Have a language expert improve your writing
Run a free plagiarism check in 10 minutes, automatically generate references for free.
- Knowledge Base
- Methodology
- Correlational Research | Guide, Design & Examples

Correlational Research | Guide, Design & Examples
Published on 5 May 2022 by Pritha Bhandari . Revised on 5 December 2022.
A correlational research design investigates relationships between variables without the researcher controlling or manipulating any of them.
A correlation reflects the strength and/or direction of the relationship between two (or more) variables. The direction of a correlation can be either positive or negative.
Table of contents
Correlational vs experimental research, when to use correlational research, how to collect correlational data, how to analyse correlational data, correlation and causation, frequently asked questions about correlational research.
Correlational and experimental research both use quantitative methods to investigate relationships between variables. But there are important differences in how data is collected and the types of conclusions you can draw.
Prevent plagiarism, run a free check.
Correlational research is ideal for gathering data quickly from natural settings. That helps you generalise your findings to real-life situations in an externally valid way.
There are a few situations where correlational research is an appropriate choice.
To investigate non-causal relationships
You want to find out if there is an association between two variables, but you don’t expect to find a causal relationship between them.
Correlational research can provide insights into complex real-world relationships, helping researchers develop theories and make predictions.
To explore causal relationships between variables
You think there is a causal relationship between two variables, but it is impractical, unethical, or too costly to conduct experimental research that manipulates one of the variables.
Correlational research can provide initial indications or additional support for theories about causal relationships.
To test new measurement tools
You have developed a new instrument for measuring your variable, and you need to test its reliability or validity .
Correlational research can be used to assess whether a tool consistently or accurately captures the concept it aims to measure.
There are many different methods you can use in correlational research. In the social and behavioural sciences, the most common data collection methods for this type of research include surveys, observations, and secondary data.
It’s important to carefully choose and plan your methods to ensure the reliability and validity of your results. You should carefully select a representative sample so that your data reflects the population you’re interested in without bias .
In survey research , you can use questionnaires to measure your variables of interest. You can conduct surveys online, by post, by phone, or in person.
Surveys are a quick, flexible way to collect standardised data from many participants, but it’s important to ensure that your questions are worded in an unbiased way and capture relevant insights.
Naturalistic observation
Naturalistic observation is a type of field research where you gather data about a behaviour or phenomenon in its natural environment.
This method often involves recording, counting, describing, and categorising actions and events. Naturalistic observation can include both qualitative and quantitative elements, but to assess correlation, you collect data that can be analysed quantitatively (e.g., frequencies, durations, scales, and amounts).
Naturalistic observation lets you easily generalise your results to real-world contexts, and you can study experiences that aren’t replicable in lab settings. But data analysis can be time-consuming and unpredictable, and researcher bias may skew the interpretations.
Secondary data
Instead of collecting original data, you can also use data that has already been collected for a different purpose, such as official records, polls, or previous studies.
Using secondary data is inexpensive and fast, because data collection is complete. However, the data may be unreliable, incomplete, or not entirely relevant, and you have no control over the reliability or validity of the data collection procedures.
After collecting data, you can statistically analyse the relationship between variables using correlation or regression analyses, or both. You can also visualise the relationships between variables with a scatterplot.
Different types of correlation coefficients and regression analyses are appropriate for your data based on their levels of measurement and distributions .
Correlation analysis
Using a correlation analysis, you can summarise the relationship between variables into a correlation coefficient : a single number that describes the strength and direction of the relationship between variables. With this number, you’ll quantify the degree of the relationship between variables.
The Pearson product-moment correlation coefficient, also known as Pearson’s r , is commonly used for assessing a linear relationship between two quantitative variables.
Correlation coefficients are usually found for two variables at a time, but you can use a multiple correlation coefficient for three or more variables.
Regression analysis
With a regression analysis , you can predict how much a change in one variable will be associated with a change in the other variable. The result is a regression equation that describes the line on a graph of your variables.
You can use this equation to predict the value of one variable based on the given value(s) of the other variable(s). It’s best to perform a regression analysis after testing for a correlation between your variables.
It’s important to remember that correlation does not imply causation . Just because you find a correlation between two things doesn’t mean you can conclude one of them causes the other, for a few reasons.
Directionality problem
If two variables are correlated, it could be because one of them is a cause and the other is an effect. But the correlational research design doesn’t allow you to infer which is which. To err on the side of caution, researchers don’t conclude causality from correlational studies.
Third variable problem
A confounding variable is a third variable that influences other variables to make them seem causally related even though they are not. Instead, there are separate causal links between the confounder and each variable.
In correlational research, there’s limited or no researcher control over extraneous variables . Even if you statistically control for some potential confounders, there may still be other hidden variables that disguise the relationship between your study variables.
Although a correlational study can’t demonstrate causation on its own, it can help you develop a causal hypothesis that’s tested in controlled experiments.
A correlation reflects the strength and/or direction of the association between two or more variables.
- A positive correlation means that both variables change in the same direction.
- A negative correlation means that the variables change in opposite directions.
- A zero correlation means there’s no relationship between the variables.
A correlational research design investigates relationships between two variables (or more) without the researcher controlling or manipulating any of them. It’s a non-experimental type of quantitative research .
Controlled experiments establish causality, whereas correlational studies only show associations between variables.
- In an experimental design , you manipulate an independent variable and measure its effect on a dependent variable. Other variables are controlled so they can’t impact the results.
- In a correlational design , you measure variables without manipulating any of them. You can test whether your variables change together, but you can’t be sure that one variable caused a change in another.
In general, correlational research is high in external validity while experimental research is high in internal validity .
A correlation is usually tested for two variables at a time, but you can test correlations between three or more variables.
A correlation coefficient is a single number that describes the strength and direction of the relationship between your variables.
Different types of correlation coefficients might be appropriate for your data based on their levels of measurement and distributions . The Pearson product-moment correlation coefficient (Pearson’s r ) is commonly used to assess a linear relationship between two quantitative variables.
Cite this Scribbr article
If you want to cite this source, you can copy and paste the citation or click the ‘Cite this Scribbr article’ button to automatically add the citation to our free Reference Generator.
Bhandari, P. (2022, December 05). Correlational Research | Guide, Design & Examples. Scribbr. Retrieved 22 April 2024, from https://www.scribbr.co.uk/research-methods/correlational-research-design/
Is this article helpful?
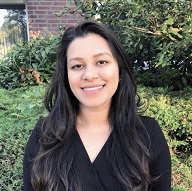
Pritha Bhandari
Other students also liked, a quick guide to experimental design | 5 steps & examples, quasi-experimental design | definition, types & examples, qualitative vs quantitative research | examples & methods.

Want to create or adapt books like this? Learn more about how Pressbooks supports open publishing practices.
7.2 Correlational Research
Learning objectives.
- Define correlational research and give several examples.
- Explain why a researcher might choose to conduct correlational research rather than experimental research or another type of nonexperimental research.
What Is Correlational Research?
Correlational research is a type of nonexperimental research in which the researcher measures two variables and assesses the statistical relationship (i.e., the correlation) between them with little or no effort to control extraneous variables. There are essentially two reasons that researchers interested in statistical relationships between variables would choose to conduct a correlational study rather than an experiment. The first is that they do not believe that the statistical relationship is a causal one. For example, a researcher might evaluate the validity of a brief extraversion test by administering it to a large group of participants along with a longer extraversion test that has already been shown to be valid. This researcher might then check to see whether participants’ scores on the brief test are strongly correlated with their scores on the longer one. Neither test score is thought to cause the other, so there is no independent variable to manipulate. In fact, the terms independent variable and dependent variable do not apply to this kind of research.
