
An official website of the United States government
The .gov means it’s official. Federal government websites often end in .gov or .mil. Before sharing sensitive information, make sure you’re on a federal government site.
The site is secure. The https:// ensures that you are connecting to the official website and that any information you provide is encrypted and transmitted securely.
- Publications
- Account settings
Preview improvements coming to the PMC website in October 2024. Learn More or Try it out now .
- Advanced Search
- Journal List
- Health Expect
- v.21(6); 2018 Dec

Public and patient involvement in quantitative health research: A statistical perspective
Ailish hannigan.
1 Public and Patient Involvement Research Unit, Graduate Entry Medical School, University of Limerick, Limerick, Ireland
2 Health Research Institute, University of Limerick, Limerick, Ireland
The majority of studies included in recent reviews of impact for public and patient involvement (PPI) in health research had a qualitative design. PPI in solely quantitative designs is underexplored, particularly its impact on statistical analysis. Statisticians in practice have a long history of working in both consultative (indirect) and collaborative (direct) roles in health research, yet their perspective on PPI in quantitative health research has never been explicitly examined.
To explore the potential and challenges of PPI from a statistical perspective at distinct stages of quantitative research, that is sampling, measurement and statistical analysis, distinguishing between indirect and direct PPI.
Conclusions
Statistical analysis is underpinned by having a representative sample, and a collaborative or direct approach to PPI may help achieve that by supporting access to and increasing participation of under‐represented groups in the population. Acknowledging and valuing the role of lay knowledge of the context in statistical analysis and in deciding what variables to measure may support collective learning and advance scientific understanding, as evidenced by the use of participatory modelling in other disciplines. A recurring issue for quantitative researchers, which reflects quantitative sampling methods, is the selection and required number of PPI contributors, and this requires further methodological development. Direct approaches to PPI in quantitative health research may potentially increase its impact, but the facilitation and partnership skills required may require further training for all stakeholders, including statisticians.
1. BACKGROUND
Public and patient involvement (PPI) in health research has been defined as research being carried out “with” or “by” members of the public rather than “to,” “about” or “for” them. 1 PPI covers a diverse range of approaches from “one off” information gathering to sustained partnerships. Tritter's conceptual framework for PPI distinguished between indirect involvement where information is gathered from patients and the public, but they do not have the power to make final decisions and direct involvement where patients and the public take part in the decision‐making. 2
A bibliometric review of the literature reported strong growth in the number of published empirical health research studies with public involvement. 3 In a systematic review of the impact of PPI on health and social care research, Brett et al 4 reported positive impacts at all stages of research from planning and undertaking the study to analysis, dissemination and implementation. The design of the majority of empirical research studies included in both reviews was qualitative (70% of studies in Brett. et al 4 and 73% in Boote et al 3 ). More significant tensions have been reported in community‐academic partnerships that use quantitative methods rather than solely qualitative methods, for example tensions with the community about having and recruiting to a “no intervention” comparison group. 5 Particular challenges for PPI have been reported in the most structured and regulated of quantitative designs, that is a randomized controlled trial (RCT), where there is little opportunity for flexibility once the trial has started 6 and Boote et al 3 concluded that researchers may find it easier to involve the public in qualitative rather than quantitative research.
If the full potential of PPI for health research is to be realized, its potential and challenges in quantitative research require more exploration, particularly the features of quantitative research which are different from qualitative research, for example, sampling, measurement and statistical analysis. Statisticians in practice have a long history of working with a variety of stakeholders in health research and have examined the difference between an indirect or consulting role for the statistician and a more direct, collaborative role, 7 yet their perspective has never been explicitly explored in health research with PPI. The objective of this study therefore was to critically reflect on the potential and challenges for PPI at distinct stages of quantitative research from a statistical perspective, distinguishing between direct and indirect approaches to PPI. 2
2. SAMPLE SIZE AND SELECTION
Quantitative research usually aims to provide precise, unbiased estimates of parameters of interest for the entire population which requires a large, randomly selected sample. Brett et al 4 reported a positive impact of PPI on recruitment in studies, but the representativeness of the sample is as important in quantitative research as sample size. Studies have shown that even when accrual targets have been met, the sample may not be fully representative of the population of interest. In cancer clinical trials, for example, those with health insurance and from higher socio‐economic backgrounds can be over‐represented, while older patients, ethnic minorities and so‐called hard‐to‐reach groups (often with higher cancer mortality rates) are under‐represented. 8 This limits the ability to generalize the results of the trials to all those with cancer. There is evidence that a direct approach to PPI with sustained partnerships between community leaders, primary care providers and clinical trial researchers can be effective in increasing awareness and participation of under‐represented groups in cancer clinical trials 9 , 10 and therefore help to achieve the goal of a population‐representative sample.
Collecting representative health data for some groups in the population may only be possible with their involvement. Marin et al 11 reports on the challenges of identifying an appropriate sampling frame for a health survey of Aboriginal adults in Southern Australia. Access to information identifying Aboriginal dwellings was not publically available, making it difficult to randomly select participants for large population household surveys. Trying to overcome this challenge involved reaching agreement on the process of research for Aboriginal adults with their local communities. An 8‐month consultation process was undertaken with representatives from multiple locations including Aboriginal owned lands in one region; however, it was ultimately agreed that it was culturally inappropriate for the research team to survey this region. The study demonstrated the opportunities for PPI in quantitative research with a representative sample of randomly chosen Aboriginal adults (excluding those resident in one region) ultimately achieved but also the challenges for PPI. The direct approach to involvement in this study, after a lengthy consultation process, resulted in a decision not to carry out the planned sampling and data collection in one region with implications for generalization of results and overall sample size.
Of course, given the importance of representativeness in quantitative research, there may be particular challenges for statisticians and quantitative researchers in accepting the term patient or public representative with some suggesting PPI contributor as a more appropriate term. 6 PPI representative may suggest to a quantitative researcher that an individual patient or member of the public is typical of an often diverse population, yet there is evidence that the opportunities and capacity to be involved as PPI contributors vary by level of education, income, cognitive skills and cultural background. 12 Dudley et al carried out a qualitative study of the impact of PPI in RCTs with patients and researchers from a cohort of RCTs. 6 The types of roles of PPI contributors described by researchers involved in the RCTs were grouped into oversight, managerial and responsive roles. Responsive PPI was described as informal and impromptu with researchers approaching multiple “responsive” PPI contributors as difficulties arose, for example advising on patient information sheets and follow‐up of patients. It was reported that contributions from responsive roles may carry more weight with the researchers in RCTs because it allowed access to a more diverse range of contributors who researchers saw as more “representative” of the target population.
3. MEASUREMENT
Measurement of quantitative data involves decisions about what to measure, how to measure it and how often to measure it with these decisions typically made by the research team. Without the involvement of patients and the public, however, important outcomes for people living with a condition have been missed or overlooked, for example fatigue for people with rheumatoid arthritis 13 or the long‐term effects of therapy for children with asthma. 14
Core outcome sets (COS) are a minimum set of agreed important outcomes to be measured in research on particular illnesses, conditions or treatments to ensure important outcomes are consistently reported and allow the results from multiple studies to be easily combined and compared. Young reported on workshops to explore what principles, methods and strategies that COS developers may need to consider when seeking patient input into the development of a COS. 15 The importance of distinguishing between an indirect role for patients in COS development where patients respond to a consensus survey or a direct role where patients are partners in planning, running and disseminating a COS study was highlighted by delegates in the workshops. While all delegates agreed that participation by patients should be meaningful and on an equal footing with other stakeholders, there was considerable uncertainty on how to achieve this, for example how many patients are needed in the COS development process or what proportion of patients relative to other stakeholders should be included. This raises the issue again of the number and selection of PPI contributors for quantitative researchers, and it was concluded that methodological work was needed to understand the COS development process from the perspective of patients and how the process may be improved for them.
Important considerations in longitudinal research are the number and timing of repeated measurements. From a statistical perspective, measurements on the same subject at different times are almost always correlated, with measurements taken close together in time being more highly correlated than measurements taken far apart in time. Unequal spacing of observation times may be more computationally challenging in statistical analysis of repeated measurements and missing data within subjects over time can be particularly challenging depending on the amount, cause and pattern of missing data. 16 There are therefore important statistical considerations to be taken into account in the design of longitudinal studies but these have to be balanced with input from PPI contributors on appropriate timing and frequency of data collection for potential participants.
Lucas et al reported on how European birth cohorts are engaging and consulting with young birth cohort members. 17 Of the 84 individual cohorts identified, only eight had a mechanism for consulting with parents and three a mechanism for consulting with young people themselves (usually “one off” consultations). Very varied follow‐up rates were reported from 13% to 84% more than 10 years after enrolment for individual data rounds of the birth cohorts. 17 Being motivated to continue to participate may be influenced by whether a participant believes the study is interesting, important, or relevant to them. 18 One of the key strategies for retention in the Australian Aboriginal Birth Cohort study was partnerships with community members with local knowledge who were involved in all phases of the follow‐up. 19 Retention rates of 86% at 11‐year follow‐up and 72% at 18‐year follow‐up were reported which demonstrates the potential of a direct approach to PPI. Ethical approval for the study involved an Aboriginal Ethical Sub‐committee which had the power of veto and a staged consent was used where participants had the right to refuse individual procedures at each wave. As with all missing data, this has implications for the statistical analysis yet only 10% of participants in this study chose to opt out of different assessments at follow‐up.
3.1. Statistical analysis
A report on the impact of PPI found that it had a positive impact at all stages of qualitative research including data analysis but that there was little evidence of its impact on quantitative data analysis. 20 It was concluded this lack of evidence may reflect a lack of involvement rather than an evidence gap. Booth et al 3 also suggested that the public may be more comfortable with interpreting interview and focus group data compared with numeric data. Low levels of numerical and statistical literacy in the general population may contribute to this.
Statistical analysis involves describing the data using appropriate graphical and numerical summaries (descriptive statistics) and using more advanced statistical methods to draw inferences about the population using the data from a sample (statistical inference). Choosing appropriate methods for statistical inference, testing the underlying assumptions and checking the adequacy of the models produced requires advanced statistical training and implementing them typically involves the use of statistical software or programming. Statisticians bring this expertise to quantitative health research and while it is important that the chosen methods are adequately communicated to all stakeholders, replicating this type of expertise in PPI contributors seems like an inefficient use of resources for PPI.
Quantitative data are, however, “not just numbers, they are numbers with a context” 21 and most practising statisticians agree that knowledge of the context is needed to carry out even a purely technical role effectively. 22 While many associate statistical analysis with objectivity, in practice, statisticians routinely use “subjective” external information to guide, for example the decision on what is a meaningful effect size; whether an outlier is an error in data entry or represents an unusual but meaningful observation; and potential issues with measurement of variables and confounding. 23 Gelman and Hennin argue that we should move beyond the discussion of objectivity and subjectivity in statistics and “replace each of them with broader collections of attributes, with objectivity replaced by transparency, consensus, impartiality and correspondence to observable reality, and subjectivity replaced by awareness of multiple perspectives and context dependence.” 23 This debate within statistics is relevant for PPI where the perceived objectivity and standardization of statistical analysis can be used as a reason for lack of involvement.
External information and context are particularly important in statistical modelling where statisticians are often faced with many potential predictors of an outcome. The “best” way of selecting a multivariable model is still unresolved from a statistical perspective, and it is generally agreed that subject matter knowledge, when available, should guide model building. 24 Even when the potential predictors are known, understanding the causal pathways of exposure on an outcome is challenging where the effect of a variable on the outcome can be direct or indirect. Christiaens et al 25 used a causal diagram to visualize the relationship between pain acceptance and personal control of women in labour and the use of pain medication during labour. Their analysis accounted for the maternal care context of the country where the women were giving birth and other characteristics such as age of the woman and duration of labour. The choice of these characteristics was underpinned by a literature review but women who have given birth also have expert knowledge on why they use pain relief and how other variables such as their personal beliefs and social context might influence that decision. 26
Collaborative or participatory modelling is an approach to scientific modelling in areas such as natural resource management which involves all stakeholders in the model building process. Participants can suggest characteristics for inclusion in the model and how they may impact on the outcome. Causal diagrams are then used to create a shared view across stakeholders. 27 Rockman et al 28 concluded, in the context of marine policy, that “participatory modelling has the potential to facilitate and structure discussions between scientists and stakeholders about uncertainties and the quality of the knowledge base. It can also contribute to collective learning, increase legitimacy and advance scientific understanding.”
There is emerging evidence that the importance of PPI in the development and application of modelling in health research is being recognized. Van Voorn 29 discussed the benefits and risks of PPI in health economic modelling of cost‐effectiveness of new drugs and treatment strategies, with public and patients described as the missing stakeholder group in the modelling process. The potential benefits included the expertise that patients could bring to the process, a greater understanding and possible acceptance by patients of the results of the models and improved model validation. The risks included potential patient bias and the increased resources required for training. The number and selection of patients to contribute to the process was also discussed with a suggestion to include patients “who were able to take a neutral view” and “at least five patients that differ significantly in their background,” again highlighting the focus of quantitative researchers on bias and sample size. The role for this type of participatory modelling in informing debate on public health problems is increasingly being recognized, drawing on the experience of its use in other areas where optimal use of limited resources is required to address complex problems in society. 30
4. CONCLUSIONS
Statistical analysis of quantitative data is underpinned by having a representative sample, and there is evidence that a direct approach to PPI can help achieve that by supporting access to and increasing participation of under‐represented groups in the population. The direct approach has also demonstrated its potential in the retention of those recruited over time, thus reducing bias caused by missing data in longitudinal studies. At all stages of statistical analysis, a statistician continuously refers back to the context of the data collected. 22 Lay knowledge of PPI contributors has an important role in providing this context, and there is evidence from other disciplines of the benefits of including this knowledge in analysis to support collective learning and advance scientific understanding.
The direct approach to PPI where patients and the public have the power to make decisions also brings challenges and the statistician needs to be able to clearly communicate the impact of each decision on the scientific rigour and validity of sampling, measurement and analysis to all stakeholders. Decisions made on participation impact on generalizability. Participatory modelling requires facilitation and partnership skills which may require further training for all stakeholders, including statisticians.
The direct and indirect role for PPI contributors mirrors what happens for statisticians in practice. Statisticians can have a consultative role, that is answering a specific statistical question or a collaborative role where a statistician works with others as equal partners to create new knowledge, with professional organizations for statisticians providing guidance and mentorship on moving from consulting to collaboration to leadership roles. 7 , 31 Statisticians therefore bring very relevant experience and understanding for PPI contributors on the ladder of participation in health research. Further exploration is required on the impact of direct compared to indirect involvement in quantitative research, drawing on the evidence base for community‐based participatory research in quantitative designs 9 and the framework for participatory health research and epidemiology. 32 , 33
CONFLICT OF INTERESTS
No conflict of interests.
ACKNOWLEDGEMENTS
Prof. Anne MacFarlane, Public and Patient Involvement Research Unit, University of Limerick, for discussion of ideas and comments on drafts.
Hannigan A. Public and patient involvement in quantitative health research: A statistical perspective . Health Expect . 2018; 21 :939–943. 10.1111/hex.12800 [ PMC free article ] [ PubMed ] [ CrossRef ] [ Google Scholar ]
- Research article
- Open access
- Published: 01 December 2006
Using quantitative and qualitative data in health services research – what happens when mixed method findings conflict? [ISRCTN61522618]
- Suzanne Moffatt 1 ,
- Martin White 1 ,
- Joan Mackintosh 1 &
- Denise Howel 1
BMC Health Services Research volume 6 , Article number: 28 ( 2006 ) Cite this article
84k Accesses
122 Citations
16 Altmetric
Metrics details
In this methodological paper we document the interpretation of a mixed methods study and outline an approach to dealing with apparent discrepancies between qualitative and quantitative research data in a pilot study evaluating whether welfare rights advice has an impact on health and social outcomes among a population aged 60 and over.
Quantitative and qualitative data were collected contemporaneously. Quantitative data were collected from 126 men and women aged over 60 within a randomised controlled trial. Participants received a full welfare benefits assessment which successfully identified additional financial and non-financial resources for 60% of them. A range of demographic, health and social outcome measures were assessed at baseline, 6, 12 and 24 month follow up. Qualitative data were collected from a sub-sample of 25 participants purposively selected to take part in individual interviews to examine the perceived impact of welfare rights advice.
Separate analysis of the quantitative and qualitative data revealed discrepant findings. The quantitative data showed little evidence of significant differences of a size that would be of practical or clinical interest, suggesting that the intervention had no impact on these outcome measures. The qualitative data suggested wide-ranging impacts, indicating that the intervention had a positive effect. Six ways of further exploring these data were considered: (i) treating the methods as fundamentally different; (ii) exploring the methodological rigour of each component; (iii) exploring dataset comparability; (iv) collecting further data and making further comparisons; (v) exploring the process of the intervention; and (vi) exploring whether the outcomes of the two components match.
The study demonstrates how using mixed methods can lead to different and sometimes conflicting accounts and, using this six step approach, how such discrepancies can be harnessed to interrogate each dataset more fully. Not only does this enhance the robustness of the study, it may lead to different conclusions from those that would have been drawn through relying on one method alone and demonstrates the value of collecting both types of data within a single study. More widespread use of mixed methods in trials of complex interventions is likely to enhance the overall quality of the evidence base.
Combining quantitative and qualitative methods in a single study is not uncommon in social research, although, 'traditionally a gulf is seen to exist between qualitative and quantitative research with each belonging to distinctively different paradigms'. [ 1 ] Within health research there has, more recently, been an upsurge of interest in the combined use of qualitative and quantitative methods, sometimes termed mixed methods research [ 2 ] although the terminology can vary. [ 3 ] Greater interest in qualitative research has come about for a number of reasons: the numerous contributions made by qualitative research to the study of health and illness [ 4 – 6 ]; increased methodological rigor [ 7 ] within the qualitative paradigm, which has made it more acceptable to researchers or practitioners trained within a predominantly quantitative paradigm [ 8 ]; and, because combining quantitative and qualitative methods may generate deeper insights than either method alone. [ 9 ] It is now widely recognised that public health problems are embedded within a range of social, political and economic contexts. [ 10 ] Consequently, a range of epidemiological and social science methods are employed to research these complex issues. [ 11 ] Further legitimacy for the use of qualitative methods alongside quantitative has resulted from the recognition that qualitative methods can make an important contribution to randomised controlled trials (RCTs) evaluating complex health service interventions. There is published work on the various ways that qualitative methods are being used in RCTs (e.g. [ 12 , 13 ] but little on how they can optimally enhance the usefulness and policy relevance of trial findings. [ 14 , 15 ]
A number of mixed methods publications outline the various ways in which qualitative and quantitative methods can be combined. [ 1 , 2 , 9 , 16 ] For the purposes of this paper with its focus on mixed methods in the context of a pilot RCT, the significant aspects of mixed methods appear to be: purpose, process and, analysis and interpretation. In terms of purpose, qualitative research may be used to help identify the relevant variables for study [ 17 ], develop an instrument for quantitative research [ 18 ], to examine different questions (such as acceptability of the intervention, rather than its outcome) [ 19 ]; and to examine the same question with different methods (using, for example participant observation or in depth interviews [ 1 ]). Process includes the priority accorded to each method and ordering of both methods which may be concurrent, sequential or iterative. [ 20 ] Bryman [ 9 ] points out that, 'most researchers rely primarily on a method associated with either quantitative or qualitative methods and then buttress their findings with a method associated with the other tradition' (p128). Both datasets may be brought together at the 'analysis/interpretation' phase, often known as 'triangulation' [ 21 ]. Brannen [ 1 ] suggests that most researchers have taken this to mean more than one type of data, but she stresses that Denzin's original conceptualisation involved methods, data, investigators or theories. Bringing different methods together almost inevitably raises discrepancies in findings and their interpretation. However, the investigation of such differences may be as illuminating as their points of similarity. [ 1 , 9 ]
Although mixed methods are now widespread in health research, quantitative and qualitative methods and results are often published separately. [ 22 , 23 ] It is relatively rare to see an account of the methodological implications of the strategy and the way in which both methods are combined when interpreting the data within a particular study. [ 1 ] A notable exception is a study showing divergence between qualitative and quantitative findings of cancer patients' quality of life using a detailed case study approach to the data. [ 13 ]
By presenting quantitative and qualitative data collected within a pilot RCT together, this paper has three main aims: firstly, to demonstrate how divergent quantitative and qualitative data led us to interrogate each dataset more fully and assisted in the interpretation process, producing a greater research yield from each dataset; secondly, to demonstrate how combining both types of data at the analysis stage produces 'more than the sum of its parts'; and thirdly, to emphasise the complementary nature of qualitative and quantitative methods in RCTs of complex interventions. In doing so, we demonstrate how the combination of quantitative and qualitative data led us to conclusions different from those that would have been drawn through relying on one or other method alone.
The study that forms the basis of this paper, a pilot RCT to examine the impact of welfare rights advice in primary care, was funded under the UK Department of Health's Policy Research Programme on tackling health inequalities, and focused on older people. To date, little research has been able to demonstrate how health inequalities can be tackled by interventions within and outside the health sector. Although living standards have risen among older people, a common experience of growing old is worsening material circumstances. [ 24 ] In 2000–01 there were 2.3 million UK pensioners living in households with below 60 per cent of median household income, after housing costs. [ 25 ] Older people in the UK may be eligible for a number of income- or disability-related benefits (the latter could be non-financial such as parking permits or adaptations to the home), but it has been estimated that approximately one in four (about one million) UK pensioner households do not claim the support to which they are entitled. [ 26 ] Action to facilitate access to and uptake of welfare benefits has taken place outside the UK health sector for many years and, more recently, has been introduced within parts of the health service, but its potential to benefit health has not been rigorously evaluated. [ 27 – 29 ]
There are a number of models of mixed methods research. [ 2 , 16 , 30 ] We adopted a model which relies of the principle of complementarity, using the strengths of one method to enhance the other. [ 30 ] We explicitly recognised that each method was appropriate for different research questions. We undertook a pragmatic RCT which aimed to evaluate the health effects of welfare rights advice in primary care among people aged over 60. Quantitative data included standardised outcome measures of health and well-being, health related behaviour, psycho-social interaction and socio-economic status ; qualitative data used semi-structured interviews to explore participants' views about the intervention, its outcome, and the acceptability of the research process.
Following an earlier qualitative pilot study to inform the selection of appropriate outcome measures [ 31 ], contemporaneous quantitative and qualitative data were collected. Both datasets were analysed separately and neither compared until both analyses were complete. The sampling strategy mirrored the embedded design; probability sampling for the quantitative study and theoretical sampling for the qualitative study, done on the basis of factors identified in the quantitative study.
Approval for the study was obtained from Newcastle and North Tyneside Joint Local Research Ethics Committee and from Newcastle Primary Care Trust.
The intervention
The intervention was delivered by a welfare rights officer from Newcastle City Council Welfare Rights Service in participants' own homes and comprised a structured assessment of current welfare status and benefits entitlement, together with active assistance in making claims where appropriate over the following six months, together with necessary follow-up for unresolved claims.
Quantitative study
The design presented ethical dilemmas as it was felt problematic to deprive the control group of welfare rights advice, since there is adequate evidence to show that it leads to significant financial gains. [ 32 ] To circumvent this dilemma, we delivered welfare rights advice to the control group six months after the intervention group. A single-blinded RCT with allocation of individuals to intervention (receipt of welfare rights consultation immediately) and control condition (welfare rights consultation six months after entry into the trial) was undertaken.
Four general practices located at five surgeries across Newcastle upon Tyne took part. Three of the practices were located in the top ten per cent of most deprived wards in England using the Index of Multiple Deprivation (two in the top one percent – ranked 30 th and 36 th most deprived); the other practice was ranked 3,774 out of a total of 8,414 in England. [ 33 ]
Using practice databases, a random sample of 100 patients aged 60 years or over from each of four participating practices was invited to take part in the study. Only one individual per household was allowed to participate in the trial, but if a partner or other adult household member was also eligible for benefits, they also received welfare rights advice. Patients were excluded if they were permanently hospitalised or living in residential or nursing care homes.
Written informed consent was obtained at the baseline interview. Structured face to face interviews were carried out at baseline, six, 12 and 24 months using standard scales covering the areas of demographics, mental and physical health (SF36) [ 34 ], Hospital Anxiety and Depression Scale (HADS) [ 35 ], psychosocial descriptors (e.g. Social Support Questionnaire [ 36 ] and the Self-Esteem Inventory, [ 37 ], and socioeconomic indicators (e.g. affordability and financial vulnerability). [ 38 ] Additionally, a short semi-structured interview was undertaken at 24 months to ascertain the perceived impact of additional resources for those who received them.
All health and welfare assessment data were entered onto customised MS Access databases and checked for quality and completeness. Data were transferred to the Statistical Package for the Social Sciences (SPSS) v11.0 [ 39 ] and STATA v8.0 for analysis. [ 40 ]
Qualitative study
The qualitative findings presented in this paper focus on the impact of the intervention. The sampling frame was formed by those (n = 96) who gave their consent to be contacted during their baseline interview for the RCT. The study sample comprised respondents from intervention and control groups purposively selected to include those eligible for the following resources: financial only; non-financial only; both financial and non financial; and, none. Sampling continued until no new themes emerged from the interviews; until data 'saturation' was reached. [ 21 ]
Initial interviews took place between April and December 2003 in participants' homes after their welfare rights assessment; follow-up interviews were undertaken in January and February 2005. The semi-structured interview schedule covered perceptions of: impact of material and/or financial benefits; impact on mental and/or physical health; impact on health related behaviours; social benefits; and views about the link between material resources and health. All participants agreed to the interview being audio-recorded. Immediately afterwards, observational field notes were made. Interviews were transcribed in full.
Data analysis largely followed the framework approach. [ 41 ] Data were coded, indexed and charted systematically; and resulting typologies discussed with other members of the research team, 'a pragmatic version of double coding'. [ 42 ] Constant comparison [ 43 ] and deviant case analysis [ 44 ] were used since both methods are important for internal validation. [ 7 , 42 ] Finally, sets of categories at a higher level of abstraction were developed.
A brief semi-structured interview was undertaken (by JM) with all participants who received additional resources. These interview data explored the impact data of additional resources on all of those who received them, not just the qualitative sub-sample. The data were independently coded by JM and SM using the same coding frame. Discrepant codes were examined by both researchers and a final code agreed.
One hundred and twenty six people were recruited into the study; there were 117 at 12 month follow-up and 109 at 24 months (five deaths, one moved, the remainder declined).
Table 1 shows the distribution of financial and non-financial benefits awarded as a result of the welfare assessments. Sixty percent of participants were awarded some form of welfare benefit, and just over 40% received a financial benefit. Some households received more than one type of benefit.
Table 2 compares the quantitative and qualitative sub-samples on a number of personal, economic, health and lifestyle factors at baseline. Intervention and control groups were comparable.
Table 3 compares outcome measures by award group, i.e. no award, non-financial and financial and shows only small differences between the mean changes across each group, none of which were statistically significant. Other analyses of the quantitative data compared the changes seen between baseline and six months (by which time the intervention group had received the welfare rights advice but the control group had not) and found little evidence of differences between the intervention and control groups of any practical importance. The only statistically significant difference between the groups was a small decrease in financial vulnerability in the intervention group after six months. [ 45 ]
There was little evidence for differences in health and social outcomes measures as a result of the receipt of welfare advice of a size that would be of major practical or clinical interest. However, this was a pilot study, with only the power to detect large differences if they were present. One reason for a lack of difference may be that the scales were less appropriate for older people and did not capture all relevant outcomes. Another reason for the lack of differences may be that insufficient numbers of people had received their benefits for long enough to allow any health outcomes to have changed when comparisons were made. Fourteen per cent of participants found to be eligible for financial benefits had not started receiving their benefits by the time of the first follow-up interview after their benefit assessment (six months for intervention, 12 months for control); and those who had, had only received them for an average of 2 months. This is likely to have diluted any impact of the intervention effect, and might account, to some extent, for the lack of observed effect.
Twenty five interviews were completed, fourteen of whom were from the intervention group. Ten participants were interviewed with partners who made active contributions. Twenty two follow-up interviews were undertaken between twelve and eighteen months later (three individuals were too ill to take part).
Table 1 (fifth column) shows that 14 of the participants in the qualitative study received some financial award. The median income gain was (€84, $101) (range £10 (€15, $18) -£100 (€148, $178)) representing a 4%-55% increase in weekly income. 18 participants were in receipt of benefit, either as a result of the current intervention or because of claims made prior to this study.
By the follow-up (FU) interviews all but one participant had been receiving their benefits for between 17 and 31 months. The intervention was viewed positively by all interviewees irrespective of outcome. However, for the fourteen participants who received additional financial resources the impact was considerable and accounts revealed a wide range of uses for the extra money. Participants' accounts revealed four linked categories, summarised on Table 4 . Firstly, increased affordability of necessities , without which maintaining independence and participating in daily life was difficult. This included accessing transport, maintaining social networks and social activities, buying better quality food, stocking up on food, paying bills, preventing debt and affording paid help for household activities. Secondly, occasional expenses such as clothes, household equipment, furniture and holidays were more affordable. Thirdly, extra income was used to act as a cushion against potential emergencies and to increase savings . Fourthly, all participants described the easing of financial worries as bringing ' peace of mind' .
Without exception, participants were of the view that extra money or resources would not improve existing health problems. The reasons behind these strongly held views about individual health conditions was generally that their poor health was attributed to specific health conditions and a combination of family history or fate, which were immune to the effects of money. Most participants had more than one chronic condition and felt that because of these conditions, plus their age, additional money would have no effect.
However, a number of participants linked the impact of the intervention with improved ways of coping with their conditions because of what the extra resources enabled them to do:
Mrs T: Having money is not going to improve his health, we could win the lottery and he would still have his health problems.
Mr T: No, but we don't need to worry if I wanted .... Well I mean I eat a lot of honey and I think it's very good, very healthful for you ... at one time we couldn't have afforded to buy these things. Now we can go and buy them if I fancy something, just go and get it where we couldn't before .
Mrs T: Although the Attendance Allowance is actually his [partners], it's made me relax a bit more ... I definitely worry less now (N15, female, 62 and partner)
Despite the fact that no-one expected their own health conditions to improve, most people believed that there was a link between resources and health in a more abstract sense, either because they experienced problems affording necessities such as healthy food or maintaining adequate heat in their homes, or because they empathised with those who lacked money. Participants linked adequate resources to maintaining health and contributing to a sense of well-being.
Money does have a lot to do with health if you are poor. It would have a lot to do with your health ... I don't buy loads and loads of luxuries, but I know I can go out and get the food we need and that sort of thing. I think that money is a big part of how a house, or how people in that house are . (N13, female, 72)
Comparing the results from the two datasets
When the separate analyses of the quantitative and qualitative datasets after the 12 month follow-up structured interviews were completed, the discrepancy in the findings became apparent. The quantitative study showed little evidence of a size that would be of practical or clinical interest, suggesting that the intervention had no impact on these outcome measures. The qualitative study found a wide-ranging impact, indicating that the intervention had a positive effect. The presence of such inter-method discrepancy led to a great deal of discussion and debate, as a result of which we devised six ways of further exploring these data.
(i) Treating the methods as fundamentally different
This process of simultaneous qualitative and quantitative dataset interrogation enables a deeper level of analysis and interpretation than would be possible with one or other alone and demonstrates how mixed methods research produces more than the sum of its parts. It is worth emphasising however, that it is not wholly surprising that each method comes up with divergent findings since each asked different, but related questions, and both are based on fundamentally different theoretical paradigms. Brannen [ 1 ] and Bryman [ 9 ] argue that it is essential to take account of these theoretical differences and caution against taking a purely technical approach to the use of mixed methods, a simple 'bolting together' of techniques. [ 17 ] Combining the two methods for crossvalidation (triangulation) purposes is not a viable option because it rests on the premise that both methods are examining the same research problem. [ 1 ] We have approached the divergent findings as indicative of different aspects of the phenomena in question and searched for reasons which might explain these inconsistencies. In the approach that follows, we have treated the datasets as complementary, rather than attempt to integrate them, since each approach reflects a different view on how social reality ought to be studied.
(ii) Exploring the methodological rigour of each component
It is standard practice at the data analysis and interpretation phases of any study to scrutinise methodological rigour. However, in this case, we had another dataset to use as a yardstick for comparison and it became clear that our interrogation of each dataset was informed to some extent by the findings of the other. It was not the case that we expected to obtain the same results, but clearly the divergence of our findings was of great interest and made us more circumspect about each dataset. We began by examining possible reasons why there might be problems with each dataset individually, but found ourselves continually referring to the results of the other study as a benchmark for comparison.
With regard to the quantitative study, it was a pilot, of modest sample size, and thus not powered to detect small differences in the key outcome measures. In addition there were three important sources of dilution effects: firstly, only 63% of intervention group participants received some type of financial award; secondly, we found that 14% of those in the trial eligible for financial benefits did not receive their money until after the follow up assessments had been carried out; and thirdly, many had received their benefits for only a short period, reducing the possibility of detecting any measurable effects at the time of follow-up. All of these factors provide some explanation for the lack of a measurable effect between intervention and control group and between those who did and did not receive additional financial resources.
The number of participants in the qualitative study who received additional financial resources as a result of this intervention was small (n = 14). We would argue that the fieldwork, analysis and interpretation [ 46 ] were sufficiently transparent to warrant the degree of methodological rigour advocated by Barbour [ 7 , 17 ] and that the findings were therefore an accurate reflection of what was being studied. However, there still remained the possibility that a reason for the discrepant findings was due to differences between the qualitative sub-sample and the parent sample, which led us to step three.
(iii) Exploring dataset comparability
We compared the qualitative and quantitative samples on a number of social and economic factors (Table 2 ). In comparison to the parent sample, the qualitative sub-sample was slightly older, had fewer men, a higher proportion with long-term limiting illness, but fewer current smokers. However, there was nothing to indicate that such small differences would account for the discrepancies. There were negligible differences in SF-36 (Physical and Mental) and HAD (Anxiety and Depression) scores between the groups at baseline, which led us to discount the possibility that those in the quantitative sub sample were markedly different to the quantitative sample on these outcome measures.
(iv) Collection of additional data and making further comparisons
The divergent findings led us to seek further funding to undertake collection of additional quantitative and qualitative data at 24 months. The quantitative and qualitative follow-up data verified the initial findings of each study. [ 45 ] We also collected a limited amount of qualitative data on the perceived impact of resources, from all participants who had received additional resources. These data are presented in figure 1 which shows the uses of additional resources at 24 month follow-up for 35 participants (N = 35, 21 previously in quantitative study only, 14 in both). This dataset demonstrates that similar issues emerged for both qualitative and quantitative datasets: transport, savings and 'peace of mind' emerged as key issues, but the data also showed that the additional money was used on a wide range of items. This follow-up confirmed the initial findings of each study and further, indicated that the perceived impact of the additional resources was the same for a larger sample than the original qualitative sub-sample, further confirming our view that the positive findings extended beyond the fourteen participants in the qualitative sub-sample, to all those receiving additional resources.
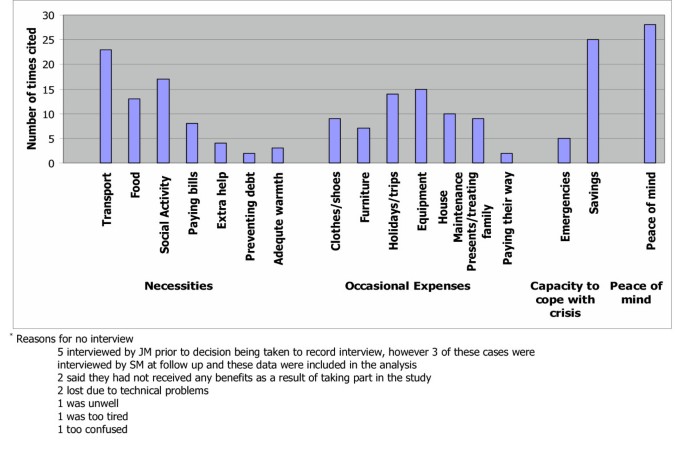
Use of additional resources at 2 year follow up (N = 35)*.
