Comparison in Scientific Research: Uncovering statistically significant relationships
by Anthony Carpi, Ph.D., Anne E. Egger, Ph.D.
Listen to this reading
Did you know that when Europeans first saw chimpanzees, they thought the animals were hairy, adult humans with stunted growth? A study of chimpanzees paved the way for comparison to be recognized as an important research method. Later, Charles Darwin and others used this comparative research method in the development of the theory of evolution.
Comparison is used to determine and quantify relationships between two or more variables by observing different groups that either by choice or circumstance are exposed to different treatments.
Comparison includes both retrospective studies that look at events that have already occurred, and prospective studies, that examine variables from the present forward.
Comparative research is similar to experimentation in that it involves comparing a treatment group to a control, but it differs in that the treatment is observed rather than being consciously imposed due to ethical concerns, or because it is not possible, such as in a retrospective study.
Anyone who has stared at a chimpanzee in a zoo (Figure 1) has probably wondered about the animal's similarity to humans. Chimps make facial expressions that resemble humans, use their hands in much the same way we do, are adept at using different objects as tools, and even laugh when they are tickled. It may not be surprising to learn then that when the first captured chimpanzees were brought to Europe in the 17 th century, people were confused, labeling the animals "pygmies" and speculating that they were stunted versions of "full-grown" humans. A London physician named Edward Tyson obtained a "pygmie" that had died of an infection shortly after arriving in London, and began a systematic study of the animal that cataloged the differences between chimpanzees and humans, thus helping to establish comparative research as a scientific method .
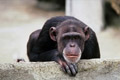
Figure 1: A chimpanzee
- A brief history of comparative methods
In 1698, Tyson, a member of the Royal Society of London, began a detailed dissection of the "pygmie" he had obtained and published his findings in the 1699 work: Orang-Outang, sive Homo Sylvestris: or, the Anatomy of a Pygmie Compared with that of a Monkey, an Ape, and a Man . The title of the work further reflects the misconception that existed at the time – Tyson did not use the term Orang-Outang in its modern sense to refer to the orangutan; he used it in its literal translation from the Malay language as "man of the woods," as that is how the chimps were viewed.
Tyson took great care in his dissection. He precisely measured and compared a number of anatomical variables such as brain size of the "pygmie," ape, and human. He recorded his measurements of the "pygmie," even down to the direction in which the animal's hair grew: "The tendency of the Hair of all of the Body was downwards; but only from the Wrists to the Elbows 'twas upwards" (Russell, 1967). Aided by William Cowper, Tyson made drawings of various anatomical structures, taking great care to accurately depict the dimensions of these structures so that they could be compared to those in humans (Figure 2). His systematic comparative study of the dimensions of anatomical structures in the chimp, ape, and human led him to state:
in the Organization of abundance of its Parts, it more approached to the Structure of the same in Men: But where it differs from a Man, there it resembles plainly the Common Ape, more than any other Animal. (Russell, 1967)
Tyson's comparative studies proved exceptionally accurate and his research was used by others, including Thomas Henry Huxley in Evidence as to Man's Place in Nature (1863) and Charles Darwin in The Descent of Man (1871).
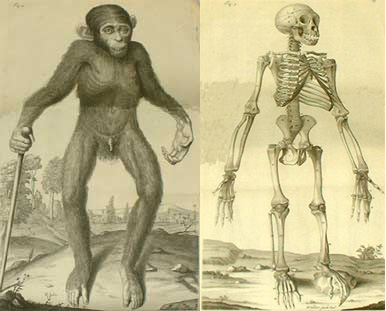
Figure 2: Edward Tyson's drawing of the external appearance of a "pygmie" (left) and the animal's skeleton (right) from The Anatomy of a Pygmie Compared with that of a Monkey, an Ape, and a Man from the second edition, London, printed for T. Osborne, 1751.
Tyson's methodical and scientific approach to anatomical dissection contributed to the development of evolutionary theory and helped establish the field of comparative anatomy. Further, Tyson's work helps to highlight the importance of comparison as a scientific research method .
- Comparison as a scientific research method
Comparative research represents one approach in the spectrum of scientific research methods and in some ways is a hybrid of other methods, drawing on aspects of both experimental science (see our Experimentation in Science module) and descriptive research (see our Description in Science module). Similar to experimentation, comparison seeks to decipher the relationship between two or more variables by documenting observed differences and similarities between two or more subjects or groups. In contrast to experimentation, the comparative researcher does not subject one of those groups to a treatment , but rather observes a group that either by choice or circumstance has been subject to a treatment. Thus comparison involves observation in a more "natural" setting, not subject to experimental confines, and in this way evokes similarities with description.
Importantly, the simple comparison of two variables or objects is not comparative research . Tyson's work would not have been considered scientific research if he had simply noted that "pygmies" looked like humans without measuring bone lengths and hair growth patterns. Instead, comparative research involves the systematic cataloging of the nature and/or behavior of two or more variables, and the quantification of the relationship between them.
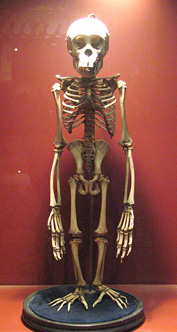
Figure 3: Skeleton of the juvenile chimpanzee dissected by Edward Tyson, currently displayed at the Natural History Museum, London.
While the choice of which research method to use is a personal decision based in part on the training of the researchers conducting the study, there are a number of scenarios in which comparative research would likely be the primary choice.
- The first scenario is one in which the scientist is not trying to measure a response to change, but rather he or she may be trying to understand the similarities and differences between two subjects . For example, Tyson was not observing a change in his "pygmie" in response to an experimental treatment . Instead, his research was a comparison of the unknown "pygmie" to humans and apes in order to determine the relationship between them.
- A second scenario in which comparative studies are common is when the physical scale or timeline of a question may prevent experimentation. For example, in the field of paleoclimatology, researchers have compared cores taken from sediments deposited millions of years ago in the world's oceans to see if the sedimentary composition is similar across all oceans or differs according to geographic location. Because the sediments in these cores were deposited millions of years ago, it would be impossible to obtain these results through the experimental method . Research designed to look at past events such as sediment cores deposited millions of years ago is referred to as retrospective research.
- A third common comparative scenario is when the ethical implications of an experimental treatment preclude an experimental design. Researchers who study the toxicity of environmental pollutants or the spread of disease in humans are precluded from purposefully exposing a group of individuals to the toxin or disease for ethical reasons. In these situations, researchers would set up a comparative study by identifying individuals who have been accidentally exposed to the pollutant or disease and comparing their symptoms to those of a control group of people who were not exposed. Research designed to look at events from the present into the future, such as a study looking at the development of symptoms in individuals exposed to a pollutant, is referred to as prospective research.
Comparative science was significantly strengthened in the late 19th and early 20th century with the introduction of modern statistical methods . These were used to quantify the association between variables (see our Statistics in Science module). Today, statistical methods are critical for quantifying the nature of relationships examined in many comparative studies. The outcome of comparative research is often presented in one of the following ways: as a probability , as a statement of statistical significance , or as a declaration of risk. For example, in 2007 Kristensen and Bjerkedal showed that there is a statistically significant relationship (at the 95% confidence level) between birth order and IQ by comparing test scores of first-born children to those of their younger siblings (Kristensen & Bjerkedal, 2007). And numerous studies have contributed to the determination that the risk of developing lung cancer is 30 times greater in smokers than in nonsmokers (NCI, 1997).

Comprehension Checkpoint
- Comparison in practice: The case of cigarettes
In 1919, Dr. George Dock, chairman of the Department of Medicine at Barnes Hospital in St. Louis, asked all of the third- and fourth-year medical students at the teaching hospital to observe an autopsy of a man with a disease so rare, he claimed, that most of the students would likely never see another case of it in their careers. With the medical students gathered around, the physicians conducting the autopsy observed that the patient's lungs were speckled with large dark masses of cells that had caused extensive damage to the lung tissue and had forced the airways to close and collapse. Dr. Alton Ochsner, one of the students who observed the autopsy, would write years later that "I did not see another case until 1936, seventeen years later, when in a period of six months, I saw nine patients with cancer of the lung. – All the afflicted patients were men who smoked heavily and had smoked since World War I" (Meyer, 1992).
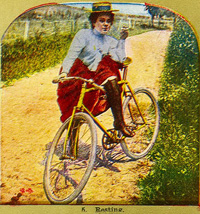
Figure 4: Image from a stereoptic card showing a woman smoking a cigarette circa 1900
The American physician Dr. Isaac Adler was, in fact, the first scientist to propose a link between cigarette smoking and lung cancer in 1912, based on his observation that lung cancer patients often reported that they were smokers. Adler's observations, however, were anecdotal, and provided no scientific evidence toward demonstrating a relationship. The German epidemiologist Franz Müller is credited with the first case-control study of smoking and lung cancer in the 1930s. Müller sent a survey to the relatives of individuals who had died of cancer, and asked them about the smoking habits of the deceased. Based on the responses he received, Müller reported a higher incidence of lung cancer among heavy smokers compared to light smokers. However, the study had a number of problems. First, it relied on the memory of relatives of deceased individuals rather than first-hand observations, and second, no statistical association was made. Soon after this, the tobacco industry began to sponsor research with the biased goal of repudiating negative health claims against cigarettes (see our Scientific Institutions and Societies module for more information on sponsored research).
Beginning in the 1950s, several well-controlled comparative studies were initiated. In 1950, Ernest Wynder and Evarts Graham published a retrospective study comparing the smoking habits of 605 hospital patients with lung cancer to 780 hospital patients with other diseases (Wynder & Graham, 1950). Their study showed that 1.3% of lung cancer patients were nonsmokers while 14.6% of patients with other diseases were nonsmokers. In addition, 51.2% of lung cancer patients were "excessive" smokers while only 19.1% of other patients were excessive smokers. Both of these comparisons proved to be statistically significant differences. The statisticians who analyzed the data concluded:
when the nonsmokers and the total of the high smoking classes of patients with lung cancer are compared with patients who have other diseases, we can reject the null hypothesis that smoking has no effect on the induction of cancer of the lungs.
Wynder and Graham also suggested that there might be a lag of ten years or more between the period of smoking in an individual and the onset of clinical symptoms of cancer. This would present a major challenge to researchers since any study that investigated the relationship between smoking and lung cancer in a prospective fashion would have to last many years.
Richard Doll and Austin Hill published a similar comparative study in 1950 in which they showed that there was a statistically higher incidence of smoking among lung cancer patients compared to patients with other diseases (Doll & Hill, 1950). In their discussion, Doll and Hill raise an interesting point regarding comparative research methods by saying,
This is not necessarily to state that smoking causes carcinoma of the lung. The association would occur if carcinoma of the lung caused people to smoke or if both attributes were end-effects of a common cause.
They go on to assert that because the habit of smoking was seen to develop before the onset of lung cancer, the argument that lung cancer leads to smoking can be rejected. They therefore conclude, "that smoking is a factor, and an important factor, in the production of carcinoma of the lung."
Despite this substantial evidence , both the tobacco industry and unbiased scientists raised objections, claiming that the retrospective research on smoking was "limited, inconclusive, and controversial." The industry stated that the studies published did not demonstrate cause and effect, but rather a spurious association between two variables . Dr. Wilhelm Hueper of the National Cancer Institute, a scientist with a long history of research into occupational causes of cancers, argued that the emphasis on cigarettes as the only cause of lung cancer would compromise research support for other causes of lung cancer. Ronald Fisher , a renowned statistician, also was opposed to the conclusions of Doll and others, purportedly because they promoted a "puritanical" view of smoking.
The tobacco industry mounted an extensive campaign of misinformation, sponsoring and then citing research that showed that smoking did not cause "cardiac pain" as a distraction from the studies that were being published regarding cigarettes and lung cancer. The industry also highlighted studies that showed that individuals who quit smoking suffered from mild depression, and they pointed to the fact that even some doctors themselves smoked cigarettes as evidence that cigarettes were not harmful (Figure 5).
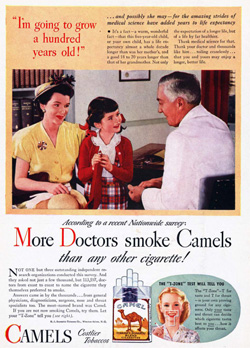
Figure 5: Cigarette advertisement circa 1946.
While the scientific research began to impact health officials and some legislators, the industry's ad campaign was effective. The US Federal Trade Commission banned tobacco companies from making health claims about their products in 1955. However, more significant regulation was averted. An editorial that appeared in the New York Times in 1963 summed up the national sentiment when it stated that the tobacco industry made a "valid point," and the public should refrain from making a decision regarding cigarettes until further reports were issued by the US Surgeon General.
In 1951, Doll and Hill enrolled 40,000 British physicians in a prospective comparative study to examine the association between smoking and the development of lung cancer. In contrast to the retrospective studies that followed patients with lung cancer back in time, the prospective study was designed to follow the group forward in time. In 1952, Drs. E. Cuyler Hammond and Daniel Horn enrolled 187,783 white males in the United States in a similar prospective study. And in 1959, the American Cancer Society (ACS) began the first of two large-scale prospective studies of the association between smoking and the development of lung cancer. The first ACS study, named Cancer Prevention Study I, enrolled more than 1 million individuals and tracked their health, smoking and other lifestyle habits, development of diseases, cause of death, and life expectancy for almost 13 years (Garfinkel, 1985).
All of the studies demonstrated that smokers are at a higher risk of developing and dying from lung cancer than nonsmokers. The ACS study further showed that smokers have elevated rates of other pulmonary diseases, coronary artery disease, stroke, and cardiovascular problems. The two ACS Cancer Prevention Studies would eventually show that 52% of deaths among smokers enrolled in the studies were attributed to cigarettes.
In the second half of the 20 th century, evidence from other scientific research methods would contribute multiple lines of evidence to the conclusion that cigarette smoke is a major cause of lung cancer:
Descriptive studies of the pathology of lungs of deceased smokers would demonstrate that smoking causes significant physiological damage to the lungs. Experiments that exposed mice, rats, and other laboratory animals to cigarette smoke showed that it caused cancer in these animals (see our Experimentation in Science module for more information). Physiological models would help demonstrate the mechanism by which cigarette smoke causes cancer.
As evidence linking cigarette smoke to lung cancer and other diseases accumulated, the public, the legal community, and regulators slowly responded. In 1957, the US Surgeon General first acknowledged an association between smoking and lung cancer when a report was issued stating, "It is clear that there is an increasing and consistent body of evidence that excessive cigarette smoking is one of the causative factors in lung cancer." In 1965, over objections by the tobacco industry and the American Medical Association, which had just accepted a $10 million grant from the tobacco companies, the US Congress passed the Federal Cigarette Labeling and Advertising Act, which required that cigarette packs carry the warning: "Caution: Cigarette Smoking May Be Hazardous to Your Health." In 1967, the US Surgeon General issued a second report stating that cigarette smoking is the principal cause of lung cancer in the United States. While the tobacco companies found legal means to protect themselves for decades following this, in 1996, Brown and Williamson Tobacco Company was ordered to pay $750,000 in a tobacco liability lawsuit; it became the first liability award paid to an individual by a tobacco company.
- Comparison across disciplines
Comparative studies are used in a host of scientific disciplines, from anthropology to archaeology, comparative biology, epidemiology , psychology, and even forensic science. DNA fingerprinting, a technique used to incriminate or exonerate a suspect using biological evidence , is based on comparative science. In DNA fingerprinting, segments of DNA are isolated from a suspect and from biological evidence such as blood, semen, or other tissue left at a crime scene. Up to 20 different segments of DNA are compared between that of the suspect and the DNA found at the crime scene. If all of the segments match, the investigator can calculate the statistical probability that the DNA came from the suspect as opposed to someone else. Thus DNA matches are described in terms of a "1 in 1 million" or "1 in 1 billion" chance of error.
Comparative methods are also commonly used in studies involving humans due to the ethical limits of experimental treatment . For example, in 2007, Petter Kristensen and Tor Bjerkedal published a study in which they compared the IQ of over 250,000 male Norwegians in the military (Kristensen & Bjerkedal, 2007). The researchers found a significant relationship between birth order and IQ, where the average IQ of first-born male children was approximately three points higher than the average IQ of the second-born male in the same family. The researchers further showed that this relationship was correlated with social rather than biological factors, as second-born males who grew up in families in which the first-born child died had average IQs similar to other first-born children. One might imagine a scenario in which this type of study could be carried out experimentally, for example, purposefully removing first-born male children from certain families, but the ethics of such an experiment preclude it from ever being conducted.
- Limitations of comparative methods
One of the primary limitations of comparative methods is the control of other variables that might influence a study. For example, as pointed out by Doll and Hill in 1950, the association between smoking and cancer deaths could have meant that: a) smoking caused lung cancer, b) lung cancer caused individuals to take up smoking, or c) a third unknown variable caused lung cancer AND caused individuals to smoke (Doll & Hill, 1950). As a result, comparative researchers often go to great lengths to choose two different study groups that are similar in almost all respects except for the treatment in question. In fact, many comparative studies in humans are carried out on identical twins for this exact reason. For example, in the field of tobacco research , dozens of comparative twin studies have been used to examine everything from the health effects of cigarette smoke to the genetic basis of addiction.
- Comparison in modern practice
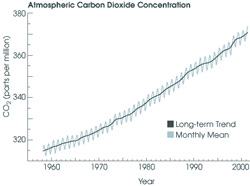
Figure 6: The "Keeling curve," a long-term record of atmospheric CO 2 concentration measured at the Mauna Loa Observatory (Keeling et al.). Although the annual oscillations represent natural, seasonal variations, the long-term increase means that concentrations are higher than they have been in 400,000 years. Graphic courtesy of NASA's Earth Observatory.
Despite the lessons learned during the debate that ensued over the possible effects of cigarette smoke, misconceptions still surround comparative science. For example, in the late 1950s, Charles Keeling , an oceanographer at the Scripps Institute of Oceanography, began to publish data he had gathered from a long-term descriptive study of atmospheric carbon dioxide (CO 2 ) levels at the Mauna Loa observatory in Hawaii (Keeling, 1958). Keeling observed that atmospheric CO 2 levels were increasing at a rapid rate (Figure 6). He and other researchers began to suspect that rising CO 2 levels were associated with increasing global mean temperatures, and several comparative studies have since correlated rising CO 2 levels with rising global temperature (Keeling, 1970). Together with research from modeling studies (see our Modeling in Scientific Research module), this research has provided evidence for an association between global climate change and the burning of fossil fuels (which emits CO 2 ).
Yet in a move reminiscent of the fight launched by the tobacco companies, the oil and fossil fuel industry launched a major public relations campaign against climate change research . As late as 1989, scientists funded by the oil industry were producing reports that called the research on climate change "noisy junk science" (Roberts, 1989). As with the tobacco issue, challenges to early comparative studies tried to paint the method as less reliable than experimental methods. But the challenges actually strengthened the science by prompting more researchers to launch investigations, thus providing multiple lines of evidence supporting an association between atmospheric CO 2 concentrations and climate change. As a result, the culmination of multiple lines of scientific evidence prompted the Intergovernmental Panel on Climate Change organized by the United Nations to issue a report stating that "Warming of the climate system is unequivocal," and "Carbon dioxide is the most important anthropogenic greenhouse gas (IPCC, 2007)."
Comparative studies are a critical part of the spectrum of research methods currently used in science. They allow scientists to apply a treatment-control design in settings that preclude experimentation, and they can provide invaluable information about the relationships between variables . The intense scrutiny that comparison has undergone in the public arena due to cases involving cigarettes and climate change has actually strengthened the method by clarifying its role in science and emphasizing the reliability of data obtained from these studies.
Table of Contents
Activate glossary term highlighting to easily identify key terms within the module. Once highlighted, you can click on these terms to view their definitions.
Activate NGSS annotations to easily identify NGSS standards within the module. Once highlighted, you can click on them to view these standards.
3. Comparative Research Methods
This chapter examines the ‘art of comparing’ by showing how to relate a theoretically guided research question to a properly founded research answer by developing an adequate research design. It first considers the role of variables in comparative research, before discussing the meaning of ‘cases’ and case selection. It then looks at the ‘core’ of the comparative research method: the use of the logic of comparative inquiry to analyse the relationships between variables (representing theory), and the information contained in the cases (the data). Two logics are distinguished: Method of Difference and Method of Agreement. The chapter concludes with an assessment of some problems common to the use of comparative methods.
- Related Documents
3. Comparative research methods
This chapter examines the ‘art of comparing’ by showing how to relate a theoretically guided research question to a properly founded research answer by developing an adequate research design. It first considers the role of variables in comparative research before discussing the meaning of ‘cases’ and case selection. It then looks at the ‘core’ of the comparative research method: the use of the logic of comparative inquiry to analyse the relationships between variables (representing theory) and the information contained in the cases (the data). Two logics are distinguished: Method of Difference and Method of Agreement. The chapter concludes with an assessment of some problems common to the use of comparative methods.
Rethinking Comparison
Qualitative comparative methods – and specifically controlled qualitative comparisons – are central to the study of politics. They are not the only kind of comparison, though, that can help us better understand political processes and outcomes. Yet there are few guides for how to conduct non-controlled comparative research. This volume brings together chapters from more than a dozen leading methods scholars from across the discipline of political science, including positivist and interpretivist scholars, qualitative methodologists, mixed-methods researchers, ethnographers, historians, and statisticians. Their work revolutionizes qualitative research design by diversifying the repertoire of comparative methods available to students of politics, offering readers clear suggestions for what kinds of comparisons might be possible, why they are useful, and how to execute them. By systematically thinking through how we engage in qualitative comparisons and the kinds of insights those comparisons produce, these collected essays create new possibilities to advance what we know about politics.
PERAN PEMUDA RELAWAN DEMOKRASI DALAM MENINGKATKAN PARTISIPASI POLITIK MASYARAKAT PADA PEMILIHAN UMUM LEGISLATIF TAHUN 2014 DAN IMPLIKASINYA TERHADAP KETAHANAN POLITIK WILAYAH (STUDI PADA RELAWAN DEMOKRASI BANYUMAS, JAWA TENGAH)
This research was going to described the role of Banyumas Democracy Volunteer ( Relawan Demokrasi Banyumas) in increasing political public partitipation in Banyumas’s legislative election 2014 and its implication to Banyumas’s political resilience. This research used qualitative research design as a research method. Data were collected by in depth review, observation and documentation. This research used purpossive sampling technique with stakeholder sampling variant to pick informants. The research showed that Banyumas Democracy Volunteer had a positive role in developing political resilience in Banyumas. Their role was gave political education and election education to voters in Banyumas. In the other words, Banyumas Democracy Volunteer had a vital role in developing ideal political resilience in Banyumas.Keywords: Banyumas Democracy Volunteer, Democracy, Election, Political Resilience of Region.
Ezer Kenegdo: Eksistensi Perempuan dan Perannya dalam Keluarga
AbstractPurpose of this study was to describe the meaning of ezer kenegdo and to know position and role of women in the family. The research method used is qualitative research methods (library research). The term of “ ezer kenegdo” refer to a helper but her position withoutsuperiority and inferiority. “The patner model” between men and women is uderstood in relation to one another as the same function, where differences are complementary and mutually beneficial in all walks of life and human endeavors.Keywords: Ezer Kenegdo; Women; Family.AbstrakTujuan penulisan artikel ini adalah untuk mendeskripsikan pengertian ezer kenegdo dan mengetahui kedudukan dan peran perempuan dalam keluarga. Metode yang digunakan adalah metode kualitatif library research. Ungkapan “ezer kenegdo” menunjuk pada seorang penolong namun kedudukannya adalah setara tanpa ada superioritas dan inferioritas. “Model kepatneran” antara laki-laki dan perempuan dipahami dengan hubungan satu dengan yang lain sebagai fungsi yang sama, yang mana perbedaan adalah saling melengkapi dan saling menguntungkan dalam semua lapisan kehidupan dan usaha manusia.Kata Kunci: Ezer Kenegdo, Prerempuan, Keluarga.
Commentary on ‘Opportunities and Challenges of Engaged Indigenous Scholarship’ (Van de Ven, Meyer, & Jing, 2018)
The mission ofManagement and Organization Review, founded in 2005, is to publish research about Chinese management and organizations, foreign organizations operating in China, or Chinese firms operating globally. The aspiration is to develop knowledge that is unique to China as well as universal knowledge that may transcend China. Articulated in the first editorial published in the inaugural issue of MOR (2005) and further elaborated in a second editorial (Tsui, 2006), the question of contextualization is framed, discussing the role of context in the choices of the research question, theory, measurement, and research design. The idea of ‘engaged indigenous research’ by Van de Ven, Meyer, and Jing (2018) describes the highest level of contextualization, with the local context serving as the primary factor guiding all the decisions of a research project. Tsui (2007: 1353) refers to it as ‘deep contextualization’.
PERAN DINAS KESEHATAN DALAM MENANGGULANGI GIZI BURUK ANAK DI KECAMATAN NGAMPRAH KABUPATEN BANDUNG BARAT
The title of this research is "The Role of the Health Office in Tackling Child Malnutrition in Ngamprah District, West Bandung Regency". The problem in this research is not yet optimal implementation of the Health Office in Overcoming Malnutrition in Children in Ngamprah District, West Bandung Regency. The research method that researchers use is descriptive research methods with a qualitative approach. The technique of determining the informants used was purposive sampling technique. The results showed that in carrying out its duties and functions, the health office, the Health Office had implemented a sufficiently optimal role seen from the six indicators of success in overcoming malnutrition, namely: All Posyandu carry out weighing operations at least once a month All toddlers are weighed, All cases of malnutrition are referred to the Puskemas Nursing or Home Sick, all cases of malnutrition are treated at the health center. Nursing or hospitalization is handled according to the management of malnutrition. All post-treatment malnourished toddlers receive assistance.
Jazz jem sessions in the aspect of listener perception
The purpose of the article is to identify the characteristic features ofjazz jam sessions as creative and concert events. The research methods arebased on the use of a number of empirical approaches. The historicalmethod has characterized the periodization of the emergence andpopularity of jam session as an artistic phenomenon. The use of themethod of comparison of jazz jam sessions and jazz concert made itpossible to determine the characteristic features of jams. An appeal toaxiological research methods has identified the most strikingimprovisational solos of leading jazz artists. Of particular importance inthe context of the article are the methods of analysis and synthesis,observation and generalization. It is important to pay attention to the use ofa structural-functional scientific-research method that indicates theeffectiveness of technological and execution processes on jams. Scientificinnovation. The article is about discovering the peculiarities of the jamsession phenomenon and defining the role of interaction between theaudience of improviser listeners and musicians throughout the jams. Theprocesses of development of jazz concerts and improvisations at jamsessions are revealed. Conclusions. The scientific research providedconfirms the fact that system of interactions between musicians amongthemselves and the audience, as well as improvisation of the performers atthe jam sessions is immense and infinite. That is why modern jazz singersand the audience will always strive for its development and understanding.This way is worth starting with repeated listening to improvisation, in theimmediate presence of the jam sessions (both participant and listener).
THE ROLE OF TECHNOLOGY INFORMATION SYSTEMS AND APPLICATION OF SAK ETAP ON DEVELOPMENT MODEL FINANCIAL POSITION REPORT
Bina Siswa SMA Plus Cisarua addressing in Jl. colonel canal masturi no. 64. At the time of document making, record-keeping of transaction relating to account real or financial position report account especially, Bina Siswa SMA Plus Cisarua has applied computer that is by using the application of Microsoft Office Word 2007 and Microsoft Excel 2007, in practice of control to relative financial position report account unable to be added with the duration process performed within financial statement making. For the problems then writer takes title: “The Role Of Technology Information Systems And Aplication Of SAK ETAP On Development Model Financial Position Report”. Research type which writer applies is research type academy, data type which writer applies is qualitative data and quantitative data, research design type which writer applies is research design deskriptif-analistis, research method which writer applies is descriptive research method, survey and eksperiment, data collecting technique which writer applies is field researcher what consisted of interview and observation library research, system development method which writer applies is methodologies orienting at process, data and output. System development structure applied is Iterasi. Design of information system applied is context diagram, data flow diagram, and flowchart. Design of this financial position report accounting information system according to statement of financial accounting standard SAK ETAP and output consisted of information of accumulated fixed assets, receivable list, transaction summary of cash, transaction summary of bank and financial position report.
Dilema Hakim Pengadilan Agama dalam Menyelesaikan Perkara Hukum Keluarga Melalui Mediasi
This article aims to determine the role of judges in resolving family law cases through mediation in the Religious Courts, where judges have the position as state officials as regulated in Law Number 43 of 1999 concerning Basic Personnel, can also be a mediator in the judiciary. as regulated in Supreme Court Regulation Number 1 of 2016 concerning Mediation Procedures where judges have the responsibility to seek peace at every level of the trial and are also involved in mediation procedures. The research method used in this article uses normative legal research methods. Whereas until now judges still have a very important role in resolving family law cases in the Religious Courts due to the fact that there are still many negotiating processes with mediation assisted by judges, even though on the one hand the number of non-judge mediators is available, although in each region it is not evenly distributed in terms of number and capacity. non-judge mediator.
Anime affection on human IQ and behavior in Saudi Arabia
The present study attempted to determine the effects of watching anime and understanding if watching anime could affect the mental and social aspects of kids or other group of ages, and also to decide that the teenagers and children should watch anime or not. The research design used in this study is the descriptive research method and observational where in data and facts from direct observations and online questionnaires were used to answer the research question. The finding of this study suggested that anime viewers has higher level of general knowledge comparing with the non- anime viewers and as well as higher IQ level significantly in a specific group, besides anime can be used to spread a background about any culture and plays a role in increase the economy.
Export Citation Format
Share document.

An official website of the United States government
The .gov means it’s official. Federal government websites often end in .gov or .mil. Before sharing sensitive information, make sure you’re on a federal government site.
The site is secure. The https:// ensures that you are connecting to the official website and that any information you provide is encrypted and transmitted securely.
- Publications
- Account settings
Preview improvements coming to the PMC website in October 2024. Learn More or Try it out now .
- Advanced Search
- Journal List
- J Clin Oncol

Methods in Comparative Effectiveness Research
Katrina armstrong.
From the Perelman School of Medicine, University of Pennsylvania, Philadelphia, PA.
Comparative effectiveness research (CER) seeks to assist consumers, clinicians, purchasers, and policy makers to make informed decisions to improve health care at both the individual and population levels. CER includes evidence generation and evidence synthesis. Randomized controlled trials are central to CER because of the lack of selection bias, with the recent development of adaptive and pragmatic trials increasing their relevance to real-world decision making. Observational studies comprise a growing proportion of CER because of their efficiency, generalizability to clinical practice, and ability to examine differences in effectiveness across patient subgroups. Concerns about selection bias in observational studies can be mitigated by measuring potential confounders and analytic approaches, including multivariable regression, propensity score analysis, and instrumental variable analysis. Evidence synthesis methods include systematic reviews and decision models. Systematic reviews are a major component of evidence-based medicine and can be adapted to CER by broadening the types of studies included and examining the full range of benefits and harms of alternative interventions. Decision models are particularly suited to CER, because they make quantitative estimates of expected outcomes based on data from a range of sources. These estimates can be tailored to patient characteristics and can include economic outcomes to assess cost effectiveness. The choice of method for CER is driven by the relative weight placed on concerns about selection bias and generalizability, as well as pragmatic concerns related to data availability and timing. Value of information methods can identify priority areas for investigation and inform research methods.
INTRODUCTION
The desire to determine the best treatment for a patient is as old as the medical field itself. However, the methods used to make this determination have changed substantially over time, progressing from the humoral model of disease through the Oslerian application of clinical observation to the paradigm of experimental, evidence-based medicine of the last 40 years. Most recently, the field of comparative effectiveness research (CER) has taken center stage 1 in this arena, driven, at least in part, by the belief that better information about which treatment a patient should receive is part of the answer to addressing the unsustainable growth in health care costs in the United States. 2 , 3
The emergence of CER has galvanized a re-examination of clinical effectiveness research methods, both among researchers and policy organizations. New definitions have been created that emphasize the necessity of answering real-world questions, where patients and their clinicians have to pick from a range of possible options, recognizing that the best choice may vary across patients, settings, and even time periods. 4 The long-standing emphasis on double-blinded, randomized controlled trials (RCTs) is increasingly seen as impractical and irrelevant to many of the questions facing clinicians and policy makers today. The importance of generating information that will “assist consumers, clinicians, purchasers, and policy makers to make informed decisions” 1 (p29) is certainly not a new tenet of clinical effectiveness research, but its primacy in CER definitions has important implications for research methods in this area.
CER encompasses both evidence generation and evidence synthesis. 5 Generation of comparative effectiveness evidence uses experimental and observational methods. Synthesis of evidence uses systematic reviews and decision and cost-effectiveness modeling. Across these methods, CER examines a broad range of interventions to “prevent, diagnose, treat, and monitor a clinical condition or to improve the delivery of care.” 1 (p29)
EXPERIMENTAL METHODS
RCTs became the gold standard for clinical effectiveness research soon after publication of the first RCT in 1948. 6 An RCT compares outcomes across groups of participants who are randomly assigned to different interventions, often including a placebo or control arm ( Fig 1 ). RCTs are widely revered for their ability to address selection bias, the correlation between the type of intervention received and other factors associated with the outcome of interest. RCTs are fundamental to the evaluation of new therapeutic agents that are not available outside of a trial setting, and phase III RCT evidence is required for US Food and Drug Administration approval. RCTs are also important for evaluating new technology, including imaging and devices. Increasingly, RCTs are also used to shed light on biology through correlative mechanistic studies, particularly in oncology.

Experimental and observational study designs. In a randomized controlled trial, a population of interest is screened for eligibility, randomly assigned to alternative interventions, and observed for outcomes of interest. In an observational study, the population of interest is assigned to alternative interventions based on patient, provider, and system factors and observed for outcomes of interest.
However, traditional approaches to RCTs are increasingly seen as impractical and irrelevant to many of the questions facing clinicians and policy makers today. RCTs have long been recognized as having important limitations in real-world decision making, 7 including: one, RCTs often have restrictive enrollment criteria so that the participants do not resemble patients in practice, particularly in clinical characteristics such as comorbidity, age, and medications or in sociodemographic characteristics such as race, ethnicity, and socioeconomic status; two, RCTs are often not feasible, either because of expense, ethical concerns, or patient acceptance; and three, given their expense and enrollment restrictions, RCTs are rarely able to answer questions about how the effect of the intervention may vary across patients or settings.
Despite these limitations, there is little doubt that RCTs will be a major component of CER. 8 Furthermore, their role is likely to grow with new approaches that increase their relevance in clinical practice. 9 Adaptive trials use accumulating evidence from the trials to modify trial design of the trial to increase efficiency and the probability that trial participants benefit from participation. 10 These adaptations can include changing the end of the trial, changing the interventions or intervention doses, changing the accrual rate, or changing the probability of being randomly assigned to the different arms. One example of an adaptive clinical trial in oncology is the multiarm I-Spy2 trial, which is evaluating multiple agents for neoadjuvant breast cancer treatment. 11 The I-Spy2 trial uses an adaptive approach to assigning patients to treatment arms (where patients with a tumor profile are more likely to be assigned to the arm with the best outcomes for that profile), and data safety monitoring board decisions are guided by Bayesian predicted probabilities of pathologic complete response. 12 , 13 Other examples of adaptive clinical trials in oncology include a randomized trial of four regiments in metastatic prostate cancer, where patients who did not respond to their initial regimen (selected based on randomization) were then randomly assigned to the remaining three regimens, 14 and the CALGB (Cancer and Leukemia Group B) 49907 trial, which used Bayesian predictive probabilities of inferiority to determine the final sample size needed for the comparison of capecitabine and standard chemotherapy in elderly women with early-stage breast cancer. 15 Pragmatic trials relax some of the traditional rules of RCTs to maximize the relevance of the results for clinicians and policy makers. These changes may include expansion of eligibility criteria, flexibility in the application of the intervention and in the management of the control group, and reduction in the intensity of follow-up or procedures for assessing outcomes. 16
OBSERVATIONAL METHODS
The emergence of comparative effectiveness has led to a renewed interest in the role of observational studies for assessing the benefits and harms of alternative interventions. Observational studies compare outcomes between patients who receive different interventions through some process other than investigator randomization. Most commonly, this process is the natural variation in clinical care, although observational studies also can take advantage of natural experiments, where higher-level changes in care delivery (eg, changes in state policy or changes in hospital unit structure) lead to changes in intervention exposure between groups. Observational studies can enroll patients by exposure (eg, type of intervention) using a cohort design or outcome using a case-control design. Cohort studies can be performed prospectively, where participants are recruited at the time of exposure, or retrospectively, where the exposure occurred before participants are identified.
The strengths and limitations of observational studies for clinical effectiveness research have been debated for decades. 7 , 17 Because the incremental cost of including an additional participant is generally low, observational studies often have relatively large numbers of participants who are more representative of the general population. Large, diverse study populations make the results more generalizable to real-world practice and enable the examination of variation in effect across patient subgroups. This advantage is particularly important for understanding effectiveness among vulnerable populations, such as racial minorities, who are often underrepresented in RCT participants. Observational studies that take advantage of existing data sets are able to provide results quickly and efficiently, a critical need for most CER. Currently, observational data already play an important role in influencing guidelines in many areas of oncology, particularly around prevention (eg, nutritional guidelines, management of BRCA1/2 mutation carriers) 18 , 19 and the use of diagnostic tests (eg, use of gene expression profiling in women with node-negative, estrogen receptor–positive breast cancer). 20 However, observational studies also have important limitations. Observational studies are only feasible if the intervention of interest is already being used in clinical practice; they are not possible for evaluation of new drugs or devices. Observational studies are subject to bias, including performance bias, detection bias, and selection bias. 17 , 21 Performance bias occurs when the delivery of one type of intervention is associated with generally higher levels of performance by the health care unit (ie, health care quality) than the delivery of a different type of intervention, making it difficult to determine if better outcomes are the result of the intervention or the accompanying higher-quality health care. Detection bias occurs when the outcomes of interest are more easily detected in one group than another, generally because of differential contact with the health care system between groups. Selection bias is the most important concern in the validity of observational studies and occurs when intervention groups differ in characteristics that are associated with the outcome of interest. These differences can occur because a characteristic is part of the decision about which treatment to recommend (ie, disease severity), which is often termed confounding by indication, or because it is correlated with both intervention and outcome for another reason. A particular concern for CER of therapies is that some new agents may be more likely to be used in patients for whom established therapies have failed and who are less likely to be responsive to any therapy.
There are two main approaches for addressing bias in observational studies. First, important potential confounders must be identified and included in the data collection. Measured confounders can be addressed through multivariate and propensity score analysis. A telling example of the importance of adequate assessment of potential confounders was found through examination of the observational studies of hormone replacement therapy (HRT) and coronary heart disease (CHD). Meta-analyses of observational studies had long estimated a substantial reduction in CHD risk with the use of postmenopausal HRT. However, the WHI (Women's Health Initiative) trial, a large, double-blind RCT of postmenopausal HRT, found no difference in CHD risk between women assigned to HRT or placebo. Although this apparent contradiction is often used as general evidence against the validity of observational studies, a re-examination of the observational studies demonstrated that studies that adjusted for measures of socioeconomic status (a clear confounder between HRT use and better health outcomes) had results similar to those of the WHI, whereas studies that did not adjust for socioeconomic status found a protective effect with HRT 22 ( Fig 2 ). The use of administrative data sets for observational studies of comparative effectiveness is likely to become increasingly common as health information technology spreads, and data become more accessible; however, these data sets may be particularly limiting in their ability to include data on potential confounders. In some cases, the characteristics that influence the treatment decision may not be available in the data (eg, performance status, tumor gene expression), making concerns about confounding by indication too high to proceed without adjusting data collection or considering a different question.

