ACM Transactions on Cyber-Physical Systems

Volume 8, Issue 3

- Computation Abstractions
- System Modeling and Languages
- System Compositionality and Integration
- Design Automation and Tool Chains
- Trustworthy System Designs
- Resilient and Robust System Designs
- Human in the Loop


Subject Areas
Announcements.
ACM Updates Its Peer Review Policy
ACM is pleased to announce that its Publications Board has approved an updated Peer Review Policy . If you have any questions regarding the update, the associated FAQ addresses topics such as confidentiality, the use of large language models in the peer review process, conflicts of interest, and several other relevant concerns. If there are any issues that are not addressed in the FAQ, please contact ACM’s Director of Publications, Scott Delman .
New ACM Policy on Authorship ACM has a new Policy on Authorship , covering a range of key topics, including the use of generative AI tools. Please familiarize yourself with the new policy and the associated list of Frequently Asked Questions .
Most Frequent Affiliations
Most cited authors, latest issue.
- Volume 8, Issue 3 July 2024 ISSN: 2378-962X EISSN: 2378-9638 View Table of Contents
DistressNet-NG: A Resilient Data Storage and Sharing Framework for Mobile Edge Computing in Cyber-Physical Systems
Department of Computer Science and Engineering, Texas A&M University, College Station, USA
Department of Computer Science and Software Engineering, Miami University, Oxford, USA
Department of Computer Engineering, University of Sharjah, Sharjah, UAE
National Institute of Standards and Technology (NIST), Boulder, USA
Experimentation and Implementation of the BFT++ Cyber-Attack Resilience Mechanism for Cyber-Physical Systems
MITRE, McLean, USA
CSAFA Labs, SCP, Georgia Institute of Technology, Atlanta, USA
Recent Award Winners
Most popular, other acm journals.

Volume 2, Issue 2

Volume 3, Issue 2
April-June 2024

Volume 56, Issue 12
December 2024

Volume 5, Issue 2

Volume 36, Issue 2
Export Citations
- Please download or close your previous search result export first before starting a new bulk export. Preview is not available. By clicking download, a status dialog will open to start the export process. The process may take a few minutes but once it finishes a file will be downloadable from your browser. You may continue to browse the DL while the export process is in progress. Download
- Download citation
- Copy citation
We are preparing your search results for download ...
We will inform you here when the file is ready.
Your file of search results citations is now ready.
Your search export query has expired. Please try again.
Thank you for visiting nature.com. You are using a browser version with limited support for CSS. To obtain the best experience, we recommend you use a more up to date browser (or turn off compatibility mode in Internet Explorer). In the meantime, to ensure continued support, we are displaying the site without styles and JavaScript.
- View all journals
- Explore content
- About the journal
- Publish with us
- Sign up for alerts
- Open access
- Published: 03 February 2022
Cyber-physical defense in the quantum Era
- Michel Barbeau 1 &
- Joaquin Garcia-Alfaro 2
Scientific Reports volume 12 , Article number: 1905 ( 2022 ) Cite this article
3318 Accesses
6 Citations
7 Altmetric
Metrics details
- Computer science
- Information technology
Networked-Control Systems (NCSs), a type of cyber-physical systems, consist of tightly integrated computing, communication and control technologies. While being very flexible environments, they are vulnerable to computing and networking attacks. Recent NCSs hacking incidents had major impact. They call for more research on cyber-physical security. Fears about the use of quantum computing to break current cryptosystems make matters worse. While the quantum threat motivated the creation of new disciplines to handle the issue, such as post-quantum cryptography, other fields have overlooked the existence of quantum-enabled adversaries. This is the case of cyber-physical defense research, a distinct but complementary discipline to cyber-physical protection. Cyber-physical defense refers to the capability to detect and react in response to cyber-physical attacks. Concretely, it involves the integration of mechanisms to identify adverse events and prepare response plans, during and after incidents occur. In this paper, we assume that the eventually available quantum computer will provide an advantage to adversaries against defenders, unless they also adopt this technology. We envision the necessity for a paradigm shift, where an increase of adversarial resources because of quantum supremacy does not translate into a higher likelihood of disruptions. Consistently with current system design practices in other areas, such as the use of artificial intelligence for the reinforcement of attack detection tools, we outline a vision for next generation cyber-physical defense layers leveraging ideas from quantum computing and machine learning. Through an example, we show that defenders of NCSs can learn and improve their strategies to anticipate and recover from attacks.
Similar content being viewed by others
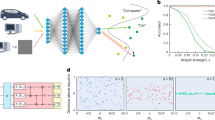
Towards quantum enhanced adversarial robustness in machine learning
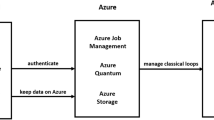
Stabilized quantum-enhanced SIEM architecture and speed-up through Hoeffding tree algorithms enable quantum cybersecurity analytics in botnet detection
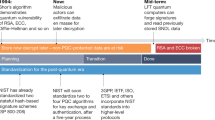
Transitioning organizations to post-quantum cryptography
Introduction.
Networked-Control Systems (NCSs) integrate computation, communications and physical processes. Their design involves fields such as computer science, automatic control, networking and distributed systems. Physical resources are orchestrated building upon concepts and technologies from these domains. In a Networked-Control System (NCS), the focus is on remote control, which means steering at distance a dynamical system according to requirements. Determined according to a target behavior, feedback and corrective control actions are transported over a communication network.
In a NCS, networks and systems represent observable and controllable physical resources. The sensors correspond to observation apparatus. The actuators represent an abstraction of devices enabling the control of the networked system. Using signals produced by the sensors, the controller generates commands to the actuators. The coupling of the controller with actuators and sensors happens through a communications network. In contrast to a classical feedback-control system, NCS provide remote control.
NCS are flexible, but vulnerable to computer and network attacks. Adversaries build upon their knowledge about dynamics, feedback predictability and countermeasures, to perpetrate attacks with severe implications 1 , 2 , 3 . When industrial systems and national infrastructures are victimized, consequences are catastrophic for businesses, governments and society 4 . A growing number of incidents have been documented. Representative instances are listed in Online Supplementary Material A .
Attacks can be looked into from several point of views 5 . We can consider attacks in relation to an adversary knowledge about a system and its defenses. In addition, we can consider attacks with respect to the criticality of disrupted resources. For example, a denial-of-service (DoS) attack targeting an element that is crucial to operation 6 . Besides, we can take into account the ability of an adversary to analyze signals, such as sensor outputs. This may enable sophisticated attacks impacting system integrity or availability. Moreover, there are incidents caused by human adversarial actions. They may forge feedback for disruption purposes. NCSs must be capable of handling security beyond breach. In other words, they must assume that cyber-physical attacks will happen. They should be equipped with cyber-physical defense tools. Response management tools must assure that crucial operational functionality is properly accomplished and cannot be stopped. For example, the cooling service of a nuclear plant reactor or safety control of an autonomous navigation system are crucial functionalities. Other less important functionalities may be temporarily stopped or partially completed, such as a printing service. It is paramount to assure that defensive tools provide appropriate responses, to rapidly take back control when incidents occur.
That being said, the quantum paradigm will render obsolete a number of cyber-physical security technologies. Solutions that are assumed to be robust today will be deprecated by quantum-enabled adversaries. Adversaries can get capable of brute-forcing and taking advantage of the upcoming quantum computing power. Disciplines, such as cryptography, are addressing this issue. Novel post-quantum cryptosystems are facing the quantum threat. Other fields, however, have overlooked the eventual existence of quantum-enabled adversaries. Cyber-physical defense, a discipline complementary to cryptography, is a proper example. It uses artificial intelligence mainly to detect anomalies and anticipate adversaries. Hence, it enables NCSs with capabilities to detect and react in response to cyber-physical attacks. More concretely, it involves the integration of machine learning to identify adverse events and prepare response plans, while and after incidents occur. An interesting question is the following. How a defender will face a quantum-enabled adversary? How can a defender use the quantum advantage to anticipate response plans? How to ensure cyber-physical defense in the quantum era? In this paper, we investigate these questions. We develop foundations of a quantum machine learning defense framework. Through an illustrative example, we show that a defender can leverage quantum machine learning to address the quantum challenge. We also highlight some recent methodological and technological progress in the domain and remaining issues.
The paper is organized as follows. Section “ Related work ” reviews related work. Section “ Cyber-physical defense using quantum machine learning ” develops our approach, exemplified with a proof-of-concept. Section “ Discussion ” discusses the generalization of the approach and open problems. Section “ Conclusion ” concludes the paper.
Related work
Protection is one of the most important branches of cybersecurity. It mainly relies on the implementation of state-of-the-art cryptographic protocols. They mainly comprise the use of encryption, digital signatures and key agreement. The security of some cryptographic families are based on computational complexity assumptions. For instance, public key cryptography builds upon factorization and discrete logarithm problems. They assume the lack of efficient solutions that break them in polynomial time. However, quantum enabled adversaries can invalidate these assumptions. They put those protocols at risk 7 , 8 . At the same time, the availability of quantum computers from research to general purpose applications led to the creation of new cybersecurity disciplines. The most prominent one is Post-Quantum Cryptography (PQC). It is a fast growing research topic aiming to develop new public key cryptosystems resistant to quantum enabled adversaries.
The core idea of PQC is to design cryptosystems whose security rely on computational problems that cannot be resolved by quantum adversaries in admissible time. Candidate PQC families include code-based 9 , hash-based 10 , multivariate 11 , lattice-based 12 , 13 and supersingular isogeny-based 14 cryptosystems. Their security is all based on mathematical problems that are believed to be hard, even with quantum computation and communications resources 15 . Furthermore, PQC has led to new research directions driven by different quantum attacks. For instance, quantum-resistant routing aims at achieving a secure and sustainable quantum-safe Internet 16 .
Besides, quantum-enabled adversaries can disrupt the operation of classical systems. For example, they can jeopardize availability properties by perpetrating brute-force attacks. Solidifying the integrity and security of the quantum Internet is of chief importance. Solutions to these challenges are being developed and published in the quantum security literature using multilevel security stacks. They involve the combination of quantum and classical security tools 17 . Cybersecurity researchers emphasized the need for more works on approaches mitigating the impact of such attacks 18 . Following their detection, adequate response to attacks is a problem that seems to have received little attention. Specially when we are dealing with quantum enabled adversaries. Intrusion detection, leveraging artificial intelligence and machine learning, is the most representative category of the detection and reaction paradigm.
The detection and reaction paradigm uses adversarial risk analysis methodologies, such as attack trees 20 and graphs 21 . Attacks are represented as sequences of incremental steps. The last steps of sequences correspond to events detrimental to the system. In other words, an attack is considered successful when the adversary reaches the last step. The cost for the adversary is quantified in terms of resource investment. It is generally assumed that with infinite resources, an adversary reaches an attack probability success of one. For instance, infinite resources can mean usage of brute-force 22 . An adversary that increases investment, such as time, computational power or memory, also increases the success probability of reaching the last step of an attack. Simultaneously, this reduces the likelihood of detection by defenders. Analysis tools may help to explore the relation between adversary investment and attack success probability 23 . Figure 1 schematically depicts the idea. The horizontal axis represents the cost of the adversary in terms of resource investment. The vertical axis represents the success probability of the attack. We depict three scenarios. The blue curve involves a classical adversary with classical resources and a relatively low probability of attack success. The red curve corresponds to a quantum-enabled adversary, classical defender scenario. The adversary has the quantum advantage with relatively high probability of attack success. The black curve represents a balanced situation, where both the adversary and defender have quantum resources. Every curve models a Cumulative Distribution Function (CDF) corresponding to the probability of success versus the adversary resource investment. Distribution functions such as Rayleigh 24 and Rician 25 are commonly used in the intrusion detection literature for this purpose. Their parameters can be estimated via empirical penetration testing tools 26 . Without empowering defenders with the same quantum capabilities, an increase of adversarial resources always translate into a higher likelihood of system disruption. In the sequel, we discuss how to equip defenders with quantum resources such that a high attack success probability is not attainable anymore.
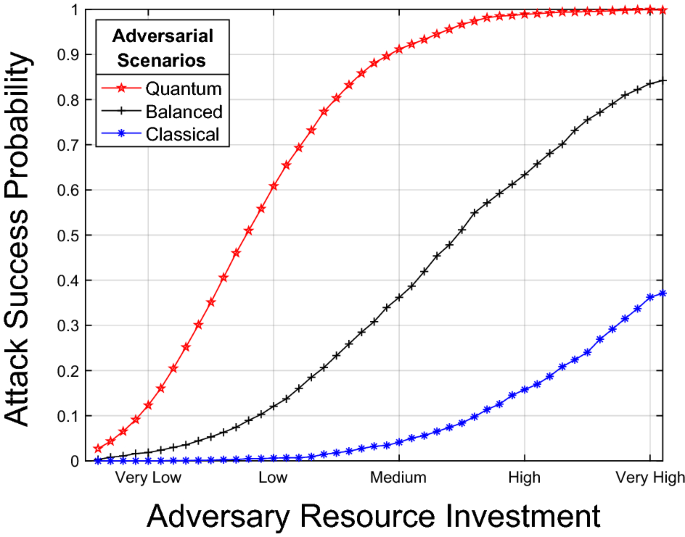
Attack success probability vs. adversary investment. We consider three adversarial scenarios. Classical (blue curve), where the resources of the adversary are lower than the resources of the defender. Balanced (black), where the resources of the defender are proportional to those of the adversary. Quantum (red), where the resources of the adversary are higher than those of the defender. Simulation code is available at the companion github repository, in the Matlab code folder 19 .
Cyber-physical defense using quantum machine learning
Machine Learning (ML) is about data and, together with clever algorithms, building experience such that next time the system does better. The relevance of ML to computer security in general has already been given consideration. Chio and Freeman 27 demonstrated general applications of ML to enhance security. A success story is the use of ML to control spam emails metadata (e.g., source reputation, user feedback and pattern recognition) to filter out junk emails. Furthermore, there is an evolution capability. The filter gets better with time. This way of thinking is relevant to Cyber-Physical System (CPS) security because its defense can learn from attacks and make the countermeasures evolve. Focusing on CPS-specific threats, as an example pattern recognition can be used to extract from data the characteristics of attacks and to prevent them in the future. Because of its ability to generalize, ML can deal with adversaries hiding by varying the exact form taken by their attacks. Note that perpetrators can adopt as well the ML paradigm to learn defense strategies and evolve attack methods. The full potential of ML for CPS security has not been fully explored. The way is open for the application of ML in several scenarios. Hereafter, we focus on using Quantum Machine Learning (QML) for cyber-physical defense.
QML, i.e., the use of quantum computing for ML 28 , has potential because the time complexity of tasks such as classification is independent of the number of data points. Quantum search techniques are data size independent. There is also the hope that the quantum computer can learn things that the classical computer is incapable of, due to the fact that the former has properties that the latter does not have, notably entanglement. At the outset, however, we must admit that a lot remains to be discovered.
QML is mainly building on the traditional quantum circuit model. Schuld and Killoran investigated the use of kernel methods 29 , employed for system identification, for quantum ML. Encoding of classical data into a quantum format is involved. A similar approach has been proposed by Havlíček et al. 30 . Schuld and Petruccione 31 discuss in details the application of quantum ML over classical data generation and quantum data processing. A translation procedure is required to map the classical data, i.e., the data points, to quantum data, enabling quantum data processing, such as quantum classification. However, there is a cost associated with translating classical data into the quantum form, which is comparable to the cost of classical ML classification. This is right now the main barrier. The approach resulting in real gains is quantum data generation and quantum data processing, since there is no need to translate from classical to quantum data. Quantum data generation requires quantum sensing. Successful implementation of this approach will grant a quantum advantage, to the adversary or CPS defenders. There are alternatives to doing QML with traditional quantum circuits. Use of tensor networks 32 , a general graph model, is one of them 33 . Next, we develop an example that illustrates the potential and current limitations of quantum ML, using variational quantum circuits 31 , 34 , 35 , for solving cyber-physical defense issues.
Let us consider the adversarial model represented in Fig. 2 . There is a controller getting data and sending control signals through networked sensors and actuators to a system. An adversary can intercept and tamper signals exchanged between the environment and controller, in both directions. Despite the perpetration of attacks, the controller may still have the ability to monitor and steer the system. This is possible using redundant sensors and actuators attack detection techniques. This topic has been addressed in related work 36 . Furthermore, we assume that:
The controller has options and can independently make choices,
The adversaries have options and can independently make choices and
The consequences of choices made by the controller, in conjunction with those made by adversaries, can be quantified, either by a penalty or a reward.
To capture these three key assumptions, we use the Markov Decision Process (MDP) model 37 , 38 . The controller is an agent evolving in a world comprising everything else, including the network, system and adversaries. At every step of its evolution, the agent makes a choice among a number of available actions, observes the outcome by sensing the state of the world and quantifies the quality of the decision with a numerical score, called reward. Several cyber-physical security and resilience issues lend themselves well to this way of seeing things.
The agent and its world are represented with the MDP model. The quantum learning part builds upon classical Reinforcement Learning (RL). The work on QML uses the feature Hilbert spaces of Schuld and Killoran 29 , relying on classical kernel methods. Classical RL, such as Q-learning 39 , 40 , assumes that the agent, i.e., the learner entity, evolves in a deterministic world. The evolution of the agent and its world is also formally modeled by the MDP. A RL algorithm trains the agent to make decisions such that a maximum reward is obtained. RL aims at optimizing the expected return of a MDP. The objectives are the same with QML. We explain MDP modeling and the quantum RL part in the sequel.
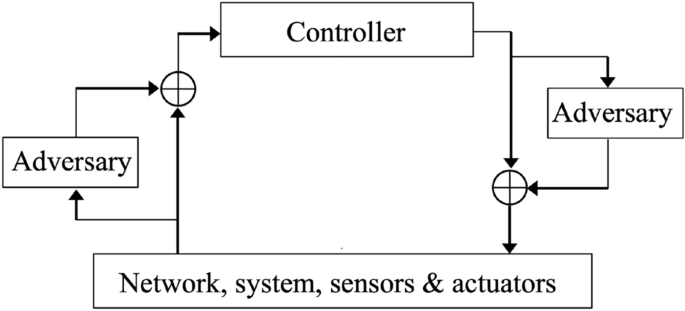
Adversarial model.
A MDP is a discrete time finite state-transition model that captures random state changes, action-triggered transitions and states-dependent rewards. A MDP is a four tuple \((S,A,P_a,R_a)\) comprising a set of n states \(S=\{ 0, 1,\ldots ,n-1 \}\) , a set of m actions \(A=\{ 0,1,\ldots ,m-1 \}\) , a transition probability function \(P_a\) and a reward function \(R_a\) . The evolution of a MDP is paced by a discrete clock. At time t , the MDP is in state \(s_t\) , such that \(t=0,1,2,\ldots\) . The MDP model starts in an initial state \(s_0=0\) . The transition probability function, denoted as
defines the probability of making a transition to state \(s_{t+1}\) equal to \(s'\) at time \(t+1\) , when at time t the state is \(s_t = s\) and action a is performed. The reward function \(R_a(s,s')\) defines the immediate reward associated with the transition from state s to \(s'\) and action a . It has domain \(S \times S\times A\) and co-domain \({\mathbb {R}}\) .
Quantum reinforcement learning
In this section, we present our cyber-physical defense approach. A reader unfamiliar with quantum computing may first read Online Supplementary Material B and C , for a short introduction to the topic. At the heart of the approach is the concept of variational circuit. Bergholm et al. 41 interpret such a circuit as the quantum implementation of a function \(f(\psi ,\Theta ): {\mathbb {R}}^m\rightarrow {\mathbb {R}}^n\) . That is, a two argument function from a dimension m real vector space to a dimension n real vector space, where m and n are two positive integers. The first argument \(\psi\) denotes an input quantum state to the variational circuit. The second argument \(\Theta\) is the variable parameter of the variational circuit. Typically, it is a matrix of real numbers. During the training, the numbers in the matrix are progressively tuned, via optimization, such that the behavior of the variational circuit eventually approaches a target function. In our cases, this function is the optimal policy \(\pi\) , in the terminology of Q-learning (see Online Supplementary Material D ).
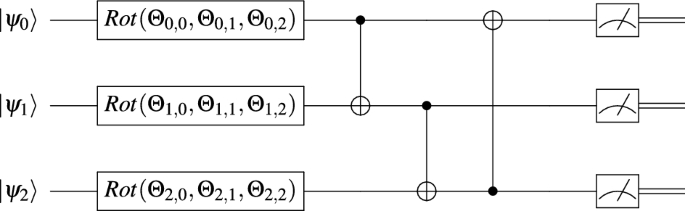
Three-qubit variational circuit layer \(W(\Theta )\) , where \(\Theta\) is a three by three matrix of rotation angles.
As an example, an instance of the variational circuit design of Farhi and Neven 42 is pictured in Fig. 3 . In this example, both m and n are three. It is a m -qubit circuit. A typical variational circuit line comprises three stages: an initial state, a sequence of gates and a measurement device. In this case, for \(i=0,1,2\) , the initial state is \(\vert {\psi _i}\rangle\) . The gates are a parameterized rotation and a CNOT. The measurement device is represented on the extreme right box, with a symbolic measuring dial. The circuit variable parameter \(\Theta\) is a three by three matrix of rotation angles. For \(i=0,1,\ldots ,m-1\) , the gate \(Rot(\Theta _{i,0},\Theta _{i,1},\Theta _{i,2})\) applies the x , y and z -axis rotations \(\Theta _{i,0}\) , \(\Theta _{i,1}\) and \(\Theta _{i,2}\) to qubit \(\vert {\psi _i}\rangle\) on the Bloch sphere (see Online Supplementary Material C for an introduction to the Bloch sphere concept). The three rotations can take qubit \(\vert {\psi _i}\rangle\) from any state to any state. To create entanglement between qubits, qubit with index i is connected to qubit with index \(i + 1\) , modulo m , using a CNOT gate. A CNOT gate can be interpreted as a controlled XOR operation. The qubit connected to the solid dot end, of the vertical line, controls the qubit connected to the circle embedding a plus sign. When the control qubit is one, the controlled qubit is XORed.
In our approach, quantum RL uses and train a variational circuit. The variational circuit maps quantum states to quantum actions, or action superpositions. The output of the variational circuit is a superposition of actions. During learning, the parameter \(\Theta\) of the variational circuit is tuned such that the output of that variational collapses to actions that are proportional to their goodness, that is, the rewards they provide to the agent.
The training process can be explained in reference to Q-learning. For a brief introduction to Q-learning, see Online Supplementary Material D . The variational circuit is a representation of the policy \(\pi\) . Let \(W(\Theta )\) be the variational circuit. W is called a variational circuit because it is parameterized with the matrix of rotation angles \(\Theta\) . The RL process tunes the rotation angles in \(\Theta\) . Given a state \(s \in S\) , an action \(a \in A\) and epoch t , the probability of measuring value a in the quantum state \(\mathrm {A}\) that is the output of the system
is proportional to the ratio
The matrix \(\Theta\) is initialized with arbitrary rotations \(\Theta _{0}\) . Starting from the initial state \(s_0\) , the following procedure is repeatedly executed. At the t th epoch, random action a is chosen from set A . In current state s , the agent applies action a causing the world to make a transition. World state \(s'\) is observed. Using a , s and \(s'\) , the Q-values ( \(Q_{t}\) ) are updated. For every other action pair ( s , a ), where \(s\in S\) is not the current state or \(a\in A\) is not the executed action, probability \(p_{t,s,a}\) is also updated according to Eq. ( 2 ). Using \(\Theta _{t-1}\) and the \(p_{t,s,a}\) probabilities, the variational circuit parameter is updated and yields \(\Theta _{t}\) .
The variational circuit is trained such that under input state \(\vert {s}\rangle\) , the measured output in the system \(\mathrm {A} = W(\Theta ) \vert {s}\rangle\) is a with probability \(p_{t,s,a}\) . Training of the circuit can be done with a gradient descent optimizer 41 . Step-by-step, the optimizer minimizes the distance between the probability of measuring \(\vert {a}\rangle\) and the ratio \(p_{t,s,a}\) , for a in A .
The variational circuit \(W(\Theta )\) is trained on the probabilities of the computational basis members of A , in a state s . Quantum RL repeatedly updates \(\Theta\) such that the evaluation of \(\mathrm {A} = W(\Theta )\vert {s}\rangle\) yields actions with probabilities proportional to the rewards. That is, the action action recommended by the policy is \(\arg \max _{a\in A} \mathrm {A}\) , i.e., the row-index of the element with highest probability amplitude.
Since \(W(\Theta )\) is a circuit, once trained it can be used multiple times. Furthermore, with this scheme the learned knowledge \(\Theta\) , which are rotations, can be easily stored or shared with other parties. This RL scheme can be implemented using the resources of the PennyLane software 41 . An illustrative example is discussed in the next subsection.
Illustrative example
In this section, we illustrate our approach with an example. We model the agent and its world with the MDP model. We define the attack model. We explain the quantum representation of the problem. We demonstrate enhancement of resilience leveraging quantum RL.
Agent and its world
Let us consider the discrete two-train configuration of Fig. 4 a. Tracks are broken into sections. We assume a scenario where Train 1 is the agent and Train 2 is part of its world. There is an outer loop visiting points 3, 4 and 5, together with a bypass from point 2, visiting point 8 to point 6. Traversal time is uniform across sections. The normal trajectory of Train 1 is the outer loop, while maintaining a Train 2-Train 1 distance greater than one empty section. For example, if Train 1 is at point 0 while Train 2 is at point 7, then the separation distance constraint is violated. The goal of the adversary is to steer the system in a state where the separation distance constraint is violated. When a train crosses point 0, it has to make a choice: either traverse the outer loop or take the bypass. Both trains can follow any path and make independent choices, when they are at point 0.
In the terms of RL, Train 1 has two actions available: take loop and take bypass. The agent gets k reward points for a relative Train 2-Train 1 distance increase of k sections with Train 2. It gets \(-k\) reward points, i.e., a penalty, for a relative Train 2-Train 1 distance decrease of k sections with Train 2. For example, let us assume that Train 1 is at point 0 and that Train 2 is at point 7. If both trains, progressing at the same speed, take the loop or both decide to take the bypass, then there is no relative distance change. The agent gets no reward. When Train 1 decides to take the bypass and Train 2 decides to take the loop, the agent gets two reward points, at return to point zero (Train 2 is at point five). When Train 1 decides to take the loop and Train 2 decides to take the bypass, the agent gets four reward points, at return to point zero (Train 2 is at point one, Train 2-Train 1 distance is five sections).
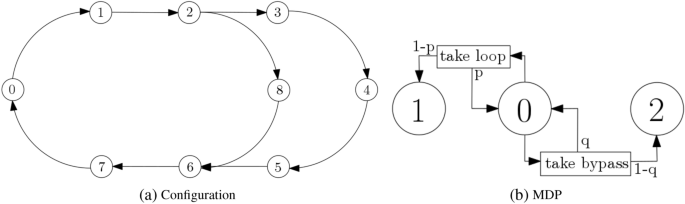
( a ) Discrete two-train configuration. ( b ) MDP representation of the agent in its world. Circles represent states. Arrows represent action-triggered transitions.
The corresponding MDP model is shown in Fig. 4 b. The state set is \(S=\{ 0, 1,2 \}\) . The action set is \(A=\{ a_0=\hbox {take loop}, a_1=\hbox {take bypass} \}\) . The transition probability function is defined as \(P_{a_0}(0,0)=p\) , \(P_{a_0}(0,1)=1-p\) , \(P_{a_1}(0,0)=q\) and \(P_{a_1}(0,2)=1-q\) . The reward function is defined as \(R_{a_0}(0,0)=0\) , \(R_{a_0}(0,1)=4\) , \(R_{a_1}(0,0)=0\) and \(R_{a_1}(0,2)=2\) . This is interpreted as follows. In the initial state 0 with a one-section separation distance, the agent selects an action to perform: take loop or take bypass. Train 1 performs the selected action. When selecting take loop, with probability p the environment goes back to state 0 (no reward) or with probability \(1-p\) it moves to state 1, with a five-section separation distance (reward is four). When selecting take bypass, with probability q the environment goes back to state 0 (no reward) or with probability \(1-q\) it moves state 2, with a three-section separation distance (reward is two). The agent memorizes how good it has been to perform a selected action.
As shown in this example, multiple choices might be available in a given state. A MDP is augmented with a policy. At any given time, the policy tells the agent which action to pick such that the expected return is maximized. The objective of RL is finding a policy maximizing the return. Q-learning captures the optimal policy into a state-action value function Q ( s , a ), i.e., an estimate of the expected discounted reward for executing action a in state s 39 , 40 . Q-learning is an iterative process. \(Q_t(s,a)\) is the state-action at the t th episode of learning.
Figure 5 plots side by side the Q-values for actions \(a_0\) and \(a_1\) , for values of probabilities p and q ranging from zero to one, in steps of 0.1. As a function of p and q , on which the agent has no control, the learned policy is that in state zero should pick the action among \(a_0\) and \(a_1\) that fields the maximum Q-value, which can be determined from Fig. 5 . This figure highlights the usefulness of RL, even for such a simple example the exact action choice is by far not always obvious. However, RL tells what this choice should be.
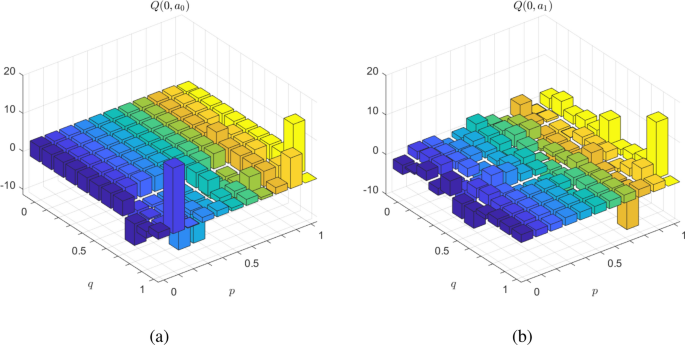
Q-values for actions \(a_0\) and \(a_1\) , for values of probabilities p and q ranging from zero to one, in steps of 0.1.
The example is simple enough so that a certain number of cases can be highlighted. When probabilities p and q tend to one, it means that the adversary is more likely to behave as the agent. Inversely, when p and q tend to null, the adversary is likely to make a different choice from that of the agent. Such a bias can be explained by the existence of an insider that leaks information to the adversary when the agent makes its choice at point 0. In the former case, the agent is trapped in a risky condition. In the latter case, the adversary is applying its worst possible strategy. When p and q are both close to 50%, the adversary is behaving arbitrarily. On the long term, the most rewarding action for the agent is to take the loop. It is of course possible to update the policy according to a varying adversarial behavior, i.e., changing values for p and q . In following, we address this RL problem with a quantum approach.
Quantum representation
The problem in the illustrative example of Fig. 4 comprises only one state (0) where choices are available. A binary decision is taken in that state. The problem can be solved by a single qubit variational quantum circuit. The output of the circuit is a single qubit with the simple following interpretation. \(\vert 0 \rangle\) is action take loop, while \(\vert 1 \rangle\) is action take bypass.
For this example, we use the variational quantum circuit pictured in Fig. 6 . The input of the circuit is ground state \(\vert {0}\rangle\) . Two rotation gates and a measurement gate are used. The circuit consists of two quantum gates: an X gate and a Y gate, parameterized with rotations \(\Theta [0]\) and \(\Theta [1]\) about the x -axis and y -axis, on the Bloch sphere. There is a measurement gate at the very end, converting the output qubit into a classical binary value. This value is an action index. The variational circuit is tuned by training such that it outputs the probably most rewarding choice.

