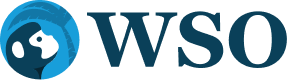
- Recently Active
- Top Discussions
- Best Content

By Industry
- Investment Banking
- Private Equity
- Hedge Funds
- Real Estate
- Venture Capital
- Asset Management
- Equity Research
- Investing, Markets Forum
- Business School
- Fashion Advice
- Financial Modeling Resources
Arises when a financial model measures any company's quantitative information, such as its market risk or Value of transactions
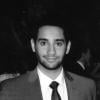
Prior to becoming a Founder for Curiocity, Hassan worked for Houlihan Lokey as an Investment Banking Analyst focusing on sellside and buyside M&A , restructurings, financings and strategic advisory engagements across industry groups.
Hassan holds a BS from the University of Pennsylvania in Economics.
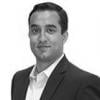
Osman started his career as an investment banking analyst at Thomas Weisel Partners where he spent just over two years before moving into a growth equity investing role at Scale Venture Partners , focused on technology. He's currently a VP at KCK Group, the private equity arm of a middle eastern family office. Osman has a generalist industry focus on lower middle market growth equity and buyout transactions.
Osman holds a Bachelor of Science in Computer Science from the University of Southern California and a Master of Business Administration with concentrations in Finance, Entrepreneurship, and Economics from the University of Chicago Booth School of Business.
- What Is Model Risk?
- Sources Of Model Risk
- Types Of Model Risk
- Quantitative Approaches In Model Risk
- Model Risk Management (MRM) Framework
- Processes In The Lifecycle Of MRM Framework
- Examples Of Model Risks
What is Model Risk?
Model risk arises when a financial model measures any company's quantitative information, such as its market risk or Value of transactions. As a result, the model fails or performs poorly, resulting in adverse effects for the company.
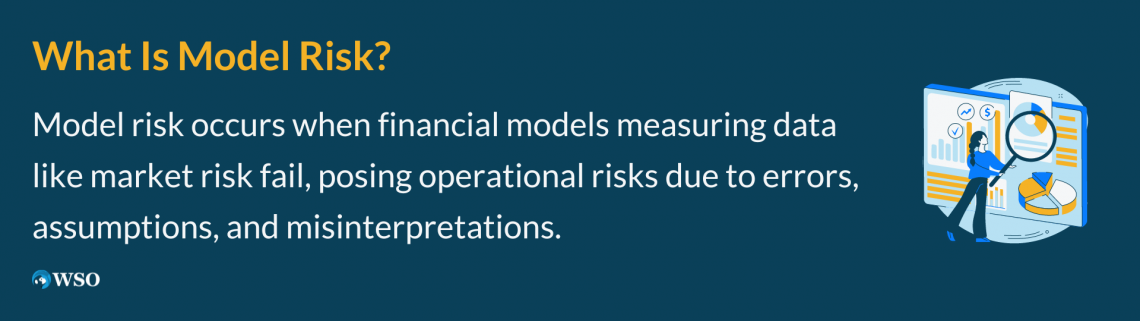
A model is a system, quantitative method, or approach based on
- Statistical
- Mathematical
- Financial techniques and theoretical assumptions
Any model-derived process data inputs into a structured output. Financial institutions and investors usually use models to determine the theoretical Value of stock prices and identify trading opportunities.
While models can be valuable tools in investment analysis, they are also subject to various risks that can arise from incorrect data use, programming, technical, and errors resulting in misinterpretation of model results.
Model risk is considered a subset of operational risk because it primarily affects the business that creates and uses the model.
Traders or other investors using a given model may not fully understand its assumptions and limitations, which limits the usefulness and application of the model itself.
In financial firms, pattern risk can affect the outcome of a prudential valuation , but it is also a factor in other industries. For example, a model may not accurately predict the likelihood that an airline passenger is a terrorist or the possibility of a fraudulent credit card transaction.
It can be due to incorrect assumptions, programming or technical errors, and other factors that increase the risk of a bad outcome.
Key Takeaways
- Model risk refers to the potential adverse effects that arise when financial models fail or perform poorly in measuring quantitative information for a company, such as market risk or transaction value.
- A Model Risk Management (MRM) framework should include regulatory-compliant minimum risk management standards, a clear statement of the Board's modeling risk appetite, and a risk identification process.
- Model risk can manifest in three main forms: specification risk, implementation risks, and application risk.
Sources of Model Risk
It can originate from different sources. A primary source is the incorrect model specification, which can manifest as missing risk factors, misidentification of the model's underlying stochastic processes, or a lack of knowledge regarding the crucial variables.
