

10 Case Study Advantages and Disadvantages
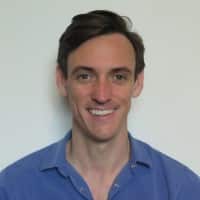
Chris Drew (PhD)
Dr. Chris Drew is the founder of the Helpful Professor. He holds a PhD in education and has published over 20 articles in scholarly journals. He is the former editor of the Journal of Learning Development in Higher Education. [Image Descriptor: Photo of Chris]
Learn about our Editorial Process
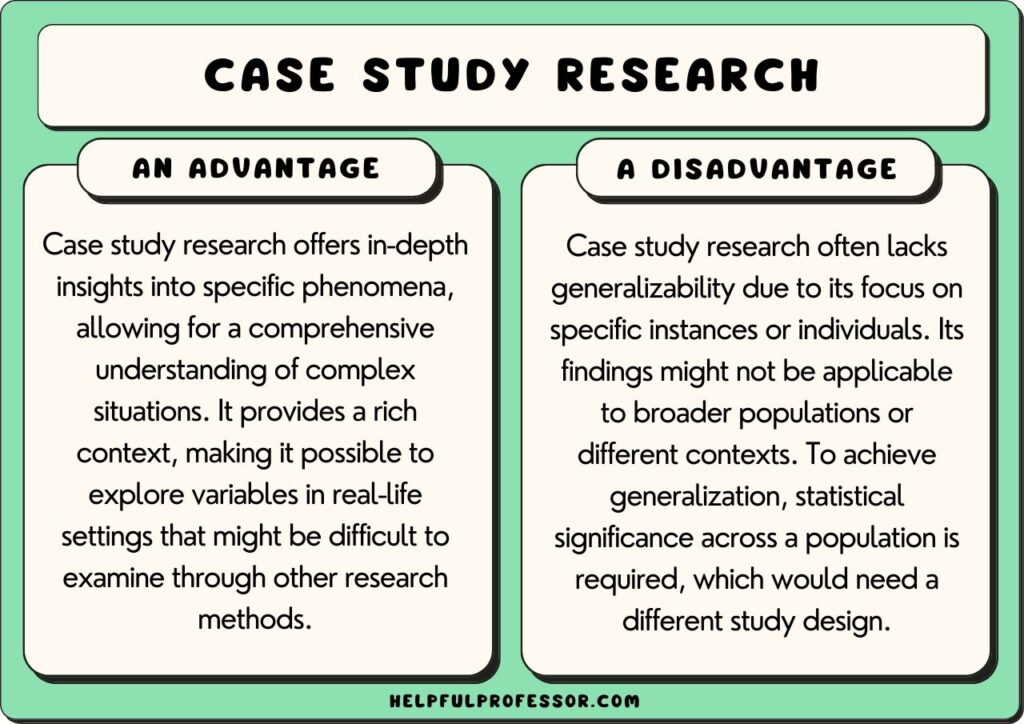
A case study in academic research is a detailed and in-depth examination of a specific instance or event, generally conducted through a qualitative approach to data.
The most common case study definition that I come across is is Robert K. Yin’s (2003, p. 13) quote provided below:
“An empirical inquiry that investigates a contemporary phenomenon within its real-life context, especially when the boundaries between phenomenon and context are not clearly evident.”
Researchers conduct case studies for a number of reasons, such as to explore complex phenomena within their real-life context, to look at a particularly interesting instance of a situation, or to dig deeper into something of interest identified in a wider-scale project.
While case studies render extremely interesting data, they have many limitations and are not suitable for all studies. One key limitation is that a case study’s findings are not usually generalizable to broader populations because one instance cannot be used to infer trends across populations.
Case Study Advantages and Disadvantages
1. in-depth analysis of complex phenomena.
Case study design allows researchers to delve deeply into intricate issues and situations.
By focusing on a specific instance or event, researchers can uncover nuanced details and layers of understanding that might be missed with other research methods, especially large-scale survey studies.
As Lee and Saunders (2017) argue,
“It allows that particular event to be studies in detail so that its unique qualities may be identified.”
This depth of analysis can provide rich insights into the underlying factors and dynamics of the studied phenomenon.
2. Holistic Understanding
Building on the above point, case studies can help us to understand a topic holistically and from multiple angles.
This means the researcher isn’t restricted to just examining a topic by using a pre-determined set of questions, as with questionnaires. Instead, researchers can use qualitative methods to delve into the many different angles, perspectives, and contextual factors related to the case study.
We can turn to Lee and Saunders (2017) again, who notes that case study researchers “develop a deep, holistic understanding of a particular phenomenon” with the intent of deeply understanding the phenomenon.
3. Examination of rare and Unusual Phenomena
We need to use case study methods when we stumble upon “rare and unusual” (Lee & Saunders, 2017) phenomena that would tend to be seen as mere outliers in population studies.
Take, for example, a child genius. A population study of all children of that child’s age would merely see this child as an outlier in the dataset, and this child may even be removed in order to predict overall trends.
So, to truly come to an understanding of this child and get insights into the environmental conditions that led to this child’s remarkable cognitive development, we need to do an in-depth study of this child specifically – so, we’d use a case study.
4. Helps Reveal the Experiences of Marginalzied Groups
Just as rare and unsual cases can be overlooked in population studies, so too can the experiences, beliefs, and perspectives of marginalized groups.
As Lee and Saunders (2017) argue, “case studies are also extremely useful in helping the expression of the voices of people whose interests are often ignored.”
Take, for example, the experiences of minority populations as they navigate healthcare systems. This was for many years a “hidden” phenomenon, not examined by researchers. It took case study designs to truly reveal this phenomenon, which helped to raise practitioners’ awareness of the importance of cultural sensitivity in medicine.
5. Ideal in Situations where Researchers cannot Control the Variables
Experimental designs – where a study takes place in a lab or controlled environment – are excellent for determining cause and effect . But not all studies can take place in controlled environments (Tetnowski, 2015).
When we’re out in the field doing observational studies or similar fieldwork, we don’t have the freedom to isolate dependent and independent variables. We need to use alternate methods.
Case studies are ideal in such situations.
A case study design will allow researchers to deeply immerse themselves in a setting (potentially combining it with methods such as ethnography or researcher observation) in order to see how phenomena take place in real-life settings.
6. Supports the generation of new theories or hypotheses
While large-scale quantitative studies such as cross-sectional designs and population surveys are excellent at testing theories and hypotheses on a large scale, they need a hypothesis to start off with!
This is where case studies – in the form of grounded research – come in. Often, a case study doesn’t start with a hypothesis. Instead, it ends with a hypothesis based upon the findings within a singular setting.
The deep analysis allows for hypotheses to emerge, which can then be taken to larger-scale studies in order to conduct further, more generalizable, testing of the hypothesis or theory.
7. Reveals the Unexpected
When a largescale quantitative research project has a clear hypothesis that it will test, it often becomes very rigid and has tunnel-vision on just exploring the hypothesis.
Of course, a structured scientific examination of the effects of specific interventions targeted at specific variables is extermely valuable.
But narrowly-focused studies often fail to shine a spotlight on unexpected and emergent data. Here, case studies come in very useful. Oftentimes, researchers set their eyes on a phenomenon and, when examining it closely with case studies, identify data and come to conclusions that are unprecedented, unforeseen, and outright surprising.
As Lars Meier (2009, p. 975) marvels, “where else can we become a part of foreign social worlds and have the chance to become aware of the unexpected?”
Disadvantages
1. not usually generalizable.
Case studies are not generalizable because they tend not to look at a broad enough corpus of data to be able to infer that there is a trend across a population.
As Yang (2022) argues, “by definition, case studies can make no claims to be typical.”
Case studies focus on one specific instance of a phenomenon. They explore the context, nuances, and situational factors that have come to bear on the case study. This is really useful for bringing to light important, new, and surprising information, as I’ve already covered.
But , it’s not often useful for generating data that has validity beyond the specific case study being examined.
2. Subjectivity in interpretation
Case studies usually (but not always) use qualitative data which helps to get deep into a topic and explain it in human terms, finding insights unattainable by quantitative data.
But qualitative data in case studies relies heavily on researcher interpretation. While researchers can be trained and work hard to focus on minimizing subjectivity (through methods like triangulation), it often emerges – some might argue it’s innevitable in qualitative studies.
So, a criticism of case studies could be that they’re more prone to subjectivity – and researchers need to take strides to address this in their studies.
3. Difficulty in replicating results
Case study research is often non-replicable because the study takes place in complex real-world settings where variables are not controlled.
So, when returning to a setting to re-do or attempt to replicate a study, we often find that the variables have changed to such an extent that replication is difficult. Furthermore, new researchers (with new subjective eyes) may catch things that the other readers overlooked.
Replication is even harder when researchers attempt to replicate a case study design in a new setting or with different participants.
Comprehension Quiz for Students
Question 1: What benefit do case studies offer when exploring the experiences of marginalized groups?
a) They provide generalizable data. b) They help express the voices of often-ignored individuals. c) They control all variables for the study. d) They always start with a clear hypothesis.
Question 2: Why might case studies be considered ideal for situations where researchers cannot control all variables?
a) They provide a structured scientific examination. b) They allow for generalizability across populations. c) They focus on one specific instance of a phenomenon. d) They allow for deep immersion in real-life settings.
Question 3: What is a primary disadvantage of case studies in terms of data applicability?
a) They always focus on the unexpected. b) They are not usually generalizable. c) They support the generation of new theories. d) They provide a holistic understanding.
Question 4: Why might case studies be considered more prone to subjectivity?
a) They always use quantitative data. b) They heavily rely on researcher interpretation, especially with qualitative data. c) They are always replicable. d) They look at a broad corpus of data.
Question 5: In what situations are experimental designs, such as those conducted in labs, most valuable?
a) When there’s a need to study rare and unusual phenomena. b) When a holistic understanding is required. c) When determining cause-and-effect relationships. d) When the study focuses on marginalized groups.
Question 6: Why is replication challenging in case study research?
a) Because they always use qualitative data. b) Because they tend to focus on a broad corpus of data. c) Due to the changing variables in complex real-world settings. d) Because they always start with a hypothesis.
Lee, B., & Saunders, M. N. K. (2017). Conducting Case Study Research for Business and Management Students. SAGE Publications.
Meir, L. (2009). Feasting on the Benefits of Case Study Research. In Mills, A. J., Wiebe, E., & Durepos, G. (Eds.). Encyclopedia of Case Study Research (Vol. 2). London: SAGE Publications.
Tetnowski, J. (2015). Qualitative case study research design. Perspectives on fluency and fluency disorders , 25 (1), 39-45. ( Source )
Yang, S. L. (2022). The War on Corruption in China: Local Reform and Innovation . Taylor & Francis.
Yin, R. (2003). Case Study research. Thousand Oaks, CA: Sage.
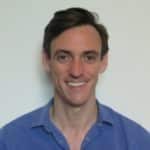
- Chris Drew (PhD) https://helpfulprofessor.com/author/chris-drew-phd/ 101 Hidden Talents Examples
- Chris Drew (PhD) https://helpfulprofessor.com/author/chris-drew-phd/ 15 Green Flags in a Relationship
- Chris Drew (PhD) https://helpfulprofessor.com/author/chris-drew-phd/ 15 Signs you're Burnt Out, Not Lazy
- Chris Drew (PhD) https://helpfulprofessor.com/author/chris-drew-phd/ 15 Toxic Things Parents Say to their Children
Leave a Comment Cancel Reply
Your email address will not be published. Required fields are marked *
- Business Essentials
- Leadership & Management
- Credential of Leadership, Impact, and Management in Business (CLIMB)
- Entrepreneurship & Innovation
- Digital Transformation
- Finance & Accounting
- Business in Society
- For Organizations
- Support Portal
- Media Coverage
- Founding Donors
- Leadership Team
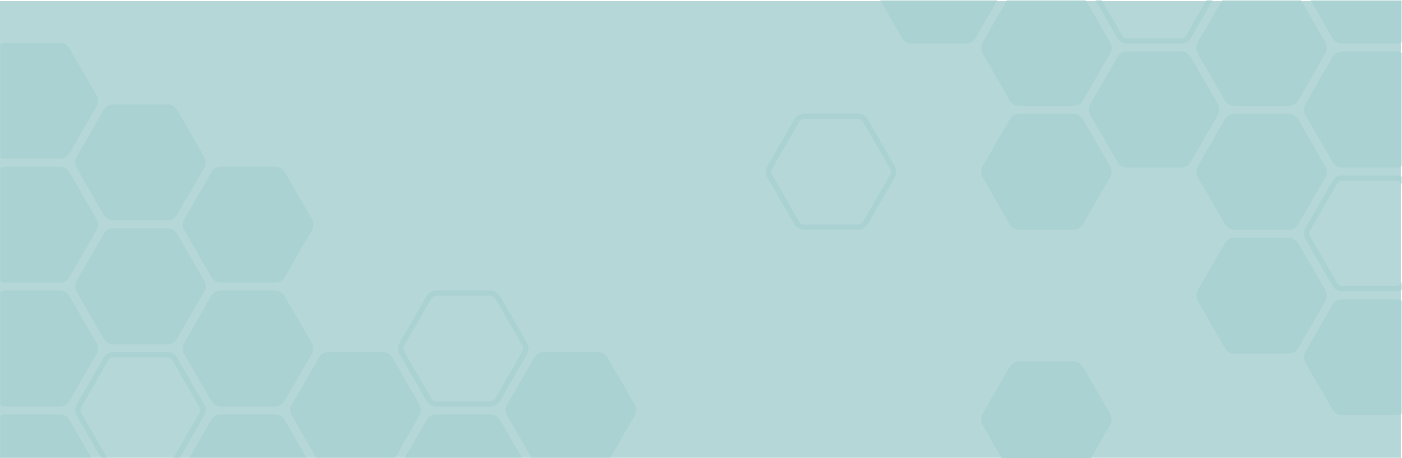
- Harvard Business School →
- HBS Online →
- Business Insights →
Business Insights
Harvard Business School Online's Business Insights Blog provides the career insights you need to achieve your goals and gain confidence in your business skills.
- Career Development
- Communication
- Decision-Making
- Earning Your MBA
- Negotiation
- News & Events
- Productivity
- Staff Spotlight
- Student Profiles
- Work-Life Balance
- AI Essentials for Business
- Alternative Investments
- Business Analytics
- Business Strategy
- Business and Climate Change
- Creating Brand Value
- Design Thinking and Innovation
- Digital Marketing Strategy
- Disruptive Strategy
- Economics for Managers
- Entrepreneurship Essentials
- Financial Accounting
- Global Business
- Launching Tech Ventures
- Leadership Principles
- Leadership, Ethics, and Corporate Accountability
- Leading Change and Organizational Renewal
- Leading with Finance
- Management Essentials
- Negotiation Mastery
- Organizational Leadership
- Power and Influence for Positive Impact
- Strategy Execution
- Sustainable Business Strategy
- Sustainable Investing
- Winning with Digital Platforms
5 Benefits of Learning Through the Case Study Method
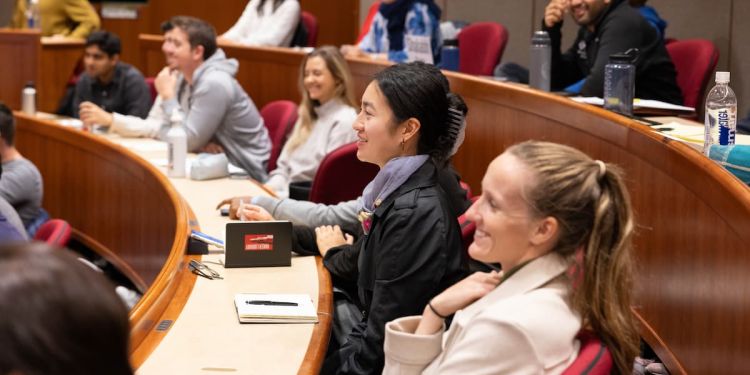
- 28 Nov 2023
While several factors make HBS Online unique —including a global Community and real-world outcomes —active learning through the case study method rises to the top.
In a 2023 City Square Associates survey, 74 percent of HBS Online learners who also took a course from another provider said HBS Online’s case method and real-world examples were better by comparison.
Here’s a primer on the case method, five benefits you could gain, and how to experience it for yourself.
Access your free e-book today.
What Is the Harvard Business School Case Study Method?
The case study method , or case method , is a learning technique in which you’re presented with a real-world business challenge and asked how you’d solve it. After working through it yourself and with peers, you’re told how the scenario played out.
HBS pioneered the case method in 1922. Shortly before, in 1921, the first case was written.
“How do you go into an ambiguous situation and get to the bottom of it?” says HBS Professor Jan Rivkin, former senior associate dean and chair of HBS's master of business administration (MBA) program, in a video about the case method . “That skill—the skill of figuring out a course of inquiry to choose a course of action—that skill is as relevant today as it was in 1921.”
Originally developed for the in-person MBA classroom, HBS Online adapted the case method into an engaging, interactive online learning experience in 2014.
In HBS Online courses , you learn about each case from the business professional who experienced it. After reviewing their videos, you’re prompted to take their perspective and explain how you’d handle their situation.
You then get to read peers’ responses, “star” them, and comment to further the discussion. Afterward, you learn how the professional handled it and their key takeaways.
HBS Online’s adaptation of the case method incorporates the famed HBS “cold call,” in which you’re called on at random to make a decision without time to prepare.
“Learning came to life!” said Sheneka Balogun , chief administration officer and chief of staff at LeMoyne-Owen College, of her experience taking the Credential of Readiness (CORe) program . “The videos from the professors, the interactive cold calls where you were randomly selected to participate, and the case studies that enhanced and often captured the essence of objectives and learning goals were all embedded in each module. This made learning fun, engaging, and student-friendly.”
If you’re considering taking a course that leverages the case study method, here are five benefits you could experience.
5 Benefits of Learning Through Case Studies
1. take new perspectives.
The case method prompts you to consider a scenario from another person’s perspective. To work through the situation and come up with a solution, you must consider their circumstances, limitations, risk tolerance, stakeholders, resources, and potential consequences to assess how to respond.
Taking on new perspectives not only can help you navigate your own challenges but also others’. Putting yourself in someone else’s situation to understand their motivations and needs can go a long way when collaborating with stakeholders.
2. Hone Your Decision-Making Skills
Another skill you can build is the ability to make decisions effectively . The case study method forces you to use limited information to decide how to handle a problem—just like in the real world.
Throughout your career, you’ll need to make difficult decisions with incomplete or imperfect information—and sometimes, you won’t feel qualified to do so. Learning through the case method allows you to practice this skill in a low-stakes environment. When facing a real challenge, you’ll be better prepared to think quickly, collaborate with others, and present and defend your solution.
3. Become More Open-Minded
As you collaborate with peers on responses, it becomes clear that not everyone solves problems the same way. Exposing yourself to various approaches and perspectives can help you become a more open-minded professional.
When you’re part of a diverse group of learners from around the world, your experiences, cultures, and backgrounds contribute to a range of opinions on each case.
On the HBS Online course platform, you’re prompted to view and comment on others’ responses, and discussion is encouraged. This practice of considering others’ perspectives can make you more receptive in your career.
“You’d be surprised at how much you can learn from your peers,” said Ratnaditya Jonnalagadda , a software engineer who took CORe.
In addition to interacting with peers in the course platform, Jonnalagadda was part of the HBS Online Community , where he networked with other professionals and continued discussions sparked by course content.
“You get to understand your peers better, and students share examples of businesses implementing a concept from a module you just learned,” Jonnalagadda said. “It’s a very good way to cement the concepts in one's mind.”
4. Enhance Your Curiosity
One byproduct of taking on different perspectives is that it enables you to picture yourself in various roles, industries, and business functions.
“Each case offers an opportunity for students to see what resonates with them, what excites them, what bores them, which role they could imagine inhabiting in their careers,” says former HBS Dean Nitin Nohria in the Harvard Business Review . “Cases stimulate curiosity about the range of opportunities in the world and the many ways that students can make a difference as leaders.”
Through the case method, you can “try on” roles you may not have considered and feel more prepared to change or advance your career .
5. Build Your Self-Confidence
Finally, learning through the case study method can build your confidence. Each time you assume a business leader’s perspective, aim to solve a new challenge, and express and defend your opinions and decisions to peers, you prepare to do the same in your career.
According to a 2022 City Square Associates survey , 84 percent of HBS Online learners report feeling more confident making business decisions after taking a course.
“Self-confidence is difficult to teach or coach, but the case study method seems to instill it in people,” Nohria says in the Harvard Business Review . “There may well be other ways of learning these meta-skills, such as the repeated experience gained through practice or guidance from a gifted coach. However, under the direction of a masterful teacher, the case method can engage students and help them develop powerful meta-skills like no other form of teaching.”

How to Experience the Case Study Method
If the case method seems like a good fit for your learning style, experience it for yourself by taking an HBS Online course. Offerings span eight subject areas, including:
- Business essentials
- Leadership and management
- Entrepreneurship and innovation
- Digital transformation
- Finance and accounting
- Business in society
No matter which course or credential program you choose, you’ll examine case studies from real business professionals, work through their challenges alongside peers, and gain valuable insights to apply to your career.
Are you interested in discovering how HBS Online can help advance your career? Explore our course catalog and download our free guide —complete with interactive workbook sections—to determine if online learning is right for you and which course to take.

About the Author
- Privacy Policy

Home » Case Study – Methods, Examples and Guide
Case Study – Methods, Examples and Guide
Table of Contents

A case study is a research method that involves an in-depth examination and analysis of a particular phenomenon or case, such as an individual, organization, community, event, or situation.
It is a qualitative research approach that aims to provide a detailed and comprehensive understanding of the case being studied. Case studies typically involve multiple sources of data, including interviews, observations, documents, and artifacts, which are analyzed using various techniques, such as content analysis, thematic analysis, and grounded theory. The findings of a case study are often used to develop theories, inform policy or practice, or generate new research questions.
Types of Case Study
Types and Methods of Case Study are as follows:
Single-Case Study
A single-case study is an in-depth analysis of a single case. This type of case study is useful when the researcher wants to understand a specific phenomenon in detail.
For Example , A researcher might conduct a single-case study on a particular individual to understand their experiences with a particular health condition or a specific organization to explore their management practices. The researcher collects data from multiple sources, such as interviews, observations, and documents, and uses various techniques to analyze the data, such as content analysis or thematic analysis. The findings of a single-case study are often used to generate new research questions, develop theories, or inform policy or practice.
Multiple-Case Study
A multiple-case study involves the analysis of several cases that are similar in nature. This type of case study is useful when the researcher wants to identify similarities and differences between the cases.
For Example, a researcher might conduct a multiple-case study on several companies to explore the factors that contribute to their success or failure. The researcher collects data from each case, compares and contrasts the findings, and uses various techniques to analyze the data, such as comparative analysis or pattern-matching. The findings of a multiple-case study can be used to develop theories, inform policy or practice, or generate new research questions.
Exploratory Case Study
An exploratory case study is used to explore a new or understudied phenomenon. This type of case study is useful when the researcher wants to generate hypotheses or theories about the phenomenon.
For Example, a researcher might conduct an exploratory case study on a new technology to understand its potential impact on society. The researcher collects data from multiple sources, such as interviews, observations, and documents, and uses various techniques to analyze the data, such as grounded theory or content analysis. The findings of an exploratory case study can be used to generate new research questions, develop theories, or inform policy or practice.
Descriptive Case Study
A descriptive case study is used to describe a particular phenomenon in detail. This type of case study is useful when the researcher wants to provide a comprehensive account of the phenomenon.
For Example, a researcher might conduct a descriptive case study on a particular community to understand its social and economic characteristics. The researcher collects data from multiple sources, such as interviews, observations, and documents, and uses various techniques to analyze the data, such as content analysis or thematic analysis. The findings of a descriptive case study can be used to inform policy or practice or generate new research questions.
Instrumental Case Study
An instrumental case study is used to understand a particular phenomenon that is instrumental in achieving a particular goal. This type of case study is useful when the researcher wants to understand the role of the phenomenon in achieving the goal.
For Example, a researcher might conduct an instrumental case study on a particular policy to understand its impact on achieving a particular goal, such as reducing poverty. The researcher collects data from multiple sources, such as interviews, observations, and documents, and uses various techniques to analyze the data, such as content analysis or thematic analysis. The findings of an instrumental case study can be used to inform policy or practice or generate new research questions.
Case Study Data Collection Methods
Here are some common data collection methods for case studies:
Interviews involve asking questions to individuals who have knowledge or experience relevant to the case study. Interviews can be structured (where the same questions are asked to all participants) or unstructured (where the interviewer follows up on the responses with further questions). Interviews can be conducted in person, over the phone, or through video conferencing.
Observations
Observations involve watching and recording the behavior and activities of individuals or groups relevant to the case study. Observations can be participant (where the researcher actively participates in the activities) or non-participant (where the researcher observes from a distance). Observations can be recorded using notes, audio or video recordings, or photographs.
Documents can be used as a source of information for case studies. Documents can include reports, memos, emails, letters, and other written materials related to the case study. Documents can be collected from the case study participants or from public sources.
Surveys involve asking a set of questions to a sample of individuals relevant to the case study. Surveys can be administered in person, over the phone, through mail or email, or online. Surveys can be used to gather information on attitudes, opinions, or behaviors related to the case study.
Artifacts are physical objects relevant to the case study. Artifacts can include tools, equipment, products, or other objects that provide insights into the case study phenomenon.
How to conduct Case Study Research
Conducting a case study research involves several steps that need to be followed to ensure the quality and rigor of the study. Here are the steps to conduct case study research:
- Define the research questions: The first step in conducting a case study research is to define the research questions. The research questions should be specific, measurable, and relevant to the case study phenomenon under investigation.
- Select the case: The next step is to select the case or cases to be studied. The case should be relevant to the research questions and should provide rich and diverse data that can be used to answer the research questions.
- Collect data: Data can be collected using various methods, such as interviews, observations, documents, surveys, and artifacts. The data collection method should be selected based on the research questions and the nature of the case study phenomenon.
- Analyze the data: The data collected from the case study should be analyzed using various techniques, such as content analysis, thematic analysis, or grounded theory. The analysis should be guided by the research questions and should aim to provide insights and conclusions relevant to the research questions.
- Draw conclusions: The conclusions drawn from the case study should be based on the data analysis and should be relevant to the research questions. The conclusions should be supported by evidence and should be clearly stated.
- Validate the findings: The findings of the case study should be validated by reviewing the data and the analysis with participants or other experts in the field. This helps to ensure the validity and reliability of the findings.
- Write the report: The final step is to write the report of the case study research. The report should provide a clear description of the case study phenomenon, the research questions, the data collection methods, the data analysis, the findings, and the conclusions. The report should be written in a clear and concise manner and should follow the guidelines for academic writing.
Examples of Case Study
Here are some examples of case study research:
- The Hawthorne Studies : Conducted between 1924 and 1932, the Hawthorne Studies were a series of case studies conducted by Elton Mayo and his colleagues to examine the impact of work environment on employee productivity. The studies were conducted at the Hawthorne Works plant of the Western Electric Company in Chicago and included interviews, observations, and experiments.
- The Stanford Prison Experiment: Conducted in 1971, the Stanford Prison Experiment was a case study conducted by Philip Zimbardo to examine the psychological effects of power and authority. The study involved simulating a prison environment and assigning participants to the role of guards or prisoners. The study was controversial due to the ethical issues it raised.
- The Challenger Disaster: The Challenger Disaster was a case study conducted to examine the causes of the Space Shuttle Challenger explosion in 1986. The study included interviews, observations, and analysis of data to identify the technical, organizational, and cultural factors that contributed to the disaster.
- The Enron Scandal: The Enron Scandal was a case study conducted to examine the causes of the Enron Corporation’s bankruptcy in 2001. The study included interviews, analysis of financial data, and review of documents to identify the accounting practices, corporate culture, and ethical issues that led to the company’s downfall.
- The Fukushima Nuclear Disaster : The Fukushima Nuclear Disaster was a case study conducted to examine the causes of the nuclear accident that occurred at the Fukushima Daiichi Nuclear Power Plant in Japan in 2011. The study included interviews, analysis of data, and review of documents to identify the technical, organizational, and cultural factors that contributed to the disaster.
Application of Case Study
Case studies have a wide range of applications across various fields and industries. Here are some examples:
Business and Management
Case studies are widely used in business and management to examine real-life situations and develop problem-solving skills. Case studies can help students and professionals to develop a deep understanding of business concepts, theories, and best practices.
Case studies are used in healthcare to examine patient care, treatment options, and outcomes. Case studies can help healthcare professionals to develop critical thinking skills, diagnose complex medical conditions, and develop effective treatment plans.
Case studies are used in education to examine teaching and learning practices. Case studies can help educators to develop effective teaching strategies, evaluate student progress, and identify areas for improvement.
Social Sciences
Case studies are widely used in social sciences to examine human behavior, social phenomena, and cultural practices. Case studies can help researchers to develop theories, test hypotheses, and gain insights into complex social issues.
Law and Ethics
Case studies are used in law and ethics to examine legal and ethical dilemmas. Case studies can help lawyers, policymakers, and ethical professionals to develop critical thinking skills, analyze complex cases, and make informed decisions.
Purpose of Case Study
The purpose of a case study is to provide a detailed analysis of a specific phenomenon, issue, or problem in its real-life context. A case study is a qualitative research method that involves the in-depth exploration and analysis of a particular case, which can be an individual, group, organization, event, or community.
The primary purpose of a case study is to generate a comprehensive and nuanced understanding of the case, including its history, context, and dynamics. Case studies can help researchers to identify and examine the underlying factors, processes, and mechanisms that contribute to the case and its outcomes. This can help to develop a more accurate and detailed understanding of the case, which can inform future research, practice, or policy.
Case studies can also serve other purposes, including:
- Illustrating a theory or concept: Case studies can be used to illustrate and explain theoretical concepts and frameworks, providing concrete examples of how they can be applied in real-life situations.
- Developing hypotheses: Case studies can help to generate hypotheses about the causal relationships between different factors and outcomes, which can be tested through further research.
- Providing insight into complex issues: Case studies can provide insights into complex and multifaceted issues, which may be difficult to understand through other research methods.
- Informing practice or policy: Case studies can be used to inform practice or policy by identifying best practices, lessons learned, or areas for improvement.
Advantages of Case Study Research
There are several advantages of case study research, including:
- In-depth exploration: Case study research allows for a detailed exploration and analysis of a specific phenomenon, issue, or problem in its real-life context. This can provide a comprehensive understanding of the case and its dynamics, which may not be possible through other research methods.
- Rich data: Case study research can generate rich and detailed data, including qualitative data such as interviews, observations, and documents. This can provide a nuanced understanding of the case and its complexity.
- Holistic perspective: Case study research allows for a holistic perspective of the case, taking into account the various factors, processes, and mechanisms that contribute to the case and its outcomes. This can help to develop a more accurate and comprehensive understanding of the case.
- Theory development: Case study research can help to develop and refine theories and concepts by providing empirical evidence and concrete examples of how they can be applied in real-life situations.
- Practical application: Case study research can inform practice or policy by identifying best practices, lessons learned, or areas for improvement.
- Contextualization: Case study research takes into account the specific context in which the case is situated, which can help to understand how the case is influenced by the social, cultural, and historical factors of its environment.
Limitations of Case Study Research
There are several limitations of case study research, including:
- Limited generalizability : Case studies are typically focused on a single case or a small number of cases, which limits the generalizability of the findings. The unique characteristics of the case may not be applicable to other contexts or populations, which may limit the external validity of the research.
- Biased sampling: Case studies may rely on purposive or convenience sampling, which can introduce bias into the sample selection process. This may limit the representativeness of the sample and the generalizability of the findings.
- Subjectivity: Case studies rely on the interpretation of the researcher, which can introduce subjectivity into the analysis. The researcher’s own biases, assumptions, and perspectives may influence the findings, which may limit the objectivity of the research.
- Limited control: Case studies are typically conducted in naturalistic settings, which limits the control that the researcher has over the environment and the variables being studied. This may limit the ability to establish causal relationships between variables.
- Time-consuming: Case studies can be time-consuming to conduct, as they typically involve a detailed exploration and analysis of a specific case. This may limit the feasibility of conducting multiple case studies or conducting case studies in a timely manner.
- Resource-intensive: Case studies may require significant resources, including time, funding, and expertise. This may limit the ability of researchers to conduct case studies in resource-constrained settings.
About the author
Muhammad Hassan
Researcher, Academic Writer, Web developer
You may also like

Transformative Design – Methods, Types, Guide

Observational Research – Methods and Guide

One-to-One Interview – Methods and Guide

Triangulation in Research – Types, Methods and...

Correlational Research – Methods, Types and...

Exploratory Research – Types, Methods and...
Have a language expert improve your writing
Run a free plagiarism check in 10 minutes, generate accurate citations for free.
- Knowledge Base
Methodology
- What Is a Case Study? | Definition, Examples & Methods
What Is a Case Study? | Definition, Examples & Methods
Published on May 8, 2019 by Shona McCombes . Revised on November 20, 2023.
A case study is a detailed study of a specific subject, such as a person, group, place, event, organization, or phenomenon. Case studies are commonly used in social, educational, clinical, and business research.
A case study research design usually involves qualitative methods , but quantitative methods are sometimes also used. Case studies are good for describing , comparing, evaluating and understanding different aspects of a research problem .
Table of contents
When to do a case study, step 1: select a case, step 2: build a theoretical framework, step 3: collect your data, step 4: describe and analyze the case, other interesting articles.
A case study is an appropriate research design when you want to gain concrete, contextual, in-depth knowledge about a specific real-world subject. It allows you to explore the key characteristics, meanings, and implications of the case.
Case studies are often a good choice in a thesis or dissertation . They keep your project focused and manageable when you don’t have the time or resources to do large-scale research.
You might use just one complex case study where you explore a single subject in depth, or conduct multiple case studies to compare and illuminate different aspects of your research problem.
Research question | Case study |
---|---|
What are the ecological effects of wolf reintroduction? | Case study of wolf reintroduction in Yellowstone National Park |
How do populist politicians use narratives about history to gain support? | Case studies of Hungarian prime minister Viktor Orbán and US president Donald Trump |
How can teachers implement active learning strategies in mixed-level classrooms? | Case study of a local school that promotes active learning |
What are the main advantages and disadvantages of wind farms for rural communities? | Case studies of three rural wind farm development projects in different parts of the country |
How are viral marketing strategies changing the relationship between companies and consumers? | Case study of the iPhone X marketing campaign |
How do experiences of work in the gig economy differ by gender, race and age? | Case studies of Deliveroo and Uber drivers in London |
Here's why students love Scribbr's proofreading services
Discover proofreading & editing
Once you have developed your problem statement and research questions , you should be ready to choose the specific case that you want to focus on. A good case study should have the potential to:
- Provide new or unexpected insights into the subject
- Challenge or complicate existing assumptions and theories
- Propose practical courses of action to resolve a problem
- Open up new directions for future research
TipIf your research is more practical in nature and aims to simultaneously investigate an issue as you solve it, consider conducting action research instead.
Unlike quantitative or experimental research , a strong case study does not require a random or representative sample. In fact, case studies often deliberately focus on unusual, neglected, or outlying cases which may shed new light on the research problem.
Example of an outlying case studyIn the 1960s the town of Roseto, Pennsylvania was discovered to have extremely low rates of heart disease compared to the US average. It became an important case study for understanding previously neglected causes of heart disease.
However, you can also choose a more common or representative case to exemplify a particular category, experience or phenomenon.
Example of a representative case studyIn the 1920s, two sociologists used Muncie, Indiana as a case study of a typical American city that supposedly exemplified the changing culture of the US at the time.
While case studies focus more on concrete details than general theories, they should usually have some connection with theory in the field. This way the case study is not just an isolated description, but is integrated into existing knowledge about the topic. It might aim to:
- Exemplify a theory by showing how it explains the case under investigation
- Expand on a theory by uncovering new concepts and ideas that need to be incorporated
- Challenge a theory by exploring an outlier case that doesn’t fit with established assumptions
To ensure that your analysis of the case has a solid academic grounding, you should conduct a literature review of sources related to the topic and develop a theoretical framework . This means identifying key concepts and theories to guide your analysis and interpretation.
There are many different research methods you can use to collect data on your subject. Case studies tend to focus on qualitative data using methods such as interviews , observations , and analysis of primary and secondary sources (e.g., newspaper articles, photographs, official records). Sometimes a case study will also collect quantitative data.
Example of a mixed methods case studyFor a case study of a wind farm development in a rural area, you could collect quantitative data on employment rates and business revenue, collect qualitative data on local people’s perceptions and experiences, and analyze local and national media coverage of the development.
The aim is to gain as thorough an understanding as possible of the case and its context.
In writing up the case study, you need to bring together all the relevant aspects to give as complete a picture as possible of the subject.
How you report your findings depends on the type of research you are doing. Some case studies are structured like a standard scientific paper or thesis , with separate sections or chapters for the methods , results and discussion .
Others are written in a more narrative style, aiming to explore the case from various angles and analyze its meanings and implications (for example, by using textual analysis or discourse analysis ).
In all cases, though, make sure to give contextual details about the case, connect it back to the literature and theory, and discuss how it fits into wider patterns or debates.
If you want to know more about statistics , methodology , or research bias , make sure to check out some of our other articles with explanations and examples.
- Normal distribution
- Degrees of freedom
- Null hypothesis
- Discourse analysis
- Control groups
- Mixed methods research
- Non-probability sampling
- Quantitative research
- Ecological validity
Research bias
- Rosenthal effect
- Implicit bias
- Cognitive bias
- Selection bias
- Negativity bias
- Status quo bias
Cite this Scribbr article
If you want to cite this source, you can copy and paste the citation or click the “Cite this Scribbr article” button to automatically add the citation to our free Citation Generator.
McCombes, S. (2023, November 20). What Is a Case Study? | Definition, Examples & Methods. Scribbr. Retrieved August 26, 2024, from https://www.scribbr.com/methodology/case-study/
Is this article helpful?
Shona McCombes
Other students also liked, primary vs. secondary sources | difference & examples, what is a theoretical framework | guide to organizing, what is action research | definition & examples, get unlimited documents corrected.
✔ Free APA citation check included ✔ Unlimited document corrections ✔ Specialized in correcting academic texts

