Information
- Author Services

Initiatives
You are accessing a machine-readable page. In order to be human-readable, please install an RSS reader.
All articles published by MDPI are made immediately available worldwide under an open access license. No special permission is required to reuse all or part of the article published by MDPI, including figures and tables. For articles published under an open access Creative Common CC BY license, any part of the article may be reused without permission provided that the original article is clearly cited. For more information, please refer to https://www.mdpi.com/openaccess .
Feature papers represent the most advanced research with significant potential for high impact in the field. A Feature Paper should be a substantial original Article that involves several techniques or approaches, provides an outlook for future research directions and describes possible research applications.
Feature papers are submitted upon individual invitation or recommendation by the scientific editors and must receive positive feedback from the reviewers.
Editor’s Choice articles are based on recommendations by the scientific editors of MDPI journals from around the world. Editors select a small number of articles recently published in the journal that they believe will be particularly interesting to readers, or important in the respective research area. The aim is to provide a snapshot of some of the most exciting work published in the various research areas of the journal.
Original Submission Date Received: .
- Active Journals
- Find a Journal
- Proceedings Series
- For Authors
- For Reviewers
- For Editors
- For Librarians
- For Publishers
- For Societies
- For Conference Organizers
- Open Access Policy
- Institutional Open Access Program
- Special Issues Guidelines
- Editorial Process
- Research and Publication Ethics
- Article Processing Charges
- Testimonials
- Preprints.org
- SciProfiles
- Encyclopedia
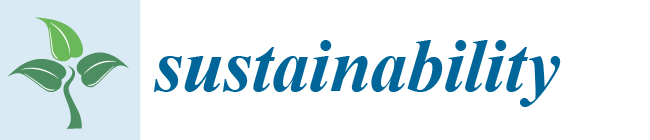
Article Menu