The other reason that researchers would choose to use a correlational study rather than an experiment is that the statistical relationship of interest is thought to be causal, but the researcher cannot manipulate the independent variable because it is impossible, impractical, or unethical. For example, Allen Kanner and his colleagues thought that the number of “daily hassles” (e.g., rude salespeople, heavy traffic) that people experience affects the number of physical and psychological symptoms they have (Kanner, Coyne, Schaefer, & Lazarus, 1981). But because they could not manipulate the number of daily hassles their participants experienced, they had to settle for measuring the number of daily hassles—along with the number of symptoms—using self-report questionnaires. Although the strong positive relationship they found between these two variables is consistent with their idea that hassles cause symptoms, it is also consistent with the idea that symptoms cause hassles or that some third variable (e.g., neuroticism) causes both.
A common misconception among beginning researchers is that correlational research must involve two quantitative variables, such as scores on two extraversion tests or the number of hassles and number of symptoms people have experienced. However, the defining feature of correlational research is that the two variables are measured—neither one is manipulated—and this is true regardless of whether the variables are quantitative or categorical. Imagine, for example, that a researcher administers the Rosenberg Self-Esteem Scale to 50 American college students and 50 Japanese college students. Although this “feels” like a between-subjects experiment, it is a correlational study because the researcher did not manipulate the students’ nationalities. The same is true of the study by Cacioppo and Petty comparing college faculty and factory workers in terms of their need for cognition. It is a correlational study because the researchers did not manipulate the participants’ occupations.
Figure 7.2 “Results of a Hypothetical Study on Whether People Who Make Daily To-Do Lists Experience Less Stress Than People Who Do Not Make Such Lists” shows data from a hypothetical study on the relationship between whether people make a daily list of things to do (a “to-do list”) and stress. Notice that it is unclear whether this is an experiment or a correlational study because it is unclear whether the independent variable was manipulated. If the researcher randomly assigned some participants to make daily to-do lists and others not to, then it is an experiment. If the researcher simply asked participants whether they made daily to-do lists, then it is a correlational study. The distinction is important because if the study was an experiment, then it could be concluded that making the daily to-do lists reduced participants’ stress. But if it was a correlational study, it could only be concluded that these variables are statistically related. Perhaps being stressed has a negative effect on people’s ability to plan ahead (the directionality problem). Or perhaps people who are more conscientious are more likely to make to-do lists and less likely to be stressed (the third-variable problem). The crucial point is that what defines a study as experimental or correlational is not the variables being studied, nor whether the variables are quantitative or categorical, nor the type of graph or statistics used to analyze the data. It is how the study is conducted.
Figure 7.2 Results of a Hypothetical Study on Whether People Who Make Daily To-Do Lists Experience Less Stress Than People Who Do Not Make Such Lists

Data Collection in Correlational Research
Again, the defining feature of correlational research is that neither variable is manipulated. It does not matter how or where the variables are measured. A researcher could have participants come to a laboratory to complete a computerized backward digit span task and a computerized risky decision-making task and then assess the relationship between participants’ scores on the two tasks. Or a researcher could go to a shopping mall to ask people about their attitudes toward the environment and their shopping habits and then assess the relationship between these two variables. Both of these studies would be correlational because no independent variable is manipulated. However, because some approaches to data collection are strongly associated with correlational research, it makes sense to discuss them here. The two we will focus on are naturalistic observation and archival data. A third, survey research, is discussed in its own chapter.
Naturalistic Observation
Naturalistic observation is an approach to data collection that involves observing people’s behavior in the environment in which it typically occurs. Thus naturalistic observation is a type of field research (as opposed to a type of laboratory research). It could involve observing shoppers in a grocery store, children on a school playground, or psychiatric inpatients in their wards. Researchers engaged in naturalistic observation usually make their observations as unobtrusively as possible so that participants are often not aware that they are being studied. Ethically, this is considered to be acceptable if the participants remain anonymous and the behavior occurs in a public setting where people would not normally have an expectation of privacy. Grocery shoppers putting items into their shopping carts, for example, are engaged in public behavior that is easily observable by store employees and other shoppers. For this reason, most researchers would consider it ethically acceptable to observe them for a study. On the other hand, one of the arguments against the ethicality of the naturalistic observation of “bathroom behavior” discussed earlier in the book is that people have a reasonable expectation of privacy even in a public restroom and that this expectation was violated.
Researchers Robert Levine and Ara Norenzayan used naturalistic observation to study differences in the “pace of life” across countries (Levine & Norenzayan, 1999). One of their measures involved observing pedestrians in a large city to see how long it took them to walk 60 feet. They found that people in some countries walked reliably faster than people in other countries. For example, people in the United States and Japan covered 60 feet in about 12 seconds on average, while people in Brazil and Romania took close to 17 seconds.
Because naturalistic observation takes place in the complex and even chaotic “real world,” there are two closely related issues that researchers must deal with before collecting data. The first is sampling. When, where, and under what conditions will the observations be made, and who exactly will be observed? Levine and Norenzayan described their sampling process as follows:
Male and female walking speed over a distance of 60 feet was measured in at least two locations in main downtown areas in each city. Measurements were taken during main business hours on clear summer days. All locations were flat, unobstructed, had broad sidewalks, and were sufficiently uncrowded to allow pedestrians to move at potentially maximum speeds. To control for the effects of socializing, only pedestrians walking alone were used. Children, individuals with obvious physical handicaps, and window-shoppers were not timed. Thirty-five men and 35 women were timed in most cities. (p. 186)
Precise specification of the sampling process in this way makes data collection manageable for the observers, and it also provides some control over important extraneous variables. For example, by making their observations on clear summer days in all countries, Levine and Norenzayan controlled for effects of the weather on people’s walking speeds.
The second issue is measurement. What specific behaviors will be observed? In Levine and Norenzayan’s study, measurement was relatively straightforward. They simply measured out a 60-foot distance along a city sidewalk and then used a stopwatch to time participants as they walked over that distance. Often, however, the behaviors of interest are not so obvious or objective. For example, researchers Robert Kraut and Robert Johnston wanted to study bowlers’ reactions to their shots, both when they were facing the pins and then when they turned toward their companions (Kraut & Johnston, 1979). But what “reactions” should they observe? Based on previous research and their own pilot testing, Kraut and Johnston created a list of reactions that included “closed smile,” “open smile,” “laugh,” “neutral face,” “look down,” “look away,” and “face cover” (covering one’s face with one’s hands). The observers committed this list to memory and then practiced by coding the reactions of bowlers who had been videotaped. During the actual study, the observers spoke into an audio recorder, describing the reactions they observed. Among the most interesting results of this study was that bowlers rarely smiled while they still faced the pins. They were much more likely to smile after they turned toward their companions, suggesting that smiling is not purely an expression of happiness but also a form of social communication.
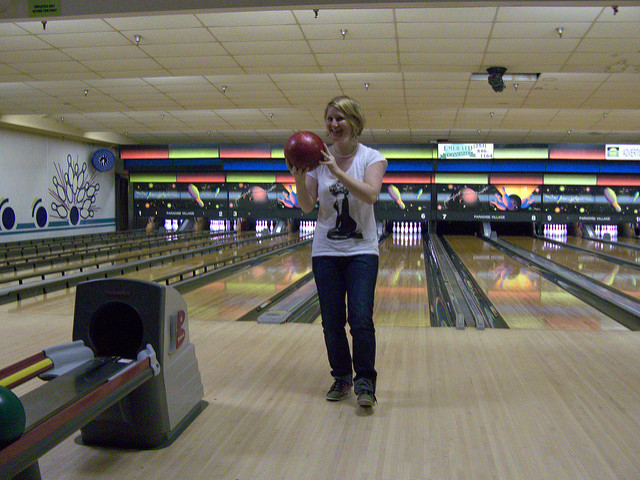
Naturalistic observation has revealed that bowlers tend to smile when they turn away from the pins and toward their companions, suggesting that smiling is not purely an expression of happiness but also a form of social communication.
sieneke toering – bowling big lebowski style – CC BY-NC-ND 2.0.
