- DOI: 10.55041/ijsrem26979
- Corpus ID: 265488889

Inventory Management Systems: A Comprehensive Review and Analysis
- Kirti Pandey , Aadyant Tripathi , +2 authors Jatin Abrol
- Published in INTERANTIONAL JOURNAL OF… 1 November 2023
Related Papers
Showing 1 through 3 of 0 Related Papers
Applications of Artificial Intelligence in Inventory Management: A Systematic Review of the Literature
- Review Article
- Published: 07 February 2023
- Volume 30 , pages 2605–2625, ( 2023 )
Cite this article
- Özge Albayrak Ünal ORCID: orcid.org/0000-0001-7798-8799 1 ,
- Burak Erkayman ORCID: orcid.org/0000-0002-9551-2679 1 &
- Bilal Usanmaz ORCID: orcid.org/0000-0003-0531-4618 2
6759 Accesses
14 Citations
Explore all metrics
Today, companies that want to keep up with technological development and globalization must be able to effectively manage their supply chains to achieve high quality, increased efficiency, and low costs. Diversified customer needs, global competitors, and market competition have led companies to pay more attention to inventory management. This article provides a comprehensive and up-to-date review of Artificial Intelligence (AI) applications used in inventory management through a systematic literature review. As a result of this analysis, which focused on research articles in two scientific databases published between 2012 and 2022 for detailed study, 59 articles were identified. Furthermore, the current situation is summarized and possible future aspects of inventory management are identified. The results show that the interest in AI methods has increased in recent years and machine learning algorithms are the most commonly used methods. This study is meticulously and comprehensively conducted so it will probably make significant contributions to the further studies in this field.
This is a preview of subscription content, log in via an institution to check access.
Access this article
Subscribe and save.
- Get 10 units per month
- Download Article/Chapter or eBook
- 1 Unit = 1 Article or 1 Chapter
- Cancel anytime
Price includes VAT (Russian Federation)
Instant access to the full article PDF.
Rent this article via DeepDyve
Institutional subscriptions
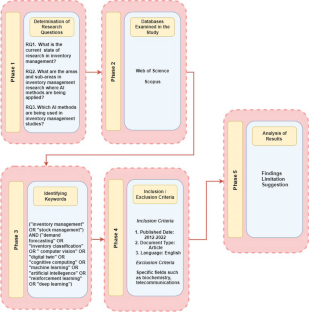
Similar content being viewed by others
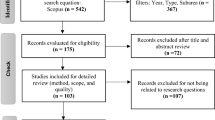
Benefits, challenges, and limitations of inventory control using machine learning algorithms: literature review
Inventory Control with Machine Learning Approach: A Bibliometric Analysis
Machine Learning Applications for Demand Driven in Supply Chain: Literature Review
Explore related subjects.
- Artificial Intelligence
Perez HD et al (2021) Algorithmic approaches to inventory management optimization. Processes 9(1):102
Article Google Scholar
Singh D, Verma A (2018) Inventory management in supply chain. Mater Today 5(2):3867–3872
MathSciNet Google Scholar
Haberleitner H, Meyr H, Taudes A (2010) Implementation of a demand planning system using advance order information. Int J Prod Econ 128(2):518–526
Abbasimehr H, Shabani M, Yousefi M (2020) An optimized model using LSTM network for demand forecasting. Comput Ind Eng 143:106435
Beutel A-L, Minner S (2012) Safety stock planning under causal demand forecasting. Int J Prod Econ 140(2):637–645
Sridhar P, Vishnu C, Sridharan R (2021) Simulation of inventory management systems in retail stores: a case study. Mater Today 47:5130–5134
Google Scholar
Granillo-Macías R (2020) Inventory management and logistics optimization: a data mining practical approach. LogForum 16(4):535–547
Nallusamy S (2021) Performance measurement on inventory management and logistics through various forecasting techniques. Int J Perform Eng 17(2):216–228
Acosta ICG et al (2018) Design of an inventory management system in an agricultural supply chain considering the deterioration of the product: the case of small citrus producers in a developing country. J Appl Eng Sci 16(4):523–537
Varghese V et al (2012) Applying actual usage inventory management best practice in a health care supply chain. Int J Supply Chain Manage 1(2):1–10
Xie C, Wang L, Yang C (2021) Robust inventory management with multiple supply sources. Eur J Oper Res 295(2):463–474
Article MathSciNet MATH Google Scholar
Tranfield D, Denyer D, Smart P (2003) Towards a methodology for developing evidence-informed management knowledge by means of systematic review. Br J Manag 14(3):207–222
Evangelista P, Durst S (2015) Knowledge management in environmental sustainability practices of third-party logistics service providers. Vine 45(4):509–529
Bryman A (2007) The research question in social research: what is its role? Int J Soc Res Methodol 10(1):5–20
Nguyen KTP, Medjaher K (2019) A new dynamic predictive maintenance framework using deep learning for failure prognostics. Reliab Eng Syst Saf 188:251–262
Tabernik D, Skocaj D (2020) Deep learning for large-scale traffic-sign detection and recognition. IEEE Trans Intell Transp Syst 21(4):1427–1440
Mohammaditabar D, Ghodsypour SH, O’Brien C (2012) Inventory control system design by integrating inventory classification and policy selection. Int J Prod Econ 140(2):655–659
Liu JP et al (2016) A classification approach based on the outranking model for multiple criteria ABC analysis. Omega-International J Manage Sci 61:19–34
Kartal H et al (2016) An integrated decision analytic framework of machine learning with multi-criteria decision making for multi-attribute inventory classification. Comput Ind Eng 101:599–613
Aggarwal SC (1974) A review of current inventory theory and its applications. Int J Prod Res 12(4):443–482
Giannoccaro I, Pontrandolfo P (2002) Inventory management in supply chains: a reinforcement learning approach. Int J Prod Econ 78(2):153–161
Cachon GP, Fisher M (2000) Supply chain inventory management and the value of shared information. Manage Sci 46(8):1032–1048
Article MATH Google Scholar
Partovi FY, Anandarajan M (2002) Classifying inventory using an artificial neural network approach. Comput Ind Eng 41(4):389–404
Giannoccaro I, Pontrandolfo P, Scozzi B (2003) A fuzzy echelon approach for inventory management in supply chains. Eur J Oper Res 149(1):185–196
Yang L, Li H, Campbell JF (2020) Improving order fulfillment performance through integrated inventory management in a multi-item finished goods system. J Bus Logistics 41(1):54–66
Wang Z, Mersereau AJ (2017) Bayesian inventory management with potential change-points in demand. Prod Oper Manage 26(2):341–359
Calle M et al (2016) Integrated management of inventory and production systems based on floating decoupling point and real-time information: a simulation based analysis. Int J Prod Econ 181:48–57
KP ASR, Nayak N (2017) A study on the effectiveness of inventory management and control system in a milk producer organisation. Int J Logistics Syst Manage 28(2):253–266
Rana R, Oliveira FS (2015) Dynamic pricing policies for interdependent perishable products or services using reinforcement learning. Expert Syst Appl 42(1):426–436
Pirayesh Neghab D, Khayyati S, Karaesmen F (2022) An integrated data-driven method using deep learning for a newsvendor problem with unobservable features. Eur J Operational Res 302(2):482–496
Bandaru S et al (2015) Generalized higher-level automated innovization with application to inventory management. Eur J Oper Res 243(2):480–496
Kara A, Dogan I (2018) Reinforcement learning approaches for specifying ordering policies of perishable inventory systems. Expert Syst Appl 91:150–158
Zwaida TA, Pham C, Beauregard Y (2021) Optimization of inventory management to prevent drug shortages in the hospital supply chain. Appl Sci 11(6):2726
Katanyukul T (2014) Ruminative reinforcement learning: improve intelligent inventory control by ruminating on the past. J Comput 9(7):1530–1535
De Moor BJ, Gijsbrechts J, Boute RN (2021) Reward shaping to improve the performance of deep reinforcement learning in perishable inventory management. Eur J Operational Res 301(2):535–545
Boute RN et al (2021) Deep reinforcement learning for inventory control: a roadmap. Eur J Operational Res 298(2):401–412
Gijsbrechts J et al (2021) Can deep reinforcement learning improve inventory management? Performance on lost sales, dual-sourcing, and multi-echelon problems. M SOM-Manuf Serv Operations Manag. https://doi.org/10.1287/msom.2021.1064
Kegenbekov Z, Jackson I (2021) Adaptive supply chain: demand–supply synchronization using deep reinforcement learning. Algorithms 14(8):240
Meisheri H et al (2022) Scalable multi-product inventory control with lead time constraints using reinforcement learning. Neural Comput Appl 34(3):1735–1757
Priore P et al (2019) Applying machine learning to the dynamic selection of replenishment policies in fast-changing supply chain environments. Int J Prod Res 57(11):3663–3677
Demey YT, Wolff M (2017) SIMISS: a model-based searching strategy for inventory management systems. IEEE Internet Things J 4(1):172–182
Merrad Y et al (2020) A real-time mobile notification system for inventory stock out detection using SIFT and RANSAC. Int J Interactive Mobile Technol. https://doi.org/10.3991/ijim.v14i05.13315
Kalinov I et al (2020) WareVision: CNN barcode detection-based uav trajectory optimization for autonomous warehouse stocktaking. IEEE Rob Autom Lett 5(4):6647–6653
Giaconia C, Chamas A (2022) GAIA: great-distribution artificial intelligence-based algorithm for advanced large-scale commercial store management. Appl Sci-Basel 12(9):4798
