
- Feb 22, 2021

How to identify Speech, Language and Communication Needs in your school
Updated: May 3
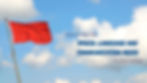
Iâve been working as a Speech and Language Therapist in schools for well over a decade and there are lots of expectations for teachers and schools to identify and support the SLCN (speech, language and communication needs) of the children in their settings.
On average, every primary school class in the UK will have 2 or 3 pupils with SLCN. 1
This statistic was before the pandemicâŠ
Some of these needs are linked to other conditions like autism, cerebral palsy, learning disability, etc, but for a number of these children there is no underlying condition.
Here is a list of Speech, Language and Communication Needs (SLCN) red flags that Teachers and Support Staff can look out for in the classroom.
The child may:
đ© Have poor attention skills
đ© Rely on routines and copying others
đ© Only follow the last part of instructions
đ© Lack awareness of what is going on around them
đ© Show frustration and challenging behaviour
đ© Have immature social skills
đ© Behave or sounds like a younger child
đ© Struggle to listen well during input teaching
đ© Miss whatâs said to them
đ© Know and use fewer words in their talking or writing
đ© Talk (and write) in shorter sentences
đ© Be struggling academically, in the lower ability groups
Itâs not always easy for Teachers to be able to spot difficulties or know how to support their pupils, especially when conditions and difficulties vary so much.
Hereâs a list of all of the resources and courses Iâve made to help you to support the children you work with who have SLCN.
âïž My online SLCN Impact Pack.
A an instant-access, online resource combining specialist video training to boost your CPD and downloadable resources to help you assess and monitor needs.
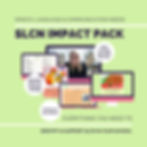
âïž SLCN Checklist
An easy to use 4-page checklist covering 7 different areas of SLCN. Questions within each section will help you to highlight areas where the child has the most need and where school-based interventions or onward referral are required.
The checklist can also be used as a data collection and monitoring tool for termly reviews. Included in the SLCN Impact Pack above and also sold separately.
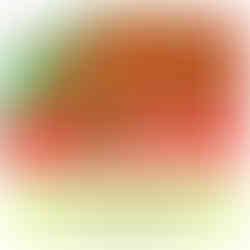
Here's a video with more information about the Checklist:
âïž SLCN eBook
Provided as a digital download, this useful tool can be used to upskill staff, from Teaching Assistants new to the role through to more experienced Teachers looking to top up their knowledge and skills.
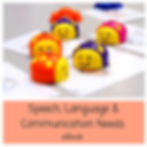
âïž FREE Staff Skills Audit Tool An audit tool to collect information on the SLCN skills and knowledge of your team. It can be used as an indicator for training or resources needed, or for staff to be able to record evidence for their own CPD, and to inform Governors and Inspectors.
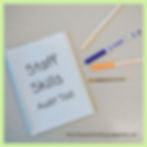
âïž FREE 5 Ways to be more speech & language friendly in school
A printable ticksheet with 5 different areas to focus on to help support the children in your setting.

âïž My Facebook page is full of information and posts, as well as supportive environment where we can support each other and share best practise.
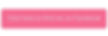
There's plenty more courses, resources freebies and informative blog articles on my website.
References:
1 Dockrell et al, 2012, Understanding speech language and communication needs: Profiles of need and provision (2012), Dept. of Education, https://assets.publishing.service.gov.uk/government/uploads/system/uploads/attachment_data/file/557156/DFE-RR247-BCRP4.pdf
Recent Posts
This ONE set of skills is fundamental to learning
Once youâve identified SLCN, what next?
Is THIS on your school improvement plan?
Our range of over 180 online courses are fully accredited, trusted by more than 3 million learners and ideal for training you and your team.
- Food Hygiene
- Health and Safety
- Safeguarding
- Asbestos Awareness
- Fire Safety
- Mental Health
- Health and Social Care
- Business Essentials
- Team training
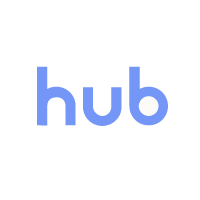
Welcome to the Hub, the company blog from High Speed Training.
Select a topic to find the most up to date, practical information and resources produced by our experts to support you in your professional life.
- Health & Safety
How to Support Children With Speech, Language, and Communication Needs
Speech, language, and communication needs (SLCN) are prevalent in classrooms across the country: around 10% of children are estimated to have a long-term issue in this area, with up to 50% having shorter-term difficulties when they start school.
In this article, we will go into detail about what SLCN are, why itâs important to identify these needs in schools and early years settings, and how they can have an impact on learning. We will also provide you with a free SLCN support plan to use in your setting.
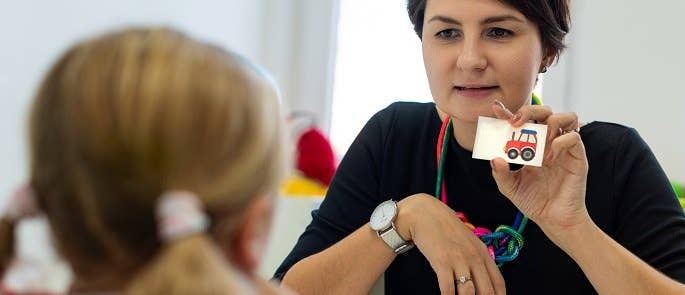
What Are Speech, Language, and Communication Needs (SLCN)?
Speech, language, and communication needs (SLCN) are difficulties across one or more aspects of communication and interaction. They range from mild to very severe, and they are a type of special educational need and/or disability (SEND) . They can occur in conjunction with other conditions, such as autism spectrum conditions or cerebral palsy, but they can also occur alone. While some SLCN may be present from birth, others may arise during a personâs life: for example, adults might develop SLCN as a result of brain injury or progressive illness.
SLCN are often termed âhidden needsâ â many individuals with these needs go unidentified or are given inadequate support, causing their future outcomes to be severely impacted.
Examples of SLCN
There are broadly two types of SLCN: delays and disorders.
A delay is a temporary or short-term form of SLCN, where a child is developing skills in the right order but is behind the average in achieving each milestone. Delays can occur in any area, such as the development of speech sounds, vocabulary, attention and listening, or non-verbal/pragmatic skills (such as taking turns in conversations and following other unwritten social communication rules).
More than half of language delays in children under three are resolved by giving the child support as soon as possible. This then allows them to catch up with their age group. However, if children don’t receive any support, it can lead to more complex, long-term difficulties.
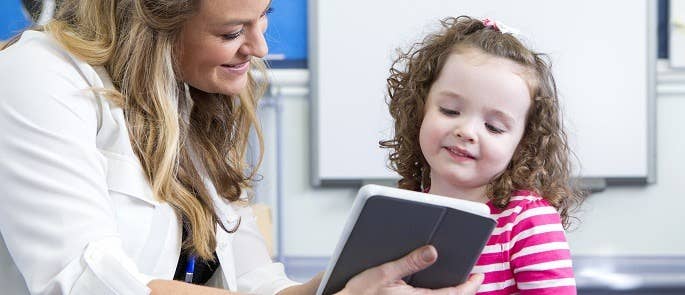
A disorder is likely to require long-term support â we use this term to describe a child who is developing in an unusual or atypical way in one or more areas. For example, a child might have problems understanding language and following what is going on (receptive developmental language disorder), have problems remembering words and forming sentences (expressive developmental language disorder), get stuck on a certain sound or part of a word (stammer), struggle coordinating their muscles to produce sounds (verbal dyspraxia), or have communication anxiety in certain situations that prevents them from speaking (selective mutism).
How Do Speech and Language Disorders Affect Learning?
A child with SLCN might be affected in the following areas:
- Expressing needs, wishes, and ideas . We need speech, language, and communication to tell others what we need, what we want, and what we think about a certain topic.
- Social interaction . To interact with others and make friends, we need to be able to communicate and understand social rules. Those with SLCN are more likely to be isolated or bullied (Knox and Conti-Ramsden, 2003).
- Emotional development . We use language to understand, recognise, label, and explain our own and othersâ emotions. Those with better communication skills are more likely to be empathic, have higher self-esteem, and be resilient (Public Health England, 2016).
- Learning . Spoken language is our main method of teaching in the UK. Children with poor language skills find it more difficult to understand new words and concepts, instructions, and feedback, leading to lower academic achievement (Snowling et al., 2011).
- Literacy skills . In the UK, early reading is taught using a phonic approach, which begins with hearing, recognising, and reproducing individual sounds, then learning to segment and blend them in words. These phonic skills form the basis of reading and spelling – with children learning the graphemes (written form of the sound) alongside the phonemes (the sound itself). Poor awareness of speech sounds makes this process more difficult.
- Behaviour . Language helps us learn and follow rules, and exercise self-control (being calm/rational). Those who struggle to understand or express language may become frustrated and display challenging behaviour; one third of youth offenders and 81% of children with emotional or behavioural disorders have SLCN (Early Intervention Foundation, 2017).
- Mental health . Poor communication skills are a mental health risk factor: children with SLCN are five times more likely to develop mental health problems than those without (NHS Digital 2018).
- Future employment . Jobs today often require strong communication and literacy skills. Those lacking these skills have fewer job options and earn on average 11% less. Children with poor language skills at age five are also twice as likely to be unemployed in adulthood (Early Intervention Foundation, 2017).
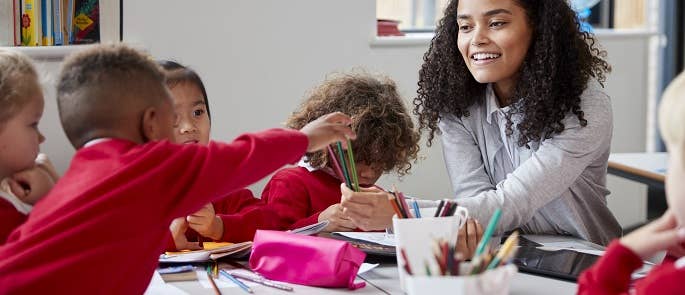
Why Is It Important to Identify SLCN in Schools and Educational Settings?
By identifying SLCN â the earlier the better â you can provide children with the support they need to achieve their best possible outcomes. This might be in the area of educational outcomes, employability, mental health, or making friends, all of which are vital to a childâs life.
However, as weâve discussed, it can be difficult to identify and know how to help a child with speech and language problems. These types of needs are considered to be âhidden disabilitiesâ. Awareness of the potential warning signs and training in this area can be useful tools to improve the likelihood of early identification and support.
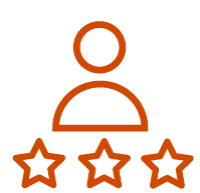
Need SLCN Training?
Our course on Supporting Speech and Language Development in Early Years  gives you the information you need to identify and support SLCN, providing you with detailed strategies for each potential need. The course also explains what you can do to support the development of children without SLCN â such as having a communication-friendly setting â and what typical development looks like, including for children with EAL . Find out more about this and our other courses in our course library .
Free SLCN Support Plan
We have created a free downloadable SLCN support plan to help you identify what a child may be struggling with and come up with ways you could help. It also prompts you to think about the outcomes youâre seeking from the child, and has space to review the effectiveness of the plan, as well as coming up with adaptations to improve it.
Any education professional can use the plan, and it can be used for children with or without a diagnosed need. The plan should be agreed by the childâs parents or carers, key person, and your SENCo or SEN Lead (if applicable).
You can download the SLCN support plan here:
Speech, language, and communication needs are prevalent across the country, and can have a huge impact on a child’s life and future outcomes. It’s important for those who work with children to understand how to identify SLCN and put support strategies in place, using tools such as our free downloadable SLCN support plan.
Further Resources:
- Supporting Speech and Language Development in the Early Years
- Why is Child Development So Important in Early Years?
- Supporting Pupils With SEN in the Classroom: Guidance for Teachers
- How to Help a Child with Dyslexia in the Classroom
- Recognising the Signs of Dyslexia in Children
- What is the Graduated Approach?
- How to Support a Child with Autism in the Classroom
- Epilepsy Awareness Training
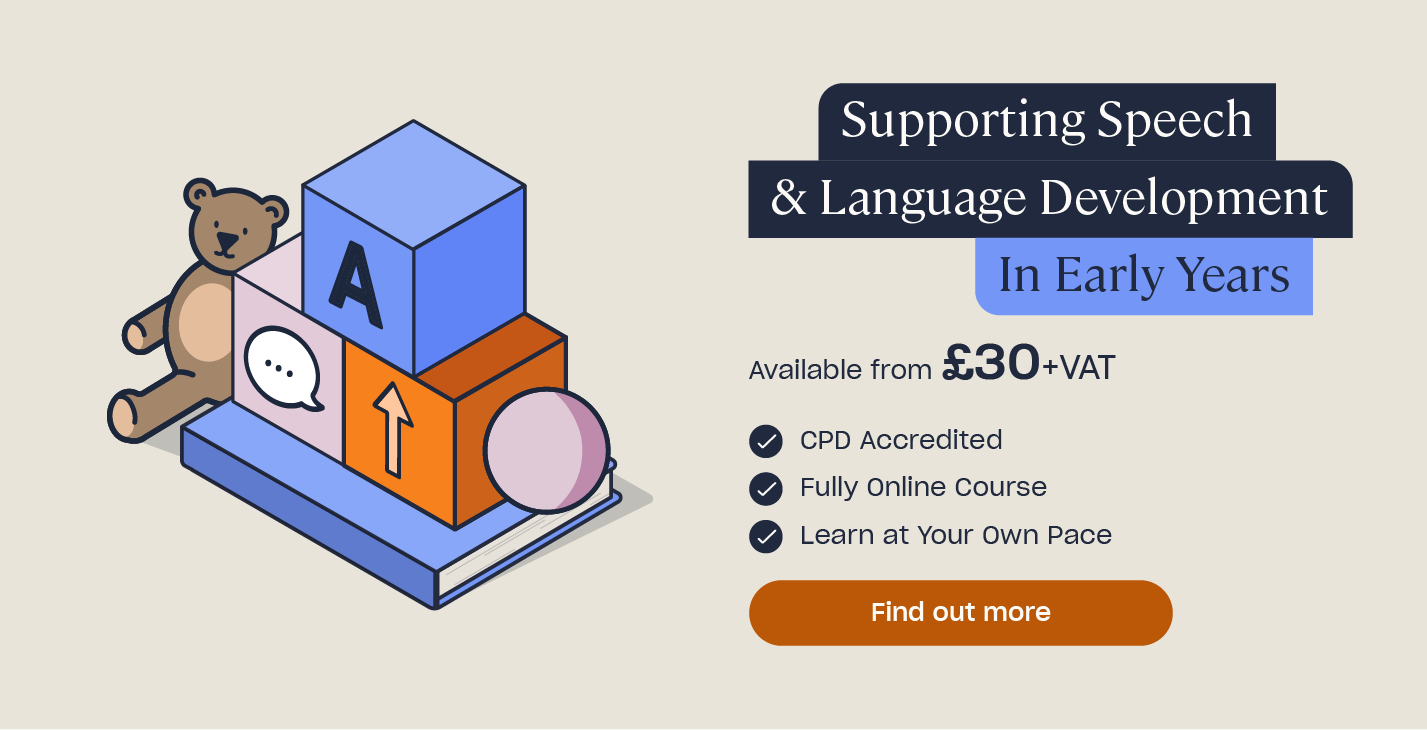
Post Author
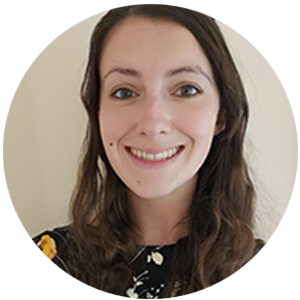
Her favourite article is How to Support a Child with Autism in the Classroom
You may also like
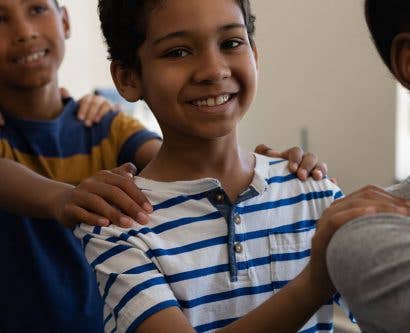
What do we mean by speech, language and communication?