(v) Exploring whether the intervention under study worked as expected
The qualitative study revealed that many participants had received welfare benefits via other services prior to this study, revealing the lack of a 'clean slate' with regard to the receipt of benefits, which we had not anticipated. We investigated this further in the quantitative dataset and found that 75 people (59.5%) had received benefits prior to the study; if the first benefit was on health grounds, a later one may have been because their health had deteriorated further.
(vi) Exploring whether the outcomes of the quantitative and qualitative components match
'Probing certain issues in greater depth' as advocated by Bryman (p134) [ 1 ] focussed our attention on the outcome measures used in the quantitative part of the study and revealed several challenges. Firstly, the qualitative study revealed a number of dimensions not measured by the quantitative study, such as, 'maintaining independence' which included affording paid help, increasing and improving access to facilities and managing better within the home. Secondly, some of the measures used with the intention of capturing dimensions of mental health did not adequately encapsulate participants' accounts of feeling 'less stressed' and 'less depressed' by financial worries. Probing both datasets also revealed congruence along the dimension of physical health. No differences were found on the SF36 physical scale and participants themselves did not expect an improvement in physical health (for reasons of age and chronic health problems). The real issue would appear to be measuring ways in which older people are better able to cope with existing health problems and maintain their independence and quality of life, despite these conditions.
Qualitative study results also led us to look more carefully at the quantitative measures we used. Some of the standardised measures were not wholly applicable to a population of older people. Mallinson [ 47 ] also found this with the SF36 when she demonstrated some of its limitations with this age group, as well as how easy it is to, 'fall into the trap of using questionnaires like a form of laboratory equipment and forget that ... they are open to interpretation'. The data presented here demonstrate the difficulties of trying to capture complex phenomena quantitatively. However, they also demonstrate the usefulness of having alternative data forms on which to draw whether complementary (where they differ but together generate insights) or contradictory (where the findings conflict). [ 30 ] In this study, the complementary and contradictory findings of the two datasets proved useful in making recommendations for the design of a definitive study.
Many researchers understand the importance, indeed the necessity, of combining methods to investigate complex health and social issues. Although quantitative research remains the dominant paradigm in health services research, qualitative research has greater prominence than before and is no longer, as Barbour [ 42 ] points out regarded as the 'poor relation to quantitative research that it has been in the past' (p1019). Brannen [ 48 ] argues that, despite epistemological differences there are 'more overlaps than differences'. Despite this, there is continued debate about the authority of each individual mode of research which is not surprising since these different styles, 'take institutional forms, in relation to cultures of and markets for knowledge' (p168). [ 49 ] Devers [ 50 ] points out that the dominance of positivism, especially within the RCT method, has had an overriding influence on the criteria used to assess research which has had the inevitable result of viewing qualitative studies unfavourably. We advocate treating qualitative and quantitative datasets as complementary rather than in competition for identifying the true version of events. This, we argue, leads to a position which exploits the strengths of each method and at the same time counters the limitations of each. The process of interpreting the meaning of these divergent findings has led us to conclude that much can be learned from scientific realism [ 51 ]which has 'sought to position itself as a model of scientific explanation which avoids the traditional epistemological poles of positivism and relativism' (p64). This stance enables investigators to take account of the complexity inherent in social interventions and reinforces, at a theoretical level, the problems of attempting to measure the impact of a social intervention via experimental means. However, the current focus on evidence based health care [ 52 ] now includes public health [ 53 , 54 ] and there is increased attention paid to the results of trials of public health interventions, attempting as they do, to capture complex social phenomena using standardised measurement tools. We would argue that at the very least, the inclusion of both qualitative and quantitative elements in such studies, is essential and ultimately more cost-effective, increasing the likelihood of arriving at a more thoroughly researched and better understood set of results.
The findings of this study demonstrate how the use of mixed methods can lead to different and sometimes conflicting accounts. This, we argue, is largely due to the outcome measures in the RCT not matching the outcomes emerging from the qualitative arm of the study. Instead of making assumptions about the correct version, we have reported the results of both datasets together rather than separately, and advocate six steps to interrogate each dataset more fully. The methodological strategy advocated by this approach involves contemporaneous qualitative and quantitative data collection, analysis and reciprocal interrogation to inform interpretation in trials of complex interventions. This approach also indicates the need for a realistic appraisal of quantitative tools. More widespread use of mixed methods in trials of complex interventions is likely to enhance the overall quality of the evidence base.
Brannen J: Mixing Methods: qualitative and quantitative research. 1992, Aldershot, Ashgate
Google Scholar
Tashakkori A, Teddlie C: Handbook of Mixed Methods in Social and Behavioural Research. 2003, London, Sage
Morgan DL: Triangulation and it's discontents: Developing pragmatism as an alternative justification for combining qualitative and quantitative methods. Cambridge, 11-12 July.. 2005.
Pill R, Stott NCH: Concepts of illness causation and responsibility: some preliminary data from a sample of working class mothers. Social Science and Medicine. 1982, 16: 43-52. 10.1016/0277-9536(82)90422-1.
Article CAS PubMed Google Scholar
Scambler G, Hopkins A: Generating a model of epileptic stigma: the role of qualitative analysis. Social Science and Medicine. 1990, 30: 1187-1194. 10.1016/0277-9536(90)90258-T.
Townsend A, Hunt K, Wyke S: Managing multiple morbidity in mid-life: a qualitative study of attitudes to drug use. BMJ. 2003, 327: 837-841. 10.1136/bmj.327.7419.837.
Article PubMed PubMed Central Google Scholar
Barbour RS: Checklists for improving rigour in qualitative research: the case of the tail wagging the dog?. British Medical Journal. 2001, 322: 1115-1117.
Article CAS PubMed PubMed Central Google Scholar
Pope C, Mays N: Qualitative Research in Health Care. 2000, London, BMJ Books
Bryman A: Quantity and Quality in Social Research. 1995, London, Routledge
Ashton J: Healthy cities. 1991, Milton Keynes, Open University Press
Baum F: Researching Public Health: Behind the Qualitative-Quantitative Methodological Debate. Social Science and Medicine. 1995, 40: 459-468. 10.1016/0277-9536(94)E0103-Y.
Donovan J, Mills N, Smith M, Brindle L, Jacoby A, Peters T, Frankel S, Neal D, Hamdy F: Improving design and conduct of randomised trials by embedding them in qualitative research: (ProtecT) study. British Medical Journal. 2002, 325: 766-769.
Cox K: Assessing the quality of life of patients in phase I and II anti-cancer drug trials: interviews versus questionnaires. Social Science and Medicine. 2003, 56: 921-934. 10.1016/S0277-9536(02)00100-4.
Article PubMed Google Scholar
Lewin S: Mixing methods in complex health service randomised controlled trials: research 'best practice'. Mixed Methods in Health Services Research Conference 23rd November 2004, Sheffield University.
Cresswell JW: Mixed methods research and applications in intervention studies.: ; Cambridge, July 11-12. 2005.
Cresswell JW: Research Design. Qualitative, Quantitative and Mixed Methods Approaches. 2003, London, Sage
Barbour RS: The case for combining qualitative and quanitative approaches in health services research. Journal of Health Services Research and Policy. 1999, 4: 39-43.
Gabriel Z, Bowling A: Quality of life from the perspectives of older people. Ageing & Society. 2004, 24: 675-691. 10.1017/S0144686X03001582.
Article Google Scholar
Koops L, Lindley RL: Thrombolysis for acute ischaemic stroke: consumer involvement in design of new randomised controlled trial. BMJ. 2002, 325: 415-418. 10.1136/bmj.325.7361.415.
O'Cathain A, Nicholl J, Murphy E: Making the most of mixed methods. Mixed Methods in Health Services Research Conference 23rd November 2004, Sheffield University.
Denzin NK: The Research Act. 1978, New York, McGraw-Hill Book Company
Roberts H, Curtis K, Liabo K, Rowland D, DiGuiseppi C, Roberts I: Putting public health evidence into practice: increasing the prevalance of working smoke alarms in disadvantaged inner city housing. Journal of Epidemiology & Community Health. 2004, 58: 280-285. 10.1136/jech.2003.007948.
Article CAS Google Scholar
Rowland D, DiGuiseppi C, Roberts I, Curtis K, Roberts H, Ginnelly L, Sculpher M, Wade A: Prevalence of working smoke alarms in local authority inner city housing: randomised controlled trial. British Medical Journal. 2002, 325: 998-1001.
Vincent J: Old Age. 2003, London, Routledge
Chapter Google Scholar
Department for Work and Pensions: Households below average income statistics 2000/01. 2002, London, Department for Work and Pensions
National Audit Office: Tackling Pensioner Pverty: Encouraging take-up of entitlements. 2002, London, National Audit Office
Paris JAG, Player D: Citizens advice in general practice. British Medical Journal. 1993, 306: 1518-1520.
Abbott S: Prescribing welfare benefits advice in primary care: is it a health intervention, and if so, what sort?. Journal of Public Health Medicine. 2002, 24: 307-312. 10.1093/pubmed/24.4.307.
Harding R, Sherr L, Sherr A, Moorhead R, Singh S: Welfare rights advice in primary care: prevalence, processes and specialist provision. Family Practice. 2003, 20: 48-53. 10.1093/fampra/20.1.48.
Morgan DL: Practical strategies for combining qualitative and quantitative methods: applications to health research. Qualitative Health Research. 1998, 8: 362-376.
Moffatt S, White M, Stacy R, Downey D, Hudson E: The impact of welfare advice in primary care: a qualitative study. Critical Public Health. 2004, 14: 295-309. 10.1080/09581590400007959.
Thomson H, Hoskins R, Petticrew M, Ogilvie D, Craig N, Quinn T, Lindsey G: Evaluating the health effects of social interventions. British Medical Journal. 2004, 328: 282-285.
Department for the Environment TR: Measuring Multiple Deprivation at the Small Area Level: The Indices of Deprivation. 2000, London, Department for the Envrionment, Trransport and the Regions
Ware JE, Sherbourne CD: The MOS 36 item short form health survey (SF-36). Conceptual framework and item selection. Medical Care. 1992, 30: 473-481.
Snaith RP, Zigmond AS: The hospital anxiety and depression scale. Acta Psychiatrica Scandinivica. 1983, 67: 361-370.
Sarason I, Carroll C, Maton K: Assessing social support: the social support questionnaire. Journal of Personality and Social Psychology. 1983, 44: 127-139. 10.1037//0022-3514.44.1.127.
Ward R: The impact of subjective age and stigma on older persons. Journal of Gerontology. 1977, 32: 227-232.
Ford G, Ecob R, Hunt K, Macintyre S, West P: Patterns of class inequality in health through the lifespan: class gradients at 15, 35 and 55 years in the west of Scotland. Social Science and Medicine. 1994, 39: 1037-1050. 10.1016/0277-9536(94)90375-1.
SPSS.: v. 11.0 for Windows [program]. 2003, Chicago, Illinois.
STATA.: Statistical Software [program]. 8.0 version. 2003, Texas, College Station
Ritchie J, Lewis J: Qualitative Research Practice. A Guide for Social Scientists. 2003, London, Sage
Barbour RS: The Newfound Credibility of Qualitative Research? Tales of Technical Essentialism and Co-Option. Qualitative Health Research. 2003, 13: 1019-1027. 10.1177/1049732303253331.
Silverman D: Doing qualitative research. 2000, London, Sage
Clayman SE, Maynard DW: Ethnomethodology and conversation analysis. Situated Order: Studies in the Social Organisation of Talk and Embodied Activities. Edited by: Have PT and Psathas G. 1994, Washington, D.C., University Press of America
White M, Moffatt S, Mackintosh J, Howel D, Sandell A, Chadwick T, Deverill M: Randomised controlled trial to evaluate the health effects of welfare rights advice in primary health care: a pilot study. Report to the Department of Health, Policy Research Programme. 2005, Newcastle upon Tyne, University of Newcastle upon Tyne
Moffatt S: "All the difference in the world". A qualitative study of the perceived impact of a welfare rights service provided in primary care. 2004, , University College London
Mallinson S: Listening to reposndents: a qualitative assessment of the Short-Form 36 Health Status Questionnaire. Social Science and Medicine. 2002, 54: 11-21. 10.1016/S0277-9536(01)00003-X.
Brannen J: Mixing Methods: The Entry of Qualitative and Quantitative Approaches into the Research Process. International Journal of Social Research Methodology. 2005, 8: 173-184. 10.1080/13645570500154642.
Green A, Preston J: Editorial: Speaking in Tongues- Diversity in Mixed Methods Research. International Journal of Social Research Methodology. 2005, 8: 167-171. 10.1080/13645570500154626.
Devers KJ: How will we know "good" qualitative research when we see it? Beginning the dialogue in Health Services Research. Health Services Research. 1999, 34: 1153-1188.
CAS PubMed PubMed Central Google Scholar
Pawson R, Tilley N: Realistic Evaluation. 2004, London, Sage
Miles A, Grey JE, Polychronis A, Price N, Melchiorri C: Current thinking in the evidence-based health care debate. Journal of Evaluation in Clinical Practice. 2003, 9: 95-109. 10.1046/j.1365-2753.2003.00438.x.
Pencheon D, Guest C, Melzer D, Gray JAM: Oxford Handbook of Public Health Practice. 2001, Oxford, Oxford University Press
Wanless D: Securing Good Health for the Whole Population. 2004, London, HMSO
Pre-publication history
The pre-publication history for this paper can be accessed here: http://www.biomedcentral.com/1472-6963/6/28/prepub
Download references
Acknowledgements
We wish to thank: Rosemary Bell, Jenny Dover and Nick Whitton from Newcastle upon Tyne City Council Welfare Rights Service; all the participants and general practice staff who took part; and for their extremely helpful comments on earlier drafts of this paper, Adam Sandell, Graham Scambler, Rachel Baker, Carl May and John Bond. We are grateful to referees Alicia O'Cathain and Sally Wyke for their insightful comments. The views expressed in this paper are those of the authors and not necessarily those of the Department of Health.
Author information
Authors and affiliations.
Public Health Research Group, School of Population & Health Sciences, Faculty of Medical Sciences, William Leech Building, Framlington Place, Newcastle upon Tyne, NE2 4HH, UK
Suzanne Moffatt, Martin White, Joan Mackintosh & Denise Howel
You can also search for this author in PubMed Google Scholar
Corresponding author
Correspondence to Suzanne Moffatt .
Additional information
Competing interests.
The author(s) declare that they have no competing interests.
Authors' contributions
SM and MW had the original idea for the study, and with the help of DH, Adam Sandell and Nick Whitton developed the proposal and gained funding. JM collected the data for the quantitative study, SM designed and collected data for the qualitative study. JM, DH and MW analysed the quantitative data, SM analysed the qualitative data. All authors contributed to interpretation of both datasets. SM wrote the first draft of the paper, JM, MW and DH commented on subsequent drafts. All authors have read and approved the final manuscript.
Authors’ original submitted files for images
Below are the links to the authors’ original submitted files for images.
Authors’ original file for figure 1
Rights and permissions.
Open Access This article is published under license to BioMed Central Ltd. This is an Open Access article is distributed under the terms of the Creative Commons Attribution License ( https://creativecommons.org/licenses/by/2.0 ), which permits unrestricted use, distribution, and reproduction in any medium, provided the original work is properly cited.
Reprints and permissions
About this article
Cite this article.
Moffatt, S., White, M., Mackintosh, J. et al. Using quantitative and qualitative data in health services research – what happens when mixed method findings conflict? [ISRCTN61522618]. BMC Health Serv Res 6 , 28 (2006). https://doi.org/10.1186/1472-6963-6-28
Download citation
Received : 29 September 2005
Accepted : 08 March 2006
Published : 01 December 2006
DOI : https://doi.org/10.1186/1472-6963-6-28
Share this article
Anyone you share the following link with will be able to read this content:
Sorry, a shareable link is not currently available for this article.
Provided by the Springer Nature SharedIt content-sharing initiative
- Mixed Method
- Welfare Benefit
- Mixed Method Research
- Divergent Finding
- Financial Vulnerability
BMC Health Services Research
ISSN: 1472-6963
- General enquiries: [email protected]
- Research article
- Open access
- Published: 03 February 2021
A review of the quantitative effectiveness evidence synthesis methods used in public health intervention guidelines
- Ellesha A. Smith ORCID: orcid.org/0000-0002-4241-7205 1 ,
- Nicola J. Cooper 1 ,
- Alex J. Sutton 1 ,
- Keith R. Abrams 1 &
- Stephanie J. Hubbard 1
BMC Public Health volume 21 , Article number: 278 ( 2021 ) Cite this article
4057 Accesses
5 Citations
3 Altmetric
Metrics details
The complexity of public health interventions create challenges in evaluating their effectiveness. There have been huge advancements in quantitative evidence synthesis methods development (including meta-analysis) for dealing with heterogeneity of intervention effects, inappropriate ‘lumping’ of interventions, adjusting for different populations and outcomes and the inclusion of various study types. Growing awareness of the importance of using all available evidence has led to the publication of guidance documents for implementing methods to improve decision making by answering policy relevant questions.
The first part of this paper reviews the methods used to synthesise quantitative effectiveness evidence in public health guidelines by the National Institute for Health and Care Excellence (NICE) that had been published or updated since the previous review in 2012 until the 19th August 2019.The second part of this paper provides an update of the statistical methods and explains how they address issues related to evaluating effectiveness evidence of public health interventions.
The proportion of NICE public health guidelines that used a meta-analysis as part of the synthesis of effectiveness evidence has increased since the previous review in 2012 from 23% (9 out of 39) to 31% (14 out of 45). The proportion of NICE guidelines that synthesised the evidence using only a narrative review decreased from 74% (29 out of 39) to 60% (27 out of 45).An application in the prevention of accidents in children at home illustrated how the choice of synthesis methods can enable more informed decision making by defining and estimating the effectiveness of more distinct interventions, including combinations of intervention components, and identifying subgroups in which interventions are most effective.
Conclusions
Despite methodology development and the publication of guidance documents to address issues in public health intervention evaluation since the original review, NICE public health guidelines are not making full use of meta-analysis and other tools that would provide decision makers with fuller information with which to develop policy. There is an evident need to facilitate the translation of the synthesis methods into a public health context and encourage the use of methods to improve decision making.
Peer Review reports
To make well-informed decisions and provide the best guidance in health care policy, it is essential to have a clear framework for synthesising good quality evidence on the effectiveness and cost-effectiveness of health interventions. There is a broad range of methods available for evidence synthesis. Narrative reviews provide a qualitative summary of the effectiveness of the interventions. Meta-analysis is a statistical method that pools evidence from multiple independent sources [ 1 ]. Meta-analysis and more complex variations of meta-analysis have been extensively applied in the appraisals of clinical interventions and treatments, such as drugs, as the interventions and populations are clearly defined and tested in randomised, controlled conditions. In comparison, public health studies are often more complex in design, making synthesis more challenging [ 2 ].
Many challenges are faced in the synthesis of public health interventions. There is often increased methodological heterogeneity due to the inclusion of different study designs. Interventions are often poorly described in the literature which may result in variation within the intervention groups. There can be a wide range of outcomes, whose definitions are not consistent across studies. Intermediate, or surrogate, outcomes are often used in studies evaluating public health interventions [ 3 ]. In addition to these challenges, public health interventions are often also complex meaning that they are made up of multiple, interacting components [ 4 ]. Recent guidance documents have focused on the synthesis of complex interventions [ 2 , 5 , 6 ]. The National Institute for Health and Care Excellence (NICE) guidance manual provides recommendations across all topics that are covered by NICE and there is currently no guidance that focuses specifically on the public health context.
Research questions
A methodological review of NICE public health intervention guidelines by Achana et al. (2014) found that meta-analysis methods were not being used [ 3 ]. The first part of this paper aims to update and compare, to the original review, the meta-analysis methods being used in evidence synthesis of public health intervention appraisals.
The second part of this paper aims to illustrate what methods are available to address the challenges of public health intervention evidence synthesis. Synthesis methods that go beyond a pairwise meta-analysis are illustrated through the application to a case study in public health and are discussed to understand how evidence synthesis methods can enable more informed decision making.
The third part of this paper presents software, guidance documents and web tools for methods that aim to make appropriate evidence synthesis of public health interventions more accessible. Recommendations for future research and guidance production that can improve the uptake of these methods in a public health context are discussed.
Update of NICE public health intervention guidelines review
Nice guidelines.
The National Institute for Health and Care Excellence (NICE) was established in 1999 as a health authority to provide guidance on new medical technologies to the NHS in England and Wales [ 7 ]. Using an evidence-based approach, it provides recommendations based on effectiveness and cost-effectiveness to ensure an open and transparent process of allocating NHS resources [ 8 ]. The remit for NICE guideline production was extended to public health in April 2005 and the first recommendations were published in March 2006. NICE published ‘Developing NICE guidelines: the manual’ in 2006, which has been updated since, with the most recent in 2018 [ 9 ]. It was intended to be a guidance document to aid in the production of NICE guidelines across all NICE topics. In terms of synthesising quantitative evidence, the NICE recommendations state: ‘meta-analysis may be appropriate if treatment estimates of the same outcome from more than 1 study are available’ and ‘when multiple competing options are being appraised, a network meta-analysis should be considered’. The implementation of network meta-analysis (NMA), which is described later, as a recommendation from NICE was introduced into the guidance document in 2014, with a further update in 2018.
Background to the previous review
The paper by Achana et al. (2014) explored the use of evidence synthesis methodology in NICE public health intervention guidelines published between 2006 and 2012 [ 3 ]. The authors conducted a systematic review of the methods used to synthesise quantitative effectiveness evidence within NICE public health guidelines. They found that only 23% of NICE public health guidelines used pairwise meta-analysis as part of the effectiveness review and the remainder used a narrative summary or no synthesis of evidence at all. The authors argued that despite significant advances in the methodology of evidence synthesis, the uptake of methods in public health intervention evaluation is lower than other fields, including clinical treatment evaluation. The paper concluded that more sophisticated methods in evidence synthesis should be considered to aid in decision making in the public health context [ 3 ].
The search strategy used in this paper was equivalent to that in the previous paper by Achana et al. (2014)[ 3 ]. The search was conducted through the NICE website ( https://www.nice.org.uk/guidance ) by searching the ‘Guidance and Advice List’ and filtering by ‘Public Health Guidelines’ [ 10 ]. The search criteria included all guidance documents that had been published from inception (March 2006) until the 19th August 2019. Since the original review, many of the guidelines had been updated with new documents or merged. Guidelines that remained unchanged since the previous review in 2012 were excluded and used for comparison.
The guidelines contained multiple documents that were assessed for relevance. A systematic review is a separate synthesis within a guideline that systematically collates all evidence on a specific research question of interest in the literature. Systematic reviews of quantitative effectiveness, cost-effectiveness evidence and decision modelling reports were all included as relevant. Qualitative reviews, field reports, expert opinions, surveillance reports, review decisions and other supporting documents were excluded at the search stage.
Within the reports, data was extracted on the types of review (narrative summary, pairwise meta-analysis, network meta-analysis (NMA), cost-effectiveness review or decision model), design of included primary studies (randomised controlled trials or non-randomised studies, intermediate or final outcomes, description of outcomes, outcome measure statistic), details of the synthesis methods used in the effectiveness evaluation (type of synthesis, fixed or random effects model, study quality assessment, publication bias assessment, presentation of results, software). Further details of the interventions were also recorded, including whether multiple interventions were lumped together for a pairwise comparison, whether interventions were complex (made up of multiple components) and details of the components. The reports were also assessed for potential use of complex intervention evidence synthesis methodology, meaning that the interventions that were evaluated in the review were made up of components that could potentially be synthesised using an NMA or a component NMA [ 11 ]. Where meta-analysis was not used to synthesis effectiveness evidence, the reasons for this was also recorded.
Search results and types of reviews
There were 67 NICE public health guidelines available on the NICE website. A summary flow diagram describing the literature identification process and the list of guidelines and their reference codes are provided in Additional files 1 and 2 . Since the previous review, 22 guidelines had not been updated. The results from the previous review were used for comparison to the 45 guidelines that were either newly published or updated.
The guidelines consisted of 508 documents that were assessed for relevance. Table 1 shows which types of relevant documents were available in each of the 45 guidelines. The median number of relevant articles per guideline was 3 (minimum = 0, maximum = 10). Two (4%) of the NICE public health guidelines did not report any type of systematic review, cost-effectiveness review or decision model (NG68, NG64) that met the inclusion criteria. 167 documents from 43 NICE public health guidelines were systematic reviews of quantitative effectiveness, cost-effectiveness or decision model reports and met the inclusion criteria.
Narrative reviews of effectiveness were implemented in 41 (91%) of the NICE PH guidelines. 14 (31%) contained a review that used meta-analysis to synthesise the evidence. Only one (1%) NICE guideline contained a review that implemented NMA to synthesise the effectiveness of multiple interventions; this was the same guideline that used NMA in the original review and had been updated. 33 (73%) guidelines contained cost-effectiveness reviews and 34 (76%) developed a decision model.
Comparison of review types to original review
Table 2 compares the results of the update to the original review and shows that the types of reviews and evidence synthesis methodologies remain largely unchanged since 2012. The proportion of guidelines that only contain narrative reviews to synthesise effectiveness or cost-effectiveness evidence has reduced from 74% to 60% and the proportion that included a meta-analysis has increased from 23% to 31%. The proportion of guidelines with reviews that only included evidence from randomised controlled trials and assessed the quality of individual studies remained similar to the original review.
Characteristics of guidelines using meta-analytic methods
Table 3 details the characteristics of the meta-analytic methods implemented in 24 reviews of the 14 guidelines that included one. All of the reviews reported an assessment of study quality, 12 (50%) reviews included only data from randomised controlled trials, 4 (17%) reviews used intermediate outcomes (e.g. uptake of chlamydia screening rather than prevention of chlamydia (PH3)), compared to the 20 (83%) reviews that used final outcomes (e.g. smoking cessation rather than uptake of a smoking cessation programme (NG92)). 2 (8%) reviews only used a fixed effect meta-analysis, 19 (79%) reviews used a random effects meta-analysis and 3 (13%) did not report which they had used.
An evaluation of the intervention information reported in the reviews concluded that 12 (50%) reviews had lumped multiple (more than two) different interventions into a control versus intervention pairwise meta-analysis. Eleven (46%) of the reviews evaluated interventions that are made up of multiple components (e.g. interventions for preventing obesity in PH47 were made up of diet, physical activity and behavioural change components).
21 (88%) of the reviews presented the results of the meta-analysis in the form of a forest plot and 22 (92%) presented the results in the text of the report. 20 (83%) of the reviews used two or more forms of presentation for the results. Only three (13%) reviews assessed publication bias. The most common software to perform meta-analysis was RevMan in 14 (58%) of the reviews.
Reasons for not using meta-analytic methods
The 143 reviews of effectiveness and cost effectiveness that did not use meta-analysis methods to synthesise the quantitative effectiveness evidence were searched for reasons behind this decision. 70 reports (49%) did not give a reason for not synthesising the data using a meta-analysis and 164 reasons were reported which are displayed in Fig. 1 . Out of the remaining reviews, multiple reasons for not using a meta-analysis were given. 53 (37%) of the reviews reported at least one reason due to heterogeneity. 30 (21%) decision model reports did not give a reason and these are categorised separately. 5 (3%) reviews reported that meta-analysis was not applicable or feasible, 1 (1%) reported that they were following NICE guidelines and 5 (3%) reported that there were a lack of studies.
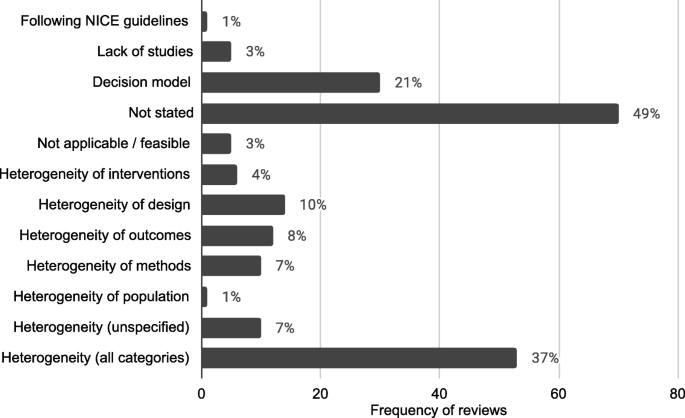
Frequency and proportions of reasons reported for not using statistical methods in quantitative evidence synthesis in NICE PH intervention reviews
The frequency of reviews and guidelines that used meta-analytic methods were plotted against year of publication, which is reported in Fig. 2 . This showed that the number of reviews that used meta-analysis were approximately constant but there is some suggestion that the number of meta-analyses used per guideline increased, particularly in 2018.
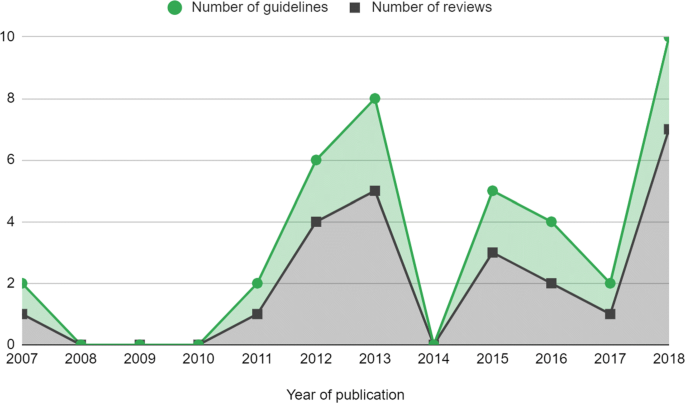
Number of meta-analyses in NICE PH guidelines by year. Guidelines that were published before 2012 had been updated since the previous review by Achana et al. (2014) [ 3 ]
Comparison of meta-analysis characteristics to original review
Table 4 compares the characteristics of the meta-analyses used in the evidence synthesis of NICE public health intervention guidelines to the original review by Achana et al. (2014) [ 3 ]. Overall, the characteristics in the updated review have not much changed from those in the original. These changes demonstrate that the use of meta-analysis in NICE guidelines has increased but remains low. Lumping of interventions still appears to be common in 50% of reviews. The implications of this are discussed in the next section.
Application of evidence synthesis methodology in a public health intervention: motivating example
Since the original review, evidence synthesis methods have been developed and can address some of the challenges of synthesising quantitative effectiveness evidence of public health interventions. Despite this, the previous section shows that the uptake of these methods is still low in NICE public health guidelines - usually limited to a pairwise meta-analysis.
It has been shown in the results above and elsewhere [ 12 ] that heterogeneity is a common reason for not synthesising the quantitative effectiveness evidence available from systematic reviews in public health. Statistical heterogeneity is the variation in the intervention effects between the individual studies. Heterogeneity is problematic in evidence synthesis as it leads to uncertainty in the pooled effect estimates in a meta-analysis which can make it difficult to interpret the pooled results and draw conclusions. Rather than exploring the source of the heterogeneity, often in public health intervention appraisals a random effects model is fitted which assumes that the study intervention effects are not equivalent but come from a common distribution [ 13 , 14 ]. Alternatively, as demonstrated in the review update, heterogeneity is used as a reason to not undertake any quantitative evidence synthesis at all.
Since the size of the intervention effects and the methodological variation in the studies will affect the impact of the heterogeneity on a meta-analysis, it is inappropriate to base the methodological approach of a review on the degree of heterogeneity, especially within public health intervention appraisal where heterogeneity seems inevitable. Ioannidis et al. (2008) argued that there are ‘almost always’ quantitative synthesis options that may offer some useful insights in the presence of heterogeneity, as long as the reviewers interpret the findings with respect to their limitations [ 12 ].
In this section current evidence synthesis methods are applied to a motivating example in public health. This aims to demonstrate that methods beyond pairwise meta-analysis can provide appropriate and pragmatic information to public health decision makers to enable more informed decision making.
Figure 3 summarises the narrative of this part of the paper and illustrates the methods that are discussed. The red boxes represent the challenges in synthesising quantitative effectiveness evidence and refers to the section within the paper for more detail. The blue boxes represent the methods that can be applied to investigate each challenge.
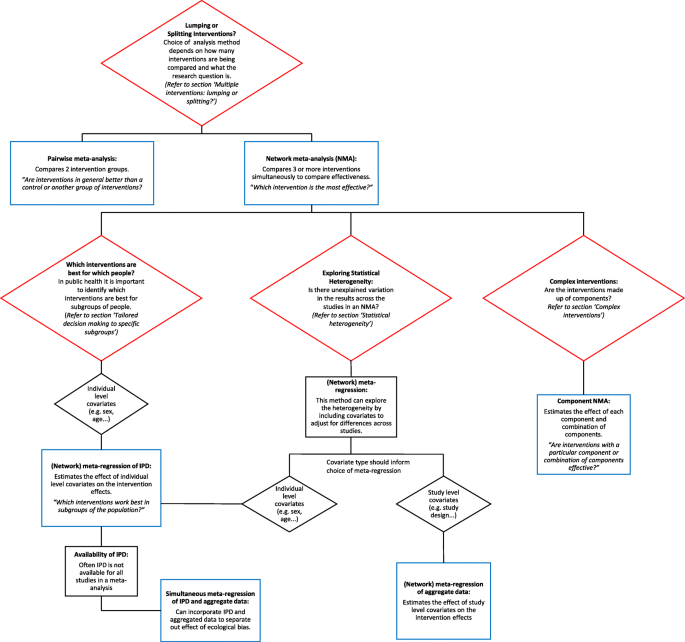
Summary of challenges that are faces in the evidence synthesis of public health interventions and methods that are discussed to overcome these challenges
Evaluating the effect of interventions for promoting the safe storage of cleaning products to prevent childhood poisoning accidents
To illustrate the methodological developments, a motivating example is used from the five year, NIHR funded, Keeping Children Safe Programme [ 15 ]. The project included a Cochrane systematic review that aimed to increase the use of safety equipment to prevent accidents at home in children under five years old. This application is intended to be illustrative of the benefits of new evidence synthesis methods since the previous review. It is not a complete, comprehensive analysis as it only uses a subset of the original dataset and therefore the results are not intended to be used for policy decision making. This example has been chosen as it demonstrates many of the issues in synthesising effectiveness evidence of public health interventions, including different study designs (randomised controlled trials, observational studies and cluster randomised trials), heterogeneity of populations or settings, incomplete individual participant data and complex interventions that contain multiple components.
This analysis will investigate the most effective promotional interventions for the outcome of ‘safe storage of cleaning products’ to prevent childhood poisoning accidents. There are 12 studies included in the dataset, with IPD available from nine of the studies. The covariate, single parent family, is included in the analysis to demonstrate the effect of being a single parent family on the outcome. In this example, all of the interventions are made up of one or more of the following components: education (Ed), free or low cost equipment (Eq), home safety inspection (HSI), and installation of safety equipment (In). A Bayesian approach using WinBUGS was used and therefore credible intervals (CrI) are presented with estimates of the effect sizes [ 16 ].
The original review paper by Achana et al. (2014) demonstrated pairwise meta-analysis and meta-regression using individual and cluster allocated trials, subgroup analyses, meta-regression using individual participant data (IPD) and summary aggregate data and NMA. This paper firstly applies NMA to the motivating example for context, followed by extensions to NMA.
Multiple interventions: lumping or splitting?