The Ultimate Guide to Qualitative Research - Part 1: The Basics
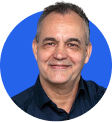
- Introduction and overview
- What is qualitative research?
- What is qualitative data?
- Examples of qualitative data
- Qualitative vs. quantitative research
- Mixed methods
- Qualitative research preparation
- Theoretical perspective
- Theoretical framework
- Literature reviews
Research question
- Conceptual framework
- Conceptual vs. theoretical framework
Data collection
- Qualitative research methods
- Focus groups
- Observational research
What is a case study?
Applications for case study research, what is a good case study, process of case study design, benefits and limitations of case studies.
- Ethnographical research
- Ethical considerations
- Confidentiality and privacy
- Power dynamics
- Reflexivity
Case studies
Case studies are essential to qualitative research , offering a lens through which researchers can investigate complex phenomena within their real-life contexts. This chapter explores the concept, purpose, applications, examples, and types of case studies and provides guidance on how to conduct case study research effectively.
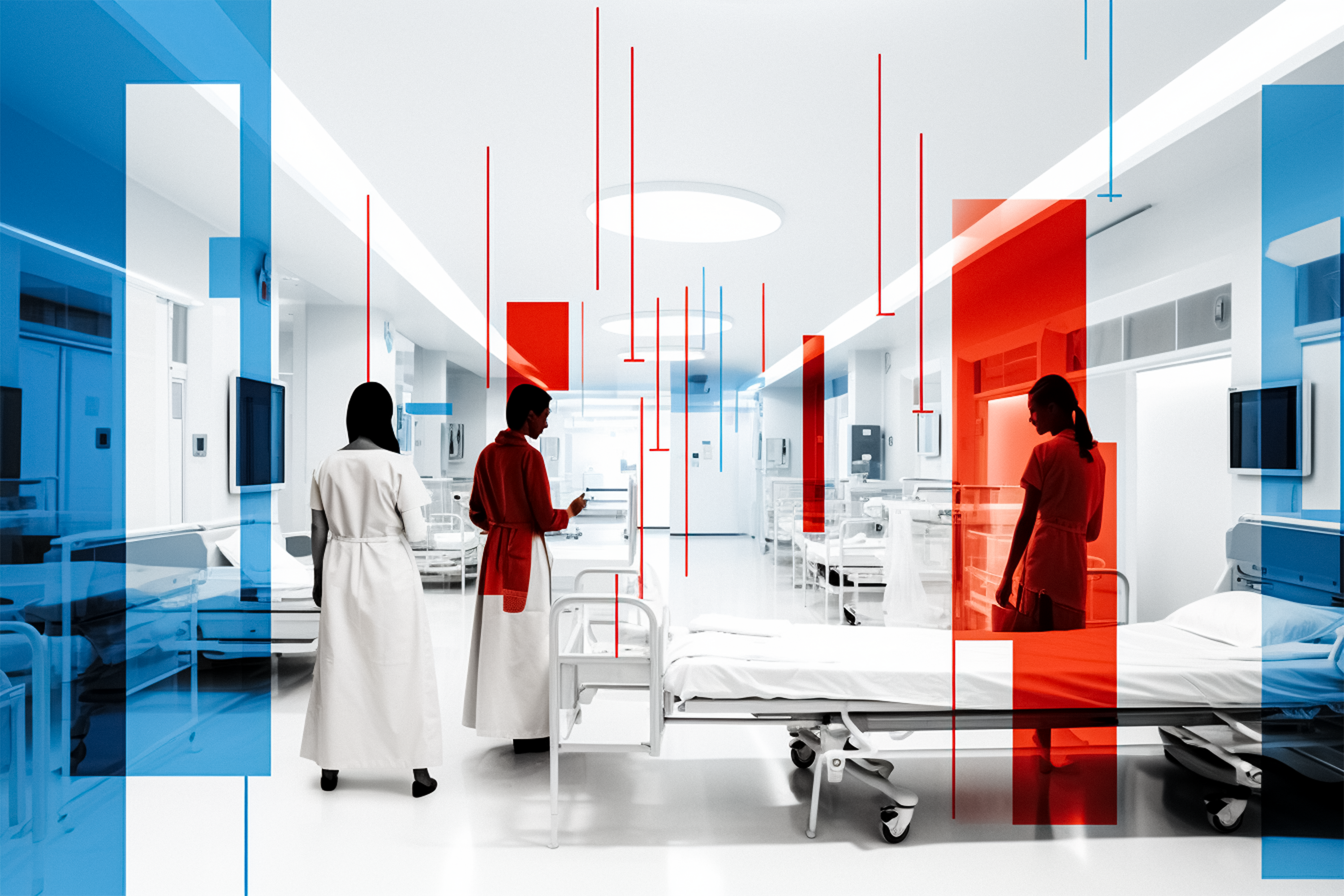
Whereas quantitative methods look at phenomena at scale, case study research looks at a concept or phenomenon in considerable detail. While analyzing a single case can help understand one perspective regarding the object of research inquiry, analyzing multiple cases can help obtain a more holistic sense of the topic or issue. Let's provide a basic definition of a case study, then explore its characteristics and role in the qualitative research process.
Definition of a case study
A case study in qualitative research is a strategy of inquiry that involves an in-depth investigation of a phenomenon within its real-world context. It provides researchers with the opportunity to acquire an in-depth understanding of intricate details that might not be as apparent or accessible through other methods of research. The specific case or cases being studied can be a single person, group, or organization – demarcating what constitutes a relevant case worth studying depends on the researcher and their research question .
Among qualitative research methods , a case study relies on multiple sources of evidence, such as documents, artifacts, interviews , or observations , to present a complete and nuanced understanding of the phenomenon under investigation. The objective is to illuminate the readers' understanding of the phenomenon beyond its abstract statistical or theoretical explanations.
Characteristics of case studies
Case studies typically possess a number of distinct characteristics that set them apart from other research methods. These characteristics include a focus on holistic description and explanation, flexibility in the design and data collection methods, reliance on multiple sources of evidence, and emphasis on the context in which the phenomenon occurs.
Furthermore, case studies can often involve a longitudinal examination of the case, meaning they study the case over a period of time. These characteristics allow case studies to yield comprehensive, in-depth, and richly contextualized insights about the phenomenon of interest.
The role of case studies in research
Case studies hold a unique position in the broader landscape of research methods aimed at theory development. They are instrumental when the primary research interest is to gain an intensive, detailed understanding of a phenomenon in its real-life context.
In addition, case studies can serve different purposes within research - they can be used for exploratory, descriptive, or explanatory purposes, depending on the research question and objectives. This flexibility and depth make case studies a valuable tool in the toolkit of qualitative researchers.
Remember, a well-conducted case study can offer a rich, insightful contribution to both academic and practical knowledge through theory development or theory verification, thus enhancing our understanding of complex phenomena in their real-world contexts.
What is the purpose of a case study?
Case study research aims for a more comprehensive understanding of phenomena, requiring various research methods to gather information for qualitative analysis . Ultimately, a case study can allow the researcher to gain insight into a particular object of inquiry and develop a theoretical framework relevant to the research inquiry.
Why use case studies in qualitative research?
Using case studies as a research strategy depends mainly on the nature of the research question and the researcher's access to the data.
Conducting case study research provides a level of detail and contextual richness that other research methods might not offer. They are beneficial when there's a need to understand complex social phenomena within their natural contexts.
The explanatory, exploratory, and descriptive roles of case studies
Case studies can take on various roles depending on the research objectives. They can be exploratory when the research aims to discover new phenomena or define new research questions; they are descriptive when the objective is to depict a phenomenon within its context in a detailed manner; and they can be explanatory if the goal is to understand specific relationships within the studied context. Thus, the versatility of case studies allows researchers to approach their topic from different angles, offering multiple ways to uncover and interpret the data .
The impact of case studies on knowledge development
Case studies play a significant role in knowledge development across various disciplines. Analysis of cases provides an avenue for researchers to explore phenomena within their context based on the collected data.
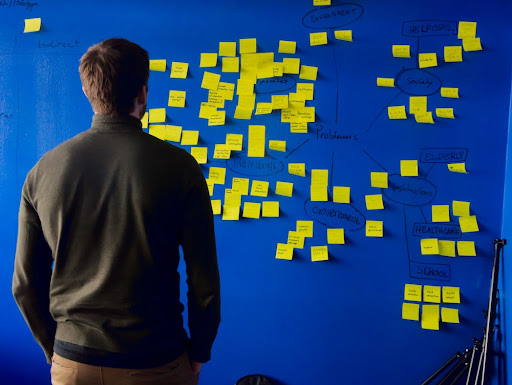
This can result in the production of rich, practical insights that can be instrumental in both theory-building and practice. Case studies allow researchers to delve into the intricacies and complexities of real-life situations, uncovering insights that might otherwise remain hidden.
Types of case studies
In qualitative research , a case study is not a one-size-fits-all approach. Depending on the nature of the research question and the specific objectives of the study, researchers might choose to use different types of case studies. These types differ in their focus, methodology, and the level of detail they provide about the phenomenon under investigation.
Understanding these types is crucial for selecting the most appropriate approach for your research project and effectively achieving your research goals. Let's briefly look at the main types of case studies.
Exploratory case studies
Exploratory case studies are typically conducted to develop a theory or framework around an understudied phenomenon. They can also serve as a precursor to a larger-scale research project. Exploratory case studies are useful when a researcher wants to identify the key issues or questions which can spur more extensive study or be used to develop propositions for further research. These case studies are characterized by flexibility, allowing researchers to explore various aspects of a phenomenon as they emerge, which can also form the foundation for subsequent studies.
Descriptive case studies
Descriptive case studies aim to provide a complete and accurate representation of a phenomenon or event within its context. These case studies are often based on an established theoretical framework, which guides how data is collected and analyzed. The researcher is concerned with describing the phenomenon in detail, as it occurs naturally, without trying to influence or manipulate it.
Explanatory case studies
Explanatory case studies are focused on explanation - they seek to clarify how or why certain phenomena occur. Often used in complex, real-life situations, they can be particularly valuable in clarifying causal relationships among concepts and understanding the interplay between different factors within a specific context.
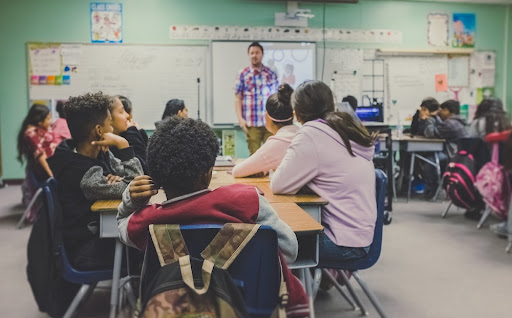
Intrinsic, instrumental, and collective case studies
These three categories of case studies focus on the nature and purpose of the study. An intrinsic case study is conducted when a researcher has an inherent interest in the case itself. Instrumental case studies are employed when the case is used to provide insight into a particular issue or phenomenon. A collective case study, on the other hand, involves studying multiple cases simultaneously to investigate some general phenomena.
Each type of case study serves a different purpose and has its own strengths and challenges. The selection of the type should be guided by the research question and objectives, as well as the context and constraints of the research.
The flexibility, depth, and contextual richness offered by case studies make this approach an excellent research method for various fields of study. They enable researchers to investigate real-world phenomena within their specific contexts, capturing nuances that other research methods might miss. Across numerous fields, case studies provide valuable insights into complex issues.
Critical information systems research
Case studies provide a detailed understanding of the role and impact of information systems in different contexts. They offer a platform to explore how information systems are designed, implemented, and used and how they interact with various social, economic, and political factors. Case studies in this field often focus on examining the intricate relationship between technology, organizational processes, and user behavior, helping to uncover insights that can inform better system design and implementation.
Health research
Health research is another field where case studies are highly valuable. They offer a way to explore patient experiences, healthcare delivery processes, and the impact of various interventions in a real-world context.
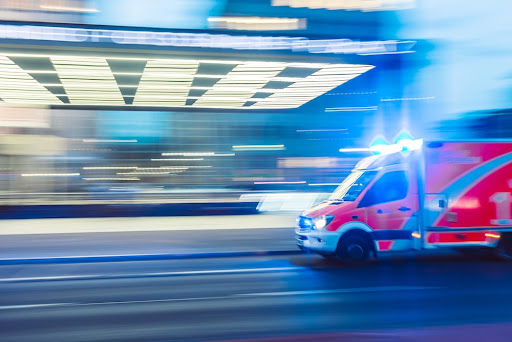
Case studies can provide a deep understanding of a patient's journey, giving insights into the intricacies of disease progression, treatment effects, and the psychosocial aspects of health and illness.
Asthma research studies
Specifically within medical research, studies on asthma often employ case studies to explore the individual and environmental factors that influence asthma development, management, and outcomes. A case study can provide rich, detailed data about individual patients' experiences, from the triggers and symptoms they experience to the effectiveness of various management strategies. This can be crucial for developing patient-centered asthma care approaches.
Other fields
Apart from the fields mentioned, case studies are also extensively used in business and management research, education research, and political sciences, among many others. They provide an opportunity to delve into the intricacies of real-world situations, allowing for a comprehensive understanding of various phenomena.
Case studies, with their depth and contextual focus, offer unique insights across these varied fields. They allow researchers to illuminate the complexities of real-life situations, contributing to both theory and practice.
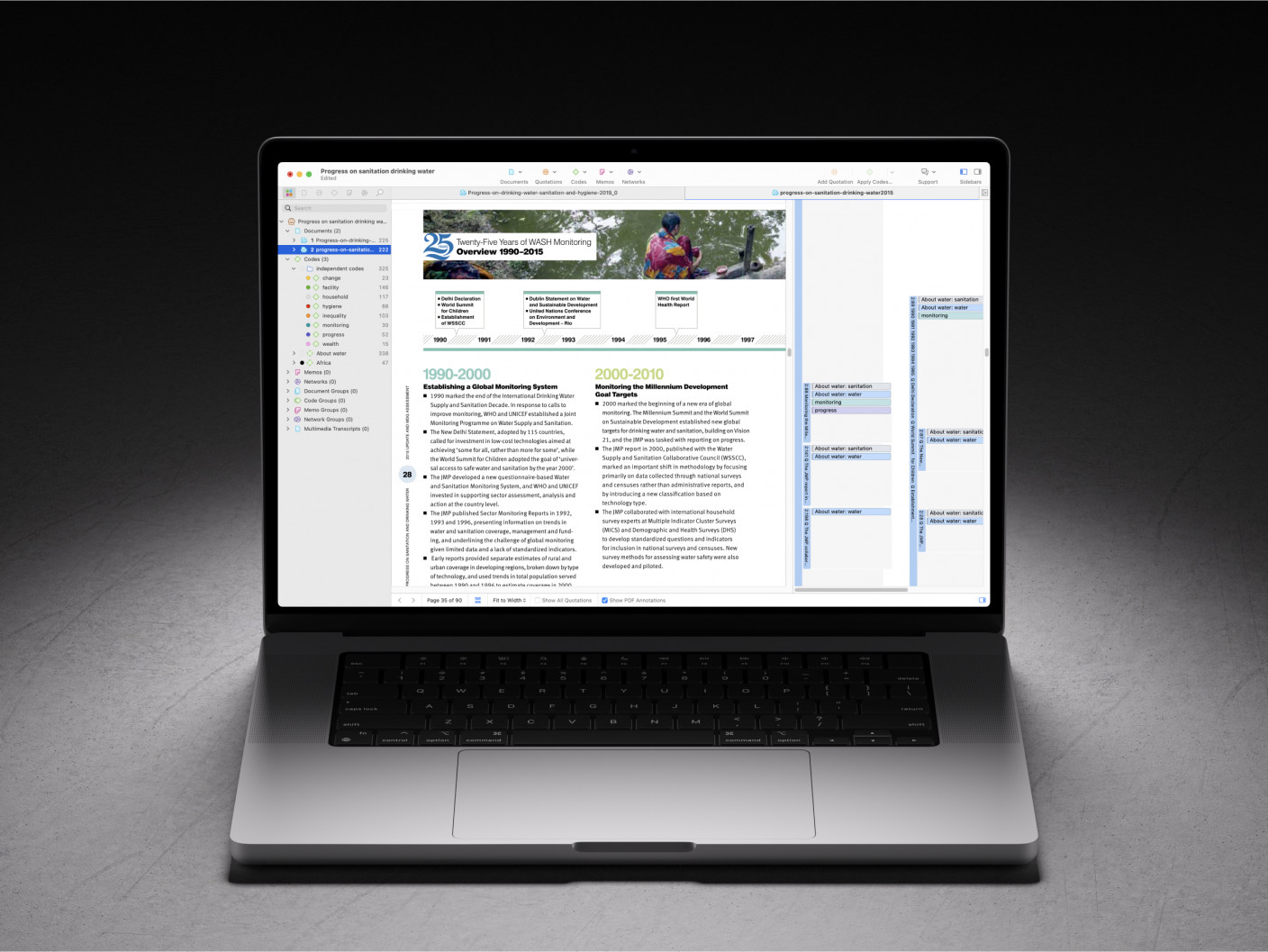
Whatever field you're in, ATLAS.ti puts your data to work for you
Download a free trial of ATLAS.ti to turn your data into insights.
Understanding the key elements of case study design is crucial for conducting rigorous and impactful case study research. A well-structured design guides the researcher through the process, ensuring that the study is methodologically sound and its findings are reliable and valid. The main elements of case study design include the research question , propositions, units of analysis, and the logic linking the data to the propositions.
The research question is the foundation of any research study. A good research question guides the direction of the study and informs the selection of the case, the methods of collecting data, and the analysis techniques. A well-formulated research question in case study research is typically clear, focused, and complex enough to merit further detailed examination of the relevant case(s).
Propositions
Propositions, though not necessary in every case study, provide a direction by stating what we might expect to find in the data collected. They guide how data is collected and analyzed by helping researchers focus on specific aspects of the case. They are particularly important in explanatory case studies, which seek to understand the relationships among concepts within the studied phenomenon.
Units of analysis
The unit of analysis refers to the case, or the main entity or entities that are being analyzed in the study. In case study research, the unit of analysis can be an individual, a group, an organization, a decision, an event, or even a time period. It's crucial to clearly define the unit of analysis, as it shapes the qualitative data analysis process by allowing the researcher to analyze a particular case and synthesize analysis across multiple case studies to draw conclusions.
Argumentation
This refers to the inferential model that allows researchers to draw conclusions from the data. The researcher needs to ensure that there is a clear link between the data, the propositions (if any), and the conclusions drawn. This argumentation is what enables the researcher to make valid and credible inferences about the phenomenon under study.
Understanding and carefully considering these elements in the design phase of a case study can significantly enhance the quality of the research. It can help ensure that the study is methodologically sound and its findings contribute meaningful insights about the case.
Ready to jumpstart your research with ATLAS.ti?
Conceptualize your research project with our intuitive data analysis interface. Download a free trial today.
Conducting a case study involves several steps, from defining the research question and selecting the case to collecting and analyzing data . This section outlines these key stages, providing a practical guide on how to conduct case study research.
Defining the research question
The first step in case study research is defining a clear, focused research question. This question should guide the entire research process, from case selection to analysis. It's crucial to ensure that the research question is suitable for a case study approach. Typically, such questions are exploratory or descriptive in nature and focus on understanding a phenomenon within its real-life context.
Selecting and defining the case
The selection of the case should be based on the research question and the objectives of the study. It involves choosing a unique example or a set of examples that provide rich, in-depth data about the phenomenon under investigation. After selecting the case, it's crucial to define it clearly, setting the boundaries of the case, including the time period and the specific context.
Previous research can help guide the case study design. When considering a case study, an example of a case could be taken from previous case study research and used to define cases in a new research inquiry. Considering recently published examples can help understand how to select and define cases effectively.
Developing a detailed case study protocol
A case study protocol outlines the procedures and general rules to be followed during the case study. This includes the data collection methods to be used, the sources of data, and the procedures for analysis. Having a detailed case study protocol ensures consistency and reliability in the study.
The protocol should also consider how to work with the people involved in the research context to grant the research team access to collecting data. As mentioned in previous sections of this guide, establishing rapport is an essential component of qualitative research as it shapes the overall potential for collecting and analyzing data.
Collecting data
Gathering data in case study research often involves multiple sources of evidence, including documents, archival records, interviews, observations, and physical artifacts. This allows for a comprehensive understanding of the case. The process for gathering data should be systematic and carefully documented to ensure the reliability and validity of the study.
Analyzing and interpreting data
The next step is analyzing the data. This involves organizing the data , categorizing it into themes or patterns , and interpreting these patterns to answer the research question. The analysis might also involve comparing the findings with prior research or theoretical propositions.
Writing the case study report
The final step is writing the case study report . This should provide a detailed description of the case, the data, the analysis process, and the findings. The report should be clear, organized, and carefully written to ensure that the reader can understand the case and the conclusions drawn from it.
Each of these steps is crucial in ensuring that the case study research is rigorous, reliable, and provides valuable insights about the case.
The type, depth, and quality of data in your study can significantly influence the validity and utility of the study. In case study research, data is usually collected from multiple sources to provide a comprehensive and nuanced understanding of the case. This section will outline the various methods of collecting data used in case study research and discuss considerations for ensuring the quality of the data.
Interviews are a common method of gathering data in case study research. They can provide rich, in-depth data about the perspectives, experiences, and interpretations of the individuals involved in the case. Interviews can be structured , semi-structured , or unstructured , depending on the research question and the degree of flexibility needed.
Observations
Observations involve the researcher observing the case in its natural setting, providing first-hand information about the case and its context. Observations can provide data that might not be revealed in interviews or documents, such as non-verbal cues or contextual information.
Documents and artifacts
Documents and archival records provide a valuable source of data in case study research. They can include reports, letters, memos, meeting minutes, email correspondence, and various public and private documents related to the case.
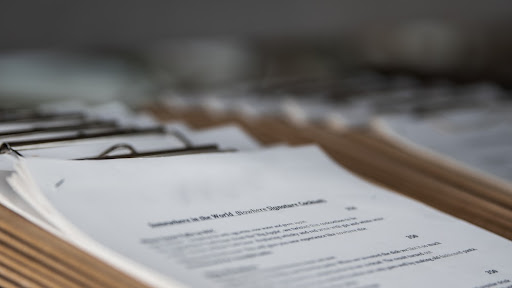
These records can provide historical context, corroborate evidence from other sources, and offer insights into the case that might not be apparent from interviews or observations.
Physical artifacts refer to any physical evidence related to the case, such as tools, products, or physical environments. These artifacts can provide tangible insights into the case, complementing the data gathered from other sources.
Ensuring the quality of data collection
Determining the quality of data in case study research requires careful planning and execution. It's crucial to ensure that the data is reliable, accurate, and relevant to the research question. This involves selecting appropriate methods of collecting data, properly training interviewers or observers, and systematically recording and storing the data. It also includes considering ethical issues related to collecting and handling data, such as obtaining informed consent and ensuring the privacy and confidentiality of the participants.
Data analysis
Analyzing case study research involves making sense of the rich, detailed data to answer the research question. This process can be challenging due to the volume and complexity of case study data. However, a systematic and rigorous approach to analysis can ensure that the findings are credible and meaningful. This section outlines the main steps and considerations in analyzing data in case study research.
Organizing the data
The first step in the analysis is organizing the data. This involves sorting the data into manageable sections, often according to the data source or the theme. This step can also involve transcribing interviews, digitizing physical artifacts, or organizing observational data.
Categorizing and coding the data
Once the data is organized, the next step is to categorize or code the data. This involves identifying common themes, patterns, or concepts in the data and assigning codes to relevant data segments. Coding can be done manually or with the help of software tools, and in either case, qualitative analysis software can greatly facilitate the entire coding process. Coding helps to reduce the data to a set of themes or categories that can be more easily analyzed.
Identifying patterns and themes
After coding the data, the researcher looks for patterns or themes in the coded data. This involves comparing and contrasting the codes and looking for relationships or patterns among them. The identified patterns and themes should help answer the research question.
Interpreting the data
Once patterns and themes have been identified, the next step is to interpret these findings. This involves explaining what the patterns or themes mean in the context of the research question and the case. This interpretation should be grounded in the data, but it can also involve drawing on theoretical concepts or prior research.
Verification of the data
The last step in the analysis is verification. This involves checking the accuracy and consistency of the analysis process and confirming that the findings are supported by the data. This can involve re-checking the original data, checking the consistency of codes, or seeking feedback from research participants or peers.
Like any research method , case study research has its strengths and limitations. Researchers must be aware of these, as they can influence the design, conduct, and interpretation of the study.
Understanding the strengths and limitations of case study research can also guide researchers in deciding whether this approach is suitable for their research question . This section outlines some of the key strengths and limitations of case study research.
Benefits include the following:
- Rich, detailed data: One of the main strengths of case study research is that it can generate rich, detailed data about the case. This can provide a deep understanding of the case and its context, which can be valuable in exploring complex phenomena.
- Flexibility: Case study research is flexible in terms of design , data collection , and analysis . A sufficient degree of flexibility allows the researcher to adapt the study according to the case and the emerging findings.
- Real-world context: Case study research involves studying the case in its real-world context, which can provide valuable insights into the interplay between the case and its context.
- Multiple sources of evidence: Case study research often involves collecting data from multiple sources , which can enhance the robustness and validity of the findings.
On the other hand, researchers should consider the following limitations:
- Generalizability: A common criticism of case study research is that its findings might not be generalizable to other cases due to the specificity and uniqueness of each case.
- Time and resource intensive: Case study research can be time and resource intensive due to the depth of the investigation and the amount of collected data.
- Complexity of analysis: The rich, detailed data generated in case study research can make analyzing the data challenging.
- Subjectivity: Given the nature of case study research, there may be a higher degree of subjectivity in interpreting the data , so researchers need to reflect on this and transparently convey to audiences how the research was conducted.
Being aware of these strengths and limitations can help researchers design and conduct case study research effectively and interpret and report the findings appropriately.
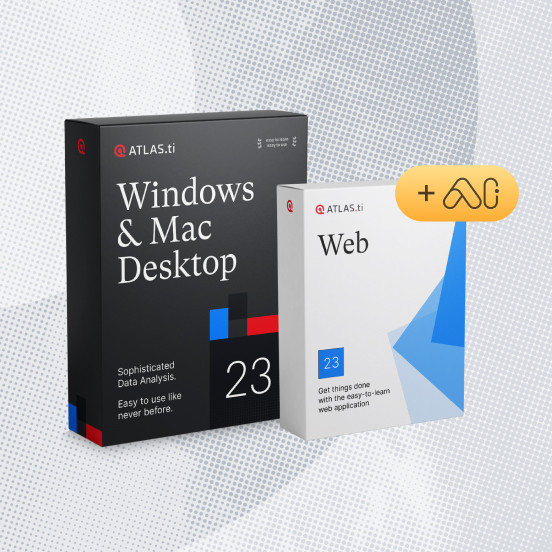
Ready to analyze your data with ATLAS.ti?
See how our intuitive software can draw key insights from your data with a free trial today.
- Open access
- Published: 27 June 2011
The case study approach
- Sarah Crowe 1 ,
- Kathrin Cresswell 2 ,
- Ann Robertson 2 ,
- Guro Huby 3 ,
- Anthony Avery 1 &
- Aziz Sheikh 2
BMC Medical Research Methodology volume 11 , Article number: 100 ( 2011 ) Cite this article
796k Accesses
1111 Citations
42 Altmetric
Metrics details
The case study approach allows in-depth, multi-faceted explorations of complex issues in their real-life settings. The value of the case study approach is well recognised in the fields of business, law and policy, but somewhat less so in health services research. Based on our experiences of conducting several health-related case studies, we reflect on the different types of case study design, the specific research questions this approach can help answer, the data sources that tend to be used, and the particular advantages and disadvantages of employing this methodological approach. The paper concludes with key pointers to aid those designing and appraising proposals for conducting case study research, and a checklist to help readers assess the quality of case study reports.
Peer Review reports
Introduction
The case study approach is particularly useful to employ when there is a need to obtain an in-depth appreciation of an issue, event or phenomenon of interest, in its natural real-life context. Our aim in writing this piece is to provide insights into when to consider employing this approach and an overview of key methodological considerations in relation to the design, planning, analysis, interpretation and reporting of case studies.
The illustrative 'grand round', 'case report' and 'case series' have a long tradition in clinical practice and research. Presenting detailed critiques, typically of one or more patients, aims to provide insights into aspects of the clinical case and, in doing so, illustrate broader lessons that may be learnt. In research, the conceptually-related case study approach can be used, for example, to describe in detail a patient's episode of care, explore professional attitudes to and experiences of a new policy initiative or service development or more generally to 'investigate contemporary phenomena within its real-life context' [ 1 ]. Based on our experiences of conducting a range of case studies, we reflect on when to consider using this approach, discuss the key steps involved and illustrate, with examples, some of the practical challenges of attaining an in-depth understanding of a 'case' as an integrated whole. In keeping with previously published work, we acknowledge the importance of theory to underpin the design, selection, conduct and interpretation of case studies[ 2 ]. In so doing, we make passing reference to the different epistemological approaches used in case study research by key theoreticians and methodologists in this field of enquiry.
This paper is structured around the following main questions: What is a case study? What are case studies used for? How are case studies conducted? What are the potential pitfalls and how can these be avoided? We draw in particular on four of our own recently published examples of case studies (see Tables 1 , 2 , 3 and 4 ) and those of others to illustrate our discussion[ 3 – 7 ].
What is a case study?
A case study is a research approach that is used to generate an in-depth, multi-faceted understanding of a complex issue in its real-life context. It is an established research design that is used extensively in a wide variety of disciplines, particularly in the social sciences. A case study can be defined in a variety of ways (Table 5 ), the central tenet being the need to explore an event or phenomenon in depth and in its natural context. It is for this reason sometimes referred to as a "naturalistic" design; this is in contrast to an "experimental" design (such as a randomised controlled trial) in which the investigator seeks to exert control over and manipulate the variable(s) of interest.
Stake's work has been particularly influential in defining the case study approach to scientific enquiry. He has helpfully characterised three main types of case study: intrinsic , instrumental and collective [ 8 ]. An intrinsic case study is typically undertaken to learn about a unique phenomenon. The researcher should define the uniqueness of the phenomenon, which distinguishes it from all others. In contrast, the instrumental case study uses a particular case (some of which may be better than others) to gain a broader appreciation of an issue or phenomenon. The collective case study involves studying multiple cases simultaneously or sequentially in an attempt to generate a still broader appreciation of a particular issue.
These are however not necessarily mutually exclusive categories. In the first of our examples (Table 1 ), we undertook an intrinsic case study to investigate the issue of recruitment of minority ethnic people into the specific context of asthma research studies, but it developed into a instrumental case study through seeking to understand the issue of recruitment of these marginalised populations more generally, generating a number of the findings that are potentially transferable to other disease contexts[ 3 ]. In contrast, the other three examples (see Tables 2 , 3 and 4 ) employed collective case study designs to study the introduction of workforce reconfiguration in primary care, the implementation of electronic health records into hospitals, and to understand the ways in which healthcare students learn about patient safety considerations[ 4 – 6 ]. Although our study focusing on the introduction of General Practitioners with Specialist Interests (Table 2 ) was explicitly collective in design (four contrasting primary care organisations were studied), is was also instrumental in that this particular professional group was studied as an exemplar of the more general phenomenon of workforce redesign[ 4 ].
What are case studies used for?
According to Yin, case studies can be used to explain, describe or explore events or phenomena in the everyday contexts in which they occur[ 1 ]. These can, for example, help to understand and explain causal links and pathways resulting from a new policy initiative or service development (see Tables 2 and 3 , for example)[ 1 ]. In contrast to experimental designs, which seek to test a specific hypothesis through deliberately manipulating the environment (like, for example, in a randomised controlled trial giving a new drug to randomly selected individuals and then comparing outcomes with controls),[ 9 ] the case study approach lends itself well to capturing information on more explanatory ' how ', 'what' and ' why ' questions, such as ' how is the intervention being implemented and received on the ground?'. The case study approach can offer additional insights into what gaps exist in its delivery or why one implementation strategy might be chosen over another. This in turn can help develop or refine theory, as shown in our study of the teaching of patient safety in undergraduate curricula (Table 4 )[ 6 , 10 ]. Key questions to consider when selecting the most appropriate study design are whether it is desirable or indeed possible to undertake a formal experimental investigation in which individuals and/or organisations are allocated to an intervention or control arm? Or whether the wish is to obtain a more naturalistic understanding of an issue? The former is ideally studied using a controlled experimental design, whereas the latter is more appropriately studied using a case study design.
Case studies may be approached in different ways depending on the epistemological standpoint of the researcher, that is, whether they take a critical (questioning one's own and others' assumptions), interpretivist (trying to understand individual and shared social meanings) or positivist approach (orientating towards the criteria of natural sciences, such as focusing on generalisability considerations) (Table 6 ). Whilst such a schema can be conceptually helpful, it may be appropriate to draw on more than one approach in any case study, particularly in the context of conducting health services research. Doolin has, for example, noted that in the context of undertaking interpretative case studies, researchers can usefully draw on a critical, reflective perspective which seeks to take into account the wider social and political environment that has shaped the case[ 11 ].
How are case studies conducted?
Here, we focus on the main stages of research activity when planning and undertaking a case study; the crucial stages are: defining the case; selecting the case(s); collecting and analysing the data; interpreting data; and reporting the findings.
Defining the case
Carefully formulated research question(s), informed by the existing literature and a prior appreciation of the theoretical issues and setting(s), are all important in appropriately and succinctly defining the case[ 8 , 12 ]. Crucially, each case should have a pre-defined boundary which clarifies the nature and time period covered by the case study (i.e. its scope, beginning and end), the relevant social group, organisation or geographical area of interest to the investigator, the types of evidence to be collected, and the priorities for data collection and analysis (see Table 7 )[ 1 ]. A theory driven approach to defining the case may help generate knowledge that is potentially transferable to a range of clinical contexts and behaviours; using theory is also likely to result in a more informed appreciation of, for example, how and why interventions have succeeded or failed[ 13 ].
For example, in our evaluation of the introduction of electronic health records in English hospitals (Table 3 ), we defined our cases as the NHS Trusts that were receiving the new technology[ 5 ]. Our focus was on how the technology was being implemented. However, if the primary research interest had been on the social and organisational dimensions of implementation, we might have defined our case differently as a grouping of healthcare professionals (e.g. doctors and/or nurses). The precise beginning and end of the case may however prove difficult to define. Pursuing this same example, when does the process of implementation and adoption of an electronic health record system really begin or end? Such judgements will inevitably be influenced by a range of factors, including the research question, theory of interest, the scope and richness of the gathered data and the resources available to the research team.
Selecting the case(s)
The decision on how to select the case(s) to study is a very important one that merits some reflection. In an intrinsic case study, the case is selected on its own merits[ 8 ]. The case is selected not because it is representative of other cases, but because of its uniqueness, which is of genuine interest to the researchers. This was, for example, the case in our study of the recruitment of minority ethnic participants into asthma research (Table 1 ) as our earlier work had demonstrated the marginalisation of minority ethnic people with asthma, despite evidence of disproportionate asthma morbidity[ 14 , 15 ]. In another example of an intrinsic case study, Hellstrom et al.[ 16 ] studied an elderly married couple living with dementia to explore how dementia had impacted on their understanding of home, their everyday life and their relationships.
For an instrumental case study, selecting a "typical" case can work well[ 8 ]. In contrast to the intrinsic case study, the particular case which is chosen is of less importance than selecting a case that allows the researcher to investigate an issue or phenomenon. For example, in order to gain an understanding of doctors' responses to health policy initiatives, Som undertook an instrumental case study interviewing clinicians who had a range of responsibilities for clinical governance in one NHS acute hospital trust[ 17 ]. Sampling a "deviant" or "atypical" case may however prove even more informative, potentially enabling the researcher to identify causal processes, generate hypotheses and develop theory.
In collective or multiple case studies, a number of cases are carefully selected. This offers the advantage of allowing comparisons to be made across several cases and/or replication. Choosing a "typical" case may enable the findings to be generalised to theory (i.e. analytical generalisation) or to test theory by replicating the findings in a second or even a third case (i.e. replication logic)[ 1 ]. Yin suggests two or three literal replications (i.e. predicting similar results) if the theory is straightforward and five or more if the theory is more subtle. However, critics might argue that selecting 'cases' in this way is insufficiently reflexive and ill-suited to the complexities of contemporary healthcare organisations.
The selected case study site(s) should allow the research team access to the group of individuals, the organisation, the processes or whatever else constitutes the chosen unit of analysis for the study. Access is therefore a central consideration; the researcher needs to come to know the case study site(s) well and to work cooperatively with them. Selected cases need to be not only interesting but also hospitable to the inquiry [ 8 ] if they are to be informative and answer the research question(s). Case study sites may also be pre-selected for the researcher, with decisions being influenced by key stakeholders. For example, our selection of case study sites in the evaluation of the implementation and adoption of electronic health record systems (see Table 3 ) was heavily influenced by NHS Connecting for Health, the government agency that was responsible for overseeing the National Programme for Information Technology (NPfIT)[ 5 ]. This prominent stakeholder had already selected the NHS sites (through a competitive bidding process) to be early adopters of the electronic health record systems and had negotiated contracts that detailed the deployment timelines.
It is also important to consider in advance the likely burden and risks associated with participation for those who (or the site(s) which) comprise the case study. Of particular importance is the obligation for the researcher to think through the ethical implications of the study (e.g. the risk of inadvertently breaching anonymity or confidentiality) and to ensure that potential participants/participating sites are provided with sufficient information to make an informed choice about joining the study. The outcome of providing this information might be that the emotive burden associated with participation, or the organisational disruption associated with supporting the fieldwork, is considered so high that the individuals or sites decide against participation.
In our example of evaluating implementations of electronic health record systems, given the restricted number of early adopter sites available to us, we sought purposively to select a diverse range of implementation cases among those that were available[ 5 ]. We chose a mixture of teaching, non-teaching and Foundation Trust hospitals, and examples of each of the three electronic health record systems procured centrally by the NPfIT. At one recruited site, it quickly became apparent that access was problematic because of competing demands on that organisation. Recognising the importance of full access and co-operative working for generating rich data, the research team decided not to pursue work at that site and instead to focus on other recruited sites.
Collecting the data
In order to develop a thorough understanding of the case, the case study approach usually involves the collection of multiple sources of evidence, using a range of quantitative (e.g. questionnaires, audits and analysis of routinely collected healthcare data) and more commonly qualitative techniques (e.g. interviews, focus groups and observations). The use of multiple sources of data (data triangulation) has been advocated as a way of increasing the internal validity of a study (i.e. the extent to which the method is appropriate to answer the research question)[ 8 , 18 – 21 ]. An underlying assumption is that data collected in different ways should lead to similar conclusions, and approaching the same issue from different angles can help develop a holistic picture of the phenomenon (Table 2 )[ 4 ].
Brazier and colleagues used a mixed-methods case study approach to investigate the impact of a cancer care programme[ 22 ]. Here, quantitative measures were collected with questionnaires before, and five months after, the start of the intervention which did not yield any statistically significant results. Qualitative interviews with patients however helped provide an insight into potentially beneficial process-related aspects of the programme, such as greater, perceived patient involvement in care. The authors reported how this case study approach provided a number of contextual factors likely to influence the effectiveness of the intervention and which were not likely to have been obtained from quantitative methods alone.
In collective or multiple case studies, data collection needs to be flexible enough to allow a detailed description of each individual case to be developed (e.g. the nature of different cancer care programmes), before considering the emerging similarities and differences in cross-case comparisons (e.g. to explore why one programme is more effective than another). It is important that data sources from different cases are, where possible, broadly comparable for this purpose even though they may vary in nature and depth.
Analysing, interpreting and reporting case studies
Making sense and offering a coherent interpretation of the typically disparate sources of data (whether qualitative alone or together with quantitative) is far from straightforward. Repeated reviewing and sorting of the voluminous and detail-rich data are integral to the process of analysis. In collective case studies, it is helpful to analyse data relating to the individual component cases first, before making comparisons across cases. Attention needs to be paid to variations within each case and, where relevant, the relationship between different causes, effects and outcomes[ 23 ]. Data will need to be organised and coded to allow the key issues, both derived from the literature and emerging from the dataset, to be easily retrieved at a later stage. An initial coding frame can help capture these issues and can be applied systematically to the whole dataset with the aid of a qualitative data analysis software package.
The Framework approach is a practical approach, comprising of five stages (familiarisation; identifying a thematic framework; indexing; charting; mapping and interpretation) , to managing and analysing large datasets particularly if time is limited, as was the case in our study of recruitment of South Asians into asthma research (Table 1 )[ 3 , 24 ]. Theoretical frameworks may also play an important role in integrating different sources of data and examining emerging themes. For example, we drew on a socio-technical framework to help explain the connections between different elements - technology; people; and the organisational settings within which they worked - in our study of the introduction of electronic health record systems (Table 3 )[ 5 ]. Our study of patient safety in undergraduate curricula drew on an evaluation-based approach to design and analysis, which emphasised the importance of the academic, organisational and practice contexts through which students learn (Table 4 )[ 6 ].
Case study findings can have implications both for theory development and theory testing. They may establish, strengthen or weaken historical explanations of a case and, in certain circumstances, allow theoretical (as opposed to statistical) generalisation beyond the particular cases studied[ 12 ]. These theoretical lenses should not, however, constitute a strait-jacket and the cases should not be "forced to fit" the particular theoretical framework that is being employed.
When reporting findings, it is important to provide the reader with enough contextual information to understand the processes that were followed and how the conclusions were reached. In a collective case study, researchers may choose to present the findings from individual cases separately before amalgamating across cases. Care must be taken to ensure the anonymity of both case sites and individual participants (if agreed in advance) by allocating appropriate codes or withholding descriptors. In the example given in Table 3 , we decided against providing detailed information on the NHS sites and individual participants in order to avoid the risk of inadvertent disclosure of identities[ 5 , 25 ].
What are the potential pitfalls and how can these be avoided?
The case study approach is, as with all research, not without its limitations. When investigating the formal and informal ways undergraduate students learn about patient safety (Table 4 ), for example, we rapidly accumulated a large quantity of data. The volume of data, together with the time restrictions in place, impacted on the depth of analysis that was possible within the available resources. This highlights a more general point of the importance of avoiding the temptation to collect as much data as possible; adequate time also needs to be set aside for data analysis and interpretation of what are often highly complex datasets.
Case study research has sometimes been criticised for lacking scientific rigour and providing little basis for generalisation (i.e. producing findings that may be transferable to other settings)[ 1 ]. There are several ways to address these concerns, including: the use of theoretical sampling (i.e. drawing on a particular conceptual framework); respondent validation (i.e. participants checking emerging findings and the researcher's interpretation, and providing an opinion as to whether they feel these are accurate); and transparency throughout the research process (see Table 8 )[ 8 , 18 – 21 , 23 , 26 ]. Transparency can be achieved by describing in detail the steps involved in case selection, data collection, the reasons for the particular methods chosen, and the researcher's background and level of involvement (i.e. being explicit about how the researcher has influenced data collection and interpretation). Seeking potential, alternative explanations, and being explicit about how interpretations and conclusions were reached, help readers to judge the trustworthiness of the case study report. Stake provides a critique checklist for a case study report (Table 9 )[ 8 ].
Conclusions
The case study approach allows, amongst other things, critical events, interventions, policy developments and programme-based service reforms to be studied in detail in a real-life context. It should therefore be considered when an experimental design is either inappropriate to answer the research questions posed or impossible to undertake. Considering the frequency with which implementations of innovations are now taking place in healthcare settings and how well the case study approach lends itself to in-depth, complex health service research, we believe this approach should be more widely considered by researchers. Though inherently challenging, the research case study can, if carefully conceptualised and thoughtfully undertaken and reported, yield powerful insights into many important aspects of health and healthcare delivery.
Yin RK: Case study research, design and method. 2009, London: Sage Publications Ltd., 4
Google Scholar
Keen J, Packwood T: Qualitative research; case study evaluation. BMJ. 1995, 311: 444-446.
Article CAS PubMed PubMed Central Google Scholar
Sheikh A, Halani L, Bhopal R, Netuveli G, Partridge M, Car J, et al: Facilitating the Recruitment of Minority Ethnic People into Research: Qualitative Case Study of South Asians and Asthma. PLoS Med. 2009, 6 (10): 1-11.
Article Google Scholar
Pinnock H, Huby G, Powell A, Kielmann T, Price D, Williams S, et al: The process of planning, development and implementation of a General Practitioner with a Special Interest service in Primary Care Organisations in England and Wales: a comparative prospective case study. Report for the National Co-ordinating Centre for NHS Service Delivery and Organisation R&D (NCCSDO). 2008, [ http://www.sdo.nihr.ac.uk/files/project/99-final-report.pdf ]
Robertson A, Cresswell K, Takian A, Petrakaki D, Crowe S, Cornford T, et al: Prospective evaluation of the implementation and adoption of NHS Connecting for Health's national electronic health record in secondary care in England: interim findings. BMJ. 2010, 41: c4564-
Pearson P, Steven A, Howe A, Sheikh A, Ashcroft D, Smith P, the Patient Safety Education Study Group: Learning about patient safety: organisational context and culture in the education of healthcare professionals. J Health Serv Res Policy. 2010, 15: 4-10. 10.1258/jhsrp.2009.009052.
Article PubMed Google Scholar
van Harten WH, Casparie TF, Fisscher OA: The evaluation of the introduction of a quality management system: a process-oriented case study in a large rehabilitation hospital. Health Policy. 2002, 60 (1): 17-37. 10.1016/S0168-8510(01)00187-7.
Stake RE: The art of case study research. 1995, London: Sage Publications Ltd.
Sheikh A, Smeeth L, Ashcroft R: Randomised controlled trials in primary care: scope and application. Br J Gen Pract. 2002, 52 (482): 746-51.
PubMed PubMed Central Google Scholar
King G, Keohane R, Verba S: Designing Social Inquiry. 1996, Princeton: Princeton University Press
Doolin B: Information technology as disciplinary technology: being critical in interpretative research on information systems. Journal of Information Technology. 1998, 13: 301-311. 10.1057/jit.1998.8.
George AL, Bennett A: Case studies and theory development in the social sciences. 2005, Cambridge, MA: MIT Press
Eccles M, the Improved Clinical Effectiveness through Behavioural Research Group (ICEBeRG): Designing theoretically-informed implementation interventions. Implementation Science. 2006, 1: 1-8. 10.1186/1748-5908-1-1.
Article PubMed Central Google Scholar
Netuveli G, Hurwitz B, Levy M, Fletcher M, Barnes G, Durham SR, Sheikh A: Ethnic variations in UK asthma frequency, morbidity, and health-service use: a systematic review and meta-analysis. Lancet. 2005, 365 (9456): 312-7.
Sheikh A, Panesar SS, Lasserson T, Netuveli G: Recruitment of ethnic minorities to asthma studies. Thorax. 2004, 59 (7): 634-
CAS PubMed PubMed Central Google Scholar
Hellström I, Nolan M, Lundh U: 'We do things together': A case study of 'couplehood' in dementia. Dementia. 2005, 4: 7-22. 10.1177/1471301205049188.
Som CV: Nothing seems to have changed, nothing seems to be changing and perhaps nothing will change in the NHS: doctors' response to clinical governance. International Journal of Public Sector Management. 2005, 18: 463-477. 10.1108/09513550510608903.
Lincoln Y, Guba E: Naturalistic inquiry. 1985, Newbury Park: Sage Publications
Barbour RS: Checklists for improving rigour in qualitative research: a case of the tail wagging the dog?. BMJ. 2001, 322: 1115-1117. 10.1136/bmj.322.7294.1115.
Mays N, Pope C: Qualitative research in health care: Assessing quality in qualitative research. BMJ. 2000, 320: 50-52. 10.1136/bmj.320.7226.50.
Mason J: Qualitative researching. 2002, London: Sage
Brazier A, Cooke K, Moravan V: Using Mixed Methods for Evaluating an Integrative Approach to Cancer Care: A Case Study. Integr Cancer Ther. 2008, 7: 5-17. 10.1177/1534735407313395.
Miles MB, Huberman M: Qualitative data analysis: an expanded sourcebook. 1994, CA: Sage Publications Inc., 2
Pope C, Ziebland S, Mays N: Analysing qualitative data. Qualitative research in health care. BMJ. 2000, 320: 114-116. 10.1136/bmj.320.7227.114.
Cresswell KM, Worth A, Sheikh A: Actor-Network Theory and its role in understanding the implementation of information technology developments in healthcare. BMC Med Inform Decis Mak. 2010, 10 (1): 67-10.1186/1472-6947-10-67.
Article PubMed PubMed Central Google Scholar
Malterud K: Qualitative research: standards, challenges, and guidelines. Lancet. 2001, 358: 483-488. 10.1016/S0140-6736(01)05627-6.
Article CAS PubMed Google Scholar
Yin R: Case study research: design and methods. 1994, Thousand Oaks, CA: Sage Publishing, 2
Yin R: Enhancing the quality of case studies in health services research. Health Serv Res. 1999, 34: 1209-1224.
Green J, Thorogood N: Qualitative methods for health research. 2009, Los Angeles: Sage, 2
Howcroft D, Trauth E: Handbook of Critical Information Systems Research, Theory and Application. 2005, Cheltenham, UK: Northampton, MA, USA: Edward Elgar
Book Google Scholar
Blakie N: Approaches to Social Enquiry. 1993, Cambridge: Polity Press
Doolin B: Power and resistance in the implementation of a medical management information system. Info Systems J. 2004, 14: 343-362. 10.1111/j.1365-2575.2004.00176.x.
Bloomfield BP, Best A: Management consultants: systems development, power and the translation of problems. Sociological Review. 1992, 40: 533-560.
Shanks G, Parr A: Positivist, single case study research in information systems: A critical analysis. Proceedings of the European Conference on Information Systems. 2003, Naples
Pre-publication history
The pre-publication history for this paper can be accessed here: http://www.biomedcentral.com/1471-2288/11/100/prepub
Download references
Acknowledgements
We are grateful to the participants and colleagues who contributed to the individual case studies that we have drawn on. This work received no direct funding, but it has been informed by projects funded by Asthma UK, the NHS Service Delivery Organisation, NHS Connecting for Health Evaluation Programme, and Patient Safety Research Portfolio. We would also like to thank the expert reviewers for their insightful and constructive feedback. Our thanks are also due to Dr. Allison Worth who commented on an earlier draft of this manuscript.
Author information
Authors and affiliations.
Division of Primary Care, The University of Nottingham, Nottingham, UK
Sarah Crowe & Anthony Avery
Centre for Population Health Sciences, The University of Edinburgh, Edinburgh, UK
Kathrin Cresswell, Ann Robertson & Aziz Sheikh
School of Health in Social Science, The University of Edinburgh, Edinburgh, UK
You can also search for this author in PubMed Google Scholar
Corresponding author
Correspondence to Sarah Crowe .
Additional information
Competing interests.
The authors declare that they have no competing interests.
Authors' contributions
AS conceived this article. SC, KC and AR wrote this paper with GH, AA and AS all commenting on various drafts. SC and AS are guarantors.
Rights and permissions
This article is published under license to BioMed Central Ltd. This is an Open Access article distributed under the terms of the Creative Commons Attribution License ( http://creativecommons.org/licenses/by/2.0 ), which permits unrestricted use, distribution, and reproduction in any medium, provided the original work is properly cited.
Reprints and permissions
About this article
Cite this article.
Crowe, S., Cresswell, K., Robertson, A. et al. The case study approach. BMC Med Res Methodol 11 , 100 (2011). https://doi.org/10.1186/1471-2288-11-100
Download citation
Received : 29 November 2010
Accepted : 27 June 2011
Published : 27 June 2011
DOI : https://doi.org/10.1186/1471-2288-11-100
Share this article
Anyone you share the following link with will be able to read this content:
Sorry, a shareable link is not currently available for this article.
Provided by the Springer Nature SharedIt content-sharing initiative
- Case Study Approach
- Electronic Health Record System
- Case Study Design
- Case Study Site
- Case Study Report
BMC Medical Research Methodology
ISSN: 1471-2288
- General enquiries: [email protected]
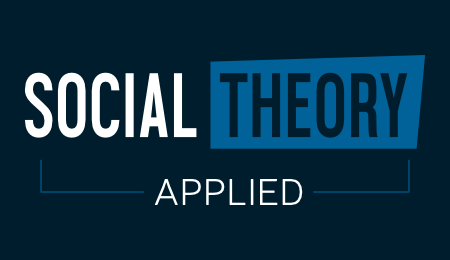
- About This Site
- What is social theory?
- Habermas/Parsons
- Frankfurt School
- Inequalities
- Research Students
- Dirty Looks
- Latest Posts
- Pedagogy & Curriculum
- Contributors
- Publications
Select Page
What are the benefits and drawbacks of case study research?
Posted by Mark Murphy | May 24, 2014 | Method , Research Students | 0
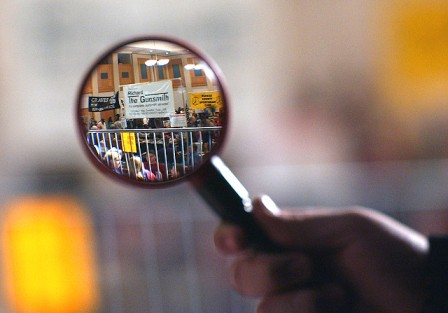
There should be no doubt that with case studies what you gain in depth you lose in breadth – this is the unavoidable compromise that needs to be understood from the beginning of the research process. So this is neither an advantage nor a disadvantage as one aspect cancels out the benefits/drawbacks of the other – there are other benefits and drawbacks that need attention however …
- Their flexibility: case studies are popular for a number of reasons, one being that they can be conducted at various points in the research process. Researchers are known to favour them as a way to develop ideas for more extensive research in the future – pilot studies often take the form of case studies. They are also effective conduits for a broad range of research methods; in that sense they are non-prejudicial against any particular type of research – focus groups are just as welcome in case study research as are questionnaires or participant observation.
- Capturing reality: One of their key benefits is their ability to capture what Hodkinson and Hodkinson call ‘lived reality’ (2001: 3). As they put it, case studies have the potential, when applied successfully, to ‘retain more of the “noise” of real life than many other types of research’ (Hodkinson and Hodkinson, 2001: 3). The importance of ‘noise’ and its place in research is especially important in contexts such as education, for example in schools where background noise is unavoidable. Educational contexts are always complex, and as a result it is difficult to exclude other unwanted variables, ‘some of which may only have real significance for one of their students’ (Hodkinson and Hodkinson, 2001, 4).
- The challenge of generality: At the same time, given their specificity, care needs to be taken when attempting to generalise from the findings. While there’s no inherent flaw in case study design that precludes its broader application, it is preferable that researchers choose their case study sites carefully, while also basing their analysis within existing research findings that have been generated via other research designs. No design is infallible but so often has the claim against case studies been made, that some of the criticism (unwarranted and unfair in many cases) has stuck.
- Suspicion of amateurism: Less partisan researchers might wonder whether the case study offers the time and finance-strapped researcher a convenient and pragmatic source of data, providing findings and recommendations that, given the nature of case studies, can neither be confirmed nor denied, in terms of utility or veracity. Who is to say that case studies offer anything more than a story to tell, and nothing more than that?
- But alongside this suspicion is another more insiduous one – a notion that ‘stories’ are not what social science research is about. This can be a concern for those who favour case study research, as the political consequences can be hard to ignore. That said, so much research is based either on peoples’ lives or the impact of other issues (poverty, institutional policy) on their lives, so the stories of what actually occurs in their lives or in professional environments tend to be an invaluable source of evidence. The fact is that stories (individual, collective, institutional) have a vital role to play in the world of research. And to play the specific v. general card against case study design suggests a tendency towards forms of research fundamentalism as opposed to any kind of rational and objective take on case study’s strengths and limitations.
- Preciousness: Having said that, researchers should not fall into the trap (surprising how often this happens) of assuming that case study data speaks for itself – rarely is this ever the case, an assumption that is as patronising to research subjects as it is false. The role of the researcher is both to describe social phenomena and also to explain – i.e., interpret. Without interpretation the research findings lack meaningful presentation – they present themselves as fact when of course the reality of ‘facts’ is one of the reasons why such research is carried out.
- Conflation of political/research objectives: Another trap that case study researchers sometimes fall into is presenting research findings as if they were self-evidently true, as if the stories were beyond criticism. This is often accompanied by a vague attachment to the notion that research is a political process – one that is performed as a form of liberation against for example policies that seek to ignore the stories of those who ‘suffer’ at the hands of overbearing political or economic imperatives. Case study design should not be viewed as a mechanism for providing a ‘local’ bulwark against the ‘global’ – bur rather as a mechanism for checking the veracity of universalist claims (at least one of its objectives). The valorisation of particularism can only get you so far in social research.
[This post is adapted from material in ‘Research and Education’ (Curtis, Murphy and Shields , Routledge 2014), pp. 80-82].
Reference: Hodkinson, P. and H. Hodkinson (2001). The strengths and limitations of case study research. Paper presented to the Learning and Skills Development Agency conference, Making an impact on policy and practice , Cambridge, 5-7 December 2001, downloaded from h ttp://education.exeter.ac.uk/tlc/docs/publications/LE_PH_PUB_05.12.01.rtf.26.01.2013
About The Author

