- Digital Marketing
- IT Staff Augmentation
- Data & AI
- E-commerce Development


The Most Impactful IoT Case Studies of the Decade

Get Free SEO Audit Report
Boost your website's performance with a free SEO audit report. Don't miss out on the opportunity to enhance your SEO strategy for free!
- Key Takeaways
Global IoT market is projected to reach $1.5 trillion by 2024. Source: Gartner
IoT adoption in manufacturing has increased by 30% in the past year. Source: Statista
85% of retailers have reported increased sales after implementing IoT solutions. Source: SEMrush
IoT is reshaping industries with real-world applications and significant market growth.
The future of IoT promises enhanced efficiency, improved customer experiences, and a more connected world.
In the last ten years, IoT ( Internet of Things ) tech has changed many industries a lot. It made businesses work smarter and connect better. From hospitals to farms, IoT helps things run smoother and use data better. Looking at the best IoT stories of the decade, we see how they changed industries and made a more connected and efficient world.
Introduction to IoT Case Studies
In the past decade, many businesses have started using IoT (Internet of Things) to work smarter and better. These stories, called IoT case studies, show how connected devices and smart technology are changing industries like healthcare, manufacturing, transportation, and cities. They prove that IoT isn’t just a fancy word but a real game-changer that’s making things easier and more efficient for companies and communities everywhere.
- Overview of IoT Case Studies:
IoT case studies show how IoT is used in healthcare, manufacturing, transport, and cities. They use devices that talk to each other, analyze data, and do tasks automatically to solve big problems. For instance, they help monitor patients from afar in healthcare, predict when machines need fixing in factories, and manage energy better in cities. These studies help other companies see how they can use IoT to be more innovative.
- Significance of IoT Case Studies:
- IoT case studies provide tangible evidence of the benefits and ROI of IoT adoption.
- They inspire confidence among decision-makers by showcasing successful implementations and best practices.
- Case studies foster knowledge sharing and collaboration within industries, accelerating digital transformation .
- They validate the potential of IoT to drive meaningful change, improve efficiency, and create value for stakeholders.
Healthcare Industry:
The healthcare sector has seen a significant transformation due to the integration of IoT technologies, particularly in the areas of remote patient monitoring and real-time health data analysis .
- Pfizer’s IoT Healthcare Solutions:
Remote Patient Monitoring:
- Pfizer has developed IoT-enabled devices for remote patient monitoring, allowing healthcare providers to track patients’ vital signs and health metrics in real time.
- These devices include wearable sensors, smart medical devices, and remote monitoring systems that provide continuous data on patients’ conditions outside traditional clinical settings.
- The ability to monitor patients remotely enables early detection of health issues, proactive interventions, and improved overall patient care.
Medication Adherence Solutions:
Pfizer uses smart pill dispensers and medication tracking apps with IoT tech to help people take their medicines on time. These tools remind patients when to take their pills and track if they’ve taken them.
When patients stick to their medication schedules, it helps them get better treatment results, reduces hospital visits, and saves money on healthcare for problems caused by not taking medicines as prescribed.
- Philips’ Connected Health Devices:
Patient Monitoring Devices:
- Philips offers a range of IoT-enabled patient monitoring devices that capture and transmit real-time data on patients’ vital signs.
- These devices include smart monitors, wearable sensors, and mobile health apps that enable continuous monitoring of patients’ health conditions.
- Healthcare providers can access and analyze this data remotely, facilitating early intervention, personalized treatment plans, and improved patient outcomes.
Telehealth Platforms:
- Philips’ IoT-based telehealth platforms facilitate virtual consultations, remote patient monitoring, and telemedicine services.
- These platforms integrate data from connected health devices, electronic health records (EHRs), and other sources to provide comprehensive healthcare services.
- Telehealth solutions enhance healthcare accessibility, reduce healthcare costs, and enable patients to receive quality care from anywhere, improving overall healthcare delivery.
Manufacturing Sector
The manufacturing industry changed a lot because of IoT. It helps make things work better and faster. One big way it helps is with Predictive Maintenance . This means using sensors and data to know when machines might break down. Companies like General Electric (GE) use this to fix things before they break. It saves time, money, and keeps production running smoothly.
- General Electric’s IoT in Manufacturing:
Predictive Maintenance:
- GE utilizes IoT sensors and data analytics to implement predictive maintenance strategies in manufacturing machinery.
- Real-time monitoring of equipment performance helps detect anomalies and predict potential failures before they occur.
- This proactive approach minimizes unplanned downtime, reduces maintenance costs, and improves overall equipment reliability.
Inventory Management and Supply Chain Optimization:
- IoT-enabled tracking systems allow GE to monitor inventory levels, track shipments, and streamline logistics operations.
- Real-time visibility into inventory helps in better planning, reducing stockouts, and improving order fulfillment.
- Optimizing supply chain processes through IoT leads to increased efficiency and cost savings.
Energy Usage Optimization:
- GE collects and analyzes IoT data from equipment and processes to optimize energy usage in manufacturing facilities.
- Identifying energy-saving opportunities and efficiency improvements reduces environmental impact and lowers operational costs.
- Sustainable practices lead to a more eco-friendly and cost-effective manufacturing ecosystem.
- Siemens’ Industrial IoT Solutions:
MindSphere IoT Platform:
- Siemens offers MindSphere, a cloud-based IoT platform that connects machines, devices, and systems for data gathering and analysis.
- Manufacturers can monitor equipment performance, predict maintenance needs, and optimize production processes in real time.
- The platform provides actionable insights for improved decision-making and operational efficiency.
Digital Twins for Simulation and Optimization:
Siemens uses digital twins, which are like virtual copies of real things, to help understand and improve stuff.
Companies can use digital twins to test out different ideas, make things work better, and make smarter choices.
By using digital twins to predict problems, find faults early, and make things run smoother, companies can save time and money.
State of Technology 2024
Humanity's Quantum Leap Forward
Explore 'State of Technology 2024' for strategic insights into 7 emerging technologies reshaping 10 critical industries. Dive into sector-wide transformations and global tech dynamics, offering critical analysis for tech leaders and enthusiasts alike, on how to navigate the future's technology landscape.
- Data and AI Services
With a Foundation of 1,900+ Projects, Offered by Over 1500+ Digital Agencies, EMB Excels in offering Advanced AI Solutions. Our expertise lies in providing a comprehensive suite of services designed to build your robust and scalable digital transformation journey.
Applications in Asset Management and Quality Control:
- Siemens’ IoT solutions encompass asset management, production optimization, and quality control applications.
- IoT data and analytics enable higher production yields, better product quality, and faster time-to-market for manufacturers.
- Siemens’ expertise in industrial automation and IoT integration provides comprehensive solutions for manufacturing excellence.
Transportation and Logistics
Transportation and logistics have changed a lot because of IoT. One big change is in tracking things. For example, FedEx now uses IoT to track packages. This means they can see where packages are in real time and know if they’re okay. It helps FedEx work better and makes customers happier because they can know exactly where their packages are and when they’ll arrive, reducing problems with lost or late deliveries.
- FedEx’s IoT Logistics Solutions
Package Tracking and Monitoring:
- Implementation of IoT-enabled sensors on packages for real-time tracking.
- Monitoring of location, temperature, and condition of shipments throughout the delivery process.
- Ensuring timely deliveries and proactive problem-solving for route deviations or delays.
Data Analytics for Operational Insights:
- Utilization of IoT-powered analytics for valuable operational insights.
- Streamlining delivery routes based on data-driven analysis.
- Reducing fuel consumption, optimizing routes, and minimizing overall costs.
Smart Fleet Maintenance:
- Integration of smart sensors in FedEx’s fleet of vehicles.
- Monitoring vehicle performance metrics such as fuel consumption and engine health.
- Proactive scheduling of maintenance to enhance fleet reliability and reduce downtime.
Improved Operational Efficiency:
- Benefits of IoT solutions in improving operational efficiency.
- Enhanced visibility into delivery patterns for better decision-making.
- Ensuring reliable and on-time deliveries, ultimately improving customer satisfaction.
- UPS’s Smart Fleet Management
Telematics Devices for Data Collection:
- Use of telematics devices in UPS vehicles to collect data on driver behavior and vehicle performance.
- Monitoring of route efficiency, idling time, and fuel consumption for optimization.
- Data-driven insights for improving fleet management and reducing operational costs.
Asset Tracking and Monitoring:
- Implementation of IoT-enabled asset tracking systems for packages.
- Real-time monitoring of package location and condition throughout the delivery process.
- Providing customers with accurate and timely updates on their shipments.
Cargo Condition Monitoring:
- Utilization of IoT sensors in delivery vehicles to monitor cargo conditions.
- Monitoring temperature, humidity, and other parameters for sensitive goods.
- Ensuring safe and secure transport of packages, especially for temperature-sensitive items.
Enhanced Customer Experience:
- Benefits of IoT solutions in improving the overall customer experience.
- Increased transparency with real-time package tracking and updates.
- Ensuring the safe and secure delivery of goods, leading to higher customer satisfaction.
Agriculture
IoT is changing farming by helping farmers know when to water, use fertilizers, and manage pests using sensors in their tools. Companies like John Deere and Monsanto make smart tools that use IoT, so farmers can make better decisions and grow more crops while using fewer resources and taking care of the environment.
- John Deere’s Precision Farming IoT:
- Technology Integration: John Deere’s Precision Farming IoT integrates a range of cutting-edge technologies such as sensors, GPS, drones, and data analytics to provide comprehensive insights into agricultural operations.
- Real-time Monitoring: The IoT sensors deployed across fields continuously monitor crucial parameters like soil moisture levels, nutrient content, and environmental conditions in real time.
- Data Analytics: The collected data is processed and analyzed using advanced analytics algorithms, allowing farmers to gain valuable insights into crop health, growth patterns, and potential risks.
- Precision Application: Armed with precise data, farmers can apply fertilizers, pesticides, and water resources with unparalleled accuracy, optimizing resource usage and minimizing environmental impact.
- Decision Support: John Deere’s IoT platform provides farmers with decision support tools that offer recommendations for crop management strategies, irrigation scheduling, and pest control measures based on real-time data analysis.
- Monsanto’s Agricultural IoT Solutions:
- Monsanto’s Agricultural IoT Solutions use data science, satellite images, and weather predictions to help farmers make smart decisions.
- Farm Monitoring: Sensors in fields check soil health, crop growth, and pests. This helps spot problems early and plan ahead.
- Predictive Tools: By studying past and current data, Monsanto’s system predicts how crops will do, when to plant, and how diseases might spread.
- Efficient Use: The system suggests just enough water, fertilizers, and pesticides based on data. This cuts waste and helps the environment.
- Less Risk: Farmers get alerts about bad weather, market changes, and pests. This helps them avoid problems and make better choices.
Retail Sector
Retail has changed a lot lately because of IoT. It’s now better at making customers happy. Smart shelves with RFID tags help stores know what’s in stock all the time. This means no empty shelves and happier customers who find what they want when they come to shop.
- Amazon Go’s IoT Retail Experience
- Seamless Shopping Experience: Amazon Go redefines retail experiences by integrating IoT technologies seamlessly. Customers enter the store, scan their Amazon Go app, and proceed to shop without the need for traditional checkout processes.
- IoT Devices and Sensors: The store is equipped with a network of IoT devices and sensors that track customer movements and product selections in real time. Each item picked up by a customer is automatically added to their virtual cart.
- Cashier-Less Transactions: With the help of IoT, Amazon Go enables cashier-less transactions. Customers simply leave the store, and their total bill is automatically charged to their Amazon account, eliminating the need for physical checkout counters.
- Enhanced Convenience: The IoT-driven retail experience at Amazon Go enhances convenience for customers. They can shop without waiting in long checkout lines, making the entire process faster and more efficient.
- Frictionless Shopping: By removing friction points in the shopping journey, such as waiting in lines or manually scanning items, Amazon Go provides a frictionless shopping experience that prioritizes speed and ease of use.
- Walmart’s IoT Inventory Management
- Real-Time Inventory Tracking: Walmart utilizes IoT-enabled devices like RFID tags and sensors to track inventory levels in real time across its stores. This technology helps monitor stock levels, identify low inventory, and optimize restocking processes.
- Shelf Life Monitoring: IoT in Walmart’s inventory management system also includes monitoring the shelf life of perishable items. This ensures that products are sold before expiration, reducing waste and improving profitability.
- Optimized Restocking: By leveraging IoT data, Walmart can optimize restocking processes based on demand patterns and inventory levels. This results in shelves consistently being stocked with popular items, reducing out-of-stock situations.
- Data-Driven Decisions: Walmart’s IoT-driven inventory management approach is data-driven. The insights gathered from IoT devices enable informed decision-making regarding inventory management strategies, pricing, and product placement.
- Improved Customer Experience: The efficient inventory management facilitated by IoT translates into an improved customer experience. Customers can find products they need readily available, leading to higher satisfaction levels and repeat business.
Smart Cities
Smart cities use IoT technology to make cities better. They save energy and make services like electricity better. For example, in Barcelona, they use smart grids and systems to save energy and give power to people more efficiently. This helps save money and makes the environment cleaner.
- Barcelona’s Smart City Initiatives:
Smart Lighting Systems:
- Barcelona has deployed IoT-enabled smart lighting systems that adjust brightness levels based on real-time data.
- These systems not only save energy but also enhance public safety by ensuring well-lit streets and public spaces.
Smart Waste Management:
- The city has implemented smart waste bins equipped with sensors to optimize waste collection routes.
- This initiative has improved waste management efficiency, reduced operational costs, and increased recycling rates.
Smart Transportation Solutions:
- Barcelona utilizes IoT technologies for smart traffic management and parking solutions.
- Commuters benefit from reduced congestion, shorter commute times, and real-time updates on public transportation options.
Environmental Impact:
- By integrating IoT into various city services, Barcelona has reduced its environmental footprint.
- Initiatives like smart energy usage and waste management contribute to a more sustainable urban environment.
- Singapore’s IoT Urban Solutions:
Smart Energy Grid:
- Singapore has implemented a smart energy grid that leverages IoT for real-time monitoring and predictive analytics.
- This has led to reduced energy consumption, lower carbon emissions, and increased energy efficiency.
Water Management Systems:
- IoT sensors are used for monitoring water quality, detecting leaks, and optimizing water distribution.
- These smart water management systems contribute to sustainable water usage and conservation efforts.
Smart Mobility Solutions:
- Singapore’s smart transportation initiatives include autonomous vehicles and IoT-enabled public transit systems.
- Commuters experience improved accessibility, safety, and efficiency in their daily travels.
Digital Infrastructure:
- The city’s robust digital infrastructure supports the seamless integration of IoT technologies across sectors.
- This infrastructure backbone facilitates data-driven decision-making and enhances overall urban management.
Citizen Engagement:
- Singapore actively involves citizens in shaping smart city initiatives through digital platforms and participatory programs.
- This engagement fosters a sense of ownership, collaboration, and innovation in building a smart and livable city.
Energy Sector:
The energy sector has changed a lot with IoT tech, making things work better and greener. One big change is smart grids. These grids use IoT sensors and data to watch how we use energy, find problems in the grid, and send power where it’s needed most. Tesla is one company leading this change, helping us use more renewable energy and less fossil fuels.
- Tesla’s Renewable Energy IoT:
Powerwall for Home Energy Storage:
- The Powerwall is an IoT-enabled home battery system designed to store solar energy.
- It integrates IoT technology to optimize energy usage based on real-time data.
- Users can monitor their energy consumption and production through a mobile app.
Solar Roof with IoT Sensors:
- Tesla’s Solar Roof tiles incorporate IoT sensors to monitor energy production and efficiency.
- These sensors provide real-time data on solar energy generation and consumption.
- Homeowners can track their energy usage patterns and make adjustments for energy conservation.
Smart Grid Integration:
- Tesla’s renewable energy solutions are integrated with smart grid technology for efficient energy management.
- IoT devices enable communication between renewable energy sources, energy storage systems, and the grid.
- This integration allows for dynamic load balancing, grid stability, and reduced reliance on fossil fuels.
- Shell’s IoT in Oil & Gas Industry:
Remote Monitoring of Oil Wells:
- Shell utilizes IoT sensors to remotely monitor oil well performance and detect anomalies.
- Real-time data collection enables predictive maintenance and proactive interventions.
- This IoT-driven approach reduces downtime and optimizes oil production efficiency.
Predictive Maintenance for Equipment:
- IoT sensors on equipment such as pumps, turbines, and valves provide insights into performance.
- Predictive analytics algorithms analyze data to forecast potential equipment failures.
- Shell can schedule maintenance activities proactively, minimizing downtime and costly repairs.
Safety Enhancement with IoT:
- Shell integrates IoT devices for safety monitoring in hazardous environments.
- Sensors detect gas leaks, temperature fluctuations, and other safety hazards.
- Real-time alerts and automated response systems improve safety protocols and mitigate risks.
Data Analytics for Operational Efficiency:
- Shell leverages IoT-generated data for advanced analytics and operational insights.
- Data-driven decision-making optimizes production processes and resource allocation.
- IoT-enabled analytics contribute to cost savings, energy efficiency, and environmental sustainability.
In conclusion, over the last ten years, many industries have changed a lot by using IoT tech. This includes healthcare, manufacturing, transportation, agriculture, retail, smart cities, and energy. Companies like Pfizer, Siemens, FedEx, John Deere, Amazon, Barcelona, Tesla, and Shell showed how IoT can make things better. They used IoT to work smarter, make customers happier, and create new ideas. This shows that in the future, IoT will keep making life and business better for everyone.
- Q. What are IoT case studies?
IoT case studies showcase real-world applications of Internet of Things technologies in various industries.
- Q. How can IoT benefit businesses?
IoT can optimize operations, enhance efficiency, improve customer experiences, and drive innovation.
- Q. Which industries are leveraging IoT?
Healthcare, manufacturing, transportation, agriculture, retail, smart cities, and energy sectors are adopting IoT solutions.
- Q. What are some notable IoT companies?
Companies like Pfizer, Siemens, FedEx, John Deere, Amazon, Barcelona, Tesla, and Shell are leading the IoT revolution.
- Q. What does the future hold for IoT?
The future of IoT promises a more connected, efficient, and technologically empowered world across industries.

Related Post
What is file management things to know, a beginner’s guide to issue tracking, what is web filtering essential things to know, all you should know about it strategy, what is bot management and how does it work, understanding human-computer interaction (hci), table of contents.
Expand My Business is Asia's largest marketplace platform which helps you find various IT Services like Web and App Development, Digital Marketing Services and all others.
Article Categories
- Technology 871
- Business 417
- Digital Marketing 348
- Social Media Marketing 135
- E-Commerce 134
Sitemap / Glossary
Copyright © 2024 Mantarav Private Limited. All Rights Reserved.

- Privacy Overview
- Strictly Necessary Cookies
This website uses cookies so that we can provide you with the best user experience possible. Cookie information is stored in your browser and performs functions such as recognising you when you return to our website and helping our team to understand which sections of the website you find most interesting and useful.
Strictly Necessary Cookie should be enabled at all times so that we can save your preferences for cookie settings.
If you disable this cookie, we will not be able to save your preferences. This means that every time you visit this website you will need to enable or disable cookies again.
- There are no suggestions because the search field is empty.
SUGGESTED CONTENT:
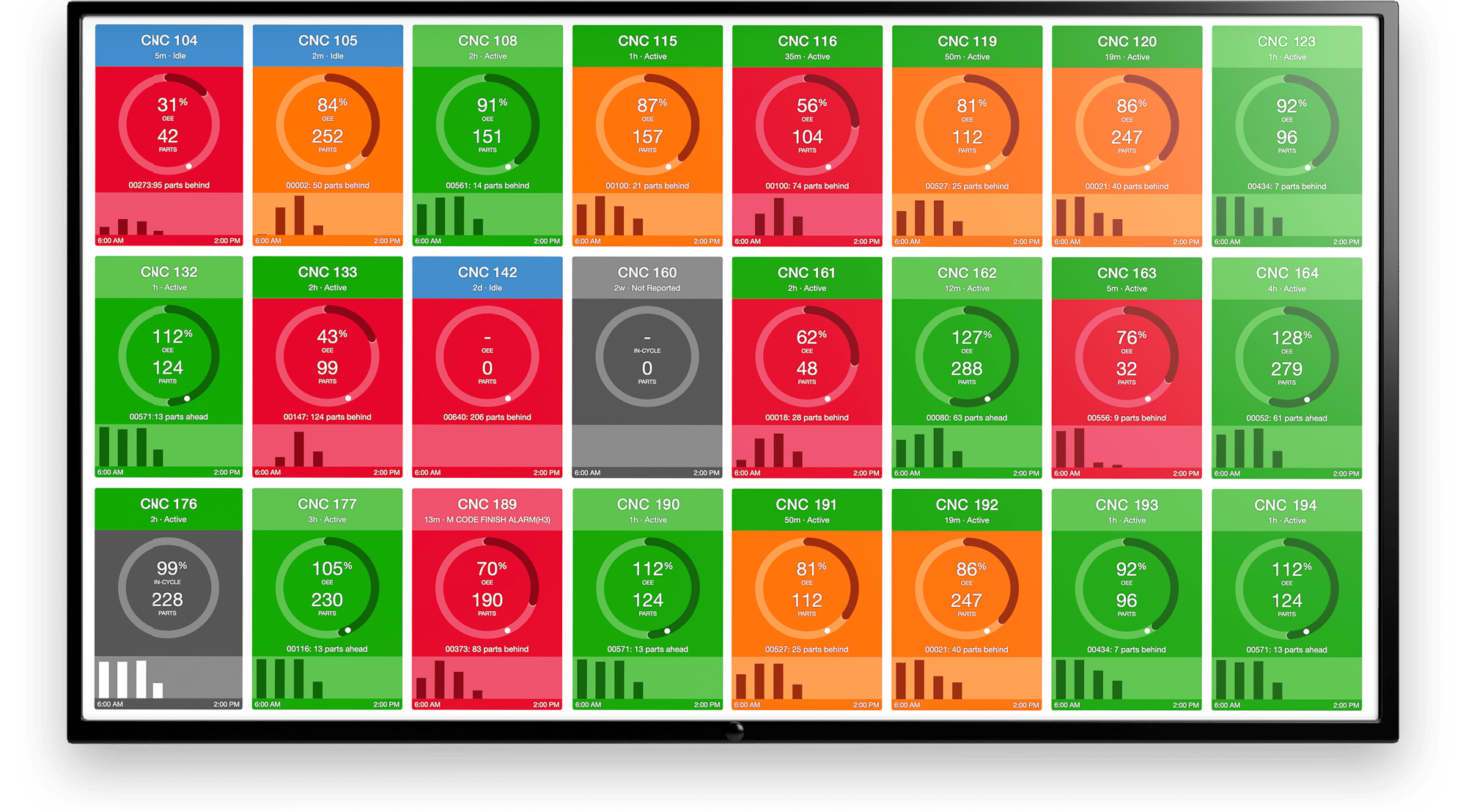
Book a Demo of MachineMetrics
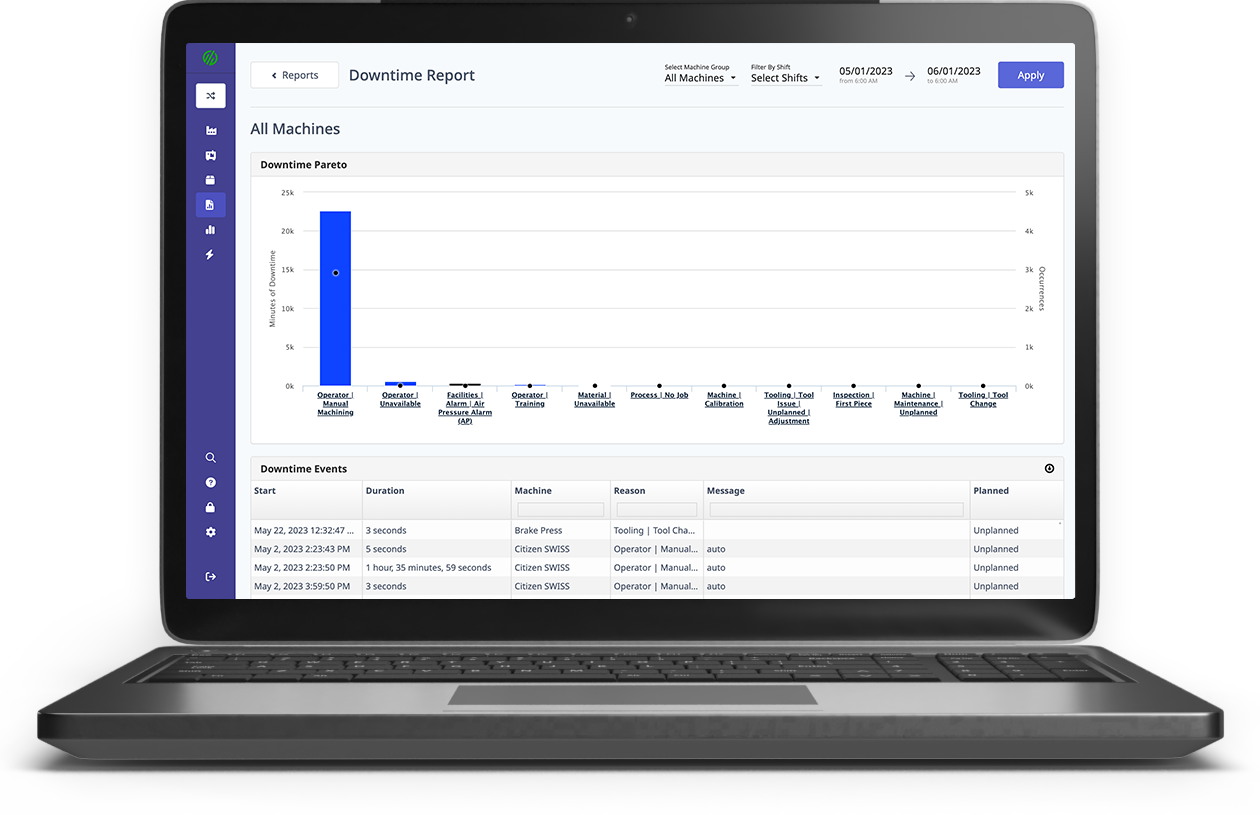
See the Platform in Action [Video]
.png?width=1960&height=1300&name=01_comp_Downtime-%26-Quality_laptop%20(1).png)
Learn How to Run a Downtime Analysis
Machinemetrics blog, machinemetrics unveils revolutionary automated schedule..., how manufacturing production scheduling software tackles an..., the challenges of manufacturing scheduling and how modern..., a definitive guide to manufacturing execution systems (mes).
Ready to empower your shop floor?
- MachineMetrics
- Product Updates
- Data Science
- Lean Manufacturing
Machine Monitoring
IoT in Manufacturing: Top Use Cases and Case Studies
Updated May 17, 2021
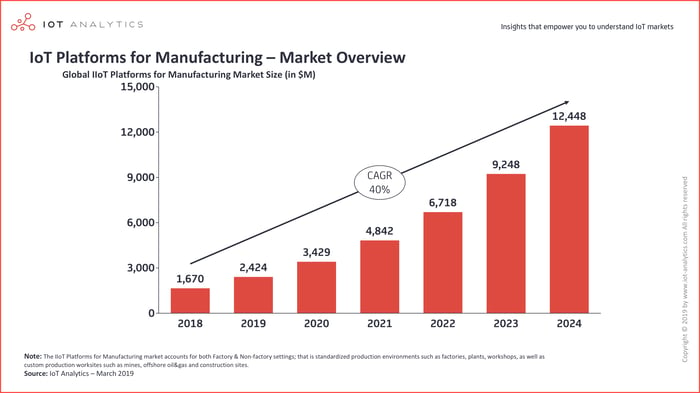
Within this article, we’ll be discussing practical IoT applications in manufacturing and use cases of industrial IoT technology in manufacturing
What is IoT?
What is iiot, the benefits of iot in manufacturing.
IoT represents a digital transformation in manufacturing processes and business operations. Using it alongside an advanced machine data platform can be transformational. And there are many benefits of IoT in manufacturing:
Process Optimization
Inventory Management
Predictive Maintenance
IoT in Manufacturing Use Cases [+Case Studies]
Remote monitoring.
Learn more about remote monitoring for machine builders and OEMs.
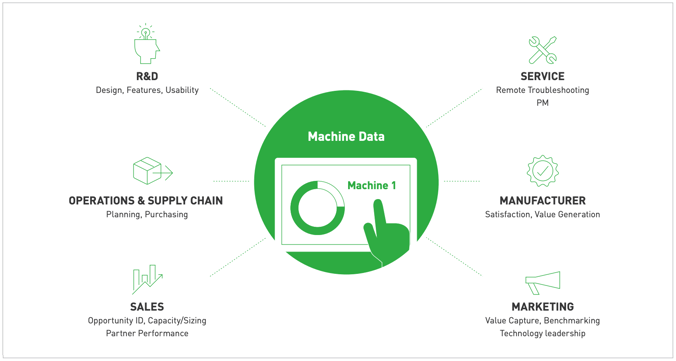
Supply Chain Management and Optimization
- Real-time tracking of assets and products
- Automation of warehouse tasks
- Digitized paperwork management
- Forecasting accuracy improvement
- Greater control of inventory
Digital Twins
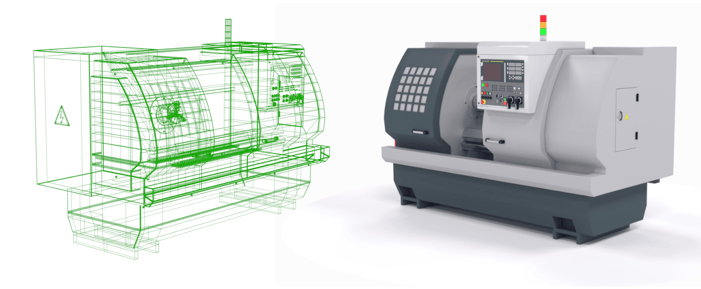
Real-Time Machine Monitoring
Production visibility.
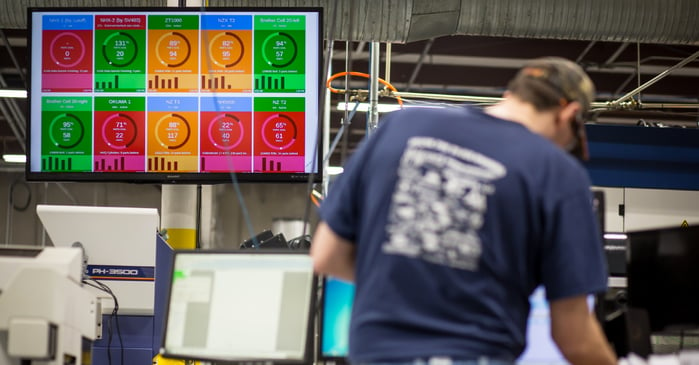
Integrating Systems
Compiling kpis.
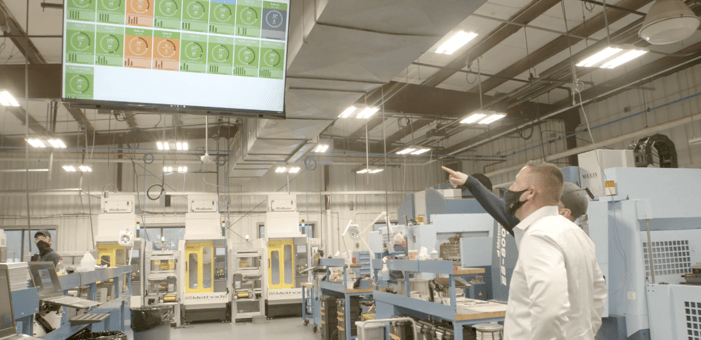
Asset Utilization
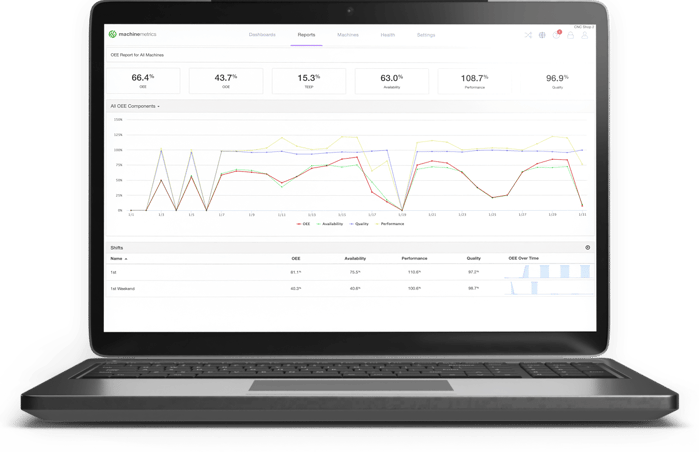
Difficulties of Adopting IoT in Manufactur ing
1. large investments are required and the roi is questionable, 2. concerns about data security, 3. employees who aren’t qualified, 4. integration with operational technology and older systems, how to use iot and machine data for remote operations, boosting your operational efficiency with iiot, subscribe to our mailing list, related posts, read the latest.
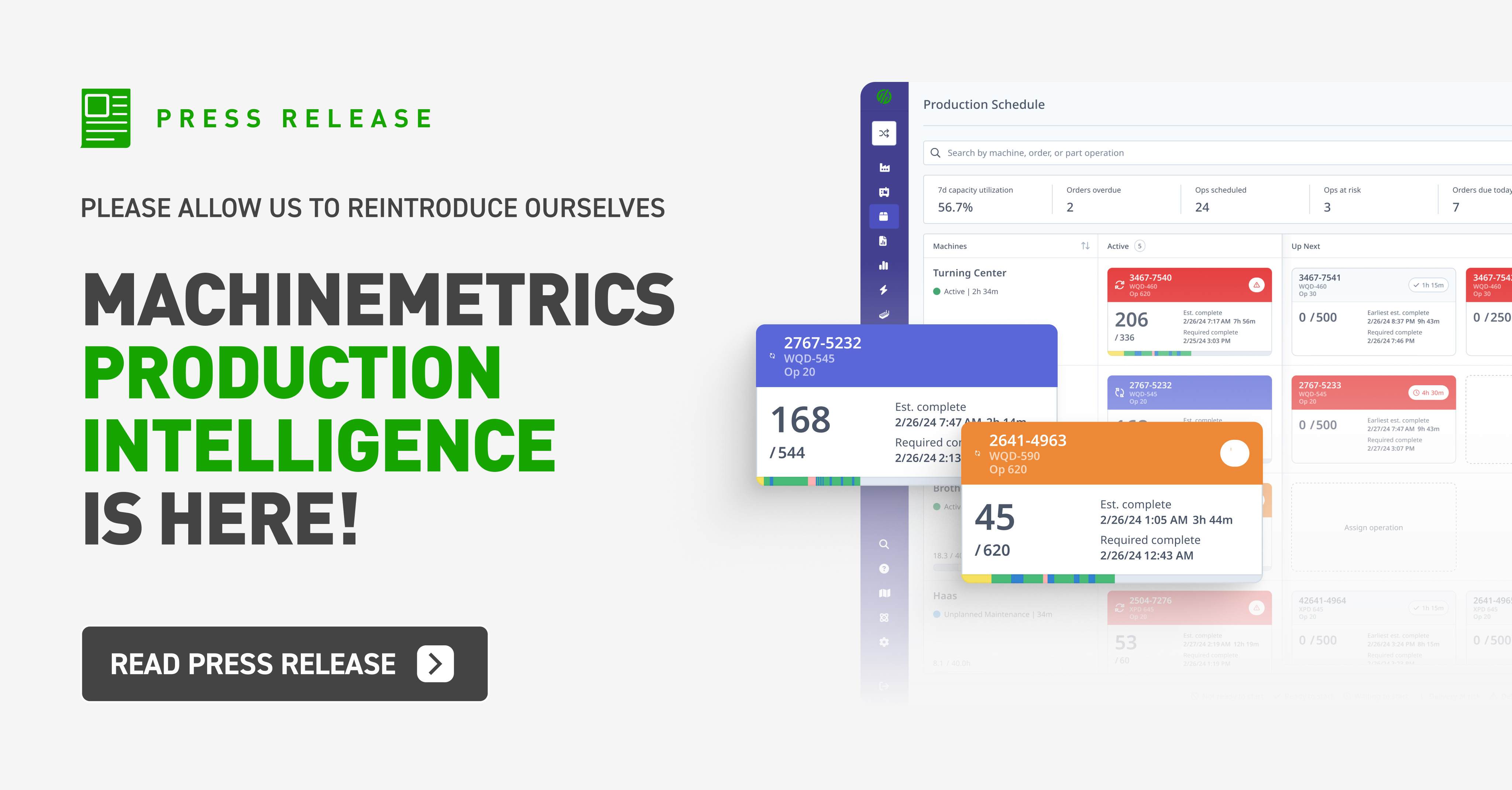
Lists by Topic
- MachineMetrics (386)
- Lean Manufacturing (72)
- Industry 4.0 (50)
- Manufacturing data (23)
- Manufacturing News (20)
- Data Science (15)
- data collection (14)
- Product Updates (12)
- Connected Factory (10)
- Machine Monitoring (10)
- manufacturing analytics (10)
- Smart Manufacturing (9)
- industrial iot (9)
- CNC Machines (8)
- Productivity (8)
- Downtime (7)
- Industrial Automation (7)
- Tool Monitoring (7)
- Big Data (6)
- Data Visualisation (5)
- Edge Computing (5)
- Maintenance (5)
- Process Optimization (5)
- Shop Floor (5)
- digital manufacturing (5)
- Automotive (4)
- Death of MES (4)
- MES software (4)
- Machine Learning (4)
- Quality Assurance (4)
- Aerospace and Defense (3)
- Contract Manufacturing (3)
- Data Cleaning (3)
- Heavy Machinery Manufacturing (3)
- Medical Device Manufacturing (3)
- OEE Software (3)
- Oil and Gas Manufacturing (3)
- Production Scheduling (3)
- capacity (3)
- continuous improvement (3)
- inustrial IOT (3)
- press release (3)
- preventative maintenance (3)
- security (3)
- 8 wastes (2)
- Condition Monitoring (2)
- Dashboards (2)
- Internet of things (2)
- Production Monitoring (2)
- Strategic Partnerships (2)
- management (2)
- real-time analytics (2)
- Digital Transformation (1)
- Downtime Categorization (1)
- FANUC FOCAS (1)
- Line Balancing (1)
- Machine Tool Distributors (1)
- Machinery (1)
- Manufacturing Innovation (1)
- Quality Control (1)
- Supply Chain (1)
- Turnkey contracts (1)
- coaching (1)
- elon musk (1)
- employee engagement (1)
- link roundup (1)
- manufacturing (1)
- manufacturing software (1)
- networks (1)
- release notes (1)
844-822-0664
Easthampton Office
116 Pleasant St, Suite 316, Easthampton, MA 01027
Edge Platform
Cloud Platform
APIs & Applications
Industrial IoT Platform
Production Monitoring
Condition Monitoring
For Machine Builders and Distributors
For Developers
Production Schedule Intelligence
Aerospace & Defense
Contract Manufacturers
Heavy Machinery
Medical devices
Oil & Gas
Precision Metalworking
ERP Integration
Metal Stamping & Fabrication
Tool, Die & Mold CNC
ROI Calculator
Waste Calculator
Connectivity Hub
Partner Program
Privacy Policy
Data Processing Addendum
Service Level Agreement
Website Terms
- +49 (0) 40 639 118 91
- info(at)iot-analytics.com
- Contact Form

The top 10 IoT Use Cases
- Adoption of various Internet of Things (IoT) use cases is on the rise, according to the latest 2021 IoT Use Case Adoption report .