Incorrect model applications frequently result in this risk. It can be because you either use the wrong model for the problem or employ a model that is no longer the recommended practice. Another type of risk metric is the implementation risk.
Let's discuss the significant sources briefly:
The data used in a model may be inaccurate, incomplete, or distorted. Nevertheless, it is essential for developing an effective model; inaccurate data can affect the whole model.
2. Model Execution
Incorrect or incomplete model implementation can lead to erroneous results, negatively affecting model outcomes and the organization's decision-making.
3. Inconsistency over time
Bruschi and Corelli formalize the concept of "time inconsistency" for non- arbitrage models that allow for a perfect fit to the term structure of interest rates.
In these models, the current yield curve is the input so that new observations on the yield curve can be used to update the model at regular intervals. In addition, they explore the question of time-appropriate and self-funding strategies in this model.
Risk modeling affects the three main phases of risk management: specification, estimation, and implementation.
4. Uncertain correlation
Trading options that depend on the performance of a basket, known as a rainbow option, are more susceptible to model uncertainty than index options, according to Cont and Digest.
Uncertainty in correlation parameters is another vital source of model risk for multi-asset derivatives.
Gennheimer argues that investors must be very confident of the dependency structure governing the basket of assets.
Default products should calculate prices according to alternative copula specifications and check their simulation error estimates for the least amount of model risk they incur.
5. Parameters and assumptions:
Bruschi and Corelli formalize the concept of "time inconsistency" for non-arbitrage models that allow for a perfect fit to the term structure of interest rates.
6. Complication
The complexity of a financial model or contract can be a source of risk metrics, leading to poor identification of its risk factors. For example, this factor was considered a significant source of risk for mortgage -backed securities portfolios during the 2007 crisis.
7. Liquidity and risk metrics
Risk metrics do not exist only for complex financial contracts. Frey (2000) presents a study on how illiquidity is marketed as a source of model risk.
Convertible, mortgage-backed, and high-yield bonds can often be illiquid and difficult to Value.
Types of Model Risk
We define model risk as the risk that a model will be misdefined, improperly implemented, or used inappropriately.
Consider the swap assignment.
- A financial engineer can use economic theory to develop a model.
- A programmer can execute the model as a computer program.
- A trader can use this implementation to price a swap.
In this example, there is a risk that the financial engineer specifies the wrong model, that the programmer may implement the model incorrectly, or that the trader uses it in a way that is not intended, possibly by pricing a non-standard swap for which the model does not fit.
Here we have three types of risk metrics:
- Type A: model specification risk,
- Type B: model implementation risks
- Type C: model application risk.
Each quantitative model has three components:
To evaluate such risk, we need to assess the probability for each type of model risk in each component of the model.
Type A: Model Specification Risk
Model risk is the risk that the model is not correctly specified. The question is not whether the model is "correct" but whether it is "useful."
All meaningful models make predictions. A well-specified model makes generally useful predictions when properly implemented and used. It is true regardless of whether the model is used for earthquake forecasting, weather forecasting, or market risk assessment.
The risk of model specifications arises primarily when the model is designed. For example, if the inputs are operationally defined, mathematical calculations and outputs are specified, and everything is preferably done in a formal design document.
Inadvertent mistakes, such as formula typing errors, are always a problem. Still, the more common problem is that certain combinations of inputs and calculations can produce misleading or useless results.
Experienced modelers can review design documentation and provide estimates of model performance, but the final test is to implement the model and evaluate its performance empirically.
Model specification errors also occur after the model is designed. Finally, the model itself is subject to change from time to time, perhaps to address new products being traded, to address issues that are perceived in its performance, or to catch up with industry practices.
It can also occur without apparent changes to the Value of at-risk measurements. For example, historical values for a particularly crucial factor can be obtained from a particular time series maintained by the data provider.
How data providers calculate this time-series value or simple quality control can adversely affect the performance of value-at-risk measurements.
Type B: Model Implementation Risks
Model implementation risk is when the model deviates from what is specified in the design document during model implementation. For example, inputs may vary when historical data for essential elements comes from sources other than those specified in the design document.
The formula may be different. For example, it may be due to a simple typing error. Alternatively, the programmer may implement the procedure in a way that accidentally modifies it.
Results can be misrepresented. The two numbers may appear side by side in the risk report, or the results may be confusing or misleading.
Implementation errors can cause an excellent model to be implemented as a wrong model, but that's only one part of the problem.
If the implemented model deviates from its design, whether it works or not, it is not what the designer or user believes. They think there is one model when there is another, due to which the results may be unpredictable.
Implementation errors are usually due to human error. Coding and logic errors are almost inevitable in large software projects. Implementation risks also arise if a rogue programmer decides on a trade-off.
