
An official website of the United States government
The .gov means it’s official. Federal government websites often end in .gov or .mil. Before sharing sensitive information, make sure you’re on a federal government site.
The site is secure. The https:// ensures that you are connecting to the official website and that any information you provide is encrypted and transmitted securely.
- Publications
- Account settings
Preview improvements coming to the PMC website in October 2024. Learn More or Try it out now .
- Advanced Search
- Journal List
- Emerg (Tehran)
- v.5(1); 2017


Sampling methods in Clinical Research; an Educational Review
Mohamed elfil.
1 Faculty of Medicine, Alexandria University, Egypt.
Ahmed Negida
2 Faculty of Medicine, Zagazig University, Egypt.
Clinical research usually involves patients with a certain disease or a condition. The generalizability of clinical research findings is based on multiple factors related to the internal and external validity of the research methods. The main methodological issue that influences the generalizability of clinical research findings is the sampling method. In this educational article, we are explaining the different sampling methods in clinical research.
Introduction
In clinical research, we define the population as a group of people who share a common character or a condition, usually the disease. If we are conducting a study on patients with ischemic stroke, it will be difficult to include the whole population of ischemic stroke all over the world. It is difficult to locate the whole population everywhere and to have access to all the population. Therefore, the practical approach in clinical research is to include a part of this population, called “sample population”. The whole population is sometimes called “target population” while the sample population is called “study population. When doing a research study, we should consider the sample to be representative to the target population, as much as possible, with the least possible error and without substitution or incompleteness. The process of selecting a sample population from the target population is called the “sampling method”.
Sampling types
There are two major categories of sampling methods ( figure 1 ): 1; probability sampling methods where all subjects in the target population have equal chances to be selected in the sample [ 1 , 2 ] and 2; non-probability sampling methods where the sample population is selected in a non-systematic process that does not guarantee equal chances for each subject in the target population [ 2 , 3 ]. Samples which were selected using probability sampling methods are more representatives of the target population.

Sampling methods.
Probability sampling method
Simple random sampling
This method is used when the whole population is accessible and the investigators have a list of all subjects in this target population. The list of all subjects in this population is called the “sampling frame”. From this list, we draw a random sample using lottery method or using a computer generated random list [ 4 ].
Stratified random sampling
This method is a modification of the simple random sampling therefore, it requires the condition of sampling frame being available, as well. However, in this method, the whole population is divided into homogeneous strata or subgroups according a demographic factor (e.g. gender, age, religion, socio-economic level, education, or diagnosis etc.). Then, the researchers select draw a random sample from the different strata [ 3 , 4 ]. The advantages of this method are: (1) it allows researchers to obtain an effect size from each strata separately, as if it was a different study. Therefore, the between group differences become apparent, and (2) it allows obtaining samples from minority/under-represented populations. If the researchers used the simple random sampling, the minority population will remain underrepresented in the sample, as well. Simply, because the simple random method usually represents the whole target population. In such case, investigators can better use the stratified random sample to obtain adequate samples from all strata in the population.
Systematic random sampling (Interval sampling)
In this method, the investigators select subjects to be included in the sample based on a systematic rule, using a fixed interval. For example: If the rule is to include the last patient from every 5 patients. We will include patients with these numbers (5, 10, 15, 20, 25, ...etc.). In some situations, it is not necessary to have the sampling frame if there is a specific hospital or center which the patients are visiting regularly. In this case, the researcher can start randomly and then systemically chooses next patients using a fixed interval [ 4 ].
Cluster sampling (Multistage sampling)
It is used when creating a sampling frame is nearly impossible due to the large size of the population. In this method, the population is divided by geographic location into clusters. A list of all clusters is made and investigators draw a random number of clusters to be included. Then, they list all individuals within these clusters, and run another turn of random selection to get a final random sample exactly as simple random sampling. This method is called multistage because the selection passed with two stages: firstly, the selection of eligible clusters, then, the selection of sample from individuals of these clusters. An example for this, if we are conducting a research project on primary school students from Iran. It will be very difficult to get a list of all primary school students all over the country. In this case, a list of primary schools is made and the researcher randomly picks up a number of schools, then pick a random sample from the eligible schools [ 3 ].
Non-probability sampling method
Convenience sampling
Although it is a non-probability sampling method, it is the most applicable and widely used method in clinical research. In this method, the investigators enroll subjects according to their availability and accessibility. Therefore, this method is quick, inexpensive, and convenient. It is called convenient sampling as the researcher selects the sample elements according to their convenient accessibility and proximity [ 3 , 6 ]. For example: assume that we will perform a cohort study on Egyptian patients with Hepatitis C (HCV) virus. The convenience sample here will be confined to the accessible population for the research team. Accessible population are HCV patients attending in Zagazig University Hospital and Cairo University Hospitals. Therefore, within the study period, all patients attending these two hospitals and meet the eligibility criteria will be included in this study.
Judgmental sampling
In this method, the subjects are selected by the choice of the investigators. The researcher assumes specific characteristics for the sample (e.g. male/female ratio = 2/1) and therefore, they judge the sample to be suitable for representing the population. This method is widely criticized due to the likelihood of bias by investigator judgement [ 5 ].
Snow-ball sampling
This method is used when the population cannot be located in a specific place and therefore, it is different to access this population. In this method, the investigator asks each subject to give him access to his colleagues from the same population. This situation is common in social science research, for example, if we running a survey on street children, there will be no list with the homeless children and it will be difficult to locate this population in one place e.g. a school/hospital. Here, the investigators will deliver the survey to one child then, ask him to take them to his colleagues or deliver the surveys to them.
Conflict of interest:
Thank you for visiting nature.com. You are using a browser version with limited support for CSS. To obtain the best experience, we recommend you use a more up to date browser (or turn off compatibility mode in Internet Explorer). In the meantime, to ensure continued support, we are displaying the site without styles and JavaScript.
- View all journals
- My Account Login
- Explore content
- About the journal
- Publish with us
- Sign up for alerts
- Open access
- Published: 23 February 2022
A simulated comparison of behavioural observation sampling methods
- James Edward Brereton 1 ,
- Jonathan Tuke 2 &
- Eduardo J. Fernandez 3
Scientific Reports volume 12 , Article number: 3096 ( 2022 ) Cite this article
8744 Accesses
14 Citations
2 Altmetric
Metrics details
- Behavioural methods
- Psychophysics
Behavioural research requires the use of sampling methods to document the occurrence of responses observed. Sampling/recording methods include ad libitum, continuous, pinpoint (instantaneous), and one-zero (interval) sampling. Researchers have questioned the utility of each sampling method under different contexts. Our study compared computerized simulations of both pinpoint and one-zero sampling to continuous recordings. Two separate computer simulations were generated, one for response frequency and one for response duration, with three different response frequencies (high, medium, or low) and response durations (short, medium, and long) in each simulation, respectively. Similarly, three different observation intervals (5, 50, and 500 s) were used to record responses as both pinpoint and one-zero sampling methods in the simulations. Under both simulations, pinpoint sampling outperformed one-zero sampling, with pinpoint sampling producing less statistical bias in error rates under all frequencies, durations, and observation intervals. As observation intervals increased, both mean error rates and variability in error rates increased for one-zero sampling, while only variability in error rate increased for pinpoint sampling. The results suggest that pinpoint sampling techniques are effective for measuring both frequency (event) and duration (state) behaviours, and that pinpoint sampling is a less statistically biased behavioural observation method than one-zero sampling.
Similar content being viewed by others
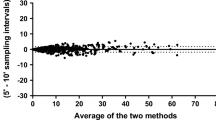
New approaches to selecting a scan-sampling method for chicken behavioral observations and their practical implications
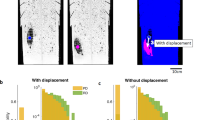
The relevance of a right scale for sampling when studying high-resolution behavioral dynamics
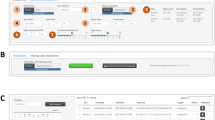
Automated analysis of activity, sleep, and rhythmic behaviour in various animal species with the Rtivity software
Introduction.
Behavioural studies are a valuable tool for the scientific study of both human and non-human animal performance. In animal research, behavioural observations are used more often than other welfare indicators such as glucocorticoid analysis 1 , 2 . Behavioural research may also be used to investigate the prevalence of positive behaviours, like foraging, or negative behaviours, such as stereotypies 3 , 4 , 5 . Studies of behaviour are also frequently conducted for wild animal populations and to better understand natural histories or investigate the impact of human disturbance 2 , 6 . Research on animal behaviour is now so well recognised that there are numerous journals dedicated to its study, for instance: Animal Behaviour , Applied Animal Behaviour Science , and Ethology.
The methods used in behavioural research can be traced back to laboratory studies. Scientists during the mid-twentieth Century often used a mixture of both human and animal models to answer questions in the field of behavioural psychology 7 , 8 . Based on the range of different techniques that were generated by earlier studies, Altmann 9 summarised the methods available. This paper became fundamentally important to those interested in behavioural research and remains a keystone paper for researchers. Other authors, such as Bateson and Martin 10 further refined the behavioural methods and their definitions. Bateson and Martin 10 distinguish between sampling and recording rules, which detail differences between the number of subjects observed (i.e. sampling rules; focal [1 subject] vs. scan [> 1 subjects]), or the observation method used to sample behaviour (i.e. recording rules; see below). However, little distinction is made between sampling and recording rules outside of this text, and for the purposes of our paper, we will refer to recording rules as sampling methods or techniques.
Types of sampling (recording) methods
Since Altmann’s 9 review, some behavioural sampling techniques have become increasingly popular in the research literature, whereas others are rarely used. Several behaviour measurement techniques have received criticism in terms of their repeatability 11 . For example, ad libitum (qualitative) sampling may be useful for developing ethograms and for pilot studies but has methodological flaws with regards to its lack of standardisation 12 , 13 . However , ad libitum sampling is still used in studies of behaviour, with a review by Mann 14 identifying that between 53 and 59% of cetacean studies published in Marine Mammal Science used this sampling technique.
Continuous recording is considered the gold standard for behavioural sampling, as this method records all occurrences of behaviour and their durations 6 , 15 . In the past, this made continuous recording often challenging for researchers: for instance, an active animal that rapidly changed behaviour would have been difficult to observe and record 16 . Similarly, measurement of multiple animals using a continuous method would have been incredibly challenging to document accurately, hence the method is often considered synonymous with the focal sampling of one individual 9 , 12 . Use of modern technology has in part ameliorated some of these issues by allowing behaviour to be recorded and analysed later 17 . However, continuous recording may remain a challenge, especially where large amounts of data are being recorded or direct comparisons of response frequencies and durations are made, and as such there is a need for alternative methods. As a result, several sampling (recording) methods have been developed that allow multiple animals and behaviours to be measured at one time (scan sampling), as well in a non-continuous fashion.
The use of pinpoint sampling, also referred to as instantaneous or momentary time sampling, is a commonly used method for observational study 6 , 18 , 19 , 20 . With pinpoint sampling, one or more responses are recorded at preselected moments in time (e.g., every 15 s for an hour). The benefits of pinpoint sampling are that it is less intensive than continuous sampling, and therefore may be more feasible for researchers to conduct 12 , 21 , 22 . The methods are also more versatile, allowing researchers to make decisions as to how long intervals should be spaced. For example, some researchers might choose to use 15-s intervals, particularly when studying an active animal or when conducting observations of key times, such as when enrichment is provided 23 , 24 . On the other hand, observers might choose to use much longer intervals, such as one-, two- or five-minute intervals when their subjects are inactive or if they are observing for long time periods 25 , 26 . Shorter intervals tend to result in values that match more closely the continuous behaviour scores but require more recording effort 27 .
One-zero or interval sampling involves choosing specific intervals of time, like pinpoint sampling, but instead recording whether one or more responses occur (or conversely, do not occur) within that interval of time 6 , 28 , 29 . While popular with both human and non-human primate research, one-zero sampling seems to receive less representation than pinpoint sampling in most animal behaviour studies and has been criticised by previous researchers 9 , 30 . However, one-zero sampling has some of the same benefits of instantaneous sampling in that interval length can be tailored in line with the requirements of the study. Additionally, one-zero sampling has the potential to collect more behaviours during a predefined period, as multiple behaviours can be recorded during each interval 9 . Leger 31 identified good agreement with continuous behaviour measures when using one-zero sampling at 15-s intervals for chimpanzees ( Pan troglodytes ). Likewise, Rhine and Flanigon 32 found similar levels of occurrence when comparing continuous, pinpoint, and one-zero sampling methods with a colony group of stumptail macaques ( Macaca arctoides ). As noted above, one-zero (interval) sampling is also frequently used in studies on human behaviour, for example in the classroom 33 , 34 .
Both pinpoint and one-zero sampling overcome some of the issues associated with continuous recording by reducing the amount of input required by the researcher, while still aiming to keep the sample representative of the subject’s behavioural repertoire 35 , 36 , 37 , 38 . However, one key question is how closely these techniques correlate with continuous recording? A major concern focuses on distinguishing between the frequency vs duration of some response, with behaviours of short duration typically referred to as “events”, while behaviours of long durations are called “states”. Pinpoint sampling loses information in terms of the duration of any response and is potentially less likely to pick up any behaviours of short duration (events) 12 , 39 . By contrast, one-zero sampling is better at recording all observable behaviours, but both behavioural frequency and duration could be easily misrepresented: there is no way to identify whether a behaviour recorded as present for one interval was seen once or thirty times during that time period 40 .
Sampling method simulations
Researchers in various fields have compared differences between pinpoint and one-zero sampling methods. Early simulations lacked the precision and/or ability to run extensive repetitions of their simulations to accurately assess sampling method differences 41 , 42 , 43 , 44 . Other researchers have attempted to make similar methodological comparisons via the data collection of actual behavioural occurrences 31 , 32 , 45 , 46 , 47 , 48 , 49 . While the results of differences in sampling methods for real occurrences of behaviour varied, most studies found pinpoint sampling to be more accurate than one-zero sampling, at least with respect to duration (state) behaviours. Nonetheless, caution should be used in making determinations of the validity of any result based on specific examples, as exceptions to any rule can and do occur.
Only three recent studies, all conducted by behaviour analysts interested in observations for applied, behaviour change purposes with human populations, have attempted to simulate data sets and compare some aspect of pinpoint and one-zero sampling methods 50 , 51 , 52 . In two of these studies 50 , 51 , limited simulations were produced via the rolling of die and pinpoint sampling was compared to a type of one-zero sampling, Partial Interval Recording (PIR), in which the response only need occur at any point during an observation interval to be recorded. In both studies, pinpoint sampling generally outperformed one-zero sampling for the detection of duration responses, with some variation in the ability of PIR to accurately detect frequency responses compared to pinpoint sampling and continuous recordings. Wirth et al. 52 is the only study to date to use extensive computer-generated simulations to examine differences between pinpoint and one-zero sampling methods. Their study utilised both PIR and Whole Interval Recording (WIR), where the duration response must occur during the entire observation interval to be recorded. Overall, they found that pinpoint sampling outperformed one-zero sampling methods on most measures.
The following study proposes to compare computer simulated occurrences of both low/short, medium, and high/long frequency/duration behaviours, as well as similar observation intervals for pinpoint and one-zero sampling methods. Different durations of behaviour were used to provide generalised situations researchers may encounter: some behaviours are normally short (e.g. sneezing), medium (e.g. feeding) or long (e.g. resting) in their duration. We hypothesised that: (1) one-zero sampling would be more accurate (less statistically biased) for detecting the occurrence of low frequency (event) behaviours, particularly when comparing less frequent pinpoint and one-zero observation methods (e.g., 500 s observation intervals), and (2) pinpoint sampling would provide a more accurate representation of percentages of occurrence for both low, medium, and high duration (state) behaviours than one-zero sampling.
Response frequency
The mean error rate for both pinpoint and one-zero sampling was calculated for each interval length and each of the three behavioural frequencies (see Fig. 1 ).
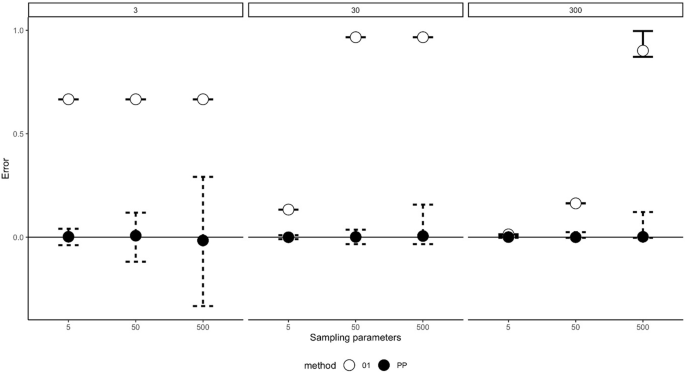
Mean error rates (with 95% percentile intervals of the errors bars) for the response frequency simulation and the pinpoint and one-zero sampling methods for the 5 s, 50 s and 500 s observation intervals. PP Pinpoint sampling, closed circles, 01 One-zero sampling, open circles.
The mean error for pinpoint sampling was minimal for all interval lengths and behavioural frequencies. However, variance for the pinpoint sampling increased as interval length increased. For one-zero sampling, error rates increased as the interval length increased, with the 500 s interval showing the largest error rates irrespective of behavioural frequency.
Overall, mean error rates were consistently lower for the pinpoint sampling method in comparison to the one-zero sampling method (χ 2 = 9, df = 1, p = 0.0027, W = 1) (see Table 1 ). Post-hoc tests for all 9 comparisons (3 frequencies × 3 recording intervals) were p < 0.001.
Response duration
The accuracy of both pinpoint and one-zero sampling was calculated for each interval length and all three behavioural durations (short, medium, and long) (see Fig. 2 ).
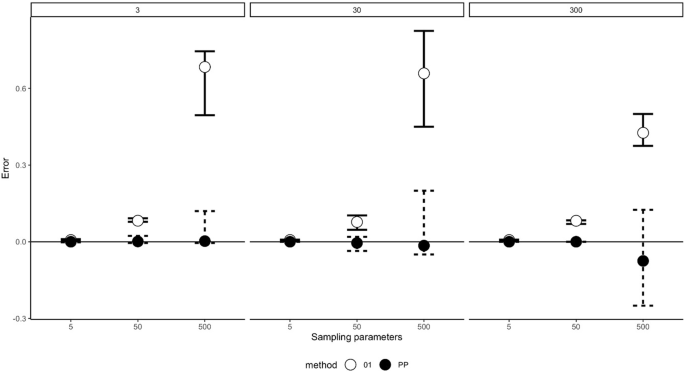
Mean error rates (with 95% percentile intervals of the errors bars) for the response duration simulation and the one-zero and pinpoint sampling methods for the 5 s, 50 s and 500 s observation intervals. PP Pinpoint sampling, closed circles, 01 One-zero sampling, open circles.