Meta-analysis of observational studies of hormone replacement therapy (HRT) and coronary artery disease incidence comparing studies that did and did not adjust for socioeconomic status (SES). Data adapted. 22
Second, several analytic approaches can be used to address differences between groups in observational studies. The standard analytic approach involves the use of multivariable adjustment through regression models. Regression allows the estimation of the change in the outcome of interest from the difference in intervention, holding the other variables in the model (covariates) constant. Although regression remains the standard approach to analysis of observational data, regression can be misleading if there is insufficient overlap in the covariates between groups or if the functional forms of the variables are incorrectly specified. 23 Furthermore, the number of covariates that can be included is limited by the number of participants with the outcome of interest in the data set.
Propensity score analysis is another approach to the estimation of an intervention effect in observational data that enables the inclusion of a large number of covariates and a transparent assessment of the balance of covariates after adjustment. 23 – 26 Propensity score analysis uses a two-step process, first estimating the probability of receiving a particular intervention based on the observed covariates (the propensity score) and estimating the effect of the intervention within groups of patients who had a similar probability of receiving the intervention (often grouped as quintiles of propensity score). The degree to which the propensity score is able to represent the differences in covariates between intervention groups is assessed by examining the balance in covariates across propensity score categories. In an ideal situation, after participants are grouped by their propensity for being treated, those who receive different interventions have similar clinical and sociodemographic characteristics—at least for the characteristics that are measured ( Table 1 ). Rates of the outcomes of interest are then compared between intervention groups within each propensity score category, paying attention to whether the intervention effect differs across patients with a different propensity for receiving the intervention. In addition, the propensity score itself can be included in a regression model estimating the effect of the intervention on the outcome, a method that also allows for additional adjustment for covariates that were not sufficiently balanced across intervention groups within propensity score categories.
Hypothetic Example of Propensity Score Analysis Comparing Two Intervention Groups, A and B
Characteristic | Overall Sample | Quintiles of Propensity Score | ||||||||||
---|---|---|---|---|---|---|---|---|---|---|---|---|
1 | 2 | 3 | 4 | 5 | ||||||||
A | B | A | B | A | B | A | B | A | B | A | B | |
Mean age, years | 45.3 | 56.9 | 58.9 | 59.0 | 56.2 | 56.1 | 50.4 | 50.4 | 46.9 | 46.7 | 43.0 | 43.2 |
No. of comorbidities | ||||||||||||
0 | 54.0 | 26.5 | 60.8 | 60.4 | 51.7 | 51.8 | 43.6 | 43.4 | 38.9 | 39 | 24.3 | 24.5 |
1-2 | 34.7 | 28.8 | 36.8 | 36.9 | 34.4 | 34.4 | 32 | 32.1 | 29.7 | 29.5 | 26.4 | 26.5 |
> 3 | 11.3 | 44.7 | 2.4 | 2.7 | 13.9 | 13.8 | 24.4 | 24.5 | 31.4 | 31.5 | 49.3 | 49 |
The use of propensity scores for oncology clinical effectiveness research has become increasingly popular over the last decade, with six articles published in Journal of Clinical Oncology in 2011 alone. 27 – 32 However, propensity score analysis has limitations, the most important of which is that it can only include the variables that are in the available data. If a factor that influences the intervention assignment is not included or measured accurately in the data, it cannot be adequately addressed by a propensity score. For example, in a prior propensity score analysis of the association between active treatment and prostate cancer mortality among elderly men, we were able to include only the variables available in Surveillance, Epidemiology, and End Results–Medicare linked data in our propensity score. 33 The data included some of the factors that influence treatment decisions (eg, age, comorbidities, tumor grade, and size) but not others (eg, functional status, prostate-specific antigen score). Furthermore, the measurement of some of the available factors was imperfect—for example, assessment of comorbidities was based on billing codes, which can underestimate actual comorbidity burden and provide no information about the severity of the comorbidity. Thus, although the final result demonstrating a fairly strong association between active treatment and reduced mortality was quite robust based on the data that were available, it is still possible that the association represents unaddressed selection factors where healthier men underwent active treatment. 34
Instrumental variable methods are a third analytic approach that estimate the effect of an intervention in observational data without requiring the factors that differ between the intervention groups to be available in the data, thereby addressing both measured and unmeasured confounders. 35 The goal underlying instrumental variable analysis is to identify a characteristic (called the instrument) that strongly influences the assignment of patients to intervention but is not associated with the outcomes of interest (except through the intervention). In essence, an instrumental variable approach is an attempt to replicate an RCT, where the instrument is randomization. 36 Common instruments include the patterns of treatment across geographic areas or health care providers, the distance to a health care facility able to provide the intervention of interest, or structural characteristics of the health care system that influence what interventions are used, such as the density of certain types of providers or facilities. The analysis involves two stages: first, the probability of receiving the intervention of interest is estimated as a function of the instrument variable and other covariates; second, a model is built predicting the outcome of interest based on the instrument-based intervention probability and the residual from the first model.
Instrumental variable analysis is commonly used in economics 37 and has increasingly been applied to health and health care. In oncology, instrumental variable approaches have been used to examine the effectiveness of treatments for lung, prostate, bladder, and breast cancers, with the most common instruments being area-level treatment patterns. 38 – 42 One recent analysis of prostate cancer treatment found that multivariable regression and propensity score methods resulted in essentially the same estimate of effect for radical prostatectomy, but an instrumental variable based on the treatment pattern of the previous year found no benefit from radical prostatectomy, similar to the estimate from a recently published trial. 41 , 43 However, concerns also exist about the validity of instrumental variable results, particularly if the instrument is not strongly associated with the intervention, or if there are other potential pathways by which the instrument may influence the outcome. Although the strength of the association between the instrument and the intervention assignment can be tested in the analysis, alternative pathways by which the instrument may be associated with the outcome are often not identified until after publication. A recent instrumental variable analysis used annual rainfall as the instrument to demonstrate an association between television watching and autism, arguing that annual rainfall is associated with the amount of time children watch television but is not otherwise associated with the risk of autism. 44 The findings generated considerable controversy after publication, with the identification of several other potential links between rainfall and autism. 45 Instrumental variable methods have traditionally been unable to examine differences in effect between patient subgroups, but new approaches may improve their utility in this important component of CER. 46 , 47
SYSTEMATIC REVIEWS
For some decisions faced by clinicians and policy makers, there is insufficient evidence to inform decision making, and new studies to generate evidence are needed. However, for other decisions, evidence exists but is sufficiently complex or controversial that it must be synthesized to inform decision making. Systematic reviews are an important form of evidence synthesis that brings together the available evidence using an organized and evaluative approach. 48 Systematic reviews are frequently used for guideline development and generally include four major steps. 49 First, the clinical decision is identified, and the analytic framework and key questions are determined. Sometimes the decision may be straightforward and involve a single key question (eg, Does drug A reduce the incidence of disease B?), but other times the question may be more complicated (eg, Should gene expression profiling be used in early-stage breast cancer?) and involve multiple key questions. 50 Second, the literature is searched to identify the relevant studies using inclusion and exclusion criteria that may include the timing of the study, the study design, and the location of the study. Third, the identified studies are graded on quality using established criteria such as the CONSORT criteria for RCTs 51 and the STROBE (Strengthening the Reporting of Observational Studies in Epidemiology) criteria for observational studies. 52 Studies that do not meet a minimum quality threshold may be excluded because of concern about the validity of the results. Fourth, the results of all the studies are collated in evidence tables, often including key characteristics of the study design or population that might influence the results. Meta-analytic techniques may be used to combine results across studies when there is sufficient homogeneity to make a single-point estimate statistically valid. Alternatively, models may be used to identify the study or population factors that are associated with different results.
Although systematic reviews are a key component of evidence-based medicine, their role in CER is still uncertain. The traditional approach to systematic reviews has often excluded observational studies because of concerns about internal validity, but such exclusions may greatly limit the evidence available for many important comparative effectiveness questions. CER is designed to inform real-world decisions between available alternatives, which may include multiple tradeoffs. Inclusion of information about harms in comparative effectiveness systematic reviews is desirable but often challenging because of limited data. Finally, systematic reviews are rarely able to examine differences in intervention effects across patient characteristics, another important step for achieving the goals of CER.
DECISION AND COST-EFFECTIVENESS ANALYSIS
Another evidence synthesis method that is gaining increasing traction in CER is decision modeling. Decision modeling is a quantitative approach to evidence synthesis that brings together data from a range of sources to estimate expected outcomes of different interventions. 53 The first step in a decision model is to lay out the structure of the decision, including the alternative choices and the clinical and economic outcomes of those alternatives. 54 Ensuring that the structure of the model holds true to the clinical scenario of interest without becoming overwhelmed by minor possible variations is critical for the eventual impact of the model. 55 Once the decision structure is determined, a decision tree or simulation model is created that incorporates the probabilities of different outcomes over time and the change in those probabilities from the use of different interventions. 56 , 57 To calculate the expected outcomes, a hypothetic cohort of patients is run through each of the decision alternatives in the model. Estimated outcomes are generally assessed as a count of events in the cohort (eg, deaths, cancers) or as the mean or median life expectancy among the cohort. 58
Decision models can also include information about the value placed on each of the outcomes (often referred to as utility) as well as the health care costs incurred by the interventions and the health outcomes. A decision model that includes cost and utility is often referred to as a cost-benefit or cost-effectiveness model and is used in some settings to compare value across interventions. The types of costs that are included depend on the perspective of the model, with a model from the societal perspective including both direct and indirect medical costs (eg, loss of productivity), a model from a payer (ie, insurer) perspective including only direct medical costs, and a model from a patient perspective including the costs experienced by the patient. Future costs are discounted to address the change in monetary value over time. 59 Sensitivity analyses are used to explore the impact of different assumptions on the model results, a critical step for understanding how the results should be used in clinical and policy decisions and for the development of future evidence-generation research. These sensitivity analyses often use a probabilistic approach, where a distribution is entered for each of the inputs and the computer samples from those distributions across a large number of simulations, thereby creating a confidence interval around the estimated outcomes of the alternative choices.
Decision models have several strengths in CER. They can link multiple sources of information to estimate the effect of different interventions on health outcomes, even when there are no studies that directly assess the effect of interest. Because they can examine the effect of variation in different probability estimates, they are particularly useful for understanding how patient characteristics will affect the expected outcomes of different interventions. Decision models can also estimate the impact of an intervention across a population, including the effect on economic outcomes. Decision and cost-effectiveness analyses have been used frequently in oncology, particularly for decisions with options that include the use of a diagnostic or screening test (eg, bone mineral density testing for management of osteoporosis risk), 60 involve significant tradeoffs (eg, adjuvant chemotherapy), 61 or have only limited empirical evidence (eg, management strategies in BRCA mutation carriers). 62
However, decision models also have several limitations that have limited their impact on clinical and policy decision making in the United States to date and are likely to constrain their role in future CER. Often, model results are highly sensitive to the assumptions of the model, and removing bias from these assumptions is difficult. The potential impact of conflicts of interest is high. Decision models require data inputs. For many decisions, data are insufficient for key inputs, requiring the use of educated guesses (ie, expert opinion). The measurement of utility has proven particularly challenging and can lead to counterintuitive results. In the end, decision analysis is similar to other comparative effectiveness methods—useful for the right question as long as results are interpreted with an understanding of the methodologic limitations.
SELECTION OF CER METHODS
The choice of method for a comparative effectiveness study involves the consideration of multiple factors. The Patient-Centered Outcomes Research Institute Methods Committee has identified five intrinsic and three extrinsic factors ( Table 2 ), including internal validity, generalizability, and variation across patient subgroups as well as the feasibility and time urgency. 63 The importance of these factors will vary across the questions being considered. For some questions, the concern about selection bias will be too great for observational studies, particularly if a strong instrument cannot be identified. Many questions about aggressive versus less aggressive treatments may fall into this category, because the decision is often correlated with patient characteristics that predict survival but are rarely found in observational data sets (eg, functional status, social support). For other questions, concern about selection bias will be less pressing than the need for rapid and efficient results. This scenario may be particularly relevant for the comparison of existing therapies that differ in cost or adverse outcomes, where the use of the therapy is largely driven by practice style. In many cases, the choice will be pragmatic based on what data are available and the feasibility of conducting an RCT. These choices will increasingly be informed by the value of information methods 64 – 66 that use economic modeling to provide guidance about where and how investment in CER should be made.
Factors That Influence Selection of Study Design for Patient-Centered Outcome Research
Factor |
---|
In reality, the questions of CER are not new but are simply more important than ever. Nearly 50 years ago, Sir Austin Bradford Hill spoke about the importance of a broad portfolio of methods in clinical research, saying “To-day … there are many drugs that work and work potently. We want to know whether this one is more potent than that, what dose is right and proper, for what kind of patient.” 7 (p109) This call has expanded beyond drugs to become the charge for CER. To fulfill this charge, investigators will need to use a range of methods, extending the experience in effectiveness research of the last decades “to assist consumers, clinicians, purchasers, and policy makers to make informed decisions that will improve health care at both the individual and population levels.” 1 (p29)
Supported by Award No. UC2CA148310 from the National Cancer Institute.
The content is solely the responsibility of the author and does not necessarily represent the official views of the National Cancer Institute or the National Institutes of Health.
Author's disclosures of potential conflicts of interest and author contributions are found at the end of this article.
AUTHOR'S DISCLOSURES OF POTENTIAL CONFLICTS OF INTEREST
The author(s) indicated no potential conflicts of interest.
Issue 13 | Summer 1995 |
Social Research Update is published quarterly by the Department of Sociology, University of Surrey, Guildford GU2 7XH, England. Subscriptions for the hardcopy version are free to researchers with addresses in the UK. Apply by email to [email protected] .
Comparative Research Methods
Linda Hantrais is Director of the European Research Centre, Loughborough University. She is convenor of the Cross-National Research Group and series editor of Cross-National Research Papers . The main focus of her research is cross-national theory, method and practice, particularly with reference to social policy. She has conducted a number of comparative studies, including ESRC/CNAF/European Commission-funded collaborative projects on women in professional occupations in Britain and France and on families and family policies in Europe. Her recent publications include a co-edited book, with Steen Mangen, on Cross-National Research Methods in the Social Sciences (Pinter, 1996).
Social Research Update is published by:
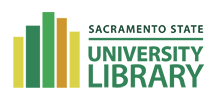
- My Library Account
- Articles, Books & More
- Course Reserves
- Site Search
- Advanced Search
- Sac State Library
- Research Guides
Research Methods Simplified
Comparative method/quasi-experimental.
- Quantitative Research
- Qualitative Research
- Primary, Seconday and Tertiary Research and Resources
- Definitions
- Sources Consulted
Comparative method or quasi-experimental ---a method used to describe similarities and differences in variables in two or more groups in a natural setting, that is, it resembles an experiment as it uses manipulation but lacks random assignment of individual subjects. Instead it uses existing groups. For examples see http://www.education.com/reference/article/quasiexperimental-research/#B
- << Previous: Qualitative Research
- Next: Primary, Seconday and Tertiary Research and Resources >>
- Last Updated: Jul 3, 2024 2:35 PM
- URL: https://csus.libguides.com/res-meth
Comparative Studies
- January 2018
- In book: Global Encyclopedia of Public Administration, Public Policy, and Governance (pp.1-7)

- Italian National Research Council

Discover the world's research
- 25+ million members
- 160+ million publication pages
- 2.3+ billion citations
- Construct Innovat

- Ali Al-Otaibi

- Coccia Mario

- Martini Martini

- Ts Ummu Hani Binti Yusof
- Noora Almansoori

- Aisha Alteneiji

- J Comp Pol Anal
- B. Guy Peters

- R W Cooksey
- F J Thompson
- Recruit researchers
- Join for free
- Login Email Tip: Most researchers use their institutional email address as their ResearchGate login Password Forgot password? Keep me logged in Log in or Continue with Google Welcome back! Please log in. Email · Hint Tip: Most researchers use their institutional email address as their ResearchGate login Password Forgot password? Keep me logged in Log in or Continue with Google No account? Sign up
Characteristics of a Comparative Research Design
Hannah richardson, 28 jun 2018.
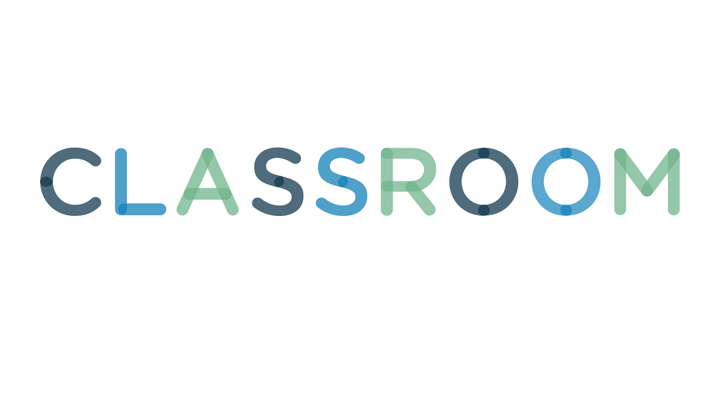
Comparative research essentially compares two groups in an attempt to draw a conclusion about them. Researchers attempt to identify and analyze similarities and differences between groups, and these studies are most often cross-national, comparing two separate people groups. Comparative studies can be used to increase understanding between cultures and societies and create a foundation for compromise and collaboration. These studies contain both quantitative and qualitative research methods.
Explore this article
- Comparative Quantitative
- Comparative Qualitative
- When to Use It
- When Not to Use It
1 Comparative Quantitative
Quantitative, or experimental, research is characterized by the manipulation of an independent variable to measure and explain its influence on a dependent variable. Because comparative research studies analyze two different groups -- which may have very different social contexts -- it is difficult to establish the parameters of research. Such studies might seek to compare, for example, large amounts of demographic or employment data from different nations that define or measure relevant research elements differently.
However, the methods for statistical analysis of data inherent in quantitative research are still helpful in establishing correlations in comparative studies. Also, the need for a specific research question in quantitative research helps comparative researchers narrow down and establish a more specific comparative research question.
2 Comparative Qualitative
Qualitative, or nonexperimental, is characterized by observation and recording outcomes without manipulation. In comparative research, data are collected primarily by observation, and the goal is to determine similarities and differences that are related to the particular situation or environment of the two groups. These similarities and differences are identified through qualitative observation methods. Additionally, some researchers have favored designing comparative studies around a variety of case studies in which individuals are observed and behaviors are recorded. The results of each case are then compared across people groups.
3 When to Use It
Comparative research studies should be used when comparing two people groups, often cross-nationally. These studies analyze the similarities and differences between these two groups in an attempt to better understand both groups. Comparisons lead to new insights and better understanding of all participants involved. These studies also require collaboration, strong teams, advanced technologies and access to international databases, making them more expensive. Use comparative research design when the necessary funding and resources are available.
4 When Not to Use It
Do not use comparative research design with little funding, limited access to necessary technology and few team members. Because of the larger scale of these studies, they should be conducted only if adequate population samples are available. Additionally, data within these studies require extensive measurement analysis; if the necessary organizational and technological resources are not available, a comparative study should not be used. Do not use a comparative design if data are not able to be measured accurately and analyzed with fidelity and validity.
- 1 San Jose State University: Selected Issues in Study Design
- 2 University of Surrey: Social Research Update 13: Comparative Research Methods
About the Author
Hannah Richardson has a Master's degree in Special Education from Vanderbilt University and a Bacheor of Arts in English. She has been a writer since 2004 and wrote regularly for the sports and features sections of "The Technician" newspaper, as well as "Coastwach" magazine. Richardson also served as the co-editor-in-chief of "Windhover," an award-winning literary and arts magazine. She is currently teaching at a middle school.
Related Articles
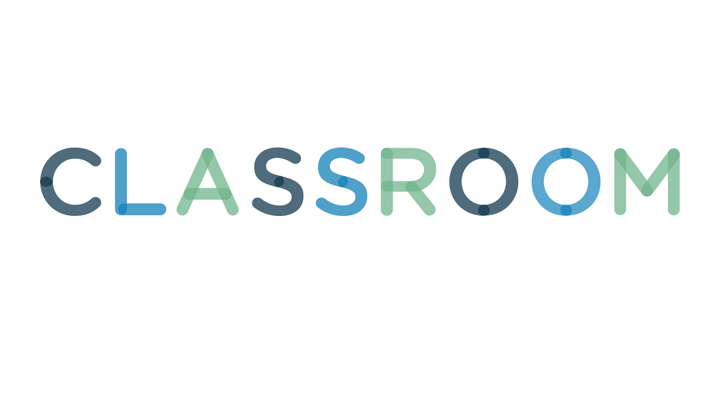
Research Study Design Types
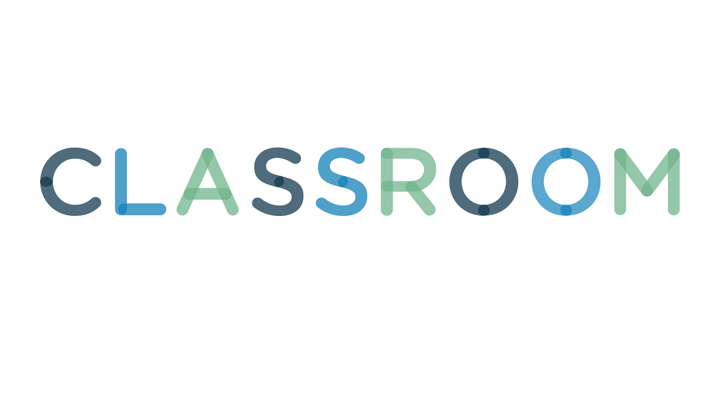
Correlational Methods vs. Experimental Methods
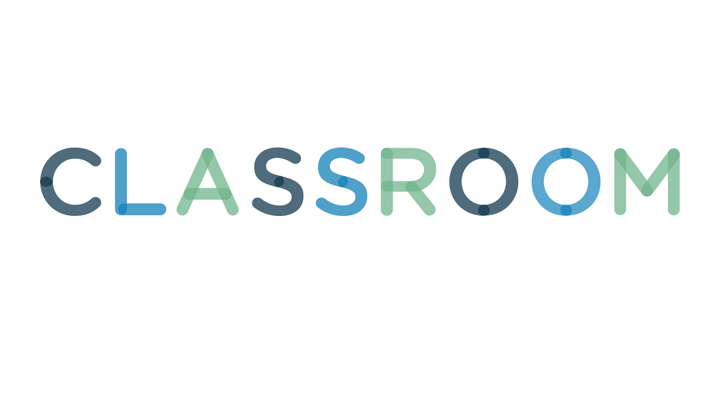
Different Types of Methodologies
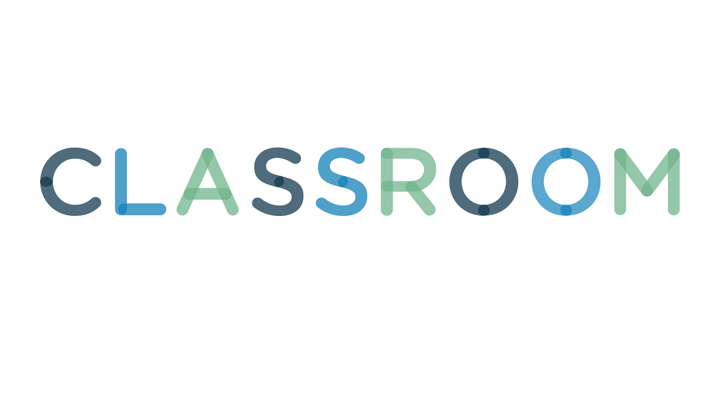
Quasi-Experiment Advantages & Disadvantages
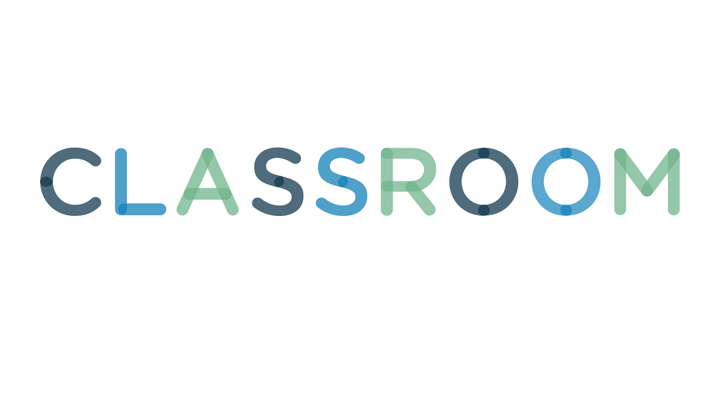
What Are the Advantages & Disadvantages of Non-Experimental...
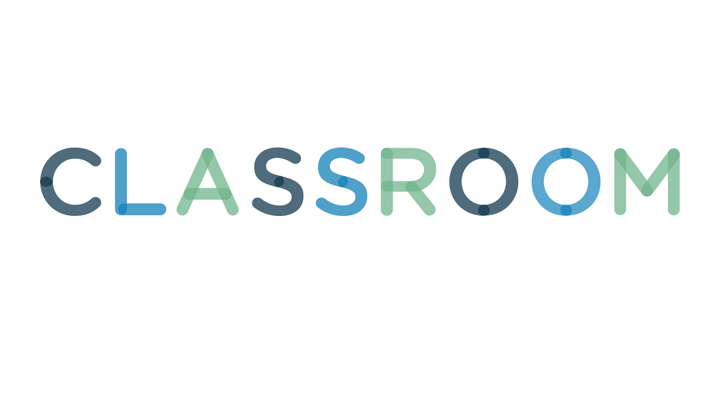
Independent vs. Dependent Variables in Sociology
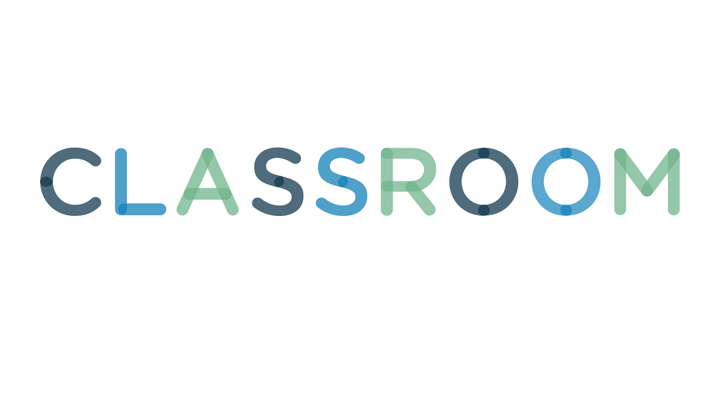
Methods of Research Design
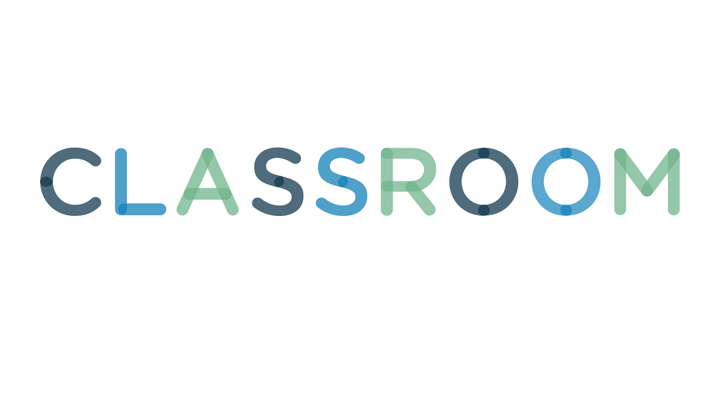
Qualitative Research Pros & Cons
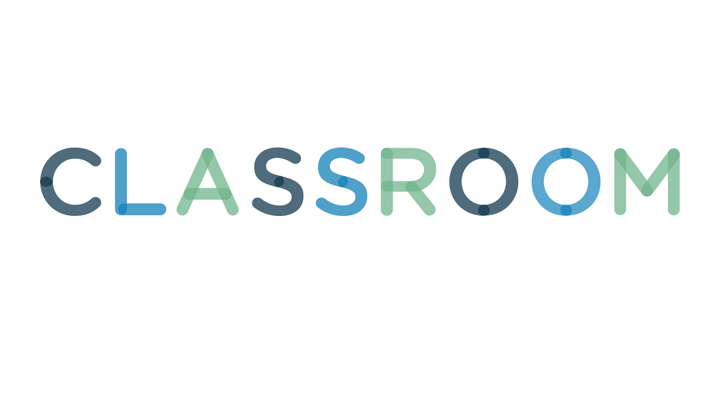
How to Form a Theoretical Study of a Dissertation
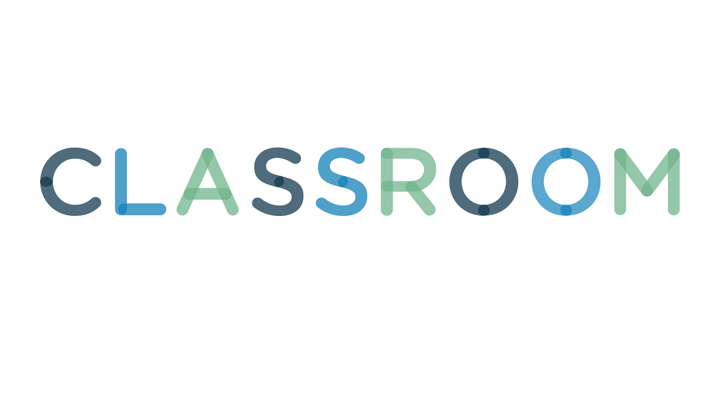
What Is the Difference Between Internal & External...
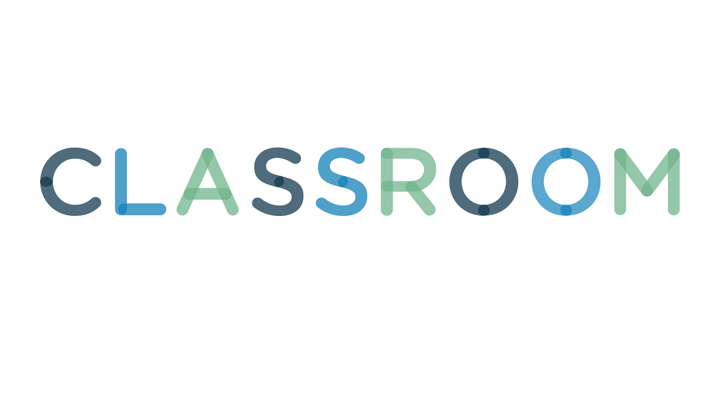
Difference Between Conceptual & Theoretical Framework
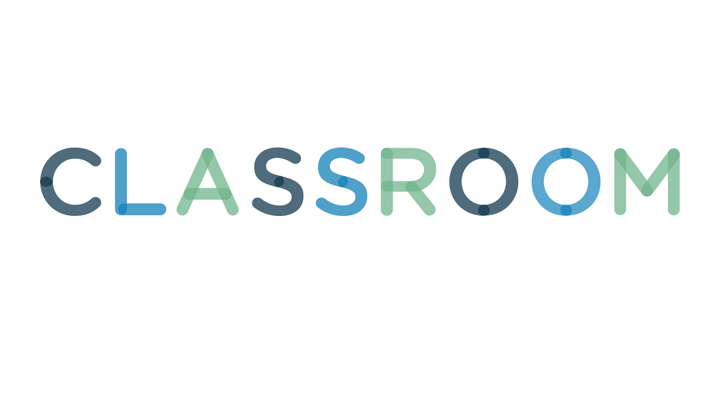
The Advantages of Exploratory Research Design
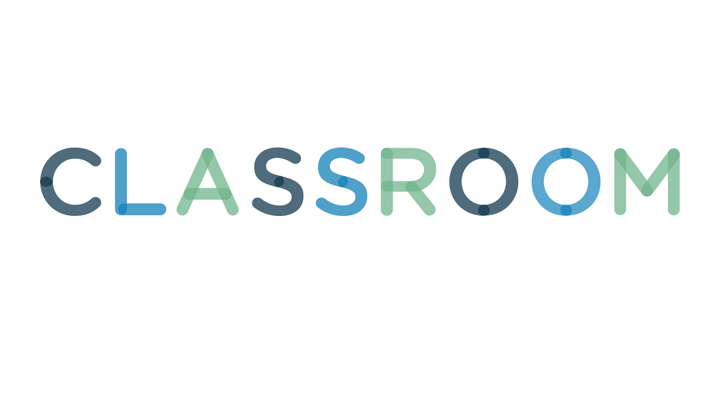
What Is Quantitative Research?
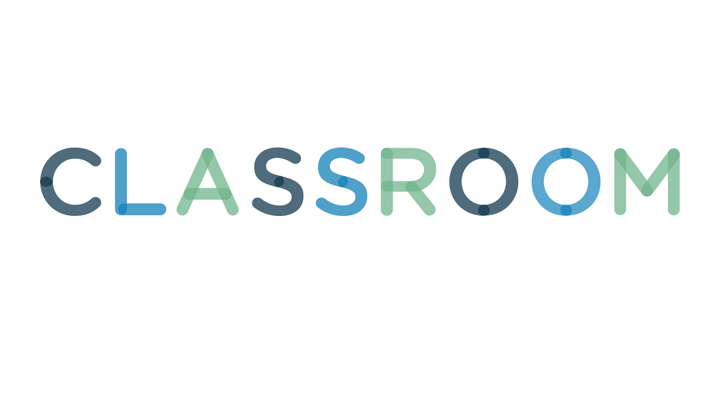
What is a Dissertation?
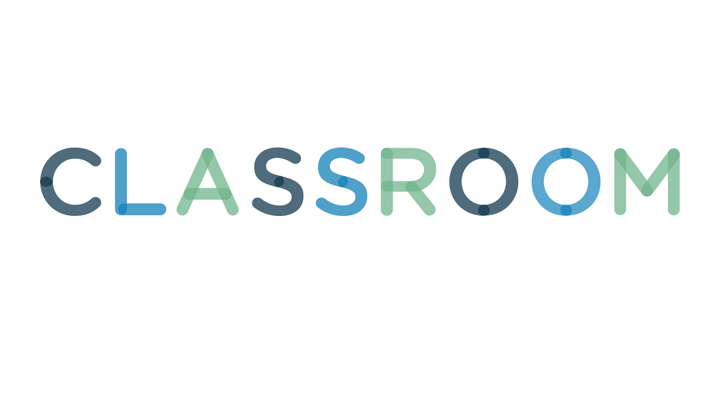
What Are the Advantages & Disadvantages of Correlation...
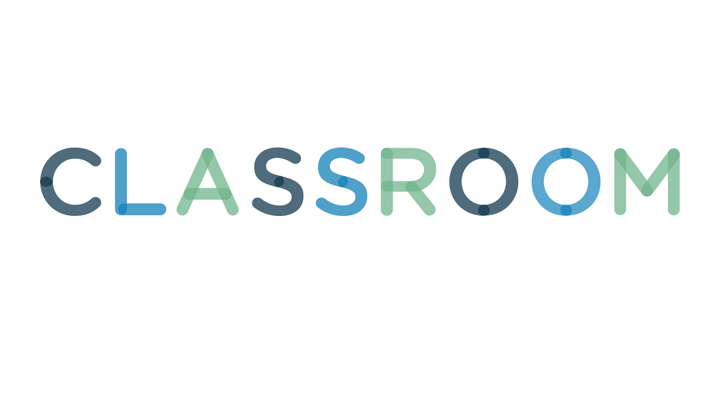
What Is the Meaning of the Descriptive Method in Research?
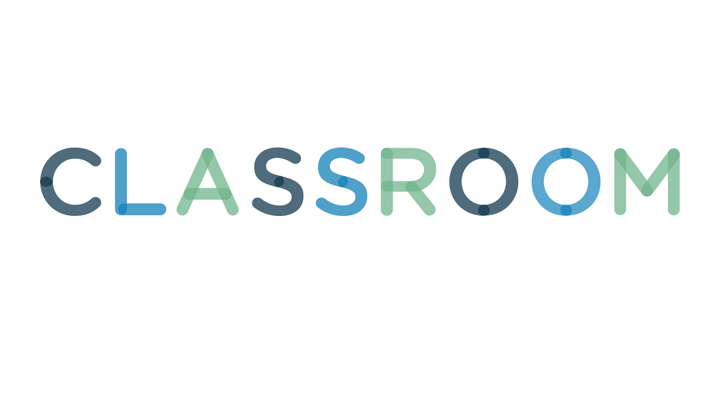
How to Use Qualitative Research Methods in a Case Study...
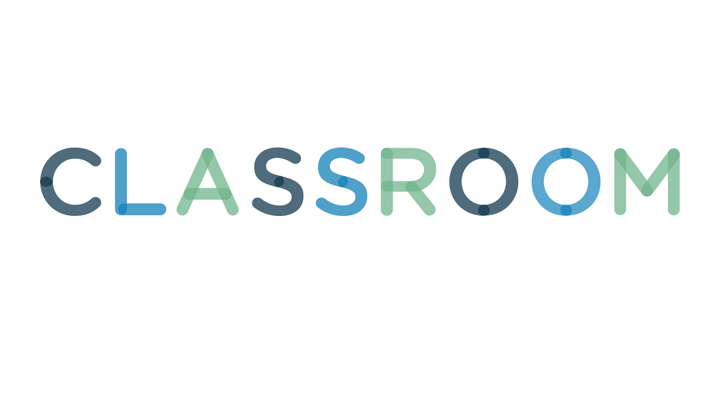
How to Tabulate Survey Results
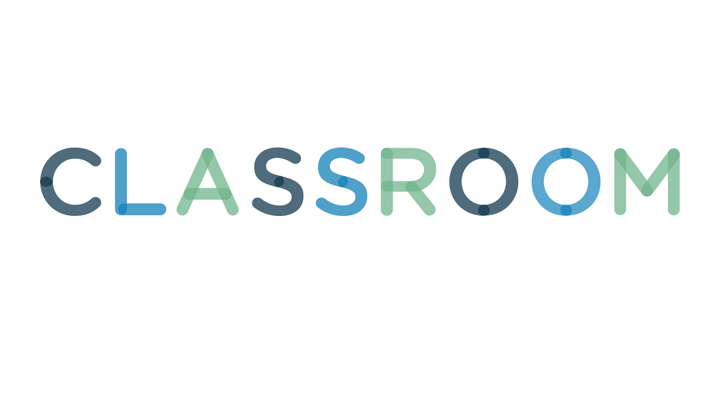
How to Cross Validate Qualitative Research Results
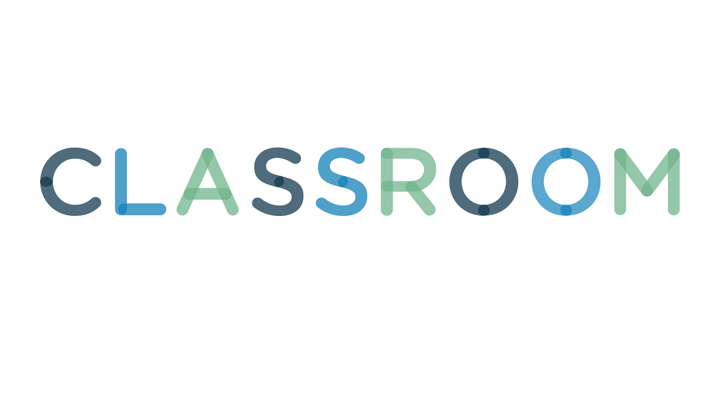
Types of Descriptive Research Methods
Regardless of how old we are, we never stop learning. Classroom is the educational resource for people of all ages. Whether you’re studying times tables or applying to college, Classroom has the answers.
- Accessibility
- Terms of Use
- Privacy Policy
- Copyright Policy
- Manage Preferences
© 2020 Leaf Group Ltd. / Leaf Group Media, All Rights Reserved. Based on the Word Net lexical database for the English Language. See disclaimer .

