
An official website of the United States government
The .gov means it’s official. Federal government websites often end in .gov or .mil. Before sharing sensitive information, make sure you’re on a federal government site.
The site is secure. The https:// ensures that you are connecting to the official website and that any information you provide is encrypted and transmitted securely.
- Publications
- Account settings
Preview improvements coming to the PMC website in October 2024. Learn More or Try it out now .
- Advanced Search
- Journal List
- HHS Author Manuscripts


The Physical Aspects of Vocal Health
Associated data.
For most people, not much conscious thought or effort is needed to produce a voice with the desired pitch, loudness, and voice quality. However, voice disorders are quite common. When disorders occur, the voice may require more effort to produce, be too weak to be heard, or have undesired quality changes that draw unwanted attention. Such changes can affect a speaker’s personal identity and the ability to effectively communicate, thus limiting the ability to participate in educational, occupational, or social activities.
Most people have experienced difficulty with their voice after screaming at a sports event or after an upper respiratory infection such as the cold or flu. For teachers, singers, and other professional voice users, voice problems occur more often and the symptoms are often severe. For these people, the voice may get tired toward the end of the day. Sometimes the voice is no longer able to meet the higher expectations and greater demands of one’s profession and those individuals have to make career changes.
This article focuses on voice disorders that are related to the production of sound by vocal fold vibration. Voice disorders are often grouped into three major categories based on their etiology. The first category includes organic voice disorders arising from structural changes to the larynx (e.g., inflammation due to an infection or voice overuse) that interfere with the vocal mechanisms.
The second category, neurogenic voice disorders, is related to neurological dysfunctions due to either paralysis, paresis, or neurological disease (e.g., Parkinson’s disease) that impact neurological control of the vocal system.
The third category has been characterized in many ways, including as “functional” voice disorders. This category includes voice disorders with no known underlying organic or neurological origins that are presumably related to the improper use of vocal mechanisms and are thus “functional” in some aspect. A widely held assumption is that these disorders may have psychological origins, but more often they are adaptations to transient tissue changes (e.g., laryngitis) or compromised vocal mechanisms (e.g., paresis or paralysis).
The purpose of this article is not to discuss every voice disorder or category of disorders (but for more information, see Boone et al., 2010 ; Colton et al., 2011 ). Instead, it provides an updated review of the physical aspects of vocal health. The focus is on the physical components involved in healthy voice production, the major pathophysiology of voice disorders, and clinical care of common voice problems. The article ends by briefly discussing the existing knowledge gaps between current scientific understanding and the practice of clinical voice care.
Physiology of Voice Production
The human voice is produced in the larynx ( Figure 1A ), which houses the two opposing vocal folds. Each vocal fold consists of a soft membranous cover layer folded around an inner muscular layer. The vocal folds are connected together anteriorly but slightly separated posteriorly, forming a triangular-shaped airway (the glottis) ( Figure 1B ). At rest, the glottis remains open and allows airflow in and out of the lungs during breathing. During voice production (also known as phonation), the two vocal folds are brought together to close the glottis ( Figure 1C ). When the lung pressure is high enough (about 200 Pa), the vocal folds will be excited into a self-sustained vibration, which periodically opens and closes the glottis. This modulates airflow through the glottis and produces sound, which then propagates through the vocal tract and radiates from the mouth and nasal opening into the voice we hear.

A: computed tomography image of the head showing the airway and the larynx. B: top view of the larynx. The vocal folds are far apart at rest. C: vocal folds are brought together to close the glottis during phonation.
An important feature of normal voice production is that the glottis remains closed for an extended duration within each cycle of vocal fold vibration (see Multimedia 1 ), which interrupts the glottal flow. The rapid decline of the glottal flow during the glottal closing phase is the main mechanism for harmonic sound production, by which voices of different quality are produced and differentiated. An abrupt cessation of the glottal flow produces a voice with strong harmonic excitation at high frequencies and a bright voice quality that often carries well in a room or open space. On the other hand, a sinusoidal-like shape of the glottal flow with a gradual flow decline, often in the presence of an incomplete glottal closure, produces a voice with a limited number of higher order harmonics in the voice spectrum and a weak voice quality.
The glottal closure pattern during voice production is controlled by adductory laryngeal muscles that bring the two folds together (vocal fold approximation) to reduce the glottal gap. Indeed, phonation is impossible if the glottal gap is too large. Vocal folds that are insufficiently approximated tend to vibrate without complete glottal closure. This produces a breathy voice quality with weak excitation of harmonics and strong noise in the voice spectrum. Increasing approximation of the vocal folds leads to increased vocal fold contact and glottal closure, reducing air leakage through the glottis and increasing harmonic sound generation.
Activation of the adductory laryngeal muscles also modifies vocal fold shape and, particularly, the vertical thickness of the vocal fold medial surface. The medial surface vertical thickness plays an important role in regulating the duration of glottal closure and the produced voice quality. Increasing the vertical thickness allows the vocal folds to better maintain their position against the subglottal pressure. This is essential to achieve complete glottal closure at high lung pressure while producing a loud voice where vocal fold approximation alone is insufficient to ensure glottal closure during phonation ( Zhang, 2016 ).
In general, thicker vocal folds tend to close the glottis for a longer duration during phonation than thinner vocal folds. Thus, changes in vertical thickness are essential to producing voice qualities ranging from breathy (see Multimedia 2 ) to normal (see Multimedia 3 ) to pressed (see Multimedia 4 ). In the extreme case of very large vocal fold thickness due to strong vocal fold adduction, the folds often exhibit subharmonic or irregular vibration, producing a rough voice quality ( Zhang, 2018 ), known as creak in the linguistic literature and more colloquially as vocal fry (see Multimedia 5 ).
Pitch is controlled by elongating and shortening the vocal folds, which regulates the tension and stiffness of the vocal folds. This is possible because the cover layer of each vocal fold consists of collagen and elastin fibers aligned along the anterior-posterior (front-back) direction. These fibers are in a wavy, crimped state at rest but are gradually straightened with elongation and thus become load bearing. As more fibers are gradually straightened with vocal fold elongation, the vocal folds become increasingly stiff, thus increasing pitch.
Because the laryngeal muscles that control the vocal fold length also regulate the vocal fold vertical thickness, changes in pitch are often accompanied by changes in voice quality. For example, a pitch glide is often accompanied by changes in vocal registers. Vocal fry, produced often with increased vertical thickness and a long period of glottal closure, occurs at the lower end of the pitch range, whereas the voice at the high end of the pitch range is often in a falsetto register, produced with a reduced vertical thickness and a brief duration of glottal closure. The modal voice, which is used in conversational speech, is produced with an intermediate thickness of the vocal fold at the intermediate pitch range.
Vocal Fold Contact Pressure and Risk of Vocal Fold Injury
During voice production, the vocal folds experience repeated mechanical stress. In particular, the contact pressure sustained by the vocal folds during repeated collision poses the greatest risk of tissue damage because this pressure acts perpendicular to the load-bearing collagen and elastin fibers within the vocal folds ( Titze, 1994 ). For a loud voice such as screaming, the contact pressure can be as high as 20 kPa locally for extreme voicing conditions as reported in recent numerical simulations ( Zhang, 2020 ).
Although the vocal folds evolved to withstand the repeated contact pressure during phonation, when the contact pressure exceeds a certain level (e.g., due to talking loudly or screaming) or is sustained over an extended period (e,g., due to excessive talking or singing), it will cause injury to the vocal folds, triggering an initial inflammation response with fluid accumulation. This often results in degraded voice quality and difficulty in producing or modulating the voice. The threshold contact pressure triggering the inflammation response appears to vary individually depending on the daily vocal load, overall health condition of the speaker, and, possibly, the microstructural composition of the vocal fold tissues. If this hyperfunction behavior (loud voice for a prolonged period) persists, there may be permanent vocal fold lesions such as vocal fold nodules ( Figure 2 ).

A: vocal hyperfunction can lead to vocal fold nodules on the medial edge of the vocal folds ( left ), which prevents complete glottal closure during phonation ( right ). B: vocal fold nodules almost disappear post-voice therapy ( left ), which significantly improves glottal closure during phonation (right ).
The magnitude of the peak contact pressure depends primarily on the subglottal pressure used to produce the voice and, to a lesser degree, the cover layer stiffness of the vocal folds ( Zhang, 2020 ). Soft vocal folds subject to high subglottal pressure will vibrate with a large vibration amplitude and vocal fold speed at contact, and thus a high contact pressure is required to stop the vocal folds during collision. In general, thinner vocal folds (as, e.g., in a falsetto register) tend to produce lower vocal fold contact pressure ( Zhang, 2020 ). Although the effect of the glottal gap on the contact pressure is generally small, the contact pressure becomes excessively high when the vocal folds are tightly compressed against each other (hyperadduction).
Because the subglottal pressure has a dominant effect on both vocal fold contact pressure and vocal intensity, the risk of vocal fold injury can be significantly reduced by lowering the vocal intensity or completely eliminated by vocal rest. However, vocal rest or reduced loudness is often not socially practical due to communication needs in everyday life. A more practical strategy is to adopt laryngeal and vocal tract adjustments to minimize the subglottal pressure required to produce voice of desired loudness, thus minimizing vocal fold contact pressure. At the laryngeal level, this can be achieved by adopting a barely abducted (with the vocal folds just touching each other), thin vocal fold configuration ( Berry et al., 2001 ; Zhang, 2020 ). This barely abducted configuration is often targeted in voice therapy (e.g., the resonant voice therapy; Verdolini-Marston et al., 1995 ). In voice training, register balancing between thick and thin vocal folds in singing is often promoted to minimize subglottal pressure and purportedly laryngeal pathologies over time (e.g., the Bel Canto technique).
Vocal fold contact pressure can also be lowered by vocal tract adjustments. For example, when targeting a desired loudness, vocal fold contact pressure can be lowered by constricting the epilarynx (the part of the upper airway immediately above the vocal folds) or increasing the mouth opening whenever possible. Epilaryngeal narrowing often leads to clustering of vocal tract resonances in the 2- to 3-kHz range, which is known as the singer’s formant, and amplifies voice harmonics in this frequency range. Increasing the mouth opening increases the efficiency of sound radiation from the mouth. Both adjustments reduce the subglottal pressure required to produce a desired loudness, thus reducing vocal fold contact pressure ( Zhang, 2021 ).
Unfortunately, untrained speakers often increase vocal fold adduction when attempting to increase vocal intensity ( Isshiki, 1964 ), especially in an emotional situation. This is particularly the case of speakers who habitually squeeze the larynx during talking. Hyperadduction of the vocal folds may also develop as an adaptive behavior in response to transient vocal fold tissue changes. Hyperadducted vocal folds are not vocally efficient, meaning that a higher subglottal pressure is required to produce a desired loudness than that needed for barely abducted vocal folds. Because hyperadduction is often accompanied by reduced stiffness and increased thickness in the cover layer, the risk of vocal fold injury is excessively high due to the combination of the high subglottal pressure required, tightly compressed vocal folds, and low cover layer stiffness. Tightly compressed vocal folds also have the tendency to exhibit irregular vocal fold vibration with large cycle-to-cycle variations, resulting in a rough voice quality. Whenever possible, this vocal fold configuration should be avoided in loud voice production by making the appropriate adjustments at the larynx and within the vocal tract.
Glottal Insufficiency and Adaptive Compensations
Although voice production with tightly compressed vocal folds is unhealthy, voice production with the vocal folds too far apart is also undesired. Whereas the latter vocal configuration requires the least laryngeal effort and poses the lowest risk to vocal fold injury at a low subglottal pressure, voice production is extremely inefficient due to the lack of glottal closure. Thus, attempting to talk loudly in this configuration would require excessively high subglottal pressures, resulting in a high respiratory effort and, potentially, a high vocal fold contact pressure. The produced voice is breathy in nature due to the large airflow escaping through the glottis. With the high lung volume expenditure, one may also feel short of breath and need to take another breath in the middle of an utterance, particularly when attempting to increase loudness. As a result, such a configuration is not ideal for conversational communication or loud voice production.
However, the ability to sufficiently adduct the vocal folds may be lost or weakened due to changes in vocal fold physiology, a condition known medically as glottal insufficiency. Such insufficiency may occur as a result of vocal fold paralysis or paresis due to trauma to the laryngeal nerves, vocal fold atrophy with aging, or changes in the membranous cover layer (e.g., vocal fold swelling or scarring). Under such conditions, one may develop adaptive vocal behaviors in an attempt to increase vocal efficiency and conserve air expenditure. This can be achieved by increasing activation of the adductory muscles to improve glottal closure if the neuromuscular mechanism is still intact. One may also adduct supraglottal structures such as the false folds and epiglottis ( Figure 3 ), as often observed in muscle tension dysphonia. Although supraglottal adduction does not improve glottal closure, it may enhance source-tract interaction and thus increase vocal efficiency in addition to air conservation. Such adaptive behaviors often lead to increased laryngeal effort, vocal fatigue over time, and a strained voice quality. If such adaptation persists, it may lead to long-term voice disorders.

