
- Paper Archives
- Journal Indexing
- Research Conference
- Add Journal

Searching By
- Search More ...

Global Journal of Science Frontier Research (ISSN: 0975-5896)
ISSN-L 0975-5896
ISSN 0975-5896
IF(Impact Factor) 2024 Evaluation Pending
Website http://www.globaljournals.org
Description
Last modified: 2010-07-21 13:19:54
- No Archives
Advertisement
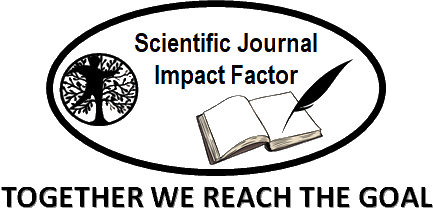
in a scored and prestigious database of scientific journals. This section allows you to place information about the journal, editors, and publisher, etc. from all over the world. | Area: Evaluated version: online | |||
The journal is indexed in: | ||||
SJIFactor.com | ||||
Basic information | ||||
Main title | ||||
ISSN | 2249-4626 (E) / 0975-5896 (P) | |||
URL | ||||
Country | Scientific | --!>|||
Frequency | Bimonthly | |||
License | Free for non-commercial use | |||
Texts availability | Free |

Global Journal of Science Frontier Research
Current issue.

ASEAN Legal Mechanism in Geopolitical Conflict of the South China Sea
- Article PDF
Beyond Inevitability: Manila as a Capital and Maritime Hub in the Contemporary Period
Advancing oceanic studies with hyperocr sensors and non-negative matrix factorization: a cost-effective, data-driven approach for analyzing light in marine water column.
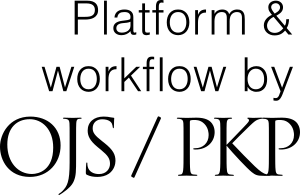
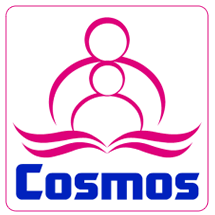
Journal Detail
Journal Name | --> |
ISSN (P) | 2249-4626 |
ISSN (O) | |
Country | Un |
Frequency | Bi-Monthly |
Journal Discipline | |
Area of specialization | |
Organization | |
Journal Language | |
Year of First Publication | 2001 |
Web Site | |
Editor | Dr. R. K. Dixit |
Indexed | |
[email protected] | |
Phone No. | 1-888-839-7392 |
Cosmos Impact Factor | 2018 : 5.784 |
Journal Description |

An official website of the United States government
The .gov means it’s official. Federal government websites often end in .gov or .mil. Before sharing sensitive information, make sure you’re on a federal government site.
The site is secure. The https:// ensures that you are connecting to the official website and that any information you provide is encrypted and transmitted securely.
- Publications
- Account settings
Preview improvements coming to the PMC website in October 2024. Learn More or Try it out now .
- Advanced Search
- Journal List
- Front Endocrinol (Lausanne)
- PMC11361993
Non-linear relationship between TyG index and the risk of prediabetes in young people: a 5-year retrospective cohort study in Chinese young adults
Jianhui xiao.
1 Department of Geriatrics, Shenzhen Hospital of Integrated Traditional Chinese and Western Medicine, Shenzhen, China
2 Department of Emergency Medicine, the First Affiliated Hospital of Shenzhen University, Shenzhen Second People’s Hospital, Shenzhen, China
Zhenhua Huang
Xiang Hu, First Affiliated Hospital of Wenzhou Medical University, China
Associated Data
Publicly available datasets were analyzed in this study. This data can be found here: https://bmjopen.bmj.com/content/8/9/e021768 .
Given the limited evidence on the relationship between the triglyceride-glucose (TyG) index and the risk of prediabetes among young adults, our study aimed to investigate the potential impact of the TyG index on the future development of prediabetes in young individuals.
This retrospective cohort study included 125,327 healthy adults aged 20 to 45 years. We utilized Cox proportional hazards regression models, combined with cubic spline functions and smooth curve fitting, to assess the relationship between baseline TyG index and the risk of prediabetes among young adults, exploring its non-linear association. A series of sensitivity analyses and subgroup analyses were conducted to ensure the robustness of our findings.
After adjusting for covariates, the study found a positive correlation between the TyG index and the risk of prediabetes (HR=1.81, 95%CI: 1.54–2.13, p<0.0001). The risk of prediabetes increased progressively across quartiles of the TyG index (Q1 to Q4), with Q4 showing a significantly higher risk compared to Q1 (adjusted HR=2.33, 95% CI=1.72–3.16). Moreover, a non-linear relationship was identified between the TyG index and the risk of prediabetes, with an inflection point at 9.39. To the left of the inflection point, the HR was 2.04 (95% CI: 1.69 to 2.46), while to the right, the HR was 0.89 (95% CI: 0.48 to 1.65).
Our study reveals a non-linear relationship and a saturation effect between the TyG index and the development of prediabetes among young individuals in China, with an inflection point at 9.39. Understanding this non-linear relationship can assist clinicians in identifying young individuals at high risk and implementing targeted interventions to reduce their risk of progressing to diabetes.
Introduction
Prediabetes is characterized by elevated blood glucose levels below the diagnostic threshold for diabetes but is associated with a higher risk of developing diabetes ( 1 ). The standardized prevalence of prediabetes in Chinese adults was 35.2% (33.5% to 37.0%) ( 2 ). Approximately 70% of prediabetes patients will progress to diabetes within 10 years, and the incidence of diabetes exceeds 90% within 20 years ( 3 ). Prediabetes is also associated with a high burden of cardiometabolic risk factors and poor outcomes ( 4 ). In a large meta-analysis of prospective studies (53 studies, 1.6 million individuals, median follow-up duration 9.5 years) examining the risks of cardiovascular disease and death in persons with prediabetes compared with normal glycemia ( 5 ), prediabetes was associated with an increased risk of cardiovascular disease and all-cause mortality. Several cohort studies have shown that individuals with prediabetes have a higher all-cause mortality rate compared to those with normal glycemia ( 6 – 12 ). Similarly, individuals with prediabetes have a high risk of hospitalization ( 11 ). Age is a significant risk factor for prediabetes, with evidence showing a strong association between the increase in prediabetes and age. In a 2011–2012 National Health and Nutrition Examination Survey (NHANES) analysis, in adults, the prevalence of prediabetes was 38.0%, while among young individuals aged 20–44 years, it reached up to 28.2% ( 13 ). Prediabetes is commonly considered a warning sign; nevertheless, many prediabetic patients, particularly younger individuals, tend to neglect this metabolic abnormality and underestimate its importance.
The triglyceride-glucose (TyG) index is calculated as the product of fasting plasma glucose (FPG) levels and triglyceride (TG) ( 14 ). Due to its convenience and ease of calculation, the TyG index has been widely used in various clinical settings ( 15 – 20 ). Insulin resistance (IR) is a key factor in metabolic disorders such as metabolic syndrome, non-alcoholic fatty liver disease (NAFLD), diabetes, and obesity ( 21 , 22 ). IR is a major contributor to the progression from normal glucose resistance to prediabetes and then to diabetes. The TyG index is considered a novel marker of IR that accurately and reliably reflects the extent of IR ( 23 , 24 ). Evidence suggests an association between the TyG index and the prevalence of prediabetes in middle-aged and older adults, but it is unclear whether this association exists in young adults ( 25 ).
Due to economic growth, lifestyle, and diet changes, diabetes rates are rising, with younger people increasingly affected. Young diabetics may show unusual symptoms and be overlooked, making early risk identification and intervention critical ( 26 , 27 ). This study used existing data to examine the TyG index and prediabetes risk in individuals aged 20–45, aiming to support its clinical use for early prediabetes detection.
Study design
This study utilized data from a previous retrospective cohort study conducted by Chinese researchers (Chen et al.) ( 28 ). The target independent variable was the TyG at baseline. The outcome variable was the development from normoglycemia to prediabetes at follow-up.
Data source
Access to the original dataset was granted at no cost through the DATADRYAD platform ( www.datadryad.org ), courtesy of Ying Chen et al. In accordance with Dryad’s usage policy, the data is available for academic and research purposes, allowing users to share, adapt, alter, and build upon the material, provided it is not for commercial use and proper attribution is given to the original authors and source. The dataset was sourced from a publicly accessible study published in 2018 titled “Association of body mass index and age with diabetes onset in Chinese adults: a population-based cohort study,” which can be found at http://dx.doi.org/10.1136/bmjopen-2018–021768 . For those interested, the dataset can be retrieved from the following link: https://doi.org/10.5061/dryad.ft8750v ( 28 ). Given that the current study involves a secondary analysis of existing data, there was no need for obtaining informed consent or additional ethical approval.
Research population
The primary research included 685,277 healthy individuals aged 20 years and above, all of whom had undergone a minimum of two health assessments. The study focused on participants who, during follow-up, had FPG levels ranging from 110 to 125 mg/dL without any prior diagnosis of diabetes. The initial selection excluded participants based on several factors (1): lack of detailed information regarding weight, height, or gender (2); BMI values outside the normal range (<15 kg/m 2 or >55 kg/m 2 ) (3); intervals between visits shorter than 2 years (4); missing fasting plasma glucose readings (5); individuals diagnosed with diabetes at the start or with uncertain diabetes status at the time of follow-up. Following these criteria, the study retained 211,833 participants.
Further analysis led to the exclusion of an additional 86,506 participants for reasons including: 1) absence of follow-up FPG readings, 2) baseline FPG levels ≥100 mg/dL, 3) diabetes diagnosis at follow-up, 4) had no available TG value, and 5) lack of TG values or being over 45 years of age. Young people are defined as those aged 45 years old or younger ( 29 ). Ultimately, the study included 125,327 healthy participants. The process of selecting participants for this study is depicted in Figure 1 .

Flowchart illustrating the selection process of study participants.
Data collection
In this study, data collection included demographic information such as age, systolic blood pressure (SBP), diastolic blood pressure (DBP), height, and weight, from which body mass index (BMI) was calculated. To ensure consistency in data collection, staff received specialized training focusing on demographic data and key measurements, including blood pressure. Tests were uniformly conducted in a standardized laboratory environment for FPG, serum creatinine (Scr), TG, total cholesterol (TC), blood urea nitrogen (BUN), alanine aminotransferase (ALT), low-density lipoprotein cholesterol (LDL-c), and high-density lipoprotein cholesterol (HDL-c). Additionally, the study collected information on the patients’ smoking and drinking histories, defining current drinking as 1, former drinking as 2, never drinking as 3. Similarly, current smoking was coded as 1, former smoking as 2, never smoking as 3.
Outcome measures
Our primary outcome was the occurrence of prediabetes, defined by FPG levels in the range of 100–125 mg/dL at follow-up without reported incident diabetes ( 30 ).
