IEEE Account
- Change Username/Password
- Update Address

Purchase Details
- Payment Options
- Order History
- View Purchased Documents
Profile Information
- Communications Preferences
- Profession and Education
- Technical Interests
- US & Canada: +1 800 678 4333
- Worldwide: +1 732 981 0060
- Contact & Support
- About IEEE Xplore
- Accessibility
- Terms of Use
- Nondiscrimination Policy
- Privacy & Opting Out of Cookies
A not-for-profit organization, IEEE is the world's largest technical professional organization dedicated to advancing technology for the benefit of humanity. © Copyright 2024 IEEE - All rights reserved. Use of this web site signifies your agreement to the terms and conditions.

An official website of the United States government
The .gov means it’s official. Federal government websites often end in .gov or .mil. Before sharing sensitive information, make sure you’re on a federal government site.
The site is secure. The https:// ensures that you are connecting to the official website and that any information you provide is encrypted and transmitted securely.
- Publications
- Account settings
- My Bibliography
- Collections
- Citation manager
Save citation to file
Email citation, add to collections.
- Create a new collection
- Add to an existing collection
Add to My Bibliography
Your saved search, create a file for external citation management software, your rss feed.
- Search in PubMed
- Search in NLM Catalog
- Add to Search
Deep learning for chest X-ray analysis: A survey
Affiliations.
- 1 Radboud University Medical Center, Institute for Health Sciences, Department of Medical Imaging, Nijmegen, the Netherlands. Electronic address: [email protected].
- 2 Radboud University Medical Center, Institute for Health Sciences, Department of Medical Imaging, Nijmegen, the Netherlands.
- PMID: 34171622
- DOI: 10.1016/j.media.2021.102125
Recent advances in deep learning have led to a promising performance in many medical image analysis tasks. As the most commonly performed radiological exam, chest radiographs are a particularly important modality for which a variety of applications have been researched. The release of multiple, large, publicly available chest X-ray datasets in recent years has encouraged research interest and boosted the number of publications. In this paper, we review all studies using deep learning on chest radiographs published before March 2021, categorizing works by task: image-level prediction (classification and regression), segmentation, localization, image generation and domain adaptation. Detailed descriptions of all publicly available datasets are included and commercial systems in the field are described. A comprehensive discussion of the current state of the art is provided, including caveats on the use of public datasets, the requirements of clinically useful systems and gaps in the current literature.
Keywords: Chest X-ray analysis; Chest radiograph; Deep learning; Survey.
Copyright © 2021 The Authors. Published by Elsevier B.V. All rights reserved.
PubMed Disclaimer
Conflict of interest statement
Declaration of Competing Interest The authors declare that they have no known competing financial interests or personal relationships that could have appeared to influence the work reported in this paper.
Similar articles
- Chest X-ray analysis empowered with deep learning: A systematic review. Meedeniya D, Kumarasinghe H, Kolonne S, Fernando C, Díez IT, Marques G. Meedeniya D, et al. Appl Soft Comput. 2022 Sep;126:109319. doi: 10.1016/j.asoc.2022.109319. Epub 2022 Jul 18. Appl Soft Comput. 2022. PMID: 36034154 Free PMC article.
- Chest x-ray automated triage: A semiologic approach designed for clinical implementation, exploiting different types of labels through a combination of four Deep Learning architectures. Mosquera C, Diaz FN, Binder F, Rabellino JM, Benitez SE, Beresñak AD, Seehaus A, Ducrey G, Ocantos JA, Luna DR. Mosquera C, et al. Comput Methods Programs Biomed. 2021 Jul;206:106130. doi: 10.1016/j.cmpb.2021.106130. Epub 2021 May 2. Comput Methods Programs Biomed. 2021. PMID: 34023576
- Using Radiomics as Prior Knowledge for Thorax Disease Classification and Localization in Chest X-rays. Han Y, Chen C, Tang L, Lin M, Jaiswal A, Wang S, Tewfik A, Shih G, Ding Y, Peng Y. Han Y, et al. AMIA Annu Symp Proc. 2022 Feb 21;2021:546-555. eCollection 2021. AMIA Annu Symp Proc. 2022. PMID: 35308939 Free PMC article.
- Deep Learning Applications in Chest Radiography and Computed Tomography: Current State of the Art. Lee SM, Seo JB, Yun J, Cho YH, Vogel-Claussen J, Schiebler ML, Gefter WB, van Beek EJR, Goo JM, Lee KS, Hatabu H, Gee J, Kim N. Lee SM, et al. J Thorac Imaging. 2019 Mar;34(2):75-85. doi: 10.1097/RTI.0000000000000387. J Thorac Imaging. 2019. PMID: 30802231 Review.
- Advances in Deep Learning for Tuberculosis Screening using Chest X-rays: The Last 5 Years Review. Santosh KC, Allu S, Rajaraman S, Antani S. Santosh KC, et al. J Med Syst. 2022 Oct 15;46(11):82. doi: 10.1007/s10916-022-01870-8. J Med Syst. 2022. PMID: 36241922 Free PMC article. Review.
- Deep Learning for Pneumonia Detection in Chest X-ray Images: A Comprehensive Survey. Siddiqi R, Javaid S. Siddiqi R, et al. J Imaging. 2024 Jul 23;10(8):176. doi: 10.3390/jimaging10080176. J Imaging. 2024. PMID: 39194965 Free PMC article. Review.
- MedCLIP: Contrastive Learning from Unpaired Medical Images and Text. Wang Z, Wu Z, Agarwal D, Sun J. Wang Z, et al. Proc Conf Empir Methods Nat Lang Process. 2022 Dec;2022:3876-3887. doi: 10.18653/v1/2022.emnlp-main.256. Proc Conf Empir Methods Nat Lang Process. 2022. PMID: 39144675 Free PMC article.
- Ensemble of Deep Learning Architectures with Machine Learning for Pneumonia Classification Using Chest X-rays. Vyas R, Khadatkar DR. Vyas R, et al. J Imaging Inform Med. 2024 Aug 13. doi: 10.1007/s10278-024-01201-y. Online ahead of print. J Imaging Inform Med. 2024. PMID: 39138748
- Two-stage deep neural network for diagnosing fungal keratitis via in vivo confocal microscopy images. Li CP, Dai W, Xiao YP, Qi M, Zhang LX, Gao L, Zhang FL, Lai YK, Liu C, Lu J, Chen F, Chen D, Shi S, Li S, Zeng Q, Chen Y. Li CP, et al. Sci Rep. 2024 Aug 8;14(1):18432. doi: 10.1038/s41598-024-68768-y. Sci Rep. 2024. PMID: 39117709 Free PMC article.
- Anatomy-specific Progression Classification in Chest Radiographs via Weakly Supervised Learning. Yu K, Ghosh S, Liu Z, Deible C, Poynton CB, Batmanghelich K. Yu K, et al. Radiol Artif Intell. 2024 Sep;6(5):e230277. doi: 10.1148/ryai.230277. Radiol Artif Intell. 2024. PMID: 39046325 Free PMC article.
Publication types
- Search in MeSH
LinkOut - more resources
Full text sources.
- Elsevier Science
- Ovid Technologies, Inc.
Other Literature Sources
- The Lens - Patent Citations
Research Materials
- NCI CPTC Antibody Characterization Program
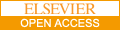
- Citation Manager
NCBI Literature Resources
MeSH PMC Bookshelf Disclaimer
The PubMed wordmark and PubMed logo are registered trademarks of the U.S. Department of Health and Human Services (HHS). Unauthorized use of these marks is strictly prohibited.
Advertisement
Chest X-ray Images for Lung Disease Detection Using Deep Learning Techniques: A Comprehensive Survey
- Survey article
- Published: 19 February 2024
- Volume 31 , pages 3267–3301, ( 2024 )
Cite this article
- Mohammed A. A. Al-qaness ORCID: orcid.org/0000-0002-6956-7641 1 , 8 ,
- Jie Zhu 1 ,
- Dalal AL-Alimi 2 ,
- Abdelghani Dahou 3 , 12 ,
- Saeed Hamood Alsamhi 4 , 5 ,
- Mohamed Abd Elaziz 6 , 9 , 10 , 11 , 13 &
- Ahmed A. Ewees 7
1296 Accesses
Explore all metrics
In medical imaging, the last decade has witnessed a remarkable increase in the availability and diversity of chest X-ray (CXR) datasets. Concurrently, there has been a significant advancement in deep learning techniques, noted for their escalating accuracy. These developments have catalyzed a surge in the application of deep learning in various medical studies, particularly in detecting and classifying lung diseases. This study delves into an extensive compilation of over 200 studies from the recent five years (2018–2023), employing advanced machine learning, including deep learning methodologies to analyze CXR images. Our exploration is twofold: it categorizes these studies based on the methods used and the types of lung diseases addressed. It also presents an in-depth examination of the current limitations and prospective trajectories in this rapidly evolving field. Our findings underscore the transformative impact and continual progress of deep learning models in enhancing the accuracy and efficiency of lung disease detection using CXR images. This survey culminates by emphasizing the critical need for further technological advancement in this domain, aiming to bridge gaps in healthcare provision and improve patient outcomes. The overarching goal is to pave the way for more precise, efficient, and accessible diagnostic tools in the battle against lung diseases, reinforcing the indispensable role of technology in modern healthcare.
This is a preview of subscription content, log in via an institution to check access.
Access this article
Subscribe and save.
- Get 10 units per month
- Download Article/Chapter or eBook
- 1 Unit = 1 Article or 1 Chapter
- Cancel anytime
Price includes VAT (Russian Federation)
Instant access to the full article PDF.
Rent this article via DeepDyve
Institutional subscriptions
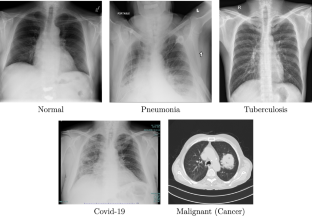
Similar content being viewed by others
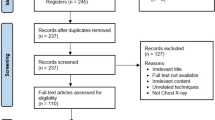
A Systematic Review: Classification of Lung Diseases from Chest X-Ray Images Using Deep Learning Algorithms
Detecting Pulmonary Lesions in Low-Prevalence Real-World Settings Using Deep Learning
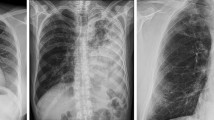
Advances in Deep Learning for Tuberculosis Screening using Chest X-rays: The Last 5 Years Review
Explore related subjects.
- Medical Imaging
Chandrasekar KS (2022) Exploring the deep-learning techniques in detecting the presence of coronavirus in the chest X-ray images: a comprehensive review. Arch Comput Methods Eng 29:5381–5395
Google Scholar
Kieu STH, Bade A, Hijazi MHA, Kolivand H (2020) A survey of deep learning for lung disease detection on medical images: state-of-the-art, taxonomy, issues and future directions. J Imaging 6:131
Kaplan A, Cao H, FitzGerald JM, Iannotti N, Yang E, Kocks JW, Kostikas K, Price D, Reddel HK, Tsiligianni I et al (2021) Artificial intelligence/machine learning in respiratory medicine and potential role in asthma and copd diagnosis J Allergy Clin Immunol Pract 9:2255–2261
M. Jasmine Pemeena Priyadarsini, G. Rajini, K. Hariharan, K. Utkarsh Raj, K. Bhargav Ram, V. Indragandhi, V. Subramaniyaswamy, S. Pandya, et al (2023) Lung diseases detection using various deep learning algorithms. J Healthcare Eng 2023:3563696
Ahmed ST, Kadhem SM (2021) Using machine learning via deep learning algorithms to diagnose the lung disease based on chest imaging: a survey. Int J Interact Mob Technol 15:95
Sun J, Liao X, Yan Y, Zhang X, Sun J, Tan W, Liu B, Wu J, Guo Q, Gao S et al (2022) Detection and staging of chronic obstructive pulmonary disease using a computed tomography-based weakly supervised deep learning approach. Eur Radiol 32:5319–5329
Bharati S, Podder P, Mondal MRH (2020) Hybrid deep learning for detecting lung diseases from X-ray images. Inf Med Unlocked 20:100391
Feng Y, Wang Y, Zeng C, Mao H (2021) Artificial intelligence and machine learning in chronic airway diseases: focus on asthma and chronic obstructive pulmonary disease. Int J Med Sci 18:2871
Kumar S, Bhagat V, Sahu P, Chaube MK, Behera AK, Guizani M, Gravina R, Di Dio M, Fortino G, Curry E et al (2023) A novel multimodal framework for early diagnosis and classification of copd based on CT scan images and multivariate pulmonary respiratory diseases. Comput Methods Progr Biomed 243:107911
Saygili A (2021) Analysis and segmentation of X-ray images of Covid-19 patients using the k-means algorithm. Veri Bilimi 4:1–6
Gunjan VK, Shaik F, Kashyap A (2020) Detection and analysis of pulmonary tb using bounding box and k-means algorithm. In: ICCCE 2020: proceedings of the 3rd international conference on communications and cyber physical engineering, Springer, pp 1587–1595
Sumarti H, Sabrina Q, Triana D, Septiani F, Rahmani TPD (2023) Identification of Covid-19 based on features texture histogram and gray level co-occurrence matrix (glcm) using k-means clustering methods in chest X-ray digital images. J Penelitian Fisika Apli 13:51–66
Manickam M, Siva R, Prabakeran S, Geetha K, Indumathi V, Sethukarasi T (2022) Pulmonary disease diagnosis using african vulture optimized weighted support vector machine approach. Int J Imaging Syst Technol 32:843–856
Oliver AS, Anuradha M, Justus JJ, Bellam K, Jayasankar T (2020) An efficient coding network based feature extraction with support vector machine based classification model for CT lung images. J Med Imaging Health Inf 10:2628–2633
Zheng H, Hu Y, Dong L, Shu Q, Zhu M, Li Y, Chen C, Gao H, Yang L (2021) Predictive diagnosis of chronic obstructive pulmonary disease using serum metabolic biomarkers and least-squares support vector machine. J Clin Lab Anal 35
Saikia T, Hansdah M, Singh KK, Bajpai MK (2022) Classification of lung nodules based on transfer learning with k-nearest neighbor (knn). In: 2022 IEEE international conference on imaging systems and techniques (IST), IEEE, pp 1–6
Kamdar A, Sharma V, Sonawane S, Patil N (2021) Lung cancer detection by classifying CT scan images using grey level co-occurrence matrix (glcm) and k-nearest neighbours. In: Innovations in computational intelligence and computer vision: proceedings of ICICV 2021, Springer, 2022, pp 293–301
Khan MU, Farman A, Rehman AU, Israr N, Ali MZH, Gulshan ZA (2021) Automated system design for classification of chronic lung viruses using non-linear dynamic system features and k-nearest neighbour. In: 2021 Mohammad Ali Jinnah University International Conference on Computing (MAJICC), IEEE, pp 1–8
Liu C, Zhao R, Pang M (2020) A fully automatic segmentation algorithm for CT lung images based on random forest. Med Phys 47:518–529
Shi Y, Wong WK, Goldin JG, Brown MS, Kim GHJ (2019) Prediction of progression in idiopathic pulmonary fibrosis using CT scans at baseline: aquantum particle swarm optimization-random forest approach. Artif Intell Med 100:101709
Koureas M, Kirgou P, Amoutzias G, Hadjichristodoulou C, Gourgoulianis K, Tsakalof A (2020) Target analysis of volatile organic compounds in exhaled breath for lung cancer discrimination from other pulmonary diseases and healthy persons. Metabolites 10:317
Ghiasi MM, Zendehboudi S, Mohsenipour AA (2020) Decision tree-based diagnosis of coronary artery disease: cart model. Comput Methods Programs Biomed 192:105400
Hsu C-H, Manogaran G, Panchatcharam P, Vivekanandan S (2018) A new approach for prediction of lung carcinoma using back propagation neural network with decision tree classifiers. In: 2018 IEEE 8th international symposium on cloud and service computing (SC2), IEEE, pp 111–115
Bhuvaneswari C, Aruna P, Loganathan D (2014) A new fusion model for classification of the lung diseases using genetic algorithm. Egypt Inf J 15:69–77
Huang Z-Y, Lin S, Long L-L, Cao J-Y, Luo F, Qin W-C, Sun D-M, Gregersen H (2020) Predicting the morbidity of chronic obstructive pulmonary disease based on multiple locally weighted linear regression model with k-means clustering. Int J Med Informatics 139:104141
Bhattacharjee A, Murugan R, Goel T (2022) A hybrid approach for lung cancer diagnosis using optimized random forest classification and k-means visualization algorithm. Heal Technol 12:787–800
Rustam Z, Hartini S, Pratama RY, Yunus RE, Hidayat R (2020) Analysis of architecture combining convolutional neural network (CNN) and kernel k-means clustering for lung cancer diagnosis. Int. J. Adv. Sci. Eng. Inf. Technol 10:1200–1206
Park B, Park H, Lee SM, Seo JB, Kim N (2019) Lung segmentation on hrct and volumetric CT for diffuse interstitial lung disease using deep convolutional neural networks. J Digit Imaging 32:1019–1026
Toğaçar M, Ergen B, Cömert Z (2020) Detection of lung cancer on chest CT images using minimum redundancy maximum relevance feature selection method with convolutional neural networks, Biocybern Biomed Eng 40:23–39
Golan R, Jacob C, Denzinger J (2016) Lung nodule detection in CT images using deep convolutional neural networks. In: 2016 international joint conference on neural networks (IJCNN), IEEE, pp 243–250
Gunjan VK, Singh N, Shaik F, Roy S (2022) Detection of lung cancer in CT scans using grey wolf optimization algorithm and recurrent neural network. Heal Technol 12:1197–1210
Shankar K, Perumal E, Díaz VG, Tiwari P, Gupta D, Saudagar AKJ, Muhammad K (2021) An optimal cascaded recurrent neural network for intelligent Covid-19 detection using chest X-ray images. Appl Soft Comput 113
Mhaske D, Rajeswari K, Tekade R (2019) Deep learning algorithm for classification and prediction of lung cancer using CT scan images. In: 2019 5th international conference on computing, communication, control and automation (ICCUBEA), IEEE, pp 1–5
Naeem H, Bin-Salem AA (2021) A CNN-lstm network with multi-level feature extraction-based approach for automated detection of coronavirus from CT scan and X-ray images. Appl Soft Comput 113:107918
Demir F (2021) Deepcoronet: a deep lstm approach for automated detection of Covid-19 cases from chest X-ray images. Appl Soft Comput 103:107160
Tan J, Jing L, Huo Y, Li L, Akin O, Tian Y (2021) Lgan: lung segmentation in CT scans using generative adversarial network. Comput Med Imaging Graph 87:101817
Li X, Zhang R, Wang Q, Duan X, Sun Y, Wang J (2023) Sar-cgan: Improved generative adversarial network for eit reconstruction of lung diseases. Biomed Signal Process Control 81:104421
Onishi Y, Teramoto A, Tsujimoto M, Tsukamoto T, Saito K, Toyama H, Imaizumi K, Fujita H (2020) Multiplanar analysis for pulmonary nodule classification in CT images using deep convolutional neural network and generative adversarial networks. Int J Comput Assist Radiol Surg 15:173–178
Sutha P, Shenbagavalli. A survey of machine learning techniques for lung disease detection on medical images. In: AIP conference proceedings, volume 2618, AIP
El-Rashidy N, Abdelrazik S, Abuhmed T, Amer E, Ali F, Hu J-W, El-Sappagh S (2021) Comprehensive survey of using machine learning in the Covid-19 pandemic. Diagnostics 11:1155
Bruzadin A, Boaventura M, Colnago M, Negri RG, Casaca W (2023) Learning label diffusion maps for semi-automatic segmentation of lung CT images with Covid-19. Neurocomputing 522:24–38
Chen Y, Feng L, Zheng C, Zhou T, Liu L, Liu P, Chen Y (2023) Ldanet: automatic lung parenchyma segmentation from CT images. Comput Biol Med 155:106659
Lee RC, Sood N, Deva S, Macedo M, Soto DW, Unger JB (2023) Evaluation of a Covid-19 rapid antigen testing program among student athletes in a public high school district. Eval Program Plann 98:102280
Ogane K, Imai K, Orihara Y, Kodana M, Tezuka M, Matsuzaki N, Takahashi R, Ichimura S, Tokano M, Sakai J et al (2023) Clinical evaluation of anterior nasal cavity swab specimens by a rapid antigen test using a gline-2019-ncov ag kit to diagnose Covid-19. J Infect Chemother 29:825–828
Toft CJ, Bourquin RA, Sorenson AE, Horwood PF, Druce JD, Schaeffer PM (2023) Analytical sensitivity of Covid-19 rapid antigen tests: a case for a robust reference standard. Talanta Open 7:100187
Ghose P, Alavi M, Tabassum M, Uddin A, Biswas M, Mahbub K, Gaur L, Mallik S, Zhao Z et al (2022) Detecting Covid-19 infection status from chest X-ray and CT scan via single transfer learning-driven approach. Front Genet 13:980338
Han Z, Jian M, Wang G-G (2022) Convunext: an efficient convolution neural network for medical image segmentation. Knowl-Based Syst 253:109512