When the observations require a judgment on the part of the observers—as in Kraut and Johnston’s study—this process is often described as coding . Coding generally requires clearly defining a set of target behaviors. The observers then categorize participants individually in terms of which behavior they have engaged in and the number of times they engaged in each behavior. The observers might even record the duration of each behavior. The target behaviors must be defined in such a way that different observers code them in the same way. This is the issue of interrater reliability. Researchers are expected to demonstrate the interrater reliability of their coding procedure by having multiple raters code the same behaviors independently and then showing that the different observers are in close agreement. Kraut and Johnston, for example, video recorded a subset of their participants’ reactions and had two observers independently code them. The two observers showed that they agreed on the reactions that were exhibited 97% of the time, indicating good interrater reliability.
Archival Data
Another approach to correlational research is the use of archival data , which are data that have already been collected for some other purpose. An example is a study by Brett Pelham and his colleagues on “implicit egotism”—the tendency for people to prefer people, places, and things that are similar to themselves (Pelham, Carvallo, & Jones, 2005). In one study, they examined Social Security records to show that women with the names Virginia, Georgia, Louise, and Florence were especially likely to have moved to the states of Virginia, Georgia, Louisiana, and Florida, respectively.
As with naturalistic observation, measurement can be more or less straightforward when working with archival data. For example, counting the number of people named Virginia who live in various states based on Social Security records is relatively straightforward. But consider a study by Christopher Peterson and his colleagues on the relationship between optimism and health using data that had been collected many years before for a study on adult development (Peterson, Seligman, & Vaillant, 1988). In the 1940s, healthy male college students had completed an open-ended questionnaire about difficult wartime experiences. In the late 1980s, Peterson and his colleagues reviewed the men’s questionnaire responses to obtain a measure of explanatory style—their habitual ways of explaining bad events that happen to them. More pessimistic people tend to blame themselves and expect long-term negative consequences that affect many aspects of their lives, while more optimistic people tend to blame outside forces and expect limited negative consequences. To obtain a measure of explanatory style for each participant, the researchers used a procedure in which all negative events mentioned in the questionnaire responses, and any causal explanations for them, were identified and written on index cards. These were given to a separate group of raters who rated each explanation in terms of three separate dimensions of optimism-pessimism. These ratings were then averaged to produce an explanatory style score for each participant. The researchers then assessed the statistical relationship between the men’s explanatory style as college students and archival measures of their health at approximately 60 years of age. The primary result was that the more optimistic the men were as college students, the healthier they were as older men. Pearson’s r was +.25.
This is an example of content analysis —a family of systematic approaches to measurement using complex archival data. Just as naturalistic observation requires specifying the behaviors of interest and then noting them as they occur, content analysis requires specifying keywords, phrases, or ideas and then finding all occurrences of them in the data. These occurrences can then be counted, timed (e.g., the amount of time devoted to entertainment topics on the nightly news show), or analyzed in a variety of other ways.
Key Takeaways
- Correlational research involves measuring two variables and assessing the relationship between them, with no manipulation of an independent variable.
- Correlational research is not defined by where or how the data are collected. However, some approaches to data collection are strongly associated with correlational research. These include naturalistic observation (in which researchers observe people’s behavior in the context in which it normally occurs) and the use of archival data that were already collected for some other purpose.
Discussion: For each of the following, decide whether it is most likely that the study described is experimental or correlational and explain why.
- An educational researcher compares the academic performance of students from the “rich” side of town with that of students from the “poor” side of town.
- A cognitive psychologist compares the ability of people to recall words that they were instructed to “read” with their ability to recall words that they were instructed to “imagine.”
- A manager studies the correlation between new employees’ college grade point averages and their first-year performance reports.
- An automotive engineer installs different stick shifts in a new car prototype, each time asking several people to rate how comfortable the stick shift feels.
- A food scientist studies the relationship between the temperature inside people’s refrigerators and the amount of bacteria on their food.
- A social psychologist tells some research participants that they need to hurry over to the next building to complete a study. She tells others that they can take their time. Then she observes whether they stop to help a research assistant who is pretending to be hurt.
Kanner, A. D., Coyne, J. C., Schaefer, C., & Lazarus, R. S. (1981). Comparison of two modes of stress measurement: Daily hassles and uplifts versus major life events. Journal of Behavioral Medicine, 4 , 1–39.
Kraut, R. E., & Johnston, R. E. (1979). Social and emotional messages of smiling: An ethological approach. Journal of Personality and Social Psychology, 37 , 1539–1553.
Levine, R. V., & Norenzayan, A. (1999). The pace of life in 31 countries. Journal of Cross-Cultural Psychology, 30 , 178–205.
Pelham, B. W., Carvallo, M., & Jones, J. T. (2005). Implicit egotism. Current Directions in Psychological Science, 14 , 106–110.
Peterson, C., Seligman, M. E. P., & Vaillant, G. E. (1988). Pessimistic explanatory style is a risk factor for physical illness: A thirty-five year longitudinal study. Journal of Personality and Social Psychology, 55 , 23–27.
Research Methods in Psychology Copyright © 2016 by University of Minnesota is licensed under a Creative Commons Attribution-NonCommercial-ShareAlike 4.0 International License , except where otherwise noted.
Research Design
- Open Access
- First Online: 02 March 2023
Cite this chapter
You have full access to this open access chapter
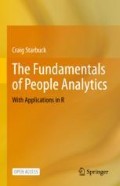
- Craig Starbuck 2
6433 Accesses
This chapter provides an overview of the elements of research, including research questions, hypotheses, methods, and designs. Factors influencing internal and external validity are also discussed.
You have full access to this open access chapter, Download chapter PDF
The importance of appropriate research methods and designs cannot be overstated. Research methods and designs help us achieve an accurate understanding of various phenomena and ensure conclusions are justified.
Research Questions
Research questions are fundamental to all research projects. Research questions help focus the study, determine the appropriate methodology, and guide each stage of inquiry, analysis, and reporting. Some examples of research questions germane to people analytics include:
Q 1 : Why has there been an increase in attrition over the past quarter?
Q 2 : How equitable are promotion nominations across the organization?
Q 3 : Are there meaningful differences in the favorability of experiences for remote vs. non-remote employees?
Q 4 : Do new joiners have the training and resources they need to be successful?
Q 5 : What portion of team performance is attributable to leadership effectiveness?
Research Hypotheses
Research hypotheses are testable statements about the expected outcome of a research project or experiment.
H 1 : Manager satisfaction is a significant predictor of voluntary attrition.
H 2 : Promotion nomination rates are not significantly different by gender and ethnicity.
H 3 : Employee experience favorability is not significantly different between remote and non-remote workers.
H 4 : New hire training perceptions are positively associated with onboarding experience favorability.
H 5 : Leadership effectiveness perceptions explain significant variation in team performance.
Internal and External Validity
Internal validity refers to the extent to which confounding variables are controlled. In other words, internal validity reflects the robustness of the study.
For example, if a study finds a significant relationship between work location and attrition but considers no other factors or explanations, this would not be a robust study. Work location may emerge significant because certain roles for which attrition is higher are more concentrated in one or more geographies. It could also be the case that the company has made acquisitions in new geographies, and the acquired employees have significantly different experiences (and attrition rates) relative to non-acquired employees.
Confounding variables are critically important in the context of internal validity. A confounding variable is an extraneous variable whose presence impacts the variables being studied such that results do not reflect the actual relationships. Studies with weak internal validity often result in spurious associations that confound the true relationship between two variables, leading to invalid conclusions and recommendations.
External validity refers to the extent to which study conclusions will hold in other contexts (for other people, in other places, at other times). Randomization is fundamental to our ability to generalize and apply findings to other groups or contexts.