Statistical analysis
The TyG index, the primary exposure variable in this study, is defined as follows: TyG index = ln [FPG (mg/dL) × TG (mg/dL)/2] ( 31 ). We divided it into four quartiles and considered it as a continuous variable. For continuous variables following a normal distribution, we reported the mean and standard deviation; for non-normally distributed data, we provided the median. For categorical variables, we presented the frequency and proportion. To compare differences between TyG index groups, we used the Kruskal-Wallis H test (for skewed distributed data), one-way analysis of variance (for normally distributed data), or chi-square test (for categorical variables).
We constructed several models to assess the relationship between the TyG index and prediabetes risk: a baseline model without any adjustments, a simplified model adjusting for gender and age only (Model I), and a comprehensive model adjusting for multiple covariates (Model II: including age, gender, BMI, systolic blood pressure, diastolic blood pressure, alanine aminotransferase, urea nitrogen, aspartate aminotransferase, total cholesterol, high-density lipoprotein cholesterol, low-density lipoprotein cholesterol, serum creatinine, family history of diabetes, alcohol consumption, and smoking status). We recorded the effect size (hazard ratio, HR) and its 95% confidence interval (CI) for each model.
We adjusted for potential confounding factors based on clinical experience, literature review, and univariate analysis results. Additionally, we used a multivariable Cox proportional hazards model and introduced cubic spline functions and smooth curve fitting to explore the possible nonlinear relationship between the TyG index and prediabetes risk. We also used a segmented Cox proportional hazards model to further clarify this nonlinear relationship. Unmeasured confounding between TyG and Prediabetes risk was assessed by calculating E-values.
To validate our findings, we conducted a series of sensitivity analyses. By incorporating continuous variables into a generalized additive model (GAM) in curve form, we further confirmed the robustness of the results. Additionally, we conducted analyses using stratified Cox proportional hazards models in different subgroups (such as age, gender, blood pressure, smoking, and drinking status). Finally, we used likelihood ratio tests to examine whether there were interactions in the model, both in models including interaction terms and those without. All analyses were performed using Empower Stats (X&Y Solutions, Inc., Boston, MA, http://www.empowerstats.com ), with a statistical significance level set at a two-sided P value less than 0.05.
The characteristics of participants
We conducted a comprehensive analysis of the baseline characteristics of participants to investigate the relationship between the TyG index and the risk of prediabetes. The results are summarized in Table 1 , demonstrating significant differences between normal and prediabetes groups across various parameters. The median follow-up duration of our study was 3.00 years, ranging from 2.00 to 6.20 years. The prediabetes group exhibited a slightly higher mean age (35.70 years) compared to the normal group (34.31 years), along with increased height, weight, and BMI. SBP and DBP were also notably elevated in the prediabetes group. The prediabetes group displayed higher levels of FPG, TG, and the TyG index, indicating a potential link between these parameters and the development of prediabetes. Additionally, AST, ALT, BUN, and Scr levels were higher in the prediabetes group. In addition, higher percentage of males, current smokers, and current drinkers were observed in the prediabetes group, along with a greater prevalence of family history of diabetes. Furthermore, the average follow-up duration was slightly longer in the prediabetes group compared to the normal group. As illustrated in Figure 2 , a detailed Distribution of TyG index was conducted. It presented a normal distribution, ranging from 4.65 to 11.78, with a mean of 8.23.
Table 1
The baseline characteristics of participants.
TyG index | Normal | prediabetes | P-value |
---|---|---|---|
Participants | 114,999 | 10,328 | |
Age (years) | 34.31 ± 5.31 | 35.70 ± 5.32 | <0.001 |
Height (cm) | 166.98 ± 8.30 | 168.75 ± 8.13 | <0.001 |
Weight (kg) | 63.15 ± 12.39 | 68.90 ± 13.03 | <0.001 |
BMI (kg/m ) | 22.52 ± 3.27 | 24.08 ± 3.57 | <0.001 |
SBP (mmHg) | 114.74 ± 13.85 | 120.30 ± 14.59 | <0.001 |
DBP (mmHg) | 71.66 ± 9.77 | 75.11 ± 10.50 | <0.001 |
FPG at baseline (mg/dL) | 84.96 ± 8.59 | 90.10 ± 7.65 | <0.001 |
TG (mg/dL) | 102.15 ± 73.75 | 130.38 ± 98.60 | <0.001 |
TyG index | 8.21 ± 0.56 | 8.48 ± 0.61 | <0.001 |
ALT (U/L) | 16.50 (12.00–26.00) | 22.00 (14.10–35.80) | <0.001 |
AST (U/L) | 21.00 (18.00–25.20) | 22.30 (19.00–28.00) | <0.001 |
BUN (mmol/L) | 4.46 ± 1.11 | 4.65 ± 1.12 | <0.001 |
Scr (μmol/L) | 68.90 ± 15.10 | 72.65 ± 14.81 | <0.001 |
TC (mmol/L) | 4.52 ± 0.83 | 4.65 ± 0.85 | <0.001 |
HDL-c (mmol/L) | 1.38 ± 0.30 | 1.32 ± 0.29 | <0.001 |
LDL-c (mmol/L) | 2.63 ± 0.63 | 2.72 ± 0.63 | <0.001 |
Sex | <0.001 | ||
Male | 59,197 (51.48%) | 7,039 (68.15%) | |
Female | 55,802 (48.52%) | 3,289 (31.85%) | |
Smoking status | <0.001 | ||
Current smoker | 4,684 (14.19%) | 620 (19.40%) | |
Ever smoker | 1,448 (4.39%) | 169 (5.29%) | |
Never | 26,882 (81.43%) | 2,407 (75.31%) | |
Drinking status | <0.001 | ||
Current drinker | 410 (1.24%) | 60 (1.88%) | |
Ever drinker | 4,801 (14.54%) | 602 (18.84%) | |
Never | 27,803 (84.22%) | 2,534 (79.29%) | |
Family history of diabetes | <0.001 | ||
No | 112,693 (97.99%) | 10,031 (97.12%) | |
Yes | 2306 (2.01%) | 297 (2.88%) | |
Follow-up (year) | 3.12 ± 0.93 | 3.23 ± 0.96 | <0.001 |
Continuous variables were summarized as mean (SD) or medians (quartile interval); categorical variables were displayed as percentage (%).
BMI, body mass index; SBP, systolic blood pressure; DBP; diastolic blood pressure; TG triglyceride; TC, total cholesterol; HDL-c, high-density lipoprotein cholesterol; LDL-c, low-density lipoprotein cholesterol; AST aspartate aminotransferase; ALT, alanine aminotransferase; BUN, blood urea nitrogen; Scr, serum creatinine; FPG, fasting plasma glucose; TyG index, triglyceride glucose index.

Distribution of TyG index. It presented a normal distribution, ranging from4.65 to 11.78, with a mean of 8.23.
Incidence of prediabetes in participants
Table 2 and Figure 3 describe the incidence rates of prediabetes. Among the participants, 10,328 (8.24%) developed prediabetes. Participants were divided into subgroups based on the quartiles of the TyG index. The incidence rates of prediabetes per 1000 person-years were 14.426, 20.012, 27.066, and 44.490 for each TyG index quartile. The incidence rates of prediabetes in each TyG index quartile were as follows: Q1: 4.68%, Q2: 6.27%, Q3: 8.33%, and Q4: 13.69%. Participants with the highest TyG index (Q4) had a higher risk of developing prediabetes compared to those with the lowest TyG index (Q1) (trend p < 0.001).
Table 2
The Incidence rate of prediabetes (% or Per 1,000 person-year).
TyG index (quartile) | Participants (n) | Prediabetes events (n) | Incidence rate (95%CI) (%) | Per 1,000 person-year |
---|---|---|---|---|
Total | 125327 | 10328 | 8.24 (8.09–8.39) | 26.355 |
Q1 | 31,328 | 1466 | 4.68 (4.45–4.91) | 14.426 |
Q2 | 31,317 | 1962 | 6.27 (6.00–6.53) | 20.012 |
Q3 | 31,349 | 2611 | 8.33 (8.02–8.64) | 27.066 |
Q4 | 31,332 | 4289 | 13.69 (13.31–14.07) | 44.490 |
P for trend | <0.001 |

Kaplan–Meier event-free survival curve. Kaplan–Meier analysis of incident prediabetes based on two group (log-rank, P < 0.0001).
Multivariable analysis using Cox proportional hazards regression model
In Table 3 , the relationship between the TyG index and the risk of prediabetes is presented across different models. The results are as follows: In the crude model, the HR for the TyG index was 2.17 (95%CI 2.11–2.24, P < 0.0001). After adjusting for age and sex (Model I), the HR decreased to 1.94 (95%CI 1.88–2.00, P < 0.0001). Further adjustments in Model II, which included age, sex, SBP, DBP, BMI, ALT, AST, BUN, Scr, TC, LDL-C, HDL-c, family history of diabetes, drinking status, and smoking status, resulted in an HR of 1.81 (95%CI 1.54–2.13, P < 0.0001).
Table 3
Relationship between TyG index and risk of prediabetes in different models.
Exposure | Crude model (HR,95%CI) P | Model I (HR,95%CI) P | Model II (HR,95%CI) P | Model III (HR,95%CI) P |
---|---|---|---|---|
TyG index | 2.17 (2.11, 2.24) <0.0001 | 1.94 (1.88, 2.00) <0.0001 | 1.81 (1.54, 2.13) <0.0001 | 1.86 (1.57, 2.19) <0.0001 |
(TyG index quartiles) | ||||
Q1 | Ref | Ref | Ref | Ref |
Q2 | 1.49 (1.39, 1.59) <0.0001 | 1.40 (1.31, 1.50) <0.0001 | 1.29 (0.95, 1.75) 0.1065 | 1.30 (0.95, 1.77) 0.0998 |
Q3 | 2.11 (1.98, 2.25) <0.0001 | 1.86 (1.74, 1.99) <0.0001 | 1.75 (1.30, 2.34) 0.0002 | 1.81 (1.35, 2.44) <0.0001 |
Q4 | 3.56 (3.36, 3.78) <0.0001 | 2.90 (2.72, 3.09) <0.0001 | 2.33 (1.72, 3.16) <0.0001 | 2.44 (1.78, 3.34) <0.0001 |
P for trend | <0.0001 | <0.0001 | <0.0001 | <0.0001 |
Crude model: we did not adjust other covariates.
Model I: we adjusted age, sex.
Model II: we adjusted age, sex, SBP, DBP, BMI, ALT, AST, BUN, Scr, TC, LDL-C, HDL-c, family history of diabetes, drinking status, and smoking status.
Model III: we adjusted age(smooth), sex, SBP (smooth), DBP (smooth), BMI (smooth), BUN (smooth), Scr (smooth), ALT (smooth), AST (smooth), TC (smooth), LDL-C(smooth), HDL-c(smooth), smoking status, drinking status, family history of diabetes.
HR, Hazard ratios; CI, confidence, Ref, reference.
After adjustments, similar trends were observed across all quartiles, indicating a significant association between the TyG index quartiles and the risk of prediabetes (P for trend < 0.0001 for all models).