For all simulation frequencies, pinpoint sampling was less statistically biased, with minimal error rates. By contrast, mean error rates were much higher for one-zero sampling, and these increased as interval length increased. For both pinpoint and one-zero sampling, the variance in error increased with interval length.
The pinpoint sampling method consistently produced lower error rates than the one-zero method (χ 2 = 9, df = 1, p = 0.0027, W = 1) (see Table 2 ). Post-hoc tests for all 9 comparisons (3 durations × 3 recording intervals) were p < 0.001.
Our study attempted to answer two hypotheses: (1) one-zero sampling would be more accurate (less statistical error or bias) for detecting the occurrence of low frequency (event) behaviours, particularly when comparing less frequent pinpoint and one-zero observation methods, and (2) pinpoint sampling would provide a more accurate representation of percentages of occurrence for both low, medium, and high duration (state) behaviours than one-zero sampling. The first hypothesis was not supported, as pinpoint sampling was better able to detect frequency responses than one-zero sampling, even when events occurred less frequently, and when recording intervals were longer. The second hypothesis was supported in that pinpoint sampling had lower error margins than one-zero sampling for detecting duration behaviours. One-zero sampling was similarly capable at detecting duration behaviours of any length at low (5 s) or medium (50 s) recording intervals. At longer recording intervals (500 s), pinpoint sampling substantially outperformed one-zero sampling for the detection of duration (state) behaviours. Finally, for both sampling methods, increasing the interval recording length appeared to increase the variability in error rates for both frequency and duration responses. As the recording interval increased, one-zero sampling became less accurate (more statistically biased), as observed by an increase in mean error rate. Increased recording intervals also increased variability in the mean error rate for one-zero sampling of duration responses. Pinpoint sampling maintained low error rates regardless of the recording interval length, however, as the recording interval increased, pinpoint sampling showed greater variability in the mean error rate for both frequency and duration responses.
As noted in the Introduction, Wirth et al. 52 is the only other study to date to use extensive computer-generated simulations to examine differences between pinpoint and one-zero sampling methods, in their case both partial interval recording (PIR) and whole interval recording (WIR) methods. Like our study, they generated 100 simulations, and found pinpoint sampling to be more accurate (less statistically biased) than PIR or WIR, which overestimated and underestimated cumulative event durations, respectively. One limitation of their simulation was that it used a truly randomized rather than block structure for the simulated responses, as ours did, which more directly limits the applicability of their simulation to real-world behaviours (behaviour is rarely, if ever, truly random). Regardless, their results were similar to our study in that pinpoint sampling was generally more accurate than one-zero sampling methods.
Taken together, the results of our study and previous simulations suggest that pinpoint sampling is more accurate in detecting responses than one-zero sampling. Below we consider these implications, as well as factors that should influence the selection of behavioural sampling methods.
Which sampling method is most appropriate for my study?
Pinpoint sampling has not been recommended for measuring frequency (event) responses, particularly those of low occurrence 6 , 9 . However, in our simulation this method was accurately able to detect low occurrence (< 1%) frequencies. Therefore, the use of pinpoint sampling to measure any event responses, regardless of their frequency of occurrence, appears to be a viable option if large amounts of behavioural data are collected.
One-zero sampling methods are often preferred as an observational method because of the ease with which behaviours can be observed, recorded, and assessed for Interobserver Agreement (IOA) 53 , 54 . The same can also be said for pinpoint sampling, which provides an equally user-friendly research method when compared to continuous (focal) recordings. In addition, researchers attempting to account for under- or over-estimates of one-zero recordings have devised different sampling methods, including partial, whole, occurrence, and non-occurrence interval (one-zero) recordings. Still, the difficulty here is that, if pinpoint sampling provides a more accurate representation of behavioural occurrence, then the solution should be to adopt this method rather than adjusting to a less accurate one-zero recording method.
An added benefit of using either pinpoint or one-zero sampling methods over continuous recordings are that frequency (event) versus duration (state) behaviours can be compared more clearly. For instance, if a researcher were assessing the impact of pacing on the welfare of an animal, measuring pacing as an event or state would result in different data being generated. Lehner 6 suggests that the former could be assessed as a bout of event responses, but it is still not clear how to evaluate the difference between about of responses to less frequent but longer duration behaviours. Pinpoint and one-zero sampling methods avoid this problem by only recording whether the response occurred during some observation period, regardless of the frequency or duration of the recorded response. This makes these observation methods valuable in circumstances where presence or absence of a particular behaviour is more important than the measurement of its frequency or duration, such as in studies of courtship or reproduction 10 , 12 .
There may remain several valuable uses for one-zero sampling as a tool for researchers. For example, one-zero sampling may still be the most useful technique when a specific, important behaviour occurs very rarely and is of short duration. The value of one-zero sampling would be further enhanced in studies where smaller amounts of data are collected. Examples could include courtship displays, where the behaviours may occur only a handful of times per individual per year for some species 12 . The chance of the behaviour being recorded by pinpoint sampling may be minimal, yet the value of identifying the behaviour may be disproportionately high. However, caution is still warranted in the application of one-zero sampling methods to record rare, short duration responses, as it is not clear whether such interval recording methods would produce an accurate representation of such low occurrence responses.
Sampling method selection and laboratory lore
Historically, a major factor in determining behavioural observation methodology has been the prevalence of that sampling method within some field or observational species. For instance, Mann 14 found that over half of all cetacean studies in their review used ad libitum sampling, even though such sampling methods are recognized to be both less quantitative and systematic. Likewise, one-zero sampling methods are typically used by primatologists and behaviour analysts for the study of non-human primate and human behaviour, respectively 30 , 31 , 32 , 33 , 34 , 35 , 36 , 49 , 53 , 54 , 55 , 56 , 57 . The concept of using methodology passed down from previous studies and labs has been referred to as “laboratory lore” and is an asset to the cultural transmission of scientific knowledge 58 , 59 . Nonetheless, the selection of behavioural observation methods, like all aspects of scientific research, should be based on the efficacy of the methodology used. In the case of selecting between pinpoint or one-zero sampling methods to estimate behavioural occurrences, our study indicates that pinpoint sampling outperforms one-zero sampling on all frequency (event) and duration (state) measures simulated. Thus, laboratory lore aside, pinpoint sampling seems to be the better option for measuring some aspect of behavioural prevalence when compared to one-zero sampling methods.
For all simulations, patterns of behaviour were computer generated for both frequency of occurrence (how often the behaviour appeared) and percentage of occurrence (the percentage of time that the behaviour occurred). On these simulated patterns of behaviour, two different non-continuous sampling methods were directly compared: pinpoint (instantaneous) and one-zero (interval) sampling. Two sets of simulations were produced: response frequency (to measure the ability of both behaviour methods to detect short, event behaviours at different rates of occurrence) and response duration (to measure the ability of the methods in assessing state behaviours of different lengths). Three levels for response frequency and response duration were determined, based on a level of frequency/duration: 3 s, 30 s, and 300 s. These three durations were selected because they are reflective of different durations of behaviour in published studies 10 , 12 . The interval lengths for both pinpoint and one-zero sampling were set at 5 s, 50 s, and 500 s, in order to compare the effect of interval length on sample accuracy. These three interval lengths were chosen to reflect some of the common sampling lengths (frequent, regular and infrequent) used in human and animal research 10 , 12 .
Simulations
All the simulations were done in the R computing language version 3.6.3 using the GUI RStudio (code publicly available at https://github.com/jonotuke/animal_simulation_2020 ) 60 . For both sets of simulations, observation periods were set to a length of one hour, or 3600 s, as this time length is often set in observational studies 61 ,. A total of 1800 h of simulated data were generated across the response frequency and duration conditions.
This simulation focused on the recording of event behaviours: behaviours of short duration 10 . For the simulation, the duration of all event behaviours was set to exactly one second. Next, three different frequencies of event behaviour were selected: high (3 s), medium (30 s) and low (300 s) frequency of occurrence, in order to reflect different types of behaviour that occur very frequently, less frequently, or infrequently 62 (Fig. 3 ). The observation period was one-hour in length (3600 s). A total of 100 simulated data sets were generated for each of the three response frequencies. The exact time that each event occurred within the 3, 30 or 300 s period was randomised within the predefined blocks (e.g. the behaviour exactly once within its 3, 30 or 300 s period).
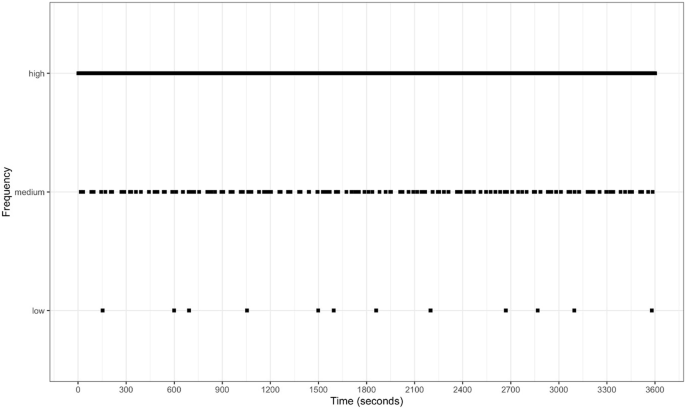
Example of simulated data for response frequency for high (3 s), medium (30 s), and low (300 s) frequency behaviours. This results in the frequency (event) occurring for exactly 33, 3.3, and 0.3% of the one-hour simulation, respectively. The high frequency occurs so often that it appears as a solid line, but the behaviour is in fact occurring once in each 3 s period.
The real (continuous) occurrence of each simulated response frequency was determined by calculating the number of seconds of each event that were possible in a simulated hour of data (observation period divided by frequency of occurrence; high frequency = 1200 s; medium frequency = 120 s; and low frequency = 12 s). The event behaviour seconds were then transformed into a percentage of total time (as is often shown in behaviour studies in the form of an activity budget), as well as frequency of occurrence. Thus, high frequency (3 s) responses occurred 33% of the hour, medium frequency (30 s) responses occurred 3.3%, and the low frequency (300 s) responses occurred 0.3% of the time.
To compare against this real (continuous) measurement, pinpoint and one-zero sampling were used on the simulated data sets. One-zero sampling recorded an event if it occurred at any point during the observation period, also commonly referred to as partial interval recording (PIR). The three interval lengths (5, 50, and 500 s) were used for both pinpoint and one-zero sampling. This resulted in nine-hundred data sets (nine combinations of simulation parameters and sampling parameters, each combination simulated 100 times) being developed.
This simulation was developed for longer duration or state behaviours. In the literature, state behaviours can be of variable length, lasting anywhere from seconds (e.g. scratching) to minutes (e.g. preening) or hours (e.g. resting). To accommodate this, three levels of behavioural duration were selected. These durations were set as short (3 s), medium (30 s) and long (300 s) durations of occurrence (Fig. 4 ). Each of these states were treated separately (only short, medium, or long behaviours occurred in each simulation). As per the Response Frequency investigation, the observation period was set to one-hour in length (3600 s). Each duration simulation was repeated 100 times.
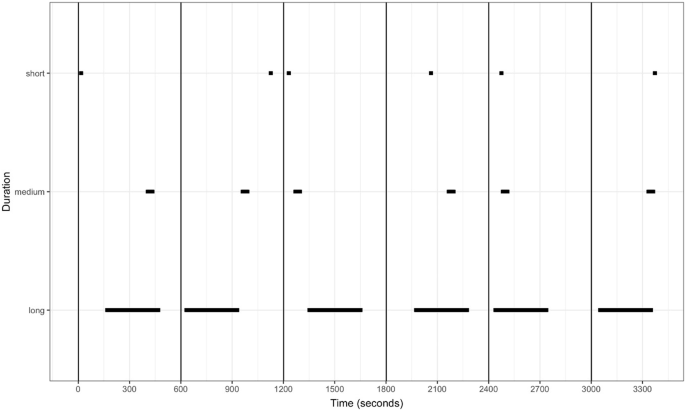
Example of simulated data for response duration for short (3 s), medium (30 s), and long (300 s) duration behaviours. The location of each state was selected at random within its 600 s period. This results in the duration (state) lasting for exactly 0.5, 5, and 50% of the one-hour observation in total respectively.
The chosen behaviour occurred once per 600 s period. The exact time that each behaviour occurred within its respective 600 s period was selected at random (though the behaviour was not allowed to slip into the next period of 600 s). Continuous data sets were developed by using the raw, simulated data and transforming this into percentages. This meant that each behaviour occurred six times during each one-hour simulation, with the short duration (3 s) responses occurring 0.5% of the hour, the medium duration (30 s) responses occurring 5%, and the long duration (300 s) responses occurring 50% of the time.
Each of the three behaviour durations (short, medium, and long) were measured using one-zero (PIR) and pinpoint sampling. Three interval lengths were recorded, again consisting of 5 s, 50 s and 500 s, as had been selected for the Response Frequency investigations. These interval lengths were used for both the pinpoint and one-zero sampling. Once complete, the results were then transformed into percentages and compared to the continuous data to determine the level of error.
Statistical analysis
Statistical analyses were conducted on the mean error scores for pinpoint and one-zero sampling at each respective interval length. The Friedman test was used to investigate whether there was a statistically significant effect of sampling method on the estimation error. The sampling/simulation combination was used as a blocking factor. The non-parametric Friedman test was used due to the non-normality of the errors and the observed heteroscedascity. When significant differences were found, paired Wilcoxon tests were used to compare the treatments. To compensate for multiple comparisons, we used an FDR adjustment. The method is statistically not-biased, meaning the process is giving an estimate of the true parameters that are correct (i.e. not over- nor under-estimated).
Fraser, D. Animal behaviour, animal welfare and the scientific study of affect. Appl. Anim. Behav. Sci. 118 , 108–117. https://doi.org/10.1016/j.applanim.2009.02.020 (2009).
Article Google Scholar
Sands, J. & Creel, S. Social dominance, aggression and faecal glucocorticoid levels in a wild population of wolves, Canis lupus . Anim. Behav. 67 , 387–396. https://doi.org/10.1016/j.anbehav.2003.03.019 (2004).
Carlstead, K., Seidensticker, J. & Baldwin, R. Environmental enrichment for zoo bears. Zoo Biol. 10 , 3–16. https://doi.org/10.1002/zoo.1430100103 (1991).
Fernandez, E. J. & Timberlake, W. Mutual benefits of research collaborations between zoos and academic institutions. Zoo Biol. 27 , 470–487. https://doi.org/10.1002/zoo.20215 (2008).
Article PubMed Google Scholar
Ward, S. J., Sherwen, S. & Clark, F. E. Advances in applied zoo animal welfare science. J. Appl. Anim. Welf. Sci. 21 , 23–33. https://doi.org/10.1016/0003-3472(79)90016-2 (2018).
Article CAS PubMed Google Scholar
Lehner, P. N. Handbook of Ethological Methods (Cambridge University Press, 1998).
Google Scholar
Domjan, M. The Principles of Learning and Behavior (Nelson Education, 2014).
Pierce, W. D. & Cheney, C. D. Behavior Analysis and Learning (Psychology Press, 2013).
Book Google Scholar
Altmann, J. Observational study of behavior: Sampling methods. Behavior 49 , 227–266. https://doi.org/10.1163/156853974X00534 (1974).
Article CAS Google Scholar
Bateson, M. & Martin, P. Measuring Behaviour: An Introductory Guide 4th edn. (Cambridge University Press, 2021).
Bernstein, I. S. An empirical comparison of focal and ad libitum scoring with commentary on instantaneous scans, all occurrence and one-zero techniques. Anim. Behav. 42 , 721–728. https://doi.org/10.1016/S0003-3472(05)80118-6 (1991).
Martin, P. & Bateson, P. Recording methods. In ‘Measuring Behaviour: An Introductory Guide’ (Cambridge University Press, 2007).
Rhine, R. J. & Ender, P. B. Comparability of methods used in the sampling of primate behavior. Am. J. Primatol. 5 , 1–15. https://doi.org/10.1002/ajp.1350050102 (1983).
Mann, J. Behavioral sampling methods for cetaceans: A review and critique. Mar. Mamm. Sci. 15 , 102–122. https://doi.org/10.1111/j.1748-7692.1999.tb00784.x (1999).
Hämäläinen, W. et al. Measuring behaviour accurately with instantaneous sampling: A new tool for selecting appropriate sampling intervals. Appl. Anim. Behav. Sci. 180 , 166–173. https://doi.org/10.1016/j.applanim.2016.04.006 (2016).
Tyler, S. Time-sampling: A matter of convention. Anim. Behav. 27 , 801–810. https://doi.org/10.1016/0003-3472(79)90016-2 (1979).
Amato, K. R., Van Belle, S. & Wilkinson, B. A comparison of scan and focal sampling for the description of wild primate activity, diet and intragroup spatial relationships. Folia Primatol. 84 , 87–101. https://doi.org/10.1159/000348305 (2013).
Fernandez, E. J., Kinley, R. C. & Timberlake, W. Training penguins to interact with enrichment devices for lasting effects. Zoo Biol. 38 , 43–49. https://doi.org/10.1002/zoo.21510 (2019).
Sanders, K. & Fernandez, E. J. Behavioral implications of enrichment for golden lion tamarins: A tool for ex situ conservation. J. Appl. Anim. Welf. Sci. 1 , 1–10 (2020).
Stevens, J., Thyssen, A., Laevens, H. & Vervaecke, H. The influence of zoo visitor numbers on the behaviour of harbour seals ( Phoca vitulina ). J. Zoo Aquat. Res. 1 , 31–34. https://doi.org/10.19227/jzar.v1i1.20 (2013).
Grenier, D., Barrette, C. & Crête, M. Food access by white-tailed deer ( Odocoileus virginianus ) at winter feeding sites in Eastern Québec. Appl. Anim. Behav. Sci. 63 , 323–337. https://doi.org/10.1016/S0168-1591(99)00017-9 (1999).
Gilby, I. C., Pokempner, A. A. & Wrangham, R. W. A direct comparison of scan and focal recording rules for measuring wild chimpanzee feeding behaviour. Folia Primatol. 81 , 254–264. https://doi.org/10.1159/000322354 (2010).
Fernandez, E. J., Ramirez, M. & Hawkes, N. C. Activity and pool use in relation to temperature and water changes in zoo hippopotamuses ( Hippopotamus amphibious ). Animals 10 , 1022 (2020).
Fernandez, E. J. & Timberlake, W. Foraging devices as enrichment in captive walruses ( Odobenus rosmarus ). Behav. Proc. 168 , 103943. https://doi.org/10.1016/j.beproc.2019.103943 (2019).
Shora, J. A., Myhill, M. N. G. & Brereton, J. E. Should zoo foods be coati chopped. J. Zoo Aquar. Res. 6 , 22–25. https://doi.org/10.19227/jzar.v6i1.309 (2021).