If we survey employees to understand sentiments about recent changes in business strategy but exclude groups for which there may be different impacts or perceptions, conclusions about the collective sentiment would be suspect at best.
Research Methods
There are three major categories of research methods: (1) quantitative , (2) qualitative , and (3) mixed methods .
Quantitative
Addresses what questions
Utilizes numerical data (e.g., surveys, systems)
Primarily deductive
Used to test hypotheses
Involves statistical analyses
More objective
More generalizable
Qualitative
Addresses how and why questions
Utilizes text data (e.g., focus groups, interviews, open-ended feedback)
Primarily inductive
Used to formulate theory or hypotheses
Involves organizing data into categories or themes
More subjective
Less generalizable
Mixed Methods
Integrates the strengths of both quantitative and qualitative methods within a single study, often leading with qualitative approaches to build theory and hypotheses followed by quantitative methods to test hypotheses
Research Designs
In addition to determining whether a quantitative, qualitative, or mixed methods study is most appropriate, researchers also need to decide on the type of study within each of these three. Research designs are the types of inquiry within quantitative, qualitative, and mixed methods approaches that issue specific direction for the research procedures (Creswell & Creswell, 2018 ). There are multiple taxonomies for research designs, and we will simplify to the most common types.
Within the quantitative category, there are three types of designs: (a) experimental , (b) quasi-experimental , and (c) non-experimental . As shown in Fig. 1 , it is important to understand the centrality of randomization in this decision.
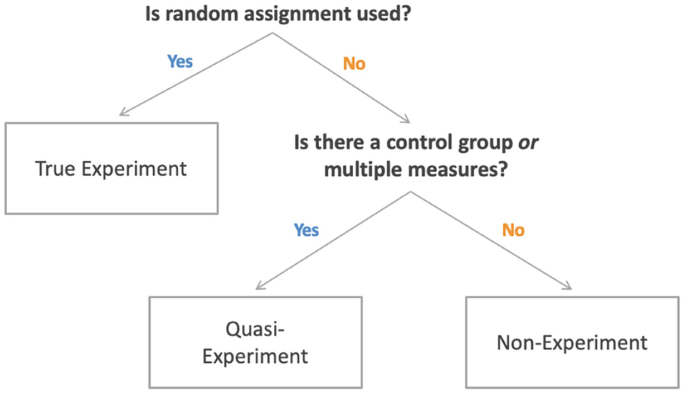
Quantitative research designs
Experimental Research
Experimental research is concerned with casual (internal) validity. Randomized experimental designs provide the most rigor with regard to causal validity. However, in social science research contexts, true experiments often are not possible due to ethical considerations.
For example, if we were interested in understanding the causal effect leadership quality has on employee engagement, based on a hypothesis that poor leadership decreases employee engagement, we would need to randomly assign employees to one of two groups that are identical on the basis of all variables that could theoretically explain why employees vary in their levels of engagement. Then, we would need to manipulate the variable of interest (leadership quality) to evaluate if the group of employees subjected to poor leadership (treatment group) reports significantly different levels of engagement relative to the group of employees for whom leadership quality has not been manipulated (control group). In a practical setting, it would of course be unethical to purposefully subject employees to poor leadership with the expectation of reducing engagement—and consequently, productivity, retention, and impact to the organization.
Clinical trials are a common setting for true experiments, as isolating the effects of an experimental drug can be a matter of life or death. In a randomized clinical trial, patients are randomly assigned to an experimental group (patients who receive the drug) or control group (patients who receive a placebo). To protect against placebo effects biasing the results, patients do not know if they receive the experimental treatment or the placebo. Done correctly, these experiments have the highest level of internal validity.
Another example of an experimental design is A/B testing . A/B testing is often performed in the context of website optimization, in which two or more versions of the site are shown to customers to identify which version impacts key success metrics more positively. In a people analytics context, we may create two versions of a dashboard and randomly assign the permissioned users to each. We could then assess whether utilization rates, average usage time, repeat usage, among other success measures are significantly different between the two groups of users to inform which design is most effective.
In experimental research, it is important to consider the potential influence of the Hawthorne Effect , which refers to the tendency of some individuals to modify their behavior in response to the awareness that they are being observed. This term was coined during experiments at Western Electric’s factory in the Hawthorne suburb of Chicago in the late 1920s and early 1930s. One of many studies conducted to understand how work environments effect productivity was known as the “Illumination Experiment.” During this study, researchers experimented with a number of lighting levels in a warehouse in which workers made electrical relays. The researchers found that any change in the lighting—even when introducing poor lighting—led to favorable changes in output. However, these productivity gains disappeared once the attention faded (Roethlisberger and Dickson, 1939 ).
In a people analytics context, if we inform employees that we are going to monitor productivity over a period of time, it is likely that at least some employees will attempt to modify their behavior in order to increase productivity levels. After all, higher productivity is generally regarded as an ideal across companies and industries. In this case, manipulating some aspect of the typical work context to study a treatment effect, such as flexible work arrangements, may be impacted by this phenomenon; that is, observed differences in productivity may not be attributable to flexible work arrangements but merely due to employees knowing they are being observed.
Quasi-Experimental Research
Quasi-experimental research is an experiment in which participants cannot be randomly assigned.
In the case of our leadership quality example, a quasi-experiment may examine engagement differences between two groups of employees who rate their leader either favorably (Group A) or unfavorably (Group B). A key limitation of this approach is that the groups may be different in important ways beyond leader perception incongruities. For example, Group A employees may be concentrated within a single department, whereas Group B employees may span all other departments. This would indicate that the difference in leadership—and presumably engagement—is driven by factors unique to the department, making it more challenging to isolate the effects of leadership quality on engagement. Perhaps the department with unfavorable leader perceptions has seen significant attrition, or the department is largely first-time people leaders in need of coaching and support.
Another example of quasi-experiments is a pretest-posttest setting in which there are multiple measures. Random assignment could be used in pretest-posttest contexts, in which case this would be characterized as a true experiment, but often this approach is implemented without random assignment. For example, we could test the hypothesized effect of leadership quality on engagement via a pretest-posttest approach. If leaders are selected for a leadership development workshop, we could survey the leaders’ teams and collect data on leader effectiveness perceptions and self-reported engagement prior to (baseline) and after the workshop. It is unlikely that leaders were selected for this workshop by a random process; there were likely criteria driving the selection, such as leaders who were identified as critical talent or who achieved a certain performance level. If this study finds that improvements in leadership effectiveness correlate with improvements in engagement, there would be some evidence favoring investments in leadership development; however, this would not be sufficient evidence for a causal effect.
Though quasi-experiments are not as robust as true experiments, they are usually more feasible in a people analytics context. True experiments control for confounding variables by way of the research design (randomization ensures equivalent groups), while these factors must be controlled statistically in quasi-experimental contexts. In chapter “Linear Regression”, we will discuss how to model relationships among multiple variables in order to study how one variable influences another while holding constant variables that may influence the outcome but are not the primary focus of the research.
Non-Experimental Research
Unlike experimental and quasi-experimental designs, non-experimental research does not involve the manipulation of a variable. The goal of non-experiments is not to provide evidence for causal effects, but to study measured variables as they naturally occur and disentangle patterns in the data.
Given the potential for alternative explanations of any observed differences or relationships, non-experimental research tends to have lower internal validity than experimental and quasi-experimental designs. As we have discussed, it is often not possible or ethical to manipulate aspects of a person’s work context or to randomly assign people to groups. In addition, the nature of research questions does not always warrant experiments. In these cases, one of three non-experimental approaches may be considered: (a) cross-sectional , (b) correlational , and (c) observational .