Adduction of the supraglottal structures may lead to medial-lateral ( A: left to right ) or anterior-posterior ( B: front to back ) constriction of the airway immediately above the vocal folds, as often observed in muscle tension dysphonia.
For example, vocal fold swelling often occurs after extensive shouting or screaming in a sports event or giving a lecture for a longer than the normal period. Extremely high subglottal pressures and, even more so, vocal fold hyperadduction in these situations readily lead to vocal fold swelling. This swelling may also occur following an upper respiratory infection (such as the cold or flu), chemical exposure of the vocal folds due to laryngopharyngeal reflux (stomach acid reflux into the throat), or smoking. Vocal fold swelling makes it difficult to completely close the glottis along the length of the vocal folds, allowing air to escape through gaps around the swollen portion of the vocal folds. When vocal fold inflammation leads to an irregular medial edge of the vocal folds, irregular glottal closure may ensue, resulting in hoarse voice quality.
Vocal fold swelling is often transient and will resolve over time with vocal rest or when the underlying medical conditions have cleared. However, if one were to talk through these voice changes, one often has to increase lung pressure, tighten adduction of the vocal folds, and possibly adduct the false folds and epiglottis. This adaptation may lead to increased contact pressure between the vocal folds, further exacerbating the underlying vocal fold inflammation. If this adaptive behavior persists after the triggering conditions are resolved, the vocal fold inflammation may further develop into vocal fold lesions such as vocal fold nodules, polyps, and contact ulcers, with a more permanent change in voice quality ( Hillman et al., 1989 ). For voice professionals, particularly singers, it is often recommended that they reduce voice use in the presence of vocal fold inflammation and avoid adaptive changes in vocal behavior.
Muscular Tension Around the Larynx
Voice disorders may also occur from increased tension in the perilaryngeal muscles that support the larynx (muscles connecting the larynx to other structures around the neck). This is often due to adaptive behaviors to compensate for glottal insufficiency but may also result from psychological stress ( Dietrich and Verdolini Abbott, 2012 ).
Tension in the perilaryngeal muscles often raises the vertical position of the larynx. This results in increased adduction of the vocal folds and the squeezing of supraglottal structures such as the false vocal folds and epiglottis ( Figure 3 ) ( Vilkman et al., 1996 ), allowing a speaker to compensate for glottal insufficiency. However, in the absence of glottal insufficiency, such increased vocal fold adduction often leads to excessively high contact forces between the vocal folds and poses a high risk of vocal fold injury. Due to the high tension in the perilaryngeal muscles, the speaker often experiences vocal fatigue after an extended period of talking and may even feel pain around the neck.
Although voice production is primarily controlled by activities of the intrinsic laryngeal muscles (muscles with origin and insertion within the larynx), these muscles act on the laryngeal framework that is supported and stabilized by the perilaryngeal muscles. Excessive tension in the perilaryngeal muscles acting on the laryngeal cartilages makes it more difficult to adjust the relative position among the thyroid, cricoid, and arytenoid cartilages to which the vocal folds are attached. This may interfere with the delicate control of vocal fold geometry and mechanical properties by the intrinsic muscles and limit the range of vocal fold posturing. Tension in the perilaryngeal muscles may also lead to undesired relative positions between laryngeal cartilages, which often require compensation by increased activity of the intrinsic laryngeal muscles to maintain pitch or adductory positions. This may change the relative balance between the intrinsic laryngeal muscles, resulting in increased laryngeal effort.
Involvement of the Respiratory System
Adaptive behavior to tighten the larynx may also result from laryngeal-respiratory compensation. The respiratory system is responsible for providing and maintaining the subglottal pressure desired for speech production. In breathing at rest, the respiratory muscles are actively engaged during inspiration, whereas expiration often relies on a passive elastic recoil of the lungs and thorax, known as the relaxation pressure. The amount of relaxation pressure increases with the lung volume and is positive (i.e., pushes air out of the lungs) at a high lung volume and becomes negative (draws air into the lungs) at a very low lung volume. Speech production occurs during the expiration phase of breathing and takes advantage of the relaxation pressure in supplying and maintaining the desired subglottal pressure. By taking a breath to start speech at the appropriate lung volume, the desired subglottal pressure can be mostly supplied and maintained by the relaxation pressure for the entire breath group duration, without much extra respiratory muscle effort. In this sense, speech is often considered “effortless.”
However, when starting speech at either too high or too low lung volumes, extra expiratory muscle effort would be required to either overcome or supplement the relaxation pressure. This additional muscle activation increases rapidly as the lung volume approaches the lower or upper end of the lung capacity. In the extreme case of starting speech at a very low lung volume, in addition to this extra expiratory muscle activation required to maintain the desired subglottal pressure, the level of vocal fold adduction must also be increased to conserve airflow and prevent running out of air before completing an utterance. Thus, speakers who habitually start their speech at a low lung volume often produce a voice with hyperadducted vocal folds and possibly adduction of supraglottal structures ( Desjardins et al., 2021 ), leading to vocal fatigue and undesired voice changes.
A tight laryngeal configuration at a low lung volume may also result from a reduced tracheal pull effect. Tracheal pull is a downward force exerted by the trachea and the respiratory system on the larynx. This force applies to the cricoid cartilage and tends to reduce the degree of vocal fold adduction. Tracheal pull increases as the diaphragm descends. That is, the tracheal pull is strong when speaking at a high lung volume and decreases as the lung volume decreases ( Sundberg, 1993 ). Thus, when speaking at a very low lung volume, vocal fold adduction may increase naturally due to reduced tracheal pull.
Hydration and Environmental Acoustic Support
Hydration is another important factor in maintaining vocal health. The vocal fold surface is lined by a mucous layer that functions as lubrication to reduce the contact pressure during vocal fold collision. When the speaker is dehydrated, the mucus becomes thick and sticky instead of thin and watery, a condition that deteriorates the lubrication effect in reducing vocal fold contact pressure ( Colton et al., 2011 ). Dehydration may also increase vocal fold stiffness and viscosity, thus increasing the lung pressure required to produce voice. Thus, maintaining good systemic hydration is essential to voice professionals who use their voice extensively in their daily life.
Voice production is mediated through auditory feedback and thus is subject to changes in the speaker’s acoustic environment. For example, with increasing background noise, we often increase vocal intensity to maintain the sufficient speech-to-noise ratio desired for communication. The increase in vocal intensity is often accompanied by a boost of high-frequency harmonic energy with respect to low-frequency harmonic energy, indicating increased vocal fold adduction.
Similar voice changes are also observed when speaking in rooms with different reverberation characteristics. Speakers produce voice with a higher vocal intensity in rooms with a shorter reverberation time compared with rooms with a longer reverberation time in which acoustic reflections of their own voice provide strong auditory feedback and acoustic support ( Brunskog et al., 2009 ). Thus, speaking for an extended period in a noisy environment or an acoustically “dead” environment with a very short reverberation time is likely to require an increased vocal effort and the speaker is prone to vocal fatigue and risk of vocal fold injury.
Clinical Voice Care
Clinical voice care attempts to restore the voice through medical, behavioral, and/or surgical interventions. When the voice disorder is triggered by an underlying medical condition, such as vocal fold swelling due to an upper respiratory infection, reflux, or smoking, medical treatment is necessary to clear the medical condition. Due to the delicate structure of the vocal folds, particularly within the membranous cover layer, the initial treatment is often behavioral or voice therapy, particularly for nonorganic voice disorders but also for some organic voice disorders such as vocal fold nodules ( Figure 2 ). The goal of voice therapy is to restore the best voice possible, something that is often achieved through vocal health education and modification of vocal behavior using different vocal techniques and exercises. Even for patients who eventually require surgery, pre- and postoperative voice therapy is essential to achieve an optimal voice outcome and prevent recurrence of the voice disorder. For organic voice disorders or conditions of glottic insufficiency, surgical intervention is often more effective.
One of the most common voice disorders in the clinic is muscle tension dysphonia. It involves too extensive an effort in producing the voice, with excessive muscle force and a tight larynx configuration. Some patients may also present with vocal fold lesions such as nodules, due to the chronic exposure to excessively high vocal fold contact pressure. Voice therapy is often effective in improving voice in these patients. For example, external circumlaryngeal massage is often used to relax the larynx in patients with notable tension in the musculature around the neck. Some techniques take advantage of tasks such as yawning or sighing that are naturally produced with a reduced laryngeal muscle tension and a less adducted glottal configuration, often with a lowered vertical position of the larynx. By starting with such tasks and gradually transitioning into speech, the speaker can be trained to produce voice with the same relaxed laryngeal configuration, thus reducing vocal fold contact pressure and the risk of vocal fold injury.
Various vocal exercises are also used to train speakers to produce voice with a focus on vibratory sensations around the lips and cheek and along the alveolar ridge of the palate (e.g., resonant voice therapy), thus avoiding a tight sensation at the larynx. In some exercises, the speaker is instructed to perform pitch or loudness glides with a semi-occluded vocal tract configuration, producing either nasal sounds, trills, or phonating into a narrow tube such as a drinking straw. It is generally believed that by focusing on vibratory sensations in certain parts of the vocal tract, the speaker may adopt a vocal configuration that improves vocal efficiency and minimizes vocal fold contact pressure.
An important component of voice therapy is to reestablish the balance between respiration, phonation, and articulation. For example, for voice disorders resulting from weakened respiratory function or improper respiratory behavior, voice therapy often focuses on respiration strength training to improve respiratory function or training the speaker to begin speaking at an appropriate lung volume to ensure sufficient air supply required for speech ( Desjardins et al., 2021 ).
For vocal fold mass lesions that are large in size, such as vocal fold polyps, cysts, and sometimes even nodules, voice therapy may have little effect and surgical removal is necessary. Because the membranous cover layer of the vocal folds is the vibrating component, it is critical that surgery remove as little tissue as possible and avoid significantly altering the delicate structure and mechanical properties of the vocal fold cover layer. Vocal fold scarring after surgery, particularly on the vocal fold medial surface where vocal fold vibration modulates airflow most effectively, often negatively impacts the patient’s voice and vocal capabilities.
For patients who are unable to sufficiently adduct the vocal folds due to vocal fold paralysis, paresis, atrophy, or aging, vocal fold adduction can be improved through an office-based injection augmentation procedure in which fat or another material is injected into the vocal folds to displace the medial edge of the vocal folds toward the glottal midline. A more permanent solution is medialization laryngoplasty, in which an implant is inserted laterally to the vocal folds to permanently displace and reposition the vocal folds toward the glottal midline ( Isshiki, 1989 ). These procedures are often able to significantly improve glottal closure and voice quality and reduce vocal effort.
In addition to adjusting the vocal fold position, vocal fold surgery also allows manipulation of vocal pitch. One way to achieve this is to adjust vocal fold tension by surgically modifying the relative positions between laryngeal cartilages. However, this often reduces the vocal range and the amount of pitch change is relatively small. In feminization voice surgery in which a large pitch increase is desired, surgery is often performed to not only adjust vocal fold length but also to reduce the vibrating length of the vocal folds by surgically merging the anterior portions of the two vocal folds or reducing vocal fold mass. Because pitch is only one of many aspects of gender perception, voice therapy is necessary in these patients to adjust other aspects of voice use such as vowel quality, stress, inflection, choice of words and conversational style.
Surgical intervention is also effective in treating some neurological voice disorders. For example, spasmodic dysphonia is a neurological voice disorder that results from involuntary spasms in laryngeal muscle activity, which interferes with normal vocal fold vibration and leads to intermittent voice breaks and strained or breathy voice quality. Current treatment aims to weaken the affected laryngeal muscles through botulinum toxin injection or surgically denervating the affected laryngeal nerves, both of which can significantly alleviate the symptoms.
Bridging the Gap Between Science and Clinical Practice
Current clinical voice care is often quite effective in at least partially improving voice production and quality. However, the voice outcome is often variable and relies heavily on the clinician’s experience. Sometimes the voice still remains unsatisfactory after intervention, and the underlying reasons are often unclear. In this sense, clinical voice care is more art than science. The translation of findings from basic science voice research can play an important role in further improving clinical management of voice disorders and reducing variability in voice outcomes. For example, although vocal fold medial surface shape in the vertical dimension has been shown to be important to voice production ( Zhang, 2016 ), it is often not monitored or targeted in current clinical voice examination and intervention, which focus on vocal fold position and glottal closure from a superior, endoscopic view. Targeting the medial surface shape in addition to other intervention goals may improve voice outcomes in patients whose voice remains unsatisfactory after intervention.
Many voice therapy techniques currently used in the clinic were modified from vocal training methods. Although many of them are effective, the underlying scientific principles often remain unclear. For example, semi-occluded vocal tract exercises are widely used in the clinic. Although some theoretical hypotheses have been put forward, they are not always consistent with the observed changes in the laryngeal and vocal tract configuration during such exercises ( Vampola et al., 2011 ). Voice therapy and vocal training often emphasize vibratory sensations in certain parts of the airway. However, it remains unclear what laryngeal and vocal tract adjustments are elicited in patients by voice therapy and which of them are responsible for improvement in voice outcomes. A better understanding of the scientific rationale would allow clinicians to better monitor the progress of voice therapy or even adapt voice therapy toward patient-specific vocal behavior to further improve voice therapy outcomes.
Each individual voice is unique. Although some individuals are prone to vocal fold injury, others can talk loudly for an extended duration without experiencing vocal fatigue or noticeable voice changes. Little is known about the physiological and behavioral factors responsible for individual differences in vocal capabilities and vocal health. A mathematical model of voice production allowing manipulation of the voice in a physiologically realistic way would provide insights into why and how each individual voice is different ( Wu and Zhang, 2019 ), which may lead to interesting applications both inside and outside the clinic.
Supplementary Material
Multimedia 1.
Multimedia1: Vocal fold vibration during normal phonation from a top view. An important feature of normal phonation is that the glottis remains closed for a considerable duration within one cycle of vocal fold vibration, which interrupts the glottal flow. This periodic flow interruption is the main mechanism for harmonic sound production and regulation of voice quality.
Multimedia 2
Multimedia2: Audio of a breathy voice.
Multimedia 3
Multimedia3: Audio of a normal sounding voice.
Multimedia 4
Multimedia4: Audio of a pressed voice.
Multimedia 5
Multimedia5: Audio of a creaky voice / vocal fry.
Acknowledgments
I thank Maude Desjardins, Bruce Gerratt, Katherine Verdolini Abbott, Lisa Bolden, and Arthur Popper for their constructive comments on an earlier draft of this paper. I also acknowledge support from the National Institute on Deafness and Other Communication Disorders (NIDCD), National Institutes of Health (NIH), Bethesda, MD.
Technical Committee: Speech Communication
- Berry D, Verdolini K, Montequin DW, Hess MM, Chan RW, and Titze IR (2001). A quantitative output-cost ratio in voice production . Journal of Speech, Language, and Hearing Research 44 , 29–37. [ PubMed ] [ Google Scholar ]
- Boone DR, McFarlane SC, Von Berg SL, and Zraick RI (2010). The Voice and Voice Therapy , 8th ed. Allyn & Bacon, Boston, MA. [ Google Scholar ]
- Brunskog J, Gade AC, Bellester GP, and Calbo LR (2009). Increase in voice level and speaker comfort in lecture rooms . The Journal of the Acoustical Society of America 125 , 2072–2082. [ PubMed ] [ Google Scholar ]
- Colton RH, Casper JK, and Leonard R (2011). Understanding Voice Problems: A Physiological Perspective for Diagnosis and Treatment . Lippincott Williams & Wilkins, Baltimore, MD. [ Google Scholar ]
- Desjardins M, Verdolini Abbott K, and Zhang Z (2021). Computational simulations of respiratory-laryngeal interactions and their effects on lung volume termination during phonation: Considerations for hyperfunctional voice disorders . The Journal of the Acoustical Society of America 149 , 3988–3999. [ PMC free article ] [ PubMed ] [ Google Scholar ]
- Dietrich M, and Verdolini Abbott K (2012). Vocal function in introverts and extraverts during a psychological stress reactivity protocol . Journal of Speech, Language, and Hearing Research 55 , 973–987. [ PubMed ] [ Google Scholar ]
- Hillman RE, Holmberg EB, Perkell JS, Walsh M, and Vaughan C (1989). Objective assessment of vocal hyperfunction: An experimental framework and initial results . Journal of Speech, Language, and Hearing Research 32 , 373–392. [ PubMed ] [ Google Scholar ]
- Isshiki N (1964). Regulatory mechanism of voice intensity variation . Journal of Speech, Language, and Hearing Research 7 , 17–29. [ PubMed ] [ Google Scholar ]
- Isshiki N (1989). Phonosurgery: Theory and Practice . Springer, Tokyo, Japan. [ Google Scholar ]
- Sundberg J (1993). Breathing behavior during singing . The NATS Journal 49 , 4–51. [ Google Scholar ]
- Titze IR (1994). Mechanical stress in phonation . Journal of Voice 8 , 99–105. [ PubMed ] [ Google Scholar ]
- Vampola T, Laukkanen A, Horáček J, & Švec JG (2011). Vocal tract changes caused by phonation into a tube: a case study using computer tomography and finite-element modeling . The Journal of the Acoustical Society of America 129 , 310–315. [ PubMed ] [ Google Scholar ]
- Verdolini-Marston K, Burke MK, Lessac A, Glaze L, and Caldwell E (1995). Preliminary study on two methods of treatment for laryngeal nodules . Journal of Voice 9 , 74–85. [ PubMed ] [ Google Scholar ]
- Vilkman E, Sonninen A, Hurme P, and Korkko P (1996). External laryngeal frame function in voice production revisited: A review . Journal of Voice 10 , 78–92. [ PubMed ] [ Google Scholar ]
- Wu L, and Zhang Z (2019). Voice production in a MRI-based subject-specific vocal fold model with parametrically controlled medial surface shape . The Journal of the Acoustical Society of America 146 , 4190–4198. [ PMC free article ] [ PubMed ] [ Google Scholar ]
- Zhang Z (2016). Mechanics of human voice production and control . The Journal of the Acoustical Society of America 140 , 2614–2635. [ PMC free article ] [ PubMed ] [ Google Scholar ]
- Zhang Z (2018). Vocal instabilities in a three-dimensional body-cover phonation model . The Journal of the Acoustical Society of America 144 , 1216–1230. [ PMC free article ] [ PubMed ] [ Google Scholar ]
- Zhang Z (2020). Laryngeal strategies to minimize vocal fold contact pressure and their effect on voice production . The Journal of the Acoustical Society of America 148 , 1039–1050. [ PMC free article ] [ PubMed ] [ Google Scholar ]
- Zhang Z (2021). Interaction between epilaryngeal and laryngeal adjustments in regulating vocal fold contact pressure . JASA Express Letters 1 , 025201. [ PMC free article ] [ PubMed ] [ Google Scholar ]
Automatic Speech Recognition: Systematic Literature Review
Ieee account.
- Change Username/Password
- Update Address
Purchase Details
- Payment Options
- Order History
- View Purchased Documents
Profile Information
- Communications Preferences
- Profession and Education
- Technical Interests
- US & Canada: +1 800 678 4333
- Worldwide: +1 732 981 0060
- Contact & Support
- About IEEE Xplore
- Accessibility
- Terms of Use
- Nondiscrimination Policy
- Privacy & Opting Out of Cookies
A not-for-profit organization, IEEE is the world's largest technical professional organization dedicated to advancing technology for the benefit of humanity. © Copyright 2024 IEEE - All rights reserved. Use of this web site signifies your agreement to the terms and conditions.
Thank you for visiting nature.com. You are using a browser version with limited support for CSS. To obtain the best experience, we recommend you use a more up to date browser (or turn off compatibility mode in Internet Explorer). In the meantime, to ensure continued support, we are displaying the site without styles and JavaScript.
- View all journals
- My Account Login
- Explore content
- About the journal
- Publish with us
- Sign up for alerts
- Open access
- Published: 19 April 2023
Voice assistants in private households: a conceptual framework for future research in an interdisciplinary field
- Bettina Minder ORCID: orcid.org/0000-0002-5874-4999 1 ,
- Patricia Wolf 2 , 3 ,
- Matthias Baldauf 4 &
- Surabhi Verma 2 , 5
Humanities and Social Sciences Communications volume 10 , Article number: 173 ( 2023 ) Cite this article
2392 Accesses
1 Citations
1 Altmetric
Metrics details
- Business and management
- Criminology
- Information systems and information technology
- Science, technology and society
The present study identifies, organizes, and structures the available scientific knowledge on the recent use and the prospects of Voice Assistants (VA) in private households. The systematic review of the 207 articles from the Computer, Social, and Business and Management research domains combines bibliometric with qualitative content analysis. The study contributes to earlier research by consolidating the as yet dispersed insights from scholarly research, and by conceptualizing linkages between research domains around common themes. We find that, despite advances in the technological development of VA, research largely lacks cross-fertilization between findings from the Social and Business and Management Sciences. This is needed for developing and monetizing meaningful VA use cases and solutions that match the needs of private households. Few articles show that future research is well-advised to make interdisciplinary efforts to create a common understanding from complementary findings—e.g., what necessary social, legal, functional, and technological extensions could integrate social, behavioral, and business aspects with technological development. We identify future VA-based business opportunities and propose integrated future research avenues for aligning the different disciplines’ scholarly efforts.
Similar content being viewed by others
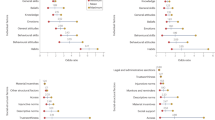
Determinants of behaviour and their efficacy as targets of behavioural change interventions
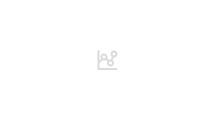
The impact of artificial intelligence on employment: the role of virtual agglomeration
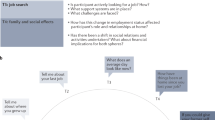
Interviews in the social sciences
Introduction.
Scholarly research across disciplines agrees that technological advancement is one of the important drivers of economic development because it brings about efficiency gains for all players of an economic system (Grossman and Helpman, 1991 ; Kortum, 1997 ; Dercole et al., 2008 ). Digitization and emerging technologies thus usually draw intense scholarly interest and are studied with the hope that their adoption will enable companies to generate “new capabilities, new products, and new markets” (Bhat, 2005 , p. 457) based on new business models, specifically designed for digitalized life spheres (Chao et al., 2007 ; Sestino et al., 2020 ; Antonopoulou and Begkos, 2020 ).
One of the recent emergent digital technologies promising companies substantial future revenues from innovative user services is voice assistants (VAs). They are “speech-driven interaction systems” (Ammari et al., 2019 , p. 3) that offer new interaction modalities (Rzepka et al., 2022 ).
Partly based on the integration of complementary Artificial Intelligence (AI) technology, they allow users’ speech to be processed, interpreted, and responded to in a meaningful way. In private households, we witness a rapid adoption rate of VAs in the form of smart speakers such as Amazon Echo, Apple Homepod, and Google Home (Pridmore and Mols, 2020 ) which, particularly in combination with customization of IoT home systems, provide a higher level of control over the smart home experience compared to a traditional setting (Papagiannidis and Davlembayeva, 2022 ). Available in the United States (US) since 2014 and in Europe since September 2016 (Trenholm, 2016 ; Hern, 2017 ), by 2018, already 15.4% of the US and 5.9% of the German population owned an Amazon Echo (Brandt, 2018 ). Overall, private household purchases already grew to 116% in the third quarter of 2018 compared to 2017 (Tung, 2018 ) and, according to a recent research report from the IoT analyst firm Berg Insight (Berg Insight, 2022 ), the number smart homes in Europe and North America reached 105 million in 2021. We realize that, at present, VAs represent an emergent technology that has its challenges (Clark et al., 2022 ), similar to the Internet of Things (IoT) or big data analytics technology. It has triggered an enormous amount of diverse scholarly research resulting “in a mass of disorganized knowledge” (Sestino et al., 2020 , p. 1). For both scholars and managers, the sheer quantity of disorganized information is making it hard to predict the characteristics of future technology use cases that fit users’ needs or to use this information for strategy development processes (Brem et al., 2019 ; Antonopoulou and Begkos, 2020 ). While Computer Science scholars already debate the technological feasibility of specific and complex VA applications, Social Science research points to VA-related market acceptance risks resulting, for example, from biased choices offered by VA (Rabassa et al., 2022 ) or from not identifying and implementing the privacy protection measures required by younger people (Shin et al., 2018 ), motivated by frequent user privacy leaks (Fathalizadeh et al., 2022 ) and worries about adverse incidents (Shank et al., 2022 ). Recent studies also specifically emphasize the need to shift the focus to user-centric product value (Nguyen et al., 2022 ) in the pursuit of the most beneficial solutions in terms of social acceptance and legal requirements (Clemente et al., 2022 ). For the most beneficial solutions, a collaboration between companies or even industries is likely to be necessary (Struckell et al., 2021 ).
There are, to our best knowledge, no systematic review papers focusing on VAs from a single discipline’s perspective that we could draw from. We did find an exploration of recent papers about the use of virtual assistants in healthcare that highlights some critical points (e.g., VA limitations concerning the ability to maintain continuity over multiple conversations (Clemente et al., 2022 ) or a review focusing on different interactions modalities in the ear of 4.0 industry—highlighting the need for strong voice recognition algorithm and coded voice commands (Kumar and Lee, 2022 ). In sum, the research that might allow for strategizing around VA solutions that match the needs of private households is scattered and needs to be organized and made sense of from an interdisciplinary perspective to shed “light on current challenges and opportunities, with the hope of informing future research and practice.” (Sestino et al., 2020 ). This paper thus sets out to identify, organize, and structure the available scientific knowledge on the recent use and the prospects of VAs in private households and propose integrated future research avenues for aligning the different disciplines’ scholarly efforts and leading research on consistent, interdisciplinary informed paths. We use a systematic literature review approach that combines bibliometric and qualitative content analysis to gain an overview of the still dispersed insights from scholarly research in different disciplines and to conceptualize topical links and common themes. Research on emerging technologies acknowledges that the adoption of these technologies depends on more factors than just technological maturity. Also, social aspects (e.g., social norms) and economic maturity (e.g., can a product be produced and sold so that it is cost-effective) play an important role (Birgonul and Carrasco, 2021 ; Xi and Hamari, 2021 ). Research particularly emphasizes that emerging technologies need to not only be creatively and economically explored—but also grounded in the user’s perspectives (Grossman-Kahn and Rosensweig, 2012 ) and serve longer enduring needs (Patnaik and Becker, 1999 ). IDEO conceptualized these requirements into the three dimensions of feasibility, viability, and desirability (IDEO, 2009 ).
Feasibility covers all aspects of VA innovation management that assures that the solution will be technically feasible and scalable. This also includes insuring that legal and regulatory requirements are met (Brenner et al., 2021 ). The viability lens focuses on economic success. “Desirability” ensures that the solutions and services are accepted by the target groups and, more generally, desired by society (Brenner et al., 2021 ). While IDEO and their focus on innovation development processes relate to a different context, the main reasoning about the relevance of these three dimensions (technical, social, and management) is also applicable when looking for research literature that helps find strategies around VA solutions that correspond to people’s needs in private homes. To cover these three dimensions, we focus on studies from Computer Science (CS), Social Science (SS), and Business and Management Science (BMS) to advance our knowledge of the still dispersed insights from scholarly research and highlight shared topics and common themes.
With this conceptual approach, we contribute an in-depth analysis and systematic overview of interdisciplinary scholarly work that allows cross-fertilization between different disciplines’ findings. Based on our findings, we develop several propositions and a framework for future research in the interest of aligning the various scholarly efforts and leading research on consistent, interdisciplinarily informed paths. This will help realize VA’s potential in people’s everyday lives. We moreover identify potential future VA-based business opportunities.
This paper is structured as follows: the section “Business opportunities related to VA use in private households” summarizes the research on potential business opportunities related to the use of VAs in private households. The research methodology, i.e., our approach of combining a bibliometric literature analysis with qualitative content analysis in a literature review, is presented in the section “Methods”. Section “Thematic clusters in recent VA research” identifies nine thematic clusters in recent VA research, and section “Analysis and conceptualization of research streams” analyzes and conceptually integrates them into four interdisciplinary research streams. Section “Discussion: Propositions and a framework for future research, and related business opportunities” identify future business opportunities and proposes future directions for integrated research, and section “Conclusion” concludes with contributions that should help both scholars and managers use this research to predict the characteristics of future technology, use cases that fit users’ needs, and use this information for their strategy development processes around VA.
Business opportunities related to VA use in private households
Sestino et al. ( 2020 , p. 7) argue that when new technologies emerge, “companies will need to assess the positives and negatives of adopting these technologies”. The positives of VA adoption lay mainly in the projection of large new consumer markets offering products and services where text-based human–computer interaction will be replaced by voice-activated input (Pridmore and Mols, 2020 :1), checkout-free stores such as Amazon Go, and the use of VA (Batat, 2021 ). Marketing studies predict high adoption rates in private households due to potential efficiency gains from managing household systems and devices by voice commands anytime from anywhere (Celebre et al., 2015 ; Chan and Shum, 2018 ; Jabbar et al., 2019 ; Vishwakarma et al., 2019 ), as well as the high potential of health check app for improving communication with patients (Abdel-Basset et al., 2021 ) or realize self-care solutions (Clemente et al., 2022 ). A study by Microsoft and Bing (Olson and Kemery, 2019 ) substantiates that claim for smart homes by revealing that, already today, 54% of the 5000 responding US users use their smart speakers to manage their homes, especially for controlling lighting and thermostats. In surveys, users state that they envision a future in which they will increasingly use voice commands to control household appliances from the microwave to the bathtub or from curtains to toilet controls (Kunath et al., 2019 ). CS scholars discuss how to design complementary Internet of Things (IoT) technology features and systems to bring about such benefits (Hamill, 2006 ; Druga et al., 2017 ; Pradhan et al., 2018 ; Gnewuch et al., 2018 ; Tsiourti et al., 2018a / b ; Azmandian et al., 2019 ; Lee et al., 2019 ; Pyae and Scifleet, 2019 ; Sanders and Martin-Hammond, 2019 ). BMS research additionally debates how companies should proceed to capture, organize, and analyze the (big) user data that become potentially available once VA is commonly used in private households, and to identify new business opportunities (Krotov, 2017 ; Sestino et al., 2020 ) and future VA applications, such as communication and monitoring services in pandemics (Abdel-Basset et al. 2021 ).
However, many recent studies also mention the negatives of VA usage, like worrying trends emerging from the so-called surveillance economy (Zuboff, 2019 ) or, instead, debate future questions, such as what happens when technology fails or what the rights of fully automated technological beings would be (Harwood and Eaves, 2020 ). 2050 out of the 5000 respondents to the Microsoft and Bing study reported concerns related to voice-enabled technology, especially about data security (52%) and passive listening (41%). The “significant new production of situated and sensitive data” (Pridmore and Mols, 2020 , p. 1) in private environments and the unclear legal situation related to the usage of these data seem to act as one of the inhibitors to the adoption of more complex VA applications by users. Thus, many of the imaginable future use cases, such as advanced smart home controls (Lopatovska and Oropeza, 2018 ; Lopatovska et al., 2019 ) or personal virtual shopping assistance (Omale, 2020 ; Sestino et al., 2020 ), are still a long way off. Although technologically feasible and partly already available, today’s users use VAs for simple tasks, such as “searching for a quick fact, playing music, looking for directions, getting the news and weather” (Olson and Kemery, 2019 ). Therefore, companies are warned against too high expectations of fast returns. Moreover, there are also some technical issues, and only the not-yet-mature integration of further AI-enabled services in VA is expected to be a game changer leading to growth in the deployment of voice-based solutions (Gartner, 2019 ; Columbus, 2020 ).
At a meta-level, BMS research advises companies to explore and implement new technologies in their products, services, or business processes, because that might result in a considerable competitive first-mover advantage (Drucker, 1988 ; Porter, 1990 ; Carayannis and Turner, 2006 ; Hofmann and Orr, 2005 ; Bhat, 2005 ). At the same time, Macdonald and Jinliang ( 1994 ) have shown that in industrial gestation (or the impact of science on society), the evolution in the demand for technology, and a set of competitors go hand in hand. Consequently, the adoption of an emergent technology by “the ultimate affected customer base” (Bhat, 2005 , p. 462) becomes of utmost importance when looking at how company investments pay off (Pridmore and Mols, 2020 ). This is particularly the case for VAs where companies are greatly dependent on the adoption of respective hardware—typically the aforementioned smart speakers (Herring and Roy, 2007 )—or of new services, such as the envisioned digital assistants (Sestino et al., 2020 , p. 7), by private users. VAs differ from other emergent technologies that allow companies to reap the benefits by implementing them in their own organization and reorganizing business or production processes, like RFID technology (Chao et al., 2007 ), nanotechnology (Bhat, 2005 ) or IoT-based business process (re)engineering (Sestino et al., 2020 ). Hence—although it is one of the most prominent emerging technologies discussed in current mass media—this might be one of the reasons for why there is yet very limited BMS research studying VA-related challenges and opportunities that could inform companies.
High-tech companies striving to develop VA-related business models need to consider and integrate scholarly knowledge from disciplines as different as CS, SS, and BMS to meet the requirements of “a secure conversational landscape where users feel safe” (Olson and Kemery, 2019 , p. 24). However, such interdisciplinary perspectives are yet hardly available—instead, we see a large amount of scattered disciplinary scholarly knowledge. This situation makes it difficult to assess opportunities for future VA-related services and to develop sustainable business models that offer a potential competitive advantage. In this paper, we set out to contribute to such an assessment by organizing and making sense of the scholarly knowledge from CS, SS, and BMS. We follow earlier research on the assumption that assessing the state of emergent technologies and making sense of available knowledge on new phenomena requires an interdisciplinary perspective (Bhat, 2005 ; Melkers and Xiao, 2010 ; Sestino et al., 2020 ) to pin down and forecast the technology’s future impact and to advise companies in their technology adoption decisions (Leahey et al., 2017 ; Demidova, 2018 ; McLean and Osei-Frimpong, 2019 ). The literature review we present here is therefore additionally aimed at substantiating the call for interdisciplinarity of research into emerging technologies that aim to offer insights about business opportunities.
Our aim of making sense of a large amount of disorganized scholarly knowledge on VAs, assessing challenges and opportunities for businesses, and identifying avenues for future interdisciplinary research, made a systematic literature review appear to be the most appropriate research strategy: Literature reviews enable systematic in-depth analyses about the theoretical advancement of an area (Callahan, 2014 ). Earlier research with similar aims that studied other emerging technologies found the method “useful for making sense of the noise” (Sestino et al., 2020 :1) in a fast-growing body of scholarly literature (Fig. 1 ).
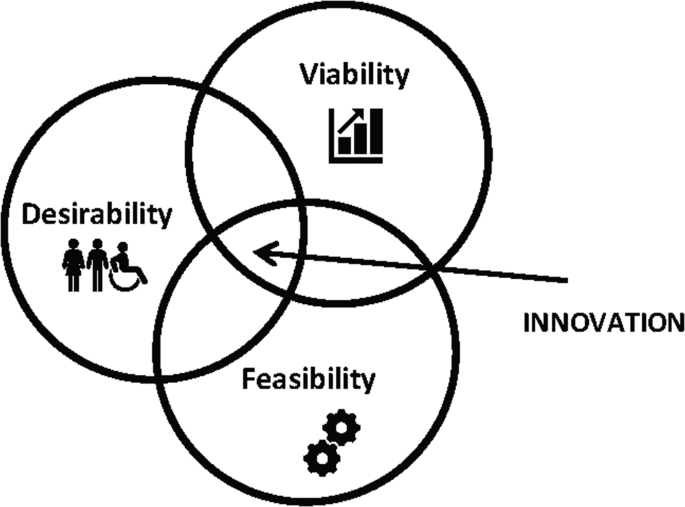
Innovation dimensions by IDEO: feasibility-viability-desirability (after IDEO, 2009 ).
For our research, we decided to combine a conventional literature review that applies qualitative content analysis, with bibliometric analysis. The bibliometric analysis provides an overview of connections between research articles and the intersection of different research areas (Singh et al., 2020 ). The qualitative content analysis-based literature review offers a more in-depth overview of the current state of the literature (Petticrew and Roberts, 2006 ). Earlier scholarly work indicates that such a combination is particularly useful for analyzing the current state of technology trends and the significance of forecasts (Chao et al., 2007 ; Li et al., 2019 ). Figure 2 depicts the methodological research approach of this study.
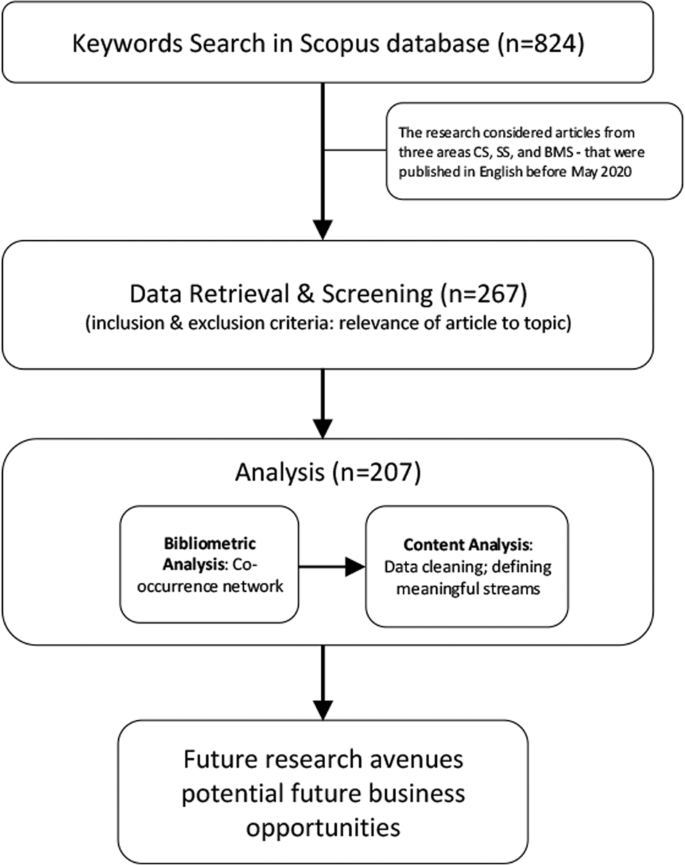
Overview of the methodological research approach of this study.
In the following, we describe the methodological approach in detail.
Article identification and screening
The literature search employed the Scopus database, as the coverage for the Scopus and Web of Science databases is similar (Harzing and Alakangas, 2016 ). In the literature search, we employed the keywords “voice assistant” and synonyms of it (“Voice assistant” OR “Virtual assistant” OR “intelligent personal assistant” OR “voice-activated personal assistant” OR “conversational agent” OR “SIRI” OR “Alexa” OR “Google Assistant” OR “Bixby” OR “Smart Loudspeaker” OR “Echo” OR “Smart Speaker”) and “home” and synonyms of it (“home” OR “house” OR “household”). The automated bibliometric analysis scanned titles, abstracts, and keywords of the article for these terms. We used the search field “theme” including title, abstract, and keywords (compare 3.2). Due to the focus of the research, the search was restricted to articles published in the CS, SS, and BMS areas, written in English, and published before May 2020.
We adopted the Preferred Reporting Items for Systematic Reviews and Meta-Analysis (PRISMA) guide proposed by Moher et al. ( 2009 ) for the bibliometric literature review. The initial search yielded 428 articles in the CS, 356 articles in the SS, and 40 articles in BMS. After scanning the abstracts of all documents in the list of each field, further articles were excluded based on their relevance to our topic. The most frequent reason for excluding an article was that it was not about VAs—e.g., articles found with the keyword “echo” referred to acoustic phenomena. Table 1 displays the descriptive results of the bibliometric literature review.
The final dataset included 267 articles in CS, 52 articles in SS, and 20 articles in BMS.
Tables 2 and 3 display the most frequent countries of origin for SS and CS.
Tables 2 and 3 present the top countries of origin of the articles from CS and SS. There was no information related to the countries of origin of the bms articles. In view of the many (regionally differing) legal questions and regulatory issues, it is important to see that, while the US is leading the list by a large margin, the discussion is also spread over countries from different continents.
Data analysis step 1: Bibliometric literature review
The final dataset consisted of bibliometric information including the author names, affiliations, titles, abstracts, publication dates, and citation information. The bibliometric analysis was conducted in each discipline separately using the VOSviewer software. For each discipline, we visualized common knowledge patterns through co-occurrence networks in the VA literature. A co-occurrence network contains keywords with similar meanings that can distort the analyses. Therefore, synonyms were grouped into topics using the VOSviewer thesaurus to ensure a rigorous analysis. For example, the keywords „voice assistant“, „virtual assistant“, „intelligent personal assistant“, „voice-activated personal assistant“, „conversational agent“, „SIRI“, „Alexa“, „Google Assistant“, „Bixby“, „smart loudspeaker“, „Echo“, „smart speaker“ were replaced with the main term “voice assistant”. Also, keywords were standardized to ensure uniformity and consistency (e.g., singular and plural). Further, a few keywords were also deleted from the thesaurus to ensure the focus of the review around the research questions of this study.
Scopus provides Subject Areas—we used these areas to generate the bibliometric analysis (e.g., select CS to analyze all papers from that area). When cleaning the data set—e.g., excluding non-relevant papers—some papers could be assigned to more than one area by checking the author’s affiliation. The co-occurrence networks (Figs. 3 – 5 ) of the keywords were obtained automatically from scanning titles, abstracts, and keywords of the articles in the final cleaned datasets. The networks present similarities between frequently co-occurring keywords (themes or topics) in the literature (Van Eck and Waltman, 2010 ). The co-occurrence number of two keywords is the number of articles that contain both keywords (Van Eck and Waltman, 2014 ). VOSviewer places these keywords in the network and identifies clusters with similar themes, and with each color representing one cluster (Van Eck and Waltman, 2010 ). The colors, therefore, reflect topical links and common themes. Boundaries between these clusters are fluid: ‘affordance’ for example (in Fig. 4 ) is in the light green cluster denoting research on VA systems—but it is also connected to the red cluster, discussing security issues (compare Fig. 4 ). The assignment to the ‘green cluster happens based on more frequent links to this topic. The co-occurrence networks for our three scholarly disciplines are displayed in Figs. 3 – 5 . By discussing the clusters, nine topic themes for our research emerged (compare next section).
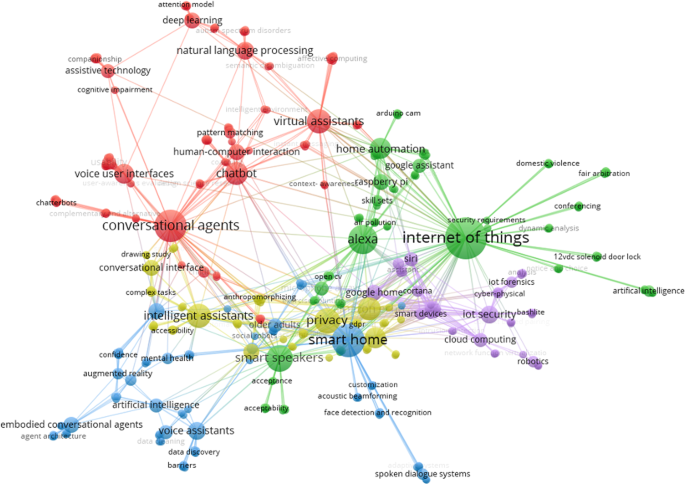
The frequently co-occurring keywords, themes, or topics in research in the CS field on VAs in private households.
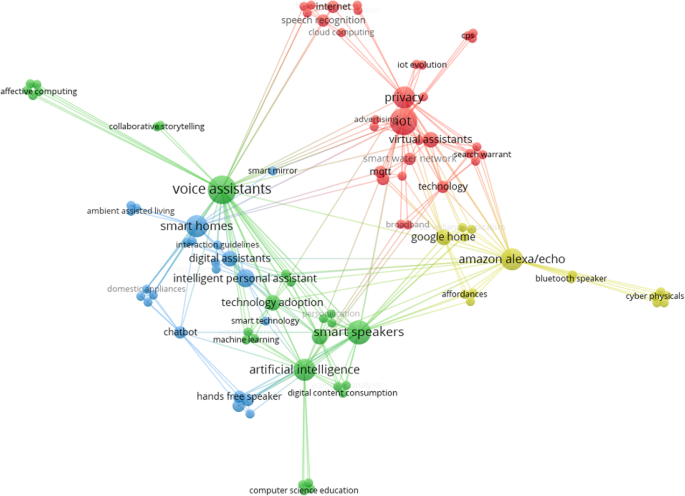
The frequently co-occurring keywords, topics, or themes in research in the SS field on VAs in private households.
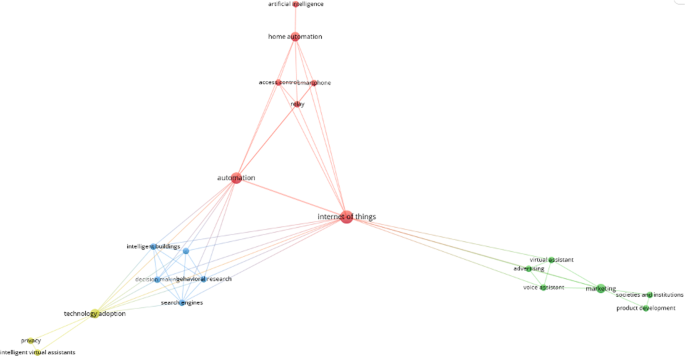
The frequently co-occurring keywords, topics, or themes in research in the BMS field on VAs in private households.
We can see that the networks and the topics covered differ in the three scientific areas. By studying and grouping the research topics that were revealed in the co-occurrence analysis within and across scientific areas, we identified nine thematic clusters in VA research. We labeled these clusters as “Smart devices” (cluster 1), “Human–computer interaction (HCI) and user experience (UX)” (cluster 2), “Privacy and technology adoption” (cluster 3), “VA marketing strategies” (cluster 4), “Technical challenges in VA applications development” (cluster 5), “Potential future VAs and augmented reality (AR) applications and developments” (cluster 6), “Efficiency increase by VA use” (cluster 7), “VAs providing legal evidence” (cluster 8) and “VAs supporting assisted living” (cluster 9). The clusters emerged from discussing the different research areas displayed in Figs. 3 – 5 in relation to our research question on strategies around VA solutions in private households. Essentially, the process of finding appropriate clusters for our research involved scanning the research areas, listening, and discussing possible grouping until the four researchers of this paper agreed on a final set of nine clusters. The nine clusters encompass different areas and terms in the figures—e.g., cluster 1 (smart devices) covered the areas ‚virtual assistants‘, ‚conversational agents‘, ‚intelligent assistants‘, ‚home automation‘, and ‚smart speakers‘, ‚smart technology‘. Cluster 2 (HCI and UX) includes areas such as ‘voice user interfaces’, ‘chatbots’, ‘human–computer interaction’, ‘hand-free speakers’. Some of the clusters we identified in this process contained only a small number of areas, such as cluster 4 (marketing strategies), which essentially covers the research areas ‘marketing’ and ‘advertising’.
Data analysis step 2: Qualitative content analysis
It can be difficult to derive qualitative conclusions from quantitative data, which is why, in this study, we additionally conducted a qualitative content analysis of the 267 articles in the cleaned dataset. The objective of this second step was to rigorously assess the results from the bibliometric review, ensuring that the identified nine themes identified in stage 1 are in accordance with the main tenets presented in the literature. Any qualitative content analysis of literature suffers, to a certain extent, from the subjective opinions of the authors. However, the benefits of this method are indisputable and follow a well-established approach used in past studies of a similar kind. To counter the risk of subjectivity in data analysis, we involved three researchers in it, thereby triangulating investigators (Denzin, 1989 ; Flick, 2009 ). We adopted Krippendorff’s ( 2013 ) content analysis methodology to ensure a robust analysis and help with the contextual dimensions of each research field.
In the first step, the nine clusters identified by using VOSViewer were evaluated by the three researchers independently by assigning each of the 267 articles to one of the nine thematic clusters. During this process, it became apparent that the qualitative content analysis confirmed the bibliometric analysis to a large extent, i.e., that most of the articles belonged to the clusters proposed in the bibliometric analysis. However, we excluded 60 articles in this process step, since many of the less obvious thematic mismatches of the articles can only be found in a more in-depth cleaning of the data set: 5 were duplicates (4 allocated to CS, 1 to SS) and 55 papers (46 from CS, 2 from SS and 6 from BMS) were not about VAs in private households. This left us with an overall sample of 207 articles (see the list in the Appendix).
Moreover, we identified articles that belonged to other clusters than suggested in the bibliometric analysis, and assigned them, after discussions with the research team, to the correct cluster. For example, the bibliometric analyses had originally not classified any of the articles in cluster 2 (“HCI and UX”) as belonging to the BMS area, while we identified such articles during the qualitative content analysis. Table 4 below displays the distribution of articles in the final dataset.
After having accomplished this data cleaning, we developed short summary descriptions summarizing the content of the research in each of the nine clusters (see section “Thematic clusters in recent VA research”).
In a final step, we condensed the nine clusters into four meaningful streams, representing distinguishable VA research topics that can support the emergence of interdisciplinary perspectives in research that studies VAs in private households. We applied the following procedure to obtain clusters and allocate papers from the clusters to the streams: First, three researchers independently conceptualized topical research streams. Then, all researchers discussed these streams and agreed on topical headlines reflecting the terminology used in the respective research. Next, they allocated—again first working independently and later together—papers to the four research streams presented in chapter 5. Our aim of finding meaningful streams that can support the emergence of interdisciplinary research on VA in private households made a qualitative procedure appear to be the most appropriate strategy for this step in the analysis. Qualitative analysis helps organize data in meaningful units (Miles and Huberman, 1994 ).
Thematic clusters in recent VA research
From our analysis, recent research on VA in private households can be divided into nine thematic clusters. In the following, we briefly present these clusters and elaborate on connections between the contributions from the three research areas we considered.
Cluster 1: Smart device solutions
Cluster 1 comprises publications on smart device solutions in smart home settings and their potential in orchestrating various household devices (Amit et al., 2019 ). Many CS papers present prototypes of web-based smart home solutions that can be controlled with voice commands, like household devices enabling location-independent access to IoT-based systems (Thapliyal et al., 2018 ; Amit et al., 2019 ; Jabbar et al., 2019 ). A research topic that appears in both the CS and SS areas relates to users’ choices, decisions, and concerns (Pridmore and Mols, 2020 ). Concerns studied relate to privacy issues (Burns and Igou, 2019 ) or the impact of VA use on different age groups of children (Sangal and Bathla, 2019 ).
A topic researched in all three scientific domains is the potential of VAs for overcoming the limitations of home automation systems. CS papers typically cover suggestions for resolving mainly technical limitations, such as those concerning language options (Pyae and Scifleet, 2019 ), wireless transmission range (Jabbar et al., 2019 ), security (Thapliyal et al., 2018 ; Parkin et al., 2019 ), learning from training with humans (Demidova, 2018 ), or sound-based context information (Alrumayh et al., 2019 ). SS research mostly investigates the limitations of VAs in acting as an interlocutor and social contact for humans (Lopatovska and Oropeza, 2018 ; Hoy, 2018 ; Pridmore and Mols, 2020 ), or identifies requirements for more user-friendly and secure systems (Vishwakarma et al., 2019 ). Finally, BMS papers focus on studying efficiency gains from using VAs, for example in the context of saving energy (Vishwakarma et al., 2019 ).
Cluster 2: Human–computer interaction and user experience
Cluster 2 contains human–computer interaction (HCI) research on the users’ experience of VA technology. Researchers investigate user challenges that result from unmet expectations concerning VA-enabled services (Santos-Pérez et al., 2011 ; Han et al., 2018 ; Komatsu and Sasayama, 2019 ). Papers from the SS area are typically discussing language issues (Principi et al., 2013 ; King et al., 2017 ).
A central topic covered both in the CS and BMS publications is trust in and user acceptance of VAs (e.g., Hamill, 2006 ; Hashemi et al., 2018 ; Lackes et al., 2019 ). From the BMS perspective, researchers find that trust and perceived (dis)advantages are factors influencing user decisions on buying or utilizing VAs (Lackes et al., 2019 ). Complementary, CS researchers find that the usefulness of human-VA interactions and access to one’s own household data impacts the acceptance of VAs (e.g., Pridmore and Mols, 2020 ). The combination of these two scientific disciplines discussing a topic without SS entering the debate is unique in our data material.
‘Humanized VAs’ is a topic discussed both in CS and SS research. In CS, this includes quasi-human voice-enabled assistants acting as buddies or companions for older adults living alone (Tsiourti et al., 2018a , b ) or technical challenges with implementing human characteristics (Hamill, 2006 ; Lopatovska and Oropeza, 2018 ; Jacques et al., 2019 ). Two papers from both CS and SS contributed to the theory of anthropomorphism in the VA context (Lopatovska and Oropeza, 2018 ; Pradhan et al., 2019 ). SS additionally offers findings about user needs, like the preferred level of autonomy and anthropomorphism for VAs (Hamill, 2006 ).
Cluster 3: Privacy and technology adoption
Cluster 3 consists predominantly of CS research into privacy-related aspects like the security risks of VA technology and corresponding technical solutions to minimize them (e.g., Dörner, 2017 ; Furey and Blue, 2018 ; Pradhan et al., 2019 ; Sudharsan et al., 2019 ). An exception concerns the user-perceived privacy risks and concerns that are studied in all three scientific domains. Related papers discuss these topics with a focus on user attitudes towards VA technology, resulting in technology adoption, and identify factors motivating VA application (e.g., Demidova, 2018 ; Fruchter and Liccardi, 2018 ; Lau et al., 2018 ; Pridmore and Mols, 2020 ): Perceived privacy risks are found to negatively influence user adoption rates (McLean and Osei-Frimpong, 2019 ). In CS studies, researchers predominantly propose solutions for more efficient VA solutions that users would want to bring into their homes (Seymour, 2018 ; Parkin et al., 2019 ; Vishwakarma et al., 2019 ). These should be equipped with standardized frameworks for data collection and processing (Bytes et al., 2019 ), or with technological countermeasures and detection features to establish IoT security and privacy protection (Stadler et al., 2012 ; Sudharsan et al., 2019 ; Javed and Rajabi, 2020 ). Complementary, SS researchers investigate measures for protecting the privacy of VA users beyond technical approaches, such as legislation ensuring privacy protection (Pfeifle, 2018 ; Dunin-Underwood, 2020 ).
Cluster 4: VA marketing strategies
Cluster 4 comprises research developing strategies for advertising the use of VAs in private households. We find here articles exclusively from BMS. Scholars address various aspects of VA marketing strategies, such as highlighting security improvements or enhanced user-friendliness and intelligence of the devices (e.g., Burns and Igou, 2019 ; Vishwakarma et al., 2019 ). Others study how to measure user satisfaction with VA technology (e.g., Hashemi et al., 2018 ).
Cluster 5: Technical challenges in VA applications development
Cluster 5 contains predominantly CS research papers investigating and proposing solutions for technical challenges in VA application development. Recent work focuses on extensions and improvements for the technologically relatively mature mass-market VAs (e.g., Liciotti et al., 2014 ; Azmandian et al., 2019 ; Jabbar et al., 2019 ; Mavropoulos et al., 2019 ). Some research investigates ways to overcome the technical challenges of VAs in household environments: For example, King et al. ( 2017 ) work on more robust speech recognition, and Ito ( 2019 ) proposes an audio watermarking technique to avoid the misdetection of utterances from other VAs in the same room. Further research on technological improvements includes work on knowledge graphs (Dong, 2019 ), on cross-lingual dialog scenarios (Liu et al., 2020 ), on fog computing for detailed VA data analysis (Zschörnig et al., 2019 ), and on the automated integration of new services based on formal specifications and error handling via follow-up questions (Stefanidi et al., 2019 ).
We identify a complementarity between CS and SS research within the research topic of “affective computing”. In both research domains, researchers strive to identify ways to create more empathic VAs. For example, Tao et al., ( 2018 ) propose a framework that conceptualizes several dimensions of emotion and VA use. SS research contributes to a virtual caregiver prototype aware of the patient’s emotional state (Tironi et al., 2019 ). However, scholarly contributions in the two areas are not related to each other.
Cluster 6: Potential future VA applications and developments
Cluster 6 investigates the future of VAs research, particularly technological advancements we can expect and suggestions for future research avenues. Most CS papers introduce prospective potential technical applications in many different areas, such as medical treatment and therapy (Shamekhi et al., 2017 ; Pradhan et al., 2018 ; Patel and Bhalodiya, 2019 ) or VA content creation and retrieval (Martin, 2017 ; Kita et al., 2019 ). A sub-group of papers also proposes functional prototypes (e.g., Yaghoubzadeh et al., 2015 ; Freed et al., 2016 ; Tielman et al., 2017 ).
We identify three topics that are discussed in both SS and CS publications. The first focuses on language and VAs and represents an area where CS research relates to SS findings: While SS identifies open language issues in dialogs with VAs (Martin, 2017 ; Ong et al., 2018 ; Huxohl, et al., 2019 ), CS researchers investigate how to approach them - not only at the technological level of speech recognition but also in terms of what it means to have a conversation with a machine (Yaghoubzadeh et al., 2015 ; Ong et al., 2018 ; Santhanaraj and Barkathunissa, 2020 ). A second focus is on near-future use scenarios (Hoy, 2018 ; Seymour, 2018 ; Tsiourti et al., 2019 ; Burns and Igou, 2019 ) such as VA library services, VA services for assisted living or support VAs for emergency detection and handling. The third common topic is about identifying future differences between the use of VAs in private households and in other environments like public spaces (Lopatovska and Oropeza, 2018 ; Robinson et al., 2018 ).
Cluster 7: Efficiency increase by VA use
Cluster 7 consists of papers about efficiency increase through VA use—with a focus on smart home automation systems. Papers in BMS discuss the increasing efficiency of home automation systems through the use of VAs (Vishwakarma et al., 2019 ). CS papers study and appraise the efficiency of home automation solutions and use cases, more efficient VA automation systems, interface device solutions (Liciotti et al., 2014 ; Jabbar et al., 2019 ; Jacques et al., 2019 ), effective activity assistance (Freed et al., 2016 ; Palumbo et al., 2016 ; Tielman et al., 2017 ), care for elderly people (Donaldson et al., 2005 ; Wallace and Morris, 2018 ; Tsiourti et al., 2019 ) , and smart assistive user interfaces and systems of the future (Shamekhi et al., 2017 ; Pradhan et al., 2018 ; Mokhtari et al., 2019 ). SS has not yet contributed to this cluster.
Cluster 8: VAs providing legal evidence
Cluster 8 addresses the rather novel topic of digital forensics in papers from the CS and SS domains. The research studies how VA activities can inform court cases. Researchers investigate which information can be gathered, derived, or inferred from IoT-collected data, and what approaches and tools are available and required to analyze them (Shin et al., 2018 ; Yildirim et al., 2019 ).
Cluster 9: VAs supporting assisted living
Cluster 9 comprises papers on VAs supporting assisted living. CS papers explore and describe technical solutions for the application of VAs in households and everyday task planning (König et al., 2016 ; Tsiourti et al., 2018a ; Sanders and Martin-Hammond, 2019 ), for improving aspects of companionship (Donaldson et al., 2005 ), for stress management in relation to chronic pain (Shamekhi et al., 2017 ), and for the recognition of distress calls (Principi et al., 2013 ; Liciotti et al., 2014 ). CS scholars also study user acceptance and the usability of VA for elderly people (Kowalski et al., 2019 ; Purao and Meng, 2019 ).
CS and SS both share a research focus on VAs helping people maintain a self-determined lifestyle (Yaghoubzadeh et al., 2015 ; Mokhtari et al., 2019 ) and on their potential and limitations for home care-therapy (Lopatovska and Oropeza, 2018 ; Kowalski et al., 2019 ; Turner-Lee, 2019 ), but without relating findings to each other.
Analysis and conceptualization of research streams
When comparing the bibliometric and the qualitative content analysis, the clusters found in the bibliometric analysis were confirmed to a large extent. The comparison did, however, also lead to the allocation of some articles to different areas. The content analysis particularly helped subsume the nine clusters in four principal research streams. The overview that we gained based on the four streams points to interdisciplinary research topics that need to be studied by scholars wanting to help realize VA potential through applications perceived as safe by users.
What all research domains share to a certain extent is a focus on users’ perceived privacy risks and concerns and a focus on the impact of perceived risks or concerns on the adoption of VA technology. At the same time, our findings confirm our assumption that these complementarities are generally not well used for advancing the field: In CS, researchers predominantly study future application development and technological advancements, but—except for language issues (cluster 6)—they do not relate this much to solving challenges identified in SS and BMS research. In the following, we first present an overview of the four deduced research streams and, in the next section, propositions and the conceptual model for future interdisciplinary research that we developed based on our analysis.
