Have a language expert improve your writing
Run a free plagiarism check in 10 minutes, generate accurate citations for free.
- Knowledge Base

The Beginner's Guide to Statistical Analysis | 5 Steps & Examples
Statistical analysis means investigating trends, patterns, and relationships using quantitative data . It is an important research tool used by scientists, governments, businesses, and other organizations.
To draw valid conclusions, statistical analysis requires careful planning from the very start of the research process . You need to specify your hypotheses and make decisions about your research design, sample size, and sampling procedure.
After collecting data from your sample, you can organize and summarize the data using descriptive statistics . Then, you can use inferential statistics to formally test hypotheses and make estimates about the population. Finally, you can interpret and generalize your findings.
This article is a practical introduction to statistical analysis for students and researchers. We’ll walk you through the steps using two research examples. The first investigates a potential cause-and-effect relationship, while the second investigates a potential correlation between variables.
Table of contents
Step 1: write your hypotheses and plan your research design, step 2: collect data from a sample, step 3: summarize your data with descriptive statistics, step 4: test hypotheses or make estimates with inferential statistics, step 5: interpret your results, other interesting articles.
To collect valid data for statistical analysis, you first need to specify your hypotheses and plan out your research design.
Writing statistical hypotheses
The goal of research is often to investigate a relationship between variables within a population . You start with a prediction, and use statistical analysis to test that prediction.
A statistical hypothesis is a formal way of writing a prediction about a population. Every research prediction is rephrased into null and alternative hypotheses that can be tested using sample data.
While the null hypothesis always predicts no effect or no relationship between variables, the alternative hypothesis states your research prediction of an effect or relationship.
- Null hypothesis: A 5-minute meditation exercise will have no effect on math test scores in teenagers.
- Alternative hypothesis: A 5-minute meditation exercise will improve math test scores in teenagers.
- Null hypothesis: Parental income and GPA have no relationship with each other in college students.
- Alternative hypothesis: Parental income and GPA are positively correlated in college students.
Planning your research design
A research design is your overall strategy for data collection and analysis. It determines the statistical tests you can use to test your hypothesis later on.
First, decide whether your research will use a descriptive, correlational, or experimental design. Experiments directly influence variables, whereas descriptive and correlational studies only measure variables.
- In an experimental design , you can assess a cause-and-effect relationship (e.g., the effect of meditation on test scores) using statistical tests of comparison or regression.
- In a correlational design , you can explore relationships between variables (e.g., parental income and GPA) without any assumption of causality using correlation coefficients and significance tests.
- In a descriptive design , you can study the characteristics of a population or phenomenon (e.g., the prevalence of anxiety in U.S. college students) using statistical tests to draw inferences from sample data.
Your research design also concerns whether you’ll compare participants at the group level or individual level, or both.
- In a between-subjects design , you compare the group-level outcomes of participants who have been exposed to different treatments (e.g., those who performed a meditation exercise vs those who didn’t).
- In a within-subjects design , you compare repeated measures from participants who have participated in all treatments of a study (e.g., scores from before and after performing a meditation exercise).
- In a mixed (factorial) design , one variable is altered between subjects and another is altered within subjects (e.g., pretest and posttest scores from participants who either did or didn’t do a meditation exercise).
- Experimental
- Correlational
First, you’ll take baseline test scores from participants. Then, your participants will undergo a 5-minute meditation exercise. Finally, you’ll record participants’ scores from a second math test.
In this experiment, the independent variable is the 5-minute meditation exercise, and the dependent variable is the math test score from before and after the intervention. Example: Correlational research design In a correlational study, you test whether there is a relationship between parental income and GPA in graduating college students. To collect your data, you will ask participants to fill in a survey and self-report their parents’ incomes and their own GPA.
Measuring variables
When planning a research design, you should operationalize your variables and decide exactly how you will measure them.
For statistical analysis, it’s important to consider the level of measurement of your variables, which tells you what kind of data they contain:
- Categorical data represents groupings. These may be nominal (e.g., gender) or ordinal (e.g. level of language ability).
- Quantitative data represents amounts. These may be on an interval scale (e.g. test score) or a ratio scale (e.g. age).
Many variables can be measured at different levels of precision. For example, age data can be quantitative (8 years old) or categorical (young). If a variable is coded numerically (e.g., level of agreement from 1–5), it doesn’t automatically mean that it’s quantitative instead of categorical.
Identifying the measurement level is important for choosing appropriate statistics and hypothesis tests. For example, you can calculate a mean score with quantitative data, but not with categorical data.
In a research study, along with measures of your variables of interest, you’ll often collect data on relevant participant characteristics.
Here's why students love Scribbr's proofreading services
Discover proofreading & editing
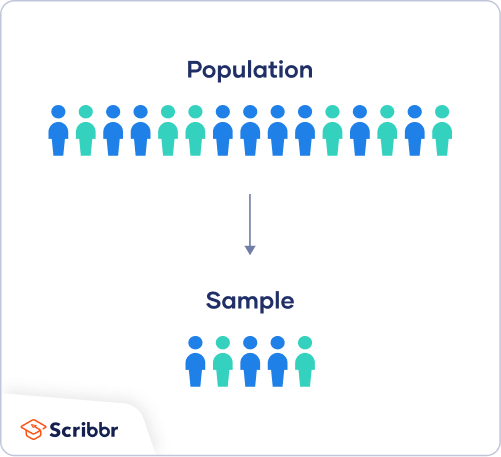
In most cases, it’s too difficult or expensive to collect data from every member of the population you’re interested in studying. Instead, you’ll collect data from a sample.
Statistical analysis allows you to apply your findings beyond your own sample as long as you use appropriate sampling procedures . You should aim for a sample that is representative of the population.
Sampling for statistical analysis
There are two main approaches to selecting a sample.
- Probability sampling: every member of the population has a chance of being selected for the study through random selection.
- Non-probability sampling: some members of the population are more likely than others to be selected for the study because of criteria such as convenience or voluntary self-selection.
In theory, for highly generalizable findings, you should use a probability sampling method. Random selection reduces several types of research bias , like sampling bias , and ensures that data from your sample is actually typical of the population. Parametric tests can be used to make strong statistical inferences when data are collected using probability sampling.
But in practice, it’s rarely possible to gather the ideal sample. While non-probability samples are more likely to at risk for biases like self-selection bias , they are much easier to recruit and collect data from. Non-parametric tests are more appropriate for non-probability samples, but they result in weaker inferences about the population.
If you want to use parametric tests for non-probability samples, you have to make the case that:
- your sample is representative of the population you’re generalizing your findings to.
- your sample lacks systematic bias.
Keep in mind that external validity means that you can only generalize your conclusions to others who share the characteristics of your sample. For instance, results from Western, Educated, Industrialized, Rich and Democratic samples (e.g., college students in the US) aren’t automatically applicable to all non-WEIRD populations.
If you apply parametric tests to data from non-probability samples, be sure to elaborate on the limitations of how far your results can be generalized in your discussion section .
Create an appropriate sampling procedure
Based on the resources available for your research, decide on how you’ll recruit participants.
- Will you have resources to advertise your study widely, including outside of your university setting?
- Will you have the means to recruit a diverse sample that represents a broad population?
- Do you have time to contact and follow up with members of hard-to-reach groups?
Your participants are self-selected by their schools. Although you’re using a non-probability sample, you aim for a diverse and representative sample. Example: Sampling (correlational study) Your main population of interest is male college students in the US. Using social media advertising, you recruit senior-year male college students from a smaller subpopulation: seven universities in the Boston area.
Calculate sufficient sample size
Before recruiting participants, decide on your sample size either by looking at other studies in your field or using statistics. A sample that’s too small may be unrepresentative of the sample, while a sample that’s too large will be more costly than necessary.
There are many sample size calculators online. Different formulas are used depending on whether you have subgroups or how rigorous your study should be (e.g., in clinical research). As a rule of thumb, a minimum of 30 units or more per subgroup is necessary.
To use these calculators, you have to understand and input these key components:
- Significance level (alpha): the risk of rejecting a true null hypothesis that you are willing to take, usually set at 5%.
- Statistical power : the probability of your study detecting an effect of a certain size if there is one, usually 80% or higher.
- Expected effect size : a standardized indication of how large the expected result of your study will be, usually based on other similar studies.
- Population standard deviation: an estimate of the population parameter based on a previous study or a pilot study of your own.
Once you’ve collected all of your data, you can inspect them and calculate descriptive statistics that summarize them.
Inspect your data
There are various ways to inspect your data, including the following:
- Organizing data from each variable in frequency distribution tables .
- Displaying data from a key variable in a bar chart to view the distribution of responses.
- Visualizing the relationship between two variables using a scatter plot .
By visualizing your data in tables and graphs, you can assess whether your data follow a skewed or normal distribution and whether there are any outliers or missing data.
A normal distribution means that your data are symmetrically distributed around a center where most values lie, with the values tapering off at the tail ends.
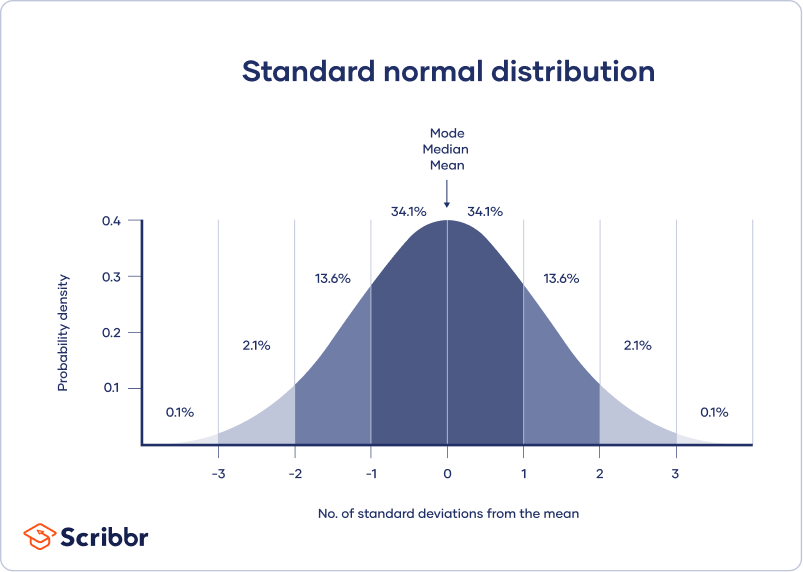
In contrast, a skewed distribution is asymmetric and has more values on one end than the other. The shape of the distribution is important to keep in mind because only some descriptive statistics should be used with skewed distributions.
Extreme outliers can also produce misleading statistics, so you may need a systematic approach to dealing with these values.
Calculate measures of central tendency
Measures of central tendency describe where most of the values in a data set lie. Three main measures of central tendency are often reported:
- Mode : the most popular response or value in the data set.
- Median : the value in the exact middle of the data set when ordered from low to high.
- Mean : the sum of all values divided by the number of values.
However, depending on the shape of the distribution and level of measurement, only one or two of these measures may be appropriate. For example, many demographic characteristics can only be described using the mode or proportions, while a variable like reaction time may not have a mode at all.
