Have a language expert improve your writing
Run a free plagiarism check in 10 minutes, generate accurate citations for free.
- Knowledge Base
Methodology
- Questionnaire Design | Methods, Question Types & Examples

Questionnaire Design | Methods, Question Types & Examples
Published on July 15, 2021 by Pritha Bhandari . Revised on June 22, 2023.
A questionnaire is a list of questions or items used to gather data from respondents about their attitudes, experiences, or opinions. Questionnaires can be used to collect quantitative and/or qualitative information.
Questionnaires are commonly used in market research as well as in the social and health sciences. For example, a company may ask for feedback about a recent customer service experience, or psychology researchers may investigate health risk perceptions using questionnaires.
Table of contents
Questionnaires vs. surveys, questionnaire methods, open-ended vs. closed-ended questions, question wording, question order, step-by-step guide to design, other interesting articles, frequently asked questions about questionnaire design.
A survey is a research method where you collect and analyze data from a group of people. A questionnaire is a specific tool or instrument for collecting the data.
Designing a questionnaire means creating valid and reliable questions that address your research objectives , placing them in a useful order, and selecting an appropriate method for administration.
But designing a questionnaire is only one component of survey research. Survey research also involves defining the population you’re interested in, choosing an appropriate sampling method , administering questionnaires, data cleansing and analysis, and interpretation.
Sampling is important in survey research because you’ll often aim to generalize your results to the population. Gather data from a sample that represents the range of views in the population for externally valid results. There will always be some differences between the population and the sample, but minimizing these will help you avoid several types of research bias , including sampling bias , ascertainment bias , and undercoverage bias .
Prevent plagiarism. Run a free check.
Questionnaires can be self-administered or researcher-administered . Self-administered questionnaires are more common because they are easy to implement and inexpensive, but researcher-administered questionnaires allow deeper insights.
Self-administered questionnaires
Self-administered questionnaires can be delivered online or in paper-and-pen formats, in person or through mail. All questions are standardized so that all respondents receive the same questions with identical wording.
Self-administered questionnaires can be:
- cost-effective
- easy to administer for small and large groups
- anonymous and suitable for sensitive topics
But they may also be:
- unsuitable for people with limited literacy or verbal skills
- susceptible to a nonresponse bias (most people invited may not complete the questionnaire)
- biased towards people who volunteer because impersonal survey requests often go ignored.
Researcher-administered questionnaires
Researcher-administered questionnaires are interviews that take place by phone, in-person, or online between researchers and respondents.
Researcher-administered questionnaires can:
- help you ensure the respondents are representative of your target audience
- allow clarifications of ambiguous or unclear questions and answers
- have high response rates because it’s harder to refuse an interview when personal attention is given to respondents
But researcher-administered questionnaires can be limiting in terms of resources. They are:
- costly and time-consuming to perform
- more difficult to analyze if you have qualitative responses
- likely to contain experimenter bias or demand characteristics
- likely to encourage social desirability bias in responses because of a lack of anonymity
Your questionnaire can include open-ended or closed-ended questions or a combination of both.
Using closed-ended questions limits your responses, while open-ended questions enable a broad range of answers. You’ll need to balance these considerations with your available time and resources.
Closed-ended questions
Closed-ended, or restricted-choice, questions offer respondents a fixed set of choices to select from. Closed-ended questions are best for collecting data on categorical or quantitative variables.
Categorical variables can be nominal or ordinal. Quantitative variables can be interval or ratio. Understanding the type of variable and level of measurement means you can perform appropriate statistical analyses for generalizable results.
Examples of closed-ended questions for different variables
Nominal variables include categories that can’t be ranked, such as race or ethnicity. This includes binary or dichotomous categories.
It’s best to include categories that cover all possible answers and are mutually exclusive. There should be no overlap between response items.
In binary or dichotomous questions, you’ll give respondents only two options to choose from.
White Black or African American American Indian or Alaska Native Asian Native Hawaiian or Other Pacific Islander
Ordinal variables include categories that can be ranked. Consider how wide or narrow a range you’ll include in your response items, and their relevance to your respondents.
Likert scale questions collect ordinal data using rating scales with 5 or 7 points.
When you have four or more Likert-type questions, you can treat the composite data as quantitative data on an interval scale . Intelligence tests, psychological scales, and personality inventories use multiple Likert-type questions to collect interval data.
With interval or ratio scales , you can apply strong statistical hypothesis tests to address your research aims.
Pros and cons of closed-ended questions
Well-designed closed-ended questions are easy to understand and can be answered quickly. However, you might still miss important answers that are relevant to respondents. An incomplete set of response items may force some respondents to pick the closest alternative to their true answer. These types of questions may also miss out on valuable detail.
To solve these problems, you can make questions partially closed-ended, and include an open-ended option where respondents can fill in their own answer.
Open-ended questions
Open-ended, or long-form, questions allow respondents to give answers in their own words. Because there are no restrictions on their choices, respondents can answer in ways that researchers may not have otherwise considered. For example, respondents may want to answer “multiracial” for the question on race rather than selecting from a restricted list.
- How do you feel about open science?
- How would you describe your personality?
- In your opinion, what is the biggest obstacle for productivity in remote work?
Open-ended questions have a few downsides.
They require more time and effort from respondents, which may deter them from completing the questionnaire.
For researchers, understanding and summarizing responses to these questions can take a lot of time and resources. You’ll need to develop a systematic coding scheme to categorize answers, and you may also need to involve other researchers in data analysis for high reliability .
Question wording can influence your respondents’ answers, especially if the language is unclear, ambiguous, or biased. Good questions need to be understood by all respondents in the same way ( reliable ) and measure exactly what you’re interested in ( valid ).
Use clear language
You should design questions with your target audience in mind. Consider their familiarity with your questionnaire topics and language and tailor your questions to them.
For readability and clarity, avoid jargon or overly complex language. Don’t use double negatives because they can be harder to understand.
Use balanced framing
Respondents often answer in different ways depending on the question framing. Positive frames are interpreted as more neutral than negative frames and may encourage more socially desirable answers.
Use a mix of both positive and negative frames to avoid research bias , and ensure that your question wording is balanced wherever possible.
Unbalanced questions focus on only one side of an argument. Respondents may be less likely to oppose the question if it is framed in a particular direction. It’s best practice to provide a counter argument within the question as well.
Avoid leading questions
Leading questions guide respondents towards answering in specific ways, even if that’s not how they truly feel, by explicitly or implicitly providing them with extra information.
It’s best to keep your questions short and specific to your topic of interest.
- The average daily work commute in the US takes 54.2 minutes and costs $29 per day. Since 2020, working from home has saved many employees time and money. Do you favor flexible work-from-home policies even after it’s safe to return to offices?
- Experts agree that a well-balanced diet provides sufficient vitamins and minerals, and multivitamins and supplements are not necessary or effective. Do you agree or disagree that multivitamins are helpful for balanced nutrition?
Keep your questions focused
Ask about only one idea at a time and avoid double-barreled questions. Double-barreled questions ask about more than one item at a time, which can confuse respondents.
This question could be difficult to answer for respondents who feel strongly about the right to clean drinking water but not high-speed internet. They might only answer about the topic they feel passionate about or provide a neutral answer instead – but neither of these options capture their true answers.
Instead, you should ask two separate questions to gauge respondents’ opinions.
Strongly Agree Agree Undecided Disagree Strongly Disagree
Do you agree or disagree that the government should be responsible for providing high-speed internet to everyone?
Receive feedback on language, structure, and formatting
Professional editors proofread and edit your paper by focusing on:
- Academic style
- Vague sentences
- Style consistency
See an example
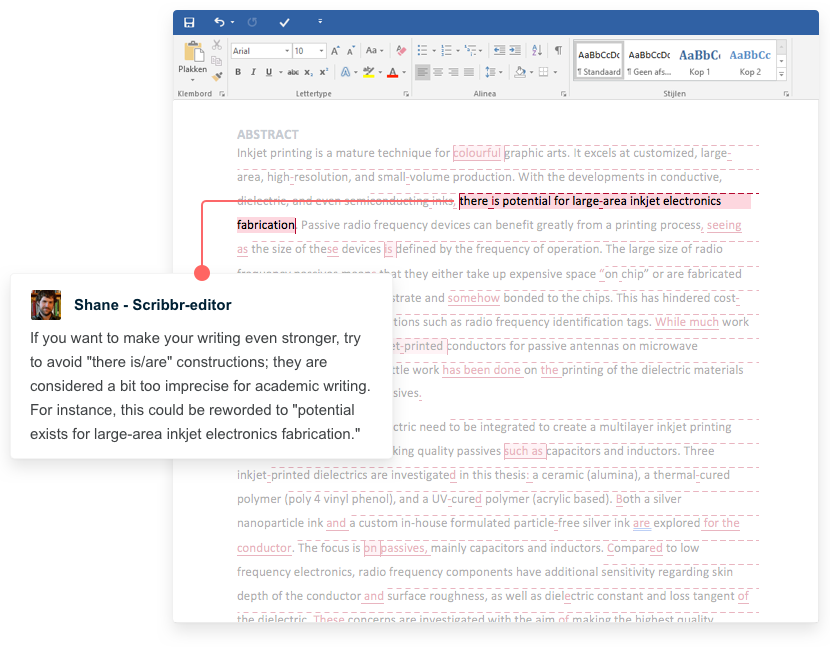
You can organize the questions logically, with a clear progression from simple to complex. Alternatively, you can randomize the question order between respondents.
Logical flow
Using a logical flow to your question order means starting with simple questions, such as behavioral or opinion questions, and ending with more complex, sensitive, or controversial questions.
The question order that you use can significantly affect the responses by priming them in specific directions. Question order effects, or context effects, occur when earlier questions influence the responses to later questions, reducing the validity of your questionnaire.
While demographic questions are usually unaffected by order effects, questions about opinions and attitudes are more susceptible to them.
- How knowledgeable are you about Joe Biden’s executive orders in his first 100 days?
- Are you satisfied or dissatisfied with the way Joe Biden is managing the economy?
- Do you approve or disapprove of the way Joe Biden is handling his job as president?
It’s important to minimize order effects because they can be a source of systematic error or bias in your study.
Randomization
Randomization involves presenting individual respondents with the same questionnaire but with different question orders.
When you use randomization, order effects will be minimized in your dataset. But a randomized order may also make it harder for respondents to process your questionnaire. Some questions may need more cognitive effort, while others are easier to answer, so a random order could require more time or mental capacity for respondents to switch between questions.
Step 1: Define your goals and objectives
The first step of designing a questionnaire is determining your aims.
- What topics or experiences are you studying?
- What specifically do you want to find out?
- Is a self-report questionnaire an appropriate tool for investigating this topic?
Once you’ve specified your research aims, you can operationalize your variables of interest into questionnaire items. Operationalizing concepts means turning them from abstract ideas into concrete measurements. Every question needs to address a defined need and have a clear purpose.
Step 2: Use questions that are suitable for your sample
Create appropriate questions by taking the perspective of your respondents. Consider their language proficiency and available time and energy when designing your questionnaire.
- Are the respondents familiar with the language and terms used in your questions?
- Would any of the questions insult, confuse, or embarrass them?
- Do the response items for any closed-ended questions capture all possible answers?
- Are the response items mutually exclusive?
- Do the respondents have time to respond to open-ended questions?
Consider all possible options for responses to closed-ended questions. From a respondent’s perspective, a lack of response options reflecting their point of view or true answer may make them feel alienated or excluded. In turn, they’ll become disengaged or inattentive to the rest of the questionnaire.
Step 3: Decide on your questionnaire length and question order
Once you have your questions, make sure that the length and order of your questions are appropriate for your sample.
If respondents are not being incentivized or compensated, keep your questionnaire short and easy to answer. Otherwise, your sample may be biased with only highly motivated respondents completing the questionnaire.
Decide on your question order based on your aims and resources. Use a logical flow if your respondents have limited time or if you cannot randomize questions. Randomizing questions helps you avoid bias, but it can take more complex statistical analysis to interpret your data.
Step 4: Pretest your questionnaire
When you have a complete list of questions, you’ll need to pretest it to make sure what you’re asking is always clear and unambiguous. Pretesting helps you catch any errors or points of confusion before performing your study.
Ask friends, classmates, or members of your target audience to complete your questionnaire using the same method you’ll use for your research. Find out if any questions were particularly difficult to answer or if the directions were unclear or inconsistent, and make changes as necessary.
If you have the resources, running a pilot study will help you test the validity and reliability of your questionnaire. A pilot study is a practice run of the full study, and it includes sampling, data collection , and analysis. You can find out whether your procedures are unfeasible or susceptible to bias and make changes in time, but you can’t test a hypothesis with this type of study because it’s usually statistically underpowered .
If you want to know more about statistics , methodology , or research bias , make sure to check out some of our other articles with explanations and examples.
- Student’s t -distribution
- Normal distribution
- Null and Alternative Hypotheses
- Chi square tests
- Confidence interval
- Quartiles & Quantiles
- Cluster sampling
- Stratified sampling
- Data cleansing
- Reproducibility vs Replicability
- Peer review
- Prospective cohort study
Research bias
- Implicit bias
- Cognitive bias
- Placebo effect
- Hawthorne effect
- Hindsight bias
- Affect heuristic
- Social desirability bias
A questionnaire is a data collection tool or instrument, while a survey is an overarching research method that involves collecting and analyzing data from people using questionnaires.
Closed-ended, or restricted-choice, questions offer respondents a fixed set of choices to select from. These questions are easier to answer quickly.
Open-ended or long-form questions allow respondents to answer in their own words. Because there are no restrictions on their choices, respondents can answer in ways that researchers may not have otherwise considered.