The four major research streams into which we consolidated the identified nine thematic clusters from our literature review are labeled as “Conceptual foundation of VA research” (stream 1), ”Systemic challenges, enabling technologies and implementation” (stream 2),” Efficiency” (stream 3) and “VA applications and (potential) use cases” (stream 4). The streams were obtained in a qualitative procedure, where three researchers conceptualized streams independently and discussed potentially meaningful streams together (compare 3.3). Table 5 provides an overview of the four main streams identified in VA literature and presents selected publications for each of the streams.
The streams systematize the scattered body of VA research in a way that offers clearly distinguishable interdisciplinary research avenues to assist in strategizing around and realizing VA technology potential with applications that are perceived as safe and make a real difference in the everyday life of users. The first stream includes all papers offering theoretical and conceptual knowledge. Papers, for example, conceptualize challenges for VA user perceptions or develop security and privacy protection concepts. Systemic challenges and enabling technologies to form a second stream in VA research. This particularly includes systemic security and UX challenges, and legal issues. Efficiency presents the third research stream, in which scholars particularly investigate private people’s awareness of how VA can make their homes more efficient and asks how VA can be advertised to private households. Finally, VA applications and potential use cases form a fourth research stream. It investigates user expectations and presents prototypes for greater VA use in future home automation systems, medical care, or IOT forensics.
The overview that we gain based on the four streams enables us to frame the contributions of the research domains to VA research more clearly than based on the nine clusters. We find that all research areas contribute publications in all streams. However, the number of contributions varies: CS acts as the main driver of current developments with most publications in all research streams. CS research predominantly addresses systemic challenges, enabling technologies and technology implementation. We recognize increasing scholarly attention on user-oriented VA applications and on VA systems for novel applications beyond their originally intended usage—such as exploiting the microphone array for sensing a user’s gestures and tracking exercises (Agarwal et al., 2018 ; Tsiourti et al., 2018a / b ), or using VA data for forensics (Dorai et al., 2018 ; Shin et al., 2018 )—which indicates that the fundamental technical challenges in the development of this emergent technology are solved. SS so far mainly contributed to the theoretical foundation of VA design principles and use affordance (Yusri et al., 2017 ), and with the theory that supports developing concrete applications. It also conceptualizes the potential or desirable impact of VA in real-life settings, such as increasing the comfort and quality of life through low-cost smart home automation systems combining VA and smartphones (Kodali et al., 2019 ), or VA adding to content creation (Martin, 2017 ). The contributions by BMS scholars are mainly aimed at researching and promoting efficiency increases from using VAs.
Discussion: Propositions and a framework for future research, and related business opportunities
In this paper, we used a systematic literature review approach combining a bibliometric and qualitative content analysis to structure the dispersed insights from scholarly research on VAs in CS, SS, and BMS, and to conceptualize linkages and common themes between them. We identified four major research streams and specified the contributions of researchers from the different disciplines to them in a conceptual overview. Our research allows us to confirm advances in the technological foundations of VAs (Pyae and Joelsson, 2018 ; Lee et al., 2019 ; McLean and Osei-Frimpong, 2019 ), and some concrete VAs like Alexa, Google, and Siri have already arrived in the mass market. Still, more technologically robust and user-friendly solutions that meet their legal requirements for data security will be needed to spark broader user interest (Kuruvilla, 2019 ; Pridmore and Mols, 2020 ).
Propositions for future research
We find that recent research from the three domains contributes to the challenges that literature identified as hindering a broader user adaption of VA in different ways, and with different foci. Table 6 summarizes the identified challenges and domain-specific research contributions.
However, to advance VA’s adoption in private households. more complex VA solutions will need to convince users that the perceived privacy risks are solved (Kowalczuk, 2018 ; Lackes et al., 2019 ). To this end, all three research domains will need to contribute: CS is required to come up with defining comprehensible frameworks for data collection and processing (Bytes et al., 2019 ), and solutions to ensure data safety (Mirzamohammadi et al., 2017 ; Sudharsan et al., 2019 ; Javed and Rajabi, 2020 ). Complementary, SS should identify the social and legal conditions which users perceive as safe environments for VA use in private households (Pfeifle, 2018 ; Dunin-Underwood, 2020 ). Finally, BMS is urged to identify user advantages that go beyond simple efficiency gains, investigate the benefits of accessing one’s own data and find metrics for user trust in technology applications (Lackes et al., 2019 ). Particularly, SS research is providing potentially valuable insights into users’ perceptions and use case areas such as home medical care or assisted living that would be worth to be taken into account by CS scholars developing advanced solutions, and vice versa benefit from taking available technical solutions into consideration. Similarly, BMS scholarly research exhibits a rather narrow focus on increasing the efficiency of activities by using VA applications, and on how to market these solutions to private households. CS scholars complement this focus with technical solutions aimed at increasing the efficiency of automated home systems, but the research efforts from the two domains are not well aligned. VA security-related issues and solutions, limitations of VA applications for assisted living, and effects of humanization and anthropomorphism seem to be under-investigated topics in BMS.
Thus, our first proposition reads as follows:
Proposition 1 : To advance users' adoption of complex VA applications in private households, domain-specific disciplinary efforts of CS, SS, and BMS need to be integrated by interdisciplinary research .
Our study has shown that this is particularly important to arrive at the necessary insights into how to overcome VA security issues and VA technological development constraints CS works on and, at the same time, deal with the effects of VA humanization (SS research) and develop VA-related business opportunities (BMS research) in smart home systems, assisted living, medical home therapy, and digital forensic. Therefore, we define the following three sub-propositions:
Proposition 1.1 : In order to realize VA potential for medical care solutions that are perceived as safe by users, research insights from studies on VA perception and on perceived security issues from SS need to be integrated with CS research aimed at resolving the technical constraints of VA applications and with BMS research about the development of use cases desirable for private households and related business models .
Proposition 1.2 : To advance smart home system efficiency and arrive at regulations that make users perceive the usage of more complex applications as safe , research insights from studies on systemic integration, and security-related technical solutions from CS need to be studied and developed .
Proposition 1.3 : In order to increase our knowledge of social and economic conditions for VA adoption in private households, BMS and SS research needs to integrate insights from research with users with VA prototypes and research about near-future scenarios of VA use to model and test valid business cases that are not based on mere assumptions of efficiency gains .
In our four streams, we moreover recognize a common interest in studying VAs beyond isolated voice-enabled ‘butlers’. In essence, VAs are increasingly investigated as gateways to smart home systems which are enabling interaction with entire ecosystems. This calls, next to the development of more complex technical applications in CS, mainly for more future research into the social (SS) and economical (BMS) conditions enabling the emergence of such ecosystems—from the necessary changes in regulations to insurance and real estate issues to designing marketing strategies for VA health applications in the home (Olson and Kemery, 2019 ; Bhat, 2005 ; Melkers and Xiao, 2010 ; Sestino et al., 2020 ). The above is not only true for the three scientific domains which we looked at, but also calls for the integration of complementary VA-related research in adjacent disciplines, such as law, policy, or real estate. Our second proposition thus reads as follows:
Proposition 2: To advance users’ adoption of complex VA applications in private households, research needs to perform interdisciplinary efforts to study and develop ways to overcome ecosystem-related technology adoption challenges .
Conceptual framework for future research
As outlined above, future research wishing to contribute to increasing user acceptance and awareness and to generate use cases that make sense for private households in everyday life is urged to make interdisciplinary efforts to integrate complementary findings.
The conceptual framework (Fig. 6 ) presents avenues for future research. The figure highlights Propositions 1 and 2 that emphasize the need to advance user adaptation through interdisciplinary research that can help overcome challenges from complex VA applications (Proposition 1) and ecosystem-related technology adoption challenges (Proposition 2), to advance users’ adoption of complex VA applications. Furthermore, the figure reflects three sub-propositions that summarize relevant avenues for interdisciplinary work that can help solve VA-related security issues, generate security and privacy protection concepts, and advance frameworks for legal regulations. The first sub-propositions is research that helps find solutions for home medical care where VA limitations and security issues are solved. Sub-proposition 2 consists of research needed to advance systemic integration and security-related solutions for efficiency and the regulation of smart home systems. The third sub-proposition involves research that can help define social and economic conditions for VA and create business opportunities by including insights from user research with VA prototypes and from research with near-future scenarios that can model and test valid business cases that are not based on mere assumptions of efficiency gains.
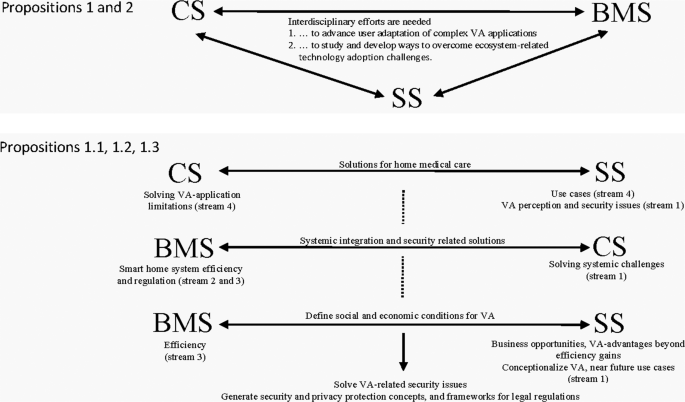
The framework highlights the focus of propositions 1 and 2 and reflecting propositions 1.1, 1,2, and 1.3.
Identified business opportunities that will help realize VA potential
Overall, we confirm that VA is not a technology that enables companies to profit from implementing it in their own organizations or make business processes more efficient like other technological innovations (Bhat, 2005 ; Chao et al., 2007 ; Sestino et al., 2020 ). Instead, we find that companies need to build business models around VA-related products and services that users perceive as safe and beneficial. Table 7 below provides an overview of potential areas providing such business opportunities, the technology maturity of these areas, and social and business-related challenges, which need to be solved to fully access VA potential for the everyday life of users.
As shown, the three areas where we identified business opportunities from literature, i.e. smart home systems (Freed et al., 2016 ; Thapliyal et al., 2018 ; Jabbar et al., 2019 ), assisted living and medical home therapy (König et al., 2016 ; Tsiourti et al., 2018a / b ; Sanders and Martin-Hammond, 2019 ), and digital forensics (Shin et al., 2018 ; Yildirim et al., 2019 ) exhibit different technology, social system conditions, and business model maturity models. It is relevant to say that, although in our review, cluster 8 ‘digital forensics’ consisted of only two papers, we can expect this to be an increasingly salient cluster in the next few years due to the importance of the topic for governmental bodies and society.
Designing appropriate business models will require companies, in the first step, to develop a deep understanding of the potential design of future ecosystems, i.e. of “the evolving set of actors, activities, and artifacts, including complementary and substitute relations, that are important for the innovative performance of an actor or a population of actors.” (Granstrand and Holgersson, 2020 , p. 3). We here call for interdisciplinary research that develops and integrates the necessary insights in a thorough and, for companies, comprehensible manner.
Methodology
In this paper, we used a relatively new approach to a literature review: We combined an automated bibliometric analysis with qualitative content analysis to gain holistic insights into a multi-faceted research topic and to structure the available body of knowledge across three scientific domains. In doing so, we followed the advice in recent research that found the classical, purely content-based literature reviews to be time-consuming, lacking rigor, and prone to be affected by the researchers’ biases (Caputo et al., 2018 ; Verma and Gustafsson, 2020 ). Overall, we can confirm that automating literature research through VOSviewer turned out to be a time-saver regarding the actual search across (partly domain-specific) sources and the collection of scientific literature, and it allowed us to relatively quickly identify meaningful research clusters based on keywords in an enormous body of data (Verma, 2017 ; Van Eck and Waltman, 2014 ). However, we also found that several additional steps were necessary to assuring the quality of the review: Despite the careful selection of keywords, the initial literature list contained several irrelevant articles (i.e., not addressing VA-related topics, yet involving the keywords ‘echo’ and ‘home’).
Thus, manual cleaning of the literature lists was required before meaningful graphs could be generated by VOSviewer. The consequent step of identifying research clusters from the graphs demanded broad topical expertise. We found this identification of clusters to be—as described by Krippendorff ( 2013 )—a necessarily iterative process, not only to continuously refine meaningful clusters but also to reach a common understanding and interpretation in an interdisciplinary team. In a similar vein, deriving higher-level categories, i.e. the research streams, turned out to require iterative refinements.
Retrospectively, the quantitative bibliometric analysis helped in recognizing both core topics and gaps in VA-related research with comprehensive reach. The complementary content analysis yielded insights into intersections and overlaps in research by the different areas considered and enabled the identification of further promising avenues for interdisciplinary research.
Conclusions
From our study, we conclude that research into VA-based services is not taking advantage of the potential synergies across disciplines. Business opportunities can specifically be found in spaces that require the combination of research domains that are still disconnected. This should be taken into account when looking for information that can help predict the service value of smart accommodation (Papagiannidis and Davlembayeva, 2022 ) or characteristics of future technology use cases that can fit users’ needs (Nguyen et al., 2022 ). This can also support scholars and managers in strategizing about future business opportunities (Brem et al., 2019 ; Antonopoulou and Begkos, 2020 ).
In consequence, our framework and the propositions we developed highlight the fact that more interdisciplinary research is needed and what type of research is needed to advance the development and application of VA in private households and, by implication, inform companies about future business opportunities.
The study also provides concrete future characteristics of VA use cases technology: Constant development in research on VAs, e.g., on novel devices and complementary technology like artificial intelligence and virtual reality, suggests that future VAs will no longer be limited to audio-only devices, but increasingly feature screens and built-in cameras, and offer more advanced use cases. Accordingly, embodied VAs in the form of for example social robots, require further technology advancement and integration, and studies on user perception.
Implications for managers
Our research enabled us to identify and describe the most promising areas for business opportunities while highlighting related technological, social, and business challenges. From this, it became obvious that managers need to take all three dimensions and related types of challenges into account in order to successfully predict characteristics of future technology use cases that fit users’ needs, and use this information for their strategy development processes (Brem et al., 2019 ; Antonopouloua and Begkos, 2020). This requires not just the design of new services and business models, but of complete business ecosystems, and the establishment of partnerships from the private sector. We moreover found that establishing trust in the safe and transparent treatment of privacy and data is key in getting users to buy and use services involving VA, while pure efficiency-based arguments are not enough to dispel current worries of potential users, like the data security of technology used to improve the tracking and monitoring of patients or viruses (Abdel-Basset et al., 2021 ).
Although our study investigated VAs in private households, with the growing acceptance of working from home, not the least due to the experiences made in the COVID-19 pandemic, our findings also have implications for organizing homework environments. While, for example, the Alexa “daily check” and Apple health check app can provide a community-based AI technology that can support self-testing and virus tracking efforts (Abdel-Basset et al., 2021 ), managers will need to ensure that company data is safe, and this will require them to consider how their employees use VA hardware at home.
Limitations
As with most research, this study has its limitations. While we see value in the combined approach taken in this research, as it allows insights around strategies for VA solutions that match the needs of private households, limitations can be seen in the qualitative approach of our methodology, which is subject to a certain degree of author subjectivity. Limitations of our work also relate to the fact that we included only articles from the Scopus database in this review. Thus, future research should consider articles published in other databases like EBSCO, Web of Science, or Google Scholar. Also, the study focused on only three scientific domains up to May 2020. This review paper does not offer a discussion of the consequences of the ongoing changes triggered by the Covid-19 pandemic for the use of VA solutions in private households. The impact of this disruptive pandemic experience on the use of VA is not yet well understood. More research will be necessary to obtain a complete account of how Covid-19 transformed the use of VA in private homes today and to help understand the linkages and intersections between further research areas using the same methodology.
The combined bibliometric and qualitative content analysis provided an overview of connections and intersections, and an in-depth overview of current research streams. Future research could conduct co-citation and/or bibliographic coupling analyses of authors, institutions, countries, references, etc. to complement our research.
Data availability
Datasets were derived from public resources. Data sources for this article are provided in the Methods section of this article. Data analysis documents are not publicly available as researchers have moved on to other institutions.
Abdel-Basset M, Chang V, Nabeeh NA (2021) An intelligent framework using disruptive technologies for COVID-19 analysis. Technol Forecast Soc Change 163:120431. https://doi.org/10.1016/j.techfore.2020.120431
Agarwal A, Jain M, Kumar P, Patel S (2018) Opportunistic sensing with MIC arrays on smart speakers for distal interaction and exercise tracking. In: IEEE Press (ed), 2018 IEEE International Conference on Acoustics, Speech and Signal Processing (ICASSP). pp. 6403–6407
Alrumayh AS, Lehman SM, Tan CC (2019) ABACUS: audio based access control utility for smarthomes. In: Association for Computing Machinery (ACM) (ed), Proceedings of the 4th ACM/IEEE Symposium on Edge Computing. pp. 395–400
Amit S, Koshy AS, Samprita S, Joshi S, Ranjitha N (2019) Internet of Things (IoT) enabled sustainable home automation along with security using solar energy. In: Institute of Electrical and Electronics Engineers (IEEE) (ed), 2019 International Conference on Communication and Electronics Systems (ICCES). pp. 1026–1029
Ammari T, Kaye J, Tsai J, Bentley F (2019) Music, search, and IoT: how people (really) use voice assistants. ACM Trans Comput–Hum Interact 26:1–28. https://doi.org/10.1145/3311956
Article Google Scholar
Antonopoulou K, Begkos C (2020) Strategizing for digital innovations: value propositions for transcending market boundaries. Technol Forecast Soc Change 156:120042
Aylett MP, Cowan BR, Clark L (2019) Siri, echo and performance: you have to suffer darling. In: Association for Computing Machinery (ACM) (ed), Extended Abstracts of the 2019 CHI Conference on Human Factors in Computing Systems. pp. 1–10
Azmandian M, Arroyo-Palacios J, Osman S (2019) Guiding the behavior design of virtual assistants. In: Association for Computing Machinery (ACM) (ed), Proceedings of the 19th ACM international conference on intelligent virtual agents. pp. 16–18
Batat W (2021) How augmented reality (AR) is transforming the restaurant sector: Investigating the impact of “Le Petit Chef” on customers’ dining experiences. Technol Forecast Soc Change 172:121013
Bhat JSA (2005) Concerns of new technology based industries—the case of nanotechnology. Technovation 25(5):457–462. https://doi.org/10.1016/j.technovation.2003.09.001
Berg Insight (2022) The number of smart homes in Europe and North America reached 105 million in 2021, Press Releases, 20 April 2022. https://www.berginsight.com/the-number-of-smart-homes-in-europe-and-north-america-reached-105-million-in-2021
Birgonul Z, Carrasco O (2021) The adoption of multidimensional exploration methodology to the design-driven innovation and production practices in AEC industry. J Constr Eng Manag Innov 4(2):92–10. https://doi.org/10.31462/jcemi.2021.02092105
Brandt M (2018) Wenig echo in Deutschland. Statista
Brasser F, Frassetto T, Riedhammer K, Sadeghi A-R, Schneider T, Weinert C (2018) VoiceGuard: secure and private speech processing. In: International Speech Communication Association (ISCA) (ed), Proceedings of the annual conference of the International Speech Communication Association, INTERSPEECH. pp. 1303–1307
Brause SR, Blank G (2020) Externalized domestication: smart speaker assistants, networks and domestication theory. Inf Commun Soc 23(5):751–763. https://doi.org/10.1080/1369118X.2020.1713845
Brem A, Bilgram V, Marchuk A (2019) How crowdfunding platforms change the nature of user innovation–from problem solving to entrepreneurship. Technol Forecast Soc Change 144:348–360
Brenner W, Giffen BV, Koehler J (2021) Management of artificial intelligence: feasibility, desirability and viability. In: Aier S et al. (eds), Engineering the transformation of the enterprise. pp. 15–36
Burns MB, Igou A (2019) “Alexa, write an audit opinion”: adopting intelligent virtual assistants in accounting workplaces. J Emerg Technol Account 16(1):81–92. https://doi.org/10.2308/jeta-52424
Bytes A, Adepu S, Zhou J (2019) Towards semantic sensitive feature profiling of IoT devices. IEEE Internet Things J 6(5):8056–8064. https://doi.org/10.1109/JIOT.2019.2903739
Calaça J, Nóbrega L, Baras K (2019) Smartly water: Interaction with a smart water network. In: Institute of Electrical and Electronics Engineers (IEEE) (ed), Proceedings of the 2019 5th Experiment International Conference (Exp. at’19). pp. 233–234
Callahan JL (2014) Writing literature reviews: a reprise and update. Hum Resour Dev Rev 13(3):271–275. https://doi.org/10.1177/1534484314536705
Caputo A, Ayoko OB, Amoo N (2018) The moderating role of cultural intelligence in the relationship between cultural orientations and conflict management styles. J Bus Res 89:10–20. https://doi.org/10.1016/j.jbusres.2018.03.042
Carayannis EG, Turner E (2006) Innovation diffusion and technology acceptance: the case of PKI technology. Technovation 26(7):847–855. https://doi.org/10.1016/j.technovation.2005.06.013
Celebre AMD, Dubouzet AZD, Medina IBA, Surposa ANM, Gustilo RC (2015) Home automation using raspberry Pi through Siri enabled mobile devices. In: Institute of Electrical and Electronics Engineers (IEEE) (ed), 2015 International Conference on Humanoid, Nanotechnology, Information Technology, Communication and Control, Environment and Management (HNICEM). pp. 1–6
Chan ZY, Shum P (2018) Smart office: a voice-controlled workplace for everyone. In: Association for Computing Machinery (ACM) (ed), Proceedings of the 2nd international symposium on computer science and intelligent control. pp. 1–5
Chao C-C, Yang J-M, Jen W-Y (2007) Determining technology trends and forecasts of RFID by a historical review and bibliometric analysis from 1991 to 2005. Technovation 27(5):268–279. https://doi.org/10.1016/j.technovation.2006.09.003
Clark M, Newman MW, Dutta P (2022) ARticulate: one-shot interactions with intelligent assistants in unfamiliar smart spaces using augmented reality. Proc ACM Interact Mob Wearable Ubiquitous Technol 6(1):1–24
Clemente C, Greco E, Sciarretta E, Altieri L (2022) Alexa, how do i feel today? Smart speakers for healthcare and wellbeing: an analysis about uses and challenges. Sociol Soc Work Rev 6(1):6–24
Google Scholar
Columbus, L (2020) What’s new in Gartner’s hype cycle for emerging technologies, 2020. Forbes. https://www.forbes.com/sites/louiscolumbus/2020/08/23/whats-new-in-gartners-hype-cycle-for-emerging-technologies-2020/?sh=6363286fa46a
Demidova E (2018) Can children teach AI? Towards expressive human–AI dialogs. In: Vrandečić D, Bontcheva K, Suárez-Figueroa MC, Presutti V, Celino I, Sabou M, Kaffee L-A, Simperl E (eds), International Semantic Web Conference Proceedings (P&D/Industry/BlueSky). p. 2180
Denzin NK (1989) Interpretive biography, vol. 17. SAGE
Dercole F, Dieckmann U, Obersteiner M, Rinaldi S (2008) Adaptive dynamics and technological change. Technovation 28(6):335–348. https://doi.org/10.1016/j.technovation.2007.11.004
Deshpande NG, Itole DA (2019) Personal assistant based home automation using Raspberry Pi. Int J Recent Technol Eng
Donaldson J, Evnin J, Saxena S (2005) ECHOES: encouraging companionship, home organization, and entertainment in seniors. In: Association for Computing Machinery (ACM) (ed), Proceedings of the CHI’05 extended abstracts on human factors in computing systems. pp. 2084–2088
Dong XL (2019) Building a broad knowledge graph for products. In: Institute of Electrical and Electronics Engineers (IEEE) (ed), Proceedings of the 2019 IEEE 35th International Conference on Data Engineering (ICDE), 2019-April. pp. 25–25
Dorai G, Houshmand S, Baggili I (2018) I know what you did last summer: Your smart home internet of things and your iPhone forensically ratting you out. In: Association for Computing Machinery (ACM) (ed), Proceedings of the 13th international conference on availability, reliability and security. Article 3232814
Dörner R (2017) Smart assistive user interfaces in private living environments. In: Gesellschaft für Informatik e.V. (GI) (ed), Lecture notes in informatics (LNI), proceedings—series of the gesellschaft fur informatik (GI). pp. 923–930
Drucker PF (1988) The coming of the new organization. Reprint Harvard Business Review, 88105. https://ams-forschungsnetzwerk.at/downloadpub/the_coming-of_the_new_organization.pdf . Accessed 10 Jul 2022
Druga S, Williams R, Breazeal C, Resnick M (2017) “Hey Google is it OK if I eat you?” Initial explorations in child-agent interaction. In: Blikstein P, Abrahamson D (eds), Proceedings of the 2017 conference on Interaction Design and Children (IDC ’17). pp. 595–600
Dunin-Underwood A (2020) Alexa, can you keep a secret? Applicability of the third-party doctrine to information collected in the home by virtual assistants. Inf Commun Technol Law 29(1):101–119. https://doi.org/10.1080/13600834.2020.1676956
Elahi H, Wang G, Peng T, Chen J (2019) On transparency and accountability of smart assistants in smart cities. Appl Sci 9(24):5344. https://doi.org/10.3390/app9245344
Fathalizadeh A, Moghtadaiee V, Alishahi M (2022) On the privacy protection of indoor location dataset using anonymization. Comput Secur 117:102665
Flick U (2009) An introduction to qualitative research, 4th edn. SAGE
Freed M, Burns B, Heller A, Sanchez D, Beaumont-Bowman S (2016) A virtual assistant to help dysphagia patients eat safely at home. IJCAI 2016:4244–4245
Fruchter N, Liccardi I (2018) Consumer attitudes towards privacy and security in home assistants. In: Association for Computing Machinery (ACM) (ed), Extended Abstracts of the 2018 CHI conference on human factors in computing systems, 2018-April. pp. 1–6
Furey E, Blue J (2018) She knows too much—voice command devices and privacy. In: Institute of Electrical and Electronics Engineers (IEEE) (ed), Proceedings of 2018 29th Irish Signals and Systems Conference (ISSC). pp. 1–6
Gartner (2019) Gartner predicts 25 percent of digital workers will use virtual employee assistants daily by 2021. Gartner https://www.gartner.com/en/newsroom/press-releases/2019-01-09-gartner-predicts-25-percent-of-digital-workers-will-u
Giorgi R, Bettin N, Ermini S, Montefoschi F, Rizzo A (2019) An iris+voice recognition system for a smart doorbell. In: Institute of Electrical and Electronics Engineers (IEEE) (ed), 2019 8th Mediterranean Conference on Embedded Computing (MECO). pp. 1–4
Gnewuch U, Morana S, Heckmann C, Maedche A (2018) Designing conversational agents for energy feedback. In: Chatterjee S, Dutta K, Sundarraj RP (eds), Proceedings of the International conference on design science research in information systems and technology, vol 10844. pp. 18–33
Gong Y, Yatawatte H, Poellabauer C, Schneider S, Latham S (2018) Automatic autism spectrum disorder detection using everyday vocalization captured by smart devices. In: Association for Computing Machinery (ACM) (ed), Proceedings of the 2018 ACM international conference on bioinformatics, computational biology, and health informatics. pp. 465–473
Goud N, Sivakami A (2019) Spectate home appliances by internet of things using MQTT and IFTTT through Google Assistant. Int J Sci Technol Res 8(10):1852–1857
Granstrand O, Holgersson M (2020) Innovation ecosystems: a conceptual review and a new definition. Technovation 90:102098
Grossman GM, Helpman E (1991) Innovation and growth in the global economy. MIT Press
Grossman-Kahn B, Rosensweig R (2012) Skip the silver bullet: driving innovation through small bets and diverse practices. Lead Through Design 18:815
Hamill L (2006) Controlling smart devices in the home. Inf Soc 22(4):241–249. https://doi.org/10.1080/01972240600791382
Han J, Chung AJ, Sinha MK, Harishankar M, Pan S, Noh HY, Zhang P, Tague P (2018) Do you feel what I hear? Enabling autonomous IoT device pairing using different sensor types. In: Institute of Electrical and Electronics Engineers (IEEE) (ed), 2018 IEEE symposium on Security and Privacy (SP). pp. 836–852
Harzing A-W, Alakangas S (2016) Google Scholar, Scopus and the Web of Science: a longitudinal and cross-disciplinary comparison. Scientometrics 106(2):787–804. https://doi.org/10.1007/s11192-015-1798-9
Hashemi SH, Williams K, El Kholy A, Zitouni I, Crook PA (2018) Measuring user satisfaction on smart speaker intelligent assistants using intent sensitive query embeddings. In: Association for Computing Machinery (ACM) (ed), Proceedings of the 27th ACM international conference on information and knowledge management. pp. 1183–1192
Hern A (2017) Google Home smart speaker brings battle of living rooms to UK. The Guardian. https://www.theguardian.com/technology/2017/mar/28/google-home-smart-speaker-launch-uk
Herring H, Roy R (2007) Technological innovation, energy efficient design and the rebound effect. Technovation 27(4):194–203. https://doi.org/10.1016/j.technovation.2006.11.004
Hofmann C, Orr S (2005) Advanced manufacturing technology adoption—the German experience. Technovation 25(7):711–724. https://doi.org/10.1016/j.technovation.2003.12.002
Hoy MB (2018) Alexa, Siri, Cortana, and more: an introduction to voice assistants. Med Ref Serv Q 37(1):81–88. https://doi.org/10.1080/02763869.2018.1404391
Article PubMed Google Scholar
Hu J, Tu X, Zhu G, Li Y, Zhou Z (2013) Coupling suppression in human target detection via impulse through wall radar. In: Institute of Electrical and Electronics Engineers (IEEE) (ed), Proceedings of the 2013 14th International Radar Symposium (IRS), vol 2. pp. 1008–1012
Huxohl T, Pohling M, Carlmeyer B, Wrede B, Hermann T (2019) Interaction guidelines for personal voice assistants in smart homes. In: Institute of Electrical and Electronics Engineers (IEEE) (ed), 2019 international conference on Speech Technology and Human–Computer Dialogue (SpeD). pp. 1–10
IDEO.org (2009) Human-centred design toolkit. IDEO.org
Ichikawa J, Mitsukuni K, Hori Y, Ikeno Y, Alexandre L, Kawamoto T, Nishizaki Y, Oka N (2019) Analysis of how personality traits affect children’s conversational play with an utterance-output device. In: Institute of Electrical and Electronics Engineers (IEEE) (ed), 2019 Joint IEEE 9th International Conference on Development and Learning and Epigenetic Robotics (ICDL-EpiRob). pp. 215–220
Ilievski A, Dojchinovski D, Ackovska N, Kirandziska V (2018) The application of an air pollution measuring system built for home living. In: Kalajdziski S, Ackovska N (eds) ICT innovations 2018. Engineering and life sciences. Springer, pp. 75–89
Ito A (2019) Muting machine speech using audio watermarking. In: Pan J-S, Ito A, Tsai P-W, Jain LC (eds) Recent advances in intelligent information hiding and multimedia signal processing. Springer, pp. 74–81
Jabbar WA, Kian TK, Ramli RM, Zubir SN, Zamrizaman NS, Balfaqih M, Shepelev V, Alharbi S (2019) Design and fabrication of smart home with internet of things enabled automation system. IEEE Access 7:144059–144074. https://doi.org/10.1109/ACCESS.2019.2942846
Jacques R, Følstad A, Gerber E, Grudin J, Luger E, Monroy-Hernández A, Wang D (2019) Conversational agents: acting on the wave of research and development. In: Association for Computing Machinery (ACM) (ed), Extended Abstracts of the 2019 CHI conference on human factors in computing systems. pp. 1–8
Javed Y, Rajabi N (2020) Multi-Layer perceptron artificial neural network based IoT botnet traffic classification. In: Arai K, Bhatia R, Kapoor S (eds) Proceedings of the Future Technologies Conference (FTC) 2019. Springer, pp. 973–984
Jones VK (2018) Voice-activated change: marketing in the age of artificial intelligence and virtual assistants. J Brand Strategy 7(3):233–245
Kandlhofer M, Steinbauer G, Hirschmugl-Gaisch S, Huber P (2016) Artificial intelligence and computer science in education: from kindergarten to university. In: Institute of Electrical and Electronics Engineers (IEEE) (ed), 2016 IEEE Frontiers in Education Conference (FIE). pp. 1–9
Kerekešová V, Babič F, Gašpar V (2019) Using the virtual assistant Alexa as a communication channel for road traffic situation. In: Choroś K, Kopel M, Kukla E, Siemiński A (eds) Multimedia and network information systems, vol 833. Springer, pp. 35–44
Khattar S, Sachdeva A, Kumar R, Gupta R (2019) Smart home with virtual assistant using Raspberry Pi. In: Institute of Electrical and Electronics Engineers (IEEE) (ed), 2019 9th International conference on cloud computing, data science & engineering (Confluence). pp. 576–579
King B, Chen I-F, Vaizman Y, Liu Y, Maas R, Parthasarathi SHK, Hoffmeister B (2017) Robust speech recognition via anchor word representations. In: International Speech Communication Association (ISCA) (ed), Proceedings of the Interspeech 2017. pp. 2471–2475
Kita T, Nagaoka C, Hiraoka N, Dougiamas M (2019) Implementation of voice user interfaces to enhance users’ activities on Moodle. In: Institute of Electrical and Electronics Engineers (IEEE) (ed), Proceedings of 2019 4th international conference on information technology. pp. 104–107
Kodali RK, Rajanarayanan SC, Boppana L, Sharma S, Kumar A (2019) Low cost smart home automation system using smart phone. In: Institute of Electrical and Electronics Engineers (IEEE) (ed), 2019 IEEE R10 Humanitarian Technology Conference (R10-HTC)(47129). pp. 120–125
Komatsu S, Sasayama M (2019) Speech error detection depending on linguistic units. In: Association for Computing Machinery (ACM) (ed), Proceedings of the 2019 3rd international conference on natural language processing and information retrieval. pp. 75–79
König A, Francis LE, Malhotra A, Hoey J (2016) Defining Affective Identities in elderly nursing home residents for the design of an emotionally intelligent cognitive assistant. In: Favela J, Matic A, Fitzpatrick G, Weibel N, Hoey J (eds) Proceedings of the 10th EAI International Conference on Pervasive Computing Technologies for Healthcare. ICST, pp. 206–210
Kortum SS (1997) Research, patenting, and technological change. Econometrica 1389–1419. https://doi.org/10.2307/2171741
Kowalczuk P (2018) Consumer acceptance of smart speakers: a mixed methods approach. J Res Interact Mark 12(4):418–431. https://doi.org/10.1108/JRIM-01-2018-0022
Kowalski J, Jaskulska A, Skorupska K, Abramczuk K, Biele C, Kopeć W, Marasek K (2019) Older adults and voice interaction: a pilot study with Google Home. In: Extended abstracts of the 2019 CHI conference on human factors in computing systems. pp. 1–6
Krippendorff K (2013) Content analysis: an introduction to its methodology. SAGE
Krotov V (2017) The Internet of Things and new business opportunities. Gener Potential Emerg Technol 60(6):831–841. https://doi.org/10.1016/j.bushor.2017.07.009
Kumar A (2018) AlexaPi3—an economical smart speaker. In: Institute of Electrical and Electronics Engineers (IEEE) (ed), 2018 IEEE Punecon. pp. 1–4
Kumar N, Lee SC (2022) Human–machine interface in smart factory: a systematic literature review. Technol Forecast Soc Change 174:121284
Kunath G, Hofstetter R, Jörg D, Demarchi D (2019) Voice first barometer Schweiz 2018. Universität Luzern, pp. 1–25
Kuruvilla R (2019) Between you, me, and Alexa: on the legality of virtual assistant devices in two-party consent states. Wash Law Rev 94(4):2029–2055
Lackes R, Siepermann M, Vetter G (2019). Can I help you?—the acceptance of intelligent personal assistants. In: Pańkowska M, Sandkuhl K (eds) Perspectives in business informatics research. Springer, pp. 204–218
Lau J, Zimmerman B, Schaub F (2018) Alexa, are you listening?: Privacy perceptions, concerns and privacy-seeking behaviors with smart speakers. Proc ACM Hum–Comput Interact 2:1–31. https://doi.org/10.1145/3274371 . (CSCW)
Leahey E, Beckman CM, Stanko TL (2017) Prominent but less productive: The impact of interdisciplinarity on scientists’ research. Adm Sci Q 62(1):105–139. https://doi.org/10.1177/0001839216665364
Lee I, Kinney CE, Lee B, Kalker AA (2009) Solving the acoustic echo cancellation problem in double-talk scenario using non-gaussianity of the near-end signal. In: Association for Computing Machinery (ACM) (ed), International conference on independent component analysis and signal separation. pp. 589–596
Lee S, Kim S, Lee S (2019) “What does your agent look like?” A drawing study to understand users’ perceived persona of conversational agent. In: Association for Computing Machinery (ACM) (ed), Extended abstracts of the 2019 CHI conference on human factors in computing systems. pp. 1–6
Li S, Garces E, Daim T (2019) Technology forecasting by analogy-based on social network analysis: the case of autonomous vehicles. Technol Forecast Soc Change 148:119731. https://doi.org/10.1016/j.techfore.2019.119731
Li W, Chen Y, Hu H, Tang C (2020) Using granule to search privacy preserving voice in home IoT systems. IEEE Access 8:31957–31969. https://doi.org/10.1109/ACCESS.2020.2972975
Liciotti D, Ferroni G, Frontoni E, Squartini S, Principi E, Bonfigli R, Zingaretti P, Piazza F (2014) Advanced integration of multimedia assistive technologies: a prospective outlook. In: Institute of Electrical and Electronics Engineers (IEEE) (ed), Proceedings of the 2014 IEEE/ASME 10th international conference on Mechatronic and Embedded Systems and Applications (MESA). pp. 1–6
Liu Z, Shin J, Xu Y, Winata GI, Xu P, Madotto A, Fung P (2020) Zero-shot cross-lingual dialogue systems with transferable latent variables. ArXiv. https://arxiv.org/pdf/1911.04081.pdf
Lopatovska I, Oropeza H (2018) User interactions with “Alexa” in public academic space. Proceedings of the Association for Information Science and Technology 55(1):309–318. https://doi.org/10.1002/pra2.2018.14505501034
Lopatovska I, Rink K, Knight I, Raines K, Cosenza K, Williams H, Sorsche P, Hirsch D, Li Q, Martinez A (2019) Talk to me: exploring user interactions with the Amazon Alexa. J Librariansh Inf Sci 51(4):984–997. https://doi.org/10.1177/0961000618759414
Lovato SB, Piper AM, Wartella EA (2019) Hey Google, do unicorns exist? Conversational agents as a path to answers to children’s questions. In: Association for Computing Machinery (ACM) (ed), Proceedings of the 18th ACM international conference on interaction design and children. pp. 301–313
Miles MB, Huberman AM (1994) Qualitative data analysis. A source book of new methods, 2nd edn. Sage
Macdonald RJ, Jinliang W (1994) Time, timeliness of innovation, and the emergence of industries. Technovation 14(1):37–53. https://doi.org/10.1016/0166-4972(94)90069-8
Malik KM, Malik H, Baumann R (2019) Towards vulnerability analysis of voice-driven interfaces and countermeasures for replay attacks. In: Institute of Electrical and Electronics Engineers (IEEE) (ed), 2019 IEEE conference on Multimedia Information Processing and Retrieval (MIPR). pp. 523–528
Martin EJ (2017) How Echo, Google Home, and other voice assistants can change the game for content creators. EContent. http://www.econtentmag.com/Articles/News/News-Feature/How-Echo-Google-Home-and-Other-Voice-Assistants-Can-Change-the-Game-for-Content--Creators-116564.htm
Masutani O, Nemoto S, Hideshima Y (2019) Toward a better IPA experience for a connected vehicle by means of usage prediction. In: Institute of Electrical and Electronics Engineers (IEEE) (ed), Qualitative data analysis. A source book of new methods, 2nd edn2019 IEEE International Conference on Pervasive Computing and Communications Workshops (PerCom Workshops). pp. 681–686
Mavropoulos T, Meditskos G, Symeonidis S, Kamateri E, Rousi M, Tzimikas D, Papageorgiou L, Eleftheriadis C, Adamopoulos G, Vrochidis S, Kompatsiaris I (2019) A context-aware conversational agent in the rehabilitation domain. Futur Internet 11(11):231. https://doi.org/10.3390/fi11110231 . Article
McLean G, Osei-Frimpong K (2019) Hey Alexa… examine the variables influencing the use of artificial intelligent in-home voice assistants. Comput Hum Behav 99:28–37. https://doi.org/10.1016/j.chb.2019.05.009
McReynolds E, Hubbard S, Lau T, Saraf A, Cakmak M, Roesner F (2017) Toys that listen: a study of parents, children, and internet-connected toys. In: Association for Computing Machinery (ACM) (ed), Proceedings of the 2017 CHI conference on human factors in computing systems. pp. 5197–5207
Melkers J, Xiao F (2010) Boundary-spanning in emerging technology research: determinants of funding success for academic scientists. J Technol Transf 37(3):251–270. https://doi.org/10.1007/s10961-010-9173-8
Mirzamohammadi S, Chen JA, Sani AA, Mehrotra S, Tsudik G (2017) Ditio: trustworthy auditing of sensor activities in mobile and IoT devices. In: Association for Computing Machinery (ACM) (ed), Proceedings of the 15th ACM conference on embedded network sensor systems
Moher D, Liberati A, Tetzlaff J, Altman DG, Prisma Group (2009) Preferred reporting items for systematic reviews and meta-analyses: the PRISMA statement. PLoS Med 6(7):e1000097. https://doi.org/10.1371/journal.pmed.1000097 . Article
Article PubMed PubMed Central Google Scholar
Mokhtari M, de Marassé A, Kodys M, Aloulou H (2019) Cities for all ages: Singapore use case. In: Stephanidis C, Antona M (eds) HCI international 2019—late breaking posters. Springer, pp. 251–258
Nguyen TH, Waizenegger L, Techatassanasoontorn AA (2022) “Don’t Neglect the User!”–Identifying Types of Human-Chatbot Interactions and their Associated Characteristics. Inf Syst Front 24(3):797–838
Oh S-R, Kim Y-G (2017) Security requirements analysis for the IoT. In: Institute of Electrical and Electronics Engineers (IEEE) (ed), 2017 International conference on Platform Technology and Service (PlatCon). pp. 1–6
Olson C, Kemery K (2019) 2019 Voice report: from answers to action: Customer adoption of voice technology and digital assistants. Technical report. Microsoft
Omale G (2020) Customer service and support leaders can use this Gartner Hype Cycle to assess the maturity and risks of customer service and support technologies. Gartner. https://www.gartner.com/smarterwithgartner/5-trends-drive-the-gartner-hype-cycle-for-customer-service-and-support-technologies-2020/
Ong DT, De Jesus CR, Gilig LK, Alburo JB, Ong E (2018) A dialogue model for collaborative storytelling with children. In: Yang JC, Chang M, Wong L-H, Rodrigo MM (eds), 26th International conference on computers in education workshop on innovative technologies for enhancing interactions and learning. pp. 205–210
Palumbo F, Gallicchio C, Pucci R, Micheli A (2016) Human activity recognition using multisensor data fusion based on reservoir computing. J Ambient Intell Smart Environ 8(2):87–107. https://doi.org/10.3233/AIS-160372
Papagiannidis S, Davlembayeva D (2022) Bringing Smart Home Technology to Peer-to-Peer Accommodation: Exploring the Drivers of Intention to Stay in Smart Accommodation. Inf Syst Front 24(4):1189–1208
Parkin S, Patel T, Lopez-Neira I, Tanczer L (2019) Usability analysis of shared device ecosystem security: Informing support for survivors of IoT-facilitated tech-abuse. In: Association for Computing Machinery (ACM) (ed), Proceedings of the new security paradigms workshop. pp. 1–15
Patel D, Bhalodiya P (2019) 3D holographic and interactive artificial intelligence system. In: Institute of Electrical and Electronics Engineers (IEEE) (ed), 2019 International Conference on Smart Systems and Inventive Technology (ICSSIT). pp. 657–662
Patnaik D, Becker R (1999) Needfinding: the why and how of uncovering people’s needs. Design Manag J (Former Ser) 10(2):37–43
Petticrew M, Roberts H (2006) Systematic reviews in the social sciences: a practical guide. John Wiley & Sons
Pfeifle A (2018) Alexa, what should we do about privacy: protecting privacy for users of voice-activated devices. Wash Law Rev 93:421
Porter ME (1990) Competitive advantage of nations. Competitive Intell Rev 1(1):14
Portillo CD, Lituchy TR (2018) An examination of online repurchasing behavior in an IoT environment. In: Simmers CA, Anandarajan M (eds) The Internet of People, Things and Services: workplace tranformations. Routledge, pp. 225–241
Pradhan A, Findlater L, Lazar A (2019) “Phantom friend” or “just a box with information”: personification and ontological categorization of smart speaker-based voice assistants by older adults. In: Association for Computing Machinery (ACM) (ed), Proceedings of the ACM on Human–Computer Interaction, 3(CSCW)
Pradhan A, Mehta K, Findlater L (2018) “Accessibility came by accident”: use of voice-controlled intelligent personal assistants by people with disabilities. In: Association for Computing Machinery (ACM) (ed), Proceedings of the 2018 CHI conference on human factors in computing systems. pp. 1–13
Pridmore J, Mols A (2020) Personal choices and situated data: privacy negotiations and the acceptance of household intelligent personal assistants. Big Data Soc 7(1):205395171989174. https://doi.org/10.1177/2053951719891748 . Article
Principi E, Squartini S, Piazza F, Fuselli D, Bonifazi M (2013) A distributed system for recognizing home automation commands and distress calls in the Italian language. INTERSPEECH, pp. 2049–2053
Purao S, Meng C (2019) Data capture and analyses from conversational devices in the homes of the elderly. In: Guizzardi G, Gailly F, Suzana R, Pitangueira Maciel (eds) Lecture notes in computer science, vol 11787. Springer, pp. 157–166
Purington A, Taft JG, Sannon S, Bazarova NN, Taylor SH (2017) “Alexa is my new BFF”: social roles, user satisfaction, and personification of the Amazon Echo. In: Association for Computing Machinery (ACM) (ed), Proceedings of the 2017 CHI conference extended abstracts on human factors in computing systems. pp. 2853–2859
Pyae A, Joelsson TN (2018) Investigating the usability and user experiences of voice user interface: a case of Google home smart speaker. In: Association for Computing Machinery (ACM) (ed), Proceedings of the 20th international conference on human-computer interaction with mobile devices and services adjunct. pp. 127–131
Pyae A, Scifleet P (2019) Investigating the role of user’s English language proficiency in using a voice user interface: a case of Google Home smart speaker. In: Association for Computing Machinery (ACM) (ed), (Extended Abstracts of the 2019 CHI Conference on Human Factors in Computing Systems—CHI EA ’19. pp. 1–6
Rabassa V, Sabri O, Spaletta C (2022) Conversational commerce: do biased choices offered by voice assistants’ technology constrain its appropriation? Technol Forecast Soc Change 174:121292
Robinson S, Pearson J, Ahire S, Ahirwar R, Bhikne B, Maravi N, Jones M (2018) Revisiting “hole in the wall” computing: private smart speakers and public slum settings. In: Association for Computing Machinery (ACM) (ed), Proceedings of the 2018 CHI conference on human factors in computing systems. pp. 1–11
Robledo-Arnuncio E, Wada TS, Juang B-H (2007) On dealing with sampling rate mismatches in blind source separation and acoustic echo cancellation. In: Institute of Electrical and Electronics Engineers (IEEE) (ed), 2007 IEEE workshop on applications of signal processing to audio and acoustics. pp. 34–37
Rzepka C, Berger B, Hess T (2022) Voice assistant vs. Chatbot–examining the fit between conversational agents’ interaction modalities and information search tasks. Inf Syst Front 24(3):839–856
Saadaoui FZ, Mahmoudi C, Maizate A, Ouzzif M (2019) Conferencing-Ng protocol for Internet of Things. In: Institute of Electrical and Electronics Engineers (IEEE) (ed), 2019 Third international conference on Intelligent Computing in Data Sciences (ICDS). pp. 1–5
Samarasinghe N, Mannan M (2019a) Towards a global perspective on web tracking. Comput Secur 87:101569. https://doi.org/10.1016/j.cose.2019.101569
Samarasinghe N, Mannan M (2019b) Another look at TLS ecosystems in networked devices vs. web servers. Comput Secur 80:1–13. https://doi.org/10.1016/j.cose.2018.09.001
Sanders J, Martin-Hammond A (2019) Exploring autonomy in the design of an intelligent health assistant for older adults. In: Association for Computing Machinery (ACM) (ed), Proceedings of the 24th International conference on intelligent user interfaces: companion. pp. 95–96
Sangal S, Bathla R (2019) Implementation of restrictions in smart home devices for safety of children. In: Institute of Electrical and Electronics Engineers (IEEE) (ed), 2019 4th International Conference on Information Systems and Computer Networks (ISCON). pp. 139–143
Santhanaraj K, Barkathunissa A (2020) A study on the factors affecting usage of voice assistants and the interface transition from touch to voice. Int J Adv Sci Technol 29(5):3084–3102
Santos-Pérez M, González-Parada E, Cano-García JM (2011) AVATAR: an open source architecture for embodied conversational agents in smart environments. In: Bravo J, Hervás R, Villarreal V (eds) Ambient Assisted living. Springer, pp. 109–115
Sestino A, Prete MI, Piper L, Guido G (2020) Internet of Things and Big Data as enablers for business digitalization strategies. Technovation 98:102173. https://doi.org/10.1016/j.technovation.2020.102173 . Article
Article PubMed Central Google Scholar
Seymour W (2018) How loyal is your Alexa? Imagining a respectful smart assistant. In: Association for Computing Machinery (ACM) (ed), Extended abstracts of the 2018 CHI conference on human factors in computing systems. pp. 1–6
Shamekhi A, Bickmore T, Lestoquoy A, Gardiner P (2017) Augmenting group medical visits with conversational agents for stress management behavior change. In: de Vries PW, Oinas-Kukkonen H, Siemons L, Beerlage-de Jong N, van Gemert-Pijnen L (eds) Persuasive technology: development and implementation of personalized technologies to change attitudes and behaviors. Springer, pp. 55–67
Shank DB, Wright D, Nasrin S, White M (2022) Discontinuance and restricted acceptance to reduce worry after unwanted incidents with smart home technology. Int J Hum–Comput Interact 1–14. https://doi.org/10.1080/10447318.2022.2085406
Shin C, Chandok P, Liu R, Nielson SJ, Leschke TR (2018) Potential forensic analysis of IoT data: an overview of the state-of-the-art and future possibilities. In: Institute of Electrical and Electronics Engineers (IEEE) (ed), 2017 IEEE International Conference on Internet of Things (IThings) and IEEE Green Computing and Communications (GreenCom) and IEEE Cyber, Physical and Social Computing (CPSCom) and IEEE Smart Data (SmartData). pp. 705–710
Singh V, Verma S, Chaurasia SS (2020) Mapping the themes and intellectual structure of corporate university: co-citation and cluster analyses. Scientometrics 122(3):1275–1302. https://doi.org/10.1007/s11192-019-03328-0
Solorio JA, Garcia-Bravo JM, Newell BA (2018) Voice activated semi-autonomous vehicle using off the shelf home automation hardware. IEEE Internet Things J 5(6):5046–5054. https://doi.org/10.1109/JIOT.2018.2854591
Souden M, Liu Z (2009) Optimal joint linear acoustic echo cancelation and blind source separation in the presence of loudspeaker nonlinearity. In: Institute of Electrical and Electronics Engineers (IEEE) (ed), 2009 IEEE international conference on multimedia and expo. pp. 117–120
Srikanth S, Saddamhussain SK, Siva Prasad P (2019) Home anti-theft powered by Alexa. In: Institute of Electrical and Electronics Engineers (IEEE) (ed), 2019 International conference on Vision Towards Emerging Trends in Communication and Networking (ViTECoN). pp. 1–6
Stefanidi Z, Leonidis A, Antona M (2019) A multi-stage approach to facilitate interaction with intelligent environments via natural language. In: Stephanidis C, Antona M (eds) HCI International 2019—Late Breaking Posters, vol 1088. Springer, pp. 67–77
Struckell E, Ojha D, Patel PC, Dhir A (2021) Ecological determinants of smart home ecosystems: A coopetition framework. Technol Forecast Soc Change 173:121147. https://doi.org/10.1016/j.techfore.2021.121147
Sudharsan B, Corcoran P, Ali MI (2019) Smart speaker design and implementation with biometric authentication and advanced voice interaction capability. In: Curry E, Keane M, Ojo A, Salwala D (eds), Proceedings for the 27th AIAI Irish Conference on Artificial Intelligence and Cognitive Science, NUI Galway, vol 2563. pp. 305–316
Tao F, Liu G, Zhao Q (2018) An ensemble framework of voice-based emotion recognition system. In: Institute of Electrical and Electronics Engineers (IEEE) (ed), 2018 First Asian Conference on Affective Computing and Intelligent Interaction (ACII Asia). pp. 1–6
Thapliyal H, Ratajczak N, Wendroth O, Labrado C (2018) Amazon Echo enabled IoT home security system for smart home environment. In: Institute of Electrical and Electronics Engineers (IEEE) (ed), 2018 IEEE International Symposium on Smart Electronic Systems (ISES) (Formerly INiS). pp. 31–36
Tielman ML, Neerincx MA, Bidarra R, Kybartas B, Brinkman W-P (2017) A therapy system for post-traumatic stress disorder using a virtual agent and virtual storytelling to reconstruct traumatic memories. Journal of Medical Systems 41(8):125. https://doi.org/10.1007/s10916-017-0771-y
Tironi A, Mainetti R, Pezzera M, Borghese AN (2019) An empathic virtual caregiver for assistance in exer-game-based rehabilitation therapies. In: Institute of Electrical and Electronics Engineers (IEEE) (ed), 2019 IEEE 7th International Conference on Serious Games and Applications for Health (SeGAH). pp. 1–6
Trenholm R (2016) Amazon Echo (and Alexa) arrive in Europe, and Echo comes in white now too. CNET. https://www.cnet.com/news/amazon-echo-and-alexa-arrives-in-europe/
Tsiourti C, Weiss A, Wac K, Vincze M (2019) Multimodal integration of emotional signals from voice, body, and context: effects of (in)congruence on emotion recognition and attitudes towards robots. Int J Soc Robot 11(4):555–573. https://doi.org/10.1007/s12369-019-00524-z
Tsiourti C, Quintas J, Ben-Moussa M, Hanke S, Nijdam NA, Konstantas D (2018a) The CaMeLi framework—a multimodal virtual companion for older adults. In: Kapoor S, Bhatia R, Bi Y (eds) Studies in computational intelligence, vol 751. Springer, pp. 196–217
Tsiourti C, Ben-Moussa M, Quintas J, Loke B, Jochem I, Lopes JA, Konstantas D (2018b) A virtual assistive companion for older adults: design implications for a real-world application. In: Sharma H, Shrivastava V, Bharti KK, Wang L (eds), Lecture notes in networks and systems, vol 15. Springer, pp. 1014-1033
Tung L (2018) Amazon Echo, Google Home: how Europe fell in love with smart speakers. ZDnet. https://www.zdnet.com/article/amazon-echo-google-home-how-europe-fell-in-love-with-smart-speakers
Turner-Lee N (2019) Can emerging technologies buffer the cost of in-home care in rural America? Generations 43(2):88–93. http://web.a.ebscohost.com/ehost/pdfviewer/pdfviewer?vid=2&sid=0aaaf704-d3bd-42ab-ad26-ecd36c0a059b%40sdc-v-sessmgr02
Vaca K, Gajjar A, Yang X (2019) Real-time automatic music transcription (AMT) with Zync FPGA. In: Institute of Electrical and Electronics Engineers (IEEE) (ed), 2019 IEEE Computer Society Annual Symposium on VLSI (ISVLSI). pp. 378–384
Van Eck NJ, Waltman L (2014) Visualizing bibliometric networks. In: Ding Y, Roussea R, Wolfram D (eds) Measuring scholarly impact: methods and practice. Springer, pp. 285–320
Van Eck NJ, Waltman L (2010) Software survey: VOSviewer, a computer program for bibliometric mapping. Scientometrics 84(2):523–538
Verma S, Gustafsson A (2020) Investigating the emerging COVID-19 research trends in the field of business and management: a bibliometric analysis approach. J Bus Res 118:253–261
Verma S (2017) The adoption of big data services by manufacturing firms: an empirical investigation in India. J Inf Syst Technol Manag 14(1):39–68
Vishwakarma SK, Upadhyaya P, Kumari B, Mishra AK (2019) Smart energy efficient home automation system using IoT. In: Institute of Electrical and Electronics Engineers (IEEE) (ed), 2019 4th International Conference on Internet of Things: smart Innovation and Usages (IoT-SIU). pp. 1–4
Vora J, Tanwar S, Tyagi S, Kumar N, Rodrigues JJPC (2017) Home-based exercise system for patients using IoT enabled smart speaker. In: Institute of Electrical and Electronics Engineers (IEEE) (ed), 2017 IEEE 19th International Conference on E-Health Networking, Applications and Services (Healthcom). pp. 1–6
Wakefield CC (2019) Achieving position 0: optimising your content to rank in Google’s answer box. J Brand Strategy 7(4):326–336
Wallace T, Morris J (2018) Identifying barriers to usability: smart speaker testing by military veterans with mild brain injury and PTSD. In: Langdon P, Lazar J, Heylighen A, Dong H (eds) Breaking down barriers. Springer, pp. 113–122
Xi N, Hamari J (2021) Shopping in virtual reality: a literature review and future agenda. J Bus Res 134:37–58. https://doi.org/10.1016/j.jbusres.2021.04.075
Yaghoubzadeh R, Pitsch K, Kopp S (2015) Adaptive grounding and dialogue management for autonomous conversational assistants for elderly users. In: Brinkman W-P, Broekens J, Heylen D (eds) Intelligent virtual agents, vol 9238. Springer, pp. 28–38
Yildirim İ, Bostancı E, Güzel MS (2019) Forensic analysis with anti-forensic case studies on Amazon Alexa and Google Assistant build-in smart home speakers. In: Institute of Electrical and Electronics Engineers (IEEE) (ed), 2019 4th International conference on computer science and engineering (UBMK). pp. 271–273
Yusri MM, Kasim S, Hassan R, Abdullah Z, Ruslai H, Jahidin K, Arshad MS (2017) Smart mirror for smart life. In: Institute of Electrical and Electronics Engineers (ed), 2017 6th ICT International Student Project Conference (ICT-ISPC) 2017 6th ICT International Student Project Conference (ICT-ISPC). pp. 1–5
Zschörnig T, Wehlitz R, Franczyk B (2019) A fog-enabled smart home analytics platform. In: Brodsky A, Hammoudi S, Filipe J, Smialek M (eds) Proceedings of the 21st International Conference on Enterprise Information Systems (ICEIS 2019), vol 1. SciTePress, pp. 604–610
Zuboff S (2019) The age of surveillance capitalism: the fight for a human future at the new frontier of power. Profile Books
Harwood S, Eaves S (2020) Conceptualising technology, its development and future: The six genres of technology. Technol Forecast Soc Change 160:120174
Stadler S, Riegler S, Hinterkörner S (2012) Bzzzt: When mobile phones feel at home. Conference on Human Factors in Computing Systems – Proceedings, 1297-1302. https://doi.org/10.1145/2212776.2212443
Download references
Acknowledgements
This research was funded by the Swiss National Science Foundation (SNSF) as part of the project “VA-People, Experiences, Practices and Routines” (VA-PEPR) (Grant Nr. CRSII5_189955). We are grateful for the support from the wider project team from Lucerne University of Applied Sciences and Arts, Eastern Switzerland University of Applied Sciences, and Northumbria University. We would also like to thank Bjørn S. Cience for his support while working on this paper.
Author information
Authors and affiliations.
Lucerne School of Information Technology and Computer Sciences, Lucerne University of Applied Sciences and Arts, Lucerne, Switzerland
Bettina Minder
Department of Business & Management, University of Southern Denmark, Odense, Denmark
Patricia Wolf & Surabhi Verma
Department of Management, Lucerne University of Applied Sciences and Arts, Lucerne, Switzerland
Patricia Wolf
Institute for Information and Process Management, Eastern Switzerland University of Applied Sciences, St.Gallen, Switzerland
Matthias Baldauf
Department of Economics and Business Economics, Aarhus University, Aarhus, Denmark
Surabhi Verma
You can also search for this author in PubMed Google Scholar
Corresponding author
Correspondence to Patricia Wolf .
Ethics declarations
Competing interests.
The authors declare no competing interests.
Ethical approval
The article does not contain any studies with human participants performed by any of the authors.
Informed consent
Additional information.
Publisher’s note Springer Nature remains neutral with regard to jurisdictional claims in published maps and institutional affiliations.
Supplementary information
Supplemental material file #1, rights and permissions.
Open Access This article is licensed under a Creative Commons Attribution 4.0 International License, which permits use, sharing, adaptation, distribution and reproduction in any medium or format, as long as you give appropriate credit to the original author(s) and the source, provide a link to the Creative Commons license, and indicate if changes were made. The images or other third party material in this article are included in the article’s Creative Commons license, unless indicated otherwise in a credit line to the material. If material is not included in the article’s Creative Commons license and your intended use is not permitted by statutory regulation or exceeds the permitted use, you will need to obtain permission directly from the copyright holder. To view a copy of this license, visit http://creativecommons.org/licenses/by/4.0/ .
Reprints and permissions
About this article
Cite this article.
Minder, B., Wolf, P., Baldauf, M. et al. Voice assistants in private households: a conceptual framework for future research in an interdisciplinary field. Humanit Soc Sci Commun 10 , 173 (2023). https://doi.org/10.1057/s41599-023-01615-z
Download citation
Received : 19 May 2022
Accepted : 14 March 2023
Published : 19 April 2023
DOI : https://doi.org/10.1057/s41599-023-01615-z
Share this article
Anyone you share the following link with will be able to read this content:
Sorry, a shareable link is not currently available for this article.
Provided by the Springer Nature SharedIt content-sharing initiative
Quick links
- Explore articles by subject
- Guide to authors
- Editorial policies