Londono J, Ghasmi S, Shah AH, Fahimipour A, Ghadimi N, Hashemi S, Sultan ZK, Dashti M (2023) Accuracy of machine learning and convolutional neural network algorithms on detecting and prediction of anatomical landmarks on 2d lateral cephalometric images-a systematic review and meta-analysis, Saudi Dent J
Terzi S, De Angeli S, Miozzo D, Massucchielli LS, Szarzynski J, Carturan F, Boni G (2022) Learning from the Covid-19 pandemic in Italy to advance multi-hazard disaster risk management. Progr Disast Sci 16:100268
Mărgărint MC, Kovačić S, Albulescu A-C, Miljković D (2023) Natural multi-hazard risk perception and educational insights among geography and tourism students and graduates amid the Covid-19 pandemic. Int J Disast Risk Reduct 86:103549
Kamalrathne T, Amaratunga D, Haigh R, Kodituwakku L (2023) Need for effective detection and early warnings for epidemic and pandemic preparedness planning in the context of multi-hazards: lessons from the Covid-19 pandemic. Int J Diast Risk Reduct 92:103724
Kumar S, Chaube MK, Alsamhi SH, Gupta SK, Guizani M, Gravina R, Fortino G (2022) A novel multimodal fusion framework for early diagnosis and accurate classification of Covid-19 patients using X-ray images and speech signal processing techniques. Comput Methods Programs Biomed 226:107109
Aggarwal A, Chakradar M, Bhatia MS, Kumar M, Stephan T, Gupta SK, Alsamhi S, Al-Dois H et al (2022) Covid-19 risk prediction for diabetic patients using fuzzy inference system and machine learning approaches. J Healthcare Eng 2022:4096950
Alghamdi HS, Amoudi G, Elhag S, Saeedi K, Nasser J (2021) Deep learning approaches for detecting Covid-19 from chest X-ray images: a survey. IEEE Access 9:20235–20254
Gu Y, Chi J, Liu J, Yang L, Zhang B, Yu D, Zhao Y, Lu X (2021) A survey of computer-aided diagnosis of lung nodules from CT scans using deep learning. Comput Biol Med 137:104806
Jiang H, Diao Z, Shi T, Zhou Y, Wang F, Hu W, Zhu X, Luo S, Tong G, Yao Y-D (2023) A review of deep learning-based multiple-lesion recognition from medical images: classification, detection and segmentation. Comput Biol Med 157:106726
Çallı E, Sogancioglu E, van Ginneken B, van Leeuwen KG, Murphy K (2021) Deep learning for chest X-ray analysis: a survey. Med Image Anal 72:102125
Rajaselvi M, Sanjith J, Koshy S, Niranjan G (2022) A survey on lung disease diagnosis using machine learning techniques. In: 2022 2nd international conference on advance computing and innovative technologies in engineering (ICACITE), IEEE, pp 01–04
Hosny A, Parmar C, Quackenbush J, Schwartz LH, Aerts HJ (2018) Artificial intelligence in radiology. Nat Rev Cancer 18:500–510
Syed AB, Zoga AC. Artificial intelligence in radiology: current technology and future directions. In: Seminars in musculoskeletal radiology, vol 22, Thieme Medical Publishers, pp 540–545
Richardson ML, Garwood ER, Lee Y, Li MD, Lo HS, Nagaraju A, Nguyen XV, Probyn L, Rajiah P, Sin J et al (2021) Noninterpretive uses of artificial intelligence in radiology. Acad Radiol 28:1225–1235
Çinar A, Yildirim M (2020) Detection of tumors on brain MRI images using the hybrid convolutional neural network architecture. Med Hypotheses 139:109684
Deepak S, Ameer P (2021) Automated categorization of brain tumor from MRI using CNN features and SVM, J Ambient Intell Hum Comput 12:8357–8369
Zhao X, Liu L, Qi S, Teng Y, Li J, Qian W (2018) Agile convolutional neural network for pulmonary nodule classification using CT images. Int J Comput Assist Radiol Surg 13:585–595
Tong C, Liang B, Su Q, Yu M, Hu J, Bashir AK, Zheng Z (2020) Pulmonary nodule classification based on heterogeneous features learning. IEEE J Sel Areas Commun 39:574–581
Zhang J, Qin Q, Ye Q, Ruan T (2023) ST-UNET: swin transformer boosted u-net with cross-layer feature enhancement for medical image segmentation. Comput Biol Med 153:106516
Manzari ON, Ahmadabadi H, Kashiani H, Shokouhi SB, Ayatollahi A (2023) Medvit: a robust vision transformer for generalized medical image classification. Comput Biol Med 157:106791
Liu Z, Shen L. CECT: controllable ensemble CNN and transformer for Covid-19 image classification by capturing both local and global image features. arXiv preprint arXiv:2302.02314 (2023)
Chen C-FR, Fan Q, Panda R. Crossvit: cross-attention multi-scale vision transformer for image classification. In: Proceedings of the IEEE/CVF international conference on computer vision, pp 357–366
Heidari M, Kazerouni A, Soltany M, Azad R, Aghdam EK, Cohen-Adad J, Merhof D. Hiformer: hierarchical multi-scale representations using transformers for medical image segmentation. In: Proceedings of the IEEE/CVF Winter conference on applications of computer vision, pp 6202–6212
Lin A, Chen B, Xu J, Zhang Z, Lu G, Zhang D (2022) DS-TRANSUNET: dual swin transformer u-net for medical image segmentation. IEEE Trans Instrum Meas 71:1–15
Almalik F, Yaqub M, Nandakumar K. Self-ensembling vision transformer (sevit) for robust medical image classification. In: International conference on medical image computing and computer-assisted intervention. Springer, pp 376–386
Dosovitskiy A, Beyer L, Kolesnikov A, Weissenborn D, Zhai X, Unterthiner T, Dehghani M, Minderer M, Heigold G, Gelly S et al (2020) An image is worth 16x16 words: transformers for image recognition at scale. arXiv preprint arXiv:2010.11929
Li Z, Li Y, Li Q, Wang P, Guo D, Lu L, Jin D, Zhang Y, Hong Q (2023) Lvit: language meets vision transformer in medical image segmentation. In: IEEE transactions on medical imaging
Dalmaz O, Yurt M, Çukur T (2022) Resvit: Residual vision transformers for multimodal medical image synthesis. IEEE Trans Med Imaging 41:2598–2614
Rajinikanth V, Kadry S, Damaševičius R, Gnanasoundharam J, Mohammed MA, Devadhas GG (2022) UNET with two-fold training for effective segmentation of lung section in chest X-ray. In: 2022 third international conference on intelligent computing instrumentation and control technologies (ICICICT), IEEE, pp 977–981
Han X, Qi L, Yu Q, Zhou Z, Zheng Y, Shi Y, Gao Y (2021) Deep symmetric adaptation network for cross-modality medical image segmentation. IEEE Trans Med Imaging 41:121–132
Wang S, Kang B, Ma J, Zeng X, Xiao M, Guo J, Cai M, Yang J, Li Y, Meng X et al (2021) A deep learning algorithm using CT images to screen for corona virus disease (Covid-19). Eur Radiol 31:6096–6104
Tang H, Chen X, Liu Y, Lu Z, You J, Yang M, Yao S, Zhao G, Xu Y, Chen T et al (2019) Clinically applicable deep learning framework for organs at risk delineation in CT images, Nature. Mach Intell 1:480–491
Frid-Adar M, Diamant I, Klang E, Amitai M, Goldberger J, Greenspan H (2018) Gan-based synthetic medical image augmentation for increased CNN performance in liver lesion classification. Neurocomputing 321:321–331
Yi X, Walia E, Babyn P (2019) Generative adversarial network in medical imaging: a review. Med Image Anal 58:101552
Jiang Y, Chen H, Loew M, Ko H (2020) Covid-19 CT image synthesis with a conditional generative adversarial network. IEEE J Biomed Health Inform 25:441–452
Jin D, Xu Z, Tang Y, Harrison AP, Mollura DJ (2018) CT-realistic lung nodule simulation from 3d conditional generative adversarial networks for robust lung segmentation. In: Medical image computing and computer assisted intervention–MICCAI 2018: 21st international conference, Granada, Spain, September 16–20, 2018, proceedings, Part II 11, Springer, pp 732–740
Anirudh R, Thiagarajan JJ, Bremer T, Kim H (2016) Lung nodule detection using 3d convolutional neural networks trained on weakly labeled data. In: Medical imaging 2016: computer-aided diagnosis, vol 9785, SPIE, pp 791–796
Chen H, Zhang Y, Zhang W, Liao P, Li K, Zhou J, Wang G (2017) Low-dose CT via convolutional neural network. Biomed Opt Express 8:679–694
Li Y, Sixou B, Peyrin F (2021) A review of the deep learning methods for medical images super resolution problems. IRBM 42:120–133
Ahmad W, Ali H, Shah Z, Azmat S (2022) A new generative adversarial network for medical images super resolution. Sci Rep 12:9533
Zhu L, He Q, Huang Y, Zhang Z, Zeng J, Lu L, Kong W, Zhou F (2022) Dualmmp-gan: dual-scale multi-modality perceptual generative adversarial network for medical image segmentation. Comput Biol Med 144:105387
Gao J, Zhao W, Li P, Huang W, Chen Z (2022) Legan: a light and effective generative adversarial network for medical image synthesis. Comput Biol Med 148:105878
Nandhini Abirami R, Durai Raj Vincent P, Srinivasan K, Manic KS et al (2022) Multimodal medical image fusion of positron emission tomography and magnetic resonance imaging using generative adversarial networks, Behav Neurol 2022:1
Topol EJ (2019) High-performance medicine: the convergence of human and artificial intelligence. Nat Med 25:44–56
Bhatt H, Shah M (2023) A convolutional neural network ensemble model for pneumonia detection using chest X-ray images. Healthcare Anal 3:100176
Sharma S, Guleria K (2023) A deep learning based model for the detection of pneumonia from chest X-ray images using vgg-16 and neural networks. Proc Comput Sci 218:357–366
Mittal V, Kumar A (2023) Covinet: a hybrid model for classification of covid and non-covid pneumonia in CT and X-ray imagery. Int J Cogn Comput Eng 4:149–159
Kumar A (2022) Ryolo v4-tiny: adeep learning based detector for detection of covid and non-covid pneumonia in CT scans and X-ray images. Optik 268:169786
Wu Y, Qi Q, Qi S, Yang L, Wang H, Yu H, Li J, Wang G, Zhang P, Liang Z et al (2023) Classification of Covid-19 from community-acquired pneumonia: bosting the performance with capsule network and maximum intensity projection image of CT scans. Comput Biol Med 154:106567
Kiliçarslan S, Közkurt C, Baş S, Elen A (2023) Detection and classification of pneumonia using novel superior exponential (supex) activation function in convolutional neural networks. Expert Syst Appl 217:119503
Siddiqui EA, Chaurasia V, Shandilya M (2023) Detection and classification of lung cancer computed tomography images using a novel improved deep belief network with gabor filters. Chemom Intell Lab Syst 235:104763
Wankhade S, Vigneshwari S (2023) A novel hybrid deep learning method for early detection of lung cancer using neural networks. Healthcare Anal 3:100195
Iqbal A, Usman M, Ahmed Z (2023) Tuberculosis chest X-ray detection using CNN-based hybrid segmentation and classification approach. Biomed Signal Process Control 84:104667
Zhao D, Liu Y, Yin H, Wang Z (2023) An attentive and adaptive 3d CNN for automatic pulmonary nodule detection in CT image. Expert Syst Appl 211:118672
Huang Y-S, Wang T-C, Huang S-Z, Zhang J, Chen H-M, Chang Y-C, Chang R-F (2023) An improved 3-d attention CNN with hybrid loss and feature fusion for pulmonary nodule classification. Comput Methods Programs Biomed 229:107278
Xu J, Ren H, Cai S, Zhang X (2023) An improved faster r-CNN algorithm for assisted detection of lung nodules. Comput Biol Med 153:106470
Tang T-W, Lin W-Y, Liang J-D, Li K-M (2023) Artificial intelligence aided diagnosis of pulmonary nodules segmentation and feature extraction. Clin Radiol 78:437–443
Sengodan P, Srinivasan K, Pichamuthu R, Matheswaran S (2023) Early detection and classification of malignant lung nodules from CT images: an optimal ensemble learning. Expert Syst Appl 229:120361
Alshahrni MM, Ahmad MA, Abdullah M, Omer N, Aziz M (2023) An intelligent deep convolutional network based Covid-19 detection from chest X-rays. Alex Eng J 64:399–417
de Jesus Silva LF, Cortes OAC, Diniz JOB (2023) A novel ensemble CNN model for Covid-19 classification in computerized tomography scans. Results Control Optim 11:100215
Duong LT, Nguyen PT, Iovino L, Flammini M (2023) Automatic detection of Covid-19 from chest X-ray and lung computed tomography images using deep neural networks and transfer learning. Appl Soft Comput 132:109851
Ying X, Liu H, Huang R (2023) Covid-19 chest X-ray image classification in the presence of noisy labels. Displays 77:102370
George GS, Mishra PR, Sinha P, Prusty MR (2023) Covid-19 detection on chest X-ray images using homomorphic transformation and vgg inspired deep convolutional neural network. Biocybern Biomed Eng 43:1–16
Celik G (2023) Detection of Covid-19 and other pneumonia cases from CT and X-ray chest images using deep learning based on feature reuse residual block and depthwise dilated convolutions neural network. Appl Soft Comput 133:109906
Motwani A, Shukla PK, Pawar M, Kumar M, Ghosh U, Alnumay W, Nayak SR (2023) Enhanced framework for Covid-19 prediction with computed tomography scan images using dense convolutional neural network and novel loss function. Comput Electr Eng 105:108479
Dixit A, Mani A, Bansal R (2021) Cov2-detect-net: design of Covid-19 prediction model based on hybrid de-pso with SVM using chest X-ray images. Inf Sci 571:676–692
MathSciNet Google Scholar
Li X, Li L, Ma W, Wang W (2022) Two-phase flow patterns identification in porous media using feature extraction and SVM. Int J Multiph Flow 156:104222
Hu R, Gan J, Zhu X, Liu T, Shi X (2022) Multi-task multi-modality SVM for early Covid-19 diagnosis using chest CT data. Inf Process Manag 59:102782
Verma SS, Prasad A, Kumar A (2022) Covxmlc: high performance Covid-19 detection on X-ray images using multi-model classification. Biomed Signal Process Control 71:103272
Nanglia P, Kumar S, Mahajan AN, Singh P, Rathee D (2021) A hybrid algorithm for lung cancer classification using SVM and neural networks. ICT Express 7:335–341
Shah SNA, Parveen R (2023) An extensive review on lung cancer diagnosis using machine learning techniques on radiological data: state-of-the-art and perspectives. Arch Comput Methods Eng 1:1–14
Romero-del Castillo J, Mendoza-Hurtado M, Ortiz-Boyer D, García-Pedrajas N (2022) Local-based k values for multi-label k-nearest neighbors rule. Eng Appl Artif Intell 116:105487
Kaneko H (2023) Local interpretation of nonlinear regression model with k-nearest neighbors. Digit Chem Eng 6:100078
Sejuti ZA, Islam MS (2023) A hybrid CNN-knn approach for identification of Covid-19 with 5-fold cross validation. Sens Int 4:100229
Vommi AM, Battula TK (2023) A hybrid filter-wrapper feature selection using fuzzy knn based on bonferroni mean for medical datasets classification: a Covid-19 case study. Expert Syst Appl 218:119612
Maleki N, Zeinali Y, Niaki STA (2021) A k-nn method for lung cancer prognosis with the use of a genetic algorithm for feature selection. Expert Syst Appl 164:113981
Avinash S, Naveen Kumar H, Guru Prasad M, Mohan Naik R, Parveen G (2023) Early detection of malignant tumor in lungs using feed-forward neural network and k-nearest neighbor classifier. SN Comput Sci 4:195
Rezaeijo SM, Abedi-Firouzjah R, Ghorvei M, Sarnameh S (2021) Screening of Covid-19 based on the extracted radiomics features from chest CT images. J Xray Sci Technol 29:229–243
Sarailidis G, Wagener T, Pianosi F (2023) Integrating scientific knowledge into machine learning using interactive decision trees. Comput Geosci 170:105248
Alves MA, Castro GZ, Oliveira BAS, Ferreira LA, Ramírez JA, Silva R, Guimarães FG (2021) Explaining machine learning based diagnosis of Covid-19 from routine blood tests with decision trees and criteria graphs. Comput Biol Med 132:104335
Shirole U, Joshi M, Bagul P (2019) Cardiac, diabetic and normal subjects classification using decision tree and result confirmation through orthostatic stress index. Inf Med Unlocked 17:100252
Hosseinpour M, Ghaemi S, Khanmohammadi S, Daneshvar S (2022) A hybrid high-order type-2 fcm improved random forest classification method for breast cancer risk assessment. Appl Math Comput 424:127038
Shiri I, Salimi Y, Pakbin M, Hajianfar G, Avval AH, Sanaat A, Mostafaei S, Akhavanallaf A, Saberi A, Mansouri Z et al (2022) Covid-19 prognostic modeling using CT radiomic features and machine learning algorithms: analysis of a multi-institutional dataset of 14,339 patients. Comput Biol Med 145:105467
Mostafiz R, Uddin MS, Reza MM, Rahman MM et al (2022) Covid-19 detection in chest X-ray through random forest classifier using a hybridization of deep CNN and dwt optimized features. J King Saud Univ 34:3226–3235
Nahiduzzaman M, Goni MOF, Islam MR, Sayeed A, Anower MS, Ahsan M, Haider J, Kowalski M (2023) Detection of various lung diseases including Covid-19 using extreme learning machine algorithm based on the features extracted from a lightweight CNN architecture. Biocybern Biomed Eng 1:1
Wu X, Chen C, Chen X, Luo C, Lv X, Shi Y, Yang J, Meng X, Chen C, Su J et al (2022) Raman spectroscopy combined with machine learning algorithms for rapid detection primary Sjögren’s syndrome associated with interstitial lung disease. Photodiagn Photodyn Ther 40:103057
Talukder MA, Islam MM, Uddin MA, Akhter A, Hasan KF, Moni MA (2022) Machine learning-based lung and colon cancer detection using deep feature extraction and ensemble learning. Expert Syst Appl 205:117695
Tamal M, Alshammari M, Alabdullah M, Hourani R, Alola HA, Hegazi TM (2021) An integrated framework with machine learning and radiomics for accurate and rapid early diagnosis of Covid-19 from chest X-ray. Expert Syst Appl 180:115152
Novitasari DCR, Hendradi R, Caraka RE, Rachmawati Y, Fanani NZ, Syarifudin A, Toharudin T, Chen RC (2020) Detection of Covid-19 chest X-ray using support vector machine and convolutional neural network. Commun Math Biol Neurosci 2020:Article-ID
Islam MR, Nahiduzzaman M (2022) Complex features extraction with deep learning model for the detection of covid19 from CT scan images using ensemble based machine learning approach. Expert Syst Appl 195:116554
Sethy PK, Behera SK (2020) Detection of coronavirus disease (Covid-19) based on deep features
Nour M, Cömert Z, Polat K (2020) A novel medical diagnosis model for Covid-19 infection detection based on deep features and bayesian optimization. Appl Soft Comput 97:106580
Turkoglu M (2021) Covidetectionet: Covid-19 diagnosis system based on X-ray images using features selected from pre-learned deep features ensemble. Appl Intell 51:1213–1226
Latif G, Morsy H, Hassan A, Alghazo J (2022) Novel coronavirus and common pneumonia detection from CT scans using deep learning-based extracted features. Viruses 14:1667
Hasoon JN, Fadel AH, Hameed RS, Mostafa SA, Khalaf BA, Mohammed MA, Nedoma J (2021) Covid-19 anomaly detection and classification method based on supervised machine learning of chest X-ray images. Results Phys 31:105045
Dhiman G, Vinoth Kumar V, Kaur A, Sharma A (2021) Don: deep learning and optimization-based framework for detection of novel coronavirus disease using X-ray images, Interdisciplinary Sciences: Comput Life Sci 13:260–272
Bhargava A, Bansal A, Goyal V (2022) Machine learning-based automatic detection of novel coronavirus (Covid-19) disease. Multimed Tools Appl 81:13731–13750
Farahat IS, Sharafeldeen A, Elsharkawy M, Soliman A, Mahmoud A, Ghazal M, Taher F et al (2022) The role of 3d CT imaging in the accurate diagnosis of lung function in coronavirus patients. Diagnostics 12:696
Tuncer T, Dogan S, Ozyurt F (2020) An automated residual exemplar local binary pattern and iterative relieff based Covid-19 detection method using chest X-ray image. Chemom Intell Lab Syst 203:104054
Allen B, Molokie R, Royston TJ (2020) Early detection of acute chest syndrome through electronic recording and analysis of auscultatory percussion. IEEE J Transl Eng Health Med 8:1–8
Chong DY, Kim HJ, Lo P, Young S, McNitt-Gray MF, Abtin F, Goldin JG, Brown MS (2015) Robustness-driven feature selection in classification of fibrotic interstitial lung disease patterns in computed tomography using 3d texture features. IEEE Trans Med Imaging 35:144–157
Jothi ESJ, Justin J, Vanithamani R, Varsha R (2023) On-mask sensor network for lung disease monitoring. Biomed Signal Process Control 83:104655
