanzees in Africa ( Figure 2.8 ). As an illustration of the types of concerns that a researcher might encounter in naturalistic observation, some scientists criticized Goodall for giving the chimps names instead of referring to them by numbers—using names was thought to undermine the emotional detachment required for the objectivity of the study (McKie, 2010).
The greatest benefit of naturalistic observation is the validity , or accuracy, of information collected unobtrusively in a natural setting. Having individuals behave as they normally would in a given situation means that we have a higher degree of ecological validity, or realism, than we might achieve with other research approaches. Therefore, our ability to generalize the findings of the research to real-world situations is enhanced. If done correctly, we need not worry about people or animals modifying their behavior simply because they are being observed. Sometimes, people may assume that reality programs give us a glimpse into authentic human behavior. However, the principle of inconspicuous observation is violated as reality stars are followed by camera crews and are interviewed on camera for personal confessionals. Given that environment, we must doubt how natural and realistic their behaviors are.
The major downside of naturalistic observation is that they are often difficult to set up and control. In our restroom study, what if you stood in the restroom all day prepared to record people’s hand washing behavior and no one came in? Or, what if you have been closely observing a troop of gorillas for weeks only to find that they migrated to a new place while you were sleeping in your tent? The benefit of realistic data comes at a cost. As a researcher you have no control of when (or if) you have behavior to observe. In addition, this type of observational research often requires significant investments of time, money, and a good dose of luck.
Sometimes studies involve structured observation. In these cases, people are observed while engaging in set, specific tasks. An excellent example of structured observation comes from Strange Situation by Mary Ainsworth (you will read more about this in the chapter on lifespan development). The Strange Situation is a procedure used to evaluate attachment styles that exist between an infant and caregiver. In this scenario, caregivers bring their infants into a room filled with toys. The Strange Situation involves a number of phases, including a stranger coming into the room, the caregiver leaving the room, and the caregiver’s return to the room. The infant’s behavior is closely monitored at each phase, but it is the behavior of the infant upon being reunited with the caregiver that is most telling in terms of characterizing the infant’s attachment style with the caregiver.
Another potential problem in observational research is observer bias . Generally, people who act as observers are closely involved in the research project and may unconsciously skew their observations to fit their research goals or expectations. To protect against this type of bias, researchers should have clear criteria established for the types of behaviors recorded and how those behaviors should be classified. In addition, researchers often compare observations of the same event by multiple observers, in order to test inter-rater reliability : a measure of reliability that assesses the consistency of observations by different observers.
Often, psychologists develop surveys as a means of gathering data. Surveys are lists of questions to be answered by research participants, and can be delivered as paper-and-pencil questionnaires, administered electronically, or conducted verbally ( Figure 2.9 ). Generally, the survey itself can be completed in a short time, and the ease of administering a survey makes it easy to collect data from a large number of people.
Surveys allow researchers to gather data from larger samples than may be afforded by other research methods . A sample is a subset of individuals selected from a population , which is the overall group of individuals that the researchers are interested in. Researchers study the sample and seek to generalize their findings to the population. Generally, researchers will begin this process by calculating various measures of central tendency from the data they have collected. These measures provide an overall summary of what a typical response looks like. There are three measures of central tendency: mode, median, and mean. The mode is the most frequently occurring response, the median lies at the middle of a given data set, and the mean is the arithmetic average of all data points. Means tend to be most useful in conducting additional analyses like those described below; however, means are very sensitive to the effects of outliers, and so one must be aware of those effects when making assessments of what measures of central tendency tell us about a data set in question.
There is both strength and weakness of the survey in comparison to case studies. By using surveys, we can collect information from a larger sample of people. A larger sample is better able to reflect the actual diversity of the population, thus allowing better generalizability. Therefore, if our sample is sufficiently large and diverse, we can assume that the data we collect from the survey can be generalized to the larger population with more certainty than the information collected through a case study. However, given the greater number of people involved, we are not able to collect the same depth of information on each person that would be collected in a case study.
Another potential weakness of surveys is something we touched on earlier in this chapter: People don't always give accurate responses. They may lie, misremember, or answer questions in a way that they think makes them look good. For example, people may report drinking less alcohol than is actually the case.
Any number of research questions can be answered through the use of surveys. One real-world example is the research conducted by Jenkins, Ruppel, Kizer, Yehl, and Griffin (2012) about the backlash against the US Arab-American community following the terrorist attacks of September 11, 2001. Jenkins and colleagues wanted to determine to what extent these negative attitudes toward Arab-Americans still existed nearly a decade after the attacks occurred. In one study, 140 research participants filled out a survey with 10 questions, including questions asking directly about the participant’s overt prejudicial attitudes toward people of various ethnicities. The survey also asked indirect questions about how likely the participant would be to interact with a person of a given ethnicity in a variety of settings (such as, “How likely do you think it is that you would introduce yourself to a person of Arab-American descent?”). The results of the research suggested that participants were unwilling to report prejudicial attitudes toward any ethnic group. However, there were significant differences between their pattern of responses to questions about social interaction with Arab-Americans compared to other ethnic groups: they indicated less willingness for social interaction with Arab-Americans compared to the other ethnic groups. This suggested that the participants harbored subtle forms of prejudice against Arab-Americans, despite their assertions that this was not the case (Jenkins et al., 2012).
Archival Research
Some researchers gain access to large amounts of data without interacting with a single research participant. Instead, they use existing records to answer various research questions. This type of research approach is known as archival research . Archival research relies on looking at past records or data sets to look for interesting patterns or relationships.
For example, a researcher might access the academic records of all individuals who enrolled in college within the past ten years and calculate how long it took them to complete their degrees, as well as course loads, grades, and extracurricular involvement. Archival research could provide important information about who is most likely to complete their education, and it could help identify important risk factors for struggling students ( Figure 2.10 ).
In comparing archival research to other research methods, there are several important distinctions. For one, the researcher employing archival research never directly interacts with research participants. Therefore, the investment of time and money to collect data is considerably less with archival research. Additionally, researchers have no control over what information was originally collected. Therefore, research questions have to be tailored so they can be answered within the structure of the existing data sets. There is also no guarantee of consistency between the records from one source to another, which might make comparing and contrasting different data sets problematic.
Longitudinal and Cross-Sectional Research
Sometimes we want to see how people change over time, as in studies of human development and lifespan. When we test the same group of individuals repeatedly over an extended period of time, we are conducting longitudinal research. Longitudinal research is a research design in which data-gathering is administered repeatedly over an extended period of time. For example, we may survey a group of individuals about their dietary habits at age 20, retest them a decade later at age 30, and then again at age 40.
Another approach is cross-sectional research. In cross-sectional research , a researcher compares multiple segments of the population at the same time. Using the dietary habits example above, the researcher might directly compare different groups of people by age. Instead of studying a group of people for 20 years to see how their dietary habits changed from decade to decade, the researcher would study a group of 20-year-old individuals and compare them to a group of 30-year-old individuals and a group of 40-year-old individuals. While cross-sectional research requires a shorter-term investment, it is also limited by differences that exist between the different generations (or cohorts) that have nothing to do with age per se, but rather reflect the social and cultural experiences of different generations of individuals that make them different from one another.
To illustrate this concept, consider the following survey findings. In recent years there has been significant growth in the popular support of same-sex marriage. Many studies on this topic break down survey participants into different age groups. In general, younger people are more supportive of same-sex marriage than are those who are older (Jones, 2013). Does this mean that as we age we become less open to the idea of same-sex marriage, or does this mean that older individuals have different perspectives because of the social climates in which they grew up? Longitudinal research is a powerful approach because the same individuals are involved in the research project over time, which means that the researchers need to be less concerned with differences among cohorts affecting the results of their study.
Often longitudinal studies are employed when researching various diseases in an effort to understand particular risk factors. Such studies often involve tens of thousands of individuals who are followed for several decades. Given the enormous number of people involved in these studies, researchers can feel confident that their findings can be generalized to the larger population. The Cancer Prevention Study-3 (CPS-3) is one of a series of longitudinal studies sponsored by the American Cancer Society aimed at determining predictive risk factors associated with cancer. When participants enter the study, they complete a survey about their lives and family histories, providing information on factors that might cause or prevent the development of cancer. Then every few years the participants receive additional surveys to complete. In the end, hundreds of thousands of participants will be tracked over 20 years to determine which of them develop cancer and which do not.
Clearly, this type of research is important and potentially very informative. For instance, earlier longitudinal studies sponsored by the American Cancer Society provided some of the first scientific demonstrations of the now well-established links between increased rates of cancer and smoking (American Cancer Society, n.d.) ( Figure 2.11 ).
As with any research strategy, longitudinal research is not without limitations. For one, these studies require an incredible time investment by the researcher and research participants. Given that some longitudinal studies take years, if not decades, to complete, the results will not be known for a considerable period of time. In addition to the time demands, these studies also require a substantial financial investment. Many researchers are unable to commit the resources necessary to see a longitudinal project through to the end.
Research participants must also be willing to continue their participation for an extended period of time, and this can be problematic. People move, get married and take new names, get ill, and eventually die. Even without significant life changes, some people may simply choose to discontinue their participation in the project. As a result, the attrition rates, or reduction in the number of research participants due to dropouts, in longitudinal studies are quite high and increase over the course of a project. For this reason, researchers using this approach typically recruit many participants fully expecting that a substantial number will drop out before the end. As the study progresses, they continually check whether the sample still represents the larger population, and make adjustments as necessary.
As an Amazon Associate we earn from qualifying purchases.
This book may not be used in the training of large language models or otherwise be ingested into large language models or generative AI offerings without OpenStax's permission.
Want to cite, share, or modify this book? This book uses the Creative Commons Attribution License and you must attribute OpenStax.
Access for free at https://openstax.org/books/psychology-2e/pages/1-introduction
- Authors: Rose M. Spielman, William J. Jenkins, Marilyn D. Lovett
- Publisher/website: OpenStax
- Book title: Psychology 2e
- Publication date: Apr 22, 2020
- Location: Houston, Texas
- Book URL: https://openstax.org/books/psychology-2e/pages/1-introduction
- Section URL: https://openstax.org/books/psychology-2e/pages/2-2-approaches-to-research
© Jan 6, 2024 OpenStax. Textbook content produced by OpenStax is licensed under a Creative Commons Attribution License . The OpenStax name, OpenStax logo, OpenStax book covers, OpenStax CNX name, and OpenStax CNX logo are not subject to the Creative Commons license and may not be reproduced without the prior and express written consent of Rice University.
- Introduction
- The Challenge in Drawing Causal Inferences From Observational Studies
- Limitations of the Randomization-Centered Criterion for Determining the Appropriateness of Causal Language and Interpretation
- An Alternative Framework for Causal Inference for Medical and Health Policy Research
- What This Framework Aims to Accomplish
- What the Framework Does Not Do
- Implications for Authors, Reviewers, Editors, and Readers
- Article Information
eText. Causal and Statistical Estimands, Identification Analysis, and Data Analysis
eFigure 1. Schematic of the Relationship Between Causal Estimands, Statistical Estimands, and Statistical Analysis Methods Applied to Data
Example 1. Analysis of Data From a Randomized Clinical Trial
eFigure 2. Relationship Between Causal Estimands, Statistical Estimands, and Statistical Analysis Methods/Estimators Applied to Data – Randomized Clinical Trial
Example 2. Analysis of Data From an Observational Study
eFigure 3. Relationship Between Causal Estimands, Statistical Estimands, and Statistical Analysis Methods/Estimators Applied to Data – Observational Study
eReferences
- Meaning of Proposed Causal Inference Framework for the JAMA Network JAMA Editor's Note May 9, 2024 Annette Flanagin, RN, MA; Roger J. Lewis, MD, PhD; Christopher C. Muth, MD; Gregory Curfman, MD
See More About
Select your interests.
Customize your JAMA Network experience by selecting one or more topics from the list below.
- Academic Medicine
- Acid Base, Electrolytes, Fluids
- Allergy and Clinical Immunology
- American Indian or Alaska Natives
- Anesthesiology
- Anticoagulation
- Art and Images in Psychiatry
- Artificial Intelligence
- Assisted Reproduction
- Bleeding and Transfusion
- Caring for the Critically Ill Patient
- Challenges in Clinical Electrocardiography
- Climate and Health
- Climate Change
- Clinical Challenge
- Clinical Decision Support
- Clinical Implications of Basic Neuroscience
- Clinical Pharmacy and Pharmacology
- Complementary and Alternative Medicine
- Consensus Statements
- Coronavirus (COVID-19)
- Critical Care Medicine
- Cultural Competency
- Dental Medicine
- Dermatology
- Diabetes and Endocrinology
- Diagnostic Test Interpretation
- Drug Development
- Electronic Health Records
- Emergency Medicine
- End of Life, Hospice, Palliative Care
- Environmental Health
- Equity, Diversity, and Inclusion
- Facial Plastic Surgery
- Gastroenterology and Hepatology
- Genetics and Genomics
- Genomics and Precision Health
- Global Health
- Guide to Statistics and Methods
- Hair Disorders
- Health Care Delivery Models
- Health Care Economics, Insurance, Payment
- Health Care Quality
- Health Care Reform
- Health Care Safety
- Health Care Workforce
- Health Disparities
- Health Inequities
- Health Policy
- Health Systems Science
- History of Medicine
- Hypertension
- Images in Neurology
- Implementation Science
- Infectious Diseases
- Innovations in Health Care Delivery
- JAMA Infographic
- Law and Medicine
- Leading Change
- Less is More
- LGBTQIA Medicine
- Lifestyle Behaviors
- Medical Coding
- Medical Devices and Equipment
- Medical Education
- Medical Education and Training
- Medical Journals and Publishing
- Mobile Health and Telemedicine
- Narrative Medicine
- Neuroscience and Psychiatry
- Notable Notes
- Nutrition, Obesity, Exercise
- Obstetrics and Gynecology
- Occupational Health
- Ophthalmology
- Orthopedics
- Otolaryngology
- Pain Medicine
- Palliative Care
- Pathology and Laboratory Medicine
- Patient Care
- Patient Information
- Performance Improvement
- Performance Measures
- Perioperative Care and Consultation
- Pharmacoeconomics
- Pharmacoepidemiology
- Pharmacogenetics
- Pharmacy and Clinical Pharmacology
- Physical Medicine and Rehabilitation
- Physical Therapy
- Physician Leadership
- Population Health
- Primary Care
- Professional Well-being
- Professionalism
- Psychiatry and Behavioral Health
- Public Health
- Pulmonary Medicine
- Regulatory Agencies
- Reproductive Health
- Research, Methods, Statistics
- Resuscitation
- Rheumatology
- Risk Management
- Scientific Discovery and the Future of Medicine
- Shared Decision Making and Communication
- Sleep Medicine
- Sports Medicine
- Stem Cell Transplantation
- Substance Use and Addiction Medicine
- Surgical Innovation
- Surgical Pearls
- Teachable Moment
- Technology and Finance
- The Art of JAMA
- The Arts and Medicine
- The Rational Clinical Examination
- Tobacco and e-Cigarettes
- Translational Medicine
- Trauma and Injury
- Treatment Adherence
- Ultrasonography
- Users' Guide to the Medical Literature
- Vaccination
- Venous Thromboembolism
- Veterans Health
- Women's Health
- Workflow and Process
- Wound Care, Infection, Healing
Others Also Liked
- Download PDF
- X Facebook More LinkedIn
- CME & MOC
Dahabreh IJ , Bibbins-Domingo K. Causal Inference About the Effects of Interventions From Observational Studies in Medical Journals. JAMA. Published online May 09, 2024. doi:10.1001/jama.2024.7741
Manage citations:
© 2024
- Permissions
Causal Inference About the Effects of Interventions From Observational Studies in Medical Journals
- 1 CAUSALab, Harvard T.H. Chan School of Public Health, Boston, Massachusetts
- 2 Department of Epidemiology, Harvard T.H. Chan School of Public Health, Boston, Massachusetts
- 3 Department of Biostatistics, Harvard T.H. Chan School of Public Health, Boston, Massachusetts
- 4 Smith Center for Outcomes Research in Cardiology, Beth Israel Deaconess Medical Center, Boston, Massachusetts
- 5 Statistical Editor, JAMA
- 6 Department of Medicine, University of California, San Francisco
- 7 Department of Epidemiology and Biostatistics, University of California, San Francisco
- 8 Editor in Chief, JAMA and JAMA Network
- Editor's Note Meaning of Proposed Causal Inference Framework for the JAMA Network Annette Flanagin, RN, MA; Roger J. Lewis, MD, PhD; Christopher C. Muth, MD; Gregory Curfman, MD JAMA
Importance Many medical journals, including JAMA, restrict the use of causal language to the reporting of randomized clinical trials. Although well-conducted randomized clinical trials remain the preferred approach for answering causal questions, methods for observational studies have advanced such that causal interpretations of the results of well-conducted observational studies may be possible when strong assumptions hold. Furthermore, observational studies may be the only practical source of information for answering some questions about the causal effects of medical or policy interventions, can support the study of interventions in populations and settings that reflect practice, and can help identify interventions for further experimental investigation. Identifying opportunities for the appropriate use of causal language when describing observational studies is important for communication in medical journals.
Observations A structured approach to whether and how causal language may be used when describing observational studies would enhance the communication of research goals, support the assessment of assumptions and design and analytic choices, and allow for more clear and accurate interpretation of results. Building on the extensive literature on causal inference across diverse disciplines, we suggest a framework for observational studies that aim to provide evidence about the causal effects of interventions based on 6 core questions: what is the causal question; what quantity would, if known, answer the causal question; what is the study design; what causal assumptions are being made; how can the observed data be used to answer the causal question in principle and in practice; and is a causal interpretation of the analyses tenable?
Conclusions and Relevance Adoption of the proposed framework to identify when causal interpretation is appropriate in observational studies promises to facilitate better communication between authors, reviewers, editors, and readers. Practical implementation will require cooperation between editors, authors, and reviewers to operationalize the framework and evaluate its effect on the reporting of empirical research.
Many medical journals, including JAMA, restrict the use of causal language to describing studies in which the intervention is randomly assigned. Indeed, randomized clinical trials are widely viewed as the preferred way of answering questions about the causal effects of interventions. Yet it is not feasible to answer all such questions with trials due to limitations including cost, follow-up duration, or ethical considerations. When such limitations preclude the conduct of trials, carefully designed analyses of observational (nonexperimental) data offer an alternative source of evidence on the effects of interventions (eg, treatment strategies, policies, or changes in behavior). Furthermore, observational studies can serve as a data-driven approach for identifying interventions that merit further experimental investigation and for examining the effects of interventions in populations and settings that reflect practice.
The potential of observational studies to contribute evidence about the causal effects of interventions is actively being examined across medicine, epidemiology, biostatistics, economics, and other social sciences. In this Special Communication, we examine a framework that might be used by medical journals as they move away from the current approach prohibiting the use of any causal language for observational studies and toward a more comprehensive approach for causal inference that reflects a synthesis of extensive prior work spanning multiple, diverse disciplines. We undertake this examination now for 3 main reasons. First, decision-makers are increasingly seeking timely answers to complex research questions about the effects of interventions that are challenging or impossible to address with randomized trials. For example, questions about long-term or rare effects of treatment, heterogeneity of treatment effects, or the effects of health care policies can be difficult to answer by relying exclusively on trials. Second, there has been wide dissemination of frameworks for posing causal questions and elaborating the assumptions needed to answer them. 1 - 41 These frameworks have supported the refinement of existing methods and the development of new methods that promise to deliver results that have a causal interpretation, provided strong assumptions are met. 42 - 138 Third, observational data from multiple sources (eg, registries, health care claims, electronic health records) are increasingly available for research purposes. Analyses from different sources can facilitate the evaluation of robustness by using data with different measurement characteristics from populations that may have different underlying causal structures.