Teixeira, D. L., Machado Filho, L. C. P., Hötzel, M. J. & Enríquez-Hidalgo, D. Effects of instantaneous stocking rate, paddock shape and fence with electric shock on dairy cows’ behaviour. Livestock Sci. 198 , 170–173. https://doi.org/10.1016/j.livsci.2017.01.007 (2017).
Pullin, A. N. et al. Instantaneous sampling intervals validated from continuous video observation for behavioral recording of feedlot lambs. J. Anim. Sci. 95 , 703–4707. https://doi.org/10.2527/jas2017.1835 (2017).
Bailey, J. S. & Burch, M. R. Research Methods in Applied Behavior Analysis (Routledge, 2017).
Bakeman, R. & Quera, V. Behavioral observation. In APA Handbooks in Psychology APA Handbook of Research Methods in Psychology. Foundations Planning Measures and Psychometrics Vol. 1 (eds Cooper, P. M. et al. ) 207–225 (American Psychological Association, 2012). https://doi.org/10.1037/13619-013 .
Chapter Google Scholar
Kraemer, H. C. One-zero sampling in the study of primate behavior. Primates 20 , 237–244 (1997).
Brecht, K. F. et al. The status and value of replications in animal behavior science. Anim. Behav. Cogn. 8 , 97–106. https://doi.org/10.26451/abc.08.02.01.2021 (2021).
Rhine, R. J. & Flanigon, M. An empirical comparison of one-zero, focal-animal, and instantaneous methods of sampling spontaneous primate social behavior. Primates 19 , 353–361. https://doi.org/10.1007/BF02382803 (1978).
Dunkerton, J. Should classroom observation be quantitative?. Educ. Res. 23 , 144–151. https://doi.org/10.1080/0013188810230208 (1981).
Omark, D. R., Fiedler, M. L. & Marvin, R. S. Dominance hierarchies: Observational techniques applied to the study of children at play. Instruct. Sci. 5 , 403–423. https://doi.org/10.1007/BF00051807 (1976).
Rhine, R. J. & Linville, A. K. Properties of one-zero scores in observational studies of primate social behavior: The effect of assumptions on empirical analyses. Primates 21 , 111–122. https://doi.org/10.1007/BF02383828 (1980).
Rhine, R. J., Norton, G. W., Wynn, G. M. & Wynn, R. D. Weaning of free-ranging infant baboons ( Papio cynocephalus ) as indicated by one-zero and instantaneous sampling of feeding. Int. J. Primatol. 6 , 491–499. https://doi.org/10.1007/BF02735572 (1985).
Mitlöhner, F. M. et al. Behavioral sampling techniques for feedlot cattle. J. Anim. Sci. 79 , 1189–1193. https://doi.org/10.1016/S0168-1591(99)00017-9 (2001).
Simpson, M. J. A. & Simpson, A. E. One-zero and scan methods for sampling behaviour. Anim. Behav. 25 , 726–731. https://doi.org/10.1016/0003-3472(77)90122-1 (1977).
Xiao, J., Wang, K. & Wang, D. Diurnal changes of behavior and respiration of Yangtze finless porpoises ( Neophocaena phocaenoides asiaeorientalis ) in captivity. Zoo Biol. 24 , 531–541. https://doi.org/10.1002/zoo.20070 (2005).
Saibaba, P., Sales, G. D., Stodulski, G. & Hau, J. Behaviour of rats in their home cages: Daytime variations and effects of routine husbandry procedures analysed by time sampling techniques. Lab. Anim. 30 , 13–21. https://doi.org/10.1258/002367796780744875 (1996).
Griffin, B. & Adams, R. A parametric model for estimating prevalence, incidence, and mean bout duration from point sampling. Am. J. Primatol. 4 , 261–271 (1983).
Harrop, A. & Daniels, M. Methods of time sampling: A reappraisal of momentary time sampling and partial interval recording. J. Appl. Anim. Behav. Anal. 19 , 73–77 (1986).
Repp, A. C. et al. A comparison of frequency, interval, and time-sampling methods of data collection. J. Appl. Behav. Anal. 9 , 501–508. https://doi.org/10.1901/jaba.1976.9-501 (1976).
Article CAS PubMed PubMed Central Google Scholar
Suen, H. K. & Ary, D. Variables influencing one-zero and instantaneous time sampling outcomes. Primates 25 , 89–94. https://doi.org/10.1007/BF02382298 (1984).
Gardenier, N. C., MacDonald, R. & Green, G. Comparison of direct observational methods for measuring stereotypic behavior in children with autism spectrum disorders. Res. Devel. Disabilit. 25 , 99–118 (2004).
Meany-Daboul, M. G., Roscoe, E. M., Bourret, J. C. & Ahearn, W. H. A comparison of momentary time sampling and partial-interval recording for evaluating functional relations. J. Appl. Behav. Anal. 40 , 501–514 (2007).
Murphy, M. J. & Harrop, A. Observer error in the use of momentary time sampling and partial interval recording. Br. J. Psych. 85 , 169–179. https://doi.org/10.1111/j.2044-8295.1994.tb02517.x (1994).
Radley, K. C., O’Handley, R. D. & Labrot, Z. C. A comparison of momentary time sampling and partial-interval recording for assessment of effects of social skills training. Psych. Schools 52 , 363–378 (2015).
Rapp, J. T. et al. Interval recording for duration events: A re-evaluation. Behav. Interv. 22 , 319–345 (2007).
Devine, S. L. et al. Detecting changes in simulated events using partial-interval recording and momentary time sampling III: Evaluating sensitivity as a function of session length. Behav. Interv. 26 , 103–124 (2011).
Rapp, J. T. et al. Detecting changes in simulated events using partial-interval recording and momentary time sampling. Behav. Interv. 23 , 237–269 (2008).
Wirth, O., Slaven, J. & Taylor, M. A. Interval sampling methods and measurement error: A computer simulation. J. Appl. Behav. Anal. 47 , 83–100. https://doi.org/10.1002/jaba.93| (2014).
Cooper, J. O., Heron, T. E. & Heward, W. L. Applied Behavior Analysis 3rd edn. (Pearson Publishing, 2020).
Poling, A., Methot, L. L. & LeSage, M. G. Fundamentals of Behavior Analytic Research (Springer, 1995).
Doran, D. M. Comparison of instantaneous and locomotor bout sampling methods: A case study of adult male chimpanzee locomotor behavior and substrate use. Am. J. Phys. Anthropol. 89 , 85–99. https://doi.org/10.1002/ajpa.1330890108| (1992).
Merrell, K. Assessment of children’s social skills: Recent developments, best practices, and new directions. Exceptionality 9 , 3–18 (2001).
Seyfarth, R. M., Cheney, D. L. & Marler, P. Vervet monkey alarm calls: Semantic communication in a free-ranging primate. Anim. Behav. 28 , 1070–1094 (1980).
Buskist, W. & Johnston, J. M. Laboratory lore and research practices in the experimental analysis of human behavior. The Behavior Analyst 11 , 41–42 (1988).
Johnston, J. M. & Pennypacker, H. S. Strategies and Tactics of Behavioral Research (Routledge, 2010).
R Core Team. R: A Language and Environment for Statistical Computing . (R Foundation for Statistical Computing, 2020). https://www.R-project.org/ .
Leger, D. W. An empirical evaluation of instantaneous and one-zero sampling of chimpanzee behavior. Primates 18 , 387–393. https://doi.org/10.1007/BF02383116 (1977).
Farrar, B. G., Voudouris, K. & Clayton, N. S. Replications, comparisons, sampling and the problem of representativeness in animal cognition research. Anim. Behav. Cogn. 8 , 273–295. https://doi.org/10.26451/abc.08.02.14.2021 (2021).
Article PubMed PubMed Central Google Scholar
Download references
Acknowledgements
The authors would like to thank Mrs S Brereton for proofreading the manuscript. As noted in the text, the code has been made publicly accessible. This allows anyone to adjust all variables and run the simulations and comparisons themselves: https://github.com/jonotuke/animal_simulation_2020 .
Author information
Authors and affiliations.
University Centre Sparsholt, Sparsholt College, Westley Lane, Sparsholt, Winchester, SO21 2NF, Hampshire, UK
James Edward Brereton
School of Mathematical Sciences, The University of Adelaide, Adelaide, SA, 5005, Australia
Jonathan Tuke
School of Animal and Veterinary Sciences, The University of Adelaide, Adelaide, SA, 5005, Australia
Eduardo J. Fernandez
You can also search for this author in PubMed Google Scholar
Contributions
E.F. and J.E.B. wrote the main manuscript text and S.T. ran the simulations and statistical tests. All authors reviewed the manuscript.
Corresponding author
Correspondence to James Edward Brereton .
Ethics declarations
Competing interests.
The authors declare no competing interests.
Additional information
Publisher's note.
Springer Nature remains neutral with regard to jurisdictional claims in published maps and institutional affiliations.
Rights and permissions
Open Access This article is licensed under a Creative Commons Attribution 4.0 International License, which permits use, sharing, adaptation, distribution and reproduction in any medium or format, as long as you give appropriate credit to the original author(s) and the source, provide a link to the Creative Commons licence, and indicate if changes were made. The images or other third party material in this article are included in the article's Creative Commons licence, unless indicated otherwise in a credit line to the material. If material is not included in the article's Creative Commons licence and your intended use is not permitted by statutory regulation or exceeds the permitted use, you will need to obtain permission directly from the copyright holder. To view a copy of this licence, visit http://creativecommons.org/licenses/by/4.0/ .
Reprints and permissions
About this article
Cite this article.
Brereton, J.E., Tuke, J. & Fernandez, E.J. A simulated comparison of behavioural observation sampling methods. Sci Rep 12 , 3096 (2022). https://doi.org/10.1038/s41598-022-07169-5
Download citation
Received : 05 May 2021
Accepted : 21 January 2022
Published : 23 February 2022
DOI : https://doi.org/10.1038/s41598-022-07169-5
Share this article
Anyone you share the following link with will be able to read this content:
Sorry, a shareable link is not currently available for this article.
Provided by the Springer Nature SharedIt content-sharing initiative
This article is cited by
- Alice Cartoni Mancinelli
- Angela Trocino
- Cesare Castellini
Scientific Reports (2023)
Social touch in the age of computational ethology: Embracing as a multidimensional and complex behaviour
- Sebastian Ocklenburg
- Julian Packheiser
- Guillermo Hidalgo-Gadea
Current Psychology (2023)
By submitting a comment you agree to abide by our Terms and Community Guidelines . If you find something abusive or that does not comply with our terms or guidelines please flag it as inappropriate.
Quick links
- Explore articles by subject
- Guide to authors
- Editorial policies
Sign up for the Nature Briefing newsletter — what matters in science, free to your inbox daily.

- Research article
- Open access
- Published: 23 July 2020
COVID-19 prevalence estimation by random sampling in population - optimal sample pooling under varying assumptions about true prevalence
- Ola Brynildsrud ORCID: orcid.org/0000-0001-7566-4133 1 , 2
BMC Medical Research Methodology volume 20 , Article number: 196 ( 2020 ) Cite this article
39k Accesses
15 Citations
47 Altmetric
Metrics details
The number of confirmed COVID-19 cases divided by population size is used as a coarse measurement for the burden of disease in a population. However, this fraction depends heavily on the sampling intensity and the various test criteria used in different jurisdictions, and many sources indicate that a large fraction of cases tend to go undetected.
Estimates of the true prevalence of COVID-19 in a population can be made by random sampling and pooling of RT-PCR tests. Here I use simulations to explore how experiment sample size and degrees of sample pooling impact precision of prevalence estimates and potential for minimizing the total number of tests required to get individual-level diagnostic results.
Sample pooling can greatly reduce the total number of tests required for prevalence estimation. In low-prevalence populations, it is theoretically possible to pool hundreds of samples with only marginal loss of precision. Even when the true prevalence is as high as 10% it can be appropriate to pool up to 15 samples. Sample pooling can be particularly beneficial when the test has imperfect specificity by providing more accurate estimates of the prevalence than an equal number of individual-level tests.
Sample pooling should be considered in COVID-19 prevalence estimation efforts.
Peer Review reports
It is widely accepted that a large fraction of COVID-19 cases go undetected. A crude measure of population prevalence is the fraction of positive tests at any given date. However, this is subject to large ascertainment bias since tests are typically only ordered from symptomatic cases, whereas a large proportion of infected might show little to no symptoms [ 1 , 2 ]. Non-symptomatic infections can still shed the Severe acute respiratory syndrome coronavirus 2 (SARS-CoV-2) virus and are therefore detectable by reverse transcriptase polymerase chain reaction (RT-PCR)-based tests. It is therefore possible to test randomly selected individuals to estimate the true disease prevalence in a population. However, if the disease prevalence is low, very little information is garnered from each individual test. Under such situations it can be advantageous to pool individual patient samples into a single pool [ 3 , 4 , 5 ]. Pooling strategies, also called group testing, effectively increase the test capacity and reduces the required number of RT-PCR-based tests. For SARS-CoV-2 pooling has been estimated to potentially reduce costs by 69% [ 6 ], use ten-fold fewer tests [ 7 ] and clearing 20 times the number of people from isolation with the same number of tests [ 8 ]. Note that I will not discuss pooling of SARS-CoV-2 antibody-based tests, since there is currently not enough information about how pooling affects test parameters. However, sample pooling has been successfully used for seroprevalence studies for other diseases such as human immunodeficiency virus (HIV) [ 9 , 10 , 11 ].
I simulated the effect sample pooling had on prevalence estimates under five different settings for true prevalence, p . I started by generating a population of 500,000 individuals and then let each individual have p probability of being infected at sampling time. The number of patient samples collected from the population is denoted by n , and the number of patient samples that are pooled into a single well is denoted by k . The total number of pools are thus \( \frac{n}{k} \) , hereby called m . The number of positive pools in an experiment is termed x . I calculated the estimated prevalence \( \hat{p} \) at each parameter combination by replicating the experiment 100,000 times and report here the 2.5 and 97.5% quantiles of the distribution of \( \hat{p} \) .
Explored parameter options:
I considered the specificity ( θ ) of a PCR-based test to be 1.0 but include simulations with the value set to 0.99. Test sensitivity ( η ) depends on a range of uncontrollable factors such as virus quantity, sample type, time from sampling, laboratory standard and the skill of personnel [ 12 ]. There have also been reports of it varying with pooling level [ 13 ]. For the purposes of this study, I fixed the sensitivity first at 0.95, then at 0.7, irrespective of the level of pooling. These estimates are rather low, which would suggest that I am somewhat overestimating the uncertainty of \( \hat{p} \) . However, since it is possible that tests will be carried out under suboptimal and non-standardized conditions I prefer to err on the side of caution.
A central point of pooled testing is that the number of positive pools, x , divided by the total number of pools, m , can be used as a proxy to measure the true prevalence when the test sensitivity and specificity is known. Note that the number of positive pools, x , can be approximated in infinite populations as a stochastic variable subject to a binomial distribution with parameters m and P , where the latter is the probability that a single pool will test positive. A positive pool can arise from two different processes: There can be one or more true positive samples in the pool, and they are detected, or there can be no true positive samples in the pool, but the test gives a false positive result. These two possibilities are represented by the first and second part of the following equation [ 14 ], respectively:
Closer inspection of the above formula reveals something disheartening: When p approaches zero, P converges towards 1 − θ . Thus, in low-prevalence scenarios, and for typical values of test sensitivity and specificity, most positive test results will be false positives. Nevertheless, with appropriate levels of sample pooling it is possible to get decent estimates of the true prevalence because the probability of having no positive samples in a pool decreases with k .
We can modify eq. 1 for finite populations by replacing P with \( \frac{x}{m} \) , p with \( \hat{p} \) , and then solving for \( \hat{p} \) . This gives us the formula of Cowling et al., 1999 [ 15 ], which is used in the following to calculate \( \hat{p} \) from a single sample:
Note that the formula incorporates the test parameters and thus gives an unbiased estimate of \( \hat{p} \) even in low-prevalence settings. In this formula, x is a stochastic variable with a binomial distribution. It depends on the number of truly positive samples in a pool, another stochastic variable with a binomial distribution. As a final layer of complexity, we can take samples from a finite population. For these reasons I will use Monte Carlo simulations to get estimates for \( \hat{p} \) rather than evaluating some closed-form mathematical expression.
An algorithm for patient-level diagnosis
A crucial objective of testing is to identify which patients have active COVID-19 infections. This information is not readily apparent from pooled tests, and in order to get diagnostic results at the patient level, some samples will need to be retested. The methodologically simplest algorithm is to consider all samples from negative pools as true negatives, but re-test every sample from a positive pool individually. This is also called Dorfman’s method [ 4 ]. This strategy is estimated to increase testing capabilities by at least 69% [ 6 ]. In this work I use an algorithm that conserves testing resources even more than this, but which might be more difficult to implement in practice: I remove all samples from negative pools, considering them true negatives. All positive pools are split into two equally large sub-pools, and then the process is repeated. Positive patient-level diagnosis is only made from sub-pools of size 1. The algorithm is illustrated in Fig. 1 . Note that this is a sub-optimal version of the generalized binary splitting (GBS) algorithm presented in the context of COVID-19 in [ 16 ]. My version is sub-optimal in the number of reactions because I am always running a test on both sub-pools when a parent pool has tested positive. It is possible to run an even lower number of reactions by not testing a sub-pool if the other sub-pool from the same parent pool has been run first and tested negative. (The positive result from the parent pool implies that the second sub-pool must be positive.) However, for practical reasons such as the ability to run multiple tests simultaneously and the fact the tests are imperfect, I have used the algorithm in Fig. 1 . A thorough discussion on group testing algorithms and their merit in testing for SARS-CoV-2 is available in [ 7 ].