On Evaluating Curricular Effectiveness: Judging the Quality of K-12 Mathematics Evaluations (2004)
Chapter: 5 comparative studies, 5 comparative studies.
It is deceptively simple to imagine that a curriculum’s effectiveness could be easily determined by a single well-designed study. Such a study would randomly assign students to two treatment groups, one using the experimental materials and the other using a widely established comparative program. The students would be taught the entire curriculum, and a test administered at the end of instruction would provide unequivocal results that would permit one to identify the more effective treatment.
The truth is that conducting definitive comparative studies is not simple, and many factors make such an approach difficult. Student placement and curricular choice are decisions that involve multiple groups of decision makers, accrue over time, and are subject to day-to-day conditions of instability, including student mobility, parent preference, teacher assignment, administrator and school board decisions, and the impact of standardized testing. This complex set of institutional policies, school contexts, and individual personalities makes comparative studies, even quasi-experimental approaches, challenging, and thus demands an honest and feasible assessment of what can be expected of evaluation studies (Usiskin, 1997; Kilpatrick, 2002; Schoenfeld, 2002; Shafer, in press).
Comparative evaluation study is an evolving methodology, and our purpose in conducting this review was to evaluate and learn from the efforts undertaken so far and advise on future efforts. We stipulated the use of comparative studies as follows:
A comparative study was defined as a study in which two (or more) curricular treatments were investigated over a substantial period of time (at least one semester, and more typically an entire school year) and a comparison of various curricular outcomes was examined using statistical tests. A statistical test was required to ensure the robustness of the results relative to the study’s design.
We read and reviewed a set of 95 comparative studies. In this report we describe that database, analyze its results, and draw conclusions about the quality of the evaluation database both as a whole and separated into evaluations supported by the National Science Foundation and commercially generated evaluations. In addition to describing and analyzing this database, we also provide advice to those who might wish to fund or conduct future comparative evaluations of mathematics curricular effectiveness. We have concluded that the process of conducting such evaluations is in its adolescence and could benefit from careful synthesis and advice in order to increase its rigor, feasibility, and credibility. In addition, we took an interdisciplinary approach to the task, noting that various committee members brought different expertise and priorities to the consideration of what constitutes the most essential qualities of rigorous and valid experimental or quasi-experimental design in evaluation. This interdisciplinary approach has led to some interesting observations and innovations in our methodology of evaluation study review.
This chapter is organized as follows:
Study counts disaggregated by program and program type.
Seven critical decision points and identification of at least minimally methodologically adequate studies.
Definition and illustration of each decision point.
A summary of results by student achievement in relation to program types (NSF-supported, University of Chicago School Mathematics Project (UCSMP), and commercially generated) in relation to their reported outcome measures.
A list of alternative hypotheses on effectiveness.
Filters based on the critical decision points.
An analysis of results by subpopulations.
An analysis of results by content strand.
An analysis of interactions among content, equity, and grade levels.
Discussion and summary statements.
In this report, we describe our methodology for review and synthesis so that others might scrutinize our approach and offer criticism on the basis of
our methodology and its connection to the results stated and conclusions drawn. In the spirit of scientific, fair, and open investigation, we welcome others to undertake similar or contrasting approaches and compare and discuss the results. Our work was limited by the short timeline set by the funding agencies resulting from the urgency of the task. Although we made multiple efforts to collect comparative studies, we apologize to any curriculum evaluators if comparative studies were unintentionally omitted from our database.
Of these 95 comparative studies, 65 were studies of NSF-supported curricula, 27 were studies of commercially generated materials, and 3 included two curricula each from one of these two categories. To avoid the problem of double coding, two studies, White et al. (1995) and Zahrt (2001), were coded within studies of NSF-supported curricula because more of the classes studied used the NSF-supported curriculum. These studies were not used in later analyses because they did not meet the requirements for the at least minimally methodologically adequate studies, as described below. The other, Peters (1992), compared two commercially generated curricula, and was coded in that category under the primary program of focus. Therefore, of the 95 comparative studies, 67 studies were coded as NSF-supported curricula and 28 were coded as commercially generated materials.
The 11 evaluation studies of the UCSMP secondary program that we reviewed, not including White et al. and Zahrt as previously mentioned, benefit from the maturity of the program, while demonstrating an orientation to both establishing effectiveness and improving a product line. For these reasons, at times we will present the summary of UCSMP’s data separately.
The Saxon materials also present a somewhat different profile from the other commercially generated materials because many of the evaluations of these materials were conducted in the 1980s and the materials were originally developed with a rather atypical program theory. Saxon (1981) designed its algebra materials to combine distributed practice with incremental development. We selected the Saxon materials as a middle grades commercially generated program, and limited its review to middle school studies from 1989 onward when the first National Council of Teachers of Mathematics (NCTM) Standards (NCTM, 1989) were released. This eliminated concerns that the materials or the conditions of educational practice have been altered during the intervening time period. The Saxon materials explicitly do not draw from the NCTM Standards nor did they receive support from the NSF; thus they truly represent a commercial venture. As a result, we categorized the Saxon studies within the group of studies of commercial materials.
At times in this report, we describe characteristics of the database by
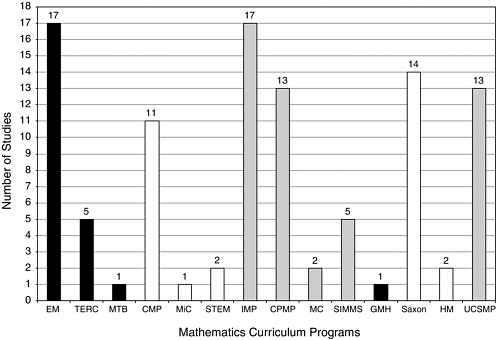
FIGURE 5-1 The distribution of comparative studies across programs. Programs are coded by grade band: black bars = elementary, white bars = middle grades, and gray bars = secondary. In this figure, there are six studies that involved two programs and one study that involved three programs.
NOTE: Five programs (MathScape, MMAP, MMOW/ARISE, Addison-Wesley, and Harcourt) are not shown above since no comparative studies were reviewed.
particular curricular program evaluations, in which case all 19 programs are listed separately. At other times, when we seek to inform ourselves on policy-related issues of funding and evaluating curricular materials, we use the NSF-supported, commercially generated, and UCSMP distinctions. We remind the reader of the artificial aspects of this distinction because at the present time, 18 of the 19 curricula are published commercially. In order to track the question of historical inception and policy implications, a distinction is drawn between the three categories. Figure 5-1 shows the distribution of comparative studies across the 14 programs.
The first result the committee wishes to report is the uneven distribution of studies across the curricula programs. There were 67 coded studies of the NSF curricula, 11 studies of UCSMP, and 17 studies of the commercial publishers. The 14 evaluation studies conducted on the Saxon materials compose the bulk of these 17-non-UCSMP and non-NSF-supported curricular evaluation studies. As these results suggest, we know more about the
evaluations of the NSF-supported curricula and UCSMP than about the evaluations of the commercial programs. We suggest that three factors account for this uneven distribution of studies. First, evaluations have been funded by the NSF both as a part of the original call, and as follow-up to the work in the case of three supplemental awards to two of the curricula programs. Second, most NSF-supported programs and UCSMP were developed at university sites where there is access to the resources of graduate students and research staff. Finally, there was some reported reluctance on the part of commercial companies to release studies that could affect perceptions of competitive advantage. As Figure 5-1 shows, there were quite a few comparative studies of Everyday Mathematics (EM), Connected Mathematics Project (CMP), Contemporary Mathematics in Context (Core-Plus Mathematics Project [CPMP]), Interactive Mathematics Program (IMP), UCSMP, and Saxon.
In the programs with many studies, we note that a significant number of studies were generated by a core set of authors. In some cases, the evaluation reports follow a relatively uniform structure applied to single schools, generating multiple studies or following cohorts over years. Others use a standardized evaluation approach to evaluate sequential courses. Any reports duplicating exactly the same sample, outcome measures, or forms of analysis were eliminated. For example, one study of Mathematics Trailblazers (Carter et al., 2002) reanalyzed the data from the larger ARC Implementation Center study (Sconiers et al., 2002), so it was not included separately. Synthesis studies referencing a variety of evaluation reports are summarized in Chapter 6 , but relevant individual studies that were referenced in them were sought out and included in this comparative review.
Other less formal comparative studies are conducted regularly at the school or district level, but such studies were not included in this review unless we could obtain formal reports of their results, and the studies met the criteria outlined for inclusion in our database. In our conclusions, we address the issue of how to collect such data more systematically at the district or state level in order to subject the data to the standards of scholarly peer review and make it more systematically and fairly a part of the national database on curricular effectiveness.
A standard for evaluation of any social program requires that an impact assessment is warranted only if two conditions are met: (1) the curricular program is clearly specified, and (2) the intervention is well implemented. Absent this assurance, one must have a means of ensuring or measuring treatment integrity in order to make causal inferences. Rossi et al. (1999, p. 238) warned that:
two prerequisites [must exist] for assessing the impact of an intervention. First, the program’s objectives must be sufficiently well articulated to make
it possible to specify credible measures of the expected outcomes, or the evaluator must be able to establish such a set of measurable outcomes. Second, the intervention should be sufficiently well implemented that there is no question that its critical elements have been delivered to appropriate targets. It would be a waste of time, effort, and resources to attempt to estimate the impact of a program that lacks measurable outcomes or that has not been properly implemented. An important implication of this last consideration is that interventions should be evaluated for impact only when they have been in place long enough to have ironed out implementation problems.
These same conditions apply to evaluation of mathematics curricula. The comparative studies in this report varied in the quality of documentation of these two conditions; however, all addressed them to some degree or another. Initially by reviewing the studies, we were able to identify one general design template, which consisted of seven critical decision points and determined that it could be used to develop a framework for conducting our meta-analysis. The seven critical decision points we identified initially were:
Choice of type of design: experimental or quasi-experimental;
For those studies that do not use random assignment: what methods of establishing comparability of groups were built into the design—this includes student characteristics, teacher characteristics, and the extent to which professional development was involved as part of the definition of a curriculum;
Definition of the appropriate unit of analysis (students, classes, teachers, schools, or districts);
Inclusion of an examination of implementation components;
Definition of the outcome measures and disaggregated results by program;
The choice of statistical tests, including statistical significance levels and effect size; and
Recognition of limitations to generalizability resulting from design choices.
These are critical decisions that affect the quality of an evaluation. We further identified a subset of these evaluation studies that met a set of minimum conditions that we termed at least minimally methodologically adequate studies. Such studies are those with the greatest likelihood of shedding light on the effectiveness of these programs. To be classified as at least minimally methodologically adequate, and therefore to be considered for further analysis, each evaluation study was required to:
Include quantifiably measurable outcomes such as test scores, responses to specified cognitive tasks of mathematical reasoning, performance evaluations, grades, and subsequent course taking; and
Provide adequate information to judge the comparability of samples. In addition, a study must have included at least one of the following additional design elements:
A report of implementation fidelity or professional development activity;
Results disaggregated by content strands or by performance by student subgroups; and/or
Multiple outcome measures or precise theoretical analysis of a measured construct, such as number sense, proof, or proportional reasoning.
Using this rubric, the committee identified a subset of 63 comparative studies to classify as at least minimally methodologically adequate and to analyze in depth to inform the conduct of future evaluations. There are those who would argue that any threat to the validity of a study discredits the findings, thus claiming that until we know everything, we know nothing. Others would claim that from the myriad of studies, examining patterns of effects and patterns of variation, one can learn a great deal, perhaps tentatively, about programs and their possible effects. More importantly, we can learn about methodologies and how to concentrate and focus to increase the likelihood of learning more quickly. As Lipsey (1997, p. 22) wrote:
In the long run, our most useful and informative contribution to program managers and policy makers and even to the evaluation profession itself may be the consolidation of our piecemeal knowledge into broader pictures of the program and policy spaces at issue, rather than individual studies of particular programs.
We do not wish to imply that we devalue studies of student affect or conceptions of mathematics, but decided that unless these indicators were connected to direct indicators of student learning, we would eliminate them from further study. As a result of this sorting, we eliminated 19 studies of NSF-supported curricula and 13 studies of commercially generated curricula. Of these, 4 were eliminated for their sole focus on affect or conceptions, 3 were eliminated for their comparative focus on outcomes other than achievement, such as teacher-related variables, and 19 were eliminated for their failure to meet the minimum additional characteristics specified in the criteria above. In addition, six others were excluded from the studies of commercial materials because they were not conducted within the grade-
level band specified by the committee for the selection of that program. From this point onward, all references can be assumed to refer to at least minimally methodologically adequate unless a study is referenced for illustration, in which case we label it with “EX” to indicate that it is excluded in the summary analyses. Studies labeled “EX” are occasionally referenced because they can provide useful information on certain aspects of curricular evaluation, but not on the overall effectiveness.
The at least minimally methodologically adequate studies reported on a variety of grade levels. Figure 5-2 shows the different grade levels of the studies. At times, the choice of grade levels was dictated by the years in which high-stakes tests were given. Most of the studies reported on multiple grade levels, as shown in Figure 5-2 .
Using the seven critical design elements of at least minimally methodologically adequate studies as a design template, we describe the overall database and discuss the array of choices on critical decision points with examples. Following that, we report on the results on the at least minimally methodologically adequate studies by program type. To do so, the results of each study were coded as either statistically significant or not. Those studies
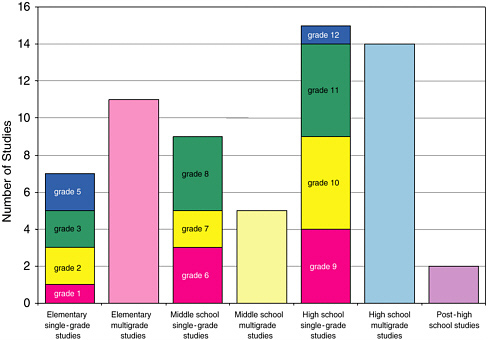
FIGURE 5-2 Single-grade studies by grade and multigrade studies by grade band.
that contained statistically significant results were assigned a percentage of outcomes that are positive (in favor of the treatment curriculum) based on the number of statistically significant comparisons reported relative to the total number of comparisons reported, and a percentage of outcomes that are negative (in favor of the comparative curriculum). The remaining were coded as the percentage of outcomes that are non significant. Then, using seven critical decision points as filters, we identified and examined more closely sets of studies that exhibited the strongest designs, and would therefore be most likely to increase our confidence in the validity of the evaluation. In this last section, we consider alternative hypotheses that could explain the results.
The committee emphasizes that we did not directly evaluate the materials. We present no analysis of results aggregated across studies by naming individual curricular programs because we did not consider the magnitude or rigor of the database for individual programs substantial enough to do so. Nevertheless, there are studies that provide compelling data concerning the effectiveness of the program in a particular context. Furthermore, we do report on individual studies and their results to highlight issues of approach and methodology and to remain within our primary charge, which was to evaluate the evaluations, we do not summarize results of the individual programs.
DESCRIPTION OF COMPARATIVE STUDIES DATABASE ON CRITICAL DECISION POINTS
An experimental or quasi-experimental design.
We separated the studies into experimental and quasiexperimental, and found that 100 percent of the studies were quasiexperimental (Campbell and Stanley, 1966; Cook and Campbell, 1979; and Rossi et al., 1999). 1 Within the quasi-experimental studies, we identified three subcategories of comparative study. In the first case, we identified a study as cross-curricular comparative if it compared the results of curriculum A with curriculum B. A few studies in this category also compared two samples within the curriculum to each other and specified different conditions such as high and low implementation quality.
A second category of a quasi-experimental study involved comparisons that could shed light on effectiveness involving time series studies. These studies compared the performance of a sample of students in a curriculum
| One study, by Peters (1992), used random assignment to two classrooms, but was classified as quasi-experimental with its sample size and use of qualitative methods. |
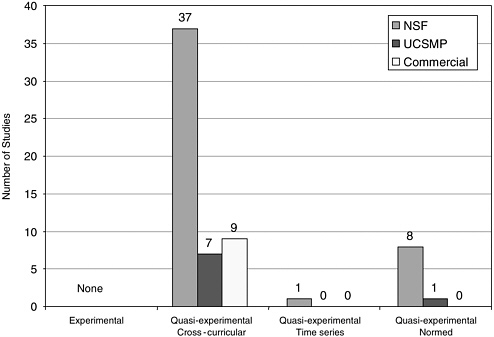
FIGURE 5-3 The number of comparative studies in each category.
under investigation across time, such as in a longitudinal study of the same students over time. A third category of comparative study involved a comparison to some form of externally normed results, such as populations taking state, national, or international tests or prior research assessment from a published study or studies. We categorized these studies and divided them into NSF, UCSMP, and commercial and labeled them by the categories above ( Figure 5-3 ).
In nearly all studies in the comparative group, the titles of experimental curricula were explicitly identified. The only exception to this was the ARC Implementation Center study (Sconiers et al., 2002), where three NSF-supported elementary curricula were examined, but in the results, their effects were pooled. In contrast, in the majority of the cases, the comparison curriculum is referred to simply as “traditional.” In only 22 cases were comparisons made between two identified curricula. Many others surveyed the array of curricula at comparison schools and reported on the most frequently used, but did not identify a single curriculum. This design strategy is used often because other factors were used in selecting comparison groups, and the additional requirement of a single identified curriculum in
these sites would often make it difficult to match. Studies were categorized into specified (including a single or multiple identified curricula) and nonspecified curricula. In the 63 studies, the central group was compared to an NSF-supported curriculum (1), an unnamed traditional curriculum (41), a named traditional curriculum (19), and one of the six commercial curricula (2). To our knowledge, any systematic impact of such a decision on results has not been studied, but we express concern that when a specified curriculum is compared to an unspecified content which is a set of many informal curriculum, the comparison may favor the coherency and consistency of the single curricula, and we consider this possibility subsequently under alternative hypotheses. We believe that a quality study should at least report the array of curricula that comprise the comparative group and include a measure of the frequency of use of each, but a well-defined alternative is more desirable.
If a study was both longitudinal and comparative, then it was coded as comparative. When studies only examined performances of a group over time, such as in some longitudinal studies, it was coded as quasi-experimental normed. In longitudinal studies, the problems created by student mobility were evident. In one study, Carroll (2001), a five-year longitudinal study of Everyday Mathematics, the sample size began with 500 students, 24 classrooms, and 11 schools. By 2nd grade, the longitudinal sample was 343. By 3rd grade, the number of classes increased to 29 while the number of original students decreased to 236 students. At the completion of the study, approximately 170 of the original students were still in the sample. This high rate of attrition from the study suggests that mobility is a major challenge in curricular evaluation, and that the effects of curricular change on mobile students needs to be studied as a potential threat to the validity of the comparison. It is also a challenge in curriculum implementation because students coming into a program do not experience its cumulative, developmental effect.
Longitudinal studies also have unique challenges associated with outcome measures, a study by Romberg et al. (in press) (EX) discussed one approach to this problem. In this study, an external assessment system and a problem-solving assessment system were used. In the External Assessment System, items from the National Assessment of Educational Progress (NAEP) and Third International Mathematics and Science Survey (TIMSS) were balanced across four strands (number, geometry, algebra, probability and statistics), and 20 items of moderate difficulty, called anchor items, were repeated on each grade-specific assessment (p. 8). Because the analyses of the results are currently under way, the evaluators could not provide us with final results of this study, so it is coded as EX.
However, such longitudinal studies can provide substantial evidence of the effects of a curricular program because they may be more sensitive to an
TABLE 5-1 Scores in Percentage Correct by Everyday Mathematics Students and Various Comparison Groups Over a Five-Year Longitudinal Study
| Sample Size | 1st Grade | 2nd Grade | 3rd Grade | 4th Grade | 5th Grade |
EM | n=170-503 | 58 | 62 | 61 | 71 | 75 |
Traditional U.S. | n=976 | 43 | 53.5 |
|
| 44 |
Japanese | n=750 | 64 | 71 |
|
| 80 |
Chinese | n=1,037 | 52 |
|
|
| 76 |
NAEP Sample | n=18,033 |
|
| 44 | 44 |
|
NOTE: 1st grade: 44 items; 2nd grade: 24 items; 3rd grade: 22 items; 4th grade: 29 items; and 5th grade: 33 items. SOURCE: Adapted from Carroll (2001). |
accumulation of modest effects and/or can reveal whether the rates of learning change over time within curricular change.
The longitudinal study by Carroll (2001) showed that the effects of curricula may often accrue over time, but measurements of achievement present challenges to drawing such conclusions as the content and grade level change. A variety of measures were used over time to demonstrate growth in relation to comparison groups. The author chose a set of measures used previously in studies involving two Asian samples and an American sample to provide a contrast to the students in EM over time. For 3rd and 4th grades, where the data from the comparison group were not available, the authors selected items from the NAEP to bridge the gap. Table 5-1 summarizes the scores of the different comparative groups over five years. Scores are reported as the mean percentage correct for a series of tests on number computation, number concepts and applications, geometry, measurement, and data analysis.
It is difficult to compare performances on different tests over different groups over time against a single longitudinal group from EM, and it is not possible to determine whether the students’ performance is increasing or whether the changes in the tests at each grade level are producing the results; thus the results from longitudinal studies lacking a control group or use of sophisticated methodological analysis may be suspect and should be interpreted with caution.
In the Hirsch and Schoen (2002) study, based on a sample of 1,457 students, scores on Ability to Do Quantitative Thinking (ITED-Q) a subset of the Iowa Tests of Education Development, students in Core-Plus showed increasing performance over national norms over the three-year time period. The authors describe the content of the ITED-Q test and point out
that “although very little symbolic algebra is required, the ITED-Q is quite demanding for the full range of high school students” (p. 3). They further point out that “[t]his 3-year pattern is consistent, on average, in rural, urban, and suburban schools, for males and females, for various minority groups, and for students for whom English was not their first language” (p. 4). In this case, one sees that studies over time are important as results over shorter periods may mask cumulative effects of consistent and coherent treatments and such studies could also show increases that do not persist when subject to longer trajectories. One approach to longitudinal studies was used by Webb and Dowling in their studies of the Interactive Mathematics Program (Webb and Dowling, 1995a, 1995b, 1995c). These researchers conducted transcript analyses as a means to examine student persistence and success in subsequent course taking.
The third category of quasi-experimental comparative studies measured student outcomes on a particular curricular program and simply compared them to performance on national tests or international tests. When these tests were of good quality and were representative of a genuine sample of a relevant population, such as NAEP reports or TIMSS results, the reports often provided one a reasonable indicator of the effects of the program if combined with a careful description of the sample. Also, sometimes the national tests or state tests used were norm-referenced tests producing national percentiles or grade-level equivalents. The normed studies were considered of weaker quality in establishing effectiveness, but were still considered valid as examples of comparing samples to populations.
For Studies That Do Not Use Random Assignment: What Methods of Establishing Comparability Across Groups Were Built into the Design
The most fundamental question in an evaluation study is whether the treatment has had an effect on the chosen criterion variable. In our context, the treatment is the curriculum materials, and in some cases, related professional development, and the outcome of interest is academic learning. To establish if there is a treatment effect, one must logically rule out as many other explanations as possible for the differences in the outcome variable. There is a long tradition on how this is best done, and the principle from a design point of view is to assure that there are no differences between the treatment conditions (especially in these evaluations, often there are only the new curriculum materials to be evaluated and a control group) either at the outset of the study or during the conduct of the study.
To ensure the first condition, the ideal procedure is the random assignment of the appropriate units to the treatment conditions. The second condition requires that the treatment is administered reliably during the length of the study, and is assured through the careful observation and
control of the situation. Without randomization, there are a host of possible confounding variables that could differ among the treatment conditions and that are related themselves to the outcome variables. Put another way, the treatment effect is a parameter that the study is set up to estimate. Statistically, an estimate that is unbiased is desired. The goal is that its expected value over repeated samplings is equal to the true value of the parameter. Without randomization at the onset of a study, there is no way to assure this property of unbiasness. The variables that differ across treatment conditions and are related to the outcomes are confounding variables, which bias the estimation process.
Only one study we reviewed, Peters (1992), used randomization in the assignment of students to treatments, but that occurred because the study was limited to one teacher teaching two sections and included substantial qualitative methods, so we coded it as quasi-experimental. Others report partially assigning teachers randomly to treatment conditions (Thompson, et al., 2001; Thompson et al., 2003). Two primary reasons seem to account for a lack of use of pure experimental design. To justify the conduct and expense of a randomized field trial, the program must be described adequately and there must be relative assurance that its implementation has occurred over the duration of the experiment (Peterson et al., 1999). Additionally, one must be sure that the outcome measures are appropriate for the range of performances in the groups and valid relative to the curricula under investigation. Seldom can such conditions be assured for all students and teachers and over the duration of a year or more.
A second reason is that random assignment of classrooms to curricular treatment groups typically is not permitted or encouraged under normal school conditions. As one evaluator wrote, “Building or district administrators typically identified teachers who would be in the study and in only a few cases was random assignment of teachers to UCSMP Algebra or comparison classes possible. School scheduling and teacher preference were more important factors to administrators and at the risk of losing potential sites, we did not insist on randomization” (Mathison et al., 1989, p. 11).
The Joint Committee on Standards for Educational Evaluation (1994, p. 165) committee of evaluations recognized the likelihood of limitations on randomization, writing:
The groups being compared are seldom formed by random assignment. Rather, they tend to be natural groupings that are likely to differ in various ways. Analytical methods may be used to adjust for these initial differences, but these methods are based upon a number of assumptions. As it is often difficult to check such assumptions, it is advisable, when time and resources permit, to use several different methods of analysis to determine whether a replicable pattern of results is obtained.
Does the dearth of pure experimentation render the results of the studies reviewed worthless? Bias is not an “either-or” proposition, but it is a quantity of varying degrees. Through careful measurement of the most salient potential confounding variables, precise theoretical description of constructs, and use of these methods of statistical analysis, it is possible to reduce the amount of bias in the estimated treatment effect. Identification of the most likely confounding variables and their measurement and subsequent adjustments can greatly reduce bias and help estimate an effect that is likely to be more reflective of the true value. The theoretical fully specified model is an alternative to randomization by including relevant variables and thus allowing the unbiased estimation of the parameter. The only problem is realizing when the model is fully specified.
We recognized that we can never have enough knowledge to assure a fully specified model, especially in the complex and unstable conditions of schools. However, a key issue in determining the degree of confidence we have in these evaluations is to examine how they have identified, measured, or controlled for such confounding variables. In the next sections, we report on the methods of the evaluators in identifying and adjusting for such potential confounding variables.
One method to eliminate confounding variables is to examine the extent to which the samples investigated are equated either by sample selection or by methods of statistical adjustments. For individual students, there is a large literature suggesting the importance of social class to achievement. In addition, prior achievement of students must be considered. In the comparative studies, investigators first identified participation of districts, schools, or classes that could provide sufficient duration of use of curricular materials (typically two years or more), availability of target classes, or adequate levels of use of program materials. Establishing comparability was a secondary concern.
These two major factors were generally used in establishing the comparability of the sample:
Student population characteristics, such as demographic characteristics of students in terms of race/ethnicity, economic levels, or location type (urban, suburban, or rural).
Performance-level characteristics such as performance on prior tests, pretest performance, percentage passing standardized tests, or related measures (e.g., problem solving, reading).
In general, four methods of comparing groups were used in the studies we examined, and they permit different degrees of confidence in their results. In the first type, a matching class, school, or district was identified.
Studies were coded as this type if specified characteristics were used to select the schools systematically. In some of these studies, the methodology was relatively complex as correlates of performance on the outcome measures were found empirically and matches were created on that basis (Schneider, 2000; Riordan and Noyce, 2001; and Sconiers et al., 2002). For example, in the Sconiers et al. study, where the total sample of more than 100,000 students was drawn from five states and three elementary curricula are reviewed (Everyday Mathematics, Math Trailblazers [MT], and Investigations [IN], a highly systematic method was developed. After defining eligibility as a “reform school,” evaluators conducted separate regression analyses for the five states at each tested grade level to identify the strongest predictors of average school mathematics score. They reported, “reading score and low-income variables … consistently accounted for the greatest percentage of total variance. These variables were given the greatest weight in the matching process. Other variables—such as percent white, school mobility rate, and percent with limited English proficiency (LEP)—accounted for little of the total variance but were typically significant. These variables were given less weight in the matching process” (Sconiers et al., 2002, p. 10). To further provide a fair and complete comparison, adjustments were made based on regression analysis of the scores to minimize bias prior to calculating the difference in scores and reporting effect sizes. In their results the evaluators report, “The combined state-grade effect sizes for math and total are virtually identical and correspond to a percentile change of about 4 percent favoring the reform students” (p. 12).
A second type of matching procedure was used in the UCSMP evaluations. For example, in an evaluation centered on geometry learning, evaluators advertised in NCTM and UCSMP publications, and set conditions for participation from schools using their program in terms of length of use and grade level. After selecting schools with heterogeneous grouping and no tracking, the researchers used a match-pair design where they selected classes from the same school on the basis of mathematics ability. They used a pretest to determine this, and because the pretest consisted of two parts, they adjusted their significance level using the Bonferroni method. 2 Pairs were discarded if the differences in means and variance were significant for all students or for those students completing all measures, or if class sizes became too variable. In the algebra study, there were 20 pairs as a result of the matching, and because they were comparing three experimental conditions—first edition, second edition, and comparison classes—in the com-
| The Bonferroni method is a simple method that allows multiple comparison statements to be made (or confidence intervals to be constructed) while still assuring that an overall confidence coefficient is maintained. |
parison study relevant to this review, their matching procedure identified 8 pairs. When possible, teachers were assigned randomly to treatment conditions. Most results are presented with the eight identified pairs and an accumulated set of means. The outcomes of this particular study are described below in a discussion of outcome measures (Thompson et al., 2003).
A third method was to measure factors such as prior performance or socio-economic status (SES) based on pretesting, and then to use analysis of covariance or multiple regression in the subsequent analysis to factor in the variance associated with these factors. These studies were coded as “control.” A number of studies of the Saxon curricula used this method. For example, Rentschler (1995) conducted a study of Saxon 76 compared to Silver Burdett with 7th graders in West Virginia. He reported that the groups differed significantly in that the control classes had 65 percent of the students on free and reduced-price lunch programs compared to 55 percent in the experimental conditions. He used scores on California Test of Basic Skills mathematics computation and mathematics concepts and applications as his pretest scores and found significant differences in favor of the experimental group. His posttest scores showed the Saxon experimental group outperformed the control group on both computation and concepts and applications. Using analysis of covariance, the computation difference in favor of the experimental group was statistically significant; however, the difference in concepts and applications was adjusted to show no significant difference at the p < .05 level.
A fourth method was noted in studies that used less rigorous methods of selection of sample and comparison of prior achievement or similar demographics. These studies were coded as “compare.” Typically, there was no explicit procedure to decide if the comparison was good enough. In some of the studies, it appeared that the comparison was not used as a means of selection, but rather as a more informal device to convince the reader of the plausibility of the equivalence of the groups. Clearly, the studies that used a more precise method of selection were more likely to produce results on which one’s confidence in the conclusions is greater.
Definition of Unit of Analysis
A major decision in forming an evaluation design is the unit of analysis. The unit of selection or randomization used to assign elements to treatment and control groups is closely linked to the unit of analysis. As noted in the National Research Council (NRC) report (1992, p. 21):
If one carries out the assignment of treatments at the level of schools, then that is the level that can be justified for causal analysis. To analyze the results at the student level is to introduce a new, nonrandomized level into
the study, and it raises the same issues as does the nonrandomized observational study…. The implications … are twofold. First, it is advisable to use randomization at the level at which units are most naturally manipulated. Second, when the unit of observation is at a “lower” level of aggregation than the unit of randomization, then for many purposes the data need to be aggregated in some appropriate fashion to provide a measure that can be analyzed at the level of assignment. Such aggregation may be as simple as a summary statistic or as complex as a context-specific model for association among lower-level observations.
In many studies, inadequate attention was paid to the fact that the unit of selection would later become the unit of analysis. The unit of analysis, for most curriculum evaluators, needs to be at least the classroom, if not the school or even the district. The units must be independently responding units because instruction is a group process. Students are not independent, the classroom—even if the teachers work together in a school on instruction—is not entirely independent, so the school is the unit. Care needed to be taken to ensure that an adequate numbers of units would be available to have sufficient statistical power to detect important differences.
A curriculum is experienced by students in a group, and this implies that individual student responses and what they learn are correlated. As a result, the appropriate unit of assignment and analysis must at least be defined at the classroom or teacher level. Other researchers (Bryk et al., 1993) suggest that the unit might be better selected at an even higher level of aggregation. The school itself provides a culture in which the curriculum is enacted as it is influenced by the policies and assignments of the principal, by the professional interactions and governance exhibited by the teachers as a group, and by the community in which the school resides. This would imply that the school might be the appropriate unit of analysis. Even further, to the extent that such decisions about curriculum are made at the district level and supported through resources and professional development at that level, the appropriate unit could arguably be the district. On a more practical level, we found that arguments can be made for a variety of decisions on the selection of units, and what is most essential is to make a clear argument for one’s choice, to use the same unit in the analysis as in the sample selection process, and to recognize the potential limits to generalization that result from one’s decisions.
We would argue in all cases that reports of how sites are selected must be explicit in the evaluation report. For example, one set of evaluation studies selected sites by advertisements in a journal distributed by the program and in NCTM journals (UCSMP) (Thompson et al., 2001; Thompson et al., 2003). The samples in their studies tended to be affluent suburban populations and predominantly white populations. Other conditions of inclusion, such as frequency of use also might have influenced this outcome,
but it is important that over a set of studies on effectiveness, all populations of students be adequately sampled. When a study is not randomized, adjustments for these confounding variables should be included. In our analysis of equity, we report on the concerns about representativeness of the overall samples and their impact on the generalizability of the results.
Implementation Components
The complexity of doing research on curricular materials introduces a number of possible confounding variables. Due to the documented complexity of curricular implementation, most comparative study evaluators attempt to monitor implementation in some fashion. A valuable outcome of a well-conducted evaluation is to determine not only if the experimental curriculum could ideally have a positive impact on learning, but whether it can survive or thrive in the conditions of schooling that are so variable across sites. It is essential to know what the treatment was, whether it occurred, and if so, to what degree of intensity, fidelity, duration, and quality. In our model in Chapter 3 , these factors were referred to as “implementation components.” Measuring implementation can be costly for large-scale comparative studies; however, many researchers have shown that variation in implementation is a key factor in determining effectiveness. In coding the comparative studies, we identified three types of components that help to document the character of the treatment: implementation fidelity, professional development treatments, and attention to teacher effects.
Implementation Fidelity
Implementation fidelity is a measure of the basic extent of use of the curricular materials. It does not address issues of instructional quality. In some studies, implementation fidelity is synonymous with “opportunity to learn.” In examining implementation fidelity, a variety of data were reported, including, most frequently, the extent of coverage of the curricular material, the consistency of the instructional approach to content in relation to the program’s theory, reports of pedagogical techniques, and the length of use of the curricula at the sample sites. Other less frequently used approaches documented the calendar of curricular coverage, requested teacher feedback by textbook chapter, conducted student surveys, and gauged homework policies, use of technology, and other particular program elements. Interviews with teachers and students, classroom surveys, and observations were the most frequently used data-gathering techniques. Classroom observations were conducted infrequently in these studies, except in cases when comparative studies were combined with case studies, typically with small numbers of schools and classes where observations
were conducted for long or frequent time periods. In our analysis, we coded only the presence or absence of one or more of these methods.
If the extent of implementation was used in interpreting the results, then we classified the study as having adjusted for implementation differences. Across all 63 at least minimally methodologically adequate studies, 44 percent reported some type of implementation fidelity measure, 3 percent reported and adjusted for it in interpreting their outcome measures, and 53 percent recorded no information on this issue. Differences among studies, by study type (NSF, UCSMP, and commercially generated), showed variation on this issue, with 46 percent of NSF reporting or adjusting for implementation, 75 percent of UCSMP, and only 11 percent of the other studies of commercial materials doing so. Of the commercial, non-UCSMP studies included, only one reported on implementation. Possibly, the evaluators for the NSF and UCSMP Secondary programs recognized more clearly that their programs demanded significant changes in practice that could affect their outcomes and could pose challenges to the teachers assigned to them.
A study by Abrams (1989) (EX) 3 on the use of Saxon algebra by ninth graders showed that concerns for implementation fidelity extend to all curricula, even those like Saxon whose methods may seem more likely to be consistent with common practice. Abrams wrote, “It was not the intent of this study to determine the effectiveness of the Saxon text when used as Saxon suggests, but rather to determine the effect of the text as it is being used in the classroom situations. However, one aspect of the research was to identify how the text is being taught, and how closely teachers adhere to its content and the recommended presentation” (p. 7). Her findings showed that for the 9 teachers and 300 students, treatment effects favoring the traditional group (using Dolciani’s Algebra I textbook, Houghton Mifflin, 1980) were found on the algebra test, the algebra knowledge/skills subtest, and the problem-solving test for this population of teachers (fixed effect). No differences were found between the groups on an algebra understanding/applications subtest, overall attitude toward mathematics, mathematical self-confidence, anxiety about mathematics, or enjoyment of mathematics. She suggests that the lack of differences might be due to the ways in which teachers supplement materials, change test conditions, emphasize
| Both studies referenced in this section did not meet the criteria for inclusion in the comparative studies, but shed direct light on comparative issues of implementation. The Abrams study was omitted because it examined a program at a grade level outside the specified grade band for that curriculum. Briars and Resnick (2000) did not provide explicit comparison scores to permit one to evaluate the level of student attainment. |
and deemphasize topics, use their own tests, vary the proportion of time spent on development and practice, use calculators and group work, and basically adapt the materials to their own interpretation and method. Many of these practices conflict directly with the recommendations of the authors of the materials.
A study by Briars and Resnick (2000) (EX) in Pittsburgh schools directly confronted issues relevant to professional development and implementation. Evaluators contrasted the performance of students of teachers with high and low implementation quality, and showed the results on two contrasting outcome measures, Iowa Test of Basic Skills (ITBS) and Balanced Assessment. Strong implementers were defined as those who used all of the EM components and provided student-centered instruction by giving students opportunities to explore mathematical ideas, solve problems, and explain their reasoning. Weak implementers were either not using EM or using it so little that the overall instruction in the classrooms was “hardly distinguishable from traditional mathematics instruction” (p. 8). Assignment was based on observations of student behavior in classes, the presence or absence of manipulatives, teacher questionnaires about the programs, and students’ knowledge of classroom routines associated with the program.
From the identification of strong- and weak-implementing teachers, strong- and weak-implementation schools were identified as those with strong- or weak-implementing teachers in 3rd and 4th grades over two consecutive years. The performance of students with 2 years of EM experience in these settings composed the comparative samples. Three pairs of strong- and weak-implementation schools with similar demographics in terms of free and reduced-price lunch (range 76 to 93 percent), student living with only one parent (range 57 to 82 percent), mobility (range 8 to 16 percent), and ethnicity (range 43 to 98 percent African American) were identified. These students’ 1st-grade ITBS scores indicated similarity in prior performance levels. Finally, evaluators predicted that if the effects were due to the curricular implementation and accompanying professional development, the effects on scores should be seen in 1998, after full implementation. Figure 5-4 shows that on the 1998 New Standards exams, placement in strong- and weak-implementation schools strongly affected students’ scores. Over three years, performance in the district on skills, concepts, and problem solving rose, confirming the evaluator’s predictions.
An article by McCaffrey et al. (2001) examining the interactions among instructional practices, curriculum, and student achievement illustrates the point that distinctions are often inadequately linked to measurement tools in their treatment of the terms traditional and reform teaching. In this study, researchers conducted an exploratory factor analysis that led them to create two scales for instructional practice: Reform Practices and Tradi-
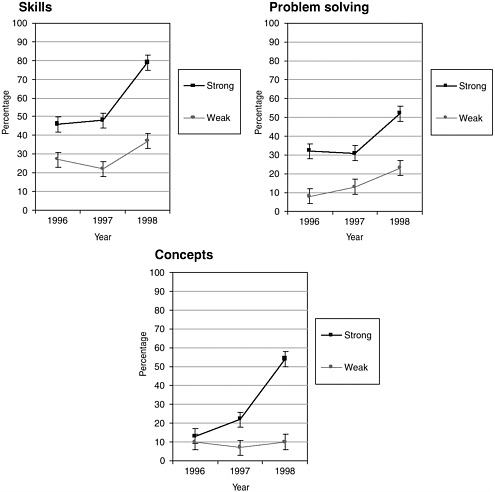
FIGURE 5-4 Percentage of students who met or exceeded the standard. Districtwide grade 4 New Standards Mathematics Reference Examination (NSMRE) performance for 1996, 1997, and 1998 by level of Everyday Mathematics implementation. Percentage of students who achieved the standard. Error bars denote the 99 percent confidence interval for each data point.
SOURCE: Re-created from Briars and Resnick (2000, pp. 19-20).
tional Practices. The reform scale measured the frequency, by means of teacher report, of teacher and student behaviors associated with reform instruction and assessment practices, such as using small-group work, explaining reasoning, representing and using data, writing reflections, or performing tasks in groups. The traditional scale focused on explanations to whole classes, the use of worksheets, practice, and short-answer assessments. There was a –0.32 correlation between scores for integrated curriculum teachers. There was a 0.27 correlation between scores for traditional
curriculum teachers. This shows that it is overly simplistic to think that reform and traditional practices are oppositional. The relationship among a variety of instructional practices is rather more complex as they interact with curriculum and various student populations.
Professional Development
