- Reference Manager
- Simple TEXT file

People also looked at
Systematic review article, a review of the application of virtual reality technology in higher education based on web of science literature data as an example.
- Department of Educational Technology, Faculty of Education, Fujian Normal University, Fuzhou, China
In recent years, with the rapid development of information technology, the visualization and interaction of virtual reality technology has developed, making the application of VR technology in education more and more attractive to scholars. This paper adopts the literature analysis method, focusing on the application of VR technology in the field of higher education, selects 80 empirical studies in the Web of Science literature database, conducts in-depth reading and analysis of the papers, and summarizes the experience of applying VR technology in the field of higher education. In order to deepen the application of VR in higher education. The research results show that the research objects of VR application in higher education are mainly undergraduates, the main majors of application are science, engineering and medical related majors, and the application of humanities and social sciences is relatively rare. At present, the devices used for VR in higher education are mainly computers and headsets, which are not portable enough. In addition, students lack guidance and training in the use of VR equipment before class. Compared with traditional education, most of the studies show that the application of VR to higher education and teaching has positive effects, mainly by affecting students' behaviors to affect students' learning results. The researchers mainly use traditional evaluation methods to evaluate teaching effects, use questionnaires and tests to collect data, and use data analysis methods mainly difference analysis and descriptive analysis. Based on the research results, the researchers put forward some suggestions at the end of the paper.
Introduction
VR is also called artificial environment. The definition of modern VR technology is using complex technology to form synthetic stimulation to replace real-world sensory information, which means that users enter the virtual scene and use special helmets, data gloves, or input devices such as keyboards and mice to interact with the virtual environment in real-time. VR can make the user feel like they are in a real environment ( Shin, 2018 ). The core VR originated in the 1960s. In the early 1990s, the Interactive
Systems Project Working Group funded by the National Science Foundation of the United States made a systematic discussion of VR. But due to the underdeveloped technical equipment at that time and the high cost of VR-related equipment, VR technology has not been developed for a long time. Later, with the rapid development of technology and the emergence of affordable VR headsets and other devices for games and entertainment, VR ushered in the second spring. At present, a new generation of information technology represented by VR technology has been widely used in the field of education. VR can provide students with teaching aids that are closer to real life, rich and diverse personalized learning environments, and change the boring traditional classrooms. Students are also provided with opportunities for active exploration and interactive communication to promote active learning. Additionally, VR has been described as a learning aid for the 21st century ( Rogers, 2019 ), with a study showing that students retain more information and are better able to apply what they learn after engaging in VR exercises ( Krokos et al., 2019 ). With the use of VR to enhance potential learning, it is understandable why researchers, organizations and educators are now paying close attention to the technology.
Although the application of VR technology in education is not new, the development of VR technology in visualization and interaction in recent years has made the application of VR technology in education more and more attractive to scholars, especially in higher education field. The core characteristics of VR are immersion, interactivity, and imagination, and these three characteristics make VR a huge advantage in the field of higher education ( Ryan, 2015 ). First of all, VR has immersion, using this technology can build a realistic virtual environment, students are immersed in it. Second, VR is interactive. In the VR environment, when students perform operations, the environment will give students corresponding feedback, and interaction can deepen students' impression of the classroom and master knowledge more efficiently. Third, VR has imagination, students can deeply understand related issues according to their own senses, cognitive methods and cognitive ability in the simulated VR environment, which also can expand students' innovative thinking awareness and effectively enhance students' creativity and imagination.
Due to the increasing attention of the academic community to VR technology, there have been some comprehensive overviews and systematic reviews of VR educational applications, but the research of many scholars has the following problems. First, many scholars have put together virtual reality, augmented reality and mixed reality to study the application of education in education ( Alalwan et al., 2020 ; Duarte et al., 2020 ). Second, most of the research on the application of virtual reality technology to higher education starts from a theoretical perspective and studies the possibility of virtual reality technology in certain disciplines in the field of higher education, and there are few empirical studies ( Moore, 1995 ; Hoffman and Vu, 1997 ). Finally, most of the current research reviews are aimed at a certain discipline or a certain course of higher education, and few researchers have comprehensively analyzed the overall application of VR technology in higher education.
This study focuses on the application of VR technology in the field of higher education. Through the analysis of the Web of Science database literature, it comprehensively analyzes the application and existing problems of VR technology in higher education, and summarizes the application of VR technology in higher education. Finally, some suggestions are put forward to deepen the application of VR in higher education. This research mainly focuses on the following questions:
Q1: Which majors in the field of higher education are VR mainly applied to, and what are the trends or characteristics?
Q2: What are the main VR devices currently used in higher education?
Q3: Compared with traditional education, what is the teaching effect of VR in higher education and what aspects mainly affect the teaching effect? What methods do scholars mainly use to evaluate the teaching effect of VR in higher education?
Q4: What research methods are used to study the application of VR in higher education, and what data collection methods and data analysis methods are mainly used by researchers?
Research methods
This paper's research method the literature analysis method. The literature analysis method refers to an analysis method through which the collected literature data of a specific subject is studied to find out the nature or situation of the research object, and draw the researcher's point of view from it. This research method is the most commonly used research method in literature review. The main steps of this research are: first, determine the topic of application of virtual reality technology in higher education; second step, collect and screen relevant literature according to the literature inclusion rules of this study; third step, carry out historical analysis and research context of the literature Analysis, analysis of research results and analysis of research methods; Finally, write a review.
Data sources and screening methods
This study reviewed the literature on the application of VR technology in higher education in the past 10 years (2012–2021), and the main database used in this study is Web of Science, and IEEE and Google Scholar are supplementary databases. The reason for choosing Web of Science as the main database is that the database has a strong interdisciplinary nature, which is consistent with the theme of this study. Two groups of keywords are used in literature retrieval. Keywords related to virtual reality technology are virtual reality, virtual teaching environment, virtual classroom. Keywords related to higher education is higher education. The keywords of the two groups are combined as search characters. The time span of the literature was set from January 1, 2012 to December 31, 2021, and 2590 related literatures were retrieved. During the analysis of this study, the following criteria were used for the inclusion and exclusion of literature: (1) Only empirical research on the application of VR in advanced fields was included, and research results such as review papers and conference abstracts were excluded. (2) The technology used in the research must be VR, and the literature on augmented reality and mixed reality is excluded. (3) The object of the research must be the object of higher education, which means that including junior college students, undergraduate students, master students, and doctoral students. According to the above principles, a total of five researchers participated in the literature screening. After three rounds of screening, the researchers finally took out the uncertain literature and discussed them together, and finally got 80 relevant literatures. The literature screening flowchart is shown in Figure 1 .
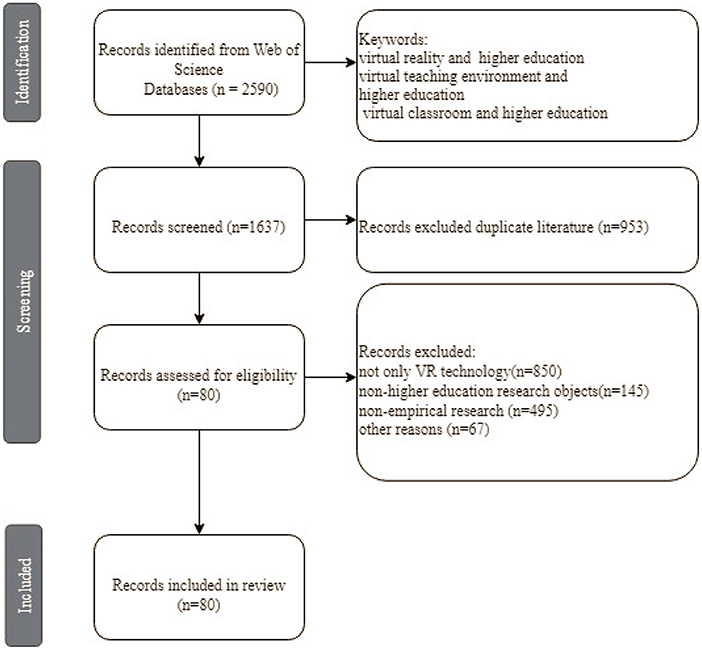
Figure 1 . Literature inclusion process.
Code analysis method
This paper uses the coding table shown in Table 1 to quantify the 80 documents obtained by screening. Table 1 is divided into three first-level dimensions, namely research context, related technology and teaching effect evaluation. Each first-level dimension is divided into 2–4 second-level dimensions.
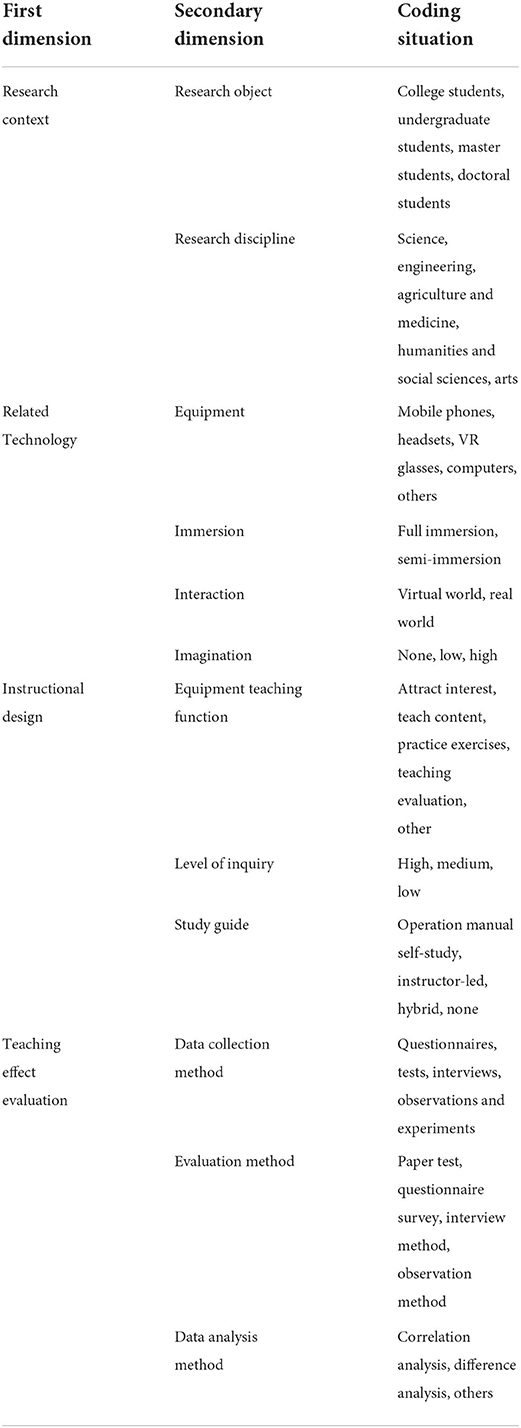
Table 1 . Coding table.
Analysis of the annual distribution of literature
Statistical statistics of the 80 selected literatures were carried out, and the trend graph of the number of empirical literatures with years was obtained as shown in Figure 2 . The horizontal axis represents the year, and the vertical axis represents the number of articles per year. Observing Figure 2 , we can see that from 2012 to 2017, researchers were not enthusiastic about the application of VR in higher education, but from 2018, the number of papers published showed a clear upward trend. The number of papers published in 2020 has reached its peak in the past decade, reaching ten times the number of papers published in 2012. To analyze the reasons, the first is the development of VR technology. After 2018, VR technology has matured, and the price of related equipment has become relatively cheap, and many colleges and universities have been able to purchase equipment. Second, since the outbreak of the new crown epidemic from the end of 2019, many college students have been unable to enter the school to study due to other reasons such as being in a risk area or the school is in a risk area, but the practical skills of college students must be mastered in the syllabus, so the researchers turned their attention to VR technology. Its 3I characteristics can just solve the problem, allowing students to complete related skills training without returning to school.
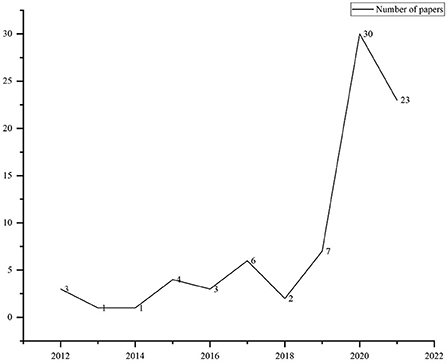
Figure 2 . Annual distribution of empirical literature on VR applications in higher education.
Analysis of the research context
The empirical research papers are classified to obtain a statistical graph of VR applied to related majors in the field of higher education, as shown in Figure 3 . Observing Figure 3 , it is obvious that VR technology is widely used in medical education in the field of higher education. Among the 80 literatures, there are 53 empirical studies on medicine, accounting for 66%, followed by engineering, Science, Physical Education, Art, History and others. In addition, VR technology can also be applied to the teaching of higher education open courses and other skills training, such as ideological and political teaching, English teaching and speech training. After classifying the research objects, it is found that the research objects of VR technology application and higher education are mainly undergraduates. Among the 80 literatures, 77 research objects are undergraduates, 3 are graduate students. The number of empirical studies taking junior college students and doctors as research objects is 0. Higher medical education is an important part of higher education and shoulders the important mission of cultivating medical talents and maintaining and promoting human health. Using VR technology can help medical students to repeatedly train their skills without considering the issue of experimental resources. For example, Jung et al. integrated VR technology to learn basic skills of laparoscopy, students repeatedly learned the basic skills of operation through the laparoscopic simulator ( Jung et al., 2012 ). In addition, VR technology can also help medical students improve their empathy skills, so that they can treat patients in a more appropriate way. For example, some researchers have applied VR technology to elderly nursing teaching, and the results show that students show higher understanding and empathy for elderly people with aging diseases such as macular degeneration and hearing loss ( Dyer et al., 2018 ). Immersive VR courses have improved medical students' ability to assess patients with dyspnea ( Zackoff et al., 2020 ), allowing timely escalation of care for patients with signs of respiratory failure. There are some necessary skills that need to be mastered in the teaching objectives of science and engineering majors in higher education. Researchers can use VR technology to conduct virtual training or virtual experiments. Virtual practical training is skill training in a virtual environment, such as applying VR technology to welding courses. The research results show that most students' final exam scores for welding practice are significantly higher than their mid-term exam scores, and students' learning effect on VR-assisted welding courses is also high. Expressed an obvious affirmation ( Huang et al., 2020 ); Applying VR to machine tool training, the results show that the effect of machine tool training in virtual environment is greatly improved ( Chen et al., 2019 ). Compared with the training room in the real environment, virtual training can not only save teaching costs and avoid safety risks, but also stimulate students' interest in learning, turn students into subjects, and promote students' active learning. Virtual experiment is to imitate the virtual environment to conduct experiments, such as physics and chemistry experimental teaching due to site restrictions or dangers cannot carry out practical operations, students can use VR equipment to conduct experiments. It can be seen from the research results that the main target of VR application in the field of higher education is undergraduates, the majors of application are mainly science, engineering and medicine-related majors, and the application of humanities and social sciences such as history and art is relatively rare.
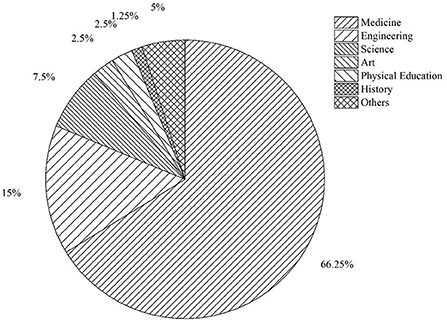
Figure 3 . The professional statistics situation of VR application in higher education.
Research on VR equipment
The main devices for VR applications in the field of higher education include computers, headsets, VR simulators, mobile phones and so on. Computers are the main equipment for VR applications in higher education. Through computers, virtual laboratories can be made, VR games can be developed, and so on. The VR head-mounted device uses the head-mounted display device to block people's vision and hearing of the external environment, and guide the user to have a feeling of being in a virtual environment. Head-mounted virtual reality devices can bring an excellent real experience, making the content in the book touchable, interactive, and perceptible. VR headsets can be divided into three categories: external headsets, all-in-one headsets, and mobile headsets. VR simulators are the main equipment for VR applications in higher education. The latest VR head-mounted displays, the HTC Vive and Oculus Rift, not only allow users to experience a high degree of immersion, but also provide interactivity. VR headsets generally have a Bluetooth connection function, and users can experience
interaction by operating Bluetooth devices such as external controllers. The advantage of this type of interaction is that the handle operation is more familiar to the user. In addition, the range of motion of such equipment is small, and the comfort level will be increased. However, the current type of external Bluetooth handle device also has some disadvantages. For example, there are too many buttons, and many buttons in the device cannot be used by the user; the operation is unreal. In the real world, if you want to pick up something on the ground, the user needs to squat down and open his palm, but in the virtual world, it can only be achieved by moving a finger and pressing a button, which is a bit contrary to the immersion of VR equipment. VR equipment is divided into three categories: non-immersive - desktop VR such as some 3D interactive animation; immersive - cave-based VR such as immersive experience under the surround wall screen in a closed room and fully immersive - full Immersive VR systems such as Google Cardboard. This study compares VR devices according to the classification of VR device immersion in the literature.
In this study, VR simulators mainly refer to medical simulators, which are mainly used for basic teaching and skill training in medical education. Typical simulators include laparoscopic simulators, oral simulators, clinical puncture simulators, and so on. Traditional medical training is based on see one, do one, teach one model. However, this traditional model does not work in the world of modern medical technology. For example, laparoscopic VR simulators allow medical students to perform training in laparoscopic and gynecological surgery in a hyper-realistic and risk-free environment ( Mulla et al., 2012 ); Oral simulator Moog simodon allows medical students to conduct repeated training of dental restoration skills in the event of accidental injury ( Murbay et al., 2020 ). Scientific studies have proven that the application of simulators greatly improves training results compared to traditional methods. A high-quality simulation experience allows trainees to lessen their doubts and immerse themselves in the training scenarios. For example, if a virtual patient bleeds suddenly during training, its performance can be visually and sensory enough for the doctor to feel real enough for the trainee to engage in the training to find a quick solution. The laparoscopic simulator can also record trainee behavior with millimeter precision, which can provide valuable feedback not only on how the trainee is performing during the procedure, but also on the potential behavioral outcomes of the simulated patient.
Compared with VR headsets, mobile phones are more portable. The combination of mobile phones and VR glasses creates a VR environment, and students can access the system through mobile terminals for learning. For example, Kader et al. created a VR crime scene activity through Web VR software such as Uptale, where students can participate remotely through mobile devices to investigate virtual reality crime scenes and collect evidence for subsequent analysis ( Kader et al., 2020 ). This paper conducts statistics and analysis on the VR devices used in the obtained 80 literatures, and the statistical results are shown in Figure 4 . Looking at Figure 4 , it can be seen that the number of VR devices in 2017–2021 has increased significantly compared to 2012–2016. In the 5 years from 2012 to 2016, the main device for VR application in higher education was the computer, followed by simulators, head-mounted devices, etc. However, in the 5 years from 2017 to 2021, headsets have become the main equipment for VR applications in higher education, and the proportion of teaching using VR simulators has also been greatly reduced. Analyzing the reasons, due to the rapid development of science and technology, researchers are more inclined to use devices with lower prices and higher interactivity, which can make users highly immersed in the virtual environment. In addition, according to the results of literature analysis, it is found that many studies are biased toward practical application, and lack relevant standards for technical index evaluation and operating environment evaluation. This part of the content deserves in-depth attention of researchers in the future.
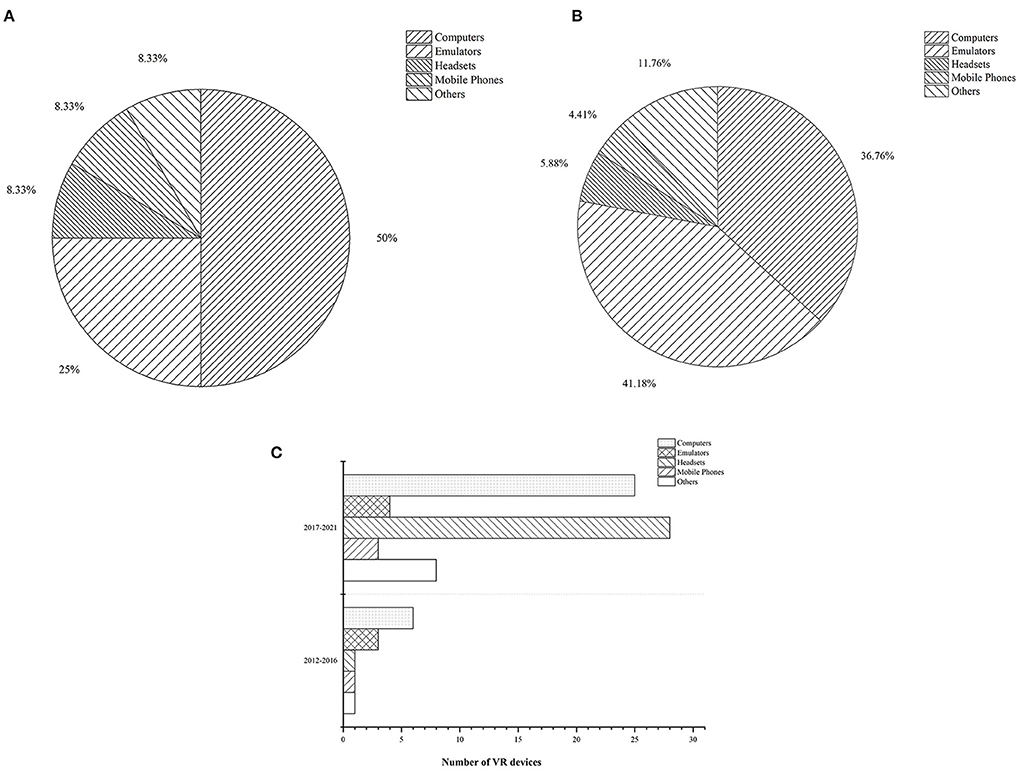
Figure 4 . Statistics of VR teaching equipment. (A) VR Equipment (2012-2016). (B) VR Equipment (2017-2021). (C) Number of VR devices.