- Of the 200+ companies from various industries interviewed, the average company has adopted eight different IoT use cases to date (out of a total of 48).
- IoT use cases related to the smart operations of a company are the most adopted, followed by smart supply chain use cases and use cases involving connected IoT products.
- Read-only remote asset monitoring is the most widely adopted use case (34% of companies interviewed have adopted it).
Why it matters?
- With various IoT topics prioritized by organizations post-COVID, getting a feel for what is hot and what is not is important for both vendors and IoT adopters.
- Vendors should prioritize those use cases that yield a high return on investment (ROI) and those that are on the investment priority list in the coming years.
- IoT adopters can learn from the best practices and examples of existing users to prevent costly mistakes.
The IoT Use Case Adoption Report 2021
In 2021, the average large manufacturing, healthcare, automotive, retail, or energy company has rolled out eight different IoT use cases, according to IoT Analytics’ latest IoT Use Case Adoption Report .
The 430-page report, which is part of IoT Analytics’ ongoing market coverage of IoT applications, is the first such in-depth report and is based on 200+ interviews with IoT end users who have rolled out more than 1,600 IoT projects over the last few years across 48 different IoT use cases. The report shows where companies have been investing and are planning to invest, which industries and regions are ahead, and which use cases promise the highest ROI.
Oil and gas companies and energy companies are ahead of others. They have rolled out an average of 15 use cases. Make no mistake: For many companies, IoT is still a small fraction of their business. The average company we interviewed had $9.6 billion in revenue and only currently spends $33 million on IoT use cases (0.34% of the revenue). The fact that the top use case today has only been adopted by 34% of respondents (but with a predominantly positive ROI) shows how much bigger the IoT opportunity is.
Note: The report looks at IoT use cases that are applicable to most organizations and does not consider industry-specific use cases such as smart heating, ventilation, and air conditioning (HVAC) (applicable only to buildings) or smart transportation systems (applicable to cities). The analysis also does not consider consumer IoT use cases such as Smart Home devices or wearables. For a broader analysis of enterprise IoT applications, see this analysis from 2020. For a deep dive on Smart City IoT use cases, see this an a lysis . A deep dive on Smart Building use cases will be published later this year.
The 10 most adopted IoT Use Cases
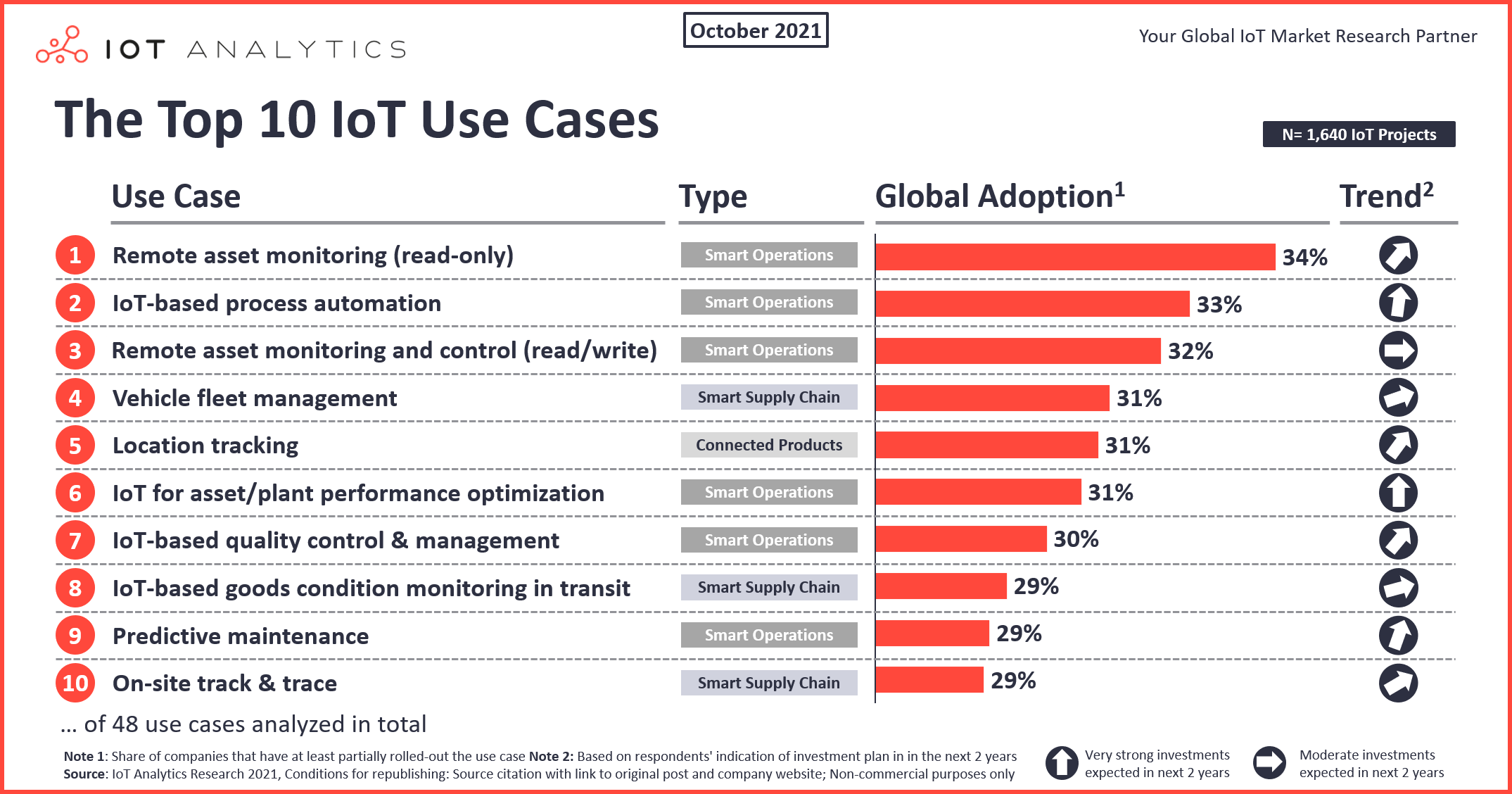
Six of the top 10 IoT use cases today (ranked by adoption) aim at making operations smart, thus improving companies’ production processes for manufacturing, enhancing maintenance operations, or advancing any other operations (e.g., energy generation in the case of an energy company, running healthcare operations in the case of a hospital, or running store operations in the case of a retail company). Three of the top 10 use cases are related to smart supply chains, and only one is related to smart products in the field.
Here are the 10 most adopted use cases, ranked by their adoption rate:
1. Read-only Remote Asset Monitoring
Not surprisingly, the simplest IoT use case is also the most adopted. Read-only remote asset monitoring refers to assets that are connected from afar in a read-only manner (i.e., one can visualize the asset data, but one cannot send back any commands to the asset itself). This use case is one of the easiest and cheapest to set up due to its simplicity. In many cases, remote asset monitoring supersedes the error-prone and costly manual task of checking and documenting asset states in person. Adoption in 2020 was clearly accelerated by the pandemic and is expected to grow even further, as 36% of interviewees say they plan to invest significantly in this use case in the next two years.
Adopter’s quote
“In the future, our engineers and plant operations staff will not need to sit in cars to go from their office location to another sub-station or satellite equipment room to perform daily routine activities. Routine read-only activities could be done more frequently, which could indirectly contribute to the reliability of control systems.” Senior operations manager of a mining company in Qatar
Implementation example
Hindustan Coca-Cola Beverages Pvt. Ltd. , located near Pune, India, adopted remote asset monitoring for its PET and can-bottling lines. T https://aiplindia.com/case-study/ he real-time and continuous monitoring of critical parameters (e.g., syrup flow rate, can-rinse pressure, and water temperature) replaced its MS Excel-based manual reporting. In this specific case, the company combined the remote connectivity of its assets with a condition-monitoring use case that generates alarms based on predefined conditions.
| Use case definition (by IoT Analytics)
Remote Asset Monitoring (read-only) = Real-time, one-way data streaming from machinery/equipment to an offsite location (e.g., cloud), making data accessible from anywhere.
2. IoT-based Process Automation
IoT-based process automation has been rolled out by 33% of companies. This type of process automation describes operational processes that were either entirely manual in the past or relied on antiquated, industrial-automation setups but have now been upgraded with state-of-the-art hardware and software. Companies that introduce this use case to upgrade their existing setups often do so to add flexibility and agility in the operations process so that specific process steps can be changed in the future. This becomes important because companies are increasingly interested in aligning their manufacturing and operations processes to ever-changing customer demands.
“Our manufacturing processes used to have many paper-based steps involved. IoT process automation has reduced many of these paper-based processes, resulting in a reduction in the number of errors, labour cost and real-time access to data.” CIO of a food and beverage manufacturer in Canada
An Australian farmer , reported he was able to use 20% less water thanks to a new IoT-based irrigation system supplied by Lindsay , a US-based manufacturer of pivot-irrigation systems. Farmers increasingly adopt smart IoT-based irrigation systems to automate the process of irrigation and thereby enhance their crop yields.
IoT-based Process Automation = The use of real-time data from connected assets to improve an entire operational process (e.g., a manufacturing process).
3. Remote Asset Monitoring and Control (read/write)
“Read/Write remote asset monitoring and control” is the extension of “Read-only remote asset monitoring.” On top of “reading” the assets data, with this setup one can also communicate back and thereby influence asset control from afar (i.e., “writing” data back).
Just like the read-only asset monitoring use case, this use case was accelerated by the COVID -19 pandemic as service teams, engineers, and other staff needed to find ways to reach an asset that needed their attention while being in a different location.
Because of the added complexity and security risk of controlling an asset, on top of just simply monitoring, this use case comes with significantly higher costs of installation and maintenance. However, it has been proven that such solutions pay off in a relatively short time: Of those decision makers whom IoT Analytics interviewed, 51% reported amortization in less than 24 months.
“Remote monitoring and control of freezers has saved costly materials before being ruined.” Research and development (R&D) manager of a manufacturing company in UK
Schlumberger , an oilfield service company, recently adopted a monitoring and control solution from Advantech and ReStream . These companies had partnered up to supply and develop a new oilfield fluid-monitoring system based on LTE connectivity. The project helped to achieve flow assurance, ensure asset integrity, and optimize production. It also helped to increase labor safety. Oilfield companies suffer from a dynamic chemical environment that, if unchecked, can lead to premature wear, lost productivity, and the release of lethal poisonous gases.
Remote Asset Monitoring and Control (read/write) = Real-time, two-way data streaming of machinery/equipment from/to an offsite location, enabling data access and the sending of commands from anywhere.
4. Vehicle Fleet Management (track/trace)
The management of vehicles in a fleet is the number one IoT supply chain use case right now. The larger the fleet of trucks (or other means of transport) to manage, the higher the complexity. Cross-border fleet management can be especially overwhelming, which is why 31% of companies have rolled out a professional vehicle fleet management solution to gather real-time information.
Today, most fleet management solutions are reliant on wide-area connectivity, such as cellular (2G, 3G, 4G). Several new, low-cost IoT satellite technologies have recently been launched and promise to add another option to this use case so that vehicle fleets are always truly connected at a reasonable price. New companies include Hiber (IoT-focused satellite network) and Starlink (part of SpaceX). Both plan to send thousands of new satellites into space in the coming years to provide low-latency, high-bandwidth connectivity globally.
“Now we know virtually in real time where our wagons are located, which routes we can optimize, and where there is potential for savings for our customers.” Suzy Verachten, project leader, Lineas (European Rail Freight Operator)
Lineas , the largest private rail freight operator in Europe, managed to increase capacity utilization of its fleet by more than 40 percent by implementing the Bosch fleet management solution.
Vehicle Fleet Management (track/trace) = Tracking and tracing the location of individual vehicles either on site while in transit or as general fleet management.
5. Location Tracking (e.g., GPS)
Not every company sells smart-connected IoT products, but of those that do, tracking the location of the asset is the number one use case (31% of companies in our interview set have adopted it). The results from this interview series are in line with results from the IoT Commercialization & Business Model Report 2020 , which found that geolocalization was also the number one use case in terms of customer adoption when original equipment manufacturers (OEMs) were asked which smart product features their end customers were adopting as part of their IoT-enabled assets. Location tracking is therefore important for developing a successful IoT business model .
Tracking the location of an asset can be beneficial to both the vendor of the products (e.g., by understanding usage patterns) and to the user (e.g., by finding a lost item or by mitigating theft).
“Connectivity means we can monitor distance covered, send service alerts, or lock the bike remotely. Things become even more interesting when we mesh this data with other transport data. If we can map the routes of thousands of cyclists, could we help urban planners develop better cycling infrastructure?” Taco Carlier, co-founder, VanMoof
VanMoof, an Amsterdam-based e-bike manufacturer, offers an anti-theft service to its customers that is based on the location tracking of a bike. For a monthly fee, the company sends its “bike hunters” if a customer’s bike is stolen. The bikes are connected and tracked by a combination of Bluetooth and cellular networks. This allows the company to keep its promise of recovering the bike in up to 70% of cases.
Location Tracking (e.g., GPS) = Tracking product location to determine its movement and geographic position.
6. IoT for Asset/Plant Performance Optimization
Asset performance management (APM) is a term in manufacturing to describe methods of capturing and integrating data, visualizing it, and analyzing it to improve the reliability and availability of physical plant–floor assets. IoT for asset/plant performance optimization is the modern version of APM. It integrates state-of-the-art data-capture and integration tools (like IoT gateways) and software tools (like IoT platforms) to analyze how assets can be run and maintained at optimum levels (e.g., optimized asset speed settings, optimized material input settings, or optimized maintenance intervals).
More than 30% of companies in our interview set have adopted IoT for asset/plant performance optimization. With 42% of companies planning to invest significantly in IoT for this purpose, it is among the use cases with the highest expected growth rate.
“The use of IoT for plant performance optimization has been a success since it now allows us to run our CHP power utilities at optimal levels based on variable fuel mix and plant loads for steam and power.” CEO, manufacturing: chemicals and chemical products , India
A beverage manufacturer that was running mostly older equipment used an IoT solution provided by Relayr in one of its bottling plants, which produces 1.2 million bottles per day per production line. It achieved improvements of 11% in performance and 8% in quality. Implementing an IoT retrofit kit and an IoT gateway, the company was able to identify the processes responsible for 90% of the plant’s downtime. Based on the IoT data collected from the legacy machinery of different manufacturers, the company was able to address several issues (e.g., changing the control logic of the machines).
IoT for Asset/Plant Performance Optimization = The use of real-time data from connected assets to optimize individual asset health for better operations performance at a site.
7. IoT- based Quality Control & Management
IoT-based quality control and management involves the use of machine vision or other IoT sensor data to detect quality issues in real time during operations. The use case pays off particularly quickly: Of the 30% of companies that have implemented this IoT use case, two-thirds report amortization in fewer than 24 months.
“We can now tell quickly if the environmental parameters are not quite right at some point, either within the paint shop or in one of the buffer areas. It takes a lot of data to do this, which we collect throughout the process, evaluate historically and analyse in real time” Martin Hilt, innovation and digitalization officer at BMW
A leading global automobile manufacturer automated the testing of the electronic functionality of its cars during the manufacturing process. As the company was adding greater electronic functionality to the cars to stay current with technological developments, it was looking to find ways to optimize its time-consuming, manual method of checking the operation of electronic parts. With the help of Cognizant , the company was able to completely automate vehicle testing and also implement a mixed-mode validation to simulate missing parts that would arrive late due to unforeseen supply chain issues. The solution realized a positive return on investment in four months.
IoT- based Quality Control & Management = The use of IoT sensor data or machine vision to monitor operational process parameters and detect faults (in real time) with the goal of reducing scrap/rework.
8. IoT-based goods condition monitoring in transit
Monitoring the conditions of goods is essential in industries such as pharmaceuticals or food and beverage and can even be seen as a way to help tackle the global food shortage. Temperature sensor data is one of the most important sensor values for this use case; it guarantees the safety of the final product by close monitoring throughout the entire supply chain, both in transit and in storage.
Condition-monitoring solutions were used by 29% of the interviewed decision makers as part of their digital supply chain initiatives.
“The assessment [of goods in transit] was an eye-opener, because we never looked at supply chain risk holistically before. Now we know where the risks are.” An D’haenens, logistics manager EMEA, DuPont
A global life sciences company worked with DHL to enable pallets with smart sensors. The sensors are equipped with low-power wide-area (LPWA) connectivity and track geographic location, movement, delay, shock, and temperature. This ability has enabled the life science company to reduce its life-cycle costs by 6 to 7%, with reported reliability of nearly 100%.
IoT-based goods condition monitoring in transit = The use of IoT sensor data to monitor the condition of transported goods and ensure they remain safe and are not exposed to the wrong temperatures or shocks.
9. Predictive Maintenance
To forecast the remaining useful life of assets and ensure they are repaired before they fail, 29% of all companies have invested in solutions at the intersection of artificial intelligence and maintenance. As IoT Analytics highlighted in a recent blog post, predictive maintenance (PdM) is an en vogue topic, with 40% of interviewed decision makers planning to invest significant amounts of money in the coming two years.
“PdM enabled us to actively monitor highly critical assets, provided us with multiple feeds of various data points (temp, vibration, throughput), allowed [us] to perform analysis of the asset health in real time, and helped us to prevent the need [for] reactive maintenance actions for critical assets.” IT director at an Oil&Gas company in the United States
Colgate-Palmolive Company , a US-based consumer goods manufacturer, leveraged a predictive maintenance solution to optimize its manufacturing capacity and prevent costly downtime. It installed wireless sensors on 2,000 machines (including tube makers and liquid machines) to collect machine data. The predictive maintenance solution detected increased temperatures and alerted staff, thereby preventing nearly 200 hours of downtime and a missing output of 2.8 million tubes of toothpaste.
Predictive Maintenance = The use of real-time IoT sensor data and artificial intelligence (AI) techniques to determine when maintenance should be performed on specific equipment.
10. On-Site Track and Trace
The availability of low-cost sensors and trackers has made it easy and relatively cheap to track goods and tools at construction sites, ports, and even inside buildings (e.g., factories). Of the experts interviewed, 29% have implemented such a solution. The median spending for a fully functional, on-site track-and-trace solution (in our interview set) is only $25,000—one of the lowest in the data set. Costs can scale up, however, as more and more assets get connected.
“Having On-Site Track & Trace has improved our efficiency [and] reduced labor time for tracking items (we have almost 1,000 stock-keeping units). We are now also able to check stock balances in real time.” Head of IT at a retailer in Singapore
Continental , a German automotive supplier, implemented KINEXON’S real-time locating solution at its logistics center and production site in Regensburg. More than 50 transportation vehicles and more than 3,000 load carriers are tracked live inside the 30,000 m² building sites, allowing Continental to locate each of these items within a precision of several centimeters (inches), thereby creating a transparent material flow in the supply chain and increasing overall throughput.
On-Site Track and Trace = Tracking and tracing goods while on site (e.g., at production sites, ports, logistics centers, or warehouses).
More information and further reading
Are you interested in learning more about iot use cases.
The IoT Use Case Adoption Report 2021 is a comprehensive 430-page report examining the adoption of 48 IoT use cases across 4 types: smart operations, smart supply chain, connected products, and connected transport. It includes the adoption (by region/country, industry), a detailed breakdown of spending, ROI per use case, key vendors & vendor satisfaction, as well as best practices and lessons learnt. It is part of IoT Analytics’ ongoing coverage of IoT in general .
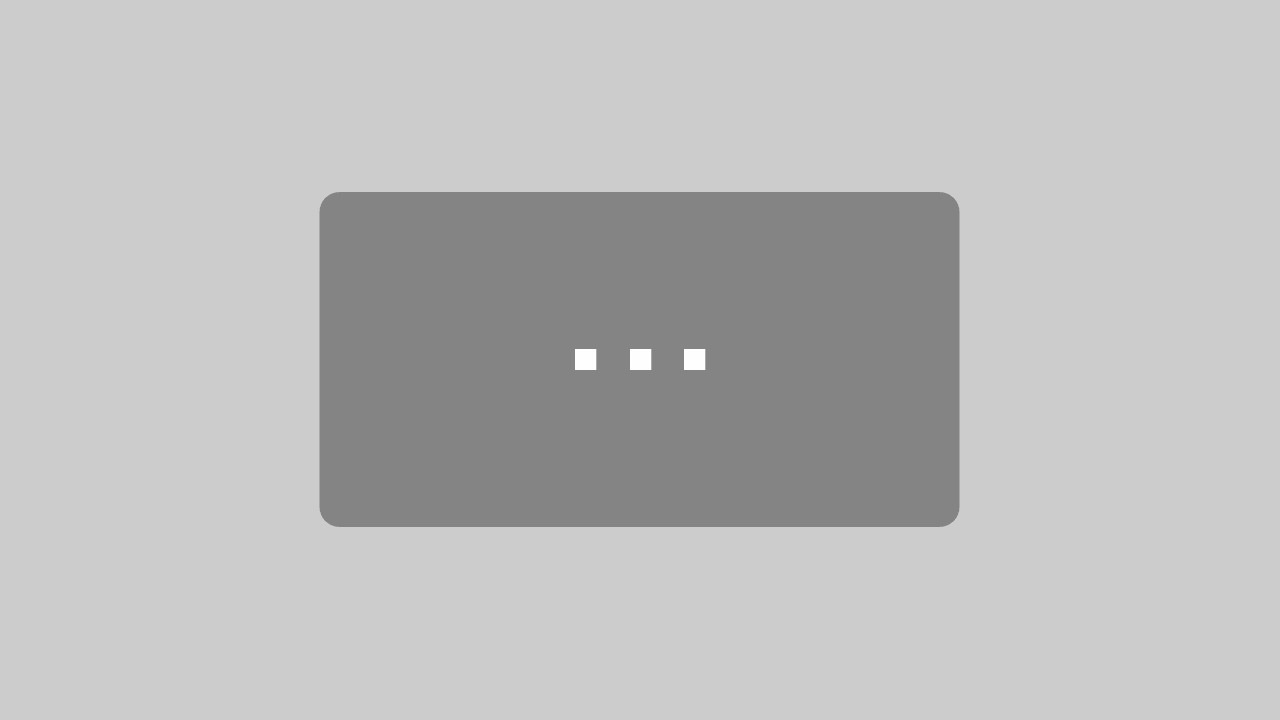
By loading the video, you agree to YouTube’s privacy policy. Learn more
Always unblock YouTube
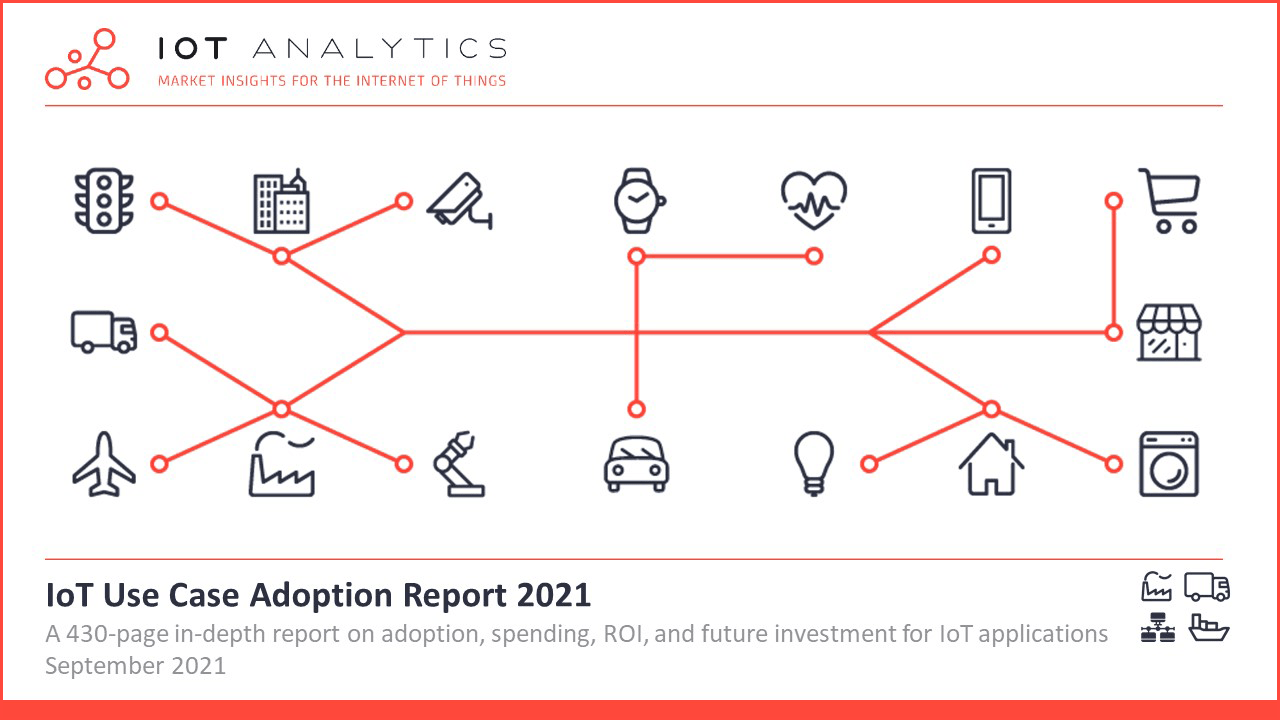
This report provides answers to the following questions (among others):
- What are the most adopted IoT use cases (out of a list of 48 use cases)?
- Which IoT use cases have the highest ROI?
- How much do companies spend to implement IoT use cases?
- How much do they spend on hardware, software and services respectively?
- Which IoT use cases do companies plan to invest in in the next 2 years?
- Who are the leading vendors for IoT use cases and how satisfied are end-users?
- What supporting technologies do IoT end-users implement as part of their IoT use case projects?
- What are the best practices and lessons learned of companies that have adopted the IoT?
- And more…
The sample of the report gives you a holistic overview of the available analysis (outline, key slides). The sample also provides additional context on the topic and describes the methodology of the analysis. You can download the sample here :
Related articles
You may also be interested in the following recent articles:
- State of IoT 2021: Number of connected IoT devices growing 9% to 12.3 billion globally, cellular IoT now surpassing 2 billion
- IoT technology market attractiveness: Where to invest going into 2022
- Top 10 IoT applications in 2020
- The top 10 Smart City use cases that are being prioritized now
- How to create a successful IoT business model – insights from early innovators
- Predictive Maintenance Market: The Evolution from Niche Topic to High ROI Application
Are you interested in continued IoT coverage and updates?
Subscribe to our newsletter and follow us on LinkedIn and Twitter to stay up-to-date on the latest trends shaping the IoT markets. For complete enterprise IoT coverage with access to all of IoT Analytics’ paid content & reports including dedicated analyst time check out Enterprise subscription .
Share this with others:
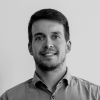
Philipp Wegner
Iot research newsletter.
Sign up for our exclusive email updates today, and receive the latest market insights before others.
Most Recent Articles
- Benchmarking IoT mobile operator pricing: MNOs vs. MVNOs
- IoT Startup Landscape 2024: 7 notable insights
- The top 6 paradigms shaping the factory of the future—insights from 500 manufacturers
- Global cellular IoT connectivity market reached $15B in 2023, 5G set to drive further growth
- IoT market update: Enterprise IoT market size reached $269 billion in 2023, with growth deceleration in 2024
Search the Blog
Blog categories.
- Industrial IoT
- Non-industrial IoT applications
- IoT Platforms & Software
- IoT Connectivity & Hardware
- General IoT
IoT Analytics, founded and operating out of Germany, is a leading provider of strategic IoT market insights and a trusted advisor for 1000+ corporate partners worldwide.
Learn more about how we can help you achieve your goals faster with the right data-driven insights and intelligence.
We need your consent before you can continue on our website. We use cookies and other technologies on our website. Some of them are essential, while others help us to improve this website and your experience. You can find more information about the use of your data in our privacy policy .
- External Media
Accept only essential cookies
Individual Privacy Preferences
Cookie Details Privacy Policy Legal Notice
We use cookies and other technologies on our website. Some of them are essential, while others help us to improve this website and your experience. You can find more information about the use of your data in our privacy policy . Here you will find an overview of all cookies used. You can give your consent to whole categories or display further information and select certain cookies.
Accept all Save Accept only essential cookies
Essential cookies enable basic functions and are necessary for the proper function of the website.
Show Cookie Information Hide Cookie Information
Name | |
---|---|
Provider | Owner of this website, |
Purpose | Saves the visitors preferences selected in the Cookie Box of Borlabs Cookie. |
Cookie Name | borlabs-cookie |
Cookie Expiry | 1 Year |
Statistics cookies collect information anonymously. This information helps us to understand how our visitors use our website.
Accept | |
---|---|
Name | |
Provider | Google Ireland Limited, Gordon House, Barrow Street, Dublin 4, Ireland |
Purpose | Cookie by Google used for website analytics. Generates statistical data on how the visitor uses the website. |
Privacy Policy | |
Cookie Name | _ga,_gat,_gid |
Cookie Expiry | 2 Months |
Marketing cookies are used by third-party advertisers or publishers to display personalized ads. They do this by tracking visitors across websites.
Accept | |
---|---|
Name | |
Provider | Crisp IM SAS |
Purpose | Cookie by Crisp used for live chat and messaging. |
Privacy Policy | |
Cookie Name | crisp-client* |
Accept | |
---|---|
Name | |
Provider | HubSpot Inc., 25 First Street, 2nd Floor, Cambridge, MA 02141, USA |
Purpose | HubSpot is a user database management service provided by HubSpot, Inc. We use HubSpot on this website for our online marketing activities. |
Privacy Policy | |
Host(s) | *.hubspot.com, hubspot-avatars.s3.amazonaws.com, hubspot-realtime.ably.io, hubspot-rest.ably.io, js.hs-scripts.com |
Cookie Name | __hs_opt_out, __hs_d_not_track, hs_ab_test, hs-messages-is-open, hs-messages-hide-welcome-message, __hstc, hubspotutk, __hssc, __hssrc, messagesUtk |
Cookie Expiry | Session / 30 Minutes / 1 Day / 1 Year / 13 Months |
Accept | |
---|---|
Name | |
Provider | OneSignal, Inc. |
Purpose | Cookie by OneSignal used for in-browser push notifications. |
Privacy Policy | |
Cookie Name | __cf_bm |
Content from video platforms and social media platforms is blocked by default. If External Media cookies are accepted, access to those contents no longer requires manual consent.
Accept | |
---|---|
Name | |
Provider | Meta Platforms Ireland Limited, 4 Grand Canal Square, Dublin 2, Ireland |
Purpose | Used to unblock Facebook content. |
Privacy Policy | |
Host(s) | .facebook.com |
Accept | |
---|---|
Name | |
Provider | Google Ireland Limited, Gordon House, Barrow Street, Dublin 4, Ireland |
Purpose | Used to unblock Google Maps content. |
Privacy Policy | |
Host(s) | .google.com |
Cookie Name | NID |
Cookie Expiry | 6 Month |
Accept | |
---|---|
Name | |
Provider | Meta Platforms Ireland Limited, 4 Grand Canal Square, Dublin 2, Ireland |
Purpose | Used to unblock Instagram content. |
Privacy Policy | |
Host(s) | .instagram.com |
Cookie Name | pigeon_state |
Cookie Expiry | Session |
Accept | |
---|---|
Name | |
Provider | Openstreetmap Foundation, St John’s Innovation Centre, Cowley Road, Cambridge CB4 0WS, United Kingdom |
Purpose | Used to unblock OpenStreetMap content. |
Privacy Policy | |
Host(s) | .openstreetmap.org |
Cookie Name | _osm_location, _osm_session, _osm_totp_token, _osm_welcome, _pk_id., _pk_ref., _pk_ses., qos_token |
Cookie Expiry | 1-10 Years |
Accept | |
---|---|
Name | |
Provider | Twitter International Company, One Cumberland Place, Fenian Street, Dublin 2, D02 AX07, Ireland |
Purpose | Used to unblock Twitter content. |
Privacy Policy | |
Host(s) | .twimg.com, .twitter.com |
Cookie Name | __widgetsettings, local_storage_support_test |
Cookie Expiry | Unlimited |
Accept | |
---|---|
Name | |
Provider | Vimeo Inc., 555 West 18th Street, New York, New York 10011, USA |
Purpose | Used to unblock Vimeo content. |
Privacy Policy | |
Host(s) | player.vimeo.com |
Cookie Name | vuid |
Cookie Expiry | 2 Years |
Accept | |
---|---|
Name | |
Provider | Google Ireland Limited, Gordon House, Barrow Street, Dublin 4, Ireland |
Purpose | Used to unblock YouTube content. |
Privacy Policy | |
Host(s) | google.com |
Cookie Name | NID |
Cookie Expiry | 6 Month |
Privacy Policy Legal Notice
- Internet of things platform
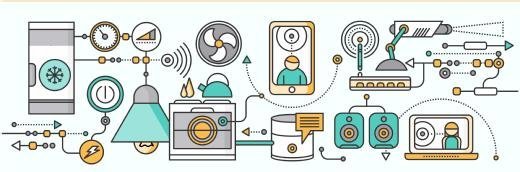
Use cases and benefits of smart sensors for IoT
Smart sensors are key to developing iot applications, as they help modernize and streamline analytics and connectivity. catch up on the types of iot sensors, their use cases and more..