Professional development and teacher effects were separated in our analysis from implementation fidelity. We recognized that professional development could be viewed by the readers of this report in two ways. As indicated in our model, professional development can be considered a program element or component or it can be viewed as part of the implementation process. When viewed as a program element, professional development resources are considered mandatory along with program materials. In relation to evaluation, proponents of considering professional development as a mandatory program element argue that curricular innovations, which involve the introduction of new topics, new types of assessment, or new ways of teaching, must make provision for adequate training, just as with the introduction of any new technology.
For others, the inclusion of professional development in the program elements without a concomitant inclusion of equal amounts of professional development relevant to a comparative treatment interjects a priori disproportionate treatments and biases the results. We hoped for an array of evaluation studies that might shed some empirical light on this dispute, and hence separated professional development from treatment fidelity, coding whether or not studies reported on the amount of professional development provided for the treatment and/or comparison groups. A study was coded as positive if it either reported on the professional development provided on the experimental group or reported the data on both treatments. Across all 63 at least minimally methodologically adequate studies, 27 percent reported some type of professional development measure, 1.5 percent reported and adjusted for it in interpreting their outcome measures, and 71.5 percent recorded no information on the issue.
A study by Collins (2002) (EX) 4 illustrates the critical and controversial role of professional development in evaluation. Collins studied the use of Connected Math over three years, in three middle schools in threat of being classified as low performing in the Massachusetts accountability system. A comparison was made between one school (School A) that engaged
| The Collins study lacked a comparison group and is coded as EX. However, it is reported as a case study. |
substantively in professional development opportunities accompanying the program and two that did not (Schools B and C). In the CMP school reports (School A) totals between 100 and 136 hours of professional development were recorded for all seven teachers in grades 6 through 8. In School B, 66 hours were reported for two teachers and in School C, 150 hours were reported for eight teachers over three years. Results showed significant differences in the subsequent performance by students at the school with higher participation in professional development (School A) and it became a districtwide top performer; the other two schools remained at risk for low performance. No controls for teacher effects were possible, but the results do suggest the centrality of professional development for successful implementation or possibly suggest that the results were due to professional development rather than curriculum materials. The fact that these two interpretations cannot be separated is a problem when professional development is given to one and not the other. The effect could be due to textbook or professional development or an interaction between the two. Research designs should be adjusted to consider these issues when different conditions of professional development are provided.
Teacher Effects
These studies make it obvious that there are potential confounding factors of teacher effects. Many evaluation studies devoted inadequate attention to the variable of teacher quality. A few studies (Goodrow, 1998; Riordan and Noyce, 2001; Thompson et al., 2001; and Thompson et al., 2003) reported on teacher characteristics such as certification, length of service, experience with curricula, or degrees completed. Those studies that matched classrooms and reported by matched results rather than aggregated results sought ways to acknowledge the large variations among teacher performance and its impact on student outcomes. We coded any effort to report on possible teacher effects as one indicator of quality. Across all 63 at least minimally methodologically adequate studies, 16 percent reported some type of teacher effect measure, 3 percent reported and adjusted for it in interpreting their outcome measures, and 81 percent recorded no information on this issue.
One can see that the potential confounding factors of teacher effects, in terms of the provision of professional development or the measure of teacher effects, are not adequately considered in most evaluation designs. Some studies mention and give a subjective judgment as to the nature of the problem, but this is descriptive at the most. Hardly any of the studies actually do anything analytical, and because these are such important potential confounding variables, this presents a serious challenge to the efficacy of these studies. Figure 5-5 shows how attention to these factors varies
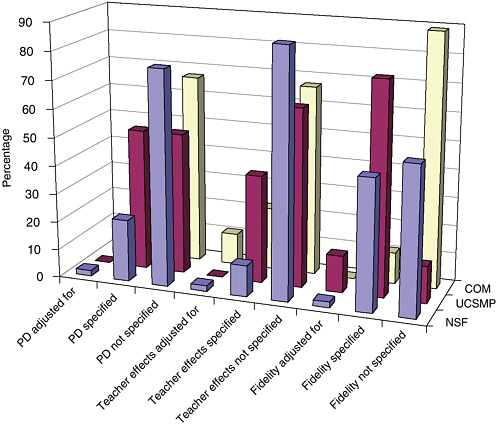
FIGURE 5-5 Treatment of implementation components by program type.
NOTE: PD = professional development.
across program categories among NSF-supported, UCSMP, and studies of commercial materials. In general, evaluations of NSF-supported studies were the most likely to measure these variables; UCSMP had the most standardized use of methods to do so across studies; and commercial material evaluators seldom reported on issues of implementation fidelity.
Identification of a Set of Outcome Measures and Forms of Disaggregation
Using the selected student outcomes identified in the program theory, one must conduct an impact assessment that refers to the design and measurement of student outcomes. In addition to selecting what outcomes should be measured within one’s program theory, one must determine how these outcomes are measured, when those measures are collected, and what
purpose they serve from the perspective of the participants. In the case of curricular evaluation, there are significant issues involved in how these measures are reported. To provide insight into the level of curricular validity, many evaluators prefer to report results by topic, content strand, or item cluster. These reports often present the level of specificity of outcome needed to inform curriculum designers, especially when efforts are made to document patterns of errors, distribution of results across multiple choices, or analyses of student methods. In these cases, whole test scores may mask essential differences in impact among curricula at the level of content topics, reporting only average performance.
On the other hand, many large-scale assessments depend on methods of test equating that rely on whole test scores and make comparative interpretations of different test administrations by content strands of questionable reliability. Furthermore, there are questions such as whether to present only gain scores effect sizes, how to link pretests and posttests, and how to determine the relative curricular sensitivity of various outcome measures.
The findings of comparative studies are reported in terms of the outcome measure(s) collected. To describe the nature of the database with regard to outcome measures and to facilitate our analyses of the studies, we classified each of the included studies on four outcome measure dimensions:
Total score reported;
Disaggregation of content strands, subtest, performance level, SES, or gender;
Outcome measure that was specific to curriculum; and
Use of multiple outcome measures.
Most studies reported a total score, but we did find studies that reported only subtest scores or only scores on an item-by-item basis. For example, in the Ben-Chaim et al. (1998) evaluation study of Connected Math, the authors were interested in students’ proportional reasoning proficiency as a result of use of this curriculum. They asked students from eight seventh-grade classes of CMP and six seventh-grade classes from the control group to solve a variety of tasks categorized as rate and density problems. The authors provide precise descriptions of the cognitive challenges in the items; however, they do not explain if the problems written up were representative of performance on a larger set of items. A special rating form was developed to code responses in three major categories (correct answer, incorrect answer, and no response), with subcategories indicating the quality of the work that accompanied the response. No reports on reliability of coding were given. Performance on standardized tests indicated that control students’ scores were slightly higher than CMP at the beginning of the
year and lower at the end. Twenty-five percent of the experimental group members were interviewed about their approaches to the problems. The CMP students outperformed the control students (53 percent versus 28 percent) overall in providing the correct answers and support work, and 27 percent of the control group gave an incorrect answer or showed incorrect thinking compared to 13 percent of the CMP group. An item-level analysis permitted the researchers to evaluate the actual strategies used by the students. They reported, for example, that 82 percent of CMP students used a “strategy focused on package price, unit price, or a combination of the two; those effective strategies were used by only 56 of 91 control students (62 percent)” (p. 264).
The use of item or content strand-level comparative reports had the advantage that they permitted the evaluators to assess student learning strategies specific to a curriculum’s program theory. For example, at times, evaluators wanted to gauge the effectiveness of using problems different from those on typical standardized tests. In this case, problems were drawn from familiar circumstances but carefully designed to create significant cognitive challenges, and assess how well the informal strategies approach in CMP works in comparison to traditional instruction. The disadvantages of such an approach include the use of only a small number of items and the concerns for reliability in scoring. These studies seem to represent a method of creating hybrid research models that build on the detailed analyses possible using case studies, but still reporting on samples that provide comparative data. It possibly reflects the concerns of some mathematicians and mathematics educators that the effectiveness of materials needs to be evaluated relative to very specific, research-based issues on learning and that these are often inadequately measured by multiple-choice tests. However, a decision not to report total scores led to a trade-off in the reliability and representativeness of the reported data, which must be addressed to increase the objectivity of the reports.
Second, we coded whether outcome data were disaggregated in some way. Disaggregation involved reporting data on dimensions such as content strand, subtest, test item, ethnic group, performance level, SES, and gender. We found disaggregated results particularly helpful in understanding the findings of studies that found main effects, and also in examining patterns across studies. We report the results of the studies’ disaggregation by content strand in our reports of effects. We report the results of the studies’ disaggregation by subgroup in our discussions of generalizability.
Third, we coded whether a study used an outcome measure that the evaluator reported as being sensitive to a particular treatment—this is a subcategory of what was defined in our framework as “curricular validity of measures.” In such studies, the rationale was that readily available measures such as state-mandated tests, norm-referenced standardized tests, and
college entrance examinations do not measure some of the aims of the program under study. A frequently cited instance of this was that “off the shelf” instruments do not measure well students’ ability to apply their mathematical knowledge to problems embedded in complex settings. Thus, some studies constructed a collection of tasks that assessed this ability and collected data on it (Ben-Chaim et al., 1998; Huntley et al., 2000).
Finally, we recorded whether a study used multiple outcome measures. Some studies used a variety of achievement measures and other studies reported on achievement accompanied by measures such as subsequent course taking or various types of affective measures. For example, Carroll (2001, p. 47) reported results on a norm-referenced standardized achievement test as well as a collection of tasks developed in other studies.
A study by Huntley et al. (2000) illustrates how a variety of these techniques were combined in their outcome measures. They developed three assessments. The first emphasized contextualized problem solving based on items from the American Mathematical Association of Two-Year Colleges and others; the second assessment was on context-free symbolic manipulation and a third part requiring collaborative problem solving. To link these measures to the overall evaluation, they articulated an explicit model of cognition based on how one links an applied situation to mathematical activity through processes of formulation and interpretation. Their assessment strategy permitted them to investigate algebraic reasoning as an ability to use algebraic ideas and techniques to (1) mathematize quantitative problem situations, (2) use algebraic principles and procedures to solve equations, and (3) interpret results of reasoning and calculations.
In presenting their data comparing performance on Core-Plus and traditional curriculum, they presented both main effects and comparisons on subscales. Their design of outcome measures permitted them to examine differences in performance with and without context and to conclude with statements such as “This result illustrates that CPMP students perform better than control students when setting up models and solving algebraic problems presented in meaningful contexts while having access to calculators, but CPMP students do not perform as well on formal symbol-manipulation tasks without access to context cues or calculators” (p. 349). The authors go on to present data on the relationship between knowing how to plan or interpret solutions and knowing how to carry them out. The correlations between these variables were weak but significantly different (0.26 for control groups and 0.35 for Core-Plus). The advantage of using multiple measures carefully tied to program theory is that they can permit one to test fine content distinctions that are likely to be the level of adjustments necessary to fine tune and improve curricular programs.
Another interesting approach to the use of outcome measures is found in the UCSMP studies. In many of these studies, evaluators collected infor-
TABLE 5-2 Mean Percentage Correct on the Subject Tests
Treatment Group | Geometry—Standard | Geometry—UCSMP | Advanced Algebra—UCSMP |
UCSMP | 43.1, 44.7, 50.5 | 51.2, 54.5 | 56.1, 58.8, 56.1 |
Comparison | 42.7, 45.5, 51.5 | 36.6, 40.8 | 42.0, 50.1, 50.0 |
“43.1, 44.7, 50.5” means students were correct on 43.1 percent of the total items, 44.7 percent of the fair items for UCSMP, and 50.5 percent of the items that were taught in both treatments. Too few items to report data. SOURCES: Adapted from Thompson et al. (2001); Thompson et al. (2003). |
mation from teachers’ reports and chapter reviews as to whether topics for items on the posttests were taught, calling this an “opportunity to learn” measure. The authors reported results from three types of analyses: (1) total test scores, (2) fair test scores (scores reported by program but only on items on topics taught), and (3) conservative test scores (scores on common items taught in both). Table 5-2 reports on the variations across the multiple- choice test scores for the Geometry study (Thompson et al., 2003) on a standardized test, High School Subject Tests-Geometry Form B , and the UCSMP-constructed Geometry test, and for the Advanced Algebra Study on the UCSMP-constructed Advanced Algebra test (Thompson et al., 2001). The table shows the mean scores for UCSMP classes and comparison classes. In each cell, mean percentage correct is reported first by whole test, then by fair test, and then by conservative test.
The authors explicitly compare the items from the standard Geometry test with the items from the UCSMP test and indicate overlap and difference. They constructed their own test because, in their view, the standard test was not adequately balanced among skills, properties, and real-world uses. The UCSMP test included items on transformations, representations, and applications that were lacking in the national test. Only five items were taught by all teachers; hence in the case of the UCSMP geometry test, there is no report on a conservative test. In the Advanced Algebra evaluation, only a UCSMP-constructed test was viewed as appropriate to cover the treatment of the prior material and alignment to the goals of the new course. These data sets demonstrate the challenge of selecting appropriate outcome measures, the sensitivity of the results to those decisions, and the importance of full disclosure of decision-making processes in order to permit readers to assess the implications of the choices. The methodology utilized sought to ensure that the material in the course was covered adequately by treatment teachers while finding ways to make comparisons that reflected content coverage.
Only one study reported on its outcomes using embedded assessment items employed over the course of the year. In a study of Saxon and UCSMP, Peters (1992) (EX) studied the use of these materials with two classrooms taught by the same teacher. In this small study, he randomly assigned students to treatment groups and then measured their performance on four unit tests composed of items common to both curricula and their progress on the Orleans-Hanna Algebraic Prognosis Test.
Peters’ study showed no significant difference in placement scores between Saxon and UCSMP on the posttest, but did show differences on the embedded assessment. Figure 5-6 (Peters, 1992, p. 75) shows an interesting display of the differences on a “continuum” that shows both the direction and magnitude of the differences and provides a level of concept specificity missing in many reports. This figure and a display ( Figure 5-7 ) in a study by Senk (1991, p. 18) of students’ mean scores on Curriculum A versus Curriculum B with a 10 percent range of differences marked represent two excellent means to communicate the kinds of detailed content outcome information that promises to be informative to curriculum writers, publishers, and school decision makers. In Figure 5-7 , 16 items listed by number were taken from the Second International Mathematics Study. The Functions, Statistics, and Trigonometry sample averaged 41 percent correct on these items whereas the U.S. precalculus sample averaged 38 percent. As shown in the figure, differences of 10 percent or less fall inside the banded area and greater than 10 percent fall outside, producing a display that makes it easy for readers and designers to identify the relative curricular strengths and weaknesses of topics.
While we value detailed outcome measure information, we also recognize the importance of examining curricular impact on students’ standardized test performance. Many developers, but not all, are explicit in rejecting standardized tests as adequate measures of the outcomes of their programs, claiming that these tests focus on skills and manipulations, that they are overly reliant on multiple-choice questions, and that they are often poorly aligned to new content emphases such as probability and statistics, transformations, use of contextual problems and functions, and process skills, such as problem solving, representation, or use of calculators. However, national and state tests are being revised to include more content on these topics and to draw on more advanced reasoning. Furthermore, these high-stakes tests are of major importance in school systems, determining graduation, passing standards, school ratings, and so forth. For this reason, if a curricular program demonstrated positive impact on such measures, we referred to that in Chapter 3 as establishing “curricular alignment with systemic factors.” Adequate performance on these measures is of paramount importance to the survival of reform (to large groups of parents and
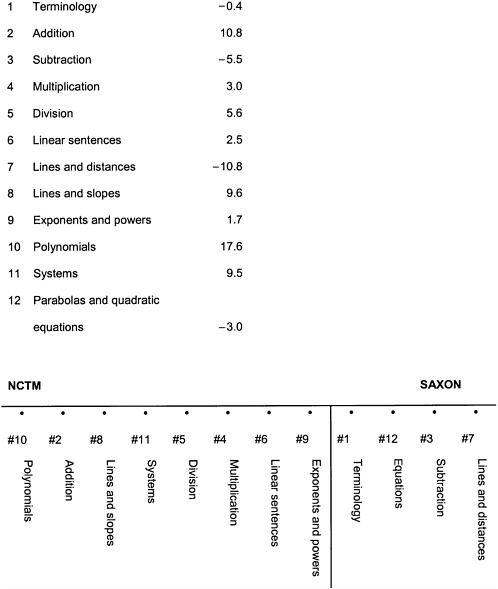
FIGURE 5-6 Continuum of criterion score averages for studied programs.
SOURCE: Peters (1992, p. 75).
school administrators). These examples demonstrate how careful attention to outcomes measures is an essential element of valid evaluation.
In Table 5-3 , we document the number of studies using a variety of types of outcome measures that we used to code the data, and also report on the types of tests used across the studies.
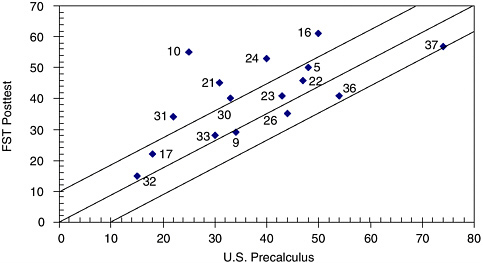
FIGURE 5-7 Achievement (percentage correct) on Second International Mathematics Study (SIMS) items by U.S. precalculus students and functions, statistics, and trigonometry (FST) students.
SOURCE: Re-created from Senk (1991, p. 18).
TABLE 5-3 Number of Studies Using a Variety of Outcome Measures by Program Type
| Total Test | Content Strands | Test Match to Program | Multiple Test | ||||
| Yes | No | Yes | No | Yes | No | Yes | No |
NSF | 43 | 3 | 28 | 18 | 26 | 20 | 21 | 25 |
Commercial | 8 | 1 | 4 | 5 | 2 | 7 | 2 | 7 |
UCSMP | 7 | 1 | 7 | 1 | 7 | 1 | 7 | 1 |
A Choice of Statistical Tests, Including Statistical Significance and Effect Size
In our first review of the studies, we coded what methods of statistical evaluation were used by different evaluators. Most common were t-tests; less frequently one found Analysis of Variance (ANOVA), Analysis of Co-
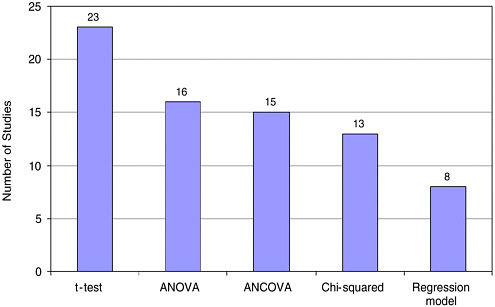
FIGURE 5-8 Statistical tests most frequently used.
variance (ANCOVA), and chi-square tests. In a few cases, results were reported using multiple regression or hierarchical linear modeling. Some used multiple tests; hence the total exceeds 63 ( Figure 5-8 ).
One of the difficult aspects of doing curriculum evaluations concerns using the appropriate unit both in terms of the unit to be randomly assigned in an experimental study and the unit to be used in statistical analysis in either an experimental or quasi-experimental study.
For our purposes, we made the decision that unless the study concerned an intact student population such as the freshman at a single university, where a student comparison was the correct unit, we believed that for statistical tests, the unit should be at least at the classroom level. Judgments were made for each study as to whether the appropriate unit was utilized. This question is an important one because statistical significance is related to sample size, and as a result, studies that inappropriately use the student as the unit of analysis could be concluding significant differences where they are not present. For example, if achievement differences between two curricula are tested in 16 classrooms with 400 students, it will always be easier to show significant differences using scores from those 400 students than using 16 classroom means.
Fifty-seven studies used students as the unit of analysis in at least one test of significance. Three of these were coded as correct because they involved whole populations. In all, 10 studies were coded as using the
TABLE 5-4 Performance on Applied Algebra Problems with Use of Calculators, Part 1
Treatment | n | M (0-100) | SD |
Control | 273 | 34.1 | 14.8 |
CPMP | 320 | 42.6 | 21.3 |
NOTE: t = -5.69, p < .001. All sites combined SOURCE: Huntley et al. (2000). Reprinted with permission. |
TABLE 5-5 Reanalysis of Algebra Performance Data
| Site Mean | Independent Samples Dependent | Difference Sample Difference | |
Site | Control | CPMP | ||
1 | 31.7 | 35.5 |
| 3.8 |
2 | 26.0 | 49.4 |
| 23.4 |
3 | 36.7 | 25.2 |
| -11.5 |
4 | 41.9 | 47.7 |
| 5.8 |
5 | 29.4 | 38.3 |
| 8.9 |
6 | 30.5 | 45.6 |
| 15.1 |
Average | 32.7 | 40.3 | 7.58 | 7.58 |
Standard deviation | 5.70 | 9.17 | 7.64 | 11.75 |
Standard error |
|
| 4.41 | 4.80 |
|
| t | 1.7 | 1.6 |
|
| p | 0.116 | 0.175 |
SOURCE: Huntley et al. (2000). |
correct unit of analysis; hence, 7 studies used teachers or classes, or schools. For some studies where multiple tests were conducted, a judgment was made as to whether the primary conclusions drawn treated the unit of analysis adequately. For example, Huntley et al. (2000) compared the performance of CPMP students with students in a traditional course on a measure of ability to formulate and use algebraic models to answer various questions about relationships among variables. The analysis used students as the unit of analysis and showed a significant difference, as shown in Table 5-4 .
To examine the robustness of this result, we reanalyzed the data using an independent sample t-test and a matched pairs t-test with class means as the unit of analysis in both tests ( Table 5-5 ). As can be seen from the analyses, in neither statistical test was the difference between groups found to be significantly different (p < .05), thus emphasizing the importance of using the correct unit in analyzing the data.
Reanalysis of student-level data using class means will not always result
TABLE 5-6 Mean Percentage Correct on Entire Multiple-Choice Posttest: Second Edition and Non-UCSMP
School | Pair | UCSMP Second Edition | |||
Code | ID | n | Mean | SD | OTL |
J | 18 | 18 | 60.8 | 9.0 | 100 |
J | 19 | 11 | 58.8 | 13.5 | 100 |
K | 20 | 22 | 63.8 | 13.0 | 94 |
K | 21 | 16 | 64.8 | 14.0 | 94 |
L | 22 | 19 | 57.6 | 16.9 | 92 |
L | 23 | 13 | 44.7 | 11.2 | 92 |
M | 24 | 29 | 58.4 | 12.7 | 92 |
M | 25 | 22 | 39.6 | 13.5 | 92 |
Overall |
| 150 | 56.1 | 15.4 |
|
NOTE: The mean is the mean percentage correct on a 36-item multiple-choice posttest. The OTL is the percentage of the items for which teachers reported their students had the opportunity to learn the needed content. Underline indicates statistically significant differences between the mean percentage correct for each pair. |
in a change in finding. Furthermore, using class means as the unit of analysis does not suggest that significant differences will not be found. For example, a study by Thompson et al. (2001) compared the performance of UCSMP students with the performance of students in a more traditional program across several measures of achievement. They found significant differences between UCSMP students and the non-UCSMP students on several measures. Table 5-6 shows results of an analysis of a multiple-choice algebraic posttest using class means as the unit of analysis. Significant differences were found in five of eight separate classroom comparisons, as shown in the table. They also found a significant difference using a matched-pairs t-test on class means.
The lesson to be learned from these reanalyses is that the choice of unit of analysis and the way the data are aggregated can impact study findings in important ways including the extent to which these findings can be generalized. Thus it is imperative that evaluators pay close attention to such considerations as the unit of analysis and the way data are aggregated in the design, implementation, and analysis of their studies.
Non-UCSMP | |||||||
n | Mean | SD | OTL | SE | t | df | p |
14 | 55.2 | 10.2 | 69 | 3.40 | 1.65 | 30 | 0.110 |
15 | 53.7 | 11.0 | 69 | 4.81 | 1.06 | 24 | 0.299 |
24 | 45.9 | 10.0 | 72 | 3.41 | 5.22 | 44 |
|
23 | 43.0 | 11.9 | 72 | 4.16 | 5.23 | 37 |
|
20 | 38.8 | 9.1 | 75 | 4.32 | 4.36 | 37 |
|
15 | 38.3 | 11.0 | 75 | 4.20 | 1.52 | 26 | 0.140 |
22 | 37.8 | 13.8 | 47 | 3.72 | 5.56 | 49 |
|
23 | 30.8 | 9.9 | 47 | 3.52 | 2.51 | 43 |
|
156 | 42.0 | 13.1 |
|
|
|
|
|
A matched-pairs t-test indicates that the differences between the two curricula are significant.
SOURCE: Thompson et al. (2001). Reprinted with permission. |
Second, effect size has become a relatively common and standard way of gauging the practical significance of the findings. Statistical significance only indicates whether the main-level differences between two curricula are large enough to not be due to chance, assuming they come from the same population. When statistical differences are found, the question remains as to whether such differences are large enough to consider. Because any innovation has its costs, the question becomes one of cost-effectiveness: Are the differences in student achievement large enough to warrant the costs of change? Quantifying the practical effect once statistical significance is established is one way to address this issue. There is a statistical literature for doing this, and for the purposes of this review, the committee simply noted whether these studies have estimated such an effect. However, the committee further noted that in conducting meta-analyses across these studies, effect size was likely to be of little value. These studies used an enormous variety of outcome measures, and even using effect size as a means to standardize units across studies is not sensible when the measures in each
study address such a variety of topics, forms of reasoning, content levels, and assessment strategies.
We note very few studies drew upon the advances in methodologies employed in modeling, which include causal modeling, hierarchical linear modeling (Bryk and Raudenbush, 1992; Bryk et al., 1993), and selection bias modeling (Heckman and Hotz, 1989). Although developing detailed specifications for these approaches is beyond the scope of this review, we wish to emphasize that these methodological advances should be considered within future evaluation designs.
Results and Limitations to Generalizability Resulting from Design Constraints
One also must consider what generalizations can be drawn from the results (Campbell and Stanley, 1966; Caporaso and Roos, 1973; and Boruch, 1997). Generalization is a matter of external validity in that it determines to what populations the study results are likely to apply. In designing an evaluation study, one must carefully consider, in the selection of units of analysis, how various characteristics of those units will affect the generalizability of the study. It is common for evaluators to conflate issues of representativeness for the purpose of generalizability (external validity) and comparativeness (the selection of or adjustment for comparative groups [internal validity]). Not all studies must be representative of the population served by mathematics curricula to be internally valid. But, to be generalizable beyond restricted communities, representativeness must be obtained by the random selection of the basic units. Clearly specifying such limitations to generalizability is critical. Furthermore, on the basis of equity considerations, one must be sure that if overall effectiveness is claimed, that the studies have been conducted and analyzed with reference of all relevant subgroups.
Thus, depending on the design of a study, its results may be limited in generalizability to other populations and circumstances. We identified four typical kinds of limitations on the generalizability of studies and coded them to determine, on the whole, how generalizable the results across studies might be.
First, there were studies whose designs were limited by the ability or performance level of the students in the samples. It was not unusual to find that when new curricula were implemented at the secondary level, schools kept in place systems of tracking that assigned the top students to traditional college-bound curriculum sequences. As a result, studies either used comparative groups who were matched demographically but less skilled than the population as a whole, in relation to prior learning, or their results compared samples of less well-prepared students to samples of students
with stronger preparations. Alternatively, some studies reported on the effects of curricula reform on gifted and talented students or on college-attending students. In these cases, the study results would also limit the generalizability of the results to similar populations. Reports using limited samples of students’ ability and prior performance levels were coded as a limitation to the generalizability of the study.
For example, Wasman (2000) conducted a study of one school (six teachers) and examined the students’ development of algebraic reasoning after one (n=100) and two years (n=73) in CMP. In this school, the top 25 percent of the students are counseled to take a more traditional algebra course, so her experimental sample, which was 61 percent white, 35 percent African American, 3 percent Asian, and 1 percent Hispanic, consisted of the lower 75 percent of the students. She reported on the student performance on the Iowa Algebraic Aptitude Test (IAAT) (1992), in the subcategories of interpreting information, translating symbols, finding relationships, and using symbols. Results for Forms 1 and 2 of the test, for the experimental and norm group, are shown in Table 5-7 for 8th graders.
In our coding of outcomes, this study was coded as showing no significant differences, although arguably its results demonstrate a positive set of
TABLE 5-7 Comparing Iowa Algebraic Aptitude Test (IAAT) Mean Scores of the Connected Mathematics Project Forms 1 and 2 to the Normative Group (8th Graders)
| Interpreting Information | Translating Symbols | Finding Relationships | Using Symbols | Total |
CMP: Form 1 | 9.35 | 8.22 | 9.90 | 8.65 | 36.12 |
7th (n=51) | (3.36) | (3.44) | (3.26) | (3.12) | (11.28) |
CMP: Form 1 | 9.76 | 8.56 | 9.41 | 8.27 | 36.00 |
8th (n=41) | (3.89) | (3.64) | (4.13) | (3.74) | (13.65) |
Norm: Form 1 | 10.03 | 9.55 | 9.14 | 8.87 | 37.59 |
(n=2,467) | (3.35) | (2.89) | (3.59) | (3.19) | (10.57) |
CMP: Form 2 | 9.41 | 7.82 | 9.29 | 7.65 | 34.16 |
7th (n=49) | (4.05) | (3.03) | (3.57) | (3.35) | (11.47) |
CMP: Form 2 | 11.28 | 8.66 | 10.94 | 9.81 | 40.69 |
8th (n=32) | (3.74) | (3.81) | (3.79) | (3.64) | (12.94) |
Norm: Form 2 | 10.63 | 8.58 | 8.67 | 9.19 | 37.07 |
(n=2,467) | (3.78) | (2.91) | (3.84) | (3.17) | (11.05) |
NOTE: Parentheses indicate standard deviation. SOURCE: Adapted from Wasman (2000). |
outcomes as the treatment group was weaker than the control group. Had the researcher used a prior achievement measure and a different statistical technique, significance might have been demonstrated, although potential teacher effects confound interpretations of results.
A second limitation to generalizability was when comparative studies resided entirely at curriculum pilot site locations, where such sites were developed as a means to conduct formative evaluations of the materials with close contact and advice from teachers. Typically, pilot sites have unusual levels of teacher support, whether it is in the form of daily technical support in the use of materials or technology or increased quantities of professional development. These sites are often selected for study because they have established cooperative agreements with the program developers and other sources of data, such as classroom observations, are already available. We coded whether the study was conducted at a pilot site to signal potential limitations in generalizability of the findings.
Third, studies were also coded as being of limited generalizability if they failed to disaggregate their data by socioeconomic class, race, gender, or some other potentially significant sources of restriction on the claims. We recorded the categories in which disaggregation occurred and compiled their frequency across the studies. Because of the need to open the pipeline to advanced study in mathematics by members of underrepresented groups, we were particularly concerned about gauging the extent to which evaluators factored such variables into their analysis of results and not just in terms of the selection of the sample.
Of the 46 included studies of NSF-supported curricula, 19 disaggregated their data by student subgroup. Nine of 17 studies of commercial materials disaggregated their data. Figure 5-9 shows the number of studies that disaggregated outcomes by race or ethnicity, SES, gender, LEP, special education status, or prior achievement. Studies using multiple categories of disaggregation were counted multiple times by program category.
The last category of restricted generalization occurred in studies of limited sample size. Although such studies may have provided more indepth observations of implementation and reports on professional development factors, the smaller numbers of classrooms and students in the study would limit the extent of generalization that could be drawn from it. Figure 5-10 shows the distribution of sizes of the samples in terms of numbers of students by study type.
Summary of Results by Student Achievement Among Program Types
We present the results of the studies as a means to further investigate their methodological implications. To this end, for each study, we counted across outcome measures the number of findings that were positive, nega-
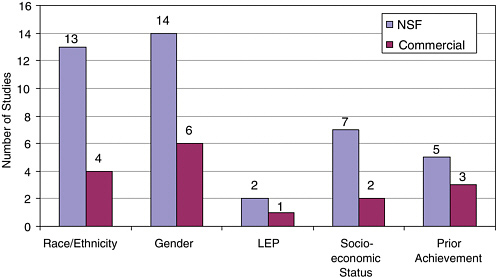
FIGURE 5-9 Disaggregation of subpopulations.
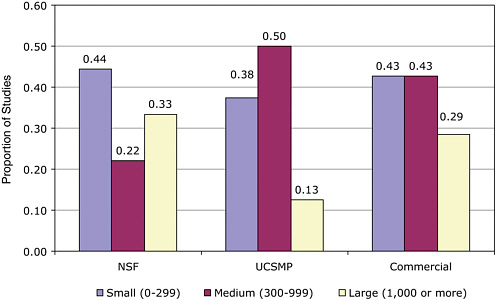
FIGURE 5-10 Proportion of studies by sample size and program.
tive, or indeterminate (no significant difference) and then calculated the proportion of each. We represented the calculation of each study as a triplet (a, b, c) where a indicates the proportion of the results that were positive and statistically significantly stronger than the comparison program, b indicates the proportion that were negative and statistically significantly weaker than the comparison program, and c indicates the proportion that showed no significant difference between the treatment and the comparative group. For studies with a single outcome measure, without disaggregation by content strand, the triplet is always composed of two zeros and a single one. For studies with multiple measures or disaggregation by content strand, the triplet is typically a set of three decimal values that sum to one. For example, a study with one outcome measure in favor of the experimental treatment would be coded (1, 0, 0), while one with multiple measures and mixed results more strongly in favor of the comparative curriculum might be listed as (.20, .50, .30). This triplet would mean that for 20 percent of the comparisons examined, the evaluators reported statistically significant positive results, for 50 percent of the comparisons the results were statistically significant in favor of the comparison group, and for 30 percent of the comparisons no significant difference were found. Overall, the mean score on these distributions was (.54, .07, .40), indicating that across all the studies, 54 percent of the comparisons favored the treatment, 7 percent favored the comparison group, and 40 percent showed no significant difference. Table 5-8 shows the comparison by curricular program types. We present the results by individual program types, because each program type relies on a similar program theory and hence could lead to patterns of results that would be lost in combining the data. If the studies of commercial materials are all grouped together to include UCSMP, their pattern of results is (.38, .11, .51). Again we emphasize that due to our call for increased methodological rigor and the use of multiple methods, this result is not sufficient to establish the curricular effectiveness of these programs as a whole with adequate certainty.
We caution readers that these results are summaries of the results presented across a set of evaluations that meet only the standard of at least
TABLE 5-8 Comparison by Curricular Program Types
Proportion of Results That Are: | NSF-Supported n=46 | UCSMP n=8 | Commercially Generated n=9 |
In favor of treatment | .591 | .491 | .285 |
In favor of comparison | .055 | .087 | .130 |
Show no significant difference | .354 | .422 | .585 |
minimally methodologically adequate . Calculations of statistical significance of each program’s results were reported by the evaluators; we have made no adjustments for weaknesses in the evaluations such as inappropriate use of units of analysis in calculating statistical significance. Evaluations that consistently used the correct unit of analysis, such as UCSMP, could have fewer reports of significant results as a consequence. Furthermore, these results are not weighted by study size. Within any study, the results pay no attention to comparative effect size or to the established credibility of an outcome measure. Similarly, these results do not take into account differences in the populations sampled, an important consideration in generalizing the results. For example, using the same set of studies as an example, UCSMP studies used volunteer samples who responded to advertisements in their newsletters, resulting in samples with disproportionately Caucasian subjects from wealthier schools compared to national samples. As a result, we would suggest that these results are useful only as baseline data for future evaluation efforts. Our purpose in calculating these results is to permit us to create filters from the critical decision points and test how the results change as one applies more rigorous standards.
Given that none of the studies adequately addressed all of the critical criteria, we do not offer these results as definitive, only suggestive—a hypothesis for further study. In effect, given the limitations of time and support, and the urgency of providing advice related to policy, we offer this filtering approach as an informal meta-analytic technique sufficient to permit us to address our primary task, namely, evaluating the quality of the evaluation studies.
This approach reflects the committee’s view that to deeply understand and improve methodology, it is necessary to scrutinize the results and to determine what inferences they provide about the conduct of future evaluations. Analogous to debates on consequential validity in testing, we argue that to strengthen methodology, one must consider what current methodologies are able (or not able) to produce across an entire series of studies. The remainder of the chapter is focused on considering in detail what claims are made by these studies, and how robust those claims are when subjected to challenge by alternative hypothesis, filtering by tests of increasing rigor, and examining results and patterns across the studies.
Alternative Hypotheses on Effectiveness
In the spirit of scientific rigor, the committee sought to consider rival hypotheses that could explain the data. Given the weaknesses in the designs generally, often these alternative hypotheses cannot be dismissed. However, we believed that only after examining the configuration of results and
alternative hypotheses can the next generation of evaluations be better informed and better designed. We began by generating alternative hypotheses to explain the positive directionality of the results in favor of experimental groups. Alternative hypotheses included the following:
The teachers in the experimental groups tended to be self-selecting early adopters, and thus able to achieve effects not likely in regular populations.
Changes in student outcomes reflect the effects of professional development instruction, or level of classroom support (in pilot sites), and thus inflate the predictions of effectiveness of curricular programs.
Hawthorne effect (Franke and Kaul, 1978) occurs when treatments are compared to everyday practices, due to motivational factors that influence experimental participants.
The consistent difference is due to the coherence and consistency of a single curricular program when compared to multiple programs.
The significance level is only achieved by the use of the wrong unit of analysis to test for significance.
Supplemental materials or new teaching techniques produce the results and not the experimental curricula.
Significant results reflect inadequate outcome measures that focus on a restricted set of activities.
The results are due to evaluator bias because too few evaluators are independent of the program developers.
At the same time, one could argue that the results actually underestimate the performance of these materials and are conservative measures, and their alternative hypotheses also deserve consideration:
Many standardized tests are not sensitive to these curricular approaches, and by eliminating studies focusing on affect, we eliminated a key indicator of the appeal of these curricula to students.
Poor implementation or increased demands on teachers’ knowledge dampens the effects.
Often in the experimental treatment, top-performing students are missing as they are advised to take traditional sequences, rendering the samples unequal.
Materials are not well aligned with universities and colleges because tests for placement and success in early courses focus extensively on algebraic manipulation.
Program implementation has been undercut by negative publicity and the fears of parents concerning change.
There are also a number of possible hypotheses that may be affecting the results in either direction, and we list a few of these:
Examining the role of the teacher in curricular decision making is an important element in effective implementation, and design mandates of evaluation design make this impossible (and the positives and negatives or single- versus dual-track curriculum as in Lundin, 2001).
Local tests that are sensitive to the curricular effects typically are not mandatory and hence may lead to unpredictable performance by students.
Different types and extent of professional development may affect outcomes differentially.
Persistence or attrition may affect the mean scores and are often not considered in the comparative analyses.
One could also generate reasons why the curricular programs produced results showing no significance when one program or the other is actually more effective. This could include high degrees of variability in the results, samples that used the correct unit of analysis but did not obtain consistent participation across enough cases, implementation that did not show enough fidelity to the measures, or outcome measures insensitive to the results. Again, subsequent designs should be better informed by these findings to improve the likelihood that they will produce less ambiguous results and replication of studies could also give more confidence in the findings.
It is beyond the scope of this report to consider each of these alternative hypotheses separately and to seek confirmation or refutation of them. However, in the next section, we describe a set of analyses carried out by the committee that permits us to examine and consider the impact of various critical evaluation design decisions on the patterns of outcomes across sets of studies. A number of analyses shed some light on various alternative hypotheses and may inform the conduct of future evaluations.
Filtering Studies by Critical Decision Points to Increase Rigor
In examining the comparative studies, we identified seven critical decision points that we believed would directly affect the rigor and efficacy of the study design. These decision points were used to create a set of 16 filters. These are listed as the following questions:
Was there a report on comparability relative to SES?
Was there a report on comparability of samples relative to prior knowledge?
Was there a report on treatment fidelity?
Was professional development reported on?
Was the comparative curriculum specified?
Was there any attempt to report on teacher effects?
Was a total test score reported?
Was total test score(s) disaggregated by content strand?
Did the outcome measures match the curriculum?
Were multiple tests used?
Was the appropriate unit of analysis used in their statistical tests?
Did they estimate effect size for the study?
Was the generalizability of their findings limited by use of a restricted range of ability levels?
Was the generalizability of their findings limited by use of pilot sites for their study?
Was the generalizability of their findings limited by not disaggregating their results by subgroup?
Was the generalizability of their findings limited by use of small sample size?
The studies were coded to indicate if they reported having addressed these considerations. In some cases, the decision points were coded dichotomously as present or absent in the studies, and in other cases, the decision points were coded trichotomously, as description presented, absent, or statistically adjusted for in the results. For example, a study may or may not report on the comparability of the samples in terms of race, ethnicity, or socioeconomic status. If a report on SES was given, the study was coded as “present” on this decision; if a report was missing, it was coded as “absent”; and if SES status or ethnicity was used in the analysis to actually adjust outcomes, it was coded as “adjusted for.” For each coding, the table that follows reports the number of studies that met that condition, and then reports on the mean percentage of statistically significant results, and results showing no significant difference for that set of studies. A significance test is run to see if the application of the filter produces changes in the probability that are significantly different. 5
In the cases in which studies are coded into three distinct categories—present, absent, and adjusted for—a second set of filters is applied. First, the studies coded as present or adjusted for are combined and compared to those coded as absent; this is what we refer to as a weak test of the rigor of the study. Second, the studies coded as present or absent are combined and compared to those coded as adjusted for. This is what we refer to as a strong test. For dichotomous codings, there can be as few as three compari-
| The significance test used was a chi-square not corrected for discontinuity. |
sons, and for trichotomous codings, there can be nine comparisons with accompanying tests of significance. Trichotomous codes were used for adjustments for SES and prior knowledge, examining treatment fidelity, professional development, teacher effects, and reports on effect sizes. All others were dichotomous.
NSF Studies and the Filters
For example, there were 11 studies of NSF-supported curricula that simply reported on the issues of SES in creating equivalent samples for comparison, and for this subset the mean probabilities of getting positive, negative, or results showing no significant difference were (.47, .10, .43). If no report of SES was supplied (n= 21), those probabilities become (.57, .07, .37), indicating an increase in positive results and a decrease in results showing no significant difference. When an adjustment is made in outcomes based on differences in SES (n=14), the probabilities change to (.72, .00, .28), showing a higher likelihood of positive outcomes. The probabilities that result from filtering should always be compared back to the overall results of (.59, .06, .35) (see Table 5-8 ) so as to permit one to judge the effects of more rigorous methodological constraints. This suggests that a simple report on SES without adjustment is least likely to produce positive outcomes; that is, no report produces the outcomes next most likely to be positive and studies that adjusted for SES tend to have a higher proportion of their comparisons producing positive results.
The second method of applying the filter (the weak test for rigor) for the treatment of the adjustment of SES groups compares the probabilities when a report is either given or adjusted for compared to when no report is offered. The combined percentage of a positive outcome of a study in which SES is reported or adjusted for is (.61, .05, .34), while the percentage for no report remains as reported previously at (.57, .07, .37). A final filter compares the probabilities of the studies in which SES is adjusted for with those that either report it only or do not report it at all. Here we compare the percentage of (.72, .00, .28) to (.53, .08, .37) in what we call a strong test. In each case we compared the probability produced by the whole group to those of the filtered studies and conducted a test of the differences to determine if they were significant. These differences were not significant. These findings indicate that to date, with this set of studies, there is no statistically significant difference in results when one reports or adjusts for changes in SES. It appears that by adjusting for SES, one sees increases in the positive results, and this result deserves a closer examination for its implications should it prove to hold up over larger sets of studies.
We ran tests that report the impact of the filters on the number of studies, the percentage of studies, and the effects described as probabilities
for each of the three study categories, NSF-supported and commercially generated with UCSMP included. We claim that when a pattern of probabilities of results does not change after filtering, one can have more confidence in that pattern. When the pattern of results changes, there is a need for an explanatory hypothesis, and that hypothesis can shed light on experimental design. We propose that this “filtering process” constitutes a test of the robustness of the outcome measures subjected to increasing degrees of rigor by using filtering.
Results of Filtering on Evaluations of NSF-Supported Curricula
For the NSF-supported curricular programs, out of 15 filters, 5 produced a probability that differed significantly at the p<.1 level. The five filters were for treatment fidelity, specification of control group, choosing the appropriate statistical unit, generalizability for ability, and generalizability based on disaggregation by subgroup. For each filter, there were from three to nine comparisons, as we examined how the probabilities of outcomes change as tests were more stringent and across the categories of positive results, negative results, and results with no significant differences. Out of a total of 72 possible tests, only 11 produced a probability that differed significantly at the p < .1 level. With 85 percent of the comparisons showing no significant difference after filtering, we suggest the results of the studies were relatively robust in relation to these tests. At the same time, when rigor is increased for the five filters just listed, the results become generally more ambiguous and signal the need for further research with more careful designs.