Calculate measures of variability
Measures of variability tell you how spread out the values in a data set are. Four main measures of variability are often reported:
- Range : the highest value minus the lowest value of the data set.
- Interquartile range : the range of the middle half of the data set.
- Standard deviation : the average distance between each value in your data set and the mean.
- Variance : the square of the standard deviation.
Once again, the shape of the distribution and level of measurement should guide your choice of variability statistics. The interquartile range is the best measure for skewed distributions, while standard deviation and variance provide the best information for normal distributions.
Using your table, you should check whether the units of the descriptive statistics are comparable for pretest and posttest scores. For example, are the variance levels similar across the groups? Are there any extreme values? If there are, you may need to identify and remove extreme outliers in your data set or transform your data before performing a statistical test.
From this table, we can see that the mean score increased after the meditation exercise, and the variances of the two scores are comparable. Next, we can perform a statistical test to find out if this improvement in test scores is statistically significant in the population. Example: Descriptive statistics (correlational study) After collecting data from 653 students, you tabulate descriptive statistics for annual parental income and GPA.
It’s important to check whether you have a broad range of data points. If you don’t, your data may be skewed towards some groups more than others (e.g., high academic achievers), and only limited inferences can be made about a relationship.
A number that describes a sample is called a statistic , while a number describing a population is called a parameter . Using inferential statistics , you can make conclusions about population parameters based on sample statistics.
Researchers often use two main methods (simultaneously) to make inferences in statistics.
- Estimation: calculating population parameters based on sample statistics.
- Hypothesis testing: a formal process for testing research predictions about the population using samples.
You can make two types of estimates of population parameters from sample statistics:
- A point estimate : a value that represents your best guess of the exact parameter.
- An interval estimate : a range of values that represent your best guess of where the parameter lies.
If your aim is to infer and report population characteristics from sample data, it’s best to use both point and interval estimates in your paper.
You can consider a sample statistic a point estimate for the population parameter when you have a representative sample (e.g., in a wide public opinion poll, the proportion of a sample that supports the current government is taken as the population proportion of government supporters).
There’s always error involved in estimation, so you should also provide a confidence interval as an interval estimate to show the variability around a point estimate.
A confidence interval uses the standard error and the z score from the standard normal distribution to convey where you’d generally expect to find the population parameter most of the time.
Hypothesis testing
Using data from a sample, you can test hypotheses about relationships between variables in the population. Hypothesis testing starts with the assumption that the null hypothesis is true in the population, and you use statistical tests to assess whether the null hypothesis can be rejected or not.
Statistical tests determine where your sample data would lie on an expected distribution of sample data if the null hypothesis were true. These tests give two main outputs:
- A test statistic tells you how much your data differs from the null hypothesis of the test.
- A p value tells you the likelihood of obtaining your results if the null hypothesis is actually true in the population.
Statistical tests come in three main varieties:
- Comparison tests assess group differences in outcomes.
- Regression tests assess cause-and-effect relationships between variables.
- Correlation tests assess relationships between variables without assuming causation.
Your choice of statistical test depends on your research questions, research design, sampling method, and data characteristics.
Parametric tests
Parametric tests make powerful inferences about the population based on sample data. But to use them, some assumptions must be met, and only some types of variables can be used. If your data violate these assumptions, you can perform appropriate data transformations or use alternative non-parametric tests instead.
A regression models the extent to which changes in a predictor variable results in changes in outcome variable(s).
- A simple linear regression includes one predictor variable and one outcome variable.
- A multiple linear regression includes two or more predictor variables and one outcome variable.
Comparison tests usually compare the means of groups. These may be the means of different groups within a sample (e.g., a treatment and control group), the means of one sample group taken at different times (e.g., pretest and posttest scores), or a sample mean and a population mean.
- A t test is for exactly 1 or 2 groups when the sample is small (30 or less).
- A z test is for exactly 1 or 2 groups when the sample is large.
- An ANOVA is for 3 or more groups.
The z and t tests have subtypes based on the number and types of samples and the hypotheses:
- If you have only one sample that you want to compare to a population mean, use a one-sample test .
- If you have paired measurements (within-subjects design), use a dependent (paired) samples test .
- If you have completely separate measurements from two unmatched groups (between-subjects design), use an independent (unpaired) samples test .
- If you expect a difference between groups in a specific direction, use a one-tailed test .
- If you don’t have any expectations for the direction of a difference between groups, use a two-tailed test .
The only parametric correlation test is Pearson’s r . The correlation coefficient ( r ) tells you the strength of a linear relationship between two quantitative variables.
However, to test whether the correlation in the sample is strong enough to be important in the population, you also need to perform a significance test of the correlation coefficient, usually a t test, to obtain a p value. This test uses your sample size to calculate how much the correlation coefficient differs from zero in the population.
You use a dependent-samples, one-tailed t test to assess whether the meditation exercise significantly improved math test scores. The test gives you:
- a t value (test statistic) of 3.00
- a p value of 0.0028
Although Pearson’s r is a test statistic, it doesn’t tell you anything about how significant the correlation is in the population. You also need to test whether this sample correlation coefficient is large enough to demonstrate a correlation in the population.
A t test can also determine how significantly a correlation coefficient differs from zero based on sample size. Since you expect a positive correlation between parental income and GPA, you use a one-sample, one-tailed t test. The t test gives you:
- a t value of 3.08
- a p value of 0.001
Prevent plagiarism. Run a free check.
The final step of statistical analysis is interpreting your results.
Statistical significance
In hypothesis testing, statistical significance is the main criterion for forming conclusions. You compare your p value to a set significance level (usually 0.05) to decide whether your results are statistically significant or non-significant.
Statistically significant results are considered unlikely to have arisen solely due to chance. There is only a very low chance of such a result occurring if the null hypothesis is true in the population.
This means that you believe the meditation intervention, rather than random factors, directly caused the increase in test scores. Example: Interpret your results (correlational study) You compare your p value of 0.001 to your significance threshold of 0.05. With a p value under this threshold, you can reject the null hypothesis. This indicates a statistically significant correlation between parental income and GPA in male college students.
Note that correlation doesn’t always mean causation, because there are often many underlying factors contributing to a complex variable like GPA. Even if one variable is related to another, this may be because of a third variable influencing both of them, or indirect links between the two variables.
Effect size
A statistically significant result doesn’t necessarily mean that there are important real life applications or clinical outcomes for a finding.
In contrast, the effect size indicates the practical significance of your results. It’s important to report effect sizes along with your inferential statistics for a complete picture of your results. You should also report interval estimates of effect sizes if you’re writing an APA style paper .
With a Cohen’s d of 0.72, there’s medium to high practical significance to your finding that the meditation exercise improved test scores. Example: Effect size (correlational study) To determine the effect size of the correlation coefficient, you compare your Pearson’s r value to Cohen’s effect size criteria.
Decision errors
Type I and Type II errors are mistakes made in research conclusions. A Type I error means rejecting the null hypothesis when it’s actually true, while a Type II error means failing to reject the null hypothesis when it’s false.
You can aim to minimize the risk of these errors by selecting an optimal significance level and ensuring high power . However, there’s a trade-off between the two errors, so a fine balance is necessary.
Frequentist versus Bayesian statistics
Traditionally, frequentist statistics emphasizes null hypothesis significance testing and always starts with the assumption of a true null hypothesis.
However, Bayesian statistics has grown in popularity as an alternative approach in the last few decades. In this approach, you use previous research to continually update your hypotheses based on your expectations and observations.
Bayes factor compares the relative strength of evidence for the null versus the alternative hypothesis rather than making a conclusion about rejecting the null hypothesis or not.
If you want to know more about statistics , methodology , or research bias , make sure to check out some of our other articles with explanations and examples.
- Student’s t -distribution
- Normal distribution
- Null and Alternative Hypotheses
- Chi square tests
- Confidence interval
Methodology
- Cluster sampling
- Stratified sampling
- Data cleansing
- Reproducibility vs Replicability
- Peer review
- Likert scale
Research bias
- Implicit bias
- Framing effect
- Cognitive bias
- Placebo effect
- Hawthorne effect
- Hostile attribution bias
- Affect heuristic
Is this article helpful?
Other students also liked.
- Descriptive Statistics | Definitions, Types, Examples
- Inferential Statistics | An Easy Introduction & Examples
- Choosing the Right Statistical Test | Types & Examples
More interesting articles
- Akaike Information Criterion | When & How to Use It (Example)
- An Easy Introduction to Statistical Significance (With Examples)
- An Introduction to t Tests | Definitions, Formula and Examples
- ANOVA in R | A Complete Step-by-Step Guide with Examples
- Central Limit Theorem | Formula, Definition & Examples
- Central Tendency | Understanding the Mean, Median & Mode
- Chi-Square (Χ²) Distributions | Definition & Examples
- Chi-Square (Χ²) Table | Examples & Downloadable Table
- Chi-Square (Χ²) Tests | Types, Formula & Examples
- Chi-Square Goodness of Fit Test | Formula, Guide & Examples
- Chi-Square Test of Independence | Formula, Guide & Examples
- Coefficient of Determination (R²) | Calculation & Interpretation
- Correlation Coefficient | Types, Formulas & Examples
- Frequency Distribution | Tables, Types & Examples
- How to Calculate Standard Deviation (Guide) | Calculator & Examples
- How to Calculate Variance | Calculator, Analysis & Examples
- How to Find Degrees of Freedom | Definition & Formula
- How to Find Interquartile Range (IQR) | Calculator & Examples
- How to Find Outliers | 4 Ways with Examples & Explanation
- How to Find the Geometric Mean | Calculator & Formula
- How to Find the Mean | Definition, Examples & Calculator
- How to Find the Median | Definition, Examples & Calculator
- How to Find the Mode | Definition, Examples & Calculator
- How to Find the Range of a Data Set | Calculator & Formula
- Hypothesis Testing | A Step-by-Step Guide with Easy Examples
- Interval Data and How to Analyze It | Definitions & Examples
- Levels of Measurement | Nominal, Ordinal, Interval and Ratio
- Linear Regression in R | A Step-by-Step Guide & Examples
- Missing Data | Types, Explanation, & Imputation
- Multiple Linear Regression | A Quick Guide (Examples)
- Nominal Data | Definition, Examples, Data Collection & Analysis
- Normal Distribution | Examples, Formulas, & Uses
- Null and Alternative Hypotheses | Definitions & Examples
- One-way ANOVA | When and How to Use It (With Examples)
- Ordinal Data | Definition, Examples, Data Collection & Analysis
- Parameter vs Statistic | Definitions, Differences & Examples
- Pearson Correlation Coefficient (r) | Guide & Examples
- Poisson Distributions | Definition, Formula & Examples
- Probability Distribution | Formula, Types, & Examples
- Quartiles & Quantiles | Calculation, Definition & Interpretation
- Ratio Scales | Definition, Examples, & Data Analysis
- Simple Linear Regression | An Easy Introduction & Examples
- Skewness | Definition, Examples & Formula
- Statistical Power and Why It Matters | A Simple Introduction
- Student's t Table (Free Download) | Guide & Examples
- T-distribution: What it is and how to use it
- Test statistics | Definition, Interpretation, and Examples
- The Standard Normal Distribution | Calculator, Examples & Uses
- Two-Way ANOVA | Examples & When To Use It
- Type I & Type II Errors | Differences, Examples, Visualizations
- Understanding Confidence Intervals | Easy Examples & Formulas
- Understanding P values | Definition and Examples
- Variability | Calculating Range, IQR, Variance, Standard Deviation
- What is Effect Size and Why Does It Matter? (Examples)
- What Is Kurtosis? | Definition, Examples & Formula
- What Is Standard Error? | How to Calculate (Guide with Examples)
What is your plagiarism score?