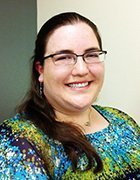
- Sharon Shea, Executive Editor
The internet of things couldn't exist without smart sensors.
While the use of sensor technology has long affected how manufacturers, industrial organizations and utilities operate, the addition of IoT to sensors has expanded their impact and use cases, bringing more connectivity and analytics to processes than ever before. Today, smart factories, smart cities, smart buildings and connected individuals are using an array of smart sensors to collect real-time data for real-time insights and decision-making.
However, these aren't your grandfather's sensors. They may collect some of the same data as the sensors of yesteryear, but today's smart sensors are internet-enabled and more sophisticated than ever.
Let's dive into how smart sensors work and their use cases across various verticals.
What is a smart sensor?
In general, a smart sensor has three major components: a sensor that captures data from an environment; a microprocessor, which computes on the output of the sensor via programming; and communications capabilities that enable the sensor to communicate the microprocessor's output for action.
A smart sensor may include multiple types of sensors (see sidebar), as well as transducers, transceivers, amplifiers, analog filters and a power source, among other components.
To be most effective, internet of things sensors should include wireless communications, be smart enough to compute data remotely and be programmable to accommodate new capabilities as needed, said Institute of Electrical and Electronics Engineers senior member Shawn Chandler.
Types of IoT sensors
"Sensors for use in IoT may be purposed for nearly any form of measurement, providing a mechanism that links desired observations from the field to an application," Chandler said.
To categorize sensors, he said, think of them in terms of the physics domains.
- Light and electromagnetism sensors. These include RFID sensors for retail and logistics applications, imaging and identity sensors for security applications, light sensors for automation in smart buildings, and radioactivity sensors for safety and health applications.
- Thermal sensors. For example, room temperature monitoring in smart buildings, environmental monitoring or material temperature monitoring in industrial process management.
- Vibration and sound sensors. For example, seismic sensors for regional safety; sound pressure for health monitoring and industrial process automation; specific sound monitoring for civil management applications, such as gunshot noise detection; personal health applications, such as fitness and health wearables; or vibration sensors for reliability-based maintenance of equipment in plants or for industrial organizations.
- Matter and materials sensors. Many applications for environmental, safety, health, agricultural and environmental automation require specific fluid and gas sensors, such as those to detect a chemical presence or oxygen, carbon dioxide, humidity or water levels.
- Time and space sensors. For example, Geographic Information System location sensors for logistics management; personal applications; or location, velocity and acceleration sensors for vehicle and traffic management.
IoT sensor use cases
Smart sensors serve three broad purposes. First, they provide greater visibility into existing processes and workflows, identifying items, locating them and determining their environmental conditions. For example, smart sensors in industrial IoT environments can keep track of temperature and humidity, can log data for historical records and quality management, or can be used as triggers for alarms or process management in plants or warehouses.
Second, IoT sensors can be embedded into products to improve processes or the products themselves. In the manufacturing process, sensors can monitor, control and improve operations or be added to logistics to streamline how products are delivered. When embedded in devices, smart products can create new revenue possibilities.
Third, the lower cost and more advanced capabilities of sensors enable wider and more effective use cases. The cost of physical sensors and RFID tags has dropped considerably, as have the costs of smart sensor software applications, connectivity options and deployments.
Smart sensor benefits
The three use cases of IoT sensors outlined above lead to three categories of benefits, said Paul Miller, a senior analyst at Forrester Research.
First, the increased visibility IoT sensors provide into existing workflows and processes enable companies to see where things are thanks to real-time data. For example, logistics companies can outfit their fleet of vehicles with sensors to detect the location of each truck.
As companies begin to mine data from those sensors, Miller said, the second benefit emerges: predicting what comes next. In the same logistics example, collecting real-time data about where a fleet has been and how long trucks take to complete their routes can enable the company to predict when drivers will deliver packages. Smart sensors in logistics can also gather information about a driver's driving style and the size and weight of packages, as well as external data, such as traffic or weather conditions that may affect delivery.
"Now you build a rich and, hopefully, accurate model that can be used to improve communication with customers, optimize loading and routing, or reduce costs and emissions," Miller said.
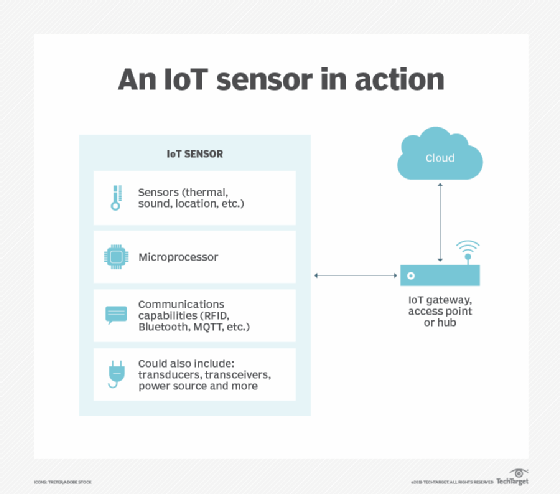
In another example, smart sensors in manufacturing can collect historical machine data that companies can use to build models to predict when machine components are likely to fail.
"Instead of facing the potentially huge cost of unplanned downtime for a production line or factory, suspect components can be proactively replaced during scheduled periods of time," Miller said.
The third benefit of smart sensors relates to revenue generation ; IoT creates a number of new business models. With data about how a machine operates, how it is used and how it wears out, manufacturers can offer outcome-based services to customers, Miller said. Manufacturers can now include consumables, servicing and even replacement parts in the price of a machine.
"Companies like Kaeser Compressor in Germany, which traditionally made and sold compressed air machines, are now able to offer compressed air as a service and charge customers by the liter of compressed air consumed," Miller said. "Consumables, spare parts, servicing and even the machine itself are only paid for when they're needed and used."
RFID and IoT
RFID tags are IoT sensors that collect data about the specific object to which a tag is attached. Commonly used in supply chain management, ID badging and access control, RFID tagging involves sending data using specific radio wave emissions.
RFID has two forms, Chandler said: active and passive. An active RFID tag contains an onboard power supply, enabling a tag to communicate data at any time and over larger distances. A passive RFID tag may not communicate unless it is activated by a radio frequency emission. It then uses the radio wave captured by the antenna in the tag to activate data collection and power the return communication path.
The future of smart sensors
Forrester Research predicts that smart sensor-based, track-and-trace and smart products will be the fastest-growing use cases, with 24.2% and 24% compound annual growth rates respectively. But the applications won't stop there.
"Sensors are increasingly being built into products at the point of manufacture," Miller said. "Industrial equipment, trains, planes and buildings increasingly arrive with hundreds or even thousands of sensors already connected."
However, internet of things sensors aren't limited to only new products.
"In markets like industrial manufacturing, where machines may have a life span measured in decades, there's also a healthy market in retrofitting sensors to older equipment," Miller said, noting that lowered sensor costs combined with the growing prevalence of infrastructure that can support IoT data flows will result in companies deploying sensors in greater numbers.
"Where today, a logistics company might install sensors in a truck, tomorrow it may be cost-effective to install sensors in every pallet or even every package," Miller said.
The arrival of 5G, he added, will eventually make it easier to work with a large number of sensors, even in environments where data latency causes problems today.
Also of note is the increased use of edge computing. As more analytics, including the use of machine learning and artificial intelligence, is run at the point of data collection , organizations -- or the sensors themselves or the IoT edge gateways the sensors connect to -- will be able to make decisions faster and more effectively.
Chandler noted that the continued miniaturization of sensors will change the future sensor landscape.
"Sensors will continue to get smaller over time and more capable in terms of computational capability," he said.
Sensors will also offer more functions in the same platform, serving different sensing categories from the same devices.
"Sensors will be installed in more and more devices, with everything from wearables to special-purpose sensors linking observations from our environment to a computing platform," Chandler said. "Estimates from pundits in the industry expect that the total number of IoT devices -- and, thus, possible sensors -- will triple in the next six years, with roughly 25 billion devices today, growing to 75 billion devices in 2025."
Additional reporting by Jim O'Donnell.
Explore how smart sensors in IoT affect manufacturing, construction and parking
Learn the top seven challenges to IoT adoption
Read how pervasive sensing affects security
An overview of IoT sensor types and challenges
Dig Deeper on Internet of things platform
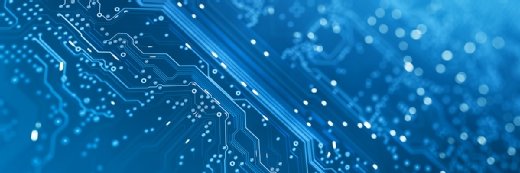
Testing IoT devices helps ensure deployment success
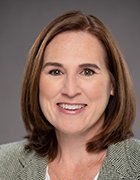
internet of medical things (IoMT) or healthcare IoT
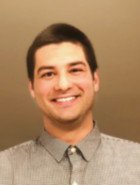
thing (in the internet of things)
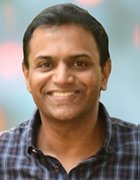
RFID vs. IoT: What are the differences?
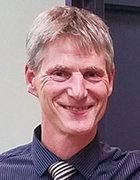
The next U.S. president will set the tone on tech issues such as AI regulation, data privacy and climate tech. This guide breaks ...
Financial services firm JPMorgan Chase seeks to balance technology modernization and cost optimization as it pursues initiatives...
Businesses nationwide will be able to forego the U.S. Federal Trade Commission's Sept. 4 deadline for compliance with the ...
The Department of Justice joined a whistleblower lawsuit against Georgia Tech for allegedly misleading the Department of Defense ...
Lumen Technologies researchers have observed exploitation of CVE-2024-39717 against four U.S. organizations in the ISP, MSP and ...
While SecOps has become easier in some ways, enterprises still struggle with areas such as data volumes, threat intelligence ...
Cloud and on-premises subnets use IP ranges, subnet masks or prefixes, and security policies. But cloud subnets are simpler to ...
Satellite connectivity lets Broadcom offer the VeloCloud SD-WAN as an option for linking IoT devices to the global network from ...
Enterprises have been slow to adopt Wi-Fi 7, as they find previous generations suit their needs. However, experts predict ...
New capabilities for VMware VCF can import and manage existing VMware services through a single console interface for a private ...
Due to rapid AI hardware advancement, companies are releasing advanced products yearly to keep up with the competition. The new ...
AMD plans to acquire AI systems designer and manufacturer ZT Systems for $5 billion. AMD CEO Lisa Su said hyperscalers want more ...
The observability specialist's latest financing, along with strong recurring revenue and customer growth, help set the vendor up ...
Serverless, first launched on AWS, is now available on all three major public clouds in a move aimed at enabling customers to ...
Implementing a data governance strategy requires a roadmap to keep everyone on track and overcome challenges. Follow eight key ...
14 Types of IoT Sensors Available Today
These IoT sensors are the glue keeping the Internet of Things connected.

When it comes to the Internet of Things , or the network of smart devices, IoT sensors play a critical role. Essentially, these sensors are integrated into devices like smartphones, doorbells, thermostats and even cars and refrigerators to capture important data that can be shared in real time with users.
Increasingly, cities, municipalities and buildings are also using devices with IoT sensors to monitor, analyze and manage resources, weather, traffic and heating and cooling systems.
Top IoT Sensors
Accelerometers .
- Air quality sensors
- Biomedical sensors
- Chemical sensors
- Electric current sensors
- Flow sensors
- Humidity sensors
- Level sensors
- Motion sensors
- Pressure sensors
- Proximity sensors
- Temperature sensors
What Is an IoT sensor?
An IoT sensor is any sort of mechanism or tool, such as a camera or air quality monitor, integrated into a device. These sensors gather information — like water levels, air temperature and traffic patterns — related to the environments in which they’re deployed and transmit it to the cloud via Wi-Fi, bluetooth, 5G or other mobile network.
Take smart thermostats, for instance, which are equipped with temperature sensors that measure fluctuations in a home’s temperature. Because these thermostats are connected to the internet, users can log in via an app to monitor the temperature of their home and control the thermostat remotely.
Explore the Future of IoT How Will the Internet of Things (IoT) Evolve?
What Are IoT Sensors Used For?
IoT sensors gather information so devices can be used remotely and data can be shared in real time. The data gathered by IoT sensors and sent to the cloud is analyzed by software that can make sense of the information and then sent to users. This data is used to track trends and gather insights about everything from efficiency and energy use in factories to athletic performance and a user’s health.
Types of IoT Sensors
These are some of the most popular IoT sensors found in smart devices like wearables , smartphones and other monitoring tools used by individuals, organizations and cities.
Accelerometers measure acceleration — basically the change in speed, direction and intensity of movement.
How is the data from these IoT sensors used? Accelerometers are used to collect data from connected devices such as wearables, medical alert devices, cameras and cars to track activity. Tablets and smartphones also rely on accelerometers to know when to rotate displays based on the device’s physical orientation.
Air Quality Sensors
Air quality sensors measure levels of pollution, carbon dioxide and other particulates in the air.
How is the data from these IoT sensors used? Air quality sensors gather data about what’s in the air and transmit their findings to the cloud. Cities typically use devices with air quality sensors so they can access real-time air quality conditions to issue warnings to residents if needed and track trends for study. Air quality sensors are being used in homes as well, detecting harmful particulates that could be indoors from appliances like gas stoves , which could warn residents to open a window or turn on an exhaust fan.
Biomedical Sensors
Biomedical sensors measure a person’s vital signs, like heart rate and oxygen level.
How is the data from these IoT sensors used? Wearable devices like smartwatches and arm bands can be equipped with biomedical sensors. These devices communicate the data they collect back to the wearer so they can monitor their own health, or to a caregiver or medical staff, who is monitoring a patient or loved one remotely.
Cameras and image sensors capture visual data and images.
How is the data from these IoT sensors used? Everything from smart refrigerators to autonomous vehicles rely on cameras to detect objects and monitor their surroundings. Cameras are also critical to smart cities as well as home and business security — think Ring cameras notifying a homeowner when someone is at their door, even if they aren’t there themselves. Cameras also play a role in factory and warehouse automation, helping perform tasks like product inspections.
Chemical Sensors
Chemical sensors detect chemical changes like radiation and pH levels in air or liquids.
How is the data from these IoT sensors used? Chemical sensors are used for environmental monitoring, like detecting chemical leaks in factories. They’re also being integrated into wearable devices to monitor the chemical makeup of sweat, saliva and even tears for more timely treatment and therapeutic care.
Electric Current Sensors
These sensors measure the amount of electricity running through a wire. They do so by monitoring the magnetic field the current generates along the wire.
How is the data from these IoT sensors used? Electric current sensors are often used to track energy consumption and for remote and real-time monitoring of power systems to manage energy needs in places like data centers.
More on IoT Innovation 24 Industrial Internet of Things Examples to Know
Flow Sensors
Flow sensors measure how quickly a liquid or gas flows past a certain point in a tube or pipe. A flow rate that’s too fast or too slow could indicate a problem, like a leak.
How is the data from these IoT sensors used? Cities typically use flow sensors to help manage water systems and detect leaks. These sensors are also a key component of smart metering devices, which monitor how water or other resources like natural gas are used and consumed.
Gyroscopes sense a device’s angular velocity, the speed of rotation around an axis.
How is the data from these IoT sensors used? In industrial settings, gyroscopes can be used to detect and identify safety issues, like careless forklift driving inside a warehouse or speeding delivery vehicles out on the road. They’re typically used in conjunction with accelerometers for display orientation on smartphones, tablets and other connected devices like gaming consoles.
Humidity Sensors
Humidity sensors monitor humidity, or the amount of water vapor, in the air.
How is the data from these IoT sensors used? These sensors are often used in smart building management and industrial settings to better manage comfort and energy use. They are typically paired with temperature sensors and other sensors like water sensors for remote monitoring of the environment.
Level Sensors
Level sensors monitor the level of liquids in a contained environment.
How is the data from these IoT sensors used? The data these sensors gather and report are critical when it comes to leak detection in industrial settings.
Motion Sensors
These sensors use infrared radiation or ultrasonic waves to detect motion.
How is the data from these IoT sensors used? Safety and surveillance is a common use case as motion sensors can detect the presence of an object or person from the heat it emits or by bouncing ultrasonic waves off the object.
Pressure Sensors
Pressure sensors measure the amount of pressure applied to a liquid or gas.
How is the data from these IoT sensors used? Pressure sensors, which are used to detect the rise and fall of pressure, are used for leak detection and used to maintain water and heating systems in real time.
Proximity Sensors
These sensors bounce infrared radiation or ultrasonic waves off objects to detect their presence.
How is the data from these IoT sensors used? Proximity sensors, which are similar to motion sensors, also use infrared radiation or ultrasonic waves to measure the distance between objects, which is used in vehicles, smart cities for monitoring traffic, and industrial settings to track how parts, people and other objects move through a factory.
More on IoT Applications We Were Promised Smart Cities. What Happened?
Temperature Sensors
Temperature sensors detect changes in temperature, measuring the amount of heat there is in an environment.
How is the data from these IoT sensors used? Temperature sensors are used to monitor air or water temperature and are common in smart buildings and smart thermostats. Increasingly, food transport companies use these sensors to monitor any rise or fall in temperature of food storage areas. They’re also used in agriculture to monitor the temperature of soil and can provide real-time updates to farmers.
Recent Hardware Articles
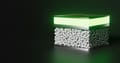
Information
- Author Services
Initiatives
You are accessing a machine-readable page. In order to be human-readable, please install an RSS reader.
All articles published by MDPI are made immediately available worldwide under an open access license. No special permission is required to reuse all or part of the article published by MDPI, including figures and tables. For articles published under an open access Creative Common CC BY license, any part of the article may be reused without permission provided that the original article is clearly cited. For more information, please refer to https://www.mdpi.com/openaccess .
Feature papers represent the most advanced research with significant potential for high impact in the field. A Feature Paper should be a substantial original Article that involves several techniques or approaches, provides an outlook for future research directions and describes possible research applications.
Feature papers are submitted upon individual invitation or recommendation by the scientific editors and must receive positive feedback from the reviewers.
Editor’s Choice articles are based on recommendations by the scientific editors of MDPI journals from around the world. Editors select a small number of articles recently published in the journal that they believe will be particularly interesting to readers, or important in the respective research area. The aim is to provide a snapshot of some of the most exciting work published in the various research areas of the journal.
Original Submission Date Received: .
- Active Journals
- Find a Journal
- Proceedings Series
- For Authors
- For Reviewers
- For Editors
- For Librarians
- For Publishers
- For Societies
- For Conference Organizers
- Open Access Policy
- Institutional Open Access Program
- Special Issues Guidelines
- Editorial Process
- Research and Publication Ethics
- Article Processing Charges
- Testimonials
- Preprints.org
- SciProfiles
- Encyclopedia
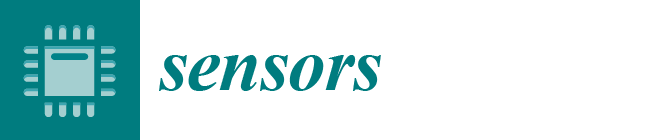
Article Menu
- Subscribe SciFeed
- PubMed/Medline
- Google Scholar
- on Google Scholar
- Table of Contents
Find support for a specific problem in the support section of our website.
Please let us know what you think of our products and services.
Visit our dedicated information section to learn more about MDPI.
JSmol Viewer
Low-cost iot-based sensor system: a case study on harsh environmental monitoring.
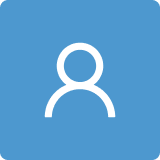
1. Introduction
2. methods and measurement principles, 2.1. sensing principle and circuitry, 2.2. sensor integration, 2.2.1. sensor selection, 2.2.2. power management, 2.2.3. communication method and display, 2.3. sensor calibration, 3. experimental setup.
- Scenario 1: Hot air flow test—pressure, gas, humidity, and temperature change inside and outside the stainless steel box under a hot air flow to monitor the temperature variation;
- Scenario 2: Hydrogen flow test—pressure, gas, humidity, and temperature change when a hydrogen flow is introduced into the stainless steel box to sense the hydrogen concentration.
4. Results and Discussion
4.1. scenario 1: hot air flow test, 4.2. scenario 2: hydrogen flow test, 5. conclusions and future work, author contributions, institutional review board statement, informed consent statement, data availability statement, acknowledgments, conflicts of interest.
- Nuclear Decommissioning Authority: Annual Report and Accounts 2019 to 2020. Available online: https://www.gov.uk/government/publications/nuclear-decommissioning-authority-annual-report-and-accounts-2019-to-2020 (accessed on 16 September 2020).
- Ewing, R.C.; Von Hippel, F.N. Nuclear Waste Management in the United States—Starting Over. Science 2009 , 325 , 151–152. [ Google Scholar ] [ CrossRef ] [ PubMed ] [ Green Version ]
- Atzori, L.; Lera, A.; Morabito, G. The Internet of Things: A Survey. Comput. Netw. 2010 , 54 , 2787–2805. [ Google Scholar ] [ CrossRef ]
- Da Xu, L.; He, W.; Li, S. Internet of Things in Industries: A Survey. IEEE Trans. Ind. Inform. 2014 , 10 , 2233–2243. [ Google Scholar ]
- Maitra, S.; Abdelgawad, A.; Yelamarthi, K. Lab in a Box: A Rapidly Deployable Environmental Monitoring IoT System. In Proceedings of the 2019 IEEE 62nd International Midwest Symposium on Circuits and Systems (MWSCAS), Dallas, TX, USA, 4–7 August 2019. [ Google Scholar ]
- Maurizio, R.; Brunelli, D. Ultra Low Power Wireless Gas Sensor Network for Environmental Monitoring Applications. In Proceedings of the 2012 IEEE Workshop on Environmental Energy and Structural Monitoring Systems (EESMS), Perugia, Italy, 28 September 2012. [ Google Scholar ]
- Wang, D.; Agrawal, D.P.; Toruksa, W.; Chaiwatpongsakorn, C.; Lu, M.; Keener, T.C. Monitoring Ambient Air Quality with Carbon Monoxide Sensor-Based Wireless Network. Commun. ACM 2010 , 53 , 138–141. [ Google Scholar ] [ CrossRef ]
- Tang, K.-T.; Chiu, S.-W.; Hao, H.-C.; Wei, S.-C.; Lin, T.-H.; Yang, C.-M.; Yao, D.-J.; Yeh, W.-C. An Electronic-Nose Sensor Node Based on Polymer-Coated Surface Acoustic Wave Array for Environmental Monitoring. In Proceedings of the 2010 International Conference on Green Circuits and Systems, Shanghai, China, 21–23 June 2010. [ Google Scholar ]
- Vana, J.; Magno, M.; Paci, G.; Brunelli, D.; Benini, L. Design, Characterization and Management of a Wireless Sensor Network for Smart Gas Monitoring. In Proceedings of the 2011 4th IEEE International Workshop on Advances in Sensors and Interfaces (IWASI), Savelletri di Fasano, Italy, 28–29 June 2011. [ Google Scholar ]
- Vana, J.; Magno, M.; Brunelli, D.; Paci, G.; Benini, L. Context-Adaptive Multimodal Wireless Sensor Network for Energy-Efficient Gas Monitoring. IEEE Sens. J. 2012 , 13 , 328–338. [ Google Scholar ]
- Sunny, A.I.; Zhang, J.; Tian, G.Y.; Tang, C.; Rafique, W.; Zhao, A.; Fan, M. Temperature Independent Defect Monitoring Using Passive Wireless RFID Sensing System. IEEE Sens. J. 2019 , 19 , 1525–1532. [ Google Scholar ] [ CrossRef ] [ Green Version ]
- Sunny, A.I.; Tian, G.Y. Enhanced Sensitivity of Low Frequency (LF) RFID Sensor Signal for Structural Health Monitoring (SHM) in High Temperature Environment. In Proceedings of the 19 World Conference on Non-Destructive Testing, Munich, Germany, 13–17 June 2016. [ Google Scholar ]
- Tang, C.; Rashvand, H.F.; Tian, G.Y.; Hu, P.; Sunny, A.I.; Wang, H. Structural Health Monitoring with WSNs. In Wireless Sensor Systems for Extreme Environments: Space, Underwater, Underground, and Industrial ; Wiley: London, UK, 2017; pp. 383–408. [ Google Scholar ]
- Sunny, A.I.; Tian, G.Y.; Zhang, J.; Pal, M. Low frequency (LF) RFID sensors and selective transient feature extraction for corrosion characterisation. Sens. Actuators A Phys. 2016 , 241 , 34–43. [ Google Scholar ] [ CrossRef ] [ Green Version ]
- Jun, Z.; Tian, G.Y.; Marindra, A.M.J.; Sunny, A.I.; Zhao, A.B. A Review of Passive Rfid Tag Antenna-Based Sensors and Systems for Structural Health Monitoring Applications. Sensors 2017 , 17 , 265. [ Google Scholar ]
- Flammable Gas Sensor (Model: MQ-8) Manual. Available online: https://cdn.sparkfun.com/datasheets/Sensors/Biometric/MQ-8%20Ver1.3%20-%20Manual.pdf (accessed on 13 September 2020).
- BME680-Low Power Gas, Pressure, Temperature & Humidity Sensor. Available online: https://www.bosch-sensortec.com/media/boschsensortec/downloads/datasheets/bst-bme680-ds001.pdf (accessed on 13 September 2020).
- Thingspeak for Iot Projects. Available online: https://thingspeak.com/ (accessed on 14 September 2020).
- Ghee, O.K.; Zeng, K.; Grimes, C.A. A Wireless, Passive Carbon Nanotube-Based Gas Sensor. IEEE Sens. J. 2002 , 2 , 82–88. [ Google Scholar ]
- Daniel, D.; Hnatiuc, M.; Lazar, I. Acquisition and Calibration Interface for Gas Sensors. In Proceedings of the 2018 IEEE 24th International Symposium for Design and Technology in Electronic Packaging (SIITME), Iasi, Romania, 25–28 October 2018. [ Google Scholar ]
- Banos, A.; Hallam, K.R.; McEwan, C.; Whittaker, D.; Hughes, P.; Scott, T. Proof of concept trials for in-situ testing of filter performance on Sellafield Self Shielded boxes. Prog. Nucl. Energy 2019 , 116 , 10–20. [ Google Scholar ] [ CrossRef ]
Click here to enlarge figure
Gas | |
---|---|
MDPI stays neutral with regard to jurisdictional claims in published maps and institutional affiliations. |
Share and Cite
Sunny, A.I.; Zhao, A.; Li, L.; Kanteh Sakiliba, S. Low-Cost IoT-Based Sensor System: A Case Study on Harsh Environmental Monitoring. Sensors 2021 , 21 , 214. https://doi.org/10.3390/s21010214
Sunny AI, Zhao A, Li L, Kanteh Sakiliba S. Low-Cost IoT-Based Sensor System: A Case Study on Harsh Environmental Monitoring. Sensors . 2021; 21(1):214. https://doi.org/10.3390/s21010214
Sunny, Ali Imam, Aobo Zhao, Li Li, and Sambu Kanteh Sakiliba. 2021. "Low-Cost IoT-Based Sensor System: A Case Study on Harsh Environmental Monitoring" Sensors 21, no. 1: 214. https://doi.org/10.3390/s21010214
Article Metrics
Article access statistics, further information, mdpi initiatives, follow mdpi.
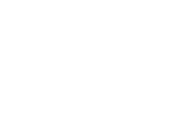
Subscribe to receive issue release notifications and newsletters from MDPI journals
About • Careers • News Support: +44 1624 639437 • Sales: +44 1624 777837
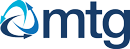
Industry Applications & IoT Case Studies
Smart agriculture.
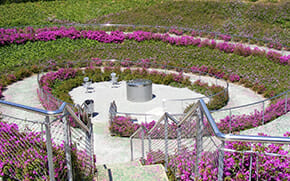
Saving water with Smart Irrigation System in Barcelona
Challenge: Controlling water flow and soil moisture in the parks and gardens of Barcelona. Developing a Smart Irrigation system based on wireless sensor networks to conserve and optimise water use, and implement an effective irrigation system.
Solutions: The solution comprised a remotely monitored irrigating system in Barcelona using IoT technologies from Libelium . The IoT solution helped Barcelona build upon its Smart City status. An integral part of the solution included soil moisture monitoring with probes utilising Libelium technology. The deployment uses remote monitoring of sensors that trigger and control the irrigating system, enabling the intelligent management of the water network.
Technologies: Smart Agriculture Libelium Plug & Sense

Project in Italy to control fertilizer, irrigation and monitor leaf growth
Challenge: Monitoring external factors that affect crop yields to forecast and prevent from diseases, saving water and using less pesticides
Solutions: Plug and Sense technologies from Libelium were installed to monitor environmental parameters such as temperature, humidity, air pressure, luminosity ans leaf wetness. This network of sensors allowed the farmers to remotely observe the conditions of the crops, by capturing data connected to a central system. Analysis preformed on the data captured, resulted in decease in plant diseases, improved health of the crop and lowered the use of fertilisers and chemicals in agricultural yields.
Smart Cities

Meshlium Scanner to monitor passengers’ activity at Manchester Airport
Challenge: Smartphone detection technology to ensure security in one of the busiest airports in Europe
Solutions: Libelium’s Meshlium Scanner were used to detect smart devices, measuring people’s movements inside the airport. Six devices were installed across the terminals in order to understand flows, detect and prevent eventual bottlenecks and especially ensure people’s security. The Meshlium Scanner is able to detect any device working with WiFi or Bluetooth interfaces . These devices can be detected without the need of being connected to any specific Access Point, as a result, 90% of individuals where identified.
Technologies: Meshlium Scanner
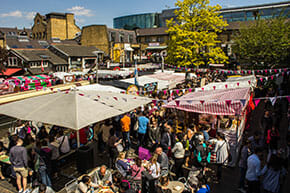
Smartphone detection to measure attendee numbers and behaviours at UK trade fair
Challenge: Detecting volume of visitors and popular locations with Bluetooth and WiFi smartphone detection in a food festival
Solutions: Each year over 267,000 visitors attend the Bolton Food and Drink Festival. To measure the visitor’s activity Libelium’s Meshlium Scanners were deployed around the festival to create a network covering three key locations. The data collected included, duration of stay, visitor volume per location, visitor volume per day, most popular locations, most popular individual and group routes, total project visitors. This data was then sent back to a central system which allowed for real-time analysis.
Smart Environment
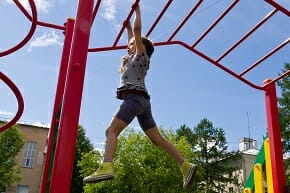
Preventing asthma attacks in children, monitor the air quality of play areas
Challenge: Use of air pollutants sensors in urban areas, focusing on play areas/parks
Solutions: Asthma is one of the most frequent causes of absences in schools. Libelium’s Plug & Sense was chosen to collect, detect and analyse data regarding particle matter, dust and air quality (SO2 and NO). Multiple sensors were deployed in local parks and playgrounds to build up a network and understanding of the local air pollution. Each sensor collected measurements/readings every 10 minutes providing a detailed collection of data.
Technologies: Smart Environment PRO Libelium Plug & Sense
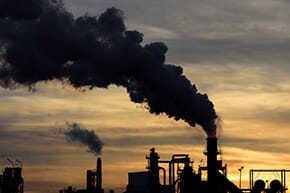
EU project uses Libelium sensors to monitor ozone levels in volunteer homes
Challenge: A network of sensors measure tropospheric ozone, a contaminant that mainly affects rural areas, in Italy, Austria and Spain
Solutions: A network of Libelium Plug & Sense sensors were deployed measuring polluting gases levels, controlling particle pollution, light intensity levels and noise pollution from road traffic, industry and construction. The data captured was then viewed via a mobile and web app.
Technologies: Smart Cites PRO Smart Environment PRO Libelium Plug & Sense
Smart Water

Controlling fish farms water quality with smart sensors in Iran
Challenge: Monitoring water quality to improve fish farms productivity, diminishing casualties, and reducing costs and time
Solutions: Libelium’s Plug & Sense sensors were deployed with the main objective to control water quality in order to increase quality and productivity as well as to reduce costs and increase profits. The sensors recorded the following values, water temperature, dissolved Oxygen, pH levels, Ammonium and Nitrite. The data collected allowed for real-time control over the fish ponds ensuring the utmost efficiency. The result of deploying the sensors, reduce production costs, saved time, reduced fish fatalities and increased productivity.
Technologies: Smart Water Smart Water Ions Libelium Plug & Sense
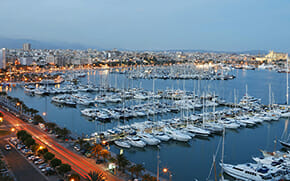
Smart marina: monitoring mooring berths, sea level and weather conditions
Challenge: Modernize marinas by monitoring mooring berths, measuring the sea level, water quality and observing weather conditions.
Solutions: A collection of different Libelium Plug & Sense sensors were deployed. These sensors measured the pH levels, Oxidation, sea level, temperature and berth occupation using ultrasound. The data collected can then be viewed on a mobile app providing users with a complete overview of the marina.
Technologies: Smart Water Smart Security Smart Cities Libelium Plug Sense
Smart Parking
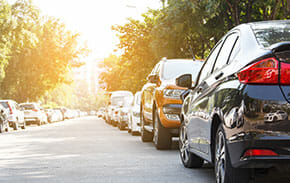
Smart Parking project in Montpellier to relieve traffic congestion and reduce car parking search
Challenge: Controlling car park areas to take actions in order to reduce traffic congestion in the centre of the city.
Challenge: Libelium Parking Sensors were distributed to help members of the public quickly find free parking spaces, to help reduce congestion and journey times. The sensors also collect road temperature measurements, which helps to identify adverse road conditions.