Often in public health there are multiple intervention options. However, interventions are often lumped together in a pairwise meta-analysis. Pairwise meta-analysis is a useful tool for two interventions or, alternatively in the presence of lumping interventions, for answering the research question: ‘are interventions in general better than a control or another group of interventions?’. However, when there are multiple interventions, this type of analysis is not appropriate for informing health care providers which intervention should be recommended to the public. ‘Lumping’ is becoming less frequent in other areas of evidence synthesis, such as for clinical interventions, as the use of sophisticated synthesis techniques, such as NMA, increases (Achana et al. 2014) but lumping is still common in public health.
NMA is an extension of the pairwise meta-analysis framework to more than two interventions. Multiple interventions that are lumped into a pairwise meta-analysis are likely to demonstrate high statistical heterogeneity. This does not mean that quantitative synthesis could not be undertaken but that a more appropriate method, NMA, should be implemented. Instead the statistical approach should be based on the research questions of the systematic review. For example, if the research question is ‘are any interventions effective for preventing obesity?’, it would be appropriate to perform a pairwise meta-analysis comparing every intervention in the literature to a control. However, if the research question is ‘which intervention is the most effective for preventing obesity?’, it would be more appropriate and informative to perform a network meta-analysis, which can compare multiple interventions simultaneously and identify the best one.
NMA is a useful statistical method in the context of public health intervention appraisal, where there are often multiple intervention options, as it estimates the relative effectiveness of three or more interventions simultaneously, even if direct study evidence is not available for all intervention comparisons. Using NMA can help to answer the research question ‘what is the effectiveness of each intervention compared to all other interventions in the network?’.
In the motivating example there are six intervention options. The effect of lumping interventions is shown in Fig. 4 , where different interventions in both the intervention and control arms are compared. There is overlap of intervention and control arms across studies and interpretation of the results of a pairwise meta-analysis comparing the effectiveness of the two groups of interventions would not be useful in deciding which intervention to recommend. In comparison, the network plot in Fig. 5 illustrates the evidence base of the prevention of childhood poisonings review comparing six interventions that promote the use of safety equipment in the home. Most of the studies use ‘usual care’ as a baseline and compare this to another intervention. There are also studies in the evidence base that compare pairs of the interventions, such as ‘Education and equipment’ to ‘Equipment’. The plot also demonstrates the absence of direct study evidence between many pairs of interventions, for which the associated treatment effects can be indirectly estimated using NMA.
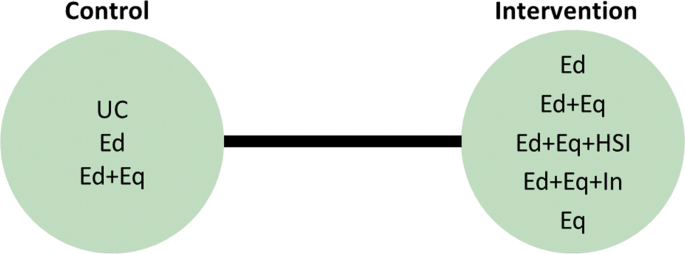
Network plot to illustrate how pairwise meta-analysis groups the interventions in the motivating dataset. Notation UC: Usual care, Ed: Education, Ed+Eq: Education and equipment, Ed+Eq+HSI: Education, equipment, and home safety inspection, Ed+Eq+In: Education, equipment and installation, Eq: Equipment
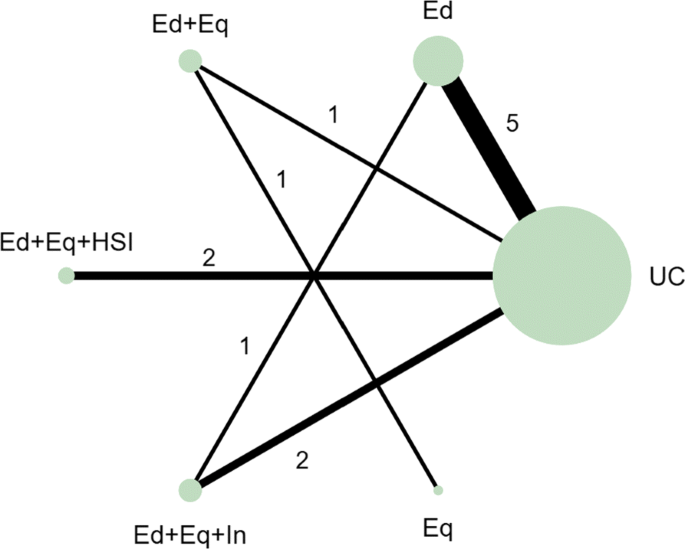
Network plot for the safe storage of cleaning products outcome. Notation UC: Usual care, Ed: Education, Ed+Eq: Education and equipment, Ed+Eq+HSI: Education, equipment, and home safety inspection, Ed+Eq+In: Education, equipment and installation, Eq: Equipment
An NMA was fitted to the motivating example to compare the six interventions in the studies from the review. The results are reported in the ‘triangle table’ in Table 5 [ 17 ]. The top right half of the table shows the direct evidence between pairs of the interventions in the corresponding rows and columns by either pooling the studies as a pairwise meta-analysis or presenting the single study results if evidence is only available from a single study. The bottom left half of the table reports the results of the NMA. The gaps in the top right half of the table arise where no direct study evidence exists to compare the two interventions. For example, there is no direct study evidence comparing ‘Education’ (Ed) to ‘Education, equipment and home safety inspection’ (Ed+Eq+HSI). The NMA, however, can estimate this comparison through the direct study evidence as an odds ratio of 3.80 with a 95% credible interval of (1.16, 12.44). The results suggest that the odds of safely storing cleaning products in the Ed+Eq+HSI intervention group is 3.80 times the odds in the Ed group. The results demonstrate a key benefit of NMA that all intervention effects in a network can be estimated using indirect evidence, even if there is no direct study evidence for some pairwise comparisons. This is based on the consistency assumption (that estimates of intervention effects from direct and indirect evidence are consistent) which should be checked when performing an NMA. This is beyond the scope of this paper and details on this can be found elsewhere [ 18 ].
NMA can also be used to rank the interventions in terms of their effectiveness and estimate the probability that each intervention is likely to be the most effective. This can help to answer the research question ‘which intervention is the best?’ out of all of the interventions that have provided evidence in the network. The rankings and associated probabilities for the motivating example are presented in Table 6 . It can be seen that in this case the ‘education, equipment and home safety inspection’ (Ed+Eq+HSI) intervention is ranked first, with a 0.87 probability of being the best intervention. However, there is overlap of the 95% credible intervals of the median rankings. This overlap reflects the uncertainty in the intervention effect estimates and therefore it is important that the interpretation of these statistics clearly communicates this uncertainty to decision makers.
NMA has the potential to be extremely useful but is underutilised in the evidence synthesis of public health interventions. The ability to compare and rank multiple interventions in an area where there are often multiple intervention options is invaluable in decision making for identifying which intervention to recommend. NMA can also include further literature in the analysis, compared to a pairwise meta-analysis, by expanding the network to improve the uncertainty in the effectiveness estimates.
Statistical heterogeneity
When heterogeneity remains in the results of an NMA, it is useful to explore the reasons for this. Strategies for dealing with heterogeneity involve the inclusion of covariates in a meta-analysis or NMA to adjust for the differences in the covariates across studies [ 19 ]. Meta-regression is a statistical method developed from meta-analysis that includes covariates to potentially explain the between-study heterogeneity ‘with the aim of estimating treatment-covariate interactions’ (Saramago et al. 2012). NMA has been extended to network meta-regression which investigates the effect of trial characteristics on multiple intervention effects. Three ways have been suggested to include covariates in an NMA: single covariate effect, exchangeable covariate effects and independent covariate effects which are discussed in more detail in the NICE Technical Support Document 3 [ 14 ]. This method has the potential to assess the effect of study level covariates on the intervention effects, which is particularly relevant in public health due to the variation across studies.
The most widespread method of meta-regression uses study level data for the inclusion of covariates into meta-regression models. Study level covariate data is when the data from the studies are aggregated, e.g. the proportion of participants in a study that are from single parent families compared to dual parent families. The alternative to study level data is individual participant data (IPD), where the data are available and used as a covariate at the individual level e.g. the parental status of every individual in a study can be used as a covariate. Although IPD is considered to be the gold standard for meta-analysis, aggregated level data is much more commonly used as it is usually available and easily accessible from published research whereas IPD can be hard to obtain from study authors.
There are some limitations to network meta-regression. In our motivating example, using the single parent covariate in a meta-regression would estimate the relative difference in the intervention effects of a population that is made up of 100% single parent families compared to a population that is made up of 100% dual parent families. This interpretation is not as useful as the analysis that uses IPD, which would give the relative difference of the intervention effects in a single parent family compared to a dual parent family. The meta-regression using aggregated data would also be susceptible to ecological bias. Ecological bias is where the effect of the covariate is different at the study level compared to the individual level [ 14 ]. For example, if each study demonstrates a relationship between a covariate and the intervention but the covariate is similar across the studies, a meta-regression of the aggregate data would not demonstrate the effect that is observed within the studies [ 20 ].
Although meta-regression is a useful tool for investigating sources of heterogeneity in the data, caution should be taken when using the results of meta-regression to explain how covariates affect the intervention effects. Meta-regression should only be used to investigate study characteristics, such as the duration of intervention, which will not be susceptible to ecological bias and the interpretation of the results (the effect of intervention duration on intervention effectiveness) would be more meaningful for the development of public health interventions.
Since the covariate of interest in this motivating example is not a study characteristic, meta-regression of aggregated covariate data was not performed. Network meta-regression including IPD and aggregate level data was developed by Samarago et al. (2012) [ 21 ] to overcome the issues with aggregated data network meta-regression, which is discussed in the next section.
Tailored decision making to specific sub-groups
In public health it is important to identify which interventions are best for which people. There has been a recent move towards precision medicine. In the field of public health the ‘concept of precision prevention may [...] be valuable for efficiently targeting preventive strategies to the specific subsets of a population that will derive maximal benefit’ (Khoury and Evans, 2015). Tailoring interventions has the potential to reduce the effect of inequalities in social factors that are influencing the health of the population. Identifying which interventions should be targeted to which subgroups can also lead to better public health outcomes and help to allocate scarce NHS resources. Research interest, therefore, lies in identifying participant level covariate-intervention interactions.
IPD meta-analysis uses data at the individual level to overcome ecological bias. The interpretation of IPD meta-analysis is more relevant in the case of using participant characteristics as covariates since the interpretation of the covariate-intervention interaction is at the individual level rather than the study level. This means that it can answer the research question: ‘which interventions work best in subgroups of the population?’. IPD meta-analyses are considered to be the gold standard for evidence synthesis since it increases the power of the analysis to identify covariate-intervention interactions and it has the ability to reduce the effect of ecological bias compared to aggregated data alone. IPD meta-analysis can also help to overcome scarcity of data issues and has been shown to have higher power and reduce the uncertainty in the estimates compared to analysis including only summary aggregate data [ 22 ].
Despite the advantages of including IPD in a meta-analysis, in reality it is often very time consuming and difficult to collect IPD for all of the studies [ 21 ]. Although data sharing is becoming more common, it remains time consuming and difficult to collect IPD for all studies in a review. This results in IPD being underutilised in meta-analyses. As an intermediate solution, statistical methods have been developed, such as the NMA in Samarago et al. (2012), that incorporates both IPD and aggregate data. Methods that simultaneously include IPD and aggregate level data have been shown to reduce uncertainty in the effect estimates and minimise ecological bias [ 20 , 21 ]. A simulation study by Leahy et al. (2018) found that an increased proportion of IPD resulted in more accurate and precise NMA estimates [ 23 ].
An NMA including IPD, where it is available, was performed, based on the model presented in Samarago et al. (2012) [ 21 ]. The results in Table 7 demonstrates the detail that this type of analysis can provide to base decisions on. More relevant covariate-intervention interaction interpretations can be obtained, for example the regression coefficients for covariate-intervention interactions are the individual level covariate intervention interactions or the ‘within study interactions’ that are interpreted as the effect of being in a single parent family on the effectiveness of each of the interventions. For example, the effect of Ed+Eq compared to UC in a single parent family is 1.66 times the effect of Ed+Eq compared to UC in a dual parent family but this is not an important difference as the credible interval crosses 1. The regression coefficients for the study level covariate-intervention interactions or the ‘between study interactions’ can be interpreted as the relative difference in the intervention effects of a population that is made up of 100% single parent families compared to a population that is made up of 100% dual parent families.
- Complex interventions
In many public health research settings the complex interventions are comprised of a number of components. An NMA can compare all of the interventions in a network as they are implemented in the original trials. However, NMA does not tell us which components of the complex intervention are attributable to this effect. It could be that particular components, or the interacting effect of multiple components, are driving the effectiveness and other components are not as effective. Often, trials have not directly compared every combination of components as there are so many component combination options, it would be inefficient and impractical. Component NMA was developed by Welton et al. (2009) to estimate the effect of each component of the complex interventions and combination of components in a network, in the absence of direct trial evidence and answers the question: ‘are interventions with a particular component or combination of components effective?’ [ 11 ]. For example, for the motivating example, in comparison to Fig. 5 , which demonstrates the interventions that an NMA can estimate effectiveness, Fig. 6 demonstrates all of the possible interventions of which the effectiveness can be estimated in a component NMA, given the components present in the network.
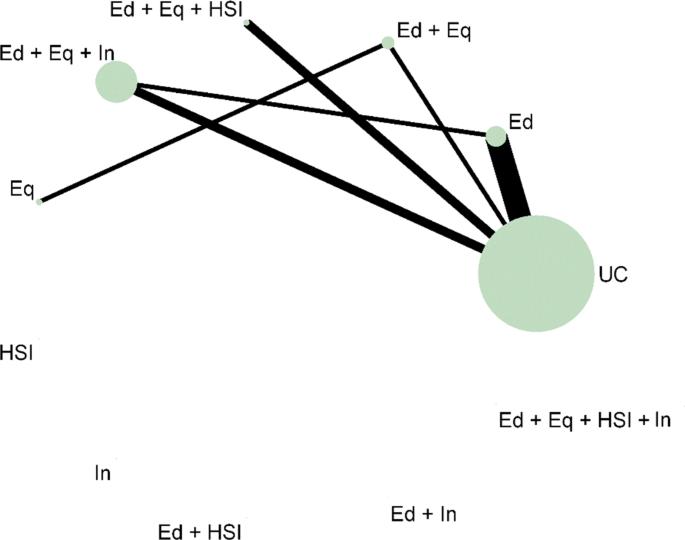
Network plot that illustrates how component network meta-analysis can estimate the effectiveness of intervention components and combinations of components, even when they are not included in the direct evidence. Notation UC: Usual care, Ed: Education, Eq: Equipment, Installation, Ed+Eq: Education and equipment, Ed+HSI: Education and home safety inspection, Ed+In: Education and installation, Eq+HSI: Equipment and home safety inspection, Eq+In: equipment and installation, HSI+In: Home safety inspection and installation, Ed+Eq+HSI: Education, equipment, and home safety inspection, Ed+Eq+In: Education, equipment and installation, Eq+HSI+In: Equipment, home safety inspection and installation, Ed+Eq+HSI+In: Education, equipment, home safety inspection and installation
The results of the analyses of the main effects, two way effects and full effects models are shown in Table 8 . The models, proposed in the original paper by Welton et al. (2009), increase in complexity as the assumptions regarding the component effects relax [ 24 ]. The main effects component NMA assumes that the components in the interventions each have separate, independent effects and intervention effects are the sum of the component effects. The two-way effects models assumes that there are interactions between pairs of the components, so the effects of the interventions are more than the sum of the effects. The full effects model assumes that all of the components and combinations of the components interact. Component NMA did not provide further insight into which components are likely to be the most effective since all of the 95% credible intervals were very wide and overlapped 1. There is a lot of uncertainty in the results, particularly in the 2-way and full effects models. A limitation of component NMA is that there are issues with uncertainty when data is scarce. However, the results demonstrate the potential of component NMA as a useful tool to gain better insights from the available dataset.
In practice, this method has rarely been used since its development [ 24 – 26 ]. It may be challenging to define the components in some areas of public health where many interventions have been studied. However, the use of meta-analysis for planning future studies is rarely discussed and component NMA would provide a useful tool for identifying new component combinations that may be more effective [ 27 ]. This type of analysis has the potential to prioritise future public health research, which is especially useful where there are multiple intervention options, and identify more effective interventions to recommend to the public.
Further methods / other outcomes
The analysis and methods described in this paper only cover a small subset of the methods that have been developed in meta-analysis in recent years. Methods that aim to assess the quality of evidence supporting a NMA and how to quantify how much the evidence could change due to potential biases or sampling variation before the recommendation changes have been developed [ 28 , 29 ]. Models adjusting for baseline risk have been developed to allow for different study populations to have different levels of underlying risk, by using the observed event rate in the control arm [ 30 , 31 ]. Multivariate methods can be used to compare the effect of multiple interventions on two or more outcomes simultaneously [ 32 ]. This area of methodological development is especially appealing within public health where studies assess a broad range of health effects and typically have multiple outcome measures. Multivariate methods offer benefits over univariate models by allowing the borrowing of information across outcomes and modelling the relationships between outcomes which can potentially reduce the uncertainty in the effect estimates [ 33 ]. Methods have also been developed to evaluate interventions with classes or different intervention intensities, known as hierarchical interventions [ 34 ]. These methods were not demonstrated in this paper but can also be useful tools for addressing challenges of appraising public health interventions, such as multiple and surrogate outcomes.
This paper only considered an example with a binary outcome. All of the methods described have also been adapted for other outcome measures. For example, the Technical Support Document 2 proposed a Bayesian generalised linear modelling framework to synthesise other outcome measures. More information and models for continuous and time-to-event data is available elsewhere [ 21 , 35 – 38 ].
Software and guidelines
In the previous section, meta-analytic methods that answer more policy relevant questions were demonstrated. However, as shown by the update to the review, methods such as these are still under-utilised. It is suspected from the NICE public health review that one of the reasons for the lack of uptake of methods in public health could be due to common software choices, such as RevMan, being limited in their flexibility for statistical methods.
Table 9 provides a list of software options and guidance documents that are more flexible than RevMan for implementing the statistical methods illustrated in the previous section to make these methods more accessible to researchers.
In this paper, the network plot in Figs. 5 and 6 were produced using the networkplot command from the mvmeta package [ 39 ] in Stata [ 61 ]. WinBUGS was used to fit the NMA in this paper by adapting the code in the book ‘Evidence Synthesis for Decision Making in Healthcare’ which also provides more detail on Bayesian methods and assessing convergence of Bayesian models [ 45 ]. The model for including IPD and summary aggregate data in an NMA was based on the code in the paper by Saramago et al. (2012). The component NMA in this paper was performed in WinBUGS through R2WinBUGS, [ 47 ] using the code in Welton et al. (2009) [ 11 ].
WinBUGS is a flexible tool for fitting complex models in a Bayesian framework. The NICE Decision Support Unit produced a series of Evidence Synthesis Technical Support Documents [ 46 ] that provide a comprehensive technical guide to methods for evidence synthesis and WinBUGS code is also provided for many of the models. Complex models can also be performed in a frequentist framework. Code and commands for many models are available in R and STATA (see Table 9 ).
The software, R2WinBUGS, was used in the analysis of the motivating example. Increasing numbers of researchers are using R and so packages that can be used to link the two softwares by calling BUGS models in R, packages such as R2WinBUGS, can improve the accessibility of Bayesian methods [ 47 ]. The new R package, BUGSnet, may also help to facilitate the accessibility and improve the reporting of Bayesian NMA [ 48 ]. Webtools have also been developed as a means of enabling researchers to undertake increasingly complex analyses [ 52 , 53 ]. Webtools provide a user-friendly interface to perform statistical analyses and often help in the reporting of the analyses by producing plots, including network plots and forest plots. These tools are very useful for researchers that have a good understanding of the statistical methods they want to implement as part of their review but are inexperienced in statistical software.
This paper has reviewed NICE public health intervention guidelines to identify the methods that are currently being used to synthesise effectiveness evidence to inform public health decision making. A previous review from 2012 was updated to see how method utilisation has changed. Methods have been developed since the previous review and these were applied to an example dataset to show how methods can answer more policy relevant questions. Resources and guidelines for implementing these methods were signposted to encourage uptake.
The review found that the proportion of NICE guidelines containing effectiveness evidence summarised using meta-analysis methods has increased since the original review, but remains low. The majority of the reviews presented only narrative summaries of the evidence - a similar result to the original review. In recent years, there has been an increased awareness of the need to improve decision making by using all of the available evidence. As a result, this has led to the development of new methods, easier application in standard statistical software packages, and guidance documents. Based on this, it would have been expected that their implementation would rise in recent years to reflect this, but the results of the review update showed no such increasing pattern.
A high proportion of NICE guideline reports did not provide a reason for not applying quantitative evidence synthesis methods. Possible explanations for this could be time or resource constraints, lack of statistical expertise, being unaware of the available methods or poor reporting. Reporting guidelines, such as the Preferred Reporting Items for Systematic Reviews and Meta-Analyses (PRISMA), should be updated to emphasise the importance of documenting reasons for not applying methods, as this can direct future research to improve uptake.
Where it was specified, the most common reported reason for not conducting a meta-analysis was heterogeneity. Often in public health, the data is heterogeneous due to the differences between studies in population, design, interventions or outcomes. A common misconception is that the presence of heterogeneity implies that it is not possible to pool the data. Meta-analytic methods can be used to investigate the sources of heterogeneity, as demonstrated in the NMA of the motivating example, and the use of IPD is recommended where possible to improve the precision of the results and reduce the effect of ecological bias. Although caution should be exercised in the interpretation of the results, quantitative synthesis methods provide a stronger basis for making decisions than narrative accounts because they explicitly quantify the heterogeneity and seek to explain it where possible.
The review also found that the most common software to perform the synthesis was RevMan. RevMan is very limited in its ability to perform advanced statistical analyses, beyond that of pairwise meta-analysis, which might explain the above findings. Standard software code is being developed to help make statistical methodology and application more accessible and guidance documents are becoming increasingly available.
The evaluation of public health interventions can be problematic due to the number and complexity of the interventions. NMA methods were applied to a real Cochrane public health review dataset. The methods that were demonstrated showed ways to address some of these issues, including the use of NMA for multiple interventions, the inclusion of covariates as both aggregated data and IPD to explain heterogeneity, and the extension to component network meta-analysis for guiding future research. These analyses illustrated how the choice of synthesis methods can enable more informed decision making by allowing more distinct interventions, and combinations of intervention components, to be defined and their effectiveness estimated. It also demonstrated the potential to target interventions to population subgroups where they are likely to be most effective. However, the application of component NMA to the motivating example has also demonstrated the issues around uncertainty if there are a limited number of studies observing the interventions and intervention components.
The application of methods to the motivating example demonstrated a key benefit of using statistical methods in a public health context compared to only presenting a narrative review – the methods provide a quantitative estimate of the effectiveness of the interventions. The uncertainty from the credible intervals can be used to demonstrate the lack of available evidence. In the context of decision making, having pooled estimates makes it much easier for decision makers to assess the effectiveness of the interventions or identify when more research is required. The posterior distribution of the pooled results from the evidence synthesis can also be incorporated into a comprehensive decision analytic model to determine cost-effectiveness [ 62 ]. Although narrative reviews are useful for describing the evidence base, the results are very difficult to summarise in a decision context.
Although heterogeneity seems to be inevitable within public health interventions due to their complex nature, this review has shown that it is still the main reported reason for not using statistical methods in evidence synthesis. This may be due to guidelines that were originally developed for clinical treatments that are tested in randomised conditions still being applied in public health settings. Guidelines for the choice of methods used in public health intervention appraisals could be updated to take into account the complexities and wide ranging areas in public health. Sophisticated methods may be more appropriate in some cases than simpler models for modelling multiple, complex interventions and their uncertainty, given the limitations are also fully reported [ 19 ]. Synthesis may not be appropriate if statistical heterogeneity remains after adjustment for possible explanatory covariates but details of exploratory analysis and reasons for not synthesising the data should be reported. Future research should focus on the application and dissemination of the advantages of using more advanced methods in public health, identifying circumstances where these methods are likely to be the most beneficial, and ways to make the methods more accessible, for example, the development of packages and web tools.
There is an evident need to facilitate the translation of the synthesis methods into a public health context and encourage the use of methods to improve decision making. This review has shown that the uptake of statistical methods for evaluating the effectiveness of public health interventions is slow, despite advances in methods that address specific issues in public health intervention appraisal and the publication of guidance documents to complement their application.
Availability of data and materials
The dataset supporting the conclusions of this article is included within the article.
Abbreviations
National institute for health and care excellence
- Network meta-analysis
Individual participant data
Home safety inspection
Installation
Credible interval
Preferred reporting items for systematic reviews and meta-analyses
Dias S, Welton NJ, Sutton AJ, Ades A. NICE DSU Technical Support Document 2: A Generalised Linear Modelling Framework for Pairwise and Network Meta-Analysis of Randomised Controlled Trials: National Institute for Health and Clinical Excellence; 2011, p. 98. (Technical Support Document in Evidence Synthesis; TSD2).
Higgins JPT, López-López JA, Becker BJ, et al.Synthesising quantitative evidence in systematic reviews of complex health interventions. BMJ Global Health. 2019; 4(Suppl 1):e000858. https://doi.org/10.1136/bmjgh-2018-000858 .
Article PubMed PubMed Central Google Scholar
Achana F, Hubbard S, Sutton A, Kendrick D, Cooper N. An exploration of synthesis methods in public health evaluations of interventions concludes that the use of modern statistical methods would be beneficial. J Clin Epidemiol. 2014; 67(4):376–90.
Article PubMed Google Scholar
Craig P, Dieppe P, Macintyre S, Michie S, Nazareth I, Petticrew M. Developing and evaluating complex interventions: the new medical research council guidance. Int J Nurs Stud. 2013; 50(5):587–92.
Caldwell DM, Welton NJ. Approaches for synthesising complex mental health interventions in meta-analysis. Evidence-Based Mental Health. 2016; 19(1):16–21.
Melendez-Torres G, Bonell C, Thomas J. Emergent approaches to the meta-analysis of multiple heterogeneous complex interventions. BMC Med Res Methodol. 2015; 15(1):47.
Article CAS PubMed PubMed Central Google Scholar
NICE. NICE: Who We Are. https://www.nice.org.uk/about/who-we-are . Accessed 19 Sept 2019.
Kelly M, Morgan A, Ellis S, Younger T, Huntley J, Swann C. Evidence based public health: a review of the experience of the national institute of health and clinical excellence (NICE) of developing public health guidance in England. Soc Sci Med. 2010; 71(6):1056–62.
NICE. Developing NICE Guidelines: The Manual. https://www.nice.org.uk/process/pmg20/chapter/introduction-and-overview . Accessed 19 Sept 2019.
NICE. Public Health Guidance. https://www.nice.org.uk/guidance/published?type=ph . Accessed 19 Sept 2019.
Welton NJ, Caldwell D, Adamopoulos E, Vedhara K. Mixed treatment comparison meta-analysis of complex interventions: psychological interventions in coronary heart disease. Am J Epidemiol. 2009; 169(9):1158–65.
Ioannidis JP, Patsopoulos NA, Rothstein HR. Reasons or excuses for avoiding meta-analysis in forest plots. BMJ. 2008; 336(7658):1413–5.
Higgins JP, Thompson SG. Quantifying heterogeneity in a meta-analysis. Stat Med. 2002; 21(11):1539–58.
Article Google Scholar
Dias S, Sutton A, Welton N, Ades A. NICE DSU Technical Support Document 3: Heterogeneity: Subgroups, Meta-Regression, Bias and Bias-Adjustment: National Institute for Health and Clinical Excellence; 2011, p. 76.
Kendrick D, Ablewhite J, Achana F, et al.Keeping Children Safe: a multicentre programme of research to increase the evidence base for preventing unintentional injuries in the home in the under-fives. Southampton: NIHR Journals Library; 2017.
Google Scholar
Lunn DJ, Thomas A, Best N, et al.WinBUGS - A Bayesian modelling framework: Concepts, structure, and extensibility. Stat Comput. 2000; 10:325–37. https://doi.org/10.1023/A:1008929526011 .
Dias S, Caldwell DM. Network meta-analysis explained. Arch Dis Child Fetal Neonatal Ed. 2019; 104(1):8–12. https://doi.org/10.1136/archdischild-2018-315224. http://arxiv.org/abs/https://fn.bmj.com/content/104/1/F8.full.pdf.
Dias S, Welton NJ, Sutton AJ, Caldwell DM, Lu G, Ades A. NICE DSU Technical Support Document 4: Inconsistency in Networks of Evidence Based on Randomised Controlled Trials: National Institute for Health and Clinical Excellence; 2011. (NICE DSU Technical Support Document in Evidence Synthesis; TSD4).
Cipriani A, Higgins JP, Geddes JR, Salanti G. Conceptual and technical challenges in network meta-analysis. Ann Intern Med. 2013; 159(2):130–7.
Riley RD, Steyerberg EW. Meta-analysis of a binary outcome using individual participant data and aggregate data. Res Synth Methods. 2010; 1(1):2–19.
Saramago P, Sutton AJ, Cooper NJ, Manca A. Mixed treatment comparisons using aggregate and individual participant level data. Stat Med. 2012; 31(28):3516–36.
Lambert PC, Sutton AJ, Abrams KR, Jones DR. A comparison of summary patient-level covariates in meta-regression with individual patient data meta-analysis. J Clin Epidemiol. 2002; 55(1):86–94.
Article CAS PubMed Google Scholar
Leahy J, O’Leary A, Afdhal N, Gray E, Milligan S, Wehmeyer MH, Walsh C. The impact of individual patient data in a network meta-analysis: an investigation into parameter estimation and model selection. Res Synth Methods. 2018; 9(3):441–69.
Freeman SC, Scott NW, Powell R, Johnston M, Sutton AJ, Cooper NJ. Component network meta-analysis identifies the most effective components of psychological preparation for adults undergoing surgery under general anesthesia. J Clin Epidemiol. 2018; 98:105–16.
Pompoli A, Furukawa TA, Efthimiou O, Imai H, Tajika A, Salanti G. Dismantling cognitive-behaviour therapy for panic disorder: a systematic review and component network meta-analysis. Psychol Med. 2018; 48(12):1945–53.
Rücker G, Schmitz S, Schwarzer G. Component network meta-analysis compared to a matching method in a disconnected network: A case study. Biom J. 2020. https://doi.org/10.1002/bimj.201900339 .
Efthimiou O, Debray TP, van Valkenhoef G, Trelle S, Panayidou K, Moons KG, Reitsma JB, Shang A, Salanti G, Group GMR. GetReal in network meta-analysis: a review of the methodology. Res Synth Methods. 2016; 7(3):236–63.
Salanti G, Del Giovane C, Chaimani A, Caldwell DM, Higgins JP. Evaluating the quality of evidence from a network meta-analysis. PLoS ONE. 2014; 9(7):99682.
Article CAS Google Scholar
Phillippo DM, Dias S, Welton NJ, Caldwell DM, Taske N, Ades A. Threshold analysis as an alternative to grade for assessing confidence in guideline recommendations based on network meta-analyses. Ann Intern Med. 2019; 170(8):538–46.
Dias S, Welton NJ, Sutton AJ, Ades AE. NICE DSU Technical Support Document 5: Evidence Synthesis in the Baseline Natural History Model: National Institute for Health and Clinical Excellence; 2011, p. 29. (NICE DSU Technical Support Document in Evidence Synthesis; TSD5).
Achana FA, Cooper NJ, Dias S, Lu G, Rice SJ, Kendrick D, Sutton AJ. Extending methods for investigating the relationship between treatment effect and baseline risk from pairwise meta-analysis to network meta-analysis. Stat Med. 2013; 32(5):752–71.
Riley RD, Jackson D, Salanti G, Burke DL, Price M, Kirkham J, White IR. Multivariate and network meta-analysis of multiple outcomes and multiple treatments: rationale, concepts, and examples. BMJ (Clinical research ed.) 2017; 358:j3932. https://doi.org/10.1136/bmj.j3932 .
Achana FA, Cooper NJ, Bujkiewicz S, Hubbard SJ, Kendrick D, Jones DR, Sutton AJ. Network meta-analysis of multiple outcome measures accounting for borrowing of information across outcomes. BMC Med Res Methodol. 2014; 14(1):92.
Owen RK, Tincello DG, Keith RA. Network meta-analysis: development of a three-level hierarchical modeling approach incorporating dose-related constraints. Value Health. 2015; 18(1):116–26.
Jansen JP. Network meta-analysis of individual and aggregate level data. Res Synth Methods. 2012; 3(2):177–90.
Donegan S, Williamson P, D’Alessandro U, Garner P, Smith CT. Combining individual patient data and aggregate data in mixed treatment comparison meta-analysis: individual patient data may be beneficial if only for a subset of trials. Stat Med. 2013; 32(6):914–30.
Saramago P, Chuang L-H, Soares MO. Network meta-analysis of (individual patient) time to event data alongside (aggregate) count data. BMC Med Res Methodol. 2014; 14(1):105.
Thom HH, Capkun G, Cerulli A, Nixon RM, Howard LS. Network meta-analysis combining individual patient and aggregate data from a mixture of study designs with an application to pulmonary arterial hypertension. BMC Med Res Methodol. 2015; 15(1):34.
Gasparrini A, Armstrong B, Kenward MG. Multivariate meta-analysis for non-linear and other multi-parameter associations. Stat Med. 2012; 31(29):3821–39.
Chaimani A, Higgins JP, Mavridis D, Spyridonos P, Salanti G. Graphical tools for network meta-analysis in stata. PLoS ONE. 2013; 8(10):76654.
Rücker G, Schwarzer G, Krahn U, König J. netmeta: Network meta-analysis with R. R package version 0.5-0. 2014. R package version 0.5-0. Availiable: http://CRAN.R-project.org/package=netmeta .
van Valkenhoef G, Kuiper J. gemtc: Network Meta-Analysis Using Bayesian Methods. R package version 0.8-2. 2016. Available online at: https://CRAN.R-project.org/package=gemtc .
Lin L, Zhang J, Hodges JS, Chu H. Performing arm-based network meta-analysis in R with the pcnetmeta package. J Stat Softw. 2017; 80(5):1–25. https://doi.org/10.18637/jss.v080.i05 .
Rücker G, Schwarzer G. Automated drawing of network plots in network meta-analysis. Res Synth Methods. 2016; 7(1):94–107.
Welton NJ, Sutton AJ, Cooper N, Abrams KR, Ades A. Evidence Synthesis for Decision Making in Healthcare, vol. 132. UK: Wiley; 2012.
Book Google Scholar
Dias S, Welton NJ, Sutton AJ, Ades AE. Evidence synthesis for decision making 1: introduction. Med Decis Making Int J Soc Med Decis Making. 2013; 33(5):597–606. https://doi.org/10.1177/0272989X13487604 .
Sturtz S, Ligges U, Gelman A. R2WinBUGS: a package for running WinBUGS from R. J Stat Softw. 2005; 12(3):1–16.
Béliveau A, Boyne DJ, Slater J, Brenner D, Arora P. Bugsnet: an r package to facilitate the conduct and reporting of bayesian network meta-analyses. BMC Med Res Methodol. 2019; 19(1):196.
Neupane B, Richer D, Bonner AJ, Kibret T, Beyene J. Network meta-analysis using R: a review of currently available automated packages. PLoS ONE. 2014; 9(12):115065.
White IR. Multivariate random-effects meta-analysis. Stata J. 2009; 9(1):40–56.
Chaimani A, Salanti G. Visualizing assumptions and results in network meta-analysis: the network graphs package. Stata J. 2015; 15(4):905–50.