In what follows, we first lay out the challenges inherent in drawing causal inferences about the effects of interventions from observational studies. We then discuss limitations of the current approach to determining the appropriateness of causal language for observational studies. Finally, we propose an alternative framework for causal inference in medical and health policy research and examine its implications for authors, reviewers, editors, and readers of clinical journals.
Increasing use of observational studies to address questions about the causal effects of interventions poses a challenge to journals that primarily serve clinical audiences. These observational studies depend more heavily on causal and statistical modeling assumptions compared with large, well-conducted randomized trials. Therefore, all other study aspects being equal, drawing causal inferences from observational studies is inherently more speculative. But, as noted earlier, all other study aspects are often not equal. Randomized trials cannot address all causal questions of importance in medicine and health policy and may have limited generalizability; thus, investigators may need to use observational studies as a source of evidence to address causal questions. The challenge, then, is to balance the importance of addressing the causal questions for which observational studies are needed with caution regarding the reliance on strong assumptions to support causal conclusions.
When researchers are confronted with this challenge, one response is to retreat from causal goals and pursue purely descriptive or predictive goals for observational studies. This approach often amounts to applying a randomization-centered criterion for determining whether causal language is allowed, resulting in exclusively associational language for any investigation using observational data. With this approach, a single study design element essentially dictates the language that can be used to describe goals, methods, and interpretations. For example, current Instructions for Authors in JAMA and the JAMA Network journals state that “[c]ausal language (including use of terms such as effect and efficacy) should be used only for randomized clinical trials. For all other study designs…, methods and results should be described in terms of association or correlation and should avoid cause-and-effect wording.” This recommendation is also included in the AMA Manual of Style . 139 Nevertheless, rare ad hoc exceptions have been made by JAMA and JAMA Network journals in allowing causal interpretations for observational analyses in which necessary assumptions were articulated and deemed plausible. 140 , 141 Furthermore, articles in the JAMA Guide to Statistics and Methods series have discussed various causal inference methods. 142 - 151
The use of a binary, randomization-centered criterion for allowing the use of causal language or interpretation is not problematic when applied to large, well-conducted randomized trials with near-perfect adherence to the study protocol and limited missing outcomes, wherein a causal interpretation is warranted. However, for many other studies, the approach based on this criterion is inadequate and does not accommodate precise descriptions of goals, research questions, methods, assumptions, and interpretations, and can result in lack of clarity during interactions among authors, editors, reviewers, and readers. The prohibition impedes the presentation and critique of study methods and risks misinterpretation of results both by allowing inappropriately drawn implicit causal inferences and by obscuring appropriate causal conclusions.
Prohibiting causal language when describing observational studies does not allow authors to communicate their research goals clearly and fully. 152 - 154 Causal goals require causal assumptions (eg, the assumption of no uncontrolled confounding). These assumptions are almost never possible to verify with the data alone, and their plausibility can best be assessed within an explicit causal framework. Without causal language, the description and critique of research methods becomes challenging because the connection between ends (causal goals) and means (research methods) is obscured. 154 Furthermore, when causal goals, assumptions, and methods cannot be explicitly discussed, assessing the choice of study design and analytic approaches and interpreting results become difficult, if not impossible. In fact, avoidance of causal language precludes effective criticism grounded in causal considerations. For example, if a manuscript purports to present only descriptive or predictive associations between some exposure (or treatment) and outcomes, there is little room for discussing confounding in the sense of comparability between intervention groups. 153 , 154 Yet such discussion is often necessary to uncover the reported study’s limitations if a causal interpretation is under consideration. In other words, restricting causal discourse is undesirable because authors and readers often hope that the estimated associations have a tenable causal interpretation and are interested to know when and why such interpretation may not be valid.
In addition, using a single study design element (randomization) as the sole criterion of whether causal conclusions can be drawn risks giving the impression of complacency about potential weaknesses that can affect both randomized trials and observational studies. Editors, reviewers, and readers would not draw causal conclusions based on simple between-treatment-group comparisons from a randomized trial with poor data collection practices, differential outcome ascertainment, or a high dropout rate, but these issues are not given the same weight as (lack of) randomization when the current approach to the use of causal language is applied. Arguably, an approach based on the randomization-centered criterion without directly confronting the difficulties listed earlier would be possible only if randomized trials with no major flaws were the only experimental studies under consideration, in which case cautions about causal interpretation could be reserved only for observational studies. Randomization strengthens the plausibility of a causal interpretation of study results, but randomization alone is not sufficient. Conversely, the absence of randomization does not on its own render a causal interpretation completely untenable. For observational studies, the blanket prohibition of causal language skirts the difficult but necessary work of judging whether a causal interpretation of any specific observational analysis is tenable. This judgment cannot rest on simply noting the absence of randomization 155 ; it requires context-informed examination of all relevant aspects of design, conduct, and analysis.
The extensive literature on causal inference across diverse disciplines 26 , 30 , 156 - 158 suggests an alternative framework for observational studies that aim to answer questions about the causal effects of interventions. This framework avoids the limitations discussed earlier and can help editors and readers determine whether a particular observational study provides valid and reliable evidence about the effects of interventions in a target population. Such a framework can be summarized in terms of several core questions that need to be considered to understand and interpret observational studies:
What is the causal question? If the goal of the research is to provide evidence about the effects of medical or health policy interventions, the research question is best explicitly framed in causal terms, comparing 2 or more well-defined alternatives with respect to clearly defined outcomes of interest, for a specific target population during a period of follow-up. 159 , 160
What quantity would, if known, answer the causal question? After stating the causal question, one can specify the quantity that could, if known, serve as the answer to the question; this quantity is the causal estimand (eg, the causal effect of interest). 161 , 162 The precise specification of the causal estimand requires describing the population of interest, the interventions or strategies to be compared, details of outcome definitions and the timing of outcome ascertainment, and the choice of effect measure (eg, risk difference, relative risk). The causal estimand can be formally specified using mathematical causal models (eg, closely related counterfactual, potential outcome, or structural models 3 , 5 , 26 , 163 - 169 ). In many cases, specification can be aided by describing the (hypothetical) target trial that could address the research question. 144 , 170 - 172
What is the study design? The approach for collecting new data or using existing data—including choosing among data sources, sampling individuals and their follow-up experience, and collecting treatment covariate and outcome information over time—determines whether the data can be used to answer the causal question. For example, in cohort studies comparing different treatment strategies, the choice of the start of follow-up (time zero) and the alignment of that time with the time at which eligibility is determined can affect the validity of observational analyses. 173 More broadly, the key goal of study design is to make the causal assumptions more plausible and to facilitate learning about the causal estimand.
What causal assumptions are being made? Drawing causal inferences from observational studies requires causal assumptions that allow investigators to learn about the causal estimand by using data. For example, many observational studies require an assumption that, given the variables that have been measured and accounted for (via study design or analysis), there remains no uncontrolled confounding. 174 Other approaches, such as instrumental variable analyses, difference-in-differences analyses, or regression discontinuity analyses, require different sets of assumptions. Typically, causal assumptions are untestable in the sense that they cannot be fully evaluated with the data alone; instead, they have to be examined on the basis of background knowledge (eg, clinical knowledge of the treatment selection process). 175 , 176
How can the observed data be used to answer the causal question in principle and in practice? Using the study design and causal assumptions, investigators can determine how analyses of observed data could, at least in principle (eg, if, hypothetically, all causal assumptions held and sampling variability were absent), provide information about the causal estimand. The formal examination of whether the observed data can in principle be used to learn about the causal estimand is referred to as identification analysis . In some cases, the assumptions suffice only to place bounds around the causal estimand. 45 , 177 - 179 Most studies aiming to estimate causal estimands using observational data rely on well-understood identification strategies (ie, the results from prior identification analyses) 180 , 181 and apply statistical methods to data for estimation and statistical inference. We offer a more detailed description of the relationship between causal estimands, identification analysis, and the use of data and statistical methods in the eText; eFigure 1, eFigure 2, and eFigure 3; and Example 1 and Example 2 in the Supplement .
The statistical methods for observational studies should have good statistical performance (eg, acceptably low bias, high precision) and support the valid quantification of uncertainty (eg, producing valid CIs). The challenges of drawing statistical inferences using data and models are, if anything, accentuated in nonexperimental research. 182 Furthermore, issues related to missing data and measurement error often arise in observational studies and require additional assumptions (typically untestable using the data alone) about the structure of missingness or measurement error, additional data (eg, validation studies), and specialized methods to address these issues and properly quantify uncertainty.
Is a causal interpretation of the analyses tenable? Evaluating the appropriateness of endowing the results of an observational analysis with a causal interpretation typically requires untestable assumptions. Determining whether such interpretation is tenable, therefore, involves subjective judgments informed by background knowledge and an understanding of the research context, drawing on multiple sources of evidence. These judgments can be informed by triangulation of results across different analyses (eg, using different assumptions or other data sources) 183 ; attempts to falsify the causal assumptions with the data, when possible (eg, negative control analyses 80 , 184 ); and quantitative bias/sensitivity analyses and other methods to examine assumption violations. 19 , 185 - 191
This framework maintains the distinction between causation and association while addressing the limitations of approaches that rely on randomization as the sole criterion: it differentiates between causal ends and the statistical means to achieve them; supports the alignment between causal questions and the analyses used to answer them; increases transparency to facilitate scientific conversations; acknowledges that subjective judgments, informed by background clinical or policy knowledge, are unavoidable in observational studies; and aims to instill intellectual humility. Disagreements regarding the appropriate interpretation of observational studies among different stakeholders are always possible. This framework clarifies such disagreements by making the relevant considerations explicit and facilitates reasoning and debate.
Far from being a list of separate items, the framework highlights that multiple interrelated components are needed to report, evaluate, and interpret observational studies. For example, investigators will select study designs that are tailored to answer the causal question of interest and that support the plausibility of the causal assumptions needed to answer it. Similarly, study design and data analysis aspects can be arranged to facilitate the conduct of quantitative bias/sensitivity and falsification analyses, providing for the rigorous evaluation of assumptions. Background knowledge and understanding of the medical or policy context of the investigation is needed in all steps of the framework, from framing the research question to evaluating the plausibility of assumptions and evaluating whether a causal interpretation is tenable.
Interpreted practically, the framework allows the use of causal language to specify research questions and study goals (eg, in a manuscript’s Introduction section); to describe study methods, assumptions under which the methods produce results that have a causal interpretation, and approaches for examining assumptions (eg, in the Methods section); and to reason about the plausibility of assumptions and the degree to which a causal interpretation is tenable in view of background knowledge while acknowledging the potential limitations of such an interpretation (eg, in the Discussion section). Two elements are central to this proposal for presenting observational studies: first, being explicit about the “if-then” (conditional) structure needed for their interpretation (eg, if certain assumptions hold, then a causal interpretation of the findings is tenable); and second, acknowledging that careful context-informed judgments are necessary to evaluate whether assumptions are plausible and a causal interpretation is tenable.
Last, although not the focus of this communication, the framework can also be applied to randomized trials and may be particularly helpful for pragmatic trials with baseline randomization that otherwise share many characteristics of observational studies (eg, trials with nonstandardized follow-up protocols and limited systematic efforts to enhance adherence to the assigned treatment). 192 , 193
The framework does not imply that all, or even most, observational studies merit a causal interpretation. For some observational studies that start with causal goals, causal inference may prove impossible; in these cases, estimates retain only associational interpretations. In addition, many important descriptive and predictive research questions can be answered by observational studies that do not require causal notions.
Furthermore, when addressing causal questions, our proposal does not single out any of the currently popular frameworks, empirical research strategies, or statistical methods for causal inference from observational studies (eg, structural approaches 27 , 167 ; identification strategies 180 , 181 ; the target trial framework 144 , 170 ; the causal roadmap and targeted learning 32 , 156 ; any specific statistical, epidemiologic, or econometric method), nor does it single out any philosophy of statistical inference (eg, frequentist, bayesian). There is room for creativity in approaching practical causal questions, and investigators should have the freedom to select the approaches that best suit their research questions, provided they follow the norms for reporting described earlier. Without delving into the details of a specific research question, perhaps the most that can be recommended is to use the simplest methods that are adequate for the study’s causal goals. 194 , 195
The framework does not address the broader issue of how to determine whether some general causal claim is warranted (eg, whether some exposure is a “cause” of some outcome). Instead, it focuses on whether observational studies can contribute independent credible evidence about causal effects of interventions in a particular target population, time, and place. Reports of such studies are the core publication type in most medical and health policy journals; more important, they are a key input to the process of evidence synthesis that can support general causal claims. This process combines information from multiple sources, including—in addition to trials and observational studies comparing interventions—basic science investigations, case reports, noncomparative studies, meta-analyses, and simulation modeling studies, as well as background knowledge.
Last, the framework does not cover other important issues that apply broadly to empirical investigations regardless of study design, such as prespecifying and preregistering analyses, following the principles of reproducible science, and sharing research materials.
Adoption and further elaboration of the framework outlined earlier by medical journals offer the promise of facilitating communication between authors, reviewers, editors, and readers, but come with challenges in operationalization and implementation.
For authors, the framework provides more freedom to express causal goals and assumptions of observational studies, but also entails the responsibility to explicitly discuss and evaluate assumptions and openly acknowledge limitations (eg, violations of assumptions) and may require additional work (eg, to report technical details; to conduct triangulation, falsification, and bias analyses).
For reviewers, the framework should aid in the assessment of manuscripts that report observational studies. It requires familiarity with causal inference methods, as well as background knowledge to judge the appropriateness of the methods in the context of applied work.
Adoption of the framework should facilitate communication between authors, reviewers, and editors by encouraging the transparent reporting and critique of methods and results of observational studies of medical interventions. Implementation at scale will require retaining expert reviewers and increasing the cooperation between editors, authors, and reviewers to operationalize the framework for use with different analyses and specific clinical applications and to evaluate whether it improves the reporting of empirical research. Furthermore, the complex judgments that the framework entails require vigilance to mitigate cognitive biases and distortions that may influence the presentation and interpretation of observational studies, particularly those using technically complex methods. 196
For readers, the framework should facilitate the clear communication of causal questions and methods. As usual, detailed technical descriptions may be appropriately placed in supplemental appendices to allow for the inclusion of the necessary detail and to maintain the readability and accessibility of the published study. Although our proposal suggests that complex concepts and more elaborate methodological descriptions may be needed to fully report and evaluate observational studies, adoption of the framework promises to improve the value of applied research that can support medical and policy decisions.
We look forward to readers’ reactions to the framework. In future communications, we plan to explore its application in the context of concrete examples of specific types of observational analyses typically encountered in medical journals such as JAMA and the JAMA Network journals.
Accepted for Publication: April 15, 2024.
Published Online: May 9, 2024. doi:10.1001/jama.2024.7741
Corresponding Author: Issa J. Dahabreh, MD, ScD, CAUSALab, Department of Epidemiology, Harvard T.H. Chan School of Public Health, 677 Huntington Ave, Room 816c, Boston, MA 02115 ( [email protected] ).
Conflict of Interest Disclosures: Dr Dahabreh reported receiving grants from Sanofi as principal investigator of a research agreement between Harvard and Sanofi for causal inference methods for transportability analyses; and reported receiving consulting fees from Moderna for trial and observational analyses outside the submitted work. No other disclosures were reported.
Additional Contributions: We thank Caroline Sietmann, MALIS, JAMA and JAMA Network, for assistance with soliciting and organizing comments on earlier versions of this article. We thank the following individuals for comments on earlier versions: Heather Gwynn Allore, MS, PhD, Yale University and JAMA Internal Medicine ; Joshua D. Angrist, PhD, Massachusetts Institute of Technology; Michael Berkwits, MD; Jesse Berlin, ScD, Rutgers University and JAMA Network Open ; Isabelle Boutron, MD, PhD, Université de Paris, CRESS, Inserm; Stephen R. Cole, PhD, University of North Carolina at Chapel Hill; John Concato, MD, MS, MPH, US Food and Drug Administration and Yale School of Medicine; Gregory Curfman, MD, JAMA and JAMA Network; Annette Flanagin, RN, MA, JAMA and JAMA Network; Maria Glymour, ScD, MS, Boston University; Deborah Grady, MD, MPH, University of California, San Francisco, and JAMA Internal Medicine ; Sander Greenland, DrPH, University of California, Los Angeles; Gordon Guyatt, MD, MSc, McMaster University; Sebastien Haneuse, PhD, Harvard University and JAMA Network Open ; Frank E. Harrell Jr, PhD, Vanderbilt University; Robert A. Harrington, MD, Weill Cornell Medicine and JAMA Cardiology ; Laura Hatfield, PhD, Harvard University and JAMA ; Miguel A. Hernán, MD, PhD, Harvard University; Guido Imbens, PhD, Stanford University; Nina Joyce, PhD, Brown University; Amy H. Kaji, MD, PhD, University of California, Los Angeles, and JAMA Surgery ; Jay S. Kaufman, PhD, McGill University; Dhruv Kazi, MD, MSc, MS, Beth Israel Deaconess Medical Center; Kenneth S. Kendler, MD, Virginia Commonwealth University; Daniel B. Kramer, MD, MPH, Beth Israel Deaconess Medical Center; Timothy Lash, DSc, MPH, Emory University; Roger J. Lewis, MD, PhD, University of California, Los Angeles, and JAMA ; Charles F. Manski, PhD, Northwestern University; M. Hassan Murad, MD, Mayo Clinic; Christopher Muth, MD, JAMA ; Sharon-Lise Normand, PhD, Harvard University; Neil Pearce, PhD, London School of Hygiene and Tropical Medicine; Maya Petersen, MD, PhD, University of California, Berkeley; Romain Pirracchio, MD, MPH, PhD, University of California, San Francisco, and JAMA ; Stuart J. Pocock, PhD, London School of Hygiene and Tropical Medicine; James M. Robins, MD, Harvard University; Sherri Rose, PhD, Stanford University; Paul R. Rosenbaum, PhD, University of Pennsylvania; Kenneth J. Rothman, DrPH, Boston University; Jeffrey Saver, MD, University of California, Los Angeles, and JAMA ; Stephen Schenkel, MD, MPP, University of Maryland Medical Center and JAMA ; David Schriger, MD, MPH, University of California, Los Angeles, and JAMA ; Ian Shrier, MD, PhD, McGill University; Dylan S. Small, PhD, University of Pennsylvania; George Davey Smith, DSc, University of Bristol; Zirui Song, MD, PhD, Harvard University and JAMA Health Forum ; Jonathan A. C. Sterne, PhD, University of Bristol; Elizabeth A. Stuart, PhD, Johns Hopkins University and JAMA Health Forum ; Sonja A. Swanson, ScD, University of Pittsburgh and JAMA Psychiatry ; Eric Tchetgen Tchetgen, PhD, University of Pennsylvania; Linda Valeri, PhD, Columbia University and JAMA Psychiatry ; Tyler J. VanderWeele, PhD, Harvard University and JAMA Psychiatry ; Rishi Wadhera, MD, MPP, MPhil, Beth Israel Deaconess Medical Center; Robert Yeh, MD, MS, MBA, Beth Israel Deaconess Medical Center; and Alan M. Zaslavsky, PhD, Harvard Medical School.