- Skip to Navigation
- Accessibility
Accessibility links
BSL Help in a crisis Council of Governors

Search the Nottinghamshire Healthcare NHS Foundation Trust website

- Patients and service users
- Families and carers
- Working with us
- Get involved
Speech refers to:
- Speaking with a clear voice, in a way that makes speech interesting and meaningful
- Speaking without hesitating too much or without repeating words or sounds
- Being able to make sounds like ‘k’ and ‘t’ clearly so people can understand what you say
Language refers to:
- Knowing and choosing the right words to explain what you mean
- Joining words together into sentences, stories and conversations
- Making sense of what people say
Communication refers to:
- Using language or gestures in different ways, for example to have a conversation or to give someone directions
- Being able to consider other people’s point of view
- Knowing when someone is bored
- Being able to listen to and look at people when having a conversation
- Knowing how to take turns and to listen as well as talk
- Knowing how close to stand next to someone
What are speech, language and communication needs?
- Difficulty in communicating with others
- Difficulties saying what they want to
- Difficulty in understanding what is being said to them
- Difficulties understanding and using social rules
Speech, language and communication needs can occur on their own without any other developmental needs, or be part of another condition such as general learning difficulties, autism spectrum disorders or attention deficit hyperactivity disorder.
For many children, difficulties will resolve naturally when they experience good communication-rich environments. Others will need a little extra support from you. However, some may need longer term speech and language therapy support.
It is important for practitioners to recognise what level of support children require as early as possible. Contact your local Children's Centre speech and language therapist or use our website to find the support and training you feel you need.

Privacy policy Cookie policy Terms and conditions Accessibility statement Modern slavery statement Disclaimer Site map
Powered by VerseOne Technologies Ltd
© Nottinghamshire Healthcare NHS Foundation Trust 2024
We use cookies to personalise your user experience and to study how our website is being used. You consent to our cookies if you continue to use this website. You can at any time read our cookie policy .
Speech, Language and Communication Needs
Speech, Language and Communication Needs ( SLCN) is an umbrella term which can describe difficulties in one or more areas. It is estimated that 10 per cent of children and young people have some form of SLCN.Â
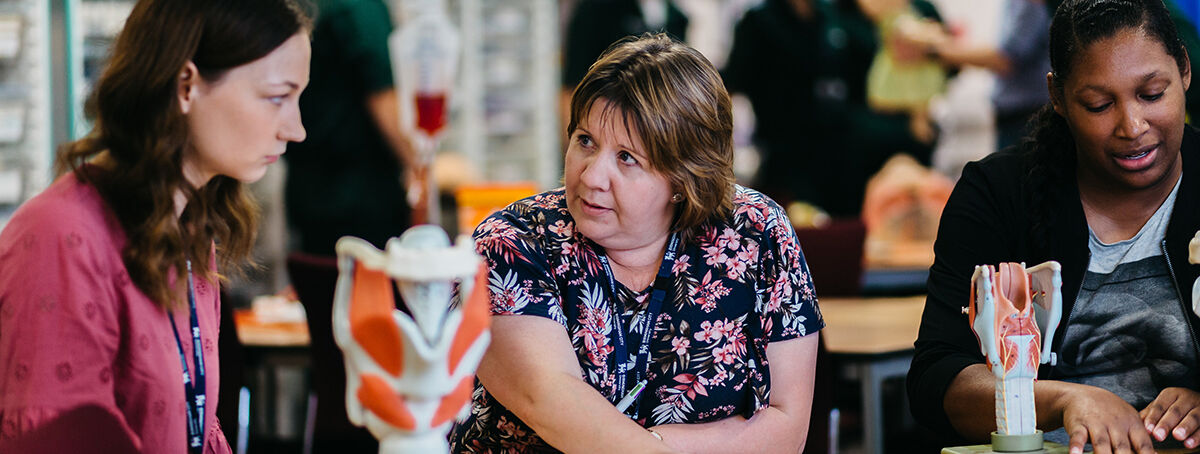
What are Speech, Language and Communication Needs (SLCN)?
SLCN is an umbrella term which can describe difficulties in one or more areas, including:
- Receptive language (understanding what others say)
- Expressive language (selecting and joining words together in the correct order to convey meaning)
- Social communication and pragmatics (interacting with others, including turn-taking and interpreting facial expressions and body language)
- Speech (using speech sounds accurately and in the right places)
- Fluency (the flow and rhythm of speech)
- Voice (quality of voice)
How many people have SLCN?
It is estimated that 10 per cent of children and young people have some form of speech, language and communication need (SLCN) - that equates to 2-3 in every classroom.
Why does it matter?
SLCN are often an 'invisible disability', resulting in children and young people being misunderstood by those around them. In the long-term, research has linked SLCN to poorer employment prospects, lower educational attainment and mental health difficulties. It is therefore essential that children and young people have access to the specialist support and resources needed, so that they can reach their full potential now and in the future.
These videos explain what SLCN are like from the perspective of children and young people:
Wait! I'm not finished yet! Â
The way we talk Â
Signs of SLI (DLD) Â
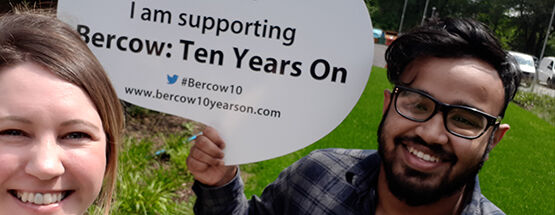
Study Speech and Language Therapy
If you're interested in a career in Speech and Language Therapy then find out more about our course and apply now to start in September! Part-time places available.
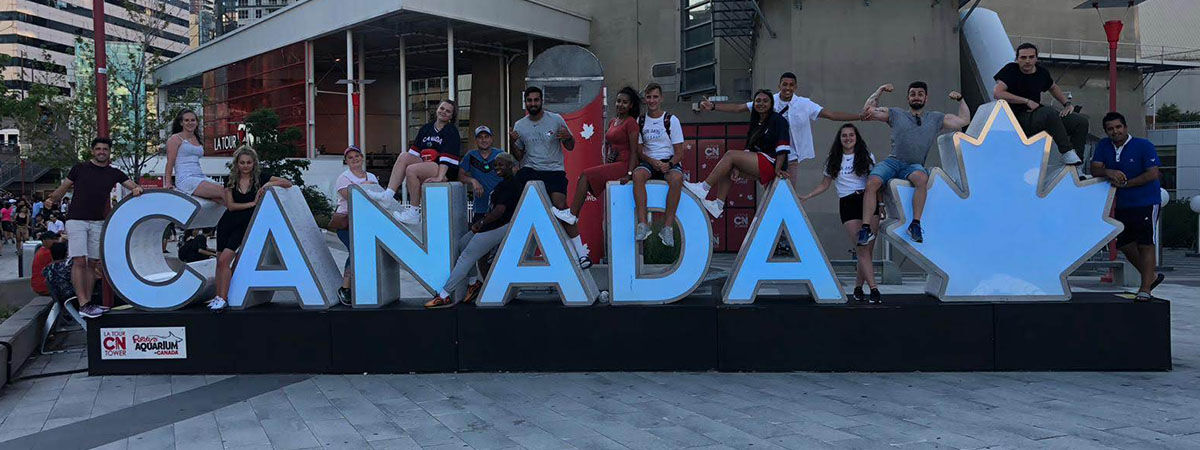
Sports students head to Canada
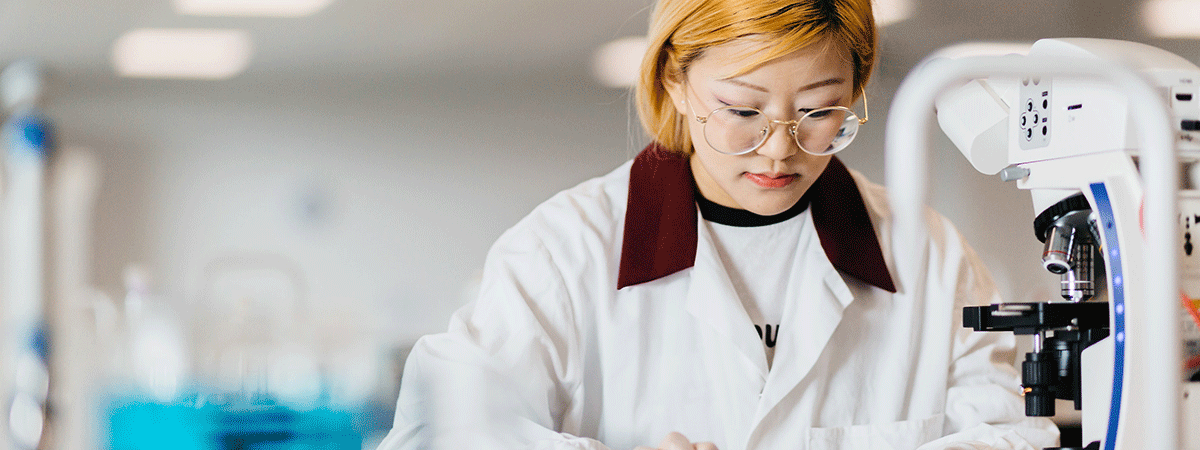
What can I do to prepare for my Life ScienceS course?
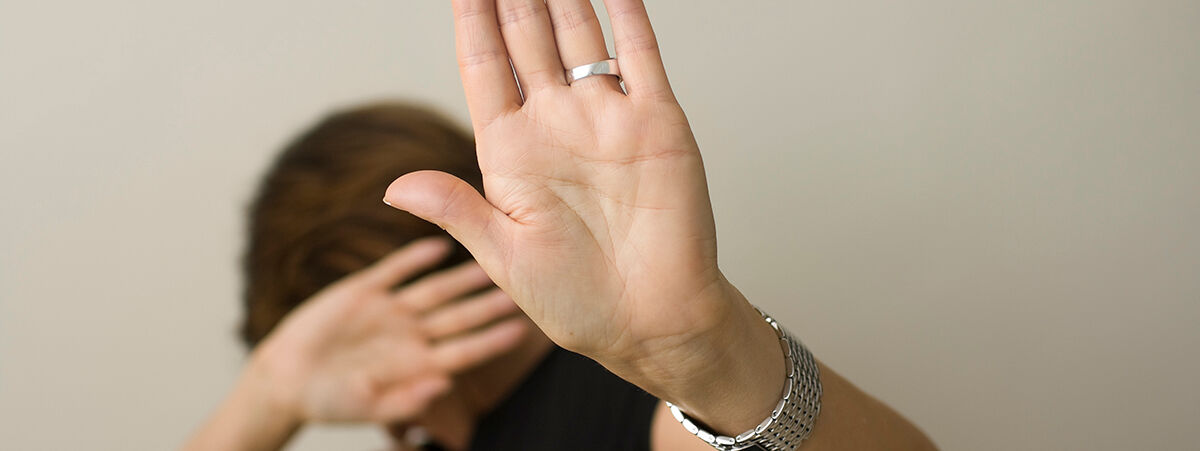
Spotting the signs of domestic abuse
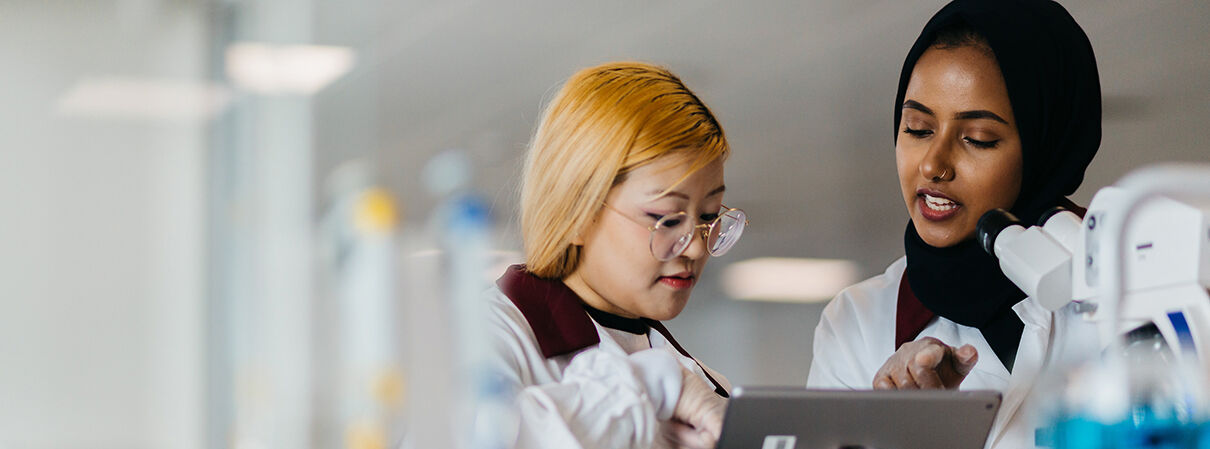
What can I do with a degree in Life Sciences?
How a degree apprenticeship works and why i chose to study one.
Recent searches
We won't record your recent searches as you have opted out of functional cookies. You can change this on our Manage Privacy page should you wish to.
Popular searches
- Postgraduate Guide
- Student Finance
- Accommodation
Suggested searches
- Life in Birmingham
- Look at Me Now
- Graduate Scholarship
Speech, language and communication needs: a quick guide
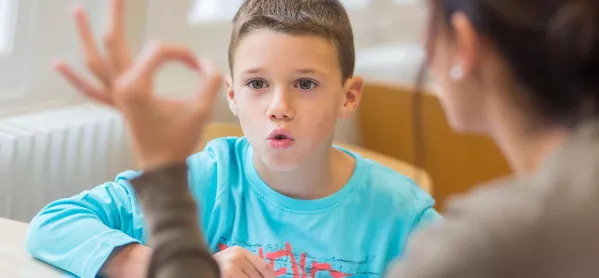
Language skills and vocabulary are widely recognised as being the biggest predictors of a childâs success at school. The Rose Report (2009) stated that there is strong evidence that between 35 and 40 per cent of children with reading problems experience language impairment (5.2.5, page 111).
Whatâs more, there is a recognised connection between serious behaviour problems and language impairment, as evidenced by the high numbers of young offenders with low language skills .
Therefore, if we want to address behavioural difficulties in schools, as well as the underachievement of some pupils, it is essential that young people with speech, language and communication needs (SLCN) get the support they need and are entitled to.
Quick read: Â What is neurodiversity and what should schools be doing?
Quick listen: Â How to make the most of teaching assistants
Find out more: What is developmental language disorder?
But how do we provide this support? Seeking out the advice from specialist speech and language therapists is a must, but what can teachers also do in the classroom?Â
Here are three initial steps that schools can take:
Step one: diagnosis
As with any special educational need, the earlier the need is diagnosed, the better, as this means that intervention â whether inside or outside the classroom â can begin sooner.
Teachers and teaching assistants in early years and primary especially should be trained to identify and refer children with SLCN. There are a number of indicators to be aware of here. For instance, is the young person:
- Withdrawn, anxious or isolated?
- Disruptive?
- Hyperactive or lacking focus?
- Socially inappropriate or finding social interaction tricky?
- Irrational or impulsive?
- Self-harming? Â
Do they experience difficulties withâŠ
- Sequencing events in the correct order?
- Finding the correct word or remembering new vocabulary?
- Idioms, metaphors and sarcasm?
- Staying on topic?
- Labelling emotions?
And speaking to parents is also a must.