- Subscribe SciFeed
- Recommended Articles
- Google Scholar
- on Google Scholar
- Table of Contents
Find support for a specific problem in the support section of our website.
Please let us know what you think of our products and services.
Visit our dedicated information section to learn more about MDPI.
JSmol Viewer
What is polluting delhi’s air a review from 1990 to 2022.
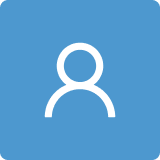
1. Introduction
2. geography and meteorology, 3. ambient air quality, 3.1. ground measurements, 3.1.1. pre-2006 period, 3.1.2. 2006–2018 period, 3.1.3. post-2018 period, 3.2. satellite observations and reanalysis, 4. receptor, source, and other modelling studies, 4.1. source apportionment, 4.2. air quality forecasting and alert systems.
- The Copernicus Atmosphere Monitoring Service (CAMS) forecasting system is a service provided by the European Union ( https://atmosphere.copernicus.eu/data , accessed 22 February 2023) that uses a combination of mathematical models and satellite data to provide air quality forecasts at 40 km spatial resolution globally and at 10–12 km spatial resolution for select regions. It is designed to provide reliable forecasts of air quality across Europe with the use of the ESA’s geostationary satellite data. The global data can be visualised at https://www.windy.com (accessed 22 February 2023). The CAMS reanalysis archives from 1990 are also available for studying long-term trends;
- The Early Warning System (EWS) for Delhi by IITM is hosted at https://ews.tropmet.res.in (accessed 22 February 2023) and includes results from the WRF-Chem regional model and GEOS and WACCM global modelling systems as a combination of national, region, and cit- level hourly maps, time series, and comparison with data from the CPCB’s monitoring network. The system also includes the forecast of fog onset and visibility for Delhi and a summary of air quality forecasts for other cities;
- The NASA-GEOS system is operated by the Global Modelling and Assimilation Office (GMAO) to support a wide range of applications, including air, weather, and climate modelling ( https://gmao.gsfc.nasa.gov , accessed 22 February 2023). A 10-day air quality forecast for Delhi from the GEOS-5 model is included on the EWS portal. Like CAMS, the GEOS system also includes a data assimilation system (GEOS-DAS) with reanalysis archives from 1990 for studying long-term trends (like MERRA-2— https://giovanni.gsfc.nasa.gov/giovanni , accessed 22 February 2023);
- SAFAR ( https://safar.tropmet.res.in , accessed 22 February 2023) uses a combination of on-ground measurements, emission inventories, and mathematical models to predict air quality for the next three days. Since its inception for Delhi, the model has been replicated for the cities of Mumbai, Pune, and Ahmedabad;
- SILAM (System for Integrated modeLing of Atmospheric composition) is a global chemical transport model developed and maintained by the Finnish Meteorological Institute (FMI). As part of a memorandum of understanding, FMI shares air quality forecasts customised for the NCR Delhi region with the Indian Meteorological Department (IMD). These results are also included on the EWS portal;
- The Urban Emissions program (by the authors) uses the WRF-CAMx modelling system covering the Indian Subcontinent and Delhi’s airshed as a nest. The city results are shared at https://www.delhiairquality.info (accessed 22 February 2023) in the form of hourly and daily average maps, city-level hourly and daily average PM 2.5 source apportionment, district-level concentration and source apportionment time series, and real-time (updated every 6 h) comparison of results with data from CPCB’s monitoring network.
5. Sectoral History
5.1. transport sector, 5.1.1. vehicle and fuel standards, 5.1.2. pollution-under-check (puc) programme, 5.1.3. public transportation and cng introduction, 5.1.4. bus rapid transit (brt) system, 5.1.5. metrorail system, 5.1.6. para-transit system, 5.1.7. odd–even experiment, 5.1.8. electric vehicle (ev) promotion, 5.1.9. new expressways, 5.2. agricultural waste burning, 5.3. residential emissions, 5.4. waste management, 5.5. construction sector, 5.6. road dust, 5.7. electricity consumption and load sharing, 5.8. diwali firecrackers, 6. judicial and institutional engagement, 6.1. role of the judicial system, 6.1.1. environment pollution (prevention and control) authority (epca), 6.1.2. diesel to cng conversion, 6.1.3. diwali firecracker ban, 6.1.4. leapfrogging from bs4 to bs6 vehicle emission and fuel standards, 6.1.5. petcoke ban, 6.1.6. installation of smog towers, 6.1.7. national green tribunal (ngt).
- Vardhaman Kaushik vs. Union of India case [ 140 ]—the NGT ordered de-registration of all diesel vehicles older than 10 years and all petrol vehicles older than 15 years.
- Smt. Ganga Lalwani vs. Union of India and Ors. case [ 141 ]—the NGT took cognisance of crop burning as a significant cause of Delhi’s air pollution and ordered various steps to reduce crop burning in adjoining states. These include converting crop waste into organic manure, use of ISRO’s services to alter lice on crop burning incidents, etc.
- Almitra H. Patel and Ors. vs. Union of India [ 142 ]—the NGT prohibited open burning of waste and directed all states to implement the solid waste management rules. Using its authority, the NGT under the polluter pays principle, in October 2022, imposed an environment compensation fee of INR 9,000,000,000 on the Delhi government for undisposed waste in its landfills.
- Mayank Manohar and Paras Singh vs. Government of Delhi and Ors. [ 143 ]—the NGT directed the government to immediately shut down 4770 industrial units running illegally in the residential areas of Delhi and directed it to adopt coercive measures to recover compensation for illegal operation of such units in accordance with law apart from prosecution.
6.2. Role of Union Government
6.2.1. graded response action plan (grap).
- When AQI conditions land in the poor category, actions include ensuring strict enforcement of controls on garbage burning, brick kilns, power plants, ash ponds, construction sites, fireworks, and periodic wet sweeping of roads; vigilance on polluting vehicles, vehicles touting PUC norms and out of state trucks; deploying more traffic police; and posting information on social media.
- When AQI conditions land in the very poor category, actions include banning diesel generator sets, increasing parking fees, increasing bus services, stopping coal and wood burning at hotels, opening eateries and stationing guards at markets in residential areas, and increasing public awareness.
- When AQI conditions land in the severe category, actions include shutting down brick kilns, hot-mix plants, stone crushers, and power plants, intensifying public transport services, and wet-sweeping roads more frequently.
- Under emergency conditions, actions include closing entry of non-commodity trucks, closing all construction activities, introducing the odd–even formula, and additional measures as the authority sees fit (for example, in January 2023, all coal use was banned in the NCR region).
6.2.2. National Clean Air Programme (NCAP)
6.2.3. commission for air quality management (caqm), 6.2.4. fifteenth finance commission grant (xvfc), 7. final remarks, supplementary materials, author contributions, institutional review board statement, informed consent statement, data availability statement, conflicts of interest.
- CPCB. White Paper on Pollution in Delhi ; Government of India: New Delhi, India, 1997.
- CPCB. Air Quality Monitoring, Emission Inventory and Source Apportionment Study for Indian Cities ; Government of India: New Delhi, India, 2011.
- Guttikunda, S.K.; Goel, R.; Pant, P. Nature of air pollution, emission sources, and management in the Indian cities. Atmos. Environ. 2014 , 95 , 501–510. [ Google Scholar ] [ CrossRef ]
- Guttikunda, S. Air pollution in Delhi. Econ. Political Wkly. 2012 , 47 , 24–27. [ Google Scholar ]
- Gani, S.; Bhandari, S.; Seraj, S.; Wang, D.S.; Patel, K.; Soni, P.; Arub, Z.; Habib, G.; Hildebrandt Ruiz, L.; Apte, J.S. Submicron aerosol composition in the world’s most polluted megacity: The Delhi Aerosol Supersite study. Atmos. Chem. Phys. 2019 , 19 , 6843–6859. [ Google Scholar ] [ CrossRef ] [ Green Version ]
- Chowdhury, S.; Dey, S.; Tripathi, S.N.; Beig, G.; Mishra, A.K.; Sharma, S. “Traffic intervention” policy fails to mitigate air pollution in megacity Delhi. Environ. Sci. Policy 2017 , 74 , 8–13. [ Google Scholar ] [ CrossRef ]
- Talukdar, S.; Tripathi, S.N.; Lalchandani, V.; Rupakheti, M.; Bhowmik, H.S.; Shukla, A.K.; Murari, V.; Sahu, R.; Jain, V.; Tripathi, N.; et al. Air Pollution in New Delhi during Late Winter: An Overview of a Group of Campaign Studies Focusing on Composition and Sources. Atmosphere 2021 , 12 , 1432. [ Google Scholar ] [ CrossRef ]
- Yadav, S.; Tripathi, S.N.; Rupakheti, M. Current status of source apportionment of ambient aerosols in India. Atmos. Environ. 2022 , 274 , 118987. [ Google Scholar ] [ CrossRef ]
- Cusworth, D.H.; Mickley, L.J.; Sulprizio, M.P.; Liu, T.; Marlier, M.E.; DeFries, R.S.; Guttikunda, S.K.; Gupta, P. Quantifying the influence of agricultural fires in northwest India on urban air pollution in Delhi, India. Environ. Res. Lett. 2018 , 13 , 044018. [ Google Scholar ] [ CrossRef ] [ Green Version ]
- Chowdhury, S.; Dey, S.; Guttikunda, S.; Pillarisetti, A.; Smith, K.R.; Di Girolamo, L. Indian annual ambient air quality standard is achievable by completely mitigating emissions from household sources. Proc. Natl. Acad. Sci. USA 2019 , 116 , 10711–10716. [ Google Scholar ] [ CrossRef ] [ Green Version ]
- Singh, A.; Pant, P.; Pope, F.D. Air quality during and after festivals: Aerosol concentrations, composition and health effects. Atmos. Res. 2019 , 227 , 220–232. [ Google Scholar ] [ CrossRef ]
- Adhikary, R.; Patel, Z.B.; Srivastava, T.; Batra, N.; Singh, M.; Bhatia, U.; Guttikunda, S. Vartalaap: What drives# airquality discussions: Politics, pollution or pseudo-science? Proc. ACM Human-Comput. Interact. 2021 , 5 , 1–29. [ Google Scholar ]
- Patel, K.; Adhikary, R.; Patel, Z.B.; Batra, N.; Guttikunda, S. Samachar: Print News Media on Air Pollution in India. In Proceedings of the ACM SIGCAS/SIGCHI Conference on Computing and Sustainable Societies (COMPASS), Seattle, WA, USA, 8 June 2022; pp. 401–413. [ Google Scholar ]
- Guttikunda, S.K.; Calori, G. A GIS based emissions inventory at 1 km × 1 km spatial resolution for air pollution analysis in Delhi, India. Atmos. Environ. 2013 , 67 , 101–111. [ Google Scholar ] [ CrossRef ]
- Lalchandani, V.; Srivastava, D.; Dave, J.; Mishra, S.; Tripathi, N.; Shukla, A.K.; Sahu, R.; Thamban, N.M.; Gaddamidi, S.; Dixit, K.; et al. Effect of Biomass Burning on PM2.5 Composition and Secondary Aerosol Formation During Post-Monsoon and Winter Haze Episodes in Delhi. J. Geophys. Res. Atmos. 2022 , 127 , e2021JD035232. [ Google Scholar ] [ CrossRef ]
- Sahu, S.K.; Beig, G.; Parkhi, N.S. Emissions inventory of anthropogenic PM2.5 and PM10 in Delhi during Commonwealth Games 2010. Atmos. Environ. 2011 , 45 , 6180–6190. [ Google Scholar ] [ CrossRef ]
- Gurjar, B.R.; van Aardenne, J.A.; Lelieveld, J.; Mohan, M. Emission estimates and trends (1990-2000) for megacity Delhi and implications. Atmos. Environ. 2004 , 38 , 5663–5681. [ Google Scholar ] [ CrossRef ]
- Guttikunda, S.K.; Goel, R. Health impacts of particulate pollution in a megacity—Delhi, India. Environ. Dev. 2013 , 6 , 8–20. [ Google Scholar ] [ CrossRef ]
- van Donkelaar, A.; Hammer, M.S.; Bindle, L.; Brauer, M.; Brook, J.R.; Garay, M.J.; Hsu, N.C.; Kalashnikova, O.V.; Kahn, R.A.; Lee, C.; et al. Monthly Global Estimates of Fine Particulate Matter and Their Uncertainty. Environ. Sci. Technol. 2021 , 55 , 15287–15300. [ Google Scholar ] [ CrossRef ]
- van Donkelaar, A.; Martin, R.V.; Li, C.; Burnett, R.T. Regional Estimates of Chemical Composition of Fine Particulate Matter Using a Combined Geoscience-Statistical Method with Information from Satellites, Models, and Monitors. Environ. Sci. Technol. 2019 , 53 , 2595–2611. [ Google Scholar ] [ CrossRef ] [ Green Version ]
- DPCC. Real-Time Advanced Air Source Management Network (R-AASMAN) ; Delhi Pollution Control Committee, Government of Delhi: New Delhi, India, 2023. Available online: http://raasman.com (accessed on 1 February 2023).
- Bhandari, S.; Gani, S.; Patel, K.; Wang, D.S.; Soni, P.; Arub, Z.; Habib, G.; Apte, J.S.; Hildebrandt Ruiz, L. Sources and atmospheric dynamics of organic aerosol in New Delhi, India: Insights from receptor modeling. Atmos. Chem. Phys. 2020 , 20 , 735–752. [ Google Scholar ] [ CrossRef ] [ Green Version ]
- Rai, P.; Furger, M.; El Haddad, I.; Kumar, V.; Wang, L.; Singh, A.; Dixit, K.; Bhattu, D.; Petit, J.-E.; Ganguly, D.; et al. Real-time measurement and source apportionment of elements in Delhi’s atmosphere. Sci. Total Environ. 2020 , 742 , 140332. [ Google Scholar ] [ CrossRef ]
- CPCB. Continuous Ambient Ait Quality Monitoring System (CAAQMS) under the National Ambient Monitoring Programme (NAMP) ; Central Pollution Control Board, Government of India: New Delhi, India, 2022.
- Pant, P.; Lal, R.M.; Guttikunda, S.K.; Russell, A.G.; Nagpure, A.S.; Ramaswami, A.; Peltier, R.E. Monitoring particulate matter in India: Recent trends and future outlook. Air Qual. Atmos. Health 2018 , 12 , 45–58. [ Google Scholar ] [ CrossRef ]
- Ganguly, T.; Selvaraj, K.L.; Guttikunda, S.K. National Clean Air Programme (NCAP) for Indian cities: Review and outlook of clean air action plans. Atmos. Environ. X 2020 , 8 , 100096. [ Google Scholar ] [ CrossRef ]
- Guttikunda, S.; Ka, N. Evolution of India’s PM2.5 pollution between 1998 and 2020 using global reanalysis fields coupled with satellite observations and fuel consumption patterns. Environ. Sci. Atmos. 2022 , 2 , 1502–1515. [ Google Scholar ] [ CrossRef ]
- HEI. State of Global Air (SOGA). A Special Report on Global Exposure to Air Pollution and its Health Impacts ; Health Effects Institute: Boston, MA, USA, 2022. [ Google Scholar ]
- Balakrishnan, K.; Dey, S.; Gupta, T.; Dhaliwal, R.S.; Brauer, M.; Cohen, A.J.; Stanaway, J.D.; Beig, G.; Joshi, T.K.; Aggarwal, A.N.; et al. The impact of air pollution on deaths, disease burden, and life expectancy across the states of India: The Global Burden of Disease Study 2017. Lancet Planet. Health 2019 , 3 , e26–e39. [ Google Scholar ] [ CrossRef ] [ PubMed ] [ Green Version ]
- Dandona, L.; Dandona, R.; Kumar, G.A.; Shukla, D.K.; Paul, V.K.; Balakrishnan, K.; Prabhakaran, D.; Tandon, N.; Salvi, S.; Dash, A.P.; et al. Nations within a nation: Variations in epidemiological transition across the states of India, 1990–2016 in the Global Burden of Disease Study. Lancet 2017 , 390 , 2437–2460. [ Google Scholar ] [ CrossRef ] [ PubMed ] [ Green Version ]
- Pandey, A.; Brauer, M.; Cropper, M.L.; Balakrishnan, K.; Mathur, P.; Dey, S.; Turkgulu, B.; Kumar, G.A.; Khare, M.; Beig, G.; et al. Health and economic impact of air pollution in the states of India: The Global Burden of Disease Study 2019. Lancet Planet. Health 2021 , 5 , e25–e38. [ Google Scholar ] [ CrossRef ] [ PubMed ]
- WHO. Health Aspects of Air Pollution with Particulate Matter, Ozone and Nitrogen Dioxide ; WHO Regional Office for Europe: Copenhagen, Denmark, 2003. [ Google Scholar ]
- Shi, L.; Wu, X.; Danesh Yazdi, M.; Braun, D.; Abu Awad, Y.; Wei, Y.; Liu, P.; Di, Q.; Wang, Y.; Schwartz, J.; et al. Long-term effects of PM2·5 on neurological disorders in the American Medicare population: A longitudinal cohort study. Lancet Planet. Health 2020 , 4 , e557–e565. [ Google Scholar ] [ CrossRef ] [ PubMed ]
- McDuffie, E.E.; Martin, R.V.; Spadaro, J.V.; Burnett, R.; Smith, S.J.; O’Rourke, P.; Hammer, M.S.; van Donkelaar, A.; Bindle, L.; Shah, V.; et al. Source sector and fuel contributions to ambient PM2.5 and attributable mortality across multiple spatial scales. Nat. Commun. 2021 , 12 , 3594. [ Google Scholar ] [ CrossRef ]
- IQair. World’s Most Polluted Cities in 2021. Available online: https://www.iqair.com/world-most-polluted-cities (accessed on 13 December 2022).
- Guttikunda, S.K.; Gurjar, B.R. Role of meteorology in seasonality of air pollution in megacity Delhi, India. Environ. Monit Assess 2012 , 184 , 3199–3211. [ Google Scholar ] [ CrossRef ]
- Ahmed, M.; Das, B.; Lotus, S.; Ali, M. A study on frequency of western disturbances and precipitation trends over Jammu & Kashmir, India: 1980-2019. Mausam 2022 , 73 , 283–294. [ Google Scholar ]
- Gautam, R.; Singh, M.K. Urban Heat Island Over Delhi Punches Holes in Widespread Fog in the Indo-Gangetic Plains. Geophys. Res. Lett. 2018 , 45 , 1114–1121. [ Google Scholar ] [ CrossRef ]
- Singh, M.K.; Gautam, R. Developing a long-term high-resolution winter fog climatology over south Asia using satellite observations from 2002 to 2020. Remote Sens. Environ. 2022 , 279 , 113128. [ Google Scholar ] [ CrossRef ]
- Kulkarni, R.; Jenamani, R.K.; Pithani, P.; Konwar, M.; Nigam, N.; Ghude, S.D. Loss to Aviation Economy Due to Winter Fog in New Delhi during the Winter of 2011–2016. Atmosphere 2019 , 10 , 198. [ Google Scholar ] [ CrossRef ] [ Green Version ]
- Hakkim, H.; Sinha, V.; Chandra, B.P.; Kumar, A.; Mishra, A.K.; Sinha, B.; Sharma, G.; Pawar, H.; Sohpaul, B.; Ghude, S.D.; et al. Volatile organic compound measurements point to fog-induced biomass burning feedback to air quality in the megacity of Delhi. Sci. Total Environ. 2019 , 689 , 295–304. [ Google Scholar ] [ CrossRef ] [ PubMed ]
- Pesaresi, M.; Ehrilch, D.; Florczyk, A.J.; Freire, S.; Julea, A.; Kemper, T.; Soille, P.; Syrris, V. GHS Built-Up Grid, Derived from Landsat, Multitemporal (1975, 1990, 2000, 2014) ; European Commission, Joint Research Centre, JRC Data Catalogue: Brussels, Belgium, 2015. [ Google Scholar ]
- DTE. The Supreme Court not to Budge on CNG Issue ; DTE: New Delhi, India, 2002. [ Google Scholar ]
- Bell, R.G.; Mathur, K.; Narain, U.; Simpson, D. Clearing the Air: How Delhi Broke the Logjam on Air Quality Reforms. Environ. Sci. Policy Sustain. Dev. 2004 , 46 , 22–39. [ Google Scholar ] [ CrossRef ]
- Balachandran, S.; Meena, B.R.; Khillare, P.S. Particle size distribution and its elemental composition in the ambient air of Delhi. Environ. Int. 2000 , 26 , 49–54. [ Google Scholar ] [ CrossRef ] [ PubMed ]
- Narain, U.; Bell, R. Who Changed Delhi’s Air? The Roles of the Court and the Executive in Environmental Policymaking ; Resources for the Future: Washington, DC, USA, 2005. [ Google Scholar ]
- Goel, R.; Guttikunda, S.K. Evolution of on-road vehicle exhaust emissions in Delhi. Atmos. Environ. 2015 , 105 , 78–90. [ Google Scholar ] [ CrossRef ]
- Goel, R.; Guttikunda, S.K. Role of urban growth, technology, and judicial interventions on vehicle exhaust emissions in Delhi for 1991–2014 and 2014–2030 periods. Environ. Dev. 2015 , 14 , 6–21. [ Google Scholar ] [ CrossRef ]
- Krelling, C.; Badami, M.G. Cost-effectiveness analysis of compressed natural gas implementation in the public bus transit fleet in Delhi, India. Transp. Policy 2022 , 115 , 49–61. [ Google Scholar ] [ CrossRef ]
- SAFAR. System of Air Quality and Weather Forecasting And Research, Indian Institute of Tropical Meteorology, Pune, India. Available online: http://safar.tropmet.res.in/ (accessed on 1 February 2023).
- Tibrewal, K.; Venkataraman, C. COVID-19 lockdown closures of emissions sources in India: Lessons for air quality and climate policy. J. Environ. Manag. 2022 , 302 , 114079. [ Google Scholar ] [ CrossRef ]
- Faridi, S.; Yousefian, F.; Janjani, H.; Niazi, S.; Azimi, F.; Naddafi, K.; Hassanvand, M.S. The effect of COVID-19 pandemic on human mobility and ambient air quality around the world: A systematic review. Urban Clim. 2021 , 38 , 100888. [ Google Scholar ] [ CrossRef ]
- Venter, Z.S.; Aunan, K.; Chowdhury, S.; Lelieveld, J. COVID-19 lockdowns cause global air pollution declines. Proc. Natl. Acad. Sci. USA 2020 , 117 , 18984–18990. [ Google Scholar ] [ CrossRef ] [ PubMed ]
- Sathe, Y.; Gupta, P.; Bawase, M.; Lamsal, L.; Patadia, F.; Thipse, S. Surface and satellite observations of air pollution in India during COVID-19 lockdown: Implication to air quality. Sustain. Cities Soc. 2021 , 66 , 102688. [ Google Scholar ] [ CrossRef ] [ PubMed ]
- Gani, S.; Pant, P.; Sarkar, S.; Sharma, N.; Dey, S.; Guttikunda, S.K.; AchutaRao, K.M.; Nygard, J.; Sagar, A.D. Systematizing the approach to air quality measurement and analysis in low and middle income countries. Environ. Res. Lett. 2022 , 17 , 021004. [ Google Scholar ] [ CrossRef ]
- Edwards, M.R.; Holloway, T.; Pierce, R.B.; Blank, L.; Broddle, M.; Choi, E.; Duncan, B.N.; Esparza, Á.; Falchetta, G.; Fritz, M.; et al. Satellite Data Applications for Sustainable Energy Transitions. Front. Sustain. 2022 , 3 , 910924. [ Google Scholar ] [ CrossRef ]
- Holloway, T.; Miller, D.; Anenberg, S.; Diao, M.; Duncan, B.; Fiore, A.M.; Henze, D.K.; Hess, J.; Kinney, P.L.; Liu, Y.; et al. Satellite Monitoring for Air Quality and Health. Annu. Rev. Biomed. Data Sci. 2021 , 4 , 417–447. [ Google Scholar ] [ CrossRef ]
- Dey, S.; Purohit, B.; Balyan, P.; Dixit, K.; Bali, K.; Kumar, A.; Imam, F.; Chowdhury, S.; Ganguly, D.; Gargava, P.; et al. A Satellite-Based High-Resolution (1-km) Ambient PM2.5 Database for India over Two Decades (2000–2019): Applications for Air Quality Management. Remote Sens. 2020 , 12 , 3872. [ Google Scholar ] [ CrossRef ]
- Kurinji, L.; Ganguly, T. Managing India’s Air Quality Through an Eye in the Sky ; Council for Energy Environment and Water: New Delhi, India, 2020. [ Google Scholar ]
- Baek, K.; Kim, J.H.; Bak, J.; Haffner, D.P.; Kang, M.; Hong, H. Evaluation of total ozone measurements from Geostationary Environmental Monitoring Satellite (GEMS). EGUsphere 2022 , 2022 , 1–23. [ Google Scholar ] [ CrossRef ]
- Ghude, S.D.; Pfister, G.G.; Jena, C.; van der A, R.J.; Emmons, L.K.; Kumar, R. Satellite constraints of nitrogen oxide (NOx) emissions from India based on OMI observations and WRF-Chem simulations. Geophys. Res. Lett. 2013 , 40 , 423–428. [ Google Scholar ] [ CrossRef ]
- Hammer, M.S.; van Donkelaar, A.; Li, C.; Lyapustin, A.; Sayer, A.M.; Hsu, N.C.; Levy, R.C.; Garay, M.J.; Kalashnikova, O.V.; Kahn, R.A.; et al. Global Estimates and Long-Term Trends of Fine Particulate Matter Concentrations (1998–2018). Environ. Sci. Technol. 2020 , 54 , 7879–7890. [ Google Scholar ] [ CrossRef ]
- Guttikunda, S.K.; Jawahar, P. Atmospheric emissions and pollution from the coal-fired thermal power plants in India. Atmos. Environ. 2014 , 92 , 449–460. [ Google Scholar ] [ CrossRef ]
- Roozitalab, B.; Carmichael, G.R.; Guttikunda, S.K.; Abdi-Oskouei, M. Elucidating the impacts of COVID-19 lockdown on air quality and ozone chemical characteristics in India. Environ. Sci. Atmos. 2022 , 2 , 1183–1207. [ Google Scholar ] [ CrossRef ]
- Nussbaumer, C.M.; Pozzer, A.; Tadic, I.; Röder, L.; Obersteiner, F.; Harder, H.; Lelieveld, J.; Fischer, H. Tropospheric ozone production and chemical regime analysis during the COVID-19 lockdown over Europe. Atmos. Chem. Phys. 2022 , 22 , 6151–6165. [ Google Scholar ] [ CrossRef ]
- Dubash, N.; Guttikunda, S.K. Delhi has a Complex air Pollution Problem, Hindustan Times. Available online: https://tinyurl.com/ybrtw9e7 (accessed on 1 February 2023).
- Banerjee, T.; Murari, V.; Kumar, M.; Raju, M.P. Source apportionment of airborne particulates through receptor modeling: Indian scenario. Atmos. Res. 2015 , 164–165 , 167–187. [ Google Scholar ] [ CrossRef ]
- Pant, P.; Harrison, R.M. Critical review of receptor modelling for particulate matter: A case study of India. Atmos. Environ. 2012 , 49 , 1–12. [ Google Scholar ] [ CrossRef ] [ Green Version ]
- Johnson, T.M.; Guttikunda, S.K.; Wells, G.; Bond, T.; Russell, A.; West, J.; Watson, J. Tools for Improving Air Quality Management. A Review of Top-Down Source Apportiontment Techniques and Their Application in Developing Countries ; ESMAP Publication Series; The World Bank: Washington, DC, USA, 2011. [ Google Scholar ]
- Amann, M.; Purohit, P.; Bhanarkar, A.D.; Bertok, I.; Borken-Kleefeld, J.; Cofala, J.; Heyes, C.; Kiesewetter, G.; Klimont, Z.; Liu, J.; et al. Managing future air quality in megacities: A case study for Delhi. Atmos. Environ. 2017 , 161 , 99–111. [ Google Scholar ] [ CrossRef ]
- TERI. Source Apportionment of PM2.5 & PM10 of Delhi NCR for Identification of Major Sources ; The Energy Research Institute: New Delhi, India, 2018. [ Google Scholar ]
- Gupta, L.; Bansal, M.; Nandi, P.; Habib, G.; Sunder Raman, R. Source apportionment and potential source regions of size-resolved particulate matter at a heavily polluted industrial city in the Indo-Gangetic Plain. Atmos. Environ. 2023 , 298 , 119614. [ Google Scholar ] [ CrossRef ]
- Tobler, A.; Bhattu, D.; Canonaco, F.; Lalchandani, V.; Shukla, A.; Thamban, N.M.; Mishra, S.; Srivastava, A.K.; Bisht, D.S.; Tiwari, S.; et al. Chemical characterization of PM2.5 and source apportionment of organic aerosol in New Delhi, India. Sci. Total Environ. 2020 , 745 , 140924. [ Google Scholar ] [ CrossRef ]
- Lalchandani, V.; Kumar, V.; Tobler, A.; Thamban, N.M.; Mishra, S.; Slowik, J.G.; Bhattu, D.; Rai, P.; Satish, R.; Ganguly, D.; et al. Real-time characterization and source apportionment of fine particulate matter in the Delhi megacity area during late winter. Sci. Total Environ. 2021 , 770 , 145324. [ Google Scholar ] [ CrossRef ]
- Guttikunda, S.K.; Calori, G.; Velay-Lasry, F.; Ngo, R. Air Quality Forecasting System for Cities: Modeling Architecture for Delhi ; SIM-22-2009; ResearchGate GmbH.: New Delhi, India, 2011. [ Google Scholar ]
- Goel, R.; Gani, S.; Guttikunda, S.K.; Wilson, D.; Tiwari, G. On-road PM2.5 pollution exposure in multiple transport microenvironments in Delhi. Atmos. Environ. 2015 , 123 , 129–138. [ Google Scholar ] [ CrossRef ]
- Apte, J.S.; Kirchstetter, T.W.; Reich, A.H.; Deshpande, S.J.; Kaushik, G.; Chel, A.; Marshall, J.D.; Nazaroff, W.W. Concentrations of fine, ultrafine, and black carbon particles in auto-rickshaws in New Delhi, India. Atmos. Environ. 2011 , 45 , 4470–4480. [ Google Scholar ] [ CrossRef ]
- MoRTH. Road Transport Yearbook and Statistics Reports for 2000 to 2020 ; Minister of Road Transport and Highways, the Government of India: New Delhi, India, 2022.
- Goel, R.; Guttikunda, S.K.; Mohan, D.; Tiwari, G. Benchmarking vehicle and passenger travel characteristics in Delhi for on-road emissions analysis. Travel Behav. Soc. 2015 , 2 , 88–101. [ Google Scholar ] [ CrossRef ]
- EPCA. Report of Assessment of Pollution Under Control (PUC) Programme in Delhi and NCR: Recommendations for Improvement to Ensure Pollution from In-Use Vehicles is Under Control ; EPCA Report No. 73; Environment Pollution (Prevention and Control) Authority for Delhi NCR: New Delhi, India, 2017. [ Google Scholar ]
- Suman, H.K.; Bolia, N.B.; Tiwari, G. Analysis of the Factors Influencing the Use of Public Buses in Delhi. J. Urban Plan. Dev. 2016 , 142 , 04016003. [ Google Scholar ] [ CrossRef ]
- World-Bank. Urban Bus Toolkit—Tools and Options for Reforming Urban Bus Systems ; The World Bank: Washington, DC, USA, 2006. [ Google Scholar ]
- Gadepalli, R.; Gumireddy, S.; Bansal, P. Cost Drivers of Electric Bus Contracts: Analysis of 33 Indian Cities. Transp. Res. Rec. 2022 , 2676 , 03611981221088593. [ Google Scholar ] [ CrossRef ]
- Hidalgo, D.; Pai, M. Delhi Bus Corridor: An Evaluation ; EMBARQ: Overland Park, KS, USA, 2009. [ Google Scholar ]
- Badami, M.G.; Haider, M. An analysis of public bus transit performance in Indian cities. Transp. Res. Part A Policy Pract. 2007 , 41 , 961–981. [ Google Scholar ] [ CrossRef ]
- Nikitas, D.A.; Karlsson, P.M. A Worldwide State-of-the-Art Analysis for Bus Rapid Transit: Looking for the Success Formula. J. Public Transp. 2015 , 18 , 1–33. [ Google Scholar ] [ CrossRef ] [ Green Version ]