Advertisement
A voice-based real-time emotion detection technique using recurrent neural network empowered feature modelling
- 1174: Futuristic Trends and Innovations in Multimedia Systems Using Big Data, IoT and Cloud Technologies (FTIMS)
- Open access
- Published: 22 June 2022
- Volume 81 , pages 35173–35194, ( 2022 )
Cite this article
You have full access to this open access article
- Sadil Chamishka 1 ,
- Ishara Madhavi 1 ,
- Rashmika Nawaratne 2 ,
- Damminda Alahakoon 1 ,
- Daswin De Silva 2 ,
- Naveen Chilamkurti ORCID: orcid.org/0000-0002-5396-8897 3 &
- Vishaka Nanayakkara 1
9215 Accesses
23 Citations
Explore all metrics
The advancements of the Internet of Things (IoT) and voice-based multimedia applications have resulted in the generation of big data consisting of patterns, trends and associations capturing and representing many features of human behaviour. The latent representations of many aspects and the basis of human behaviour is naturally embedded within the expression of emotions found in human speech. This signifies the importance of mining audio data collected from human conversations for extracting human emotion. Ability to capture and represent human emotions will be an important feature in next-generation artificial intelligence, with the expectation of closer interaction with humans. Although the textual representations of human conversations have shown promising results for the extraction of emotions, the acoustic feature-based emotion detection from audio still lags behind in terms of accuracy. This paper proposes a novel approach for feature extraction consisting of Bag-of-Audio-Words (BoAW) based feature embeddings for conversational audio data. A Recurrent Neural Network (RNN) based state-of-the-art emotion detection model is proposed that captures the conversation-context and individual party states when making real-time categorical emotion predictions. The performance of the proposed approach and the model is evaluated using two benchmark datasets along with an empirical evaluation on real-time prediction capability. The proposed approach reported 60.87% weighted accuracy and 60.97% unweighted accuracy for six basic emotions for IEMOCAP dataset, significantly outperforming current state-of-the-art models.
Similar content being viewed by others
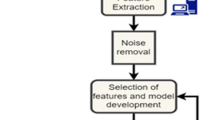
Speech based emotion recognition by using a faster region-based convolutional neural network
Emotion Recognition from Speech Using Deep Neural Network
Speech Emotion Recognition Based on a Recurrent Neural Network Classification Model
Avoid common mistakes on your manuscript.
1 Introduction
Real-time multimedia applications and services including video conferencing, telepresence, real-time content delivery, telemedicine, voice-controls on wearables and online-gaming, have contributed to the exponential growth of the Internet multimedia traffic [ 7 ]. Multimedia systems are rich sources of integrated audio, text and video streams which facilitate capturing, processing and transmission of multimedia information. This rapidly growing internet traffic of human conversations contains a massive volume of information, especially the voice-related attributes that help characterize human behaviour and embedded emotions. Emotions impact the way individuals think and act in real-life situations. Humans have unique ways to express themselves, sometimes even combining multiple emotions together as a mix [ 21 ]. These basic and complex emotion-swings influence the human physical movements, perceptions, cognition, actions and personality [ 22 ]. The ability to detect, capture and utilize human emotions from digital footprints left in multimedia has become a very important research direction.
Identification of emotions has a great value in multiple aspects. It allows us to understand the people we communicate with as decisions people make differ based on their emotions [ 22 ]. Although a precise and a concrete interpretation of how emotions are provoked in human minds is not yet available, scientists and psychologists have concentrated effort in defining and interpreting emotion generation in different perspectives including cognitive sciences, neurology, psychology and social sciences [ 22 ]. On one hand, the emotion generation can be viewed as a joint function of a physiologically arousing condition and the way a person tends to evaluate or appraise the situation. In terms of neurology, the emotions are regarded as activations caused by the changes in the density of neural stimulations or firings per unit time. In addition, emotions have been categorized as positive and negative emotions. However, the sufficiency of this segregation is questionable as the valence tag or the positivity or negativity of emotions depend on the situation encountered, and in most situations require deeper and more granular interpretation.
Recent studies have recognized the role of significant low-level acoustic features including spectrograms, Mel-Frequency Cepstral Coefficients (MFCC), fundamental frequency (F0) analysed via high-level statistical functions and other deep learnt features for emotion detection [ 29 , 40 ]. In addition, deep neural networks and sequence modelling techniques are developed and evaluated for emotion detection from audio. The Recurrent Neural Network models have been widely used, due to its ability to model sequential information while preserving more complex interactions. The LSTM and GRU based neural architectures are followed by additional attention layers to precisely detect the emotional content embedded in human speech. As an embedding mechanism, the Bag-of-Audio-Words (BoAW) feature embeddings are known to perform well for detecting dimensional emotions (arousal and valence) in literature, yet its robustness is not experimented for detecting more granular categorical emotions i.e., happy, sad, angry, etc. [ 38 ]. Latest research includes the development of model architectures which are capable of utilizing the context of conversations to enhance the emotion prediction strength.
Despite the significant amount of research conducted focusing on emotion detection from audio conversations, a number of key issues are still to be addressed. The following Table 1 summarises the key limitations in emotion detection from audio conversations.
In this context, our research presents four major contributions to the state-of-the-art models in constructing a novel feature based approach for detecting emotion categories from audio conversations.
First, we propose a Natural Language Processing (NLP) inspired Bag of Audio Words (BoAW) approach to represent rich audio embeddings in distinguishing six basic emotion categories, i.e., happy, sad, neutral, angry, frustrated and excited.
Second, alongside the BoAW feature embeddings, we propose an appropriate attention mechanism that best aligns with the feature representations input to the emotion detection model.
Third, we explore and evaluate the robustness and the effectiveness of this feature extraction and embedding process followed by an emotion detection model which utilizes conversation context information for emotion class predictions.
Fourth, we evaluate the performance of each component in the proposed approach in terms of delivering emotion predictions in real-time to validate the usefulness of the novel approach to be integrated with real-time applications or systems. This validation demonstrates the benefit of capturing emotion variations of the participants in a conversation such as in human or machine-driven (automated) call-centers and health care systems.
The rest of the paper is organized as follows. The second section briefly discusses the conceptual background of the theories adapted. Section three presents the proposed approach followed by section four on experiments and results. The last section concludes the paper, discussing the implications of the results, limitations, and potential for future work.
2 Conceptual background
Emotion detection research has developed with collaborative research contributions from psychology, cognitive science, machine learning and Natural Language Processing (NLP) [ 34 ]. Emotional intelligence (or an artificial counterpart) is important for machines when interacting with humans, as emotions and the ability to sense emotion play an important role in maintaining productive social interactions [ 35 ]. A significant volume of research has been conducted in the area of detection of emotionally relevant information from different sources, offering positive as well as compelling evidence of impact on multiple fields covering healthcare, human resource management and Artificial Intelligence [ 1 , 2 , 3 , 4 , 6 ]. The traditional process of emotion prediction includes frame-based feature extraction (low-level descriptors - LLDs), followed by utterance-level information collection, and input to a classification or a regression technique [ 36 ]. This includes and highlights the existing feature extraction techniques and the robustness of various models used for emotion predictions. Existing research attempts focus on audio feature extraction techniques via handcrafted methods, and deep-learnt features together with emotion detection models experimented in multimodality (text transcripts, audio, visual) aspects, utterance level or attentive (contextual) emotion predictors based on classifiers of Support Vector Machines (SVM), variations of deep learning networks, etc. Nevertheless, significant potential for further improvements exist in the accuracy and the interpretability of the proposed approaches due to the current limited capability in predicting higher variation of basic emotions [ 40 , 41 ], lack of conversational context utilization [ 40 ] and inferior performance reported in acoustic-based emotion detection compared to text [ 32 ].
Prevailing feature extraction techniques either extract shallow handcrafted features and apply statistical functions including mean, variance, range, quartiles, linear regression coefficients, etc. to determine the temporal variations of feature patterns or allow deep neural networks to unveil useful feature representations. The work elaborated in [ 29 ] utilizes deep learnt features for emotion detection. The related studies attempt to find the suitability of pitch information in determining the emotions from audio segments. As Mel-scale spectrograms cause loss of pitch information, the research work in [ 27 , 36 ] better utilizes pitch information by extracting linearly spaced spectrogram features. It has been reported that the usage of statistical learning of the layers in a deep neural network for feature extraction, yields better results in contrast to the handcrafted low-level features [ 36 ]. Inspired by the domain of Natural Language Processing, Bag-of-Audio-Words (BoAW) feature representations have been successfully used for classifying audio events and detecting other acoustic activities [ 38 ]. The work in [ 38 ] utilizes Mel-Frequency Cepstral Coefficients (MFCC) as low-level features for the codebook creation using a random sampling of audio words. The feature embeddings are input to a Support Vector Regression model to retrieve predictions for emotions in the dimensions of valence and arousal which respectively refer to the positive or negative affectivity and the degree of excitement of the emotion. Regardless of the rich interpretability of this feature representation, the evaluations have been conducted only in the dimensional aspects (arousal and valence) of emotions.
Most of the existing research studies focus on constructing emotion detection models. Due to the lack of publicly available datasets, most of the researchers have conducted their study on the rich Interactive Emotional Dyadic Motion Capture (IEMOCAP) dataset using sequence modelling techniques for audio and text analysis. The datasets including the Multimodal EmotionLines Dataset (MELD) in which an average of five participants were engaged in conversations have been more challenging to evaluate when compared to the dyadic conversations found in the IEMOCAP dataset. There are different groupings of existing models, including multimodal emotion detection models, classifiers involving deep neural networks and context-independent or dependent predictors. A considerable number of these attempts utilize multimodal information to construct robust solutions. In literature, the treatment of multimodal features, i.e., transcripts, audio and visual modalities, provide the potentiality to construct rich feature representations induced by their complementary information. The research conducted in [ 40 ] studies the impact of speech and text transcriptions from a speech for emotion detection through multiple CNN based architectures. This work expresses the fitness of Mel-Frequency Cepstral Coefficients (MFCC) over spectrogram features in terms of acoustic modality and the low representational capability of text embeddings due to the possible loss of information in the speech-to-text translation process. Furthermore, a considerable accuracy gain has been attained in this research from combining text and audio modalities. A state-of-the-art multimodal emotion detection technique is proposed in [ 20 ] in which the textual features are extracted from pre-trained word embeddings via a single layer Convolutional Neural Network (CNN), the audio features being extracted from openSMILE [ 16 ] toolkit and visual modality from a deep 3D-CNN architecture. Although the multimodal emotion detection performs better than the unimodal approaches, a considerable accuracy gap prevails between the text modality and the audio modality based feature representations [ 32 ].
The current main emotion detection models are based on distance or tree-based machine learning algorithms as well as variants of deep neural networks. The research work in [ 25 ] implements a hierarchical decision tree classifier which utilizes prior knowledge (i.e., acoustic features can differentiate between high and low activated emotions) for the initial, top-level split and performing a series of classifier-results (Gaussian Mixture Models, Linear Discriminant Analysis, Support Vector Machine) for the cascading splits outperforming Support Vector Machine (SVM) baseline accuracies. Several variations of deep neural networks such as recurrent neural networks and convolutional networks have been reported [ 36 ]. The research described in [ 18 ] has proposed a Deep Neural Network (DNN) based segment level predictor on emotion probability distribution which is input to a succeeding Extreme Learning Machine (ELM), a single-hidden-layer neural network, for utterance level emotion detection. This DNN and ELM stacked approach is efficient and outperforms Support Vector Machine (SVM) based emotion detection for small scale training partitions. Not only the sequence modelling techniques but also the Convolutional Neural Network (CNN) based techniques [ 40 ] have been proposed using a combined feature flow of Mel-Frequency Cepstral Coefficients (MFCC) and spectrograms. The work in [ 24 ] proposes a novel approach to combine CNN based feature extraction from sequential data and feed to an RNN.
While most of the studies conducted focus on acquiring emotionally relevant information independently from utterances, RNN based memory networks with attention mechanisms have been proposed and successfully utilized to capture historical aspects of the conversation and query the memory bank to get relevant information needed to detect emotions [ 23 , 33 ]. Due to the recurrent structure of RNN models that handle input data, RNN models have been the first choice among researchers recently, for sequence modelling tasks such as speech recognition and emotion prediction when compared to conventional Hidden Markov Models (HMM). Furthermore, the improved variations of RNN including LSTM and GRU cells have proven to be capable of pertaining short and long term context information over a conversation [ 26 ]. Thus, the ability inherent in RNN models with memory cells to track memory states in sequential data, provide valid reasons to incorporate RNN models in emotion recognition of multi-party conversations to better utilize contextual information in conversations. As human nature is naturally influenced by various emotions which arise from the context of a conversation, it is essential for a model to exhibit the capability to detect complex emotions in considerable accuracies, with better utilization of the context information in conversations. Poria et al. [ 14 ] use contextual information from neighbouring utterances of the same speaker to predict emotions. Recent emergence in the research community for filtering emotionally salient information from utterances and infusing conversational context has gained significant accuracy gains. The research work in [ 29 ] focuses on performing emotion detection by combining a bidirectional LSTM with a weighted pooling strategy using an attention mechanism which enables the network to focus on emotionally salient parts of a sentence. The approach in [ 20 ] utilizes an RNN-based memory network, including multi-hop attention mechanism which injects self and interpersonal influences into the global memory of the conversation to acquire affective summaries of the context. The DialogueRNN and its variants (BiDialogRNN, BiDialogRNN with attention) are recently constructed state-of-the-art models used to retrieve the emotion predictions from conversations considering the context information considering the global context of the conversation, speaker states, and emotion states using three separate Gated Recurrent Units (GRU) [ 28 ]. Although the performance of the model has been investigated in terms of textual modality and trimodal scenario (text, audio, visual), no work has been reported on the individual performance of the audio modality.
Major limitations identified in the study of existing work include the low accuracy of audio modality based feature representations compared to the text modality and lack of consideration towards the capability of Natural Language Processing (NLP) influenced audio embedding techniques (BoAW) for predicting emotion categories. Furthermore, the literature review reveals the existing possibilities of utilizing contextual information in a conversation flow via RNN models and appropriate attention mechanisms to yield enriched emotion predictions. Addressing the aforementioned limitations, we design and evaluate a new audio feature extraction approach consisting of the BoAW approach and a state-of-the-art recurrent neural network to uplift the performance of emotion detection from human conversations.
3 Proposed method
The proposed methodology is empowered by the Bag of Audio Words (BoAW) based novel feature extraction approach and a state-of-the-art Recurrent Neural Network model. The method aims to highlight the applicability of the Bag of Words (BoW) feature representation techniques extensively used in the NLP domain, to represent audio features and evaluate the performance improvement of emotion classification in audio conversations utilizing one of the state-of-the-art emotion detection models. Adhering to dependencies present in human conversations, the adopted RNN model captures the long term and short term contextual information in the conversation. In a typical conversation between two or more parties, each individual implicitly contributes to the context of the conversation and the emotions of each speaker vary over time. Therefore, the feature encodings from the BoAW are combined with the contextual information derived along the conversation using the RNN, to improve the predictive power of the model. Figure 1 illustrates the proposed approach consisting of the proposed audio feature representation mechanism, the BoAW approach, from which the feature embeddings are input to the RNN model. The low-level descriptors (LLDs) related to emotional classification tasks are extracted from audio streams using the openSMILE toolkit. As the number of extracted LLD feature vectors for different utterances can vary in lengths depending on the utterance lengths, an encoding mechanism is required to represent the extracted LLDs prior to present as input to an RNN model at each step of the conversation. The BoAW approach outputs fixed-size audio embeddings for each utterance with the support of a codebook generated from the LLD features from the training partition. The codebook consists of frequently occurring distinct feature vectors from the audio segments, leading to a compact and rich feature encoding while reducing the impact of infrequent noisy audio segments. At the final stage, a Bidirectional Recurrent Neural Network model with attention is selected to retrieve the respective emotion predictions from the utterances of the conversation.
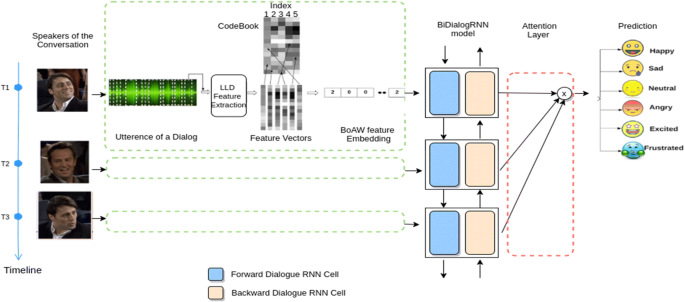
Bag of Audio Words based Recurrent Neural Network model for emotion detection from audio
The model functions in three phases. (1) Feature extraction, (2) Bag of Audio words (BoAW) feature embeddings, and (3) Emotion extraction. The feature extraction phase yields 130 low-level features per every 10 milliseconds of an utterance. The BoAW component creates rich feature embeddings from the extracted low-level features, by generating a term frequency matrix which denotes the frequency of each index of the codebook within the extracted low-level features from audio data. These rich feature embeddings contribute in reducing the existing accuracy gap between text and audio feature representations. The emotion extraction is achieved through a recurrent neural network architecture and utilizes the rich feature embeddings to predict 6 basic emotion categories. We describe each of the phases in the following subsections.
3.1 Feature extraction
Extracting prominent feature sets for emotion detection is important for constructing a precise emotion detection model. As a pre-processing step, the audio conversations have to be segmented into utterances and the respective speakers should be identified. Manual speaker diarization or Automatic speaker diarization techniques can be utilized to segment and label each utterance of the speakers. Then the audio files of utterances are formatted in the form of 16-bit PCM WAV files and use the available, open-sourced openSMILE toolkit [ 16 ] to extract acoustic low-level descriptors (LLDs). The LLDs include Energy related features such as root mean square energy, zero-crossing rate and spectral features including MFCC 1–14, spectral flux along with voicing related features like fundamental frequency, logHNR, jitter, shimmer as provided in the ComParE_2016 feature set [ 39 ] which are known to be promising features which carry emotional contents in human voice. The extracted feature vector comprises 65 low-level audio descriptors and their respective first order derivatives. Altogether, 130 low level features are extracted from each 25 milliseconds with 10 milliseconds frame rate, assuming the emotion related features are stationary during the aforementioned interval. After the feature extraction phase, a rich feature corpus consisting of time-varying feature sequences is available for the downstream feature engineering tasks. In the next step, the variable-length time series feature vectors consisting of low-level features of the audio signal are put through a BoAW based rich encoding mechanism prior to feeding into prediction models.
3.2 Bag of Audio Words (BoAW) feature embeddings
The approach has its roots in NLP where the documents are represented as Bag-of-Words representations, and this approach provides Bag-of-Audio-Words as an encoding mechanism for audio data. The overall BoAW feature embedding approach is illustrated in Fig. 2 . The first stage creates an indexed codebook consisting of different feature patterns from the training audio data which were generated from a set of low-level features extracted from audio. The algorithm can achieve this by randomly selecting patterns or by kmeans + + clustering algorithm [ 8 ]. The codebook vectors are iteratively selected randomly with higher dissimilarity within codebook vectors based on the Euclidean distance measure. At the presence of an unseen (test) low level feature pattern, the best matching codebook pattern, having the least distance to the pattern at hand, is selected and the term frequency of the respective index is increased. Once all the term frequencies are found in utterance levels (bag/histogram of audio words), a term frequency matrix is created, of which the entries act as fixed size encodings that can be input to a sequence to sequence modelling approach for predicting the latent emotion categories. As in the standard NLP BoW approach in document classification, the decimal logarithm is taken to shrink term frequency range as shown in Eq. ( 1 ) where \(TF\) and \(w\) denote term-frequency and audio-word respectively. The complete BoAW framework, namely the openXBOW has been implemented in Java and available in open source [ 37 ].
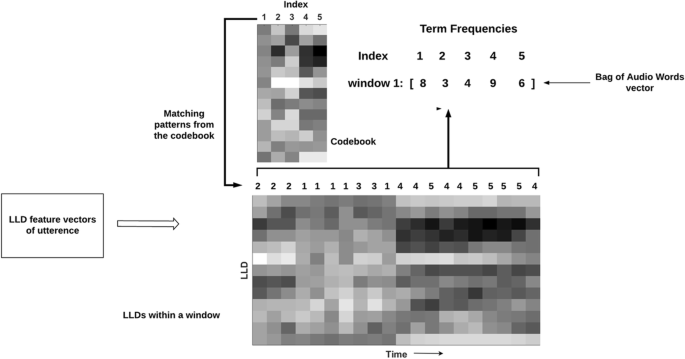
Bag of Audio Words based feature embedding approach
3.3 Recurrent neural network model
BiDialogueRNN [ 28 ] is a recently developed model used to retrieve the emotion predictions from a conversation utilizing the context information by taking into account both the past and the future utterances. Three major factors that help understand the emotion variations in conversations are elaborated in [ 28 ], in particular, the global context of the conversation ( \({G}_{t}\) ), speaker’s state ( \({P}_{t}\) ) and emotion of the utterance ( \({E}_{t}\) ) which are modeled using three separate recurrent neural networks. According to Chung et al. [ 12 ], GRU cells have been used to capture the long-term dependencies of sequences which leads to identifying the dynamics of the conversation while preserving the inter-party relationships by maintaining the context of the conversation. Each GRU cell computes a hidden state defined as \({h}_{t}\) = GRU( \({h}_{t-1}\) , \({x}_{t}\) ), where \({x}_{t}\) is the current input and \({h}_{t-1}\) is the previous GRU state. The state \({h}_{t}\) also serves as the current GRU output.
3.3.1 Global state GRU
When the speakers are engaged in a conversation by taking turns, at each turn of the speaker, the context of the conversation has to be updated. Further, the most recent-past context of the conversation has a relatively high impact on the emotional state of the speaker. Therefore, it is crucial to keep track of the information of the previous states of the conversation which is achieved by encoding the utterance and speaker’s previous state which are concatenated and fed to the global state GRU at each time step. The captured inter-speaker and inter-utterance dependencies convey more reliable contextual representations of the conversation as the current utterance \({u}_{t}\) updates the previous state \({q}_{s \left( {u}_{t }\right) , t-1}\) of the speaker to \({q}_{s \left( {u}_{t }\right) , t}\) . This information is captured through the GRU cell as shown in Eq. ( 2 ) along with \({g}_{t-1 }\) which is the previous context of the conversation to generate the current context of the conversation \({g}_{t }\in {R}^{\varrho }\) where \(\varrho\) is the size of the global state vector and \(\oplus\) is the concatenation. This is illustrated in Fig. 3 .
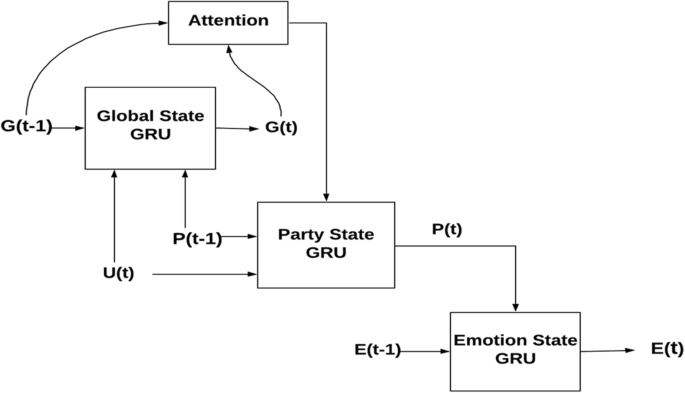
BiDialogRNN with the improved attention mechanism
3.3.2 Party state GRU
The states of the individual participants change over time during the conversation and informative qualities embedded in participant states can be used to detect the emotions of the speaker. Party state GRU has proposed a computational model to capture the speaker’s state throughout the conversation by updating corresponding current states of the speakers at the presence of an utterance, as shown in Eq. ( 3 ). Generally, the response of a speaker depends on the previous global states of the conversation. To cope with this feature, a speaker’s previous state \({q}_{s \left( {u}_{t }\right) , t-1}\) is modified to \({q}_{s \left( {u}_{t }\right) , t}\) based on the current utterance \({u}_{t}\) of the speaker and the context \({c}_{t}\) as shown in Eq. ( 3 ). The attention mechanism proposed for the model, as an extension when utilizing BoAW approach has improved the performance, which has the ability to come up with more reliable party states form RNNs by considering the effects of hidden states. The emotionally relevant states of the conversations get high attention scores and provide contextually enriched representation \({c}_{t}\) . In Eqs. ( 4 ) and ( 6 ), the \({ g}_{1}, {g}_{2} \dots { g}_{t}\) are the preceding states \(( {g}_{t }\in {R}^{\varrho }\) ) of the conversation, \(\alpha\) denotes the attention score over the previous global states and \(W\alpha \in {R}^{1 \times \varrho }\) denotes a weight vector for the softmax layer.
3.3.3 Proposed attention mechanism
As the speaker is influenced by previous states of the conversation, it is important to pay attention to the emotionally relevant segments of the conversation to determine the speaker’s next state. We propose an attention mechanism as the most appropriate attention when coping with BoAW based feature representations. The proposed attention mechanism considers the transposed vector of the current global state \({ g}_{t}\) as shown in Eq. ( 4 ), in contrast to obtaining the transpose of the current utterance \({ u}_{t}\) as previously in [ 28 ]. Results indicate this proposed attention to be more relevant to relate to the attention weights based on \({ g}_{t}\) , as \({ g}_{t}\) contains compact (dense) information than the high dimensional (2000-length) sparse utterance encodings resulted from encoded \({ u}_{t}\) .
3.3.4 Emotion state GRU
In order to predict the emotional state \({e}_{t}\) at timestamp t, the emotionally relevant features embedded in the party states \({q}_{s \left( {u}_{t }\right) , t}\) are input to the emotion state GRU along with the previous emotion state \({e}_{t-1 }\) of the speaker as indicated in Eq. ( 7 ). It can be identified as speaker GRU and global GRU in combination act similar to an encoder, whereas emotion GRU serves as a decoder. The forward and backward passes of the Bidirectional Emotion state RNN provide emotional representations of the speakers throughout the conversation. The forward and backward emotion states are concatenated, and a separate attention-based mechanism is applied to capture emotionally relevant parts to provide a more intuitive emotion classification process. A feedforward neural network with one hidden layer with a final SoftMax classifier is used to classify 6 emotion-class probabilities from the emotion representation \({e^\sim}_t\) derived via attention mechanism for each respective utterance \({u}_{t}\) . Here, \({W}_{\beta }\) denotes a weight vector for the softmax layer and \({\beta }_{t}\) is the attention score over the previous emotion states.
3.3.5 Real-time emotion recognition
A conversation proceeds with t number of sessions shared among n number of participants, along with the utterance sequence { \({u}_{1}\) , \({u}_{2}\) ,., \({u}_{t}\) }. The emotion corresponding to the utterance at index \(t\) is queried using historical utterances of the conversation up to the timestamp \(t\) . The prediction time is crucial for the real-time application and the performance measurement of the proposed approach is explained in the experiment section.
4 Experiments
The proposed emotion detection pathway is evaluated using two datasets: Interactive Emotional Dyadic Motion Capture (IEMOCAP) dataset and the Multimodal Emotion Lines Dataset (MELD) datasets of IEMOCAP and MELD. Table 2 shows the distribution of train and test samples for both datasets. For the IEMOCAP dataset, the proposed emotion detection methodology is also evaluated by varying the number of emotions facilitating appropriate comparison with existing research studies. When evaluating performance for five emotions, the predictions of happy and excited emotions are combined to represent the happy-emotion category. Furthermore, when evaluating the model performance for four emotions, the angry and frustrated emotion predictions are combined to reproduce the angry-emotion category. With this experiment, we demonstrate the high representational strength of BoAW based feature embeddings proposed in our approach by comparing accuracies (weighted and unweighted) in classifying 6, 5 and 4 categorical emotions derived from human conversations. By comparing the results with text modality based approaches, we further justify the reduction of the accuracy gap between text modality and audio modality. The performance of the novel pathway is evaluated using the MELD for 7 categorical emotion prediction. In addition, the ability of the novel feature pipeline to provide real-time predictions for online conversations is evaluated.
4.1 Datasets
IEMOCAP [ 10 ] dataset is collected at Signal Analysis and Interpretation Laboratory (SAIL) at the University of Southern California (USC) which consists of videos of two-way conversations conducted for five sessions by ten unique speakers where two speakers contribute per session for a scripted or an improvisation act to evoke the specified emotions. The corpus contains dyadic conversations and the dialogues segmented into utterances. Each utterance is labelled by 3 human annotators using categorical and dimensional (arousal and valence) labels and the majority voted emotion is taken as the label. We use 6 of the categorical labels in our study (happy, sad, neutral, angry, excited, frustrated) and train the model in a speaker-independent manner, i.e., the first four sessions (8 speakers) belong to the training partition while the last session (2 speakers) is taken as the testing partition. The residual utterances having miscellaneous labels are removed from the dialogue by assuming that the context of the conversation is not adversely impacted as 75% of the utterances fall under the six basic emotion categories.
The MELD [ 32 ] is provided with multiple modalities and consists of multiparty conversations extracted from the Friends TV series for emotion detection tasks. Compared to dyadic conversations, multiparty conversations are more challenging for emotion detection. The majority voting of five annotators was taken by looking at the video clip of each utterance. The utterances are labelled with the 7 emotion classes according to Ekman’s six universal emotions (fear, anger, surprise, sadness, joy and disgust) [ 15 ] extended with neutral emotion. The four emotions i.e., happy, sad, angry, neutral cover 96% percent of the emotion distribution in the training partition.
4.2 Experiment setup
We conduct experiments mainly on the IEMOCAP dataset and conduct an additional evaluation of the proposed approach using the MELD, despite a few facts related to its inherent weaknesses including constant background noise (laugh) and unrealistic, swift emotion switching. The audio files of the given utterances in the IEMOCAP dataset are directly used as input to the proposed pipeline. As only the conversational videos are present in the MELD, related audio streams were extracted from the videos and converted to the audio format (of 16-bit PCM WAV files) required by the openSMILE tool using ffmpeg [ 13 ]. The remainder of the experiment setup is common to both the datasets.
By specifically using the openSMILE configuration file ComParE_2016 , for each speech of duration 25 milliseconds, 130 LLDs are extracted with a frame rate of 10ms. The low-level patterns are extracted from 10 milliseconds of speech segments in which the audio-related properties are assumed to be stationary. The utterances have variable lengths with an average time of 4.5 s. The applied bag of audio word approach is able to generate fixed size feature encodings by generating a term frequency matrix by taking the audio features into account irrespective of the duration of the utterance. The openXBOW open-source bag-of-words toolkit is a Java application which supports the generation of bag-of-audio-word representations from numerical feature sequences. We have used openXBOW toolkit to generate a ‘bag of audio words’ representation by providing the acoustic LLDs extracted from the audio data. The LLD feature vectors of the train partition are split into two sets to create two codebooks with 1000 indices yielding 2000 indices in total. Each codebook acts as the vocabulary for train and test feature vectors of 65 LLDs. A given 10-millisecond frame of an utterance, represented by a 130 length LLD vector is compared against existing patterns in the codebook by calculating Euclidean distances and the term frequency (TF) of the highest matching pattern’s (least distant pattern) index is increased. In our approach, we use 5 as a parameter for the number of index matchings to be considered when comparing with the respective feature vector and update the indices of 5 highest similar term frequencies in the matrix. At the end of the process feature embeddings of 2000 dimensions are obtained for each utterance. The term frequency of the matrix of size 2000 is log-transformed before feeding to the emotion detection model.
In our experiment, the dimensions inside RNN are as follows: encoded utterance \({u}_{t }\in {R}^{2000}\) , the global state of the conversation \({g}_{t}\in {R}^{150}\) , speaker’s state \({p}_{t }\in {R}^{150}\) and emotional state of the speaker \({e}_{t }\in {R}^{100}.\) In the emotion detection model, the Negative log-likelihood loss with L2 regularization is used and weights are assigned to each class to compensate for the data imbalance in the training partition. Stochastic gradient descent based Adam optimizer is used for optimization with a learning rate of 0.0001 and weight decay of 0.0001. The model is trained for 60 epochs with a batch size of 2 dialogue sequences.
4.3 Results
First, we present the results obtained in the IEMOCAP dataset with a comparison to the available state-of-the-art emotion detection models. Second, the result achieved from the experiment on MELD is discussed. Since the datasets are imbalanced, we measure the overall accuracy (weighted accuracy, WA) as well as average recall (unweighted accuracy, UA) over the different emotional categories.
Our new approach resulted in achieving a weighted accuracy of 60.87% and an unweighted accuracy of 60.97%. To the best of our knowledge, this is the highest reported result for the 6 basic emotion classification using the audio modality for the IEMOCAP dataset. As shown in Table 3 , we evaluated the performance improvements gained by the integrations of the proposed components, in particular the BoAW and attention mechanism. Initially, we construct a baseline emotion detection pipeline by extracting 6373 features from each utterance using IS13 ComParE configuration script available in openSMILE and feeding it to the BiDialogRNN emotion detection model (Baseline). Then the performance of the proposed BoAW integration to the BiDialogRNN is evaluated, which outperforms the baseline by 10% showing the applicability of the novel feature pipeline. This architecture is further enhanced by the proposed attention mechanism and this outperforms the baseline by 13%. The confusion matrix shown in Fig. 4 shows the performance of the proposed novel feature pipeline of emotion detection. The confusion matrix indicates that the misclassifications occur often among similar emotion classes which can be misinterpreted even in human-intervened classification. A set of happy emotions are misclassified as excited as the two emotions are close in nature. Some of the frustrated emotions are misclassified as angry as the roots for both the emotions are similar. Furthermore, most of the other emotion categories are frequently misclassified and tagged as neutral. The naive reasoning would be the centred-location of the neutral emotion on the activation-valence space [ 41 ] which attracts other emotions which are not clearly separated in terms of deviation on either side (positive, negative).
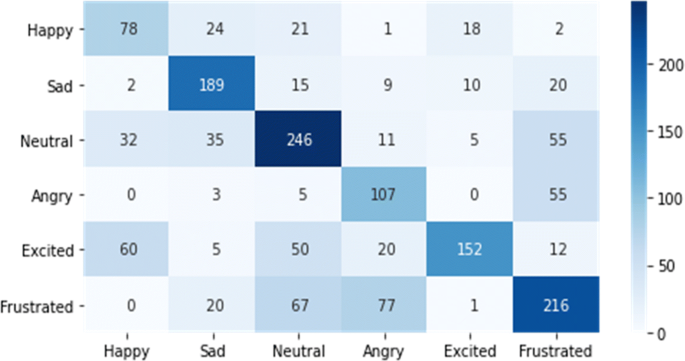
Confusion Matrix for Emotion detection on IEMOCAP Dataset
We evaluated the performance of the proposed novel approach, which utilizes the Bag-of-Audio-Words (BOAW) embeddings as input to a BiDialogRNN (with attention) model by comparing major state-of-the-art models in Table 4 . As most of the emotion detection models are limited to a few basic emotions including happy, angry, neutral and sad, we aggregate happy with excited and angry with frustrated for convenient comparison with models which cater to a lower number of emotions. The work in [ 20 ] proposed a multimodal emotion detection framework updating only the global conversational context could achieve good accuracy, but the performance of the audio modality is lower compared to its text modality. The model fusion of audio and text for identifying 4 emotions in [ 40 ] has improved the weighted accuracy to 76%, but the unweighted accuracy of 69% is still less than our results. The work in [ 29 ] proposed RNN-weighted pool approach with attention, which improves their accuracy. As most of the reported work is based on utterance level predictions without considering contextual information of the conversation, it highlights the importance of conversational modeling. It can be seen from the comparison that the proposed combination of bag-of-audio-words audio embedding and the recurrent network yields state-of-the-art results.
Compared with the IEMOCAP dataset, MELD dataset is more challenging. One reason is that the average number of speaker sessions is 10 (shorter conversations) in the MELD dataset, whereas it is 50 in the IEMOCAP dataset. In addition, there exists a rapid emotion switching with an average of three emotion categories per dialogue in MELD which adversely affects the attention-based context capturing mechanism in the RNN. This issue is exacerbated by having more than 5 speakers present in the majority of the conversations resulting in less information to keep track of the speaker states. In addition, the average length of an utterance is lower (3.59 s) compared to the IEMOCAP dataset (4.59 s), resulting in reduced emotion detection ability. Although all seven emotion categories are input for the training phase, the dataset is severely imbalanced with 47% of neutral data while the fear and disgust classes include 2% of the training samples. The background noise of laughing in a majority of utterances leads to increased incorrect classifications. This has resulted in MELD dataset is hardly used for emotion classification with audio in recent literature. Thereby, in our work, we compared our audio based emotion classification approach with recent text based emotion classification approaches with MELD dataset. Comparison of accuracy with the baselines provided by MELD dataset are shown in Table 5 . As such, we achieved the highest F1-score of identifying disgust, joy and sadness emotions outperforming the baselines provided by the MELD dataset. Due to the limitations highlighted, emotion detection accuracies are lower than other IEMOCAP dataset. Considering the comparable performance with the state of the art, we believe it is important to present these outcomes as these will inform interested researchers to further explore and take the work forward.
4.4 Comparison with text based emotion detection
The results from the MELD dataset are compared with the text modality based existing state-of-the-art emotion models in order to highlight the representational ability and the robustness of the audio embeddings of the proposed pipeline. The previous attempts for emotion detection using the textual features have reported higher accuracies compared to audio feature feature-based techniques. The proposed model successfully utilizes audio features to predict 6 basic emotions for the MELD dataset achieving comparable average accuracy and F1 scores (Table 6 ) achieving on par accuracies with textual modality based state-of-the-art models.
4.5 Impact of proposed attention mechanism
The attention mechanism is used to capture the emotionally relevant segments of the conversation to enrich the emotion detection process. When predicting the emotion for each utterance, in addition to the utterance, weighted conversation-contexts from the preceding utterances are considered by means of attention scores. The current global state of the conversation is the query parameter to retrieve the attention scores. In order to elaborate on the impact of the proposed attention mechanism, the utterance sequence of the dialog “Ses05F_impro03” in the IEMOCAP test partition is examined. This is a conversation between two parties, continuing an excited and happy emotion mixed conversation in which one person initially announces her marriage proposal. The excitement generated from this major occurrence at the beginning of the conversation continues forward during the conversation. Figure 5 illustrates snapshots of attention scores given by both the forward and backward RNNs of the model, which extract information from the past and future states of the conversation respectively. The attention scores elicited at the presence of the utterance at index 20 in the conversation is shown in Fig. 5(a) which indicates that the utterances residing in the range 5 to 12 have gained more attention indicating the initial excited state of the actual conversation. When predicting emotion for the utterance at the index 40 of the conversation (Fig. 5(b) ), it denotes that attention has been influenced by not only the neighbouring utterances in the index range from 24 to 30, but also the significant incident captured in the 4 to 12 utterance range. Similarly, the residual plots for the utterances at indices 60 and 80 of the conversation (Fig. 5(d) ) preserve the context. The illustration demonstrates that the attention mechanism has the ability to capture the emotionally salient segments, unlike in the independent utterance-wise emotion detection methodologies. The proposed attention mechanism provides better interpretability and context preservation to the emotion detection model.
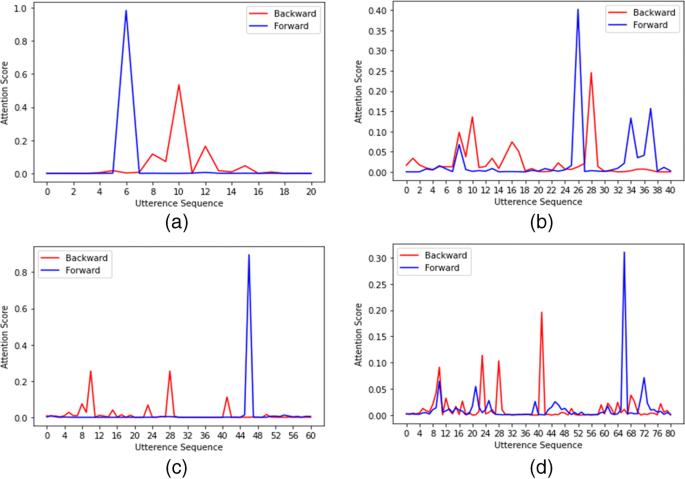
The attention scores for the preceding utterances at each quarter of the conversation “Ses05F_impro03”, taken from the IEMOCAP test set. ( a ) After 20 utterances, ( b ) after 40 utterances, ( c ) after 60 utterances and ( d ) after 80 utterances of the conversation
4.6 Real time emotion detection
Latency during real-time emotion prediction is measured by deploying the proposed emotion detection approach. The sub-modules of the emotion detection approach are tested separately, and the results shown in Table 7 show the applicability of the proposed approach in real-time emotion prediction settings. The evaluation is conducted for the test partition of the IEMOCAP dataset, including 31 dialogs consisting of 1623 utterances. At each session of the speaker utterance, the openSMILE toolkit extracts features and generates audio-relevant embeddings via the openXBOW tool. For the task of emotion detection, with the audio encoding of the current utterance, the previous speaker utterances are also provided as input to the model in order to yield context-influenced emotion predictions. As a byproduct, the time consumed for deriving emotion predictions increases with the length of the conversation, i.e., the time for retrieving the emotion prediction for the final utterance is equal in value to the time to retrieve the emotion sequence of the full conversation. As IEMOCAP dataset contains 50 utterances in average per conversation, in the worst possible scenario it is justified to assume the time taken for retrieving an emotion for an arbitrary utterance, is the same as the time spent to retrieve emotion predictions for a conversation having 50 utterances on average. The results suggest approximately 0.7 s of latency per utterance for passing through the complete pipeline.
5 Discussion and conclusion
Emotion detection from human conversations in audio form is a key challenge which can provide significant benefits if successfully overcome. The proposed research has contributed multiple theoretical contributions to the research community. Although the Bag-of-Audio-Words (BoAW) based embeddings have been used for the detection of the arousal and valence of emotions, to the best of our knowledge, this is the first study which highlights the potential of the BoAW based feature representations for basic and complex human emotion detection tasks. The evaluation of the proposed novel feature pipeline conducted upon the IEMOCAP dataset yields promising results of 60.87% weighted accuracy (approximately 20% improvement) and 60.97% unweighted accuracy in recognizing the 6 basic emotions outperforming current state-of-the-art models using the audio modality. In a multi-modal setting where audio, text and video streams are present, emotion recognition can be done with higher accuracy due to the richness of a wide variety of features. However, when only the audio modality is available for analysis, such as in a customer care call centre, presence of an emotion detection modal which is trained only using audio modality and can provide reasonable level of accuracy is very useful. Although the accuracies shown are approx. 61%, these far exceed the current state of the art (by 20%) highlighting the potential of the novel emotion recognition pipeline for audio/acoustic data. As potential future work, the proposed pathway can be experimented on tracking emotions of multiparty scenarios as the adapted emotion detection model is scalable enough to be evaluated for multiple parties. Going beyond the basic emotion categories, the research can be extended to detect mixed emotions by adding a final mixed-emotion classifier by utilizing the probability values yielded from the proposed RNN model [ 5 ]. This mixed-emotion classification can benefit from the theoretical backgrounds of the plutchik’s emotion wheel [ 30 ]. As another future direction, the feature extraction can be strengthened by augmenting the extracted auditory features by textual features from Bag-of-Words (BoW) by utilizing automatic speech detection techniques resulting in the potential utilization of big audio data. Beyond the traditional BoAW based feature encoding, the Convolution Neural Network-based deep learnt feature extraction techniques utilizing sequential information such as wave2vec 2.0 have emerged in the arena of speech recognition which could be further experimented on downstream tasks including emotion recognition from human conversations [ 9 ]. During the research, a limiting factor identified for deploying the modal in a real-world setting is the lack of devised state-of-the-art solutions for real-time automatic speaker diarization. With the availability of such speaker diarization, a fully automated emotion recognition pipeline from human conversations could be developed based upon the research contributions described in this paper. It is anticipated such input the accuracy will improve significantly. The proposed feature pipeline is simulated for real-time emotion detection from human conversations via manually diarized conversations due to the non-existence of a robust real-time technique. The proposed system can be implemented with high commercial use by integrating with a real-time speaker diarising methodology. A mechanism to generate speech embeddings from the bag of audio words from a large corpus of speech data can be more reliable as the bag of audio word encodings have a high dimension with high sparsity where the generated embeddings represent rich information.
The proposed research contributes a number of practical innovations to the modern tech-driven world. If machines become capable of identifying complex human emotions, various systems including elderly care agents, virtual call centre agents and specially designed AI robots can provide an improved customized service. Organizations which consider customer satisfaction as a major driving force of their businesses can benefit by analyzing how the agents manage the customers via exploring the emotion variations throughout the agent-customer conversations. Although understanding emotions from daily conversations is natural for human beings, the detection of emotions only through audio conversations could be difficult without use of facial expressions. In this context, empowering machines to understand human emotions via audio conversations is a significant step advancing the field of human-computer interaction by enhanced leveraging of big audio data.
Abeysinghe S et al. (2018) Enhancing decision making capacity in tourism domain using social media analytics. 2018 18th International Conference on Advances in ICT for Emerging Regions (ICTer), pp 369–375. https://doi.org/10.1109/ICTER.2018.8615462
Adikari A, Alahakoon D (2021) Understanding citizens’ emotional pulse in a smart city using artificial intelligence. IEEE Trans Ind Inf 17(4):2743–2751. https://doi.org/10.1109/TII.2020.3009277
Adikari A, Burnett D, Sedera D, de Silva D, Alahakoon D (2021) Value co-creation for open innovation: An evidence-based study of the data driven paradigm of social media using machine learning. Int J Inf Manag Data Insights 1(2):100022
Adikari A, Nawaratne R, De Silva D, Ranasinghe S, Alahakoon O, Alahakoon D (2021) Emotions of COVID-19: Content analysis of self-reported information using artificial intelligence. J Med Internet Res 23(4):e27341
Article Google Scholar
Adikari A, Gamage G, de Silva D, Mills N, Wong S, Alahakoon D (2021) A self structuring artificial intelligence framework for deep emotions modeling and analysis on the social web. Futur Gener Comput Syst 116:302–315
Alahakoon D, Nawaratne R, Xu Y, De Silva D, Sivarajah U, Gupta B (2020)Self-building artificial intelligence and machine learning to empower big data analytics in smart cities. Inform Syst Front. https://doi.org/10.1007/s10796-020-10056-x
Alvi S, Afzal B, Shah G, Atzori L, Mahmood W (2015) Internet of multimedia things: Vision and challenges. Ad Hoc Networks 33:87–111
Arthur D, Vassilvitskii S (2007) k-means++: The advantages of careful seeding. In: Proc. of the 18th annual ACM-SIAM symposium on Discrete algorithms. Society for Industrial and Applied Mathematics, pp 1027–1035
Baevski A, Zhou H, Mohamed A, Auli M (2021) wav2vec 2.0: A framework for self-supervised learning of speech representations. arXiv.org
Busso C, Bulut M, Lee C-C, Kazemzadeh A, Mower E, Kim S, Chang JN, Lee S, Narayanan SS (2008) IEMOCAP: Interactive emotional dyadic motion capture database. Lang Resour Eval 42(4):335
Chen M, He X, Yang J, Zhang H (2018)3-D Convolutional recurrent neural networks with attention model for speech emotion recognition. IEEE Signal Process Lett 25(10):1440–1444. https://doi.org/10.1109/LSP.2018.2860246
Chung J, Gulcehre C, Cho K, Bengio Y (2014) Empirical evaluation of gated recurrent neural networks on sequence modeling. https://doi.org/10.48550/arXiv.1412.3555
Converting Video (2020) Formats with FFmpeg | Linux Journal. Linuxjournal.com
Devamanyu Hazarika S, Poria A, Zadeh E, Cambria L-P, Morency, Zimmermann R (2018) Conversational memory network for emotion recognition in dyadic dialogue videos. In: Proceedings of the 2018 Conference of the North American Chapter of the Association for Computational Linguistics: Human Language Technologies, Volume1 (Long Papers), vol 1, pp 2122–2132
Ekman P (1992) An argument for basic emotions. Cognit Emot 6(3–4):169–200. https://doi.org/10.1080/02699939208411068
Florian Eyben F, Weninger F, Gross B (2013) Schuller: Recent Developments in open SMILE, the Munich Open-Source Multimedia Feature Extractor. In: Proc. ACM Multimedia (MM), Barcelona, Spain, ACM, ISBN 978-1-4503-2404-5, pp 835–838. https://doi.org/10.1145/2502081.2502224
Ghosal D, Majumder N, Poria S, Chhaya N, Gelbukh A (2019) Dialoguegcn: A graph convolutional neural network for emotion recognition in conversation. arXiv preprint arXiv:1908.11540
Han K, Yu D, Tashev I (2020) Speech emotion recognition using deep neural network and extreme learning machine. Microsoft Research
Hazarika D, Poria S, Zadeh A, Cambria E, Morency L-P, Zimmermann R (2018) Conversational memory network for emotion recognition in dyadic dialogue videos. In: Proceedings of the Conference of the North American Chapter of the Association for Computational Linguistics: Human Language Technologies, vol 1 (Long Papers), pp 2122–2132
Hazarika D, Poria S, Mihalcea R, Cambria E, Zimmermann R (2020) ICoN: Interactive conversational memory network for multimodal emotion detection. Proc. 2018 Conf. Empir. Methods Nat. Lang. Process. EMNLP 2018, pp 2594–2604. https://doi.org/10.18653/v1/d18-1280
De Barros PVA (2016) Modeling affection mechanisms using deep and self-organizing neural networks. Staats-und Universitätsbibliothek Hamburg Carl von Ossietzky
Izard C (2013) Human emotions. Springer, New York, pp 1–4
Google Scholar
Jiao W, Lyu MR, King I (2019)Real-time emotion recognition via attention gated hierarchical memory network. arXiv preprint arXiv:1911.09075
Keren G, Schuller B (2016) Convolutional RNN: An enhanced model for extracting features from sequential data. Proc. Int. Jt. Conf. Neural Networks, vol. 2016-October, pp 3412–3419. https://doi.org/10.1109/IJCNN.2016.7727636
Lee C-C, Mower E, Busso C, Lee S, Narayanan S (2011) Emotion recognition using a hierarchical binary decision tree approach. Speech Commun 53(9–10):1162–1171
Lieskovská E, Jakubec M, Jarina R, Chmulík M (2021) A review on speech emotion recognition using deep learning and attention mechanism. Electronics 10(10):1163
Madhavi I, Chamishka S, Nawaratne R, Nanayakkara V, Alahakoon D, De Silva D (2020) A deep learning approach for work related stress detection from audio streams in cyber physical environments. 2020 25th IEEE International Conference on Emerging Technologies and Automation F (ETFA), pp 929–936. https://doi.org/10.1109/ETFA46521.2020.9212098
Majumder N, Poria S, Hazarika D, Mihalcea R, Gelbukh A, Cambria E (2019) DialogueRNN: An Attentive RNN for Emotion Detection in Conversations. Proceedings of the AAAI Conference on Artificial Intelligence, vol 33, pp 6818–6825. Available: https://doi.org/10.1609/aaai.v33i01.33016818
Mirsamadi S, Barsoum E, Zhang C (2017) Automatic speech emotion recognition using recurrent neural networks with local attention center for robust speech systems. The University of Texas at Dallas, Richardson, TX 75080, USA Microsoft Research, One Microsoft Way, Redmond, WA 98052, USA. IEEE Int. Conf. Acoust. Speech, Signal Process, pp 2227–2231. https://doi.org/10.1109/ICASSP.2017.7952552
Plutchik R (2001) The Nature of Emotions: Human emotions have deep evolutionary roots, a fact that may explain their complexity and provide tools for clinical practice. Am Sci 89(4):344–350
Poria S, Cambria E, Hazarika D, Majumder N, Zadeh A, Morency L-P(2017)Context-dependent sentiment analysis in user-generated videos. In: Proceedings of the 55th Annual Meeting of the Association for Computational Linguistics (vol 1: Long Papers), pp 873– 883
Poria S, Hazarika D, Majumder N, Naik G, Cambria E, Mihalcea R (2019) MELD: A multimodal multi-party dataset for emotion recognition in conversations. ACL, pp 527–536
Rathnayaka P, Abeysinghe S, Samarajeewa C, Manchanayake I, Walpola M, Nawaratne R, Bandaragoda T, Alahakoon D (2019) Gated recurrent neural network approach for multilabel emotion detection in microblogs. 2012:2012–2017. http://arxiv.org/abs/1907.07653
Rosalind WP (2010) Affective computing: from laughter to IEEE. IEEE Trans Affect Comput 1(1):11–17
Ruusuvuori J (2013) Emotion, affect and conversation. The handbook of conversation analysis, pp 330–349
Satt A, Rozenberg S, Hoory R (2017) Efficient emotion recognition from speech using deep learning on spectrograms,. Proc. Annu. Conf. Int. Speech Commun. Assoc. INTERSPEECH, vol 2017-August, pp 1089–1093. https://doi.org/10.21437/Interspeech.2017-200
Schmitt M, Schuller B (2017) openXBOW - Introducing the passau open-source crossmodal bag-of-words toolkit. J Mach Learn Res 18(96):1–5
MathSciNet Google Scholar
Schmitt F, Ringeval, Schuller B (2016) At the border of acous-tics and linguistics: Bag-of-audio-words for the recognition of emotions in speech. Proc of Interspeech, pp 495–499
Schuller B, Steidl S, Batliner A, Epps J, Eyben F, Ringeval F, Marchi E, Zhang Y (2014) The INTERSPEECH 2014 Computational Paralinguistics Challenge: Cognitive & Physical Load. In: Proceedings INTERSPEECH 2014. 15th Annual Conference of the International Speech Communication Association, (Singapore, Singapore), ISCA, ISCA
Tripathi S, Kumar A, Ramesh A, Singh C, Yenigalla P (2019) Deep learning based emotion recognition system using speech features and transcriptions, pp 1–12
Yoon S, Byun S, Jung K (2019) Multimodal speech emotion recognition using audio and text. 2018 IEEE Spok. Lang. Technol. Work. SLT 2018 - Proc., no. December, pp 112–118. https://doi.org/10.1109/SLT.2018.8639583
Yoon S, Byun S, Dey S, Jung K (2019) Speech emotion recognition using multi-hop attention mechanism. In ICASSP 2019-2019 IEEE International Conference on Acoustics, Speech and Signal Processing (ICASSP), pp. 2822–2826
Download references
Open Access funding enabled and organized by CAUL and its Member Institutions.
Author information
Authors and affiliations.
Computer Science and Engineering, University of Moratuwa, Moratuwa, Sri Lanka
Sadil Chamishka, Ishara Madhavi, Damminda Alahakoon & Vishaka Nanayakkara
Research Centre for Data Analytics and Cognition, La Trobe University, Victoria, Australia
Rashmika Nawaratne & Daswin De Silva
Computer Science and Computer Engineering, La Trobe University, Victoria, Australia
Naveen Chilamkurti
You can also search for this author in PubMed Google Scholar
Corresponding author
Correspondence to Naveen Chilamkurti .