Single-qubit variational circuit \(W(\Theta )\) .
A detailed implementation of the example is available as supplementary material in a companion github repository 19 . Figure 7 provides graphical interpretations of the two-train example. In all the plots, the x -axis represents epoch (time). Part (a) shows the Train 2-Train 1 separation distance (in sections) as a function of the epoch, when the agent is doing the normal behavior, i.e., do action take loop, and the adversary is behaving arbitrarily, p and q are equal to 0.5. The average distance (three sections) indicates that more often the separation distance constraint is not violated. Part (b) also shows the Train 2-Train 1 distance as a function of the epoch, but this time the adversary figured out the behavior of the agent. The average distance (less than two sections) indicates that the separation distance constraint is often violated. Part (c) plots the value of state zero, in Fig. 4 b, versus epoch. The adversary very likely learns the choices made by the agent, when at point 0. There is an insider leaking the information. Train 2 is likely to mimic Train 1. The probabilities of p and q are equal to 0.9. In such a case, for Train 1 the most rewarding choice is to take the loop. Part (d) shows the evolution of the probabilities of the actions, as the training of the quantum variational circuit pictured in Fig. 6 progresses. They evolve consistently with the value of state zero (learning rate \(\alpha\) is 0.01). The y -axis represents probabilities of selecting the actions take loop (square marker) and take bypass (triangle marker). Under this condition, quantum RL infers that the maximum reward is obtained selecting the take loop action. It has indeed higher probability than the take bypass action.
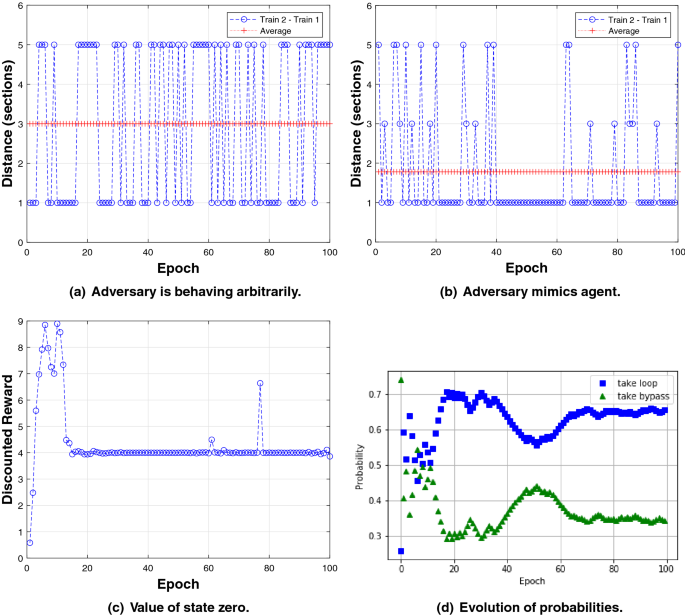
( a ) The adversary randomly alternates between take loop and take bypass, with equal probabilities. ( b ) The agent choices are leaked, e.g., due to the presence of an insider. With high probability, the adversary is mimicking the agent. ( c ) Evolution of the value of state zero. ( d ) Evolution of quantum variational circuit probabilities, with learning rate \(\alpha\) equal to 0.01.
Section “ Illustrative example ” detailed an illustrative example. Of course, it can be enriched. The successors of states 1 and 2 can be expanded. More elaborate railways can be represented. More sophisticated attack models can be studied. For example, let \(v_i\) denote the velocity of Train i , where i is equal to 1 or 2. Hijacking control signals, the adversary may slowly change the velocity of one of the trains until the separation distance is not greater than a threshold \(\tau\) . Mathematically, the velocity of the victimized train is represented as
The launched time of the attack is t . \(v_i(t)\) is the train velocity at time t , while \(v_i(t+\Delta )\) is the speed after a delay \(\Delta\) . Symbols \(\alpha\) and \(\beta\) are constants. During normal operation, the two trains are moving at equal constant velocities. During an attack on the velocity of a train, the separation distance slowly shrinks down to a value equal to or lower than a threshold. The safe-distance constraint is violated. While an attack is being perpetrated, the state of the system must be recoverable 43 , i.e., the velocities and compromised actuators or sensors can be determined using redundant sensing resources.
The approach can easily be generalized to other applications. For instance, let us consider switching control strategies used to mitigate DoS attacks 6 or input and output signal manipulation attacks 44 . States are controller configurations, actions are configuration-to-configuration transitions and rewards are degrees of attack mitigation. The variational circuit is trained such that the agent is steered in an attack mitigation condition. This steering strategy is acquired through RL.
In Section “ Quantum reinforcement learning ”, quantum RL is explained referring to Q-learning. Table 1 compares Q-learning and quantum RL. The first column list the RL concepts. The second column define their implementation in Q-learning 45 . The third column lists their analogous in quantum RL. The core concept is a data structure used to represent the expected future rewards for action at each state. Q-learning uses a table while quantum RL employs a variational circuit. The following line quantifies the amount of resources needed in every case. For Q-learning, n times m expected reward numbers need to be stored, where n is the number of states and m the number of actions of the MDP. For quantum RL, \(k \log n\) quantum gates are required, where k is the number of gates used for each variational circuit line. Note that deep learning 45 , 46 , 47 and quantum RL can be used to approximate the Q-value function, with respectively, a neural network or a variational quantum circuit. The second line compares tuneable parameters, which are neural network weight for the classical model and variational circuit rotations for the quantum model. For both models, gradient descent optimization method is used to tune iteratively the model, the neural network or variational circuit. Chen et al. 34 did a comparison of Deep learning and quantum RL. According to their analysis, similar results can be obtained with similar order quantities of resources. While there is no neural network computer in the works, apart for hardware accelerators, there are considerable efforts being deployed to develop the quantum computer 48 . The eventually available quantum computer will provide an incomparable advantage to the ones who will have access to the technology, in particular the defender or adversary.
There are a few options for quantum encoding of states, including computational basis encoding, single-qubit unitary encoding and probability encoding. They all have a time complexity cost proportional to the number of states. Computational basis encoding is the simplest to grasp. States are indexed \(i=0, \ldots ,m-1\) . In the quantum format, the state is represented as \(\vert {i}\rangle\) .
Amplitude encoding works particularly well for supervised machine learning 31 , 49 . For example, let \(\vec {\psi }=(\psi _0,\ldots ,\psi _7)\) be such a unit vector. Amplitude encoding means that the data is encoded in the probability amplitudes of quantum states. Vector \(\vec {\psi }\) is mapped to the following three-qubit register
The term \(\vert {i}\rangle\) is one of the eight computational basis members for a three-qubit register. Every feature-vector component \(\psi _i\) becomes the probability amplitude of computational basis member \(\vert {i}\rangle\) . The value \(\psi ^2\) corresponds to the probability of measuring the quantum register in state \(\vert {i}\rangle\) . The summation operation is interpreted as the superposition of the quantum states \(\vert {i}\rangle\) , \(i=0,\ldots ,7\) . Superposition means that the quantum state \(\vert {\psi }\rangle\) assumes all the values of i at the same time. In this representation exercise, there is a cost associated with coding the feature vectors in the quantum format, linear in their number. The time complexity of an equivalent classical computing classifier is linear as well. However, in the quantum format the time taken to do classification is data-size independent. The coding overhead, although, makes quantum ML performance comparable to classical NL performance. Ideally, data should be directly represented in the quantum format, bypassing the classical to quantum data translation step and enabling gains in performance. Further research in quantum sensing is needed to enable this 50 .
There are also other RL training alternatives. Dong et al. have developed a quantum RL approach 51 . In the quantum format, a state \(i \in S\) of the MDP is mapped to quantum state \(\vert {i}\rangle\) . Similarly, an action \(j \in A\) is mapped to quantum state \(\vert {j}\rangle\) . In state i , the action space is represented by the quantum state
where the probability amplitudes \(\psi _i\) ’s, initially all equal, are modulated, using Grover iteration by the RL procedure. In state i , selecting an action amounts to observing the quantum state \(\vert {A_i}\rangle\) . According to the non-cloning theorem, it can be done just once, which is somewhat limited.
By far, not all QML issues have been resolved. More research on encoding and training is required. Variational circuit optimization experts 41 highlight the need for more research to determine what works best, among the available variational circuit designs, versus the type of problem considered.
We have presented our vision of a next generation cyber-physical defense in the quantum era. In the same way that nobody thinks about system protection making abstraction of the quantum threat, we claim that in the future nobody will think about cyber-physical defense without using quantum resources. When available, adversaries will use quantum resources to support their strategies. Defenders must be equipped as well with the same resources to face quantum adversaries and achieve security beyond breach. ML and quantum computing communities will play very important roles in the design of such resources. This way, the quantum advantage will be granted to defenders rather than solely to adversaries. The essence of the war between defenders and adversaries is knowledge. RL can be used by an adversary for the purpose of system identification, an enabler for cyber-physical attacks. The paper has clearly demonstrated the plausibility of using quantum technique to search defense strategies and counter adversaries. Furthermore, the design of new defense techniques can leverage quantum ML to speedup decision making and support networked control systems. These benefits of QML will although materialize when the quantum computer will be available. These ideas have been explored in this article, highlighting capabilities and limitations which resolution requires further research.
Ding, D., Han, Q.-L., Ge, X. & Wang, J. Secure state estimation and control of cyber-physical systems: a survey. IEEE Trans. Syst. Man Cybern. Syst. 51 , 176–190 (2020).
Article Google Scholar
Ge, X., Han, Q.-L., Zhang, X.-M., Ding, D. & Yang, F. Resilient and secure remote monitoring for a class of cyber-physical systems against attacks. Inf. Sci. 512 , 1592–1605 (2020).
Article MathSciNet Google Scholar
Ding, D., Han, Q.-L., Xiang, Y., Ge, X. & Zhang, X.-M. A survey on security control and attack detection for industrial cyber-physical systems. Neurocomputing 275 , 1674–1683 (2018).
Courtney, S. & Riley, M. Biden rushes to protect power grid as hacking threats grow (2021). Bloomberg. https://j.mp/3fyZcQE . Accessed June 2021.
Teixeira, A., Shames, I., Sandberg, H. & Johansson, K. H. A secure control framework for resource-limited adversaries. Automatica 51 , 135–148 (2015).
Zhu, Y. & Zheng, W. X. Observer-based control for cyber-physical systems with periodic dos attacks via a cyclic switching strategy. IEEE Trans. Autom. Control 65 , 3714–3721 (2020).
Shor, P. Polynomial-time algorithms for prime factorization and discrete logarithms on a quantum computer. SIAM J. Comput. 26 , 1484–1509 (1997).
Shor, P. W. & Preskill, J. Simple proof of security of the bb84 quantum key distribution protocol. Phys. Rev. Lett. 441–444 (2000).
McEliece, R. J. A public-key cryptosystem based on algebraic. Codin. Thv. 4244 , 114–116 (1978).
Google Scholar
Merkle, R. Secrecy, Authentication, and Public Key Systems . Computer Science Series (UMI Research Press, 1982).
Patarin, J. Hidden fields equations (hfe) and isomorphisms of polynomials (ip): Two new families of asymmetric algorithms. In International Conference on the Theory and Applications of Cryptographic Techniques , 33–48 (1996).
Hoffstein, J., Pipher, J. & Silverman, J. H. Ntru: a ring-based public key cryptosystem. In International Algorithmic Number Theory Symposium , 267–288 (Springer, 1998).
Regev, O. On lattices, learning with errors, random linear codes, and cryptography. J. ACM (JACM) 56 , 34 (2009).
Jao, D. & De Feo, L. Towards quantum-resistant cryptosystems from supersingular elliptic curve isogenies. PQCrypto 7071 , 19–34 (2011).
MathSciNet MATH Google Scholar
Nielsen, M. A. & Chuang, I. Quantum computation and quantum information (2002).
Satoh, T. et al. Attacking the quantum internet. IEEE Trans. Quant. Eng. 2 , 1–17 (2021).
Iwakoshi, T. Security evaluation of y00 protocol based on time-translational symmetry under quantum collective known-plaintext attacks. IEEE Access 9 , 31608–31617 (2021).
Giraldo, J., Sarkar, E., Cardenas, A. A., Maniatakos, M. & Kantarcioglu, M. Security and privacy in cyber-physical systems: a survey of surveys. IEEE Des. Test 34 , 7–17 (2017).
Barbeau, M. & Garcia-Alfaro, J. Supplementary material to: Cyber-physical defense in the quantum Era. https://github.com/jgalfaro/DL-PoC (2021).
Schneier, B. Modelling security threats. Dr. Dobb’s Journal (1999).
Lallie, H. S., Debattista, K. & Bal, J. A review of attack graph and attack tree visual syntax in cyber security. Comput. Sci. Rev. 35 , 100219 (2020).
Heule, M. J. & Kullmann, O. The science of brute force. Commun. ACM 60 , 70–79 (2017).
Arnold, F., Hermanns, H., Pulungan, R. & Stoelinga, M. Time-dependent analysis of attacks. In International Conference on Principles of Security and Trust , 285–305 (Springer, 2014).
Hoffman, D. & Karst, O. J. The theory of the rayleigh distribution and some of its applications. J. Ship Res. 19 , 172–191 (1975).
Gudbjartsson, H. & Patz, S. The rician distribution of noisy mri data. Magn. Reson. Med. 34 , 910–914 (1995).
Article CAS Google Scholar
Arnold, F., Pieters, W. & Stoelinga, M. Quantitative penetration testing with item response theory. In 2013 9th International Conference on Information Assurance and Security (IAS) , 49–54 (IEEE, 2013).
Chio, C. & Freeman, D. Machine Learning and Security: Protecting Systems with Data and Algorithms (O’Reilly Media, 2018).
Biamonte, J. et al. Quantum machine learning. Nature 549 , 195–202 (2017).
Article ADS CAS Google Scholar
Schuld, M. & Killoran, N. Quantum machine learning in feature Hilbert spaces. Phys. Rev. Lett. 122 , 040504 (2019).
Havlíček, V. et al. Supervised learning with quantum-enhanced feature spaces. Nature 567 , 209–212 (2019).
Article ADS Google Scholar
Schuld, M. & Petruccione, F. Supervised Learning with Quantum Computers . Quantum science and technology (Springer, 2018).
Montangero, S. Introduction to Tensor Network Methods: Numerical Simulations of Low-Dimensional Many-Body Quantum Systems (Springer International Publishing, 2018).
Book Google Scholar
Huggins, W., Patil, P., Mitchell, B., Whaley, K. B. & Stoudenmire, E. M. Towards quantum machine learning with tensor networks. Quant. Sci. Technol. 4 , 024001 (2019).
Yen-Chi Chen, S. et al. Variational quantum circuits for deep reinforcement learning. IEEE Access 141007–141024 (2020).
Lockwood, O. & Si, M. Reinforcement learning with quantum variational circuit. Proc. AAAI Conf. Artif. Intell. Interact. Digit. Entertain. 16 , 245–251 (2020).
Barbeau, M., Cuppens, F., Cuppens, N., Dagnas, R. & Garcia-Alfaro, J. Resilience estimation of cyber-physical systems via quantitative metrics. IEEE Access 9 , 46462–46475 (2021).
Bellman, R. A Markovian decision process. J. Math. Mech. 6 , 679–684 (1957).
Puterman, M. Markov Decision Processes: Discrete Stochastic Dynamic Programming . Wiley Series in Probability and Statistics (Wiley, 2014).
Watkins, C. J. C. H. Learning from delayed rewards. PhD thesis, King’s College, University of Cambridge (1989).
Watkins, C. J. C. H. & Dayan, P. Q-learning. Machine Learning 8 , 279–292 (1992).
Article MATH Google Scholar
Bergholm, V. et al. Pennylane: Automatic differentiation of hybrid quantum-classical computations. arXiv preprint arXiv:1811.04968 (2020).
Farhi, E. & Neven, H. Classification with quantum neural networks on near term processors (2018). arXiv:1802.06002 .
Weerakkody, S. et al. Resilient control in cyber-physical systems: countering uncertainty, constraints, and adversarial behavior. Found. Trends Syst. Control 7 , 1–252 (2019).
Segovia-Ferreira, M., Rubio-Hernan, J., Cavalli, R. & Garcia-Alfaro, J. Switched-based resilient control of cyber-physical systems. IEEE Access 8 , 212194–212208 (2020).
LeCun, Y., Bengio, Y. & Hinton, G. Deep learning. Nature 521 , 436–444 (2015).
Mnih, V. et al. Human-level control through deep reinforcement learnin. Nature 518 , 529–533 (2015).
Mnih, V. et al. Asynchronous methods for deep reinforcement learning. In International conference on machine learning , 1928–1937 (PMLR, 2016).
Barbeau, M. et al. The quantum what? advantage, utopia or threat? Digitale Welt 4 , 34–39 (2021).
Barbeau, M. Recognizing drone swarm activities: Classical versus quantum machine learning. Digitale Welt 3 , 45–50 (2019).
Degen, C. L., Reinhard, F. & Cappellaro, P. Quantum sensing. Rev. Mod. Phys. 89 , 035002 (2017).
Article ADS MathSciNet Google Scholar
Dong, D., Chen, C., Li, H. & Tarn, T. Quantum reinforcement learning. IEEE Trans. Syst. Man Cybern. Part B (Cybernetics) 38 , 1207–1220 (2008).
Download references
Acknowledgements
We acknowledge the financial support from the Natural Sciences and Engineering Research Council of Canada (NSERC) and the European Commission (H2020 SPARTA project, under Grant Agreement 830892).
Author information
Authors and affiliations.
School of Computer Science, Carleton University, Ottawa, Canada
- Michel Barbeau
Télécom SudParis, Institut Polytechnique de Paris, Palaiseau, France
- Joaquin Garcia-Alfaro
You can also search for this author in PubMed Google Scholar
Contributions
All authors contributed equally. All authors reviewed the manuscript.
Corresponding authors
Correspondence to Michel Barbeau or Joaquin Garcia-Alfaro .
Ethics declarations
Competing interests.
The authors declare no competing interests.
Additional information
Publisher's note.
Springer Nature remains neutral with regard to jurisdictional claims in published maps and institutional affiliations.
Supplementary Information
Supplementary information 1., supplementary information 2., supplementary information 3., supplementary information 4., rights and permissions.
Open Access This article is licensed under a Creative Commons Attribution 4.0 International License, which permits use, sharing, adaptation, distribution and reproduction in any medium or format, as long as you give appropriate credit to the original author(s) and the source, provide a link to the Creative Commons licence, and indicate if changes were made. The images or other third party material in this article are included in the article's Creative Commons licence, unless indicated otherwise in a credit line to the material. If material is not included in the article's Creative Commons licence and your intended use is not permitted by statutory regulation or exceeds the permitted use, you will need to obtain permission directly from the copyright holder. To view a copy of this licence, visit http://creativecommons.org/licenses/by/4.0/ .
Reprints and permissions
About this article
Cite this article.
Barbeau, M., Garcia-Alfaro, J. Cyber-physical defense in the quantum Era. Sci Rep 12 , 1905 (2022). https://doi.org/10.1038/s41598-022-05690-1
Download citation
Received : 14 June 2021
Accepted : 03 November 2021
Published : 03 February 2022
DOI : https://doi.org/10.1038/s41598-022-05690-1
Share this article
Anyone you share the following link with will be able to read this content:
Sorry, a shareable link is not currently available for this article.
Provided by the Springer Nature SharedIt content-sharing initiative
This article is cited by
Jamming precoding in af relay-aided plc systems with multiple eavessdroppers.
- Zhengmin Kong
- Jiaxing Cui
Scientific Reports (2024)
Scientific Reports (2022)
By submitting a comment you agree to abide by our Terms and Community Guidelines . If you find something abusive or that does not comply with our terms or guidelines please flag it as inappropriate.
Quick links
- Explore articles by subject
- Guide to authors
- Editorial policies
Sign up for the Nature Briefing: AI and Robotics newsletter — what matters in AI and robotics research, free to your inbox weekly.