- Register for email alerts with links to free full-text articles
- Access PDFs of free articles
- Manage your interests
- Save searches and receive search alerts
- Open access
- Published: 15 May 2024
Learning together for better health using an evidence-based Learning Health System framework: a case study in stroke
- Helena Teede 1 , 2 na1 ,
- Dominique A. Cadilhac 3 , 4 na1 ,
- Tara Purvis 3 ,
- Monique F. Kilkenny 3 , 4 ,
- Bruce C.V. Campbell 4 , 5 , 6 ,
- Coralie English 7 ,
- Alison Johnson 2 ,
- Emily Callander 1 ,
- Rohan S. Grimley 8 , 9 ,
- Christopher Levi 10 ,
- Sandy Middleton 11 , 12 ,
- Kelvin Hill 13 &
- Joanne Enticott ORCID: orcid.org/0000-0002-4480-5690 1
BMC Medicine volume 22 , Article number: 198 ( 2024 ) Cite this article
228 Accesses
1 Altmetric
Metrics details
In the context of expanding digital health tools, the health system is ready for Learning Health System (LHS) models. These models, with proper governance and stakeholder engagement, enable the integration of digital infrastructure to provide feedback to all relevant parties including clinicians and consumers on performance against best practice standards, as well as fostering innovation and aligning healthcare with patient needs. The LHS literature primarily includes opinion or consensus-based frameworks and lacks validation or evidence of benefit. Our aim was to outline a rigorously codesigned, evidence-based LHS framework and present a national case study of an LHS-aligned national stroke program that has delivered clinical benefit.
Current core components of a LHS involve capturing evidence from communities and stakeholders (quadrant 1), integrating evidence from research findings (quadrant 2), leveraging evidence from data and practice (quadrant 3), and generating evidence from implementation (quadrant 4) for iterative system-level improvement. The Australian Stroke program was selected as the case study as it provides an exemplar of how an iterative LHS works in practice at a national level encompassing and integrating evidence from all four LHS quadrants. Using this case study, we demonstrate how to apply evidence-based processes to healthcare improvement and embed real-world research for optimising healthcare improvement. We emphasize the transition from research as an endpoint, to research as an enabler and a solution for impact in healthcare improvement.
Conclusions
The Australian Stroke program has nationally improved stroke care since 2007, showcasing the value of integrated LHS-aligned approaches for tangible impact on outcomes. This LHS case study is a practical example for other health conditions and settings to follow suit.
Peer Review reports
Internationally, health systems are facing a crisis, driven by an ageing population, increasing complexity, multi-morbidity, rapidly advancing health technology and rising costs that threaten sustainability and mandate transformation and improvement [ 1 , 2 ]. Although research has generated solutions to healthcare challenges, and the advent of big data and digital health holds great promise, entrenched siloes and poor integration of knowledge generation, knowledge implementation and healthcare delivery between stakeholders, curtails momentum towards, and consistent attainment of, evidence-and value-based care [ 3 ]. This is compounded by the short supply of research and innovation leadership within the healthcare sector, and poorly integrated and often inaccessible health data systems, which have crippled the potential to deliver on digital-driven innovation [ 4 ]. Current approaches to healthcare improvement are also often isolated with limited sustainability, scale-up and impact [ 5 ].
Evidence suggests that integration and partnership across academic and healthcare delivery stakeholders are key to progress, including those with lived experience and their families (referred to here as consumers and community), diverse disciplines (both research and clinical), policy makers and funders. Utilization of evidence from research and evidence from practice including data from routine care, supported by implementation research, are key to sustainably embedding improvement and optimising health care and outcomes. A strategy to achieve this integration is through the Learning Health System (LHS) (Fig. 1 ) [ 2 , 6 , 7 , 8 ]. Although there are numerous publications on LHS approaches [ 9 , 10 , 11 , 12 ], many focus on research perspectives and data, most do not demonstrate tangible healthcare improvement or better health outcomes. [ 6 ]
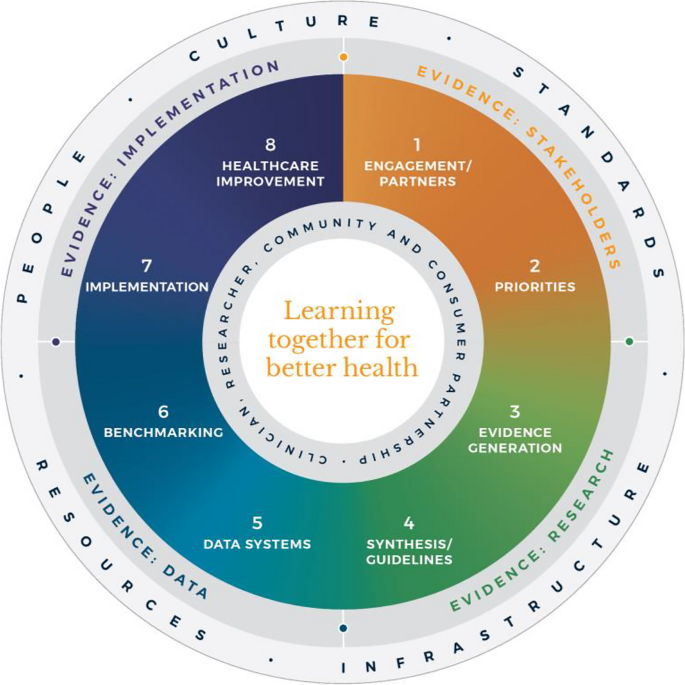
Monash Learning Health System: The Learn Together for Better Health Framework developed by Monash Partners and Monash University (from Enticott et al. 2021 [ 7 ]). Four evidence quadrants: Q1 (orange) is evidence from stakeholders; Q2 (green) is evidence from research; Q3 (light blue) is evidence from data; and, Q4 (dark blue) is evidence from implementation and healthcare improvement
In developed nations, it has been estimated that 60% of care provided aligns with the evidence base, 30% is low value and 10% is potentially harmful [ 13 ]. In some areas, clinical advances have been rapid and research and evidence have paved the way for dramatic improvement in outcomes, mandating rapid implementation of evidence into healthcare (e.g. polio and COVID-19 vaccines). However, healthcare improvement is challenging and slow [ 5 ]. Health systems are highly complex in their design, networks and interacting components, and change is difficult to enact, sustain and scale up. [ 3 ] New effective strategies are needed to meet community needs and deliver evidence-based and value-based care, which reorients care from serving the provider, services and system, towards serving community needs, based on evidence and quality. It goes beyond cost to encompass patient and provider experience, quality care and outcomes, efficiency and sustainability [ 2 , 6 ].
The costs of stroke care are expected to rise rapidly in the next decades, unless improvements in stroke care to reduce the disabling effects of strokes can be successfully developed and implemented [ 14 ]. Here, we briefly describe the Monash LHS framework (Fig. 1 ) [ 2 , 6 , 7 ] and outline an exemplar case in order to demonstrate how to apply evidence-based processes to healthcare improvement and embed real-world research for optimising healthcare. The Australian LHS exemplar in stroke care has driven nationwide improvement in stroke care since 2007.
An evidence-based Learning Health System framework
In Australia, members of this author group (HT, AJ, JE) have rigorously co-developed an evidence-based LHS framework, known simply as the Monash LHS [ 7 ]. The Monash LHS was designed to support sustainable, iterative and continuous robust benefit of improved clinical outcomes. It was created with national engagement in order to be applicable to Australian settings. Through this rigorous approach, core LHS principles and components have been established (Fig. 1 ). Evidence shows that people/workforce, culture, standards, governance and resources were all key to an effective LHS [ 2 , 6 ]. Culture is vital including trust, transparency, partnership and co-design. Key processes include legally compliant data sharing, linkage and governance, resources, and infrastructure [ 4 ]. The Monash LHS integrates disparate and often siloed stakeholders, infrastructure and expertise to ‘Learn Together for Better Health’ [ 7 ] (Fig. 1 ). This integrates (i) evidence from community and stakeholders including priority areas and outcomes; (ii) evidence from research and guidelines; (iii) evidence from practice (from data) with advanced analytics and benchmarking; and (iv) evidence from implementation science and health economics. Importantly, it starts with the problem and priorities of key stakeholders including the community, health professionals and services and creates an iterative learning system to address these. The following case study was chosen as it is an exemplar of how a Monash LHS-aligned national stroke program has delivered clinical benefit.
Australian Stroke Learning Health System
Internationally, the application of LHS approaches in stroke has resulted in improved stroke care and outcomes [ 12 ]. For example, in Canada a sustained decrease in 30-day in-hospital mortality has been found commensurate with an increase in resources to establish the multifactorial stroke system intervention for stroke treatment and prevention [ 15 ]. Arguably, with rapid advances in evidence and in the context of an ageing population with high cost and care burden and substantive impacts on quality of life, stroke is an area with a need for rapid research translation into evidence-based and value-based healthcare improvement. However, a recent systematic review found that the existing literature had few comprehensive examples of LHS adoption [ 12 ]. Although healthcare improvement systems and approaches were described, less is known about patient-clinician and stakeholder engagement, governance and culture, or embedding of data informatics into everyday practice to inform and drive improvement [ 12 ]. For example, in a recent review of quality improvement collaborations, it was found that although clinical processes in stroke care are improved, their short-term nature means there is uncertainty about sustainability and impacts on patient outcomes [ 16 ]. Table 1 provides the main features of the Australian Stroke LHS based on the four core domains and eight elements of the Learning Together for Better Health Framework described in Fig. 1 . The features are further expanded on in the following sections.
Evidence from stakeholders (LHS quadrant 1, Fig. 1 )
Engagement, partners and priorities.
Within the stroke field, there have been various support mechanisms to facilitate an LHS approach including partnership and broad stakeholder engagement that includes clinical networks and policy makers from different jurisdictions. Since 2008, the Australian Stroke Coalition has been co-led by the Stroke Foundation, a charitable consumer advocacy organisation, and Stroke Society of Australasia a professional society with membership covering academics and multidisciplinary clinician networks, that are collectively working to improve stroke care ( https://australianstrokecoalition.org.au/ ). Surveys, focus groups and workshops have been used for identifying priorities from stakeholders. Recent agreed priorities have been to improve stroke care and strengthen the voice for stroke care at a national ( https://strokefoundation.org.au/ ) and international level ( https://www.world-stroke.org/news-and-blog/news/world-stroke-organization-tackle-gaps-in-access-to-quality-stroke-care ), as well as reduce duplication amongst stakeholders. This activity is built on a foundation and culture of research and innovation embedded within the stroke ‘community of practice’. Consumers, as people with lived experience of stroke are important members of the Australian Stroke Coalition, as well as representatives from different clinical colleges. Consumers also provide critical input to a range of LHS activities via the Stroke Foundation Consumer Council, Stroke Living Guidelines committees, and the Australian Stroke Clinical Registry (AuSCR) Steering Committee (described below).
Evidence from research (LHS quadrant 2, Fig. 1 )
Advancement of the evidence for stroke interventions and synthesis into clinical guidelines.
To implement best practice, it is crucial to distil the large volume of scientific and trial literature into actionable recommendations for clinicians to use in practice [ 24 ]. The first Australian clinical guidelines for acute stroke were produced in 2003 following the increasing evidence emerging for prevention interventions (e.g. carotid endarterectomy, blood pressure lowering), acute medical treatments (intravenous thrombolysis, aspirin within 48 h of ischemic stroke), and optimised hospital management (care in dedicated stroke units by a specialised and coordinated multidisciplinary team) [ 25 ]. Importantly, a number of the innovations were developed, researched and proven effective by key opinion leaders embedded in the Australian stroke care community. In 2005, the clinical guidelines for Stroke Rehabilitation and Recovery [ 26 ] were produced, with subsequent merged guidelines periodically updated. However, the traditional process of periodic guideline updates is challenging for end users when new research can render recommendations redundant and this lack of currency erodes stakeholder trust [ 27 ]. In response to this challenge the Stroke Foundation and Cochrane Australia entered a pioneering project to produce the first electronic ‘living’ guidelines globally [ 20 ]. Major shifts in the evidence for reperfusion therapies (e.g. extended time-window intravenous thrombolysis and endovascular clot retrieval), among other advances, were able to be converted into new recommendations, approved by the Australian National Health and Medical Research Council within a few months of publication. Feedback on this process confirmed the increased use and trust in the guidelines by clinicians. The process informed other living guidelines programs, including the successful COVID-19 clinical guidelines [ 28 ].
However, best practice clinical guideline recommendations are necessary but insufficient for healthcare improvement and nesting these within an LHS with stakeholder partnership, enables implementation via a range of proven methods, including audit and feedback strategies [ 29 ].
Evidence from data and practice (LHS quadrant 3, Fig. 1 )
Data systems and benchmarking : revealing the disparities in care between health services. A national system for standardized stroke data collection was established as the National Stroke Audit program in 2007 by the Stroke Foundation [ 30 ] following various state-level programs (e.g. New South Wales Audit) [ 31 ] to identify evidence-practice gaps and prioritise improvement efforts to increase access to stroke units and other acute treatments [ 32 ]. The Audit program alternates each year between acute (commencing in 2007) and rehabilitation in-patient services (commencing in 2008). The Audit program provides a ‘deep dive’ on the majority of recommendations in the clinical guidelines whereby participating hospitals provide audits of up to 40 consecutive patient medical records and respond to a survey about organizational resources to manage stroke. In 2009, the AuSCR was established to provide information on patients managed in acute hospitals based on a small subset of quality processes of care linked to benchmarked reports of performance (Fig. 2 ) [ 33 ]. In this way, the continuous collection of high-priority processes of stroke care could be regularly collected and reviewed to guide improvement to care [ 34 ]. Plus clinical quality registry programs within Australia have shown a meaningful return on investment attributed to enhanced survival, improvements in quality of life and avoided costs of treatment or hospital stay [ 35 ].
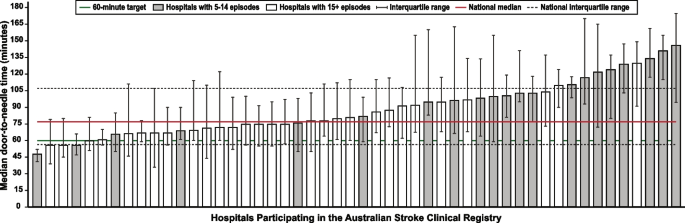
Example performance report from the Australian Stroke Clinical Registry: average door-to-needle time in providing intravenous thrombolysis by different hospitals in 2021 [ 36 ]. Each bar in the figure represents a single hospital
The Australian Stroke Coalition endorsed the creation of an integrated technological solution for collecting data through a single portal for multiple programs in 2013. In 2015, the Stroke Foundation, AuSCR consortium, and other relevant groups cooperated to design an integrated data management platform (the Australian Stroke Data Tool) to reduce duplication of effort for hospital staff in the collection of overlapping variables in the same patients [ 19 ]. Importantly, a national data dictionary then provided the common data definitions to facilitate standardized data capture. Another important feature of AuSCR is the collection of patient-reported outcome surveys between 90 and 180 days after stroke, and annual linkage with national death records to ascertain survival status [ 33 ]. To support a LHS approach, hospitals that participate in AuSCR have access to a range of real-time performance reports. In efforts to minimize the burden of data collection in the AuSCR, interoperability approaches to import data directly from hospital or state-level managed stroke databases have been established (Fig. 3 ); however, the application has been variable and 41% of hospitals still manually enter all their data.
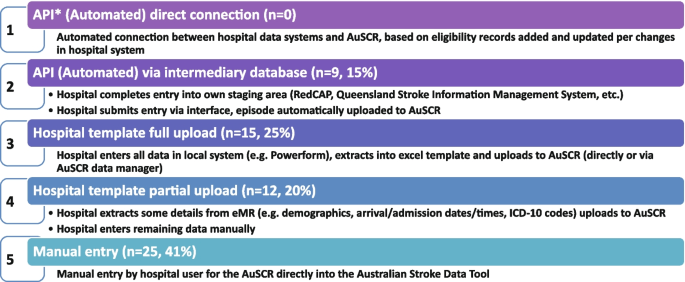
Current status of automated data importing solutions in the Australian Stroke Clinical Registry, 2022, with ‘ n ’ representing the number of hospitals. AuSCR, Australian Stroke Clinical Registry; AuSDaT, Australian Stroke Data Tool; API, Application Programming Interface; ICD, International Classification of Diseases; RedCAP, Research Electronic Data Capture; eMR, electronic medical records
For acute stroke care, the Australian Commission on Quality and Safety in Health Care facilitated the co-design (clinicians, academics, consumers) and publication of the national Acute Stroke Clinical Care Standard in 2015 [ 17 ], and subsequent review [ 18 ]. The indicator set for the Acute Stroke Standard then informed the expansion of the minimum dataset for AuSCR so that hospitals could routinely track their performance. The national Audit program enabled hospitals not involved in the AuSCR to assess their performance every two years against the Acute Stroke Standard. Complementing these efforts, the Stroke Foundation, working with the sector, developed the Acute and Rehabilitation Stroke Services Frameworks to outline the principles, essential elements, models of care and staffing recommendations for stroke services ( https://informme.org.au/guidelines/national-stroke-services-frameworks ). The Frameworks are intended to guide where stroke services should be developed, and monitor their uptake with the organizational survey component of the Audit program.
Evidence from implementation and healthcare improvement (LHS quadrant 4, Fig. 1 )
Research to better utilize and augment data from registries through linkage [ 37 , 38 , 39 , 40 ] and to ensure presentation of hospital or service level data are understood by clinicians has ensured advancement in the field for the Australian Stroke LHS [ 41 ]. Importantly, greater insights into whole patient journeys, before and after a stroke, can now enable exploration of value-based care. The LHS and stroke data platform have enabled focused and time-limited projects to create a better understanding of the quality of care in acute or rehabilitation settings [ 22 , 42 , 43 ]. Within stroke, all the elements of an LHS culminate into the ready availability of benchmarked performance data and support for implementation of strategies to address gaps in care.
Implementation research to grow the evidence base for effective improvement interventions has also been a key pillar in the Australian context. These include multi-component implementation interventions to achieve behaviour change for particular aspects of stroke care, [ 22 , 23 , 44 , 45 ] and real-world approaches to augmenting access to hyperacute interventions in stroke through the use of technology and telehealth [ 46 , 47 , 48 , 49 ]. The evidence from these studies feeds into the living guidelines program and the data collection systems, such as the Audit program or AuSCR, which are then amended to ensure data aligns to recommended care. For example, the use of ‘hyperacute aspirin within the first 48 h of ischemic stroke’ was modified to be ‘hyperacute antiplatelet…’ to incorporate new evidence that other medications or combinations are appropriate to use. Additionally, new datasets have been developed to align with evidence such as the Fever, Sugar, and Swallow variables [ 42 ]. Evidence on improvements in access to best practice care from the acute Audit program [ 50 ] and AuSCR is emerging [ 36 ]. For example, between 2007 and 2017, the odds of receiving intravenous thrombolysis after ischemic stroke increased by 16% 9OR 1.06 95% CI 1.13–1.18) and being managed in a stroke unit by 18% (OR 1.18 95% CI 1.17–1.20). Over this period, the median length of hospital stay for all patients decreased from 6.3 days in 2007 to 5.0 days in 2017 [ 51 ]. When considering the number of additional patients who would receive treatment in 2017 in comparison to 2007 it was estimated that without this additional treatment, over 17,000 healthy years of life would be lost in 2017 (17,786 disability-adjusted life years) [ 51 ]. There is evidence on the cost-effectiveness of different system-focussed strategies to augment treatment access for acute ischemic stroke (e.g. Victorian Stroke Telemedicine program [ 52 ] and Melbourne Mobile Stroke Unit ambulance [ 53 ]). Reciprocally, evidence from the national Rehabilitation Audit, where the LHS approach has been less complete or embedded, has shown fewer areas of healthcare improvement over time [ 51 , 54 ].