Share this page
This program has a rich tradition of creating groundbreaking statistical methods and conducting innovative applied statistics, bridging theory and practice and supporting knowledge discovery and decision-making through meaningful data extraction and analysis. Statistics is an indispensable pillar of modern science, including data science and artificial intelligence.
You can take advantage of the department’s flexible research options and work with your faculty of choice. You can leverage cross-department collaboration with biology, chemistry, medical sciences, economics, computer science, government, and public health to pursue your intellectual interests. You will become part of a close-knit, friendly department that offers many extra learning opportunities both inside and outside the program.
Examples of student projects include developing statistical methods to forecast infectious diseases from online search data, delineating causality from association, building a software package for evaluating redistricting plans in 50 states, leveraging machine learning algorithms for model-free inference, and employing a randomization-based inference framework to study peer effects.
Graduates have secured faculty positions in institutions such as Stanford University; University of Pennsylvania; University of California, Berkeley; Johns Hopkins University; Carnegie Mellon University; Columbia University; and Georgia Institute of Technology. Others have begun careers at organizations such as Google, Apple, Etsy, Citadel, and the Boston Red Sox.
Additional information on the graduate program is available from the Department of Statistics , and requirements for the degree are detailed in Policies .
Admissions Requirements
Please review admissions requirements and other information before applying. You can find degree program-specific admissions requirements below and access additional guidance on applying from the Department of Statistics .
Academic Background
Applicants should understand what the discipline of statistics entails and show evidence of involvement in applications or a strong theoretical interest.
The minimum mathematical preparation for admission is linear algebra and advanced calculus. Ideally, each student’s preparation should include at least one term each of mathematical probability and mathematical statistics. Additional study in statistics and related mathematical areas, such as analysis and measure theory, is helpful. In the initial stages of graduate study, students should give high priority to acquiring the mathematical level required to satisfy their objectives.
As statistics is so intimately connected with computation, computation is an important part of almost all courses and research projects in the department. Preferably, students should have programming experience relevant for statistical computation and simulation.
Standardized Tests
GRE General: Optional GRE Subject: Optional
Theses & Dissertations
Theses & Dissertations for Statistics
See list of Statistics faculty
APPLICATION DEADLINE
Questions about the program.
Statistics and R
An introduction to basic statistical concepts and R programming skills necessary for analyzing data in the life sciences.
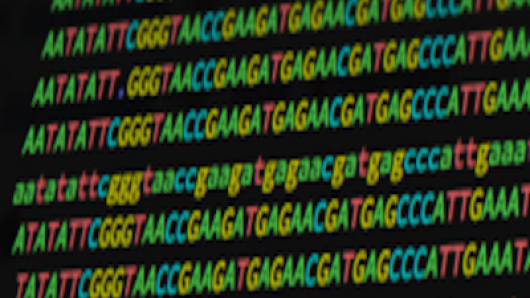
Associated Schools
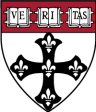
Harvard T.H. Chan School of Public Health
What you'll learn.
Random variables
Distributions
Inference: p-values and confidence intervals
Exploratory Data Analysis
Non-parametric statistics
Course description
We will learn the basics of statistical inference in order to understand and compute p-values and confidence intervals, all while analyzing data with R. We provide R programming examples in a way that will help make the connection between concepts and implementation. Problem sets requiring R programming will be used to test understanding and ability to implement basic data analyses. We will use visualization techniques to explore new data sets and determine the most appropriate approach. We will describe robust statistical techniques as alternatives when data do not fit assumptions required by the standard approaches. By using R scripts to analyze data, you will learn the basics of conducting reproducible research.
Given the diversity in educational background of our students we have divided the series into seven parts. You can take the entire series or individual courses that interest you. If you are a statistician you should consider skipping the first two or three courses, similarly, if you are biologists you should consider skipping some of the introductory biology lectures. Note that the statistics and programming aspects of the class ramp up in difficulty relatively quickly across the first three courses. By the third course will be teaching advanced statistical concepts such as hierarchical models and by the fourth advanced software engineering skills, such as parallel computing and reproducible research concepts.

Instructors
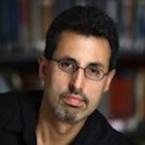
Rafael Irizarry
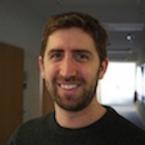
Michael Love
You may also like.
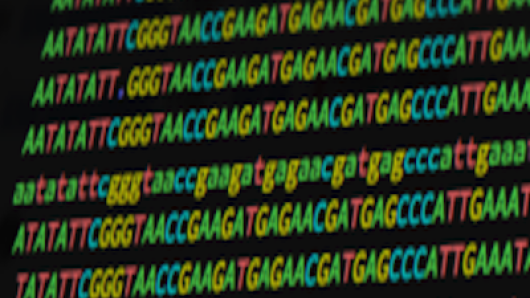
Introduction to Linear Models and Matrix Algebra
Learn to use R programming to apply linear models to analyze data in life sciences.
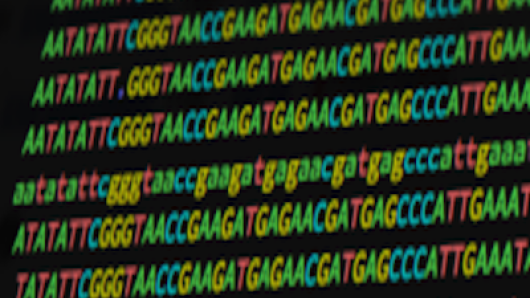
Case Studies in Functional Genomics
Perform RNA-Seq, ChIP-Seq, and DNA methylation data analyses, using open source software, including R and Bioconductor.
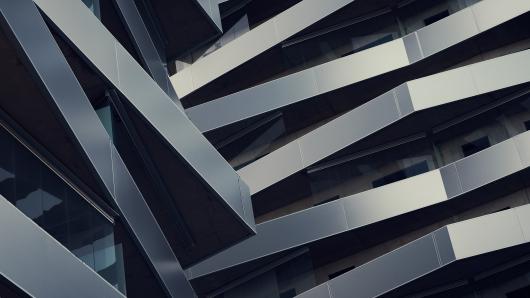
Data Science: Productivity Tools
Keep your projects organized and produce reproducible reports using GitHub, git, Unix/Linux, and RStudio.

Statistics Made Easy
The Importance of Statistics in Research (With Examples)
The field of statistics is concerned with collecting, analyzing, interpreting, and presenting data.
In the field of research, statistics is important for the following reasons:
Reason 1 : Statistics allows researchers to design studies such that the findings from the studies can be extrapolated to a larger population.
Reason 2 : Statistics allows researchers to perform hypothesis tests to determine if some claim about a new drug, new procedure, new manufacturing method, etc. is true.
Reason 3 : Statistics allows researchers to create confidence intervals to capture uncertainty around population estimates.
In the rest of this article, we elaborate on each of these reasons.
Reason 1: Statistics Allows Researchers to Design Studies
Researchers are often interested in answering questions about populations like:
- What is the average weight of a certain species of bird?
- What is the average height of a certain species of plant?
- What percentage of citizens in a certain city support a certain law?
One way to answer these questions is to go around and collect data on every single individual in the population of interest.
However, this is typically too costly and time-consuming which is why researchers instead take a sample of the population and use the data from the sample to draw conclusions about the population as a whole.

There are many different methods researchers can potentially use to obtain individuals to be in a sample. These are known as sampling methods .
There are two classes of sampling methods:
- Probability sampling methods : Every member in a population has an equal probability of being selected to be in the sample.
- Non-probability sampling methods : Not every member in a population has an equal probability of being selected to be in the sample.
By using probability sampling methods, researchers can maximize the chances that they obtain a sample that is representative of the overall population.
This allows researchers to extrapolate the findings from the sample to the overall population.
Read more about the two classes of sampling methods here .
Reason 2: Statistics Allows Researchers to Perform Hypothesis Tests
Another way that statistics is used in research is in the form of hypothesis tests .
These are tests that researchers can use to determine if there is a statistical significance between different medical procedures or treatments.
For example, suppose a scientist believes that a new drug is able to reduce blood pressure in obese patients. To test this, he measures the blood pressure of 30 patients before and after using the new drug for one month.
He then performs a paired samples t- test using the following hypotheses:
- H 0 : μ after = μ before (the mean blood pressure is the same before and after using the drug)
- H A : μ after < μ before (the mean blood pressure is less after using the drug)
If the p-value of the test is less than some significance level (e.g. α = .05), then he can reject the null hypothesis and conclude that the new drug leads to reduced blood pressure.
Note : This is just one example of a hypothesis test that is used in research. Other common tests include a one sample t-test , two sample t-test , one-way ANOVA , and two-way ANOVA .
Reason 3: Statistics Allows Researchers to Create Confidence Intervals
Another way that statistics is used in research is in the form of confidence intervals .
A confidence interval is a range of values that is likely to contain a population parameter with a certain level of confidence.
For example, suppose researchers are interested in estimating the mean weight of a certain species of turtle.
Instead of going around and weighing every single turtle in the population, researchers may instead take a simple random sample of turtles with the following information:
- Sample size n = 25
- Sample mean weight x = 300
- Sample standard deviation s = 18.5
Using the confidence interval for a mean formula , researchers may then construct the following 95% confidence interval:
95% Confidence Interval: 300 +/- 1.96*(18.5/√ 25 ) = [292.75, 307.25]
The researchers would then claim that they’re 95% confident that the true mean weight for this population of turtles is between 292.75 pounds and 307.25 pounds.
Additional Resources
The following articles explain the importance of statistics in other fields:
The Importance of Statistics in Healthcare The Importance of Statistics in Nursing The Importance of Statistics in Business The Importance of Statistics in Economics The Importance of Statistics in Education

Published by Zach
Leave a reply cancel reply.
Your email address will not be published. Required fields are marked *
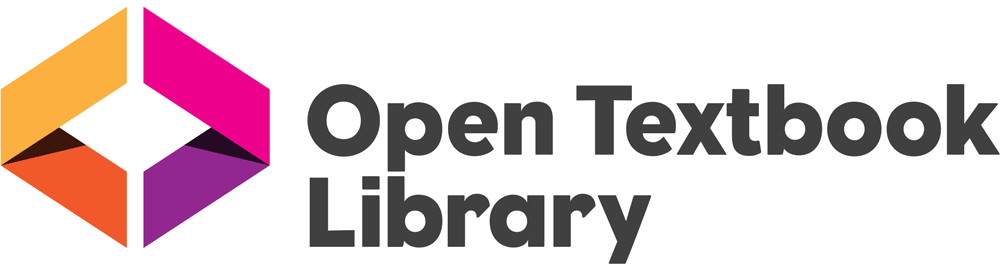
Introduction to Statistics
(15 reviews)

David Lane, Rice University
Copyright Year: 2003
Publisher: David Lane
Language: English
Formats Available
Conditions of use.