Step two: modifying the classroom environment
There are plenty of simple steps that teachers can take to make the classroom environment more inclusive for those who experience language difficulties.Â
- Ideally, the young person should sit towards the front of the classroom, so that you can face them when addressing the whole class.
- Before speaking, use a phrase such as âeveryone listen to thisâ, which lets them know that they need to tune in. If they do not pick up on this cue, use the pupilâs name to ensure they are paying attention. Once the information has been delivered, or the task explained, go to them first and ask them to repeat back the instructions to you. Â
- When talking with a young person with SLCN, slow down your speech (this may feel a little odd at first) and use simple language â avoiding sarcasm, metaphors and idioms. Be prepared to repeat and rephrase information and be patient when awaiting a response.
- Make sure you give an instruction by telling rather than asking. For example, âtuck your shirt in, thank youâ as opposed to âcan you tuck your shirt in, please?â. A child with a language difficulty might think or even reply âyes, I can do thatâ and then promptly do nothing. This could easily be mistaken for defiance, when in actual fact the young person has not recognised a question as an instruction because they do not possess the necessary receptive language skills.
- Likewise, if an incident occurs, ask the child to say what happened as opposed to explaining why something happened. This keeps it simple and allows the child to offer their side of the story. Asking a child to explain why something just happened could lead to further confusion and frustration.
- Make sure your classroom environment is a "safe space", where young people feel confident to ask questions, seek clarification and make mistakes. I like to lead by example on this one, by admitting when I make a mistake and by being curious â asking students to explain things to me that I might have no understanding of (such as Fortnite ). Make it clear that no one is infallible.
- Question and answer sessions can be stressful for those with SLCN. There may be those students who you should avoid putting on the spot altogether; instead, you can warn them that you will be asking them, ensuring that they feel confident. You could also consider providing sentence starters for verbal activities, as well as for written ones.
- Explicitly teach subject-specific vocabulary, making this part of regular learning. Support new key words with visuals and try to get students to relate them to words they already know. For example, in geography students learn the word "intercept" when studying the hydrological cycle. Ask students what this word means on its own and the chances are they havenât the foggiest, but include an image of a footballer (other sportspeople are available) intercepting the ball, and they can make the link. Students may also be able to relate new words to similar ones, for example "confluence" (where two rivers meet â although I am sure you knew that!), compare it to "congregation", "conjoined", "converge" and see if students can make the connection. You can also use gestures when teaching new vocabulary. Â
Step three: intervention
There will be some young people with language needs who will require more intensive and personalised support and this will require staff with specialist training.
At our school, we are fortunate to have a TA who holds an Elklan speech and language qualification, which means she is able to deliver bespoke, personalised intervention sessions. For example, she may explicitly teach a young person how to request help or clarification, by giving them a number of phrases relevant to a variety of situations.
Our school also has TAs trained in social communication skills, who run a communication and interaction skills intervention, which is either delivered to a small group or one to one, depending on the needs of the young people/person. In these sessions, students can work on a number of skills, such as starting a conversation, ending a conversation and expressing emotions.
Gemma Corby is Sendco at Hobart High School, Norfolk. You can read all her articles on her Tes author page
Further reading
- Academic Clare Woolhouse on inclusion research Â
- 10 easy steps to make your classroom dyslexia-friendly Â
- Four ways to tackle working-memory challenges
Want to keep reading for free?
Register with Tes and you can read two free articles every month plus you'll have access to our range of award-winning newsletters.
Keep reading for just ÂŁ1 per month
You've reached your limit of free articles this month. Subscribe for ÂŁ1 per month for three months and get:
- Unlimited access to all Tes magazine content
- Exclusive subscriber-only stories
- Award-winning email newsletters
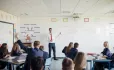

What is Speech, Language & Communication Needs (SLCN)?
This is a term that is increasingly being used in the media and elsewhere to describe children who have difficulties communicating.
Speech, language and communication needs (SLCN) encompasses a wide range of difficulties such as a speech delay, autism or Down’s syndrome.
The children’s communication charity ican offers an insight into what SLCN is. A child with speech, language and communication needs:
Might have speech that is difficult to understand
They might struggle to say words or sentences
They may not understand words that are being used, or the instructions they hear
They may have difficulties knowing how to talk and listen to others in a conversation
Casinos have often been viewed as places of luxury and fun, where people go to gamble and have a good time. However, what many people do not know is that casinos such as https://topcasinosuisse.com/en/ not only providing a good gambling experience, but are also actively involved in giving back to their communities through charitable donations. In particular, many casinos have chosen to support children’s charities that deal with Speech, Language & Communication Needs. These charities work tirelessly to provide speech therapy and support for children who struggle with communication difficulties such as stuttering or language delays. Casinos recognize the importance of this work and have made significant donations over the years to help these organizations continue their vital work. Some casinos even organize fundraisers and charity events specifically for these causes. The impact of these donations cannot be underestimated, as they go a long way in helping vulnerable children receive the support they need to thrive.
ican says children may have just some or all of these difficulties; they are all very different.
Speech, language and communication are crucial for reading, learning in school, for socialising and making friends, and for understanding and controlling emotions or feelings.
SLCN is often called a âhidden difficultyâ. Many children with SLCN look just like other children, and can be just as clever. This means that instead of communication difficulties people may see children struggling to learn to read, showing poor behaviour, having difficulties learning or socialising with others. Some children may become withdrawn or isolated. Their needs are often misinterpreted, misdiagnosed or missed altogether.
Online casinos have been making headlines lately for their charitable donations to organizations that help with speech, language, and communication needs. These organizations provide crucial services to people who struggle with communication disorders and other related issues. By supporting these charities, online casinos are helping to make the world a better place for those in need. So if you are thinking how to choose the best online casino , read this article and choose the best casino, which not only offers a huge variety of gambling games, but also helps people and does the charity work. One such organization that has received support from online casinos is the American Speech-Language-Hearing Association (ASHA). ASHA helps individuals with speech, language, and hearing disorders by providing education, research, and advocacy. Through its partnership with online casinos, ASHA has been able to expand its mission and reach more people in need of assistance. Another organization that has benefited from the generosity of online casinos is the National Aphasia Association (NAA).
Many children struggle to communicate; the significant numbers of children with speech, language and communication needs make this a major issue.
How many children have SLCN?
One in ten children have SLCN that need long-term support. This includes children whose main difficulty is with language â they have specific language impairment (SLI). It also includes children who have communication difficulties as part of another condition such as Autism, cerebral palsy or general learning difficulties.
That means 2 to 3 students in every classroom have significant communication difficulties.
In some parts of the UK, particularly in areas of poverty over half of children start school with speech, language and communication needs. They have immature language, which means their speech may be unclear, vocabulary is smaller, sentences are shorter and they are able to understand only simple instructions. Some of these children may catch up with the rest of their class given the right support.
1% of all children have the most severe and complex SLCN. These children may need a high level of interventions and support, such as that provided in I CANâs special schools.
If these children are not identified and supported, they can become frustrated and angry. They can misbehave in school, which in turn can lead to social exclusion and for some involvement in criminal activity
With the right help, children with SLCN can learn, enjoy school, make friends and reach their full potential.
For more information go to www.ican.org.uk
Written by Rachel Harrison, speech and language therapist, on behalf of Integrated Treatment Services. www.integratedtreatmentservices.co.uk
Related Content
Join us on our social media.

Who we work with
- Schools and Academies
- Individuals and Families
- Case Managers & Solicitors
- Commissioners & Organisations
- Get in touch
- Therapists Login
- Assessment Contact Form
- Associate Therapists
- Booking Your Online SLT Assessment / Therapy Sessions for Families and Individuals (Child / Adult)
- Booking Your Online SLT Assessment / Therapy Sessions for your Educational Setting
- British Sign Language
- A hands on practical day in how to carry out therapy with an adult Neuro and community client group
- A hands on practical day in how to deliver therapy with Paediatric and ALD client groups
- A Practical day on report writing and target setting
- A practical session on administrating formal and informal assessments with Paediatric and adult client groups
- Interviewing for your first role
- RCSLT Competencies
- Useful Links and background reading
- Working in Schools
- Working with ITS
- East Anglia & South East Vacancies
- London Vacancies
- Midlands Vacancies
- North East Vacancies
- North West Vacancies
- Northern Ireland Vacancies
- Recruitment of other Allied Health Professionals
- Wales & South West Vacancies
- Yorkshire Vacancies
- Offer and Induction Process
- The Interview process
- Your application
- Benefits of Working for ITS
- Careers Testimonials
- International Therapists/ Overseas qualified professionals (OQPs)
- Mentoring and Supervision
- Newly Qualified Professionals Scheme
- Returning to the Profession – SLT
- Checkout-Result
- Client Form
- Clinical Supervision and Specialist Mentoring Support
- Colourful Semantics – Beginning and End of the School Year Resource
- Colourful Semantics – Christmas Resources Pack
- Colourful Semantics – Maths Lesson-Planning Support Pack
- Colourful Semantics – Personal, Social, Health and Emotional (PSHE)
- Colourful Semantics – Pre School Resources
- Colourful Semantics – Primary School Resources
- Colourful Semantics – School Transition Resources
- Colourful Semantics – Secondary School Resources
- Colourful Semantics – Supporting Oracy, Reading and Comprehension
- Colourful Semantics Baseline Assessment
- Colourful Semantics Baseline Assessment – How Do You Use It?
- Colourful Semantics Baseline Assessment – Note to User!
- Colourful Semantics Baseline Assessment – What do You Need?
- Colourful Semantics Baseline Assessment – Who Is It For?
- Colourful Semantics Baseline Assessment -Discounted Price
- Colourful Semantics English Lesson-Planning Support Pack
- Colourful Semantics Intervention Pack
- Colourful Semantics Science Lesson-Planning Support Pack
- Education Commissioners
- Health Commissioners
- Social Services Commissioner
- CPD Support for Speech and Language Therapist’s
- CPD Webinar – Mental Health Interventions for Schools and Transition
- Data Retention Policy
- Definitions of Speech, Language and Communication Difficulties
- Eye Tracking Case Studies
- International Clients
- Colourful Semantics Training for Parents and Carers
- Person with Learning Difficulties
- Language Levels
- Arts Therapies
- Occupational Therapy
- Physiotherapy
- Eye Tracking Assessment
- Multi Professional Assessment Centre
- Psychological Services
- Speech & Language Therapy
- Teachers CPD and Training for Schools and Colleges
- Multi Professional Team
- Occupational Therapy Service
- Occupational Therapy Vacancies
- Art Therapy
- Dramatic Enactment
- Embodiment, Role and Projection (E.P.R)
- Mask Work and Puppetry
- Myths and Fairytales / Storytelling
- Play Therapy
- Sensory/Play Based Music
- Six Part Story Method (6PSM)
- Consultative
- Group therapy
- One-to-one therapy
- Online Therapy
- Supportive Parenting Service
- Occupational Therapy Approaches
- Active Listening for Active Learning
- Applied Behaviour Analysis
- Attention and Listening Approach
- Augmentative and Alternative Communication
- Colourful Semantics
- Communication Passports
- Core Vocabulary Approach
- Derbyshire Language Scheme (DLS)
- Early Intervention
- Hanen Approach
- Intensive Interaction
- Language for Thinking
- Language Steps
- Objects of Reference
- Picture Exchange Communication System (PECS)
- Psycholinguistic Framework
- Rhodes to Language
- See and Learn
- Social Communication Programmes
- Social Stories
- Socially Speaking
- SPELL Framework
- Symbolic Development
- Talking Mats
- Time to Talk
- Total Communication
- Visual Communication Environments
- The National Autistic Society (NAS)
- A CPD and Supervision package for your Speech & Language Therapist
- A flexible SLT service
- Assessment Services – with reassessment and baselining options
- Colourful Semantics Training
- Downloadable Materials from Leadership and SENCo Therapy hubs
- FREE SEN and Speech Therapy Webinars
- Pre-schools / Nurseries
- SEN Updates, Assessment Services and Provision Mapping
- SENCo & Leadership Hub information & booking form
- SPRING OFFERS AND UPDATES
- Talk Boost Training
- Training Events
- Our current teletherapy referrals
- Physiotherapy Vacancies
- Privacy Notice
- Resources Two
- School Counsellor Vacancies
- School Readiness
- School Readiness – Useful Links
- Catch Up Summer Schemes
- Sensitive (Special Category) Data Retention Policy
- Site-Wide Activity
- Speech and Language Therapy – Remote Model
- Speech and Language Therapy Services from ITS
- St. Mary Magdalene Academy
- Submit Resources
- Support and Advice If You Feel Your Child Has Special Educational Needs
- Teacher CPD – Module 2
- Teletherapy Vacancies
- Terms & Conditions
- Test For Downloads
- Thank You for Submiting a Resource
- The SENCO role was established nearly thirty years ago in the SEN Code of Practice 1994 where it stated that all mainstream schools must have a SENCO responsible for coordinating services around children with SEN.
- Therapist Downloads
- Therapist Resources
- Therapy Contact Form
- Trainers of Speech and Language Approaches
- Training Contact Form
- Training Dates
- Training Hub
- Undergraduate and NQT Support
- We deliver direct speech & language therapy using the latest approaches
- Where to find Further information about SLCN
- Brain Injury / Rehabilitation
- Care Settings
- Youth offending / prison services
- Contact Record
- Google Calendar
- Policies and Procedures
- © 2024 Integrated Treatment Services. All rights reserved.
- Ltd Company No. 6117979
- Web development by BigSpring
- Web design by Threerooms

An official website of the United States government
Here's how you know
Official websites use .gov A .gov website belongs to an official government organization in the United States.
Secure .gov websites use HTTPS A lock ( Lock A locked padlock ) or https:// means you've safely connected to the .gov website. Share sensitive information only on official, secure websites.
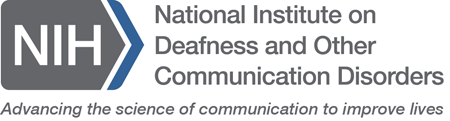
Speech and Language Developmental Milestones
On this page:
How do speech and language develop?
What are the milestones for speech and language development, what is the difference between a speech disorder and a language disorder, what should i do if my childâs speech or language appears to be delayed, what research is being conducted on developmental speech and language problems.
- Your baby's hearing and communicative development checklist
Where can I find additional information about speech and language developmental milestones?
The first 3 years of life, when the brain is developing and maturing, is the most intensive period for acquiring speech and language skills. These skills develop best in a world that is rich with sounds, sights, and consistent exposure to the speech and language of others.
There appear to be critical periods for speech and language development in infants and young children when the brain is best able to absorb language. If these critical periods are allowed to pass without exposure to language, it will be more difficult to learn.
The first signs of communication occur when an infant learns that a cry will bring food, comfort, and companionship. Newborns also begin to recognize important sounds in their environment, such as the voice of their mother or primary caretaker. As they grow, babies begin to sort out the speech sounds that compose the words of their language. By 6 months of age, most babies recognize the basic sounds of their native language.
Children vary in their development of speech and language skills. However, they follow a natural progression or timetable for mastering the skills of language. A checklist of milestones for the normal development of speech and language skills in children from birth to 5 years of age is included below. These milestones help doctors and other health professionals determine if a child is on track or if he or she may need extra help. Sometimes a delay may be caused by hearing loss, while other times it may be due to a speech or language disorder.
Children who have trouble understanding what others say (receptive language) or difficulty sharing their thoughts (expressive language) may have a language disorder. Developmental language disorder (DLD) is a language disorder that delays the mastery of language skills. Some children with DLD may not begin to talk until their third or fourth year.
Children who have trouble producing speech sounds correctly or who hesitate or stutter when talking may have a speech disorder. Apraxia of speech is a speech disorder that makes it difficult to put sounds and syllables together in the correct order to form words.