Technologies: Smart Parking Sensors
IoT Insights and Blog Posts
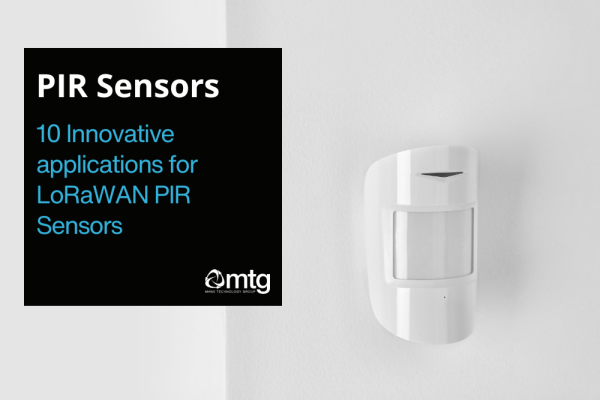
6 x Innovative Applications for IoT PIR Sensors
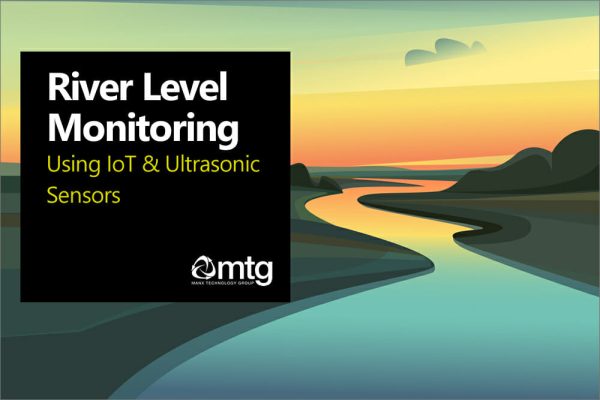
IoT & Ultrasonic Sensors: Revolutionising River Level Monitoring
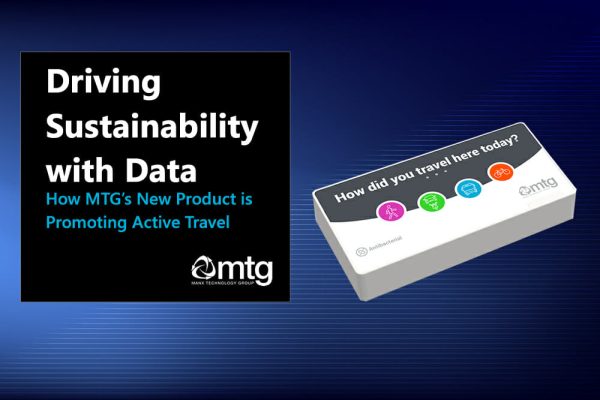
Driving Sustainability with Data: How MTG’s New Product is Promoting Active Travel
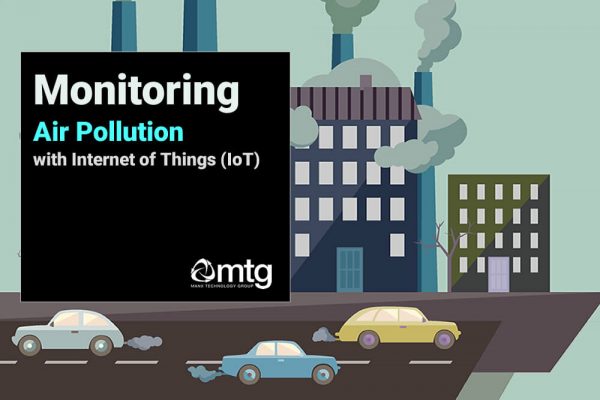
Monitoring air pollution with Internet of Things (IoT)
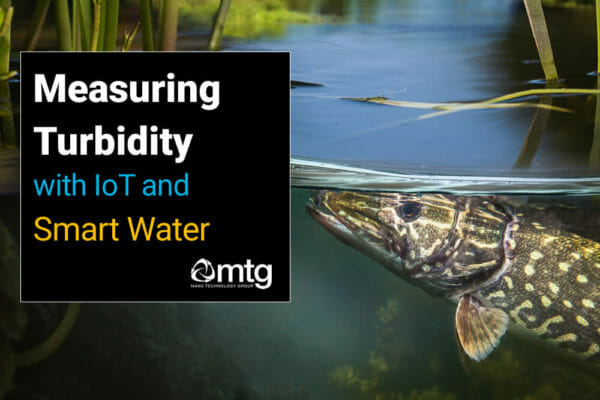
Measuring Turbidity with IoT – Water Quality
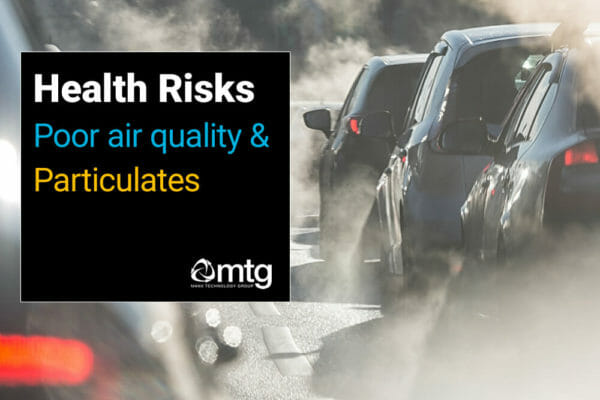
Health Risks – Poor air quality and particulates
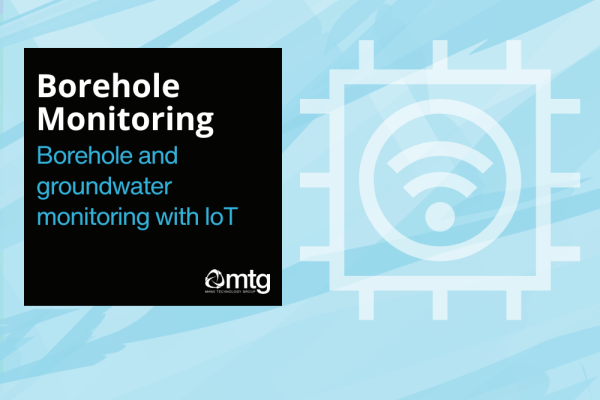
Borehole and groundwater monitoring with IoT
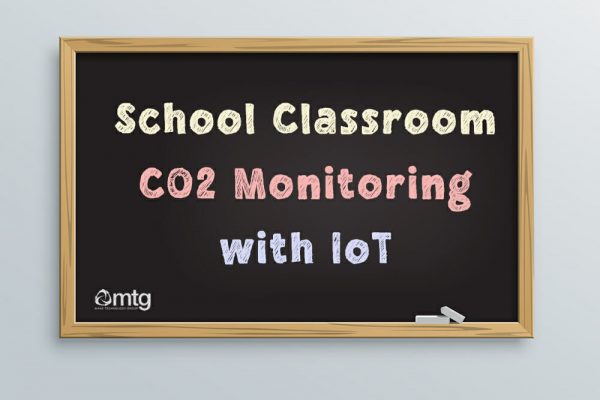
College and School classroom CO2 monitoring
Request quote.
- Name First Last
- Company Name *
- Service of Interest Select the service or product of interest -- Technical Services -- IT Support 24x7 Helpdesk Field Services / Hardware Support / Logistics Firewalls / Network Security -- Software -- Bespoke Software Development Web Design / Web Development -- Internet of Things -- Libelium hardware supply IoT Solutions IoT Consultancy Is there a specific service or product you are interested in?
- Additional Information
- Consent I would like to receive news and insights from Manx Technology Group You can unsubscribe at any time. Our privacy policy is available at www.manxtechgroup.com/legal
- Comments This field is for validation purposes and should be left unchanged.
IoT-Based Environmental Monitoring: Types and Use Cases
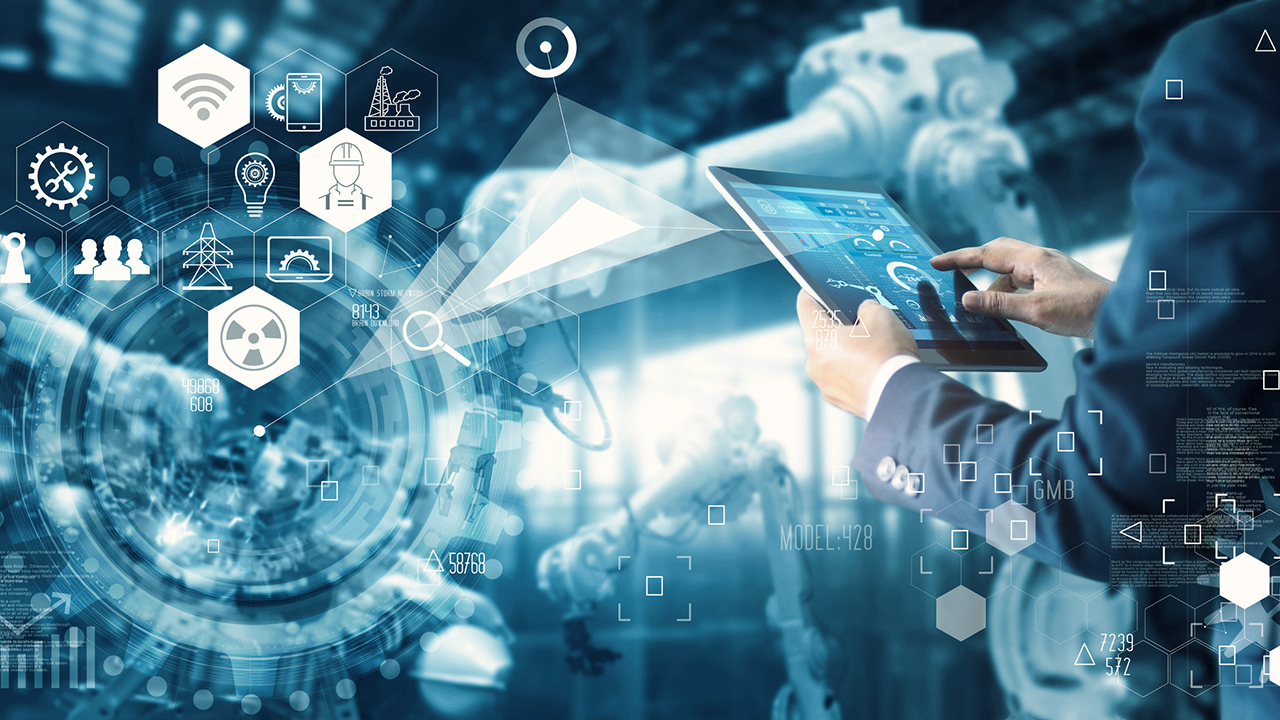
What Is IoT-Based Environmental Monitoring?
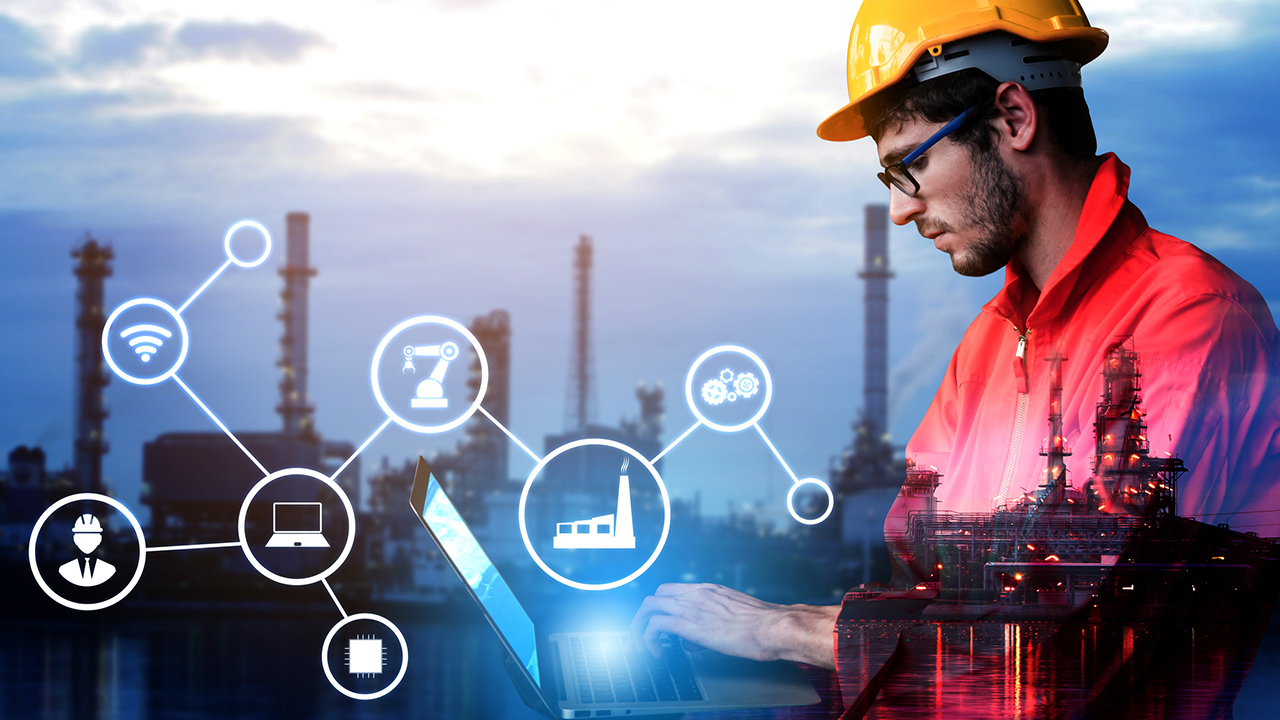
How IoT Technology Helps Enterprises to Go Green
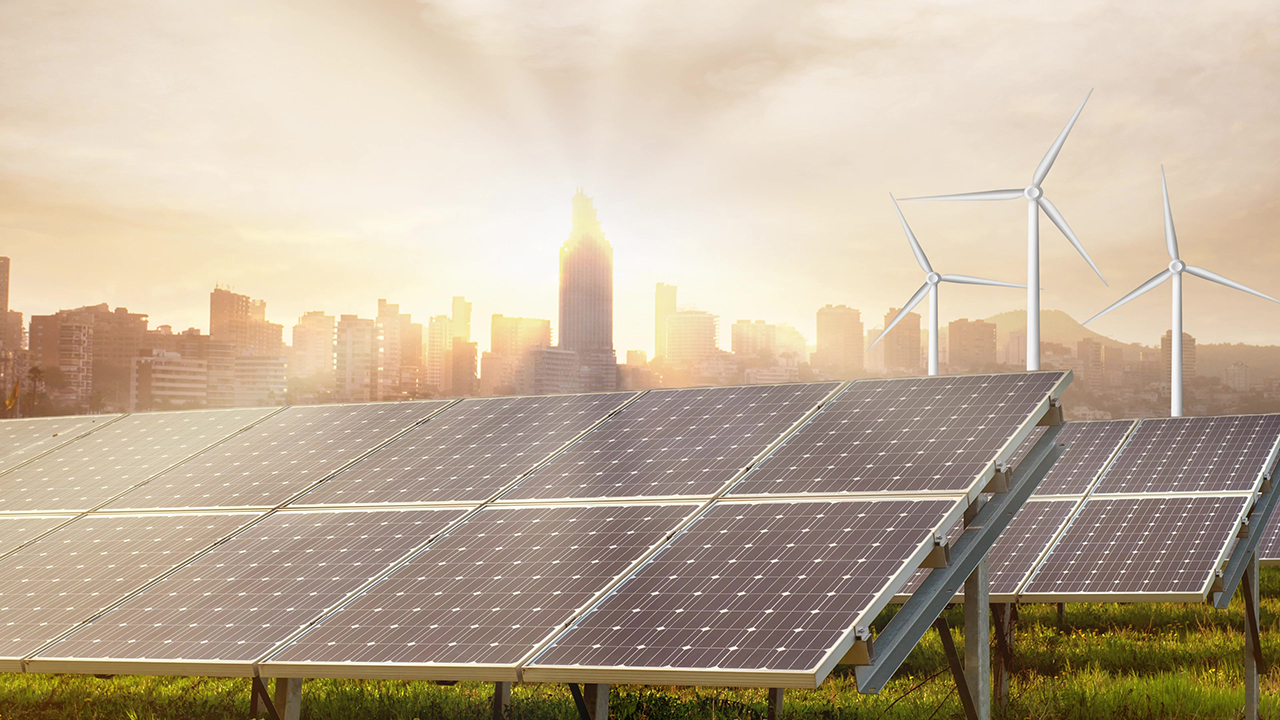
Download our Industrial IoT White Paper
Set up your field devices to be configured, maintained, and diagnosed from a central location.
Download PDF
IoT for Environmental Monitoring: 4 Essential Components
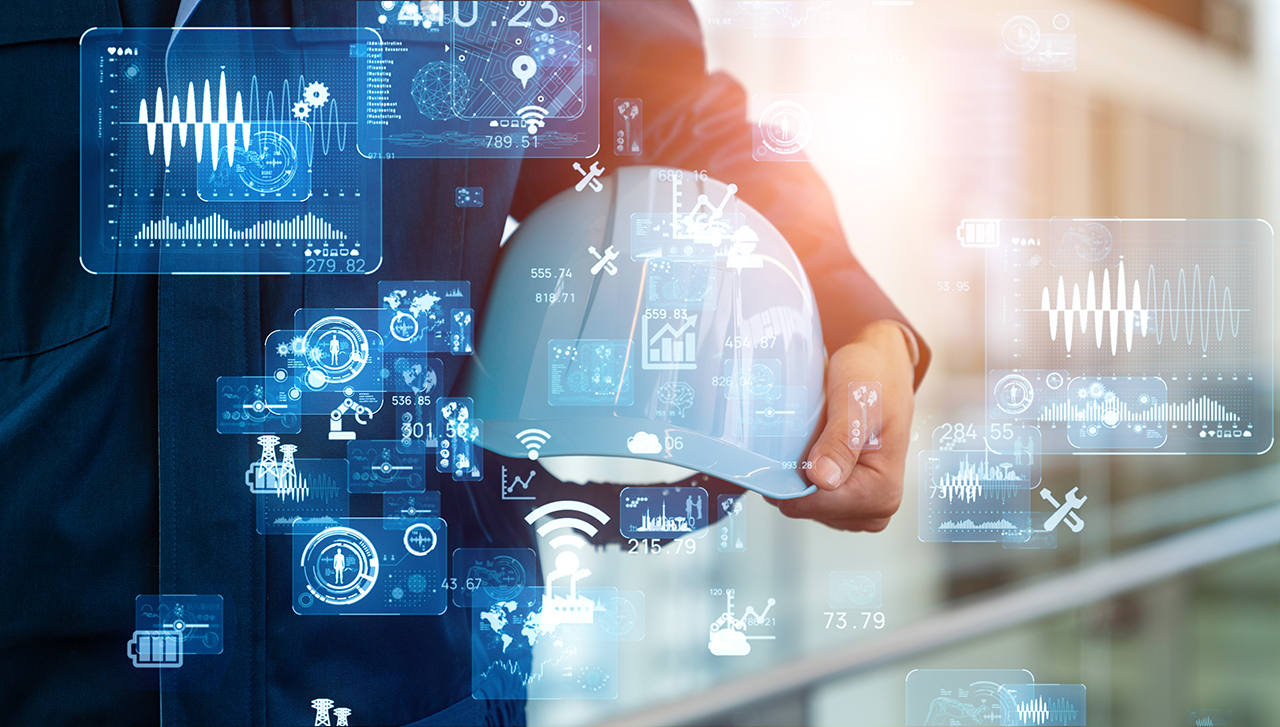
- Monitor the Environment : Environmental condition monitors across fields, industrial sites and water management systems require installed sensors as well as an information delivery system, such as Digi XBee wireless communication modules and sensor connectivity gateways . These connected devices gather and deliver critical information exactly where it is needed.
- Measure Data : To measure environmental impact, these systems must make it possible to evaluate key data points that can indicate everything from water and chemical leaks to critical equipment failures. This data can be used by industrial operators and municipalities to measure their environmental footprint and take action to reduce waste, increase sustainability, manage valuable resources like water, and prevent environmental disasters.
- Catalog Data : The massive amounts of data collected from environmental monitoring stations around the globe cannot be overstated. There are global databases that catalog an enormous range of environmental data, such as the Microsoft Planetary Computer . Industrial sites and other enterprises, similarly, must utilize cloud and data center storage to catalog the gathered data for accessibility by business applications.
- Provide Actionable Insights from the Data and Analysis : The critical end game is actionable insights from data. Digi’s IoT solutions , integrated with cloud applications like Microsoft Azure and Amazon Web Services, deliver data into complex software systems that enable personnel to gain those insights, get alerts and notifications, and take action.
Use Cases for IoT Environment Monitoring
With IoT systems, we can connect and manage wireless devices across an industrial field or across the globe for environmental monitoring and management to support better decision making, environmental sustainability and a circular economy . Let’s explore some use cases that demonstrate how IoT can help the environment .
Water Quality Monitoring
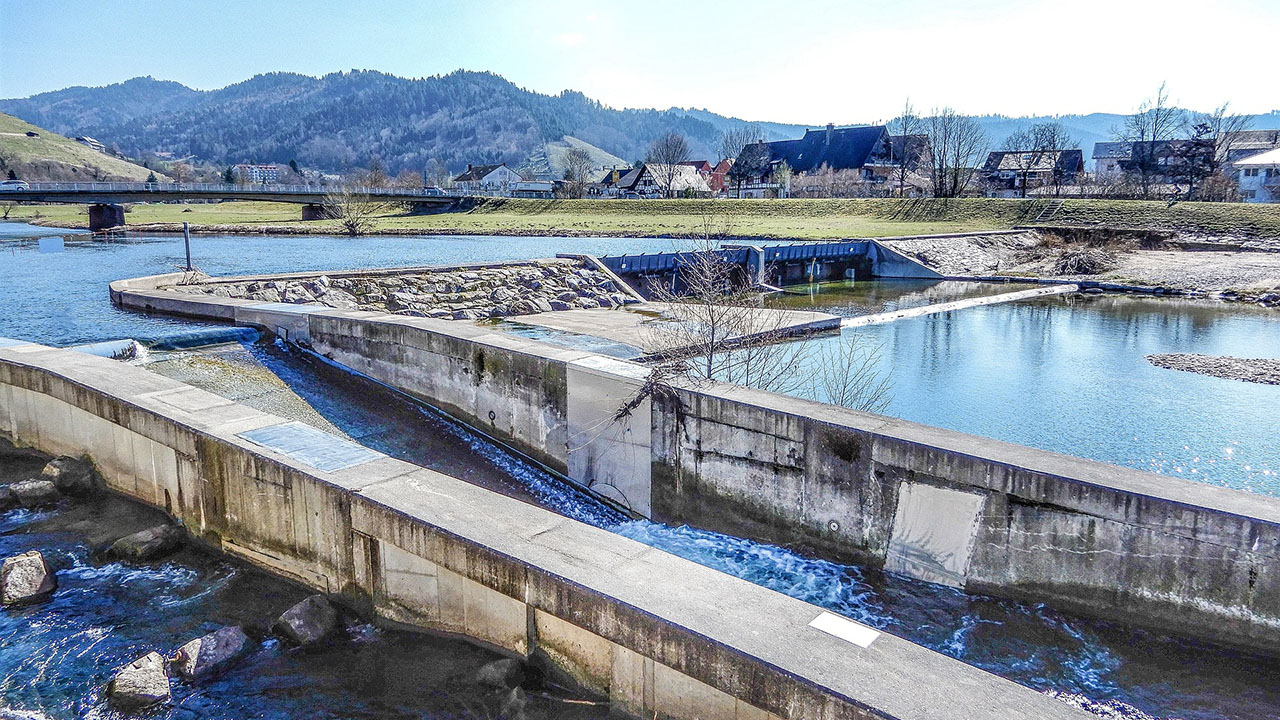
- Municipal water treatment monitoring
- Stormwater and groundwater monitoring
- Agricultural irrigation monitoring and control
- City water and drinking water quality monitoring
Air Quality Monitoring
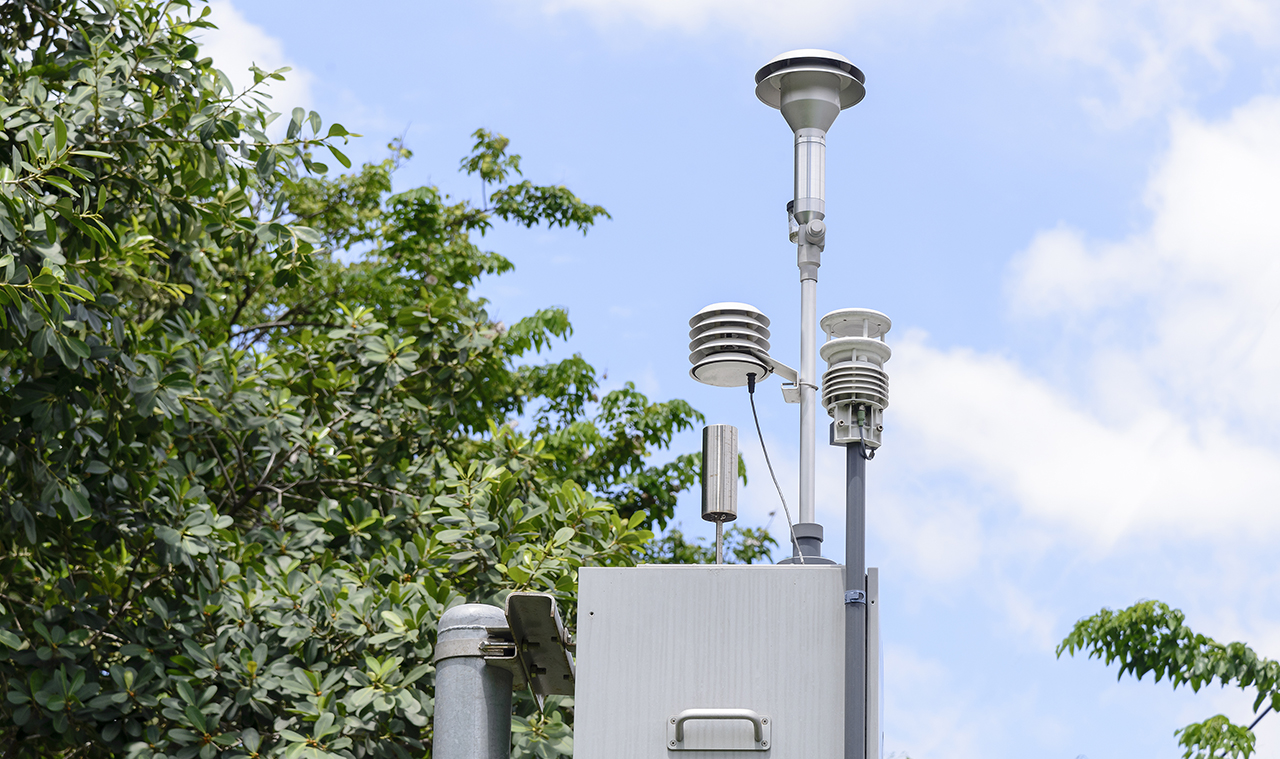
- Carbon monoxide monitoring in homes and buildings
- Methane monitoring in agriculture and waste management
- Ambient air quality monitoring for pollutants, lead and toxic particulates
Energy Monitoring
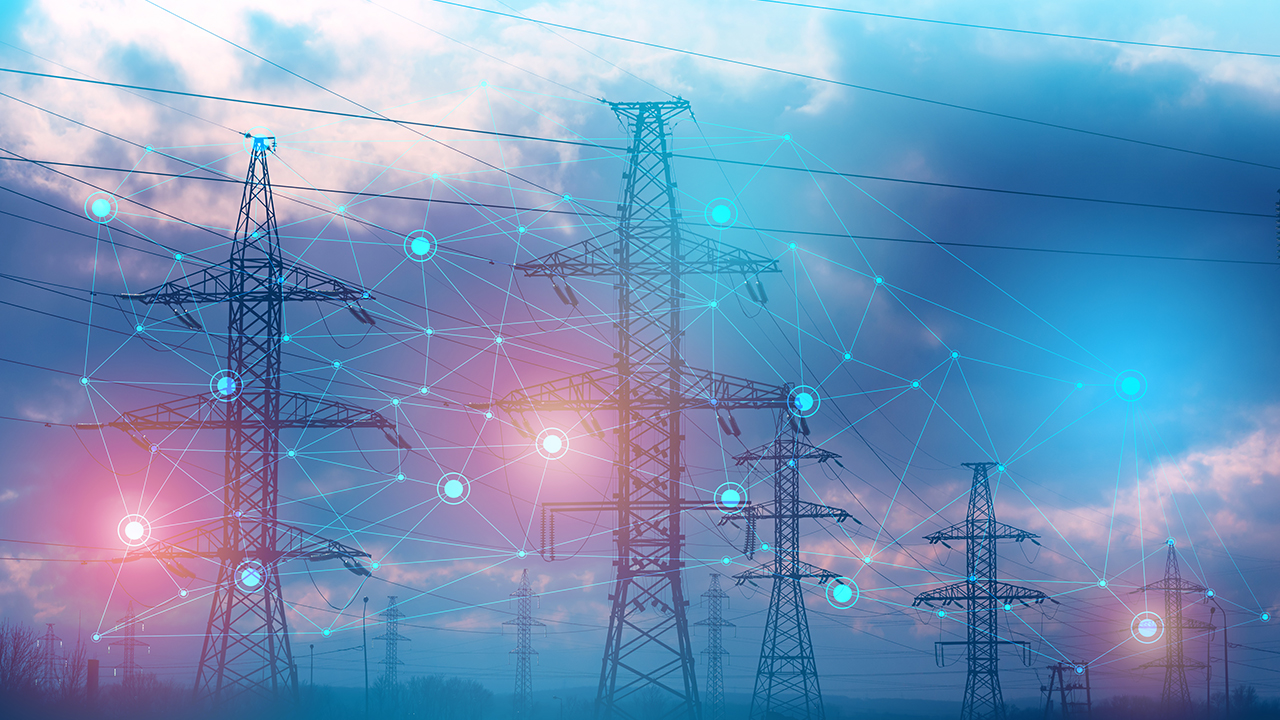
- Reduction in the use of fossil fuels in homes and businesses
- Stabilizing the power grid
- Preventing spikes in energy usage, and associated equipment failures and service disruption
Toxic Gas Detection
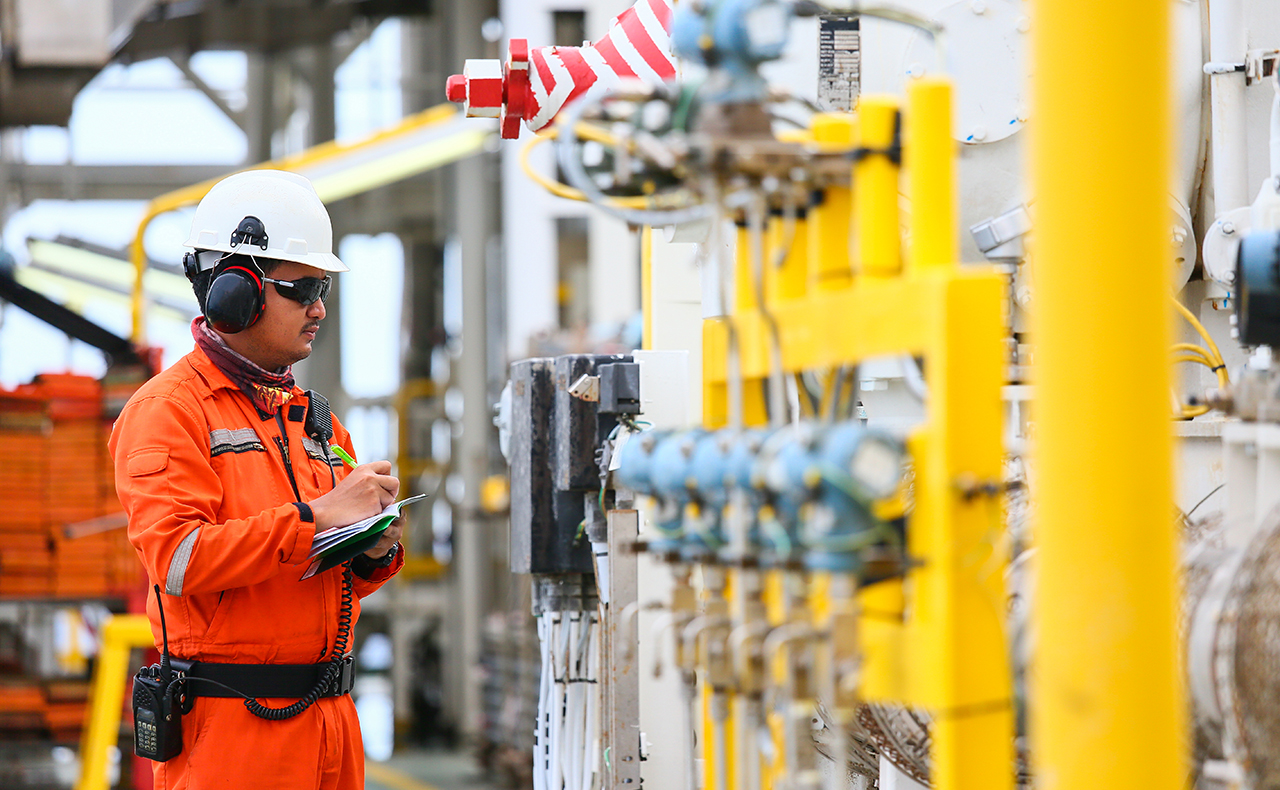
- Gas monitoring for safe management of toxic, flammable, or pyrophoric (spontaneously combustible in air) gases
- Monitoring of H2S or CO in refinery and petrochemical applications as well as parking garages and enclosed spaces such as warehouses
- Toxic gas detection for workplaces, to protect workers from toxic and corrosive gas exposure anywhere these substances may be present
- Monitoring for harmful gases in mining operations
Explore IoT-Based Environmental Monitoring Sensors and Solutions at Digi
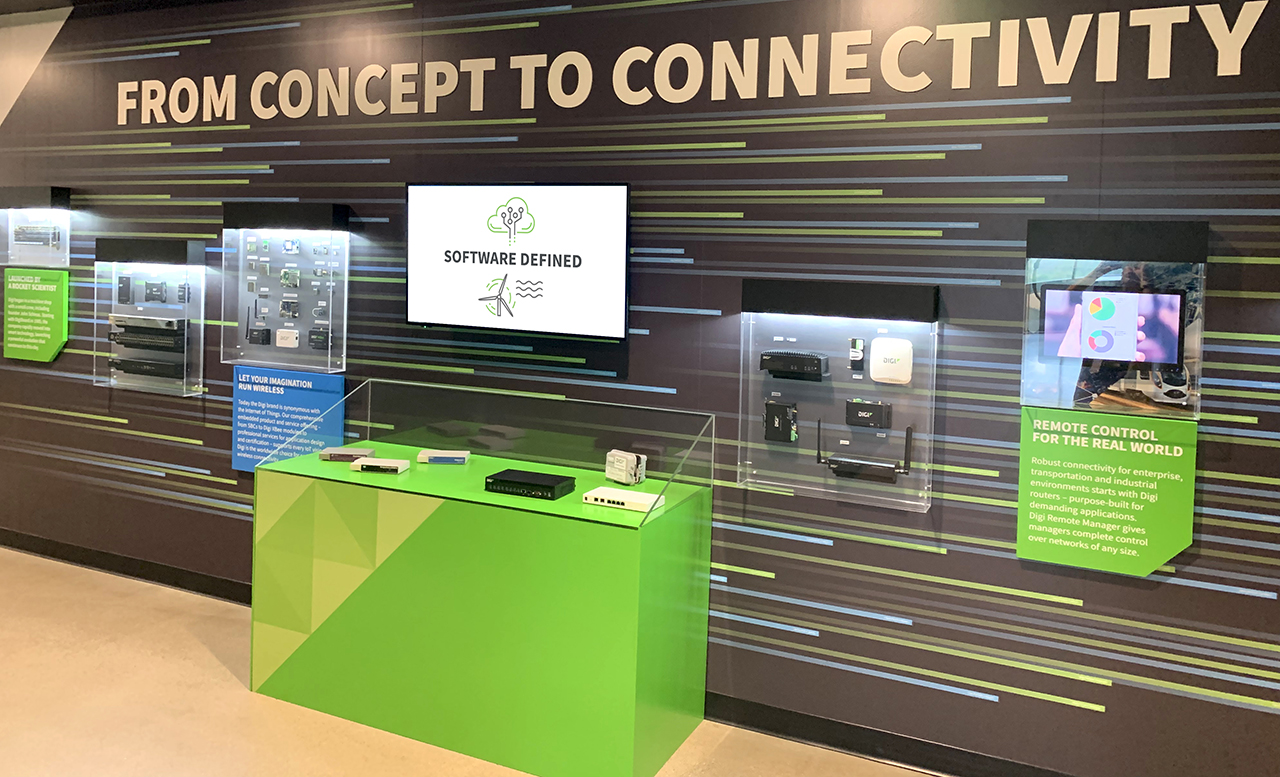
Related Content
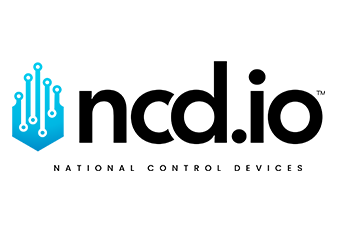
Contact Information
Packaged solutions, managed services, technologies, technical support.

May 12, 2023
IoT Predictive Maintenance Explained with Case Studies
In this article, you can learn more about IoT predictive maintenance and its significance in modern industries
Table of contents
Brief explanation of predictive maintenance and iot, the importance of predictive maintenance in modern industries, understanding iot and predictive maintenance, advantages of iot-enabled predictive maintenance, key components of iot-based predictive maintenance systems, implementing iot-enabled predictive maintenance, challenges and considerations in iot-based predictive maintenance, real-world examples of iot-enabled predictive maintenance, future trends in iot and predictive maintenance, benefits for businesses to explore iot-based predictive maintenance, choosing the right msp, interested in exploring the possibilities of iot predictive maintenance for your business.
Looking to integrate IoT predictive maintenance into your operations? Our team is here to help! Reach out to us now for a consultation and let's discuss how we can tailor a solution for your business.
Predictive maintenance is a type of maintenance strategy that uses data analytics techniques to predict when a machine or equipment is likely to fail. This approach is used to reduce unplanned downtime and improve maintenance efficiency by allowing maintenance teams to perform maintenance activities proactively, based on data-driven insights.
Internet of Things (IoT) refers to the network of interconnected devices that are able to collect and exchange data with each other. IoT devices can include sensors, wearables, and other smart devices that are embedded with technology that allows them to connect to the internet and transmit data. In the context of predictive maintenance, IoT devices can be used to collect real-time data from machines and equipment, which can then be used to predict when maintenance is required. By combining predictive maintenance with IoT, organizations can improve their maintenance efficiency and reduce the risk of downtime. Now when we’ve talked about predictive maintenance meaning and IoT, let’s look further at the importance of IoT preventative maintenance.
