Time Series Forecasting with Recurrent Neural Networks
In this post, we’ll review three advanced techniques for improving the performance and generalization power of recurrent neural networks. We’ll demonstrate all three concepts on a temperature-forecasting problem, where you have access to a time series of data points coming from sensors installed on the roof of a building.
In this post, we’ll review three advanced techniques for improving the performance and generalization power of recurrent neural networks. By the end of the section, you’ll know most of what there is to know about using recurrent networks with Keras. We’ll demonstrate all three concepts on a temperature-forecasting problem, where you have access to a time series of data points coming from sensors installed on the roof of a building, such as temperature, air pressure, and humidity, which you use to predict what the temperature will be 24 hours after the last data point. This is a fairly challenging problem that exemplifies many common difficulties encountered when working with time series.
We’ll cover the following techniques:
- Recurrent dropout — This is a specific, built-in way to use dropout to fight overfitting in recurrent layers.
- Stacking recurrent layers — This increases the representational power of the network (at the cost of higher computational loads).
- Bidirectional recurrent layers — These present the same information to a recurrent network in different ways, increasing accuracy and mitigating forgetting issues.

A temperature-forecasting problem
Until now, the only sequence data we’ve covered has been text data, such as the IMDB dataset and the Reuters dataset. But sequence data is found in many more problems than just language processing. In all the examples in this section, you’ll play with a weather timeseries dataset recorded at the Weather Station at the Max Planck Institute for Biogeochemistry in Jena, Germany.
In this dataset, 14 different quantities (such air temperature, atmospheric pressure, humidity, wind direction, and so on) were recorded every 10 minutes, over several years. The original data goes back to 2003, but this example is limited to data from 2009–2016. This dataset is perfect for learning to work with numerical time series. You’ll use it to build a model that takes as input some data from the recent past (a few days’ worth of data points) and predicts the air temperature 24 hours in the future.
Download and uncompress the data as follows:
Let’s look at the data.
Here is the plot of temperature (in degrees Celsius) over time. On this plot, you can clearly see the yearly periodicity of temperature.

Here is a more narrow plot of the first 10 days of temperature data (see figure 6.15). Because the data is recorded every 10 minutes, you get 144 data points per day.

On this plot, you can see daily periodicity, especially evident for the last 4 days. Also note that this 10-day period must be coming from a fairly cold winter month.
If you were trying to predict average temperature for the next month given a few months of past data, the problem would be easy, due to the reliable year-scale periodicity of the data. But looking at the data over a scale of days, the temperature looks a lot more chaotic. Is this time series predictable at a daily scale? Let’s find out.
Preparing the data
The exact formulation of the problem will be as follows: given data going as far back as lookback timesteps (a timestep is 10 minutes) and sampled every steps timesteps, can you predict the temperature in delay timesteps? You’ll use the following parameter values:
- lookback = 1440 — Observations will go back 10 days.
- steps = 6 — Observations will be sampled at one data point per hour.
- delay = 144 — Targets will be 24 hours in the future.
To get started, you need to do two things:
- Preprocess the data to a format a neural network can ingest. This is easy: the data is already numerical, so you don’t need to do any vectorization. But each time series in the data is on a different scale (for example, temperature is typically between -20 and +30, but atmospheric pressure, measured in mbar, is around 1,000). You’ll normalize each time series independently so that they all take small values on a similar scale.
- Write a generator function that takes the current array of float data and yields batches of data from the recent past, along with a target temperature in the future. Because the samples in the dataset are highly redundant (sample N and sample N + 1 will have most of their timesteps in common), it would be wasteful to explicitly allocate every sample. Instead, you’ll generate the samples on the fly using the original data.
NOTE: Understanding generator functions A generator function is a special type of function that you call repeatedly to obtain a sequence of values from. Often generators need to maintain internal state, so they are typically constructed by calling another yet another function which returns the generator function (the environment of the function which returns the generator is then used to track state).
For example, the sequence_generator() function below returns a generator function that yields an infinite sequence of numbers:
The current state of the generator is the value variable that is defined outside of the function. Note that superassignment ( <<- ) is used to update this state from within the function.
Generator functions can signal completion by returning the value NULL . However, generator functions passed to Keras training methods (e.g. fit_generator() ) should always return values infinitely (the number of calls to the generator function is controlled by the epochs and steps_per_epoch parameters).
First, you’ll convert the R data frame which we read earlier into a matrix of floating point values (we’ll discard the first column which included a text timestamp):
You’ll then preprocess the data by subtracting the mean of each time series and dividing by the standard deviation. You’re going to use the first 200,000 timesteps as training data, so compute the mean and standard deviation for normalization only on this fraction of the data.
The code for the data generator you’ll use is below. It yields a list (samples, targets) , where samples is one batch of input data and targets is the corresponding array of target temperatures. It takes the following arguments:
- data — The original array of floating-point data, which you normalized in listing 6.32.
- lookback — How many timesteps back the input data should go.
- delay — How many timesteps in the future the target should be.
- min_index and max_index — Indices in the data array that delimit which timesteps to draw from. This is useful for keeping a segment of the data for validation and another for testing.
- shuffle — Whether to shuffle the samples or draw them in chronological order.
- batch_size — The number of samples per batch.
- step — The period, in timesteps, at which you sample data. You’ll set it 6 in order to draw one data point every hour.
The i variable contains the state that tracks next window of data to return, so it is updated using superassignment (e.g. i <<- i + length(rows) ).
Now, let’s use the abstract generator function to instantiate three generators: one for training, one for validation, and one for testing. Each will look at different temporal segments of the original data: the training generator looks at the first 200,000 timesteps, the validation generator looks at the following 100,000, and the test generator looks at the remainder.
A common-sense, non-machine-learning baseline
Before you start using black-box deep-learning models to solve the temperature-prediction problem, let’s try a simple, common-sense approach. It will serve as a sanity check, and it will establish a baseline that you’ll have to beat in order to demonstrate the usefulness of more-advanced machine-learning models. Such common-sense baselines can be useful when you’re approaching a new problem for which there is no known solution (yet). A classic example is that of unbalanced classification tasks, where some classes are much more common than others. If your dataset contains 90% instances of class A and 10% instances of class B, then a common-sense approach to the classification task is to always predict “A” when presented with a new sample. Such a classifier is 90% accurate overall, and any learning-based approach should therefore beat this 90% score in order to demonstrate usefulness. Sometimes, such elementary baselines can prove surprisingly hard to beat.
In this case, the temperature time series can safely be assumed to be continuous (the temperatures tomorrow are likely to be close to the temperatures today) as well as periodical with a daily period. Thus a common-sense approach is to always predict that the temperature 24 hours from now will be equal to the temperature right now. Let’s evaluate this approach, using the mean absolute error (MAE) metric:
Here’s the evaluation loop.
This yields an MAE of 0.29. Because the temperature data has been normalized to be centered on 0 and have a standard deviation of 1, this number isn’t immediately interpretable. It translates to an average absolute error of 0.29 x temperature_std degrees Celsius: 2.57˚C.
That’s a fairly large average absolute error. Now the game is to use your knowledge of deep learning to do better.
A basic machine-learning approach
In the same way that it’s useful to establish a common-sense baseline before trying machine-learning approaches, it’s useful to try simple, cheap machine-learning models (such as small, densely connected networks) before looking into complicated and computationally expensive models such as RNNs. This is the best way to make sure any further complexity you throw at the problem is legitimate and delivers real benefits.
The following listing shows a fully connected model that starts by flattening the data and then runs it through two dense layers. Note the lack of activation function on the last dense layer, which is typical for a regression problem. You use MAE as the loss. Because you evaluate on the exact same data and with the exact same metric you did with the common-sense approach, the results will be directly comparable.
Let’s display the loss curves for validation and training.

Some of the validation losses are close to the no-learning baseline, but not reliably. This goes to show the merit of having this baseline in the first place: it turns out to be not easy to outperform. Your common sense contains a lot of valuable information that a machine-learning model doesn’t have access to.
You may wonder, if a simple, well-performing model exists to go from the data to the targets (the common-sense baseline), why doesn’t the model you’re training find it and improve on it? Because this simple solution isn’t what your training setup is looking for. The space of models in which you’re searching for a solution – that is, your hypothesis space – is the space of all possible two-layer networks with the configuration you defined. These networks are already fairly complicated. When you’re looking for a solution with a space of complicated models, the simple, well-performing baseline may be unlearnable, even if it’s technically part of the hypothesis space. That is a pretty significant limitation of machine learning in general: unless the learning algorithm is hardcoded to look for a specific kind of simple model, parameter learning can sometimes fail to find a simple solution to a simple problem.
A first recurrent baseline
The first fully connected approach didn’t do well, but that doesn’t mean machine learning isn’t applicable to this problem. The previous approach first flattened the time series, which removed the notion of time from the input data. Let’s instead look at the data as what it is: a sequence, where causality and order matter. You’ll try a recurrent-sequence processing model – it should be the perfect fit for such sequence data, precisely because it exploits the temporal ordering of data points, unlike the first approach.