Studies of Commercial Materials and the Filters
To ensure enough studies to conduct the analysis (n=17), our filtering analysis of the commercially generated studies included UCSMP (n=8). In this case, there were six filters that produced a probability that differed significantly at the p < .1 level. These were treatment fidelity, disaggregation by content, use of multiple tests, use of effect size, generalizability by ability, and generalizability by sample size. In this case, because there were no studies in some possible categories, there were a total of 57 comparisons, and 9 displayed significant differences in the probabilities after filtering at the p < .1 level. With 84 percent of the comparisons showing no significant difference after filtering, we suggest the results of the studies were relatively robust in relation to these tests. Table 5-9 shows the cases in which significant differences were recorded.
Impact of Treatment Fidelity on Probabilities
A few of these differences are worthy of comment. In the cases of both the NSF-supported and commercially generated curricula evaluation studies, studies that reported treatment fidelity differed significantly from those that did not. In the case of the studies of NSF-supported curricula, it appeared that a report or adjustment on treatment fidelity led to proportions with less positive effects and more results showing no significant differences. We hypothesize that this is partly because larger studies often do not examine actual classroom practices, but can obtain significance more easily due to large sample sizes.
In the studies of commercial materials, the presence or absence of measures of treatment fidelity worked differently. Studies reporting on or adjusting for treatment fidelity tended to have significantly higher probabilities in favor of experimental treatment, less positive effects in fewer of the comparative treatments, and more likelihood of results with no significant differences. We hypothesize, and confirm with a separate analysis, that this is because UCSMP frequently reported on treatment fidelity in their designs while study of Saxon typically did not, and the change represents the preponderance of these different curricular treatments in the studies of commercially generated materials.
Impact of Identification of Curricular Program on Probabilities
The significant differences reported under specificity of curricular comparison also merit discussion for studies of NSF-supported curricula. When the comparison group is not specified, a higher percentage of mean scores in favor of the experimental curricula is reported. In the studies of commercial materials, a failure to name specific curricular comparisons also produced a higher percentage of positive outcomes for the treatment, but the difference was not statistically significant. This suggests the possibility that when a specified curriculum is compared to an unspecified curriculum, reports of impact may be inflated. This finding may suggest that in studies of effectiveness, specifying comparative treatments would provide more rigorous tests of experimental approaches.
When studies of commercial materials disaggregate their results of content strands or use multiple measures, their reports of positive outcomes increase, the negative outcomes decrease, and in one case, the results show no significant differences. Percentage of significant difference was only recorded in one comparison within each one of these filters.
TABLE 5-9 Cases of Significant Differences
Test | Type of Comparison | Category Code | N= | Probabilities Before Filter | p= |
| |||||
Treatment fidelity | Simple compare | Specified | 21 | .51, .02, .47* | *p =.049 |
| Not specified |
| 24 | .68, .09, .23* |
|
| Adjusted for |
| 1 | .25, .00, .75 |
|
Treatment fidelity | Strong test | Adjusted for | 22 | .49*, .02, .49** | *p=.098 |
| Reported or not specified |
| 24 | .68*, .09, .23** | **p=.019 |
Control group specified | Simple compare | Specified | 8 | .33*, .00, .66** | *p=.033 |
|
| Not specified | 38 | .65*, .07, .29** | **p=.008 |
Appropriate unit of analysis | Simple compare | Correct | 5 | .30*, .40**, .30 | *p=.069 |
|
| Incorrect | 41 | .63*, .01**, .36 | **p=.000 |
Generalizability by ability | Simple compare | Limited | 5 | .22*, .41**, .37 | *p=.019 |
|
| Not limited | 41 | .64*, .01**, .35 | **p=.000 |
Generalizability by disaggregated subgroup | Simple compare | Limited | 28 | .48*, .09, .43** | *p=.013 |
| Not limited | 18 | .76*, .00, .24** | **p=.085 |
| |||||
Treatment fidelity | Simple compare | Reported | 7 | .53, .37*, .20 | *p=.032 |
|
| Not specified | 9 | .26, .67*, .11 |
|
|
| Adjusted for | 1 | .45, .00*, .55 |
|
Treatment fidelity | Weak test | Adjusted for or | 8 | .52, .33, .25* | *p=.087 |
|
| Reported versus | 9 | .26, .67, .11* |
|
|
| Not specified |
|
|
|
Outcomes disaggregated by content strand | Simple compare | Reported | 11 | .50, .37, .22* | *p=.052 |
| Not reported | 6 | .17, .77, .10* |
| |
Outcomes using multiple tests | Simple compare | Yes | 9 | .55*, .35, .19 | *p=.076 |
|
| No | 8 | .20*, .68, .20 |
|
Effect size reported | Simple compare | Yes | 3 | .72, .05, .29* | *p=.029 |
|
| No | 14 | .31, .61, .16* |
|
Generalization by ability | Simple compare | Limited | 4 | .23, .41*, .32 | *p=.004 |
|
| Not limited | 14 | .42, .53, .09 |
|
Generalization by sample size | Simple compare | Limited | 6 | .57, .23, .27* | *p=.036 |
|
| Not limited | 11 | .28, .66, .10* |
|
NOTE: In the comparisons shown, only the comparisons marked by an asterisk showed significant differences at p<.1. Probabilitie s are estimated for each significant difference. |
Impact of Units of Analysis on Probabilities 6
For the evaluations of the NSF-supported materials, a significant difference was reported on the outcomes for the studies that used the correct unit of analysis compared to those that did not. The percentage for those with the correct unit were (.30, .40, .30) compared to (.63, .01, .36) for those that used the incorrect result. These results suggest that our prediction that using the correct unit of analysis would decrease the percentage of positive outcomes is likely to be correct. It also suggests that the most serious threat to the apparent conclusions of these studies comes from selecting an incorrect unit of analysis. It causes a decrease in favorable results, making the results more ambiguous, but never reverses the direction of the effect. This is a concern that merits major attention in the conduct of further studies.
For the commercially generated studies, most of the ones coded with the correct unit of analysis were UCSMP studies. Because of the small number of studies involved, we could not break out from the overall filtering of studies of commercial materials, but report this issue to assist readers in interpreting the relative patterns of results.
Impact of Generalizability on Probabilities
Both types of studies yielded significant differences for some of the comparisons coded as restrictions to generalizability. Investigating these is important in order to understand the effects of these curricular programs on different subpopulations of students. In the case of the studies of commercially generated materials, significantly different results occurred in the categories of ability and sample size. In the studies of NSF-supported materials, the significant differences occurred in ability and disaggregation by subgroups.
In relation to generalizability, the studies of NSF-supported curricula reported significantly more positive results in favor of the treatment when they included all students. Because studies coded as “limited by ability” were restricted either by focusing only on higher achieving students or on lower achieving students, we sorted these two groups. For higher performing students (n=3), the probabilities of effects were (.11, .67, .22). For lower
| It should be noted that of the five studies in which the correct unit of analysis was used, two of these were population studies of freshmen entering college, and these reported few results in favor of the experimental treatments. However, the high proportion of these studies involving college students may skew this particular result relative to the preponderance of other studies involving K-12 students. |
performing students (n=2), the probabilities were (.39, .025, .59). The first two comparisons are significantly different at p < .05. These findings are based on only a total of five studies, but they suggest that these programs may be serving the weaker ability students more effectively than the stronger ability students, serving both less well than they serve whole heterogeneous groups. For the studies of commercial materials, there were only three studies that were restricted to limited populations. The results for those three studies were (.23, .41, .32) and for all students (n=14) were (.42, .53, .09). These studies were significantly different at p = .004. All three studies included UCSMP and one also included Saxon and was limited by serving primarily high-performing students. This means both categories of programs are showing weaker results when used with high-ability students.
Finally, the studies on NSF-supported materials were disaggregated by subgroups for 28 studies. A complete analysis of this set follows, but the studies that did not report results disaggregated by subgroup generated probabilities of results of (.48, .09, .43) whereas those that did disaggregate their results reported (.76, 0, .24). These gains in positive effects came from significant losses in reporting no significant differences. Studies of commercial materials also reported a small decrease in likelihood of negative effects for the comparison program when disaggregation by subgroup is reported offset by increases in positive results and results with no significant differences, although these comparisons were not significantly different. A further analysis of this topic follows.
Overall, these results suggest that increased rigor seems to lead in general to less strong outcomes, but never reports of completely contrary results. These results also suggest that in recommending design considerations to evaluators, there should be careful attention to having evaluators include measures of treatment fidelity, considering the impact on all students as well as one particular subgroup; using the correct unit of analysis; and using multiple tests that are also disaggregated by content strand.
Further Analyses
We conducted four further analyses: (1) an analysis of the outcome probabilities by test type; (2) content strands analysis; (3) equity analysis; and (4) an analysis of the interactions of content and equity by grade band. Careful attention to the issues of content strand, equity, and interaction is essential for the advancement of curricular evaluation. Content strand analysis provides the detail that is often lost by reporting overall scores; equity analysis can provide essential information on what subgroups are adequately served by the innovations, and analysis by content and grade level can shed light on the controversies that evolve over time.
Analysis by Test Type
Different studies used varied combinations of outcome measures. Because of the importance of outcome measures on test results, we chose to examine whether the probabilities for the studies changed significantly across different types of outcome measures (national test, local test). The most frequent use of tests across all studies was a combination of national and local tests (n=18 studies), a local test (n=16), and national tests (n=17). Other uses of test combinations were used by three studies or less. The percentages of various outcomes by test type in comparison to all studies are described in Table 5-10 .
These data ( Table 5-11 ) suggest that national tests tend to produce less positive results, and with the resulting gains falling into results showing no significant differences, suggesting that national tests demonstrate less curricular sensitivity and specificity.
TABLE 5-10 Percentage of Outcomes by Test Type
Test Type | National/Local | Local Only | National Only | All Studies |
All studies | (.48, .18, .34) n=18 | (.63, .03, .34) n=16 | (.31, .05, .64) n= 3 | (.54, .07, .40) n=63 |
NOTE: The first set of numbers in the parenthesis represent the percentage of outcomes that are positive, the second set of numbers in the parenthesis represent the percentage of outcomes that are negative, and the third set of numbers represent the percentage of outcomes that are nonsignificant. |
TABLE 5-11 Percentage of Outcomes by Test Type and Program Type
Test Type | National/Local | Local Only | National Only | All Studies |
NSF effects | (.52, .15, .34) n=14 | (.57, .03, .39) n=14 | (.44, .00, .56) n=4 | (.59, .06, .35) n=46 |
UCSMP effects | (.41, .18, .41) n=3 | *** | *** | (.49, .09, .42) n=8 |
Commercial effects | ** | ** | (.29, .08, .63) n=8 | (.29, .13, .59) n=9 |
NOTE: The first set of numbers in the parenthesis represent the percentage of outcomes that are positive, the second set of numbers represent the percentage of outcomes that are negative, and the third set of numbers represent the percentage of outcomes that are nonsignificant. |
TABLE 5-12 Number of Studies That Disaggregated by Content Strand
Program Type | Elementary | Middle | High School | Total |
NSF-supported | 14 | 6 | 9 | 29 |
Commercially generated | 0 | 4 | 5 | 9 |
Content Strand
Curricular effectiveness is not an all-or-nothing proposition. A curriculum may be effective in some topics and less effective in others. For this reason, it is useful for evaluators to include an analysis of curricular strands and to report on the performance of students on those strands. To examine this issue, we conducted an analysis of the studies that reported their results by content strand. Thirty-eight studies did this; the breakdown is shown in Table 5-12 by type of curricular program and grade band.
To examine the evaluations of these content strands, we began by listing all of the content strands reported across studies as well as the frequency of report by the number of studies at each grade band. These results are shown in Figure 5-11 , which is broken down by content strand, grade level, and program type.
Although there are numerous content strands, some of them were reported on infrequently. To allow the analysis to focus on the key results from these studies, we separated out the most frequently reported on strands, which we call the “major content strands.” We defined these as strands that were examined in at least 10 percent of the studies. The major content strands are marked with an asterisk in the Figure 5-11 . When we conduct analyses across curricular program types or grade levels, we use these to facilitate comparisons.
A second phase of our analysis was to examine the performance of students by content strand in the treatment group in comparison to the control groups. Our analysis was conducted across the major content strands at the level of NSF-supported versus commercially generated, initially by all studies and then by grade band. It appeared that such analysis permitted some patterns to emerge that might prove helpful to future evaluators in considering the overall effectiveness of each approach. To do this, we then coded the number of times any particular strand was measured across all studies that disaggregated by content strand. Then, we coded the proportion of times that this strand was reported as favoring the experimental treatment, favoring the comparative curricula, or showing no significant difference. These data are presented across the major content strands for the NSF-supported curricula ( Figure 5-12 ) and the commercially generated curricula, ( Figure 5-13 ) (except in the case of the elemen-
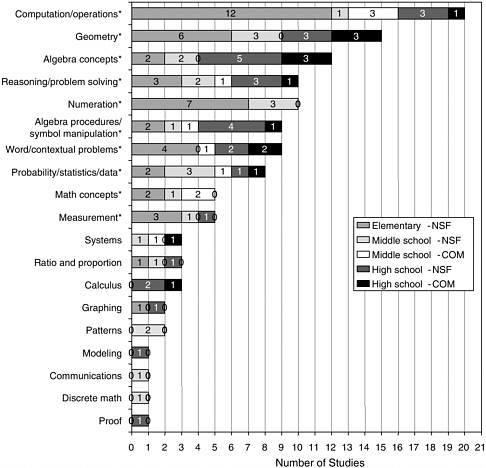
FIGURE 5-11 Study counts for all content strands.
tary curricula where no data were available) in the forms of percentages, with the frequencies listed in the bars.
The presentation of results by strands must be accompanied by the same restrictions as stated previously. These results are based on studies identified as at least minimally methodologically adequate. The quality of the outcome measures in measuring the content strands has not been examined. Their results are coded in relation to the comparison group in the study and are indicated as statistically in favor of the program, as in favor of the comparative program, or as showing no significant differences. The results are combined across studies with no weighting by study size. Their results should be viewed as a means for the identification of topics for potential future study. It is completely possible that a refinement of methodologies may affect the future patterns of results, so the results are to be viewed as tentative and suggestive.
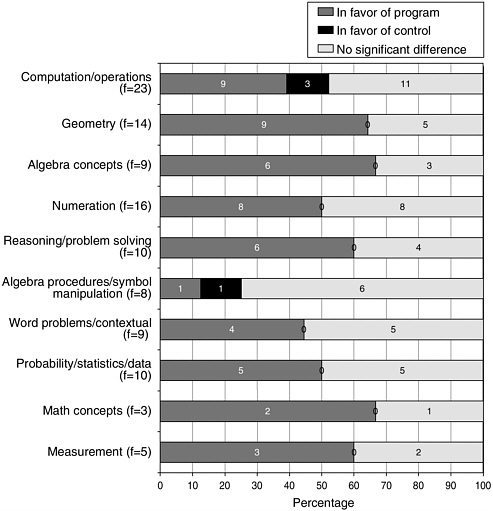
FIGURE 5-12 Major content strand result: All NSF (n=27).
According to these tentative results, future evaluations should examine whether the NSF-supported programs produce sufficient competency among students in the areas of algebraic manipulation and computation. In computation, approximately 40 percent of the results were in favor of the treatment group, no significant differences were reported in approximately 50 percent of the results, and results in favor of the comparison were revealed 10 percent of the time. Interpreting that final proportion of no significant difference is essential. Some would argue that because computation has not been emphasized, findings of no significant differences are acceptable. Others would suggest that such findings indicate weakness, because the development of the materials and accompanying professional development yielded no significant difference in key areas.
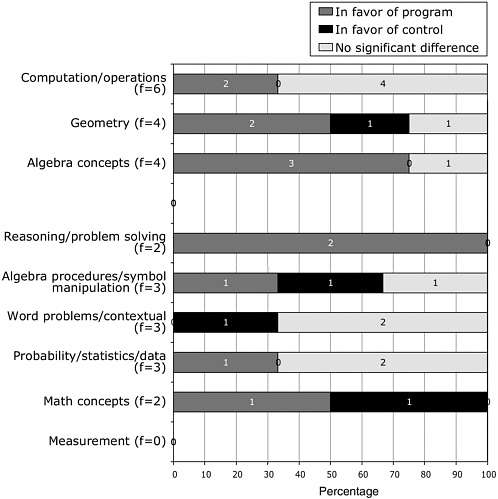
FIGURE 5-13 Major content strand result: All commercial (n=8).
From Figure 5-13 of findings from studies of commercially generated curricula, it appears that mixed results are commonly reported. Thus, in evaluations of commercial materials, lack of significant differences in computations/operations, word problems, and probability and statistics suggest that careful attention should be given to measuring these outcomes in future evaluations.
Overall, the grade band results for the NSF-supported programs—while consistent with the aggregated results—provide more detail. At the elementary level, evaluations of NSF-supported curricula (n=12) report better performance in mathematics concepts, geometry, and reasoning and problem solving, and some weaknesses in computation. No content strand analysis for commercially generated materials was possible. Evaluations
(n=6) at middle grades of NSF-supported curricula showed strength in measurement, geometry, and probability and statistics and some weaknesses in computation. In the studies of commercial materials, evaluations (n=4) reported favorable results in reasoning and problem solving and some unfavorable results in algebraic procedures, contextual problems, and mathematics concepts. Finally, at the high school level, the evaluations (n=9) by content strand for the NSF-supported curricula showed strong favorable results in algebra concepts, reasoning/problem solving, word problems, probability and statistics, and measurement. Results in favor of the control were reported in 25 percent of the algebra procedures and 33 percent of computation measures.
For the studies of commercial materials (n=4), only the geometry results favor the control group 25 percent of the time, with 50 percent having favorable results. Algebra concepts, reasoning, and probability and statistics also produced favorable results.
Equity Analysis of Comparative Studies
When the goal of providing a standards-based curriculum to all students was proposed, most people could recognize its merits: the replacement of dull, repetitive, largely dead-end courses with courses that would lead all students to be able, if desired and earned, to pursue careers in mathematics-reliant fields. It was clear that the NSF-supported projects, a stated goal of which was to provide standards-based courses to all students, called for curricula that would address the problem of too few students persisting in the study of mathematics. For example, as stated in the NSF Request for Proposals (RFP):
Rather than prematurely tracking students by curricular objectives, secondary school mathematics should provide for all students a common core of mainstream mathematics differentiated instructionally by level of abstraction and formalism, depth of treatment and pace (National Science Foundation, 1991, p. 1). In the elementary level solicitation, a similar statement on causes for all students was made (National Science Foundation, 1988, pp. 4-5).
Some, but not enough attention has been paid to the education of students who fall below the average of the class. On the other hand, because the above average students sometimes do not receive a demanding education, it may be incorrectly assumed they are easy to teach (National Science Foundation, 1989, p. 2).
Likewise, with increasing numbers of students in urban schools, and increased demographic diversity, the challenges of equity are equally significant for commercial publishers, who feel increasing pressures to demonstrate the effectiveness of their products in various contexts.
The problem was clearly identified: poorer performance by certain subgroups of students (minorities—non-Asian, LEP students, sometimes females) and a resulting lack of representation of such groups in mathematics-reliant fields. In addition, a secondary problem was acknowledged: Highly talented American students were not being provided adequate challenge and stimulation in comparison with their international counterparts. We relied on the concept of equity in examining the evaluation. Equity was contrasted to equality, where one assumed all students should be treated exactly the same (Secada et al., 1995). Equity was defined as providing opportunities and eliminating barriers so that the membership in a subgroup does not subject one to undue and systematically diminished possibility of success in pursuing mathematical study. Appropriate treatment therefore varies according to the needs of and obstacles facing any subgroup.
Applying the principles of equity to evaluate the progress of curricular programs is a conceptually thorny challenge. What is challenging is how to evaluate curricular programs on their progress toward equity in meeting the needs of a diverse student body. Consider how the following questions provide one with a variety of perspectives on the effectiveness of curricular reform regarding equity:
Does one expect all students to improve performance, thus raising the bar, but possibly not to decrease the gap between traditionally well-served and under-served students?
Does one focus on reducing the gap and devote less attention to overall gains, thus closing the gap but possibly not raising the bar?
Or, does one seek evidence that progress is made on both challenges—seeking progress for all students and arguably faster progress for those most at risk?
Evaluating each of the first two questions independently seems relatively straightforward. When one opts for a combination of these two, the potential for tensions between the two becomes more evident. For example, how can one differentiate between the case in which the gap is closed because talented students are being underchallenged from the case in which the gap is closed because the low-performing students improved their progress at an increased rate? Many believe that nearly all mathematics curricula in this country are insufficiently challenging and rigorous. Therefore achieving modest gains across all ability levels with evidence of accelerated progress by at-risk students may still be criticized for failure to stimulate the top performing student group adequately. Evaluating curricula with regard to this aspect therefore requires judgment and careful methodological attention.
Depending on one’s view of equity, different implications for the collection of data follow. These considerations made examination of the quality of the evaluations as they treated questions of equity challenging for the committee members. Hence we spell out our assumptions as precisely as possible:
Evaluation studies should include representative samples of student demographics, which may require particular attention to the inclusion of underrepresented minority students from lower socioeconomic groups, females, and special needs populations (LEP, learning disabled, gifted and talented students) in the samples. This may require one to solicit participation by particular schools or districts, rather than to follow the patterns of commercial implementation, which may lead to an unrepresentative sample in aggregate.
Analysis of results should always consider the impact of the program on the entire spectrum of the sample to determine whether the overall gains are distributed fairly among differing student groups, and not achieved as improvements in the mean(s) of an identifiable subpopulation(s) alone.
Analysis should examine whether any group of students is systematically less well served by curricular implementation, causing losses or weakening the rate of gains. For example, this could occur if one neglected the continued development of programs for gifted and talented students in mathematics in order to implement programs focused on improving access for underserved youth, or if one improved programs solely for one group of language learners, ignoring the needs of others, or if one’s study systematically failed to report high attrition affecting rates of participation of success or failure.
Analyses should examine whether gaps in scores between significantly disadvantaged or underperforming subgroups and advantaged subgroups are decreasing both in relation to eliminating the development of gaps in the first place and in relation to accelerating improvement for underserved youth relative to their advantaged peers at the upper grades.
In reviewing the outcomes of the studies, the committee reports first on what kinds of attention to these issues were apparent in the database, and second on what kinds of results were produced. Some of the studies used multiple methods to provide readers with information on these issues. In our report on the evaluations, we both provide descriptive information on the approaches used and summarize the results of those studies. Developing more effective methods to monitor the achievement of these objectives may need to go beyond what is reported in this study.
Among the 63 at least minimally methodologically adequate studies, 26 reported on the effects of their programs on subgroups of students. The
TABLE 5-13 Most Common Subgroups Used in the Analyses and the Number of Studies That Reported on That Variable
Identified Subgroup | Number of Studies of NSF-Supported | Number of Studies of Commercially Generated | Total |
Gender | 14 | 5 | 19 |
Race and ethnicity | 14 | 2 | 16 |
Socioeconomic status | 8 | 2 | 10 |
Achievement levels | 5 | 3 | 8 |
English as a second language (ESL) | 2 | 1 | 3 |
Total | 43 | 13 | 56 |
Achievement levels: Outcome data are reported in relation to categorizations by quartiles or by achievement level based on independent test. |
other 37 reported on the effects of the curricular intervention on means of whole groups and their standard deviations, but did not report on their data in terms of the impact on subpopulations. Of those 26 evaluations, 19 studies were on NSF-supported programs and 7 were on commercially generated materials. Table 5-13 reports the most common subgroups used in the analyses and the number of studies that reported on that variable. Because many studies used multiple categories for disaggregation (ethnicity, SES, and gender), the number of reports is more than double the number of studies. For this reason, we report the study results in terms of the “frequency of reports on a particular subgroup” and distinguish this from what we refer to as “study counts.” The advantage of this approach is that it permits reporting on studies that investigated multiple ways to disaggregate their data. The disadvantage is that in a sense, studies undertaking multiple disaggregations become overrepresented in the data set as a result. A similar distinction and approach were used in our treatment of disaggregation by content strands.
It is apparent from these data that the evaluators of NSF-supported curricula documented more equity-based outcomes, as they reported 43 of the 56 comparisons. However, the same percentage of the NSF-supported evaluations disaggregated their results by subgroup, as did commercially generated evaluations (41 percent in both cases). This is an area where evaluations of curricula could benefit greatly from standardization of ex-
pectation and methodology. Given the importance of the topic of equity, it should be standard practice to include such analyses in evaluation studies.
In summarizing these 26 studies, the first consideration was whether representative samples of students were evaluated. As we have learned from medical studies, if conclusions on effectiveness are drawn without careful attention to representativeness of the sample relative to the whole population, then the generalizations drawn from the results can be seriously flawed. In Chapter 2 we reported that across the studies, approximately 81 percent of the comparative studies and 73 percent of the case studies reported data on school location (urban, suburban, rural, or state/region), with suburban students being the largest percentage in both study types. The proportions of students studied indicated a tendency to undersample urban and rural populations and oversample suburban schools. With a high concentration of minorities and lower SES students in these areas, there are some concerns about the representativeness of the work.
A second consideration was to see whether the achievement effects of curricular interventions were achieved evenly among the various subgroups. Studies answered this question in different ways. Most commonly, evaluators reported on the performance of various subgroups in the treatment conditions as compared to those same subgroups in the comparative condition. They reported outcome scores or gains from pretest to posttest. We refer to these as “between” comparisons.
Other studies reported on the differences among subgroups within an experimental treatment, describing how well one group does in comparison with another group. Again, these reports were done in relation either to outcome measures or to gains from pretest to posttest. Often these reports contained a time element, reporting on how the internal achievement patterns changed over time as a curricular program was used. We refer to these as “within” comparisons.
Some studies reported both between and within comparisons. Others did not report findings by comparing mean scores or gains, but rather created regression equations that predicted the outcomes and examined whether demographic characteristics are related to performance. Six studies (all on NSF-supported curricula) used this approach with variables related to subpopulations. Twelve studies used ANCOVA or Multiple Analysis of Variance (MANOVA) to study disaggregation by subgroup, and two reported on comparative effect sizes. In the studies using statistical tests other than t-tests or Chi-squares, two were evaluations of commercially generated materials and the rest were of NSF-supported materials.
Of the studies that reported on gender (n=19), the NSF-supported ones (n=13) reported five cases in which the females outperformed their counterparts in the controls and one case in which the female-male gap decreased within the experimental treatments across grades. In most cases, the studies
present a mixed picture with some bright spots, with the majority showing no significant difference. One study reported significant improvements for African-American females.
In relation to race, 15 of 16 reports on African Americans showed positive effects in favor of the treatment group for NSF-supported curricula. Two studies reported decreases in the gaps between African Americans and whites or Asians. One of the two evaluations of African Americans, performance reported for the commercially generated materials, showed significant positive results, as mentioned previously.
For Hispanic students, 12 of 15 reports of the NSF-supported materials were significantly positive, with the other 3 showing no significant difference. One study reported a decrease in the gaps in favor of the experimental group. No evaluations of commercially generated materials were reported on Hispanic populations. Other reports on ethnic groups occurred too seldom to generalize.
Students from lower socioeconomic groups fared well, according to reported evaluations of NSF-supported materials (n=8), in that experimental groups outperformed control groups in all but one case. The one study of commercially generated materials that included SES as a variable reported no significant difference. For students with limited English proficiency, of the two evaluations of NSF-supported materials, one reported significantly more positive results for the experimental treatment. Likewise, one study of commercially generated materials yielded a positive result at the elementary level.
We also examined the data for ability differences and found reports by quartiles for a few evaluation studies. In these cases, the evaluations showed results across quartiles in favor of the NSF-supported materials. In one case using the same program, the lower quartiles showed the most improvement, and in the other, the gains were in the middle and upper groups for the Iowa Test of Basic Skills and evenly distributed for the informal assessment.
Summary Statements
After reviewing these studies, the committee observed that examining differences by gender, race, SES, and performance levels should be examined as a regular part of any review of effectiveness. We would recommend that all comparative studies report on both “between” and “within” comparisons so that the audience of an evaluation can simply and easily consider the level of improvement, its distribution across subgroups, and the impact of curricular implementation on any gaps in performance. Each of the major categories—gender, race/ethnicity, SES, and achievement level—contributes a significant and contrasting view of curricular impact. Further-
more, more sophisticated accounts would begin to permit, across studies, finer distinctions to emerge, such as the effect of a program on young African-American women or on first generation Asian students.
In addition, the committee encourages further study and deliberation on the use of more complex approaches to the examination of equity issues. This is particularly important due to the overlaps among these categories, where poverty can show itself as its own variable but also may be highly correlated to prior performance. Hence, the use of one variable can mask differences that should be more directly attributable to another. The committee recommends that a group of measurement and equity specialists confer on the most effective design to advance on these questions.
Finally, it is imperative that evaluation studies systematically include demographically representative student populations and distinguish evaluations that follow the commercial patterns of use from those that seek to establish effectiveness with a diverse student population. Along these lines, it is also important that studies report on the impact data on all substantial ethnic groups, including whites. Many studies, perhaps because whites were the majority population, failed to report on this ethnic group in their analyses. As we saw in one study, where Asian students were from poor homes and first generation, any subgroup can be an at-risk population in some setting, and because gains in means may not necessarily be assumed to translate to gains for all subgroups or necessarily for the majority subgroup. More complete and thorough descriptions and configurations of characteristics of the subgroups being served at any location—with careful attention to interactions—is needed in evaluations.
Interactions Among Content and Equity, by Grade Band
By examining disaggregation by content strand by grade levels, along with disaggregation by diverse subpopulations, the committee began to discover grade band patterns of performance that should be useful in the conduct of future evaluations. Examining each of these issues in isolation can mask some of the overall effects of curricular use. Two examples of such analysis are provided. The first example examines all the evaluations of NSF-supported curricula from the elementary level. The second examines the set of evaluations of NSF-supported curricula at the high school level, and cannot be carried out on evaluations of commercially generated programs because they lack disaggregation by student subgroup.
Example One
At the elementary level, the findings of the review of evaluations of data on effectiveness of NSF-supported curricula report consistent patterns of
benefits to students. Across the studies, it appears that positive results are enhanced when accompanied by adequate professional development and the use of pedagogical methods consistent with those indicated by the curricula. The benefits are most consistently evidenced in the broadening topics of geometry, measurement, probability, and statistics, and in applied problem solving and reasoning. It is important to consider whether the outcome measures in these areas demonstrate a depth of understanding. In early understanding of fractions and algebra, there is some evidence of improvement. Weaknesses are sometimes reported in the areas of computational skills, especially in the routinization of multiplication and division. These assertions are tentative due to the possible flaws in designs but quite consistent across studies, and future evaluations should seek to replicate, modify, or discredit these results.
The way to most efficiently and effectively link informal reasoning and formal algorithms and procedures is an open question. Further research is needed to determine how to most effectively link the gains and flexibility associated with student-generated reasoning to the automaticity and generalizability often associated with mastery of standard algorithms.
The data from these evaluations at the elementary level generally present credible evidence of increased success in engaging minority students and students in poverty based on reported gains that are modestly higher for these students than for the comparative groups. What is less well documented in the studies is the extent to which the curricula counteract the tendencies to see gaps emerge and result in long-term persistence in performance by gender and minority group membership as they move up the grades. However, the evaluations do indicate that these curricula can help, and almost never do harm. Finally, on the question of adequate challenge for advanced and talented students, the data are equivocal. More attention to this issue is needed.
Example Two
The data at the high school level produced the most conflicting results, and in conducting future evaluations, evaluators will need to examine this level more closely. We identify the high school as the crucible for curricular change for three reasons: (1) the transition to postsecondary education puts considerable pressure on these curricula; (2) the criteria outlined in the NSF RFP specify significant changes from traditional practice; and (3) high school freshmen arrive from a myriad of middle school curricular experiences. For the NSF-supported curricula, the RFP required that the programs provide a core curriculum “drawn from statistics/probability, algebra/functions, geometry/trigonometry, and discrete mathematics” (NSF, 1991, p. 2) and use “a full range of tools, including graphing calculators
and computers” (NSF, 1991, p. 2). The NSF RFP also specified the inclusion of “situations from the natural and social sciences and from other parts of the school curriculum as contexts for developing and using mathematics” (NSF, 1991, p. 1). It was during the fourth year that “course options should focus on special mathematical needs of individual students, accommodating not only the curricular demands of the college-bound but also specialized applications supportive of the workplace aspirations of employment-bound students” (NSF, 1991, p. 2). Because this set of requirements comprises a significant departure from conventional practice, the implementation of the high school curricula should be studied in particular detail.
We report on a Systemic Initiative for Montana Mathematics and Science (SIMMS) study by Souhrada (2001) and Brown et al. (1990), in which students were permitted to select traditional, reform, and mixed tracks. It became apparent that the students were quite aware of the choices they faced, as illustrated in the following quote:
The advantage of the traditional courses is that you learn—just math. It’s not applied. You get a lot of math. You may not know where to use it, but you learn a lot…. An advantage in SIMMS is that the kids in SIMMS tell me that they really understand the math. They understand where it comes from and where it is used.
This quote succinctly captures the tensions reported as experienced by students. It suggests that student perceptions are an important source of evidence in conducting evaluations. As we examined these curricular evaluations across the grades, we paid particular attention to the specificity of the outcome measures in relation to curricular objectives. Overall, a review of these studies would lead one to draw the following tentative summary conclusions:
There is some evidence of discontinuity in the articulation between high school and college, resulting from the organization and emphasis of the new curricula. This discontinuity can emerge in scores on college admission tests, placement tests, and first semester grades where nonreform students have shown some advantage on typical college achievement measures.
The most significant areas of disadvantage seem to be in students’ facility with algebraic manipulation, and with formalization, mathematical structure, and proof when isolated from context and denied technological supports. There is some evidence of weakness in computation and numeration, perhaps due to reliance on calculators and varied policies regarding their use at colleges (Kahan, 1999; Huntley et al., 2000).
There is also consistent evidence that the new curricula present
strengths in areas of solving applied problems, the use of technology, new areas of content development such as probability and statistics and functions-based reasoning in the use of graphs, using data in tables, and producing equations to describe situations (Huntley et al., 2000; Hirsch and Schoen, 2002).
Despite early performance on standard outcome measures at the high school level showing equivalent or better performance by reform students (Austin et al., 1997; Merlino and Wolff, 2001), the common standardized outcome measures (Preliminary Scholastic Assessment Test [PSAT] scores or national tests) are too imprecise to determine with more specificity the comparisons between the NSF-supported and comparison approaches, while program-generated measures lack evidence of external validity and objectivity. There is an urgent need for a set of measures that would provide detailed information on specific concepts and conceptual development over time and may require use as embedded as well as summative assessment tools to provide precise enough data on curricular effectiveness.
The data also report some progress in strengthening the performance of underrepresented groups in mathematics relative to their counterparts in the comparative programs (Schoen et al., 1998; Hirsch and Schoen, 2002).
This reported pattern of results should be viewed as very tentative, as there are only a few studies in each of these areas, and most do not adequately control for competing factors, such as the nature of the course received in college. Difficulties in the transition may also be the result of a lack of alignment of measures, especially as placement exams often emphasize algebraic proficiencies. These results are presented only for the purpose of stimulating further evaluation efforts. They further emphasize the need to be certain that such designs examine the level of mathematical reasoning of students, particularly in relation to their knowledge of understanding of the role of proofs and definitions and their facility with algebraic manipulation as we as carefully document the competencies taught in the curricular materials. In our framework, gauging the ease of transition to college study is an issue of examining curricular alignment with systemic factors, and needs to be considered along with those tests that demonstrate a curricular validity of measures. Furthermore, the results raising concerns about college success need replication before secure conclusions are drawn.
Also, it is important that subsequent evaluations also examine curricular effects on students’ interest in mathematics and willingness to persist in its study. Walker (1999) reported that there may be some systematic differences in these behaviors among different curricula and that interest and persistence may help students across a variety of subgroups to survive entry-level hurdles, especially if technical facility with symbol manipulation
can be improved. In the context of declines in advanced study in mathematics by American students (Hawkins, 2003), evaluation of curricular impact on students’ interest, beliefs, persistence, and success are needed.
The committee takes the position that ultimately the question of the impact of different curricula on performance at the collegiate level should be resolved by whether students are adequately prepared to pursue careers in mathematical sciences, broadly defined, and to reason quantitatively about societal and technological issues. It would be a mistake to focus evaluation efforts solely or primarily on performance on entry-level courses, which can clearly function as filters and may overly emphasize procedural competence, but do not necessarily represent what concepts and skills lead to excellence and success in the field.
These tentative patterns of findings indicate that at the high school level, it is necessary to conduct individual evaluations that examine the transition to college carefully in order to gauge the level of success in preparing students for college entry and the successful negotiation of majors. Equally, it is imperative to examine the impact of high school curricula on other possible student trajectories, such as obtaining high school diplomas, moving into worlds of work or through transitional programs leading to technical training, two-year colleges, and so on.
These two analyses of programs by grade-level band, content strand, and equity represent a methodological innovation that could strengthen the empirical database on curricula significantly and provide the level of detail really needed by curriculum designers to improve their programs. In addition, it appears that one could characterize the NSF programs (and not the commercial programs as a group) as representing a particular approach to curriculum, as discussed in Chapter 3 . It is an approach that integrates content strands; relies heavily on the use of situations, applications, and modeling; encourages the use of technology; and has a significant dose of mathematical inquiry. One could ask the question of whether this approach as a whole is “effective.” It is beyond the charge and scope of this report, but is a worthy target of investigation if one uses proper care in design, execution, and analysis. Likewise other approaches to curricular change should be investigated at the aggregate level, using careful and rigorous design.
The committee believes that a diversity of curricular approaches is a strength in an educational system that maintains local and state control of curricular decision making. While “scientifically established as effective” should be an increasingly important consideration in curricular choice, local cultural differences, needs, values, and goals will also properly influence curricular choice. A diverse set of effective curricula would be ideal. Finally, the committee emphasizes once again the importance of basing the studies on measures with established curricular validity and avoiding cor-
ruption of indicators as a result of inappropriate amounts of teaching to the test, so as to be certain that the outcomes are the product of genuine student learning.
CONCLUSIONS FROM THE COMPARATIVE STUDIES
In summary, the committee reviewed a total of 95 comparative studies. There were more NSF-supported program evaluations than commercial ones, and the commercial ones were primarily on Saxon or UCSMP materials. Of the 19 curricular programs reviewed, 23 percent of the NSF-supported and 33 percent of the commercially generated materials selected had programs with no comparative reviews. This finding is particularly disturbing in light of the legislative mandate in No Child Left Behind (U.S. Department of Education, 2001) for scientifically based curricular programs and materials to be used in the schools. It suggests that more explicit protocols for the conduct of evaluation of programs that include comparative studies need to be required and utilized.
Sixty-nine percent of NSF-supported and 61 percent of commercially generated program evaluations met basic conditions to be classified as at least minimally methodologically adequate studies for the evaluation of effectiveness. These studies were ones that met the criteria of including measures of student outcomes on mathematical achievement, reporting a method of establishing comparability among samples and reporting on implementation elements, disaggregating by content strand, or using precise, theoretical analyses of the construct or multiple measures.
Most of these studies had both strengths and weaknesses in their quasi-experimental designs. The committee reviewed the studies and found that evaluators had developed a number of features that merit inclusions in future work. At the same time, many had internal threats to validity that suggest a need for clearer guidelines for the conduct of comparative evaluations.
Many of the strengths and innovations came from the evaluators’ understanding of the program theories behind the curricula, their knowledge of the complexity of practice, and their commitment to measuring valid and significant mathematical ideas. Many of the weaknesses came from inadequate attention to experimental design, insufficient evidence of the independence of evaluators in some studies, and instability and lack of cooperation in interfacing with the conditions of everyday practice.
The committee identified 10 elements of comparative studies needed to establish a basis for determining the effectiveness of a curriculum. We recognize that not all studies will be able to implement successfully all elements, and those experimental design variations will be based largely on study size and location. The list of elements begins with the seven elements
corresponding to the seven critical decisions and adds three additional elements that emerged as a result of our review:
A better balance needs to be achieved between experimental and quasi-experimental studies. The virtual absence of large-scale experimental studies does not provide a way to determine whether the use of quasi-experimental approaches is being systematically biased in unseen ways.
If a quasi-experimental design is selected, it is necessary to establish comparability. When quasi-experimentation is used, it “pertains to studies in which the model to describe effects of secondary variables is not known but assumed” (NRC, 1992, p. 18). This will lead to weaker and potentially suspect causal claims, which should be acknowledged in the evaluation report, but may be necessary in relation to feasibility (Joint Committee on Standards for Educational Evaluation, 1994). In general, to date, studies have assumed prior achievement measures, ethnicity, gender, and SES, are acceptable variables on which to match samples or on which to make statistical adjustments. But there are often other variables in need of such control in such evaluations including opportunity to learn, teacher effectiveness, and implementation (see #4 below).
The selection of a unit of analysis is of critical importance to the design. To the extent possible, it is useful to randomly assign the unit for the different curricula. The number of units of analysis necessary for the study to establish statistical significance depends not on the number of students, but on this unit of analysis. It appears that classrooms and schools are the most likely units of analysis. In addition, the development of increasingly sophisticated means of conducting studies that recognize that the level of the educational system in which experimentation occurs affects research designs.
It is essential to examine the implementation components through a set of variables that include the extent to which the materials are implemented, teaching methods, the use of supplemental materials, professional development resources, teacher background variables, and teacher effects. Gathering these data to gauge the level of implementation fidelity is essential for evaluators to ensure adequate implementation. Studies could also include nested designs to support analysis of variation by implementation components.
Outcome data should include a variety of measures of the highest quality. These measures should vary by question type (open ended, multiple choice), by type of test (international, national, local) and by relation of testing to everyday practice (formative, summative, high stakes), and ensure curricular validity of measures and assess curricular alignment with systemic factors. The use of comparisons among total tests, fair tests, and
conservative tests, as done in the evaluations of UCSMP, permits one to gain insight into teacher effects and to contrast test results by items included. Tests should also include content strands to aid disaggregation, at a level of major content strands (see Figure 5-11 ) and content-specific items relevant to the experimental curricula.
Statistical analysis should be conducted on the appropriate unit of analysis and should include more sophisticated methods of analysis such as ANOVA, ANCOVA, MACOVA, linear regression, and multiple regression analysis as appropriate.
Reports should include clear statements of the limitations to generalization of the study. These should include indications of limitations in populations sampled, sample size, unique population inclusions or exclusions, and levels of use or attrition. Data should also be disaggregated by gender, race/ethnicity, SES, and performance levels to permit readers to see comparative gains across subgroups both between and within studies.
It is useful to report effect sizes. It is also useful to present item-level data across treatment program and show when performances between the two groups are within the 10 percent confidence interval of each other. These two extremes document how crucial it is for curricula developers to garner both precise and generalizable information to inform their revisions.
Careful attention should also be given to the selection of samples of populations for participation. These samples should be representative of the populations to whom one wants to generalize the results. Studies should be clear if they are generalizing to groups who have already selected the materials (prior users) or to populations who might be interested in using the materials (demographically representative).
The control group should use an identified comparative curriculum or curricula to avoid comparisons to unstructured instruction.
In addition to these prototypical decisions to be made in the conduct of comparative studies, the committee suggests that it would be ideal for future studies to consider some of the overall effects of these curricula and to test more directly and rigorously some of the findings and alternative hypotheses. Toward this end, the committee reported the tentative findings of these studies by program type. Although these results are subject to revision, based on the potential weaknesses in design of many of the studies summarized, the form of analysis demonstrated in this chapter provides clear guidance about the kinds of knowledge claims and the level of detail that we need to be able to judge effectiveness. Until we are able to achieve an array of comparative studies that provide valid and reliable information on these issues, we will be vulnerable to decision making based excessively on opinion, limited experience, and preconceptions.
This book reviews the evaluation research literature that has accumulated around 19 K-12 mathematics curricula and breaks new ground in framing an ambitious and rigorous approach to curriculum evaluation that has relevance beyond mathematics. The committee that produced this book consisted of mathematicians, mathematics educators, and methodologists who began with the following charge:
- Evaluate the quality of the evaluations of the thirteen National Science Foundation (NSF)-supported and six commercially generated mathematics curriculum materials;
- Determine whether the available data are sufficient for evaluating the efficacy of these materials, and if not;
- Develop recommendations about the design of a project that could result in the generation of more reliable and valid data for evaluating such materials.
The committee collected, reviewed, and classified almost 700 studies, solicited expert testimony during two workshops, developed an evaluation framework, established dimensions/criteria for three methodologies (content analyses, comparative studies, and case studies), drew conclusions on the corpus of studies, and made recommendations for future research.
READ FREE ONLINE
Welcome to OpenBook!
You're looking at OpenBook, NAP.edu's online reading room since 1999. Based on feedback from you, our users, we've made some improvements that make it easier than ever to read thousands of publications on our website.
Do you want to take a quick tour of the OpenBook's features?
Show this book's table of contents , where you can jump to any chapter by name.
...or use these buttons to go back to the previous chapter or skip to the next one.
Jump up to the previous page or down to the next one. Also, you can type in a page number and press Enter to go directly to that page in the book.
Switch between the Original Pages , where you can read the report as it appeared in print, and Text Pages for the web version, where you can highlight and search the text.
To search the entire text of this book, type in your search term here and press Enter .
Share a link to this book page on your preferred social network or via email.
View our suggested citation for this chapter.
Ready to take your reading offline? Click here to buy this book in print or download it as a free PDF, if available.
Get Email Updates
Do you enjoy reading reports from the Academies online for free ? Sign up for email notifications and we'll let you know about new publications in your areas of interest when they're released.