Learn more about reviews.
Reviewed by Terri Torres, professor, Oregon Institute of Technology on 8/17/23
This author covers all the topics that would be covered in an introductory statistics course plus some. I could imagine using it for two courses at my university, which is on the quarter system. I would rather have the problem of too many topics... read more
Comprehensiveness rating: 5 see less
This author covers all the topics that would be covered in an introductory statistics course plus some. I could imagine using it for two courses at my university, which is on the quarter system. I would rather have the problem of too many topics rather than too few.
Content Accuracy rating: 5
Yes, Lane is both thorough and accurate.
Relevance/Longevity rating: 5
What is covered is what is usually covered in an introductory statistics book. The only topic I may, given sufficient time, cover is bootstrapping.
Clarity rating: 5
The book is clear and well-written. For the trickier topics, simulations are included to help with understanding.
Consistency rating: 5
All is organized in a way that is consistent with the previous topic.
Modularity rating: 5
The text is organized in a way that easily enables navigation.
Organization/Structure/Flow rating: 5
The text is organized like most statistics texts.
Interface rating: 5
Easy navigation.
Grammatical Errors rating: 5
I didn't see any grammatical errors.
Cultural Relevance rating: 5
Nothing is included that is culturally insensitive.
The videos that accompany this text are short and easy to watch and understand. Videos should be short enough to teach, but not so long that they are tiresome. This text includes almost everything: videos, simulations, case studies---all nicely organized in one spot. In addition, Lane has promised to send an instructor's manual and slide deck.
Reviewed by Professor Sandberg, Professor, Framingham State University on 6/29/21
This text covers all the usual topics in an Introduction to Statistics for college students. In addition, it has some additional topics that are useful. read more
This text covers all the usual topics in an Introduction to Statistics for college students. In addition, it has some additional topics that are useful.
I did not find any errors.
Some of the examples are dated. And the frequent use of male/female examples need updating in terms of current gender splits.
I found it was easy to read and understand and I expect that students would also find the writing clear and the explanations accessible.
Even with different authors of chapter, the writing is consistent.
The text is well organized into sections making it easy to assign individual topics and sections.
The topics are presented in the usual order. Regression comes later in the text but there is a difference of opinions about whether to present it early with descriptive statistics for bivariate data or later with inferential statistics.
I had no problem navigating the text online.
The writing is grammatical correct.
I saw no issues that would be offensive.
I did like this text. It seems like it would be a good choice for most introductory statistics courses. I liked that the Monty Hall problem was included in the probability section. The author offers to provide an instructor's manual, PowerPoint slides and additional questions. These additional resources are very helpful and not always available with online OER texts.
Reviewed by Emilio Vazquez, Associate Professor, Trine University on 4/23/21
This appears to be an excellent textbook for an Introductory Course in Statistics. It covers subjects in enough depth to fulfill the needs of a beginner in Statistics work yet is not so complex as to be overwhelming. read more
This appears to be an excellent textbook for an Introductory Course in Statistics. It covers subjects in enough depth to fulfill the needs of a beginner in Statistics work yet is not so complex as to be overwhelming.
I found no errors in their discussions. Did not work out all of the questions and answers but my sampling did not reveal any errors.
Some of the examples may need updating depending on the times but the examples are still relevant at this time.
This is a Statistics text so a little dry. I found that the derivation of some of the formulas was not explained. However the background is there to allow the instructor to derive these in class if desired.
The text is consistent throughout using the same verbiage in various sections.
The text dose lend itself to reasonable reading assignments. For example the chapter (Chapter 3) on Summarizing Distributions covers Central Tendency and its associated components in an easy 20 pages with Measures of Variability making up most of the rest of the chapter and covering approximately another 20 pages. Exercises are available at the end of each chapter making it easy for the instructor to assign reading and exercises to be discussed in class.
The textbook flows easily from Descriptive to Inferential Statistics with chapters on Sampling and Estimation preceding chapters on hypothesis testing
I had no problems with navigation
All textbooks have a few errors but certainly nothing glaring or making text difficult
I saw no issues and I am part of a cultural minority in the US
Overall I found this to be a excellent in-depth overview of Statistical Theory, Concepts and Analysis. The length of the textbook appears to be more than adequate for a one-semester course in Introduction to Statistics. As I no longer teach a full statistics course but simply a few lectures as part of our Research Curriculum, I am recommending this book to my students as a good reference. Especially as it is available on-line and in Open Access.
Reviewed by Audrey Hickert, Assistant Professor, Southern Illinois University Carbondale on 3/29/21
All of the major topics of an introductory level statistics course for social science are covered. Background areas include levels of measurement and research design basics. Descriptive statistics include all major measures of central tendency and... read more
All of the major topics of an introductory level statistics course for social science are covered. Background areas include levels of measurement and research design basics. Descriptive statistics include all major measures of central tendency and dispersion/variation. Building blocks for inferential statistics include sampling distributions, the standard normal curve (z scores), and hypothesis testing sections. Inferential statistics include how to calculate confidence intervals, as well as conduct tests of one-sample tests of the population mean (Z- and t-tests), two-sample tests of the difference in population means (Z- and t-tests), chi square test of independence, correlation, and regression. Doesn’t include full probability distribution tables (e.g., t or Z), but those can be easily found online in many places.
I did not find any errors or issues of inaccuracy. When a particular method or practice is debated in the field, the authors acknowledge it (and provide citations in some circumstances).
Relevance/Longevity rating: 4
Basic statistics are standard, so the core information will remain relevant in perpetuity. Some of the examples are dated (e.g., salaries from 1999), but not problematic.
Clarity rating: 4
All of the key terms, formulas, and logic for statistical tests are clearly explained. The book sometimes uses different notation than other entry-level books. For example, the variance formula uses "M" for mean, rather than x-bar.
The explanations are consistent and build from and relate to corresponding sections that are listed in each unit.
Modularity is a strength of this text in both the PDF and interactive online format. Students can easily navigate to the necessary sections and each starts with a “Prerequisites” list of other sections in the book for those who need the additional background material. Instructors could easily compile concise sub-sections of the book for readings.
The presentation of topics differs somewhat from the standard introductory social science statistics textbooks I have used before. However, the modularity allows the instructor and student to work through the discrete sections in the desired order.
Interface rating: 4
For the most part the display of all images/charts is good and navigation is straightforward. One concern is that the organization of the Table of Contents does not exactly match the organizational outline at the start of each chapter in the PDF version. For example, sometimes there are more detailed sub-headings at the start of chapter and occasionally slightly different section headings/titles. There are also inconsistencies in section listings at start of chapters vs. start of sub-sections.
The text is easy to read and free from any obvious grammatical errors.
Although some of the examples are outdated, I did not review any that were offensive. One example of an outdated reference is using descriptive data on “Men per 100 Women” in U.S. cities as “useful if we are looking for an opposite-sex partner”.
This is a good introduction level statistics text book if you have a course with students who may be intimated by longer texts with more detailed information. Just the core basics are provided here and it is easy to select the sections you need. It is a good text if you plan to supplement with an array of your own materials (lectures, practice, etc.) that are specifically tailored to your discipline (e.g., criminal justice and criminology). Be advised that some formulas use different notation than other standard texts, so you will need to point that out to students if they differ from your lectures or assessment materials.
Reviewed by Shahar Boneh, Professor, Metropolitan State University of Denver on 3/26/21, updated 4/22/21
The textbook is indeed quite comprehensive. It can accommodate any style of introductory statistics course. read more
The textbook is indeed quite comprehensive. It can accommodate any style of introductory statistics course.
The text seems to be statistically accurate.
It is a little too extensive, which requires instructors to cover it selectively, and has a potential to confuse the students.
It is written clearly.
Consistency rating: 4
The terminology is fairly consistent. There is room for some improvement.
By the nature of the subject, the topics have to be presented in a sequential and coherent order. However, the book breaks things down quite effectively.
Organization/Structure/Flow rating: 3
Some of the topics are interleaved and not presented in the order I would like to cover them.
Good interface.
The grammar is ok.
The book seems to be culturally neutral, and not offensive in any way.
I really liked the simulations that go with the book. Parts of the book are a little too advanced for students who are learning statistics for the first time.
Reviewed by Julie Gray, Adjunct Assistant Professor, University of Texas at Arlington on 2/26/21
The textbook is for beginner-level students. The concept development is appropriate--there is always room to grow to high higher level, but for an introduction, the basics are what is needed. This is a well-thought-through OER textbook project by... read more
The textbook is for beginner-level students. The concept development is appropriate--there is always room to grow to high higher level, but for an introduction, the basics are what is needed. This is a well-thought-through OER textbook project by Dr. Lane and colleagues. It is obvious that several iterations have only made it better.
I found all the material accurate.
Essentially, statistical concepts at the introductory level are accepted as universal. This suggests that the relevance of this textbook will continue for a long time.
The book is well written for introducing beginners to statistical concepts. The figures, tables, and animated examples reinforce the clarity of the written text.
Yes, the information is consistent; when it is introduced in early chapters it ties in well in later chapters that build on and add more understanding for the topic.
Modularity rating: 4
The book is well-written with attention to modularity where possible. Due to the nature of statistics, that is not always possible. The content is presented in the order that I usually teach these concepts.
The organization of the book is good, I particularly like the sample lecture slide presentations and the problem set with solutions for use in quizzes and exams. These are available by writing to the author. It is wonderful to have access to these helpful resources for instructors to use in preparation.
I did not find any interface issues.
The book is well written. In my reading I did not notice grammatical errors.
For this subject and in the examples given, I did not notice any cultural issues.
For the field of social work where qualitative data is as common as quantitative, the importance of giving students the rationale or the motivation to learn the quantitative side is understated. To use this text as an introductory statistics OER textbook in a social work curriculum, the instructor will want to bring in field-relevant examples to engage and motivate students. The field needs data-driven decision making and evidence-based practices to become more ubiquitous than not. Preparing future social workers by teaching introductory statistics is essential to meet that goal.
Reviewed by Mamata Marme, Assistant Professor, Augustana College on 6/25/19
This textbook offers a fairly comprehensive summary of what should be discussed in an introductory course in Statistics. The statistical literacy exercises are particularly interesting. It would be helpful to have the statistical tables... read more
Comprehensiveness rating: 4 see less
This textbook offers a fairly comprehensive summary of what should be discussed in an introductory course in Statistics. The statistical literacy exercises are particularly interesting. It would be helpful to have the statistical tables attached in the same package, even though they are available online.
The terminology and notation used in the textbook is pretty standard. The content is accurate.
The statistical literacy example are up to date but will need to be updated fairly regularly to keep the textbook fresh. The applications within the chapter are accessible and can be used fairly easily over a couple of editions.
The textbook does not necessarily explain the derivation of some of the formulae and this will need to be augmented by the instructor in class discussion. What is beneficial is that there are multiple ways that a topic is discussed using graphs, calculations and explanations of the results. Statistics textbooks have to cover a wide variety of topics with a fair amount of depth. To do this concisely is difficult. There is a fine line between being concise and clear, which this textbook does well, and being somewhat dry. It may be up to the instructor to bring case studies into the readings we are going through the topics rather than wait until the end of the chapter.