Instead of the LSTM layer introduced in the previous section, you’ll use the GRU layer , developed by Chung et al. in 2014. Gated recurrent unit (GRU) layers work using the same principle as LSTM, but they’re somewhat streamlined and thus cheaper to run (although they may not have as much representational power as LSTM). This trade-off between computational expensiveness and representational power is seen everywhere in machine learning.
The results are plotted below. Much better! You can significantly beat the common-sense baseline, demonstrating the value of machine learning as well as the superiority of recurrent networks compared to sequence-flattening dense networks on this type of task.

The new validation MAE of ~0.265 (before you start significantly overfitting) translates to a mean absolute error of 2.35˚C after denormalization. That’s a solid gain on the initial error of 2.57˚C, but you probably still have a bit of a margin for improvement.
Using recurrent dropout to fight overfitting
It’s evident from the training and validation curves that the model is overfitting: the training and validation losses start to diverge considerably after a few epochs. You’re already familiar with a classic technique for fighting this phenomenon: dropout, which randomly zeros out input units of a layer in order to break happenstance correlations in the training data that the layer is exposed to. But how to correctly apply dropout in recurrent networks isn’t a trivial question. It has long been known that applying dropout before a recurrent layer hinders learning rather than helping with regularization. In 2015, Yarin Gal, as part of his PhD thesis on Bayesian deep learning, determined the proper way to use dropout with a recurrent network: the same dropout mask (the same pattern of dropped units) should be applied at every timestep, instead of a dropout mask that varies randomly from timestep to timestep. What’s more, in order to regularize the representations formed by the recurrent gates of layers such as layer_gru and layer_lstm , a temporally constant dropout mask should be applied to the inner recurrent activations of the layer (a recurrent dropout mask). Using the same dropout mask at every timestep allows the network to properly propagate its learning error through time; a temporally random dropout mask would disrupt this error signal and be harmful to the learning process.
Yarin Gal did his research using Keras and helped build this mechanism directly into Keras recurrent layers. Every recurrent layer in Keras has two dropout-related arguments: dropout , a float specifying the dropout rate for input units of the layer, and recurrent_dropout , specifying the dropout rate of the recurrent units. Let’s add dropout and recurrent dropout to the layer_gru and see how doing so impacts overfitting. Because networks being regularized with dropout always take longer to fully converge, you’ll train the network for twice as many epochs.
The plot below shows the results. Success! You’re no longer overfitting during the first 20 epochs. But although you have more stable evaluation scores, your best scores aren’t much lower than they were previously.

Stacking recurrent layers
Because you’re no longer overfitting but seem to have hit a performance bottleneck, you should consider increasing the capacity of the network. Recall the description of the universal machine-learning workflow: it’s generally a good idea to increase the capacity of your network until overfitting becomes the primary obstacle (assuming you’re already taking basic steps to mitigate overfitting, such as using dropout). As long as you aren’t overfitting too badly, you’re likely under capacity.
Increasing network capacity is typically done by increasing the number of units in the layers or adding more layers. Recurrent layer stacking is a classic way to build more-powerful recurrent networks: for instance, what currently powers the Google Translate algorithm is a stack of seven large LSTM layers – that’s huge.
To stack recurrent layers on top of each other in Keras, all intermediate layers should return their full sequence of outputs (a 3D tensor) rather than their output at the last timestep. This is done by specifying return_sequences = TRUE .
The figure below shows the results. You can see that the added layer does improve the results a bit, though not significantly. You can draw two conclusions:
- Because you’re still not overfitting too badly, you could safely increase the size of your layers in a quest for validation-loss improvement. This has a non-negligible computational cost, though.
- Adding a layer didn’t help by a significant factor, so you may be seeing diminishing returns from increasing network capacity at this point.

Using bidirectional RNNs
The last technique introduced in this section is called bidirectional RNNs . A bidirectional RNN is a common RNN variant that can offer greater performance than a regular RNN on certain tasks. It’s frequently used in natural-language processing – you could call it the Swiss Army knife of deep learning for natural-language processing.
RNNs are notably order dependent, or time dependent: they process the timesteps of their input sequences in order, and shuffling or reversing the timesteps can completely change the representations the RNN extracts from the sequence. This is precisely the reason they perform well on problems where order is meaningful, such as the temperature-forecasting problem. A bidirectional RNN exploits the order sensitivity of RNNs: it consists of using two regular RNNs, such as the layer_gru and layer_lstm you’re already familiar with, each of which processes the input sequence in one direction (chronologically and antichronologically), and then merging their representations. By processing a sequence both ways, a bidirectional RNN can catch patterns that may be overlooked by a unidirectional RNN.
Remarkably, the fact that the RNN layers in this section have processed sequences in chronological order (older timesteps first) may have been an arbitrary decision. At least, it’s a decision we made no attempt to question so far. Could the RNNs have performed well enough if they processed input sequences in antichronological order, for instance (newer timesteps first)? Let’s try this in practice and see what happens. All you need to do is write a variant of the data generator where the input sequences are reverted along the time dimension (replace the last line with list(samples[,ncol(samples):1,], targets) ). Training the same one-GRU-layer network that you used in the first experiment in this section, you get the results shown below.

The reversed-order GRU underperforms even the common-sense baseline, indicating that in this case, chronological processing is important to the success of your approach. This makes perfect sense: the underlying GRU layer will typically be better at remembering the recent past than the distant past, and naturally the more recent weather data points are more predictive than older data points for the problem (that’s what makes the common-sense baseline fairly strong). Thus the chronological version of the layer is bound to outperform the reversed-order version. Importantly, this isn’t true for many other problems, including natural language: intuitively, the importance of a word in understanding a sentence isn’t usually dependent on its position in the sentence. Let’s try the same trick on the LSTM IMDB example from section 6.2.
You get performance nearly identical to that of the chronological-order LSTM. Remarkably, on such a text dataset, reversed-order processing works just as well as chronological processing, confirming the hypothesis that, although word order does matter in understanding language, which order you use isn’t crucial. Importantly, an RNN trained on reversed sequences will learn different representations than one trained on the original sequences, much as you would have different mental models if time flowed backward in the real world – if you lived a life where you died on your first day and were born on your last day. In machine learning, representations that are different yet useful are always worth exploiting, and the more they differ, the better: they offer a new angle from which to look at your data, capturing aspects of the data that were missed by other approaches, and thus they can help boost performance on a task. This is the intuition behind ensembling , a concept we’ll explore in chapter 7.
A bidirectional RNN exploits this idea to improve on the performance of chronological-order RNNs. It looks at its input sequence both ways, obtaining potentially richer representations and capturing patterns that may have been missed by the chronological-order version alone.

To instantiate a bidirectional RNN in Keras, you use the bidirectional() function, which takes a recurrent layer instance as an argument. The bidirectional() function creates a second, separate instance of this recurrent layer and uses one instance for processing the input sequences in chronological order and the other instance for processing the input sequences in reversed order. Let’s try it on the IMDB sentiment-analysis task.
It performs slightly better than the regular LSTM you tried in the previous section, achieving over 89% validation accuracy. It also seems to overfit more quickly, which is unsurprising because a bidirectional layer has twice as many parameters as a chronological LSTM. With some regularization, the bidirectional approach would likely be a strong performer on this task.
Now let’s try the same approach on the temperature prediction task.
This performs about as well as the regular layer_gru . It’s easy to understand why: all the predictive capacity must come from the chronological half of the network, because the antichronological half is known to be severely underperforming on this task (again, because the recent past matters much more than the distant past in this case).
Going even further
There are many other things you could try, in order to improve performance on the temperature-forecasting problem:
- Adjust the number of units in each recurrent layer in the stacked setup. The current choices are largely arbitrary and thus probably suboptimal.
- Adjust the learning rate used by the RMSprop optimizer.
- Try using layer_lstm instead of layer_gru .
- Try using a bigger densely connected regressor on top of the recurrent layers: that is, a bigger dense layer or even a stack of dense layers.
- Don’t forget to eventually run the best-performing models (in terms of validation MAE) on the test set! Otherwise, you’ll develop architectures that are overfitting to the validation set.
As always, deep learning is more an art than a science. We can provide guidelines that suggest what is likely to work or not work on a given problem, but, ultimately, every problem is unique; you’ll have to evaluate different strategies empirically. There is currently no theory that will tell you in advance precisely what you should do to optimally solve a problem. You must iterate.
Wrapping up
Here’s what you should take away from this section:
- As you first learned in chapter 4, when approaching a new problem, it’s good to first establish common-sense baselines for your metric of choice. If you don’t have a baseline to beat, you can’t tell whether you’re making real progress.