Mark Murphy
Mark Murphy is a Reader in Education and Public Policy at the University of Glasgow. He previously worked as an academic at King’s College, London, University of Chester, University of Stirling, National University of Ireland, Maynooth, University College Dublin and Northern Illinois University. Mark is an active researcher in the fields of education and public policy. His research interests include educational sociology, critical theory, accountability in higher education, and public sector reform.
Related Posts
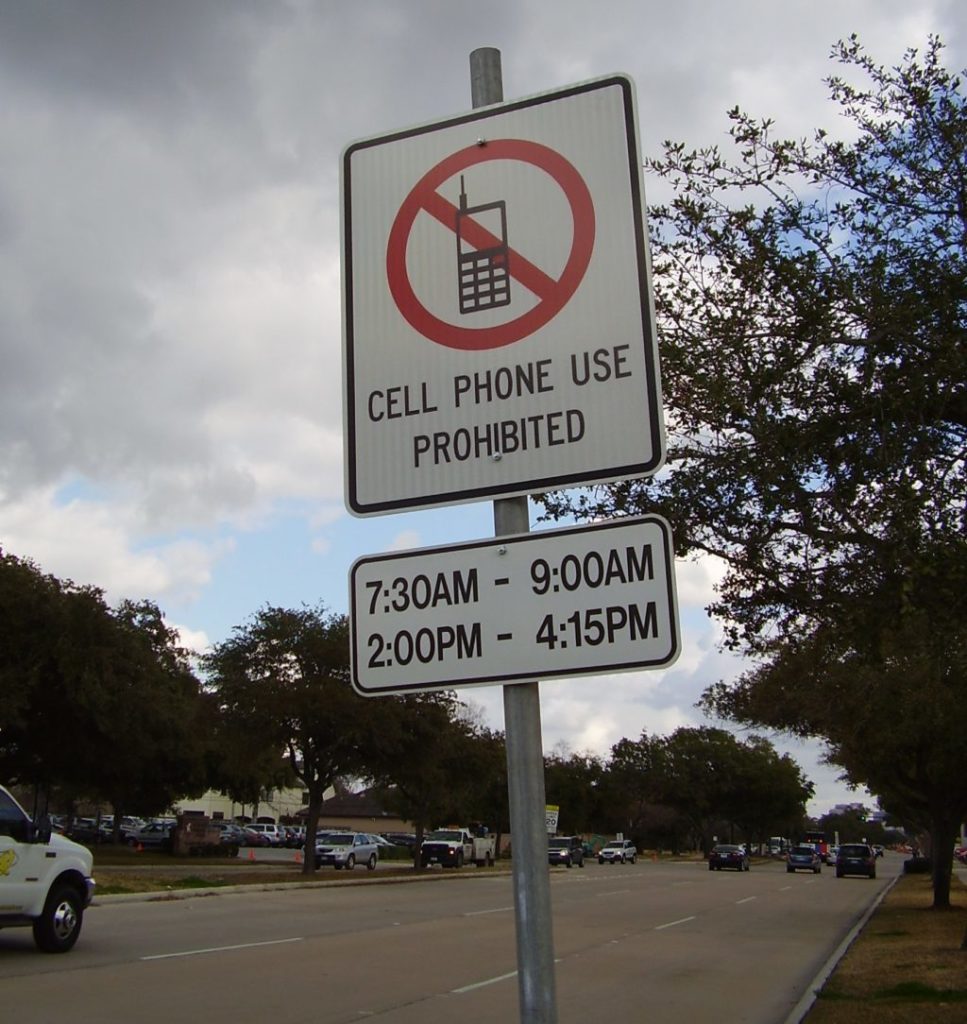
Should mobile phones be banned from Scottish Schools?
August 16, 2017

Foucault and academic leadership in Taiwan: A BERA2103 blog post
May 29, 2013

Positionality and my PhD
April 15, 2016
![benefits of a case study research design Dramatic encounters: My methodological engagement with Foucault [PART 2]](https://socialtheoryapplied.com/wp-content/blogs.dir/3/files/2016/01/control-yourself.jpg)
Dramatic encounters: My methodological engagement with Foucault [PART 2]
January 30, 2016
Recent Posts
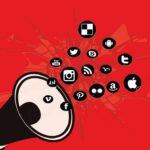
- Open access
- Published: 10 November 2020
Case study research for better evaluations of complex interventions: rationale and challenges
- Sara Paparini ORCID: orcid.org/0000-0002-1909-2481 1 ,
- Judith Green 2 ,
- Chrysanthi Papoutsi 1 ,
- Jamie Murdoch 3 ,
- Mark Petticrew 4 ,
- Trish Greenhalgh 1 ,
- Benjamin Hanckel 5 &
- Sara Shaw 1
BMC Medicine volume 18 , Article number: 301 ( 2020 ) Cite this article
19k Accesses
46 Citations
35 Altmetric
Metrics details
The need for better methods for evaluation in health research has been widely recognised. The ‘complexity turn’ has drawn attention to the limitations of relying on causal inference from randomised controlled trials alone for understanding whether, and under which conditions, interventions in complex systems improve health services or the public health, and what mechanisms might link interventions and outcomes. We argue that case study research—currently denigrated as poor evidence—is an under-utilised resource for not only providing evidence about context and transferability, but also for helping strengthen causal inferences when pathways between intervention and effects are likely to be non-linear.
Case study research, as an overall approach, is based on in-depth explorations of complex phenomena in their natural, or real-life, settings. Empirical case studies typically enable dynamic understanding of complex challenges and provide evidence about causal mechanisms and the necessary and sufficient conditions (contexts) for intervention implementation and effects. This is essential evidence not just for researchers concerned about internal and external validity, but also research users in policy and practice who need to know what the likely effects of complex programmes or interventions will be in their settings. The health sciences have much to learn from scholarship on case study methodology in the social sciences. However, there are multiple challenges in fully exploiting the potential learning from case study research. First are misconceptions that case study research can only provide exploratory or descriptive evidence. Second, there is little consensus about what a case study is, and considerable diversity in how empirical case studies are conducted and reported. Finally, as case study researchers typically (and appropriately) focus on thick description (that captures contextual detail), it can be challenging to identify the key messages related to intervention evaluation from case study reports.
Whilst the diversity of published case studies in health services and public health research is rich and productive, we recommend further clarity and specific methodological guidance for those reporting case study research for evaluation audiences.
Peer Review reports
The need for methodological development to address the most urgent challenges in health research has been well-documented. Many of the most pressing questions for public health research, where the focus is on system-level determinants [ 1 , 2 ], and for health services research, where provisions typically vary across sites and are provided through interlocking networks of services [ 3 ], require methodological approaches that can attend to complexity. The need for methodological advance has arisen, in part, as a result of the diminishing returns from randomised controlled trials (RCTs) where they have been used to answer questions about the effects of interventions in complex systems [ 4 , 5 , 6 ]. In conditions of complexity, there is limited value in maintaining the current orientation to experimental trial designs in the health sciences as providing ‘gold standard’ evidence of effect.
There are increasing calls for methodological pluralism [ 7 , 8 ], with the recognition that complex intervention and context are not easily or usefully separated (as is often the situation when using trial design), and that system interruptions may have effects that are not reducible to linear causal pathways between intervention and outcome. These calls are reflected in a shifting and contested discourse of trial design, seen with the emergence of realist [ 9 ], adaptive and hybrid (types 1, 2 and 3) [ 10 , 11 ] trials that blend studies of effectiveness with a close consideration of the contexts of implementation. Similarly, process evaluation has now become a core component of complex healthcare intervention trials, reflected in MRC guidance on how to explore implementation, causal mechanisms and context [ 12 ].
Evidence about the context of an intervention is crucial for questions of external validity. As Woolcock [ 4 ] notes, even if RCT designs are accepted as robust for maximising internal validity, questions of transferability (how well the intervention works in different contexts) and generalisability (how well the intervention can be scaled up) remain unanswered [ 5 , 13 ]. For research evidence to have impact on policy and systems organisation, and thus to improve population and patient health, there is an urgent need for better methods for strengthening external validity, including a better understanding of the relationship between intervention and context [ 14 ].
Policymakers, healthcare commissioners and other research users require credible evidence of relevance to their settings and populations [ 15 ], to perform what Rosengarten and Savransky [ 16 ] call ‘careful abstraction’ to the locales that matter for them. They also require robust evidence for understanding complex causal pathways. Case study research, currently under-utilised in public health and health services evaluation, can offer considerable potential for strengthening faith in both external and internal validity. For example, in an empirical case study of how the policy of free bus travel had specific health effects in London, UK, a quasi-experimental evaluation (led by JG) identified how important aspects of context (a good public transport system) and intervention (that it was universal) were necessary conditions for the observed effects, thus providing useful, actionable evidence for decision-makers in other contexts [ 17 ].
The overall approach of case study research is based on the in-depth exploration of complex phenomena in their natural, or ‘real-life’, settings. Empirical case studies typically enable dynamic understanding of complex challenges rather than restricting the focus on narrow problem delineations and simple fixes. Case study research is a diverse and somewhat contested field, with multiple definitions and perspectives grounded in different ways of viewing the world, and involving different combinations of methods. In this paper, we raise awareness of such plurality and highlight the contribution that case study research can make to the evaluation of complex system-level interventions. We review some of the challenges in exploiting the current evidence base from empirical case studies and conclude by recommending that further guidance and minimum reporting criteria for evaluation using case studies, appropriate for audiences in the health sciences, can enhance the take-up of evidence from case study research.
Case study research offers evidence about context, causal inference in complex systems and implementation
Well-conducted and described empirical case studies provide evidence on context, complexity and mechanisms for understanding how, where and why interventions have their observed effects. Recognition of the importance of context for understanding the relationships between interventions and outcomes is hardly new. In 1943, Canguilhem berated an over-reliance on experimental designs for determining universal physiological laws: ‘As if one could determine a phenomenon’s essence apart from its conditions! As if conditions were a mask or frame which changed neither the face nor the picture!’ ([ 18 ] p126). More recently, a concern with context has been expressed in health systems and public health research as part of what has been called the ‘complexity turn’ [ 1 ]: a recognition that many of the most enduring challenges for developing an evidence base require a consideration of system-level effects [ 1 ] and the conceptualisation of interventions as interruptions in systems [ 19 ].
The case study approach is widely recognised as offering an invaluable resource for understanding the dynamic and evolving influence of context on complex, system-level interventions [ 20 , 21 , 22 , 23 ]. Empirically, case studies can directly inform assessments of where, when, how and for whom interventions might be successfully implemented, by helping to specify the necessary and sufficient conditions under which interventions might have effects and to consolidate learning on how interdependencies, emergence and unpredictability can be managed to achieve and sustain desired effects. Case study research has the potential to address four objectives for improving research and reporting of context recently set out by guidance on taking account of context in population health research [ 24 ], that is to (1) improve the appropriateness of intervention development for specific contexts, (2) improve understanding of ‘how’ interventions work, (3) better understand how and why impacts vary across contexts and (4) ensure reports of intervention studies are most useful for decision-makers and researchers.
However, evaluations of complex healthcare interventions have arguably not exploited the full potential of case study research and can learn much from other disciplines. For evaluative research, exploratory case studies have had a traditional role of providing data on ‘process’, or initial ‘hypothesis-generating’ scoping, but might also have an increasing salience for explanatory aims. Across the social and political sciences, different kinds of case studies are undertaken to meet diverse aims (description, exploration or explanation) and across different scales (from small N qualitative studies that aim to elucidate processes, or provide thick description, to more systematic techniques designed for medium-to-large N cases).
Case studies with explanatory aims vary in terms of their positioning within mixed-methods projects, with designs including (but not restricted to) (1) single N of 1 studies of interventions in specific contexts, where the overall design is a case study that may incorporate one or more (randomised or not) comparisons over time and between variables within the case; (2) a series of cases conducted or synthesised to provide explanation from variations between cases; and (3) case studies of particular settings within RCT or quasi-experimental designs to explore variation in effects or implementation.
Detailed qualitative research (typically done as ‘case studies’ within process evaluations) provides evidence for the plausibility of mechanisms [ 25 ], offering theoretical generalisations for how interventions may function under different conditions. Although RCT designs reduce many threats to internal validity, the mechanisms of effect remain opaque, particularly when the causal pathways between ‘intervention’ and ‘effect’ are long and potentially non-linear: case study research has a more fundamental role here, in providing detailed observational evidence for causal claims [ 26 ] as well as producing a rich, nuanced picture of tensions and multiple perspectives [ 8 ].
Longitudinal or cross-case analysis may be best suited for evidence generation in system-level evaluative research. Turner [ 27 ], for instance, reflecting on the complex processes in major system change, has argued for the need for methods that integrate learning across cases, to develop theoretical knowledge that would enable inferences beyond the single case, and to develop generalisable theory about organisational and structural change in health systems. Qualitative Comparative Analysis (QCA) [ 28 ] is one such formal method for deriving causal claims, using set theory mathematics to integrate data from empirical case studies to answer questions about the configurations of causal pathways linking conditions to outcomes [ 29 , 30 ].
Nonetheless, the single N case study, too, provides opportunities for theoretical development [ 31 ], and theoretical generalisation or analytical refinement [ 32 ]. How ‘the case’ and ‘context’ are conceptualised is crucial here. Findings from the single case may seem to be confined to its intrinsic particularities in a specific and distinct context [ 33 ]. However, if such context is viewed as exemplifying wider social and political forces, the single case can be ‘telling’, rather than ‘typical’, and offer insight into a wider issue [ 34 ]. Internal comparisons within the case can offer rich possibilities for logical inferences about causation [ 17 ]. Further, case studies of any size can be used for theory testing through refutation [ 22 ]. The potential lies, then, in utilising the strengths and plurality of case study to support theory-driven research within different methodological paradigms.
Evaluation research in health has much to learn from a range of social sciences where case study methodology has been used to develop various kinds of causal inference. For instance, Gerring [ 35 ] expands on the within-case variations utilised to make causal claims. For Gerring [ 35 ], case studies come into their own with regard to invariant or strong causal claims (such as X is a necessary and/or sufficient condition for Y) rather than for probabilistic causal claims. For the latter (where experimental methods might have an advantage in estimating effect sizes), case studies offer evidence on mechanisms: from observations of X affecting Y, from process tracing or from pattern matching. Case studies also support the study of emergent causation, that is, the multiple interacting properties that account for particular and unexpected outcomes in complex systems, such as in healthcare [ 8 ].
Finally, efficacy (or beliefs about efficacy) is not the only contributor to intervention uptake, with a range of organisational and policy contingencies affecting whether an intervention is likely to be rolled out in practice. Case study research is, therefore, invaluable for learning about contextual contingencies and identifying the conditions necessary for interventions to become normalised (i.e. implemented routinely) in practice [ 36 ].
The challenges in exploiting evidence from case study research
At present, there are significant challenges in exploiting the benefits of case study research in evaluative health research, which relate to status, definition and reporting. Case study research has been marginalised at the bottom of an evidence hierarchy, seen to offer little by way of explanatory power, if nonetheless useful for adding descriptive data on process or providing useful illustrations for policymakers [ 37 ]. This is an opportune moment to revisit this low status. As health researchers are increasingly charged with evaluating ‘natural experiments’—the use of face masks in the response to the COVID-19 pandemic being a recent example [ 38 ]—rather than interventions that take place in settings that can be controlled, research approaches using methods to strengthen causal inference that does not require randomisation become more relevant.
A second challenge for improving the use of case study evidence in evaluative health research is that, as we have seen, what is meant by ‘case study’ varies widely, not only across but also within disciplines. There is indeed little consensus amongst methodologists as to how to define ‘a case study’. Definitions focus, variously, on small sample size or lack of control over the intervention (e.g. [ 39 ] p194), on in-depth study and context [ 40 , 41 ], on the logic of inference used [ 35 ] or on distinct research strategies which incorporate a number of methods to address questions of ‘how’ and ‘why’ [ 42 ]. Moreover, definitions developed for specific disciplines do not capture the range of ways in which case study research is carried out across disciplines. Multiple definitions of case study reflect the richness and diversity of the approach. However, evidence suggests that a lack of consensus across methodologists results in some of the limitations of published reports of empirical case studies [ 43 , 44 ]. Hyett and colleagues [ 43 ], for instance, reviewing reports in qualitative journals, found little match between methodological definitions of case study research and how authors used the term.
This raises the third challenge we identify that case study reports are typically not written in ways that are accessible or useful for the evaluation research community and policymakers. Case studies may not appear in journals widely read by those in the health sciences, either because space constraints preclude the reporting of rich, thick descriptions, or because of the reported lack of willingness of some biomedical journals to publish research that uses qualitative methods [ 45 ], signalling the persistence of the aforementioned evidence hierarchy. Where they do, however, the term ‘case study’ is used to indicate, interchangeably, a qualitative study, an N of 1 sample, or a multi-method, in-depth analysis of one example from a population of phenomena. Definitions of what constitutes the ‘case’ are frequently lacking and appear to be used as a synonym for the settings in which the research is conducted. Despite offering insights for evaluation, the primary aims may not have been evaluative, so the implications may not be explicitly drawn out. Indeed, some case study reports might properly be aiming for thick description without necessarily seeking to inform about context or causality.
Acknowledging plurality and developing guidance
We recognise that definitional and methodological plurality is not only inevitable, but also a necessary and creative reflection of the very different epistemological and disciplinary origins of health researchers, and the aims they have in doing and reporting case study research. Indeed, to provide some clarity, Thomas [ 46 ] has suggested a typology of subject/purpose/approach/process for classifying aims (e.g. evaluative or exploratory), sample rationale and selection and methods for data generation of case studies. We also recognise that the diversity of methods used in case study research, and the necessary focus on narrative reporting, does not lend itself to straightforward development of formal quality or reporting criteria.
Existing checklists for reporting case study research from the social sciences—for example Lincoln and Guba’s [ 47 ] and Stake’s [ 33 ]—are primarily orientated to the quality of narrative produced, and the extent to which they encapsulate thick description, rather than the more pragmatic issues of implications for intervention effects. Those designed for clinical settings, such as the CARE (CAse REports) guidelines, provide specific reporting guidelines for medical case reports about single, or small groups of patients [ 48 ], not for case study research.
The Design of Case Study Research in Health Care (DESCARTE) model [ 44 ] suggests a series of questions to be asked of a case study researcher (including clarity about the philosophy underpinning their research), study design (with a focus on case definition) and analysis (to improve process). The model resembles toolkits for enhancing the quality and robustness of qualitative and mixed-methods research reporting, and it is usefully open-ended and non-prescriptive. However, even if it does include some reflections on context, the model does not fully address aspects of context, logic and causal inference that are perhaps most relevant for evaluative research in health.
Hence, for evaluative research where the aim is to report empirical findings in ways that are intended to be pragmatically useful for health policy and practice, this may be an opportune time to consider how to best navigate plurality around what is (minimally) important to report when publishing empirical case studies, especially with regards to the complex relationships between context and interventions, information that case study research is well placed to provide.
The conventional scientific quest for certainty, predictability and linear causality (maximised in RCT designs) has to be augmented by the study of uncertainty, unpredictability and emergent causality [ 8 ] in complex systems. This will require methodological pluralism, and openness to broadening the evidence base to better understand both causality in and the transferability of system change intervention [ 14 , 20 , 23 , 25 ]. Case study research evidence is essential, yet is currently under exploited in the health sciences. If evaluative health research is to move beyond the current impasse on methods for understanding interventions as interruptions in complex systems, we need to consider in more detail how researchers can conduct and report empirical case studies which do aim to elucidate the contextual factors which interact with interventions to produce particular effects. To this end, supported by the UK’s Medical Research Council, we are embracing the challenge to develop guidance for case study researchers studying complex interventions. Following a meta-narrative review of the literature, we are planning a Delphi study to inform guidance that will, at minimum, cover the value of case study research for evaluating the interrelationship between context and complex system-level interventions; for situating and defining ‘the case’, and generalising from case studies; as well as provide specific guidance on conducting, analysing and reporting case study research. Our hope is that such guidance can support researchers evaluating interventions in complex systems to better exploit the diversity and richness of case study research.
Availability of data and materials
Not applicable (article based on existing available academic publications)
Abbreviations
Qualitative comparative analysis
Quasi-experimental design
Randomised controlled trial
Diez Roux AV. Complex systems thinking and current impasses in health disparities research. Am J Public Health. 2011;101(9):1627–34.
Article Google Scholar
Ogilvie D, Mitchell R, Mutrie N, M P, Platt S. Evaluating health effects of transport interventions: methodologic case study. Am J Prev Med 2006;31:118–126.
Walshe C. The evaluation of complex interventions in palliative care: an exploration of the potential of case study research strategies. Palliat Med. 2011;25(8):774–81.
Woolcock M. Using case studies to explore the external validity of ‘complex’ development interventions. Evaluation. 2013;19:229–48.
Cartwright N. Are RCTs the gold standard? BioSocieties. 2007;2(1):11–20.
Deaton A, Cartwright N. Understanding and misunderstanding randomized controlled trials. Soc Sci Med. 2018;210:2–21.
Salway S, Green J. Towards a critical complex systems approach to public health. Crit Public Health. 2017;27(5):523–4.
Greenhalgh T, Papoutsi C. Studying complexity in health services research: desperately seeking an overdue paradigm shift. BMC Med. 2018;16(1):95.
Bonell C, Warren E, Fletcher A. Realist trials and the testing of context-mechanism-outcome configurations: a response to Van Belle et al. Trials. 2016;17:478.
Pallmann P, Bedding AW, Choodari-Oskooei B. Adaptive designs in clinical trials: why use them, and how to run and report them. BMC Med. 2018;16:29.
Curran G, Bauer M, Mittman B, Pyne J, Stetler C. Effectiveness-implementation hybrid designs: combining elements of clinical effectiveness and implementation research to enhance public health impact. Med Care. 2012;50(3):217–26. https://doi.org/10.1097/MLR.0b013e3182408812 .
Moore GF, Audrey S, Barker M, Bond L, Bonell C, Hardeman W, et al. Process evaluation of complex interventions: Medical Research Council guidance. BMJ. 2015 [cited 2020 Jun 27];350. Available from: https://www.bmj.com/content/350/bmj.h1258 .
Evans RE, Craig P, Hoddinott P, Littlecott H, Moore L, Murphy S, et al. When and how do ‘effective’ interventions need to be adapted and/or re-evaluated in new contexts? The need for guidance. J Epidemiol Community Health. 2019;73(6):481–2.
Shoveller J. A critical examination of representations of context within research on population health interventions. Crit Public Health. 2016;26(5):487–500.
Treweek S, Zwarenstein M. Making trials matter: pragmatic and explanatory trials and the problem of applicability. Trials. 2009;10(1):37.
Rosengarten M, Savransky M. A careful biomedicine? Generalization and abstraction in RCTs. Crit Public Health. 2019;29(2):181–91.
Green J, Roberts H, Petticrew M, Steinbach R, Goodman A, Jones A, et al. Integrating quasi-experimental and inductive designs in evaluation: a case study of the impact of free bus travel on public health. Evaluation. 2015;21(4):391–406.
Canguilhem G. The normal and the pathological. New York: Zone Books; 1991. (1949).
Google Scholar
Hawe P, Shiell A, Riley T. Theorising interventions as events in systems. Am J Community Psychol. 2009;43:267–76.
King G, Keohane RO, Verba S. Designing social inquiry: scientific inference in qualitative research: Princeton University Press; 1994.
Greenhalgh T, Robert G, Macfarlane F, Bate P, Kyriakidou O. Diffusion of innovations in service organizations: systematic review and recommendations. Milbank Q. 2004;82(4):581–629.
Yin R. Enhancing the quality of case studies in health services research. Health Serv Res. 1999;34(5 Pt 2):1209.
CAS PubMed PubMed Central Google Scholar
Raine R, Fitzpatrick R, Barratt H, Bevan G, Black N, Boaden R, et al. Challenges, solutions and future directions in the evaluation of service innovations in health care and public health. Health Serv Deliv Res. 2016 [cited 2020 Jun 30];4(16). Available from: https://www.journalslibrary.nihr.ac.uk/hsdr/hsdr04160#/abstract .
Craig P, Di Ruggiero E, Frohlich KL, E M, White M, Group CCGA. Taking account of context in population health intervention research: guidance for producers, users and funders of research. NIHR Evaluation, Trials and Studies Coordinating Centre; 2018.
Grant RL, Hood R. Complex systems, explanation and policy: implications of the crisis of replication for public health research. Crit Public Health. 2017;27(5):525–32.
Mahoney J. Strategies of causal inference in small-N analysis. Sociol Methods Res. 2000;4:387–424.
Turner S. Major system change: a management and organisational research perspective. In: Rosalind Raine, Ray Fitzpatrick, Helen Barratt, Gywn Bevan, Nick Black, Ruth Boaden, et al. Challenges, solutions and future directions in the evaluation of service innovations in health care and public health. Health Serv Deliv Res. 2016;4(16) 2016. https://doi.org/10.3310/hsdr04160.
Ragin CC. Using qualitative comparative analysis to study causal complexity. Health Serv Res. 1999;34(5 Pt 2):1225.
Hanckel B, Petticrew M, Thomas J, Green J. Protocol for a systematic review of the use of qualitative comparative analysis for evaluative questions in public health research. Syst Rev. 2019;8(1):252.
Schneider CQ, Wagemann C. Set-theoretic methods for the social sciences: a guide to qualitative comparative analysis: Cambridge University Press; 2012. 369 p.
Flyvbjerg B. Five misunderstandings about case-study research. Qual Inq. 2006;12:219–45.
Tsoukas H. Craving for generality and small-N studies: a Wittgensteinian approach towards the epistemology of the particular in organization and management studies. Sage Handb Organ Res Methods. 2009:285–301.
Stake RE. The art of case study research. London: Sage Publications Ltd; 1995.
Mitchell JC. Typicality and the case study. Ethnographic research: A guide to general conduct. Vol. 238241. 1984.
Gerring J. What is a case study and what is it good for? Am Polit Sci Rev. 2004;98(2):341–54.
May C, Mort M, Williams T, F M, Gask L. Health technology assessment in its local contexts: studies of telehealthcare. Soc Sci Med 2003;57:697–710.
McGill E. Trading quality for relevance: non-health decision-makers’ use of evidence on the social determinants of health. BMJ Open. 2015;5(4):007053.
Greenhalgh T. We can’t be 100% sure face masks work – but that shouldn’t stop us wearing them | Trish Greenhalgh. The Guardian. 2020 [cited 2020 Jun 27]; Available from: https://www.theguardian.com/commentisfree/2020/jun/05/face-masks-coronavirus .
Hammersley M. So, what are case studies? In: What’s wrong with ethnography? New York: Routledge; 1992.
Crowe S, Cresswell K, Robertson A, Huby G, Avery A, Sheikh A. The case study approach. BMC Med Res Methodol. 2011;11(1):100.
Luck L, Jackson D, Usher K. Case study: a bridge across the paradigms. Nurs Inq. 2006;13(2):103–9.
Yin RK. Case study research and applications: design and methods: Sage; 2017.
Hyett N, A K, Dickson-Swift V. Methodology or method? A critical review of qualitative case study reports. Int J Qual Stud Health Well-Being. 2014;9:23606.
Carolan CM, Forbat L, Smith A. Developing the DESCARTE model: the design of case study research in health care. Qual Health Res. 2016;26(5):626–39.
Greenhalgh T, Annandale E, Ashcroft R, Barlow J, Black N, Bleakley A, et al. An open letter to the BMJ editors on qualitative research. Bmj. 2016;352.
Thomas G. A typology for the case study in social science following a review of definition, discourse, and structure. Qual Inq. 2011;17(6):511–21.
Lincoln YS, Guba EG. Judging the quality of case study reports. Int J Qual Stud Educ. 1990;3(1):53–9.
Riley DS, Barber MS, Kienle GS, Aronson JK, Schoen-Angerer T, Tugwell P, et al. CARE guidelines for case reports: explanation and elaboration document. J Clin Epidemiol. 2017;89:218–35.
Download references
Acknowledgements
Not applicable
This work was funded by the Medical Research Council - MRC Award MR/S014632/1 HCS: Case study, Context and Complex interventions (TRIPLE C). SP was additionally funded by the University of Oxford's Higher Education Innovation Fund (HEIF).
Author information
Authors and affiliations.
Nuffield Department of Primary Care Health Sciences, University of Oxford, Oxford, UK
Sara Paparini, Chrysanthi Papoutsi, Trish Greenhalgh & Sara Shaw
Wellcome Centre for Cultures & Environments of Health, University of Exeter, Exeter, UK
Judith Green
School of Health Sciences, University of East Anglia, Norwich, UK
Jamie Murdoch
Public Health, Environments and Society, London School of Hygiene & Tropical Medicin, London, UK
Mark Petticrew
Institute for Culture and Society, Western Sydney University, Penrith, Australia
Benjamin Hanckel
You can also search for this author in PubMed Google Scholar
Contributions
JG, MP, SP, JM, TG, CP and SS drafted the initial paper; all authors contributed to the drafting of the final version, and read and approved the final manuscript.
Corresponding author
Correspondence to Sara Paparini .
Ethics declarations
Ethics approval and consent to participate, consent for publication, competing interests.
The authors declare that they have no competing interests.
Additional information
Publisher’s note.
Springer Nature remains neutral with regard to jurisdictional claims in published maps and institutional affiliations.
Rights and permissions
Open Access This article is licensed under a Creative Commons Attribution 4.0 International License, which permits use, sharing, adaptation, distribution and reproduction in any medium or format, as long as you give appropriate credit to the original author(s) and the source, provide a link to the Creative Commons licence, and indicate if changes were made. The images or other third party material in this article are included in the article's Creative Commons licence, unless indicated otherwise in a credit line to the material. If material is not included in the article's Creative Commons licence and your intended use is not permitted by statutory regulation or exceeds the permitted use, you will need to obtain permission directly from the copyright holder. To view a copy of this licence, visit http://creativecommons.org/licenses/by/4.0/ . The Creative Commons Public Domain Dedication waiver ( http://creativecommons.org/publicdomain/zero/1.0/ ) applies to the data made available in this article, unless otherwise stated in a credit line to the data.
Reprints and permissions
About this article
Cite this article.
Paparini, S., Green, J., Papoutsi, C. et al. Case study research for better evaluations of complex interventions: rationale and challenges. BMC Med 18 , 301 (2020). https://doi.org/10.1186/s12916-020-01777-6
Download citation
Received : 03 July 2020
Accepted : 07 September 2020
Published : 10 November 2020
DOI : https://doi.org/10.1186/s12916-020-01777-6
Share this article
Anyone you share the following link with will be able to read this content:
Sorry, a shareable link is not currently available for this article.
Provided by the Springer Nature SharedIt content-sharing initiative
- Qualitative
- Case studies
- Mixed-method
- Public health
- Health services research
- Interventions
BMC Medicine
ISSN: 1741-7015
- General enquiries: [email protected]
Case Study Research Method in Psychology
Saul McLeod, PhD
Editor-in-Chief for Simply Psychology
BSc (Hons) Psychology, MRes, PhD, University of Manchester
Saul McLeod, PhD., is a qualified psychology teacher with over 18 years of experience in further and higher education. He has been published in peer-reviewed journals, including the Journal of Clinical Psychology.
Learn about our Editorial Process
Olivia Guy-Evans, MSc
Associate Editor for Simply Psychology
BSc (Hons) Psychology, MSc Psychology of Education
Olivia Guy-Evans is a writer and associate editor for Simply Psychology. She has previously worked in healthcare and educational sectors.
On This Page:
Case studies are in-depth investigations of a person, group, event, or community. Typically, data is gathered from various sources using several methods (e.g., observations & interviews).
The case study research method originated in clinical medicine (the case history, i.e., the patient’s personal history). In psychology, case studies are often confined to the study of a particular individual.
The information is mainly biographical and relates to events in the individual’s past (i.e., retrospective), as well as to significant events that are currently occurring in his or her everyday life.
The case study is not a research method, but researchers select methods of data collection and analysis that will generate material suitable for case studies.
Freud (1909a, 1909b) conducted very detailed investigations into the private lives of his patients in an attempt to both understand and help them overcome their illnesses.
This makes it clear that the case study is a method that should only be used by a psychologist, therapist, or psychiatrist, i.e., someone with a professional qualification.
There is an ethical issue of competence. Only someone qualified to diagnose and treat a person can conduct a formal case study relating to atypical (i.e., abnormal) behavior or atypical development.
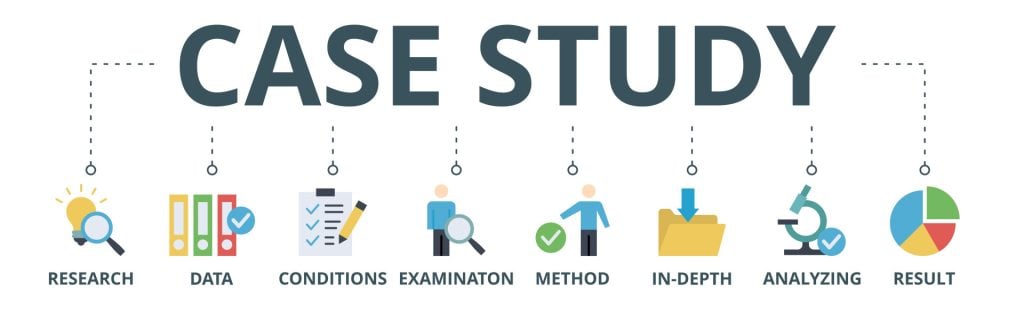
Famous Case Studies
- Anna O – One of the most famous case studies, documenting psychoanalyst Josef Breuer’s treatment of “Anna O” (real name Bertha Pappenheim) for hysteria in the late 1800s using early psychoanalytic theory.
- Little Hans – A child psychoanalysis case study published by Sigmund Freud in 1909 analyzing his five-year-old patient Herbert Graf’s house phobia as related to the Oedipus complex.
- Bruce/Brenda – Gender identity case of the boy (Bruce) whose botched circumcision led psychologist John Money to advise gender reassignment and raise him as a girl (Brenda) in the 1960s.
- Genie Wiley – Linguistics/psychological development case of the victim of extreme isolation abuse who was studied in 1970s California for effects of early language deprivation on acquiring speech later in life.
- Phineas Gage – One of the most famous neuropsychology case studies analyzes personality changes in railroad worker Phineas Gage after an 1848 brain injury involving a tamping iron piercing his skull.

Clinical Case Studies
- Studying the effectiveness of psychotherapy approaches with an individual patient
- Assessing and treating mental illnesses like depression, anxiety disorders, PTSD
- Neuropsychological cases investigating brain injuries or disorders
Child Psychology Case Studies
- Studying psychological development from birth through adolescence
- Cases of learning disabilities, autism spectrum disorders, ADHD
- Effects of trauma, abuse, deprivation on development
Types of Case Studies
- Explanatory case studies : Used to explore causation in order to find underlying principles. Helpful for doing qualitative analysis to explain presumed causal links.
- Exploratory case studies : Used to explore situations where an intervention being evaluated has no clear set of outcomes. It helps define questions and hypotheses for future research.
- Descriptive case studies : Describe an intervention or phenomenon and the real-life context in which it occurred. It is helpful for illustrating certain topics within an evaluation.
- Multiple-case studies : Used to explore differences between cases and replicate findings across cases. Helpful for comparing and contrasting specific cases.
- Intrinsic : Used to gain a better understanding of a particular case. Helpful for capturing the complexity of a single case.
- Collective : Used to explore a general phenomenon using multiple case studies. Helpful for jointly studying a group of cases in order to inquire into the phenomenon.
Where Do You Find Data for a Case Study?
There are several places to find data for a case study. The key is to gather data from multiple sources to get a complete picture of the case and corroborate facts or findings through triangulation of evidence. Most of this information is likely qualitative (i.e., verbal description rather than measurement), but the psychologist might also collect numerical data.
1. Primary sources
- Interviews – Interviewing key people related to the case to get their perspectives and insights. The interview is an extremely effective procedure for obtaining information about an individual, and it may be used to collect comments from the person’s friends, parents, employer, workmates, and others who have a good knowledge of the person, as well as to obtain facts from the person him or herself.
- Observations – Observing behaviors, interactions, processes, etc., related to the case as they unfold in real-time.
- Documents & Records – Reviewing private documents, diaries, public records, correspondence, meeting minutes, etc., relevant to the case.
2. Secondary sources
- News/Media – News coverage of events related to the case study.
- Academic articles – Journal articles, dissertations etc. that discuss the case.
- Government reports – Official data and records related to the case context.
- Books/films – Books, documentaries or films discussing the case.
3. Archival records
Searching historical archives, museum collections and databases to find relevant documents, visual/audio records related to the case history and context.
Public archives like newspapers, organizational records, photographic collections could all include potentially relevant pieces of information to shed light on attitudes, cultural perspectives, common practices and historical contexts related to psychology.
4. Organizational records
Organizational records offer the advantage of often having large datasets collected over time that can reveal or confirm psychological insights.
Of course, privacy and ethical concerns regarding confidential data must be navigated carefully.
However, with proper protocols, organizational records can provide invaluable context and empirical depth to qualitative case studies exploring the intersection of psychology and organizations.
- Organizational/industrial psychology research : Organizational records like employee surveys, turnover/retention data, policies, incident reports etc. may provide insight into topics like job satisfaction, workplace culture and dynamics, leadership issues, employee behaviors etc.
- Clinical psychology : Therapists/hospitals may grant access to anonymized medical records to study aspects like assessments, diagnoses, treatment plans etc. This could shed light on clinical practices.
- School psychology : Studies could utilize anonymized student records like test scores, grades, disciplinary issues, and counseling referrals to study child development, learning barriers, effectiveness of support programs, and more.
How do I Write a Case Study in Psychology?
Follow specified case study guidelines provided by a journal or your psychology tutor. General components of clinical case studies include: background, symptoms, assessments, diagnosis, treatment, and outcomes. Interpreting the information means the researcher decides what to include or leave out. A good case study should always clarify which information is the factual description and which is an inference or the researcher’s opinion.
1. Introduction
- Provide background on the case context and why it is of interest, presenting background information like demographics, relevant history, and presenting problem.
- Compare briefly to similar published cases if applicable. Clearly state the focus/importance of the case.
2. Case Presentation
- Describe the presenting problem in detail, including symptoms, duration,and impact on daily life.
- Include client demographics like age and gender, information about social relationships, and mental health history.
- Describe all physical, emotional, and/or sensory symptoms reported by the client.
- Use patient quotes to describe the initial complaint verbatim. Follow with full-sentence summaries of relevant history details gathered, including key components that led to a working diagnosis.
- Summarize clinical exam results, namely orthopedic/neurological tests, imaging, lab tests, etc. Note actual results rather than subjective conclusions. Provide images if clearly reproducible/anonymized.
- Clearly state the working diagnosis or clinical impression before transitioning to management.
3. Management and Outcome
- Indicate the total duration of care and number of treatments given over what timeframe. Use specific names/descriptions for any therapies/interventions applied.
- Present the results of the intervention,including any quantitative or qualitative data collected.
- For outcomes, utilize visual analog scales for pain, medication usage logs, etc., if possible. Include patient self-reports of improvement/worsening of symptoms. Note the reason for discharge/end of care.
4. Discussion
- Analyze the case, exploring contributing factors, limitations of the study, and connections to existing research.
- Analyze the effectiveness of the intervention,considering factors like participant adherence, limitations of the study, and potential alternative explanations for the results.
- Identify any questions raised in the case analysis and relate insights to established theories and current research if applicable. Avoid definitive claims about physiological explanations.
- Offer clinical implications, and suggest future research directions.
5. Additional Items
- Thank specific assistants for writing support only. No patient acknowledgments.
- References should directly support any key claims or quotes included.
- Use tables/figures/images only if substantially informative. Include permissions and legends/explanatory notes.
- Provides detailed (rich qualitative) information.
- Provides insight for further research.
- Permitting investigation of otherwise impractical (or unethical) situations.
Case studies allow a researcher to investigate a topic in far more detail than might be possible if they were trying to deal with a large number of research participants (nomothetic approach) with the aim of ‘averaging’.
Because of their in-depth, multi-sided approach, case studies often shed light on aspects of human thinking and behavior that would be unethical or impractical to study in other ways.
Research that only looks into the measurable aspects of human behavior is not likely to give us insights into the subjective dimension of experience, which is important to psychoanalytic and humanistic psychologists.
Case studies are often used in exploratory research. They can help us generate new ideas (that might be tested by other methods). They are an important way of illustrating theories and can help show how different aspects of a person’s life are related to each other.
The method is, therefore, important for psychologists who adopt a holistic point of view (i.e., humanistic psychologists ).
Limitations
- Lacking scientific rigor and providing little basis for generalization of results to the wider population.
- Researchers’ own subjective feelings may influence the case study (researcher bias).
- Difficult to replicate.
- Time-consuming and expensive.
- The volume of data, together with the time restrictions in place, impacted the depth of analysis that was possible within the available resources.
Because a case study deals with only one person/event/group, we can never be sure if the case study investigated is representative of the wider body of “similar” instances. This means the conclusions drawn from a particular case may not be transferable to other settings.
Because case studies are based on the analysis of qualitative (i.e., descriptive) data , a lot depends on the psychologist’s interpretation of the information she has acquired.
This means that there is a lot of scope for Anna O , and it could be that the subjective opinions of the psychologist intrude in the assessment of what the data means.
For example, Freud has been criticized for producing case studies in which the information was sometimes distorted to fit particular behavioral theories (e.g., Little Hans ).
This is also true of Money’s interpretation of the Bruce/Brenda case study (Diamond, 1997) when he ignored evidence that went against his theory.
Breuer, J., & Freud, S. (1895). Studies on hysteria . Standard Edition 2: London.
Curtiss, S. (1981). Genie: The case of a modern wild child .
Diamond, M., & Sigmundson, K. (1997). Sex Reassignment at Birth: Long-term Review and Clinical Implications. Archives of Pediatrics & Adolescent Medicine , 151(3), 298-304
Freud, S. (1909a). Analysis of a phobia of a five year old boy. In The Pelican Freud Library (1977), Vol 8, Case Histories 1, pages 169-306
Freud, S. (1909b). Bemerkungen über einen Fall von Zwangsneurose (Der “Rattenmann”). Jb. psychoanal. psychopathol. Forsch ., I, p. 357-421; GW, VII, p. 379-463; Notes upon a case of obsessional neurosis, SE , 10: 151-318.
Harlow J. M. (1848). Passage of an iron rod through the head. Boston Medical and Surgical Journal, 39 , 389–393.
Harlow, J. M. (1868). Recovery from the Passage of an Iron Bar through the Head . Publications of the Massachusetts Medical Society. 2 (3), 327-347.
Money, J., & Ehrhardt, A. A. (1972). Man & Woman, Boy & Girl : The Differentiation and Dimorphism of Gender Identity from Conception to Maturity. Baltimore, Maryland: Johns Hopkins University Press.
Money, J., & Tucker, P. (1975). Sexual signatures: On being a man or a woman.
Further Information
- Case Study Approach
- Case Study Method
- Enhancing the Quality of Case Studies in Health Services Research
- “We do things together” A case study of “couplehood” in dementia
- Using mixed methods for evaluating an integrative approach to cancer care: a case study

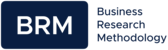
Case Studies
Case studies are a popular research method in business area. Case studies aim to analyze specific issues within the boundaries of a specific environment, situation or organization.
According to its design, case studies in business research can be divided into three categories: explanatory, descriptive and exploratory.
Explanatory case studies aim to answer ‘how’ or ’why’ questions with little control on behalf of researcher over occurrence of events. This type of case studies focus on phenomena within the contexts of real-life situations. Example: “An investigation into the reasons of the global financial and economic crisis of 2008 – 2010.”
Descriptive case studies aim to analyze the sequence of interpersonal events after a certain amount of time has passed. Studies in business research belonging to this category usually describe culture or sub-culture, and they attempt to discover the key phenomena. Example: “Impact of increasing levels of multiculturalism on marketing practices: A case study of McDonald’s Indonesia.”
Exploratory case studies aim to find answers to the questions of ‘what’ or ‘who’. Exploratory case study data collection method is often accompanied by additional data collection method(s) such as interviews, questionnaires, experiments etc. Example: “A study into differences of leadership practices between private and public sector organizations in Atlanta, USA.”
Advantages of case study method include data collection and analysis within the context of phenomenon, integration of qualitative and quantitative data in data analysis, and the ability to capture complexities of real-life situations so that the phenomenon can be studied in greater levels of depth. Case studies do have certain disadvantages that may include lack of rigor, challenges associated with data analysis and very little basis for generalizations of findings and conclusions.
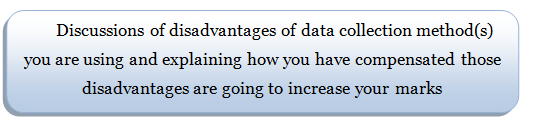
John Dudovskiy