Unfortunately, such errors do occur, but they are not common in large-scale value-at-risk implementations, which are subject to rigorous testing and validation.
Type C: Model Application Risk
Model application risk is the risk that the model will be misinterpreted or misused. Misunderstandings can lead to false assurance and adverse actions.
Modelers tend to have different backgrounds than traders and senior management. Model designers understand the meaning of the output at a technical level, but users may find it less accurate and more intuitive.
Modelers generally understand the reliability of the model's results better than users who may have unrealistic expectations. If the user doesn't like what the model is saying, it is more likely that user emotions will cloud the evaluation of the model's Value.
A common misconception about Value at risk is that it is the most significant loss. However, in the scheme of things, the loss quantile is more straightforward to grasp than other PMMRs, such as B—the standard deviation of expected tail loss or return.
A common form of model application risk is to use a value-at-risk indicator that is otherwise inadequate for the portfolio.
It can happen as an organization's trading activities evolve, but its risk value measurements have not been updated to reflect new products or trading strategies.
Alternatively, the model's environment may evolve, making its predictions useless. The model may be specified appropriately for the old domain but is irrelevant when applied to the new environment.
For example, value-at-risk indicators must consider critical factors associated with liquid and actively traded commodities. But the market is evolving, and liquidity can be exhausted.
Quantitative Approaches in Model Risk
They are techniques that help quantify the modeling risk inherent in each source, using a methodology based on the sensitivity of the model output to potential variations that characterize the uncertainty of the head.
Risk mitigation models are identified and quantified by applying appropriate measures, depending on the nature of each source.
Even when modeling risk cannot be eliminated, an approach combining a rigorous risk management structure with the sensitive and detailed quantification described can be an effective strategy to reduce risk.
Let's discuss some fundamental quantitative approaches to mitigating these risks:
The model mean and worst-case approach
Rantala (2006) mentions, "When faced with model risk, instead of basing decisions on a single selected 'best' model, modelers can base their inferences on a complete set of models using the mean of the models." This approach avoids the "default of the mean."
Another approach to model risk is the worst-case approach, or maximum approach, advocated by Gilboa and Schmeidler in decision theory. This approach considers a series of models, minimizing the worst-case loss.
Jokhadze and Schmidt (2018) propose risk measurement models using the Bayesian method. They introduce layered risk measures that combine risk metrics and enable consistent model and market risk management.
In addition, they provide the hypothesis of these risk measures and identify some real-world examples of overlapping risk metric measures in the context of financial risk management and potential loss pricing.
Quantify the model's level of risk
Measure the risk posed by a model. It must be compared with an alternative model or a set of alternative reference models. The question is how to choose these reference models.
In the context of derivatives valuation , Cont (2006) offers a quantitative approach to measuring the model risk of derivative portfolios, where a set of reference models are specified and adjusted to the market price of the liquid product model.
Risk measurements arise from the difference between the current portfolio and worst-case valuation within the benchmark model framework. In addition, such indicators can determine the risk metrics reserved for derivative portfolios .
Position limits and valuation reserves
Jokhadze and Schmidt (2018) introduce financial market risk measures that cover the loss of risk metrics.
Their methodology harmonizes market and risk management, allowing them to define risk position limits and capital requirements. Kato and Yoshiba discuss qualitative and quantitative methods for managing risk metrics.
They write: "From a quantitative point of view, you can create a reserve in the price model to explain the difference in estimation from the alternative model. Cont (2006) advocates using risk metrics to calculate such accounts.
The risk measurement model provides scenario analysis for various volatility patterns. You can do this. You can set risk factors or location limits based on the information obtained from the scenario analysis.
Model Risk Management Framework
Model-based risk management aims to identify and reduce the potential negative consequences of decisions based on incorrect or misused models. This risk management uses techniques and practices to identify, measure, and reduce risk metrics, i.e., the possibility of model failure or misuse.
In finance, it is the risk of loss resulting from using models that are not accurate enough for decision-making.
It is becoming more common in financial services sectors, which include consumer credit scoring, predicting real-time probabilities of fraudulent credit card transactions, and money laundering .
Financial institutions rely heavily on credit, market, and behavioral models because modeling risk has become central to risk management and performance.
These institutions make money by taking risks - they leverage models to assess risk, understand client behavior, evaluate capital adequacy , make investment decisions, and manage data risk analysis.
Implementing a practical model-based risk management framework is a prerequisite for organizations that rely heavily on quantitative models for operations and decision-making.
Processes in the Lifecycle of MRM Framework
A good Model Risk Management (MRM) framework should be designed around industry best practices and in compliance with regulatory guidelines.