- Try simple models before expensive ones, to justify the additional expense. Sometimes a simple model will turn out to be your best option.
- When you have data where temporal ordering matters, recurrent networks are a great fit and easily outperform models that first flatten the temporal data.
- To use dropout with recurrent networks, you should use a time-constant dropout mask and recurrent dropout mask. These are built into Keras recurrent layers, so all you have to do is use the dropout and recurrent_dropout arguments of recurrent layers.
- Stacked RNNs provide more representational power than a single RNN layer. They’re also much more expensive and thus not always worth it. Although they offer clear gains on complex problems (such as machine translation), they may not always be relevant to smaller, simpler problems.
- Bidirectional RNNs, which look at a sequence both ways, are useful on natural-language processing problems. But they aren’t strong performers on sequence data where the recent past is much more informative than the beginning of the sequence.
NOTE: Markets and machine learning Some readers are bound to want to take the techniques we’ve introduced here and try them on the problem of forecasting the future price of securities on the stock market (or currency exchange rates, and so on). Markets have very different statistical characteristics than natural phenomena such as weather patterns. Trying to use machine learning to beat markets, when you only have access to publicly available data, is a difficult endeavor, and you’re likely to waste your time and resources with nothing to show for it.
Always remember that when it comes to markets, past performance is not a good predictor of future returns – looking in the rear-view mirror is a bad way to drive. Machine learning, on the other hand, is applicable to datasets where the past is a good predictor of the future.
Comment on this article Share:
For attribution, please cite this work as
BibTeX citation
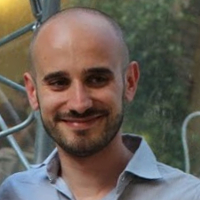
A Step-by-Step Walkthrough Neural Networks for Time-series Forecasting
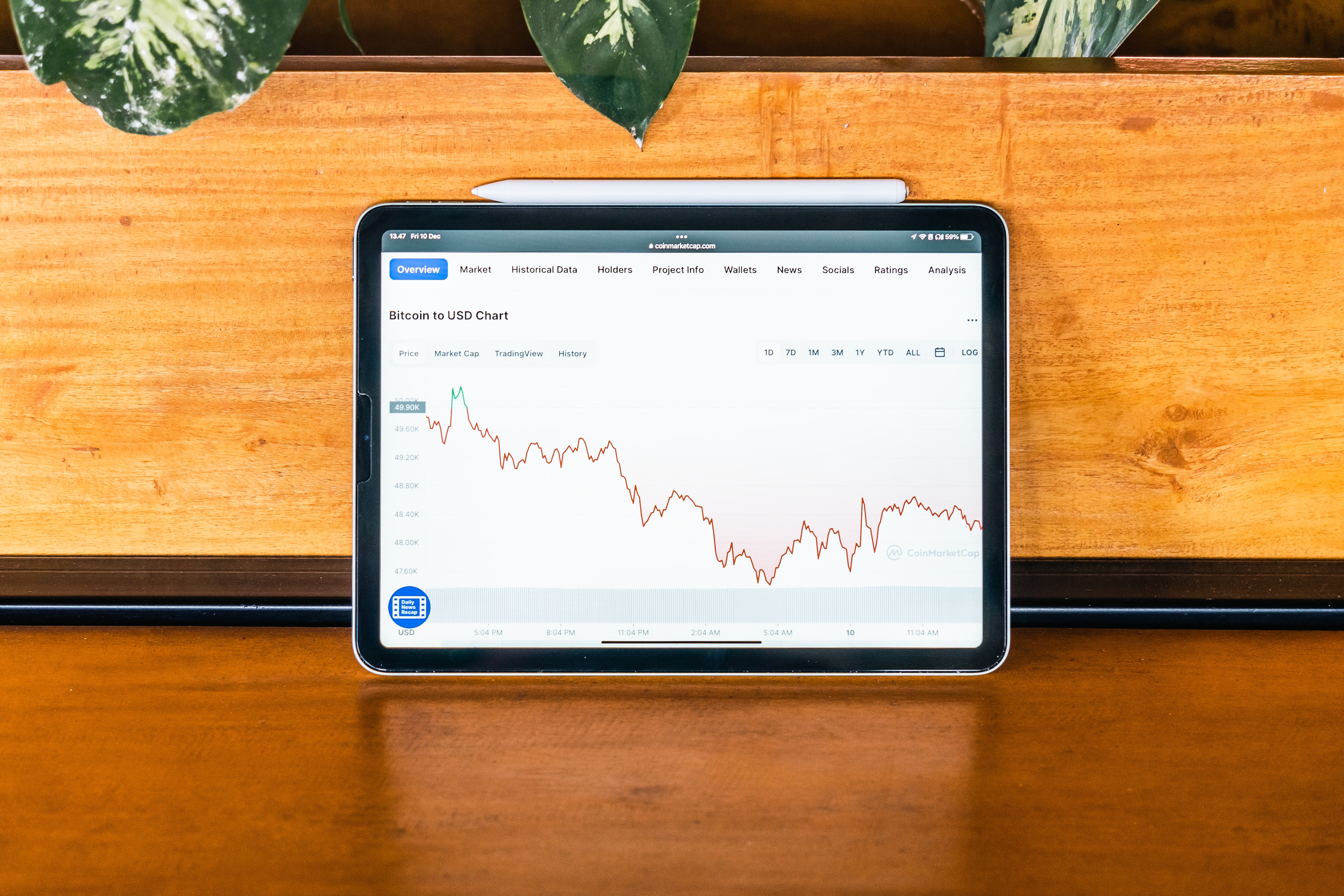
So you want to forecast your sales? Or maybe you would like to know the future price of bitcoin?
In both cases, you are trying to solve a problem known as “time-series forecasting”. A time-series is a sorted set of values that varies depending on time.
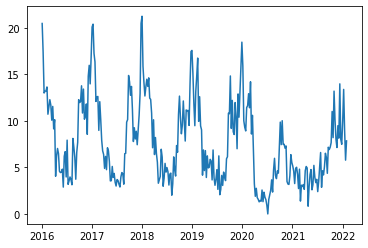
No one can predict the future, but one can search in the past looking for patterns, and hope that those are going to repeat.
Guess what is very good in finding patterns? Neural networks (NNs from now on).
But which type of NN? I am going to test different kind of models on some artificially generated time-series . Each time-series will present a different combination of patterns, so that I can compare the different NN results.
After reading, you will know:
- how to choose a NN for time-series forecasting;
- how many past samples are needed to discover a pattern;
- which is the impact of noise on the prediction quality;
It is easy to say “Neural Networks”
There exist different kind of NN that can be applied to this use case.
- Multi-Layer Perceptron (MLP): the most common and simple. More about it here .
- Recurrent Neural Network (RNN): in literature, the most suited to time-series forecasting. They combine the information of the current observation, with the information of the previous observations. More about it here .
- Convolutional Neural Network (CNN): usually applied for Computer Vision, they are raising also for time-series forecasting. More about it here
It is not the purpose of this article going deep about each kind of network. Anyway, useful links are left for the reader that want to.
There are also different kinds of time-series, classifiable by the patterns that they present. It may happen that NNs perform differently depending on the time-series features.
Patterns and composition of time-series
Time-series presents mainly two types of patterns.
- Seasonality : the values periodically repeat.
- Trend : the values continue to increase or decrease.
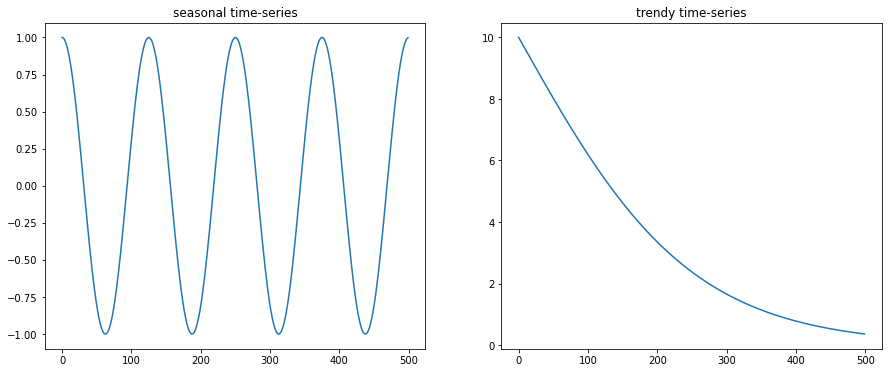
A time-series forms from a non-linear combination of one or more trends , one ore more seasonalities and some noise .
Trends and seasonalities are auto-correlated : future values depend on past values. The noise component may be instead totally random, or it could present a correlation with some feature external to the time-series.
I will conduct this analysis to test which NN is the best in finding seasonalities, trends and non-linearities.
During the experiment, the noise will be generated randomly . This means that there is nothing to discover that could predict the noise. It goes that our best models will present an average error very close to the amount of noise.