Home » Pros and Cons » 12 Case Study Method Advantages and Disadvantages
12 Case Study Method Advantages and Disadvantages
A case study is an investigation into an individual circumstance. The investigation may be of a single person, business, event, or group. The investigation involves collecting in-depth data about the individual entity through the use of several collection methods. Interviews and observation are two of the most common forms of data collection used.
The case study method was originally developed in the field of clinical medicine. It has expanded since to other industries to examine key results, either positive or negative, that were received through a specific set of decisions. This allows for the topic to be researched with great detail, allowing others to glean knowledge from the information presented.
Here are the advantages and disadvantages of using the case study method.
List of the Advantages of the Case Study Method
1. it turns client observations into useable data..
Case studies offer verifiable data from direct observations of the individual entity involved. These observations provide information about input processes. It can show the path taken which led to specific results being generated. Those observations make it possible for others, in similar circumstances, to potentially replicate the results discovered by the case study method.
2. It turns opinion into fact.
Case studies provide facts to study because you’re looking at data which was generated in real-time. It is a way for researchers to turn their opinions into information that can be verified as fact because there is a proven path of positive or negative development. Singling out a specific incident also provides in-depth details about the path of development, which gives it extra credibility to the outside observer.
3. It is relevant to all parties involved.
Case studies that are chosen well will be relevant to everyone who is participating in the process. Because there is such a high level of relevance involved, researchers are able to stay actively engaged in the data collection process. Participants are able to further their knowledge growth because there is interest in the outcome of the case study. Most importantly, the case study method essentially forces people to make a decision about the question being studied, then defend their position through the use of facts.
4. It uses a number of different research methodologies.
The case study method involves more than just interviews and direct observation. Case histories from a records database can be used with this method. Questionnaires can be distributed to participants in the entity being studies. Individuals who have kept diaries and journals about the entity being studied can be included. Even certain experimental tasks, such as a memory test, can be part of this research process.
5. It can be done remotely.
Researchers do not need to be present at a specific location or facility to utilize the case study method. Research can be obtained over the phone, through email, and other forms of remote communication. Even interviews can be conducted over the phone. That means this method is good for formative research that is exploratory in nature, even if it must be completed from a remote location.
6. It is inexpensive.
Compared to other methods of research, the case study method is rather inexpensive. The costs associated with this method involve accessing data, which can often be done for free. Even when there are in-person interviews or other on-site duties involved, the costs of reviewing the data are minimal.
7. It is very accessible to readers.
The case study method puts data into a usable format for those who read the data and note its outcome. Although there may be perspectives of the researcher included in the outcome, the goal of this method is to help the reader be able to identify specific concepts to which they also relate. That allows them to discover unusual features within the data, examine outliers that may be present, or draw conclusions from their own experiences.
List of the Disadvantages of the Case Study Method
1. it can have influence factors within the data..
Every person has their own unconscious bias. Although the case study method is designed to limit the influence of this bias by collecting fact-based data, it is the collector of the data who gets to define what is a “fact” and what is not. That means the real-time data being collected may be based on the results the researcher wants to see from the entity instead. By controlling how facts are collected, a research can control the results this method generates.
2. It takes longer to analyze the data.
The information collection process through the case study method takes much longer to collect than other research options. That is because there is an enormous amount of data which must be sifted through. It’s not just the researchers who can influence the outcome in this type of research method. Participants can also influence outcomes by given inaccurate or incomplete answers to questions they are asked. Researchers must verify the information presented to ensure its accuracy, and that takes time to complete.
3. It can be an inefficient process.
Case study methods require the participation of the individuals or entities involved for it to be a successful process. That means the skills of the researcher will help to determine the quality of information that is being received. Some participants may be quiet, unwilling to answer even basic questions about what is being studied. Others may be overly talkative, exploring tangents which have nothing to do with the case study at all. If researchers are unsure of how to manage this process, then incomplete data is often collected.
4. It requires a small sample size to be effective.
The case study method requires a small sample size for it to yield an effective amount of data to be analyzed. If there are different demographics involved with the entity, or there are different needs which must be examined, then the case study method becomes very inefficient.
5. It is a labor-intensive method of data collection.
The case study method requires researchers to have a high level of language skills to be successful with data collection. Researchers must be personally involved in every aspect of collecting the data as well. From reviewing files or entries personally to conducting personal interviews, the concepts and themes of this process are heavily reliant on the amount of work each researcher is willing to put into things.
These case study method advantages and disadvantages offer a look at the effectiveness of this research option. With the right skill set, it can be used as an effective tool to gather rich, detailed information about specific entities. Without the right skill set, the case study method becomes inefficient and inaccurate.
Related Posts:
- 25 Best Ways to Overcome the Fear of Failure
- Monroe's Motivated Sequence Explained [with Examples]
- 21 Most Effective Bundle Pricing Strategies with Examples
- Force Field Analysis Explained with Examples
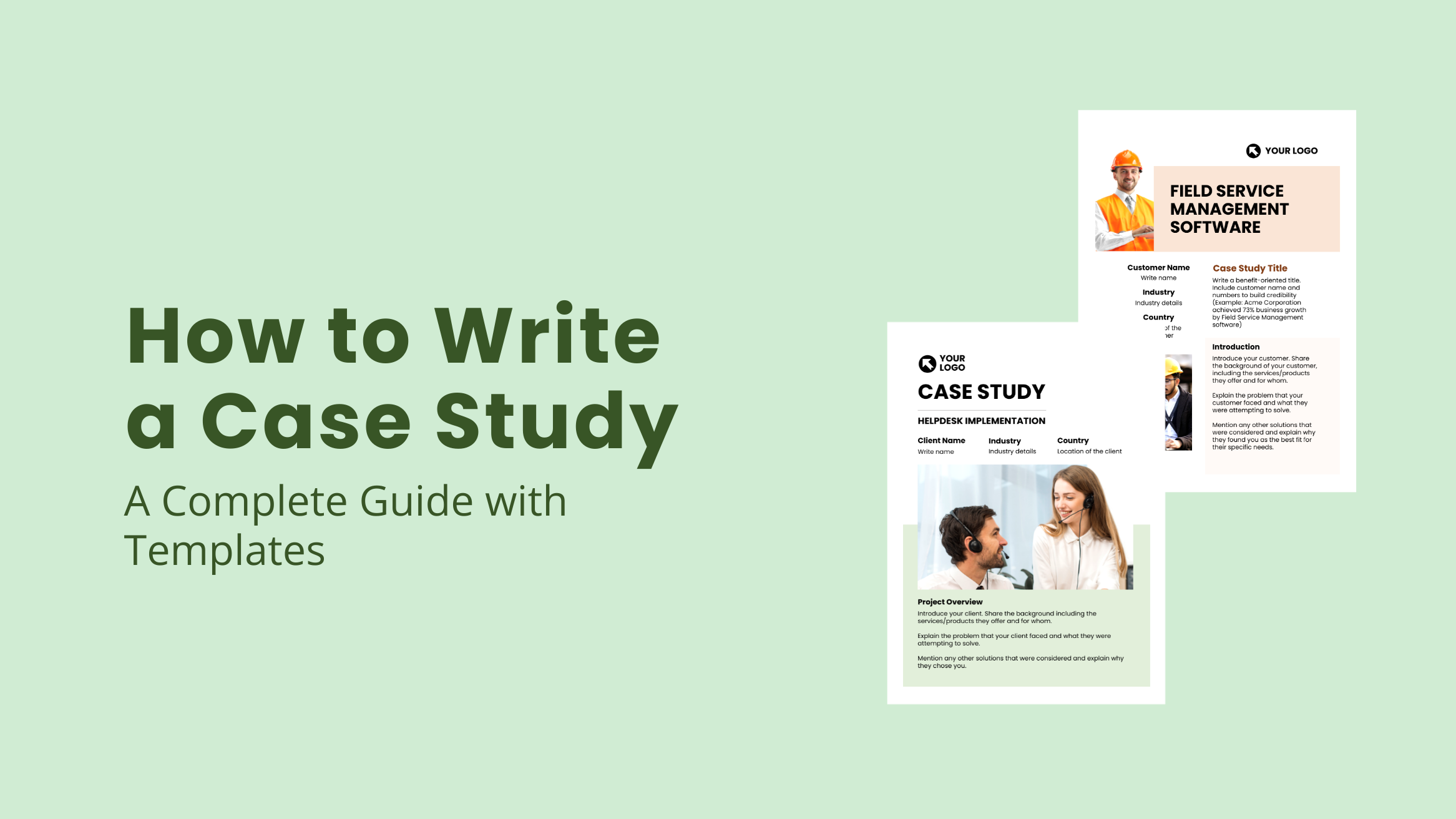
How to Write a Case Study: A Complete Guide with Templates

Writing compelling and insightful case studies is a marketer’s biggest job, yet most get frustrated with this content. The challenge? Figuring out how to write a case study that not only highlights the company’s strongest suit but engages new clients with strategic information. If you often struggle with making case studies as more than just dry facts and figures, you’re leading your efforts to missed opportunities.
How to Write a Case Study Step-by-Step
- Craft a Compelling Headline: Highlight the main success with a clear, direct title.
- Start with a Strong Introduction: Provide a broad overview and hook the reader.
- Discuss Unique Client Challenges: Highlight specific industry-related challenges.
- Highlight the Solution: Showcase your strategies and key results.
- Present Quantifiable Results: Use data and visuals to demonstrate impact.
- Be Clear and Concise: Stick to the point and support claims with data.
- Treat Your Case Study Like a Story: Focus on the customer’s journey and success.
- Use Direct Quotes from the Client: Add authenticity with client testimonials.
- Make the Key Takeaway Clear: Reinforce your expertise and the solution’s value.
- Include a Call to Action (CTA): Guide the reader on what to do next.
- Make It Readable: Use simple language, short paragraphs, and bullet points.
- Finalize and Proofread: Review for errors and ensure a smooth flow.
In this blog, you’ll discover a step-by-step guide that simplifies the process, making it easier to create interesting case studies. From planning to writing, I’ve got you covered. So, let’s start with some basics.
Table of Contents
What is the format of a case study.
- How to Plan a Case Study
How to Write a Case Study
How to summarize a case study, how to cite a case study.
A well-structured case study isn’t just a collection of facts—it’s a powerful marketing tool that tells a compelling story. Using the right format for a case study ensures that your message is clear, engaging, and impactful.
The proper format guides readers through the narrative with hierarchy and scannability, helping them connect with your brand on a deeper level. Most importantly, it empowers you as a marketer to set clear goals for presenting your case studies and ensures you deliver the correct information effectively!
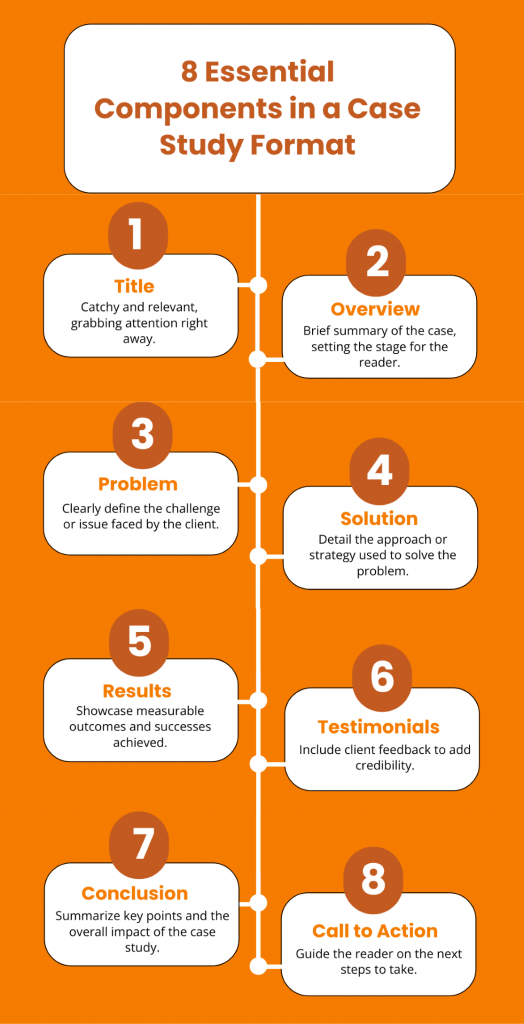
Case studies format helps you to plan and write the case study for your clients. With this outline in mind, you can create steps to complete the process of writing and publishing your case study research. There are eight components of a case study that are essential for building a layout of information in the correct order that makes sense to the viewers.
Start with a catchy “Title” that grabs attention and an “Overview” that sets the stage. Clearly define the “Problem” your client faced, and then showcase your “Solution” in detail. Highlight the success with “Results” that are measurable and impactful. Add authenticity with “Testimonials and Quotes” from satisfied clients. Wrap it up with a firm “Conclusion” and a compelling “Call to Action” in the “About Us” section that guides the reader on what to do next.
By following this format, you create a case study design that resonates with your audience and effectively showcases your brand’s value.
Check out the marketing case study template I’ve included below—it has a clear outline that makes it easy to see how sticking to a format can help you plan and write the entire thing.
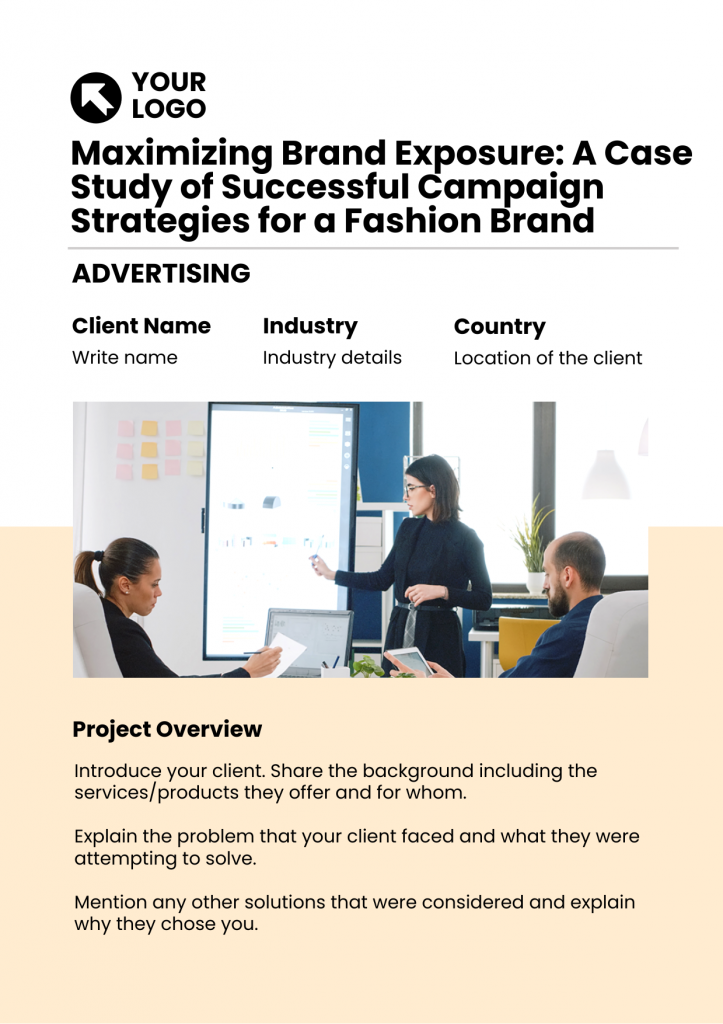
How to Plan a Case Study
Now comes the big part! Understanding what to include in a case study outline is just the starting point for beginners. The real challenge lies in creating a step-by-step plan to craft that outline and filling it in with the right information!
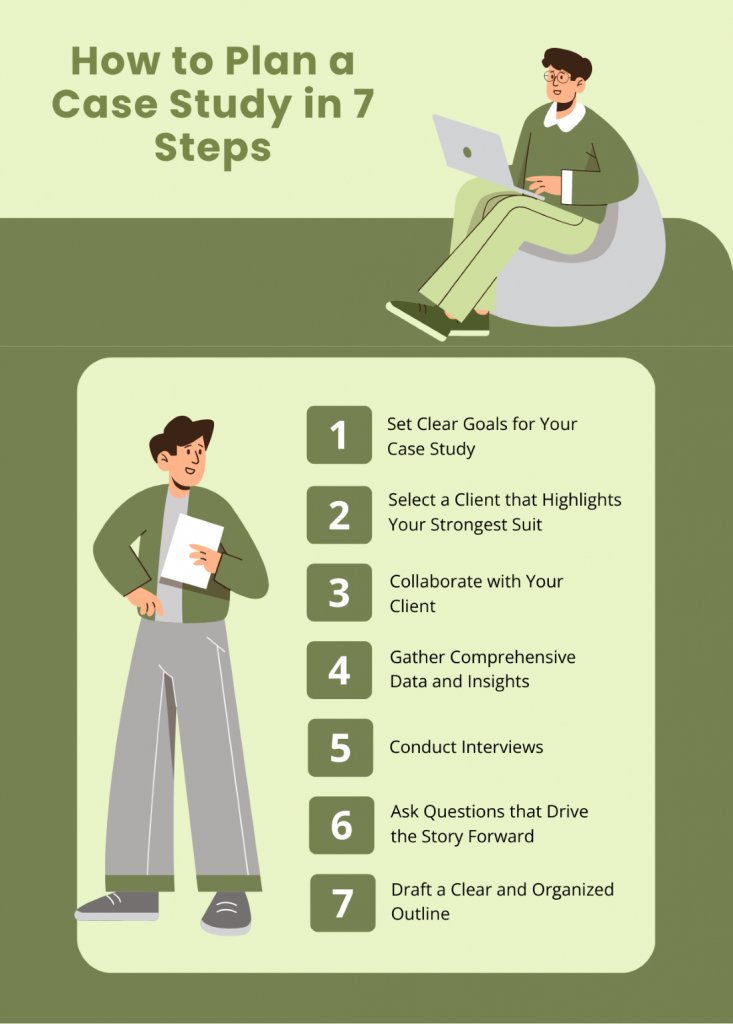
1. Set Clear Goals for Your Case Study
Before diving into how to write a case study, defining your ultimate objective is essential. Think about it—what do you want your audience to take away from this case study? For example, your goal is to showcase how your SEO strategies boosted a client’s organic traffic by 150% in just six months. This clear goal will shape your entire narrative and ensure that your case study is laser-focused on demonstrating your expertise and the value you bring.
2. Select a Client that Highlights Your Strongest Suit
Choosing the right client or subject is vital while creating case studies. Imagine you’ve worked with a small e-commerce brand struggling to rank for competitive keywords. Your strategies helped them rank on the first page and increase conversions. This is the perfect client for your case study because their success story directly showcases your SEO prowess.
By picking a client whose experience aligns with your goals, you’ll create a case study that resonates with your target audience.
3. Reach Out to Your Client for Collaboration
Now that you’ve identified the ideal client, it’s time to reach out. Let’s say you contact your client and explain how a case study can highlight their remarkable success story. It’s a great way to spotlight a mutual collaboration based on credibility. Their buy-in is crucial; their insights and data will authenticate your case study.
4. Gather Comprehensive Data and Insights
Data is the lifeblood of any compelling case study. For instance, in your SEO case study, you’ll need to gather data on key metrics like keyword rankings, organic traffic, and conversion rates before and after implementing your strategies. Let’s say your client saw a 50% increase in organic traffic within three months of optimizing their website. Collecting this data will help you build a robust, evidence-based narrative highlighting your impact.
It’s essential to monitor the before-and-after data to track the effectiveness of implementing your strategies.
5. Prepare Insightful Questions and Conduct Interviews
It would be best to ask the right questions to get the most out of your client interviews. Imagine asking your client, “What specific challenges were you facing with your organic search rankings before we started working together?” or “How did our SEO strategies help you achieve your business goals?” These questions will lead to detailed responses that add depth to your case study, making it more than just numbers on a page.
Always ask questions that uncover the key challenges your clients face. This way, your prospects will know when to turn to you to navigate or overcome similar obstacles in their business.
Since I’m giving an example of an SEO case study in marketing, you can try these questions to interview your existing client. Obviously, you can modify the sentences according to your industry basics, but these types of questions are fundamental for collecting structured data from your clients.
- What were your business’s main SEO challenges before we started working together?
- Can you describe your initial expectations for implementing our SEO strategies?
- What specific SEO tactics did we implement that you found most effective?
- How did you monitor and measure the impact of these strategies on your organic traffic?
- What were the key metrics or results that stood out to you after the first three months?
6. Ask Questions That Drive the Story Forward
Impactful questions are the backbone of a strong case study. They allow you to highlight the unique value you delivered to your clients. You can effortlessly showcase your USPs within the case study by asking the right questions.
Focus on inquiring about the effectiveness of your services and strategies, their impact, and which aspects of the solution were most beneficial. This insight will be your key to demonstrating the tangible benefits you offer your clients.
Consider asking questions like:
- Can you share a moment when you first noticed a significant improvement in your website’s organic traffic?
- How did the increase in organic traffic impact other business areas, such as lead generation or sales?
- What feedback did your team or customers receive regarding the changes in your site’s performance?
- Looking back, what do you believe was the most critical factor in achieving these results?
- How has this success with SEO influenced your overall marketing strategy moving forward?
These types of questions encourage clients to share their experiences in a way that paints a vivid picture for your readers, making the case study more relatable and engaging.
7. Draft a Clear and Organized Outline
With all the data and insights gathered, it’s time to create a well-structured case study outline. Let’s say you start with a brief overview of your client’s business and its challenges, followed by a detailed account of the SEO strategies you implemented. Then, you showcase the results with hard data and close with client testimonials and a solid call to action.
As mentioned above, organizing your content in a logical, easy-to-follow format will help you write a case study that not only informs but also captivates your audience.
These steps are the cornerstones of designing a case study. Once you complete this checklist, you can proceed to the next step, which is writing a case study. Since I discussed planning an SEO case study extensively, here is a case study template that perfectly illustrates the process.
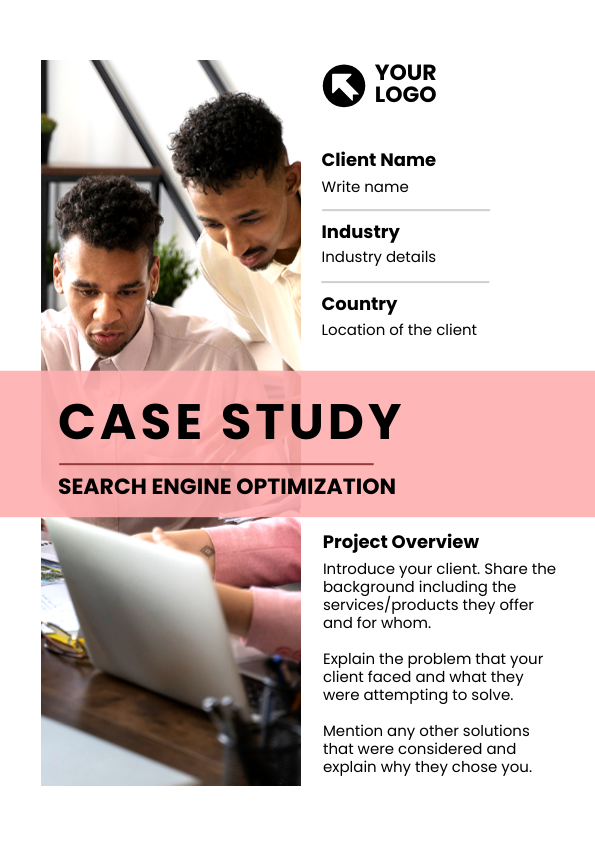
You want to create an informative case study for your prospects. But how do you make sure it’s done right? Here’s a step-by-step guide on how to write a case study that drives results.
1. Craft a Compelling Headline
Your headline is the first thing readers see, so make it count! It should grab attention and hint at the success story you’re about to share.
How to Write a Case Study Title:
1. Highlight the Result: Showcase the critical success, like “Increased Sales by 200%.”
2. Be Clear and Direct: Make sure the headline is straightforward to grasp.
3. Use Action Words: Start with strong verbs like “How We” or “Achieved.”
4. Mention Client or Industry: Include relevant details for specificity.
5. Keep It Short: Make it concise and attention-grabbing.
2. Start with a Strong Introduction
Kick off your case study with a broad overview that sets the stage. Provide the big picture and construct a clear narrative that draws readers in, making them eager to learn more about how you solved a significant challenge.
Look at the consulting case study template , which includes a stunning overview description and precise instructions for writing a short and compelling introduction. You can add every little detail to hook the reader.
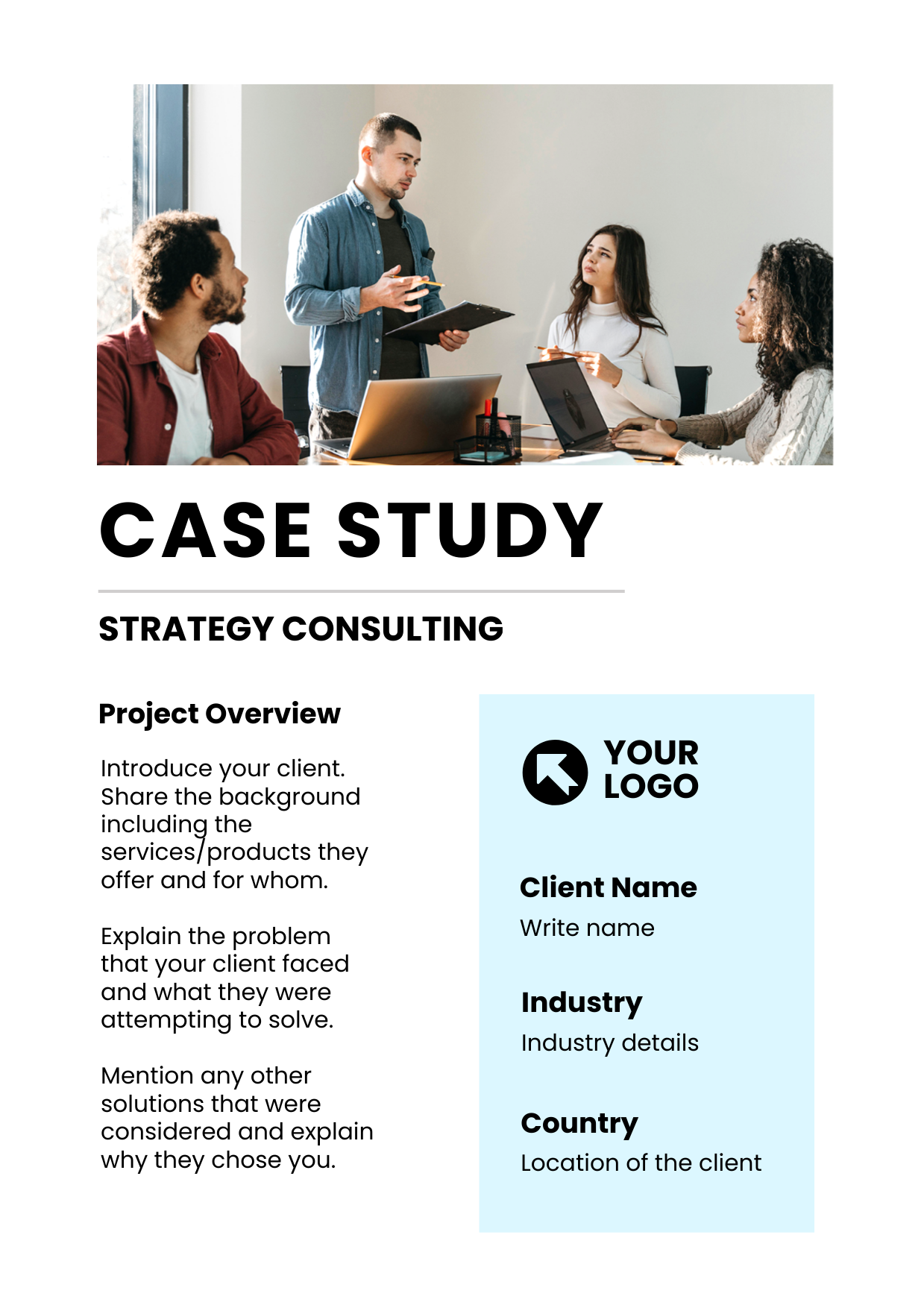
Get This Template and More
3. Discuss Specific Challenges of Your Client
This is where your prospect can truly connect. By highlighting unique yet specific challenges to their industry, you give them insight into issues they might not have encountered yet—or ones they’ve already faced. This way, they’ll know exactly who to turn to when similar challenges arise.
The following financial case study template provides a brief flow of the company’s common challenges in the financial analysis process. The template is almost ready to use with this domain-specific content, requiring minimal adjustments to design your case study.
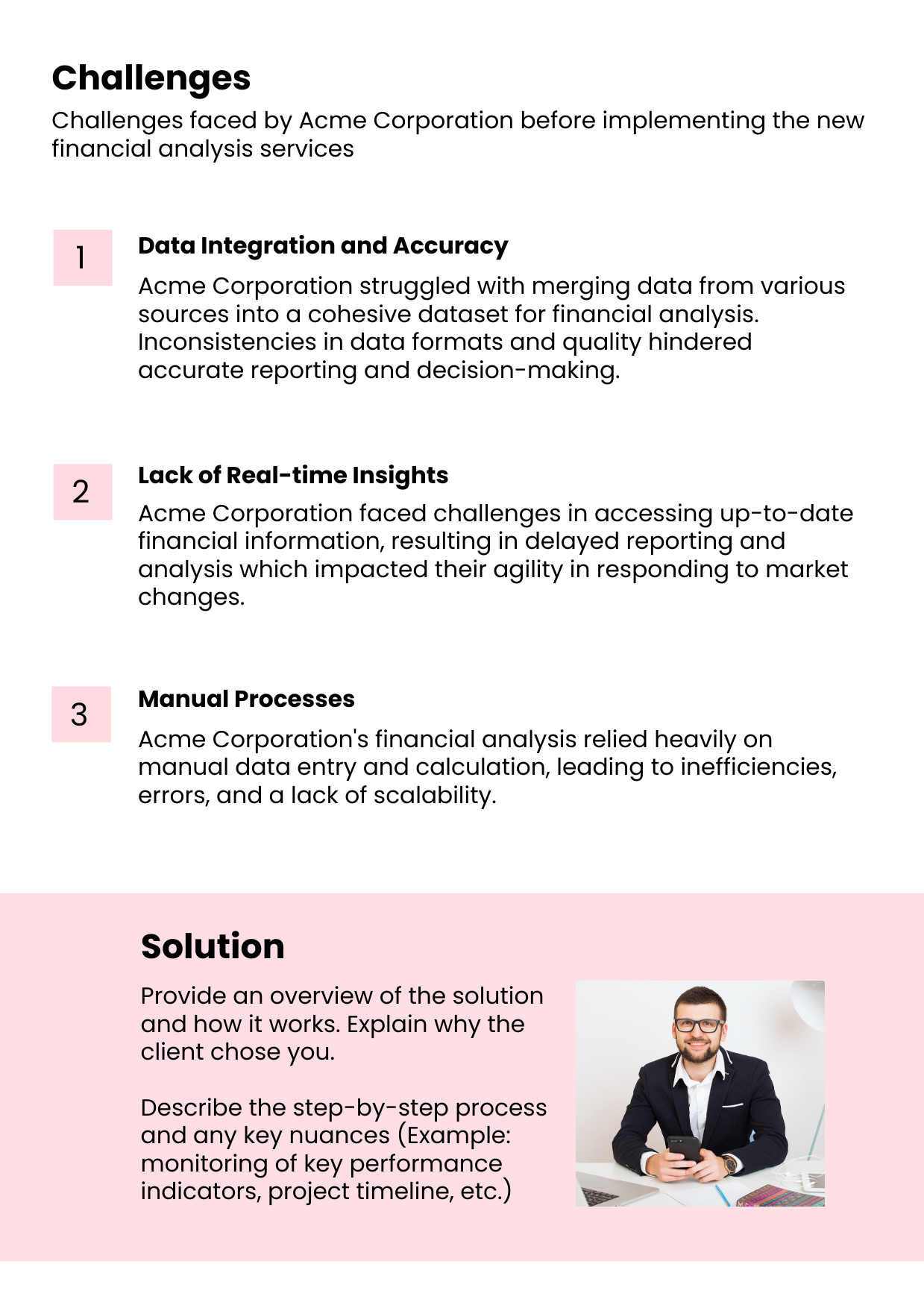
4. Highlight the Solution
Now, dive into the heart of the story. Highlight the solution you provided, and make sure to include a notable achievement or key result. This is your chance to shine!
Check out the format for presenting the implications of your service on your client’s business. The benefits should be well-written and data-driven to convince your upcoming clients. This graphic design case study format helps you understand the specific impacts a company seeks from a reputable graphic design firm.
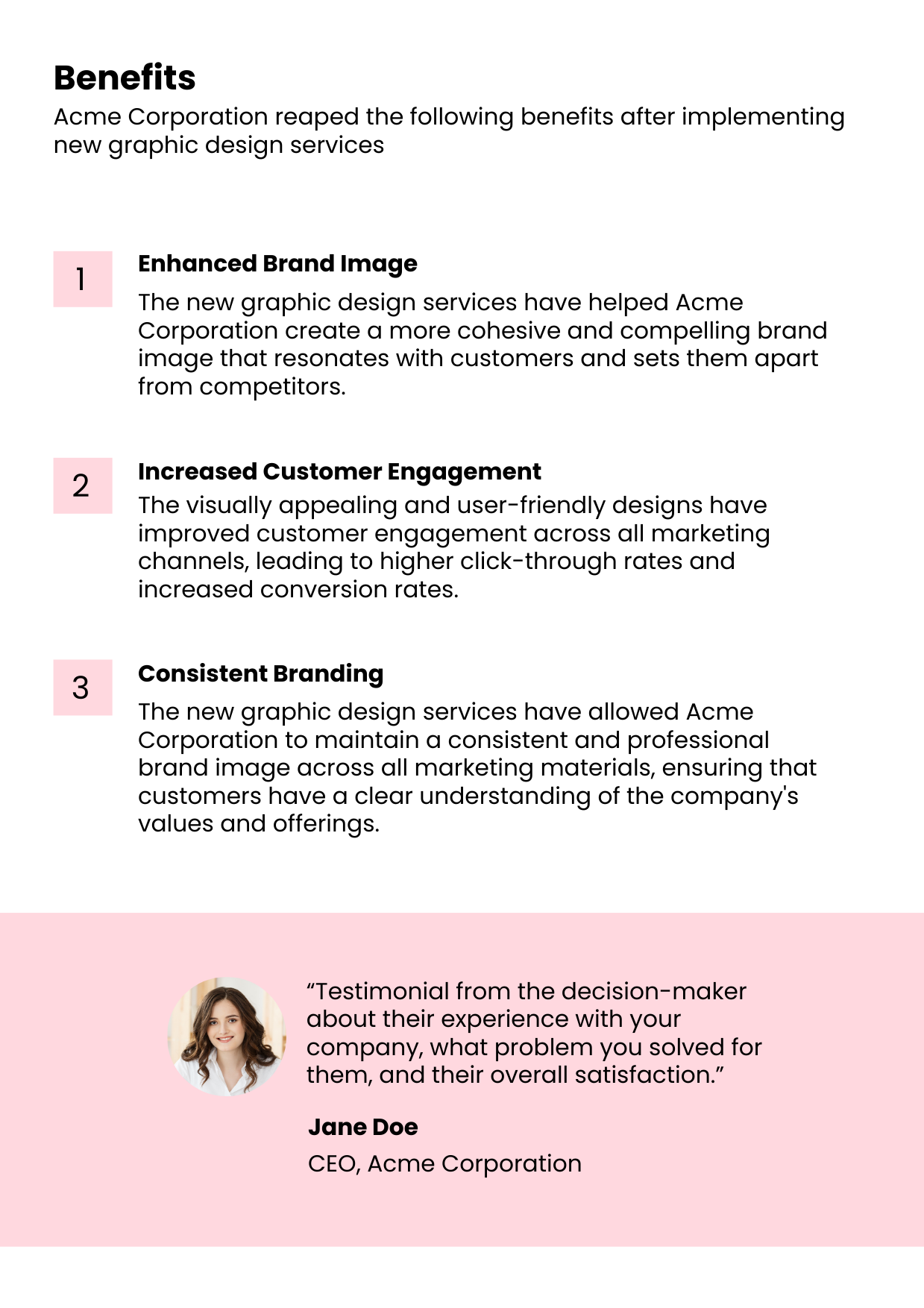
5. Present Quantifiable Results
When sharing the outcome, numbers speak louder than words. Present quantifiable results that clearly demonstrate the impact of your solution. Use graphs or charts to make the data easy to digest and visually appealing.
6. Be Clear and Concise
Less is more. Stick to the point and offer just the right amount of detail to keep your readers engaged. Include data that supports your claims, but avoid overwhelming them with too much information.
Here’s a stunning sales consulting case study that uses a simple case study layout and details written in readable, plain language to gauge more utility.
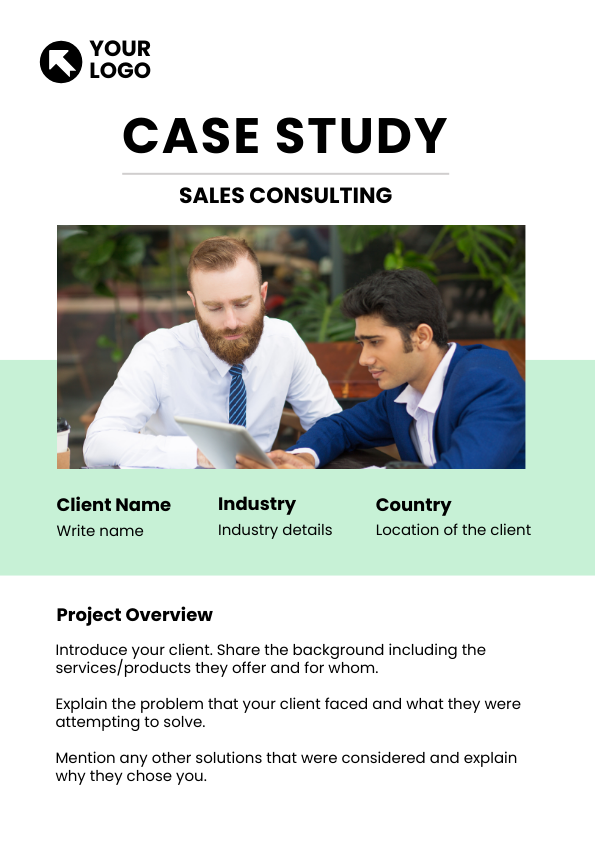
7. Treat Your Case Study Like a Story
Focus on your customer’s journey. Think of your case study as a story in which your client is the hero, and your solution is the tool that helped them succeed. This approach will make your case study relatable and compelling.
8. Be as Specific as Possible
Don’t be vague—details matter. Mention the specific company and its industry to let your audience know that the challenge and solution are relevant to them. The more precise you are, the more credible and trustworthy your case study will be.
Check out the sample case study below for payroll accounting. The details are clearly organized and grouped to emphasize the type of case study.
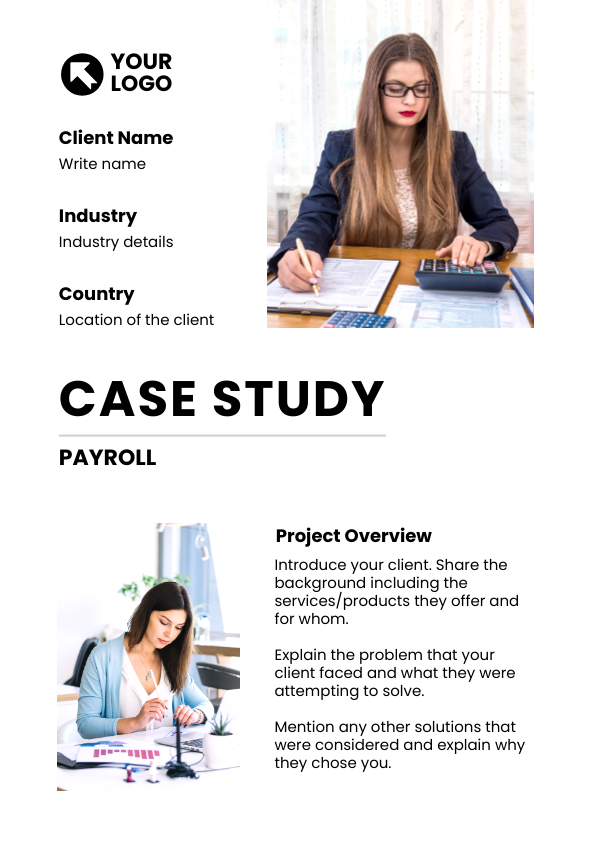
Also, the next case study template displays very specific problems that a company faces when it lacks digital marketing expertise.
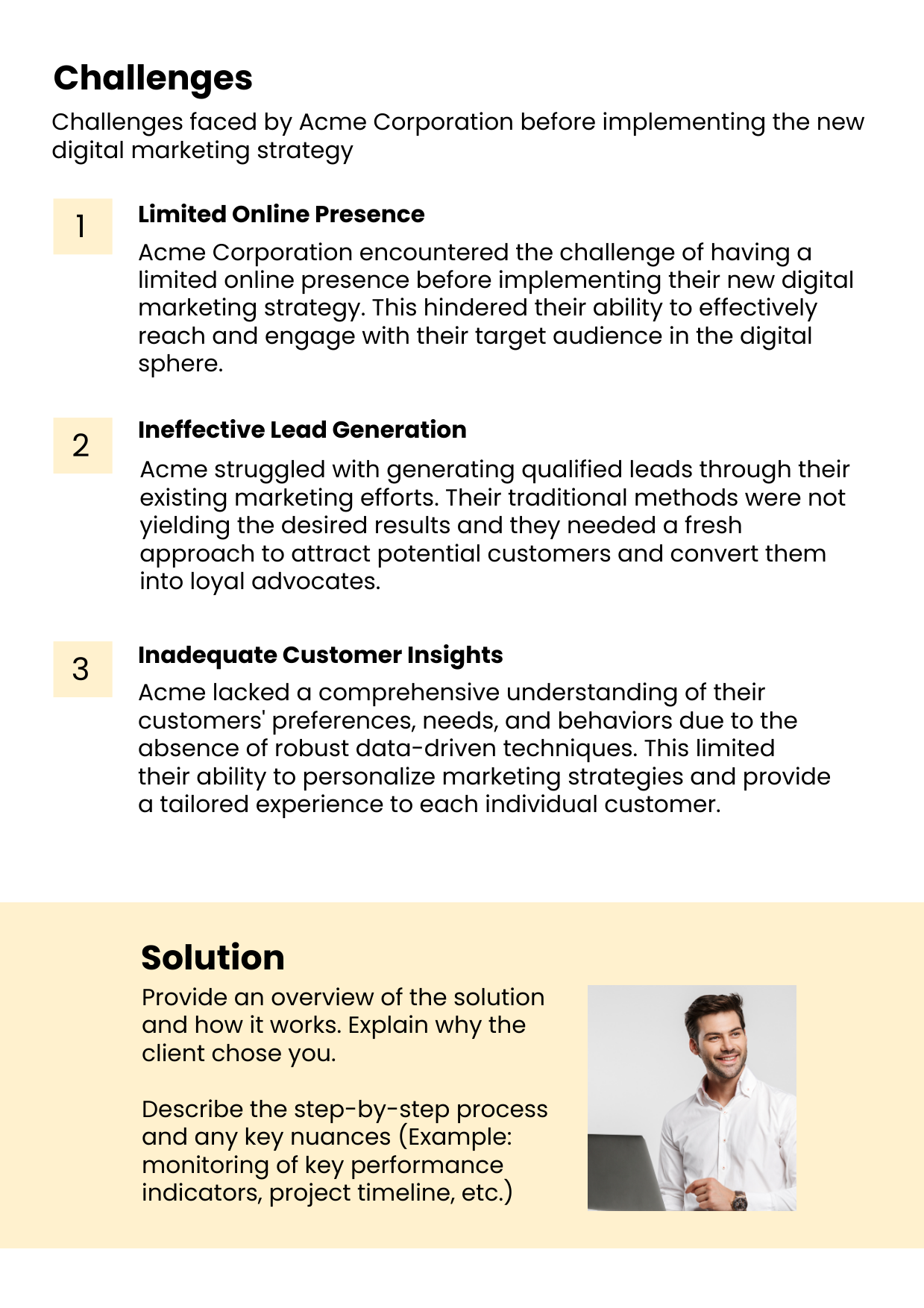
These templates make it a breeze to craft a case study that’s perfect for your niche.
9. Use Direct Quotes from the Client
Quotes from your client add authenticity and credibility. They give readers insight into the client’s perspective and make your case study more relatable. Plus, a glowing testimonial is always a nice touch!
The following inbound marketing case study has a prominent client testimonial. With the brief instructions on this template, it’s easier for you to understand how to capture the golden words of your client and use them as a word-of-mouth strategy within the case study.
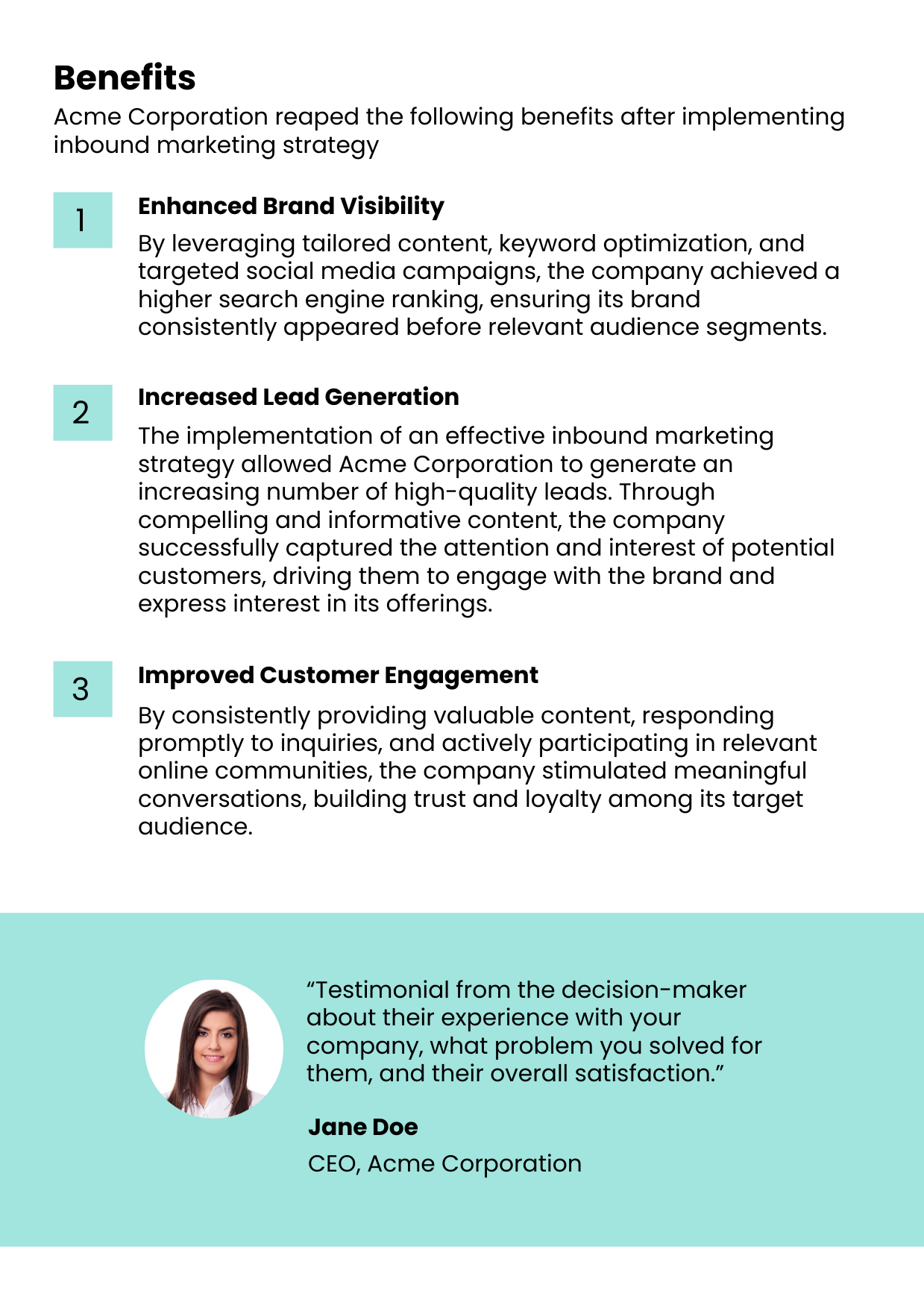
10. Pick an Interesting Angle
Find a unique angle that makes your case study stand out. Maybe it’s an unexpected challenge you overcame, or perhaps it’s a particularly innovative solution. Whatever it is, make it intriguing.
11. Make the Key Takeaway Crystal Clear
Your readers should walk away with a clear understanding of the main point of your case study. This takeaway should reinforce your expertise and the value of your solution.
12. Include a Call to Action (CTA)
Don’t leave your readers hanging—tell them what to do next! Include a compelling summary about your company, showcase your happy client base, and conclude the journey with a strong CTA, whether to contact you for a consultation, download a related resource, or learn more about your services on social media, like the following case study template design.
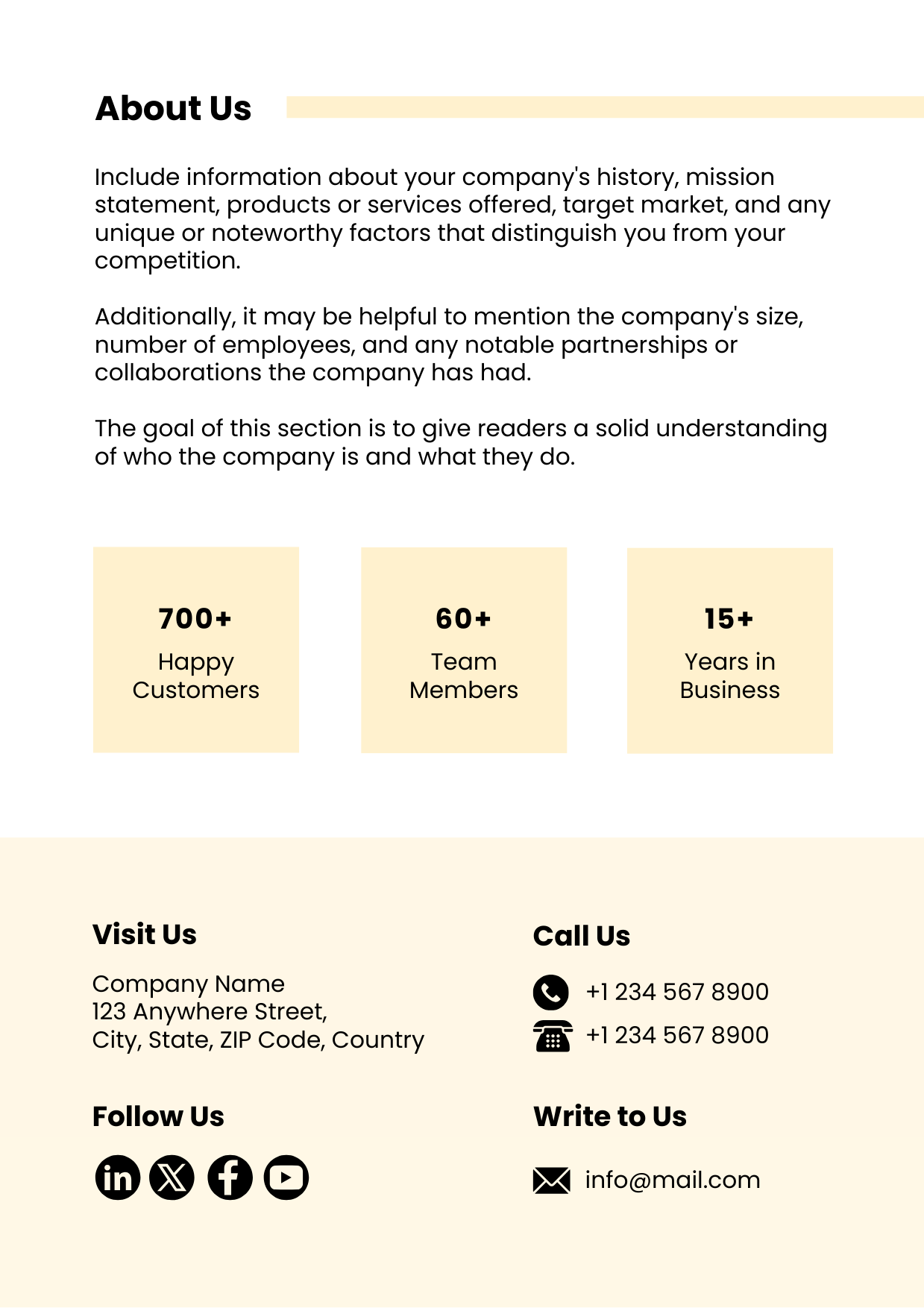
12. Format Professionally
The design of the case study is just as important as the content. A well-formatted, visually appealing document makes a great impression and enhances readability. With ready-to-use niche-oriented templates, you can easily create a professional-looking case study that impresses and converts. Here is an eye-catching template for an AI assistant software case study that displays a sleek and well-navigated format.
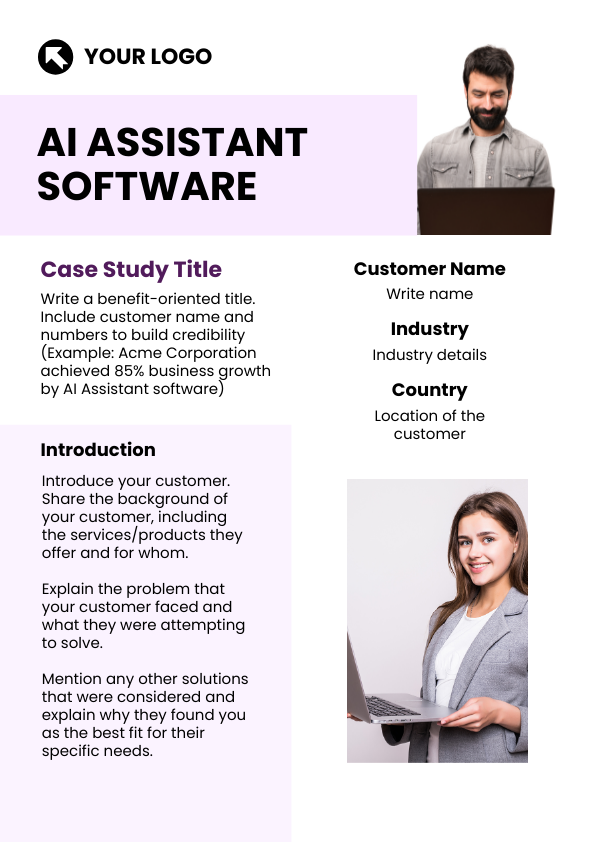
13. Make It Readable
Easy readability is key. Use simple language, short paragraphs, and bullet points where appropriate. Your case study should be easy to scan and digest. Follow the thirteen design principles to create a standout piece that enhances your marketing efforts.
To understand this, take a look at the following consulting case study template.
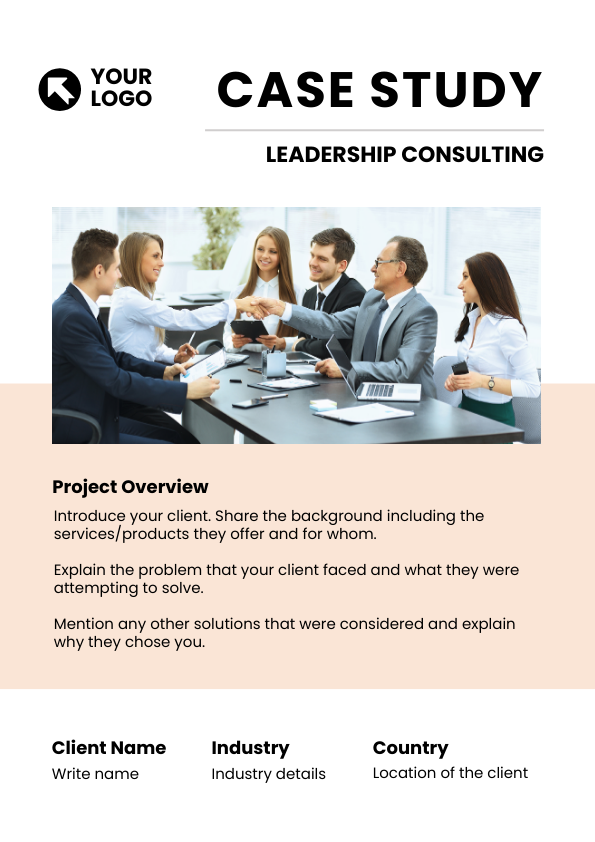
14. Finalize and Proofread Your Case Study
In order to excel in how to write case studies, give your case study a final review before you hit publish. Proofread carefully to catch any typos or errors, and make sure everything flows smoothly. A polished case study reflects your attention to detail and professionalism.
To effectively summarize a case study, start by completing all sections, including the introduction, challenges, solutions, and results. This approach helps marketers identify key points to highlight, making it easier to craft a succinct and engaging summary.
One tricky thing is the length of the case study summary. So, how long should a case study summary be?
The length of a case study summary can vary depending on the details you’re covering. Generally, it should be kept concise, usually spanning a couple of lines or up to a single page with several paragraphs. If you’re crafting a customer case study and want to flex your storytelling muscles, it’s perfectly fine for the summary to stretch to a full page.
If summarizing a case study seems daunting, try DocHipo’s advanced AI Writer tool, which effortlessly creates a crisp and concise summary.
Watch this short video to use it.
This is the last step in writing a case study analysis. Citation in a case study is the practice of giving proper credit to the sources you reference or use in your research. It helps validate your work, shows the depth of your research, and avoids plagiarism. Follow the below steps to cite a case study:
- Identify the Source: Gather details like the author, title, publication year, and where the case study was published.
- Choose a Citation Style: Follow the specific formatting style (APA, MLA, Chicago, etc.) required for your work.
- Format the Citation: Arrange the details according to the chosen citation style.
- Include In-Text Citations: Place citations within the text or paragraphs for the case study.
- Create a References List: At the end of your case study, compile all your sources in a bibliography or reference list.
For case studies, citations in APA and MLA styles are very common. If you are just beginning, then you might be confused about these case study citation formats.
Hence, take a look at the picture below, which easily comprehends the APA vs MLA citation features.
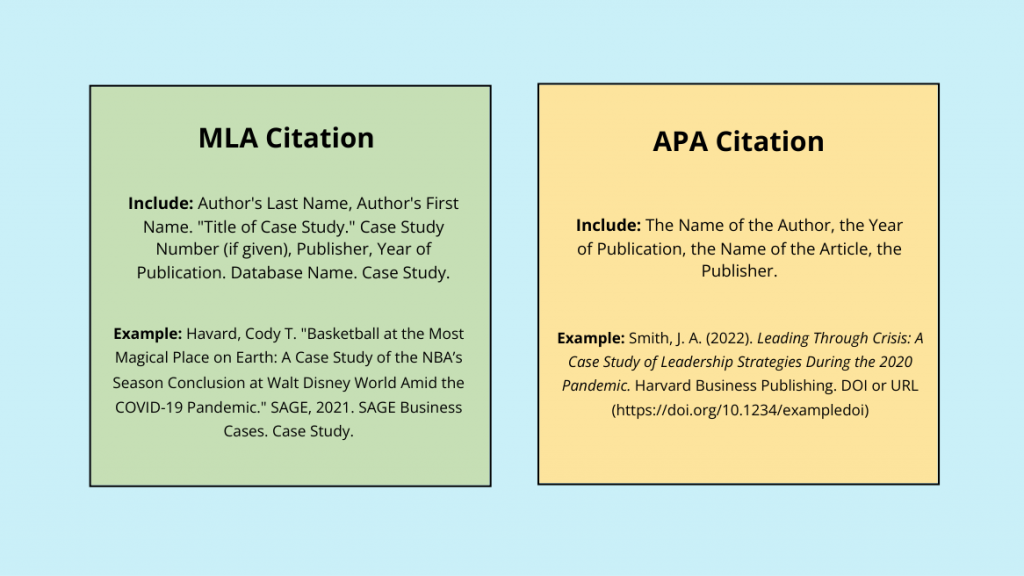
Still feeling overwhelmed about case studies? Be stress-free with the most convenient case study maker, which saves time and allows you to present data in the most attention-grabbing way.
Watch the video to create case studies in minutes with DocHipo’s case study maker.
Conclusion
To summarize, if you want to write a case study, start with a proper case study format, plan the case study, and finally write it with all the information in hand. Then, write a summary to provide an overview of your case study, and finally, add citations for reference.
Meanwhile, if you want to design a case study, Try DocHipo templates. Sign up to explore all the case study templates.
What is the structure of a case study?
A case study typically includes the following sections: Title, Introduction, Background, Problem Statement, Solutions, Results, and Conclusion. Each section serves to tell a comprehensive story of the business, from the issue at hand to the resolution and outcomes.
What are the 5 essential elements of a great case study?
The 5 essential elements are: 1) Clear Objective, 2) Detailed Background, 3) Specific Challenges, 4) Effective Solutions, and 5) Measurable Results. These components provide a compelling narrative that highlights the value delivered.
How to begin a case study?
Start a case study by defining the purpose and scope of the study. Introduce the subject, outline the problem, and provide background information to give readers context. This sets the stage for the detailed analysis that follows.
How to make an introduction in case study?
To craft a compelling introduction, briefly describe the subject, outline the problem they faced, and explain why the study is relevant. This section should grab the reader’s attention and make them interested in the rest of the study.
How to make a business case study?
A business case study should begin with a clear objective and background information. Identify the problem, explain the solutions implemented, and conclude with the results achieved. Use real data and quotes from stakeholders to enhance credibility.
How to write a case study step by step?
To write a case study step by step, start by identifying the case you want to explore and gathering relevant data on the subject. Outline the structure of your case study, then craft an engaging Introduction to set the context. Next, detail the Background and Challenges faced, followed by the Solutions applied. Share the Results and Conclusion to highlight the impact. Finally, edit and proofread your case study to ensure clarity and accuracy.
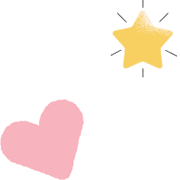
Turn your ideas into beautiful design
No prior design skill required
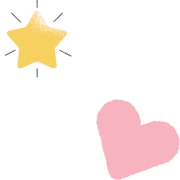
Talk to Sales
Wherever you are on your Dochipo journey, you can always get in touch.