Bhattacharjee S, Saha B, Bhattacharyya P, Saha S (2022) Classification of obstructive and non-obstructive pulmonary diseases on the basis of spirometry using machine learning techniques. J Comput Sci 63:101768
Guo S, Xiu J, Chen W, Ji T, Wang F, Liu H (2023) Precise diagnosis of lung cancer enabled by improved ftir-based machine learning. Infrared Phys Technol 132:104732
Saygılı A (2021) A new approach for computer-aided detection of coronavirus (Covid-19) from CT and X-ray images using machine learning methods. Appl Soft Comput 105:107323
Mahdy LN, Ezzat KA, ElmoUSAlami HH, Ella HA, Hassanien AE (2020) Automatic X-ray Covid-19 lung image classification system based on multi-level thresholding and support vector machine. MedRxiv 2020-03
Barstugan M, Ozkaya U, Ozturk S (2020) Coronavirus (Covid-19) classification using CT images by machine learning methods. arXiv preprint arXiv:2003.09424
Gayathri J, Abraham B, Sujarani M, Ramachandran S (2023) A novel CNN framework for the detection of Covid-19 using manta ray optimization and knn classifier in lus images. Int J Intell Syst Appl Eng 11:55–63
Rao DS, Nair SAH, Rao TVN, Kumar KS (2022) Classification of pneumonia from chest X-ray image using machine learning models. Int J Intell Syst Appl Eng 10:399–408
Krizhevsky A, Sutskever I, Hinton GE (2017) Imagenet classification with deep convolutional neural networks. Commun ACM 60:84–90
Krizhevsky A, Sutskever I, Hinton GE (2012) Imagenet classification with deep convolutional neural networks. Adv Neural Inf Process Syst 25:2012
Xu Y, Wang Y, Razmjooy N (2022) Lung cancer diagnosis in CT images based on alexnet optimized by modified bowerbird optimization algorithm. Biomed Signal Process Control 77:103791
Simonyan K, Zisserman A (2014) Very deep convolutional networks for large-scale image recognition. arXiv preprint https://arxiv.org/abs/1409.1556
Ikechukwu AV, Murali S, Deepu R, Shivamurthy R (2021) Resnet-50 vs vgg-19 vs training from scratch: a comparative analysis of the segmentation and classification of pneumonia from chest X-ray images. Glob Trans Proc 2:375–381
Chouat I, Echtioui A, Khemakhem R, Zouch W, Ghorbel M, Hamida AB (2022) Lung disease detection in chest X-ray images using transfer learning. In: 2022 6th international conference on advanced technologies for signal and image processing (ATSIP), IEEE, pp 1–6
Khan MA, Rajinikanth V, Satapathy SC, Taniar D, Mohanty JR, Tariq U, Damaševičius R (2021) Vgg19 network assisted joint segmentation and classification of lung nodules in CT images. Diagnostics 11:2208
Szegedy C, Liu W, Jia Y, Sermanet P, Reed S, Anguelov D, Erhan D, Vanhoucke V, Rabinovich A. Going deeper with convolutions. In: Proceedings of the IEEE conference on computer vision and pattern recognition, pp 1–9
More P, Ratre S, Ligade S, Bhise R (2022) Design of an efficient approach for performance enhancement of Covid-19 detection using auxiliary googlenet by using chest CT scan images. In: 2022 IEEE Bombay Section Signature Conference (IBSSC), IEEE, pp 1–6
He K, Zhang X, Ren S, Sun J. Deep residual learning for image recognition. In: Proceedings of the IEEE conference on computer vision and pattern recognition, pp 770–778
Showkat S, Qureshi S (2022) Efficacy of transfer learning-based resnet models in chest X-ray image classification for detecting Covid-19 pneumonia. Chemom Intell Lab Syst 224:104534
Chollet F. Xception: deep learning with depthwise separable convolutions. In: Proceedings of the IEEE conference on computer vision and pattern recognition, pp 1251–1258
Rahimzadeh M, Attar A (2020) A modified deep convolutional neural network for detecting Covid-19 and pneumonia from chest X-ray images based on the concatenation of xception and resnet50v2. Inf Med Unlocked 19:100360
Rajinikanth V, Kadry S, Damaševičius R, Pandeeswaran C, Mohammed MA, Devadhas GG (2022) Pneumonia detection in chest X-ray using inceptionv3 and multi-class classification. In: 2022 Third International Conference on Intelligent Computing Instrumentation and Control Technologies (ICICICT), IEEE, pp 972–976
Hu J, Shen L, Sun G. Squeeze-and-excitation networks. In: Proceedings of the IEEE conference on computer vision and pattern recognition, pp 7132–7141
Rehman A, Naz S, Khan A, Zaib A, Razzak I. Improving coronavirus (Covid-19) diagnosis using deep transfer learning. In: Proceedings of international conference on information technology and applications: ICITA 2021, Springer, pp 23–37
Huang G, Liu Z, Van Der Maaten L, Weinberger KQ. Densely connected convolutional networks. In: Proceedings of the IEEE conference on computer vision and pattern recognition, pp 4700–4708
Lanjewar MG, Panchbhai KG, Charanarur P (2023) Lung cancer detection from CT scans using modified densenet with feature selection methods and ml classifiers. Expert Syst Appl 224:119961
Albahli S, Ayub N, Shiraz M (2021) Coronavirus disease (Covid-19) detection using X-ray images and enhanced densenet. Appl Soft Comput 110:107645
Verma D, Bose C, Tufchi N, Pant K, Tripathi V, Thapliyal A (2020) An efficient framework for identification of tuberculosis and pneumonia in chest X-ray images using neural network. Proc Comput Sci 171:217–224
Yu X, Wang S-H, Zhang Y-D (2021) Cgnet: a graph-knowledge embedded convolutional neural network for detection of pneumonia. Inf Process Manag 58:102411
Jain R, Nagrath P, Kataria G, Kaushik VS, Hemanth DJ (2020) Pneumonia detection in chest X-ray images using convolutional neural networks and transfer learning. Measurement 165:108046
Kavya NS, Veeranjaneyulu N, Priya DD et al (2022) Detecting covid19 and pneumonia from chest X-ray images using deep convolutional neural networks. Mater Today 64:737–743
Szepesi P, Szilágyi L (2022) Detection of pneumonia using convolutional neural networks and deep learning, Biocybern Biomed Eng 42:1012–1022
Nahiduzzaman M, Islam MR, Hassan R (2023) Chest X-ray 6: prediction of multiple diseases including Covid-19 from chest X-ray images using convolutional neural network. Expert Syst Appl 211:118576
Ghose P, Uddin MA, Acharjee UK, Sharmin S (2022) Deep viewing for the identification of Covid-19 infection status from chest X-ray image using CNN based architecture. Intell Syst Appl 16:200130
Chauhan T, Palivela H, Tiwari S (2021) Optimization and fine-tuning of densenet model for classification of Covid-19 cases in medical imaging. Int J Inf Manag Data Insights 1:100020
Reis HC, Turk V (2022) Covid-dsnet: a novel deep convolutional neural network for detection of coronavirus (sars-cov-2) cases from CT and chest X-ray images. Artif Intell Med 134:102427
Mothkur R, Veerappa B (2023) Classification of lung cancer using lightweight deep neural networks. Proc Comput Sci 218:1869–1877
Rajasekar V, Vaishnnave M, Premkumar S, Sarveshwaran V, Rangaraaj V (2023) Lung cancer disease prediction with CT scan and histopathological images feature analysis using deep learning techniques. Results Eng 18:101111
Alshmrani GMM, Ni Q, Jiang R, Pervaiz H, Elshennawy NM (2023) A deep learning architecture for multi-class lung diseases classification using chest X-ray (CXR) images. Alex Eng J 64:923–935
Mamalakis M, Swift AJ, Vorselaars B, Ray S, Weeks S, Ding W, Clayton RH, Mackenzie LS, Banerjee A (2021) Denrescov-19: a deep transfer learning network for robust automatic classification of Covid-19, pneumonia, and tuberculosis from X-rays. Comput Med Imaging Graph 94:102008
Mahbub MK, Biswas M, Gaur L, Alenezi F, Santosh K (2022) Deep features to detect pulmonary abnormalities in chest X-rays due to infectious diseasex: Covid-19, pneumonia, and tuberculosis. Inf Sci 592:389–401
Bhandari M, Shahi TB, Siku B, Neupane A (2022) Explanatory classification of CXR images into Covid-19, pneumonia and tuberculosis using deep learning and xai. Comput Biol Med 150:106156
Rahman M, Cao Y, Sun X, Li B, Hao Y (2021) Deep pre-trained networks as a feature extractor with xgboost to detect tuberculosis from chest X-ray. Comput Electr Eng 93:107252
Sherstinsky A (2020) Fundamentals of recurrent neural network (rnn) and long short-term memory (lstm) network. Physica D 404:1
Graves A, Graves A (2012) Long short-term memory. Supervised sequence labelling with recurrent neural networks. Springer, Berlin, pp 37–45
Chung J, Gulcehre C, Cho K, Bengio Y (2014) Empirical evaluation of gated recurrent neural networks on sequence modeling. arXiv preprint https://arxiv.org/abs/1412.3555
Muñoz-Organero M, Callejo P, Hombrados-Herrera MÁ (2023) A new rnn based machine learning model to forecast Covid-19 incidence, enhanced by the use of mobility data from the bike-sharing service in madrid. Heliyon 9:e17625
Shouman MA, El-Fiky A, Hamada S, El-Sayed A, Karar ME (2022) Computer-assisted lung diseases detection from pediatric chest radiography using long short-term memory networks. Comput Electr Eng 103:108402
Liang G, Zheng L (2020) A transfer learning method with deep residual network for pediatric pneumonia diagnosis. Comput Methods Programs Biomed 187:104964
ArunKumar K, Kalaga DV, Kumar CMS, Kawaji M, Brenza TM (2021) Forecasting of Covid-19 using deep layer recurrent neural networks (rnns) with gated recurrent units (grus) and long short-term memory (lstm) cells. Chaos Solitons Fract 146:110861
Indumathi V, Siva R (2023) An efficient lung disease classification from X-ray images using hybrid mask-rCNN and bidlstm. Biomed Signal Process Control 81:104340
Shin H-C, Roth HR, Gao M, Lu L, Xu Z, Nogues I, Yao J, Mollura D, Summers RM (2016) Deep convolutional neural networks for computer-aided detection: CNN architectures, dataset characteristics and transfer learning. IEEE Trans Med Imaging 35:1285–1298
Zhao D, Che N, Song Z, Liu C, Wang L, Shi H, Dong Y, Lin H, Mu J, Ying L et al (2020) Pathological diagnosis of lung cancer based on deep transfer learning, Chin. J Pathol 49:1120–1125
Da Nóbrega RVM, Peixoto SA, da Silva SPP, Rebouças Filho PP (2018) Lung nodule classification via deep transfer learning in CT lung images. In: 2018 IEEE 31st international symposium on computer-based medical systems (CBMS), IEEE, pp 244–249
Saveri PL, Kumar S (2022) Classification of cancerous lung images by using transfer learning. In: 2022 8th international conference on signal processing and communication (ICSC), IEEE, pp 298–303
Shamrat FJM, Azam S, Karim A, Ahmed K, Bui FM, De Boer F (2023) High-precision multiclass classification of lung disease through customized mobilenetv2 from chest X-ray images. Comput Biol Med 155:106646
Vieira P, SoUSA O, Magalhães D, Rabêlo R, Silva R (2021) Detecting pulmonary diseases using deep features in X-ray images. Pattern Recogn 119:108081
Islam MK, Habiba SU, Khan TA, Tasnim F (2022) Cov-radnet: a deep convolutional neural network for automatic detection of Covid-19 from chest X-rays and CT scans. Comput Methods Program Biomed Update 2:100064
Karaddi SH, Sharma LD (2023) Automated multi-class classification of lung diseases from CXR-images using pre-trained convolutional neural networks. Expert Syst Appl 211:2023
Bhattacharyya A, Bhaik D, Kumar S, Thakur P, Sharma R, Pachori RB (2022) A deep learning based approach for automatic detection of Covid-19 cases using chest X-ray images. Biomed Signal Process Control 71:103182
Gilanie G, Bajwa UI, Waraich MM, Asghar M, KoUSAr R, Kashif A, Aslam RS, Qasim MM, Rafique H (2021) Coronavirus (Covid-19) detection from chest radiology images using convolutional neural networks. Biomed Signal Process Control 66:102490
Hassantabar S, Ahmadi M, Sharifi A (2020) Diagnosis and detection of infected tissue of Covid-19 patients based on lung X-ray image using convolutional neural network approaches. Chaos Solitons Fract 140:110170
Jain R, Gupta M, Taneja S, Hemanth DJ (2021) Deep learning based detection and analysis of Covid-19 on chest X-ray images. Appl Intell 51:1690–1700
Thakur S, Kumar A (2021) X-ray and ct-scan-based automated detection and classification of Covid-19 using convolutional neural networks (CNN). Biomed Signal Process Control 69:102920
Jia G, Lam H-K, Xu Y (2021) Classification of Covid-19 chest X-ray and CT images using a type of dynamic CNN modification method. Comput Biol Med 134:104425
Ibrahim AU, Ozsoz M, Serte S, Al-Turjman F, Yakoi PS (2021) Pneumonia classification using deep learning from chest X-ray images during Covid-19. Cogn Comput 1:1–13
Minaee S, Kafieh R, Sonka M, Yazdani S, Soufi GJ (2020) Deep-covid: predicting Covid-19 from chest X-ray images using deep transfer learning. Med Image Anal 65:101794
Apostolopoulos ID, Mpesiana TA (2020) Covid-19: automatic detection from X-ray images utilizing transfer learning with convolutional neural networks. Phys Eng Sci Med 43:635–640
Sheykhivand S, MoUSAvi Z, Mojtahedi S, Rezaii TY, Farzamnia A, Meshgini S, Saad I (2021) Developing an efficient deep neural network for automatic detection of Covid-19 using chest X-ray images. Alex Eng J 60:2885–2903
Kong L, Cheng J (2022) Classification and detection of Covid-19 X-ray images based on densenet and vgg16 feature fusion. Biomed Signal Process Control 77:103772
Liu J, Sun W, Zhao X, Zhao J, Jiang Z (2022) Deep feature fusion classification network (dffcnet): towards accurate diagnosis of Covid-19 using chest X-rays images. Biomed Signal Process Control 76:103677
Gopatoti A, Vijayalakshmi P (2022) Cxgnet: a tri-phase chest X-ray image classification for Covid-19 diagnosis using deep CNN with enhanced grey-wolf optimizer. Biomed Signal Process Control 77:103860
de Moura J, Novo J, Ortega M (2022) Fully automatic deep convolutional approaches for the analysis of Covid-19 using chest X-ray images. Appl Soft Comput 115:108190
Hemdan EE-D, Shouman MA, Karar MME (2020) Covidx-net: a framework of deep learning classifiers to diagnose Covid-19 in X-ray images. arXiv preprint http://arxiv.org/abs/2003.11055
Garstka J, Strzelecki M (2020) Pneumonia detection in X-ray chest images based on convolutional neural networks and data augmentation methods. In: 2020 Signal processing: algorithms, architectures, arrangements, and applications (SPA), IEEE, pp 18–23
Sahu P, Yu D, Dasari M, Hou F, Qin H (2018) A lightweight multi-section CNN for lung nodule classification and malignancy estimation. IEEE J Biomed Health Inform 23:960–968
Kalaivani S, Seetharaman K (2022) A three-stage ensemble boosted convolutional neural network for classification and analysis of Covid-19 chest X-ray images. Int J Cogn Comput Eng 3:35–45
Shakibapour E, Cunha A, Aresta G, Mendonça AM, Campilho A (2019) An unsupervised metaheuristic search approach for segmentation and volume measurement of pulmonary nodules in lung CT scans. Expert Syst Appl 119:415–428
Liu L, Zhao D, Yu F, Heidari AA, Li C, Ouyang J, Chen H, Mafarja M, Turabieh H, Pan J (2021) Ant colony optimization with Cauchy and greedy levy mutations for multilevel covid 19 X-ray image segmentation. Comput Biol Med 136:104609
Hao S, Huang C, Heidari AA, Xu Z, Chen H, Alabdulkreem E, Elmannai H, Wang X (2023) Multi-threshold image segmentation using an enhanced fruit fly optimization for Covid-19 X-ray images. Biomed Signal Process Control 1:10517
Poap D, Wozniak M, Damaševičius R, Wei W (2018) Chest radiographs segmentation by the use of nature-inspired algorithm for lung disease detection. In: 2018 IEEE symposium series on computational intelligence (SSCI), IEEE, pp.2298–2303
Yousri D, Abd Elaziz M, Abualigah L, Oliva D, Al-Qaness MA, Ewees AA (2021) Covid-19 X-ray images classification based on enhanced fractional-order cuckoo search optimizer using heavy-tailed distributions. Appl Soft Comput 101:107052
Abugabah A, AlZubi AA, Al-Obeidat F, Alarifi A, Alwadain A (2020) Data mining techniques for analyzing healthcare conditions of urban space-person lung using meta-heuristic optimized neural networks. Clust Comput 23:1781–1794
Prabukumar M, Agilandeeswari L, Ganesan K (2019) An intelligent lung cancer diagnosis system using cuckoo search optimization and support vector machine classifier. J Ambient Intell Humaniz Comput 10:267–293
Vijh S, Gaur D, Kumar S (2020) An intelligent lung tumor diagnosis system using whale optimization algorithm and support vector machine. Int J Syst Assur Eng Manag 11:374–384
Johnson DS, Johnson DLL, Elavarasan P, Karunanithi A (2020) Feature selection using flower pollination optimization to diagnose lung cancer from CT images. In: Advances in information and communication: proceedings of the 2020 future of information and communication conference (FICC), Volume 2, Springer, pp 604–620
Mukherjee J, Sikdar B, Chakrabarti A, Kar M, Das S (2018) A novel technique for contrast enhancement of chest X-ray images based on bio-inspired meta-heuristics. Adv Comput Syst Secur 5:71–93
Jaszcz A, Połap D, Damaševičius R (2022) Lung X-ray image segmentation using heuristic red fox optimization algorithm. Sci Program 2022:1–8
Jalali SMJ, Ahmadian M, Ahmadian S, Hedjam R, Khosravi A, Nahavandi S (2022) X-ray image based Covid-19 detection using evolutionary deep learning approach. Expert Syst Appl 201:116942
Jalali SMJ, Ahmadian M, Ahmadian S, Khosravi A, Alazab M, Nahavandi S (2021) An oppositional-Cauchy based gsk evolutionary algorithm with a novel deep ensemble reinforcement learning strategy for Covid-19 diagnosis. Appl Soft Comput 111:107675
Sabzalian MH, Kharajinezhadian F, Tajally A, Reihanisaransari R, Alkhazaleh HA, Bokov D (2023) New bidirectional recurrent neural network optimized by improved ebola search optimization algorithm for lung cancer diagnosis. Biomed Signal Process Control 84:104965
Altan A, Karasu S (2020) Recognition of Covid-19 disease from X-ray images by hybrid model consisting of 2d curvelet transform, chaotic salp swarm algorithm and deep learning technique. Chaos Solitons Fract 140:110071
Harshavardhan A, Cheerla S, Parkavi A, Latha Mary SA, Qureshi K, Mhaske HR (2023) Deep learning modified neural networks with chicken swarm optimization-based lungs disease detection and severity classification. J Electron Imaging 32:062603–062603
Rajagopal R, Karthick R, Meenalochini P, Kalaichelvi T (2023) Deep convolutional spiking neural network optimized with arithmetic optimization algorithm for lung disease detection using chest X-ray images. Biomed Signal Process Control 79:104197
Mohammed SN, Alkinani FS, Hassan YA (2020) Automatic computer aided diagnostic for Covid-19 based on chest X-ray image and particle swarm intelligence. Int J Intell Eng Syst 13:63–73
Canayaz M (2021) MH-covidnet: diagnosis of Covid-19 using deep neural networks and meta-heuristic-based feature selection on X-ray images. Biomed Signal Process Control 64:102257
Farhan AMQ, Yang S (2023) Automatic lung disease classification from the chest X-ray images using hybrid deep learning algorithm. Multimed Tools Appl 1:1–27
Chakraborty S, Murali B, Mitra AK (2022) An efficient deep learning model to detect Covid-19 using chest X-ray images. Int J Environ Res Public Health 19:2013
Annamalai B, Saravanan P, Varadharajan I (2023) Aboa-CNN: auction-based optimization algorithm with convolutional neural network for pulmonary disease prediction. Neural Comput Appl 35:7463–7474
Agarwal M, Gupta SK, Biswas K (2023) Development of a compressed fcn architecture for semantic segmentation using particle swarm optimization. Neural Comput Appl 1:1–14
Izumi T, Shaw R (2022) A multi-country comparative analysis of the impact of Covid-19 and natural hazards in India, Japan, the Philippines, and USA. Int J Disas Risk Reduct 73:102899
Hamood Alsamhi S, Hawbani A, Shvetsov AV, Kumar S et al (2023) Advancing pandemic preparedness in healthcare 50: a survey of federated learning applications. Adv Hum Comput Interact 2023:9992393
Qi L, Gao Y, Yang J, Ding X-B, Xiong Y, Su K, Liu T, Li Q, Tang W-G, Liu Q-Y (2020) The burden of influenza and pneumonia mortality attributable to absolute humidity among elderly people in Chongqing, China, 2012–2018. Sci Total Environ 716:136682