A Likert scale is a rating scale that quantitatively assesses opinions, attitudes, or behaviors. It is made up of 4 or more questions that measure a single attitude or trait when response scores are combined.
To use a Likert scale in a survey , you present participants with Likert-type questions or statements, and a continuum of items, usually with 5 or 7 possible responses, to capture their degree of agreement.
You can organize the questions logically, with a clear progression from simple to complex, or randomly between respondents. A logical flow helps respondents process the questionnaire easier and quicker, but it may lead to bias. Randomization can minimize the bias from order effects.
Questionnaires can be self-administered or researcher-administered.
Researcher-administered questionnaires are interviews that take place by phone, in-person, or online between researchers and respondents. You can gain deeper insights by clarifying questions for respondents or asking follow-up questions.
Cite this Scribbr article
If you want to cite this source, you can copy and paste the citation or click the “Cite this Scribbr article” button to automatically add the citation to our free Citation Generator.
Bhandari, P. (2023, June 22). Questionnaire Design | Methods, Question Types & Examples. Scribbr. Retrieved April 10, 2024, from https://www.scribbr.com/methodology/questionnaire/
Is this article helpful?
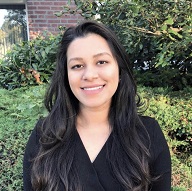
Pritha Bhandari
Other students also liked, survey research | definition, examples & methods, what is a likert scale | guide & examples, reliability vs. validity in research | difference, types and examples, unlimited academic ai-proofreading.
✔ Document error-free in 5minutes ✔ Unlimited document corrections ✔ Specialized in correcting academic texts

An official website of the United States government
The .gov means it’s official. Federal government websites often end in .gov or .mil. Before sharing sensitive information, make sure you’re on a federal government site.
The site is secure. The https:// ensures that you are connecting to the official website and that any information you provide is encrypted and transmitted securely.
- Publications
- Account settings
Preview improvements coming to the PMC website in October 2024. Learn More or Try it out now .
- Advanced Search
- Journal List
- J Educ Eval Health Prof
Assessing study skills among a sample of university students: an Iranian survey
Alireza didarloo.
1 Social Determinants of Health Research Center, Faculty of Medicine, Urmia University of Medical Sciences, Urmia, Iran
Hamid Reza Khalkhali
2 Department of Biostatistics, Inpatient Safety Research Center, Faculty of Medicine, Urmia University of Medical Sciences, Urmia, Iran
Associated Data
Numerous studies have revealed that study skills have a constructive role on the academic performance of students, in addition to educational quality, students’ intelligence, and their affective characteristics. This study aims to examine study skills and the factors influencing them among the health sciences students of Urmia University of Medical Sciences in Iran.
This was a cross-sectional study carried out from May to November 2013. A total of 340 Urmia health sciences students were selected using a simple sampling method. Data were collected using the Study Skills Assessment Questionnaire of Counseling Center of Houston University and analyzed with descriptive and analytical statistics.
The mean and standard deviation of the students’ study skills were 172.5±23.2, out of a total score of 240. Around 1.2% of the study skills were weak; 86.8%, moderate; and 12%, good. Among the study skills, the scores of time management, and memory and concentration were better than the others. Also, there was a significant positive correlation between study skills scores and the students’ family housing status and academic level (P<0.05).
Conclusion:
Although the majority of the participants had moderate study skills, these were not sufficient and far from good. Improving and promoting the study skills of university students require the designing and implementing of education programs for study strategies. Therefore, decision makers and planners in the educational areas of universities should consider the topic described above.
INTRODUCTION
One of the most important necessities in higher education systems is the development and reinforcement of the study skills of students. In recent decades, extensive research has been conducted on students’ study skills and strategies, but only in developed countries. Hence, examining the study skills of students who have grown up in different cultures is essential. Accordingly, the present investigation aims to evaluate study skills of health sciences students in Urmia University of Medical Sciences, Iran, and identify the factors influencing them. On the one hand, such research increases the existing knowledge about study skills and helps generalize the findings of other investigators. On the other hand, through a precise understanding of students’ study skills, appropriate educational interventions can be designed to address defects in study skills.
This project is a descriptive and analytic research design that was carried out from May to November 2013 to examine study skills and related factors among health sciences students in Urmia University of Medical Sciences. A total 340 students were selected and entered in the study using the census method. The subjects were divided into two groups: (1) discontinuous undergraduates, that is, students who already had two years of college teaching experience and were pursuing further university education; and (2) continuous undergraduates, that is, university students who had not yet completed their first degree.
The data-gathering instrument was a two-part questionnaire. The first part was used to obtain the demographic characteristics of the participants and the second part, a Study Skills Assessment Questionnaire of Counseling Center of Houston University (SSAQ-CCHU), was used to measure the students’ study skills [ 1 ]. The original instrument, which had 64 items, was translated into the Persian language and tested for validity and reliability by a panel of experts. The panel found some items incompatible with Iranian cultural factors; thus, these were either omitted or integrated, bringing down the total to 48. The adjusted SSAQ-CCHU had eight domains: time management and procrastination, concentration and memory, study aids and note taking, test strategies and test anxiety, organizing and processing information, motivation and attitude, reading and selecting the main idea, and writing. Each domain was examined by six items. The study skills instrument employed a five-point Likert scale (always, often, sometimes, rarely, never), and its score range was from 5 to 1. The minimum and maximum scores for the total scale were 48 and 240, and for each domain, 6 and 30. Scoring less than 50% in each domain or less than 120 in all domains indicated poor study skills; 50 to 75% in each domain or 120 to 180 in all domains, moderate study skills; and more than 75% in each domain or more than 180 in all domains, good study skills.
To determine the validity of the study instrument content, we applied the Banville method [ 2 ]. The original questionnaire was translated into the Persian language, then evaluated by a panel of experts. Some items in the translated instrument were deleted or merged, in accordance with the experts’ opinions. In the item analysis stage, some of questions were restricted because of the decreasing instrument reliability coefficient. Finally, after the required revisions had been applied, the adjusted questionnaire was developed. The Persian questionnaire was then translated back into English by two English language teachers and compared with the original version. The two instruments were similar in content. The internal consistency approach was utilized to evaluate the reliability of the Persian questionnaire. In a pilot study, the instrument was completed by 20 students who were similar to the main research subjects. The questionnaire had a Cronbach’s alpha coefficient of 93% and was approved. After obtaining permission from university authorities and the oral consent of the students, and in coordination with the classroom teachers, the questionnaires were filled out by the study subjects. The data were analyzed by descriptive statistics (frequency tables, measures of central tendency and dispersion for displaying frequency, percentage, and mean and standard deviation [SD]) and inferential tests (independent t-test for comparing means) using SPSS ver. 16 (SPSS Inc., Chicago, IL, USA).
All questionnaires distributed were analyzed; therefore, the response rate was 100%. The responses showed that 66.5% of the subjects were male (226) and 33.5%, female (114). The majority (288, or 84.7%) were single, of whom 259 (76.2%) lived in student dormitories. As to occupation, the fathers of 133 students (39.1%) worked in the government, and the rest had other jobs; the mothers of 324 subjects (95.3%) were housewives. More than half of the parents of samples (54.6%) were illiterate or of low literacy. The mean and SD of the students’ age were 22.56±3.9, and ranged from 18 to 41 years. The academic average of the students was 15.15, and their average scores ranged from 10 to 19. The mean and SD score of the students’ total study skills were 172.5±23.2. Five of the participants (1.2%) had good study skills; 295 (86.8%), moderate; and 40 (12%), poor. Among the areas of study skills, the highest scores were in time management, and concentration and memory; and those have placed in good, moderate, or weak skill ( Table 1 ).
Frequency, mean, and standard deviation of subcategories of students'study skills
The findings indicated that there was a significant difference of total skills according to the subjects’ family housing. The mean and SD scores of total study skills were 164.00±26.21 for students living in dormitories and 173.36±22.71 for those living in private housing (t=2.15, P=0.02) ( Table 2 ). Also, the results showed that there was a significant difference of total score of the students’ study skills according to their academic degree. The discontinuous undergraduates had better scores than the continuous undergraduates (P<0.05). The results showed that students’ residence is a significant variable to the reading skill of the participants (P<0.01), and non-significant to the other study skills. Moreover, the students’ family housing is a significant variable to the total score of their study skills (P<0.05) ( Table 2 ).
Comparison of the mean score of students’ study skills in different areas, according to demographic variables
The results of the study revealed that the students from Urmia University of Medical Sciences did not possess favorable study skills, as their mean score was merely moderate. This finding is consistent with those of other studies. The study of Fereidouni Moghadam and Cheraghian [ 3 ] also concluded that university students had poor-to-moderate study habits [ 3 ]. Meanwhile, the study of Hosseini et al. coincides with the present results in that it suggested the importance of organized and continuous educational courses to improve study skills. The factors that influenced their studies were time management, readiness to take exams, concentration, reading, and taking notes [ 4 ].
Above results showed that among the different subcategories of study skills, the participants were better in time management, and concentration and memory than in the other areas. This means that if students properly manage the time for studying and learning science topics, or concentrate on studying, they will succeed in acquiring information and learning. Some studies reinforce and support this part of the study’s findings. Nourian et al. [ 5 ] revealed that the mean score for time management was higher than that of other study skills; this was perfectly compatible and consistent with the results of the present investigation.
It revealed that there exists a statistically significant difference of the students’ study skills according to their family housing status and to academic degree. Students with private housing had remarkable study skills compared to those who were living in dormitories or rented housing. It seems that compared to the other participants, students with personal housing had better facilities, greater prosperity, and mental peace, all of which contributed to better study skills. In contrast, those living in dormitories and rented places often found it difficult to concentrate because of the noise, presence of roommates, or an uncomfortable environment. Knowing where to find a quiet, comfortable, and distraction-free place to study in is one of the simplest and most effective means of facilitating concentration. Above findings also indicated that discontinuous undergraduates had better study skills than continuous undergraduate. Although the literature review revealed no study that supported and confirmed this section of the results, educational researchers and specialists in research centers and universities can nevertheless use this as a basis for their studies.
Like other surveys, this project has limitations. First, results cannot be generalized beyond the study sample and, therefore, can be generalized only in populations with similar features. Second, the data of this study were collected using a self-reported questionnaire. Participants may have underestimated or overestimated their study skills behavior, and thus, the findings may have been affected. Third, a cross-sectional design was used to describe the relationship between variables. The main characteristic of cross-sectional design is that all data are collected at one time, thereby limiting the ability to identify cause-and-effect relationships between variables.
From the results above, it was concluded that the total study skills of students of Health Sciences at the Urmia University of Medical Sciences in Iran were moderate, that is, far from good. This trend could jeopardize students’ academic performance. It seems that improving the housing status of students, preparing the necessary equipment in their dormitories, and increasing their awareness about study skills and their different domains can be helpful. Therefore, measures such as designing and implementing study skills educational programs for students and considering study skills as a course for them in university educational curriculums are recommended and emphasized.
Acknowledgments
The authors would like to thank Dr. Gamini Premadasa for his suggestions about editing this article. We would like to thank the students who participated in this study and all the people who kindly helped us in conducting this research, especially in collecting the data. In addition, we acknowledge the official support of Urmia University of Medical Sciences.
CONFLICT OF INTEREST
No potential conflict of interest relevant to this article was reported.
SUPPLEMENTARY MATERIAL
Audio recording of the abstract.
- Open access
- Published: 10 April 2024
Medical students’ AI literacy and attitudes towards AI: a cross-sectional two-center study using pre-validated assessment instruments
- Matthias Carl Laupichler 1 ,
- Alexandra Aster 1 ,
- Marcel Meyerheim 2 ,
- Tobias Raupach 1 &
- Marvin Mergen 2
BMC Medical Education volume 24 , Article number: 401 ( 2024 ) Cite this article
Metrics details
Artificial intelligence (AI) is becoming increasingly important in healthcare. It is therefore crucial that today’s medical students have certain basic AI skills that enable them to use AI applications successfully. These basic skills are often referred to as “AI literacy”. Previous research projects that aimed to investigate medical students’ AI literacy and attitudes towards AI have not used reliable and validated assessment instruments.
We used two validated self-assessment scales to measure AI literacy (31 Likert-type items) and attitudes towards AI (5 Likert-type items) at two German medical schools. The scales were distributed to the medical students through an online questionnaire. The final sample consisted of a total of 377 medical students. We conducted a confirmatory factor analysis and calculated the internal consistency of the scales to check whether the scales were sufficiently reliable to be used in our sample. In addition, we calculated t-tests to determine group differences and Pearson’s and Kendall’s correlation coefficients to examine associations between individual variables.
The model fit and internal consistency of the scales were satisfactory. Within the concept of AI literacy, we found that medical students at both medical schools rated their technical understanding of AI significantly lower ( M MS1 = 2.85 and M MS2 = 2.50) than their ability to critically appraise ( M MS1 = 4.99 and M MS2 = 4.83) or practically use AI ( M MS1 = 4.52 and M MS2 = 4.32), which reveals a discrepancy of skills. In addition, female medical students rated their overall AI literacy significantly lower than male medical students, t (217.96) = -3.65, p <.001. Students in both samples seemed to be more accepting of AI than fearful of the technology, t (745.42) = 11.72, p <.001. Furthermore, we discovered a strong positive correlation between AI literacy and positive attitudes towards AI and a weak negative correlation between AI literacy and negative attitudes. Finally, we found that prior AI education and interest in AI is positively correlated with medical students’ AI literacy.
Conclusions
Courses to increase the AI literacy of medical students should focus more on technical aspects. There also appears to be a correlation between AI literacy and attitudes towards AI, which should be considered when planning AI courses.