The authority for comparing MRM frameworks is the US Federal Reserve 's Supervision Guidelines for Model Risk Management (SR 11-07).
An organization must create a framework for managing risk metrics to model risk effectively. The MRM Framework shows how to manage modeling risk.
Regardless of the size and structure of the organization, regulators require that business model risk management frameworks cover all essential aspects of the MRM lifecycle with roles and responsibilities assigned.
Like other generic risk management frameworks, modeled risk management provides a comprehensive explanation of the four pillars below:
1. Risk modeling standards
Minimum standards must have come for model development and must be followed and adhered to. Internal standards should be at the same level or higher than regulatory standards, such as the Model Risk Management Monitoring Guide (SR 11-07).
These standards should include data quality, model modification, model usage, expert judgment, model methodology, model validation, documentation, model data external, and model reporting, among other standards.
Risk appetite pattern: After establishing the risk policy, it is prudent that the board's statement of the MR appetite is clearly articulated to manage it effectively. Risk appetite is the amount of risk an organization is prepared for and can accept to achieve its desired objectives.
The model's risk preference for risk will depend on the objective to which the model is applied. The most express the model's risk appetite in terms of risk tolerance and related metrics such as overall quantitative risk level, number of high-risk models, etc.
2. Risk Metrics Determination
It is necessary to identify the specific risks affecting the organization. An inventory of existing models should be conducted to determine fundamental model changes. Model inventory should categorize features as follows (among others):
- Describe the purpose of the model
- How to use the model
- How often to use it
- Assumptions or inputs of the model
3. Model risk assessment and measurement
It should perform quantitative and qualitative risk assessments to assess each model's risk. Both approaches will lead to an enterprise-wide risk assessment framework.
The risk quantifiers use different modeling risk measures, or they may use operational risk modeling approaches. There are three main techniques for quantifying risk, namely:
- Sensitivity Analysis - Changes in the model's assumptions and parameters and monitoring the evolution of the results
- Backtesting - Test the model using historical data and compare outputs with past results
- Challenge Model - Compare the results of one model with the results of another alternative model using the same data
The quantitative assessment will measure and aggregate each quantitative risk assessment separately through appropriate correlation factors. Qualitative risk assessment involves looking at the model's fit to the objective.
The results will indicate how robust the model is, impacting the model's risk rating.
The qualitative assessment uses qualitative measures to measure risk in a model - including model fit to standards, cumulative model error, degree of risk assessment, model risk, and other qualitative factors.
4. Risk Mitigation Model
Possible risk reduction strategies may include the following:
- Changes during model development.
- Conduct additional validation of the model, taking into account changes in the nature and structure of existing risks and the emergence of new threats the organization faces.
- Use independent expert judgment to interpret model results due to model uncertainty.
- The Compliance models and applicability to new risk regulations.
- Measures to improve model efficiency and applicability to reduce risk, such as additional capital, can help mitigate risk.
5. Risk monitoring and reporting model
The model's risk monitoring and reporting functionality aim to identify the following issues:
- Monitor whether the policy follows the risk metrics policy and risk appetite. The process will recommend whether management intervention is needed in the event of a discrepancy.
- A material model inventory should be performed on each model to measure whether the MRM policy framework is using the model.
- The model's risk assessment and validation results should be analyzed, and corrective actions should be taken for any identified weaknesses.
- An overview of new trends in modeling risk management and other related topics.
Examples of Model Risks
Models can fail due to technical errors, such as a lack of mathematical rigor, data problems, and implementation errors. These types of model-risk failures can be considered part of operational risk.
However, the models produce inaccurate results simply because of the inherent uncertainty associated with the mathematical model. Of course, this is a feature of modeling, but failure to properly recognize this level of uncertainty is a major element of risk metrics.
Failure of a model can result in financial loss, regulatory or legal penalties, or damage to an organization's reputation due to the use of a model that contains data or assumptions that do not fit that model.
Some examples of model risk failures witnessed over the past years are as follows:
1. Long-term capital management
Long-term capital management (LTCM) failure in 1998 was attributed to risk metrics. In this case, a small error in the company's computer models was magnified by some large orders due to the high-leverage trading strategy used by LTCM.
At its peak, the hedge fund managed more than $100 billion in assets and posted annual returns of over 40%.
LTCM had two economics Nobel laureates as major shareholders, but the company collapsed due to its financial model failing in this market environment.
2. JPMorgan Chase
JPMorgan Chase ( JPM ) suffered massive trading losses from its Value at Risk ( VaR ) model that contained formulas and operational errors. Risk managers use VaR models to estimate a portfolio's future losses.