Talk to Support
Federated Learning of XAI Models in Healthcare: A Case Study on Parkinson’s Disease
- Open access
- Published: 28 August 2024
Cite this article
You have full access to this open access article
- Pietro Ducange 1 ,
- Francesco Marcelloni 1 ,
- Alessandro Renda 1 &
- Fabrizio Ruffini 1
Artificial intelligence (AI) systems are increasingly used in healthcare applications, although some challenges have not been completely overcome to make them fully trustworthy and compliant with modern regulations and societal needs. First of all, sensitive health data, essential to train AI systems, are typically stored and managed in several separate medical centers and cannot be shared due to privacy constraints, thus hindering the use of all available information in learning models. Further, transparency and explainability of such systems are becoming increasingly urgent, especially at a time when “opaque” or “black-box” models are commonly used. Recently, technological and algorithmic solutions to these challenges have been investigated: on the one hand, federated learning (FL) has been proposed as a paradigm for collaborative model training among multiple parties without any disclosure of private raw data; on the other hand, research on eXplainable AI (XAI) aims to enhance the explainability of AI systems, either through interpretable by-design approaches or post-hoc explanation techniques. In this paper, we focus on a healthcare case study, namely predicting the progression of Parkinson’s disease, and assume that raw data originate from different medical centers and data collection for centralized training is precluded due to privacy limitations. We aim to investigate how FL of XAI models can allow achieving a good level of accuracy and trustworthiness. Cognitive and biologically inspired approaches are adopted in our analysis: FL of an interpretable by-design fuzzy rule-based system and FL of a neural network explained using a federated version of the SHAP post-hoc explanation technique. We analyze accuracy, interpretability, and explainability of the two approaches, also varying the degree of heterogeneity across several data distribution scenarios. Although the neural network is generally more accurate, the results show that the fuzzy rule-based system achieves competitive performance in the federated setting and presents desirable properties in terms of interpretability and transparency.
Explore related subjects
- Artificial Intelligence
- Medical Ethics
Avoid common mistakes on your manuscript.
Introduction and Motivations
The extensive reliance on artificial intelligence (AI) and machine learning (ML) tools in the healthcare sector poses significant challenges, especially concerning the concept of trust . Any AI system must meet the requirements of robustness, fairness, and transparency throughout its whole life cycle. Furthermore, sensitive health-related data hold an intrinsic value and become a lucrative target for cyber attacks.
The concept of trustworthy AI has recently been considered also by government entities: European Union, for example, is at the forefront for AI regulation as witnessed by the proposal of the “AI ACT” Footnote 1 (2021), which is often referred to as the first law on AI and is conceived for introducing a common regulatory and legal framework for AI. The European Commission had previously promoted the definition of the “Ethics guidelines for trustworthy AI” [ 1 ], which identifies lawfulness, ethics, and robustness as key pillars for trustworthiness and describes the requirements for an AI system to be deemed trustworthy. The ethical aspects are pivotal in the healthcare domain given the sensitive nature of patient data, the disclosure of which poses serious risks. For example, discrimination based on such data can occur in the insurance field: insurance companies could decide to charge different fees depending on the individual health status. Likewise, securing the non-discrimination in financial services is nowadays perceived as an important matter, as witnessed for example by the regulations on the “right to be forgotten” for cancer survivors [ 2 ]. Finally, special attention should be paid to specific domains (e.g., mental health), due to the stigmatized nature of some types of illness.
In the pursuit of trustworthiness, data privacy and transparency emerge as pivotal enablers, especially in the healthcare domain. While data privacy is considered an invaluable right, it somehow clashes with the creation of accurate ML models that, to date, require large amounts of data in their training phase. The common scenario is in fact that many different entities (be they individuals, medical centers, or hospitals) have few or limited amounts of data and are often reluctant to share their assets and sensitive information with other parties. The processes of data mining and knowledge extraction are therefore hampered by the unfeasibility of data collection for centralized processing. The requirement of transparency encompasses the traceability of the learning process, beginning from the data gathering phase, and the ability to comprehend the structure and the functioning of the ML model itself. The latter challenge is the central focus of a branch of AI named Explainable AI (XAI) [ 3 , 4 , 5 , 6 ]. The right for explanation is explicitly mentioned both in the “Ethics guidelines for trustworthy AI" [ 1 ], “ [...] AI systems and their decisions should be explained in a manner adapted to the stakeholder concerned ”, and in the recital 71 of the General Data Protection Regulation (GDPR) [ 2 ] “ [...] the processing should be subject to suitable safeguards, which should include specific information to the data subject and the right to obtain human intervention, to express his or her point of view, to obtain an explanation of the decision reached after such assessment and to challenge the decision.” Indeed, the ability to understand the inner working of an AI system represents a cornerstone of trust and holds particular significance in high-stakes applications in the healthcare domain.
In this work, we embrace the challenge of enhancing trustworthiness of AI systems in medicine investigating technical enablers for the requirements of data privacy and explainability.
Data access limitations, driven by privacy requirements and by the need to prevent ethical risks associated with the disclosure of sensitive data, have prompted the development of new paradigms for training ML models, including federated learning (FL) [ 7 , 8 ]. FL enables multiple parties to collaboratively train an ML model without any disclosure of private raw data. Essentially, a shared global model is learned through proper aggregation of locally computed updates from remote data owners, thus removing the need of centralizing data for training purposes.
The requirement of explainability is typically addressed through two main categories of approaches [ 3 , 4 ]: the exploitation of interpretable by-design models and the adoption of post-hoc explainability techniques. Interpretability and transparency refer to inherent properties of a model and consist in the ability to understand how decisions have been taken and what is the structure of the model itself, respectively. Post-hoc explanation techniques, instead, typically address the goal of explaining why a model provides a decision. It follows that an interpretable model results to be also explainable. The property of interpretability is generally attributed to models such as decision trees (DTs) and rule-based systems (RBSs): in fact, they consist of (or can be traced back to) collection of “IF antecedent THEN consequent ” rules. As a consequence, the inference process turns out to be highly understandable. Understandability can be defined as “ the characteristic of a model to make a human understand its function (that is, how the model works) without any need for explaining its internal structure or the algorithmic means by which the model processes data internally ” [ 3 ]. It is worth emphasizing that the concept of understandability is strongly related to the targeted audience in terms of their a-priori knowledge and cognitive skills. For example, a rule-based inference requires familiarity with logic and, possibly, an adequate training depending on the specific implementation adopted in the antecedent and consequent parts of the rules.
Post-hoc techniques are applied on models which are referred to as opaque or “black boxes,” such as Neural Networks (NNs) and ensemble models, to explain their outcomes. The vast majority of existing post-hoc methods can be roughly ascribed to the following categories, which emulate different nuances of human reasoning: feature importance explanations, rule-based explanations, prototypes explanations, contrastive/counterfactual explanations, and textual or visual explanations [ 3 , 9 ]. Notably, since the field of research is constantly evolving, the list should not be considered exhaustive; in addition, different post-hoc strategies can be specifically tailored based on the different kinds of data (e.g., images or text) and based on the specific models involved [ 9 ]. In the context of XAI, a further distinction is also made between local and global explanations: the former refers to the inference process and focuses on how/why the decision is taken for any single input instance. The latter refers to structural properties of the models (thus pertaining to the concept of transparency) or to aggregated information computed over the entire dataset.
The awareness of the importance of explainability and privacy preservation has greatly increased in recent years. While FL inherently tackles the challenge of preserving data privacy in decentralized ML, it typically lacks integrated solutions for the issue of explainability [ 10 ]. Actually, FL was originally conceived for models optimized through stochastic gradient descent (SGD) (e.g., Deep Neural Networks (DNNs)), and the application of post-hoc techniques is not straightforward in the federated setting.
This work lies at the intersection between FL and XAI and contributes to a research area named Fed-XAI (acronym for fed erated learning of XAI models) [ 11 , 12 , 13 ]. We explore the adoption of Fed-XAI approaches within the healthcare domain for predicting the progression of Parkinson’s disease (PD), formulated as a regression task. We consider a plausible scenario where sensitive raw data originate from different medical centers, making centralized learning unfeasible. In particular, the task is to predict one of the most commonly used indicators for the severity of PD symptoms, namely the Unified PD Rating Scale (UPDRS, firstly introduced in 1987 [ 14 ]), by exploiting real-world voice recordings. The analysis extends a recent work [ 15 ] and encompasses two approaches for Fed-XAI in order to explore the trade-off between accuracy and trustworthiness. The first approach adopts an interpretable by-design model, learned in a federated fashion. The second one employs an opaque model, where both training and post-hoc explanation are compliant with the federated setting. On one hand, we can assess the generalization capability of the models in the regression problem by exploiting a real, publicly available dataset; on the other hand, trustworthiness is meant here as the concurrent attainment of explainability in all its nuances, from both local and global perspectives, and privacy preservation through the adoption of FL.
As for the interpretable by-design model, we employ the Takagi-Sugeno-Kang Fuzzy Rule-Based system (TSK-FRBS) [ 16 ] which is considered as a transparent and interpretable model: its inference method mimics a cognitive process typical of human reasoning in the form of if-then rules . The partitioning of numerical variables into fuzzy sets, which is one of the defining aspects of TSK-FRBS, has proven to enable competitive levels of performance for classification and regression tasks [ 17 ] and has a twofold implication. First, fuzziness in rule-based systems enhances semantic interpretability through linguistic representation of numerical variables. Second, a fuzzy set can be interpreted as a formal representation of an information granule, intended as a generic and conceptually meaningful entity [ 18 , 19 ]: in this context, a fuzzy set allows any number in the real unit interval to represent the membership degree of a feature value to the information granule. As a consequence, the adopted TSK-FRBS fits into the paradigm of granular computing and makes use of information granules in the explainable decision-making pipeline.
As for the opaque model, we employ a well-known biologically inspired model, namely Multi Layer Perceptron Neural Network (MLP-NN). FL is performed exploiting the popular federated averaging (FedAvg) aggregation strategy [ 7 ]. Furthermore, the SHAP explainer [ 20 ], purposely adapted to comply with the federated setting based on a recently proposed approach [ 21 ], is used for explaining the output of the MLP-NN by attributing the contribution (i.e., importance) of each feature to each prediction.
The main contributions of this work can be summarized as follows:
We simulate a scenario in which several medical institutions cooperate in creating a PD progression prediction model pursuing the requirements of explainability and privacy preservation;
To achieve this goal, we implement and exploit two Fed-XAI approaches, based on TSK-FRBS, and MLP-NN plus SHAP, which represent state-of-art techniques for by-design interpretability and post-hoc explainability, respectively, in the federated setting;
We discuss the accuracy of the approaches under several data distribution scenarios, considering the independent and identically distributed (i.i.d.) case and three different non-i.i.d. cases;
For each scenario, we compare the FL scheme with two baselines, namely centralized learning and local learning, to verify the suitability of the federated approach;
We discuss the explainability of MLP-NN and the interpretability of TSK-FRBS, both from a local and a global perspective;
We discuss about the consistency of explanations provided by the two approaches in the federated setting. Here, consistency is achieved when different participants in the FL process obtain the same explanation given the same input information.
The rest of the paper is organized as follows: in Section 2 , we provide a brief overview of recent works that adopt XAI and FL tools in healthcare and more specifically in the context of PD. Furthermore, we describe recent advances in the field of Fed-XAI. Section 3 describes the background related to FL, detailing the approaches for FL of TSK-FRBS and FL of MLP-NN. Furthermore, SHAP is introduced as post-hoc explainability technique, and a recent approach for exploiting SHAP in the federated setting is presented. Section 4 describes the PD progression prediction case study, providing details about the experimental setup: we outline the different data distribution scenarios, the evaluation strategies, and the configuration of the two approaches. In Section 5 , we report and discuss the experimental results. The considerations regarding interpretability and explainability are given in Section 6 . Finally, in Section 7 , we draw some conclusions.
Related Works
The adoption of AI techniques in healthcare has been widely investigated. In this section, we first discuss the most relevant works concerning the adoption of FL and XAI in this application domain. Then, we discuss existing works related to XAI in Parkinson’s disease studies. Finally, we discuss recent algorithmic efforts for combining FL paradigm and XAI approaches.
Federated Learning and XAI in Healthcare Scenarios
The opportunities and the practical utility of FL in the healthcare domain have been recently acknowledged in the specialized literature [ 22 , 23 , 24 ], with applications mainly in the fields of medical imaging [ 25 ] and precision medicine [ 26 ]. FL is presented as a solution to protect sensitive data for privacy concerns and ethical constraints [ 27 ] and also in relation to cyber attacks [ 28 ]. At the same time, the interest in XAI is increasingly widespread, especially in the attempt to “open” the so-called black-boxes [ 29 , 30 ], which have enabled unprecedented performance in the field of deep learning (DL) in medicine. The surveys on XAI for healthcare applications usually delve into the problem of how to present the AI results and their explanations to physicians, medical staff, patients, and caregivers: the explanations should be a tool to understand the outcomes of an AI system, but also a way to allow interaction and enhance stakeholders’ trust in AI (human-centered AI). The XAI goal is usually achieved through the adoption of post-hoc methods for opaque models, often concerning image data analysis (e.g., X-rays and CT scans).
Authors of a recently published survey [ 10 ] provide a review of clinical cases where post-hoc methods and interpretable by-design models are applied to more than 20 different medical case studies, spanning from COVID-19 diagnosis and early detection to diagnostic for breast cancer. Different data types are exploited, depending on the application: images (e.g., EEG, MRI) are often involved, and SHAP is among the most used post-hoc methods. Example case studies include prediction of depressive symptoms from texts with adoption of a post-hoc method for the estimation of word importance [ 31 ] and Alzheimer classification using Random Forest and SHAP [ 32 ].
In the same survey [ 10 ], the practical utility of FL in healthcare applications is discussed, especially considering DL approaches, horizontal data partitioning, and FedAvg optimization strategy. Example case studies include the detection of COVID-19 from decentralized medical data, with Convolutional Neural Networks applied on anterior and posterior chest X-rays [ 33 ]. Authors in [ 34 ] exploit tabular electronic health records (demographics, past medical history, vital signs, lab tests results) from five hospitals to predict mortality in patients diagnosed with COVID-19 within a week of hospital admission. However, it is worth noticing that the applications of FL and XAI are treated separately, emphasizing the substantial lack of works that simultaneously address the requirements of privacy through FL and transparency through XAI in the healthcare domain.
XAI in Parkinson’s Disease Studies
PD is diagnosed in about 10 million people worldwide [ 35 ]: after the Alzheimer, it is one of the most prevalent neurodegenerative diseases. Given its socioeconomic relevance, several AI methods have been proposed for supporting diagnosis and monitoring [ 35 , 36 ]. The most commonly used data types exploited for PD studies include images and speech signals [ 37 , 38 ].
A few works discuss the topic of explainability in the context of PD studies supported by AI techniques: for instance, authors in [ 39 ] apply the LIME [ 40 ] post-hoc method on a DNN used to classify healthy from not-healthy subjects using images from SPECT scanning. Authors in [ 41 ] provide explanations for different ML model outcomes using three post-hoc methods, namely LIME, SHAP, and SHAPASH (a tool for making ML models more understandable and interpretable for general audience), on a multiclass classification task. Since the aspect of data privacy holds high relevance in this context, few recent works elaborate upon the exploitation of the FL paradigm for PD-related applications [ 42 , 43 , 44 ].
In this work, we consider the Parkinson Telemonitoring dataset, which has been analyzed in several recent works for both classification [ 45 , 46 ] and regression [ 47 , 48 , 49 ] tasks. None of the works mentioned above, however, considers the aspects of privacy and explainability simultaneously.
The primary goal of our analysis is to understand the potentialities of the Fed-XAI paradigm in a PD-related application. In the following, we provide a brief overview of the most relevant approaches for Fed-XAI proposed in the literature, relaxing the constraint on the application domain.
Federated Learning of XAI Models
Explainability in FL has been pursued either using post-hoc [ 20 , 50 , 51 , 52 , 53 , 54 , 55 ] or ex-ante [ 13 , 56 , 57 , 58 , 59 ] approaches. A thorough review of such approaches has been provided in several recent works [ 11 , 12 , 60 ]. Here, we describe the most recent advances on the topic.
Bogdanova et al. [ 60 ] have proposed a novel approach (named DC-SHAP) for consistent explainability over both horizontally (different instances, same features) and vertically (different features, same instances) partitioned data for the Data Collaboration (DC) paradigm. This paradigm consists of two stages: first, participants obtain intermediate representations of data through irreversible transformations and transmit them to a central server (unlike FL, which typically shares models rather than data). Then, the server combines such intermediate representations into a single dataset, trains an ML model, and distributes it back to the participants. Unlike other ex-ante [ 58 ] and post-hoc [ 50 , 61 ] explainability approaches tailored for the decentralized setting, DC-SHAP ensures consistency of explanations: In this context, the property of consistency is met if the explanations of the same data instance for a global model are the same for different participants. As underlined by the authors in [ 60 ], model-agnostic post-hoc explainability methods are prone to misalignment of client-side explanations, since they rely on probing the global model with various inputs generated from the local data distribution (typically referred to as background or reference dataset ). In their proposal for horizontally partitioned data, they use a set of auxiliary synthetic data shared among the participants to solve the issue of different background datasets and show how this allows the mitigation of feature attribution discrepancies among the participants. The approach proposed in [ 51 ] is conceived to obtain a consistent global feature attribution score for horizontal FL. A model-specific post-hoc explainability method, namely Integrated Gradients (IGs) [ 62 ], has been adopted for computing feature relevance. The integrated gradients get averaged and thus unified among the clients; however, local explainability is not addressed.
The issue of Fed-XAI for PD has been recently discussed in [ 63 ], with the aim of identifying digital bio-markers for the progress of the disease. Three assumptions constitute the privacy model, considering a scenario with multiple hospitals, each with its own patients: (i) input records and corresponding labels are isolated; (ii) the raw inputs are isolated between patients; (iii) the target labels are isolated between hospitals.
A hierarchical framework is adopted to build the FL model: local FL processes allow to collaboratively train a model among patients in the same hospital, whereas a global FL process aggregates models from each hospital for generating the complete model. An adaptation of SHAP is then adopted as post-hoc method for feature importance explanation. To address the issue of misalignment of client-side explanations, background datasets are generated sampling from a Gaussian distribution: the parameters of such Gaussian distribution (mean and variance) are estimated for each feature in a hierarchical way, by combining the parameters estimated intra- and inter-hospitals. It is shown that the average feature importance computed in the federated fashion is qualitatively similar to but quantitatively different from that obtained in the centralized fashion, where the union of the participants’ training sets can be used as background dataset. Although the proposed method theoretically allows for it, the aspect of local explainability/interpretability is not however discussed.
Authors in [ 21 ] have proposed an approach for obtaining SHAP explanations [ 20 ] in horizontal FL. Specifically, the explanation of an instance prediction made by the federated ML model is obtained by aggregating the explanation of the participants. Such an approach ensures consistency of explanations and is shown to be a faithful approximation of the SHAP explanations obtained in a centralized setting. However, the approach requires that test instances are available to all participants, which may be undesirable or unfeasible in real-world applications where privacy must be guaranteed also at inference time.
In the framework of FL of interpretable-by-design models, TSK-FRBSs [ 13 , 57 , 59 ] and DTs [ 56 , 58 ] have been considered as XAI models to be learnt in a federated fashion. Approaches proposed in [ 57 , 59 ] for federated TSK-FRBS rely on a clustering procedure for the structure identification stage and on a federated adaptation of classical gradient-based learning schemes for adjusting the parameters of the consequent part of the rules. In this work, we consider the approach introduced in [ 13 ], which leads to more interpretable TSK-FRBSs compared to the ones considered in [ 57 ] and [ 59 ]. Additional details on such an approach are reported in Section 3.1 .
As for DTs, the IBM FL framework [ 56 ] supports, among others, a federated adaptation of the ID3 algorithm for horizontally partitioned data. Specifically, an orchestrating server grows a single decision tree by exploiting client contributions based on their local data, in an iterative, round-based, procedure. Similarly, the approach proposed in [ 58 ] allows multiple clients to collaborate in the generation of a global DT by transmitting encrypted statistics, but it refers to the vertical data partitioning scenario. Finally, Polato et al. [ 64 ] have proposed a federated version of the AdaBoost algorithm, posing minimal constraints on the learning settings of the clients, enabling a federation of DTs, and without relying on gradient-based methods.
The categorization of FL approaches is typically based on the data partitioning scheme and the scale of federation. Data partitioning can be broadly categorized into horizontal and vertical settings. In the horizontal setting, training instances from different participants refer to the same set of features, whereas in the vertical setting, the feature set itself is partitioned among participants. The scale of federation refers to the number of participants and is typically classified into cross-silo FL, involving a low number of participants with ample data and computational power, and cross-device FL, where a large number of participants, often represented by smartphones or personal equipment, may feature a relatively small amount of data and computational power.
The PD progress prediction case study discussed in this work pertains to a cross-silo horizontal FL setting. This section reports background information for the two approaches adopted to address this task, which can be ascribed to the Fed-XAI research field: federated TSK-FRBS and federated MLP-NN with post-hoc explainability.
Federated Learning of TSK-FRBS
Let \(\textbf{X}=\left\{ X_{1}, X_{2}, \dots , X_{M}\right\} \) and Y be the set of M input variables and the output variable, respectively. A generic input instance is in the form \(\textbf{x}_{i} = [x_{i,1}, x_{i,2}, \dots , x_{i,M}]^T\) and has an associated output value \(y_i\) . Let \(U_{j}\) be the universe of discourse of variable \(X_j\) and \(P_{j} =\left\{ A_{j,1}, A_{j,2}, \dots , A_{j, T_{j}} \right\} \) be a fuzzy partition over \(U_j\) with \(T_{j}\) fuzzy sets, each labeled with a linguistic term. The term \(A_{j,t}\) indicates the \(t^{th}\) fuzzy set of the fuzzy partition over the \(j^{th}\) input variable \(X_j\) . A TSK-FRBS consists of a collection of fuzzy if-then rules, where the antecedent part of each rule is a conjunction of fuzzy propositions and the consequent part implements a regression model. In case of the commonly used first-order regression model, the generic \(r^{th}\) rule is expressed as follows:
where \(\gamma _{r,j}\) (with \(j=0,\dots ,M\) ) are the coefficients of the linear model that evaluates the output prediction \(y_r\) .
The parameters of the rules are determined through a data-driven approach. The if part (antecedent) is generated either using grid-partitioning or fuzzy clustering over the input space. Once the antecedent is determined, the then part (consequent) estimation consists of local linear models obtained, for instance, through the least squares method.
At inference time, TSK-FRBS exploits the rule base as follows. Given an input instance, first the strength of activation of each rule is computed as
where \(\mu _{j, t_{r,j}}(x_{i,j})\) is the membership degree of \(x_{i,j}\) to the fuzzy set \(A_{j,t_{r,j}}\) . Then, the final output can be evaluated with either weighted average or maximum matching policy. In the former case, the TSK-FRBS output is computed as the average of the outputs of all the activated rules weighted by their strengths of activation. In the latter case, the output corresponds to the output of the rule with the maximum strength of activation.
The maximum matching policy enhances the interpretability of TSK-FRBS, since a single rule explains a predicted output for an input instance. Furthermore, the fuzzy linguistic representation of numerical variables fosters the semantic interpretability of the model itself, whose operation, based on the evaluation of rules, turns out to be highly intuitive.
From an algorithmic perspective, FL of TSK-FRBS, as well as of other families of highly interpretable models, requires ad-hoc strategies. In this work, we rely on the approach for building TSK-FRBSs in a federated fashion recently proposed in [ 13 ]. We consider horizontally partitioned data: every participant produces a local TSK-FRBS and sends it to the server. Subsequently, the server consolidates the received rule bases by juxtaposing the rules received from the participants and by resolving potential conflicts. A conflict occurs when rules from different local TSK-FRBSs have the same antecedent, thus identifying the same specific region of the input space, but they have different consequents. In this case, the federated TSK-FRBS summarizes conflicting rules in a single rule with the same antecedent as the conflicting rules and with the consequent obtained by computing the weighted average of the regression model coefficients in the consequents of the conflicting rules. Such average takes into account the weight associated with each rule, which is estimated on the local training set as the harmonic mean of its support (how many instances activate the rule), and confidence (average quality of the prediction of the rule).
With the aim of ensuring the consistency of the rules among participants and increasing the system interpretability, the input variables are partitioned by using a strong uniform fuzzy partition with triangular fuzzy sets An example of strong uniform fuzzy partition with five triangular fuzzy sets is shown in Fig. 1 . Here, each fuzzy set is associated with a meaningful label that is used to express linguistically the rules.
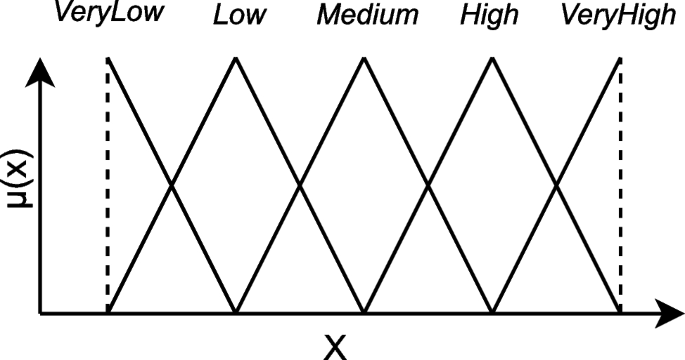
An example of strong uniform fuzzy partition with five triangular fuzzy sets
It should be noted that building a fuzzy system requires a careful design especially regarding the choice of its hyperparameters (e.g., number, shape, and position of fuzzy sets for each partition), also considering their impact on interpretability [ 65 ]. Uniform fuzzy partitions with triangular fuzzy sets are generally deemed highly interpretable since they satisfy the criteria of coverage, completeness, distinguishability, and complementarity [ 66 ]. However, in practical applications, a meaningful partition should be agreed with the human users who are expected to interact with the AI system and to interpret the given rules. An interesting future development of this work would consist in examining the choice of the uniform partitioning together with domain experts (e.g., physicians).
Federated Learning of MLP-NN
Models optimized through Stochastic Gradient Descent (SGD), such as NNs, can be learned in a federated fashion by exploiting an aggregation strategy based on, or derived from, the popular federated averaging (FedAvg) procedure. FedAvg is an iterative, round-based procedure, in which each round encompasses the following steps: the server sends the global model to the participants; each participant locally updates the model through SGD on its local training set and sends the updated model to the server; the server obtains an updated global model by computing the weighted average of the locally updated models, where weights are based on the local training set cardinality. Several extensions of FedAvg have been proposed in the literature, mostly aimed at addressing FL in heterogeneous settings [ 67 , 68 ]. In this paper, we focus on classical FedAvg and deal in more detail with the issue of explainability of MLP-NN learned in a federated fashion. To this end, in the following, we first describe a popular post-hoc method for explainability, namely SHAP (SHapley Additive exPlanations) method [ 20 ], and then an approach for the adoption of SHAP in the federated setting.
Post-hoc Explainability: The SHAP Method
One of the most popular post-hoc strategies used to explain a model prediction is to assess the importance of each feature in producing the output. In general, given a model f , an input instance \(\textbf{x}_{i}\) , and a predicted output \(\hat{y_i} =f(\textbf{x}_{i})\) , the explainer assigns to each input component \(x_{i,j}\) a value that reflects how much that particular feature is important for the prediction. These values are interpreted in terms of sign and magnitude: if the sign is positive (negative), then that feature contributes positively (negatively) to the prediction output; as per the magnitude, the larger it is, the higher the impact of the corresponding feature on the output.
In this work, we adopt the SHAP method [ 20 ] which is one of the most widely used approaches to assess the feature importance for both regression and classification tasks. SHAP provides local explainability, that is, it explains individual predictions. Global explainability insights can be obtained by aggregating the individual explanations over a set of data.
SHAP computes the importance of the individual features using the optimal Shapley values introduced by L. Shapley in 1953 [ 69 ] in game theory. In SHAP, the connection between game theory and explainability is that a prediction for an individual instance \(\textbf{x}_{i}\) can be explained by conceiving the features \(X_i\) as the “players” of a “game” where the prediction \(\hat{y_i}\) is the game “payout.” Intuitively, the different M players of the game (features) receive different rewards, called Shapley values \(\phi _j\) , depending on their contribution to the total prediction, i.e., \(\hat{y_i} = \phi _0 + \sum _{j=1}^M \phi _j\) where \(\phi _0\) is a reference value (baseline) computed as the average of output values. In this game-explanation analogy, the player who contributes with the larger \(\phi _j\) to the total prediction is the most important feature in the explanation.
Since the computation of the Shapley values involves testing all the possible combinations of the features ( coalitions of the players in the game theory) by perturbating the instance \(\textbf{x}_i\) , the time increases exponentially with the number of features [ 70 ]. Thus, various approaches have been proposed to estimate them efficiently, including SHAP. There are several kinds of SHAP methods, corresponding to different ways of approximating the Shapely values. In this work, we consider the widely adopted KernelExplainer variant of SHAP (KernelSHAP), as it is model-agnostic [ 21 ]. Indeed, other methods, such as TreeExplainer, result to be more efficient but are model-specific. Algorithm 1 describes the KernelSHAP procedure.
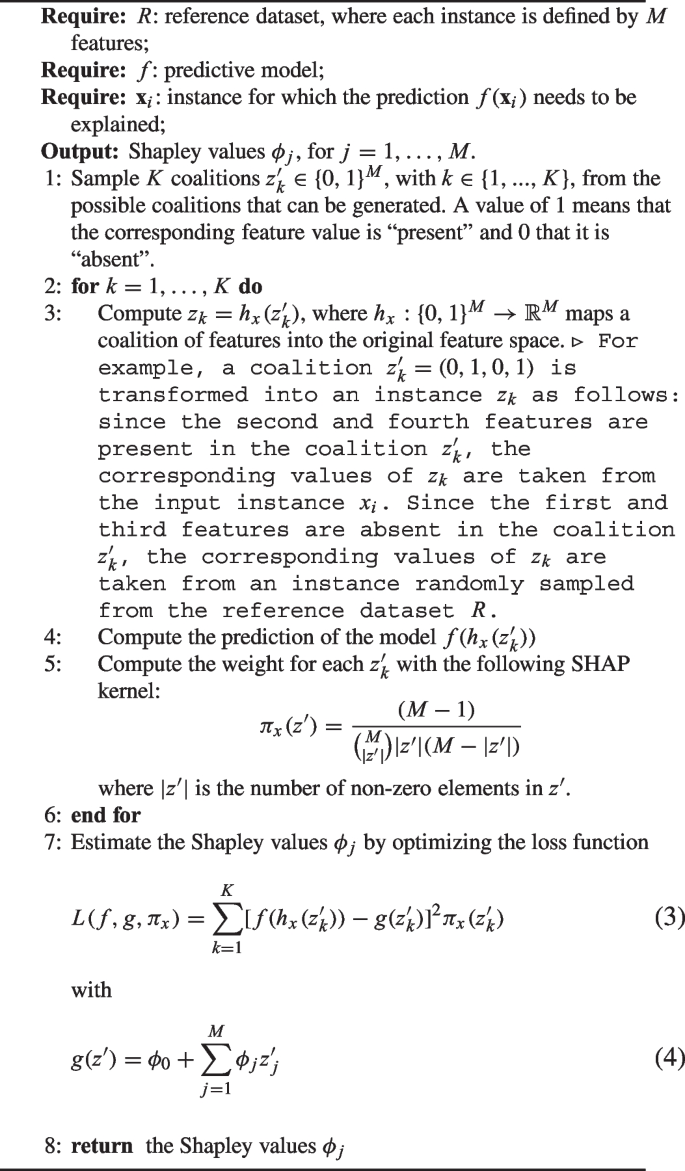
KernelSHAP algorithm, from [ 70 ].
Notably, KernelSHAP requires a background dataset that serves as a reference: whenever a feature is excluded from a coalition, its value is replaced using an instance randomly sampled from such dataset. The choice of a representative background dataset is crucial for obtaining accurate estimates of the Shapley values. For this reason, the training set is typically adopted for this purpose. However, it is not the unique possible choice: a different, generally smaller, dataset can be used at the condition of being representative of the data distribution of the training set. In the literature, representative objects such as medoids or centroids of clusters generated by applying a clustering algorithm on the training set have allowed faster estimations of the Shapley values. Additional details on SHAP and Shapley values can be found in [ 70 ].
Federated SHAP
Let H be the number of clients involved in the federation, \(\textbf{x}_{i} { aninputinstance},\,{ and}f(\textbf{x}_{i}){ thepredictiontobeexplained}.{ Inthiswork},\,{ themodel} f(\cdot ) \) is an MLP-NN learnt in a federated fashion. Following the setup proposed in [ 21 ], the goal is to achieve a federated explanation for \(f(\textbf{x}_{i}){ consideringtheinputinstancesimultaneouslyavailabletoalltheclients}.{ Itisworthunderliningthatthismaybeundesirableorunfeasibleiftheinstance}\textbf{x}_{i} { issubjecttotheprivacyconstraint}.{ Notably},\,{ insomeparticularscenarios},\,{ forexample},\,{ ifthepatientsrequiremultiplemedicalconsultations},\,{ theinstance}\textbf{x}_{i} \) could, under specific agreements, be shared to the other clients. Another scenario is represented by the presence of a benchmark dataset available to research entities, with the objective of comparing the goodness of the explainability produced by different methods.
As discussed in Section 3.2.1 , the application of the SHAP method requires a background dataset. Typically, this is the dataset used to train the prediction model. However, in the federated setting, local training sets belong to different entities and cannot be shared due to privacy issues. Therefore, the server has no reference data to be used as background. Federated SHAP proposed in [ 21 ] represents a possible strategy to overcome this issue and to achieve federated explanations by exploiting the additive property of the Shapley values.
In the federated SHAP procedure, first of all, each client estimates the Shapley values using the local dataset as background dataset; then the values are transmitted to the server that evaluates their average. In this way, the data privacy is preserved, since the raw data are never shared. Furthermore, it is shown that the average can be considered a good approximation of the Shapley values calculated if the union of the local training sets was available to the server.
Schematically, the overall procedure for FL with post-hoc explainability technique entails the following steps: (i) each participant \(h ({ with}h=1, \dots , H){ contributestothecreationofanFLmodel}.{ Attheendofthefederatedprocedure},\,{ thefederatedmodelismadeavailabletoeachparticipant};\,({ ii}){ givenanunseeninstance}\textbf{x}_{i},\,{ eachparticipant}h{ computestheShapelyvalues}\phi ^{(i,h)}_j{ with}j = 1, \dots , M,\,{ evaluatingKernelSHAPlocally},\,{ byexploitingtheprivatetrainingsetasbackgrounddataset};\,({ iii}){ theShapelyvalues}\phi ^{(i,h)}_j{ estimatedatparticipantlevelaretransmittedtotheserver},\,{ whichperformssimpleaveragingtoobtainthefederatedestimationoftheShapleyvalues}\phi ^{(i)}_j{ forexplainingtheprediction}f(\textbf{x}_i)\) .
Case Study: Federated Learning for Parkinson’s Disease Progress Prediction
In this section, we describe the case study for the evaluation of the Fed-XAI approaches. First, details about the PD telemonitoring dataset are provided. Then, we describe the experimental setup in terms of data partitioning scenarios and evaluation strategies. Finally, we give the configurations of the ML models adopted in the two Fed-XAI approaches based on MLP-NN and TSK-FRBS, respectively.
The Parkinson Telemonitoring Dataset
The Parkinson Telemonitoring dataset is a well-known regression dataset available within the UCI Machine Learning Repository [ 71 ]. The dataset is composed of 5875 instances of biomedical voice measurements from 42 patients with early-stage PD. Data are acquired remotely during a 6-month trial. Each instance corresponds to one voice recording, characterized by 22 features as reported in Table 1 . The regression task consists in predicting the total Unified PD Rating Scale score ( total_UPDRS ) associated with a given voice recording. Differently from motor_UPDRS , which is related only to the motor symptoms, total_UPDRS is related to the overall set of symptoms.
Federated Learning Scenarios
In this paper, extending the preliminary analysis performed in [ 15 ], we consider the challenging setting in which the raw dataset is not available on a single node for centralized processing, as in traditional ML, but it is instead scattered over multiple physical locations, e.g., hospitals or healthcare institutions. Specifically, we simulate several scenarios featuring 10 hospitals (cross-silo FL setting), in order to evaluate the performance of two Fed-XAI approaches under different horizontal data partitioning schemes that could be encountered in real-world situations.
In the following, we formally define the four scenarios considered in our experimental analysis. Let \(P_h(\textbf{x},y){ bethelocaldistributionofinputdata}\textbf{x} { andassociatedtargetvalues}y\) ( total_UPDRS ) for the hospital \(h,\,{ and}P(\textbf{x},y)\) the overall data distribution.
Scenario IID It is a simple independent and identically distributed ( i.i.d. ) setting; formally,
The training data of the ten hospitals follow the same distribution, with about 500 instances each.
Scenario NIID-Q (acronym for n on- i . i . d . q uantity skew). It is a non-i.i.d. setting with quantity skew [ 72 ]: different hospitals can hold different amounts of training data, which follow the same overall distribution.
Scenario NIID-F (acronym for n on- i . i . d . f eature skew). It is a non-i.i.d. setting with feature distribution skew [ 72 ] based on the \(age\) feature; formally,
Each hospital contains training data from only a specific range of ages (e.g., 56 to 57, 58 to 59, \(\dots \) , more than 75 years old). In this scenario, we aim to have training sets with as similar amount of data as possible.
Scenario NIID-FQ (acronym for n on- i . i . d . f eature and q uantity skew). It is a non-i.i.d. setting with both quantity skew and feature distribution skew based on the \(age\) feature. Each hospital contains training data from only a specific range of ages; furthermore, different hospitals can hold different amounts of data.
The four scenarios concern different partitioning schemes for training data. As for the testing data, we consider the case of an external publicly available test set, valid for all the scenarios. The test set follows the overall data distribution (i.e., representative of all age groups) and has 588 instances. The distribution of the training data in the four scenarios is summarized in Table 2 and in Fig. 2 .
As for the NIID-F and NIID-FQ scenarios, it is worth underlining that other features besides age may be affected by bias or skewness. However, this contingency still meets the definition of feature distribution skew. Therefore, the four scenarios enable a thorough and extensive evaluation of the performance of the two Fed-XAI approaches based, respectively, on MLP-NN and TSK-FRBS.
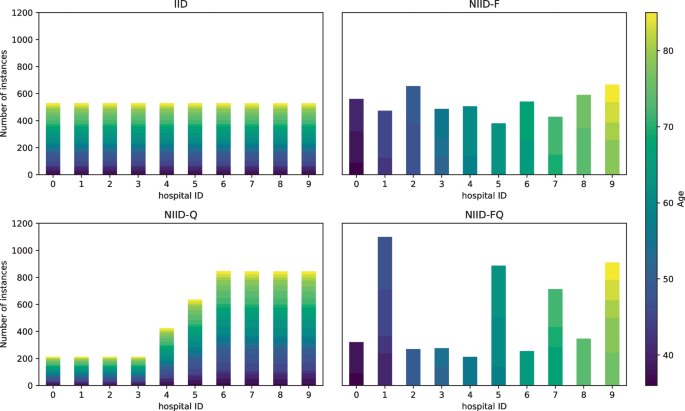
Barplot of the training data partitioning scheme over ten hospitals in the four scenarios. Data for different ages are represented in different colors
Evaluation Settings
Typically, the performance evaluation of a model in the federated setting is performed not only in absolute terms, but also comparatively against two baseline settings [ 15 , 73 , 74 ]: local learning and centralized learning. Figure 3 provides a schematic overview of the three learning settings.
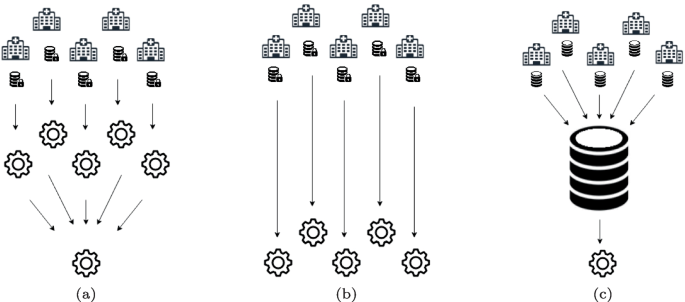
Schematized representation of the three learning settings: a federated learning (FL), b local learning (LL), c centralized learning (CL)
Federated learning (FL), local learning (LL), and centralized learning (CL) can be summarized for the dataset under consideration as follows:
FL: the hospitals collaborate in obtaining a single federated model without sharing their raw data. The privacy of sensitive data is preserved.
LL: each hospital locally learns a model from its private training data. As a consequence, the privacy of sensitive data is preserved, as in the FL case, but there is no collaboration among different hospitals.
CL: training data from all hospitals are collected in a single central repository in the server and exploited to learn a global model. CL implies indeed maximum collaboration among hospitals, but violates privacy, as private sensitive data are moved from their owner to the server.
A model learned in the FL setting is expected to be more accurate than the ones learned in the LL setting. On the other hand, a model learned in the CL setting can outperform the other models (both LL and FL), in terms of accuracy, because it can rely on the union of the training datasets. The CL approach, however, is not viable in real applications where privacy protection is a mandatory constraint.
Regression Problem and Fed-XAI Models
The PD progress prediction is formulated as a regression problem where the target variable is the Total_UPDRS score. In our experiments, we replicated the preprocessing steps adopted in [ 15 ], namely, (i) a robust scaling using 0.025 and 0.975 quantiles is applied to the input features to remove outliers and clip the distribution in the range [0,1], and (ii) the output variable is normalized in the range [0,1].
Unlike in [ 15 ], the univariate feature selection procedure is carried out independently for the three learning settings, for a fair comparison of the entire regression pipelines. We select the \(G=4\) best features in terms of Mutual Information (MI) [ 75 ] with the target variable. The estimate of MI and the subsequent feature selection is done individually by each participant in the LL setting, based on the local training sets, and globally in the CL setting, based on the union of the training sets. As for the FL setting, the federated feature selection procedure is schematized in Fig. 4 , considering the example of the IID scenario. Each participant computes the MI score for all the features and transmits such information to the server. The server computes the average MI score for each feature and communicates the \(G\) best features to each participant. Thereafter, the FL process starts considering only the selected subset of features. In the example of Fig. 4 concerning the IID setting, the federated feature selection procedure selects the following features: age , test_time , DFA , and HNR . Note that the feature importance scores of each participant may change depending on the data distribution scenario: thus, the selected features may vary and may generally differ from the CL setting.
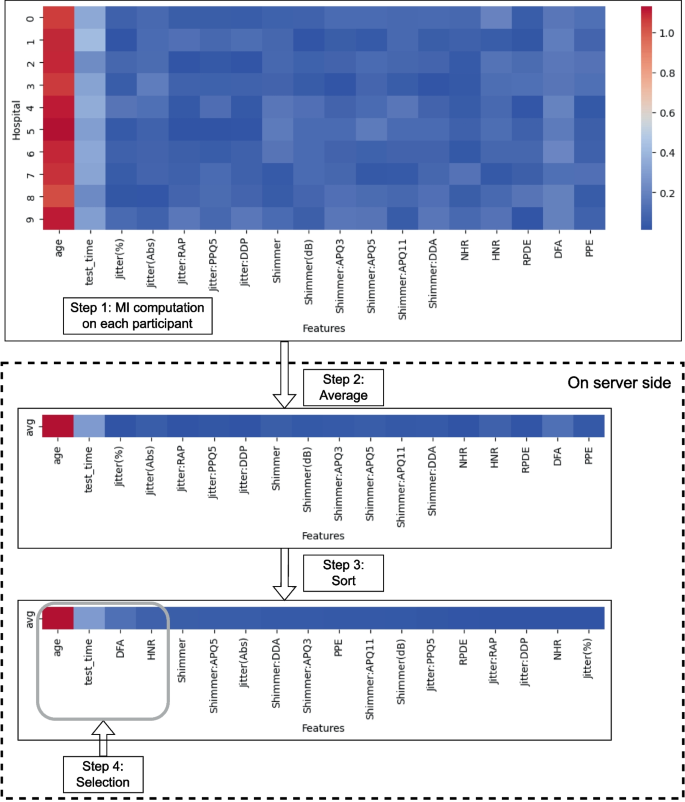
A schematic overview of the federated feature selection procedure. The example concerns the IID scenario
The choice of the \(G\) value is guided by the following considerations: a reduced number of features generally improves the explainability task, both for post-hoc and interpretable by-design approaches. In addition, TSK-FRBSs struggle to handle high dimensional datasets [ 76 ]: the set of candidate rules grows exponentially with the number of features, thus jeopardizing the accuracy and the interpretability of the system. We have verified that \(G=4\) ensures a good generalization capability for both models and an increase in the number of features does not lead to a significant improvement in performance.
In our experiments, for each data distribution scenario, we trained a TSK-FRBS and an MLP-NN according to FL, LL, and CL settings. The experimental analysis is approached from a twofold perspective: model accuracy and model explainability . We assess the accuracy of the predictions obtained by the regression models as in [ 15 ] by using two popular metrics, namely Root Mean Squared Error (RMSE) and Pearson correlation coefficient ( \(r\) ). They are defined as follows:
where \(N{ isthenumberofsamplesconsideredfortheevaluation},\,{ and}y_i{ and}\hat{y}_i{ arethegroundtruthvalueandthepredictedvalueassociatedwiththe}i\) -th instance, respectively. Finally, \(\bar{y} { isthemeanofgroundtruthvalues},\,{ and}\hat{\bar{y}} { isthemeanofthepredictedvalues}.{ Obviously},\,{ thegoalistominimizeRMSEandmaximize}r\) .
It is worth underlining that the evaluation of an FL system typically covers other aspects besides accuracy such as computation and communication efficiency. These aspects, however, represent often crucial requirements or potential bottlenecks in cross-device FL, with many devices featuring limited computational resources [ 77 ]. In a cross-silo FL scenario, as the one considered in this work, such aspects are generally deemed less critical.
Interpretable By-design Fed-XAI: TSK-FRBS Configuration
As in [ 15 ], we employ a first order TSK-FRBS model (described in Section 3.1 ). We adopt a strong uniform fuzzy partition on the features with five triangular fuzzy sets, as shown in Fig. 1 . The choice of five fuzzy set is driven by the indication of the specialized literature and by the pursuit of a reasonable trade-off between model complexity and generalization capability. The number of linguistic terms associated with a linguistic variable should be below the limit of \(7\pm 2\) [ 78 ]. Indeed, it has been shown that this represents a threshold for information processing capability, and thus exceeding it undermines the interpretability of the system [ 79 ]. With the aim of describing linguistically a given rule, the five fuzzy sets can be labeled with the following linguistic terms: VeryLow , Low , Medium , High , and VeryHigh . Furthermore, it should be noted that different features may be partitioned using a different number of fuzzy sets, e.g., by exploiting domain knowledge to enhance understandability. Although this represents an interesting future development, we did not conduct extensive hyperparameters optimization. Rather, we verified in the CL setting that beyond 5 fuzzy sets, there is a substantial increase in model complexity, without significant improvement in terms of performance metrics. The choice of 5 fuzzy sets ensures a high linguistic interpretability and represents a reasonable trade-off between model complexity and generalization capability.
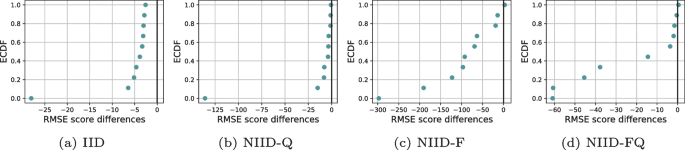
TSK-FRBS: empirical cumulative distribution function (ECDF) of the differences of RMSE scores between FL and LL for the four data partitioning schemes
Post-hoc Explainable Fed-XAI: MLP-NN Configuration
The MLP-NN consists of two hidden layers with 128 neurons, each with the ReLu activation function. The Mean Squared Error (MSE) is adopted as loss function and Adam as optimizer. The minibatch size is set to 64. The overall number of epochs is set to 100 in the CL and LL settings. In the FL setting, we set the number of local epochs and the number of rounds as 5 and 20, respectively. We have not performed a thorough optimization of hyperparameters for each learning setting and each data distribution scenario individually; however, we have empirically observed that a further increase in the capacity of the models in terms of number of layers, number of neurons, and training epochs does not lead to a significant increase in the generalization capability of the MLP-NN.
Analysis of the Experimental Results
Table 3 presents the RMSEs and \(r\) coefficients obtained by the TSK-FRBS and MLP-NN models for all the learning settings and the data distribution scenarios. As regards the LL setting, we report the average values \(\pm \) standard deviation obtained by the models learned locally in each participant. In the table, we have highlighted in bold the best results for each row, considering the comparison between TSK-FRBS and MLP-NN. Notably, in certain cases, the result is obtained from a distribution of values and is expressed in terms of mean and standard deviation: this occurs in the LL setting when ten local models are evaluated on the test sets and, regardless of the learning setting, when performance metrics are evaluated on ten training sets from as many hospitals. In such cases, we highlighted in bold the best result only if there exists a statistical difference in metrics values between TSK-FRBS and MLP-NN. The statistical significance has been assessed through a pairwise Wilcoxon signed-rank test [ 80 ] with confidence level \(\alpha =0.05.{ Ingeneral},\,{ federatedmodelsoutperformthelocalcounterparts},\,{ bothintermsofRMSEand}r\) . The benefit of FL over the LL setting is particularly evident for the TSK-FRBS and especially in the non-i.i.d. settings.
Federated TSK-FRBS and federated MLP-NN achieve comparable performances. The most noticeable difference occurs in the NIID-F setting, in which the two metrics provide diverse insights: on one hand, RMSE indicates that the deviation of predictions from true values is lower for the MLP-NN (10.268) compared to the TSK-FRBS (16.848); on the other hand, predictions and true values are more correlated for TSK-FRBS ( \(r=0.461\) ) than for MLP-NN ( \(r=0.205\) ).
The non-i.i.d. setting with quantity skew (NIID-Q) does not harm particularly the performance of the models: RMSE and \(r\) values are comparable to those of the IID setting, for both TSK-FRBS and MLP-NN. In the case of TSK-FRBS, the simple average of the performances measured on the training sets shows poor performance for the LL setting, where some models likely suffer from low data availability. The aggregation strategy based on the rule weight (defined as a combination of confidence and support) ensures that this unfavorable situation is mitigated in the FL setting.
Scenarios with feature distribution skew (NIID-F and NIID-FQ) turn out to be the most challenging for both models. The generalization capability of models built in the LL setting is rather poor, due to exposure to data from a limited age range during training: both TSK-FRBS and MLP-NN perfectly model the training data (resulting in low RMSE values and an \(r\) coefficient around 0.9 on training sets) but fail in properly predicting the total_UPDRS score of the test instances (resulting in high RMSE and low \(r).{ DiscrepancyinRMSEand}r\) values between training and test sets is noticeable in the LL setting, whereas it is limited or negligible in the FL setting.
It is worth highlighting that the performances in the FL setting are always worse than those obtained in the CL setting, both for TSK-FRBS and MLP-NN. In general, the centralized MLP-NN is able to achieve the best performance with a slight improvement in terms of RMSE and \(r\) over the centralized TSK-FRBS. The superior performance of the centralized model can be attributed to the utilization of all data for conventional training. However, it is deemed unfeasible when privacy preservation represents a critical requirement.
The results in Table 3 provide an aggregate view of the LL setting. A better understanding of the outcomes can be gained by analyzing the specific performance obtained in each hospital: such detailed results are illustrated in Figs. 5 and 6 through the empirical cumulative distribution function (ECDF) for the RMSE metric.