Sensitivity analysis
We performed a series of sensitivity analyses to confirm the reliability of our conclusions. Initially, the generalized additive model (GAM) in Model III, which included additional smooth terms for various variables, showed an HR of 1.86 (95%CI 1.57–2.19, P < 0.0001) ( Table 3 ). Secondly, participants with a BMI ≥ 28 kg/m 2 were excluded ( 32 ). After controlling for confounding variables, the results demonstrated a persistent positive association between the TyG index and the risk of prediabetes (HR= 1.82, 95% CI: 1.52–2.17, p < 0.0001). Furthermore, we performed a sensitivity analysis excluding patients aged ≥ 40 years. After adjusting for confounding variables, the results continued to demonstrate a positive association between the TyG index and the risk of prediabetes (HR = 1.99, 95% CI: 1.64–2.42, p < 0.0001). Additionally, we conducted an analysis on participants without a family history of diabetes, revealing a risk ratio of 1.83 (95% confidence interval: 1.54–2.16, p < 0.0001). Based on all sensitivity analyses, we concluded that our findings are reliable ( Table 4 ).
Table 4
Relationship between TyG index and the risk of prediabetes in different sensitivity analyses.
Exposure | Model I (HR,95%CI) P | Model II (HR,95%CI) P | Model III (HR,95%CI) P |
---|---|---|---|
TyG index | 1.82 (1.52, 2.17) <0.0001 | 1.99 (1.64, 2.42) <0.0001 | 1.83 (1.54, 2.16) <0.0001 |
(TyG index quartiles) | |||
Q1 | Ref | Ref | Ref |
Q2 | 1.33 (0.97, 1.82) 0.0792 | 1.18 (0.84, 1.65) 0.3502 | 1.21 (0.88, 1.67) 0.2386 |
Q3 | 1.77 (1.31, 2.40) 0.0002 | 1.59 (1.15, 2.20) 0.0053 | 1.67 (1.23, 2.26) 0.0010 |
Q4 | 2.39 (1.73, 3.29) <0.0001 | 2.14 (1.52, 3.01) <0.0001 | 2.25 (1.64, 3.09) <0.0001 |
P for trend | <0.0001 | <0.0001 | <0.0001 |
Model I was a sensitivity analysis performed after excluding participants with BMI≥ 28 kg/m 2 (N= 8,595). we adjusted age, sex, BMI, SBP, DBP, ALT, AST, BUN, Scr, TC, LDL-C, HDL-c, family history of diabetes, drinking status, and smoking status.
Model II was a sensitivity analysis performed after excluding participants with age≥ 40 years (N= 25,255). we adjusted age, sex, BMI, SBP, DBP, ALT, AST, BUN, Scr, TC, LDL-C, HDL-c, family history of diabetes, drinking status, and smoking status.
Model III was a sensitivity analysis performed on participants without family of diabetes (N= 2,603). We adjusted age, sex, SBP, DBP, BMI, ALT, AST, BUN, Scr, TC, LDL-C, HDL-c, drinking status, and smoking status. HR, Hazard ratios; CI, confidence, Ref, reference.
Results of the two-piece Cox proportional hazards regression model
We identified a non-linear relationship between the TyG index and the risk of prediabetes ( Figure 4 and Table 5 ). Firstly, we used a Cox proportional hazards regression model with cubic spline functions to evaluate the relationship between the TyG index and prediabetes risk. The result showed that the relationship between the TyG index and prediabetes risk was non-linear. We employed a two-piecewise Cox proportional hazards regression model to investigate the relationship between the TyG index and the risk of developing prediabetes. The standard Cox regression model revealed a HR of 1.81 (95% CI: 1.54, 2.13) with a P value of <0.0001, indicating a significant association between the TyG index and prediabetes risk. In addition, we identified an inflection point in the TyG index at 9.39. Below this inflection point (<9.39), there is a significant positive correlation between the TyG index and the risk of prediabetes (HR: 2.04, 95% CI: 1.69, 2.46, P <0.0001). Conversely, Conversely, when the TyG index is 9.39 or higher, their relationship is not statistically significant (HR: 0.89, 95% CI: 0.48, 1.65, P=0.7019).

The non-linear relationship between TyG index and the risk of prediabetes in participants. We used a Cox proportional hazards regression model with cubic spline functions to evaluate the relationship between the TyG index and prediabetes risk. We adjusted age, sex, SBP, DBP, BMI, ALT, AST, BUN, Scr, TC, LDL-C, HDL-c, family history of diabetes, drinking status, and smoking status. The result showed that the relationship between the TyG index and prediabetes risk was non-linear, with the inflection point of TyG index being 9.39.

Table 5
The result of the two-piecewise Cox proportional hazards regression model.
Outcome: prediabetes | HR, 95% CI | P-value |
---|---|---|
Fitting model by standard Cox regression | 1.81 (1.54, 2.13) | <0.0001 |
Fitting model by two-piecewise Cox regression | ||
Inflection points of TyG index | 9.39 | |
<9.39 | 2.04 (1.69, 2.46) | <0.0001 |
≥9.39 | 0.89 (0.48, 1.65) | 0.7019 |
P for log likelihood ratio test | 0.011 |
We adjusted age, sex, SBP, DBP, BMI, ALT, AST, BUN, Scr, TC, LDL-C, HDL-c, family history of diabetes, drinking status, and smoking status.
Subgroup analysis results
As illustrated in Table 6 , a detailed subgroup analysis was conducted. Gender, age, BMI, systolic and diastolic blood pressures, smoking and drinking habits, and a family history of diabetes did not alter the association between the TyG index and the risk of prediabetes. Thus, no significant interactions were observed between these variables and the TyG index (all interaction P > 0.05).
Table 6
Effect size of TyG index on prediabetes in prespecified and exploratory subgroups.
Variable | HR (95% CI) | P-value | P for interaction |
---|---|---|---|
0.4260 | |||
20–30 | 2.14 (1.40, 3.25) | 0.0004 | |
30–40 | 1.90 (1.57, 2.30) | <0.0001 | |
>40 | 1.66 (1.32, 2.08) | <0.0001 | |
0.7626 | |||
<18 | 2.73 (0.55, 13.53) | 0.2186 | |
18–24 | 1.95 (1.56, 2.42) | <0.0001 | |
24–28 | 1.71 (1.38, 2.14) | <0.0001 | |
≥28 | 1.95 (1.39, 2.76) | 0.0001 | |
0.1758 | |||
Male | 1.74 (1.46, 2.06) | <0.0001 | |
Female | 2.19 (1.59, 3.03) | <0.0001 | |
0.660 | |||
<140 | 1.79 (1.52, 2.12) | <0.0001 | |
≥140 | 2.00 (1.25, 3.20) | 0.0040 | |
0.3484 | |||
<90 | 1.79 (1.52, 2.11) | <0.0001 | |
≥90 | 2.22 (1.42, 3.47) | 0.0005 | |
0.3930 | |||
Current drinker | 2.93 (1.20, 7.14) | 0.0183 | |
Ever drinker | 1.66 (1.30, 2.12) | <0.0001 | |
Never | 1.87 (1.55, 2.24) | <0.0001 | |
0.2937 | |||
Current smoker | 1.58 (1.22, 2.06) | 0.0006 | |
Ever smoker | 2.38 (1.43, 3.96) | 0.0008 | |
Never | 1.86 (1.55, 2.23) | <0.0001 | |
0.2497 | |||
Yes | 1.86 (1.57, 2.19) | <0.0001 | |
No | 1.49 (1.03, 2.16) | 0.0360 |
Above model adjusted for age, sex, SBP, DBP, BMI, ALT, AST, BUN, Scr, TC, LDL-C, HDL-c, family history of diabetes, drinking status, and smoking status. In each case, the model is not adjusted for the stratification variable when the stratification variable was a categorical variable.
This retrospective cohort study revealed that a higher TyG index independently predicts an increased risk of prediabetes in Chinese adults aged 20–45. Furthermore, a nonlinear relationship was observed, indicating that the risk of prediabetes tends to rise with an increasing TyG index. Individuals in the highest TyG index quartile had a 2.33-fold increased risk of developing prediabetes compared to those in the lowest quartile. Additionally, a threshold effect curve was identified, revealing varying relationships between the TyG index and prediabetes risk across the inflection point.
Initially, the TyG index has been widely validated as an indicator of IR in various epidemiological studies. A cross-sectional study conducted in Mexico involving 99 individuals identified the TyG index as an optimal tool for assessing IR, showing a high sensitivity (96.5%) and good specificity (85.0%) compared to the gold standard, HIEC ( 23 ). Research involving 82 Brazilian subjects corroborated the TyG index as a more precise predictor of IR compared to HOMA-IR ( 33 ). Numerous studies have indicated an association between the TyG index and cardiovascular disease (CVD) risk ( 34 – 37 ). A study involving 4,340 American patients under the age of 65 with prediabetes or diabetes found that an increase in the TyG index is associated with a higher incidence of CVD, including congestive heart failure (CHF), coronary heart disease (CHD), atherosclerotic cardiovascular disease (ASCVD), heart attack, angina, and stroke ( 34 ). Additionally, several studies have found a close relationship between the TyG index and coronary artery disease ( 35 – 37 ). Furthermore, recent studies have identified a significant correlation between the TyG index and both cognitive and physical impairments in elderly individuals with prefrail hypertension ( 38 ).
Recently, there has been a growing body of research exploring the relationship between the TyG index and the risk of diabetes ( 39 – 42 ). A prospective cohort study conducted in rural China suggests that an increasing TyG index is associated with a higher cumulative risk of developing incident T2DM among individuals with normal weight ( 43 ). Another retrospective cohort study demonstrated a link between elevated TyG index levels and an augmented risk of diabetes within the Chinese population ( 44 ). Additionally, recent studies have indicated that there is a positive correlation between the TyG index and the risk of diabetes ( 45 ). It is noteworthy that prediabetes is a critical period for promoting, preventing, or delaying the development of diabetes mellitus (DM).
But research on the TyG index’s association with prediabetes is limited. An initial study demonstrated that the TyG index is comparable to HbA1C as a diagnostic marker for prediabetes ( 46 ). Findings from a case-control study conducted in Palembang, Indonesia, involving 570 participants without a family history of T2DM (265 prediabetes cases and an equal number of age-matched controls), indicated that the TyG index was the primary risk predictor for prediabetes ( 47 ). A prospective cohort study in China indicated that the predictive capacity of the TyG index in forecasting prediabetes surpassed that of obesity, lipid profiles, and other non-insulin-based IR indices ( 48 ). A cross-sectional study conducted in the United States, involving 25,159 participants, found that 23.88% had prediabetes and 16.22% were diagnosed with diabetes. The study revealed a positive relationship between the TyG index and the prevalence of prediabetes and diabetes ( 49 ). Interestingly, a cohort study conducted in China indicated that, among prediabetic patients, an elevated TyG-BMI might reduce the likelihood of returning to normal glucose levels in the future ( 50 ). However, no specific studies have investigated the association between TyG index and prediabetes in a young population.