In 2012, CEO Jamie Dimon declared a "storm in the teapot" that turned out to be a $6.2 billion loss from erroneous transactions in his General Credit Portfolio (SCP). As a result, a trader established prominent derivative locations indicated by the VaR pattern.
The bank's chief investment officer responded by adjusting the VaR model. However, owing to a spreadsheet flaw in the program, trading losses might accrue without notice from the model.
It is not the first time that VaR models have failed. For example, in 2007 and 2008, the VaR model was criticized for failing to predict many banks' significant losses during the global financial crisis .
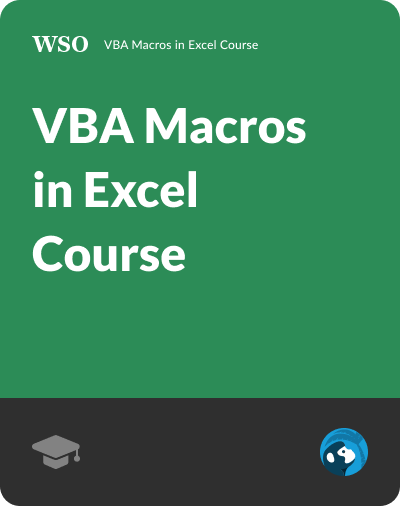
Everything You Need To Master Financial Statement Modeling
To Help you Thrive in the Most Prestigious Jobs on Wall Street.
Researched and authored by Kavya Sharma | Linkedin
Reviewed and edited by Priyansh Singal
Free Resources
- Discount Factor
- 3 Statement Model
- LBO Modeling
- Payback Period
- Scenario Analysis
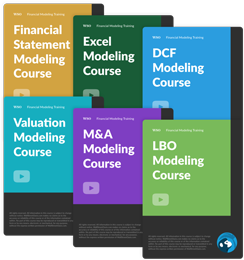
Get instant access to lessons taught by experienced private equity pros and bulge bracket investment bankers including financial statement modeling, DCF, M&A, LBO, Comps and Excel Modeling.
or Want to Sign up with your social account?
The evolution of model risk management
The number of models is rising dramatically—10 to 25 percent annually at large institutions—as banks utilize models for an ever-widening scope of decision making. More complex models are being created with advanced-analytics techniques, such as machine learning, to achieve higher performance standards. A typical large bank can now expect the number of models included within its model risk management (MRM) framework to continue to increase substantially.
Among the model types that are proliferating are those designed to meet regulatory requirements, such as capital provisioning and stress testing . But importantly, many of the new models are designed to achieve business needs, including pricing, strategic planning, and asset-liquidity management. Big data and advanced analytics are opening new areas for more sophisticated models—such as customer relationship management or anti-money laundering and fraud detection.
Insights from benchmarking and MRM best practices
Model risk management (MRM) was addressed as a top-of-mind concern by leading global banks in recent surveys and roundtables conducted in Europe and the United States by McKinsey and Risk Dynamics. The overall number of models varied widely, ranging from 100 to 3,000 per bank; the number of full-time equivalents (FTEs) dedicated to MRM and validation is also highly variable, with European banks dedicating an average of 8 FTEs per €100 billion of assets, while for US banks this average is 19. MRM groups have grown considerably in recent years, and that growth is expected to continue. Most banks said they still rely heavily on the support of external consultants for validation. The time period for validation varies, depending on model intensity. For European banks, model validation can take anywhere from a few days to 30 weeks, whereas in the United States, we found that variation takes between one and 17 weeks. For both US and EU banks, pass/fail rates vary widely by model. The scope of MRM activities varies widely as well, especially for ongoing model monitoring and model implementation. With respect to governance, most of the MRM groups report directly to the chief risk officer (CRO), or to his or her direct report; the boards of these banks typically discuss MRM in at least six meetings per bank.
In probing the model risk management terrain more closely, our research identified important trends and defined a model life cycle, from planning and development through model use, risk appetite, and policies. 1 1. The research was performed by McKinsey Risk Dynamics, which specializes in model risk and validation. Our research also revealed the key questions on the agenda of chief risk officers (exhibit), and the extent to which these questions are being addressed in some of the most important areas.
Model planning and development
Model planning should be well coordinated across the whole bank. While taking great care to maintain the independence of validation, the model-development group should work closely with validation, an approach that controls costs by reducing the number of iterations and overall development time.
Banks are increasingly centralizing model planning and development, with best-practice institutions setting up “centers of excellence”—advanced-analytics centers acting as service providers to business units. They have created three location models: a local model with the bulk of the work close to model owners, each of them with dedicated teams; a hybrid model; and a centralized model, with the bulk of the work performed in the dedicated corporate center.