Palacios-Ceña D, Hernández-Barrera V, López-de Andrés A, Fernández-de Las-Peñas C, Palacios-Ceña M, de Miguel-Díez J, Carrasco-Garrido P, Jiménez-García R (2017) Time trends in incidence and outcomes of hospitalizations for aspiration pneumonia among elderly people in Spain (2003- 2013). Eur J Internal Med 38:61–67
Ahmad S, Singh V, Gautam HK, Raza K (2023) Multisampling-based docking reveals imidazolidinyl urea as a multitargeted inhibitor for lung cancer: an optimisation followed multi-simulation and in-vitro study. J Biomol Struct Dyn 1:1–18
Ahmad S, Raza K (2023) Identification of 5-nitroindazole as a multitargeted inhibitor for cdk and transferase kinase in lung cancer: a multisampling algorithm-based structural study. Mol Divers 1:1–14
Malkova A, Starshinova A, Zinchenko Y, Gavrilova N, Kudryavtsev I, Lapin S, Mazing A, Surkova E, Pavlova M, Belaeva E et al (2021) New laboratory criteria of the autoimmune inflammation in pulmonary sarcoidosis and tuberculosis. Clin Immunol 227:108724
Rolo M, González-Blanco B, Reyes C, Rosillo N, López-Roa P (2023) Epidemiology and factors associated with extra-pulmonary tuberculosis in a low-prevalence area. J Clin Tubercul Mycobact Dis 32:100377
Kermany DS, Goldbaum M, Cai W, Valentim CC, Liang H, Baxter SL, McKeown A, Yang G, Wu X, Yan F et al (2018) Identifying medical diagnoses and treatable diseases by image-based deep learning. Cell 172:1122–1131
Jaeger S, Candemir S, Antani S, Wáng Y-XJ, Lu P-X, Thoma G (2014) Two public chest X-ray datasets for computer-aided screening of pulmonary diseases. Quant Imaging Med Surg 4:475
Cohen JP, Morrison P, Dao L (2020) Covid-19 image data collection. arXiv preprint https://arxiv.org/abs/2003.11597
Rahman T, Khandakar A, Qiblawey Y, Tahir A, Kiranyaz S, Kashem SBA, Islam MT, Al Maadeed S, Zughaier SM, Khan MS et al (2021) Exploring the effect of image enhancement techniques on Covid-19 detection using chest X-ray images. Comput Biol Med 132:104319
Rahman T, Khandakar A, Kadir MA, Islam KR, Islam KF, Mazhar R, Hamid T, Islam MT, Kashem S, Mahbub ZB et al (2020) Reliable tuberculosis detection using chest X-ray with deep learning, segmentation and visualization. IEEE Access 8:191586–191601
Kermany D, Zhang K, Goldbaum M et al (2018) Labeled optical coherence tomography (oct) and chest X-ray images for classification. Mendeley Data 2:651
Hatano H, Tsujimoto Y, Watanabe H, Tsukada A, Izumi S, Nagasaka S, Igari T, Suzuki M, Iikura M, Hojo M (2023) A case of idiopathic multicentric castleman disease with pulmonary hyalinizing granuloma-like multiple pulmonary nodules. Respir Investig 61:405–408
Cai J, Vonder M, Heuvelmans MA, Pelgrim GJ, Rook M, Kramer G, Sidorenkov G, Groen HJ, de Bock GH, Vliegenthart R (2022) CT characteristics of solid pulmonary nodules of never smokers versus smokers: a population-based study. Eur J Radiol 154:110410
Huang H, Li Y, Wu R, Li Z, Zhang J (2022) Benign-malignant classification of pulmonary nodule with deep feature optimization framework. Biomed Signal Process Control 76:103701
Armato SG III, McLennan G, Bidaut L, McNitt-Gray MF, Meyer CR, Reeves AP, Zhao B, Aberle DR, Henschke CI, Hoffman EA et al (2011) The lung image database consortium (lidc) and image database resource initiative (idri): a completed reference database of lung nodules on CT scans. Med Phys 38:915–931
Lv W, Wang Y, Zhou C, Yuan M, Pang M, Fang X, Zhang Q, Huang C, Li X, Zhou Z et al (2021) Development and validation of a clinically applicable deep learning strategy (honors) for pulmonary nodule classification at CT: a retrospective multicentre study. Lung Cancer 155:78–86
Liang C-H, Liu Y-C, Wu M-T, Garcia-Castro F, Alberich-Bayarri A, Wu F-Z (2020) Identifying pulmonary nodules or masses on chest radiography using deep learning: external validation and strategies to improve clinical practice. Clin Radiol 75:38–45
Alqudah AM, Qazan S, Alqudah A (2020) Automated systems for detection of Covid-19 using chest X-ray images and lightweight convolutional neural networks
Castiglioni I, Ippolito D, Interlenghi M, Monti CB, Salvatore C, Schiaffino S, Polidori A, Gandola D, Messa C, Sardanelli F (2021) Machine learning applied on chest X-ray can aid in the diagnosis of Covid-19: a first experience from Lombardy, Italy . Eur Radiol Exp 5:1–10
Wu T, Yang N, Chen L, Xiao X, Xian X, Liu J, Qiao S, Cui C (2022) Ergcn: Data enhancement-based robust graph convolutional network against adversarial attacks. Inf Sci 617:234–253
Tan, C, Sun, T, Kong T, Zhang W, Yang C, Liu C (2018) A survey on deep transfer learning. In: Artificial neural networks and machine learning–ICANN 2018: 27th international conference on artificial neural networks, Rhodes, Greece, October 4-7, 2018, Proceedings, Part III 27. Springer, Berlin. pp 270–279
Heaton J (2018) Ian goodfellow, yoshua bengio, and aaron courville: deep learning. Genet Program Evol Mach 19:305–307
Ghoshal B, Tucker A (2020) Estimating uncertainty and interpretability in deep learning for coronavirus (Covid-19) detection. arXiv preprint https://arxiv.org/abs/2003.10769
Rea G, Sverzellati N, Bocchino M, Lieto R, Milanese G, M. DAlto, G. Bocchini, M. Maniscalco, T. Valente, G. Sica (2023) Beyond visual interpretation: quantitative analysis and artificial intelligence in interstitial lung disease diagnosis expanding horizons in radiology. Diagnostics 13:2333
Siala H, Wang Y (2022) Shifting artificial intelligence to be responsible in healthcare: a systematic review. Soc Sci Med 296:114782
Jasmine Pemeena Priyadarsini M, Rajini G, Hariharan K, Utkarsh Raj K, Bhargav Ram K, Indragandhi V, Subramaniyaswamy V, Pandya S et al (2023) Lung diseases detection using various deep learning algorithms. J Healthcare Eng 2023:3563696
Teng Q, Liu Z, Song Y, Han K, Lu Y (2022) A survey on the interpretability of deep learning in medical diagnosis. Multimed Syst 28:2335–2355
Cifci MA (2023) A deep learning-based framework for uncertainty quantification in medical imaging using the dropweak technique: an empirical study with baresnet. Diagnostics 13:800
Salehin I, Islam MS, Saha P, Noman S, Tuni A, Hasan MM, Baten MA (2023) Automl: a systematic review on automated machine learning with neural architecture search. J Inf Intell 2:6
Jiang X, Wang N, Xin J, Xia X, Yang X, Gao X (2021) Learning lightweight super-resolution networks with weight pruning. Neural Netw 144:21–32
Sahal R, Alsamhi SH, Brown KN, D. OShea, B. Alouffi, et al (2022) Blockchain-based digital twins collaboration for smart pandemic alerting: decentralized Covid-19 pandemic alerting use case. Comput Intell Neurosci 2022:7786441
Alsamhi SH, Lee B, Guizani M, Kumar N, Qiao Y, Liu X (2021) Blockchain for decentralized multi-drone to combat Covid-19 and future pandemics: framework and proposed solutions. Trans Emerg Telecommun Technol 32:e4255
Alsamhi SH, Lee B (2020) Blockchain-empowered multi-robot collaboration to fight Covid-19 and future pandemics. IEEE Access 9:44173–44197
Keith JA, Vassilev-Galindo V, Cheng B, Chmiela S, Gastegger M, Muller K-R, Tkatchenko A (2021) Combining machine learning and computational chemistry for predictive insights into chemical systems. Chem Rev 121:9816–9872
Rasheed K, Qayyum A, Ghaly M, Al-Fuqaha A, Razi A, Qadir J (2022) Explainable, trustworthy, and ethical machine learning for healthcare: a survey. Comput Biol Med 149:106043
Ali S, Abuhmed T, El-Sappagh S, Muhammad K, Alonso-Moral JM, Confalonieri R, Guidotti R, Del Ser J, Díaz-Rodríguez N, Herrera F (2023) Explainable artificial intelligence (xai): What we know and what is left to attain trustworthy artificial intelligence. Inf Fusion 99:10805
Asan O, Bayrak AE, Choudhury A (2020) Artificial intelligence and human trust in healthcare: focus on clinicians. J Med Internet Res 22:e15154
Giuffrè M, Shung DL (2023) Harnessing the power of synthetic data in healthcare: innovation, application, and privacy. NPJ Digit Med 6:186
Tahri Sqalli M, Aslonov B, Gafurov M, Nurmatov S (2023) Humanizing ai in medical training: ethical framework for responsible design. Front Artif Intell 6:1189914
Solanki P, Grundy J, Hussain W (2023) Operationalising ethics in artificial intelligence for healthcare: a framework for ai developers. AI Ethics 3:223–240
Gerke S, Minssen T, Cohen G (2020) Ethical and legal challenges of artificial intelligence-driven healthcare. Artificial intelligence in healthcare. Elsevier, Amsterdam, pp 295–336
Zhang J, Zhang Z-M (2023) Ethics and governance of trustworthy medical artificial intelligence. BMC Med Inform Decis Mak 23:7
Klimova B, Pikhart M, Kacetl J (2023) Ethical issues of the use of ai-driven mobile apps for education. Front Public Health 10:1118116
Naik N, Hameed B, Shetty DK, Swain D, Shah M, Paul R, Aggarwal K, Ibrahim S, Patil V, Smriti K et al (2022) Legal and ethical consideration in artificial intelligence in healthcare: who takes responsibility? Front Surg 9:266
Najjar R (2023) Redefining radiology: a review of artificial intelligence integration in medical imaging. Diagnostics 13:2760
González-Gonzalo C, Thee EF, Klaver CC, Lee AY, Schlingemann RO, Tufail A, Verbraak F, Sánchez CI (2022) Trustworthy ai: closing the gap between development and integration of ai systems in ophthalmic practice. Prog Retin Eye Res 90:101034
van Leeuwen KG, de Rooij M, Schalekamp S, van Ginneken B, Rutten MJ (2021) How does artificial intelligence in radiology improve efficiency and health outcomes? Pediatr Radiol 52:1–7
Hong G-S, Jang M, Kyung S, Cho K, Jeong J, Lee GY, Shin K, Kim KD, Ryu SM, Seo JB et al (2023) Overcoming the challenges in the development and implementation of artificial intelligence in radiology: a comprehensive review of solutions beyond supervised learning. Korean J Radiol 24:1061
Bhatt AB, Bae J (2023) Collaborative intelligence to catalyze the digital transformation of healthcare. NPJ Digit Med 6:177
Download references
Author information
Authors and affiliations.
College of Physics and Electronic Information Engineering, Zhejiang Normal University, Jinhua, 321004, China
Mohammed A. A. Al-qaness & Jie Zhu
School of Computer Science, China University of Geosciences, Wuhan, 430074, China
Dalal AL-Alimi
Faculty of Computer Sciences and Mathematics, Ahmed Draia University, Adrar, 01000, Algeria
Abdelghani Dahou
Insight Centre for Data Analytics, University of Galway, Galway, Ireland
Saeed Hamood Alsamhi
Faculty of Engineering, IBB University, IBB, Yemen
Department of Mathematics, Faculty of Science, Zagazig University, Zagazig, 44519, Egypt
Mohamed Abd Elaziz
Department of Computer, Damietta University, Damietta, 34517, Egypt
Ahmed A. Ewees
Zhejiang Optoelectronics Research Institute, 321004, Jinhua, China
Mohammed A. A. Al-qaness
Faculty of Computer Science & Engineering, Galala University, Galala University, 435611, Suze, Egypt
Artificial Intelligence Research Center (AIRC), Ajman University, 346, Ajman, United Arab Emirates
Department of Electrical and Computer Engineering, Lebanese American University, 13-5053, Byblos , Lebanon
School of Computer Science and Technology, Zhejiang Normal University, 321004, Jinhua, China
MEU Research Unit, Middle East University, 11831, Amman, Jordan
You can also search for this author in PubMed Google Scholar
Corresponding author
Correspondence to Mohammed A. A. Al-qaness .
Ethics declarations
Conflict of interest.
All authors declare that they have no conflict of interest.
Ethical Approval
This article does not contain any studies with human participants or animals performed by the authors.
Informed Consent
This article does not contain any studies with human participants or animals performed by any of the authors.
Additional information
Publisher's note.
Springer Nature remains neutral with regard to jurisdictional claims in published maps and institutional affiliations.
Rights and permissions
Springer Nature or its licensor (e.g. a society or other partner) holds exclusive rights to this article under a publishing agreement with the author(s) or other rightsholder(s); author self-archiving of the accepted manuscript version of this article is solely governed by the terms of such publishing agreement and applicable law.
Reprints and permissions
About this article
Al-qaness, M.A.A., Zhu, J., AL-Alimi, D. et al. Chest X-ray Images for Lung Disease Detection Using Deep Learning Techniques: A Comprehensive Survey. Arch Computat Methods Eng 31 , 3267–3301 (2024). https://doi.org/10.1007/s11831-024-10081-y
Download citation
Received : 20 October 2023
Accepted : 17 January 2024
Published : 19 February 2024
Issue Date : August 2024
DOI : https://doi.org/10.1007/s11831-024-10081-y
Share this article
Anyone you share the following link with will be able to read this content:
Sorry, a shareable link is not currently available for this article.
Provided by the Springer Nature SharedIt content-sharing initiative
- Find a journal
- Publish with us
- Track your research
An official website of the United States government
Official websites use .gov A .gov website belongs to an official government organization in the United States.
Secure .gov websites use HTTPS A lock ( Lock Locked padlock icon ) or https:// means you've safely connected to the .gov website. Share sensitive information only on official, secure websites.
- Publications
- Account settings
- Advanced Search
- Journal List

Efficient Pneumonia Detection in Chest Xray Images Using Deep Transfer Learning
Mohammad farukh hashmi, satyarth katiyar, avinash g keskar, neeraj dhanraj bokde, zong woo geem.
- Author information
- Article notes
- Copyright and License information
Correspondence: [email protected] ; Tel.: +82-31-750-5586
These authors contributed equally to this work.
Received 2020 May 17; Accepted 2020 Jun 16; Collection date 2020 Jun.
Licensee MDPI, Basel, Switzerland. This article is an open access article distributed under the terms and conditions of the Creative Commons Attribution (CC BY) license ( http://creativecommons.org/licenses/by/4.0/ ).
Pneumonia causes the death of around 700,000 children every year and affects 7% of the global population. Chest X-rays are primarily used for the diagnosis of this disease. However, even for a trained radiologist, it is a challenging task to examine chest X-rays. There is a need to improve the diagnosis accuracy. In this work, an efficient model for the detection of pneumonia trained on digital chest X-ray images is proposed, which could aid the radiologists in their decision making process. A novel approach based on a weighted classifier is introduced, which combines the weighted predictions from the state-of-the-art deep learning models such as ResNet18, Xception, InceptionV3, DenseNet121, and MobileNetV3 in an optimal way. This approach is a supervised learning approach in which the network predicts the result based on the quality of the dataset used. Transfer learning is used to fine-tune the deep learning models to obtain higher training and validation accuracy. Partial data augmentation techniques are employed to increase the training dataset in a balanced way. The proposed weighted classifier is able to outperform all the individual models. Finally, the model is evaluated, not only in terms of test accuracy, but also in the AUC score. The final proposed weighted classifier model is able to achieve a test accuracy of 98.43% and an AUC score of 99.76 on the unseen data from the Guangzhou Women and Children’s Medical Center pneumonia dataset. Hence, the proposed model can be used for a quick diagnosis of pneumonia and can aid the radiologists in the diagnosis process.
Keywords: pneumonia, chest X-ray images, convolution neural network (CNN), deep learning, transfer learning, computer-aided diagnostics
1. Introduction
Pneumonia is an acute respiratory infection that affects the lungs. It is a fatal illness in which the air sacs get filled with pus and other liquid [ 1 ]. There are mainly two types of pneumonia: bacterial and viral. Generally, it is observed that bacterial pneumonia causes more acute symptoms. The most significant difference between bacterial and viral pneumonia is the treatment. Treatment of bacterial pneumonia is done using antibiotic therapy, while viral pneumonia will usually get better on its own [ 2 ]. It is a prevalent disease all across the globe. Its principal cause includes a high level of pollution. Pneumonia is ranked eight in the list of the top 10 causes of death in the United States [ 3 ]. Due to pneumonia, every year, 3.7 lakh children die in India, which constitutes a total of fifty percent of the pneumonia deaths that occur in India [ 4 ]. The disease frequently goes overlooked and untreated until it has reached a fatal point, especially in the case of old patients. It is the single largest cause of death in children (especially under the age of five) worldwide [ 5 ]. According to the WHO, “Every year, it kills an estimated 1.4 million children under the age of five years, accounting for 18% of all deaths of children under five years old worldwide. Pneumonia affects children and families everywhere but is most prevalent in South Asia and sub-Saharan Africa. Children can be protected from pneumonia. It can be prevented with simple interventions and treated with low-cost, low-tech medication and care” [ 2 ]. Therefore, there is an urgent need to do research and development on computer-aided diagnosis so that the pneumonia-related mortality, especially in children, can be reduced.
One of the following tests can be done for pneumonia diagnosis: chest X-rays, CT of the lungs, ultrasound of the chest, needle biopsy of the lung, and MRI of the chest [ 6 ]. Currently, chest X-rays are one of the best methods for the detection of pneumonia [ 7 ]. X-ray imaging is preferred over CT imaging because CT imaging typically takes considerably more time than X-ray imaging, and sufficient high-quality CT scanners may not be available in many underdeveloped regions. In contrast, X-rays are the most common and widely available diagnostic imaging technique, playing a crucial role in clinical care and epidemiological studies [ 8 , 9 ]. There are several regions across the globe where there is a scarce availability of practiced healthcare workers and radiologists whose prediction on such diseases matter greatly [ 10 , 11 , 12 ]. Computer-aided diagnosis using artificial intelligence based solutions is becoming increasingly popular these days [ 13 , 14 ]. This facility can be made available to a large population at a minimal cost. Another issue with this disease is that sometimes, the features that describe the very existence of the disease often get mixed with other diseases, and hence, radiologists find it challenging to diagnose this disease. Deep learning techniques solve all these problems, and their accuracy in the prediction of the disease is the same and sometimes even greater than an average radiologist [ 15 ]. Among the deep learning techniques, convolutional neural networks (CNNs) have shown great promise in image classification and segmentation and therefore are widely adopted by the research community. Biomedical image diagnosis that uses the techniques of deep learning and computer vision has proven to be very helpful to provide a quick and accurate diagnosis of the disease that matches the accuracy of a reliable radiologist [ 16 ]. Currently, deep learning based methods cannot replace trained clinicians in medical diagnosis, and they aim to supplement clinical decision making. In this paper, a model is presented based on the applications of deep learning and convolutional neural networks that are capable of classifying automatically that the patient has pneumonia or not. The proposed methodology uses a deep transfer learning algorithm that extracts the features from the X-ray image that describes the presence of disease automatically and reports whether it is a case of pneumonia.