Research on the effect of VR teaching
The teaching effect is a common concern of researchers. As shown in Figure 5 , the researchers used a variety of evaluation methods to evaluate the teaching effect of VR in higher education, including traditional evaluation, embedded evaluation and hybrid evaluation. The traditional evaluation includes questionnaires, test papers and various tests, while the embedded evaluation can be completed in the VR environment. The mixed evaluation includes both traditional evaluation and embedded evaluation. Observing Figure 5 , it can be found that when studying the application of VR in higher education, researchers mostly use traditional evaluation to evaluate the teaching effect, a total of 64 papers, accounting for 80% of the research literature. For example, Samosorn et al. used questionnaires and tests to understand the effect of the intervention after training nursing students in the use of VR intervention for difficult airway management ( Samosorn et al., 2020 ). Followed by the embedded evaluation, a total of 9, accounting for 11.25% of the research literature. Four studies used mixed assessment to evaluate the teaching effect, and three studies did not evaluate the teaching effect. In the 80 literatures, the teaching effect of most of the research is positive. For example, the research results of Arif et al. show that in the virtual reality environment, students show stronger concentration ( Arif, 2021 ); However, some research results show that the teaching effect of VR in higher education is not so ideal. For example, Harrison et al. research results showed that in the teaching of surgical preparation, the application of VR technology did not show a perceptible advantage compared with traditional video teaching ( Harrison et al., 2017 ). In addition, this paper also studies the impact of VR technology teaching on students' learning results, and analyzes 80 literatures that were finally screened. The application of VR technology in higher education mainly affects students' learning results by affecting students' behavior ( n = 35), followed by affecting students' cognition ( n = 22), and finally affecting students' learning outcomes ( n = 6) by affecting students' emotional attitudes.
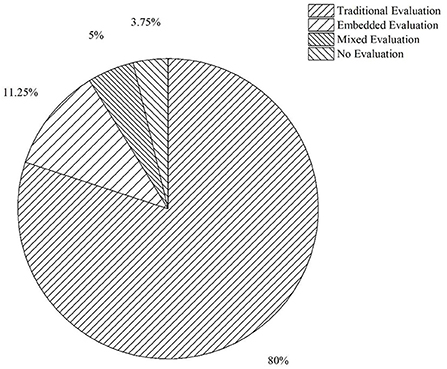
Figure 5 . Statistics of evaluation methods.
VR research methods
This paper also sorts out and categorizes the research methods of related literature. The number of these articles is larger than the total number of research literature after measurement, because an article may contain more than one research design category, one data collection method and one data analysis method. The research results show that there are 63 empirical quantitative studies, 13 empirical qualitative studies, 29 design-oriented studies, and 5 without methods in the literature studied. The data collection methods used in the research literature mainly include questionnaires, tests, interviews, observations and experiments. The main data collection methods are questionnaires ( n = 39) and tests ( n = 39). Semi-structured questionnaires are between structured questionnaires and unstructured questionnaires. The answers to the questions include both pre-set, fixed, and standard options types, as well as questions that the respondents can answer freely. Therefore, semi-structured questionnaires have the advantages of both structured and unstructured questionnaires, and such questionnaires are widely used in research surveys. In addition to questionnaires and tests, the data collection methods in some literatures are the interview method ( n = 8) and the observation method ( n = 5), most of which are used for qualitative research. The data analysis methods used in the research literature mainly include descriptive analysis ( n = 25), difference analysis ( n = 57) and correlation analysis ( n = 2). Difference analysis mainly includes t -test, ANOVA, ANCOVA, chi-square test, Mann-Whitney U -test and other analysis methods, correlation analysis methods include correlation coefficient, regression model, factor analysis and other methods. Among all the 57 articles that used the method of difference analysis, the t -test ( n = 24) was the most commonly used method for quantitative data analysis, and the other analysis methods averaged only 10–12 articles. Most of these findings are that VR-generated interventions are positive and improve teachers' teaching effectiveness.
Answer to questions
This paper organizes and analyzes the relevant literature on the application of VR technology in the field of higher education. The research results show that the main objects of VR application in the field of higher education are undergraduates, and the majors of application are mainly related to science, engineering and medicine, history, art and others. Humanities and social science majors have relatively few applications. The main devices for VR applications in the field of higher education include computers, headsets, VR simulators, mobile phones and so on. Due to the development of technology, head-mounted devices have become the main equipment for VR applications in higher education. Because researchers are more inclined to use devices that are less expensive and more interactive, allowing users to be highly immersed in a virtual environment. Compared with traditional education, the vast majority of studies show that the application of VR to higher education and teaching has positive effects, mainly by affecting students' behaviors to affect students' learning results, secondly by affecting students' cognition, and finally by affecting students' learning. Affect students' emotional attitudes to affect students' learning results. Analysis of the reasons, due to the immersion and interactivity of the VR environment, VR is often used in higher operability courses in higher education, such as medical anatomy courses, dental and dental repair courses, machine tool operation training courses, and so on. L In addition, scholars mainly use objective test questions and subjective questionnaires to evaluate the teaching effect of VR, and a small number of researchers use embedded evaluation to evaluate the teaching effect. The research results show that the main research method of the researchers to study VR application and higher education is quantitative research, the main data collection methods selected are questionnaires and tests, and most of the questionnaire surveys are mainly semi-structured questionnaires. The data analysis methods used in the research literature mainly include descriptive analysis and variance analysis. Among all 57 articles using variance analysis methods, t -test is the most commonly used quantitative data analysis method.
Suggestions
Based on the relevant findings of this study, researchers put forward the following suggestions, hoping to provide some references for the application of VR in the field of higher education. First of all, VR is mainly applied to undergraduates in higher education, and the applied majors are mainly science, engineering and medical related majors. The application of history, art and other humanities and social science majors is relatively less, so researchers can turn their attention to the teaching of humanities and social science majors. It is hoped that future researchers will make full use of these advantages and deepen the application of VR in the overall field of higher education.
Secondly, it can be seen from the review results that the current VR related devices applied in the field of higher education are mainly computers and head-mounted displays. Compared with the past, the immersion and interaction of devices are improved, but there is still a lot of room for development. The portability of the device is not enough, and students cannot use the device to study anytime, anywhere. Mobile devices such as mobile phones, although portable, must be combined with other devices such as VR glasses to simulate the VR environment. The immersion and interaction of this VR environment Sex will be greatly reduced, and it is expected that researchers in the future can break through the technical difficulties and solve this problem. Third, in the literature studied, only a few researchers have conducted VR training and teaching guidance for students before class. The lack of guidance will cause students to operate equipment irregularly and cause some unnecessary problems. First of all, if students do not receive relevant guidance in advance, they will aimlessly explore VR equipment in class, which not only wastes teaching time, but also has a negative impact on teaching effectiveness. In addition, the screen of the VR equipment covers most of the student's field of vision, and the immersion brought by the equipment is very strong, which is easy to cause 3D vertigo. Therefore, in the process of VR teaching, it is very necessary to provide training or guidance to students. Finally, most researchers need to develop, test, and continuously improve VR educational resources before applying them to teaching, which is time-consuming and labor-intensive. In the future, researchers can develop more professional and mature VR educational resources according to the teaching objectives of different majors in higher education, and directly apply them to the teaching of higher education, promoting the deep integration of the entire higher education teaching field and VR technology.
Limitations
Due to the nature of the review, selection, and filtering process, our work has several limitations. First of all, this paper does not deeply explore the application of VR technology to the various majors in higher education mentioned in the text, but only elaborates on the application of some representative majors. Secondly, this study does not focus on some obstacles when VR is applied in teaching, such as adverse reactions after students use VR equipment, and network conditions of VR equipment. But these obstacles are related to technology, and we believe that the development of technology will solve these problems soon.
Conclusion and future research
In this paper, the researchers focus on the application of VR technology in higher education. The teaching contexts, VR devices, teaching effects, and research methods used in the recent literature on educational VR applications are studied. The results of the review show that people are very interested in the application of VR technology in higher education. Many researchers believe that VR technology is a very useful teaching tool, and they have conducted many experimental studies. But the maturity of VR use in higher education is still uncertain, including the VR technology used and the teaching resources developed by educators. In addition, most researchers use VR technology to teach teaching practice, which also implies that VR technology is mostly used in higher operability majors. This paper also points out the problems and puts forward some suggestions. Our work will continue with an analysis of VR technologies available for higher education, followed by an in-depth survey of higher education workers to gain a more detailed understanding of the current state of adoption. Our goal is to deepen the application of VR in higher education.
Data availability statement
The original contributions presented in the study are included in the article/supplementary material, further inquiries can be directed to the corresponding author.
Author contributions
XD and ZL contributed to conception and design of the study. XD organized the database, performed the statistical analysis, and wrote the manuscript. All authors contributed to manuscript revision, read, and approved the submitted version.
This paper was supported by Fujian Province Educational Science Plan 2021 key special project for high-quality development of basic education, project name is Fujian Province Robot Education Application Research under the National Intelligent Manufacturing Industry Policy (Grant No. FJWTZD21-06). This paper was supported by 2022 Fujian Provincial Social Science Fund, project title is Research on the construction of foreign language teaching model based on educational robots (Grant No. FJ2022BF015).
Acknowledgments
We thank ZL for his guidance on the manuscript.
Conflict of interest
The authors declare that the research was conducted in the absence of any commercial or financial relationships that could be construed as a potential conflict of interest.
Publisher's note
All claims expressed in this article are solely those of the authors and do not necessarily represent those of their affiliated organizations, or those of the publisher, the editors and the reviewers. Any product that may be evaluated in this article, or claim that may be made by its manufacturer, is not guaranteed or endorsed by the publisher.
Alalwan, N., Cheng, L., Al-Samarraie, H., Yousef, R., Alzahrani, A. I., and Sarsam, S. M. (2020). Challengeand prospects of virtual reality and augmented reality utilization among primary school teachers: a developing country perspective. Stud. Edu. Evaluat. 66, 100876. doi: 10.1016/j.stueduc.2020.100876
CrossRef Full Text | Google Scholar
Arif, F. (2021). Application of virtual reality for infrastructure management education in civil engineering. Edu. Inform. Technol. 26, 3607–3627. doi: 10.1007/s10639-021-10429-y
Chen, L. W., Tsai, J. P., Kao, Y. C., and Wu, Y. X. (2019). Investigating the learning performances between sequence-and context-based teaching designs for virtual reality (VR)-based machine tool operation training. Comput. Appl. Eng. Edu. 27, 1043–1063. doi: 10.1002/cae.22133
Duarte, M. L., Santos, L. R., Júnior, J. G., and Peccin, M. S. (2020). Learning anatomy by virtual reality and augmented reality. A scope review. Morphologie 104, 254–266. doi: 10.1016/j.morpho.2020.08.004
PubMed Abstract | CrossRef Full Text | Google Scholar
Dyer, E., Swartzlander, B. J., and Gugliucci, M. R. (2018). Using virtual reality in medical education to teach empathy. J. Med. Library Assoc. JMLA 106, 498. doi: 10.5195/jmla.2018.518
Harrison, B., Oehmen, R., Robertson, A., Robertson, B., De Cruz, P., Khan, R., et al. (2017). “Through the eye of the master: the use of virtual reality in the teaching of surgical hand preparation,” in 2017 IEEE 5th International Conference on Serious Games and Applications for Health (SeGAH: IEEE). p. 1–6. doi: 10.1109/SeGAH.2017.7939269
Hoffman, H., and Vu, D. (1997). Virtual reality: teaching tool of the twenty-first century?. Acad. Med. J. Assoc. Am. Med. Colleges 72, 1076–1081. doi: 10.1097/00001888-199712000-00018
Huang, C. Y., Lou, S. J., Cheng, Y. M., and Chung, C. C. (2020). Research on teaching a welding implementation course assisted by sustainable virtual reality technology. Sustainability 12, 10044. doi: 10.3390/su122310044
Jung, E. Y., Park, D. K., Lee, Y. H., Jo, H. S., Lim, Y. S., and Park, R. W. (2012). Evaluation of practical exercises using an intravenous simulator incorporating virtual reality and haptics device technologies. Nurse Edu. Today 32, 458–463. doi: 10.1016/j.nedt.2011.05.012
Kader, S. N., Ng, W. B., Tan, S. W. L., and Fung, F. M. (2020). Building an interactive immersive virtual reality crime scene for future chemists to learn forensic science chemistry. J. Chem. Edu. 97, 2651–2656. doi: 10.1021/acs.jchemed.0c00817
Krokos, E., Plaisant, C., and Varshney, A. (2019). Virtual memory palaces: immersion aids recall. Virtual reality 23, 1–15. doi: 10.1007/s10055-018-0346-3
Moore, P. (1995). Learning and teaching in virtual worlds: implications of virtual reality for education. Aust. J. Edu. Technol. 11:92–102. doi: 10.14742/ajet.2078
Mulla, M., Sharma, D., Moghul, M., Kailani, O., Dockery, J., Ayis, S., et al. (2012). Learning basic laparoscopic skills: a randomized controlled study comparing box trainer, virtual reality simulator, and mental training. J. Surg. Edu. 69, 190–195. doi: 10.1016/j.jsurg.2011.07.011
Murbay, S., Chang, J. W. W., Yeung, S., and Neelakantan, P. (2020). Evaluation of the introduction of a dental virtual simulator on the performance of undergraduate dental students in the pre-clinical operative dentistry course. Eur. J. Dental Edu. 24, 5–16. doi: 10.1111/eje.12453
Rogers, S. (2019). “Virtual reality: the learning aid of the 21st century” in Secondary Virtual Reality: The Learning Aid of the 21st Century , New York.
PubMed Abstract | Google Scholar
Ryan, M. L. (2015). Narrative as Virtual Reality 2: Revisiting Immersion and Interactivity in Literature and Electronic Media . Baltimore: JHU press.
Google Scholar
Samosorn, A. B., Gilbert, G. E., Bauman, E. B., Khine, J., and McGonigle, D. (2020). Teaching airway insertion skills to nursing faculty and students using virtual reality: a pilot study. Clin. Simulat. Nursing 39, 18–26. doi: 10.1016/j.ecns.2019.10.004
Shin, D. (2018). Empathy and embodied experience in virtual environment: To what extent can virtual reality stimulate empathy and embodied experience? Comput. Hum. Behav. 78, 64–73. doi: 10.1016/j.chb.2017.09.012
Zackoff, M. W., Real, F. J., Sahay, R. D., Fei, L., Guiot, A., Lehmann, C., et al. (2020). Impact of an immersive virtual reality curriculum on medical students' clinical assessment of infants with respiratory distress. Pediatric Critical Care Med. 21, 477–485. doi: 10.1097/PCC.0000000000002249
Keywords: virtual reality, higher education, empirical research, virtual environment, immersion learning
Citation: Ding X and Li Z (2022) A review of the application of virtual reality technology in higher education based on Web of Science literature data as an example. Front. Educ. 7:1048816. doi: 10.3389/feduc.2022.1048816
Received: 20 September 2022; Accepted: 02 November 2022; Published: 17 November 2022.
Reviewed by:
Copyright © 2022 Ding and Li. This is an open-access article distributed under the terms of the Creative Commons Attribution License (CC BY) . The use, distribution or reproduction in other forums is permitted, provided the original author(s) and the copyright owner(s) are credited and that the original publication in this journal is cited, in accordance with accepted academic practice. No use, distribution or reproduction is permitted which does not comply with these terms.
*Correspondence: Zhe Li, lizheritetu@163.com
This article is part of the Research Topic
Education and Innovative Perspectives in Higher Education

An official website of the United States government
The .gov means it’s official. Federal government websites often end in .gov or .mil. Before sharing sensitive information, make sure you’re on a federal government site.
The site is secure. The https:// ensures that you are connecting to the official website and that any information you provide is encrypted and transmitted securely.
- Publications
- Account settings
Preview improvements coming to the PMC website in October 2024. Learn More or Try it out now .
- Advanced Search
- Journal List
- Front Psychol
- PMC11040080
The impact of virtual reality on student engagement in the classroom–a critical review of the literature
Xiao ping lin.
1 Faculty of Education, Silpakorn University, Nakhon Pathom, Thailand
2 Melbourne Graduate School of Education, The University of Melbourne, Melbourne, VIC, Australia
Zhen Ning Yao
3 Graduate Department, Xi’an Physical Education University, Xi’an, China
Mingshu Zhang
4 College of Commerce and Tourism, Hunan Vocational College for Nationalities, Yueyang, China
5 Graduate Department, Sehan University, Yeongam County, Republic of Korea
José Manuel de Amo Sánchez-Fortún, University of Almeria, Spain
The purpose of this review is to identify the impact of virtual reality (VR) technology on student engagement, specifically cognitive engagement, behavioral engagement, and affective engagement.
A comprehensive search of databases such as Google, Scopus, and Elsevier was conducted to identify English-language articles related to VR and classroom engagement for the period from 2014 to 2023. After systematic screening, 33 articles were finally reviewed.
The use of VR in the classroom is expected to improve student engagement and learning outcomes, and is particularly effective for students with learning disabilities. However, introducing VR into middle school education poses several challenges, including difficulties in the education system to keep up with VR developments, increased demands on students’ digital literacy, and insufficient proficiency of teachers in using VR.
To effectively utilize VR to increase student engagement, we advocate for educational policymakers to provide training and technical support to teachers to ensure that they can fully master and integrate VR to increase student engagement and instructional effectiveness.
Introduction
In recent years, virtual reality (VR) has emerged as a transformative technology in education, providing new avenues for immersive and interactive learning experiences ( Pottle, 2019 ). At its core, VR offers a departure from the tangible, allowing users to delve into an environment transcending conventional reality ( Brooks, 1999 ; Jeong et al., 2019 ). VR’s essence is captured in three pillars: presence, interactivity, and immersion ( Lee et al., 2017 ). Presence grants users access to previously unreachable 3D landscapes, facilitating a unique, experiential insight ( Poux et al., 2020 ). Interactivity kindles user curiosity, enabling dynamic engagements within the virtual milieu ( Steuer et al. 1995 ; Huvila, 2013 ; Song et al., 2023 ). Immersion pushes the boundaries of conventional experiences, reviving or manifesting phenomena outside the realm of everyday life ( Sanchez-Vives and Slater, 2005 ; Poux et al., 2020 ).
The introduction of VR in education might increase student engagement, which is closely related to the cognitive, behavioral, and affective dimensions of the engagement model ( Wang and Degol, 2014 ). Cognitive engagement underscores the depth of students’ attention, comprehension, and retention ( Wang and Degol, 2014 ). Behavioral engagement is observable, characterized by consistent attendance and active classroom participation ( Wang and Degol, 2014 ). Affective engagement delves into the emotional realm, encompassing motivation, passion, and learning efficacy ( Wang and Degol, 2014 ).
Existing literature emphasizes the importance of virtual reality technology in promoting full student engagement in cognitive, behavioral, and affective dimensions, and states that the application of virtual reality technology in education has become a trend ( Mystakidis et al., 2021 ). Some literature shows that higher education institutions are increasingly adopting VR, with adoption rates as high as 46% at US universities and 96% at United Kingdom universities ( United Kingdom Authority, 2019 ; Agbo et al., 2021 ). In addition, the establishment of dedicated VR laboratories at leading universities such as Harvard University and Colorado State University underscores the commitment to using VR for educational innovation and advancement ( Reid, 1987 ; Leidner and Jarvenpaa, 1995 ). This literature shows that the widespread use of VR in education has attracted the attention of a growing number of researchers and educators, with a particular interest in the impact of VR in the classroom in terms of students’ cognitive, behavioral, and affective engagement.
It is worth noting that although existing literature begins to discuss the impact of VR on student engagement, there are still shortcomings in determining the impact of VR on various dimensions of student engagement, which may limit our overall understanding of the topic. Therefore, further discussion is needed to more specifically identify the impact of VR on the various dimensions of student engagement to gain a more comprehensive and concrete understanding. To accomplish this, this review is guided by the following three questions: (1) What are the positive impacts of VR in education? (2) What are the challenges of VR in education? (3) What interventions can address these challenges? With this in mind, the article will first discuss the positive impact of VR on students’ cognitive, behavioral, and affective engagement to help readers understand its potential in education. It will then discuss the challenges facing VR to make constructive recommendations to address the problems in education.
Searching strategy
In our methods, we used critical review. According to Grant and Booth (2009) “an effective critical review presents, analyses and synthesizes material from diverse sources”(p.93). Critical perspectives were used to assess the potential of VR in reforming educational practices and improving teaching and learning outcomes. The purpose of this article was to collect literature on the impact of VR on student engagement. Therefore, this article summarizes the previous studies as follows. First, information was obtained from Google, Scopus, and Elsevier databases: “virtual reality,” “cognitive engagement,” “affective engagement,” “behavioral engagement” and “learning outcomes.” The search was limited to articles published between January 2014 and December 2023 in English. The first search used all combinations of the above keywords and, after an initial review, produced 97 potentially relevant articles (Google: 92, Scopus: 3, Elsevier: 2).
In the second phase, secondary terms such as “affect,” “challenge,” and “education” were added, reducing the number of studies to 63 (Google:60, Scopus:1, Elsevier:2). Of these, 34 did not meet the criteria and were excluded. They were excluded because their target audience was teachers and did not discuss the impact of VR on student engagement from the student’s perspective. In the final stage, another 53 articles were excluded because they were repetitive and their purpose was to discuss either technology or engagement, or both. Finally, their full texts were reviewed to determine if their work fits the focus of this article 20 articles (Google: 17, Scopus: 1, Elsevier: 2) qualified for final review, covered a sample on the impact of VR on student engagement, and were included in the analysis.
Inclusion and exclusion criteria
To ensure the quality of the literature, we selected only peer-reviewed journal articles published in English in the last decade. The main purpose of this article was to review the impact of VR on student engagement. Therefore, we selected only review articles on the impact of VR on student engagement in educational settings. Articles that were not written in English did not discuss the impact on engagement from a student perspective, and were published beyond the previously established time and language were excluded. In addition, a selection of articles was identified and assessed by manually searching the references of articles related to the topic, of which 13 met the eligibility criteria. Therefore, 13 additional articles were added to the 20 identified. In total, 33 articles that met these eligibility criteria were included and reviewed here. Full-text versions of the articles were obtained, with each article being reviewed and confirmed as appropriate by the authors. Finally, to maximize transparency and traceability, we list the rationale and relevant evidence for all articles included (see Table 1 ). The process of article selection followed the Preferred Reporting of Items for Systematic Reviews and Meta-Analyses (PRISMA) Statement ( Moher et al., 2009 ; see Figure 1 ). Figure 1 illustrates the process of article selection.