Within the field of stroke in Australia, there is indirect evidence that the collective efforts that align to establishing the components of a LHS have had an impact. Overall, the age-standardised rate of stroke events has reduced by 27% between 2001 and 2020, from 169 to 124 events per 100,000 population. Substantial declines in mortality rates have been reported since 1980. Commensurate with national clinical guidelines being updated in 2007 and the first National Stroke Audit being undertaken in 2007, the mortality rates for men (37.4 deaths per 100,000) and women (36.1 deaths per 100,0000 has declined to 23.8 and 23.9 per 100,000, respectively in 2021 [ 55 ].
Underpinning the LHS with the integration of the four quadrants of evidence from stakeholders, research and guidelines, practice and implementation, and core LHS principles have been addressed. Leadership and governance have been important, and programs have been established to augment workforce training and capacity building in best practice professional development. Medical practitioners are able to undertake courses and mentoring through the Australasian Stroke Academy ( http://www.strokeacademy.com.au/ ) while nurses (and other health professionals) can access teaching modules in stroke care from the Acute Stroke Nurses Education Network ( https://asnen.org/ ). The Association of Neurovascular Clinicians offers distance-accessible education and certification to develop stroke expertise for interdisciplinary professionals, including advanced stroke co-ordinator certification ( www.anvc.org ). Consumer initiative interventions are also used in the design of the AuSCR Public Summary Annual reports (available at https://auscr.com.au/about/annual-reports/ ) and consumer-related resources related to the Living Guidelines ( https://enableme.org.au/resources ).
The important success factors and lessons from stroke as a national exemplar LHS in Australia include leadership, culture, workforce and resources integrated with (1) established and broad partnerships across the academic-clinical sector divide and stakeholder engagement; (2) the living guidelines program; (3) national data infrastructure, including a national data dictionary that provides the common data framework to support standardized data capture; (4) various implementation strategies including benchmarking and feedback as well as engagement strategies targeting different levels of the health system; and (5) implementation and improvement research to advance stroke systems of care and reduce unwarranted variation in practice (Fig. 1 ). Priority opportunities now include the advancement of interoperability with electronic medical records as an area all clinical quality registry’s programs needs to be addressed, as well as providing more dynamic and interactive data dashboards tailored to the need of clinicians and health service executives.
There is a clear mandate to optimise healthcare improvement with big data offering major opportunities for change. However, we have lacked the approaches to capture evidence from the community and stakeholders, to integrate evidence from research, to capture and leverage data or evidence from practice and to generate and build on evidence from implementation using iterative system-level improvement. The LHS provides this opportunity and is shown to deliver impact. Here, we have outlined the process applied to generate an evidence-based LHS and provide a leading exemplar in stroke care. This highlights the value of moving from single-focus isolated approaches/initiatives to healthcare improvement and the benefit of integration to deliver demonstrable outcomes for our funders and key stakeholders — our community. This work provides insight into strategies that can both apply evidence-based processes to healthcare improvement as well as implementing evidence-based practices into care, moving beyond research as an endpoint, to research as an enabler, underpinning delivery of better healthcare.
Availability of data and materials
Not applicable
Abbreviations
Australian Stroke Clinical Registry
Confidence interval
- Learning Health System
World Health Organization. Delivering quality health services . OECD Publishing; 2018.
Enticott J, Braaf S, Johnson A, Jones A, Teede HJ. Leaders’ perspectives on learning health systems: A qualitative study. BMC Health Serv Res. 2020;20:1087.
Article PubMed PubMed Central Google Scholar
Melder A, Robinson T, McLoughlin I, Iedema R, Teede H. An overview of healthcare improvement: Unpacking the complexity for clinicians and managers in a learning health system. Intern Med J. 2020;50:1174–84.
Article PubMed Google Scholar
Alberto IRI, Alberto NRI, Ghosh AK, Jain B, Jayakumar S, Martinez-Martin N, et al. The impact of commercial health datasets on medical research and health-care algorithms. Lancet Digit Health. 2023;5:e288–94.
Article CAS PubMed PubMed Central Google Scholar
Dixon-Woods M. How to improve healthcare improvement—an essay by Mary Dixon-Woods. BMJ. 2019;367: l5514.
Enticott J, Johnson A, Teede H. Learning health systems using data to drive healthcare improvement and impact: A systematic review. BMC Health Serv Res. 2021;21:200.
Enticott JC, Melder A, Johnson A, Jones A, Shaw T, Keech W, et al. A learning health system framework to operationalize health data to improve quality care: An Australian perspective. Front Med (Lausanne). 2021;8:730021.
Dammery G, Ellis LA, Churruca K, Mahadeva J, Lopez F, Carrigan A, et al. The journey to a learning health system in primary care: A qualitative case study utilising an embedded research approach. BMC Prim Care. 2023;24:22.
Foley T, Horwitz L, Zahran R. The learning healthcare project: Realising the potential of learning health systems. 2021. Available from https://learninghealthcareproject.org/wp-content/uploads/2021/05/LHS2021report.pdf . Accessed Jan 2024.
Institute of Medicine. Best care at lower cost: The path to continuously learning health care in America. Washington: The National Academies Press; 2013.
Google Scholar
Zurynski Y, Smith CL, Vedovi A, Ellis LA, Knaggs G, Meulenbroeks I, et al. Mapping the learning health system: A scoping review of current evidence - a white paper. 2020:63
Cadilhac DA, Bravata DM, Bettger J, Mikulik R, Norrving B, Uvere E, et al. Stroke learning health systems: A topical narrative review with case examples. Stroke. 2023;54:1148–59.
Braithwaite J, Glasziou P, Westbrook J. The three numbers you need to know about healthcare: The 60–30-10 challenge. BMC Med. 2020;18:1–8.
Article Google Scholar
King D, Wittenberg R, Patel A, Quayyum Z, Berdunov V, Knapp M. The future incidence, prevalence and costs of stroke in the UK. Age Ageing. 2020;49:277–82.
Ganesh A, Lindsay P, Fang J, Kapral MK, Cote R, Joiner I, et al. Integrated systems of stroke care and reduction in 30-day mortality: A retrospective analysis. Neurology. 2016;86:898–904.
Lowther HJ, Harrison J, Hill JE, Gaskins NJ, Lazo KC, Clegg AJ, et al. The effectiveness of quality improvement collaboratives in improving stroke care and the facilitators and barriers to their implementation: A systematic review. Implement Sci. 2021;16:16.
Australian Commission on Safety and Quality in Health Care. Acute stroke clinical care standard. 2015. Available from https://www.safetyandquality.gov.au/our-work/clinical-care-standards/acute-stroke-clinical-care-standard . Accessed Jan 2024.
Australian Commission on Safety and Quality in Health Care. Acute stroke clinical care standard. Sydney: ACSQHC; 2019. Available from https://www.safetyandquality.gov.au/publications-and-resources/resource-library/acute-stroke-clinical-care-standard-evidence-sources . Accessed Jan 2024.
Ryan O, Ghuliani J, Grabsch B, Hill K, G CC, Breen S, et al. Development, implementation, and evaluation of the Australian Stroke Data Tool (AuSDaT): Comprehensive data capturing for multiple uses. Health Inf Manag. 2022:18333583221117184.
English C, Bayley M, Hill K, Langhorne P, Molag M, Ranta A, et al. Bringing stroke clinical guidelines to life. Int J Stroke. 2019;14:337–9.
English C, Hill K, Cadilhac DA, Hackett ML, Lannin NA, Middleton S, et al. Living clinical guidelines for stroke: Updates, challenges and opportunities. Med J Aust. 2022;216:510–4.
Cadilhac DA, Grimley R, Kilkenny MF, Andrew NE, Lannin NA, Hill K, et al. Multicenter, prospective, controlled, before-and-after, quality improvement study (Stroke123) of acute stroke care. Stroke. 2019;50:1525–30.
Cadilhac DA, Marion V, Andrew NE, Breen SJ, Grabsch B, Purvis T, et al. A stepped-wedge cluster-randomized trial to improve adherence to evidence-based practices for acute stroke management. Jt Comm J Qual Patient Saf. 2022.
Elliott J, Lawrence R, Minx JC, Oladapo OT, Ravaud P, Jeppesen BT, et al. Decision makers need constantly updated evidence synthesis. Nature. 2021;600:383–5.
Article CAS PubMed Google Scholar
National Stroke Foundation. National guidelines for acute stroke management. Melbourne: National Stroke Foundation; 2003.
National Stroke Foundation. Clinical guidelines for stroke rehabilitation and recovery. Melbourne: National Stroke Foundation; 2005.
Phan TG, Thrift A, Cadilhac D, Srikanth V. A plea for the use of systematic review methodology when writing guidelines and timely publication of guidelines. Intern Med J . 2012;42:1369–1371; author reply 1371–1362
Tendal B, Vogel JP, McDonald S, Norris S, Cumpston M, White H, et al. Weekly updates of national living evidence-based guidelines: Methods for the Australian living guidelines for care of people with COVID-19. J Clin Epidemiol. 2021;131:11–21.
Grimshaw JM, Eccles MP, Lavis JN, Hill SJ, Squires JE. Knowledge translation of research findings. Implement Sci. 2012;7:50.
Harris D, Cadilhac D, Hankey GJ, Hillier S, Kilkenny M, Lalor E. National stroke audit: The Australian experience. Clin Audit. 2010;2:25–31.
Cadilhac DA, Purvis T, Kilkenny MF, Longworth M, Mohr K, Pollack M, et al. Evaluation of rural stroke services: Does implementation of coordinators and pathways improve care in rural hospitals? Stroke. 2013;44:2848–53.
Cadilhac DA, Moss KM, Price CJ, Lannin NA, Lim JY, Anderson CS. Pathways to enhancing the quality of stroke care through national data monitoring systems for hospitals. Med J Aust. 2013;199:650–1.
Cadilhac DA, Lannin NA, Anderson CS, Levi CR, Faux S, Price C, et al. Protocol and pilot data for establishing the Australian Stroke Clinical Registry. Int J Stroke. 2010;5:217–26.
Ivers N, Jamtvedt G, Flottorp S, Young J, Odgaard-Jensen J, French S, et al. Audit and feedback: Effects on professional practice and healthcare outcomes. Cochrane Database Syst Rev . 2012
Australian Commission on Safety and Quality in Health Care. Economic evaluation of clinical quality registries. Final report. . 2016:79
Cadilhac DA, Dalli LL, Morrison J, Lester M, Paice K, Moss K, et al. The Australian Stroke Clinical Registry annual report 2021. Melbourne; 2022. Available from https://auscr.com.au/about/annual-reports/ . Accessed 6 May 2024.
Kilkenny MF, Kim J, Andrew NE, Sundararajan V, Thrift AG, Katzenellenbogen JM, et al. Maximising data value and avoiding data waste: A validation study in stroke research. Med J Aust. 2019;210:27–31.
Eliakundu AL, Smith K, Kilkenny MF, Kim J, Bagot KL, Andrew E, et al. Linking data from the Australian Stroke Clinical Registry with ambulance and emergency administrative data in Victoria. Inquiry. 2022;59:469580221102200.
PubMed Google Scholar
Andrew NE, Kim J, Cadilhac DA, Sundararajan V, Thrift AG, Churilov L, et al. Protocol for evaluation of enhanced models of primary care in the management of stroke and other chronic disease (PRECISE): A data linkage healthcare evaluation study. Int J Popul Data Sci. 2019;4:1097.
CAS PubMed PubMed Central Google Scholar
Mosalski S, Shiner CT, Lannin NA, Cadilhac DA, Faux SG, Kim J, et al. Increased relative functional gain and improved stroke outcomes: A linked registry study of the impact of rehabilitation. J Stroke Cerebrovasc Dis. 2021;30: 106015.
Ryan OF, Hancock SL, Marion V, Kelly P, Kilkenny MF, Clissold B, et al. Feedback of aggregate patient-reported outcomes (PROs) data to clinicians and hospital end users: Findings from an Australian codesign workshop process. BMJ Open. 2022;12:e055999.
Grimley RS, Rosbergen IC, Gustafsson L, Horton E, Green T, Cadigan G, et al. Dose and setting of rehabilitation received after stroke in Queensland, Australia: A prospective cohort study. Clin Rehabil. 2020;34:812–23.
Purvis T, Middleton S, Craig LE, Kilkenny MF, Dale S, Hill K, et al. Inclusion of a care bundle for fever, hyperglycaemia and swallow management in a national audit for acute stroke: Evidence of upscale and spread. Implement Sci. 2019;14:87.
Middleton S, McElduff P, Ward J, Grimshaw JM, Dale S, D’Este C, et al. Implementation of evidence-based treatment protocols to manage fever, hyperglycaemia, and swallowing dysfunction in acute stroke (QASC): A cluster randomised controlled trial. Lancet. 2011;378:1699–706.
Middleton S, Dale S, Cheung NW, Cadilhac DA, Grimshaw JM, Levi C, et al. Nurse-initiated acute stroke care in emergency departments. Stroke. 2019:STROKEAHA118020701.
Hood RJ, Maltby S, Keynes A, Kluge MG, Nalivaiko E, Ryan A, et al. Development and pilot implementation of TACTICS VR: A virtual reality-based stroke management workflow training application and training framework. Front Neurol. 2021;12:665808.
Bladin CF, Kim J, Bagot KL, Vu M, Moloczij N, Denisenko S, et al. Improving acute stroke care in regional hospitals: Clinical evaluation of the Victorian Stroke Telemedicine program. Med J Aust. 2020;212:371–7.
Bladin CF, Bagot KL, Vu M, Kim J, Bernard S, Smith K, et al. Real-world, feasibility study to investigate the use of a multidisciplinary app (Pulsara) to improve prehospital communication and timelines for acute stroke/STEMI care. BMJ Open. 2022;12:e052332.
Zhao H, Coote S, Easton D, Langenberg F, Stephenson M, Smith K, et al. Melbourne mobile stroke unit and reperfusion therapy: Greater clinical impact of thrombectomy than thrombolysis. Stroke. 2020;51:922–30.
Purvis T, Cadilhac DA, Hill K, Reyneke M, Olaiya MT, Dalli LL, et al. Twenty years of monitoring acute stroke care in Australia from the national stroke audit program (1999–2019): Achievements and areas of future focus. J Health Serv Res Policy. 2023.
Cadilhac DA, Purvis T, Reyneke M, Dalli LL, Kim J, Kilkenny MF. Evaluation of the national stroke audit program: 20-year report. Melbourne; 2019.
Kim J, Tan E, Gao L, Moodie M, Dewey HM, Bagot KL, et al. Cost-effectiveness of the Victorian Stroke Telemedicine program. Aust Health Rev. 2022;46:294–301.
Kim J, Easton D, Zhao H, Coote S, Sookram G, Smith K, et al. Economic evaluation of the Melbourne mobile stroke unit. Int J Stroke. 2021;16:466–75.
Stroke Foundation. National stroke audit – rehabilitation services report 2020. Melbourne; 2020.
Australian Institute of Health and Welfare. Heart, stroke and vascular disease: Australian facts. 2023. Webpage https://www.aihw.gov.au/reports/heart-stroke-vascular-diseases/hsvd-facts/contents/about (accessed Jan 2024).
Download references
Acknowledgements
The following authors hold National Health and Medical Research Council Research Fellowships: HT (#2009326), DAC (#1154273), SM (#1196352), MFK Future Leader Research Fellowship (National Heart Foundation #105737). The Funders of this work did not have any direct role in the design of the study, its execution, analyses, interpretation of the data, or decision to submit results for publication.
Author information
Helena Teede and Dominique A. Cadilhac contributed equally.
Authors and Affiliations
Monash Centre for Health Research and Implementation, 43-51 Kanooka Grove, Clayton, VIC, Australia
Helena Teede, Emily Callander & Joanne Enticott
Monash Partners Academic Health Science Centre, 43-51 Kanooka Grove, Clayton, VIC, Australia
Helena Teede & Alison Johnson
Stroke and Ageing Research, Department of Medicine, School of Clinical Sciences at Monash Health, Monash University, Level 2 Monash University Research, Victorian Heart Hospital, 631 Blackburn Rd, Clayton, VIC, Australia
Dominique A. Cadilhac, Tara Purvis & Monique F. Kilkenny
Stroke Theme, The Florey Institute of Neuroscience and Mental Health, University of Melbourne, Heidelberg, VIC, Australia
Dominique A. Cadilhac, Monique F. Kilkenny & Bruce C.V. Campbell
Department of Neurology, Melbourne Brain Centre, Royal Melbourne Hospital, Parkville, VIC, Australia
Bruce C.V. Campbell
Department of Medicine, Faculty of Medicine, Dentistry and Health Sciences, University of Melbourne, Victoria, Australia
School of Health Sciences, Heart and Stroke Program, University of Newcastle, Hunter Medical Research Institute, University Drive, Callaghan, NSW, Australia
Coralie English
School of Medicine and Dentistry, Griffith University, Birtinya, QLD, Australia
Rohan S. Grimley
Clinical Excellence Division, Queensland Health, Brisbane, Australia
John Hunter Hospital, Hunter New England Local Health District and University of Newcastle, Sydney, NSW, Australia
Christopher Levi
School of Nursing, Midwifery and Paramedicine, Australian Catholic University, Sydney, NSW, Australia
Sandy Middleton
Nursing Research Institute, St Vincent’s Health Network Sydney and and Australian Catholic University, Sydney, NSW, Australia
Stroke Foundation, Level 7, 461 Bourke St, Melbourne, VIC, Australia
Kelvin Hill
You can also search for this author in PubMed Google Scholar
Contributions
HT: conception, design and initial draft, developed the theoretical formalism for learning health system framework, approved the submitted version. DAC: conception, design and initial draft, provided essential literature and case study examples, approved the submitted version. TP: revised the manuscript critically for important intellectual content, approved the submitted version. MFK: revised the manuscript critically for important intellectual content, provided essential literature and case study examples, approved the submitted version. BC: revised the manuscript critically for important intellectual content, provided essential literature and case study examples, approved the submitted version. CE: revised the manuscript critically for important intellectual content, provided essential literature and case study examples, approved the submitted version. AJ: conception, design and initial draft, developed the theoretical formalism for learning health system framework, approved the submitted version. EC: revised the manuscript critically for important intellectual content, approved the submitted version. RSG: revised the manuscript critically for important intellectual content, provided essential literature and case study examples, approved the submitted version. CL: revised the manuscript critically for important intellectual content, provided essential literature and case study examples, approved the submitted version. SM: revised the manuscript critically for important intellectual content, provided essential literature and case study examples, approved the submitted version. KH: revised the manuscript critically for important intellectual content, provided essential literature and case study examples, approved the submitted version. JE: conception, design and initial draft, developed the theoretical formalism for learning health system framework, approved the submitted version. All authors read and approved the final manuscript.