Predictive maintenance is a proactive approach to maintenance that involves using data analysis techniques to predict when equipment is likely to fail and scheduling maintenance activities accordingly. This approach can help modern industries save time and money by reducing unplanned downtime, improving equipment reliability, and extending the lifespan of assets.
Here are some key reasons why predictive maintenance is important in modern industries:
Reduced downtime
By predicting when equipment is likely to fail, organizations can schedule maintenance activities during planned downtime periods, minimizing the impact of equipment failure on production.
Improved equipment reliability
Predictive maintenance can help organizations identify and address issues before they become major problems, preventing equipment breakdowns and extending the lifespan of assets.
Increased safety
Regular maintenance can help ensure that equipment is functioning safely, reducing the risk of accidents and injuries.
Cost savings
Predictive maintenance can help organizations save money by reducing the need for emergency repairs, lowering maintenance costs, and extending the lifespan of assets.
Enhanced efficiency
By optimizing maintenance schedules, organizations can reduce the amount of time and resources needed for maintenance activities, allowing them to focus on other areas of the business.
Predictive maintenance is an approach that aims to optimize maintenance activities by using data analysis techniques to predict when equipment or machinery is likely to fail. Instead of relying on fixed maintenance schedules or waiting for a breakdown, predictive maintenance leverages data from sensors, equipment logs, and other sources to identify patterns, anomalies, and early signs of failure.
By monitoring the condition of equipment in real-time and analyzing historical data, predictive maintenance algorithms can detect potential issues before they cause major problems. This allows maintenance teams to schedule repairs or replacements proactively, reducing downtime, optimizing maintenance costs, and increasing overall operational efficiency.
IoT plays a crucial role in enabling predictive maintenance. IoT devices embedded in machinery and equipment collect real-time data on factors like temperature, vibration, pressure, and usage. This data is then analyzed using machine learning algorithms and predictive models to identify patterns that indicate impending failures or anomalies.
Definition and key features of IoT
The key features of IoT include:
Connectivity
IoT devices are equipped with various communication technologies such as Wi-Fi, Bluetooth, cellular networks, or even specialized low-power protocols to enable seamless data transmission and interaction with other devices or systems.
Sensing and Actuation
IoT devices incorporate sensors and actuators to monitor and collect data from their surrounding environment. Sensors can include temperature sensors, motion sensors, light sensors, and more. Actuators enable the devices to interact with their environment by performing actions based on received data.
Data Analytics
IoT generates a massive amount of data, and analytics play a crucial role in extracting valuable insights from this data. Advanced analytics techniques, including machine learning and artificial intelligence, are applied to analyze the collected data and derive meaningful information.
Automation and Control
IoT devices often aim to automate processes and control systems remotely. By leveraging connectivity and data analytics, they enable remote monitoring, management, and control of various devices and systems, offering enhanced convenience and efficiency.
Scalability and Flexibility
IoT systems can be scaled up or down easily to accommodate a growing number of devices and users. They are designed to be flexible, allowing for the addition of new devices or the modification of existing ones without disrupting the overall system.
Security and Privacy
Given the sensitive nature of the data collected and transmitted by IoT devices, ensuring security and privacy is critical. IoT systems need robust measures to protect against cyber threats, data breaches, and unauthorized access, both at the device level and during data transmission.
These key features collectively enable IoT to create a network of smart, interconnected devices that can improve various aspects of our lives, such as home automation, industrial processes, healthcare monitoring, environmental monitoring, transportation systems, and more.
At A-Team Global, we offer custom IoT solutions that can significantly improve business operational efficiency.

Explanation of predictive maintenance
Predictive maintenance is a proactive maintenance strategy that uses data analysis techniques and advanced technologies to predict when equipment or machinery is likely to fail and requires maintenance. It aims to prevent unexpected breakdowns, reduce downtime, and optimize maintenance activities.
Traditional maintenance approaches typically involve scheduled maintenance at fixed intervals, regardless of the actual condition of the equipment. This can result in unnecessary maintenance or, conversely, equipment failures if the scheduled maintenance is insufficient. Predictive maintenance, on the other hand, relies on real-time monitoring, data collection, and analysis to determine the optimal time for maintenance.
The process of predictive maintenance involves several key steps:
Data collection
Sensors, meters, and other monitoring devices are used to collect various types of data from the equipment. This can include temperature, pressure, vibration, noise, fluid levels, and other relevant parameters. The data is typically collected at regular intervals or continuously.
Data analysis
The collected data is analyzed using advanced analytics techniques, such as machine learning algorithms. Patterns, trends, and anomalies are identified to detect any deviations from normal operating conditions. The analysis aims to find correlations between the collected data and equipment failures or performance degradation.
Fault detection and diagnosis
By comparing the real-time data with historical data and established thresholds, predictive maintenance systems can detect the early signs of equipment faults or failures. This allows for timely intervention before the situation worsens.
Maintenance planning
Once a potential issue is identified, maintenance planners can schedule the appropriate maintenance activities. This can involve ordering replacement parts, allocating resources, and coordinating maintenance personnel. Predictive maintenance enables maintenance to be performed only when necessary, minimizing the disruption to operations and reducing costs.
Proactive maintenance
With the knowledge gained from predictive maintenance, proactive measures can be taken to address potential issues. This can include adjusting operating parameters, implementing temporary workarounds, or applying preventive measures to mitigate the risk of failure.
The benefits of predictive maintenance include increased equipment uptime, optimized maintenance schedules, reduced maintenance costs, improved safety, and enhanced overall operational efficiency. By identifying and addressing maintenance needs in a timely manner, organizations can avoid unplanned downtime, extend equipment lifecycles, and optimize their maintenance resources.
The synergy between IoT and predictive maintenance
The synergy between the Internet of Things (IoT) and predictive maintenance is significant and has the potential to revolutionize maintenance practices in various industries. IoT refers to the network of interconnected devices and sensors that can collect and exchange data over the internet. Predictive maintenance, on the other hand, involves using data and analytics to predict equipment failures or maintenance needs before they occur.
So with IoT preventative maintenance, organizations can achieve several benefits:
Real-time monitoring
IoT sensors can continuously monitor equipment and collect data on various parameters such as temperature, vibration, pressure, and more. This real-time monitoring allows for early detection of anomalies or deviations from normal operating conditions.
Data-driven insights
The vast amount of data collected by IoT devices can be processed and analyzed using advanced analytics techniques. By applying machine learning algorithms to this data, patterns, trends, and potential failure signatures can be identified. This enables maintenance teams to make data-driven decisions and prioritize maintenance activities effectively.
Reduced downtime and costs
Predictive maintenance allows for proactive and preventive maintenance actions. By identifying and addressing potential issues before they lead to failures, organizations can avoid unplanned downtime and costly repairs. This approach helps optimize maintenance schedules and reduce overall maintenance costs.
Remote monitoring and diagnostics
IoT facilitates remote monitoring of equipment, enabling maintenance teams to monitor and diagnose issues from a central location. This capability is particularly valuable for geographically dispersed assets or inaccessible locations, as it reduces the need for physical inspections and improves the speed of response.
Enhanced safety and risk mitigation
Predictive maintenance helps identify potential safety risks or hazards associated with equipment failures. By addressing these issues proactively, organizations can improve workplace safety and reduce the likelihood of accidents or incidents.
You can also check out the article on IoT-based GPS tracking software - https://a-team.global/blog/iot-based-gps-tracking-software/
Now let’s look at some advantages of IoT-enabled predictive maintenance.
Increased equipment lifespan
One of the significant advantages of IoT-enabled predictive maintenance is the increased lifespan of equipment. By utilizing IoT sensors, data analytics, and machine learning algorithms, organizations can monitor the health and performance of their equipment in real-time. This proactive approach allows them to identify potential issues and perform necessary maintenance actions before major failures occur. Here's how it contributes to increased equipment lifespan:
Early fault detection
IoT sensors continuously collect data from the equipment, including temperature, vibration, pressure, and other relevant parameters. By analyzing this data in real-time, anomalies and patterns indicative of potential faults or malfunctions can be detected early.
Condition-based maintenance
Instead of following a fixed maintenance schedule or waiting for breakdowns to occur, predictive maintenance relies on the actual condition of the equipment. By monitoring critical parameters and performance metrics, organizations can schedule maintenance activities based on the equipment's real-time health status.
Improved maintenance accuracy
IoT-enabled predictive maintenance utilizes data-driven insights and predictive models to optimize maintenance activities. Maintenance technicians receive accurate information about the condition and potential issues of the equipment, allowing them to perform targeted repairs or replacements.
Enhanced equipment performance
By continuously monitoring equipment performance, organizations can identify opportunities for optimization. IoT sensors can detect deviations from optimal performance, enabling organizations to make necessary adjustments and fine-tune equipment settings. This proactive approach not only increases the equipment's lifespan but also enhances its overall performance, leading to improved productivity and efficiency.
Reduced downtime and maintenance costs
Here's how IoT-enabled predictive maintenance can help reduce downtime and maintenance costs.:
IoT sensors and devices deployed in machinery and equipment can continuously monitor their operational parameters, such as temperature, vibration, pressure, and performance metrics. By collecting real-time data, anomalies or deviations from normal operating conditions can be detected early on.
Predictive analytics
The collected data from IoT devices is processed and analyzed using advanced analytics techniques, such as machine learning algorithms. By analyzing historical and real-time data patterns, predictive models can be built to forecast potential equipment failures. This allows maintenance teams to schedule maintenance activities based on predicted failure probabilities and prioritize resources accordingly.
Instead of following a fixed schedule for maintenance activities, IoT-enabled predictive maintenance allows for condition-based maintenance. By monitoring the actual health and performance of equipment in real-time, maintenance can be performed when necessary, based on the condition and usage of the asset. This approach minimizes unnecessary maintenance and optimizes the utilization of maintenance resources.
Optimized maintenance planning
IoT-enabled predictive maintenance provides actionable insights into the health and performance of assets. Maintenance teams can leverage this information to optimize maintenance planning and scheduling. This leads to more efficient maintenance operations and minimizes the impact on regular production activities.
Enhanced operational efficiency
Enhanced operational efficiency is a significant advantage of IoT-enabled predictive maintenance. By leveraging the power of the Internet of Things (IoT) and predictive analytics, organizations can optimize their maintenance practices, leading to improved efficiency across various operational processes.
By implementing IoT-enabled predictive maintenance, organizations can achieve several operational efficiency benefits. First, they can significantly reduce unplanned downtime by addressing issues before they escalate into major failures. This leads to improved productivity and uninterrupted operations, resulting in higher overall equipment effectiveness.
Second, maintenance activities can be streamlined and optimized. Instead of conducting routine inspections and maintenance on all assets, organizations can focus their efforts on those that require attention based on predictive analytics. This targeted approach optimizes the allocation of maintenance resources, reduces costs associated with unnecessary maintenance, and extends the lifespan of critical assets.
Third, IoT-enabled predictive maintenance enables organizations to optimize their inventory management. With accurate predictions of failure probabilities and maintenance needs, spare parts and supplies can be stocked strategically. This prevents overstocking or stockouts, reduces inventory carrying costs, and ensures timely availability of necessary components when maintenance is required.
Improved safety and risk management
Improved safety and risk management is a significant advantage of IoT-enabled predictive maintenance. By harnessing the power of the Internet of Things (IoT) and advanced analytics, organizations can proactively identify and address potential equipment failures or safety hazards before they escalate into major incidents.
With IoT-enabled predictive maintenance, sensors embedded in machines continuously monitor their performance and collect real-time data. This data is then analyzed using sophisticated algorithms to detect patterns, anomalies, and potential failure indicators. By identifying early warning signs, maintenance teams can take timely action to address issues, reducing the risk of accidents, breakdowns, and other safety hazards.
This proactive approach to maintenance enables organizations to move away from reactive and time-based maintenance practices, where equipment is repaired or replaced only after a failure occurs. By predicting and preventing failures, businesses can significantly enhance workplace safety by minimizing the occurrence of sudden breakdowns or malfunctions that could pose risks to employees, customers, or the environment.
Furthermore, IoT-enabled predictive maintenance enables organizations to optimize their maintenance schedules and resource allocation. Instead of conducting routine maintenance activities on fixed schedules, maintenance can be performed when it is truly needed. This not only increases operational efficiency but also reduces the chances of human error or oversight, which can contribute to safety incidents.
Ready to harness the power of IoT predictive maintenance and stay ahead of the competition? Reach out to us now and let's discuss how our solutions can transform your maintenance practices and drive your business forward.
Let’s look at the major components of IoT-based predictive maintenance systems
IoT sensors and devices
IoT sensors and devices play a crucial role as key components in IoT-based predictive maintenance systems. Predictive maintenance refers to the practice of using data and analytics to predict when and how equipment or machinery might fail. By leveraging IoT sensors and devices, organizations can monitor the health and performance of their assets in real-time, enabling them to optimize maintenance activities, reduce downtime, and improve operational efficiency.
IoT sensors are small, intelligent devices that collect and transmit data from the physical world to the digital realm. They are capable of measuring various parameters such as temperature, pressure, vibration, humidity, and more. These sensors can be attached to different types of equipment, ranging from industrial machinery to vehicles, appliances, and infrastructure components.
In an IoT-based predictive maintenance system, these sensors are strategically placed on critical components or areas of the equipment to capture relevant data points. The sensors can be integrated into the existing infrastructure or added as retrofitted components. They are designed to be power-efficient, with long battery life or energy harvesting capabilities, ensuring continuous data collection without disrupting the equipment's operation.
Besides sensors, IoT devices such as gateways or edge devices are also essential components of predictive maintenance systems. These devices act as intermediaries between the sensors and the central data processing system. They aggregate the data from multiple sensors, perform basic analytics or preprocessing tasks locally, and transmit the relevant information to the cloud or a centralized server.
IoT devices often incorporate edge computing capabilities, which allow for real-time analysis and decision-making at the edge of the network. This helps reduce latency, bandwidth usage, and dependency on cloud connectivity. Edge computing enables immediate response to critical events or triggers, making it ideal for time-sensitive predictive maintenance scenarios.
Connectivity and communication protocols
Connectivity and communication protocols play a crucial role in IoT-based predictive maintenance systems. These systems leverage the power of the Internet of Things (IoT) to enable real-time monitoring, analysis, and proactive maintenance of various assets and equipment. By utilizing a network of interconnected sensors and devices, organizations can optimize maintenance practices, minimize downtime, and improve operational efficiency.
One of the fundamental requirements of IoT-based predictive maintenance systems is reliable and seamless connectivity. These systems involve the deployment of numerous sensors and devices across a wide area, including factories, industrial plants, or even smart cities. These devices generate a vast amount of data related to the health, performance, and condition of the assets they monitor. To make effective use of this data, it must be transmitted in a timely and secure manner.
Various connectivity options are available for IoT-based predictive maintenance systems, depending on the specific use case and environment. Some commonly used connectivity technologies include: Wireless Connectivity, Cellular Connectivity, LPWAN (Low Power Wide Area Network).
Data storage and processing
Data storage and processing are key components of IoT-based predictive maintenance systems that rely on IoT sensors and devices.
In IoT-based predictive maintenance systems, data storage plays a crucial role in ensuring the availability and accessibility of sensor-generated data. As IoT devices continuously generate a massive volume of data, it is important to have robust and scalable storage solutions capable of handling the influx of information. Cloud-based storage platforms are often utilized, providing flexible and virtually limitless storage capacity. These platforms enable organizations to store and manage their sensor data efficiently, without the need for significant on-premises infrastructure investments.
Furthermore, data processing is essential for extracting valuable insights from the collected data. The raw data obtained from IoT sensors often needs to be transformed, aggregated, and analyzed to derive meaningful information. Data processing techniques, such as data cleansing, filtering, and feature extraction, are applied to ensure data quality and relevance
To facilitate data storage and processing in IoT-based predictive maintenance systems, edge computing is often utilized. Edge computing involves performing data processing and analysis closer to the data source, at the network edge, rather than sending all the data to a centralized cloud infrastructure. This approach reduces latency, optimizes bandwidth usage, and enables real-time or near-real-time decision-making. By deploying edge computing capabilities, organizations can process and store critical data locally, while still leveraging the scalability and computational power of cloud platforms when needed.
Predictive analytics and machine learning algorithms
Two key components that enable the effectiveness of IoT-based predictive maintenance systems are predictive analytics and machine learning algorithms.
In the context of IoT-based predictive maintenance systems, predictive analytics plays a crucial role in processing the vast amount of sensor data collected from IoT devices. It involves analyzing historical data, detecting patterns, and identifying potential anomalies or abnormalities that could indicate equipment failure or maintenance needs.
Machine learning algorithms are an integral part of predictive analytics in IoT-based predictive maintenance systems. These algorithms can be trained using historical data to recognize patterns, correlations, and anomalies that indicate the likelihood of future failures or maintenance requirements. As new data is continuously collected from IoT sensors and devices, machine learning algorithms adapt and improve their predictive capabilities over time, allowing them to make more accurate predictions.
Visualization and reporting tools
IoT-based predictive maintenance systems rely on the continuous monitoring of equipment and machinery through the use of IoT sensors and devices. These systems generate vast amounts of data, providing valuable insights into the performance and health of various assets. However, to make this data actionable and facilitate informed decision-making, visualization and reporting tools play a crucial role.
These predictive maintenance technologies help transform raw data into meaningful visual representations. They allow users to analyze and interpret complex data sets by presenting them in intuitive and interactive formats such as charts, graphs, dashboards, and heat maps. By visualizing data, operators and maintenance personnel can quickly identify patterns, trends, anomalies, and correlations that might indicate potential equipment failures or maintenance requirements.
Reporting tools complement visualization by offering summarized and detailed reports based on the data collected and analyzed. These reports can be generated automatically on a scheduled basis or on-demand. They provide insights into the overall equipment performance, maintenance activities, and key trends over a specific period. Reports may include information on equipment downtime, failure rates, mean time between failures (MTBF), maintenance costs, and other relevant metrics.
Let’s look at the steps for implementing IoT-enabled predictive maintenance.
Identifying critical assets and processes
Implementing IoT-enabled predictive maintenance involves identifying critical assets and processes to optimize maintenance activities and minimize downtime. Here are some key steps to identify critical assets and processes in this context:
Asset Inventory
Begin by creating an inventory of all assets and equipment that are relevant to your organization's operations. This includes machinery, vehicles, infrastructure, or any other physical components that are vital to the business.
Historical Data Analysis
Analyze historical maintenance records and performance data of the assets. Look for patterns and trends related to breakdowns, failures, and maintenance requirements. This analysis helps identify assets that frequently require maintenance or have a significant impact on production when they malfunction.
Risk Assessment
Conduct a risk assessment to evaluate the potential consequences of asset failures. Consider the impact on safety, productivity, operational efficiency, customer satisfaction, and regulatory compliance. Assets that pose higher risks or have a significant impact on critical processes should be prioritized.
Sensor and Data Analysis
Determine the type of sensors or monitoring devices required to collect real-time data from the assets. These sensors could measure variables such as temperature, vibration, pressure, or other relevant parameters. Analyze the data collected from the sensors to identify anomalies, patterns, or indicators of potential failures.
Feedback from Maintenance Team
Involve your maintenance team in the identification process. They possess valuable domain expertise and can provide insights based on their experience. The maintenance team can help identify assets that frequently require repairs, cause bottlenecks, or have a history of unexpected failures.
Continuous Improvement
Implement an iterative approach where you continually refine your asset and process identification based on ongoing data collection, analysis, and feedback. As you gather more data and insights, you can optimize your maintenance strategies and adapt to changing conditions.
Selecting and installing appropriate sensors and devices
Implementing IoT-enabled predictive maintenance involves selecting and installing the appropriate sensors and devices to collect and transmit data from various assets and equipment. The selection of sensors and devices depends on the specific maintenance requirements and the assets being monitored. Here are some common sensors and devices used in IoT-enabled predictive maintenance:
- Vibration sensors: Vibration sensors detect abnormal vibrations in machinery, which can indicate potential faults or failures. These sensors measure and transmit vibration data for analysis.
- Temperature sensors: Temperature sensors monitor the operating temperature of equipment and machinery. Deviations from normal temperature ranges can indicate potential issues.
- Pressure sensors: Pressure sensors measure fluid pressure in systems such as hydraulic or pneumatic equipment. Unusual pressure fluctuations can be a sign of problems.
- GPS trackers: GPS trackers provide location information for mobile assets or vehicles. They can be used to track the usage patterns, maintenance history, and predict maintenance needs based on location data.
- Data loggers: Data loggers record and store data over time. They can be used to capture and analyze trends, patterns, or events that may affect maintenance requirements.
During the installation process, it is essential to ensure proper sensor placement and calibration, reliable power supply, and secure data transmission. Integration with a robust IoT platform or a predictive maintenance software solution enables data processing, analytics, and generating actionable insights for maintenance decision-making.
Establishing connectivity and data transmission
Implementing IoT-enabled predictive maintenance requires establishing connectivity and data transmission between various components. Here are the key steps involved in the process:
- Identify sensors and devices: Determine the sensors and IoT devices required to collect relevant data for predictive maintenance. These can include sensors for temperature, pressure, vibration, humidity, or any other relevant parameters based on the equipment or system being monitored.
- Select communication protocols: Choose appropriate communication protocols to facilitate data transmission between the sensors/devices and the central monitoring system. Commonly used protocols include Wi-Fi, Bluetooth, Zigbee, cellular networks (2G/3G/4G/5G), or specialized industrial protocols like MQTT or OPC-UA.
- Establish connectivity: Set up the necessary infrastructure to enable connectivity between the sensors/devices and the central monitoring system. This typically involves deploying gateways, access points, or network infrastructure that supports the chosen communication protocols.
- Data collection and aggregation: Configure the sensors/devices to collect data at regular intervals or in response to specific events. The collected data is then aggregated and packaged for transmission to the central monitoring system. This step may involve preprocessing and filtering the data to reduce noise or extract relevant features.
- Secure data transmission: Implement appropriate security measures to protect the data during transmission. This includes encrypting the data, implementing authentication mechanisms, and ensuring the integrity and confidentiality of the data as it moves through the network.
- Data transmission protocols: Define the protocols for transmitting data from the sensors/devices to the central monitoring system. This could involve establishing a real-time streaming connection or using periodic data uploads based on the available network bandwidth and latency requirements.
- Cloud or edge processing: Decide whether the data should be processed at the edge (near the sensors/devices) or in the cloud. Edge processing involves performing initial data analysis and filtering on the local devices, reducing the amount of data transmitted and minimizing latency.
- Central monitoring system: Develop or deploy a central monitoring system that receives, stores, and analyzes the collected data. This system should have the necessary capabilities to process the incoming data, apply predictive analytics models, and generate maintenance alerts or recommendations based on the analyzed data.
- Continuous improvement: Regularly review the performance of the IoT-enabled predictive maintenance system, analyze the collected data, and refine the algorithms or models used for predictive maintenance. This iterative process helps improve the accuracy of predictions and optimize maintenance schedules.
By following these steps, organizations can establish connectivity and ensure efficient data transmission in an IoT-enabled predictive maintenance system.
Developing and fine-tuning predictive models
Implementing IoT-Enabled Predictive Maintenance involves developing and fine-tuning predictive models that leverage data from IoT devices to proactively identify and prevent potential equipment failures. Here are the key steps involved in this process:
- Define the Problem: Clearly define the maintenance problem you want to address. Identify the equipment or systems you want to monitor and the specific failure modes or performance degradation you want to predict.
- Data Collection: Install appropriate sensors and IoT devices to collect relevant data from the equipment.
- Feature Selection: Analyze the collected data and select the most informative features for predictive modeling.
- Model Selection: Choose an appropriate predictive modeling technique based on the nature of the problem and the available data.
- Model Evaluation: Assess the performance of the trained model using the validation set.
- Model Deployment: Once the model performance is satisfactory, deploy it to a production environment.
- Ongoing Model Maintenance: Continuously monitor the performance of the deployed predictive model.
Remember that successful implementation of IoT-enabled predictive maintenance requires a multidisciplinary approach involving domain expertise, data engineering, machine learning, and continuous collaboration between maintenance teams and data scientists.
Integrating insights into maintenance workflows
Implementing IoT-enabled predictive maintenance involves leveraging data from connected devices to gain insights and optimize maintenance workflows. By integrating these insights into maintenance workflows, organizations can improve equipment reliability, reduce downtime, and minimize maintenance costs.
By integrating insights from IoT-enabled predictive maintenance into maintenance workflows, organizations can transform reactive maintenance into proactive and data-driven approaches. This integration optimizes resource allocation, improves equipment reliability, and ultimately enhances operational efficiency.
Ready to take the next step towards implementing IoT predictive maintenance? Get in touch with us now and discover how our solutions can drive your maintenance strategy forward.
As we mentioned, IoT-based predictive maintenance have lots of benefits. However, amidst the benefits and opportunities, there are significant challenges and considerations. Let’s now discuss the main challenges.
Data privacy and security
Data privacy refers to the protection of an individual's personal information and ensuring that it is collected, stored, and used in a manner that respects their rights. In the context of IoT-based predictive maintenance, several privacy challenges arise due to the extensive data collection and processing involved. These challenges include:
Data Collection
Predictive maintenance systems rely on vast amounts of data collected from various IoT devices, such as sensors, actuators, and equipment. This data often includes sensitive information about individuals, such as location, usage patterns, and maintenance history. It is crucial to establish clear consent mechanisms and transparent data collection practices to ensure individuals' privacy rights are respected.
Data Storage and Transmission
The massive volume of data generated by IoT devices requires robust storage and transmission mechanisms. Organizations must implement secure data storage practices, including encryption and access controls, to safeguard data against unauthorized access or breaches during transit.
Data Ownership and Control
Determining ownership and control over the data collected through IoT devices can be complex, especially when multiple stakeholders are involved. Clear data ownership policies and agreements must be established to avoid disputes and ensure that individuals' privacy rights are protected.
Third-Party Involvement
IoT-based predictive maintenance often involves partnerships and collaborations with third-party service providers, data analytics companies, and cloud platforms. Sharing data with these entities introduces additional privacy risks. Organizations must carefully assess the privacy practices and security measures of third-party providers to ensure compliance with privacy regulations and protect sensitive data.
User Consent and Transparency
Organizations should obtain explicit user consent for data collection and clearly communicate how the collected data will be used. Users should have control over their data, including the ability to access, modify, and delete their personal information. Transparent privacy policies and practices are essential for building trust with users.
Integration with existing systems and processes
Integration with existing systems and processes is a crucial aspect when implementing IoT-based predictive maintenance solutions. While predictive maintenance offers numerous benefits, such as reduced downtime, improved asset performance, and cost savings, it also presents several challenges and considerations when it comes to integrating with existing systems and processes.
Data compatibility
IoT-based predictive maintenance relies on collecting and analyzing large volumes of data from various sources, including sensors, machines, and other connected devices. One of the primary challenges is ensuring data compatibility between different systems and devices.
Scalability and infrastructure
Integrating IoT-based predictive maintenance solutions into existing systems requires careful consideration of the infrastructure and scalability aspects. Legacy systems may not have the necessary capacity or capabilities to handle the increased data influx from IoT devices.
Legacy system constraints
Existing systems and processes may have limitations or constraints that pose challenges during integration. For example, legacy systems might not provide the necessary interfaces or APIs for direct integration with IoT devices. In such cases, developing custom adapters or middleware to bridge the gap between the legacy systems and IoT infrastructure becomes necessary.
Change management
Integrating IoT-based predictive maintenance solutions often requires changes in processes, workflows, and personnel responsibilities. This change management aspect can be challenging, as it involves training and educating employees, revising standard operating procedures, and ensuring smooth transitions without disrupting ongoing operations.
Ensuring sensor accuracy and reliability
In IoT-based predictive maintenance, ensuring sensor accuracy and reliability is crucial for the overall effectiveness and success of the system. Sensors play a vital role in collecting data from various equipment and assets, enabling predictive analytics and maintenance strategies. However, there are several challenges and considerations involved in ensuring the accuracy and reliability of these sensors. Let's explore some of them:
Sensor Selection
Choosing the right sensors is the first step in ensuring accuracy and reliability. Different types of sensors are available for monitoring different parameters such as temperature, pressure, vibration, humidity, etc. It is essential to select sensors that are suitable for the specific application and can provide accurate measurements consistently.
Calibration and Maintenance
Regular calibration of sensors is necessary to maintain their accuracy. Calibration involves comparing the sensor's measurements with a known reference to identify and correct any deviations. Additionally, routine maintenance procedures should be implemented to keep the sensors in optimal working condition and prevent drift or degradation over time.
Environmental Considerations
The environment in which sensors operate can significantly impact their accuracy and reliability. Factors such as temperature variations, humidity, electromagnetic interference, and physical obstructions can introduce errors or affect sensor performance. Proper shielding, insulation, and environmental monitoring can help mitigate these challenges.
Data Validation and Filtering
Sensor data can be prone to noise, outliers, or intermittent failures. It is crucial to implement data validation and filtering techniques to identify and discard erroneous or inconsistent data points. Statistical methods, signal processing algorithms, and machine learning techniques can be employed to detect and filter out unreliable data, ensuring the accuracy of the overall predictive maintenance system.
Managing the volume and complexity of data
Managing the volume and complexity of data is a critical challenge in IoT-based predictive maintenance systems. With the proliferation of IoT devices and the continuous monitoring of assets, vast amounts of data are generated at an unprecedented rate. This data includes sensor readings, equipment logs, maintenance records, and other relevant information. Effectively handling and processing this data is essential to extract actionable insights and enable proactive maintenance strategies.
The following are key challenges and considerations in managing the volume and complexity of data in IoT-based predictive maintenance:
Data Acquisition and Integration
IoT devices generate massive amounts of data from diverse sources. Ensuring seamless integration and consolidation of this data from various sensors, devices, and systems is a complex task. It requires standardized data formats, protocols, and interfaces to capture and aggregate data efficiently
Data Storage and Management
Storing and managing large volumes of data pose significant challenges. Traditional databases may not be suitable due to their limited scalability and performance. Instead, organizations need to adopt scalable storage solutions like distributed file systems or NoSQL databases.
Data Quality and Preprocessing
Ensuring the quality and reliability of the data is paramount. Data collected from IoT devices may be noisy, incomplete, or subject to anomalies. Preprocessing techniques such as data cleaning, filtering, and outlier detection are necessary to enhance the quality and integrity of the data.
Scalability and Real-time Processing
IoT-based predictive maintenance systems must handle the increasing scale and velocity of data generated in real-time. The architecture should support horizontal scalability, enabling the system to handle a growing number of devices and data sources
Let’s look at some examples of how businesses use IoT-enabled predictive maintenance
Case Study 1: Optimizing Fleet Management in a Transportation Company
A logistics company experienced increased maintenance costs and vehicle downtime. These factors impacted business in a negative way, and it was important to find the solution and reduce maintenance costs.
Implementation: the company implemented an IoT-based predictive maintenance system to monitor vehicle health and performance, which helped spot any problems immediately.
Results and Benefits: as a result of IoT-based predictive maintenance system, the company received:
- Reduced maintenance costs and downtime;
- Improved fleet utilization and efficiency;
- Enhanced driver safety and customer satisfaction.
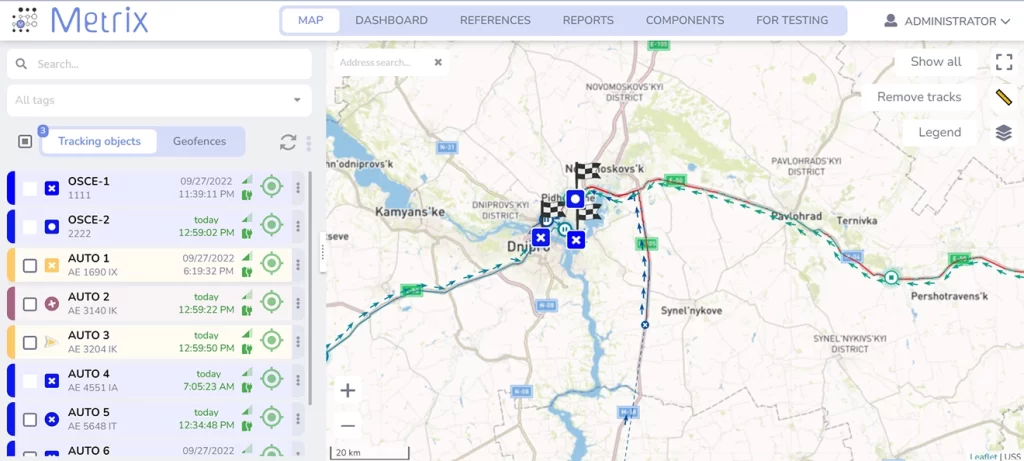
Case Study 2: Enhancing Production Efficiency in a Manufacturing Plant
A manufacturing plant at some point faced frequent equipment breakdowns and, as a result, lost production time. It was necessary to address the problem as soon as possible.
Implementation: the company implemented an IoT-enabled predictive maintenance system to monitor machine conditions and performance.
Results and Benefits: as a result of implementation of IoT-enabled predictive maintenance, the company received the following benefits:
- Increased equipment lifespan and reduced maintenance costs;
- Improved production efficiency and reduced downtime;
- Enhanced decision-making and resource allocation.
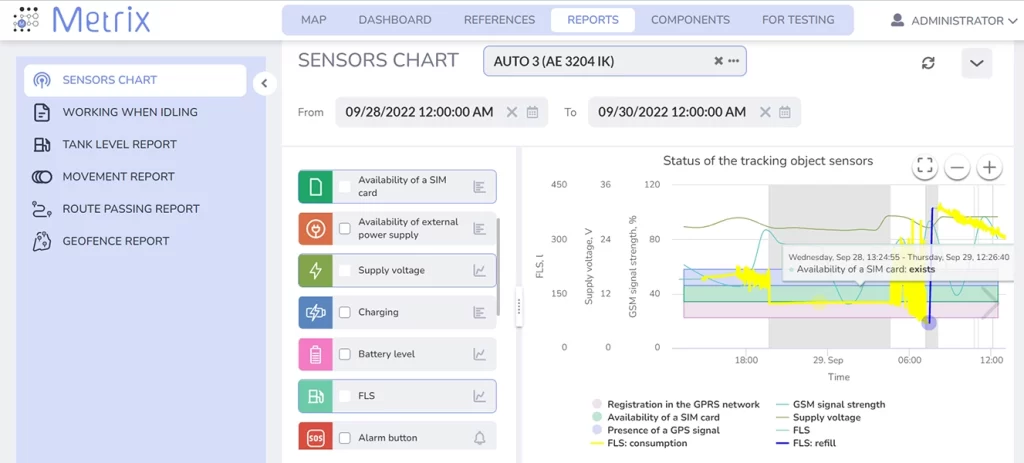
What is the future of predictive maintenance IoT? Here are some future trends
Integration of advanced analytics and AI technologies
Predictive maintenance, a key application of advanced analytics in IoT, involves the proactive monitoring of equipment and systems to detect potential failures or malfunctions before they occur.
Artificial Intelligence (AI) technologies, such as machine learning, deep learning, and natural language processing, complement advanced analytics by enabling automated decision-making and the ability to learn from data. AI algorithms can analyze vast volumes of IoT data in real-time, identify patterns and trends, and make predictions or recommendations. This capability is particularly beneficial in predictive maintenance scenarios, where AI algorithms can identify early warning signs of equipment failure, recommend maintenance actions, and optimize maintenance schedules to minimize downtime and maximize asset performance.
The integration of advanced analytics and AI technologies with IoT in predictive maintenance offers several advantages. Firstly, it enables organizations to move from a reactive maintenance approach to a proactive or even predictive one. By continuously monitoring and analyzing IoT data, organizations can anticipate equipment failures, schedule maintenance activities in advance, and avoid costly unplanned downtime.
The rise of digital twins and simulations
The integration of digital twins and simulations into IoT and predictive maintenance has several implications and benefits. Firstly, it enables organizations to gain deep insights into the performance of their assets and predict maintenance requirements accurately. By continuously monitoring the digital twin, companies can detect anomalies, anticipate failures, and plan maintenance activities proactively. This approach helps minimize downtime, optimize resource allocation, and extend the lifespan of assets, leading to significant cost savings.
Secondly, digital twins and simulations enhance the ability to optimize operations and improve overall efficiency. By running virtual scenarios, organizations can identify the optimal settings, configurations, and parameters for their systems. This iterative process enables them to fine-tune operations, reduce energy consumption, optimize supply chains, and streamline production processes. As a result, organizations can achieve higher productivity, reduce waste, and enhance their competitive advantage.