- Nguyen, M.H.; Pojani, D. Chapter Two—Why Do Some BRT Systems in the Global South Fail to Perform or Expand. In Advances in Transport Policy and Planning ; Shiftan, Y., Kamargianni, M., Eds.; Academic Press: Cambridge, MA, USA, 2018; Volume 1, pp. 35–61. [ Google Scholar ]
- Hodgson, P.; Potter, S.; Warren, J.; Gillingwater, D. Can bus really be the new tram? Res. Transp. Econ. 2013 , 39 , 158–166. [ Google Scholar ] [ CrossRef ] [ Green Version ]
- Goel, R.; Tiwari, G. Access-egress and other travel characteristics of metro users in Delhi and its satellite cities. IATSS Res. 2016 , 39 , 164–172. [ Google Scholar ] [ CrossRef ] [ Green Version ]
- Rajagopal, D.; Sawant, V.; Bauer, G.S.; Phadke, A.A. Benefits of electrifying app-taxi fleet—A simulation on trip data from New Delhi. Transp. Res. Part D Transp. Environ. 2022 , 102 , 103113. [ Google Scholar ] [ CrossRef ]
- Tirachini, A. Ride-hailing, travel behaviour and sustainable mobility: An international review. Transportation 2020 , 47 , 2011–2047. [ Google Scholar ] [ CrossRef ]
- Kumar, P.; Gulia, S.; Harrison, R.M.; Khare, M. The influence of odd–even car trial on fine and coarse particles in Delhi. Environ. Pollut. 2017 , 225 , 20–30. [ Google Scholar ] [ CrossRef ]
- Beiser-McGrath, L.F.; Bernauer, T.; Prakash, A. Do policy clashes between the judiciary and the executive affect public opinion? Insights from New Delhi’s odd–even rule against air pollution. J. Public Policy 2021 , 42 , 185–200. [ Google Scholar ] [ CrossRef ]
- Davis, L.W. The Effect of Driving Restrictions on Air Quality in Mexico City. J. Political Econ. 2008 , 116 , 38–81. [ Google Scholar ] [ CrossRef ] [ Green Version ]
- Delhi-Govt. Delhi Electric Vehicles Policy 2020 ; Government of Delhi: New Delhi, India, 2020. Available online: https://ev.delhi.gov.in (accessed on 1 February 2023).
- RMI. Accelerating Electric Mobility in Delhi: Journey and Insights from Implementing the Delhi Electric Vehicles Policy ; Rocky Mountain Institute: New Delhi, India, 2022. Available online: https://ev.delhi.gov.in/files/Accelerating-Electric-Mobility-in-Delhi8497bf.pdf (accessed on 1 February 2023).
- CSE. Debunking Official Numbers of Trucks Entering Delhi ; Center for Science and Environment: New Delhi, India, 2015. [ Google Scholar ]
- Shyamsundar, P.; Springer, N.P.; Tallis, H.; Polasky, S.; Jat, M.L.; Sidhu, H.S.; Krishnapriya, P.P.; Skiba, N.; Ginn, W.; Ahuja, V.; et al. Fields on fire: Alternatives to crop residue burning in India. Science 2019 , 365 , 536–538. [ Google Scholar ] [ CrossRef ] [ PubMed ]
- Singha, M.; Dong, J.; Ge, Q.; Metternicht, G.; Sarmah, S.; Zhang, G.; Doughty, R.; Lele, S.; Biradar, C.; Zhou, S.; et al. Satellite evidence on the trade-offs of the food-water–air quality nexus over the breadbasket of India. Glob. Environ. Change 2021 , 71 , 102394. [ Google Scholar ] [ CrossRef ]
- Jethva, H.; Chand, D.; Torres, O.; Gupta, P.; Lyapustin, A.; Patadia, F. Agricultural Burning and Air Quality over Northern India: A Synergistic Analysis using NASA’s A-train Satellite Data and Ground Measurements. Aerosol Air Qual. Res. 2018 , 18 , 1756–1773. [ Google Scholar ] [ CrossRef ] [ Green Version ]
- Jethva, H.; Torres, O.; Field, R.D.; Lyapustin, A.; Gautam, R.; Kayetha, V. Connecting Crop Productivity, Residue Fires, and Air Quality over Northern India. Sci. Rep. 2019 , 9 , 16594. [ Google Scholar ] [ CrossRef ] [ Green Version ]
- Wooster, M.J.; Roberts, G.J.; Giglio, L.; Roy, D.P.; Freeborn, P.H.; Boschetti, L.; Justice, C.; Ichoku, C.; Schroeder, W.; Davies, D.; et al. Satellite remote sensing of active fires: History and current status, applications and future requirements. Remote Sens. Environ. 2021 , 267 , 112694. [ Google Scholar ] [ CrossRef ]
- Wiedinmyer, C.; Akagi, S.K.; Yokelson, R.J.; Emmons, L.K.; Al-Saadi, J.A.; Orlando, J.J.; Soja, A.J. The Fire INventory from NCAR (FINN): A high resolution global model to estimate the emissions from open burning. Geosci. Model Dev. 2011 , 4 , 625–641. [ Google Scholar ] [ CrossRef ] [ Green Version ]
- Jethva, H. Assessing predictability of post-monsoon crop residue fires in Northwestern India. Front. Earth Sci. 2022 , 10 , 1047278. [ Google Scholar ] [ CrossRef ]
- Beig, G.; Sahu, S.K.; Singh, V.; Tikle, S.; Sobhana, S.B.; Gargeva, P.; Ramakrishna, K.; Rathod, A.; Murthy, B.S. Objective evaluation of stubble emission of North India and quantifying its impact on air quality of Delhi. Sci. Total Environ. 2020 , 709 , 136126. [ Google Scholar ] [ CrossRef ]
- Kanawade, V.P.; Srivastava, A.K.; Ram, K.; Asmi, E.; Vakkari, V.; Soni, V.K.; Varaprasad, V.; Sarangi, C. What caused severe air pollution episode of November 2016 in New Delhi? Atmos. Environ. 2020 , 222 , 117125. [ Google Scholar ] [ CrossRef ]
- GBD-MAPS. Global Burden of Disease—Mapping Air Pollution Sources in India ; Health Effects Institute: Boston, MA, USA, 2018. [ Google Scholar ]
- PIB. Agricultural Mechanization for In-Situ Management of Crop Residue by MoAFW ; Release ID: 1707021; Press Information Bureau, Government of India: New Delhi, India, 2021.
- PIB. Measures to Reduce Pollution Due to Stubble Burning by MoEFCC ; Release ID: 1779712; Press Information Bureau, Government of India: New Delhi, India, 2021.
- Mukherjee, A.; Tripathi, S.N.; Ram, K.; Saha, D. New Delhi Air Potentially Chokes from Groundwater Conservation Policies in Adjoining Regions. Environ. Sci. Technol. Lett. 2023 , 10 , 3–5. [ Google Scholar ] [ CrossRef ]
- Chowdhury, S.; Chafe, Z.A.; Pillarisetti, A.; Lelieveld, J.; Guttikunda, S.K.; Dey, S. The Contribution of Household Fuels to Ambient Air Pollution in India—A Comparison of Recent Estimates ; Policy Brief No. CCAPC/2019/01; Collaborative Clean Air Policy Centre: New Delhi, India, 2019. [ Google Scholar ]
- Census-India. Census of India, 2011 ; The Governement of India: New Delhi, India, 2011.
- Harish, S.; Smith, K.R. (Eds.) Ujjwala 2.0: From Access to Sustained Usage ; Policy Brief No. CCAPC/2019/03; Collaborative Clean Air Policy Centre: New Delhi, India, 2019. [ Google Scholar ]
- Tripathi, A.; Sagar, A.D.; Smith, K.R. Promoting clean and affordable cooking: Smarter subsidies for LPG. Econ. Political Wkly. 2015 , 50 , 81–84. [ Google Scholar ]
- Gill-Wiehl, A.; Brown, T.; Smith, K. The need to prioritize consumption: A difference-in-differences approach to analyze the total effect of India’s below-the-poverty-line policies on LPG use. Energy Policy 2022 , 164 , 112915. [ Google Scholar ] [ CrossRef ]
- Gupta, A.; Vyas, S.; Hathi, P.; Khalid, N.; Srivastav, N.; Spears, D.; Coffey, D. Persistence of solid fuel use in rural North India. Econ. Political Wkly. 2020 , 55 , 55. [ Google Scholar ]
- Guttikunda, S.; Jawahar, P. Can We Vacuum Our Air Pollution Problem Using Smog Towers? Atmosphere 2020 , 11 , 922. [ Google Scholar ] [ CrossRef ]
- DPCC. Quarterly Report on Solid Waste Management, March 2022 ; Delhi Pollution Control Committee, Government of Delhi: New Delhi, India, 2022.
- Nagpure, A.S.; Ramaswami, A.; Russell, A. Characterizing the Spatial and Temporal Patterns of Open Burning of Municipal Solid Waste (MSW) in Indian Cities. Environ. Sci. Technol. 2015 , 49 , 12911–12912. [ Google Scholar ] [ CrossRef ]
- Randhawa, P.; Marshall, F.; Kushwaha, P.K.; Desai, P. Pathways for Sustainable Urban Waste Management and Reduced Environmental Health Risks in India: Winners, Losers, and Alternatives to Waste to Energy in Delhi. Front. Sustain. Cities 2020 , 2 , 14. [ Google Scholar ] [ CrossRef ]
- DPCC. Annual Report 2021-22 on Implementation of Construction and Demolition Waste Management Rules 2016 ; Delhi Pollution Control Committee, Government of Delhi: New Delhi, India, 2022.
- CPCB. Graded Response Action Plan (GRAP) ; Central Pollution Control Board, Government of India: New Delhi, India, 2017. Available online: https://cpcb.nic.in (accessed on 1 February 2023).
- Maithel, S.; Uma, R.; Bond, T.; Baum, E.; Thao, V.T.K. Brick Kilns Performance Assessment, Emissions Measurements, & A Roadmap for Cleaner Brick Production in India ; CATF: Boston, MA, USA, 2012. [ Google Scholar ]
- Weyant, C.; Athalye, V.; Ragavan, S.; Rajarathnam, U.; Lalchandani, D.; Maithel, S.; Baum, E.; Bond, T.C. Emissions from South Asian Brick Production. Environ. Sci. Technol. 2014 , 48 , 6477–6483. [ Google Scholar ] [ CrossRef ]
- Pant, P.; Shukla, A.; Kohl, S.D.; Chow, J.C.; Watson, J.G.; Harrison, R.M. Characterization of ambient PM2.5 at a pollution hotspot in New Delhi, India and inference of sources. Atmos. Environ. 2015 , 109 , 178–189. [ Google Scholar ] [ CrossRef ]
- Pant, P.; Baker, S.J.; Shukla, A.; Maikawa, C.; Godri Pollitt, K.J.; Harrison, R.M. The PM10 fraction of road dust in the UK and India: Characterization, source profiles and oxidative potential. Sci. Total Environ. 2015 , 530–531 , 445–452. [ Google Scholar ] [ CrossRef ] [ PubMed ]
- Delhi-Govt. Economic Survey of Delhi 2021-22, Chapter 11: Energy ; Delhi Planning Commission: New Delhi, India, 2022.
- Cropper, M.; Cui, R.; Guttikunda, S.; Hultman, N.; Jawahar, P.; Park, Y.; Yao, X.; Song, X.-P. The mortality impacts of current and planned coal-fired power plants in India. Proc. Natl. Acad. Sci. USA 2021 , 118 , e2017936118. [ Google Scholar ] [ CrossRef ] [ PubMed ]
- Liu, J.; Chen, Y.; Chao, S.; Cao, H.; Zhang, A. Levels and health risks of PM2.5-bound toxic metals from firework/firecracker burning during festival periods in response to management strategies. Ecotoxicol. Environ. Saf. 2019 , 171 , 406–413. [ Google Scholar ] [ CrossRef ] [ PubMed ]
- Ghosh, S. Chapter: Environment. In Regulation in India: Design, Capacity, Performance ; Bloomsbury Publishers: London, UK, 2018. [ Google Scholar ]
- Dutta, R. Twenty Years of EPCA: Lessons for the New EPCA ; Legal Initiative for Forests and Environment: New Delhi, India, 2018. [ Google Scholar ]
- Supreme-Court. Arjun Gopal vs Union Of India, Writ Petition(Civil) No. 728/2015 ; The Supreme Court: New Delhi, India, 2015. Available online: https://indiankanoon.org/doc/83069161/ (accessed on 1 February 2023).
- Supreme-Court. Arjun Gopal vs Union Of India, Writ Petition(Civil) No. 728/2015 ; The Supreme Court: New Delhi, India, 7 November 2016. Available online: https://indiankanoon.org/doc/54446501/ (accessed on 1 February 2023).
- Supreme-Court. Arjun Gopal vs Union Of India, Writ Petition(Civil) No. 728/2015 ; The Supreme Court: New Delhi, India, 11 November 2016. Available online: https://indiankanoon.org/doc/150260580/ (accessed on 1 February 2023).
- Supreme-Court. Arjun Gopal vs Union Of India, Writ Petition(Civil) No. 728/2015 ; The Supreme Court: New Delhi, India, 2021. Available online: https://indiankanoon.org/doc/199698532/ (accessed on 1 February 2023).
- PIB. Government Decides to Directly Shift from BS-IV to BS-VI Emission Norms ; Release ID: 134232; Press Information Bureau, Government of India: New Delhi, India, 2016.
- Supreme-Court. M.C. Mehta vs Union Of India, Writ Petition(Civil) No. 13029/1985 ; The Supreme Court: New Delhi, India, 2018. Available online: https://indiankanoon.org/doc/73307198/ (accessed on 1 February 2023).
- Supreme-Court. M.C. Mehta vs Union Of India, Writ Petition(Civil) No. 13029/1985 ; The Supreme Court: New Delhi, India, 2017. Available online: https://indiankanoon.org/doc/174022915/ (accessed on 1 February 2023).
- Supreme-Court. M.C. Mehta vs Union Of India, Writ Petition(Civil) No. 13029/1985 ; The Supreme Court: New Delhi, India, 2019. Available online: https://indiankanoon.org/doc/40459213/ (accessed on 1 February 2023).
- NGT. Vardhaman Kaushik vs Union of India Case on De-Registering Older Vehicles ; The National Green Tribunal: New Delhi, India, 2018. Available online: https://greentribunal.gov.in/caseDetails/DELHI/0701102000092014 (accessed on 1 February 2023).
- NGT. Smt. Ganga Lalwani vs. Union of India and Ors Case to Reduce Crop-Burning in Neighbouring States ; The National Green Tribunal: New Delhi, India, 2018. Available online: https://greentribunal.gov.in/sites/default/files/all_documents/GANGA%20LALWANI.PDF (accessed on 1 February 2023).
- NGT. Almitra H. Patel & Ors. vs. Union of India Case on Waste Management in Delhi ; The National Green Tribunal: New Delhi, India, 2014. Available online: https://www.dpcc.delhigovt.nic.in/uploads/sitedata/almrita_H_UOI_1.pdf (accessed on 1 February 2023).
- NGT. Mayank Manohar & Paras Singh vs. Government of Delhi & Ors Case to Shutdown illegal Industries in Delhi ; The National Green Tribunal: New Delhi, India, 2018. Available online: https://greentribunal.gov.in (accessed on 1 February 2023).
- CPCB. Swachh Vayu Survekshan ; Central Pollution Control Board, Government of India: New Delhi, India, 2022. Available online: https://prana.cpcb.gov.in/assets/pdf/Resources/Swachh_Vayu_Survekshan_15_aug_2022.pdf (accessed on 1 February 2023).
- Finance-Commission. Fifteen Finance Commission Report for the Year 2020–2021. Available online: https://fincomindia.nic.in (accessed on 1 February 2023).
- CREA. Tracing the Hazy Air 2023. Progress Report on National Clean Air Programme (NCAP) ; Centre for Research on Energy and Clean Air: New Delhi, India, 2023. [ Google Scholar ]
Click here to enlarge figure
Characteristic | Data |
---|---|
Total area | 1500 km |
Green cover (2019) | 21% |
Number of districts | 11 |
Number of sub-districts | 27 |
Number of municipal corporations | 3 |
Total state population | 19 million |
Net migrant population (2011 census) | 2 million |
Population density | 12,000 persons/km |
Urbanization (state) | 86% |
GDP (per capita, 2020) | US$4600 |
Road density | 2100 km/100 km |
Total registered vehicles (2021) | 14 million |
Metro rail length | 350 km |
Landfills | 3 |
Landfill capacity | 7000 tons/day |
PM pollution rank (2021) | 4 (among world cities) [ ] |
PM pollution rank (2021) | 1 (among world capital cities) [ ] |
MH-AD | MH-DT | MH-NT | T-DT | T-NT | WS-AD | |
---|---|---|---|---|---|---|
JAN | 298 (58) | 557 (118) | 39 (8) | 18.9 (1.8) | 9.9 (1.5) | 2.7 (0.7) |
FEB | 516 (94) | 974 (187) | 57 (56) | 24.2 (2.8) | 15.3 (2.5) | 2.8 (0.9) |
MAR | 926 (198) | 1801 (393) | 51 (18) | 30.5 (2.6) | 19.6 (1.8) | 3.1 (0.6) |
APR | 1075 (254) | 2066 (501) | 84 (45) | 35.5 (2.4) | 26.3 (2.2) | 3.8 (0.8) |
MAY | 1243 (307) | 2377 (640) | 109 (60) | 39.4 (2.7) | 31.1 (1.8) | 3.7 (0.9) |
JUN | 1054 (244) | 1855 (485) | 254 (124) | 39.0 (3.2) | 34.0 (2.3) | 4.5 (1.2) |
JUL | 573 (240) | 994 (450) | 153 (85) | 33.9 (2.9) | 30.6 (2.1) | 3.1 (0.7) |
AUG | 505 (152) | 906 (269) | 104 (58) | 33.0 (2.1) | 29.3 (1.3) | 2.7 (0.7) |
SEP | 462 (123) | 827 (239) | 97 (105) | 31.4 (2.2) | 26.5 (1.1) | 2.8 (0.9) |
OCT | 501 (91) | 959 (184) | 43 (13) | 30.0 (1.6) | 21.8 (1.8) | 2.5 (0.5) |
NOV | 350 (73) | 651 (129) | 50 (33) | 25.3 (1.5) | 17.5 (1.9) | 2.7 (0.7) |
DEC | 286 (71) | 534 (140) | 38 (8) | 18.8 (2.2) | 11.1 (2.8) | 2.4 (0.6) |
Year | JAN | FEB | MAR | APR | MAY | JUN | JUL | AUG | SEP | OCT | NOV | DEC | Annual |
---|---|---|---|---|---|---|---|---|---|---|---|---|---|
2006 | 74 | 168 | 217 | 203 | 171.0 | ||||||||
2007 | 253 | 146 | 88 | 109 | 73 | 89 | 45 | 32 | 52 | 145 | 188 | 111.1 | |
2008 | 156 | 178 | 136 | 90 | 73 | 50 | 51 | 22 | 23 | 174 | 226 | 189 | 138.6 |
2009 | 145 | 123 | 91 | 68 | 65 | 73 | 55 | 43 | 37 | 163 | 91.5 | ||
2010 | 69 | 116 | 82 | 115 | 117 | 61 | 60 | 65 | 187 | 292 | 267 | 144.1 | |
2011 | 217 | 161 | 123 | 127 | 98 | 70 | 69 | 53 | 61 | 164 | 258 | 277 | 140.1 |
2012 | 205 | 141 | 139 | 101 | 122 | 69 | 58 | 70 | 147 | 263 | 179 | 140.3 | |
2013 | 190 | 128 | 148 | 105 | 129 | 126 | 66 | 85 | 95 | 145 | 212 | 106 | 125.3 |
2014 | 193 | 123 | 69 | 98 | 120 | 137 | 114 | 87 | 74 | 137 | 166 | 161 | 117.2 |
2015 | 179 | 124 | 96 | 101 | 120 | 97 | 67 | 58 | 90 | 153 | 243 | 177 | 125.3 |
2016 | 249 | 151 | 126 | 120 | 92 | 73 | 56 | 42 | 61 | 152 | 258 | 217 | 133.3 |
2017 | 164 | 134 | 96 | 96 | 122 | 60 | 36 | 37 | 58 | 135 | 268 | 200 | 117.2 |
2018 | 205 | 143 | 104 | 94 | 95 | 86 | 42 | 43 | 45 | 139 | 209 | 236 | 120.0 |
2019 | 190 | 123 | 83 | 81 | 88 | 63 | 46 | 34 | 39 | 114 | 187 | 203 | 105.0 |
2020 | 148 | 120 | 57 | 44 | 54 | 46 | 34 | 24 | 47 | 132 | 199 | 190 | 93.0 |
2021 | 187 | 150 | 96 | 86 | 53 | 53 | 39 | 41 | 32 | 74 | 230 | 191 | 106.0 |
2022 | 150 | 103 | 98 | 106 | 78 | 62 | 35 | 32 | 40 | 106 | 178 | 171 | 99.7 |
Study | Study Year | Year of Publication | Source Information and Other Remarks |
---|---|---|---|
CPCB white paper [ ] | 1970–71 1980–81 1990–91 | 1997 | Industrial–vehicular–domestic source contributions were reported as 56%–23%–21%, 40%–42%–18%, and 29%–64%–7% respectively. This is the first known official account of source contributions in Delhi. The industrial sources include power plants, and no other sources were mentioned as part of the source apportionment. There is no mention of the technique utilised for this assessment. From the description provided in the report, this apportionment is likely for ambient PM concentrations. |
CPCB six-city study [ ] | 2006 | 2011 | For Delhi’s PM samples, average contributions were all dust (45.5%), domestic cooking and heating (7%), garbage burning (16.6%), and industries + diesel gensets (17.2%). However, the published results for PM were difficult to accept with domestic use of LPG resulting in 45.4% of the total mass. Relevant pages from the official report are included in the for reference. PM and PM samples were collected at residential, industrial, and kerbside locations for multiple seasons. The study also included establishing a gridded emissions inventory and a database of emission factors for other cities to adapt. The total emission loads were calculated for a representative grid of 2 km × 2 km and extrapolated to the city size of 32 km × 32 km. The estimated PM emission load is 53.6 kt/year. |
SAFAR | 2010 & 2018 | 2011 | SAFAR was developed by IITM for the 2010 CWG. The reports did not include any apportionment for ambient concentrations. The program conducted a series of surveys and on-ground measurements to develop an emission inventory at 1.67 km × 1.67 km and updated to 400 m × 400 m resolution in 2018. Total PM emission loads in 2010 and 2018 were 94 kt and 108 kt, respectively. The % shares of key sectors were 32% and 39% for transport, 17% and 23% for industries, 28% and 18% for all dust, 18% and 6% for residential cooking and heating, 3% and 3% for power plants, 2% and 12% for others, respectively. |
Urban Emissions (authors) [ , ] | 2010 | 2013 | Emission and ATMoS Lagrangian model-based source contributions for PM ranged 16–30% for vehicle exhaust, 8–14% for road dust + construction dust, 20–27% for diffused sources including cooking, heating, and open waste burning, 3–17% for diesel generator sets, and 34–41% for industries including brick kilns and power plants. Emissions inventory covered an airshed of 52 km × 52 km around Delhi. The estimated PM emission load is 63 kt/year. The inventory was extended to an area covering 80 km × 80 km ( ) and utilised for short-term (3-day) air quality forecasting and validating against monitoring data every hour. |
DPCC—IIT Kanpur | Winter 2013–14 and Summer 2014 | 2016 | Receptor-model-based source contributions for PM in winter–summer months were 25–9% for vehicle exhaust, 6–31% for all dust, 8–7% for open waste burning, 5–26% for industrial coal and fly ash, 26–12% for biomass burning, and 30–15% for secondary PM component, respectively. The estimated PM emission load was 21.5 kt/year for Delhi city. Emission and CTM model-based simulations were conducted, but no contribution shares were published. |
GAINS [ ] | 2015 | 2017 | Emissions and CTM-based source contributions for PM were 8.7% for vehicle exhaust, 17.4% for cooking, 19.1% for all dust, 16.5% for industries including power plants, open waste burning 6.1%, agricultural waste burning 4.3%, Diwali fireworks 1–2%, and 24.3% for secondary PM. The study also estimated that 60% of the estimated PM originates outside Delhi administrative limits. |
TERI [ ] | Summer and Winter 2016 | 2018 | Receptor-model-based source contributions for PM in summer–winter months were 18–23% for all transport, 34–15% for all dust, 15–22% for all biomass, 11–10% for industry, 5–4% other sources, and 17–23% secondary PM component, respectively. Emission and CTM model-based source contributions for PM in summer–winter months were 17–28% for all transport, 38–17% for all dust, 8–10% for residential cooking, 7–4% for agricultural waste burning, 22–30% for industry, and 8–10% for other sources. Total estimated PM emission load is 32 kt/year for Delhi and 528 kt/year for the NCR Delhi. For receptor modelling, 24 h PM and PM samples were collected at 20 representative locations in Delhi and its satellite cities. For emissions modelling, the study included updates to activity levels, source profiles and emission factors. |
GBD-MAPS [ , ] | 2017 | 2021 | Global emission and CTM model-based source contributions for PM were 29% for all residential cooking and heating, 7% for vehicle exhaust, 25% for industry including power plants, 15% all dust, 3% open waste burning, 2% agricultural waste burning, and 19% others. The Global Burden of Disease (GBD) study (since 1990) quantifies impacts of over 300 diseases and risk factors by age and sex [ ] ( , accessed 22 February 2023). An extension to the program is GBD-MAPS (mapping air pollution sources), which uses the same global chemical transport model to apportion sources. Because of the coarse nature of the model, the data were extracted for the grid covering the Delhi city. |
Gupta et al., 2023 [ ] | 2018–19 | 2023 | Receptor-model-based source contributions for PM were 17–28% for all transport, 16–30% for all dust, 14–31% for mixed combustion including biomass, 12–25% for industries, and 17–33% secondary PM component, respectively. PM and PM 457 samples were collected at two locations in Ghaziabad (one of the prominent satellite cities of Delhi) for one year from June 2018 to May 2019. |
Gani et al., 2020 [ ]; Bhandari et al., 2021 [ ]; Rai et al., 2020 [ ]; Tobler et al., 2020 [ ] | 2019–20 | 2020–21 | These studies used new techniques, equipment, and analytical platforms that allow for real-time sampling, metal and ion speciation, and receptor modelling to ascertain source contributions. Applications in Delhi used aerosol chemical speciation monitors (ACSMs), scanning mobility particle sizers (SMPSs), aerosol mass spectrometers (AMSs) and Xact ambient metals monitors (XACTs). These systems provide information at a higher temporal resolution and avoid the risk of contamination that is associated with offline measurements, storage, and analysis of filters. However, this approach was limited to only one (IIT-Delhi campus) location, PM fractions, and chemical speciation, and continues to be mostly academic in nature due to higher equipment costs and unique expertise required to operate them. |
DPCC [ ] | 2023 | 2023 | This real-time source apportionment system was launched in January 2023 ( , accessed 22 February 2023). No long-term data were available at the time of the review. Receptor-model-based source contributions for PM for three days in January 2023 were 4–24% for all transport, 10–18% for all dust, 13–30% for all biomass, 5–7% for coal combustion, 2–6% open waste burning, and 30–34% secondary PM component. |
Sector/Source Category | % Annual Contribution Range |
---|---|
Vehicle exhaust from petrol, diesel, and gas combustion | 10–30% |
Dust from roads and construction activities | 10–30% |
Industrial sources, including power plants | 10–30% |
Residential cooking and heating activities | Under 10% in summer and under 30% in winter |
Open waste burning | 5–15% |
Power plants (mostly outside city limits) | Under 7% |
Dust storms as a seasonal regional source | Under 5% |
Agricultural residue burning as a seasonal, regional short-term source | Under 3% |
Diwali firecrackers as a 2-day extreme event source | Under 1% |
Plant | MW | 2013 | 2014 | 2015 | 2016 | 2017 | 2018 | 2019 | 2020 | 2021 | 2022 |
---|---|---|---|---|---|---|---|---|---|---|---|
Badarpur TPS | 705 | 4317 (71%) | 3768 (62%) | 2359 (39%) | 2087 (34%) | 1559 (26%) | 1400 (23%) | ||||
Dadri NCTPP | 1820 | 13,007 (83%) | 12,786 (81%) | 10,319 (66%) | 9936 (63%) | 8880 (56%) | 10,870 (69%) | 7411 (47%) | 3494 (22%) | 5824 (37%) | 8671 (60%) |
Dadri CCPP | 830 | 3404 (47%) | 2645 (37%) | 2960 (41%) | 2620 (37%) | 1741 (24%) | 1491 (21%) | 1771 (25%) | 2107 (29%) | 885 (12%) | 659 (10%) |
Faridabad CCPP | 432 | 1679 (45%) | 1586 (43%) | 1360 (36%) | 986 (26%) | 839 (22%) | 560 (15%) | 648 (17%) | 849 (23%) | 355 (10%) | |
Indraprastha CCPP | 270 | 1070 (46%) | 975 (42%) | 593 (25%) | 622 (27%) | 621 (27%) | 607 (26%) | 525 (22%) | 462 (20%) | 224 (10%) | 309 (14%) |
Indira Gandhi STPP | 1500 | 5272 (41%) | 6657 (51%) | 6178 (48%) | 5808 (45%) | 6702 (52%) | 7638 (59%) | 4712 (36%) | 2594 (20%) | 6916 (53%) | 7684 (65%) |
Mahatma Gandhi TPS | 1320 | 5735 (50%) | 6256 (55%) | 5764 (51%) | 3163 (28%) | 5823 (51%) | 7181 (63%) | 6222 (55%) | 4706 (41%) | 7889 (69%) | 7055 (67%) |
Panipat TPS | 1360 | 6234 (53%) | 4421 (38%) | 1890 (16%) | 2241 (26%) | 2404 (30%) | 3372 (42%) | 2337 (29%) | 916 (14%) | 2310 (38%) | 4562 (81%) |
Pragati CCGT-III | 1500 | 999 (11%) | 1612 (12%) | 2041 (16%) | 1948 (15%) | 2819 (22%) | 3698 (29%) | 3765 (29%) | 3374 (26%) | 3340 (26%) | 2558 (22%) |
Pragati CCPP | 330 | 2469 (86%) | 2061 (72%) | 1695 (59%) | 1716 (60%) | 1791 (63%) | 1782 (62%) | 1391 (49%) | 1555 (54%) | 1547 (54%) | 1074 (41%) |
Rajghat TPS | 135 | 555 (48%) | 367 (31%) | 130 (11%) | |||||||
Rajiv Gandhi TPS | 1200 | 4577 (44%) | 4940 (48%) | 4637 (45%) | 4431 (43%) | 3828 (37%) | 5216 (50%) | 2081 (20%) | 1528 (15%) | 2286 (22%) | 5450 (57%) |
Rithala CCPP | 108 | 7 (1%) | |||||||||
Yamuna Nagar TPS | 600 | 3291 (63%) | 3610 (70%) | 3812 (74%) | 3889 (75%) | 3362 (65%) | 2975 (57%) | 3380 (65%) | 2032 (39%) | 2543 (49%) | 4019 (85%) |
The statements, opinions and data contained in all publications are solely those of the individual author(s) and contributor(s) and not of MDPI and/or the editor(s). MDPI and/or the editor(s) disclaim responsibility for any injury to people or property resulting from any ideas, methods, instructions or products referred to in the content. |
Share and Cite
Guttikunda, S.K.; Dammalapati, S.K.; Pradhan, G.; Krishna, B.; Jethva, H.T.; Jawahar, P. What Is Polluting Delhi’s Air? A Review from 1990 to 2022. Sustainability 2023 , 15 , 4209. https://doi.org/10.3390/su15054209
Guttikunda SK, Dammalapati SK, Pradhan G, Krishna B, Jethva HT, Jawahar P. What Is Polluting Delhi’s Air? A Review from 1990 to 2022. Sustainability . 2023; 15(5):4209. https://doi.org/10.3390/su15054209
Guttikunda, Sarath K., Sai Krishna Dammalapati, Gautam Pradhan, Bhargav Krishna, Hiren T. Jethva, and Puja Jawahar. 2023. "What Is Polluting Delhi’s Air? A Review from 1990 to 2022" Sustainability 15, no. 5: 4209. https://doi.org/10.3390/su15054209
Article Metrics
Article access statistics, supplementary material.
- Externally hosted supplementary file 1 Doi: 10.5281/zenodo.7595761 Link: https://doi.org/10.5281/zenodo.7595761 Description: Data: Ambient Air Quality, Reanalysis Fields, Satellite Retrievals, and Emissions Support Information for Delhi
Further Information
Mdpi initiatives, follow mdpi.
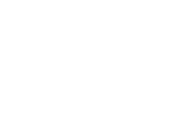
Subscribe to receive issue release notifications and newsletters from MDPI journals
Delhi, the world’s most air polluted capital fights back
Subscribe to global connection, vinod thomas and vinod thomas distinguished fellow - asian institute of management, manila, former senior vice president - world bank chitranjali tiwari ct chitranjali tiwari associate fellow - jk lakshmipat university, jaipur.
November 25, 2020
After an unexpected respite as coronavirus lockdowns stalled economic activity, air pollution has returned to pre-COVID-19 levels in Delhi, the world’s most air polluted capital city (Figure 1).