As talent demands rise, the highly specialized skills needed to develop and validate models are becoming increasingly scarce. Nearly three-quarters of banks said they are understaffed in MRM, so the importance of adjusting the model risk function to favor talent acquisition and retention has become pronounced. Banks are now developing talent solutions combining flexible and scalable resourcing with an outsourcing component.
Best-practice institutions are classifying models (model “tiering”) using a combination of quantitative and qualitative criteria, including materiality and risk exposure (potential financial loss), and regulatory impact. Models are typically prioritized for validation based on complexity and risk associated with model failure or misuse. Model risk is defined according to potential impact (materiality), uncertainty of model parameters, and what the model is used for. The level of validation is located along a continuum, with high-risk models prioritized for full validation and models of low risk assigned light validation. In the majority of banks we surveyed, validation is highly centralized and situated in the risk organization. Outsourcing is increasing at both European and US institutions, as a result of talent constraints.
Most US banks have strengthened the independence of validation, with the head reporting directly to the CRO. In the United States, material models have to be validated in great detail, with systematic replication and the use of challenger models. This approach is not uniformly applied in Europe, where “conceptual” validations are still accepted in many cases. Likewise, model implementation (in operational and production systems) is not validated consistently across EU banks.
Control and monitoring
In the United States, the Federal Reserve is strict about proper deployment of the three lines of defense, with all stakeholders playing their roles: model developers need to continuously monitor their models; validation must make periodic reviews and audits, relying on the right level of rigor and skills. In Europe, implementation of the three lines remains less defined. The regulatory focus is mainly on regulatory models, as opposed to the US approach, where proper control is expected for all material models, whatever their type. Consequently, in the European Union, few banks have a control and governance unit in charge of MRM policies and appetite; in the United States, nearly all banks have an MRM unit.
Model use, risk appetite, and policies
In accordance with best practices, approximately half the surveyed banks have integrated model risk within their risk-appetite statement, either as a separate element or within nonfinancial risks. Only around 20 percent, however, use specific key performance indicators for model risk, mainly based on model performance and open validation findings on models.
All banks have a model governance framework in place, but 60 percent of the group uses it for the main models only (such as internal ratings based or stress testing). Half of the survey group has a model risk policy. For 60 percent of the group, model ownership is held by users, representing the preferred option for institutions that are more advanced in model management, allowing a better engagement of business on data and modeling assumptions. Risk committees authorize model-use exceptions in around 70 percent of cases.
The promise and wider application of models have brought into focus the need for an efficient MRM function, to ensure the development and validation of high-quality models across the whole organization—eventually beyond risk itself. Financial institutions have already invested millions in developing and deploying sophisticated MRM frameworks. In analyzing these investments, we have discovered the ways that MRM is evolving and the best practices for building a systematically value-based MRM function (see sidebar, “Insights from benchmarking and MRM best practices”). This article summarizes our findings.
Model risk and regulatory scrutiny
The stakes in managing model risk have never been higher. When things go wrong, consequences can be severe. With digitization and automation, more models are being integrated into business processes, exposing institutions to greater model risk and consequent operational losses. The risk lies equally in defective models and model misuse. A defective model caused one leading financial institution to suffer losses of several hundred million dollars when a coding error distorted the flow of information from the risk model to the portfolio-optimization process. Incorrect use of models can cause as much (or greater) harm. A global bank misused a risk-hedging tool in a highly aggressive manner and, as a result, passed its value-at-risk limits for nearly a week. The bank eventually detected the risk, but because the risk model it used was inadequately governed and validated, it only adjusted control parameters rather than change its investment strategy. The consequent loss ran into the billions. Another global bank was found in violation of European banking rules and fined hundreds of millions of dollars after it misused a calculation model for counterparty-risk capital requirements.
Stay current on your favorite topics
Events like these at top institutions have focused financial-industry attention on model risk. Supervisors on both sides of the Atlantic decided that additional controls were needed and began applying specific requirements for model risk management on banks and insurers. In April 2011, the US Board of Governors of the Federal Reserve System published the Supervisory Guidance on Model Risk Management (SR 11-7). This document provided an early definition of model risk that subsequently became standard in the industry: “The use of models invariably presents model risk, which is the potential for adverse consequences from decisions based on incorrect or misused model outputs and reports.” SR 11-7 explicitly addresses incorrect model outputs, taking account of all errors at any point from design through implementation. It also requires that decision makers understand the limitations of a model and avoid using it in ways inconsistent with the original intent. The European Banking Authority’s Supervisory Review and Evaluation Process , meanwhile, requires that model risk be identified, mapped, tested, and reviewed. Model risk is assessed as a material risk to capital, and institutions are asked to quantify it accordingly. If the institution is unable to calculate capital needs for a specific risk, then a comprehensible lump-sum buffer must be fixed.