Therefore, we hypothesize that the TyG index is positively correlated with the risk of prediabetes in young populations. Therefore, our study investigates the TyG index’s role in identifying prediabetes risk factors among young individuals, aiming to enhance prevention strategies for diabetes onset and progression within this demographic. Initially, our findings indicate the TyG index as a reliable predictor of prediabetes and an independent risk factor, consistent with existing research [45–46]. Furthermore, our study has shown a positive correlation between the TyG index and prediabetes, consistent with our research hypothesis. That is for every 1-unit increase in the TyG index, the risk of prediabetes will increase by 81% (HR: 1.81, 95% CI 1.54–2.13, P < 0.0001). Similarly, a cohort study conducted in China also identified a positive correlation between TyG and the risk of prediabetes after adjusting for variables ( 48 ). However, there are significant differences between the two studies in terms of subjects, outcomes, and adjusted variables. Their study sample is relatively small compared to ours. They also adjusted for different variables, such as age, cigarette smoking, alcohol consumption, education level, family history of diabetes, hypertension, and cardiovascular disease history, which differs slightly from our study. Additionally, they did not use the Cox proportional hazards regression model combined with cubic splines and smoothing curve fits to examine the nonlinear association between the TyG index and the risk of prediabetes. A study in the United States also found a nonlinear relationship between the TyG index and the prevalence of prediabetes and diabetes, identifying an inflection point at 8 ( 49 ), which is very similar to our conclusion but with some notable differences. Firstly, their study was cross-sectional, which has limitations such as a smaller sample size, unclear causality, and selection bias. Secondly, there are differences in the age distribution of the study populations between the two studies. Additionally, variations in the adjusted variables between the two studies may impact the research outcomes. Although both studies confirm a nonlinear relationship, we identified a critical threshold of 9.39. Our study focused on prediabetes as the outcome, while their study included both prediabetes and diabetes, which could be the primary reason for the earlier inflection point in their findings.
Our study has significant clinical implications. We have identified a non-linear relationship with a saturation effect between the TyG index and the risk of prediabetes in young individuals. Monitoring TyG index values can facilitate early interventions in young individuals to decrease the occurrence of prediabetes. Specifically, when the TyG index is below 9.39, by lowering FBG and TG, we can significantly reduce the risk of prediabetes. However, when the TyG index exceeds 9.39, merely controlling FBG and TG is insufficient to reduce the risk of prediabetes. Thus, it is necessary to manage other related risk factors such as BMI, smoking, and alcohol consumption to better prevent the onset of the disease. Consequently, it is advisable for young people to start making lifestyle changes early, including reducing the intake of high-fat foods, increasing physical activity, and controlling blood sugar levels. These measures can effectively help reduce the risk of developing prediabetes.
This study has some advantages. Firstly, we first identified a nonlinear relationship between the TyG index and prediabetes risk in young individuals. Furthermore, this study included a large sample of 125,327 young adults, minimizing potential biases. Sensitivity analysis was conducted to ensure the reliability and robustness of the results, and a two-stage Cox proportional hazards regression model was applied to determine the inflection point of the TyG index. These findings underscore the critical threshold between the TyG index and diabetes risk, emphasizing the importance of TyG index monitoring and early intervention strategies in diabetes prevention efforts. Additionally, subgroup analysis and interaction tests were performed. The results revealed variations in the impact of the TyG index on prediabetes risk across different subgroups, yet interaction analysis did not indicate significant interactions between the TyG index and factors such as drinking status, smoking status, or family history of diabetes. This further validates the stability of the results.
This study has several limitations. Firstly, as all participants were of Chinese descent, further research is needed to determine the relationship between the TyG index and the risk of prediabetes in individuals with different genetic backgrounds. Secondly, since this large-scale cohort study only focused on young adults in China, these findings may not be applicable to other racial groups and specific populations, such as children and the elderly. Thirdly, the average follow-up time for participants was relatively short. Future research could benefit from extending follow-up duration to mitigate potential misinterpretations or chance findings from short-term observations. Fourthly, there was no data available on serum insulin levels to compare the predictive value of the TyG index and HOMA-IR; additionally, there was also no data on the use of lipid-lowering medications, which could potentially impact the results. Fively, this report represents a secondary analysis of existing databases. Although adjustments were made for many confounding factors, variables not included in the database were not adjusted for. However, we calculated the E value to quantify the potential impact of unmeasured confounders and found that unmeasured confounders were unlikely to explain the results. sixly, The current diagnostic criteria for prediabetes have some limitations, primarily relying on impaired fasting glucose and not considering oral glucose tolerance tests, glycated hemoglobin, and multiple fasting glucose measurements. This single-index approach may lead to underdiagnosis of prediabetes. Therefore, future studies evaluating the incidence of prediabetes should consider measuring additional variables, including oral glucose tolerance tests, glycated hemoglobin, and multiple fasting glucose measurements, to improve diagnostic accuracy and comprehensiveness. Additionally, this retrospective observational study provides an associative inference rather than establishing a causal relationship between the TyG index and the risk of prediabetes. Lastly, this study only measured the TyG index and other parameters at baseline and did not consider changes in the TyG index over time. Therefore, in the future, we should collect as much information as possible, including information on changes in the TyG index.
This study reveals a nonlinear relationship and a saturation effect between the TyG index and the risk of prediabetes among individuals aged 20 to 45 in China. There is a significant positive correlation between the TyG index and the risk of prediabetes to the left of the inflection point at a TyG index of 9.39. Reducing FBG and TG levels can significantly decrease the risk of prediabetes. Conversely, when the TyG index reaches or exceeds 9.39, its relationship with the risk of prediabetes is no longer statistically significant; merely lowering the TyG index is not enough to reduce the risk of prediabetes. It is also necessary to comprehensively control other factors such as BMI, smoking, and alcohol consumption. Understanding this non-linear relationship can help clinicians identify high-risk young individuals and implement focused interventions to reduce the risk of developing diabetes.
Funding Statement
The author(s) declare financial support was received for the research, authorship, and/or publication of this article. The Guangdong Provincial Medical Science and Technology Research Fund project (NO: A2024429).
Data availability statement
Ethics statement.
The studies involving humans were approved by The Rich Healthcare Group Review Board. The ethics committee waived the need for written informed consent. The studies were conducted in accordance with the local legislation and institutional requirements. Written informed consent for participation in this study was provided by the participants’ legal guardians/next of kin.
Author contributions
JX: Data curation, Writing – original draft, Writing – review & editing. LZ: Formal analysis, Writing – original draft, Writing – review & editing. CL: Data curation, Formal analysis, Writing – review & editing. YH: Formal analysis, Writing – review & editing. ZH: Formal analysis, Writing – original draft, Writing – review & editing.
Conflict of interest
The authors declare that the research was conducted in the absence of any commercial or financial relationships that could be construed as a potential conflict of interest.
Publisher’s note
All claims expressed in this article are solely those of the authors and do not necessarily represent those of their affiliated organizations, or those of the publisher, the editors and the reviewers. Any product that may be evaluated in this article, or claim that may be made by its manufacturer, is not guaranteed or endorsed by the publisher.
Climate change and irrigation expansion in Northwest Minas Gerais, Brazil: the need for hydroclimatic monitoring
- Original Paper
- Published: 05 September 2024
Cite this article
- M. L. Ferreira ORCID: orcid.org/0000-0002-8106-2793 1 ,
- G. P. Tormen ORCID: orcid.org/0000-0002-8404-0178 2 &
- A. M. De Andrade ORCID: orcid.org/0000-0003-3502-7847 1
The Northwest Minas Gerais is a significant grain-producing region in Brazil. Center pivot irrigation in the region increased by 84% from 2010 to 2020, and records of conflicts over water use have occurred since 2000. This study carries out a hydroclimatic analysis of water resources in the Northwest Minas Gerais, using orbital and field data related to 567 pivots, considering the expansion of the irrigated area in the region over the years, the rainfall regime, and the flow of rivers. The time series of precipitation from 1982 to 2022 and river flows from 1978 to 2015 show a significant decrease in rainfall of up to 14.4% since 2012 and a reduction in river flow in all sub-basins analyzed. Furthermore, there was a 99.4% growth in irrigated areas in the last ten years. Due to this scenario, using outdated information to define water allocation rights can escalate conflicts in the use of water resources in the Northwest Minas Gerais region. The results show the need to implement sustainable and contemporary water use management in the Northwest Minas Gerais region. Implementing effective policies, advocating the efficient use of water in agriculture, and promoting collaboration between various sectors are vital to facing current and future challenges.
This is a preview of subscription content, log in via an institution to check access.
Access this article
Subscribe and save.
- Get 10 units per month
- Download Article/Chapter or eBook
- 1 Unit = 1 Article or 1 Chapter
- Cancel anytime
Price includes VAT (Russian Federation)
Instant access to the full article PDF.