This is ok because I want to test how good is a model in discovering the patterns hidden by the noise.
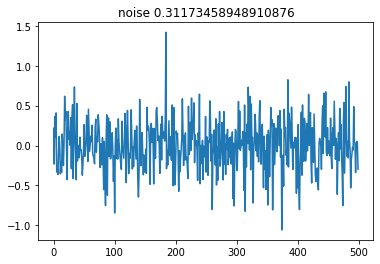
Neural networks benefits over statistical techniques.
Time-series forecasting is traditionally approached with statistical techniques, like ARMA (Auto-Regressive Moving Average), ARIMA (Auto-Regressive Integrated Moving Average), SARIMA (Seasonal Auto-Regressive Integrated Moving Average) or Facebook Prophet models. These require that you have some a-priori knowledge about the series.
- Is it the series stationary or not? (More about stationarity here )
- How many different seasonalities are present in the series ( SARIMA ).
- The differentiation order value to make the series stationary ( ARIMA ).
Also, if you plan to predict only one next value, given a set of past values ( many-to-one prediction ), then the statical models need to be retrained every time a new value is added to the series.
In contrast, NNs don’t need to be retrained so frequently and don’t require any a-priori knowledge . In addition, it is quite straightforward to add external information that may correlate to the noise generation (multi-variate input).
Experiments
You can find the entire notebook here
You can also download and alternative version from here . Install this requirements.txt if you want to run it locally.
Let’s start by defining a function to generate a wave, and use it to plot a wave of period 10 with 520 samples and amplitude 1.
I will start from the most simple MLP with one hidden layer of 5 neurons, an output layer of 1 neuron, and an input layer of the same size as the input. Which input?
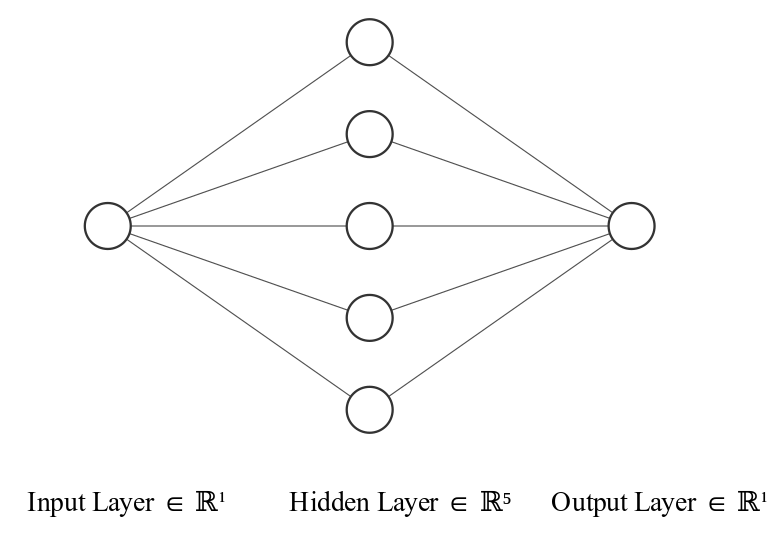
I want to forecast the value of the series at time t , let’s call it y(t) . Then I will input to the NN the values y(t-N)...y(t-1) for N <= t and N > 1 . I will call this input “lags” . I want to see how the network performs, feeding it with only the first lag y(t-1) .
Data preparation
NNs better perform with dataset values ranging between [0, 1] (as explained here ). Then let’s apply a scaling function.
After, let’s define a function to prepare our dataset. It will output a pandas DataFrame where each row is an input sample, and the columns are the lags together with the actual output value.
The last operation is to split the dataset into train and test sets. I will use the first 60% of the dataset to train, and the left 40% to test.
Model Hyper-parameters and evaluation environment
I will use Keras backed by Tensorflow .
Let’s consider some of the hyper-parameters that I adopt for training. I will use Adam as the gradient descent optimizer and mean squared error for measuring the training error. The batch size will be the default value of 32 (empirically choosen). The model will train for a maximum of 200 epochs , early stopped if no further improvement is observed for 30 consecutive epochs.
I choose the Elu activation function because it makes the models training more stable . I experienced the improved stability during tests, not reported here for brevity. For the convolutional layer I use instead the Relu activation function, because I empirically observed better performance. The output has a linear activation function .
The prediction is going to be compared with the actual test values, measuring the error with the root mean squared error .
I will also compare the NN prediction, with two naive predictors. The average value of the test set (not usable as a predictor, as it uses future values) and the test set shifted by 1 time lag (i.e. y'(t+1) = y(t) ).
There is always a certain level of randomness when training a model. This makes it very difficult to understand the effects of changing the hyper-parameters.
One countermeasure is to train the same model many times and then average the results . In this experiment, I will train the models 5 times.
The quality of the model is given by it’s average RMSE and the Standard Deviation (std) of the errors. A high quantity of std means that the model is not stable in its training. (i.e. Different trainings may result in models that performs very differently.)
Model training (finally 😅)
Let’s train our simple MLP
The result is not satisfying. The predictions are just the test series shifted by one lag.
I can think of changing 3 things to improve the prediction:
- the number of hidden neurons;
- the number of training epochs;
- the number of lags.
Let’s reason for a while .
It is straightforward to exclude the number of epochs, looking at the above graphics of the training losses. All of the 5 trainings stopped early, after a consistent number of epochs with an error close to 0 and no improvements.
Now zoom-in our sinusoid, near one of the higher peaks.
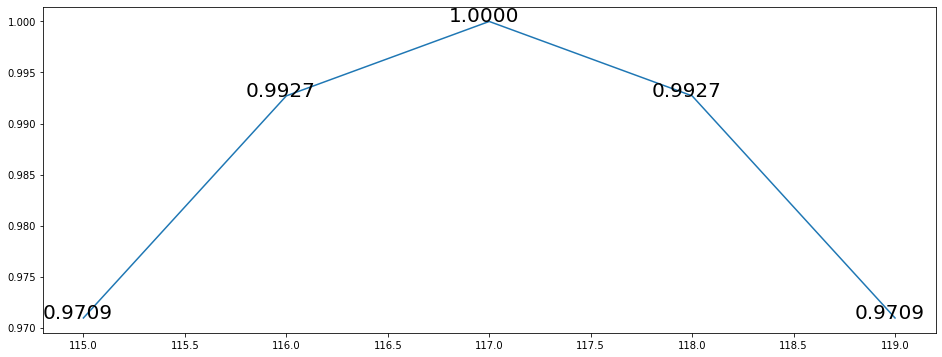
You can notice that the same values repeat both ascending and descending. The same input y(t) can output two different y(t+1) values. It doesn’t exist a unique relation between inputs and outputs.
We can conclude that we need at least two input lags to learn the function.
We observed that a simple MLP with one hidden layer, can learn a sinusoidal function, with a minimum input of 2 lags.
Let’s do some noise!
What happens if I introduce noise?
We should not be surprised to see that the prediction looks again laggy. Indeed, the RMSE is even worst than the shifted baseline. Given a noise with an std value 0.1, we should expect an RMSE value as close as possible to 0.1.
How many lags do we need to discover the pattern hidden by the noise?
Let’s try to see what happens with 4 lags.
Better, but no pattern looks to have been discovered. Let’s now try again with 10 and 20 lags.
With 10 lags the prediction gets better but it is still a bit noisy.
With 20 lags it finally looks like the model found our pattern again. Also the average is very close to the target value of 0.1.
You promised MOAR networks!!
Yes, I did. So, let’s define a helper function to test many different models together. Namely:
- Our simple MLP
- A deeper MLP with 3 hidden layers
- The same as 2, but with a Dropout layer , to see if it helps
- A Simple RNN
- A model with 2 stacked RNN layers
- A Long-Short Term Memory ( LSTM ). A special kind of RNN that can keep information more distant in the past.
- A Gated Recurrent Unit ( GRU ). Has similar properties of an LSTM, but requires less computation effort.
- A Convolutional NN
- The same as 5 but with a Dropout layer.
And here are the results.
Looks like complicating the model doesn’t actually improve.
Will it be the same also with more complicated patterns?
Experiments with different time-series.
I am going to observe the NN behavior, against series that present the following features:
- Fading Wave : a wave that changes its amplitude as time passes. With some noise.
- Complex series : a series that is composed of 3 waves with different frequencies and amplitude, one trend, and a significative amount of noise.
- Realistic series : a series that is composed of many waves, one trend, and a very high amount of noise. I call it “realistic” because it is built looking at the spectrum of its Fourier Transform. The purpose is that it presents many components, with no one clearly prevailing over the others (similar peaks). This should mimic the FT Spectrum of a real time-series of the sales of a product.