Kosanoglu F, Atmis M, Turan HH (2022) A deep reinforcement learning assisted simulated annealing algorithm for a maintenance planning problem. Annals of Operations Research 15:1–32
Deutsch J, He D (2017) Using deep learning-based approach to predict remaining useful life of rotating components. IEEE Trans Syst Man Cybernetics: Syst 48(1):11–20
Tao F et al (2019) Digital twin-driven product design framework. Int J Prod Res 57(12):3935–3953
Fuller A et al (2020) Digital twin: enabling technologies, challenges and open research. IEEE Access 8:108952–108971
Abosuliman SS, Almagrabi AO (2021) Computer vision assisted human computer interaction for logistics management using deep learning. Comput Electr Eng 96:107555
Mao J et al (2020) The importance of public support in the implementation of green transportation in the smart cities. Computational Intell. https://doi.org/10.1111/coin.12326
Tian X, Wang H, Erjiang E (2021) Forecasting intermittent demand for inventory management by retailers: a new approach. J Retailing Consumer Serv 62:102662
do Rego JR, De Mesquita MA (2015) Demand forecasting and inventory control: a simulation study on automotive spare parts. Int J Prod Econ 161:1–16
Tangtisanon P (2018) Web service based food additive inventory management with forecasting system. in 2018 3rd International Conference on Computer and Communication Systems (ICCCS). IEEE
Yu Q et al (2017) Application of long short-term memory neural network to sales forecasting in retail—a case study. in International Workshop of Advanced Manufacturing and Automation. Springer
Kumar A, Shankar R, Aljohani NR (2020) A big data driven framework for demand-driven forecasting with effects of marketing-mix variables. Ind Mark Manage 90:493–507
Seyedan M, Mafakheri F, Wang C (2022) Cluster-based demand forecasting using bayesian model averaging: an ensemble learning approach. Decis Anal J 3:100033
Feizabadi J (2022) Machine learning demand forecasting and supply chain performance. Int J Logistics Res Appl 25(2):119–142
Deng CN, Liu YJ (2021) A deep learning-based inventory management and demand prediction optimization method for anomaly detection. Wirel Commun Mobile Comput. https://doi.org/10.1155/2021/9969357
Kack M, Freitag M (2021) Forecasting of customer demands for production planning by local k-nearest neighbor models. Int J Prod Econ 231:107837
Bala PK (2012) Improving inventory performance with clustering based demand forecasts. J Modelling Manage 7(1):23–37
Lee CY, Liang CL (2018) Manufacturer’s printing forecast, reprinting decision, and contract design in the educational publishing industry. Comput Ind Eng 125:678–687
Abbasi B et al (2020) Predicting solutions of large-scale optimization problems via machine learning: a case study in blood supply chain management. Comput Operations Res 119:104941
van Steenbergen RM, Mes MRK (2020) Forecasting demand profiles of new products. Decis Support Syst 139:113401
Zhu XD et al (2021) Demand forecasting with supply-chain information and machine learning: evidence in the Pharmaceutical Industry. Prod Oper Manage 30(9):3231–3252
Benhamida FZ et al (2021) Demand forecasting tool for inventory control smart systemsy. J Commun Softw Syst 17(2):185–196
Zhang P et al (2021) Pharmaceutical cold chain management based on blockchain and deep learning. J Internet Technol 22(7):1531–1542
Article MathSciNet Google Scholar
Ulrich M et al (2021) Distributional regression for demand forecasting in e-grocery. Eur J Oper Res 294(3):831–842
Li N et al (2021) A decision integration strategy for short-term demand forecasting and ordering for red blood cell components. Oper Res Health Care 29:100290
Ran H (2021) Construction and optimization of inventory management system via cloud-edge collaborative computing in supply chain environment in the internet of things era. PLoS ONE 16:e0259284
Sun X et al (2021) RBC inventory-management system based on XGBoost model. Indian J Hematol Blood Transfus 37(1):126–133
Aktepe A, Yanik E, Ersoz S (2021) Demand forecasting application with regression and artificial intelligence methods in a construction machinery company. J Intell Manuf 32(6):1587–1604
Galli L et al (2021) Prescriptive analytics for inventory management in health. J Oper Res Soc 72(10):2211–2224
Eljaouhari A et al (2022) Demand forecasting application with regression and iot based inventory management system: a case study of a semiconductor manufacturing company. Int J Eng Res Afr 60:189–210
Sucharitha RS, Lee S (2022) GMM clustering for in-depth food accessibility pattern exploration and prediction model of food demand behavior. Socio-Economic Plan Sci. https://doi.org/10.48550/arXiv.2202.01347
Wang Z et al (2022) Development and validation of a machine learning method to predict intraoperative red blood cell transfusions in cardiothoracic surgery. Sci Rep 12(1):1–9
Ulrich M et al (2022) Classification-based model selection in retail demand forecasting. Int J Forecast 38(1):209–223
Ji S et al (2019) An application of a three-stage XGboost-based model to sales forecasting of a cross-border e-commerce enterprise. Math Probl Eng. https://doi.org/10.1155/2019/8503252
Wang S, Yang Y (2021) M-GAN-XGBOOST model for sales prediction and precision marketing strategy making of each product in online stores. Data Technol Appl 55(5):749–770
Kim M et al (2022) Framework of 2D KDE and LSTM-based forecasting for cost-effective inventory management in smart manufacturing. Appl Sci-Basel 12(5):2380
Ntakolia C et al (2021) An explainable machine learning model for material backorder prediction in inventory management. Sensors 21(23):7926
Islam S, Amin SH (2020) Prediction of probable backorder scenarios in the supply chain using distributed random forest and gradient boosting machine learning techniques. J Big Data 7(1):1–22
O’Neil S et al (2016) Newsvendor problems with demand shocks and unknown demand distributions. Decis Sci 47(1):125–156
Lee CKM et al (2017) Design and development of inventory knowledge discovery system. Enterp Inform Syst 11(8):1262–1282
Van Belle J, Guns T, Verbeke W (2021) Using shared sell-through data to forecast wholesaler demand in multi-echelon supply chains. Eur J Oper Res 288(2):466–479
Tang Y-M et al (2022) Integrated smart warehouse and manufacturing management with demand forecasting in small-scale cyclical industries. Machines 10(6):472
Ecemiş O, Irmak S (2018) Paslanmaz çelik sektörü satış tahmininde veri madenciliği yöntemlerinin karşılaştırılması. Kilis 7 Aralık Üniversitesi Sosyal Bilimler Dergisi. 8:148–16915
Aktepe A et al (2018) An inventory classification approach combining expert systems, clustering, and fuzzy logic with the abc method, and an application. S Afr J Ind Eng 29(1):49–62
Huang B, Gan W, Li Z (2021) Application of medical material inventory model under deep learning in supply planning of public emergency. IEEE Access 9:44128–44138
Wang A, Gao XD (2021) A variable-scale dynamic clustering method. Comput Commun 171:163–172
Kaabi H, Jabeur K, Ladhari T (2018) A genetic algorithm-based classification approach for multicriteria ABC analysis. Int J Inform Technol Decis Mak 17(6):1805–1837
Maathavan KSK, Venkatraman S (2022) A secure encrypted classified electronic healthcare data for public cloud environment. Intell Autom Soft Comput 32(2):765–779
García-Barrios D et al (2021) A machine learning based method for managing multiple impulse purchase products: an inventory management approach. J Eng Sci Technol Rev 14(1):25–37
Yang K et al (2021) Multi-criteria spare parts classification using the deep convolutional neural network method. Appl Sci 11(15):7088
Zhang S et al (2020) Importance degree evaluation of spare parts based on clustering algorithm and back-propagation neural network. Math Problems Eng. https://doi.org/10.1155/2020/6161825
Balali V, Ashouri Rad A, Golparvar-Fard M (2015) Detection, classification, and mapping of U.S. traffic signs using google street view images for roadway inventory management. Vis Eng 3(1):1–18
Balali V, Golparvar-Fard M (2016) Evaluation of multiclass traffic sign detection and classification methods for us roadway asset inventory management. J Comput Civil Eng 30(2):04015022
Van Eck NJ et al (2010) A comparison of two techniques for bibliometric mapping: multidimensional scaling and VOS. J Am Soc Inform Sci Technol 61(12):2405–2416
Breiman L (2001) Random forests. Mach Learn 45(1):5–32
Bai J et al (2022) Multinomial random forest. Pattern Recogn 122:108331
Liu Y, Wang Y, Zhang J (2012) New machine learning algorithm: Random forest. International Conference on Information Computing and Applications. Springer, Berlin
Hong JS, Lie CH (1993) Joint reliability-importance of two edges in an undirected network. IEEE Trans Reliab 42(1):17–23
Ma M et al (2021) XGBoost-based method for flash flood risk assessment. J Hydrol 598:126382
Jawad J, Hawari AH, Zaidi SJ (2021) Artificial neural network modeling of wastewater treatment and desalination using membrane processes: a review. Chem Eng J 419:129540
Download references
This research did not receive any specific grant from funding agencies in the public, commercial, or not-for-profit sectors.
Author information
Authors and affiliations.
Department of Industrial Engineering, Engineering Faculty, Ataturk University, 25240, Erzurum, Turkey
Özge Albayrak Ünal & Burak Erkayman
Department of Computer Engineering, Engineering Faculty, Ataturk University, 25240, Erzurum, Turkey
Bilal Usanmaz
You can also search for this author in PubMed Google Scholar
Corresponding author
Correspondence to Burak Erkayman .