Rent this article via DeepDyve
Institutional subscriptions
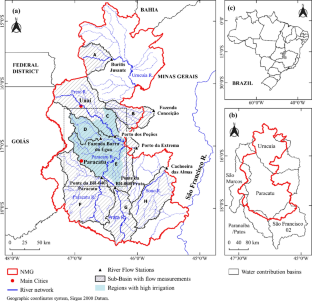
Explore related subjects
- Environmental Chemistry
Abraham T, Liu Y, Tekleab S, Hartmann A (2022) Prediction at ungauged catchments through parameter optimization and uncertainty estimation to quantify the regional water balance of the ethiopian rift valley lake basin. Hydrology 9(8):150. https://doi.org/10.3390/hydrology9080150
Article Google Scholar
Agência Nacional de Águas – ANA (2021) Atlas irrigação: uso da água na agricultura irrigada. ANA, Brasília
Agência Nacional de Águas – ANA (2019) Outorga dos direitos de uso de recursos hídricos. ANA, Brasília
Agência Nacional de Águas – ANA (2020) Polos nacionais de agricultura irrigada: mapeamento de áreas irrigadas com imagens de satélite. ANA, Brasília
Ahansal Y, Bouziani M, Yaagoubi R, Sebari I, Sebari K, Kenny L (2022) Towards smart irrigation: a literature review on the use of geospatial technologies and machine learning in the management of water resources in arboriculture. Agronomy 12(2):297. https://doi.org/10.3390/agronomy12020297
Bettencourt P, de Oliveira RP, Fulgêncio C, Canas Â, Wasserman JC (2022) Prospective water balance scenarios (2015–2035) for the management of São Francisco river basin. Eastern Brazil Water 14:2283. https://doi.org/10.3390/w14152283
Chan SS, Seidenfaden IK, Jensen KH, Sonnenborg TO (2021) Climate change impacts and uncertainty on spatiotemporal variations of drought indices for an irrigated catchment. J Hydrol 601:126814. https://doi.org/10.1016/j.jhydrol.2021.126814
Chen J, Wang Z, Wu X, Lai C, Chen X (2021) Evaluation of TMPA 3B42-V7 product on extreme precipitation estimates. Remote Sensing 13(2):209. https://doi.org/10.3390/rs13020209
Coelho CAS, de Oliveira CP, Ambrizzi T et al (2016) The 2014 southeast Brazil austral summer drought: regional scale mechanisms and teleconnections. Clim Dyn 46:3737–3752. https://doi.org/10.1007/s00382-015-2800-1
Da Silva MVM, Silveira CDS, Costa JMFD, Martins ESPR, Vasconcelos Júnior FDC (2021) Projection of climate change and consumptive demands projections impacts on hydropower generation in the São Francisco River basin. Brazil Water 13:332. https://doi.org/10.3390/w13030332
Silva VP, Santos França GL (2018) Percepções de mudanças do clima, impactos e adaptação para sertanejos do semiárido. Rev Brasileira de Climatol, 22
Deusdará-leal K, Mohor GS, Cuartas LA, Seluchi ME, Marengo JA, Zhang R, Broedel E, Amore DDJ, Alvalá RCS, Cunha APMA, Gonçalves JAC (2022) Trends and climate elasticity of streamflow in South-Eastern Brazil basins. Water 14(14):2245. https://doi.org/10.3390/w14142245
Duan W, Maskey S, Chaffe PLB, Luo P, He B, Wu Y, Hou J (2021) Recent advancement in remote sensing technology for hydrology analysis and water resources management. Remote Sens 13(6):1097. https://doi.org/10.3390/rs13061097
Dyba K, Ermida S, Ptak M, Piekarczyk J, Sojka M (2022) Evaluation of methods for estimating lake surface water temperature using landsat 8. Remote Sens 14(15):3839. https://doi.org/10.3390/rs14153839
Ebodé VB, Braun JJ, Nnomo BN, Mahé G, Nkiaka E, Riotte J (2022) Impact of rainfall variability and land use change on river discharge in South Cameroon. Water 14(6):941. https://doi.org/10.3390/w14060941
Empresa de Pesquisa Agropecuária de Minas Gerais – EPAMIG (2014) Solos e avaliação do potencial agrossilvipastoril das microrregiões Paracatu e Unaí - Minas Gerais. EPAMIG, Belo Horizonte
Ersi C, Bayaer T, Bao Y, Bao Y, Yong M, Zhang X (2022) Temporal and spatial changes in evapotranspiration and its potential driving factors in Mongolia over the past 20 years. Remote Sens 14(8):1856. https://doi.org/10.3390/rs14081856
Escobar GCJ, Reboita MS (2021) Relationship between daily atmospheric circulation patterns and South Atlantic Convergence Zone (SACZ) events. Atmósfera 35(1):1–25. https://doi.org/10.20937/ATM.52936
Ferrant S, Selles A, Le Page M, AlBitar A, Mermoz S, Gascoin S, Bouvet A, Ahmed S, Kerr Y (2019) Sentinel-1&2 for near real-time cropping pattern monitoring in drought prone areas. Application to irrigation water needs in Telangana, South India. Photogramm. Remote Sens Spatial Inf Sci. XLII-3/W6: 285–292. 10.5194/isprs-archives-XLII-3-W6-285-2019
Ferrarini ADSF, Ferreira Filho JBDS, Cuadra SV, Victoria DDC (2020) Water demand prospects for irrigation in the São Francisco River: Brazilian public policy. Water Policy 22(3):449–467. https://doi.org/10.2166/wp.2020.215
Finke K, Jiménez-Esteve B, Taschetto AS, Ummenhofer CC, Bumke K, Domeisen DIV (2020) Revisiting remote drivers of the 2014 drought in Southeastern Brazil. Clim Dyn 55:3197–3211. https://doi.org/10.1007/s00382-020-05442-9
Freitas AA, Drumond A, Carvalho VSB, Reboita MS, Silva BC, Uvo CB (2022) Drought assessment in São Francisco River Basin, Brazil: characterization through SPI and associated anomalous climate patterns. Atmosphere 13(1):41. https://doi.org/10.3390/atmos13010041
Funk C, Peterson P, Landsfeld M, Pedreros D, Verdin J, Shukla S, Michaelsen J (2015) The climate hazards infrared precipitation with stations: a new environmental record for monitoring extremes. Scientific Data 2(1):150066. https://doi.org/10.1038/sdata.2015.66
Gozzo LP, Palma DS, Custódio MS, Drumond A (2021) Padrões Climatológicos Associados a Eventos de Seca no Leste do Estado de São Paulo. Rev Brasileira De Climatol 17(28):321–341. https://doi.org/10.5380/rbclima.v28i0.76268
Guimarães DP, Landau EC (2020) Georreferenciamento dos pivôs centrais de irrigação no Brasil: ano base 2020. Portal Embrapa, Sete Lagoas
Instituto Mineiro de Gestão das Águas – IGAM (2020) Áreas de Conflito Declaradas em Minas Gerais - 2020. IGAM Web. http://www.repositorioigam.meioambiente.mg.gov.br/handle/123456789/3064?mode=full . Accessed 20 June 2023
Instituto Mineiro de Gestão das Águas – IGAM (2019) Outorgas de direito de uso de recursos hídricos. DE-Sisema Web. https://idesisema.meioambiente.mg.gov.br/geonetwork/srv/por/catalog.search#/metadata/45589b8c-4f82-4d42-9ec8-351c65f3ea18 . Accessed 20 June 2023
Instituto Mineiro de Gestão das Águas – IGAM (2023) Portaria IGAM N o 48, de 04 de outubro de 2019. SIAM Web. http://www.siam.mg.gov.br/sla/download.pdf?idNorma=49719 . Accessed 20 June 2023
Instituto Nacional de Meteorologia – INMET (2023) Dados de estações. INMET Web: https://tempo.inmet.gov.br . Accessed 20 June 2023
Jalilvand E, Tajrishy M, Hashemi SAGZ, Brocca L (2019) Quantification of irrigation water using remote sensing of soil moisture in a semi-arid region. Remote Sens Environ 231:111226. https://doi.org/10.1016/j.rse.2019.111226
Jha G, Nicolas F, Schmidt R, Suvočarev K, Diaz D, Kisekka I, Scow K, Nocco MA (2022) Irrigation decision support systems (IDSS) for California’s water–nutrient–energy nexus. Agronomy 12(8):1962. https://doi.org/10.3390/agronomy12081962
Article CAS Google Scholar
Jimenez JC, Marengo JA, Alves LM, Sulca JC, Takahashi K, Ferrett S, Collins M (2021) The role of ENSO flavors and TNA on recent droughts over Amazon forests and the Northeast Brazil region. Int J Climatol 41(7):3761–3780. https://doi.org/10.1002/joc.6453
Kendall MG (1975) Rank correlation methods, 4th edn. Charles Griffin, London
Google Scholar
Köppen W (1948) Climatologia: con un studio de los climas de la tierra. 1° edición em español. Fundo de Cultura Económica, México
Lima CES, da Silva MVM, Rocha SMG, Silveira CdS (2022) Anthropic changes in land use and land cover and their impacts on the hydrological variables of the São Francisco river basin. Brazil Sustain 14(19):12176. https://doi.org/10.3390/su141912176
Lima EP, Andrade RG, Sediyama GC, Bof LHN (2019) Temporal-spatial control of the difference between precipitation and evapotranspiration in Paracatu sub-basins. Int J Adv Eng Res Sci 6(6):617–622. https://doi.org/10.22161/ijaers.6.6.70
Liu H, Song D, Kong J, Mu Z, Wang X, Jiang Y, Zhang J (2022) Complementarity characteristics of actual and potential evapotranspiration and spatiotemporal changes in evapotranspiration drought index over Ningxia in the upper reaches of the Yellow River in China. Remote Sens 14(23):5953. https://doi.org/10.3390/rs14235953
Liu W, Sun F, Lim WH, Zhang J, Shiogama WH, Zhang Y (2018) Global drought and severe drought-affected populations in 1.5 and 2 °C warmer worlds. Earth Syst Dyn 9:267–283. https://doi.org/10.5194/esd-9-267-2018
Lucas MC, Kublik N, Rodrigues DBB, Meira Neto AA, Almagro A, Melo DdCD, Zipper SC, Oliveira PTS (2021) Significant baseflow reduction in the Sao Francisco River basin. Water 13(1):2. https://doi.org/10.3390/w13010002
Ma Z, Wu B, Yan N, Zhu W, Zeng H, Xu J (2021) Spatial allocation method from coarse evapotranspiration data to agricultural fields by quantifying variations in crop cover and soil moisture. Remote Sens 13(3):343. https://doi.org/10.3390/rs13030343
Mallakpour I, Villarini G (2016) A simulation study to examine the sensitivity of the Pettitt test to detect abrupt changes in mean. Hydrol Sci J 61(2):245–254. https://doi.org/10.1080/02626667.2015.1008482
Mapbiomas Brasil (2023). MapBiomas Web. https://mapbiomas.org/o-projeto . Accessed 20 May 2023
Marengo JA, Jimenez JC, Espinoza JC, Cunha AP, Aragão LEO (2022) Increased climate pressure on the agricultural frontier in the Eastern Amazonia-Cerrado transition zone. Sci Rep 12:457. https://doi.org/10.1038/s41598-021-04241-4
Marengo JA, Torres RR, Alves LM (2017) Drought in Northeast Brazil—past, present, and future. Theoret Appl Climatol 129:1189–1200. https://doi.org/10.1007/s00704-016-1840-8
Marques EA, Junior GCS, Eger GZ, Ilambwetsi AM, Raphael P, Generoso TN, Oliveira J, Júnior JN (2020) Analysis of groundwater and river stage fluctuations and their relationship with water use and climate variation effects on Alto Grande watershed, Northeastern Brazil. J S Am Earth Sci 103:102723. https://doi.org/10.1016/j.jsames.2020.102723
Martins AP, Galvani E (2020) Performance of the SEBAL algorithm for flow estimation in watersheds of Brazilian Savannah. Sociedade Natureza 32:687–698. https://doi.org/10.14393/SN-v32-2020-53169
Maselli F, Battista P, Chiesi M, Rapi B, Angeli L, Fibbi L, Magno R, Gozzini B (2020) Use of Sentinel-2 MSI data to monitor crop irrigation in Mediterranean areas. Int J Ppl Earth Obs Geoinformation 93:102216. https://doi.org/10.1016/j.jag.2020.102216
Mokhtari S, Sharafati A, Raziei T (2022) Satellite-based streamflow simulation using CHIRPS satellite precipitation product in Shah Bahram Basin. Iran Acta Geophysica 70:385–398. https://doi.org/10.1007/s11600-021-00724-0
Montaldo N, Gaspa A, Corona R (2022) Multiscale assimilation of sentinel and landsat data for soil moisture and leaf area index predictions using an ensemble-Kalman-filter-based assimilation approach in a heterogeneous ecosystem. Remote Sens 14(14):3458. https://doi.org/10.3390/rs14143458