Furthermore, digital twins and simulations facilitate the development and testing of new products and services. Organizations can create virtual prototypes and simulate their performance under different conditions, allowing for rapid iteration and design improvements. This approach accelerates the innovation cycle, reduces development costs, and enhances the quality of final products or services.
However, the widespread adoption of digital twins and simulations also presents challenges. Firstly, it requires significant investments in infrastructure, sensors, connectivity, and data management systems. Organizations need to ensure the availability, reliability, and security of data to maintain accurate digital replicas and perform reliable simulations. Additionally, interoperability and standardization across different systems and platforms are essential for seamless integration and collaboration between stakeholders.
Increased adoption of edge computing and 5G networks
The integration of 5G networks with IoT systems is another transformative trend in the field. 5G offers significantly higher data transfer speeds, lower latency, and increased network capacity compared to its predecessors. These capabilities make it an ideal communication infrastructure for IoT devices, particularly in scenarios that demand ultra-reliable and low-latency connectivity.
By leveraging 5G networks, IoT devices can transmit vast amounts of data in near real-time, enabling faster and more accurate predictive maintenance. For instance, in a smart city environment, 5G-enabled sensors can continuously monitor the condition of critical infrastructure such as bridges, roads, and utility systems. The high-speed connectivity provided by 5G networks ensures that the data from these sensors reaches the analytics platforms rapidly, allowing for proactive maintenance and timely interventions. This not only enhances the safety and reliability of the infrastructure but also minimizes disruptions and improves the overall quality of life for citizens.
Don't miss out on the opportunity to leverage IoT predictive maintenance for your organization. Contact us today and let our knowledgeable team guide you towards a more efficient and cost-effective maintenance approach.
Embracing IoT-based predictive maintenance can bring numerous benefits to businesses, including enhanced efficiency and cost savings. Predictive maintenance helps reduce both planned and unplanned maintenance costs. By adopting a proactive approach, businesses can avoid unnecessary scheduled maintenance, thereby saving on labor, spare parts, and associated expenses. By leveraging IoT data for predictive maintenance, businesses can optimize their operational processes. Among other benefits are:
Improved website performance and customer experience
IoT devices integrated into the digital infrastructure can continuously monitor various components of a website, such as servers, databases, networks, and applications. These devices collect real-time data on performance metrics. By analyzing this data using predictive analytics, businesses can identify patterns, detect anomalies, and predict potential failures or bottlenecks.
With this information, organizations can take proactive measures to address issues before they impact website performance. For example, predictive maintenance can alert IT teams about an impending server overload and enable them to allocate additional resources or optimize server configurations in advance.
A fast, reliable, and user-friendly website is crucial for providing an exceptional customer experience. IoT-based predictive maintenance helps businesses achieve this by minimizing service disruptions, reducing response times, and preventing performance issues that can frustrate customers.
Increased sales due to reduced downtime
IoT-based predictive maintenance offers a range of benefits, and reduced downtime stands out as a key factor contributing to increased sales. By leveraging real-time monitoring, accurate analytics, and proactive maintenance strategies, businesses can optimize equipment uptime, allocate resources efficiently, satisfy customers, save costs, and gain a competitive advantage. Embracing this transformative approach not only improves operational efficiency but also drives sales growth and enhances business performance in the long run.
Enhanced ability to focus on core business operations and growth
One of the significant benefits of utilizing IoT-based predictive maintenance is the enhanced ability to focus on core business operations and growth. By leveraging IoT sensors and data analytics, companies can proactively monitor and predict equipment failures or maintenance needs. This proactive approach minimizes unplanned downtime, reduces maintenance costs, and optimizes overall operational efficiency.
It might not be easy to choose the right MSP. Here are some things to consider while choosing the right MSP.
Factors to consider when selecting an MSP
When selecting a Managed Service Provider (MSP), it's essential to consider several factors to ensure you choose the right provider that aligns with your business needs. Here are some key factors to consider:
Expertise and Services
Assess the MSP's expertise and range of services. Ensure they have experience in managing the specific technologies, systems, or infrastructure your organization uses.
Evaluate the MSP's ability to scale their services according to your business growth and evolving needs.
Service Level Agreements (SLAs)
Review the SLAs offered by the MSP. Assess their commitments regarding uptime, response times, resolution times, and support availability
Security and Compliance
Consider the MSP's approach to security and compliance. Determine if they have robust security measures, such as data encryption, access controls, and threat detection and response protocols.
Cost and Value
Compare the costs and value proposition of different MSPs. Request detailed pricing proposals and consider the value they provide relative to their pricing.
Importance of cultural fit and communication
A strong cultural fit facilitates smoother collaboration, promotes a shared vision, and enhances teamwork. When the MSP understands your organization's culture, it becomes easier for them to adapt to your processes, engage with your employees, and provide services that align with your company's goals. This alignment leads to increased productivity, satisfaction, and overall success.
Effective communication is another vital aspect to consider when choosing an MSP. Communication serves as the foundation for a healthy working relationship. Clear and open communication channels allow for seamless information exchange, enabling both parties to understand expectations, goals, and requirements. Regular and transparent communication ensures that the MSP is updated on any changes in your business, allowing them to respond promptly and provide the necessary support.
Assessing MSPs through references and testimonials
When evaluating MSPs, requesting references allows you to connect with their existing or previous clients. By speaking directly with these clients, you can gain firsthand knowledge about the MSP's capabilities, strengths, and weaknesses. It's essential to ask specific questions related to the services provided, responsiveness, problem resolution, and overall experience working with the MSP. References can offer a realistic perspective on the MSP's reliability, professionalism, and adherence to service level agreements (SLAs).
Testimonials are another valuable source of information when assessing MSPs. These are typically statements or reviews provided by clients who have had positive experiences with the MSP's services. Testimonials often highlight the MSP's ability to meet client expectations, deliver high-quality solutions, and provide excellent customer support. Reading testimonials can give you an overview of the MSP's strengths and the benefits they bring to their clients.
In conclusion, predictive maintenance with the Internet of Things (IoT) has emerged as a powerful solution for optimizing maintenance practices in various industries. By harnessing real-time data from connected devices, predictive maintenance enables organizations to monitor the condition of equipment, identify potential failures before they occur, and schedule maintenance activities proactively.
The integration of IoT devices allows for the collection of vast amounts of sensor data, including temperature, vibration, pressure, and other relevant parameters. Advanced analytics and machine learning algorithms can then analyze this data to identify patterns, anomalies, and potential signs of equipment degradation or failure. By leveraging these insights, maintenance teams can make informed decisions, prioritize resources, and perform necessary repairs or replacements at the right time, reducing downtime, minimizing costs, and optimizing asset performance.
Let us help you take your business to the next level with our innovative IoT solutions. Contact us today, and we’ll be happy to answer all your inquiries
you may also want to read
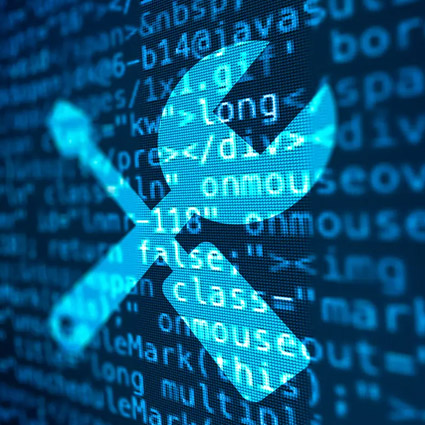
Hire Laravel Framework Developer: Tips and Offers
Do you dream of a dynamic website, a secure eCommerce store, or a new game but lack the resources for...
AI in HR: Transforming Human Resources
AI in HR is promised to change the recruitment operational activities dramatically. Despite the changes expected to be grasped by...
Python Telematic Solutions: Unfolding Capabilities for Fleet Management
The success of transportation and logistics companies is defined by how efficiently their fleet operates. This includes vehicle tracking, route...
* Yes, I understand and agree to the Terms and Conditions and the Privacy Policy .
This website uses cookies to ensure you get the best experience on our website. By continuing to use this site, you consent to our use of cookies. For more information, please read our Privacy Policy .
IoT Applications in 2024: 30+ Use Cases
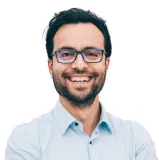
Internet of Things (IoT) applications are becoming more widespread. According to Statista , worldwide expenditure on IoT in 2022 is expected to be $1B.
IoT enables a myriad of different business applications. Knowing those IoT examples and use cases can help businesses integrate IoT technologies into their future investment decisions. That is why we set out to create the most comprehensive list of IoT use cases in industries.
The image below shows the potential impact of IoT technologies in various industries in 2025.
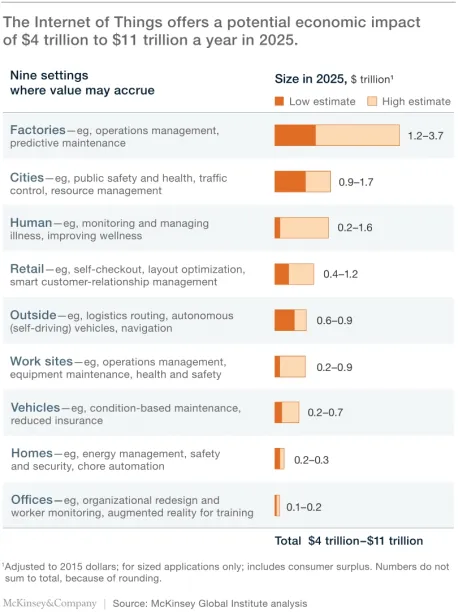
We have compiled 33 IoT applications for business leaders to select the correct use case for their IoT implementation . Use cases include:
Smart Factories
1. enterprise asset management.
Enterprise asset management involves work management, asset maintenance, planning and scheduling, supply chain management, and environmental, health, and safety (EHS) initiatives. Businesses collect real-time data from an asset with IoT sensors.
Businesses are rapidly adopting smart asset management systems into their businesses. Due to their asset-intensive environments, we mostly encounter IoT asset monitoring in industries such as logistics, retail, and manufacturing.
IoT-powered asset management increases real-time visibility of assets and helps businesses optimize their resource while providing benefits such as:
- Increased operational efficiency
- Better control over the sales lifecycle
- More efficient safety and compliance checks
- More responsive smart environment.
2. Predictive maintenance
Maintenance is conducted to prevent predicted problems. So over the lifetime of a machine, some components may never be checked if they are not predicted to cause problems.
For example, Fanuc is a robotics company that is working on reducing the downtime of machines with IoT technology. Fanuc uses sensors to predict when the failure of the component will happen.
3. Industrial process automation/optimization
Organizations can keep a real-time record of the metrics of all the machines inside a plant using IoT and IP networks. Manufacturers can use this data to automate workflows and optimize production systems. Automation and optimization support industrial companies to reduce costs and increase the quality and volume of output.
The market for automated industrial robots is proliferating. The market size was 41 billion U.S. dollars in 2017 and is expected to reach 73 billion U.S. dollars in 2023.
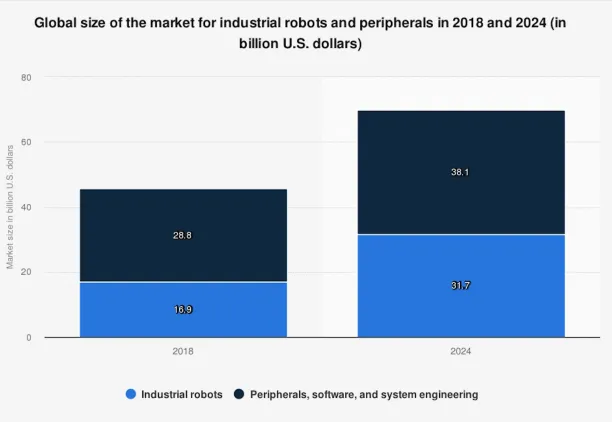
4. Energy management
Energy can be a costly input for industrial businesses. With fluctuating energy costs and strict government requirements for efficiency, managing energy distribution becomes important.
IoT devices can help manufacturers manage energy consumption based on real-time data collected from devices. Intelligent energy management systems reduce energy bills, operational expenditures, and the carbon footprint of the factory while increasing energy efficiency. WebNMS is an IoT platform that provides IoT applications including energy management to optimize the energy consumption of businesses.
Smart Cities
Kay Sharpington from Gartner, states “The COVID-19 pandemic is slowing down spending, however, governments across the globe continue to use IoT technologies and solutions to improve citizen safety. At the same time, the falling endpoint and connectivity costs make smart city initiatives more viable”.
Gartner estimated that the global government Internet of Things (IoT) endpoint electronics and communications market totaled $15 billion in 2020, an increase of 6% from 2019. Same study also reveals the top 5 government IoT applications and revenue generated by each use case as seen below:
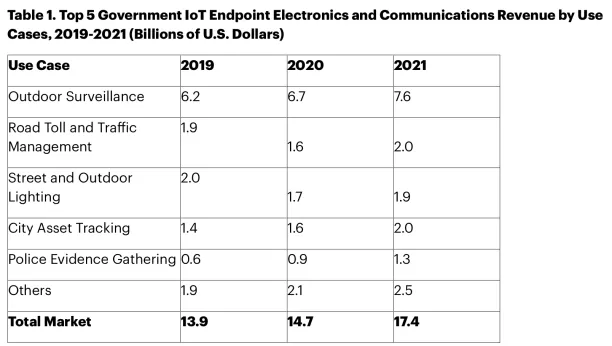
5. Outdoor surveillance
When IoT CCTV cameras are combined with artificial intelligence and machine vision, governments can automate the surveillance of streets through cameras. As IoT enables connectivity of machines, they can record and analyze video data in real-time, and they can provide police officers with insights instead of single pieces of images.
However, outdoor surveillance processes personal information and there is potential for abuse in the use of such technologies. Therefore appropriate checks and balances need to be implemented in such systems to ensure that personal information is not abused while the risk of crime is minimized.
6. Smart lighting
According to the 2018 Gartner IoT hype cycle report, smart lighting is the fourth-most mature IoT tech application. Smart lighting aims to optimize energy management.
Smart lighting is made up of street lighting with IoT sensors. Sensors collect data about the condition of traffic and pedestrians. With that data, street lights provide optimum lighting so that street lighting systems can save up to 80% of the energy.
Smart lighting can also be applied to factories or homes.
7. Electronic road toll collection and traffic management
Traffic engineers augmented by smart systems at a central traffic management center (TMC) can analyze data from IoT sensors and then optimize the timing of traffic lights throughout the day. This can help divide the traffic more evenly over roads as traffic volume fluctuates.
8. Smart parking
In cities like San Francisco, parking is a big problem. With IoT sensors, parking problems in a city can be minimized.
The working principle of smart parking is:
- Sensors are attached to parking lots to detect parked cars
- Measurements are periodically sent to the cloud by microcontrollers
- Mobile Apps use cloud data to identify empty parking spaces,
- Drivers check mobile apps to identify vacant parking spaces close to the location they aim to go to.
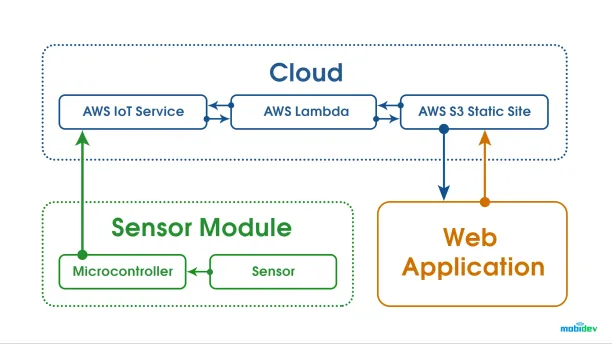
Noise Monitoring
In smart cities, sound monitoring systems can monitor noise levels warn companies that violate limits, and help manage noise levels.
9. Structural health monitoring
IoT allows remote collection of architectural data to monitor events such as vibrations and changes in material conditions, predict structural damage, and prepare action plans for structures such as bridges, buildings, stadiums, ships, airplanes, etc.
10. Waste management
Traditional waste collections are complicated and costly since a fleet of trucks drives along busy streets using inefficient routes. Fill levels of garbage containers differ for each container: ranging from overflowing, partially filled, and empty. IoT sensors can monitor fill levels for conventional bins and send the data to the relevant department of the city hall. With that information, the garbage truck routes can be optimized for trash collection.
Machine learning methods can also be implemented in IoT sensors (i.e. edge analytics ) so that sensors can predict the fill levels of containers by learning from historical data.
Below the video, you can find how Proximus, an IoT solutions vendor, uses IoT to manage waste:
Water Management
Due to the drastic increase in urbanization levels and the importance of water quality in human health, water management is a key topic for cities. A water management system is based on real-time data collected from sensors. Water management can provide the following applications:
11. Water conservation
Sensors detect the water level in tanks and alert when the water level is lower than the threshold. Well™, a smart home water conservation system developed by Mindtribe , uses IoT sensors to monitor water usage.
12. Smart irrigation
IoT sensors determine the weather conditions and the soil moisture, which will help in getting the appropriate amount of water that the soil needs. For instance, greenIQ is a vendor that offers a sprinkler sensor that optimizes its functionality based on the dryness of the soil.
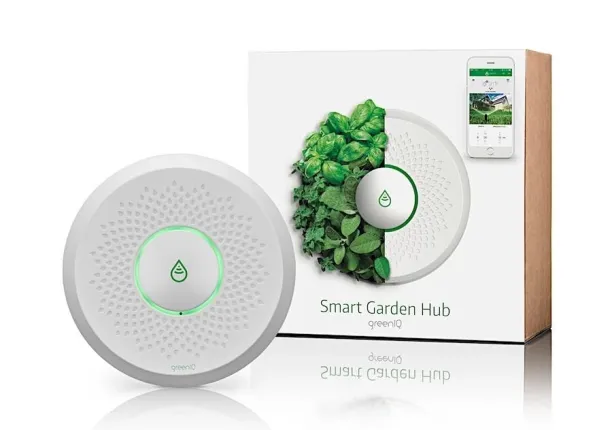
13. Leakage management
IoT sensors can detect temperature changes, water leakage, chemical leakage, and pressure levels in water tanks.
14. Water quality management
IoT sensors determine what kind of chemicals are in the water. They also identify metrics such as total dissolved solids (TDS), bacteria, chlorine, electrical conductivity, etc.
Learn more about IoT in agriculture.
Digital Health
15. ultraviolet radiation monitoring.
Sunlight consists of three major components:
- Visible light: Wavelengths between 0.4 and 0.8 micrometers,
- Ultraviolet light: Wavelengths shorter than 0.4 micrometers,
- Infrared light: Wavelengths longer than 0.8 micrometers.
Ultraviolet (UV) rays are electromagnetic waves that account for about 10% of solar light. When overexposed, UV rays have harmful effects such as skin cancer, premature aging, cataracts, and immune system suppression. IoT sensors measure UV sun rays to warn people not to be exposed during certain hours.
16. Fall detection
Falling to the ground and not being able to get up or request help can be a scary experience for senior citizens. IoT sensors can detect falls using geolocation data and summon help so that it reduces the time the elderly remain on the floor after a fall which could lead to lethal consequences.
The below video is an example of how fall detection systems work from a vendor called Walabot:
17. Companion robots
A companion robot is a robot that is designed to create companionship mostly for elderly and single children. IoT sensors are essential for robotics and it is the same for companion robots as well. Sensors detect objects that surround the robot and enable the robot to move.
Researchers claim that people have become more receptive to companion robots during the pandemic. Social isolation may lead people to loneliness, anxiety, and frustration, especially the elderly.
18. Medical fridges
Medical fridges monitor the temperature of vaccines, medicines, and organic elements for clinics and health centers. Medical fridges provide an opportunity to follow all safety standards and national regulations of the pharmaceutical market using IoT sensors. They prevent medicines and vaccines from spoiling.
Efento is an IoT sensor and IoT platform vendor that has a variety of temperature measurement products along with wireless monitoring of temperature in medical refrigerators.
19. Patient surveillance/remote patient monitoring
20% of patients who had surgery are readmitted to the hospital within just 30 days. Remote patient monitoring (RPM) systems use wearables to monitor the condition of patients who are resting at home after surgery. RPM enables real-time data collection about patients’ body temperature which is the main indicator of infections. With RPM, doctors can observe patients’ data and provide early diagnoses without requiring patients to be physically present at the hospital.
Telit is an IoT solution vendor and offers its customers a remote patient monitoring (RPM) solution that enhances patient monitoring capabilities and patient satisfaction. Telit’s offering can reduce:
- Patients hospital stay duration thanks to early diagnosis of complications
- Hospital readmissions
Smart Retail
20. supply chain control.
IoT devices have transformed supply chain management. Sensors, which are attached to storage containers or products themselves,
- show the location of goods using GPS,
- track the speed of movement providing an accurate estimated time of arrival (ETA) for goods,
- monitor warehouse conditions such as temperature, humidity, light intensity, and other environmental factors
21. Near field communication (NFC) payment
NFC enables contactless payments. POS vendors include NFC support in their systems, and customers are adopting contactless payments via their smartphones.
22. Layout optimization
Sensors in the store collect data like voice, image, or video to better understand customer habits and preferences. Retailers can get insights to redesign the layout of their stores. The optimized layout can enhance sales.
23. Smart product management
IoT sensors enable retailers to control the rotation of products on shelves and warehouses to automate merchandising decisions. We have already written about retail analytics use cases, feel free to check it out if you want to learn more.
Smart Workplace
24. sociometric badges.
Sociometric sensors are wearable IoT devices that measure the amount of face-to-face interaction, conversational time, physical proximity to other people, and physical activity levels using social signals derived from vocal features, body motion, and relative location.
For example, Humanyze is a vendor that uses sociometric sensors to perform people analytics. The company helps organizations understand how their teams interact to increase performance.
Smart Homes
25. remote control appliances.
IoT-powered home appliances let residents remotely switch on and off devices using smartphone apps to avoid incidents and save energy. Additionally, these devices can make autonomous decisions based on sensor inputs such as preparing fresh coffee when a resident is identified to wake up. Other examples of autonomous or remote-controlled actions include:
- Turning on lights,
- Starting the coffee maker,
- Setting temperature,
- Open up a music playlist,
- And locking doors.
Home Intrusion Detection Systems:
IoT-based home security applications give users capabilities such as smart locks and security cameras that detect motions and send alerts to their smartphones so that they can monitor the safety conditions of their homes from anywhere.
26. Smart locks
Eyelock is a security provider vendor that offers its clients an iris-based authentication solution.
27. Motion detection
Manything is another vendor in IoT IoT-based home security market. It streams home/office videos and lets users receive alerts when it detects any activity.
Smart Logistics
28. fleet tracking.
IoT fleet tracking systems improve security and provide precise and complete reports that give the fleet managers full transparency regarding the fleet’s activities. Through GPS monitoring and geo-location tools, companies can track the location of their trucks, optimize routes, and monitor their fleet utilization in detail.
For instance, Canadian delivery service Sure Track Courier saved 6-10% per month on fuel costs by optimizing routes using IoT data from trucks.
29. Platooning
Platooning involves a group of self-driving trucks that follow a lead truck at high speed safely and efficiently. Trucks use IoT sensors so that each truck communicates with the other trucks to adapt its speed and braking accordingly.
30. Connected vehicles
Sensors are enhancing vehicles along with AI and analytical capabilities. These sensors provide communication with the driver to supply useful information about other cars on the road and roadside infrastructure to the driver to help the driver make safer or more informed decisions. For example, these vehicles provide GPS-enabled location detection feature that helps them detect traffic congestion.
Autonomous vehicles are also an application of IoT devices. Though it is not commonly used in logistics yet, we will witness this approach soon. For instance, the Mercedes-Benz prototype of the semi-autonomous truck is scheduled for release in 2025.
Smart Metering
31. smart grid.
With the increasing attention regarding climate change and carbon emissions, utilities focus on reducing energy consumption. For utility companies, IoT enables remote data management and monitoring capabilities to manage better power flows into and out of their grids, and give users the insights needed to understand their energy infrastructure investments.
32. Digital Twins
A digital twin is a virtual replica of physical entities such as devices, people, processes, or systems that help businesses make model-driven decisions. With the help of IoT sensors, businesses collect data that is needed to create a digital twin.
Digital twins enable businesses to gain a deeper understanding of real-world conditions so that they make necessary adjustments to their products & services.
33. IoT devices in healthcare
In healthcare , the ecosystem of IoT-enabled devices such as wearables (smartwatches, rings, vests, etc.), monitors (heart monitors, sleep monitors, temperature monitors, etc.), and trackers (medication refill reminder, drug effectiveness tracking, etc.) can be used for:
- Constant monitoring of patients’ vitals (blood pressure, heart rate, temperature rate, etc.),
- And more accurate diagnoses of patients, thanks to real-time data from IoT devices, thus reducing the possibility of readmission and rising hospital bills.
Read more on IoT
We have written articles about IoT technologies before, feel free to read our other articles on:
- Increase Speed to Market Using an IoT Platform
- Edge Analytics in 2022: What it is, Why it Matters & Use Cases
- IoT Testing: Framework, Challenges, Case Studies & Tools 2022
We also have some in-depth articles on other use cases of IoT in different industries:
- Top 6 Use Cases of IoT in Manufacturing
- 5 Use Cases of IoT in Automotive in 2022
- 7 Ways IoT Will Improve Your Banking Experience in 2022
Finally, if you believe your business could benefit from implementing an IoT solution, we have a data-driven list of vendors prepared .
We will help you choose the best one for your business:
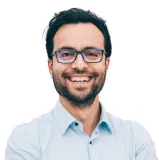
Next to Read
How to use iot & process mining together in '24, iot analytics: benefits, challenges, use cases & vendors [2024].
Your email address will not be published. All fields are required.
Related research
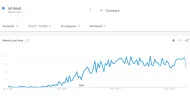
IoT Cloud in 2024: Benefits, Challenges, Platforms&Functionality
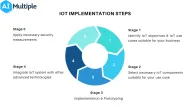
IoT Implementation: Steps & Best Practices in 2024
IEEE Account
- Change Username/Password
- Update Address
Purchase Details
- Payment Options
- Order History
- View Purchased Documents
Profile Information
- Communications Preferences
- Profession and Education
- Technical Interests
- US & Canada: +1 800 678 4333
- Worldwide: +1 732 981 0060
- Contact & Support
- About IEEE Xplore
- Accessibility
- Terms of Use
- Nondiscrimination Policy
- Privacy & Opting Out of Cookies
A not-for-profit organization, IEEE is the world's largest technical professional organization dedicated to advancing technology for the benefit of humanity. © Copyright 2024 IEEE - All rights reserved. Use of this web site signifies your agreement to the terms and conditions.
- How It Works
- Request a quote
- Get in touch
IoT in Agriculture: 9 Technology Use Cases for Smart Farming (and Challenges to Consider)
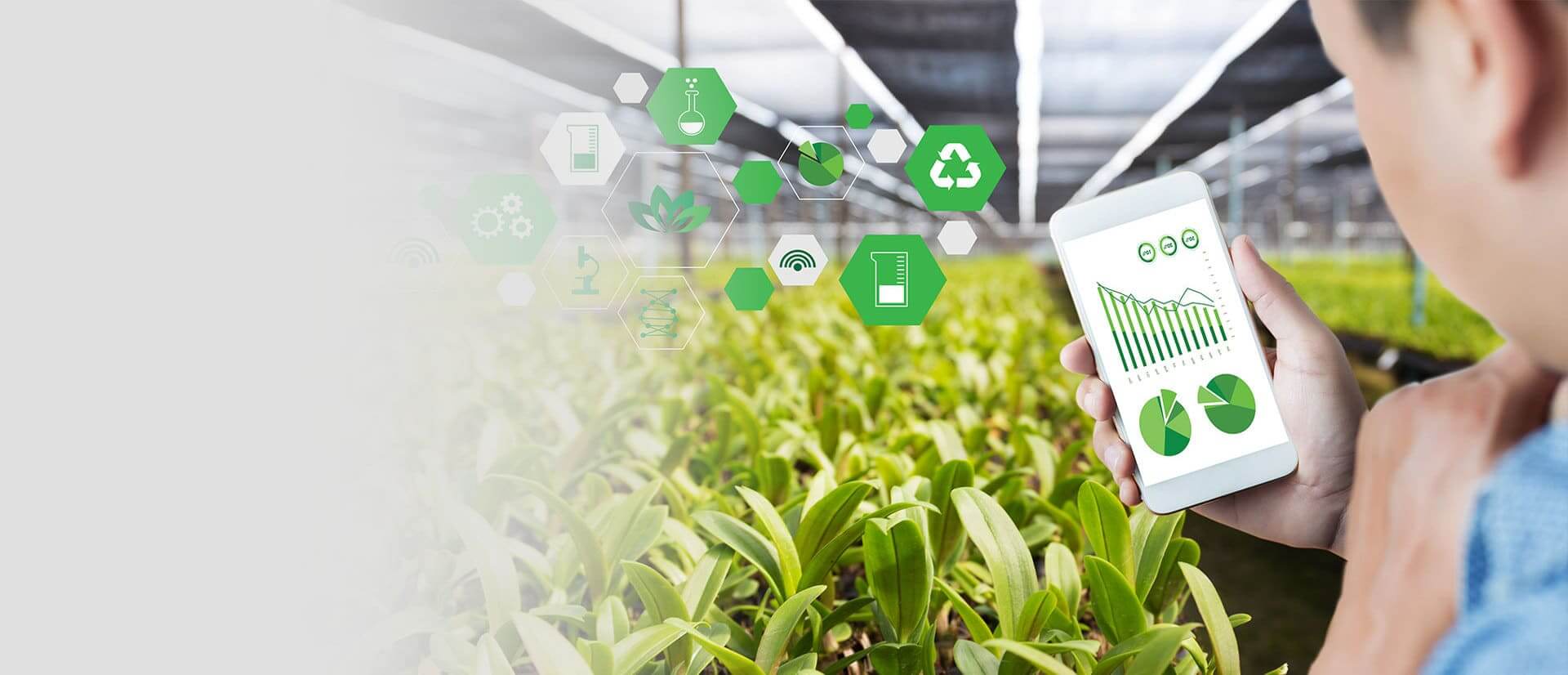
The article was updated on March 1, 2023.
With the growing adoption of the Internet of Things (IoT), connected devices have penetrated every aspect of our life , from health and fitness, home automation, automotive and logistics, to smart cities and industrial IoT.
Thus, it is only logical that IoT, connected devices, and automation would find its application in agriculture, and as such, tremendously improve nearly every facet of it. How could one still rely on horses and plows when self-driving cars and virtual reality are no longer a sci-fi fantasy but an everyday occurrence?
Farming has seen a number of technological transformations in the last decades, becoming more industrialized and technology-driven. By using various smart agriculture gadgets, farmers have gained better control over the process of raising livestock and growing crops, making it more predictable and improving its efficiency.
This, along with the growing consumer demand for agriculture products, has contributed to the increased proliferation of smart farming technologies worldwide. In 2022, the market share for IoT in agriculture reached $13.76 billion.
In this article, we will explore the IoT use cases in agriculture and examine their benefits. So, if you are considering investing into smart farming, or are planning to build an IoT solution for agriculture, dive right in.
- What is smart agriculture? The definition and market size
There are many ways to refer to modern agriculture. For example, AgriTech refers to the application of technology in agriculture in general.
Smart agriculture , on the other hand, is mostly used to denote the application of IoT solutions in agriculture. So what is smart agriculture using IoT? By using IoT sensors to collect environmental and machine metrics, farmers can make informed decisions, and improve just about every aspect of their work – from livestock to crop farming.
For example, by using smart agriculture sensors to monitor the state of crops, farmers can define exactly how many pesticides and fertilizers they have to use to reach optimal efficiency. The same applies to the smart farming definition.
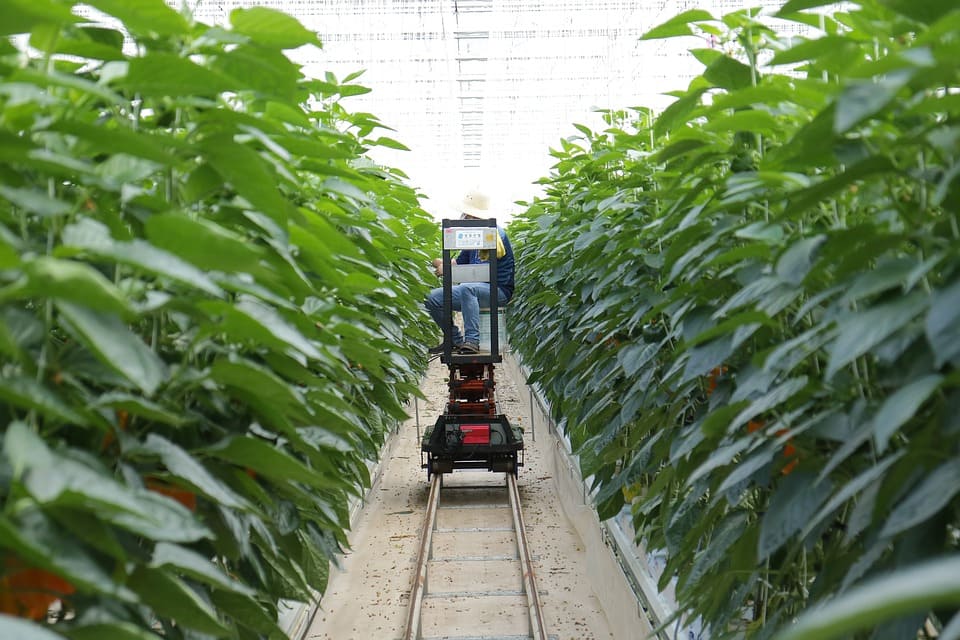
Although smart agriculture IoT, as well as industrial IoT in general, aren’t as popular as consumer connected devices; yet the market is still very dynamic. The adoption of IoT solutions for agriculture is constantly growing.
Namely, COVID-19 has had a positive impact on IoT in the agriculture market share. Disruptions in the supply chain, and the shortage of qualified workers, has propelled its CAGR to 9,9%. In fact, as per recent reports, the smart framing market share is set to reach $28.56 billion by 2030.
At the same time, the global smart agriculture market size is expected to triple by 2025, reaching $15.3 billion (compared to being slightly over $5 billion back in 2016).
Because the market is still developing, there is still ample opportunity for businesses willing to join in. Building IoT products for agriculture within the coming years can set you apart as an early adopter, and as such, help you pave the way to success.
But why should you consider building an IoT application for agriculture in the first place?
The Benefits of smart farming: How’s IoT shaping agriculture
Technologies and IoT have the potential to transform agriculture in many aspects. Namely, there are 6 ways IoT can improve agriculture :
- Data, tons of data, collected by smart agriculture sensors, e.g. weather conditions, soil quality, crop’s growth progress or cattle’s health. This data can be used to track the state of your business in general as well as staff performance, equipment efficiency, etc.
- Better control over the internal processes and, as a result, lower production risks . The ability to foresee the output of your production allows you to plan for better product distribution. If you know exactly how much crops you are going to harvest, you can make sure your product won’t lie around unsold.
- Cost management and waste reduction thanks to the increased control over the production . Being able to see any anomalies in the crop growth or livestock health, you will be able to mitigate the risks of losing your yield.
- Increased business efficiency through process automation . By using smart devices, you can automate multiple processes across your production cycle, e.g. irrigation, fertilizing, or pest control.
- Enhanced product quality and volumes . Achieve better control over the production process and maintain higher standards of crop quality and growth capacity through automation.
- Reduced environmental footprint. Automation also carries environmental benefits. Smart farming technologies can cut down on the use of pesticides and fertilizer by offering more precise coverage, and thus, reduce greenhouse gas emissions.
As a result, all of these factors can eventually lead to higher revenue .