Peer Review reports
The rise of artificial intelligence in medicine
The potential benefits of using artificial intelligence (AI) for the healthcare sector have been discussed for decades [ 1 , 2 , 3 ]. However, while in the past the focus was predominantly on theoretical considerations and ambitious future scenarios, AI and its most important subfield, machine learning, have now become an integral part of healthcare [ 4 ]. In addition to clinical practice, AI applications have reached medical schools and are being used by students, educators and administrators alike to improve teaching and learning [ 5 – 6 ].
At the same time, a “consensus on what and how to teach AI” [ 7 , p1] in the medical curriculum appears to be lacking, and although there are individual elective courses attempting to foster AI competencies [ 8 – 9 ], the majority of medical students still receive very little AI education [ 10 – 11 ]. However, learning basic AI skills will be critical for all future physicians to fulfill their roles as professionals, communicators, collaborators, leaders, healthcare advocates, and scholars, as all of these roles will be increasingly permeated by AI [ 12 ].
Medical student’s “AI literacy” and related constructs
In recent years, basic AI skills have often been referred to as AI literacy [ 13 ]. AI literacy can be defined as “a set of competencies that enables individuals to critically evaluate AI technologies; communicate and collaborate effectively with AI; and use AI as a tool online, at home, and in the workplace” [13, p2]. Thus, AI literacy for medical professionals is less about the ability to develop AI programs or to conduct clinical research with AI, but rather about the ability to interact with AI and use AI applications in the day-to-day provision of healthcare services.
Despite the large number of studies investigating the attitudes and feelings of medical students towards AI (i.e., the affective component of AI interaction [ 14 , 15 , 16 ]),, research projects have rarely focused on AI knowledge (i.e., conceptual understanding of AI) or even AI skills (i.e., ability to identify, use, and scrutinize AI applications reasonably). Mousavi Baigi et al. [ 17 ] found that all 38 studies they included in their literature review reported some kind of investigation on healthcare students’ “attitudes towards AI” (ATAI), while only 26 of the included studies stated that they had asked participants about their AI knowledge. However, a closer look at the studies showed that most of them assessed AI knowledge superficially and focused more on familiarity with AI. Furthermore, only six of the included studies looked at the AI skills of medical students. However, since the concept of AI literacy not only encompasses AI knowledge, but also includes practical AI competencies (such as the ability to recognize the use of AI applications in technical systems), this empirical foundation is not sufficient to make reliable statements about the AI literacy of medical students.
Karaca et al. [ 18 ] were among the few who took a systematic approach to studying a closely related but not identical concept to AI literacy. They developed the so-called MAIRS-MS questionnaire instrument specifically designed to assess the “AI readiness” of medical students. AI readiness can be interpreted as a link between attitudes towards AI and knowledge and skills for dealing with AI. Aboalshamat et al. [ 19 ] used the MAIRS-MS instrument and found that medical students in a Saudi Arabian sample rated their AI readiness rather poorly with an average score of 2.5 on a Likert scale of 1 (negative) to 5 (positive). Due to the influence of socio-cultural differences and the country-specific characteristics of the medical curricula on the data, these results can only be transferred to other countries to a limited extent.
While the assessment of medical students’ AI readiness is an important endeavor, only few studies are currently dealing with competence-focused AI literacy. Evaluating these competences, however, could provide a sufficient baseline to identify knowledge gaps and, if necessary, to revise the medical curricula by developing and implementing appropriate AI courses.
The importance of validated assessment instruments
A major disadvantage of the few available studies on the AI literacy of medical students is the attempt to assess AI literacy with self-developed and non-validated questionnaires. Thus, accuracy and reliability of their measures have not been established. In this study, we therefore used the “Scale for the assessment of non-experts’ AI literacy” (SNAIL), which was validated in several peer-reviewed studies. In a pilot study, the scale’s items were generated, refined, and subsequently evaluated for their relevance through a Delphi expert survey. As a result, a set of content-valid items covering the entire breadth of AI literacy was available to researchers and practitioners alike [ 20 ]. Subsequently, the itemset was presented to a large sample of non-experts who assessed their individual AI literacy. Based on this dataset, an exploratory factor analysis was conducted, which firstly identified the three subscales “Technical Understanding” (TU), “Critical Appraisal” (CA), and “Practical Application” (PA), and secondly excluded some redundant items [ 21 ]. In another study, it was demonstrated that the final SNAIL questionnaire is also suitable for assessing AI literacy among university students who have just completed an AI course [ 22 ].
Even though medical students’ ATAI has been assessed in multiple instances (as described above), very few studies have attempted to investigate the correlative (let alone causal) relationship between medical students’ AI literacy and ATAI. Furthermore, to our knowledge, the studies that have recorded both constructs did not use validated and standardized measurement instruments to investigate ATAI. In this study, the ATAI construct was therefore assessed using the “Attitudes towards Artificial Intelligence” scale [ 23 ], which has been validated in several languages. This scale was also developed in a systematic way, using principal component analysis and multiple samples. In addition, the reliability of the ATAI scale was evaluated and found to be acceptable. A major advantage of the scale is its efficiency, since the instrument comprises only 5 items that load on two factors (“fear” and “acceptance” of AI) in total.
Research objective
With this study we wanted to answer five research questions (RQs). RQ1 deals with medical students’ assessment of their individual AI literacy. In particular, we aimed to assess the AI literacy sub-constructs described above (TU, CA, PA), as the identification of literacy gaps is paramount for the development of appropriate medical education programs.
RQ1: How do medical students rate their individual AI literacy overall and for the factors “Technical Understanding”, “Critical Appraisal”, and “Practical Application”?
Regarding RQ2, we wanted to investigate the extent to which the assessment of one’s own AI literacy is associated with factors such as gender, age or semester. It is conceivable, for example, that older medical students would rate their AI skills lower than younger students, as younger students might consider themselves to be more technically adept. On the contrary, older medical students might generally consider themselves to be more competent across various competence areas, as they have already acquired extensive knowledge and skills during their academic training.
RQ2: Are there statistically significant differences in AI literacy self-assessment between (a) older and younger, (b) male or female and (c) less and more advanced students?
Furthermore, the medical students’ ATAI is covered by RQ3. It is important to know whether medical students have a positive or negative attitude towards AI, as this can have a decisive influence on the acceptance of teaching programs designed to foster AI literacy.
RQ3: How do medical students rate their individual attitudes towards AI?
RQ4 follows from the ideas presented in RQ3, as it is possible that the two constructs AI literacy and ATAI are related. In addition to efforts to increase AI literacy, interventions might be required to change attitudes towards AI.
RQ4: Are the two constructs AI literacy and attitudes towards AI and their respective sub-constructs significantly correlated?
The last RQ deals with previous education and interest in AI, since both aspects might increase AI literacy. We asked if the medical students had attended courses on AI in the past or if they had already educated themselves on the topic independently. In addition, interest in the subject area of AI was surveyed.
RQ5: Is there a correlative relationship between AI education or interest in AI and the AI literacy of medical students?
Questionnaires
We used the “Scale for the assessment of non-experts’ AI literacy” (SNAIL) by Laupichler et al. [ 20 ] to assess the AI literacy of medical students. The SNAIL instrument assesses AI literacy on three latent factors: Technical Understanding (14 items focusing on basic machine learning methods, the difference between narrow and strong AI, the interplay between computer sensors and AI, etc.), Critical Appraisal (10 items focusing on data privacy and data security, ethical issues, risks and weaknesses, etc.), and Practical Application (7 items focusing on AI in daily life, examples of technical applications supported by AI, etc.). Each item represents a statement on one specific AI literacy aspect (e.g., “I can give examples from my daily life (personal or professional) where I might be in contact with artificial intelligence.”), which is rated on a 7-point Likert scale from 1 (“strongly disagree”) to 7 (“strongly agree”). Furthermore, we integrated the “Attitudes towards Artificial Intelligence” scale (ATAI scale) by Sindermann et al. [ 23 ]. The ATAI scale assesses participants’ “acceptance” of AI with three items and the “fear” of AI with two items. Although an eleven-point Likert scale was used in the original study, we decided to use a 7-point scale (as in SNAIL) to ensure that the items were presented as uniformly as possible. Since the sample described here consisted of German medical students, the validated German questionnaire version was used for both SNAIL [ 22 ] and ATAI [ 23 ]. All SNAIL and ATAI items were presented in random order.
We included an attention control item (“mark box 3 here.”) and a bogus item for identifying nonsensical responses (“I consider myself among the top 10 AI researchers in the world.”), which were randomly presented. Additionally, we used 4-point Likert scales to gather information on whether the students had previously taken AI courses or had educated themselves about AI through other sources. The values ranged from 1 (“I have never attended a course on AI.” and “I haven’t used other ways to learn about AI yet.”) to 4 (“I have already attended AI courses with a workload of more than 120 hours.” and “I have informed myself very extensively about AI in other ways.”). In addition, we used a 7-point Likert scale to assess students’ interest in the field of AI, with lower values indicating less interest in AI. Finally, we inquired about the participants’ age, gender, and the total number of semesters they were enrolled in their study program.
The study was conducted at two German medical schools (MS1 and MS2) between October and December 2023 after receiving positive ethical approval from the local ethics committees (file number 151/23-EP at medical school 1 and 244/21 at medical school 2). Invitations to participate in the study were distributed via university-exclusive social media groups and online education platforms, mailing lists, and advertisements in lectures. Medical students who were at least 18 years old were eligible for the study and could access the online questionnaire after giving their informed consent to participate. The questionnaire was accessible via a QR code on their mobile device and participants received no financial incentive to take part in the study. The average time it took respondents to complete the questionnaire was 05:52 min (SD = 02:27 min).
Data analysis
The data were analyzed using RStudio (Posit Software, Version 2023). The visual presentation of the results was carried out using Microsoft Excel (Microsoft, Version 2016). Significance level was set at α = 0.05 for all statistical tests.
Independent two-sample t-tests were carried out to evaluate differences between groups (e.g., differences in AI literacy between MS1 and MS2). To check the requirements of t-tests, the data were examined for outliers, Shapiro-Wilk tests were carried out to check for normal distribution and Levene tests were run to check for variance homogeneity. In case of variance heterogeneity, Welch’s t-test was used. To check for differences considering age and semester distribution between MS1 and MS2, the Mann-Whitney-Wilcoxon-Test was used. Fisher’s test served to examine if there was a difference in the gender ratio.
Pearson’s correlation was calculated to determine the correlative relationship between continuous variables and Kendall’s τ coefficient was computed for ordinal variables. In addition, the factor structure of the two validated instruments (SNAIL and ATAI) was analyzed using a confirmatory factor analysis (CFA). We checked the prerequisites for conducting a confirmatory factor analysis, including univariate and multivariate skewness and kurtosis (using Mardia’s test for the multivariate analyses), the number and distribution of missing values, and whether the data differed significantly between the two medical schools, which would necessitate separate CFAs for each subsample. Due to the ordinal scaled variables and multivariate non-normality, we used polychoric correlation matrices to perform the CFA. We calculated the Comparative Fit Index (CFI), the Tucker-Lewis Index (TLI), the Root Mean Square Error of Approximation (RMSEA) and the Standardized Root Mean Square Residual (SRMR) as measures of model fit. As part of this analysis, the internal consistency, represented as the reliability measure Cronbach’s alpha, was also calculated for the overall scales as well as for the corresponding subscales.
Participant characteristics
Of 444 completed questionnaires, 28 (6%) participants had to be excluded since they omitted more than 3 (10%) of the SNAIL items. In addition, 8 (2%) participants were excluded because they indicated that they did not study medicine. Furthermore, 24 (5%) participants were excluded since they did not answer or answered incorrectly to the attention control item. Finally, 7 (2%) participants had to be excluded because they agreed, at least in part, to the bogus item (i.e., counting themselves among the “Top 10 AI researchers”). Accordingly, the final sample consisted of a total of 377 (85%) subjects, of which 142 (38% of the final sample) came from MS1 and 235 (62% of the final sample) from MS2.
The participants were on average 22.5 years old ( Mdn = 22, Min = 18, Max = 36, SD = 3.2) and on average in their 5th semester ( M = 4.7, Mdn = 5, Min = 1, Max = 13, SD = 2.6). Of the participants, 259 (69%) identified as female, 114 (30%) as male and one person as diverse. A Mann-Whitney-Wilcoxon test showed that the two medical schools differed significantly from each other in terms of the age of the participants, U = 13658.00, Z = -2.63, p <.01. The participants in MS1 were on average 0.9 years younger than the participants in MS2. There was no significant difference regarding participants’ semesters between the two medical schools, and according to a Fisher’s test, the gender distribution was similar.
Most participants stated that they had received little or no AI training. Of all participants, 342 (91%) stated that they had never attended an AI course. Only 28 (7%) had attended a course of up to 30 h and 6 (2%) people had attended a course of more than 30 h. In addition, a total of 338 (90%) of the participants stated that they never ( n = 177; 47%) or only irregularly ( n = 161; 43%) educated themselves on AI using other sources (such as videos, books, etc.). Only 32 (8%) respondents stated that they regularly educated themselves on AI with the help of other sources, and only 5 (1%) participants stated that they had already educated themselves in great detail on AI.
SNAIL and ATAI model fit
The univariate skewness and kurtosis values for the SNAIL were − 1.06 to 1.50 and − 1.08 to 1.73, which is in the acceptable range of -2.0 and + 2.0 for skewness and − 7.0 and + 7.0 for kurtosis, respectively [ 24 ]. The univariate skewness and kurtosis for the ATAI scale was also acceptable, with skewness values between − 0.45 and 0.56 and kurtosis values between − 0.68 and 0.77. Mardia’s test for multivariate skewness and kurtosis were both significant ( p <.001), which is why multivariate non-normality had to be assumed. Due to the non-normality and the fact that the values were ordinal (because of the 7-point Likert scale), we used a polychoric correlation matrix instead of the usual Pearson correlation matrix [ 25 ]. The polychoric correlation matrix is robust against a violation of the normal distribution assumption. Since participants with a high number of missing answers were excluded before analyzing the data (see Sect. 3.1), the final data set only had an average of 1.1 missing values per variable (0.3%), which is why no data imputation was necessary.