Authors’ Twitter handles
@HelenaTeede
@DominiqueCad
@Coralie_English
@EmilyCallander
@EnticottJo
Corresponding authors
Correspondence to Helena Teede or Dominique A. Cadilhac .
Ethics declarations
Ethics approval and consent to participate, consent for publication, competing interests, additional information, publisher's note.
Springer Nature remains neutral with regard to jurisdictional claims in published maps and institutional affiliations.
Rights and permissions
Open Access This article is licensed under a Creative Commons Attribution 4.0 International License, which permits use, sharing, adaptation, distribution and reproduction in any medium or format, as long as you give appropriate credit to the original author(s) and the source, provide a link to the Creative Commons licence, and indicate if changes were made. The images or other third party material in this article are included in the article's Creative Commons licence, unless indicated otherwise in a credit line to the material. If material is not included in the article's Creative Commons licence and your intended use is not permitted by statutory regulation or exceeds the permitted use, you will need to obtain permission directly from the copyright holder. To view a copy of this licence, visit http://creativecommons.org/licenses/by/4.0/ . The Creative Commons Public Domain Dedication waiver ( http://creativecommons.org/publicdomain/zero/1.0/ ) applies to the data made available in this article, unless otherwise stated in a credit line to the data.
Reprints and permissions
About this article
Cite this article.
Teede, H., Cadilhac, D.A., Purvis, T. et al. Learning together for better health using an evidence-based Learning Health System framework: a case study in stroke. BMC Med 22 , 198 (2024). https://doi.org/10.1186/s12916-024-03416-w
Download citation
Received : 23 July 2023
Accepted : 30 April 2024
Published : 15 May 2024
DOI : https://doi.org/10.1186/s12916-024-03416-w
Share this article
Anyone you share the following link with will be able to read this content:
Sorry, a shareable link is not currently available for this article.
Provided by the Springer Nature SharedIt content-sharing initiative
- Evidence-based medicine
- Person-centred care
- Models of care
- Healthcare improvement
BMC Medicine
ISSN: 1741-7015
- Submission enquiries: [email protected]
- General enquiries: [email protected]
Log in using your username and password
- Search More Search for this keyword Advanced search
- Latest content
- For authors
- Browse by collection
- BMJ Journals More You are viewing from: Google Indexer
You are here
- Volume 14, Issue 5
- Exploring the influence of health system factors on adaptive capacity in diverse hospital teams in Norway: a multiple case study approach
- Article Text
- Article info
- Citation Tools
- Rapid Responses
- Article metrics

- http://orcid.org/0000-0002-4689-8376 Birte Fagerdal 1 ,
- http://orcid.org/0000-0001-7107-4224 Hilda Bø Lyng 1 ,
- http://orcid.org/0000-0002-9124-1664 Veslemøy Guise 1 ,
- Janet E Anderson 2 ,
- http://orcid.org/0000-0003-0296-4957 Jeffrey Braithwaite 3 ,
- http://orcid.org/0000-0003-0186-038X Siri Wiig 1
- 1 SHARE, Faculty of Health Sciences , University of Stavanger , Stavanger , Norway
- 2 Anaesthesiology and Perioperative Medicine , Monash University Faculty of Medicine Nursing and Health Sciences , Melbourne , Victoria , Australia
- 3 Australian Institute of Health Innovation , Macquarie University , North Ryde , New South Wales , Australia
- Correspondence to Mrs Birte Fagerdal; birte.fagerdal{at}uis.no
Objectives Understanding flexibility and adaptive capacities in complex healthcare systems is a cornerstone of resilient healthcare. Health systems provide structures in the form of standards, rules and regulation to healthcare providers in defined settings such as hospitals. There is little knowledge of how hospital teams are affected by the rules and regulations imposed by multiple governmental bodies, and how health system factors influence adaptive capacity in hospital teams. The aim of this study is to explore the extent to which health system factors enable or constrain adaptive capacity in hospital teams.
Design A qualitative multiple case study using observation and semistructured interviews was conducted between November 2020 and June 2021. Data were analysed through qualitative content analysis with a combined inductive and deductive approach.
Setting Two hospitals situated in the same health region in Norway.
Participants Members from 8 different hospital teams were observed during their workday (115 hours) and were subsequently interviewed about their work (n=30). The teams were categorised as structural, hybrid, coordinating and responsive teams.
Results Two main health system factors were found to enable adaptive capacity in the teams: (1) organisation according to regulatory requirements to ensure adaptive capacity, and (2) negotiation of various resources provided by the governing authorities to ensure adaptive capacity. Our results show that aligning to local context of these health system factors affected the team’s adaptive capacity.
Conclusions Health system factors should create conditions for careful and safe care to emerge and provide conditions that allow for teams to develop both their professional expertise and systems and guidelines that are robust yet sufficiently flexible to fit their everyday work context.
- Health & safety
- Organisation of health services
- Quality in health care
- Protocols & guidelines
- QUALITATIVE RESEARCH
Data availability statement
Data are available upon reasonable request.
This is an open access article distributed in accordance with the Creative Commons Attribution Non Commercial (CC BY-NC 4.0) license, which permits others to distribute, remix, adapt, build upon this work non-commercially, and license their derivative works on different terms, provided the original work is properly cited, appropriate credit is given, any changes made indicated, and the use is non-commercial. See: http://creativecommons.org/licenses/by-nc/4.0/ .
https://doi.org/10.1136/bmjopen-2023-076945
Statistics from Altmetric.com
Request permissions.
If you wish to reuse any or all of this article please use the link below which will take you to the Copyright Clearance Center’s RightsLink service. You will be able to get a quick price and instant permission to reuse the content in many different ways.
STRENGTHS AND LIMITATIONS OF THIS STUDY
Data for this study were collected during the COVID-19 pandemic, which enabled the research team to observe how novel national policy measures affected the frontline.
The study contributes to resilient healthcare as there have been few multilevel studies looking at how macrolevel factors affect microlevel adaptive capacity.
The combination of observations and interviews provided a substantial amount of data which were then triangulated.
Data collected at the national level are limited as our study focused on the hospital team level.
Introduction
Healthcare systems provide the formal healthcare delivery structures for a defined population, whose funding, management, scope and content are defined by laws, policies and regulations. They provide services to people, aiming to contribute to their health and well-being. Services are usually delivered in defined settings, such as homes, nursing homes and hospitals. Healthcare systems are complex and adaptive and continuously responsive to multiple factors including patients’ needs, innovations, pressures, pandemics and funding structures. 1 Understanding flexibility and adaptive capacities in these complex healthcare systems is a key focus of investigators of resilient healthcare. 2 3 Resilience in healthcare can briefly be defined as ‘the capacity to adapt to challenges and changes at different system levels, to maintain high quality care’ p6. 4
To date, research on resilient healthcare has paid most attention to work as done at the sharp end of the system. Less is therefore known about how actions, strategies and practices enacted by regulatory bodies and policy-makers affect every day work at the microlevel, such as hospital teams. 5 While regulations in the form of standards, rules and protocols are known to be key drivers in the structuring of healthcare activities and in the design of healthcare organisations, the interfaces between policy-making, regulation and resilience are subtle and nuanced, and regulatory strategies to improve quality and safety are therefore complex and multifarious. 6 7 However, the relationship between governmental bodies and adaptive capacity at the sharp end of the system has received insufficient attention and is thus in need of closer examination. 2 8 9
In this study, we define macrolevel healthcare system actors as governmental bodies, regulators and national and regional bodies, who act or intend to shape, monitor, control and modify practices within organisations in order to achieve an identifiable, desirable state of affairs. 10 They aim to constrain action, optimise performance and attempt to prevent error.
In complex systems like hospitals, much work is performed in teams. 11–13 Understanding the nature of teams and team performance is important to promote team effectiveness. The few studies that have been undertaken are limited in scope as they have not considered how teams are defined and structured, what their functions are or differences across healthcare teams. 11 14 Most research on teams in healthcare has focused on the dynamic domains in healthcare, such as emergency medicine or operating rooms, and teams that are similar to the teams in other industries, for instance in aviation. 15 16 However, not all teams in hospitals operate in an emergency setting. Teams in hospitals differ depending on their goals, tasks, structure, membership and situation, affecting how they adapt to a multitude of contingencies that are encountered in everyday work. 17 Hence, their requirements for support could differ depending on these attributes but this question has not been addressed sufficiently in previous research. Knowledge of these differences may enable optimisation of support and better function for the different teams. This study will address these knowledge gaps.
Aim and research question
This study aims to explore whether and how health system factors enable adaptive capacity in different types of hospital teams in Norway. We asked: What kind of health system factors enable adaptive capacity in hospital teams, and how do these factors affect adaptive capacity?
Design and setting
A qualitative exploratory methodology was chosen, using a multiple-embedded case study design. 11 18 A case was defined as one hospital containing four different types of teams. Two case hospitals were recruited to the study, featuring a total of eight teams. The study’s design was in line with that of an international comparative study, involving six countries (The Netherlands, Japan, Australia, England, Switzerland and Norway), where this article reports partial findings from the Norwegian case (see protocol of Anderson et al ). 11 The two Norwegian hospitals and the four team types were recruited in line with the study protocol. Findings from each of the countries will be written up as country case reports following an agreed on template. Furthermore, an international cross-case comparative analysis will be performed using the Qualitative Comparative Analysis method 19 with the aim of exploring how multilevel system factors interact to support or hinder adaptive capacity in different types of hospital teams in different countries, and how this leads to performance variability. This international comparative analysis is currently in progress. This article stands alone and uses Norwegian data only.
Recruitment and study context
The Norwegian health system is a semidecentralised system with the Norwegian Parliament as its highest decision-making body. The municipalities are responsible for providing primary care for their citizens, mainly through nursing homes, homecare, general practitioners and rehabilitation services. The hospitals are mainly state owned and administered by four Regional Health Authorities. The Norwegian Board of Health Supervision is a national regulatory body, organised under the Ministry of Health and Care Services. County Governors at the regional level oversee services within primary and specialised healthcare. Norway has a comprehensive set of legislation governing the health services, including requirements for the quality of services, regulations for authorised healthcare personnel and service users’ rights. These legislated requirements are subject to supervision and investigation by the Norwegian Board of Health Supervision and the County Governors. 20 21
The two hospitals in this study were selected and recruited based on their size and role in teaching provision. 11 Both hospitals are situated in the same health region in Norway. Hospital 1 is a large teaching hospital and hospital 2 is a middle-sized local hospital which is also responsible for educating healthcare professionals. The four different team types were structural, hybrid, responsive and coordinating, and are displayed in table 1 . See Fagerdal et al 22 for further descriptions of the teams.
- View inline
Descriptions of the four different teams studied in each hospital
Data were collected through observation, interviews and document analysis, all undertaken between December 2020 and June 2021. Researcher BF and HBL conducted the observations, which entailed following one or more team members for two workdays using an observation guide. Both researchers wrote their own individual fields notes which were both included in the data material. Using the observation guide enabled a structuring of the text in line with the central concepts used in resilience literature. 23 During observations, we looked for various types of demands from the different levels of the organisations, the teams’ capacities to meet the demands and types of adaptations that were performed by the teams and team members. The observed teams differed in how they work together and consequently our undertaking of the observations had to align with those differences. The structural and hybrid teams were observed during two shifts, including evening and dayshifts. With the responsive teams, we followed one team member during their workday and their response to acute alarms. The coordinating teams meet for 10 min every weekday, and the researchers attended all their meetings during a 14-day period. Due to the COVID-19 pandemic, one of the coordinating teams held their meetings digitally, which we also attended. The observations totalled 115 hours (see table 2 ).
Overview of data collection methods and data material according to team types and case sites
All interviews were undertaken post observation by researcher BF using a semistructured interview guide based on content from the Concepts for Applying Resilience Engineering (CARE) model, that is, demand, capacity, misalignments and adaptations, 24 and the four potentials of resilience; monitoring, anticipating, responding and learning. 23 Team members and one leader from each team were interviewed, resulting in 30 interviews (see table 3 ). Participants comprised 27 females and 3 males and their ages ranged between 24 and 56. The interview length varied from 40 to 90 min with a median of 55 min. All participants signed a written consent form and were given the opportunity to withdraw without any negative implications; all invited participants accepted the invitation to interview.
Overview of the interviewed participants in the study
Patient and public involvement statement
A coresearcher employed in the overall Resilience in Healthcare project, of which this study is a part, 11 collaborated in the planning and design of the study, and access to teams at hospital 1. In hospital 2, we used a local coordinator to help identify and facilitate access to the different teams.
All interviews were audio recorded and transcribed verbatim by researcher BF. Observation notes were included in the analysis, and all notes and interview transcripts were grouped according to hospital and team types to streamline the analysis work. We conducted a within-case analysis of each hospital and a cross-case analysis to identify patterns and themes in our overall material. 18 The data material was first read through in full by all the researchers to get a sense of the whole. The analysis was then done using a combined deductive and inductive approach. 25 The CARE model 24 was used as a framework to assist the deductive part of the analysis as visualised in figure 1 .
- Download figure
- Open in new tab
- Download powerpoint
Concepts for Applying Resilience Engineering model after Anderson et al 24 visualising the study’s focus on team adaptation.
Data were organised using three of the four key concepts in the CARE model matrix: capacities, misalignments and adaptations. The capacities were defined as health system factors in this analysis and represent the factors that influence teams’ ability to adapt. All data were in addition coded for team type and hospital which allowed for a cross hospital and cross-team analysis. After the data material had been divided into three parts of text, to enable further analysis, we proceeded with an inductive content analysis approach. 25 The categories were inductively reviewed and recoded and further developed into latent themes across the four teams. This process resulted in overarching themes representing health system factors, that influence teams’ adaptive capacity (see table 4 ).
Inductive coding structure
The national and regional health authorities set the scene for how the hospitals prioritises and arrange their work. System-level decisions filter down through the organisation and influence the team’s everyday work. Our analysis shows that the effect of system factors on teams’ everyday work and adaptive capacity can be divided into two main themes, each with associated subthemes: (1) organisation according to regulatory requirements to ensure adaptive capacity and (2) negotiation of various resources provided by the governing authorities to ensure adaptive capacity. In table 4 , we present the themes along with their subthemes, codes and examples of quotes from the participants or description from the observation.
Organising according to regulatory requirements to ensure adaptive capacity
National and regional guidelines, financial governance and regulatory inspections by the health supervision authorities all shaped the organisation of the hospitals.
Context and organisational structure
The organisational context was important. It affected how the teams enacted and performed patient care. For instance, the smaller hospital 2 had restrictions and limitations regarding both the types of diagnoses and the number of patients they were able to treat due to regional regulations. These regulations had a large impact on the smaller hospital and their teams in how they organised their work, their competence requirements and what kind of learning opportunities were available to the team members. For instance, since hospital provided an acute function for surgical patients, it could continue to be an educational institution for healthcare personnel, which also meant that healthcare professionals in the structural and hybrid teams could maintain and develop their skills in acute care. In addition, it also impacted the hybrid and structural teams in how they arranged their work by always being prepared for the admission of acute surgical patients during their workday. Furthermore, the regional health authority maintained overall flexibility in acute care provision by having this function in both hospitals.
Both the coordinating teams in our study had been established by the hospitals in response to a government policy of preventing corridor beds in hospitals as a means of improving patients’ safety. The teams were set up to include all ward managers cooperating to manage patient flow, and with a goal of evening out the overall strain across the hospital. These teams’ main assignment was to allocate patients to free beds within the hospital. In addition, a positive consequence of having these teams was that the team members got a better mutual understanding of the overall situation within the hospitals and an improved understanding of each other’s challenges across the hospital. This provided them with a greater range of solutions to use when making adaptations to avoid patients in the corridors. The coordinating team in hospital 2 also functioned as an arena for the team members to exchange advice and suggest solutions to other challenges in their work. This was to a certain extent also valid for the team in hospital 1, but due to the comparatively larger size of the team there, it was more difficult for the team members to get well acquainted. In addition to better patient flow and avoiding corridor patients, the hospitals aimed for the teams to focus on building a culture of helping each other across their respective hospitals and to foster a feeling of joint responsibility for the betterment of the hospital overall (see table 4 ). Similar to the responsive teams, the coordinating teams had been enabled to make quick decisions spanning hospital units, allowing for a wider range of alternative solutions to the problems encountered than if they were to make decisions on their own. Also, team members felt more of a responsibility to help each other and found that it was more difficult to say no to requests for free beds when meeting face to face with colleagues. Both the individual team members and the hospital organisation as a whole were thus found to have widened their adaptive capacities after establishing these teams.
Aligning with national and regional guidelines
The use of clinical guidelines provided teams with direction in the different treatment courses offered to patients. National guidelines were translated and aligned to work practices within the organisation to fit the current work in the teams. This gave the team a standard to maintain, a structure for their work and also brought them a sense of safety in knowing their boundaries and priorities for adaptation. For instance, the national guideline for sepsis treatment recommends starting antibiotics treatment within 1 hour of the start of symptoms and also lists early important diagnostic signs to look for in patients who are deteriorating. Early intervention and treatment improve the overall survival of these patients and both hospitals needed to ensure proper alignment to these standards (see table 4 ). The hybrid and structural teams were well aware of this, due to guidelines and information campaigns. The teams thus adapted their work to meet the national demands imposed here, prioritising this work over what were considered other less important tasks, such as helping patients with personal hygiene.
Another example of how guidelines shaped the organisation of hospital teams and how teams acted was seen in the work of both the responsive teams in the study. The two hospitals had to comply with the national requirements of diagnostic and treatment guidelines for cerebral infarction, and both hospitals had created responsive stroke teams to allow for quick diagnostics and treatment. Tailoring the responsive teams to fit the requirements of the national guidelines, reduced the ‘door to needle time’ in both hospitals significantly. This was accomplished by providing and designing equipment, procedures, role descriptions and facilities along with the right competent personnel. The responsive teams frequently made adaptations to the clinical procedure to fit with the patient’s condition, the proximity of the competent team members and the tailored equipment and location enabled for quick decision-making within the team, instead of encountering communication via phones or waiting for each other to finish other tasks.
Negotiating various resources provided by the governing authorities to ensure adaptive capacity
Financial incentives.
Incentives like the national funding model which generates income for the hospitals impacted both what kind of and how the hospitals prioritised treatment. Governing authorities use financial incentives to orient the hospitals towards planned direction. Budget cuts and other financial restraints imposed on hospitals demanded that both hospitals adapt their priorities, which consequently affected the teams’ delivery of treatment and care in the sharp end of the system. The government requirements for increased efficiency in the healthcare system, such as financial incentives for reducing beds, increased the pace of work and often required development of new work practices to cope with these demands. For instance, in both hospitals, there had been a decrease in hospital beds, and a shift towards outpatient treatment due to governing authorities funding schemes. To cope with this, both the hybrid and structural teams in both hospitals treated patients for a shorter amount of time. For example, the structural teams no longer admitted patients overnight preoperatively and discharged patients earlier postoperatively to primary healthcare service or the home. The teams coped with this by planning the discharge of the patient already at admittance to facilitate a safe and good-quality discharge. However, they often adapted their plans by not discharging patients due to either lack of capacity in primary care services, or disagreement and concern with the level of care offered in the municipalities. This example shows that the teams in practice negotiated the consequences of government funding restrictions to suit the patients’ needs.