2. Related Work
Deep learning based methods are already being used in various fields [ 17 , 18 , 19 , 20 , 21 ]. Different authors have already proposed several biomedical image detection techniques. M.I.Razaak [ 22 ] discussed the challenges and the future of medical image processing. Much work has already been done for the detection of numerous diseases by using deep learning based techniques, as stated by Dinggang Shen [ 23 ]. Andre [ 24 ] presented a deep learning model for dermatologist-level classification of skin cancer, and F.Milletari [ 25 ] also proposed a methodology for the depiction of prostrate in MRI volumes using CNN. Grewal [ 26 ] used the technique of deep learning for brain hemorrhage detection in CT scans, and Varun [ 27 ] proposed a method for detecting diabetic retinopathy in retinal fundus photographs. Y. Bar [ 28 ] also discussed chest pathology detection by the techniques based on deep learning. Methods regarding the examination of the detection of disease by chest X-ray have also been worked on earlier by performing various examination techniques [ 29 , 30 , 31 ]. The chest X-ray images are passed through the evaluation process of scan line optimization such that it eliminates all the other body parts to avoid any error in diagnosis. The algorithm was described by S. Hermann [ 32 ]. Nasrullah et al. [ 33 ] used two deep three-dimensional (3D) customized mixed link network (CMixNet) architectures for lung nodule detection and classification. Yao L et al. [ 34 ] combined DenseNet and long-short term memory networks (LSTM) to exploit the dependencies between abnormalities. Several authors also have worked on pneumonia classification. Khatri et al. [ 35 ] proposed the use of EMD (earth mover’s distance) to identify infected pneumonia lungs from normal non-infected lungs. Rahib et al. [ 36 ] and Okeke et al. [ 37 ] used a CNN model for pneumonia classification. Some researchers have shown assuring results such as Cohen et al. [ 38 ] and Rajaraman et al. [ 39 ]. Rajaraman et al. [ 39 ] tried to explain the performance of customized CNNs to detect pneumonia and further differentiate between bacterial and viral types in pediatric CXRs. Sirazitdinov et al. [ 40 ] used a region based convolutional neural network for segmenting the pulmonary images along with image augmentation for pneumonia identification. Lakhani and Sundaram [ 41 ] used the AlexNet and GoogLeNet neural networks with data augmentation and without any pre-training to obtain an area under the curve (AUC) of 0.94–0.95. Rajpurkar et al. [ 42 ] used CheXNeXt, a very deep CNN with 121 layers, to detect 14 different pathologies, including pneumonia, in frontal-view chest X-rays. A localization approach based on pre-trained DenseNet-121, along with feature extraction, was used to identify 14 thoracic diseases in [ 43 ]. Saraiva et al. [ 44 ], Ayan et al. [ 45 ], and Rahman et al. [ 46 ] used deep learning based methods for pneumonia classification. Xiao et al. [ 47 ] proposed a novel multi-scale heterogeneous three-dimensional (3D) convolutional neural network (MSH-CNN) based on chest computed tomography (CT) images. Xu et al. [ 48 ] used a hierarchical convolutional neural network (CNN) structure and a novel loss function, sin-loss, for pneumonia detection. Jaiswal et al. [ 49 ] used Mask-RCNN, utilizing both global and local features for pulmonary image segmentation, with dropout and L2 regularization, for pneumonia identification. Jung et al. [ 50 ] used a 3D deep CNN (3D DCNN), which had shortcut connections. Vikash et al. [ 51 ] combined the outputs of different neural networks and reached the final prediction using majority voting. None of the above-mentioned approaches except that of Vikash et al. [ 51 ] tried to combine predictions from different neural networks.
The main contribution is a weighted classifier that integrates five deep learning models. The weights for each model are based on each model’s performance on the testing dataset.
This paper is structured as follows: Section 3 deals with the methods used. A brief description of the methods used in this paper is given. The experimental dataset is introduced in Section 4 . In Section 5 , and the proposed methodology is discussed. In Section 6 , the results obtained are discussed concerning different parameters. This section is followed by Section 7 , containing the discussion, and Section 8 , containing the conclusion of the paper.
3. Background of Deep Learning Methods
3.1. convolutional neural network.
LeCun et al. [ 52 ] first used CNN, in 1989, for handwritten zip code recognition. This is a type of feed-forward network. The main advantage of CNN compared to its predecessors is that it is capable of detecting the relevant features without any human supervision.
A series of convolution and pooling operations is performed on the input image, which is followed by a single or multiple fully connected layers, as shown in Figure 1 . The output layer depends on the operations being performed. For multiclass classification, the output layer is a softmax layer. The main disadvantage with deeper CNNs is vanishing gradients, which can be solved by using residual networks introduced in the following section.
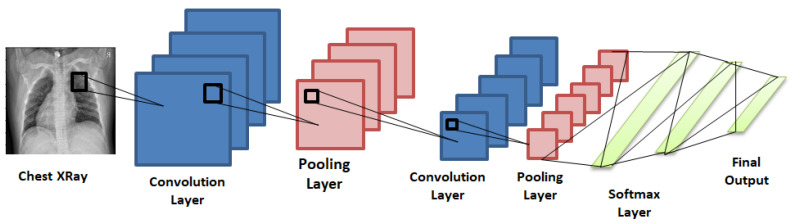
Convolutional neural network consisting of convolution and pooling layers and fully connected Softmax layers at the end to give the final prediction.
3.2. Transfer Learning
In transfer learning, a model that is trained for a particular task is employed as the starting point for solving another task. Therefore, in transfer learning, pre-trained models are used as the starting point for some specific tasks, instead of going through the long process of training with randomly initialized weights. Hence, it helps with saving the substantial computer resources needed to develop neural network models to solve these problems.
Pan and Yang [ 53 ] used domain, task, and marginal probabilities to propose a framework for better understanding the transfer learning. The domain D was defined as a two-element tuple consisting of the feature space, χ , with a marginal probability, P ( X ) , where X is a sample data point. Hence, mathematically, domain D can be defined as,
(1) |
Here, χ is the space of all term vectors, x i is the i th term vector corresponding to some documents, and X is a particular learning sample ( X = x 1 , ⋯ , x n , ∈ χ ) .
For a given domain D , the given task T is defined as:
(2) |
where γ is the label space. η is a predictive function learned from the feature vector/label pairs ( x i , y i ), where x i ∈ χ and y i ∈ γ .
(3) |
Here, η predicts a label for each feature vector.
Due to the lack of a sufficient dataset, training a deep learning based model for medical diagnosis related problems is computationally expensive, and the results achieved are also not up to the mark. Hence, pre-trained deep learning models, which were previously trained on ImageNet [ 54 ] dataset, were used in this paper. Further, all these pre-trained models were fine-tuned for pneumonia classification. All the layers of the architectures used were trainable. Further details, related to fine-tuning, are discussed in Section 5.2 .
3.3. Pre-Trained Neural Networks
Five state-of-the-art deep learning networks, ResNet18, DenseNet121, InceptionV3, Xception, and MobileNetV2, were used in this study. They are briefly discussed in Appendix A at the end of the paper.
3.4. Performance Metrics for Classification
All the models were tested on the test dataset after the completion of the training phase. Their performance was validated using the accuracy, recall, precision, F1, and area under the curve (AUC) score. All the performance metrics used in this paper are discussed below.
In the below-mentioned definitions and equations, while classifying healthy and pneumonia patients, true positive (TP) denotes the number of pneumonia images identified as pneumonia, true negative (TN) denotes the number of normal images identified as normal (healthy), false positive (FP) denotes the number of normal images incorrectly identified as pneumonia images, and false negative (FN) denotes the number of pneumonia images incorrectly identified as normal.
(4) |
(5) |
(6) |
(7) |
AUC Score and ROC Curve: ROC (receiver operating characteristics) is a probability curve, and AUC (area under curve) represents the degree of separability. The ROC curve is the plot of sensitivity (true positive rate) against specificity (false positive rate).
4. Materials
Experimental dataset.
The dataset [ 55 ] comprised a total of 5836 images ( Table 1 ) segmented into two main parts, a training set and a test set. Both bacterial and viral pneumonia were considered as a single category, pneumonia infected. The dataset used in this study did not include any case of viral and bacterial co-infection. All chest X-ray images were taken during the routine clinical care of the patients. Two expert physicians then graded the diagnoses for the images before being cleared for training the AI system. The evaluation set was also checked by a third expert to account for any grading errors. The proportion of data assigned to training and testing was highly imbalanced. Therefore, the dataset was shuffled and arranged into training and test sets only. Finally, there were 5136 images in the training set and 700 images in the test set. Eleven-point-nine-five percent of the complete dataset was used as the testing dataset. Figure 2 shows two chest X-ray images, one of a healthy person and the other of a person suffering from pneumonia.
Description of the experimental dataset.
Category | Training Set | Test Set |
---|---|---|
Normal (Healthy) | 1283 | 300 |
Pneumonia (Viral + Bacteria) | 3873 | 400 |
Total | 5156 | 700 |
Percentage | 88.05% | 11.95% |
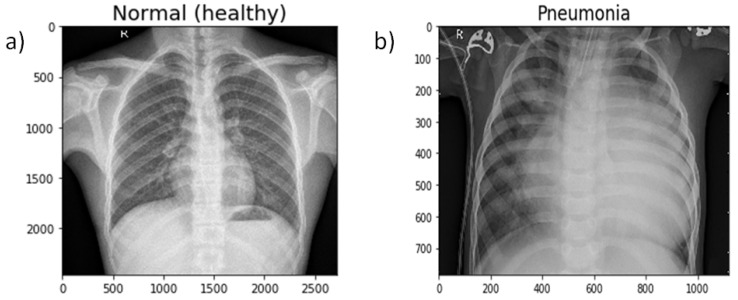
Chest Xray of ( a ) a healthy person and ( b ) a person suffering from pneumonia.
5. Proposed Methodology
An optimum solution for the detection of pneumonia from chest X-rays is proposed in this paper. Data augmentation was used to address the problem of the limited dataset, and then, state-of-the-art deep learning models, as discussed in Section 3 , were fine-tuned for pneumonia classification. Then, predictions from these models were combined, using a weighted classifier (discussed afterward in this section), to compute the final prediction. The complete block diagram of the proposed methodology can be seen in Figure 3 .
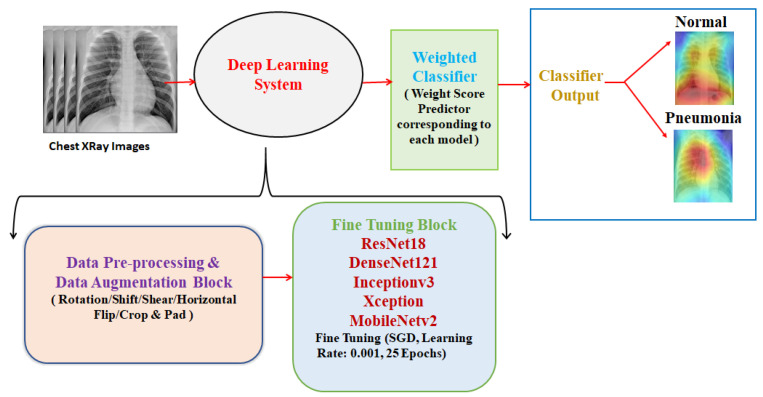
Block diagram of the proposed methodology (deep learning system consists of the data pre-processing and data augmentation block and the fine-tuning block; the weighted classifier gives the final prediction).
5.1. Data Preprocessing and Augmentation
Each image had to be preprocessed according to the deep neural network used. There were two important steps involved: resizing and normalization. Different neural networks require images of different sizes according to their defined architecture. ResNet18, DenseNet121, and MobileNetV2 expect images of size 224 × 224, while InceptionV3 and Xception require images of size 229 × 229. All the images were also normalized according to the respective architectures.
Adequate training of a neural net requires big data. With less data availability, parameters are undermined, and learned networks generalize poorly. Data augmentation solves this problem by utilizing existing data more efficiently. It aids in increasing the size of the existing training dataset and helps the model not to overfit this dataset. In this case, there were a total of 1283 images of the normal (healthy) case and 3873 images of the pneumonia case in the training dataset. Out of these, four-hundred images were reserved for optimizing the weighted classifier. This dataset was highly imbalanced. There were already enough images in the pneumonia case. Therefore, each image of only the normal (healthy) case was augmented twice. Finally, after augmentation, there were 3399 healthy chest X-ray images and 3623 pneumonia chest X-ray images.
The settings utilized in image augmentation are shown below in Table 2 . The images after performing various augmentation techniques are shown below ( Figure 4 ). Only one of these techniques was used to generate the augmented image.
Augmentation techniques used in the proposed methodology.
Technique | Setting |
---|---|
Rotation | 45 |
Vertical Shift | 0.2 |
Horizontal Shift | 0.15 |
Shear | 16 |
Crop and Pad | 0.25 |
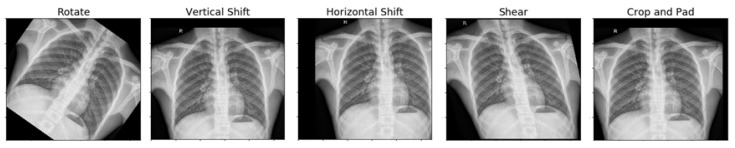
Resultant image after performing the augmentation technique.
5.2. Fine-Tuning the Architectures
All the architecture details used in this paper are discussed in Appendix A . Raw chest X-ray images, after being pre-processed and normalized, were used to train the network. Then, data augmentation techniques were used to process the dataset more efficiently. All the layers of the networks used were trainable, and these layers extracted the features from the images. Some parameters must be set to train the network. An interesting paper from UC Berkeley [ 56 ] came out, and according to it, stochastic gradient descent (SGD) had better generalization than adaptive optimizers. Therefore, SGD as the optimizer was used, and the model was trained for 25 epochs. The learning rate, the momentum, and the weight decay were set to 0.001, 0.9, and 0.0001, respectively ( Table 3 ). These configurations were to make sure that the networks were fine-tuned for pneumonia diagnosis.
Hyper-parameters used while fine-tuning the deep learning models.
Architecture | Image Size | Epochs | Optimizer | Learning Rate | Momentum | Weight Decay |
---|---|---|---|---|---|---|
ResNet18 | 224 × 224 | |||||
DenseNet121 | 224 × 224 | |||||
InceptionV3 | 229 × 229 | 25 | Stochastic Gradient Descent | 0.001 | 0.9 | 0.0001 |
Xception | 229 × 229 | |||||
MobileNetV2 | 224 × 224 |
5.3. Weighted Classifier
In this module of the proposed methodology, a weight ( W k ) corresponding to each model was estimated. W k can be defined as the belief in the k th model, with k being equal to 5 as 5 pre-trained models were used in this paper. W k has values between 0 and 1, and the sum of all weights is 1 (Equation ( 9 )). Each model, after it was fine-tuned, returned the probabilities for each class label, i.e., 2 classes in the form of a matrix ( P k ) . A weighted sum of all these predictions arrays was calculated (Equation ( 8 )).
(8) |
(9) |
(10) |
P k is the prediction matrix, with shape: number of optimization images * class labels (400*2), corresponding to each architecture. In Equation ( 8 ), the contribution of each model is weighted by a coefficient ( W k ) , which indicates the trust in the model. First, we obtained the P k for every model for an unseen image set (400 images). Then, Equation ( 8 ) was optimized such that the classification error was minimized and Equation ( 9 ) was also satisfied. We used differential evolution [ 57 ] for global optimization of Equation ( 8 ). Differential evolution is a stochastic global search algorithm. It optimized Equation ( 8 ) by iteratively refining a candidate solution with regard to Equation ( 9 ). Hence, optimizing Equation ( 8 ) would provide the W k values corresponding to each model. The value of W k for the k th model depended on the respective models’ performance on the test dataset. The maximum iterations for differential evolution algorithms were kept to be 1000. With the help of P f , the prediction of a class label could be computed. Classification loss corresponding to this P f was reduced while optimizing Equation ( 8 ). Log loss (Equation ( 10 ), also known as logistic loss or cross-entropy loss, was used as the loss function. In Equation ( 10 ), N denotes the size of the image set (400) and p denotes the probability that the given image is pneumonia infected. Figure 5 shows the process followed to find the optimal weight corresponding to each model. Figure 6 shows the weighted classifier used in the proposed methodology.
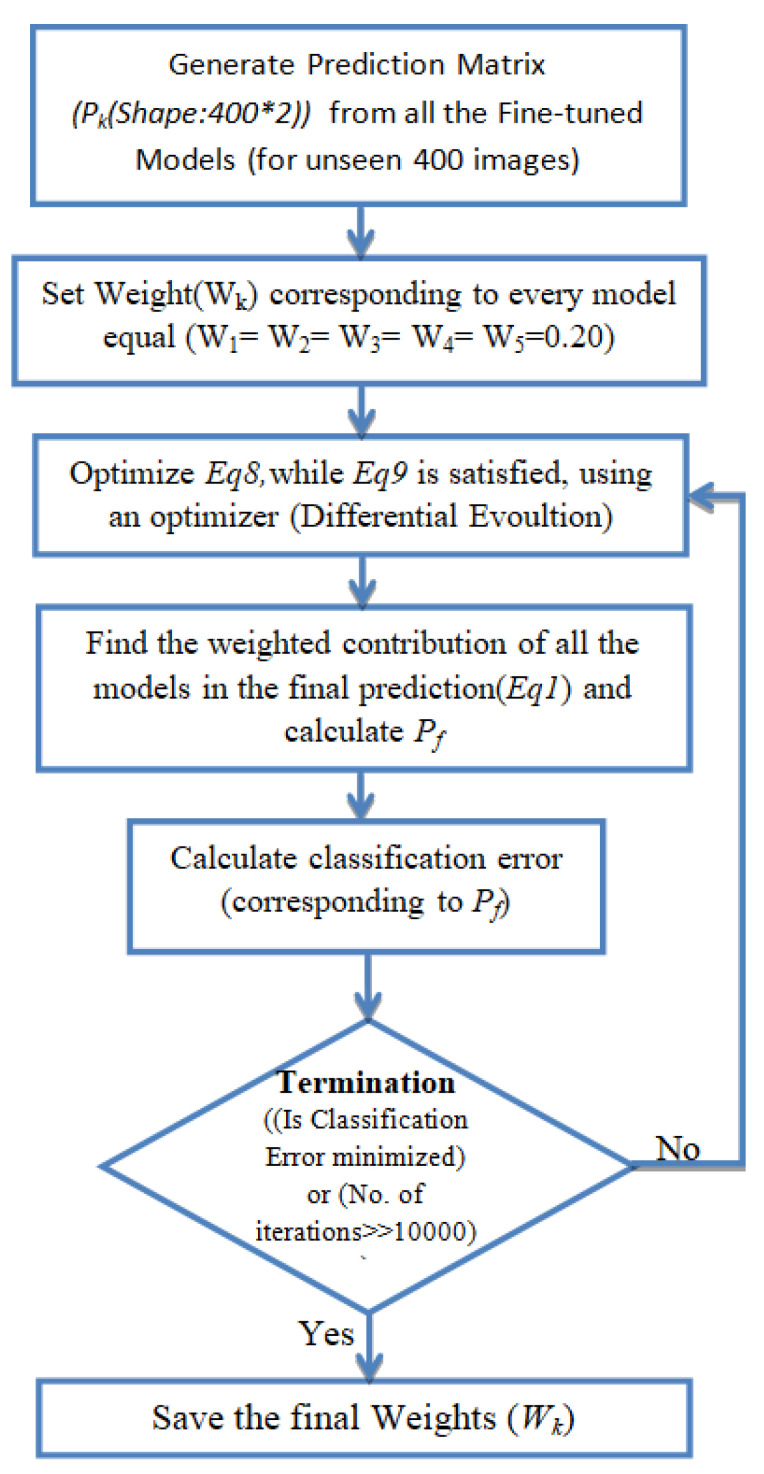
Procedure followed while calculating the optimal weight corresponding to every model.
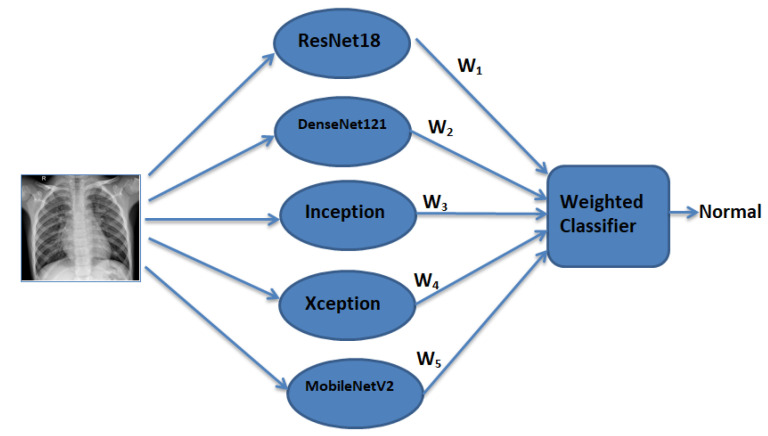
Weighted classifier module used in this paper (weighted predictions from all the models are passed to the weighted classifier, which gives the final weighted prediction).
5.4. Class Activation Maps
Class activation maps (CAMs) [ 58 ] can help in demystifying the results of deep learning models. Traditionally, deep learning based methods are considered to be a black-box approach. For clinical decision making, it is necessary that the results of the deep learning model can be interpreted. CAMs can help in identifying the parts of the image on which the model was focusing while making the final prediction and hence can provide insights into the working of the model. Such an analysis can further help in hyperparameter tuning and gain understanding of the reason behind the failure of the model. For obtaining the class activation map, the network needed to be trained with the global average pooling (GAP) layer. After the GAP layer, a fully connected network was maintained, which was followed by the softmax layer, providing the class, such as pneumonia, as shown in Figure 7 . CAMS class activation maps were generated for both bacterial and viral pneumonia for all the fine-tuned model and are discussed in detail in the results section.