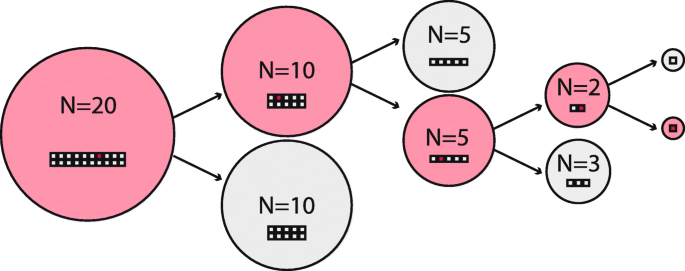
Algorithm used to minimize the number of RT-PCR reactions in pooled sampling. Negative pools regard all constituent patient samples as negative, whereas positive pools are split in two, and the process repeated. Red circle = Pool testing positive. Grey circle = Pool testing negative. Red/grey squares = Patient samples in pool, with color indicating diseased/non-diseased status
Estimates of prevalence
In the following, I use simulations to calculate the central 95% estimates of \( \hat{p} \) using tests with varying sensitivity (0.7 and 0.95) and specificity (0.99 and 1.0) (Figs. 2 , 3 , 4 , 5 ). These estimates are based on the initial pooled tests only, not the follow-up tests on sub-pools that allow for patient-level diagnosis. (Including results from these samples would allow the precision from the pooled test estimates to approach those of testing individually.) More samples are associated with a distribution of \( \hat{p} \) more narrowly centered around the true value, while higher levels of pooling are generally associated with higher variance in the \( \hat{p} \) estimates. The latter effect is less pronounced in populations with low prevalence. For example, if the true population prevalence is 0.001 and a total of 500 samples are taken from the population, the expected distribution of \( \hat{p} \) is nearly identical whether samples are run individually ( k = 1) or whether they are run in pools of 25 (Figs. 2 or 4 , panel A). Thus, it is possible to economize lab efforts by reducing the required number of pools to be run from 500 to 20 (500 divided by 25) without any significant alteration to the expected distribution of \( \hat{p} \) . At this prevalence and with this pooling level, 40 tests are sufficient to get a correct patient-level diagnosis for all 500 individuals 97.5% of the time (Supplementary Table 1 ). With 5000 total samples, the central estimates of \( \hat{p} \) vary little between individual samples (95% interval 0.00021–0.0021) and a pooling level of 200 (95% interval 0.0022–0.0021). 145 reactions is enough to get patient-level diagnosis 97.5% of the time, in other words a reduction in the number of separate RT-PCR setups by a factor of 34.5. (Supplementary Table 1 ).
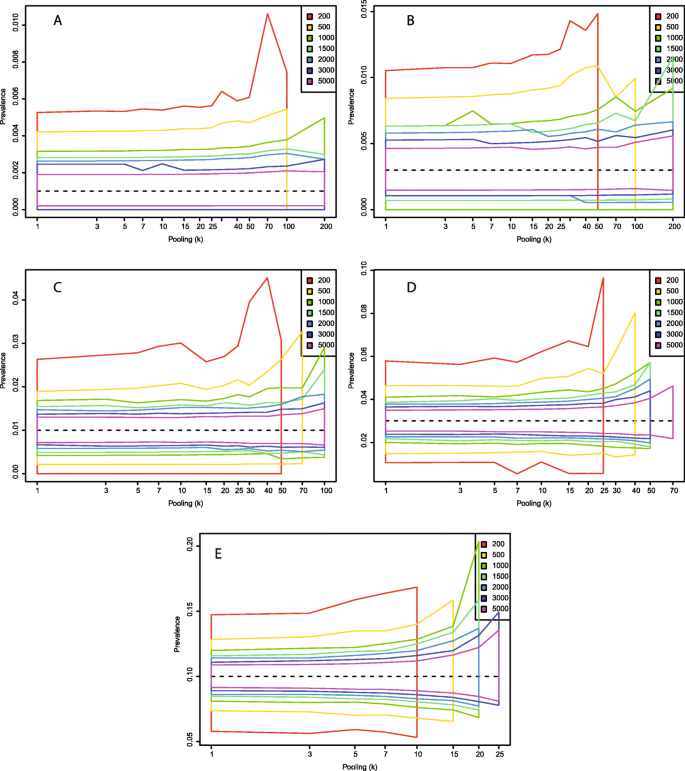
Central 95% estimates of \( \hat{p} \) with a test with sensitivity ( η ) 0.95 and perfect specificity ( θ = 1) under different combinations of total number of samples and level of sample pooling. a : p = 0.001; b : p = 0.003; c : p = 0.01; d : p = 0.03; e : p = 0.10
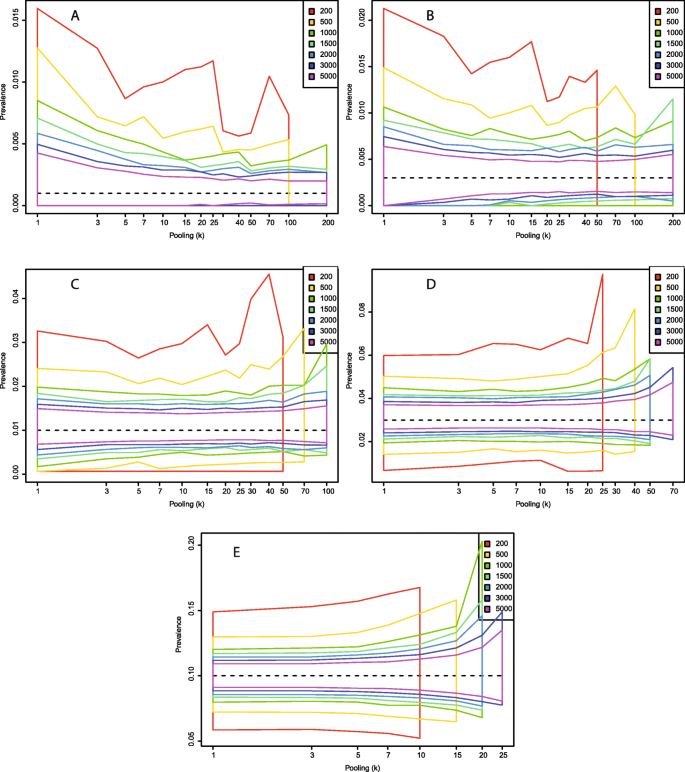
Central 95% estimates of \( \hat{p} \) with a test with sensitivity ( η ) 0.95 and a specificity ( θ ) of 0.99 under different combinations of total number of samples and level of sample pooling. a : p = 0.001; b : p = 0.003; c : p = 0.01; d : p = 0.03; e : p = 0.10
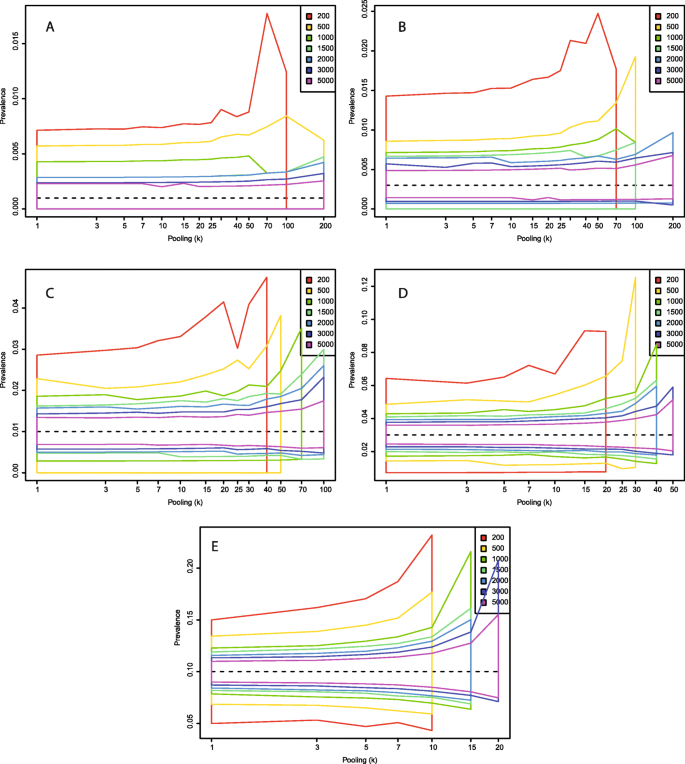
Central 95% estimates of \( \hat{p} \) with a test with sensitivity ( η ) 0.70 and perfect specificity ( θ = 1) under different combinations of total number of samples and level of sample pooling. a : p = 0.001; b : p = 0.003; c : p = 0.01; d : p = 0.03; e : p = 0.10
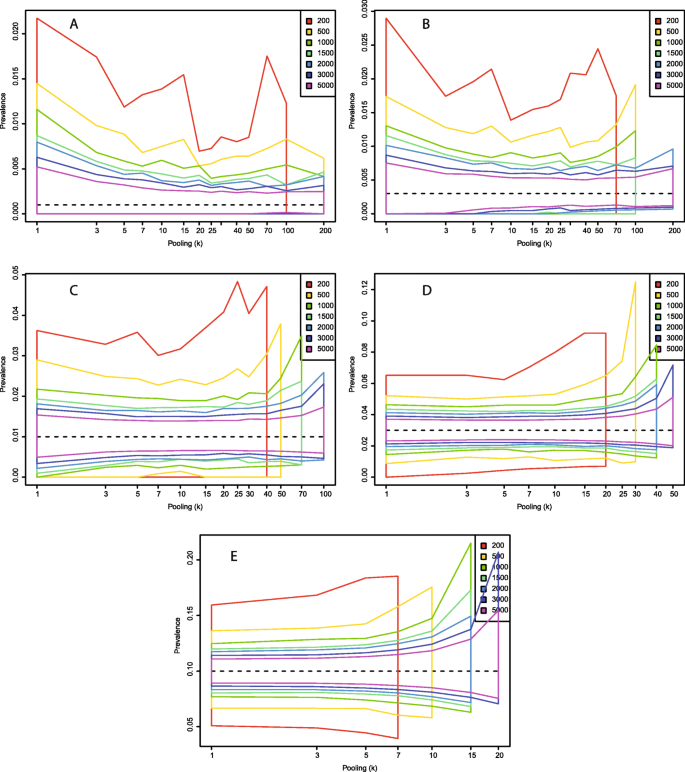
Central 95% estimates of \( \hat{p} \) with a test with sensitivity ( η ) 0.70 and a specificity ( θ ) of 0.99 under different combinations of total number of samples and level of sample pooling. a : p = 0.001; b : p = 0.003; c : p = 0.01; d : p = 0.03; e : p = 0.10
The situation changes when the test specificity ( θ ) is set to 0.99, that is, allowing for false positive test results (Figs. 3 , 5 ). This could theoretically occur from PCR cross-reactivity between COVID-19 and other viruses, or from human errors in the lab. A problem with imperfect specificity tests are that false positives typically outnumber true positives when the true prevalence is low. This creates a seemingly paradoxical situation in which higher levels of sample pooling often leads to prevalence estimates that are more accurate. This is because many pools test positive without containing a single true positive sample, leading to inflated estimates of the prevalence. When the level of pooling goes up, the probability that a positive pool contains at least one true positive sample increases, which increases the total precision. The trends about appropriate levels of pooling for different sample numbers and levels of true population prevalence are similar as for the perfect specificity scenario, but with imperfect specificity, we have an added incentive for sample pooling in that prevalence estimates are closer to the true value with higher levels of pooling. Even with a moderately accurate test (sensitivity 0.7 and specificity 0.99), when the prevalence is 1%, pooling 50 together lets us diagnose 5000 individuals at the patient-level with a median of 282 tests, a 17-fold reduction in the number of tests. This has virtually no influence on our estimate of \( \hat{p} \) , and no significant effect on the number of wrongly diagnosed patients, which in both cases is about 1%.
The relationship between true prevalence, total sample number and level of pooling is not always intuitive. Some combinations of parameters have serrated patterns for \( \hat{p} \) , which looks like Monte Carlo errors (Figs. 2 , 3 , 4 , and 5 ). This is particularly true for the lower sample counts. However, this is not due to stochasticity, but due to the discrete nature of each estimate of \( \hat{p} \) . That is, \( \hat{p} \) is not continuous and for small pool sizes miniscule changes in the number of positive pools can affect the estimate quite a bit.
For example, if we take 200 samples and go with a pool size of 100, there are only three potential outcomes: First, both pools are negative, in which case we believe the prevalence is 0. Second, one pool is positive and the other negative, in which case we estimate \( \hat{p} \) as approximately 0.007 if the test sensitivity is 0.95. Finally, both pools are positive, in which case the formula of Cowling et al. does not provide an answer because the fraction of positive pools is higher than the test sensitivity. This formula is only intended to be used when the fraction of positive pools is much lower than the test sensitivity.
In general, very high levels of pooling are not appropriate since, depending on the true prevalence, the probability that every single pool has at least one positive sample approaches 1. (Indicated by “NA” in Supplementary Table 1 ). In low prevalence settings however, it can be appropriate to pool hundreds of samples, but the total number of samples required to get a precise estimate of the prevalence is much higher. Thus, decisions about the level of pooling need to be informed by the prior assumptions about prevalence in the population, and there is a prevalence-dependent sweet spot to be found in the tradeoff between precision and workload.
It is worth noting that the strategy I have outlined here does present some logistical challenges. Firstly, samples must be allocated to pools in a random manner. This rules out some practical approaches such as sampling a particular sub-district and pooling these, then sampling another district the next day. Secondly, binary testing of sub-pools might be more cumbersome than it’s worth, in which case Dorfman’s method should be preferred. Finally, there are major organizational challenges related to planning and conducting such experiments across different testing sites and jurisdictions.
Attempts to estimate the true current prevalence of COVID-19 by PCR tests can benefit from sample pooling strategies. Such strategies have the potential to greatly reduce the required number of tests with only slight decreases in the precision of prevalence estimates. If the prevalence is low, it is generally appropriate to pool even hundreds of samples, but the total sample count needs to be high in order to get reasonably precise estimates of the true prevalence. On the other hand, if the prevalence is high there is little to be gained by pooling more than 15 samples. Pooling strategies makes it possible to get patient-level diagnostic information with only a fraction of the number of tests as individual testing. For a prevalence of 10%, pooling cut the required number of tests by about two thirds, while for a prevalence of 0.1%, the number of required tests could on average be lowered by a factor of 50.
Availability of data and materials
Code written for this project is available at https://github.com/admiralenola/pooledsampling-covid-simulation . All simulations and plots were created in R version 3.2.3 [ 17 ].
Abbreviations
Human immunodeficiency virus
Reverse transcriptase polymerase chain reaction
Severe acute respiratory syndrome coronavirus 2
Mizumoto K, Kagaya K, Zarebski A, Chowell G. Estimating the asymptomatic proportion of coronavirus disease 2019 (COVID-19) cases on board the diamond princess cruise ship, Yokohama, Japan, 2020. Eurosurveillance. 2020. https://doi.org/10.2807/1560-7917.es.2020.25.10.2000180 .
Q&A: Similarities and differences – COVID-19 and influenza. Available: https://www.who.int/news-room/q-a-detail/q-a-similarities-and-differences-covid-19-and-influenza . [cited 17 Apr 2020].
Hogan CA, Sahoo MK, Pinsky BA. Sample pooling as a strategy to detect community transmission of SARS-CoV-2. JAMA. 2020. https://doi.org/10.1001/jama.2020.5445 .
Dorfman R. The detection of defective members of large populations. Ann Math Stat. 1943;14(4):436–40.
Article Google Scholar
Cherif A, Grobe N, Wang X, Kotanko P. Simulation of Pool Testing to Identify Patients With Coronavirus Disease 2019 Under Conditions of Limited Test Availability. JAMA Network Open. 2020;3(6):e2013075.
Abdalhamid B, Bilder CR, McCutchen EL, Hinrichs SH, Koepsell SA, Iwen PC. Assessment of specimen pooling to conserve SARS CoV-2 testing resources. Am J Clin Pathol. 2020;153(6):715–8.
Article CAS Google Scholar
Verdun CM, Fuchs T, Harar P, Elbrächter D, Fischer DS, Berner J, Grohs P, Theis FJ, Krahmer F. Group testing for SARS-CoV-2 allows for up to 10-fold efficiency increase across realistic scenarios and testing strategies. medRxiv. 2020. https://doi.org/10.1101/2020.04.30.20085290 .
Gollier C, Gossner O. Group testing against Covid-19. Covid Econ. 2020;8:2.
Google Scholar
Cahoon-Young B, Chandler A, Livermore T, Gaudino J, Benjamin R. Sensitivity and specificity of pooled versus individual sera in a human immunodeficiency virus antibody prevalence study. J Clin Microbiol. 1989;27(8):1893–5.
Kline RL, Brothers TA, Brookmeyer R, Zeger S, Quinn TC. Evaluation of human immunodeficiency virus seroprevalence in population surveys using pooled sera. J Clin Microbiol. 1989;27(7):1449–52.
Behets F, Bertozzi S, Kasali M, Kashamuka M, Atikala L, Brown C, Ryder RW, Quinn TC. Successful use of pooled sera to determine HIV-1 seroprevalence in Zaire with development of cost-efficiency models. Aids. 1990;4(8):737–42.
Corman VM, Landt O, Kaiser M, Molenkamp R, Meijer A, Chu DK, et al. Detection of 2019 novel coronavirus (2019-nCoV) by real-time RT-PCR. Euro Surveill. 2020;25. https://doi.org/10.2807/1560-7917.ES.2020.25.3.2000045 .
Yelin I, Aharony N, Shaer-Tamar E, Argoetti A, Messer E, Berenbaum D, et al. Evaluation of COVID-19 RT-qPCR test in multi-sample pools. Clin Infect Dis. 2020;ciaa531. https://doi.org/10.1093/cid/ciaa531 .
Tu XM, Litvak E, Pagano M. Screening tests: can we get more by doing less? Stat Med. 1994;13(19–20):1905–19.
Cowling DW, Gardner IA, Johnson WO. Comparison of methods for estimation of individual-level prevalence based on pooled samples. Prev Vet Med. 1999;39:211–25.
Theagarajan LN. Group Testing for COVID-19: How to Stop Worrying and Test More. arXiv preprint arXiv:2004.06306. 2020 Apr 14.
R Core Team. R: a language and environment for statistical computing. Vienna: R Foundation for Statistical Computing; 2013.
Download references
Acknowledgements
Not applicable.
Author information
Authors and affiliations.
Norwegian Institute of Public Health, Oslo, Norway
Ola Brynildsrud
Norwegian University of Life Science, Ås, Norway
You can also search for this author in PubMed Google Scholar
Contributions
All work was done by OB. The author(s) read and approved the final manuscript.
Corresponding author
Correspondence to Ola Brynildsrud .
Ethics declarations
Ethics approval and consent to participate, consent for publication, competing interests, additional information, publisher’s note.
Springer Nature remains neutral with regard to jurisdictional claims in published maps and institutional affiliations.
Supplementary information
Additional file 1 : supplementary table 1.
. Table containing prevalence estimates and, the estimated required number of tests, and the expected proportion incorrectly classified patients for all parameter combinations. Se = sensitivity. Sp = specificity. N = number of samples. k = pooling level. P = true prevalence. p 2.5%, p 50.0%, p 97.5% = 2.5, 50 and 97.5 quantile of estimated prevalence. T 2.5%, T 50.0%, T 97.5% = 2.5, 50 and 97.5 quantile of estimated number of tests required to get individual-level diagnoses. E(S) = Expected number of tests saved when compared to testing individually for this N. E(inc) = Expected percentage of patients that are diagnosed incorrectly at this parameter combination. [Excel file].