A t-test was performed for the SNAIL overall score, the TU, CA, and PA subscores, as well as the ATAI subscores (fear and acceptance) to check whether the data sets of the two medical schools differed significantly from each other. As the group size was much larger than n = 30, it could be assumed that the normal distribution assumption was not violated following the central limit theorem. A Levene test for variance homogeneity was performed for all SNAIL and ATAI scores. Since the Levene test was significant ( p <.05) for the TU factor of the SNAIL instrument and the fear factor of the ATAI instrument, Welch’s t-test was used. Welch’s t-test showed that the overall SNAIL score, t (277.15) = 2.32, p =.02, the TU subscore, t (260.14) = 2.60, p <.01, and the fear subscore, t (331.36) = -2.06, p =.04, differed statistically significantly between the two medical schools (see Fig. 1 ). It was therefore decided that a separate CFA had to be carried out for the data sets of the two medical schools.
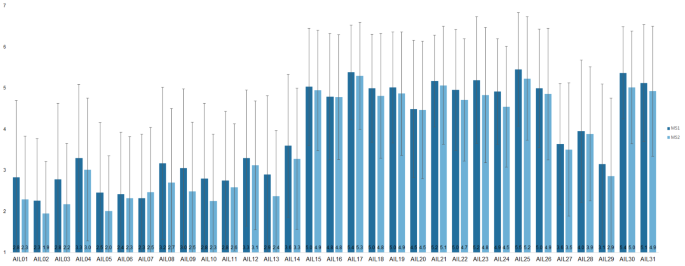
Mean score for each SNAIL item for both medical schools. Note Number of participants in MS1 = 142, number of participants in MS2 = 235, total N = 377
We found an equally acceptable to good model fit of the three factor model proposed by [ 20 ] for both medical schools. For MS1, the Comparative Fit Index (CFI) and Tucker-Lewis Index (TLI) were both 0.994, the Root Mean Square Error of Approximation (RMSEA) was 0.059 and the Standardized Root Mean Square Residual (SRMR) was 0.071. Accordingly, the three-factor solution fitted slightly better than a one-factor solution (i.e., a single latent factor “AI literacy”), as the latter had the following values: CFI = 0.988, TLI = 0.987, RMSEA = 0.084, SRMR = 0.083. The CFA of the MS2 data set led to comparable results. The 3-factor structure seemed to fit better with CFI = 0.994, TLI = 0.994, RMSEA = 0.059, SRMR = 0.071 than the 1-factor structure with CFI = 0.959, TLI = 0.956, RMSEA = 0.130, SRMR = 0.112. However, as expected, there is a high interfactor correlation of 0.81 between TU and CA, 0.90 between TU and PA and 0.93 between CA and PA.
Regarding ATAI, the two-factor solution proposed by Sindermann et al. [ 23 ] appears to have an excellent model fit. The following fit indices were found for MS1: CFI = 1.000, TLI = 1.012, RMSEA < 0.001, SRMR = 0.027. Excellent values were also found for MS2: CFI = 1.000, TLI = 1.016, RMSEA < 0.001, SRMR = 0.008. We found a negative interfactor correlation between “fear” and “acceptance” of − 0.83.
The internal consistency of the SNAIL subscales, expressed by the reliability measure Cronbach’s α, was good to excellent in both samples (MS1 and MS2). In the MS1 sample, the subscales had the following internal consistencies: TU, α = 0.94 [CI 0.93, 0.96]; CA, α = 0.89 [CI 0.86, 0.92], and PA, α = 0.83 [CI 0.78, 0.87]. In the MS2 sample, a Cronbach’s α of α = 0.93 [CI 0.91, 0.94] was found for the TU subscale, α = 0.89 [CI 0.87, 0.91] for the CA subscale, and α = 0.81 [CI 0.77, 0.85] for the PA subscale. However, the internal consistency of the ATAI subscales was rather low, with α = 0.53 [CI 0.35, 0.67] for the “acceptance” subscale and α = 0.61 [CI 0.48, 0.71] for the “fear” subscale in the MS1 sample and α = 0.60 [CI 0.48, 0.69] for the “acceptance” subscale and α = 0.64 [CI 0.56, 0.72] for the “fear” subscale in the MS2 sample.
Medical students’ AI literacy (RQ1)
To determine how medical students rated their overall AI literacy, the average score of each participant was calculated for each factor as well as for the overall SNAIL scale (see Table 1 ). The mean TU score was 2.26 points lower than the mean CA score, t (734.68) = -27.26, p <.001, and 1.77 points lower than the mean PA score, t (744) = -20.86, p <.001. The mean CA score was 0.49 points higher than the mean PA score, t (750.08) = 6.28, p <.001. Thus, the differences between the mean values of the subscales are all statistically significant. The results of the individual analyses of the two medical schools were very similar to the overall analysis (see Fig. 2 ), which is why they are not reported in more detail. In the further course of this paper, the results of the individual medical schools are only given if the values differ significantly between the schools.
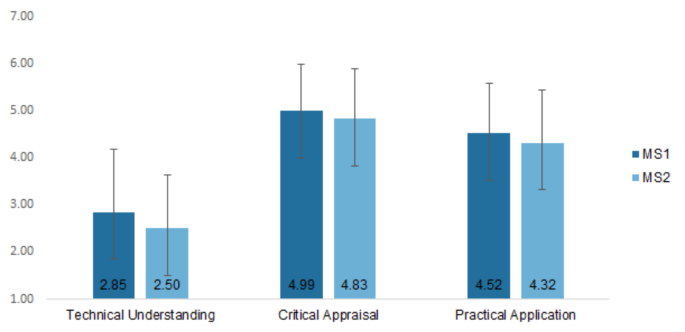
Mean score for each SNAIL factor for both medical schools. Note Number of participants in MS1 = 142, number of participants in MS2 = 235, total N = 377. MS = medical school
Differences in medical students’ AI literacy due to moderator variables (RQ2)
There was no statistically significant association between the age and the average SNAIL score of participants. This applies both to the overall sample, r =.07, p =.16, as well as to the MS1 and MS2 sample, r =.05, p =.59 and r =.12, p =.07, respectively. In the overall sample, women rated their AI literacy on average 0.413 points lower than men, t (217.96) = -3.65, p <.001. There were no differences within the separate samples of the two medical schools in this respect (i.e., in both medical schools, male participants rated themselves as more AI literate). The association between the general SNAIL score and medical students’ current semester was statistically significant for the overall sample, τ c = 0.08, p <.05. However, there was a notable difference between the two medical schools: In MS1, the association between SNAIL score and semester was not statistically significant, τ c = 0.04, p =.52, while it was significant in MS2, τ c = 0.13, p <.01.
Medical students’ attitudes towards artificial intelligence (RQ3)
The participants rated their “acceptance” of AI 0.83 points higher than their “fear” of AI, t (745.42) = 11.72, <.001. The calculations for the MS1 and MS2 subsets led to very similar results (see Table 2 ).
Relationship between medical students’ AI literacy and attitudes towards AI (RQ4)
The SNAIL total score and the TU, CA and PA factor scores were all significantly correlated (all correlations r =.64 to r =.92, p <.001; see Table 3 ). This result indicated that the 31 items of the SNAIL questionnaire measure a common main construct, namely AI literacy.
In addition, the “acceptance” subscale of the ATAI questionnaire was also significantly positively correlated with the subscales of the SNAIL questionnaire and with the total SNAIL score. The correlation between the ATAI subscale “fear” and the SNAIL scales, on the other hand, was lower and negative. “fear” correlated strongly negatively with the TU score and weakly (but still significantly) negatively with the SNAIL total score and the PA score. However, the correlation between “fear” and the CA score was not significant. Lastly, the “fear” factor of the ATAI scale correlated strongly negatively with the “acceptance” factor.
Effect of AI education and interest on medical students’ AI literacy (RQ5)
Medical students who had attended at least one shorter AI course of up to 30 h rated their AI literacy on average 1.47 points higher than medical students’ who stated that they had never attended an AI course, t (42.492) = 9.90, p <.001. The association between the two variables “Time spent attending AI courses” (ordinally scaled) and the SNAIL total score was significant, τ c = 0.31, p <.001. In addition, students who at least irregularly used other ways to educate themselves about AI rated their AI literacy on average 0.92 points higher than students who never did so, t (373) = 9.70, p <.001. As expected, the association between the two variables “Regularity with which students train themselves on AI” (ordinally scaled) and the SNAIL total score was significant, τ c = 0.43, p <.001. Finally, medical students’ interest in AI also appeared to be a good predictor of their AI literacy (although the causal direction of this association is not clear). Students who rated their interest in AI as rather high (5 to 7 on a 7-point Likert scale) rated their AI literacy on average 0.94 points higher than students who were less interested in AI (1 to 3 on a 7-point Likert scale), t (373) = 8.68, p <.001. The association between “Interest in AI” and the SNAIL total score was significant, τ c = 0.37, p <.001 (see Fig. 3 ).
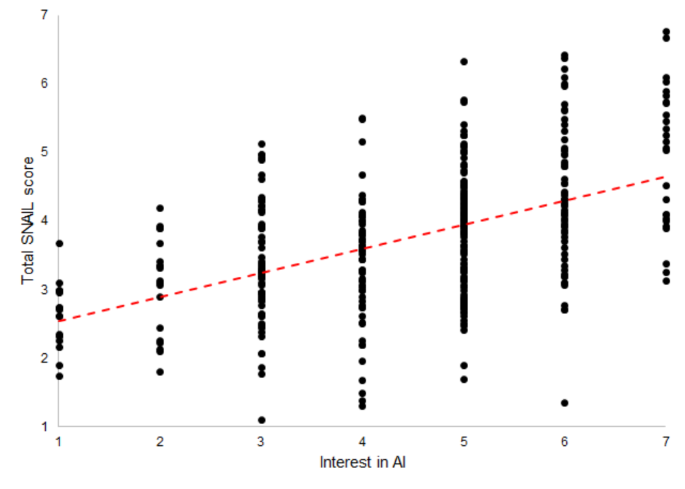
Scatterplot of Kendall’s rank correlation between the total SNAIL score and medical students’ interest in AI. Note The associations shown in the figure are based on the total sample ( N = 377)
In this study, we assessed AI literacy and attitudes towards AI among medical students at two German medical schools using validated assessment instruments. Remarkably, medical students rated their ability to critically appraise AI and to use AI in practice as relatively high, while they rated their technical understanding of AI as rather low. In addition, although both positive and negative attitudes towards AI were evident, positive attitudes (acceptance of AI) seemed to outweigh negative attitudes (fear of AI). While the correlation between medical students’ AI literacy and acceptance of AI was clearly positive, the link between AI literacy and negative attitudes appears to be more complex.
Interpretation and implications of the results
By using the CFA, we were able to show that the SNAIL questionnaire instrument was suitable for assessing the three latent AI literacy factors TU, CA and PA. This is evident from the good model fit of the three-factor model, but also by the excellent Cronbach’s α values for the three subscales. While the model fit was even better for the ATAI measuring instrument, Cronbach’s α of that scale was rather low, although this does not necessarily question the usefulness of the ATAI scale [ 26 ]. The low alpha values of the ATAI scale are somewhat unsurprising, considering that scales with a very small number of items also tend to have low internal consistency [ 27 ]. While the small number of items ensured good questionnaire efficiency, we could not conclusively clarify whether the five ATAI items were able to reliably assess medical students’ ATAI in our sample. Finally, we wonder whether the model fit of the ATAI model is not artificially increased, as the two subscales “acceptance” and “fear” measure practically opposite constructs. In future studies, it might therefore be advisable to recode one of the two subscales and conduct a CFA again to determine whether the two-factor structure still results in a good model fit.
RQ1 addressed the level of AI literacy and the AI literacy subconstructs TU, CA and PA of medical students. While the values of all three subscales differ statistically significantly from each other, the difference between TU and the other two factors is particularly interesting. Considering that the midpoint of a 7-point Likert scale is 4, it is surprising that the participants rated their CA and PA skills higher but their TU skills lower than the midpoint. This difference is particularly interesting because it could be assumed that a certain level of technical understanding is crucial for the practical use of AI applications. One possible explanation for the lower self-assessment score of the TU scale could be that aspects such as AI ethics, data security in connection with AI, or the recent AI hype are discussed in popular media, while technical aspects of AI, such as the function of machine learning or the difference between strong and weak AI are rather neglected.
While the age of the medical students did not appear to have any effect on their AI literacy, gender in particular had an important influence on the self-assessment of AI literacy. This is in line with a wealth of evidence suggesting that women rate themselves more negatively than men in self-assessments [ 28 ]. This effect appears to be even more pronounced for technical or scientific subjects, and negative self-assessment may even be associated with objectively lower performance [ 29 ]. Nevertheless, it is advisable to use objective AI literacy tests in addition to pure self-assessment scales in order to avoid response biases as far as possible. Furthermore, the semester also seemed to have had an influence on the self-assessment of participants’ AI literacy. The correlative relationship between the SNAIL overall score and the participants’ semester was particularly pronounced in MS2. However, a closer look reveals that in the MS2 sample, 120 participants (51% of the MS2 sample) were in semester 3 and 67 participants (29% of the MS2 sample) were in semester 7. Since 80% of the MS2 sample therefore stems from one of these two semesters, the association between semester and SNAIL score could be attributed to a sample effect.