Cross-sectional research compares two or more natural groups of people. For example, we may examine differences in engagement between employees in the Engineering department relative to employees in the Product department. In this case, we would neither manipulate one’s department to determine how department influences engagement nor randomly assign employees to these departments. Department membership exists apart from the research, so these naturally occurring groups can be leveraged for comparisons. There are of course many examples of naturally occurring groups that we would not manipulate, such as gender, ethnicity, generation, education, job family, job level, location, and tenure band. When participant characteristics are used to create groups, these variables are sometimes referred to as experimenter-selected —rather than experimenter-manipulated .
Correlational research involves studying the statistical relationship between two variables without manipulating some aspect of a person’s natural context. The relationship between leadership quality and engagement could be evaluated using correlational research. However, we would be unable to leverage a correlational design to test a hypothesis positing a causal effect of leadership quality on engagement. We would be limited to understanding how leadership quality and engagement covary; that is, to what extent a change in one variable is associated with a change in the other. Engagement may tend to increase as leadership quality increases, but a correlational design does not lend to understanding the direction of causal influence—if such an effect exists.
Observational research refers to studies in which the researcher gathers information without research subjects being explicitly involved in the recording of data. Collecting data from the company’s Human Capital Management (HCM) system could be an observational research method. For example, if we access data on terminations to determine the rate of attrition over a specified period, we would not need to interfere by asking past or present employees for this information. We would also do so without manipulating any aspect of the ordinary environment, tagging people to naturally occurring or artificially created groups, or evaluating the association of attrition with another variable. The reality is that such an approach would not be too actionable, however, as this would offer no understanding of what may be influencing attrition.
Review Questions
What type of research method and design would be best suited for a study aiming to understand the effect of stay interviews on employee attrition?
Why are quasi-experiments less rigorous than true experiments?
When evaluating the effectiveness of a new program, what are some reasons an experimental design would not be implemented?
What is the role of research questions?
What is the role of research hypotheses?
What is the difference between internal and external validity, and why are these concepts important in research?
What is an example of a mixed methods study?
What is the key difference between experimental and non-experimental research designs?
What are the differences between cross-sectional, correlational, and observational non-experimental designs?
How can the Hawthorne Effect impact the integrity of an experiment?
Bibliography
Creswell, J. W., & Creswell, J. D. (2018). Research design: qualitative, quantitative, and mixed methods approaches (5th ed.). Los Angeles: Sage.
MATH Google Scholar
Roethlisberger, F. J., & Dickson, W. J. (1939). Management and the worker . Harvard University Press.
Google Scholar
Download references
Author information
Authors and affiliations.
St. Louis, USA
Craig Starbuck
You can also search for this author in PubMed Google Scholar
Corresponding author
Correspondence to Craig Starbuck .
Rights and permissions
Open Access This chapter is licensed under the terms of the Creative Commons Attribution 4.0 International License ( http://creativecommons.org/licenses/by/4.0/ ), which permits use, sharing, adaptation, distribution and reproduction in any medium or format, as long as you give appropriate credit to the original author(s) and the source, provide a link to the Creative Commons license and indicate if changes were made.
The images or other third party material in this chapter are included in the chapter's Creative Commons license, unless indicated otherwise in a credit line to the material. If material is not included in the chapter's Creative Commons license and your intended use is not permitted by statutory regulation or exceeds the permitted use, you will need to obtain permission directly from the copyright holder.
Reprints and permissions
Copyright information
© 2023 The Author(s)
About this chapter
Starbuck, C. (2023). Research Design. In: The Fundamentals of People Analytics. Springer, Cham. https://doi.org/10.1007/978-3-031-28674-2_4

Download citation
DOI : https://doi.org/10.1007/978-3-031-28674-2_4
Published : 02 March 2023
Publisher Name : Springer, Cham
Print ISBN : 978-3-031-28673-5
Online ISBN : 978-3-031-28674-2
eBook Packages : Mathematics and Statistics Mathematics and Statistics (R0)
Share this chapter
Anyone you share the following link with will be able to read this content:
Sorry, a shareable link is not currently available for this article.
Provided by the Springer Nature SharedIt content-sharing initiative
- Publish with us
Policies and ethics
- Find a journal
- Track your research
- Privacy Policy
Buy Me a Coffee

Home » Correlational Research – Methods, Types and Examples
Correlational Research – Methods, Types and Examples
Table of Contents

Correlational Research
Correlational Research is a type of research that examines the statistical relationship between two or more variables without manipulating them. It is a non-experimental research design that seeks to establish the degree of association or correlation between two or more variables.
Types of Correlational Research
There are three types of correlational research:
Positive Correlation
A positive correlation occurs when two variables increase or decrease together. This means that as one variable increases, the other variable also tends to increase. Similarly, as one variable decreases, the other variable also tends to decrease. For example, there is a positive correlation between the amount of time spent studying and academic performance. The more time a student spends studying, the higher their academic performance is likely to be. Similarly, there is a positive correlation between a person’s age and their income level. As a person gets older, they tend to earn more money.
Negative Correlation
A negative correlation occurs when one variable increases while the other decreases. This means that as one variable increases, the other variable tends to decrease. Similarly, as one variable decreases, the other variable tends to increase. For example, there is a negative correlation between the number of hours spent watching TV and physical activity level. The more time a person spends watching TV, the less physically active they are likely to be. Similarly, there is a negative correlation between the amount of stress a person experiences and their overall happiness. As stress levels increase, happiness levels tend to decrease.
Zero Correlation
A zero correlation occurs when there is no relationship between two variables. This means that the variables are unrelated and do not affect each other. For example, there is zero correlation between a person’s shoe size and their IQ score. The size of a person’s feet has no relationship to their level of intelligence. Similarly, there is zero correlation between a person’s height and their favorite color. The two variables are unrelated to each other.
Correlational Research Methods
Correlational research can be conducted using different methods, including:
Surveys are a common method used in correlational research. Researchers collect data by asking participants to complete questionnaires or surveys that measure different variables of interest. Surveys are useful for exploring the relationships between variables such as personality traits, attitudes, and behaviors.
Observational Studies
Observational studies involve observing and recording the behavior of participants in natural settings. Researchers can use observational studies to examine the relationships between variables such as social interactions, group dynamics, and communication patterns.
Archival Data
Archival data involves using existing data sources such as historical records, census data, or medical records to explore the relationships between variables. Archival data is useful for investigating the relationships between variables that cannot be manipulated or controlled.
Experimental Design
While correlational research does not involve manipulating variables, researchers can use experimental design to establish cause-and-effect relationships between variables. Experimental design involves manipulating one variable while holding other variables constant to determine the effect on the dependent variable.
Meta-Analysis
Meta-analysis involves combining and analyzing the results of multiple studies to explore the relationships between variables across different contexts and populations. Meta-analysis is useful for identifying patterns and inconsistencies in the literature and can provide insights into the strength and direction of relationships between variables.
Data Analysis Methods
Correlational research data analysis methods depend on the type of data collected and the research questions being investigated. Here are some common data analysis methods used in correlational research:
Correlation Coefficient
A correlation coefficient is a statistical measure that quantifies the strength and direction of the relationship between two variables. The correlation coefficient ranges from -1 to +1, with -1 indicating a perfect negative correlation, +1 indicating a perfect positive correlation, and 0 indicating no correlation. Researchers use correlation coefficients to determine the degree to which two variables are related.
Scatterplots
A scatterplot is a graphical representation of the relationship between two variables. Each data point on the plot represents a single observation. The x-axis represents one variable, and the y-axis represents the other variable. The pattern of data points on the plot can provide insights into the strength and direction of the relationship between the two variables.
Regression Analysis
Regression analysis is a statistical method used to model the relationship between two or more variables. Researchers use regression analysis to predict the value of one variable based on the value of another variable. Regression analysis can help identify the strength and direction of the relationship between variables, as well as the degree to which one variable can be used to predict the other.
Factor Analysis
Factor analysis is a statistical method used to identify patterns among variables. Researchers use factor analysis to group variables into factors that are related to each other. Factor analysis can help identify underlying factors that influence the relationship between two variables.