Additional file 2 : Supplementary document 1
. Testing for freedom from disease and distinguishing a disease-free population from a low-prevalence one.
Additional file 3 : Figure S1
. Testing for freedom of disease with a test with perfect specificity. The x-axis represents different true levels of p , and the colored lines represent the number of samples associated with 95% probability of having at least one positive sample at that prevalence level. For perfect specificity tests this is commonly interpreted as meaning that we can be 95% certain that the true prevalence is lower. The effects of sample pooling are explored with different color lines. Panel A: Test specificity = 1.0; Panel B: Test specificity = 0.99.
Additional file 4 : Figure S2
. Using a test with specificity of 0.99 to discriminate a disease-free population from a population with p = 0.005 with 2743 samples from both populations. Panel A: The expected number of positive samples from the disease-free and the low-prevalence populations; Panel B: The probability mass function of the difference in the number of positive samples between the low-prevalence and the disease-free population. With 2743 samples from both populations, there is a 5% probability of getting more positive tests from the disease-free population.
Rights and permissions
Open Access This article is licensed under a Creative Commons Attribution 4.0 International License, which permits use, sharing, adaptation, distribution and reproduction in any medium or format, as long as you give appropriate credit to the original author(s) and the source, provide a link to the Creative Commons licence, and indicate if changes were made. The images or other third party material in this article are included in the article's Creative Commons licence, unless indicated otherwise in a credit line to the material. If material is not included in the article's Creative Commons licence and your intended use is not permitted by statutory regulation or exceeds the permitted use, you will need to obtain permission directly from the copyright holder. To view a copy of this licence, visit http://creativecommons.org/licenses/by/4.0/ . The Creative Commons Public Domain Dedication waiver ( http://creativecommons.org/publicdomain/zero/1.0/ ) applies to the data made available in this article, unless otherwise stated in a credit line to the data.
Reprints and permissions
About this article
Cite this article.
Brynildsrud, O. COVID-19 prevalence estimation by random sampling in population - optimal sample pooling under varying assumptions about true prevalence. BMC Med Res Methodol 20 , 196 (2020). https://doi.org/10.1186/s12874-020-01081-0
Download citation
Received : 27 May 2020
Accepted : 19 July 2020
Published : 23 July 2020
DOI : https://doi.org/10.1186/s12874-020-01081-0
Share this article
Anyone you share the following link with will be able to read this content:
Sorry, a shareable link is not currently available for this article.
Provided by the Springer Nature SharedIt content-sharing initiative
BMC Medical Research Methodology
ISSN: 1471-2288
- General enquiries: [email protected]

Physical Chemistry Chemical Physics
Application of molecular dynamics-based pharmacophore and machine learning approaches to identify novel mcl1 inhibitors through drug repurposing and mechanics research †.

* Corresponding authors
a Key Laboratory of Structure-Based Drug Design & Discovery, Ministry of Education, Shenyang Pharmaceutical University, Shenyang 110016, Liaoning, P. R. China E-mail: [email protected] , [email protected] Tel: +86-13804014230
b School of Pharmaceutical Engineering, Shenyang Pharmaceutical University, 103 Wenhua Road, Shenhe District, Shenyang, Liaoning, P. R. China E-mail: [email protected] Tel: +86-24-43520227
Myeloid cell leukemia 1 (Mcl1), a critical protein that regulates apoptosis, has been considered as a promising target for antitumor drugs. The conventional pharmacophore screening approach has limitations in conformation sampling and data mining. Here, we offered an innovative solution to identify Mcl1 inhibitors with molecular dynamics-refined pharmacophore and machine learning methods. Considering the safety and druggability of FDA-approved drugs, virtual screening of the database was performed to discover Mcl1 inhibitors, and the hit was subsequently validated via TR-FRET, cytotoxicity, and flow cytometry assays. To reveal the binding characteristics shared by the hit and a typical Mcl1 selective inhibitor, we employed quantum mechanics and molecular mechanics (QM/MM) calculations, umbrella sampling, and metadynamics in this work. The combined studies suggested that fluvastatin had promising cell inhibitory potency and was suitable for further investigation. We believe that this research will shed light on the discovery of novel Mcl1 inhibitors that can be used as a supplemental treatment against leukemia and provide a possible method to improve the accuracy of drug repurposing with limited computational resources while balancing the costs of experimentation well.
Supplementary files
- Supplementary information PDB (215K)

Article information
Download citation, permissions.

Application of molecular dynamics-based pharmacophore and machine learning approaches to identify novel Mcl1 inhibitors through drug repurposing and mechanics research
H. Wang, Z. Qi, W. Lian, L. Ma, S. Wang, H. Liu, Y. Jin, H. Yang, J. Wang and M. Cheng, Phys. Chem. Chem. Phys. , 2024, Advance Article , DOI: 10.1039/D4CP00576G
To request permission to reproduce material from this article, please go to the Copyright Clearance Center request page .
If you are an author contributing to an RSC publication, you do not need to request permission provided correct acknowledgement is given.
If you are the author of this article, you do not need to request permission to reproduce figures and diagrams provided correct acknowledgement is given. If you want to reproduce the whole article in a third-party publication (excluding your thesis/dissertation for which permission is not required) please go to the Copyright Clearance Center request page .
Read more about how to correctly acknowledge RSC content .
Social activity
Search articles by author.
This article has not yet been cited.
Advertisements
Stroke and frailty index: a two-sample Mendelian randomisation study
- Original Article
- Open access
- Published: 22 May 2024
- Volume 36 , article number 114 , ( 2024 )
Cite this article
You have full access to this open access article
- Jiangnan Wei 1 ,
- Jiaxian Wang 1 ,
- Jiayin Chen 1 ,
- Kezhou Yang 1 &
- Ning Liu 2
Introduction
Previous observational studies have found an increased risk of frailty in patients with stroke. However, evidence of a causal relationship between stroke and frailty is scarce. The aim of this study was to investigate the potential causal relationship between stroke and frailty index (FI).
Pooled data on stroke and debility were obtained from genome-wide association studies (GWAS).The MEGASTROKE Consortium provided data on stroke (N = 40,585), ischemic stroke (IS,N = 34,217), large-vessel atherosclerotic stroke (LAS,N = 4373), and cardioembolic stroke (CES,N = 7 193).Summary statistics for the FI were obtained from the most recent GWAS meta-analysis of UK BioBank participants and Swedish TwinGene participants of European ancestry (N = 175,226).Two-sample Mendelian randomization (MR) analyses were performed by inverse variance weighting (IVW), weighted median, MR-Egger regression, Simple mode, and Weighted mode, and heterogeneity and horizontal multiplicity of results were assessed using Cochran’s Q test and MR-Egger regression intercept term test.
The results of the current MR study showed a significant correlation between stroke gene prediction and FI (odds ratio 1.104, 95% confidence interval 1.064 − 1.144, P < 0.001). In terms of stroke subtypes, IS (odds ratio 1.081, 95% confidence interval 1.044 − 1.120, P < 0.001) and LAS (odds ratio 1.037, 95% confidence interval 1.012 − 1.062, P = 0.005). There was no causal relationship between gene-predicted CES and FI. Horizontal multidimensionality was not found in the intercept test for MR Egger regression (P > 0.05), nor in the heterogeneity test (P > 0.05).
Conclusions
This study provides evidence for a causal relationship between stroke and FI and offers new insights into the genetic study of FI.
Avoid common mistakes on your manuscript.
Stroke is a cerebrovascular lesion caused by sudden cerebrovascular injury with high morbidity, disability, mortality and recurrence rates [ 1 ]. Stroke is the second leading cause of death worldwide, with an annual death rate of about 5.5 million [ 2 ]. Studies have shown that the incidence of stroke increases dramatically with age, with about three-quarters of all strokes occurring in people over the age of 65 [ 3 ]. The United Nations Population Prospects predicts that the number of people aged 60 years and older will reach 2.1 billion globally by 2050 and 3.2 billion by 2100 [ 4 ]. Elderly stroke patients suffer from long-term sequelae such as incapacitation, emotional deficits, and cognitive disorders [ 5 ], and because of the long duration of illness, high medical costs, and poor adherence to treatment, elderly stroke patients impose a heavy burden on society, families, and patients [ 6 ].
Frailty, characterized by age-related multisystem dysfunction, is a major public health problem in older adults [ 7 , 8 ]. Studies show that frailty is common in strokes, with at least a quarter of stroke victims being physically frail [ 9 ]. Understanding the potential association between frailty and senescence-related diseases and the underlying mechanisms may facilitate the individualized management and early interventions of frail patients.FI reflects the accumulation of physiological deficits in various systems of the body, and assesses frailty by calculating the number of deficits in the health variables, taking into account the effects of physical, psychosocial, and social factors on the human body [ 10 ]. Studies have shown that stroke frailty can be assessed by physicians or researchers in the early stages of stroke by means of a scale survey, and studies have confirmed the applicability of the FI in stroke patients [ 11 ].
Randomized controlled trials (RCTs) are the gold standard of clinical evidence and are widely used to infer causality, mainly by eliminating confounding bias through randomized grouping. However, randomized controlled trials require a great deal of time, money, and human resources; therefore, RCT studies are difficult to perform in many medical studies. Mendelian randomization (MR) is a genetic epidemiology study design method that allows for the exploration of causal relationships between exposures and outcomes through the use of genetic variation as an instrumental variable (IV) [ 12 ]. Due to the ability to overcome the effects of potential confounding and reverse causation, MR methods have been increasingly used in observational studies in recent years [ 13 ]. MR research has been facilitated by the discovery in biology of a large number of genetic variants that are strongly associated with specific traits, and by the public release of hundreds of thousands of pooled data on the association of exposures and diseases with genetic variants from many large-sample genome-wide association studies (GWAS),which have allowed researchers to estimate genetic associations in large-sample data.The method of MR, with its use of genetic variables, allows for the avoidance of reverse causality and minimizes the interference of environmental factors in a manner similar to that of RCTs [ 14 ].
In this study, we aimed to investigate the causal relationship between stroke and frailty index using MR methods.
Materials and methods
Mendelian randomization assumptions.
The MR method is an instrumental variables analysis that uses genetic variants as proxies for exposure. As in Fig. 1 , the MR analysis relies on 3 important assumptions: (1) instrumental variables are closely related to exposure factors; (2) instrumental variables are independent of confounding factors; and (3) instrumental variables affect outcome only through exposure and not through other means [ 15 ].
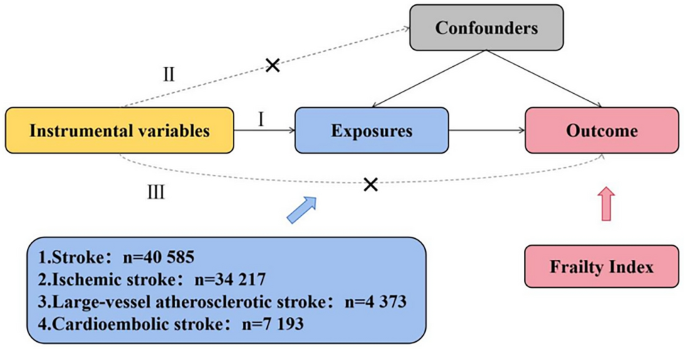
Design and main assumptions of our Mendelian randomization study
Data sources and SNP selection for stroke
The exposure factor for this study was defined as stroke, and data were obtained from the MEGASTROKE consortium, including 446,696 individuals of European ancestry (406,111 non-stroke cases and 40,585 stroke cases); the total number of ischemic stroke cases was 34,217, including 4373 large-vessel atherosclerotic stroke (LAS) cases, 5386 small-vessel stroke cases, and 7193 cardiac stroke cases.
Data sources and SNP selection for frailty index
Frailty is commonly defined using the Frailty Index (FI) [ 16 ]. In this MR study, frailty was measured according to the FI, which was calculated based on the accumulation of 44–49 self-reported health deficits over the life course.Summary statistics for the FI were obtained from the most recent GWAS meta-analysis of UK BioBank participants and Swedish TwinGene participants of European ancestry (N = 175,226).
Genetic instrumental variable selection
To meet the above hypotheses, the specific instrumental variables screening criteria were: (1) the association of single nucleotide polymorphism(SNPs) with single amino acids was genome-wide significant (P < 5 × 10 −8 ); (2) the linkage disequilibrium (LD) between SNPs was calculated using the European population genome of 1000 individuals as the reference template, and SNPs with r 2 < 0.001 and physical genetic distance > 10,000 kb were screened. (3) remove SNPs with minor allele frequencies < 0.01;(4) exclude SNPs with F values < 10 to avoid weak instrumental bias,and the following formula was used to calculate the F statistic [ 17 ]: F statistic = R 2 (N–2)/(1–R 2 ). R 2 = 2 × EAF × (1–EAF) × β 2 .The F values were all > 10, which indicated that our IVs were not biased by weak instrument. (5) apply MR Steiger [ 18 ] to assess the causal direction of each SNP on exposure and outcome, and exclude SNPs with reverse causality.
Statistical analysis
Two-sample mendelian randomization analysis.
We performed two-sample MR analyses using the inverse-variance-weighted method as the primary approach.Four other MR methods based on different model assumptions were also used for the analysis: weighted median method [ 19 ], MR-Egger regression [ 20 ], Weighted mode and Simple mode. According to the MR-Egger regression method, intercepts different from the origin can be used to assess potential pleiotropic effects [ 21 ]. These various MR methods can test the stability and reliability of association under different assumptions.
Sensitivity and power analysis
In this study, MR-Egger intercept was used to detect horizontal multiplicity, and if the intercept term in MR-Egger intercept analysis was statistically significant compared to 0, it indicated that the study had horizontal multiplicity [ 22 ]; Cochran Q test was applied to determine the heterogeneity of SNPs [ 23 ], and if the Cochran Q statistic test is statistically significant and proves that the analysis results have significant heterogeneity, then focus on the results of the random effects IVW method; using the Leave-one-out sensitivity test for sensitivity analysis [ 24 ], each SNP is eliminated in turn, and the remaining The MR results are robust if the remaining SNPs are not significantly different from the total results.The above methods were implemented using the TwoSampleMR package in the R 4. 2. 3 software with a test level of α= 0. 05.
Instrumental variable
According to the screening criteria of the instrumental variables in this study, all strokes, ischemic strokes, atherosclerotic strokes of large arteries, and cerebral embolic strokes were finally screened for 17 SNP stroke , 18 SNP IS , 4 SNP LAS , and 4 SNP CES , respectively. Table 1 shows 18 SNPs significantly associated with ischemic stroke.The MR-Egger regression intercepts were b stroke = 0.0179 (P = 0.8888), b IS = 0.1234 (P = 0.2288), b LAS = − 0.0121 (P = 0.8912), and b CES = 0.0306 (P = 0.3773), respectively. That is, there was no genetic pleiotropy between the screened SNPs and the outcome frailty index, and thus the Mendelian randomization method was a valid method for causal inference in this study.
Mendelian randomization analysis
The results of the ivw method showed a significant relationship between stroke (OR = 1.104, 95% CI 1.064–1.144, p < 0.001), IS (OR = 1.081, 95% CI 1.044–1.120, p < 0.001), LAS (OR = 1.037, 95% CI 1.012–1.062, p = 0.005), and FI There was a causal relationship between. In Weighted median method analysis, stroke (OR = 1.085, 95% CI 1.037–1.135, p < 0.001), IS (OR = 1.081, 95% CI 1.034–1.129, p < 0.001), LAS (OR = 1.035, 95% CI 1.005–1.066, and p = 0.026) and FI were also causally related. However, there was no evidence to support a causal relationship between CES and FI. MR estimates and efficacy analyses for stroke and FI are shown in Table 2 , and scatter plots of MR analyses for the 5 methods are shown in Fig. 2 .
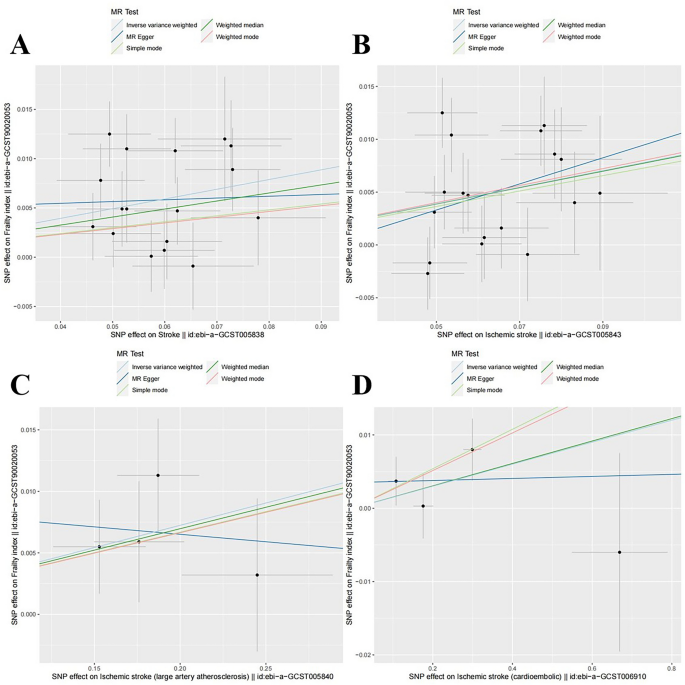
Scatter plots of the results of 5 MR methods: A Stroke; B IS; C LAS; and D CES
In this study Cochran’s Q test was used to assess the heterogeneity of the results which was done by both IVW and MR-Egger’s analyses.The results of CochranQ test for IVW method showed Q stroke = 22.193, Q IS = 26.370, Q LAS = 1.813, Q CES = 1.208.MR-Egger’s CochranQ test showed Q stroke = 21.590, Q IS = 26.016, Q LAS = 1.421, Q CES = 1.084.Regarding the results of the horizontal multivariate test, it was assessed using MR Egger’s intercept term. The p-value of heterogeneity and horizontal polytropy was greater than 0.05, so there was no heterogeneity and horizontal polytropy in this study, so the results had a weak risk of bias and high reliability. In addition, we performed Leave-one-out analysis, and the results showed that after removing each SNP in turn, the b-values of the remaining SNPs were (0.089–0.105), (0.694–0.841), (0.012–0.150), and (0.012–0.027), respectively, with p < 0.01. The b-values were all > 0, and the directions were all the same, indicating that removing either SNP had little effect on the results, and a positive causal association between stroke and frailty index was still observed. Sensitivity analyses and forest plots of the association between gene-predicted stroke and FI are shown in Fig. 3 .