The analyses conducted regarding RQ3 showed that medical students’ AI literacy is significantly positively correlated with their acceptance of AI, and significantly negatively correlated with their fear of AI. Thus, either AI literate medical students are more likely to accept (and less likely to fear) AI applications than AI illiterate students, or medical students who accept AI are more likely to be AI literate than students who do not accept AI. This finding complements the literature review published by Mousavi Baigi et al. [ 17 ], which found that 76% of studies reported positive attitudes towards AI among healthcare students. However, the scale midpoint of 4 should be emphasized again at this point. The medical students only “accept” AI with an average of 4.32 (MS1) and 4.12 (MS2) points and “fear” AI with 3.27 (MS1) and 3.49 (MS2) points. Although we found a statistically significant difference, it is obvious that both the negative and positive attitudes towards AI are relatively close to the midpoint. This may indicate that medical students have nuanced attitudes towards AI.
The investigation of the correlation between AI literacy and ATAI (RQ4) yielded interesting results. In the past, it has been shown for various constructs such as financial literacy [ 30 ] or scientific literacy [ 31 ] that there is a positive correlation between knowledge about a topic and positive attitudes towards it. A comparable effect was found in our study for the relationship between AI literacy and ATAI. Medical students who had a higher AI literacy were more likely to have a positive attitude towards AI (and vice versa). However, it should be mentioned again that the causality cannot be evaluated in this cross-sectional study. It is possible that medical students with a positive attitude are more willing to inform themselves about AI, resulting in a higher AI literacy. Nevertheless, it is also possible that students who are well versed in AI are better able to assess the real benefits and risks of AI, which leads to a more critical perception of exaggeratedly negative portrayals of AI.
The results regarding RQ5 indicate that courses and programs to increase AI literacy do indeed appear to have a positive effect on the AI literacy of medical students. This is an important finding as it illustrates that even relatively short AI courses (up to 30 h) are associated with higher AI literacy scores. This is particularly important in the very tightly scheduled medical curriculum, as medical AI education might be perceived as an additional burden by medical students and medical educators alike. Finally, our results indicate that the further development of curricula should arouse medical students’ interest in AI. As depicted in Fig. 3 , interest in AI seems to have a strong influence on the AI literacy of medical students.
Limitations
We have identified three main limitations: Firstly, this study was designed as a cross-sectional study which serves well to provide an initial picture of the AI literacy and ATAI of medical students. However, the correlative relationships presented here cannot provide any information about the causality of the effects. Secondly, the data was collected from two different medical schools in order to prevent sampling effects from influencing the validity of the results. Nevertheless, it is not possible to draw conclusions from the results of the two medical schools to all medical schools in Germany or even internationally, as various location factors can have an influence on AI literacy and ATAI, e.g. the current status of AI education in the medical curricula. Thirdly, all the instruments used were self-assessment questionnaires. It is conceivable that medical students’ self-assessment was subject to response biases that shifted the response behavior in one direction or the other. A bias that is particularly significant in this context is social desirability, which “refers to the tendency of research subjects to choose responses they believe are more socially desirable or acceptable rather than choosing responses that are reflective of their true thoughts or feelings” [ 32 ] (Grimm, 2010, p.1). Given that AI is a hyped topic due to recent developments such as the release of OpenAI’s ChatGPT, medical students may feel that they have at least somewhat engaged with the topic, which could potentially positively bias their response tendency. Another potential bias is the so-called acquiescence bias, which “describes the general tendency of a person to provide affirmative answers” [ 33 ]. This bias might be particularly problematic in the case of the SNAIL, as this scale has only “positive” items (i.e., higher self-assessment ratings equal higher AI literacy). However, at least the latter bias is mitigated by the fact that the SNAIL items are worded neutrally (i.e., not suggestively), which should mitigate the acquiescence tendency to some extent.
We also presented the SNAIL and ATAI items in random order and used a 7-point Likert scale for all items, as opposed to the 11-point Likert scale used by Sindermann et al. [ 23 ]. However, we believe that these adjustments to the original scales do not limit the ability of the scales to capture AI literacy and ATAI.
Future research directions
Future studies should firstly attempt to overcome the limitations of this study and secondly continue research on AI literacy and ATAI of medical students to contribute to their better acquisition of such crucial skills.
In order to determine the causal relationships between AI literacy and ATAI or other variables (such as interest in AI), experiments should be conducted that manipulate the ATAI of medical students while establishing a control group. Longitudinal studies or randomized controlled trials would also be suitable for investigating the direction of these effects. In addition, the study should be conducted at other locations and in other countries in order to verify the generalizability of the results considering different medical curricula. Objective testing of medical students’ AI literacy [ 34 ] would also be desirable for future research projects, as objective performance measurements using knowledge or skill tests are subject to significantly less response bias. Last but not least, the development of AI education programs for medical students should be further supported and their effectiveness measured using validated scales. In this way, courses could be continuously improved to ensure that all medical students have a chance to reach a certain level of AI literacy which is required given the technological advancements. The difference between voluntary elective courses on AI and AI education as part of medical schools’ compulsory curricula would also be an important research endeavor. We call for the implementation of AI education for all medical students and believe that in the future all medical students should have a certain level of AI literacy in order to continue to fulfill their various professional roles in an effective and safe manner. However, this theory should be empirically tested.
To our knowledge, we were the first to use validated questionnaire instruments to assess the AI literacy and ATAI of medical students. We found that medical students’ technical understanding of AI in particular was still relatively low compared to their confidence in critically evaluating and practically using AI applications. This study sheds crucial light on the AI literacy landscape among medical students, emphasizing the necessity for tailored programs. These initiatives should accentuate the technical facets of AI while accommodating students’ attitudes towards AI.

Data availability
The datasets used and analyzed during the current study are available from the corresponding author on reasonable request.
Abbreviations
Artificial Intelligence
- Attitudes towards AI
Critical Appraisal
Confirmatory Factor Analysis
Comparative Fit Index
Confidence Interval
Medical Artificial Intelligence Readiness Scale for Medical Students
Medical School
Practical Application
Quick-response
Root Mean Square Error of Approximation
Research Question
Scale for the assessment of non-experts’ AI literacy
Standardized Root Mean Square Residual
Tucker-Lewis Index
Technical Understanding
Schwartz WB, Patil RS, Szolovits P. Artificial Intelligence in Medicine. N Engl J Med. 1987;316(11):685–8. https://doi.org/10.1056/NEJM198703123161109 .
Article Google Scholar
Ramesh AN, Kambhampati C, Monson JRT, Drew PJ. Artificial intelligence in medicine. Ann R Coll Surg Engl. 2004;86(5):334–8. https://doi.org/10.1308/147870804290 .
Hamet P, Tremblay J. Artificial intelligence in medicine. Metab Clin Exp. 2017;69:36–40. https://doi.org/10.1016/j.metabol.2017.01.011 .
Haug CJ, Drazen JM. (2023). Artificial Intelligence and Machine Learning in Clinical Medicine, 2023. New England Journal of Medicine , 388 (13), 1201–1208. https://doi.org/10.1056/nejmra2302038 .
Chan KS, Zary N. Applications and Challenges of Implementing Artificial Intelligence in Medical Education: integrative review. JMIR Med Educ. 2019;5(1):e13930. https://doi.org/10.2196/13930 .
Mergen M, Junga A, Risse B, Valkov D, Graf N, Marschall B, medical.training.consortium. Immersive training of clinical decision making with AI driven virtual patients - a new VR platform called medical. GMS J Med Educ. 2023;40(2). https://doi.org/10.3205/zma001600 .
Lee J, Wu AS, Li D, Kulasegaram K, Mahan. Artificial Intelligence in Undergraduate Medical Education: a scoping review. Acad Med. 2021;96(11):62–70. https://doi.org/10.1097/ACM.0000000000004291 .
Laupichler MC, Hadizadeh DR, Wintergerst MWM, von der Emde L, Paech D, Dick EA, Raupach T. Effect of a flipped classroom course to foster medical students’ AI literacy with a focus on medical imaging: a single group pre-and post-test study. BMC Med Educ. 2022;22(1). https://doi.org/10.1186/s12909-022-03866-x .
Hu R, Fan KY, Pandey P, Hu Z, Yau O, Teng M, Wang P, Li T, Ashraf M, Singla R. Insights from teaching artificial intelligence to medical students in Canada. Commun Med. 2022;2(1). https://doi.org/10.1038/s43856-022-00125-4 .
Frommeyer TC, Fursmidt RM, Gilbert MM, Bett ES. (2022). The Desire of Medical Students to Integrate Artificial Intelligence Into Medical Education: An Opinion Article. Frontiers in Digital Health , 4 . https://doi.org/10.3389/fdgth.2022.831123 .
Sit C, Srinivasan R, Amlani A, Muthuswamy K, Azam A, Monzon L, Poon DS. Attitudes and perceptions of UK medical students towards artificial intelligence and radiology: a multicentre survey. Insights into Imaging. 2020;11(1). https://doi.org/10.1186/s13244-019-0830-7 .
Rampton V, Mittelman M, Goldhahn J. Implications of artificial intelligence for medical education. Lancet Digit Health. 2020;2(3):111–2. https://doi.org/10.1016/S2589-7500(20)30023-6 .
Long D, Magerko B. (2020). What is AI Literacy? Competencies and Design Considerations. Proceedings of the 2020 CHI Conference on Human Factors in Computing Systems , 1–16. https://doi.org/10.1145/3313831.3376727 .
dos Pinto D, Giese D, Brodehl S, Chon SH, Staab W, Kleinert R, Maintz D, Baeßler B. Medical students’ attitude towards artificial intelligence: a multicentre survey. Eur Radiol. 2019;29(4):1640–6. https://doi.org/10.1007/s00330-018-5601-1 .
Stewart J, Lu J, Gahungu N, Goudie A, Fegan PG, Bennamoun M, Sprivulis P, Dwivedi G. Western Australian medical students’ attitudes towards artificial intelligence in healthcare. PLoS ONE. 2023;18(8):e0290642. https://doi.org/10.1371/journal.pone.0290642 .
Kimmerle J, Timm J, Festl-Wietek T, Cress U, Herrmann-Werner A. Medical students’ attitudes toward AI in Medicine and their expectations for Medical Education. J Med Educ Curric Dev. 2023;10. https://doi.org/10.1177/23821205231219346 .
Mousavi Baigi SF, Sarbaz M, Ghaddaripouri K, Ghaddaripouri M, Mousavi AS, Kimiafar K. Attitudes, knowledge, and skills towards artificial intelligence among healthcare students: a systematic review. Health Sci Rep. 2023;6(3). https://doi.org/10.1002/hsr2.1138 .
Karaca O, Çalışkan SA, Demir K. Medical artificial intelligence readiness scale for medical students (MAIRS-MS)– development, validity and reliability study. BMC Med Educ. 2021;21(1). https://doi.org/10.1186/s12909-021-02546-6 .
Aboalshamat K, Alhuzali R, Alalyani A, Alsharif S, Qadhi H, Almatrafi R, Ammash D, Alotaibi S. Medical and Dental professionals readiness for Artificial Intelligence for Saudi Arabia Vision 2030. Int J Pharm Res Allied Sci. 2022;11(4):52–9. https://doi.org/10.51847/nu8y6y6q1m .
Laupichler MC, Aster A, Raupach T. (2023). Delphi study for the development and preliminary validation of an item set for the assessment of non-experts’ AI literacy. Computers and Education: Artificial Intelligence , 4 . https://doi.org/10.1016/j.caeai.2023.100126 .
Laupichler MC, Aster A, Haverkamp N, Raupach T. (2023). Development of the Scale for the assessment of non-experts’ AI literacy– An exploratory factor analysis. Computers in Human Behavior Reports , 12 . https://doi.org/10.1016/j.chbr.2023.100338 .
Laupichler MC, Aster A, Perschewski JO, Schleiss J. Evaluating AI courses: a Valid and Reliable Instrument for assessing Artificial-Intelligence Learning through Comparative Self-Assessment. Educ Sci. 2023;13(10). https://doi.org/10.3390/educsci13100978 .
Sindermann C, Sha P, Zhou M, Wernicke J, Schmitt HS, Li M, Sariyska R, Stavrou M, Becker B, Montag C. Assessing the attitude towards Artificial Intelligence: introduction of a short measure in German, Chinese, and English Language. KI - Kuenstliche Intelligenz. 2021;35(1):109–18. https://doi.org/10.1007/s13218-020-00689-0 .
Curran PJ, West SG, Finch JF. The robustness of test statistics to nonnormality and specification error in confirmatory factor analysis. Psychol Methods. 1996;1(1):16–29. https://doi.org/10.1037/1082-989X.1.1.16 .
Wang WC, Cunningham EG. Comparison of alternative estimation methods in confirmatory factor analyses of the General Health Questionnaire. Psychol Rep. 2005;97(1):3–10.
Taber KS. The Use of Cronbach’s alpha when developing and Reporting Research Instruments in Science Education. Res Sci Educ. 2018;48(6):1273–96. https://doi.org/10.1007/s11165-016-9602-2 .
Kopalle PK, Lehmann DR. Alpha inflation? The impact of eliminating scale items on Cronbach’s alpha. Organ Behav Hum Decis Process. 1997;70(3):189–97. https://doi.org/10.1006/obhd.1997.2702 .
Torres-Guijarro S, Bengoechea M. Gender differential in self-assessment: a fact neglected in higher education peer and self-assessment techniques. High Educ Res Dev. 2017;36(5):1072–84. https://doi.org/10.1080/07294360.2016.1264372 .