Ethics declarations
Conflicts of interests.
The Authors declare that there is no conflict of interest.
Additional information
Publisher’s note.
Springer Nature remains neutral with regard to jurisdictional claims in published maps and institutional affiliations.
Rights and permissions
Open Access This article is licensed under a Creative Commons Attribution 4.0 International License, which permits use, sharing, adaptation, distribution and reproduction in any medium or format, as long as you give appropriate credit to the original author(s) and the source, provide a link to the Creative Commons licence, and indicate if changes were made. The images or other third party material in this article are included in the article's Creative Commons licence, unless indicated otherwise in a credit line to the material. If material is not included in the article's Creative Commons licence and your intended use is not permitted by statutory regulation or exceeds the permitted use, you will need to obtain permission directly from the copyright holder. To view a copy of this licence, visit http://creativecommons.org/licenses/by/4.0/ .
Reprints and permissions
About this article
Chamishka, S., Madhavi, I., Nawaratne, R. et al. A voice-based real-time emotion detection technique using recurrent neural network empowered feature modelling. Multimed Tools Appl 81 , 35173–35194 (2022). https://doi.org/10.1007/s11042-022-13363-4
Download citation
Received : 04 October 2020
Revised : 09 March 2022
Accepted : 03 June 2022
Published : 22 June 2022
Issue Date : October 2022
DOI : https://doi.org/10.1007/s11042-022-13363-4
Share this article
Anyone you share the following link with will be able to read this content:
Sorry, a shareable link is not currently available for this article.
Provided by the Springer Nature SharedIt content-sharing initiative
- Bag-of-audio-words
- Machine learning
- Artificial intelligence
- Emotion analysis
- Find a journal
- Publish with us
- Track your research
Help | Advanced Search
Electrical Engineering and Systems Science > Audio and Speech Processing
Title: robust singing voice transcription serves synthesis.
Abstract: Note-level Automatic Singing Voice Transcription (AST) converts singing recordings into note sequences, facilitating the automatic annotation of singing datasets for Singing Voice Synthesis (SVS) applications. Current AST methods, however, struggle with accuracy and robustness when used for practical annotation. This paper presents ROSVOT, the first robust AST model that serves SVS, incorporating a multi-scale framework that effectively captures coarse-grained note information and ensures fine-grained frame-level segmentation, coupled with an attention-based pitch decoder for reliable pitch prediction. We also established a comprehensive annotation-and-training pipeline for SVS to test the model in real-world settings. Experimental findings reveal that ROSVOT achieves state-of-the-art transcription accuracy with either clean or noisy inputs. Moreover, when trained on enlarged, automatically annotated datasets, the SVS model outperforms its baseline, affirming the capability for practical application. Audio samples are available at this https URL .
Submission history
Access paper:.
- HTML (experimental)
- Other Formats