Cyber-Physical Systems Research Center
The mission of the Cyber-Physical Systems Research Center (CPSRC) is to promote and facilitate collaborative research activities within UCSC as well as with its academic and industrial partners on key cyber-physical systems topics. CPSRC will foster novel foundational and applied research to support applications such as smart cities and buildings, power grids, agriculture, manufacturing, transportation, and connected health.
What are Cyber-Physical Systems?
Cyber-physical systems (CPSs) are built from, and depend upon, the seamless integration of computational algorithms and physical components. These systems combine digital and analog devices, interfaces, sensors, networks, actuators, and computers with the natural environment and with human-made objects and structures. Just as the Internet has transformed the way people interact with information, cyber-physical systems are transforming the way people interact with the physical world. At the same time, the scale and inherent heterogeneity of these systems pose tremendous engineering challenges. New technological approaches are needed to formalize their design, manage and control them in a scalable, efficient, and secure way, and ensure their usability.
CPSRC Reseach Areas
Autonomous systems.
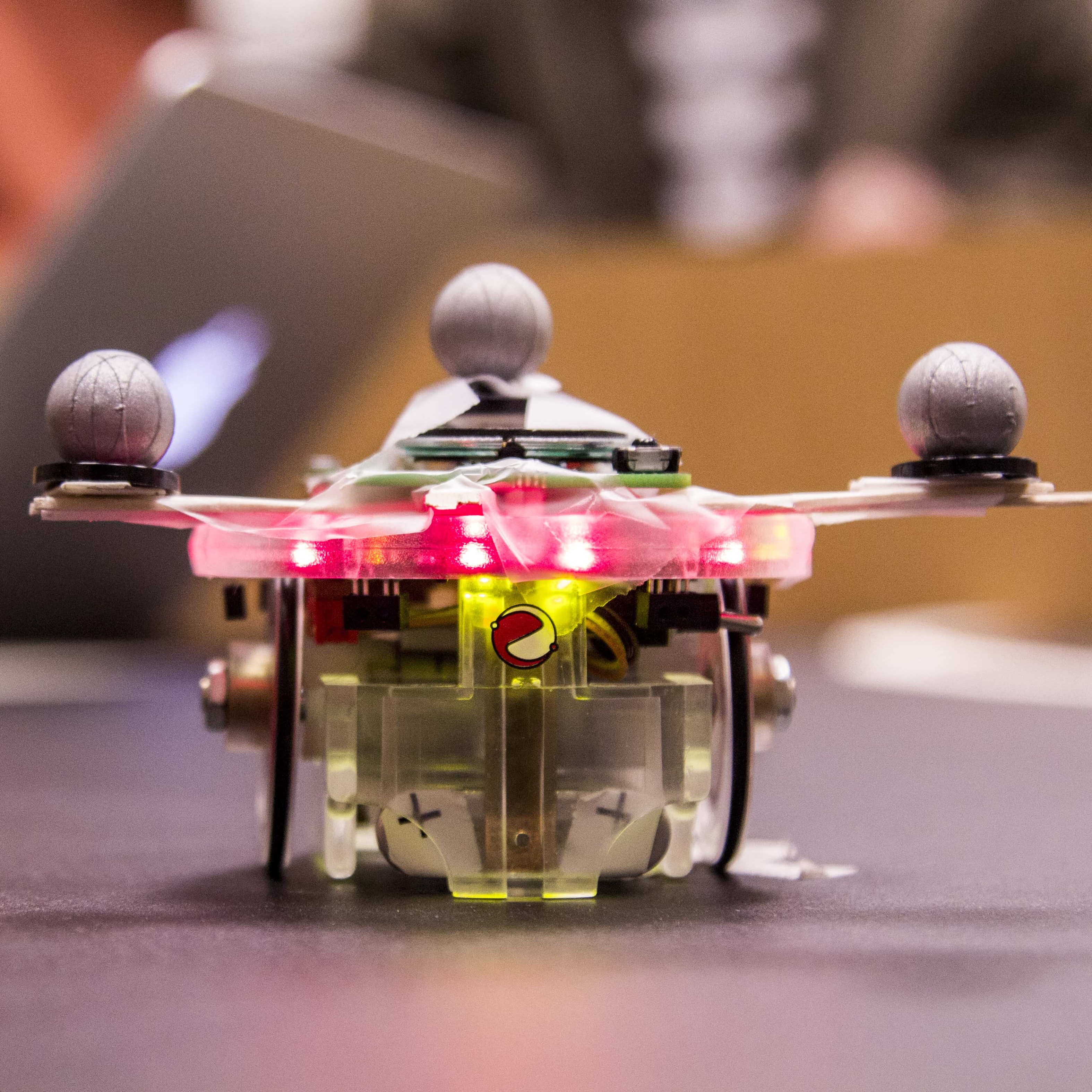
Human sensing
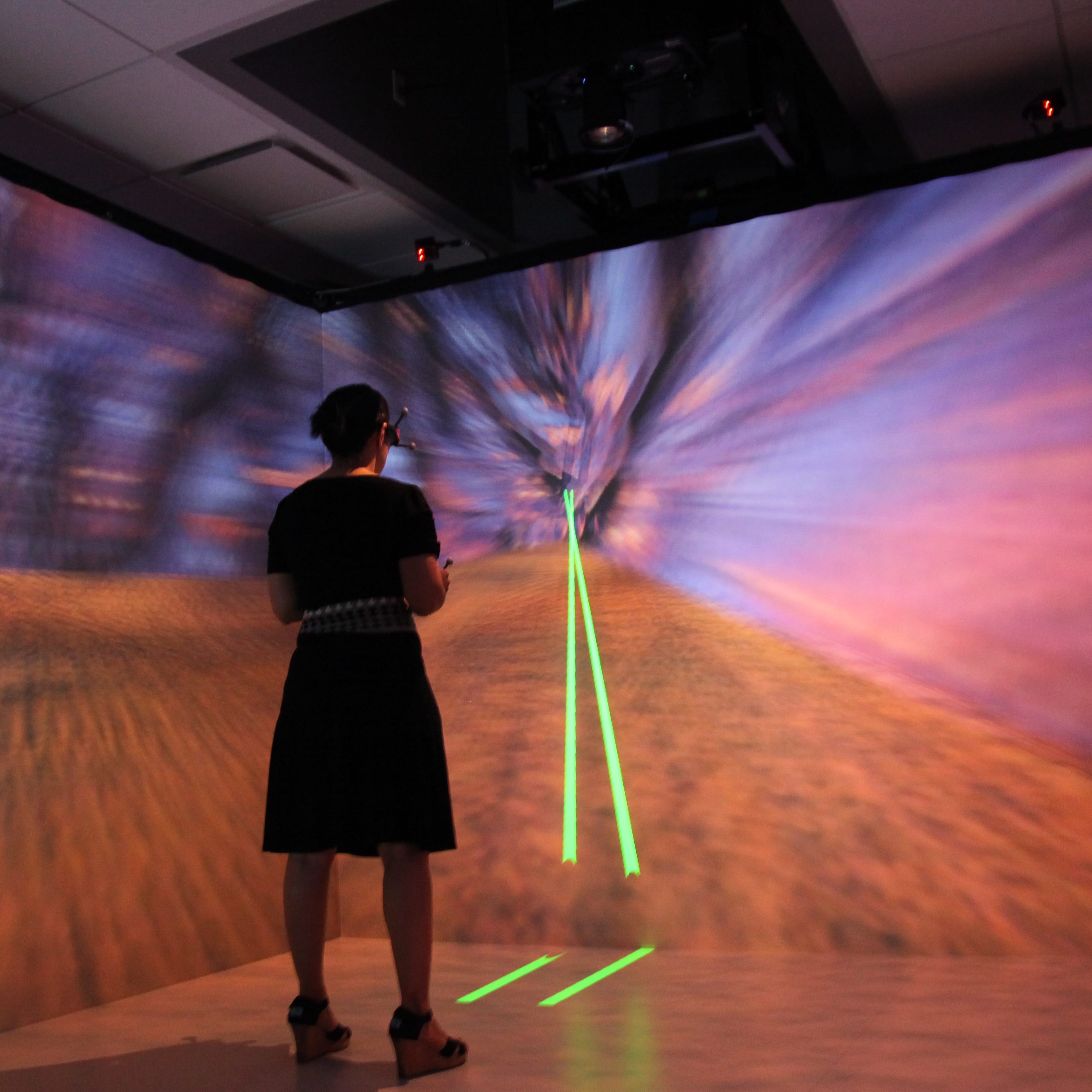
Interconnected Things
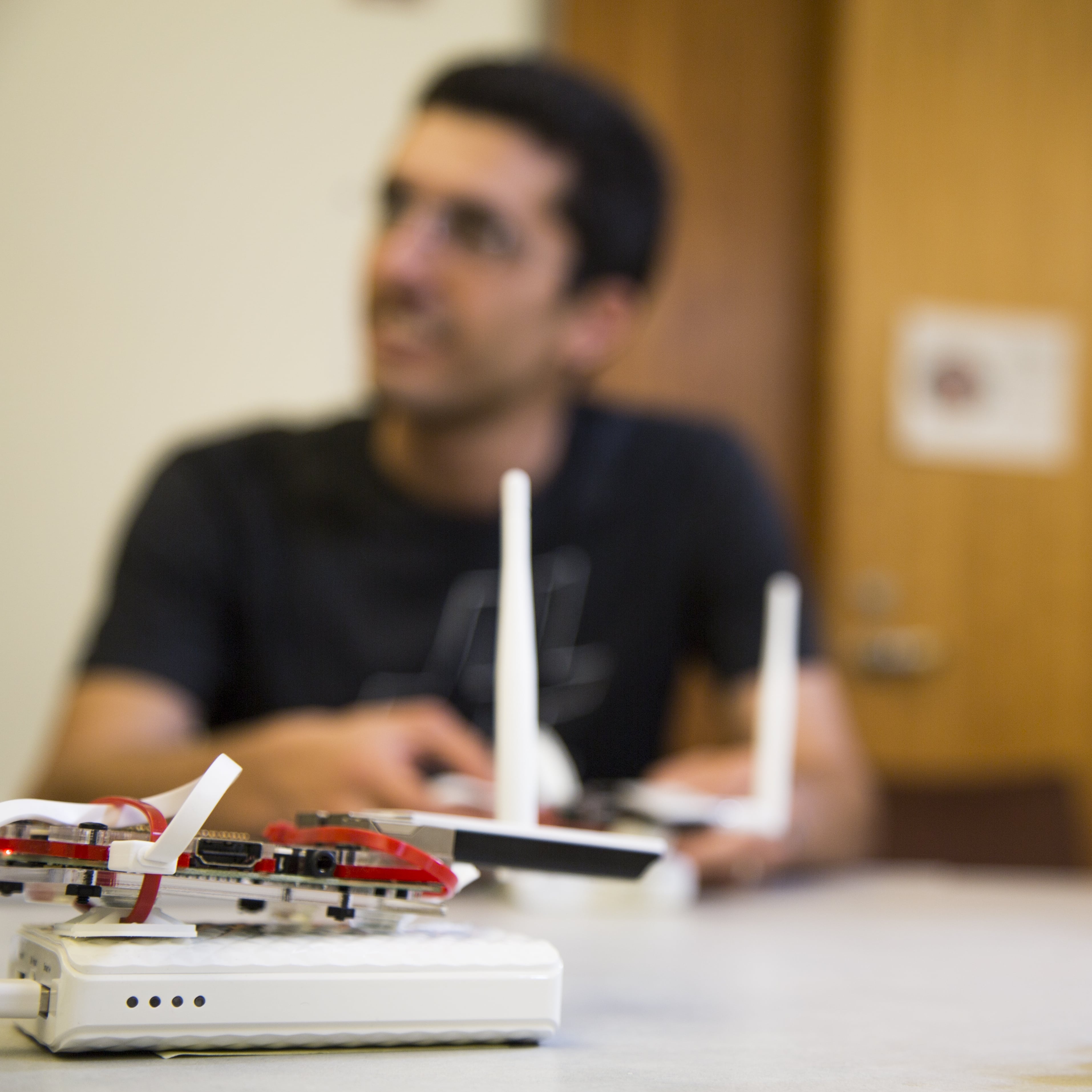
Copyright © 2024,
Designed by Zymphonies
Cyber-physical systems: a bibliometric analysis of literature
- Published: 29 April 2024
Cite this article
- Nitin Singh 1 ,
- Prabin Kumar Panigrahi 2 ,
- Zuopeng Zhang 3 &
- Sajjad M. Jasimuddin ORCID: orcid.org/0000-0003-2627-9241 4
357 Accesses
2 Citations
Explore all metrics
Recently, there is a significant growth in the use of the Cyber-Physical System (CPS). New technologies such as Internet of Things (IoT), Industry 4.0, and Analytics have become enablers of CPS implementation. Study of the development and application of CPS in the supply chain context is valuable to operations management and information systems research and practice; especially, a focus on IoT-enabled CPS in production/manufacturing is highly relevant. Knowledge about the research trends of the development and use of CPS for supply chain management supported by new innovations in IT is very limited in the extant literature. The aim of this research is to investigate the research trends of applying CPS in manufacturing. The study encompasses a scientometric analysis of research on deploying the CPS in production systems. Based on a systematic selection process, we collect a total of 245 articles from the Web of Science (WoS) database as the sample for analysis. Using appropriate software, we conduct bibliometric analyses of the sample articles that include citation, cocitation analysis, centrality co-occurrence analysis, and co-authorship analysis. From the bibliometric analysis, we discover major themes of CPS in manufacturing and their evolutions in the extant literature.
This is a preview of subscription content, log in via an institution to check access.
Access this article
Subscribe and save.
- Get 10 units per month
- Download Article/Chapter or eBook
- 1 Unit = 1 Article or 1 Chapter
- Cancel anytime
Price includes VAT (Russian Federation)
Instant access to the full article PDF.
Rent this article via DeepDyve
Institutional subscriptions
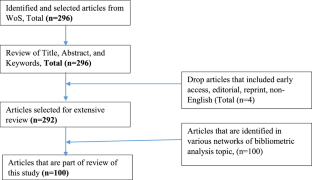
Similar content being viewed by others
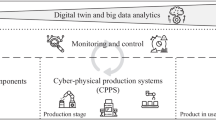
Cyber-Physical Systems in the Context of Industry 4.0: A Review, Categorization and Outlook
Systematic Review on How the Internet of Things will Impact Management in the Manufacturing Industry
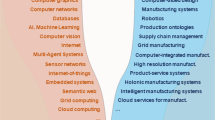
Trends in intelligent manufacturing research: a keyword co-occurrence network based review
Explore related subjects.
- Artificial Intelligence
Ahmad, S., Mehmood, F., Mehmood, A. and Kim, D., (2019). Design and implementation of decoupled IoT application store: A novel prototype for virtual objects sharing and discovery. Electronics , 8(3), 285.
Ali, S., Qaisar, S., Saeed, H., Khan, M., Naeem, M., & Anpalagan, A. (2015). Network challenges for cyber physical systems with tiny wireless devices: A case study on reliable pipeline condition monitoring. Sensors, 15 (4), 7172–7205. https://doi.org/10.3390/s150407172
Article Google Scholar
Andronie, M., Lăzăroiu, G., Ștefănescu, R., Uță, C., & Dijmărescu, I. (2021). Sustainable, smart, and sensing technologies for cyber-physical manufacturing systems: A systematic literature review. Sustainability, 13 (10), 5495. https://doi.org/10.3390/su13105495
Ao, W., Song, Y., & Wen, C. (2020). Distributed secure state estimation and control for CPSs under sensor attacks. IEEE Transactions on Cybernetics, 50 (1), 259–269. https://doi.org/10.1109/tcyb.2018.2868781
Ardanuy, J. (2013). Sixty years of citation analysis studies in the humanities (1951–2010). Journal of the American Society for Information Science and Technology, 64 (8), 1751–1755.
Babiceanu, R., & Seker, R. (2016). Big data and virtualization for manufacturing cyber-physical systems: A survey of the current status and future outlook. Computers in Industry, 81 , 128–137. https://doi.org/10.1016/j.compind.2016.02.004
Bar-Ilan, J. (2010). Introduction to informetrics. Scientometrics, 82 (3), 495–506.
Batagelj, V. & Mrvar, A. (2020). Pajek, Retreived September 2020, from http://vlado.fmf.uni-lj.si/pub/networks/pajek/ .
Bi, Z., Xu, L. D., & Wang, C. (2014). Internet of things for enterprise systems of modern manufacturing. IEEE Transactions on Industrial Informatics, 10 (2), 1537–1546. https://doi.org/10.1109/tii.2014.2300338
Bi, Z., Da Xu, L. and Wang, C., (2014). Internet of things for enterprise systems of modern manufacturing. IEEE Transactions on Industrial Informatics , 10(2), 1537–1546.
Bibby, L., & Dehe, B. (2018). Defining and assessing industry 4.0 maturity levels—case of the defence sector. Production Planning & Control, 29 (12), 1030–1043. https://doi.org/10.1080/09537287.2018.1503355
Blanco-Novoa, O., Fernandez-Carames, T., Fraga-Lamas, P., & Vilar-Montesinos, M. (2018). A practical evaluation of commercial industrial augmented reality systems in an industry 4.0 shipyard. IEEE Access, 6 , 8201–8218. https://doi.org/10.1109/access.2018.2802699
Bonilla, S., Silva, H., Terra da Silva, M., Franco Gonçalves, R., & Sacomano, J. (2018). Industry 4.0 and sustainability implications: A scenario-based analysis of the impacts and challenges. Sustainability, 10 (10), 3740. https://doi.org/10.3390/su10103740
Borgatti, S., & Halgin, D. (2011). On network theory. Organization Science, 22 (5), 1168–1181. https://doi.org/10.1287/orsc.1100.0641
Borgatti, S., Mehra, A., Brass, D., & Labianca, G. (2009). Network analysis in the social sciences. Science, 323 (5916), 892–895. https://doi.org/10.1126/science.1165821
Buer, S., Strandhagen, J., & Chan, F. (2018). The link between Industry 4.0 and lean manufacturing: Mapping current research and establishing a research agenda. International Journal of Production Research, 56 (8), 2924–2940. https://doi.org/10.1080/00207543.2018.1442945
Chaari, R., Ellouze, F., Koubaa, A., Qureshi, B., Pereira, N., Youssef, H., & Tovar, E. (2016). Cyber-physical systems clouds: A survey. Computer Networks, 108 , 260–278.
Checa, D., Urbikain, G., Beranoagirre, A., Bustillo, A., & de Lacalle, L. N. L. (2022). Using machine-learning techniques and virtual reality to design cutting tools for energy optimization in milling operations. International Journal of Computer Integrated Manufacturing, 35 (9), 951–971. https://doi.org/10.1080/0951192X.2022.2027020
Chen, Y. (2017). Integrated and intelligent manufacturing: perspectives and enablers. Engineering, 3 (5), 588–595. https://doi.org/10.1016/j.eng.2017.04.009
Chen, B., Ho, D., Hu, G., & Yu, L. (2018a). Secure fusion estimation for bandwidth constrained cyber-physical systems under replay attacks". IEEE Transactions on Cybernetics, 48 (6), 1862–1876. https://doi.org/10.1109/tcyb.2017.2716115
Chen, B., Wan, J., Shu, L., Li, P., Mukherjee, M., & Yin, B. (2018b). Smart factory of industry 4.0: Key technologies application case, and challenges. IEEE Access, 6 , 6505–6519. https://doi.org/10.1109/access.2017.2783682
Cheng, J., Chen, W., Tao, F., & Lin, C. (2018). Industrial IoT in 5G environment towards smart manufacturing. Journal of Industrial Information Integration, 10 , 10–19. https://doi.org/10.1016/j.jii.2018.04.001
Choi, S., Woo, J., Kim, J., & Lee, J. (2022). Digital twin-based integrated monitoring system: Korean application cases. Sensors, 22 (14), 5450. https://doi.org/10.3390/s22145450
Dalpe, R. (2002). Bibliometric analysis of biotechnology. Scientometrics, 55 (2), 189–213.
Ebadpour, M., Jamshidi, M., Talla, J., Hashemi-Dezaki, H., & Peroutka, Z. (2023). Digital twin model of electric drives empowered by EKF. Sensors, 23 (4), 2006. https://doi.org/10.3390/s23042006
Egghe, L., & Rousseau, R. (1990). Introduction to informetrics: Quantitative methods in library, documentation and information science (p. 19). Elsevier Science Publishers.
Google Scholar
Fang, C., Liu, X., Pardalos, P., & Pei, J. (2015). Optimization for a three-stage production system in the internet of things: Procurement, production and product recovery, and acquisition. The International Journal of Advanced Manufacturing Technology, 83 (5–8), 689–710. https://doi.org/10.1007/s00170-015-7593-1
Fernandez-Carames, T., & Fraga-Lamas, P. (2018). A review on human-centered iot-connected smart labels for the industry 4.0. IEEE Access, 6 , 25939–25957. https://doi.org/10.1109/access.2018.2833501
Fernández-Caramés, T., Fraga-Lamas, P., Suárez-Albela, M., & Díaz-Bouza, M. (2018). A fog computing based cyber-physical system for the automation of pipe-related tasks in the industry 4.0 shipyard. Sensors, 18 (6), 1961. https://doi.org/10.3390/s18061961
Fraga-Lamas, P., & Fernandez-Carames, T. (2019). A review on blockchain technologies for an advanced and cyber-resilient automotive industry. IEEE Access, 7 , 17578–17598. https://doi.org/10.1109/access.2019.2895302
Fraga-Lamas, P., Noceda-Davila, D., Fernández-Caramés, T., Díaz-Bouza, M., & Vilar-Montesinos, M. (2016). Smart pipe system for a shipyard 4. 0. Sensors . https://doi.org/10.3390/s16122186
Frank, A., Dalenogare, L., & Ayala, N. (2019). Industry 4.0 technologies: Implementation patterns in manufacturing companies. International Journal of Production Economics, 210 , 15–26. https://doi.org/10.1016/j.ijpe.2019.01.004
Freeman, L. (1978). Centrality in social networks conceptual clarification. Social Networks, 1 (3), 215–239. https://doi.org/10.1016/0378-8733(78)90021-7
Garfieldm, E. (1983). Citation indexing . ISI Press.
Giraldo, J., Sarkar, E., Cardenas, A., Maniatakos, M., & Kantarcioglu, M. (2017). Security and privacy in cyber-physical systems: A survey of surveys. IEEE Design & Test, 34 (4), 7–17. https://doi.org/10.1109/mdat.2017.2709310
Gorecky, D., Khamis, M., & Mura, K. (2015). Introduction and establishment of virtual training in the factory of the future. International Journal of Computer Integrated Manufacturing, 30 (1), 1–9. https://doi.org/10.1080/0951192x.2015.1067918
Gruner, S., Pfrommer, J., & Palm, F. (2016). RESTful industrial communication with OPC UA. IEEE Transactions on Industrial Informatics, 12 (5), 832–1841. https://doi.org/10.1109/tii.2016.2530404
H. Lasi, P, Fettke, H-G. Kemper, T. Feld, M. Hoffmann. (2014) Industrie 4.0. Wirtschaftsinformatik, 56(4), 261–264.
Hehenberger, P., Vogel-Heuser, B., Bradley, D., Eynard, B., Tomiyama, T., & Achiche, S. (2016). Design, modelling, simulation and integration of cyber physical systems: Methods and applications. Computers in Industry, 82 , 273–289. https://doi.org/10.1016/j.compind.2016.05.006
Hofmann, E., & Rüsch, M. (2017). Industry 4.0 and the current status as well as future prospects on logistics. Computers in Industry, 89 , 23–34. https://doi.org/10.1016/j.compind.2017.04.002
Hsieh, F. (2021). A dynamic context-aware workflow management scheme for cyber-physical systems based on multi-agent system architecture. Applied Sciences, 11 (5), 2030. https://doi.org/10.3390/app11052030
Huang, X., & Dong, J. (2018). Reliable control policy of cyber-physical systems against a class of frequency-constrained sensor and actuator attacks. IEEE Transactions on Cybernetics, 48 (12), 3432–3439. https://doi.org/10.1109/tcyb.2018.2815758
Humayed, A., Lin, J., Li, F., & Luo, B. (2017). Cyber-physical systems security—a survey". IEEE Internet of Things Journal, 4 (6), 1802–1831. https://doi.org/10.1109/jiot.2017.2703172
J. Lee, H. D. Ardakani, S. Yang, B. Bagheri. (2015) Industrial big data analytics and cyber-physical systems for future mainte- nance & service innovation. Procedia CIRP , Proceedings of the 4th International Conference on Through-life Engineering Services, 38, 3–7.
J. Sztipanovits, S. Ying, I. Cohen, D. Corman, J. Davis, H. Khurana, P. J. Mosterman, V. Prasad, & L. Stormo, (2012). Strategic R&D opportunities for 21st century cyber-physical systems, Technical report, steering committee for foundation in innovation for cyber-physical systems. Chicago, IL, USA
Jamwal, A., Agrawal, R., Sharma, M., & Giallanza, A. (2021). Industry 4.0 technologies for manufacturing sustainability: A systematic review and future research directions. Applied Sciences, 11 (12), 5725. https://doi.org/10.3390/app11125725
Jirkovsky, V., Obitko, M., & Marik, V. (2017). Understanding data heterogeneity in the context of cyber-physical systems integration. IEEE Transactions on Industrial Informatics, 13 (2), 660–667. https://doi.org/10.1109/tii.2016.2596101
Kamble, S., Gunasekaran, A., & Gawankar, S. (2018b). Sustainable industry 4.0 framework: A systematic literature review identifying the current trends and future perspectives. Process Safety and Environmental Protection, 117 , 408–425. https://doi.org/10.1016/j.psep.2018.05.009
Kamble, S., Gunasekaran, A., & Sharma, R. (2018a). Analysis of the driving and dependence power of barriers to adopt industry 4.0 in Indian manufacturing industr. Computers in Industry, 101 , 107–119. https://doi.org/10.1016/j.compind.2018.06.004
Kang, H., Lee, J. Y., Choi, S., Kim, H., Park, J. H., Son, J. Y., Kim, B. H., & Noh, S. D. (2016). Smart manufacturing: Past research, present findings, and future directions. International Journal of Precision Engineering and Manufacturing-Green Technology, 3 (1), 111–128. https://doi.org/10.1007/s40684-016-0015-5
Khalaj, O., Jamshidi, M., Hassas, P., Mašek, B., Štadler, C., & Svoboda, J. (2023). Digital twinning of a magnetic forging holder to enhance productivity for industry 4.0 and metaverse. Processes, 11 (6), 1703. https://doi.org/10.3390/pr11061703
Kim, J., Seo, D., Moon, J., Kim, H., Jeong, J., Kim, J., & Lee, J. (2022). Design and implementation of an HCPS-based PCB smart factory system for next-generation intelligent manufacturing. Applied Sciences, 12 (15), 7645. https://doi.org/10.3390/app12157645
Kusiak, A. (2017). Smart manufacturing. International Journal of Production Research, 56 (1–2), 508–517. https://doi.org/10.1080/00207543.2017.1351644
Labucay, I. (2022). Is there a smart sustainability transition in manufacturing? Tracking externalities in machine tools over three decades. Sustainability, 14 (2), 838. https://doi.org/10.3390/su14020838
Landherr, A., Friedl, B., & Heidemann, J. (2010). A critical review of centrality measures in social networks. Business & Information Systems Engineering, 2 (6), 371–385. https://doi.org/10.1007/s12599-010-0127-3
Lee, H. (2017). Framework and development of fault detection classification using IoT device and cloud environment. Journal of Manufacturing Systems, 43 , 257–270. https://doi.org/10.1016/j.jmsy.2017.02.007
Lee, G., Kim, M., Quan, Y. J., Kim, M. S., Kim, T. I. J., Yoon, H. S., Min, S., Kim, D. H., Mun, J. W., Oh, J. W., & Choi, I. G. (2018). Machine health management in smart factory: A review. Journal of Mechanical Science and Technology, 32 (3), 987–1009. https://doi.org/10.1007/s12206-018-0201-1
Lee, J., Noh, S., Kim, H., & Kang, Y. (2018). Implementation of cyber-physical production systems for quality prediction and operation control in metal casting. Sensors, 18 (5), 1428. https://doi.org/10.3390/s18051428
Lee, J., Davari, H., Singh, J. and Pandhare, V., (2018). Industrial artificial intelligence for industry 4.0-based manufacturing systems. Manufacturing Letters , 18, 20–23.
Lee, J., Kao, H.A. and Yang, S., (2014). Service innovation and smart analytics for industry 4.0 and big data environment. Procedia Cirp , 16, 3–8.
Lee, J., Ardakani, H.D., Yang, S. and Bagheri, B., (2015). Industrial big data analytics and cyber-physical systems for future maintenance & service innovation. Procedia Cirp , 38, 3-7.
Lee, E. (2008). Cyber physical systems: Design challenges, In 11th IEEE International Symposium on Object and Component-Oriented Real-Time Distributed Computing, 363–369.
Li, L. (2018). China’s manufacturing locus in 2025: With a comparison of “made-in-China 2025” and “industry 4.0.” Technological Forecasting and Social Change, 135 , 66–74. https://doi.org/10.1016/j.techfore.2017.05.028
Li, J., Burnham, J. F., Lemley, T., & Britton, R. M. (2010). Citation analysis: Comparison of web of science, scopus, scifinder, and google scholar. Journal of Electronic Resources in Medical Libraries, 7 (3), 196–217.
Li, F., & Tang, Y. (2020). False data injection attack for cyber-physical systems with resource constraint. IEEE Transactions on Cybernetics, 50 (2), 729–738. https://doi.org/10.1109/tcyb.2018.2871951
Li, D., Tang, H., Wang, S., & Liu, C. (2017). A big data enabled load-balancing control for smart manufacturing of Industry 4.0. Cluster Computing, 20 (2), 1855–1864. https://doi.org/10.1007/s10586-017-0852-1
Li, X., Wang, Z., Chen, C., & Zheng, P. (2021a). A data-driven reversible framework for achieving sustainable smart product-service systems. Journal of Cleaner Production . https://doi.org/10.1016/j.jclepro.2020.123618
Li, X., Wang, Z., Chen, C. H., & Zheng, P. (2021b). A data-drivenreversible framework for achieving sustainable smart product-service systems. Journal of Cleaner Production, 279 , 123618.
Liao, Y., Deschamps, F., Loures, E., & Ramos, L. (2017). Past, present and future of Industry 4. 0—a systematic literature review and research agenda proposal. International Journal of Production Research, 55 (12), 3609–3629. https://doi.org/10.1080/00207543.2017.1308576
Lin, W. Y. C. (2012). Research status and characteristics of library and information science in Taiwan: A bibliometric analysis. Scientometrics, 92 (1), 7–21.
Lin, Y., Hung, M. H., Huang, H. C., Chen, C. C., Yang, H. C., Hsieh, Y. S., & Cheng, F. T. (2017). Development of advanced manufacturing cloud of things (AMCoT)—a smart manufacturing platform. IEEE Robotics and Automation Letters, 2 (3), 1809–1816. https://doi.org/10.1109/lra.2017.2706859
Lin, Z., Shi-long, W., Jun-wei, TFC, Xiao-dan, J., Cao, J.W., Jiang, X.D., Song, X. Chai, X.D., (2010). Cloud manufacturing: A new service-oriented networked manufacturing model. Computer Integrated Manufacturing System , 16(01), 0.
Liu, G., Yang, H., Fu, H., Mao, C., Xu, P., Hong, J., & Li, R. (2020). Cyber-physical system-based real-time monitoring and visualization of greenhouse gas emissions of prefabricated construction. Journal of Cleaner Production, 246 , 119059. https://doi.org/10.1016/j.jclepro.2019.119059
Liu, Y., Zhang, Y., Ren, S., Yang, M., Wang, Y., & Huisingh, D. (2020a). How can smart technologies contribute to sustainable product lifecycle management? Journal of Cleaner Production, 249 , 119423. https://doi.org/10.1016/j.jclepro.2019.119423
Liu, Y., Zhang, Y., Ren, S., Yang, M., Wang, Y., & Huisingh, D. (2020b). How can smart technologies contribute to sustainable product lifecycle management? Journal of Cleaner Production, 249 , 119423. https://doi.org/10.1016/j.jclepro.2019.119423
Magalhães, L. C., Magalhães, L. C., Ramos, J. B., Moura, L. R., de Moraes, R. E. N., Gonçalves, J. B., Hisatugu, W. H., Souza, M. T., de Lacalle, L. N. L., & Ferreira, J. C. E. (2022). Conceiving a digital twin for a flexible manufacturing system. Applied Sciences, 12 (19), 9864. https://doi.org/10.3390/app12199864
Masood, T., & Egger, J. (2019). Augmented reality in support of industry 4.0—implementation challenges and success factors. Robotics and Computer-Integrated Manufacturing, 58 , 181–195. https://doi.org/10.1016/j.rcim.2019.02.003
Masood, T., & Egger, J. (2020). Adopting augmented reality in the age of industrial digitalisation. Computers in Industry, 115 , 103112. https://doi.org/10.1016/j.compind.2019.07.002
Masood, T., Egger, J., & Kern, M. (2018). Future-proofing the through-life engineering service systems. Procedia Manufacturing, 16 , 179–186. https://doi.org/10.1016/j.promfg.2018.10.162
Meier, H., Roy, R. Seliger, G., (2010). Industrial product-service systems—IPS2. CIRP Annals , 59(2), 607–627.
Merton, R. K. (1968). Social theory and social structure . The Free Press.
Miorandi, D., Sicari, S., De Pellegrini, F. and Chlamtac, I., (2012). Internet of things: Vision, applications and research challenges. Ad Hoc Networks , 10(7), 1497-1516.
Moghaddam, M., Cadavid, M., Kenley, C., & Deshmukh, A. (2018). Reference architectures for smart manufacturing: A critical review. Journal of Manufacturing Systems, 49 , 215–225. https://doi.org/10.1016/j.jmsy.2018.10.006
Moghaddam, M., & Nof, S. (2017). Collaborative service-component integration in cloud manufacturing. International Journal of Production Research, 56 (1–2), 677–691. https://doi.org/10.1080/00207543.2017.1374574
Monostori, L. (2014). )Cyber-physical production systems: Roots, expectations and R&D challenge". Procedia CIRP, 17 , 9–13. https://doi.org/10.1016/j.procir.2014.03.115
Monostori, L., Kádár, B., Bauernhansl, T., Kondoh, S., Reinhart, G., Sauer, O., Schuh, G., Sihn, W., & Ueda, K. (2016). Cyber-physical systems in manufacturing. CIRP Annals—Manufacturing Technology, 65 (2), 621–641.
Moral-Muñoz, J., Herrera-Viedma, E., Santisteban-Espejo, A., & Cobo, M. J. (2020). Software tools for conducting bibliometric analysis in science: An up-to-date review. El Profesional De La Información, 29 (1), e290103.
Mourtzis, D., & Vlachou, E. (2018). A cloud-based cyber-physical system for adaptive shop-floor scheduling and condition-based maintenance. Journal of Manufacturing Systems, 47 , 179–198. https://doi.org/10.1016/j.jmsy.2018.05.008
Mousavinejad, E., Yang, F., Han, Q., & Vlacic, L. (2018). A novel cyber attack detection method in networked control systems". IEEE Transactions on Cybernetics, 48 (11), 3254–3264. https://doi.org/10.1109/tcyb.2018.2843358
Moustafa, N., Adi, E., Turnbull, B., & Hu, J. (2018). A new threat intelligence scheme for safeguarding industry 4.0 systems. IEEE Access, 6 , 32910–32924. https://doi.org/10.1109/access.2018.2844794
Nagy, J., Oláh, J., Erdei, E., Máté, D., & Popp, J. (2018). The role and impact of industry 4.0 and the internet of things on the business strategy of the value chain—the case of Hungary. Sustainability, 10 (10), 3491. https://doi.org/10.3390/su10103491
Nalimov, V. V. (1966). Quantitative methods of research of scientific evolution. Voprosy Filisofii, 12 , 38–47.
Oks, S. J., Jalowski, M., Lechner, M., Mirschberger, S., Merklein, M., Vogel-Heuser, B., & Möslein, K. M. (2022). Cyber-physical systems in the context of industry 4.0: A review, categorization and outlook. Information Systems Frontiers . https://doi.org/10.1007/s10796-022-10252-x
del Olmo, A., López de Lacalle, L. N., Martínez de Pissón, G., Pérez-Salinas, C., Ealo, J. A., Sastoque, L., Fernandes, M. H., & Posada, J. (2022). Tool wear monitoring of high-speed broaching process with carbide tools to reduce production errors. Mechanical Systems and Signal Processing, 172 , 109003. https://doi.org/10.1016/j.ymssp.2022.109003
Oxman, A. D., & Guyatt, G. H. (1993). The science of reviewing research. Annals of the New York Academy of Sciences . https://doi.org/10.1111/j.1749-6632.1993.tb26342.x
Petrillo, A., Pescape, A., & Santini, S. (2021). A secure adaptive control for cooperative driving of autonomous connected vehicles in the presence of heterogeneous communication delays and cyberattacks. IEEE Transactions on Cybernetics, 51 (3), 1134–1149. https://doi.org/10.1109/tcyb.2019.2962601
Pinzone, M., Pinzone, M., Albè, F., Orlandelli, D., Barletta, I., Berlin, C., Johansson, B., & Taisch, M. (2020). A framework for operative and social sustainability functionalities in human-centric cyber-physical production systems. Computers and Industrial Engineering . https://doi.org/10.1016/j.cie.2018.03.028
Pivoto, D., Fernandes, L., Righi, R., Rodrigues, J., Lugli, A., & Alberti, A. (2020). Cyber-physical systems architectures for industrial internet of things applications in industry 4.0: A literature review. Journal of Manufacturing Systems, 58 , 176. https://doi.org/10.1016/j.jmsy.2020.11.017
Preuveneers, D., & Ilie-Zudor, E. (2017). The intelligent industry of the future: A survey on emerging trends, research challenges and opportunities in Industry 4.0. Journal of Ambient Intelligence and Smart Environments, 9 (3), 287–298. https://doi.org/10.3233/ais-170432
Ramasubramanian, A., Mathew, R., Kelly, M., Hargaden, V., & Papakostas, N. (2022). Digital twin for human-robot collaboration in manufacturing: Review and outlook. Applied Sciences, 12 (10), 4811. https://doi.org/10.3390/app12104811
Rehman, M. H., Yaqoob, U. R., Salah, I., Imran, K., Jayaraman, M., & Perera, P. (2019). The role of big data analytics in industrial internet of things. Future Generation Computer Systems, 99 , 247–259. https://doi.org/10.1016/j.future.2019.04.020
Rho, S., Vasilakos, A., & Chen, W. (2016). Cyber physical systems technologies and applications. Future Generation Computer Systems, 56 , 436–437. https://doi.org/10.1016/j.future.2015.10.019
Riel, A., Kreiner, C., Macher, G., & Messnarz, R. (2017). Integrated design for tackling safety and security challenges of smart products and digital manufacturing. CIRP Annals, 66 (1), 177–180. https://doi.org/10.1016/j.cirp.2017.04.037
Scopus. Retrieved February, 2023, from https://www.scopus.com/search/form.uri?display=basic#basic .
Sharma, H., Srivastava, P., Jasimuddin, S. M., Zhang, Z., & Jebabli, I. (2023). Privacy concerns in tourism: A bibliometric analysis. Tourism Review . https://doi.org/10.1108/TR-10-2022-0517
Small, H. (2007). Co-citation in the scientific literature: A new measure of the relationship between two documents. Journal of the American Society for Information Science, 24 (4), 265–269. https://doi.org/10.1002/asi.4630240406
Song, Z., & Moon, Y. (2016). Assessing sustainability benefits of cybermanufacturing systems. The International Journal of Advanced Manufacturing Technology, 90 (5–8), 1365–1382. https://doi.org/10.1007/s00170-016-9428-0
Srivastava, P., Zhang, Z., Eachempati, P., Trivedi, S., & Jasimuddin, S. M. (2022). The response of the scientific community to a global crisis: A systematic review of COVID-19 research in 2020. British Journal of Healthcare Management, 28 (2), 1–7.
Stock, T. Seliger, G., (2016). Opportunities of sustainable manufacturing in industry 4.0. Procedia CIRP , 40, 536-541.
Tang, H., Li, D., Wang, S., & Dong, Z. (2018). CASOA: An architecture for agent-based manufacturing system in the context of industry 4.0. IEEE Access, 6 , 12746–12754. https://doi.org/10.1109/access.2017.2758160
Tang, Y., Zhang, D., Ho, D., & Qian, F. (2019). Tracking control of a class of cyber-physical systems via a flexray communication network. IEEE Transactions on Cybernetics, 49 (4), 1186–1199. https://doi.org/10.1109/tcyb.2018.2794523
Tao, F., Cheng, J., Cheng, Y., Gu, S., Zheng, T., & Yang, H. (2017). SDMSim: A manufacturing service supply–demand matching simulator under cloud environment. Robotics and Computer-Integrated Manufacturing, 45 , 34–46. https://doi.org/10.1016/j.rcim.2016.07.001
Tao, F., Cheng, J., & Qi, Q. (2018). IIHub: An industrial internet-of-things hub toward smart manufacturing based on cyber-physical system. IEEE Transactions on Industrial Informatics, 14 (5), 2271–2280. https://doi.org/10.1109/tii.2017.2759178
Tao, H., Cheng, L., Qiu, J., & Stojanovic, V. (2022). Few shot cross equipment fault diagnosis method based on parameter optimization and feature metric. Measurement Science and Technology . https://doi.org/10.1088/1361-6501/ac8368
Tao, F., Cheng, Y., Xu, L., Zhang, D., & Li, B. H. (2014). CCIoT-CMfg: Cloud computing and internet of things-based cloud manufacturing service system. IEEE Transactions on Industrial Informatics, 10 (2), 1435–1442. https://doi.org/10.1109/tii.2014.2306383
Tao, F., Liu, W. R., Zhang, M., Hu, T., Qi, Q., & Zhang, H. (2019). Five dimension digital twin model and its ten applications. Computer Integrated Manufacturing Systems, 25 (1), 1–18.
Tao, F., & Qi, Q. (2019). New IT driven service-oriented smart manufacturing: framework and characteristics. IEEE Transactions on Systems, Man, and Cybernetics: Systems, 49 (1), 81–91. https://doi.org/10.1109/tsmc.2017.2723764
Tao, F., & Zhang, M. (2017). Digital twin shop-floor: A new shop-floor paradigm towards smart manufacturing. IEEE Access, 5 , 20418–20427. https://doi.org/10.1109/access.2017.2756069
Thakral, P., Srivastava, P., Dash, S., Jasimuddin, S. M., & Zhang, Z. (2023). Trends in the thematic landscape of HR analytics research: A structural topic modeling approach. Management Decision . https://doi.org/10.1108/MD-01-2023-0080
Thramboulidis, K., & Christoulakis, F. (2016). UML4IoT—A UML-based approach to exploit IoT in cyber-physical manufacturing systems". Computers in Industry, 82 , 259–272. https://doi.org/10.1016/j.compind.2016.05.010
Tu, M., Lim, M., & Yang, M. (2018). IoT-based production logistics and supply chain system—part 1. Industrial Management & Data Systems, 118 (1), 65–95. https://doi.org/10.1108/imds-11-2016-0503
van Eck, N., & Waltman, L. (2009). Software survey: VOSviewer, a computer program for bibliometric mapping. Scientometrics, 84 (2), 523–538. https://doi.org/10.1007/s11192-009-0146-3
van Eck, N., & Waltman, L. (2017). Itation-based clustering of publications using citnetexplorer and VOSviewer. Scientometrics, 111 (2), 1053–1070. https://doi.org/10.1007/s11192-017-2300-7
Wan, J., Chen, B., Imran, M., Tao, F., Li, D., Liu, C., & Ahmad, S. (2018). Toward dynamic resources management for IoT-based manufacturing. IEEE Communications Magazine, 56 (2), 52–59. https://doi.org/10.1109/mcom.2018.1700629
Wan, J., Tang, S., Shu, Z., Li, D., Wang, S., Imran, M., & Vasilakos, A. V. (2016). Software-defined industrial internet of things in the context of industry 4. 0. IEEE Sensors Journal, 16 (20), 1–1. https://doi.org/10.1109/jsen.2016.2565621
Wang, X., Ong, S., & Nee, A. (2017). A comprehensive survey of ubiquitous manufacturing research. International Journal of Production Research, 56 (1–2), 604–628. https://doi.org/10.1080/00207543.2017.1413259
Wang, Y., Song, Y., Hill, D., & Krstic, M. (2019). Prescribed-time consensus and containment control of networked multiagent systems". IEEE Transactions on Cybernetics, 49 (4), 1138–1147. https://doi.org/10.1109/tcyb.2017.2788874
Wang, Y., Song, Y., & Krstic, M. (2017). Collectively rotating formation and containment deployment of multiagent systems: A polar coordinate-based finite time approach. IEEE Transactions on Cybernetics, 47 (8), 2161–2172. https://doi.org/10.1109/tcyb.2016.2624307
Wang, L., Törngren, M., & Onori, M. (2015). Current status and advancement of cyber-physical systems in manufacturing. Journal of Manufacturing Systems, 37 , 517–527. https://doi.org/10.1016/j.jmsy.2015.04.008
Wang, S., Wan, J., Li, D., & Zhang, C. (2016). Implementing smart factory of industrie 4.0: An outlook. International Journal of Distributed Sensor Networks, 12 (1), 3159805. https://doi.org/10.1155/2016/3159805
Wang, J., & Yang, G. (2019). Data-driven methods for stealthy attacks on TCP/IP-based networked control systems equipped with attack detectors. IEEE Transactions on Cybernetics, 49 (8), 3020–3031. https://doi.org/10.1109/tcyb.2018.2837874
Waschull, S., Bokhorst, J., Molleman, E., & Wortmann, J. (2020). Work design in future industrial production: Transforming towards cyber-physical systems. Computers & Industrial Engineering, 139 , 105679. https://doi.org/10.1016/j.cie.2019.01.053
Web of Science Retrieved February, 2023, from https://www.webofscience.com/wos/woscc/basic-search
Weizhong, Y., Mestha, L., & Abbaszadeh, M. (2019). Attack detection for securing cyber physical systems. IEEE Internet of Things Journal . https://doi.org/10.1109/JIOT.2019.2919635
White, H. D., & McCain, K. W. (1998). Visualizing a discipline: An author co-citation analysis of information science, 1972–1995. Journal of the American Society for Information Science, 49 (4), 327–355.
Winkelhaus, S., & Grosse, E. (2019). Logistics 4 0: A systematic review towards a new logistics system. International Journal of Production Research, 58 (1), 18–43. https://doi.org/10.1080/00207543.2019.1612964
Wu, G., Sun, J., & Chen, J. (2018). Optimal data injection attacks in cyber-physical systems. IEEE Transactions on Cybernetics, 48 (12), 3302–3312. https://doi.org/10.1109/tcyb.2018.2846365
Wu, D., Terpenny, J. and Gentzsch, W., (2015). Economic benefit analysis of cloud-based design, engineering analysis, and manufacturing. Journal of Manufacturing Science and Engineering , 137(4), 040903.
Xu, X. (2012). From cloud computing to cloud manufacturing. Robotics and Computer-Integrated Manufacturing, 28 (1), 75–86. https://doi.org/10.1016/j.rcim.2011.07.002
Xu, L., He, W., & Li, S. (2014). Internet of things in industries: A survey". IEEE Transactions on Industrial Informatics, 10 (4), 2233–2243. https://doi.org/10.1109/tii.2014.2300753
Xu, Z., Li, X., & Stojanovic, V. (2021). Exponential stability of nonlinear state-dependent delayed impulsive systems with applications. Nonlinear Analysis: Hybrid Systems . https://doi.org/10.1016/j.nahs.2021.101088
Xu, L., Xu, E., & Li, L. (2018). Industry 4. 0: State of the art and future trends. International Journal of Production Research, 56 (8), 2941–2962. https://doi.org/10.1080/00207543.2018.1444806
Yang, T. (2001). Impulsive control theory. Lecture Notes in Control and Information Sciences . https://doi.org/10.1007/3-540-47710-1
Yang, C., Shi, Z., Zhang, H., Wu, J., & Shi, X. (2020). Multiple attacks detection in cyber-physical systems using random finite set theory. IEEE Transactions on Cybernetics, 50 (9), 4066–4075. https://doi.org/10.1109/tcyb.2019.2912939
Yao, X. F., Lian, Z. T., Yang, Y., Zhang, Y., & Jin, H. (2014). Wisdom manufacturing: New humans-computers-things collaborative manufacturing model. Computer Integrated Manufacturing Systems, 20 (6), 1490.
Yao, X., & Yi, L. (2019). On big data driving manufacturing from “internet plus” to “AI plus.” China Mechanical Engineering, 30 (2), 134–142.
Yao, X., Zhou, J., Lin, Y., Li, Y., Yu, H., & Liu, Y. (2017b). Smart manufacturing based on cyber-physical systems and beyond. Journal of Intelligent Manufacturing, 30 (8), 2805–2817. https://doi.org/10.1007/s10845-017-1384-5
Yao, X., Zhou, J., Zhang, C., & Liu, M. (2017a). Proactive manufacturing-a big-data driven emerging manufacturing paradigm. Computer Integrated Manufacturing Systems, 23 (1), 172–185.
Ye, D., & Zhang, T. (2020). Summation detector for false data-injection attack in cyber-physical systems. IEEE Transactions on Cybernetics, 50 (6), 2338–2345. https://doi.org/10.1109/tcyb.2019.2915124
Zhang, Y., Guo, Z., Lv, J., & Liu, Y. (2018). A framework for smart production-logistics systems based on CPS and industrial IoT. IEEE Transactions on Industrial Informatics, 14 (9), 4019–4032. https://doi.org/10.1109/tii.2018.2845683
Zhang, Q., Liu, K., Xia, Y., & Ma, A. (2020). Optimal stealthy deception attack against cyber-physical systems. IEEE Transactions on Cybernetics, 50 (9), 3963–3972. https://doi.org/10.1109/tcyb.2019.2912622
Zhang, T., & Ye, D. (2020). Distributed secure control against denial-of-service attacks in cyber-physical systems based on K-connected communication topology. IEEE Transactions on Cybernetics, 50 (7), 3094–3103. https://doi.org/10.1109/tcyb.2020.2973303
Zhao, Y., & Zhao, R. (2016). An evolutionary analysis of collaboration networks in scientometrics. Scientometrics, 107 (2), 759–772. https://doi.org/10.1007/s11192-016-1857-x
Zheng, P., Lin, T., Chen, C., & Xu, X. (2018). A systematic design approach for service innovation of smart product-service systems. Journal of Cleaner Production, 201 , 657–667. https://doi.org/10.1016/j.jclepro.2018.08.101
Zheng, P., Wang, H., Sang, Z., Zhong, R. Y., Liu, Y., Liu, C., & Xu, X. (2018). Smart manufacturing systems for Industry 4. 0: Conceptual framework, scenarios, and future perspectives. Frontiers of Mechanical Engineering, 13 (2), 137–150. https://doi.org/10.1007/s11465-018-0499-5
Zhong, H., & Nof, S. (2015). The dynamic lines of collaboration model: Collaborative disruption response in cyber–physical systems. Computers & Industrial Engineering, 87 , 370–382. https://doi.org/10.1016/j.cie.2015.05.019
Zhong, R., Xu, X., Klotz, E., & Newman, S. (2017). Intelligent manufacturing in the context of industry 4.0: A review. Engineering, 3 (5), 616–630. https://doi.org/10.1016/j.eng.2017.05.015
Zhou, J., Li, P., Zhou, Y., Wang, B., Zang, J., & Meng, L. (2018). Toward new-generation intelligent manufacturing. Engineering, 4 (1), 11–20. https://doi.org/10.1016/j.eng.2018.01.002
Zhuang, Z., Tao, H., Chen, Y., Stojanovic, V., & Paszke, W. (2022). Iterative learning control for repetitive tasks with randomly varying trial lengths using successive projection. International Journal of Adaptive Control and Signal Processing . https://doi.org/10.1002/acs.3396
Zhuge, H. (2014). Cyber-physical society—the science and engineering for future society. Future Generation Computer Systems, 32 , 180–186. https://doi.org/10.1016/j.future.2013.10.008
Download references
Acknowledgements
The authors would like to thank Professor Andrew Kusiak (Editor in Chief), the Associate Editor and the anonymous reviewers for their valuable comments and suggestions which helped to significantly improve the quality and presentation of this paper.
Author information
Authors and affiliations.
Indian Institute of Management, Ranchi, India
Nitin Singh
Indian Institute of Management, Indore, India
Prabin Kumar Panigrahi
Department of Management, University of North Florida, Jacksonville, FL, 32224, USA
Zuopeng Zhang
Kedge Business School, Marseille, France
Sajjad M. Jasimuddin
You can also search for this author in PubMed Google Scholar
Corresponding author
Correspondence to Sajjad M. Jasimuddin .
Additional information
Publisher's note.
Springer Nature remains neutral with regard to jurisdictional claims in published maps and institutional affiliations.
Rights and permissions
Springer Nature or its licensor (e.g. a society or other partner) holds exclusive rights to this article under a publishing agreement with the author(s) or other rightsholder(s); author self-archiving of the accepted manuscript version of this article is solely governed by the terms of such publishing agreement and applicable law.
Reprints and permissions
About this article
Singh, N., Panigrahi, P.K., Zhang, Z. et al. Cyber-physical systems: a bibliometric analysis of literature. J Intell Manuf (2024). https://doi.org/10.1007/s10845-024-02380-9
Download citation
Received : 08 September 2022
Accepted : 18 March 2024
Published : 29 April 2024
DOI : https://doi.org/10.1007/s10845-024-02380-9
Share this article
Anyone you share the following link with will be able to read this content:
Sorry, a shareable link is not currently available for this article.
Provided by the Springer Nature SharedIt content-sharing initiative
- Cyber physical systems
- Industry 4.0
- Smart factory
- Intelligent manufacturing
- Internet of things
- Find a journal
- Publish with us
- Track your research