Igbo JN, Onu VC, Obiyo NO. Impact of gender stereotype on secondary school students’ self-concept and academic achievement. SAGE Open. 2015;5(1). https://doi.org/10.1177/2158244015573934 .
Dewi V, Febrian E, Effendi N, Anwar M. Financial literacy among the millennial generation: relationships between knowledge, skills, attitude, and behavior. Australasian Acc Bus Finance J. 2020;14(4):24–37. https://doi.org/10.14453/aabfj.v14i4.3 .
Evans G, Durant J. The relationship between knowledge and attitudes in the public understanding of science in Britain. Public Underst Sci. 1995;4(1):57–74. https://doi.org/10.1088/0963-6625/4/1/004 .
Grimm P. Social desirability bias. Wiley international encyclopedia of marketing; 2010.
Hinz A, Michalski D, Schwarz R, Herzberg PY. (2007). The acquiescence effect in responding to a questionnaire. Psychosocial Medicine, 4 . PMID: 19742288.
Hornberger M, Bewersdorff A, Nerdel C. What do university students know about Artificial Intelligence? Development and validation of an AI literacy test. Computers Education: Artif Intell. 2023. https://doi.org/10.1016/j.caeai.2023.100165 . 5 .
Download references
Acknowledgements
The authors express their gratitude to everyone who contributed to the execution of the research project, with special appreciation for the medical educators who encouraged participation in our study.
M.C.L., A.A. and T.R. received no financial funding to conduct this study. M.Mey. and M.Mer. were funded by the German Federal Ministry of Education and Research (research grant: 16DHBKI080).
Open Access funding enabled and organized by Projekt DEAL.
Author information
Authors and affiliations.
Institute of Medical Education, University Hospital Bonn, Venusberg Campus 1, 53127, Bonn, Germany
Matthias Carl Laupichler, Alexandra Aster & Tobias Raupach
Department of Pediatric Oncology and Hematology, Faculty of Medicine, Saarland University, Homburg, Germany
Marcel Meyerheim & Marvin Mergen
You can also search for this author in PubMed Google Scholar
Contributions
M.C.L. analyzed the data and wrote the first draft of the manuscript. A.A., M.Mey. and M.Mer. co-wrote the manuscript. M.Mer. was significantly involved in the planning, organization and execution of the study. A.A. and M.Mey. assisted with the data analysis. T.R. provided feedback on the manuscript’s content and assisted with the linguistic refinement of the manuscript. All authors read and approved the final manuscript.
Corresponding author
Correspondence to Matthias Carl Laupichler .
Ethics declarations
Ethics approval and consent to participate.
The study was approved by the Research Ethics Committee of the University of Bonn (Reference 194/22) and of Saarland University (Reference 244/21). Medical students who were at least 18 years old were eligible for the study and could access the online questionnaire after giving their informed consent to participate.
Consent for publication
Not applicable.
Competing interests
The authors declare that they have no competing interests.
Additional information
Publisher’s note.
Springer Nature remains neutral with regard to jurisdictional claims in published maps and institutional affiliations.
Rights and permissions
Open Access This article is licensed under a Creative Commons Attribution 4.0 International License, which permits use, sharing, adaptation, distribution and reproduction in any medium or format, as long as you give appropriate credit to the original author(s) and the source, provide a link to the Creative Commons licence, and indicate if changes were made. The images or other third party material in this article are included in the article’s Creative Commons licence, unless indicated otherwise in a credit line to the material. If material is not included in the article’s Creative Commons licence and your intended use is not permitted by statutory regulation or exceeds the permitted use, you will need to obtain permission directly from the copyright holder. To view a copy of this licence, visit http://creativecommons.org/licenses/by/4.0/ . The Creative Commons Public Domain Dedication waiver ( http://creativecommons.org/publicdomain/zero/1.0/ ) applies to the data made available in this article, unless otherwise stated in a credit line to the data.
Reprints and permissions
About this article
Cite this article.
Laupichler, M.C., Aster, A., Meyerheim, M. et al. Medical students’ AI literacy and attitudes towards AI: a cross-sectional two-center study using pre-validated assessment instruments. BMC Med Educ 24 , 401 (2024). https://doi.org/10.1186/s12909-024-05400-7
Download citation
Received : 15 January 2024
Accepted : 08 April 2024
Published : 10 April 2024
DOI : https://doi.org/10.1186/s12909-024-05400-7
Share this article
Anyone you share the following link with will be able to read this content:
Sorry, a shareable link is not currently available for this article.
Provided by the Springer Nature SharedIt content-sharing initiative
- Artificial intelligence
- AI literacy
- Confirmatory factor analysis
- Medical students
- Questionnaire
BMC Medical Education
ISSN: 1472-6920
- Submission enquiries: [email protected]
- General enquiries: [email protected]
- Open access
- Published: 08 April 2024
Development and implementation of a worksite-based intervention to improve mothers’ knowledge, attitudes, and skills in sharing information with their adolescent daughters on preventing sexual violence: lessons learned in a developing setting, Sri Lanka
- Dilini Mataraarachchi 1 ,
- P.K. Buddhika Mahesh 2 ,
- T.E.A. Pathirana 3 &
- P.V.S.C. Vithana 1
BMC Public Health volume 24 , Article number: 983 ( 2024 ) Cite this article
21 Accesses
Metrics details
Sexual violence among adolescents has become a major public health concern in Sri Lanka. Lack of sexual awareness is a major reason for adverse sexual health outcomes among adolescents in Sri Lanka. This study was intended to explore the effectiveness of a worksite-based parent-targeted intervention to improve mothers’ knowledge, and attitudes on preventing sexual violence among their adolescent female offspring and to improve mother-daughter communication of sexual violence prevention with the family.
“My mother is my best friend” is an intervention designed based on previous research and behavioral theories, to help parents to improve their sexual communication skills with their adolescent daughters. A quasi-experimental study was conducted from August 2020 to March 2023 in randomly selected two Medical Officer of Health (MOH)areas in Kalutara district, Sri Lanka. Pre and post-assessments were conducted among a sample of 135 mothers of adolescent girls aged 14–19 years in both intervention and control areas.
Out of the 135 mothers who participated in the baseline survey, 127 mothers (94.1%) from the intervention area (IA) physically participated in at least one session of the intervention. The worksite-based intervention was effective in improving mothers’ knowledge about adolescent sexual abuse prevention (Difference in percentage difference of pre and post intervention scores in IA and CA = 4.3%, p = 0.004), mother’s attitudes in communicating sexual abuse prevention with adolescent girls (Difference in percentage difference of pre and post intervention scores in IA and CA = 5.9%, p = 0.005), and the content of mother-daughter sexual communication (Difference in percentage difference of pre and post intervention scores in IA and CA = 27.1%, p < 0.001).
Conclusions and recommendations
Worksite-based parenting program was effective in improving mothers’ knowledge about sexual abuse prevention among adolescent daughters and in improving the content of mother-daughter communication about sexual abuse prevention. Developing appropriate sexual health programs for mothers of different ethnicities, and cultures using different settings is important. Conduction of need assessment programs to identify the different needs of mothers is recommended.
Peer Review reports
Sexual violence and coercion involving children are seen in every part of society occurring in homes, workplaces, communities, and public spaces. World Health Organization defines sexual violence as “any unwanted sexual act, comment or advance encompassing various forms of abuse and exploitation” [ 1 ].
The UNICEF reports depict that worldwide, at least one in ten girls under the age of twenty are being forced into some form of sexual activity [ 2 ]. However, cases of sexual abuse and violence among children and adolescents in Sri Lanka are often under-reported due to stigma and ignorance [ 3 ]. A study reported that 14% of both male and female students in Sri Lanka suffered some form of sexual abuse [ 4 ]. A significant 23% of sexually active school adolescents experienced non-consensual sexual intercourse [ 5 ].
The literature shows that lack of awareness and poor life skills are the root causes of the higher incidence of sexual abuse among adolescents [ 6 ]. Despite more than three decades of school sexual health education, Sri Lankan adolescents lack adequate sexual health knowledge and receive conflicting messages [ 7 ].
Global research advocates family-based sexuality education, as this will create an open and comfortable environment for discussion about sexuality, relationships, and other related topics [ 8 , 9 ]. Family-based sex education enables children to have early access to quality information on sexual matters, develop healthy attitudes towards sex and relationships, reduce risky behaviors, and make informed decisions about their sexual health.
In a family, the role of the mother is more pronounced and can have an impact on the health of other members [ 10 ]. Literature indicates that well-informed and prepared mothers are one of the best sources of sexual health information for adolescent girls [ 11 ].
In Sri Lanka, the stigma around the topic, coupled with various religious and mythological beliefs, discourages the implementation of school or community-based sex education for children. Nevertheless, the ability to tailor sexual health messages to suit one’s family values, religious beliefs highlights family-based sex education as a suitable approach for South Asian settings like Sri Lanka [ 12 ]. However, parental discomfort and lack of awareness of adolescent sexual health and lack of skills on sexual health communication, pose significant challenges hindering the opportunity to family based sexual education in the country [ 12 ].
It was discovered that 67% of Sri Lankan parents lack knowledge about child sexual abuse [ 13 ].The objective of this study was to develop a comprehensive intervention for mothers of adolescent girls, to enhance their understanding and enable them to convey crucial information to their daughters about preventing child sexual. The study aimed to evaluate the potential for family-based sex education in the study context. This is the first ever study to explore the potential for family-based sex education in this study context.
Setting for the intervention
Intervention was carried out in seven randomly selected government work-sites in IA and CA in Kalutara district. A spacious room or the auditorium in the work place was chosen as the setting for the intervention.
Study period
The intervention was conducted in August 2020 while the baseline assessment was carried out one week prior to the intervention. Post-interventional assessment was carried out in March 2021.
Target audience
Target audience for the intervention was mothers of adolescent girls aged 14–19 years.
Planning of the intervention
The intervention development process involved technical experts, policymakers, practitioners, and representatives from the target population [ 14 ]. The intervention was based on the views and concerns of its users. Results of a descriptive-cross sectional study that was conducted among the adolescents in the study setting [ 15 ] and findings of a qualitative study carried out among mothers of adolescent girls to explore their views on providing sexual health information to their children [ 12 ] were taken into account during the intervention development.
The intervention in the present study was inspired by the information-motivation and behavioral skills model. Further, previous literature on similar parenting interventions to improve mothers’ knowledge, attitudes, and communication skills on adolescent sexual health matters was looked into.
Piloting the intervention
The intervention was piloted at a government work site in Colombo, involving fifteen eligible mothers. Two public health specialists and an adolescent psychiatrist supervised the sessions. Practical issues regarding the timing of each session and logistics were identified. Participant feedback was collected to pinpoint areas needing improvement. The intervention content, lecture timing, and interactive sessions were adjusted based on participant suggestions. Handbooks and materials were distributed, with follow-ups conducted after two weeks to assess participant’s engagement. Mothers were inquired about the material readability and clarity. Post-pilot experts discussed identified issues and made necessary corrections.
Intervention implementation
“My mother is my best friend” was carried out as two sessions one week apart, followed by a one-hour follow-up session six weeks apart. Trainer guide and all materials to be used during the session and material to be distributed among mothers following the session were developed with the expert opinion, while content and consensus generation among the experts on the content of the program was done using the Modified Delphi technique using electronic mail service.
The take-home materials were left at the workplace for the mothers who could not participate in the second session of the intervention. An online training program was carried out for the mothers who missed the sessions intervention. The online program was conducted in two separate sessions and the mothers who missed at least one session of the intervention were invited to participate.
Program content
The intervention for the mothers included;
Activity I - A short lecture presentation on physiological changes during adolescence.
Activity II - A short lecture presentation on parent guide to adolescent sexual violence prevention.
Activity III - A lecture presentation on how to communicate with your teen about protecting herself from sexual violence.
Activity IV - Two video presentations that showed how to catch up with teachable moments and initiate a sexual conversation with an adolescent girl, and the techniques that can be used when responding to difficult questions forwarded during such discussion.
Activity V - A handbook on preventing sexual violence among adolescent girls, to refer to after the program.
Activity VI - Case scenarios for role play.
All participant mothers were allocated into three small groups. Each group was provided with a case scenario. Each group was asked to narrate a brief role-play between a mother and a daughter about the given case scenario. Feedback from the audience was obtained after each role-play.
Activity VII - Table topics–
The table topics consisted of some questions, including general questions and a few sex and relationship-related questions. Mothers were expected to discuss each of the topics randomly with the children as a family game.
Activity VIII - Checklist–
A checklist was developed and given to all mothers for the self-assessment of the level of communication with adolescent daughters on preventing sexual violence. The checklist was assessed by the PI during the follow-up session, six weeks following the intervention.
Implementation fidelity of the intervention
All programs were facilitated by the principal investigator and one other medical officer, who had special training in adolescent health and experience in working with adolescents and their parents. The facilitators adhered to the trainer’s guide developed with expert opinion, to preserve the uniformity of the program when conducting each session.
Monitoring of the intervention
Three months following the implementation of the intervention, the PI carried out an online follow-up session with the mother participants where they were inquired about the progress of the communication with daughters and for any problems they had encountered while practicing communication at home. Those who were not available at the online follow-up session were followed up over the phone.
Control area - Intervention was not implemented in the control area until the post-interventional assessment was done. Following the post-interventional survey, one hour lecture on sexual violence prevention among adolescent girls was carried out with the distribution of IEC material.
Evaluation of the effectiveness of the intervention
Study design.
Quasi experimental study design was used.
Sample size calculation
Pre and post-interventional evaluation of the intervention was carried out among a sample of 135 mothers working in selected government worksites in both IA and CA. Pocock’s formula, a standard formula for sample size calculation of intervention studies was used to determine the sample size [ 16 ].