In addition, they could to some extent handle some demands by determining how they could change procedures to fit certain requirements. For instance, one of the changes the structural team in hospital 2 made to manage earlier discharge was to have the nightshift staff remove the postoperative urine catheter from patients. The clinical procedure stated that for the patient to be discharged, they had to be able to urinate spontaneously after catheter removal. Catheter removal later in the day regularly meant that the patient had to stay an extra night, so by changing the timing of its removal staff still managed to provide care within the frame of guidelines given.
Physical surroundings
Both the hybrid and responsive teams in both hospitals had been placed in new premises designed specifically to accommodate their way of working, with well-designed spaces to facilitate their workday with proximity to necessary equipment, and a nearness to each other that enabled team members to easily assist if needed. Similarly, the structural team in hospital 2 had new premises, with a uniform design across the new hospital building making it easy for personnel to change teams and wards since their premises were already familiar to them. This uniformity in building design improved the teams’ overall adaptive capacity in peak situations, or when there was an absence of key personnel across wards and teams. Staff could easily assist personnel from other wards as they knew where equipment was stored and how the different facilities in the ward functioned (patient rooms, nurses’ stations, etc). The structural team in hospital 1, however, worked in old premises with narrow hallways and few physical meeting arenas for the team members, which hampered their workflow in that they had to spend time looking for each other, and otherwise had few opportunities to engage in direct communication with each other during their workday. The physical surroundings of the two coordinating teams differed. Due to the size of the team in hospital 1, the team there used digital software to manage the overall patient flow in the hospital. The smaller team in hospital 2 managed the same using a paper form that each member completed. However, both of the teams used the meeting to elaborate on their numbers with additional information as the numbers alone did not provide a sufficient representation of the overall situation on the wards.
Training and development resources
Training and development resources were crucial for a team’s adaptive capacity. The national attention on patient safety in recent decades has led to improved treatment courses and changed the focus on how healthcare personnel can learn from adverse events to avoid similar incidents in the future. Consequently, this has led to innovative solutions in how hospital managers organise learning activities for their employees. In accordance with a growing focus on simulation-based training and learning from regulatory bodies and policy-makers, all the teams in the study apart from the coordinating teams increasingly used simulation training (see table 4 ). Often, the teams would make simulation scenario cases based on adverse events or incidents that had happened on their ward and used them in their training. For the responsive teams, this type of training was mandatory and part of regulatory requirements for the teams. Also, for these teams that only worked together for limited episodes and had changing membership and different professional cultures, these simulation trainings were their only chance to practice and improve their team communication. During the period of our observation, they developed new cases with COVID-19 themes and used them to train and learn before they received actual COVID-19 patients. This improved their performance, as they had found several shortcomings in their COVID-19 procedure and thus changed it accordingly. For instance, they made efforts to prevent unnecessary contamination of team members and had detected a lack in the procedure of personal protective equipment. This shows that these types of prescribed training exercises enable teams to adapt procedures to fit their everyday work conditions.
Quality improvement resources
Quality improvement resources outside the hospital organisation supported team’s adaptive capacity. The national and regional healthcare authorities arrange various conferences and campaigns for hospitals and other healthcare institutions. Here, policy-makers, leaders and healthcare professionals meet and create reflexive spaces. As part of such efforts, the best practices are displayed and workshops are provided to encourage and translate quality and safety improvement into practice in different ways, alongside guidelines, learning tools and other materials for the different organisations to use and implement in their quality improvement work. Having this competence base within the health regions and at the national level to support teams added knowledge and increased adaptive capacity as it required knowledge transfer and new ideas anchored in research and practice. Moreover, the patient safety focus within the wards and teams like the safe care screening programme and safety huddles, launched by the Norwegian Directorate of Health and implemented through the regional health authorities, increased the team’s awareness of patient safety culture. The increased amount of quality measures the clinicians had to undertake and report on in their daily work were generally seen as good quality measures from both the organisations and the team’s point of view. However, it sometimes felt counterproductive constantly having to cope with balancing patients’ needs with the requirements of screening procedures, especially if staff felt they had little room for autonomous clinical assessment. For instance, the safe care screening programme where every patient over the age of 18 had to be screened for their risk of falling, bedsores and possible malnutrition within 24 hours was questioned. Screening young patients for this felt unnecessary and if there were other more pressing tasks that were seen as more important, they adapted the way they prioritised.
This study investigated the relationship between health system factors and adaptive capacity in hospital teams. Our results have shown that health system-level factors influence adaptive capacity in the teams through the provision of guidelines and resources, and how the teams align these to their current demands and capacity situation. Their effects on different teams are not uniform; some are advantageous to one team but disadvantageous to another. 5 6 We argue that it is the team’s opportunity to align these factors to context that are key for enabling adaptive capacity, as illustrated in figure 2 .
Illustrating the teams aligning system-levels factors to context for adaptive capacity.
All levels of a health system can influence each other, especially in an integrated and tightly coupled system. Higher system levels can affect lower levels through, for example, explicit instructions, by the provision or limitation of resources, or by establishing incentive systems. 26–28 On the other hand, lower system levels may use discretion when they interpret and implement directives from higher levels, and they may control the information flow to higher levels. 26 Our results show that decisions made at one level of the system can support or hinder adaptive capacity at other lower hierarchical levels of the system. 29–31 Accordingly, the system-level governing factors affect adaptive capacity at the sharp end by setting the framework and boundaries within which activity can take place. Regulatory bodies have system-wide responsibilities and must respond to system-wide disturbances, without detailed knowledge of how work is done in practice at the sharp end. Consequently, the sharp end must adapt to respond appropriately to disturbances within its own field of responsibility. 32
This study has operationalised adaptation using the CARE model 24 to see how different teams at the sharp end work in practice to negotiate system-level factors, such as regulations and guidelines. The findings show that factors at the macrolevel required different forms of adaptations within different team types to managing everyday work. Enabling adaptation at the team level by taking action at the macrolevel to attempt to reconcile work as imagined with work as done ( figure 1 ). The system-level factors also represent long-term planning and transformation of practices rather than short-term adaptations or adjustments in the system. 33 They envisage setting up the processes that design, produce and circulate resources that underpin safety, and prevent errors through standardisation, regulation and training. 32 How the teams negotiate these long-term transformations to their everyday work determines their adaptive capacity as our results have shown. Adaptation and adjustments to local context are inevitable in healthcare. 9 11 34 35 However, the vast number of protocols, policies, checklists, standards, guidelines, pathways and other regulatory requirements may lead those working at the sharp end to feel overwhelmed. 6 If not aligned with goals, tasks and current challenges, these governing factors may end up being counterproductive. 5 The teams studied talked about their everyday work and their primary focus on patient care along with their willingness to act in the best interest of the patients. 36 They talked about feeling a compound pressure in order to align system-level demands with their context and patients’ wishes and needs. 37 38 Taking the perspective of the patient into account was important to the teams. 39 40 Consequently, different teams had to align system-level demands differentially to ensure quality care for patients.
Our study showed that teams must balance continuous efficiency with thoroughness assessments 32 41–43 in everyday work (eg, making the nightshift prepare discharge adding more work to reduce corridor patients). Ways that the teams in our study continuously adapted regulatory requirements to their work context illuminated how resilient systems must have robust yet flexible structures to assist the system to deal with both everyday work and unexpected events. 8 30 44 45 System-level factors must therefore provide flexibility to fit different situations and types of teams, as teams differ in how they cooperate and function in everyday work. To ensure alignment of perspectives between macrolevel and microlevel actors, common arenas and structures for mutual feedback and reflections between stakeholders are crucial. 7 Furthermore, system factors need to entail robustness in the directions they provide to practice and the implementation of improvement efforts. 33
The findings show that for the responsive and coordinating teams the size of the hospital played a significant role in their ability to adapt. These two team types operated in part at the mesolevel of the hospital organisation, spanning hospital departments. Their work was characteristically ad hoc, dynamically changing team memberships and members who work primarily in other teams. The large size of hospital 1 hampered development of relationships between the team members in both the responsive and the coordinating team, whereas in the smaller hospital 2 it was easier to develop close relationships between colleagues. This implies that ad hoc teams, and especially large ones, need to have structure and guidelines in place that direct their work, and support to adapt their work based on the team members understanding of the tasks and their roles. The structural and hybrid teams were colocated and this seemed to allow for the development of long-term collegial relationships, better cooperation between team members, more flexible adaptation of their work and also seemed to allow for working with greater levels of independence and a larger room for self-organisation. Their work is influenced by system-level demands, but the size of the organisation does not affect their day-to-day work to the same degree as for the coordination and responsive teams.
Strengths and limitations
A strength of the study is that by combining observation and interviews we have gathered in-depth data of the team’s everyday work.
Data collection during COVID-19 pandemic could hamper everyday work practice; however, we collaborated closely with the sites to avoid any problems for the involved teams and units. Only two hospitals contributed to the data collection and including additional hospitals could add more than we have from two hospitals. However, the inclusion of eight teams, the total amount of data gave rich information to analyse our research questions.
Interview data from the macrolevel could have added additional perspectives from the regulators and policy-makers. We suggest further studies to integrate this in their activities to uncover the role of system factors seen from the policy-makers’ and regulators’ perspectives.
Conclusions and implications
This study illuminated how teams negotiate the health system factors that shape their work to provide as much adaptive capacity as possible and attempt to align system-level regulation and guidelines with everyday work demands. The results show that the size of both the organisation and team had an effect on adaptive capacity. Our findings imply that healthcare systems need to facilitate conditions that allow for teams to develop their professional expertise and develop systems that are robust and flexible to fit the context. Teams should be enabled to adapt to the functions and structure of the health system to carry out their everyday work in a changing environment.
Ethics statements
Patient consent for publication.
Not applicable.
Ethics approval
This study involves human participants and was approved by Regional committe for Medical and Health Research Ethics, ref.nr. 166280. Participants gave informed consent to participate in the study before taking part.
Acknowledgments
The authors would like to thank all participating teams and their leaders at the two hospitals who shared their valuable knowledge and reflections.
- Hollnagel E ,
- Braithwaite J
- Anderson JE ,
- Macrae C , et al
- Billett S , et al
- Smaggus A ,
- Ellis LA , et al
- Bal R , et al
- Christian JS ,
- Christian MS ,
- Pearsall MJ , et al
- Roberts APJ ,
- Webster LV ,
- Salmon PM , et al
- Lavelle M ,
- Cross S , et al
- Ballangrud R ,
- Husebø SE ,
- Aase K , et al
- Salas E , et al
- Saunes IS ,
- Karanikolos M ,
- Saunes, I.S., M. Karanikolos, and A. Sagan, Norway
- Fagerdal B ,
- Guise V , et al
- Hollnagel E
- Back J , et al
- Rasmussen J
- Bergström J ,
- Blanchet K ,
- Ramalingam B , et al
- Schubert CC
- Johannessen T ,
- Krystallidou D ,
- Deveugele M , et al
- Dillon EC ,
- Tai-Seale M ,
- Meehan A , et al
- Hofmeyer A ,
- Svensson I ,
- von Knorring M ,
- Hagerman H , et al
- Churruca K ,
- Clay-Williams R , et al
- Glette MK ,
X @fagerbirte
Contributors The study design was developed in collaboration with the whole research team. BF and HBL conducted the data collection. BF conducted and transcribed all the interviews. The analysis and interpretation of data were conducted in close collaboration between BF, HBL, VG, JEA and SW. SW is the guarantor of this study. All authors contributed with writing, critical revision and approval of the final version.
Funding This project is part of the Resilience in Healthcare Research program which has received funding from the Research Council of Norway from the FRIPRO TOPPFORSK program, grant agreement no. 275367. The University of Stavanger, Norway, NTNU Gjøvik, Norway supports the study with kind funding.
Competing interests None declared.
Patient and public involvement Patients and/or the public were not involved in the design, or conduct, or reporting, or dissemination plans of this research.
Provenance and peer review Not commissioned; externally peer reviewed.
Read the full text or download the PDF:
- - Google Chrome
Intended for healthcare professionals
- Access provided by Google Indexer
- My email alerts
- BMA member login
- Username * Password * Forgot your log in details? Need to activate BMA Member Log In Log in via OpenAthens Log in via your institution

Search form
- Advanced search
- Search responses
- Search blogs
- Effect of the HPV...
Effect of the HPV vaccination programme on incidence of cervical cancer and grade 3 cervical intraepithelial neoplasia by socioeconomic deprivation in England: population based observational study
Linked editorial.
HPV vaccine: the key to eliminating cervical cancer inequities
- Related content
- Peer review
- Milena Falcaro , senior statistician 1 ,
- Kate Soldan , scientist and epidemiologist 2 ,
- Busani Ndlela , cancer information analyst 3 ,
- Peter Sasieni , professor of cancer epidemiology 1
- 1 Centre for Cancer Screening, Prevention and Early Diagnosis, Wolfson Institute of Population Health, Queen Mary University of London, London EC1M 6BQ, UK
- 2 Blood Safety, Hepatitis, Sexually Transmitted Infections and HIV Division, UK Health Security Agency (UKHSA), London, UK
- 3 National Disease Registration Service (NDRS), NHS England, London, UK
- Correspondence to: P Sasieni p.sasieni{at}qmul.ac.uk (or @petersasieni on X)
- Accepted 27 March 2024
Objectives To replicate previous analyses on the effectiveness of the English human papillomavirus (HPV) vaccination programme on incidence of cervical cancer and grade 3 cervical intraepithelial neoplasia (CIN3) using 12 additional months of follow-up, and to investigate effectiveness across levels of socioeconomic deprivation.
Design Observational study.
Setting England, UK.
Participants Women aged 20-64 years resident in England between January 2006 and June 2020 including 29 968 with a diagnosis of cervical cancer and 335 228 with a diagnosis of CIN3. In England, HPV vaccination was introduced nationally in 2008 and was offered routinely to girls aged 12-13 years, with catch-up campaigns during 2008-10 targeting older teenagers aged <19 years.
Main outcome measures Incidence of invasive cervical cancer and CIN3.
Results In England, 29 968 women aged 20-64 years received a diagnosis of cervical cancer and 335 228 a diagnosis of CIN3 between 1 January 2006 and 30 June 2020. In the birth cohort of women offered vaccination routinely at age 12-13 years, adjusted age standardised incidence rates of cervical cancer and CIN3 in the additional 12 months of follow-up (1 July 2019 to 30 June 2020) were, respectively, 83.9% (95% confidence interval (CI) 63.8% to 92.8%) and 94.3% (92.6% to 95.7%) lower than in the reference cohort of women who were never offered HPV vaccination. By mid-2020, HPV vaccination had prevented an estimated 687 (95% CI 556 to 819) cervical cancers and 23 192 (22 163 to 24 220) CIN3s. The highest rates remained among women living in the most deprived areas, but the HPV vaccination programme had a large effect in all five levels of deprivation. In women offered catch-up vaccination, CIN3 rates decreased more in those from the least deprived areas than from the most deprived areas (reductions of 40.6% v 29.6% and 72.8% v 67.7% for women offered vaccination at age 16-18 and 14-16, respectively). The strong downward gradient in cervical cancer incidence from high to low deprivation in the reference unvaccinated group was no longer present among those offered the vaccine.
Conclusions The high effectiveness of the national HPV vaccination programme previously seen in England continued during the additional 12 months of follow-up. HPV vaccination was associated with a substantially reduced incidence of cervical cancer and CIN3 across all five deprivation groups, especially in women offered routine vaccination.
Introduction
Human papillomavirus (HPV) comprises a family of viruses, a subset of which are responsible for virtually all cervical and some anogenital and oropharyngeal cancers. 1 More than 100 countries worldwide have introduced prophylactic HPV vaccination as part of routine immunisation schedules. 2 One important outcome yet to be reported is whether vaccination has reduced or increased the inequalities seen for cervical disease in the UK and elsewhere.
In England, the national HPV vaccination programme started in 2008 using the bivalent Cervarix vaccine to prevent infections due to HPV types 16 and 18, which are estimated to cause around 80% of all cervical cancers in the UK. 3 Vaccination was offered routinely to 12-13 year old (school year 8) girls and as part of a catch-up campaign to those aged <19 years. 4 In September 2012 the programme switched to the quadrivalent vaccine (Gardasil), which additionally protects against HPV types 6 and 11 (responsible for genital warts), and in 2019 the programme was extended to 12-13 year old boys. Those who are eligible but not vaccinated can receive the vaccine free of charge from their general practitioner until their 25th birthday. 5
The introduction and implementation of HPV immunisation in this way means that noticeable discontinuities exist in the proportion of women vaccinated by date of birth, enabling a rigorous evaluation of the effectiveness of the programme. 6 For example, women born in August 1990 are unlikely to have received HPV vaccination, whereas among those born in the year from 1 September 1990 nearly 70% have received at least one dose of the vaccine.
Findings on the early effect of national HPV vaccination programmes have been encouraging. A wealth of real world evidence for the effect of vaccination on HPV prevalence exists 7 8 9 10 11 and evidence is growing for its effectiveness in reducing high grade cervical intraepithelial neoplasia (CIN) 12 13 14 15 and cervical cancer in vaccinated women. 14 16 17 18 19 For instance, we found that in England rates of grade 3 CIN (CIN3) and of cervical cancer were greatly reduced among those who were offered HPV vaccination, and that the magnitude of the reduction was greatest in the cohorts with the highest uptake and younger age at vaccination. 14 We estimated that by mid-2019 the immunisation programme had prevented cervical cancer in nearly 450 women and CIN3 in around 17 000 women.
Along with preventing ill health, a key aim of the NHS is to reduce health inequalities. 20 To this end, we investigated whether the effect of immunisation against HPV has resulted in a reduction in inequalities in cervical disease or a widening. Concern has been expressed that if the uptake of HPV vaccination is lower in those at greatest risk of cervical cancer, as has been seen in the US, 21 this could accentuate health inequalities. One study found that the introduction of HPV immunisation in England might initially have increased inequities in HPV related cancer incidence among ethnic minority groups because of the differential effect of herd protection in subpopulations with dissimilar vaccination coverage. 22 Previous studies have suggested that white people have a higher awareness of HPV and acceptance of the immunisation 23 and that vaccination uptake is lower in women from ethnic minority groups and more deprived areas. 24 Using data on HPV vaccination coverage by local area, however, a study found little variation by deprivation score in women offered routine vaccination (83% v 86% for most and least deprived areas, respectively) and only a small negative correlation between deprivation and vaccine uptake in those offered catch-up vaccination (47% v 53% for most and least deprived areas, respectively). 25 A full understanding of the effect of HPV vaccination across different socioeconomic groups is complicated by the poor uptake of cervical screening observed among younger women in the most deprived areas, leading to lower rates of screen detected cervical cancer and CIN3 at age 25 years compared with women in less deprived areas. 26 27
We replicated results from an analysis of population based cancer registry data to evaluate if the high vaccination effectiveness seen previously continued during an additional year of follow-up. The combined data were also used to investigate the effect of the vaccination programme by socioeconomic deprivation.
To represent socioeconomic deprivation, we used the index of multiple deprivation, a small area measure based on several domains of deprivation, such as income, employment, and health. The index is determined by using a standard statistical geographical unit, called lower super output area, which divides England into small areas of similar sized populations (on average about 1500 residents, or 650 households). 28 The lower super output areas are then ranked from the most to the least deprived and divided into five equal groups. The first and fifth groups correspond to the 20% most deprived and 20% least deprived lower super output areas in England, respectively.