Muñoz AA, Klock-Barría K, Alvarez-Garreton C, Aguilera-Betti I, González-Reyes Á, Lastra JA, Chávez RO, Barría P, Christie D, Rojas-Badilla M, LeQuesne C (2020) Water crisis in Petorca Basin, Chile: the combined effects of a mega-drought and water management. Water 12:648. https://doi.org/10.3390/w12030648
Pacific Institute (2022) Water Conflict Chronology. Pacific Institute, Oakland, CA. https://www.worldwater.org/water-conflict/ . Accessed 01 Jan 2023
Parajuli B, Zhang X, Deuja S, Liu Y (2022) Regional and seasonal precipitation and drought trends in Ganga-Brahmaputra Basin. Water 13(16):2218. https://doi.org/10.3390/w13162218
Paredes-Trejo F, Barbosa HA, Giovannettone J, Kumar TVL, Thakur MK, Buriti CdO, Uzcátegui-Briceño C (2021) Drought assessment in the São Francisco River basin using satellite-based and ground-based indices. Remote Sens 13(19):3921. https://doi.org/10.3390/rs13193921
Pettitt AN (1979) A non-parametric approach to the change-point problem. J R Stat Soc 28(2):126–135
Pousa R, Costa MH, Pimenta FM, Fontes VC, Brito VFAD, Castro M (2019) Climate change and intense irrigation growth in Western Bahia, Brazil: The urgent need for hydroclimatic monitoring. Water 11(5):933. https://doi.org/10.3390/w11050933
Reboita MS, Kuki CAC, Marrafon VH, de Souza CA, Ferreira GWS, Teodoro T, Lima JWM (2022) South America climate change revealed through climate indices projected by GCMs and Eta-RCM ensembles. Clim Dyn 58(1–2):459–485. https://doi.org/10.1007/s00382-021-05918-2
Reboita MS, Rodrigues M, Silva LF, Alves MA (2015) Climate aspects in Minas Gerais state. Rev Brasileira De Climatol 17:2237–8642. https://doi.org/10.5380/abclima.v17i0.41493
Running S, Mu Q, Zhao M, Moreno A (2021) MODIS/terra net evapotranspiration gap-filled 8-Day L4 Global 500m SIN Grid V061 . NASA EOSDIS Land Processes DAAC. Accessed 01 July 2023 from https://doi.org/10.5067/MODIS/MOD16A2GF.061
Safi AR, Karimi P, Mul M, Chukalla A, Fraiture C (2022) Translating open-source remote sensing data to crop water productivity improvement actions. Agric Water Manag 261:107373. https://doi.org/10.1016/j.agwat.2021.107373
Santos AB, Heil Costa M, Chartuni Mantovani E, Boninsenha I, Castro M (2020) A remote sensing diagnosis of water use and water stress in a Region with intense irrigation growth in Brazil. Remote Sensing 12(22):3725. https://doi.org/10.3390/rs12223725
Sarsour A, Nagabhatla N (2022) Options and strategies for planning water and climate security in the occupied Palestinian Territories. Water 14:3418. https://doi.org/10.3390/w14213418
Schmidt DM, Lima KC, Jesus ES (2018) Variability climatic of water availability in the semi-arid of the State of Rio Grande do Norte. Anuário do Instituto de Geociências – UFRJ 41(3):483–491. https://doi.org/10.11137/2018_3_483_491
Schwatke C, Dettmering D, Seitz F (2020) Volume variations of small inland water bodies from a combination of satellite altimetry and optical imagery. Remote Sens 12(10):1606. https://doi.org/10.3390/rs12101606
Sen PK (1968) Estimates of the regression coefficient based on Kendall’s Tau. J Am Stat Assoc 63(324):1379–1389. https://doi.org/10.1080/01621459.1968.10480934
Silva C, Manzione RL, Caldas MM (2023) Net water flux and land use shifts across the Brazilian Cerrado between 2000 and 2019. Reg Environ Change 23:151. https://doi.org/10.1007/s10113-023-02127-x
Silva VP, França SGL (2018) Percepções de mudanças do clima, impactos e adaptação para sertanejos do semiárido. Rev Brasileira de Climatol 22(14). https://doi.org/10.5380/abclima.v22i0.55958
Silveira CDS, Souza Filho FDAD, Martins ESPR, Oliveira JL, Costa AC, Nobrega MT et al (2016) Climate change in the São Francisco river basin: analysis of precipitation and temperature. Rev Brasileira De Recursos Hídricos 21:416–428. https://doi.org/10.21168/rbrh.v21n2.p416-428
Sousa HT, Pruski FF, Bof LHN, et al . (2009) SisCaH 1.0-Sistema Computacional para Análises Hidrológicas. ANA, Viçosa
Sun T, Ferreira VG, He X, Andam-Akorful SA (2016) Water availability of São Francisco River Basin based on a space-borne geodetic sensor. Water 8(5):213. https://doi.org/10.3390/w8050213
Tao L, Ryu D, Western A, Lee SG (2022) Comparison of KOMPSAT-5 and sentinel-1 radar data for soil moisture estimations using a new semi-empirical model. Remote Sens 14(16):4042. https://doi.org/10.3390/rs14164042
United Nations (2019) World population prospects 2019. Probabilistic Population Projections. UN, New York
United Nations Educational, Scientific and Cultural Organization – UNESCO (2019) The United Nations World Water Development Report 2019: Leaving No One Behind. UNESCO, Paris
Verhoeven E, Wardle GM, Roth GW, Greenville AC (2022) Characterising the spatiotemporal dynamics of drought and wet events in Australia. Sci Total Environ 846:157480. https://doi.org/10.1016/j.scitotenv.2022.157480
Vervoort RW, Fuentes I, Brombacher J, Degen J, Chambel-Leitão P, Santos F (2022) Progress in developing scale-able approaches to field-scale water accounting based on remote sensing. Sustainability 14(5):2732. https://doi.org/10.3390/su14052732
Vogels MF, de Jong SM, Sterk G, Wanders N, Bierkens MF, Addink EA (2020) An object-based image analysis approach to assess irrigation-water consumption from MODIS products in Ethiopia. Int J Appl Earth Obs Geoinf 88:102067. https://doi.org/10.1016/j.jag.2020.102067
Walker D, Shutler JD, Morrison EHJ, Harper DM, Hoedjes JCB, Laing CG (2022) Quantifying water storage within the north of lake naivasha using sonar remote sensing and landsat satellite data. Ecohydrology & Hydrobiology 22(1):12–20. https://doi.org/10.1016/j.ecohyd.2021.07.011
Williams J, Grafton RQ (2019) Missing in action: possible effects of water recovery on stream and river flows in the Murray-Darling Basin, Australia. Australasian J Water Resour 23:78–87. https://doi.org/10.1080/13241583.2019.1579965
World Economic Forum - WEF (2021) The Global Risks Report 2021, 16th Edition. Cologny, Switzerland
Yang Y, Wu J, Bai L, Wang B (2020) Reliability of gridded precipitation products in the Yellow River Basin, China. Remote Sens 12(13):374. https://doi.org/10.3390/rs12030374
Zappa L, Schlaffer S, Bauer-Marschallinger B, Nendel C, Zimmerman B, Dorigo W (2021) Detection and quantification of irrigation water amounts at 500 m using sentinel-1 surface soil moisture. Remote Sens 13(9):1727. https://doi.org/10.3390/rs13091727
Zhang K, Zhu C, Ma X, Zhang X, Yang D, Shao Y (2023) Spatiotemporal variation characteristics and dynamic persistence analysis of carbon sources/sinks in the Yellow River Basin. Remote Sens 15(2):323. https://doi.org/10.3390/rs15020323
Download references
Acknowledgements
This research was supported by the Foundation for Research Support of the State of Minas Gerais—FAPEMIG (APQ-00241-22) and the Universidade Federal dos Vales Jequitinhonha e Mucuri (UFVJM), Unaí Campus, Minas Gerais, Brazil.
Fundação de Amparo à Pesquisa do Estado de Minas Gerais, APQ-00241-22.
Author information
Authors and affiliations.
Instituto de Ciências Agrárias, Universidade Federal Dos Vales Jequitinhonha E Mucuri, Unaí, Minas Gerais, Brazil
M. L. Ferreira & A. M. De Andrade
Instituto Federal de Minas Gerais, Campus Bambuí, Minas Gerais, Brazil
G. P. Tormen
You can also search for this author in PubMed Google Scholar
Corresponding author
Correspondence to M. L. Ferreira .
Ethics declarations
Conflict of interests.
The authors state that they have no competing financial interests or personal relationships that may have influenced the work reported in this article.
Ethical approval
This article does not contain any studies with human participants or animals performed by any of the authors.
Additional information
Editorial responsibility: Samareh Mirkia.
Rights and permissions
Springer Nature or its licensor (e.g. a society or other partner) holds exclusive rights to this article under a publishing agreement with the author(s) or other rightsholder(s); author self-archiving of the accepted manuscript version of this article is solely governed by the terms of such publishing agreement and applicable law.
Reprints and permissions
About this article
Ferreira, M.L., Tormen, G.P. & De Andrade, A.M. Climate change and irrigation expansion in Northwest Minas Gerais, Brazil: the need for hydroclimatic monitoring. Int. J. Environ. Sci. Technol. (2024). https://doi.org/10.1007/s13762-024-05994-x
Download citation
Received : 30 September 2023
Revised : 08 July 2024
Accepted : 19 August 2024
Published : 05 September 2024
DOI : https://doi.org/10.1007/s13762-024-05994-x
Share this article
Anyone you share the following link with will be able to read this content:
Sorry, a shareable link is not currently available for this article.
Provided by the Springer Nature SharedIt content-sharing initiative
- Water conflict
- Water crisis
- Water management
- Water resources
- Water scarcity
- Find a journal
- Publish with us
- Track your research
Global Journal of Engineering Education
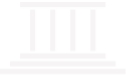
Subject Area and Category
- Computer Science (miscellaneous)
World Institute for Engineering and Technology Education (WIETE)
Publication type
Information.
How to publish in this journal
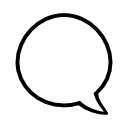
The set of journals have been ranked according to their SJR and divided into four equal groups, four quartiles. Q1 (green) comprises the quarter of the journals with the highest values, Q2 (yellow) the second highest values, Q3 (orange) the third highest values and Q4 (red) the lowest values.
Category | Year | Quartile |
---|---|---|
Computer Science (miscellaneous) | 2011 | Q4 |
Computer Science (miscellaneous) | 2012 | Q3 |
Computer Science (miscellaneous) | 2013 | Q2 |
Computer Science (miscellaneous) | 2014 | Q3 |
Computer Science (miscellaneous) | 2015 | Q3 |
Computer Science (miscellaneous) | 2016 | Q3 |
Computer Science (miscellaneous) | 2017 | Q2 |
Computer Science (miscellaneous) | 2018 | Q1 |
Computer Science (miscellaneous) | 2019 | Q1 |
Computer Science (miscellaneous) | 2020 | Q1 |
Computer Science (miscellaneous) | 2021 | Q2 |
Computer Science (miscellaneous) | 2022 | Q1 |
Computer Science (miscellaneous) | 2023 | Q3 |
Education | 2011 | Q4 |
Education | 2012 | Q4 |
Education | 2013 | Q2 |
Education | 2014 | Q3 |
Education | 2015 | Q3 |
Education | 2016 | Q4 |
Education | 2017 | Q3 |
Education | 2018 | Q2 |
Education | 2019 | Q2 |
Education | 2020 | Q2 |
Education | 2021 | Q2 |
Education | 2022 | Q1 |
Education | 2023 | Q3 |
The SJR is a size-independent prestige indicator that ranks journals by their 'average prestige per article'. It is based on the idea that 'all citations are not created equal'. SJR is a measure of scientific influence of journals that accounts for both the number of citations received by a journal and the importance or prestige of the journals where such citations come from It measures the scientific influence of the average article in a journal, it expresses how central to the global scientific discussion an average article of the journal is.