Last month, ahead of the usual spike in winter, the Delhi administration launched an antipollution campaign. But to win, nothing short of sustained action on multiple fronts will suffice. Other Asian capitals too have faced pollution crises. But Delhi’s is extreme because of a combination of smoke from thermal plants and brick kilns in the capital region, effluents from a congested transportation network, stubble or biomass burning by farmers in neighboring states, and the lack of cleansing winds that causes air pollution to hang over the city. Even as technical solutions are within reach, the campaign must overcome the poor policy coordination among central, city, and local governments.
Delhi’s toxic haze is a deadly health risk to its residents, particularly children, the elderly, and the ill. Particulate matter—PM2.5 and PM10—far exceeds national and World Health Organization limits and is the main culprit for Delhi’s high incidence of cardiovascular damage. The city’s toxic air also contains high quantities of sulfur dioxide, nitrogen oxide, and carbon monoxide, putting people at higher risk of strokes, heart attacks, and high blood pressure, and worsening the respiratory complications from COVID-19.
The main sources of Delhi’s particulate emissions are, in equal measure, particles from large power plants and refineries, vehicles, and stubble burning. The experiences of Bangkok, Beijing, and Singapore suggest that an ambitious but feasible goal is to cut air pollution by one-third by 2025, which, if sustained, could extend people’s lives by two to three years. The current effort is designed to confront all three sources, but strong implementation is needed.
Delhi is moving simultaneously on three fronts: energy, transport, and agriculture. In each case, East Asia offers valuable lessons.
- Coal-fired plants. Delhi’s environment minister has called for the closure of 11 coal-fired power plants operating within 300 kilometers of Delhi. But policy implementation must improve: All the plants have missed two deadlines to install flue-gas desulfurization units to reduce sulfur dioxide emissions. Last year, 10 coal-fired power plants missed a December deadline to install pollution control devices. Beijing provides valuable lessons in cutting concentrations of PM2.5 more than 40 percent since 2013. Beijing substituted its four major coal-fired stations with natural gas plants. The city government ordered 1,200 factories to shut with stricter controls and inspections of emitters. Bangkok had success with its inspection and maintenance program.
- Cleaner transport . Delhi has tried pollution checking of vehicles by mobile enforcement teams, public awareness campaigns , investment in mass rapid transport systems, and phasing out old commercial vehicles. The Delhi government’s recent push for electric vehicles shows promise, while the response of industry and the buy-in from customers will be key. Overall results in cutting pollution have been weak because of poor governance at every level. Better outcomes will be predicated on investment in public transportation, including integration of transport modes and last-mile connectivity. Unfortunately, Delhi Transport Corporation’s fleet shrank from 6,204 buses in 2013 to 3,796 buses in 2019, with most of the bus fleet aging. Delhi should look at Singapore’s regulation on car ownership and use; its improved transit systems; and promotion of pedestrian traffic and nonmotorized transport.
- Better farming practices . Burning of crop stubble in Delhi’s neighboring states has become a serious source of pollution in the past decade. In 2019, India’s Supreme Court ordered a complete halt to the practice of stubble burning and reprimanded authorities in two of these states, Punjab and Haryana, for allowing this illegal practice to continue. Needed is the political will to act , as poor farmers complain that they receive no financial support to dispose of post-harvest stubble properly. Delhi’s “Green War Room” signaling the fight against the smog, is analyzing satellite data on farm fires from Punjab and Haryana to identify and deal with the culprits. The Indian Agricultural Research Institute has proposed a low-cost way to deal with the problem of stubble burning by spraying a chemical solution to decompose the crop residue and turn it into manure. Better coordination is needed. In 2013, when Singapore faced a record-breaking haze due to agricultural waste burning in neighboring countries, the Environment Agency and ministries of education and manpower together issued guidelines based on a Pollution Standards Index to minimize the health impacts of haze. Stubble burning has been banned or discouraged in China, the United Kingdom, and Australia.
Delhi, projected to be the world’s most populous city by 2030, is motivated by a sense of urgency. Facing a growing environmental and health calamity, antipollution efforts are being strengthened. But to succeed, the different levels of government must harness the political will to invest more, coordinate across boundaries, and motivate businesses and residents to do their bit.
Related Content
Massimiliano Cali, Nicola Cantore, Leonardo Iacovone, Mariana Pereira-Lopez, Giorgio Presidente, Niki Rodousakis
November 19, 2020
Amar Bhattacharya
November 17, 2020
Global Economy and Development
India South Asia
Caren Grown, Junjie Ren
August 19, 2024
Keon L. Gilbert, Calvin Bell
Manann Donoghoe, Andre M. Perry
July 17, 2024
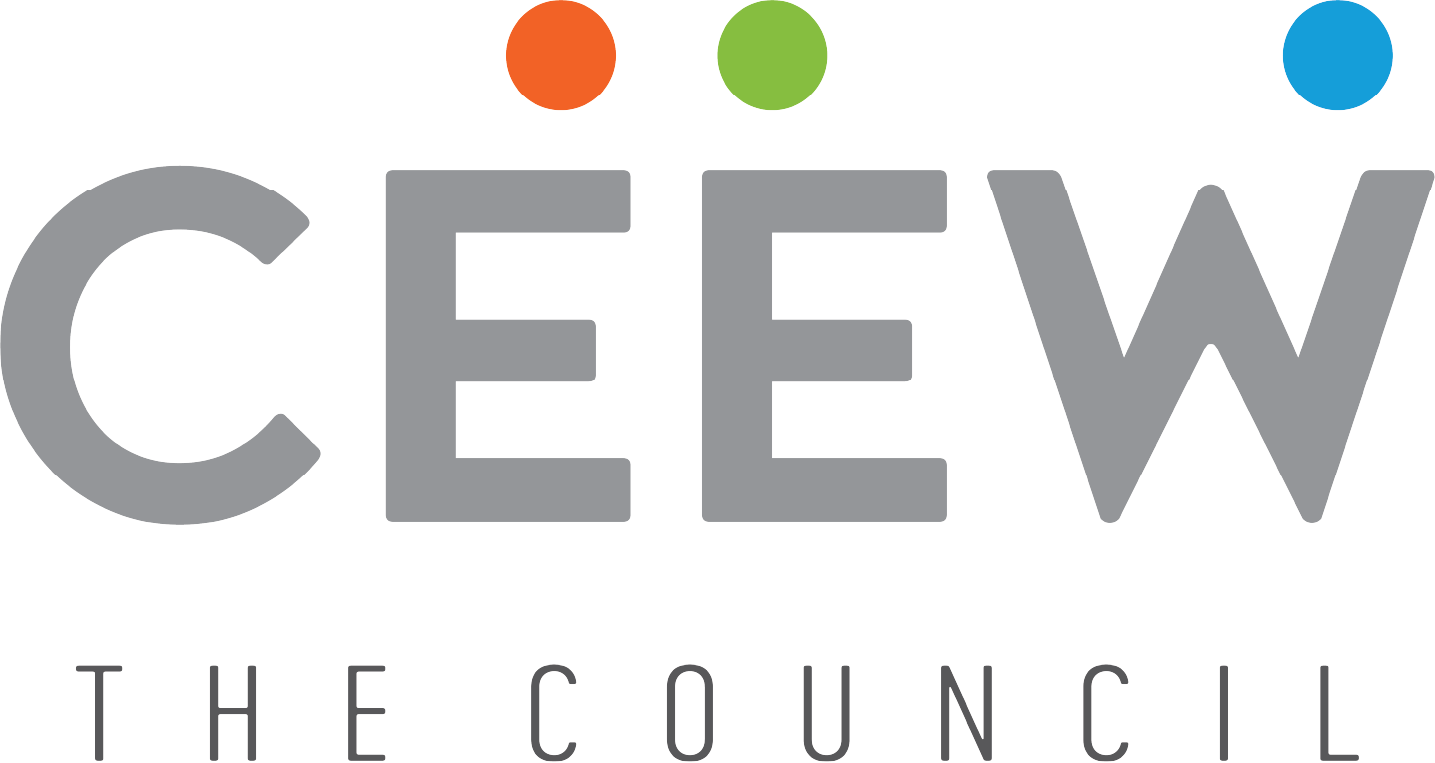
Suggested citation: Kurinji, L. S, Adeel Khan , and Tanushree Ganguly . 2021. Bending Delhi’s Pollution Curve: Learnings from 2020 to Improve 2021 . New Delhi: Council on Energy, Environment and Water.
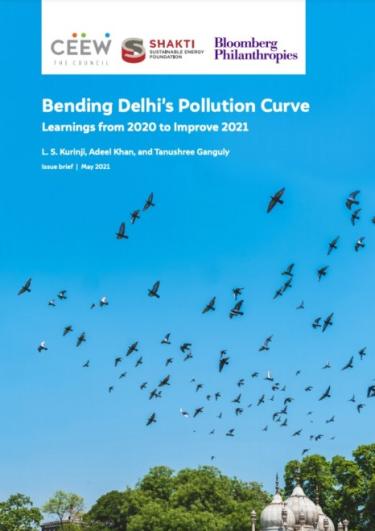
The study intends to support the Delhi government, the Central Pollution Control Board (CPCB), and the Delhi Pollution Control Committee (DPCC) in identifying priority areas of interventions needed in controlling air pollution in Delhi during the winter season of 2021. It establishes the primary drivers of Delhi's winter air pollution during the different phases of the season. It considers meteorological parameters, source activity levels, and its contributions to investigate how winter 2020 was, if at all, different in the pandemic circumstances compared to the previous years.
Key Highlights
- Delhi residents were exposed to air that does not meet the National Ambient Air Quality Standards (60 µg/m3) for more than half of 2020 despite low economic activity levels for close to eight months (March to November) due to the pandemic-induced lockdown.
- Delhi’s air quality in winter 2020 was worse than winter 2019 with 92 severe and very poor air quality days in the winter of 2020 compared to 80 such days in 2019.
- Relative contribution from farm fires was the highest (~30 per cent) between 15 October and 15 November 2020. In the following months, the contribution from household emissions (including domestic cooking, space heating, water heating, and lighting) primarily drove poor air quality in Delhi.
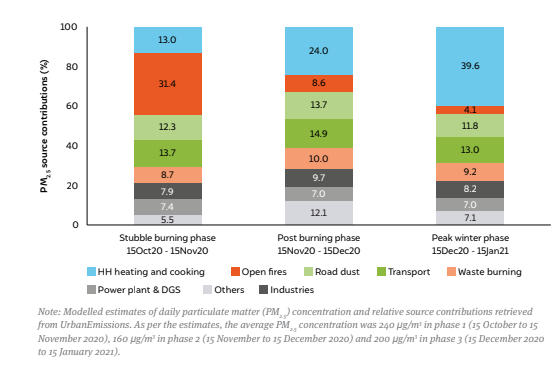
Source: Author’s analysis
- Calmer winds in October and November amplified the impact of farm fires on Delhi’s air quality. The stubble burning phase (15 October to 15 November) in 2020 experienced 172 hours (70 per cent higher) of calm and light winds (<5 km/h) compared to 101 hours in 2019.
- Activity levels were low at the start of the winter. But most activities, including vehicular traffic and power generation, bounced back to the previous year’s levels (proxied by indicators such as congestion and electricity generation levels) as the season progressed.
- Adverse meteorological conditions in Delhi intensified the impact of local and regional emissions on Delhi’s air quality. While meteorological conditions cannot be controlled, sustained air quality gains can be realised only by steeper emission cuts across sectors.
HAVE A QUERY?
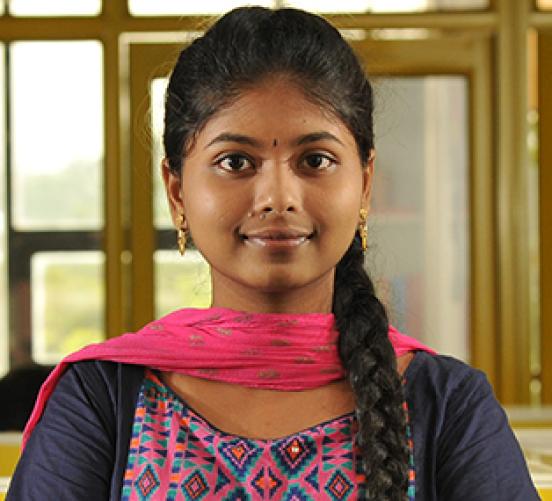
Executive Summary
Delhi is among the most polluted cities in the world (IQ Air 2021). In 2019 alone, air pollution caused over 17,000 premature deaths and an economic loss of 1,207 million USD in the national capital (Pandey et al. 2021). With regards to air quality, 2020 was an aberration. The pandemic-induced lockdown measures provided temporary respite from the year-round poor air quality.
Despite the lockdown, Delhi’s annual average PM 2.5 in 2020 was more than 2-times its permissible limit
Barring a few days in April and May, Delhi experienced National Ambient Air Quality Standard (NAAQS)1 compliant air quality on most days during the lockdown. Yet, the annual average PM 2.5 concentration in 2020 was 93 µg/m3, which is more than twice the permissible limit for PM 2.5 in India. Despite low activity levels for close to eight months (March to November) in 2020, Delhi residents were exposed to NAAQS non-compliant air for more than half of the year (Figure ES1).
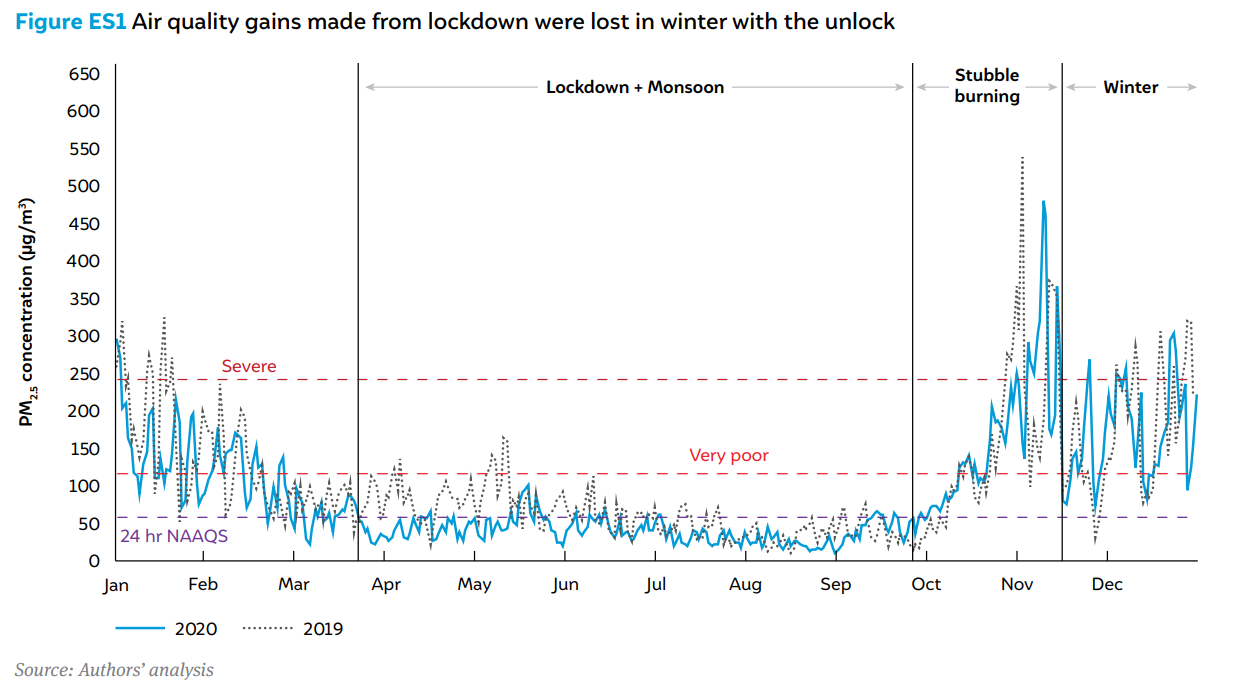
Winters saw poor quality despite proactive measures by the government
While the NAAQS non-compliant air quality in Delhi is not a new phenomenon, the winter of 2020 witnessed proactive measures from the State Government in the wake of COVID and evidence pointing at the association between high air pollution and COVID mortality (Petroni et al. 2020; Cole, Ozgen, and Strobl 2020; Wu et al. 2020). This includes the Yuddh Pradushan Ke Virudh (war against pollution) campaign and a seven-point action plan to combat air pollution in Delhi which listed measures ranging from combating dust and mitigating hotspots to a mobile application called Green Delhi for complaints and a ‘war room’ for monitoring air pollution control activities (PTI 2020a). Similar to 2019, the Graded Response Action Plan (GRAP), also came into force on 15th October 2020 and the Environmental Pollution Control Authority (EPCA)2 oversaw its implementation until the announcement of its dissolution on 28th October 2020 (Koshy 2020; EPCA 2020). However, despite these measures, the PM 2.5 levels remained almost three times higher than the NAAQS on an average between October 2020 and January 2021. We also observe that PM 2.5 levels in winter 2020 were higher than those in 2019. To explain this end, we analyse meteorological parameters, source activity levels, and contributions to establish primary drivers of pollution during different phases of the winter season. Through this brief, we intend to help the Delhi government, the Central Pollution Control Board (CPCB) and the Delhi Pollution Control Committee (DPCC) to identify priority areas of intervention for the year 2021. We summarise key highlights as follows
Air quality in winter 2020 was worse than winter 2019
Delhi observed 92 severe and very poor air quality days in the winter of 2020 compared to 80 such days in 2019. Compared to an average PM 2.5 concentration of 161 μg/m3 in 2019, between October and November 2020, this value was 172 μg/m3. It further shot up to an average level of 192 μg/m3 in the period between December 2020 to January 2021 compared to 178 μg/m3 during the same period previous year.
Contributions from stubble burning and household emissions from cooking and space heating were significant fractions of the pollution pie
Modelled source contribution estimates of particulate matter (PM 2.5 ) by UrbanEmissions suggest that relative contribution from farm fires was the highest (~30 per cent) in the period between 15 October and 15 November 2020 (Figure ES2). We find that compared to the stubble burning period in 2019, a longer harvesting season in 2020 led to a significant increase in the number of fires. In the following months, contribution from household emissions (including domestic cooking, space heating, water heating, and lighting) primarily drove poor air quality in Delhi. It is worth highlighting that these values are modelled estimates and are subject to the sector-specific assumptions used in the model.
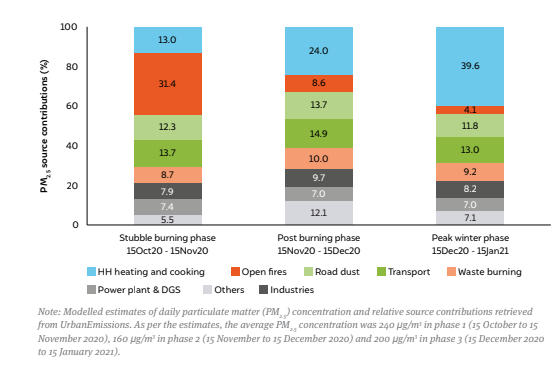
Calmer winds in October and November amplified the impact of farm fires on Delhi’s air quality
The stubble burning phase (15 October to 15 November) in 2020 experienced 172 hours (70 per cent higher) of calm and light winds (<5 km/h) compared to 101 hours in 2019. Winds predominantly from the north-west direction facilitated the transport of smoke emanating from farm fires and calm winds in Delhi further intensified its adverse impact on air quality. Interestingly, for brief periods in the season, even when high fire counts were reported in Punjab and Haryana, Delhi’s air quality was not affected due to favourable meteorological conditions (Easterly and southerly winds) (Figure ES3). Unfavourable meteorological conditions include low wind speeds and shallow mixing height height3 .
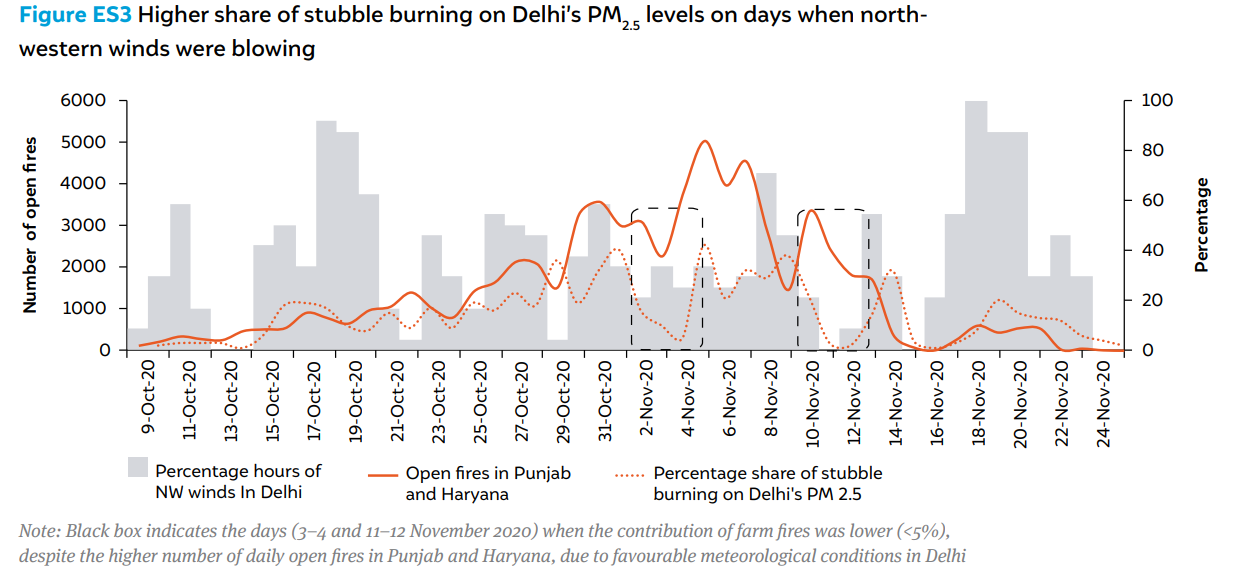
Lowered activity levels at the start of winter due to lockdown bounced back to the previous year’s levels as the winter progressed
While Delhi’s average PM 2.5 concentration during the stubble burning period (October’20 and November’20) was 172 µg/m3, it increased to 192 µg/m3 during peak winter (December’20 and January’21). The higher PM 2.5 levels in December 2020 and January 2021 were primarily caused by locally emitted pollutants and added burden of household emissions from space heating. Activity levels were low at the start of the season, but most activities, including vehicular traffic and power generation, bounced back to the previous year’s levels (proxied by indicators such as congestion and electricity generation levels in Figures ES4 and ES5) as the season progressed.
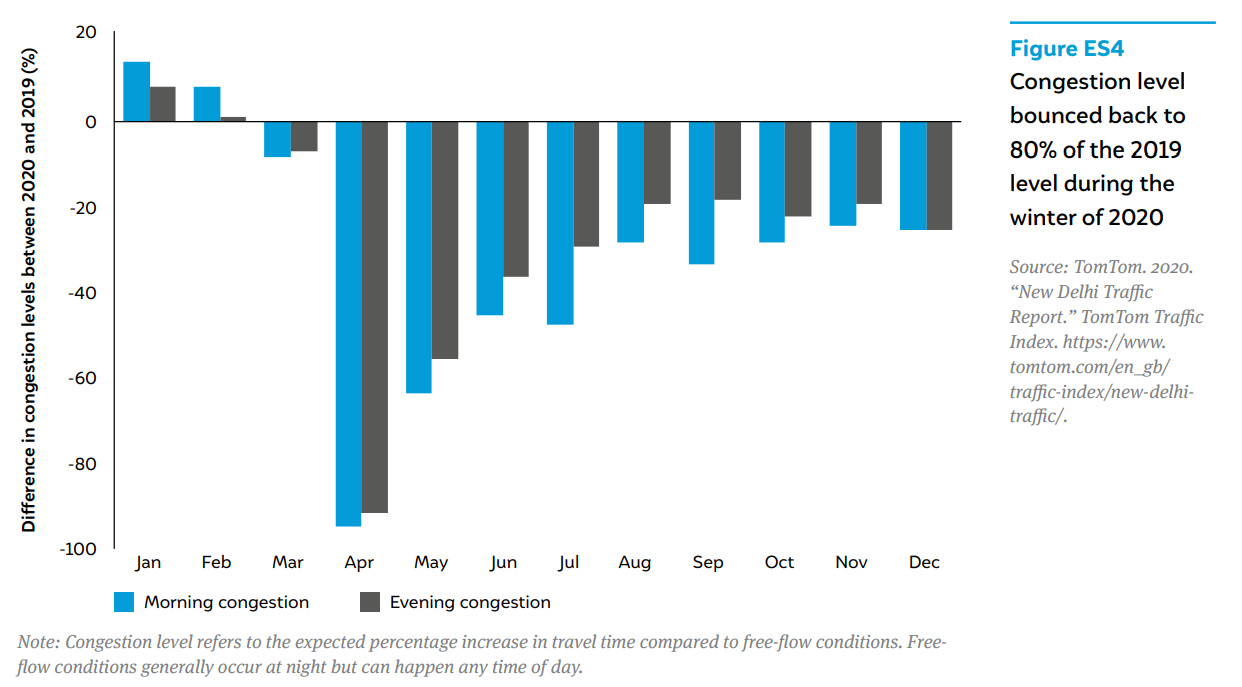
Delhi needs a dedicated air quality forecasting cell to facilitate roll out of preventive measures
Delhi needs a dedicated air quality forecasting cell to facilitate the rollout of preventive measures.
“I see skies so blue and clouds so white … What a wonderful world,” wrote Bob Thiele and George David Weiss in 1967. I t took a pandemic and complete shutdown of activities for blue skies and white clouds to appear in Delhi. This respite from year-round air pollution was short-lived and the gains from cessation of activities were lost with the unlock of economic activities and the arrival of winter in Delhi. Despite the reduced activity levels for close to eight months (March to November) in 2020, Delhi residents were exposed to NAAQS non-compliant air for more than half of the year. Our analysis compared the anthropogenic activity levels and meteorological conditions in 2020 with those in 2019. We also explain how these factors influenced air quality in the winter of 2020. We find that air quality in the winter of 2020 was worse than in the winter of 2019. Lower vehicular congestion and power generation levels in October and November 2020 are indicative of reduced emissions from these two activities. A relatively longer stubble burning period, colder and drier winter conditions, and calmer winds in October and November 2020 were primarily responsible for the worsening Delhi’s air quality that year. As the winter season progressed, most anthropogenic activities such as power generation and vehicular levels bounced back to previous year’s levels. Household heating and cooking contributed to a significant share (40 per cent) to the pollution burden in December 2020 and January 2021. We stress that the interplay of meteorological conditions on Delhi’s air quality cannot be discounted, but there is need for steeper cuts in emissions across sectors. The GRAP presents the state government with an opportunity to constitute an air quality forecasting cell that can advise the government to take necessary measures to prevent severe air quality episodes in the capital city. We recommend that in addition to supporting source identification studies, the government should also encourage air quality modelling and forecasting efforts. Augmenting the existing monitoring infrastructure would help air quality modellers validate their forecasts. The state government and the city administration could also work collaboratively with the modellers in developing necessary databases to track emissions from local anthropogenic sources.
Sign up for the latest on our pioneering research
- News, Stories & Speeches
- Get Involved
- Structure and leadership
- Committee of Permanent Representatives
- UN Environment Assembly
- Funding and partnerships
- Policies and strategies
- Evaluation Office
- Secretariats and Conventions
- Asia and the Pacific
- Latin America and the Caribbean
- New York Office
- North America
- Climate action
- Nature action
- Chemicals and pollution action
- Digital Transformations
- Disasters and conflicts
- Environment under review
- Environmental law and governance
- Extractives
- Fresh Water
- Green economy
- Ocean, seas and coasts
- Resource efficiency
- Sustainable Development Goals
- Youth, education and environment
- Publications & data
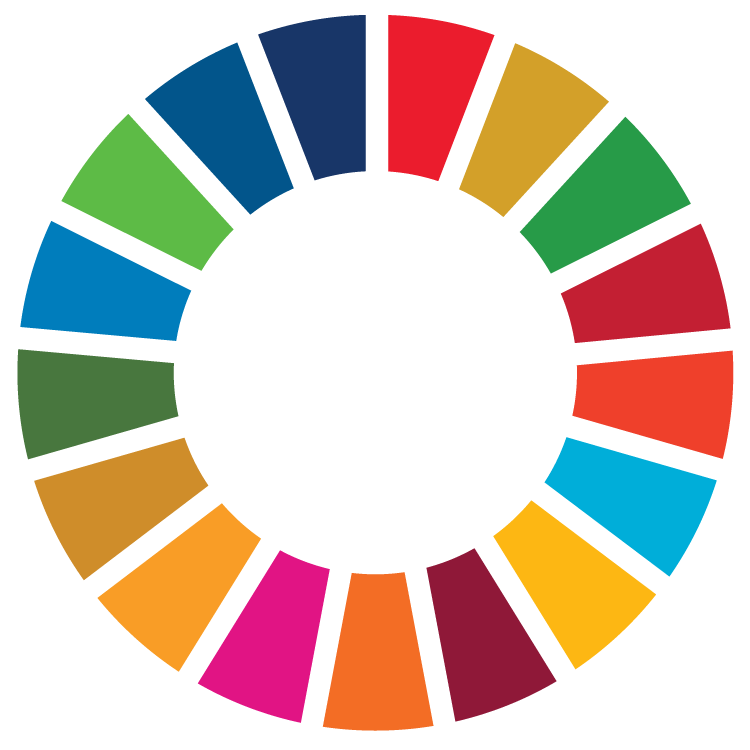
A vision to tackle Delhi’s dirty air
When thirty-eight-year-old Jasmine Shah moved to Delhi in 2013 and found himself engulfed in the city’s toxic winter smog, everything changed for him.
“This scale of air pollution in Delhi, and the fact that it is among the top ten most polluted cities in the world, resulted in my urgent call to tackle the issue,” said Shah.
Targeting public transport seemed like the most logical way to start, with potential for bigger impact than tackling individuals, and Shah began by working with the government, creating momentum for policy change and more electric buses.
From UN Environment, Valentin Foltescu, Senior Programme and Science Officer of the Climate & Clean Air Coalition, added:
“If current policies aimed at reducing air pollution are effectively enforced, air quality will be no worse in 2030 than now, despite population growth, rapid urbanization and an ever-increasing demand for goods and services, but neither will air quality be better.
“What do we need then to make the air breathable amid rapid economic development? We must move quickly to low and zero emissions solutions and comprehensive transformations. We should aim for full electrification of sectors in combination with clean energy.”
Today, as Vice Chairperson of the Dialogue and Development Commission of Delhi, an internal think tank of the Delhi government, we asked Shah what advice he has for young people interested in working alongside government towards effective policy change.