Additional information
Publisher’s note.
Springer Nature remains neutral with regard to jurisdictional claims in published maps and institutional affiliations.
Full names of the algorithms used in the study.
Rights and permissions
Springer Nature or its licensor (e.g. a society or other partner) holds exclusive rights to this article under a publishing agreement with the author(s) or other rightsholder(s); author self-archiving of the accepted manuscript version of this article is solely governed by the terms of such publishing agreement and applicable law.
Reprints and permissions
About this article
Albayrak Ünal, Ö., Erkayman, B. & Usanmaz, B. Applications of Artificial Intelligence in Inventory Management: A Systematic Review of the Literature. Arch Computat Methods Eng 30 , 2605–2625 (2023). https://doi.org/10.1007/s11831-022-09879-5
Download citation
Received : 13 August 2022
Accepted : 23 December 2022
Published : 07 February 2023
Issue Date : May 2023
DOI : https://doi.org/10.1007/s11831-022-09879-5
Share this article
Anyone you share the following link with will be able to read this content:
Sorry, a shareable link is not currently available for this article.
Provided by the Springer Nature SharedIt content-sharing initiative
- Inventory Management
- Machine Learning
- Deep Learning
- Systematic Literature Review
- Find a journal
- Publish with us
- Track your research
Information
- Author Services
Initiatives
You are accessing a machine-readable page. In order to be human-readable, please install an RSS reader.
All articles published by MDPI are made immediately available worldwide under an open access license. No special permission is required to reuse all or part of the article published by MDPI, including figures and tables. For articles published under an open access Creative Common CC BY license, any part of the article may be reused without permission provided that the original article is clearly cited. For more information, please refer to https://www.mdpi.com/openaccess .
Feature papers represent the most advanced research with significant potential for high impact in the field. A Feature Paper should be a substantial original Article that involves several techniques or approaches, provides an outlook for future research directions and describes possible research applications.
Feature papers are submitted upon individual invitation or recommendation by the scientific editors and must receive positive feedback from the reviewers.
Editor’s Choice articles are based on recommendations by the scientific editors of MDPI journals from around the world. Editors select a small number of articles recently published in the journal that they believe will be particularly interesting to readers, or important in the respective research area. The aim is to provide a snapshot of some of the most exciting work published in the various research areas of the journal.
Original Submission Date Received: .
- Active Journals
- Find a Journal
- Proceedings Series
- For Authors
- For Reviewers
- For Editors
- For Librarians
- For Publishers
- For Societies
- For Conference Organizers
- Open Access Policy
- Institutional Open Access Program
- Special Issues Guidelines
- Editorial Process
- Research and Publication Ethics
- Article Processing Charges
- Testimonials
- Preprints.org
- SciProfiles
- Encyclopedia
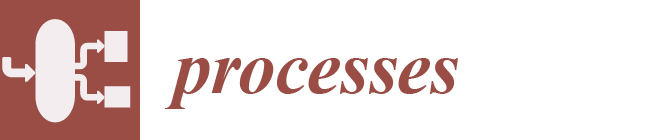
Article Menu
- Subscribe SciFeed
- Recommended Articles
- Google Scholar
- on Google Scholar
- Table of Contents
Find support for a specific problem in the support section of our website.
Please let us know what you think of our products and services.
Visit our dedicated information section to learn more about MDPI.
JSmol Viewer
Algorithmic approaches to inventory management optimization.
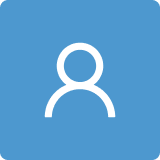
1. Introduction
- A deterministic linear programming model (DLP) that uses either the rolling horizon or shrinking horizon technique in order to determine optimal re-order quantities for each time period at each node in the supply network. Customer demand is modeled at its expectation value throughout the rolling/shrinking horizon time window.
- A multi-stage stochastic program (MSSP) with a simplified scenario tree, as described in Section 2.7 . Shrinking and rolling horizon for the MSSP model are both implemented to decide the reorder quantity at each time period.
- A reinforcement learning model (RL) that makes re-order decisions based on the current state of the entire network.
2. Materials and Methods
2.1. problem statement, 2.2. sequence of events.
- Main network nodes (retailer, distributors, and producers) place replenishment orders to their respective suppliers. Replenishment orders are filled according to available production capacity and available feedstock inventory at the respective suppliers. The supply network is assumed to be centralized, such that replenishment orders never exceed what can be provided by the suppliers to each node.
- The main network nodes receive incoming feedstock inventory replenishment shipments that have made it down the product pipeline (after the associated lead times have transpired). The lead times between stages include both production times and transportation times.
- Single-product customer demand occurs at the retail node and it is filled according to the available inventory at that stage.
- One of the following occurs at the retailer node, (a) Unfulfilled sales are backlogged at a penalty. Backlogged sales take priority in the following period. (b) Unfulfilled sales are lost and a goodwill loss penalty is levied.
- Surplus inventory is held at each node at a holding cost. Inventory holding capacity limits are not included in the present formulation, but they can be easily added to the model, if needed. The IMP that is presented here is capacitated in the sense that manufacturing at production nodes is limited by both the production capacity and the availability of feedstock inventory at each node. Because the supply network operates as a make-to-order system, only feedstock inventories are held at the nodes. All of the product inventory is immediately shipped to the downstream nodes upon request, becoming feedstock inventory to those nodes (or simply inventory if the downstream node is a distributor/retailer). A holding (e.g., transportation) cost is also placed on any pipeline inventory (in-transit inventory).
- Any inventory remaining at the end of the last period (period 30 in the base case) is lost, which means that it has no salvage value.
2.3. Key Variables
2.4. objective function, 2.5. imp model, 2.5.1. network profit, 2.5.2. inventory balances, 2.5.3. inventory requests, 2.5.4. market sales, 2.5.5. variable domains, 2.6. scenario tree for multistage stochastic programming, 2.7. approximation for the multistage scenario tree, 2.8. perfect information and deterministic model, 2.9. reinforcement learning model, 2.10. case study, 4. discussion, 5. conclusions, author contributions, acknowledgments, conflicts of interest, abbreviations.
LP | Linear Programming |
DLP | Deterministic Linear Programming |
MILP | Mixed-integer Linear Programming |
2SSP | Two-stage Stochastic Programming |
MSSP | Multi-stage Stochastic Programming |
RL | Reinforcement Learning |
AI | Artificial Intelligence |
PPO | Proximal Policy Optimization |
MDP | Markov Decision Process |
IMP | Inventory Management Problem |
- Lee, H.L.; Padmanabhan, V.; Whang, S. Information distortion in a supply chain: The bullwhip effect. Manag. Sci. 1997 , 43 , 546–558. [ Google Scholar ] [ CrossRef ]
- Eruguz, A.S.; Sahin, E.; Jemai, Z.; Dallery, Y. A comprehensive survey of guaranteed-service models for multi-echelon inventory optimization. Int. J. Prod. Econ. 2016 , 172 , 110–125. [ Google Scholar ] [ CrossRef ]
- Simchi-Levi, D.; Zhao, Y. Performance Evaluation of Stochastic Multi-Echelon Inventory Systems: A Survey. Adv. Oper. Res. 2012 , 2012 , 126254. [ Google Scholar ] [ CrossRef ] [ Green Version ]
- Glasserman, P.; Tayur, S. Sensitivity analysis for base-stock levels in multiechelon production-inventory systems. Manag. Sci. 1995 , 41 , 263–281. [ Google Scholar ] [ CrossRef ]
- Chu, Y.; You, F.; Wassick, J.M.; Agarwal, A. Simulation-based optimization framework for multi-echelon inventory systems under uncertainty. Comput. Chem. Eng. 2015 , 73 , 1–16. [ Google Scholar ] [ CrossRef ]
- Dillon, M.; Oliveira, F.; Abbasi, B. A two-stage stochastic programming model for inventory management in the blood supply chain. Int. J. Prod. Econ. 2017 , 187 , 27–41. [ Google Scholar ] [ CrossRef ]
- Fattahi, M.; Mahootchi, M.; Moattar Husseini, S.M.; Keyvanshokooh, E.; Alborzi, F. Investigating replenishment policies for centralised and decentralised supply chains using stochastic programming approach. Int. J. Prod. Res. 2015 , 53 , 41–69. [ Google Scholar ] [ CrossRef ]
- Pauls-Worm, K.G.; Hendrix, E.M.; Haijema, R.; Van Der Vorst, J.G. An MILP approximation for ordering perishable products with non-stationary demand and service level constraints. Int. J. Prod. Econ. 2014 , 157 , 133–146. [ Google Scholar ] [ CrossRef ]
- Zahiri, B.; Torabi, S.A.; Mohammadi, M.; Aghabegloo, M. A multi-stage stochastic programming approach for blood supply chain planning. Comput. Ind. Eng. 2018 , 122 , 1–14. [ Google Scholar ] [ CrossRef ]
- Bertsimas, D.; Thiele, A. A robust optimization approach to inventory theory. Oper. Res. 2006 , 54 , 150–168. [ Google Scholar ] [ CrossRef ]
- Govindan, K.; Cheng, T.C. Advances in stochastic programming and robust optimization for supply chain planning. Comput. Oper. Res. 2018 , 100 , 262–269. [ Google Scholar ] [ CrossRef ]