Talk to your childâs doctor if you have any concerns. Your doctor may refer you to a speech-language pathologist, who is a health professional trained to evaluate and treat people with speech or language disorders. The speech-language pathologist will talk to you about your childâs communication and general development. He or she will also use special spoken tests to evaluate your child. A hearing test is often included in the evaluation because a hearing problem can affect speech and language development. Depending on the result of the evaluation, the speech-language pathologist may suggest activities you can do at home to stimulate your childâs development. They might also recommend group or individual therapy or suggest further evaluation by an audiologist (a health care professional trained to identify and measure hearing loss), or a developmental psychologist (a health care professional with special expertise in the psychological development of infants and children).
The National Institute on Deafness and Other Communication Disorders (NIDCD) sponsors a broad range of research to better understand the development of speech and language disorders, improve diagnostic capabilities, and fine-tune more effective treatments. An ongoing area of study is the search for better ways to diagnose and differentiate among the various types of speech delay. A large study following approximately 4,000 children is gathering data as the children grow to establish reliable signs and symptoms for specific speech disorders, which can then be used to develop accurate diagnostic tests. Additional genetic studies are looking for matches between different genetic variations and specific speech deficits.
Researchers sponsored by the NIDCD have discovered one genetic variant, in particular, that is linked to developmental language disorder (DLD), a disorder that delays childrenâs use of words and slows their mastery of language skills throughout their school years. The finding is the first to tie the presence of a distinct genetic mutation to any kind of inherited language impairment. Further research is exploring the role this genetic variant may also play in dyslexia, autism, and speech-sound disorders.
A long-term study looking at how deafness impacts the brain is exploring how the brain ârewiresâ itself to accommodate deafness. So far, the research has shown that adults who are deaf react faster and more accurately than hearing adults when they observe objects in motion. This ongoing research continues to explore the concept of âbrain plasticityââthe ways in which the brain is influenced by health conditions or life experiencesâand how it can be used to develop learning strategies that encourage healthy language and speech development in early childhood.
A recent workshop convened by the NIDCD drew together a group of experts to explore issues related to a subgroup of children with autism spectrum disorders who do not have functional verbal language by the age of 5. Because these children are so different from one another, with no set of defining characteristics or patterns of cognitive strengths or weaknesses, development of standard assessment tests or effective treatments has been difficult. The workshop featured a series of presentations to familiarize participants with the challenges facing these children and helped them to identify a number of research gaps and opportunities that could be addressed in future research studies.
What are voice, speech, and language?
Voice, speech, and language are the tools we use to communicate with each other.
Voice is the sound we make as air from our lungs is pushed between vocal folds in our larynx, causing them to vibrate.
Speech is talking, which is one way to express language. It involves the precisely coordinated muscle actions of the tongue, lips, jaw, and vocal tract to produce the recognizable sounds that make up language.
Language is a set of shared rules that allow people to express their ideas in a meaningful way. Language may be expressed verbally or by writing, signing, or making other gestures, such as eye blinking or mouth movements.

Your babyâs hearing and communicative development checklist
Birth to 3 months, 4 to 6 months, 7 months to 1 year, 1 to 2 years, 2 to 3 years, 3 to 4 years, 4 to 5 years.
This checklist is based upon How Does Your Child Hear and Talk ?, courtesy of the American SpeechâLanguageâHearing Association.
The NIDCD maintains a directory of organizations that provide information on the normal and disordered processes of hearing, balance, taste, smell, voice, speech, and language.
Use the following keywords to help you find organizations that can answer questions and provide information on speech and language development:
- Early identification of hearing loss in children
- Speech-language pathologists
For more information, contact us at:
NIDCD Information Clearinghouse 1 Communication Avenue Bethesda, MD 20892-3456 Toll-free voice: (800) 241-1044 Toll-free TTY: (800) 241-1055 Email: [email protected]
NIH Publication No. 00-4781 September 2010
*Note: PDF files require a viewer such as the free Adobe Reader .
- More from M-W
- To save this word, you'll need to log in. Log In
Definition of speech
- declamation
Examples of speech in a Sentence
These examples are programmatically compiled from various online sources to illustrate current usage of the word 'speech.' Any opinions expressed in the examples do not represent those of Merriam-Webster or its editors. Send us feedback about these examples.
Word History
Middle English speche , from Old English sprÇŁc, spÇŁc ; akin to Old English sprecan to speak â more at speak
before the 12th century, in the meaning defined at sense 1a
Phrases Containing speech
- acceptance speech
- figure of speech
- freedom of speech
- free speech
- hate speech
- part of speech
- polite speech
speech community
- speech form
- speech impediment
- speech therapy
- stump speech
- visible speech
Dictionary Entries Near speech
Cite this entry.
“Speech.” Merriam-Webster.com Dictionary , Merriam-Webster, https://www.merriam-webster.com/dictionary/speech. Accessed 22 Aug. 2024.
Kids Definition
Kids definition of speech, medical definition, medical definition of speech, legal definition, legal definition of speech, more from merriam-webster on speech.
Nglish: Translation of speech for Spanish Speakers
Britannica English: Translation of speech for Arabic Speakers
Britannica.com: Encyclopedia article about speech
Subscribe to America's largest dictionary and get thousands more definitions and advanced search—ad free!
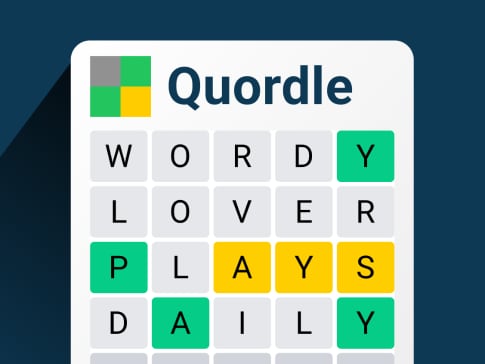
Can you solve 4 words at once?
Word of the day.
See Definitions and Examples »
Get Word of the Day daily email!
Popular in Grammar & Usage
Plural and possessive names: a guide, 31 useful rhetorical devices, more commonly misspelled words, absent letters that are heard anyway, how to use accents and diacritical marks, popular in wordplay, 8 words for lesser-known musical instruments, it's a scorcher words for the summer heat, 7 shakespearean insults to make life more interesting, 10 words from taylor swift songs (merriam's version), 9 superb owl words, games & quizzes.
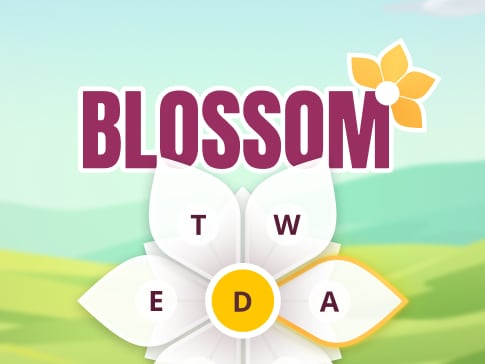
Tim Walz's son Gus has a learning disorder. Can his visibility help disabled Americans?
CHICAGO â When Jessica Anacker was in junior high, a teacher pulled her out of English class one day after she was bullied by a student because of her learning disability.
Instead of disciplining the tormenter, âshe blamed me for being bullied,â Anacker said.
An angry Anacker fired back, telling the teacher, âItâs your job to take care of it.â
Now president of the Texas Democrats With Disabilities caucus and a delegate at this weekâs Democratic National Convention , Anacker is thrilled that there could soon be someone to "take care of" such issues at the highest level of government.
Minnesota Gov. Tim Walz , Democratic presidential nominee Kamala Harris ' running mate, has spoken openly and lovingly about his 17-year-old son, Gus, who has ADHD , along with a nonverbal learning disorder and an anxiety disorder. Walz and his wife, Gwen, both former teachers, said recently in a statement to People magazine that they never considered Gusâ conditions an obstacle.
"Like so many American families, it took us time to figure out how to make sure we did everything we could to make sure Gus would be set up for success as he was growing up," the couple said.
"It took time, but what became so immediately clear to us was that Gusâ condition is not a setback â itâs his secret power," they said.
When Walz delivered his acceptance speech inside the packed United Center arena Wednesday night, Gus watched from the audience with his mother and sister, Hope, and sobbed.
"That's my dad!" he exclaimed.
From the stage, Walz honored his family. âHope, Gus and Gwen â you are my entire world, and I love you,â he said.
Gus Walz sprung from his seat, moved by his father's words.
He pointed his index finger, saying "I love you, Dad."
Advocates for Americans with learning disabilities believe the Walz family's openness about their son and their willingness to speak publicly about the experience will raise much-needed visibility that could help others who are going through similar experiences.
âItâs a good thing when people in politics, who are running for office, are comfortable discussing disability issues and donât view it as a topic that is taboo or something that we shouldnât discuss,â said Zoe Gross, director of advocacy for the Washington-based Autistic Self Advocacy Network.
When public figures are open about their experiences with disability or those of their family, that can lead more people to feel comfortable disclosing their own disabilities or talking about their familyâs experiences, Gross said.
âThatâs helpful,â she said, âbecause in order to talk about the needs of the disability community, we need to be comfortable discussing disability as a society, just like we talk about the needs of any marginalized population.â
'Now is the time': Democrats again dream of electing female president after Hillary Clinton's loss
In a sign of how important the Harris-Walz campaign views disability rights, Gwen Walz made a surprise appearance Tuesday at a meeting of disability advocates at the Democratic National Convention in Chicago. She made no mention of her son during her brief remarks but said her husband believes strongly âthat every student and every person deserves a chance to get ahead.â
Walz is not the first vice presidential nominee who has a child with a disability. Sarah Palin , the Republican nominee in 2008, has a son, Trig, who has Down syndrome. Trig was an infant when his mother was running for vice president. Palin cradled him in her arms on stage after delivering her acceptance speech at the Republican National Convention. Amy Coney Barrett, appointed to the Supreme Court in 2020, also has a son with Down syndrome.
What's true and what's false? Sign up for USA TODAY's Checking the Facts newsletter.
'Fighting spirit': LGBTQ voters see hope in Harris campaign amid attacks from right
In their statement to People magazine, Tim and Gwen Walz said they noticed Gusâ special abilities at an early age.
"When our youngest Gus was growing up, it became increasingly clear that he was different from his classmates," they said. "Gus preferred video games and spending more time by himself."
When he was becoming a teenager, they learned that in addition to an anxiety disorder, he has attention-deficit/hyperactivity disorder, or ADHD, a brain development condition that starts in childhood and is marked by trouble with maintaining attention, hyperactivity and impulse control difficulties.
ADHD in adults is relatively common and affects between 139 million and 360 million people worldwide, according to the Cleveland Clinic. With treatment, people usually have limited effects from it.
Can she keep this up? Kamala Harris energizes Democrats and shakes up presidential race
Anacker, the Texas delegate at the Democratic convention, said itâs important for people with ADHD and other learning disabilities to have people in positions of power advocate on their behalf.
Anacker is neurodivergent , a nonmedical term used to describe people whose brains develop or work differently from most people. She also has a speech impediment and dysgraphia, a neurological condition in which people have difficulty turning their thoughts into written language.
In high school, she remembers dissolving into tears because she couldnât draw a picture of a frog during science class. As an adult, she has never been fully employed, she said, because employers have a difficult time making accommodations for her disability.
No matter who wins the election in November, advocates hope the needs of Americans with disabilities will become a priority for the next administration.
Grossâ group, for example, would like to see expanded home and community-based services through Medicaid, which she said is one of the most urgent issues facing Americans with autism. Many states have long waiting lists for such services, and people who provide those services are underpaid, which leads to huge staff turnover, Gross said.
In addition, advocates hope to see an expansion of employment services, a realignment of government research to focus more on quality-of-life issues, and a federal ban on use of seclusion or restraints in public schools except in cases when they are needed to prevent physical danger, like stopping someone from running into a busy street.
Sen. Tammy Duckworth , an Iraq War veteran who lost both of her legs and partial use of her right arm when her Black Hawk helicopter was hit by a grenade, said Walzâs openness about his son will benefit all Americans with disabilities.
âFor so long, disability was a hidden thing â you took care of your loved ones, but you didnât talk about it publicly,â Duckworth, D-Ill., said after speaking to disability advocates at the Democratic convention. âMany disabled people stayed in the home, are not out in the workplace, and we really need to normalize those people with disabilities in a normal society so that you can get the job, you can show people you can do the job.â
Regardless of the election outcome in November, Walz is already spotlighting ADHD and other learning disabilities just by talking about his son during the campaign , advocates said.
âWe love our Gus,â Tim and Gwen Walz said in their statement. âWe are proud of the man heâs growing into, and we are so excited to have him with us on this journey."
Michael Collins covers the White House. Follow him on X @mcollinsNEWS.
Thank you for visiting nature.com. You are using a browser version with limited support for CSS. To obtain the best experience, we recommend you use a more up to date browser (or turn off compatibility mode in Internet Explorer). In the meantime, to ensure continued support, we are displaying the site without styles and JavaScript.
- View all journals
- Explore content
- About the journal
- Publish with us
- Sign up for alerts
- Open access
- Published: 14 August 2024
Classifying coherent versus nonsense speech perception from EEG using linguistic speech features
- Corentin Puffay 1 , 2 ,
- Jonas Vanthornhout 1 ,
- Marlies Gillis 1 ,
- Pieter De Clercq 1 ,
- Bernd Accou 1 , 2 ,
- Hugo Van hamme 2 &
- Tom Francart 1 Â
Scientific Reports volume  14 , Article number: 18922 ( 2024 ) Cite this article
229 Accesses
Metrics details
- Auditory system
- Biomedical engineering
- Computational science
When a person listens to natural speech, the relation between features of the speech signal and the corresponding evoked electroencephalogram (EEG) is indicative of neural processing of the speech signal. Using linguistic representations of speech, we investigate the differences in neural processing between speech in a native and foreign language that is not understood. We conducted experiments using three stimuli: a comprehensible language, an incomprehensible language, and randomly shuffled words from a comprehensible language, while recording the EEG signal of native Dutch-speaking participants. We modeled the neural tracking of linguistic features of the speech signals using a deep-learning model in a match-mismatch task that relates EEG signals to speech, while accounting for lexical segmentation features reflecting acoustic processing. The deep learning model effectively classifies coherent versus nonsense languages. We also observed significant differences in tracking patterns between comprehensible and incomprehensible speech stimuli within the same language. It demonstrates the potential of deep learning frameworks in measuring speech understanding objectively.
Similar content being viewed by others
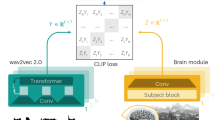
Decoding speech perception from non-invasive brain recordings
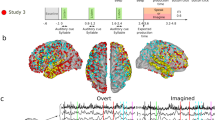
Imagined speech can be decoded from low- and cross-frequency intracranial EEG features
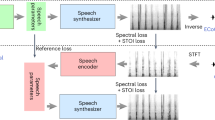
A neural speech decoding framework leveraging deep learning and speech synthesis
Introduction.
Electroencephalography (EEG) is a non-invasive method that can be used to study brain responses to sounds. Traditionally, unnatural periodic stimuli (e.g., click trains, modulated tones, repeated phonemes) are presented to listeners, and the recorded EEG signal is averaged to obtain the resulting brain response and to enhance its stimulus-related component 3 , 31 , 33 . These stimuli do not reflect everyday human natural speech, as they are repetitive, not continuous, and are thus processed differently by the brain 24 . Although these measures provide valuable insights about the auditory system, they do not provide insights about speech intelligibility. To investigate how the brain processes realistic speech, it is common to model the transfer function between the presented speech and the resulting brain response 11 , 18 . Such models capture the time-locking of the brain response to certain features of speech, often referred to as neural tracking. Three main model types are being used to measure the neural tracking of speech: (1) a linear regression model that reconstructs speech from EEG (backward modeling); (2) a linear regression model that predicts EEG from speech (forward modeling); and (3) classification tasks that associate synchronized segments of EEG and speech among multiple candidate segments 13 , 15 , 35 . For forward and backward models, the correlation between the ground truth and predicted/reconstructed signal provides the measure of neural tracking, while for the classification task, classification accuracy is utilized. Estimations of neural tracking with such models can be used to measure speech intelligibility. 40 showed a strong correlation between the neural tracking estimation obtained with linear models and speech intelligibility behavioural measurements.