Inclusion criteria
Eligible participant were Sinhala mothers having an adolescent daughter aged 14–19 years, and is living with the daughter in the same household for at least two days per week.
Exclusion criteria
The following groups were excluded from the pre and post intervenion surveys, although they were allowed to participate in the intervention.
Mothers diagosed with any severe mental disability at the time of the intervention would prevent them from effectively engaging with the daughter.
Mothers with adolescent daughters who had cognitive or communication disabilities that would prevent her from effectively engaging with her mother.
Sampling procedure
Out of the 13 MOH areas in Kalutara district, two MOH areas that share similar socio-demographic characteristics were selected as IA and CA. A list of government working places with more than fifty female employees in both IA and CA were taken from the relevant Divisional Secretariat office. From each list 9 government working places were randomly selected. From each worksite fifteen female employees meeting the eligibility criteria were included in to the study.
Data collection
Data collectors visited the worksite one week prior to the intervention and six months after the implementation of the worksite intervention. Data was collected using an interviewer administered questionnaire. Trained interviewers made pre and post interventional visits to the worksites to collect data from study participants.
Data Analysis
All data were coded and entered into a database using a standard statistical package (SPSS 25). Data cleaning and checking were done by the PI. Socio-demographic information of the participants was presented in numbers and percentages.
The evaluation of the effectiveness of the intervention was carried out in 3 stages;
Comparison of pre-interventional scores between intervention and control areas.
Comparison of post-interventional scores between intervention and control areas.
Comparison of pre and post interventional scores within intervention and control area.
The total percentage scores were calculated for each subscale. Since the total percentage scores were not normally distributed (Shapiro-Wilk test p < 0.05, Kolmogov-Smirnov test < 0.05), the analysis was carried-out using non-parametric tests. Between area comparison of knowledge, attitudes and communication practices of the mothers was carried out using Mann- Whitney U test, while Pre and post-test comparison within IA and CA area was done using Wilcoxon Signed Rank test.
The effect sizes were computed to determine the strength of the association. Since the outcomes were not normally distributed, the effect sizes were calculated using non-parametric effect size estimator, Cohen’s r.
Interpretation of effect size.
Effect size < 0.1 = no effect
Effect size 0.1–0.3 = Small effect
Effect size 0.3 = 0.5 = Medium effect
Effect size > 0.5 = Large effect
The percentage difference in pre and post intervention scores in IA and CA was calculated. Percentage Increase = [(Post-Intervention Score - Pre-Intervention Score) / Pre-Intervention Score] * 100
The response rate for the pre-intervention was 100% for the mothers in both IA and CA. Out of the mothers who participated in the baseline survey 127 mothers (94.1%) from the intervention area (IA), physically participated in at least one session of the intervention. Five out of eight participants who missed both sessions participated in the online session. Participants who did not participate in the intervention ( n = 3) were considered lost-to-follow-ups (Fig. 1 ).
For the post-interventional assessment, 122 mothers from the IA, and 125 mothers from the Control area (CA) participated giving a final response rate of 90.3% (122/135) and 92.6% (125/135) respectively.
The reasons for loss-to-follow-up among IA mothers were later change of mind ( n = 6),
the husband was against participating in the study ( n = 4), and change of workplaces during the six months follow-up period ( n = 3). Among the CA mothers, five changed their minds later in the course, while four mothers said their husbands were against the idea. One mother had shifted workplaces due to a change of residence.
Out of all 135 mothers recruited to the study in IA and CA, who participated in the baseline assessment, the number of mothers who responded to the six months post-interventional survey was 122 and 125 in IA and CA, respectively. Therefore, the loss to follow-up rate calculated was 9.6% ( n = 13) for the mothers in IA and 7.4% ( n = 10) for the mothers in CA (Fig. 1 ).
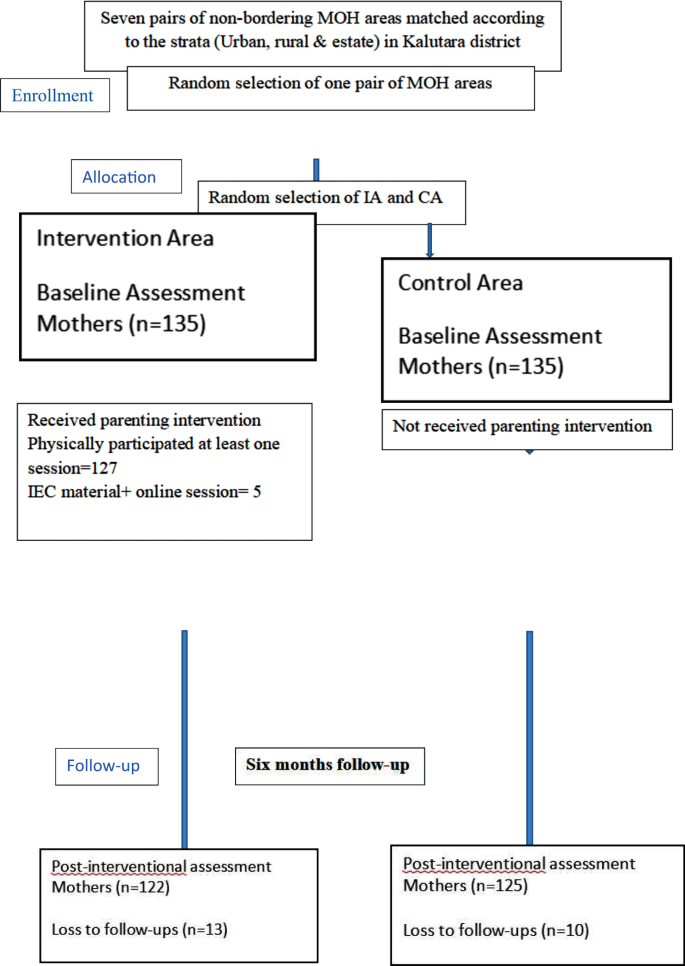
CONSORT diagram graphically describes the participant’s disposition throughout the study
At 95% confidence level, there was no significant difference between the participants who completed the study and the drop-outs, in their age, educational status, civil status, or the age of the adolescent daughter, which suggested random dropouts (Table 1 ).
Preliminary effects of the intervention
There was no significant difference between the IA and CA mothers in their knowledge, attitudes about adolescent sexual violence prevention or in the content and frequency of communication with their daughters on sexual violence prevention (Table 2 ).
According to the results mothers’ post-intervention knowledge, content, and frequency of communication with their daughters on sexual violence prevention was significantly higher compared to the baseline in the IA. In the IA the strength of association for mother’s knowledge improvement (0.3) and the content of communication (0.47) was moderate, while it was high for the frequency of communication. However, no such improvement could be seen in the control area. (Table 3 ).
The percentage increase in scores for mother’s knowledge, attitudes and communication on adolescent sexual abuse prevention pre and post intervention in IA and CA is shown in Table 4 .
Comparison of post-interventional scores between IA and CA indicated that there is a significant difference in the mother’s knowledge about preventing sexual violence in adolescent girls and in the content of mother-daughter communication on sexual violence prevention. However, no significant difference was observed in mother’s attitudes or in the frequency of communication (Table 5 ).
The intervention in this study was inspired by the information-motivation and behavioral skills model, a framework that has consistently demonstrated empirical support in the sexual and reproductive health risk reduction in various key populations including adolescents and youth [ 17 , 18 ]. The program structure was adapted from ‘Talking parents-healthy teens’, a successful worksite-based parenting program conducted in the US, which significantly increased parent-teen sexual health [ 19 ].
The intervention primarily focused on preventing sexual violence among adolescent girls. This emphasis stemmed from the previous research conducted among mothers of adolescent girls in the study setting, which revealed that mothers were more concerned about protecting their daughters from sexual violence [ 12 , 20 ]. To enhance engagement and effectiveness, the intervention incorporated interactive sessions such as role-plays, video presentations, and take-home activities as suggested by previous literature [ 21 , 22 , 23 ]. Additionally, interactive games were incorporated to enhance easy communication between mothers and adolescents about SRH matters [ 23 ].
The study recognized the efficacy of utilizing worksite settings as platforms to implement health programs for working mothers. The support received from the worksite administration in conducting the program affirmed the potential to expand health promoting initiatives at worksites, moving beyond routine health assessments. Unlike challenges reported in previous literature concerning recruiting and retaining parents in parenting interventions [ 24 ], the work setting in this study facilitated the parent participation without additional effort. The enthusiasm of the worksite management to implement the program was partly driven by the growing number of sexual violence cases in the study setting and their interest in employee-assistance programs as work-life balance initiatives. Similar health-promoting interventions such as weight reduction programs or smoking cessation programs had been proven effective when conducted in worksite setting [ 25 ]. Moreover, evidence suggests that interventions aimed at facilitating parent-child sexual communication and reducing sexual risk behaviors among adolescents, conducted at parent’s workplaces, can be effective [ 26 ]. For instance, Talking Parents-Healthy Teens, a parenting intervention conducted at thirteen worksites in Southern California led to significant positive outcomes [ 27 ].
The study revealed a significant improvement in mother’s knowledge about preventing adolescent sexual abuse and in the content and frequency of sexual communication with their daughters among IA mothers six months following the intervention compared to baseline. However, when comparing areas, the intervention proved more effective in improving mother’s knowledge about preventing adolescent sexual abuse and the content of mother-daughter sexual communication among IA mothers compared to CA but no significant difference was observed in the frequency of communication. This disparity could be attributed to the fact that CA mothers reported higher frequency of sexual health communication with their daughters even at the baseline compared to the IA, which can be due to some unknown external factors. The finding aligns with a US study, “Talking parent-healthy teens”, where intervention area mothers reported more discussion of new topics ( p < 0.001) and more frequency of conversations about sex compared to the control group [ 27 ].
Although there was no significant difference between the pre and post interventional scores for mother’s attitudinal change in IA, the percentage score increase in IA was significantly higher compared to the CA. A review of studies that have been carried out between 1980 and 2010 to evaluate the effectiveness of parenting interventions to improve sex communication indicated that very few interventions successfully influenced mothers’ attitudes [ 28 ]. Adopting different socio-psychological approaches would be essential to unfreeze mothers’ attitudes, which is crucial for ensuing the long-term sustainability of the intervention’s impact.
Public Health implications of the study findings
According to the present study findings, parent-targeted interventions are an effective way of delivering sexual and reproductive health information to adolescents in Sri Lanka. The present intervention could be adopted with necessary modifications to the existing public health system to reduce sexual abuse among adolescent girls in the country.
Strengths and limitations
The parenting intervention was developed considering the views of both the mothers and daughters in the same study setting, giving a more comprehensive approach to the study problem. The quasi-experimental study design enabled identifying the actual effect of the parenting intervention on the mothers and adolescent girls. The use of government worksites as the setting for the study, was an advantage since as it improved parent participation, reducing attrition bias. Carrying out face-to-face intervention was a challenge due to the COVID-19 pandemic situation in the country. Hence, we were unable to gain the full effect of a face-to-face intervention and follow-up sessions.
Furthermore, the pandemic resulted in both the mothers and daughters to work from home and spend more time together than usual. This may limit the generalization of the findings to the population during the COVID-19 free times.
The study highlights the effectiveness of mother targeted interventions in enhancing mothers’ knowledge and communication with their adolescent daughters regarding sexual health. Mothers’ enthusiasm to learn about adolescent health, indicated the necessity for future parent-targeted programs. The success of worksite setting in engaging mothers suggests its effectiveness for parenting programs.
The study calls for policymakers to recognize parents as a primary source of sexual health information for adolescent girls. It recommends implementing parent awareness and skill-building sessions alongside the existing school-based sexual health education programs. Additionally, education sector is encouraged to conduct such sessions in parallel to the current school sexuality education, promoting mothers’ role as early sexual health educators for their children even before adolescence.
Future research
Future research should assess the effectiveness of a parenting intervention in improving mother-daughter sexual communication among unemployed mothers, considering potential difference between employed and unemployed groups. Additionally, it is crucial to include women who are employed in the private sector in these studies.
Furthermore, there is a need to develop interventions targeting fathers. The present intervention mainly focused on communicating sexual violence prevention with adolescents. Future interventions should encourage parent-adolescent communication on various sexual health topics.
The present intervention was implemented solely at large government worksites. Research should be conducted to evaluate the intervention’s effectiveness in small workplaces and private sector work settings.
Data availability
Data presented in this study are available with the corresponding author and can be produced on request.
Jewkes R, Dartnall E. Sexual violence. Int Encycl Public Heal. 2016;491–8.
UNICEF. Sexual violence against children [Internet]. 2021. Available from: https://www.unicef.org/protection/sexual-violence-against-children .
Immigration and Refugee board C. Responses to information requests (RIRs). Sri Lanka Sex Domest violence, Incl Legis state Prot Serv available Vict. 2012;1–8.
Perera B. Prevalence and correlates of sexual abuse reported by late adolescent school children in Sri Lanka. 2009;21(2):203–11.
Rajapaksa-hewageegana N, Piercy H, Salway S, Samarage S. Sexual & reproductive healthcare sexual and reproductive knowledge, attitudes and behaviours in a school going population of Sri Lankan adolescents. Sex Reprod Healthc [Internet]. 2015;6(1):3–8. https://doi.org/10.1016/j.srhc.2014.08.001 .
Udigwe I, Ofiaeli O, Ebenebe J, Nri-Ezedi C, Ofora V, Nwaneli E. Sexual abuse among adolescents. Ann Heal Res. 2021;7:50–8.
Article Google Scholar
Hettiarachchi D. The place of sexuality education in preventing child pregnancies in Sri Lanka. Sri Lanka J Child Heal. 2022;51(1):4–7.