Owen RK, Bradbury N, Xin Y, Cooper N, Sutton A. MetaInsight: An interactive web-based tool for analyzing, interrogating, and visualizing network meta-analyses using R-shiny and netmeta. Res Synth Methods. 2019; 10(4):569–81. https://doi.org/10.1002/jrsm.1373 .
Freeman SC, Kerby CR, Patel A, Cooper NJ, Quinn T, Sutton AJ. Development of an interactive web-based tool to conduct and interrogate meta-analysis of diagnostic test accuracy studies: MetaDTA. BMC Med Res Methodol. 2019; 19(1):81.
Nikolakopoulou A, Higgins JPT, Papakonstantinou T, Chaimani A, Del Giovane C, Egger M, Salanti G. CINeMA: An approach for assessing confidence in the results of a network meta-analysis. PLoS Med. 2020; 17(4):e1003082. https://doi.org/10.1371/journal.pmed.1003082 .
Viechtbauer W. Conducting meta-analyses in R with the metafor package. J Stat Softw. 2010; 36(3):1–48.
Freeman SC, Carpenter JR. Bayesian one-step ipd network meta-analysis of time-to-event data using royston-parmar models. Res Synth Methods. 2017; 8(4):451–64.
Riley RD, Lambert PC, Staessen JA, Wang J, Gueyffier F, Thijs L, Boutitie F. Meta-analysis of continuous outcomes combining individual patient data and aggregate data. Stat Med. 2008; 27(11):1870–93.
Debray TP, Moons KG, van Valkenhoef G, Efthimiou O, Hummel N, Groenwold RH, Reitsma JB, Group GMR. Get real in individual participant data (ipd) meta-analysis: a review of the methodology. Res Synth Methods. 2015; 6(4):293–309.
Tierney JF, Vale C, Riley R, Smith CT, Stewart L, Clarke M, Rovers M. Individual Participant Data (IPD) Meta-analyses of Randomised Controlled Trials: Guidance on Their Use. PLoS Med. 2015; 12(7):e1001855. https://doi.org/10.1371/journal.pmed.1001855 .
Stewart LA, Clarke M, Rovers M, Riley RD, Simmonds M, Stewart G, Tierney JF. Preferred reporting items for a systematic review and meta-analysis of individual participant data: the prisma-ipd statement. JAMA. 2015; 313(16):1657–65.
StataCorp. Stata Statistical Software: Release 16. College Station: StataCorp LLC; 2019.
Cooper NJ, Sutton AJ, Abrams KR, Turner D, Wailoo A. Comprehensive decision analytical modelling in economic evaluation: a bayesian approach. Health Econ. 2004; 13(3):203–26.
Download references
Acknowledgements
We would like to acknowledge Professor Denise Kendrick as the lead on the NIHR Keeping Children Safe at Home Programme that originally funded the collection of the evidence for the motivating example and some of the analyses illustrated in the paper.
ES is funded by a National Institute for Health Research (NIHR), Doctoral Research Fellow for this research project. This paper presents independent research funded by the National Institute for Health Research (NIHR). The views expressed are those of the authors and not necessarily those of the NHS, the NIHR or the Department of Health and Social Care. The funding bodies played no role in the design of the study and collection, analysis, and interpretation of data and in writing the manuscript.
Author information
Authors and affiliations.
Department of Health Sciences, University of Leicester, Lancaster Road, Leicester, UK
Ellesha A. Smith, Nicola J. Cooper, Alex J. Sutton, Keith R. Abrams & Stephanie J. Hubbard
You can also search for this author in PubMed Google Scholar
Contributions
ES performed the review, analysed the data and wrote the paper. SH supervised the project. SH, KA, NC and AS provided substantial feedback on the manuscript. All authors have read and approved the manuscript.
Corresponding author
Correspondence to Ellesha A. Smith .
Ethics declarations
Ethics approval and consent to participate.
Not applicable.
Consent for publication
Competing interests.
KA is supported by Health Data Research (HDR) UK, the UK National Institute for Health Research (NIHR) Applied Research Collaboration East Midlands (ARC EM), and as a NIHR Senior Investigator Emeritus (NF-SI-0512-10159). The views expressed are those of the author(s) and not necessarily those of the NIHR or the Department of Health and Social Care. KA has served as a paid consultant, providing unrelated methodological advice, to; Abbvie, Amaris, Allergan, Astellas, AstraZeneca, Boehringer Ingelheim, Bristol-Meyers Squibb, Creativ-Ceutical, GSK, ICON/Oxford Outcomes, Ipsen, Janssen, Eli Lilly, Merck, NICE, Novartis, NovoNordisk, Pfizer, PRMA, Roche and Takeda, and has received research funding from Association of the British Pharmaceutical Industry (ABPI), European Federation of Pharmaceutical Industries & Associations (EFPIA), Pfizer, Sanofi and Swiss Precision Diagnostics. He is a Partner and Director of Visible Analytics Limited, a healthcare consultancy company.
Additional information
Publisher’s note.
Springer Nature remains neutral with regard to jurisdictional claims in published maps and institutional affiliations.
Supplementary Information
Additional file 1.
Key for the Nice public health guideline codes. Available in NICEGuidelinesKey.xlsx .
Additional file 2
NICE public health intervention guideline review flowchart for the inclusion and exclusion of documents. Available in Flowchart.JPG .
Rights and permissions
Open Access This article is licensed under a Creative Commons Attribution 4.0 International License, which permits use, sharing, adaptation, distribution and reproduction in any medium or format, as long as you give appropriate credit to the original author(s) and the source, provide a link to the Creative Commons licence, and indicate if changes were made. The images or other third party material in this article are included in the article’s Creative Commons licence, unless indicated otherwise in a credit line to the material. If material is not included in the article’s Creative Commons licence and your intended use is not permitted by statutory regulation or exceeds the permitted use, you will need to obtain permission directly from the copyright holder. To view a copy of this licence, visit http://creativecommons.org/licenses/by/4.0/ . The Creative Commons Public Domain Dedication waiver ( http://creativecommons.org/publicdomain/zero/1.0/ ) applies to the data made available in this article, unless otherwise stated in a credit line to the data.
Reprints and permissions
About this article
Cite this article.
Smith, E.A., Cooper, N.J., Sutton, A.J. et al. A review of the quantitative effectiveness evidence synthesis methods used in public health intervention guidelines. BMC Public Health 21 , 278 (2021). https://doi.org/10.1186/s12889-021-10162-8
Download citation
Received : 22 September 2020
Accepted : 04 January 2021
Published : 03 February 2021
DOI : https://doi.org/10.1186/s12889-021-10162-8
Share this article
Anyone you share the following link with will be able to read this content:
Sorry, a shareable link is not currently available for this article.
Provided by the Springer Nature SharedIt content-sharing initiative
- Meta-analysis
- Systematic review
- Public health
- Decision making
- Evidence synthesis
BMC Public Health
ISSN: 1471-2458
- General enquiries: [email protected]
Thank you for visiting nature.com. You are using a browser version with limited support for CSS. To obtain the best experience, we recommend you use a more up to date browser (or turn off compatibility mode in Internet Explorer). In the meantime, to ensure continued support, we are displaying the site without styles and JavaScript.
- View all journals
- My Account Login
- Explore content
- About the journal
- Publish with us
- Sign up for alerts
- Open access
- Published: 28 February 2018
Quantitative account of social interactions in a mental health care ecosystem: cooperation, trust and collective action
- Anna Cigarini 1 , 2 ,
- Julián Vicens ORCID: orcid.org/0000-0003-0643-0469 1 , 2 , 3 ,
- Jordi Duch ORCID: orcid.org/0000-0003-2639-6333 3 , 4 ,
- Angel Sánchez 5 , 6 , 7 , 8 &
- Josep Perelló ORCID: orcid.org/0000-0001-8533-6539 1 , 2
Scientific Reports volume 8 , Article number: 3794 ( 2018 ) Cite this article
9470 Accesses
10 Citations
51 Altmetric
Metrics details
- Applied mathematics
- Human behaviour
- Psychology and behaviour
- Public health
An Author Correction to this article was published on 26 September 2018
This article has been updated
Mental disorders have an enormous impact in our society, both in personal terms and in the economic costs associated with their treatment. In order to scale up services and bring down costs, administrations are starting to promote social interactions as key to care provision. We analyze quantitatively the importance of communities for effective mental health care, considering all community members involved. By means of citizen science practices, we have designed a suite of games that allow to probe into different behavioral traits of the role groups of the ecosystem. The evidence reinforces the idea of community social capital, with caregivers and professionals playing a leading role. Yet, the cost of collective action is mainly supported by individuals with a mental condition - which unveils their vulnerability. The results are in general agreement with previous findings but, since we broaden the perspective of previous studies, we are also able to find marked differences in the social behavior of certain groups of mental disorders. We finally point to the conditions under which cooperation among members of the ecosystem is better sustained, suggesting how virtuous cycles of inclusion and participation can be promoted in a ‘care in the community’ framework.
Similar content being viewed by others
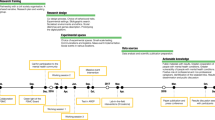
Reformulating computational social science with citizen social science: the case of a community-based mental health care research
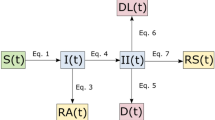
Investigation of turning points in the effectiveness of Covid-19 social distancing
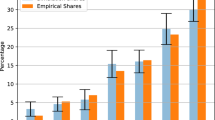
An agent-based model of social care provision during the early stages of Covid-19
Introduction.
Approximately one fifth of the world population will suffer some mental disorder (MD) at some point in their lives, such as anxiety or depression 1 . The direct economic costs of MD, including care and indirect effects, is estimated to reach $6 trillion in 2030, which is more than cancer, diabetes, and respiratory diseases combined 2 . As part of a global effort to scale up services and bring down costs, reliance is increasingly made upon informal social networks 3 . A holistic approach to mental health promotion and care provision is then necessary, and emphasis is placed on the idea of individuals-in-community: individuals with MD are defined not just alone but in relationship to others 4 . Such a paradigm shift implies superseding the traditional physician-patient dyad to include caregivers, relatives, social workers, and the community as a whole, recognizing their crucial role in the recovery process.
A key aspect in the definition and aetiology of MD has to do with social behavior 5 : behavioral symptoms, or consequences at the behavioral level, characterize most MD. For instance, autism, social phobia, or personality disorders are determined by the presence of impairments in social interaction. Other disorders result in significant difficulties in the social domain, such as depression or psychotic disorders. Further, conditions that are intrinsically behavioral (as for eating disorders or substance abuse) seem to be exacerbated by the influence of social peers. A large body of research has therefore looked at the neural basis of social decision-making among individuals with MD to identify objective biomarkers that may prove useful for its diagnosis, therapy evaluation, and understanding 6 , 7 , 8 . However, such a methodology does not well fit into the individuals-in-community paradigm. We argue that an agent-based approach which draws upon experimental game theory might prove insightful and ecologically valid for the study of behavior in a given social environment.
Within the mental health literature, the use of game theory as a way to understand the multi-faceted dimensions of behavior has received already quite some attention 9 , 10 . Most research addressed the issue of behavioral differences between individuals with MD and healthy populations 6 , 7 , 11 , 12 , 13 , 14 , 15 , 16 . These works, that point to cognitive and affective processing impairments 6 , 16 , 17 , further support the idea that MDs are associated with significant and pervasive difficulties in social cognition and altered decision-making at various levels. Yet, despite these studies are of very much interest, they are primarly concerned with dyadic interactions among people with specific MDs. That is, they lack insights into the complexity of individual behaviors of MD within a specific social context.
Here we adopt a novel community perspective. Our objective is twofold: First, we aim to develop a thorough taxonomy of the behavioral traits of role groups within the collective. We thus account for both the heterogeneity of actors, and for multiple types of social interactions. We strongly believe that to predict and understand behavior is necessary to consider the relationship context in which individuals are embedded. Therefore diversity of roles, motivations or capabilities, must be taken into account. Also, real life social interactions occur in different forms; sometimes people must work together, some others they have to coordinate or anti-coordinate their behaviors, yet in other situations they find themselves in more or less disadvantaged positions. It is therefore of crucial importance to encompass a comprehensive range of strategic situations if we are to appreciate behavior. That is, traits such as trust, altruism, or reciprocity, along with the person’s own expectations, all play a role in the process of decision making in social contexts. This calls for an experimental approach in which participants face several strategic settings. Our second objective is to provide quantitative accounts of social capital within the mental health community, bringing the notion of social capital into the forefront of mental health care. Far from being universally defined, its core contention is that social networks are a valuable asset, providing a basis for social cohesion and cooperation towards a common goal 18 (which is, in our case, mental care provision). It thus encompasses those norms and forces that shape social interactions, serving as the glue that holds society together 19 .
For these purposes, we have designed an experimental setup that probes into the complexity of the interdependencies at play within the mental health ecosystem. Accordingly, our experiments take place in a socialized, lab-in-the-field setting 20 , in order to be as close as possible to the dynamic and unique nature of real-life social interactions. The design of our socialized setup is based on a participatory process and citizen science practices 20 which counted on the collaboration of all stakeholders of the mental health ecosystem. By combining all these ingredients, we have developed a framework that, as will be shown below, allows to capture some difficult-to-observe aspects of behavior and social capital within mental health ecosystems as a way to understand how communities contribute to care and resocialization.
A full description of the games we implemented can be found in the Methods section below, but for clarity we briefly describe here the games we used. We had participants play two dyadic games, namely the Trust game, in which they had to lend money to another player who then obtains a return, and has the option to send some money back to the lender; players played in both roles. They also played the well known Prisoner’s Dilemma, in which they had to choose to cooperate or to try to benefit from the other’s cooperation. Finally, they played a collective risk dilemma, in which the whole group had to reach a common goal to avert a catastrophe that most likely would wipe out their money. Participants belonging to the mental health ecosystem played with each other in group of six players. However, they could by no means guess with whom they were actually playing.
We begin the presentation of our results from the dyadic games of our suite of strategic interactions. Aggregate behavioral measures point to systematic deviations from self-interested predictions which are in line with previous literature on experimental game play 21 . In the Prisoner’s Dilemma (PD), the average cooperation rate across all individuals is c = 0.61 ± 0.03 (standard error of the mean), which is notoriously well above the Nash equilibrium prediction of c = 0. Participants behavior in the PD is also significantly associated with their estimates about the likely cooperation of the partner ( \({\chi }^{2}=32.48\) , p = 1.2 · 10 8 ), with 44% of all participants expecting the partner to cooperate, and thus cooperating themselves. This points to the crucial role of positive expectations on cooperative behavior 22 . Further, participants trust and reciprocate positive amounts in the Trust Game (5.79 ± 0.15 monetary units (MU) and 41.3 ± 1.37% of the amount available to return, respectively), again departing largely from Nash equilibrium conjectures of 0 MU transferred. The results also suggest that in considering the mental health community in its whole, thus accounting for the diversity of actors and roles, the global picture does not substantially differ from society at large.
Sectorial and dyadic behavior
As we stated above, our main interest is to delve into the behavior of the different actors who make up the mental health ecosystem Fig. 1 summarizes the results for the five groups of individuals concerned. The heatmap yields several insights that are worth commenting upon.
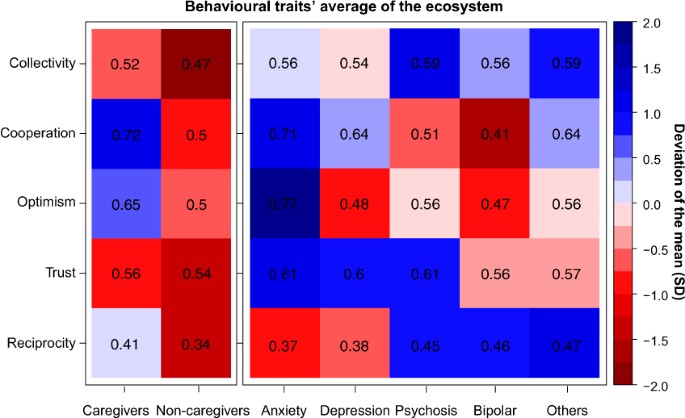
Heatmap of behavioural traits’ average and deviation of the mean across games. Collectivity refers to the ratio of contribution in the Collective-Risk Social Dilemma. Cooperation and Optimism refers to the ratio of cooperation and expected cooperation, respectively, in Prisoner’s Dilemma. Trust and Reciprocity refers to the ratio of capital trusted and reciprocated in Trust Game. The left part shows the ratio of individuals without mental conditions: caregivers (professionals and relatives with caregiving tasks) and non-caregivers (relatives without caregiving tasks, friends and others). The right part shows the actions of individuals with mental conditions. Therefore, the number in each cell indicates the ratio of social preferences per subjects in each social dilemma and the color scale shows the deviation of the mean measured in SD units.
In one-shot dyadic interactions some marked differences in the frequency of cooperative behaviors (PD) arise within the collective formed by affected with MD, caregivers, non-caregivers (Kruskal-Wallis rank sum test, \(H=6.04,df=2,p=0.0488\) ). Further pairwise comparisons (see Supplementary Table S1 ) show that participants with anxiety and caregivers are more likely to opt for the cooperative strategy compared to participants with bipolar disorder, psychosis or other members of the collective. Participants with anxiety are also the ones with the most positive expectations about the partner’s behavior compared to all but caregivers (see Supplementary Table S2 ). Also, relatives, friends and other members with no MD defect more than caregivers (Mann-Whitney U test, \(U=1352,p=0.02839\) ), being relatives remarkably less cooperative than the rest of the collective c = 0.33 ± 0.16. This suggests that cooperation among members of the mental health ecosystem is contextually based, depending on the role that actors play in the recovery process. It also varies across diagnostics, revealing a marked cooperativeness and optimism of individuals with anxiety disorders.
On the other hand, in sequential dyadic interactions (TG) all participants trust more than half of their endowment, being the distribution of initial transfers similar across groups. No variation is indeed found in trust levels between participants with MD, caregivers and non caregivers (Kruskal-Wallis rank sum test, \(H=2.75,df=2,p=0.25\) ). Yet, at the time of reciprocating the partner’s behavior, participants with anxiety and depression return the least (37.5 ± 3.3%). The difference is significant if compared to return transfers of participants with psychosis or other diagnostics (see Supplementary Table S4 ).
Group interaction
Our experimental setup has proven extremely informative in its most novel section, namely the analysis of group interactions framed within the Collective Risk Dilemma (CRD), with no prior result within the mental health literature. In global terms, the average amount contributed to the public good (22.6 MUs) is much more than the fair contribution of 20 MUs, where by fair we understand sharing equally the total amount needed for the threshold (120 MUs) among all six participants. Here it is important to keep in mind that participants were told that all money contributed would go to reforestation projects, so it is not irrational to keep contributing beyond the threshold as many of our subjects did. The key result in the CRD is that large, significant differences (t-test, \(t=2.85,df=242,p=0.0047\) ) are found between participants with and without mental disorders. The former contribute with 22.95 ± 0.63 MUs compared to 20.34 ± 0.68 MUs from the latter, and therefore it appears that when repeated interaction and sustained teamwork (CRD) are required, people with MD contribute much more to the common goal (See Supplementary Section 1.6.2).
Contribution dynamics vary according to group composition in terms of number of participants with mental disorder conditions and other actors involved in the recovery process. All groups successfully reach the target collecting on average 135.64 ± 1.75 MUs (see Supplementary Section 1.6.1). Similarly to other public good experiments, contributions decrease over time 23 . While in the first round participants contribute around 56.3% of the allowed contribution per round (2.2 ± 0.07 MUs, where the social optimum is 2 MU), contributions drop when the endgame effect sets in. A Spearman’s rank-order correlation of contributions over rounds corroborates this negative time trend ( \(\rho =-0.757,p < 0.05\) ). Both patients and actors involved in the recovery process reduce their contributions by the end of the game. However, in almost all rounds, participants with a mental condition contribute more than caregivers and non caregivers, for whom motivations to contribute decline steadily (see Fig. 2 ).
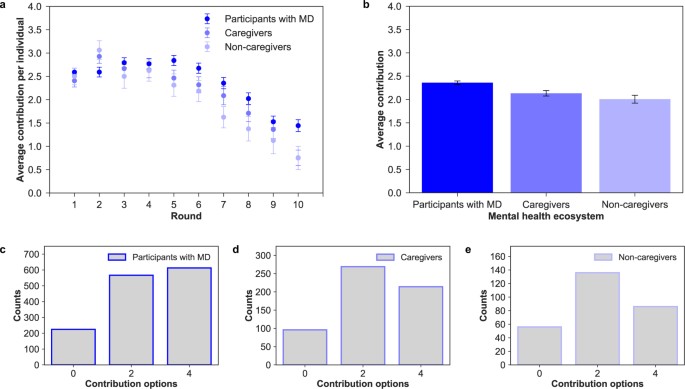
(a) Individual contribution over rounds. Evolution of contributions (mean and standard error of the mean) during the game between participants with mental disorder conditions, caregivers and non-caregivers. We can see that all groups behave similarly and in an identical way to a previous experiment run outside the mental health ecosystem 40 . (b) Average individual contribution per round. Average contribution and standard error of the mean in the mental health ecosystem. There are significant differences between participant with MD and the rest of actors, caregivers (t-test, \(t=2.107,df=155,p < 0.0294\) ) and non-caregivers (t-test, \(t=2.499,df=48,p=0.01588\) ). Distribution of choices by participants with MD ( c ), caregivers (d) and non-caregivers ( e ). The most of participants with MD (43.6%) selected the maximum contribution (4), while the caregivers (46.5%) and non-caregivers (48.9%) mostly selected the fair contribution (2).
In terms of the group composition, groups where individuals with MD conditions constitute half or the majority of the group (n = 36) do much better in sustaining cooperation compared to groups where firsthand affected are the minority (n = 9). It is here worth to mention that participants may see who the rest of the members are but ignore who is exactly making the choice in the game (see Methods for further details). As Fig. 3b shows, while average individual contributions are similar in the last periods (rounds 6–10 t-test, t = 0.19, p = 0.85), groups with half or more individuals with MD contribute significantly more at the beginning of the game (rounds 1–5 t-test, t = 2.79, p = 0.0054). Hence, the presence of three or more individuals with a mental condition in the group has a positive and stabilizing effect on average individual contributions. Likewise, in games with a low proportion of participants affected with MD the group achieved the goal, on average, later than in games with more than 50% of participants affected with MD (see Fig. 3a ).
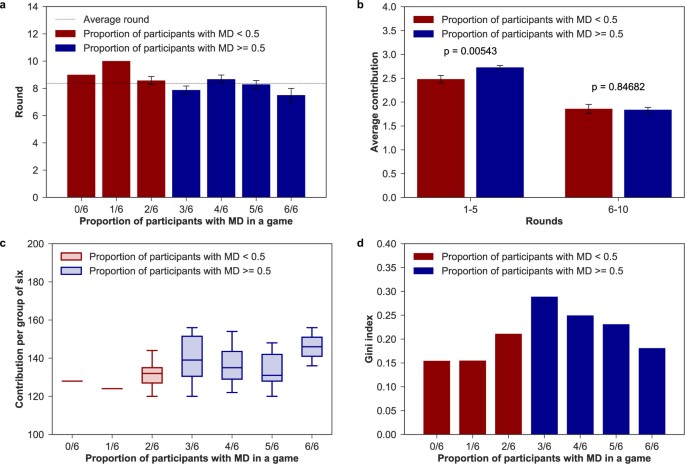
(a) Average round of achievement. Round (mean and standard error of the mean) in which the group of six achieved the target. (b) Aggregated contributions per group composition. Contributions (mean and standard error of the mean) in the first and last five-rounds per number of individuals with MD in a group. There are significant differences (t-test p < 0.01) in contributions in the first part of the game. (c ) Contributions per group of six. Total group contributions by number of individuals with mental conditions in the group. (d ) Gini index of final payoff within groups. Level of inequality in final payoff based on the number of individuals with MD in each group.
If we then break down the analysis by group type, we find that group members contribute and benefit differently from cooperation (see Fig. 3c ). Indeed, final payoffs within groups are far from being equally distributed (see Fig. 3d ), with the highest inequality found in the group where the number of patients equals the number of actors involved in the recovery process (Gini coefficient = 0.289). We thus see clearly that the cost of collective action is mainly supported by individuals with a mental disorder. Given that they contribute the most within all groups, lower investments are needed for other members of the collective to reach the common target. Yet, in 4/6 and 5/6 groups caregivers reduce average individual contributions while non-caregivers pay more than their fair share. In 1/6 and 2/6 groups, on the other hand, caregivers are the ones who compensate the unfair contributions of other members. These last groups are the ones that ensure the lowest inequality in final payoffs. Therefore, while our results are unambiguous about the larger readiness for collective action among people with MD, we cannot claim nothing about the rest of the collective.
Let us now turn to the discussion of the above results and their implications (see Table 1 for a summary of the key findings). As a first general remark, through our lab-in-the field experiment we found that an ecosystem approach to mental health care brings with it a quite complex scenario with several interesting insights. To begin with, participants with anxiety symptoms display a markedly different behavior compared to other diagnostics: they are more likely to opt for the cooperative strategy compared to individuals with bipolar disorder or depression, and return significantly less than participants with psychosis or other disorders. Since the current study is the first to investigate social decision-making within a heterogeneous population of individuals diagnosed with MD, a comparison with previous research is only possible referring to studies focusing on specific clinical and quite homogeneous populations. Several experiments have demonstrated deficits in cooperative behavior among individuals with anxiety or depression when playing iterated versions of the PD 11 , 17 , 24 , 25 , but results about altruism (Ultimatum Game) and trust are inconsistent between studies 6 , 7 , 11 , 12 , 17 , 26 . Individuals with major depressive disorders (which include anxiety and depressive symptoms) have also been found to systematically differ when their emotional responses to fairness are compared 6 , 17 , showing higher levels of negative feelings when faced with unfair treatments. One of the hypothesis advanced to explain the systematic behavioral differences of individuals with anxiety relates to a potentiated sensitivity to negative stimuli as well as a tendency to treat neutral or ambiguous stimuli as negative or as less positive 6 , 12 , 17 , 27 . This hypothesis might find support in our results as for the low returns in the Trust Game, despite displaying relatively high trust in the partner’s behavior and very high expectations. Indeed, participants with depressive or anxiety symptoms in our experiment significantly over-punish trustee transfers, but the low returns are independent of the amount received. This seems to imply that participants with mood disorders respond negatively to their partner behavior, as if they interpret their partner’s choice in a negative sense. Alternatively, fairness considerations may be playing a role: low returns of participants with mood disorders might therefore be due to different fairness perceptions 6 , 12 , 17 , which result in a bias towards negative reactions rather than positive rewarding.
Deficits in economic game play have also been documented for individuals with bipolar disorder. Studies report low and decreasing trust levels over sequential interactions, skeptical beliefs about the partner’s behavior and a tendency to break cooperative interactions 28 , 29 . Again, this is partly supported by our results. Negative expectations of participants with bipolar disorder indeed agree with a low frequency of cooperative choices, little amounts of money sent to trustees, and low contributions to collective action. In line with King-Casas et al . results 29 , while individuals with depression trust in the cooperativeness of other people, those with bipolar personality disorders do not. Cognitive dysfunctions (insula response) might possibly reflect an atypical social norm in this group 29 . Consequently, defection by partners might not violate the social expectations of individuals with BPD. In contrast, in our experiment, participants with bipolar disorder return the most within the group of individuals with a mental disorder. That is, they report a strong willingness to positively respond to a norm of trust as to signal their partner trustworthiness. Therefore, conditioned on the previous action of the partner, it seems that individuals with BPD are willing to show cooperative behavior. Considering now individuals with high levels of psychopathy, they have been found to make less fair offers, accept less fair offers, and show very high levels of defection 15 , 16 , 30 . Major explanations for such behavior point to deficits in emotion regulations (amygdala dysfunctions), which would lead to lack of anxiety, empathy, and guilt, coupled with exaggerated levels of anger and frustration 30 and to the absence of prepotent biases toward minimizing the distress of others 16 . In this case, our experiments do not confirm those previous results: Indeed, participants with psychosis are the ones who trust, contribute the most to the public good, and are willing to take costly actions to reciprocate their partner’s behavior. It could be possible that, as psychopathic disorders are in fact a large group of different ones, behavioral differences among subgroups may lead to this discrepancy. In connection with these results, it is interesting to note that recent results on a large population of patients with paranoia suggest that distrust is not the best explanation for reduced cooperation and alternative explanations incorporating self-interest might be more relevant 31 , 32 . This calls for further research into this particular family of MD to clarify whether or not the behavioral characterization applies to all or to a subclass of them.
However, pointing to deficits in social cognition can only account for a partial explanation of individual behavior, and does not contribute to community care narratives. The fact that nothing in this direction has been reported before also reinforces the need to adopt a more holistic view on the interdependencies at play within the mental health collective. Indeed, if statistically relevant differences in cooperative behavior are found across diagnostics, they also depend on the role that actors play in the recovery process. That is, caregivers display exceedingly large degrees of cooperativeness and optimism in one-shot interactions. Caregivers can be thus considered the strong ties of the mental health ecosystem, of particular value when one seeks emotional support. With the de-institutionalization of health systems, caregivers have indeed become key players in care provision. Taking into account their behavior and expectations is therefore of particular interest to extend the support tailored to their needs. These actions should improve the effectiveness of their role by guiding them 33 . Yet, relatives who do not strictly contribute to caregiving practices turn out to be the weak links. It is thus likely that interventions designed to increase their participation in the community might help improve the recovery process.
Also, members of the mental health ecosystem do not equally contribute and benefit from collective action. Rather, systematic behavioral differences arise as the number of social interactions increase, i.e., when teamwork is required for the collective to benefit as a whole. This suggests that considering repeated games may prove extremely insightful for the purpose of the research. Indeed, our experiments show that individuals with MD are the ones who contribute the most to the public good: they make larger efforts towards reaching the collective goal, thus playing a leading role for the functioning of the ecosystem. As a consequence, groups with half or more participants with MD do better in sustaining cooperation in the first rounds, which implies that a community care setting might prove successful for capability building. Yet, large proportions of individuals with MD in a group result in higher inequalities in final gains, which reach the maximum when the number of individuals with MD equals the number of caregivers or relatives. This means that community care perspectives might also take account of group composition to deal with potential inequalities arising from differential capabilities. In summary, we have explored the behavior of all individuals and role groups who make up the mental health ecosystem through an extensive suite of games that simulate strategic social situations. Overall, the results point to the availability of large social capital in the mental health community that can make a difference in the welfare and recovery process of firsthand affected, and suggest that the community-centered approach to mental care may turn out to be very beneficial. Indeed, the behavior of individuals with MD can be better explained by examining not only their cognitive abilities, but also the web of relationships in which they are embedded. Yet, that web of relationships presents opportunities and imposes constraints.
Though we depicted some behavioral differences in dyadic interactions, most importantly we found that individuals with MD show a remarkably larger disposition towards sustaining cooperation within groups. The larger readiness of individuals with MD to contribute to the collective action problem can thus be seen as a way to claim their place in the community. By having participants unaware of their partner’s identity, we could indeed measure participants decisions based solely on the value they placed on the group’s welfare, independently of its composition or other factors. Yet, the fact that participants with MD contribute the most implies for other members of the group lower investments to reach the common target. This, on the other hand, unveils the vulnerability of individuals with a diagnosis of MD. Repeated or periodic and more situated experiments with digital platforms 34 , in the future, can surely provide further valuable insights into the effect of participants prior knowledge of and relation with the partner on their behavior. We are indeed sure that our experimental setup can prove helpful in complementing the diagnostic process of physicians and health professionals and even to evaluate care service providers. On the other hand, other possible application of this approach arises in the realm of behavior change interventions 35 , that should focus on the aspects that are more specific of every disorder.
In conclusion, the results reinforce the idea of community social capital as a key approach to the recovery process based on an ecosystem paradigm (see also the recent results in ref. 36 about the role and impact of family and community social capital on MD in children and adolescents). Also, if on the one hand the fact that the results of our dyadic games are in general agreement with previous studies validates our procedure; on the other hand it supports the validity and contributions of neuroeconomics and experimental approaches to the study of MD. Finally, given that our work has been carried out in a fully socialized context, this approach can be applied to any similar’ ‘care in the community’ initiative. The adoption of our setup could lead to the identification of core groups that can boost and sustain cooperation within a given community. It can also help in discriminating among different communities in order to identify best practices and optimize resource allocation 37 .
All participants were fully informed about the purpose, methods and intended uses of the research. No participant could approach any experimental station without having signed a written informed consent. The use of pseudonyms ensured the anonymity of participants’ identity, in agreement with the Spanish Law for Personal Data Protection. No association was ever made between the participants’ real names and the results. The whole procedure was approved by the Ethics Committee of Universitat de Barcelona. All methods were performed in accordance with the relevant guidelines and regulations.
Experimental design
As indicated in the main text, the dialogue with the main stakeholders of the mental health ecosystem was at the centre of the project. Around 20 representatives including members of the Catalonia Federation of Mental Health (Federació Salut Mental Catalunya), firsthand affected, relatives, caregivers, and other professionals related to both the health and social sector, informed and validated the whole research through focus groups and further discussions, leading to the largest experiment of this kind ever carried out. Citizen science principles guided the whole experimental design process in order to raise concerns grounded in the daily life of mental health professionals and service users, and to increase public awareness. The experimental dilemmas being proposed served both to advance in knowledge on the social dynamics at play within ‘care in the community’ settings and as a self-reflection experience for all participants. The experimental design process developed in four main phases: (i) identification of the behavioral traits perceived as of fundamental importance within the community, (ii) operationalization of those same behavioral traits thorugh game theoretical paradigms and literature reviews, (iii) definition of the socio-demographic information relevant for the analysis, and (iv) a beta testing of the digital interface (including contents, time duration, and language used). The locations where the experiments took place were accorded with the Catalonia Federation of Mental Health in an attempt to explore the functioning of some communities of interest for inclusive and effective policy making. The Federation provided a fundamental support throughout the whole experiments’ implementation, serving as a crucial intermediaire between the scientists and different mental health collectives. It also provided valuable insights to better interpret the data obtained.
Participants and procedure
To our knowledge, experimental work on this issue has been conducted only recently and on specific collectives of orders of magnitude smaller. A total of 270 individuals participated in the experiments, that were run over 45 sessions between October 2016 and March 2017. The experiments were carried out in Girona (n = 60), Lleida (n = 120), Sabadell (n = 48) and Valls (n = 42). Participants were either diagnosed with a mental condition (n = 169) or members of the broader mental health ecosystem (n = 101), including professionals of the health and social sector (n = 52), formal and informal caregivers (n = 17), relatives (n = 9), friends (n = 4) and other members of the collective (n = 19). Individuals with a mental condition had to self-assess their diagnosis selecting one from a spectrum of options agreed upon with representatives of the mental health ecosystem during the co-design phases of the experiment. Those participants who had received more than one diagnosis had to select the one they considered to be the most relevant. Overall, they had received a diagnosis of psychosis (n = 63), depression (n = 33), anxiety (n = 31), bipolar disorder (n = 17) or other unspecified diagnosis (n = 25). They ranged in age from 21 to 77 years old (these are weighted values since for ethical and privacy reasons participants were only asked to choose among different age ranges) with 47.2 years on average. Further, 55.6% were men and 44.4% were women. Yet, actors involved in the recovery process were predominantly women (76.2%), and up to 21.8% of them was over 60 years old (see Supplementary Section 1.1). Participants were told that they would play against each others a set of games meant to explore human decision-making processes. They played in random groups of six players through a web interface specifically developed for the research. They were informed that they had to make a decision under different conditions and against different opponents in every round. Every game represented an interactive situation requiring the participants to make a decision, the result of which depended also on the opponent’s behavior. To incentivize the participation, they would earn a voucher worth their final score (the experimental settings and instructions, can be found in the Supplementary Section 1.2 and 1.3 respectively). First, participants participated in a Collective Risk Dilemma 23 against five opponents. Briefly, the game is a public goods game with threshold: If the participants’ total contribution after 10 rounds is lower than a given threshold, they loose all the money they kept with a probability of 90%. Otherwise, they are told that the money collected in the common fund are spent in reforesting land plots in Catalonia, where the experimental sessions took place, and each participants earns the money left in the personal account. After completing the task, participants played one round of the Trust Game 38 in both roles: as trustors and as trustees. They played against different partners in each role. Finally, they played one round of a Prisoner Dilemma 39 with (unincentivized) belief elicitation about their counterpart’s behavior prior to playing. Before starting the games, participants had to complete a brief survey covering some key dimensions of their sociodemographic background. The assignment of players’ partners in the dyadic games was completely random and every action was made with a different partner. The average (standard error of the mean) time for completing the three experiments (CRD, PD and TG and tutorials) is around 12 minutes, 705.86 ± 17.93 s. At the end of each session, participants received a gift card worth their earnings. The average individual earning is 46.84 ± 0.77 MUs equivalent to a 4.04 ± 0.077 EUR voucher. The behavioral patterns that emerged do not reveal significant variation across the different experiments, which may suggest that our results are robust to generalizations (see Supplementary Section 1.7).