Year | SJR |
---|---|
2011 | 0.114 |
2012 | 0.172 |
2013 | 0.346 |
2014 | 0.215 |
2015 | 0.192 |
2016 | 0.165 |
2017 | 0.297 |
2018 | 0.549 |
2019 | 0.509 |
2020 | 0.580 |
2021 | 0.480 |
2022 | 0.839 |
2023 | 0.327 |
Evolution of the number of published documents. All types of documents are considered, including citable and non citable documents.
Year | Documents |
---|---|
2010 | 8 |
2011 | 22 |
2012 | 36 |
2013 | 28 |
2014 | 23 |
2015 | 22 |
2016 | 39 |
2017 | 48 |
2018 | 34 |
2019 | 36 |
2020 | 31 |
2021 | 37 |
2022 | 23 |
2023 | 38 |
This indicator counts the number of citations received by documents from a journal and divides them by the total number of documents published in that journal. The chart shows the evolution of the average number of times documents published in a journal in the past two, three and four years have been cited in the current year. The two years line is equivalent to journal impact factor ™ (Thomson Reuters) metric.
Cites per document | Year | Value |
---|---|---|
Cites / Doc. (4 years) | 2010 | 0.000 |
Cites / Doc. (4 years) | 2011 | 0.250 |
Cites / Doc. (4 years) | 2012 | 0.433 |
Cites / Doc. (4 years) | 2013 | 0.303 |
Cites / Doc. (4 years) | 2014 | 0.245 |
Cites / Doc. (4 years) | 2015 | 0.422 |
Cites / Doc. (4 years) | 2016 | 0.404 |
Cites / Doc. (4 years) | 2017 | 0.545 |
Cites / Doc. (4 years) | 2018 | 1.311 |
Cites / Doc. (4 years) | 2019 | 0.853 |
Cites / Doc. (4 years) | 2020 | 1.261 |
Cites / Doc. (4 years) | 2021 | 1.309 |
Cites / Doc. (4 years) | 2022 | 1.348 |
Cites / Doc. (4 years) | 2023 | 1.622 |
Cites / Doc. (3 years) | 2010 | 0.000 |
Cites / Doc. (3 years) | 2011 | 0.250 |
Cites / Doc. (3 years) | 2012 | 0.433 |
Cites / Doc. (3 years) | 2013 | 0.303 |
Cites / Doc. (3 years) | 2014 | 0.256 |
Cites / Doc. (3 years) | 2015 | 0.425 |
Cites / Doc. (3 years) | 2016 | 0.329 |
Cites / Doc. (3 years) | 2017 | 0.560 |
Cites / Doc. (3 years) | 2018 | 1.422 |
Cites / Doc. (3 years) | 2019 | 0.917 |
Cites / Doc. (3 years) | 2020 | 1.415 |
Cites / Doc. (3 years) | 2021 | 1.475 |
Cites / Doc. (3 years) | 2022 | 1.433 |
Cites / Doc. (3 years) | 2023 | 1.659 |
Cites / Doc. (2 years) | 2010 | 0.000 |
Cites / Doc. (2 years) | 2011 | 0.250 |
Cites / Doc. (2 years) | 2012 | 0.433 |
Cites / Doc. (2 years) | 2013 | 0.345 |
Cites / Doc. (2 years) | 2014 | 0.281 |
Cites / Doc. (2 years) | 2015 | 0.235 |
Cites / Doc. (2 years) | 2016 | 0.400 |
Cites / Doc. (2 years) | 2017 | 0.574 |
Cites / Doc. (2 years) | 2018 | 1.529 |
Cites / Doc. (2 years) | 2019 | 1.012 |
Cites / Doc. (2 years) | 2020 | 1.629 |
Cites / Doc. (2 years) | 2021 | 1.701 |
Cites / Doc. (2 years) | 2022 | 1.221 |
Cites / Doc. (2 years) | 2023 | 1.800 |
Evolution of the total number of citations and journal's self-citations received by a journal's published documents during the three previous years. Journal Self-citation is defined as the number of citation from a journal citing article to articles published by the same journal.
Cites | Year | Value |
---|---|---|
Self Cites | 2010 | 0 |
Self Cites | 2011 | 1 |
Self Cites | 2012 | 4 |
Self Cites | 2013 | 4 |
Self Cites | 2014 | 3 |
Self Cites | 2015 | 0 |
Self Cites | 2016 | 1 |
Self Cites | 2017 | 10 |
Self Cites | 2018 | 25 |
Self Cites | 2019 | 25 |
Self Cites | 2020 | 13 |
Self Cites | 2021 | 30 |
Self Cites | 2022 | 26 |
Self Cites | 2023 | 28 |
Total Cites | 2010 | 0 |
Total Cites | 2011 | 2 |
Total Cites | 2012 | 13 |
Total Cites | 2013 | 20 |
Total Cites | 2014 | 22 |
Total Cites | 2015 | 37 |
Total Cites | 2016 | 24 |
Total Cites | 2017 | 47 |
Total Cites | 2018 | 155 |
Total Cites | 2019 | 111 |
Total Cites | 2020 | 167 |
Total Cites | 2021 | 149 |
Total Cites | 2022 | 149 |
Total Cites | 2023 | 151 |
Evolution of the number of total citation per document and external citation per document (i.e. journal self-citations removed) received by a journal's published documents during the three previous years. External citations are calculated by subtracting the number of self-citations from the total number of citations received by the journal’s documents.
Cites | Year | Value |
---|---|---|
External Cites per document | 2010 | 0 |
External Cites per document | 2011 | 0.125 |
External Cites per document | 2012 | 0.300 |
External Cites per document | 2013 | 0.242 |
External Cites per document | 2014 | 0.221 |
External Cites per document | 2015 | 0.425 |
External Cites per document | 2016 | 0.315 |
External Cites per document | 2017 | 0.440 |
External Cites per document | 2018 | 1.193 |
External Cites per document | 2019 | 0.711 |
External Cites per document | 2020 | 1.305 |
External Cites per document | 2021 | 1.178 |
External Cites per document | 2022 | 1.183 |
External Cites per document | 2023 | 1.352 |
Cites per document | 2010 | 0.000 |
Cites per document | 2011 | 0.250 |
Cites per document | 2012 | 0.433 |
Cites per document | 2013 | 0.303 |
Cites per document | 2014 | 0.256 |
Cites per document | 2015 | 0.425 |
Cites per document | 2016 | 0.329 |
Cites per document | 2017 | 0.560 |
Cites per document | 2018 | 1.422 |
Cites per document | 2019 | 0.917 |
Cites per document | 2020 | 1.415 |
Cites per document | 2021 | 1.475 |
Cites per document | 2022 | 1.433 |
Cites per document | 2023 | 1.659 |
International Collaboration accounts for the articles that have been produced by researchers from several countries. The chart shows the ratio of a journal's documents signed by researchers from more than one country; that is including more than one country address.
Year | International Collaboration |
---|---|
2010 | 12.50 |
2011 | 9.09 |
2012 | 13.89 |
2013 | 17.86 |
2014 | 4.35 |
2015 | 9.09 |
2016 | 10.26 |
2017 | 6.25 |
2018 | 14.71 |
2019 | 13.89 |
2020 | 9.68 |
2021 | 5.41 |
2022 | 13.04 |
2023 | 13.16 |
Not every article in a journal is considered primary research and therefore "citable", this chart shows the ratio of a journal's articles including substantial research (research articles, conference papers and reviews) in three year windows vs. those documents other than research articles, reviews and conference papers.
Documents | Year | Value |
---|---|---|
Non-citable documents | 2010 | 0 |
Non-citable documents | 2011 | 1 |
Non-citable documents | 2012 | 4 |
Non-citable documents | 2013 | 7 |
Non-citable documents | 2014 | 9 |
Non-citable documents | 2015 | 9 |
Non-citable documents | 2016 | 8 |
Non-citable documents | 2017 | 9 |
Non-citable documents | 2018 | 7 |
Non-citable documents | 2019 | 8 |
Non-citable documents | 2020 | 7 |
Non-citable documents | 2021 | 6 |
Non-citable documents | 2022 | 4 |
Non-citable documents | 2023 | 2 |
Citable documents | 2010 | 0 |
Citable documents | 2011 | 7 |
Citable documents | 2012 | 26 |
Citable documents | 2013 | 59 |
Citable documents | 2014 | 77 |
Citable documents | 2015 | 78 |
Citable documents | 2016 | 65 |
Citable documents | 2017 | 75 |
Citable documents | 2018 | 102 |
Citable documents | 2019 | 113 |
Citable documents | 2020 | 111 |
Citable documents | 2021 | 95 |
Citable documents | 2022 | 100 |
Citable documents | 2023 | 89 |
Ratio of a journal's items, grouped in three years windows, that have been cited at least once vs. those not cited during the following year.
Documents | Year | Value |
---|---|---|
Uncited documents | 2010 | 0 |
Uncited documents | 2011 | 6 |
Uncited documents | 2012 | 21 |
Uncited documents | 2013 | 52 |
Uncited documents | 2014 | 72 |
Uncited documents | 2015 | 72 |
Uncited documents | 2016 | 56 |
Uncited documents | 2017 | 55 |
Uncited documents | 2018 | 57 |
Uncited documents | 2019 | 72 |
Uncited documents | 2020 | 52 |
Uncited documents | 2021 | 42 |
Uncited documents | 2022 | 41 |
Uncited documents | 2023 | 30 |
Cited documents | 2010 | 0 |
Cited documents | 2011 | 2 |
Cited documents | 2012 | 9 |
Cited documents | 2013 | 14 |
Cited documents | 2014 | 14 |
Cited documents | 2015 | 15 |
Cited documents | 2016 | 17 |
Cited documents | 2017 | 29 |
Cited documents | 2018 | 52 |
Cited documents | 2019 | 49 |
Cited documents | 2020 | 66 |
Cited documents | 2021 | 59 |
Cited documents | 2022 | 63 |
Cited documents | 2023 | 61 |
Evolution of the percentage of female authors.
Year | Female Percent |
---|---|
2010 | 0.00 |
2011 | 28.21 |
2012 | 36.76 |
2013 | 27.78 |
2014 | 36.36 |
2015 | 36.84 |
2016 | 32.81 |
2017 | 40.96 |
2018 | 42.25 |
2019 | 32.43 |
2020 | 42.86 |
2021 | 41.94 |
2022 | 51.85 |
2023 | 47.69 |
Evolution of the number of documents cited by public policy documents according to Overton database.