An official website of the United States government
Here’s how you know
Official websites use .gov A .gov website belongs to an official government organization in the United States.
Secure .gov websites use HTTPS A lock ( Lock A locked padlock ) or https:// means you’ve safely connected to the .gov website. Share sensitive information only on official, secure websites.
https://www.nist.gov/cyberphysical-systems
Cyberphysical systems
Cyber-physical systems topics.
- Smart cities
News and Updates
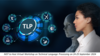
NIST to Host Technical Language Processing Virtual Workshop on 24-25 September 2024
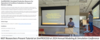
NIST Researchers Present Tutorial on Discrete-Event Manufacturing Simulation Using Open-Source SimPROCESD Environment
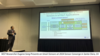
NIST Researcher Provides Insights on Interoperability Testing Methods of Smart Sensors at 2024 Sensor Converge
Projects and programs.
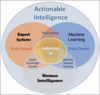
Industrial Artificial Intelligence Management and Metrology (IAIMM)
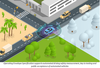
Automated Vehicles and AV Communications
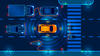
NIST Automated Vehicles Program
Reliable, high performance wireless systems for factory automation, publications, development of a simulation tool for the intelligent building agents project, scalable hla co-simulations of connected and automated vehicles using aggregation of virtual federates, an overarching quality evaluation framework for additive manufacturing digital twin, enhancing digital twins with advances in simulation and artificial intelligence: opportunities and challenges.
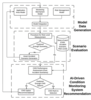