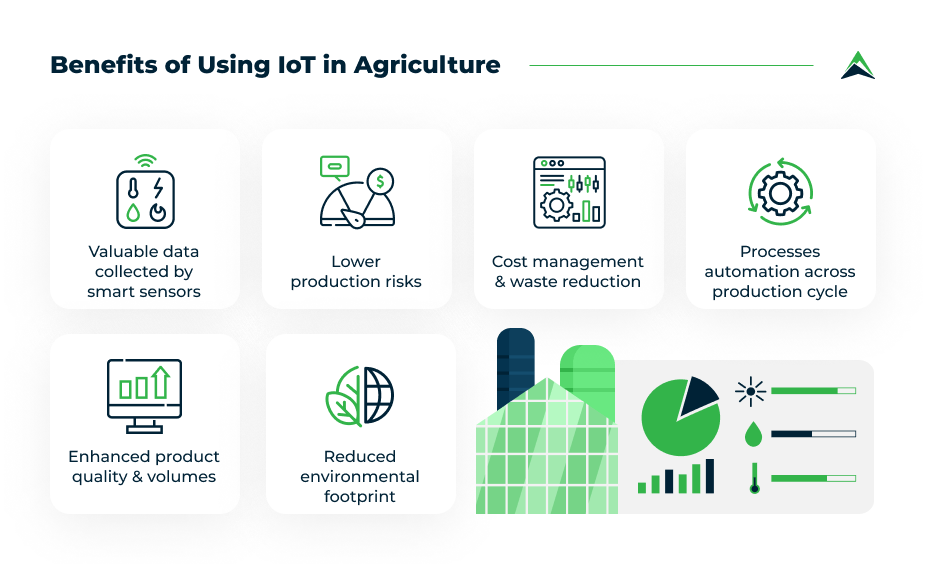
Now that we have outlined how IoT can be advantageously applied in the sphere of agriculture, let’s take a look at how the listed benefits can find their application in real life.
- IoT use cases in agriculture (with examples)
There are many types of IoT sensors for agriculture as well as IoT applications in agriculture in general:
1. Monitoring of climate conditions
Probably the most popular smart agriculture gadgets are weather stations, combining various smart farming sensors. Located across the field, they collect various data from the environment and send it to the cloud. The provided measurements can be used to map the climate conditions, choose the appropriate crops, and take the required measures to improve their capacity (i.e. precision farming).
Some examples of such agriculture IoT devices are allMETEO , Smart Elements , and Pycno .
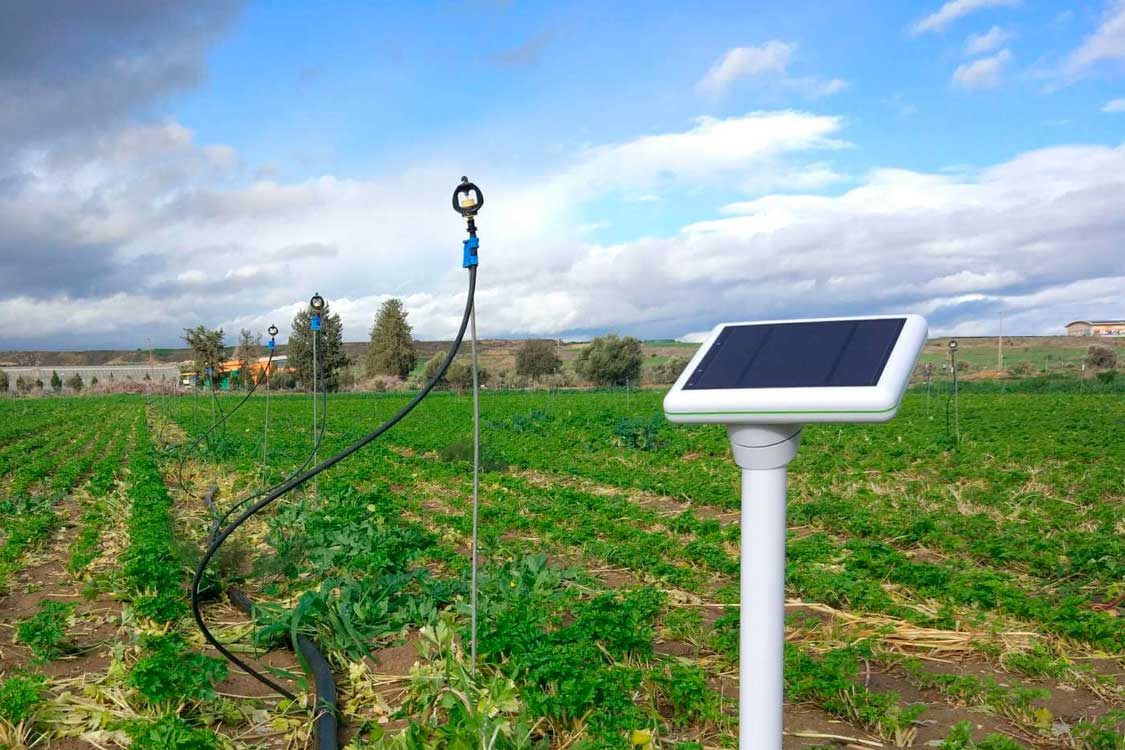
2. Greenhouse automation
Typically, farmers use manual intervention to control the greenhouse environment. The use of IoT sensors enables them to get accurate real-time information on greenhouse conditions such as lighting, temperature, soil condition, and humidity.
In addition to sourcing environmental data, weather stations can automatically adjust the conditions to match the given parameters. Specifically, greenhouse automation systems use a similar principle.
For instance, Farmapp and Growlink are also IoT agriculture products offering such capabilities among others.
3. Crop management
One more type of IoT product in agriculture and another element of precision farming are crop management devices. Just like weather stations, they should be placed in the field to collect data specific to crop farming; from temperature and precipitation to leaf water potential and overall crop health.
Thus, you can monitor your crop growth and any anomalies to effectively prevent any diseases or infestations that can harm your yield. Arable and Semios can serve as good representations of how this use case can be applied in real life.
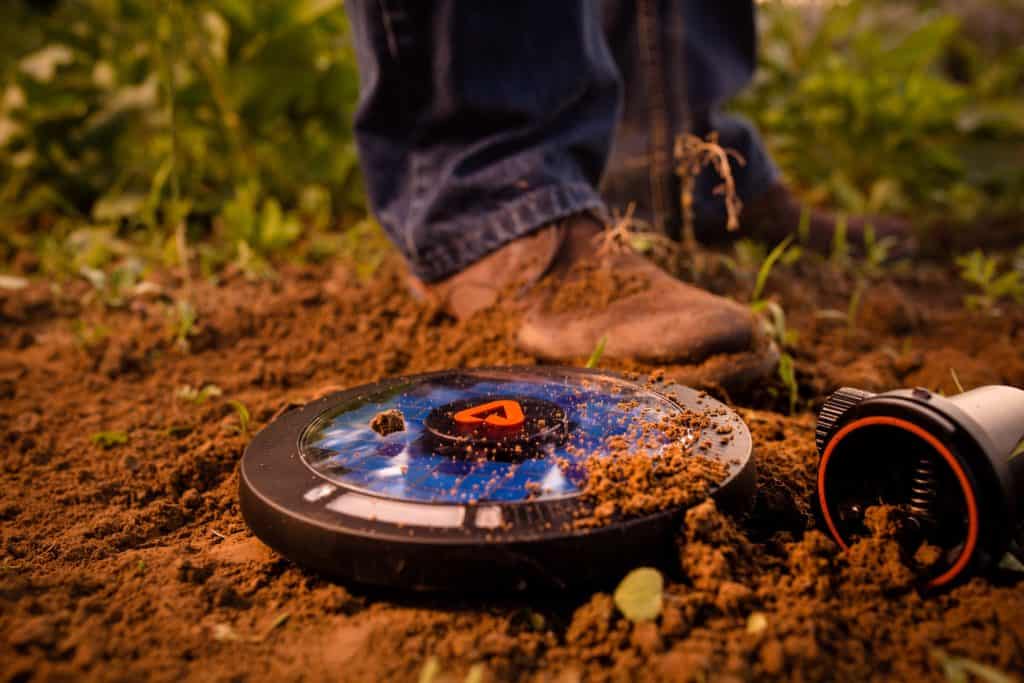
4. Cattle monitoring and management
Just like crop monitoring, there are IoT agriculture sensors that can be attached to the animals on a farm to monitor their health and log performance. Livestock tracking and monitoring help collect data on stock health, well-being, and physical location.
For example, such sensors can identify sick animals so that farmers can separate them from the herd and avoid contamination. Using drones for real-time cattle tracking also helps farmers reduce staffing expenses. This works similarly to IoT devices for petcare .
For example, SCR by Allflex and Cowlar use smart agriculture sensors (collar tags) to deliver temperature, health, activity, and nutrition insights on each individual cow as well as collective information about the herd.
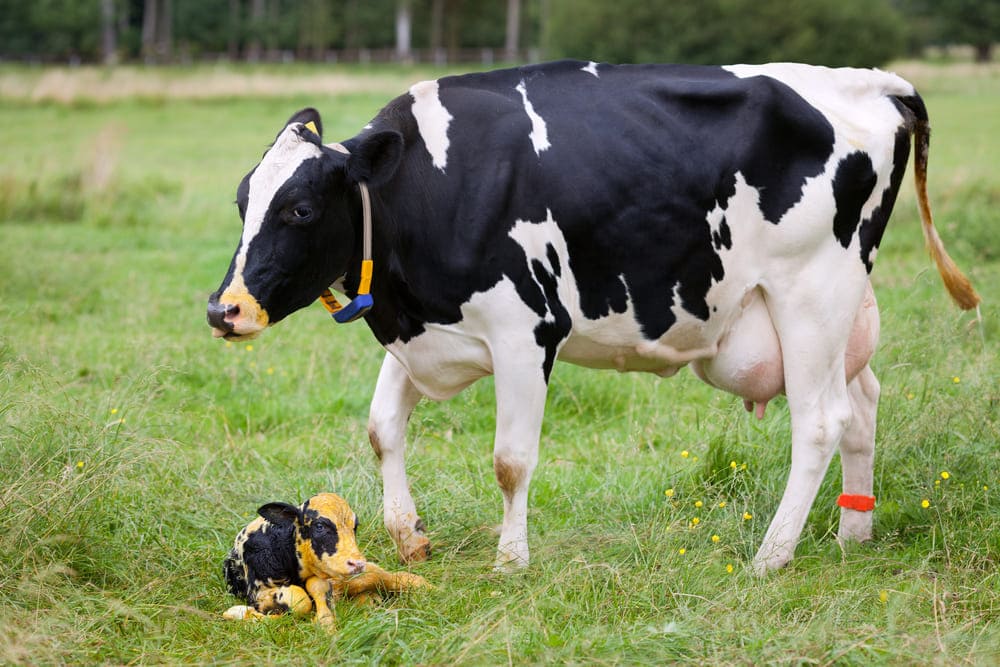
5. Precision farming
Also known as precision agriculture, precision farming is all about efficiency and making accurate data-driven decisions. It’s also one of the most widespread and effective applications of IoT in agriculture.
By using IoT sensors, farmers can collect a vast array of metrics on every facet of the field microclimate and ecosystem: lighting, temperature, soil condition, humidity, CO2 levels, and pest infections. This data enables farmers to estimate optimal amounts of water, fertilizers, and pesticides that their crops need, reduce expenses, and raise better and healthier crops.
For example, CropX builds IoT soil sensors that measure soil moisture, temperature, and electric conductivity enabling farmers to approach each crop’s unique needs individually. Combined with geospatial data, this technology helps create precise soil maps for each field. Mothive offers similar services, helping farmers reduce waste, improve yields, and increase farm sustainability.
6. Agricultural drones
Perhaps one of the most promising agritech advancements is the use of agricultural drones in smart farming. Also known as UAVs (unmanned aerial vehicles), drones are better equipped than airplanes and satellites to collect agricultural data. Apart from surveillance capabilities, drones can also perform a vast number of tasks that previously required human labor: planting crops, fighting pests and infections, agriculture spraying, crop monitoring, etc.
Read more: Why Use Agriculture Drones? Main Benefits and Best Practices
DroneSeed , for example, builds drones for planting trees in deforested areas. The use of such drones is 6 times more effective than human labor. A Sense Fly agriculture drone eBee SQ uses multispectral image analyses to estimate the health of crops and comes at an affordable price.
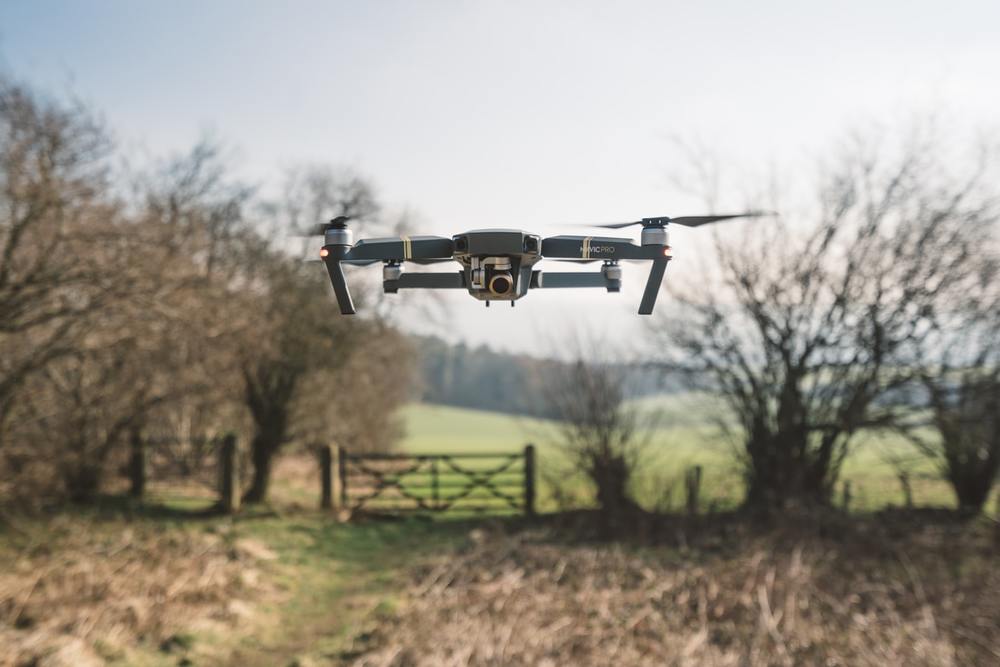
7. Predictive analytics for smart farming
Precision agriculture and predictive data analytics go hand in hand. While IoT and smart sensor technology are a goldmine for highly relevant real-time data, the use of data analytics helps farmers make sense of it and come up with important predictions: crop harvesting time, the risks of diseases and infestations, yield volume, etc. Data analytics tools help make farming, which is inherently highly dependent on weather conditions, more manageable, and predictable.
For example, the Crop Performance platform helps farmers access the volume and quality of yields in advance, as well as their vulnerability to unfavorable weather conditions, such as floods and drought. It also enables farmers to optimize the supply of water and nutrients for each crop and even select yield traits to improve quality.
Applied in agriculture, solutions like SoilScout enable farmers to save up to 50% irrigation water, reduce the loss of fertilizers caused by overwatering, and deliver actionable insights regardless of season or weather conditions.
8. End-to-end farm management systems
A more complex approach to IoT products in agriculture can be represented by the so-called farm productivity management systems. They usually include a number of agriculture IoT devices and sensors, installed on the premises as well as a powerful dashboard with analytical capabilities and in-built accounting/reporting features.
This offers remote farm monitoring capabilities and allows you to streamline most of the business operations. Similar solutions are represented by FarmLogs and Cropio .
In addition to the listed IoT agriculture use cases, some prominent opportunities include vehicle tracking (or even automation), storage management, logistics, etc.
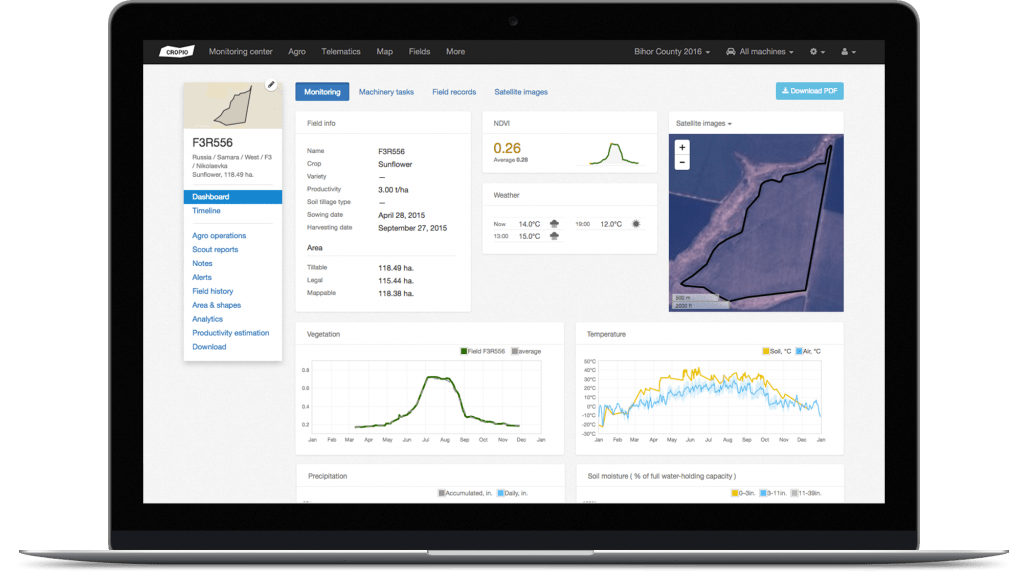
9. Robots and autonomous machines
Robotic innovations also offer a promising future in the field of autonomous machines for agricultural purposes. Some farmers already use automated harvesters, tractors, and other machines and vehicles that can operate without a human controlling it. Such robots can complete repetitive, challenging, and labor-intensive tasks.
For instance, modern agrobots include automated tractors that can work on assigned routes, send notifications, start work at planned hours, etc. Such tractors are driverless and cut farmers’ labor costs. Bear Flag Robotics is one company that works on such technology at the moment.
In addition, smart farming also uses robots for planting seeds, weeding, and watering. The given jobs are very demanding and labor-intensive. Yet, robots, such as ones from Eco Robotics , can detect weeds or plant seeds using computer vision and AI technology. These agricultural robots work delicately, drastically reducing harm to the plants and the environment.
- Things to consider before developing your smart farming solution
As we can see, the use cases for IoT in agriculture are endless. There are many ways smart devices can help you increase your farm’s performance and revenue. However, agriculture IoT apps development is no easy task.
There are certain challenges you need to be aware of if you are considering investing into smart farming.
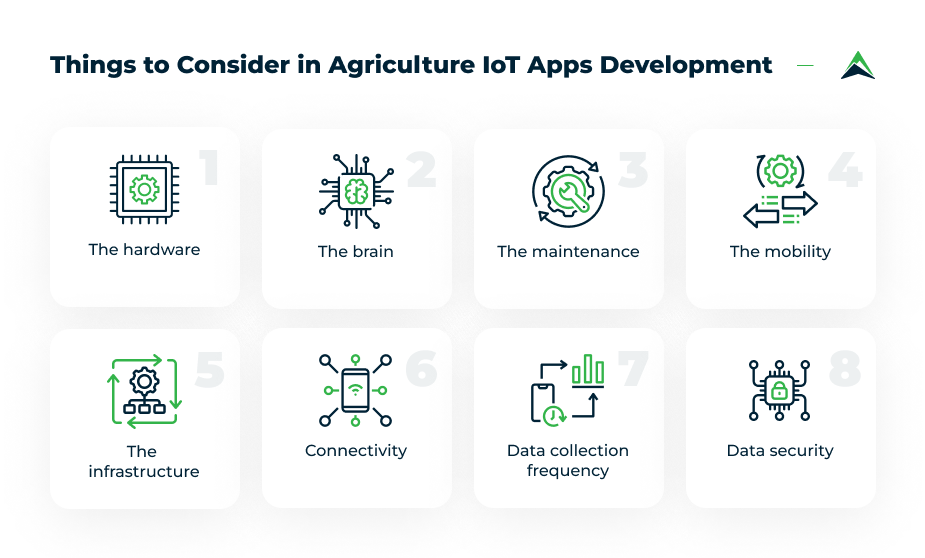
1. The hardware
To build an IoT solution for agriculture, you need to choose the sensors for your device (or create a custom one). Your choice will depend on the types of information you want to collect and the purpose of your solution in general.
In any case, the quality of your sensors is crucial to the success of your product: it will depend on the accuracy of the collected data and its reliability.
2. The brain
Data analytics should be at the core of every smart agriculture solution. The collected data itself will be of little help if you cannot make sense of it.
Thus, you need to have powerful data analytics capabilities and apply predictive algorithms and machine learning in order to obtain actionable insights based on the collected data.
3. The maintenance
Maintenance of your hardware is a challenge that is of primary importance for IoT products in agriculture, as the sensors are typically used in the field and can be easily damaged.
Thus, you need to make sure your hardware is durable and easy to maintain. Otherwise you will need to replace your sensors more often than you would like.
4. The mobility
Smart farming applications should be tailored for use in the field. A business owner or farm manager should be able to access the information on site or remotely via a smartphone or desktop computer.
Plus, each connected device should be autonomous and have enough wireless range to communicate with the other devices and send data to the central server.
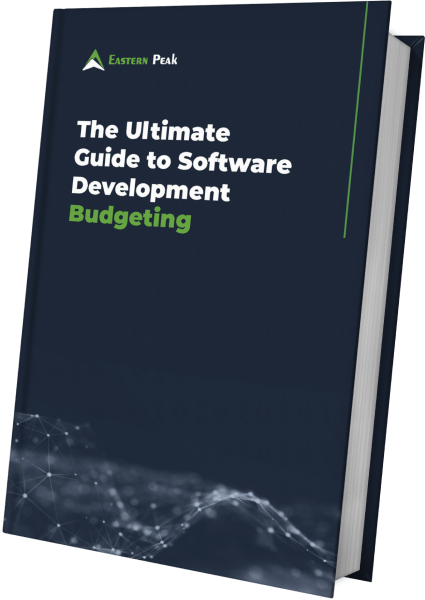
Thank you for downloading the guide!
Free guide to your project budgeting.
Explore real-life examples and discover the ways to stay within your budget.
5. The infrastructure
To ensure that your smart farming application performs well (and to make sure it can handle the data load), you need a solid internal infrastructure.
Furthermore, your internal systems have to be secure. Failing to properly secure your system only increases the likeliness of someone breaking into it, stealing your data or even taking control of your autonomous tractors.
6. Connectivity
The need to transmit data between many agricultural facilities still poses a challenge for the adoption of smart farming. Needless to say, the connection between these facilities should be reliable enough to withstand bad weather conditions and to ensure non-disruptive operations.
Today, IoT devices still use varying connection protocols, although the efforts to develop unified standards in this area are currently underway. The advent of 5G and technologies like space-based Internet will, hopefully, help find a solution to this problem.
7. Data collection frequency
Because of the high variety of data types in the agricultural industry, ensuring the optimal data collection frequency can be problematic. The data from field-based, aerial and environmental sensors, apps, machinery, and equipment, as well as processed analytical data, can be a subject of restriction and regulations.
Today, the safe and timely delivery, and sharing of this data is one of the current smart farming challenges.
8. Data security in the agriculture industry
Precision agriculture and IoT technology imply working with large sets of data, which increases the number of potential security loopholes that perpetrators can use for data theft and hacking attacks. Unfortunately, data security in agriculture is still, to a large extent, an unfamiliar concept.
Many farms, for example, use drones that transmit data to farm machinery. This machinery connects to the Internet but has little to zero security protection, such as user passwords or remote access authentications.
Some of the basic IoT security recommendations include monitoring data traffic, using encryption methods to protect sensitive data, leveraging AI-based security tools to detect traces of suspicious activity in real-time, and storing data in the blockchain to ensure its integrity.
To fully benefit from IoT, farmers will have to get familiar with the data security concept, set up internal security policies, and adhere to them.
- Our work case of IoT solutions for agriculture
Our team at Eastern Peak has also contributed to the progress of IoT applications in agriculture. The IoT-powered irrigation application, GreenIQ, helps gardeners reduce water usage by 50%, monitor humidity levels, and predict the best timing for irrigation. GreenIQ uses smart sensors to analyze meteorological conditions and soil types, creating the perfect irrigation strategy and adapting to new environments.
The GreenIQ application also integrates with the most well-known home automation platforms. This app is another valuable contribution to eco-friendly gardening and one of many examples of how smart farming products can change the future of agriculture.
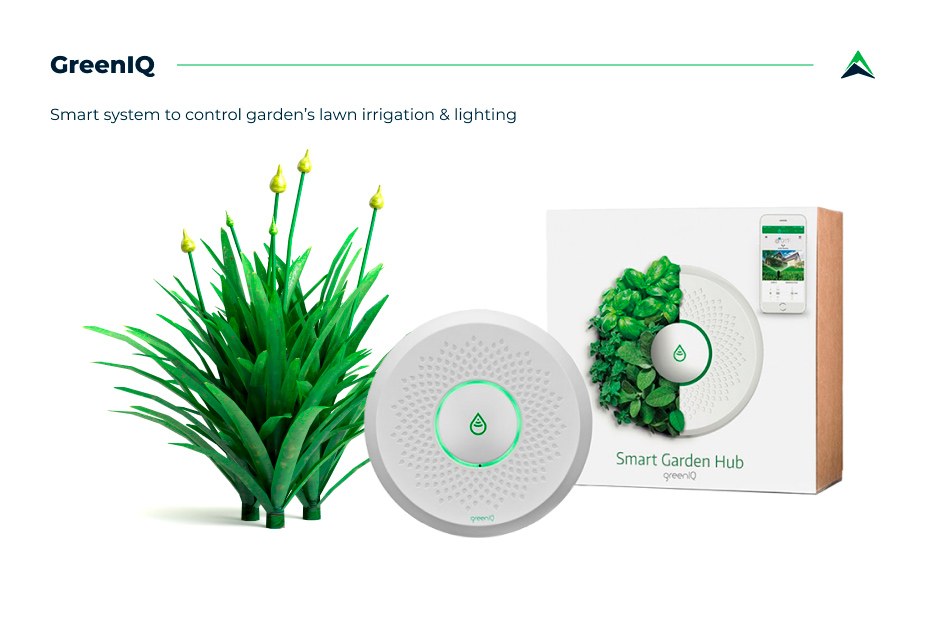
- Grow your agriculture business with smart IoT solutions from Eastern Peak
According to the UN Food and Agriculture Organization (FAO) , the global population is expected to surpass 9 billion people by 2050. To produce enough food for the given population, agriculture production volumes have to increase by 50%.
As the resources for agricultural operations are limited (most of the lands suitable for farming are already in use), the only way to increase volume is to improve production efficiency. There is no doubt as to the extent with which smart farming can help tackle this challenge; in fact, it seems that it is not possible without it. Here at Eastern Peak we develop custom IoT solutions for agriculture, tailored to your particular needs.
How to get started?
From cattle tracking to advanced field mapping, IoT applications in smart agriculture vary from farm to farm depending on your market segment, climate, and region. In many instances, out-of-the-box tools won’t be relevant, and you may need a tailored smart farming IoT solution. At Eastern Peak we approach each customer individually to meet their unique needs.
The product discovery phase is the best first step you can take to lay a solid foundation for the development of your app. It includes a functional specification, UX/UI design, and a visual prototype that will give you a clear vision of the end product. On average, this phase takes 4-6 weeks.
The product discovery phase can help you:
- define a full scope of work and develop a roadmap for the project
- set a realistic budget for your MVP and plan your resources
- test the waters with your audience using a visual prototype
- craft a convincing investment pitch
- get to know your team
We at Eastern Peak have already helped many startups and Fortune 500 companies digitize and streamline their operations with the help of technologies. We provide end-to-end services building IoT solutions across a number of business domains, from hardware design to software development, testing, and integration.
To receive professional consultation from our experts, get in touch with us using our contact form .
- Smart Farming: How Automation Is Transforming Agriculture
- 3 Edge Computing Use Cases for Smart Farming
Smart Agriculture Monitoring Solutions to Optimize Farming Productivity
- 6 Cool Examples of Internet of Things Applications and How to Develop One
About the author:
Alexey Shalimov, CEO at Eastern Peak
As CEO at Eastern Peak, a professional software consulting and development company, Alexey ensures top quality and cost-effective services to clients from all over the world. Alexey is also a founder and technology evangelist at several technology companies. Previously, as a CEO of the Gett (GetTaxi) technology company, Alexey was in charge of developing the revolutionary Gett service from ground up and deploying the operation across the globe from New York to London and Tel Aviv.
- The Benefits of smart farming: How’s IoT shaping agriculture
Enjoyed the read?
Don't miss our next article!
MORE ARTICLES
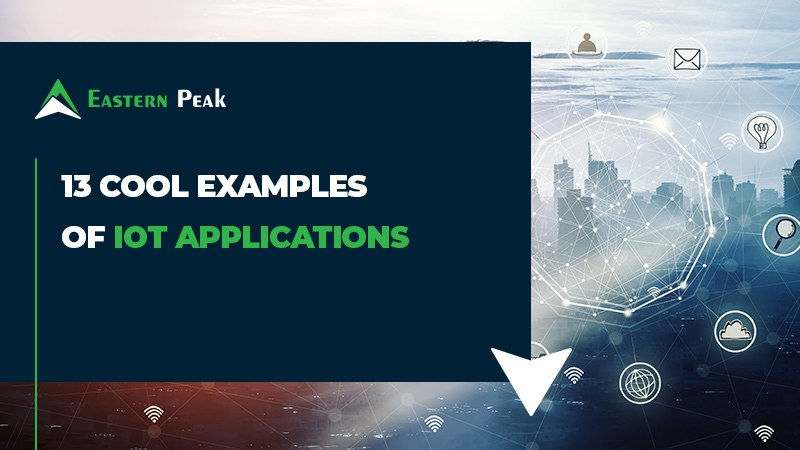
13 Cool Examples of Internet of Things Applications and How to Develop One
The article was updated on February 28, 2023. The number of IoT-connected devices is predicted to triple in just a ...
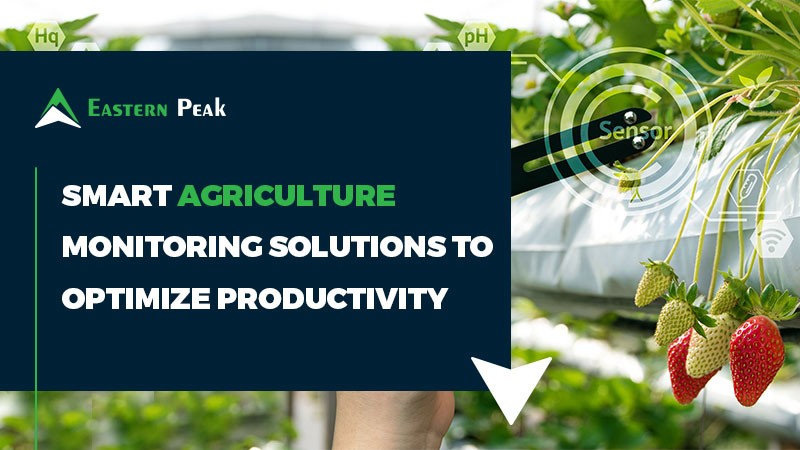
The article was updated on March 2, 2023. Of all the IoT-enabled solutions, smart agriculture systems unmistakably stand out. Being ...
Cookies help us enhance your experience and navigation. By continuing to browse, you agree to the storing of cookies on your device. We do not collect your personal information unless you explicitly ask us to do so. Please see our Privacy policy for more details.
New invention harvests ambient Wi-Fi and Bluetooth signals to power small devices
Wasted radio signals can be converted into electricity using a new kind of antenna rooted in how electrons behave at a quantum level.
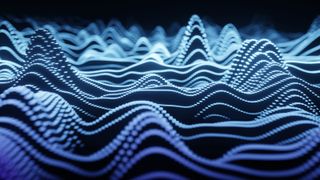
Small devices like light sensors or network components could soon harvest power from background Wi-Fi and Bluetooth signals — using a sophisticated new component that can turn even the faintest electromagnetic waves into electricity.
Researchers have created a highly sensitive "rectenna," or rectifying antenna, a component that exploits quirks of quantum physics to efficiently convert electromagnetic energy into direct current (DC) electricity. The researchers used this novel approach of capturing electrons to power a commercial thermometer.
In a study published July 24 in the journal Nature Electronics , the scientists suggested this technology could be scaled up to power Internet of Things (IoT) devices and sensors using a small proportion of the excess radiofrequency (RF) signals they use to communicate with one another.
Rectennas receive electromagnetic waves as found in radiofrequency (RF) signals like Wi-Fi and Bluetooth, or different wavelengths of light, and capture them as alternating current (AC) electricity via the antenna. The device then converts this to DC electricity through its rectifier circuit.
It's long been known that rectennas can be used to generate low levels of electricity; researchers have demonstrated this by wirelessly powering model vehicles and similar experiments since the 1960s. For example, in 1964 the weapons manufacturer Raytheon ran a television broadcast in which it demonstrated a remote-controlled helicopter powered by microwaves .
Related: Wireless tech could replace Bluetooth at short distances and boost battery life 5-fold
But in these cases, power was beamed directly at the device in the form of microwave energy. Ambient RF signals are far weaker and are not aimed directly at the devices.
Sign up for the Live Science daily newsletter now
Get the world’s most fascinating discoveries delivered straight to your inbox.
In the paper, the researchers said that ambient RF signals may register well below minus 20 decibel-milliwatts, a unit of measurement used to express signal strength. To put it in perspective, the average smartphone transmits signals at 27 dBm while a microwave oven operates at 60 dBm.
To harness the very weak ambient signals produced by Wi-Fi and Bluetooth networks, the researchers turned to a relatively obscure corner of quantum research.
Known as "spintronics," it studies the quantum spin of electrons and how this relates to magnetic fields. For their demonstration, the researchers leaned on the properties of magnetic tunnel junctions (MTJs), a component consisting of a very thin layer of insulating material sandwiched between two magnetic layers. MTJs are most commonly used in hard disk drives and have been utilized in other types of computing memory .
— Quantum data beamed alongside 'classical data' in the same fiber-optic connection for the 1st time
— Future electric cars could go more than 600 miles on a single charge thanks to battery-boosting gel
— New solar cell technology could ditch batteries in gadgets for good by harvesting ambient room light
RF signals can exert a shift on MTJs, in which the current of the signal affects the spin of the electrons within the construct. This can be harnessed to produce electricity.
The team created a series of nanoscale "'spin rectifiers"' (SRs) formed from MTJs, with full dimensions of 40 x 100 nanometers squared and 80 x 200 nm2, sensitive to the frequencies of common ambient electromagnetic signals such as Wi-Fi (2.4 gigahertz frequencies), 4G (2.3 to 2.6 GHz), and 5G (3.5 GHz).
Having demonstrated the effectiveness of their component on its own, the researchers created an SR array that could power a commercially available temperature sensor using only minus 27 dBm of ambient RF.
In the future, the team hopes this method could be used to lower the carbon cost of running wireless networks by reducing battery dependency and energy consumption in sensors and other small devices.
Rory Bathgate is a freelance writer for Live Science and Features and Multimedia Editor at ITPro, overseeing all in-depth content and case studies. Outside of his work for ITPro, Rory is keenly interested in how the tech world intersects with our fight against climate change. This encompasses a focus on the energy transition, particularly renewable energy generation and grid storage as well as advances in electric vehicles and the rapid growth of the electrification market. In his free time, Rory enjoys photography, video editing and science fiction. He joined ITPro in 2022 as a graduate, after completing an MA (Hons) in Eighteenth-Century Studies at King’s College London. You can contact Rory at [email protected].
Ultra-thin solar 'coating' can turn phone cases and EVs into mini power generators
Unique 'fan-on-a-chip' could prevent AI smartphones from overheating — with 1st devices launching in 2026
200 meteorites on Earth traced to 5 craters on Mars
Most Popular
- 2 Scientists collect high-resolution images of the North Star's surface for 1st time
- 3 Supercharged 'cocoon of energy' may power the brightest supernovas in the universe
- 4 AI and brain implant enables ALS patient to easily converse with family 'for 1st time in years'
- 5 Fallout from NASA's asteroid-smashing DART mission could hit Earth — potentially triggering 1st human-caused meteor shower
Conceptual modelling of sensor-based geographic data: interoperable approach with real-time air quality index (AQI) dashboard
- Published: 24 August 2024
Cite this article
- Rabia Bovkir 1 &
- Arif Cagdas Aydinoglu 2
11 Accesses
Explore all metrics
The rapid and uncontrolled development in the urban environment leads to significant problems, negatively affecting the quality of life in many areas. Smart Sustainable City concept has emerged to solve these problems and enhance the quality of life for its citizens. A smart city integrates the physical, digital and social system in order to provide a sustainable and comfortable future by the help of the Information and Communication Technologies (ICT) and Spatial Data Infrastructures (SDI). However, the integrated management of urban data requires the inclusion of ICT enabled SDI that can be applied as a decision support element in different urban problems by giving a comprehensive understanding of city dynamics; an interoperable and integrative conceptual data modelling, essential for smart sustainable cities and successful management of big urban data. The main purpose of this study is to propose an integrated data management approach in accordance with international standards for sustainable management of smart cities. Thematic data model designed within the scope of quality of life, which is one of the main purposes of smart cities, offers an exemplary approach to overcome the problems arising from the inability to manage and analyse big and complex urban data for sustainability. In this aspect, it is aimed to provide a conceptual methodology for successful implementation of smart sustainable city applications within the international and national SDIs with environmental quality of life theme. With this object, firstly, the literature on smart sustainable cities was examined together with the scope of quality and sustainability of urban environment along with all related components. Secondly, the big data and its management was examined within the concept of the urban SDI. In this perspective, new trends and standards related to sensors, internet of things (IoT), real-time data, online services and application programming interfaces (API) were investigated. After, thematic conceptual models for the integrated management of sensor-based data were proposed and a real time Air Quality Index (AQI) dashboard was designed in Istanbul, Türkiye as the thematic case application of proposed models.