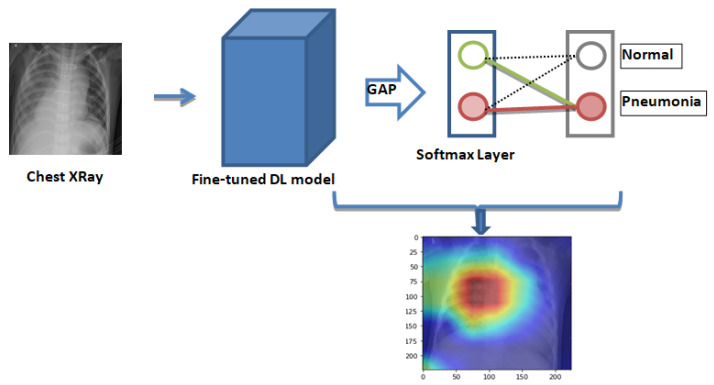
Generation of the class activation map for fine-tuned deep learning models. The layers of deep learning models are followed by the global average pooling layer (GAP) (⇒) and the softmax layer to give the final prediction. Features that are used for pneumonia detection get highlighted in the class activation map.
6. Experimental Results
In this section, the experiments and evaluation techniques used in the paper to test the efficiency of the proposed model are presented. The chest X-ray image dataset, proposed in [ 55 ], was used. The Keras open-source deep learning framework with TensorFlow as the backend was used, first to load the pre-trained architectures on the ImageNet Dataset [ 54 ] and then fine-tune them for the task at hand. All the computation work was done on a Standard PC with8 GB RAM, NVIDIA GeForce GTX 1060 6 GB GPU, and Intel i7, seventh-generation processor.
6.1. Result in Terms of Testing Accuracy and Testing Loss
To test and evaluate the performance of the proposed network, each experiment was conducted five times. Parameters and hyperparameters were tuned during the training. Figure 8 shows the training accuracy and training loss curves obtained while training the models for 25 epochs. The training accuracy for all the models exceeded 99%, and the training loss for all the models was below 0.03. Except for Xception, all the other models had similar training accuracy and training loss curves. Table 4 summarizes the testing accuracy and testing loss for different networks and the final weighted classifier. DenseNet121 was able to attain the maximum testing accuracy and the minimum testing loss. Initially, all the weights of the weighted classifier were kept equal ( W 1 = W 2 ⋯ W 5 = 0.20 ) . Hence, every model contributed equally towards the final prediction. A test accuracy of 97.45 and a loss of 0.087 was obtained. Then, the optimum weights were estimated for every model. The value of these estimated weights is shown in Table 5 . With these weights, the final weighted classifier was able to achieve a testing accuracy of 98.43, and the testing loss was 0.062.
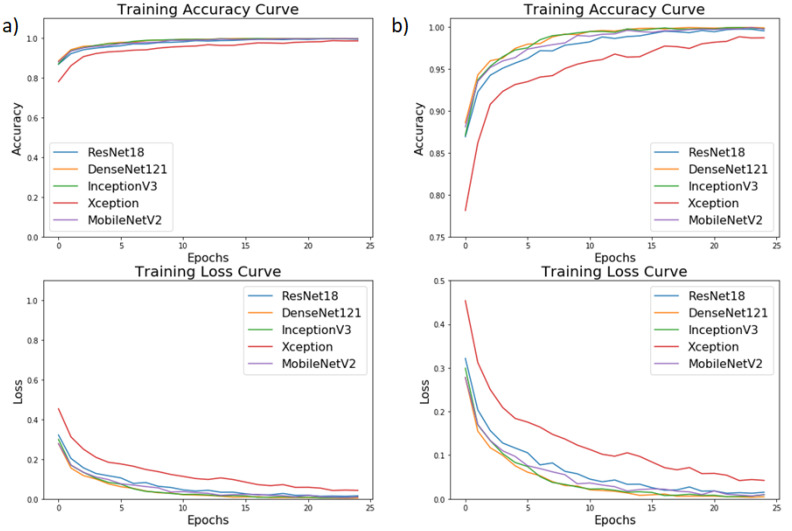
( a ) Training accuracy and training loss curves for different architectures over the training dataset while the models were trained for 25 epochs, ( b ) Zoom-in version of ( a ).
Final testing accuracy and testing loss achieved by all the architectures and the weighted classifier.
Architecture | Testing Accuracy | Testing Loss |
---|---|---|
ResNet18 | 97.29 | 0.096 |
DenseNet121 | 98.00 | 0.064 |
Inception | 97.00 | 0.098 |
Xception | 96.57 | 0.101 |
MobileNetV2 | 96.71 | 0.096 |
Weighted Classifier (With Equal Weights) | 97.45 | 0.087 |
Weighted Classifier (With Optimized Weights) | 98.43 | 0.062 |
Weight value (belief or trust value) corresponding to every architecture.
Architecture | Weight |
---|---|
ResNet18 (W1) | 0.25 |
DenseNet121 (W2) | 0.30 |
Inception (W3) | 0.18 |
Xception (W4) | 0.08 |
MobileNetV2 (W5) | 0.19 |
In Table 4 , it can be seen that when equal weights were assigned to every model, the testing accuracy of the weighted classifier was less than that of DenseNet121. This could be attributed to the fact that even the models with less testing accuracy were assigned the same weights as that to the models with higher testing accuracy. Finally, when optimum weights were calculated, the testing accuracy of the weighted classifier showed an improvement of 0.98%. Table 5 shows that the weight assigned to every model depended on its performance on the test dataset. Hence, it could be said that the weight assigned to a model represented the belief or trust in that model. The maximum weight was assigned to DenseNet121, which was followed by ResNet18.
All the test images were pre-processed similarly as the training images and hence had the same size as required by the respective architecture. The test images were of size 224 × 224 for ResNet18, DenseNet121, and MobileNetV2, while for InceptionV3 and Xception, they were of size 229 × 229. The testing was also done on the same system on which training was done. The average inference time for all the models was 0.045 s (while the GPU was used), and for the weighted classifier, it was 0.203 s.
6.2. Performance Analysis
To further test the robustness of the proposed methodology, the accuracy, recall, precision score, F1 score, and AUC score for all the models and the proposed weighted classifier were calculated. To calculate the mentioned scores, confusion matrices for all the architectures were obtained ( Figure 9 ). With the help of the confusion matrix, the number of true positives, true negatives, false positives, and false negatives could be calculated, which further helped in checking the efficacy of the model.
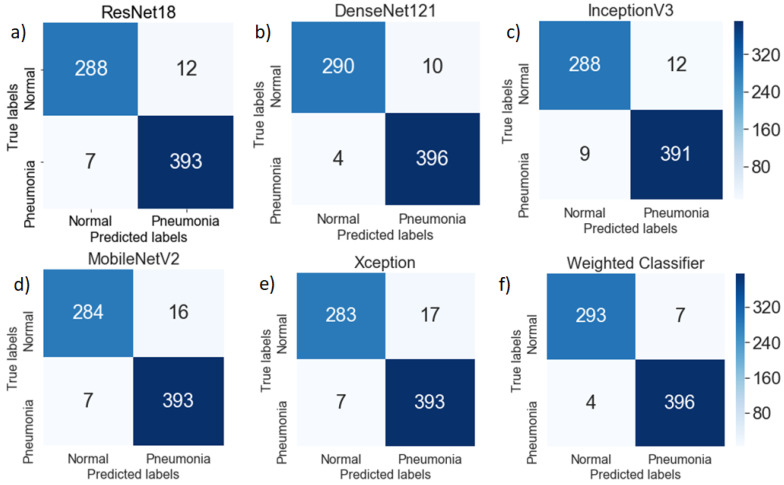
Confusion matrix for ( a ) ResNet18, ( b ) DenseNet121, ( c ) InceptionV3, ( d ) MobileNetV2, ( e ) Xception, and ( f ) Weighted Classifier architectures and the weighted classifier over the testing dataset. False positives were greater than the false negatives for all the models.
As the recall was increased, the precision decreased, and vice versa. In medical applications, all the patients who had the disease needed to be identified, and hence, the recall could be maximized. A low recall could be accepted if the cost of a follow-up medical examination was not high. Hence, the F1 score could be used to find the optimal blend of precision and recall.
In the plotted confusion matrices ( Figure 9 ), it can be seen that the proposed weighted classifier outperformed all the individual models. The generic image features, learned by the deep learning models from ImageNet, served as a good initialization of the weights. The misclassification error for normal (healthy) images as pneumonia images was greater compared to pneumonia images as healthy images. This might be because the number of chest X-ray images of the normal (healthy) case was significantly lower compared to the pneumonia cases.
Figure 10 shows the ROC curves for different architectures and the proposed classifier. The maximum AUC score (99.76) was achieved by the proposed classifier. All the models had a similar AUC/ROC curve. All the results are tabulated in Table 6 . After analyzing the results, it can be said the weighted classifier gave the best results with an AUC score of 99.75, F1 score of 98.63, and test accuracy of 98.43. Hence, the proposed weighted classifier was able to combine the predictions from all the individual architectures in an optimum manner. The differences in the performance of other models were not significant. This might be because all the models used in this paper were deep learning based and were fine tuned on the same insufficient dataset.
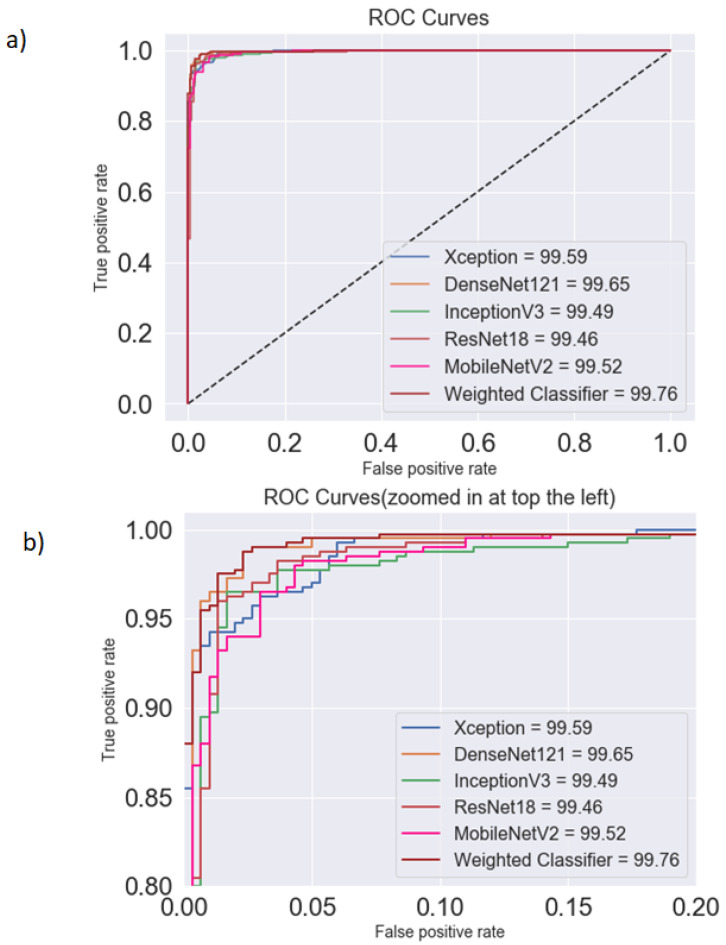
Comparison between ( a ) the AUC (area under the curve) and ( b ) ROC (receiver operating characteristics) curves for different architectures and the weighted classifier for the testing dataset. The weighted classifier (brown line), followed by DenseNet121 (yellow line), had the highest AUC.
Accuracy, precision, recall, F1 score, and AUC score corresponding to different architectures.
Architecture | Accuracy | Precision | Recall | F1 Score | AUC Score |
---|---|---|---|---|---|
ResNet18 | 97.29 | 97.03 | 98.25 | 97.63 | 99.46 |
DenseNet121 | 98.00 | 97.53 | 99.00 | 98.26 | 99.65 |
InceptionV3 | 97.00 | 97.02 | 97.75 | 97.39 | 99.49 |
Xception | 96.57 | 95.85 | 98.25 | 97.03 | 99.59 |
MobileNetV2 | 96.71 | 96.08 | 98.25 | 97.15 | 99.52 |
Weighted Classifier | 98.43 | 98.26 | 99.00 | 98.63 | 99.76 |
6.3. Explanation of the Results Using Heat Maps
The activation maps were plotted for every individual network. These activation maps helped in localizing areas in the image most indicative of pneumonia. The activation maps were obtained for the last convolutional layer of each network. In the case of bacterial pneumonia ( Figure 11 ), all the networks detected the abnormal lung to predict the presence of pneumonia correctly. Viral pneumonia manifested with a more diffuse “interstitial” pattern in both lungs, which was detected by all the fine-tuned architectures [ 59 ] ( Figure 12 ).
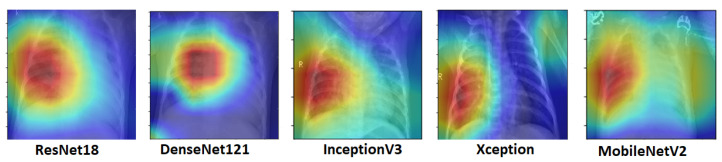
Activation maps for chest X-rays having bacterial pneumonia corresponding to different architectures. Abnormal lungs, in the case of bacterial pneumonia, were detected by the deep learning models.
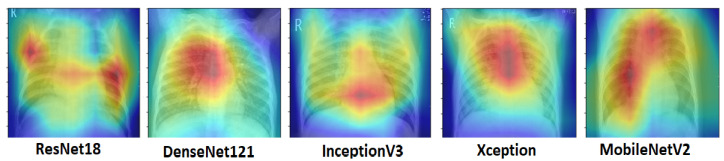
Activation maps for chest X-rays having viral pneumonia corresponding to different architectures. Viral pneumonia, with a more diffused “interstitial” pattern in both lungs, was detected by the deep learning models.
6.4. Comparative Analysis of Various Existing Methods
The accuracy of various existing methods and the proposed methodology were compared. All the results mentioned in this section are reported by the authors in their respective studies. Rahib H. Abiyey et al. [ 36 ] used CNN and achieved a validation accuracy of 92.4%. The test dataset used was smaller compared to this paper. Okeke Stephen et al. [ 37 ] achieved a validation accuracy of 93.73% with their own CNN model. No other metric was published in either of these works. Cohen et al. [ 38 ] used a model based on DenseNet-121. They reported an AUC score of 98.4%. Unfortunately, the other metrics were not reported in the paper. Rajaraman et al. [ 39 ] used customized CNNs to detect pneumonia and reported a test accuracy of 96.2%. M.Togacar et al. [ 60 ] combined features from different deep learning models for pneumonia classification and achieved an accuracy of 96.84%. Vikash et al. [ 51 ] combined the outputs of different neural networks and reached the final prediction using majority voting. They achieved an AUC score of 99.34. Saraiva et al. [ 44 ], Ayan et al. [ 45 ], and Rahman et al. [ 46 ] used deep learning based methods and achieved an accuracy of 94.4%, 84.5%, and 98.0%, respectively. In all of these papers, the dataset used was of a similar size. All the studies other than Rahib H.Abiyey et al. [ 36 ] used image augmentation techniques. All the above-discussed results are summarized in Table 7 .
Comparison of the proposed methodology with different existing methods.
Model | No. of Images | Precision | Recall | Accuracy | AUC |
---|---|---|---|---|---|
Rahib H.Abiyey et al. [ ] | 1000 | - | - | 92.4 | - |
Okeke Stephen et al. [ ] | 5856 | - | - | 93.73 | - |
Cohen et al. [ ] | 5232 | 90.1 | 93.2 | 92.8 | 99.0 |
Rajaraman et al. [ ] | 5856 | 97.0 | 99.5 | 96.2 | 99.0 |
M.Togacar et al. [ ] | 5849 | 96.88 | 96.83 | 96.84 | 96.80 |
Saraiva et al. [ ] | 5840 | 94.3 | 94.5 | 94.4 | 94.5 |
Ayan et al. [ ] | 5856 | 91.3 | 89.1 | 84.5 | 87.0 |
Rahman et al. [ ] | 5247 | 97.0 | 99.0 | 98.0 | 98.0 |
Vikash et al. [ ] | 5232 | 93.28 | 96.39 | 99.34 | |
Proposed Methodology | 99.00 |
The numbers are bold to show the best performance.
It can be seen in the above table that the proposed methodology surpassed the other approaches in terms of precision, recall, accuracy, and the AUC score. The weighted classifier showed the best accuracy of all the recent studies to the best of our knowledge.
7. Discussion
The high test accuracy (98.43) and AUC score (99.76) showed that the proposed method could be used as a supplement in clinical decision making. It can only aid the radiologists in the decision making process; the final decision has to be made by an expert. The proposed weighted classifier, with optimum weights, showed an improvement of 0.98%, in terms of the testing accuracy, over the case in which equal weights were assigned to every model. The false positives were greater than the false negatives, and hence, the classification error of pneumonia suffering patients as healthy was comparatively lesser, which is ideally required in medical diagnosis. Further, the activation maps plotted in this paper showed that the deep learning based models used were able to identify pneumonia affected regions in the chest X-rays. When compared to DenseNet121, the proposed weighted classifier showed an improvement of 0.43% in terms of testing accuracy, which in the real world on a large test dataset would be a significant number.
One of the limitations of this approach was the scarcity of available data. Usually, deep learning models are trained over thousands of images. Training deep neural networks with limited data might lead to overfitting and restricts the models’ generalization ability. Unlike large datasets like ImageNet, the variability in the chest X-ray data was several orders of magnitude smaller. The performance of the proposed methodology would only increase with the availability of more data.
Another limitation was that the results of the deep learning models could not be properly explained. A deep understanding of the radiological features visible in chest X-rays is required for the diagnosis of the disease from the X-rays. The proper explanation of the final prediction of the model is also required, and this is one of the drawbacks of the deep learning based models. To this end, the activation maps were plotted, but further work is required. In the future, with better annotated datasets available, deep learning based methods might be able to solve this problem.
8. Conclusions and Future Scope
Pneumonia constitutes a significant cause of morbidity and mortality. It accounts for a considerable number of adult hospital admissions, and a significant number of those patients ultimately die (with a mortality rate of 24.8% for patients over 75 years) [ 61 ]. According to the WHO, pneumonia can be prevented with a simple intervention and early diagnosis and treatment [ 4 ]. Nevertheless, the majority of the global population lacks access to radiology diagnostics [ 62 ]. Even when there is the availability of imaging equipment, there is a shortage of experts who can examine X-rays. Through this paper, the automatic detection of pneumonia in chest X-ray images using deep transfer learning techniques was proposed. The deep networks, which were used in our methodology, had more complex structures, but fewer parameters and, hence, required less computation power, but achieved higher accuracy. Transfer learning and data augmentation were used to solve the problem of overfitting, which is seen when there is insufficient training data, as in the case of medical image processing. Further, to combine different architectures efficiently, a weighted classifier was proposed. The experiments were performed, and the different scores obtained, such as the accuracy, recall, precision, and AUC score, proved the robustness of the model. The proposed model was able to achieve an accuracy of 98.857%, and further, a high F1 score of 99.002 and AUC score of 99.809 affirmed the efficacy of the proposed model. Though many methods have been developed to work on this dataset, the proposed methodology achieved better results. In the future, it would be interesting to see approaches in which the weights corresponding to different models can be estimated more efficiently and a model that takes into account the patient’s history while making predictions.
Abbreviations
The following abbreviations are used in this manuscript:
2D | 2-dimensional |
3D | 3-dimensional |
AI | Artificial intelligence |
AUC | Area under the curve |
BPNN | Back propagation neural network |
CNN | Convolutional neural network |
CpNN | Competitive neural network |
CT | Computed tomography |
DDR | Double data rate |
GPU | General processing unit |
DNN | Deep neural network |
PC | Personal computer |
SGD | Stochastic gradient descent |
UNICEF | United Nations Children’s Fund |
WHO | World Health Organization |
Appendix A. Pre-Trained Neural Networks Used in the Paper
Appendix a.1. resnet18.
With deeper neural networks, the degradation problem is exposed, and with network depth increasing, accuracy gets saturated and then degrades rapidly. Kaiming He et al. first proposed residual networks at the Microsoft Research Institute [ 63 ] to solve this problem. They explained the architecture of residual networks in their paper. Here, the incoming eigenvalues are processed by both the network parameter layers, and their original values are also taken into account as a part of the output obtaining the result. Bypass connections are an integral part of the architecture and can skip one or more layers. Here, bypass connections are used to implement identity mapping, and their outputs are summed with the outputs of the stacked layers. The complete network consists of five convolution blocks and can be easily trained using SGD with backpropagation. Lastly, Figure A1 shows the ResNet18 architecture used in the paper.