Path Analysis
Path analysis is a statistical method used to model the relationship between multiple variables. Researchers use path analysis to test causal models and identify direct and indirect effects between variables.
Applications of Correlational Research
Correlational research has many practical applications in various fields, including:
- Psychology : Correlational research is commonly used in psychology to explore the relationships between variables such as personality traits, behaviors, and mental health outcomes. For example, researchers may use correlational research to examine the relationship between anxiety and depression, or the relationship between self-esteem and academic achievement.
- Education : Correlational research is useful in educational research to explore the relationships between variables such as teaching methods, student motivation, and academic performance. For example, researchers may use correlational research to examine the relationship between student engagement and academic success, or the relationship between teacher feedback and student learning outcomes.
- Business : Correlational research can be used in business to explore the relationships between variables such as consumer behavior, marketing strategies, and sales outcomes. For example, marketers may use correlational research to examine the relationship between advertising spending and sales revenue, or the relationship between customer satisfaction and brand loyalty.
- Medicine : Correlational research is useful in medical research to explore the relationships between variables such as risk factors, disease outcomes, and treatment effectiveness. For example, researchers may use correlational research to examine the relationship between smoking and lung cancer, or the relationship between exercise and heart health.
- Social Science : Correlational research is commonly used in social science research to explore the relationships between variables such as socioeconomic status, cultural factors, and social behavior. For example, researchers may use correlational research to examine the relationship between income and voting behavior, or the relationship between cultural values and attitudes towards immigration.
Examples of Correlational Research
- Psychology : Researchers might be interested in exploring the relationship between two variables, such as parental attachment and anxiety levels in young adults. The study could involve measuring levels of attachment and anxiety using established scales or questionnaires, and then analyzing the data to determine if there is a correlation between the two variables. This information could be useful in identifying potential risk factors for anxiety in young adults, and in developing interventions that could help improve attachment and reduce anxiety.
- Education : In a correlational study in education, researchers might investigate the relationship between two variables, such as teacher engagement and student motivation in a classroom setting. The study could involve measuring levels of teacher engagement and student motivation using established scales or questionnaires, and then analyzing the data to determine if there is a correlation between the two variables. This information could be useful in identifying strategies that teachers could use to improve student motivation and engagement in the classroom.
- Business : Researchers might explore the relationship between two variables, such as employee satisfaction and productivity levels in a company. The study could involve measuring levels of employee satisfaction and productivity using established scales or questionnaires, and then analyzing the data to determine if there is a correlation between the two variables. This information could be useful in identifying factors that could help increase productivity and improve job satisfaction among employees.
- Medicine : Researchers might examine the relationship between two variables, such as smoking and the risk of developing lung cancer. The study could involve collecting data on smoking habits and lung cancer diagnoses, and then analyzing the data to determine if there is a correlation between the two variables. This information could be useful in identifying risk factors for lung cancer and in developing interventions that could help reduce smoking rates.
- Sociology : Researchers might investigate the relationship between two variables, such as income levels and political attitudes. The study could involve measuring income levels and political attitudes using established scales or questionnaires, and then analyzing the data to determine if there is a correlation between the two variables. This information could be useful in understanding how socioeconomic factors can influence political beliefs and attitudes.
How to Conduct Correlational Research
Here are the general steps to conduct correlational research:
- Identify the Research Question : Start by identifying the research question that you want to explore. It should involve two or more variables that you want to investigate for a correlation.
- Choose the research method: Decide on the research method that will be most appropriate for your research question. The most common methods for correlational research are surveys, archival research, and naturalistic observation.
- Choose the Sample: Select the participants or data sources that you will use in your study. Your sample should be representative of the population you want to generalize the results to.
- Measure the variables: Choose the measures that will be used to assess the variables of interest. Ensure that the measures are reliable and valid.
- Collect the Data: Collect the data from your sample using the chosen research method. Be sure to maintain ethical standards and obtain informed consent from your participants.
- Analyze the data: Use statistical software to analyze the data and compute the correlation coefficient. This will help you determine the strength and direction of the correlation between the variables.
- Interpret the results: Interpret the results and draw conclusions based on the findings. Consider any limitations or alternative explanations for the results.
- Report the findings: Report the findings of your study in a research report or manuscript. Be sure to include the research question, methods, results, and conclusions.
Purpose of Correlational Research
The purpose of correlational research is to examine the relationship between two or more variables. Correlational research allows researchers to identify whether there is a relationship between variables, and if so, the strength and direction of that relationship. This information can be useful for predicting and explaining behavior, and for identifying potential risk factors or areas for intervention.
Correlational research can be used in a variety of fields, including psychology, education, medicine, business, and sociology. For example, in psychology, correlational research can be used to explore the relationship between personality traits and behavior, or between early life experiences and later mental health outcomes. In education, correlational research can be used to examine the relationship between teaching practices and student achievement. In medicine, correlational research can be used to investigate the relationship between lifestyle factors and disease outcomes.
Overall, the purpose of correlational research is to provide insight into the relationship between variables, which can be used to inform further research, interventions, or policy decisions.
When to use Correlational Research
Here are some situations when correlational research can be particularly useful:
- When experimental research is not possible or ethical: In some situations, it may not be possible or ethical to manipulate variables in an experimental design. In these cases, correlational research can be used to explore the relationship between variables without manipulating them.
- When exploring new areas of research: Correlational research can be useful when exploring new areas of research or when researchers are unsure of the direction of the relationship between variables. Correlational research can help identify potential areas for further investigation.
- When testing theories: Correlational research can be useful for testing theories about the relationship between variables. Researchers can use correlational research to examine the relationship between variables predicted by a theory, and to determine whether the theory is supported by the data.
- When making predictions: Correlational research can be used to make predictions about future behavior or outcomes. For example, if there is a strong positive correlation between education level and income, one could predict that individuals with higher levels of education will have higher incomes.
- When identifying risk factors: Correlational research can be useful for identifying potential risk factors for negative outcomes. For example, a study might find a positive correlation between drug use and depression, indicating that drug use could be a risk factor for depression.
Characteristics of Correlational Research
Here are some common characteristics of correlational research:
- Examines the relationship between two or more variables: Correlational research is designed to examine the relationship between two or more variables. It seeks to determine if there is a relationship between the variables, and if so, the strength and direction of that relationship.
- Non-experimental design: Correlational research is typically non-experimental in design, meaning that the researcher does not manipulate any variables. Instead, the researcher observes and measures the variables as they naturally occur.
- Cannot establish causation : Correlational research cannot establish causation, meaning that it cannot determine whether one variable causes changes in another variable. Instead, it only provides information about the relationship between the variables.
- Uses statistical analysis: Correlational research relies on statistical analysis to determine the strength and direction of the relationship between variables. This may include calculating correlation coefficients, regression analysis, or other statistical tests.
- Observes real-world phenomena : Correlational research is often used to observe real-world phenomena, such as the relationship between education and income or the relationship between stress and physical health.
- Can be conducted in a variety of fields : Correlational research can be conducted in a variety of fields, including psychology, sociology, education, and medicine.
- Can be conducted using different methods: Correlational research can be conducted using a variety of methods, including surveys, observational studies, and archival studies.
Advantages of Correlational Research
There are several advantages of using correlational research in a study:
- Allows for the exploration of relationships: Correlational research allows researchers to explore the relationships between variables in a natural setting without manipulating any variables. This can help identify possible relationships between variables that may not have been previously considered.
- Useful for predicting behavior: Correlational research can be useful for predicting future behavior. If a strong correlation is found between two variables, researchers can use this information to predict how changes in one variable may affect the other.
- Can be conducted in real-world settings: Correlational research can be conducted in real-world settings, which allows for the collection of data that is representative of real-world phenomena.
- Can be less expensive and time-consuming than experimental research: Correlational research is often less expensive and time-consuming than experimental research, as it does not involve manipulating variables or creating controlled conditions.