Statistical analysis
Results were analyzed at two levels: first, we tested for behavioral differences between the whole group of individuals with mental condition compared to members of the mental health ecosystem; we then checked for systematic behavioral variation across diagnostics and role played in the recovery process. In one shot, two-person dyadic interactions we performed Mann-Whitney-U tests for independent groups to compare the distributions of cooperative choices (PD), and initial and back transfers (TG), between individuals with and without a mental condition. We then checked for marginal differences within groups using Kruskal-Wallis tests, and post-hoc comparisons were run with Mann-Whitney-U tests adjusting for p-values with the Holm-Bonferroni method. Welch’s two-tailed t-tests were performed to check for differences in average contributions (CRD) between participants with and without a MD, controlling for unequal variances and sample sizes. Finally, ANOVA and further Tukey HSD post-hoc comparisons served to check for differences in average contributions over round across diagnostics and members of the mental health community.
Accession codes
Data is available in an structured way at Zenodo public repository with DOI 10.5281/zenodo.1175627.
Change history
26 september 2018.
A correction to this article has been published and is linked from the HTML and PDF versions of this paper. The error has not been fixed in the paper.
Steel, Z. et al . The global prevalence of common mental disorders: A systematic review and meta-analysis 1980–2013. Int. J. Epidemiol. 43 , 476–493 (2014).
Article Google Scholar
Insel, T. R., Collins, P. Y. & Hyman, S. E. Darkness invisible: The hidden global costs of mental illness. Foreign Affairs 94 (2015).
White, R. G., Jain, S., Orr, D. M. R. & Read, U. M. The Palgrave handbook of sociocultural perspectives on global mental health. The Palgrave handbook of sociocultural perspectives on global mental health. (2017).
Chapter Google Scholar
World Health Organisation. The World Health Report 2001: mental health, new understanding, new hope. World Health Report 1–169 (2001).
American Psychiatric Association. Diagnostic and statistical manual of mental disorders. Diagnostic and Statistical Manual of Mental Disorders 4th edition TR. (2000).
Gradin, V. B. et al . Abnormal brain responses to social fairness in depression: an fMRI study using the Ultimatum Game. Psychol. Med. 45 , 1241–51 (2015).
Article CAS Google Scholar
Shao, R., Zhang, H. & Lee, T. M. C. The neural basis of social risky decision making in females with major depressive disorder. Neuropsychologia 67 , 100–10 (2015).
Guroglu, B., van den Bos, W., Rombouts, S. A. R. B. & Crone, E. A. Unfair? It depends: Neural correlates of fairness in social context. Soc. Cogn. Affect. Neurosci. 5 , 414–423 (2010).
Wang, Y., Yang, L. –Q., Li, S. & Zhou, Y. Game Theory Paradigm: A New Tool for Investigating Social Dysfunction in Major Depressive Disorders. Front. Psychiatry 6 , 128 (2015).
PubMed PubMed Central Google Scholar
King–Casas, B. & Chiu, P. H. Understanding interpersonal function in psychiatric illness through multiplayer economic games. Biol. Psychiatry 72 , 119–125 (2012).
Pulcu, E. et al . Social–economical decision making in current and remitted major depression. Psychol. Med. 45 , 1–13 (2015).
Wang, Y. et al . Impaired social decision making in patients with major depressive disorder. BMC Psychiatry 14 , 18 (2014).
Csukly, G., Polgár, P., Tombor, L., Réthelyi, J. & Kéri, S. Are patients with schizophrenia rational maximizers? Evidence from an ultimatum game study. Psychiatry Res. 187 , 11–17 (2011).
Agay, N., Kron, S., Carmel, Z., Mendlovic, S. & Levkovitz, Y. Ultimatum bargaining behavior of people affected by schizophrenia. Psychiatry Res. 157 , 39–46 (2008).
Mokros, A. et al . Diminished cooperativeness of psychopaths in a prisoner’s dilemma game yields higher rewards. J. Abnorm. Psychol. 117 , 406–413 (2008).
Rilling, J. K. et al . Neural Correlates of Social Cooperation and Non-Cooperation as a Function of Psychopathy. Biol. Psychiatry 61 , 1260–1271 (2007).
Scheele, D., Mihov, Y., Schwederski, O., Maier, W. & Hurlemann, R. A negative emotional and economic judgment bias in major depression. Eur. Arch. Psychiatry Clin. Neurosci. 263 , 675–683 (2013).
Putnam, R. D. Bowling Aalone: The Collapse and Revival of American Community. J. Democr. 6 , 1–18 (2000).
Google Scholar
Ostrom, E. In Handbook of Social Capital: The troika of sociology, political science and economics, pp. 17–35 (2009).
Sagarra, O., Gutiérrez-Roig, M., Bonhoure, I. & Perelló, J. Citizen science practices for computational social science research: The conceptualization of pop-up experiments. Front. Phys. 3 , 93 (2016).
Camerer, C.F., Behavioral Game Theory: Experiments in Strategic Interaction (Princeton University Press, 2003).
Brañas-Garza, P., Rodríguez-Lara, I. & Sánchez, A. Humans expect generosity. Sci. Rep. 7 , 42446 (2017).
Article ADS Google Scholar
Milinski, M., Sommerfeld, R. D., Krambeck, H.-J., Reed, F. A. & Marotzke, J. The collective-risk social dilemma and the prevention of simulated dangerous climate change. Proc. Nat’l. Acad. Sci. USA 105 , 2291–2294 (2008).
Article ADS CAS Google Scholar
Surbey, M. K. Adaptive significance of low levels of self-deception and cooperation in depression. Evol. Hum. Behav. 32 , 29–40 (2011).
Clark, C. B., Thorne, C. B., Hardy, S. & Cropsey, K. L. Cooperation and depressive symptoms. J. Affect. Disord. 150 , 1184–1187 (2013).
Destoop, M., Schrijvers, D., De Grave, C., Sabbe, B. & De Bruijn, E. R. A. Better to give than to take? Interactive social decision–making in severe major depressive disorder. J. Affect. Disord. 137 , 98–105 (2012).
Harlé, K. M., Allen, J. J. B., Sanfey, A. G., Harlé, K. M. & Sanfey, A. G. The impact of depression on social economic decision making. J. Abnorm. Psychol. 119 , 440–446 (2010).
Unoka, Z., Seres, I., Aspán, N., Bódi, N. & Kéri, S. Trust game reveals restricted interpersonal transactions in patients with borderline personality disorder. J. Pers. Disord. 23 , 399–409 (2009).
King-Casas, B. et al . The rupture and repair of cooperation in borderline personality disorder. Science 321 , 806–810 (2008).
Koenigs, M., Kruepke, M. & Newman, J. P. Economic decision-making in psychopathy: A comparison with ventromedial prefrontal lesion patients. Neuropsychologia 48 , 2198–2204 (2010).
Raihani, N. J. & Bell, V. Paranoia and the social representation of others: a large-scale game theory approach. Scientific Reports 7 , 4544, https://doi.org/10.1038/s41598-017-04805-3 (2017).
Article ADS CAS PubMed PubMed Central Google Scholar
Raihani, N. J. & Bell, V. Conflict and cooperation in paranoia: a large-scale behavioural experiment. Psychological Medicine 1–11, https://doi.org/10.1017/S0033291717003075 (2017).
Collins, L. G. & Swartz, K. Caregiver care. American family physician 83 , 1309–1317 (2011).
PubMed Google Scholar
Aledwood, T. et al . Data collection for mental health studies through digital platforms: requirements and design of a prototype. JMIR research protocols 6 (6), 1–11 (2017).
Blaga, O. M., Vasilescu, L. & Chereches, R. M. Use and effectiveness of behavioural economics in interventions for lifestyle risk factors of non-communicable diseases: a systematic review with policy implications. Perspectives in Public Health 20 (10), 1–11 (2017).
McPherson, K. E. et al . The association between social capital and mental health and behavioural problems in children and adolescents: an integrative systematic review. BMC psychology 2 , 7, https://doi.org/10.1186/2050-7283-2-7 (2014).
Article PubMed PubMed Central Google Scholar
Almedom, A. M. Social capital and mental health: An interdisciplinary review of primary evidence. Social Science and Medicine 61 , 943–964 (2005).
Berg, J., Dickhaut, J. & McCabe, K. Trust, reciprocity, and social history. Games Econ. Behav. 10 , 122–142 (1995).
Rapoport, A. & Chammah, A. M. Prisoner’s Dilemma (University of Michigan Press, 1965).
Vicens, J. et al . Resource heterogeneity leads to unjust effort distribution in climate change mitigation. Preprint at https://arxiv.org/abs/1709.02857 (2017).
Download references
Acknowledgements
We thank the community of patients, caregivers and families working within the Federació de Salut Mental Catalunya (Catalonia Mental Health Federation) for the enthusiasm and for their invaluable help in the design and realization of the experiments. We are also especially thankful to I Bonhoure for the necessary logistics to make the experiments possible, to F Español for contributing in the first steps in the experimental design, to M Poll for always giving us the institutional support from inside the Federation, to both E Ferrer and F Muñoz for building the bridge between us and the mental health ecosystem and to X Trabado for encouraging us to run this research. This work was partially supported by Federació de Salut Mental Catalunya; by MINEICO (Spain), Agencia Estatal de Investigación (AEI) and Fondo Europeo de Desarrollo Regional (FEDER) through grants FIS2013-47532-C3-1-P (JD), FIS2016-78904-C3-1-P (JD), FIS2013-47532-C3-2-P (JP), FIS2016-78904-C3-2-P (JP, AC); by Generalitat de Catalunya (Spain) through Complexity Lab Barcelona (contract no. 2014 SGR 608, JP) and through Secretaria d’Universitats i Recerca (contract no. 2013 DI 49, JD, JV); and by the EU through FET Open Project IBSEN (contract no. 662725, AS) and FET-Proactive Project DOLFINS (contract no. 640772, AS).
Author information
Authors and affiliations.
Departament de Física de la Matèria Condensada, Universitat de Barcelona, 08028, Barcelona, Spain
Anna Cigarini, Julián Vicens & Josep Perelló
Universitat de Barcelona Institute of Complex Systems UBICS, 08028, Barcelona, Spain
Departament d’Enginyeria Informàtica i Matemàtiques, Universitat Rovira i Virgili, 43007, Tarragona, Spain
Julián Vicens & Jordi Duch
Northwestern Institute on Complex Systems (NICO), Northwestern University, 60208, Evanston, IL, USA
Grupo Interdisciplinar de Sistemas Complejos (GISC), Unidad de Matemática, Modelización y Ciencia Computacional, Universidad Carlos III de Madrid, 28911, Leganés, Spain
Angel Sánchez
Unidad Mixta Interdisciplinar de Comportamiento y Complejidad Social (UMICCS) UC3M-UV-UZ, Universidad Carlos III de Madrid, 28911, Leganés, Spain
Institute UC3M-BS of Financial Big Data, Universidad Carlos III de Madrid, 28903, Getafe, Spain
Instituto de Biocomputación y Física de Sistemas Complejos (BIFI), Universidad de Zaragoza, 50009, Zaragoza, Spain
You can also search for this author in PubMed Google Scholar
Contributions
J.D., A.S., and J.P. conceived the original idea for the experiment; J.V. and J.D. prepared the software for the final experimental setup; A.C. and J.V. analyzed the data; and all authors carried out the experiments, discussed the analysis results, and wrote the paper.
Corresponding author
Correspondence to Josep Perelló .
Ethics declarations
Competing interests.
The authors declare no competing interests.
Additional information
Publisher's note: Springer Nature remains neutral with regard to jurisdictional claims in published maps and institutional affiliations.
Electronic supplementary material
Supplementary information, rights and permissions.
Open Access This article is licensed under a Creative Commons Attribution 4.0 International License, which permits use, sharing, adaptation, distribution and reproduction in any medium or format, as long as you give appropriate credit to the original author(s) and the source, provide a link to the Creative Commons license, and indicate if changes were made. The images or other third party material in this article are included in the article’s Creative Commons license, unless indicated otherwise in a credit line to the material. If material is not included in the article’s Creative Commons license and your intended use is not permitted by statutory regulation or exceeds the permitted use, you will need to obtain permission directly from the copyright holder. To view a copy of this license, visit http://creativecommons.org/licenses/by/4.0/ .
Reprints and permissions
About this article
Cite this article.
Cigarini, A., Vicens, J., Duch, J. et al. Quantitative account of social interactions in a mental health care ecosystem: cooperation, trust and collective action. Sci Rep 8 , 3794 (2018). https://doi.org/10.1038/s41598-018-21900-1
Download citation
Received : 15 November 2017
Accepted : 01 February 2018
Published : 28 February 2018
DOI : https://doi.org/10.1038/s41598-018-21900-1
Share this article
Anyone you share the following link with will be able to read this content:
Sorry, a shareable link is not currently available for this article.
Provided by the Springer Nature SharedIt content-sharing initiative
- Mental Health
- Community Social Capital
- Group Roles
- Dyadic Games
- Average Individual Contribution
This article is cited by
Gender-based pairings influence cooperative expectations and behaviours.
- Anna Cigarini
- Julián Vicens
- Josep Perelló
Scientific Reports (2020)
By submitting a comment you agree to abide by our Terms and Community Guidelines . If you find something abusive or that does not comply with our terms or guidelines please flag it as inappropriate.
Quick links
- Explore articles by subject
- Guide to authors
- Editorial policies
Sign up for the Nature Briefing: AI and Robotics newsletter — what matters in AI and robotics research, free to your inbox weekly.

Cookies on GOV.UK
We use some essential cookies to make this website work.
We’d like to set additional cookies to understand how you use GOV.UK, remember your settings and improve government services.
We also use cookies set by other sites to help us deliver content from their services.
You have accepted additional cookies. You can change your cookie settings at any time.
You have rejected additional cookies. You can change your cookie settings at any time.

- Health and social care
- Public health
Evaluation methods: evaluation in health and wellbeing
Helping public health practitioners conducting evaluations – choosing evaluation methods.
Choosing methods for evaluation
A wide variety of research methods and data collection tools are available for use in evaluation: qualitative and quantitative. Different methods are suitable for answering different types of evaluation questions. This section provides an overview of the most common methods. The resources referred to at the end of this section give more detail on different methods and how to use them.
Qualitative research
Qualitative research encompasses a variety of methods, but is often defined in terms of using words and text in data collection and analysis, rather than using numerical measurement and quantification (Bryman, A (2015), ‘Social research methods’ (fifth edition) Oxford University Press, Oxford). It commonly investigates how people make sense of the world and experience events. For example, it might explore how it feels to be a patient with a long-term condition or what it is like to live with someone with a terminal illness, and aim to gain insight into how people make sense of and manage these situations.
Qualitative research is usually ‘bottom up’ rather than ‘top down’. That is, a theory or explanation is developed from the data rather than data being collected to test a theory or hypothesis. Qualitative research often aims to describe and explain rather than predict or identify cause and effect. It can be used to enrich our understanding of an issue or to improve services (Willig, C (2008), ‘Introducing qualitative research in psychology: adventures in theory and method’ Open University Press, Oxford).
Qualitative research is not concerned with representing a population, in the way that quantitative research is, but with investigating topics in depth (Willig, 2008). Usually, a relatively small number of people are included in a sample because of the richness of the data collected and the time it takes to collect and analyse information. Sometimes, simple qualitative research methods are combined with quantitative research, for example, via the inclusion of a comments section within a questionnaire.
Benefits of qualitative research
The benefits to using qualitative methods include that they:
- are useful for investigating the perspectives and interpretations of participants in a holistic fashion
- are less dependent than quantitative methods on the pre-conceived ideas of the researchers
- facilitate new or deeper insights into, and understanding of, a phenomenon (this can be very useful where little is known about a topic and exploratory research is required)
- can be used to investigate unusual cases from which valuable insights can be gained (in quantitative research these ‘outliers’ may be discarded)
- can usually be modified on an ongoing basis as new insights are gained – for example, to explore if unexpected findings emerge
- is adaptable – for example, the order and style of questions in an interview or focus group can be altered to fit or accommodate the needs of different participants or groups
- can be coded and summarised in a quantitative way, if desired
Limitations of qualitative research
The limitations of qualitative methods include:
- they are generally time-consuming to use – it can take a lot of time to arrange and undertake data collection (such as via interviews and focus groups), transcribe discussions, analyse data, and interpret and present findings in a meaningful way
- data analysis can be challenging and relies on having the necessary knowledge and skills, such as in qualitative data analysis approaches, techniques, analysis software
- they produce data and findings which may vary depending on the skills and theoretical perspectives of the researchers collecting, analysing and interpreting the data – however, the reporting of qualitative research should make this process transparent to allow the reader to judge findings in light of this
- they usually collect and analyse data from relatively small numbers of participants, meaning that the generalisability of findings is not addressed and trends or distributions of views within a population are not ascertained
- the anonymity and confidentiality of participants is more difficult to maintain
Qualitative research tools
Interviews are frequently used to collect data in qualitative research. They are usually based on a topic guide. In evaluation studies, interviews are commonly semi-structured where questions or themes are decided in advance, but the interviewer has flexibility to re-order the questions and follow-up with further questions, if necessary.
Interviews allow topics to be explored in depth. These topics can be adapted to take account of participant’s needs (for example, if there are language or disability challenges) or experiences (for example, skipping questions which are not relevant). An interviewee might also discuss a topic without being prompted or the interviewer might change the order of the questions depending on the situation.
In semi-structured interviews, questions are usually relatively ‘open’ – that is, they encourage a detailed answer from the interviewee rather than a ‘yes’ or ‘no’ response. Generally, the interview topic guide or schedule would contain prompts in case a topic doesn’t naturally arise. Leading questions or otherwise indicating to an interviewee that a certain type of answer is the ‘right’ one should be avoided as this would bias the data.
Interviews can be conducted by telephone, through an online video resource or in person. There are benefits and drawbacks of using the telephone or online video compared to face-to-face interviews, summarised below.
Advantages of telephone or online video interviews are they:
- are cheaper in terms of staff time and travel
- avoid wasted time if the sample population has a high rate of ‘no-shows’ for interviews
- are more practical if the interviewee lives far away, or if they have problems travelling
- allow more people to be interviewed due to reduced cost and time
Limitations of telephone or online video interviews are they:
- make it more difficult to establish a rapport with the interviewee
- make it difficult to assess how interviewees are reacting to questions because their body language cannot be observed
- tend to be shorter and are less suitable for sensitive topics
- rely on having access to online video packages or good phone connection or reception, which may be problematic for some harder-to-reach participants
Advantages of face-to-face interviews are they:
- capture verbal and non-verbal cues and responses to questions
- observe emotions and body language
- establish a rapport with the interviewee
Limitations of face-to-face video interviews are:
- they require a number of people to conduct the interviews, which means there will be personnel costs
- quality of data you receive will often depend on the ability of the interviewer
- size of the sample can be limited to the size of your interviewing staff, the area in which the interviews are conducted, and the number of qualified respondents within that area
- they are more expensive and require time for travelling
In advance of the interview, researchers should decide if: the interview should be recorded, how it will be transcribed and analysed and how excerpts will be presented in any write up. In line with research ethics, it is good practice to discuss confidentiality issues and ask interviewees to read and sign a consent form to confirm that they are happy with the arrangements. Complete transcription of recordings is very time-consuming and partial transcriptions or summaries are sometimes used, depending on the type of evaluation.
Interviews can be tiring for interviewers, as they have to listen carefully to determine which topic to go to next and to ensure understanding. If the interviews are lengthy, it is generally a good idea not to arrange more than 2 in a day.
Focus groups
Focus groups bring together a small number of selected participants to discuss a specific topic and are usually facilitated by someone who does not know the group. The participants might be strangers or people who meet together already. In general, it is best not to include people with more power than others in the same group, such as teachers and pupils or junior and senior staff, as this can stop participants being open with their views or opinions. It takes time to set up a focus group and to ensure that everyone attends. Set up can be easier if the group is run alongside a regular meeting. For example, if you are interested in the views of men with an alcohol problem, you might try to get permission to conduct a focus group before or after an alcohol support group session.
Focus groups have similarities to interviews in that a facilitator uses a topic guide or scenario (see below) to ask particular questions and steer the discussion. The person who facilitates the focus group should be experienced, and there is training available to support development of relevant skills. Facilitators must ensure that one person does not dominate the discussion and that everyone gets the opportunity to have their views heard. The facilitator should not have a particular vested interest in the findings, so they do not lead the discussion or impose their own views. The facilitator should always be neutral.
It is useful to record a focus group or at least have a second person taking notes of the discussion as it is difficult to do this alongside facilitating the group. As with interviews, issues of consent and confidentiality should be discussed with participants.
Advantages of focus groups are that they:
- are useful when the interactions and contributions within the group may prompt additional, interesting themes to arise
- are useful when time is short and there is a need to get a range of people’s views quickly
- tend to work well for existing groups where people are comfortable with speaking to each other and are confident in expressing their opinion in a group situation
- can be useful for understanding how and why different groups or types of participants have differing views (for example, men versus women, patients versus health professionals)
Disadvantages of focus groups are that:
- they are generally less useful for recording the responses of individual participants, as it can be difficult to note this down accurately during a discussion, or to identify individuals from a recording during transcription
- they are less suitable to use with some participants – especially if the topic to be discussed is sensitive
- people with hearing problems might also find a group discussion difficult
- they can be challenging and require training to facilitate, especially if there are disagreements amongst participants, dominant participants, or discussions get side-tracked away from the main topics of interest
- they produce complex data which can be particularly difficult and time-consuming to transcribe, summarise, and analyse
Topic guide
Topic guides include questions and prompts used when conducting a focus group or interview. They are called guides because they are meant to be adapted to the needs and experiences of the interviewee or focus group and used in a flexible way. The content of a guide would be decided based on the evaluation questions and objectives as well as after discussions with any oversight or steering group and/or with the type of people you will be interviewing. It is a good idea to test questions with a pilot group to ensure they are understandable and appropriate for the type of participants in the sample.
For example, if you were interviewing smokers about resources and services for quitting smoking in Manchester, you may wish to meet with a group of smokers in Liverpool (to talk to smokers in a similar area who are likely to have similar experiences) to discuss what topics should be included and what language should be used. It is most useful to ask open-ended questions to encourage participants to speak, and it is usual to start with some generic questions at the beginning to relax the participants and build a rapport.
For example, you might want to start with a question which asks for some background and gauges the participants’ knowledge of the area. This will help with adapting later topics and deciding in which order to ask questions. The opening questions also give insight into how forthcoming participants are likely to be. Some people speak readily and at length whereas others can be more reluctant to express their views and need prompts to explore issues in depth. This is particularly important if the topic is of a sensitive nature.
Scenarios can also be used in interviews or focus groups to depersonalise an issue. If a topic is sensitive and people might be uncomfortable talking about their own experiences, they could be asked to give advice to someone else in a similar situation or to imagine a person and what their responses would be.
For example, if you wanted to interview someone about addictions and their views on the services available, you could ask: ‘If a friend or neighbour wished to access addiction services in this area what advice would you give them? What problems might you highlight? Where would you suggest that they start?’
Alternatively, if you wanted to gain insight into the concerns of someone with terminal illness regarding hospices, you might say: ‘Mr McKenzie is moving to a hospice 20 miles from his home after a long illness. His wife is elderly and 2 of his 4 children have moved away from the area. What do you think his concerns might be? What about those of his wife and children?’
This would allow participants to discuss a topic in a more general way without feeling that the questions are intrusive or require sharing too much personal information.
Other qualitative methods
There are many more qualitative research methods such as naturalistic or ethnographic observations, video recordings of behaviour, open-ended questions on questionnaires, and analysis of text (for example, in advertising or educational materials) or photographs.
Analysing qualitative data
In-depth qualitative analysis requires training and experience. It can be conducted in different ways but usually proceeds in the following stages.
- It is usually helpful to thoroughly familiarise yourself with the data by reading and re-reading transcripts or notes, or by listening to recordings.
- Next, segments of data are labelled through ‘coding’, and then higher level, more interpretive themes are developed from these codes in one or more further stages.
- Later stages often involve organising themes (for example, into groups), exploring their relationships with each other and observing patterns in the data.
In this way, qualitative analysis goes beyond merely summarising data, to drawing out underlying themes that provide in-depth insight into an issue.
There are computer packages that can help with analysis of qualitative data. The most common of these are NVivo, Atlas and MAXQDA. These programmes allow large amounts of qualitative data to be stored, analysed and summarised in a systematic way. Use of these packages requires training and a good understanding of qualitative methods.
Quantitative methods
Quantitative methods are used to investigate things that can be measured or quantified to generate numerical data. For example, what percentage of people hold a particular view, does use of a service vary by gender and age, what has changed after a particular intervention? Quantitative research aims to be objective and usually collects data in a pre-defined, structured way (Black, T R (1999), ‘Doing quantitative research in the social sciences: an integrated approach to research design, measurement and statistics’ Sage Publishing). It is used to describe general trends or distributions, or to test a theory or hypothesis about relationships between things, including cause and effect.
Quantitative research methods usually aim to tell you something about a population (for example, smokers or older adults) based on a representative sample selected for a study. However, evaluation studies do not always make claims for the population at large, and may only provide more specific information about the effects of an intervention in a specific group of people or context.
Often, the aim of an evaluation is to find out how things have changed after an intervention. For example, are clinicians more knowledgeable about correct hand washing techniques after a training session? Alternatively, an evaluation may assess whether a service achieves a pre-existing standard. For example, have young people with mental health problems been offered support within a defined time period? See the section on outcome evaluation.
When planning an evaluation, you should identify what data is needed, from whom and when. This will be influenced by the questions being addressed and the most suitable study design for answering these questions within the resources available. It is important to determine if the required data are already available and accessible, or whether the data will need to be collected as this will have time and resource implications. It may be possible to directly observe (for example, to produce counts) or measure (for example, using specialist equipment or monitoring to assess physiological markers) the phenomena of interest. However, evaluation often relies on self-reported or pre-existing data, and various tools are available to support collection of this type of data. See the section on evaluation planning.
Quantitative research tools
Surveys and questionnaires.
A common and quick way of collecting quantitative data from large samples of people is through surveys and questionnaires. Data collected from questionnaires and surveys allow comparisons between groups and subgroups to decide if there are differences between them, and to explore potential reasons for these differences.
Questionnaires are less useful when you wish to conduct more exploratory research (for example, where you might not have enough understanding of something to know what questions to include). They are also unsuitable for understanding aspects such as the meaning of participant experiences or for exploring issues in-depth. In these cases, qualitative methods are usually more suitable.
Questionnaire administration
There are different ways of administering surveys and questionnaires. The advantages and limitations of some of the more common methods are outlined below.
Advantages of administering surveys in person:
- you can lead participant through appropriate sections, explaining what is required, if necessary
- high response rate and minimal errors or missing responses
- good for overcoming disability and language problems
Limitations of administering surveys in person:
- time consuming
- geographically limited
- problematic for sensitive topics
Advantages of administering surveys online:
- wide geographical reach
- can ‘force’ responses and lead people through questions to reduce missing data
- people can do in own time
- can ensure anonymity
Limitations of administering surveys online:
- response rate may be low
- internet access may be a problem and exclude certain groups (for example, those with sensory impairments or older people)
- requires technical knowledge to set up
Advantages of mailing out surveys:
Limitations of mailing out surveys:
- risk of very low response rates
- may need to build in time and budget for reminders or incentives
- need to be very clearly designed and self-explanatory
Designing a questionnaire
Questionnaires are usually accompanied by some demographic questions about age, gender, employment, place of residence and so on. For evaluations, specific questions are then based on what the intervention to be evaluated aims to achieve. If possible, it is better to use or adapt a questionnaire that has been used for similar purposes or in a similar sample and, ideally, has been demonstrated to be reliable and valid – see outcome evaluation section.
When selecting or designing a questionnaire, these issues may be considered:
- who is your sample; who do you need to answer the questions; will they complete the questionnaire
- where or how can you best reach these people
- how can you ensure a good response rate
- if the answers are confidential, how to ensure this
- are the questions simple and clear and only ask about one issue at a time
- avoid jargon in questions - this can be tested by piloting the questionnaire
- avoid leading questions (for example you would not ask ‘why did you like the service?’; ask ‘did you like the service? If so can you say why?’) – exclude any questions that are not necessary for the study
- exclude any questions to which the respondent might not know the answer
- include an ‘other’ option when necessary and provide space for respondents to write or add their own answers
- keep the questionnaire as short as possible
- leave any sensitive or confidential questions to the end to avoid putting people off at an early stage
- check whether the questionnaire is reliable and valid for the sample to be studied
- plan how the data generated by the questionnaire will be captured and stored (for example, entered into a spreadsheet), summarised (for example, to produce overall scores) and analysed
Secondary data collection
It is not always necessary to collect new data as data relevant to an evaluation may already exist – for example, data that’s been collected for administrative purposes or added to patient records. Certain permissions (for example ethics approval, letters of access) may need to be obtained to allow access to these data. A data collection ‘pro forma’ which specifies the data required is sometimes designed and can then be populated using the existing data sources. Alternatively, a request can be made for data or reports for a sample of patients to be transferred directly to the evaluator.
Secondary data collection, however, can also have its own challenges. Some data may be confidential or stored in a way that makes the data hard to access or to use for the desired purpose. Sometimes, data should be available in theory, but, in practice, they are missing, misfiled or not collected consistently.
Analysing quantitative data
Some quantitative analyses required for evaluation will be quite simple – for example, describing the number or percentage of people with a certain characteristic, achieving a goal or expressing a particular opinion. This analysis can usually be managed in Excel. More complex analyses (for example, comparing groups, looking at difference between subgroups, or controlling for extraneous factors) will require knowledge of statistical analyses and associated software packages (for example, SPSS, Stata, SAS). Support for statistical training or knowledge of the relevant software packages will need be to identified when planning an evaluation.
Combining qualitative and quantitative research
Qualitative and quantitative methods can usefully be combined in ‘mixed methods’ research to provide more comprehensive information for an evaluation (Andrew, S and Halcomb, E J (2009), ‘Mixed methods research for nursing and the health sciences’ Blackwell, West Sussex). You may wish to use qualitative research to find out how people feel and follow this with quantitative research to find out if these feelings are commonly experienced.
For example, if you want to explore pregnant mothers’ understanding and knowledge of antenatal care and their compliance with recommendations, you could start by conducting a focus group of mothers from different areas and backgrounds. This qualitative research would give you an insight into their knowledge and other the main topics that are important. You could then use these topics to develop a questionnaire to discover what is happening across the population of expecting mothers as a whole.
Alternatively, you may have obtained findings from a questionnaire and wish to discuss these with a focus group to get a deeper understanding of what the results mean. For example, if data from a survey or questionnaire shows that one hospital has more infections than others, you may wish to follow this up by interviewing staff or visiting the hospital and undertaking observations to get a more in-depth understanding of why that might be and how it could be resolved.
Andrew, S and Halcomb, E J (2009): ‘Mixed methods research for nursing and the health sciences’. Blackwell, West Sussex.
Black, T R (1999): ‘Doing quantitative research in the social sciences: an integrated approach to research design, measurement and statistics’. Sage Publishing.
Bryman, A (2015): ‘Social research methods’ (fifth edition). Oxford University Press, Oxford.
Guest, G. and Namely, E E (2014): ‘Public health research methods’. Sage Publishing.
Willig, C (2008): ‘Introducing qualitative research in psychology: adventures in theory and method’. Open University Press, Oxford.
Acknowledgements
Written by Sarah Denford, Jane Smith, Sarah Morgan Trimmer, Charles Abraham, and Krystal Warmoth, Psychology Applied to Health, University of Exeter Medical School.
This work was partially funded by the UK National Institute for Health Research ( NIHR ) School for Public Health Research, the NIHR Collaboration for Leadership in Applied Health Research and Care of the South West Peninsula (PenCLAHRC) and by Public Health England. However, the views expressed are those of the authors.
Related content
Is this page useful.
- Yes this page is useful
- No this page is not useful
Help us improve GOV.UK
Don’t include personal or financial information like your National Insurance number or credit card details.
To help us improve GOV.UK, we’d like to know more about your visit today. Please fill in this survey (opens in a new tab) .

Quantitative Research in Healthcare Simulation: An Introduction and Discussion of Common Pitfalls
- First Online: 14 November 2019
Cite this chapter
- Aaron W. Calhoun 6 ,
- Joshua Hui 7 &
- Mark W. Scerbo 8
2507 Accesses
In contrast to qualitative research, quantitative research focuses primarily on the testing of hypotheses using variables that are measured numerically and analyzed using statistical procedures. If appropriately designed, quantitative approaches provide the ability to establish causal relationships between variables. Hypothesis testing is a critical component of quantitative methods, and requires appropriately framed research questions, knowledge of the appropriate literature, and guidance from relevant theoretical frameworks. Within the field of simulation, two broad categories of quantitative research exist: studies that investigate the use of simulation as a variable and studies using simulation to investigate other questions and issues. In this chapter we review common study designs and introduce some key concepts pertaining to measurement and statistical analysis. We conclude the chapter with a survey of common errors in quantitative study design and implementation.
This is a preview of subscription content, log in via an institution to check access.
Access this chapter
- Available as EPUB and PDF
- Read on any device
- Instant download
- Own it forever
- Compact, lightweight edition
- Dispatched in 3 to 5 business days
- Free shipping worldwide - see info
Tax calculation will be finalised at checkout
Purchases are for personal use only
Institutional subscriptions
Similar content being viewed by others
Simulation modelling in healthcare: an umbrella review of systematic literature reviews.
Applications of Simulation within the Healthcare Context
An analysis of the academic literature on simulation and modeling in health care.
Babbie ER. The practice of social research. 12th ed. Belmont: Wadsworth Cengage; 2010.
Google Scholar
Mujis D. Doing quantitative research in education with SPSS. 2nd ed. London: SAGE Publications; 2010.
Vogt WP. Dictionary of statistics and methodology. 2nd ed. London: SAGE Publications; 1999.
Kessler D, Pusic M, Chang TP, Fein DM, Grossman D, Mehta R, et al. Impact of just-in-time and just-in-place simulation on intern success with infant lumbar puncture. Pediatrics. 2015;135(5):e1237–46.