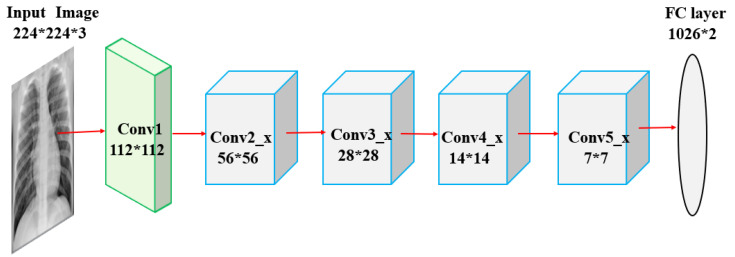
ResNet18 architecture comprised of a convolutional (112*112) layer at the beginning and five convolutional blocks.
Appendix A.2. DenseNet121
Studies have shown that the connections between layers close to the input and those close to the output help in increasing the performance of convolutional networks. This idea was implemented in residual networks [ 63 ] and also in dense convolutional networks (DenseNet) [ 64 ]. DenseNet requires lesser parameters compared to conventional convolutional networks as it does not require relearning excessive feature maps because of its dense connectivity pattern. The network is divided into dense blocks, with the dimensions of the feature maps remaining constant within a block and the number of filters changing between them. DenseNet has several advantages such as the number of parameters is significantly reduced, the features are reused, and the vanishing gradient is mitigated. Figure A2 depicts the pre-trained DenseNet architecture used in the paper.
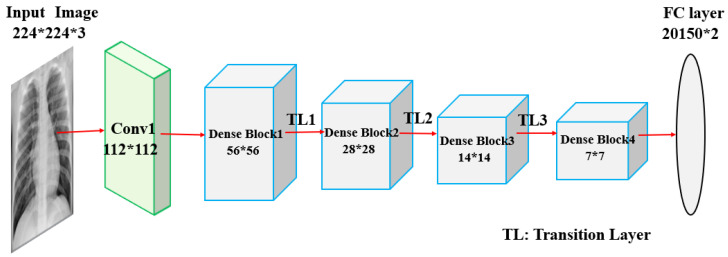
Densenet121 architecture with the convolutional (112*112) layer at the beginning and five dense blocks.
Appendix A.3. InceptionV3
The bigger the deep learning model is, the more prone it is to overfitting, and increasing the number of parameters also means the need to increase the computational resources. In the Inception model [ 65 ] These problems are addressed, which allows increasing the depth and width of the deep learning model and also keeping the computational cost constant. A sparsely connected network architecture is introduced in this model. One by one, 3*3, and 5*5 convolutions are computed, and an auxiliary classifier is used as a regularizer in the architecture. With only 42 layers, it is more efficient compared to VGGNet [ 66 ]. This model was trained on the ImageNet dataset. Figure A3 shows the pre-trained InceptionV3 model used in this paper.
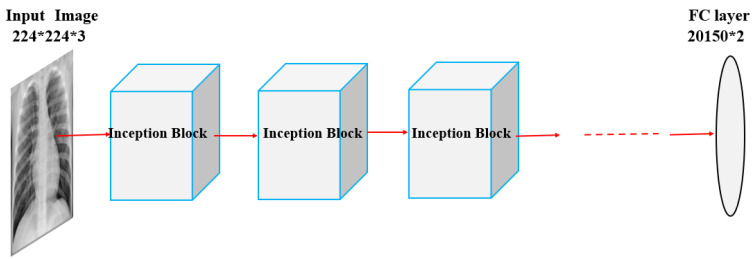
InceptionV3 architecture with Inception blocks comprising 1*1, 3*3, and 5*5 convolutions.
Appendix A.4. Xception
The Xception model was proposed by Francois Chollet [ 67 ]. Xception is an extension of the Inception architecture where the standard Inception modules are replaced by modified depthwise separable convolutions, which makes it better for both the ImageNet ILSVRCand JFTdatasets. The modified depthwise separable convolution is the pointwise convolution, which is followed by a depthwise convolution. There are also residual/skip connections as proposed in the ResNet architecture [ 63 ]. The Xception architecture can be divided into three blocks: entry flow, middle flow, and exit flow. The middle flow block is repeated eight times. There is no intermediate non-linearity in modified depthwise separable convolution in the architecture. The pre-trained model of Xception used in the paper is shown in Figure A4 .
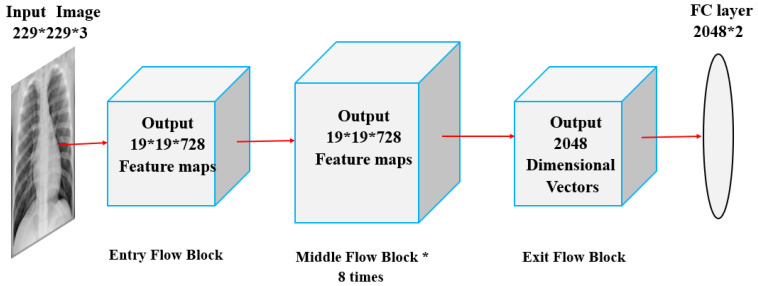
Xception architecture divided into three blocks: entry flow block, middle flow block, and exit flow block.
Appendix A.5. MobileNetV2
MobileNets are small and low power models developed by a group of researchers from Google [ 68 ]. MobileNetV2 is an upgrade to MobileNetV1 and uses depth-wise separable convolutions as efficient building blocks. There are two types of blocks in the architecture: one is a residual block with a stride of one, and the other one is a block with a stride of two for downsizing. Other than this, it has linear bottlenecks between the layers where the use of linear layers is essential, as it blocks nonlinearities from damaging too much information. These bottlenecks help the model in encoding the intermediate inputs and outputs. The inner layer helps in transforming lower-level concepts such as pixels to higher-level descriptors such as image categories. There are also shortcut connections between the bottlenecks. A pre-trained MobileNetV2 was used in this paper ( Figure A5 ). Transfer learning was used to optimize the model for pneumonia classification.
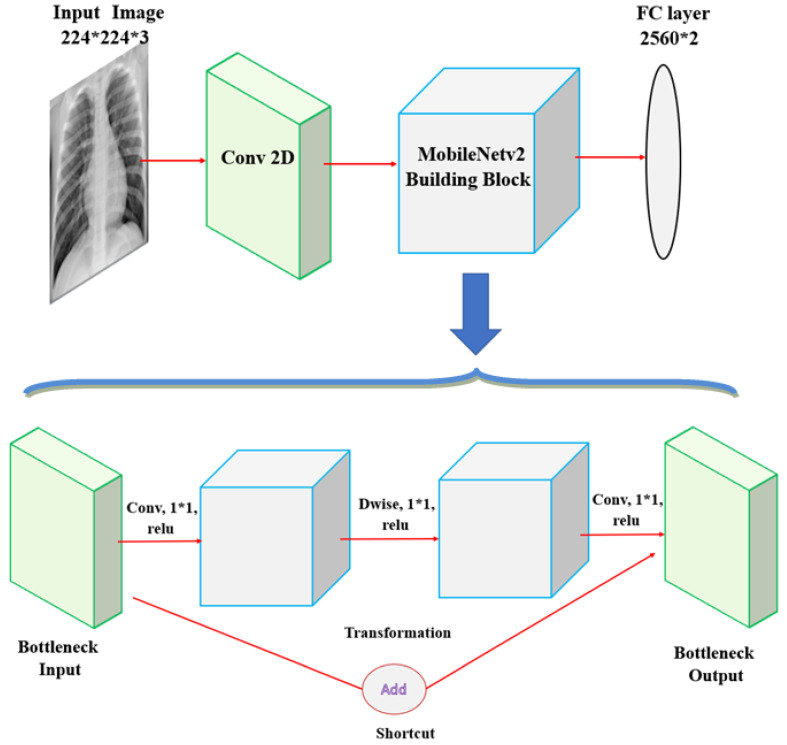
MobileNetV2 architecture with the MobileNetv2 building block comprising bottlenecks and depth-wise separable convolutions.
Author Contributions
Conceptualization, S.K. and M.F.H.; methodology, S.K. and M.F.H.; software, S.K., M.F.H., and N.D.B.; validation, S.K., M.F.H., A.G.K., and N.D.B.; formal analysis, S.K., M.F.H., and A.G.K.; investigation, S.K. and M.F.H.; resources, S.K., M.F.H., and A.G.K.; data curation, S.K. and M.F.H.; writing, original draft preparation, S.K., M.F.H., and N.D.B.; writing, review and editing, S.K., M.F.H., A.G.K., N.D.B., and Z.W.G.; visualization, M.F.H. and N.D.B.; supervision, A.G.K., N.D.B., M.F.H., and Z.W.G.; project administration, M.F.H., N.D.B., and Z.W.G.; funding acquisition, Z.W.G. and N.D.B. All authors read and agreed to the published version of the manuscript.
This work was supported by the National Research Foundation of Korea (NRF) grant funded by the Korea government (MSIT) (2020R1A2C1A01011131). This research was also supported by the Energy Cloud R&D Program through the National Research Foundation of Korea (NRF) funded by the Ministry of Science, ICT (2019M3F2A1073164).
Conflicts of Interest
The authors declare no conflict of interest.
- 1. Johns Hopkins Medicine Pneumonia. [(accessed on 31 December 2019)]; Available online: https://www.hopkinsmedicine.org/health/conditions-and-diseases/pneumonia .
- 2. Johnson S., Wells D., Healthline Viral Pneumonia: Symptoms, Risk Factors, and More. [(accessed on 31 December 2019)]; Available online: https://www.healthline.com/health/viral-pneumonia .
- 3. Healthcare, University of Utah. Pneumonia Makes List for Top 10 Causes of Death. [(accessed on 31 December 2019)];2016 Available online: https://healthcare.utah.edu/the-scope/shows.php?shows=0_riw4wti7 .
- 4. WHO Pneumonia is the Leading Cause of Death in Children. [(accessed on 31 December 2019)];2011 Available online: https://www.who.int/maternal_child_adolescent/news_events/news/2011/pneumonia/en .
- 5. Rudan I., Tomaskovic L., Boschi-Pinto C., Campbell H. Global estimate of the incidence of clinical pneumonia among children under five years of age. Bull. World Health Organ. 2004;82:895–903. [ PMC free article ] [ PubMed ] [ Google Scholar ]
- 6. Pneumonia. [(accessed on 31 December 2019)]; Available online: https://www.radiologyinfo.org/en/info.cfm?pg=pneumonia .
- 7. World Health Organization . Standardization of Interpretation of Chest Radiographs for the Diagnosis of Pneumonia in Children. World Health Organization; Geneva, Switzerland: 2001. Technical Report. [ Google Scholar ]
- 8. Cherian T., Mulholland E.K., Carlin J.B., Ostensen H., Amin R., Campo M.D., Greenberg D., Lagos R., Lucero M., Madhi S.A., et al. Standardized interpretation of paediatric chest radiographs for the diagnosis of pneumonia in epidemiological studies. Bull. World Health Organ. 2005;83:353–359. [ PMC free article ] [ PubMed ] [ Google Scholar ]
- 9. Franquet T. Imaging of pneumonia: Trends and algorithms. Eur. Respir. J. 2001;18:196–208. doi: 10.1183/09031936.01.00213501. [ DOI ] [ PubMed ] [ Google Scholar ]
- 10. Tahir A.M., Chowdhury M.E., Khandakar A., Al-Hamouz S., Abdalla M., Awadallah S., Reaz M.B.I., Al-Emadi N. A systematic approach to the design and characterization of a smart insole for detecting vertical ground reaction force (vGRF) in gait analysis. Sensors. 2020;20:957. doi: 10.3390/s20040957. [ DOI ] [ PMC free article ] [ PubMed ] [ Google Scholar ]
- 11. Chowdhury M.E., Alzoubi K., Khandakar A., Khallifa R., Abouhasera R., Koubaa S., Ahmed R., Hasan A. Wearable real-time heart attack detection and warning system to reduce road accidents. Sensors. 2019;19:2780. doi: 10.3390/s19122780. [ DOI ] [ PMC free article ] [ PubMed ] [ Google Scholar ]
- 12. Chowdhury M.E.H., Khandakar A., Alzoubi K., Mansoor S., Tahir A.M., Reaz M.B.I., Al-Emadi N. Real-time smart-digital stethoscope system for heart diseases monitoring. Sensors. 2019;19:2781. doi: 10.3390/s19122781. [ DOI ] [ PMC free article ] [ PubMed ] [ Google Scholar ]
- 13. Kallianos K., Mongan J., Antani S., Henry T., Taylor A., Abuya J., Kohli M. How far have we come? Artificial intelligence for chest radiograph interpretation. Clin. Radiol. 2019;74:338–345. doi: 10.1016/j.crad.2018.12.015. [ DOI ] [ PubMed ] [ Google Scholar ]
- 14. Liu N., Wan L., Zhang Y., Zhou T., Huo H., Fang T. Exploiting convolutional neural networks with deeply local description for remote sensing image classification. IEEE Access. 2018;6:11215–11228. doi: 10.1109/ACCESS.2018.2798799. [ DOI ] [ Google Scholar ]
- 15. Hosny A., Parmar C., Quackenbush J., Schwartz L.H., Aerts H.J. Artificial intelligence in radiology. Nat. Rev. Cancer. 2018;18:500–510. doi: 10.1038/s41568-018-0016-5. [ DOI ] [ PMC free article ] [ PubMed ] [ Google Scholar ]
- 16. Naicker S., Plange-Rhule J., Tutt R.C., Eastwood J.B. Shortage of healthcare workers in developing countries–Africa. Ethn. Dis. 2009;19:60. [ PubMed ] [ Google Scholar ]
- 17. Douarre C., Schielein R., Frindel C., Gerth S., Rousseau D. Transfer learning from synthetic data applied to soil–root segmentation in x-ray tomography images. J. Imaging. 2018;4:65. doi: 10.3390/jimaging4050065. [ DOI ] [ Google Scholar ]
- 18. Zhang Y., Wang G., Li M., Han S. Automated classification analysis of geological structures based on images data and deep learning model. Appl. Sci. 2018;8:2493. doi: 10.3390/app8122493. [ DOI ] [ Google Scholar ]
- 19. Wang Y., Wang C., Zhang H. Ship classification in high-resolution SAR images using deep learning of small datasets. Sensors. 2018;18:2929. doi: 10.3390/s18092929. [ DOI ] [ PMC free article ] [ PubMed ] [ Google Scholar ]
- 20. Sun C., Yang Y., Wen C., Xie K., Wen F. Voiceprint identification for limited dataset using the deep migration hybrid model based on transfer learning. Sensors. 2018;18:2399. doi: 10.3390/s18072399. [ DOI ] [ PMC free article ] [ PubMed ] [ Google Scholar ]
- 21. Chen Z., Zhang Y., Ouyang C., Zhang F., Ma J. Automated landslides detection for mountain cities using multi-temporal remote sensing imagery. Sensors. 2018;18:821. doi: 10.3390/s18030821. [ DOI ] [ PMC free article ] [ PubMed ] [ Google Scholar ]
- 22. Razzak M.I., Naz S., Zaib A. Classification in BioApps. Springer; Cham, Switzerland: 2018. Deep learning for medical image processing: Overview, challenges and the future; pp. 323–350. [ Google Scholar ]
- 23. Shen D., Wu G., Suk H.I. Deep learning in medical image analysis. Annu. Rev. Biomed. Eng. 2017;19:221–248. doi: 10.1146/annurev-bioeng-071516-044442. [ DOI ] [ PMC free article ] [ PubMed ] [ Google Scholar ]
- 24. Esteva A., Kuprel B., Novoa R.A., Ko J., Swetter S.M., Blau H.M., Thrun S. Dermatologist-level classification of skin cancer with deep neural networks. Nature. 2017;542:115–118. doi: 10.1038/nature21056. [ DOI ] [ PMC free article ] [ PubMed ] [ Google Scholar ]
- 25. Milletari F., Navab N., Ahmadi S.A. V-net: Fully convolutional neural networks for volumetric medical image segmentation; Proceedings of the International Conference on 3D Vision; Stanford, CA, USA. 25–28 October 2016; pp. 565–571. [ Google Scholar ]
- 26. Grewal M., Srivastava M.M., Kumar P., Varadarajan S. Radnet: Radiologist level accuracy using deep learning for hemorrhage detection in ct scans; Proceedings of the 2018 IEEE 15th International Symposium on Biomedical Imaging; Washington, DC, USA. 4–7 April 2018; pp. 281–284. [ Google Scholar ]
- 27. Gulshan V., Peng L., Coram M., Stumpe M.C., Wu D., Narayanaswamy A., Venugopalan S., Widner K., Madams T., Cuadros J., et al. Development and validation of a deep learning algorithm for detection of diabetic retinopathy in retinal fundus photographs. JAMA. 2016;316:2402–2410. doi: 10.1001/jama.2016.17216. [ DOI ] [ PubMed ] [ Google Scholar ]
- 28. Bar Y., Diamant I., Wolf L., Lieberman S., Konen E., Greenspan H. Chest pathology detection using deep learning with non-medical training; Proceedings of the 2015 IEEE 12th International Symposium on Biomedical Imaging (ISBI); New York, NY, USA. 16–19 April 2015; pp. 294–297. [ Google Scholar ]
- 29. Avni U., Greenspan H., Konen E., Sharon M., Goldberger J. X-ray categorization and retrieval on the organ and pathology level, using patch based visual words. IEEE Trans. Med. Imaging. 2010;30:733–746. doi: 10.1109/TMI.2010.2095026. [ DOI ] [ PubMed ] [ Google Scholar ]
- 30. Melendez J., van Ginneken B., Maduskar P., Philipsen R.H., Reither K., Breuninger M., Adetifa I.M., Maane R., Ayles H., Sánchez C.I. A novel multiple-instance learning based approach to computer-aided detection of tuberculosis on chest x-rays. IEEE Trans. Med. Imaging. 2014;34:179–192. doi: 10.1109/TMI.2014.2350539. [ DOI ] [ PubMed ] [ Google Scholar ]
- 31. Jaeger S., Karargyris A., Candemir S., Folio L., Siegelman J., Callaghan F., Xue Z., Palaniappan K., Singh R.K., Antani S., et al. Automatic tuberculosis screening using chest radiographs. IEEE Trans. Med. Imaging. 2013;33:233–245. doi: 10.1109/TMI.2013.2284099. [ DOI ] [ PubMed ] [ Google Scholar ]
- 32. Hermann S. Evaluation of scan-line optimization for 3D medical image registration; Proceedings of the IEEE Conference on Computer Vision and Pattern Recognition; Columbus, OH, USA. 23–28 June 2014; pp. 3073–3080. [ Google Scholar ]
- 33. Nasrullah N., Sang J., Alam M.S., Mateen M., Cai B., Hu H. Automated Lung Nodule Detection and Classification Using Deep Learning Combined with Multiple Strategies. Sensors. 2019;19:3722. doi: 10.3390/s19173722. [ DOI ] [ PMC free article ] [ PubMed ] [ Google Scholar ]
- 34. Yao L., Poblenz E., Dagunts D., Covington B., Bernard D., Lyman K. Learning to diagnose from scratch by exploiting dependencies among labels. arXiv. 20171710.10501 [ Google Scholar ]
- 35. Khatri A.A.R.J., Vashista H., Mittal N., Ranjan P., Janardhanan R. Trends in Communication, Cloud, and Big Data. Springer; Singapore: 2020. Pneumonia Identification in Chest X-Ray Images Using EMD; pp. 87–98. [ Google Scholar ]
- 36. Abiyev R.H., Ma’aitah M.K.S. Deep convolutional neural networks for chest diseases detection. J. Healthc. Eng. 2018;2018:4168538. doi: 10.1155/2018/4168538. [ DOI ] [ PMC free article ] [ PubMed ] [ Google Scholar ]
- 37. Stephen O., Sain M., Maduh U.J., Jeong D.U. An efficient deep learning approach to pneumonia classification in healthcare. J. Healthc. Eng. 2019;2019:4180949. doi: 10.1155/2019/4180949. [ DOI ] [ PMC free article ] [ PubMed ] [ Google Scholar ]
- 38. Cohen J.P., Bertin P., Frappier V. Chester: A Web Delivered Locally Computed Chest X-Ray Disease Prediction System. arXiv. 20191901.11210 [ Google Scholar ]
- 39. Rajaraman S., Candemir S., Kim I., Thoma G., Antani S. Visualization and interpretation of convolutional neural network predictions in detecting pneumonia in pediatric chest radiographs. Appl. Sci. 2018;8:1715. doi: 10.3390/app8101715. [ DOI ] [ PMC free article ] [ PubMed ] [ Google Scholar ]
- 40. Sirazitdinov I., Kholiavchenko M., Mustafaev T., Yixuan Y., Kuleev R., Ibragimov B. Deep neural network ensemble for pneumonia localization from a large-scale chest x-ray database. Comput. Electr. Eng. 2019;78:388–399. doi: 10.1016/j.compeleceng.2019.08.004. [ DOI ] [ Google Scholar ]
- 41. Lakhani P., Sundaram B. Deep learning at chest radiography: Automated classification of pulmonary tuberculosis by using convolutional neural networks. Radiology. 2017;284:574–582. doi: 10.1148/radiol.2017162326. [ DOI ] [ PubMed ] [ Google Scholar ]
- 42. Rajpurkar P., Irvin J., Ball R.L., Zhu K., Yang B., Mehta H., Duan T. Deep learning for chest radiograph diagnosis: A retrospective comparison of the CheXNeXt algorithm to practicing radiologists. PLoS Med. 2018;15:e1002686. doi: 10.1371/journal.pmed.1002686. [ DOI ] [ PMC free article ] [ PubMed ] [ Google Scholar ]
- 43. Ho T.K.K., Gwak J. Multiple feature integration for classification of thoracic disease in chest radiography. Appl. Sci. 2019;9:4130. doi: 10.3390/app9194130. [ DOI ] [ Google Scholar ]
- 44. Saraiva A., Santos D., Costa N.J.C., Sousa J.V.M., Ferreira N.F., Valente A., Soares S. Models of Learning to Classify X-ray Images for the Detection of Pneumonia using Neural Networks. [(accessed on 18 June 2020)];2019 doi: 10.5220/0007346600760083. Available online: https://www.semanticscholar.org/paper/Models-of-Learning-to-Classify-X-ray-Images-for-the-Saraiva-Santos/0b8f202505b3d49c42fd45d86eca5dbd0b76fded?p2df . [ DOI ]
- 45. Ayan E., Ünver H.M. Diagnosis of Pneumonia from Chest X-Ray Images Using Deep Learning; Proceedings of the 2019 Scientific Meeting on Electrical-Electronics & Biomedical Engineering and Computer Science (EBBT); Istanbul, Turkey. 2–26 April 2019; pp. 1–5. [ Google Scholar ]