Publications reviewed in full text with reasons for inclusion or exclusion.

PRISMA flow diagram for article selection.
The review found that the number of publications increased each year from 2014 to 2023, indicating the continued interest of researchers in exploring the impact of VR on student engagement. When reviewing the impact of VR on student engagement, Wang and Degol’s (2014) article had the most citations at 450, suggesting that the article had a strong impact in the area of student use of VR in the classroom. The majority of articles had only 10 or fewer citations, which may have indicated that these articles were relatively new or had less impact in the field. It was worth noting that more recently published articles, such as Rzanova et al. (2023) , did not have enough time to accumulate citations, so their impact on the field may not have been fully reflected in current citations.
To summarize, the differences in the number of citations for these articles highlighted their different levels of influence in the area of VR’s impact on student engagement. However, there were some limitations to the review methods. For example, some articles might not have fully reflected their impact on the field in the current citations due to their short time frames, which might have resulted in less comprehensive findings. Furthermore, the literature included was small, and in the future consideration would be given to expanding the search of literature and databases, such as PubMed and Web of Science databases, as well as expanding the search with keywords, such as “students’ attitudes toward VR.” In addition, the inclusion and exclusion criteria might have limited the generalizability of the results of the review, and therefore more caution was needed when generalizing the results of the review.
The positive impact of VR on education
This section will discuss the impact of VR on students’ cognitive, behavioral, and affective engagement participation. It is important in the field of education. Radianti et al. (2020) noted that student engagement in educational settings was critical to learning outcomes and classroom climate. Yuan and Wang (2021) further noted that the combined effects of cognitive, behavioral, and affective engagement could directly impact student learning outcomes and classroom contextual experiences. Therefore, a deeper understanding of the impact of VR on these three dimensions of engagement can provide valuable insights into educational practices and help educators better optimize classroom environments and teaching methods.
First, Papanastasiou et al. (2019) noted that VR immersive learning experiences promoted students’ cognitive engagement and aided in understanding complex and abstract knowledge. That is, through immersive learning, students can understand and remember what they have learned in greater depth and increase cognitive engagement. Pellas (2016) also found that VR encouraged students to learn through self-directed inquiry and move away from traditional teacher-centered instruction. Pellas (2016) further explained that, through VR scenario reenactments and simulations, students could engage in real-world unavailable learning experiences such as exploring historical sites and visiting distant planets. This means that such learning experiences enable students to explore knowledge in deeper and more varied ways, thus increasing cognitive engagement. Similarly, Maples-Keller et al. (2017) showed that VR was beneficial in engaging different types of students in learning, particularly for at-risk students, including those with learning difficulties, anxiety disorders, and other mental illnesses. VR provided personalized and adaptive learning environments that helped students improve cognitive engagement and achievement ( Maples-Keller et al., 2017 ). In summary, VR facilitates understanding of complex knowledge and promotes cognitive engagement for different types of students through immersive learning experiences and self-directed inquiry learning.
Secondly, Pirker and Dengel (2021) demonstrated that VR could promote student behavioral engagement. They discussed the potential of immersive VR in education through an in-depth analysis of 64 articles. They showed that “learning tasks in 3-D VLEs can foster intrinsic motivation for and engagement with the learning content” (p.77). Sun and Peng (2020) also suggested that by combining classical educational concepts with VR, such as Confucianism’s promotion of teaching for fun, students were better able to engage in learning activities. For example, Rzanova et al. (2023) found that the use of VR in the teaching of poetry to create the scenarios depicted in the verses enabled students to actively participate in classroom activities. Similarly, Freina and Ott (2015) also found that by simulating real school escape scenarios in VR, students could take on different roles to perform escape drills, and this sense of behavioral engagement can help students better master escape techniques and enhance safety awareness. These articles seem to echo that VR helps to enhance student behavioral engagement.
It is worth noting that there is debate about whether VR has a positive impact on student behavioral engagement. Proponents noted that students’ hands-on experience and exploration in virtual environments stimulated interest and behavioral engagement ( Wong et al., 2010 ; Allcoat and Von Mühlenen, 2018 ). This view suggests that VR provides an immersive learning experience that enhances students’ motivation and promotes deeper engagement in classroom activities. However, contrary findings exist, suggesting that the use of VR may have some negative effects. For example, students might have become addicted to the virtual world and neglected their real-life tasks and responsibilities, thus affecting their behavior in the classroom ( Cheng et al., 2015 ; Greenwald et al., 2018 ; Makransky et al., 2019 ). In addition, some other scholars noted that there might have been a gap between learning experiences in virtual environments and real-world learning experiences, which might have affected students’ ability to acquire and apply knowledge ( Makransky and Petersen, 2021 ). These conflicting results remind us that these complexities and diversities need to be taken into account when evaluating the role of VR technology in improving student engagement in the classroom.
Finally, scholars such as Wu et al. (2013) , Schutte and Stilinović (2017) , and Yuen et al. (2011) found that VR helped to promote student affective engagement. For example, Schutte and Stilinović (2017) found that contexts provided by VR for children with emotional impairments or disabilities taught them skills in communicating with people and managing their emotions, thus fostering empathy. This implies that VR may stimulate affective engagement. Wu et al. (2013) and Yuen et al. (2011) also found that VR provided opportunities for affective interaction, enabling students to interact with characters in the virtual environment. In language learning, for example, practicing through conversations with virtual characters could help students improve their oral expression ( Dhimolea et al., 2022 ). This means that affective interactions may increase students’ affective engagement with the learning content. Similarly, Misak (2018) noted that VR allowed students to role-play in virtual literature and experience the affective portrayed in the story. In other words, affective experiences may deepen students’ understanding of literary works and increase affective engagement. This literature seems to reflect that VR can promote student affective engagement.
In general, VR positively impacts students’ cognitive, behavioral, and affective engagement. In terms of cognitive engagement, VR can facilitate students’ cognitive engagement with learning materials and better understanding of abstract and complex knowledge by creating immersive situations. In terms of behavioral engagement, VR stimulates active student engagement and action through interactive learning. Although there is debate about whether VR has a positive impact on student behavioral engagement, literature has demonstrated the positive impact of VR on student behavioral engagement. In terms of affective engagement, VR promotes students’ emotional engagement by triggering affective resonance through affective experience and affective interaction. This full engagement helps students improve their learning and develop empathy.
The following section discusses the challenges faced when introducing VR in education. Through understanding these challenges, we can better understand the problems in the education system and make some constructive suggestions to help address them.
The challenge of VR in education
Despite the positive impact of VR on students’ cognitive, behavioral, and affective engagement, there are still two challenges to introducing VR into middle education, namely the difficulty of the educational system in keeping up with VR developments and the lack of teacher proficiency in VR use ( Islam et al., 2015 ; Zhong, 2017 ; Abich et al., 2021 ). For example, Islam et al. (2015) observed that the pace of technological advancement, including VR, outpaced the ability of the education system to adapt. This phenomenon is due to the slow reform of the education system, which takes time for the acceptance and adoption of emerging technologies ( Islam et al., 2015 ). To this end, the education sector may take longer to standardize the syllabus, resulting in students not having immediate access to VR ( Zhong, 2017 ). In other words, students may not have the opportunity to experience VR in the classroom until the education department completes the standardization process. Sahlberg (2016) further stated that while reform and standardization in the education sector took time, once VR and the education system evolved in tandem, students benefited from an education that matched the VR of the day.
Other scholars observed that VR education faced several challenges in developing digital literacy in students ( Aviram and Eshet-Alkalai, 2006 ; Sahlberg, 2016 ). According to Reddy et al. (2020) , “digital literacy is a set of skills required by 21st Century individuals to use digital tools to support the achievement of goals in their life situations” (p. 66). Digital literacy encompasses the assessment of digital technologies, critical thinking, and the ability to create and express oneself digitally ( Reddy et al., 2020 ). For example, Tsivitanidou et al. (2021) and Necci et al. (2015) emphasized the need for students to identify the differences between the results of simulation experiments and real experiments and to assess the reliability and accuracy of simulation experiments. In other words, students need to judge the plausibility of the results of simulation experiments and interpret and evaluate those results in real-world situations.
Similarly, Farmer and Farmer (2023) found that digital literacy required students to master VR painting and sculpting tools to create art. This involved learning to select appropriate colors and textures and creating three-dimensional effects with VR tools ( Skulmowski et al., 2021 ). Meanwhile, Andone et al. (2018) further noted that students also needed to learn to share and present their work to others in virtual reality. This observation seems to reflect the high demand for students’ creativity, technical skills, and expressive abilities when introducing VR into education. In sum, while the development of VR education benefits students’ learning in conjunction with VR, there are challenges to students’ digital literacy and the technological adaptability of the education system.
In addition, teachers’ lack of proficiency in the use of VR is another major challenge in introducing VR into middle education. For example, Abich et al. (2021) found that teachers might lack proficiency in the operation and application of VR, which might result in teachers not being able to fully utilize VR to supplement instruction. Jensen and Konradsen (2018) claimed that “for HMDs to become a relevant tool for instructors they must have the ability to produce and edit their content” (p.1525). This means that teachers need to spend time familiarizing themselves with HMDs and related software to create, edit, and customize content to meet their specific instructional needs. Similarly, Fransson et al. (2020) discussed the challenges of teachers operating VR equipment and software. They interviewed 28 teachers to understand teachers’ challenges with implementing helmet display VR in educational settings. Fransson et al. (2020) indicated that there might be a technological threshold and learning curve for teachers in controlling and operating VR devices, which might affect the effective use of VR for teaching and learning.
While teachers may lack familiarity with VR, there are solutions to this challenge. For example, Alfalah (2018) noted that proper training and support could help teachers make the most of VR to supplement instruction. That is, teacher training can provide teachers with the technical knowledge and operational skills they need to familiarize themselves with how VR equipment and software work. To this end, Alfalah (2018) found the impact of providing teachers with VR training in schools. They used a quantitative approach by distributing a questionnaire online to 30 IT teachers. Alfalah (2018) indicated that “technology training may be maximized for the integration of VR technology” (P.2634). This finding seems to reflect that proper teacher training and support can be effective in helping teachers overcome the operational and application of VR technology’s difficulties.
In sum, prior literature has shown that introducing VR into middle school education faces several challenges. First, the rapid development of technology makes the educational system keep up with VR, resulting in a disconnect between the educational curriculum and VR. Second, there may be a lack of proficiency in students’ digital literacy and teachers’ handling and application of VR. However, these challenges are not insurmountable. With proper training and support, teachers can make full use of VR to supplement their teaching and learning to realize the potential of VR in education. It is worth noting that through the literature we have found that in practice, due to the rapid development of technology and the limitations of the educational system, achieving a complete balance may take some time and effort. Therefore, considering how to address the gap between the speed of VR development and the education system to better integrate and apply VR in education makes sense.
This article describes the impact of VR on student cognitive, behavioral, and affective engagement and the challenges posed by VR education. The literature review finds that using VR in the classroom can positively impact student engagement and learning outcomes. An interesting finding is that VR can be a promising tool for providing education to students with learning disabilities. For example, the previous literature review section describes how for students with learning difficulties, anxiety disorders, and other mental illnesses, VR can provide personalized and adaptive learning environments that can help students improve cognitive engagement and academic performance. And, for children with emotional disorders or disabilities, VR provides contexts that can teach them skills for communicating with others and managing their emotions, thereby developing empathy and stimulating affective engagement.
However, the potential problems with incorporating VR in middle education are the difficulty of the education system in keeping up with VR developments, the higher demands of student digital literacy, and the lack of teacher proficiency in the use of VR. These challenges require educational policymakers to provide training and technical support to teachers to ensure that they can fully master and integrate VR to improve student engagement and teaching effectiveness.
Author contributions
XL: Writing – original draft, Writing – review & editing. BL: Conceptualization, Writing – original draft, Writing – review & editing. ZNY: Writing – original draft, Writing – review & editing. ZY: Funding acquisition, Supervision, Writing – original draft, Writing – review & editing. MZ: Funding acquisition, Writing – original draft, Writing – review & editing, Supervision.
Acknowledgments
We are deeply appreciative of the editors and reviewers of this journal for their unwavering dedication and contributions that have shaped the publication of this article. Their constructive feedback and invaluable insights were instrumental in bringing this piece to fruition. We extend our heartfelt thanks to the readers with a keen interest in virtual reality technology. It is our sincere hope that this article will inspire enriched discussions within the academic community about the potential and nuances of using virtual reality in educational contexts.
Funding Statement
The author(s) declare financial support was received for the research, authorship, and/or publication of this article. This work was supported by the General Topics of China’s Hunan Province Social Science Achievement Evaluation Committee Fund [Grant no. XSP2023JYC123].
Conflict of interest
The authors declare that the research was conducted in the absence of any commercial or financial relationships that could be construed as a potential conflict of interest.
Publisher’s note
All claims expressed in this article are solely those of the authors and do not necessarily represent those of their affiliated organizations, or those of the publisher, the editors and the reviewers. Any product that may be evaluated in this article, or claim that may be made by its manufacturer, is not guaranteed or endorsed by the publisher.
- Abich J., Parker J., Murphy J. S., Eudy M. (2021). A review of the evidence for training effectiveness with virtual reality technology . Virtual Reality 25 , 919–933. doi: 10.1007/s10055-020-00498-8 [ CrossRef ] [ Google Scholar ]
- Agbo F. J., Sanusi I. T., Oyelere S. S., Suhonen J. (2021). Application of virtual reality in computer science education: a systemic review based on bibliometric and content analysis methods . Educ. Sci. 11 , 1–23. doi: 10.3390/educsci11030142 [ CrossRef ] [ Google Scholar ]
- Alfalah S. F. (2018). Perceptions toward adopting virtual reality as a teaching aid in information technology . Educ. Inf. Technol. 23 , 2633–2653. doi: 10.1007/s10639-018-9734-2 [ CrossRef ] [ Google Scholar ]
- Allcoat D., Von Mühlenen A. (2018). Learning in virtual reality: effects on performance, emotion, and engagement . Res. Learn. Technol. 26 , 1–13. doi: 10.25304/rlt.v26.2140 [ CrossRef ] [ Google Scholar ]
- Andone D., Vert S., Frydenberg M., Vasiu R. (2018). Open virtual reality project to improve students’ skills . In 2018 IEEE 18th International Conference on Advanced Learning Technologies (ICALT) , 6–10. doi: 10.1109/ICALT.2018.00008 [ CrossRef ] [ Google Scholar ]
- Aviram A., Eshet-Alkalai Y. (2006). Towards a theory of digital literacy: three scenarios for the next steps . Eur. J. Open Distance E Learn 9 , 1–11. [ Google Scholar ]
- Brooks F. P. (1999). What's real about virtual reality? Institute of Electrical and Electronic Engineers (IEEE) . Comput. Graph. Appl. 19 , 16–27. doi: 10.1109/38.799723 [ CrossRef ] [ Google Scholar ]
- Cheng M.-T., Chen J.-H., Chu S.-J., Chen S.-Y. (2015). The use of serious games in science education: a review of selected empirical research from 2002 to 2013 . J. Comput. Educ. 2 , 353–375. doi: 10.1007/s40692-015-0039-9 [ CrossRef ] [ Google Scholar ]
- Dhimolea T. K., Kaplan-Rakowski R., Lin L. (2022). A systematic review of research on high-immersion virtual reality for language learning . TechTrends 66 , 810–824. doi: 10.1007/s11528-022-00717-w [ CrossRef ] [ Google Scholar ]
- Fransson G., Holmberg J., Westelius C. (2020). The challenges of using head mounted virtual reality in K-12 schools from a teacher perspective . Educ. Inf. Technol. 25 , 3383–3404. doi: 10.1007/s10639-020-10119-1 [ CrossRef ] [ Google Scholar ]
- Freina L., Ott M. (2015). A literature review on immersive virtual reality in education: state of the art and perspectives . Int. Sci. Conf. E Learn. Softw. Educ. 1 , 10–1007. doi: 10.12753/2066-026x-15-020 [ CrossRef ] [ Google Scholar ]
- Grant M. J., Booth A. (2009). A typology of reviews: an analysis of 14 review types and associated methodologies . Health Inf. Libr. J. 26 , 91–108. doi: 10.1111/j.1471-1842.2009.00848.x, PMID: [ PubMed ] [ CrossRef ] [ Google Scholar ]
- Greenwald S. W., Corning W., Funk M., Maes P. (2018). Comparing learning in virtual reality with learning on a 2D screen using electrostatics activities . J. Comput. Sci. 24 , 220–245. doi: 10.3217/jucs-024-02-0220 [ CrossRef ] [ Google Scholar ]
- Huvila I. (2013). Sorting out the metaverse and how the metaverse is sorting us out . London: Palgrave Macmillan. [ Google Scholar ]
- Islam N., Beer M., Slack F. (2015). E-learning challenges faced by academics in higher education . J. Educ. Train. Stud. 3 , 102–112. doi: 10.11114/jets.v3i5.947 [ CrossRef ] [ Google Scholar ]
- Jensen L., Konradsen F. (2018). A review of the use of virtual reality head-mounted displays in education and training . Educ. Inf. Technol. 23 , 1515–1529. doi: 10.1007/s10639-017-9676-0 [ CrossRef ] [ Google Scholar ]
- Jeong K., Kim J., Kim M., Lee J., Kim C. (2019). Asymmetric interface: user interface of asymmetric virtual reality for new presence and experience . Symmetry 12 , 1–25. doi: 10.3390/sym12010053 [ CrossRef ] [ Google Scholar ]
- Lee J., Kim M., Kim J. (2017). A study on immersion and VR sickness in walking interaction for immersive virtual reality applications . Symmetry 9 , 1–17. doi: 10.3390/sym9050078 [ CrossRef ] [ Google Scholar ]
- Leidner D. E., Jarvenpaa S. L. (1995). The use of information technology to enhance management school education: a theoretical view . Manag. Inf. Serv. Q. 19 , 265–291. doi: 10.2307/249596 [ CrossRef ] [ Google Scholar ]
- Makransky G., Petersen G. B. (2021). The cognitive-affective model of immersive learning: a theoretical research-based model of learning in immersive virtual reality . Educ. Psychol. Rev. 33 , 937–958. doi: 10.1007/s10648-020-09586-2 [ CrossRef ] [ Google Scholar ]
- Makransky G., Terkildsen T. S., Mayer R. E. (2019). Adding immersive virtual reality to a science lab simulation causes more presence but less learning . Learn. Instr. 60 , 225–236. doi: 10.1016/j.learninstruc.2017.12.007 [ CrossRef ] [ Google Scholar ]
- Maples-Keller J. L., Bunnell B. E., Kim S.-J., Rothbaum B. O. (2017). The use of virtual reality technology in the treatment of anxiety and other psychiatric disorders . Harv. Rev. Psychiatry 25 , 103–113. doi: 10.1097/HRP.0000000000000138, PMID: [ PMC free article ] [ PubMed ] [ CrossRef ] [ Google Scholar ]
- Misak J. (2018). A (virtual) bridge not too far: teaching narrative sense of place with virtual reality . Comput. Compos. 50 , 39–52. doi: 10.1016/j.compcom.2018.07.007 [ CrossRef ] [ Google Scholar ]
- Moher D., Liberati A., Tetzlaff J., Altman D. G., PRISMA Group* (2009). Preferred reporting items for systematic reviews and meta-analyses: the PRISMA statement . Ann. Intern. Med. 151 , 264–269. doi: 10.7326/0003-4819-151-4-200908180-00135 [ PubMed ] [ CrossRef ] [ Google Scholar ]
- Mystakidis S., Berki E., Valtanen J. P. (2021). Deep and meaningful e-learning with social virtual reality environments in higher education: a systematic literature review . Appl. Sci. 11 , 1–25. doi: 10.3390/app11052412 [ CrossRef ] [ Google Scholar ]
- Necci A., Cozzani V., Spadoni G., Khan F. (2015). Assessment of domino effect: state of the art and research needs . Reliab. Eng. Syst. Saf. 143 , 3–18. doi: 10.1016/j.ress.2015.05.017 [ CrossRef ] [ Google Scholar ]
- Papanastasiou G., Drigas A., Skianis C., Lytras M., Papanastasiou E. (2019). Virtual and augmented reality effects on K-12, higher, and tertiary education students’twenty-first-century skills . Virtual Reality 23 , 425–436. doi: 10.1007/s10055-018-0363-2 [ CrossRef ] [ Google Scholar ]
- Pellas N. (2016). “ Unraveling a progressive inquiry script in persistent virtual worlds: theoretical foundations and decision processes for constructing a socio-cultural learning framework ” in Web design and development: Concepts, methodologies, tools, and applications (Pennsylvania, US: IGI Global; ), 610–647. [ Google Scholar ]
- Pirker J., Dengel A. (2021). The potential of 360 virtual reality videos and real VR for education—a literature review . IEEE Comput. Graph. Appl. 41 , 76–89. doi: 10.1109/MCG.2021.3067999, PMID: [ PubMed ] [ CrossRef ] [ Google Scholar ]
- Pottle J. (2019). Virtual reality and the transformation of medical education . Future Healthcare J. 6 , 181–185. doi: 10.7861/fhj.2019-0036, PMID: [ PMC free article ] [ PubMed ] [ CrossRef ] [ Google Scholar ]
- Poux F., Valembois Q., Mattes C., Kobbelt L., Billen R. (2020). Initial user-centered design of a virtual reality heritage system: applications for digital tourism . Remote Sens. 12 , 1–25. doi: 10.3390/rs12162583 [ CrossRef ] [ Google Scholar ]
- Radianti J., Majchrzak T. A., Fromm J., Wohlgenannt I. (2020). A systematic review of immersive virtual reality applications for higher education: design elements, lessons learned, and research agenda . Comput. Educ. 147 , 103778–103729. doi: 10.1016/j.compedu.2019.103778 [ CrossRef ] [ Google Scholar ]
- Reddy P., Sharma B., Chaudhary K. (2020). Digital literacy: a review of literature . Int. J. Technoethics (IJT) 11 , 65–94. doi: 10.4018/IJT.20200701.oa1 [ CrossRef ] [ Google Scholar ]
- Reid J. M. (1987). The learning style preferences of English as a second language (ESL) students . Teach. Engl. Speakers Other Lang. Q. 21 , 87–111. doi: 10.2307/3586356 [ CrossRef ] [ Google Scholar ]
- Rzanova S., Yushchik E., Markova S., Sergeeva A. (2023). Impact of virtual reality technologies in the context of the case method on engineering students’ competencies . Educ. Inf. Technol. 7 , 1–19. doi: 10.56028/aetr.7.1.7.2023 [ CrossRef ] [ Google Scholar ]
- Sahlberg P. (2016). The global educational reform movement and its impact on schooling . In: Mundy K., Green A., Lingard B., Verger A. The Handbook of Global Education Policy . Hoboken, NJ: John Wiley and Sons, 128–144. [ Google Scholar ]
- Sanchez-Vives M. V., Slater M. (2005). From presence to consciousness through virtual reality . Nat. Rev. Neurosci. 6 , 332–339. doi: 10.1038/nrn1651 [ PubMed ] [ CrossRef ] [ Google Scholar ]
- Schutte N. S., Stilinović E. J. (2017). Facilitating empathy through virtual reality . Motiv. Emot. 41 , 708–712. doi: 10.1007/s11031-017-9641-7 [ CrossRef ] [ Google Scholar ]
- Skulmowski A., Nebel S., Remmele M., Rey G. D. (2021). Is a preference for realism really naive after all? A cognitive model of learning with realistic visualizations . Educ. Psychol. Rev. 34 , 1–27. doi: 10.1007/s10648-021-09638-1 [ CrossRef ] [ Google Scholar ]
- Song C., Shin S. Y., Shin K. S. (2023). Optimizing foreign language learning in virtual reality: a comprehensive theoretical framework based on constructivism and cognitive load theory . Appl. Sci. 13 , 1–31. doi: 10.3390/app132312557 [ CrossRef ] [ Google Scholar ]
- Steuer J., Biocca F., Levy M. R. (1995). Communication in the age of virtual reality , New York: Routledge [ Google Scholar ]
- Sun S. Y., Peng L. H. (2020). Study of the virtual reality education and digitalization in China . J. Physics 1456 , 012042–012047. doi: 10.1088/1742-6596/1456/1/012042 [ CrossRef ] [ Google Scholar ]
- Tsivitanidou O. E., Georgiou Y., Ioannou A. (2021). A learning experience in inquiry-based physics with immersive virtual reality: student perceptions and an interaction effect between conceptual gains and attitudinal profiles . J. Sci. Educ. Technol. 30 , 841–861. doi: 10.1007/s10956-021-09924-1 [ CrossRef ] [ Google Scholar ]
- United Kingdom Authority . (2019). VR and AR attract education sector interest . Available at: https://www.ukauthority.com/articles/vr-and-ar-attract-education-sector-interest/ .