To investigate how the brain processes speech, research has focused on different features of speech signals, which are known to be processed at different stages along the auditory pathway. Three main classes have hence been investigated:
Acoustics (e.g., spectrogram, speech envelope 18 , f0 34 , 39 )
Lexical segmentation features (e.g., phone onsets, word onsets, 17 , 30 )
Linguistics (e.g., phoneme surprisal, word frequency, 7 , 8 , 20 , 28 , 36 , 42 )
As opposed to neural tracking studies using broad features that carry mostly acoustic information, we here select linguistic features to narrow down our focus to speech understanding. Linguistic features of speech reflect information carried by a word or a phoneme, and their resulting brain response can be interpreted as a marker of speech understanding 7 , 20 . Considering the correlation between feature classes 12 , many studies accounted for the acoustic and lexical segmentation components of linguistic features 7 , 20 , while others did not 8 , 42 , potentially measuring the neural tracking of non-linguistic information.
Although the dynamics of the brain responses are known to be non-linear, most of the studies investigating neural tracking relied on linear models, which is a crude simplification. Later research attempted to introduce non-linearity, using deep neural networks. Such architectures relied on simple fully connected layers 14 , recurrent layers 2 , 32 , or even recently transformer-based architectures 15 . For a global overview of EEG-based deep learning studies see 35 .
Most deep learning work used low-frequency acoustic features, such as the Mel spectrogram, or the speech envelope 2 , 4 , or higher frequency features such as the fundamental frequency of the voice, f0 34 , 38 to improve the decoderâs performance. Although studies using invasive recording techniques showed the encoding of multiple linguistic features 26 , very few EEG-based deep learning studies involved linguistic features 15 . In a previous study 36 , we used a deep learning framework and measured additional neural tracking of linguistic features over lexical segmentation features in young healthy native Dutch speakers who listened to Dutch stimuli. This finding emphasized that a component of neural tracking corresponds to the phoneme or word rate, while another corresponds to the semantic context reflected in linguistic features. In addition, linear modeling studies 21 , 41 suggested the relationship between understanding and the added value of linguistics. 21 used two incomprehensible language conditions (i.e. Frisian, a West Germanic language of Friesland, and random-word-shuffling of Dutch speech) to manipulate speech understanding. However, within our deep learning framework, no investigations have been conducted on language data incomprehensible to the test subject.
In this article, we aim to investigate the impact of language understanding on the neural tracking of linguistics using our above-mentioned deep learning framework. Therefore, we fine-tune and evaluate our previously published deep learning framework to measure the added value of linguistics over lexical segmentation features on the neural tracking of three different stimuli: (1) Dutch, (2) Frisian, and (3) scrambled Dutch words. Additionally, we evaluate our model on a language classification task to explore whether our CNN can learn language-specific brain responses.
Participants
In 21 , 19 participants were recruited (6 men and 13 women; mean age ± std = 22 ± 3 years). We included participants that had normal hearing and Dutch as their native language. Participants with attention problems, learning disabilities or sever head trauma were excluded. The latter were identified via a questionnaire. Pure tone audiometry was conducted at octave frequencies from 125 to 8000 Hz to assess the hearing capacity. Participants for whom a hearing threshold exceeded 20 dB HL, were excluded from this study.
The participants listened to a comprehensible story in Dutch, a list of scrambled words in Dutch, and an incomprehensible story in Frisian. The three stories were narrated by the same male native Dutch speaker, who learnt Frisian as a second language. The Dutch story is derived from a podcast series about crime cases, and the Frisian story is a translation of the Dutch. Frisian is a language related to Dutch but poorly understood by Dutch native participants who have no prior knowledge of it. The list of scrambled words consists of randomly shuffled words from the Dutch story. This story plays the role of intermediary comprehension as words are in Dutch (understood), however there is no sentence structure.
The duration of the Dutch, scrambled Dutch and Frisian stories, from now on referred to as ânguage conditionsâare 10, 9, and 7Â min, respectively. For the Dutch story, the participants listened to it entirely without any break, and had to answer a content question to make sure they paid attention. The Frisian and the scrambled Dutch story were presented in fragments of 2Â min, with a word identification task at the end of each fragment to ensure focus. For more details, see 21 .
For the pre-training of our model, we use an additional dataset from 36 , containing EEG of 60 young healthy native Dutch participants listening to 8 to 10 audiobooks of 14Â min each.
Speech features
This study relates 4 features from EEG signals only including the linguistic features that showed a benefit over lexical segmentation features 36 .
The investigated lexical segmentation features are: the onset of any phoneme (PO) and of any word (WO). We then tested the added value of the following linguistic features:
Cohort entropy (CE), over PO
Word frequency (WF), over WO
on our modelâs performance, measuring the neural tracking of speech.
Example phoneme-level features are depicted in Figure 1 a, and word-level features in Figure 1 b.
Lexical segmentation features: Time-aligned sequences of phonemes and words were extracted by performing a forced alignment of the identified phonemes 19 . PO and WO are the resulting one-dimensional arrays with pulses on the onsets of, respectively, phonemes and words. Silence onsets were set to 0 for both phonemes and words.
Active cohort of words: Before introducing cohort entropy, the active cohort of words must be defined. Following previous studiesâ definition 7 , 20 , it is a set of words that starts with the same acoustic input at any point in the word. For example, should we find cohorts in English, the active cohort of words for the phoneme / n / in âbanâ corresponds to the ensemble of words that exist in that language starting with âbanâ (e.g., âbannedâ,âbandwidthâ etc.). For each phoneme, the active cohort was determined by taking word segments that started with the same phoneme sequence from the lexicon.
Lexicon: For the Dutch language, the lexicon for determining the active cohort was based on a custom word-to-phoneme dictionary (9082 words). As some linguistic features are based on the word frequency in Dutch, the prior probability for each word was computed, based on its frequency in the SUBTLEX-NL database 27 .
For the Frisian language, the word-to-phoneme dictionary (75036 words) and the word frequencies were taken from 43 .
Cohort entropy: CE reflects the degree of competition among possible words that can be created from the active cohort including the current phoneme. It is defined as the Shannon entropy of the active cohort of words at each phoneme as explained in 7 (see Equation 1 ). \(CE_{i}\) is the entropy at phoneme i and \(p_{word}\) is the probability of the given word in the language. The sum iterates over words from the active cohort \(cohort_{i}\) .
Word frequency: For the Dutch language, the prior probability for each word was based on its frequency in the SUBTLEX-NL database 27 . Values corresponding to words not found in the SUBTLEX-NL were set to 0.
For the Frisian language, the word probabilities were used from 43 . WF is a measure of how frequently a word occurs in the language and is defined in Equation 2 .
More details about their implementation can be found in previous studies 6 , 20 , 36 .
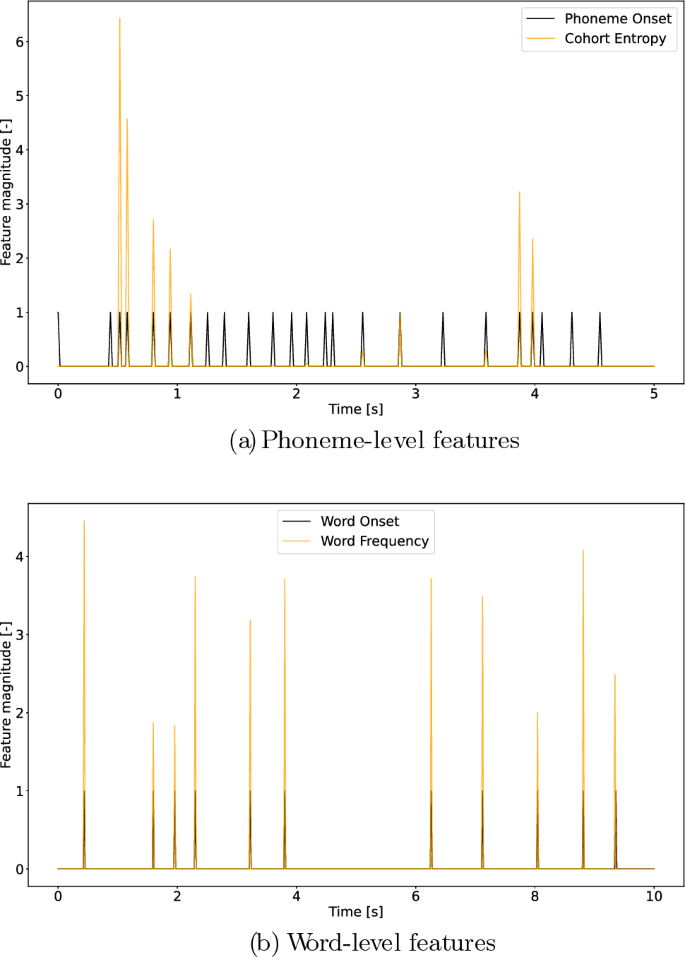
Visualization of word- and phoneme-level lexical segmentation and linguistic features. ( a ) Cohort entropy is depicted in yellow, phoneme onset in black, over a 5Â s window. ( b ) Word frequency is depicted in yellow, word onset in black, over a 10Â s window.
Preprocessing
The EEG was initially downsampled using an anti-aliasing filter from 8192 to 128Â Hz to decrease the processing time. A multi-channel Wiener filter 37 was then used to remove eyeblink artifacts, and re-referencing was performed to the average of all electrodes. The resulting signal was band-pass filtered between 0.5 and 25Â Hz using a Least Squares filter of order 5000 for the high-pass filter, and 500 for the low-pass filter, with 10% transition bands (transition of frequencies 10% above the lowpass filter and 10% below the highpass) and compensation for the group delay. We then downsampled the signal to 64Â Hz.
Lexical segmentation and linguistic features are discrete representation, namely vectors of zero and nonzero values. They were calculated at 64Â Hz and no further pre-processing was needed.
The match-mismatch task
In this study, we use the performance of the match-mismatch (MM) classification task 13 to measure the neural tracking of different speech features (Figure 2 ). We use the same paradigm as 36 . The model is trained to associate the EEG segment with the matched speech segment among two presented speech segments. The matched speech segment is synchronized with the EEG, while the mismatched speech segment occurs 1 second after the end of the matched segment. These segments are of fixed length, namely 10Â s for word-based features and 5Â s for phoneme-based features, to provide enough context to the models as hypothesized by 36 . This task is supervised since the matched and mismatched segments are labeled. The evaluation metric is classification accuracy.
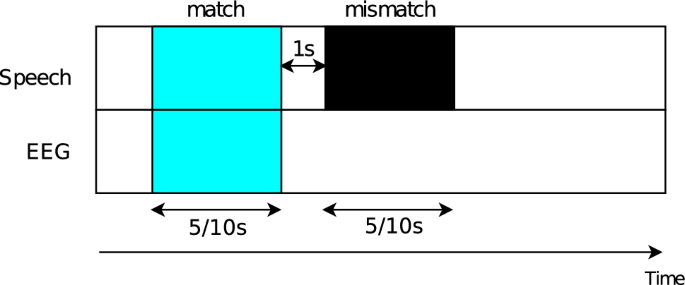
Match-mismatch classification task. The match-mismatch task is a binary classification paradigm that associates the herewith blue EEG and speech segments. The matched speech segment is synchronized with the EEG (blue segment), while the mismatched speech occurs 1 second after the end of the matched segment (black segment). The figure depicts segments of 5Â s and 10Â s, which will be lengths used in our studies for the phoneme and word levels respectively.
Multi-input features convolutional neural network 36
In 36 , we developed a multi-input convolutional neural network (MICNN) model that aims to relate different features of the presented speech to the resulting EEG. The MICNN model has 127k parameters and is trained using binary cross-entropy as its loss function (Adam optimizer, 50 epochs, learning rate: \(10^-{3}\) ). We used early stopping as regularization. It is trained to perform well on the MM task presented in "The match-mismatch task ". Through the MM task, the MICNN model learns to measure the neural tracking of speech features, which we can thereafter use to quantify the added value of one speech feature over another. By inputting multiple features, we make sure to account for redundancies and correlations between them and enable interpretation of what information makes the model better on the MM task. In our case, our models enable us to quantify the added value of a given linguistic feature (WF or CE) over their corresponding lexical segmentation feature (WO or PO, respectively)
To ensure that the model has enough data to identify a typical neural response to Dutch linguistic features, we always train the MICNN model on the dataset used in 36 as a first step. We use an identical training procedure.
Fine-tuning and evaluation on validation datasets
We performed two fine-tuning conditions: one subject-independent (language fine-tuning) and one subject-dependent (subject fine-tuning). For both, we keep the training parameters mentioned in " Multi-input features convolutional neural network ", and solely change the data used for training and evaluation.
For the language condition fine-tuning, we trained a separate model for each subject, including data from the other 25 subjects, for each of the three language conditions (i.e., Dutch, Frisian, and scrambled Dutch): We exclude a selected subject and separate the data from the 25 other subjects in an 60%/20%/20% training/validation/test split. For the 25 other subjects, the first and last 30% of their recording segment were used for training. The first half of the remaining 40% was used for validation (i.e., for regularization) and the second half to get an estimate of the accuracy of unseen speech data. Once the model is fine-tuned, we evaluate it on the selected subject.
For the subject-specific fine-tuning, a 25%/25%/50% training/validation/test split was performed. Compared to the language condition fine-tuning, selecting the data of a single subject divides the amount of data by a factor of 26 (i.e., the number of subjects) for training validation and testing. For the Dutch, Frisian and scrambled Dutch stories, the total amount of data is thus 10Â min, 7Â min and 9Â min respectively. We thus modified the split ratio to increase the amount of data in the validation set to enable keeping the batch size constant across fine-tuning conditions. We used the validation set for regularization. The first and last 12.5% of the recording segment were used for training. The first third of the remaining 75% was used for validation and the two remaining thirds for testing. For each set (training, validation, and testing), each EEG channel was then normalized by subtracting the mean and dividing by the standard deviation.
Language condition classification
Inspired by the support vector machine (SVM) utilization for aphasia classification from 10 , we use the MM accuracy obtained with four models to classify the language presented to the participant. The four models are the following: the control (word onset or phoneme onset) and linguistic (cohort entropy or word frequency) models for both fine-tuning conditions. We chose to use only the fine-tuned conditions as the non-fine-tuned one was biased towards giving better performance on the Dutch condition. These four MM accuracy values constitute the features provided to the SVM to solve a one-vs-one classification: did the person listen to one or another selected language condition. We consider three language conditions: Dutch, scrambled Dutch, and Frisian, which in total leads to three binary classification tasks.
We used a radial basis function kernel SVM and performed a nested cross-validation approach. In the inner cross-validation, the C-hyperparameter (determining the margin) and pruning were optimized (accuracy-based) and tested in a validation set using 5-fold cross-validation. Predictions were made on the test set in the outer loop using leave-one-subject-out cross-validation. We computed the receiver operating characteristic (ROC) curve and calculated the area under the curve (AUC), and further reported the accuracy, F1-score, sensitivity, and specificity of the classifier.
Impact of the language on the neural tracking of linguistic features
We only depict results with language or subject fine-tuning as pure evaluation would potentially give a performance advantage to the model on Dutch because of the pre-training on Dutch stimuli. We still show the non-fine-tuned results in Appendix A.