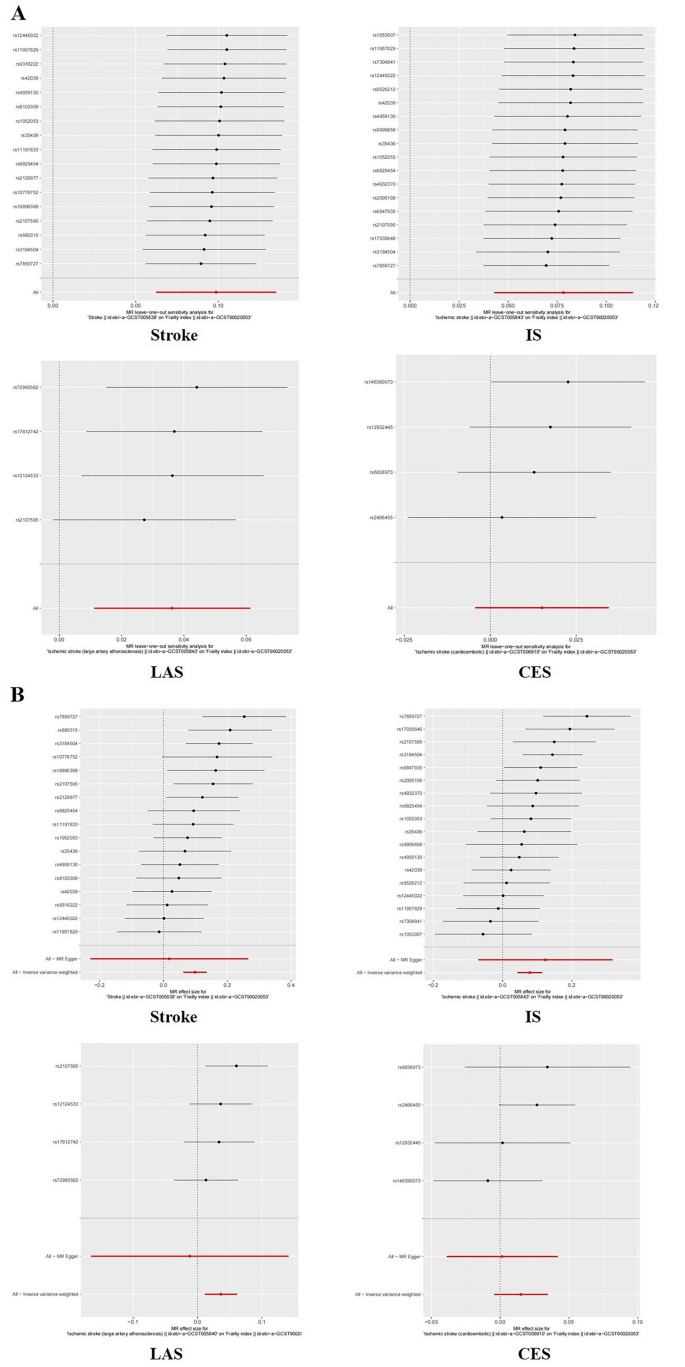
Sensitivity analysis of the association between genetically predicted Stroke and FI. A “Leave-one-out” sensitivity analysis results; B Forest plot.IS,ischaemic stroke;LAS,large artery atherosclerosis stroke;CES, cardioembolism stroke
In this study, based on large-scale GWAS pooled data, a two-sample MR method was used to analyze the causal association between stroke, IS, LAS, CES and FI. The results showed that stroke, IS, LAS, and CES were risk factors for FI. Further sensitivity analyses showed the consistency and reliability of these results.
Our finding that stroke may cause frailty provides evidence for early observational studies.Previous studies have reported an association between stroke and frailty [ 25 ]. The research results show that 12.8% of ischemic stroke patients and 10.3% of hemorrhagic stroke patients are already in a weakened state before stroke, and the degree of weakness worsens after stroke [ 26 ]. Stroke may accelerate the occurrence and development of physical weakness. In a large-scale assessment of debility, Hanlon et al. found that debility was common among stroke survivors. Rodriguez et al. showed an overall prevalence of frailty of 15.2% in older adults and a positive correlation between frailty and stroke in a survey of eight urban and four rural areas in eight countries, including Cuba, the Dominican Republic, Puerto Rico, Venezuela, Peru, Mexico, China, and India.Palmer et al. [ 27 ] found that the incidence of frailty was twice as high in stroke patients as in those who did not have a stroke [ 28 ]. Rowan et al. found frailty in about a quarter of acute stroke patients through a cross-sectional survey [ 11 ]. Stroke increases the risk of debilitation, and the prevalence of debilitation varies widely by region between countries, while debilitation imposes a serious burden on stroke patients and reduces their quality of life.
Exploring the causal relationship between these two comorbidities may be difficult because they share the same risk factors, such as hyperglycemia and Dyslipidemia. In addition,Evans et al showed that neurological deficits after stroke may exacerbate the phenotypic features of frailty, that hemodynamic changes in central and peripheral vasculature occur with age, and that frailty is associated with impaired brain self-regulation. And that a history of previous stroke is an important factor in the transition from robust to debilitated patients and in the worsening of the debilitating trajectory [ 29 ]. Hanotier et al. pointed out that prolonged malnutrition can easily lead to electrolyte disorders in the elderly. Once a stroke occurs, due to insufficient nutrition intake, electrolyte disorders worsen, and body mass sharply decreases, ultimately leading to frailty in the elderly [ 30 ]. Stroke patients have varying degrees of neurological dysfunction, which can affect the number of skeletal muscles to a certain extent [ 31 ], leading to the occurrence of sarcopenia, thereby reducing limb muscle strength and grip strength, and increasing the risk of physical weakness.
Zhu et al. [ 8 ] conducted a two-way Mendelian randomisation study of FI and stroke and found that FI was significantly associated with stroke in both directions of the outcome, but stroke-related subgroup analyses were not performed, which somewhat supports our findings. Liu et al. [ 32 ] found an implied association between FI and any stroke, and FI was associated with a high risk of LAS, but there was no causal association between FI and IS and small-artery stroke. There was no causal relationship.However, our study found that stroke, IS, and LAS were all causally related to FI, which may be due to the fact that our study was conducted in a different direction than theirs.
The main strengths of this study include the use of MR for causal inference and analyzing a large sample group. MR methods can effectively avoid the drawbacks of uncertain residual confounding and reverse causality that exist in traditional observational study methods [ 21 ]. Data on stroke and frailty are derived from existing large GWAS, which allows for more precise assessment of effect sizes than individual-level data or results from studies with limited sample sizes. Inevitably, there are some limitations. First, the two-sample Mendelian randomization method assumes a correlation between the exposure factor and the outcome, and the MR method is not applicable if the relationship is nonlinear. Second, the results of the analysis in this study are based only on populations of European origin, so further research and validation are needed for generalization to other populations. Finally, database statistics are difficult to analyze stratified by sex or age, which may lead to biased findings.
In summary, we found a causal relationship between stroke and its subtypes and debility by two-sample MR analysis. Further studies are needed to elucidate the potential mechanisms underlying the various causal relationships between stroke subtypes and debility.
Data availability
All data are publicly available. Detailed information for these datasets is summarized in supplementary material.
Collaborators GBDS (2021) Global, regional, and national burden of stroke and its risk factors, 1990–2019: a systematic analysis for the global burden of disease study 2019. Lancet Neurol 20:795–820
Article Google Scholar
Paul S, Candelario-Jalil E (2021) Emerging neuroprotective strategies for the treatment of ischemic stroke: an overview of clinical and preclinical studies. Exp Neurol 335:113518
Article CAS PubMed Google Scholar
Myint PK, Sinha S, Luben RN et al (2008) Risk factors for first-ever stroke in the EPIC-Norfolk prospective population-based study. Eur J Cardiovasc Prev Rehabil 15:663–9
Article PubMed Google Scholar
Sanuade OA, Dodoo FN, Koram K et al (2019) Prevalence and correlates of stroke among older adults in Ghana: evidence from the study on global AGEing and adult health (SAGE). PLoS ONE 14:e0212623
Article CAS PubMed PubMed Central Google Scholar
Nguyen TV, Le D, Tran KD et al (2019) Frailty in older patients with acute coronary syndrome in Vietnam. Clin Interv Aging 14:2213–22
Article PubMed PubMed Central Google Scholar
Blanco S, Ferrieres J, Bongard V et al (2017) Prognosis impact of frailty assessed by the edmonton frail scale in the setting of acute coronary syndrome in the elderly. Can J Cardiol 33:933–9
Proietti M, Cesari M (2020) Frailty: what is it? Adv Exp Med Biol 1216:1–7
Zhu J, Zhou D, Wang J et al (2022) Frailty and cardiometabolic diseases: a bidirectional Mendelian randomisation study. Age Ageing 51:afac256
PubMed Google Scholar
Burton JK, Stewart J, Blair M et al (2022) Prevalence and implications of frailty in acute stroke: systematic review & meta-analysis. Age Ageing 51:afac064
Sonny A, Kurz A, Skolaris LA et al (2020) Deficit accumulation and phenotype assessments of frailty both poorly predict duration of hospitalization and serious complications after noncardiac surgery. Anesthesiology 132:82–94
Taylor-Rowan M, Cuthbertson G, Keir R et al (2019) The prevalence of frailty among acute stroke patients, and evaluation of method of assessment. Clin Rehabil 33:1688–96
Davies NM, Holmes MV, Davey Smith G (2018) Reading Mendelian randomisation studies: a guide, glossary, and checklist for clinicians. BMJ 362:k601
Burgess S, Timpson NJ, Ebrahim S et al (2015) Mendelian randomization: where are we now and where are we going? Int J Epidemiol 44:379–88
Larsson SC, Traylor M, Markus HS (2019) Homocysteine and small vessel stroke: a mendelian randomization analysis. Ann Neurol 85:495–501
Zheng J, Baird D, Borges MC et al (2017) Recent developments in Mendelian randomization studies. Curr Epidemiol Rep 4:330–45
Atkins JL, Jylhava J, Pedersen NL et al (2021) A genome-wide association study of the frailty index highlights brain pathways in ageing. Aging Cell 20:e13459
Gao L, Di X, Gao L et al (2023) The Frailty Index and colon cancer: a 2-sample Mendelian-randomization study. J Gastrointest Oncol 14:798–805
Hemani G, Tilling K, Davey Smith G (2017) Orienting the causal relationship between imprecisely measured traits using GWAS summary data. PLoS Genet 13:e1007081
Bowden J, Davey Smith G, Haycock PC et al (2016) Consistent estimation in Mendelian randomization with some invalid instruments using a weighted median estimator. Genet Epidemiol 40:304–14
Bowden J, Davey Smith G, Burgess S (2015) Mendelian randomization with invalid instruments: effect estimation and bias detection through Egger regression. Int J Epidemiol 44:512–25
Zhao H, Zhu J, Ju L et al (2022) Osteoarthritis & stroke: a bidirectional mendelian randomization study. Osteoarthr Cartil 30:1390–7
Article CAS Google Scholar
Martin S, Tyrrell J, Thomas EL et al (2022) Disease consequences of higher adiposity uncoupled from its adverse metabolic effects using Mendelian randomisation. Elife 11:e72452
Bowden J, Spiller W, Del Greco MF et al (2018) Improving the visualization, interpretation and analysis of two-sample summary data Mendelian randomization via the radial plot and radial regression. Int J Epidemiol 47:2100
Gronau QF, Wagenmakers EJ (2019) Limitations of bayesian leave-one-out cross-validation for model selection. Comput Brain Behav 2:1–11
Calado LB, Ferriolli E, Moriguti JC et al (2016) Frailty syndrome in an independent urban population in Brazil (FIBRA study): a cross-sectional populational study. Sao Paulo Med J 134:385–392
Article PubMed Central Google Scholar
Kanai M, Noguchi M, Kubo H et al (2020) Pre-stroke frailty and stroke severity in elderly patients with acute stroke. J Stroke Cerebrovasc Dis 29:105346
Llibre Rodriguez JJ, Prina AM, Acosta D et al (2018) The prevalence and correlates of frailty in urban and rural populations in latin America, China, and India: a 10/66 population-based survey. J Am Med Dir Assoc 19:287–95.e4
Palmer K, Vetrano DL, Padua L et al (2019) Frailty syndromes in persons with cerebrovascular disease: a systematic review and meta-analysis. Front Neurol 10:1255
Evans NR, Todd OM, Minhas JS et al (2022) Frailty and cerebrovascular disease: concepts and clinical implications for stroke medicine. Int J Stroke 17:251–9
Hanotier P (2015) Hyponatremia in the elderly: its role in frailty. Rev Med Brux 36:475–84
CAS PubMed Google Scholar
Jung H, Kim M, Lee Y et al (2020) Prevalence of physical frailty and its multidimensional risk factors in Korean community-dwelling older adults: findings from Korean frailty and aging cohort study. Int J Environ Res Public Health 17:7883
Liu W, Zhang L, Fang H et al (2022) Genetically predicted frailty index and risk of stroke and Alzheimer’s disease. Eur J Neurol 29:1913–21
Download references
This study was supported by the National Natural Science Foundation of China (Project No. 82260281) and the Science and Technology Fund Project of Guizhou Provincial Health Commission (gzwkj2023-588);Scientific study on the Construction of shared Medical Model and Optimization Mechanism for patients with Stroke Depression in low Resource area. Guizhou Science and Technology Plan Project, Guizhou Science and Technology Cooperation (Qiankehe) Foundation(NO:. ZK [2024] key Project 069).
Author information
Authors and affiliations.
Department of Nursing, Zhuhai Campus of Zunyi Medical University, No. 368 Jinwan Road, Zhuhai, Guangdong, China
Jiangnan Wei, Jiaxian Wang, Jiayin Chen & Kezhou Yang
Department of Fundamentals, Department of Basic Teaching and Research in General Medicine, Zunyi Medical University Zhuhai Campus, Zhuhai, Guangdong, China
You can also search for this author in PubMed Google Scholar
Contributions
Jiangnan Wei wrote the main manuscript text, Jiaxian Wang drew the images and tables, Jiayin Chen performed the data collection, Kezhou Yang organized the information, and Ning Liu revised the manuscript. All authors reviewed the manuscript.
Corresponding author
Correspondence to Ning Liu .
Ethics declarations
Conflict of interest.
The authors declare no conflict of interest.
Ethical approval
For this study, publicly available summarized data from the GWAS database were used and the data were approved by the ethical review board.
Informed consent
Informed consent was obtained from all participants.
Additional information
Publisher's note.
Springer Nature remains neutral with regard to jurisdictional claims in published maps and institutional affiliations.
Supplementary Information
Below is the link to the electronic supplementary material.
Supplementary file 1 (DOCX 432 KB)
Rights and permissions.
Open Access This article is licensed under a Creative Commons Attribution 4.0 International License, which permits use, sharing, adaptation, distribution and reproduction in any medium or format, as long as you give appropriate credit to the original author(s) and the source, provide a link to the Creative Commons licence, and indicate if changes were made. The images or other third party material in this article are included in the article's Creative Commons licence, unless indicated otherwise in a credit line to the material. If material is not included in the article's Creative Commons licence and your intended use is not permitted by statutory regulation or exceeds the permitted use, you will need to obtain permission directly from the copyright holder. To view a copy of this licence, visit http://creativecommons.org/licenses/by/4.0/ .
Reprints and permissions
About this article
Wei, J., Wang, J., Chen, J. et al. Stroke and frailty index: a two-sample Mendelian randomisation study. Aging Clin Exp Res 36 , 114 (2024). https://doi.org/10.1007/s40520-024-02777-9
Download citation
Received : 29 November 2023
Accepted : 15 May 2024
Published : 22 May 2024
DOI : https://doi.org/10.1007/s40520-024-02777-9
Share this article
Anyone you share the following link with will be able to read this content:
Sorry, a shareable link is not currently available for this article.
Provided by the Springer Nature SharedIt content-sharing initiative
- Mendelian randomization
- Genetic analyses
- Find a journal
- Publish with us
- Track your research
- Introduction
- Article Information
eTable. Baseline Factors Considered for Association With Any Melatonin Use and Newly Initiated Melatonin Use
eReferences
Data Sharing Statement
See More About
Sign up for emails based on your interests, select your interests.
Customize your JAMA Network experience by selecting one or more topics from the list below.
- Academic Medicine
- Acid Base, Electrolytes, Fluids
- Allergy and Clinical Immunology
- American Indian or Alaska Natives
- Anesthesiology
- Anticoagulation
- Art and Images in Psychiatry
- Artificial Intelligence
- Assisted Reproduction
- Bleeding and Transfusion
- Caring for the Critically Ill Patient
- Challenges in Clinical Electrocardiography
- Climate and Health
- Climate Change
- Clinical Challenge
- Clinical Decision Support
- Clinical Implications of Basic Neuroscience
- Clinical Pharmacy and Pharmacology
- Complementary and Alternative Medicine
- Consensus Statements
- Coronavirus (COVID-19)
- Critical Care Medicine
- Cultural Competency
- Dental Medicine
- Dermatology
- Diabetes and Endocrinology
- Diagnostic Test Interpretation
- Drug Development
- Electronic Health Records
- Emergency Medicine
- End of Life, Hospice, Palliative Care
- Environmental Health
- Equity, Diversity, and Inclusion
- Facial Plastic Surgery
- Gastroenterology and Hepatology
- Genetics and Genomics
- Genomics and Precision Health
- Global Health
- Guide to Statistics and Methods
- Hair Disorders
- Health Care Delivery Models
- Health Care Economics, Insurance, Payment
- Health Care Quality
- Health Care Reform
- Health Care Safety
- Health Care Workforce
- Health Disparities
- Health Inequities
- Health Policy
- Health Systems Science
- History of Medicine
- Hypertension
- Images in Neurology
- Implementation Science
- Infectious Diseases
- Innovations in Health Care Delivery
- JAMA Infographic
- Law and Medicine
- Leading Change
- Less is More
- LGBTQIA Medicine
- Lifestyle Behaviors
- Medical Coding
- Medical Devices and Equipment
- Medical Education
- Medical Education and Training
- Medical Journals and Publishing
- Mobile Health and Telemedicine
- Narrative Medicine
- Neuroscience and Psychiatry
- Notable Notes
- Nutrition, Obesity, Exercise
- Obstetrics and Gynecology
- Occupational Health
- Ophthalmology
- Orthopedics
- Otolaryngology
- Pain Medicine
- Palliative Care
- Pathology and Laboratory Medicine
- Patient Care
- Patient Information
- Performance Improvement
- Performance Measures
- Perioperative Care and Consultation
- Pharmacoeconomics
- Pharmacoepidemiology
- Pharmacogenetics
- Pharmacy and Clinical Pharmacology
- Physical Medicine and Rehabilitation
- Physical Therapy
- Physician Leadership
- Population Health
- Primary Care
- Professional Well-being
- Professionalism
- Psychiatry and Behavioral Health
- Public Health
- Pulmonary Medicine
- Regulatory Agencies
- Reproductive Health
- Research, Methods, Statistics
- Resuscitation
- Rheumatology
- Risk Management
- Scientific Discovery and the Future of Medicine
- Shared Decision Making and Communication
- Sleep Medicine
- Sports Medicine
- Stem Cell Transplantation
- Substance Use and Addiction Medicine
- Surgical Innovation
- Surgical Pearls
- Teachable Moment
- Technology and Finance
- The Art of JAMA
- The Arts and Medicine
- The Rational Clinical Examination
- Tobacco and e-Cigarettes
- Translational Medicine
- Trauma and Injury
- Treatment Adherence
- Ultrasonography
- Users' Guide to the Medical Literature
- Vaccination
- Venous Thromboembolism
- Veterans Health
- Women's Health
- Workflow and Process
- Wound Care, Infection, Healing
Get the latest research based on your areas of interest.