- Wang M. T., Degol J. (2014). Staying engaged: knowledge and research need in student engagement . Child Dev. Perspect. 8 , 137–143. doi: 10.1111/cdep.12073, PMID: [ PMC free article ] [ PubMed ] [ CrossRef ] [ Google Scholar ]
- Wong B. M., Etchells E. E., Kuper A., Levinson W., Shojania K. G. (2010). Teaching quality improvement and patient safety to trainees: a systematic review . Acad. Med. 85 , 1425–1439. doi: 10.1097/ACM.0b013e3181e2d0c6, PMID: [ PubMed ] [ CrossRef ] [ Google Scholar ]
- Wu H.-K., Lee S. W.-Y., Chang H.-Y., Liang J.-C. (2013). Current status, opportunities and challenges of augmented reality in education . Comput. Educ. 62 , 41–49. doi: 10.1016/j.compedu.2012.10.024 [ CrossRef ] [ Google Scholar ]
- Yuan H., Wang Z. (2021). A review of research on technology enhancing Chinese learning . 2021 international conference on internet, education and information technology (IEIT), pp. 462–467.
- Yuen S. C.-Y., Yaoyuneyong G., Johnson E. (2011). Augmented reality: an overview and five directions for augmented reality (AR) in education . J. Educ. Technol. Dev. Exchange 4 , 119–140. doi: 10.18785/jetde.0401.10 [ CrossRef ] [ Google Scholar ]
- Zhong L. (2017). Indicators of digital leadership in the context of K-12 education . J. Educ. Technol. Dev. Exchange 10 , 27–40. doi: 10.18785/jetde.1001.03 [ CrossRef ] [ Google Scholar ]
DigitalCommons@University of Nebraska - Lincoln
- < Previous Article
- Next Article >
Home > Biological Systems Engineering > Biological Systems Engineering Papers > 899
Biological Systems Engineering
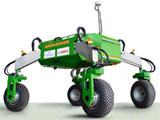
Biological Systems Engineering: Papers and Publications
Framework for the use of extended reality modalities in aec education.
Barbara Oliveira Spitzer , Georgia Institute of Technology Jae Hoon Ma , Georgia Institute of Technology - Main Campus Ece Erdogmus , Georgia Institute of Technology Follow Ben Kreimer , Emerging Media Consulting, Lincoln, NE Erica Ryherd , University of Nebraska-Lincoln Follow Heidi Diefes-Dux , University of Nebraska-Lincoln Follow
Ece Erdogmus
Heidi Diefes-Dux
Date of this Version
Buildings 12 (2022), 2169.
doi: 10.3390/buildings12122169
Copyright 2022 Barbara Oliveira Spitzer, Jae Hoon Ma, Ece Erdogmus, Ben Kreimer, Erica Ryherd, and Heidi Deifes-Dux.
Open access
The educational applications of extended reality (XR) modalities, including virtual reality (VR), augmented reality (AR), and mixed reality (MR), have increased significantly over the last ten years. Many educators within the Architecture, Engineering, and Construction (AEC) related degree programs see student benefits that could be derived from bringing these modalities into classrooms, which include but are not limited to: a better understanding of each of the subdisciplines and the coordination necessary between them, visualizing oneself as a professional in AEC, and visualization of difficult concepts to increase engagement, self-efficacy, and learning. These benefits, in turn, help recruitment and retention efforts for these degree programs. However, given the number of technologies available and the fact that they quickly become outdated, there is confusion about the definitions of the different XR modalities and their unique capabilities. This lack of knowledge, combined with limited faculty time and lack of financial resources, can make it overwhelming for educators to choose the right XR modality to accomplish particular educational objectives. There is a lack of guidance in the literature for AEC educators to consider various factors that affect the success of an XR intervention. Grounded in a comprehensive literature review and the educational framework of the Model of Domain Learning, this paper proposes a decision-making framework to help AEC educators select the appropriate technologies, platforms, and devices to use for various educational outcomes (e.g., learning, interest generation, engagement) considering factors such as budget, scalability, space/equipment needs, and the potential benefits and limitations of each XR modality. To this end, a comprehensive review of the literature was performed to decipher various definitions of XR modalities and how they have been previously utilized in AEC Education. The framework was then successfully validated at a summer camp in the School of Building Construction at Georgia Institute of Technology, highlighting the importance of using appropriate XR technologies depending on the educational context.
Since May 09, 2024
Included in
Bioresource and Agricultural Engineering Commons , Environmental Engineering Commons , Instructional Media Design Commons , Other Civil and Environmental Engineering Commons , Science and Mathematics Education Commons
Advanced Search
Search Help
- Notify me via email or RSS
- Administrator Resources
- How to Cite Items From This Repository
- Copyright Information
- Collections
- Disciplines
Author Corner
- Guide to Submitting
- Submit your paper or article
- Biological Systems Engineering Website
Home | About | FAQ | My Account | Accessibility Statement
Privacy Copyright
Thank you for visiting nature.com. You are using a browser version with limited support for CSS. To obtain the best experience, we recommend you use a more up to date browser (or turn off compatibility mode in Internet Explorer). In the meantime, to ensure continued support, we are displaying the site without styles and JavaScript.
- View all journals
- My Account Login
- Explore content
- About the journal
- Publish with us
- Sign up for alerts
- Open access
- Published: 11 March 2024
Twitter users perceptions of AI-based e-learning technologies
- Luisa Stracqualursi 1 &
- Patrizia Agati 1
Scientific Reports volume 14 , Article number: 5927 ( 2024 ) Cite this article
1521 Accesses
15 Altmetric
Metrics details
- Human behaviour
- Machine learning
- Psychology and behaviour
Today, teaching and learning paths increasingly intersect with technologies powered by emerging artificial intelligence (AI).This work analyses public opinions and sentiments about AI applications that affect e-learning, such as ChatGPT, virtual and augmented reality, microlearning, mobile learning, adaptive learning, and gamification. The way people perceive technologies fuelled by artificial intelligence can be tracked in real time in microblog messages promptly shared by Twitter users, who currently constitute a large and ever-increasing number of individuals. The observation period was from November 30, 2022, the date on which ChatGPT was launched, to March 31, 2023. A two-step sentiment analysis was performed on the collected English-language tweets to determine the overall sentiments and emotions. A latent Dirichlet allocation model was built to identify commonly discussed topics in tweets. The results show that the majority of opinions are positive. Among the eight emotions of the Syuzhet package, ‘trust’ and ‘joy’ are the most common positive emotions observed in the tweets, while ‘fear’ is the most common negative emotion. Among the most discussed topics with a negative outlook, two particular aspects of fear are identified: an ‘apocalyptic-fear’ that artificial intelligence could lead the end of humankind, and a fear for the ‘future of artistic and intellectual jobs’ as AI could not only destroy human art and creativity but also make the individual contributions of students and researchers not assessable. On the other hand, among the topics with a positive outlook, trust and hope in AI tools for improving efficiency in jobs and the educational world are identified. Overall, the results suggest that AI will play a significant role in the future of the world and education, but it is important to consider the potential ethical and social implications of this technology. By leveraging the positive aspects of AI while addressing these concerns, the education system can unlock the full potential of this emerging technology and provide a better learning experience for students.
Similar content being viewed by others
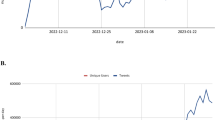
ChatGPT in education: global reactions to AI innovations
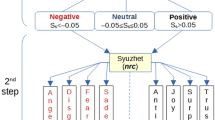
Tweet topics and sentiments relating to distance learning among Italian Twitter users
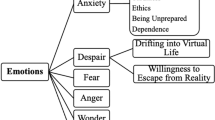
A perspective from Turkey on construction of the new digital world: analysis of emotions and future expectations regarding Metaverse on Twitter
Introduction, ai-powered e-learning technologies.
Current technology continues to advance, continuously transforming the way we live and the way we learn. Over the past few years, e-learning has become increasingly popular, as more people turn to online platforms for education and training. The COVID-19 pandemic has further accelerated this trend, as traditional classroom-based learning has become difficult, if not impossible, in many parts of the world.
Some of the key trends that we are also likely to see in the future of e-learning are as follows:
Adaptive learning. This method uses AI technology to personalize the learning experience for each student. This method adjusts the presentation of educational material according to an individual learner’s needs, preferences, and progress. By analysing students’ performance, it is possible to track their progress, and identify areas where they need more support or challenge. 1 , 2 , 3
Immersive learning. This refers to an educational approach that involves deeply engaging learners in a simulated or interactive environment. It seeks to create a sense of immersion, in which individuals feel fully involved in the learning process through various sensory experiences. The primary goal is to enhance the understanding, retention, and application of knowledge or skills. The key components of immersive learning include virtual and augmented reality (VR/AR). These technologies are becoming increasingly advanced and accessible, and we are likely to see more e-learning platforms using these technologies. 4 , 5 For example, a medical student could use VR to practice surgical techniques in a simulated operating room, 6 or an engineering student could use AR to visualize complex machinery and processes. 7
Microlearning. This refers to short, bite-sized learning modules that are designed to be completed in a few minutes or less. These modules are ideal for learners who have limited time or attention spans, and they can be easily accessed on mobile devices. In the future, we are likely to see more e-learning platforms using microlearning to deliver targeted, on-demand learning experiences. 8 , 9
Gamification. This refers to the use of game-like elements, such as badges, points, and leaderboards, to increase engagement and motivation among learners. Through the addition of game-like features to e-learning courses, learners can be incentivized to complete assignments and reach learning goals; thus, the learning experience becomes more engaging and enjoyable. 10 , 11 , 12
Mobile learning. With the widespread use of smartphones and tablets, e-learning is becoming more mobile-friendly, allowing learners to access course materials and complete assignments on the go. This makes learning more convenient and accessible, as learners can fit their learning into their busy schedules and on-the-go lifestyles. 13 , 14
Social learning. Social media and collaborative tools can enable learners to connect and learn from each other in online communities. 15 Learners can share their experiences, ask questions, and receive feedback from their peers, creating a sense of community and collaboration that can enhance the learning experience. 16
Generative AI. This refers to a subset of artificial intelligence focused on creating new content or information. It involves algorithms and models that are capable of generating novel content that can resemble human-generaed data. In February, 2022, an AI generative system named AlphaCode was launched. This system has been trained to ‘understand’ natural language, design algorithms to solve problems, and then implement them in code. At the end of November 2022, a new generative artificial intelligence chatbot that was developed by OpenAI and named ChatGPT was launched. ChatGPT has a wide range of potential applications due to its ability to generate human-like responses to natural language input. Some of its potentialities include text generation, summarization of long texts into shorter summaries, language translation, question answering, text completion, text correction, programming code generation, equation solutions and so on. ChatGPT evolved into OpenAI’s most widely used product to date, leading to the launch of ChatGPT Plus, a pilot paid subscription, in March 2023. Overall, the potential of AlphaCode and ChatGPT are vast, and these tools are likely to be used in many applications in the future of e-learning, as their capabilities will continue to improve through further research and development. ChatGPT can be integrated as a conversational AI within e-learning platforms. It can provide real-time responses to queries, clarify doubts, offer explanations, and guide learners through course materials. It is a promising tool for language lessons since it can translate text from one language to another. 17 To assist students and improve their writing abilities, ChatGPT may check for grammatical and structural problems in their work and provide valuable comments. 18 Students can explore many things with the help of ChatGPT, such as developing a computer program, writing an essay and solving a mathematical problem. 19 AlphaCode can aid e-learning platforms focused on programming or coding courses. It can provide code suggestions, explanations, and debugging assistance, helping learners better understand coding concepts. 20
How people perceive AI-based technologies: general and e-learning-focused literature overview
In the literature, people’s perceptions of technologies fuelled by artificial intelligence (AI) can vary depending on various factors such as their personal experiences and cultural backgrounds, and the way in which AI is portrayed in the media. The following are some common perceptions of AI technologies:
Fear of Job Loss. One of the most common fears associated with AI technologies is that they will take over jobs previously performed by humans. This fear is especially prominent in industries such as manufacturing, customer service, translation 21 , 22 , 23 and teaching 24 .
Improved Efficiency. Many people view AI technologies as a way to improve efficiency and accuracy in various fields. For example, AI-powered tools can improve students’ performance, 25 AI-powered software can help doctors diagnose diseases, 26 and chatbots can help students 27 and customer service representatives answer queries more quickly. 28
Ethical Concerns. There are concerns about the ethical implications of AI, such as bias in decision-making, 29 invasion of privacy, 30 and the potential for the development of AI-powered weapons. 31 For example, schools and institutions use AI-powered technologies to analyse student academic performance and collect a large amount of personal identity data; if these data are leaked or misused, this will seriously affect students’ personal privacy and security. In addition, students have difficulty controlling their own data and understanding how it is being used and shared, which may also lead to concerns and mistrust regarding personal privacy. 32 , 33
Excitement for Innovation. Some people are excited about the potential of AI to bring about new and innovative solutions to long-standing problems. For example, AI is being used to develop autonomous vehicles, which could revolutionize transportation, 34 and new methods for teaching and learning music. 35
Lack of Trust. Many people are still sceptical about the reliability and safety of AI technologies, especially given recent high-profile incidents of AI systems making mistakes 36 or being manipulated. 37 The lack of trust in the current application of generative AI in education mainly involves two aspects: opacity and reliability. When AI gives a result, it is difficult to explain the decision-making process, which makes it difficult for students to understand why they obtain a particular answer and how to improve their mistakes (opacity). Moreover, generative AI needs to be trained on a large dataset to ensure its effectiveness and reliability. For example, to train an effective automatic grading model, a large dataset of student essays and high-quality labelled data, such as scores for grammar, spelling, and logic, is needed. Insufficient datasets or low-quality labelled data may cause an automatic grading model to make mistakes and miss important aspects and affect its accuracy and application effectiveness. 38
Overall, people’s perceptions of AI technologies are complex and multifaceted, are influenced by a range of factors and are likely to continue evolving as AI becomes more integrated into our lives.
Purpose and outline of the paper
Considering what has been said thus far, it could be interesting to explore sentiments and major topics in the tweets about the new AI-based technologies.
Social media are indeed a major and rich data source for research in many domains due to their 4.8 billion active users 39 across the globe. For instance, researchers analyse user comments extracted from social media platforms (such as Facebook, 40 Twitter, 40 and Instagram 41 ) to uncover insights into social issues such as health, politics and business. Among these platforms, Twitter is one of the most immediate; tweets flow nonstop on the bulletin boards of users. Twitter allows users to express and spread opinions, thoughts and emotions as concisely and quickly as possible. Therefore, researchers have often preferred to analyse user comments on Twitter to immediately uncover insights into social issues during the COVID-19 pandemic (e.g., conspiracy theories, 42 why people oppose wearing a mask, 43 experiences in health care 44 and vaccinations 45 ) or distance learning. 46 , 47 , 48
Furthermore, we chose Twitter for its ability to immediately capture and spread people’s opinions and emotions on any topic, as well as for its ability to provide plentiful data, even in a short amount of time. Moreover, the people who have more direct experience with e-learning and AI technologies are students, teachers and researchers, i.e., persons of school or working age; that is, people who, by age, make up approximately 83% of Twitter users. 49
The text content of a tweet is a short microblog message containing at most 280 characters. This feature makes tweets particularly suitable for natural language processing (NLP) techniques, which are widely used to extract insights from unstructured texts and can then be used to explore sentiments and major topics of tweets. Unlike traditional methods, which use surveys and samples to evaluate these frameworks and are expensive and time-consuming, NLP techniques are economical and fast and provide immediate results.
In this paper, we aim to answer three main questions related to the first months following the launch of ChatGPT:
What has been the dominant sentiment towards AI-powered technologies? We responded through a sentiment analysis of related tweets. We used VADER as a sentiment analysis tool. 50
Which emotions about AI-powered technologies are prevalent? In this regard, we explored the emotions to the tweets using the Syuzhet package. 51
What are the most discussed topics among those who have positive feelings and those who have negative feelings? With respect to this problem, we used the latent Dirichlet allocation (LDA) model. 52
The findings from this study could aid in reimagining education in the postpandemic era to exploit technology and emerging strategies as benefits for educational institutions rather than as preparation for a new possible increase in infections. To this end, we decided to use only the technologies listed in “ AI-powered e-learning technologies ” as keywords for extracting tweets.

Methodology
Twitter was chosen as the data source. It is one of the world’s major social media platforms, with 237.8 million active users in July 2022, 53 and it is also a common source of text for sentiment analyses. 54 , 55 , 56
To collect AI-related tweets, we used ‘Academic Account for Twitter API V2’, which provides historical data and allows for the data to be filtered by language and geolocation. 57
For our study, we chose geolocated English-tweets only, posted from November 30, 2022 - March 31, 2023, with one or more of the following keywords: ‘ChatGPT’, ‘AlphaCode’, ‘virtual reality’, ‘augmented reality’, ‘micro-learning’, ‘mobile learning’, ‘adaptive learning’, ‘social leaning’, ‘AI’, ‘AI learning’ and ‘gamification’. A total of 31,147 tweets were collected.
Data preprocessing
In order to prepare the data for sentiment analysis, we employed various preprocessing techniques using NLP tools in Python. The steps we followed are as follows:
Eliminated mentions, URLs, and hashtags from the text,
Substituted HTML characters with their respective Unicode equivalents (e.g., replacing ‘ &’ with ‘ &’),
Removed HTML tags such as<br>,<p>, and others,
Eliminated unnecessary line breaks,
Removed special characters and punctuation except for exclamation points (the exclamation point is the only punctuation marks to which the used VADER lexicon is sensitive),
Excluded words that consist of only numbers.
For the second part, a high-quality dataset was required for the topic model. To achieve this, we removed duplicate tweets. In addition to the general data cleaning methods, we employed tokenization and lemmatization techniques to enhance the model’s performance.
We used the Gensim library 58 to tokenize the text, converting all the content to lowercase to ensure uniformity in word representation. Next, we pruned the vocabulary by removing stop words and terms unrelated to the topic. Additionally, we created a ‘ bigrams ' model to capture meaningful word combinations.
Finally, we employed the ‘ spaCy ' library from NLTK 59 to carry out lemmatization, which helped simplify words to their base form.
Sentiment and emotions analysis
To conduct sentiment analysis, we utilized the Valence Aware Dictionary for Sentiment Reasoning (VADER) algorithm, developed by Hutto et al. 50 VADER is a sentiment analysis tool that uses a sentiment lexicon, a dictionary specifically designed for sentiment analysis, to determine the emotion intensity of sentiment expressed in a text. The lexicon consists of words or phrases with their accompanying sentiment ratings. It allows for efficient sentiment analysis of social media content and exhibits remarkable accuracy comparable to that of humans.
Using VADER, we assigned sentiment scores to the preprocessed text data of each tweet. We followed the classification method recommended by the authors, categorizing the sentiment scores into three main categories: positive, negative, and neutral (see Fig. 1 —1st Step).