The potential value in mature MRM
The value of sophisticated MRM extends well beyond the satisfaction of regulatory regimes. But how can banks ensure that their MRM frameworks are capturing this value thoroughly? To find the answer, we must first look more closely at the value at stake. Effective MRM can improve an institution’s earnings through cost reduction, loss avoidance, and capital improvement. Cost reduction and loss avoidance come mainly from increased operational and process efficiency in model development and validation, including the elimination of defective models.
Capital improvement comes mainly from the reduction of undue capital buffers and add-ons. When supervisors feel an institution’s MRM is inadequate, they request add-ons. An improved MRM function that puts regulators in a more comfortable position leads to a reduction of these penalties. (The benefit is similar to remediation for noncompliance.) Capital inefficiency is also the result of excessive modeler conservatism. To deal with uncertainty, modelers tend to make conservative assumptions at different points in the models. The assumptions and attending conservatism are often implicit and not well documented or justified. The opacity leads to haphazard application of conservatism across several components of the model and can be costly. Good MRM and proper validation increases model transparency (on model uncertainties and related assumptions) and allows for better judgments from senior management on where and how much conservatism is needed.
Would you like to learn more about our Risk Practice ?
This approach typically leads to the levels of conservatism being presented explicitly, at precise and well-defined locations in models, in the form of overlays subject to management oversight. As a result, the total level of conservatism is usually reduced, as end users better understand model uncertainties and the dynamics of model outcomes. They can then more clearly define the most relevant mitigation strategies, including revisions of policies governing model use.
Profit and loss
With respect to improvement in profit and loss (P&L), MRM reduces rising modeling costs, addressing fragmented model ownership and processes caused by high numbers of complex models. This can save millions. At one global bank, the capital budget for models increased sevenfold in four years, rising from €7 million to €51 million. By gaining a better understanding of the model landscape, banks are able to align model investments with business risks and priorities. By reducing model risk and managing its impact, MRM can also reduce some P&L volatility. The overall effect heightens model transparency and institutional risk culture. The resources released by cost reductions can then be reallocated to high-priority decision-making models.
Systematic cost reduction can only be achieved with an end-to-end approach to MRM. Such an approach seeks to optimize and automate key modeling processes, which can reduce model-related costs by 20 to 30 percent. To take one example, banks are increasingly seeking to manage the model-validation budget, which has been rising because of larger model inventories, increasing quality and consistency requirements, and higher talent costs. A pathway has been found in the industrialization of validation processes, which use lean fundamentals and an optimized model-validation approach.
- Prioritization (savings: 30 percent). Models for validation are prioritized based on factors such as their importance in business decisions. Validation intensity is customized by model tiers to improve speed and efficiency. Likewise, model tiers are used to define the resource strategy and governance approach.
- Portfolio-management office and supporting tools (savings: 25 percent). Inefficiency can be reduced at each stage of the validation process, with predefined processes, tools, and governance mechanisms. These include development and submission standards as well as validation plans and playbooks.
- Testing and coding (savings: 25 percent). Automation of well-defined and repetitive validation tasks, such as standardized testing or model replication, can further lower costs.
The evolution toward capturing value systematically
To manage the P&L, capital, and regulatory challenges to their institutions’ advantage, leading banks are moving toward a robust MRM framework that deploys all available tools to capture efficiencies and value. The path to sophisticated model risk management is evolutionary—it can be usefully discussed as having three stages: building the elements of the foundation, implementing a robust MRM program, and capturing the value from it (Exhibit 1).
Building the foundational elements
The initial phase is mainly about setting up the basic infrastructure for model validation. This includes the policies for MRM objectives and scope, the models themselves, and the management of model risk through the model life cycle. Further policies determine model validation and annual review. Model inventory is also determined, based on the defined characteristics of the model to be captured and a process to identify all models and nonmodels used in the bank. Reports for internal and external stakeholders can then be generated from the inventory. It is important to note, however, that the industry still has no standard of what should be defined as a model. Since banks differ on this basic definition, there are large disparities in model-inventory statistics.
Governance and standards are also part of the MRM infrastructure. Two levels of governance are set up: one covering the steps of the model life cycle and one for the board and senior management. At this point, the MRM function will mainly consist of a small governance team and a team of validators. The governance team defines and maintains standards for model development, inventory, and validation. It also defines stakeholder roles, including skills, responsibilities, and the people who will fill them. The validation team conducts technical validation of the models. Most institutions build an MRM work-flow tool for the MRM processes.