MLP-NN: empirical cumulative distribution function (ECDF) of the differences of RMSE scores between FL and LL for the four data partitioning schemes
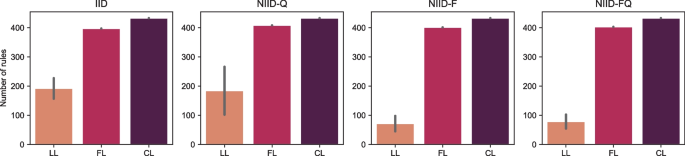
Number of rules of the TSK-FRBS for each learning setting and each data partitioning scheme. Error bar represents the standard deviation
For both models, the ECDF is reported for the values of the difference, for each hospital, of the RMSE achieved in the FL setting and the 10 locally computed values of RMSE obtained in the LL setting: each plot, therefore, is made up of 10 points. The plot can be interpreted as follows: if a point lies in the negative half-plane (negative RMSE difference), then the RMSE value of the FL model is lower (and therefore the FL model is better) than the RMSE value of the LL model. The fine-grained analysis shown in Figs. 5 and 6 confirms that the FL setting generally outperforms the LL setting.
Finally, we report on the overall complexity of the models, which will be further discussed in Section 6 . In the case of the MLP-NN, the network architecture is fixed: the complexity, intended as the number of parameters, does not change with the learning setting. In the case of TSK-FRBS, the complexity can be assessed in terms of the number of rules in the rule base. Figure 7 shows the complexity for each data distribution scenario and each learning setting.
The number of rules of the federated TSK-FRBS never exceeds, by construction, that of the centralized TSK-FRBS, which in any case is limited (433 rules). As expected, the federated TSK-FRBS is more complex than locally learned ones. In the IID and NIID-Q scenarios (in which each hospital has data representative of the entire distribution), the 10 local models have approximately half the number of rules of the federated ones, indicating that common antecedents are often found in the rule aggregation phase. The complexity of locally learned TSK-FRBSs in the presence of feature distribution skew (NIID-F and NIID-FQ) is significantly lower than the one in IID setting, and the gain in accuracy provided by the federated models comes at a cost in terms of number of rules, which is approximately five times higher.
Explainability Analysis
The extensive adoption of AI systems in the healthcare field depends not only on the achievement of adequate levels of accuracy, but also on how much they are perceived to be trustable. In particular, the ability to explain how the outcomes have been produced by the models is more and more required and represents the main driver of XAI. This section discusses the aspect of transparency of AI systems, focusing on how it is defined for the two Fed-XAI approaches analyzed in this paper. First, we analyze the explainability of the MLP-NN model, in which SHAP is used as post-hoc method. Then, we discuss the interpretability by-design of the TSK-FRBS. We consider models built in a federated fashion according to the IID data partitioning scheme: the discussion of the outcomes is limited to such case, but the pipeline for explainability analysis can be easily replicated for any data distribution scenario. Furthermore, we recall that all the input variables and the output variable are normalized in the unit interval \([0,1]\) : the considerations in this section refer to the predicted values before inverse transformation.
Post-hoc Explainability of MLP-NN
In this section, we discuss the explainability of the MLP-NN after the application of the agnostic post-hoc method SHAP. We recall that, given an input instance, the Shapley value associated with each feature represents the contribution given by such feature to the predicted value. In this sense, for each prediction, SHAP explains why the model produces a particular output.
We adopt the Federated SHAP approach proposed in [ 21 ] and introduced in Section 3.2.2 . KernelSHAP is employed in each hospital to estimate the Shapley values considering the full local training set as background dataset.
It is worth underlining a first crucial aspect concerning the explainability of MLP-NN: unlike interpretable-by-design Fed-XAI approaches, the post-hoc method affects the overall efficiency of the systems, both from a computation and a communication point of view. As for the former aspect, the estimation of the Shapley values with KernelShap is time consuming and the runtime increases with the number of features and the size of the adopted background dataset [ 70 ]. As for the latter aspect, Federated SHAP introduces a communication overhead, as Shapley values need to be transmitted by the participants for central aggregation. Conversely, the interpretable-by-design TSK-FRBS has no computation and communication overhead for generating the explanations.
MLP-NN: Global Insights
Globally, an MLP-NN is generally considered “opaque,” due to the presence of several layers of non-linear information processing. In our case, the structure consists of two hidden layers with 128 neurons, resulting in 17,281 trainable parameters. The high number of parameters and the relations among these parameters make very hard to provide a global explanation of the model. Thus, indirect methods based, for instance, on feature importance are typically used to provide global explainability information [ 3 ].
As Shapley values represent additive feature importance scores for each particular prediction, the overall feature importance can be assessed by computing the average of the absolute Shapely values across the data [ 70 ]. Of course, the larger the average absolute value of the contribution given by a feature, the greater the importance of that feature. The assessment of the feature importance of a model is typically independent of the test data. In the case of the MLP-NN, it can be estimated as follows. First, each hospital \(h{ evaluatestheimportance}I{ ofthefeature}i\) on its training data:
where \(\phi _j^{(i,h)} { istheShapelyvalueforafeature}j{ andatraininginstance}i{ athospital}h,\,{ and}N_h{ isthesizeofthetrainingsetathospital}h\) . Then, each client can transmit locally computed features importance to the server, and the overall features importance for the federated model can be computed by the server as follows:
where \(H{ isthenumberofhospitalsand}N = \sum _{h=1}^{H}N_h\) .
Figure 8 shows the global feature importance scores for the MLP-NN, as per ( 10 ): in the IID setting, the most relevant feature is age , while test_time seems to be less relevant than vocal features, namely DFA and HNR , which in turn are of similar relevance.
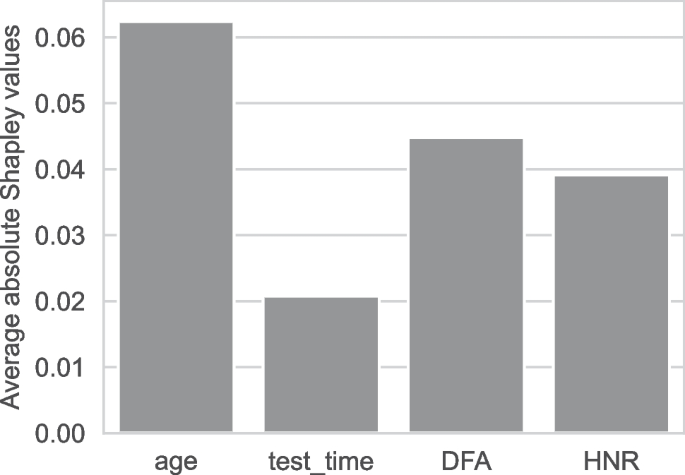
Feature importance scores for the MLP-NN
MLP-NN: Local Insights
Figure 9 reports the SHAP values for two instances of the test set; they correspond to two cases where both models (MLP-NN and TSK-FRBS) obtain high and low errors, respectively. The absolute error (AE) made by the MLP-NN is around 0.55 for instance #2496 and around 0 for instance #2323.
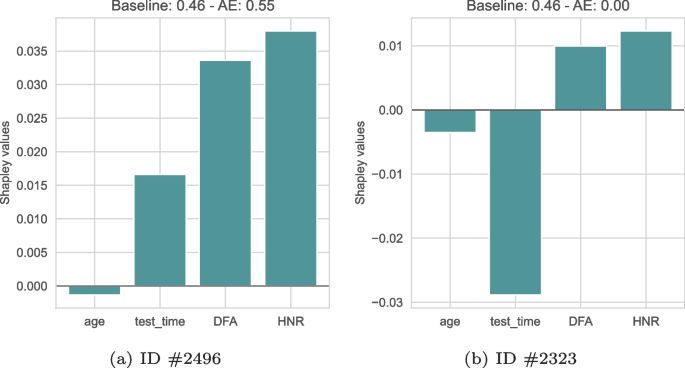
MLP-NN local explainability: Shapley values for two instances in the test set. The absolute error for each instance is reported along with the baseline value
The Shapley values reveal, a-posteriori, the relevance of the corresponding features in the prediction performed by the model: as expected, they are different when considering different instances. In the former case (Fig. 9 a), age has little negative influence, while the other features have a large and positive impact on the output value. In the latter case (Fig. 9 b), the most influential feature is test_time , which has a negative impact on the output. Notably, the SHAP values for individual features are evaluated, for both instances, with respect to the same baseline value \(\phi _0 = 0.46\) .
Interpretability By-design of TSK-FRBS
TSK-FRBSs are often considered as “ light gray box” models [ 81 ]: their operation is highly interpretable, since they consist of a collection of linguistic, fuzzy, if-then rules. However, in the first-order TSK-FRBSs used in this paper, the adoption of a linear model in the consequent part, which certainly improves the accuracy with respect to the zero-order TSK-FRBS, makes the interpretation of a single rule less intuitive than the zero-order counterpart.
A substantial difference with respect to the MLP-NN model analyzed in Section 6.1 is that interpretability information is given without additional overhead in terms of computation and communication (as it is the case for the calculation of Shapley values on the MLP-NN model).
The following analysis aims to characterize both global and local interpretability of TSK-FRBS learnt in a federated fashion.
TSK-FRBS: Global Insights
The global interpretability of TSK-FRBSs can be quantitatively assessed by measuring the complexity of the system in terms of the number of rules and/or parameters. Less complex models, i.e., those with fewer rules, can be generally considered more interpretable [ 66 ]. Figure 7 reports the number of rules for each learning setting and each data partitioning scenario. As underlined in Section 5 for the IID case, the number of rules in the FL setting is rather limited (i.e., 397) and just double that in the LL case (despite the presence of 10 participants).
The model is therefore comprehensively described by the rule base, which can be represented in the intelligible form reported in the following.
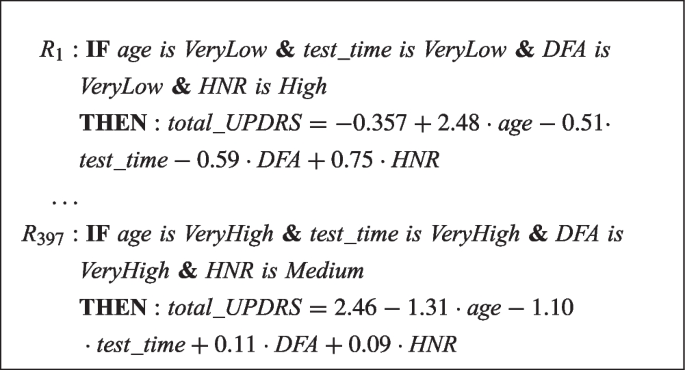
The number of parameters of a rule in a TSK-FRBS can be estimated as follows, considering the presence of four input variables: it is given by the sum of four parameters for the antecedent part (one for each input variable, to identify a fuzzy set of the a-priori partitioning) and five parameters for the linear model of the consequent part. Ultimately, the considered TSK-FRBS has 3573 parameters overall.
In the case of TSK-FRBS, a measure of feature importance can be obtained by averaging the absolute values of the coefficients of the linear models in the rule base. Formally, the importance \(I{ ofthefeature}j\) is evaluated as follows:
where \(\gamma _{r,j} { isthecoefficientforthevariable}j{ intherule}r\) .
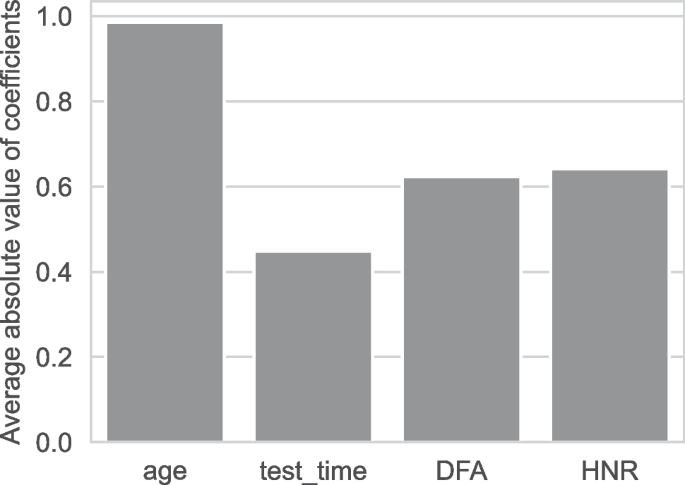
Feature importance scores for the TSK-FRBS
Figure 10 suggests that feature importance computed for the TSK-FRBS model is consistent with the one computed for the MLP-NN by the SHAP method in the IID setting. Age and test_time are identified as the most and the least relevant features, respectively. Furthermore, the importance of HNR and DFA is similar, consistently with what is observed for the MLP-NN.
A direct comparison of the importance values between Fig. 8 (MLP-NN) and 10 (TSK-FRBS) is not meaningful. Indeed, in the case of MLP-NN, Fig. 8 shows the average absolute contribution for each feature with respect to the baseline value. In case of TSK-FRBS, Fig. 10 represents the average absolute value of the coefficients of the linear models used in the case of TSK-FRBS.
It is worth underlining that model-agnostic nature of SHAP can be exploited to compute post-hoc explanations also on the TSK-FRBS model. Thus, we can directly compare the feature importance obtained by averaging the absolute values of the coefficients of the linear models in the rule base and reported in Fig. 10 , with that obtained by averaging the absolute Shapely values across the data for TSK-FRBS ( 9 ) and ( 10 ). The latter approach results in the scores reported in Fig. 11 .
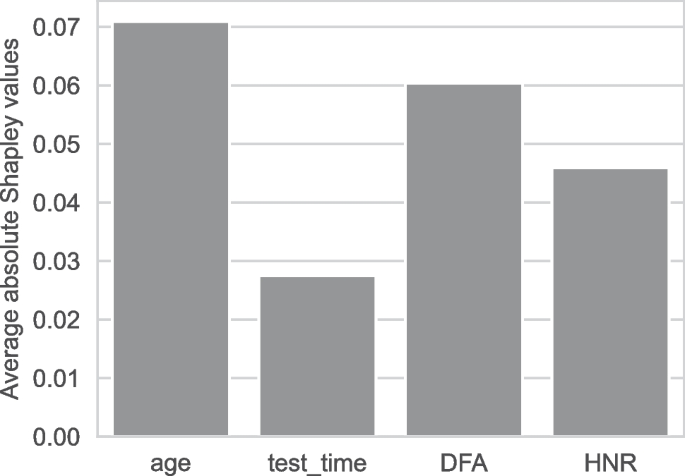
Feature importance scores for the TSK-FRBS evaluated in terms of Shapley values
It is interesting to note that age and test_time are still identified as the most and least important features, indicating a summary agreement among the results obtained with different important attribution methods in the IID scenario. There is a discrepancy, on the other hand, between the relative values of DFA and HNR , possibly because the two approaches estimate importance values with different criteria. Furthermore, it is worth noting that feature importance scores computed for TSK-FRBS using SHAP are consistent with those computed for MLP-NN and shown in Fig. 8 , also in terms of range of values.
The identification of age as the most important feature regardless of the model and the attribution method adopted is not surprising. First, in the IID scenario, each hospital can have data for all age ranges and therefore the feature entails a high variability. Second, such evidence is reflected in the specialized literature, which indicates that age is the best predictor of the progression of Parkinson’s disease and the most important risk factor for the development of the disease [ 82 ].
TSK-FRBS: Local Insights
The interpretability of the TSK-FRBS derives from its structure and the type of inference strategy we use. Indeed, for any given input instance, the predicted output depends on a single rule: the antecedent part isolates a region (a hypercube) of the input space, where its consequent part defines a local linear model. The coefficients of this model indicate how the input features contribute to form the TSK-FRBS output in that region: a positive (negative) coefficient for a given feature indicates that the output increases (decreases) with that feature. Notably, all instances belonging to that region will refer to the same linear model.
Given an instance \(\textbf{x}_{i} { andarule}r,\,{ whichistheonewiththemaximumstrengthofactivationfor}\textbf{x}_{i} \) , the actual contribution of each feature to the prediction \(\hat{y_{i}} { isobtainedas}\gamma _{r,j}\cdot x_{i,j} \) . In other words, contributions are obtained as the element-wise product between the coefficients of the linear model and the feature values of an instance. Figure 12 reports both the feature contributions and the coefficients of the linear model of the TSK-FRBS for the same two instances of the test set analyzed in the case of the MLP-NN (instances #2496 and #2323).
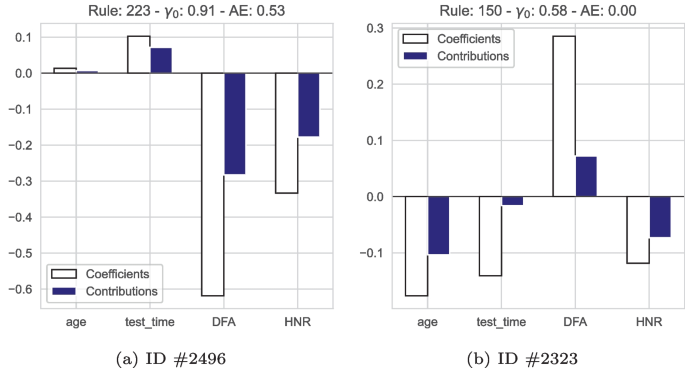
TSK-FRBS local explainability: coefficients of the linear model and actual feature contributions for two instances in the test set. The absolute error for each instance is reported above the relevant plot along with the ID of the rule considered for the prediction and the term \(\gamma _0\) of the linear model
The two instances activate different rules: as a consequence, the contributions are significantly different. Furthermore, in general, the contributions are reduced compared to the coefficients, since each feature is normalized in the unit interval.
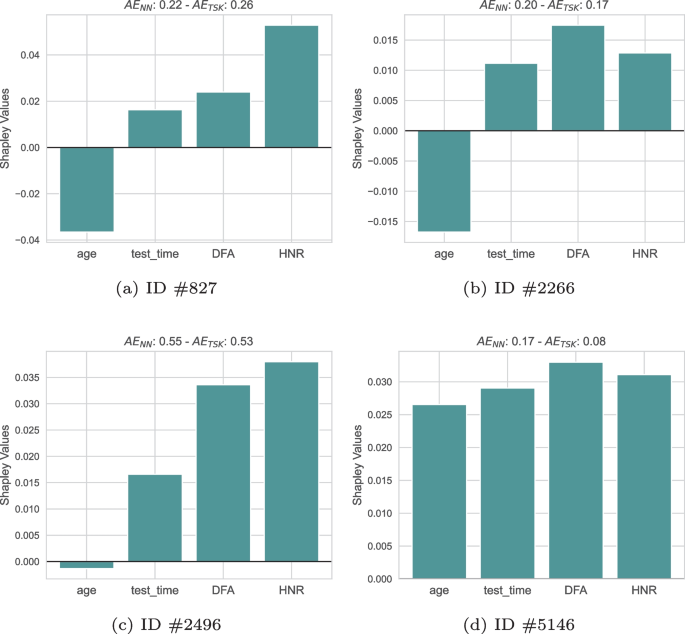
Shapley values calculated for the MLP-NN model on the instances in the test set that activate rule \(R_{233} \) of the TSK-FRBS. The absolute error (AE) for each instance and for each model is reported above the relevant plot
Local Explanations: Comparison Between TSK-FRBS and MLP-NN
A relevant outcome can be drawn by comparing the barplots of Figs. 9 and 12 : although the absolute errors are similar (we have verified that the predicted values are similar as well), the two models “reason” differently and assign different—sometimes diametrically opposed—contributions to the features, also because the term \(\gamma _0\) is different from the baseline value of SHAP.
To better examine this aspect, we focus on a set of instances and analyze the explanations provided by the two models. Specifically, we consider the instance resulting in a high AE for both models (ID #2496) and all the instances of the test set that activate the same rule (namely, \(R_{223} \) ) of the TSK-FRBS. In this way, we isolate four instances (ID #827, ID #2266, ID #2496, ID #5146) which are inevitably close to each other in the input space.
The local interpretability of the TSK-FRBS is straightforward: predictions are obtained by applying the following rule:
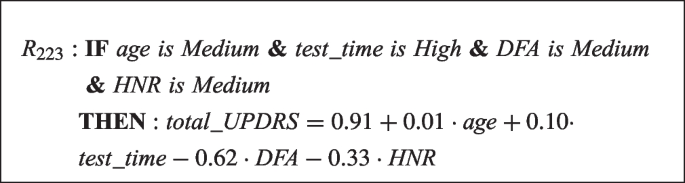
In the case of MLP-NN, the prediction is explained through the Shapley values: Fig. 13 shows the contributions for the MLP-NN considering the four instances of the test set that activate rule \(R_{233} \) of the TSK-FRBS system.
It is evident that the Shapley values for the MLP-NN vary greatly even though the instances are fairly close in the input space: as an example, age has a negative contribution for ID #827 and a positive one for ID #5146. For this reason, it is equally evident that a correspondence cannot be found between the explanations offered by SHAP for the MLP-NN and the interpretation of the linear model of the TSK-FRBS. For example, SHAP always assigns a positive contribution to DFA , while the relevant coefficient is negative for TSK-FRBS.
As noted above, the divergence in explanations between TSK-FRBS and MLP-NN in the IID scenario does not correspond to a divergence in output values. We have verified that the predicted outputs are similar (and indeed the reported AE values are similar): different models, which achieve similar results, lead to different explanations from a local point of view. This is not to be considered odd: our analysis entails different feature importance methods (inherent and post-hoc) and different models (TSK-FRBS and MLP-NN, respectively). Actually, it has been empirically shown that the feature importance score may suffer from numerical instability (when model, instance and attribution method are the same), solution diversity (if different models are considered, but on the same instance with the same attribution methods), or disagreement problem (if different attribution methods are considered, but on the same instance and the same model) [ 83 ]. These scenarios are due to the so-called Rashomon effect [ 84 ], whereby for a given dataset there may exist many models with equally good performance but with different solution strategies.
Consistency of Explanations
As mentioned in Section 2.3 , the property of consistency in the FL setting, introduced in [ 60 ], is met if different participants receive the same explanation of an output obtained with the federated model given the same data instance.
Evidently, for all the different operative scenarios discussed in this paper, the explanations for the federated TSK-FRBS are consistent: any local explanation obtained in a given hospital depends only on the input instance and on the activated rule of the federated model.
Conversely, the approach proposed in [ 21 ] and adopted in this work as post-hoc technique for the MLP-NN explainability does ensure the consistency of the explanations only in the situation where the test instances are shareable to all the clients. The local explanation obtained in a given hospital, in fact, depends not only on the input instance and the federated MLP-NN, but also on the background dataset used for estimating the Shapley values. Since each hospital has its own private dataset, the Shapley values for the same input instance may differ, in general, from one hospital to another. The Federated SHAP approach allows obtaining an explanation for each test instance by averaging the local explanations from different hospitals. On one hand, this ensures that a unique and unambiguous explanation is obtained. On the other hand, this requires that any test instance is shared with other hospitals at inference time, which may be problematic due to privacy and/or latency issues.
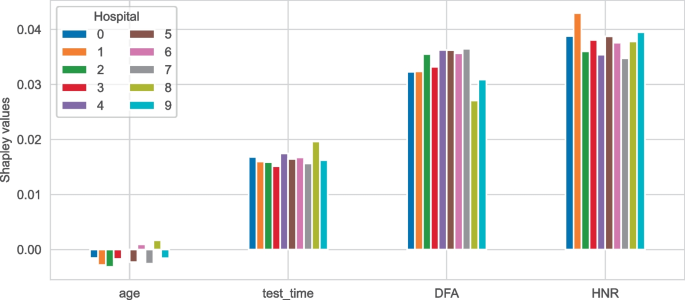
Shapley values for the MLP-NN for instance ID #2496 for each hospital. IID Scenario
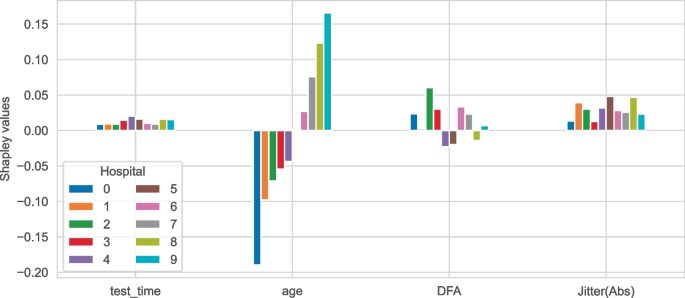
Shapley values for the MLP-NN for instance ID #2496 for each hospital. NIID-FQ scenario
In the following, we quantitatively assess the misalignment of client-side explanations obtained with SHAP for the MLP-NN, before applying the averaging operation that characterizes Federated SHAP. We consider an input test instance (ID# 2496, discussed also in the previous examples) and suppose that it is available at every hospital. Specifically, we evaluate how the prediction for such instance would be explained on different clients, in case that sharing Shapley values for averaging is precluded for privacy reason.
Figure 14 reports the Shapley values for each hospital and each feature in the IID scenario.
The barplot suggests that the explanations are consistent in the IID scenario, albeit showing some slight variability, which is reasonable since the background datasets are identically distributed. Indeed, explanations are in line with the average pattern reported in Fig. 14 c.
The variability among client-side explanations turns out to be substantial in non-i.i.d. scenarios. Figure 15 shows the Shapley values for the same instance for each hospital and each feature when considering the NIID-FQ scenario. We recall that such scenario entails both a quantity skew and a feature skew on the age feature. Furthermore, it is worth mentioning that the feature selection process is part of the FL pipeline. As a consequence, the set of selected features depends on the data distribution scenario: this explains the presence of different features compared to the IID case, with Jitter(Abs) replacing HNR .
Figure 15 suggests that the misalignment of explanations is severe, especially for the contribution assigned to the age feature by different hospitals. The relevant Shapley value goes from negative in hospitals with younger patients to positive in hospitals with older patients. Probing the global model with data from heterogeneous distributions results in a difference also in the importance assigned to the DFA feature. In summary, the same instance, analyzed with the same model, is explained in very different ways on different hospitals. Thus, the property of consistency among explanations is not achieved.
The consistency of SHAP explanations in the FL setting can be achieved by avoiding the use of private training data as background: Chen et al. [ 63 ], for example, propose to use synthetic background datasets generated sampling from a Gaussian distribution whose parameters are estimated on the server side based on the contributions of all participants. However, such explanations may be different from those obtained using actual training data. Ensuring both consistency and accuracy of explanations, intended as agreement with the centralized case, is one of the interesting future developments of this work.
Conclusions
In this paper, we have addressed the problem of developing a trustworthy AI system for a healthcare application, with specific focus on a Parkinson’s disease (PD) progression prediction task. For this purpose, we designed two approaches that simultaneously meet the requirements of data privacy preservation and explainability, which are usually deemed crucial for enabling trustworthiness. The first approach adopts a Takagi-Sugeno-Kang Fuzzy Rule-Based system (TSK-FRBS), which is interpretable by-design. TSK-FRBSs make use of fuzzy sets as information granules, thus guaranteeing high semantic interpretability. The second approach employs a Multi Layer Perceptron Neural Network (MLP-NN): as a “black-box” model, it requires the adoption of a post-hoc technique for explainability purposes. In this paper, we have adopted SHAP, which is considered as a state of art feature importance explainability method.
For both approaches, the federated learning (FL) paradigm has been exploited as it inherently enables privacy preservation during global model training procedures in decentralized settings. In detail, we devised an experimental setting assuming that sensitive data originate from ten hospitals and cannot be shared for privacy reasons. In order to cover several real-world situations, four (one i.i.d. and three non-i.i.d.) scenarios with different degrees of heterogeneity are simulated.
The critical analysis of the two approaches has concerned the following aspects: (i) the accuracy of the models, in terms of Root Mean Squared Error (RMSE) and Pearson correlation coefficient \(r,\,{ dependingonthelearningsetting}({ federatedlearning},\,{ locallearning},\,{ centralizedlearning}){ andthefourdatadistributionscenarios},\,{ and}({ ii}){ theexplainabilityofthemodelsatglobalandlocallevels}.{ Thekeyfindingscanbesummarizedasfollows}.{ Fromtheperspectiveofperformancemetrics},\,{ resultshighlightthatthefederatedmodelsareabletooutperformtheoneslearnedbyusingonlylocaldata},\,{ bothintermsofRMSEand}r\) values, hence highlighting the benefits of the federation. This is particularly evident in the non-i.i.d. settings. Also, results suggest that federated TSK-FRBS and federated MLP-NN achieve comparable performance, within the context of the considered case study.
As regards explainability, we have presented the results of the post-hoc explainability of MLP-NN and of the by-design interpretability of TSK-FRBS, also providing a comparative analysis of the two approaches. It turns out that the two approaches can lead to different local explanations, even if the underlying models achieve similar results in terms of regression metrics. A first major difference between the two approaches lies in their nature: the TSK-FRBS model provides insights about “how” an outcome is obtained, whereas the post-hoc method provides insight about “why” an outcome is provided. Consequently, a qualitative comparison is more reasonable than a quantitative one. We have to take into account, however, that if a model is able to provide a glimpse on how an outcome has been obtained, implicitly it is also making manifest why that outcome has been computed from the inputs.
More precisely, the TSK-FRBS is a collection of linguistic if-then rules: its global interpretability is usually assessed in terms of the number of rules and/or parameters: a lower number of rules corresponds to systems considered more interpretable. An equivalent global picture is not immediately available in the case of MLP-NN: the adoption of the SHAP method enables local explainability (i.e., it explains individual predictions), and a global explainability indication can be obtained in terms of feature importance, by aggregating the individual explanations over a set of data. The comparison of the feature importance obtained for the MLP-NN (as the average of the absolute Shapley values) and for the TSK-FRBS (as the average of the absolute values of the coefficients of the linear models in the rule base) in the IID setting reveals a fair agreement: both methods identify age as the most important feature and test_time as the least important one.
Local explanations of the two approaches convey inherently different messages. For the MLP-NN, the Shapley values represent the importance of each feature to each prediction with respect to a baseline value, computed as the average output value. For the TSK-FRBS, the predicted output depends, in our setting, just on the mostly activated rule: the antecedent part isolates a region of the input space and is expressed using linguistic terms associated with fuzzy sets (information granules), whereas its consequent part defines a local linear model whose coefficients indicate how the input features contribute to form the output. Focusing on a specific region of the input space, corresponding to the one isolated by the antecedent of a rule in the TSK-FRBS, we have evaluated the explanations provided by the two models for the instances located within that region. As expected, the explanations obtained with SHAP for the MLP-NN are generally different for the different instances and do not match what is expressed by the relevant rule of the TSK-FRBS. In essence, different models, which produce similar outputs and achieve similar overall results, lead to different explanations from a local point of view.
Finally, it is worth underlining that the property of consistency holds for the federated TSK-FRBS: for the same input data, different participants get the same explanation from the common federated model. In the case of the SHAP post-hoc technique, this is not as straightforward: if participants use their local training data as background dataset for the estimation of the Shapley values, the feature importance scores are necessarily different; if the federated SHAP strategy of averaging locally computed Shapley values is adopted, a single value is obtained. This ensures consistency of explanation but requires that the privacy preservation constraint is relaxed at test time. How to achieve consistency of post-hoc explanation and simultaneously to ensure privacy preservation represents an interesting future development of this work.
Overall, the Fed-XAI approach offers remarkable potential and can have important practical implications in high-stake applications such as healthcare: in this domain, in fact, data centralization for ML model training is not only difficult to implement from a technical perspective, but it also raises ethical concerns related to privacy and is subject to limitations imposed by regulatory policies. On one hand, FL removes the need for data centralization while still allowing ML models training to benefit from ample and diverse data, which is crucial to address urgent challenges such as health disparities, under-served populations, and rare diseases [ 85 ]. On the other hand, the adoption of XAI tools endow the AI system with the capability of explaining its decisions, which is paramount in these kinds of applications.
We have discussed strengths and weaknesses of the two federated approaches for learning XAI models. In the future, we aim to enrich the comparative analysis with an additional level of assessment by collecting feedbacks on explainability from human experts (e.g., physicians) and by considering other case studies.
Data Availability
The dataset is publicly available at https://archive.ics.uci.edu/dataset/189/parkinsons+telemonitoring .
https://artificialintelligenceact.eu/the-act/ , visited May 2024.
High level expert group on AI. Ethics guidelines for trustworthy AI, Technical Report. European Commission. https://ec.europa.eu/digital-single-market/en/news/ethics-guidelines-trustworthy-ai .
: GDPR. Available from: https://gdpr-info.eu/recitals/no-71/ [cited 06.10.2022].
Barredo Arrieta A, Díaz-Rodríguez N, Del Ser J, Bennetot A, Tabik S, Barbado A, et al. Explainable Artificial Intelligence (XAI): concepts, taxonomies, opportunities and challenges toward responsible AI. Information Fusion. 2020;58:82–115. https://doi.org/10.1016/j.inffus.2019.12.012 .
Article Google Scholar
Guidotti R, Monreale A, Ruggieri S, Turini F, Giannotti F, Pedreschi D. A survey of methods for explaining black box models. ACM Comput Surv. 2018. https://doi.org/10.1145/3236009 .
Guidotti R, Monreale A, Pedreschi D, Giannotti F. In: Sayed-Mouchaweh M, editor. Principles of explainable artificial intelligence. Cham: Springer International Publishing; 2021. p. 9–31.
Ali S, Abuhmed T, El-Sappagh S, Muhammad K, Alonso-Moral JM, Confalonieri R, et al. Explainable Artificial Intelligence (XAI): what we know and what is left to attain Trustworthy Artificial Intelligence. Information Fusion. 2023;99:101805. https://doi.org/10.1016/j.inffus.2023.101805 .
McMahan B, Moore E, Ramage D, Hampson S, Arcas BAy. Communication-efficient learning of deep networks from decentralized data. In: Singh A, Zhu J, editors. Proceedings of the 20th international conference on artificial intelligence and statistics. vol. 54 of Proceedings of Machine Learning Research. PMLR; 2017. p. 1273–1282. Available from: https://proceedings.mlr.press/v54/mcmahan17a.html .
Yang Q, Liu Y, Chen T, Tong Y. Federated machine learning: concept and applications. ACM Trans Intell Syst Technol. 2019. https://doi.org/10.1145/3298981 .
Bodria F, Giannotti F, Guidotti R, Naretto F, Pedreschi D, Rinzivillo S. Benchmarking and survey of explanation methods for black box models. Data Mining and Knowledge Discovery. 2023;37(5):1719–78. https://doi.org/10.1007/s10618-023-00933-9 .
Article MathSciNet Google Scholar
Chaddad A, Lu Q, Li J, Katib Y, Kateb R, Tanougast C, et al. Explainable, domain-adaptive, and federated artificial intelligence in medicine. IEEE/CAA Journal of Automatica Sinica. 2023;10(4):859–76. https://doi.org/10.1109/JAS.2023.123123 .
Corcuera Bárcena JL, Daole M, Ducange P, Marcelloni F, Renda A, Ruffini F, et al. Fed-XAI: Federated Learning of Explainable Artificial Intelligence Models. In: XAI.it: 3rd Italian workshop on explainable artificial intelligence, co-located with AI*IA; 2022. Available from: https://ceur-ws.org/Vol-3277/paper8.pdf .
López-Blanco R, Alonso RS, González-Arrieta A, Chamoso P, Prieto J. Federated Learning of Explainable Artificial Intelligence (FED-XAI): a review. In: Ossowski S, Sitek P, Analide C, Marreiros G, Chamoso P, Rodríguez S, editors. Distributed computing and artificial intelligence, 20th international conference. Cham: Springer Nature Switzerland; 2023. p. 318–26.
Google Scholar
Corcuera Bárcena JL, Ducange P, Ercolani A, Marcelloni F, Renda A. An approach to federated learning of explainable fuzzy regression models. In: IEEE International conference on fuzzy systems (FUZZ-IEEE); 2022. p. 1–8.
Daneault JF, Carignan B, Sadikot AF, Duval C. Are quantitative and clinical measures of bradykinesia related in advanced Parkinson’s disease? [Article]. Journal of Neuroscience Methods. 2013;219(2):220–3. https://doi.org/10.1016/j.jneumeth.2013.08.009 .
Corcuera Bárcena JL, Ducange P, Marcelloni F, Renda A, Ruffini F. Federated learning of explainable artificial intelligence models for predicting Parkinson’s disease progression. In: Longo L, editor. Explainable artificial intelligence. Cham: Springer Nature Switzerland; 2023. p. 630–48.
Chapter Google Scholar
Takagi T, Sugeno M. Fuzzy identification of systems and its applications to modeling and control. IEEE Transactions on Systems, Man, and Cybernetics. 1985;SMC–15(1):116–32. https://doi.org/10.1109/TSMC.1985.6313399 .
Fernandez A, Herrera F, Cordon O, Jose del Jesus M, Marcelloni F. Evolutionary fuzzy systems for explainable artificial intelligence: why, when, what for, and where to? Comp Intell Mag. 2019;14(1):69–81. https://doi.org/10.1109/MCI.2018.2881645 .
Zadeh LA. Toward a theory of fuzzy information granulation and its centrality in human reasoning and fuzzy logic. Fuzzy Sets and Systems. 1997;90(2):111–27. https://doi.org/10.1016/S0165-0114(97)00077-8 . ( Fuzzy Sets: Where Do We Stand? Where Do We Go? ).
Yao JT, Vasilakos AV, Pedrycz W. Granular computing: perspectives and challenges. IEEE Transactions on Cybernetics. 2013;43(6):1977–89. https://doi.org/10.1109/TSMCC.2012.2236648 .
Lundberg SM, Lee SI. A unified approach to interpreting model predictions. In: Guyon I, Luxburg UV, Bengio S, Wallach H, Fergus R, Vishwanathan S, et al., editors. Advances in Neural Information Processing Systems. vol. 30. Curran Associates, Inc.; 2017. Available from: https://proceedings.neurips.cc/paper_files/paper/2017/file/8a20a8621978632d76c43dfd28b67767-Paper.pdf .
Corbucci L, Guidotti R, Monreale A. Explaining black-boxes in federated learning. In: Longo L, editor. Explainable artificial intelligence. Cham: Springer Nature Switzerland; 2023. p. 151–63.
Li H, Li C, Wang J, Yang A, Ma Z, Zhang Z, et al. Review on security of federated learning and its application in healthcare. Future Generation Computer Systems. 2023;144:271–90. https://doi.org/10.1016/j.future.2023.02.021 .
Hwang H, Yang S, Kim D, Dua R, Kim JY, Yang E, et al. Towards the practical utility of federated learning in the medical domain. In: Mortazavi BJ, Sarker T, Beam A, Ho JC, editors. Proceedings of the conference on health, inference, and learning. vol. 209 of Proceedings of Machine Learning Research. PMLR; 2023. p. 163–181. Available from: https://proceedings.mlr.press/v209/hwang23a.html .
Rahman A, Hossain MS, Muhammad G, Kundu D, Debnath T, Rahman M, et al. Federated learning-based AI approaches in smart healthcare: concepts, taxonomies, challenges and open issues. Cluster computing. 2023;26(4):2271–311. https://doi.org/10.1007/s10586-022-03658-4 .
Sohan MF, Basalamah A. A systematic review on federated learning in medical image analysis. IEEE Access. 2023;11:28628–44. https://doi.org/10.1109/ACCESS.2023.3260027 .
De Falco I, Della Cioppa A, Koutny T, Scafuri U, Tarantino E. Model-free-communication federated learning: framework and application to precision medicine. Biomedical Signal Processing and Control. 2024;87:105416. https://doi.org/10.1016/j.bspc.2023.105416 .
Nguyen DC, Pham QV, Pathirana PN, Ding M, Seneviratne A, Lin Z, et al. Federated learning for smart healthcare: a survey [article]. ACM Computing Surveys. 2022. https://doi.org/10.1145/3501296 . ( Cited by: 209; All Open Access, Green Open Access ).
Ali M, Naeem F, Tariq M, Kaddoum G. Federated learning for privacy preservation in smart healthcare systems: a comprehensive survey [article]. IEEE Journal of Biomedical and Health Informatics. 2023;27(2):778–89. https://doi.org/10.1109/JBHI.2022.3181823 . ( Cited by: 36; All Open Access, Green Open Access ).
Hassija V, Chamola V, Mahapatra A, Singal A, Goel D, Huang K, et al. Interpreting black-box models: a review on explainable artificial intelligence. Cognitive Computation. 2023. https://doi.org/10.1007/s12559-023-10179-8 .
Patrício C, JaC Neves, Teixeira LF. Explainable deep learning methods in medical image classification: a survey. ACM Comput Surv. 2023. https://doi.org/10.1145/3625287 .
Uddin MZ, Dysthe KK, Følstad A, Brandtzaeg PB. Deep learning for prediction of depressive symptoms in a large textual dataset. Neural Computing and Applications. 2022;34(1):721–44. https://doi.org/10.1007/s00521-021-06426-4 .
El-Sappagh S, Alonso JM, Islam SMR, Sultan AM, Kwak KS. A multilayer multimodal detection and prediction model based on explainable artificial intelligence for Alzheimer’s disease. Sci Rep. 2021;11(1):2660.
Dong N, Voiculescu I. Federated contrastive learning for decentralized unlabeled medical images. In: Medical Image Computing and Computer Assisted Intervention - MICCAI 2021: 24th International Conference, Strasbourg, France, September 27-October 1, 2021, Proceedings, Part III. Berlin, Heidelberg: Springer-Verlag; 2021. p. 378–87. https://doi.org/10.1007/978-3-030-87199-4_36 .
Vaid A, Jaladanki SK, Xu J, Teng S, Kumar A, Lee S, et al. Federated learning of electronic health records to improve mortality prediction in hospitalized patients with COVID-19: machine learning approach. JMIR Med Inform. 2021;9(1):e24207.
Bounsall K, Milne-Ives M, Hall A, Carroll C, Meinert E. Artificial intelligence applications for assessment, monitoring, and management of parkinson disease symptoms: protocol for a systematic review. JMIR Res Protoc. 2023;12:e46581. https://doi.org/10.2196/46581 .
El-Sappagh S, Alonso-Moral JM, Abuhmed T, Ali F, Bugarín-Diz A. Trustworthy artificial intelligence in Alzheimer’s disease: state of the art, opportunities, and challenges. Artif Intell Rev. 2023;56(10):11149–296. https://doi.org/10.1007/s10462-023-10415-5 .
Gómez-Vilda P, Rodellar-Biarge V, Nieto-Lluis V, Muñoz-Mulas C, Mazaira-Fernández LM, Martínez-Olalla R, et al. Characterizing neurological disease from voice quality biomechanical analysis. Cognitive Computation. 2013;5(4):399–425. https://doi.org/10.1007/s12559-013-9207-2 .
Arias-Vergara T, Vásquez-Correa JC, Orozco-Arroyave JR. Parkinson’s disease and aging: analysis of their effect in phonation and articulation of speech. Cognitive Computation. 2017;9(6):731–48. https://doi.org/10.1007/s12559-017-9497-x .
Magesh P, Myloth R, Tom R. An explainable machine learning model for early detection of Parkinson’s disease using lime on DaTSCAN imagery. Computers in Biology and Medicine. 2020;11(126):104041. https://doi.org/10.1016/j.compbiomed.2020.104041 .
Ribeiro MT, Singh S, Guestrin C. “Why should i trust you?”: explaining the predictions of any classifier. In: Proceedings of the 22nd ACM SIGKDD international conference on knowledge discovery and data mining. KDD ’16. New York, USA: Association for Computing Machinery; 2016. p. 1135-1144.
Junaid M, Ali S, Eid F, El-Sappagh S, Abuhmed T. Explainable machine learning models based on multimodal time-series data for the early detection of Parkinson’s disease. Computer Methods and Programs in Biomedicine. 2023;234:107495. https://doi.org/10.1016/j.cmpb.2023.107495 .
Jorge J, Barros PH, Yokoyama R, Guidoni D, Ramos HS, Fonseca N, et al. Applying federated learning in the detection of freezing of gait in Parkinson’s disease. In: IEEE/ACM 15th International conference on Utility and Cloud Computing (UCC); 2022. p. 195–200.
Sarlas A, Kalafatelis A, Alexandridis G, Kourtis MA, Trakadas P. Exploring federated learning for speech-based Parkinson’s disease detection. In: Proceedings of the 18th international conference on availability, reliability and security. ARES ’23. New York, USA: Association for Computing Machinery; 2023.
Dipro SH, Islam M, Al Nahian A, Sharmita Azad M, Chakrabarty A, Reza T. A federated learning based privacy preserving approach for detecting Parkinson’s disease using deep learning. In: 2022 25th International Conference on Computer and Information Technology (ICCIT); 2022. p. 139–144.
Grover S, Bhartia S, Akshama, Yadav A, R SK. Predicting severity of Parkinson’s disease using deep learning. Procedia Computer Science. Procedia Computer Science. 2018;132:1788–94. https://doi.org/10.1016/j.procs.2018.05.154 . ( International Conference on Computational Intelligence and Data Science ).
Gunduz H. Deep sets learning-based Parkinson’s disease classification using vocal feature. IEEE Access. 2019;7:115540–51. https://doi.org/10.1109/ACCESS.2019.2936564 .
Nilashi M, Ibrahim O, Samad S, Ahmadi H, Shahmoradi L, Akbari E. An analytical method for measuring the Parkinson’s disease progression: a case on a Parkinson’s telemonitoring dataset. Measurement. 2019;136:545–57. https://doi.org/10.1016/j.measurement.2019.01.014 .
Shahid AH, Singh MP. A deep learning approach for prediction of Parkinson’s disease progression. Biomedical Engineering Letters. 2020;10:227–39. https://doi.org/10.1007/s13534-020-00156-7 .
Xue Z, Zhang T, Lin L. Progress prediction of Parkinson’s disease based on graph wavelet transform and attention weighted random forest. Expert Systems with Applications. 2022;203: 117483. https://doi.org/10.1016/j.eswa.2022.117483 .
Chen P, Du X, Lu Z, Wu J, Hung PCK. EVFL: an explainable vertical federated learning for data-oriented Artificial Intelligence systems. Journal of Systems Architecture. 2022;126:102474. https://doi.org/10.1016/j.sysarc.2022.102474 .
Fiosina J. Explainable federated learning for taxi travel time prediction. vol. 2021-April; 2021. p. 670 - 677. Available from: https://www.scopus.com/inward/record.uri?eid=2-s2.0-85121125924&partnerID=40 &md5=9a076bc80768b97eee10c0883e2557e7 .
Fiosina J. Interpretable privacy-preserving collaborative deep learning for taxi trip duration forecasting. In: International conference on vehicle technology and intelligent transport systems, international conference on smart cities and green ICT systems. Springer; 2022. p. 392–411.
Wang G. Interpret federated learning with Shapley values. arXiv preprint arXiv:1905.04519 . 2019;.
Sidhpura J, Shah P, Veerkhare R, Godbole A. FedSpam: privacy preserving SMS spam prediction. In: Tanveer M, Agarwal S, Ozawa S, Ekbal A, Jatowt A, editors. Neural information processing. Singapore: Springer Nature Singapore; 2023. p. 52–63.
Ben Saad S, Brik B, Ksentini A. A trust and explainable federated deep learning framework in zero touch B5G networks. In: GLOBECOM - IEEE Global Communications Conference; 2022. p. 1037–1042.
Ludwig H, Baracaldo N, Thomas G, Zhou Y, Anwar A, Rajamoni S, et al.: IBM federated learning: an enterprise framework white paper V0.1. arXiv. Available from: https://arxiv.org/abs/2007.10987 .
Wilbik A, Grefen P. Towards a federated fuzzy learning system. In: IEEE International conference on fuzzy systems (FUZZ-IEEE); 2021. p. 1–6.
Wu Y, Cai S, Xiao X, Chen G, Ooi BC. Privacy preserving vertical federated learning for tree-based models. Proc VLDB Endow. 2020;13(12):2090–103. https://doi.org/10.14778/3407790.3407811 .
Zhu X, Wang D, Pedrycz W, Li Z. Horizontal federated learning of Takagi-Sugeno fuzzy rule-based models. IEEE Transactions on Fuzzy Systems. 2022;30(9):3537–47. https://doi.org/10.1109/TFUZZ.2021.3118733 .
Bogdanova A, Imakura A, Sakurai T. DC-SHAP method for consistent explainability in privacy-preserving distributed machine learning. Human-Centric Intelligent Systems. 2023;3(3):197–210. https://doi.org/10.1007/s44230-023-00032-4 .
Zheng S, Cao Y, Yoshikawa M. Secure Shapley value for cross-silo federated learning. Proc VLDB Endow. 2023, 16(7), pp. 1657–1670. https://doi.org/10.14778/3587136.3587141
Janzing D, Minorics L, Blöbaum P. Feature relevance quantification in explainable AI: a causal problem. In: International Conference on artificial intelligence and statistics. PMLR; 2020. p. 2907–2916. Available from: https://www.scopus.com/inward/record.uri?eid=2-s2.0-85122610367&partnerID=40 &md5=32e4054213ccb02f05bfba648c864fbb .
Chen Y, Yang X, He Y, Miao C, Chan P. FedDBM: federated digital biomarker for detecting Parkinson’s disease progress. In: IEEE International Conference on Multimedia and Expo (ICME); 2023. p. 678–683.
Polato M, Esposito R, Aldinucci M. Boosting the federation: cross-silo federated learning without gradient descent. In: International Joint Conference on Neural Networks (IJCNN); 2022. p. 1–10.
Alonso Moral JM, Castiello C, Magdalena L, Mencar C. In: Designing interpretable fuzzy systems. Cham: Springer International Publishing; 2021. p. 119–168. Available from: https://doi.org/10.1007/978-3-030-71098-9_5 .
Gacto MJ, Alcalá R, Herrera F. Interpretability of linguistic fuzzy rule-based systems: an overview of interpretability measures. Information Sciences. 2011;181(20):4340–60. https://doi.org/10.1016/j.ins.2011.02.021 . ( Special Issue on Interpretable Fuzzy Systems ).
Zhu H, Xu J, Liu S, Jin Y. Federated learning on non-IID data: a survey. Neurocomputing. 2021;465:371–90. https://doi.org/10.1016/j.neucom.2021.07.098 .
Morafah M, Wang W, Lin B. A practical recipe for federated learning under statistical heterogeneity experimental design. IEEE Transactions on Artificial Intelligence. 2023;p. 1–14. https://doi.org/10.1109/TAI.2023.3297090 .
Shapley LS. In: Kuhn HW, Tucker AW, editors. 17. A value for n-person games. Princeton University Press: Princeton; 1953. p. 307–18.
Molnar C.: Interpretable machine learning: a guide for making black box models explainable. Available from: https://christophm.github.io/interpretable-ml-book .
Tsanas A, Little MA, McSharry PE, Ramig LO. Accurate telemonitoring of Parkinson’s disease progression by noninvasive speech tests. IEEE Transactions on Biomedical Engineering. 2010;57(4):884–93. https://doi.org/10.1109/TBME.2009.2036000 .
Kairouz P, McMahan HB, Avent B, Bellet A, Bennis M, Bhagoji AN, et al.: Advances and open problems in federated learning. Available from: http://dx.doi.org/10.1561/2200000083 .
Bakopoulou E, Tillman B, Markopoulou A. FedPacket: a federated learning approach to mobile packet classification. IEEE Transactions on Mobile Computing. 2022;21(10):3609–28. https://doi.org/10.1109/TMC.2021.3058627 .
Corcuera Bárcena JL, Ducange P, Marcelloni F, Nardini G, Noferi A, Renda A, et al. Enabling federated learning of explainable AI models within beyond-5G/6G networks. Computer Communications. 2023;210:356–75. https://doi.org/10.1016/j.comcom.2023.07.039 .
Cover TM. Elements of information theory. John Wiley & Sons; 1999.
Cózar J, Ossa Ldl, Gámez JA. TSK-0 fuzzy rule-based systems for high-dimensional problems using the apriori principle for rule generation. In: International Conference on Rough Sets and Current Trends in Computing. Springer; 2014. p. 270–279.
Li Q, Wen Z, Wu Z, Hu S, Wang N, Li Y, et al. A survey on federated learning systems: vision, hype and reality for data privacy and protection. IEEE Transactions on Knowledge and Data Engineering. 2023;35(4):3347–66. https://doi.org/10.1109/TKDE.2021.3124599 .
Alonso JM, Magdalena L, Guillaume S. HILK: a new methodology for designing highly interpretable linguistic knowledge bases using the fuzzy logic formalism. International Journal of Intelligent Systems. 2008;23(7):761–94. https://doi.org/10.1002/int.20288 .
Miller GA. The magical number seven, plus or minus two: some limits on our capacity for processing information. Psychological Review. 1956;63(2):81–97. https://doi.org/10.1037/h0043158 .
Wilcoxon F. Individual comparisons by ranking methods. In: Breakthroughs in statistics. Springer; 1992. p. 196–202.
Fuchs C, Spolaor S, Nobile MS, Kaymak U. pyFUME: a Python package for fuzzy model estimation. In: IEEE International Conference on Fuzzy Systems (FUZZ-IEEE); 2020. p. 1–8.
Obeso JA, Rodriguez-Oroz MC, Goetz CG, Marin C, Kordower JH, Rodriguez M, et al. Missing pieces in the Parkinson’s disease puzzle [Review]. Nature Medicine. 2010;16(6):653–61. https://doi.org/10.1038/nm.2165 .
Müller S, Toborek V, Beckh K, Jakobs M, Bauckhage C, Welke P. An empirical evaluation of the Rashomon effect in explainable machine learning. In: Koutra D, Plant C, Gomez Rodriguez M, Baralis E, Bonchi F, editors. Machine learning and knowledge discovery in databases: Research Track. Cham: Springer Nature Switzerland; 2023. p. 462–78.
Breiman L. Statistical modeling: the two cultures (with comments and a rejoinder by the author). Statistical Science. 2001;16(3):199–231. https://doi.org/10.1214/ss/1009213726
Pati S, Baid U, Edwards B, Sheller M, Wang SH, Reina GA, et al. Federated learning enables big data for rare cancer boundary detection. Nature Communications. 2022. https://doi.org/10.1038/s41467-022-33407-5 .
Download references
Open access funding provided by Università di Pisa within the CRUI-CARE Agreement. This work has been partly funded by the PON 2014–2021 “Research and Innovation,” DM MUR 1062/2021, Project title: “Progettazione e sperimentazione di algoritmi di federated learning per data stream mining,” PNRR - M4C2 - Investimento 1.3, Partenariato Esteso PE00000013 - “FAIR - Future Artificial Intelligence Research” - Spoke 1 “Human-centered AI” and the PNRR “Tuscany Health Ecosystem” (THE) (Ecosistemi dell’Innovazione) - Spoke 6 - Precision Medicine & Personalized Healthcare (CUP I53C22000780001) under the NextGeneration EU programme, and by the Italian Ministry of University and Research (MUR) in the framework of the FoReLab and CrossLab projects (Departments of Excellence).
Author information
Authors and affiliations.
Department of Information Engineering, University of Pisa, Largo Lucio Lazzarino 1, Pisa, 56122, Italy
Pietro Ducange, Francesco Marcelloni, Alessandro Renda & Fabrizio Ruffini
You can also search for this author in PubMed Google Scholar
Contributions
All authors contributed to the study conception and design. Material preparation, data collection, and analysis were performed by Alessandro Renda and Fabrizio Ruffini. The first draft of the manuscript was written by Francesco Marcelloni, Alessandro Renda, and Fabrizio Ruffini, and all authors commented on previous versions of the manuscript. All authors read and approved the final manuscript.
Corresponding authors
Correspondence to Alessandro Renda or Fabrizio Ruffini .
Ethics declarations
Conflict of interest.
The authors declare no competing interests.
Additional information
Publisher's note.
Springer Nature remains neutral with regard to jurisdictional claims in published maps and institutional affiliations.
Rights and permissions
Open Access This article is licensed under a Creative Commons Attribution 4.0 International License, which permits use, sharing, adaptation, distribution and reproduction in any medium or format, as long as you give appropriate credit to the original author(s) and the source, provide a link to the Creative Commons licence, and indicate if changes were made. The images or other third party material in this article are included in the article’s Creative Commons licence, unless indicated otherwise in a credit line to the material. If material is not included in the article’s Creative Commons licence and your intended use is not permitted by statutory regulation or exceeds the permitted use, you will need to obtain permission directly from the copyright holder. To view a copy of this licence, visit http://creativecommons.org/licenses/by/4.0/ .
Reprints and permissions
About this article
Ducange, P., Marcelloni, F., Renda, A. et al. Federated Learning of XAI Models in Healthcare: A Case Study on Parkinson’s Disease. Cogn Comput (2024). https://doi.org/10.1007/s12559-024-10332-x
Download citation
Received : 15 December 2023
Accepted : 23 July 2024
Published : 28 August 2024
DOI : https://doi.org/10.1007/s12559-024-10332-x
Share this article
Anyone you share the following link with will be able to read this content:
Sorry, a shareable link is not currently available for this article.
Provided by the Springer Nature SharedIt content-sharing initiative
- Federated learning
- Explainable artificial intelligence
- Granular fuzzy models
- Parkinson’s disease
Advertisement
- Find a journal
- Publish with us
- Track your research