Downing J, Jones L, Bates G, Sumnall H, Bellis MA. A systematic review of parent and family-based intervention effectiveness on sexual outcomes in young people. Health Educ Res [Internet]. 2011;26(5):808–33. https://doi.org/10.1093/her/cyr019 .
Romo LF, Bravo M, Tschann JM. The effectiveness of a joint mother-daughter sexual health program for Latina early adolescents. J Appl Dev Psychol [Internet]. 2014;35(1):1–9. https://doi.org/10.1016/j.appdev.2013.10.001 .
Grusec JE. Socialization processes in the family: social and emotional development. Annu Rev Psychol. 2011;62:243–69.
Article PubMed Google Scholar
Shams M, Parhizkar S, Mousavizadeh A, Majdpour M. Mothers’ views about sexual health education for their adolescent daughters: a qualitative study. Reprod Health. 2017;14(1).
Mataraarachchi D, Buddhika Mahesh PK, Pathirana TEA, Ariyadasa G, Wijemanne C, Gunatilake I et al. Mother’s perceptions and concerns over sharing sexual and reproductive health information with their adolescent daughters- A qualitative study among mothers of adolescent girls aged 14–19 years in the developing world, Sri Lanka. BMC Womens Health [Internet]. 2023;23(1):223. https://doi.org/10.1186/s12905-023-02369-1 .
Rohanachandra YM, Amarakoon L, Alles PS, Amarasekera AU, Mapatunage CN. Parental knowledge and attitudes about child sexual abuse and their practices of sex education in a Sri Lankan setting. Asian J Psychiatr [Internet]. 2023;85:103623. Available from: https://www.sciencedirect.com/science/article/pii/S1876201823001788 .
Bessant J, Maher L. Developing radical service innovations in healthcare—the role of design methods. Int J Innov Manag. 2009;13(04):555–68.
Mataraarachchi D, Buddhika APTE, Vithana PKM. Mother-daughter communication of sexual and reproductive health (SRH) matters and associated factors among sinhalese adolescent girls aged 14–19 years, in Sri Lanka. BMC Womens Health. 2023;23(1):1–10.
Google Scholar
Pocock SJ. The Size of a Clinical Trial [Internet]. Clinical Trials. 2013. p. 123–41. (Wiley Online Books). https://doi.org/10.1002/9781118793916.ch9 .
Robinson WT. Adaptation of the information-motivation-behavioral skills model to needle sharing behaviors and hepatitis C risk: a structural equation model. SAGE Open. 2017;7(1).
John SA, Walsh JL, Weinhardt LS. The information–motivation–behavioral skills model revisited: A network-perspective structural equation model within a public sexually transmitted infection clinic sample of hazardous alcohol users. AIDS Behav. 2017;21(4):1208–18.
Eastman KL, Corona R, Schuster MA. Talking parents, healthy teens: a worksite-based program for parents to promote adolescent sexual health. Prevening Chronic Dis Public Heal Res Pract Policy. 2006;3(4).
Godamunne PKS. Sri Lankan parents’ attitudes towards adolescent reproductive and sexual health education needs: A qualitative study. 2008.
Whalen CK, Henker B, Hollingshead J, Burgess S. Parent–adolescent dialogues about AIDS. J Fam Psychol. 1996;10(3):343–57.
Coombs RH, Santana FO, Fawzy FI. Parent training to prevent adolescent drug use: an educational model. J Drug Issues [Internet]. 1984;14(2):393–402. https://doi.org/10.1177/002204268401400214 .
Kirby D, Miller BC. Interventions designed to promote parent-teen communication about sexuality. 2002;(97):93–110.
Mytton J, Ingram J, Manns S, Thomas J, Manns S, Hons L et al. Facilitators and barriers to engagement in parenting progrmas. Health Educ Behav. 2014;(May 2013).
Winick C, Rothacker DQ, Norman RL. Four worksite weight loss programs with high-stress occupations using a meal replacement product. Occup Med (Lond). 2002;52(1):25–30.
Article CAS PubMed Google Scholar
Bogart LM, Skinner D, Thurston IB, Toefy Y, Klein DJ, Hu CH, et al. Let’s talk! A South African worksite-based HIV prevention parenting program. J Adolesc Health. 2013;53(5):602–8.
Article PubMed PubMed Central Google Scholar
Schuster MA, Corona R, Elliott MN, Kanouse DE, Eastman KL, Zhou AJ, et al. Evaluation of talking parents, Healthy Teens, a new worksite based parenting programme to promote parent-adolescent communication about sexual health: Randomised controlled trial. BMJ. 2008;337(7664):273–7.
Akers AY, Holland CL, Bost J. Interventions to improve parental communication about sex: a systematic review. Pediatrics. 2011;127(3):494–510.
Download references
Acknowledgements
We acknowledge the support given by the administration of the worksite settings in which the interventions were taking place.
This research was not funded by any authorities.
Author information
Authors and affiliations.
Family Health Bureau, Colombo, Sri Lanka
Dilini Mataraarachchi & P.V.S.C. Vithana
Provincial Director of Health Services, Colombo, Sri Lanka
P.K. Buddhika Mahesh
Base Hospital, Panadura, Sri Lanka
T.E.A. Pathirana
You can also search for this author in PubMed Google Scholar
Contributions
A. Dilini Mataraarachchi- Study conception and design, Wrote the main manuscriptB. Buddhika Mahesh P.K- Reviewed the paperC. T.E.A.Pathirana- Supported the implementation of the intervention at the worksite settingD. P.V.S.C. Vithana- Reviewed the manuscript.
Ethics declarations
Ethical approval and consent to participate.
Ethical approval to conduct the study was obtained from the Ethical Approval Committee, University of Colombo; Ethical approval no: EC-19-115. All mothers were allowed to participate in the study, irrespective of their daughter’s age, even though only mothers having a daughter aged 14–19 years were included in the assessment. Informed, written consent was obtained from all mothers before including them in the pre and post-assessment. Participants were given the freedom to decide on their participation in the intervention. Since the intervention was carried out during the times of COVID pandemic, all measures were taken to prevent infection transmission. Online sessions were conducted for the ones who were unable to participate in physical sessions. All methods used in the study were in accordance with the relevant ethical guidelines for research with human participants.
Consent for publication
Not Applicable.
Competing interests
There are no known competing financial or non-financial interests that may affect the work related to this paper.
Additional information
Publisher’s note.
Springer Nature remains neutral with regard to jurisdictional claims in published maps and institutional affiliations.
Electronic supplementary material
Below is the link to the electronic supplementary material.
Supplementary Material 1
Rights and permissions.
Open Access This article is licensed under a Creative Commons Attribution 4.0 International License, which permits use, sharing, adaptation, distribution and reproduction in any medium or format, as long as you give appropriate credit to the original author(s) and the source, provide a link to the Creative Commons licence, and indicate if changes were made. The images or other third party material in this article are included in the article’s Creative Commons licence, unless indicated otherwise in a credit line to the material. If material is not included in the article’s Creative Commons licence and your intended use is not permitted by statutory regulation or exceeds the permitted use, you will need to obtain permission directly from the copyright holder. To view a copy of this licence, visit http://creativecommons.org/licenses/by/4.0/ . The Creative Commons Public Domain Dedication waiver ( http://creativecommons.org/publicdomain/zero/1.0/ ) applies to the data made available in this article, unless otherwise stated in a credit line to the data.
Reprints and permissions
About this article
Cite this article.
Mataraarachchi, D., Buddhika Mahesh, P., Pathirana, T. et al. Development and implementation of a worksite-based intervention to improve mothers’ knowledge, attitudes, and skills in sharing information with their adolescent daughters on preventing sexual violence: lessons learned in a developing setting, Sri Lanka. BMC Public Health 24 , 983 (2024). https://doi.org/10.1186/s12889-024-18416-x
Download citation
Received : 30 May 2023
Accepted : 22 March 2024
Published : 08 April 2024
DOI : https://doi.org/10.1186/s12889-024-18416-x
Share this article
Anyone you share the following link with will be able to read this content:
Sorry, a shareable link is not currently available for this article.
Provided by the Springer Nature SharedIt content-sharing initiative
- Adolescent sexual health
- Sexual violence
- Family-based sexuality education
BMC Public Health
ISSN: 1471-2458
- Submission enquiries: [email protected]
- General enquiries: [email protected]

IMAGES
VIDEO
COMMENTS
When reading, I can distinguish readily between important and unimportant points. _____ 2. I break assignments into manageable parts. _____ 3. I maintain a critical attitude during my study—thinking before accepting or rejecting. _____ 4. I relate material learned in one course to materials of other courses. _____ 5.
review was done. Literature in research skills development and measuring research skills was selected. To name, the literature is: Developing an Instrument to Measure Research Skills (Meerah, et al. 2011), Design and Validation of a questionnaire to Measure Research Skills (Alvarado, et al. 2016),
the assessment for Human biology IA are aimed at assisting you to develop and/or refine these essential research skills while. Background: Lit -RSD Task 1 expands upon and extends the RSD ...
Four instruments were used in more than one study - the Knowledge Attitudes and Practice of Research survey (Van Mullem et al., 1999; two studies), the BARRIERS scale (Funk et al., 1991; two studies), the Edmonton Research Orientation scale (Pain et al., 1996; three studies) and the questionnaire developed by Powell and Case-Smith (2003; two ...
Self-assessment of research knowledge and skills level This questionnaire helps you to evaluate your competency level in various areas of research knowledge and skills. Which faculty are you from? Please indicate your perception of your level of competency in each area using the scale of 1 - 5; 1 = not competent and 5 = highly competent 1.
The third part addressed the stages of research activity, the number of research participants, and the assessment of skills needed for effective research. The assessment of the participant's skills was assessed using a five-point Likert scale, ranging from "Excellent - 1", "Very Good - 2", "Good - 3", "Poor - 4", and ...
The first aspect of good research and good study skills is the ability to ask good questions. In research, a solid, interesting, open-ended and answerable question directs a student's project. The ...
The aim of this study is the validation of a questionnaire to measure research skills with engineering students. Questionnaire validation was performed by: literature review, semantic and content validation by ... (2005); self-assessment tool research skills, Rivera and Torres (2006); and an inventory of skills for university research (ICUNI ...
Questionnaire for Research Skills - Home - QuestMeraki
Questionnaires vs. surveys. A survey is a research method where you collect and analyze data from a group of people. A questionnaire is a specific tool or instrument for collecting the data.. Designing a questionnaire means creating valid and reliable questions that address your research objectives, placing them in a useful order, and selecting an appropriate method for administration.
also describes the methods developed to ensure that the assessment tasks are valid across countries, are strong at measuring relevant skills and are based on authentic life situations. Measuring Student Knowledge and Skills A New Framework for Assessment (96 1999 05 1 P) FF 150 ISBN 92-64-17053-7 9:HSTCQE=V\UZX\: OECD-
Abstract and Figures. A diagnostic instrument to identify the competencies in research knowledge and skills of doctoral student was developed. There is a need to measure student deficiencies in ...
A random selection of degree and graduate students graduating at the end of 2010/2011 was asked to fill a questionnaire to measure the outcomes in research knowledge and skills. The administration ...
This fact sheet provides a sample of instruments for assessing soft skills that can help educators identify students' strengths or challenges with different soft skills, such as teamwork or conscientiousness. Responsible Decision Making: Identifying and solving problems, analyzing situations The table lists instruments, noting each instrument ...
Self-Assessment Questionnaire . Scholarly Competencies Self Assessment Research What research theories or questions have you developed in the past year? What other related theories or questions do you need to develop? How can you continue to build on these theories or questions? What research-related skills have you acquired in the past year?
Most teachers (70-80%) determine assessment criteria, norms and passing. grades themselves. About half the teachers base their norms and passing grades on the performance of the class at hand, other classes of the same age level, and/or the. previous year's students (norm-oriented), about 40% on the subject matter and.
2 The research reported here was supported, in whole or in part, by the Institute of Education Sciences, ... cognitive skills. Questions that require judgment and application to real-world context are more ... cognitive skills assessments are administered in-person, one-on-one with a neuropsychologist, and via pencil-paper, which is both time- ...
(Stokking, Schaaf, Jaspers, & Erkens, 2004) examines the teacher's assessment of students' research skills and the quality of student research in high schools in the social and natural sciences ...
In recent decades, extensive research has been conducted on students' study skills and strategies, but only in developed countries. Hence, examining the study skills of students who have grown up in different cultures is essential. ... a Study Skills Assessment Questionnaire of Counseling Center of Houston University (SSAQ-CCHU), was used to ...
of students' IL skills in universities. IL assessments among the undergraduate students have been overlooked by many scholars [3, 18, 19]. King [21] opines that in imparting IL instruction to the students whether formally or informally, there is need to conduct an assessment. Such assessments help to determine students' mastery of skills ...
With this study we wanted to answer five research questions (RQs). RQ1 deals with medical students' assessment of their individual AI literacy. In particular, we aimed to assess the AI literacy sub-constructs described above (TU, CA, PA), as the identification of literacy gaps is paramount for the development of appropriate medical education ...
Pre and post-assessments were conducted among a sample of 135 mothers of adolescent girls aged 14-19 years in both intervention and control areas. Out of the 135 mothers who participated in the baseline survey, 127 mothers (94.1%) from the intervention area (IA) physically participated in at least one session of the intervention.
teaching and assessment of skills using more authentic assignments is already common practice. Research skills in the natural and social science subjects in upper secondary schools fall into this category and are an obvious choice. However, the meaning of the term 'research' should be clarified. Teachers in a number of subject
This paper presents the development and validation process of an online questionnaire aimed at measuring Te Pūkenga educators' self-assessment of their soft skills before and during the COVID ...