- Roy, B.V.; Bertsekas, D.P.; North, L. A neuro-dynamic programming approach to admission control in ATM networks. In Proceedings of the IEEE International Conference on Acoustics, Speech, and Signal Processing, Munich, Germany, 21–24 April 1997. [ Google Scholar ]
- Kleywegt, A.J.; Non, V.S.; Savelsbergh, M.W. Dynamic Programming Approximations for a Stochastic Inventory Routing Problem. Transport. Sci. 2004 , 38 , 42–70. [ Google Scholar ] [ CrossRef ]
- Topaloglu, H.; Kunnumkal, S. Approximate dynamic programming methods for an inventory allocation problem under uncertainty. Naval Res. Logist. 2006 , 53 , 822–841. [ Google Scholar ] [ CrossRef ] [ Green Version ]
- Kunnumkal, S.; Topaloglu, H. Using stochastic approximation methods to compute optimal base-stock levels in inventory control problems. Oper. Res. 2008 , 56 , 646–664. [ Google Scholar ] [ CrossRef ] [ Green Version ]
- Cimen, M.; Kirkbride, C. Approximate dynamic programming algorithms for multidimensional inventory optimization problems. In Proceedings of the 7th IFAC Conference on Manufacturing, Modeling, Management, and Control, Saint Petersburg, Russia, 19–21 June 2013; Volume 46, pp. 2015–2020. [ Google Scholar ] [ CrossRef ]
- Sarimveis, H.; Patrinos, P.; Tarantilis, C.D.; Kiranoudis, C.T. Dynamic modeling and control of supply chain systems: A review. Comput. Oper. Res. 2008 , 35 , 3530–3561. [ Google Scholar ] [ CrossRef ]
- Mortazavi, A.; Arshadi Khamseh, A.; Azimi, P. Designing of an intelligent self-adaptive model for supply chain ordering management system. Eng. Appl. Artif. Intell. 2015 , 37 , 207–220. [ Google Scholar ] [ CrossRef ]
- Oroojlooyjadid, A.; Nazari, M.; Snyder, L.; Takáč, M. A Deep Q-Network for the Beer Game: A Reinforcement Learning algorithm to Solve Inventory Optimization Problems. arXiv 2017 , arXiv:1708.05924. [ Google Scholar ]
- Kara, A.; Dogan, I. Reinforcement learning approaches for specifying ordering policies of perishable inventory systems. Expert Syst. Appl. 2018 , 91 , 150–158. [ Google Scholar ] [ CrossRef ]
- Sultana, N.N.; Meisheri, H.; Baniwal, V.; Nath, S.; Ravindran, B.; Khadilkar, H. Reinforcement Learning for Multi-Product Multi-Node Inventory Management in Supply Chains. arXiv 2020 , arXiv:2006.04037. [ Google Scholar ]
- Hubbs, C.D.; Perez, H.D.; Sarwar, O.; Sahinidis, N.V.; Grossmann, I.E.; Wassick, J.M. OR-Gym: A Reinforcement Learning Library for Operations Research Problems. arXiv 2020 , arXiv:2008.06319. [ Google Scholar ]
- Hochreiter, R.; Pflug, G.C. Financial scenario generation for stochastic multi-stage decision processes as facility location problems. Ann. Oper. Res. 2007 , 152 , 257–272. [ Google Scholar ] [ CrossRef ]
- Schulman, J.; Moritz, P.; Levine, S.; Jordan, M.I.; Abbeel, P. High-dimensional continuous control using generalized advantage estimation. arXiv 2016 , arXiv:1506.02438. [ Google Scholar ]
- Moritz, P.; Nishihara, R.; Wang, S.; Tumanov, A.; Liaw, R.; Liang, E.; Elibol, M.; Yang, Z.; Paul, W.; Jordan, M.I.; et al. Ray: A Distributed Framework for Emerging AI Applications. In Proceedings of the 13th USENIX Symposium on Operating Systems Design and Implementation (OSDI 18), Carlsbad, CA, USA, 8–10 October 2018. [ Google Scholar ]
- Lima, R.M.; Grossmann, I.E.; Jiao, Y. Long-term scheduling of a single-unit multi-product continuous process to manufacture high performance glass. Comput. Chem. Eng. 2011 , 35 , 554–574. [ Google Scholar ] [ CrossRef ]
Click here to enlarge figure
Variable | Description |
---|---|
The reorder quantity requested to supplier node j by node k at the beginning of period t (the amount of material sent from node j to node k) | |
The amount retailer j sells to market k in period . Note: Retail sales are indexed at the next period since these occur after demand in the current period is realized. | |
The on-hand inventory at node j just prior to when the demand is realized in period t. | |
The in-transit (pipeline) inventory between node j and node k just prior to when the demand is realized in period t. | |
The unfulfilled demand at retailer j associated with market k in period . Note: indexing is also shifted since any unfulfilled demand occurs after the uncertain demand is realized. | |
The profit (reward) in node j for period t. |
DLP-RH | DLP-SH | MSSP-RH | MSSP-SH | RL | Oracle | |
---|---|---|---|---|---|---|
Backlog | ||||||
Mean Profit | 791.6 | 825.3 | 802.7 | 847.7 | 737.2 | 861.3 |
Standard Deviation | 52.5 | 37.0 | 56.3 | 49.4 | 24.8 | 56.4 |
Performance Ratio | 1.09 | 1.04 | 1.07 | 1.02 | 1.17 | 1.00 |
Lost Sales | ||||||
Mean Profit | 735.8 | 786.9 | 790.6 | 830.6 | 757.8 | 854.9 |
Standard Deviation | 31.2 | 30.8 | 47.8 | 37.7 | 33.1 | 49.9 |
Performance Ratio | 1.16 | 1.09 | 1.08 | 1.03 | 1.13 | 1.00 |
MDPI stays neutral with regard to jurisdictional claims in published maps and institutional affiliations. |
Share and Cite
Perez, H.D.; Hubbs, C.D.; Li, C.; Grossmann, I.E. Algorithmic Approaches to Inventory Management Optimization. Processes 2021 , 9 , 102. https://doi.org/10.3390/pr9010102
Perez HD, Hubbs CD, Li C, Grossmann IE. Algorithmic Approaches to Inventory Management Optimization. Processes . 2021; 9(1):102. https://doi.org/10.3390/pr9010102
Perez, Hector D., Christian D. Hubbs, Can Li, and Ignacio E. Grossmann. 2021. "Algorithmic Approaches to Inventory Management Optimization" Processes 9, no. 1: 102. https://doi.org/10.3390/pr9010102
Article Metrics
Article access statistics, further information, mdpi initiatives, follow mdpi.
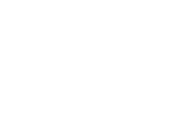
Subscribe to receive issue release notifications and newsletters from MDPI journals

IEEE Account
- Change Username/Password
- Update Address
Purchase Details
- Payment Options
- Order History
- View Purchased Documents
Profile Information
- Communications Preferences
- Profession and Education
- Technical Interests
- US & Canada: +1 800 678 4333
- Worldwide: +1 732 981 0060
- Contact & Support
- About IEEE Xplore
- Accessibility
- Terms of Use
- Nondiscrimination Policy
- Privacy & Opting Out of Cookies
A not-for-profit organization, IEEE is the world's largest technical professional organization dedicated to advancing technology for the benefit of humanity. © Copyright 2024 IEEE - All rights reserved. Use of this web site signifies your agreement to the terms and conditions.
Click through the PLOS taxonomy to find articles in your field.
For more information about PLOS Subject Areas, click here .
Loading metrics
Open Access
Peer-reviewed
Research Article
Construction and optimization of inventory management system via cloud-edge collaborative computing in supply chain environment in the Internet of Things era
Roles Writing – original draft, Writing – review & editing
* E-mail: [email protected]
Affiliation Xi’an Fanyi University, Xi’an City, China

- Published: November 3, 2021
- https://doi.org/10.1371/journal.pone.0259284
- Reader Comments
The PLOS ONE Editors retract this article [ 1 ] because it was identified as one of a series of submissions for which we have concerns about peer review integrity and similarities across articles. These concerns call into question the validity and provenance of the reported results. We regret that the issues were not identified prior to the article’s publication.
The author either did not respond directly or could not be reached.
6 Sep 2023: The PLOS ONE Editors (2023) Retraction: Construction and optimization of inventory management system via cloud-edge collaborative computing in supply chain environment in the Internet of Things era. PLOS ONE 18(9): e0291318. https://doi.org/10.1371/journal.pone.0291318 View retraction
The present work aims to strengthen the core competitiveness of industrial enterprises in the supply chain environment, and enhance the efficiency of inventory management and the utilization rate of inventory resources. First, an analysis is performed on the supply and demand relationship between suppliers and manufacturers in the supply chain environment and the production mode of intelligent plant based on cloud manufacturing. It is found that the efficient management of spare parts inventory can effectively reduce costs and improve service levels. On this basis, different prediction methods are proposed for different data types of spare parts demand, which are all verified. Finally, the inventory management system based on cloud-edge collaborative computing is constructed, and the genetic algorithm is selected as a comparison to validate the performance of the system reported here. The experimental results indicate that prediction method based on weighted summation of eigenvalues and fitting proposed here has the smallest error and the best fitting effect in the demand prediction of machine spare parts, and the minimum error after fitting is only 2.2%. Besides, the spare parts demand prediction method can well complete the prediction in the face of three different types of time series of spare parts demand data, and the relative error of prediction is maintained at about 10%. This prediction system can meet the basic requirements of spare parts demand prediction and achieve higher prediction accuracy than the periodic prediction method. Moreover, the inventory management system based on cloud-edge collaborative computing has shorter processing time, higher efficiency, better stability, and better overall performance than genetic algorithm. The research results provide reference and ideas for the application of edge computing in inventory management, which have certain reference significance and application value.