- Useful in identifying risk factors: Correlational research can be used to identify potential risk factors for negative outcomes. By identifying variables that are correlated with negative outcomes, researchers can develop interventions or policies to reduce the risk of negative outcomes.
- Useful in exploring new areas of research: Correlational research can be useful in exploring new areas of research, particularly when researchers are unsure of the direction of the relationship between variables. By conducting correlational research, researchers can identify potential areas for further investigation.
Limitation of Correlational Research
Correlational research also has several limitations that should be taken into account:
- Cannot establish causation: Correlational research cannot establish causation, meaning that it cannot determine whether one variable causes changes in another variable. This is because it is not possible to control all possible confounding variables that could affect the relationship between the variables being studied.
- Directionality problem: The directionality problem refers to the difficulty of determining which variable is influencing the other. For example, a correlation may exist between happiness and social support, but it is not clear whether social support causes happiness, or whether happy people are more likely to have social support.
- Third variable problem: The third variable problem refers to the possibility that a third variable, not included in the study, is responsible for the observed relationship between the two variables being studied.
- Limited generalizability: Correlational research is often limited in terms of its generalizability to other populations or settings. This is because the sample studied may not be representative of the larger population, or because the variables studied may behave differently in different contexts.
- Relies on self-reported data: Correlational research often relies on self-reported data, which can be subject to social desirability bias or other forms of response bias.
- Limited in explaining complex behaviors: Correlational research is limited in explaining complex behaviors that are influenced by multiple factors, such as personality traits, situational factors, and social context.
About the author
Muhammad Hassan
Researcher, Academic Writer, Web developer
You may also like

Questionnaire – Definition, Types, and Examples

Case Study – Methods, Examples and Guide

Observational Research – Methods and Guide

Quantitative Research – Methods, Types and...

Qualitative Research Methods

Explanatory Research – Types, Methods, Guide
Academia.edu no longer supports Internet Explorer.
To browse Academia.edu and the wider internet faster and more securely, please take a few seconds to upgrade your browser .
Enter the email address you signed up with and we'll email you a reset link.
- We're Hiring!
- Help Center
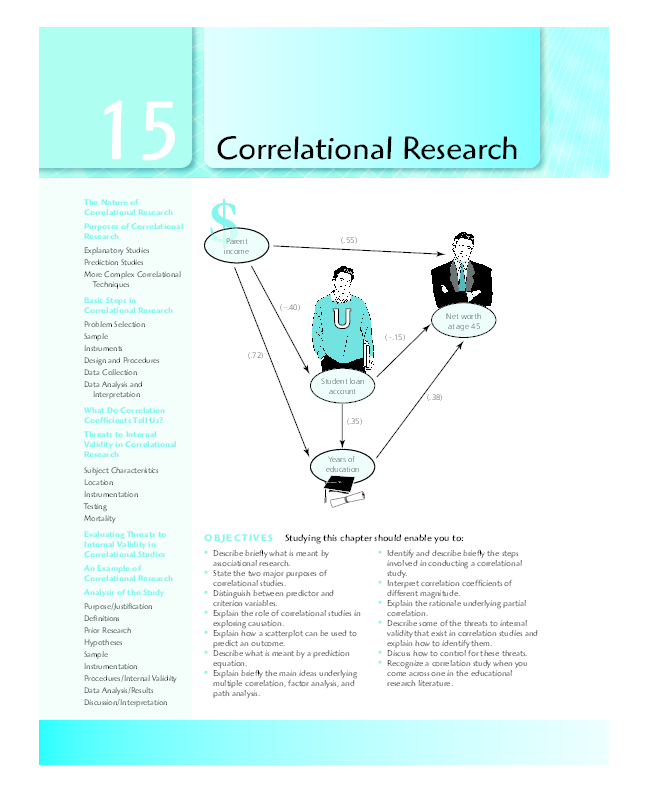
Chapter 15 Correlational Research_How to Design and Evaluate Research in Education 8th.pdf

Related Papers
Xynna Andagan
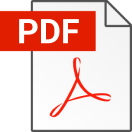
Farzad Javidanrad
Evans Okumu
Correlation - Levels of Measurement Introduction For this study, a correlation test was utilized for predictive analytics, to predict as well as estimate whether student’s self-ability to master science depend on their teacher’s intelligence (IQ). Correlation is a statistical technique used by researchers to determine the relationship between two or more variables. Variables In order to achieve the objective of the study, two variables were obtained from a university Longitudinal Study. The variables were: The first variable (dependent) is the aggregate of student’s science self-competence, whereas the second variable (independent) is the IQ score for the science lecturer. The study presumes that the high levels of science lecturer’s intelligence, the better outcome to student’s self-efficacy in the subject. The study is particularly interested in determining if lecturer’s scale of brainpower in science can influence their learner’s self-ability in the subject. The outcome of this study can be used to inform decision-maker in schools during the selection process of the science instructors. Levels of Measurement Level of measurement for the two variables is continuous. Data for students was obtained as exam scores (measured between 0-100), it’s therefore ratio. Conversely, lecturers data was captured based on their completion time (estimated in hours) for IQ scale, hence it’s measured in interval (time intervals). Therefore, the level of measurement for this study is ratio/interval. Type of Correlation Used A bivariate (Pearson) correlation test was used to determine whether there is a relationship between students’ science self-efficacy and lecturer’s IQ score (intelligence level). The test establishes the strength (strong or weak) and the direction (positive or negative) of the relationship between two variables (Salkind, 2013). The value of the Pearson correlation coefficient (r) lies between -1 to +1, and 0. A negative value implies no association (complete inverse relationship), for instance, an increase in variable X facilitates a decrease in variable Y. Hypothesis and Research Research question: Is there a significant positive correlation between lecturer’s science IQ score and student’s science self-capability? Null hypothesis: There is no significant positive correlation between lecturer’s science IQ score and students’ science self-ability. Results Output To test the study hypothesis, a bivariate Pearson Correlation test was performed as presented in Table-set 1.1 below. The analysis was performed using MS Excel, 2016 version. Table-set 1.1: Correlations (Teacher IQ scale and student’s science self-efficacy) SUMMARY OUTPUT Regression Statistics Multiple R 0.84 R Square 0.07 Adjusted R Square 0.06 Standard Error 0.07 Observations 56.00 ANOVA df SS MS F Significance F Regression 1.00 962.2 962.215 43.345 3E-06 Residual 54.00 399.6 22.199 Total 55.00 1361.8 Coefficients Standard Error t Stat P-value Lower 95% Upper 95% Intercept 7.78 0.0 5.540 0.000 5E+00 10.7 Scale of science lec IQ 0.02 0.0 6.584 0.000 1E-02 0.0 Results Interpretation The Pearson correlation results indicate a statistically significant and positive relationship between lecturer IQ score and their students’ science self-ability, r= 0.84, p=0.00. This shows that the P-value is smaller than the significance level used 0.5. Therefore, the null hypothesis is rejected. The study concludes that; there is a significant (strong) positive correlation between lecturer’s science IQ score and students’ science self-ability. The implication of the Study The study results imply that students with better aggregates in science self-efficacy must have been instructed by lecturers who have high levels of IQ (intelligence) in a science subject. What is more, the implication is that highly professional and brainy lecturers have required confidence, skills, experience, and competence to train students and make them understand so as to gain self-efficacy. The findings of this study resonate with Kunter et al., (2013) research, which advanced that professional competence of lecturers has an effect on the instructional quality and student development. References Salkind, N. J. (2013). Types of Correlation. In Statistics for people who (think they) hate statistics: Excel 2010 edition (p. 148-149). Kansas: SAGE Publications. Kunter, Mareike & Klusmann, Uta & Baumert, Jürgen & Richter. (2013). Professional Competence of Teachers: Effects on Instructional Quality and Student Development. Journal of Educational Psychology. 105. 805–820. 10.1037/a0032583.