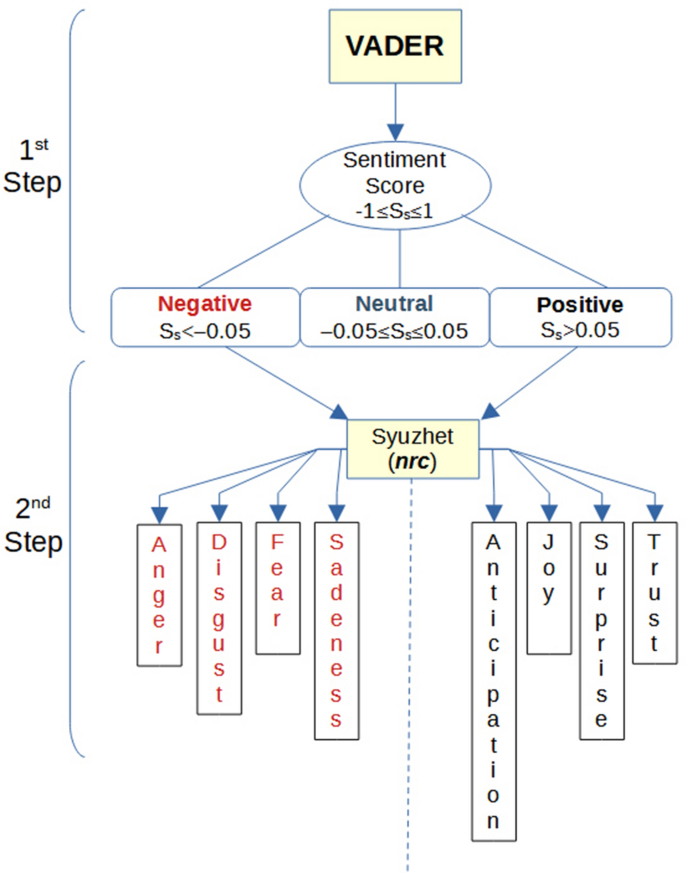
Steps in determining sentiment and emotions analysis.
VADER has demonstrated outstanding performance in analysing social media text. Comprehensive rules to consider various lexical features, including punctuation, capitalization, degree modifiers, the contrastive conjunction ’but’ and negation flipping trigrams.
Next, we employed the ‘ nrc ' algorithm, a component of the R library Syuzhet package, 51 to explore the underlying emotions associated with the tweet categories. In the ‘ nrc ' algorithm, an emotion dictionary is utilized to evaluate each tweet based on two sentiments (positive or negative) and eight emotions (anger, fear, anticipation, trust, surprise, sadness, joy, and disgust). Its purpose is to recognize the emotions conveyed within a tweet.
Whenever a tweet is connected to a specific emotion or sentiment, it receives points indicating the degree of valence in relation to that category. For instance, if a tweet includes three words associated with the ‘fear’ emotion in the word list, the tweet will receive a score of 3 in the fear category. Conversely, if a tweet does not contain any words related to a particular emotion, it will not receive a score for that specific emotion category.
When employing the ‘ nrc ’ lexicon, each tweet is assigned a score for each emotion category instead of a single algebraic score based on positive and negative words. However, this algorithm has limitations in accounting for negators and relies on a bag-of-words approach, disregarding the influence of syntax and grammar. Consequently, the VADER and ‘ nrc ’ methods are not directly comparable in terms of tweet volume and polarity categories.
Therefore, we utilized VADER for sentiment analysis and subsequently employed the ‘ nrc ' algorithm specifically for identifying positive and negative emotions. The sentiment analysis process follows a two-step procedure, as illustrated in Fig. 1 . While VADER’s neutral tweets play a valuable role in classification, they are not particularly informative for emotion analysis. Therefore we focused on tweets exhibiting positive and negative sentiments. This methodology was the original source of our previous paper. 60
The topic model
The topic model is an unsupervised machine learning method; that is, it is a text mining procedure that can be used to identify the topics or themes of documents in a large document corpus. 61 The latent Dirichlet allocation (LDA) model is one of the most popular topic modelling methods; it is a probabilistic model for expressing a corpus based on a three-level hierarchical Bayesian model. Latent Dirichlet allocation (LDA) is a generative probabilistic model of a corpus. The basic idea is that documents are represented as random mixtures over latent topics, where each topic is characterized by a distribution over words. 62 Particularly in LDA models, the generation of documents within a corpus follows the following process:
A mixture of k topics, \(\theta\) , is sampled from a Dirichlet prior, which is parameterized by \(\alpha\) ;
A topic \(z_n\) is sampled from the multinomial distribution, \(p(\theta \mid \alpha )\) that is the document topic distribution which models \(p(z_{n}=i\mid \theta )\) ;
Fixed the number of topics \(k=1...,K\) , the distribution of words for k topics is denoted by \(\phi\) ,which is also a multinomial distribution whose hyper-parameter \(\beta\) follows the Dirichlet distribution;
Given the topic \(z_n\) , a word, \(w_n\) , is then sampled via the multinomial distribution \(p(w \mid z_{n};\beta )\) .
Overall, the probability of a document (or tweet, in our case) “ \(\textbf{w}\) ” containing words can be described as:
Finally, the probability of the corpus of M documents \(D=\{\textbf{ w}_{\textbf{1}},...,{\textbf{w}}_{\textbf{M}}\}\) can be expressed as the product of the marginal probabilities of each single document \(D_m\) , as shown in ( 2 ).
An essential challenge in LDA is deterining an appropriate number of topics. Roder et al. 63 proposed coherence scores to evaluate the quality of each topic model. In particular, topic coherence is the metric used to evaluate the coherence between topics inferred by a model. As coherence measures, we used \(C_v\) which is a measure based on a sliding window that uses normalized pointwise mutual information (NPMI) and cosine similarity. 63 This value is used to emulate the relative score that a human is likely to assign to a topic and indicate how much the topic words ‘make sense’. This score is used to infer cohesiveness between ‘top’ words within a given topic. For topic visualization we used PyLDAvis, a web-based interactive visualization package that facilitates the display of the topics that were identified using the LDA approach. 52 In this package, each topic is visualized as a circle in a two-dimensional plane determined by principal components between topics and used Multidimensional scaling is used to project all the interrelated topic distances to two dimensions. 64 In the best hypothetic situation, the circles have similar dimensions and are well spaced from each other, covering the entire space made up of the 4 quadrants of the graph. An LDA model is evaluated the better the more the coherence is and the closer the pyLDAvis visualization is to the hypothetical situation.
Exploring the tweets
The word frequency of the most frequent 25 words terms are counted and visualized in Fig. 2 . The words are related to new AI tools and have positive attributes such as ‘good’ or ‘great’.
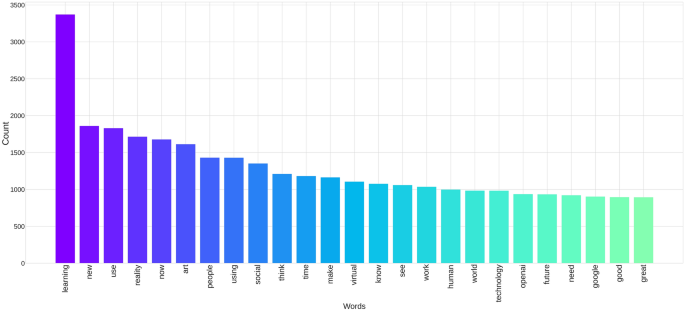
The total text word frequency. After removing irrelevant words, we counted and visualized the 25 most frequent words in our dataset.
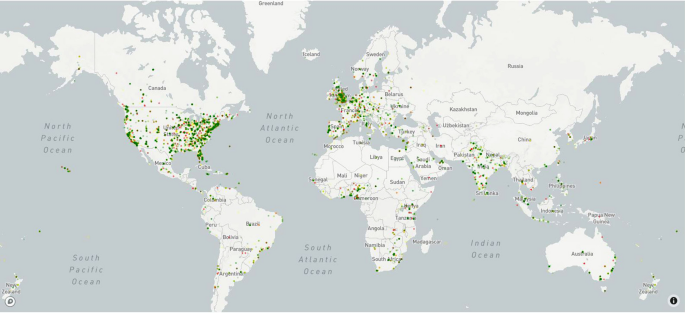
Tweets according to polarity by country.
All the tweets extracted were geolocated, but the ‘user location’ was detected in only approximately 85% of the tweets, highlighting the number of tweets that came from different counties around the world. The countries with the highest number of tweets are the United States and Great Britain (Fig. 3 ) but this result should be read considering that we have extracted tweets in the English language; therefore, it is normal that in countries where English is spoken, the number of tweets is very high. Notably, India and Europe also have a many Twitter users. In the United States, most Twitter users are located near the East and West Coasts. This figure also shows the polarity of the tweets: the colours red, yellow and green indicate negative, neutral and positive tweets, respectively.
Sentiment analysis
The sentiment score of a sentence is calculated by summing up the lexicon rates of each VADER-dictionary-listed word in the sentence. After proper normalization is applied, VADER returns a ‘compound’ sentiment score ( \(S_s\) ) in the range of \(-1\) to 1, from the most negative to the most positive. Once the score \(S_s\) is known, threshold values can be used to categorize tweets as positive, negative, or neutral (see Fig. 1 —1st Step). According to our analysis, the output of the VADER model shows a great predominance of positive public opinion (Table 1 ). As an example, three tweets with their own polarity are shown in Table 2 . Table 3 shows the number of total tweets with the related percentages and the percentages of positive, negative and neutral tweets of the various AI applications examined.
Regarding the timeline, the results showed that the number of tweets slowly increased during the observation period (Fig. 4 ). Clearly, as shown in the chart, there was a single weekly decline in the third week of March, likely due to the St. Patrick’s Day holiday, which fell close to the weekend (March 17).
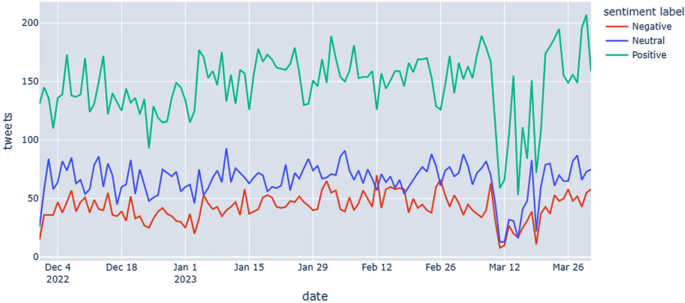
Timeline showing the sentiment of tweets.
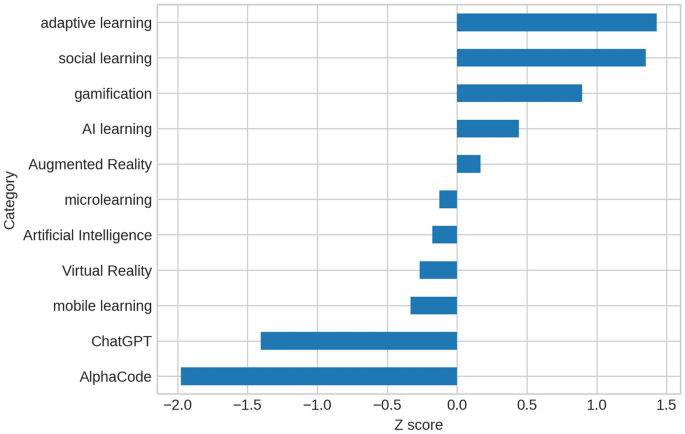
z scores of relative frequencies for positive sentiments.
The graph in Fig. 5 includes only the tweets with positive sentiment towards the 11 AI-based tools (categories). It returns the z scores of the relative frequencies \(Fr_{i}\) of positive tweets of the i -tool ( \(i=1,...,11\) ), where the z score is computed as follows:
A z score describes the position of a score (in our case \(Fr_i\) ) in terms of standard deviation units from the average. This score is grater then 0 if the relative frequency of \(Fr_i\) lies above the mean, while it is less than 0 if \(Fr_i\) lies below the mean.
We preferred z scores to relative frequencies because the different categories have different averages: the z scores highlight the position of each \(Fr_i\) with respect to the average of all the relative frequencies.
In Fig. 5 , we can see that the new generative AI ‘AlphaCode’ and ‘ChatGPT’ have scores that are much lower than and far from the average of positive sentiments. This could be due to concerns about the possible errors of such AI-based tools. Instead, ‘adaptive learning’, ‘social learning’ and ‘gamification’ lie above the mean of positive sentiments. This clearly attests to the more positive sentiment towards these tools, in our opinion largely due to their immediate feedback and to their attitude towards keeping learners engaged and motivated.
The second step of the analysis focused on identifying emotions in non-neutral tweets (see Fig. 1 —2nd Step). Among the eight basic emotions, ‘trust’ was the most common positive emotion observed in the tweets, followed by ‘joy’, while ‘fear’ was the most common negative emotion (Fig. 6 ). These results need to be interpreted in light of recent literature on the psychological dimensions of AI-based e-learning technologies (see section " How people perceive AI-based technologies: general and e-learning-focused literature overview "). In the literature, the dimension of fear includes the fear of job loss for teachers but also for the entire working world, 21 , 22 , 23 , 65 as well as concerns about AI systems making mistakes 36 or being manipulated. 37 The ‘trust’ dimension could be interpreted as the expectation that such technologies can improve the performance of students and people in general, 26 while ‘joy’ could be associated with enthusiasm for the potential of artificial intelligence in creating new and innovative solutions. 34
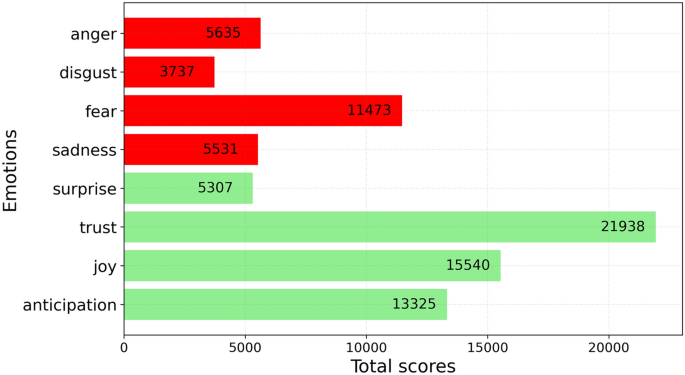
Emotion analysis of non-neutral tweets performed by Syuzhet .
To explore what concerns about AI-based tools Twitter users have, we applied the LDA model to our clean corpus of 28,259 words, which included only the following tagger components: nouns, adjectives, verbs and adverbs. Our goal was not to discover the topics discussed in the whole set of tweets but to detect the topics discussed in the positive sentiment tweets and the topics discussed in the negative sentiment tweets. Due to the large difference between the number of tweets with positive and negative sentiment polarity (57.58% vs. 16.65%), the application of the LDA model to the whole dataset would lead to not seeing the topics discussed in tweets with negative sentiment. Therefore, we chose to create two LDA models: one for tweets with positive polarity and one for those with negative polarity. For a better representation of the entire content, in each model, it is necessary to find an appropriate number of topics. By using topic numbers k ranging from 2 to 10, we initialized the LDA models and calculated the model coherence.
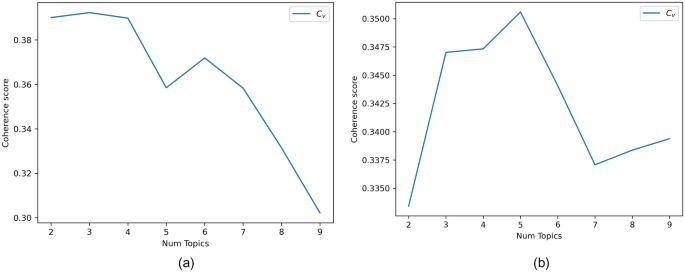
Coherence values of the LDA models.
We used \(C_v\) coherence for both models as a first reference. This value indicates the degree of ‘sense’ and ‘cohesiveness’ of the main words within a topic. 63
According to Fig. 7 a, the coherence score peaked at 3 for the negative tweet model. In contrast, in the positive tweet model, the coherence score (Fig. 7 b) peaked at 3 and 5 topics. The choice of 5 topic numbers would lead to a nonuniform distribution on Principal Component (PC) axes displayed by pyLDAvis, which means that there is not a high degree of independence for each topic (see the LDAvis map in Supplementary Information ‘ S2 ’ and ‘ S3 ’). A good model is judeged by a higher coherence and an average distribution on the principal component analysis displayed by pyLDAvis. 52 Therefore, we chose 3 as the topic number: the model has no intersections among topics, summarizes the whole word space well, and retains relatively independent topics.
The LDA analysis for negative and positive polarity tweets is shown in Table 4 .
In the negative tweets, the first theme accounts for 37.5% of the total tokens and includes tokens such as ‘chatgpt’, ‘write’,‘art’, ‘need’, ‘thing’, ‘stop’ and ‘generate’. It is rather immediate to think that this negative opinion refers to tools such as ChatGPT and expresses concerns about its use in art and writing. People think that human creativity can generate art and literature rather than generative AI; for this reason, the use of generative tools in these contexts should be stopped. The second theme accounts for 33.3% of the total tokens and includes the words ‘bad’, ‘job’, ‘technology’, ‘scary’, ‘change’ and ‘learn’. We infer people’s fear of the changes that technology will bring in the world of job and learning. Several words, such as ‘kill’, ‘war’, ‘worry’ ,‘fear’, ‘fight’, ‘robot’ and ‘dangerous’, are mentioned in the third topic. This may indicate a sort of ‘apocalyptic fear’ that artificial intelligence could lead us to a new war and the end of humankind. For a map representation of the three topics, see the Supplementary Information ‘ S1 ’.
In the positive tweets, the first theme accounts for 36.3% of the total tokens and includes tokens such as ‘learn’, ‘help’, ‘technology’, ‘student’ and ‘job’. Based on this, we inferred that people think that AI technologies have the potential to improve the job world and educational system. The second theme accounts for 34.7% of the total tokens, including the words ‘chatgpt’, ‘well’, ‘write’, ‘create’ and ‘ask’, showing people’s positive perception of AIs such as ChatGPT writing, asking questions and creating new solutions. After all, several words, such as ‘love’, ‘chatgpt’, ‘good’ ,‘answer’ and ‘believe’, are mentioned in the third topic. This indicates that people ‘believe in AI’ and trust tha AI, particularly ChatGPT provides good answers and solutions. For a map representation of the three topics, see the Supplementary Information ‘ S2 ’.
Based on the LDA outputs, the following six topics were identified:
For ‘negative polarity’ tweets:
Topic 1: Concerns about ChatGPT use in art and writing
Topic 2: Fear of changes in the world of job and learning
Topic 3: Apocalyptic-fear.
For ‘positive polarity’ tweets:
Topic 1: AI technologies can improve job and learning
Topic 2: Useful ChatGPT features
Topic 3: Belief in the ability of ChatGPT.
Limitations
This study has several limitations, which can be summarized as follows.
Limitations related to the use of keywords to extract tweets. Sometimes, keywords can be ambiguous, leading to noise-affected results. Due to the dynamic nature of social media, trends and topics change rapidly. Keywords might quickly lose relevance as new terms emerge.
Limitations related to emotion analysis . A first limitation is that the number of emotion categories was limited to 8; 51 , 66 however, emotion is a broad concept and, according to Cowen and Keltner 67 , may involve up to 27 categories. A second limitation is that misspelled words could not be identified or analysed in the algorithm. Further limitations involve the dictionary of sentiments (“lexicon”) developed by Mohammad and Turney for emotion analysis. 51 This dictionary maps a list of language features to emotion intensities, where:
Only 5 individuals were recruited to annotate a term against each of the 8 primary emotions.
The emotions associated with a term were annotated without considering the possible term context.
Although the percentages of agreement were apparently high, interrater reliability statistics were not reported.
Limitations of topic analysis. Considering that LDA is an unsupervised learning technique, the main limitation is the degree of subjectivity in defining the topic created. 45
Limitations of Twitter-based studies. Twitter data generally underestimate the opinions of people aged 50 and over, because approximately 83% of Twitter users worldwide are indeed under age 50. 49 However, in the present study bias has an almost negligible impact: the future of AI-powered e-learning technologies indeed has a greater impact on younger people than on older people.
Conclusions and future perspectives
With the aim of studying the opinions and emotions related to AI-powered e-learning technologies, we collected tweets on this issue and carried out a sentiment analysis using the VADER and Syuzhet packages in combination with a topic analysis.
There is no doubt that artificial intelligence has the potential to transform the whole education system. The results showed a predominance of positive attitudes: topics with a positive outlook indicate trust and hope in AI tools that can improve efficiency in jobs and the educational world. Indeed, among the eight emotions of the Syuzhet package, ‘trust’ and ’joy’ were the most positive emotions observed in the tweets, while ‘fear’ was the most common negative emotion. Based on the analysis, two particular aspects of fear were identified: an ‘apocalyptic fear’ that artificial intelligence could lead to the end of humankind and a fear of the ‘future of artistic and intellectual jobs’, as AI could not only destroy human art and creativity but also make individual contributions of students and researchers not assessable.
In our analysis, people with positive sentiments were directed towards ‘adaptive learning’, ‘social learning’ and ‘gamification’. Therefore, from a future perspective, we can expect an ever-increasing implementation of these aspects in e-learning. AI could help educators ‘adapt learning’ techniques to tailor them to the individual student, with his or her own interests, strengths and preferences.
By analysing data about interactions between students, AI can identify opportunities for collaboration between students and thus transform ‘social learning’ into ‘collaborative learning’. AI could help educators create more effective group work assignments, provide targeted support to struggling students, and promote positive social interactions among them.
In class, instead of administering boring tests, AI-powered ‘games and simulations’ could increasingly provide engaging and interactive learning experiences to help students develop skills and knowledge in a fun and engaging way. Moreover, gamification could be increasingly useful for providing immediate feedback and monitoring student progress over time.
Despite our analysis highlighting the great potential of and people’s expectations for AI-based technologies, there is an aspect that cannot be elucidated by examining tweets.