HVAC SIMulation PLUS other systems (HVACSIM+)
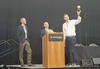
Sokwoo Rhee Receives the Portland Golden Globe Award 2019
Human-in-the-loop Cyber-Physical Systems
Loading... Editorial 14 November 2022 Editorial: Human-in-the-loop cyber-physical systems Kok-Lim Alvin Yau 1,249 views 0 citations
Original Research 26 September 2022 Research on the dynamic viseme of the lip shape based on facial motion capture technology Shengyin Zhu 1,487 views 0 citations
Loading... Original Research 18 July 2022 DeepMatch: Toward Lightweight in Point Cloud Registration Lizhe Qi , 2 more and Yuquan Sun 4,743 views 3 citations
Loading... Original Research 06 July 2022 Spatial-Temporal Attention Mechanism and Graph Convolutional Networks for Destination Prediction Cong Li , 3 more and Fei Yang 6,419 views 6 citations
Original Research 01 July 2022 An Augmented Neural Network for Sentiment Analysis Using Grammar Baohua Zhang , 2 more and Jiahao Cai 3,706 views 0 citations
Loading... Original Research 23 June 2022 Application of Intelligent Inspection Robot in Coal Mine Industrial Heritage Landscape: Taking Wangshiwa Coal Mine as an Example Yan Shen , 1 more and Zengping Li 3,305 views 5 citations
Loading... Original Research 08 April 2022 Color Constancy via Multi-Scale Region-Weighed Network Guided by Semantics Fei Wang , 2 more and Guowang Gao 3,793 views 7 citations

An official website of the United States government
The .gov means it’s official. Federal government websites often end in .gov or .mil. Before sharing sensitive information, make sure you’re on a federal government site.
The site is secure. The https:// ensures that you are connecting to the official website and that any information you provide is encrypted and transmitted securely.
- Publications
- Account settings
Preview improvements coming to the PMC website in October 2024. Learn More or Try it out now .
- Advanced Search
- Journal List
- Sensors (Basel)
- PMC10490255

A Holistic Review of Cyber–Physical–Social Systems: New Directions and Opportunities
Associated data.
No new data were created or analyzed in this study. Data sharing is not applicable to this article.
A Cyber–Physical–Social System (CPSS) is an evolving subset of Cyber–Physical Systems (CPS), which involve the interlinking of the cyber, physical, and social domains within a system-of-systems mindset. CPSS is in a growing state, which combines secure digital technologies with physical systems (e.g., sensors and actuators) and incorporates social aspects (e.g., human interactions and behaviors, and societal norms) to facilitate automated and secure services to end-users and organisations. This paper reviews the field of CPSS, especially in the scope of complexity theory and cyber security to determine its impact on CPS and social media’s influence activities. The significance of CPSS lies in its potential to provide solutions to complex societal problems that are difficult to address through traditional approaches. With the integration of physical, social, and cyber components, CPSS can realize the full potential of IoT, big data analytics, and machine learning, leading to increased efficiency, improved sustainability and better decision making. CPSS presents exciting opportunities for innovation and advancement in multiple domains, improving the quality of life for people around the world. Research challenges to CPSS include the integration of hard and soft system components within all three domains, in addition to sociological metrics, data security, processing optimization and ethical implications. The findings of this paper note key research trends in the fields of CPSS, and recent novel contributions, followed by identified research gaps and future work.
1. Introduction
Cyber–Physical Systems (CPS) are increasingly becoming integration points for computation and are a significant part of people’s daily lives in their homes, businesses, governments, and industry. CPS were developed in order to address modeling challenges for control systems and embedded computing, focusing on efficiency optimization and secure components [ 1 ]. Not only have CPS come to represent industrial control systems, but a wide variety of systems at the interface of the digital and physical worlds, including medical devices, education devices, Internet of Things (IoT), smart cities, and transportation systems, among others [ 2 , 3 , 4 ]. A CPS integrates the computational and physical capabilities of systems, enabling those embedded systems to interact with sensors and actuators that exist in the physical world [ 4 , 5 ]. A CPS is characterized by its ability to interact with the physical world through a combination of control and computation, enabled through communication [ 5 ] (p. 1) and [ 1 ]. The term is broad, spanning several generations of technological growth and application development, and has evolved with technologies such as the Internet of Things (IoT).
CPS have traditionally been focused on systems that involve purely technical and physical components, with humans existing as external entities to the system, considering cyber aspects, including confidentiality, integrity and availability [ 6 ]. A CPS architecture is closely aligned with IoT, consisting of three layers: the perception layer, the network layer, and the application layer [ 4 , 6 , 7 ]. The perception layer is concerned with sensors and actuators that measure and collect data. The network layer is responsible for the communication of data between agents. Finally, the application layer is concerned with the processing of information and decision making. As the field of CPS grows and system boundaries extend beyond traditional Cyber–Physical components, there is the potential need for new architectural paradigms to be considered, especially including a security-by-design layer to be embedded in order to safeguard these layers efficiently.
With the prevalence of social media, a Cyber–Physical–Social System (CPSS) has emerged, concerned with identifying and understanding how humans integrate within systems that encompass the cyber, physical and social space [ 6 , 8 ]. Such systems extend beyond human-in-the-loop systems, where people interact with the system in switch-case-like predefined actions or decision support scenarios [ 9 ]. Instead, CPSSs include an understanding of the social ramifications and their feedback loops within the scope of the system boundary [ 6 ]. CPSSs are designed to offer seamless communication, coordination, and integration between the cyber, physical, and social elements, resulting in more effective system performance. This integration allows systems to adapt and respond to changing conditions, optimise resource allocation, and improve overall system performance and user experiences. A CPSS was defined as a Cyber–Physical System that exhibits the coordination, conjoining and integration of both human and social characteristics within a larger CPS [ 10 ] (p. 85). This further highlighted the complexity of CPSS, being exacerbated by the social system’s inclusion in the wider system boundary. This definition would be linked with three hypotheses, where the physical, mental, and artificial worlds combine, and highlighted the internet as a vector for this integration [ 10 , 11 ]. Pervasive Intelligent Spaces (PIS) would be also added in the definition to enable the interaction of all agents in real time and heavily rely on pervasive IoT technologies later expanded [ 10 ] (p. 86). This research highlights that social media serves as the new revolution for CPSSs, changing the way that societies and industries may function [ 10 ]. Each of the domains incorporated into CPSSs—social, physical, and cyber—individually meet the definition of complex systems. The large-scale and significant numbers of interactions between each of these domains create a strong system-of-systems dynamic [ 12 ]. Considering CPSS approaches therefore requires the use of a system-of-systems mindset, and will continue to do so in the future [ 13 ].
The field of CPSS attempts to bridge the gap between traditional CPS research and social complexity research. As CPS become more integrated into society within a system-of-systems mindset, social factors have the potential to effect the functioning of Cyber–Physical components in unpredictable ways. CPSS provides researchers with the opportunity to solve complex societal problems that are difficult to address using traditional methods. These include attempts to understand and model human behavior in online social media environments in addition to modeling systems that include real-time human feedback such as seen in smart cities and smart transportation [ 14 , 15 ].
Some of the emerging trends in CPSS include the development of smart cities, intelligent transportation systems, and smart healthcare systems [ 16 , 17 , 18 , 19 ]. The significance of CPSS lies in its potential to provide solutions to complex societal problems that are difficult to address through traditional approaches. CPSS can be used in numerous domains such as healthcare, transportation, energy conservation, disaster management, and environmental monitoring [ 17 , 20 , 21 , 22 ]. With the integration of physical, social, and cyber systems, CPSS can realize the full potential of IoT, big data analytics, and machine learning, leading to increased efficiency, improved sustainability and better decision making. Therefore, CPSS presents exciting opportunities for innovation and advancement in multiple domains, improving the quality of life for people around the world. In a CPSS, the cyber component involves the seamless integration of computational algorithms, communication networks, and software applications. The physical component includes physical infrastructure, devices, and sensors that collect and provide data to the system. The social component encompasses human interactions, preferences, and behaviors, which influence the system’s operation and outcomes
In a CPSS, sensors and actuators in the physical domain are often underpinned by IoT technologies. Security challenges posed by IoT-enabling technologies include signal integrity and accurate event actuation [ 23 ]. Thapa et al. addresses concerns related to these fundamental units in a complex CPS context, proposing security solutions for autonomous vehicle case studies to enable secure design [ 24 ]. However, the perception layer of a CPSS has the potential to exist conceptually beyond the physical domain, and within the cyber and/or social ones. For example, a human agent within a social network acts as a sensor to its environment, which may then inform feedback loops into the wider CPSS [ 25 ].
A CPSS represents a field that encompasses a complex system of systems. Social factors have the potential to create emergent behaviors that can induce instability into critical CPSS networks within the world. CPSS effects exist beyond the boundaries of traditional CPS. This can be exemplified with two case examples—Non-Fungible Tokens (NFTs) and the historical events of the Arab Spring. These case studies highlight the importance of CPSSs and the need to understand their interactions within a complex system-of-systems due to their potential wide-scale consequences across multiple domains.
The economic value of Non-Fungible Tokens (NFTs) is derived through social factors. They exist within the cyber domain, but have real physical-world consequences including economic and market impacts. The value of NFTs is created based on social perception of the cost/benefit trade-off of that token, and is heavily influenced by aesthetic human psychological values [ 26 ]. Of course, NFTs and similar digital ledger technologies exist within the cyber domain, but they also have physical-world impacts through economic returns and potential changes to the labor market [ 27 , 28 , 29 ]. Thus, the existence and financial impacts of NFTs demonstrate the consequences of CPSSs on the large scale system-of-systems that effects large-scale world dynamics.
The Arab Spring [ 30 ] also highlighted how CPSSs can induce emergent behaviors that have multi-domain impacts. Protests that initially began in Tunisia spread to other countries including Libya, Egypt, Yemen, Syria, and Bahrain. This information spread was fueled through social media platforms including Facebook and Twitter, enabling real-time dissemination of information across national boundaries and organization and mobilization of protests based on shared ideologies. During the Arab Spring, features of complexity were exemplified through sensitivity to initial conditions with the initial protest suicide of Tunisian Mohamed Bouazizi, the self-organization of political groups via the internet, adaptive interaction of protests as events evolved, and emergent behaviors including the coup of the Egyptian President Mohamed Morsi [ 31 , 32 , 33 , 34 , 35 , 36 ]. The three CPSS domains were also clearly present throughout the Arab Spring. The cyber component was a key enabler to the dissemination of information between protesters via social media. The social component manifested through the sharing of ideologies and political engagement by both citizens and governments. Finally, the physical domain manifested through physical protests, the Egyptian coup, the Tremeseh massacre, the Syrian civil war, and diverse government reactions and reform.
A CPSS poses several challenges from a scientific perspective. One of the main challenges is the integration of various components of the CPS within the CPSS context. These components include sensors, network components, and actuators [ 37 ]. The nature of CPSS components further requires that resource synchronization and optimization occur for performance—a complex task [ 38 , 39 ]. The development of secure and reliable communication protocols that can safely transmit data between different components is an issue [ 4 , 40 ]. The new trends in CPSSs are characterized by the use of advanced technologies and methods such as artificial intelligence, machine learning, blockchain, and IoT [ 41 , 42 , 43 ]. These technologies allow CPSSs to interact with their environment more intelligently, accurately, and efficiently. As a result, CPSSs can improve the performance of physical and social systems, enhance decision-making capabilities, and unlock new applications.
CPSSs face a variety of cyber security challenges. Some challenges are common with those underlying the IoT, such as node capture, routing attacks and data theft [ 44 ]. Because CPSSs include the social landscape, this provides greater opportunity for social engineering attacks that exploit human vulnerabilities, using tactics such as deception, manipulation, or influence [ 45 , 46 , 47 ]. CPSSs present unique cyber security challenges, where threats may arise through any of the cyber, physical or social agents within the system. Subsequently, conscious development of CPSSs within a secure digital technology mindset is essential to assuring system operation in the face of cyber threats [ 48 ]. Ensuring the security and privacy of CPSSs is crucial because of the high volume of sensitive data being processed and shared [ 49 ]. Protecting against cyber attacks, asserting data integrity, and maintaining user privacy are significant challenges. CPSSs include various interconnected components, such as sensors, devices, and networks [ 50 ]. Ensuring seamless interoperability between these components is a challenge because of differences in protocols, standards, and technologies. CPSSs would also need to handle large amounts of data, users, and interconnected systems, ensuring scalability to accommodate such growth while maintaining system performance and efficiency, which is a problem. A CPSS should be resilient to failures, disruptions, and adverse events [ 51 ]. Ensuring robustness and fault tolerance to maintain system functionality and minimize potential damages is a significant challenge [ 52 ]. CPSSs raise ethical concerns, such as the potential amplification of social biases, discriminatory practices, and the misuse of personal data. Addressing these challenges and promoting responsible and inclusive CPSS design and usage is essential, which we attempt to highlight in this study.
The ongoing advancements in CPSSs are expected to have a significant impact on various industries and aspects of daily lives [ 52 ]. As a consequence, understanding the scope of current CPSS research within these topics is essential for the development of future work and innovation in the research field. This is enhanced by CPSS’s potential for impact in diverse and interconnected domains, ranging from politics to economics, infrastructure and algorithm design [ 18 , 51 , 53 ]. This paper aims to holistically review CPSSs from three angles—cyber, physical and social—in order to determine their impact on system performance and how they offer secure and automated services to end-users and organisations in the era of artificial intelligence (AI). We define a CPSS as a system that integrates cyber, physical, and social elements to interact and collaborate with each other.
The key contributions of this work are structured as follows:
- We determine a CPS and its interaction with social media and its components, and to what level it influences human behaviors.
- We examine the distribution and intersection of research associated with CPSS, complex systems, social media, influence and cyber security applications.
- We discuss the recent advancements of CPSSs and how they enhance human activities and system performances.
- We describe the recent challenges and lessons learned and future research directions of CPSSs.
This paper discusses Cyber–Physical–Social Systems within the larger context of Cyber–Physical Systems and the impact of social complexity on these systems in Section 2 . In Section 3 , we discuss the related studies mixed between CPS, social media, and CPSS. The recent technologies with CPSS are explained in Section 4 . This is followed by examining the most influential terms in Section 5 . Section 6 describes the role of CPSS with recent smart applications. Research challenges and lessons learned are explained in Section 7 . Finally, we conclude the paper in Section 8 .
2. Cyber Physical Social System (CPSS)
We define a CPSS in a similar way to Wang [ 10 ], but in addition, we include security-by-design to each component as an extension of a Cyber–Physical-System that includes human social systems within its holistic system boundary. A CPSS, therefore, includes social and cognitive functions not considered within traditional CPS [ 54 ]. We define a CPSS as a systematic architecture that connects social features with human and network elements, with social attributes disseminating in accordance with node relationships, considering a secure design to the elements and fractures. In this way, the social space exists beyond just people, but also within their interactions, networks, and interactions.
We do not consider any of the competing definitions of CPSS that do not model social systems within their system boundary, and instead note that these systems interface with the CPS, as seen in the work of Zhou and Lin [ 55 ]. Competing definitions include considering CPSS as battery-supplied low-energy devices [ 55 ] or systems that highly conjoin communication, sensing, computing, and C2 (Command and Control) within larger human societal contexts [ 56 ]. The application of CPSSs spans various domains, including smart cities, transportation systems, healthcare, manufacturing, and entertainment. By combining cyber, physical, and social elements, CPSSs can enable advancements such as autonomous vehicles, smart grid systems, personalized healthcare, and immersive virtual environments. Overall, CPSSs represent an interdependent and interconnected system that leverages digital technologies, physical infrastructure, and social dynamics to create intelligent and adaptive systems capable of addressing complex societal challenges.
A further distinction should be made between the concept of CPSS and Cyber–Physical–Human Systems (CPHS). CPHS include humans in the loop and focus on the interaction between people and Cyber–Physical Systems [ 9 ]. CPHS play a crucial role in various scientific fields, including robotics, artificial intelligence, and human–computer interaction. They enable the development of advanced technologies such as autonomous vehicles, wearable devices, smart homes, and interactive virtual reality platforms. By leveraging CPHS, researchers aim to enhance human productivity, safety, and well-being in various domains such as healthcare, transportation, manufacturing, and entertainment. For example, in healthcare, CPHS can be used to monitor patients’ vital signs remotely, enabling early detection of health issues and prompt intervention. In transportation, CPHS can facilitate the development of self-driving cars that optimize traffic flow and improve road safety. However, CPHS rely on people who feature cognition, predictability and motivation without considering complex social factors [ 9 ]. CPHS are suited for applications where the human element of the CPS behaves in a defined way using defined options, and has been used heavily in modelling human-in-the-loop systems such as those at the National Aeronautics and Space Administration [ 9 ].
2.1. Cyber–Physical System
The ontology of CPSSs is difficult to define for two reasons. The first is that the boundaries between these agents or sub-systems are unclear. The second reason is that a CPSS includes human social systems within its holistic system boundary, as shown in Figure 1 . Subsequently, modelling and measuring people’s social feelings and attitudes is more difficult than placing sensors in traditional Cyber–Physical Systems, because these sensors may need to exist at the interface or within the human mind itself [ 57 ]. The collection of data from social sensors is therefore a challenge within this field, as accurate metrics regarding social or emotional human states pose obstacles in terms of collection accuracy. In a traditional CPS, sensors can be implemented technically through technology paradigms such as IoT.