An official website of the United States government
The .gov means it’s official. Federal government websites often end in .gov or .mil. Before sharing sensitive information, make sure you’re on a federal government site.
The site is secure. The https:// ensures that you are connecting to the official website and that any information you provide is encrypted and transmitted securely.
- Publications
- Account settings
Preview improvements coming to the PMC website in October 2024. Learn More or Try it out now .
- Advanced Search
- Journal List
- BMC Med Res Methodol

The case study approach
Sarah crowe.
1 Division of Primary Care, The University of Nottingham, Nottingham, UK
Kathrin Cresswell
2 Centre for Population Health Sciences, The University of Edinburgh, Edinburgh, UK
Ann Robertson
3 School of Health in Social Science, The University of Edinburgh, Edinburgh, UK
Anthony Avery
Aziz sheikh.
The case study approach allows in-depth, multi-faceted explorations of complex issues in their real-life settings. The value of the case study approach is well recognised in the fields of business, law and policy, but somewhat less so in health services research. Based on our experiences of conducting several health-related case studies, we reflect on the different types of case study design, the specific research questions this approach can help answer, the data sources that tend to be used, and the particular advantages and disadvantages of employing this methodological approach. The paper concludes with key pointers to aid those designing and appraising proposals for conducting case study research, and a checklist to help readers assess the quality of case study reports.
Introduction
The case study approach is particularly useful to employ when there is a need to obtain an in-depth appreciation of an issue, event or phenomenon of interest, in its natural real-life context. Our aim in writing this piece is to provide insights into when to consider employing this approach and an overview of key methodological considerations in relation to the design, planning, analysis, interpretation and reporting of case studies.
The illustrative 'grand round', 'case report' and 'case series' have a long tradition in clinical practice and research. Presenting detailed critiques, typically of one or more patients, aims to provide insights into aspects of the clinical case and, in doing so, illustrate broader lessons that may be learnt. In research, the conceptually-related case study approach can be used, for example, to describe in detail a patient's episode of care, explore professional attitudes to and experiences of a new policy initiative or service development or more generally to 'investigate contemporary phenomena within its real-life context' [ 1 ]. Based on our experiences of conducting a range of case studies, we reflect on when to consider using this approach, discuss the key steps involved and illustrate, with examples, some of the practical challenges of attaining an in-depth understanding of a 'case' as an integrated whole. In keeping with previously published work, we acknowledge the importance of theory to underpin the design, selection, conduct and interpretation of case studies[ 2 ]. In so doing, we make passing reference to the different epistemological approaches used in case study research by key theoreticians and methodologists in this field of enquiry.
This paper is structured around the following main questions: What is a case study? What are case studies used for? How are case studies conducted? What are the potential pitfalls and how can these be avoided? We draw in particular on four of our own recently published examples of case studies (see Tables Tables1, 1 , ,2, 2 , ,3 3 and and4) 4 ) and those of others to illustrate our discussion[ 3 - 7 ].
Example of a case study investigating the reasons for differences in recruitment rates of minority ethnic people in asthma research[ 3 ]
Minority ethnic people experience considerably greater morbidity from asthma than the White majority population. Research has shown however that these minority ethnic populations are likely to be under-represented in research undertaken in the UK; there is comparatively less marginalisation in the US. |
To investigate approaches to bolster recruitment of South Asians into UK asthma studies through qualitative research with US and UK researchers, and UK community leaders. |
Single intrinsic case study |
Centred on the issue of recruitment of South Asian people with asthma. |
In-depth interviews were conducted with asthma researchers from the UK and US. A supplementary questionnaire was also provided to researchers. |
Framework approach. |
Barriers to ethnic minority recruitment were found to centre around: |
1. The attitudes of the researchers' towards inclusion: The majority of UK researchers interviewed were generally supportive of the idea of recruiting ethnically diverse participants but expressed major concerns about the practicalities of achieving this; in contrast, the US researchers appeared much more committed to the policy of inclusion. |
2. Stereotypes and prejudices: We found that some of the UK researchers' perceptions of ethnic minorities may have influenced their decisions on whether to approach individuals from particular ethnic groups. These stereotypes centred on issues to do with, amongst others, language barriers and lack of altruism. |
3. Demographic, political and socioeconomic contexts of the two countries: Researchers suggested that the demographic profile of ethnic minorities, their political engagement and the different configuration of the health services in the UK and the US may have contributed to differential rates. |
4. Above all, however, it appeared that the overriding importance of the US National Institute of Health's policy to mandate the inclusion of minority ethnic people (and women) had a major impact on shaping the attitudes and in turn the experiences of US researchers'; the absence of any similar mandate in the UK meant that UK-based researchers had not been forced to challenge their existing practices and they were hence unable to overcome any stereotypical/prejudicial attitudes through experiential learning. |
Example of a case study investigating the process of planning and implementing a service in Primary Care Organisations[ 4 ]
Health work forces globally are needing to reorganise and reconfigure in order to meet the challenges posed by the increased numbers of people living with long-term conditions in an efficient and sustainable manner. Through studying the introduction of General Practitioners with a Special Interest in respiratory disorders, this study aimed to provide insights into this important issue by focusing on community respiratory service development. |
To understand and compare the process of workforce change in respiratory services and the impact on patient experience (specifically in relation to the role of general practitioners with special interests) in a theoretically selected sample of Primary Care Organisations (PCOs), in order to derive models of good practice in planning and the implementation of a broad range of workforce issues. |
Multiple-case design of respiratory services in health regions in England and Wales. |
Four PCOs. |
Face-to-face and telephone interviews, e-mail discussions, local documents, patient diaries, news items identified from local and national websites, national workshop. |
Reading, coding and comparison progressed iteratively. |
1. In the screening phase of this study (which involved semi-structured telephone interviews with the person responsible for driving the reconfiguration of respiratory services in 30 PCOs), the barriers of financial deficit, organisational uncertainty, disengaged clinicians and contradictory policies proved insurmountable for many PCOs to developing sustainable services. A key rationale for PCO re-organisation in 2006 was to strengthen their commissioning function and those of clinicians through Practice-Based Commissioning. However, the turbulence, which surrounded reorganisation was found to have the opposite desired effect. |
2. Implementing workforce reconfiguration was strongly influenced by the negotiation and contest among local clinicians and managers about "ownership" of work and income. |
3. Despite the intention to make the commissioning system more transparent, personal relationships based on common professional interests, past work history, friendships and collegiality, remained as key drivers for sustainable innovation in service development. |
It was only possible to undertake in-depth work in a selective number of PCOs and, even within these selected PCOs, it was not possible to interview all informants of potential interest and/or obtain all relevant documents. This work was conducted in the early stages of a major NHS reorganisation in England and Wales and thus, events are likely to have continued to evolve beyond the study period; we therefore cannot claim to have seen any of the stories through to their conclusion. |
Example of a case study investigating the introduction of the electronic health records[ 5 ]
Healthcare systems globally are moving from paper-based record systems to electronic health record systems. In 2002, the NHS in England embarked on the most ambitious and expensive IT-based transformation in healthcare in history seeking to introduce electronic health records into all hospitals in England by 2010. |
To describe and evaluate the implementation and adoption of detailed electronic health records in secondary care in England and thereby provide formative feedback for local and national rollout of the NHS Care Records Service. |
A mixed methods, longitudinal, multi-site, socio-technical collective case study. |
Five NHS acute hospital and mental health Trusts that have been the focus of early implementation efforts. |
Semi-structured interviews, documentary data and field notes, observations and quantitative data. |
Qualitative data were analysed thematically using a socio-technical coding matrix, combined with additional themes that emerged from the data. |
1. Hospital electronic health record systems have developed and been implemented far more slowly than was originally envisioned. |
2. The top-down, government-led standardised approach needed to evolve to admit more variation and greater local choice for hospitals in order to support local service delivery. |
3. A range of adverse consequences were associated with the centrally negotiated contracts, which excluded the hospitals in question. |
4. The unrealistic, politically driven, timeline (implementation over 10 years) was found to be a major source of frustration for developers, implementers and healthcare managers and professionals alike. |
We were unable to access details of the contracts between government departments and the Local Service Providers responsible for delivering and implementing the software systems. This, in turn, made it difficult to develop a holistic understanding of some key issues impacting on the overall slow roll-out of the NHS Care Record Service. Early adopters may also have differed in important ways from NHS hospitals that planned to join the National Programme for Information Technology and implement the NHS Care Records Service at a later point in time. |
Example of a case study investigating the formal and informal ways students learn about patient safety[ 6 ]
There is a need to reduce the disease burden associated with iatrogenic harm and considering that healthcare education represents perhaps the most sustained patient safety initiative ever undertaken, it is important to develop a better appreciation of the ways in which undergraduate and newly qualified professionals receive and make sense of the education they receive. | |
---|---|
To investigate the formal and informal ways pre-registration students from a range of healthcare professions (medicine, nursing, physiotherapy and pharmacy) learn about patient safety in order to become safe practitioners. | |
Multi-site, mixed method collective case study. | |
: Eight case studies (two for each professional group) were carried out in educational provider sites considering different programmes, practice environments and models of teaching and learning. | |
Structured in phases relevant to the three knowledge contexts: | |
Documentary evidence (including undergraduate curricula, handbooks and module outlines), complemented with a range of views (from course leads, tutors and students) and observations in a range of academic settings. | |
Policy and management views of patient safety and influences on patient safety education and practice. NHS policies included, for example, implementation of the National Patient Safety Agency's , which encourages organisations to develop an organisational safety culture in which staff members feel comfortable identifying dangers and reporting hazards. | |
The cultures to which students are exposed i.e. patient safety in relation to day-to-day working. NHS initiatives included, for example, a hand washing initiative or introduction of infection control measures. | |
1. Practical, informal, learning opportunities were valued by students. On the whole, however, students were not exposed to nor engaged with important NHS initiatives such as risk management activities and incident reporting schemes. | |
2. NHS policy appeared to have been taken seriously by course leaders. Patient safety materials were incorporated into both formal and informal curricula, albeit largely implicit rather than explicit. | |
3. Resource issues and peer pressure were found to influence safe practice. Variations were also found to exist in students' experiences and the quality of the supervision available. | |
The curriculum and organisational documents collected differed between sites, which possibly reflected gatekeeper influences at each site. The recruitment of participants for focus group discussions proved difficult, so interviews or paired discussions were used as a substitute. |
What is a case study?
A case study is a research approach that is used to generate an in-depth, multi-faceted understanding of a complex issue in its real-life context. It is an established research design that is used extensively in a wide variety of disciplines, particularly in the social sciences. A case study can be defined in a variety of ways (Table (Table5), 5 ), the central tenet being the need to explore an event or phenomenon in depth and in its natural context. It is for this reason sometimes referred to as a "naturalistic" design; this is in contrast to an "experimental" design (such as a randomised controlled trial) in which the investigator seeks to exert control over and manipulate the variable(s) of interest.
Definitions of a case study
Author | Definition |
---|---|
Stake[ ] | (p.237) |
Yin[ , , ] | (Yin 1999 p. 1211, Yin 1994 p. 13) |
• | |
• (Yin 2009 p18) | |
Miles and Huberman[ ] | (p. 25) |
Green and Thorogood[ ] | (p. 284) |
George and Bennett[ ] | (p. 17)" |
Stake's work has been particularly influential in defining the case study approach to scientific enquiry. He has helpfully characterised three main types of case study: intrinsic , instrumental and collective [ 8 ]. An intrinsic case study is typically undertaken to learn about a unique phenomenon. The researcher should define the uniqueness of the phenomenon, which distinguishes it from all others. In contrast, the instrumental case study uses a particular case (some of which may be better than others) to gain a broader appreciation of an issue or phenomenon. The collective case study involves studying multiple cases simultaneously or sequentially in an attempt to generate a still broader appreciation of a particular issue.
These are however not necessarily mutually exclusive categories. In the first of our examples (Table (Table1), 1 ), we undertook an intrinsic case study to investigate the issue of recruitment of minority ethnic people into the specific context of asthma research studies, but it developed into a instrumental case study through seeking to understand the issue of recruitment of these marginalised populations more generally, generating a number of the findings that are potentially transferable to other disease contexts[ 3 ]. In contrast, the other three examples (see Tables Tables2, 2 , ,3 3 and and4) 4 ) employed collective case study designs to study the introduction of workforce reconfiguration in primary care, the implementation of electronic health records into hospitals, and to understand the ways in which healthcare students learn about patient safety considerations[ 4 - 6 ]. Although our study focusing on the introduction of General Practitioners with Specialist Interests (Table (Table2) 2 ) was explicitly collective in design (four contrasting primary care organisations were studied), is was also instrumental in that this particular professional group was studied as an exemplar of the more general phenomenon of workforce redesign[ 4 ].
What are case studies used for?
According to Yin, case studies can be used to explain, describe or explore events or phenomena in the everyday contexts in which they occur[ 1 ]. These can, for example, help to understand and explain causal links and pathways resulting from a new policy initiative or service development (see Tables Tables2 2 and and3, 3 , for example)[ 1 ]. In contrast to experimental designs, which seek to test a specific hypothesis through deliberately manipulating the environment (like, for example, in a randomised controlled trial giving a new drug to randomly selected individuals and then comparing outcomes with controls),[ 9 ] the case study approach lends itself well to capturing information on more explanatory ' how ', 'what' and ' why ' questions, such as ' how is the intervention being implemented and received on the ground?'. The case study approach can offer additional insights into what gaps exist in its delivery or why one implementation strategy might be chosen over another. This in turn can help develop or refine theory, as shown in our study of the teaching of patient safety in undergraduate curricula (Table (Table4 4 )[ 6 , 10 ]. Key questions to consider when selecting the most appropriate study design are whether it is desirable or indeed possible to undertake a formal experimental investigation in which individuals and/or organisations are allocated to an intervention or control arm? Or whether the wish is to obtain a more naturalistic understanding of an issue? The former is ideally studied using a controlled experimental design, whereas the latter is more appropriately studied using a case study design.
Case studies may be approached in different ways depending on the epistemological standpoint of the researcher, that is, whether they take a critical (questioning one's own and others' assumptions), interpretivist (trying to understand individual and shared social meanings) or positivist approach (orientating towards the criteria of natural sciences, such as focusing on generalisability considerations) (Table (Table6). 6 ). Whilst such a schema can be conceptually helpful, it may be appropriate to draw on more than one approach in any case study, particularly in the context of conducting health services research. Doolin has, for example, noted that in the context of undertaking interpretative case studies, researchers can usefully draw on a critical, reflective perspective which seeks to take into account the wider social and political environment that has shaped the case[ 11 ].
Example of epistemological approaches that may be used in case study research
Approach | Characteristics | Criticisms | Key references |
---|---|---|---|
Involves questioning one's own assumptions taking into account the wider political and social environment. | It can possibly neglect other factors by focussing only on power relationships and may give the researcher a position that is too privileged. | Howcroft and Trauth[ ] Blakie[ ] Doolin[ , ] | |
Interprets the limiting conditions in relation to power and control that are thought to influence behaviour. | Bloomfield and Best[ ] | ||
Involves understanding meanings/contexts and processes as perceived from different perspectives, trying to understand individual and shared social meanings. Focus is on theory building. | Often difficult to explain unintended consequences and for neglecting surrounding historical contexts | Stake[ ] Doolin[ ] | |
Involves establishing which variables one wishes to study in advance and seeing whether they fit in with the findings. Focus is often on testing and refining theory on the basis of case study findings. | It does not take into account the role of the researcher in influencing findings. | Yin[ , , ] Shanks and Parr[ ] |
How are case studies conducted?
Here, we focus on the main stages of research activity when planning and undertaking a case study; the crucial stages are: defining the case; selecting the case(s); collecting and analysing the data; interpreting data; and reporting the findings.
Defining the case
Carefully formulated research question(s), informed by the existing literature and a prior appreciation of the theoretical issues and setting(s), are all important in appropriately and succinctly defining the case[ 8 , 12 ]. Crucially, each case should have a pre-defined boundary which clarifies the nature and time period covered by the case study (i.e. its scope, beginning and end), the relevant social group, organisation or geographical area of interest to the investigator, the types of evidence to be collected, and the priorities for data collection and analysis (see Table Table7 7 )[ 1 ]. A theory driven approach to defining the case may help generate knowledge that is potentially transferable to a range of clinical contexts and behaviours; using theory is also likely to result in a more informed appreciation of, for example, how and why interventions have succeeded or failed[ 13 ].
Example of a checklist for rating a case study proposal[ 8 ]
Clarity: Does the proposal read well? | |
Integrity: Do its pieces fit together? | |
Attractiveness: Does it pique the reader's interest? | |
The case: Is the case adequately defined? | |
The issues: Are major research questions identified? | |
Data Resource: Are sufficient data sources identified? | |
Case Selection: Is the selection plan reasonable? | |
Data Gathering: Are data-gathering activities outlined? | |
Validation: Is the need and opportunity for triangulation indicated? | |
Access: Are arrangements for start-up anticipated? | |
Confidentiality: Is there sensitivity to the protection of people? | |
Cost: Are time and resource estimates reasonable? |
For example, in our evaluation of the introduction of electronic health records in English hospitals (Table (Table3), 3 ), we defined our cases as the NHS Trusts that were receiving the new technology[ 5 ]. Our focus was on how the technology was being implemented. However, if the primary research interest had been on the social and organisational dimensions of implementation, we might have defined our case differently as a grouping of healthcare professionals (e.g. doctors and/or nurses). The precise beginning and end of the case may however prove difficult to define. Pursuing this same example, when does the process of implementation and adoption of an electronic health record system really begin or end? Such judgements will inevitably be influenced by a range of factors, including the research question, theory of interest, the scope and richness of the gathered data and the resources available to the research team.
Selecting the case(s)
The decision on how to select the case(s) to study is a very important one that merits some reflection. In an intrinsic case study, the case is selected on its own merits[ 8 ]. The case is selected not because it is representative of other cases, but because of its uniqueness, which is of genuine interest to the researchers. This was, for example, the case in our study of the recruitment of minority ethnic participants into asthma research (Table (Table1) 1 ) as our earlier work had demonstrated the marginalisation of minority ethnic people with asthma, despite evidence of disproportionate asthma morbidity[ 14 , 15 ]. In another example of an intrinsic case study, Hellstrom et al.[ 16 ] studied an elderly married couple living with dementia to explore how dementia had impacted on their understanding of home, their everyday life and their relationships.
For an instrumental case study, selecting a "typical" case can work well[ 8 ]. In contrast to the intrinsic case study, the particular case which is chosen is of less importance than selecting a case that allows the researcher to investigate an issue or phenomenon. For example, in order to gain an understanding of doctors' responses to health policy initiatives, Som undertook an instrumental case study interviewing clinicians who had a range of responsibilities for clinical governance in one NHS acute hospital trust[ 17 ]. Sampling a "deviant" or "atypical" case may however prove even more informative, potentially enabling the researcher to identify causal processes, generate hypotheses and develop theory.
In collective or multiple case studies, a number of cases are carefully selected. This offers the advantage of allowing comparisons to be made across several cases and/or replication. Choosing a "typical" case may enable the findings to be generalised to theory (i.e. analytical generalisation) or to test theory by replicating the findings in a second or even a third case (i.e. replication logic)[ 1 ]. Yin suggests two or three literal replications (i.e. predicting similar results) if the theory is straightforward and five or more if the theory is more subtle. However, critics might argue that selecting 'cases' in this way is insufficiently reflexive and ill-suited to the complexities of contemporary healthcare organisations.
The selected case study site(s) should allow the research team access to the group of individuals, the organisation, the processes or whatever else constitutes the chosen unit of analysis for the study. Access is therefore a central consideration; the researcher needs to come to know the case study site(s) well and to work cooperatively with them. Selected cases need to be not only interesting but also hospitable to the inquiry [ 8 ] if they are to be informative and answer the research question(s). Case study sites may also be pre-selected for the researcher, with decisions being influenced by key stakeholders. For example, our selection of case study sites in the evaluation of the implementation and adoption of electronic health record systems (see Table Table3) 3 ) was heavily influenced by NHS Connecting for Health, the government agency that was responsible for overseeing the National Programme for Information Technology (NPfIT)[ 5 ]. This prominent stakeholder had already selected the NHS sites (through a competitive bidding process) to be early adopters of the electronic health record systems and had negotiated contracts that detailed the deployment timelines.
It is also important to consider in advance the likely burden and risks associated with participation for those who (or the site(s) which) comprise the case study. Of particular importance is the obligation for the researcher to think through the ethical implications of the study (e.g. the risk of inadvertently breaching anonymity or confidentiality) and to ensure that potential participants/participating sites are provided with sufficient information to make an informed choice about joining the study. The outcome of providing this information might be that the emotive burden associated with participation, or the organisational disruption associated with supporting the fieldwork, is considered so high that the individuals or sites decide against participation.
In our example of evaluating implementations of electronic health record systems, given the restricted number of early adopter sites available to us, we sought purposively to select a diverse range of implementation cases among those that were available[ 5 ]. We chose a mixture of teaching, non-teaching and Foundation Trust hospitals, and examples of each of the three electronic health record systems procured centrally by the NPfIT. At one recruited site, it quickly became apparent that access was problematic because of competing demands on that organisation. Recognising the importance of full access and co-operative working for generating rich data, the research team decided not to pursue work at that site and instead to focus on other recruited sites.
Collecting the data
In order to develop a thorough understanding of the case, the case study approach usually involves the collection of multiple sources of evidence, using a range of quantitative (e.g. questionnaires, audits and analysis of routinely collected healthcare data) and more commonly qualitative techniques (e.g. interviews, focus groups and observations). The use of multiple sources of data (data triangulation) has been advocated as a way of increasing the internal validity of a study (i.e. the extent to which the method is appropriate to answer the research question)[ 8 , 18 - 21 ]. An underlying assumption is that data collected in different ways should lead to similar conclusions, and approaching the same issue from different angles can help develop a holistic picture of the phenomenon (Table (Table2 2 )[ 4 ].
Brazier and colleagues used a mixed-methods case study approach to investigate the impact of a cancer care programme[ 22 ]. Here, quantitative measures were collected with questionnaires before, and five months after, the start of the intervention which did not yield any statistically significant results. Qualitative interviews with patients however helped provide an insight into potentially beneficial process-related aspects of the programme, such as greater, perceived patient involvement in care. The authors reported how this case study approach provided a number of contextual factors likely to influence the effectiveness of the intervention and which were not likely to have been obtained from quantitative methods alone.
In collective or multiple case studies, data collection needs to be flexible enough to allow a detailed description of each individual case to be developed (e.g. the nature of different cancer care programmes), before considering the emerging similarities and differences in cross-case comparisons (e.g. to explore why one programme is more effective than another). It is important that data sources from different cases are, where possible, broadly comparable for this purpose even though they may vary in nature and depth.
Analysing, interpreting and reporting case studies
Making sense and offering a coherent interpretation of the typically disparate sources of data (whether qualitative alone or together with quantitative) is far from straightforward. Repeated reviewing and sorting of the voluminous and detail-rich data are integral to the process of analysis. In collective case studies, it is helpful to analyse data relating to the individual component cases first, before making comparisons across cases. Attention needs to be paid to variations within each case and, where relevant, the relationship between different causes, effects and outcomes[ 23 ]. Data will need to be organised and coded to allow the key issues, both derived from the literature and emerging from the dataset, to be easily retrieved at a later stage. An initial coding frame can help capture these issues and can be applied systematically to the whole dataset with the aid of a qualitative data analysis software package.
The Framework approach is a practical approach, comprising of five stages (familiarisation; identifying a thematic framework; indexing; charting; mapping and interpretation) , to managing and analysing large datasets particularly if time is limited, as was the case in our study of recruitment of South Asians into asthma research (Table (Table1 1 )[ 3 , 24 ]. Theoretical frameworks may also play an important role in integrating different sources of data and examining emerging themes. For example, we drew on a socio-technical framework to help explain the connections between different elements - technology; people; and the organisational settings within which they worked - in our study of the introduction of electronic health record systems (Table (Table3 3 )[ 5 ]. Our study of patient safety in undergraduate curricula drew on an evaluation-based approach to design and analysis, which emphasised the importance of the academic, organisational and practice contexts through which students learn (Table (Table4 4 )[ 6 ].
Case study findings can have implications both for theory development and theory testing. They may establish, strengthen or weaken historical explanations of a case and, in certain circumstances, allow theoretical (as opposed to statistical) generalisation beyond the particular cases studied[ 12 ]. These theoretical lenses should not, however, constitute a strait-jacket and the cases should not be "forced to fit" the particular theoretical framework that is being employed.
When reporting findings, it is important to provide the reader with enough contextual information to understand the processes that were followed and how the conclusions were reached. In a collective case study, researchers may choose to present the findings from individual cases separately before amalgamating across cases. Care must be taken to ensure the anonymity of both case sites and individual participants (if agreed in advance) by allocating appropriate codes or withholding descriptors. In the example given in Table Table3, 3 , we decided against providing detailed information on the NHS sites and individual participants in order to avoid the risk of inadvertent disclosure of identities[ 5 , 25 ].
What are the potential pitfalls and how can these be avoided?
The case study approach is, as with all research, not without its limitations. When investigating the formal and informal ways undergraduate students learn about patient safety (Table (Table4), 4 ), for example, we rapidly accumulated a large quantity of data. The volume of data, together with the time restrictions in place, impacted on the depth of analysis that was possible within the available resources. This highlights a more general point of the importance of avoiding the temptation to collect as much data as possible; adequate time also needs to be set aside for data analysis and interpretation of what are often highly complex datasets.
Case study research has sometimes been criticised for lacking scientific rigour and providing little basis for generalisation (i.e. producing findings that may be transferable to other settings)[ 1 ]. There are several ways to address these concerns, including: the use of theoretical sampling (i.e. drawing on a particular conceptual framework); respondent validation (i.e. participants checking emerging findings and the researcher's interpretation, and providing an opinion as to whether they feel these are accurate); and transparency throughout the research process (see Table Table8 8 )[ 8 , 18 - 21 , 23 , 26 ]. Transparency can be achieved by describing in detail the steps involved in case selection, data collection, the reasons for the particular methods chosen, and the researcher's background and level of involvement (i.e. being explicit about how the researcher has influenced data collection and interpretation). Seeking potential, alternative explanations, and being explicit about how interpretations and conclusions were reached, help readers to judge the trustworthiness of the case study report. Stake provides a critique checklist for a case study report (Table (Table9 9 )[ 8 ].
Potential pitfalls and mitigating actions when undertaking case study research
Potential pitfall | Mitigating action |
---|---|
Selecting/conceptualising the wrong case(s) resulting in lack of theoretical generalisations | Developing in-depth knowledge of theoretical and empirical literature, justifying choices made |
Collecting large volumes of data that are not relevant to the case or too little to be of any value | Focus data collection in line with research questions, whilst being flexible and allowing different paths to be explored |
Defining/bounding the case | Focus on related components (either by time and/or space), be clear what is outside the scope of the case |
Lack of rigour | Triangulation, respondent validation, the use of theoretical sampling, transparency throughout the research process |
Ethical issues | Anonymise appropriately as cases are often easily identifiable to insiders, informed consent of participants |
Integration with theoretical framework | Allow for unexpected issues to emerge and do not force fit, test out preliminary explanations, be clear about epistemological positions in advance |
Stake's checklist for assessing the quality of a case study report[ 8 ]
1. Is this report easy to read? |
2. Does it fit together, each sentence contributing to the whole? |
3. Does this report have a conceptual structure (i.e. themes or issues)? |
4. Are its issues developed in a series and scholarly way? |
5. Is the case adequately defined? |
6. Is there a sense of story to the presentation? |
7. Is the reader provided some vicarious experience? |
8. Have quotations been used effectively? |
9. Are headings, figures, artefacts, appendices, indexes effectively used? |
10. Was it edited well, then again with a last minute polish? |
11. Has the writer made sound assertions, neither over- or under-interpreting? |
12. Has adequate attention been paid to various contexts? |
13. Were sufficient raw data presented? |
14. Were data sources well chosen and in sufficient number? |
15. Do observations and interpretations appear to have been triangulated? |
16. Is the role and point of view of the researcher nicely apparent? |
17. Is the nature of the intended audience apparent? |
18. Is empathy shown for all sides? |
19. Are personal intentions examined? |
20. Does it appear individuals were put at risk? |
Conclusions
The case study approach allows, amongst other things, critical events, interventions, policy developments and programme-based service reforms to be studied in detail in a real-life context. It should therefore be considered when an experimental design is either inappropriate to answer the research questions posed or impossible to undertake. Considering the frequency with which implementations of innovations are now taking place in healthcare settings and how well the case study approach lends itself to in-depth, complex health service research, we believe this approach should be more widely considered by researchers. Though inherently challenging, the research case study can, if carefully conceptualised and thoughtfully undertaken and reported, yield powerful insights into many important aspects of health and healthcare delivery.
Competing interests
The authors declare that they have no competing interests.
Authors' contributions
AS conceived this article. SC, KC and AR wrote this paper with GH, AA and AS all commenting on various drafts. SC and AS are guarantors.
Pre-publication history
The pre-publication history for this paper can be accessed here:
http://www.biomedcentral.com/1471-2288/11/100/prepub
Acknowledgements
We are grateful to the participants and colleagues who contributed to the individual case studies that we have drawn on. This work received no direct funding, but it has been informed by projects funded by Asthma UK, the NHS Service Delivery Organisation, NHS Connecting for Health Evaluation Programme, and Patient Safety Research Portfolio. We would also like to thank the expert reviewers for their insightful and constructive feedback. Our thanks are also due to Dr. Allison Worth who commented on an earlier draft of this manuscript.