The textbook uses standard notation and terminology. The heading section of each chapter is closely tied to topics that are covered. The end of chapter problems and the statistical literacy applications are closely tied to the material covered.
The authors have done a good job treating each chapter as if they stand alone. The lack of connection to a past reference may create a sense of disconnect between the topics discussed
The text's "modularity" does make the flow of the material a little disconnected. If would be better if there was accountability of what a student should already have learnt in a different section. The earlier material is easy to find but not consistently referred to in the text.
I had no problem with the interface. The online version is more visually interesting than the pdf version.
I did not see any grammatical errors.
Cultural Relevance rating: 4
I am not sure how to evaluate this. The examples are mostly based on the American experience and the data alluded to mostly domestic. However, I am not sure if that creates a problem in understanding the methodology.
Overall, this textbook will cover most of the topics in a survey of statistics course.
Reviewed by Alexandra Verkhovtseva, Professor, Anoka-Ramsey Community College on 6/3/19
This is a comprehensive enough text, considering that it is not easy to create a comprehensive statistics textbook. It is suitable for an introductory statistics course for non-math majors. It contains twenty-one chapters, covering the wide range... read more
This is a comprehensive enough text, considering that it is not easy to create a comprehensive statistics textbook. It is suitable for an introductory statistics course for non-math majors. It contains twenty-one chapters, covering the wide range of intro stats topics (and some more), plus the case studies and the glossary.
The content is pretty accurate, I did not find any biases or errors.
The book contains fairly recent data presented in the form of exercises, examples and applications. The topics are up-to-date, and appropriate technology is used for examples, applications, and case studies.
The language is simple and clear, which is a good thing, since students are usually scared of this class, and instructors are looking for something to put them at ease. I would, however, try to make it a little more interesting, exciting, or may be even funny.
Consistency is good, the book has a great structure. I like how each chapter has prerequisites and learner outcomes, this gives students a good idea of what to expect. Material in this book is covered in good detail.
The text can be easily divided into sub-sections, some of which can be omitted if needed. The chapter on regression is covered towards the end (chapter 14), but part of it can be covered sooner in the course.
The book contains well organized chapters that makes reading through easy and understandable. The order of chapters and sections is clear and logical.
The online version has many functions and is easy to navigate. This book also comes with a PDF version. There is no distortion of images or charts. The text is clean and clear, the examples provided contain appropriate format of data presentation.
No grammatical errors found.
The text uses simple and clear language, which is helpful for non-native speakers. I would include more culturally-relevant examples and case studies. Overall, good text.
In all, this book is a good learning experience. It contains tools and techniques that free and easy to use and also easy to modify for both, students and instructors. I very much appreciate this opportunity to use this textbook at no cost for our students.
Reviewed by Dabrina Dutcher, Assistant Professor, Bucknell University on 3/4/19
This is a reasonably thorough first-semester statistics book for most classes. It would have worked well for the general statistics courses I have taught in the past but is not as suitable for specialized introductory statistics courses for... read more
This is a reasonably thorough first-semester statistics book for most classes. It would have worked well for the general statistics courses I have taught in the past but is not as suitable for specialized introductory statistics courses for engineers or business applications. That is OK, they have separate texts for that! The only sections that feel somewhat light in terms of content are the confidence intervals and ANOVA sections. Given that these topics are often sort of crammed in at the end of many introductory classes, that might not be problematic for many instructors. It should also be pointed out that while there are a couple of chapters on probability, this book spends presents most formulas as "black boxes" rather than worry about the derivation or origin of the formulas. The probability sections do not include any significant combinatorics work, which is sometimes included at this level.
I did not find any errors in the formulas presented but I did not work many end-of-chapter problems to gauge the accuracy of their answers.
There isn't much changing in the introductory stats world, so I have no concerns about the book becoming outdated rapidly. The examples and problems still feel relevant and reasonably modern. My only concern is that the statistical tool most often referenced in the book are TI-83/84 type calculators. As students increasingly buy TI-89s or Inspires, these sections of the book may lose relevance faster than other parts.
Solid. The book gives a list of key terms and their definitions at the end of each chapter which is a nice feature. It also has a formula review at the end of each chapter. I can imagine that these are heavily used by students when studying! Formulas are easy to find and read and are well defined. There are a few areas that I might have found frustrating as a student. For example, the explanation for the difference in formulas for a population vs sample standard deviation is quite weak. Again, this is a book that focuses on sort of a "black-box" approach but you may have to supplement such sections for some students.
I did not detect any problems with inconsistent symbol use or switches in terminology.
Modularity rating: 3
This low rating should not be taken as an indicator of an issue with this book but would be true of virtually any statistics book. Different books still use different variable symbols even for basic calculated statistics. So trying to use a chapter of this book without some sort of symbol/variable cheat-sheet would likely be frustrating to the students.
However, I think it would be possible to skip some chapters or use the chapters in a different order without any loss of functionality.
This book uses a very standard order for the material. The chapter on regressions comes later than it does in some texts but it doesn't really matter since that chapter never seems to fit smoothly anywhere.
There are numerous end of chapter problems, some with answers, available in this book. I'm vacillating on whether these problems would be more useful if they were distributed after each relevant section or are better clumped at the end of the whole chapter. That might be a matter of individual preference.
I did not detect any problems.
I found no errors. However, there were several sections where the punctuation seemed non-ideal. This did not affect the over-all useability of the book though
I'm not sure how well this book would work internationally as many of the examples contain domestic (American) references. However, I did not see anything offensive or biased in the book.
Reviewed by Ilgin Sager, Assistant Professor, University of Missouri - St. Louis on 1/14/19
As the title implies, this is a brief introduction textbook. It covers the fundamental of the introductory statistics, however not a comprehensive text on the subject. A teacher can use this book as the sole text of an introductory statistics.... read more
As the title implies, this is a brief introduction textbook. It covers the fundamental of the introductory statistics, however not a comprehensive text on the subject. A teacher can use this book as the sole text of an introductory statistics. The prose format of definitions and theorems make theoretical concepts accessible to non-math major students. The textbook covers all chapters required in this level course.
It is accurate; the subject matter in the examples to be up to date, is timeless and wouldn't need to be revised in future editions; there is no error except a few typographical errors. There are no logic errors or incorrect explanations.
This text will remain up to date for a long time since it has timeless examples and exercises, it wouldn't be outdated. The information is presented clearly with a simple way and the exercises are beneficial to follow the information.
The material is presented in a clear, concise manner. The text is easy readable for the first time statistics student.
The structure of the text is very consistent. Topics are presented with examples, followed by exercises. Problem sets are appropriate for the level of learner.
When the earlier matters need to be referenced, it is easy to find; no trouble reading the book and finding results, it has a consistent scheme. This book is set very well in sections.
The text presents the information in a logical order.
The learner can easily follow up the material; there is no interface problem.
There is no logic errors and incorrect explanations, a few typographical errors is just to be ignored.
Not applicable for this textbook.
Reviewed by Suhwon Lee, Associate Teaching Professor, University of Missouri on 6/19/18
This book is pretty comprehensive for being a brief introductory book. This book covers all necessary content areas for an introduction to Statistics course for non-math majors. The text book provides an effective index, plenty of exercises,... read more
This book is pretty comprehensive for being a brief introductory book. This book covers all necessary content areas for an introduction to Statistics course for non-math majors. The text book provides an effective index, plenty of exercises, review questions, and practice tests. It provides references and case studies. The glossary and index section is very helpful for students and can be used as a great resource.
Content appears to be accurate throughout. Being an introductory book, the book is unbiased and straight to the point. The terminology is standard.
The content in textbook is up to date. It will be very easy to update it or make changes at any point in time because of the well-structured contents in the textbook.
The author does a great job of explaining nearly every new term or concept. The book is easy to follow, clear and concise. The graphics are good to follow. The language in the book is easily understandable. I found most instructions in the book to be very detailed and clear for students to follow.
Overall consistency is good. It is consistent in terms of terminology and framework. The writing is straightforward and standardized throughout the text and it makes reading easier.
The authors do a great job of partitioning the text and labeling sections with appropriate headings. The table of contents is well organized and easily divisible into reading sections and it can be assigned at different points within the course.
Organization/Structure/Flow rating: 4
Overall, the topics are arranged in an order that follows natural progression in a statistics course with some exception. They are addressed logically and given adequate coverage.
The text is free of any issues. There are no navigation problems nor any display issues.
The text contains no grammatical errors.
The text is not culturally insensitive or offensive in any way most of time. Some examples might need to consider citing the sources or use differently to reflect current inclusive teaching strategies.
Overall, it's well-written and good recourse to be an introduction to statistical methods. Some materials may not need to be covered in an one-semester course. Various examples and quizzes can be a great recourse for instructor.
Reviewed by Jenna Kowalski, Mathematics Instructor, Anoka-Ramsey Community College on 3/27/18
The text includes the introductory statistics topics covered in a college-level semester course. An effective index and glossary are included, with functional hyperlinks. read more
The text includes the introductory statistics topics covered in a college-level semester course. An effective index and glossary are included, with functional hyperlinks.
Content Accuracy rating: 3
The content of this text is accurate and error-free, based on a random sampling of various pages throughout the text. Several examples included information without formal citation, leading the reader to potential bias and discrimination. These examples should be corrected to reflect current values of inclusive teaching.
The text contains relevant information that is current and will not become outdated in the near future. The statistical formulas and calculations have been used for centuries. The examples are direct applications of the formulas and accurately assess the conceptual knowledge of the reader.
The text is very clear and direct with the language used. The jargon does require a basic mathematical and/or statistical foundation to interpret, but this foundational requirement should be met with course prerequisites and placement testing. Graphs, tables, and visual displays are clearly labeled.
The terminology and framework of the text is consistent. The hyperlinks are working effectively, and the glossary is valuable. Each chapter contains modules that begin with prerequisite information and upcoming learning objectives for mastery.
The modules are clearly defined and can be used in conjunction with other modules, or individually to exemplify a choice topic. With the prerequisite information stated, the reader understands what prior mathematical understanding is required to successfully use the module.
The topics are presented well, but I recommend placing Sampling Distributions, Advanced Graphs, and Research Design ahead of Probability in the text. I think this rearranged version of the index would better align with current Introductory Statistics texts. The structure is very organized with the prerequisite information stated and upcoming learner outcomes highlighted. Each module is well-defined.
Adding an option of returning to the previous page would be of great value to the reader. While progressing through the text systematically, this is not an issue, but when the reader chooses to skip modules and read select pages then returning to the previous state of information is not easily accessible.
No grammatical errors were found while reviewing select pages of this text at random.
Cultural Relevance rating: 3
Several examples contained data that were not formally cited. These examples need to be corrected to reflect current inclusive teaching strategies. For example, one question stated that “while men are XX times more likely to commit murder than women, …” This data should be cited, otherwise the information can be interpreted as biased and offensive.
An included solutions manual for the exercises would be valuable to educators who choose to use this text.