Evolution of the CPSS.
The connection between CPS IoT lies in their shared objective of connecting and exchanging data between physical devices. CPS involves the integration of sensors, actuators, and control systems, while IoT extends this concept by connecting a vast array of everyday objects or “things” through the internet. IoT acts as the backbone for CPS, providing the network infrastructure and communication protocols necessary to connect and exchange data between CPS components. This allows remote monitoring and control of physical processes in real time, enabling more efficient and autonomous decision making.
Converting human interactions and and behaviors in the social space into calculations inputted to sensors is a challenge within CPSS. Intrinsic social calculations that occur within the human mind are difficult to effectively record and calculate, and thus developing mechanisms to appropriately measure these social metrics is a field of continued CPSS research [ 57 ].
The combination of CPS with IoT leads to numerous applications across various domains, such as smart cities, transportation systems, healthcare, manufacturing, and energy management. By leveraging the capabilities of CPS and IoT, organizations can achieve improved efficiency, increased productivity, enhanced safety, and better resource management. The introduction of humans and their associated social contexts presents a unique challenge for CPSS research, as sensors to monitor these conditions need to quantify social data points [ 57 ]. An example of a CPS in this context would be a modern manufacturing facility, which would have computational sensors, actuators, and humans that would be making predefined or limited decisions based on the system state. By contrast, an example of a CPSS would be a humanitarian assistance or disaster relief effort, which would be considering multiple sensors and unstructured reports from groups of people. This latter example has a greater role for people, social systems and their interactions. This is an evolving area, as the forms of human interaction are increasing, especially as new communication platforms appear. There has been some development into ontologies for CPSS modelling, although much of this is sourced from a few limited authors [ 8 , 58 ].
2.2. Social Media Complexity
Social media exemplifies the construct of a Cyber–Physical–Social System. Social systems, regardless of technological integration, are regarded as complex systems [ 31 ] (p. 29). Complex systems are entities that consist of “many interacting parts”, and exist on the “edge of chaos” [ 59 ] (p. 1) and [ 60 ]. Social media is inherently reliant on layers of complex systems, often driven by people, and is fed by these principles [ 61 , 62 ]. Terms such as “the Internet of Minds” and “Humans as Sensors” have also contributed to understanding social interaction with CPS through an IoT perspective [ 63 , 64 ]. Furthermore, Maier et al. [ 65 ] discussed the role of humans as “neurons in a hive mind” as part of Society 5.0, enabled by the token economy. Subsequently, there is a strong link between the concept of social media and its intersection with the field of CPSS.
Complex systems are characterized by several behaviors and features. These aid in distinguishing complex systems from both chaos and complicated systems. Complicated systems contain a multitude of parts that are defined by ordered and simple rules [ 66 ]. Complex systems exist at the intersection between chaos and complicated systems, with sub-units potentially including both chaotic and complicated dynamics. Complex adaptive systems are a more specialized case of complex systems, although sometimes the terms are used interchangeably. Complex adaptive systems dynamically evolve with and exhibit adaptation within changing environments, meaning that the system will not always react consistently to the same stimuli [ 67 ] (p. 2). They are distinct from the features of systems of equilibrium or homeostasis. Complex adaptive systems contain agents within their system boundaries, which are semi-autonomous units that seek to optimize based on a schema of prescriptive actions and rules [ 68 ] (p. 18) and [ 69 ] (p. 85). The behavior of these agents can subsequently display patterns at the system level, not all of which are predictable or repeatable. The degree of complexity experienced by a system can be measured by its difficulty in description, its difficulty in creation, and its degree of organization [ 70 ]. Many complex adaptive systems change over time, with changing optimized organization depending on the current context or environment. Many human and social interactions form parts of larger complex adaptive systems, with wider environment changes influencing both individual and system behavior [ 71 ].
The features of complex systems identified in the literature are varied; however, there are some key commonalities between interpretations [ 31 , 32 , 33 , 34 ]. The boundaries between these systems and their sub-units are sometimes blurred [ 34 ]. Complex systems often contain the features of self-organization; interdependence; hierarchy and scale; evolution, feedback and adaptive interaction; unpredictability and non-linearity; sensitivity to initial conditions; and emergence [ 31 , 32 , 33 , 34 ]. Social media is clearly established in the literature as a complex system, which creates additional challenges for the conceptualization and modelling of the system within the scope of CPSS [ 72 ] (p. 374). Social media case studies have exemplified how its emergence has had far-reaching consequences on political, economic, and social outcomes.
Conceptual frameworks and analysis of historic social and economic events fueled by complex social media interactions have been studied, including such diverse events as the Arab Spring, Euromaidan, and Gamestop [ 73 , 74 , 75 , 76 , 77 ]. Furthermore, the evolution of news consumption through social media has increased opportunities for the generation of ‘Fake News’. This has been a contributing factor to real-world phenomena including politically influenced ideological polarization within the social population [ 78 , 79 , 80 ]. CPS theory is an opportunity to examine emergence within social media, and therefore model the complexity in CPSS. There are further epistemological questions regarding complex CPSS, with challenges in identifying and understanding the inputs, processes, and outputs that social agents have within these systems. Subsequently, modelling and understanding social media as a form of complex CPSS is a field warranting further investigation. This study attempts to understand the distribution of research relating to this topic and determine where research gaps and opportunities exist to further its conceptual development.
3. Current State of the Art
This study was conducted via a mixed methods approach, with both quantitative and qualitative data analysis. It involved surveying search results from the Scopus database related to the research topic, statistical analysis of those results, exporting result metadata to file for topic cluster analysis in VOSViewer, and finally a conceptual analysis of key research papers identified. VOSViewer and similar visualization tools have been widely used in the preliminary analysis and identification research opportunities in several academic domains [ 81 ], including specific IoT environments [ 82 ] and computational physical chemistry [ 83 ]. There are also several other tools designed to perform similar meta-analysis of academic media via citations, keywords and themes. These include CitNetExplorer [ 84 ] and SciMAT [ 85 ]. These tools, first developed a decade ago, are seeing increased and significant use in publication analysis, in particular for environmental scans and surveys. Visualization has become an important tool across understanding research domains as varied as deep learning in autonomous vehicles [ 86 ], understanding how COVID-19 has shaped research [ 87 ], and the links with Augmented Reality in learning [ 88 ]. This work has chosen VOSViewer for use, but does not preclude the use of other tools for future comparative analysis. Through the methods discussed, the aforementioned research questions were analyzed and able to be answered.
3.1. Distribution of Current Research
Data collection occurred by searching for key terms within the Scopus database, and analysis of the results’ bibliographic data. The search phrases utilized are detailed in Table 1 . The search query was applied to the article title, abstract and keywords. These terms were used because they related to answering the aforementioned research questions. They focus on understanding the distribution of research across these fields, whilst also highlighting the degree of intersection into the chosen research area.
Scopus search phrases.
Report Term | Scopus Search Phrase |
---|---|
Complex system | “*Complex system*” OR “*Complexity*” |
Cyber–Physical System/CPS | “*Cyber Physical System*” OR “*Cyber–Physical System*” |
Cyber–Physical–Social System/CPSS | “*Cyber Physical Social System*” OR “*Cyber–Physical Social System*” |
Social Media | “*Social media*” |
Influence | “*Influence*” |
Cyber Security | “*Cybersecurity*” OR “*Cyber security*” |
3.2. Statistical Visualization of Current Research
From the Scopus data sets the degree of research within the chosen fields is quantified and visualized. Figure 2 illustrates the comparative size of each search result. The field of complex systems is the largest and most comprehensive. This implies how this field is endemic and underlies many other research areas. As a subset of CPS, CPSS predictably returned a smaller results size. In its early stage, CPSS is mainly focused on exploring potential applications across various domains, including smart cities, healthcare, transportation, and manufacturing. The CPSS trend focuses on studying how to merge traditional physical systems with cyber intelligence, communication capabilities, and social behavior analysis.

Single search term results.
The intersection between CPS and CPSS involves exploring how technology and human behavior interact and influence each other, considering the security by design of their elements. For example, in a smart city scenario, CPS would include sensor networks and data processing systems to monitor and control infrastructure, while CPSS considers how people will interact with these systems, such as through social media platforms or mobile apps that provide information about the city’s status and encourage citizen engagement. Understanding the intersection between CPS and CPSS is important for designing more efficient and user-centric systems, as well as addressing ethical, legal, and societal implications. It involves studying complex socio-technical systems to ensure that technological advancements are aligned with human needs, values, and social structures.
CPS are systems that combine physical components with computational elements to enable data sensing, processing, and control, with applications ranging from smart homes and energy grids to autonomous vehicles and industrial automation. CPSS, on the other hand, extends CPS by considering not only the technical aspects but also the social interactions, human behavior and cyber security within these systems. Figure 3 presents the intersection of the different fields of research. The most common fields of intersection were complex systems and CPS , followed by complex systems and social media . Conversely, intersections between CPSS and other topics were among the lowest statistics. These statistics align with the general trends identified in Figure 2 , as topics that had a larger quantity of papers were more likely to have a larger intersection of multiple paper topics in Figure 3 . With CPSS being a subset of CPS, the results aligned with this assumption that the subset topic’s intersection with other fields of research would be less than the overarching parents’ topic intersection.

Dual term search results.
Table 2 shows the distribution of papers with an intersection of multiple search topics. Of note, CPSS intersection with multiple other research fields yielded no results in any combination. This indicates that there is potentially a research gap in these areas, which is worth exploring further within the literature.
Multiple term search results.
CPSS | CPS | ||
---|---|---|---|
Three Terms | Complex system + Social Media | 0 | 3 |
Three Terms | Complex system + Cyber Security | 0 | 182 |
Four Terms | Complex system + Social Media + Cyber Security | 0 | 0 |
3.3. Network Analysis of Current Research
The bibliographic data from the Scopus database results were then assessed utilizing network analysis maps in the tool VOSViewer. They were analyzed utilizing the co-occurrence method using keywords of the papers. The counting method specified was ‘full counting’ and no thesaurus file was used. Phrases were prioritized for analysis by lowering the occurrence threshold until the number of occurrences was simultaneously minimized, whilst remaining above 30. For inputs that could not meet the threshold of 30, all terms were used. This meant that most maps had approximately 30 terms. The Scopus search terms for each result set were discounted from the final data set, and any outlying data that did not connect to the main graph was also discounted.
3.4. Conceptual Analysis of Most Influential Research
The conceptual analysis involved a review of the key topics utilizing the Scopus search outputs for the CPSS search term. The intent of this analysis was to determine novel research within the field and highlight the most influential papers. Selection criteria were applied to the results to narrow the focus of the review. The following terms and criteria were excluded from the analysis: survey papers, conference proceedings/journal introductions/opening chapters, references that did not contain abstracts, papers that did not include CPSS in their abstract, references whose full text could not be found, and papers that were not written in English. After this, the top 30 highly cited papers were selected for the development of a CPSS influence concept map.
This process was then repeated with the original CPSS Scopus search results data set. In this iteration, research papers were limited to the years 2021–2023 to assist in identifying the most novel and highly influential recent papers. References were chosen that had 5 or more citations for review, with the final reference count for analysis being 25.
3.5. Analysis of Current Research
The distribution of the research topics was significantly varied. Fields such as complex systems returned over a million results, whereas newer terms such as CPSS returned less than one thousand. CPSS was also highlighted as a significantly small subset against the wider field of CPS, with approximately 500 papers compared to 25,000 in the search results. These results indicated that the field of CPSS is relatively small compared to other established related research areas, and may warrant further expansion and investigation.
When considering the intersection of multiple search terms, as highlighted in Table 2 , there were limited multi-disciplinary papers that addressed the research topics defined in Table 1 . In particular, the queries that searched for the intersection of CPSS with multiple other research topics all produced zero search results, indicating that there is a significant research gap at the overlap of these key areas. As an emerging research area, this indicates that applications and research into CPSS require increased focus and commitment in order to understand the interrelation of complex CPS in the case of social media implications for cyber security.
4. Recent Technologies with CPSS
Recent technologies, including big data, IoT, machine learning and social media platforms have been employed in conjunction with CPSS. These technologies with CPSS, have revolutionised various fields by allowing advanced data analysis and decision-making processes. Big data allow massive volumes of structured and unstructured data that cannot be effectively processed by traditional methods. With recent technological advancements, it is now possible to collect, store, and analyze these vast amounts of data. Machine learning (ML), a subset of artificial intelligence, is a methodology that allows computer systems to automatically learn and improve from experience without being explicitly programmed. By utilizing ML algorithms, they can make predictions, classify data, and discover patterns within big data sets. Social media plays a significant role in the collection and generation of big data. Platforms like Facebook, Twitter, and Instagram provide vast amounts of data generated by users worldwide. These data can be utilized to study human behavior, sentiment analysis, and societal trends.
The integration of these technologies allows monitoring and control of various systems, ranging from smart cities, transportation networks, and healthcare systems, to environmental monitoring. Figure 4 , which is the Complex system and CPS intersection map, exemplifies this trend. Every cluster map generated throughout the analysis, with the exception of the CPS and CPSS intersection map and the CPSS and social media intersection map, includes at least one of the aforementioned topics. This indicates a significant research focus across the literature on these key topics.

Complex system + Cyber–Physical System clusters (2019–2020).
When drilling down into the research topic of CPSS, the largest areas of research focused on big data and the IoT; see Figure 5 . In this model, the oldest research areas included IoT, intelligent systems, and social manufacturing. The newest areas included computational modelling, the Metaverse, and computational experiments.

CPSS research clusters (2018–2021).
When considering the intersection of topics, research that focused on both CPSS and complex systems was clustered across a linear scale. The oldest research topics were located in the center, with newer research extending to either side, as shown in Figure 6 . The oldest research was associated with knowledge automation and parallel control, with newer research associated with computational modeling, smart manufacturing, task analysis and the Metaverse.

CPSS + complex systems time overlay (2019–2022).
5. Most Influential Research Focuses
Within the field of Cyber–Physical–Social Systems, there are several areas of research focus apparent within the literature. Figure 7 highlights the top thirty influential CPSS papers, based on citations, and their topic clusters. Modelling CPSS through frameworks, simulations and data models is a key research area within the most influential CPSS papers. The complexity considerations of CPSS are identified and discussed throughout the literature. There was also a significant research focus on data processing implementations and optimization in areas such as resource allocation and capacity management.