What inspired you to tackle air pollution?
A couple of winters in Delhi brought me face to face with the toxic smog that engulfs Delhi during this period. I realized that we needed more long-term measures to tackle air pollution since what we have is a health emergency in India. Particulate matter—hazardous pollution particles suspended in the air—is highly concentrated in most Indian cities and nearly 10–15 times the World Health Organization’s safety limits. And unfortunately, the problem disproportionately affects children and the elderly. Something had to be done.
What inspired you to get involved in the transport industry specifically?
The city of Delhi is well devoted to tackling air pollution and the government had already prioritized this issue on their agenda. In 2016, Delhi became the first city in India to launch a very successful Odd-Even scheme as an emergency measure to restrict the movement of private vehicles on polluted days. But since the transport sector is a top contributor to particulate emissions, I was motivated to look for long-term solutions. In February 2018, I advised the Delhi government in preparation of a comprehensive Green Budget—a 26-point long-term action plan to tackle air pollution in Delhi, with a major focus on the transport sector. Two of the most important initiatives in this were the introduction of 1,000 electric buses in Delhi and a comprehensive electric vehicle policy.
What challenges have you faced in introducing electric buses to Delhi?
With the political will already in place, the challenges we faced in Delhi were largely infrastructural and operational. We had to undertake a detailed study of the city and its transport networks before planning the electric bus routes. We undertook site visits to China to learn from best practices, since no other city outside of China has committed to bringing such a large number of electric buses. I am happy to say that we have already initiated procurement of 385 e-buses for Delhi, which should arrive by November. By 2020, we aim to bring in 1,000 e-buses.
What is your advice to youth interested to inspire change through policy action?
I encourage all young people to be persistent, creative and innovative. We have the know-how to beat air pollution. Build networks and connect with relevant people whose vision aligns with yours, and together we can combat air pollution. I believe this is achievable but requires patience and hard work.
What is your hope for the future in terms of tackling air pollution?
Air pollution is a complex problem. There are various sources of pollutants—dust, transport, industrial emissions. A comprehensive set of strategies is required to tackle the problem, and our vision for the future in Delhi is to work on all of these. In the transport sector, we are taking many steps to work towards a zero-carbon city and to ensure 25 per cent of all new vehicles in Delhi are electric by 2024. We are developing more pedestrian walkways and cycle lanes in the city to encourage walking and cycling. My vision for the future of India is to see the political leadership at national level fully acknowledge air pollution as a health emergency and act upon it. There are many solutions to tackle air pollution but we need political commitment and specific budgets and action plans to address this rising health issue.
Air pollution is the theme for World Environment Day on 5 June 2019 hosted by China. The quality of the air we breathe depends on the lifestyle choices we make every day. Learn more about how air pollution affects you, and what is being done to clean the air. What are you doing to reduce your emissions footprint and #BeatAirPollution?
The 2019 World Environment Day is hosted by China.
- Air quality
- Clean fuels
- Young Champions
Related Content

Related Sustainable Development Goals

© 2024 UNEP Terms of Use Privacy Report Project Concern Report Scam Contact Us
- Current Issue
- Election 2024
- Arts & Culture
- Social Issues
- Science & Technology
- Environment
- World Affairs
- Data Stories
- Photo Essay
- Newsletter Sign-up
- Print Subscription
- Digital Subscription
- Digital Exclusive Stories

- CONNECT WITH US

India’s Air-pocalypse: Understanding the air pollution crisis in Delhi and beyond
A toxic spectre is haunting the nation. how shall we tackle it.
Published : Nov 06, 2023 11:55 IST - 2 MINS READ

Students wear masks amid dense smog near the India Gate in New Dellhi on November 4, 2023. | Photo Credit: PTI
As of November 6, the air quality in Delhi remains critically poor, according to the System of Air Quality and Weather Forecasting and Research (SAFAR)-India. Reports suggest that this situation is expected to deteriorate further. Pollution levels in and around Delhi have consistently been in the “critical” category, with air quality index (AQI) readings in the range of 400 at various locations for the past few days. On the morning of November 6, SAFAR recorded an overall average AQI of 471. In response, the Delhi government has implemented a “work from home” policy for 50 per cent of government employees as part of their pollution control plan.
Data from the Central Pollution Control Board reveals that Delhi’s AQI has worsened by more than 200 points since October 27. The most severe air quality was reported on November 3, surpassing the previous high of 471 recorded on November 12, 2021. There has been some improvement due to better wind speed, but a dense and toxic haze still blankets the national capital for the sixth consecutive day.
Unfortunately, the issue of air pollution is not a new one and is not limited to Delhi or the National Capital Region (NCR). Several cities in India have been grappling with air pollution for decades, with little substantial action taken beyond political and policy discussions.
In the case of Delhi, there have been numerous studies, reports, papers, seminars, monographs, speeches, and explanations on the subject. A recent study has identified Delhi as the most polluted city in the world, with residents potentially losing a significant portion of their lifespan due to pollution. The study, called the Air Quality Life Index (AQLI), was published in August 2023 by the Energy Policy Institute at the University of Chicago. It also indicated that the entire Indian population of 1.3 billion people lives in areas with an annual average particulate pollution level exceeding the WHO’s limit of 5 μg/m3.
Still, nothing much has been done to check air pollution and the issue continues to persist like a haunting spectre in India. In this context, we have selected a collection of insightful stories from our archives to help you better understand the air pollution issue and make informed decisions regarding your use of fossil fuels. Please feel free to share your comments with us at [email protected].

Delhi's air pollution: Taming a killer

An odd (-even) formula

Season of smog: Not just Delhi, many north Indian cities are suffering

Breathing space for the capital

A scripted performance in Chandigarh puts stubble burning in focus

Mumbai in a haze: Pollution hits alarming levels
Maharashtra has the second-highest number of air pollution–related deaths in the country, air pollution can affect bone density.

Greenpeace study claims that Visakhapatnam and Hyderabad air most toxic in south India

India ranks at the bottom in a list of 180 countries in the 2022 Environmental Performance Index
India in search of clean air, global call to tackle air pollution.

India is haunted by an unprecedented economic deprivation
Editor’s note: will india vote for what really matters.
- Bookmark stories to read later.
- Comment on stories to start conversations.
- Subscribe to our newsletters.
- Get notified about discounts and offers to our products.
Terms & conditions | Institutional Subscriber

Comments have to be in English, and in full sentences. They cannot be abusive or personal. Please abide to our community guidelines for posting your comment
Thank you for visiting nature.com. You are using a browser version with limited support for CSS. To obtain the best experience, we recommend you use a more up to date browser (or turn off compatibility mode in Internet Explorer). In the meantime, to ensure continued support, we are displaying the site without styles and JavaScript.
- View all journals
- Explore content
- About the journal
- Publish with us
- Sign up for alerts
- Open access
- Published: 02 September 2024
Transforming air pollution management in India with AI and machine learning technologies
- Kuldeep Singh Rautela 1 &
- Manish Kumar Goyal 1
Scientific Reports volume 14 , Article number: 20412 ( 2024 ) Cite this article
54 Accesses
3 Altmetric
Metrics details
- Engineering
- Environmental chemistry
A comprehensive approach is essential in India's ongoing battle against air pollution, combining technological advancements, regulatory reinforcement, and widespread societal engagement. Bridging technological gaps involves deploying sophisticated pollution control technologies and addressing the rural–urban disparity through innovative solutions. The review found that integrating Artificial Intelligence and Machine Learning (AI&ML) in air quality forecasting demonstrates promising results with a remarkable model efficiency. In this study, initially, we compute the PM 2.5 concentration over India using a surface mass concentration of 5 key aerosols such as black carbon (BC), dust (DU), organic carbon (OC), sea salt (SS) and sulphates (SU), respectively. The study identifies several regions highly vulnerable to PM 2.5 pollution due to specific sources. The Indo-Gangetic Plains are notably impacted by high concentrations of BC, OC, and SU resulting from anthropogenic activities. Western India experiences higher DU concentrations due to its proximity to the Sahara Desert. Additionally, certain areas in northeast India show significant contributions of OC from biogenic activities. Moreover, an AI&ML model based on convolutional autoencoder architecture underwent rigorous training, testing, and validation to forecast PM 2.5 concentrations across India. The results reveal its exceptional precision in PM 2.5 prediction, as demonstrated by model evaluation metrics, including a Structural Similarity Index exceeding 0.60, Peak Signal-to-Noise Ratio ranging from 28–30 dB and Mean Square Error below 10 μg/m 3 . However, regulatory challenges persist, necessitating robust frameworks and consistent enforcement mechanisms, as evidenced by the complexities in predicting PM 2.5 concentrations. Implementing tailored regional pollution control strategies, integrating AI&ML technologies, strengthening regulatory frameworks, promoting sustainable practices, and encouraging international collaboration are essential policy measures to mitigate air pollution in India.
Similar content being viewed by others
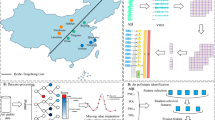
Optimized air quality management based on air quality index prediction and air pollutants identification in representative cities in China
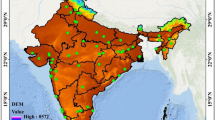
Machine learning-based country-level annual air pollutants exploration using Sentinel-5P and Google Earth Engine
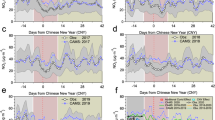
Disentangling drivers of air pollutant and health risk changes during the COVID-19 lockdown in China
Introduction.
Air pollution has emerged as a critical global environmental health issue, with 92% of the world's population exposed to pollutant levels exceeding air quality guidelines 1 , 2 . This widespread exposure poses significant health risks, including increased incidence of respiratory diseases, cardiovascular problems, and premature mortality 3 , 4 . In India specifically, ambient particulate matter (PM) exposure has been linked to an estimated 1.1 million premature deaths annually, with air pollution becoming the fourth leading cause of mortality nationwide 5 , 6 . The economic impact is also substantial, with the World Bank estimating that air pollution costs India 3–8% of its GDP due to healthcare expenses, reduced productivity, and premature deaths 7 .
Atmospheric aerosols, particularly black carbon, organic carbon, dust, sea salt, and sulfates, have been extensively researched in South and Southeast Asia over the past two decades 8 . However, the magnitude of these impacts is largely influenced by spatio-temporal variability and the composition of these aerosols 9 . Aerosols, including, are significant constituents of atmospheric PM and account for approximately 30–70% of the fine aerosol mass over urban areas in India 5 , 10 . However, in recent decades, this concern has increased notably, primarily attributed to the rapid surge in population, unplanned urban development, and the expansion of industries 11 , 12 . India, home to the world's largest population share at 17.76%, faces a significant environmental challenge, with many of its cities (eg; Delhi, Mumbai, Kolkata) ranking among the most polluted on the global scale 13 , 14 . An investigation based on World Health Organization (WHO) data from 2008–2013 brought attention to India's status among the most polluted nations 15 . India has faced alarming and extensive air pollution incidents in the last twenty years, prompting substantial concern among regulatory authorities. The Indo-Gangetic Plain (IGP) is highly susceptible to severe pollution incidents, notably prevalent in the post-monsoon and winter period 16 . Similarly, in many metropolitan cities across India, such as Delhi, air quality has deteriorated to hazardous levels. Concentrations of particulate matter (PM 2.5 and PM 10 ) have surged beyond 500 µg/m 3 , while nitrogen oxides (NO 2 ) have exceeded 10 µg/m 3 . Additionally, ozone (O 3 ) and sulfur dioxide (SO 2 ) levels have surpassed 5 µg/m 3 , alongside other pollutants 17 . The concentration of these pollutants, often surpassing 500 µg/m 3 , far exceeds WHO's safe annual limit of 10 µg/m 3 and India’s national ambient air quality standards (NAAQS) of 40 µg/m 3 during winters 18 . According to the Economic Times, 12.25 million vehicles are registered in Delhi, growing at a rate of 7% per annum, and they account for 67% of the total pollution 19 , 20 . Additionally, Coal-based thermal power plants and small-scale industries each contribute 12% to the pollution, including emissions from various industrial units followed by the agricultural and biomass burning in Delhi and surrounding areas 20 . This increased pollution level has raised considerable concern among authorities and stakeholders, prompting focused efforts towards addressing this critical issue 9 . The urgency of addressing air pollution in India is evident through compelling data illustrating its significant impact across various sectors.
AI & ML have become pivotal in addressing air pollution by harnessing big data analytics, utilising advanced computing systems, scalable storage, and parallel processing technologies 21 , 22 , 23 . These innovations enable comprehensive management and mitigation strategies for various air pollutants, bridging the gap between atmospheric and climate sciences through sophisticated data-driven approaches. Previous studies have proposed various AI&ML-based models as pivotal components for air pollution and aerosol transport 5 , 8 , 24 , 25 , 26 . Initially, researchers have introduced succinct and efficient statistical models for practical applications. These statistical models primarily encompass multiple linear regression (MLR) 27 and autoregression moving average (ARMA) 28 methods. The predominant use of linear hypotheses in developing statistical models contrasts with the inherent nonlinear properties exhibited by pollutant concentrations. Consequently, researchers have advocated for integrating data mining methods 29 and machine learning models 30 , 31 , 32 designed to accommodate nonlinear predictions in studying air pollutants. However, the notably nonlinear and non-stationary nature of pollutants poses challenges for achieving high prediction accuracy with these models. As a result, several studies have turned to various deep learning techniques 8 , 33 , 34 , 35 , 36 to enhance the prediction of air pollutant levels.
Despite numerous efforts to forecast concentration of major pollutants, comprehending the complex relationship among diverse influencing factors remains a persistently challenging task. Studies exploring the relevance of these factors in predicting pollutants have been scarce and constrained in scope 37 , 38 . Typically, researchers tend to utilize all accessible features and input them into prediction models. While it holds true that AI&ML models exhibit superior performance in scenarios with abundant data availability, the effectiveness of these models in pollutant prediction hinges on understanding and incorporating the most influential factors. Figure 1 illustrates the comprehensive AI/ML model development workflow for environmental or traffic-related predictions. The process includes data collection across various domains, preprocessing, algorithm selection, model development, training, testing, and validation. The process completes with prediction, incorporating a feedback loop for model refinement if needed, ensuring adaptability and continuous improvement in predictive accuracy.
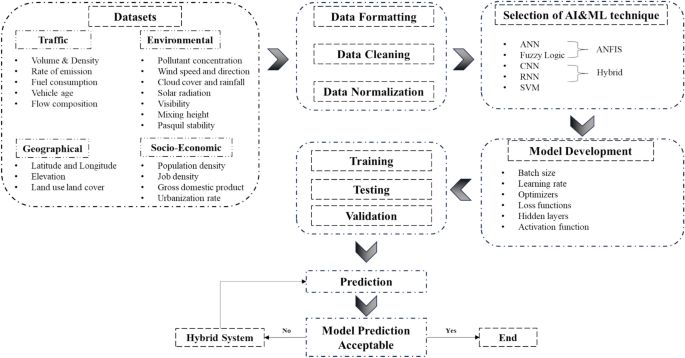
Charting the sequential steps of AI and ML involvement in predicting air pollution concentrations.
Previous studies have conducted comparative analyses between AI&ML-based methodologies for forecasting concentrations of various pollutants. Initially, Mc Kendry 39 evaluated Artificial Neural Networks (ANN) with MLR for simulating the concentrations of PM 2.5 and PM 10 . Similarly, Dutta and Jinsart 40 compared the performances of decision tree and ANN algorithms in estimating PM 10 concentrations. Other comparisons include Turias et al. 41 pitting back-propagation based ANN against ARIMA for predicting the Sulfur Dioxide (SO 2 ), concentrations of Carbon Monoxide (CO) and Suspended Particulate Matter (SPM), over an industrialized region. Shang and He 42 formulated an innovative prediction method by coupling of ANN and Random forest (RF) to forecast hourly PM 2.5 concentrations. Bozdağ et al. 43 presented a comprehensive analysis for the simulation of PM 10 concentrations by comparing various modelling approaches—ANN, KNN (K-Nearest Neighbour Algorithm), SVM (Support Vector Machine) , LASSO (Least Absolute Shrinkage and Selection Operator), RF, and xGBoost.
This study systematically explores the consequences of severe air pollution in India, focusing on contributors like PM, Organic Aerosols (OAs), BC, Water-Soluble Brown Carbon (WS-BrC), and Volatile Organic Compounds (VOCs). Remediation techniques, including legislation, NAAQS, and an Air Quality Index (AQI), are inspected alongside the evolution of emission load studies and management strategies. Additionally, the study investigates the integration of AI&ML in mitigating and predicting air pollution. It details the application of AI&ML models and underscores the potential of deep learning algorithms, exemplified through a case study predicting PM 2.5 concentrations over India. Identifying challenges like technological barriers, regulatory hurdles, public awareness gaps, agricultural practices, urbanization impacts, cross-border pollution, climate change interlinkages, and socio-economic disparities, the study emphasizes the urgency of comprehensive solutions. Looking forward, the study discusses prospects involving emerging technologies and global collaborations. The study emphasizes the imperative to address air pollution in India holistically, leveraging AI&ML advancements, global cooperation, and technological innovations to formulate effective strategies for combatting the multifaceted challenges posed by air pollution in the region.
Results and discussion
Consequences of air pollution in india.
Air pollution in India specially in metropolitan cities has dire consequences for public health, stemming from increased levels of particulate matter, nitrogen oxides, and various pollutants. This increase pollution level is consistently linked to increased respiratory diseases, particularly asthma, chronic obstructive pulmonary disease (COPD), and bronchitis 7 , 44 . Children, with developing respiratory systems, are particularly vulnerable to irreversible health issues upon prolonged exposure, while the elderly, with compromised immune systems, face pre-eminent risks, including deep lung penetration, inflammation, and enduring damage caused by PM 2.5 . Beyond respiratory implications, air pollution has severe cardiovascular consequences, with nitrogen oxides significantly contributing to an increased risk of heart attacks and strokes, leading to heightened cardiovascular mortality with prolonged exposur 7 . The significant study conducted by the CPCB in Delhi highlighted robust correlations between air quality levels and negative health effects. Comparative analysis against a rural control population in West Bengal indicated a 1.7-fold higher occurrence of respiratory symptoms in Delhi, emphasizing the direct impact of air quality on public health 20 , 45 , 46 , 47 . Odds ratios for upper and lower respiratory symptoms were 1.59 and 1.67, respectively, emphasizing the profound impact of air pollution. The study also highlighted a significantly higher prevalence of current and physician-diagnosed asthma in Delhi, with lung function notably reduced in 40.3% of Delhi's participants compared to 20.1% in the control group 20 .
In addition to respiratory effects, non-respiratory impacts were observed in the cities as compared to rural controls. The prevalence of hypertension was notably higher in cities (36% vs. 9.5% in controls), correlating positively with respirable suspended particulate matter (PM 10 ) levels in ambient air 48 . Chronic headaches, eye irritation, and skin irritation were significantly more pronounced in most of the cities. Community-based studies consistently affirm the association between air pollution and respiratory morbidity. Studies focusing on indoor air pollution reveal similar correlations with respiratory morbidity, extending to conditions such as attention-deficit hyperactivity disorder in children, increased blood levels of lead, and decreased serum concentration of vitamin D metabolites 49 . Beyond health impacts, the environmental consequences of air pollution are profound. Pollutants harm plants and animals, disrupt ecosystems, and lead to biodiversity loss 50 . The issue extends beyond health and the environment, impacting economics and society, straining healthcare, productivity, and social equity, demanding holistic strategies spanning economic, social, and environmental facets making it imperative, in this crisis, to understand the existing and potential remediation techniques 51 .
The economic and social ramifications are substantial, with healthcare costs soaring as the incidence of pollution-related illnesses rises 7 . Treating respiratory and cardiovascular diseases places a significant burden on the healthcare system, affecting both public and private healthcare expenditures 44 . Air pollution in India incurred an estimated economic toll of $95 billion in 2019, amounting to 3% of the country's GDP, attributable to decreased productivity, increased work absences, and premature fatalities 52 . The economic implications of air pollution extend beyond direct healthcare costs, affecting labor markets and overall productivity 53 . Social disparities are accentuated by air pollution, with vulnerable communities facing disproportionate exposure to pollutants. Factors such as socio-economic status, access to healthcare, and geographic location contribute to disparities in exposure and health outcomes 54 . Addressing these social dimensions is crucial for devising equitable solutions that prioritize environmental justice. As India grapples with the immediate consequences of air pollution, emerging challenges require attention. Also, climate change exacerbates existing issues, influencing weather patterns and contributing to the persistence of stagnant air masses that trap pollutants and their transportation mechanism 8 . The increasing frequency of extreme weather events further complicates pollution dynamics 55 . Moreover, the complex interplay of indoor and outdoor air pollution adds another layer of complexity, with indoor air pollution often stemming from household activities such as cooking with solid fuels, compounding the overall burden on public health 49 . However, government policies and initiatives take center stage in this exploration, with regulatory measures, such as emission standards and vehicle restrictions, scrutinized for their effectiveness and implementation challenges 12 . Sustainable urban planning, including the creation of green spaces and transportation planning for pollution reduction, is examined as a proactive approach to mitigate pollution at its source 56 . Technological solutions, ranging from air purifiers to pollution monitoring devices, are also evaluated 57 . The challenges of scalability, accessibility, and integration into existing infrastructure are dissected to discern the practicality and potential impact of these technologies. Emerging technologies and global collaborations are explored as potential catalysts for change 57 , 58 .
Contributors to air pollution in India
Air pollution in India is a complex issue with multiple sources and contributors, as highlighted by various studies conducted by Lalchandani et al. 59 , Tobler et al. 60 , Rai et al. 61 , Talukdar et al. 62 and Wang et al. 63 . The sources and contributors to air pollution can be broadly categorized into particulate matter (PM 2.5 and PM 10 ), organic aerosols (OAs) including black carbon (BC), water-soluble brown carbon (WS-BrC), and volatile organic compounds (VOCs). Each of these components plays a signifsicant role in the overall air quality of the region.
Particulate matter (PM)
Particulate matter is a key component of air pollution, and Lalchandani et al. 59 conducted studies using the Positive Matrix Factorization (PMF) model to identify and apportion different sources of PM. The sources identified included traffic-related emissions, dust transportation, solid-fuel burning emissions, and secondary factors 62 , 64 . Traffic-related emissions in metropolitan cities were found to be the significant contributor to the total concentration of PM, for example, at the IIT Delhi site, emphasizing the impact of vehicular activities on air quality. Additionally, solid fuel burning emissions, often associated with residential cooking and heating, were identified as a major contributor to PM, particularly at night 62 . Rai et al. 61 conducted source apportionment of elements in PM 10 and PM 2.5 , identifying nine source profiles/factors, including dust, non-exhaust sources, solid fuel combustion, and industrial/combustion aerosol plume events. The contribution of anthropogenic sources to elements associated with health risks, such as carcinogenic elements. The geographical origins of these sources were also determined, emphasizing the regional and local influences on element concentrations in atmosphere 65 .
Organic aerosols (OAs)
Organic aerosols are another crucial component of air pollution, and studies by Tobler et al. 60 and Lalchandani et al. 62 revealed three main components of OAs: solid fuel combustion OAs (SFC OAs), hydrocarbon-like OA (HOAs) from vehicular emissions, and oxygenated OAs (OOAs). Lalchandani et al. 65 further categorized these components into sub-factors, providing a detailed understanding of the OA composition. Emissions stemming from traffic emerged as the primary contributor to the overall OA mass, underscoring the profound influence of vehicular pollution 59 .
Black carbon (BC)
BC, a product of incomplete combustion, was studied by Using the Absorption Ångström Exponent (AAE) method, contributions from biomass burning and vehicular emissions were apportioned 66 . Vehicular emissions were found to be a dominant source of BC, contributing around 67.5% 62 , 67 . The distinction between BC and brown carbon (BrC), which absorbs light in the near-UV to visible region, was also discussed, highlighting the need to consider multiple light-absorbing aerosols in air quality assessments.
Water-soluble brown carbon (WS-BrC)
Rastogi et al. 68 performed a PMF analysis of WS-BrC spectra, identifying six factors representing specific sources of BrC. The study revealed diurnal variability in BrC absorption, with factors associated with different emission sources. The presence of secondary BrC was indicated, suggesting the importance of atmospheric processes in the formation of brown carbon. This finding adds another layer of complexity to the sources of light-absorbing aerosols in the atmosphere 69 .
Volatile organic compounds (VOCs)
Wang et al. 63 investigated the characteristics and sources of VOCs, identifying six factors related to traffic, solid fuel combustion, and secondary sources. Traffic-related emissions were found to be the dominant source of VOCs at the urban site, while at the suburban site (MRIIRS), contributions from secondary formation and solid fuel combustion were more significant. The study highlighted the major role of anthropogenic sources in VOC pollution 70 .
Current remediation techniques
India has faced escalating challenges in managing air pollution over the years, necessitating the implementation of diverse remediation techniques. Figure 2 illustrates the legislative evolution of air quality management in India across three eras: Pre-Internet (1905–89), Transition (1990–99), and Internet Era (2000 onwards). This timeline showcases key acts and regulations implemented over time to address air pollution. The bottom timeline highlights the progression of NAAQS in India, from monitoring just 3 pollutants in 1982 to 7 in 1994, and 12 in 2009. The latest phase (2019–24) involves a comprehensive review of air quality standards under the National Clean Air Programme (NCAP) in 2019, demonstrating India's ongoing commitment to improving air quality management.
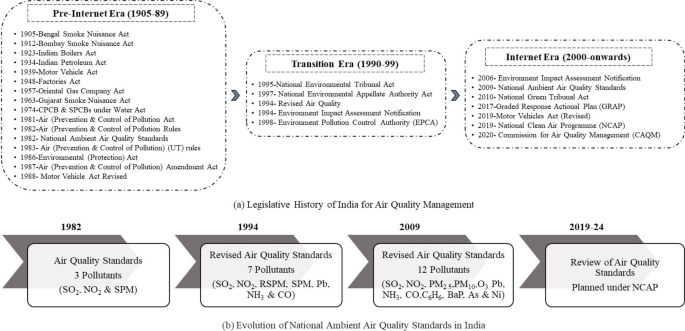
Legalisation and Evaluation of NAAQS in India 12 .
Legislation and regulatory measures
India's legislative landscape has evolved significantly to address air pollution. The introduction of key acts such as the Air (Prevention and Control of Air Pollution) Act in 1981 and subsequent amendments empowered central and state pollution control boards to handle severe air pollution emergencies 71 . The Environment (Protection) Act of 1986 served as an umbrella act for environmental protection, while the Motor Vehicles Act has been periodically amended to regulate vehicular pollution 72 . Recent developments include the Motor Vehicles (Amendment) Bill of 2019, allowing the government to recall vehicles causing environmental harm 73 . The establishment of institutions like the National Green Tribunal (NGT) and the National Environment Tribunal reflects a commitment to environmental accountability 74 .
National ambient air quality standards (NAAQS) and air quality index (AQI)
The formulation and periodic revision of National Ambient Air Quality Standards (NAAQS) have been pivotal in regulating air quality 18 . Beginning in 1982, the Central Pollution Control Board (CPCB) introduced NAAQS, initially covering SO 2 , NO 2 , and SPM 47 . Subsequent amendments expanded the list to include RSPM, Pb, NH 3 , and CO 75 . The National Air Quality Index (NAQI) was introduced to enhance public awareness, categorizing air quality into six levels from 'Good' to 'Severe' 76 . This index, based on the concentration of eight pollutants, guides interventions for improved air quality.
Air pollution monitoring network
India's air quality monitoring network has witnessed substantial growth. The initiation of the National Ambient Air Quality Monitoring (NAAQM) Network in 1984, expanded to the National Air Quality Monitoring Programme (NAMP), marked a critical step 77 . The network, comprising both manual and Continuous Ambient Air Quality Monitoring System (CAAQMS) stations, now stands at 1082 locations 78 , 79 . Real-time monitoring, as exemplified by CAAQMS, provides valuable data for prompt decision-making. The introduction of the System of Air Quality and Weather Forecasting and Research (SAFAR) further enhances forecasting capabilities 80 .
Evolution of studies on emission load
Emission inventories, critical for formulating air pollution control policies, have evolved over time. Initiatives by CSIR-NEERI and CPCB in the late twentieth century laid the foundation 12 . Emission inventory data, collected through GIS, has become integral in mapping pollution sources and understanding spatial distribution 81 . The Air Pollution Knowledge Assessments (APnA) city program and organizations like TERI contribute to city-specific inventories 82 . The emphasis on utilizing secondary data streamlines the process, enabling the creation of comprehensive databases for national and urban pollution inventories. The secondary data refers to datasets that include emission loads from various sources such as vehicular emissions, industrial outputs, construction activities, residential heating, and biomass burning 83 .
Management strategies and control policies
India's air pollution management strategies encompass a multifaceted approach, with a blend of judicial interventions and executive actions.
Judicial interventions
The judiciary, particularly through petitions filed by M.C. Mehta, has been instrumental in setting guidelines and policies 84 . For instance, interventions in the Taj Trapezium Zone and the oversight of air quality management plans for non-attainment cities by the National Green Tribunal (NGT) are notable 74 . The judiciary has played a significant role in shaping policies for better governance and legislation.
Executive actions
Several executive measures contribute to air pollution control. The Auto Fuel Policy, initiated in 2003 and updated in 2014, addresses vehicular emissions 85 . Emphasis on alternative fuels, as seen in the National Auto Fuel Policy and the Pradhan Mantri Ujwala Yojana (PMUY) for subsidized LPG connections, aligns with cleaner fuel initiatives 86 . Stricter emission standards for thermal power plants and the push for Hybrid and Electric Vehicles (EVs) under schemes like Faster Adoption and Manufacturing of Hybrid & Electric Vehicles (FAMHE) contribute to pollution reductions 87 .
AI&ML Techniques for addressing and forecasting air pollution
Overview of ai&ml models.
Various AI&ML techniques, such as ANN, Fuzzy logic (FL), Support Vector Machine (SVM), Convolutional Neural Network (CNN), Recurrence Neural Network (RNN), Long Short-Term Memory (LSTM), Convolutional Autoencoder (CA) etc., are commonly used in previous studies to predict and forecast earth and atmospheric variables 8 , 25 , 88 , 89 , 90 , 91 (Table 1 ). AI&ML models have become pivotal in processing and simulating non-linear information, with a notable focus on ANNs 92 . ANNs emulate the human nervous system, comprising interconnected neurons that collectively address a spectrum of challenges, from function approximation to clustering and optimization 93 . The three-stage process involved in ANN modelling, encompassing design, training, and validation, underscores its versatility 92 . During the design phase, crucial parameters such as architecture, layers, neurons, and learning algorithms are thoroughly chosen 94 . Training involves iterative adjustments of synaptic weights to minimize errors, while validation gauges the network's generalization performance for unknown data.
Multilayer Perceptron (MLPs), a prominent type of ANN, have proven effective in predicting atmospheric pollution events. Typically featuring input, hidden, and output layers, MLPs can adapt to complex patterns by incorporating multiple hidden layers 92 . Configuring neurons in the hidden layers is of utmost importance, as an incorrect count can lead to over-fitting or under-fitting. Techniques like thumb rule and trial and error, network reduction offer solutions to optimize neuron numbers. FL, another AI technique, operates on a different paradigm by assigning truth values in a range. Developed from fuzzy set theory, it accommodates linguistic variables, making it adept at handling uncertainty in natural language statements. Fuzzy logic's three main phases—fuzzification, inference, and defuzzification—form a robust modelling system capable of addressing nuanced problems. SVM are popular for supervised learning, excelling in classification, prediction, density estimation, and pattern recognition. SVM seeks an optimal hyperplane to segregate data into predefined classes, with kernel functions playing a pivotal role in introducing non-linearity.
Deep Neural Networks (DNNs) represent an advanced version of ANNs, characterized by structural depth and scalability 8 . DNNs, with more than three layers, can automatically extract features from raw inputs, known as feature learning. Notable architectures within DNNs, such as CA, LSTM, CNNs and RNNs have demonstrated superior performance, especially in air pollution forecasting. The training of DNNs demands significant computational power, leading to advancements in processing capabilities and the development of sophisticated algorithms. Overcoming challenges like vanishing gradient and overfitting has prompted the application of advanced algorithms like SVM, RF, Greedy layer-wise, and Dropout. The application of these models extends across various domains due to their versatility and robust performance. The modelling of complex atmospheric variables such as air pollution forecasting, LSTM, CA, and CNNs emerge as particularly effective and popular architectures.
Application of AI&ML in addressing and forecasting air pollution
The application of AI&ML models, particularly ANNs, FL, SVM and DL models, have emerged as a crucial tool in addressing and forecasting air pollution. ANNs have helped in a transformative era in air pollution forecasting, with a diverse range of applications capturing the attention of researchers. Numerous studies attest to the success of ANNs in predicting both particulate and gaseous pollutants with desired accuracy over various spatio-temporal resolution. The early forays into air pollution forecasting by Mlakar et al. 95 marked a significant milestone, employing a trained nonlinear three-layered back propagation feed forward network. This model successfully predicted the concentration of SO 2 over a thermal power plant, showcasing the potential of ANNs. Subsequent research expanded the scope and sophistication of ANN applications. Similarly, Arena et al. 96 demonstrated the efficacy of multi-layer perceptron in predicting concentration of SO 2 over an industrial area, emphasizing the model's accuracy across diverse weather conditions. Sohn et al. 97 extended the ANN approach to model multiple pollutants, including NO, SO 2 , NO 2 , CO, O 3 , CH 4 and total hydrocarbons. The results indicated reasonable accuracy within a limited prediction range, highlighting the need for further optimization by incorporating additional weather-related input parameters. The application of ANNs in gaseous pollutants forecasting continued with studies by Slini et al. 98 and Kandya 99 both emphasizing the importance of optimizing input parameters for improved accuracy. Comparative assessments with other forecasting techniques consistently positioned ANNs as superior for gaseous pollutants. Chaloulakou et al. 100 found that ANN outperformed Multiple Linear Regression (MLR) in predicting ozone concentrations, showcasing the model's superior accuracy. Similar findings were reported by Mishra and Goyal 101 , compared Principal Component Analysis (PCA)-based ANN model with MLR for estimating the concentrations of NO 2 . In the realm of particulate matter forecasting, ANNs have proven equally effective. Fernando et al. 102 successfully used multi-layered MLP to predict PM 10 concentrations, considering parameters such as hourly meteorological data, particulate, matter with statistical indicators. Grivas and Chaloulakou 103 employed an ANN model for hourly PM 10 predictions, showcasing consistent accuracy even in the presence of noisy datasets. The versatility of ANNs extends to predicting roadside contributions to PM 10 concentrations, as demonstrated by Suleiman et al. 104 . Comparative studies with other models have affirmed the efficacy of ANNs in particulate matter forecasting. Zhang et al. 105 utilized BPANN to forecast the concentrations of PM 10 and found BPANN outperforming other models in predictive accuracy. Paschalidou et al. 106 evaluated the multi-layer perceptron-based ANN those models provided superior results compared to Radial Basis Function models, establishing the former's dominance in terms of forecasting capability. Contrasting trends were observed in certain studies, such as those by Mishra et al. 107 and Moisan et al. 108 , where alternative models outperformed ANN during extreme events. This highlights the nuanced nature of model performance, with specific conditions favouring different approaches. However, recent progress has witnessed researchers utilizing ensemble methods to improve both the stability and accuracy of ANN models. Liu et al. 109 combined Wavelet Packet Decomposition (WPD), Particle Swarm Optimization (PSO), and BPNN to create an ensemble model for PM 2.5 forecasting, demonstrating superior precision compared to individual models.
FL, renowned for its capacity to manage uncertainty, enhanced fault tolerance, and adeptness in handling highly complex nonlinear functions, has garnered extensive adoption in the realm of air pollution prediction. The advantages of FL are exemplified in various studies. For example, Chen et al. 110 innovatively introduced a novel fuzzy time series model specifically for O 3 prediction, showcasing its superior performance when compared to traditional fuzzy time series models. Jain and Khare 111 applied a neuro-fuzzy model to predicts the concentration of CO in Delhi, achieving accurate estimates at complex urban levels. Carbajal-Hernández et al. 112 predicts air quality in Mexico City by utilising FL model alongside autoregression model and signal processing. The introduction of a novel algorithm, the "Sigma operator," allowed for precise evaluation of air quality variables, showcasing the effectiveness of fuzzy-based models. Moreover, Al-Shammari et al. 113 , evaluates stochastic and FL-driven models to estimate the daily maximum concentrations of O 3 . The findings indicated that the FL-based model exhibited a marginal superiority over the statistical model particularly in instances of severe pollution events. Innovative approaches like the Fuzzy Inference Ensemble (FIE), as proposed by Bougoudis et al. 114 , demonstrated high accuracy in air pollution forecasting for Athens. Another significant application was presented by Song et al. 115 , where different probability density functions were employed to enhance particulate matter (PM) forecasting. They developed an adaptive neuro-fuzzy model, emphasizing the importance of density functions in addressing uncertainty associated with future PM trends. Furthermore, Wang et al. 116 presented a hybrid model for forecasting air pollution. This model merges uncertainty analysis with fuzzy time series, demonstrating precision in predicting PM and NO 2 concentrations. Behal and Singh 117 leveraged FL within an intelligent IoT sensor framework to monitor and simulate benzene, demonstrating satisfactory statistical efficacy in recent advancements. The versatility of fuzzy logic extends to unconventional pollutants as demonstrated by Arbabsiar et al. 118 , who modelled the leakage of CH 4 and H 2 S using a fuzzy inference technique. The suggested model demonstrated satisfactory performance when evaluating these contaminants.
Support Vector Machines (SVM), when combined with other machine learning algorithms, have been helpful in forecasting diverse types of pollutants. Feng et al. 119 compared SVM with other models for forecasting daily maximum concentrations of O 3 in Beijing, highlighting its stable and accurate performance. Yeganeh et al. 120 assessed the efficacy of a forecasting model utilizing SVM integrated with Partial Least Squares (PLS) for the prediction of CO concentrations, demonstrating positive outcomes. García Nieto et al. 121 conducted a comparative analysis of various prediction models for PM 10 concentrations, determining that the SVM method exhibited superior accuracy and robustness. Luna et al. 122 utilized Principal PCA in combination with SVM and ANN for the prediction of O 3 levels in Rio de Janeiro. Their study specifically investigated the influence of meteorological parameters on the concentrations of O 3 . Wang et al. 123 proposed hybrid adaptive forecasting models combining SVM and ANN for predicting PM 10 and SO 2 , demonstrating superior performance compared to individual models. FL and SVM in the forecasting air pollution levels have proven to be highly effective in addressing the complexities and uncertainties associated with predicting pollutant concentrations.
While still in its early stages, the potential of DNNs in this domain is evident from a review of various applications such as forecasting of variables in earth and atmospheric sciences. Early on, Freeman et al. (2018) employed a combination of Long Short-Term Memory (LSTM) and Recurrent Neural Networks (RNN) to predict ozone concentrations in an urban area. While showing strong predictability in 8 h average ozone concentrations, various model runs revealed overfitting concerns, underscoring the necessity for further refinement. Wang and Song 125 introduced an ensemble method using a deep LSTM network with fuzzy c-means clustering for air quality forecasting. This ensemble approach outperformed individual models, showcasing its efficacy in both short-term and long-term predictions. Zhou et al. 126 explored the application of LSTM and deep learning algorithms for multi-step ahead forecasting of PM 2.5 , PM 10 , and NO x . Their deep learning architecture, integrating dropout neurons and L 2 regularization, demonstrated exceptional capabilities in capturing variations in the processes of air pollutant generation. Recent research highlights the growing preference for employing deep neural networks to capture dynamic spatiotemporal features from historical air quality and climatological datasets. Fan et al. 91 introduced stacked LSTM (LSTME), spatiotemporal deep learning (STDL), time delay neural network (TDNN), autoregressive moving average (ARMA), and support vector regression (SVR) for modelling of air pollutants over different spatiotemporal resolutions. The inclusion of auxiliary inputs resulted in a model with exceptional performance, outshining other machine learning techniques. Soh et al. 127 proposed a STDL integrating ANN, CNN, and LSTM for PM 2.5 prediction. The model exhibited stability over extended time periods, with noise reduction achieved through Airbox sensor source models, further enhancing prediction accuracy. Qi et al. 128 presented a novel forecasting approach employing a fusion of Graph Convolutional and LSTM (GC-LSTM) neural networks, aiming to investigate spatial interdependence within air quality data. The spatial correlation modelling highlighted the consistency of the GC-LSTM model for short-term forecasting, suggesting potential improvements for long-term predictions with enhanced spatiotemporal considerations. Fan et al. 91 developed a LSTM-based deep–RNN for predicting PM 2.5 for different spatiotemporal frames showcasing superior specificity measures compared to baseline models. In a novel approach, Li et al. 129 and Zhang et al. 130 incorporated large-scale datasets of graphical images for air pollution estimation, utilizing CNN. The models, trained on images capturing various atmospheric conditions, demonstrated improved prediction accuracy, emphasizing the adaptability of deep learning to diverse data types. These models offer robust solutions, demonstrating superior performance in various studies and showcasing their potential to contribute significantly to the field of environmental monitoring and public health.
Performance analysis
The evaluation is based on the comparison of their performances using statistical measures such as RMSE and R 2 , widely accepted metrics in air pollution forecasting studies. Previous research, utilizing a range of datasets, has yielded disparate results 134 . While certain studies advocate for ensemble methods, others find negligible disparities in the overall accuracy of the outcomes. The efficacy of AI and ML-driven methodologies relies heavily on the precise curation of influential parameters, especially when addressing various pollutants such as PM, O 3 , NO 2 , SO 2 , and CO 29 . For example, for PM forecasting, critical elements such as precipitation, pressure, humidity, land utilization, wind speed and direction, traffic flow on roads, and population density exert significant influence. Similarly, different influential parameters are identified for SO 2 , NO 2 , O 3 , and CO, emphasizing the importance of tailoring models to specific pollutants. The precision of the methods is notably impacted by the direct correlation between these factors and forecasted levels of pollutants. Additionally, the efficacy of AI&ML models hinges upon variables including network structure, intricacy, learning algorithms, correspondence between input and output information, and the presence of data interference. A comprehensive analysis shows the varying performances of DNN, SVM, ANN, and Fuzzy techniques across different pollutants. DNNs emerge as particularly effective in forecasting PM concentrations, outperforming other techniques with R 2 and mean RMSE values of 0.96 and 7.27 μg/m 3 , respectively 91 , 126 , 133 . In O 3 prediction, SVM, FL and DNN exhibit superior accuracy, with DNNs once again leading with R 2 and mean RMSE values of 0.92 and 3.51 μg/m 3 , respectively 119 , 120 . SVM excels in forecasting NO 2 concentrations, although Fuzzy and DNN techniques also demonstrate reasonable accuracy 116 , 118 , 131 . Notably, the DNN approach consistently stands out, showcasing the best statistical performance for O 3 and CO categories. For CO, DNN achieves an exceptional RMSE of 0.69 × 10 –5 ppm and an R 2 of 0.95 119 , 120 , 124 , 125 . The overall analysis represents the superiority of DNN across all pollutants, with the lowest overall RMSE score of 5.68. However, despite DNN's dominance, it is crucial to note the underdeveloped application of ensemble methodologies based on DL models for the forecasting of air pollution 131 , 135 , 136 . These approaches, involving multiscale spatiotemporal predictions, have untapped potential to further advance the field, incorporating more explanatory variables to represent air pollution episodes with robust dynamical forcing. The DNN emerges as the leading AI&ML system for the forecasting and prediction of air pollution based on statistical evidence, the exploration of ensemble approaches presents an avenue for future developments in enhancing predictive accuracy.
Prediction of PM 2.5 concentrations
The study used a convolutional autoencoder (CA) for analysing PM 2.5 concentrations. The dataset was divided into training (70%), testing (20%), and validation (10%) sets, trained over 30 epochs (Fig. 3 ). This PM 2.5 -focused CA processes sequences of ten consecutive images, using acquired features to reconstruct subsequent images. The visual representation of the model's capabilities includes sequences of 10 input images, their corresponding 11 th ground truth, and the model's predictions (Fig. 4 ). The model demonstrates promising performance in predicting PM 2.5 concentration patterns across India. Comparing the actual 11 th image with the predicted one reveals that the model successfully captures the broad spatial distribution of PM 2.5 concentrations. Key findings show that the model accurately predicts high concentration areas in the northern regions, particularly in the IGP (Fig. 4 ). It also effectively represents lower concentrations in southern and eastern coastal areas. The model captures the general gradient from northwest to southeast quite effectively. The prediction tends to slightly overestimate PM 2.5 levels in the northwestern region. Additionally, some localized high-concentration areas in central India are not fully captured in the prediction. Furthermore, the model's prediction shows a smoother distribution compared to the more granular actual data. (Fig. 4 ). Performance evaluation employed established image quality metrics: Structural Similarity Index (SSIM), Peak Signal-to-Noise Ratio (PSNR) and Mean Squared Error (MSE) (Fig. 5 ). SSIM, which assesses image similarity, predominantly ranged from 0.50 to 0.70 during training, slightly lowering to 0.45 to 0.55 during testing, and stabilizing at 0.50 to 0.60 in validation. PSNR peaked at 25 to 30 dB during training, followed by 24 to 28 dB in testing, and 28 to 30 dB in validation. Lower MSE values (10 to 15 µg/m 3 in training, 10 to 20 µg/m 3 in testing, and 8 to 11 µg/m 3 in validation) signify improved accuracy at the pixel level.
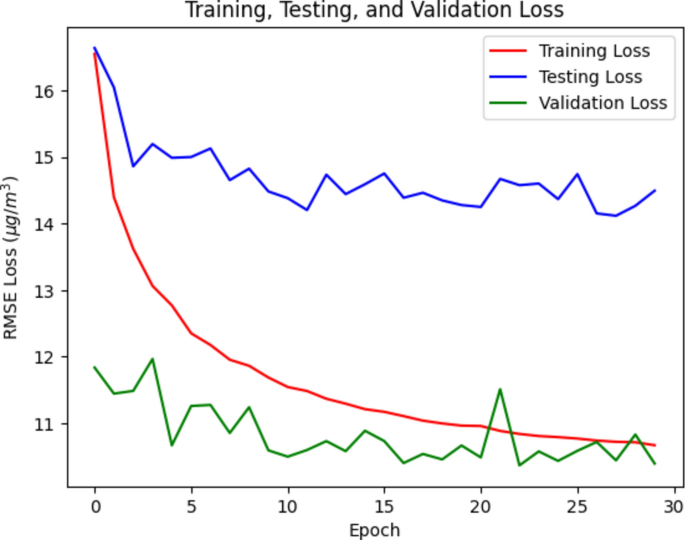
RMSE loss during the training, testing and validation phase.
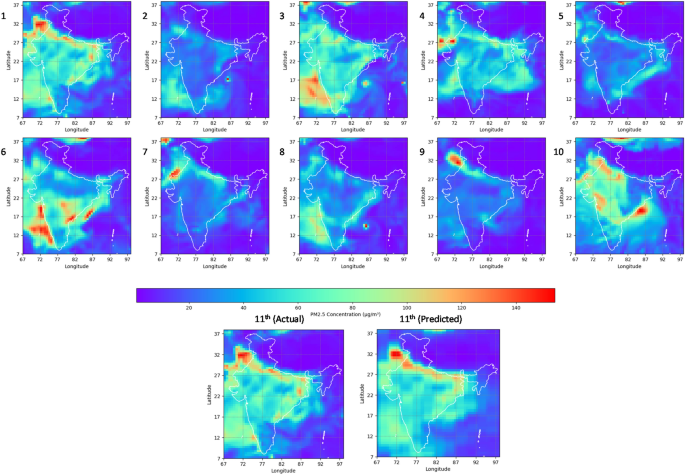
Example set for predicting the 11th image of PM 2.5 by providing a batch of 10 images of concentration and comparing with the 11th actual image. The maps were generated using Python in a Jupyter Notebook with Matplotlib (v3.3.4) and Basemap (v1.2.2) libraries ( https://matplotlib.org/ and https://matplotlib.org/basemap/ ).
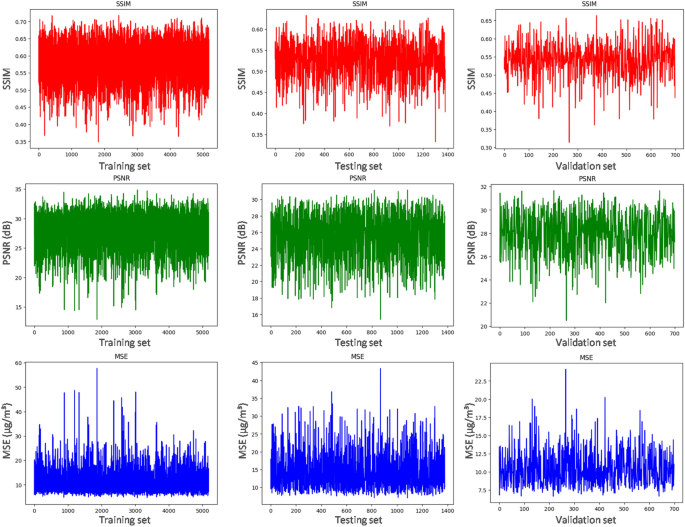
Model evaluation parameters used for prediction the PM 2.5 concentrations.
These metrics offer insights into image quality, indicating some variation between training, testing, and validation, yet within acceptable ranges. Consistently higher SSIM and PSNR values and lower MSE values highlight the model's exceptional precision compared to benchmarks. The model's excellence traces back to its ability to capture complex spatio-temporal features through Autoencoder-based models and strategic integration of Conv2d, Batch Normalization, and Upsampling layers. The model outperforms prior methodologies in predicting PM 2.5 concentrations, achieving precise and high-quality predictions across phases. Attempting to forecast PM 2.5 levels for the next 4 days led to efficiency parameter decreases (SSIM, PSNR, MSE) with increased time frames, suggesting the need for more parameters for model efficiency improvement (Fig. 6 ). Predicting PM 2.5 concentrations remains challenging due to intricate spatiotemporal features, where DL models offer promise. Leveraging deep learning architectures and transfer learning, this study fine-tuned models, achieving promising PM 2.5 prediction results. Despite ongoing challenges in precise location predictions due to PM 2.5 's dynamic nature, the model demonstrated spatial distribution prediction abilities, evident in visual comparisons between predicted and actual PM 2.5 concentration maps.
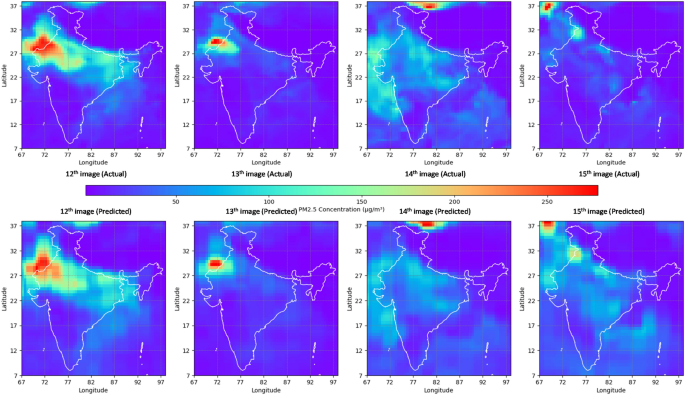
Example set of predictions of PM 2.5 for next 4 days compared with their actual images. The maps were generated using Python in a Jupyter Notebook with Matplotlib (v3.3.4) and Basemap (v1.2.2) libraries ( https://matplotlib.org/ and https://matplotlib.org/basemap/ ).
Challenges and limitations
Technological barriers.
One of the primary challenges lies in overcoming technological barriers. While advanced pollution control technologies exist, their widespread adoption is hindered by factors such as high costs and limited access to cutting-edge solutions. Many regions, particularly in rural areas, lack the infrastructure necessary to deploy and maintain sophisticated air quality monitoring and purification systems. Bridging this technological divide is essential for comprehensive pollution control.
Regulatory and enforcement challenges
India grapples with the challenge of implementing and enforcing air quality regulations consistently. While the country has established regulatory frameworks to curb emissions from industries, vehicles, and other pollution sources, enforcement remains uneven. This inconsistency is often compounded by resource constraints, bureaucratic hurdles, and the need for stronger mechanisms to penalize non-compliance. Strengthening regulatory frameworks and enhancing enforcement mechanisms are critical steps in addressing this challenge.
Public awareness and participation
Creating widespread awareness and fostering public participation are essential components of any successful pollution control strategy. However, there is a considerable gap in public awareness regarding the causes and consequences of air pollution. Engaging citizens in proactive measures, such as adopting sustainable practices and reducing individual carbon footprints, requires comprehensive educational campaigns and community involvement. Overcoming societal inertia and instigating behavioral change are significant challenges in this regard.
Agricultural practices and crop burning
Agricultural practices, particularly the prevalent practice of crop burning, contribute significantly to air pollution. The burning of crop residues releases substantial amounts of particulate matter and pollutants into the air. Farmers resort to this practice due to a lack of viable alternatives and time constraints between harvest seasons. Developing and promoting sustainable agricultural practices, coupled with providing farmers with effective alternatives to crop burning, is a complex challenge that requires a holistic approach.
Urbanization and infrastructure development
Rapid urbanization and infrastructure development, while essential for economic growth, often contribute to increased pollution levels. The construction industry, in particular, releases pollutants into the air. Balancing the need for development with sustainable and environmentally conscious practices poses a significant challenge. Implementing green building technologies, stringent emission norms for construction activities, and incorporating urban planning strategies that prioritize air quality are vital steps in addressing this challenge.
Cross-border pollution
Air pollution knows no boundaries, and India contends with the impact of cross-border pollution. Transboundary movement of pollutants, especially during crop burning seasons, contributes to elevated pollution levels in various regions. Collaborative efforts with neighbouring countries are necessary to address this challenge effectively. Developing joint strategies, sharing data, and fostering regional cooperation are imperative for tackling the transboundary dimension of air pollution.