Citation: Ran H (2021) Construction and optimization of inventory management system via cloud-edge collaborative computing in supply chain environment in the Internet of Things era. PLoS ONE 16(11): e0259284. https://doi.org/10.1371/journal.pone.0259284
Editor: Haibin Lv, Ministry of Natural Resources North Sea Bureau, CHINA
Received: September 12, 2021; Accepted: October 17, 2021; Published: November 3, 2021
Copyright: © 2021 Hailan Ran. This is an open access article distributed under the terms of the Creative Commons Attribution License , which permits unrestricted use, distribution, and reproduction in any medium, provided the original author and source are credited.
Data Availability: All relevant data are within the manuscript and its Supporting Information files.
Funding: This work was supported by Xi’an Fanyi University Research team (No. XF17KYTD202).
Competing interests: The authors have declared that no competing interests exist.
Introduction
With the rapid development of science and technology, the combination of manufacturing processes, industrial IoT (Internet of Things), advanced computing, and other technologies has become increasingly close. Meanwhile, the manufacturing mode has changed from a product-centric mode to a user-centric mode [ 1 , 2 ]. Due to the complexity of business processes in large manufacturing plants, it is necessary to coordinate the relationship between people, information system, and physical system, which causes the traditional imbalance between resource allocation and task planning [ 3 ]. Moreover, concepts such as the intelligent plant, intelligent transportation and smart city have emerged as AI (artificial intelligence) and computer technology develop fast. Lv et al. (2018) designed a new government service platform by using 3D (three-dimensional) geographic information system and cloud computing to effectively manage and use urban data. In addition, they achieved the 3D analysis and visualization of urban information through the smart city platform, which made the life of the masses more convenient [ 4 ]. This proves that the application of computer and AI technology has become a hot research topic.
The development of China’s industry in the next decade will shift from labor-intensive production to technology-intensive production, which will bring great progress in advanced technology. Correspondingly, domestic enterprises have begun to explore the transformation approach to adapt to market changes and meet government needs. The fast-growing IoT applications can produce enormous amounts of data at the network edge, effectively promoting the generation and development of edge computing. Edge computing is one of the crucial technologies to realize intelligent industry. In large manufacturing workshops, sensors, instruments, and intelligent devices can collect mass of machine data [ 5 ]. These kinds of data are the main sources of industrial big data. Moreover, it is difficult to effectively master and forecast market demands. To reduce the dependence on the accuracy of market demand forecasting and improve the efficiency of supply chain inventory management, it is necessary to improve inventory management efficiency to adapt to the changes in market demand. Besides, it is essential to use management methods to compensate for many negative impacts of market uncertainty [ 6 ]. In this case, the upstream and downstream enterprises of the supply chain must create a constant speed supply chain based on the network platform to reduce the inventory cost of the supply chain and meet the needs of customers in real time. Industrial big data is considered as a necessary means to further enlarge product profit margin. At present, industrial data platform is the paramount component of data storage, calculation and analysis for intelligent factories. With the increase in smart devices in smart factories, a large number of data such as RFID (radio frequency identification) is obtained, providing a rich data set for the manufacturing industry. As IoT applications develop rapidly, mass of data is generated at the edge of the network, effectively facilitating the emergence and development of edge computing. Consequently, in large manufacturing workshops, sensors, instruments, intelligent terminals, and other devices can collect a large amount of machine data, as the main source of industrial big data. Under the background of increasingly socialized mass production and global economic integration, all links of the supply chain, such as raw material supply, production, logistics, consumption, processing, distribution, and retail must cooperate closely. Nevertheless, the coordination and management in all links, including inventory management, are still relatively closed, significantly reducing the comprehensive benefits of the overall supply chain.
The industrial production data is investigated here based on the analysis of the related concepts and production modes of supply chain and cloud manufacturing. Then, the demand prediction method for different types of industrial spare parts and the inventory management system are proposed via cloud-edge collaborative computing. The purpose of this work is to optimize inventory management and utilization efficiency by predicting the demand for vulnerable spare parts, and improve the performance of inventory management system with the advantage of cloud-edge collaboration computing. Moreover, cloud computing and IoT technology are utilized to explore the implementation method of refining the traditional inventory management of the supply chain. The innovation of this study is that corresponding demand prediction methods are studied separately according to three demand modes of vulnerable spare parts, namely periodic demand, stationary demand, and trend demand. Specifically, the simple exponential smoothing method is used to predict demand of stationary spare parts. The quadratic exponential smoothing method is selected to predict the linear demand, and the feature synthesis method is proposed for forecasting the spare parts with periodic demand mode. On this basis, edge computing is employed to develop a cloud-edge collaborative computing architecture, to optimize the spare parts prediction algorithm and improve inventory management efficiency and pertinence.
Related theories and research methods
Overview and status of supply chain inventory management.
IoT technology is the combination of intelligent recognition technology, wireless sensor technology, ubiquitous computing technology, and network technology. The global IoT network is still in the stage of concept, demonstration, and test, many key technologies need to be further studied, and standardization norms need to be further developed. However, it has triggered the third wave of information industry development in the world after computers and the Internet, which is an impactful upgrade of the application of information technology to human production and life. Supply chain is a kind of complete and functional network chain consisting of suppliers, manufacturers, distributors, retailers and end users centering on the business center enterprise and formed through controlling feed-forward information flow and the feedback of material flow and information flow [ 7 ]. There are diversified research works about supply chain inventory management. Bornkamp (2019) emphasized the importance of supply chain in his research. The author believed that the renegotiation of the UK-EU relationship would most likely take several years, but European distributors had to assess their current inventory management to mitigate future disruptions. Moreover, with the political pattern continuing to change, the growing e-commerce market would bring trade growth, so managing availability and distribution of inventory was critical to reducing overall costs, improving cash flows and increasing flexibility in supply chain operations, in order to effectively serve the European market [ 8 ]. Aaha et al. [ 9 ] analyzed six professional education courses offered by THE Council of Supply Chain Management Professionals, including senior certified professional forecaster, certified production and inventory management professionals, certified supply management professionals, and supply chain professionals. They took personal interests and organizational interests as the two main standards, and took the professional education plan as an alternative [ 9 ]. Evidently, people have gradually realized the significance of supply chain, and delved into supply chain deeply and professionally. Fig 1 reveals the basic structure of the supply chain.
- PPT PowerPoint slide
- PNG larger image
- TIFF original image
https://doi.org/10.1371/journal.pone.0259284.g001
The supply chain not only aggerates the logistics information and funds of suppliers and users, but also forms its own value. In the distribution link of the supply chain, the appreciation in products has been achieved through packaging, processing, transportation, and delivery. Supply chain inventory management is the process of defining the overall goal of supply chain inventory management and reviewing the inventory strategy of enterprises on supply chain nodes. The supply chain inventory management aims to sustain the optimal overall supply chain inventory and reduce the total inventory to respond to changing market demands. The improvement of the cost of supply chain inventory and supply chain can enhance the rapid response of inventory to the market.
Introduction to concepts related to cloud-edge collaboration for logistics management
Cloud manufacturing includes cloud-edge collaboration technology, AI service technology, container-based platform service technology, digital twins service technology, data security, and other related technologies. It is a novel type of digital, intelligent, and smart networked manufacturing with Chinese characteristics. Fig 2 reveals the overall schematic of the system of cloud manufacturing technology.
https://doi.org/10.1371/journal.pone.0259284.g002
The foundation of intelligent cloud manufacturing is a ubiquitous and human-centered network, which integrates digital technology such as information manufacturing technology and intelligent technology comprehensively [ 10 ]. The cloud manufacturing system enables users to obtain manufacturing resources and capabilities according to their own needs anytime and anywhere through the cloud-based manufacturing service platform, and intelligently perform various activities throughout the life cycle.
In the industrial field, the IoT proactively identifies and remotely controls all physical devices in the cloud manufacturing scenario of existing network infrastructure, and obtains content in the physical world (real space) in the information world (cyberspace). The data reflects the whole life cycle of the corresponding physical equipment, and realizes the digital twins [ 11 , 12 ].
Internet technology facilitates the active and independent analysis of industrial product manufacturing process, generates intelligent perception and active prediction of the outside world, and forms a closed-loop process of automatic repair and complete feedback. With the emergence of intelligent control, industrial IoT can optimize all aspects of industrial systems, including intelligent manufacturing and business systems, real-time monitoring, supply chain collaboration, value-added services, and other business needs. The wide application of industrial IoT technology makes the production process more active and intelligent, which can accurately predict and effectively solve the potential obstacles, to effectively increase corporate profits [ 13 , 14 ].