Evaluation of the trained MICNN across languages with language fine-tuning
Although not significant, at both the word and the phoneme levels, the neural tracking when adding the linguistics on top of lexical segmentation features is typically higher at the group level. We depict in Appendix B (see Figure B1a and B1b) the models performances at the phoneme and the word levels across stimuli.
Figure 3 depicts for all three stimuli, the difference in the MM accuracy between L and C conditions for phoneme-level features. We observed no significant difference when comparing the Frisian and the Dutch conditions (Wilcoxon signed-rank test, \(W=172, \textit{p}=0.94\) ), the Dutch and the Sc. Dutch conditions (Wilcoxon signed-rank test, \(W=160, \textit{p}=0.73\) ), and the Frisian and the Sc. Dutch conditions (Wilcoxon signed-rank test, \(W=149, \textit{p}=0.51\) ).
We also depict for all three stimuli, the L-C accuracy at the word level. We observed a significant increase of the L-C accuracy of Sc. Dutch over Frisian (Wilcoxon signed-rank test, \(W=97, \textit{p}=0.046\) ), however no significant difference in the Dutch-Frisian and Dutch-Sc. Dutch comparisons (Wilcoxon signed-rank test, Dutch-Frisian: \(W=104, \textit{p}=0.07\) , Dutch-Sc. Dutch: \(W=163, \textit{p}=0.78\) ).
To see whether the model could be improved by introducing subject information, we add a subject fine-tuning step in the next Section (for details about the method, see " Fine-tuning and evaluation on validation datasets ").
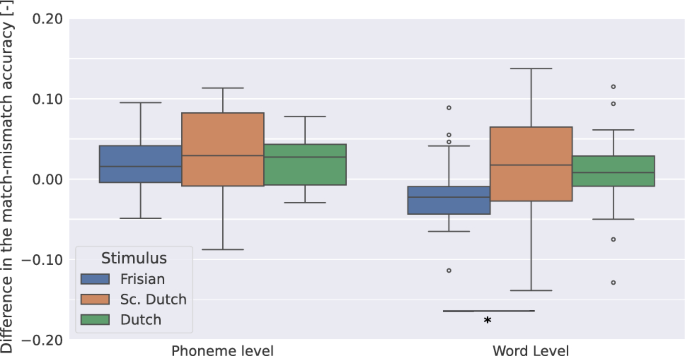
L - C accuracy for three stimuli with a language-finetuned model. L corresponds to the MM accuracy obtained by (1) the cohort entropy model at the phoneme level; (2) the word frequency model at the word level. C corresponds to the MM accuracy obtained by (1) the phoneme onset model at the phoneme level; (2) the word onset model at the word level. Significance levels : \(p<0.05\) : *.
Evaluation of the trained MICNN across languages with language and subject fine-tuning
We depict results up to half of the recording length of the shortest stimulus for each subject (i.e., 3.5Â min) as we used the other half to fine-tune the model.
Figure 4 depicts for all three stimuli, the L-C accuracy at the phoneme level. We observed no significant difference in the L-C accuracy in the Frisian-Sc. Dutch, Dutch-Sc.-Dutch and Frisian-Dutch comparisons (Wilcoxon signed-rank test, \(W=141, \textit{p}=0.39\) , and \(W=118, \textit{p}=0.15\) , and \(W=152, \textit{p}=0.78\) respectively).
We also depict for all three stimuli, the L-C accuracy at the word level. We observed a significant increase in the L-C accuracy of Dutch over Frisian (Wilcoxon signed-rank test, \(W=97, \textit{p}=0.046\) ). We observed no significant difference in the L-C accuracy for the other comparisons (Wilcoxon signed-rank test, Dutch-Frisian: Dutch-Sc.Dutch: \(W=134, \textit{p}=0.30\) , and Sc.Dutch-Frisian: \(W=144, \textit{p}=0.44\) ).
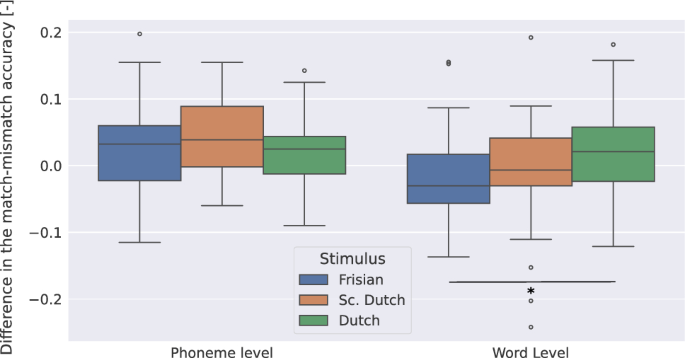
L - C accuracy across recording lengths for three stimuli with a subject-finetuned model. L corresponds to the MM accuracy obtained by (1) the cohort entropy model at the phoneme level; (2) the word frequency model at the word level. C corresponds to the MM accuracy obtained by (1) the phoneme onset model at the phoneme level; (2) the word onset model at the word level. Significance levels : \(p<0.05\) : *.
Language classification task
Figure 5 depicts the SVM classification results of the three binary classification tasks: (1) Dutch vs. Frisian, (2) Dutch vs. Scrambled Dutch, and (3) Frisian vs. Scrambled Dutch. For more details about the methods, see " Language condition classification" .
When evaluated over all subjects, our SVM classifier correctly classified the scrambled Dutch from the Frisian condition with an accuracy of 61.5% for both the FTL and FTS conditions. In addition, the classifier correctly classified the scrambled Dutch from the Dutch condition with an accuracy of 69.23% and 71.15% for the FTL and FTS conditions, respectively. We do not show the results for the Dutch vs. Frisian task, as the classifier performed close to the chance level.
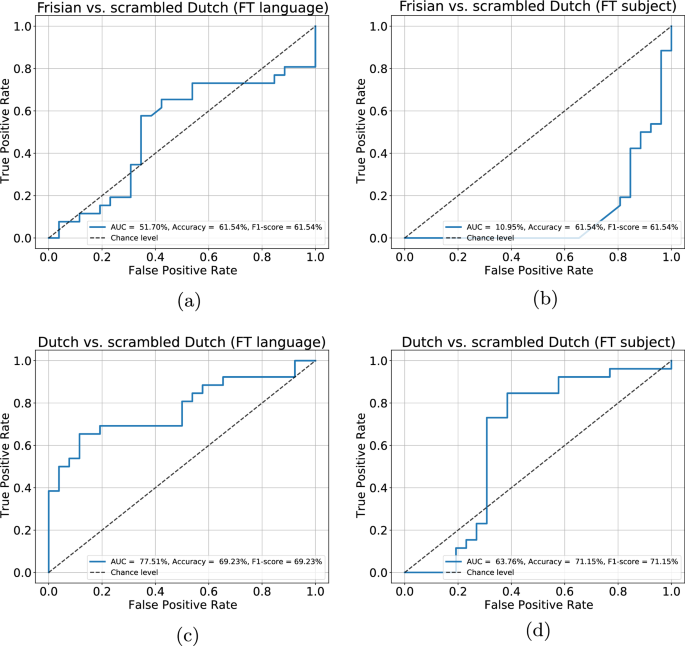
SVM performance across fine-tuning conditions. The performance is depicted for each condition as a ROC curve plotting the true positive rate as a function of the false positive rate. ( a ) Scrambled Dutch vs. Frisian classification with language fine-tuning; ( b ) with subject fine-tuning; ( c ) Scrambled Dutch vs. Dutch classification with language fine-tuning; ( d ) with subject fine-tuning.
We evaluated a deep learning framework that measures the neural tracking of linguistics on top of lexical segmentation features in different language understanding conditions. Although we used the same dataset, direct comparison with 21 is difficult, considering the difference in the models, and the features provided to the model.
As our model was trained uniquely on Dutch before, the model might not have learned the typical brain response to Frisian linguistics, or scrambled Dutch, thus leading to overfitting on Dutch, impairing the objective measure of linguistics tracking on other language conditions. To avoid this bias, we fine-tuned our model on Frisian and scrambled Dutch data before respective evaluations.
Since we are interested in the linguistics added value over lexical segmentation features, we compared the difference between the linguistic and lexical segmentation modelsâ performance across language conditions. For cohort entropy, although there is no significant difference between language conditions in the linguistics added value, the one of Frisian is systematically lower. For word frequency, we observed a significant increase in the added value of linguistics for scrambled Dutch over Frisian. In addition, although not significantly different, the linguistics added value also appeared lower for Frisian than Dutch. This finding suggests that a language that is not understood might show a lower linguistics added value. Regarding the scrambled Dutch results performing non-significantly different than Dutch, although the subjective rating of understanding was very low, individual words are still in Dutch and thus understood. Cohort entropy and word frequency are features that are independent of the order of words in the sentence, which might explain why we do not observe a drop in the neural tracking of linguistics.
Language processing in the brain is influenced by our memory, and top-down processing 23 , 29 , and might thus have a strong subject-specific component in the response to linguistic features. We therefore decided to fine-tune the models on each subject before evaluation on top of the language fine-tuning. The only significant difference we observed was for word frequency between Dutch and Frisian. This finding supports the conclusion drawn with language fine-tuning: the added value of linguistics is larger when the language is understood. We note that the subject fine-tuning diminished the data used per subject by 50% (i.e., up to  3.5 min of recording) for evaluation, which might not be sufficient to get a good estimate of the accuracy. We therefore do not interpret further the subject fine-tuning condition.
With SVM classifiers, we were able, from the match-mismatch accuracies on our different features, to classify the Frisian vs. the scrambled Dutch condition, as well as the Dutch vs. the scrambled Dutch condition. This suggests that neural tracking of linguistics and lexical segmentation features differs between continuous and scrambled speech. We expected the classifier to be able to differentiate Frisian from Dutch, as participants were not Frisian speakers. Our hypotheses to explain this phenomenon are fourfold: (1) Frisian is a language that is too similar to Dutch to measure a difference in linguistic tracking, therefore there is some understanding by the participants, as emphasized by the subjective rating from 21 (the authors reported that the speech understanding median subjective rating for the Dutch condition was 100%, while the Frisian and scrambled Dutch were 50% and 10.5%, respectively. The value for Frisian is strangely high and we believe it might in reality be lower.). On the other hand, we believe that within our framework, choosing a similar language is advisable. A very different language (e.g., Mandarin), could have caused a decrease of neural tracking for both lexical segmentation and linguistic features, obliterating our method relying on the added value of linguistics; (2) Linguistic and lexical segmentation features are too correlated, notably because their only differ in the magnitude, which might be too limited to describe the language complexity (3) the magnitude of linguistics has a distribution that tends to be skewed towards the value 1 (i.e., the magnitude of lexical segmentation features) in our three stimuli (see 21 ). A more controlled speech content (e.g., sentences with uncommon words) might make the impact of linguistics larger; (4) An additional concern can be added for word frequency: the most frequent words in the language are non-content words (e.g., âandâ, âorâ, âofâ). In addition, most of these words are short words. The model might therefore have learnt a spurious content vs. non-content words threshold from the word frequency, which can be globally narrowed-down to short vs. long words. The length of words can possibly be derived from the word onsets as well. The model could therefore simply use word onset information and omit the magnitude provided by linguistics, which would explain the low benefit of adding word frequency over word onsets.
A possible shortcoming of our training paradigm is the use of a single language for pre-training (i.e., Dutch), which might provide the fine-tuning insufficient abilities to generalize to other language conditions. To solve this issue and preserve a necessary pre-training step for complex deep learning frameworks, we could change our experimental paradigm by: (1) keeping the same language across understanding conditions to avoid biasing the model during pre-training; and (2) avoid random word-shuffling to preserve the word context in sentences. Other non-understanding conditions could involve vocoded speech or degraded-SNR speech as done in 1 . We also evaluated our framework to a speech rate paradigm 41 . However, although we observed a decreased neural tracking of linguistics in challenging listening scenarios (i.e., very high speech rates), we also observed an equivalent decreased neural tracking of lexical segmentation features. We could thus not draw any conclusions whether the nature of this decrease was acoustic or linguistic.
Another pitfall in our comparison across languages is that our linguistic features both rely on word frequencies. The word frequency values were therefore calculated for Dutch and Frisian, respectively. Our participants being Dutch speakers not speaking Frisian, have an language representation in the brain corresponding to the Dutch word frequencies, and not to the Frisian one. This might thus result in a lower neural tracking of linguistics when listening to Frisian content compared to Dutch content.
Linguistic features, as we use them now, are very constrained: they mainly give information about the word or phoneme frequency in the language. Language models are known to capture more information. As an example, the Bidirectional Transformers for Language Understanding (i.e., BERT) 16 model carries phrase-level information in its early layers, surface (e.g., sentence length) and syntactic (e.g., word order) information in the intermediate layers and semantic features (e.g., subject-verb agreement) in the late layers 25 . Such representations could contain more detailed information about the language than our current linguistic features. 15 used larger pre-trained speech encoder models, and following up on this work, we could use language model layers, providing information about the structure of language that can be related to brain responses 9 , 22 .
In this article, we investigated the impact of language understanding on the neural tracking of linguistics. We demonstrated that our previously developed deep learning framework can classify coherent from nonsense languages using the neural tracking of linguistics. We explored the ability and the limitations of state-of-the-art linguistic features to objectively measure speech understanding using lexical segmentation features as our acoustic tracking baseline. Our findings along with the current literature support the idea that, considering this framework, further work should be dedicated to (1) designing new linguistic features using recent powerful language models, and (2) using incomprehensible and comprehensible speech stimuli from the same language, to facilitate the comparison across conditions.
Data availibility
The data that support the findings of this study can be made available from the corresponding author on reasonable request, so far as this is in agreement with privacy and ethical regulations. A subset of the pretraining dataset (i.e., 60 subjects, 10 stories) was published and is available online 5 .
Accou, B., Monesi, M. J., Hamme, H. V. & Francart, T. Predicting speech intelligibility from EEG in a non-linear classification paradigm. J. Neural Eng. 18 , 066008. https://doi.org/10.1088/1741-2552/ac33e9 (2021).
Article  ADS  Google Scholar Â
Accou, B., Van Vanthornhout, J., Hamme, H. & Francart, T. Decoding of the speech envelope from EEG using the VLAAI deep neural network. Sci. Rep. 13 (1), 812. https://doi.org/10.1038/s41598-022-27332-2 (2023).
Article  ADS  CAS  PubMed  PubMed Central  Google Scholar Â
Anderson, S., Parbery-Clark, A., White-Schwoch, T. & Kraus, N. Auditory brainstem response to complex sounds predicts self-reported speech-in-noise performance. J. Speech Lang. Hear. Res. 56 (1), 31â43. https://doi.org/10.1044/1092-4388(2012/12-0043) (2013).
Article  PubMed  Google Scholar Â
Bollens, L., Francart, T., Hamme Van, H. Learning subject-invariant representations from speech-evoked eeg using variational autoencoders. In ICASSP 2022 - 2022 IEEE International Conference on Acoustics, Speech and Signal Processing (ICASSP) , pages 1256â1260, (2022). https://doi.org/10.1109/ICASSP43922.2022.9747297 .
Bollens, L., Accou, B., Van Hamme, H., Francart, T. A Large Auditory EEG decoding dataset, (2023). https://doi.org/10.48804/K3VSND
Brodbeck, C. & Simon, J. Z. Continuous speech processing. Curr. Opin. Physio. 18 , 25â31. https://doi.org/10.1016/j.cophys.2020.07.014 (2020).
Article  Google Scholar Â
Brodbeck, C., Hong, L. E. & Simon, J. Z. Rapid transformation from auditory to linguistic representations of continuous speech. Curr. Biol. 28 (24), 3976-3983.e5. https://doi.org/10.1016/j.cub.2018.10.042 (2018).