Fading Wave : Noise 0.1. RMSE 0.1914 for the mean. RMSE 0.125 for the shift:
Complex Series : Noise 3. RMSE 4.008 for the mean. RMSE 4.196 for the shift:
Realistic series : Noise 6. RMSE 8.14 for the mean. RMSE 11.04 for the shift:
Fading Wave results : Noise 0.1. RMSE 0.1914 for the mean. RMSE 0.125 for the shift
Complex series results : Noise 3. RMSE 4.008 for the mean. RMSE 4.196 for the shift
Realistic series results : Noise 6. RMSE 8.14 for the mean. RMSE 11.04 for the shift
The models do very well with the “Fading series”. Even less than the target value 0.1. We can deduce that all the models can discover that the pattern changes in time.
Looks like in presence of more complex patterns, CNN does the best job. While the RNN flavors are not performing better than the others. This is not what we would expect from the literature.
“Realistic series” presents a very large amount of noise, and all the networks have an average error higher than 7, which is quite far from the target value of 6. This suggests that in a real environment we should try to add more information external to the time-series, to search for patterns that correlate to the noise . For example, if we are analyzing sales of a product that has a categorical classification, adding the sales of other products of the same category may help.
It is surprising that even the simple and the deep MLPs always return quite good results.
The dropout level in the CNN helps in lowering the Standard Deviation.
More information from the past
Is there any information coming from the series itself that we are not yet considering?
Let’s have a look at the auto-correlation plots:
We are currently considering 20 lags, but there is some correlations with the lags older the 20. Instead of highering the number of lags, we could try to add past values in an aggregated form. Let’s add the average values of the 4 previous lags, and of the 12 previous lags.
In the “Complex series” there is a seasonality that repeats about every 25 lags. Let’s try to catch these kinds of seasonalities by adding the averaged values (1 lag, 4 lags, and 12 lags), shifted by the number of lags that corresponds to the higher auto-correlation (after the 12th lag).
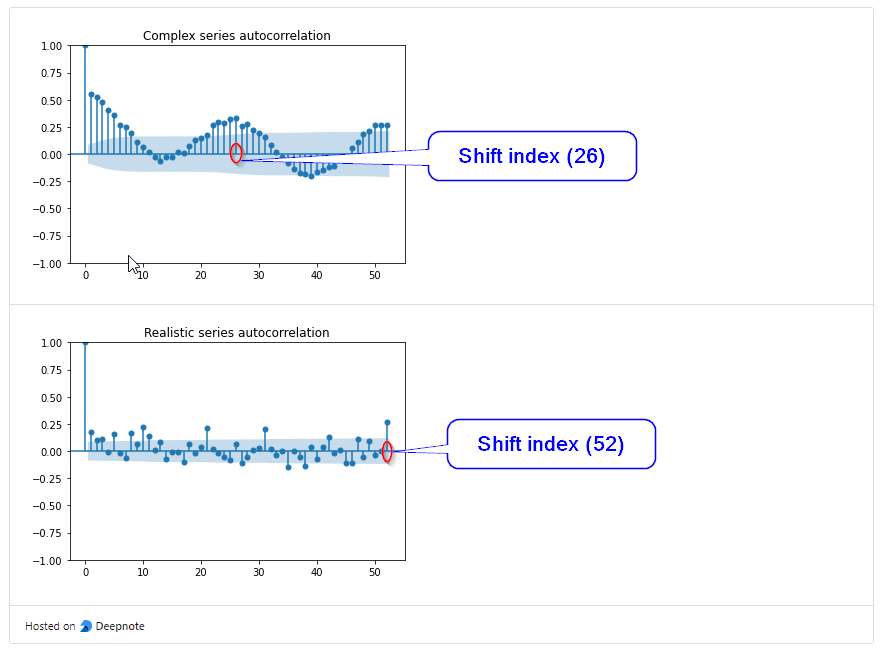
We now adapt the CNN to be 2D CNN instead of 1D, as now we are passing 20 lags for each sample, with 6 features vector each. We also add one more model: a CNN with 2 Convolutional layers, separated by one pooling layer .
Let’s have a look at the results:
All the models improve. In the “Realistic series” the RNN model improve a lot with LSTM becoming the best performer. The noise value is now closer to the target noise value 6. Also in the “Complex series” the LSTM gives a result comparable to the CNN, but more stable.
The Deep CNN model does not give any significant improvement.
During this analysis, I demonstrate that a simple MLP can learn a sinusoid, with an input of 2 lags.
When noise is introduced, we need 20 lags to learn the underlying pattern. We then observed that more complicated NN (RNN and CNN) does not improve the results for a sinusoid with noise.
We then observed that all the models can discover even more complex patterns. CNN does the best with complex patterns, and a Dropout level helps improve the result and the model stability.
CNNs start having some difficulties when the series presents too many patterns and a high amount of noise. I demonstrated that feeding the NN with aggregated information from the past improves a lot the results. Finally, LSTM become one of the best performers, as we would have expected.
If we would have to choose a model for a real-world time-series, a good idea would be to choose an ensemble of CNN with a Dropout layer and an LSTM. The model should be input with at least 20 lags and some averaged values from the past.
Another good idea would be to add more information external to the time-series values. The attempt is to find any measurable correlation with the noise.
28 Jun 2022
- Artificial Intelligence
- Data Science
- Machine Learning
- Neural Networks
- Time Series
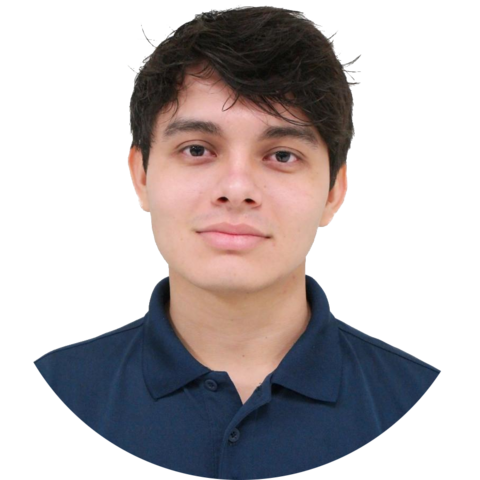

Eduardo Avelar
C4w2: predicting time series, c4w2: predicting time series #.
https-deeplearning-ai/ tensorflow-1-public /C4/W2/assignment/ C4W2_Assignment.ipynb
Commit 50a14a4 on Feb 6, 2023 - Compare
Generating the data #
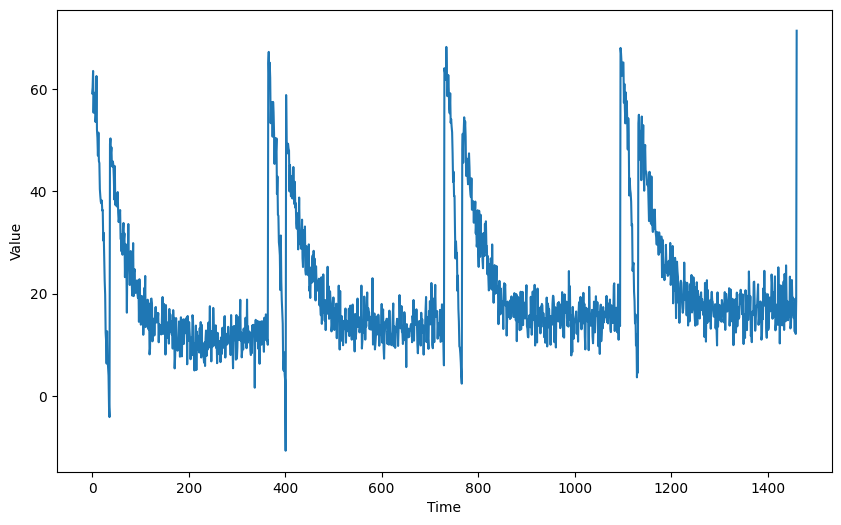
Splitting the data #
Processing the data #, defining the model architecture #, evaluating the forecast #.
Time Series Forecasting Using Artificial Neural Networks
A Model for the IBEX 35 Index
- Conference paper
- First Online: 12 September 2022
- Cite this conference paper
- Daniel González-Cortés ORCID: orcid.org/0000-0002-5170-9883 18 ,
- Enrique Onieva ORCID: orcid.org/0000-0001-9581-1823 19 ,
- Iker Pastor ORCID: orcid.org/0000-0002-3068-6248 19 &
- Jian Wu ORCID: orcid.org/0000-0002-0855-1881 18
Part of the book series: Lecture Notes in Computer Science ((LNAI,volume 13469))
Included in the following conference series:
- International Conference on Hybrid Artificial Intelligence Systems
708 Accesses
The amount of data generated daily in the financial markets is diverse and extensive; hence, creating systems that facilitate decision-making is crucial. In this paper, different intelligent systems are proposed and tested to predict the closing price of the IBEX 35 using ten years of historical data with four different neural networks architectures. The first was a multi-layer perceptron (MLP) with two different activation functions (AF) to continue with a simple recurrent neural network (RNN), a long-short-term memory (LSTM) network and a gated recurrent unit (GRU) network. The analytical results of these models have shown a strong, predictable power. Furthermore, by comparing the errors of predicted outcomes between the models, the LSTM presents the lowest error with the highest computational time in the training phase. Finally, the empirical results revealed that these models could efficiently predict financial data for trading purposes.