The continuous development of the mobile Internet has brought new convenience for people’s life and production, as well as more needs and challenges, such as higher requirement of timeliness, security, and reliability. Hence, edge computing is needed to improve cloud computing ability. Fig 3 illustrates the typical architecture of edge computing for intelligent plants.
https://doi.org/10.1371/journal.pone.0259284.g003
Many problems such as single-point faults may occur in industrial applications. In addition to the unified control of the cloud, the edge nodes have the computing ability to independently make decisions and solve problems, which can improve factory productivity, while avoiding equipment failure. In IoT scenarios, edge computing focuses on solving problems of lightweight data size closer to the user’s by transferring computing operation [ 15 ]. Therefore, it cannot completely replace cloud computing, but assists cloud computing to improve work efficiency. With the deepening of industry research and academic research, cloud collaboration is widely used in numerous fields such as medical treatment, industry, and finance. Cloud-edge collaborative architecture can balance the load and reduce the hardware requirements of edge devices, making the peripheral equipment more convenient while maintaining the capacity [ 16 , 17 ]. Fig 4 provides a manufacturing factory example based on cloud-edge collaborative computing architecture.
https://doi.org/10.1371/journal.pone.0259284.g004
Demand prediction of vulnerable spare parts in IoT supply chain environment
In the cloud manufacturing scenario, the amount of data sent by the terminal equipment deployed in each plant is different for various plant equipment and actual business needs. Therefore, it is necessary to design a scheme for the edge server equipped in different plants to effectively reduce the procurement funds of enterprises and avoid the waste of limited resources. Based on this consideration, a demand prediction method is proposed for vulnerable spare parts, and it is combined with the cloud-edge cooperative inventory management system to improve the efficiency and quality of inventory management.
Timely maintenance and supply of spare parts are two important components of the after-sales service system provided by large equipment manufacturers in the service network [ 18 ]. Among them, the efficiency of spare parts inventory management determines whether spare parts can reach the demand in time, which directly affects the market competitiveness of service systems and manufacturers. In the IoT era, many consumption data and consumption behaviors based on IoT provide sufficient data basis for market demand prediction. Shen et al. (2020) extracted knowledge from user generated content and depicted the differences between IT service companies’ use of social media and users’ expectations based on daily interaction between suppliers with customers [ 19 ]. The data analysis method is also adopted here to forecast the spare parts demand.
The purpose of inventory management is to deal with various changes and uncertainties in spare part supply to ensure the normal operation of spare part supply. According to the function and direction of spare parts, they can be divided into two categories: maintenance spare parts and service spare parts.
The function of maintenance spare parts is to ensure the normal operation of production equipment, while the function of service spare parts is to ensure the after-sales service of products. Different types of spare parts have diverse inventory management purposes and management methods [ 20 ]. In summary, in the case of low total cost of spare parts inventory, it is very practical to study how to optimize the inventory management system according to the actual situation of enterprises to achieve a significant improvement in service level. The spare parts inventory management strategy includes spare parts classification, spare parts demand analysis, spare parts shortage management, spare parts inventory mode, and inventory strategy.
The common vulnerable parts of pump trucks in industrial production are taken as the research object here to predict their needs, including conveying cylinder, concrete piston, and cutting ring, usually with relatively large demands. Through the analysis of the sales volume of concrete piston in different regions, the demand is classified into the following three categories: periodic demand time series, demand time series with rising trend, and stable demand time series [ 21 ].
The prediction based on periodic demand time series is first discussed. Spare parts with periodic changes in demand patterns include random components and periodic components in the past demand time series [ 22 , 23 ]. The proposed prediction method calculates the cycle length according to the time series of spare parts demand in the past, calculates the demand data of original spare parts according to the cycle length, and divides each segment and performs polynomial fitting. The polynomial function of each cycle is integrated to obtain a new polynomial function to extract periodic ports and remove random factors, which is used as a prediction model to predict the demand of the next period.
In Eq (1) , T is the target time series, S denotes the time series needed for similarity measurement, and n represents the length of two time series. Besides, t i or s i refers to a factor at a time in a time series.
In Eq (2) , P TS refers to the correlation coefficient of time series. Meanwhile, d ( T , S ) stands for the Euclidean distance of two time series data, and f ( T , S ) is the similarity measure function.
In Eq (4) , the value of a is 1, and the value of b is n/2. α signifies the threshold.
There are several methods to predict the continuous demand of spare parts for the non-periodic demand time series, such as the exponential smoothing method and the weighted moving average method. The exponential smoothing method is an improvement of moving average method characterized by simple form, easy implementation, and high precision, which can accurately reflect the changes in demand data and is widely used in practice. Therefore, the exponential smoothing method is selected as the spare parts demand prediction method based on aperiodic demand time series here.
Among Eqs ( 7 ) and ( 8 ), a denotes the smooth constant, and S t + 1 stands for the smooth value of (t+1) period.
For the prediction of intermittent time series, the intermittent demand time of spare parts has two characteristics. (1) There is less demand. In other words, there is no demand during this period. (2) There is great volatility in demand value. These two characteristics cause a large prediction error of intermittent time series [ 27 ]. Furthermore, the time aggregation prediction method is used to predict the demand of intermittent time series.
Inventory management system based on cloud-edge collaboration
Core competitiveness is crucial to large equipment manufacturers, because efficient management of spare parts inventory can effectively reduce costs and improve service levels. The engineering machinery and equipment usually have a complex structure and various components and parts. However, the existing spare parts inventory management is still cumbersome and unsystematic, which determines inventory according to personal experience and plans demands according to inventory proportion, bringing great pressure to the production department and other related departments. The solution of traditional cloud computing architecture is to download the sensor data of various factory equipment, and use the data analysis technique of big data. Meanwhile, it transits the downloaded data to remote cloud servers through the data acquisition module, to improve work efficiency and competitiveness [ 28 , 29 ]. Here, the cloud-edge collaborative computing in industrial IoT is proposed to solve the rapid response problem of real-time control and data fast processing in large-scale manufacturing plants. Fig 5 provides the architecture of cloud-edge collaborative computing.
https://doi.org/10.1371/journal.pone.0259284.g005
The deployment of industrial IoT in intelligent manufacturing environment mainly contains the equipment perception layer, data resource layer, service application layer, and operation and maintenance management layer, which work together to maintain all data links [ 30 , 31 ]. From the specific business point of view, the cloud components are mainly responsible for the formation of the model of the collected data, and the peripheral components are basically responsible for obtaining the model from the data dictionary, providing timely services for factory equipment in real time. Reducing the training time of models and networks can shorten the response time of the closed-loop system and improve the overall production quality of the plant equipment. OpenStpack and Starling X enable companies to build their own cloud- edge collaborative computing services using the most advanced open-source cloud computing platform and the latest distributed cloud computing platform, respectively.
The solution of traditional cloud computing architecture is to upload all kinds of sensor data from factory equipment, such as vibration, pressure, and temperature, to the cloud remote server through data acquisition module. Besides, it utilizes the popular big data analysis technology to establish the mathematical model of index data and factory equipment performance, to enhance the production quality, work efficiency, and market competitiveness of factory equipment. Taking the coal industry as an example, the mine is generally located in a remote location where it is difficult to implement network communication. Due to the characteristics of large scale, numerous varieties, low value density, and fast update and processing requirements of coal mine data, the traditional cloud computing architecture is inadequate, because it is easy to produce problems of single point faults and slow closed-loop response. Based on the above analysis, the cloud-edge collaborative computing architecture is selected for the industrial IoT to cope with the problems of fast real-time control response and fast data calculation in large manufacturing workshops. Fig 6 illustrates the workflow of cloud-edge collaborative computing architecture, where various data acquisition devices and user requests are collectively referred to as collectors. The smart endpoint simply pre-processes information from the collectors and sends it to the computing node in the edge server cluster [ 32 ]. Then, the I/O intensive virtual machine on the computing node receives the information and stores it in the database on the storage node.
https://doi.org/10.1371/journal.pone.0259284.g006
The following is the specific processing of the edge server:
- the intelligent terminal sends the collected data to the edge data storage module;
- data processing module retrieves the corresponding data from the edge data storage module according to the user’s request;
- data processing module carries out lightweight big data analysis according to the model parameters provided by the data dictionary module. Besides, the edge data dictionary module is analyzed and synchronized;
- the decision module outputs the processing results of the data processing module to the intelligent equipment and checks them accordingly.
The procedure of the remote centralized server is as follows:
- the edge server and remote centralized data storage module synchronize incremental data;
- data processing module retrieves data from the remote centralized data storage module according to user needs;
- the data processing module conducts large-scale big data analysis according to the model parameters provided by the data dictionary module.
The analysis and synchronization of the remote data dictionary module are presented as follows:
- edge server synchronizes incremental data with the remote centralized data storage module;
- the data processing module retrieves data from remote centralized data storage module according to user needs;
- the data processing module conducts large-scale big data analysis according to the model parameters provided by the data dictionary module. Meanwhile, analysis and synchronization are performed on the remote data dictionary module;
- the remote data dictionary module synchronizes data processing with edge data dictionary module according to specific requirements.
Edge servers and remote centralized servers regularly analyze and use stored data, and the data dictionary is updated to ensure the correctness of the decision message.
Simulation and experimental design
Three time series prediction methods are provided for the demand prediction of vulnerable parts based on spare parts. The demand data of high-strength circular chains in the mining industry is used here for verification. The circular chain is also a spare part of construction machinery, and the experimental data comes from the network. In the simulation experiment, the genetic algorithm is introduced as a comparative algorithm to verify the performance of the inventory management system based on cloud-edge collaborative computing architecture. Table 1 indicates the task parameters under different configurations in this experiment.
https://doi.org/10.1371/journal.pone.0259284.t001
Analysis of demand prediction results and the performance verification of inventory management system
Comparison results of the prediction method based on demand of vulnerable spare parts..