Climate change interlinkages
The interlinkages between air pollution and climate change present a complex challenge. Mitigating air pollution often aligns with climate action goals, but there are trade-offs and synergies that need careful consideration. Striking a balance between addressing immediate air quality concerns and contributing to long-term climate resilience requires integrated policies and strategic planning.
Socio-economic disparities
Air pollution disproportionately affects vulnerable communities, exacerbating existing socio-economic disparities. The challenge lies in designing interventions that address environmental concerns and promote social equity. Ensuring that pollution control measures do not inadvertently burden marginalized communities and providing equitable access to clean technologies are critical to overcoming this challenge.
Future prospects
India stands at the cusp of a pivotal moment in its battle against air pollution, with promising avenues emerging on both technological and collaborative fronts.
Emerging technoloagies
The integration of cutting-edge technologies offers hope for India's future in pollution control. Advancements in AI&ML, when coupled with sophisticated numerical weather prediction models, present a potent toolset for predicting and managing air pollution. These technologies can enhance real-time monitoring, improve predictive capabilities, and facilitate data-driven decision-making, allowing for more precise and targeted interventions. Additionally, the fusion of AI&ML with numerical weather prediction (NWP) models can refine pollution control strategies by providing a deeper understanding of atmospheric dynamics and pollutant dispersion patterns. Furthermore, exploring potential breakthroughs in sustainable energy sources offers a transformative pathway. Shifting from traditional, pollutant-intensive energy sources to sustainable alternatives is crucial for reducing the overall carbon footprint. Investments in research and development, coupled with policy incentives, can accelerate the adoption of clean and renewable energy solutions, fostering a paradigm shift in India's energy landscape.
Global collaborations
Recognizing that air pollution transcends national boundaries, India looks toward global collaborations as a key driver for progress. International efforts in combating air pollution gain significance as countries join forces to address shared challenges. Collaborative platforms provide opportunities for knowledge sharing, exchange of best practices, and collective research initiatives. India's participation in these global endeavours not only enriches its own understanding of air pollution dynamics but also contributes to the global pool of knowledge. By fostering partnerships with other nations, India can access expertise, technologies, and resources that augment its capacity to implement effective pollution control measures. Knowledge sharing and collaborative research initiatives form the cornerstone of global efforts. Platforms that facilitate the exchange of data, research findings, and innovative solutions enable nations to collectively tackle the intricate and interconnected challenges of air pollution. As India engages in these collaborative endeavours, it not only benefits from the collective wisdom of the global community but also contributes its unique insights and experiences, enriching the collective understanding of air pollution dynamics.
India's strategic focus on emerging technologies and global collaborations holds immense promise in navigating the future. By harnessing the power of advanced technologies and participating in international initiatives, India can chart a course toward a cleaner, more sustainable future where the skies are clear, and the air is a testament to the collective commitment to environmental well-being.
Materials and methods
Maintaining fresh air quality is a complex undertaking influenced by various factors over time. These elements encompass air pollutant emissions, deposition, weather patterns, traffic dynamics, and human activities, among others 8 , 64 . The complexity of these interrelated factors makes it challenging for traditional shallow models to offer precise portrayals of air quality attributes. Based on the above review, deep learning algorithms were found most suitable for predicting air quality variables without needing prior knowledge. This capability enhances the potential for more accurate predictions regarding air quality, signifying a valuable contribution to addressing the intricacies associated with sustaining optimal air quality levels.
The case study utilized MERRA-2 reanalysis data from the NASA GESDISC DATA ARCHIVE application 137 , 138 . This dataset, spanning from January 1, 2015, to December 31, 2022, features a spatial resolution of 0.5° × 0.625° and a temporal resolution of 1 h (Fig. 7 ). It includes five key variables: black carbon surface mass concentration (BCSMASS), dust surface mass concentration—PM 2.5 (DUSMASS25), organic carbon surface mass concentration (OCSMASS), sea salt surface mass concentration—PM 2.5 (SSSMASS25), and SO 4 surface mass concentration (SO 4 SMASS). These variables are analysed across three dimensions: latitude, longitude, and time. The concentration of the PM 2.5 (µg/m 3 ) for each grid cell was computed as 139 , 140 :
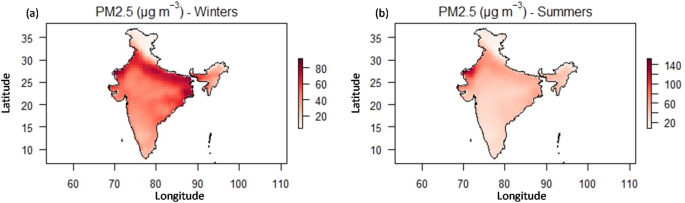
Surface PM 2.5 concentration over India during ( a ) Winters and ( b ) Summers; Maps were generated using R Studio (v4.3.3, https://www.rstudio.com/ ).
Convolutional Autoencoder model
Air quality monitoring and predicting PM 2.5 concentrations accurately stands crucial for public health and environmental management 8 . The case study explores an innovative approach employing an Autoencoder-based DL model for forecasting PM 2.5 concentrations from spatiotemporal data over India. The study begins by complexly handling the datasets, leveraging PyTorch's Dataset and data loader classes. The ATMriver Dataset class is crafted to capture the dataset, enabling sequential data handling 9 . The data, formatted into tensors and split into training, testing, and validation subsets in a ratio of 70, 20 and 10, respectively, undergoes a custom transformation via the tensor class, ensuring compatibility with the neural network model 8 , 141 . The core of this methodology lies in the architecture of the Autoencoder, a neural network comprising convolutional and transposed convolutional layers. Specifically, the model comprises convolutional layers (conv1, conv2, conv3) responsible for feature extraction and transposed convolutional layers (conv1_d, conv2_d, conv3_d) for data reconstruction (Fig. 8 ). Each convolutional layer is paired with batch normalization and dropout (set at 25%) to regularize the network and prevent overfitting. The use of five layers in this Autoencoder architecture allows for hierarchical feature extraction and reconstruction, enhancing the model's ability to learn complex representations. The learning rate, a critical hyperparameter governing the magnitude of parameter updates during optimization, is set to 0.0025 for the Adam optimizer. This value influences the convergence speed and stability of the training process. A higher learning rate might lead to faster convergence but risks overshooting the optimal parameters, while a lower rate might result in slower convergence. The chosen learning rate balances the trade-off between convergence speed and stability, aiming to facilitate efficient model training while preventing divergence or oscillation in the optimization process.
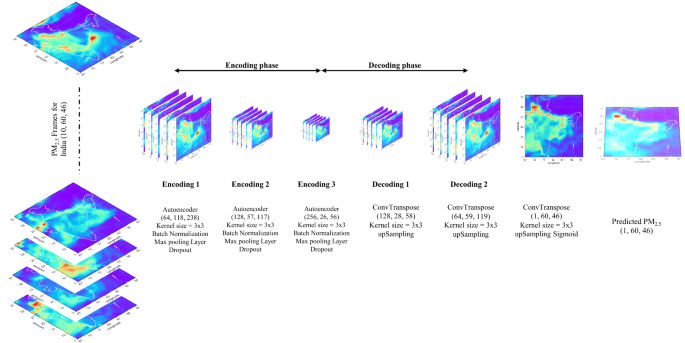
Convolution autoencoder architecture for PM 2.5 data processing with model features an encoding phase with three autoencoder stages, followed by a decoding phase with two transpose convolution stages; structure enables dimensionality reduction and subsequent reconstruction of PM 2.5 concentration maps.
To train the Autoencoder, a custom root mean squared error (RMSE) loss function is defined. This loss function quantifies the disparity between predicted and actual PM 2.5 concentrations, guiding the model toward more accurate predictions. The training process iterates through the dataset multiple times (epochs), optimizing the model parameters using the Adam optimizer. The evaluation phase of the model involves assessing its predictive capabilities on separate testing and validation sets. The model's outputs are compared against the original images PM 2.5 concentrations, and the RMSE loss is computed. The best-performing model, based on its performance on the testing set, is identified and saved for the prediction. Further the records and reports the losses incurred during training, testing, and validation across epochs, providing insights into the model's loss curve and performance stability. Additionally, the best model's loss metric is highlighted, signifying its capability to accurately predict PM 2.5 concentrations. The evaluation of the trained model's predictive capability in this study primarily relied on two widely accepted image quality metrics: Structural Similarity Index (SSIM) and Peak Signal-to-Noise Ratio (PSNR). The Structural Similarity Index (SSIM) serves as a measure to assess the similarity between the predicted and actual images 142 . SSIM evaluates the perceived change in structural information, including luminance, contrast, and structure, between the predicted and actual images. A higher SSIM score, closer to 1, indicates a greater similarity between the two images, implying better predictive performance of the model. Peak Signal-to-Noise Ratio (PSNR) is another commonly used metric for quantifying the quality of reconstructed or predicted images. PSNR measures the ratio between the maximum possible power of a signal and the power of corrupting noise that affects the fidelity of its representation. Higher PSNR values signify lower image distortion or higher image fidelity, implying better prediction accuracy in capturing the details of the actual images.
Addressing the complex challenges of air pollution in India necessitates a multifaceted and technically informed approach. The existing impediments, including technological barriers and limited access to advanced pollution control technologies, underline the urgency of bridging the technological divide, particularly in rural areas. While regulatory frameworks are in place, inconsistent enforcement due to resource constraints and bureaucratic hurdles requires strategic strengthening. Public awareness and participation, integral components of effective pollution control, demand targeted educational campaigns to instigate behavioural change. Agricultural practices, notably crop burning, pose a significant challenge, and resolving this requires not only viable alternatives but a holistic approach that integrates sustainable agricultural practices. Rapid urbanization and infrastructure development, while essential for economic growth, necessitate the incorporation of green building technologies, stringent emission norms, and urban planning strategies prioritizing air quality. Cross-border pollution adds a transboundary dimension, demanding collaborative efforts with neighbouring countries. The intricate interlinkages between air pollution and climate change underscore the need for carefully balanced policies that address immediate air quality concerns while contributing to long-term climate resilience. Moreover, the disproportionate impact of air pollution on vulnerable communities emphasizes the importance of interventions that promote social equity alongside environmental considerations. Looking towards the future, the convergence of emerging technologies offers a beacon of hope. The integration of AI&ML with numerical weather prediction models presents a potent toolset for real-time monitoring, precise predictive capabilities, and data-driven decision-making. This amalgamation not only enhances our understanding of atmospheric dynamics and pollutant dispersion patterns but also refines pollution control strategies. Exploring breakthroughs in sustainable energy sources becomes imperative for reducing the overall carbon footprint. Shifting from traditional, pollutant-intensive energy sources to clean and renewable alternatives require concerted efforts through research, development, and policy incentives.
Furthermore, global collaborations stand out as a key driver for progress, given the transboundary nature of air pollution. Participating in international efforts fosters knowledge sharing, exchange of best practices, and collective research initiatives. By engaging in these collaborative activities, India not only enriches its understanding of air pollution dynamics but contributes to the global pool of knowledge. Platforms facilitating data exchange, research findings, and innovative solutions enable nations to collectively tackle the complex challenges of air pollution. In navigating the future, India's strategic focus on emerging technologies and global collaborations holds immense promise. The careful harnessing of advanced technologies and participation in international initiatives can chart a course toward a cleaner, more sustainable future. The fusion of AI&ML with numerical weather prediction (NWP) models positions India to proactively manage air quality, with the skies serving as a testament to the collective commitment to environmental well-being. As India progresses, the synergy of technological advancements and global cooperation emerges as the cornerstone for effective, informed, and sustainable solutions to combat air pollution.
Data availability
Data will be made online on a reasonable request to the corresponding author.
Masood, A. & Ahmad, K. A review on emerging artificial intelligence (AI) techniques for air pollution forecasting: Fundamentals, application and performance. J. Clean. Prod. 322 , 129072 (2021).
Article CAS Google Scholar
WHO. Atlas on Children’s Health and the Environment . (2017).
Jiang, X. Q., Mei, X. D. & Feng, D. Air pollution and chronic airway diseases: what should people know and do?. J. Thorac. Dis. 8 , E31–E40 (2016).
PubMed PubMed Central Google Scholar
Hayes, R. B. et al. PM25 air pollution and cause-specific cardiovascular disease mortality. Int. J. Epidemiol. 49 , 25–35 (2020).
Article PubMed Google Scholar
Shakya, D., Deshpande, V., Goyal, M. K. & Agarwal, M. PM2.5 air pollution prediction through deep learning using meteorological, vehicular, and emission data: A case study of New Delhi. India. J. Clean. Prod. 427 , 139278 (2023).
Brown, P. E. et al. Mortality Associated with Ambient PM2.5 Exposure in India: Results from the Million Death Study. Environ. Health Perspect. 130 , 097004 (2022).
Article PubMed PubMed Central Google Scholar
Pandey, A. et al. Health and economic impact of air pollution in the states of India: the Global Burden of Disease Study 2019. Lancet Planet. Heal. 5 , e25–e38 (2021).
Article Google Scholar
Rautela, K. S., Singh, S. & Goyal, M. K. Characterizing the spatio-temporal distribution, detection, and prediction of aerosol atmospheric rivers on a global scale. J. Environ. Manage. 351 , 119675 (2024).
Article CAS PubMed Google Scholar
Rautela, K. S., Singh, S. & Goyal, M. K. Resilience to Air Pollution: A Novel Approach for Detecting and Predicting Aerosol Atmospheric Rivers within Earth System Boundaries. Earth Syst. Environ. https://doi.org/10.1007/s41748-024-00421-0 (2024).
Chakraborty, S. et al. Extending the Atmospheric River Concept to Aerosols: Climate and Air Quality Impacts. Geophys. Res. Lett. 48 (9), e2020GL091827 (2021).
Kapoor, M. Managing Ambient Air Quality Using Ornamental Plants-An Alternative Approach. Univers. J. Plant Sci. 5 , 1–9 (2017).
Article MathSciNet Google Scholar
Gulia, S. et al. Evolution of air pollution management policies and related research in India. Environ. Challenges 6 , 100431 (2022).
UN. Two ‘Population Billionaires’, China and India, Face Divergent Demographic Futures. Dep. Econ. Soc. Affiars 1–10 (2023).
IQAir. (Report) World Air Quality Report. 2020 World Air Qual. Rep. 1–35 (2020).
WHO. World Health Statistics . (2014).
Kumari, S., Verma, N., Lakhani, A. & Kumari, K. M. Severe haze events in the Indo-Gangetic Plain during post-monsoon: Synergetic effect of synoptic meteorology and crop residue burning emission. Sci. Total Environ. 768 , 145479 (2021).
Delhi Air Pollution: Real-time Air Quality Index. https://aqicn.org/city/delhi .
CPCB. National Ambient Air Quality Status & Trends 2019. Cent. Pollut. Control Board 53 , 1689–1699 (2020).
Google Scholar
IITK. Comprehensive study on air pollution and green house Google Scholar. A Rep. Submitt. to Gov. NCT Delhi DPCC Delhi 1–334 (2016).
Rizwan, S., Nongkynrih, B. & Gupta, S. K. Air pollution in Delhi: Its Magnitude and Effects on Health. Indian J. Community Med. 38 , 4 (2013).
Bai, L., Wang, J., Ma, X. & Lu, H. Air Pollution Forecasts: An Overview. Int. J. Environ. Res. Public Health 15 , 780 (2018).
Masood, A. & Ahmad, K. A model for particulate matter (PM2.5) prediction for Delhi based on machine learning approaches. Procedia Comput. Sci. 167 , 2101–2110 (2020).
Mo, X., Zhang, L., Li, H. & Qu, Z. A Novel Air Quality Early-Warning System Based on Artificial Intelligence. Int. J. Environ. Res. Public Health 16 , 3505 (2019).
Article CAS PubMed PubMed Central Google Scholar
Krishan, M. et al. Air quality modelling using long short-term memory (LSTM) over NCT-Delhi. India. Air Qual. Atmos. Heal. 12 , 899–908 (2019).
Singh, S. & Goyal, M. K. An innovative approach to predict atmospheric rivers: Exploring convolutional autoencoder. Atmos. Res. 289 , 106754 (2023).
Singh, S. & Goyal, M. K. Enhancing climate resilience in businesses: The role of artificial intelligence. J. Clean. Prod. 418 , 138228 (2023).
Li, C., Hsu, N. C. & Tsay, S.-C. A study on the potential applications of satellite data in air quality monitoring and forecasting. Atmos. Environ. 45 , 3663–3675 (2011).
Article ADS CAS Google Scholar
Box, G. E. P., Jenkins, G. M., Reinsel, G. C. & Ljung, G. M. Journal of time series analysis book review time series analysis: forecasting and control, 5th edition, by. J. Time. Ser. Anal 37 , 709–711 (2016).
Siwek, K. & Osowski, S. Data mining methods for prediction of air pollution. Int. J. Appl. Math. Comput. Sci. 26 , 467–478 (2016).
Fu, M., Wang, W., Le, Z. & Khorram, M. S. Prediction of particular matter concentrations by developed feed-forward neural network with rolling mechanism and gray model. Neural Comput. Appl. 26 , 1789–1797 (2015).
Sekar, C., Gurjar, B. R., Ojha, C. S. P. & Goyal, M. K. Potential Assessment of Neural Network and Decision Tree Algorithms for Forecasting Ambient PM2.5 and CO Concentrations: Case Study. J. Hazardous, Toxic, Radioact. Waste 20 , (2016).
Akhtar, A., Masood, S., Gupta, C. & Masood, A. Prediction and Analysis of Pollution Levels in Delhi Using Multilayer Perceptron (Springer, 2018).
Book Google Scholar
Li, X., Peng, L., Hu, Y., Shao, J. & Chi, T. Deep learning architecture for air quality predictions. Environ. Sci. Pollut. Res. 23 , 22408–22417 (2016).
Huang, C.-J. & Kuo, P.-H. A Deep CNN-LSTM Model for Particulate Matter (PM2.5) Forecasting in Smart Cities. Sensors 18 , 2220 (2018).
Article ADS PubMed PubMed Central Google Scholar
Ma, J., Ding, Y., Cheng, J. C. P., Jiang, F. & Wan, Z. A temporal-spatial interpolation and extrapolation method based on geographic Long Short-Term Memory neural network for PM2.5. J. Clean. Prod. 237 , 117729 (2019).
Chang, Y.-S. et al. An LSTM-based aggregated model for air pollution forecasting. Atmos. Pollut. Res. 11 , 1451–1463 (2020).
Biancofiore, F. et al. Recursive neural network model for analysis and forecast of PM10 and PM2.5. Atmos. Pollut. Res. 8 , 652–659 (2017).
Konovalov, I. B., Beekmann, M., Meleux, F., Dutot, A. & Foret, G. Combining deterministic and statistical approaches for PM10 forecasting in Europe. Atmos. Environ. 43 , 6425–6434 (2009).
McKendry, I. G. Evaluation of Artificial Neural Networks for Fine Particulate Pollution (PM 10 and PM 2.5) Forecasting. J. Air Waste Manage. Assoc. 52 , 1096–1101 (2002).
Dutta, A. & Jinsart, W. Air Pollution in Indian Cities and Comparison of MLR, ANN and CART Models for Predicting PM10 Concentrations in Guwahati. India. Asian J. Atmos. Environ. 15 , 68–93 (2021).
Turias, I. J., González, F. J., Martin, M. L. & Galindo, P. L. Prediction models of CO, SPM and SO2 concentrations in the Campo de Gibraltar Region, Spain: a multiple comparison strategy. Environ. Monit. Assess. 143 , 131–146 (2008).
Shang, Z. & He, J. Predicting Hourly <tex>$\mathbf{PM}_{2.5}$</tex> Concentrations Based on Random Forest and Ensemble Neural Network. in 2018 Chinese Automation Congress (CAC) 2341–2345 (IEEE, 2018). https://doi.org/10.1109/CAC.2018.8623175 .
Bozdağ, A., Dokuz, Y. & Gökçek, Ö. B. Spatial prediction of PM10 concentration using machine learning algorithms in Ankara. Turkey. Environ. Pollut. 263 , 114635 (2020).
Murray, C. J. L. et al. Five insights from the Global Burden of Disease Study 2019. Lancet 396 , 1135–1159 (2020).
Haque, M. & Singh, R. Air Pollution and Human Health in Kolkata, India: A Case Study. Climate 5 , 77 (2017).
Rajak, R. & Chattopadhyay, A. Short and Long Term Exposure to Ambient Air Pollution and Impact on Health in India: A Systematic Review. Int. J. Environ. Health Res. 30 , 593–617 (2020).
CPCB. Epidemiological Study on Effect of Air Pollution on Human Health (Adults) in Delhi CENTRAL POLLUTION CONTROL BOARD MINISTRY OF ENVIRONMENT & FORESTS. (2012).
‘India needs to address challenge of adult immunisation†TM - Elets eHealth. https://ehealth.eletsonline.com/2018/10/india-needs-to-address-challenge-of-adult-immunisation/ .
Raju, S., Siddharthan, T. & McCormack, M. C. Indoor Air Pollution and Respiratory Health. Clin. Chest Med. 41 , 825–843 (2020).
Manisalidis, I., Stavropoulou, E., Stavropoulos, A. & Bezirtzoglou, E. Environmental and Health Impacts of Air Pollution: A Review. Front. Public Heal. 8 , 14 (2020).
Fang, Z., Wu, P.-Y., Lin, Y.-N., Chang, T.-H. & Chiu, Y. Air Pollution’s Impact on the Economic, Social, Medical, and Industrial Injury Environments in China. Healthcare 9 , 261 (2021).
Economy and air pollution - Clean Air Fund. https://www.cleanairfund.org/theme/economics/ .
OECD. Climate-resilient Infrastructure. Policy Perspectives. OECD Environ. Policy Pap. 1–46 (2018).
EPA Research: Environmental Justice and Air Pollution | US EPA. https://www.epa.gov/ej-research/epa-research-environmental-justice-and-air-pollution .
Chakraborty, S., Fu, R., Massie, S. T. & Stephens, G. Relative influence of meteorological conditions and aerosols on the lifetime of mesoscale convective systems. Proc. Natl. Acad. Sci. 113 , 7426–7431 (2016).
Article ADS CAS PubMed PubMed Central Google Scholar
Sturiale & Scuderi. The Role of Green Infrastructures in Urban Planning for Climate Change Adaptation. Climate 7 , 119 (2019).
Gulia, S. et al. Performance evaluation of air pollution control device at traffic intersections in Delhi. Int. J. Environ. Sci. Technol. 19 , 785–796 (2022).
Allioui, H. & Mourdi, Y. Exploring the Full Potentials of IoT for Better Financial Growth and Stability: A Comprehensive Survey. Sensors 23 , 8015 (2023).
Lalchandani, V. et al. Real-time characterization and source apportionment of fine particulate matter in the Delhi megacity area during late winter. Sci. Total Environ. 770 , 145324 (2021).
Tobler, A. et al. Chemical characterization of PM2.5 and source apportionment of organic aerosol in New Delhi. India. Sci. Total Environ. 745 , 140924 (2020).
Rai, P. et al. Real-time measurement and source apportionment of elements in Delhi’s atmosphere. Sci. Total Environ. 742 , 140332 (2020).
Talukdar, S. et al. Air Pollution in New Delhi during Late Winter: An Overview of a Group of Campaign Studies Focusing on Composition and Sources. Atmosphere (Basel). 12 , 1432 (2021).
Wang, T., Wei, K. & Ma, J. Atmospheric Rivers and Mei-yu Rainfall in China: A Case Study of Summer 2020. Adv. Atmos. Sci. https://doi.org/10.1007/s00376-021-1096-9 (2020).
Sarkar, S., Chauhan, A., Kumar, R. & Singh, R. P. Impact of Deadly Dust Storms (May 2018) on Air Quality, Meteorological, and Atmospheric Parameters Over the Northern Parts of India. GeoHealth 3 , 67–80 (2019).
Wei, W. et al. Comprehensive Assessment of Pollution Sources and Health Impacts in Suburban Area of Shanghai. Toxics 11 , 552 (2023).
Blanco-Donado, E. P. et al. Source identification and global implications of black carbon. Geosci. Front. 13 , 101149 (2022).
Mangaraj, P., Sahu, S. K., Beig, G. & Yadav, R. A comprehensive high-resolution gridded emission inventory of anthropogenic sources of air pollutants in Indian megacity Kolkata. SN Appl. Sci. 4 , 117 (2022).
Rastogi, N. et al. Diurnal variability in the spectral characteristics and sources of water-soluble brown carbon aerosols over Delhi. Sci. Total Environ. 794 , 148589 (2021).
Mukherjee, A. et al. Sources and atmospheric processing of brown carbon and HULIS in the Indo-Gangetic Plain: Insights from compositional analysis. Environ. Pollut. 267 , 115440 (2020).
Tripathi, N. et al. Characteristics of VOC Composition at Urban and Suburban Sites of New Delhi, India in Winter. J. Geophys. Res. Atmos. https://doi.org/10.1029/2021JD035342 (2022).
Act, A. (Prevention and C. of A. P. Air_Act_1981. (1981).
Environment (Protection) Act. The Environment (Protection) Act, 1986 Act No. 29 OF 1986. 1–9 (1986).
Bill, M. V. Amendment. THE GAZETTE OF INDIA EXTRAORDINARY. 1988 , 4–6 (2019).
Rengarajan, S., Palaniyappan, D., Ramachandran, P. & Ramachandran, R. National Green Tribunal of India—an observation from environmental judgements. Environ. Sci. Pollut. Res. 25 , 11313–11318 (2018).
CPCB. Pollution Control Acts, Rules & Notifications Issued Thereunder. Central Pollution Control Board, Ministry of Environment, Forest and Climate Change, Government of India. https://cpcb.nic.in/7thEditionPollutionControlLawSeries2021.pdf (2021).
CPCB. National Air Quality Index. Cent. Pollut. Control Board 1–58. https://app.cpcbccr.com/ccr_docs/About_AQI.pdf (2014).
National Ambient Air Quality Monitoring. Air Quality Trends and Action For Plan. Naaqms 5. http://cpcb.nic.in/upload/NewItems/NewItem_104_airquality17cities-package-.pdf (2006).
Roychowdhury, A. & Somvanshi, A. Breathing Space: How to track and report air pollution under the National Clean Air Programme. Cent. Sci. Environ. (New Delhi, 2020).
Roychowdhury, A., Somvanshi, A. & Kaur, S. Urban Lab-Centre for Science and Environment Analysis Status of air quality monitoring in India: Spatial spread, population coverage and data completeness. https://www.cseindia.org/Note-AQM-Network-analysis.pdf (2023).
Yadav, R. et al. COVID-19 lockdown and air quality of SAFAR-India metro cities. Urban Clim. 34 , 100729 (2020).
Lestari, P., Arrohman, M. K., Damayanti, S. & Klimont, Z. Emissions and spatial distribution of air pollutants from anthropogenic sources in Jakarta. Atmos. Pollut. Res. 13 , 101521 (2022).
Guttikunda, S. K., Nishadh, K. A. & Jawahar, P. Air pollution knowledge assessments (APnA) for 20 Indian cities. Urban Clim. 27 , 124–141 (2019).
Gargava, P. & Rajagopalan, V. Source apportionment studies in six Indian cities—drawing broad inferences for urban PM10 reductions. Air Qual. Atmos. Heal. 9 , 471–481 (2016).
M.C. Mehta And Anr vs Union Of India & Ors on 20 December, 1986. https://indiankanoon.org/doc/1486949/ .
AFVP 2025. Report of the Expert Committee on Auto Fuel Vision & Policy 2025. Press Inf. Bur. 221 , 174. https://cdn.climatepolicyradar.org/navigator/IND/2014/national-auto-fuel-policy-and-auto-fuel-vision-and-policy-2025_c53488e9acdfd8095d576abd64e15892.pdf (2014).
Sahu, V. et al. Assessment of a clean cooking fuel distribution scheme in rural households of India – “Pradhan Mantri Ujjwala Yojana (PMUY)”. Energy Sustain. Dev. 81 , 101492 (2024).
Das, P. K. & Bhat, M. Y. Global electric vehicle adoption: implementation and policy implications for India. Environ. Sci. Pollut. Res. 29 , 40612–40622 (2022).
Gimeno, L. et al. Major Mechanisms of Atmospheric Moisture Transport and Their Role in Extreme Precipitation Events. Annu. Rev. Environ. Resour. 41 , 117–141. https://doi.org/10.1146/annurev-environ-110615-085558 (2016).
Thayyib, P. V. et al. State-of-the-Art of Artificial Intelligence and Big Data Analytics Reviews in Five Different Domains: A Bibliometric Summary. Sustainability 15 , 4026 (2023).
Alzubaidi, L. et al. Review of deep learning: concepts, CNN architectures, challenges, applications, future directions. J. Big Data 8 , 53 (2021).
Fan, J. et al. A Spatiotemporal Prediction Framework for Air Pollution Based on Deep RNN. ISPRS Ann. Photogramm. Remote Sens. Spat. Inf. Sci. IV - 4/W2 , 15–22 (2017).
Rautela, K. S., Kumar, D., Gandhi, B. G. R., Kumar, A. & Dubey, A. K. Application of ANNs for the modeling of streamflow, sediment transport, and erosion rate of a high-altitude river system in Western Himalaya. Uttarakhand. RBRH https://doi.org/10.1590/2318-0331.272220220045 (2022).
Sofi, M. S. et al. Modeling the hydrological response of a snow-fed river in the Kashmir Himalayas through SWAT and Artificial Neural Network. Int. J. Environ. Sci. Technol. https://doi.org/10.1007/s13762-023-05170-7 (2023).
Karagulian, F. et al. Contributions to cities’ ambient particulate matter (PM): A systematic review of local source contributions at global level. Atmos. Environ. 120 , 475–483 (2015).
Mlakar, P., Božnar, M. & Lesjak, M. Neural Networks Predict Pollution. In Air Pollution Modeling and Its Application X (ed. Millán, M. M.) (Springer, 1994).
Arena, P., Fortuna, L., Gallo, A., Nunnari, G. & Xibilia, M. G. Air pollution estimation via neural networks. IFAC Proc. 28 , 787–792 (1995).
Sohn, S. H., Oh, S. C. & Yeo, Y.-K. Prediction of air pollutants by using an artificial neural network. Korean J. Chem. Eng. 16 , 382–387 (1999).
Slini, T., Karatzas, K. & Moussiopoulos, N. Correlation of air pollution and meteorological data using neural networks. Int. J. Environ. Pollut. 20 , 218 (2003).
Kandya, A. Forecasting the Tropospheric Ozone using Artificial Neural Network Modelling Approach: A Case Study of Megacity Madras. India. J. Civ. Environ. Eng. 01 , 2 (2013).
Chaloulakou, A., Saisana, M. & Spyrellis, N. Comparative assessment of neural networks and regression models for forecasting summertime ozone in Athens. Sci. Total Environ. 313 , 1–13 (2003).
Article ADS CAS PubMed Google Scholar
Mishra, D. & Goyal, P. Development of artificial intelligence based NO 2 forecasting models at Taj Mahal. Agra. Atmos. Pollut. Res. 6 , 99–106 (2015).
Fernando, H. J. S. et al. Forecasting PM10 in metropolitan areas: Efficacy of neural networks. Environ. Pollut. 163 , 62–67 (2012).
Grivas, G. & Chaloulakou, A. Artificial neural network models for prediction of PM10 hourly concentrations, in the Greater Area of Athens. Greece. Atmos. Environ. 40 , 1216–1229 (2006).
Suleiman, A., Tight, M. R. & Quinn, A. D. Hybrid Neural Networks and Boosted Regression Tree Models for Predicting Roadside Particulate Matter. Environ. Model. Assess. 21 , 731–750 (2016).
Zhang, H., Liu, Y., Shi, R. & Yao, Q. Evaluation of PM 10 forecasting based on the artificial neural network model and intake fraction in an urban area: A case study in Taiyuan City. China. J. Air Waste Manage. Assoc. 63 , 755–763 (2013).
Paschalidou, A. K., Karakitsios, S., Kleanthous, S. & Kassomenos, P. A. Forecasting hourly PM10 concentration in Cyprus through artificial neural networks and multiple regression models: Implications to local environmental management. Environ. Sci. Pollut. Res. 18 , 316–327 (2011).
Mishra, D., Goyal, P. & Upadhyay, A. Artificial intelligence based approach to forecast PM 2.5 during haze episodes: A case study of Delhi India. Atmos. Environ. 102 , 239–248 (2015).
Moisan, S., Herrera, R. & Clements, A. A dynamic multiple equation approach for forecasting PM 2.5 pollution in Santiago. Chile. Int. J. Forecast. 34 , 566–581 (2018).
Liu, H., Jin, K. & Duan, Z. Air PM2.5 concentration multi-step forecasting using a new hybrid modeling method: Comparing cases for four cities in China. Atmos. Pollut. Res. 10 , 1588–1600 (2019).
Chen, J. et al. A comparison of linear regression, regularization, and machine learning algorithms to develop Europe-wide spatial models of fine particles and nitrogen dioxide. Environ. Int. 130 , 104934 (2019).
Jain, S. & Khare, M. Adaptive neuro-fuzzy modeling for prediction of ambient CO concentration at urban intersections and roadways. Air Qual. Atmos. Heal. 3 , 203–212 (2010).
Carbajal-Hernández, J. J., Sánchez-Fernández, L. P., Carrasco-Ochoa, J. A. & Martínez-Trinidad, J. F. Assessment and prediction of air quality using fuzzy logic and autoregressive models. Atmos. Environ. 60 , 37–50 (2012).
Article ADS Google Scholar
Al-Shammari, E. T. Public warning systems for forecasting ambient ozone pollution in Kuwait. Environ. Syst. Res. 2 , 2 (2013).
Bougoudis, I., Demertzis, K. & Iliadis, L. HISYCOL a hybrid computational intelligence system for combined machine learning: the case of air pollution modeling in Athens. Neural Comput. Appl. 27 , 1191–1206 (2016).
Song, Y., Qin, S., Qu, J. & Liu, F. The forecasting research of early warning systems for atmospheric pollutants: A case in Yangtze River Delta region. Atmos. Environ. 118 , 58–69 (2015).
Wang, J., Li, H. & Lu, H. Application of a novel early warning system based on fuzzy time series in urban air quality forecasting in China. Appl. Soft Comput. 71 , 783–799 (2018).
Behal, V. & Singh, R. Personalised healthcare model for monitoring and prediction of airpollution: machine learning approach. J. Exp. Theor. Artif. Intell. 33 , 425–449 (2021).
Arbabsiar, M. H., Ebrahimi Farsangi, M. A. & Mansouri, H. Fuzzy logic modelling to predict the level of geotechnical risks in rock tunnel boring machine (TBM) tunnelling. Rud. Zb. 35 , 1–14 (2020).
Feng, Y., Zhang, W., Sun, D. & Zhang, L. Ozone concentration forecast method based on genetic algorithm optimized back propagation neural networks and support vector machine data classification. Atmos. Environ. 45 , 1979–1985 (2011).
Yeganeh, B., Motlagh, M. S. P., Rashidi, Y. & Kamalan, H. Prediction of CO concentrations based on a hybrid Partial Least Square and Support Vector Machine model. Atmos. Environ. 55 , 357–365 (2012).
García Nieto, P. J., Combarro, E. F., del Coz Díaz, J. J. & Montañés, E. A SVM-based regression model to study the air quality at local scale in Oviedo urban area (Northern Spain): A case study. Appl. Math. Comput. 219 , 8923–8937 (2013).
Luna, A. S., Paredes, M. L. L., de Oliveira, G. C. G. & Corrêa, S. M. Prediction of ozone concentration in tropospheric levels using artificial neural networks and support vector machine at Rio de Janeiro. Brazil. Atmos. Environ. 98 , 98–104 (2014).
Wang, P., Liu, Y., Qin, Z. & Zhang, G. A novel hybrid forecasting model for PM10 and SO2 daily concentrations. Sci. Total Environ. 505 , 1202–1212 (2015).
Freeman, B. S., Taylor, G., Gharabaghi, B. & Thé, J. Forecasting air quality time series using deep learning. J. Air Waste Manage. Assoc. 68 , 866–886 (2018).
Wang, J. & Song, G. A Deep Spatial-Temporal Ensemble Model for Air Quality Prediction. Neurocomputing 314 , 198–206 (2018).
Zhou, Y., Chang, F.-J., Chang, L.-C., Kao, I.-F. & Wang, Y.-S. Explore a deep learning multi-output neural network for regional multi-step-ahead air quality forecasts. J. Clean. Prod. 209 , 134–145 (2019).
Soh, P.-W., Chang, J.-W. & Huang, J.-W. Adaptive Deep Learning-Based Air Quality Prediction Model Using the Most Relevant Spatial-Temporal Relations. IEEE Access 6 , 38186–38199 (2018).
Qi, Y., Li, Q., Karimian, H. & Liu, D. A hybrid model for spatiotemporal forecasting of PM2.5 based on graph convolutional neural network and long short-term memory. Sci. Total Environ. 664 , 1–10 (2019).
Li, Y., Huang, J. & Luo, J. Using user generated online photos to estimate and monitor air pollution in major cities. Proceedings of the 7th International Conference on Internet Multimedia Computing and Service 1–5 (ACM, New York, NY, USA). https://doi.org/10.1145/2808492.2808564 . (2015).
Zhang, L., Nan, Z., Xu, Y. & Li, S. Hydrological impacts of land use change and climate variability in the headwater region of the Heihe River Basin, northwest China. PLoS One 11 , 1–25 (2016).
Li, X. et al. Long short-term memory neural network for air pollutant concentration predictions: Method development and evaluation. Environ. Pollut. 231 , 997–1004 (2017).
Zhang, C. et al. On Estimating Air Pollution from Photos Using Convolutional Neural Network. in Proceedings of the 24th ACM international conference on Multimedia 297–301 (ACM, New York, NY, USA). https://doi.org/10.1145/2964284.2967230 . (2016).
Qiu, Y. et al. Regional aerosol forecasts based on deep learning and numerical weather prediction. Npj Clim. Atmos. Sci. 6 , 71 (2023).
Goyal, M. K. & Rautela, K. S. Aerosol Atmospheric Rivers: Detection and Spatio-Temporal Patterns. https://doi.org/10.1007/978-3-031-66758-9_2 (2024).
Du, S., Li, T., Yang, Y. & Horng, S.-J. Deep Air Quality Forecasting Using Hybrid Deep Learning Framework. IEEE Trans. Knowl. Data Eng. 33 , 2412–2424 (2021).
Araujo, L. N., Belotti, J. T., Alves, T. A., de Tadano, Y. S. & Siqueira, H. Ensemble method based on Artificial Neural Networks to estimate air pollution health risks. Environ. Model. Softw. 123 , 104567 (2020).
Randles, C. A. et al. The MERRA-2 Aerosol Reanalysis, 1980 Onward. Part I: System Description and Data Assimilation Evaluation. J. Clim. 30 , 6823–6850 (2017).
Rautela, K. S., Singh, S. & Goyal, M. K. Aerosol atmospheric rivers: patterns, impacts, and societal insights. Environ. Sci. Pollut. Res. https://doi.org/10.1007/s11356-024-34625-8 (2024).
Buchard, V. et al. Evaluation of the surface PM2.5 in Version 1 of the NASA MERRA Aerosol Reanalysis over the United States. Atmos. Environ. 125 , 100–111 (2016).
Provençal, S., Buchard, V., da Silva, A. M., Leduc, R. & Barrette, N. Evaluation of PM surface concentrations simulated by Version 1 of NASA’s MERRA Aerosol Reanalysis over Europe. Atmos. Pollut. Res. 8 , 374–382 (2017).
Singh, S., Goyal, M. K. & Jha, S. Role of large-scale climate oscillations in precipitation extremes associated with atmospheric rivers: nonstationary framework. Hydrol. Sci. J. https://doi.org/10.1080/02626667.2022.2159412 (2023).
Cheggoju, N. & Satpute, V. R. Blind quality scalable video compression algorithm for low bit-rate coding. Multimed. Tools Appl. 81 , 33715–33730 (2022).
Download references
Acknowledgements
We would like to express our sincere gratitude to the Department of Civil Engineering, Indian Institute of Technology, Indore for their support and resources, which have been instrumental in the successful completion of the present study.
Author information
Authors and affiliations.
Department of Civil Engineering, Indian Institute of Technology Indore, Simrol, Indore, 453552, Madhya Pradesh, India
Kuldeep Singh Rautela & Manish Kumar Goyal
You can also search for this author in PubMed Google Scholar
Contributions
Kuldeep Singh Rautela: Conception and design, material preparation, data collection and analysis, writing original draft -review and editing. Manish Kumar Goyal: Conception and design, provided critical feedback, writing original draft -review and editing, supervision.
Corresponding author
Correspondence to Manish Kumar Goyal .
Ethics declarations
Competing interests.
The authors declare no competing interests.
Additional information
Publisher's note.
Springer Nature remains neutral with regard to jurisdictional claims in published maps and institutional affiliations.
Rights and permissions
Open Access This article is licensed under a Creative Commons Attribution-NonCommercial-NoDerivatives 4.0 International License, which permits any non-commercial use, sharing, distribution and reproduction in any medium or format, as long as you give appropriate credit to the original author(s) and the source, provide a link to the Creative Commons licence, and indicate if you modified the licensed material. You do not have permission under this licence to share adapted material derived from this article or parts of it. The images or other third party material in this article are included in the article’s Creative Commons licence, unless indicated otherwise in a credit line to the material. If material is not included in the article’s Creative Commons licence and your intended use is not permitted by statutory regulation or exceeds the permitted use, you will need to obtain permission directly from the copyright holder. To view a copy of this licence, visit http://creativecommons.org/licenses/by-nc-nd/4.0/ .
Reprints and permissions
About this article
Cite this article.
Rautela, K.S., Goyal, M.K. Transforming air pollution management in India with AI and machine learning technologies. Sci Rep 14 , 20412 (2024). https://doi.org/10.1038/s41598-024-71269-7
Download citation
Received : 11 March 2024
Accepted : 26 August 2024
Published : 02 September 2024
DOI : https://doi.org/10.1038/s41598-024-71269-7
Share this article
Anyone you share the following link with will be able to read this content:
Sorry, a shareable link is not currently available for this article.
Provided by the Springer Nature SharedIt content-sharing initiative
- Air pollution
- Technological innovation
- Regulatory frameworks
- Societal engagement
- Global collaboration
By submitting a comment you agree to abide by our Terms and Community Guidelines . If you find something abusive or that does not comply with our terms or guidelines please flag it as inappropriate.
Quick links
- Explore articles by subject
- Guide to authors
- Editorial policies
Sign up for the Nature Briefing newsletter — what matters in science, free to your inbox daily.