Most influential research clusters in the field of CPSS.
The most influential case studies within the field related to manufacturing and industrial applications, smart cities, social media, and the smart grid. The most influential enabling technologies identified included tensor, edge computing, the IoT, and artificial societies, computational experiments, and parallel execution (ACP).
6. Role of CPSS with Recent Smart Applications
The concept of CPSS was noted in the literature to be linked regularly to several smart applications such as smart cities, smart healthcare, blockchain technologies, etc. The most regularly referenced included industrial applications and smart city implementations. These included manufacturing and Industry 4.0, smart cities, and blockchain tokenization [ 89 , 90 , 91 , 92 ]. Smart city implementations are also referred to in the highest CPSS paper citations [ 15 , 18 , 92 , 93 ]. Other CPSS implementations explored within the recent novel literature include smart wearable devices, data center cooling systems, industrial chart data processing, and electricity savings [ 22 , 94 , 95 , 96 ]. The gaming industry is additionally identified as a CPSS, with research in this space aligning with opportunities to crowd-source task allocation and optimization problems relying on the interaction of people with digital systems [ 97 ]. Future aims of this work include crowd-sensing via real user testing in order to generate incentive strategy evaluations [ 97 ].
Industrial implementations are identified in the literature as novel case studies for CPSS within the wider context of Industry 4.0. Performance monitoring is one area of development, where a CPSS framework is complimented to enable greater fidelity to detection and monitoring processes [ 90 ]. Evolving out of Industry 4.0, Industry 5.0 is an additional paradigm through which the concept of CPSS is discussed, integrating with other enabling technologies such as 6G, tokenization, robotics, tactile internet and collective intelligence [ 65 , 91 ]. These novel ideas also further link to the concept of Society 5.0, where CPSS’s aim becomes to enhance wider system harmony [ 65 , 91 ]. However, Maier’s work is challenged by the semantics of CPSS, sometimes identifying human-in-the-loop centric systems, which in some scenarios may align better with categorization as Cyber–Physical–Human Systems (CPHS) [ 91 ]. This cross-association indicates there is potentially a need for further work in defining the ontologies that separate these two sub-types of CPS, and the factors that conclusively assign a system to one definition over another.
A portion of the research related to industrial applications of CPSS appears to be more closely aligned with a traditional CPS or Industrial CPS (ICPS), instead of the social dimensions and metrics traditionally included within the CPSS construct. In some papers, this manifested in a Cyber–Physical–Human System (CPHS) context, where people’s interactions with the industrial system were considered, but frameworks did not include metrics or sensors relating to complex human social systems, and instead focused on predictable defined human-in-the-loop switch case interactions [ 9 ].
6.1. Data Processing and Resource Allocation
Innovations and novel implementations of CPSS in the recent literature often focused on improving resource allocation or optimizing data mining within large data sets as enabled by sensors and IoT. CPSS is identified as having significant data processing and analysis considerations due to its heterogeneity, growth, and dimensionality [ 98 ].
Collaboration is one research area that aims to improve CPSS data processing in order to support multi-goal optimization solutions [ 98 ]. Furthermore, Wang et al. introduced and tests the performance of algorithms in their role of processing CPSS big data in smart cities, including singular value decomposition (HOSVD), ring-based tree algorithms and tree-based tree algorithms [ 92 ]. This research identifies the need for future computational efficiency studies to improve the algorithmic processing of CPSS data in wide-scale applications [ 92 ].
Machine learning is a further tool used to build solutions to big data for CPSS processing challenges, with algorithms leveraging computing constructs such as cloud computing, edge computing, and distributed training in order to enable systematic processing [ 92 ]. Linear Regression is another machine learning technique that is applied to data analysis for CPSS, with application implications to the cyber security of data processed in untrustworthy environments, such as cloud servers or the blockchain [ 99 ]. Whilst privacy and security concerns were identified as a focus point for experimentation in CPSS data processing, the authors noted that future work in this domain would need to continue to improve data privacy, verifiability of results, and fairness [ 99 ] (p. 3966). Parallel execution is used as one way to validate AI-processed CPSS data, with future work in this space highlighting the need for real-world accurate simulation systems, and validation of outputs against read scene data [ 93 ]. Notably, the recent novel literature regarding CPSS data algorithms does not address newly emerging fields such as federated learning, where collaborative machine learning occurs across multiple decentralized clients utilizing architecture such as IoT [ 100 , 101 ]. The discussions regarding applications of such algorithms are limited, and present a further opportunity for research growth and maturity [ 96 ].
The processing of data to feed recommendation services as part of the CPSS construct is also considered in the literature, with algorithms built to process and analyze user characteristic data in diverse scenarios [ 51 ]. Future work in this area focuses on testing existing solutions with larger and more complex data sets to ensure quality of service in wide-scale applications [ 51 ] (p. 3858). Diverse scenario processing in the context of CPSS is an emerging space. Through the conceptual analysis, there were limited novel studies identified that challenge legacy machine learning algorithms with alternatives such as federated learning.
Data for CPSS processing must also be considered with a system-of-systems mindset, where multiple subsystems with varying data sets must be interconnected and processed. Within the smart city case study, this problem set is exemplified, with sub-systems including power grids, human social systems, and SCADA systems [ 102 ]. Hazard identification for safety is one research goal of these studies, where risk analytics are derived from interdependent network theory. However, future work is required in the fields of system resiliency in the case of cyber attacks in order to provide accurate hazard assessments [ 102 ] (p. 15).
Tensors are identified as one avenue for enabling heterogeneous data learning and optimization for CPSS [ 103 , 104 , 105 ]. Considerations for how to optimize the modelling of CPSS that utilize Intelligent Edge Services (IES) as part of an edge computing construct experience barriers in the form of security-efficiency compromises, energy consumption effects, and cost [ 104 ]. Future work in this optimization space needs to consider the secure optimization of multiple objectives, due to the complexity of CPSS, in order for CPSS-related price execution to become more economically viable [ 104 ] (p. 44). Additionally, the application of leading-edge machine learning technologies, such as federated learning, is a further opportunity for CPSS data processing research, especially in consideration of the joint foundational applicability of IoT to the two fields [ 101 , 106 ].
Algorithms that leverage sensor technology are identified in the literature to demonstrate more accurate classification results compared to legacy models; however, they highlight challenges to the flexibility of such models when training data constraints are introduced [ 105 ]. This is of particular concern when considered within the CPSS construct, where emergence may introduce unpredictable results to algorithm outputs, with the potential introduction of new data sets that do not nearly align with trained parameters.
The social component of human-generated information is also a data processing challenge, with sensors such as social media posts requiring analysis and comprehension in the digital space. The use of models such as Natural Language Processing needs to evolve to meet this need, as data sets may exhibit emergent or unpredictable behavior [ 63 ]. Opportunities within this sphere are posed through solutions such as Zero-Shot algorithms, that can potentially analyze data from unseen classes; however, continued development into the processing of unlabeled data is required to improve performance, especially in relation to semantics and knowledge graphs [ 63 ].
6.2. Cyber Security Implications
Cyber security and privacy concerns relating to data in IoT-enabled CPSS are of note within the literature and are explored through multiple case studies and implementations. Integration of social and IoT technologies into CPSS presents an opportunity to develop frameworks that conceptualize Enterprise Digital Transformation, such as seen in Mendhurwar and Mishra’s framework [ 107 ]. Their model specifically identifies not only the inter-dependencies between social agents and IoT sensors as part of CPSS but highlights the cyber security challenges associated with this digital transformation process [ 107 ]. They further identify that future work in this space may consider case studies that can provide insight into the nuances of specific applications and deliverables within the industry, considering factors such as entity needs and security posture [ 107 ].
Data analysis in industrial applications is also at the leading edge of research regarding CPSS, especially in conjunction with privacy considerations associated with data mining [ 89 ]. Algorithms such as the high-order Bi-Lanczos (HOBI-Lanczos) approach demonstrate an ability to securitize data and protect user privacy. Future work in this space includes exploration of energy implementations and collusion approach considerations [ 89 ]. Gati et al. [ 41 ] explored this concern regarding privacy preservation within CPSS, with their solution leveraging deep learning techniques in order to process private data. They propose a differential privacy framework that is applicable across multiple use cases such as smart agriculture, smart healthcare, and smart transportation [ 41 ]. Privacy protection and secure data considerations are also highlighted within CPSS research [ 96 , 103 ]. Human Activity Recognition (HAR) utilizing machine learning is one avenue for enabling secure processing of data framed through wearable devices [ 96 ]. Future research in this field involves investing in greater efficiency of algorithms, in addition to more personalized modelling whilst maintaining user privacy [ 96 ]. Additional challenges in this space involve the privacy preservation of data and processing, in relation to centralized models, and also the collection and integration of nonindependent and identically distributed (non-IID) data.
CPSS is also identified in the literature as an opportunity for attack vectors, which have the potential to compromise the cyber security of systems and their users. In these contexts, variations of existing attack vectors such as social engineering and malicious code execution are applied within the CPSS context, rather than a new attack class being defined. For example, Yang et al. propose the use of a neural network, called MCNN, to identify digitally manipulated ‘fake’ images in order to reduce the attack surface for anti-forensics tools [ 108 ]. Research such as this highlights the potential for CPSS to have real-world impacts; however, current works tend to focus on specific case studies rather than wider cross-domain conceptual applications. As people and technology integrate further in the Cyber–Physical–Social world, this poses additional opportunities for cyber attacks, as each edge between nodes in the CPSS presents additional potential attack vectors.
7. Research Challenges and Lessons Learned
As discussed above, the majority of novel contributions to the literature surrounding CPSS over the last three years can be categorized into one of three research fields. These areas of concern are broadly considered as case study CPSS implementations, such as smart cities; data processing considerations, such as efficiency algorithms; and cyber security implications, such as user data privacy concerns.
Through this critical survey and analysis of the literature, several significant research gaps and opportunities were identified and synthesized. Research gaps that specifically addressed the research questions included a lack of social metric inclusion in system-of-systems modelling for CPSS, a need for a CPSS ontology that includes consideration of the other intersecting research fields, and the inclusion of complex system dynamics within frameworks, including an understanding of emergent effects within the cyber, physical and social domains of CPSS. Additionally, CPSS research gaps were identified that related to comprehensive holistic case study analysis and cross-silo analysis of enabling emergent technologies including smart contracts and the blockchain, federated learning, sensor design and distribution. Furthermore, the existing research rarely addresses the impact of opaqueness within complex CPSS and how that may affect the accuracy and reliability of machine learning algorithms and decision support systems.
There is a lack of highly cited novel papers that specifically focus on developing frameworks or ontologies related to the social component of CPSS. Many papers that reference CPSS in their keywords or abstract often use this term to exemplify how a system can be considered a CPSS, without necessarily focusing on the nature of CPSS as part of their research. Multiple CPSS papers in the literature name the system in question as a CPSS, but then focus research on multidisciplinary fields such as algorithms for resource allocation or case studies such as smart grids [ 95 ]. Models and frameworks for CPSS as identified in the most recent novel literature also highlighted implementations focused on either parallel execution, or only considered the Cyber–Physical components of the system instead of including the human social metrics. Social data within the context of CPSS are focused upon little within the literature, with the outputs usually measured through methods such as social media posts or interactions with CPS system boundaries, such as interfacing with a smart city application.
There is, therefore, a research opportunity to aid in defining how these social data can be measured, and the subsequent challenges of processing such heterogeneous unlabeled data at scale. There is subsequently a need to identify how human metrics can be effectively modelled within larger CPSS frameworks, which include their social behaviors, attributes, and subsystems. Furthermore, there was evidence in the literature that CPSS was defined in broad and sometimes contradicting ways between different authors, with the comparison of CPHS to CPSS as an example of this. Analysis of the literature further highlighted some related research terms that could contribute to understanding the nature of CPSS. Terms such as persuasive computing, Society 5.0, Internet of Minds, and cybernetics also appeared to constitute part of CPSS as a concept, thus warranting further investigation and linking towards this larger CPSS ontology.
Whilst complexity is defined as an essential component of CPSS as per its originating conception, features of complexity such as emergence are rarely considered within the literature [ 10 ]. When machine learning is considered part of a CPSS application, the feature of emergence is conceptually at odds with the traditional design of training data, which tend to be predictable. Additionally, algorithm design is often considered within a data mining/processing context instead of from the context of human interaction and social media analytics. Finally, there is a lack of research focus associated with understanding multi-domain effects caused by the complexity of data within CPSS, particularly in the social space, especially when these effects are related to emergence. Social systems are inherently complex, albeit self-organizing, and subsequently CPSS research needs to consider the features of complexity within its models and frameworks. As an example, leveraging technologies such as machine learning and artificial intelligence to assist in modelling and data processing for CPSS is an opportunity. However, complications arise when considering the effects of emergence on pre-trained models, and unstructured, unlabeled human metric data sets.
There is a research need for future work that directly addresses these concerns relative to human metrics, an inclusive research ontology, and complexity considerations. This research would have to rely on the assumption that CPSS are inherently different to CPHS and that social systems are included within the CPSS boundary, instead of simply interfacing with it. The development of such a framework would then require testing against an established real-world CPSS that includes real social interactions for validation purposes, such as social media.
The process used to develop this work prioritized impact based on the number of citations individual publications have received. This approach therefore focuses on what has already been deemed impactful within the academic communities. Subsequently, there were some very recent publications that did not fall within the catchment of the analysis, but represent the leading edge of literature published within the last year. Such research still should be considered in future work in the field of CPSS.
Additionally, some of the existing novel literature focuses on fields relating to this paper’s research question. For example, Che et al.’s work into tensor factorization for fake news detection does not explicitly identify the system in question as a CPSS, but still offers valuable insight into CPSS research [ 109 ]. Other recent non-CPSS explicit literature that may contribute to CPSS future research related to this paper’s research focus include studies pertaining to modeling misinformation and bias within social networks and those that address emergence within CPS constructs [ 110 , 111 , 112 , 113 , 114 , 115 ]. Additionally, emergence in the field of cyber security is an identified area of research interest that is worth exploring within the context of CPSS and the IoT.
Ultimately, the field of Cyber–Physical–Social Systems is growing, which has the potential to underpin a multitude of cross-domain applications from healthcare to politics to algorithm optimization to Industry 4.0. Thus, building effective frameworks to understand this field and its cyber security implications is fundamental to ongoing reliance in both the cyber, physical and social spheres of influence.
8. Conclusions
The research field of CPSS is in an evolving state. Definitions, applications, and understandings of the concept are fluid and sometimes contradicting. This is exacerbated by its cross-applicability to multi-disciplinary applications, enabling silos of ontological understanding. However, the research in the field clearly points towards constructs of CPSS being a key enabler for future technological development and implementation into digitally enabled society, and thus warrants continued research and synthesis.
This work has shown that there is are several areas of CPSS that require additional research in order to reach its potential. This work has noted that the community is yet to standardize on common terms and definitions. This is not unexpected with such an emerging area. The development of structured representation mechanisms, such as formal ontologies, are one area of potential future research, that would assist in alleviating some of these issues.
Similarly, the broad nature of CPSS requires both a balance of the holistic in addition to detailed perspectives. This balance is difficult to achieve. The nature of complex systems makes this difficult, and there is more work to be carried out to understand some of the interactions of and between different domains.
Finally, this work has outlined the need for additional cybersecurity research both across both each domain of interest and at the areas that these domains integrate. The social aspect of cybersecurity is well known, but as CPSS evolves, so too will the challenges and opportunities in this area.
Social factors can heavily influence global current affairs, and thus the relationship between social agents and their Cyber–Physical counterparts presents a potentially volatile vector for influence, feedback, and emergence. Fake news, political events like the Arab Spring and Euromaidan, and economic events such as Gamestop all exemplify how Cyber–Physical Systems can have social components that affect outcomes in the real, physical world. Understanding how Cyber–Physical–Social Systems exist and operate is imperative to predict the emergence and the subsequent cyber security implications of these events.
Abbreviations
The following abbreviations are used in this manuscript:
MDPI | Multidisciplinary Digital Publishing Institute |
DOAJ | Directory of open access journals |
TLA | Three letter acronym |
LD | Linear dichroism |
IoT | Internet of Things |
CPS | Cyber–Physical System |
CPSS | Cyber–Physical–Social System |
C2 | Command and Control |
CPHS | Cyber–Physical–Human System |
HOBI-Lanczos | High-Order Bi-Lanczos |
IES | Intelligent Edge Services |
HAR | Human Activity Recognition |
Non-IID | Nonindependent and Identically Distributed |
ICPS | Industrial Cyber–Physical Systems |
ACP | Artificial Societies, Computational Experiments, and Parallel Execution |
Funding Statement
This research is funded by UNSW Canberra.
Author Contributions
Conceptualization, T.S. and B.T.; methodology, T.S. and B.T.; software, T.S.; validation, T.S., B.T. and N.M.; formal analysis, T.S.; investigation, T.S.; resources, T.S. and B.T.; data curation, T.S.; writing—original draft preparation, T.S.; writing—review and editing, T.S., B.T. and N.M.; visualization, T.S., B.T. and N.M.; supervision, B.T. and N.M.; project administration, B.T.; funding acquisition, B.T. All authors have read and agreed to the published version of the manuscript.
Institutional Review Board Statement
Not applicable
Informed Consent Statement
Data availability statement, conflicts of interest.
The authors declare no conflict of interest.
Disclaimer/Publisher’s Note: The statements, opinions and data contained in all publications are solely those of the individual author(s) and contributor(s) and not of MDPI and/or the editor(s). MDPI and/or the editor(s) disclaim responsibility for any injury to people or property resulting from any ideas, methods, instructions or products referred to in the content.
- Discovery Platform
- Innovation Scouting
- Startup Scouting
- Technology Scouting
- Tech Supplier Scouting
- Venture Clienting
- Startup Program
- Trend Intelligence
- Business Intelligence
- All Industries
- Industry 4.0
- Manufacturing
- Case Studies
- Research & Development
- Corporate Strategy
- Corporate Innovation
- Open Innovation
- New Business Development
- Product Development
- Agriculture
- Construction
- Sustainability
- All Startups
- Circularity
- All Innovation
- Business Trends
- Emerging Tech
- Innovation Intelligence
- New Companies
- Scouting Trends
- Startup Programs
- Supplier Scouting
- Tech Scouting
- Top AI Tools
- Trend Tracking
- All Reports [PDF]
- Circular Economy
- Engineering
- Oil & Gas
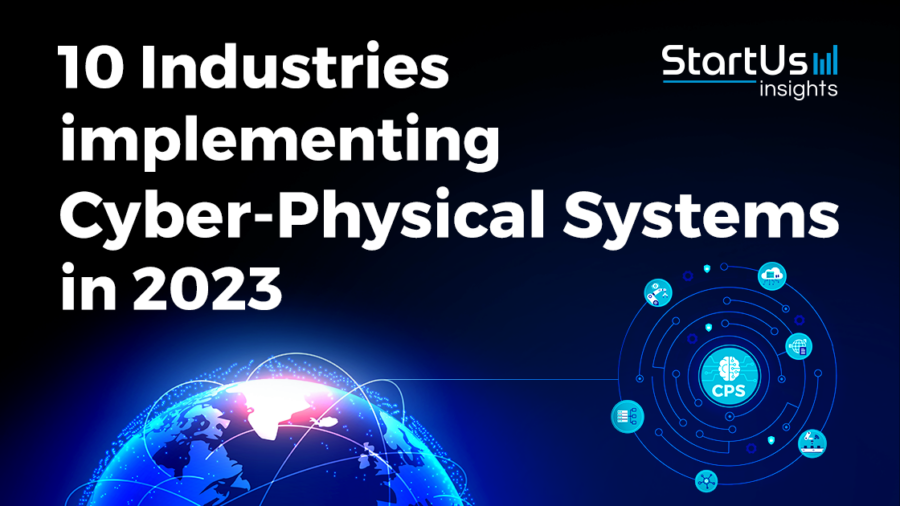
Share this:
- Click to share on Facebook (Opens in new window)
- Click to share on Twitter (Opens in new window)
- Click to share on LinkedIn (Opens in new window)
Top 10 Cyber-Physical Systems Examples in 2023 & 2024
How are businesses integrating various cyber-physical systems to increase productivity? Explore our in-depth analysis on the top examples of cyber-physical systems based on the analysis of 2300+ companies. These applications span autonomous vehicle systems, CPS security solutions, brain interfaces & more!
Cyber-physical systems (CPS) leverage computer-based algorithms to control and monitor mechanisms. They also feature intricate software-hardware interactions. Some examples include smart grids, autonomous vehicles, industrial control systems (ICS), and robotics. This research focuses on the top 10 industries advancing cyber-physical systems in 2023. For this, we analyzed 2313 emerging companies working in the field. Read more to explore the industry-specific applications of CPS and find out how they impact your business.
Tree Map reveals the Cyber-Physical Systems Examples across 10 Industries
Based on the Innovation Map, the Tree Map below illustrates the Applications of Cyber-Physical Systems in 10 industries in 2023. As digitization introduces large numbers of intelligent systems, their protection against cyber threats is very critical. That is why the cybersecurity industry is most impacted by CPS.
The manufacturing, utility, automotive, agriculture, industry 4.0, and aerospace industries integrate smarter assets, including Internet of Things (IoT) devices, to streamline operations. Further, CPS enhances the interfacing between humans and machines in the healthcare, smart city, and agriculture sectors.
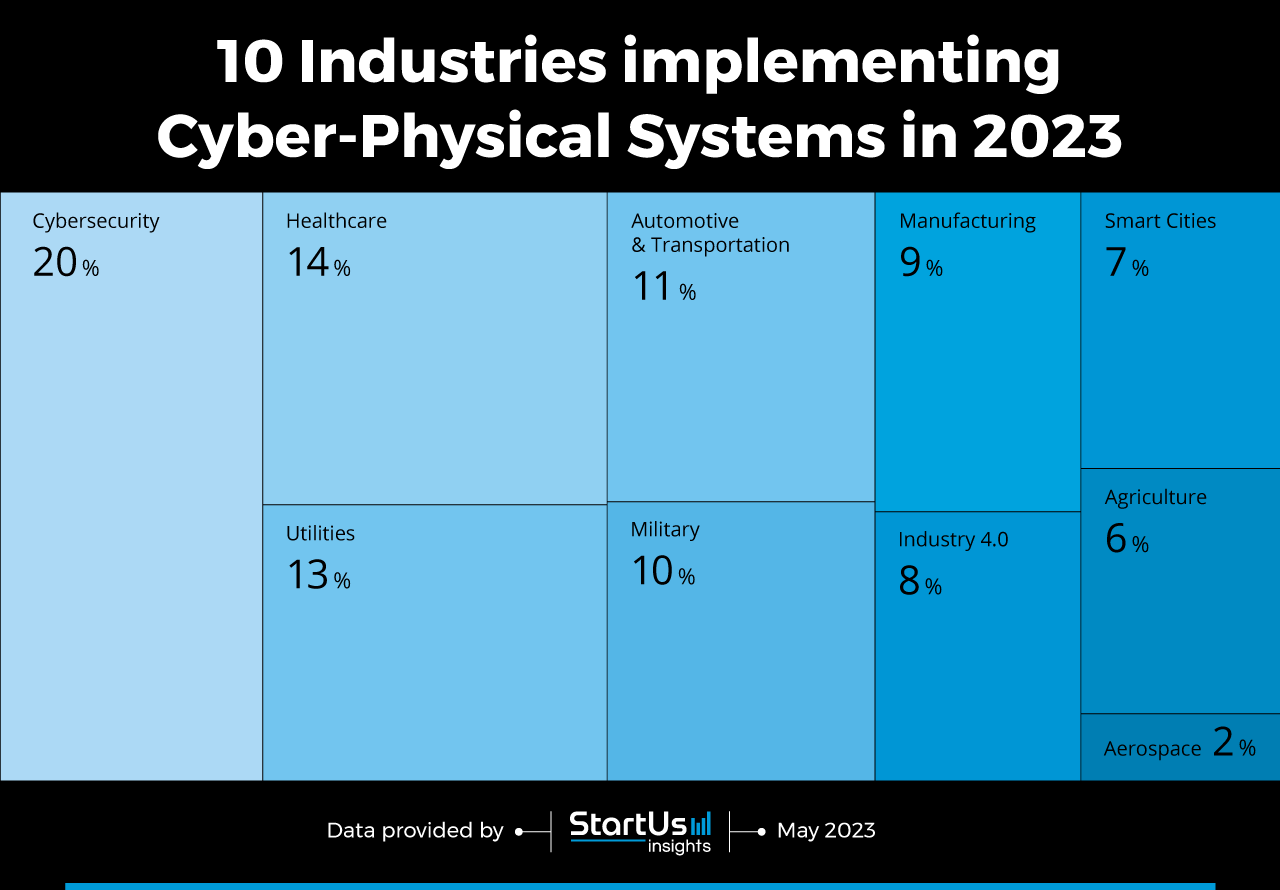
Click to download
Global Startup Heat Map covers 2313 Cyber-Physical System Startups & Scaleups
The Global Startup Heat Map below highlights the global distribution of the 2313 exemplary startups & scaleups that we analyzed for this research. Created through the StartUs Insights Discovery Platform that covers 3 790 000+ startups & scaleups globally, the Heat Map reveals that the US has a high concentration of cyber-physical system companies, followed by Western Europe and India.
Below, you get to meet 10 out of these 2313 promising startups & scaleups as well as the solutions they develop. These emerging CPS companies are hand-picked based on criteria such as founding year, location, funding raised, & more. Depending on your specific needs, your top picks might look entirely different.
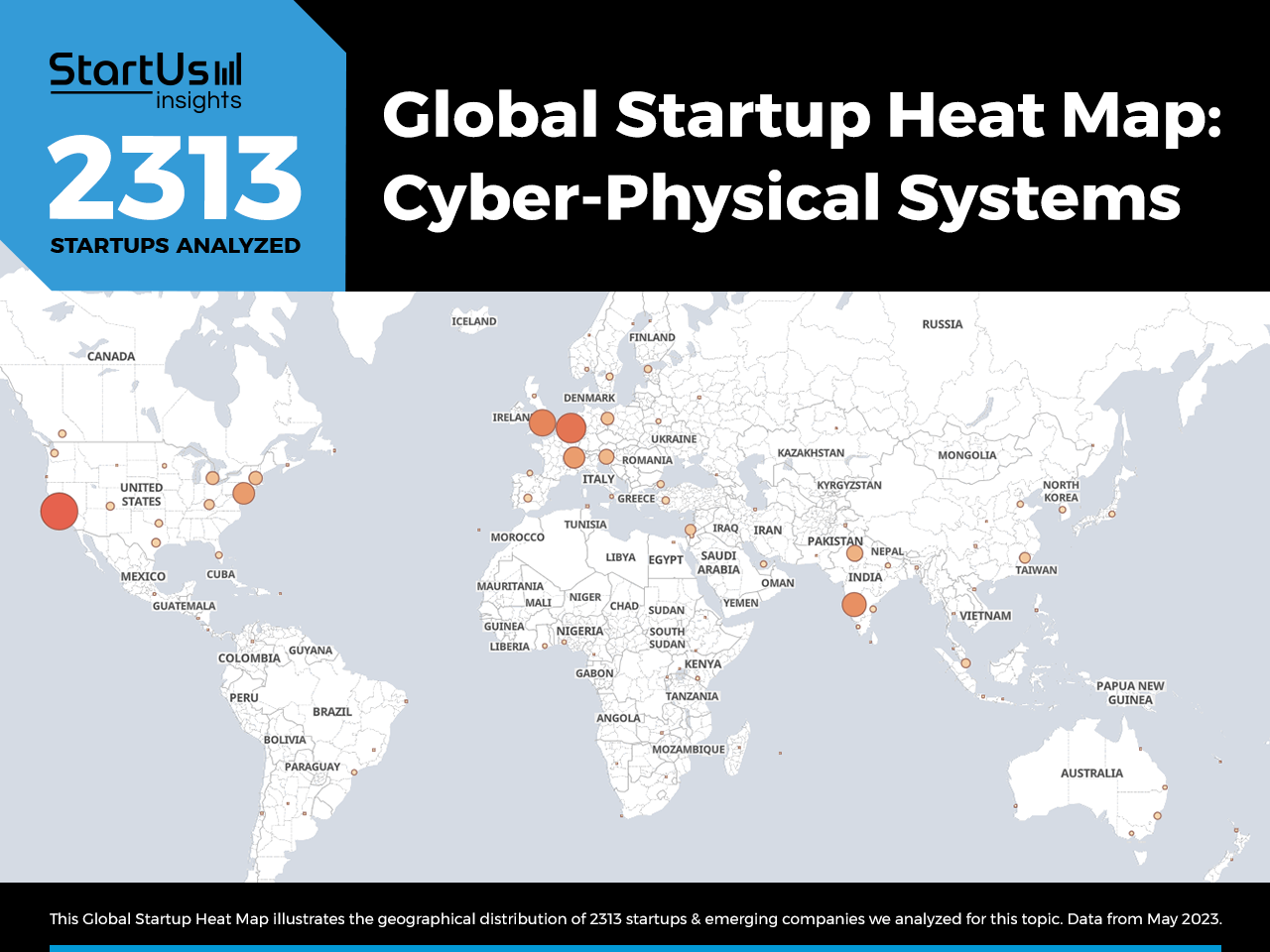
Interested to explore all 2000+ cyber-physical system startups?
Top Cyber-Physical Systems Applications across 10 Industries
1. cybersecurity.
The large-scale integration of cyber-physical systems expands the attack surface for cyber threats. Such malicious actors exploit vulnerabilities and compromise critical systems, leading to infrastructure downtime and sensitive data leaks. To tackle this challenge, the cybersecurity industry is leveraging encrypted communication systems and real-time network and device monitoring.
Further, blockchain drives business decisions and operations with immutable and tamper-resistant data while decentralizing access control. CPS developers are also transitioning to a security-first design approach to ensure data security. Other solutions to tackle cyber attacks against intelligent systems include anomaly detection, AI-assisted protection, and specialized software against ransomware.
C3i Hub enables CPS Intrusion Detection
C3i Hub is an Indian startup that facilitates intrusion detection for cyber-physical systems. The startup’s solution, RobustD , and an integrated controller are deployed in the operational technology (OT) layer. It collects data related to OT devices, calculates anomalies for newly generated measurements, and detects intrusion.
For this, the startup uses machine learning. RobustD thus aids infrastructure management in cities, smart car operations for automotive businesses, and patient tracking in healthcare.
2. Healthcare
Applications of CPS in healthcare operations lead to improved patient monitoring and medication management. Many CPS workflows also automate labor and time-intensive manual tasks while reducing costs. Telehealth solutions are extensions of such intelligent systems that improve access to healthcare. Advances in healthcare CPS include the Internet of Medical Things (IoMT), healthcare AI, machine learning, medical robotics, and more.
For example, healthcare institutions apply AI to analyze massive datasets and improve disease diagnosis as well as deliver personalized treatment recommendations. Additionally, virtual and augmented reality(VR/AR)-powered CPS solutions streamline surgical planning and pain management.
Precision Neuroscience makes a Brain-Computer Interface
Precision Neuroscience is a US-based startup that develops a brain-computer interface. Its flexible electrode arrays are implanted using a micro-slit technique and conform to the brain’s surface to maximize data collection without damage.
In clinical trials, the company’s technology has successfully mapped human brain activity. Using the technology, the startup assists patients with conditions like stroke, dementia, and other neurological conditions.
3. Utilities
Cities now integrate real-time data processing, process automation, and control systems to optimize the operations of utility companies. They improve power generation as well as energy and water distribution and consumption. For example, smart grids collect data across regions to derive insights into power consumption patterns and enable predictive maintenance. This allows energy companies to enhance demand response efforts and avoid blackouts.
Such solutions also support water and sewage infrastructure in making maintenance more streamlined while improving customer satisfaction. Further, implementing intelligent systems, like industrial IoT (IIoT) devices, predictive analytics, and blockchain, aids the integration of distributed energy resources (DERs). This, in turn, reduces the intermittency of renewable resources and accelerates the transition to a low/zero-carbon economy.
Orbis Intelligent Systems offers a Smart Hydrant
Orbis Intelligent Systems is a US-based startup that offers a smart hydrant for utilities. Its multi-sensor configuration detects various pipe and flow conditions and it uploads the data to a portal. The portal, ADARI , allows operators to access these scheduled reports and offers real-time alerts on unauthorized extraction and illegal tampering, reducing leakage and water theft.
The startup also develops a retrofit device, SmartCap , for existing fire hydrants. These solutions enable utilities and municipalities to track water pipeline networks in real time, optimize maintenance planning, and reduce non-revenue water (NRW).
4. Automotive & Transportation
Cyber-physical systems are impacting the automotive and transportation sectors in two key segments – production and post-sales. Automakers integrate IoT, AI, and more at factory floors to increase manufacturing throughput. Other solutions in the sector include advanced driver assistance systems (ADAS), autonomous driving systems, and vehicle-to-everything (V2X) connectivity technologies.
While production-ready solutions cater to the manufacturing segment, the latter elevates the driving experience for vehicle owners. Through such solutions, vehicle manufacturers and mobility service providers ensure passenger and road safety. Further, predictive analytics allows automakers to forecast potential machine and vehicle breakdowns to improve maintenance planning and safety.
Aura Intelligent Systems provides High-Definition (HD) Radar Perception
Aura Intelligent Systems is a US-based startup that delivers HD perception for vehicle radar imaging systems. The startup’s technology combines 5G, sensing, and intelligent software to enable high-resolution 5D information – 3D location, doppler, and signature.
This enables a low-false alarm perception system that enhances the performance of autonomous mobility solutions like drones and ground vehicles. With the startup’s solution, automakers and mobility providers are able to improve vehicle navigation, road safety, and traffic management.
5. Military
Real-time data acquisition, improved communication, and autonomous systems for surveillance and reconnaissance greatly increase the efficiency of military operations. Swarm technology, augmented reality (AR), satellite, edge computing, and unmanned system integrations are key enablers of such solutions. Loitering munitions, also known as kamikaze or suicide drones, and countermeasures against them are gaining interest due to their recent use in the Russo-Ukrainian war.
Through these systems, military organizations improve situational awareness, command and control, personnel training, and more. Militaires further leverage novel encryption techniques and blockchain to make data security and privacy more effective as well as tackle information warfare.
SightX manufactures Edge AI-powered Military Drones
SightX is an Israeli startup that manufactures edge AI-powered military drones. The startup’s autonomous drones leverage electro-optics-based autonomy and connectivity at low power requirements.
Its multi-object acquisition engine also aids target or personnel tracking for mission-critical applications on air, land, and maritime-based platforms. This allows militaries to improve situational awareness and safety on battlefields.
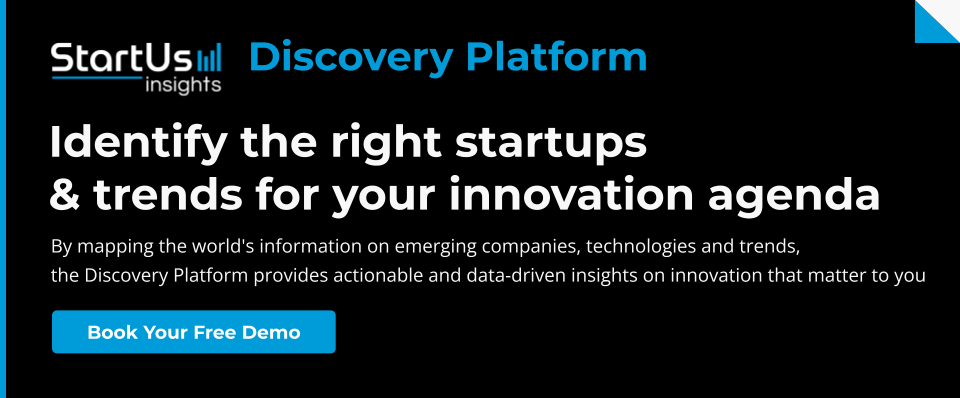
6. Manufacturing
The manufacturing industry utilizes IIoT, digital twins, 3D printers, and cobots, among other cyber-physical systems. They allow businesses to collect production data in real-time, power data processing algorithms, and improve process visualization. Further, 3D printers and robotic systems automate manufacturing workflows to increase throughput and quality control.
Such intelligent systems in manufacturing floors also increase operational flexibility and customization to enable agile workflows. Assisted maintenance is another critical application of CPS in manufacturing. Historic and real-time asset information allows floor managers to monitor equipment health and promptly detect anomalies. This proactive approach reduces unplanned downtime and extends equipment lifespan.
IN40S implements On-Demand Smart Factories
IN40S is a US-based startup that makes cyber-physical infrastructures for the manufacturing sector. The startup’s on-demand smart factory, i-Optimus , combines additive manufacturing, multi-material support, cobots, post-processing equipment, and more. It also features scalable architecture, edge and cloud-powered, AI, and digital warehouse as well as physical security and zero-trust architecture. This allows manufacturers to mitigate in-house hardware and software development while delivering manufacturing at scale.
7. Industry 4.0
Cyber-physical systems are critical factors that enable smart or connected and automated factories. To achieve this, CPS developers leverage low-latent connectivity like 5G, IoT, edge AI, and more. For instance, data-driven process automation platforms and systems allow businesses to streamline their operations while saving cost and time. Digital twins enable companies to track operations in real time while blockchain ensures transparent operations and cybersecurity.
CPS solutions in Industry 4.0 further ensure seamless connectivity across the manufacturing ecosystem and aid human-machine collaboration. For this, businesses leverage cobots and novel human-machine interfaces (HMIs), which enhance productivity and safety. Lastly, CPS enables intelligent supply chains that ensure real-time visibility and traceability while delivering demand forecasts and inventory management.
NQMCyber improves CPS Security
NQMCyber is an Irish startup that creates secure cyber-physical systems. The startup’s high-performance industrial hub features trusted platform modules (TPM), secure boot, and other hardware-based integrity checking. It also features secure storage, multiple network connectivity, AI, IoT, and edge processing to deliver advanced analytics for industries.
8. Smart Cities
Implementing intelligent systems in cities supports four key areas – mobility, infrastructure management, public safety, and energy management. Smart cities utilize IoT sensors, edge devices, AI, connected grids, and more. They enable efficient infrastructure management to track and optimize energy, waste management, and public safety systems. Additionally, intelligent transportation systems aid real-time traffic monitoring, smart traffic signal control, and optimized routing.
This reduces congestion and improves traffic flow. CPS integration further enables demand response programs, facilitates renewable integration, optimizes grids, and more. In the public safety sector, cyber-physical systems increase the performance of surveillance and emergency response systems to monitor critical areas and quickly detect potential threats. These solutions foster citizen engagement and improve the quality of life in cities.
Allas Network aids in Traffic Optimization
Allas Network is a Swedish startup that provides a traffic optimization solution. It combines hardware and software components, Allas Street and Allas Platform . Allas Street features AI-powered cameras, thermal monitoring, and various sensors. Additionally, Allas Platform offers live video feeds and environmental data securely from the distribution sites.
It also preserves citizen privacy with anonymized data, enhances access management, and utilizes an immutable database. The startup’s other scalable hardware platform, Allas Intelligence , uses AI and machine learning. It analyzes data from the Allas Platform to measure air quality and CO2 levels, count pedestrians on crossings, and improve road safety.
9. Agriculture
IoT devices, agricultural drones, blockchain-powered platforms, and smart irrigation systems are the most common cyber-physical systems in agriculture. Connected sensors provide farmers with vital plant and soil health parameters like pH and moisture levels to enable real-time and remote monitoring. Autonomous drones capture and analyze aerial imagery to assess crop health and ensure targeted interventions, also enabling precision agriculture.
Farmers are also transitioning to automated food production systems, such as automated vertical farms and controlled environment agriculture (CEA). This optimizes growing conditions, reduces resource consumption, and enables year-round food production. Moreover, agricultural robots, including autonomous tractors and robotic arms, simplify various farming operations like harvesting and weeding. Lastly, CPS in livestock management aids environmental and animal health monitoring as well as enables automated livestock handling.
Machine Eye Technology facilitates Autonomous Risk Assessment
Machine Eye Technology is a UK-based startup that offers autonomous risk assessment using off-highway vehicles. It leverages edge computer vision and deep learning to continuously assess risks in real time and identify humans.
This solution enables autonomous tractors to navigate in fields with humans and improve worker safety. For example, Agri-EPI Centre utilizes the startup’s perception system to detect people who are not within a safe distance.
10. Aerospace
Advanced flight control systems use sensor data, automation systems, and algorithms to improve flight stability, reduce pilot workload, and improve maneuverability and safety. Further, aerospace manufacturers apply digital twins to simulate and test aircraft designs and monitor aircraft parameters in real-time to enhance maintenance planning. They also leverage additive manufacturing to accelerate rapid prototyping, product/part customization, and reduce lead times.
Other cyber-physical systems in the aerospace industry include IoT devices to improve situational awareness, AI to speed up fault diagnosis, and cybersecurity systems to ensure data integrity and confidentiality. These applications of CPS further enhance operational efficiency, safety, and air traffic management while enabling remote monitoring and diagnostics.
Developair Technologies enhances Aerospace CPS Security
Developair Technologies is a Spanish startup that creates cybersecurity software for cyber-physical systems in the aerospace industry. The startup’s platform offers intelligent assistance across multiple phases of the software development cycle.
It also allows developers to define architecture, features cloud processing, and supports interaction with complementary tools. The startup’s software accelerates software validation and automatically generates tests and oracles based on specific requirements. As a result, it saves costs associated with software specification, implementation, and testing.
Discover All Cyber-Physical System Startups
AI-driven and edge or fog-hosted CPS will move data processing closer to the edge while ensuring high-performance data processing. IoT integration is accelerating across industries, powering more and more intelligent systems. Further, swarm robotics, digital twins, 5G, and explainable AI will improve the performance of cyber-physical systems while novel cybersecurity solutions are critical to keep them online. These technologies, along with other innovations in the field, will make CPS more accessible and interoperable. Get in touch to identify specific cyber-physical system startups & solutions that advance your business!
Your Name Business Email Company
Get our free newsletter on technology and startups.
Protected by reCAPTCHA and the Google Privacy Policy and Terms of Service apply.
Discover our Free Industry 4.0 Report 22 pages
Get free updates on Global Startups, Technologies & Trends!
Join 25k subscribers.
Business Email
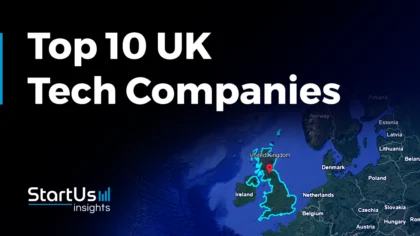
Industry 4.0 22 pages report
Manufacturing 22 pages report, energy 21 pages report, autonomous vehicles.
First & Last Name Business Email Company
Leverage our unparalleled data advantage to quickly and easily find hidden gems among 4.7M+ startups, scaleups. Access the world's most comprehensive innovation intelligence and stay ahead with AI-powered precision.
Get in touch
Your Name Business Email Company How can we support you? (optional)
Explore 30+ Emerging Tech Startups for Free
Get ahead with our interactive Innovation Map, featuring 30+ of the most promising tech startups. Download now to discover top tech companies and accelerate your innovation agenda.