Article Google Scholar
Calhoun AW, Sutton ERH, Barbee AP, McClure B, Bohnert C, Forest R, et al. Compassionate options for pediatric EMS (COPE): addressing communication skills. Prehosp Emerg Care. 2017;21(3):334–43.
Sullivan GM, Sargeant J. Qualities of qualitative research: part I. J Grad Med Educ. 2011;3(4):449–52.
Crandall SJ, Caelleigh AS, Steinecke A. Reference to the literature and documentation. Acad Med. 2001;76(9):925–7.
Picho K, Artino AR Jr. 7 deadly sins in educational research. J Grad Med Educ. 2016;8(4):483–7.
Cook DA, Beckman TJ, Bordage G. Quality of reporting of experimental studies in medical education: a systematic review. Med Educ. 2007;41(8):737–45.
Bernhard HR. Social research methods, qualitative and quantitative approaches. 2nd ed. London: SAGE Publications; 2013.
Evans BC, Coon DW, Ume E. Use of theoretical frameworks as a pragmatic guide for mixed methods studies: a methodological necessity? J Mix Methods Res. 2011;5(4):276–92.
Morgan DL. Paradigms lost and pragmatism regained: methodological implications of combining qualitative and quantitative methods. J Mixed Methods Res. 2007;1(1):48–76.
Tavakol M, Sandars J. Quantitative and qualitative methods in medical education research: AMEE Guide No 90: part II. Med Teach. 2014;36(10):838–48.
Ingham-Broomfield RA. Nurse’s guide to quantitative research. Aust J Adv Nurs. 2014;32(2):32–8.
Neuman WL. Social research methods: qualitative and quantitative approaches. 7th ed. Edinburgh Gate: Pearson Education Limited; 2014.
Lopreiato JO, editor. Healthcare simulation dictionary. Rockville: Agency for Healthcare Research and Quality; 2016.
Issenberg SB, McGaghie WC, Petrusa ER, Lee Gordon D, Scalese RJ. Features and uses of high-fidelity medical simulations that lead to effective learning: a BEME systematic review. Med Teach. 2005;27(1):10–28.
Calhoun AW, Bhanji F, Sherbino J, Hatala R. Simulation for high-stakes assessment in pediatric emergency medicine. Clin Pediatr Emerg Med. 2016;17(3):212–23.
Calhoun AW, Donoghue A, Adler M. Assessment in pediatric simulation. In: Grant V, Cheng A, editors. Comprehensive healthcare simulation: pediatrics. Cham: Springer International; 2016. p. 77–94.
Chapter Google Scholar
Cook DA, Brydges R, Ginsburg S, Hatala R. A contemporary approach to validity arguments: a practical guide to Kane’s framework. Med Educ. 2015;49(6):560–75.
Downing SM. Validity: on meaningful interpretation of assessment data. Med Educ. 2003;37(9):830–7.
Messick S. Meaning and values in test validation: the science and ethics of assessment. Educ Res. 1989;18(2):5–11.
Vetter TR. Fundamentals of research data and variables: the devil is in the details. Anesth Analg. 2017;125:1375–80.
Sullivan GM, Feinn R. Using effect size-or why the P value is not enough. J Grad Med Educ. 2012;4(3):279–82.
Sullivan GM. Is there a role for spin doctors in Med Ed research? J Grad Med Educ. 2014;6(3):405–7.
Feise RJ. Do multiple outcomes measures require P-value adjustment? BMC Med Res Methodol. 2002;2(8):1–4.
Noble WS. How does multiple testing correction work? Nat Biotechnol. 2009;27(12):1135–7.
Article CAS Google Scholar
Quertemont E. How to statistically show the absence of an effect. Psychol Belg. 2011;51(2):109–27.
Download references
Author information
Authors and affiliations.
Department of Pediatrics, University of Louisville School of Medicine, Louisville, KY, USA
Aaron W. Calhoun
Emergency Medicine, Kaiser Permanente, Los Angeles Medical Center, Los Angeles, CA, USA
Department of Psychology, Old Dominion University, Norfolk, VA, USA
Mark W. Scerbo
You can also search for this author in PubMed Google Scholar
Corresponding author
Correspondence to Aaron W. Calhoun .
Editor information
Editors and affiliations.
Monash Institute for Health and Clinical Education, Monash University, Clayton, VIC, Australia
Debra Nestel
Department of Surgery, University of Maryland, Baltimore, Baltimore, MD, USA
Kevin Kunkler
Rights and permissions
Reprints and permissions
Copyright information
© 2019 Springer Nature Switzerland AG
About this chapter
Calhoun, A.W., Hui, J., Scerbo, M.W. (2019). Quantitative Research in Healthcare Simulation: An Introduction and Discussion of Common Pitfalls. In: Nestel, D., Hui, J., Kunkler, K., Scerbo, M., Calhoun, A. (eds) Healthcare Simulation Research. Springer, Cham. https://doi.org/10.1007/978-3-030-26837-4_21
Download citation
DOI : https://doi.org/10.1007/978-3-030-26837-4_21
Published : 14 November 2019
Publisher Name : Springer, Cham
Print ISBN : 978-3-030-26836-7
Online ISBN : 978-3-030-26837-4
eBook Packages : Biomedical and Life Sciences Biomedical and Life Sciences (R0)
Share this chapter
Anyone you share the following link with will be able to read this content:
Sorry, a shareable link is not currently available for this article.
Provided by the Springer Nature SharedIt content-sharing initiative
- Publish with us
Policies and ethics
- Find a journal
- Track your research
How Has Quantitative Analysis Changed Health Care?
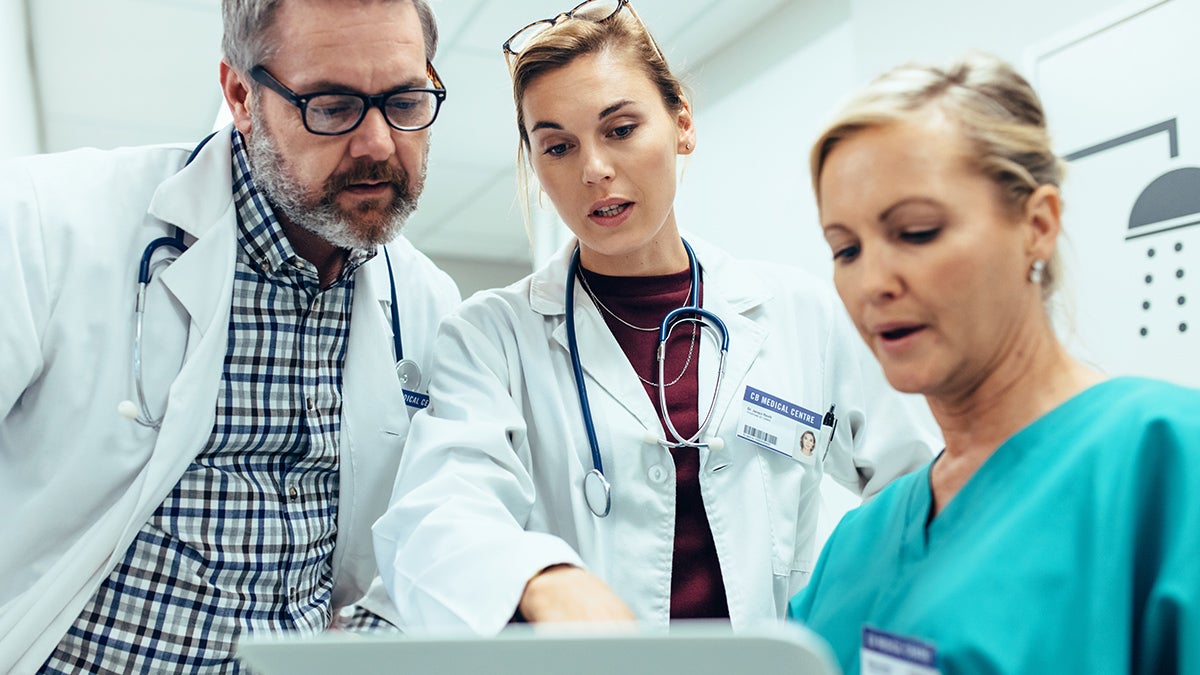
Fill out the form below and we'll email you more information about UCF's online healthcare programs.
- Name * First Last
- Degree * Autism Spectrum Disorders Executive Master of Health Administration, EMHA Forensic Science, MS Fundraising Gender Studies Health Informatics and Information Management, BS Health Services Administration, BS Healthcare Simulation Healthcare Systems Engineering Certificate Healthcare Systems Engineering, MS Integrative General Studies, BGS Interdisciplinary Studies – Diversity Studies Leadership Track, BA Interdisciplinary Studies, BA/BS Master of Public Administration, MPA Master of Science in Healthcare Informatics Master of Social Work Online Nonprofit Management Nonprofit Management, MNM Nursing Education Nursing Practice, DNP, Advanced Track Nursing Practice, DNP, Executive Track Nursing, BS Nursing, BSN to PhD Nursing, MSN Nursing, PhD Project Engineering Psychology, BS Public Administration Research Administration Certificate Research Administration, MRA Systems Engineering
Privacy Notice
In health care, groundbreaking solutions often follow a new capacity for measurement and pattern finding. For example, developing the ability to measure blood glucose levels led to better treatments of diabetes. Florence Nightingale changed nursing forever with her careful measurements of hospital care outcomes. Today, we’re in the midst of an even more significant change in the health care industry: Troves of data are mixing with technologies newly powerful enough to adequately analyze them. As a result, the unprecedented pattern-finding power of quantitative analysis is remaking the health care industry.
Quantitative analysis refers to the process of using complex mathematical or statistical modeling to make sense of data and potentially to predict behavior. Though quantitative analysis is well-established in the fields of economics and finance, cutting-edge quantitative analysis has only recently become possible in health care. Some experts insist that the unfurling of QA in health care will radically change the industry—and how all of us maintain our health and are treated when we’re sick.
It will be up to professionals in the transforming field of health care information technology to make the most of the opportunities borne from these expanding data sets. But what are a few of the specific ways in which quantitative analysis could improve health care?
Stronger Research
Dr. Richard Biehl, former education coordinator of the online Master of Science in Health Care Systems Engineering program at the University of Central Florida, explains that QA stands to change the face of research in the health care field, because, suddenly, it may become very easy to test the strength of correlations between thousands of variables with the touch of a button. In other words, no researcher will need to make the concerted decision to build a study around a question such as “Is this particular allele driving lipid metabolism?” Powerful analytical tools driven by QA will be able to point researchers in the direction of promising correlations between variables they might not have realized were linked.
“We used to get the data to support our research; now we’re getting the data to suggest our research,” Dr. Biehl says. “That’s very, very different.”
The upshot? The field of health care research will become a much more targeted and efficient space—and more likely to regularly uncover lifesaving treatments.
Saving Time, Money, and Lives Through Efficiency and Safety
New QA tools will decrease wait times and call patients into doctors’ offices only when a visit is necessary. As more and more data is crunched to determine, for example, what bodily indicators tend to precede a heart attack, the provider (who will be monitoring the patient’s vital signs via wearable devices) will be able to alert the patient when his or her indicators are trending in a worrisome direction. That means paying for fewer checkup appointments when one is healthy.
Even more importantly, QA tools will allow health care professionals to decrease the impact of human error in prescribing medication and invasive health care procedures. More data can save lives by uncovering complicated patterns (in physiology, DNA, diet, or lifestyle) that help explain why certain medications can prove dangerous for some.
Making Sure Supply Meets Demand
Certain geographic locations and clinical specialties are already facing doctor shortages as mergers and acquisitions reform the health care landscape and financial difficulties force providers to close their doors. But by filling in the picture of oversupply and undersupply around the country, QA can help providers plug holes where they need to.
“Making sure there’s an adequate supply of health care in the right places, in the right specialties, and at the right times, is a health care systems engineering challenge,” Dr. Biehl says.
Amid all the exciting possibilities, QA’s application to health care is still newer than other industries and faces challenges. This type of analysis requires that variables be recorded as numerical data so that they can be analyzed with statistical tools—a format that health care has struggled to conform with, as much of its outcome data is recorded as “positive” or “negative.”
Additionally, QA statistical tools work best when fed with huge amounts of data, as more data makes for clearer patterns and stronger conclusions. Big data analysts at corporations such as Amazon and Google—where every click is tracked and measured—have been collecting unprecedented amounts of data to feed into complex statistical tools for years. Health care has yet to catch up, but it likely will once more wearable technology options, such as expanded versions of Fitbit devices and trackers embedded in up-and-coming “internet of things” appliances, track more and more users’ every move, bite, and night of sleep.
“Once we start collecting all this personal, wearable data from people, health care will start to look more like the Googles and Amazons of the world,” Dr. Biehl says. “We’ll have hundreds of millions of people collecting tens of thousands of data points a day. We’ll finally have big data; we’re heading in that direction.”
Additional Resources
https://www.worldcat.org/wcpa/servlet/DCARead?standardNo=0787971642&standardNoType=1&excerpt=true https://bizfluent.com/info-8168865-benefits-quantitative-research-health-care.html https://www.ruralhealthinfo.org/community-health/rural-toolkit/4/quantitative-qualitative https://www.dotmed.com/news/story/37262
UCF’s Online Healthcare Degrees
- Autism Spectrum Disorders
- Executive Master of Health Administration, EMHA
- Forensic Science, MS
- Fundraising
- Gender Studies
- Health Informatics and Information Management, BS
- Health Services Administration, BS
- Healthcare Simulation
- Healthcare Systems Engineering Certificate
- Healthcare Systems Engineering, MS
- Integrative General Studies, BGS
- Interdisciplinary Studies – Diversity Studies Leadership Track, BA
- Interdisciplinary Studies, BA/BS
- Master of Public Administration, MPA
- Master of Science in Healthcare Informatics
- Master of Social Work Online
- Nonprofit Management
- Nonprofit Management, MNM
- Nursing Education
- Nursing Practice, DNP, Advanced Track
- Nursing Practice, DNP, Executive Track
- Nursing, BS
- Nursing, BSN to PhD
- Nursing, MSN
- Nursing, PhD
- Project Engineering
- Psychology, BS
- Public Administration
- Research Administration Certificate
- Research Administration, MRA
- Systems Engineering
You May Also Enjoy
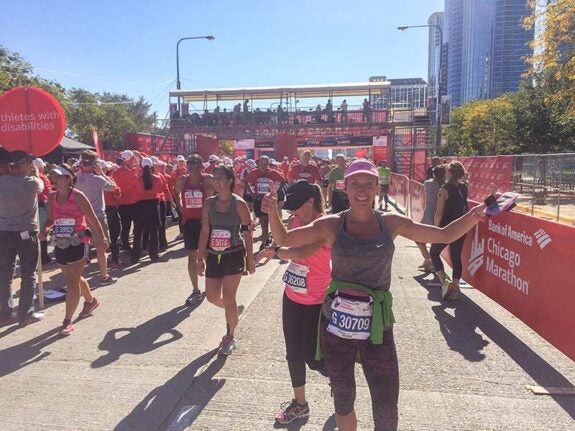
Cookies on the NHS England website
We’ve put some small files called cookies on your device to make our site work.
We’d also like to use analytics cookies. These send information about how our site is used to a service called Google Analytics. We use this information to improve our site.
Let us know if this is OK. We’ll use a cookie to save your choice. You can read more about our cookies before you choose.
Change my preferences I'm OK with analytics cookies
Maximising the benefits of research: Guidance for integrated care systems
England has a vibrant research and development ecosystem, with well-developed research infrastructure and research expertise within our health and care workforce. The value of research in transforming health and care is significant; additionally, staff satisfaction, recruitment and retention is higher among staff who are involved in research. The inception of integrated care systems (ICSs) provides the opportunity for systems to embed research within health and care for the benefit of our population. Supporting this opportunity, a clear research thread runs through ICS strategies and plans, from joint strategic needs assessments and joint health and wellbeing strategies , integrated care strategies , joint forwards plans , integrated care board (ICB) annual reports and the assessment by NHS England of the discharge of duties by ICBs.
The Health and Care Act 2022 (the 2022 Act) sets new legal duties on ICBs around the facilitation and promotion of research in matters relevant to the health service, and the use in the health service of evidence obtained from research. NHS England will assess ICBs for their discharge of these duties. The ICS design framework sets the expectation that in arranging provision of health services, ICBs will facilitate their partners in the health and care system to work together, combining expertise and resources to foster and deploy research and innovations. This guidance supports ICBs in fulfilling their research duties.
ICSs are encouraged to develop a research strategy that aligns to or could be included in their integrated care strategy. This strategy will enable the unification of research across ICS partners, and be consistently embedded to:
- identify and address local research priorities and needs, and work collaboratively to address national research priorities
- improve the quality of health and care and outcomes for all through the evidence generated by research
- increase the quality, quantity and breadth of research undertaken locally
- extend and expand research in settings such as primary care, community care, mental health services, public health and social care
- drive the use of research evidence for quality improvement and evidence-based practice
- influence the national research agenda to better meet local priorities and needs
- improve co-ordination and standardisation within and between localities for the set up and delivery of research
- harness the patient and economic benefits of commercial contract research
- co-ordinate and develop the research workforce across all settings.
1. Introduction
This guidance sets out what good research practice looks like. It supports integrated care systems (ICSs) to maximise the value of their duties around research for the benefit of their population’s health and care and, through co-ordination across ICSs, for national and international impact. It supports integrated care boards (ICBs), integrated care partnerships (ICPs) and their partners to develop a research strategy that aligns to or can be incorporated into their integrated care strategy, and helps them and their workforce to build on existing research initiatives and activities across health and social care to improve sector-wide performance and best practice
- explains the ICB legal duties and other requirements around research and the use of evidence from research, and that research is included in forward planning and reporting
- encourages system leaders to develop a footprint-wide research strategy that aligns to local and national research priorities, develops and supports their workforce, takes the opportunities offered by commercial research and includes plans to embed research in their system’s governance and leadership
- identifies best practice examples and other resources that ICBs may find useful as they develop their research strategies.
This guidance provides comprehensive information for use by:
- those with senior responsibility, including at board level, for research strategy development and/or operationalising research
- managers responsible for developing joint strategic needs assessments, integrated care strategies, joint health and wellbeing strategies, joint forward plans, other linked strategies, or reporting on ICB activities
- research managers
- research and development/innovation leads
- heads of services
- knowledge and library specialists.
It may also be useful to individuals involved in research, education, and partner organisations such as local authorities, social care services, the voluntary, community and social enterprise sector (VCSE) and other providers of healthcare services.
NHS England provides guidance on embedding research in the NHS and secure data environments, and the Office for Life Sciences (OLS ) champions research, innovation and the use of technology to transform health and care service. Other sources of guidance, support and information are signposted in this guidance to support ICSs in aligning to national visions, strategies and plans around research.
1.1 Definition of research
NHS England uses the UK Policy Framework for Health and Social Care Research definition of research:
“… the attempt to derive generalisable or transferable new knowledge to answer or refine relevant questions with scientifically sound methods. This excludes audits of practice and service evaluation. It includes activities that are carried out in preparation for or as a consequence of the interventional part of the research, such as screening potential participants for eligibility, obtaining participants’ consent and publishing results. It also includes non-interventional health and social care research (that is, projects that do not involve any change in standard treatment, care, or other services), projects that aim to generate hypotheses, methodological research and descriptive research”.
This broad definition encompasses the range of types of research:
- clinical trials and other clinical investigations into the safety and effectiveness of medicines, devices and health technologies
- public health research
- observational studies
- discovery science and experimental medicine
- translational research in which results from basic research are developed into results that directly benefit people
- applied research
- research to support policy-making and commissioning
- social care research and research in social care settings
- research into NHS services and care pathways.
1.2 Why research is important
The UK is a world leader for research and invention in healthcare, with around 25% of the world’s top 100 prescription medicines being discovered and developed in the UK ( The impact of collaboration: The value of UK medical research to EU science and health ). Research in the health and care system is important because it underpins all advances in health and care and is the basis for evidence-based practice. Engaging clinicians and healthcare organisations in research is associated with improvements in delivery of healthcare ( Does the engagement of clinicians and organisations in research improve healthcare performance: a three-stage review) . To benefit service users and the public, the NHS and local government, and achieve return on investment, it is vital that research is disseminated, shared and translated into practice.
The National Institute for Health and Care Research (NIHR) is funded by the Department of Health and Social Care (DHSC) to transform research in the health and social care system, including through support for NHS research. Research led to the first proven treatments for Covid, for example the use of dexamethasone, estimated to have saved over a million lives worldwide . This success was in part due to how research is undertaken in the unique environment of the NHS, innovative trial designs, the support provided by the NIHR, frontline staff enabling research, and the awareness and readiness of the public to support research. We need to learn from these and other successes, and translate this across all health and care settings. ICSs will play a vital role in enabling research to be embedded in evolving patient pathways across their footprints.
Example: PRINCIPLE trial – finding treatments for Covid recovery at home
The Platform Randomised Trial of Treatment in the Community for Epidemic and Pandemic Illnesses (PRINCIPLE) was a UK-wide, clinical study to find Covid treatments for recovery at home without the need to attend hospital. The study was open to all with ongoing Covid symptoms, registration was easy, and the trial was run entirely remotely by delivering ‘participant packs’ to people’s homes. It was one of the first trials in the world to show that azithromycin and doxycycline did not benefit patients with Covid and to identify the effectiveness of a commonly used drug – inhaled budesonide –in reducing time to recovery.
The PRINCIPLE study team demonstrated the integral role that primary, secondary and ambulatory care staff can play in the delivery of studies. Local collaborators were trained in good clinical practice to allow them to assess and confirm the eligibility of potential participants, and were commended specifically for their use of patient data to contact people soon after they received a positive test result. It is this network of local staff contributing to research within their healthcare setting that has enabled over 10,000 people to be recruited onto this study so far – one of the largest at home Covid treatment studies worldwide.
This is an example of a study design that incorporates the vital contributions of healthcare providers across the system.
Policy-makers and commissioners need evidence to support their decision-making around the delivery and system-wide transformation of health and care services, including how health inequalities will be reduced.
There is also evidence that:
- staff involved in research have greater job satisfaction and staff turnover is lower in research active trusts ( Academic factors in medical recruitment: evidence to support improvements in medical recruitment and retention by improving the academic content in medical posts)
- research active hospitals have lower mortality rates, and not just among research participants ( Research activity and the association with mortality )
- 83% of people believe that health research is very important ( Survey of the general public: attitudes towards health research)
- healthcare performance improvements have been seen from the creation of academic research placements ( Experiences of hospital allied health professionals in collaborative student research projects: a qualitative study )
- clinical academic research, and in particular the practice changes resulting from it, is associated with improved patient and carer experiences ( A qualitative systematic review and thematic synthesis exploring the impacts of clinical academic activity by healthcare professionals outside medicine ).
Key to having research embedded in health and care is having staff who can understand, undertake, use and generate new research, and share actionable research finding as part of a pro-research culture. Education and training are therefore critical for research to be sustainably embedded within health and care, and for people to develop careers in research and support it in their clinical or care roles.
DHSC, NHS England, the devolved administrations, NIHR and other partners expect to publish a clinical research workforce strategy in 2023/24 to help the UK realise the national clinical research vision outlined in Saving and Improving Lives: The Future of UK Clinical Research Delivery and deliver the Life Sciences Vision to see research embedded in the NHS as part of health and care pathways.
Research will support ICSs to deliver on their four key aims:
Improving outcomes
The NHS 2023/34 priorities and operational planning guidance emphasises the importance of research in improving patient care, outcomes and experience.
Research evidence will inform commissioning decisions to improve experience and outcomes. Research activities should align with the local health priorities identified through local joint strategic needs assessments, and may be best designed and delivered by collaborating with partners. Research priorities may be best addressed by collaborating with partners nationally to design and deliver research.
Tackling inequalities
Research can give a better understanding of local populations and the wider determinants of health, and with this the steps to maintain health and narrow health inequalities.
Enhancing productivity
The development of ICSs creates the opportunity to consider research delivery within the ICS and across ICS boundaries, increasing flexibility of workforce or recruitment while reducing bureaucracy and improving research productivity and value for money.
Supporting social and economic development
An active research ecosystem working in a co-ordinated way and to national standards brings revenue and jobs to regions. The NIHR Clinical Research Network (CRN) supports service users, the public and health and care organisations across England to participate in high-quality research. The 2019 impact and value report detailed the significant income and cost savings that commercial research generates for NHS trusts. Between 2016/17 and 2018/19 the NHS received on average £9,000 per patient recruited to a commercial clinical trial and saved over £5,800 in drug costs for each of these patients. This equates to income of £355 million and cost savings of £26.8 million in 2018/19.
In 2021 150 members of the Association of Medical Research Charities funded £1.55 billion of medical research, including the salaries of 20,000 researchers. Every £1 million spent by charities on medical research in the UK contributes £1.83 million to the economy.
Example: Research that cut problematic prescribing and generated cost savings in general practice – a local health priority
Analysis of routine patient data identified the need for strategies targeting clinicians and patients to curb rising opioid prescribing. From this, the Campaign to Reduce Opioid Prescription (CROP) was launched in 2016, urging GPs across West Yorkshire to ‘think-twice’ before prescribing opioids. This promoted the NICE guidance on chronic pain , which recommends reducing the use of opioids because there is little or no evidence that they make any difference to people’s quality of life, pain or psychological distress, but they can cause harm, including possible addiction.
Over a year 15,000 fewer people were prescribed opioids (a 5.63% relative reduction), a net saving to the NHS of £700,000. The biggest reduction was in people aged over 75, who are at higher risk of opioid-related falls and death, and there was no compensatory rise in the prescribing of other painkillers or referrals to musculoskeletal services.
The CROP campaign, led by researchers at the University of Leeds, has subsequently been rolled out across all ICBs in Yorkshire and the Humber, and the North East and North Cumbria ICB, and the 1,045 practices to which it has been delivered are reporting results similar to the above.
Foy R, Leaman B, McCrorie C, Petty D, House A, Bennett M, et al (2016) Prescribed opioids in primary care: cross-sectional and longitudinal analyses of influence of patient and practice characteristics | BMJ Open 69(5).
Alderson SL, Faragher TM, Willis TA, Carder P, Johnson S, Foy R (2021) The effects of an evidence- and theory-informed feedback intervention on opioid prescribing for non-cancer pain in primary care: A controlled interrupted time series analysis. PLOS Med .
2. ICS, ICP and ICB responsibilities and requirements
ICBs have legal duties and other requirements that relate to research. These are additional to the duties and responsibilities of individual providers within ICS footprints. This section sets out what these duties mean in practical terms and gives examples of how to meet them.
2.1 Legal duties relating to research in the Health and Care Act 2022
Part 1 of the 2022 Act includes specific legal duties for ICBs and NHS England in respect of research. In the Explanatory Notes to the 2022 Act, government sets out how ICBs could discharge their research duty.
Duty to facilitate or otherwise promote research
The ICB duty builds on the previous clinical commissioning group (CCG) duty to promote research, by requiring each ICB, in the exercise of its functions, to facilitate or otherwise promote research on matters relevant to the health service. This duty is intended to include a range of activities to enable research. Section 3 of this guidance outlines ways in which ICBs can do this.
The NHS Constitution also makes clear that patients should be enabled to take part in research: “the NHS pledges … to inform you of research studies in which you may be eligible to participate”.
The Provider Selection Regime (PSR) will be a new set of rules for arranging healthcare services in England, introduced by regulations made under the 2022 Act. The research component should be referred to once the PSR is published.
Duty to facilitate or otherwise promote the use in the health service of evidence obtained from research
This duty similarly builds on the CCG requirement to promote the use of evidence. ICBs must, in the exercise of their functions, facilitate or otherwise promote the use in the health service of evidence obtained from research. For example, ICBs should facilitate or otherwise promote the use of evidence in care, clinical and commissioning decisions.
Duty for ICSs to include research in their joint forward plans and annual reports
Joint forward plans are five-year plans developed by ICBs and their partner NHS trusts and foundation trusts. Systems are encouraged to use the joint forward plan as a shared delivery plan for the integrated care strategy and joint health and wellbeing strategy, aligned to the NHS’s universal commitments. The plan must explain how the ICB will discharge its duties around research, and the ICB must report on the discharge of its research duties in its annual report. These inclusions will raise the profile of research at board level and help embed research as a business-as-usual activity.
The joint forward plan and NHS Oversight Framework guidance set the minimum requirements for what needs to be included in plans and reports.
NHS England duty to include how each ICB is carrying out its duties relating to research in its annual performance assessment of each ICB
NHS England has a new legal duty to annually assess the performance of each ICB and publish a summary of its findings. For 2022/23 NHS England will complete a narrative assessment, identifying areas of good and/or outstanding performance, areas for improvement and any areas that are particularly challenged, drawing on national expertise as required and having regard to relevant guidance. This assessment will include a section considering how effectively the ICB has discharged its duties to facilitate or otherwise promote research and the use of evidence obtained from research.
This, alongside the implementation of the NHS Long Term Plan commitment to develop research metrics for NHS providers, will increase transparency across the system and enable more targeted support for research. Research metrics from NHS England, the Care Quality Commission (CQC) and NIHR will enable the monitoring of progress over time, and are under development with sector colleagues, including providers.
2.2 Legal requirement to work with people and communities
Working with people and communities is a requirement of ICBs, and statutory guidance is available to support them and their partner providers meet this legal duty. A co-ordinated approach across healthcare delivery and research will make it more likely that research reflects what matters to people and communities.
This will also help ICBs to fulfil their legal duty in the 2022 Act to reduce health inequalities in access to health services and the outcomes achieved. Section 3.9 includes links to resources to help guide engagement with underserved communities around research.
The Public Sector Equality Duty also applies and requires equality of opportunities between persons who share a relevant protected characteristic and persons who do not.
2.3 Research governance
While research can address local priorities, it typically operates across ICS boundaries and at national and international levels. Health and social care research is governed by a range of laws, policies, and international, national and professional standards.
The Health Research Authority (HRA ) is responsible for ensuring such regulation is co-ordinated and standardised across the UK to make it easier to do research that people can trust. The HRA is an executive non-departmental public body created by the Care Act 2014 to protect and promote the interests of patients and the public in health and social care research, including by co-ordinating and standardising the practice of research regulation. Local authorities and the NHS are obliged to have regard to its guidance on the management and conduct of research.
Before a research project can start in the NHS in England it must receive approval from the HRA. This includes research taking place in NHS trusts, NHS foundation trusts, ICBs or primary care providers of NHS commissioned services in England, and all research under an NHS duty of care, including that undertaken by NHS staff working in social care or other non-NHS environments.
The HRA schemes indemnify NHS organisations accepting these assurances against any claim covered by the NHS Litigation Authority arising as a result of incorrect assurances. If an NHS organisation duplicates the HRA assessments, it will be liable for any consequences of the decisions it bases on its own checks.
ICBs and partner organisations should have processes for the set up and delivery of research that comply with national laws and systems, and does not duplicate them. Such national systems include confirmation of capacity, National Contract Value Review (NCVR), management of Excess Treatment Costs (ETCs) and contracting arrangements (see section 2.4).
The UK Policy Framework for Health and Social Care sets out the roles and responsibilities of individuals and organisations involved in research.
2.4 Contractual requirements around research
NHS England mandates commissioner use of the NHS Standard Contract for all contracts for healthcare services other than primary care. The contract is updated annually. References to research in the current NHS Standard Contract and service conditions fall into three main areas.
Recruitment of service users and staff into approved research studies
The NHS Standard Contract obliges every provider of NHS-funded services to assist the recruitment of suitable subjects (whether patients or staff) into approved research studies. This requirement aligns to those in the 2022 Act that require ICBs to facilitate or otherwise promote research (see section 2.1). Section 3 considers how this requirement can best be met. Research involving people or their data requires ethical and potentially other approvals (see section 2.3).
National Directive on Commercial Contract Research Studies
Adherence to the National Directive is mandated as part of the NHS Standard Contract. The directive states that providers must:
- Use the unmodified model agreements for sponsor-to-site contracting; HRA and Health and Care Research Wales (HCRW) approval of studies will be dependent on use of these templates.
- Use the standard costing methodology to set prices for commercial contract research undertaken by NHS providers; this is currently in the NIHR interactive costing tool (NIHR iCT).
- Introduce the National Contract Value Review (NCVR) process in line with national rollout. NCVR is a standardised national approach to costing commercial contract research within the NHS. It currently covers acute, specialist and mental health trusts, but the intention is to roll it out to all NHS providers. The creation of ICSs is the ideal opportunity to explore how commercial study set up can be supported across these footprints, reducing the resource needed and time taken.
Comply with HRA/NIHR research reporting guidance
The provider must comply with HRA/NIHR research reporting guidance, as applicable.
2.5 Excess treatment costs
Patients in a research study may receive healthcare that differs from what is standard in the NHS, requires more clinician time or is delivered in a different location. The associated NHS treatment costs may exceed or be less than those of standard treatment. If greater, the difference is referred to as the NHS Excess Treatment Costs (ETCs).
In the case of commercial contract research, the commercial funder will pay the full cost of the study. In the case of non-commercial research, the commissioner of the service in which the study operates is responsible for funding the ETCs.
ICBs as commissioners of services are responsible for ETCs in services that they commission. Guidance for the management of ETCs is available.
DHSC and NIHR are piloting interim arrangements to support non-NHS ETCs for research in public health and social care (non-NHS intervention costs). Please refer to the further detail on the NIHR website .
2.6 Care Quality Commission
The CQC is currently developing its approach for ICS-level assessments, and its new assessment framework will be introduced towards the end of 2023 .
CQC inspection of NHS providers continue, with research assessed as part of the review of the trust-level Well-led framework. Providers are asked:
- Are divisional staff aware of research undertaken in and through the trust, how it contributes to improvement and the service level needed across departments to support it?
- How do senior leaders support internal investigators initiating and managing clinical studies?
- Does the vision and strategy incorporate plans for supporting clinical research activity as a key contributor to best patient care?
- Does the trust have clear internal reporting systems for its research range, volume, activity, safety and performance?
- How are service users and carers given the opportunity to participate in or become actively involved in clinical research studies in the trust?
3. Developing a research strategy
3.1 why develop a research strategy.
Like the health and care system, the research environment is complex. Developing a research strategy will help bring together the legal and other duties around research in a coherent way, and help the ICS understand its local research capability, workforce, activity and needs, set ambitions around research and maximise the benefits associated with commercial research. It will help demonstrate the benefit of research locally, nationally and internationally, and guide the production of clear plans.
Example: Value of research partnerships and integration with ICSs
Bristol Health Partners (BHP) Academic Health Science Centre (AHSC) has a fully integrated relationship as the new Research and Innovation Steering Group for the Bristol, North Somerset and South Gloucestershire (BNSSG) ICS, and reports directly to ICB chief executives.
The group provides the strategic direction and oversight for all research undertaken and delivered across the system. Membership includes directors of research, clinical strategy, public health, social care, senior innovation and education leaders from its core funding partners. It also includes public contributors and senior representatives from primary care, NIHR Applied Research Collaboration West, NIHR CRN West of England, West of England Academic Health Science Network (WEAHSN), Healthier Together ICS, university research institutes and People in Health West of England.
The group has reviewed ICS programmes, identified current and potential research and innovation connections, and begun to establish new connections. It has also supported work with the ICS Ageing Well programme and secured funding for innovative pilots to improve dementia care and increase physical activity for older adults.
Since 2016 BHP has directly contributed an estimated additional £1.1 million to support ICS priorities through Health Integration Team projects and other activities, and has attracted more than £33 million of external research, service redesign and infrastructure into the region.
3.2 General considerations
In developing its research strategy, the ICS may find it helpful to consider these overarching questions alongside the suggested focused content covered in the sections below:
- What do you hope to achieve within a given timeframe?
- Are all the right organisations involved in developing the research strategy?
- How will the health and care workforce be enabled to deliver the research strategy?