Article  CAS  PubMed  PubMed Central  Google Scholar Â
Broderick, M. P., Anderson, A. J., Di Liberto, G. M., Crosse, M. J. & Lalor, E. C. Electrophysiological correlates of semantic dissimilarity reflect the comprehension of natural, narrative speech. Curr. Biol. 28 (5), 803-809.e3. https://doi.org/10.1016/j.cub.2018.01.080 (2018).
Article  CAS  PubMed  Google Scholar Â
Caucheteux, C. & King, J.-R. Brains and algorithms partially converge in natural language processing. Commun. Biol. 5 (1), 134. https://doi.org/10.1038/s42003-022-03036-1 (2022).
Article  PubMed  PubMed Central  Google Scholar Â
De Clercq, P., Puffay, C., Kries, J., Van Hamme, H., Vandermosten, M., Francart, T, Vanthornhout, J. Detecting post-stroke aphasia via brain responses to speech in a deep learning framework, arXiv:2401.10291 (2024).
Crosse, M. J., Di Liberto, G. M., Bednar, A. & Lalor, E. C. The multivariate temporal response function (mTRF) toolbox: A MATLAB toolbox for relating neural signals to continuous stimuli. Front. Hum. Neurosci. 10 (NOV2016), 1â14. https://doi.org/10.3389/fnhum.2016.00604 (2016).
Daube, C., Ince, R. A. A. & Gross, J. Simple acoustic features can explain phoneme-based predictions of cortical responses to speech. Curr. Biol. 29 (12), 1924â19379. https://doi.org/10.1016/j.cub.2019.04.067 (2019).
de Cheveigné, A., Slaney, M., Fuglsang, S. A. & Hjortkjaer, J. Auditory stimulus-response modeling with a match-mismatch task. J. Neural Eng. 18 (4), 046040. https://doi.org/10.1088/1741-2552/abf771 (2021).
de Taillez, T., Kollmeier, B. & Meyer, B. T. Machine learning for decoding listenersâ attention from electroencephalography evoked by continuous speech. Eur. J. Neurosci. 51 (5), 1234â1241. https://doi.org/10.1111/ejn.13790 (2020).
DĂ©fossez, A., Caucheteux, C., Rapin, J., Kabeli, O. & King, J. R. Decoding speech perception from non-invasive brain recordings. Nat. Mach. Intell. 5 (10), 1097â1107. https://doi.org/10.1038/s42256-023-00714-5 (2023).
Devlin, J., Chang, M.-W., Lee, K., Toutanova, K. BERT: Pre-training of deep bidirectional transformers for language understanding. In Jill Burstein, Christy Doran, and Thamar Solorio, editors, Proceedings of the 2019 Conference of the North American Chapter of the Association for Computational Linguistics: Human Language Technologies, Volume 1 (Long and Short Papers) , pages 4171â4186, Minneapolis, Minnesota, June (2019). Association for Computational Linguistics. https://doi.org/10.18653/v1/N19-1423 .
Di Liberto, G. M., OâSullivan, J. A. & Lalor, E. C. Low-frequency cortical entrainment to speech reflects phoneme-level processing. Curr. Biol. 25 (19), 2457â2465. https://doi.org/10.1016/J.CUB.2015.08.030 (2015).
Ding, N, Simon, J.Z. Emergence of neural encoding of auditory objects while listening to competing speakers. Proceedings of the National Academy of Sciences 109 (29), 11854â11859 https://doi.org/10.1073/PNAS.1205381109 (2012).
Duchateau, J., Kong, Y., Cleuren, L., Latacz, L., Roelens, J., S., Abdurrahman, D., Kris, G., Pol, V., Werner & Van hamme, H. Developing a reading tutor : design and evaluation of dedicated speech recognition and synthesis modules, (2009). ISSN 1872-7182.
Gillis, M., Van Canneyt, J., Francart, T. & Vanthornhout, J. Neural tracking as a diagnostic tool to assess the auditory pathway. bioRxiv , (2022). https://doi.org/10.1101/2021.11.26.470129 .
Gillis, M., Vanthornhout, J. & Francart, T. Heard or understood? neural tracking of language features in a comprehensible story, an incomprehensible story and a word list. eNeuro https://doi.org/10.1523/ENEURO.0075-23.2023 (2023).
Goldstein, A. et al. Shared computational principles for language processing in humans and deep language models. Nat. Neurosci. 25 (3), 369â380. https://doi.org/10.1038/s41593-022-01026-4 (2022).
Gwilliams, L. & Davis, M. H. Extracting language content from speech sounds: The information theoretic approach 113â139 (Springer, Cham, 2022).
Google Scholar Â
Hullett, P. W., Hamilton, L. S., Mesgarani, N., Schreiner, C. E. & Chang, E. F. Human superior temporal gyrus organization of spectrotemporal modulation tuning derived from speech stimuli. J. Neurosci. 36 (6), 2014â2026 (2016).
Jawahar, G., Sagot, B., Seddah, D. What does BERT learn about the structure of language? In ACL 2019 - 57th Annual Meeting of the Association for Computational Linguistics , Florence, Italy, July (2019). https://inria.hal.science/hal-02131630 .
Keshishian, M. et al. Joint, distributed and hierarchically organized encoding of linguistic features in the human auditory cortex. Nat. Hum. Behav. 7 (5), 740â753. https://doi.org/10.1038/s41562-023-01520-0 (2023).
Keuleers, E., Brysbaert, M. & New, B. S. A new measure for Dutch word frequency based on film subtitles. Behav. Res. Methods 42 (3), 643â650 (2010).
Koskinen, M., Kurimo, M., Gross, J., HyvÀrinen, A. & Hari, R. Brain activity reflects the predictability of word sequences in listened continuous speech. Neuroimage 219 , 116936. https://doi.org/10.1016/j.neuroimage.2020.116936 (2020).
Gwilliams, D. P. L., Marantz, A. & King, J.-R. Top-down information shapes lexical processing when listening to continuous speech. Lang. Cognit. Neurosci. https://doi.org/10.1080/23273798.2023.2171072 (2023).
Lesenfants, D., Vanthornhout, J., Verschueren, E & Francart, T. Data-driven spatial filtering for improved measurement of cortical tracking of multiple representations of speech. bioRxiv , (2019). https://doi.org/10.1101/551218 .
McGee, T. J. & Clemis, J. D. The approximation of audiometric thresholds by auditory brain stem responses. Otolaryngol. Head Neck Surg. 88 (3), 295â303. https://doi.org/10.1177/019459988008800319 (1980).
Monesi, M.J., Accou, B., Montoya-Martinez, J., Francart, T., Van Hamme, H. An LSTM Based Architecture to Relate Speech Stimulus to Eeg. ICASSP, IEEE International Conference on Acoustics, Speech and Signal Processing - Proceedings 2020-May (637424): 941â945, (2020). ISSN 15206149. https://doi.org/10.1109/ICASSP40776.2020.9054000 .
Picton, T. W., Dimitrijevic, A., Perez-Abalo, M.-C. & Van Roon, P. Estimating audiometric thresholds using auditory steady-state responses. J. Am. Acad. Audiol. 16 (03), 140â156. https://doi.org/10.3766/jaaa.16.3.3 (2005).
Puffay, C., Van Canneyt, J., Vanthornhout, J., Van hamme, H. & Francart, T. 2022 Relating the fundamental frequency of speech with EEG using a dilated convolutional network. In 23rd Annual Conf. of the Int. Speech Communication Association (ISCA)âInterspeech 4038â4042 (2022).
Puffay, C. et al. Relating EEG to continuous speech using deep neural networks: A review. J. Neural Eng. 20 (4) 041003. https://doi.org/10.1088/1741-2552/ace73f (2023).
Puffay, C. et al. Robust neural tracking of linguistic speech representations using a convolutional neural network. J. Neural Eng. 20 (4), 046040. https://doi.org/10.1088/1741-2552/acf1ce (2023).
Somers, B., Francart, T. & Bertrand, A. A generic EEG artifact removal algorithm based on the multi-channel Wiener filter. J. Neural Eng. 15 (3), 036007. https://doi.org/10.1088/1741-2552/aaac92 (2018).
Article  ADS  PubMed  Google Scholar Â
Thornton, M., Mandic, D., Reichenbach, T. Relating eeg recordings to speech using envelope tracking and the speech-ffr. In ICASSP 2023 - 2023 IEEE International Conference on Acoustics, Speech and Signal Processing (ICASSP) , pages 1â2, (2023). https://doi.org/10.1109/ICASSP49357.2023.10096082 .
Van Canneyt, J., Wouters, J. & Francart, T. Neural tracking of the fundamental frequency of the voice: The effect of voice characteristics. Eur. J. Neurosci. 53 (11), 3640â3653. https://doi.org/10.1111/ejn.15229 (2021).
Vanthornhout, J., Decruy, L., Wouters, J., Simon, J. Z. & Francart, T. Speech intelligibility predicted from neural entrainment of the speech envelope. JARO - J. Assoc. Res. Otolaryngol. 19 (2), 181â191. https://doi.org/10.1007/s10162-018-0654-z (2018).
Verschueren, E., Gillis, M., Decruy, L., Vanthornhout, J. & Francart, T. Speech understanding oppositely affects acoustic and linguistic neural tracking in a speech rate manipulation paradigm. J. Neurosci. 42 (39), 7442â7453. https://doi.org/10.1523/JNEUROSCI.0259-22.2022 (2022).
Weissbart, H., Kandylaki, K. & Reichenbach, T. Cortical tracking of surprisal during continuous speech comprehension. J. Cognit. Neurosci. 32 , 1â12 (2019).
Yılmaz, E. et al. Open Source Speech and Language Resources for Frisian. Proc. Interspeech 2016 , pages 1536â1540, (2016). https://doi.org/10.21437/Interspeech.2016-48 .
Download references
Acknowledgements
The authors thank all the participants for the recordings, as well as Wendy Verheijen, Marte De Jonghe, Kyara Cloes, Amelie Algoet, Jolien Smeulders, Lore Kerkhofs, Sara Peeters, Merel Dillen, Ilham Gamgami, Amber Verhoeven, Lies Bollens, Vitor Vasconcelos and Amber Aerts for their help with data collection. Funding was provided by FWO fellowships to Bernd Accou (1S89622N), Marlies Gillis (1SA0620N; additional Internal Funds KU Leuven: PDMT1/23/011), Corentin Puffay (1S49823N), Pieter De Clercq (1S40122N), and Jonas Vanthornhout (1290821N).
Author information
Authors and affiliations.
Department Neurosciences, KU Leuven, ExpORL, Leuven, Belgium
Corentin Puffay, Jonas Vanthornhout, Marlies Gillis, Pieter De Clercq, Bernd Accou & Tom Francart
Department of Electrical engineering (ESAT), KU Leuven, PSI, Leuven, Belgium
Corentin Puffay, Bernd Accou & Hugo Van hamme
You can also search for this author in PubMed  Google Scholar
Contributions
C.P. wrote the manuscript, prepared figures and did the analyses, as well as the interpretation of the results present in the article. J.V. provided the main guidance, was heavily involved in the thinking process, and in the interpretation of the results. M.G. shared the data from her publication, provided help to C.P. in the preprocessing of data, was involved in the thinking process, and the interpretation of the results. P.DC. was involved in the thinking process and wrote the scripts for the SVM classification task. B.A. was involved in the thinking process, and provided the basis of the deep learning framework code. H.VH. and T.F. provided guidance and were involved in the interpretation of the results. All authors reviewed the manuscript.
Corresponding authors
Correspondence to Corentin Puffay or Tom Francart .
Ethics declarations
Competing interests.
The authors declare no competing interests.
Additional information
Publisher's note.
Springer Nature remains neutral with regard to jurisdictional claims in published maps and institutional affiliations.
Supplementary Information
Below is the link to the electronic supplementary material.
Supplementary Information 1.
Rights and permissions.
Open Access This article is licensed under a Creative Commons Attribution 4.0 International License, which permits use, sharing, adaptation, distribution and reproduction in any medium or format, as long as you give appropriate credit to the original author(s) and the source, provide a link to the Creative Commons licence, and indicate if changes were made. The images or other third party material in this article are included in the article's Creative Commons licence, unless indicated otherwise in a credit line to the material. If material is not included in the article's Creative Commons licence and your intended use is not permitted by statutory regulation or exceeds the permitted use, you will need to obtain permission directly from the copyright holder. To view a copy of this licence, visit http://creativecommons.org/licenses/by/4.0/ .
Reprints and permissions
About this article
Cite this article.
Puffay, C., Vanthornhout, J., Gillis, M. et al. Classifying coherent versus nonsense speech perception from EEG using linguistic speech features. Sci Rep 14 , 18922 (2024). https://doi.org/10.1038/s41598-024-69568-0
Download citation
Received : 15 April 2024
Accepted : 06 August 2024
Published : 14 August 2024
DOI : https://doi.org/10.1038/s41598-024-69568-0
Share this article
Anyone you share the following link with will be able to read this content:
Sorry, a shareable link is not currently available for this article.
Provided by the Springer Nature SharedIt content-sharing initiative
- EEG decoding
- Deep learning
- Linguistics
By submitting a comment you agree to abide by our Terms and Community Guidelines . If you find something abusive or that does not comply with our terms or guidelines please flag it as inappropriate.
Quick links
- Explore articles by subject
- Guide to authors
- Editorial policies
Sign up for the Nature Briefing: AI and Robotics newsletter â what matters in AI and robotics research, free to your inbox weekly.

Advertisement
Michelle Obama, Thrashing Trump, Suggests the Presidency Is a âBlack Jobâ
The former first lady enthralled a packed arena on Tuesday evening with one of the Democratic National Conventionâs most emphatic takedowns of Donald J. Trump.
- Share full article
Michelle Obama Speaks on Second Night of Democratic Convention
The former first lady delivered a takedown of former president donald j. trump, asking, âwhoâs going to tell him that the job heâs currently seeking might just be one of those âblack jobsââ.
For years, Donald Trump did everything in his power to try to make people fear us. See, his limited, narrow view of the world made him feel threatened by the existence of two hard-working, highly educated, successful people who happen to be Black. I want to know. I want to know whoâs going to tell him? Whoâs going to tell him that the job heâs currently seeking might just be one of those Black jobs?

By Katie Rogers
Reporting from the Democratic National Convention in Chicago
- Aug. 21, 2024
Michelle Obama, the former first lady and one of the most popular figures in the Democratic Party, delivered one of the Democratic National Conventionâs most emphatic takedowns of former President Donald J. Trump on Tuesday night and turned one of his most controversial campaign lines against him: âWhoâs going to tell him that the job heâs currently seeking might just be one of those âBlack jobsâ?â she said.
Mrs. Obama, a reluctant campaigner, enthralled a packed arena in Chicago with a convention appearance that lent firepower to Vice President Kamala Harrisâs presidential campaign. She offered support and praise for Ms. Harris, but focused much of her nearly 20-minute speech squarely on Mr. Trump, mocking his past comments, his background and his behavior, while mostly avoiding naming him.
And for a speech delivered at a political convention, her remarks struck a remarkably personal tone as she spoke of the former president, who led a multiyear campaign to question the birthplace of her husband, former President Barack Obama.
âFor years, Donald Trump did everything in his power to try to make people fear us,â she said, adding that âhis limited, narrow view of the world made him feel threatened by the existence of two hardworking, highly educated, successful people who happen to be Black.â
She zeroed in on his debate-night complaint about immigrants taking âBlack jobsâ by pointing out that the presidency of the United States has been one and might soon be again. She said that Americans like Ms. Harris understood âthat most of us will never be afforded the grace of failing forward,â a reference to Mr. Trumpâs business troubles. She noted that most Americans do not grow up with âthe affirmative action of generational wealth.â (Mr. Trump was born into a wealthy family in Queens.)
âIf we see a mountain in front of us, we donât expect there to be an escalator waiting to take us to the top,â she said. Line by line, she received thunderous applause.