Reviewed by Zaki Kuruppalil, Associate Professor, Ohio University on 2/1/18
This is a comprehensive book on statistical methods, its settings and most importantly the interpretation of the results. With the advent of computers and software’s, complex statistical analysis can be done very easily. But the challenge is the... read more
This is a comprehensive book on statistical methods, its settings and most importantly the interpretation of the results. With the advent of computers and software’s, complex statistical analysis can be done very easily. But the challenge is the knowledge of how to set the case, setting parameters (for example confidence intervals) and knowing its implication on the interpretation of the results. If not done properly this could lead to deceptive inferences, inadvertently or purposely. This book does a great job in explaining the above using many examples and real world case studies. If you are looking for a book to learn and apply statistical methods, this is a great one. I think the author could consider revising the title of the book to reflect the above, as it is more than just an introduction to statistics, may be include the word such as practical guide.
The contents of the book seems accurate. Some plots and calculations were randomly selected and checked for accuracy.
The book topics are up to date and in my opinion, will not be obsolete in the near future. I think the smartest thing the author has done is, not tied the book with any particular software such as minitab or spss . No matter what the software is, standard deviation is calculated the same way as it is always. The only noticeable exception in this case was using the Java Applet for calculating Z values in page 261 and in page 416 an excerpt of SPSS analysis is provided for ANOVA calculations.
The contents and examples cited are clear and explained in simple language. Data analysis and presentation of the results including mathematical calculations, graphical explanation using charts, tables, figures etc are presented with clarity.
Terminology is consistant. Framework for each chapter seems consistent with each chapter beginning with a set of defined topics, and each of the topic divided into modules with each module having a set of learning objectives and prerequisite chapters.
The text book is divided into chapters with each chapter further divided into modules. Each of the modules have detailed learning objectives and prerequisite required. So you can extract a portion of the book and use it as a standalone to teach certain topics or as a learning guide to apply a relevant topic.
Presentation of the topics are well thought and are presented in a logical fashion as if it would be introduced to someone who is learning the contents. However, there are some issues with table of contents and page numbers, for example chapter 17 starts in page 597 not 598. Also some tables and figures does not have a number, for instance the graph shown in page 114 does not have a number. Also it would have been better if the chapter number was included in table and figure identification, for example Figure 4-5 . Also in some cases, for instance page 109, the figures and titles are in two different pages.
No major issues. Only suggestion would be, since each chapter has several modules, any means such as a header to trace back where you are currently, would certainly help.
Grammatical Errors rating: 4
Easy to read and phrased correctly in most cases. Minor grammatical errors such as missing prepositions etc. In some cases the author seems to have the habbit of using a period after the decimal. For instance page 464, 467 etc. For X = 1, Y' = (0.425)(1) + 0.785 = 1.21. For X = 2, Y' = (0.425)(2) + 0.785 = 1.64.
However it contains some statements (even though given as examples) that could be perceived as subjective, which the author could consider citing the sources. For example from page 11: Statistics include numerical facts and figures. For instance: • The largest earthquake measured 9.2 on the Richter scale. • Men are at least 10 times more likely than women to commit murder. • One in every 8 South Africans is HIV positive. • By the year 2020, there will be 15 people aged 65 and over for every new baby born.
Solutions for the exercises would be a great teaching resource to have
Reviewed by Randy Vander Wal, Professor, The Pennsylvania State University on 2/1/18
As a text for an introductory course, standard topics are covered. It was nice to see some topics such as power, sampling, research design and distribution free methods covered, as these are often omitted in abbreviated texts. Each module... read more
As a text for an introductory course, standard topics are covered. It was nice to see some topics such as power, sampling, research design and distribution free methods covered, as these are often omitted in abbreviated texts. Each module introduces the topic, has appropriate graphics, illustration or worked example(s) as appropriate and concluding with many exercises. An instructor’s manual is available by contacting the author. A comprehensive glossary provides definitions for all the major terms and concepts. The case studies give examples of practical applications of statistical analyses. Many of the case studies contain the actual raw data. To note is that the on-line e-book provides several calculators for the essential distributions and tests. These are provided in lieu of printed tables which are not included in the pdf. (Such tables are readily available on the web.)
The content is accurate and error free. Notation is standard and terminology is used accurately, as are the videos and verbal explanations therein. Online links work properly as do all the calculators. The text appears neutral and unbiased in subject and content.
The text achieves contemporary relevance by ending each section with a Statistical Literacy example, drawn from contemporary headlines and issues. Of course, the core topics are time proven. There is no obvious material that may become “dated”.
The text is very readable. While the pdf text may appear “sparse” by absence varied colored and inset boxes, pictures etc., the essential illustrations and descriptions are provided. Meanwhile for this same content the on-line version appears streamlined, uncluttered, enhancing the value of the active links. Moreover, the videos provide nice short segments of “active” instruction that are clear and concise. Despite being a mathematical text, the text is not overly burdened by formulas and numbers but rather has “readable feel”.
This terminology and symbol use are consistent throughout the text and with common use in the field. The pdf text and online version are also consistent by content, but with the online e-book offering much greater functionality.
The chapters and topics may be used in a selective manner. Certain chapters have no pre-requisite chapter and in all cases, those required are listed at the beginning of each module. It would be straightforward to select portions of the text and reorganize as needed. The online version is highly modular offering students both ease of navigation and selection of topics.
Chapter topics are arranged appropriately. In an introductory statistics course, there is a logical flow given the buildup to the normal distribution, concept of sampling distributions, confidence intervals, hypothesis testing, regression and additional parametric and non-parametric tests. The normal distribution is central to an introductory course. Necessary precursor topics are covered in this text, while its use in significance and hypothesis testing follow, and thereafter more advanced topics, including multi-factor ANOVA.
Each chapter is structured with several modules, each beginning with pre-requisite chapter(s), learning objectives and concluding with Statistical Literacy sections providing a self-check question addressing the core concept, along with answer, followed by an extensive problem set. The clear and concise learning objectives will be of benefit to students and the course instructor. No solutions or answer key is provided to students. An instructor’s manual is available by request.
The on-line interface works well. In fact, I was pleasantly surprised by its options and functionality. The pdf appears somewhat sparse by comparison to publisher texts, lacking pictures, colored boxes, etc. But the on-line version has many active links providing definitions and graphic illustrations for key terms and topics. This can really facilitate learning as making such “refreshers” integral to the new material. Most sections also have short videos that are professionally done, with narration and smooth graphics. In this way, the text is interactive and flexible, offering varied tools for students. To note is that the interactive e-book works for both IOS and OS X.
The text in pdf form appeared to free of grammatical errors, as did the on-line version, text, graphics and videos.
This text contains no culturally insensitive or offensive content. The focus of the text is on concepts and explanation.
The text would be a great resource for students. The full content would be ambitious for a 1-semester course, such use would be unlikely. The text is clearly geared towards students with no statistics background nor calculus. The text could be used in two styles of course. For 1st year students early chapters on graphs and distributions would be the starting point, omitting later chapters on Chi-square, transformations, distribution-free and size effect chapters. Alternatively, for upper level students the introductory chapters could be bypassed with the latter chapters then covered to completion.
This text adopts a descriptive style of presentation with topics well and fully explained, much like the “Dummy series”. For this, it may seem a bit “wordy”, but this can well serve students and notably it complements powerpoint slides that are generally sparse on written content. This text could be used as the primary text, for regular lectures, or as reference for a “flipped” class. The e-book videos are an enabling tool if this approach is adopted.
Reviewed by David jabon, Associate Professor, DePaul University on 8/15/17
This text covers all the standard topics in a semester long introductory course in statistics. It is particularly well indexed and very easy to navigate. There is comprehensive hyperlinked glossary. read more
This text covers all the standard topics in a semester long introductory course in statistics. It is particularly well indexed and very easy to navigate. There is comprehensive hyperlinked glossary.
The material is completely accurate. There are no errors. The terminology is standard with one exception: the book calls what most people call the interquartile range, the H-spread in a number of places. Ideally, the term "interquartile range" would be used in place of every reference to "H-spread." "Interquartile range" is simply a better, more descriptive term of the concept that it describes. It is also more commonly used nowadays.
This book came out a number of years ago, but the material is still up to date. Some more recent case studies have been added.
The writing is very clear. There are also videos for almost every section. The section on boxplots uses a lot of technical terms that I don't find are very helpful for my students (hinge, H-spread, upper adjacent value).
The text is internally consistent with one exception that I noted (the use of the synonymous words "H-spread" and "interquartile range").
The text book is brokenly into very short sections, almost to a fault. Each section is at most two pages long. However at the end of each of these sections there are a few multiple choice questions to test yourself. These questions are a very appealing feature of the text.
The organization, in particular the ordering of the topics, is rather standard with a few exceptions. Boxplots are introduced in Chapter II before the discussion of measures of center and dispersion. Most books introduce them as part of discussion of summaries of data using measure of center and dispersion. Some statistics instructors may not like the way the text lumps all of the sampling distributions in a single chapter (sampling distribution of mean, sampling distribution for the difference of means, sampling distribution of a proportion, sampling distribution of r). I have tried this approach, and I now like this approach. But it is a very challenging chapter for students.
The book's interface has no features that distracted me. Overall the text is very clean and spare, with no additional distracting visual elements.
The book contains no grammatical errors.
The book's cultural relevance comes out in the case studies. As of this writing there are 33 such case studies, and they cover a wide range of issues from health to racial, ethnic, and gender disparity.
Each chapter as a nice set of exercises with selected answers. The thirty three case studies are excellent and can be supplement with some other online case studies. An instructor's manual and PowerPoint slides can be obtained by emailing the author. There are direct links to online simulations within the text. This text is very high quality textbook in every way.
Table of Contents
- 1. Introduction
- 2. Graphing Distributions
- 3. Summarizing Distributions
- 4. Describing Bivariate Data
- 5. Probability
- 6. Research Design
- 7. Normal Distributions
- 8. Advanced Graphs
- 9. Sampling Distributions
- 10. Estimation
- 11. Logic of Hypothesis Testing
- 12. Testing Means
- 14. Regression
- 15. Analysis of Variance
- 16. Transformations
- 17. Chi Square
- 18. Distribution-Free Tests
- 19. Effect Size
- 20. Case Studies
- 21. Glossary
Ancillary Material
- Ancillary materials are available by contacting the author or publisher .
About the Book
Introduction to Statistics is a resource for learning and teaching introductory statistics. This work is in the public domain. Therefore, it can be copied and reproduced without limitation. However, we would appreciate a citation where possible. Please cite as: Online Statistics Education: A Multimedia Course of Study (http://onlinestatbook.com/). Project Leader: David M. Lane, Rice University. Instructor's manual, PowerPoint Slides, and additional questions are available.
About the Contributors
David Lane is an Associate Professor in the Departments of Psychology, Statistics, and Management at the Rice University. Lane is the principal developer of this resource although many others have made substantial contributions. This site was developed at Rice University, University of Houston-Clear Lake, and Tufts University.
Contribute to this Page
Statistics MS Required Courses (2023-24)
The four core disciplines of the Statistics M.S. program provide students the foundational structure for building their program.