Moses Kumi Asamoah
Correlational research is a type of quantitative research method that some researchers wrongly apply in a given academic study. It is time to highlight and address this problem by the way of publication in very reputable Journal. The paper is meant to re-examine the limitations and uses of correlational studies. At the end of the day, researchers are alerted to weigh various methods of quantitative research before making decision on the method suitable for their research objectives. Desktop approach that reviewed, critiqued and synthesized representative literature on a topic in an integrated way such that new frameworks and perspectives on the topic are generated was adopted. Books and articles were used as well. The revelation was that despite the challenges associated with using correlation, it was found very useful in bi-variate data analysis method used for predictions in some cases. Complex correlational statistics such as path analysis, multiple regression and partial correla...
Social Science Research Network
Dr. S. Senthilnathan
The measure of correlation coefficient (r or R) provides information on closeness of two variables. Irrespective of non-linear correlation, this paper mainly considers the linear correlation analysis, as it is most likely applied in social science studies. Explicitly, the purpose of carrying out correlation analysis is almost the same in quantitative analytical studies, thus becoming useful to explore the association between independent and dependent variables. This paper, as an extension, attempts additionally to explain the usefulness of linear correlation coefficient between two variables in the context of identifying the level of multicollinearity and mediating/moderating status of independent variables in a model. This paper also demonstrates how the level of multicollinearity can be explored by using correlation coefficient of two independent variables in a regression model.
Proceedings, NEDSI Conference
Hershey H Friedman
In the age of analytics and big data, it is becoming easier to dredge the data and find significant correlations. It is important for educators to enhance their lectures dealing with correlation. The authors feel that the best way to accomplish this is by using actual, real-world correlations uncovered by researchers. This paper provides examples of correlations from many diverse disciplines including management, education, psychology, and health.
Australian Critical Care
Roshani Prematunga
Marlynn Griffin
Critical Care
Jonathan Ball
The most commonly used techniques for investigating the relationship between two quantitative variables are correlation and linear regression. Correlation quantifies the strength of the linear relationship between a pair of variables, whereas regression expresses the relationship ...
Cut Eka Para Samya
RELATED PAPERS
maximiliano garbarino
Arturo Flores-Martínez AFM
James Barker
Entomologia Experimentalis et Applicata
Oldřich Nedvěd
International Journal of Academic Research in Business and Social Sciences
Hanis Diyana Kamarudin
Angel Ginés Gracia
adilson machado
Adelar Fochezatto
Fuzzy Information and Engineering
Amir Mohammad Golmohammadi
EUROPEAN RESEARCH STUDIES JOURNAL
Simon Grima
Hyperfine Interactions
Diana Prada
Lecture Notes in Computer Science
nguyen tran
Computer Speech & Language
Luis Enrique Lopez Pineda
Small Business Economics
Massimiliano Vesci
Marko Bergant
مجلة علوم ذوي الاحتياجات الخاصة
Journal of immunology (Baltimore, Md. : 1950)
Peter Pioli
hjjgfh rgrgfgfd
Gusztáv Kecskés D.
wahyu nur fitriyana
médecine/sciences
Alain Prochiantz
- We're Hiring!
- Help Center
- Find new research papers in:
- Health Sciences
- Earth Sciences
- Cognitive Science
- Mathematics
- Computer Science
- Academia ©2024

IMAGES
VIDEO
COMMENTS
correlational designs. We begin this chapter with an introduction to the research design that was illustrated here: the survey research design. 8.1 An Overview of Survey Designs A nonexperimental research design used to describe an individual or a group by having participants complete a survey or questionnaire is called the survey research design.
PDF | Correlational design is a study design for examining the relationships between or among two or more variables in a single group, which can occur... | Find, read and cite all the research you ...
A correlational research design investigates relationships between variables without the researcher controlling or manipulating any of them. A correlation reflects the strength and/or direction of the relationship between two (or more) variables. The direction of a correlation can be either positive or negative. Positive correlation.
Correlational research is a quantitative method of inquiry that evaluates the relationship between two variables by determining the pattern of how the two variables covary (i.e., change together ...
A correlational research design investigates relationships between variables without the researcher controlling or manipulating any of them. A correlation reflects the strength and/or direction of the relationship between two (or more) variables. The direction of a correlation can be either positive or negative. Positive correlation.
Correlational studies, therefore, are distinct from true experimental designs where researchers randomly assign. participants to groups and provide appropriate control to hold all other variables ...
tional design from qualitative design. Although both strategies focus on naturally oc-curring patterns, qualitative research is more attentive to the holistic qualities of phenomena. (See Chapter 7.) As is typical for a correlational design, the researchers in Whyte's study employed observational tactics whereby the sheer number of people
Correlational research is a type of nonexperimental research in which the researcher measures two variables and assesses the statistical relationship (i.e., the correlation) between them with little or no effort to control extraneous variables. There are essentially two reasons that researchers interested in statistical relationships between ...
Engagement may tend to increase as leadership quality increases, but a correlational design does not lend to understanding the direction of causal influence—if such an effect exists. Observational research refers to studies in which the researcher gathers information without research subjects being explicitly involved in the recording of data ...
(Gall, Gall & Borg, 2003). This research study is non experimental in design, and as the purpose of this study is prediction, a correlational research design is used. Correlational research refers to studies in which the purpose is to discover relationships between variables through the use of correlational statistics.
In this chapter, we suggested several research questions that would be appropriate for each of the nonexperimental designs. Develop your own research question for a correlational design, an ex post facto design, a naturalistic observation, a case study, a phenomenological study, and an ethnographic study. Briefly describe the methodology for each.
Sometimes correlational research is referred as a form of descriptive research. Describes the degree to which two or more quantitative variables are related. Purposes of Correlation Research • Two basic purposes: Help explain important human behaviors (Explanatory Studies) Explanatory Study: An explanatory correlational design
Correlational research has many practical applications in various fields, including: Psychology: Correlational research is commonly used in psychology to explore the relationships between variables such as personality traits, behaviors, and mental health outcomes. For example, researchers may use correlational research to examine the ...
This chapter includes research design, population and sample, research hypothesis, data collection, the research instruments try out, and data analysis. 3.1 Research Design Since the main purpose of this research is to investigate whether there is any correlation between explicit grammatical knowledge and writing ability of EFL
3.2 Research Design This research is a correlational research design that had determined the answer the objectives of the study. Those were finding out the correlation between students' academic self concept and their writing performance. According to Fraenkel, Wallen and Hyun (2012), correlational studies investigate the possibility
The designs. in this chapter are survey design, descriptive design, correlational design, ex-. perimental design, and causal-comparative design. As we address each research. design, we will learn ...
However, correlational studies lack the components of random control trials and quasi-experimental studies to allow them to assign causality. A correlational study might look at diferences in behaviors or outcomes, but it cannot prove that a specifc factor caused the changes. Even if it looks causal it is always possible there is an unobserved ...
objective in a valid way. The essence of research design is to translate a research problem into data for analysis so as to provide relevant answers to research questions at a minimum cost. This paper investigates what research design is, the different kinds of research design and how a researcher can choose the appropriate research design for ...
The Nature of Correlational Research Correlational research, like causal-comparative research (which we discuss in Chapter 16), is an example of what is sometimes called associational research. In associational research, the relationships among two or more variables are studied without any attempt to inluence them.
The design of a study defines the study type (descriptive, correlational, semi-experimental, experimental, review, meta-analytic) and sub-type (e.g., descriptive-longitudinal case study), research ...
3.1 Research Design The design of this research is Correlational research. It is a type of nonexperimental research in which the researcher measures two variables and assesses the statistical relationship (i.e., the correlation) between them with little or no effort to control extraneous variables.This research attempts to get the empirical data