This is a preview of subscription content, log in via an institution to check access.
Access this chapter
- Available as PDF
- Read on any device
- Instant download
- Own it forever
- Available as EPUB and PDF
- Compact, lightweight edition
- Dispatched in 3 to 5 business days
- Free shipping worldwide - see info
Tax calculation will be finalised at checkout
Purchases are for personal use only
Institutional subscriptions
Abadi, M., Agarwal, A., Barham, P., Brevdo, E., Chen, Z., et al.: TensorFlow: large-scale machine learning on heterogeneous systems (2015). https://www.tensorflow.org/ . Software available from tensorflow.org
Adebiyi, A.A., Adewumi, A.O., Ayo, C.K.: Comparison of ARIMA and artificial neural networks models for stock price prediction. J. Appl. Math. 2014 , 1–7 (2014). https://doi.org/10.1155/2014/614342
Article MathSciNet Google Scholar
Alom, M.Z., et al.: A state-of-the-art survey on deep learning theory and architectures. Electronics 8 (3), 292 (2019). https://doi.org/10.3390/electronics8030292
Article Google Scholar
Baur, D.: Financial contagion and the real economy. J. Banking Financ. 36 (10), 2680–2692 (2012)
Bengio, Y.: Probabilistic neural network models for sequential data. In: Proceedings of the IEEE-INNS-ENNS International Joint Conference on Neural Networks. IJCNN 2000. Neural Computing: New Challenges and Perspectives for the New Millennium (2000). https://doi.org/10.1109/ijcnn.2000.861438
Bengio, Y., Simard, P., Frasconi, P.: Learning long-term dependencies with gradient descent is difficult. IEEE Trans. Neural Netw. 5 (2), 157–166 (1994). https://doi.org/10.1109/72.279181
Che, Z., Purushotham, S., Cho, K., Sontag, D., Liu, Y.: Recurrent neural networks for multivariate time series with missing values. Sci. Rep. 8 (1), 1–12 (2018). https://doi.org/10.1038/s41598-018-24271-9
Cho, K., et al.: Learning phrase representations using RNN encoder-decoder for statistical machine translation. In: Proceedings of the 2014 Conference on Empirical Methods in Natural Language Processing (EMNLP) (2014). https://doi.org/10.3115/v1/d14-1179
Chung, J., Gulcehre, C., Cho, K., Bengio, Y.: Empirical evaluation of gated recurrent neural networks on sequence modeling (2014)
Google Scholar
Enke, D., Thawornwong, S.: The use of data mining and neural networks for forecasting stock market returns. Expert Syst. Appl. 29 (4), 927–940 (2005). https://doi.org/10.1016/j.eswa.2005.06.024
Gocken, M., Ozcalici, M., Boru, A., Dosdogru, A.T.: Integrating metaheuristics and artificial neural networks for improved stock price prediction. Expert Syst. Appl. 44 , 320–331 (2016). https://doi.org/10.1016/j.eswa.2015.09.029
Guresen, E., Kayakutlu, G., Daim, T.U.: Using artificial neural network models in stock market index prediction. Expert Syst. Appl. 38 (8), 10389–10397 (2011). https://doi.org/10.1016/j.eswa.2011.02.068
Hochreiter, S., Schmidhuber, J.: Long short-term memory. Neural Comput. 9 (8), 1735–1780 (1997). https://doi.org/10.1162/neco.1997.9.8.1735
Kara, Y., Boyacioglu, M.A., Baykan, Ö.K.: Predicting direction of stock price index movement using artificial neural networks and support vector machines: the sample of the Istanbul stock exchange. Expert Syst. Appl. 38 (5), 5311–5319 (2011). https://doi.org/10.1016/j.eswa.2010.10.027
Kim, H.Y., Won, C.H.: Forecasting the volatility of stock price index: a hybrid model integrating LSTM with multiple GARCH-type models. Expert Syst. Appl. 103 , 25–37 (2018). https://doi.org/10.1016/j.eswa.2018.03.002
Lecun, Y., Bengio, Y., Hinton, G.: Deep learning. Nature 521 (7553), 436–444 (2015). https://doi.org/10.1038/nature14539
Lee, J., Suh, T., Roy, D., Baucus, M.: Emerging technology and business model innovation: the case of artificial intelligence. MDPI (2019). https://www.mdpi.com/2199-8531/5/3/44
Lo, A.W., MacKinlay, A.C.: Stock market prices do not follow random walks: evidence from a simple specification test. Rev. Financ. Stud. 1 (1), 41–66 (1988)
Menkhoff, L.: The use of technical analysis by fund managers international evidence. J. Banking Financ. 34 (11), 2573–2586 (2010). https://doi.org/10.1016/j.jbankfin.2010.04.014
Moghaddam, A.H., Moghaddam, M.H., Esfandyari, M.: Stock market index prediction using artificial neural network. J. Econ. Financ. Adm. Sci. 21 (41), 89–93 (2016). https://doi.org/10.1016/j.jefas.2016.07.002
Pei, S., Shen, T., Wang, X., Gu, C., Ning, Z., Ye, X., Xiong, N.: 3DACN: 3D augmented convolutional network for time series data. Inf. Sci. 513 , 17–29 (2020). https://doi.org/10.1016/j.ins.2019.11.040
Pyo, S., Lee, J., Cha, M., Jang, H.: Predictability of machine learning techniques to forecast the trends of market index prices: hypothesis testing for the Korean stock markets. PLoS One 12 , e0188107 (2017). https://doi.org/10.1371/journal.pone.0188107
Qiu, M., Song, Y., Akagi, F.: Application of artificial neural network for the prediction of stock market returns: the case of the Japanese stock market. Chaos Solitons Fractals 85 , 1–7 (2016). https://doi.org/10.1016/j.chaos.2016.01.004
Sagir, A., Sathasivan, S.: The use of artificial neural network and multiple linear regressions for stock market forecasting. Matematika 33 , 1–10 (2017)
Sezer, O.B., Gudelek, M.U., Ozbayoglu, A.M.: Financial time series forecasting with deep learning : a systematic literature review: 2005–2019. Appl. Soft Comput. 90 , 106181 (2020). https://doi.org/10.1016/j.asoc.2020.106181
Tkáč, M., Verner, R.: Artificial neural networks in business: two decades of research. Appl. Soft Computi. 38 , 788–804 (2016). https://doi.org/10.1016/j.asoc.2015.09.040
Wang, J., Zhang, Y., Yu, L.C., Zhang, X.: Contextual sentiment embeddings via bi-directional GRU language model. Knowl.-Based Syst. 235 , 107663 (2022). https://doi.org/10.1016/j.knosys.2021.107663
Download references
Author information
Authors and affiliations.
NEOMA Business School, rue du Maréchal Juin, 76825, Mont Saint Aignan Cedex, France
Daniel González-Cortés & Jian Wu
Faculty of Engineering, University of Deusto, 48007, Bilbao, Spain
Enrique Onieva & Iker Pastor
You can also search for this author in PubMed Google Scholar
Corresponding author
Correspondence to Daniel González-Cortés .
Editor information
Editors and affiliations.
University of Deusto, Bilbao, Spain
Pablo García Bringas
University of León, León, Spain
Hilde Pérez García
University of La Rioja, Logroño, La Rioja, Spain
Francisco Javier Martínez de Pisón
University of Oviedo, Oviedo, Spain
José Ramón Villar Flecha
Data Science and Big Data Analytics Lab, Pablo de Olavide University, Sevilla, Spain
Alicia Troncoso Lora
Department of Computer Science, University of Oviedo, Oviedo, Spain
Enrique A. de la Cal
Applied Computational Intelligence, University of Burgos, Burgos, Burgos, Spain
Álvaro Herrero
Universidad Pablo de Olavide, Seville, Spain
Francisco Martínez Álvarez
DIGIP, University of Bergamo, Dalmine, Bergamo, Italy
Giuseppe Psaila
Department of Industrial Engineering, University of A Coruña, Ferrol, Spain
Héctor Quintián
University of Salamanca, Salamanca, Spain
Emilio Corchado
Rights and permissions
Reprints and permissions
Copyright information
© 2022 Springer Nature Switzerland AG
About this paper
Cite this paper.