Algorithms such as ChatGPT disrupt traditional text-based assessments, as students can query the program to research a topic that results in documents authored by an algorithm and ready to submit as a graded assignment. Therefore, we need to reimagine student assessment in new ways. The current debate is whether educators should ban artificial intelligence platforms through school internet filters 38 or embrace algorithms as teaching and research tools. 68
In March, 2023, Italy was the first government to ban ChatGPT as a result of privacy concerns. The Italian data-protection authority said there were privacy concerns relating to the model and said it would investigate immediately. However, in late April, the ChatGPT chatbot was reactivated in Italy after its maker OpenAI addressed issues raised by Italy’s data protection authority.
Regardless of privacy concerns, possible data manipulations or the right answers to AI-based tools, we believe that the future cannot be stopped.
Data availability
All data generated or analyzed during this study are included in the Supplementary Information Files of this published article.
Zahabi, M. & Abdul Razak, A. M. Adaptive virtual reality-based training: A systematic literature review and framework. Virtual Real. 24 , 725–752. https://doi.org/10.1007/s10055-020-00434-w (2020).
Article Google Scholar
Raj, N. S. & Renumol, V. G. A systematic literature review on adaptive content recommenders in personalized learning environments from 2015 to 2020. J. Comput. Educ. 9 , 113–148. https://doi.org/10.1007/s40692-021-00199-4 (2022).
Al-Badi, A., Khan, A. & Eid-Alotaibi,. Perceptions of learners and instructors towards artificial intelligence in personalized learning. Proced. Comput. Sci. 201 , 445–451. https://doi.org/10.1016/j.procs.2022.03.058 (2022).
Bizami, N. A., Tasir, Z. & Kew, S. N. Innovative pedagogical principles and technological tools capabilities for immersive blended learning: A systematic literature review. Educ. Inf. Technol. 28 , 1373–1425. https://doi.org/10.1007/s10639-022-11243-w (2023).
Won, M. et al. Diverse approaches to learning with immersive virtual reality identified from a systematic review. Comput. Educ. 195 , 104701. https://doi.org/10.1016/j.compedu.2022.104701 (2023).
Tang, Y. M., Chau, K. Y., Kwok, A. P. K., Zhu, T. & Ma, X. A systematic review of immersive technology applications for medical practice and education—trends, application areas, recipients, teaching contents, evaluation methods, and performance. Educ. Res. Rev. 35 , 100429. https://doi.org/10.1016/j.edurev.2021.100429 (2022).
Wilkerson, M., Maldonado, V., Sivaraman, S., Rao, R. R. & Elsaadany, M. Incorporating immersive learning into biomedical engineering laboratories using virtual reality. J. Biol. Eng. 16 , 20. https://doi.org/10.1186/s13036-022-00300-0 (2022).
Article CAS PubMed PubMed Central Google Scholar
Taylor, A.-D. & Hung, W. The effects of microlearning: A scoping review. Educ. Technol. Res. Dev. 70 , 363–395. https://doi.org/10.1007/s11423-022-10084-1 (2022).
Wang, C., Bakhet, M., Roberts, D., Gnani, S. & El-Osta, A. The efficacy of microlearning in improving self-care capability: A systematic review of the literature. Public Health 186 , 286–296. https://doi.org/10.1016/j.puhe.2020.07.007 (2020).
Article CAS PubMed Google Scholar
Oliveira, W. et al. Tailored gamification in education: A literature review and future agenda. Educ. Inf. Technol. 28 , 373–406. https://doi.org/10.1007/s10639-022-11122-4 (2023).
Indriasari, T. D., Luxton-Reilly, A. & Denny, P. Gamification of student peer review in education: A systematic literature review. Educ. Inf. Technol. 25 , 5205–5234. https://doi.org/10.1007/s10639-020-10228-x (2020).
Liu, T., Oubibi, M., Zhou, Y. & Fute, A. Research on online teachers’ training based on the gamification design: A survey analysis of primary and secondary school teachers. Heliyon 9 , e15053. https://doi.org/10.1016/j.heliyon.2023.e15053 (2023).
Article PubMed PubMed Central Google Scholar
Widiastuti, N. L. A systematic literature review of mobile learning applications in environmental education from 2011–2021. J. Educ. Technol. Inst. 1 , 89–98 (2022).
Google Scholar
Criollo-C, S., Guerrero-Arias, A., Jaramillo-Alcázar, A. & Luján-Mora, S. Mobile learning technologies for education: Benefits and pending issues. Appl. Sci. https://doi.org/10.3390/app11094111 (2021).
Chelarescu, P. Deception in social learning: a multi-agent reinforcement learning perspective. arxiv: 2106.05402 (2021)
Gweon, H. Inferential social learning: Cognitive foundations of human social learning and teaching. Trends Cogn. Sci. 25 , 896–910. https://doi.org/10.1016/j.tics.2021.07.008 (2021).
Article PubMed Google Scholar
Javaid, M., Haleem, A., Singh, R. P., Khan, S. & Khan, I. H. Unlocking the opportunities through chatgpt tool towards ameliorating the education system. BenchCouncil Trans. Benchmarks Stand. Eval. 3 , 100115. https://doi.org/10.1016/j.tbench.2023.100115 (2023).
Sok, S. & Heng, K. ChatGPT for education and research: A review of benefits and risks. SSRN Electron. J. https://doi.org/10.2139/ssrn.4378735 (2023).
Yilmaz, R. & Karaoglan Yilmaz, F. G. Augmented intelligence in programming learning: Examining student views on the use of chatgpt for programming learning. Comput. Hum. Behav. Artif. Hum. 1 , 100005. https://doi.org/10.1016/j.chbah.2023.100005 (2023).
Becker, B. A. et al. Programming is hard—or at least it used to be: Educational opportunities and challenges of AI code generation. In Proceedings of the 54th ACM Technical Symposium on Computer Science Education V. 1 , SIGCSE 2023, 500–506, https://doi.org/10.1145/3545945.3569759 (Association for Computing Machinery, New York, 2023).
Ernst, E., Merola, R. & Samaan, D. Economics of artificial intelligence: Implications for the future of work. IZA J. Labor Policy 9 , 55. https://doi.org/10.2478/izajolp-2019-0004 (2019).
Jaiswal, A., Arun, C. J. & Varma, A. Rebooting employees: Upskilling for artificial intelligence in multinational corporations. Int. J. Hum. Resour. Manag. 33 , 1179–1208. https://doi.org/10.1080/09585192.2021.1891114 (2022).
Kirov, V. & Malamin, B. Are translators afraid of artificial intelligence?. Societies https://doi.org/10.3390/soc12020070 (2022).
Selwyn, N. Should Robots Replace Teachers?: AI and the Future of Education (John Wiley & Sons, 2019).
Baidoo-Anu, D. & Owusu Ansah, L. Education in the era of generative artificial intelligence (AI): Understanding the potential benefits of chatgpt in promoting teaching and learning. J. AI 7 , 52–62. https://doi.org/10.61969/jai.1337500 (2023).
van Leeuwen, K. G., de Rooij, M., Schalekamp, S., van Ginneken, B. & Rutten, M. J. C. M. How does artificial intelligence in radiology improve efficiency and health outcomes?. Pediatr. Radiol. 52 , 2087–2093. https://doi.org/10.1007/s00247-021-05114-8 (2022).
Shingte, K., Chaudhari, A., Patil, A., Chaudhari, A. & Desai, S. Chatbot development for educational institute. SSRN Electron. J. https://doi.org/10.2139/ssrn.3861241 (2021).
Wang, X., Lin, X. & Shao, B. How does artificial intelligence create business agility? Evidence from chatbots. Int. J. Inf. Manage. 66 , 102535. https://doi.org/10.1016/j.ijinfomgt.2022.102535 (2022).
Parikh, R. B., Teeple, S. & Navathe, A. S. Addressing bias in artificial intelligence in health care. JAMA 322 , 2377–2378. https://doi.org/10.1001/jama.2019.18058 (2019).
Mazurek, G. & Małagocka, K. Perception of privacy and data protection in the context of the development of artificial intelligence. J. Manag. Anal. 6 , 344–364 (2019).
David, W. E. A. Ai-powered lethal autonomous weapon systems in defence transformation. Impact and challenges. In Modelling and Simulation for Autonomous Systems (eds Mazal, J. et al. ) 337–350 (Springer International Publishing, 2020).
Chapter Google Scholar
May, M. & George, S. Privacy concerns in e-learning: Is UsingTracking system a threat?. Int. J. Inf. Educ. Technol. 1 , 1–8 (2011).
ADS Google Scholar
Ashman, H. et al. The ethical and social implications of personalization technologies for e-learning. Inf. Manag. 51 , 819–832. https://doi.org/10.1016/j.im.2014.04.003 (2014).
Ma, Y., Wang, Z., Yang, H. & Yang, L. Artificial intelligence applications in the development of autonomous vehicles: A survey. IEEE/CAA J. Autom. Sin. 7 , 315–329. https://doi.org/10.1109/JAS.2020.1003021 (2020).
Wei, J., Karuppiah, M. & Prathik, A. College music education and teaching based on AI techniques. Comput. Electr. Eng. 100 , 107851. https://doi.org/10.1016/j.compeleceng.2022.107851 (2022).
Mahmood, A., Fung, J. W., Won, I. & Huang, C.-M. Owning mistakes sincerely: Strategies for mitigating AI errors. In Proceedings of the 2022 CHI Conference on Human Factors in Computing Systems , CHI ’22, https://doi.org/10.1145/3491102.3517565 (Association for Computing Machinery, New York, 2022).
Carroll, M., Chan, A., Ashton, H. & Krueger, D. Characterizing manipulation from AI systems. arxiv: 2303.09387 (2023).
Yu, H. & Guo, Y. Generative artificial intelligence empowers educational reform: Current status, issues, and prospects. Front. Educ. https://doi.org/10.3389/feduc.2023.1183162 (2023).
Kemp, S. Digital 2023: Global digital overview. (Accessed April 2023); Online https://datareportal.com/reports/digital-2023-april-global-statshot (2023).
Zhan, Y., Etter, J.-F., Leischow, S. & Zeng, D. Electronic cigarette usage patterns: A case study combining survey and social media data. J. Am. Med. Inform. Assoc. 26 , 9–18. https://doi.org/10.1093/jamia/ocy140 (2019).
Hassanpour, S., Tomita, N., DeLise, T., Crosier, B. & Marsch, L. A. Identifying substance use risk based on deep neural networks and instagram social media data. Neuropsychopharmacology 44 , 487–494. https://doi.org/10.1038/s41386-018-0247-x (2019).
Rains, S. A., Leroy, G., Warner, E. L. & Harber, P. Psycholinguistic markers of COVID-19 conspiracy tweets and predictors of tweet dissemination. Health Commun. https://doi.org/10.1080/10410236.2021.1929691 (2021).
He, L. et al. Why do people oppose mask wearing? a comprehensive analysis of U.S. tweets during the COVID-19 pandemic. J. Am. Med. Inform. Assoc. 28 , 1564–1573. https://doi.org/10.1093/jamia/ocab047 (2021).
Ainley, E., Witwicki, C., Tallett, A. & Graham, C. Using twitter comments to understand people’s experiences of UK health care during the COVID-19 pandemic: Thematic and sentiment analysis. J. Med. Internet Res. https://doi.org/10.2196/31101 (2021).
Kwok, S. W. H., Vadde, S. K. & Wang, G. Tweet topics and sentiments relating to COVID-19 vaccination among Australian twitter users: Machine learning analysis. J. Med. Internet Res. 23 , e26953. https://doi.org/10.2196/26953 (2021).
Aljabri, M. et al. Sentiment analysis of Arabic tweets regarding distance learning in Saudi Arabia during the COVID-19 pandemic. Sensors (Basel) 21 , 5431. https://doi.org/10.3390/s21165431 (2021).
Article ADS CAS PubMed Google Scholar
Mujahid, M. et al. Sentiment analysis and topic modeling on tweets about online education during COVID-19. Appl. Sci. (Basel) 11 , 8438. https://doi.org/10.3390/app11188438 (2021).
Article CAS Google Scholar
Asare, A. O., Yap, R., Truong, N. & Sarpong, E. O. The pandemic semesters: Examining public opinion regarding online learning amidst COVID-19. J. Comput. Assist. Learn. 37 , 1591–1605. https://doi.org/10.1111/jcal.12574 (2021).
Statista. Distribution of twitter users worldwide as of april 2021, by age group. Statista. https://www.statista.com/statistics/283119/age-distribution-of-global-twitter-users/ (2021).
Hutto, C. & Gilbert, E. Vader: A parsimonious rule-based model for sentiment analysis of social media text. In Proceedings of the 8th International Conference on Weblogs and Social Media, ICWSM 2014 (2015).
Mohammad, S. & Turney, P. Emotions evoked by common words and phrases: Using mechanical turk to create an emotion lexicon. In Proceedings of the NAACL HLT 2010 Workshop on Computational Approaches to Analysis and Generation of Emotion in Text (LA, California, 2010).
Sievert, C. & Shirley, K. LDAvis: A method for visualizing and interpreting topics. In Proceedings of the Workshop on Interactive Language Learning, Visualization, and Interfaces , 63–70, https://doi.org/10.3115/v1/W14-3110 (Association for Computational Linguistics, https://aclanthology.org/W14-3110 , Baltimore, Maryland, 2014).
Kemp, S. Digital 2022: Twitter report (Accessed July 2022). Online https://datareportal.com/reports/digital-2023-deep-dive-the-state-of-twitter-in-april-2023 (2022).
Tumasjan, A., Sprenger, T., Sandner, P. & Welpe, I. Predicting elections with twitter: What 140 characters reveal about political sentiment. In Proc. Fourth Int. AAAI Conf. Weblogs Soc. Media Predict. , vol. 10 (2010).
Oyebode, O., Orji, R. Social. & media and sentiment analysis: The Nigeria presidential election,. In 2019 IEEE 10th Annual Information Technology. Electronics and Mobile Communication Conference (IEMCON) 2019 , https://doi.org/10.1109/IEMCON.2019.8936139 (IEEE 2019).
Budiharto, W. & Meiliana, M. Prediction and analysis of Indonesia presidential election from twitter using sentiment analysis. J. Big Data https://doi.org/10.1186/s40537-018-0164-1 (2018).
Twitter API V2 . Academic Account for Twitter API V2. https://developer.twitter.com/en/products/twitter-api/academic-research (2022).
Řehuřek, R. & Sojka, P. Software framework for topic modelling with large corpora. In Proceedings of LREC 2010 workshop New Challenges for NLP Frameworks , 46–50 (Univerity of Malta, 2010).
Bird, S., Klein, E. & Loper, E. Natural Language Processing with Python (O’Reilly Media, 2009).
Stracqualursi, L. & Agati, P. Tweet topics and sentiments relating to distance learning among Italian twitter users. Sci. Rep. 12 , 9163 (2022).
Article ADS CAS PubMed PubMed Central Google Scholar
Blei, D. M., Ng, A. Y., Jordan, M. I. & Lafferty, J. Latent dirichlet allocation. J. Mach. Learn. Res. 3 , 993–1022 (2003).
Lee, J. et al. Ensemble modeling for sustainable technology transfer. Sustainability 10 , 22–78. https://doi.org/10.3390/su10072278 (2018).
Röder, M., Both, A. & Hinneburg, A. Exploring the space of topic coherence measures. In Proceedings of the Eighth ACM International Conference on Web Search and Data Mining - WSDM ’15 (ACM Press, 2015).
Sievert, C. & Shirley, K. Package ldavis (Online) https://cran.r-project.org/web/packages/LDAvis/LDAvis.pdf (2022).
Edwards, B. I. & Cheok, A. D. Why not robot teachers: Artificial intelligence for addressing teacher shortage. Appl. Artif. Intell. 32 , 345–360. https://doi.org/10.1080/08839514.2018.1464286 (2018).
Plutchik, R. A general psychoevolutionary theory of emotion. In Theories of Emotion , 3–33, https://doi.org/10.1016/b978-0-12-558701-3.50007-7 (Elsevier, 1980).
Cowen, A. S. & Keltner, D. Self-report captures 27 distinct categories of emotion bridged by continuous gradients. Proc. Natl. Acad. Sci. U. S. A. 114 , E7900–E7909. https://doi.org/10.1073/pnas.1702247114 (2017).
Reyna, J. The potential of artificial intelligence (AI) and chatgpt for teaching, learning and research. In EdMedia+ Innovate Learning , 1509–1519 (Association for the Advancement of Computing in Education (AACE), 2023).
Download references
Author information
Authors and affiliations.
Department of Statistics, University of Bologna, 40126, Bologna, Italy
Luisa Stracqualursi & Patrizia Agati
You can also search for this author in PubMed Google Scholar
Contributions
L.S. and P.A. contributed equally to this work. Particularly, L.S. wrote the main text of the manuscript, contributed to the formal analysis, conceptualization, investigation and creation of the software in Python. P.A. contributed to the formalization of the applied methodology, to the data management, to the preparation of the figures, to the supervision and to the revision and modification of the manuscript.
Corresponding author
Correspondence to Luisa Stracqualursi .
Ethics declarations
Competing interests.
The authors declare no competing interests.
Additional information
Publisher's note.
Springer Nature remains neutral with regard to jurisdictional claims in published maps and institutional affiliations.
Supplementary Information
Supplementary figure s1., supplementary figure s2., supplementary figure s3., supplementary table s1., rights and permissions.
Open Access This article is licensed under a Creative Commons Attribution 4.0 International License, which permits use, sharing, adaptation, distribution and reproduction in any medium or format, as long as you give appropriate credit to the original author(s) and the source, provide a link to the Creative Commons licence, and indicate if changes were made. The images or other third party material in this article are included in the article’s Creative Commons licence, unless indicated otherwise in a credit line to the material. If material is not included in the article’s Creative Commons licence and your intended use is not permitted by statutory regulation or exceeds the permitted use, you will need to obtain permission directly from the copyright holder. To view a copy of this licence, visit http://creativecommons.org/licenses/by/4.0/ .
Reprints and permissions
About this article
Cite this article.
Stracqualursi, L., Agati, P. Twitter users perceptions of AI-based e-learning technologies. Sci Rep 14 , 5927 (2024). https://doi.org/10.1038/s41598-024-56284-y
Download citation
Received : 06 May 2023
Accepted : 05 March 2024
Published : 11 March 2024
DOI : https://doi.org/10.1038/s41598-024-56284-y
Share this article
Anyone you share the following link with will be able to read this content:
Sorry, a shareable link is not currently available for this article.
Provided by the Springer Nature SharedIt content-sharing initiative
By submitting a comment you agree to abide by our Terms and Community Guidelines . If you find something abusive or that does not comply with our terms or guidelines please flag it as inappropriate.
Quick links
- Explore articles by subject
- Guide to authors
- Editorial policies
Sign up for the Nature Briefing newsletter — what matters in science, free to your inbox daily.

This paper is in the following e-collection/theme issue:
Published on 8.5.2024 in Vol 10 (2024)
The Scope of Virtual Reality Simulators in Radiology Education: Systematic Literature Review
Authors of this article:

- Shishir Shetty 1 , PhD ;
- Supriya Bhat 2 , MDS ;
- Saad Al Bayatti 1 , MSc ;
- Sausan Al Kawas 1 , PhD ;
- Wael Talaat 1 , PhD ;
- Mohamed El-Kishawi 3 , PhD ;
- Natheer Al Rawi 1 , PhD ;
- Sangeetha Narasimhan 1 , PhD ;
- Hiba Al-Daghestani 1 , MSc ;
- Medhini Madi 4 , MDS ;
- Raghavendra Shetty 5 , PhD
1 Department of Oral and Craniofacial Health Sciences, College of Dental Medicine, University of Sharjah, , Sharjah, , United Arab Emirates
2 Department of Oral Medicine and Radiology, AB Shetty Memorial Institute of Dental Sciences, Nitte (Deemed to be University), , Mangalore, , India
3 Department of Preventive and Restorative Dentistry, College of Dental Medicine, University of Sharjah, , Sharjah, , United Arab Emirates
4 Department of Oral Medicine and Radiology, Manipal College of Dental Sciences, Manipal Academy of Higher Education, , Manipal, , India
5 Department of Clinical Sciences, College of Dentistry, Ajman University, , Ajman, , United Arab Emirates
Corresponding Author:
Supriya Bhat, MDS
Background: In recent years, virtual reality (VR) has gained significant importance in medical education. Radiology education also has seen the induction of VR technology. However, there is no comprehensive review in this specific area. This review aims to fill this knowledge gap.
Objective: This systematic literature review aims to explore the scope of VR use in radiology education.
Methods: A literature search was carried out using PubMed, Scopus, ScienceDirect, and Google Scholar for articles relating to the use of VR in radiology education, published from database inception to September 1, 2023. The identified articles were then subjected to a PRISMA (Preferred Reporting Items for Systematic Reviews and Meta-Analyses)–defined study selection process.
Results: The database search identified 2503 nonduplicate articles. After PRISMA screening, 17 were included in the review for analysis, of which 3 (18%) were randomized controlled trials, 7 (41%) were randomized experimental trials, and 7 (41%) were cross-sectional studies. Of the 10 randomized trials, 3 (30%) had a low risk of bias, 5 (50%) showed some concerns, and 2 (20%) had a high risk of bias. Among the 7 cross-sectional studies, 2 (29%) scored “good” in the overall quality and the remaining 5 (71%) scored “fair.” VR was found to be significantly more effective than traditional methods of teaching in improving the radiographic and radiologic skills of students. The use of VR systems was found to improve the students’ skills in overall proficiency, patient positioning, equipment knowledge, equipment handling, and radiographic techniques. Student feedback was also reported in the included studies. The students generally provided positive feedback about the utility, ease of use, and satisfaction of VR systems, as well as their perceived positive impact on skill and knowledge acquisition.
Conclusions: The evidence from this review shows that the use of VR had significant benefit for students in various aspects of radiology education. However, the variable nature of the studies included in the review reduces the scope for a comprehensive recommendation of VR use in radiology education.