Causal Comparative Research: Methods And Examples
Ritu was in charge of marketing a new protein drink about to be launched. The client wanted a causal-comparative study…

Ritu was in charge of marketing a new protein drink about to be launched. The client wanted a causal-comparative study highlighting the drink’s benefits. They demanded that comparative analysis be made the main campaign design strategy. After carefully analyzing the project requirements, Ritu decided to follow a causal-comparative research design. She realized that causal-comparative research emphasizing physical development in different groups of people would lay a good foundation to establish the product.
What Is Causal Comparative Research?
Examples of causal comparative research variables.
Causal-comparative research is a method used to identify the cause–effect relationship between a dependent and independent variable. This relationship is usually a suggested relationship because we can’t control an independent variable completely. Unlike correlation research, this doesn’t rely on relationships. In a causal-comparative research design, the researcher compares two groups to find out whether the independent variable affected the outcome or the dependent variable.
A causal-comparative method determines whether one variable has a direct influence on the other and why. It identifies the causes of certain occurrences (or non-occurrences). It makes a study descriptive rather than experimental by scrutinizing the relationships among different variables in which the independent variable has already occurred. Variables can’t be manipulated sometimes, but a link between dependent and independent variables is established and the implications of possible causes are used to draw conclusions.
In a causal-comparative design, researchers study cause and effect in retrospect and determine consequences or causes of differences already existing among or between groups of people.
Let’s look at some characteristics of causal-comparative research:
- This method tries to identify cause and effect relationships.
- Two or more groups are included as variables.
- Individuals aren’t selected randomly.
- Independent variables can’t be manipulated.
- It helps save time and money.
The main purpose of a causal-comparative study is to explore effects, consequences and causes. There are two types of causal-comparative research design. They are:
Retrospective Causal Comparative Research
For this type of research, a researcher has to investigate a particular question after the effects have occurred. They attempt to determine whether or not a variable influences another variable.
Prospective Causal Comparative Research
The researcher initiates a study, beginning with the causes and determined to analyze the effects of a given condition. This is not as common as retrospective causal-comparative research.
Usually, it’s easier to compare a variable with the known than the unknown.
Researchers use causal-comparative research to achieve research goals by comparing two variables that represent two groups. This data can include differences in opportunities, privileges exclusive to certain groups or developments with respect to gender, race, nationality or ability.
For example, to find out the difference in wages between men and women, researchers have to make a comparative study of wages earned by both genders across various professions, hierarchies and locations. None of the variables can be influenced and cause-effect relationship has to be established with a persuasive logical argument. Some common variables investigated in this type of research are:
- Achievement and other ability variables
- Family-related variables
- Organismic variables such as age, sex and ethnicity
- Variables related to schools
- Personality variables
While raw test scores, assessments and other measures (such as grade point averages) are used as data in this research, sources, standardized tests, structured interviews and surveys are popular research tools.
However, there are drawbacks of causal-comparative research too, such as its inability to manipulate or control an independent variable and the lack of randomization. Subject-selection bias always remains a possibility and poses a threat to the internal validity of a study. Researchers can control it with statistical matching or by creating identical subgroups. Executives have to look out for loss of subjects, location influences, poor attitude of subjects and testing threats to produce a valid research study.
Harappa’s Thinking Critically program is for managers who want to learn how to think effectively before making critical decisions. Learn how leaders articulate the reasons behind and implications of their decisions. Become a growth-driven manager looking to select the right strategies to outperform targets. It’s packed with problem-solving and effective-thinking tools that are essential for skill development. What more? It offers live learning support and the opportunity to progress at your own pace. Ask for your free demo today!
Explore Harappa Diaries to learn more about topics such as Objectives Of Research Methodology , Types Of Thinking , What Is Visualisation and Effective Learning Methods to upgrade your knowledge and skills.
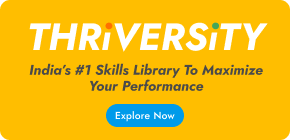
Comparative Research
- First Online: 14 August 2019
Cite this chapter
- Frank Esser 5
3709 Accesses
5 Citations
This chapter highlights the particular advantages of comparative international approaches for media policy research. It starts by explaining the basic logic and main objectives of comparative analysis. Further sections of the chapter are devoted to different design types and related methodological procedures. Finally, special attention is given to the question of how to avoid various types of cultural bias. All these points are illustrated by the concrete example of a comparative international study on media (self-) regulation.
This is a preview of subscription content, log in via an institution to check access.
Access this chapter
Subscribe and save.
- Get 10 units per month
- Download Article/Chapter or eBook
- 1 Unit = 1 Article or 1 Chapter
- Cancel anytime
- Available as PDF
- Read on any device
- Instant download
- Own it forever
- Available as EPUB and PDF
- Durable hardcover edition
- Dispatched in 3 to 5 business days
- Free shipping worldwide - see info
Tax calculation will be finalised at checkout
Purchases are for personal use only
Institutional subscriptions
Similar content being viewed by others
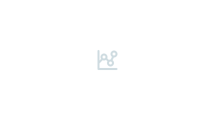
Media Use and Its Effects in a Cross-National Perspective
Methods of Media Economics
The Limits of Empirical Indicators: Media Pluralism as an Essentially Contested Concept
Downey, J., & Stanyer, J. (2010). Comparative media analysis: Why some fuzzy thinking might help. Applying fuzzy set qualitative comparative analysis to the personalization of mediated political communication. European Journal of Communication, 25 (4), 331–347.
Article Google Scholar
Eberwein, T., Fengler, S., Lauk, E., & Leppik-Bork, T. (Eds.). (2011). Mapping media accountability—In Europe and beyond. Cologne: Herbert von Halem Verlag.
Google Scholar
Esser, F. (2014). Methodological challenges in comparative communication research: Advancing cross-national research in times of globalization. In M. J. Canel & K. Voltmer (Eds.), Comparing political communication across time and space (pp. 17–33). Basingstoke: Palgrave Macmillan.
Esser, F., & Hanitzsch, T. (Eds.). (2012a). Handbook of comparative communication research . London: Routledge.
Esser, F., & Hanitzsch, T. (2012b). On the why and how of comparative inquiry in communication studies. In F. Esser & T. Hanitzsch (Eds.), Handbook of comparative communication research (pp. 3–22). London: Routledge.
Fengler, S., Eberwein, T., Mazzoleni, G., Porlezza, C., & Russ-Mohl, S. (Eds.). (2014). Journalists and media accountability: An international study of news people in the digital age . New York: Peter Lang.
George, A. L., & Bennett, A. (2005). Case studies and theory development in the social sciences . Cambridge, MA: MIT Press.
Gurevitch, M., & Blumler, J. G. (1990). Comparative research: The extending frontier. In D. L. Swanson & D. Nimmo (Eds.), New directions in political communication (pp. 305–328). Newbury Park: Sage.
Hallin, D., & Mancini, P. (2004). Comparing media systems: Three models of media and politics . Cambridge: Cambridge University Press.
Book Google Scholar
Humphreys, P. (2012). A political scientist’s contribution to the comparative study of media systems in Europe: A response to Hallin and Mancini. In N. Just & M. Puppis (Eds.), Trends in communication policy research: New theories, new methods, new subjects (pp. 141–158). Bristol: Intellect.
Landman, T. (2008). Issues and methods in comparative politics (3rd ed.). London: Routledge.
Livingstone, S. (2003). On the challenges of cross-national comparative media research. European Journal of Communication, 18 (4), 477–500.
Mill, J. S. (1843). A system of logic . London: Longman.
Przeworski, A., & Teune, H. (1970). The logic of comparative social inquiry . New York: Wiley.
Puppis, M., & d’Haenens, L. (2012). Comparing media policy and regulation. In F. Esser & T. Hanitzsch (Eds.), Handbook of comparative communication research (pp. 221–233).
Ragin, C. C. (1987). The comparative method: Moving beyond qualitative and quantitative strategies . Berkeley and Los Angeles, CA: University of California Press.
Ragin, C. C. (2008). Qualitative comparative analysis using fuzzy sets (fsQCA). In B. Rihoux & C. C. Ragin (Eds.), Configurational comparative methods: Qualitative comparative analysis and related techniques (pp. 87–122). Thousand Oaks, CA: Sage.
Rihoux, B. (2006). Qualitative comparative analysis (QCA) and related systematic comparative methods: Recent advances and remaining challenges for social science research. International Sociology, 21 (5), 679–706.
Roessler, P. (2012). Comparative content analysis. In F. Esser & T. Hanitzsch (Eds.), Handbook of comparative communication research (pp. 459–468). London: Routledge.
Sartori, G. (1994). Compare why and how? In M. Dogan & A. Kazancigil (Eds.), Comparing nations: Concepts, strategies, substance (pp. 14–34). Oxford: Blackwell.
Shoemaker, P. J., & Reese, S. D. (2016). A media sociology for the networked public sphere: The hierarchy of influences model. Mass Communication and Society, 19, 389–410.
van de Vijver, F., & Leung, K. (1997). Methods and data analysis of comparative research. In J. W. Berry, Y. P. Poortinga, & J. Pandey (Eds.), Handbook of cross-cultural psychology (2nd ed., Vol. 1, pp. 257–300). Needham Heights, MA: Allyn & Bacon.
Verhulst, S. G., & Price, M. E. (2008). Comparative media law research and its impact on policy. International Journal of Communication, 2, 406–420.
Vliegenthart, R. (2012). Advanced strategies for data analysis: Opportunities and challenges of comparative data. In F. Esser & T. Hanitzsch (Eds.), Handbook of comparative communication research (pp. 486–500). New York: Routledge.
Wirth, W., & Kolb, S. (2004). Designs and methods of comparative political communication research. In F. Esser & B. Pfetsch (Eds.), Comparing political communication: Theories, cases, and challenges (pp. 87–111). New York: Cambridge University Press.
Chapter Google Scholar
Wirth, W., & Kolb, S. (2012). Securing equivalence: Problems and solutions. In F. Esser & T. Hanitzsch (Eds.), The handbook of comparative communication research (pp. 469–485). London: Routledge.
Further Reading
d’Haenens, L., Sousa, H., & Trappel, J. (Eds.). (2018). Comparative media policy, regulation and governance in Europe . Chicago: University of Chicago Press.
Esser, F., & Hanitzsch, T. (Eds.). (2012). Handbook of comparative communication research . London: Routledge.
Download references
Author information
Authors and affiliations.
University of Zurich, Zurich, Switzerland
Frank Esser
You can also search for this author in PubMed Google Scholar
Editor information
Editors and affiliations.
Department of Communication, Drexel University, Philadelphia, USA
Hilde Van den Bulck
Department of Communication and Media Research, University of Fribourg, Fribourg, Switzerland
Manuel Puppis
Department of Communication Studies, Vrije Universiteit Brussel, Brussels, Belgium
Karen Donders
Leo Van Audenhove
Rights and permissions
Reprints and permissions
Copyright information
© 2019 The Author(s)
About this chapter
Esser, F. (2019). Comparative Research. In: Van den Bulck, H., Puppis, M., Donders, K., Van Audenhove, L. (eds) The Palgrave Handbook of Methods for Media Policy Research. Palgrave Macmillan, Cham. https://doi.org/10.1007/978-3-030-16065-4_5
Download citation
DOI : https://doi.org/10.1007/978-3-030-16065-4_5
Published : 14 August 2019
Publisher Name : Palgrave Macmillan, Cham
Print ISBN : 978-3-030-16064-7
Online ISBN : 978-3-030-16065-4
eBook Packages : Literature, Cultural and Media Studies Literature, Cultural and Media Studies (R0)
Share this chapter
Anyone you share the following link with will be able to read this content:
Sorry, a shareable link is not currently available for this article.
Provided by the Springer Nature SharedIt content-sharing initiative
- Publish with us
Policies and ethics
- Find a journal
- Track your research
- - Google Chrome
Intended for healthcare professionals
- My email alerts
- BMA member login
- Username * Password * Forgot your log in details? Need to activate BMA Member Log In Log in via OpenAthens Log in via your institution