References & Citations
- Google Scholar
- Semantic Scholar
BibTeX formatted citation

Bibliographic and Citation Tools
Code, data and media associated with this article, recommenders and search tools.
- Institution
arXivLabs: experimental projects with community collaborators
arXivLabs is a framework that allows collaborators to develop and share new arXiv features directly on our website.
Both individuals and organizations that work with arXivLabs have embraced and accepted our values of openness, community, excellence, and user data privacy. arXiv is committed to these values and only works with partners that adhere to them.
Have an idea for a project that will add value for arXiv's community? Learn more about arXivLabs .
- Alzheimer's disease & dementia
- Arthritis & Rheumatism
- Attention deficit disorders
- Autism spectrum disorders
- Biomedical technology
- Diseases, Conditions, Syndromes
- Endocrinology & Metabolism
- Gastroenterology
- Gerontology & Geriatrics
- Health informatics
- Inflammatory disorders
- Medical economics
- Medical research
- Medications
- Neuroscience
- Obstetrics & gynaecology
- Oncology & Cancer
- Ophthalmology
- Overweight & Obesity
- Parkinson's & Movement disorders
- Psychology & Psychiatry
- Radiology & Imaging
- Sleep disorders
- Sports medicine & Kinesiology
- Vaccination
- Breast cancer
- Cardiovascular disease
- Chronic obstructive pulmonary disease
- Colon cancer
- Coronary artery disease
- Heart attack
- Heart disease
- High blood pressure
- Kidney disease
- Lung cancer
- Multiple sclerosis
- Myocardial infarction
- Ovarian cancer
- Post traumatic stress disorder
- Rheumatoid arthritis
- Schizophrenia
- Skin cancer
- Type 2 diabetes
- Full List »
share this!
May 14, 2024
This article has been reviewed according to Science X's editorial process and policies . Editors have highlighted the following attributes while ensuring the content's credibility:
fact-checked
peer-reviewed publication
trusted source
New research challenges widespread beliefs about why we're attracted to certain voices
by McMaster University
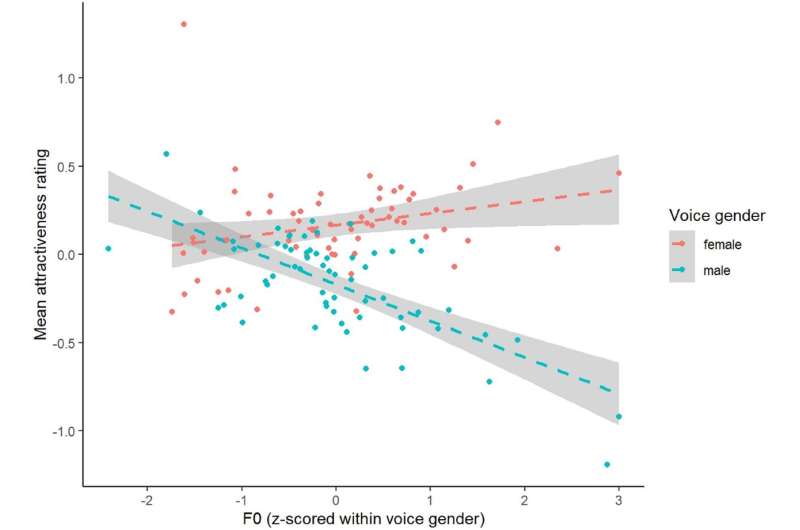
New insights into how people perceive the human voice are challenging beliefs about which voices we find attractive.
Previous studies have linked vocal averageness and attractiveness, finding that the more average a voice sounds, the higher it is rated in attractiveness.
However, McMaster researchers have found that average voice characteristics are not inherently appealing, and it may be beneficial to stick out from the crowd.
"Contrary to past studies, we discovered that averageness is not always more attractive. Pitch is a critical factor in attraction judgements, an insight that highlights the complexity of the way we perceive the human voice ," explained study lead Jessica Ostrega, who recently earned her Ph.D. in Psychology, Neuroscience, and Behavior.
"Understanding this allows us to look at how specific features of a person's voice affect the way we form impressions of others and interact with them."
The findings are outlined in a study published this month in the journal Scientific Reports . Researchers used advanced voice morphing technology to blend multiple voices together to create average-sounding voices to use in their experiments. They asked participants to rate the attractiveness of those voices.
Vocal attractiveness refers to how beautiful or handsome a voice makes someone sound to a listener. The term goes beyond simple appeal to encompass characteristics that might influence romantic or sexual interest.
"This research contributes to a deeper understanding of the complex dynamics of human communication and attraction," said David Feinberg, associate professor in the Department of Psychology, Neuroscience and Behavior, who oversaw the research, adding that the implications of the study extend beyond the academic realm into practical applications.
"Understanding the nuances of voice perception can influence practices in industries such as marketing, media, and even technology design, where voice interfaces are becoming increasingly common."
Explore further
Feedback to editors

Modular communicative leadless ICD found to be safe and exceeds performance expectations
19 hours ago

Creativity and humor shown to promote well-being in older adults via similar mechanisms

Sweet taste receptor affects how glucose is handled metabolically by humans
21 hours ago

Better medical record-keeping needed to fight antibiotic overuse, studies suggest
May 18, 2024

Repeat COVID-19 vaccinations elicit antibodies that neutralize variants, other viruses

A long-term ketogenic diet accumulates aged cells in normal tissues, new study shows
May 17, 2024

Gut bacteria enhance cancer immunotherapy in mouse study

Research finds the protein VISTA directly blocks T cells from functioning in immunotherapy

Study opens the door to designing therapies to improve lung development in growth-restricted fetuses

Researchers make strides in microbiome-based cancer therapies via iron deprivation in the tumor microenvironment
Related stories.

Higher voice pitch makes female faces appear younger
Jul 20, 2022

The magic of voices: Why we like some singers' voices and not others
Apr 25, 2024

Do you dislike your voice? You're not alone
Jul 13, 2023

Study reveals first genetic locus for voice pitch
Jun 9, 2023

DeFake tool protects voice recordings from cybercriminals
Apr 22, 2024
Low voice pitch increases standing among strangers, cross-cultural study finds
Feb 8, 2024
Recommended for you

The neural signature of subjective disgust could apply to both sensory and socio-moral experiences

Study uncovers key factors for resilience after trauma

Under stress, study finds an observer is more likely to help the victim than to punish the perpetrator
May 16, 2024

Genetics, environment and health disparities linked to increased stress and mental health challenges during adolescence

Why do we overindulge? Study explores how distraction affects 'hedonic consumption'

Scientists want to know how the smells of nature benefit our health
May 15, 2024
Let us know if there is a problem with our content
Use this form if you have come across a typo, inaccuracy or would like to send an edit request for the content on this page. For general inquiries, please use our contact form . For general feedback, use the public comments section below (please adhere to guidelines ).
Please select the most appropriate category to facilitate processing of your request
Thank you for taking time to provide your feedback to the editors.
Your feedback is important to us. However, we do not guarantee individual replies due to the high volume of messages.
E-mail the story
Your email address is used only to let the recipient know who sent the email. Neither your address nor the recipient's address will be used for any other purpose. The information you enter will appear in your e-mail message and is not retained by Medical Xpress in any form.
Newsletter sign up
Get weekly and/or daily updates delivered to your inbox. You can unsubscribe at any time and we'll never share your details to third parties.
More information Privacy policy
Donate and enjoy an ad-free experience
We keep our content available to everyone. Consider supporting Science X's mission by getting a premium account.
E-mail newsletter

- Walden University
- Faculty Portal
Scholarly Voice: Active and Passive Voice
Active and passive voice.
Active voice and passive voice are grammatical constructions that communicate certain information about an action. Specifically, APA explains that voice shows relationships between the verb and the subject and/or object (see APA 7, Section 4.13). Writers need to be intentional about voice in order to ensure clarity. Using active voice often improves clarity, while passive voice can help avoid unnecessary repetition.
Active voice can help ensure clarity by making it clear to the reader who is taking action in the sentence. In addition, the active voice stresses that the actor (or grammatical subject) precedes the verb, again, putting emphasis on the subject. Passive voice construction leaves out the actor (subject) and focuses on the relationship between the verb and object.
The order of words in a sentence with active voice is subject, verb, object.
- Active voice example : I conducted a study of elementary school teachers.
- This sentence structure puts the emphasis of the sentence on the subject, clarifying who conducted the study.
- Passive voice example : A study was conducted of elementary school teachers.
- In this sentence, it is not clear who conducted this study.
Generally, in scholarly writing, with its emphasis on precision and clarity, the active voice is preferred. However, the passive voice is acceptable in some instances, for example:
- if the reader is aware of who the actor is;
- in expository writing, where the goal of the discussion is to provide background, context, or an in-depth explanation;
- if the writer wants to focus on the object or the implications of the actor’s action; or
- to vary sentence structure.
Also, much like for anthropomorphism , different writing styles have different preferences. So, though you may see the passive voice used heavily in articles that you read for your courses and study, it does not mean that APA style advocates the same usage.
Examples of Writing in the Active Voice
Here are some examples of scholarly writing in the active voice:
- This is active voice because the subject in the sentence precedes the verb, clearly indicating who (I) will take the action (present).
Example : Teachers conducted a pilot study addressing the validity of the TAKS exam.
- Similarly, teachers (subject) clearly took the action (conducted) in this sentence.
Recognizing the Passive Voice
According to APA, writers should select verb tenses and voice carefully. Consider these examples to help determine which form of the verb is most appropriate:
Example : A study was conducted of job satisfaction and turnover.
- Here, it is not clear who did the conducting. In this case, if the context of the paragraph does not clarify who did the action, the writer should revise this sentence to clarify who conducted the study.
Example : I conducted a study of job satisfaction and turnover.
- This revised sentence clearly indicates the action taker. Using “I” to identify the writer’s role in the research process is often a solution to the passive voice and is encouraged by APA style (see APA 7, Section 4.16).
Using the past tense of the verb “to be” and the past participle of a verb together is often an indication of the passive voice. Here are some signs to look for in your paper:
- Example : This study was conducted.
- Example : Findings were distributed.
Another indication of passive voice is when the verb precedes the actor in the sentence. Even if the action taker is clearly identified in a passive voice construction, the sentence is usually wordier. Making the actor the grammatical subject that comes before the verb helps to streamline the sentence.
- Issue : Though the verb and the actor (action taker) are clearly identified here, to improve clarity and word economy, the writer could place that actor, Rogers, before the verb.
- More concise active voice revision : Rogers (2016) conducted a study on nursing and turnover.
- Issue : Here, the actor follows the verb, which reduces emphasis and clarity.
- This revised sentence is in the active voice and makes the actor the subject of the sentence.
Intentional Use of the Passive Voice
Sometimes, even in scholarly writing, the passive voice may be used intentionally and strategically. A writer may intentionally include the subject later in the sentence so as to reduce the emphasis and/or importance of the subject in the sentence. See the following examples of intentional passive voice to indicate emphasis:
Example : Schools not meeting AYP for 2 consecutive years will be placed on a “needs improvement” list by the State’s Department of Education.
- Here, all actors taking actions are identified, but this is in the passive voice as the State’s Department of Education is the actor doing the placing, but this verb precedes the actor. This may be an intentional use of the passive voice, to highlight schools not meeting AYP.
- To write this in the active voice, it would be phrased: “The State’s Department of Education will place schools not meeting AYP for 2 consecutive years on a “needs improvement” list. This sentence places the focus on the State’s Department of Education, not the schools.
Example : Participants in the study were incentivized with a $5 coffee gift card, which I gave them upon completion of their interview.
- As the writer and researcher, I may want to vary my sentence structure in order to avoid beginning several sentences with “I provided…” This example is written in the passive voice, but the meaning is clear.
Using Passive Voice in Scholarly Writing
As noted before, passive voice is allowed in APA style and can be quite appropriate, especially when writing about methods and data collection. However, students often overuse the passive voice in their writing, which means their emphasis in the sentence is not on the action taker. Their writing is also at risk of being repetitive. Consider the following paragraph in which the passive voice is used in each sentence:
A survey was administered . Using a convenience sample, 68 teachers were invited to participate in the survey by emailing them an invitation. E-mail addresses of teachers who fit the requirements for participation were provided by the principal of the school . The teachers were e-mailed an information sheet and a consent form. Responses were collected from 45 teachers… As you can see, the reader has no idea who is performing these actions, which makes the research process unclear. This is at odds with the goal of the methods discussion, which is to be clear and succinct regarding the process of data collection and analysis.
However, if translated entirely to the active voice, clearly indicating the researcher’s role, “I” becomes redundant and repetitive, interrupting the flow of the paragraph:
In this study, I administered a survey. I created a convenience sample of 68 teachers. I invited them to participate in the survey by emailing them an invitation. I obtained e-mail addresses from the principal of the school… “I” is quite redundant here and repetitive for the reader.
The Walden Writing Center suggests that students use “I” in the first sentence of the paragraph . Then, as long as it is clear to the reader that the student (writer) is the actor in the remaining sentences, use the active and passive voices appropriately to achieve precision and clarity (where applicable):
In this study, I administered a survey using a convenience sample. Sixty-eight teachers were invited to participate in the survey. The principal of the school provided me with the e-mail addresses of teachers who fit the requirements for participation. I e-mailed the teachers an information sheet and a consent form. A total of 45 teachers responded …
The use of the passive voice is complicated and requires careful attention and skill. There are no hard-and-fast rules. Using these guidelines, however, should help writers be clearer and more engaging in their writing, as well as achieving the intended purposes.
Remember, use voice strategically. APA recommends the active voice for clarity. However, the passive voice may be used, with intention, to remove the emphasis on the subject and also as a method for varying sentence structure. So, generally write in the active voice, but consider some of the above examples and some uses of the passive voice that may be useful to implement in your writing. Just be sure that the reader is always aware of who is taking the action of the verb.
- For more practice, try our Clarifying the Actor module .
Related Resources

- Principles of Writing: Active and Passive Voice (blog post) APA Style Blog post.

Didn't find what you need? Email us at [email protected] .
- Previous Page: Avoiding Bias
- Next Page: Word Choice (Diction)
- Office of Student Disability Services
Walden Resources
Departments.
- Academic Residencies
- Academic Skills
- Career Planning and Development
- Customer Care Team
- Field Experience
- Military Services
- Student Success Advising
- Writing Skills
Centers and Offices
- Center for Social Change
- Office of Academic Support and Instructional Services
- Office of Degree Acceleration
- Office of Research and Doctoral Services
- Office of Student Affairs
Student Resources
- Doctoral Writing Assessment
- Form & Style Review
- Quick Answers
- ScholarWorks
- SKIL Courses and Workshops
- Walden Bookstore
- Walden Catalog & Student Handbook
- Student Safety/Title IX
- Legal & Consumer Information
- Website Terms and Conditions
- Cookie Policy
- Accessibility
- Accreditation
- State Authorization
- Net Price Calculator
- Contact Walden
Walden University is a member of Adtalem Global Education, Inc. www.adtalem.com Walden University is certified to operate by SCHEV © 2024 Walden University LLC. All rights reserved.
MIT Technology Review
- Newsletters
OpenAI’s new GPT-4o lets people interact using voice or video in the same model
The company’s new free flagship “omnimodel” looks like a supercharged version of assistants like Siri or Alexa.
- James O'Donnell archive page
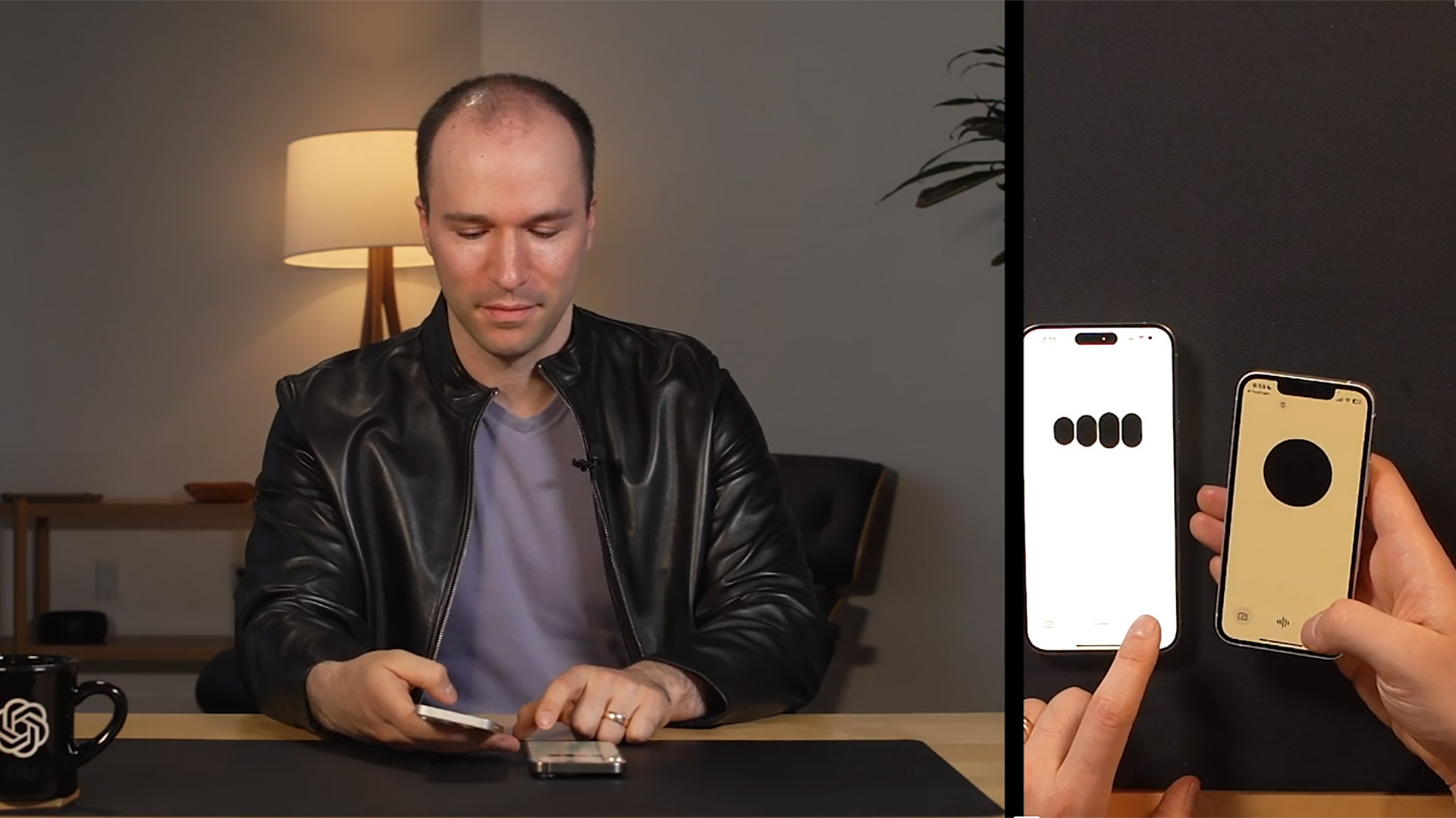
OpenAI just debuted GPT-4o, a new kind of AI model that you can communicate with in real time via live voice conversation, video streams from your phone, and text. The model is rolling out over the next few weeks and will be free for all users through both the GPT app and the web interface, according to the company. Users who subscribe to OpenAI’s paid tiers, which start at $20 per month, will be able to make more requests.
OpenAI CTO Mira Murati led the live demonstration of the new release one day before Google is expected to unveil its own AI advancements at its flagship I/O conference on Tuesday, May 14.
GPT-4 offered similar capabilities, giving users multiple ways to interact with OpenAI’s AI offerings. But it siloed them in separate models, leading to longer response times and presumably higher computing costs. GPT-4o has now merged those capabilities into a single model, which Murati called an “omnimodel.” That means faster responses and smoother transitions between tasks, she said.
The result, the company’s demonstration suggests, is a conversational assistant much in the vein of Siri or Alexa but capable of fielding much more complex prompts.
“We’re looking at the future of interaction between ourselves and the machines,” Murati said of the demo. “We think that GPT-4o is really shifting that paradigm into the future of collaboration, where this interaction becomes much more natural.”
Barret Zoph and Mark Chen, both researchers at OpenAI, walked through a number of applications for the new model. Most impressive was its facility with live conversation. You could interrupt the model during its responses, and it would stop, listen, and adjust course.
OpenAI showed off the ability to change the model’s tone, too. Chen asked the model to read a bedtime story “about robots and love,” quickly jumping in to demand a more dramatic voice. The model got progressively more theatrical until Murati demanded that it pivot quickly to a convincing robot voice (which it excelled at). While there were predictably some short pauses during the conversation while the model reasoned through what to say next, it stood out as a remarkably naturally paced AI conversation.
The model can reason through visual problems in real time as well. Using his phone, Zoph filmed himself writing an algebra equation (3 x + 1 = 4) on a sheet of paper, having GPT-4o follow along. He instructed it not to provide answers, but instead to guide him much as a teacher would.
“The first step is to get all the terms with x on one side,” the model said in a friendly tone. “So, what do you think we should do with that plus one?”
Like previous generations of GPT, GPT-4o will store records of users’ interactions with it, meaning the model “has a sense of continuity across all your conversations,” according to Murati. Other new highlights include live translation, the ability to search through your conversations with the model, and the power to look up information in real time.
As is the nature of a live demo, there were hiccups and glitches. GPT-4o’s voice might jump in awkwardly during the conversation. It appeared to comment on one of the presenters’ outfits even though it wasn’t asked to. But it recovered well when the demonstrators told the model it had erred. It seems to be able to respond quickly and helpfully across several mediums that other models have not yet merged as effectively.
Previously, many of OpenAI’s most powerful features, like reasoning through image and video, were behind a paywall. GPT-4o marks the first time they’ll be opened up to the wider public, though it’s not yet clear how many interactions you’ll be able to have with the model before being charged. OpenAI says paying subscribers will “continue to have up to five times the capacity limits of our free users.”
Additional reporting by Will Douglas Heaven.
Artificial intelligence
Sam altman says helpful agents are poised to become ai’s killer function.
Open AI’s CEO says we won’t need new hardware or lots more training data to get there.
Is robotics about to have its own ChatGPT moment?
Researchers are using generative AI and other techniques to teach robots new skills—including tasks they could perform in homes.
- Melissa Heikkilä archive page
What’s next for generative video
OpenAI's Sora has raised the bar for AI moviemaking. Here are four things to bear in mind as we wrap our heads around what's coming.
- Will Douglas Heaven archive page
An AI startup made a hyperrealistic deepfake of me that’s so good it’s scary
Synthesia's new technology is impressive but raises big questions about a world where we increasingly can’t tell what’s real.
Stay connected
Get the latest updates from mit technology review.
Discover special offers, top stories, upcoming events, and more.
Thank you for submitting your email!
It looks like something went wrong.
We’re having trouble saving your preferences. Try refreshing this page and updating them one more time. If you continue to get this message, reach out to us at [email protected] with a list of newsletters you’d like to receive.
Advertisement
Supported by
OpenAI Unveils New ChatGPT That Listens, Looks and Talks
Chatbots, image generators and voice assistants are gradually merging into a single technology with a conversational voice.
- Share full article