González-Cortés, D., Onieva, E., Pastor, I., Wu, J. (2022). Time Series Forecasting Using Artificial Neural Networks. In: García Bringas, P., et al. Hybrid Artificial Intelligent Systems. HAIS 2022. Lecture Notes in Computer Science(), vol 13469. Springer, Cham. https://doi.org/10.1007/978-3-031-15471-3_22
Download citation
DOI : https://doi.org/10.1007/978-3-031-15471-3_22
Published : 12 September 2022
Publisher Name : Springer, Cham
Print ISBN : 978-3-031-15470-6
Online ISBN : 978-3-031-15471-3
eBook Packages : Computer Science Computer Science (R0)
Share this paper
Anyone you share the following link with will be able to read this content:
Sorry, a shareable link is not currently available for this article.
Provided by the Springer Nature SharedIt content-sharing initiative
- Publish with us
Policies and ethics
- Find a journal
- Track your research
- Trending Now
- Foundational Courses
- Data Science
- Practice Problem
- Machine Learning
- System Design
- DevOps Tutorial
Time Series Forecasting using Recurrent Neural Networks (RNN) in TensorFlow
- Training of Recurrent Neural Networks (RNN) in TensorFlow
- Types of Recurrent Neural Networks (RNN) in Tensorflow
- TIme Series Forecasting using TensorFlow
- Sentiment Analysis with an Recurrent Neural Networks (RNN)
- Multivariate Time Series Forecasting with LSTMs in Keras
- Time Series and Forecasting Using R
- Artificial Neural Network in TensorFlow
- Stock Price Prediction Project using TensorFlow
- Time Series Forecasting with Support Vector Regression
- Recurrent Neural Networks in R
- Tensorflow.js tf.layers.bidirectional() Function
- Tensorflow.js tf.layers.stackedRNNCells() Function
- Time Series Forecasting using Pytorch
- Python | Creating tensors using different functions in Tensorflow
- Introduction to Recurrent Neural Network
- Difference between Recursive and Recurrent Neural Network
- Bidirectional Recurrent Neural Network
- Recurrent Neural Networks Explanation
- Inventory Demand Forecasting using Machine Learning - Python
- Removing stop words with NLTK in Python
- Decision Tree
- Linear Regression in Machine learning
- Agents in Artificial Intelligence
- Plotting Histogram in Python using Matplotlib
- One Hot Encoding in Machine Learning
- Best Python libraries for Machine Learning
- Introduction to Hill Climbing | Artificial Intelligence
- Clustering in Machine Learning
- Digital Image Processing Basics
Time Series Data: Each data point in a time series is linked to a timestamp, which shows the exact time when the data was observed or recorded. Many fields, including finance, economics, weather forecasting, and machine learning, frequently employ this kind of data.
The fact that time series data frequently display patterns or trends across time, such as seasonality or cyclical patterns, is an essential feature associated with it. To make predictions or learn more about the underlying processes or occurrences being observed, these patterns can be analyzed and modeled.
Recurrent Neural Networks (RNN) model the temporal dependencies present in the data as it contains an implicit memory of previous inputs. Hence, time series data being sequential in nature is often used in RNN. For working with time series data in RNNs, TensorFlow provides a number of APIs and tools, like tf.keras.layers.RNN API, which allows to create of unique RNN cell classes and use them with data. Several RNN cell types are also supported by this API, including Basic RNN, LSTM, and GRU.
To demonstrate the same, we’re going the run the following code snippets in Google Colaboratory which comes pre-installed with Machine Learning and Deep Learning Libraries. This example will use stock price data, the most popular type of time series data.
Step 1: Import the required libraries.
- Numpy & Pandas – For data manipulation and analysis
- Matplotlib – For data visualization.
- Yahoo Finance – Provides financial data for analysis.
- Datetime – For working with dates and times.
- Math – Provides basic mathematical functions in Python.
Step 2: This code uses the yf.download() method of the yfinance library to download historical stock data for Google from Yahoo Finance. Using the dt.datetime() method of the datetime module, the start and end dates of the time period for which the data has been obtained are given.
The downloaded data is then shown using the print() function, where the Pandas DataFrame’s display options are configured using pd.set_option().
Step 3: Next, we split the dataset into training and testing in the ratio 80:20. Only the first column of the data is chosen using iloc[:,:1] and the train_data contains the first training_data_len rows of the original data. The test_data, contains all of the remaining rows of the original data starting from training_data_len to the end.
Output:
Step 4: This code creates a numpy array called dataset_train and populates it with the “Open” pricing values from the training data. The 1-dimensional array is then transformed into a 2-dimensional array. The shape property, which returns the tuple (num_rows, num_columns) denoting the dataset_train array’s final shape.
Step 5: Normalization is a crucial step in data preprocessing to enhance the effectiveness and interpretability of machine learning models. Hence MinMaxScaler from sklearn is imported to scale the dataset from 0 to 1. Using the sklearn fit_transform() method, the training dataset is scaled.
Step 6: The same data preprocessing is done for test data.
Step 7: The time-series data must be divided into X_train and y_train from the training set and X_test and y_test from the testing set in this phase. It is done to turn time series data into a supervised learning problem that can be utilized to train the model. The loop generates input/output sequences of length 50 while iterating through the time series data. Using this method, we can forecast future values while taking into consideration the data’s temporal dependence on prior observations.
For training set:
For testing set:.
Step 8: In this step, the data is converted into a format that is suitable for input to an RNN. np.reshape(X_train, (X_train.shape[0], X_train.shape[1], 1)) transforms the X_train array, which was originally a 2-dimensional array of shape (samples, features), into a 3-dimensional array of shape (samples, time steps, features), where time steps denotes the number of time steps in the input sequence and features denotes the number of features in the input data. Size 1 is an additional dimension that serves as an indication that each time step only has a single feature.
The y_train array is transformed from a 1-dimensional array of shape (samples) into a 2-dimensional array of shape (samples, 1) by np.reshape(y_train, (y_train.shape[0], 1)) , where each row represents the output value at a certain time step.
Step 9: Three RNN models are created in this step. The libraries needed for the model is imported.
SimpleRNN Model:
Using the Keras API, this code creates a recurrent neural network (RNN) with four layers of basic RNNs and a dense output layer. It makes use of the tanh hyperbolic tangent activation function. To avoid overfitting, a dropout layer with a rate of 0.2 is introduced. It employs the optimizer as Adam, mean squared error as the loss function, and accuracy as the evaluation metric while compiling. With a batch size of 2, it fits the model to the training data for 20 epochs. The number of parameters in each layer and the overall number of parameters in the model are listed in a summary of the model architecture.
LSTM RNN Model:
This code creates a LSTM Model with three layers and a dense output layer. It employs the optimizer as Adam, mean squared error as the loss function, and accuracy as the evaluation metric while compiling. With a batch size of 1, it fits the model to the training data for 10 epochs. The number of parameters in each layer and the overall number of parameters in the model are listed in a summary of the model architecture.
GRU RNN Model:
This code defines a recurrent neural network (RNN) model using the GRU (Gated Recurrent Unit) layer in Keras. It consists of four stacked GRU layers followed by a single output layer. It makes use of the ‘tanh’ hyperbolic tangent activation function. To avoid overfitting, a dropout layer with a rate of 0.2 is introduced. It employs the optimizer as Stochastic Gradient Descent (SGD) with a learning rate of 0.01, the decay rate of 1e-7, the momentum of 0.9, and Nesterov is set to False. The mean squared error is the loss function, and accuracy is the evaluation metric while compiling. With a batch size of 2, it fits the model to the training data for 20 epochs. The number of parameters in each layer and the overall number of parameters in the model are listed in a summary of the model architecture.
Step 10: The X_test data is then used to make predictions from all three models.
Step 11: The predicted values are transformed back from the normalized state to their original scale using the inverse_transform() function.
Step 12: Visualize the predicted prices using matplotlib.