Others also liked.
- Download PDF
- X Facebook More LinkedIn
Sadikova E , Rakesh D , Tiemeier H. Patterns of Melatonin Use in a Diverse National Pediatric Sample. JAMA Netw Open. 2024;7(5):e2412502. doi:10.1001/jamanetworkopen.2024.12502
Manage citations:
© 2024
- Permissions
Patterns of Melatonin Use in a Diverse National Pediatric Sample
- 1 Department of Social and Behavioral Sciences, Harvard T.H. Chan School of Public Health, Boston, Massachusetts
- 2 Neuroimaging Department, Institute of Psychiatry, Psychology & Neuroscience, King’s College London, London, United Kingdom
Over-the-counter melatonin sales in the US more than doubled between 2017 and 2020. 1 While melatonin is generally considered safe, accidental ingestion reports and melatonin-related pediatric hospitalizations increased with greater sales between 2012 and 2021. 2 Recent analysis 3 of a demographically homogeneous convenience sample suggested that prevalence of melatonin use increases with age and may reach 19.4% by adolescence. Here, we describe longitudinal patterns of melatonin use and characterize factors associated with use in a diverse national pediatric sample.
In this cohort study, parents of 11 864 children and adolescents aged 9 to 16 years (hereafter, children) from the Adolescent Brain Cognitive Development (ABCD) Study 4 provided medications and supplements used by their children 2 weeks before each of 5 annual visits (2016-2022). The ABCD Study was approved by either site-specific or central (University of California, San Diego) institutional review boards. Written informed consent was obtained from the parents; assent, from the children. We followed the STROBE reporting guideline.
Child race and ethnicity were identified by parent report; categories are listed in Table 1 . We compared melatonin use prevalence across ages using a linear mixed effects model and any use by race and ethnicity using a χ 2 test. We compiled a list of child, family, and neighborhood characteristics (121 variables; missingness ranging from 0%-11% across variables was multiply imputed) to identify associations with any melatonin use and newly initiated melatonin use among treatment-naive participants at baseline. Single indicators of these outcomes were created at the end of follow-up. We used cross-validated extreme gradient boosting classification models, extracting Shapley Additive Explanation (SHAP) values to quantify relative importance of associations. 5 Multivariable logistic regression models included the 10 factors with the highest SHAP values. Significance was tested at a 2-sided .05 level. We used R Statistical Software, version 4.1.2 (R Project for Statistical Computing). (The eMethods in Supplement 1 provides details).
The study included 11 864 participants (mean [SD] age, 9.5 [0.5] years at baseline; 6163 males [51.9%] and 5701 females [48.1%]). Of these, 20.3% were Hispanic; 2.1%, non-Hispanic Asian; 15.0%, non-Hispanic Black; 52.0%, non-Hispanic White; and 10.5%, other race and ethnicity. During 5 years of follow-up, 1442 children (12.2%) used melatonin; 990 of 11 044 treatment-naive children (9.0%) initiated use after baseline. Prevalence increased significantly with age and was lowest among non-Hispanic Asian (3.6%), non-Hispanic Black (4.9%), and Hispanic (7.9%) children ( P < .001) ( Table 1 ). Table 2 lists the 10 factors with the highest SHAP values, given in descending order. Greater problems with initiating and maintaining sleep in children were associated with prevalent and newly initiated melatonin use (odds ratio [OR], 1.47; 95% CI, 1.39-1.56; and OR, 1.55; 95% CI, 1.47-1.64 per 1-SD change, respectively). In addition to female sex and attention problems in children, parental mental health treatment, somatic symptoms, and low parental acceptance of the child significantly predisposed to melatonin use. Melatonin use was less likely in disadvantaged neighborhoods ( Table 2 ). Newly initiated melatonin use was associated with greater child screen time (OR, 1.04; 95% CI, 1.02-1.06 per hour), symptoms of anxiety and depression (OR, 1.09; 95% CI, 1.03-1.15 per 1-SD), and lower parental aggressive behavior scores (OR, 0.89; 95% CI, 0.84-0.95 per 1-SD).
This cohort study is the first, to our knowledge, to describe longitudinal melatonin use patterns and outline factors associated with prevalent and newly initiated melatonin use in a diverse national pediatric sample. We corroborated the age trend reported by Hartstein et al, 3 but found lower melatonin use prevalence (12.2% in children aged 9-16 years vs 19.4% for children aged 10-13 years), likely due to differences in sample composition and melatonin use ascertainment. Despite poorer sleep, 6 minoritized populations living in disadvantaged neighborhoods were less likely to use melatonin. While sleep health is a factor, child and parental mental health and neighborhood environments contributed significantly to melatonin uptake. A study limitation is that melatonin use was measured annually, leaving ambiguous continuity of use between visits. These findings indicate that child, family, and neighborhood determinants of use are important to consider when assessing melatonin’s safety and efficacy in the general pediatric population.
Accepted for Publication: May 7, 2024.
Published: May 22, 2024. doi:10.1001/jamanetworkopen.2024.12502
Open Access: This is an open access article distributed under the terms of the CC-BY License . © 2024 Sadikova E et al. JAMA Network Open .
Corresponding Author: Ekaterina Sadikova, PhD, Department of Social and Behavioral Sciences, Harvard T.H. Chan School of Public Health, 677 Huntington Ave, Boston, MA 02115 ( [email protected] ).
Author Contributions: Dr Sadikova had full access to all of the data in the study and takes responsibility for the integrity of the data and the accuracy of the data analysis.
Concept and design: All authors.
Acquisition, analysis, or interpretation of data: Sadikova, Rakesh.
Drafting of the manuscript: Sadikova.
Critical review of the manuscript for important intellectual content: All authors.
Statistical analysis: Sadikova.
Obtained funding: Tiemeier.
Supervision: Rakesh, Tiemeier.
Conflict of Interest Disclosures: None reported.
Funding/Support: The work was supported by grant T32 HD 104612-3 from the National Institutes of Health’s Eunice Kennedy Shriver National Institute of Child Health and Human Development (Dr Sadikova).
Role of the Funder/Sponsor: The funder had no role in the design and conduct of the study; collection, management, analysis, and interpretation of the data; preparation, review, or approval of the manuscript; and decision to submit the manuscript for publication.
Data Sharing Statement: See Supplement 2 .
- Register for email alerts with links to free full-text articles
- Access PDFs of free articles
- Manage your interests
- Save searches and receive search alerts
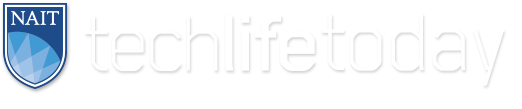
- Environment
- Science & Tech
- Subscribe to the e-newsletter
- Alumni Relations
- NAIT in the News
- Past Issues
- Unsubscribe
- Privacy Policy
- Terms of Use
NAIT team develops first-of-its-kind approach to studying microplastics in rivers
Applied research clean technologies innovation being used across north america.
Just how much microscopic plastic is flowing through the river systems that are our water sources?
That’s a question that the NAIT Applied Research Clean Technologies team set out to answer in a project focused on Edmonton’s North Saskatchewan River, in partnership with Heartland Polymers as part of the Plastics Research in Action initiative.
But to answer that question, the team first had to develop a reliable method to successfully filter waterborne particles that can be as small as 45 micrometres, or roughly the width of a human hair.
The result is a prototype produced in collaboration with NAIT's Centre for Sensors and System Integration. That work is now featured in a paper published in the June 2024 issue of MethodsX , a journal that reports on advancements in methodology in any field of science. Lead researcher Jeremiah Bryksa ( Laboratory Research and Biotechnology ’12) discusses the project in the video below.
The challenge involved identifying and refining a method that would improve upon the use of a mesh net, which can clog or rip. Bryksa’s group was one of the first to use an established method involving a series of sieves, modifying it for a flowing river.
The team’s innovations included a pump that was free of plastic, preventing possible contamination of the samples, and the addition of a battery, making the system completely portable.
“With this paper, we hope to show other microplastic researchers that you can use the standardized method to sample freshwater rivers,” says Bryksa.
Recently, researchers in the Yukon and the United States have also put the team’s method to work. The paper includes a list of components for anyone wanting to build the system for their own use, and even a wiring diagram.
Read the full paper
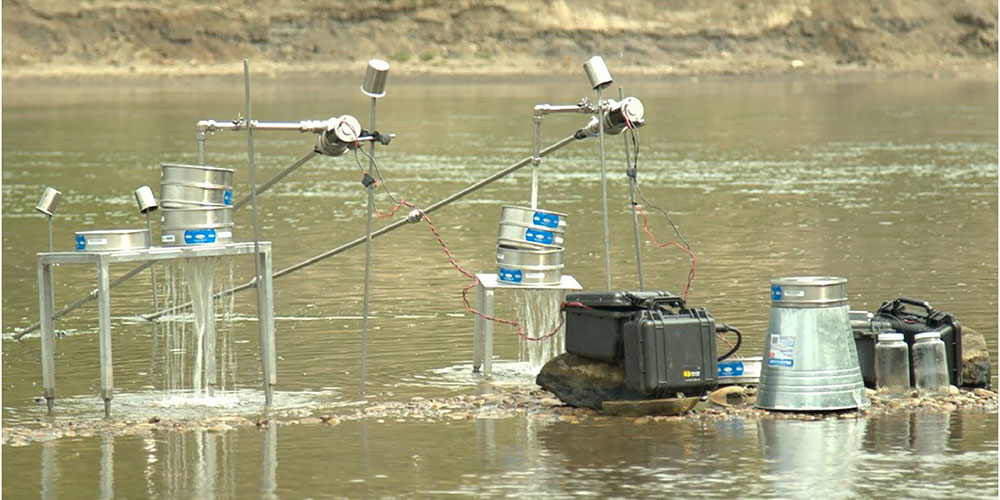
Plastics Research in Action
In the largest applied research partnership in NAIT’s history, the polytechnic has partnered with Heartland Polymers, Inter Pipeline's polypropylene brand, in a $10-million, ten-year research collaboration to advance the reuse and recycling of plastic in Canada and beyond.
The project is currently yielding solutions to transform waste plastics into valuable resources in the development of other products, while reducing their environmental impact. It is also creating practical opportunities for students and graduates to learn while contributing to impactful research.
Learn more about the project
Share this page:
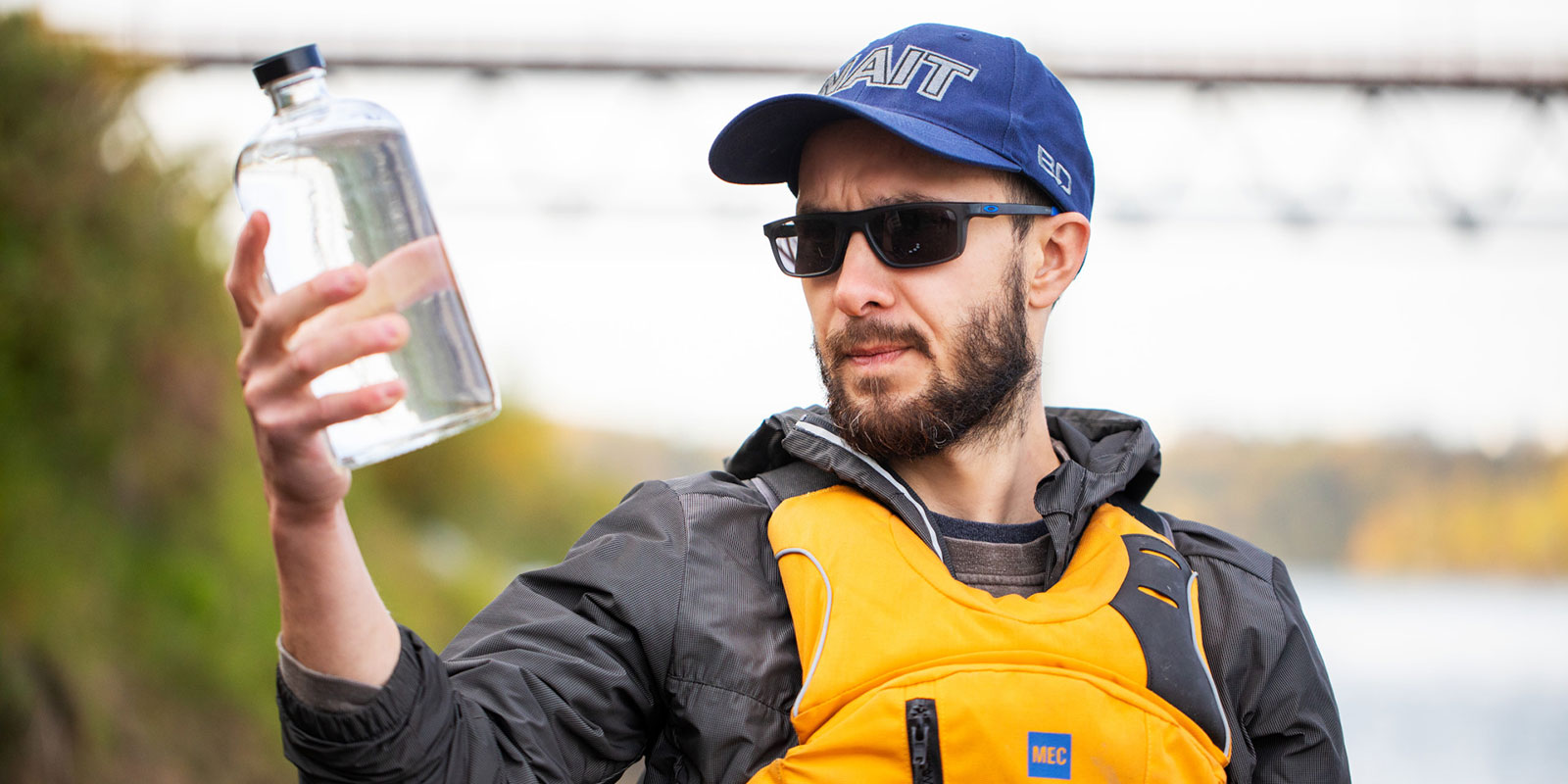
New paper details method and equipment used in North Saskatchewan River
If you enjoyed that story, try these
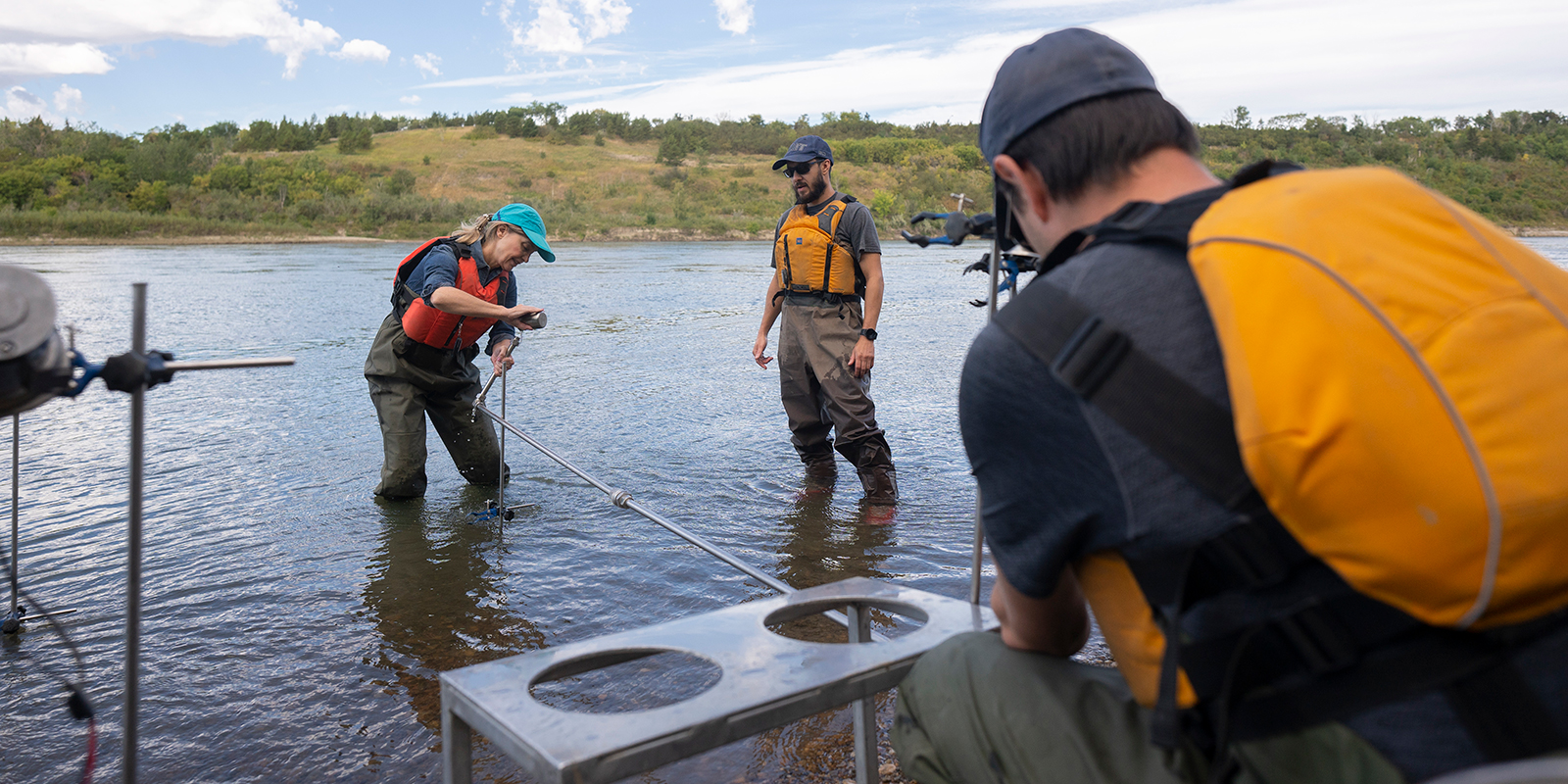
Curious President Podcast Ep. 2: How NAIT is measuring microplastics in the North Saskatchewan River
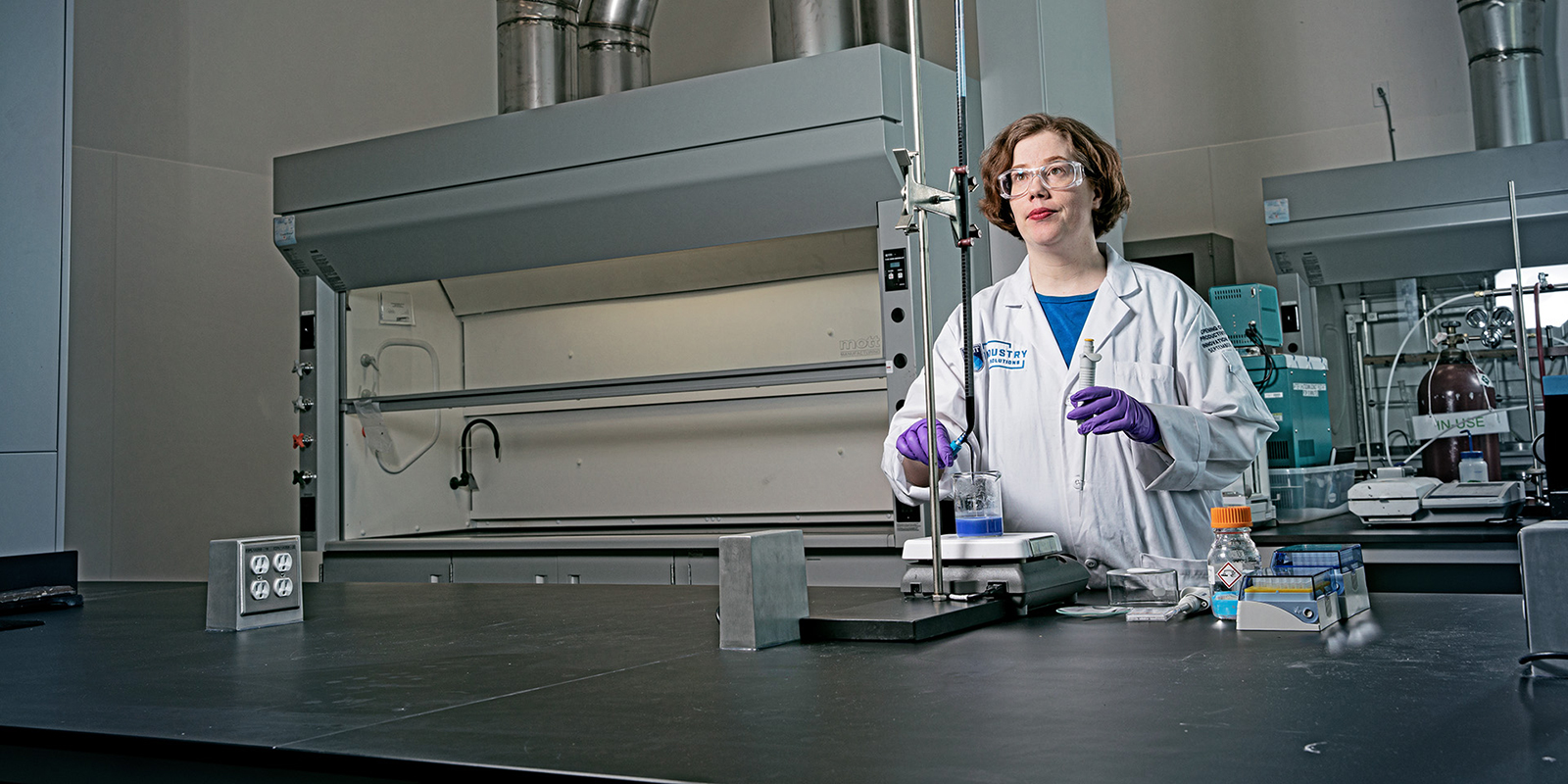
How a NAIT researcher is building community around tailings pond research
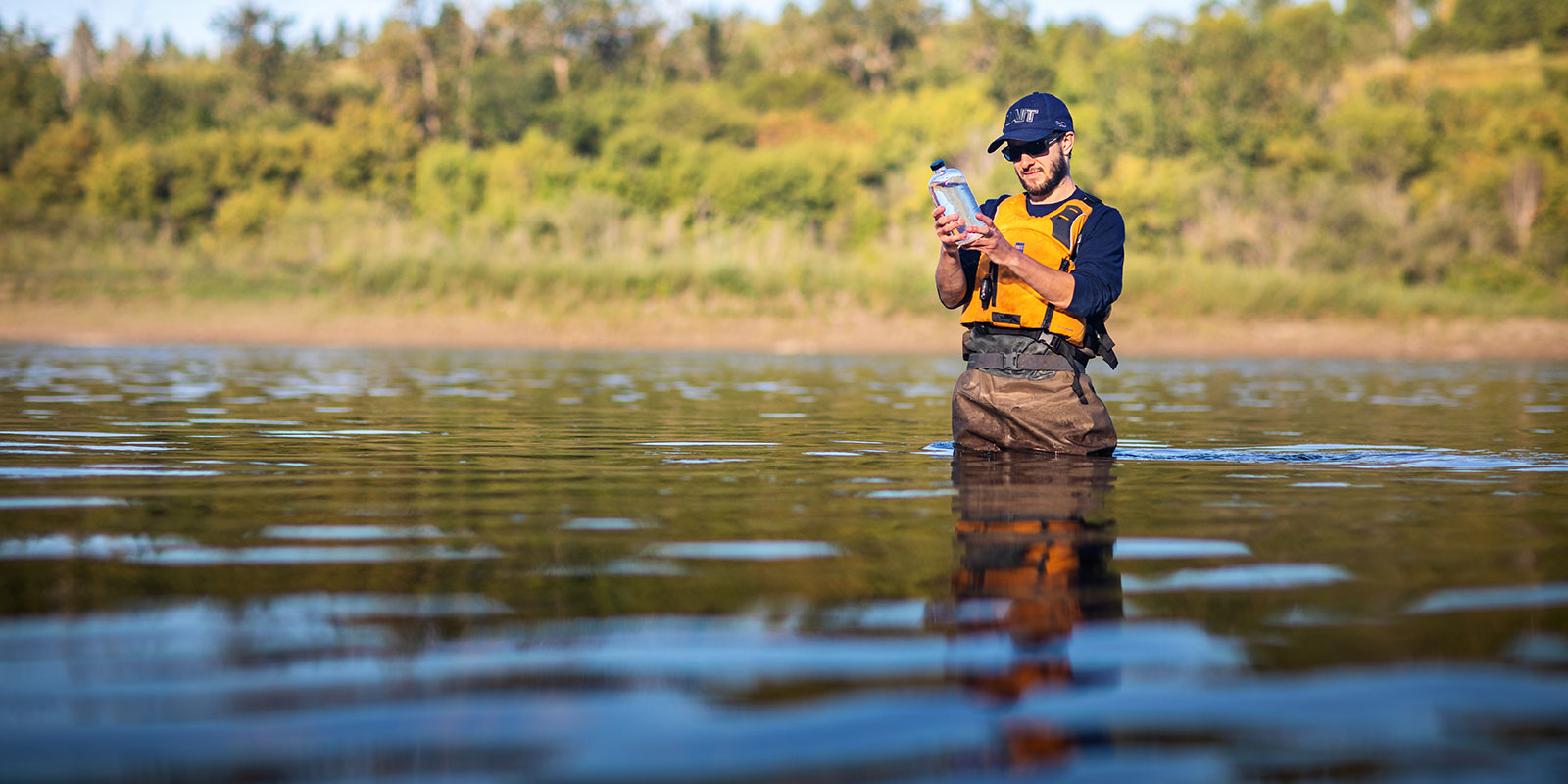
NAIT researcher named Science Fellow by TELUS World of Science
Subscribe to receive more great stories every month.
Find out more news about NAIT, stories about our alumni and their impact on their communities, and useful how-to content featuring our experts.
Northern Alberta Institute of Technology
Mailing Address: 11762 - 106 Street Edmonton , AB , Canada , T5G 2R1
View on Map
At NAIT, we honour and acknowledge that the land on which we learn, work and live is Treaty Six territory. We seek to learn from history and the lessons that have come before us, and to draw on the wisdom of the First Peoples in Canada. Only through learning can we move forward in truth and reconciliation, and to a better future together. Read more
About techlifetoday
Techlifetoday is the source for NAIT alumni and students to discover news about the polytechnic, and insights, advice and inspiration from the NAIT community to help them build successful careers and lead fulfilling lives.
- Email for Feedback or Story Ideas
Media inquiries
- Email NAIT media relations
- For more news, visit the NAIT newsroom
Connect with us
- Follow @NAIT on Instagram
- Like our Facebook
- Follow @techlifeNAIT on Twitter
- Subscribe to our YouTube Channel


Influenza H5N1 and H1N1 viruses remain infectious in unpasteurized milk on milking machinery surfaces
- Find this author on Google Scholar
- Find this author on PubMed
- Search for this author on this site
- ORCID record for Valerie Le Sage
- ORCID record for A.J. Campbell
- ORCID record for Douglass Reed
- ORCID record for W Paul Duprex
- ORCID record for Seema Lakdawala
- For correspondence: [email protected]