This is a preview of subscription content, log in via an institution to check access.
Access this article
Subscribe and save.
- Get 10 units per month
- Download Article/Chapter or eBook
- 1 Unit = 1 Article or 1 Chapter
- Cancel anytime
Price includes VAT (Russian Federation)
Instant access to the full article PDF.
Rent this article via DeepDyve
Institutional subscriptions

Similar content being viewed by others
Integration of the Multi-scale Heterogeneous Data for the Deployment of the Concept of Energy Efficiency in Buildings within an SDI Framework
A Review on Data-Driven Approach Applied for Smart Sustainable City: Future Studies
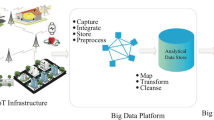
Environmentally data-driven smart sustainable cities: applied innovative solutions for energy efficiency, pollution reduction, and urban metabolism
Explore related subjects.
- Artificial Intelligence
Data availability
The datasets analysed during the current study are available at: https://data.ibb.gov.tr/en/dataset? q=air+quality.
Aazam M, Hung PP, Huh E (2014) Smart Gateway Based Communication for Cloud of Things. IEEE Ninth International Conference on Intelligence Sensors, Sensor Networks and Information Processing, Singapore
Albino V, Berardi U, Dangelico RM (2015) Smart cities: definitions, dimensions, performance, and initiatives. J Urban Technol 22(1):3–21. https://doi.org/10.1080/10630732.2014.942092
Article Google Scholar
Allam Z, Dhunny ZA (2019) On big data, artificial intelligence and smart cities. Cities 89:80–91
Aminian L (2019) Modelling and measuring quality of urban life: housing, neighbourhood, transport and job. Technische Universiteit Eindhoven
Anejionu ODD, Thakuriah P, McHugh A, Sun Y, McArthur D, Mason P, Walpole R (2019) Spatial urban data system: A cloud-enabled big data infrastructure for social and economic urban analytics. Future Generation Comput Syst 98:456–473. https://doi.org/10.1016/j.future.2019.03.052 .
Appio FP, Lima M, Paroutis S (2019) Understanding Smart cities: Innovation ecosystems, technological advancements, and societal challenges. Technol Forecast Soc Chang 142:1–14. https://doi.org/10.1016/j.techfore.2018.12.018
Aydinoglu AC (2016) Geocarto Int 31(4):446–461. https://doi.org/10.1080/10106049.2015.1054442 . Modelling, encoding and transforming of open geographic data to examine interoperability between GIS applications
Aydinoglu AC, Bovkir R, Colkesen I (2021) Implementing a mass valuation application on interoperable land valuation data model designed as an extension of the national GDI. Surv Rev 53:349–365
Badii C, Bilotta S, Cenni D, Difino A, Nesi P, Paoli I, Paolucci M (2020) High density real-Time Air Quality Derived services from IoT Networks. Sensors 20(18):5435. https://doi.org/10.3390/s20185435
Article CAS Google Scholar
Ballas D (2013) What makes a ‘Happy. City’? Cities 32(1):39–50. https://doi.org/10.1016/j.cities.2013.04.009
Behera RR, Satapathy D, Majhi R, Panda A, C., R (2021) Spatiotemporal variation of atmospheric pollution and its plausible sources in an industrial populated city, Bay of Bengal, Paradip, India. Urban Climate, 37, Article: 100860 https://doi.org/10.1016/j.uclim.2021.100860
Benites AJ, Simones AF (2021) Assessing the urban sustainable development strategy: an application of a smart city services sustainability taxonomy. Ecol Ind 127. https://doi.org/10.1016/j.ecolind.2021.107734
Berntzen L, Rohde Johannessen M, El-Gazzar R (2018) Smart Cities, Big Data and Smart Decision-making Understanding Big Data in Smart City Applications. The Twelfth International Conference on Digital Society and eGovernments (ICDS 2018), 25–28 March, Rome, Italy
Biagi B, Ladu MG, Meleddu M (2018) Urban Quality of Life and capabilities: an experimental study. Ecol Econ 150:137–152. https://doi.org/10.1016/j.ecolecon.2018.04.011
Bibri SE (2019) On the sustainability of smart and smarter cities in the era of big data: an interdisciplinary and transdisciplinary literature review. J Big Data 6(25). https://doi.org/10.1186/s40537-019-0182-7
Bibri SE (2021) Data-driven smart sustainable cities of the future: urban computing and intelligence for strategic, short-term, and joined-up planning. Comput Urban Sci 1(8). https://doi.org/10.1007/s43762-021-00008-9
Bibri SE, Krogstie J (2016) On the social shaping dimensions of smart sustainable cities: a study in science, technology, and society. Sustainable Cities Soc 29:219–246
Bibri SE, Krogstie J (2017) The core enabling technologies of big data analytics and context-aware computing for smart sustainable cities: a review and synthesis. J Big Data 4(38). https://doi.org/10.1186/s40537-017-0091-6 .
Bouder A, Markevych I, Jacquemin B, Abramson MJ, Accordini S, Forsberg B, Fuertes E, Garcia-Aymerich J, Heinrich J, Johannessen A, Leynaert B, Pin I, Siroux V (2022) Long-term air pollution exposure, greenspace and health-related quality of life in the ECRHS study. Sci Total Environ 849:157693. https://doi.org/10.1016/j.scitotenv.2022.157693
Bovkir R, Aydinoglu AC (2018) Surv Rev 78:46–60. https://doi.org/10.1016/j.landusepol.2018.06.040 . Providing land value information from geographic data infrastructure by using fuzzy logic analysis approach
Bovkir R, Aydinoglu AC (2021) Big Urban Data visualization approaches within the Smart City: GIS-Based Open-Source Dashboard Example. Int Archives Photogrammetry Remote Sens Spat Inform Sci XLVI–4/W5:125–130. https://doi.org/10.5194/isprs-archives-XLVI-4-W5-2021-125-2021
Bovkir R, Ustaoglu E, Aydinoglu AC (2023) Assessment of Urban Quality of Life Index at Local Scale with different weighting approaches. Soc Indic Res 165:655–678. https://doi.org/10.1007/s11205-022-03036-y
Cao X, Wang M, Liu X (2020) Application of Big Data Visualization in Urban Planning. IOP Conf Ser : Earth Environ Sci 440:042066
Google Scholar
Caragliu A, Del Bo C, Nijkamp P (2009) Smart cities in Europe. 3rd Central European Conference in Regional Science – CERS
Castell N, Kobernus M, Liu H-Y, Schneider P, Lahoz W, Berre AJ, Noll J (2015) Mobile technologies and services for environmental monitoring: the citi-Sense-MOB approach. Urban Clim 14(3):370–382. https://doi.org/10.1016/j.uclim.2014.08.002
CEC-Commission of the European Communities (1999) European spatial development perspective: towards Balanced and Sustainable Development of the Territory of the EU. Office for Official Publications of the European Communities, Luxembourg
Chen M, Mao S, Zhang Y, Leung VCM (2014) Big data: related technologies, challenges and future prospects. Springer Briefs in Computer Science
Choi H-S, Song S-K (2023) Direction for a transition toward Smart sustainable cities based on the diagnosis of Smart City Plans. Smart Cities 6(1):156–178. https://doi.org/10.3390/smartcities6010009
Chong M, Habib A, Evangelopoulos N, Park HW (2018) Dynamic capabilities of a smart city: an innovative approach to discovering urban problems and solutions. Government Inform Q 35(4):682–692. https://doi.org/10.1016/j.giq.2018.07.005
D’Alpaos C, Andreolli F (2020) Urban quality in the city of the future: a bibliometric multicriteria assessment model. Ecol Ind 117. https://doi.org/10.1016/j.ecolind.2020.106575
de la Durán-Sánchez A (2017) Cruz del Río-Rama M., Sereno-Ramírez A., Bredis K., Sustainability and Quality of Life in Smart Cities: Analysis of Scientific Production. In: Peris-Ortiz M., Bennett D., Pérez-Bustamante Yábar D. (eds) Sustainable Smart Cities. Innovation, Technology, and Knowledge Management. Springer, Cham. https://doi.org/10.1007/978-3-319-40895-8_12
Degbelo A, Granell C, Trilles S, Bhattacharya D, Casteleyn S, Kray C (2015) Opening up Smart cities: Citizen-Centric challenges and opportunities from GIScience. Geo-Information 5(2):16. https://doi.org/10.3390/ijgi5020016
EC - European Community (2007) Directive 2007/2/EC of the European Parliament and of the Council of 14 March 2007 establishing an infrastructure for spatial information in the European Community (INSPIRE).
EEA - European Environment Agency (2019a) Reportnet and data harvesting using INSPIRE infrastructure - report 1: data harvesting using INSPIRE network services. European Environmental Agency, Copenhagen
EEA (2019b) Reportnet and data harvesting using INSPIRE infrastructure - report 2: referencing spatial objects using INSPIRE network services. European Environmental Agency, Copenhagen
ESPON - European Spatial Planning Observation Network (2019) ESPON QoL – quality of Life Measurement and Methodology, Inception Report. ESPON EGTC, Luxembourg
EU-SPI - European Union - European Social Progress Index (2020) The Regional Dimension of Social Progress in Europe: Presenting The New EU Social Progress Index. Working Paper. Luxembourg: Publications Office of the European Union
European Union (EU) (2014) Mapping Smart cities in the EU. PE 507.480, Directorate General for Internal policies. Economic and Scientific Policy, European Parliament, Brussels, Policy Department A
Eurostat - European Statistical Office (2015) Eurostat - Quality of Life: Facts and Views. Luxembourg: Publications Office of the European Union. ISBN: 978-92-79-43616-1
Garau C, Pavan VM (2018) Evaluating Urban Quality: indicators and Assessment Tools for Smart sustainable cities. Sustainability 10:575. https://doi.org/10.3390/su10030575
GD-GIS - General Directorate of GIS (2019) TUCBS conceptual model components. Ministry of Environment, Urbanization and Climate Change. General Directorate of GIS, Ankara
Hammer S, Kamal - Chaoui L, Robert A, Plouin M (2011) Cities and Green Growth: A Conceptual Framework. OECD Regional Development Working Papers 08, OECD Publishing
Hashem IAT, Chang V, Anuar NB, Adewole K, Yaqoob I, Gani A, Ahmed E, Chiroma H (2016) The role of big data in smart city. Int J Inf Manag 36:748–758. https://doi.org/10.1016/j.ijinfomgt.2016.05.002
Hatzelhoffer L, Humboldt K, Lobeck M, Wiegandt C (2012) Smart City in Practice: converting innovative ideas into reality. JOVIS, Berlin, Germany
Ho JY, Shi Y, Lau KKL, Ng EYY, Ren C, Goggins WB (2023) Urban heat island effect-related mortality under extreme heat and non-extreme heat scenarios: a 2010–2019 case study in Hong Kong. Sci Total Environ 858(1):159791. https://doi.org/10.1016/j.scitotenv.2022.159791
Höjer M, Wangel J (2015) Smart sustainable cities: Definition and challenges. In: Hilty L, Aebischer B (eds) Editors, ICT innovations for sustainability. Advances in Intelligent systems and Computing, vol 310. Springer, Cham
Hollands RG (2008) Will the real smart city please stand up? Intelligent, progressive or entrepreneurial? City 12(3):303–319. https://doi.org/10.1080/13604810802479126
IBM- International Business Machines Corporation (2009) A vision of smarter cities - how cities can lead the way into a prosperous and sustainable future. IBM Global Business Services Executive Report, Somers, NY, USA
ICLEI – Local Governments for Sustainability (2017) Corporate report 2016–2017. Bonn, Germany
IDB - Inter-American Development Bank (2016) The Road toward Smart cities: migrating from Traditional City Management to the Smart City. IDB-MG-454
International Telecommunications Union (ITU) (2014) Agreed definition of a smart sustainable city, Focus Group on Smart Sustainable Cities, SSC-0146 version Geneva, Switzerland
ISO (2016) ISO 37101:2016 - sustainable development in communities — management system for sustainable development — requirements with guidance for use. Geneva, Switzerland
ISO (2018) ISO 37120:2018 - sustainable cities and communities — indicators for city services and quality of life. Geneva, Switzerland
ISO (2019) ISO 37122: 2019 - sustainable cities and communities — indicators for smart cities. Geneva, Switzerland
ISO-International Organization for Standardization (2011) ISO 19156:2011 - Geographic information — Observations and measurements. Geneva, Switzerland
ITU-International Telecommunication, Union (2022) Official website of ITU Telecommunication Standardization Sector (ITU-T). Accessed at: https://www.itu.int/en/ITU-T/about/Pages/default.aspx (27.03.2022)
ITU-T, (2016) Shaping smarter and more sustainable cites - striving for sustainable development goals. Switzerland, Geneva
ITU-T, (2015) Internet of Things Global Standards Initiative. Accessed at: https://www.itu.int/en/ITU-T/gsi/iot/Pages/default.aspx (Accessed: 12.07.2022)
ITU-T International Telecommunication Union-Telecommunication Standardization (2012) Y.2060: Overview of the Internet of things
Jing C, Du M, Li S, Liu S (2019) Geospatial dashboards for Monitoring Smart City performance. Sustainability 11:5648. https://doi.org/10.3390/su11205648
JRC (2020b) Architectures and Standards for Spatial Data Infrastructures and Digital Government - European Union Location Framework Guidelines. JRC121025-EUR 30336 EN. Publications Office of the European Union, Luxembourg
JRC (2021a) JRC126319-EUR 30832 EN. Publications Office of the European Union, Luxembourg, INSPIRE A Public Sector Contribution to the European Green Deal Data Space: A vision for the technological evolution of Europe’s Spatial Data Infrastructures for 2030
JRC (2021b) Establishment of sustainable data ecosystems: recommendations for the evolution of spatial data infrastructures. JRC124148-EUR 30626 EN. Publications Office of the European Union, Luxembourg
JRC (2021c) Enabling the interoperability of digital government from a location perspective. Proceedings of the ELISE Workshop at DigitALL conference, 20–22 April 2021. JRC125328, Publications Office of the European Union, Luxembourg
JRC-European Commission DG Joint Research Centre (2020a) Establishing a new baseline for monitoring the status of EU Spatial Data Infrastructure: experiences and conclusions from INSPIRE 2019 monitoring and reporting. JRC122351-EUR 30513 EN. Publications Office of the European Union, Luxembourg
Kaginalkar A, Kumar S, Gargava P, Niyogi D (2021) Review of urban computing in air quality management as smart city service: an integrated IoT, AI, and cloud technology perspective. Urban Clim 39:Article100972. https://doi.org/10.1016/j.uclim.2021.100972
Keim DA (2002) Information Visualization and Visual Data Mining. IEEE Trans Visualizations Comput Graphics 8:100–107
Kim HM, Soheil S, Kent A (2021) Smart cities as a platform for technological and social innovation in productivity, sustainability, and livability: a conceptual framework. In: Min Kim H, Sabri S, Kent A (eds) Smart Cities for Technological and Social Innovation. Academic
Kitchin R (2014) The real-time city? Big data and smart urbanism. GeoJournal 79:1–14. https://doi.org/10.1007/s10708-013-9516-8
Kitchin R, Lauriault TP, McArdle G (2015) Knowing and governing cities through urban indicators, city benchmarking, and real-time dashboards. Reg Stud Reg Sci J Reg Stud Association 1:6–28. https://doi.org/10.1080/21681376.2014.983149
Kotsev A, Peeters O, Smits P, Grothe M (2015) Building bridges: experiences and lessons learned from the implementation of INSPIRE and e-reporting of air quality data in Europe. Earth Sci Inf 8:353–365. https://doi.org/10.1007/s12145-014-0160-8
Kotsev A, Minghini M, Tomas R, Cetl V, Lutz M (2020) ISPRS Int J Geo-Information 9(3):176. https://doi.org/10.3390/ijgi9030176 . From Spatial Data Infrastructures to Data Spaces—A Technological Perspective on the Evolution of European SDIs
Leach JM, Lee SE, Hunt DV, Rogers CD (2017) Improving city-scale measures of livable sustainability: a study of urban measurement and assessment through application to the city of Birmingham. UK Cities 71:80–87. https://doi.org/10.1016/j.cities.2017.06.016
Lee JH, Hancock MG, Hu M (2014) Towards an effective framework for building smart cities: lessons from Seoul and San Francisco. Technol Forecast Soc Chang 89:80–99. https://doi.org/10.1016/j.techfore.2013.08.033
Li L, Zheng Y, Zheng S, Ke H (2020) The new smart city programme: evaluating the effect of the internet of energy on air quality in China. Sci Total Environ 714:136380. https://doi.org/10.1016/j.scitotenv.2019.136380
Liu CC, Lin TC, Yuan KY, Chiueh PT (2022) Spatio-temporal prediction and factor identification of urban air quality using support vector machine. Urban Clim 41., Article 101055, https://doi.org/10.1016/j.uclim.2021.101055
Lo W-C, Hu T-H, Hwang J-J (2023) Lifetime exposure to PM2.5 air pollution and disability-adjusted life years due to cardiopulmonary disease: a modeling study based on nationwide longitudinal data. Sci Total Environ 855:158901. https://doi.org/10.1016/j.scitotenv.2022.158901
Lombardi P, Giordano S, Farouh H, Yousef W (2012) Modelling the Smart City performance. Innovation: Eur J Social Sci Res 25(2):137–149. https://doi.org/10.1080/13511610.2012.660325
Mittal S, Chadchan J, Mishra SK (2020) Review of concepts, tools and indices for the Assessment of Urban Quality of Life. Soc Indic Res 149:87–214. https://doi.org/10.1007/s11205-019-02232-7
Mohan AS, Abraham L (2024) An ensemble deep learning approach for air quality estimation in Delhi, India. Earth Sci Inf. https://doi.org/10.1007/s12145-023-01210-5
Moshrefzadeh M, Kolbe TH (2016) Smart Data Infrastructure for Smart and Sustainable Cities. In the Proceedings of the 13th International Conference on Design & Decision Support Systems in Architecture and Urban Planning (DDSS 2016), Eindhoven, The Netherlands
Nedovic-Budic Z, Feeney M-EF, Rajabifard A, Williamson I, Computers (2004) Environ Urban Syst, 28(4), 329–351. https://doi.org/10.1016/S0198-9715(03)00042-5
Neirotti P, De Marco A, Cagliano A, Mangano G, Scorrano F (2014) Current trends in Smart City initiatives: some stylised facts. Cities 38:25–36. https://doi.org/10.1016/j.cities.2013.12.010
O’Grady M, O’Hare G (20012) How smart is your city? Science 335(3):1581–1582. https://doi.org/10.1126/science.1217637
OECD - Organization for Economic Co-operation and Development (2020) Better Life Index/Regional Well-Being Index. https://www.oecdbetterlifeindex.org/
OECD-JRC (2008) Handbook on constructing Composite indicators: methodology and user guide. OECD Publishing, Paris, France
Book Google Scholar
OGC (2015) OGC smart cities spatial information Framework. White Paper
OGC (2020) OGC SensorThings API part 1 – sensing. OGC 18–088
OGC (2022) Official website of Open Geospatial Consortium DWGs. https://www.ogc.org/projects/groups/wg (Accessed: 27.03.2022)
OGC-Open Geospatial Consortium (2011) Geographic information — Observations and measurements (O&M) - OGC 10-004r3
Pettit C, Lieske SN, Jamal M (2017) CityDash: visualising a changing City using Open Data. In: Geertman S, Allan A, Pettit C, Stillwell J (eds) Planning support science for smarter Urban futures. Springer, Cham
Pira M (2021) A novel taxonomy of smart sustainable city indicators. Humanit ans Social Sci Commun 8:197
Rajabifard A, Ho S, Sabri S (2016) Urban Analytics Data Infrastructure: critical SDI for Urban Management in Australia. In: Coleman D, Rajabifard A, Crompvoets J (eds) Spatial enablement in a Smart Word. GSDU Association
Rajabifard A, Sabri S, Chen Y, Agunbiade M, Kalantari M (2020) Urban Analytics Data Infrastructure: critical SDI for Measuring and Monitoring the National and local progress of SDGs. In: Rajabifard A (ed) Sustainable Development Goals Connectivity Dilemma Land and Geospatial Information for Urban and Rural Resilience. CRC Press Taylor & Francis Group
Rathore MM, Ahmad A, Paul A, Rho S (2016) Urban planning and building smart cities based on the internet of things using Big Data analytics. Comput Netw 101:63–80
Reddy NM, Budati AK, Islam S, Ramesh G (2023) Enhanced elliptic curve- Diffie Hellman Technique with Bigdata Analytics for Satellite Image Security Enhancement in Internet of things systems. Earth Sci Inf. https://doi.org/10.1007/s12145-023-01194-2
Ren Y, Yu G (2004) Research and development of data visualization technology. Comput Sci 31(12):92–96
Sabri S (2021) Introduction: being smarter for productivity, livability, and sustainability. In: Min Kim H, Sabri S, Kent A (eds) Smart Cities for Technological and Social Innovation. Academic
Sahil N, Sood SK Fog-cloud centric IoT-based cyber physical framework for panic oriented disaster evacuation in smart cities. Earth Sci Inf, 15, 1449–1470. https://doi.org/10.1007/s12145-020-00481-6
SSCC-CG - Smart and Sustainable Cities and Communities’ Coordination Group, 2015. SSCC-CG Final report
Stratigea A, Papadopoulou C-A, Panagiotopoulou M (2015) Tools and technologies for planning the development of Smart cities. J Urban Technol 22(2):43–62. https://doi.org/10.1080/10630732.2015.1018725
Sun X, Tian Z, Zhang Z (2023) A new decomposition-integrated air quality index prediction model. Earth Sci Inf 16:2307–2321. https://doi.org/10.1007/s12145-023-01028-1
Suziki L, Finkelstein A (2019) Data as infrastructure for Smart cities. The Institution of Engineering and Technology, London, United Kingdom
Swan M (2012) Sensor mania! The internet of things, wearable computing, objective metrics, and the quantified self 2.0. J Sens Actuator Networks 1(3):217–253. https://doi.org/10.3390/jsan1030217
Taamallah A, Khemaja M, Faiz S (2017) Strategy ontology construction and learning: insights from smart city strategies. Int J Knowledge-Based Dev 8(3):206–228. https://doi.org/10.1504/IJKBD.2017.086427
Tawalbeh LA, Basalamah A, Mehmood R, Tawalbeh H (2016) Greener and smarter phones for future cities: characterizing the impact of GPS signal strength on power consumption. IEEE Access 4:858–868. https://doi.org/10.1109/ACCESS.2016.2532745
Thakuriah P, Dirks L, Keita Y (2017) Digital infomediaries and civic hacking in emerging urban data initiatives. In: Thakuriah P, Tilahun N, Zellner M (eds) Seeing cities through Big Data. Springer Geography, Springer, Cham
Chapter Google Scholar
TKGM (2006) Turkish national GIS policy and strategy document – action 36. General Directorate of Land Registry and Cadastre, Ankara
TKGM - General Directorate of Land Registry and Cadastre (2005) A preliminary Report to Build National GIS – action 47. General Directorate of Land Registry and Cadastre, Ankara
Tonchovska R, Stanley V, De Martino S (2012) Spatial Data Infrastructure and INSPIRE. Europe and Central Asia Knowledge Brief, 55. World Bank, Washington, DC. https://openknowledge.worldbank.org/handle/10986/17082
Tong X, Wu Z (2018) Study of Chinese city portrait based on data visualization: take City Dashboard for Example. In: Marcus A, Wang W (eds) Design, user experience, and usability: Designing interactions. DUXU 2018. Lecture notes in Computer Science(), vol 10919. Springer, Cham. https://doi.org/10.1007/978-3-319-91803-7_26 .
Treude M (2021) Sustainable Smart City—opening a Black Box. Sustainability 13(2):769. https://doi.org/10.3390/su13020769
Treude M, Schüle R, Haake H (2022) Smart Sustainable Cities—Case Study Südwestfalen Ger Sustain 14(10):5957. https://doi.org/10.3390/su14105957
UN (2019) United Nations Department of Economic and Social Affairs, World Population prospects 2019 – highlights. United Nations, USA, New York
UN (2022) Sustainable Development Goals – Goal 3: Ensure healty lives and promote well-being for all at all ages. https://www.un.org/sustainabledevelopment/health/ (Accessed: 28.08.2022)
UN - HABITAT (2021) The city prosperity initiative https://unhabitat.org/programme/city-prosperity-initiative
UN-GGIM-United Nations Committee of Experts on Global Geospatial Information Management (2015) The Application of Geospatial Information – Land Administration and Management
UN-HABITAT - United Nations Human Settlements Programme (2020) World cities Report 2020: the value of sustainable urbanization. Nairobi, Kenya
UN-United Nations (2016) New Urban Agenda. United Nations – Habitat III, A/RES/71/256. ISBN: 978-92-1-132731-1
URL-1 https://www.un.org/development/desa/en/news/population/2018-revision-of-world-urbanization-prospects.html (Accessed: 18.03.2022)
URL-2 https://www.iso.org/cms/%20render/live/en/sites/isoorg/contents/data/committee/65/69/656906/x/catalogue/ (Accessed: 28.08.2022)
URL-3 https://www.iso.org/committee/45020.html (Accessed: 28.08.2022)
van der Schaaf H, Moßgraber J, Grellet S, Beaufils M, Schleidt K, Usländer T (2020) An environmental Sensor Data suite using the OGC SensorThings API. In: Athanasiadis I, Frysinger S, Schimak G, Knibbe W (eds) Environmental Software Systems. Data Science in Action. ISESS 2020. IFIP Advances in Information and Communication Technology, vol 554. Springer, Cham
Webb R, Bai X, Smith MS, Costanza R, Griggs D, Moglia M et al (2018) Sustainable urban systems: co-design and framing for transformation. Ambio 47:57–77. https://doi.org/10.1007/s13280-017-0934-6
WHO - UN World Health Organization (2012) Quality of Life (WHOQOL) Reports. WHO/HIS/HSI Rev.2012.03
WHO (2022) Health Topics – Urban Health. https://www.who.int/health-topics/urban-health#tab=tab_1 (Accessed: 28.08.2022)
Wiesli TX, Liebe U, Hammer T, Bar R (2021) Sustainable quality of life: a conceptualization that integrates the views of inhabitants of Swiss Rural regions. Sustainability 13(16):9187. https://doi.org/10.3390/su13169187
Zeng Y-R, Chang Y, Fang Y (2019) Data Visualization for Air Quality Analysis on Big data Platform. International Conference on System Science and Engineering (ICSSE) – 2019, 313–317. https://doi.org/10.1109/ICSSE.2019.8823437
Download references
Acknowledgements
The authors would like to thank Eyup Tunahan UNAL and Ismail KAHRAMAN for their contribution and support in dashboard design.
This research received no specific grant from any funding agency in the public, commercial, or not-for-profit sectors.
Author information
Authors and affiliations.
Geomatics Engineering Department, Hacettepe University, Ankara, Türkiye
Rabia Bovkir
Geomatics Engineering Department, Gebze Technical University, Kocaeli, Türkiye
Arif Cagdas Aydinoglu
You can also search for this author in PubMed Google Scholar
Contributions
Rabia Bovkir: Conceptualization, Methodology, Investigation, Writing – Original Draft; Arif Cagdas Aydinoglu: Methodology, Writing – Review & Editing, Supervision.
Corresponding author
Correspondence to Arif Cagdas Aydinoglu .
Ethics declarations
Competing interests.
The authors declare no competing interests.
Additional information
Communicated by Hassan Babaie.
Publisher’s Note
Springer Nature remains neutral with regard to jurisdictional claims in published maps and institutional affiliations.
Electronic supplementary material
Below is the link to the electronic supplementary material.
Supplementary Material 1
Rights and permissions.
Springer Nature or its licensor (e.g. a society or other partner) holds exclusive rights to this article under a publishing agreement with the author(s) or other rightsholder(s); author self-archiving of the accepted manuscript version of this article is solely governed by the terms of such publishing agreement and applicable law.
Reprints and permissions
About this article
Bovkir, R., Aydinoglu, A.C. Conceptual modelling of sensor-based geographic data: interoperable approach with real-time air quality index (AQI) dashboard. Earth Sci Inform (2024). https://doi.org/10.1007/s12145-024-01444-x
Download citation
Received : 11 September 2023
Accepted : 07 August 2024
Published : 24 August 2024
DOI : https://doi.org/10.1007/s12145-024-01444-x
Share this article
Anyone you share the following link with will be able to read this content:
Sorry, a shareable link is not currently available for this article.
Provided by the Springer Nature SharedIt content-sharing initiative
- Sensor data
- Sustainable cities
- Air quality
- Web dashboard
- Conceptual models
Advertisement
- Find a journal
- Publish with us
- Track your research

IMAGES
COMMENTS
Diversity of Types: There is a wide variety of IoT sensor types, each serving a specific purpose. Common types include temperature sensors, motion sensors, proximity sensors, light sensors and gas sensors. Applications: IoT sensors find applications across numerous industries, including agriculture, healthcare, manufacturing, transportation ...
Conclusion. In conclusion, over the last ten years, many industries have changed a lot by using IoT tech. This includes healthcare, manufacturing, transportation, agriculture, retail, smart cities, and energy. Companies like Pfizer, Siemens, FedEx, John Deere, Amazon, Barcelona, Tesla, and Shell showed how IoT can make things better.
With IoT-connected assets and IoT sensors on an industrial internet, you can monitor equipment usage and health in order to assess performance and deploy service should there be any problems. ... Case Study: Fastenal Uses Real-Time Machine Monitoring to run faster, gain production hours, take on more jobs, and produce more parts than ever ...
The IoT Use Case Adoption Report 2021. In 2021, the average large manufacturing, healthcare, automotive, retail, or energy company has rolled out eight different IoT use cases, according to IoT Analytics' latest IoT Use Case Adoption Report.. The 430-page report, which is part of IoT Analytics' ongoing market coverage of IoT applications, is the first such in-depth report and is based on ...
The internet of things couldn't exist without smart sensors. While the use of sensor technology has long affected how manufacturers, industrial organizations and utilities operate, the addition of IoT to sensors has expanded their impact and use cases, bringing more connectivity and analytics to processes than ever before. Today, smart factories, smart cities, smart buildings and connected ...
What Is an IoT sensor? An IoT sensor is any sort of mechanism or tool, such as a camera or air quality monitor, integrated into a device. These sensors gather information — like water levels, air temperature and traffic patterns — related to the environments in which they're deployed and transmit it to the cloud via Wi-Fi, bluetooth, 5G or other mobile network.
Consumer packaged goods (CPG) giant Nestlé wanted to accelerate the development of Internet of Things (IoT) solutions across its global business units, including coffee brand Nespresso and pet care brand Nestlé Purina Petcare (Purina). Rather than have each business unit build its own IoT offering, Nestlé set out to build a single centralized framework, the IoT Platform, that could be ...
Global studies on IoT and smart sensor-based smart farming have produced satisfactory results. IoT-based technology has been implemented in multiple small scale agricultural fields. However, deployment of the same on a large scale level is still pending. One of the major challenges is the financial cost that will be imposed during the ...
Wireless Sensor Networks (WSNs) are promising technologies for exploiting in harsh environments such as can be found in the nuclear industry. Nuclear storage facilities can be considered harsh environments in that, amongst other variables, they can be dark, congested, and have high gamma radiation levels, which preclude operator access. These conditions represent significant challenges to ...
By leveraging IoT Big Data, BPM can gain real-time physical world information to make faster and more accurate decisions, but there is a technical gap between IoT sensors and businesses. To bridge the gap, an event penetration mechanism from IoT sensors to business processes is proposed along a practical case study. This paper presents a concrete IoT-BPM application case dealing with seaborne ...
The IoT solution helped Barcelona build upon its Smart City status. An integral part of the solution included soil moisture monitoring with probes utilising Libelium technology. The deployment uses remote monitoring of sensors that trigger and control the irrigating system, enabling the intelligent management of the water network. Technologies:
IoT-based environmental monitoring is the consistent collection of measurements and data from our physical environment, using sensors and connected devices. Sensors embedded in irrigation systems, pipelines, tanks, weather stations, oceanic applications, and industrial equipment — anywhere on the planet — can detect temperature, moisture ...
An integration of cost-efficient IoT sensor hardware, low-power consumption wireless WAN/BLE technologies, and AWS Cloud services to gather real-time concrete data. Durable IoT sensors can get a wireless signal from within six inches in poured concrete. Concrete Sensors has a product that can go as deep as 15 feet.
IoT sensors and devices deployed in machinery and equipment can continuously monitor their operational parameters, such as temperature, vibration, pressure, and performance metrics. ... Let's look at some examples of how businesses use IoT-enabled predictive maintenance. Case Study 1: Optimizing Fleet Management in a Transportation Company ...
A smart city - as any IoT system - uses smart things equipped with sensors and actuators. The immediate goal of sensors is to collect data and pass it to a central cloud management platform. Actuators allow devices to act - alter the lights, restrict the flow of water to the pipe with leakage, etc. Gateways.
This paper looks into a case study of incorporating Arduino and Raspberry Pi for sensor networking, data transmission and enabling IoT functionalities. As a practical realization, an experimental smart home is realized with an array of sensors and a system architecture consisting of a set of Arduino and Raspberry Pi modules.
In 2021, more than 5.5 million new devices will be connected to the Internet of things every day. The discovery and access of IoT sensors are difficult to adapt to the growth rate of machines. Especially for non-smart IoT sensors, due to their inability to actively match and lack of self-description, the existing IoT technology is challenging to find and access. This paper provides a two ...
are deploying 500 smart litterbins. NB-IoT-connected sensors inside the bins can detect when they are full, enabling cleaning service providers to remove the trash at the right time, increasing efficiency and improving the urban environment. \ In Malaysia, mobile operator Maxis is using NB-IoT to connect environmental sensors in a plantation.
Gartner estimated that the global government Internet of Things (IoT) endpoint electronics and communications market totaled $15 billion in 2020, an increase of 6% from 2019. Same study also reveals the top 5 government IoT applications and revenue generated by each use case as seen below: Source: Gartner. 5.
Internet of Things (IoT) has been emerging day-to-day because of multiple technologies like embedded systems, machine learning, real-time analytics, and commodity sensors. IoT contributes more to smart home applications, it can control with devices that are associated with it. In IoT, the applications are often divided into infrastructure, consumer, industrial, commercial applications. IoT ...
A research background study is conducted as part of an objective definition. It has been observed that wireless sensor networks are widely applied in traffic management projects and have a significant role in detecting and reducing traffic congestion [16, 62, 72].Many kinds of sensors are used for real-time traffic monitoring.
The IoT-powered irrigation application, GreenIQ, helps gardeners reduce water usage by 50%, monitor humidity levels, and predict the best timing for irrigation. GreenIQ uses smart sensors to analyze meteorological conditions and soil types, creating the perfect irrigation strategy and adapting to new environments.
Experimental (case study) results are obtained from the implemented prototype by taking the readings of the sensors stored on the secure digital card under various conditions and numerous results ...
In a study published July 24 in the journal Nature Electronics, the scientists suggested this technology could be scaled up to power Internet of Things (IoT) devices and sensors using a small ...
STA used for management, storage, sharing and analysis of IoT-based sensor observation data. It provides functionality that allows multiple IoT and sensor network devices to communicate by eliminating the complicated and heterogeneous protocols used by different IoT devices, gateways, and services (van der Schaaf et al. 2020). OGC STA includes ...