Search form
- Advanced search
- Search responses
- Search blogs
- Comparative oral...
Comparative oral monotherapy of psilocybin, lysergic acid diethylamide, 3,4-methylenedioxymethamphetamine, ayahuasca, and escitalopram for depressive symptoms: systematic review and Bayesian network meta-analysis
- Related content
- Peer review
- Tien-Wei Hsu , doctoral researcher 1 2 3 ,
- Chia-Kuang Tsai , associate professor 4 ,
- Yu-Chen Kao , associate professor 5 6 ,
- Trevor Thompson , professor 7 ,
- Andre F Carvalho , professor 8 ,
- Fu-Chi Yang , professor 4 ,
- Ping-Tao Tseng , assistant professor 9 10 11 12 ,
- Chih-Wei Hsu , assistant professor 13 ,
- Chia-Ling Yu , clinical pharmacist 14 ,
- Yu-Kang Tu , professor 15 16 ,
- 1 Department of Psychiatry, E-DA Dachang Hospital, I-Shou University, Kaohsiung, Taiwan
- 2 Department of Psychiatry, E-DA Hospital, I-Shou University, Kaohsiung, Taiwan
- 3 Graduate Institute of Clinical Medicine, College of Medicine, Kaohsiung Medical University, Kaohsiung, Taiwan
- 4 Department of Neurology, Tri-Service General Hospital, National Defense Medical Centre, Taipei, Taiwan
- 5 Department of Psychiatry, National Defense Medical Centre, Taipei, Taiwan
- 6 Department of Psychiatry, Beitou Branch, Tri-Service General Hospital, Taipei, Taiwan
- 7 Centre for Chronic Illness and Ageing, University of Greenwich, London, UK
- 8 IMPACT (Innovation in Mental and Physical Health and Clinical Treatment) Strategic Research Centre, School of Medicine, Barwon Health, Deakin University, Geelong, VIC, Australia
- 9 Institute of Biomedical Sciences, National Sun Yat-sen University, Kaohsiung, Taiwan
- 10 Department of Psychology, College of Medical and Health Science, Asia University, Taichung, Taiwan
- 11 Prospect Clinic for Otorhinolaryngology and Neurology, Kaohsiung, Taiwan
- 12 Institute of Precision Medicine, National Sun Yat-sen University, Kaohsiung, Taiwan
- 13 Department of Psychiatry, Kaohsiung Chang Gung Memorial Hospital and Chang Gung University College of Medicine, Kaohsiung, Taiwan
- 14 Department of Pharmacy, Chang Gung Memorial Hospital Linkou, Taoyuan, Taiwan
- 15 Institute of Health Data Analytics and Statistics, College of Public Health, National Taiwan University, Taipei, Taiwan
- 16 Department of Dentistry, National Taiwan University Hospital, Taipei, Taiwan
- Correspondence to: C-S Liang lcsyfw{at}gmail.com
- Accepted 20 June 2024
Objective To evaluate the comparative effectiveness and acceptability of oral monotherapy using psychedelics and escitalopram in patients with depressive symptoms, considering the potential for overestimated effectiveness due to unsuccessful blinding.
Design Systematic review and Bayesian network meta-analysis.
Data sources Medline, Cochrane Central Register of Controlled Trials, Embase, PsycINFO, ClinicalTrial.gov, and World Health Organization’s International Clinical Trials Registry Platform from database inception to 12 October 2023.
Eligibility criteria for selecting studies Randomised controlled trials on psychedelics or escitalopram in adults with depressive symptoms. Eligible randomised controlled trials of psychedelics (3,4-methylenedioxymethamphetamine (known as MDMA), lysergic acid diethylamide (known as LSD), psilocybin, or ayahuasca) required oral monotherapy with no concomitant use of antidepressants.
Data extraction and synthesis The primary outcome was change in depression, measured by the 17-item Hamilton depression rating scale. The secondary outcomes were all cause discontinuation and severe adverse events. Severe adverse events were those resulting in any of a list of negative health outcomes including, death, admission to hospital, significant or persistent incapacity, congenital birth defect or abnormality, and suicide attempt. Data were pooled using a random effects model within a Bayesian framework. To avoid estimation bias, placebo responses were distinguished between psychedelic and antidepressant trials.
Results Placebo response in psychedelic trials was lower than that in antidepression trials of escitalopram (mean difference −3.90 (95% credible interval −7.10 to −0.96)). Although most psychedelics were better than placebo in psychedelic trials, only high dose psilocybin was better than placebo in antidepression trials of escitalopram (mean difference 6.45 (3.19 to 9.41)). However, the effect size (standardised mean difference) of high dose psilocybin decreased from large (0.88) to small (0.31) when the reference arm changed from placebo response in the psychedelic trials to antidepressant trials. The relative effect of high dose psilocybin was larger than escitalopram at 10 mg (4.66 (95% credible interval 1.36 to 7.74)) and 20 mg (4.69 (1.64 to 7.54)). None of the interventions was associated with higher all cause discontinuation or severe adverse events than the placebo.
Conclusions Of the available psychedelic treatments for depressive symptoms, patients treated with high dose psilocybin showed better responses than those treated with placebo in the antidepressant trials, but the effect size was small.
Systematic review registration PROSPERO, CRD42023469014.
Introduction
Common psychedelics belong to two classes: classic psychedelics, such as psilocybin, lysergic acid diethylamide (known as LSD), and ayahuasca; and entactogens, such as 3,4-methylenedioxymethamphetamine (MDMA). 1 Several randomised controlled trials have shown efficacy of psychedelics for people with clinical depression. 2 3 The proposed mechanism of its fast and persistent antidepressant effects is to promote structural and functional neuroplasticity through the activation of intracellular 5-HT 2A receptors in the cortical neurons. 4 Additionally, the increased neuroplasticity was associated with psychedelic’s high affinity directly binding to brain derived neurotrophic factor receptor TrkB, indicating a dissociation between the hallucinogenic and plasticity promoting effects of psychedelics. 5 A meta-analysis published in 2023 reported that the standardised mean difference of psychedelics for depression reduction ranged from 1.37 to 3.12, 2 which are considered large effect sizes. 6 Notably, the standardised mean difference of antidepressant trials is approximately 0.3 (a small effect size). 7 8
Although modern randomised controlled trials involving psychedelics usually use a double blinded design, the subjective effects of these substances can compromise blinding. 9 Unsuccessful blinding may lead to differing placebo effects between the active and control groups, potentially introducing bias into the estimation of relative treatment effects. 10 Concerns have arisen regarding the overestimated effect sizes of psychedelics due to the issues of blinding and response expectancy. 9 Psychedelic treatment is usually administered with psychological support or psychotherapy, and thereby the isolated pharmacological effects of psychedelics remain to be determined. 2 Surprisingly, on 1 July 2023, Australia approved psilocybin for the treatment of depression 11 ; the first country to classify psychedelics as a medicine at a national level.
To date, only one double blind, head-to-head randomised controlled trial has directly compared a psychedelic drug (psilocybin) with an antidepressant drug (escitalopram) for patients with major depressive disorder. 12 This randomised controlled trial reported that psilocybin showed a better efficacy than escitalopram on the 17 item Hamilton depression rating scale (HAMD-17).
We aimed to assess the comparative effectiveness and acceptability of oral monotherapy with psychedelics and escitalopram in patients experiencing depressive symptoms. Given that unsuccessful blinding can potentially lead to a reduced placebo response in psychedelic trials, we distinguished between the placebo responses in psychedelic and antidepressant trials. We also investigated the differences in patient responses between people who received extremely low dose psychedelics as a placebo and those who received a placebo in the form of a fake pill, such as niacin, in psilocybin trials. 13 14 Our study allowed for a relative effect assessment of psychedelics compared with placebo responses observed in antidepressant trials.
The study protocol was registered with PROSPERO (CRD42023469014). We followed the Preferred Reporting Items for Systematic Reviews and Meta-Analyses (PRISMA) extension statement for reporting systematic reviews incorporating network meta-analysis (NMA) (appendix 1). 15
Data sources and searches
A comprehensive search of the Medline, Cochrane Central Register of Controlled Trials (CENTRAL), Embase, PsycINFO, ClinicalTrial.gov, and World Health Organization’s International Clinical Trials Registry Platform databases were performed without language restrictions from database inception to 12 October 2023. We also searched the grey literature and reviewed reference lists of the included studies and related systematic reviews. 2 3
Study selection
Eligible studies were randomised controlled trials with parallel group or crossover designs. We included: (i) adults (≥18 years) with clinically diagnosed depression (eg, major depressive disorder, bipolar disorder, or other psychiatric disorders with comorbid clinical depression) or life threatening diagnoses and terminal illness with depressive symptoms; and (ii) adults with assessment of treatment response (preapplication/postapplication) using standard, validated, and internationally recognised instruments, such as HAMD-17. The outcome of interest was the change in depressive symptoms at the end of treatment compared with the controls, and we only extracted data from the first phase of crossover randomised controlled trials to avoid carry-over effects. Eligible psychedelic randomised controlled trials (including psilocybin, lysergic acid diethylamide, MDMA, and ayahuasca without dosage limit) required oral monotherapy without the concomitant use of antidepressants. For escitalopram, we included only fixed dose randomised controlled trials that compared at least two arms with different doses of oral form escitalopram (maximum dose of 20 mg/day) with placebo because psychedelic therapies usually use a fixed dose study design. We also included randomised controlled trials that evaluated psychedelic monotherapy compared with escitalopram monotherapy. We excluded follow-up studies and studies with healthy volunteers. We also excluded conference abstracts, editorials, reviews, meta-analyses, case reports, and case series, as well as publications reporting duplicate data. We did not consider ketamine because this drug is usually administered parenterally and is not a classic psychedelic. 16 Screening and selection of the studies were performed independently by two authors. Discrepancies in study inclusion were resolved by deliberation among the reviewer pairs or with input from a third author. Appendix 2 shows the complete search strategies, and appendix 3 presents the reasons for exclusion.
Definition of outcomes, data extraction, and risk of bias assessment
The primary outcome was change in depressive symptoms from baseline (continuous outcome), as measured by a validated rating scale, such as HAMD-17. When multiple measurement tools were used, they were selected in the following order: the HAMD-17, Montgomery-Åsberg depression rating scale, and Beck depression inventory (second edition). To improve interpretability, all extracted depression scores were converted to corresponding HAMD-17 scores using a validated method. 17 We used a conservative correlation coefficient of 0.5 or other statistics (eg, t statistics) to calculate the standard deviation of change from baseline when unreported. 18 The secondary outcomes were all cause discontinuation and severe adverse events (categorical outcomes). Severe adverse events were classified as those resulting in any of a list of negative health outcomes including, death, admission to hospital, significant or persistent incapacity, congenital birth defect or abnormality, and suicide attempt. Outcome data were extracted from original intention-to-treat or last observation carrying forward analysis, as well as from estimates of mixed-effect models for repeated measures.
Two authors independently extracted and reviewed the data, each being reviewed by another author. WebPlot Digitizer ( https://automeris.io/WebPlotDigitizer/ ) was used to extract numerical data from the figures. Two authors independently used the Cochrane randomised trial risk of bias tool (version 2.0) to assess the risk of bias in the included trials, and discrepancies were resolved by consensus. 19
Data synthesis
To estimate the relative effect between two interventions, we computed mean difference on the basis of change values (with 95% credible interval) for continuous outcomes (change in depressive symptoms) and odds ratios for categorical outcomes (all cause discontinuation and severe adverse event). To assess the clinical significance of the relative effect, we evaluated whether the mean difference exceeded the minimal important difference, which is estimated to be 3 points for HAMD-17. 20 We defined high, low, and extremely low doses of the included psychedelics as follows: (i) psilocybin: high dose (≥20 mg), extremely low dose (1-3 mg), low dose (other range); and (ii) MDMA: high dose (≥100 mg), extremely low dose (≤40 mg), low dose (other range). Escitalopram was divided into escitalopram 10 mg and escitalopram ≥20 mg. In previous clinical trials, a dose of 1 mg of psilocybin or a dose range of 1-3 mg/70 kg were used as an active control because these doses were believed not to produce significant psychedelic effects. 21 22 A dose of 5 mg/70 kg can produce noticeable psychedelic effects. 22 In many two arm psilocybin trials, the psilocybin dose in the active group typically falls within the range of 20-30 mg. 12 21 23 24 In a three arm trial, 25 mg was defined as high dose, and 10 mg was considered a moderate dose. 21 Another clinical trial also defined 0.215 mg/kg of psilocybin as a moderate dose for the active group. 25 Therefore, we used 20 mg and 3 mg as the boundaries for grouping psilocybin doses; when the dosage was calculated per kilogram in the study, we converted it to per 70 kg. For MDMA, in two trials with three arms, 125 mg was defined as high dose, and 30-40 mg was defined as active control. 26 27 Thus, we used 100 mg and 40 mg as the boundaries for grouping MDMA doses.
We conducted random effects network meta-analysis and meta-analysis within a Bayesian framework. 28 29 Previous meta-analyses considered all control groups as a common comparator; however, concerns have been raised regarding the overestimated effect sizes of psychedelics because of unsuccessful blinding and poor placebo response. 9 Therefore, we treated the three treatments as distinct interventions: the placebo response observed in psychedelic trials, the placebo response observed in antidepressant escitalopram trials, and extremely low dose psychedelics (ie, psilocybin and MDMA). We calculated the relative effects of all interventions compared with these three groups, indicating the following three conditions: (1) the treatment response of placebo response in the psychedelic trials is assumed to be lower than that of placebo response in antidepressant trials because of unsuccessful blinding. 9 As such, the relative effects compared with placebo response in the psychedelic trials represented potential overestimated effect sizes. (2) the placebo response in antidepressant trials is assumed to be the placebo response in antidepressant trials with adequate blinding, therefore, the relative effects compared with placebo response in antidepressant trials represents effect sizes in trials with adequate blinding. (3) Psychedelic drugs are usually administered with psychotherapy 13 or psychological support, 14 the relative effects of psychedelics compared with extremely low dose psychedelics might eliminate the concomitant effects from psychotherapeutic support, approximating so-called pure pharmacological effects.
In network meta-analysis, the validity of indirect comparison relies on transitivity assumption. 30 We assessed the transitivity assumption by comparing the distribution of potential effect modifies across treatment comparisons. In addition, we assessed whether the efficacy of escitalopram is similar in placebo controlled randomised controlled trials (escitalopram v placebo response in antidepressant trials) and in the head-to-head randomised controlled trial (psilocybin v escitalopram) using network meta-analysis. 12 Furthermore, we assessed the efficacy of the different placebo responses (placebo response in the psychedelic trials v placebo response in antidepressant trials) as additional proof of transitivity. If the placebo response in antidepressant trials was better than that in the psychedelic trials, the transitivity assumption did not hold when grouping placebo response in antidepressant trials and placebo response in the psychedelic trials together. Finally, for the primary outcome (change in depressive symptoms), network meta-regression analyses were conducted to evaluate the impact of potential effect modifiers, including proportion of men and women in the study, mean age, baseline depression severity, disorder type, and follow-up assessment period. We assumed a common effect on all treatment comparisons for each of the effect modifiers. In other words, all interactions between the treatment comparisons and the effect modifier were constrained to be identical.
We also conducted the following sensitivity analyses: analysing studies of patients with major depressive disorder; excluding studies with a high risk of bias; adjusting for baseline depression severity; and using correlation coefficient of zero (most conservative) to calculate the standard deviation of change from baseline when unreported.
Publication bias was assessed by visual inspection of a comparison adjusted funnel plots. The first funnel plot used placebo response in the psychedelic trials as the comparator. The second funnel plot used placebo response in antidepressant trials as the comparator. The third funnel plot used both placebo response in the psychedelic trials and placebo response in antidepressant trials as comparators simultaneously. Additionally, we conducted the Egger test, Begg test, and Thompson test to examine the asymmetry of the third funnel plot. A previous meta-analysis reported that the standardised mean difference of psychedelics for depression reduction ranged from 1.37 to 3.12. 2 Therefore, we also transformed the effect size of mean difference to standardised mean difference (Hedges’ g) for the primary outcome. The global inconsistency of the network meta-analysis was examined by fitting an unrelated main effects model. Local inconsistency of the network meta-analysis was examined using node splitting methods. 31 Four Markov chains were implemented. 50 000 iterations occurred per chain and the first 20 000 iterations for each chain were discarded as a warm-up. Convergence was assessed by visual inspection of the trace plots of the key parameters for each analysis. The prior settings and convergence results are shown in appendix 4. All statistical analyses were done using R version 4.3.1. The network meta-analysis and pairwise meta-analysis within a Bayesian framework were fitted using the Bayesian statistical software called Stan within the R packages multinma 28 and brms, 29 respectively. The frequentist random effects network meta-analysis, funnel plots, and tests for funnel plot asymmetry were conducted using the R package netmeta. Reasons for protocol changes are in appendix 5.
Assessment certainty of evidence for the primary outcome
The certainty of evidence produced by the network meta-analysis was evaluated using GRADE (Grading of recommendations, assessment, development and evaluation). 32 33 We used a minimally contextualised framework with the value of 3 (minimal important difference) as our decision threshold. The certainty of evidence refers to our certainty that the intervention had, relative to minimal intervention, any clinically minimal important difference. The optimal information size was calculated using a validated method. 32 33 34
Patient and public involvement
Both patients and the public are interested in research on novel depression treatments and their efficacy compared with existing antidepressants. However, due to a scarcity of available funding for recruitment and researcher training, patients and members of the public were not directly involved in the planning or writing of this manuscript. We did speak to patients about the study, and we asked a member of the public to read our manuscript after submission.
Characteristics of included study
After searching the database and excluding duplicated records, we identified 3104 unique potential studies. We then screened the titles and abstracts of these studies for eligibility and excluded 3062 of them, in which 42 studies remained. Twenty six studies were excluded after an assessment of the full text for various reasons (appendix 3). We identified three additional studies through a manual search resulting in total 19 eligible studies (efigure 1). Details of the characteristics of the included studies are shown in etable 1. Protocols of psychological support or psychotherapy with psychedelic treatment are shown in etable 2. Overall, 811 people (mean age of 42.49 years, 54.2% (440/811) were women) were included in psychedelic trials (15 trials), and 1968 participants (mean age of 39.35 years, 62.5% (1230/1968) were women) were included in escitalopram trials (five trials).
Risk of bias of the included studies
No psychedelic study (0/15) had a high overall risk of bias (efigure 2A and efigure 3A). The percentages of studies with high, some concerns, or low risk of bias in the 15 psychedelic trials were as follows: 0% (k=15), 33% (k=5), and 67% (k=10) for randomisation; 0% (k=0), 33% (k=5), and 67% (k=10) for deviations from intended interventions; 0% (k=0), 13% (k=2), and 87% (k=13) for missing outcome data; 0% (k=0), 33% (k=5), and 67% (k=10) for measurements of outcomes; 0% (k=0), 67% (k=1), and 93% (k=14) for selection of reported results. No non-psychedelic studies (0/5) were rated as high risk of bias (efigure 2B and efigure 3B). The percentages of studies with high, some concerns, and low risk of bias in the five non-psychedelic trials were as follows: 0% (k=0), 80% (k=4), and 20% (k=1) for randomisation; 0% (k=0), 100% (k=5), and 0% (k=0) for deviations from intended interventions; 0% (k=0), 80% (k=4), and 20% (k=1) for missing outcome data; 0% (k=0), 80% (k=4), and 20% (k=1) for measurements of outcomes; 0% (k=0), 20% (k=1), and 80% (k=4) for selection of reported results.
Network meta-analysis
In the network structure, all interventions were connected, with two main structures ( fig 1 ). All psychedelics were compared with placebo response in the psychedelic trials, and escitalopram was compared with placebo response in antidepressant trials. A head-to-head comparison of high dose psilocybin and 20 mg escitalopram connected the two main structures. 12

Network structure. LSD=lysergic acid diethylamide; MDMA=3,4-methylenedioxymethamphetamine
- Download figure
- Open in new tab
- Download powerpoint
In the main network meta-analysis, all interventions, except for extremely low dose and low dose MDMA, were associated with a larger mean difference exceeding the minimal important difference of 3 points on the HAMD-17 than with placebo response in the psychedelic trials ( fig 2 ). Notably, placebo response in antidepressant trials (3.79 (95% credibile interval 0.77 to 6.80)) and extremely low dose psilocybin (3.96 (0.61 to 7.17)) were better than placebo response in the psychedelic trials, with mean differences exceeding 3 and 95% credibile intervals that did not cross zero. Additionally, in comparison with placebo response in antidepressant trials ( fig 2 ), the relative effects of high dose psilocybin (6.52 (3.19 to 9.57)), escitalopram 10 mg (1.86 (0.21 to 3.50)), and escitalopram 20 mg (1.82 (0.16 to 3.43)) did not cross zero. Only high dose psilocybin resulted in a mean difference that was greater than 3. The standardised mean difference of high dose psilocybin decreased from large (0.88) to small (0.31) when the reference arm was changed from placebo response in the psychedelic trials to placebo response in antidepressant trials.

Forest plots of network meta-analytical estimates v different reference arms by observed placebo response. The dotted line represents the minimal important difference of 3 whereas the red line indicates 0. LSD=lysergic acid diethylamide; MDMA=3,4-methylenedioxymethamphetamine
When compared with extremely low dose psilocybin ( fig 2 ), only the relative effects of high dose psilocybin (6.35 (95% credibile interval 3.41 to 9.21)) and placebo response in the psychedelic trials (−3.96 (−7.17 to −0.61)) showed a larger mean difference exceeding 3, without crossing zero. All relative effects between interventions are showed in efigure 4. Importantly, the mean differences of high dose psilocybin compared with escitalopram 10 mg (4.66 (1.36 to 7.74); standardised mean difference 0.22), escitalopram 20 mg (4.69 (1.64 to 7.54); 0.24), high dose MDMA (4.98 (1.23 to 8.67); 0.32), and low dose psilocybin (4.36 (1.20 to 7.51); 0.32) all exceeded 3 and did not cross zero (efigure 4).
Transitivity assumption
The assessment of transitivity assumption is showed in efigure 5 and efigure 6. We compared the efficacy of escitalopram in the placebo controlled antidepressant trials 8 with that in the head-to-head trial (psilocybin v escitalopram) 12 using network meta-analysis and pairwise meta-analysis. The results of the network meta-analysis showed that the relative effects between these two study designs (0.64 (95% credibile interval −4.41 to 5.40), efigure 6A; 1.94 (−2.66 to 6.14), efigure 6B) included zero, and the mean differences did not exceed 3. Placebo response in antidepressant trials was better than placebo response in the psychedelic trials with a small effect size (3.79 (0.77 to 6.80), standardised mean difference 0.2), and the mean difference exceed 3 ( fig 2 ).
Sensitivity analyses
When including only patients with major depressive disorder, the relative effects of escitalopram 20 mg, escitalopram 10 mg, ayahuasca, and high dose psilocybin were better than placebo response in antidepressant trials, while placebo response in the psychedelic trials was worse than placebo response in antidepressant trials ( fig 3 ). However, only the mean differences for high dose psilocybin (6.82 (95% credibile interval 3.84 to 9.67)), ayahuasca (5.38 (0.02 to 10.61)), and placebo response in the psychedelic trials (−4.00 (−6.87 to −1.13)) exceeded 3. When compared with extremely low dose psilocybin (excluding the effects from concomitant psychotherapeutic support), only the 95% credibile intervals of the relative effects of high dose psilocybin (4.36 (0.54 to 8.27); standardised mean difference 0.30) and placebo response in the psychedelic trials (−6.46 (−10.41 to −2.32), standardised mean difference −0.46) exceeded 3 and did not cross zero ( fig 3 ). All of the relative effects between interventions are showed in efigure 7. Notably, the relative effects of high dose psilocybin compared with escitalopram 10 mg (4.96 (1.97 to 7.82)), escitalopram 20 mg (4.97 (2.19 to 7.64)), and low dose psilocybin (3.82 (0.61 to 7.04)) all exceeded 3 and did not cross zero (efigure 7).