After the prediction model is established, the predicted value of spare parts demand is calculated to be compared with the true value. The polynomial is established and fitted according to the period length. Fig 7 illustrates the relationship between fitting times and prediction errors.
https://doi.org/10.1371/journal.pone.0259284.g007
Fig 7 shows that the prediction error decreases first and then increases with the increase of fitting times. When the fitting time of the polynomial reaches 10, the prediction error begins to stabilize. After 13 times of fitting of the polynomial, the prediction error reaches a minimum of 11.7%, and then begins to increase. Based on this result, in the following simulation experiment, 13 times of fitting are used to obtain the fitting polynomial of each section when the polynomial regression model is used to fit the demand data of spare parts.
The eigenvalues and the weighted fitting process of each cycle are shown in Fig 8 .
Eigenvalues and the weighted fitting processes ((a): eigenvalues of each cycle; (b): weighted fitting processes of each cycle).
https://doi.org/10.1371/journal.pone.0259284.g008
Fig 8 displays the intermediate process of eigenvalue fitting. As can be seen from Fig 8B , the prediction error of monthly demand after weighted fitting is smaller. The mean value of the sum of eigenvalues and the weighted sum of eigenvalues shown in Fig 8A is used to synthesize new feature sets. In other words, the determination of values of a n , b n , and c n is similar to the determination of polynomial degree. Through experimental analysis, the prediction accuracy is the highest when w = 0.1, w = 0.1, and w = 0.8.
Fig 9A illustrates the mean value of the sum of eigenvalues and the weighted sum of eigenvalues shown in Fig 8A . Fig 9 provides the prediction results of spare parts demand based on the weighted synthesized eigenvalues.
Prediction results of spare parts demand based on weighted eigenvalues ((a): weighted eigenvalues; (b): prediction results based on weighted eigenvalues; (c): error comparison of two processing methods).
https://doi.org/10.1371/journal.pone.0259284.g009
According to Fig 9b , from a macro perspective, the prediction result based on the weighted sum is closer to the real value than the prediction based on the mean value of sum of the eigenvalues. From the perspective of error value, the highest prediction error based on the weighted eigenvalues is 34.9%, and the lowest is 2.2%. Through the comparison of error in Fig 9C , the average relative error based on the weighted fitting is lower than that based on the mean value of the sum of eigenvalues, the former is 11.7%, and the latter is 18.4%. Therefore, the prediction accuracy of the prediction model established by the weighted fitting method is higher. To sum up, the prediction method based on weighted fitting of eigenvalues has the smallest error and the best fitting effect in the demand prediction of machine spare parts.
Verification results of the perdition method based on vulnerable spare parts demand. The simulation experiment adopts the moving average period coefficient prediction of the prediction method based on weighted eigenvalues as a comparison with the true value. The specific results are presented in Fig 10 .
Weighted eigenvalues and prediction results ((a): weighted eigenvalues; (b): fitting processing of weighted eigenvalues; (c): comparison between prediction results and true results).
https://doi.org/10.1371/journal.pone.0259284.g010
In Fig 10A , a n refers to the first set of eigenvalues, b n denotes the second set of eigenvalues, c n represents the eigenvalues after fitting, the value range of cycle length is 1 ~ 13, and the threshold is 10% of the mean value. Polynomial fitting is carried out for the first two data segments to obtain the periodic term of the data segment, which is used to predict the true value of the third cycle segment. When n = 10, the prediction error is the smallest, so the degree of the fitting polynomial is n = 10. The fitting polynomial function of each segment is obtained. From Fig 10 , the average relative error between the actual value of spare parts demand and the predicted value is 9.4%. When the moving period coefficient method is used to predict the demand for spare parts, the average relative error between the predicted value and the actual value is 13.0%.
The proposed prediction method is also used to predict the demand of the circular chain, and the results are compared with those of the moving average period coefficient method, to further verify the advantages of this method. The comparison results are shown in Fig 11 .
https://doi.org/10.1371/journal.pone.0259284.g011
From Fig 11 , the average absolute error of the actual value and predicted value of spare parts demand based on the moving average period coefficient method is 286.8, and the average relative error is 12.8%. The average absolute error of the polynomial fitting model is 250.7, and the average relative error is 11.7%. Therefore, the proposed prediction mode has a better prediction effect.
The prediction results of exponential smoothing method and quadratic exponential smoothing method are shown in Fig 12 .
The prediction results of exponential smoothing method and quadratic exponential smoothing method ((a): the prediction results of exponential smoothing method; (b): the prediction results of quadratic exponential smoothing method).
https://doi.org/10.1371/journal.pone.0259284.g012
The simple smoothing index prediction method is used to investigate the spare parts demand data with the nonlinear trend. According to Fig 12A , the predicted value of spare parts demand by smoothing index prediction method is close to the actual value, and the average relative error is 18.0%. The quadratic smoothing index prediction method is aimed at the spare parts demand data with linear trend. From the results in Fig 12B , the predicted value of demand of the quadratic exponential smoothing method is close to the actual value, and the average relative error is 11.3%. In conclusion, the exponential smoothing method and quadratic exponential smoothing method both have high prediction accuracy in spare parts demand.
To sum up, the cycle length detection method based on similarity is adopted to calculate the cycle length. Then, the data is divided into several segments according to its cycle length, and polynomials are used to fit the data in the cycle segment. Moreover, the polynomials are synthesized to obtain a new polynomial function, which is used as the prediction model to predict the demand in the next cycle. The experimental results demonstrate that this prediction method can achieve high prediction accuracy.
Performance verification results of inventory management system based on cloud-edge collaborative computing. The algorithm of the inventory management system optimizes the resource allocation for virtual machines from the impact of virtual machines on the performance of physical machines and the impact of different configurations of virtual machines on task execution time. Table 2 and Fig 13 signify the resource allocation scheme for the best virtual machine performance obtained by the two algorithms and the comparison of the results after 100 executions of six tasks at the same time, respectively.
Performance comparison between two algorithms ((a)-(f) represent the experimental results from Task 1 to Task 6).
https://doi.org/10.1371/journal.pone.0259284.g013
https://doi.org/10.1371/journal.pone.0259284.t002
Fig 13 illustrates the performance comparison between the proposed algorithm and the genetic algorithm. Specifically, the average completion time of the six tasks executed by the genetic algorithm is 15.64 seconds, 14.92 seconds, 21.55 seconds, 21.34 seconds, 24.03 seconds, and 23.95 seconds, respectively. The average completion time of the six tasks by the proposed algorithm is 15.10 seconds, 15.00 seconds, 20.35 seconds, 20.60 seconds, 23.66 seconds, and 23.59 seconds. From the completion time point of view, the proposed virtual machine performance algorithm has shorter processing time and higher efficiency than genetic algorithm. In terms of stability, the genetic algorithm fluctuates greatly, so the proposed algorithm has higher stability.
In conclusion, in the prediction of spare parts demand with strong periodicity, the prediction method based on weighted fitting of eigenvalues has the smallest error and the optimal fitting effect in the prediction of machine spare parts demand, and the lowest error after fitting is only 2.2%. For spare parts with non-periodic linear demand and spare parts with nonlinear demand, exponential smoothing method and quadratic exponential smoothing method are used for prediction respectively, and the prediction results are close to the actual value. The spare parts demand prediction method proposed here can well complete the prediction for three different types of time series of demand data of spare parts, and the relative error of prediction is maintained at about 10%. The prediction effect can meet the basic requirements of spare parts demand prediction, and the prediction accuracy is higher than that of periodic prediction method. Compared with genetic algorithm, the cloud-edge collaborative computing algorithm for inventory management system takes less processing time and has higher efficiency. In terms of stability, genetic algorithm fluctuates greatly, but the algorithm reported here is much more stable.
Conclusions
Efficient spare parts inventory management can reduce inventory costs, improve service level, and bring huge benefits to large equipment manufacturing enterprises. There are a variety of spare parts for large-scale equipment as well as many uncertain factors in the supply process. Therefore, it is essential to continuously update relevant technologies for higher efficiency of spare parts inventory management, to save inventory costs. Based on the supply chain background, the critical role of inventory management plan and spare parts demand relationship in improving the core competitiveness of enterprises. Secondly, according to different types of spare parts demand prediction data, different spare parts and demand prediction methods for vulnerable parts are proposed. In addition, the efficiency of inventory management is improved by predicting the demand for industrial vulnerable parts. For the three spare demand models of vulnerable parts, including periodic model, stationary model, and trend model, the corresponding demand forecasting methods are studied respectively. The simple exponential smoothing method is used to predict the spare parts with stable demands, while the quadratic exponential smoothing method is used to predict the demand for spare parts with linear trend. Meanwhile, the prediction method based on weighted fitting of eigenvalues is adopted to predict the periodical demand of machine spare parts. Finally, an inventory management system based on cloud-edge collaborative computing is proposed to reasonably allocate inventory resources and improve the utilization of inventory resources. The prediction method based on weighted fitting of eigenvalues proposed here has the smallest error and the best fitting effect in the demand prediction of machine spare parts, and the lowest error after fitting is only 2.2%. Exponential smoothing method and quadratic exponential smoothing method are used for spare parts with non-periodic linear demands and spare parts with nonlinear demands, respectively, and the prediction results are close to the actual values. In terms of completion time, the virtual machine performance algorithm reported here realizes shorter processing time and higher efficiency than genetic algorithm. In terms of stability, this research algorithm is much more stable than the genetic algorithm. Despite particular outcomes achieved in this work, due to the limitations of research level and some objective factors, there are still some deficiencies. On the one hand, there remains space for improvement in the relative error of the prediction method for vulnerable spare parts proposed here. It is expected to further improve the accuracy and efficiency of prediction by introducing the deep learning algorithm in future. On the other hand, there lacks the combination of the prediction method based on vulnerable spare parts and the inventory management system based on cloud-edge collaborative computing reported here. The follow-up work will make efforts to integrate spare parts demand forecasting and inventory resource management into one intelligent system.