- How can research be embedded in existing health and care delivery and pathways?
- What mechanisms are in place to translate actionable research findings into practice and decision-making?
- What inequalities exist in different areas, communities or groups? How will you ensure planning and delivery of research aligns to CORE20plus5 priorities?
- Are you considering equality, diversity and inclusivity and the Public Sector Equality Duty in facilitating and promoting research opportunities for service users and for health and care staff?
- Is the ICS considering the opportunities of developing their commercial research portfolio?
- Is research informing or being informed by population health management?
- How will you plan and deliver research in a sustainable manner, aligning it to the Greener NHS agenda and the ICB’s duties in relation to climate change ?
Buy-in from NHS staff, patients and the public will be vital if ICBs are to discharge their research duties and deliver on their research plans. An important consideration is how to develop sustainable, routine and accessible information flows to ensure the ICB, partners, staff, patients and public can access up-to-date and appropriate information around local research activity, regional, national and international research opportunities and findings, and contact information.
3.3 Leadership and governance across the ICS
Executive leadership.
The Explanatory Notes to the 2022 Act suggest that ICBs have board-level discussions on research activity, the use of the evidence from research, the research workforce and research culture within the ICS. ICSs should refer to the NHS Leadership Competency Framework for board-level leaders at organisation and ICS level for the competencies relating to the research duties of ICSs, once published.
All ICBs are encouraged to have an executive lead responsible for fulfilling the research duties conferred by the 2022 Act. They should help give the ICB a clear understanding of research across the area, regularly reporting on progress towards agreed aims. An executive lead can take responsibility for ensuring clear research ambitions and a research strategy are developed; oversight of organisational research portfolios, diversity in research, alignment to national priorities; promotion of research skills and the need for research skills training; and succession planning.
Senior leaders could engage, consult and be supported by representatives of each registered health and social care professional group when developing strategic plans, and for oversight of training, succession planning, and equality and inclusivity. They could use the capacity and capability of the research and development leads within provider organisations, although established lead roles across social care settings are rare so extra effort may be needed to garner social care research insight.
Research steering group, board or forum
Some CCGs had research steering groups and some of these have expanded with the widening remit of ICBs. ICSs that do not have a such a group should consider adopting a model similar to one in other ICSs where research is effectively embedded in ICS governance structures.
A dedicated steering research group, board and/or forum can:
- provide dedicated time to plan, oversee and report on research
- bring a range of representatives from research infrastructure organisations, patients and the public together with representation from across the ICS, to develop a common aim and objective
- ensure board-level sight of research
- take a cross-ICS approach to research, increasing participation and diversity in research, and reducing bureaucracy.
Example: A dedicated research and innovation subgroup
East and North Hertfordshire Health Care Partnership established a formal research and innovation subgroup to support its objectives to transform services, reduce health inequalities and improve patient health and wellbeing. This subgroup is dedicated to determining and supporting local research priorities and developing an innovation agenda. With effective patient and public involvement, it is working to ensure the local population has access to more research opportunities.
Bringing together the NIHR, academia, industry and local health and care services, the subgroup develops collaborative work plans that support the design, implementation and evaluation of local transformation needs, sharing resources, staff, expertise and facilities. Its work exemplifies a sustainable approach to partnership working and supports Hertfordshire and West Essex ICS’s developing strategy.
HWE ICS Partnership Board 14 September 2021
3.4 Understanding your research activity and working with local and national research infrastructure
Research in NHS and non-NHS settings across an ICS footprint will be supported by different organisations. In some areas networks or collaboratives already exist to bring these organisations together, but in others the links are not as well formed. ICBs would benefit from having a clear map of the research infrastructure and pre-existing local or national investment into research in their area.
It may be valuable to consider:
- Who are the research leaders in your local health and care system, NIHR, higher education institutions, VCSE sector and businesses?
- Are there any pre-existing local or regional research, researcher or research engagement networks?
- What are the opportunities to inform, participate in, collaborate with or lead national and international research efforts in addition to local opportunities?
A list of organisations involved in research including NIHR-funded infrastructure and programmes is included in Annex 1 .
Much of the research undertaken in NHS and other health and care settings is funded though national calls and grants provided by funders such as NIHR, research charities , UK Research and Innovation (UKRI) , including the Medical Research Council (MRC ) and Economic and Social Research Council (ESRC) , and is aligned to national priorities. Other research may include national or international commercial or non-commercial clinical trials funders.
Partners within ICS systems can use NIHR research portfolio data to monitor and plan research activity; however, not all research is included within the NIHR’s portfolio, so this will not give a full picture of the research within the footprint. Mechanisms to map and monitor research more widely could be incorporated in ICB research strategies.
Some local needs may best be addressed through public health or social care research rather than research in primary, secondary or tertiary healthcare settings. Public health and social care research are described in Annex 2 .
Example: Mapping health and care research activity, expertise, interests and infrastructure
The Nottingham and Nottinghamshire Integrated Care System Research Partners Group meets bi-monthly and is chaired by the ICB Head of Research and Evidence. It brings together senior managers from the NHS providers, ICB, two local authorities, two universities and the NIHR CRN East Midlands, providing a forum for ICS-wide research discussions and the development of a system-wide collaborative approach to health and care research across the ICS. Among its aims, the group seeks to increase participation in research at both the organisational and population level, enable equity of access to research opportunities and generate impact on health and care pathways.
The group have mapped health and care research activity, expertise, interests and infrastructure in the constituent organisations. With this the ICS can see the research capabilities, strengths, expertise, and areas of synergy and opportunities for future collaboration that align to its needs and priorities, and also gaps for future development, recognising that organisations are at different stages of research development.
3.5 Understanding local needs
Universal NHS priorities will be reflected in local research needs, and each ICS footprint is likely to have its own specific local research needs. Joint strategic needs assessments (JSNAs) are undertaken jointly by local authorities and ICBs through health and wellbeing boards (HWBs) to identify current health and social care needs of local communities, where more information is needed to do so or to understand how best to address the need. People and communities should be directly involved in identifying local need, including by working with local charities, specific communities or groups who face inequalities in access to, experience of or outcomes from healthcare, eg to target health research at those areas and populations with greatest need.
ICPs are required to develop an integrated care strategy informed by JSNAs and the joint health and wellbeing strategy (JHWS). The integrated care strategy sets out how the assessed needs can be met through the exercise of the functions of the ICB, partner local authorities or NHS England, and is informed by research and practice-based evidence, as stated in the health and wellbeing guidance. In considering where such evidence is lacking, HWBs should identify in JSNAs those research needs that ICBs, local authorities and NHS England could meet through the exercise of their research functions.
Systems are encouraged to use their joint forward plan to develop a shared delivery plan for the Integrated Care Strategy and the JHWS that is supported by the whole system, including local authorities and VCSE partners. ICBs and trusts must also use their Joint Forward Plan to describe how the ICB will discharge its duty in respect of research.
The Explanatory Notes to the 2022 Act suggest how ICBs can discharge their duties around research. These include the articulating local research needs when assessing local needs and how they will be addressed when preparing strategies and plans, and encouraging partner organisations to play an active and collaborative role in pursuing these.
3.6 Supporting delivery of research
Once an ICS has a clear picture of its local research infrastructure it can consider how best to target and support research and the research workforce across its footprint and how research findings will be used. For this, the ICB should ensure that its approaches reflect national approaches to costing, contracting, approvals and information governance, and that they are also informed by learning from effective practices across equivalent ICBs.
As healthcare shifts into communities, ICSs should support the parallel shift in research by embedding research in health and care. Increasing access to research opportunities will give service users earlier access to new treatments, and faster research set up and delivery may provide the evidence needed to support improvements to local care sooner. Inclusive recruitment practices will be needed to ensure that all groups in society have the opportunity to help shape and take part in research, and benefit from research findings.
In developing its research strategy, an ICS has opportunities to reduce bureaucracy, and make research more efficient and effective across its own and with other ICS footprints, and across NHS and non-NHS boundaries, while meeting national regulatory guidance. ICBs will be expected to work with the HRA to co-develop, build on and implement strategies for further co-ordination and standardisation of study set-up and delivery processes. Any regional systems and processes that ICBs do establish must support consistent national practice in relation to the management and regulation of research, and should not duplicate them. The HRA will work with ICBs to address barriers to efficient and rapid study set-up, including model agreements, information governance and R&D office functions.
Other potential areas for streamlining and cross-organisational working include:
- cross-ICS research proposals to identify research needs
- research delivery – identifying how ICS-wide approaches could accelerate patient recruitment and deployment of research delivery staff
- shared data architecture, including the NHS Secure Data Environment for Research Network and its subnational secure data environments (SDEs). Subnational SDEs cover multiple ICSs to achieve access to multimodal data at a scale of approximately 5 million citizens, and over time will achieve technical and governance interoperability
- a greater focus on translation and implementation of research findings into health and care practice, supporting faster improvements
- sharing access to and funding for knowledge and library services
- shared processes and repositories for research assets.
The Explanatory Notes to the 2022 Act suggest that one way an ICB could discharge its research duty would be to have a dedicated research office or team supporting research.
3.7 Enabling cross-provider research
Health and care priorities can often only be addressed with complex, multiorganisational approaches and as such the research to inform these needs to span organisational boundaries. Organisational policies should promote cross-organisational research and dissemination of research findings, including through participation in collaborative research to address national priorities, joint staff posts, honorary contracts, and administratively easier movement of researchers between health and care organisations and other sector partners, including higher education, industry, charities and local authorities.
The HRA and ICS partners are developing national guidance to support cross-provider research.
The NIHR CRN can offer ICSs opportunities to participate in national and international research studies, including those the NIHR, industry and others commission.
3.8 Commercial research
Commercial contract research is research funded solely by industry, where NHS providers are contracted to carry out the research. Most of these research studies in the NHS are interventional clinical trials, such as the NHS-Galleri trial and Astra Zeneca’s COVID-19 vaccine development . Commercial research can give patients access to a wider range of research opportunities, earlier access to novel therapies and treatments, provide drugs free of charge to patients in trials, accelerate the development of new treatments and devices, generate income for providers, and fund NHS staff. It is vitally important for the benefit of patients, the NHS and the UK economy that we create an environment in the NHS that makes it easy and efficient for the NHS to undertake commercial research. This is particularly important when it comes to international commercial research, where companies can place their studies in a number of different countries and consideration of anticipated set up and recruitment times informs where they place trials.
Data gathered during some commercial research is specific to the study and is the property of the company, as is any Intellectual Property (IP) generated. In other cases, where the NHS contributes to the foreground IP – such as through the use of NHS data for research or where NHS expertise provides important contributions to a commercial product – it is important that the NHS shares in the value of IP generated as a consequence of its contributions.
The establishment of ICSs is an ideal opportunity for their creation of ambitions to enable, grow and benefit from commercial research. ICSs should explore how efficient commercial study set up and delivery could be streamlined across sites within their footprint, and should set ambitions around commercial research.
3.9 Involving patients, service users, carers and the public in research
In developing a research strategy ICSs should set out their approach to diverse public and patient involvement (PPI) in relation to research.
Areas where working with people and communities could add value in the context of research include:
- identification of local research needs, including through JSNAs and JHWSs
- designing research proposals in partnership with local or national experts
- raising awareness of research opportunities and recruitment of participants
- developing research outcome reports and identification of how and when participants will be able to access these
- consideration of how members of the public can access the outputs from publicly-funded research
- how volunteers should be involved and what they should be paid.
The UK Standards for Public Involvement sets out the core components of good public involvement. A guide outlining good practice in engaging underserved communities around research is available from NHS England. Resources about good practice around PPI in designing and delivering research, including around incentivisation , are also available from the HRA and NIHR .
It will be useful to link into established community involvement approaches. NIHR infrastructure organisations may have established networks of expert PPI representatives, and ICSs have extensive VCSE Alliances. A co-ordinated community engagement approach across health and care delivery and research will reduce the risk of overburdening communities with organisations wanting to work with them, and will support the identification of under-served communities.
3.10 Ensuring anyone can participate in research
Making research more visible within communities and increasing the public’s understanding of research can ensure greater diversity in research participation. Research findings will then be more generalisable to a broader range of groups or communities, or can be targeted and specific to relevant communities.
ICSs should seek mechanisms to ensure that opportunities to take part in research are available to all. They should consider encouraging patients and members of the public to register on NIHR Be Part of Research (a national registry where people can express their interest in being contacted about research that is relevant to them), widely disseminate research opportunities and make provision for inclusive access for communities to take part in research. Decentralised or virtual trials are remote access trials recruited to and delivered using electronic tools, making it easier for people to participate in some studies without needing to visit a recruiting hospital or attend appointments in person. ICBs should consider ways in which research delivery can increase access to research opportunities for people within their area. ICBs should also advise the public how they can access research outputs.
NIHR and UK Research and Innovation provide resources that help organisations address issues of equality, diversity and inclusion in research settings.
Example: RELIEVE-IBS decentralised trial
In 2020, Newcastle researchers launched RELIEVE-IBS, one of the first interventional decentralised clinical studies in the UK to trial Enterosgel, a new treatment for irritable bowel syndrome with diarrhoea (IBS-D). Decentralised trials are remote access trials that use electronic tools for trial recruitment and delivery, without the patient needing to visit a recruiting hospital site, which could be miles from their homes – a convenient option for patients with IBS-D. By running the trial remotely, researchers could reach beyond the small proportion of those with this condition who attend specialist clinics, as well as save resource for the sponsor.
Not only did this trial embrace technological developments to deliver research, but it empowered more patients to become involved regardless of where they lived. With in-depth patient input, the research team were able to shape the recruitment approach to be highly accessible to participants and were offered feedback on how to refine the trial design by the sponsors. The resulting patient-centric design ensured a good recruitment response when the trial opened.
NIHR (2020) Virtual trial recruits 67% faster led by NIHR Patient Recruitment Centre in Newcastle in collaboration with Enteromed
NIHR (2021) Pushing virtual boundaries to improve patient engagement and accessibility
NIHR (2022) RELIEVE IBS-D trial case study
3.11 Health data in research
Health data generated through care of service users in the NHS can fuel a revolution in the research and development of new diagnostics and treatments, maximising the potential to improve service user outcomes and experiences, support diversity in research, and minimise health inequalities through research. To do this, researchers need access to high quality and timely data to generate insights. The public expect data to be used legally and efficiently to conduct and support research.
National commitments around data for research can be found in Data saves lives: reshaping health and social care with data . This strategy shows how data will be used to bring benefits to all parts of health and social care. To achieve this vision, the NHS will be making a strategic move away from a system of data dissemination to one of data access when making NHS health and social care data available for research and analysis. This will be facilitated by the implementation of secure data environments (SDEs).
SDEs are data storage and access platforms with features that enable organisations to have greater control and oversight over their data. SDEs allow approved users to view and analyse data without it having to leave the environment. The SDE policy guidelines provide a clear signal to the sector that SDEs will become the default way of accessing NHS data for research.
This change is supported by major investments in digital infrastructure through the Data for Research & Development Programme, which is funding the development of national and subnational SDEs. The subnational SDEs will cover the entirety of England and individual platforms will cover several ICS.
ICBs should seek ways to promote and enable the use of these rich data sources for research and include them in their research strategy.
3.12 Using evidence for planning, commissioning and improving health and care
Evidence-based commissioning has advantages for the commissioner, workforce and service users, as it can:
- lead to innovation in service design and delivery
- enhance the quality of health and care provision
- reduce clinical variation between locations and providers
- improve equity of access to services
- improve patient and population outcomes.
As part of the commissioning process, commissioners are expected to use evidence-based clinical policies, as per the Roadmap for integrating specialised services within integrated care systems . Knowledge and library services can help source and interpret evidence.
The Provider Selection Regime will reflect the research duties of the 2022 Act and should be referred to when commissioning provider services, once it has been published.
NHS knowledge and library services provide access to evidence and support for knowledge management; they train people in searching for, handling and publishing information. The Knowledge for Healthcare strategy encourages and equips NHS knowledge and library services to support NHS organisations with the translation of knowledge for the spread and adoption of research and innovation. To fulfil their obligations under the 2022 Act, ICBs could commit to active knowledge translation.
Evidence for commissioning information is available from a number of sources:
- NHS Library and Knowledge Hub
- Health Libraries and Information Services Directory
- NICE guidance
- NIHR evidence
- NHS evidence works toolkit
- Academy of Medical Royal Colleges: Evidence-based Intervention
- A million decisions
The infographic for the role of research and evidence in commissioning also provides sources for evidence-based commissioning.
Example: Evidence mobilisation, knowledge sharing and improving outcomes
The STEMClub (Sustaining Transformation by Evidence Mobilisation) is a network in the North East and North Cumbria that brings together local policy and decision-makers with NHS knowledge and library specialists to facilitate evidence-based decision-making. The input of knowledge specialists ensures timely access to published research and provides knowledge management expertise to shape how soft intelligence is translated into knowledge assets.
As members within the STEMClub network, knowledge and library specialists are providing ongoing detailed evidence reviews and information management expertise to facilitate system-wide working , eg:
- North East North Cumbria Frailty Framework
- North East and North Cumbria Maternity Clinical Network
- a review of optimal patient transfer times in the North East and North Cumbria
- regular evidence summaries for the ICS Mental Health Evidence and Evaluation subgroup.
3.13 The health and care workforce and research
Staff involved in research have greater job satisfaction and research active trusts have lower staff turnover [3] . Clinical academic roles [7] , having research colleagues within services [8] and taking students on research placements [6] are felt to foster an increase in knowledge and skills across the wider staff workforce. The General Medical Council (GMC) and the Royal College of Physicians (RCP) and NIHR have issued position statements and recommendations around research, with additional signatories including UKRI, UKRD, the Academy of Medical Royal Colleges and the Royal College of Surgeons of England. Learning resources, including programmes for ongoing professional development of the research delivery workforce, are available through NIHR Learn.
In developing a research strategy ICSs could ensure that, as part of their people function and approach to workforce planning :
- Staff roles in leading, delivering or facilitating research and in supervising those developing research skills are recognised, supported and enabled across all staff groups and health and care settings as part of a positive research culture.
- The value of evidence is recognised, and education and training around research are facilitated. Opportunities to develop research careers or in overseeing the development of other researchers are enabled; this may include having protected time, inclusion in job plans and joint appointments across health and care providers and academic institutions.
- Ensuring that there is capacity and systems that support research through services like imaging, pathology and pharmacy, as well as finance and human resources.
- Individual organisations do not always have the necessary skills or services to support effective research and its impact, such as IP management, methodological expertise, regulatory compliance, statistical analysis, knowledge mobilisation expertise, genomics expertise, health informatics and data analytics. Mechanisms are needed to ensure that these can readily and rapidly be accessed across other health and care organisations, including from local authorities and other non-NHS care providers.
A UK Clinical Research Workforce Strategy is under development. ICSs should update their approaches to their research workforce once DHSC publishes this in 2023/24.
Example: Investing in the research workforce – developing capacity for chief investigators
Across the West Midlands NIHR CRN, an investment of approximately £750,000 to develop capacity for chief investigators returned additional research grant income of over £18 million in three years. This was achieved primarily by increasing the programme activity for consultants in areas where chief investigators were underrepresented.
The funding was provided through a competitive process and co-supported by the local NIHR CRN, with several local trusts jointly funded these scholars.
Kirk J, Willcocks J, Boyle P, Brocklehurst P, Morris K, Kearney R, et al (2022) Developing chief investigators within the NHS: the West Midlands clinical trials scholars programme. Clin Med 22(2): 149–52.
Kirk J, Reynolds F, Adey E, Boazman M, Brookes M, Brocklehurst P (2022) Developing paediatric chief investigators within the NHS: the Clinical Trials Scholars programme . Arch Dis Child Educ Pract Published online first: 22 February 2022. doi: 10.1136/archdischild-2021-322186
4. References
- Varnai P, Rentel M, Dave A, De Scalzi M, Timmerman W, Rosemberg-Mantes C, Simmonds P, Technopolis Group (2017) The impact of collaboration: The value of UK medical research to EU science and health .
- Boaz A, Hanney S, Jones T, Soper B (2015) Does the engagement of clinicians and organisations in research improve healthcare performance: a three-stage review. BMJ Open 5: e009415. doi:10.1136/ bmjopen-2015-009415 .
- Rees MR, Bracewell M (2019) Academic factors in medical recruitment: evidence to support improvements in medical recruitment and retention by improving the academic content in medical posts. Postgrad Med J 95(1124): 323-327. doi:10.1136/postgradmedj-2019-136501 .
- Ozdemir BA, Karthikesalingham A, Singha S, Poloniecki JD, Hinchliffe RJ, Thompson MM, et al (2015) Research activity and the association with mortality. PLoS ONE 10(2): doi.org/10.1371/journal.pone.0118253 .
- Hunn A (2017) Survey of the general public: attitudes towards health research . Health Research Authority.
- Angus RL, Hattingh HL, Weir KA (2022) Experiences of hospital allied health professionals in collaborative student research projects: a qualitative study. BMC Health Services Research 22(1). Available at: https://doi.org/10.1186/s12913-022-08119-7 .
- Newington L, Wells M, Adonis A, Bolten L, Bolton Saghdaoui L, Coffey M, et al (2021) A qualitative systematic review and thematic synthesis exploring the impacts of clinical academic activity by healthcare professionals outside medicine. BMC Health Serv Res 21(1). Available at: https://doi.org/10.1186/s12913-021-06354-y .
- Wenke RJ, Hickman I, Hulcombe J, Phillips R, Mickan S (2017) Allied health research positions: A qualitative evaluation of their impact. Health Res Policy Syst 15(6). Available at: https://doi.org/10.1186/s12961-016-0166-4
Annex 1 – Organisations that may be involved in regional or local research
- Clinical Research Networks (CRNs) , which will be retendered and renamed regional research delivery networks (RRDNs) from April 2024
- Applied Research Collaborations (ARCs)
- Biomedical Research Centres (BRCs)
- Experimental Cancer Medicine Centres (ECMCs) , jointly funded with Cancer Research UK
- Research Design Services (RDSs) and Clinical Trials Units (CTUs) which will be replaced by the NIHR Research Support Service from 1 October 2023
- Patient Recruitment Centres (PRCs)
- MedTech and In vitro diagnostic Co-operatives (MICs) , which will be replaced with HealthTech research centres from April 2024
- School of Public Health Research, School of Primary Care Research and School of Social Care Research
- Health Determinants Research Collaborations (HDRCs)
- Clinical Research Facilities (CRFs)
- Patient Safety Research Collaborations (PSRCs)
- Translational Research Collaborations (TRCs)
- Academic Health Science Centres (AHSCs)
- university teaching hospitals and all trusts that deliver research activity
- primary care organisations, including GP practices, that deliver research activity
- higher education institutions (HEIs)
- local authorities
- social care partners
- Local Government Association
- local and national charities that fund, collaborate in or support participation in research
- research and development offices in providers or CSUs, including primary care providers and ambulance, community and mental health trusts, and those in the VCSE sector
- UKRD members
- NHS subnational secure data environments for research
- NHS R&D Forum
- NHS Genomic Medicines Service Research Collaborative
- NHS Knowledge and Library Services
- Academic Health Science Networks (AHSNs) are often well linked with research organisations and infrastructure as part of their roles in development, adoption and spread of innovation.
Annex 2 – Public health and social care research
Public health research investigates issues that impact at a population rather than an individual level. This can be done within the NHS with system-level studies, such as secondary prevention of cardiovascular disease and examining the impact on health inequalities of changes to the NHS resource allocation formula, and outside the NHS for the wider determinants of health such as air quality, transport systems and housing. There is a substantial body of public health evidence for the clinical and cost effectiveness of prevention, health protection, health service redesign and addressing health inequalities.
Social care research aims to improve the lives of children and adults who need to draw on personal or practical care and support, and family members or other unpaid carers. It can include research around the introduction, use and impact of technologies, and changing social care interventions, policies and practice. Social care research also examines issues pertaining to the safeguarding of adults and children and workforce, commissioning of services, and questions about organisational and professional practice, including decision-making, training and the quality of care.
Publication reference: PR1662
Click through the PLOS taxonomy to find articles in your field.
For more information about PLOS Subject Areas, click here .
Loading metrics
Open Access
Study Protocol
Mapping the evidence on factors related to postpartum contraception among sub-Saharan African immigrant and refugee women in the United States of America: A scoping review protocol
Roles Conceptualization, Investigation, Methodology, Supervision, Validation, Writing – original draft
Affiliation Department of Public Health, Arcadia University, Glenside, PA, United States of America

Roles Data curation, Methodology, Writing – review & editing
Affiliation College of Public Health and Health Professions, University of Florida, Gainesville, FL, United States of America
Roles Formal analysis, Methodology, Writing – review & editing
Affiliation Department of Biological Sciences, School of Environmental Sciences, Njala University, PMB, Freetown, Sierra Leone
Affiliation Department of Health and Education, Coventry University, Scarborough, United Kingdom
Roles Investigation, Supervision, Writing – review & editing
* E-mail: [email protected]
Affiliations School of International Development and Global Studies, University of Ottawa, Ottawa, Canada, The George Institute for Global Health, Imperial College London, London, United Kingdom
- Comfort Z. Olorunsaiye,
- Mariam A. Badru,
- Augustus Osborne,
- Hannah M. Degge,
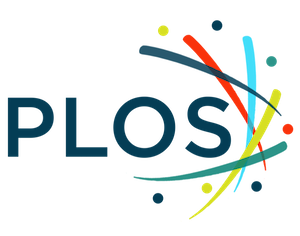
- Published: May 29, 2024
- https://doi.org/10.1371/journal.pone.0304222
- Reader Comments
Postpartum contraception is essential to sexual and reproductive health (SRH) care because it encourages healthy spacing between births, helps women avoid unwanted pregnancies, and lessens the risks of health problems for mothers and babies. Sub-Saharan African immigrant and refugee populations are rapidly increasing in the United States, and they come from a wide range of cultural, linguistic, religious, and social origins, which may pose challenges in timely access to culturally acceptable SRH care, for preventing mistimed or unwanted childbearing. The objective of this scoping review is to assess the extent of the available literature on postpartum contraception among sub-Saharan African immigrant and refugee women living in the United States.
We developed preliminary search terms with the help of an expert librarian, consisting of keywords including birth intervals, birth spacing, contraception, postpartum contraception or family planning, and USA or America, and sub-Saharan African immigrants, or emigrants. The study will include the following electronic databases: PubMed/MEDLINE, PsycINFO, CINAHL, EMBASE, and the Global Health Database. The sources will include studies on postpartum care and contraceptive access and utilization among sub-Saharan African immigrants living in the US. Citations, abstracts, and full texts will be independently screened by two reviewers. We will use narrative synthesis to analyze the data using quantitative and qualitative methods. Factors associated with postpartum contraception will be organized using the domains and constructs of the PEN-3 Model as a guiding framework.
This scoping review will map the research on postpartum contraception among sub-Saharan African immigrant and refugee women living in the US. We expect to identify knowledge gaps, and barriers and facilitators of postpartum contraception in this population. Based on the findings of the review, recommendations will be made for advocacy and program and policy development toward optimizing interpregnancy intervals in sub-Saharan African immigrants living in the US.
Trial registration
Review registration Open Science Framework: https://osf.io/s385j .
Citation: Olorunsaiye CZ, Badru MA, Osborne A, Degge HM, Yaya S (2024) Mapping the evidence on factors related to postpartum contraception among sub-Saharan African immigrant and refugee women in the United States of America: A scoping review protocol. PLoS ONE 19(5): e0304222. https://doi.org/10.1371/journal.pone.0304222
Editor: Adetayo Olorunlana, Caleb University, NIGERIA
Received: September 12, 2023; Accepted: May 7, 2024; Published: May 29, 2024
Copyright: © 2024 Olorunsaiye et al. This is an open access article distributed under the terms of the Creative Commons Attribution License , which permits unrestricted use, distribution, and reproduction in any medium, provided the original author and source are credited.
Data Availability: No datasets were generated or analysed during the current study. All relevant data from this study will be made available upon study completion.
Funding: The authors received no specific funding for this work.
Competing interests: None
Abbreviations: ACOG, American College of Obstetricians and Gynecologists; IPI, Interpregnancy interval; JBI, Joanna Briggs Institute; LAM, Lactational amenorrhea method; LARC, Long-acting reversible contraceptive; PCC, Population, Concept, Context; PRISMA-ScR, Preferred Reporting Items for Systematic Reviews and Meta-Analyses, extension for scoping review; SRH, Sexual and Reproductive Health; WHO, World Health Organization
Postpartum contraception is essential to sexual and reproductive health (SRH) care because it helps women avoid unwanted pregnancies, reduces the risks of health problems for mothers and babies, and encourages healthy spacing between births [ 1 ]. The World Health Organization’s (WHO) Postpartum Family Planning Compendium (2013) provides guidelines for postpartum contraception, with recommendations for health care providers to initiate most postpartum contraception for most women from as early as within 48 hours of childbirth to six weeks—with a few other considerations for initiating certain methods after six weeks through the first 12 months postpartum (e.g., diaphragm for all women, and progestogen-only injections in breastfeeding women)—to prevent closely spaced and unintended pregnancies [ 2 ]. Similarly, within the first six weeks after childbirth, the American College of Obstetricians and Gynecologists (ACOG) recommends that women have access to various contraception options to prevent short-interval and/or unintended pregnancy [ 3 ]. Short interpregnancy intervals (IPIs), defined as an interval of less than 18 months between pregnancies [ 4 ], is a common occurrence among American women, yet many do not use effective postpartum contraception between pregnancies. Preterm delivery, low birth weight, and early gestational age are among pregnancy complications that are more likely to occur in women with shorter IPIs [ 5 , 6 ]. Healthy People 2020 aimed to decrease the number of infant mortality rates (IMRs) by 40 percent due to postpartum contraception usage [ 4 ]. Further, Healthy People 2030 aims to reduce the prevalence of pregnancies conceived within 18 months of a previous live birth, i.e., births with short IPI [ 7 ].
African immigrant and refugee populations, specifically populations from sub-Saharan Africa, are rapidly increasing in the United States, and they come from a wide range of cultural, linguistic, religious, and social origins [ 8 ]. Immigrant and refugee populations may have trouble using postpartum contraception and other preventative SRH care because of their prior experiences with SRH in their home countries, and relocation, migration, and resettlement experiences [ 9 ]. Evidence from other high-income migrant-receiving nations in the European Union and Asia [ 10 ], and a recent systematic review of five countries [ 11 ] have revealed many factors that contribute to low rates of maternal health care use among immigrant women. Language difficulties, immigrants’ exposure to prejudice and racism from healthcare professionals, women’s familiarity with maternal health care information, and the strain of cultural adjustment are all factors that impede the use of SRH services. Inadequate or no prenatal care, increased rates of stillbirths and infant death, and financial barriers [ 12 ] are some of the maternal health challenges the United Nations (UN) research finds common among immigrants across the European Union and Asia. British studies on immigrant birth outcomes show that infants delivered to Asian mothers had lower birth weights, and those born to Caribbean and Pakistani women have higher perinatal and postnatal death rates than the general population [ 13 ]. Other high-income countries, such as Norway, Japan, and Italy, have found similar results [ 11 ]. In the United States, the few studies available have found shorter IPIs in sub-Saharan African-born women compared to US-born women [ 14 – 16 ].
Low-income immigrant women in the US are particularly vulnerable because they typically lack the resources to pay for prenatal and postnatal care [ 17 ]. Medicaid, the biggest single-payer for obstetric care in the United States [ 18 ], is a lifeline for these women since it provides coverage for their pregnancies and limited postpartum care. However, many immigrant women are not covered by Medicaid, even though prenatal care is a prioritized preventive health treatment under the program [ 19 ]. Full (conventional) Medicaid benefits are not available to undocumented immigrants or documented immigrants in the country for less than five years [ 20 ]. Emergency Medicaid coverage, available in some states, which women must qualify for, limits coverage to just the direst circumstances, including hospitalization for delivery.
Understanding the factors influencing postpartum contraceptive use among sub-Saharan African immigrant and refugee women is vital for developing targeted interventions that address their unique needs. Sub-Saharan African immigrant and refugee women may face various challenges; culture and personal beliefs largely influence decisions regarding postpartum contraception. Some cultural norms and religious beliefs discourage modern contraceptive methods, endorsing more traditional methods or no contraception at all [ 21 ]. Access to healthcare services is a significant structural factor affecting postpartum contraception use among these women. Moreover, many sub-Saharan African immigrant and refugee women in the US face structural barriers to healthcare access, such as lack of insurance, language barriers, or unfamiliarity with the US healthcare system [ 22 ]. These barriers can limit these women’s ability to receive timely contraceptive counseling and access contraceptive services.
Financial considerations further impact the use of postpartum contraception. The cost of contraceptive methods, especially long-term methods like intrauterine devices (IUDs) and implants, may be prohibitive for some women, especially those that have low incomes and/or lack insurance coverage [ 23 ]. Although safety net family planning services via Title X funding may be available for uninsured or under-insured people, these services are commonly strained and many immigrants do not use them, sometimes due to lack of awareness or fear of jeopardizing their immigration status [ 24 ]. Educational level is also associated with postpartum contraception and immigrant and refugee women, with higher levels of education are more likely to use contraceptives than those with lower levels of education [ 25 ]. Education can improve women’s knowledge about contraceptive methods, their effectiveness, and where to access them. The role of healthcare providers is paramount in influencing postpartum contraceptive use. Effective, culturally sensitive contraceptive counseling by healthcare providers can encourage contraceptive use among immigrant women. However, health worker bias and lack of culturally sensitive health care services can hinder these efforts [ 26 ].
Rationale for a scoping review
Sub-Saharan African immigrants account for the fastest growing group of Black immigrants in the US [ 27 ]. Moreover, according to recent data, sub-Saharan African immigrant women accounted for 5% of all live births in the US, and also have the highest birth rate among all immigrant groups in the US [ 28 ]. To our knowledge, there is no comprehensive review of the evidence on factors related to postpartum contraception among sub-Saharan African immigrant and refugee women in the US. Identifying the factors that contribute to lower rates of postpartum contraceptive use and higher rates of unintended and short interval pregnancies, which can have adverse health and socioeconomic consequences, is warranted. Therefore, the objective of this scoping review is to assess the extent of the available literature on postpartum contraception among sub-Saharan African immigrant and refugee women living in the United States. A scoping review is a suitable method to map the existing literature on this topic, identify knowledge gaps, and inform future research directions [ 29 ]. This review aims to build on existing research by assessing the evidence on factors related to postpartum contraception among sub-Saharan African immigrant and refugee women residing in the US. The review will identify barriers and facilitators to postpartum family planning services among immigrant and refugee people. By identifying the key factors influencing postpartum contraceptive use in this population, this study, when completed, can inform the development of interventions and policies promoting reproductive health equity for immigrant and refugee women living in the US.
The protocol for this study was systematically developed using the Joanna Briggs Institute (JBI) guidelines for conducting scoping reviews to ensure replicability [ 30 ], and has been submitted to Open Science Framework (OSF) for registration. The review, when completed, will be reported according to the guidelines of the Preferred Reporting Items for Systematic Reviews and Meta-Analyses–Extension for Scoping Review (PRISMA-ScR) ( S1 File ) [ 31 ].
Protocol design
The scoping review protocol was developed using the 2021 updated methodological guidelines of the Joanna Briggs Institute (JBI) [ 30 ]. Specifically, we followed the JBI multi-step process for protocol development, as described in the following paragraphs below.