By Cade Metz
Reporting from San Francisco
As Apple and Google transform their voice assistants into chatbots, OpenAI is transforming its chatbot into a voice assistant.
On Monday, the San Francisco artificial intelligence start-up unveiled a new version of its ChatGPT chatbot that can receive and respond to voice commands, images and videos.
The company said the new app — based on an A.I. system called GPT-4o — juggles audio, images and video significantly faster than previous versions of the technology. The app will be available starting on Monday, free of charge, for both smartphones and desktop computers.
“We are looking at the future of the interaction between ourselves and machines,” said Mira Murati, the company’s chief technology officer.
The new app is part of a wider effort to combine conversational chatbots like ChatGPT with voice assistants like the Google Assistant and Apple’s Siri. As Google merges its Gemini chatbot with the Google Assistant, Apple is preparing a new version of Siri that is more conversational.
OpenAI said it would gradually share the technology with users “over the coming weeks.” This is the first time it has offered ChatGPT as a desktop application.
The company previously offered similar technologies from inside various free and paid products. Now, it has rolled them into a single system that is available across all its products.
During an event streamed on the internet, Ms. Murati and her colleagues showed off the new app as it responded to conversational voice commands, used a live video feed to analyze math problems written on a sheet of paper and read aloud playful stories that it had written on the fly.
The new app cannot generate video. But it can generate still images that represent frames of a video.
With the debut of ChatGPT in late 2022 , OpenAI showed that machines can handle requests more like people. In response to conversational text prompts, it could answer questions, write term papers and even generate computer code.
ChatGPT was not driven by a set of rules. It learned its skills by analyzing enormous amounts of text culled from across the internet, including Wikipedia articles, books and chat logs. Experts hailed the technology as a possible alterative to search engines like Google and voice assistants like Siri.
Newer versions of the technology have also learned from sounds, images and video. Researchers call this “multimodal A.I.” Essentially, companies like OpenAI began to combine chatbots with A.I. image , audio and video generators.
(The New York Times sued OpenAI and its partner, Microsoft, in December, claiming copyright infringement of news content related to A.I. systems.)
As companies combine chatbots with voice assistants, many hurdles remain. Because chatbots learn their skills from internet data, they are prone to mistakes. Sometimes, they make up information entirely — a phenomenon that A.I. researchers call “ hallucination .” Those flaws are migrating into voice assistants.
While chatbots can generate convincing language, they are less adept at taking actions like scheduling a meeting or booking a plane flight. But companies like OpenAI are working to transform them into “ A.I. agents ” that can reliably handle such tasks.
OpenAI previously offered a version of ChatGPT that could accept voice commands and respond with voice. But it was a patchwork of three different A.I. technologies: one that converted voice to text, one that generated a text response and one that converted this text into a synthetic voice.
The new app is based on a single A.I. technology — GPT-4o — that can accept and generate text, sounds and images. This means that the technology is more efficient, and the company can afford to offer it to users for free, Ms. Murati said.
“Before, you had all this latency that was the result of three models working together,” Ms. Murati said in an interview with The Times. “You want to have the experience we’re having — where we can have this very natural dialogue.”
An earlier version of this article misstated the day when OpenAI introduced its new version of ChatGPT. It was Monday, not Tuesday.
How we handle corrections
Cade Metz writes about artificial intelligence, driverless cars, robotics, virtual reality and other emerging areas of technology. More about Cade Metz
Explore Our Coverage of Artificial Intelligence
News and Analysis
Ilya Sutskever, the OpenAI co-founder and chief scientist who in November joined three other board members to force out Sam Altman before saying he regretted the move, is leaving the company .
OpenAI has unveiled a new version of its ChatGPT chatbot that can receive and respond to voice commands, images and videos.
A bipartisan group of senators released a long-awaited legislative plan for A.I. , calling for billions in funding to propel U.S. leadership in the technology while offering few details on regulations.
The Age of A.I.
D’Youville University in Buffalo had an A.I. robot speak at its commencement . Not everyone was happy about it.
A new program, backed by Cornell Tech, M.I.T. and U.C.L.A., helps prepare lower-income, Latina and Black female computing majors for A.I. careers.
Publishers have long worried that A.I.-generated answers on Google would drive readers away from their sites. They’re about to find out if those fears are warranted, our tech columnist writes .
A new category of apps promises to relieve parents of drudgery, with an assist from A.I. But a family’s grunt work is more human, and valuable, than it seems.
Suggestions or feedback?
MIT News | Massachusetts Institute of Technology
- Machine learning
- Social justice
- Black holes
- Classes and programs
Departments
- Aeronautics and Astronautics
- Brain and Cognitive Sciences
- Architecture
- Political Science
- Mechanical Engineering
Centers, Labs, & Programs
- Abdul Latif Jameel Poverty Action Lab (J-PAL)
- Picower Institute for Learning and Memory
- Lincoln Laboratory
- School of Architecture + Planning
- School of Engineering
- School of Humanities, Arts, and Social Sciences
- Sloan School of Management
- School of Science
- MIT Schwarzman College of Computing
Using ideas from game theory to improve the reliability of language models
Press contact :.
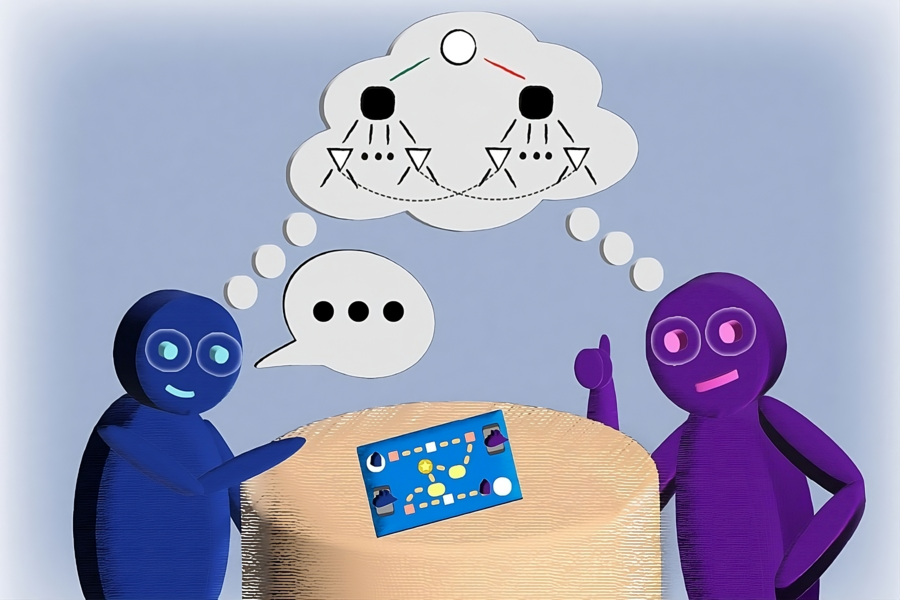
Previous image Next image
Imagine you and a friend are playing a game where your goal is to communicate secret messages to each other using only cryptic sentences. Your friend's job is to guess the secret message behind your sentences. Sometimes, you give clues directly, and other times, your friend has to guess the message by asking yes-or-no questions about the clues you've given. The challenge is that both of you want to make sure you're understanding each other correctly and agreeing on the secret message.
MIT Computer Science and Artificial Intelligence Laboratory (CSAIL) researchers have created a similar "game" to help improve how AI understands and generates text. It is known as a “consensus game” and it involves two parts of an AI system — one part tries to generate sentences (like giving clues), and the other part tries to understand and evaluate those sentences (like guessing the secret message).
The researchers discovered that by treating this interaction as a game, where both parts of the AI work together under specific rules to agree on the right message, they could significantly improve the AI's ability to give correct and coherent answers to questions. They tested this new game-like approach on a variety of tasks, such as reading comprehension, solving math problems, and carrying on conversations, and found that it helped the AI perform better across the board.
Traditionally, large language models answer one of two ways: generating answers directly from the model (generative querying) or using the model to score a set of predefined answers (discriminative querying), which can lead to differing and sometimes incompatible results. With the generative approach, "Who is the president of the United States?" might yield a straightforward answer like "Joe Biden." However, a discriminative query could incorrectly dispute this fact when evaluating the same answer, such as "Barack Obama."
So, how do we reconcile mutually incompatible scoring procedures to achieve coherent, efficient predictions?
"Imagine a new way to help language models understand and generate text, like a game. We've developed a training-free, game-theoretic method that treats the whole process as a complex game of clues and signals, where a generator tries to send the right message to a discriminator using natural language. Instead of chess pieces, they're using words and sentences," says Athul Jacob, an MIT PhD student in electrical engineering and computer science and CSAIL affiliate. "Our way to navigate this game is finding the 'approximate equilibria,' leading to a new decoding algorithm called 'equilibrium ranking.' It's a pretty exciting demonstration of how bringing game-theoretic strategies into the mix can tackle some big challenges in making language models more reliable and consistent."
When tested across many tasks, like reading comprehension, commonsense reasoning, math problem-solving, and dialogue, the team's algorithm consistently improved how well these models performed. Using the ER algorithm with the LLaMA-7B model even outshone the results from much larger models. "Given that they are already competitive, that people have been working on it for a while, but the level of improvements we saw being able to outperform a model that's 10 times the size was a pleasant surprise," says Jacob.
"Diplomacy," a strategic board game set in pre-World War I Europe, where players negotiate alliances, betray friends, and conquer territories without the use of dice — relying purely on skill, strategy, and interpersonal manipulation — recently had a second coming. In November 2022, computer scientists, including Jacob, developed “Cicero,” an AI agent that achieves human-level capabilities in the mixed-motive seven-player game, which requires the same aforementioned skills, but with natural language. The math behind this partially inspired the Consensus Game.
While the history of AI agents long predates when OpenAI's software entered the chat in November 2022, it's well documented that they can still cosplay as your well-meaning, yet pathological friend.
The consensus game system reaches equilibrium as an agreement, ensuring accuracy and fidelity to the model's original insights. To achieve this, the method iteratively adjusts the interactions between the generative and discriminative components until they reach a consensus on an answer that accurately reflects reality and aligns with their initial beliefs. This approach effectively bridges the gap between the two querying methods.
In practice, implementing the consensus game approach to language model querying, especially for question-answering tasks, does involve significant computational challenges. For example, when using datasets like MMLU, which have thousands of questions and multiple-choice answers, the model must apply the mechanism to each query. Then, it must reach a consensus between the generative and discriminative components for every question and its possible answers.
The system did struggle with a grade school right of passage: math word problems. It couldn't generate wrong answers, which is a critical component of understanding the process of coming up with the right one.
“The last few years have seen really impressive progress in both strategic decision-making and language generation from AI systems, but we’re just starting to figure out how to put the two together. Equilibrium ranking is a first step in this direction, but I think there’s a lot we’ll be able to do to scale this up to more complex problems,” says Jacob.
An avenue of future work involves enhancing the base model by integrating the outputs of the current method. This is particularly promising since it can yield more factual and consistent answers across various tasks, including factuality and open-ended generation. The potential for such a method to significantly improve the base model's performance is high, which could result in more reliable and factual outputs from ChatGPT and similar language models that people use daily.
"Even though modern language models, such as ChatGPT and Gemini, have led to solving various tasks through chat interfaces, the statistical decoding process that generates a response from such models has remained unchanged for decades," says Google Research Scientist Ahmad Beirami, who was not involved in the work. "The proposal by the MIT researchers is an innovative game-theoretic framework for decoding from language models through solving the equilibrium of a consensus game. The significant performance gains reported in the research paper are promising, opening the door to a potential paradigm shift in language model decoding that may fuel a flurry of new applications."
Jacob wrote the paper with MIT-IBM Watson Lab researcher Yikang Shen and MIT Department of Electrical Engineering and Computer Science assistant professors Gabriele Farina and Jacob Andreas, who is also a CSAIL member. They presented their work at the International Conference on Learning Representations (ICLR) earlier this month, where it was highlighted as a "spotlight paper." The research also received a “best paper award” at the NeurIPS R0-FoMo Workshop in December 2023.
Share this news article on:
Press mentions, quanta magazine.
MIT researchers have developed a new procedure that uses game theory to improve the accuracy and consistency of large language models (LLMs), reports Steve Nadis for Quanta Magazine . “The new work, which uses games to improve AI, stands in contrast to past approaches, which measured an AI program’s success via its mastery of games,” explains Nadis.
Previous item Next item
Related Links
- Article: "Game Theory Can Make AI More Correct and Efficient"
- Jacob Andreas
- Athul Paul Jacob
- Language & Intelligence @ MIT
- Computer Science and Artificial Intelligence Laboratory (CSAIL)
- Department of Electrical Engineering and Computer Science
- MIT-IBM Watson AI Lab
Related Topics
- Computer science and technology
- Artificial intelligence
- Human-computer interaction
- Natural language processing
- Game theory
- Electrical Engineering & Computer Science (eecs)
Related Articles
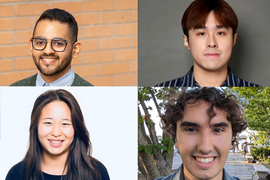
Reasoning and reliability in AI
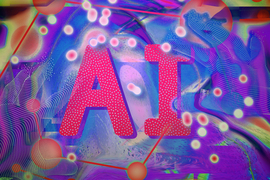
Explained: Generative AI
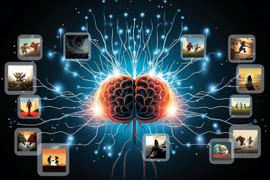
Synthetic imagery sets new bar in AI training efficiency
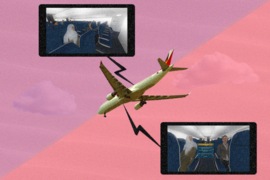
Simulating discrimination in virtual reality
More mit news.
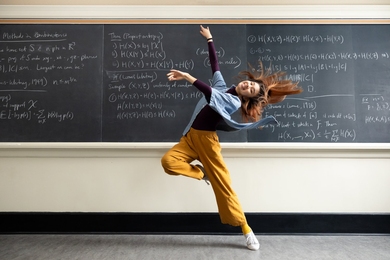
Janabel Xia: Algorithms, dance rhythms, and the drive to succeed
Read full story →
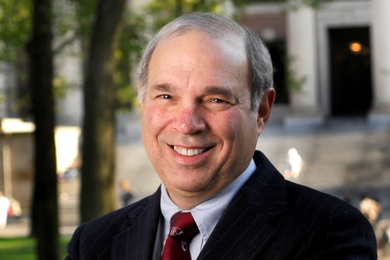
Jonathan Byrnes, MIT Center for Transportation and Logistics senior lecturer and visionary in supply chain management, dies at 75
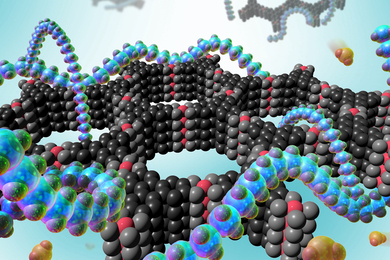
Researchers develop a detector for continuously monitoring toxic gases
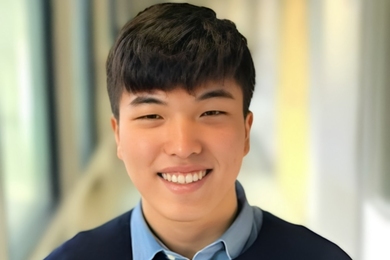
The beauty of biology
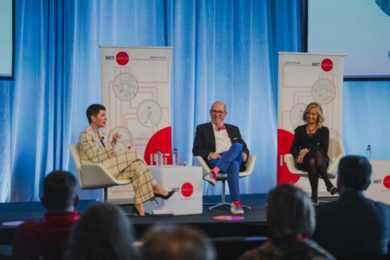
Navigating longevity with industry leaders at MIT AgeLab PLAN Forum
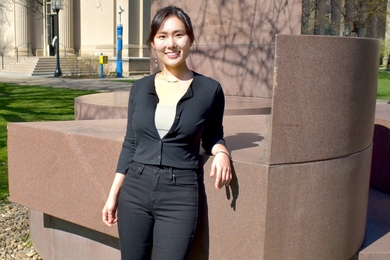
Jeong Min Park earns 2024 Schmidt Science Fellowship
- More news on MIT News homepage →
Massachusetts Institute of Technology 77 Massachusetts Avenue, Cambridge, MA, USA
- Map (opens in new window)
- Events (opens in new window)
- People (opens in new window)
- Careers (opens in new window)
- Accessibility
- Social Media Hub
- MIT on Facebook
- MIT on YouTube
- MIT on Instagram