Supporting information
https://doi.org/10.1371/journal.pone.0259284.s001
- View Article
- Google Scholar
- PubMed/NCBI
To read this content please select one of the options below:
Please note you do not have access to teaching notes, a review of inventory management research in major logistics journals: themes and future directions.
The International Journal of Logistics Management
ISSN : 0957-4093
Article publication date: 15 August 2008
The purpose of this paper is to provide a review of inventory management articles published in major logistics outlets, identify themes from the literature and provide future direction for inventory management research to be published in logistics journals.
Design/methodology/approach
Articles published in major logistics articles, beginning in 1976, which contribute to the inventory management literature are reviewed and cataloged. The articles are segmented based on major themes extracted from the literature as well as key assumptions made by the particular inventory management model.
Two major themes are found to emerge from logistics research focused on inventory management. First, logistics researchers have focused considerable attention on integrating traditional logistics decisions, such as transportation and warehousing, with inventory management decisions, using traditional inventory control models. Second, logistics researchers have more recently focused on examining inventory management through collaborative models.
Originality/value
This paper catalogs the inventory management articles published in the major logistics journals, facilitates the awareness and appreciation of such work, and stands to guide future inventory management research by highlighting gaps and unexplored topics in the extant literature.
- Inventory management
- Supply chain management
Williams, B.D. and Tokar, T. (2008), "A review of inventory management research in major logistics journals: Themes and future directions", The International Journal of Logistics Management , Vol. 19 No. 2, pp. 212-232. https://doi.org/10.1108/09574090810895960
Emerald Group Publishing Limited
Copyright © 2008, Emerald Group Publishing Limited
Related articles
All feedback is valuable.
Please share your general feedback
Report an issue or find answers to frequently asked questions
Contact Customer Support
Impact of Inventory Management on Firm’s Efficiency – A Quantitative Research Study on Departmental Stores Operating in Karachi
Khan, F. and Siddiqui, D. A. (2019). Impact of Inventory Management on Firm’s Efficiency – A Quantitative Research Study on Departmental Stores Operating in Karachi. Social Science and Humanities Journal, 3(4), 964-980
17 Pages Posted: 6 Jun 2019 Last revised: 14 Apr 2022
Karachi University Business School, Students
Danish Ahmed Siddiqui
University of Karachi - Karachi University Business School
Date Written: May 31, 2019
This study investigated the effect of various inventory management factors on firm’s efficiency. These factors included Capacity Utilization, Inventory Accuracy, Lean Inventory, and Stock Availability. Data was collected by the use of liker scale questionnaire from 250 individuals from different departmental stores in Karachi. Data was analysed using Structural Equation Modelling. The results showed Inventory Accuracy, Lean Inventory, and Stock Availability has positive and significant impact on efficiency. However, Capacity Utilization doesn’t seem to affect efficiency. Hence, main indicator of inventory control comes out to be inventory accuracy which allows having an effective control of the outputs of the different goods.
Keywords: Supply Chain Management, Capacity Utilization, Inventory Accuracy, Lean Inventory, Stock Availability, and Inventory Management
Suggested Citation: Suggested Citation
Karachi University Business School, Students ( email )
Karachi Pakistan
Danish Ahmed Siddiqui (Contact Author)
University of karachi - karachi university business school ( email ).
University Road Karachi, Sindh 75270 Pakistan 3333485884 (Phone)
Do you have a job opening that you would like to promote on SSRN?
Paper statistics, related ejournals, io: firm structure, purpose, organization & contracting ejournal.
Subscribe to this fee journal for more curated articles on this topic
Manufacturing, Service, & Supply Chain Operations eJournal
Supply chain management ejournal, microeconomics: production, market structure & pricing ejournal, technology, operations management & production ejournal, inventory management ejournal, organizations & markets: policies & processes ejournal, development economics: microeconomic issues in developing economies ejournal.
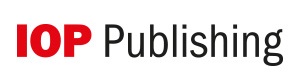
We apologize for the inconvenience...
To ensure we keep this website safe, please can you confirm you are a human by ticking the box below.
If you are unable to complete the above request please contact us using the below link, providing a screenshot of your experience.
https://ioppublishing.org/contacts/

IMAGES
COMMENTS
This research reviews inventory management concepts and implementations in the face of increasingly demanding human need.
PDF | Inventory management is a challenging problem area in supply chain management. Companies need to have inventories in warehouses in order to fulfil... | Find, read and cite all the research ...
Management Systems (IMS) that can pro vide better control and. handling of product stock, customer order, customer service. and order delivery that relates to company inventory. information. The t ...
This study aimed to empirically examine the impact of inventory management practice on firms' competitiveness and organizational performance. Data for the study were collected from 188 micro and sm...
This research selects level 2 of the SCOR sourcing process "source make-to-order product (S2)" to modeling, evaluation, and analysis of the performance of our inventory management systems.
This paper reviews part of the work carried out, dealing with the optimization of the IM problem. The main objective of this study is to analyze different parameters of existing mathematical models of IMO, to deliver an overview describing the main factors of the problem. Download conference paper PDF.
This research paper introduces an innovative Inventory Management System (IMS) designed to address common inventory challenges. The IMS leverages advanced technology, including barcode scanning and real-time analytics, to optimize stock levels, improve order management, and reduce carrying costs. Implementation of the IMS in real-world ...
The aim of this article is to provide a comprehensive overview of the current and future research potential of inventory management through a systematic literature review of articles from 2012 to 2022. Inventory management and related AI techniques are categorized and presented in a way that facilitates orientation for researchers in the field.
Despite the widespread adoption of technology in inventory management, empirical research that examines the effect of these technologies is scarce. Does IT capability really have a positive effect on the inventory management? This paper empirically studies the usage of information technology by the definition of IT capability. Our unique panel data set allows us to explore the relationship ...
In addition, when referring to inventory management in this research, the term 'inventory' refers only to the raw material inventory of the case company. Furthermore, the research's scope is restricted to inventory items recorded in the case company's enterprise resource planning system, with project-specific items excluded from the analysis.
An inventory management problem is addressed for a make-to-order supply chain that has inventory holding and/or manufacturing locations at each node. The lead times between nodes and production capacity limits are heterogeneous across the network. This study focuses on a single product, a multi-period centralized system in which a retailer is subject to an uncertain stationary consumer demand ...
In recent years, the correct management of inventories has become a fundamental pillar for achieving success in enterprises. Unfortunately, studies suggesting the investment and adoption of advanced inventory management and control systems are not easy to find. In this context, this article aims to analyze and present an extensive literature concerning inventory management, containing multiple ...
This research paper introduces an innovative Inventory Management System (IMS) designed to address common inventory challenges. The IMS leverages advanced technology, including barcode scanning ...
Literature review suggests that simulation models are the most appropriate methodology to analyze different inventory management policies [5]. Simulation models enable the investigator to analyse various inventory policies, possible results and their implications [6]. In the present research, demand and inventory characteristics of a regular product in a retail outlet is simulated using the ...
The proposed framework may guide researchers and practitioners dealing with similar kinds of problems to improve the system. Lastly, the review paper recommends some important future research directions in the field of inventory management systems in healthcare. The structure of the remaining paper is as follows.
The present work aims to strengthen the core competitiveness of industrial enterprises in the supply chain environment, and enhance the efficiency of inventory management and the utilization rate of inventory resources. First, an analysis is performed on the supply and demand relationship between suppliers and manufacturers in the supply chain environment and the production mode of intelligent ...
The research benefits to industry by suggesting the importance of information systems and human resources to inventory management. As for academics, this research enriches inventory management literature.
This paper catalogs the inventory management articles published in the major logistics journals, facilitates the awareness and appreciation of such work, and stands to guide future inventory management research by highlighting gaps and unexplored topics in the extant literature.
This paper delves into the multifaceted role of Artificial Intelligence (AI) in inventory management, encompassing its applications, challenges, and future opportunities. AI's integration into ...
The results of this research showed that to improve the availability of pharmaceutical products and avoid shortages, inventory control processes need to be streamlined toward customer needs and demands by implementing a proper technique for inventory level control practices and improving inventory management policy.
Impact of Inventory Management on Firm's Efficiency - A Quantitative Research Study on Departmental Stores Operating in Karachi Khan, F. and Siddiqui, D. A. (2019). Impact of Inventory Management on Firm's Efficiency - A Quantitative Research Study on Departmental Stores Operating in Karachi. Social Science and Humanities Journal, 3 (4), 964-980 17 Pages Posted: 6 Jun 2019 Last revised ...
Effects of Inventory Management System on Firm Performance An Empirical StudyEff. ty of MombasaLecturer, School of Business, Technical University of MombasaAbstract:- The study was guided by the following objectives: to find out how the systematic application production in data processing (SAP), just i.
The research benefits to industry by suggesting the importance of information systems and human resources to inventory management. As for academics, this research enriches inventory management literature.