We retrieved the records of all women aged 20-64 years resident in England with a diagnosis of invasive cervical cancer (ICD-10 (international classification of diseases, 10th revision) code C53) or CIN3 (ICD-10 code D06) between 1 January 2006 and 30 June 2020. These records are stored in the database managed by NHS England’s National Disease Registration Service, 29 and for each patient included information on index of multiple deprivation derived from the patient’s home postcode at the time of diagnosis. To convert these counts into rates, we used mid-year estimates of the female population for England by single year of age, calendar year (January 2006 to June 2020), and index of multiple deprivation (five groups). These estimates were retrieved from multiple tables publicly available on the website of the UK’s Office for National Statistics (ONS). 30 The supplementary material provides more details about the index of multiple deprivation versions used by the National Disease Registration Service and ONS, along with information on how we derived the population estimates required in our statistical analysis.
Statistical analysis
We separately analysed incidence rates of cervical cancer and CIN3 by using extensions of our previously described age-period-cohort Poisson model. 14 31 32 Data on women with cancer or CIN3 were aggregated by single month of age, calendar time (period), and date of birth (cohort). We derived the corresponding population risk time by subdividing the mid-year ONS population estimates into one month intervals for age, period, and cohort. For the analysis of the effectiveness by deprivation, we further split both the data on women with cancer or CIN3 and the population estimates by deprivation group (fifths). We then used the population risk time as the denominator for calculating rates (formally, the subdivided population estimates were log transformed and included in the Poisson regression model as an offset). Confidence intervals were computed using robust standard errors. 33 34
The code for the analysis was written and tested on synthetic data (extending the Simulacrum dataset) 35 by a statistician (MF) at King’s College London and then run on the real dataset by an analyst (BN) at the National Disease Registration Service.
We started by considering a core model where we included the main effects for age, period, and birth cohort, along with selected age by cohort and age by period interactions (see supplementary table S1). The interaction terms were included to account for variations in screening policy and historical events that affected cervical cancer rates. Specifically, we defined seven birth cohorts to capture differences in the age at first invitation to screening and the school years in which HPV vaccination was offered (see table 1 ). We added terms for seasonality and for events that may have affected registrations for cervical cancer and CIN3, such as the covid-19 lockdown, the “Jade Goody effect,” 36 37 and the 2019 cervical screening awareness campaign. In our previous paper, 14 we used several similar regression models to study the sensitivity of results to the precise way in which we adjusted for potential confounding factors. Because we found that the estimates of the cohort specific incidence rate ratios changed little across the various models, here we report on only a single model adjustment for confounders.
Characteristics of the birth cohorts
- View inline
Using the core model described, we investigated if the high effectiveness of the HPV immunisation programme reported previously 14 continued during an additional 12 months of follow-up. To do this we split the main effect of each cohort offered vaccination into two subgroup effects depending on whether the data related to the periods 1 January 2006 to 30 June 2019 or 1 July 2019 to 30 June 2020; this approach corresponded to adding three cohort by period interaction terms.
To evaluate the impact of socioeconomic deprivation on incidences of cervical cancer and CIN3, we extended the core model by adding main effects for deprivation and deprivation by cohort interactions. Specifically, we allowed the effect of each deprivation level to vary between unvaccinated women (cohorts 1-4) and those offered vaccination (cohorts 5-7), but we assumed it was otherwise constant within these two groups. We did not include further interactions between deprivation and other covariates as they were not of primary interest in this analysis. Using the fitted Poisson regression models, we made “what if” predictions by changing the value of one or more predictors and by leaving the others as observed. In this way it was possible to compare what happened (factual scenario) with what would have happened under an alternative (counterfactual) scenario.
We also carried out a sensitivity analysis where the effects of these deprivation by cohort interactions were allowed to vary across the three different groups offered vaccination (ie, we used 15 terms instead of five). For cervical cancer, owing to small numbers in cohort 7, we fitted a reduced model where the effects of these interactions were constrained to be the same for cohorts 6 and 7.
All analyses were performed in Stata, version 17. 38
Patient and public involvement
Patient and public involvement contributors were not formally involved in this research. We did, however, engage with Cancer Research UK (CRUK), Jo’s Cervical Cancer Trust, and the HPV Coalition on the importance of these analyses and the dissemination of the results. This included taking part in a video produced by ITN Business for World Cancer Day 2023, writing a piece for the 20th anniversary of the creation of CRUK, and engaging with international media about our research findings on the effect of the English HPV vaccination programme. We have also discussed the research and a draft of this paper with individual patients, journalists, and patient and public involvement representatives linked to broader research programmes.
Table 1 lists the characteristics of the birth cohorts included in the study. We defined the different cohorts so that each cohort is homogeneous in terms of the age women would have been offered HPV vaccination (if at all) and the age at which they would have first been invited for cervical screening.
Overall, there were 231.1 million women years of observation between 1 January 2006 and 30 June 2020 on women aged 20-64 years in England. During this time, 29 968 women received a diagnosis of invasive cervical cancer and 335 228 a diagnosis of CIN3 ( table 2 ). Observations between 1 July 2019 and 30 June 2020 have not been reported previously. With these additional 12 months of follow-up, there are, in the routine vaccination group (cohort 7), about twice the number of diagnoses compared with the same group in our previous study (we now have 13 v 7 previously for cervical cancer, 109 v 49 for CIN3; see supplementary table S2).
Summary statistics of study population
Our previously published findings on the effect of the national HPV vaccination were largely confirmed with the new data ( table 3 , also see supplementary table S3). The analysis showed that the previously observed low rates of disease and the estimated high effectiveness of the immunisation programme continued during the additional 12 months of follow-up (diagnoses in July 2019 to June 2020) among women born since 1 September 1990. In particular, the estimated effects of vaccination for that later period in cohort 7 (those born since 1 September 1995) imply a reduction in incidence of 83.9% (95% confidence interval (CI) 63.8% to 92.8%) for cervical cancer and 94.3% (92.6% to 95.7%) for CIN3 ( table 3 ). The relative risk reduction estimates for the earlier period are not identical to those reported previously because we also had new data for the unvaccinated cohorts that affected the baseline rates.
Estimated relative risk reductions (percentages) in incidence of invasive cervical cancer and CIN3 in the three cohorts offered HPV vaccination compared with the most recent unvaccinated cohort
Supplementary table S4 shows the full estimates from modelling the effects of vaccination in different levels of socioeconomic deprivation, with summary results reported in table 4 , table 5 , and table 6 . The highest incidence rates for invasive cervical cancer were observed among women living in the most deprived areas (first fifth) but, while in the reference unvaccinated group there was a strong downward gradient moving from women in the most deprived areas to those in the least deprived, little difference was found between the second and fifth fifths of deprivation in the groups offered vaccination. In both the reference and the vaccination cohorts the highest rates of CIN3 occurred in those from the most deprived areas, but no clear trend was observed among the other four fifths of deprivation (see supplementary tables S5 and S6).
Estimated number of invasive cervical cancers and CIN3s predicted and prevented by mid-2020 in the three cohorts of women offered HPV vaccination
Estimated cohort specific numbers of invasive cervical cancers predicted and prevented by mid-2020 among women in the least and most deprived areas
Estimated cohort specific numbers of CIN3 predicted and prevented by mid-2020 among women in the least and most deprived areas
Overall, our model estimated that 687 (95% CI 556 to 819) cervical cancers and 23 192 (22 163 to 24 220) CIN3s had been prevented by the vaccination programme up to mid-2020 among young women in England ( table 4 ). The greatest numbers for cervical cancer were prevented in women in the most deprived areas (192 and 199 for first and second fifths, respectively) and the fewest in women in the least deprived fifth (61 cancers prevented). The number of women with CIN3 prevented was high across all deprivation groups but greatest among women living in the more deprived areas: 5121 and 5773 for first and second fifths, respectively, compared with 4173 and 3309 in the fourth and fifth fifths, respectively. When we looked at the corresponding cohort specific figures ( table 5 and table 6 ), we noticed differences between the cohorts, particularly for CIN3. In all three cohorts offered vaccination the numbers and rates of prevented cervical cancers were much higher in women from the most deprived areas than least deprived areas ( table 5 ). The proportion of women with prevented cervical cancer in each cohort was, however, similar between the first and fifth fifths of deprivation. For CIN3 ( table 6 ), the results were more complicated. In women offered vaccination at age 16-18 years (cohort 5), the proportion of cervical cancers prevented was substantially less in those from the most deprived areas (29.6%) compared with those from the least deprived areas (40.6%). An inequality still existed in cohorts 6 and 7, but it was greatly reduced (67.7% v 72.8% in cohort 6 and 95.3% v 96.1% in cohort 7).
In England, the social-class gradient for cervical cancer is one of the steepest of any cancers: women in the most deprived fifth have had double the risk of those in the least deprived fifth. 39 40 Some of this results from differences in exposure to HPV and risk of an infection becoming persistent, 41 but differential uptake of cervical screening has also been an important factor. Previous research has highlighted the need for new engagement strategies to improve attendance for cervical screening among young women living in more socially deprived areas. 42 Encouragingly, the coverage of HPV vaccination has been (at least for the routine campaign and before the covid-19 pandemic) uniformly high. 43 It is, however, important to investigate whether immunisation—including the indirect effects achieved by high uptake—is helping to reduce health inequalities.
Using population based cancer registrations updated to mid-2020, which provided information on about twice the expected number of cancers in women offered HPV vaccination aged 12-13 years than in our previous analysis, we were able to show that the high vaccination effectiveness seen previously was confirmed with more recent data. The largest differences between the old and the new data were found for cohort 6 (the catch-up group offered the vaccine at age 14-16 years): for cervical cancer the estimated effectiveness increased, whereas for CIN3 it decreased. The reasons behind these differences are unclear. The results for cohorts 6 and 7 in the new data are more in keeping with what we would have expected given that the proportion of disease caused by HPV types 16 and 18 is greater for invasive cancer than for CIN3.
We also investigated the effect of the HPV immunisation programme by socioeconomic deprivation. Overall, we found that the programme was associated with a substantial reduction in the expected number of women with cervical cancers and CIN3 in all fifths of deprivation. For cervical cancer before vaccination, the downward gradient with decreasing deprivation was strong. In all cohorts offered vaccination, the highest rate was still seen among women living in the most deprived areas, but little difference was observed between women living in the second to fifth deprived areas. For CIN3, similar patterns were observed for the reference unvaccinated group and the three cohorts offered vaccination, but rates were greatly reduced in all fifths of deprivation in the latter. When we compared women in the most deprived areas with those in the least deprived areas in terms of percentage of disease averted, we observed differences across the cohorts for CIN3, with women in the least deprived areas in the older catch-up cohort (vaccine offered at age 16-18 years) having a greater proportion of averted CIN3s after HPV immunisation than women in the most deprived area (40.6% v 29.6%). The same, although to a much less extent, was observed for the younger catch-up cohort (72.8% v 67.7%). For invasive cervical cancer, we found no evidence of a less beneficial impact (in terms of percentage of cases averted) of the vaccination in women living in the most deprived areas; in fact, especially for the older catch-up cohort, the percentage was slightly higher in women in the most deprived areas compared with those in the least deprived areas.
The observed incidences of cervical cancer and CIN3 depend on three key factors: the intensity of exposure to HPV infections (including age at first exposure), the uptake of cervical screening, and HPV vaccination coverage. It is therefore difficult to disentangle the effects of these three drivers on the index of multiple deprivation specific rates with the data at hand. The health inequality in CIN3 in cohort 5 might result from the lower vaccination coverage among women in the most deprived areas since at age 16-18 years when they became eligible for vaccination more of those from the most deprived fifth may not have been in school or, for other reasons, may have missed the offer of HPV immunisation. These observations are consistent with previous understanding that higher uptake of catch-up vaccination was associated, although not as strongly as in some countries, with lower deprivation. 25 It is, however, reassuring that cohorts 6 and 7 showed little inequality in relative reductions in cancer (as in vaccination coverage).
However, since the UK has recently announced a change to a one dose schedule for routine HPV vaccination, ensuring this change achieves high coverage (including in the birth cohorts currently with lower coverage owing to covid-19 related interruption to schooling, and to immunisation services) is important to maintain the effects we have seen on cervical disease and on inequalities. Further investigations could be carried out in the future to check for any effect on cancer incidence caused by covid-19, gender neutral vaccination (since 2019), a change in the type of vaccine used, or reduced dose schedules.
Strengths and limitations of this study
Our analysis has several strengths. Our study provides direct evidence for the effect of a public health intervention (such as HPV vaccination) on cancer rates by deprivation. We used high quality data from population based cancer registries and were able to investigate the extent of socioeconomic inequalities in cohorts offered vaccination and whether the effectiveness of the HPV immunisation continued in an additional year of follow-up. The code for the analysis was written and tested using simulated data and an independent analyst later ran the code on the real dataset, guaranteeing reliable and robust results and preserving patient confidentiality.
The main limitations of our study are that it was observational and individual level data on vaccination status were not available. However, previous published research 14 provided detailed information on potential confounding factors and the best way to adjust for these in the analysis. Additionally, the discontinuities in vaccine uptake with date of birth makes this study powerful and less prone to biases from unobserved confounders than an analysis based on individual level data on HPV vaccination status.
Women born after 1 September 1999 were offered the Gardasil vaccine from 1 September 2012. As these women were at most aged 20 years and 10 months at the end of the study follow-up (30 June 2020), it is not yet possible with the data available to compare the effectiveness of the programme among those offered Cervarix and those offered Gardasil. This additional comparative analysis will become feasible with a longer follow-up on the recipients of Gardasil.
Policy implications
We found that the high effectiveness of the national HPV immunisation continued in the additional year of follow-up (July 2019 to June 2020). This is encouraging as it validates the previously published results and further supports consideration of more limited cervical screening for cohorts with high vaccination coverage aged 12-13 years. Moreover, although women living in the most deprived areas are still at higher risk of cervical cancer than those in less deprived areas, the HPV vaccination programme is associated with substantially lowered rates of disease across all fifths of socioeconomic deprivation. For cervical cancer, this has led to the levelling-up of the rates across the second to fifth fifths of deprivation so that the strong downward gradient observed in the reference unvaccinated cohort is no longer present in the cohorts offered vaccination. For CIN3, in the older catch-up cohorts women living in the least deprived areas seem to have benefited more from vaccination than those living in the most deprived areas, but the rates were still greatly reduced in all socioeconomic groups. Cervical screening strategies for women offered vaccination should carefully consider the differential effect both on rates of disease and on inequalities that are evident among women offered catch-up vaccination.
Conclusions
The HPV vaccination programme in England has not only been associated with a substantial reduction in incidence of cervical neoplasia in targeted cohorts, but also in all socioeconomic groups. This shows that well planned and executed public health interventions can both improve health and reduce health inequalities.
What is already known on this topic
In England, immunisation against human papillomavirus (HPV) has been associated with greatly reduced incidence rates of cervical cancer and grade 3 cervical intraepithelial neoplasia (CIN3) up to June 2019, especially among women offered routine vaccination at age 12-13 years
The social-class gradient for cervical cancer incidence has been one of the steepest of any cancers
Concern has been raised that HPV vaccination could least benefit those at highest risk of cervical cancer
What this study adds
The high effectiveness of vaccination against HPV seen previously continued during an additional year of follow-up, from July 2019 to June 2020
The English HPV vaccination programme was associated with substantially lower rates of cervical cancer and CIN3 in all fifths of socioeconomic deprivation, although the highest rates remained among women in the most deprived areas
For cervical cancer, the strong downward gradient from high to low deprivation observed in the reference unvaccinated cohort was no longer present among those offered vaccination
Ethics statements
Ethical approval.
Not required as the study used aggregated data from the National Disease Registration Service as well as publicly available information from the Office for National Statistics website.
Data availability statement
The cancer registry data analysed for this paper are securely held by the National Disease Registration Service (NDRS). Requests to access the data can be made through NHS England’s DARS service ( https://digital.nhs.uk/services/data-access-request-service-dars ). The Simulacrum ( https://simulacrum.healthdatainsight.org.uk/ ) is a synthetic dataset developed by Health Data Insight and derived from anonymous cancer data provided by NHS England’s NDRS. Mid-year population estimates are freely downloadable from the Office for National Statistics website ( https://www.ons.gov.uk/ ).
Acknowledgments
We thank Alejandra Castañon (LCP Health Analytics), Marta Checchi (UK Health Security Agency), and Lucy Elliss-Brookes (NHS England) for helpful comments on the study protocol, and Kwok Wong (NHS England) for contributing to the quality assurance of the data extraction code.
Contributors: PS had the original idea. He is the guarantor. MF and PS conceptualised the study and prepared the study protocol, which was subsequently reviewed by the other co-authors. MF wrote and tested the Stata code (checked by PS) for the data analysis and drafted the manuscript. BN extracted the dataset and ran the Stata code on it. All authors critically reviewed and approved the final submitted version. The corresponding author attests that all listed authors meet authorship criteria and that no others meeting the criteria have been omitted.
Funding: This work was supported by Cancer Research UK (grant No C8162/A27047). The funder had no role in the study design or in the collection, analysis, interpretation of data, writing of the report or decision to submit the article for publication.
Competing interests: All authors have completed the ICMJE uniform disclosure form at www.icmje.org/disclosure-of-interest/ and declare support from Cancer Research UK for the submitted work; no financial relationships with any organisations that might have an interest in the submitted work in the previous three years; no other relationships or activities that could appear to have influenced the submitted work.
Transparency: The lead author (the manuscript’s guarantor) affirms that the manuscript is an honest, accurate, and transparent account of the study being reported; that no important aspects of the study have been omitted; and that any discrepancies from the study as planned (and, if relevant, registered) have been explained.
Dissemination to participants and related patient and public communities: The results of this research will be disseminated through the media, blogs and scientific meetings and will inform the design and implementation of interventions to reduce health inequalities. We will also work with others to produce information for the public to support human papillomavirus immunisation and cervical screening programmes and, if the opportunity arises, to contribute summary data for an international meta-analysis of similar studies.
Provenance and peer review: Not commissioned; externally peer reviewed.
This is an Open Access article distributed in accordance with the terms of the Creative Commons Attribution (CC BY 4.0) license, which permits others to distribute, remix, adapt and build upon this work, for commercial use, provided the original work is properly cited. See: http://creativecommons.org/licenses/by/4.0/ .
- ↵ IARC. Human papillomaviruses. IARC Monographs on the Evaluation of Carcinogenic Risks to Humans, Volume 90. 2007.
- ↵ World Health Organization (WHO). Global Market Study: HPV 2022 https://cdn.who.int/media/docs/default-source/immunization/mi4a/who-mi4a-global-market-study-hpv.pdf?sfvrsn=649561b3_1&download=true .
- Cuschieri K ,
- Hibbitts S ,
- ↵ Public Health England (PHE). Human Papillomavirus (HPV) vaccine coverage in England, 2008/09 to 2013/14. A review of the full six years of the three-dose schedule: Public Health England (PHE); 2015. https://www.gov.uk/government/publications/human-papillomavirus-hpv-immunisation-programme-review-2008-to-2014 ; accessed 6 January 2021.
- ↵ UK Health Security Agency. HPV vaccination: guidance for healthcare practitioners (version 6) 2022 [updated April 2022]. https://www.gov.uk/government/publications/hpv-universal-vaccination-guidance-for-health-professionals ; accessed 24 August 2022.