We are having trouble retrieving the article content.
Please enable JavaScript in your browser settings.
Thank you for your patience while we verify access. If you are in Reader mode please exit and log into  your Times account, or subscribe  for all of The Times.
Thank you for your patience while we verify access.
Already a subscriber? Log in .
Want all of The Times? Subscribe .
Harris' plan to fight price gouging: what is the legal framework?
- Medium Text
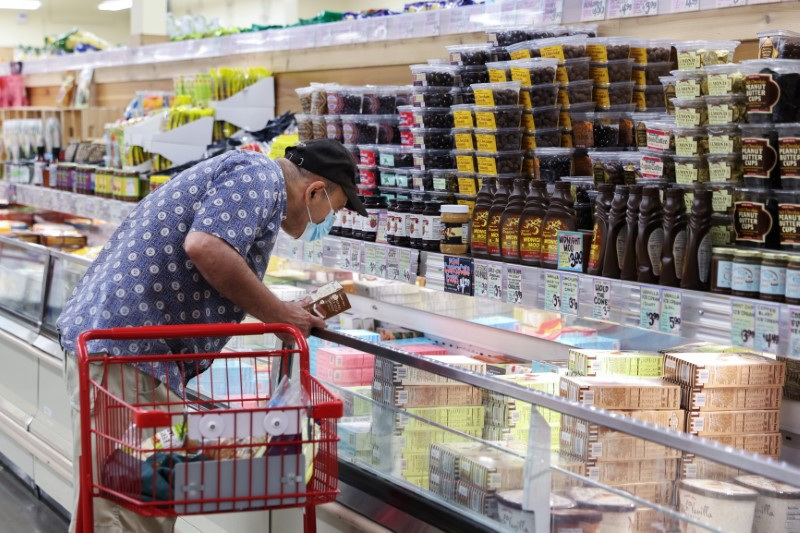
Sign up here.
Reporting by Jody Godoy in New York; editing by Chris Sanders and Jonathan Oatis
Our Standards: The Thomson Reuters Trust Principles. , opens new tab

Thomson Reuters
Jody Godoy reports on tech policy and antitrust enforcement, including how regulators are responding to the rise of AI. Reach her at [email protected]
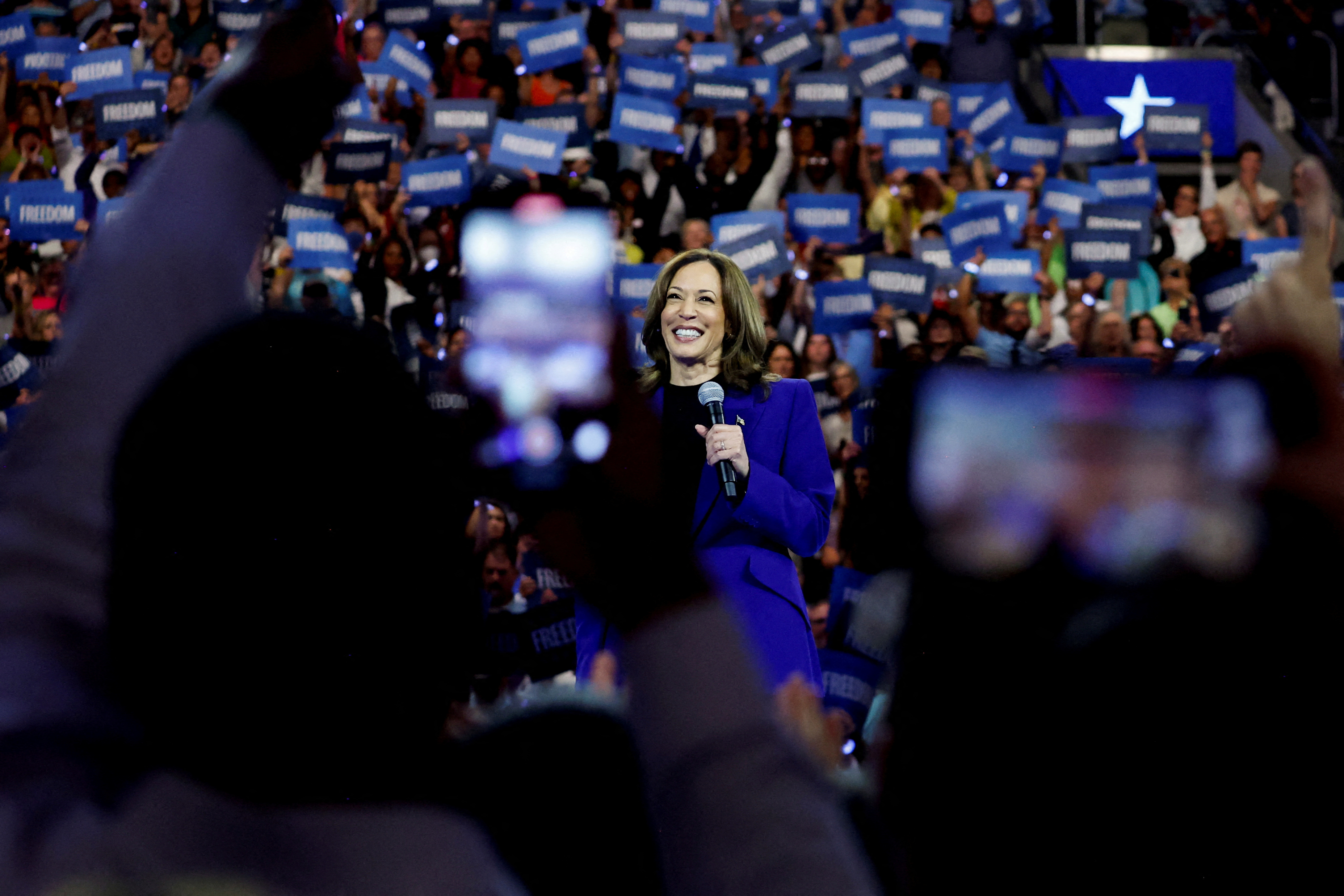
NASA says decision on Boeing Starliner crew's path home coming Saturday
NASA said on Thursday it expects to announce on Saturday its decision on whether the two astronauts who rode Boeing's glitchy Starliner spacecraft to the International Space Station will need a SpaceX vehicle to return to Earth.
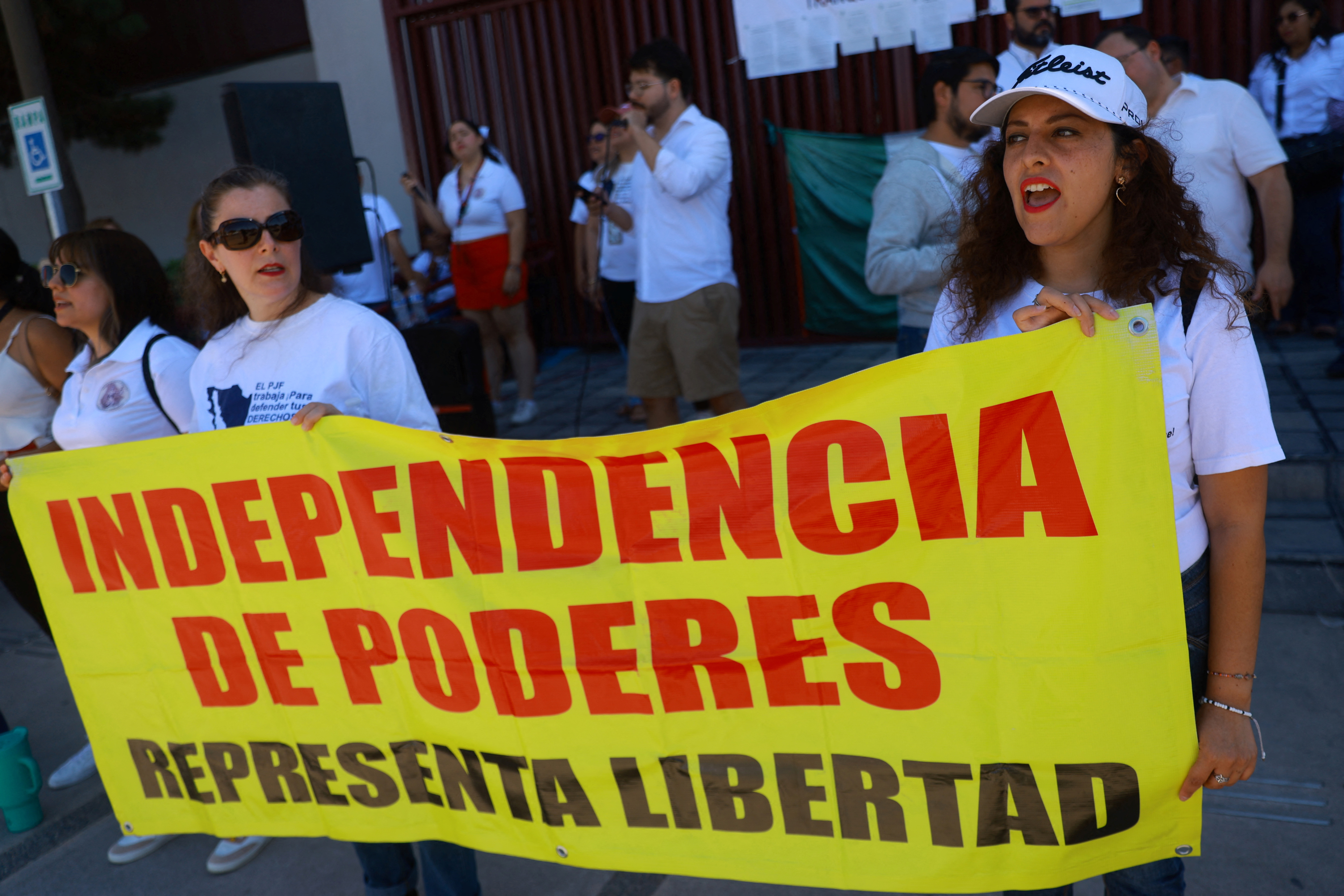

IMAGES
COMMENTS
Speech, language and communication needs can occur in childhood as primary difficulties with speech, language and communication or secondary to other developmental conditions such as autism. They can also be acquired in adulthood. Children Speech, language and communication needs are some of the most common childhood disabilities:
Communication refers to how we interact with others: Using spoken language, and, signs, symbols and gestures to have a conversation or give directions. Being able to start and close a conversation, keep the conversation flowing, and repair the conversation if there's a misunderstanding. Being able to consider other people's points of view.
Some children and young people find it difficult to listen, understand and communicate with others and may need support to develop the surprising number of skills involved. SLCN is the umbrella term most commonly used to describe these difficulties. It stands for Speech, Language and Communication Needs. Children with SLCN.
Speech, language and communication needs are difficulties using intelligible speech; understanding and using language; talking fluently; and using a clear voice. Oral language is much more than speaking and listening. We think and learn through language - oral language is the basis for all thought and communication.
Here is a list of Speech, Language and Communication Needs (SLCN) red flags that Teachers and Support Staff can look out for in the classroom. The child may: đ© Have poor attention skills. đ© Rely on routines and copying others. đ© Only follow the last part of instructions. đ© Lack awareness of what is going on around them.
Speech, language, and communication needs (SLCN) are difficulties across one or more aspects of communication and interaction. They range from mild to very severe, and they are a type of special educational need and/or disability (SEND). They can occur in conjunction with other conditions, such as autism spectrum conditions or cerebral palsy ...
Speech, language and communication needs can occur on their own without any other developmental needs, or be part of another condition such as general learning difficulties, autism spectrum disorders or attention deficit hyperactivity disorder. For many children, difficulties will resolve naturally when they experience good communication-rich ...
Speech language and communication needs Speech, language and communication needs are associated with a number of factors: Gender is associated with the greatest increase in risk for both SLCN and ASD, with boys overrepresented relative to girls 2.5:1 for SLCN and over 6:1 for ASD. Birth season effects are strong for SLCN but not ASD.
Speech, Language and Communication Needs (SLCN) is an umbrella term which can describe difficulties in one or more areas. It is estimated that 10 per cent of children and young people have some form of SLCN. What are Speech, Language and Communication Needs (SLCN)? SLCN is an umbrella term which can describe difficulties in one or more areas, including:
Speech is how we say sounds and words. Speech includes: How we make speech sounds using the mouth, lips, and tongue. For example, we need to be able to say the "r" sound to say "rabbit" instead of "wabbit.". How we use our vocal folds and breath to make sounds. Our voice can be loud or soft or high- or low-pitched.
Communication disability can impact on interactions at home, at work and socially. Speech and language difficulties can affect learning at school including literacy, numeracy and interacting socially with other children. Long term implications of speech and language difficulty include reduced academic achievement, risk to mental health, reduced ...
Detailed Findings. The term 'speech, language and commun ication needs' (SLCN) The term speech, language and communication n eeds is problematic because. The term is used in different ways by ...
Speech, language and communication needs (SLCN) is the term used to describe difficulties with: producing speech sounds accurately. stammering. voice problems, such as hoarseness and loss of voice. understanding language (making sense of what people say) using language (words and sentences) interacting with others, for example, difficulties ...
Sendco Gemma Corby shares her advice for working with pupils with speech, language and communication needs. Language skills and vocabulary are widely recognised as being the biggest predictors of a child's success at school. The Rose Report (2009) stated that there is strong evidence that between 35 and 40 per cent of children with reading ...
Communication refers to how we interact with others and is sometimes referred to as pragmatics. Communication skills can include: D being able to use and demonstrate good listening and attention. D looking at people when in a conversation. D knowing how to talk to others and take turns.
Speech, language and communication needs (SLCN) encompasses a wide range of difficulties such as a speech delay, autism or Down's syndrome. The children's communication charity ican offers an insight into what SLCN is. A child with speech, language and communication needs: Might have speech that is difficult to understand.
A checklist of milestones for the normal development of speech and language skills in children from birth to 5 years of age is included below. These milestones help doctors and other health professionals determine if a child is on track or if he or she may need extra help. Sometimes a delay may be caused by hearing loss, while other times it ...
Definition of Communication. Communication is the active process of exchanging information and ideas. Communication involves both understanding and expression. Forms of expression may include personalized movements, gestures, objects, vocalizations, verbalizations, signs, pictures, symbols, printed words, and output from augmentative and ...
Speech therapy is the assessment and treatment of various speech and language disorders and communication problems. It may be needed for speech disorders that develop in childhood or speech impairments in adults caused by an illness or injury, such as stroke or brain injury.
Speech is the use of the human voice as a medium for language. Spoken language combines vowel and consonant sounds to form units of meaning like words, which belong to a language's lexicon.There are many different intentional speech acts, such as informing, declaring, asking, persuading, directing; acts may vary in various aspects like enunciation, intonation, loudness, and tempo to convey ...
speech: [noun] the communication or expression of thoughts in spoken words. exchange of spoken words : conversation.
When Walz delivered his acceptance speech inside the packed United Center arena Wednesday night, Gus watched from the audience with his mother and sister, Hope, and sobbed.
A woman holding a mobile phone. "Connections" has become an online sensation since it was released in June 2023. Davidovici/Getty Images. The uniting themes can come from various categoriesâbe ...
Electroencephalography (EEG) is a non-invasive method that can be used to study brain responses to sounds. Traditionally, unnatural periodic stimuli (e.g., click trains, modulated tones, repeated ...
Michelle Obama, Thrashing Trump, Suggests the Presidency Is a 'Black Job' The former first lady enthralled a packed arena on Tuesday evening with one of the Democratic National Convention's ...
The most comprehensive solution to manage all your complex and ever-expanding tax and compliance needs. Checkpoint , opens new tab The industry leader for online information for tax, accounting ...
By definition, to be demure it to be "reserved, modest, and shy." But this month the term has become the internet's latest buzzword, aesthetic and mentality, with many referring to the upcoming ...