Probability Theory
Provides the framework of probability to quantify uncertainty and update beliefs given the right evidence; learn how to use a variety of strategies to calculate probabilities and expectations, both conditional and unconditional, as well as how to understand the generative stories for discrete and continuous distributions and recognize when they are appropriate for real-world scenarios.
Stochastic Processes
A stochastic process is a set of random variables indexed by time or space; gain the theoretical knowledge and practical skills necessary for the analysis of stochastic systems; including basic concepts of the theory of stochastic processes and explore different types of stochastic processes including Markov chains, Poisson processes and birth-and-death processes.
Applied Statistics
Survey of regression techniques from both a theoretical and applied perspective. Numerical reasoning and predictive data modeling, with an emphasis on conceptual rather than theoretical understanding. Modeling and interpretation of observational and experimental data using linear and nonlinear regression methods. Model building and selection methods. Multivariable analysis. Fixed and random effects models. Experimental design. Practice of linear regression; Interactions and qualitative variables; analysis of variance; transformations and weighted least squares.
Statistical Theory
Statistical concepts and methods developed in a mathematical framework: Hypothesis testing, point estimation, confidence intervals. Neyman-Pearson theory, maximum likelihood estimation, likelihood ratio tests, Bayesian analysis. Asymptotic theory and simulation-based methods.
Statistics Core Courses
Students must complete all four courses to provide depth in the field of statistics. Students with prior background may replace each course with a more advanced course from the same area. All must be taken for a letter grade.
STATS 116 Explore Courses Schedule
Textbook: A First Course in Probability, 10th ed. by Sheldon Ross
This course is a prerequisite for Introduction to Statistical Inference (Stats 200), Stochastic Process (Stats 217) and Introduction to Regression Models and Analysis of Variances (Stats 203).
As stated in the Stanford bulletin, enrollment in STATS 116 after successfully completing STATS 217, 218, and/or 219 may not be used to fulfill degree requirements, including as an elective.”
STATS 217 Explore Courses Schedule
- Essentials of Stochastic Processes by Durrett
- An Introduction to Stochastic Modeling by Pinsky and Karlin
- Introduction to Stochastic Processes by Ross
- Stochastic Processes by Ross
STATS 200 Explore Courses Schedule
Mathematical Statistics and Data Analysis, third edition (2009) by John Rice.
STATS 191 Explore Courses Schedule
Students with prior background may replace each course with a more advanced course from the same area:
When replacing STATS116, students must take two courses in stochastic processing or probability theory taught by the Statistics department.
- When replacing STATS 116 with 217, students do not need to replace STATS 217 however, students must take an additional course in probability or stochastic processes taught by the department when replacing STATS 116.
and/or one of the following:
- STATS 218 Intro to Stochastic Processes II,
- STATS 219 Stochastic Processes, or
- STATS 310A Theory of Probability I
- STATS 317 Stochastic Processes
- STATS 221 Random Processes on Graphs and Lattices
When replacing STATS 217, students must take either
- STATS 218 Intro to Stochastic Processes II, or
- STATS 219 Stochastic Processes
When replacing STATS 200, students may take either:
- STATS 300A Theory of Statistics I
- STATS 300B Theory of Statistics II
- STAT 270 A Course in Bayesian Statistics (STATS 370)
In this order, students may replace:
- STATS 203 Introduction to Regression Models and Analysis of Variance
- STATS 305A Applied Statistics I
Statistics Depth
At least five additional Statistics courses must be taken from graduate offerings in the department. All must be taken for a letter grade (with the exception of courses offered satisfactory/no credit only).
STATS 202 through 376A
except for the following courses that may only be used to fulfill elective credit :
Literature study of topics in statistics and probability culminating in oral and written reports.
Linear Algebra requirement
Students who have had linear algebra may take a more advanced mathematics course, such as from the following, or other math course with program advisor's approval. Must be taken for a letter grade .
Substitution of more advanced courses in Mathematics, that provide similar skills, may be made with consent of the advisor. All must be taken for a letter grade, with the exception of courses offered satisfactory/no credit only.
Select one of the following:
offered A,S,Su
Programming requirement
CS 106A/B, CS 107, CS 140 - 182, or other course with the faculty advisor's approval. Students who have these skills may elect a more advanced CS course. Must be taken for a letter grade .
Substitution of other courses in Computer Science may be made with consent of the advisor. All must be taken for a letter grade, with the exception of courses offered satisfactory/no credit only.
offered A,W,S,Su
offered A,W,S
Introduction to Scientific Computing Numerical computation for mathematical, computational, physical sciences and engineering: error analysis, floating-point arithmetic, nonlinear equations, numerical solution of systems of algebraic equations, banded matrices, least squares, unconstrained optimization, polynomial interpolation, numerical differentiation and integration, numerical solution of ordinary differential equations, truncation error, numerical stability for time dependent problems and stiffness. Implementation of numerical methods in MATLAB programming assignments. Prerequisites: CME 100 , 102 or MATH 51 , 52, 53; prior programming experience (MATLAB or other language at level of CS 106A or higher).
offered W,Su
Breadth/General Electives
Elective courses in the area of statistics, computational mathematics and engineering, programming (Python and C/C++ programming languages), biomedical data science, economics, operations management, electrical engineering, machine learning, etc.
Up to 6 units of the following courses may be used to fulfill breadth/elective credit:
- STATS 260 series: Workshop in Biostatistics (1-2 units)
- STATS 242: NeuroTech Training Seminar (1 unit)
- STATS 352: Topics in Computing for Data Science (1 unit)
- STATS 298/398: Industrial research of statisticians (1 unit)
- STATS 299/399: Independent study/research (1 unit)
- STATS 319: Literature of Statistics (1 unit)
- STATS 390: Statistical Consulting (1 unit)

Graduate Student Handbook (Coming Soon: New Graduate Student Handbook)
Phd program overview.
The PhD program prepares students for research careers in probability and statistics in academia and industry. Students admitted to the PhD program earn the MA and MPhil along the way. The first year of the program is spent on foundational courses in theoretical statistics, applied statistics, and probability. In the following years, students take advanced topics courses. Research toward the dissertation typically begins in the second year. Students also have opportunities to take part in a wide variety of projects involving applied probability or applications of statistics.
Students are expected to register continuously until they distribute and successfully defend their dissertation. Our core required and elective curricula in Statistics, Probability, and Machine Learning aim to provide our doctoral students with advanced learning that is both broad and focused. We expect our students to make Satisfactory Academic Progress in their advanced learning and research training by meeting the following program milestones through courseworks, independent research, and dissertation research:
By the end of year 1: passing the qualifying exams;
By the end of year 2: fulfilling all course requirements for the MA degree and finding a dissertation advisor;
By the end of year 3: passing the oral exam (dissertation prospectus) and fulfilling all requirements for the MPhil degree
By the end of year 5: distributing and defending the dissertation.
We believe in the Professional Development value of active participation in intellectual exchange and pedagogical practices for future statistical faculty and researchers. Students are required to serve as teaching assistants and present research during their training. In addition, each student is expected to attend seminars regularly and participate in Statistical Practicum activities before graduation.
We provide in the following sections a comprehensive collection of the PhD program requirements and milestones. Also included are policies that outline how these requirements will be enforced with ample flexibility. Questions on these requirements should be directed to ADAA Cindy Meekins at [email protected] and the DGS, Professor John Cunningham at [email protected] .
Applications for Admission
- Our students receive very solid training in all aspects of modern statistics. See Graduate Student Handbook for more information.
- Our students receive Fellowship and full financial support for the entire duration of their PhD. See more details here .
- Our students receive job offers from top academic and non-academic institutions .
- Our students can work with world-class faculty members from Statistics Department or the Data Science Institute .
- Our students have access to high-speed computer clusters for their ambitious, computationally demanding research.
- Our students benefit from a wide range of seminars, workshops, and Boot Camps organized by our department and the data science institute .
- Suggested Prerequisites: A student admitted to the PhD program normally has a background in linear algebra and real analysis, and has taken a few courses in statistics, probability, and programming. Students who are quantitatively trained or have substantial background/experience in other scientific disciplines are also encouraged to apply for admission.
- GRE requirement: Waived for Fall 2024.
- Language requirement: The English Proficiency Test requirement (TOEFL) is a Provost's requirement that cannot be waived.
- The Columbia GSAS minimum requirements for TOEFL and IELTS are: 100 (IBT), 600 (PBT) TOEFL, or 7.5 IELTS. To see if this requirement can be waived for you, please check the frequently asked questions below.
- Deadline: Jan 8, 2024 .
- Application process: Please apply by completing the Application for Admission to the Columbia University Graduate School of Arts & Sciences .
- Timeline: P.hD students begin the program in September only. Admissions decisions are made in mid-March of each year for the Fall semester.
Frequently Asked Questions
- What is the application deadline? What is the deadline for financial aid? Our application deadline is January 5, 2024 .
- Can I meet with you in person or talk to you on the phone? Unfortunately given the high number of applications we receive, we are unable to meet or speak with our applicants.
- What are the required application materials? Specific admission requirements for our programs can be found here .
- Due to financial hardship, I cannot pay the application fee, can I still apply to your program? Yes. Many of our prospective students are eligible for fee waivers. The Graduate School of Arts and Sciences offers a variety of application fee waivers . If you have further questions regarding the waiver please contact gsas-admissions@ columbia.edu .
- How many students do you admit each year? It varies year to year. We finalize our numbers between December - early February.
- What is the distribution of students currently enrolled in your program? (their background, GPA, standard tests, etc)? Unfortunately, we are unable to share this information.
- How many accepted students receive financial aid? All students in the PhD program receive, for up to five years, a funding package consisting of tuition, fees, and a stipend. These fellowships are awarded in recognition of academic achievement and in expectation of scholarly success; they are contingent upon the student remaining in good academic standing. Summer support, while not guaranteed, is generally provided. Teaching and research experience are considered important aspects of the training of graduate students. Thus, graduate fellowships include some teaching and research apprenticeship. PhD students are given funds to purchase a laptop PC, and additional computing resources are supplied for research projects as necessary. The Department also subsidizes travel expenses for up to two scientific meetings and/or conferences per year for those students selected to present. Additional matching funds from the Graduate School Arts and Sciences are available to students who have passed the oral qualifying exam.
- Can I contact the department with specific scores and get feedback on my competitiveness for the program? We receive more than 450 applications a year and there are many students in our applicant pool who are qualified for our program. However, we can only admit a few top students. Before seeing the entire applicant pool, we cannot comment on admission probabilities.
- What is the minimum GPA for admissions? While we don’t have a GPA threshold, we will carefully review applicants’ transcripts and grades obtained in individual courses.
- Is there a minimum GRE requirement? No. The general GRE exam is waived for the Fall 2024 admissions cycle.
- Can I upload a copy of my GRE score to the application? Yes, but make sure you arrange for ETS to send the official score to the Graduate School of Arts and Sciences.
- Is the GRE math subject exam required? No, we do not require the GRE math subject exam.
- What is the minimum TOEFL or IELTS requirement? The Columbia Graduate School of Arts and Sciences minimum requirements for TOEFL and IELTS are: 100 (IBT), 600 (PBT) TOEFL, or 7.5 IELTS
- I took the TOEFL and IELTS more than two years ago; is my score valid? Scores more than two years old are not accepted. Applicants are strongly urged to make arrangements to take these examinations early in the fall and before completing their application.