Title and review questions
This scoping review seeks to synthesize and summarize the available research on postpartum family planning among sub-Saharan African immigrant and refugee populations living in the United States, and to identify knowledge gaps in this area of research. The title and review questions were clarified and aligned with the purpose of the scoping review. Specifically, we ensured that key elements of the inclusion criteria, i.e., the population, concept, and context (PCC) are reflected in the title to help readers more easily identify the study [ 30 , 31 ]. The primary question that will guide the review is: What is the evidence on postpartum contraception among sub-Saharan African immigrant and refugee women living in the United States? In addition, three sub questions that will enable the research team to further explore specific attributes of the review’s population, concept, and context will be explored, as outlined below:
- What sub-Saharan African immigrant populations have been studied in the literature on postpartum contraception among sub-Saharan African immigrant and refugee women living in the US?
- What are the barriers to and facilitators of postpartum contraception from the perspectives of sub-Saharan African immigrant and refugee women and health care providers in the US?
- What is the evidence linking postpartum contraceptive counseling, access, and use to the prevention of short-interval births or unintended pregnancy among sub-Saharan African immigrant and refugee populations in the US?
Inclusion criteria
As noted earlier, the eligibility criteria for this scoping review consist of three key elements: participants, concept, and context (PCC) [ 30 ].
Participants.
The study particiants includes sub-Saharan African immigrant and refugee women of reproductive age (15–49 years) living in the US, and with at least one live birth. This age range aligns with the range used in population-based SRH surveys and databases [ 32 ]. Specifically, eligible studies will include participants that identify as immigrant or refugee individuals, born in a country in sub-Saharan Africa, and living in the US at the time of the study. Further, to better identify gaps in the literature, this protocol will also include sources that contain data on health care providers such as nurses, physicians, midwives, doulas, social workers, community health workers, and refugee resettlement staff, to provide relevant perspectives and information about the barriers and facilitators of postpartum contraceptive access and use within this population.
The development of the review questions was guided by the World Health Organization’s definition of postpartum family planning, as “services for the prevention of unintended pregnancy and closely spaced pregnancies through the first 12 months following childbirth” [ 2 ]. This definition is similar to that used by the ACOG: “using a birth control method in the weeks after [having a baby] to avoid an unintended pregnancy…experts recommend waiting at least 18 months between pregnancies before having another baby” [ 33 ]. Data will include postpartum family planning and contraceptive information and counseling, patterns of use, and contraceptive methods. There will be no restriction on the types of contraceptive methods reported in eligible studies. Thus, eligible sources will include studies on very effective methods such as sterilization, long acting reversible contraceptives (LARCs) such as IUDs and implants; effective methods such as short-acting hormonal contraceptives, including the pill, injectable, emergency contraception, and lactational amenorrhea method (LAM); moderately effective methods such as barrier methods, for example, the female and male condoms, and fertility awareness methods; and less effective methods, such as, withdrawal or coitus interruptus [ 34 ]. We will also identify key barriers, facilitators, and social determinants, of postpartum contraception access and utilization in sub-Saharan African immigrant and refugee women. Some of these include, access to insurance coverage, literacy levels, language, patient-provider factors, childcare, transportation, stigma, and knowledge/awareness of available/preferred methods [ 9 , 24 , 35 ].
Only studies conducted in, and on participants currently residing in the United States will be included in this review. Studies of sub-Saharan African immigrant and refugee women residing outside the United States will be excluded. Only full-text peer-reviewed studies will be included in this review. Further, only papers written in English will be included. Conference abstracts will be excluded.
Exclusion criteria
We will exclude studies that do not include sub-Saharan African immigrant and refugee women of reproductive age (i.e., 15–49 years). Sources that include immigrant and refugee populations born in countries outside of the sub-Saharan Africa region will also be excluded. Studies that are conducted with populations living outside the United States, studies that were published prior to 2000, and in a language other than English will also be excluded. Finally, sources that do not include postpartum contraception will also be excluded from this scoping review.
Types of studies
All types of study designs will be considered in this review, including randomized controlled trials (RCTs), non-RCTs, pre/post intervention and time series designs. Observational studies, including prospective, retrospective, cross-sectional, descriptive, and case studies will also be included in the review. We will include systematic and other types of reviews. Grey literature such as technical reports and program evaluation reports will also be considered. We will include opinion papers in this scoping review. Across all the aforementioned types of sources, we will include quantitative, qualitative, and mixed methods designs in this review.
Theoretical framework
The PEN-3 Model, which centers culture in the understanding of health behaviors and outcomes, [ 36 ], will be used as a guiding framework for this study. The PEN-3 Model uses a strength-based approach to identify individual, family and community values and assets, as well as social and cultural norms, and factors that may facilitate or hinder behaviors that affect health outcomes [ 36 , 37 ]. Since its introduction in the context of studying the impact of the sociocultural context on health behaviors in sub-Saharan Africa, the model has been used extensively in other areas of research and programs, including SRH, adolescent health, family planning, and chronic disease prevention and management in several countries [ 36 , 38 – 40 ]. The model has three domains, each with three constructs, namely: 1) cultural identity (constructs: person, extended family, neighborhood); 2) relationships and expectations (constructs: perceptions, enablers, and nurturers; and 3) cultural empowerment (constructs: positive, unique/existential, and negative factors).
Search strategy
A public health research librarian helped the authors with identifying keywords and developing the preliminary search terms, including Boolean search terms, and relevant databases for an exploratory database search in PubMed/MEDLINE ( Table 1 ). We will search the following databases: PubMed/MEDLINE, PsycINFO, CINAHL, EMBASE, and the Global Health Database. In addition, we will search the grey literature, including the webpages of multilateral organizations such as the World Health Organization and United Nations agencies, as well as the Migration Policy Institute, the American College of Obstetricians and Gynecologists, and the Centers for Disease Control and Prevention. We will also conduct a manual search of article reference lists in Google Scholar to ensure we capture the breadth of the available research on the topic. Further, we will manually search the publications of key reproductive health journals for relevant sources that may not be retrieved in our database search. Prior to the implementation of the review, we will work with the professioanal librarian to update and refine the search terms as needed. All changes to the protocol will be highlighted in the reporting of the review, when completed.
- PPT PowerPoint slide
- PNG larger image
- TIFF original image
https://doi.org/10.1371/journal.pone.0304222.t001
Study screening and selection process
The search will cover the period from January 1, 2000, to June 30, 2023. We selected this period for the search because sub-Saharan African immigration to the US has increased rapidly since 2000 [ 8 ]. When the database searches are completed, we will review the reference lists of selected articles to identify additional, relevant sources. The final list of relevant sources will be imported into Covidence, which will automatically identify duplicates for removal. Covidence will be used to manage all steps of the title/abstract and full-text screening. We will review the titles and abstracts of the retrieved results and exclude those that do not meet the inclusion criteria. Abstract and title screening will be carried out independently by two reviewers. Where there are disagreements between both reviewers, these will be resolved by discussion. In a second screening step, full texts of the retained articles will be imported into Covidence, via Zotero, a bibliography management software, [ 41 ]. Detailed assessment of the full texts of articles will be independently carried out by two reviewers. Full texts not meeting inclusion criteria will be deleted in this step. Where there are disagreements among the two reviewers, these will be resolved by consensus or a third reviewer if warranted. The full-text articles to be included in the data synthesis and analysis will be finalized. We will develop a PRISMA flowchart to document the bases for inclusion or exclusion of articles [ 42 ].
Data extraction and charting
Using the JBI (Joanna Briggs Institute) data extraction manual as a guide [ 30 ], we developed a draft data extraction tool ( Table 2 ). The data extraction tool will be modified and revised as needed during the data extraction process, to to capture pertinent information from each included source of evidence To pilot the data extraction tool, two reviewers will independently screen a subset of the full-text sources, using the draft data extraction tool to record key information from the subset of studies. We will include 10% of the total number of sources meeting the review includsion criteria in this pilot [ 43 ]. Upon finalization of the data extraction tool, each of the two reviewers will be assigned a subset from the remaining articles to review. The data extracted will include details about the participants’ sociodemographic information (e.g., age, education, parity, country of birth, immigration status), concept (e.g., postpartum contraceptive method use), and context (e.g., state or region of residence in the US) (PCC). We will also extract data about study details specific to each included source, such as authors’ names, article title, year of publication, study aims/objectives, study design, study population (country of birth), sample size, and key findings related to the review questions. The two reviewers will discuss discrepancies and necessary amendments to the data extraction tool. If needed, a subject matter expert will serve as a third reviewer to help resolve discrepancies.
https://doi.org/10.1371/journal.pone.0304222.t002
Summarizing, analyzing, synthesizing, and reporting the data
The extracted data will be tabulated, accompanied by a narrative summary of the characteristics of the included studies, for example, publication date, study participants’ country of origin, and study design. Table 2 outlines the key information that will be extracted. We will present the results quantitatively using frequencies, and qualitatively using thematic analysis. The results will be further analyzed using narrative synthesis, which is a suitable approach for systematically synthesizing evidence from multiple sources using words and text to summarize and explain the findings [ 44 ]. We will identify social and structural factors that may influence postpartum contraception and family planning. These findings will be organized into perceptions, enabling, and nurturing factors, and will then, be categorized into positive, unique/existential, and/or negative factors, using the PEN-3 Model domain and constructs [ 37 , 38 ]. The data will be presented in tables and charts, accompanied by narrative descriptions of each table and chart.
Quality assessment and risk of bias
Consistent with the JBI Manual for conducting scoping reviews [ 30 ], the risk of bias or methodologic quality of included studies will not assessed. To minimize the risk of bias, we have developed a plan for at least two reviewers to independently conduct the title/abstract and full text screening of retrieved sources. The exclusion of articles published in a language other than English is not expected to be a source of bias since the focus of this review is on the US, where the official language is English. The exclusion of studies published prior to 2000 may eliminate some sources; however, we expect this to cause minimal bias since sub-Saharan African immigration to the US only started to rise sharply in the 2000’s.
This paper presents a protocol for a scoping review aimed at mapping the existing research on postpartum contraception among sub-Saharan African immigrant and refugee women living in the US. It has been observed that foreign-born Black women exhibit a less favorable attitude towards contraceptive uptake compared to their US-born counterparts [ 45 ]; hence it would be meaningful to assess if this correlation extends to contraceptive method use during the postpartum period. Additionally, contraceptive care for resettled refugees is reported to be limited due to the absence of epidemiological data on their distinctive needs [ 35 ].
The primary objective of this review is to identify knowledge gaps and utilize existing evidence to support policies, programs, and advocacy for reducing the prevalence of short-interval births among sub-Saharan African immigrants. Findings will be discussed in the context of previous research. We will also discuss the results of this scoping review in relation to future research, policy, and service delivery to support African-born migrant and refugee women living in the US, to prevent unintended pregnancy and achieve healthy birth intervals and reproductive autonomy.
Short interpregnancy interval is associated with adverse maternal outcomes, such as increased risks of subsequent obesity, gestational diabetes, precipitous labor, and placental abruption, as well as decreased risks of preeclampsia and labor dystocia. Furthermore, a previous caesarean delivery followed by a short interval pregnancy of less than 6 months has been linked to increased risks of uterine rupture, blood transfusions, and other birthing complications [ 46 ].
Limitations and strengths
As a scoping review’s focus is to provide a broad overview of the subject matter, a limitation is that an in-depth analysis may be hampered. We also acknowledge the challenge in ensuring that the search strategy is neither too narrow nor too broad. The wide range of study designs and methodologies that will be included may lead to heterogeneity among the studies, making it challenging to synthesize the findings. This limitation will be mitigated by using a narrative synthesis approach for analyzing the retrieved data [ 44 ]. Additionally, the methodological quality of the included studies will not be assessed. It should be noted that, consistent with guidelines [ 30 ], quality assessment of retrieved studies is not a focus of scoping reviews.
Despite these limitations, scoping reviews play a vital role in research by offering an overview of existing literature, identifying knowledge gaps that may warrant a systematic review, and outlining areas for future research. The findings of this scoping review will be disseminated through a peer-reviewed journal and presented at national/international conferences. This scoping review will contribute to the existing knowledge on, and aid in the development of evidence-based interventions for promoting postpartum contraception among African immigrant and refugee women living in the US.
Potential contributions of this scoping review
Through this review and data synthesis, we aim to propose a working understanding of the concept of postpartum contraception among sub-Saharan African immigrant and refugee women. The review will provide insights into various aspects, including postpartum contraceptive information and counseling, patterns of use, and the range of contraceptive methods utilized. Additionally, we will identify barriers and facilitators related to postpartum contraceptive education and counseling, and contraceptive access (e.g., insurance, childcare, transportation,), as well as health system and structural barriers/facilitators (e.g., preferred/available methods, language, provider factors, etc.). This review, when completed, will be published in a peer-reviewed journal e.g., PLoS ONE . Findings will also be disseminated to knowledge users via scientific conference presentations and seminars.
Supporting information
S1 file. prisma 2020 checklist..
https://doi.org/10.1371/journal.pone.0304222.s001
Acknowledgments
We express our appreciation to Jon Drucker, Assistant Professor and Collections and Technical Services Librarian, Landman Library, Arcadia University, who helped with the development of the search strategy.
- 1. World Health Organization, Medical Eligibility Criteria for Contraceptive Use . World Health Organization, 2010.
- 2. World Health Organization and United States. Agency for International Development, Programming strategies for postpartum family planning . Geneva: World Health Organization, 2013. Accessed: Jul. 12, 2023. [Online]. Available: https://apps.who.int/iris/handle/10665/93680 .
- View Article
- PubMed/NCBI
- Google Scholar
- 7. “Healthy People 2030 | health.gov.” Accessed: Aug. 01, 2023. [Online]. Available: https://health.gov/healthypeople .
- 12. “Maternal health.” Accessed: Aug. 01, 2023. [Online]. Available: https://www.who.int/health-topics/maternal-health .
- 20. “Trends in Emergency Medicaid Expenditures for Recent and Undocumented Immigrants | Emergency Medicine | JAMA | JAMA Network.” Accessed: Aug. 01, 2023. [Online]. Available: https://jamanetwork.com/journals/jama/article-abstract/206014 .
- 33. “Postpartum Birth Control.” Accessed: Aug. 01, 2023. [Online]. Available: https://www.acog.org/womens-health/faqs/postpartum-birth-control .
- 34. “Family planning/contraception methods.” Accessed: Aug. 31, 2023. [Online]. Available: https://www.who.int/news-room/fact-sheets/detail/family-planning-contraception .
- Smart Assessor
- Safeguarding
- Accessibility
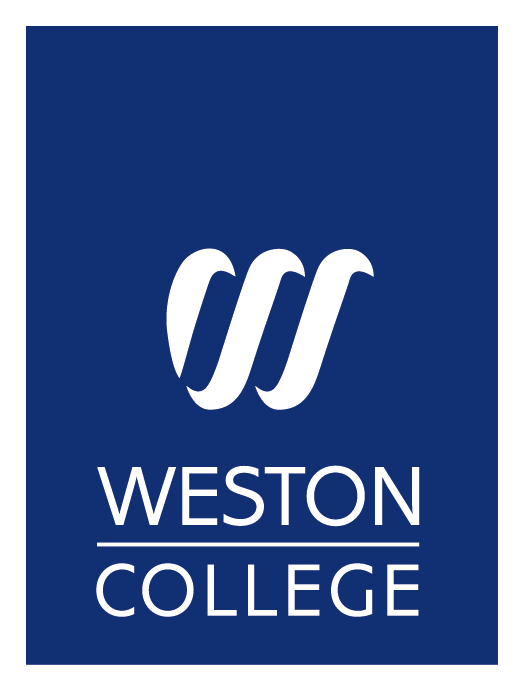
Level 3
Access to he: nursing, midwifery and allied health fast-track (blended).
This is a fast course, which starts in January and ends in time for learners who join it to progress to university in September 2025. It is designed for people aged 18 or over and is perfect for anyone looking to start a career in nursing, midwifery or any of the allied health professions, or considering changing careers to work in these fields.
A career in any nursing and midwifery-focussed profession empowers you to help people, working in a variety of positions and a wide choice of specialties.
We have a highly skilled and experienced teaching team, and this is one of the reasons we see success and progression to university rates significantly above the national average.
The course is well-established and widely accepted by universities, providing you with UCAS tariff points that are equivalent to three A Levels.
Subjects/Topics Covered
The Access to HE Diploma in Nursing and Midwifery is designed to prepare students for entry into a range of undergraduate programs in the allied health professions. The subjects covered in this course typically include:
Human Biology: students will learn about the structure and function of the human body, including the cardiovascular, respiratory, digestive, and nervous systems.
Psychology: within this topic you will explore a range of studies relating to human behaviour and to mental health conditions and the range of treatments and therapies which are successful in supporting recovery from them.
Research and Referencing: students will learn about research methods used in medical and healthcare fields, including qualitative and quantitative research. Study Skills: This module covers communication skills that are essential for success in the social sciences, including verbal and written communication, presentation skills, and teamwork.
Assessment Method
The Access to HE Diploma is designed to reflect the rigor and demands of undergraduate study. It includes a range of assessment methods such as essays, reports, exams, presentations, and portfolios. The assessments will be spread throughout the year, and you will receive regular feedback to help you progress and develop your skills.
You will study in college for 12 hours (two days) a week and complete another 6 hours of study using online self-directed learning at times to suit you. If you are prepared to study hard, this is a great flexible course to fit around your lifestyle and working commitments - voluntary or paid work within related fields will strengthen your university application and is recommended.
The assessment submission deadlines on this course are more regular than the September start courses, so if you wish to enrol, you must be in a position to commit a lot of time to studying. In addition to the 6 hours of self-directed learning at home, Access courses require at least one day per week of further research and independent study.
Progression Opportunities
This course will help you progress onto a variety of degree courses within science pathways, including University Centre Weston’s:
- Assistant Practitioner (Health and Social Care) Higher Apprenticeship
- Assistant Practitioner (Mental Health) Higher Apprenticeship
- Nursing Associate FdSc (Higher Apprenticeship)
- SEND and Inclusive Practice BA (Hons)
Plus a range of degrees at universities across the country, including:
- Adult Nursing
- Mental Health Nursing
- Learning Disabilities Nursing
- Children’s Nursing
- Paramedic Science
- Radiography
- Chiropractic
- Physiotherapy
Entry Requirements
GSCE English grade 4/C and maths grade 4/Cis mandatory.
We will consider equivalent qualifications, but the applicant must check that their equivalents will be accepted for entry to their chosen university.
Please note, that if you wish to progress to UWE Bristol, they will accept maths units which are studied within the Access programme as equivalent to GCSE maths.
Additional Support
Our dedicated team of experts is on hand to provide you with tailored advice and support to help you make the right decisions about your future career and course choices. Whether you need help developing your employability skills, exploring your options, or deciding which course to take, we're here to help you achieve your goals.
Plus, as a Microsoft College, all of our students and apprentices get FREE access to LinkedIn Learning. This is an amazing online platform that offers expert-led courses to help you develop your business, technology-related, and creative skills. At Weston College, we're dedicated to helping you achieve success in your career, whatever your goals may be. So why not take that first step today and join our adult community of learners? We can't wait to help you unlock your full potential!
Financing Your Course
At Weston College, we believe that learning should be accessible to everyone. That's why we offer a range of adult courses that could be free or significantly discounted. Apply online today and our friendly team will be in touch to see how we can ensure you can enrol and get the best price for your course. Don't let finances hold you back from pursuing your passion and advancing your career. Apply now and let us help you achieve your goals.
Category & Subject Area
i An interview is required for this course.
Financial Information
Most people qualify for FREE tuition and material fees due to discounts we offer. Click on the icon(s) below to find out what you’ll need to pay.
Full Fee: £3,384.00 ?
Supported fee: £3,384.00 , fees information, level 2 qualifications.
This course may be Free* if you live in the South West of England and:
- receive Jobseeker’s Allowance (JSA), including those receiving National Insurance credits only
- receive Employment and Support Allowance (ESA)
- receive Universal Credit and your take home pay, as recorded on your Universal Credit statement (disregarding Universal Credit payment and other benefits) is less than £345 a month (learner is sole adult in the benefit claim) or £552 a month (learner has a joint benefit claim with their partner)
- are released on temporary licence, studying outside a prison environment, and not funded by the Ministry of Justice
- You are employed, or self-employed, and earn less than £18,525.00 annual gross salary.
Level 3 Qualifications
This course is Free* if:
The qualification you have chosen to study is part of the Legal Entitlements list and you are aged 19-23 at the start of your course and you do not already have a qualification at Level 3 or higher. From April 2021, any adult aged 24 and over who wants to achieve their first full level 3 qualification will be able to access certain courses we run for free.
The full fee is payable if:
- this is not your first full level 3
- the qualification you wish to study isn’t on the approved list of level 3 qualifications the College can run for free.
However, you may qualify for an Advanced Learner Loan .
Apprenticeships - Information for Employers
The cost of apprenticeships will be split into 30 funding bands ranging from £1,500 to £27,000. The prices will depend on the type of apprenticeship and the level it is taught at, and are negotiable with your training provider.
For 98% of employers, the reforms mean that employing an apprentice will be better value for money than ever before. Small businesses will only have to pay 5% of the training costs, with the Government picking up the remaining 95%. In some cases, the Government will fully fund apprenticeships for small businesses.
Click here for more information about how the reforms and levy will affect your company.
Advanced Learner Loans
You may be eligible to apply for an Advanced Learner Loan from Student Finance England, to fund the cost of your course. For further information and to check eligibility, please follow the links below:
- www.weston.ac.uk/supporting-you/financial-support
Spreading the Costs
If the cost you have to pay for a course is over £120 and lasts for 24 weeks or more, you may be able to spread the cost.
To do this you will be asked for a 25% deposit at enrolment, plus any examination fees payable. You will then be invoiced for the remaining fee and provided with information on taking the instalment option where applicable.
Enhanced Learning Credits
Weston College is an approved provider of courses under the Ministry of Defence's Enhanced Learning Credits (ELC) scheme. Enhanced Learning Credits are designed to promote lifelong learning amongst members of the Armed Forces and ex-service personnel. ELCs provide a financial contribution towards a first full Level 3 qualification (Certificates and Diplomas), a first Foundation Degree, a first full undergraduate degree or national equivalents.
Sorry, no help exists for Hinkley Point Courses.
Please see here for more details about Hinkley Point.
Other Important information
While every care has been made to ensure the accuracy of our prospectuses and website, errors may occur from time to time resulting in incorrect information / fees being shown / printed.
Fees quoted online and in our prospectuses are for the current academic year only, and are subject to change – two and three-year courses will have fees to pay again in subsequent years.
You may still need to buy a uniform/kit which is your professional start-up kits or pay professional/personal memberships and all students will need to provide a National Insurance number and up-to-date proof of benefits, if applicable, at the time of enrolment.
Please click here for our current term dates.
If you are 16-18 years old on the 31st August in the current academic year, this course is usually Free.
Students who are EU/EEA or Swiss Citizens who have lived in the UK before 1 January 2021 who do not have indefinite leave to enter or remain in the UK or haven’t applied for the EU Settlement Scheme and been granted pre-settled or settled status – please contact the College Registrar on 01934 411 531 for advice on appropriate UKVI student visa fees before you enrol.
Full refunds will be payable if we need to cancel a course.
Tuition fee refunds are payable if you change your mind within 14 days of enrolling on your course and before the start of the course (10% of tuition fee or £25 administration fee whichever is larger will be charged).
If you change your mind about a course before it starts or during the first three weeks, we will happily transfer you to an alternative course - you may need to pay any difference in fees or have a credit note issued to you if the course is cheaper.
If you wish to withdraw from a course you can request a credit note for the remaining proportion of the tuition fees to a maximum of 50% of tuition fees paid (a £25 administration fee will be charged). Credit notes are valid for one calendar year.
There are different terms and conditions for Higher Education courses - please call 01934 411 409 for further details.
Discretionary Learner Support Fund
This is a College-administered fund which can support some costs associated with attending qualification courses. It is mean-tested against gross household income and limited in its amount per learner. For further information please contact Student Services on 01934 411 571.
Got a question?
Whatever you'd like to know, we're here to help...
Register your interest
Marketing Permissions
We will contact you when this course becomes available, we would also love to keep you informed of other/similar courses you may be interested in. You can unsubscribe at any time. Your personal information will be processed in line with our privacy notice available on our policy page . Is this ok (please tick)?
By submitting this form you are consenting to Weston College recording and processing the personal information on this form, for any purposes of the college associated with the provision of advice and guidance to potential students on the range of courses available to them, college facilities and any other associated information. In accordance with the Data Protection Act 2018, the information will only be used for the above mentioned purposes.
You will need to complete a full enrolment form prior to starting your course.
Apply or find out more
Once we have your booking we would love to keep you informed of other, similar events you may be interested in. You can unsubscribe at any time. Your personal information will be processed in line with our privacy notice available on our policy page . Is this ok (please tick)?

A .gov website belongs to an official government organization in the United States.
A lock ( ) or https:// means you've safely connected to the .gov website. Share sensitive information only on official, secure websites.
- Test for Fentanyl
- if You Think Someone is Overdosing
- Stop Overdose
- Naloxone FAQs
- Stigma Reduction
About Stop Overdose
- Through preliminary research and strategic workshops, CDC identified four areas of focus to address the evolving drug overdose crisis.
- Stop Overdose resources speak to the reality of drug use, provide practical ways to prevent overdoses, educate about the risks of illegal drug use, and show ways to get help.
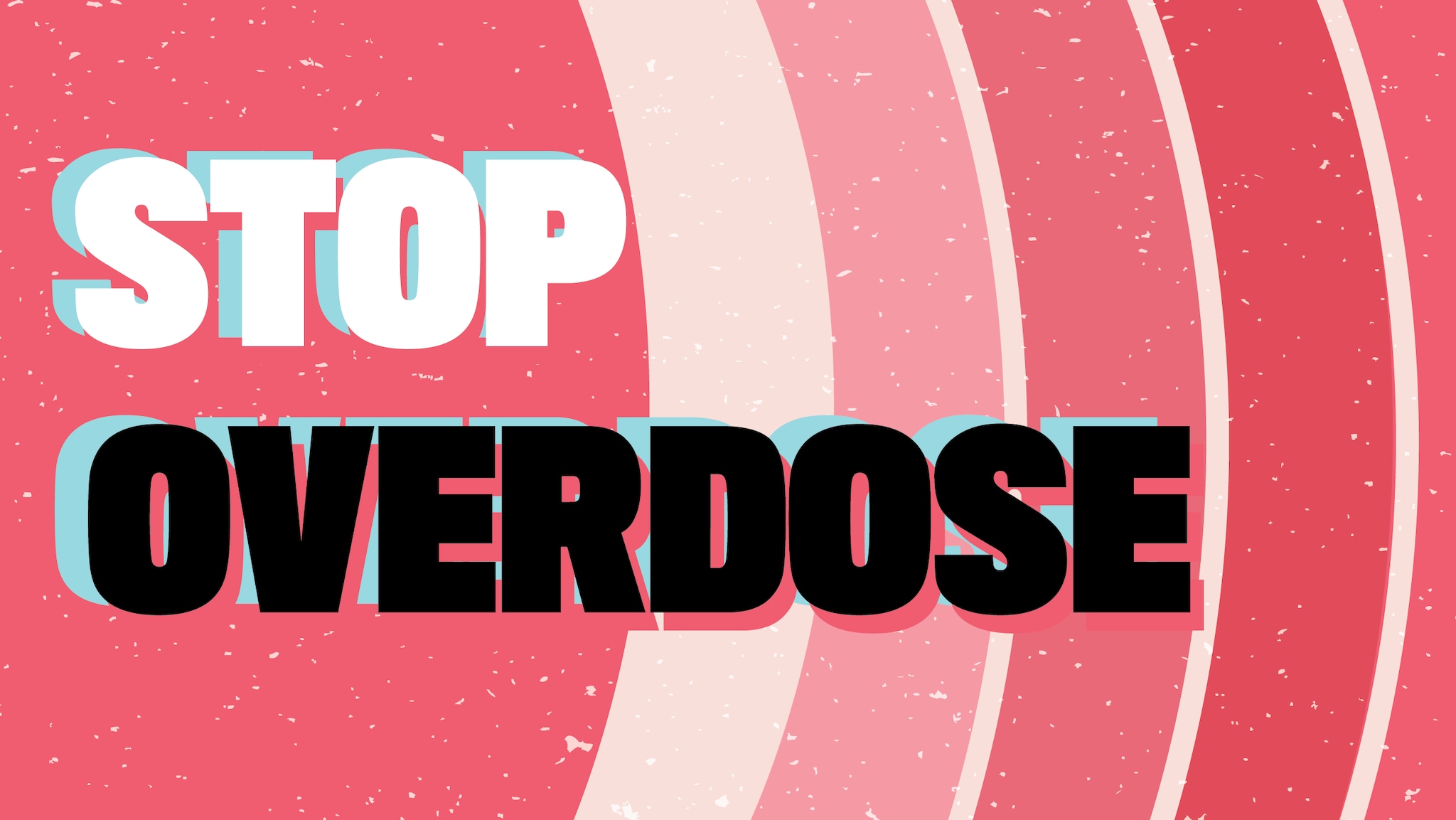
Drugs take nearly 300 lives every day. 1 To address the increasing number of overdose deaths related to both prescription opioids and illegal drugs, we created a website to educate people who use drugs about the dangers of illegally manufactured fentanyl, the risks and consequences of mixing drugs, the lifesaving power of naloxone, and the importance of reducing stigma around recovery and treatment options. Together, we can stop drug overdoses and save lives.
What you can do
- Get the facts on fentanyl
- Learn about lifesaving naloxone
- Understand the risks of polysubstance use
- Reduce stigma around recovery and treatment
Explore and download Stop Overdose and other educational materials on CDC's Overdose Resource Exchange .
- Centers for Disease Control and Prevention, National Center for Health Statistics. National Vital Statistics System, Mortality 2018-2021 on CDC WONDER Online Database, released in 2023. Data are from the Multiple Cause of Death Files, 2018-2021, as compiled from data provided by the 57 vital statistics jurisdictions through the Vital Statistics Cooperative Program. Accessed at http://wonder.cdc.gov/mcd-icd10-expanded.html on Mar 5, 2024
Every day, drugs claim hundreds of lives. The Stop Overdose website educates drug users on fentanyl, naloxone, polysubstance use, and dealing with stigma.

IMAGES
VIDEO
COMMENTS
A bibliometric review of the literature reported strong growth in the number of published empirical health research studies with public involvement. 3 In a systematic review of the impact of PPI on health and social care research, Brett et al 4 reported positive impacts at all stages of research from planning and undertaking the study to ...
Background Identifying determinants of health and understanding their role in health production constitutes an important research theme. We aimed to document the state of recent multi-country research on this theme in the literature. Methods We followed the PRISMA-ScR guidelines to systematically identify, triage and review literature (January 2013—July 2019). We searched for studies that ...
Background In this methodological paper we document the interpretation of a mixed methods study and outline an approach to dealing with apparent discrepancies between qualitative and quantitative research data in a pilot study evaluating whether welfare rights advice has an impact on health and social outcomes among a population aged 60 and over. Methods Quantitative and qualitative data were ...
KA is supported by Health Data Research (HDR) UK, the UK National Institute for Health Research (NIHR) Applied Research Collaboration East Midlands (ARC EM), and as a NIHR Senior Investigator Emeritus (NF-SI-0512-10159). The views expressed are those of the author(s) and not necessarily those of the NIHR or the Department of Health and Social Care.
Chronic diseases have an impact on and change patients' lives, and the way they experience their bodies alters. Patients may struggle with identity and self-esteem, a shrinking lifeworld and a challenging reality. 1 The chronic diseases become part of the patients' lives, whether they affect their physical health and functions, autonomy, freedom and identity, or threaten their life. 2 The ...
Abstract. Quantitative research is the foundation for evidence-based global health practice and interventions. Preparing health research starts with a clear research question to initiate the study, careful planning using sound methodology as well as the development and management of the capacity and resources to complete the whole research cycle.
This is the first textbook to show how research using a range of qualitative and quantitative methods relates to improving health and social care practice. The book shows how different research approaches are undertaken in practice and the challenges and strengths of different methodologies, thus facilitating students to make informed decisions ...
Reformulating computational social science with citizen social science: the case of a community-based mental health care research Article Open access 04 March 2023
The movement towards blending qualitative and quantitative methods in health services research is now well established. Models for blending the two include a hierarchical approach, in which one or other approach dominates, and a partnership model featuring more equal (albeit differing) roles for each.
The use of research questionnaires or surveys in nursing is a long standing tradition, dating back to the 1960s (Logan, 1966) and 1970s (Oberst, 1978), when the scientific discipline emerged.This type of tool enables nursing researchers to gather primary data from a specific population, whether it is patients, carers, nurses, or other stakeholders to address gaps in the existing evidence base ...
Editorial: Detailed guidelines for reporting quantitative research in Health & Social Care in the Community
Health and social care; Public health; ... Black, T R (1999): 'Doing quantitative research in the social sciences: an integrated approach to research design, measurement and statistics'. Sage ...
Quantitative research methods focus on variables that can be measured numerically and analyzed using statistical techniques [1,2,3].The primary advantage of this approach is its ability to investigate relationships between variables within a sample that can then be inferred to a larger population of interest while more consistently controlling for threats to validity.
Quantitative research objectives can be to establish the incidence or prevalence of a health problem; the health personnel degree of adherence to a new intervention; or, the users' level of ...
Quantitative research methods are often used in public health research and evaluation to determine the needs of a community or population, examine associations among multiple factors, and compare outcomes across subpopulations based on demographic characteristics (e.g., age, gender, race, education, income). Quantitative data and analysis can ...
Quantitative analysis refers to the process of using complex mathematical or statistical modeling to make sense of data and potentially to predict behavior. Though quantitative analysis is well-established in the fields of economics and finance, cutting-edge quantitative analysis has only recently become possible in health care.
It is just as applicable to clinical trials as it is to health services research, enabling an in depth understanding of the problem that quantitative research alone cannot deliver. 1 It is imperative, however, that qualitative studies are well-designed with pre-determined methods to ensure reduction in research bias. While quantitative research ...
Health and social care research is governed by a range of laws, policies, and international, national and professional standards. The Health Research Authority (HRA) is responsible for ensuring such regulation is co-ordinated and standardised across the UK to make it easier to do research that people can trust. The HRA is an executive non ...
The Journal of Clinical Nursing publishes research and developments relevant to all areas of nursing practice- community, geriatric, mental health, pediatric & more. Abstract Aims To identify the multiple mediating effects of resilience and depression between social support and self-care ability among patients with breast cancer during ...
Strengthening the evidence base and research capacity on public health and social measures (PHSM) The unprecedented implementation of PHSM during the COVID-19 pandemic has exposed knowledge gaps about the effectiveness of PHSM, factors influencing people's acceptance of and adherence to measures and their unintended health and socio-economic consequences.
Background Postpartum contraception is essential to sexual and reproductive health (SRH) care because it encourages healthy spacing between births, helps women avoid unwanted pregnancies, and lessens the risks of health problems for mothers and babies. Sub-Saharan African immigrant and refugee populations are rapidly increasing in the United States, and they come from a wide range of cultural ...
Research and Referencing: students will learn about research methods used in medical and healthcare fields, including qualitative and quantitative research. Study Skills: This module covers communication skills that are essential for success in the social sciences, including verbal and written communication, presentation skills, and teamwork.
Through preliminary research and strategic workshops, CDC identified four areas of focus to address the evolving drug overdose crisis. ... National Center for Health Statistics. National Vital Statistics System, Mortality 2018-2021 on CDC WONDER Online Database, released in 2023. Data are from the Multiple Cause of Death Files, 2018-2021, as ...
Get daily stock ideas from top-performing Wall Street analysts. Get short term trading ideas from the MarketBeat Idea Engine. View which stocks are hot on social media with MarketBeat's trending stocks report. Advanced Stock Screeners and Research Tools. Identify stocks that meet your criteria using seven unique stock screeners.