People without an inner voice have poorer verbal memory
Between 5-10 per cent of the population do not experience an inner voice.
The vast majority of people have an ongoing conversation with themselves, an inner voice, that plays an important role in their daily lives. But between 5-10 per cent of the population do not have the same experience of an inner voice, and they find it more difficult to perform certain verbal memory tasks, new research shows.
Previously, it was commonly assumed that having an inner voice had to be a human universal. But in recent years, researchers have become aware that not all people share this experience.
According to postdoc and linguist Johanne Nedergård from the University of Copenhagen, people describe the condition of living without an inner voice as time-consuming and difficult because they must spend time and effort translating their thoughts into words:
"Some say that they think in pictures and then translate the pictures into words when they need to say something. Others describe their brain as a well-functioning computer that just does not process thoughts verbally, and that the connection to loudspeaker and microphone is different from other people's. And those who say that there is something verbal going on inside their heads will typically describe it as words without sound."
Harder to remember words and rhymes
Johanne Nedergård and her colleague Gary Lupyan from the University of Wisconsin-Madison are the first researchers in the world to investigate whether the lack of an inner voice, or anendophasia as they have coined the condition, has any consequences for how these people solve problems, for example how they perform verbal memory tasks.
People who reported that they either experienced a high degree of inner voice or very little inner voice in everyday life were subjected to one experiment that aimed to determine whether there was a difference in their ability to remember language input and one about their ability to find rhyme words. The first experiment involved the participants remembering words in order -- words that were similar, either phonetically or in spelling, e.g. "bought," "caught," "taut" and "wart."
"It is a task that will be difficult for everyone, but our hypothesis was that it might be even more difficult if you did not have an inner voice because you have to repeat the words to yourself inside your head in order to remember them," Johanne Nedergård explains and continues:
"And this hypothesis turned out to be true: The participants without an inner voice were significantly worse at remembering the words. The same applied to an assignment in which the participants had to determine whether a pair of pictures contained words that rhyme, e.g. pictures of a sock and a clock. Here, too, it is crucial to be able to repeat the words in order to compare their sounds and thus determine whether they rhyme."
In two other experiments, in which Johanne Nedergård and Gary Lupyan tested the role of the inner voice in switching quickly between different tasks and distinguishing between figures that are very similar, they did not find any differences between the two groups. Despite the fact that previous studies indicate that language and the inner voice play a role in these types of experiments.
"Maybe people who don't have an inner voice have just learned to use other strategies. For example, some said that they tapped with their index finger when performing one type of task and with their middle finger when it was another type of task," Johanne Nedergård says.
The results of the two researchers' study have just been published in the article "Not everybody has an inner voice: Behavioural consequences of anendophasia" in the scientific journal Psychological Science .
Does it make a difference?
According to Johanne Nedergård, the differences in verbal memory that they have identified in their experiments will not be noticed in ordinary everyday conversations. And the question is, does not having an inner voice hold any practical or behavioural significance?
"The short answer is that we just don't know because we have only just begun to study it. But there is one field where we suspect that having an inner voice plays a role, and that is therapy; in the widely used cognitive behavioural therapy, for example, you need to identify and change adverse thought patterns, and having an inner voice may be very important in such a process. However, it is still uncertain whether differences in the experience of an inner voice are related to how people respond to different types of therapy," says Johanne Nedergård, who would like to continue her research to find out whether other language areas are affected if you do not have an inner voice.
"The experiments in which we found differences between the groups were about sound and being able to hear the words for themselves. I would like to study whether it is because they just do not experience the sound aspect of language, or whether they do not think at all in a linguistic format like most other people," she concludes.
About the study
Johanne Nedergård's and Gary Lupyan's study comprised almost a hundred participants, half of whom experienced having very little inner voice and the other half very much inner voice.
The participants were subjected to four experiments, e.g. remembering words in sequence and switching between different tasks. The study has been published in the scientific journal Psychological Science.
Johanne Nedergård and Gary Lupyan have dubbed the condition of having no inner voice anendophasia, which means without an inner voice.
- Language Acquisition
- Child Development
- Infant and Preschool Learning
- Intelligence
- Limbic system
- Left-handed
- Consumerism
- Memory-prediction framework
- Obsessive-compulsive personality disorder
- Double blind
Story Source:
Materials provided by University of Copenhagen - Faculty of Humanities . Note: Content may be edited for style and length.
Journal Reference :
- Johanne S. K. Nedergaard, Gary Lupyan. Not Everybody Has an Inner Voice: Behavioral Consequences of Anendophasia . Psychological Science , 2024; DOI: 10.1177/09567976241243004
Cite This Page :
Explore More
- High-Efficiency Photonic Integrated Circuit
- Life Expectancy May Increase by 5 Years by 2050
- Toward a Successful Vaccine for HIV
- Highly Efficient Thermoelectric Materials
- Toward Human Brain Gene Therapy
- Whale Families Learn Each Other's Vocal Style
- AI Can Answer Complex Physics Questions
- Otters Use Tools to Survive a Changing World
- Monogamy in Mice: Newly Evolved Type of Cell
- Sustainable Electronics, Doped With Air
Trending Topics
Strange & offbeat.
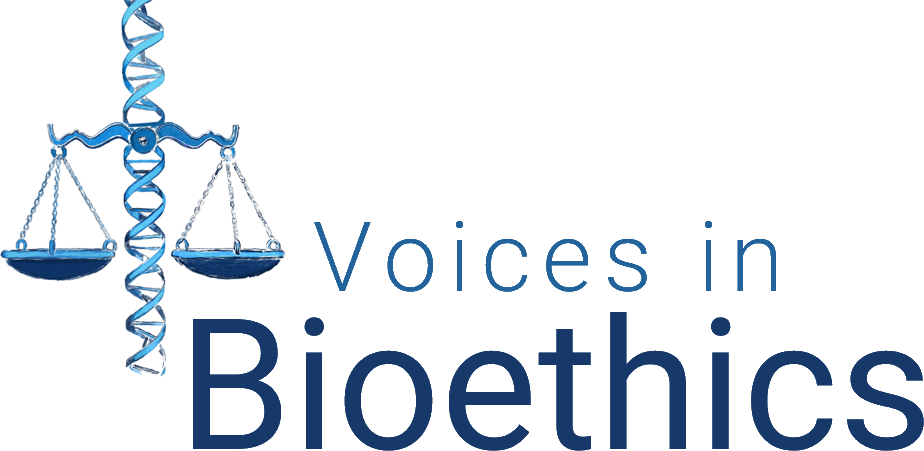
Cultural Relativity and Acceptance of Embryonic Stem Cell Research
Article sidebar.
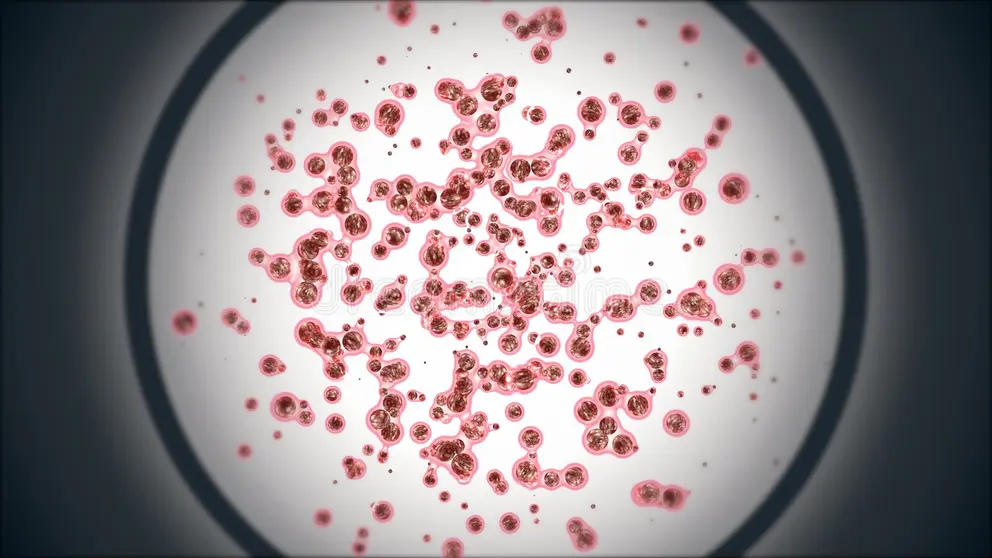
Main Article Content
There is a debate about the ethical implications of using human embryos in stem cell research, which can be influenced by cultural, moral, and social values. This paper argues for an adaptable framework to accommodate diverse cultural and religious perspectives. By using an adaptive ethics model, research protections can reflect various populations and foster growth in stem cell research possibilities.
INTRODUCTION
Stem cell research combines biology, medicine, and technology, promising to alter health care and the understanding of human development. Yet, ethical contention exists because of individuals’ perceptions of using human embryos based on their various cultural, moral, and social values. While these disagreements concerning policy, use, and general acceptance have prompted the development of an international ethics policy, such a uniform approach can overlook the nuanced ethical landscapes between cultures. With diverse viewpoints in public health, a single global policy, especially one reflecting Western ethics or the ethics prevalent in high-income countries, is impractical. This paper argues for a culturally sensitive, adaptable framework for the use of embryonic stem cells. Stem cell policy should accommodate varying ethical viewpoints and promote an effective global dialogue. With an extension of an ethics model that can adapt to various cultures, we recommend localized guidelines that reflect the moral views of the people those guidelines serve.
Stem cells, characterized by their unique ability to differentiate into various cell types, enable the repair or replacement of damaged tissues. Two primary types of stem cells are somatic stem cells (adult stem cells) and embryonic stem cells. Adult stem cells exist in developed tissues and maintain the body’s repair processes. [1] Embryonic stem cells (ESC) are remarkably pluripotent or versatile, making them valuable in research. [2] However, the use of ESCs has sparked ethics debates. Considering the potential of embryonic stem cells, research guidelines are essential. The International Society for Stem Cell Research (ISSCR) provides international stem cell research guidelines. They call for “public conversations touching on the scientific significance as well as the societal and ethical issues raised by ESC research.” [3] The ISSCR also publishes updates about culturing human embryos 14 days post fertilization, suggesting local policies and regulations should continue to evolve as ESC research develops. [4] Like the ISSCR, which calls for local law and policy to adapt to developing stem cell research given cultural acceptance, this paper highlights the importance of local social factors such as religion and culture.
I. Global Cultural Perspective of Embryonic Stem Cells
Views on ESCs vary throughout the world. Some countries readily embrace stem cell research and therapies, while others have stricter regulations due to ethical concerns surrounding embryonic stem cells and when an embryo becomes entitled to moral consideration. The philosophical issue of when the “someone” begins to be a human after fertilization, in the morally relevant sense, [5] impacts when an embryo becomes not just worthy of protection but morally entitled to it. The process of creating embryonic stem cell lines involves the destruction of the embryos for research. [6] Consequently, global engagement in ESC research depends on social-cultural acceptability.
a. US and Rights-Based Cultures
In the United States, attitudes toward stem cell therapies are diverse. The ethics and social approaches, which value individualism, [7] trigger debates regarding the destruction of human embryos, creating a complex regulatory environment. For example, the 1996 Dickey-Wicker Amendment prohibited federal funding for the creation of embryos for research and the destruction of embryos for “more than allowed for research on fetuses in utero.” [8] Following suit, in 2001, the Bush Administration heavily restricted stem cell lines for research. However, the Stem Cell Research Enhancement Act of 2005 was proposed to help develop ESC research but was ultimately vetoed. [9] Under the Obama administration, in 2009, an executive order lifted restrictions allowing for more development in this field. [10] The flux of research capacity and funding parallels the different cultural perceptions of human dignity of the embryo and how it is socially presented within the country’s research culture. [11]
b. Ubuntu and Collective Cultures
African bioethics differs from Western individualism because of the different traditions and values. African traditions, as described by individuals from South Africa and supported by some studies in other African countries, including Ghana and Kenya, follow the African moral philosophies of Ubuntu or Botho and Ukama , which “advocates for a form of wholeness that comes through one’s relationship and connectedness with other people in the society,” [12] making autonomy a socially collective concept. In this context, for the community to act autonomously, individuals would come together to decide what is best for the collective. Thus, stem cell research would require examining the value of the research to society as a whole and the use of the embryos as a collective societal resource. If society views the source as part of the collective whole, and opposes using stem cells, compromising the cultural values to pursue research may cause social detachment and stunt research growth. [13] Based on local culture and moral philosophy, the permissibility of stem cell research depends on how embryo, stem cell, and cell line therapies relate to the community as a whole . Ubuntu is the expression of humanness, with the person’s identity drawn from the “’I am because we are’” value. [14] The decision in a collectivistic culture becomes one born of cultural context, and individual decisions give deference to others in the society.
Consent differs in cultures where thought and moral philosophy are based on a collective paradigm. So, applying Western bioethical concepts is unrealistic. For one, Africa is a diverse continent with many countries with different belief systems, access to health care, and reliance on traditional or Western medicines. Where traditional medicine is the primary treatment, the “’restrictive focus on biomedically-related bioethics’” [is] problematic in African contexts because it neglects bioethical issues raised by traditional systems.” [15] No single approach applies in all areas or contexts. Rather than evaluating the permissibility of ESC research according to Western concepts such as the four principles approach, different ethics approaches should prevail.
Another consideration is the socio-economic standing of countries. In parts of South Africa, researchers have not focused heavily on contributing to the stem cell discourse, either because it is not considered health care or a health science priority or because resources are unavailable. [16] Each country’s priorities differ given different social, political, and economic factors. In South Africa, for instance, areas such as maternal mortality, non-communicable diseases, telemedicine, and the strength of health systems need improvement and require more focus. [17] Stem cell research could benefit the population, but it also could divert resources from basic medical care. Researchers in South Africa adhere to the National Health Act and Medicines Control Act in South Africa and international guidelines; however, the Act is not strictly enforced, and there is no clear legislation for research conduct or ethical guidelines. [18]
Some parts of Africa condemn stem cell research. For example, 98.2 percent of the Tunisian population is Muslim. [19] Tunisia does not permit stem cell research because of moral conflict with a Fatwa. Religion heavily saturates the regulation and direction of research. [20] Stem cell use became permissible for reproductive purposes only recently, with tight restrictions preventing cells from being used in any research other than procedures concerning ART/IVF. Their use is conditioned on consent, and available only to married couples. [21] The community's receptiveness to stem cell research depends on including communitarian African ethics.
c. Asia
Some Asian countries also have a collective model of ethics and decision making. [22] In China, the ethics model promotes a sincere respect for life or human dignity, [23] based on protective medicine. This model, influenced by Traditional Chinese Medicine (TCM), [24] recognizes Qi as the vital energy delivered via the meridians of the body; it connects illness to body systems, the body’s entire constitution, and the universe for a holistic bond of nature, health, and quality of life. [25] Following a protective ethics model, and traditional customs of wholeness, investment in stem cell research is heavily desired for its applications in regenerative therapies, disease modeling, and protective medicines. In a survey of medical students and healthcare practitioners, 30.8 percent considered stem cell research morally unacceptable while 63.5 percent accepted medical research using human embryonic stem cells. Of these individuals, 89.9 percent supported increased funding for stem cell research. [26] The scientific community might not reflect the overall population. From 1997 to 2019, China spent a total of $576 million (USD) on stem cell research at 8,050 stem cell programs, increased published presence from 0.6 percent to 14.01 percent of total global stem cell publications as of 2014, and made significant strides in cell-based therapies for various medical conditions. [27] However, while China has made substantial investments in stem cell research and achieved notable progress in clinical applications, concerns linger regarding ethical oversight and transparency. [28] For example, the China Biosecurity Law, promoted by the National Health Commission and China Hospital Association, attempted to mitigate risks by introducing an institutional review board (IRB) in the regulatory bodies. 5800 IRBs registered with the Chinese Clinical Trial Registry since 2021. [29] However, issues still need to be addressed in implementing effective IRB review and approval procedures.
The substantial government funding and focus on scientific advancement have sometimes overshadowed considerations of regional cultures, ethnic minorities, and individual perspectives, particularly evident during the one-child policy era. As government policy adapts to promote public stability, such as the change from the one-child to the two-child policy, [30] research ethics should also adapt to ensure respect for the values of its represented peoples.
Japan is also relatively supportive of stem cell research and therapies. Japan has a more transparent regulatory framework, allowing for faster approval of regenerative medicine products, which has led to several advanced clinical trials and therapies. [31] South Korea is also actively engaged in stem cell research and has a history of breakthroughs in cloning and embryonic stem cells. [32] However, the field is controversial, and there are issues of scientific integrity. For example, the Korean FDA fast-tracked products for approval, [33] and in another instance, the oocyte source was unclear and possibly violated ethical standards. [34] Trust is important in research, as it builds collaborative foundations between colleagues, trial participant comfort, open-mindedness for complicated and sensitive discussions, and supports regulatory procedures for stakeholders. There is a need to respect the culture’s interest, engagement, and for research and clinical trials to be transparent and have ethical oversight to promote global research discourse and trust.
d. Middle East
Countries in the Middle East have varying degrees of acceptance of or restrictions to policies related to using embryonic stem cells due to cultural and religious influences. Saudi Arabia has made significant contributions to stem cell research, and conducts research based on international guidelines for ethical conduct and under strict adherence to guidelines in accordance with Islamic principles. Specifically, the Saudi government and people require ESC research to adhere to Sharia law. In addition to umbilical and placental stem cells, [35] Saudi Arabia permits the use of embryonic stem cells as long as they come from miscarriages, therapeutic abortions permissible by Sharia law, or are left over from in vitro fertilization and donated to research. [36] Laws and ethical guidelines for stem cell research allow the development of research institutions such as the King Abdullah International Medical Research Center, which has a cord blood bank and a stem cell registry with nearly 10,000 donors. [37] Such volume and acceptance are due to the ethical ‘permissibility’ of the donor sources, which do not conflict with religious pillars. However, some researchers err on the side of caution, choosing not to use embryos or fetal tissue as they feel it is unethical to do so. [38]
Jordan has a positive research ethics culture. [39] However, there is a significant issue of lack of trust in researchers, with 45.23 percent (38.66 percent agreeing and 6.57 percent strongly agreeing) of Jordanians holding a low level of trust in researchers, compared to 81.34 percent of Jordanians agreeing that they feel safe to participate in a research trial. [40] Safety testifies to the feeling of confidence that adequate measures are in place to protect participants from harm, whereas trust in researchers could represent the confidence in researchers to act in the participants’ best interests, adhere to ethical guidelines, provide accurate information, and respect participants’ rights and dignity. One method to improve trust would be to address communication issues relevant to ESC. Legislation surrounding stem cell research has adopted specific language, especially concerning clarification “between ‘stem cells’ and ‘embryonic stem cells’” in translation. [41] Furthermore, legislation “mandates the creation of a national committee… laying out specific regulations for stem-cell banking in accordance with international standards.” [42] This broad regulation opens the door for future global engagement and maintains transparency. However, these regulations may also constrain the influence of research direction, pace, and accessibility of research outcomes.
e. Europe
In the European Union (EU), ethics is also principle-based, but the principles of autonomy, dignity, integrity, and vulnerability are interconnected. [43] As such, the opportunity for cohesion and concessions between individuals’ thoughts and ideals allows for a more adaptable ethics model due to the flexible principles that relate to the human experience The EU has put forth a framework in its Convention for the Protection of Human Rights and Dignity of the Human Being allowing member states to take different approaches. Each European state applies these principles to its specific conventions, leading to or reflecting different acceptance levels of stem cell research. [44]
For example, in Germany, Lebenzusammenhang , or the coherence of life, references integrity in the unity of human culture. Namely, the personal sphere “should not be subject to external intervention.” [45] Stem cell interventions could affect this concept of bodily completeness, leading to heavy restrictions. Under the Grundgesetz, human dignity and the right to life with physical integrity are paramount. [46] The Embryo Protection Act of 1991 made producing cell lines illegal. Cell lines can be imported if approved by the Central Ethics Commission for Stem Cell Research only if they were derived before May 2007. [47] Stem cell research respects the integrity of life for the embryo with heavy specifications and intense oversight. This is vastly different in Finland, where the regulatory bodies find research more permissible in IVF excess, but only up to 14 days after fertilization. [48] Spain’s approach differs still, with a comprehensive regulatory framework. [49] Thus, research regulation can be culture-specific due to variations in applied principles. Diverse cultures call for various approaches to ethical permissibility. [50] Only an adaptive-deliberative model can address the cultural constructions of self and achieve positive, culturally sensitive stem cell research practices. [51]
II. Religious Perspectives on ESC
Embryonic stem cell sources are the main consideration within religious contexts. While individuals may not regard their own religious texts as authoritative or factual, religion can shape their foundations or perspectives.
The Qur'an states:
“And indeed We created man from a quintessence of clay. Then We placed within him a small quantity of nutfa (sperm to fertilize) in a safe place. Then We have fashioned the nutfa into an ‘alaqa (clinging clot or cell cluster), then We developed the ‘alaqa into mudgha (a lump of flesh), and We made mudgha into bones, and clothed the bones with flesh, then We brought it into being as a new creation. So Blessed is Allah, the Best of Creators.” [52]
Many scholars of Islam estimate the time of soul installment, marked by the angel breathing in the soul to bring the individual into creation, as 120 days from conception. [53] Personhood begins at this point, and the value of life would prohibit research or experimentation that could harm the individual. If the fetus is more than 120 days old, the time ensoulment is interpreted to occur according to Islamic law, abortion is no longer permissible. [54] There are a few opposing opinions about early embryos in Islamic traditions. According to some Islamic theologians, there is no ensoulment of the early embryo, which is the source of stem cells for ESC research. [55]
In Buddhism, the stance on stem cell research is not settled. The main tenets, the prohibition against harming or destroying others (ahimsa) and the pursuit of knowledge (prajña) and compassion (karuna), leave Buddhist scholars and communities divided. [56] Some scholars argue stem cell research is in accordance with the Buddhist tenet of seeking knowledge and ending human suffering. Others feel it violates the principle of not harming others. Finding the balance between these two points relies on the karmic burden of Buddhist morality. In trying to prevent ahimsa towards the embryo, Buddhist scholars suggest that to comply with Buddhist tenets, research cannot be done as the embryo has personhood at the moment of conception and would reincarnate immediately, harming the individual's ability to build their karmic burden. [57] On the other hand, the Bodhisattvas, those considered to be on the path to enlightenment or Nirvana, have given organs and flesh to others to help alleviate grieving and to benefit all. [58] Acceptance varies on applied beliefs and interpretations.
Catholicism does not support embryonic stem cell research, as it entails creation or destruction of human embryos. This destruction conflicts with the belief in the sanctity of life. For example, in the Old Testament, Genesis describes humanity as being created in God’s image and multiplying on the Earth, referencing the sacred rights to human conception and the purpose of development and life. In the Ten Commandments, the tenet that one should not kill has numerous interpretations where killing could mean murder or shedding of the sanctity of life, demonstrating the high value of human personhood. In other books, the theological conception of when life begins is interpreted as in utero, [59] highlighting the inviolability of life and its formation in vivo to make a religious point for accepting such research as relatively limited, if at all. [60] The Vatican has released ethical directives to help apply a theological basis to modern-day conflicts. The Magisterium of the Church states that “unless there is a moral certainty of not causing harm,” experimentation on fetuses, fertilized cells, stem cells, or embryos constitutes a crime. [61] Such procedures would not respect the human person who exists at these stages, according to Catholicism. Damages to the embryo are considered gravely immoral and illicit. [62] Although the Catholic Church officially opposes abortion, surveys demonstrate that many Catholic people hold pro-choice views, whether due to the context of conception, stage of pregnancy, threat to the mother’s life, or for other reasons, demonstrating that practicing members can also accept some but not all tenets. [63]
Some major Jewish denominations, such as the Reform, Conservative, and Reconstructionist movements, are open to supporting ESC use or research as long as it is for saving a life. [64] Within Judaism, the Talmud, or study, gives personhood to the child at birth and emphasizes that life does not begin at conception: [65]
“If she is found pregnant, until the fortieth day it is mere fluid,” [66]
Whereas most religions prioritize the status of human embryos, the Halakah (Jewish religious law) states that to save one life, most other religious laws can be ignored because it is in pursuit of preservation. [67] Stem cell research is accepted due to application of these religious laws.
We recognize that all religions contain subsets and sects. The variety of environmental and cultural differences within religious groups requires further analysis to respect the flexibility of religious thoughts and practices. We make no presumptions that all cultures require notions of autonomy or morality as under the common morality theory , which asserts a set of universal moral norms that all individuals share provides moral reasoning and guides ethical decisions. [68] We only wish to show that the interaction with morality varies between cultures and countries.
III. A Flexible Ethical Approach
The plurality of different moral approaches described above demonstrates that there can be no universally acceptable uniform law for ESC on a global scale. Instead of developing one standard, flexible ethical applications must be continued. We recommend local guidelines that incorporate important cultural and ethical priorities.
While the Declaration of Helsinki is more relevant to people in clinical trials receiving ESC products, in keeping with the tradition of protections for research subjects, consent of the donor is an ethical requirement for ESC donation in many jurisdictions including the US, Canada, and Europe. [69] The Declaration of Helsinki provides a reference point for regulatory standards and could potentially be used as a universal baseline for obtaining consent prior to gamete or embryo donation.
For instance, in Columbia University’s egg donor program for stem cell research, donors followed standard screening protocols and “underwent counseling sessions that included information as to the purpose of oocyte donation for research, what the oocytes would be used for, the risks and benefits of donation, and process of oocyte stimulation” to ensure transparency for consent. [70] The program helped advance stem cell research and provided clear and safe research methods with paid participants. Though paid participation or covering costs of incidental expenses may not be socially acceptable in every culture or context, [71] and creating embryos for ESC research is illegal in many jurisdictions, Columbia’s program was effective because of the clear and honest communications with donors, IRBs, and related stakeholders. This example demonstrates that cultural acceptance of scientific research and of the idea that an egg or embryo does not have personhood is likely behind societal acceptance of donating eggs for ESC research. As noted, many countries do not permit the creation of embryos for research.
Proper communication and education regarding the process and purpose of stem cell research may bolster comprehension and garner more acceptance. “Given the sensitive subject material, a complete consent process can support voluntary participation through trust, understanding, and ethical norms from the cultures and morals participants value. This can be hard for researchers entering countries of different socioeconomic stability, with different languages and different societal values. [72]
An adequate moral foundation in medical ethics is derived from the cultural and religious basis that informs knowledge and actions. [73] Understanding local cultural and religious values and their impact on research could help researchers develop humility and promote inclusion.
IV. Concerns
Some may argue that if researchers all adhere to one ethics standard, protection will be satisfied across all borders, and the global public will trust researchers. However, defining what needs to be protected and how to define such research standards is very specific to the people to which standards are applied. We suggest that applying one uniform guide cannot accurately protect each individual because we all possess our own perceptions and interpretations of social values. [74] Therefore, the issue of not adjusting to the moral pluralism between peoples in applying one standard of ethics can be resolved by building out ethics models that can be adapted to different cultures and religions.
Other concerns include medical tourism, which may promote health inequities. [75] Some countries may develop and approve products derived from ESC research before others, compromising research ethics or drug approval processes. There are also concerns about the sale of unauthorized stem cell treatments, for example, those without FDA approval in the United States. Countries with robust research infrastructures may be tempted to attract medical tourists, and some customers will have false hopes based on aggressive publicity of unproven treatments. [76]
For example, in China, stem cell clinics can market to foreign clients who are not protected under the regulatory regimes. Companies employ a marketing strategy of “ethically friendly” therapies. Specifically, in the case of Beike, China’s leading stem cell tourism company and sprouting network, ethical oversight of administrators or health bureaus at one site has “the unintended consequence of shifting questionable activities to another node in Beike's diffuse network.” [77] In contrast, Jordan is aware of stem cell research’s potential abuse and its own status as a “health-care hub.” Jordan’s expanded regulations include preserving the interests of individuals in clinical trials and banning private companies from ESC research to preserve transparency and the integrity of research practices. [78]
The social priorities of the community are also a concern. The ISSCR explicitly states that guidelines “should be periodically revised to accommodate scientific advances, new challenges, and evolving social priorities.” [79] The adaptable ethics model extends this consideration further by addressing whether research is warranted given the varying degrees of socioeconomic conditions, political stability, and healthcare accessibilities and limitations. An ethical approach would require discussion about resource allocation and appropriate distribution of funds. [80]
While some religions emphasize the sanctity of life from conception, which may lead to public opposition to ESC research, others encourage ESC research due to its potential for healing and alleviating human pain. Many countries have special regulations that balance local views on embryonic personhood, the benefits of research as individual or societal goods, and the protection of human research subjects. To foster understanding and constructive dialogue, global policy frameworks should prioritize the protection of universal human rights, transparency, and informed consent. In addition to these foundational global policies, we recommend tailoring local guidelines to reflect the diverse cultural and religious perspectives of the populations they govern. Ethics models should be adapted to local populations to effectively establish research protections, growth, and possibilities of stem cell research.
For example, in countries with strong beliefs in the moral sanctity of embryos or heavy religious restrictions, an adaptive model can allow for discussion instead of immediate rejection. In countries with limited individual rights and voice in science policy, an adaptive model ensures cultural, moral, and religious views are taken into consideration, thereby building social inclusion. While this ethical consideration by the government may not give a complete voice to every individual, it will help balance policies and maintain the diverse perspectives of those it affects. Embracing an adaptive ethics model of ESC research promotes open-minded dialogue and respect for the importance of human belief and tradition. By actively engaging with cultural and religious values, researchers can better handle disagreements and promote ethical research practices that benefit each society.
This brief exploration of the religious and cultural differences that impact ESC research reveals the nuances of relative ethics and highlights a need for local policymakers to apply a more intense adaptive model.
[1] Poliwoda, S., Noor, N., Downs, E., Schaaf, A., Cantwell, A., Ganti, L., Kaye, A. D., Mosel, L. I., Carroll, C. B., Viswanath, O., & Urits, I. (2022). Stem cells: a comprehensive review of origins and emerging clinical roles in medical practice. Orthopedic reviews , 14 (3), 37498. https://doi.org/10.52965/001c.37498
[2] Poliwoda, S., Noor, N., Downs, E., Schaaf, A., Cantwell, A., Ganti, L., Kaye, A. D., Mosel, L. I., Carroll, C. B., Viswanath, O., & Urits, I. (2022). Stem cells: a comprehensive review of origins and emerging clinical roles in medical practice. Orthopedic reviews , 14 (3), 37498. https://doi.org/10.52965/001c.37498
[3] International Society for Stem Cell Research. (2023). Laboratory-based human embryonic stem cell research, embryo research, and related research activities . International Society for Stem Cell Research. https://www.isscr.org/guidelines/blog-post-title-one-ed2td-6fcdk ; Kimmelman, J., Hyun, I., Benvenisty, N. et al. Policy: Global standards for stem-cell research. Nature 533 , 311–313 (2016). https://doi.org/10.1038/533311a
[4] International Society for Stem Cell Research. (2023). Laboratory-based human embryonic stem cell research, embryo research, and related research activities . International Society for Stem Cell Research. https://www.isscr.org/guidelines/blog-post-title-one-ed2td-6fcdk
[5] Concerning the moral philosophies of stem cell research, our paper does not posit a personal moral stance nor delve into the “when” of human life begins. To read further about the philosophical debate, consider the following sources:
Sandel M. J. (2004). Embryo ethics--the moral logic of stem-cell research. The New England journal of medicine , 351 (3), 207–209. https://doi.org/10.1056/NEJMp048145 ; George, R. P., & Lee, P. (2020, September 26). Acorns and Embryos . The New Atlantis. https://www.thenewatlantis.com/publications/acorns-and-embryos ; Sagan, A., & Singer, P. (2007). The moral status of stem cells. Metaphilosophy , 38 (2/3), 264–284. http://www.jstor.org/stable/24439776 ; McHugh P. R. (2004). Zygote and "clonote"--the ethical use of embryonic stem cells. The New England journal of medicine , 351 (3), 209–211. https://doi.org/10.1056/NEJMp048147 ; Kurjak, A., & Tripalo, A. (2004). The facts and doubts about beginning of the human life and personality. Bosnian journal of basic medical sciences , 4 (1), 5–14. https://doi.org/10.17305/bjbms.2004.3453
[6] Vazin, T., & Freed, W. J. (2010). Human embryonic stem cells: derivation, culture, and differentiation: a review. Restorative neurology and neuroscience , 28 (4), 589–603. https://doi.org/10.3233/RNN-2010-0543
[7] Socially, at its core, the Western approach to ethics is widely principle-based, autonomy being one of the key factors to ensure a fundamental respect for persons within research. For information regarding autonomy in research, see: Department of Health, Education, and Welfare, & National Commission for the Protection of Human Subjects of Biomedical and Behavioral Research (1978). The Belmont Report. Ethical principles and guidelines for the protection of human subjects of research.; For a more in-depth review of autonomy within the US, see: Beauchamp, T. L., & Childress, J. F. (1994). Principles of Biomedical Ethics . Oxford University Press.
[8] Sherley v. Sebelius , 644 F.3d 388 (D.C. Cir. 2011), citing 45 C.F.R. 46.204(b) and [42 U.S.C. § 289g(b)]. https://www.cadc.uscourts.gov/internet/opinions.nsf/6c690438a9b43dd685257a64004ebf99/$file/11-5241-1391178.pdf
[9] Stem Cell Research Enhancement Act of 2005, H. R. 810, 109 th Cong. (2001). https://www.govtrack.us/congress/bills/109/hr810/text ; Bush, G. W. (2006, July 19). Message to the House of Representatives . National Archives and Records Administration. https://georgewbush-whitehouse.archives.gov/news/releases/2006/07/20060719-5.html
[10] National Archives and Records Administration. (2009, March 9). Executive order 13505 -- removing barriers to responsible scientific research involving human stem cells . National Archives and Records Administration. https://obamawhitehouse.archives.gov/the-press-office/removing-barriers-responsible-scientific-research-involving-human-stem-cells
[11] Hurlbut, W. B. (2006). Science, Religion, and the Politics of Stem Cells. Social Research , 73 (3), 819–834. http://www.jstor.org/stable/40971854
[12] Akpa-Inyang, Francis & Chima, Sylvester. (2021). South African traditional values and beliefs regarding informed consent and limitations of the principle of respect for autonomy in African communities: a cross-cultural qualitative study. BMC Medical Ethics . 22. 10.1186/s12910-021-00678-4.
[13] Source for further reading: Tangwa G. B. (2007). Moral status of embryonic stem cells: perspective of an African villager. Bioethics , 21(8), 449–457. https://doi.org/10.1111/j.1467-8519.2007.00582.x , see also Mnisi, F. M. (2020). An African analysis based on ethics of Ubuntu - are human embryonic stem cell patents morally justifiable? African Insight , 49 (4).
[14] Jecker, N. S., & Atuire, C. (2021). Bioethics in Africa: A contextually enlightened analysis of three cases. Developing World Bioethics , 22 (2), 112–122. https://doi.org/10.1111/dewb.12324
[15] Jecker, N. S., & Atuire, C. (2021). Bioethics in Africa: A contextually enlightened analysis of three cases. Developing World Bioethics, 22(2), 112–122. https://doi.org/10.1111/dewb.12324
[16] Jackson, C.S., Pepper, M.S. Opportunities and barriers to establishing a cell therapy programme in South Africa. Stem Cell Res Ther 4 , 54 (2013). https://doi.org/10.1186/scrt204 ; Pew Research Center. (2014, May 1). Public health a major priority in African nations . Pew Research Center’s Global Attitudes Project. https://www.pewresearch.org/global/2014/05/01/public-health-a-major-priority-in-african-nations/
[17] Department of Health Republic of South Africa. (2021). Health Research Priorities (revised) for South Africa 2021-2024 . National Health Research Strategy. https://www.health.gov.za/wp-content/uploads/2022/05/National-Health-Research-Priorities-2021-2024.pdf
[18] Oosthuizen, H. (2013). Legal and Ethical Issues in Stem Cell Research in South Africa. In: Beran, R. (eds) Legal and Forensic Medicine. Springer, Berlin, Heidelberg. https://doi.org/10.1007/978-3-642-32338-6_80 , see also: Gaobotse G (2018) Stem Cell Research in Africa: Legislation and Challenges. J Regen Med 7:1. doi: 10.4172/2325-9620.1000142
[19] United States Bureau of Citizenship and Immigration Services. (1998). Tunisia: Information on the status of Christian conversions in Tunisia . UNHCR Web Archive. https://webarchive.archive.unhcr.org/20230522142618/https://www.refworld.org/docid/3df0be9a2.html
[20] Gaobotse, G. (2018) Stem Cell Research in Africa: Legislation and Challenges. J Regen Med 7:1. doi: 10.4172/2325-9620.1000142
[21] Kooli, C. Review of assisted reproduction techniques, laws, and regulations in Muslim countries. Middle East Fertil Soc J 24 , 8 (2020). https://doi.org/10.1186/s43043-019-0011-0 ; Gaobotse, G. (2018) Stem Cell Research in Africa: Legislation and Challenges. J Regen Med 7:1. doi: 10.4172/2325-9620.1000142
[22] Pang M. C. (1999). Protective truthfulness: the Chinese way of safeguarding patients in informed treatment decisions. Journal of medical ethics , 25(3), 247–253. https://doi.org/10.1136/jme.25.3.247
[23] Wang, L., Wang, F., & Zhang, W. (2021). Bioethics in China’s biosecurity law: Forms, effects, and unsettled issues. Journal of law and the biosciences , 8(1). https://doi.org/10.1093/jlb/lsab019 https://academic.oup.com/jlb/article/8/1/lsab019/6299199
[24] Wang, Y., Xue, Y., & Guo, H. D. (2022). Intervention effects of traditional Chinese medicine on stem cell therapy of myocardial infarction. Frontiers in pharmacology , 13 , 1013740. https://doi.org/10.3389/fphar.2022.1013740
[25] Li, X.-T., & Zhao, J. (2012). Chapter 4: An Approach to the Nature of Qi in TCM- Qi and Bioenergy. In Recent Advances in Theories and Practice of Chinese Medicine (p. 79). InTech.
[26] Luo, D., Xu, Z., Wang, Z., & Ran, W. (2021). China's Stem Cell Research and Knowledge Levels of Medical Practitioners and Students. Stem cells international , 2021 , 6667743. https://doi.org/10.1155/2021/6667743
[27] Luo, D., Xu, Z., Wang, Z., & Ran, W. (2021). China's Stem Cell Research and Knowledge Levels of Medical Practitioners and Students. Stem cells international , 2021 , 6667743. https://doi.org/10.1155/2021/6667743
[28] Zhang, J. Y. (2017). Lost in translation? accountability and governance of Clinical Stem Cell Research in China. Regenerative Medicine , 12 (6), 647–656. https://doi.org/10.2217/rme-2017-0035
[29] Wang, L., Wang, F., & Zhang, W. (2021). Bioethics in China’s biosecurity law: Forms, effects, and unsettled issues. Journal of law and the biosciences , 8(1). https://doi.org/10.1093/jlb/lsab019 https://academic.oup.com/jlb/article/8/1/lsab019/6299199
[30] Chen, H., Wei, T., Wang, H. et al. Association of China’s two-child policy with changes in number of births and birth defects rate, 2008–2017. BMC Public Health 22 , 434 (2022). https://doi.org/10.1186/s12889-022-12839-0
[31] Azuma, K. Regulatory Landscape of Regenerative Medicine in Japan. Curr Stem Cell Rep 1 , 118–128 (2015). https://doi.org/10.1007/s40778-015-0012-6
[32] Harris, R. (2005, May 19). Researchers Report Advance in Stem Cell Production . NPR. https://www.npr.org/2005/05/19/4658967/researchers-report-advance-in-stem-cell-production
[33] Park, S. (2012). South Korea steps up stem-cell work. Nature . https://doi.org/10.1038/nature.2012.10565
[34] Resnik, D. B., Shamoo, A. E., & Krimsky, S. (2006). Fraudulent human embryonic stem cell research in South Korea: lessons learned. Accountability in research , 13 (1), 101–109. https://doi.org/10.1080/08989620600634193 .
[35] Alahmad, G., Aljohani, S., & Najjar, M. F. (2020). Ethical challenges regarding the use of stem cells: interviews with researchers from Saudi Arabia. BMC medical ethics, 21(1), 35. https://doi.org/10.1186/s12910-020-00482-6
[36] Association for the Advancement of Blood and Biotherapies. https://www.aabb.org/regulatory-and-advocacy/regulatory-affairs/regulatory-for-cellular-therapies/international-competent-authorities/saudi-arabia
[37] Alahmad, G., Aljohani, S., & Najjar, M. F. (2020). Ethical challenges regarding the use of stem cells: Interviews with researchers from Saudi Arabia. BMC medical ethics , 21 (1), 35. https://doi.org/10.1186/s12910-020-00482-6
[38] Alahmad, G., Aljohani, S., & Najjar, M. F. (2020). Ethical challenges regarding the use of stem cells: Interviews with researchers from Saudi Arabia. BMC medical ethics , 21(1), 35. https://doi.org/10.1186/s12910-020-00482-6
Culturally, autonomy practices follow a relational autonomy approach based on a paternalistic deontological health care model. The adherence to strict international research policies and religious pillars within the regulatory environment is a great foundation for research ethics. However, there is a need to develop locally targeted ethics approaches for research (as called for in Alahmad, G., Aljohani, S., & Najjar, M. F. (2020). Ethical challenges regarding the use of stem cells: interviews with researchers from Saudi Arabia. BMC medical ethics, 21(1), 35. https://doi.org/10.1186/s12910-020-00482-6), this decision-making approach may help advise a research decision model. For more on the clinical cultural autonomy approaches, see: Alabdullah, Y. Y., Alzaid, E., Alsaad, S., Alamri, T., Alolayan, S. W., Bah, S., & Aljoudi, A. S. (2022). Autonomy and paternalism in Shared decision‐making in a Saudi Arabian tertiary hospital: A cross‐sectional study. Developing World Bioethics , 23 (3), 260–268. https://doi.org/10.1111/dewb.12355 ; Bukhari, A. A. (2017). Universal Principles of Bioethics and Patient Rights in Saudi Arabia (Doctoral dissertation, Duquesne University). https://dsc.duq.edu/etd/124; Ladha, S., Nakshawani, S. A., Alzaidy, A., & Tarab, B. (2023, October 26). Islam and Bioethics: What We All Need to Know . Columbia University School of Professional Studies. https://sps.columbia.edu/events/islam-and-bioethics-what-we-all-need-know
[39] Ababneh, M. A., Al-Azzam, S. I., Alzoubi, K., Rababa’h, A., & Al Demour, S. (2021). Understanding and attitudes of the Jordanian public about clinical research ethics. Research Ethics , 17 (2), 228-241. https://doi.org/10.1177/1747016120966779
[40] Ababneh, M. A., Al-Azzam, S. I., Alzoubi, K., Rababa’h, A., & Al Demour, S. (2021). Understanding and attitudes of the Jordanian public about clinical research ethics. Research Ethics , 17 (2), 228-241. https://doi.org/10.1177/1747016120966779
[41] Dajani, R. (2014). Jordan’s stem-cell law can guide the Middle East. Nature 510, 189. https://doi.org/10.1038/510189a
[42] Dajani, R. (2014). Jordan’s stem-cell law can guide the Middle East. Nature 510, 189. https://doi.org/10.1038/510189a
[43] The EU’s definition of autonomy relates to the capacity for creating ideas, moral insight, decisions, and actions without constraint, personal responsibility, and informed consent. However, the EU views autonomy as not completely able to protect individuals and depends on other principles, such as dignity, which “expresses the intrinsic worth and fundamental equality of all human beings.” Rendtorff, J.D., Kemp, P. (2019). Four Ethical Principles in European Bioethics and Biolaw: Autonomy, Dignity, Integrity and Vulnerability. In: Valdés, E., Lecaros, J. (eds) Biolaw and Policy in the Twenty-First Century. International Library of Ethics, Law, and the New Medicine, vol 78. Springer, Cham. https://doi.org/10.1007/978-3-030-05903-3_3
[44] Council of Europe. Convention for the protection of Human Rights and Dignity of the Human Being with regard to the Application of Biology and Medicine: Convention on Human Rights and Biomedicine (ETS No. 164) https://www.coe.int/en/web/conventions/full-list?module=treaty-detail&treatynum=164 (forbidding the creation of embryos for research purposes only, and suggests embryos in vitro have protections.); Also see Drabiak-Syed B. K. (2013). New President, New Human Embryonic Stem Cell Research Policy: Comparative International Perspectives and Embryonic Stem Cell Research Laws in France. Biotechnology Law Report , 32 (6), 349–356. https://doi.org/10.1089/blr.2013.9865
[45] Rendtorff, J.D., Kemp, P. (2019). Four Ethical Principles in European Bioethics and Biolaw: Autonomy, Dignity, Integrity and Vulnerability. In: Valdés, E., Lecaros, J. (eds) Biolaw and Policy in the Twenty-First Century. International Library of Ethics, Law, and the New Medicine, vol 78. Springer, Cham. https://doi.org/10.1007/978-3-030-05903-3_3
[46] Tomuschat, C., Currie, D. P., Kommers, D. P., & Kerr, R. (Trans.). (1949, May 23). Basic law for the Federal Republic of Germany. https://www.btg-bestellservice.de/pdf/80201000.pdf
[47] Regulation of Stem Cell Research in Germany . Eurostemcell. (2017, April 26). https://www.eurostemcell.org/regulation-stem-cell-research-germany
[48] Regulation of Stem Cell Research in Finland . Eurostemcell. (2017, April 26). https://www.eurostemcell.org/regulation-stem-cell-research-finland
[49] Regulation of Stem Cell Research in Spain . Eurostemcell. (2017, April 26). https://www.eurostemcell.org/regulation-stem-cell-research-spain
[50] Some sources to consider regarding ethics models or regulatory oversights of other cultures not covered:
Kara MA. Applicability of the principle of respect for autonomy: the perspective of Turkey. J Med Ethics. 2007 Nov;33(11):627-30. doi: 10.1136/jme.2006.017400. PMID: 17971462; PMCID: PMC2598110.
Ugarte, O. N., & Acioly, M. A. (2014). The principle of autonomy in Brazil: one needs to discuss it ... Revista do Colegio Brasileiro de Cirurgioes , 41 (5), 374–377. https://doi.org/10.1590/0100-69912014005013
Bharadwaj, A., & Glasner, P. E. (2012). Local cells, global science: The rise of embryonic stem cell research in India . Routledge.
For further research on specific European countries regarding ethical and regulatory framework, we recommend this database: Regulation of Stem Cell Research in Europe . Eurostemcell. (2017, April 26). https://www.eurostemcell.org/regulation-stem-cell-research-europe
[51] Klitzman, R. (2006). Complications of culture in obtaining informed consent. The American Journal of Bioethics, 6(1), 20–21. https://doi.org/10.1080/15265160500394671 see also: Ekmekci, P. E., & Arda, B. (2017). Interculturalism and Informed Consent: Respecting Cultural Differences without Breaching Human Rights. Cultura (Iasi, Romania) , 14 (2), 159–172.; For why trust is important in research, see also: Gray, B., Hilder, J., Macdonald, L., Tester, R., Dowell, A., & Stubbe, M. (2017). Are research ethics guidelines culturally competent? Research Ethics , 13 (1), 23-41. https://doi.org/10.1177/1747016116650235
[52] The Qur'an (M. Khattab, Trans.). (1965). Al-Mu’minun, 23: 12-14. https://quran.com/23
[53] Lenfest, Y. (2017, December 8). Islam and the beginning of human life . Bill of Health. https://blog.petrieflom.law.harvard.edu/2017/12/08/islam-and-the-beginning-of-human-life/
[54] Aksoy, S. (2005). Making regulations and drawing up legislation in Islamic countries under conditions of uncertainty, with special reference to embryonic stem cell research. Journal of Medical Ethics , 31: 399-403.; see also: Mahmoud, Azza. "Islamic Bioethics: National Regulations and Guidelines of Human Stem Cell Research in the Muslim World." Master's thesis, Chapman University, 2022. https://doi.org/10.36837/ chapman.000386
[55] Rashid, R. (2022). When does Ensoulment occur in the Human Foetus. Journal of the British Islamic Medical Association , 12 (4). ISSN 2634 8071. https://www.jbima.com/wp-content/uploads/2023/01/2-Ethics-3_-Ensoulment_Rafaqat.pdf.
[56] Sivaraman, M. & Noor, S. (2017). Ethics of embryonic stem cell research according to Buddhist, Hindu, Catholic, and Islamic religions: perspective from Malaysia. Asian Biomedicine,8(1) 43-52. https://doi.org/10.5372/1905-7415.0801.260
[57] Jafari, M., Elahi, F., Ozyurt, S. & Wrigley, T. (2007). 4. Religious Perspectives on Embryonic Stem Cell Research. In K. Monroe, R. Miller & J. Tobis (Ed.), Fundamentals of the Stem Cell Debate: The Scientific, Religious, Ethical, and Political Issues (pp. 79-94). Berkeley: University of California Press. https://escholarship.org/content/qt9rj0k7s3/qt9rj0k7s3_noSplash_f9aca2e02c3777c7fb76ea768ba458f0.pdf https://doi.org/10.1525/9780520940994-005
[58] Lecso, P. A. (1991). The Bodhisattva Ideal and Organ Transplantation. Journal of Religion and Health , 30 (1), 35–41. http://www.jstor.org/stable/27510629 ; Bodhisattva, S. (n.d.). The Key of Becoming a Bodhisattva . A Guide to the Bodhisattva Way of Life. http://www.buddhism.org/Sutras/2/BodhisattvaWay.htm
[59] There is no explicit religious reference to when life begins or how to conduct research that interacts with the concept of life. However, these are relevant verses pertaining to how the fetus is viewed. (( King James Bible . (1999). Oxford University Press. (original work published 1769))
Jerimiah 1: 5 “Before I formed thee in the belly I knew thee; and before thou camest forth out of the womb I sanctified thee…”
In prophet Jerimiah’s insight, God set him apart as a person known before childbirth, a theme carried within the Psalm of David.
Psalm 139: 13-14 “…Thou hast covered me in my mother's womb. I will praise thee; for I am fearfully and wonderfully made…”
These verses demonstrate David’s respect for God as an entity that would know of all man’s thoughts and doings even before birth.
[60] It should be noted that abortion is not supported as well.
[61] The Vatican. (1987, February 22). Instruction on Respect for Human Life in Its Origin and on the Dignity of Procreation Replies to Certain Questions of the Day . Congregation For the Doctrine of the Faith. https://www.vatican.va/roman_curia/congregations/cfaith/documents/rc_con_cfaith_doc_19870222_respect-for-human-life_en.html
[62] The Vatican. (2000, August 25). Declaration On the Production and the Scientific and Therapeutic Use of Human Embryonic Stem Cells . Pontifical Academy for Life. https://www.vatican.va/roman_curia/pontifical_academies/acdlife/documents/rc_pa_acdlife_doc_20000824_cellule-staminali_en.html ; Ohara, N. (2003). Ethical Consideration of Experimentation Using Living Human Embryos: The Catholic Church’s Position on Human Embryonic Stem Cell Research and Human Cloning. Department of Obstetrics and Gynecology . Retrieved from https://article.imrpress.com/journal/CEOG/30/2-3/pii/2003018/77-81.pdf.
[63] Smith, G. A. (2022, May 23). Like Americans overall, Catholics vary in their abortion views, with regular mass attenders most opposed . Pew Research Center. https://www.pewresearch.org/short-reads/2022/05/23/like-americans-overall-catholics-vary-in-their-abortion-views-with-regular-mass-attenders-most-opposed/
[64] Rosner, F., & Reichman, E. (2002). Embryonic stem cell research in Jewish law. Journal of halacha and contemporary society , (43), 49–68.; Jafari, M., Elahi, F., Ozyurt, S. & Wrigley, T. (2007). 4. Religious Perspectives on Embryonic Stem Cell Research. In K. Monroe, R. Miller & J. Tobis (Ed.), Fundamentals of the Stem Cell Debate: The Scientific, Religious, Ethical, and Political Issues (pp. 79-94). Berkeley: University of California Press. https://escholarship.org/content/qt9rj0k7s3/qt9rj0k7s3_noSplash_f9aca2e02c3777c7fb76ea768ba458f0.pdf https://doi.org/10.1525/9780520940994-005
[65] Schenker J. G. (2008). The beginning of human life: status of embryo. Perspectives in Halakha (Jewish Religious Law). Journal of assisted reproduction and genetics , 25 (6), 271–276. https://doi.org/10.1007/s10815-008-9221-6
[66] Ruttenberg, D. (2020, May 5). The Torah of Abortion Justice (annotated source sheet) . Sefaria. https://www.sefaria.org/sheets/234926.7?lang=bi&with=all&lang2=en
[67] Jafari, M., Elahi, F., Ozyurt, S. & Wrigley, T. (2007). 4. Religious Perspectives on Embryonic Stem Cell Research. In K. Monroe, R. Miller & J. Tobis (Ed.), Fundamentals of the Stem Cell Debate: The Scientific, Religious, Ethical, and Political Issues (pp. 79-94). Berkeley: University of California Press. https://escholarship.org/content/qt9rj0k7s3/qt9rj0k7s3_noSplash_f9aca2e02c3777c7fb76ea768ba458f0.pdf https://doi.org/10.1525/9780520940994-005
[68] Gert, B. (2007). Common morality: Deciding what to do . Oxford Univ. Press.
[69] World Medical Association (2013). World Medical Association Declaration of Helsinki: ethical principles for medical research involving human subjects. JAMA , 310(20), 2191–2194. https://doi.org/10.1001/jama.2013.281053 Declaration of Helsinki – WMA – The World Medical Association .; see also: National Commission for the Protection of Human Subjects of Biomedical and Behavioral Research. (1979). The Belmont report: Ethical principles and guidelines for the protection of human subjects of research . U.S. Department of Health and Human Services. https://www.hhs.gov/ohrp/regulations-and-policy/belmont-report/read-the-belmont-report/index.html
[70] Zakarin Safier, L., Gumer, A., Kline, M., Egli, D., & Sauer, M. V. (2018). Compensating human subjects providing oocytes for stem cell research: 9-year experience and outcomes. Journal of assisted reproduction and genetics , 35 (7), 1219–1225. https://doi.org/10.1007/s10815-018-1171-z https://www.ncbi.nlm.nih.gov/pmc/articles/PMC6063839/ see also: Riordan, N. H., & Paz Rodríguez, J. (2021). Addressing concerns regarding associated costs, transparency, and integrity of research in recent stem cell trial. Stem Cells Translational Medicine , 10 (12), 1715–1716. https://doi.org/10.1002/sctm.21-0234
[71] Klitzman, R., & Sauer, M. V. (2009). Payment of egg donors in stem cell research in the USA. Reproductive biomedicine online , 18 (5), 603–608. https://doi.org/10.1016/s1472-6483(10)60002-8
[72] Krosin, M. T., Klitzman, R., Levin, B., Cheng, J., & Ranney, M. L. (2006). Problems in comprehension of informed consent in rural and peri-urban Mali, West Africa. Clinical trials (London, England) , 3 (3), 306–313. https://doi.org/10.1191/1740774506cn150oa
[73] Veatch, Robert M. Hippocratic, Religious, and Secular Medical Ethics: The Points of Conflict . Georgetown University Press, 2012.
[74] Msoroka, M. S., & Amundsen, D. (2018). One size fits not quite all: Universal research ethics with diversity. Research Ethics , 14 (3), 1-17. https://doi.org/10.1177/1747016117739939
[75] Pirzada, N. (2022). The Expansion of Turkey’s Medical Tourism Industry. Voices in Bioethics , 8 . https://doi.org/10.52214/vib.v8i.9894
[76] Stem Cell Tourism: False Hope for Real Money . Harvard Stem Cell Institute (HSCI). (2023). https://hsci.harvard.edu/stem-cell-tourism , See also: Bissassar, M. (2017). Transnational Stem Cell Tourism: An ethical analysis. Voices in Bioethics , 3 . https://doi.org/10.7916/vib.v3i.6027
[77] Song, P. (2011) The proliferation of stem cell therapies in post-Mao China: problematizing ethical regulation, New Genetics and Society , 30:2, 141-153, DOI: 10.1080/14636778.2011.574375
[78] Dajani, R. (2014). Jordan’s stem-cell law can guide the Middle East. Nature 510, 189. https://doi.org/10.1038/510189a
[79] International Society for Stem Cell Research. (2024). Standards in stem cell research . International Society for Stem Cell Research. https://www.isscr.org/guidelines/5-standards-in-stem-cell-research
[80] Benjamin, R. (2013). People’s science bodies and rights on the Stem Cell Frontier . Stanford University Press.
Mifrah Hayath
SM Candidate Harvard Medical School, MS Biotechnology Johns Hopkins University
Olivia Bowers
MS Bioethics Columbia University (Disclosure: affiliated with Voices in Bioethics)
Article Details

This work is licensed under a Creative Commons Attribution 4.0 International License .

IMAGES
VIDEO
COMMENTS
Future research should evaluate the effect of voice recognition, social presence and social image on consumers' cognitive load. Second, our sample size was limited. Future research should also explore personality traits associated the smart speakers, including Amazon's Show or Apple's Home. Further, we suggest examining time distortion to find ...
work is a systematic review of the literature on V oice assistants (V A). Innovative mode of interaction, the V oice. Assistant definition is derived from advances in artificial intelligence ...
The translation of findings from basic science voice research can play an important role in further improving clinical management of voice disorders and reducing variability in voice outcomes. ... Lisa Bolden, and Arthur Popper for their constructive comments on an earlier draft of this paper. I also acknowledge support from the National ...
1. ABSTRACT. Voice assistants have become an integral part of our daily lives, enabling natura l and seamless. interactions with technology. Recent advancements in natural language processing (NLP ...
A huge amount of research has been done in the field of speech signal processing in recent years. In particular, there has been increasing interest in the automatic speech recognition (ASR) technology field. ASR began with simple systems that responded to a limited number of sounds and has evolved into sophisticated systems that respond fluently to natural language. This systematic review of ...
The present study identifies, organizes, and structures the available scientific knowledge on the recent use and the prospects of Voice Assistants (VA) in private households. The systematic review ...
The 21 papers that comprise this two-part issue highlight the breadth and diversity of research on voice modulation. Beyond extensive scientific applications within the human voice sciences and animal communication more broadly, research in this area also has crucial practical applications, from societal to technological.
The idea of voice as an expression of authenticity, truth or socially significant meaning is fundamental to the qualitative research paradigm (St Pierre, 2008).Underlying the edifice of much of what we do as qualitative researchers is the belief that the talk that happens in research encounters - the concentrated and collective practice of putting (researcher solicited) perspectives and ...
The "so what": adding robust stage violence training to musical theatre is a unique perspective. In "Voice Dosimetry in an Elementary Music Student Teacher," Bryan E. Nichols, Kay Piña, and Scott-Lee Atchison pilot a study with a new voice dosimeter device applied to a K-12 music student teacher. The "so what": no research ...
Voice conversion is a process where the essence of a speaker's identity is seamlessly transferred to another speaker, all while preserving the content of their speech. This usage is accomplished using algorithms that blend speech processing techniques, such as speech analysis, speaker classification, and vocoding. The cutting-edge voice conversion technology is characterized by deep neural ...
View a PDF of the paper titled Voice Cloning: a Multi-Speaker Text-to-Speech Synthesis Approach based on Transfer Learning, by Giuseppe Ruggiero and 3 other authors. View PDF Abstract: Deep learning models are becoming predominant in many fields of machine learning. Text-to-Speech (TTS), the process of synthesizing artificial speech from text ...
The advancements of the Internet of Things (IoT) and voice-based multimedia applications have resulted in the generation of big data consisting of patterns, trends and associations capturing and representing many features of human behaviour. The latent representations of many aspects and the basis of human behaviour is naturally embedded within the expression of emotions found in human speech ...
View PDF Abstract: There are growing implications surrounding generative AI in the speech domain that enable voice cloning and real-time voice conversion from one individual to another. This technology poses a significant ethical threat and could lead to breaches of privacy and misrepresentation, thus there is an urgent need for real-time detection of AI-generated speech for DeepFake Voice ...
Negotiating the voice of the researcher with the voice of the participant in the dissemination process is an important part of qualitative research. Lincoln, Lynham, and Guba (2011) explained, "Today . . . voice can mean not only having a real researcher—and a researcher's voice—in the text, but also letting research participants speak ...
Leveraging Python's robust capabilities in. natural language processi ng and artificial intelli gence, o ur. voice assistant sy stem stands as a testa ment to the po tential o f. open-source ...
Note-level Automatic Singing Voice Transcription (AST) converts singing recordings into note sequences, facilitating the automatic annotation of singing datasets for Singing Voice Synthesis (SVS) applications. Current AST methods, however, struggle with accuracy and robustness when used for practical annotation. This paper presents ROSVOT, the first robust AST model that serves SVS ...
This paper advocates for a universal understanding of research that positions itself as 'voice' research to (1) engage with and be transparent about, its power and participatory positioning; (2) articulate potential or actual action and influence of the views that are reported on; and (3) recognise and position participants as experts in ...
Academic voice means to meet the writing expectations of academic writing. It is different than writing to a friend, writing for business purposes, or writing to the general public. Your audience for academic writing includes other academic writers and learners. Academic writing includes: using credible evidence to support ideas, adding your ...
While a research paper will be based on your opinion on a topic, it will be an opinion based on evidence (from your research) and one that has been argued in a rational manner in your paper. You use the academic voice because your opinion is based on thinking; in your paper you're revealing your thought process to your reader. Because you ...
New research challenges widespread beliefs about why we're attracted to certain voices. by McMaster University. The significant relationships between fundamental frequency (F0) and attractiveness ...
Using "I" to identify the writer's role in the research process is often a solution to the passive voice and is encouraged by APA style (see APA 7, Section 4.16). Using the past tense of the verb "to be" and the past participle of a verb together is often an indication of the passive voice. Here are some signs to look for in your paper:
The model can reason through visual problems in real time as well. Using his phone, Zoph filmed himself writing an algebra equation (3x + 1 = 4) on a sheet of paper, having GPT-4o follow along. He ...
On Monday, the San Francisco artificial intelligence start-up unveiled a new version of its ChatGPT chatbot that can receive and respond to voice commands, images and videos. The company said the ...
MIT researchers' "consensus game" is a game-theoretic approach for language model decoding. The equilibrium-ranking algorithm harmonizes generative and discriminative querying to enhance prediction accuracy across various tasks, outperforming larger models and demonstrating the potential of game theory in improving language model consistency and truthfulness.
But between 5-10 per cent of the population do not have the same experience of an inner voice, and they find it more difficult to perform certain verbal memory tasks, new research shows. The vast ...
3 mins. The active voice refers to a sentence format that emphasizes the doer of an action. For example, in the sentence "The mice inhaled the tobacco-infused aerosol," the doer, i.e., "the mice" seem important. On the other hand, in the passive voice, the action being performed is emphasized, and the doer may be omitted, e.g.,
The terms active and passive voice refer to the way subjects and verbs are used in sentence construction. In scientific writing, we use both voices to write clear and coherent research articles. Although many scientists overuse the passive voice, most scientific journals (e.g. Science and Nature) actually encourage active voice.
Prior to GPT-4o, you could use Voice Mode to talk to ChatGPT with latencies of 2.8 seconds (GPT-3.5) and 5.4 seconds (GPT-4) on average. To achieve this, Voice Mode is a pipeline of three separate models: one simple model transcribes audio to text, GPT-3.5 or GPT-4 takes in text and outputs text, and a third simple model converts that text back to audio.
Voices in Bioethics is currently seeking submissions on philosophical and practical topics, both current and timeless. Papers addressing access to healthcare, the bioethical implications of recent Supreme Court rulings, environmental ethics, data privacy, cybersecurity, law and bioethics, economics and bioethics, reproductive ethics, research ethics, and pediatric bioethics are sought.