Introduction
The use of technology in education helps students achieve improved acquisition of professional knowledge and practical skills [ 1 - 3 ]. Virtual reality (VR) is a modern technology that simulates experience by producing 3D interactive situations and presenting objects in a virtual world with spatial dimensions [ 4 , 5 ]. VR technology can be classified as nonimmersive or immersive [ 6 ]. In a nonimmersive VR, the simulated 3D environment is experienced through a computer monitor [ 6 ]. On the other hand, an immersive VR provides a sense of presence in a computer-generated environment, created by producing realistic sights, sounds, and other sensations that replicate a user’s physical presence in a virtual environment [ 6 , 7 ]. Using VR technology, a person can look about the artificial world, navigate around in it, and interact with simulated objects or items [ 5 , 8 ]. Due to the broad nature of VR technology, it has many applications, some of which are in the field of medicine [ 9 , 10 ].
The use of VR in medicine started in the 1990s when medical researchers were trying to create 3D models of patients’ internal organs [ 11 - 13 ]. Since then, VR use in the field of medicine and general health care has increased substantially to cover many areas including medical education. Radiology education has also come to see the use of VR technology in the recent past [ 14 ]. The use of VR in radiology education enables students to practice radiography in a virtual environment, which is radiation free [ 15 ]. Additionally, the use of VR enables effective and repeatable training. This allows trainees to recognize and correct errors as they occur [ 16 , 17 ]. The aim of this review is to explore the scope of VR in radiology education.
This systematic review has been performed using the PRISMA (Preferred Reporting Items for Systematic Review and Meta-Analysis) guidelines [ 18 ] [ Checklist 1 ]).
Information Sources and Study Selection
The bibliographic databases used were PubMed, Scopus, ScienceDirect, and Google Scholar. A systematic literature search was conducted for articles published from database inception to September 1, 2023. Topic keywords were used to generate search strings. The search strings that were used are provided in Table 1 . Only the first 10 pages of Google Scholar results were exported. The identified studies were then subjected to a study selection process. The search string for ScienceDirect was shorter because the database only allows a maximum of 8 Boolean operators, hence the sting had to be shortened. The search in PubMed was limited to the title and abstract. The searches in Scopus and ScienceDirect were limited to title, abstract, and keywords.
Inclusion and Exclusion Criteria
Original research articles written in the English language were included in the review. Studies conducted on medical, dental, and allied health sciences students (undergraduate and postgraduate) from any part of the world were included in the review. Studies exploring the use of VR learning in radiology education were included.
Narrative reviews, scoping reviews, systematic reviews, meta-analyses, editorials, and commentaries were excluded. Studies that did not align with the required study objective were excluded.
Method of Quality Assessment
Randomized controlled trials (RCTs) and randomized experimental studies were appraised using the RoB 2 tool from the Cochrane Collaboration [ 19 ]. A visualization of the risk-of-bias assessment was done using the web-based robvis tool [ 20 ]. Cross-sectional studies were appraised using the appraisal checklist for analytical cross-sectional studies from the Joanna Briggs Institute [ 21 ].
Data Extraction
Each article included in the review was summarized in a table, including basic study characteristics. The extracted attributes were study author(s), publication year, study design, type and number of participants, type of radiology education under study, and the outcome being assessed. The extracted data are provided in Table 2 .
a RCT: randomized controlled trial.
b CT: computed tomography.
Search Results
The database search identified a total of 2877 studies; 374 (13%) studies were from PubMed, 2169 (75.4%) were from Scopus, 234 (8.1%) were from ScienceDirect, and 100 (3.5%) were from Google Scholar. Before the screening procedure, 37 duplicates were removed. During title and abstract screening, 2808 articles were excluded since they did not align with the eligibility criteria. The remaining 32 articles were then subjected to a full-text review, and 15 were excluded for reasons provided in Figure 1 , which shows the study selection process [ 38 ]. At the end of the process, 17 studies were found eligible for inclusion in the review.

Characteristics of Included Studies
Among the 17 studies, 3 (18%) RCTs, 7 (41%) randomized experimental trials, and 7 (41%) cross-sectional studies were included. The studies encompassed various aspects of radiology education, including dental radiology [ 28 , 29 ], diagnostic radiology [ 22 , 24 ], and interventional radiology [ 25 , 31 ].
Results of Quality Assessment
Among the 7 cross-sectional studies, 2 (29%) scored “good” in overall quality and the remaining 5 (71%) scored “fair.” The results for the quality appraisal of cross-sectional studies are shown in Table 3 . Studies were appraised using the checklist for analytical cross-sectional studies from the Joanna Briggs Institute [ 21 ].
Among the 10 randomized trials, 3 (30%) had a low risk of bias, 5 (50%) showed some concerns, and 2 (20%) had a high risk of bias. These results are shown in Table 4 . RCTs were appraised using the RoB 2 tool from the Cochrane Collaboration [ 19 ]. A risk-of-bias graph ( Figure 2 ) and a risk-of-bias summary ( Figure 3 ) are also provided.
a Item 1: were the criteria for inclusion in the sample clearly defined?
b Item 2: were the study subjects and the setting described in detail?
c Item 3: was the exposure measured in a valid and reliable way?
d Item 4: were objective, standard criteria used for measurement of the condition?
e Item 5: were confounding factors identified?
f Item 6: were strategies to deal with confounding factors stated?
g Item 7: were the outcomes measured in a valid and reliable way?
h Item 8: was appropriate statistical analysis used?
i N/A: not assessable.
a D1: risk of bias arising from the randomization process.
b D2: risk of bias due to deviations from the intended interventions (effect of assignment to intervention).
c D3: risk of bias due to missing outcome data.
d D4: risk of bias in measurement of the outcome.
e D5: risk of bias in selection of the reported result.

Type of VR Hardware and Software Used in the Studies
The studies used a wide range of VR software and hardware. Some of the studies used 3D simulation software packages displayed on 2D desktop computers [ 22 , 24 , 25 , 36 ], whereas others used headsets for an immersive VR environment [ 15 , 23 , 26 , 35 , 37 ]. The most used VR teaching software were the CETSOL VR Clinic software [ 33 , 35 ], Virtual Medical Coaching VR software [ 15 , 30 , 32 ], Projection VR (Shaderware) software [ 36 ], SieVRt VR system (Luxsonic Technologies) [ 37 ], medical imaging training immersive environment software [ 23 ], VR CT Sim software [ 25 ], VitaSim ApS software [ 26 ], VR X-Ray (Skilitics and Virtual Medical Coaching) software [ 27 ], and radiation dosimetry VR software (Virtual Medical Coaching Ltd) [ 31 ].
Effect of VR Teaching on Skill Acquisition
Ahlqvist et al [ 22 ] looked at how virtual simulation can be used as an effective tool to teach quality assessment of radiographic images. They also compared how it faired in comparison to traditional teaching. The study reported a statistically significant improvement in proficiency from before training to after training. Additionally, the study reported that the proficiency score improvement for the VR-trained students was higher than that for the students trained using conventional method.
In the study conducted by Sapkaroski et al [ 34 ], students in the VR group demonstrated significantly better patient positioning skills compared to those in the conventional role-play group. The positioning parameters that were assessed were digit separation and palm flatness (the VR group scored 11% better), central ray positioning onto the third metacarpophalangeal joint (the VR group scored 23% better), and a control position projection of an oblique hand. The results for the control position projection indicated no significant difference in positioning between the 2 groups [ 34 ].
Bridge et al [ 23 ] also performed a performance comparison between students trained by VR and traditional methods. They assessed skills about patient positioning, equipment positioning, and time taken to complete a performative role-play. Students in the VR group performed better than those in the control group, with 91% of them receiving an overall score of above average (>3). The difference in mean group performance was statistically significant ( P =.0366). Similarly, Gunn et al [ 24 ] reported improved and higher role-play skill scores for students trained using VR software simulation compared to those trained on traditional laboratory simulation. The mean role-play score for the VR group was 30.67 and that for the control group was 28.8 [ 24 ].
Another study reported that students trained using VR performed significantly better (ranked as “very good” or “excellent”) than the control group (conventional learning) in skills such as patient positioning, selecting exposure factors, centering and collimating the x-ray beam, placing the anatomical marker, appraisal of image quality, equipment positioning, and procedure explanation to the patient [ 30 ]. Another recently conducted study found that the VR-taught group achieved better test duration and fewer errors in moving equipment and positioning a patient. There was no significant difference in the frequency of errors in the radiographic exposure setting such as source-to-image distance between the VR and the physical simulation groups [ 32 ].
Nilsson et al [ 28 ] developed a test to evaluate the student’s ability to interpret 3D information in radiographs using parallax. This test was applied to students before and after training. There was a significantly larger ( P <.01) pre-post intervention mean score for the VR group (3.11 to 4.18) compared to the control group (3.24 to 3.72). A subgroup analysis was also performed, and students with low visuospatial ability in the VR group had a significantly higher improvement in the proficiency test compared to those in the control group. The same authors conducted another follow-up study to test skill retention [ 29 ]. Net skill improvement was calculated as the difference in test scores after 8 months. The results from the proficiency test showed that the ability to interpret spatial relations in radiographs 8 months after the completion of VR training was significantly better than before VR training. The students who trained conventionally showed almost the same positive trend in improvement. The group difference was smaller and not statistically significant. This meant that, 8 months after training, the VR group and the traditionally trained group had the same skill level [ 29 ].
Among the included studies, only 1 reported that the VR group had lower performance in proficiency tests and radiographic skill tests, compared to a conventionally trained group. The study, conducted in 2022, showed that the proficiency of the VR group was significantly lower than that of the conventional technique group in performing lateral elbow and posterior-anterior chest radiography [ 27 ]. An itemized rubric evaluation used in the study revealed that the VR group also had lower performance in most of the radiographic skills, such as locating and centering of the x-ray beam, side marker placement, positioning the x-ray image detector, patient interaction, and process control and safety [ 27 ]. The study concluded that VR simulation can be less effective than real-world training in radiographic techniques, which requires palpation and patient interaction. These results may be different from those of other studies due to different outcome evaluation methods and since they used head-mounted display VR coaching, whereas the other studies, except O’Connor et al [ 15 ], used VR on a PC monitor.
All of the studies except Kato et al [ 27 ] agreed that VR use was more effective for students in developing radiographic and radiologic skills. Despite this general agreement, there were slight in-study variations in learning outcomes, which made some of the studies look at factors that may influence skill and knowledge acquisition during VR use. In studies such as Bridge et al [ 23 ], it was noted that the arrangement of equipment had the greatest influence on the overall score. After performing a multivariable analysis, Gunn et al [ 24 ] reported that there was no effect of age, gender, and gaming skills or activity on the outcome of VR learning. In the study by Shanahan [ 36 ], a few students (19/84, 23%) had previously used VR simulation software. This had no bearing on the learning outcomes. Another observation in the same study was that student age was found to significantly affected the student’s confidence about skill acquisition after VR training [ 36 ].
Students’ Perception of VR Uses for Learning
The findings from the study by Gunn et al [ 25 ] revealed that 68% of students agreed or strongly agreed that VR simulation was significantly helpful in learning about computed tomography (CT) scanning. In another study by Jensen et al [ 26 ], 90% of the students strongly agreed that VR simulators could contribute to learning radiography, with 90% reporting that the x-ray equipment in the VR simulation was realistic. In the study by Wu et al [ 37 ], most of the students (55.6%) agreed or somewhat agreed that VR use was useful in radiology education. Similarly, 83% of the students in Shanahan’s [ 36 ] study regarded VR learning with an ease of use. In the same study, students also reported that one of the major benefits of VR learning include using the simulation to repeat activities until being satisfied with the results (95% of respondents). Students also stated that VR enabled them to quickly see images and understand if changes needed to be made (94%) [ 36 ]. In the study by Gunn et al [ 25 ], 75% of medical imaging students agreed on the ease of use and software enjoyment in VR simulated learning. In the same study, 57% of the students reported a positive perceived usefulness of VR. Most respondents (80%) in the study by Rainford et al [ 31 ] favored the in-person VR experience over web-based VR. Similarly, 58% of the respondents in the study conducted by O’Connor et al [ 15 ] reported enjoying learning using VR simulation. In the study by Wu et al [ 37 ], 83.3% of students agreed or strongly agreed that they enjoyed using VR for learning. Similarly, the studies by Rainford et al [ 31 ] and O’Connor et al [ 15 ] reported student recommendation of 87% and 94%, respectively, for VR as a learning tool.
Students’ Perceived Skill and Knowledge Acquisition
In the study by Bridge et al [ 23 ], students who trained using VR reported an increase in perceived skill acquisition and high levels of satisfaction. The study authors attributed this feedback to the availability of “gold standards” that showed correct positioning techniques, as well as instant feedback provided by the VR simulators. Gunn et al [ 25 ] examined students’ confidence in performing a CT scan in a real clinical environment after using VR simulations as a learning tool. The study reported an increase (from before to after training) in the students’ perceived confidence in performing diagnostic CT scans. Similarly, the study by Jensen et al [ 26 ] reported that the use of VR had influenced students’ self-perceived readiness to perform wrist x-ray radiographs. The study, however, found no significant difference in pre- and posttraining (perceived preparedness) scores. The pre- and posttraining scores were 75 (95% CI 54-96) and 77 (95% CI 59-95), respectively. The study by O’Connor et al [ 15 ] looked at the effect of VR on perceived skill adoption. Most of the students in the study reported high levels of perceived knowledge acquisition in the areas of beam collimation, anatomical marker placement, centering of the x-ray tube, image evaluation, anatomical knowledge, patient positioning, and exposure parameter selection to their VR practice. However, most students felt that VR did not contribute to their knowledge of patient dose tracking and radiation safety [ 15 ]. In the study by Rainford et al [ 31 ], 73% of radiography and medical students felt that VR learning increased their confidence across all relevant learning outcomes. The biggest increase in confidence level was regarding their understanding of radiation safety matters [ 31 ]. Sapkaroski et al [ 33 ] performed a self-perception test to see how students viewed their clinical and technical skills after using VR for learning. In their study, students reported a perceived improvement in their hand and patient positioning skills. Their study also compared 2 software, CETSOL VR Clinic and Shaderware. The cohort who used CETSOL VR Clinic had higher scores on perceived improvement [ 33 ]. Sapkaroski et al [ 35 ] compared the student’s perception scores on the educational enhancement of their radiographic hand positioning skills, after VR or clinical role-play scenario training. Although the VR group scored higher, there was no significant difference between the scores for the 2 groups [ 35 ]. In the study by Shanahan [ 36 ], when the perception of skill development was evaluated, most of the students reported that the simulation positively developed their technical (78%), radiographic image evaluation (85%), problem-solving (85%), and self-evaluation (88%) abilities. However, in the study by Kato et al [ 27 ], there was no difference in the perceived acquisition of knowledge among students using traditional teaching and VR-based teaching.
Principal Findings
The results presented in this review reveal strong evidence for the effectiveness of VR teaching in radiology education, particularly in the context of skill acquisition and development [ 22 , 24 , 27 , 30 , 32 , 34 ].
In this review, quality appraisal of the cross-sectional studies revealed that the strategies for deal with confounding factors was one of the factors directly affecting the reliability of the results. Similarly, the appraisal of the randomized trials revealed that the bias arising due to missing outcome data was one of the factors directly affecting the reliability of the results.
All the studies found that VR-based teaching had a positive impact on various areas of radiographic and radiologic skill development. In comparison to the traditional way of teaching, only 1 study by Kato et al [ 27 ] reported VR teaching as inferior to traditional teaching. The studies consistently reported better improvements in proficiency, patient positioning outcomes, equipment handling, and radiographic techniques among students trained using VR. According to Nilsson et al [ 29 ], O’Connor et al [ 15 ], and Wu et al [ 37 ], the improvements were due to the immersive and interactive nature of VR simulations, which allowed learners to engage with radiological scenarios in a dynamic and hands-on manner. The studies also revealed that VR learning has the ability to easily and effectively introduce students to new skills. It was also found that existing skills could be improved, mainly through simulation feedback that happens in real time during training [ 22 , 24 , 28 , 30 , 36 ].
The improvement of skills after VR training have been noted in different domains, including patient positioning, equipment positioning, equipment knowledge, assessment of radiographic image quality, and patient interaction. Improvement was also observed in other skills such as as central ray positioning, source-to-image distance, image receptor placement, and side marker placement [ 22 , 24 , 30 , 32 , 34 ]. Two studies, Nilsson et al [ 28 ] and Nilsson et al [ 29 ], looked at how VR affected the students’ ability to interpret 3D information in radiographs using parallax. They both reported a positive effect. Nilsson et al [ 29 ] also gave insights into the long-term benefits of VR training in radiology. Eight months after training, the control (traditionally taught) group in Nilsson et al [ 29 ] showed a slight increase in skills, but the VR-trained group still maintained a significantly higher skill level. This finding shows the enduring impact of VR-based education on skill acquisition in radiology. Although most studies supported the effectiveness of VR in radiology education, 1 study reported contrasting results [ 27 ]. VR-trained students were found to perform worse than traditionally trained students in conducting lateral elbow and posterior-anterior chest radiography in Kato et al [ 27 ]. This difference in results was, according to the authors, attributed to the use of a different rubric evaluation method and the use of a head-mounted display–based immersive VR system, which was not used in other studies. These 2 reasons may be the reason for the variation in study findings.
A wide range of VR software with different functions were used in the studies. In addition to acquiring radiographic images, the CETSOL VR Clinic software facilitated students to interact with their learning environment [ 33 , 35 ]. Students using the Virtual Medical Coaching VR software performed imaging exercise on a virtual patient with VR headsets and hand controllers [ 15 , 30 , 32 ]. The SieVRt VR system displayed Digital Imaging and Communications in Medicine format images in a virtual environment, thus facilitating teaching [ 37 ]. The medical imaging training immersive environment simulation software provided automated feedback to the learners including a rerun of procedures, thus highlighting procedural errors [ 23 ]. The VR CT Sim software allowed the student virtually to perform the complete CT workflow [ 25 ]. Students could manipulate patient positioning and get feedback from the VitaSim ApS software [ 26 ]. The VR X-Ray software allowed students to manipulate radiographic equipment and patient’s position with a high level of immersive experience [ 27 ]. Radiation dosimetry VR software facilitated virtual movement of the staff and equipment to radiation-free areas, thus optimizing radiation protection [ 31 ].
The included studies also looked at factors that could influence skill acquisition when VR is used in radiology education. Bridge et al [ 23 ], Gunn et al [ 24 ], Kato et al [ 27 ], and Shanahan [ 36 ] investigated factors such as age, gender, prior gaming experience, and familiarity with VR technology. However, these factors were shown to have no significant effect on VR learning outcomes. This shows that VR education can equally accommodate a wide range of learners, regardless of experience or existing attributes.
Across several studies, positive feedback emerged regarding the utility, ease of use, enjoyment, and perceived impact on skill and knowledge acquisition. The included studies consistently reported positive perceptions of VR use among students [ 25 , 26 , 37 ]. Gunn et al [ 25 ] reported that a significant proportion of medical imaging and radiation therapy students found the use of VR simulation to be significantly helpful in learning about CT scanning. Similarly, Jensen et al [ 26 ] and Wu et al [ 37 ] reported that a majority of students agreed on the usefulness of VR in radiology education. Another aspect that received positive feedback was the ease of use. Students liked the ability to repeat tasks until they were satisfied with the results and the ability to quickly visualize radiographs to determine the need for revisions [ 36 ]. Rainford et al [ 31 ] and O’Connor and Rainford [ 30 ] found that most students would recommend VR as a learning tool to other students.
Several studies investigated student’s perceptions of skill and knowledge acquisition when using VR for radiology education. Bridge et al [ 15 ] and O’Connor et al [ 23 ] discovered an increase in students’ perceived acquisition of radiographic skills. Gunn et al [ 25 ] reported an increase in students’ perceived confidence to perform CT scans after learning using VR simulations. According to Rainford et al [ 31 ], a large percentage of radiography and medical students felt that VR learning boosted their confidence across all relevant learning outcomes, with the highest levels of confidence recorded in radiation safety. Sapkaroski et al [ 33 ] discovered that after using VR for learning, students experienced an improvement in their hand and patient placement skills. In summary, the positive feedback from the students shows that VR use in radiology education is a useful, engaging, and effective teaching tool. This perceived acquisition of skills is backed by the results from the proficiency tests.
The VR modalities used in some of the studies allowed remote assistance from an external agent (teacher), as the VR training is conducted in front of a screen while being part of a team, with the teacher making constant corrections and indications [ 22 , 24 , 27 ]. However, researchers are looking into VR systems with artificial intelligence–supported tutoring, which includes the assessment of learners, generation of learning content, and automated feedback [ 39 ].
Findings from the included studies show that VR-based teaching offers substantial benefits in various aspects of radiographic and radiologic skill development. The studies consistently reported that students educated using VR systems improved significantly in overall proficiency, patient positioning, equipment knowledge, equipment handling, and radiographic techniques. However, the variable nature of the studies included in the review reduces the scope for a comprehensive recommendation of VR use in radiology education. A key contributing factor to relatively better learning outcomes was the immersive and interactive nature of VR systems, which provided real-time feedback and dynamic learning experiences to students. Factors such as age, gender, gaming experience, and familiarity with VR systems did not significantly influence learning outcomes. This shows that VR can be used for diverse groups of students when teaching radiology. Students generally provided positive feedback about the utility, ease of use, and satisfaction of VR, as well as its perceived impact on skill and knowledge acquisition. These students’ reports show the value of VR as an important, interesting, and effective tool in radiology education.
Conflicts of Interest
None declared.
PRISMA (Preferred Reporting Items for Systematic Reviews and Meta-Analyses) checklist.