An official website of the United States government
The .gov means it’s official. Federal government websites often end in .gov or .mil. Before sharing sensitive information, make sure you’re on a federal government site.
The site is secure. The https:// ensures that you are connecting to the official website and that any information you provide is encrypted and transmitted securely.
- Publications
- Account settings
Preview improvements coming to the PMC website in October 2024. Learn More or Try it out now .
- Advanced Search
- Journal List

Air pollution in Delhi, India: It’s status and association with respiratory diseases
Abhishek dutta.
Department of Environmental Science, Faculty of Science, Chulalongkorn University, Pathumwan, Bangkok, Thailand
Wanida Jinsart
Associated data.
Data Availability: Air quality data of Delhi that support the findings of this study are owned by the Central Pollution Control Board (CPCB). For further information about the air quality data please visit https://cpcb.nic.in/real-time-air-qulity-data/ or https://app.cpcbccr.com/ccr/#/dashboard-emergency-stats . Meteorological data of Delhi can be obtained from the Regional Meteorological Centre, India Meteorological Department ( https://rmcnewdelhi.imd.gov.in/ ). Both for data and permission to use the data, please contact the Deputy Director General of Meteorology (DDGM), Regional Meteorological Centre, Lodi Road, New Delhi – 110003 via E-mail: moc.liamg@ihledwencfwr . Daily hospital visit data between the years 2016 and 2018 for respiratory diseases (ICD-10) J00-J99, used in this study, were collected from Vardhman Mahavir Medical College Safdarjung hospital, Ansari Nagar East, New Delhi. For data and permission to use data please contact the Medical Superintendent M.S. Office, New OPD Building, Safdarjung Hospital, New Delhi-110 029.Tel (011-26190763), e mail: ni.cin.hjs-cmmv@eciffosm .
The policymakers need research studies indicating the role of different pollutants with morbidity for polluted cities to install a strategic air quality management system. This study critically assessed the air pollution of Delhi for 2016–18 to found out the role of air pollutants in respiratory morbidity under the ICD-10, J00-J99. The critical assessment of Delhi air pollution was done using various approaches. The mean PM 2.5 and PM 10 concentrations during the measurement period exceeded both national and international standards by a wide margin. Time series charts indicated the interdependence of PM 2.5 and PM 10 and connection with hospital visits due to respiratory diseases. Violin plots showed that daily respiratory disease hospital visits increased during the winter and autumn seasons. The winter season was the worst from the city’s air pollution point of view, as revealed by frequency analyses. The single and multi-pollutant GAM models indicated that short-term exposure to PM 10 and SO 2 led to increased hospital visits due to respiratory diseases. Per 10 units increase in concentrations of PM 10 brought the highest increase in hospital visits of 0.21% (RR: 1.00, 95% CI: 1.001, 1.002) at lag0-6 days. This study found the robust effect of SO 2 persisted in Delhi from lag0 to lag4 days and lag01 to lag06 days for single and cumulative lag day effects, respectively. While every 10 μg m -3 increase of SO 2 concentrations on the same day (lag0) led to 32.59% (RR: 1.33, 95% CI: 1.09, 1.61) rise of hospital visits, the cumulative concentration of lag0-1 led to 37.21% (RR: 1.37, 95% CI:1.11, 1.70) rise in hospital visits which further increased to even 83.33% (RR: 1.83, 95% CI:1.35, 2.49) rise at a lag0-6 cumulative concentration in Delhi. The role of SO 2 in inducing respiratory diseases is worrying as India is now the largest anthropogenic SO 2 emitter in the world.
1. Introduction
Time and again, the policymakers felt the requirements of understanding the status of air pollution in growing cities and association of short-term air pollution exposures spanning one or a few days on morbidity. This is particularly more relevant for the world’s fast-growing cities to accrue benefits of sustainable development. Epidemiological studies conducted in the past in cities held air pollution responsible for inducing different health hazards. The quasi-poison regression model within over-dispersed Generalized Additive Model (GAM) has been very handy for many researchers for exploring the association of air pollution with different morbidity and mortality [ 1 – 6 ]. In a time series where the respondent variable depends on the nonlinear relationship of independent variables, GAM model finds its best applicability. In GAM, the nonlinear confounders can be controlled using smooth functions to correctly estimate the best connection between dependent and independent variables [ 7 – 12 ]. Accordingly, researchers used the GAM model extensively to indicate the role of air pollution in causing health effects for US and European cities [ 13 , 14 ].
Chinese and Indian cities frequently grabbed the world’s attention because of increasing air pollution and reported health effects on city dwellers. Indian cities were in the limelight because of the uncontrollable nature of air pollution in already declared polluted cities. Different Chinese cities have been put under strict scanners by the researchers who continuously reported or updated the policymakers on air pollution and health hazards so that policy-level initiatives may defuse the situation. Recently Lu et al. [ 15 ] reported that research ably supported the polluted Chinese cities to progress in air pollution control and place the much-needed strategic air quality management system. Another recent article indicated that out of 31 research papers published during 2010–2020 investigating the role of different air pollutants on the health of city dwellers using the GAM model, the majority, i.e., 17 were in the backdrop of Chinese cities and 3 for Indian cities [ 16 ]. GAM successfully explored the role of different pollutants in establishing their relationships with different types of respiratory morbidity/mortality for 21 cities of China, India, Iran, Brazil. Denmark and Kuwait ( S1 Table ). Zhao et al. [ 17 ], using GAM, reported that Dongguan city dwellers in China faced the threat of enhanced respiratory diseases due to short term exposure to CO. Song et al. [ 18 ] found respiratory diseases amongst the children of Shijiazhuang city of China due to PM 10 , SO 2 , NO 2 presence in the air. Cai et al. [ 19 ], studied the total respiratory diseases mortality of Shenzhen, China, and linked them with PM 2.5 presence in ambient air through GAM modelling. Liang et al. [ 20 ] used GAM model to indicate a direct relationship between pulmonary disease in Beijing with air pollution. Very recently Wang et al. [ 21 ] confirmed the role of particulate matter (PM) with pneumonia hospitalizations of children in Hefei, China.
Delhi, the capital city of India, is the second most populated and one of the most polluted cities in the world and should be the obvious choice for pollution and health hazard research. The recent air quality report of IQ Air has ranked Delhi first out of the air-polluted capital cities of 106 countries based on PM 2.5 concentration [ 22 ]. According to WHO, Delhi is the sixth-worst polluted city amongst 13 notable other Indian cities. Indeed, the city-dwellers had terrible times when PM 2.5 of Delhi stood at 440 μg m -3 during October 2019, i.e., 12 times the US recommended level. Past studied blamed the huge transport sector with the largest vehicle stock of the country as the critical emission source [ 23 – 27 ]. Chen et al. [ 28 ] demonstrated that local transport emissions and neighboring states contributed dominantly to PM 2.5 and O 3 concentration strengthening in Delhi. Sreekanth et al. [ 29 ] found high PM 2.5 pollution persists across all the seasons in Delhi despite pollution control efforts in vogue. In the pan-Indian context, air pollution significantly contributed to morbidity and premature mortality in India for a long time [ 30 ]. Sharma et al. [ 31 ] reviewed 234 journal papers and noted the knowledge gaps in connecting hospital admissions of patients with air pollution of Delhi. Balyan et al. [ 32 ] also noted that a deeper understanding of ambient pollutants at the city level and their effect on morbidity was lacking.
Against the background above, the primary objective of this paper to explore the environmental data of Delhi for confirming the poor air quality status of the city and, after that, assess the role of air pollutants with morbidity (respiratory diseases) through the application of the GAM model. A more profound grasp of the city air quality and influences of ambient air pollution on respiratory diseases is much needed. Such studies may provide all critical information for initiating actions to curb air pollution, health risk, developing public health policies, and above all, a strategic environmental management system for Delhi.
2. Study location
As a highly populated and polluted city, Delhi provides an opportunity to apply the GAM model for ascertaining how much the prevailing air pollution is responsible for respiratory diseases of the city dwellers. Delhi has spread over 1,483 km 2 and a population size of about 11 million per the 2011 census study. With time Delhi emerged as a significant city of the country so far as commerce, industry, medical service, and education are concerned. As per Köppen’s climate classification, Delhi’s climate is extreme with five seasons. The summer is scorching (April–June), while winter is freezing (December-January). The average temperature range during the summer is between 25°C to 45°C, while the winter temperature range is between 22°C to 5°C [ 33 ]. The comfortable season spring prevails from February to March, and autumn runs from mid-September to late November. The rainy monsoon season spans almost three months, starting from July. Air pollution varies across seasons due to the influence of climatic conditions [ 34 ].
3. Materials and methods
3.1. air pollution data.
Daily average data for three years, January 2016 to December 2018, (1096 data points) of key air pollutants were collected from the State Pollution Control Board (SPCB), Delhi. The pollutants were sulfur dioxide (SO 2 ), nitrogen dioxide (NO 2 ), carbon monoxide (CO), particulate meter 10 micrometers or less (PM 10 ), and particulate meter 2.5 micrometers or less (PM 2.5 ) as recorded by 11 NAMP (National Air Quality Monitoring Programme) stations of the city as shown in Fig 1 and S2 Table .

3.2. Meteorological data
Time series meteorological data for 1 January 2016–31 December 2018 were collected from Regional Meteorological Department located in Delhi. The data were of a total of 1096 days and included daily average temperature (T), daily average relative humidity (RH), daily average wind speed (WS), and daily rainfall (RF). The collected meteorological and air monitoring data will be adequate to estimate the confounding effect of meteorological conditions on morbidity related to respiratory diseases using GAM model.
3.3. Hospital visit data
We considered respiratory diseases covered by J00-J99 under the ICD-10 classification system. Data related to daily hospital outpatient visits of patients for respiratory diseases under International Classification of Diseases-10 (ICD-10), J00-J99 for 2016–2018 (1096 days) were collected from Safdarjung Medical College and Hospital (SMCH) of Delhi. The SMCH had its existence from pre-independence days of India and now functioning under the Ministry of Health and Family Welfare, Government of India. SMCH has many different specialties and super specialty departments, and Respiratory Medicine (RM) is one. Fig 1 shows that all the 11 air pollution monitoring stations considered in this study are located within a road distance of 12 km from SMCH. The hospital records contained information related visit date of patients, age, gender, and final medical diagnosis for each patient. The patient data were grouped age-wise under three categories (i) elderly people (more than or equal to 65 years), (ii) middle-aged (45–64 years), and (iii) young (less than or equal to 44 years). For hospital data collection formal request letter was submitted to the hospital authority. As the data were old data without identifiers and not having any possibility of ascertaining the identities of the individuals to whom the data belong, the hospital waived IRB approval.
3.4. Methods of analysis
3.4.1 summary statistics and analysis of time series.
Summary statistics of climatic variables, air pollutants, and hospital visits of the patients such as mean, standard deviation, maximum, minimum, and different quartiles were computed using the SPSS 25 version of the software. Daily hospital visit counts for three years (2016–2018) in SMCH were structured based on the patient’s age, sex, and visit dates. Violin plots were developed for three air pollutants (PM 10 , PM 2.5 , and CO), two climatic variables (T, RH), and hospital visits of patients regarding five seasons of Delhi, indicating the distribution of data prevailing in the city during different seasons. Violin plots have been drawn with XLSTAT statistical software. Time series plots were developed using the SPSS 25 version of the software with time dimensions on the horizontal axis and hospital visits, pollutants and, meteorological variables on the vertical coordinate axes to shed light on the data distribution for three years.
3.4.2 Frequency analysis
The seasonal distribution of PM 2.5 and PM 10 concentrations in Delhi during 2016–18 has been done by frequency analysis [ 35 ]. Under frequency analysis, first, the city level average concentrations of PM per day were calculated by averaging the concentration of 11 monitoring stations. Then, PM concentrations (both for PM 10 and PM 2.5 ), i.e., number of per day observations for the period 2016–18 falling under six categories like 0–25, 25–50, 50–100, 100–200, 200–300, and more than 300 μg m -3 worked out. So, the three-year period (2016–18) data or 1096 observations were segregated session-wise for each of the six categories, and the frequency of their appearances was then expressed in percentage terms. The calculations were done with the help of data analysis ’ToolPak’ of excel. As per the air quality index (AQI) Of India, the range 0–100 is considered a good category, 100–200 as moderate, 200–300 as poor, and above 300 as very poor or severe.
3.4.3 Correlation analysis
To understand the interrelationship between climatic variables and air pollutants data for Delhi (2016–2018), we executed Pearson correlation analysis using SPSS version 25.0 (SPSS Inc., Chicago, IL, USA) software. The coefficients of correlations were established between daily meteorological variables and air pollutants for Delhi. The correlation coefficients at p < .01 were accepted as statistically significant [ 36 ]. For better visualization, correlation matrix plots have been drawn with R software’s ’corrplot’ package.
3.4.4 Generalized Additive Models (GAM)
The nonlinear associations of various independent variables (climatic variables and criteria pollutants) and the outcome variable (hospital visits due to respiratory diseases) of Delhi can be better explained by (GAM) model. GAM explicitly allows the relationship between outcome variables independent variables to be developed based on the smooth functions fitted to some independent variables, thereby bringing the flavor of parametric relationships of the covariates in a regression model [ 37 , 38 ]. Accordingly, in this study, the potential confounding effects of few independent variables that entered the regression model were controlled with non-parametric smoothening splines. Smoothening splines of 7 degrees of freedom (df) per year were fitted to calendar time (time since 1 January 1970) to control long-term trends and possible calendar effects [ 39 , 40 ]. In line with Wei [ 41 ] smoothening splines with 7 df were also applied to mean RH and mean temperature (T) to control their respective confounding effects on the regression model. A linear term of mean wind speed (WS) was allowed to prevail. A dummy variable as the day of the week (DOW) was additionally introduced in the categorical form to control for week effects. As per Peng et al. and Zheng et al. [ 39 , 42 ], the dfs for smoothing splines were allowed to be determined by the generalized cross-validation (GCV) scores. Finally, based on the description of the regression model formation above, we formed the following GAM model ( Eq 1 ) in our present study with usual notations and applied.
where i denotes the day of observation; E ( Y i ) denotes the daily hospital visits expected due to respiratory diseases; β denotes regression coefficient; X i denotes the daily mean concentration of pollutants; s stands for the smoothing spline applied, and α is the intercept. Once the basic GAM model is set with the smoothing splines for RH, T, and time variables, the independent variables like PM 2.5 , PM 10 , NO 2 , SO 2 , and CO (per day concentrations) were added to the basic model to make it the multi-pollutant GAM model. We also constructed two single pollutant models for PM 2.5 and PM 10 , respectively, to understand their respective sole effects on respiratory diseases related to hospital visits in the city under study. In the single-pollutant model, PM 2.5 and PM 10 concentrations, in turn, were entered as independent variables in the base model. Generally, single pollutant models do not reflect the synergistic effect of pollutants on morbidity, but in consideration with the multi-pollutant models, they provide crucial complementary understanding.
The respective coefficients of pollutants of the multi-pollutant and single-pollutant GAM models, found out as regression model output, were the inputs in deriving the relative risk (RR) of hospital visits due to one unit rise of each modelled air pollutants in the ambient air.
Past studies have shown that the air pollutants remain in the ambient air and create lingering effects on morbidity. Accordingly, we have considered pollutant concentrations for a single day and multiple days in the study. We tested the lingering effects of air pollution for single-day lags and cumulative lag days. Single-day lag (lag0) means air pollutant concentrations on the same day of the hospital visit, while lag6 indicates air pollutant concentrations of 6 days before the hospital visit. Similarly, for cumulative concentrations of pollutants lag0-1indicate the mean of pollutant concentration of the current day and previous day of the hospital visit (i.e., 2 days mean). Similarly, lag 0–2 indicates the mean of current day pollutant concentration, 1 day before and 2 days before the visit (i.e. 3 days mean). In the same way, lag0-3, lag0-4, lag0-5, and lag0-6 means 4 days, 5 days, 6 days, and 7 days mean pollutant concentrations, respectively. We used single lags of 0, 1, 2, 3, 5, and 5 days (lag0–lag 5) and cumulative lags of 0–1, 0–2, 0–3, 0–4, 0–5, and 0–6 days (lag 0–1 to lag0-6) to explore the lag pattern of health effects in the multi pollutants and single pollutant models. The R software with "mgcv" package (version 4.0.2) was applied to construct the GAM models. For visualizations of GAM models developed in this study, we have used visual tools of the mgcViz R package.
3.5. Relative Risk (RR)
Relative risk (RR), often used in epidemiological studies, helps understand the risk of the outcome of an intervened event with non-intervened events. Thus, RR compares one group with another group. In this study, the exposure-response coefficient β of pollutants obtained from the GAM models under different lag conditions have been used to estimate RR and their 95% confidence intervals (95% CIs). RR for the i th predictor variable and its confidence intervals were calculated using the following Eqs 2 , 3 and 4 .
where Δ C i is the rise of the i th pollutant concentration in air and S.E i is the standard error of i th pollutants. Here, Δ C will be 1 unit increase in CO and 10 units increase in all other pollutants. RR provides information on the rise of hospital visits due to each unit increase of a pollutant’s concentration level. To make the RR estimates of daily hospital visits due to air pollution more expressive, we also calculated the percentage change (PC, %,) at 95% CI in the following way ( Eq 5 ).
PC = Percentage change of daily hospital visits due to air pollution
In all analyses p-value < 0.05 considered significant.
4. Results and discussion
4.1 data distribution and time-series analyses.
The distribution of criteria pollutants, climatic variables (T and RH), and daily counts of hospital visits in Delhi are placed in Table 1 for 2016–18. Table 1 indicates that the mean value of PM 2.5 and PM 10 concentrations exceeded the guidelines of NAAQS and WHO both by a wide margin. They shoot to as high as 693.08 μg m - ³ for PM 10 and 478.25 μg m - ³ for PM 2.5 during 2016–2018. The mean RH value of 58.5% (range, 98.3% to 12.5%) in Delhi indicates the city’s humid condition higher than the ideal level relative humidity for health and comfort of 30–50%. The three years mean temperature of 25.63 ± 7.65 °C with a maximum as high as 45°C and a minimum of 0.5°C along with a higher level of RH indicates the extreme climate of Delhi. Daily mean hospital visits of patients for respiratory diseases during 2016–18 was 20±23.52.
Variable | Mean ± SD | Maximum | Minimum | Percentile | IQR | ||
---|---|---|---|---|---|---|---|
25th | 50th | 75th | |||||
Temperature (°C) | 25.63 ± 7.65 | 45.00 | 0.56 | 19.44 | 28.06 | 31.39 | 11.94 |
Relative humidity (%) | 58.5 ± 18.76 | 98.3 | 12.5 | 45.98 | 60.5 | 72.3 | 26.33 |
PM (μg m ) | 107.32±71.06 | 478.25 | 18.53 | 54.83 | 85.93 | 142.80 | 87.97 |
PM (μg m ) | 210.61±95.90 | 693.08 | 38.65 | 140.79 | 203.28 | 262.57 | 121.78 |
NO (ppb) | 44.60±14.82 | 101.15 | 18.13 | 32.19 | 43.82 | 53.93 | 21.74 |
SO (ppb) | 14.65±4.35 | 32.26 | 6.84 | 11.43 | 13.76 | 16.86 | 5.43 |
CO (ppm) | 1.40±0.54 | 5.96 | 0.54 | 1.03 | 1.285 | 1.62 | 0.59 |
Daily Hospital admission | 20±23.52 | 176 | 0 | 6 | 11 | 28 | 22 |
Table 2 reveals that a total of 22,253 patients visited SMCH, Delhi, either for outpatient consultation or admission for respiratory diseases during 2016–2018, as retrieved from hospital records. The maximum number of people who visited the hospital for respiratory ailments for a day was 176, and the minimum 0 patients. Out of the total patients, 63.5% were female, and 30% had ≥65 years of age. Similarly, out of male patients, 52% were aged ≥65 years, as shown in Table 2 .
Variables | Total (N) | Mean | Standard Deviation | Minimum | Maximum |
---|---|---|---|---|---|
Patients visited | 22253 | 20.30 | 23.52 | 0 | 176 |
Gender distribution | |||||
Male | 8125 | 7.41 | 12.04 | 0 | 103 |
Female | 14128 | 12.89 | 17.37 | 0 | 175 |
Age distribution (Male) | |||||
≥65 | 4218 | 72.15 | 5.15 | 65 | 91 |
45–64 | 3000 | 53.23 | 6.28 | 45 | 64 |
≤44 | 907 | 37.61 | 6.50 | 4 | 44 |
Age female (Female) | |||||
≥65 | 8009 | 73.23 | 6.12 | 65 | 93 |
45–64 | 5678 | 56.12 | 6.30 | 45 | 64 |
≤44 | 441 | 37.33 | 6.23 | 7 | 44 |
Time series charts in ( Fig 2A–2F ) depict behaviors of meteorological variables (RH, temperature), air pollutants (PM 2.5 , PM 10 , and CO), hospital visits, and their interrelationship during 2016–2018 for Delhi. PM 2.5 and PM 10 were positively correlated in Delhi during 2016–18, indicating the interdependency ( Fig 2A ) while maintaining a positive correlation with hospital visits due to respiratory diseases ( Fig 2B and 2C ). Fig 2D–2E shows that hospital visits tended to negatively correlate with RH and temperature. Fig 2(F) shows a positive correlation of hospital visits with CO concentration too in the city’s environment.

The time series of Delhi from 2016–2018 (A) PM 2.5 Vs Hospital visit, (B) PM 10 Vs Hospital visit, (C) RH Vs Hospital visit, (D) T Vs Hospital visit, (E) CO Vs Hospital visit, (F) PM 2.5 Vs PM 10 .
Violin plots of three air pollutants (PM 10 , PM 2.5 , and CO), two meteorological variables (T, RH), and hospital visits of patients were drawn for the five distinct seasons of Delhi have been provided in ( Fig 3A–3F ) below. Fig 3A indicates that PM 2.5 dominates the city environment during winter and autumn. Fig 3B indicates that PM 10 dominates the city air during the winter and summer seasons, but the median value of PM 10 concentrations was higher during winter. The concentration of CO in the air remains high during winter and low during the monsoon season ( Fig 3C ). Fig 3D clearly shows that the city experiences comparatively higher RH during summer and monsoon, with the highest median value during monsoon. Fig 3E indicates that the city experiences the hottest season during summer and autumn. From Fig 3F , it can be observed that during the winter and autumn season’s daily hospital visits due to respiratory diseases increased. The rectangles within the violin plots indicate finishing points of the first and third quartile of data distribution with central dots as medians. The upper and lower whiskers show data spread.

(A) PM 2.5 , (B) PM 10 , (C) CO, (D) RH, (E) Temperature, (F) Hospital visit.
4.2 Seasonal distribution of PM 2.5 and PM 10 in Delhi
The frequency distribution of PM 2.5 and PM 10 concentrations for five Delhi seasons are shown in Fig 4 . Fig 4 indicates that the winter season was terrible from the air pollution point of view as almost 95.2% of the time, the ambient PM 2.5 concentrations recorded to be more than 100 μg m -3 . Alarmingly, 100% of the time, the ambient PM 10 concentrations crossed the 100 μg m -3 benchmark during winter, indicating very harsh wintertime for the city dwellers. The spring season brought some relief for the city dwellers when 42.2% of the time PM 2.5 concentrations crossed 100 μg m -3 benchmark, but PM 10 remained very strong with 99.4% of the time crossing the 100 μg m -3 benchmark. During summer, about 76.9% of the time PM 2.5 concentrations were under the ’good’ category, and 15.8% of the time PM 2.5 concentrations were more than the 100 μg m -3 benchmark. During summer PM 2.5 concentrations improved considerably with only 15.8% of the time, its concentrations were more than the 100 μg m -3 benchmark, but PM 10 remained razing with 97.8% time crossing 100 μg m -3 benchmark. However, two and half months of monsoon (July, August, and mid-September) brought relief from PM 2.5 pollution. Almost 100% of the time, PM 2.5 concentrations remained under the ’good’ category, but PM 10 remained 51.1% crossing the 100 μg m -3 benchmark during monsoon. From autumn (mid-September to late November), PM pollution built up with 97.8% of the time PM 2.5 concentrations crossing 100 μg m -3 benchmark, as shown in Fig 4 . In summary, the frequency distribution of PM 2.5 and PM 10 concentrations indicates that except winter, the PM concentrations remained very high, which could be a possible cause of health hazards for the city dwellers.

4.3 Correlation between pollutants and meteorological variables
Positive correlation existed between two important gaseous pollutants SO 2 and NO 2 (r = 0.341), while PM 10 maintained a mild positive correlation with SO 2 (r = 0.281). PM 10 almost had linear positive correlation both with NO 2 (r = 0.783) and CO (r = 0.733) as shown in Table 3 and Fig 5 . PM 2.5 also had positive correlation with SO 2 (r = 0.137), and positive linear correlation with NO 2 (r = 0.673) and CO (r = 0.757). Also, PM 10 and PM 2.5 maintained positive linear correlation.

Blue, red, and while indicate positive, negative, and no correlation respectively.
Variables | RF | T | DP | RH | WS | PM | NO | SO | CO | PM |
---|---|---|---|---|---|---|---|---|---|---|
RF | 1 | |||||||||
T | .097 | 1 | ||||||||
DP | .342 | .556 | 1 | |||||||
RH | .299 | -.351 | .482 | 1 | ||||||
WS | 0.047 | .347 | .220 | -.154 | 1 | |||||
PM | -.240 | -.612 | -.600 | 0.024 | -.438 | 1 | ||||
NO | -.227 | -.348 | -.626 | -.299 | -.262 | .673 | 1 | |||
SO | -.185 | 0.031 | -.540 | -.647 | .106 | .137 | .341 | 1 | ||
CO | -.173 | -.418 | -.501 | -.064 | -.391 | .757 | .721 | .150 | 1 | |
PM | -.285 | -.412 | -.611 | -.225 | -.289 | .897 | .738 | .281 | .733 | 1 |
**. Correlation is significant at the 0.01 level (2-tailed).
*. Correlation is significant at the 0.05 level (2-tailed).
4.4 Association of criteria pollutants with respiratory diseases, Delhi
Multi-pollutant and single pollutant GAM models were formed for Delhi to understand the impact of air pollutants on hospital visits due to respiratory diseases. Multi pollutant models indicate combined effects of the involved pollutants on the hospital visits, whereas single pollutant GAM models cast light on the sole effect of pollutants. The models were tested with different lag concentrations to comprehensively understand the impact of short-term exposure of pollutants on hospital visit counts due to respiratory diseases.
4.4.1. Association of criteria pollutants with respiratory diseases in Delhi (multi-pollutant models)
In the multi-pollutant model, criteria pollutants for 2016–18 were included in the base GAM model. Table 4 and Fig 6 indicate the relative risks (RR) of hospital visits due to a rise of 1 unit increase in CO and 10 units for all other pollutant concentrations for different single lag days. The RR patterns in Table 4 indicate synergistic effects of criteria pollutants on respiratory diseases related hospital visits in the city. Table 4 reveals that both PM 2.5 and PM 10 concentrations of all the 6 single lag days had no significant effect on respiratory disease-related hospital visits. The effect of NO 2 on hospital visits was there during lag1 day concentrations only but without any positive acceleration. The effect of SO 2 on respiratory diseases-related hospital visits was found to be robust instantaneously, i.e., the increase of every 10 ppb SO 2 on the same day (lag 0) resulted in a 32.6% (RR: 1.326, 95% CI: 1.089, 1.614) rise in hospital visits. The effect of SO 2 on hospital visits persisted throughout the lag days from lag0 up lag4. The increase in CO on hospital visits throughout the different lag days (lag0 to lag6) was found to be non-significant for respiratory diseases.

Lag 0 | Lag 1 | Lag 2 | Lag 3 | Lag 4 | Lag 5 | Lag 6 | |||||||||
---|---|---|---|---|---|---|---|---|---|---|---|---|---|---|---|
0.978 (-2.17) | 0.993 (-0.71) | 0.989 (-1.11) | 0.990 (-1.02) | 0.993 (-0.66) | 0.996 (-0.43) | 0.988 (-1.21) | |||||||||
0.957 | 1.000 | 0.971 | 1.015 | 0.968 | 1.010 | 0.968 | 1.012 | 0.973 | 1.014 | 0.975 | 1.017 | 0.967 | 1.009 | ||
0.05 | 0.52 | 0.31 | 0.36 | 0.53 | 0.70 | 0.26 | |||||||||
1.012 (1.21) | 1.007 (0.71) | 1.007 (0.707) | 1.007 (0.736) | 1.006 (0.628) | 1.008 (0.806) | 1.008 (0.847) | |||||||||
0.997 | 1.027 | 0.992 | 1.022 | 0.992 | 1.022 | 0.992 | 1.023 | 0.992 | 1.021 | 0.993 | 1.023 | 0.994 | 1.023 | ||
0.12 | 0.36 | 0.35 | 0.34 | 0.40 | 0.28 | 0.26 | |||||||||
0.958 (-4.213) | 0.920 (-8.045) | 0.946 (-5.401) | 0.993 (-0.660) | 1.007 (0.696) | 0.986 (-1.386) | 1.004 (0.429) | |||||||||
0.904 | 1.015 | 0.866 | 0.977 | 0.892 | 1.003 | 0.937 | 1.054 | 0.949 | 1.069 | 0.929 | 1.047 | 0.946 | 1.067 | ||
0.15 | <0.001 | 0.63 | 0.83 | 0.82 | 0.65 | 0.89 | |||||||||
1.326 (32.60) | 1.278 (27.77) | 1.384 (38.42) | 1.371 (37.14) | 1.296 (29.57) | 1.119 (11.87) | 1.180 (18.03) | |||||||||
1.089 | 1.614 | 1.048 | 1.558 | 1.142 | 1.677 | 1.130 | 1.665 | 1.069 | 1.571 | 0.919 | 1.362 | 0.970 | 1.437 | ||
<0.001 | < 0.01 | <0.01 | <0.01 | <0.01 | 0.26 | 0.10 | |||||||||
0.971 (-2.85) | 1.286 (28.58) | 2.521 (152.13) | 0.493 (-50.73) | 0.191 (-80.93) | 0.267 (-73.31) | 0.467 (-53.25) | |||||||||
0.273 | 3.456 | 0.318 | 5.200 | 0.657 | 9.680 | 0.125 | 1.947 | 0.047 | 0.779 | 0.066 | 1.079 | 0.114 | 1.923 | ||
0.96 | 0.72 | 0.18 | 0.31 | 0.02 | 0.06 | 0.29 |
* Figs. in the brackets indicates PC (% change in hospital visits)
Note: p < 0.05, p < 0.01, and p < 0.001 considered significant
Table 5 and Fig 6 below indicate the relative risks (RR) pattern of change in hospital visits due to a rise of 1 unit increase in CO and 10 units for all other pollutant concentrations for different cumulative concentrations of pollutants. Both for PM 2.5 and PM 10 , in terms of cumulative days effect of air pollution, no significant effect could be found. NO 2 and CO were also not significantly responsible for enhancing respiratory diseases in the city. However, per 10 ppb rise in cumulative lag days, concentrations of SO 2 led to a comparatively more robust effect on respiratory diseases than single-day lag effects. At lag0-1 per 10 ppb, rise in concentrations of SO 2 was associated with the percentage change in hospital visits of 37.21% (RR: 1.372, 95% CI: 1.107, 1.701), which increased to 83.34% (RR: 1.833, 95% CI: 1.351, 2.489) during the lag0-6 day. The result indicates the robust effect of pollutants SO 2 on respiratory disease-related hospital visits in Delhi.
Lag 0–1 | Lag 0–2 | Lag 0–3 | Lag 0–4 | Lag 0–5 | Lag 0–6 | |||||||
---|---|---|---|---|---|---|---|---|---|---|---|---|
0.986 (-1.44) | 0.981 (-1.93) | 0.984 (-1.59) | 0.984 (-1.56) | 0.978 (-2.16) | 0.976 (-2.36) | |||||||
0.963 | 1.009 | 0.957 | 1.005 | 0.958 | 1.011 | 0.957 | 1.012 | 0.951 | 1.007 | 0.947 | 1.006 | |
0.22 | 0.12 | 0.24 | 0.27 | 0.14 | 0.12 | |||||||
1.009 (0.92) | 1.010 (1.03) | 1.010 (0.96) | 1.012 (1.24) | 1.016 (1.61) | 1.017 (1.67) | |||||||
0.993 | 1.025 | 0.993 | 1.028 | 0.991 | 1.028 | 0.993 | 1.032 | 0.996 | 1.037 | 0.995 | 1.039 | |
0.25 | 0.23 | 0.30 | 0.21 | 0.13 | 0.14 | |||||||
0.928 (-7.20) | 0.955 (-4.52) | 0.960 (-3.95) | 0.956 (-4.40) | 0.962 (-3.82) | 0.963 (-3.74) | |||||||
0.871 | 0.989 | 0.893 | 1.021 | 0.895 | 1.031 | 0.888 | 1.030 | 0.890 | 1.040 | 0.887 | 1.045 | |
0.02 | 0.18 | 0.27 | 0.23 | 0.33 | 0.36 | |||||||
1.372 (37.21) | 1.429 (42.87) | 1.553 (55.27) | 1.573 (57.34) | 1.684 (68.44) | 1.833 (83.34) | |||||||
1.107 | 1.701 | 1.130 | 1.806 | 1.206 | 1.999 | 1.201 | 2.061 | 1.262 | 2.248 | 1.351 | 2.489 | |
<0.01 | <0.01 | <0.01 | <0.01 | <0.01 | <0.01 | |||||||
2.447 (144.68) | 1.577 (57.68) | 1.038 (3.79) | 0.671 (-2.86) | 0.543 (-5.70) | 0.814 (-8.61) | |||||||
0.541 | 11.068 | 0.296 | 8.413 | 0.168 | 6.415 | 0.094 | 4.787 | 0.064 | 4.603 | 0.081 | 8.197 | |
0.25 | 0.59 | 0.97 | 0.69 | 0.58 | 0.86 |
Note: p < 0.05, p < 0.01, and p < 0.001 considered significant.
Figs Figs7 7 and and8 8 below, drawn with the "mgcViz" R software package (Fasiolo et al., [ 43 ], provide the visual representation of the smoothing applied to the non-parametric terms and performance of the GAM model at lag0 respectively.

4.4.2. Association of criteria pollutants with respiratory diseases in Delhi (Single-pollutant models)
Two single-pollutant models were developed with pollutants PM 2.5 and PM 10, respectively, to understand the sole effect of PM pollution on respiratory diseases. We fitted different single lag days and cumulative lag days to express the association of daily hospital visits for respiratory diseases with a 10μg m -3 increase in PM 10 or PM 2.5 in Delhi. Both PM 2.5 and PM 10 did not show any significant association with the number of respiratory disease-related hospital visits in Delhi for all the single lag days considered here, as revealed by the p values ( Table 6 and Fig 9 ). In other words, the association of PM 2.5 and PM 10 with the respiratory disease was negligible as RR was found to be less than the baseline (RR<1).