Documents | Year | Value |
---|---|---|
Overton | 2010 | 0 |
Overton | 2011 | 0 |
Overton | 2012 | 0 |
Overton | 2013 | 0 |
Overton | 2014 | 0 |
Overton | 2015 | 0 |
Overton | 2016 | 0 |
Overton | 2017 | 0 |
Overton | 2018 | 0 |
Overton | 2019 | 0 |
Overton | 2020 | 0 |
Overton | 2021 | 0 |
Overton | 2022 | 0 |
Overton | 2023 | 0 |
Evoution of the number of documents related to Sustainable Development Goals defined by United Nations. Available from 2018 onwards.
Documents | Year | Value |
---|---|---|
SDG | 2018 | 13 |
SDG | 2019 | 11 |
SDG | 2020 | 12 |
SDG | 2021 | 15 |
SDG | 2022 | 13 |
SDG | 2023 | 19 |
Leave a comment
Name * Required
Email (will not be published) * Required
* Required Cancel
The users of Scimago Journal & Country Rank have the possibility to dialogue through comments linked to a specific journal. The purpose is to have a forum in which general doubts about the processes of publication in the journal, experiences and other issues derived from the publication of papers are resolved. For topics on particular articles, maintain the dialogue through the usual channels with your editor.
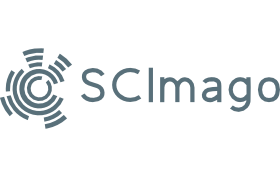
Follow us on @ScimagoJR Scimago Lab , Copyright 2007-2024. Data Source: Scopus®

Cookie settings
Cookie Policy
Legal Notice
Privacy Policy
- Sub Journals
- Peer Review Policy
- Short Guide to New Editors
- Retraction Guidelines
- Research Institute
- Recommendations for the Board of Directors
- Editor Research Audit and Service Assessments
- Guidelines for Journals Publishers
- Code of Conduct for Journal Publishers
- Commandment in Social Media
- Suggestions
- Authorship Disputes
- Rights and Responsibilities
- About the Publisher
- Call for Papers
- Editorial Team
- Submissions
Get in touch with us
Principal contact.
- Name: Global Journals Support Team
Global Headquarters Corporate Office Email: [email protected] Global Journals™ Headquarters 945th Concord Streets Framingham Massachusetts Pin: 01701 United States of America
Call to Subscribe: +1-888-839-7392, +1-508-620-4529 Subscription Fax: +1-888-839-7392
- Phone: +1-888-839-7392, +1-508-620-4529
- Email: [email protected]
Support Contact
- Name: Global Journals IT Department

COMMENTS
Global Journal of Science Frontier Research GJSFR-B Chemistry: Volume 21 Issue B2. Published 2021-09-03 : ISSN Online: 2249-4626 ISSN Print: 0975-5896: DOI: 10.17406/GJSFR GJSFR-B Chemistry: Volume 21 Issue B2. Published: 2021-09-03 Articles
The Global Journal of Science Frontier Research (GJSFR) is a journal that publishes articles which contribute new theoretical results in all the areas of Natural Science. In order to provide a timely and broad coverage of these ever-evolving fields, GJMR offers a combination of regular and special issues to its readers. The publication of ...
For the JIF Percentile, the higher the value, the higher the Impact Factor of the journal in relation to its peers. Examples: A journal is rank 104 out of 177 in a specific category. The Journal Impact Factor Percentile is (177 - 104 + 0.5) / 177 = 0.41525 = 41.525 Percentile A different journal is rank 27 out of 30 in a specific category.
Global Journal of Science Frontier Research. Since 2001, The Global Journal of Science Frontier Research (GJSFR) has been an academic, Hybrid access, peer-reviewed, interdisciplinary, refereed; journal focusing on all aspects of Science Frontier published by Global Journals, which is one of the fastest growing and leading Research Journal publishing organization in the world.
Global Journal of Science Frontier Research SJIF 2024: Under evaluation Area: Multidisciplinary Evaluated version: online Previous evaluation SJIF. 2023: 8.293 2022: 8.065 2021: 8.243 2020: 7.991. The journal is indexed in: SJIFactor.com Basic information ...
About the Journal. Global Journals is an international platform for researchers, inventors, scientists, engineers, managers, doctors, and professors involved in all streams of research with the purpose of publishing high-quality research and review papers. Global Journals Inc. offers research papers, authentic surveys, and review papers by ...
Global Journal of Science Frontier Research: A Physics and Space Science . Volume 20 Issue 2 Version 1.0 Year 2020 Type : Double Blind Peer Reviewed International Research Journal Publisher: Global Journals Online ISSN: 2249-4626 & Print ISSN: 0975-5896 A New Approach to Quantum Gravity
Global Journal of Science Frontier Research Dr. John Korstad Dr. Mazeyar Parvinzadeh Gashti Ph.D., M.S. at California State University Professor of Biology Department of Biology Oral Roberts University Ph.D, M.Sc., B.Sc. Science and Research Branch of Islamic Azad University, Tehran, Iran
Global Journal of Science Frontier Research-A: Physics and Space Science. Condensed Matter Physics. Instrumentation. Interdisciplinary Physics. Nonlinear, Statistical and Applied Physics. Nuclear and High Energy Physics. Optics with Atomic, Molecular and Plasma Physics. Physics (General) Surfaces and Interfaces.
The mass of this new particle is only 10-20 of the electron, and it is named Yizi. Through the discovery of Yizi, we will reveal the cause of gravitation and expound the essence of force ...
Global Journal of Science Frontier Research Global Journal of Science Frontier Research. Current Archives About About the Journal Submissions ... Search Register Login Current Issue Vol. 24 No. E1 (2024): GJSFR-E Marine Science: Volume 24 Issue E1 GJSFR-E Marine Science: Volume 24 Issue E1 Published: 2024 -07-24 Articles ...
Answer: The database you are referring to is the ISI Web of Science, originally owned by Thomson Reuters, now owned by Clarivate Analytics. To check if your manuscript is indexed in the ISI Web of Science, you can log on to Clarivate Analytics Master Journal List. Once you log in, you can simply search by the full journal name or the ISSN number.
One of the premier publications in the field of feminist and gender studies, Frontiers has distinguished itself for its diverse and decisively interdisciplinary publication agenda that explores the critical intersections among—to name a few dimensions—gender, race, sexuality, and transnationalism. Many landmark articles in the field have ...
The Messinian Salinity Crisis (MSC) represents a relatively recent severe environmental and landscape change (1, 2) involving the isolation and alternating hyper- and hyposalinization of the Mediterranean Sea () between 5.97 and 5.33 million years ago (Ma).The Mediterranean Sea is a large marginal sea at mid-latitudes that functions as a biodiversity hotspot refuge for hundreds of marine species.
GLOBAL JOURNAL OF SCIENCE FRONTIER RESEARCH : ISSN (P) 2249-4626: ISSN (O) Country: Un: Frequency: Bi-Monthly: Journal Discipline : General Science : Area of specialization : Organization : Journal Language : Year of First Publication ... Journal Description ...
Scopus journal percentiles are a metric used to rank journals within their respective subject areas. They provide a comparative measure of a journal's performance based on citation data. Table 7 presents an analysis of the citability rate of chitosan research in patents, categorized by journal percentiles. The data indicates that documents ...
The top three journals are the Journal of Surgical Research (IF 2.2), the American Journal of Physiology-Heart and Circulatory Physiology (IF 4.8), and Plastic and Reconstructive Surgery (IF 3.6). Nine of the top 20 journals showed a JCR quartile ranking of Q1, thus indicating that these journals have a high academic reputation in the field.
We hypothesized that strongly absorbing molecules can achieve optical transparency in live biological tissues. By applying the Lorentz oscillator model for the dielectric properties of tissue components and absorbing molecules, we predicted that dye molecules with sharp absorption resonances in the near-ultraviolet spectrum (300 to 400 nm) and blue region of the visible spectrum (400 to 500 nm ...
Global Journal of Science Frontier Research. Since 2001, Global Journal of Science Frontier Research (GJSFR): (I) Interdisciplinary, has been an academic open access, peer-reviewed, interdisciplinary, refereed journal focusing on all aspects of Engineering Research published by Global Journals, which is one of the fastest growing and leading Research Journal publishing organization in the world.
Global Journal of Science Frontier Research. We are one of the world's leading and fastest-growing research publications with the paramount objective of discovering advances by publishing insightful, double-blind, peer-reviewed scientific journals. Learn More. For Author. For Editors.
The risk of prediabetes increased progressively across quartiles of the TyG index (Q1 to Q4), with Q4 showing a significantly higher risk compared to Q1 (adjusted HR=2.33, 95% CI=1.72-3.16). Moreover, a non-linear relationship was identified between the TyG index and the risk of prediabetes, with an inflection point at 9.39.
Abbreviation of Global journal of science frontier research. The ISO4 abbreviation of Global journal of science frontier research is Glob. j. sci. front. res. . It is the standardised abbreviation to be used for abstracting, indexing and referencing purposes and meets all criteria of the ISO 4 standard for abbreviating names of scientific journals.
The Northwest Minas Gerais is a significant grain-producing region in Brazil. Center pivot irrigation in the region increased by 84% from 2010 to 2020, and records of conflicts over water use have occurred since 2000. This study carries out a hydroclimatic analysis of water resources in the Northwest Minas Gerais, using orbital and field data related to 567 pivots, considering the expansion of ...
Scope. The Global Journal of Engineering Education (GJEE) is included in the Scopus journal citation index, one of the products of Elsevier, the world's leading multinational publisher of science and health information. This represents a step forward in our persistent endeavour to make the Journal available to a wider global community.
Techniques for writing a good quality Science Frontier Research paper: 1. Choosing the topic: In most cases, the topic is selected by the interests of the author, but it can also be suggested by the guides. You can have several topics, and then judge which you are most comfortable with.
The ISSN of Global journal of science frontier research. is 0975-5896 . An ISSN is an 8-digit code used to identify newspapers, journals, magazines and periodicals of all kinds and on all media-print and electronic. Global journal of science frontier research. Key Factor Analysis
The Global Journal of Science Frontier Research is to fulfill all such demands and requirements, and functions also as an international platform. Of course, the publication of research work must be reviewed to establish its authenticity. This helps to promote research activity also. We know, great scientific research have been worked
Editor Research Audit and Service Assessments Guidelines for Journals Publishers Code of Conduct for Journal Publishers Commandment in Social Media Suggestions Authorship Disputes Rights and Responsibilities Home; ... Address Global Journals™ Headquarters 945th Concord Streets Framingham Massachusetts Pin: ...
Name: Global Journals Support Team; Address: Global Headquarters Corporate Office Email: [email protected] Global Journals™ Headquarters 945th Concord Streets Framingham Massachusetts Pin: 01701 United States of America. Call to Subscribe: +1-888-839-7392, +1-508-620-4529 Subscription Fax: +1-888-839-7392. Phone: +1-888-839-7392, +1-508-620-4529