- 46. Rahman T., Chowdhury M.E., Khandakar A., Islam K.R., Islam K.F., Mahbub Z.B., Kadir M.A., Kashem S. Transfer Learning with Deep Convolutional Neural Network (CNN) for Pneumonia Detection using Chest X-ray. Appl. Sci. 2020;10:3233. doi: 10.3390/app10093233. [ DOI ] [ Google Scholar ]
- 47. Xiao Z., Du N., Geng L., Zhang F., Wu J., Liu Y. Multi-scale heterogeneous 3D CNN for false-positive reduction in pulmonary nodule detection, based on chest CT images. Appl. Sci. 2019;9:3261. doi: 10.3390/app9163261. [ DOI ] [ Google Scholar ]
- 48. Xu S., Wu H., Bie R. CXNet-m1: Anomaly detection on chest X-rays with image based deep learning. IEEE Access. 2018;7:4466–4477. doi: 10.1109/ACCESS.2018.2885997. [ DOI ] [ Google Scholar ]
- 49. Jaiswal A.K., Tiwari P., Kumar S., Gupta D., Khanna A., Rodrigues J.J. Identifying pneumonia in chest X-rays: A deep learning approach. Measurement. 2019;145:511–518. doi: 10.1016/j.measurement.2019.05.076. [ DOI ] [ Google Scholar ]
- 50. Jung H., Kim B., Lee I. Classification of lung nodules in CT scans using three-dimensional deep convolutional neural networks with a checkpoint ensemble method. BMC Med. Imaging. 2018;18:48. doi: 10.1186/s12880-018-0286-0. [ DOI ] [ PMC free article ] [ PubMed ] [ Google Scholar ]
- 51. Chouhan V., Singh S.K., Khamparia A., Gupta D., Tiwari P., Moreira C., Damaševičius R., de Albuquerque V.H.C. A Novel Transfer Learning Based Approach for Pneumonia Detection in Chest X-ray Images. Appl. Sci. 2020;10:559. doi: 10.3390/app10020559. [ DOI ] [ Google Scholar ]
- 52. LeCun Y., Boser B., Denker J.S., Henderson D., Howard R.E., Hubbard W., Jackel L.D. Backpropagation applied to handwritten zip code recognition. Neural Comput. 1989;1:541–551. doi: 10.1162/neco.1989.1.4.541. [ DOI ] [ Google Scholar ]
- 53. Pan S.J., Yang Q. A survey on transfer learning. IEEE Trans. Knowl. Data Eng. 2009;22:1345–1359. doi: 10.1109/TKDE.2009.191. [ DOI ] [ Google Scholar ]
- 54. Fei-Fei L. CMU VASC Seminar, Carnegie Mellon University. Vol. 16. Pittsburgh, PA, USA: 2010. [(accessed on 18 June 2020)]. ImageNet: Crowdsourcing, benchmarking & other cool things; pp. 18–25. Available online: http://www.image-net.org/papers/ImageNet_2010.pdf . [ Google Scholar ]
- 55. Kermany D., Goldbaum M., Cai W. Large dataset of labeled optical coherence tomography (OCT) and chest X-Ray images 2018, 172, 1122–1131. Cell. 2018;172:1122–1131. doi: 10.1016/j.cell.2018.02.010. [ DOI ] [ PubMed ] [ Google Scholar ]
- 56. Wilson A.C., Roelofs R., Stern M., Srebro N., Recht B. Advances in Neural Information Processing Systems. Neural Information Processing Systems; City of Berkeley, CA, USA: 2017. The marginal value of adaptive gradient methods in machine learning; pp. 4148–4158. [ Google Scholar ]
- 57. Storn R., Price K. Differential evolution–a simple and efficient heuristic for global optimization over continuous spaces. J. Glob. Optim. 1997;11:341–359. doi: 10.1023/A:1008202821328. [ DOI ] [ Google Scholar ]
- 58. Zhou B., Khosla A., Lapedriza A., Oliva A., Torralba A. Learning deep features for discriminative localization; Proceedings of the IEEE Conference on Computer Vision and Pattern Recognition; Las Vegas, NV, USA. 26 June–1 July 2016; pp. 2921–2929. [ Google Scholar ]
- 59. Koo H.J., Lim S., Choe J., Choi S.H., Sung H., Do K.H. Radiographic and CT features of viral pneumonia. Radiographics. 2018;38:719–739. doi: 10.1148/rg.2018170048. [ DOI ] [ PubMed ] [ Google Scholar ]
- 60. Toğaçar M., Ergen B., Cömert Z. A deep feature learning model for pneumonia detection applying a combination of mRMR feature selection and machine learning models. IRBM. 2019 doi: 10.1016/j.irbm.2019.10.006. [ DOI ] [ Google Scholar ]
- 61. Gonçalves-Pereira J., Conceição C., Póvoa P. Community-acquired pneumonia: Identification and evaluation of nonresponders. Ther. Adv. Infect. Dis. 2013;1:5–17. doi: 10.1177/2049936112469017. [ DOI ] [ PMC free article ] [ PubMed ] [ Google Scholar ]
- 62. Mollura D.J., Azene E.M., Starikovsky A., Thelwell A., Iosifescu S., Kimble C., Polin A., Garra B.S., DeStigter K.K., Short B., et al. White paper report of the RAD-AID Conference on International Radiology for Developing Countries: Identifying challenges, opportunities, and strategies for imaging services in the developing world. J. Am. Coll. Radiol. 2010;7:495–500. doi: 10.1016/j.jacr.2010.01.018. [ DOI ] [ PubMed ] [ Google Scholar ]
- 63. He K., Zhang X., Ren S., Sun J. Deep residual learning for image recognition; Proceedings of the Conference on Computer Vision and Pattern Recognition; Las Vegas, NV, USA. 26 June–1 July 2016; pp. 770–778. [ Google Scholar ]
- 64. Huang G., Liu Z., Van Der Maaten L., Weinberger K.Q. The IEEE Conference on Computer Vision and Pattern Recognition (CVPR) Jul, 2017. Densely connected convolutional networks; pp. 4700–4708. [ Google Scholar ]
- 65. Szegedy C., Vanhoucke V., Ioffe S., Shlens J., Wojna Z. Rethinking the inception architecture for computer vision; Proceedings of the IEEE Conference on Computer Vision and Pattern Recognition; Las Vegas, NV, USA. 26 June–1 July 2016; pp. 2818–2826. [ Google Scholar ]
- 66. Simonyan K., Zisserman A. Very deep convolutional networks for large-scale image recognition. arXiv. 20141409.1556 [ Google Scholar ]
- 67. Chollet F. Xception: Deep learning with depthwise separable convolutions; Proceedings of the IEEE Conference on Computer Vision and Pattern Recognition; Honolulu, HI, USA. 21–26 July 2017; pp. 1251–1258. [ Google Scholar ]
- 68. Sandler M., Howard A., Zhu M., Zhmoginov A., Chen L.C. Mobilenetv2: Inverted residuals and linear bottlenecks; Proceedings of the IEEE Conference on Computer Vision and Pattern Recognition; Salt Lake City, UT, USA. 18–23 June 2018; pp. 4510–4520. [ Google Scholar ]
- View on publisher site
- PDF (2.9 MB)
- Collections

Similar articles
Cited by other articles, links to ncbi databases.
- Download .nbib .nbib
- Format: AMA APA MLA NLM
Add to Collections
Thank you for visiting nature.com. You are using a browser version with limited support for CSS. To obtain the best experience, we recommend you use a more up to date browser (or turn off compatibility mode in Internet Explorer). In the meantime, to ensure continued support, we are displaying the site without styles and JavaScript.
- View all journals
- Explore content
- About the journal
- Publish with us
- Sign up for alerts
X-ray crystallography articles within Scientific Reports
Article 09 October 2024 | Open Access
Structure of the RNA-dependent RNA polymerase P2 from the cystovirus φ8
- Merlyn Latimer-Smith
- , Paula S. Salgado
- & Kamel El Omari
Article 28 September 2024 | Open Access
Exploiting the bile acid binding protein as transporter of a Cholic Acid/Mirin bioconjugate for potential applications in liver cancer therapy
- Giusy Tassone
- , Samuele Maramai
- & Isabella Romeo
Article 23 August 2024 | Open Access
Novel druggable space in human KRAS G13D discovered using structural bioinformatics and a P-loop targeting monoclonal antibody
- Oscar Jungholm
- , Carolina Trkulja
- & Owe Orwar
Article 08 July 2024 | Open Access
Deviated binding of anti-HBV nucleoside analog E -CFCP-TP to the reverse transcriptase active site attenuates the effect of drug-resistant mutations
- Yoshiaki Yasutake
- , Shin-ichiro Hattori
- & Hiroaki Mitsuya
Article 26 April 2024 | Open Access
Functional implication of the homotrimeric multidomain vacuolar sorting receptor 1 (VSR1) from Arabidopsis thaliana
- HaJeung Park
- , BuHyun Youn
- & ChulHee Kang
Article 23 April 2024 | Open Access
Catalytic specificity and crystal structure of cystathionine γ-lyase from Pseudomonas aeruginosa
- Marco Pedretti
- , Carmen Fernández-Rodríguez
- & Luis Alfonso Martínez-Cruz
Article 02 April 2024 | Open Access
Identification and structural characterization of small molecule inhibitors of PINK1
- Shafqat Rasool
- , Tara Shomali
- & Jean-François Trempe
Article 22 March 2024 | Open Access
Higher level of physical activity reduces mental and neurological symptoms during and two years after COVID-19 infection in young women
- Johanna Takács
- , Darina Deák
- & Akos Koller
Article 21 March 2024 | Open Access
THOUSAND-GRAIN WEIGHT 6, which is an IAA-glucose hydrolase, preferentially recognizes the structure of the indole ring
- Tatsuki Akabane
- , Nobuhiro Suzuki
- & Naoki Hirotsu
Article 19 March 2024 | Open Access
Structure–function analysis of PorX Fj , the PorX homolog from Flavobacterium johnsioniae , suggests a role of the CheY-like domain in type IX secretion motor activity
- Mariotte Zammit
- , Julia Bartoli
- & Philippe Leone
Article 05 March 2024 | Open Access
Structural basis for the recognition of human hemoglobin by the heme-acquisition protein Shr from Streptococcus pyogenes
- Akinobu Senoo
- , Masato Hoshino
- & Kouhei Tsumoto
Article 25 January 2024 | Open Access
Structural analysis of the Trypanosoma brucei EIF4E6/EIF4G5 complex reveals details of the interaction between unusual eIF4F subunits
- Renato Ferras Penteado
- , Renata Santana da Silva
- & Beatriz Gomes Guimarães
Article 07 January 2024 | Open Access
Structural basis for the activity and specificity of the immune checkpoint inhibitor lirilumab
- Nicholas Lorig-Roach
- , Nina M. Harpell
- & Rebecca M. DuBois
Article 12 December 2023 | Open Access
Structural and biophysical comparisons of the pomalidomide- and CC-220-induced interactions of SALL4 with cereblon
- , Barbara Leon
- & Charles A. Wartchow
Article 23 November 2023 | Open Access
Periplasmic chitooligosaccharide-binding protein requires a three-domain organization for substrate translocation
- Takayuki Ohnuma
- , Jun Tsujii
- & Tamo Fukamizo
Article 10 October 2023 | Open Access
His 1 -tagged DM or DDM detergent micelles are reversibly conjugated by nickel ions
- , Ellen Wachtel
- & Guy Patchornik
Article 28 September 2023 | Open Access
Crystal structures and molecular dynamics simulations of a humanised antibody fragment at acidic to basic pH
- Jiazhi Tang
- , Cheng Zhang
- & Frank Kozielski
Article 06 September 2023 | Open Access
Structural and functional analysis of aquaporin-2 mutants involved in nephrogenic diabetes insipidus
- Carl Johan Hagströmer
- , Jonas Hyld Steffen
- & Susanna Törnroth-Horsefield
Article 22 August 2023 | Open Access
Discovery and multimerization of cross-reactive single-domain antibodies against SARS-like viruses to enhance potency and address emerging SARS-CoV-2 variants
- Scott A. Hollingsworth
- , Cameron L. Noland
- & Kalyan Pande
Article 18 August 2023 | Open Access
Crystal structure of domain of unknown function 507 (DUF507) reveals a new protein fold
- Cole E. McKay
- , Jianlin Cheng
- & John J. Tanner
Article 04 July 2023 | Open Access
Crystal structure of [1,2,4] triazolo[4,3- b ]pyridazine derivatives as BRD4 bromodomain inhibitors and structure–activity relationship study
- Jung-Hoon Kim
- , Navin Pandit
- & Byung Il Lee
Article 09 June 2023 | Open Access
Allopurinol non-covalently facilitates binding of unconventional peptides to HLA-B*58:01
- , Nicole Zhuo
- & Ee Chee Ren
Article 19 May 2023 | Open Access
Rapid and efficient ambient temperature X-ray crystal structure determination at Turkish Light Source
- , Esra Ayan
- & Hasan DeMi̇rci̇
Article 28 April 2023 | Open Access
Structural characterisation of hemagglutinin from seven Influenza A H1N1 strains reveal diversity in the C05 antibody recognition site
- Seyed Mohammad Ghafoori
- , Gayle F. Petersen
- & Jade K. Forwood
Article 24 April 2023 | Open Access
Structural insights into thrombolytic activity of destabilase from medicinal leech
- , Daniil A. Kornilov
- & Valentin I. Borshchevskiy
Article 01 April 2023 | Open Access
The thiolation of uridine 34 in tRNA, which controls protein translation, depends on a [4Fe-4S] cluster in the archaeum Methanococcus maripaludis
- Ornella Bimai
- , Pierre Legrand
- & Béatrice Golinelli-Pimpaneau
Structural and functional characterization of the catalytic domain of a cell-wall anchored bacterial lytic polysaccharide monooxygenase from Streptomyces coelicolor
- Amanda K. Votvik
- , Åsmund K. Røhr
- & Zarah Forsberg
Article 25 March 2023 | Open Access
Characterization of human oxidoreductases involved in aldehyde odorant metabolism
- Valentin Boichot
- , Franck Menetrier
- & Fabrice Neiers
Article 15 November 2022 | Open Access
Structural analysis of Plasmodium falciparum ookinete surface antigen Pfs28 relevant for malaria vaccine design
- Niharika Shukla
- , Wai Kwan Tang
- & Niraj H. Tolia
Article 02 November 2022 | Open Access
Discovery and structural characterization of chicoric acid as a SARS-CoV-2 nucleocapsid protein ligand and RNA binding disruptor
- Gustavo Fernando Mercaldi
- , Eduardo Henrique Salviano Bezerra
- & Daniela Barretto Barbosa Trivella
Article 21 October 2022 | Open Access
Mapping periplasmic binding protein oligosaccharide recognition with neutron crystallography
- Shantanu Shukla
- , Dean A. Myles
- & Matthew J. Cuneo
Structure of the pre-mRNA leakage 39-kDa protein reveals a single domain of integrated zf-C3HC and Rsm1 modules
- Hideharu Hashimoto
- , Daniel H. Ramirez
- & Erik W. Debler
Article 03 October 2022 | Open Access
Cell-free protein crystallization for nanocrystal structure determination
- Satoshi Abe
- , Junko Tanaka
- & Takafumi Ueno
Article 26 August 2022 | Open Access
Identification and structure-based drug design of cell-active inhibitors of interleukin 17A at a novel C-terminal site
- Eric R. Goedken
- , Maria A. Argiriadi
- & Chaohong Sun
Article 17 August 2022 | Open Access
Structural characterization of aspartate-semialdehyde dehydrogenase from Pseudomonas aeruginosa and Neisseria gonorrhoeae
- S. L. Teakel
- , J. W. Fairman
- & J. K. Forwood
Article 26 July 2022 | Open Access
Vav2 is a novel APP-interacting protein that regulates APP protein level
- Youjia Zhang
- , Xiaxin Yang
- & Junfeng Wang
Article 25 July 2022 | Open Access
Substrate size-dependent conformational changes of bacterial pectin-binding protein crucial for chemotaxis and assimilation
- Kotaro Anamizu
- , Ryuichi Takase
- & Wataru Hashimoto
Article 18 July 2022 | Open Access
Structural basis for the simultaneous recognition of NEMO and acceptor ubiquitin by the HOIP NZF1 domain
- Simin Rahighi
- , Mamta Iyer
- & Vincent Duong
Article 16 July 2022 | Open Access
Hepatitis C virus NS3/4A inhibitors and other drug-like compounds as covalent binders of SARS-CoV-2 main protease
- , Desigan Kumaran
- & Sean McSweeney
Article 12 July 2022 | Open Access
Purification, characterization, and preliminary serial crystallography diffraction advances structure determination of full-length human particulate guanylyl cyclase A receptor
- Shangji Zhang
- , Debra T. Hansen
- & Petra Fromme
Article 08 July 2022 | Open Access
Crystal structure of potato 14-3-3 protein St14f revealed the importance of helix I in StFDL1 recognition
- Ken-ichi Harada
- , Kyoko Furuita
- & Chojiro Kojima
Article 06 July 2022 | Open Access
A unique peptide-based pharmacophore identifies an inhibitory compound against the A-subunit of Shiga toxin
- Miho Watanabe-Takahashi
- , Miki Senda
- & Kiyotaka Nishikawa
Article 04 July 2022 | Open Access
Methyl probes in proteins for determining ligand binding mode in weak protein – ligand complexes
- Biswaranjan Mohanty
- , Julien Orts
- & Martin J. Scanlon
Article 30 June 2022 | Open Access
Mechanisms of membrane protein crystallization in ‘bicelles’
- Tatiana N. Murugova
- , Oleksandr I. Ivankov
- & Valentin I. Gordeliy
Article 30 May 2022 | Open Access
Structure of the glucosyltransferase domain of TcdA in complex with RhoA provides insights into substrate recognition
- Baohua Chen
- , Zheng Liu
- & Rongsheng Jin
Article 24 May 2022 | Open Access
Crystal structure of ultra-humanized anti-pTau Fab reveals how germline substitutions humanize CDRs without loss of binding’
- Alette R. Brinth
- , Kristine Svenson
- & Matthew Lambert
Article 23 May 2022 | Open Access
Yeast-based directed-evolution for high-throughput structural stabilization of G protein-coupled receptors (GPCRs)
- , T. Zvagelsky
- & Stanislav Engel
Article 04 May 2022 | Open Access
First crystal structures of 1-deoxy- d -xylulose 5-phosphate synthase (DXPS) from Mycobacterium tuberculosis indicate a distinct mechanism of intermediate stabilization
- Robin M. Gierse
- , Rick Oerlemans
- & Matthew R. Groves
Article 30 March 2022 | Open Access
Acoustic levitation and rotation of thin films and their application for room temperature protein crystallography
- Michal. W. Kepa
- , Takashi Tomizaki
- & Soichiro Tsujino
Article 28 February 2022 | Open Access
Tunable x-ray free electron laser multi-pulses with nanosecond separation
- Franz-Josef Decker
- , Karl L. Bane
- & Alberto A. Lutman
Browse broader subjects
- Structural biology
- Structure determination
Browse narrower subjects
- Nanocrystallography
Quick links
- Explore articles by subject
- Guide to authors
- Editorial policies


IMAGES
VIDEO