Forest plots of network meta-analytical estimates when considering a population with major depressive disorder
The other three sensitivity analyses showed similar findings with the main analyses: exclusion of studies with high risk of bias (efigure 8); adjustment of baseline depression severity (efigure 9); and use of most conservative correlation coefficient of zero (efigure 10).
All cause discontinuation and severe adverse event
When referencing placebo in psychedelic trials, no interventions were associated with higher risks of all cause discontinuation rate nor severe adverse event rate (efigure 11).
Network meta-regression and publication bias
In network meta-regression analyses, the 95% credibile intervals of the relative effects of the baseline depressive severity, mean age, and percentage of women, crossed zero (etable 3). The results of the statistical tests (Egger, Begg, and Thompson-Sharp tests) for funnel plot asymmetry and visual inspection of funnel plots did not show publication bias (efigure 12). The results of GRADE assessment are provided in the efigure 13. Most of the certainty of evidence for treatment comparisons was moderate or low.
Consistency assumptions
The back calculation methods for all the models (appendix 6) did not show any inconsistencies. The node splitting methods also did not show any inconsistencies (appendix 7).
Principal findings
This network meta-analysis investigated the comparative effectiveness between psychedelics and escitalopram for depressive symptoms. Firstly, we found that the placebo response observed in antidepressant trials was associated with greater effectiveness than that observed in psychedelic trials. Secondly, when compared with placebo responses in antidepressant trials, only escitalopram and high dose psilocybin were associated with greater effectiveness, and only high dose psilocybin exceeded minimal important difference of 3. Notably, the effect size of high dose psilocybin decreased from large to small. Thirdly, among the included psychedelics, only high dose psilocybin was more likely to be better than escitalopram 10 mg or 20 mg, exceeding the minimally important difference of 3. Fourthly, in patients with major depressive disorder, escitalopram, ayahuasca, and high dose psilocybin were associated with greater effectiveness than placebo responses in antidepressant trials; however, only high dose psilocybin was better than extremely low dose psilocybin, exceeding minimal important difference of 3. Taken together, our study findings suggest that among psychedelic treatments, high dose psilocybin is more likely to reach the minimal important difference for depressive symptoms in studies with adequate blinding design, while the effect size of psilocybin was similar to that of current antidepressant drugs, showing a mean standardised mean difference of 0.3. 7
Comparison with other studies
In a randomised controlled trial, treatment response was defined as the response observed in the active arm; placebo response was defined as the response observed in the control (placebo) arm. 10 Treatment response consists of non-specific effects, placebo effect, and true treatment effect; placebo response consisted of non-specific effects and placebo effect. Therefore, when the placebo effect is not the same for the active and control arms within an randomised controlled trial, the estimation of the true treatment effect is biased. For example, in a psychedelic trial, unsuccessful blinding may occur due to the profound subjective effects of psychedelics. This unblinding may lead to high placebo effect in the active arm and low placebo effect in the control arms, and the true treatment effect is overestimated. 10 Without addressing unequal placebo effects within studies, the estimation of meta-analysis and network meta-analysis are biased. 10 However, in most psychedelic trials, blinding was either reported as unsuccessful or not assessed at all. For example, two trials of lysergic acid diethylamide reported unsuccessful blinding, 35 36 whereas the trial of ayahuasca only reported that five of 10 participants misclassified the placebo as ayahuasca. 37 In trials of MDMA, participants' accuracy in guessing which treatment arm they were in ranged from approximately 60-90%. 26 27 38 39 40 In the case of most psilocybin trials, blinding was not assessed, with the exception of the study by Ross and colleagues in 2016. 13 In that study, participants were asked to guess whether the psilocybin or an active control was received, and the correct guessing rate was 97%. In our study, we established several network meta-analysis models addressing this issue, and we found that placebo response in the psychedelic trials was associated with less effectiveness than that in antidepressant trials. Therefore, the effect sizes of psychedelics compared with placebo response observed in psychedelic trials may be overestimated. All of the psychedelics’ 95% credibile intervals of the relative effects crossed zero when compared with the placebo response in antidepressant trials, except for high dose psilocybin.
The comparisons between psychedelics and escitalopram showed that high dose psilocybin was more likely to be better than escitalopram. Psilocybin was usually administered with psychotherapy or psychological support. 13 14 Therefore, the greater effectiveness of psilocybin may be from not only pharmacological effects but also psychotherapeutic support. However, we also found that high doses of psilocybin was associated greater effectiveness than extremely low doses of psilocybin. This effect also indicates that the effectiveness of psilocybin cannot be attributed only to concomitant psychotherapy or psychological support.
In patients with major depressive disorder, ayahuasca, low dose psilocybin, high dose psilocybin, escitalopram 10 mg, and escitalopram 20 mg were associated with greater effectiveness than the placebo response in antidepressant trials . However, when compared with extremely low dose psilocybin, only high dose psilocybin was associated with better effectiveness; the standardised mean difference decreased from 0.38 (compared with placebo response in antidepressant trials) to 0.30 (compared with extremely low dose psilocybin). As such, the effectiveness of psilocybin should be considered with concomitant psychotherapeutic support in people with major depressive disorder. The effect size of high dose psilocybin was similar with antidepressant trials of patients with major depressive disorder showing a mean standardised mean difference of 0.3. 7 8
Strengths and limitations of this study
This study has several strengths. We conducted separate analyses for placebo response in antidepressant trials, placebo response in psychedelic trials, and an extremely low active dose of psychedelics, thereby mitigating the effect of placebo response variations across different studies. This approach allowed us to assess the efficacy of psychedelics more impartially and make relatively unbiased comparisons than if these groups were not separated. This study supported the transitivity assumption of the efficacy of escitalopram in placebo controlled antidepressant trials with that in psilocybin versus escitalopram head-to-head trial, thereby bridging the escitalopram trials and psychedelic trials. We also performed various sensitivity analyses to ensure the validation of our statistical results.
Nevertheless, our study has several limitations. Firstly, we extracted only the acute effects of the interventions. A comparison of the long term effects of psychedelics and escitalopram remains unclear. Secondly, participants in the randomised controlled trials on MDMA were predominantly diagnosed with post-traumatic stress disorder, whereas participants in the randomised controlled trials on escitalopram were patients with major depressive disorder. However, depressive symptoms in post-traumatic stress disorder could be relatively treatment resistant, requiring high doses of psychotropic drugs. 41 Moreover, our study focused not only on major depressive disorder but also on the generalisability of psychedelic treatment for depressive symptoms. Thirdly, although all available studies were included, the sample size of the psychedelic randomised controlled trials was small (k=15). Fourthly, when using extremely low dose psychedelics as a reference group, the relative effect may also eliminate some pharmacological effects because our study found that extremely low dose psychedelics could not be considered a placebo. Fifthly, in network meta-analysis, direct evidence for one treatment comparison may serve as indirect evidence for other treatment comparisons, 42 and biases in the direct evidence might affect estimates of other treatment comparisons. Because the absolute effect of escitalopram in the head-to-head trial (high dose psilocybin v escitalopram 20 mg) 12 was lower than those of placebo controlled trials, the relative effects of high dose psilocybin might be slightly overestimated when compared with other treatments in the current study. We addressed this issue by use of a Bayesian network meta-analysis, distinguishing between placebo response in psychedelic trials and placebo response in antidepressant trials. Specifically, we only considered that the 95% credibile interval of the relative effect between two comparisons did not cross zero. Indeed, the relative effect of escitalopram 20 mg between these two study designs included zero. Finally, our network meta-analysis may not have sufficient statistical power to detect potential publication bias due to the scarcity of trials and participants.
Implications and conclusions
Serotonergic psychedelics, especially high dose psilocybin, appeared to have the potential to treat depressive symptoms. However, study designs may have overestimated the efficacy of psychedelics. Our analysis suggested that the standardised mean difference of high dose psilocybin was similar to that of current antidepressant drugs, showing a small effect size. Improved blinding methods and standardised psychotherapies can help researchers to better estimate the efficacy of psychedelics for depressive symptoms and other psychiatric conditions.
What is already known on this topic
Psychedelic treatment resulted in significant efficacy in treating depressive symptoms and alleviating distress related to life threatening diagnoses and terminal illness
Meta-analyses have reported standardised mean difference of psychedelics for depression reduction ranging from 1.37 to 3.12, while antidepressant trials were approximately 0.3
No network meta-analysis has examined comparative efficacy between psychedelics and antidepressants for depressive symptoms, and effect sizes of psychedelics might be overestimated because of unsuccessful blinding and response expectancies
What this study adds
To avoid estimation bias, placebo responses in psychedelic and antidepressant trials were separated; placebo response in psychedelic trials was lower than that in antidepressant trials
Among all psychedelics studied, only high dose psilocybin was associated with greater effectiveness than placebo response in antidepressant trials (standardised mean difference 0.31)
Among all psychedelics, only high dose psilocybin was associated with greater effectiveness than escitalopram
Ethics statements
Ethical approval.
Not required because this study is an analysis of aggregated identified clinical trial data.
Data availability statement
The data that support the findings of this study are available from the corresponding author (C-SL) upon reasonable request.
Contributors: T-WH and C-KT contributed equally to this work and are joint first authors. Y-KT and C-SL contributed equally to this work and are joint last/corresponding authors. C-SL, T-WH, and Y-KT conceived and designed the study. T-WH, C-KT, C-WH, and P-TT selected the articles, extracted the data, and assess the risk of bias. C-LY did the systemic search. T-WH and C-SL wrote the first draught of the manuscript. TT, AFC, Y-CK, F-CY, and Y-KT interpreted the data and contributed to the writing of the final version of the manuscript. C-KT and T-WH have accessed and verified the data. C-SL and Y-KT were responsible for the decision to submit the manuscript. All authors confirmed that they had full access to all the data in the study and accept responsibility to submit for publication. The corresponding author attests that all listed authors meet authorship criteria and that no others meeting the criteria have been omitted.
Funding: The study was supported by grant from the National Science and Technology Council (NSTC 112-2314-B-016−036-MY2 and NSTC 112-2314-B-002−210-MY3). The funding source had no role in any process of our study.
Competing interests: All authors have completed the ICMJE uniform disclosure form at www.icmje.org/disclosure-of-interest/ and declare: support from National Science and Technology Council for the submitted work; no financial relationships with any organisations that might have an interest in the submitted work in the previous three years; no other relationships or activities that could appear to have influenced the submitted work.
Transparency: The lead author (C-SL) affirms that the manuscript is an honest, accurate, and transparent account of the study being reported; that no important aspects of the study have been omitted; and that any discrepancies from the study as planned
Dissemination to participants and related patient and public communities: Dissemination of the work to the public and clinical community through social media and lectures is planned.
Provenance and peer review: Not commissioned; externally peer reviewed.
This is an Open Access article distributed in accordance with the terms of the Creative Commons Attribution (CC BY 4.0) license, which permits others to distribute, remix, adapt and build upon this work, for commercial use, provided the original work is properly cited. See: http://creativecommons.org/licenses/by/4.0/ .
- Tupper KW ,
- Cleare AJ ,
- Muttoni S ,
- Ardissino M ,
- Vargas MV ,
- Dunlap LE ,
- Moliner R ,
- Brunello CA ,
- Sawilowsky SS
- Cipriani A ,
- Furukawa TA ,
- Salanti G ,
- Muthukumaraswamy SD ,
- Forsyth A ,
- Nikolakopoulou A ,
- Chaimani A ,
- Carhart-Harris R ,
- Giribaldi B ,
- Raison CL ,
- Sanacora G ,
- Woolley J ,
- Caldwell DM ,
- Marcantoni WS ,
- Akoumba BS ,
- Thorlund K ,
- Walter SD ,
- Johnston BC ,
- Higgins JPT ,
- Chandler J ,
- Sterne JAC ,
- Savović J ,
- Hengartner MP ,
- Goodwin GM ,
- Aaronson ST ,
- Alvarez O ,
- Griffiths RR ,
- Johnson MW ,
- Carducci MA ,
- Barrett FS ,
- von Rotz R ,
- Schindowski EM ,
- Jungwirth J ,
- Mithoefer MC ,
- Mithoefer AT ,
- Feduccia AA ,
- Ot’alora G M ,
- Grigsby J ,
- Poulter B ,
- ↵ Phillippo DM. multinma: Bayesian network meta-analysis of individual and aggregate data. 2020.
- Bürkner P-C
- Del Giovane C ,
- Welton NJ ,
- Brignardello-Petersen R ,
- Alexander PE ,
- GRADE Working Group
- Izcovich A ,
- Mustafa RA ,
- Brignardello-Petersen R
- Guyatt GH ,
- Holstein D ,
- Dolder PC ,
- Palhano-Fontes F ,
- Barreto D ,
- Mitchell JM ,
- Bogenschutz M ,
- Lilienstein A ,
- Wagner MT ,
- Wolfson PE ,
- Andries J ,
Information
- Author Services
Initiatives
You are accessing a machine-readable page. In order to be human-readable, please install an RSS reader.
All articles published by MDPI are made immediately available worldwide under an open access license. No special permission is required to reuse all or part of the article published by MDPI, including figures and tables. For articles published under an open access Creative Common CC BY license, any part of the article may be reused without permission provided that the original article is clearly cited. For more information, please refer to https://www.mdpi.com/openaccess .
Feature papers represent the most advanced research with significant potential for high impact in the field. A Feature Paper should be a substantial original Article that involves several techniques or approaches, provides an outlook for future research directions and describes possible research applications.
Feature papers are submitted upon individual invitation or recommendation by the scientific editors and must receive positive feedback from the reviewers.
Editor’s Choice articles are based on recommendations by the scientific editors of MDPI journals from around the world. Editors select a small number of articles recently published in the journal that they believe will be particularly interesting to readers, or important in the respective research area. The aim is to provide a snapshot of some of the most exciting work published in the various research areas of the journal.
Original Submission Date Received: .
- Active Journals
- Find a Journal
- Proceedings Series
- For Authors
- For Reviewers
- For Editors
- For Librarians
- For Publishers
- For Societies
- For Conference Organizers
- Open Access Policy
- Institutional Open Access Program
- Special Issues Guidelines
- Editorial Process
- Research and Publication Ethics
- Article Processing Charges
- Testimonials
- Preprints.org
- SciProfiles
- Encyclopedia
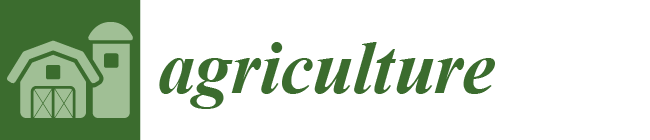
Article Menu
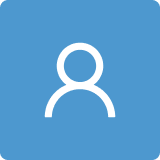
- Subscribe SciFeed
- Recommended Articles
- Google Scholar
- on Google Scholar
- Table of Contents
Find support for a specific problem in the support section of our website.
Please let us know what you think of our products and services.
Visit our dedicated information section to learn more about MDPI.
JSmol Viewer
Efficiency and driving factors of agricultural carbon emissions: a study in chinese state farms.
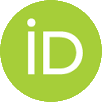
1. Introduction
- What is the current status of agricultural carbon emissions and carbon emission efficiency in Chinese state farms?
- How have the agricultural carbon emissions and the carbon emission efficiency evolved spatiotemporally?
- What are the key factors influencing carbon emissions in Chinese state farms, and how can these factors be decomposed to inform the development of low-carbon strategies?
2. Methodology
2.1. study area, 2.2. data and sample, 2.3. ipcc method, 2.4. super-sbm model and malmquist–luenberger index, 2.5. lmdi method, 3.1. temporal evolution of agricultural carbon emissions, 3.2. spatial evolution of agricultural carbon emissions, 3.3. the distribution of agricultural carbon emission efficiency, 3.4. influencing factors of agricultural carbon emission, 4. discussion, 5. conclusions, 6. limitations and future directions, author contributions, institutional review board statement, data availability statement, conflicts of interest.
- Chen, X.; Chen, Z.G. Can Green Finance Development Reduce Carbon Emissions? Empirical Evidence from 30 Chinese Provinces. Sustainability 2021 , 13 , 12137. [ Google Scholar ] [ CrossRef ]
- Wijerathna-Yapa, A.; Pathirana, R. Sustainable Agro-Food Systems for Addressing Climate Change and Food Security. Agriculture 2022 , 12 , 1554. [ Google Scholar ] [ CrossRef ]
- Vidican, R.; Malinas, A.; Ranta, O.; Moldovan, C.; Marian, O.; Ghete, A.; Ghise, C.R.; Popovici, F.; Catunescu, G.M. Using Remote Sensing Vegetation Indices for the Discrimination and Monitoring of Agricultural Crops: A Critical Review. Agronomy 2023 , 13 , 3040. [ Google Scholar ] [ CrossRef ]
- Kamyab, H.; Saberikamarposhti, M.; Hashim, H.; Yusuf, M. Carbon dynamics in agricultural greenhouse gas emissions and removals: A comprehensive review. Carbon Lett. 2024 , 34 , 265–289. [ Google Scholar ] [ CrossRef ]
- Wang, Y.; Guo, C.-H.; Chen, X.-J.; Jia, L.-Q.; Guo, X.-N.; Chen, R.-S.; Zhang, M.-S.; Chen, Z.-Y.; Wang, H.-D. Carbon peak and carbon neutrality in China: Goals, implementation path and prospects. China Geol. 2021 , 4 , 720–746. [ Google Scholar ] [ CrossRef ]
- Yang, G.; Xiang, X.; Deng, F.; Wang, F. Towards high-quality development: How does digital economy impact low-carbon inclusive development?: Mechanism and path. Environ. Sci. Pollut. Res. 2023 , 30 , 41700–41725. [ Google Scholar ] [ CrossRef ]
- Yang, L.; Guan, Z.Y.; Chen, S.Y.; He, Z.H. Re-measurement and influencing factors of agricultural eco-efficiency under the ‘dual carbon’ target in China. Heliyon 2024 , 10 , e24944. [ Google Scholar ] [ CrossRef ] [ PubMed ]
- Liu, Y.; Feng, C. What drives the decoupling between economic growth and energy-related CO<sub>2</sub> emissions in China’s agricultural sector? Environ. Sci. Pollut. Res. 2021 , 28 , 44165–44182. [ Google Scholar ] [ CrossRef ]
- Xiong, C.H.; Chen, S.; Xu, L.T. Driving factors analysis of agricultural carbon emissions based on extended STIRPAT model of Jiangsu Province, China. Growth Chang. 2020 , 51 , 1401–1416. [ Google Scholar ] [ CrossRef ]
- Liu, Z.W.; Balezentis, T.; Song, Y.Y.; Yang, G.L. Estimating Capacity Utilization of Chinese State Farms. Sustainability 2019 , 11 , 4894. [ Google Scholar ] [ CrossRef ]
- Chu, T.S.; Yu, L.; Wang, D.R.; Yang, Z.L. Carbon footprint of crop production in Heilongjiang land reclamation area, China. Int. J. Agric. Biol. Eng. 2022 , 15 , 182–191. [ Google Scholar ] [ CrossRef ]
- Liu, S.; Zhang, P.; Song, F.; Pan, X.; Wen, X. Measuring the Agricultural Modernization Level of Heilongjiang Reclamation Areas in China. Sci. Geogr. Sin. 2018 , 38 , 1051–1060. [ Google Scholar ]
- Gong, B.L. Agricultural reforms and production in China: Changes in provincial production function and productivity in 1978-2015. J. Dev. Econ. 2018 , 132 , 18–31. [ Google Scholar ] [ CrossRef ]
- Mattila, T.J.; Pakarinen, S.; Sokka, L. Quantifying the Total Environmental Impacts of an Industrial Symbiosis—A Comparison of Process-, Hybrid and Input-Output Life Cycle Assessment. Environ. Sci. Technol. 2010 , 44 , 4309–4314. [ Google Scholar ] [ CrossRef ]
- Turconi, R.; Tonini, D.; Nielsen, C.F.B.; Simonsen, C.G.; Astrup, T. Environmental impacts of future low-carbon electricity systems: Detailed life cycle assessment of a Danish case study. Appl. Energy 2014 , 132 , 66–73. [ Google Scholar ] [ CrossRef ]
- Wang, H.; Yang, Y.; Zhang, X.; Tian, G. Carbon Footprint Analysis for Mechanization of Maize Production Based on Life Cycle Assessment: A Case Study in Jilin Province, China. Sustainability 2015 , 7 , 15772–15784. [ Google Scholar ] [ CrossRef ]
- Benjaafar, S.; Li, Y.Z.; Daskin, M. Carbon Footprint and the Management of Supply Chains: Insights From Simple Models. Ieee Trans. Autom. Sci. Eng. 2013 , 10 , 99–116. [ Google Scholar ] [ CrossRef ]
- Song, R.; Liu, J.; Niu, K.; Feng, Y. Comparative Analysis of Trade’s Impact on Agricultural Carbon Emissions in China and the United States. Agriculture 2023 , 13 , 1967. [ Google Scholar ] [ CrossRef ]
- Gusmao Caiado, R.G.; Dias, R.d.F.; Mattos, L.V.; Goncalves Quelhas, O.L.; Leal Filho, W. Towards sustainable development through the perspective of eco-efficiency—A systematic literature review. J. Clean. Prod. 2017 , 165 , 890–904. [ Google Scholar ] [ CrossRef ]
- Wang, R.; Feng, Y. Research on China’s agricultural carbon emission efficiency evaluation and regional differentiation based on DEA and Theil models. Int. J. Environ. Sci. Technol. 2021 , 18 , 1453–1464. [ Google Scholar ] [ CrossRef ]
- Shang, J.; Ji, X.; Shi, R.; Zhu, M. Structure and driving factors of spatial correlation network of agricultural carbon emission efficiency in China. Chin. J. Eco-Agric. 2022 , 30 , 543–557. [ Google Scholar ]
- Zhang, X.; Zhou, X.; Liao, K. Regional differences and dynamic evolution of China’s agricultural carbon emission efficiency. Int. J. Environ. Sci. Technol. 2023 , 20 , 4307–4324. [ Google Scholar ] [ CrossRef ]
- Zhang, H.; Guo, S.D.; Qian, Y.B.; Liu, Y.; Lu, C.P. Dynamic analysis of agricultural carbon emissions efficiency in Chinese provinces along the Belt and Road. PLoS ONE 2020 , 15 , e0228223. [ Google Scholar ] [ CrossRef ]
- Guo, X.; Yang, J.; Shen, Y.; Zhang, X. Prediction of agricultural carbon emissions in China based on a GA-ELM model. Front. Energy Res. 2023 , 11 , 1245820. [ Google Scholar ] [ CrossRef ]
- Yasmeen, R.; Tao, R.; Shah, W.U.H.; Padda, I.U.H.; Tang, C. The nexuses between carbon emissions, agriculture production efficiency, research and development, and government effectiveness: Evidence from major agriculture-producing countries. Environ. Sci. Pollut. Res. 2022 , 29 , 52133–52146. [ Google Scholar ] [ CrossRef ]
- Zhu, Y.; Huo, C. The Impact of Agricultural Production Efficiency on Agricultural Carbon Emissions in China. Energies 2022 , 15 , 4464. [ Google Scholar ] [ CrossRef ]
- Yang, Y.; Tian, Y.; Peng, X.; Yin, M.; Wang, W.; Yang, H. Research on Environmental Governance, Local Government Competition, and Agricultural Carbon Emissions under the Goal of Carbon Peak. Agriculture 2022 , 12 , 1703. [ Google Scholar ] [ CrossRef ]
- Chen, Y.; Li, M.; Su, K.; Li, X. Spatial-Temporal Characteristics of the Driving Factors of Agricultural Carbon Emissions: Empirical Evidence from Fujian, China. Energies 2019 , 12 , 3102. [ Google Scholar ] [ CrossRef ]
- Shi, H.; Chang, M. How does agricultural industrial structure upgrading affect agricultural carbon emissions? Threshold effects analysis for China. Environ. Sci. Pollut. Res. 2023 , 30 , 52943–52957. [ Google Scholar ] [ CrossRef ]
- Liu, X.; Ye, Y.; Ge, D.; Wang, Z.; Liu, B. Study on the Evolution and Trends of Agricultural Carbon Emission Intensity and Agricultural Economic Development Levels-Evidence from Jiangxi Province. Sustainability 2022 , 14 , 14265. [ Google Scholar ] [ CrossRef ]
- Han, H.; Zhong, Z.; Guo, Y.; Xi, F.; Liu, S. Coupling and decoupling effects of agricultural carbon emissions in China and their driving factors. Environ. Sci. Pollut. Res. 2018 , 25 , 25280–25293. [ Google Scholar ] [ CrossRef ]
- Yao, B.; Zheng, Y.; Hu, D.; Nie, L.; Fu, S.; Hu, Q. Spatial and Temporal Variations of County Based Agricultural Carbon Emissions and Associated Effect Factors in JiangxiI Province. Resour. Environ. Yangtze Basin 2014 , 23 , 311–318. [ Google Scholar ]
- Zhang, Y.; Zou, X.J.; Xu, C.F.; Yang, Q.S. Decoupling Greenhouse Gas Emissions from Crop Production: A Case Study in the Heilongjiang Land Reclamation Area, China. Energies 2018 , 11 , 1480. [ Google Scholar ] [ CrossRef ]
- Zhang, F.S.; Wang, H.Y.; Zhao, X.Y.; Jiang, Q.S. Investigation on Zoning Management of Saline Soil in Cotton Fields in Alar Reclamation Area, Xinjiang. Agriculture 2024 , 14 , 3. [ Google Scholar ] [ CrossRef ]
- West, T.O.; Marland, G. Net carbon flux from agricultural ecosystems: Methodology for full carbon cycle analyses. Environ. Pollut. 2002 , 116 , 439–444. [ Google Scholar ] [ CrossRef ] [ PubMed ]
- Zhang, L.; Cai, C.Z. Innovative measurement, trade-off-synergy relationship and influencing factors for agricultural net carbon emissions and effective supply of agricultural products in China. Heliyon 2024 , 10 , e24621. [ Google Scholar ] [ CrossRef ]
- O’Brien, D.; Shalloo, L.; Crosson, P.; Donnellan, T.; Farrelly, N.; Finnan, J.; Hanrahan, K.; Lalor, S.; Lanigan, G.; Thorne, F.; et al. An evaluation of the effect of greenhouse gas accounting methods on a marginal abatement cost curve for Irish agricultural greenhouse gas emissions. Environ. Sci. Policy 2014 , 39 , 107–118. [ Google Scholar ] [ CrossRef ]
- Rathnayake, H.; Mizunoya, T. A study on GHG emission assessment in agricultural areas in Sri Lanka: The case of Mahaweli H agricultural region. Environ. Sci. Pollut. Res. 2023 , 30 , 88180–88196. [ Google Scholar ] [ CrossRef ]
- Yuan, Y.; Dai, X.; Wang, H.; Xu, M.; Fu, X.; Yang, F. Effects of Land-Use Conversion from Double Rice Cropping to Vegetables on Methane and Nitrous Oxide Fluxes in Southern China. PLoS ONE 2016 , 11 , e0155926. [ Google Scholar ] [ CrossRef ]
- Tian, Y.; Zhang, J.B.; He, Y.Y. Research on Spatial-Temporal Characteristics and Driving Factor of Agricultural Carbon Emissions in China. J. Integr. Agric. 2014 , 13 , 1393–1403. [ Google Scholar ] [ CrossRef ]
- Charnes, A.; Cooper, W.W.; Rhodes, E. MEASURING EFFICIENCY OF DECISION-MAKING UNITS. Eur. J. Oper. Res. 1978 , 2 , 429–444. [ Google Scholar ] [ CrossRef ]
- Aslam, M.S.; Pan, H.X.; Sohail, S.; Majeed, M.T.; Rahman, S.U.; Anees, S.A. Assessment of major food crops production-based environmental efficiency in China, India, and Pakistan. Environ. Sci. Pollut. Res. 2022 , 29 , 10091–10100. [ Google Scholar ] [ CrossRef ] [ PubMed ]
- Lu, C.C.; Lin, I.F.; Lin, T.Y.; Chiu, Y.H. Two-stage dynamic data envelopment analysis measuring the overall efficiency and productivity changes of industry and agriculture in EU countries. J. Clean. Prod. 2023 , 382 , 135332. [ Google Scholar ] [ CrossRef ]
- Wang, Z.; Liu, B.; Wang, L.; Shao, Q. Measurement and temporal & spatial variation of urban eco-efficiency in the Yellow River Basin. Phys. Chem. Earth 2021 , 122 , 102981. [ Google Scholar ] [ CrossRef ]
- Tone, K. A slacks-based measure of efficiency in data envelopment analysis. Eur. J. Oper. Res. 2001 , 130 , 498–509. [ Google Scholar ] [ CrossRef ]
- Tone, K. A slacks-based measure of super-efficiency in data envelopment analysis. Eur. J. Oper. Res. 2002 , 143 , 32–41. [ Google Scholar ] [ CrossRef ]
- Chung, Y.H.; Fare, R.; Grosskopf, S. Productivity and undesirable outputs: A directional distance function approach. J. Environ. Manag. 1997 , 51 , 229–240. [ Google Scholar ] [ CrossRef ]
- Liu, H.M.; Wen, S.B.; Zhang, Z. Agricultural production agglomeration and total factor carbon productivity: Based on NDDF-MML index analysis. China Agric. Econ. Rev. 2022 , 14 , 709–740. [ Google Scholar ] [ CrossRef ]
- Chen, X.; Shuai, C.Y.; Wu, Y.; Zhang, Y. Analysis on the carbon emission peaks of China’s industrial, building, transport, and agricultural sectors. Sci. Total Environ. 2020 , 709 , 135768. [ Google Scholar ] [ CrossRef ]
- Hossain, M.A.; Chen, S. The decoupling study of agricultural energy-driven CO 2 emissions from agricultural sector development. Int. J. Environ. Sci. Technol. 2022 , 19 , 4509–4524. [ Google Scholar ] [ CrossRef ]
- Moon, H.; Kihoon, L. An Analysis on the Change of Energy Efficiency in Korean Manufacturing Industry. Asia-Pac. J. Bus. Commer. 2020 , 12 , 74–101. [ Google Scholar ] [ CrossRef ]
- Huang, X.Q.; Xu, X.C.; Wang, Q.Q.; Zhang, L.; Gao, X.; Chen, L.H. Assessment of Agricultural Carbon Emissions and Their Spatiotemporal Changes in China, 1997–2016. Int. J. Environ. Res. Public Health 2019 , 16 , 3105. [ Google Scholar ] [ CrossRef ]
- Wang, X.; Meng, Y.; Shan, Y.; Gong, Y. Carbon Emissions and Influencing Factors of FarmersAgricultural Production—Taking Some Areas in Hubei Province as Examples. Bull. Soil Water Conserv. 2020 , 40 , 160–167, 174. [ Google Scholar ]
- Song, S.; Zhao, S.; Zhang, Y.; Ma, Y. Carbon Emissions from Agricultural Inputs in China over the Past Three Decades. Agriculture 2023 , 13 , 919. [ Google Scholar ] [ CrossRef ]
- Wang, G.F.; Liao, M.L.; Jiang, J. Research on Agricultural Carbon Emissions and Regional Carbon Emissions Reduction Strategies in China. Sustainability 2020 , 12 , 2627. [ Google Scholar ] [ CrossRef ]
- Wang, C.; Gao, Q.; Wang, X.; Yu, M. Spatially differentiated trends in urbanization, agricultural land abandonment and reclamation, and woodland recovery in Northern China. Sci. Rep. 2016 , 6 , 37658. [ Google Scholar ] [ CrossRef ]
- Wang, X.H.; Zhang, Y.L. Carbon Footprint of the Agricultural Sector in Qinghai Province, China. Appl. Sci. 2019 , 9 , 2047. [ Google Scholar ] [ CrossRef ]
- Guo, Z.D.; Zhang, X.N. Carbon reduction effect of agricultural green production technology: A new evidence from China. Sci. Total Environ. 2023 , 874 , 162483. [ Google Scholar ] [ CrossRef ]
- Yang, Q.F.; Zhang, P.Y.; Li, Y.X.; Ning, J.C.; Chu, N.C. Does the Policy of Decoupled Subsidies Improve the Agricultural Economic Resilience?-Evidence from China’s Main Corn Producing Regions. Sustainability 2023 , 15 , 10164. [ Google Scholar ] [ CrossRef ]
- Xia, Y.J.; Guo, H.P.; Xu, S.; Pan, C.L. Environmental regulations and agricultural carbon emissions efficiency: Evidence from rural China. Heliyon 2024 , 10 , e25677. [ Google Scholar ] [ CrossRef ]
- Cheng, C.M.; Li, J.Q.; Qiu, Y.Q.; Gao, C.F.; Gao, Q. Evaluating the Spatiotemporal Characteristics of Agricultural Eco-Efficiency Alongside China’s Carbon Neutrality Targets. Int. J. Environ. Res. Public Health 2022 , 19 , 15478. [ Google Scholar ] [ CrossRef ]
- Zhang, M.; Liu, X.; Peng, S.G. Effects of urban land intensive use on carbon emissions in China: Spatial interaction and multi-mediating effect perspective. Environ. Sci. Pollut. Res. 2023 , 30 , 7270–7287. [ Google Scholar ] [ CrossRef ] [ PubMed ]
- Sui, J.L.; Lv, W.Q. Crop Production and Agricultural Carbon Emissions: Relationship Diagnosis and Decomposition Analysis. Int. J. Environ. Res. Public Health 2021 , 18 , 8219. [ Google Scholar ] [ CrossRef ]
- Xiong, C.H.; Yang, D.G.; Huo, J.W.; Zhao, Y.N. The Relationship between Agricultural Carbon Emissions and Agricultural Economic Growth and Policy Recommendations of a Low-carbon Agriculture Economy. Pol. J. Environ. Stud. 2016 , 25 , 2187–2195. [ Google Scholar ] [ CrossRef ] [ PubMed ]
- Zhao, W.; Wang, X.; Chen, M.Z.; Liu, X.J.; Zhao, L.; Zhang, X.L. Forest Resource Assets Departure Audit Considering Ecological Sustainable Development: A Case Study. Land 2022 , 11 , 2156. [ Google Scholar ] [ CrossRef ]
- Zhao, J.C.; Du, Y.M.; Duan, K.F. Has industrial structure upgrading reduced agricultural carbon emissions? An empirical analysis from China. Appl. Econ. Lett. 2024 , 1–5. [ Google Scholar ] [ CrossRef ]
- Huang, X.B.; Gao, S.Q. Temporal characteristics and influencing factors of agricultural carbon emission in Jiangxi province of China. Environ. Res. Commun. 2022 , 4 , 045006. [ Google Scholar ] [ CrossRef ]
- Yang, J.Q.; Luo, P.Z.; Li, L.P. Driving factors and decoupling trend analysis between agricultural CO 2 emissions and economic development in China based on LMDI and Tapio decoupling. Math. Biosci. Eng. 2022 , 19 , 13093–13113. [ Google Scholar ] [ CrossRef ] [ PubMed ]
- Hao, Y.; Zhang, Z.Y.; Yang, C.X.; Wu, H.T. Does structural labor change affect CO 2 emissions? Theoretical and empirical evidence from China. Technol. Forecast. Soc. Chang. 2021 , 171 , 120936. [ Google Scholar ] [ CrossRef ]
- Li, N.; Wei, C.D.; Zhang, H.; Cai, C.F.; Song, M.W.; Miao, J. Drivers of the national and regional crop production-derived greenhouse gas emissions in China. J. Clean. Prod. 2020 , 257 , 120503. [ Google Scholar ] [ CrossRef ]
Click here to enlarge figure
State Farms | 2010 | 2012 | 2014 | 2016 | 2018 | 2020 | 2022 |
---|---|---|---|---|---|---|---|
Heilongjiang | 2,800,938 | 2,879,660 | 2,892,305 | 2,908,927 | 2,956,354 | 2,972,109 | 3,121,274 |
Xinjiang | 1,470,835 | 1,512,900 | 1,555,603 | 1,605,949 | 1,630,039 | 1,658,270 | 1,978,867 |
Inner Mongolia | 648,530 | 654,136 | 660,308 | 669,128 | 694,576 | 748,202 | 756,670 |
Liaoning | 146,551 | 154,932 | 154,835 | 157,661 | 158,165 | 161,369 | 161,905 |
Hubei | 136,640 | 137,617 | 135,880 | 131,993 | 142,551 | 143,851 | 144,109 |
Jilin | 114,346 | 117,478 | 123,752 | 130,138 | 103,739 | 108,780 | 109,590 |
Hebei | 89,095 | 92,820 | 98,017 | 96,726 | 95,797 | 98,236 | 101,001 |
Jiangxi | 52,116 | 52,580 | 83,154 | 83,360 | 84,384 | 79,462 | 83,877 |
Hunan | 67,271 | 67,216 | 67,146 | 67,141 | 76,187 | 77,860 | 79,137 |
Jiangsu | 73,112 | 71,428 | 71,084 | 65,526 | 64,577 | 64,053 | 64,044 |
Gansu | 56,952 | 59,270 | 65,202 | 67,288 | 68,921 | 70,594 | 72,415 |
Qinghai | 26,220 | 26,492 | 25,866 | 152,267 | 38,063 | 31,762 | 29,237 |
Ningxia | 39,708 | 39,613 | 42,346 | 43,283 | 41,201 | 43,231 | 48,443 |
Guangdong | 37,500 | 37,836 | 37,921 | 37,866 | 38,052 | 46,460 | 54,133 |
Hainan | 39,178 | 37,568 | 34,201 | 35,798 | 35,492 | 34,266 | 34,576 |
Guangxi | 32,647 | 32,805 | 33,846 | 33,959 | 33,624 | 34,660 | 35,613 |
Shanghai | 29,450 | 29,447 | 35,763 | 35,763 | 38,238 | 38,101 | 29,497 |
Anhui | 34,746 | 29,356 | 30,104 | 30,167 | 30,215 | 29,291 | 32,925 |
Henan | 26,843 | 27,605 | 30,009 | 29,623 | 28,084 | 21,874 | 21,772 |
Yunnan | 12,709 | 12,194 | 12,380 | 13,122 | 12,014 | 13,539 | 13,368 |
Shandong | 10,165 | 12,290 | 14,613 | 14,022 | 12,814 | 5,669 | 5,849 |
Shaanxi | 9081 | 8948 | 9217 | 9559 | 10,844 | 11,179 | 11,197 |
Fujian | 11,101 | 10,957 | 10,799 | 9359 | 8347 | 7079 | 6693 |
Shanxi | 6440 | 6683 | 6752 | 6588 | 6485 | 6405 | 6275 |
Zhejiang | 4102 | 4070 | 4045 | 3787 | 4260 | 3416 | 3539 |
Tianjin | 3191 | 2760 | 2612 | 2917 | 2727 | 2739 | 2289 |
Guizhou | 2014 | 1722 | 1701 | 1763 | 1145 | 1023 | 2800 |
Beijing | 1483 | 1454 | 1434 | 1439 | 1396 | 1410 | 1278 |
Sichuan | 849 | 899 | 898 | 873 | 850 | 1040 | 1054 |
Total | 5,983,813 | 6,122,736 | 6,241,793 | 6,445,992 | 6,419,139 | 6,515,930 | 6,613,427 |
Carbon Source | Measurement | Coefficient |
---|---|---|
Chemical fertilizer (kg) | Chemical fertilizer usage | 0.8956 kg (CO eq)·kg |
Pesticide (kg) | Pesticide usage | 4.9341 kg (CO eq)·kg |
Agricultural film (kg) | Agricultural plastic film usage | 5.18 kg (CO eq)·kg |
Diesel fuel (kg) | Agricultural diesel consumption | 0.5927 kg (CO eq)·kg |
Land tillage (hm ) | Crop sown area | 312.6 kg (CO eq)·hm |
Irrigation (hm ) | Effective irrigated area | 266.48 kg (CO eq)·hm |
Rice planting (hm ) | CH emissions from rice | 338 kg (CH eq)·hm |
Layer | Indicator | Measurement | Unit |
---|---|---|---|
Input | Land | Cropland area | hm |
Machine | Total power of agricultural machinery | kW | |
Labor | Quantity of agricultural employees | persons | |
Chemical fertilizer | Total fertilizer application | tons | |
Pesticide | Total pesticide application | tons | |
Agricultural film | Total film application | tons | |
Desirable output | Economic output | Total agricultural output | CNY |
Ecological output | Agricultural carbon sequestration | tons | |
Undesirable output | Environmental cost | Agricultural carbon emissions | tons |
State Farms | 2010 | 2012 | 2014 | 2016 | 2018 | 2020 | 2022 |
---|---|---|---|---|---|---|---|
Heilongjiang | 12,758.25 | 15,194.59 | 14,849.48 | 14,645.47 | 15,288.60 | 15,010.13 | 14,921.04 |
Xinjiang | 1948.78 | 2032.07 | 2479.68 | 2381.51 | 2806.80 | 2706.74 | 2764.25 |
Liaoning | 1000.03 | 973.47 | 1019.56 | 1044.85 | 946.56 | 967.26 | 952.73 |
Jiangxi | 721.95 | 730.44 | 958.27 | 950.66 | 928.62 | 963.60 | 947.54 |
Hunan | 867.19 | 886.81 | 892.27 | 902.77 | 688.69 | 745.35 | 789.21 |
Hubei | 657.17 | 688.33 | 762.55 | 778.93 | 788.39 | 739.80 | 730.73 |
Jiangsu | 627.61 | 629.58 | 675.23 | 759.32 | 775.73 | 739.19 | 706.34 |
Inner Mongolia | 387.74 | 412.08 | 457.20 | 493.05 | 508.22 | 514.22 | 519.72 |
Jilin | 396.15 | 444.12 | 471.63 | 456.52 | 327.33 | 355.21 | 343.54 |
Hainan | 373.07 | 456.71 | 335.74 | 401.50 | 367.51 | 214.71 | 198.08 |
Hebei | 233.17 | 260.88 | 269.76 | 305.03 | 389.52 | 323.47 | 301.67 |
Shanghai | 268.25 | 266.80 | 274.98 | 259.04 | 262.81 | 263.34 | 219.94 |
Anhui | 198.03 | 153.95 | 155.60 | 168.38 | 191.09 | 194.09 | 194.96 |
Ningxia | 162.20 | 170.04 | 165.50 | 153.97 | 157.22 | 150.32 | 147.22 |
Guangdong | 141.13 | 150.35 | 155.23 | 151.93 | 133.59 | 138.77 | 135.30 |
Yunnan | 119.32 | 101.26 | 109.70 | 119.78 | 68.08 | 80.51 | 75.16 |
Guangxi | 86.29 | 91.08 | 94.12 | 99.20 | 93.81 | 80.25 | 147.13 |
Fujian | 125.60 | 113.38 | 109.59 | 97.59 | 66.34 | 59.07 | 54.72 |
Gansu | 62.99 | 78.42 | 96.71 | 93.89 | 92.94 | 90.28 | 111.58 |
Henan | 53.45 | 48.30 | 59.38 | 57.43 | 53.67 | 42.79 | 42.82 |
Shandong | 19.56 | 22.33 | 59.68 | 48.46 | 67.26 | 11.21 | 11.31 |
Qinghai | 18.21 | 10.64 | 11.72 | 50.23 | 23.48 | 22.97 | 22.15 |
Shaanxi | 14.75 | 17.10 | 17.20 | 19.65 | 15.09 | 13.76 | 17.07 |
Zhejiang | 25.19 | 20.06 | 16.01 | 13.58 | 11.41 | 7.03 | 11.38 |
Tianjin | 9.36 | 9.38 | 10.42 | 10.35 | 9.68 | 18.96 | 18.36 |
Shanxi | 7.38 | 7.76 | 8.60 | 8.47 | 7.61 | 12.01 | 11.71 |
Guizhou | 7.58 | 7.82 | 7.97 | 8.64 | 2.23 | 3.64 | 3.67 |
Beijing | 4.69 | 4.72 | 3.73 | 4.74 | 4.88 | 3.41 | 15.45 |
Sichuan | 5.68 | 2.59 | 2.33 | 1.25 | 0.91 | 1.42 | 0.76 |
Total | 21,300.78 | 23,985.06 | 24,529.84 | 24,486.19 | 25,078.04 | 24,473.51 | 24,415.54 |
State Farms | 2010 | 2012 | 2014 | 2016 | 2018 | 2020 | 2022 | Mean |
---|---|---|---|---|---|---|---|---|
Heilongjiang | 1.92 | 2.08 | 2.15 | 2.34 | 2.15 | 1.94 | 1.94 | 2.12 |
Xinjiang | 1.41 | 1.28 | 1.24 | 1.89 | 1.18 | 1.55 | 1.74 | 1.45 |
Guangxi | 1.43 | 1.38 | 1.40 | 1.41 | 1.13 | 1.37 | 1.08 | 1.29 |
Shanghai | 1.18 | 1.17 | 1.23 | 1.20 | 1.33 | 1.24 | 1.51 | 1.28 |
Jiangsu | 0.91 | 0.86 | 1.18 | 1.40 | 1.50 | 1.55 | 1.32 | 1.25 |
Tianjin | 1.26 | 1.44 | 1.21 | 1.22 | 1.24 | 1.01 | 0.58 | 1.20 |
Qinghai | 1.19 | 1.14 | 1.00 | 1.20 | 1.04 | 1.13 | 1.16 | 1.14 |
Ningxia | 1.18 | 1.05 | 1.07 | 1.19 | 1.08 | 1.14 | 1.17 | 1.14 |
Guangdong | 1.04 | 1.10 | 0.61 | 1.16 | 1.21 | 0.74 | 1.29 | 1.07 |
Beijing | 0.70 | 1.36 | 1.35 | 1.12 | 1.01 | 1.17 | 1.34 | 1.06 |
Hainan | 1.02 | 0.41 | 0.72 | 1.29 | 1.34 | 1.05 | 1.25 | 1.05 |
Shaanxi | 0.77 | 0.56 | 1.10 | 1.17 | 1.28 | 1.27 | 1.21 | 1.04 |
Jilin | 1.09 | 0.56 | 1.31 | 1.28 | 0.88 | 0.58 | 1.24 | 0.94 |
Fujian | 1.05 | 1.09 | 0.28 | 1.12 | 0.64 | 1.03 | 1.16 | 0.90 |
Sichuan | 1.04 | 1.22 | 0.30 | 1.13 | 1.35 | 0.70 | 0.39 | 0.86 |
Inner Mongolia | 1.13 | 1.13 | 1.07 | 0.73 | 0.52 | 0.45 | 0.62 | 0.82 |
Zhejiang | 1.05 | 0.24 | 0.28 | 1.14 | 1.08 | 0.25 | 1.01 | 0.77 |
Guizhou | 0.36 | 0.17 | 0.22 | 1.07 | 1.32 | 1.19 | 1.30 | 0.75 |
Anhui | 1.10 | 0.61 | 0.45 | 0.50 | 1.08 | 0.60 | 0.74 | 0.75 |
Liaoning | 0.87 | 0.69 | 0.72 | 0.73 | 0.47 | 0.46 | 0.43 | 0.68 |
Shandong | 0.60 | 0.59 | 0.44 | 0.65 | 0.61 | 0.59 | 1.13 | 0.64 |
Gansu | 0.81 | 0.57 | 0.44 | 0.49 | 0.43 | 0.39 | 1.01 | 0.63 |
Yunnan | 0.62 | 0.35 | 0.40 | 0.58 | 0.49 | 0.34 | 1.04 | 0.62 |
Shanxi | 0.48 | 1.04 | 1.07 | 0.40 | 1.02 | 0.18 | 0.17 | 0.58 |
Hunan | 0.34 | 0.31 | 0.28 | 0.31 | 0.53 | 0.50 | 1.17 | 0.57 |
Hebei | 0.59 | 0.62 | 0.51 | 0.68 | 0.44 | 0.38 | 0.50 | 0.54 |
Henan | 0.55 | 0.63 | 0.42 | 1.02 | 0.47 | 0.30 | 0.38 | 0.53 |
Hubei | 0.66 | 0.64 | 0.34 | 0.69 | 0.39 | 0.31 | 0.41 | 0.51 |
Jiangxi | 0.54 | 0.45 | 0.32 | 0.47 | 0.41 | 0.31 | 0.34 | 0.45 |
Mean | 0.93 | 0.85 | 0.80 | 1.02 | 0.95 | 0.82 | 0.99 | 0.92 |
Year | Agricultural Production Efficiency (∆BI) | Agricultural Industry Structure (∆AI) | Agricultural Economic Development Level (∆EI) | Agricultural Labor Scale (∆P) | Total Effect (∆CE) |
---|---|---|---|---|---|
2010–2011 | −1708.90 | −428.51 | 4249.42 | −248.60 | 1863.35 |
2011–2012 | 6179.10 | −7710.64 | 2830.68 | −478.32 | 820.92 |
2012–2013 | −10,917.83 | 9740.51 | −1258.81 | 3188.85 | 752.66 |
2013–2014 | −840.74 | 217.21 | 5175.89 | −4760.28 | −207.88 |
2014–2015 | −1204.14 | 603.41 | 222.12 | 14.78 | −363.79 |
2015–2016 | 165.63 | 114.01 | −105.41 | 145.98 | 320.14 |
2016–2017 | −416.05 | −1275.95 | 2041.20 | 550.52 | 899.84 |
2017–2018 | −632.46 | 422.95 | 8027.58 | −8126.07 | −307.99 |
2018–2019 | 465.34 | −1125.34 | 755.11 | −500.56 | −405.49 |
2019–2020 | −2934.64 | 549.55 | 2300.12 | −54.38 | −139.45 |
2020–2021 | −2600.58 | 563.20 | 1946.44 | 317.69 | 226.76 |
2021–2022 | −167.46 | −907.73 | 1363.31 | −632.44 | −344.33 |
Cumulative effect | −14,612.73 | 762.66 | 27,547.63 | −10,582.82 | 3114.76 |
Contribution rate/% | −469.15 | 24.49 | 884.42 | −339.76 | 100 |
The statements, opinions and data contained in all publications are solely those of the individual author(s) and contributor(s) and not of MDPI and/or the editor(s). MDPI and/or the editor(s) disclaim responsibility for any injury to people or property resulting from any ideas, methods, instructions or products referred to in the content. |
Share and Cite
Han, G.; Xu, J.; Zhang, X.; Pan, X. Efficiency and Driving Factors of Agricultural Carbon Emissions: A Study in Chinese State Farms. Agriculture 2024 , 14 , 1454. https://doi.org/10.3390/agriculture14091454
Han G, Xu J, Zhang X, Pan X. Efficiency and Driving Factors of Agricultural Carbon Emissions: A Study in Chinese State Farms. Agriculture . 2024; 14(9):1454. https://doi.org/10.3390/agriculture14091454
Han, Guanghe, Jiahui Xu, Xin Zhang, and Xin Pan. 2024. "Efficiency and Driving Factors of Agricultural Carbon Emissions: A Study in Chinese State Farms" Agriculture 14, no. 9: 1454. https://doi.org/10.3390/agriculture14091454
Article Metrics
Article access statistics, further information, mdpi initiatives, follow mdpi.
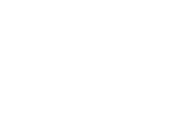
Subscribe to receive issue release notifications and newsletters from MDPI journals

COMMENTS
A comparative study is a kind of method that analyzes phenomena and then put them together. to find the points of differentiation and similarity (MokhtarianPour, 2016). A comparative perspective ...
Definition Comparative research, simply put, is the act of comparing two or more things with a view to discovering something about one or all of the things being compared. This technique often utilizes multiple disciplines in one study.
Comparative studies are based on research techniques and strategies for drawing inferences about causation and/or association of factors that are similar or different between two or more subjects/objects. In general, all investigations of cause-effect and/or of association between variables are, by nature, comparative studies.
Comparative research in communication and media studies is conventionally understood as the contrast among different macro-level units, such as world regions, countries, sub-national regions, social milieus, language areas and cultural thickenings, at one point or more points in time.
For comparative studies, the design options are experimental versus observational and prospective versus retrospective. The quality of eHealth comparative studies depends on such aspects of methodological design as the choice of variables, sample size, sources of bias, confounders, and adherence to quality and reporting guidelines.
Definition. The goal of comparative analysis is to search for similarity and variance among units of analysis. Comparative research commonly involves the description and explanation of similarities and differences of conditions or outcomes among large-scale social units, usually regions, nations, societies, and cultures.
Comparison as a scientific research method. Comparative research represents one approach in the spectrum of scientific research methods and in some ways is a hybrid of other methods, drawing on aspects of both experimental science (see our Experimentation in Science module) and descriptive research (see our Description in Science module ...
A comparative design involves studying variation by comparing a limited number of cases without using statistical probability analyses. Such designs are particularly useful for knowledge development when we lack the conditions for control through variable-centred, quasi-experimentaldesigns. Comparative designs often combine different research ...
This chapter examines the 'art of comparing' by showing how to relate a theoretically guided research question to a properly founded research answer by developing an adequate research design. It first considers the role of variables in comparative research, before discussing the meaning of 'cases' and case selection.
Comparative effectiveness research (CER) seeks to assist consumers, clinicians, purchasers, and policy makers to make informed decisions to improve health care at both the individual and population levels. CER includes evidence generation and evidence synthesis. Randomized controlled trials are central to CER because of the lack of selection ...
Comparative communication research is a combination of substance (specific objects of investigation studied in diferent macro-level contexts) and method (identification of diferences and similarities following established rules and using equivalent concepts).
A Comparative Study involves analyzing the dynamics of societies across different scales and environmental conditions to understand their emergence and functioning. The aim is to identify a common level of abstraction for describing and comparing these dynamics systematically. AI generated definition based on: Current Opinion in Environmental ...
Comparative research methods have long been used in cross-cultural studies to identify, analyse and explain similarities and differences across societies. Whatever the methods used, research that crosses national boundaries increasingly takes account of socio-cultural settings. Problems arise in managing and funding cross-national projects, in ...
A comparative study is an adequate research methodology to determine and quantify the relationships between two or more variables, observing different groups that, by choice or circumstance, are ...
Comparative method or quasi-experimental---a method used to describe similarities and differences in variables in two or more groups in a natural setting, that is, it resembles an experiment as it uses manipulation but lacks random assignment of individual subjects.
com-, it is a systematic comparison). Comparative studies are investigations to analyze and evaluate, with quantitative and qualitative methods, a phe- nomenon and/or facts among different areas ...
Comparative research essentially compares two groups in an attempt to draw a conclusion about them. Researchers attempt to identify and analyze similarities and differences between groups, and these studies are most often cross-national, comparing two separate people groups.
A third category of comparative study involved a comparison to some form of externally normed results, such as populations taking state, national, or international tests or prior research assessment from a published study or studies.
Approaches and methods in comparative education are of obvious importance, but do not always receive adequate attention. This second edition of a well-received book, containing thoroughly updated and additional material, contributes new insights within the longstanding traditions of the field.
Causal-comparative research is a method used to identify the cause-effect relationship between a dependent and independent variable. Understand causal-comparative research from Harappa to determine the consequences or causes of differences already existing between groups of people.
In the United States, comparative policy research is commonly mistaken for international policy research. The confusion may stem from the fact that early comparativists were among the few to be looking at policies abroad, either as a source for inspiration and lesson drawing or, equally widespread, as self-proclaimed proof that there was little to learn from other countries.
Introduction While there is an interest in defining longitudinal change in people with chronic illness like Parkinson's disease (PD), statistical analysis of longitudinal data is not straightforward for clinical researchers. Here, we aim to demonstrate how the choice of statistical method may influence research outcomes, (e.g., progression in apathy), specifically the size of longitudinal ...
This chapter highlights the particular advantages of comparative international approaches for media policy research. It starts by explaining the basic logic and main objectives of comparative analysis. Further sections of the chapter are devoted to different design...
Research Comparative oral monotherapy of psilocybin, lysergic acid diethylamide, 3,4-methylenedioxymethamphetamine, ayahuasca, and escitalopram for depressive symptoms: systematic review and Bayesian network meta-analysis ... including proportion of men and women in the study, mean age, baseline depression severity, disorder type, and follow-up ...
Promoting low-carbon agriculture is vital for climate action and food security. State farms serve as crucial agricultural production bases in China and are essential in reducing China's carbon emissions and boosting emission efficiency. This study calculates the carbon emissions of state farms across 29 Chinese provinces using the IPCC method from 2010 to 2022. It also evaluates emission ...