- Info/History
- Preview PDF
Spillover of highly pathogenic avian H5N1 into the cattle population poses a risk to humans through the close contact with farm workers. High viral loads of influenza viruses in the unpasteurized milk of infected lactating cows has the potential to contaminate equipment within milking parlors and create fomites for transmission to dairy workers. Cattle H5N1 and human 2009 H1N1 pandemic influenza viruses were found to remain infectious on surfaces commonly found in milking equipment materials for a few hours. The data presented here provide a compelling case for the risk of contaminated surfaces generated during milking to facilitate transmission of H5N1 from cattle-to-cattle and to dairy farm workers.
Competing Interest Statement
The authors have declared no competing interest.
Funding Statement
This project has been funded in part with Federal funds from the National Institute of Allergy and Infectious Diseases, National Institutes of Health, Department of Health and Human Services, under Contract No. 75N93021C00015 and an NIH award (UC7AI180311) from the National Institute of Allergy and Infectious Diseases (NIAID) supporting the Operations of The University of Pittsburgh Regional Biocontainment Laboratory (RBL) within the Center for Vaccine Research (CVR).
Author Declarations
I confirm all relevant ethical guidelines have been followed, and any necessary IRB and/or ethics committee approvals have been obtained.
I confirm that all necessary patient/participant consent has been obtained and the appropriate institutional forms have been archived, and that any patient/participant/sample identifiers included were not known to anyone (e.g., hospital staff, patients or participants themselves) outside the research group so cannot be used to identify individuals.
I understand that all clinical trials and any other prospective interventional studies must be registered with an ICMJE-approved registry, such as ClinicalTrials.gov. I confirm that any such study reported in the manuscript has been registered and the trial registration ID is provided (note: if posting a prospective study registered retrospectively, please provide a statement in the trial ID field explaining why the study was not registered in advance).
I have followed all appropriate research reporting guidelines, such as any relevant EQUATOR Network research reporting checklist(s) and other pertinent material, if applicable.
Data Availability
All raw data can be accessed at: https://doi.org/10.6084/m9.figshare.c.7242034.v1.
https://doi.org/10.6084/m9.figshare.c.7242034.v1
View the discussion thread.
Thank you for your interest in spreading the word about medRxiv.
NOTE: Your email address is requested solely to identify you as the sender of this article.

Citation Manager Formats
- EndNote (tagged)
- EndNote 8 (xml)
- RefWorks Tagged
- Ref Manager
- Tweet Widget
- Facebook Like
- Google Plus One
- Addiction Medicine (324)
- Allergy and Immunology (632)
- Anesthesia (168)
- Cardiovascular Medicine (2399)
- Dentistry and Oral Medicine (289)
- Dermatology (207)
- Emergency Medicine (381)
- Endocrinology (including Diabetes Mellitus and Metabolic Disease) (850)
- Epidemiology (11795)
- Forensic Medicine (10)
- Gastroenterology (705)
- Genetic and Genomic Medicine (3766)
- Geriatric Medicine (350)
- Health Economics (637)
- Health Informatics (2408)
- Health Policy (939)
- Health Systems and Quality Improvement (905)
- Hematology (342)
- HIV/AIDS (786)
- Infectious Diseases (except HIV/AIDS) (13346)
- Intensive Care and Critical Care Medicine (769)
- Medical Education (368)
- Medical Ethics (105)
- Nephrology (401)
- Neurology (3523)
- Nursing (199)
- Nutrition (528)
- Obstetrics and Gynecology (679)
- Occupational and Environmental Health (667)
- Oncology (1832)
- Ophthalmology (538)
- Orthopedics (221)
- Otolaryngology (287)
- Pain Medicine (234)
- Palliative Medicine (66)
- Pathology (447)
- Pediatrics (1037)
- Pharmacology and Therapeutics (426)
- Primary Care Research (424)
- Psychiatry and Clinical Psychology (3187)
- Public and Global Health (6178)
- Radiology and Imaging (1290)
- Rehabilitation Medicine and Physical Therapy (751)
- Respiratory Medicine (832)
- Rheumatology (380)
- Sexual and Reproductive Health (373)
- Sports Medicine (324)
- Surgery (403)
- Toxicology (50)
- Transplantation (172)
- Urology (147)

COMMENTS
Sampling types. There are two major categories of sampling methods ( figure 1 ): 1; probability sampling methods where all subjects in the target population have equal chances to be selected in the sample [ 1, 2] and 2; non-probability sampling methods where the sample population is selected in a non-systematic process that does not guarantee ...
Abstract. Knowledge of sampling methods is essential to design quality research. Critical questions are provided to help researchers choose a sampling method. This article reviews probability and non-probability sampling methods, lists and defines specific sampling techniques, and provides pros and cons for consideration.
The article provides an overview of the various sampling techniques used in research. These techniques can be broadly categorised into two types: probability sampling techniques and non ...
The article provides an overview of the various sampling techniques used in research. These techniques can be broadly categorised into two types: probability sampling techniques and non ...
Mine tends to start with a reminder about the different philosophical assumptions undergirding qualitative and quantitative research projects ( Staller, 2013 ). As Abrams (2010) points out, this difference leads to "major differences in sampling goals and strategies." (p.537). Patton (2002) argues, "perhaps nothing better captures the ...
Sampling methods are crucial for conducting reliable research. In this article, you will learn about the types, techniques and examples of sampling methods, and how to choose the best one for your study. Scribbr also offers free tools and guides for other aspects of academic writing, such as citation, bibliography, and fallacy.
Understand sampling methods in research, from simple random sampling to stratified, systematic, and cluster sampling. Learn how these sampling techniques boost data accuracy and representation, ensuring robust, reliable results. Check this article to learn about the different sampling method techniques, types and examples.
Behavioural research requires the use of sampling methods to document the occurrence of responses observed. Sampling/recording methods include ad libitum, continuous, pinpoint (instantaneous), and ...
We offer a structured process for sample development and present eight key sampling considerations. The paper contributes to research method selection, development, and use, as well as extending discussions surrounding knowledge construction, standards of reporting, and design research impact. How a research sample is defined forms a key ...
Abstract. Qualitative sampling methods differ from quantitative sampling methods. It is important that one understands those differences, as well as, appropriate qualitative sampling techniques. Appropriate sampling choices enhance the rigor of qualitative research studies. These types of sampling strategies are presented, along with the pros ...
How to choose the best sampling methods for headache research? This article provides a comprehensive overview of the advantages and disadvantages of different sampling techniques, and offers practical guidance for researchers and clinicians. Read the full text online at Wiley Online Library.
Background The number of confirmed COVID-19 cases divided by population size is used as a coarse measurement for the burden of disease in a population. However, this fraction depends heavily on the sampling intensity and the various test criteria used in different jurisdictions, and many sources indicate that a large fraction of cases tend to go undetected. Methods Estimates of the true ...
The take-home point is that although the "gold standard" for research designs that study groups is to use a randomly drawn sample and randomly assign participants to treatment and control groups, it is generally not possible to gather such large groups in the field of visual impairment because of the fact that vision loss is a low-incidence disability.
We included all adults in the sample frame because, despite young adults typically being the focus of existing research, advertising content controls are population-level measures and middle and older-aged adults may also be targeted by, or vulnerable to, the effects of alcohol marketing, including higher-risk drinkers (Critchlow & Moodie, 2021 ...
Purposeful sampling is a technique widely used in qualitative research for the identification and selection of information-rich cases for the most effective use of limited resources (Patton 2002).This involves identifying and selecting individuals or groups of individuals that are especially knowledgeable about or experienced with a phenomenon of interest (Cresswell and Plano Clark 2011).
The conventional pharmacophore screening approach has limitations in conformation sampling and data mining. Here, we offered an innovative solution to identify Mcl1 inhibitors with molecular dynamics-refined pharmacophore and machine learning methods. ... We believe that this research will shed light on the discovery of novel Mcl1 inhibitors ...
MR research has been facilitated by the discovery in biology of a large number of genetic variants that are strongly associated with specific traits, and by the public release of hundreds of thousands of pooled data on the association of exposures and diseases with genetic variants from many large-sample genome-wide association studies (GWAS ...
Over-the-counter melatonin sales in the US more than doubled between 2017 and 2020. 1 While melatonin is generally considered safe, accidental ingestion reports and melatonin-related pediatric hospitalizations increased with greater sales between 2012 and 2021. 2 Recent analysis 3 of a demographically homogeneous convenience sample suggested that prevalence of melatonin use increases with age ...
Purposive sampling has a long developmental history and there are as many views that it is simple and straightforward as there are about its complexity. The reason for purposive sampling is the better matching of the sample to the aims and objectives of the research, thus improving the rigour of the study and trustworthiness of the data and ...
The team's innovations included a pump that was free of plastic, preventing possible contamination of the samples, and the addition of a battery, making the system completely portable. "With this paper, we hope to show other microplastic researchers that you can use the standardized method to sample freshwater rivers," says Bryksa.
Google Research & Lichtman Lab/Harvard University. Next up, the team behind the project aims to create a full map of the brain of a mouse, which would require between 500 and 1,000 times the ...
Confusion about how to employ theoretical sampling in grounded theory can exist among researchers who use or who want to use the grounded theory method. We illustrate how we employed theoretical sampling in diverse grounded theory studies and answer key questions about theoretical sampling in grounded theory.
Spillover of highly pathogenic avian H5N1 into the cattle population poses a risk to humans through the close contact with farm workers. High viral loads of influenza viruses in the unpasteurized milk of infected lactating cows has the potential to contaminate equipment within milking parlors and create fomites for transmission to dairy workers. Cattle H5N1 and human 2009 H1N1 pandemic ...