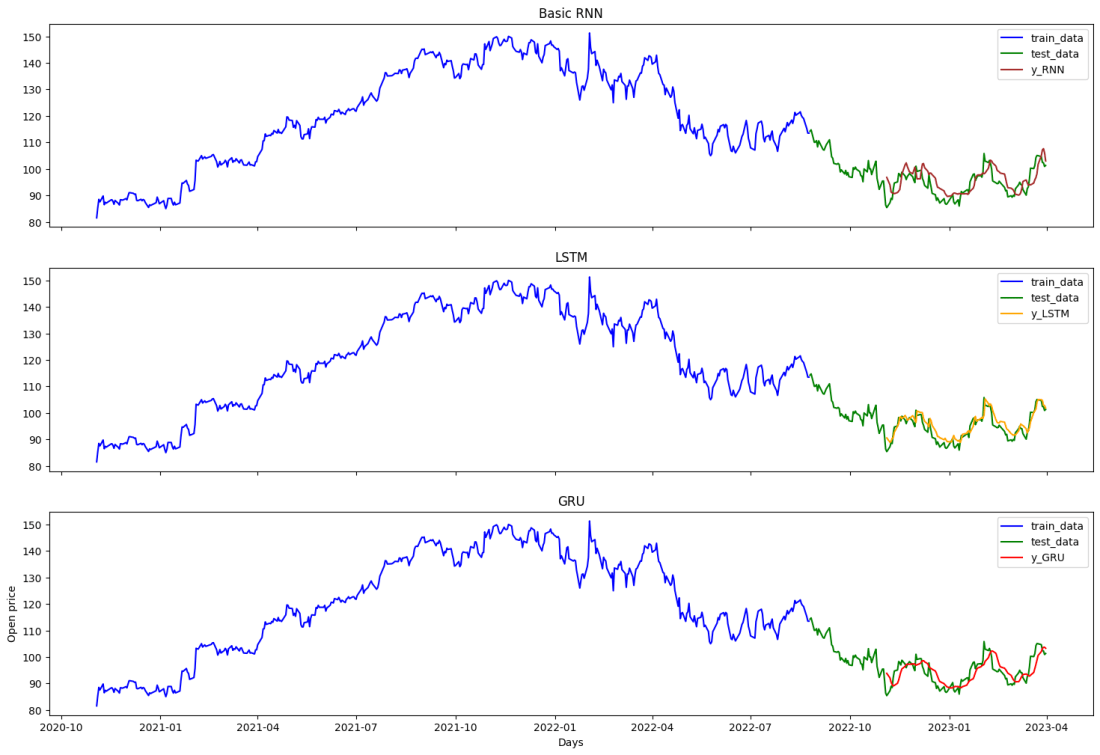
Time Series Forecasting
Please Login to comment...
Similar reads.
- AI-ML-DS With Python
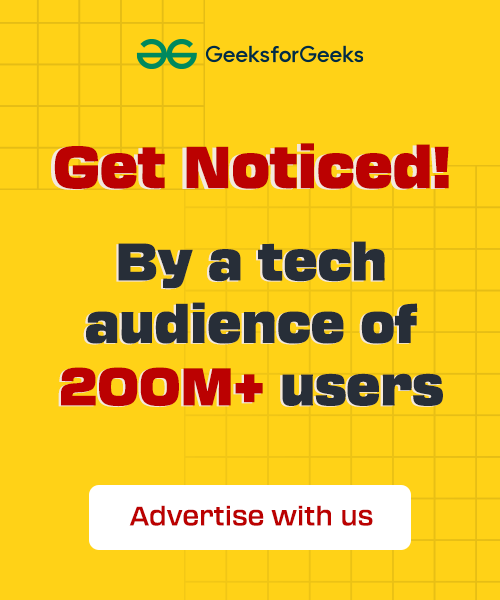
Improve your Coding Skills with Practice
What kind of Experience do you want to share?
Navigation Menu
Search code, repositories, users, issues, pull requests..., provide feedback.
We read every piece of feedback, and take your input very seriously.
Saved searches
Use saved searches to filter your results more quickly.
To see all available qualifiers, see our documentation .
- Notifications

IMAGES
VIDEO
COMMENTS
Notes, programming assignments and quizzes from all courses within the Coursera Deep Learning specialization offered by deeplearning.ai: (i) Neural Networks and Deep Learning; (ii) Improving Deep Neural Networks: Hyperparameter tuning, Regularization and Optimization; (iii) Structuring Machine Learning Projects; (iv) Convolutional Neural Networks; (v) Sequence Models - amanchadha/coursera-deep ...
Programming Assignment: Deep Neural Network Application; 2. Improving Deep Neural Networks Hyperparameter tuning, Regularization and Optimization. Details. ... Programming Assignment: Neural Machine Translation with Attention; Programming Assignment: Trigger word detection; Contributing.
Week 3: Shallow neural networks. Understand the key parameters in a neural network's architecture. Planar data classification with a hidden layer; Week 4: Deep Neural Networks. Understand the key computations underlying deep learning, use them to build and train deep neural networks, and apply it to computer vision. Course 2 - Improving Deep ...
This Specialization will teach you best practices for using TensorFlow, a popular open-source framework for machine learning. In this fourth course, you will learn how to build time series models in TensorFlow. You'll first implement best practices to prepare time series data. You'll also explore how RNNs and 1D ConvNets can be used for ...
Using Neural Networks for Content-Based Recommendation Systems Machine Learning Operations (MLOps): Getting Started ... C1W1 Assignment: Housing Prices C1W2: Implementing Callbacks in TensorFlow using the MNIST Dataset ... def model_forecast (model, series, window_size): ds = tf. data.
Time series prediction problems are a difficult type of predictive modeling problem. Unlike regression predictive modeling, time series also adds the complexity of a sequence dependence among the input variables. A powerful type of neural network designed to handle sequence dependence is called a recurrent neural network. The Long Short-Term Memory network or LSTM network is a type of ...
Model 1: DNN. A deep neural network (DNN) is an artificial neural network (ANN) with multiple layers between the input and output layers — from WikiHow to apply DNN for time-series data? The key idea here: we consider time-series as linear model: {X(i) …X(i+t)}~Y(i+t+1). In the format, it shows using t steps input time-series to predict the next step which is Y(i+t+1).
This guide will help you understand the basics of TimeSeries Forecasting. You'll learn how to pre-process TimeSeries Data and build a simple LSTM model, train it, and use it for forecasting. Consider you're dealing with data that is captured in regular intervals of time, i.e., for example, if you're using Google Stock Prices data and ...
Deep neural networks tackle forecasting problems using auto-regression. Auto-regression is a modeling technique that involves using past observations to predict future ones. Deep neural networks can be designed in different ways, such as recurrent or convolutional architectures. Recurrent neural networks are often preferred for time series data.
In this post, we'll review three advanced techniques for improving the performance and generalization power of recurrent neural networks. We'll demonstrate all three concepts on a temperature-forecasting problem, where you have access to a time series of data points coming from sensors installed on the roof of a building. Authors.
It is easy to say "Neural Networks" There exist different kind of NN that can be applied to this use case. Multi-Layer Perceptron (MLP): the most common and simple. More about it here. Recurrent Neural Network (RNN): in literature, the most suited to time-series forecasting. They combine the information of the current observation, with the ...
Custom Training with Linear, Neural Network and Deep Neural Network models Introduction Introduction to Linear Models Reading the Data Implementing Linear Models for Image Classification Neural Networks and Deep Neural Networks for Image Classification
In this paper, different intelligent systems are proposed and tested to predict the closing price of the IBEX 35 using ten years of historical data with four different neural networks architectures. The first was a multi-layer perceptron (MLP) with two different activation functions (AF) to continue with a simple recurrent neural network (RNN ...
Evaluation Metric. A forecast will be comprised of seven values, one for each day of the week ahead. It is common with multi-step forecasting problems to evaluate each forecasted time step separately. This is helpful for a few reasons: To comment on the skill at a specific lead time (e.g. +1 day vs +3 days).
Use saved searches to filter your results more quickly. Name. Query. To see all available qualifiers, see our documentation. Cancel Create saved search Sign in Sign up You signed in with another tab or window. Reload to refresh your session. You signed out in another tab or window. ...
Convolutional Neural Network models, or CNNs for short, can be applied to time series forecasting. There are many types of CNN models that can be used for each specific type of time series forecasting problem. In this tutorial, you will discover how to develop a suite of CNN models for a range of standard time series forecasting problems.
Before deep learning neural networks became popular, particularly the Recurrent Neural Networks , there were a number of classical analytical methods / algorithms used for Time Series forecast- AR ...
Step 7: Forecasting. After training the neural network on the provided data, now comes the forecasting for next month. The model predicts the future opening price and store the future values along with their corresponding dates. Using for loop, we are going to perform a rolling forecasting, the steps are as follows -
Keywords: neural networks, time series, forecasting, exchange rate, predicting Introduction ... optimum step length obtained a mathematical programming model. Data transformation in order to improve the accuracy of the forecast is used in (Proietti, 2013). The authors considered the Box-Cox power transformation and showed the forecasts are
We have presented a forecasting system for univariate time series that uses artificial neural networks. This computing devices proved themselves to be viable al- ternatives to conventional techniques. The system can be used in conjunction with other techniques for time series analysis or as a stand-alone tool.
Recurrent Neural Networks (RNN) model the temporal dependencies present in the data as it contains an implicit memory of previous inputs.Hence, time series data being sequential in nature is often used in RNN. For working with time series data in RNNs, TensorFlow provides a number of APIs and tools, like tf.keras.layers.RNN API, which allows to create of unique RNN cell classes and use them ...
Time Series Forecasting with Neural Networks. From the course Sequences, Time Series and Prediction, DeepLearning.AI, Coursera, Week 2 - Deep Neural Networks for Time Series - marcosoares-92/timeSe...
In this step, the instances are divided into two separate parts including the train and test sets. The train set is input to the LSTM network to obtain forecasting model. The test set is used for evaluating the predictive power of the built model in terms of performance metrics. 3.4. Model configuration and training.