Lag days | Pollutants and RR | PC (%) | LL | UL | p value | Lag days | Pollutants and RR | PC (%) | LL | UL | p value | ||
---|---|---|---|---|---|---|---|---|---|---|---|---|---|
Lag 0 | PM | 0.993 | -0.74 | 0.984 | 1.001 | 0.08 | Lag 0–1 | PM | 0.999 | -0.11 | 0.990 | 1.008 | 0.81 |
PM | 0.997 | -0.31 | 0.991 | 1.003 | 0.30 | PM | 1.001 | 0.08 | 0.995 | 1.007 | <0.05 | ||
Lag1 | PM | 0.998 | -0.24 | 0.989 | 1.006 | 0.60 | Lag 0–2 | PM | 0.996 | -0.36 | 0.987 | 1.006 | 0.46 |
PM | 0.999 | -0.05 | 0.993 | 1.006 | 0.86 | PM | 0.999 | -0.07 | 0.993 | 1.006 | 0.84 | ||
Lag 2 | PM | 1.001 | 0.12 | 0.993 | 1.010 | 0.79 | Lag 0–3 | PM | 0.998 | -0.21 | 0.988 | 1.008 | 0.69 |
PM | 1.002 | 0.21 | 0.996 | 1.008 | 0.50 | PM | 1.000 | 0.02 | 0.993 | 1.007 | <0.01 | ||
Lag 3 | PM | 0.998 | -0.19 | 0.989 | 1.007 | 0.67 | Lag 0–4 | PM | 0.999 | -0.06 | 0.989 | 1.010 | 0.92 |
PM | 1.000 | 0.00 | 0.994 | 1.006 | 0.99 | PM | 1.001 | 0.15 | 0.994 | 1.009 | <0.01 | ||
Lag 4 | PM | 0.996 | -0.42 | 0.987 | 1.004 | 0.34 | Lag 0–5 | PM | 0.999 | -0.14 | 0.987 | 1.010 | 0.80 |
PM | 0.998 | -0.20 | 0.992 | 1.004 | 0.53 | PM | 1.001 | 0.12 | 0.993 | 1.009 | <0.05 | ||
Lag 5 | PM | 0.999 | -0.12 | 0.990 | 1.008 | 0.79 | Lag 0–6 | PM | 1.000 | -0.03 | 0.988 | 1.012 | 0.96 |
PM | 1.000 | 0.03 | 0.994 | 1.006 | 0.93 | PM | 1.002 | 0.21 | 1.001 | 1.003 | <0.05 | ||
Lag 6 | PM | 0.997 | -0.29 | 0.989 | 1.006 | 0.51 | |||||||
PM | 0.999 | -0.06 | 0.993 | 1.005 | 0.84 |
*Note: p < 0.05, p < 0.01, and p < 0.001 considered significant
However, in cumulative exposure single-pollutant models, PM 10 was found to have persistently enhanced hospital visits of patients with the respiratory disease excepting lag 0–2 days, as shown in Table 6 . Table 6 shows that per 10 units increase in concentrations of PM 10 brought the highest increase in hospital visits of 0.21% (RR: 1.002, 95% CI: 1.001, 1.002) at lag0-6 days. PM 2.5 association with respiratory disease-related hospital visits found to be non-significant during all the cumulative lag days considered.
5. Conclusion and discussion
The study investigated first the level of air pollution in Delhi and then assessed the impact of air pollution on respiratory diseases. The result suggests that Delhi has been struggling to cope up with the increasing nature of criteria pollutants in the first place. A total of 22,253 patients visited the Delhi hospital either for outpatient consultation or admission for respiratory diseases for 2016–2018. The study found that the mean value of PM 2.5 and PM 10 concentrations for the period 2016–2018 were 107.32±71.06 μg m -3 and 210.61±95.90 μg m -3 for Delhi, respectively, which were substantially higher than the NAAQS and WHO standards. Out of the five seasons in Delhi, the winter season is hugely dominated by PM 2.5 and PM 10 pollution, as revealed by frequency analyses. Initial time series analysis revealed that PM 2.5 maintained a positive correlation with PM 10 have while PM 2.5 , PM 10 , and CO maintained a positive correlation with hospital visits during 2016–18 in Delhi. Pearson correlation analysis confirmed that PM 10 in Delhi had almost positive linear correlations with NO 2 and CO while PM 10 maintained a strong positive correlation with PM 2.5 . Interestingly, SO 2 too maintained a significant positive correlation with PM 2.5 , PM 10 , NO 2 , and CO. Previous studies in the Indian city of Mumbai highlighted the strong positive correlation of PM 2.5 with NO 2 and referred to them as a dummy indicator of air pollution due to transport-related emissions in the city [ 44 ]. In the same line, significant positive correlations between PM concentrations and gaseous pollutants, shown by air pollution data, point towards transport-related pollution, solvent evaporation, and waste disposal as sources [ 45 , 46 ].
This study shows PM 10 to have persistent enhancing effects on the number of hospital visits with the respiratory disease during all the cumulative lag days excepting lag 0–2 days. Luong et al. [ 47 ] reported PM 10 and respiratory disease-related hospital admission in polluted Hanoi city of Vietnam. Past studies confirmed the role of PM in inducing oxidative stress in the human respiratory system [ 48 ]. PM 10 impact on respiratory diseases in Delhi may be aggravated due to the road dust fraction of PM 10 that has significant oxidative potential [ 49 ]. It was interesting to note that in multi-pollutant models, the role of PM 10 causing respiratory diseases got subdued due to the combined presence of other pollutants in Delhi city.
This study found that short-term exposure to SO 2 and PM 10 led to increased hospital visits of the city dwellers due to respiratory diseases under (ICD-10) J00-J99. The present study reports the mean SO 2 in ambient air for three years (2016–18) as 14.65 ppb or 38.25 μg m -3 . SO 2 is a very critical gaseous pollutant connected with public health [ 50 ]. Past studies reported that an ordinary person could withstand only 2.62 μg m -3 of SO 2 in the ambient air without any respiratory problem [ 51 ]. However, short but higher concentration exposure to SO 2 gas can cause persistent pulmonary problems [ 52 ]. Orellano et al. [ 53 ], in a more recent and extensive review and metadata analysis, confirmed that short-term exposure to SO 2 , varying from few hours to days, can lead to an increased risk of respiratory morbidity/mortality. Our findings agree with that and found a robust effect of SO 2 on respiratory diseases hospital visits in Delhi. This study shows the robust effect of SO 2 persisted in Delhi throughout the single lag days (from lag0 up lag4) and had an instantaneous (same day, lag 0) increase of 32.6% (RR: 1.326, 95% CI: 1.089, 1.614) of hospital visits. The cumulative concentrations of SO 2 were more robust than the single lag day concentration in Delhi. While every 10 μg m -3 SO 2 concentrations on the same day (lag0) showing 32.59% (RR: 1.326, 95% CI: 1.089, 1.614) rise of hospital visits, the cumulative concentration on the day and its previous day (lag0-1) showing 37.21% (RR: 1.372, 95% CI: 1.107, 1.701) rise in hospital visits which further increased to even 83.33% (RR: 1.833, 95% CI: 1.351, 2.489) rise at a lag0-6 cumulative concentration of the pollutant in Delhi. Ren et al. [ 54 ], using the GAM model, confirmed the SO 2 effect on respiratory diseases in the fast-industrializing Chinese city of Wuhan and found that a 10 μg m -3 rise in SO 2 concentrations led to a rise of RR for respiratory disease mortality by 1.9% at lag0 day or same day. More recently, another two highly industrializing cities of Zhoushan and Hangzhou of China with the comparatively lesser presence of average SO 2 of 6.12 μg m -3 and 17.25 μg m -3 in ambient air, respectively, confirmed the active role of SO 2 in enhancing hospital visits of the patient for respiratory diseases [ 55 ]. Phosri et al. [ 56 ] also reported the effect of SO 2 for hospital admissions for respiratory diseases in industrializing Bangkok city of Thailand.
Recent COVID-19 and air pollution studies in Delhi indicated that even during the rigorous ’lockdown’ period, there was only a marginal decrease of mean SO 2 in the ambient air than in the regular times [ 33 , 57 ]. Therefore, it proves that a significant portion of ambient SO 2 in Delhi is likely to be from non-local origins like distant transfer, fossil fuel-fired thermal power plants in the bordering areas of Delhi, and biomass burning in the neighboring states. India’s recognition as the largest anthropogenic SO 2 emitter replacing China in recent times will be much more worrisome in the context of this study’s findings [ 58 , 59 ].
Suneja et al. [ 60 ], through an experimental study in Delhi, reported the seven-year (2011–2018) mean value of SO 2 level was 2.26 ppb, while this study found a much higher three-year average (2016–18) of 14.65 ppb, indicating the rise of SO concentrations in Delhi in the more recent years. The association of respiratory diseases with PM 10 and SO 2 was found stable in different lag days analyses, indicating the problem’s depth for the city dwellers. The robust and instantaneous nature of the relationship between SO 2 and respiratory morbidity indicated in this study and evidence of similar relationships found in the previous studies highlight the necessity of taking policy-level measures to reduce SO 2 in the ambient air. Limited GAM model application in Indian cities to link air pollution and health effects is not a limitation of the present study findings but rather a call for more sponsored research in the area.
Supporting information
Acknowledgments.
The authors thank the Central Pollution Control Board and the Indian Meteorological Department of Delhi city for providing air pollution and meteorological information, respectively.
Funding Statement
This study was supported by the Graduate School Thesis Grant GCUGR1225632064D, Chulalongkorn University, Bangkok, Thailand. The funders had no role in study design, data collection and analysis, decision to publish, or preparation of the manuscript.
Data Availability
10 September 2024: Due to technical disruption, we are experiencing some delays to publication. We are working to restore services and apologise for the inconvenience. For further updates please visit our website: https://www.cambridge.org/universitypress/about-us/news-and-blogs/cambridge-university-press-publishing-update-following-technical-disruption
We use cookies to distinguish you from other users and to provide you with a better experience on our websites. Close this message to accept cookies or find out how to manage your cookie settings .
Login Alert

- > Global Change and Future Earth
- > Air Pollution and Human Health Risk Reduction: The Case Study of Delhi Megacity, India
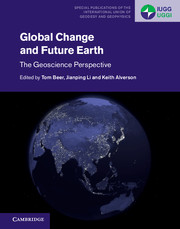
Book contents
- Global Change and Future Earth
- Special Publications of the International Union of Geodesy and Geophysics Series
- Global Change and Future Earth: The Geoscience Perspective
- Copyright page
- Contributors
- Acknowledgments
- Abbreviations
- Part I Future Earth and Planetary Issues
- Part II Future Earth and Geodetic Issues
- Part III Future Earth and the Earth’s Fluid Environment
- Part IV Future Earth and Regions
- Part V Future Earth and Urban Environments
- 15 Nutrition, Urban Environments, and Future Earth
- 16 Nutrition Science and Future Earth: Current Nutritional Policy Dilemmas
- 17 Air Pollution and Human Health Risk Reduction: The Case Study of Delhi Megacity, India
- Part VI Future Earth and Food Security
- Part VII Future Earth and Risk, Safety and Security
- Part VIII Climate Change and Global Change
- Subject Index
17 - Air Pollution and Human Health Risk Reduction: The Case Study of Delhi Megacity, India
from Part V - Future Earth and Urban Environments
Published online by Cambridge University Press: 22 October 2018
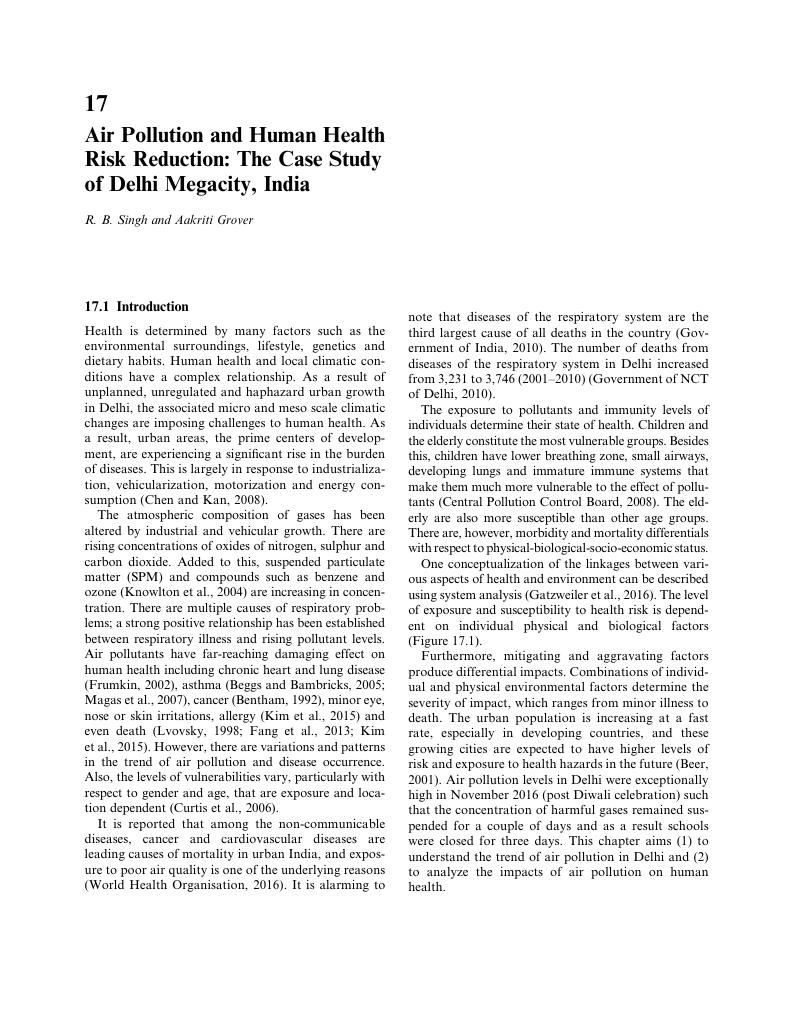
Access options
Save book to kindle.
To save this book to your Kindle, first ensure [email protected] is added to your Approved Personal Document E-mail List under your Personal Document Settings on the Manage Your Content and Devices page of your Amazon account. Then enter the ‘name’ part of your Kindle email address below. Find out more about saving to your Kindle .
Note you can select to save to either the @free.kindle.com or @kindle.com variations. ‘@free.kindle.com’ emails are free but can only be saved to your device when it is connected to wi-fi. ‘@kindle.com’ emails can be delivered even when you are not connected to wi-fi, but note that service fees apply.
Find out more about the Kindle Personal Document Service .
- Air Pollution and Human Health Risk Reduction: The Case Study of Delhi Megacity, India
- By R. B. Singh , Aakriti Grover
- Edited by Tom Beer , Jianping Li , Beijing Normal University , Keith Alverson
- Book: Global Change and Future Earth
- Online publication: 22 October 2018
Save book to Dropbox
To save content items to your account, please confirm that you agree to abide by our usage policies. If this is the first time you use this feature, you will be asked to authorise Cambridge Core to connect with your account. Find out more about saving content to Dropbox .
Save book to Google Drive
To save content items to your account, please confirm that you agree to abide by our usage policies. If this is the first time you use this feature, you will be asked to authorise Cambridge Core to connect with your account. Find out more about saving content to Google Drive .
Environmental Pollution and Control: A Case Study of Delhi Mega City
- Population and Environment 25(5):461-473
- 25(5):461-473

- International Institute for Population Sciences
Abstract and Figures

Discover the world's research
- 25+ million members
- 160+ million publication pages
- 2.3+ billion citations
- AEROBIOLOGIA
- Chetna Ugale
- Jaykiran A. Tidke
- Girish Korekar
- Anita Sheshrao Ingle
- Narsingrao Narayanswami Bandela
- Anshu Sharma

- Ravindra V. Wanjule
- H. H. Shinde

- Ritesh Kumar

- Tugba Karacocuk

- R. B. Singh
- WHO Commission on Health and Environment
- P Chatterjee
- Recruit researchers
- Join for free
- Login Email Tip: Most researchers use their institutional email address as their ResearchGate login Password Forgot password? Keep me logged in Log in or Continue with Google Welcome back! Please log in. Email · Hint Tip: Most researchers use their institutional email address as their ResearchGate login Password Forgot password? Keep me logged in Log in or Continue with Google No account? Sign up
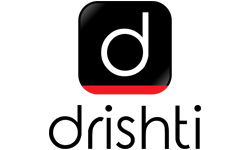
- Classroom Programme
- Interview Guidance
- Online Programme
- Drishti Store
- My Bookmarks
- My Progress
- Change Password
- From The Editor's Desk
- How To Use The New Website
- Help Centre
Achievers Corner
- Topper's Interview
- About Civil Services
- UPSC Prelims Syllabus
- GS Prelims Strategy
- Prelims Analysis
- GS Paper-I (Year Wise)
- GS Paper-I (Subject Wise)
- CSAT Strategy
- Previous Years Papers
- Practice Quiz
- Weekly Revision MCQs
- 60 Steps To Prelims
- Prelims Refresher Programme 2020
Mains & Interview
- Mains GS Syllabus
- Mains GS Strategy
- Mains Answer Writing Practice
- Essay Strategy
- Fodder For Essay
- Model Essays
- Drishti Essay Competition
- Ethics Strategy
- Ethics Case Studies
- Ethics Discussion
- Ethics Previous Years Q&As
- Papers By Years
- Papers By Subject
- Be MAINS Ready
- Awake Mains Examination 2020
- Interview Strategy
- Interview Guidance Programme
Current Affairs
- Daily News & Editorial
- Daily CA MCQs
- Sansad TV Discussions
- Monthly CA Consolidation
- Monthly Editorial Consolidation
- Monthly MCQ Consolidation
Drishti Specials
- To The Point
- Important Institutions
- Learning Through Maps
- PRS Capsule
- Summary Of Reports
- Gist Of Economic Survey
Study Material
- NCERT Books
- NIOS Study Material
- IGNOU Study Material
- Yojana & Kurukshetra
- Chhatisgarh
- Uttar Pradesh
- Madhya Pradesh
Test Series
- UPSC Prelims Test Series
- UPSC Mains Test Series
- UPPCS Prelims Test Series
- UPPCS Mains Test Series
- BPSC Prelims Test Series
- RAS/RTS Prelims Test Series
- Daily Editorial Analysis
- YouTube PDF Downloads
- Strategy By Toppers
- Ethics - Definition & Concepts
- Mastering Mains Answer Writing
- Places in News
- UPSC Mock Interview
- PCS Mock Interview
- Interview Insights
- Prelims 2019
- Product Promos
- Daily Updates
Biodiversity & Environment
Make Your Note
Battling the Winter Smog: Delhi's Pollution Predicament
- 28 Oct 2023
- 11 min read
- GS Paper - 2
- Government Policies & Interventions
- Environmental Pollution & Degradation
This editorial is based on “Delhi's battle against pollution” which was published in The Hindustan Times on 24/10/2023. It talks about the deteriorating air quality and the factors that contribute to the pollution crisis.
For Prelims: Air quality index , SAFAR , stubble burning, Smog , Temperature inversion,
For Mains: Delhi Air pollution and its causes, Government initiatives and Way Forward
Recently, Delhi got a trailer of the inevitable environmental misery that awaits it in the coming months: Air pollution. On Some day, the air quality index surpassed 300 on a scale topping out at 500, as a blanket of haze shrouded the skies and a distinct smell of dust and smoke pervaded the outdoors. Luckily, winds picked up the following day and the skies cleared up.
For the 20 million residents of the city (and millions more in neighboring states), it was a relief for the air to improve — from “very poor” to just “poor”.
Delhi’s pollution is a serious health hazard that affects millions of people every year. According to a study by the Indian Council of Medical Research , air pollution was responsible for 1.67 million deaths in India in 2019 , and Delhi had the highest per capita mortality rate due to air pollution among all states.
What are the Reasons behind Rising Pollution levels in Delhi during Winters?
- According to SAFAR , in 2021, stubble burning's contribution to Delhi pollution was 25%.
- Stubble burning emits toxic pollutants in the atmosphere containing harmful gasses like Carbon Monoxide (CO), methane (CH4), carcinogenic polycyclic aromatic hydrocarbons, volatile organic compounds (VOC).
- According to a study conducted by National Physical Laboratory, 72% of Delhi’s wind in winters comes from the northwest.
- For example, on October 25, 2023, the air quality improved marginally when the wind changed direction from north to northeast.
- Temperature inversion affects Delhi’s pollution in winter, when the weather is cold and calm. The pollutants from stubble burning, vehicle emissions, industrial emissions, and other sources accumulate in the lower atmosphere and form a thick layer of smog .
- Dry and Still Air: In winters, there is less rainfall and wind speed, which means that the pollutants do not get washed away or diluted by fresh air. The pollutants remain suspended in the air for longer periods of time.
- A study by IIT Delhi noted that vehicular emissions contribute around 25% to Delhi’s PM2.5 levels.
- A 2015 study conducted by IIT-Kanpur states that 17-26% of all particulate matter in Delhi in winters is because of biomass burning.
Government Initiatives to Control Delhi's Pollution
- Green War Room: A nine-member team that monitors the actions taken by 20 government agencies against pollution on a real-time and daily basis.
- Anti-Pollution Campaign: Delhi Government has recently launched a major anti-pollution campaign, Yuddh Pradushan Ke Viruddh, which includes a tree transplantation and other such initiatives.
- Green Delhi App: A mobile app that allows citizens to report any instances of pollution such as garbage burning, industrial emissions, or traffic congestion.
- Bio-Decomposer : A solution developed by PUSA institute that helps farmers decompose the crop residue in their fields without burning it. The government provides free spraying of bio-decomposer in Delhi’s farmlands.
- Water Sprinklers: The use of water sprinklers, mechanized road sweeping machines, anti-smog guns, and sprinkling facilities on high-rise buildings to reduce dust and particulate matter in the air.
- Industry Pollution: The monitoring of industrial sites and ensuring that they use clean and authorized fuel. The government has also extended piped natural gas (PNG) to industries and set up the country’s first e-waste eco-park in Delhi.
- PUC Certificates: The enforcement of pollution under control (PUC) certificates for vehicles and banning trucks that carry non-essential goods from entering the city. The government has also hired 1,000 private CNG vehicles to augment the public transport system.
- Smog Towers: The installation of smog towers that use large fans and filters to purify the air. The first smog tower has been set up at Connaught Place and has shown positive effects.
- Pollution Hotspots: The identification of 21 pollution hotspots in Delhi and deploying special teams to monitor and mitigate the sources of pollution in these areas.
What Measures should be taken to Control Delhi’s Pollution?
- A congestion charge is a fee that drivers have to pay to enter or use certain areas or roads that are prone to traffic congestion.
- Cap-and-Trade for Industrial Emissions: A cap-and-trade system sets a limit on industrial emissions and promotes a market-driven approach to reducing pollution. This system creates financial incentives for industries to reduce their emissions and invest in cleaner technologies, ultimately leading to a decrease in overall pollution.
- For instance, the New Engineering Education Transformation (NEET) cohort’s drone system is designed to provide real-time air quality data with a 15-meter resolution that is publicly accessible through a user-friendly interface.
- Vertical Gardens: Vertical gardens are an aesthetically pleasing and environmentally beneficial addition to urban areas. They not only enhance the visual appeal of the city but also help purify the air by absorbing carbon dioxide and releasing oxygen. Additionally, they can provide habitats for birds and insects, contributing to urban biodiversity.
- Rewards for Low-Carbon Lifestyles: Encouraging citizens to adopt low-carbon lifestyles through a rewards system is an innovative approach. By providing incentives like points or vouchers or tax benefits for eco-friendly behaviors such as using public transport or carpooling, people are more likely to make environmentally conscious choices, reducing their carbon footprint.
Analyze the key factors contributing to Delhi's pollution and suggest measures that could be taken to address the persistent problem of air pollution in Delhi. |
UPSC Civil Services Examination Previous Year Question (PYQ)
Q. In the cities of our country, which among the following atmospheric gases are normally considered in calculating the value of Air Quality Index? (2016)
- Carbon dioxide
- Carbon monoxide
- Nitrogen dioxide
- Sulfur dioxide
Select the correct answer using the code given below:
(a) 1, 2 and 3 only (b) 2, 3 and 4 only (c) 1, 4 and 5 only (d) 1, 2, 3, 4 and 5
Q. Describe the key points of the revised Global Air Quality Guidelines (AQGs) recently released by the World Health Organisation (WHO). How are these different from its last update in 2005? What changes in India’s National Clean Air Programme are required to achieve revised standards? (2021)
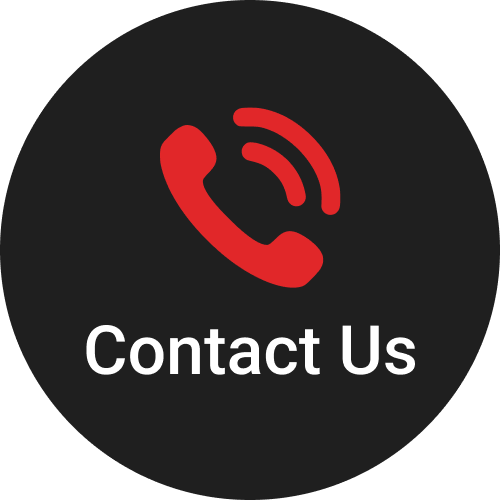
Crop residue burning in Punjab not main culprit of air pollution in Delhi: Study
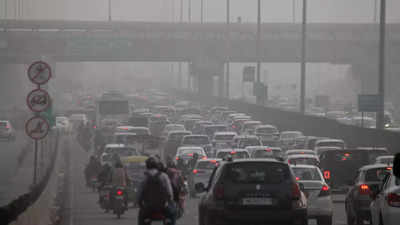
About the Author
Vinod Kumar is with The Times of India’s Punjab Bureau at Chandigarh. He covers news concerning Punjab politics, Health, Education, Employment and Environment.
Visual Stories
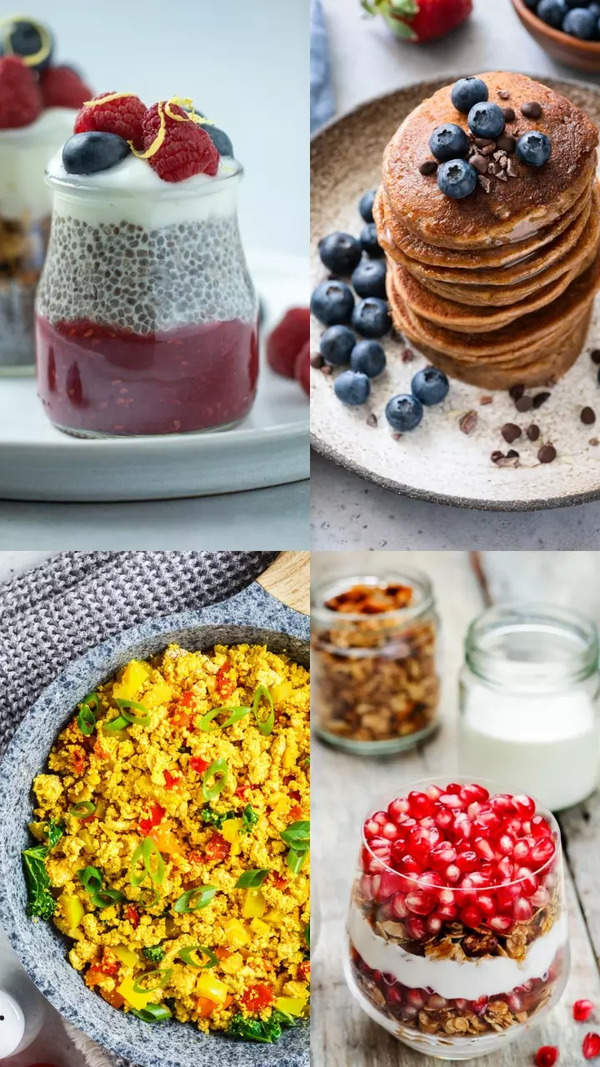

COMMENTS
This study assesses Delhi's air pollution scenario in the winter of 2021 and the actions to tackle it. Winter 2021 was unlike previous winters as the control measures mandated by the Commission of Air Quality Management (CAQM) in Delhi National Capital Region and adjoining areas were rolled out. These measures included the Graded Response ...
The answer lies in water, writes climate expert Mridula Ramesh. India loses an estimated $95bn (£70bn) to air pollution every year. From mid-March to mid-October, when Delhi's air quality varies ...
Delhi's annual average PM2.5 concentration in 2021-22 was 100 μg/m3—20 times more than the WHO guideline of 5 μg/m3. This is an improvement compared to the limited information available for the pre-CNG-conversion era (~30%), immediately before and after 2010 CWG (~28%), and the mid-2010s (~20%). These changes are a result of continuous technical and economic interventions interlaced ...
Another study estimated that on average, 20% of PM 2.5 in Delhi during the post-monsoon season was from crop fires, but that could peak to 50-75% on severe days 2.
November 25, 2020. 4 min read. After an unexpected respite as coronavirus lockdowns stalled economic activity, air pollution has returned to pre-COVID-19 levels in Delhi, the world's most air ...
We have science and the finance, but we lack a reduction-based approach," said Sunil Dahiya, from the Centre for Research on Energy and Clean Air (CREA) in New Delhi. In comparison to Beijing ...
The top court first began hearing cases on Delhi pollution in 1984, when environmentalist MC Mehta filed a clutch of pleas on three main issues: rising vehicular pollution in Delhi, the impact of ...
Through this study, we intend to examine what worked and what did not this season. As is the case every year, meteorological conditions played an important role in both aggravating and alleviating pollution levels. To assess the impact of meteorological conditions on Executive summary A Case Study of Delhi's Air Pollution of Winter 2021
The study intends to support the Delhi government, the Central Pollution Control Board (CPCB), and the Delhi Pollution Control Committee (DPCC) in identifying priority areas of interventions needed in controlling air pollution in Delhi during the winter season of 2021. It establishes the primary drivers of Delhi's winter air pollution during ...
A vision to tackle Delhi's dirty air. When thirty-eight-year-old Jasmine Shah moved to Delhi in 2013 and found himself engulfed in the city's toxic winter smog, everything changed for him. "This scale of air pollution in Delhi, and the fact that it is among the top ten most polluted cities in the world, resulted in my urgent call to ...
Air pollution can shorten lives by almost 10 years in the Indian capital, Delhi, the world's most polluted city, says a report by a US research group. The study adds that the average Indian life ...
A recent study has identified Delhi as the most polluted city in the world, with residents potentially losing a significant portion of their lifespan due to pollution. The study, called the Air Quality Life Index (AQLI), was published in August 2023 by the Energy Policy Institute at the University of Chicago.
A study funded by the World Bank Development Research Group was carried out in 1991-1994 to study the effects of air pollution. During the study period, the average total suspended particulate (TSP) level in Delhi was approximately five-times the World Health Organization's annual average standard. Furthermore, the total suspended particulate ...
Talukdar, S. et al. Air Pollution in New Delhi during Late Winter: An Overview of a Group of Campaign Studies Focusing on Composition and Sources. Atmosphere (Basel). 12 , 1432 (2021).
The policymakers need research studies indicating the role of different pollutants with morbidity for polluted cities to install a strategic air quality management system. This study critically assessed the air pollution of Delhi for 2016-18 to found out the role of air pollutants in respiratory morbidity under the ICD-10, J00-J99.
Understanding urban vehicular pollution problem vis-à-vis air quality: Case study of a megacity (Delhi, India). Environmental Monitoring and Assessment , 119 , 557 - 569 . Google Scholar
A study in 2016 measured the sources and average levels of various types of air pollution in Delhi. Of PM2.5 pollution, 38% came from road dust, 20% to vehicles, 12% to domestic fuel burning, and 11% to industrial point sources. Of PM10 pollution, 56% came from road dust, 10% from concrete batching, 10% from industrial point sources, and 9% ...
India's capital Delhi has alarmingly high levels of indoor air pollution, new research has found. The study found that the levels of PM2.5, the lung-damaging tiny particles in the air, indoors ...
Air Pollution - Monitoring and Management A case Study DelhiAi. r M P George Delhi Pollution Control CommitteeAir PollutionAir pollution is the contamination of air due to the presence. r introduction of a substance which has a poisonous effect.Air pollution is a type of environmental pollution that affects the air and is usually caused by Dust ...
Environmental pollution and its impact on the incidence of morbidity and mortality pattern: A case study of Delhi mega city. Paper presented in IASP Conference, Visakhapatnam, 22-24 February, 2001.
Around 64% of Delhi's winter pollution load comes from outside Delhi, according to the study, which used data from UrbanEmissions.Info, the Decision Support System for Air Quality Management in Delhi, and the Air Quality Early Warning System for Delhi. Local sources of PM2.5 include transport (12%), dust (7%), and domestic biomass burning (6%).
Delhi's pollution is a serious health hazard that affects millions of people every year. According to a study by the Indian Council of Medical Research, air pollution was responsible for 1.67 million deaths in India in 2019, and Delhi had the highest per capita mortality rate due to air pollution among all states.
Delhi's winter air pollution crisis prompts the government to unveil a 21-point action plan. ... A Centre for Science and Environment study established that Delhi has seen a gradual and consistent ...
A recent study shows that industrial, transport, and domestic activities in Delhi and surrounding areas contribute more to carbon monoxide levels than crop residue burning in Punjab. The research ...