Protected by reCAPTCHA and the Google Privacy Policy and Terms of Service apply.

COMMENTS
Large Scale Systems and Fuzzy Cognitive Maps: A critical overview of challenges and research opportunities. Peter P. Groumpos, in Annual Reviews in Control, 2014 6.2 Cyber-Physical Systems (CPS). A Cyber-Physical System (CPS) is a system of collaborating computational elements controlling physical entities.Today, a pre-cursor generation of cyber-physical systems can be found in areas as ...
Open issues in medical cyber physical system (MCPS) During our research, we have found a few things that need to be done in the field of Medical Cyber Physical System (MCPS). These issues can be called: 8.1.1. Security and privacy. Security and privacy issues are being talked about in MCPS, which is when medical devices can talk to each other.
Journal overview. Cyber-Physical Systems is an international interdisciplinary journal dedicated to publishing the highest quality research in the rapidly-growing field of cyber-physical systems / Internet-of-Things. Publications cover theory, algorithms, simulations, architectures, implementations, services and applications of state-of-the-art ...
Cyber-physical system (CPS) is an interdisciplinary research field that features systematic integrations of computation, communication, and control of physical processes. It is characterized by the deep complex intertwining process among the embedded cyber components and the dynamic physical components that involve mechanical components, human ...
Then, we introduce the recent trend in CPS research with an emphasis on major research topics in CPS. ... computation are tightly merged into a unified framework by carefully considering the complex interactions among all the physical and cyber parts in the system. We believe that this special section will be a meaningful step towards this end.
JOURNAL METRICS >. IET Cyber-Physical Systems: Theory & Applications is a fully open access journal that addresses the close interactions and feedback loop between cyber, and dynamic physical components, and challenges from a system perspective. Professor Shiyan Hu Elected as a Member of the European Academy of Sciences and Arts.
A Cyber-Physical System (CPS) is a spatiotemporal multi-dimensional and heterogeneous hybrid autonomous system composed of deep integration of information resources and physical systems. ... In the ADN with a high proportion of distributed generations and electrified loads in the future, it is an important research topic to strengthen the ...
ACM Transactions on Cyber-Physical Systems (TCPS) is the premier journal for the publication of high-quality original research papers and survey papers that have scientific and technological understanding of the interactions of information processing, networking and physical processes. TCPS covers the following topics: Computation Abstractions; System Modeling and Languages
In this article, we combine the system theory as well as its digital translation and the use of quantum decision networks to propose a quantum framework for Industry 4.0 cyber-physical systems ...
His research spans radio resource management, Internet of Things (IoT) services, and modeling in networked cyber-physical systems. Abd-Elhamid is a senior member of the IEEE, and a recipient of the Natural Sciences and Engineering Research Council (NSERC) Early Career Researcher Award as well as several awards at Alfaisal University for ...
This Section lays the groundwork for this research; the theoretical foundations of CPS are presented in 2.1 and additional related work is introduced that also seeks to structure industrial CPS in 2.2.. 2.1 Foundations of Cyber-Physical Systems. The term CPS was introduced by E. A. Lee in 2006; since then, the topic has been further analyzed and developed in several scientific and practical ...
They call for more research on cyber-physical security. Fears about the use of quantum computing to break current cryptosystems make matters worse. ... It is a fast growing research topic aiming ...
This relates or research on Artificial Intelligence (AI) with Cyber Physical Systems (CPS). By Cyber Physical Systems (CPS), we refer to computer-human networks, controlling physical processes, where physical processes affect computations and vice versa. One modern version of Cyber Physical Systems is the Internet of Things (IoT).
Cyber-Physical Systems (CPS) are integrations of computation with physical processes. [1] In cyber-physical systems, physical and software components are deeply intertwined, able to operate on different spatial and temporal scales, exhibit multiple and distinct behavioral modalities, and interact with each other in ways that change with context. [2] [3] CPS involves transdisciplinary ...
About the journal. Internet of Things and Cyber-Physical Systems publishes rigorously peer-reviewed, high quality original articles and authoritative reviews that focus on Internet of Things and Cyber-Physical Systems. All articles are published fully Open Access on ScienceDirect. The journal welcomes research …. View full aims & scope.
Cyber-physical system (CPS) is an integration of physical processes with computation and communication. ... mitigation for cyber-physical wireless body area Network System using social networks IEEE Transactions on Emerging Topics in Computing 2013 1 121-132. Crossref. ... A Review of Cyber-Physical System Research Relevant to the Emerging IT ...
The mission of the Cyber-Physical Systems Research Center (CPSRC) is to promote and facilitate collaborative research activities within UCSC as well as with its academic and industrial partners on key cyber-physical systems topics. CPSRC will foster novel foundational and applied research to support applications such as smart cities and ...
Recently, there is a significant growth in the use of the Cyber-Physical System (CPS). New technologies such as Internet of Things (IoT), Industry 4.0, and Analytics have become enablers of CPS implementation. Study of the development and application of CPS in the supply chain context is valuable to operations management and information systems research and practice; especially, a focus on IoT ...
Cyber-physical systems Topics. Smart cities. ... Building on a successful first-year Strategic and Emerging Research Initiatives (SERI) project NIST and Autonomous Vehicles (see 2022 NIST AV workshop and ... A digital twin goes beyond this by connecting a simulation with the physical system with the purpose of Software. SimPROCESD. August 15 ...
The purpose of this Research Topic is to promote outstanding research concerning human-in-the-loop cyber-physical systems, focusing on state-of-the-art progress, developments, and new trends. Cyber-physical systems (CPS) are connecting our physical world and cyberspace through real-time sensing, situational awareness, and intelligent control.
Such that their multi-scale interconnection and interaction is better captured by the notion of Cyber-Physical System of Systems (CPSoS), defining a large and distributed complex system of CPSs. ... To get an overview on the open research topics, the general topics are split into following categories. They only address several general topics as ...
A Cyber-Physical-Social System (CPSS) is an evolving subset of Cyber-Physical Systems (CPS), which involve the interlinking of the cyber, physical, and social domains within a system-of-systems mindset. ... The oldest research topics were located in the center, with newer research extending to either side, as shown in Figure 6. The oldest ...
This research focuses on the top 10 industries advancing cyber-physical systems in 2023. For this, we analyzed 2313 emerging companies working in the field. Read more to explore the industry-specific applications of CPS and find out how they impact your business. Tree Map reveals the Cyber-Physical Systems Examples across 10 Industries