- Ding X, Li Z. A review of the application of virtual reality technology in higher education based on Web of Science literature data as an example. Front Educ. Nov 17, 2022;7:1048816. [ CrossRef ]
- Oyelere SS, Bouali N, Kaliisa R, Obaido G, Yunusa AA, Jimoh ER. Exploring the trends of educational virtual reality games: a systematic review of empirical studies. Smart Learning Environments. Oct 19, 2020;7:31. [ CrossRef ]
- Zamyatina NV, Ushakova IA, Mandrikov VB. Medical education in digital transformation. In: Proceedings of the International Scientific Conference “Digitalization of Education: History, Trends and Prospects” (DETP 2020). Atlantis Press; 2020:324-327. [ CrossRef ]
- Kardong-Edgren S, Farra SL, Alinier G, Young HM. A call to unify definitions of virtual reality. Clin Simul Nurs. Jun 2019;31:28-34. [ CrossRef ]
- Sousa Santos B, Dias P, Pimentel A, et al. Head-mounted display versus desktop for 3D navigation in virtual reality: a user study. Multimed Tools Appl. Jan 2009;41(1):161-181. [ CrossRef ]
- Hamad A, Jia B. How virtual reality technology has changed our lives: an overview of the current and potential applications and limitations. Int J Environ Res Public Health. Sep 8, 2022;19(18):11278. [ CrossRef ] [ Medline ]
- Kaplan-Rakowski R, Meseberg K. Immersive media and their future. In: Branch RM, Lee H, Tseng S, editors. Educational Media and Technology Yearbook. Vol 42. Springer; 2019:143-153. [ CrossRef ]
- Kuliga SF, Thrash T, Dalton RC, Hölscher C. Virtual reality as an empirical research tool — exploring user experience in a real building and a corresponding virtual model. Comput Environ Urban Syst. Nov 2015;54:363-375. [ CrossRef ]
- Guan H, Xu Y, Zhao D. Application of virtual reality technology in clinical practice, teaching, and research in complementary and alternative medicine. Evid Based Complement Alternat Med. Aug 11, 2022;2022:1373170. [ CrossRef ] [ Medline ]
- Pottle J. Virtual reality and the transformation of medical education. Future Healthc J. Oct 2019;6(3):181-185. [ CrossRef ] [ Medline ]
- Satava RM, Jones SB. Current and future applications of virtual reality for medicine. Proc IEEE. Mar 1998;86(3):484-489. [ CrossRef ]
- Satava RM. Medical virtual reality. the current status of the future. Stud Health Technol Iform. 1996;29:100-106. [ Medline ]
- Rosenberg LB, Stredney D. A haptic interface for virtual simulation of endoscopic surgery. Stud Health Technol Inform. 1996;29:371-387. [ Medline ]
- Chytas D, Salmas M, Demesticha T, et al. A review of the use of virtual reality for teaching radiology in conjunction with anatomy. Cureus. Dec 5, 2021;13(12):e20174. [ CrossRef ] [ Medline ]
- O’Connor M, Stowe J, Potocnik J, Giannotti N, Murphy S, Rainford L. 3D virtual reality simulation in radiography education: the students' experience. Radiography (Lond). Feb 2021;27(1):208-214. [ CrossRef ] [ Medline ]
- Hart R, Karthigasu K. The benefits of virtual reality simulator training for laparoscopic surgery. Curr Opin Obstet Gynecol. Aug 2007;19(4):297-302. [ CrossRef ] [ Medline ]
- Thomas DJ, Singh D. Letter to the editor: virtual reality in surgical training. Int J Surg. May 2021;89:105935. [ CrossRef ] [ Medline ]
- Page MJ, McKenzie JE, Bossuyt PM, et al. The PRISMA 2020 statement: an updated guideline for reporting systematic reviews. BMJ. Mar 29, 2021;372(71):n71. [ CrossRef ] [ Medline ]
- Sterne JAC, Savović J, Page MJ, et al. RoB 2: a revised tool for assessing risk of bias in randomised trials. BMJ. Aug 28, 2019;366:l4898. [ CrossRef ] [ Medline ]
- McGuinness LA, Higgins JPT. Risk-of-bias visualization (robvis): an R package and shiny web app for visualizing risk-of-bias assessments. Res Synth Methods. Jan 2021;12(1):55-61. [ CrossRef ] [ Medline ]
- Critical appraisal tools. JBI. URL: https://jbi.global/critical-appraisal-tools [Accessed 2024-04-25]
- Ahlqvist JB, Nilsson TA, Hedman LR, et al. A randomized controlled trial on 2 simulation-based training methods in radiology: effects on radiologic technology student skill in assessing image quality. Simul Healthc. Dec 2013;8(6):382-387. [ CrossRef ] [ Medline ]
- Bridge P, Gunn T, Kastanis L, et al. The development and evaluation of a medical imaging training immersive environment. J Med Radiat Sci. Sep 2014;61(3):159-165. [ CrossRef ] [ Medline ]
- Gunn T, Jones L, Bridge P, Rowntree P, Nissen L. The use of virtual reality simulation to improve technical skill in the undergraduate medical imaging student. Interactive Learning Environments. 2018;26(5):613-620. [ CrossRef ]
- Gunn T, Rowntree P, Starkey D, Nissen L. The use of virtual reality computed tomography simulation within a medical imaging and a radiation therapy undergraduate programme. J Med Radiat Sci. Mar 2021;68(1):28-36. [ CrossRef ] [ Medline ]
- Jensen J, Graumann O, Jensen RO, et al. Using virtual reality simulation for training practical skills in musculoskeletal wrist x-ray - a pilot study. J Clin Imaging Sci. Jul 11, 2023;13:20. [ CrossRef ] [ Medline ]
- Kato K, Kon D, Ito T, Ichikawa S, Ueda K, Kuroda Y. Radiography education with VR using head mounted display: proficiency evaluation by rubric method. BMC Med Educ. Jul 28, 2022;22(1):579. [ CrossRef ] [ Medline ]
- Nilsson TA, Hedman LR, Ahlqvist JB. A randomized trial of simulation-based versus conventional training of dental student skill at interpreting spatial information in radiographs. Simul Healthc. 2007;2(3):164-169. [ CrossRef ] [ Medline ]
- Nilsson TA, Hedman LR, Ahlqvist JB. Dental student skill retention eight months after simulator-supported training in oral radiology. J Dent Educ. May 2011;75(5):679-684. [ Medline ]
- O’Connor M, Rainford L. The impact of 3D virtual reality radiography practice on student performance in clinical practice. Radiography (Lond). Jan 2023;29(1):159-164. [ CrossRef ] [ Medline ]
- Rainford L, Tcacenco A, Potocnik J, et al. Student perceptions of the use of three-dimensional (3-D) virtual reality (VR) simulation in the delivery of radiation protection training for radiography and medical students. Radiography (Lond). Jul 2023;29(4):777-785. [ CrossRef ] [ Medline ]
- Rowe D, Garcia A, Rossi B. Comparison of virtual reality and physical simulation training in first-year radiography students in South America. J Med Radiat Sci. Jun 2023;70(2):120-126. [ CrossRef ] [ Medline ]
- Sapkaroski D, Baird M, McInerney J, Dimmock MR. The implementation of a haptic feedback virtual reality simulation clinic with dynamic patient interaction and communication for medical imaging students. J Med Radiat Sci. Sep 2018;65(3):218-225. [ CrossRef ] [ Medline ]
- Sapkaroski D, Baird M, Mundy M, Dimmock MR. Quantification of student radiographic patient positioning using an immersive virtual reality simulation. Simul Healthc. Aug 2019;14(4):258-263. [ CrossRef ] [ Medline ]
- Sapkaroski D, Mundy M, Dimmock MR. Virtual reality versus conventional clinical role-play for radiographic positioning training: a students' perception study. Radiography (Lond). Feb 2020;26(1):57-62. [ CrossRef ] [ Medline ]
- Shanahan M. Student perspective on using a virtual radiography simulation. Radiography (Lond). Aug 2016;22(3):217-222. [ CrossRef ]
- Wu Y, Mondal P, Stewart M, Ngo R, Burbridge B. Bringing radiology education to a new reality: a pilot study of using virtual reality as a remote educational tool. Can Assoc Radiol J. May 2023;74(2):251-263. [ CrossRef ] [ Medline ]
- Haddaway NR, Page MJ, Pritchard CC, McGuinness LA. PRISMA2020: an R package and Shiny app for producing PRISMA 2020-compliant flow diagrams, with interactivity for optimised digital transparency and open synthesis. Campbell Syst Rev. Mar 27, 2022;18(2):e1230. [ CrossRef ] [ Medline ]
- King S, Boyer J, Bell T, Estapa A. An automated virtual reality training system for teacher-student interaction: a randomized controlled trial. JMIR Serious Games. Dec 8, 2022;10(4):e41097. [ CrossRef ] [ Medline ]
Abbreviations
Edited by A Hasan Sapci, Taiane de Azevedo Cardoso; submitted 23.09.23; peer-reviewed by FernandezHerrero Jorge, Stacey Kassutto; final revised version received 01.02.24; accepted 31.03.24; published 08.05.24.
© Shishir Shetty, Supriya Bhat, Saad Al Bayatti, Sausan Al Kawas, Wael Talaat, Mohamed El-Kishawi, Natheer Al Rawi, Sangeetha Narasimhan, Hiba Al-Daghestani, Medhini Madi, Raghavendra Shetty. Originally published in JMIR Medical Education (https://mededu.jmir.org), 8.5.2024.
This is an open-access article distributed under the terms of the Creative Commons Attribution License ( https://creativecommons.org/licenses/by/4.0/ ), which permits unrestricted use, distribution, and reproduction in any medium, provided the original work, first published in JMIR Medical Education, is properly cited. The complete bibliographic information, a link to the original publication on https://mededu.jmir.org/ , as well as this copyright and license information must be included.
The Impact of Virtual Reality in Education: A Comprehensive Research Study
- Conference paper
- First Online: 13 December 2023
- Cite this conference paper
- Shivani Vats 20 &
- Raman Joshi 20
Part of the book series: IFIP Advances in Information and Communication Technology ((IFIPAICT,volume 699))
Included in the following conference series:
- International Working Conference on Transfer and Diffusion of IT
418 Accesses
In recent years, virtual reality (VR) technologies have made significant advancements and are now being explored for their potential applications in various fields, including education. This exciting development has sparked a growing interest in investigating the use of VR technologies to enhance learning experiences. VR offers a unique opportunity to create immersive and interactive environments that can transport students to places they may never have the chance to visit otherwise. By simulating real-world scenarios, VR can provide students with hands-on experiences and facilitate active learning. From exploring historical landmarks to conducting complex scientific experiments, the possibilities are endless.
This research paper aims to investigate the use of VR technologies in the field of education, exploring their potential benefits, challenges, and implications. Through a comprehensive literature review and analysis, this study provides insights into the current state of VR in education, its applications, and its impact on learning outcomes. One of the key advantages of using VR in education is its ability to engage students on a deeper level. Traditional teaching methods often struggle to capture and maintain students’ attention, but VR has the potential to captivate learners by making education more interactive and enjoyable. The findings reveal that VR technologies have the potential to enhance student engagement, facilitate immersive learning experiences, and improve knowledge retention. However, challenges such as cost, accessibility, and technological limitations must be addressed to ensure widespread adoption and integration of VR in educational settings. Furthermore, By creating an environment where students can actively participate in their learning process, VR helps foster curiosity, critical thinking, and problem-solving skills.. The research concludes by highlighting the future prospects of VR in education and suggesting areas for further research.
This is a preview of subscription content, log in via an institution to check access.
Access this chapter
- Available as PDF
- Read on any device
- Instant download
- Own it forever
- Available as EPUB and PDF
- Durable hardcover edition
- Dispatched in 3 to 5 business days
- Free shipping worldwide - see info
Tax calculation will be finalised at checkout
Purchases are for personal use only
Institutional subscriptions
Rojas-Sánchez, M.A., Palos-Sánchez, P.R., Folgado-Fernández, J.A.: Systematic literature review and bibliometric analysis on virtual reality and education. Educ. Inf. Technol. 28 (1), 155–192 (2023)
Article Google Scholar
Radianti, J., Majchrzak, T.A., Fromm, J., Wohlgenannt, I.: A systematic review of immersive virtual reality applications for higher education: design elements, lessons learned, and research agenda. Comput. Educ.. Educ. 147 , 103778 (2020)
Fonseca, D., Sanchez-Sepulveda, M., Necchi, S., Peña, E., Marti, N., Villagrasa, S., Redondo, E., Franquesa, J., Navarro, I.: 2020, October. What is happening in the process of engaging Architectural Students and Teachers for Including Virtual and Interactive Systems in the Projects Developments? In: Eighth International Conference on Technological Ecosystems for Enhancing Multiculturality, pp. 775–783
Google Scholar
Su, Y.S., Cheng, H.W., Lai, C.F.: Study of virtual reality immersive technology enhanced mathematics geometry learning. Front. Psychol. 13 , 760418 (2022)
Liu, Y., Tan, W., Chen, C., Liu, C., Yang, J., Zhang, Y.: A review of the application of virtual reality technology in the diagnosis and treatment of cognitive impairment. Front. Aging Neurosci. 11 , 280 (2019)
Marougkas, A., Troussas, C., Krouska, A., Sgouropoulou, C.: Virtual reality in education: a review of learning theories, approaches and methodologies for the last decade. Electronics 12 (13), 2832 (2023)
Elmqaddem, N.: Augmented reality and virtual reality in education. Myth or reality? International journal of emerging technologies in learning, 14(3) (2019)
Pellas, N., Kazanidis, I., Palaigeorgiou, G.: A systematic literature review of mixed reality environments in K-12 education. Educ. Inf. Technol. 25 (4), 2481–2520 (2020)
Akçayır, M., Akçayır, G.: Advantages and challenges associated with augmented reality for education: a systematic review of the literature. Educ. Res. Rev. 20 , 1–11 (2017)
Zhang, H., et al.: Investigating high school students’ perceptions and presences under VR learning environment. Interact. Learn. Environ. 28 (5), 635–655 (2020)
Download references
Author information
Authors and affiliations.
Department of Information Technology, Jagannath Institute of Management Sciences, Rohini, New Delhi, India
Shivani Vats & Raman Joshi
You can also search for this author in PubMed Google Scholar
Corresponding author
Correspondence to Raman Joshi .
Editor information
Editors and affiliations.
IIM Nagpur, Nagpur, India
Sujeet K. Sharma
Swansea University, Swansea, UK
Yogesh K. Dwivedi
Bhimaraya Metri
University of Bradford, Bradford, UK
Royal Holloway, University of London, Egham, UK
Amany Elbanna
Rights and permissions
Reprints and permissions
Copyright information
© 2024 IFIP International Federation for Information Processing
About this paper
Cite this paper.
Vats, S., Joshi, R. (2024). The Impact of Virtual Reality in Education: A Comprehensive Research Study. In: Sharma, S.K., Dwivedi, Y.K., Metri, B., Lal, B., Elbanna, A. (eds) Transfer, Diffusion and Adoption of Next-Generation Digital Technologies. TDIT 2023. IFIP Advances in Information and Communication Technology, vol 699. Springer, Cham. https://doi.org/10.1007/978-3-031-50204-0_11
Download citation
DOI : https://doi.org/10.1007/978-3-031-50204-0_11
Published : 13 December 2023
Publisher Name : Springer, Cham
Print ISBN : 978-3-031-50203-3
Online ISBN : 978-3-031-50204-0
eBook Packages : Computer Science Computer Science (R0)
Share this paper
Anyone you share the following link with will be able to read this content:
Sorry, a shareable link is not currently available for this article.
Provided by the Springer Nature SharedIt content-sharing initiative
- Publish with us
Policies and ethics
Societies and partnerships
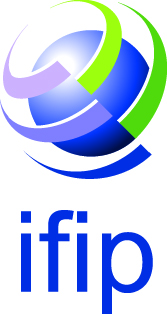
- Find a journal
- Track your research

COMMENTS
This study is a systematic review of 20 years of research on the usage of virtual reality (VR) in K-12 and higher education settings, which aims to consolidate, evaluate, and communicate evidence that can inform both the theory and practice of VR-based instruction.
This paper reports a survey on the scientific literature on the advantages and potentials in the use of Immersive Virtual Reality in Education in the last two years (2013-14).
The adoption of immersive virtual reality (I-VR) as a pedagogical method in education has challenged the conceptual definition of what constitutes a learning environment. High fidelity graphics and immersive content using head-mounted-displays (HMD) have allowed students to explore complex subjects in a way that traditional teaching methods cannot. Despite this, research focusing on learning ...
In our search for existing systematic reviews and mapping studies on VR in an educational context, the Scopus digital library returned 59 peer-reviewed articles published between 2009-2018, which included search terms "virtual reality" and "systematic review" in their titles and were linked to education, training, teaching, or ...
There has been an increasing interest in applying immersive virtual reality (VR) applications to support various instructional design methods and outcomes not only in K-12 (Primary and Secondary), but also in higher education (HE) settings. However, there is a scarcity of studies to provide the potentials and challenges of VR-supported instructional design strategies and/or techniques that can ...
During the last decade, there has been a substantial increase of interest in studies related to Virtual Reality (VR) as a learning tool. This paper presents a systematic literature review of personalization strategies utilized in immersive VR for educational objectives in the classroom. For the purposes of this review, 69 studies between 2012 and 2022 were analyzed in terms of their benefits ...
' virtual reality ' and its abbreviation ' VR '; the second included signi- fiers of educational contexts such as ' education ' , ' class* ' , ' teach* ' ,
In other words, an up-to-date systematic review with an exclusive focus on VR implementation in both K-12 and higher education is still lacking in the literature. To address such a research gap ...
This study is a systematic review of 20 years of research on the usage of virtual reality (VR) in K-12 and higher education settings, which aims to consolidate, evaluate, and communicate evidence that can inform both the theory and practice of VR-based instruction.
Literature Review Before the emergence of virtual reality technology, in 1960 there were several concepts that led to virtual reality such as cyberspace, synthetic environment, artificial reality, simulator technology and so on. but this concept continues to grow until finally a concept emerges where humans can interact with various objects ...
Immersive virtual reality as a pedagogical tool in education: A systematic literature review of quantitative learning outcomes and experimental design Journal of Computers in Education , 8 ( 1 ) ( 2021 ) , pp. 1 - 32 , 10.1007/s40692-020-00169-2
Background: While research on Virtual Reality's potential for education continues to advance, research on its support for Collaborative Learning is small in scope. With remote collaboration and distance learning becoming increasingly relevant for education (especially since the COVID-19 pandemic), an understanding of Virtual Reality's potential for Collaborative Learning is of importance ...
A survey on the scientific literature on the advantages and potentials in the use of Immersive Virtual Reality in Education in the last two years shows how VR in general, and immersive VR in particular, has been used mostly for adult training in special situations or for university students. Since the first time the term "Virtual Reality" (VR) has been used back in the 60s, VR has evolved in ...
Virtual reality has existed in the realm of education for over half a century. However, its widespread adoption is still yet to occur. This is a result of a myriad of limitations to both the technologies themselves, and the costs and logistics required to deploy them. In order to gain a better understanding of what these issues are, and what it is that educators hope to gain by using these ...
The review explores the peer-reviewed scholarly studies conducted between 2006 and May 2017, which involved the use of virtual reality (VR), augmented reality (AR) or mixed reality (MR) technologies in the instruction of students in elementary, middle or high school.
In recent years, with the rapid development of information technology, the visualization and interaction of virtual reality technology has developed, making the application of VR technology in education more and more attractive to scholars. This paper adopts the literature analysis method, focusing on the application of VR technology in the field of higher education, selects 80 empirical ...
A literature review on immersive virtual reality in education: state of the art and perspectives. Int. Sci. Conf. E Learn. Softw. Educ. 1, 10-1007. doi: 10.12753/2066-026x-15-020 [Google Scholar] Grant M. J., Booth A. (2009). A typology of reviews: an analysis of 14 review types and associated methodologies.
Abstract. The objective of this study is to identify and analyze the scientific literature with a bibliometric analysis to find the main topics, authors, sources, most cited arti-cles, and countries in the literature on virtual reality in education. Another aim is to understand the conceptual, intellectual, and social structure of the ...
We designed an interactive virtual reality (VR) application to provide a controlled and yet unpredictable environment for the development of classroom management skills. The simulated environment allows teachers in training to interact with virtual students in realistic and meaningful ways. The VR application allows rich verbal interaction by using artificial intelligence (AI). Initial ...
Spherical video-based virtual reality (SVVR) is a form of virtual reality that employs 360-degree videos to immerse learners in virtual environments. As SVVR can simulate realistic experiences, the current trend of language education is towards more use of it.
Learning in this modern era can use various kinds of technology. One of them is virtual reality. Virtual Reality is an environment generated by a computer that makes the user feel immersed with the object that is generated in their surroundings. This paper presents a previous review to understand the implementation of virtual reality in learning.
The educational applications of extended reality (XR) modalities, including virtual reality (VR), augmented reality (AR), and mixed reality (MR), have increased significantly over the last ten years. Many educators within the Architecture, Engineering, and Construction (AEC) related degree programs see student benefits that could be derived from bringing these modalities into classrooms, which ...
In the field of education, virtual reality (VR) offers learners an immersive and interactive learning experience, allowing them to comprehend challenging concepts and ideas more efficiently and ...
Practicum is regarded as a fundamental aspect of the training of prospective teachers. In addition, digital tools are increasingly used to enrich a traditional face-to-face experience. However, the technological exploitation of Augmented Reality (AR) by undergraduate students studying early childhood and primary education is low. A Systematic Literature Review (SLR) on the use of Augmented ...
A systematic review of immersive technology applications for medical practice and education—trends, application areas, recipients, teaching contents, evaluation methods, and performance. Educ. Res.
Background: In recent years Virtual reality (VR) has gained significant importance in medical education. Radiology education also has seen the induction of VR technology. However, there is no comprehensive review in this specific area. The present review aims to fill this gap in the knowledge. Objective: This systematic literature review aims to explore the scope of virtual reality (VR) use in ...
A systematic literature review of mixed reality environments in K-12 education. Educ. Inf. Technol. 25(4), 2481-2520 (2020) Article Google Scholar Akçayır, M., Akçayır, G.: Advantages and challenges associated with augmented reality for education: a systematic review of the literature. Educ. Res. Rev. 20, 1-11 (2017)
By incorporating technology, such as online education, virtual reality (VR), artificial reality (AR), and mixed reality (MR), we may enhance learning, while maintaining high standards and reducing costs. ... This literature review aims to provide insights into the effectiveness of haptic devices in fixed prosthodontics, specifically crown ...
Abstract. Virtual schooling was first employed in the mid-1990s and has become a common method of distance education used in K-12 jurisdictions. The most accepted definition of a virtual school is an entity approved by a state or governing body that offers courses through distance delivery - most commonly using the Internet.