- I am an international student and earned a master’s degree from a US university. Can I obtain a TOEFL or IELTS waiver? You may only request a waiver of the English proficiency requirement from the Graduate School of Arts and Sciences by submitting the English Proficiency Waiver Request form and if you meet any of the criteria described here . If you have further questions regarding the waiver please contact gsas-admissions@ columbia.edu .
- My transcript is not in English. What should I do? You have to submit a notarized translated copy along with the original transcript.
Can I apply to more than one PhD program? You may not submit more than one PhD application to the Graduate School of Arts and Sciences. However, you may elect to have your application reviewed by a second program or department within the Graduate School of Arts and Sciences if you are not offered admission by your first-choice program. Please see the application instructions for a more detailed explanation of this policy and the various restrictions that apply to a second choice. You may apply concurrently to a program housed at the Graduate School of Arts and Sciences and to programs housed at other divisions of the University. However, since the Graduate School of Arts and Sciences does not share application materials with other divisions, you must complete the application requirements for each school.
How do I apply to a dual- or joint-degree program? The Graduate School of Arts and Sciences refers to these programs as dual-degree programs. Applicants must complete the application requirements for both schools. Application materials are not shared between schools. Students can only apply to an established dual-degree program and may not create their own.
With the sole exception of approved dual-degree programs , students may not pursue a degree in more than one Columbia program concurrently, and may not be registered in more than one degree program at any institution in the same semester. Enrollment in another degree program at Columbia or elsewhere while enrolled in a Graduate School of Arts and Sciences master's or doctoral program is strictly prohibited by the Graduate School. Violation of this policy will lead to the rescission of an offer of admission, or termination for a current student.
When will I receive a decision on my application? Notification of decisions for all PhD applicants generally takes place by the end of March.
Notification of MA decisions varies by department and application deadlines. Some MA decisions are sent out in early spring; others may be released as late as mid-August.
Can I apply to both MA Statistics and PhD statistics simultaneously? For any given entry term, applicants may elect to apply to up to two programs—either one PhD program and one MA program, or two MA programs—by submitting a single (combined) application to the Graduate School of Arts and Sciences. Applicants who attempt to submit more than one Graduate School of Arts and Sciences application for the same entry term will be required to withdraw one of the applications.
The Graduate School of Arts and Sciences permits applicants to be reviewed by a second program if they do not receive an offer of admission from their first-choice program, with the following restrictions:
- This option is only available for fall-term applicants.
- Applicants will be able to view and opt for a second choice (if applicable) after selecting their first choice. Applicants should not submit a second application. (Note: Selecting a second choice will not affect the consideration of your application by your first choice.)
- Applicants must upload a separate Statement of Purpose and submit any additional supporting materials required by the second program. Transcripts, letters, and test scores should only be submitted once.
- An application will be forwarded to the second-choice program only after the first-choice program has completed its review and rendered its decision. An application file will not be reviewed concurrently by both programs.
- Programs may stop considering second-choice applications at any time during the season; Graduate School of Arts and Sciences cannot guarantee that your application will receive a second review.
- What is the mailing address for your PhD admission office? Students are encouraged to apply online . Please note: Materials should not be mailed to the Graduate School of Arts and Sciences unless specifically requested by the Office of Admissions. Unofficial transcripts and other supplemental application materials should be uploaded through the online application system. Graduate School of Arts and Sciences Office of Admissions Columbia University 107 Low Library, MC 4303 535 West 116th Street New York, NY 10027
- How many years does it take to pursue a PhD degree in your program? Our students usually graduate in 4‐6 years.
- Can the PhD be pursued part-time? No, all of our students are full-time students. We do not offer a part-time option.
- One of the requirements is to have knowledge of linear algebra (through the level of MATH V2020 at Columbia) and advanced calculus (through the level of MATH V1201). I studied these topics; how do I know if I meet the knowledge content requirement? We interview our top candidates and based on the information on your transcripts and your grades, if we are not sure about what you covered in your courses we will ask you during the interview.
- Can I contact faculty members to learn more about their research and hopefully gain their support? Yes, you are more than welcome to contact faculty members and discuss your research interests with them. However, please note that all the applications are processed by a central admission committee, and individual faculty members cannot and will not guarantee admission to our program.
- How do I find out which professors are taking on new students to mentor this year? Applications are evaluated through a central admissions committee. Openings in individual faculty groups are not considered during the admissions process. Therefore, we suggest contacting the faculty members you would like to work with and asking if they are planning to take on new students.
For more information please contact us at [email protected] .

For more information please contact us at [email protected]
Quick Links
- Undergraduate Programs
- M.A. Statistics Programs
- M.A. in Mathematical Finance
- M.S. in Actuarial Science
- M.A. in Quantitative Methods in the Social Sciences
- M.S. in Data Science
- PhD Program
- BA/MA Program
- Department Directory
- Faculty Positions
- Founder’s Postdoctoral Fellowship Positions
- Staff Hiring
- Joint Postdoc with Data Science Institute
- Department News
- Department Calendar
- Research Computing
Upcoming Events
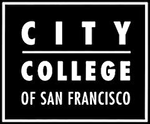
Psych Statistics
An introduction to data analysis including measurement and research design. Intended for general education and prospective behavioral science majors. The course will focus on computation, interpretation, and application of both descriptive and inferential statistics. Topics include organization of data, central tendency and variability, hypothesis testing, non-parametric statistics, analysis of variance (ANOVA), and linear regression.
Psyc 5 has no scheduled meetings and requires the use of Canvas. All coursework is completed on Canvas using an internet enabled device. This class is taught asynchronously and is not self-paced. This class is part of CityOnline and is 7 weeks long. For course details, students must check the instructor's website .
PREREQ: Intermediate algebra or higher Recommended Preparation, Readiness for college-level English or ESL 188

IMAGES
VIDEO
COMMENTS
There are 12 modules in this course. Stanford's "Introduction to Statistics" teaches you statistical thinking concepts that are essential for learning from data and communicating insights. By the end of the course, you will be able to perform exploratory data analysis, understand key principles of sampling, and select appropriate tests of ...
Table of contents. Step 1: Write your hypotheses and plan your research design. Step 2: Collect data from a sample. Step 3: Summarize your data with descriptive statistics. Step 4: Test hypotheses or make estimates with inferential statistics.
Click "ENROLL NOW" to visit Coursera and get more information on course details and enrollment. Stanford's "Introduction to Statistics" teaches you statistical thinking concepts that are essential for learning from data and communicating insights. By the end of the course, you will be able to perform exploratory data analysis, understand ...
Principles, Statistical and Computational Tools for Reproducible Data Science. Learn skills and tools that support data science and reproducible research, to ensure you can trust your own research results, reproduce them yourself, and communicate them to others. Free *. 8 weeks long.
What You'll Earn. You'll earn a Stanford Graduate Certificate in Statistics when you successfully earn a grade of B (3.0) or better in each course in the program.. With each successful completion of a course in this program, you'll receive a Stanford University transcript and academic credit, which may be applied to a relevant graduate degree program that accepts these credits.
The textbook covers all necessary areas and topics for students who want to conduct research in statistics. It includes foundational concepts, application methods, and advanced statistical techniques relevant to research methodologies. ... He currently teaches four courses in research methods and statistics. His research involves leadership, ...
Statistics is an indispensable pillar of modern science, including data science and artificial intelligence. You can take advantage of the department's flexible research options and work with your faculty of choice. You can leverage cross-department collaboration with biology, chemistry, medical sciences, economics, computer science ...
The course is divided into 11 broad sections, which include 59 lectures and 21 quizzes. Participants would benefit from the course because understanding basic research methodology and statistics is essential prior to beginning any research-related endeavor. It is also an important part of the college curriculum from undergraduate to Ph.D ...
Note that the statistics and programming aspects of the class ramp up in difficulty relatively quickly across the first three courses. By the third course will be teaching advanced statistical concepts such as hierarchical models and by the fourth advanced software engineering skills, such as parallel computing and reproducible research concepts.
This course teaches the R programming language in the context of statistical data and statistical analysis in the life sciences. We will learn the basics of statistical inference in order to understand and compute p-values and confidence intervals, all while analyzing data with R code. We provide R programming examples in a way that will help ...
The Statistics courses we offer are designed to help prepare you for a career in data analysis, research, statistical modeling, and business intelligence, equipping you with the skills to interpret data accurately and make informed, data-driven decisions.
In this section of the course, we consider the role of null hypothesis significance testing in psychological research, some objections that have been raised to that approach, and some alternative approaches that have been proposed. Conclusion; Review the main ideas from the previous module, organized by learning objectives (LOs). Course Assessment
Statistical methods have enabled us to answer some of the most pressing questions facing humanity. In the field of medicine the ability to ask the right research questions and interpret data is an essential skill, whether you are a physician, researcher, data scientist, or journalist. The Medical Statistics program uses real-world examples from medical literature and the popular press to ...
The field of statistics is concerned with collecting, analyzing, interpreting, and presenting data.. In the field of research, statistics is important for the following reasons: Reason 1: Statistics allows researchers to design studies such that the findings from the studies can be extrapolated to a larger population.. Reason 2: Statistics allows researchers to perform hypothesis tests to ...
The length of the textbook appears to be more than adequate for a one-semester course in Introduction to Statistics. As I no longer teach a full statistics course but simply a few lectures as part of our Research Curriculum, I am recommending this book to my students as a good reference. Especially as it is available on-line and in Open Access.
Learn the essentials of statistics in this complete course. This course introduces the various methods used to collect, organize, summarize, interpret and re...
Introduction to Understanding Clinical Research • 2 minutes • Preview module. About the course • 2 minutes. Observing and intervening: Observational & experimental studies • 3 minutes. Observing and describing: Case series studies • 3 minutes. Comparing groups: Case-control studies • 3 minutes.
A seminar-style course jointly supported by the Statistics department and Stanford Data Science, and suitable for doctoral students engaged in either research on data science techniques (statistical or computational, for example) or research in scientific fields relying on advanced data science to achieve its goals.
The PhD program prepares students for research careers in probability and statistics in both academia and industry. The first year of the program is devoted to training in theoretical statistics, applied statistics, and probability. In the following years, students take advanced topics courses and s
In summary, here are 10 of our most popular statistical analysis courses. Introduction to Statistics: Stanford University. Business Statistics and Analysis: Rice University. Statistical Analysis with R for Public Health: Imperial College London. Data Analysis with R Programming: Google.
Catalog Description. An introduction to data analysis including measurement and research design. Intended for general education and prospective behavioral science majors. The course will focus on computation, interpretation, and application of both descriptive and inferential statistics. Topics include organization of data, central tendency and ...
Specialization - 3 course series. This specialization is primarily aimed at first- and second-year undergraduates interested in psychology, data analysis, ethics in research, and quantitative research methods along with high school students and professionals with similar interests. Learners will develop knowledge about the fundamentals of ...
Business statistics involves the use of statistical methods and analyses to make informed decisions and solve problems in the business world. With business statistics, you might use different analytical methods to collect, analyze, and interpret your data to inform insights on market trends, manage financial data, assess performance within your ...