- Yin RK. Case study research, design and method. 4. London: Sage Publications Ltd.; 2009. [ Google Scholar ]
- Keen J, Packwood T. Qualitative research; case study evaluation. BMJ. 1995; 311 :444–446. [ PMC free article ] [ PubMed ] [ Google Scholar ]
- Sheikh A, Halani L, Bhopal R, Netuveli G, Partridge M, Car J. et al. Facilitating the Recruitment of Minority Ethnic People into Research: Qualitative Case Study of South Asians and Asthma. PLoS Med. 2009; 6 (10):1–11. [ PMC free article ] [ PubMed ] [ Google Scholar ]
- Pinnock H, Huby G, Powell A, Kielmann T, Price D, Williams S, The process of planning, development and implementation of a General Practitioner with a Special Interest service in Primary Care Organisations in England and Wales: a comparative prospective case study. Report for the National Co-ordinating Centre for NHS Service Delivery and Organisation R&D (NCCSDO) 2008. http://www.sdo.nihr.ac.uk/files/project/99-final-report.pdf
- Robertson A, Cresswell K, Takian A, Petrakaki D, Crowe S, Cornford T. et al. Prospective evaluation of the implementation and adoption of NHS Connecting for Health's national electronic health record in secondary care in England: interim findings. BMJ. 2010; 41 :c4564. [ PMC free article ] [ PubMed ] [ Google Scholar ]
- Pearson P, Steven A, Howe A, Sheikh A, Ashcroft D, Smith P. the Patient Safety Education Study Group. Learning about patient safety: organisational context and culture in the education of healthcare professionals. J Health Serv Res Policy. 2010; 15 :4–10. doi: 10.1258/jhsrp.2009.009052. [ PubMed ] [ CrossRef ] [ Google Scholar ]
- van Harten WH, Casparie TF, Fisscher OA. The evaluation of the introduction of a quality management system: a process-oriented case study in a large rehabilitation hospital. Health Policy. 2002; 60 (1):17–37. doi: 10.1016/S0168-8510(01)00187-7. [ PubMed ] [ CrossRef ] [ Google Scholar ]
- Stake RE. The art of case study research. London: Sage Publications Ltd.; 1995. [ Google Scholar ]
- Sheikh A, Smeeth L, Ashcroft R. Randomised controlled trials in primary care: scope and application. Br J Gen Pract. 2002; 52 (482):746–51. [ PMC free article ] [ PubMed ] [ Google Scholar ]
- King G, Keohane R, Verba S. Designing Social Inquiry. Princeton: Princeton University Press; 1996. [ Google Scholar ]
- Doolin B. Information technology as disciplinary technology: being critical in interpretative research on information systems. Journal of Information Technology. 1998; 13 :301–311. doi: 10.1057/jit.1998.8. [ CrossRef ] [ Google Scholar ]
- George AL, Bennett A. Case studies and theory development in the social sciences. Cambridge, MA: MIT Press; 2005. [ Google Scholar ]
- Eccles M. the Improved Clinical Effectiveness through Behavioural Research Group (ICEBeRG) Designing theoretically-informed implementation interventions. Implementation Science. 2006; 1 :1–8. doi: 10.1186/1748-5908-1-1. [ PMC free article ] [ PubMed ] [ CrossRef ] [ Google Scholar ]
- Netuveli G, Hurwitz B, Levy M, Fletcher M, Barnes G, Durham SR, Sheikh A. Ethnic variations in UK asthma frequency, morbidity, and health-service use: a systematic review and meta-analysis. Lancet. 2005; 365 (9456):312–7. [ PubMed ] [ Google Scholar ]
- Sheikh A, Panesar SS, Lasserson T, Netuveli G. Recruitment of ethnic minorities to asthma studies. Thorax. 2004; 59 (7):634. [ PMC free article ] [ PubMed ] [ Google Scholar ]
- Hellström I, Nolan M, Lundh U. 'We do things together': A case study of 'couplehood' in dementia. Dementia. 2005; 4 :7–22. doi: 10.1177/1471301205049188. [ CrossRef ] [ Google Scholar ]
- Som CV. Nothing seems to have changed, nothing seems to be changing and perhaps nothing will change in the NHS: doctors' response to clinical governance. International Journal of Public Sector Management. 2005; 18 :463–477. doi: 10.1108/09513550510608903. [ CrossRef ] [ Google Scholar ]
- Lincoln Y, Guba E. Naturalistic inquiry. Newbury Park: Sage Publications; 1985. [ Google Scholar ]
- Barbour RS. Checklists for improving rigour in qualitative research: a case of the tail wagging the dog? BMJ. 2001; 322 :1115–1117. doi: 10.1136/bmj.322.7294.1115. [ PMC free article ] [ PubMed ] [ CrossRef ] [ Google Scholar ]
- Mays N, Pope C. Qualitative research in health care: Assessing quality in qualitative research. BMJ. 2000; 320 :50–52. doi: 10.1136/bmj.320.7226.50. [ PMC free article ] [ PubMed ] [ CrossRef ] [ Google Scholar ]
- Mason J. Qualitative researching. London: Sage; 2002. [ Google Scholar ]
- Brazier A, Cooke K, Moravan V. Using Mixed Methods for Evaluating an Integrative Approach to Cancer Care: A Case Study. Integr Cancer Ther. 2008; 7 :5–17. doi: 10.1177/1534735407313395. [ PubMed ] [ CrossRef ] [ Google Scholar ]
- Miles MB, Huberman M. Qualitative data analysis: an expanded sourcebook. 2. CA: Sage Publications Inc.; 1994. [ Google Scholar ]
- Pope C, Ziebland S, Mays N. Analysing qualitative data. Qualitative research in health care. BMJ. 2000; 320 :114–116. doi: 10.1136/bmj.320.7227.114. [ PMC free article ] [ PubMed ] [ CrossRef ] [ Google Scholar ]
- Cresswell KM, Worth A, Sheikh A. Actor-Network Theory and its role in understanding the implementation of information technology developments in healthcare. BMC Med Inform Decis Mak. 2010; 10 (1):67. doi: 10.1186/1472-6947-10-67. [ PMC free article ] [ PubMed ] [ CrossRef ] [ Google Scholar ]
- Malterud K. Qualitative research: standards, challenges, and guidelines. Lancet. 2001; 358 :483–488. doi: 10.1016/S0140-6736(01)05627-6. [ PubMed ] [ CrossRef ] [ Google Scholar ]
- Yin R. Case study research: design and methods. 2. Thousand Oaks, CA: Sage Publishing; 1994. [ Google Scholar ]
- Yin R. Enhancing the quality of case studies in health services research. Health Serv Res. 1999; 34 :1209–1224. [ PMC free article ] [ PubMed ] [ Google Scholar ]
- Green J, Thorogood N. Qualitative methods for health research. 2. Los Angeles: Sage; 2009. [ Google Scholar ]
- Howcroft D, Trauth E. Handbook of Critical Information Systems Research, Theory and Application. Cheltenham, UK: Northampton, MA, USA: Edward Elgar; 2005. [ Google Scholar ]
- Blakie N. Approaches to Social Enquiry. Cambridge: Polity Press; 1993. [ Google Scholar ]
- Doolin B. Power and resistance in the implementation of a medical management information system. Info Systems J. 2004; 14 :343–362. doi: 10.1111/j.1365-2575.2004.00176.x. [ CrossRef ] [ Google Scholar ]
- Bloomfield BP, Best A. Management consultants: systems development, power and the translation of problems. Sociological Review. 1992; 40 :533–560. [ Google Scholar ]
- Shanks G, Parr A. Proceedings of the European Conference on Information Systems. Naples; 2003. Positivist, single case study research in information systems: A critical analysis. [ Google Scholar ]

An official website of the United States government
Here’s how you know
The .gov means it’s official. Federal government websites often end in .gov or .mil. Before sharing sensitive information, make sure you’re on a federal government site.
The site is secure. The https:// ensures that you are connecting to the official website and that any information you provide is encrypted and transmitted securely.

User Research and the Paperwork Reduction Act
Highlighting case studies across the government.
In 2021, the Biden-Harris Administration issued an Executive Order to improve customer experience and make service delivery simple, seamless, and secure for Americans. Since then, government agencies have been hard at work making their services, tools, and content more effective in addressing the needs of the people they serve.
User research is at the heart of transforming customer experience. The best way to ensure services are effective, efficient, easy to use, fair, and safe is to include the people they are intended for when building it.
In this piece, we’ll demonstrate:
The importance of user research and how it helps mitigate risk in agency projects.
How and when to integrate user research into a project.
Examples of agencies successfully incorporating and conducting certain types of user research without needing Paperwork Reduction Act approval.
The basics of a customer-centered approach
Before we dive into the examples, let’s discuss the basics of a customer-centered approach, and why it’s important to ensure an agency is meeting the needs of the people using its products or services.
Creating a new digital tool or making changes to a website or service can affect the experience of millions. If working in isolation, agencies can miss important details that could slow down or prevent people from using your services.
The key to any successful project is to observe how real people interact with a government service or product . Failing to prioritize user research is risky and can lead to poorly designed products and services that are difficult to access.
Conducting user research by the rules
The Paperwork Reduction Act (PRA) ensures that the Federal Government collects information in a way that reduces unnecessary burden on the public. The PRA process generally involves notifying the public about each information collection and giving them a chance to provide feedback. It also includes review of a proposed information collection by the Office of Information and Regulatory Affairs Management (OIRA) within the Office of Management and Budget. However, as discussed in regulation 5 CFR 1320.3(h) , information collection does not include items in categories that are common during user research. Agencies might not need PRA approval for most user research done on projects, depending on how the project is structured.
Specifically, there are activities common in user research that do not require PRA clearance because they do not qualify as an information collection under PRA regulations (5 C.F.R. § 1320.3). Those activities include:
Directly observing someone using a product (i.e., observing someone filling out a form or finding information on a website) or
Asking people non-standardized questions as part of a one-on-one research session (i.e., asking people questions orally, in an unstructured way, about navigating government benefits or signing up for an appointment). “Non-standardized,” in this context, means the questions asked vary from person to person, and are not drawn from a list of identical questions posed in every research session.
Unlike methods that constitute information collection and require PRA clearance (for example, surveys or large focus groups with a set of the same questions or tasks), these types of research conversations (direct observations or one-on-one sessions with non-standardized questions) do not require PRA approval, regardless of the number of users ultimately involved in the research.
Direct observations and one-on-one feedback sessions can be more valuable than focus groups or surveys , since they may provide a deeper understanding of people’s behavior rather than feelings and opinions and showcase what’s working well, what isn’t, and why. You can learn more about talking to people with tips from the Department of Homeland Security.
Incorporating user research into your project
Incorporating user research results in a better product and reduces risks. It helps address usability concerns, reduces the potential for major backlogs on call centers, increases customer satisfaction, and ensures agencies are prioritizing the right changes. Research is a continuous process, and certain project stages benefit from research, including:
Before a project
Look at comparable systems and services to learn lessons.
Gain a better understanding of the pain points people experience with this system or service.
Get to know stakeholders’ business needs or concerns, possibly using methods suggested by 18F, the digital services agency within the General Services Administration.
Do desk research, such as reading threads on social media websites and discussions on online forums. Find out where people are talking about the topic, go there, and read as much as you can.
Test high-level ideas or potential future solutions.
Start of a project
Observe people using the existing system or service.
Work with local groups, advocates, or nonprofits to identify people to talk to.
Review any comments or letters submitted by the public.
See what people who use the system or service are saying publicly online about their experience.
Read research conducted on this topic.
Middle of a project
Place the thing that your agency team is building in front of someone who will use it, and observe them using it.
- Create a few different product versions and solutions to each problem.
- Gather feedback on what people found easiest to use.
In operations
Conduct content testing to understand what people think the message means and make updates until it’s in plain language. (For example, the agency can write improvements to system messages and try to understand what people think the messages mean until the messages are plain, understandable, and straightforward. This approach could be used for emails, text messages, policy guidance, or webpage updates.)
Hold one-on-one listening sessions with people to learn about existing pain points and then prioritize new features based on feedback.
Now let’s take a look at seven government projects that have successfully incorporated user research and improved the customer experience without conducting an information collection requiring PRA clearance.
By exploring these projects, you can start thinking of ways to conduct more research or advocate for more research to enhance the customer experience. This list can generate new ideas and help you find ways to integrate user research more effectively.
Highlighting seven government projects
Case study 1: conducting user research for an informative website launch.
Reporting Unemployment Identity Theft, Department of Labor (DOL)- March 2021
In 2022, thousands of Americans received a fraudulent unemployment insurance tax form (government Form 1099-G) in the mail despite never applying for unemployment insurance (UI). These individuals were victims of unemployment identity theft, and fraudsters used their information to illegally receive unemployment benefits. For most victims, understanding what steps to take next was confusing.
The DOL met with victims of UI theft and developed a website to guide them through reporting the fraud. After the site went live, the DOL collaborated with other government agencies and organizations to incorporate the same tested language for reporting UI fraud on their sites. This created a consistent and reliable standard across all websites, fostering trustworthiness among victims.
User research
Ten unstructured, one-on-one user research sessions with victims helped the DOL learn more about the current process and what victims did when they received the fraudulent unemployment insurance form. Participants walked through their unique scenarios and researchers took detailed notes.
During the second round of research, the main focus was observing individuals as they navigated through the newly drafted website content. Participants were instructed to vocalize their thoughts as they started at the top of the page and explained what they saw. Any aspects of the website that people found confusing were revised and improved following the sessions.
Why did the PRA not cover this research?
In this project, the DOL directly observed the experiences of program applicants and participants and asked non-standardized questions on a particular process, theme, or issue without any specification of the information being sought. See 5 CFR 1320.3(h)(3).
Case study 2: Conducting user research for a new application launch
A New Digital Application for VA Health Care, Department of Veterans Affairs (VA) - July 2016
Many Veterans found the health care application process at the VA frustrating. Most weren’t able to open the fillable PDF online application because it required a certain software that wasn’t common in most browsers. As a result, over 70 percent of visitors had trouble accessing the health care application, according to USDS research.
The team developed a new, user-friendly online application that doesn’t require a certain software to use. For more details, check out the USDS blog post , “Introducing a new digital application for healthcare at VA.”
The team observed Veterans using the existing application to identify pain points and then worked on a new version. Then, they did user research sessions with the new form again to ensure it was easy and removed any previous pain points. Watch a real-life user research session, conducted by a VA employee with a Veteran at this video link .
In this project, the VA directly observed the experiences of program applicants and participants and engaged in unstructured one-on-one interactions. They asked non-standardized questions on a particular process, theme, or issue without any specification of the information being sought. See 5 CFR 1320.3(h)(3).
Case study 3: Conducting user research to inform policy and strategy
Welcome Corps, Department of State - February 2024
The Department of State launched a new program to allow Americans to sponsor refugees. The Welcome Corps program involves forming a sponsor group, completing pre-application steps, and then submitting an application. The process was burdensome to Americans seeking to sponsor a refugee, causing frustration and incomplete applications.
The team did user research to inform which steps in the process could be improved in order to reduce unnecessary burden on sponsors and increase successful application submissions. This research helped ensure any policy changes under consideration would actually support program goals. It also helped inform the agency’s roadmap.
The team met one-on-one with current sponsors to learn about their experience and met one-on-one with potential sponsors to understand what steps of the process were challenging. These research sessions used non-standardized questions. The research findings were presented to program leadership, and policy, tech, and operations teams to inform improvements to the application process.
Why the PRA did not cover this research?
In this project, the team observed the experiences of sponsors and potential sponsors and engaged in unstructured one-on-one interactions. They asked non-standardized questions on a particular process, theme, or issue without any specification of the information being sought. See 5 CFR 1320.3(h)(3).
Case study 4: Conducting user research to streamline digital experiences
My VA Dashboard for Veterans, Department of Veterans Affairs (VA) - November 2020
Many services are available to Veterans on the VA websites but it can be challenging to locate them and take action. Veterans asked for a centralized location that was relevant to their needs.
The team worked with a vendor to create My VA, a personalized dashboard for Veterans to access tools and information.
A vendor conducted user research to identify the information that Veterans expect to find in the My VA Dashboard tool and the best way to navigate it.
The contract required vendors to conduct user research to determine people’s goals, needs, and behaviors. The vendor conducted one-on-one, non-structured conversations with Veterans to inform how the agency should build the dashboard.
The contractor collected information and observed program applicants and participants by asking non-standardized questions on a particular process, theme, or issue without any specification of the information being sought. See 5 CFR 1320.3(h)(3), (h)(6).
Case study 5: Conducting user research to inform outreach strategies via text messages
Child Tax Credit Outreach, Department of Treasury and the White House - June 2021
When the American Rescue Plan Act became law in March 2021, millions of Americans were suddenly eligible for unprecedented tax relief by expanding both earned income and child tax credits. Americans who don’t make enough income to require a tax filing would benefit most from the expansion, but first, they needed to know about the credits and include them when they filed a tax return.
Outreach was key to reaching families in most need. The team learned throughout the year that:
Messages from official government entities work well, specifically, government benefits agencies.
Emails and text messages have worked when encouraging state and local governments to send messages directly to beneficiaries.
The team performed user research throughout the year by working with non-profits on the ground to identify research participants and understand what was working and not working. They partnered with Code for America to test several text messages to ensure clear language. They also joined research sessions to observe SNAP applicants engaging with a third-party app that helps them manage their SNAP benefits electronically.
In this project, the groups directly observed the experiences of program applicants and participants. They asked non-standardized questions on a particular process, theme, or issue without any specification of the information being sought. See 5 CFR 1320.3(h)(3).
Case study 6: Conducting user research with internal users of a system
Updates to the Unaccompanied Children Case Management System, Office of Refugee Resettlement (ORR), Administration for Children and Families, Department of Health and Human Services – August 2022
Case managers were updating both paper and digital forms when assessing a potential sponsor. Many duplications existed across the two forms, and filling out both caused inefficiencies.
The team changed the online form, eliminating the need for the paper form and transferred them to the digital experience, reducing burden for the sponsor and case manager. The updated digital form also included design improvements to enhance the flow of the questions.
ORR contacted case workers nationwide and observed them filling out both versions of the form to identify pain points. Another round of research was conducted later on, once they had an updated digital form. These were one-on-one conversations to confirm that the form was easy to use. Any areas where the case managers had questions or trouble were good indicators that the digital form needed tweaking. Finally, they arrived at a version that was easy to use and improved the previous safety and efficiency concerns.
In this project, the ORR directly observed case workers (not federal employees) using a form via one-on-one interviews to understand any usability concerns. They asked non-standardized questions on a particular process, theme, or issue without any specification of the information being sought. See 5 CFR 1320.3(h)(3).
Case study 7: Conducting user research with students and families
College Scorecard, Department of Education - August 2015
Deciding on a college can be an overwhelming task with limited access to reliable information on student outcomes like student earnings, graduates’ student debt, and borrowers’ repayment rates.
In September 2015, the Department of Education launched the College Scorecard, which made data transparent for the public about colleges by leveraging existing data on costs, graduation, etc. and providing new data points on earnings after attendance, student debt, and borrower repayment rates. As these data sets were published through an open application programming interface (API), researchers, policymakers, and the public could customize their analysis of college performance easily. For more information on this project, check out the Obama White House blog post , “Under the Hood: Building a College Scorecard with Students.”
The team conducted user research at every single step in the project. This user research involved one-on-one conversations with high school students in Washington, D.C.’s Anacostia neighborhood, guidance counselors, 4-H kids, parents, college advisors, and data journalists. They also conducted research and met one-on-one with a diverse set of stakeholders across the higher education community to learn about their concerns, ideas, and hopes for how they could help students and families make a more informed decision.
Based on this research, the team developed a College Scorecard prototype and then turned it into a website. The prototype was put in front of students during one-on-one sessions, to observe if the tool was easy to use. The research revealed that students were unlikely to use a mobile app and were hesitant to use government websites, so the team ensured other sites that were actually frequented by students had access to the same data.
Why did the PRA not cover this research ?
In this project, the Department of Education directly observed the program applicants and participants engaging with the College Scorecard. The Department of Education also asked non-standardized questions on a particular process, theme, or issue without any specification of the information being sought. See 5 CFR 1320.3(h)(3).
Ready to conduct user research?
Throughout this piece, we emphasized the significance of user research and described some ways to incorporate it into agency projects without conducting a PRA information collection. These examples showcase how an agency can successfully conduct user research to improve delivery of services.
Agencies should incorporate user research into their work to make well-informed decisions, minimize risk, and save time and money. These case studies are a reminder that by applying user research best practices, agencies can build trust in government and improve customer experience for all Americans.
If you’d like to work on projects like this, consider joining USDS! We’re hiring mission-driven engineers, product managers, designers, bureaucracy hackers, procurement specialists, and operations experts who want to make an impact on the lives of their fellow Americans.
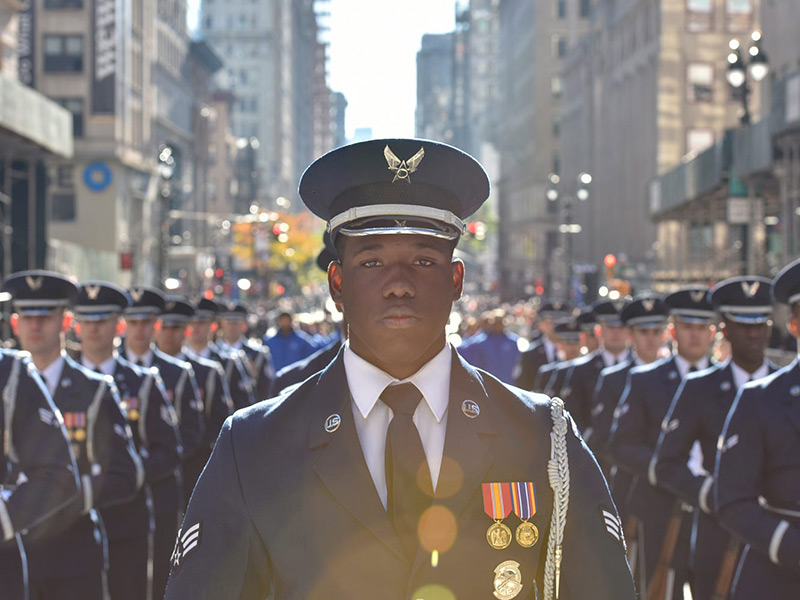
Meeting veterans where they are with accessible mobile tech
This Veterans Day, we’re honoring our current and former service members by highlighting a collaboration between the Department of Veterans Affairs and the U.S. Digital Service.
Read full post

A quick guide to inclusive design
Designing for inclusion isn’t just about coding for accessibility or section 508 compliance, it’s about providing equitable and easy-to-use websites and services for diverse populations. Because good design is design that works for everyone.
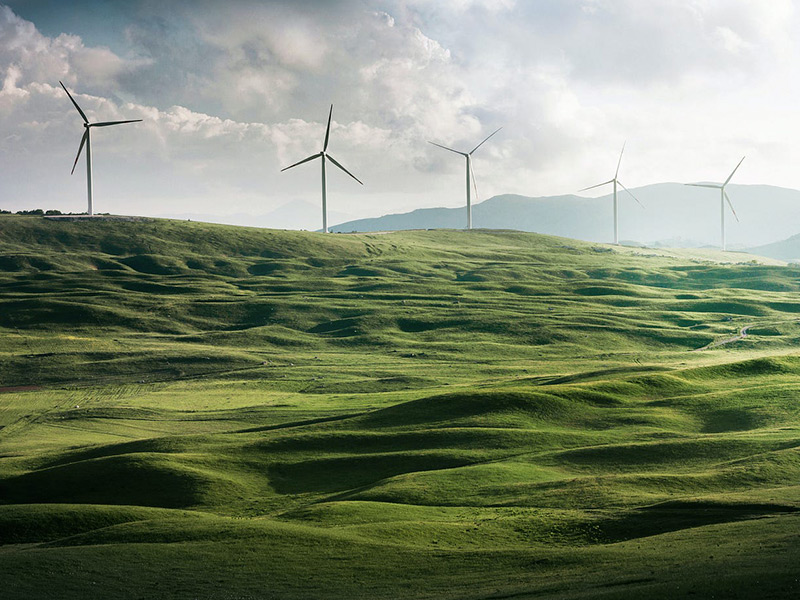
Tackling the climate crisis with open source
Every one of us has the right to breathe clean air, drink clean water, eat safe, nourishing food, and live free from the threat of climate disasters wrecking our neighborhoods and livelihoods.
We need you.
Let’s help millions of people together.
Information
- Author Services
Initiatives
You are accessing a machine-readable page. In order to be human-readable, please install an RSS reader.
All articles published by MDPI are made immediately available worldwide under an open access license. No special permission is required to reuse all or part of the article published by MDPI, including figures and tables. For articles published under an open access Creative Common CC BY license, any part of the article may be reused without permission provided that the original article is clearly cited. For more information, please refer to https://www.mdpi.com/openaccess .
Feature papers represent the most advanced research with significant potential for high impact in the field. A Feature Paper should be a substantial original Article that involves several techniques or approaches, provides an outlook for future research directions and describes possible research applications.
Feature papers are submitted upon individual invitation or recommendation by the scientific editors and must receive positive feedback from the reviewers.
Editor’s Choice articles are based on recommendations by the scientific editors of MDPI journals from around the world. Editors select a small number of articles recently published in the journal that they believe will be particularly interesting to readers, or important in the respective research area. The aim is to provide a snapshot of some of the most exciting work published in the various research areas of the journal.
Original Submission Date Received: .
- Active Journals
- Find a Journal
- Proceedings Series
- For Authors
- For Reviewers
- For Editors
- For Librarians
- For Publishers
- For Societies
- For Conference Organizers
- Open Access Policy
- Institutional Open Access Program
- Special Issues Guidelines
- Editorial Process
- Research and Publication Ethics
- Article Processing Charges
- Testimonials
- Preprints.org
- SciProfiles
- Encyclopedia
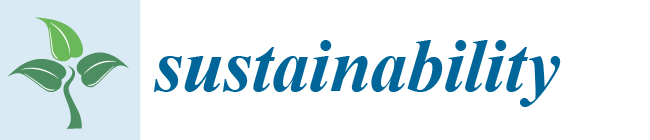
Article Menu

- Subscribe SciFeed
- Recommended Articles
- Google Scholar
- on Google Scholar
- Table of Contents
Find support for a specific problem in the support section of our website.
Please let us know what you think of our products and services.
Visit our dedicated information section to learn more about MDPI.
JSmol Viewer
Lighting design for lanna buddhist architecture: a case study of suan dok temple, chiang mai, thailand.
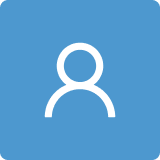
Share and Cite
Tayhuadong, L.; Inkarojrit, V. Lighting Design for Lanna Buddhist Architecture: A Case Study of Suan Dok Temple, Chiang Mai, Thailand. Sustainability 2024 , 16 , 7494. https://doi.org/10.3390/su16177494
Tayhuadong L, Inkarojrit V. Lighting Design for Lanna Buddhist Architecture: A Case Study of Suan Dok Temple, Chiang Mai, Thailand. Sustainability . 2024; 16(17):7494. https://doi.org/10.3390/su16177494
Tayhuadong, Lattapon, and Vorapat Inkarojrit. 2024. "Lighting Design for Lanna Buddhist Architecture: A Case Study of Suan Dok Temple, Chiang Mai, Thailand" Sustainability 16, no. 17: 7494. https://doi.org/10.3390/su16177494
Article Metrics
Further information, mdpi initiatives, follow mdpi.
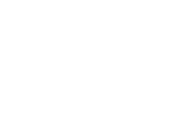
Subscribe to receive issue release notifications and newsletters from MDPI journals

IMAGES
VIDEO
COMMENTS
Advantages. 1. In-depth analysis of complex phenomena. Case study design allows researchers to delve deeply into intricate issues and situations. By focusing on a specific instance or event, researchers can uncover nuanced details and layers of understanding that might be missed with other research methods, especially large-scale survey studies.
A case study is one of the most commonly used methodologies of social research. This article attempts to look into the various dimensions of a case study research strategy, the different epistemological strands which determine the particular case study type and approach adopted in the field, discusses the factors which can enhance the effectiveness of a case study research, and the debate ...
The purpose of case study research is twofold: (1) to provide descriptive information and (2) to suggest theoretical relevance. Rich description enables an in-depth or sharpened understanding of the case. It is unique given one characteristic: case studies draw from more than one data source. Case studies are inherently multimodal or mixed ...
Through the case method, you can "try on" roles you may not have considered and feel more prepared to change or advance your career. 5. Build Your Self-Confidence. Finally, learning through the case study method can build your confidence. Each time you assume a business leader's perspective, aim to solve a new challenge, and express and ...
A case study is a research method that involves an in-depth examination and analysis of a particular phenomenon or case, such as an individual, organization, community, event, or situation. It is a qualitative research approach that aims to provide a detailed and comprehensive understanding of the case being studied.
Revised on November 20, 2023. A case study is a detailed study of a specific subject, such as a person, group, place, event, organization, or phenomenon. Case studies are commonly used in social, educational, clinical, and business research. A case study research design usually involves qualitative methods, but quantitative methods are ...
Although case studies have been discussed extensively in the literature, little has been written about the specific steps one may use to conduct case study research effectively (Gagnon, 2010; Hancock & Algozzine, 2016).Baskarada (2014) also emphasized the need to have a succinct guideline that can be practically followed as it is actually tough to execute a case study well in practice.
Case study method enables a researcher to closely examine the data within a specific context. In most cases, a case study method selects a small geograph ical area or a very li mited number. of ...
Previous research can help guide the case study design. When considering a case study, an example of a case could be taken from previous case study research and used to define cases in a new research inquiry. ... This section outlines some of the key strengths and limitations of case study research. Benefits include the following: Rich ...
Case study research is typically extensive; it draws on multiple methods of data collection and involves multiple data sources. The researcher begins by identifying a specific case or set of cases to be studied. Each case is an entity that is described within certain parameters, such as a specific time frame, place, event, and process.
Contrary to Yin who suggests a really tight and structured design for case study method, Stake (1995) argues for a flexible design which allows researchers to make major changes even after they proceed from design to research. as stated by Yazan (2015) Stake does not suggest a specific design for case study and his obvious flexibility in terms ...
A case study is a research approach that is used to generate an in-depth, multi-faceted understanding of a complex issue in its real-life context. It is an established research design that is used extensively in a wide variety of disciplines, particularly in the social sciences. A case study can be defined in a variety of ways (Table 5 ), the ...
The fact is that stories (individual, collective, institutional) have a vital role to play in the world of research. And to play the specific v. general card against case study design suggests a tendency towards forms of research fundamentalism as opposed to any kind of rational and objective take on case study's strengths and limitations.
Case study research is a type of qualitative research because it typically does not rely on numerical data and statistics to answer a research question. Case study research has both advantages and ...
Introduction. The popularity of case study research methodology in Health Services Research (HSR) has grown over the past 40 years. 1 This may be attributed to a shift towards the use of implementation research and a newfound appreciation of contextual factors affecting the uptake of evidence-based interventions within diverse settings. 2 Incorporating context-specific information on the ...
Abstract. This article presents the case study as a type of qualitative research. Its aim is to give a detailed description of a case study - its definition, some classifications, and several ...
The Design of Case Study Research in Health Care (DESCARTE) model suggests a series of questions to be asked of a case study researcher (including clarity about the philosophy underpinning their research), study design (with a focus on case definition) and analysis (to improve process). The model resembles toolkits for enhancing the quality and ...
The case study design is preferred as a research strategy when "how," "why," and "what" questions are the interest of the researcher. ... and the particular advantages and ...
Case studies are in-depth investigations of a person, group, event, or community. Typically, data is gathered from various sources using several methods (e.g., observations & interviews). The case study research method originated in clinical medicine (the case history, i.e., the patient's personal history). In psychology, case studies are ...
Case Studies. Case studies are a popular research method in business area. Case studies aim to analyze specific issues within the boundaries of a specific environment, situation or organization. According to its design, case studies in business research can be divided into three categories: explanatory, descriptive and exploratory.
A well-known template for this case study research design is the book by Yin . It is a method-orientated handbook of how to design single and multiple case studies with regard to this purpose. Such a case study research design includes: "A 'how' and 'why' question" (Yin 2014: 14). Research questions can be identified and shaped ...
Even interviews can be conducted over the phone. That means this method is good for formative research that is exploratory in nature, even if it must be completed from a remote location. 6. It is inexpensive. Compared to other methods of research, the case study method is rather inexpensive.
To summarize, if you want to write a case study, start with a proper case study format, plan the case study, and finally write it with all the information in hand. Then, write a summary to provide an overview of your case study, and finally, add citations for reference. Meanwhile, if you want to design a case study, Try DocHipo templates.
Authors of a recently published survey provide a review of clinical cases where post-hoc methods and interpretable by-design models are applied to more than 20 different medical case studies, spanning from COVID-19 diagnosis and early detection to diagnostic for breast cancer. Different data types are exploited, depending on the application ...
A case study is a research approach that is used to generate an in-depth, multi-faceted understanding of a complex issue in its real-life context. It is an established research design that is used extensively in a wide variety of disciplines, particularly in the social sciences. A case study can be defined in a variety of ways (Table 5 ), the ...
Highlighting case studies across the government. In 2021, the Biden-Harris Administration issued an Executive Order to improve customer experience and make service delivery simple, seamless, and secure for Americans. Since then, government agencies have been hard at work making their services, tools, and content more effective in addressing the needs of the people they serve.
Hierarchical linear modeling (HLM) is a promising approach that can be applied to explain variability in intervention effectiveness between participants in single-case experimental design (SCED) research. This approach allows the inclusion of participant characteristics as moderators to account for variability in intervention effectiveness.
The research underscores the importance of inclusive design in developing IoT services, ensuring higher inclusivity rates and addressing diverse learning patterns. ... The practical applications of this technology are highlighted through case studies that demonstrate how physically disabled students can benefit from interactive and immersive ...
In Chiang Mai, Thailand, there has been an increasing demand for lighting design strategies that enhance tangible and intangible values of cultural heritage destinations. This research explores the role of lighting design in enhancing the cultural experiences and promoting the cultural tourism of Lanna Buddhist architecture. Suan Dok Temple was selected as a case study due to its historical ...
The long-term effects of COVID-19 are still being studied, and the incidence rate of LC may change over time. In the UK, studies have explored LC symptoms and risk factors in non-hospitalised individuals using primary care records 4 and consolidated evidence on persistent symptoms and their associations in broader populations. 5 Additionally, there has been significant interest in Patient ...