- Lévesque LE ,
- Kaufman JS ,
- Brisson M ,
- HPV Vaccination Impact Study Group
- Thomas SL ,
- Tabrizi SN ,
- Brotherton JM ,
- Kaldor JM ,
- Markowitz LE ,
- Steinau M ,
- Hernandez-Aguado JJ ,
- Sánchez Torres DA ,
- Martínez Lamela E ,
- Lehtinen M ,
- Lagheden C ,
- Luostarinen T ,
- Falcaro M ,
- Castañon A ,
- Wallace L ,
- Pollock KG ,
- Elfström KM ,
- Skorstengaard M ,
- Thamsborg LH ,
- Dillner J ,
- Dehlendorff C ,
- Belmonte F ,
- ↵ NHS. The NHS long term plan 2019. https://www.longtermplan.nhs.uk/ ; accessed 24 August 2022.
- Johnson HC ,
- Lafferty EI ,
- Roberts SA ,
- Stretch R ,
- Sheridan A ,
- Pappas-Gogos G ,
- Douglas E ,
- McLennan D ,
- Henson KE ,
- Elliss-Brookes L ,
- Coupland VH ,
- ↵ Office for National Statistics. https://www.ons.gov.uk/ ; accessed 24 October 2022.
- Carstensen B
- Sasieni P ,
- ↵ Huber P. The behavior of maximum likelihood estimates under nonstandard conditions. Proceedings of the 5th Berkeley Symposium on Mathematical Statistics and Probability: University of California Press, 1967:221-33.
- Health Data Insight
- Lancucki L ,
- Patnick J ,
- Castanon A ,
- Thomson CS ,
- UK Association of Cancer Registries
- ↵ Cancer Research UK. Cervical Cancer Incidence Statistics 2015. https://www.cancerresearchuk.org/health-professional/cancer-statistics/statistics-by-cancer-type/cervical-cancer/incidence ; accessed 14 March 2023.
- Currin LG ,
- Linklater KM ,
- Rahman MA ,
- Paranjothy S
- ↵ UK Health Security Agency (UKHSA). HPV vaccine uptake 2023. https://www.gov.uk/government/collections/vaccine-uptake#hpv-vaccine-uptake ; accessed 12 March 2023.
In the tech world and beyond, new 5G applications are being discovered every day. From driverless cars to smarter cities, farms, and even shopping experiences, the latest standard in wireless networks is poised to transform the way we interact with information, devices and each other. What better time to take a closer look at how humans are putting 5G to use to transform their world.
What is 5G?
5G (fifth-generation mobile technology is the newest standard for cellular networks. Like its predecessors, 3G, 4G and 4G LTE, 5G technology uses radio waves for data transmission. However, due to significant improvements in latency, throughput and bandwidth, 5G is capable of faster download and upload speeds than previous networks.
Since its release in 2019, 5G broadband technology has been hailed as a breakthrough technology with significant implications for both consumers and businesses. Primarily, this is due to its ability to handle large volumes of data that is generated by complex devices that use its networks.
As mobile technology has expanded over the years, the number of data users generate every day has increased exponentially. Currently, other transformational technologies like artificial intelligence (AI), the Internet of Things (IoT ) and machine learning (ML) require faster speeds to function than 3G and 4G networks offer. Enter 5G, with its lightning-fast data transfer capabilities that allow newer technologies to function in the way they were designed to.
Here are some of the biggest differences between 5G and previous wireless networks.
- Physical footprint : The transmitters that are used in 5G technology are smaller than in predecessors’ networks, allowing for discrete placement in out-of-the-way places. Furthermore, “cells”—geographical areas that all wireless networks require for connectivity—in 5G networks are smaller and require less power to run than in previous generations.
- Error rates : 5G’s adaptive Modulation and Coding Scheme (MCS), a schematic that wifi devices use to transmit data, is more powerful than ones in 3G and 4G networks. This makes 5G’s Block Error Rate (BER)—a metric of error frequency—much lower.
- Bandwidth : By using a broader spectrum of radio frequencies than previous wireless networks, 5G networks can transmit on a wider range of bandwidths. This increases the number of devices that they can support at any given time.
- Lower latency : 5G’s low latency , a measurement of the time it takes data to travel from one location to another, is a significant upgrade over previous generations. This means that routine activities like downloading a file or working in the cloud is going to be faster with a 5G connection than a connection on a different network.
Like all wireless networks, 5G networks are separated into geographical areas that are known as cells. Within each cell, wireless devices—such as smartphones, PCs, and IoT devices—connect to the internet via radio waves that are transmitted between an antenna and a base station. The technology that underpins 5G is essentially the same as in 3G and 4G networks. But due to its lower latency, 5G networks are capable of delivering faster download speeds—in some cases as high as 10 gigabits per second (Gbps).
As more and more devices are built for 5G speeds, demand for 5G connectivity is growing. Today, many popular Internet Service Providers (ISPs), such as Verizon, Google and AT&T, offer 5G networks to homes and businesses. According to Statista, more than 200 million homes and businesses have already purchased it with that number expected to at least double by 2028 (link resides outside ibm.com).
Let’s take a look at three areas of technological improvement that have made 5G so unique.
New telecom specifications
The 5G NR (New Radio) standard for cellular networks defines a new radio access technology (RAT) specification for all 5G mobile networks. The 5G rollout began in 2018 with a global initiative known as the 3rd Generation Partnership Project (3FPP). The initiative defined a new set of standards to steer the design of devices and applications for use on 5G networks.
The initiative was a success, and 5G networks grew swiftly in the ensuing years. Today, 45% of networks worldwide are 5G compatible, with that number forecasted to rise to 85% by the end of the decade according to a recent report by Ericsson (link resides outside ibm.com).
Independent virtual networks (network slicing)
On 5G networks, network operators can offer multiple independent virtual networks (in addition to public ones) on the same infrastructure. Unlike previous wireless networks, this new capability allows users to do more things remotely with greater security than ever before. For example, on a 5G network, enterprises can create use cases or business models and assign them their own independent virtual network. This dramatically improves the user experience for their employees by adding greater customizability and security.
Private networks
In addition to network slicing, creating a 5G private network can also enhance personalization and security features over those available on previous generations of wireless networks. Global businesses seeking more control and mobility for their employees increasingly turn to private 5G network architectures rather than public networks they’ve used in the past.
Now that we better understand how 5G technology works, let’s take a closer look at some of the exciting applications it’s enabling.
Autonomous vehicles
From taxi cabs to drones and beyond, 5G technology underpins most of the next-generation capabilities in autonomous vehicles. Until the 5G cellular standard came along, fully autonomous vehicles were a bit of a pipe dream due to the data transmission limitations of 3G and 4G technology. Now, 5G’s lightning-fast connection speeds have made transport systems for cars, trains and more, faster than previous generations, transforming the way systems and devices connect, communicate and collaborate.
Smart factories
5G, along with AI and ML, is poised to help factories become not only smarter but more automated, efficient, and resilient. Today, many mundane but necessary tasks that are associated with equipment repair and optimization are being turned over to machines thanks to 5G connectivity paired with AI and ML capabilities. This is one area where 5G is expected to be highly disruptive, impacting everything from fuel economy to the design of equipment lifecycles and how goods arrive at our homes.
For example, on a busy factory floor, drones and cameras that are connected to smart devices that use the IoT can help locate and transport something more efficiently than in the past and prevent theft. Not only is this better for the environment and consumers, but it also frees up employees to dedicate their time and energy to tasks that are more suited to their skill sets.
Smart cities
The idea of a hyper-connected urban environment that uses 5G network speeds to spur innovation in areas like law enforcement, waste disposal and disaster mitigation is fast becoming a reality. Some cities already use 5G-enabled sensors to track traffic patterns in real time and adjust signals, helping guide the flow of traffic, minimize congestion, and improve air quality.
In another example, 5G power grids monitor supply and demand across heavily populated areas and deploy AI and ML applications to “learn” what times energy is in high or low demand. This process has been shown to significantly impact energy conservation and waste, potentially reducing carbon emissions and helping cities reach sustainability goals.
Smart healthcare
Hospitals, doctors, and the healthcare industry as a whole already benefit from the speed and reliability of 5G networks every day. One example is the area of remote surgery that uses robotics and a high-definition live stream that is connected to the internet via a 5G network. Another is the field of mobile health, where 5G gives medical workers in the field quick access to patient data and medical history. This enables them to make smarter decisions, faster, and potentially save lives.
Lastly, as we saw during the pandemic, contact tracing and the mapping of outbreaks are critical to keeping populations safe. 5G’s ability to deliver of volumes of data swiftly and securely allows experts to make more informed decisions that have ramifications for everyone.
5G paired with new technological capabilities won’t just result in the automation of employee tasks, it will dramatically improve them and the overall employee experience . Take virtual reality (VR) and augmented reality (AR), for example. VR (digital environments that shut out the real world) and AR (digital content that augments the real world) are already used by stockroom employees, transportation drivers and many others. These employees rely on wearables that are connected to a 5G network capable of high-speed data transfer rates that improve several key capabilities, including the following:
- Live views : 5G connectivity provides live, real-time views of equipment, events, and even people. One way in which this feature is being used in professional sports is to allow broadcasters to remotely call a sporting event from outside the stadium where the event is taking place.
- Digital overlays : IoT applications in a warehouse or industrial setting allow workers that are equipped with smart glasses (or even just a smartphone) to obtain real-time insights from an application. This includes repair instructions or the name and location of a spare part.
- Drone inspections : Right now, one of the leading causes of employee injury is inspection of equipment or project sites in remote and potentially dangerous areas. Drones, which are connected via 5G networks, can safely monitor equipment and project sites and even take readings from hard-to-reach gauges.
Edge computing , a computing framework that allows computations to be done closer to data sources, is fast becoming the standard for enterprises. According to this Gartner white paper (link resides outside ibm.com), by 2025, 75% of enterprise data will be processed at the edge (compared to only 10% today). This shift saves businesses time and money and enables better control over large volumes of data. It would be impossible without the new speed standards that are generated by 5G technology.
Ultra-reliable edge computing and 5G enable the enterprise to achieve faster transmission speeds, increased control and greater security over massive volumes of data. Together, these twin technologies will help reduce latency while increasing speed, reliability and bandwidth, resulting in faster, more comprehensive data analysis and insights for businesses everywhere.
5G solutions with IBM Cloud Satellite
5G presents significant opportunities for the enterprise, but first, you need a platform that can handle its speed. IBM Cloud Satellite® lets you deploy and run apps consistently across on-premises, edge computing and public cloud environments on a 5G network. And it’s all enabled by secure and auditable communications within the IBM Cloud®.
Get the latest tech insights and expert thought leadership in your inbox.
Get our newsletters and topic updates that deliver the latest thought leadership and insights on emerging trends.
Impact of flowering temperature on litchi yield under climate change: A case study in Taiwan
- Hwang, Ya-Wen
- Hsu, Yung-Heng
- Chen, Yung-Ming
Litchi is a subtropical fruit tree that undergoes flower bud differentiation under low-temperature conditions. However, climate change has affected litchi production in Taiwan, causing litchi farmers to experience economic losses. This study explored the influence of flowering temperature on litchi yield under climate change in Taiwan by analyzing litchi production data from 2001 to 2020 and observation data from meteorological stations in litchi-producing areas. Historical observed data were used to construct several regression models relating temperature to yield, with the performance of the models used to determine critical temperature thresholds for litchi flower bud differentiation. Analytical climate data (CMIP5) were used to project yield changes in Taiwan's litchi-producing regions under anticipated low-temperature conditions for the mid- (2036–2065) and late- (2071–2100) 21st century. The variable that exhibited the highest correlation with yield changes was the number of days with an average flowering temperature below 16 °C. The production yield, in terms of yield variation per hectare, is expected to decrease by 12 % to 35 % by the end of the 21st century (2071–2100). Given the projected decline in the number of cooler days due to climate change, existing litchi cultivars may become unsuitable for cultivation in production areas in southern Taiwan. Some fruit trees require a period of low temperature before their flowering stage. Climate change is expected to cause warming of winter temperatures in Taiwan, which is likely to lead to reduced litchi flowering. The current study assessed the potential effects of climate change on litchi flowering in the future. Historical observed data were used to establish models, and critical temperature thresholds for litchi flowering were determined on the basis of model performance. Days with average temperatures below 16 °C exhibited the highest correlation with litchi yield among the tested thresholds. According to our results, farmers can use this 16 °C threshold to evaluate the potential effects of future climate change at their current farm locations and to identify other areas with similar or more favorable conditions for litchi cultivation. For agricultural researchers, this temperature threshold could provide a target for new litchi variety breeding and a reference basis for research on optimal cultivation methods. Notably, because climate change projection data have a high degree of uncertainty, the results of this study may differ from those of studies using different databases. In this study, we used an ensemble of CMIP5 projections incorporating data from models from various research centers around the world, which can provide more robust results based on an ensemble mean than those obtainable from a single model or a few models. In addition, rainfall is a crucial factor during the flowering growth stage. Future studies should consider the effects of rainfall and temperature on yield and should consider using a model with yield being considered a function of both of these variables to improve model accuracy. To conclude, the present study provides researchers, policymakers, and other stakeholders with insights into the primary effects of climate change on litchi production. It also lays the groundwork for future climate adaptation strategies in Taiwan's litchi industry.
- Climate change;
- Litchi yield;
- Flowering temperature

IMAGES
VIDEO
COMMENTS
An observational study is used to answer a research question based purely on what the researcher observes. There is no interference or manipulation of the research subjects, and no control and treatment groups. These studies are often qualitative in nature and can be used for both exploratory and explanatory research purposes.
Observation methods have the potential to reach beyond other methods that rely largely or solely on self-report. This article describes the distinctive characteristics of case study observational research, a modified form of Yin's 2014 model of case study research the authors used in a study exploring interprofessional collaboration in primary ...
A case study protocol outlines the procedures and general rules to be followed during the case study. This includes the data collection methods to be used, the sources of data, and the procedures for analysis. Having a detailed case study protocol ensures consistency and reliability in the study.
characteristics of case study observational research, a modified form of Yin's 2014 model of case study research the authors used in a study exploring interprofessional collaboration in primary care. In this approach, observation data are positioned as the central component of the research design.
Observational studies provide critical descriptive data and information on long-term efficacy and safety that clinical trials cannot provide, at generally much less expense. Observational studies include case reports and case series, ecological studies, cross-sectional studies, case-control studies and cohort studies. ...
Cohort studies and case-control studies are two primary types of observational studies that aid in evaluating associations between diseases and exposures. In this review article, we describe these study designs, methodological issues, and provide examples from the plastic surgery literature. Keywords: observational studies, case-control study ...
Case-control study. A case-control study is an observational analytic retrospective study design [].It starts with the outcome of interest (referred to as cases) and looks back in time for exposures that likely caused the outcome of interest [13, 20].This design compares two groups of participants - those with the outcome of interest and the matched control [].
Case study research is a comprehensive method that incorporates multiple sources of data to provide detailed accounts of complex research phenomena in real-life contexts. However, current models of case study research do not particularly distinguish the unique contribution observation data can make.
Like many observational research methods, case studies tend to be more qualitative in nature. Case study methods involve an in-depth, and often a longitudinal examination of an individual. Depending on the focus of the case study, individuals may or may not be observed in their natural setting. If the natural setting is not what is of interest ...
Observational studies play a significant role in healthcare, including the study of the use and effects of medicines in large populations known as 'pharmacoepidemiology', ... Case-control studies compare the proportion of cases with a specific exposure to the proportion of controls with the same exposure (the odds ratio). Case-control ...
A case study is an in-depth examination of an individual. Sometimes case studies are also completed on social units (e.g., a cult) and events (e.g., a natural disaster). ... So, as with all observational methods, case studies do not permit determination of causation. In addition, because case studies are often of a single individual, and ...
An observational case study is a study of a real-world case without performing an intervention. Measurement may influence the measured phenomena, but as in all forms of research, the researcher tries to restrict this to a minimum. The researcher may study a sample of two or even more cases, but the goal of case study research is not to acquire ...
A case study is one of the most commonly used methodologies of social research. This article attempts to look into the various dimensions of a case study research strategy, the different epistemological strands which determine the particular case study type and approach adopted in the field, discusses the factors which can enhance the effectiveness of a case study research, and the debate ...
Case Control Observational Study. Researchers in case control studies identify individuals with an existing health issue or condition, or "cases," along with a similar group without the condition, or "controls." These two groups are then compared to identify predictors and outcomes. This type of study is helpful to generate a hypothesis ...
Case studies are in-depth investigations of a person, group, event, or community. Typically, data is gathered from various sources using several methods (e.g., observations & interviews). The case study research method originated in clinical medicine (the case history, i.e., the patient's personal history). In psychology, case studies are ...
A case study is an in-depth analysis of one individual or group. Learn more about how to write a case study, including tips and examples, and its importance in psychology. ... Direct observation: This strategy involves observing the subject, often in a natural setting. While an individual observer is sometimes used, it is more common to utilize ...
The three main types of descriptive studies are, naturalistic observation, case studies, and surveys. Try It. Naturalistic Observation. If you want to understand how behavior occurs, one of the best ways to gain information is to simply observe the behavior in its natural context. However, people might change their behavior in unexpected ways ...
Like case studies, naturalistic observation is often used to generate new ideas. Because it gives the researcher the opportunity to study the total situation, it often suggests avenues of inquiry not thought of before. The ability to capture actual behaviors as they unfold in real-time, analyze sequential patterns of interactions, measure base ...
There are seven types of observational studies. Researchers might choose to use one type of observational study or combine any of these multiple observational study approaches: 1. Cross-sectional studies. Cross-sectional studies happen when researchers observe their chosen subject at one particular point in time.
Again, case studies provide enormous amounts of information, but since the cases are so specific, the potential to apply what's learned to the average person may be very limited. Naturalistic Observation. ... This type of observational study is called naturalistic observation: observing behavior in its natural setting. To better understand ...
Importance Many medical journals, including JAMA, restrict the use of causal language to the reporting of randomized clinical trials. Although well-conducted randomized clinical trials remain the preferred approach for answering causal questions, methods for observational studies have advanced such that causal interpretations of the results of well-conducted observational studies may be ...
This LHS case study is a practical example for other health conditions and settings to follow suit. In the context of expanding digital health tools, the health system is ready for Learning Health System (LHS) models. These models, with proper governance and stakeholder engagement, enable the integration of digital infrastructure to provide ...
The aim of this study is to explore the extent to which health system factors enable or constrain adaptive capacity in hospital teams. Design A qualitative multiple case study using observation and semistructured interviews was conducted between November 2020 and June 2021.
Objectives To replicate previous analyses on the effectiveness of the English human papillomavirus (HPV) vaccination programme on incidence of cervical cancer and grade 3 cervical intraepithelial neoplasia (CIN3) using 12 additional months of follow-up, and to investigate effectiveness across levels of socioeconomic deprivation. Design Observational study. Setting England, UK. Participants ...
Of 38,353 persons interviewed, 595 were found to have undiagnosed COPD or asthma and 508 underwent randomization: 253 were assigned to the intervention group and 255 to the usual-care group.
In the tech world and beyond, new 5G applications are being discovered every day. From driverless cars to smarter cities, farms, and even shopping experiences, the latest standard in wireless networks is poised to transform the way we interact with information, devices and each other.
This study explored the influence of flowering temperature on litchi yield under climate change in Taiwan by analyzing litchi production data from 2001 to 2020 and observation data from meteorological stations in litchi-producing areas. Historical observed data were used to construct several regression models relating temperature to yield, with ...