Implementing a robust program
With foundational elements in place, banks can then build an MRM program that creates transparency for senior stakeholders on the model risk to the bank. Once model-development standards have been established, for example, the MRM program can be embedded across all development teams. Leading banks have created detailed templates for development, validation, and annual review, as well as online training modules for all stakeholders. They often use scorecards to monitor the evolution of model risk exposure across the institution.

McKinsey on Risk, Volume 2
A fundamental objective is to ensure high-quality, prioritized submissions. Model submissions missing key components such as data, feeder models, or monitoring plans reduce efficiency and increase delivery time. Efficiency can be meaningfully enhanced if all submissions adhere to standards before the validation process begins. Models are prioritized based on their importance to the business, outcome of prior validation, and potential for regulatory scrutiny.
Gaining efficiencies and extracting value
In the mature stage, the MRM function seeks efficiencies and value, reducing the cost of managing model risk while ensuring that models are of the highest quality. In our survey of leading financial institutions, most respondents (76 percent) identified incomplete or poor quality of model submissions as the largest barrier for their validation timelines. 1 1. Many fewer respondents cited a lack of sufficient resources (14 percent) and the need to validate each model comprehensively (10 percent). Model owners need to understand the models they use, as they shall be responsible for errors in decisions based on those models.
One of the best ways to improve model quality is with a center of excellence for model development, set up as an internal service provider on a pay-per-use basis. Centers of excellence enable best-practice sharing and advanced analytics across business units, capturing enterprise-wide efficiencies. The approach increases model transparency and reduces the risk of delays, as center managers apply such tools as control dashboards and checkpoints to reduce rework.
Process automation defines MRM maturity, as model development, validation, and resource management are “industrialized” (Exhibit 2). Validation is led by a project-management office setting timelines, allocating resources, and applying model-submission standards. Models are prioritized according to their importance in business decisions. An onshore “validation factory” reviews, tests, and revises models. It can be supported by an offshore group for data validation, standards tests and sensitivity analysis, initial documentation, and review of model monitoring and reporting. The industrial approach to validation ensures that models across the organization attain the highest established standards and that the greatest value is captured in their deployment.
The standards-based approach to model inventory and validation enhances transparency around model quality. Process efficiency is also monitored, as key metrics keep track of the models in validation and the time to completion. The validation work-flow system improves the model-validation factory, whose enterprise-wide reach enables efficient resource deployment, with cross-team resource sharing and a clear view of validator capabilities and model characteristics.
Consistent standards for model planning and development allow institutions to develop more accurate models with fewer resources and in less time. In our experience, up to 15 percent of MRM resources can be conserved. Similarly, streamlining the model-validation organization can save up to 25 percent in costs. With the significant regulatory spending now being demanded of institutions on both sides of the Atlantic, these savings are not only welcome but also necessary.
The contours of a mature stage of model risk management have only lately become clear. We now know where the MRM function has to go in order to create the most value amid costly and highly consequential operations. The sooner institutions get started in building value-based MRM on an enterprise-wide basis, the sooner they will be able to get ahead of the rising costs and get the most value from their models.
Ignacio Crespo is an associate partner in McKinsey’s Madrid office, Pankaj Kumar is an associate partner in the New York office , where Peter Noteboom is a partner, and Marc Taymans is a managing partner in McKinsey’s Risk Dynamics group.
Explore a career with us
Related articles.
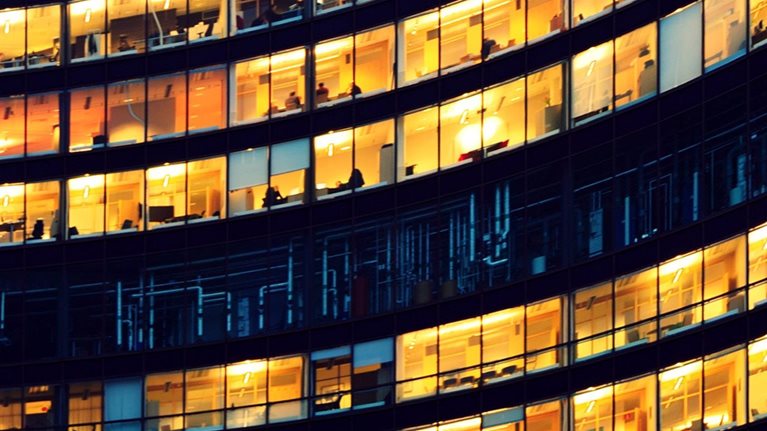
Sustainable compliance: Seven steps toward effectiveness and efficiency

The future of bank risk management
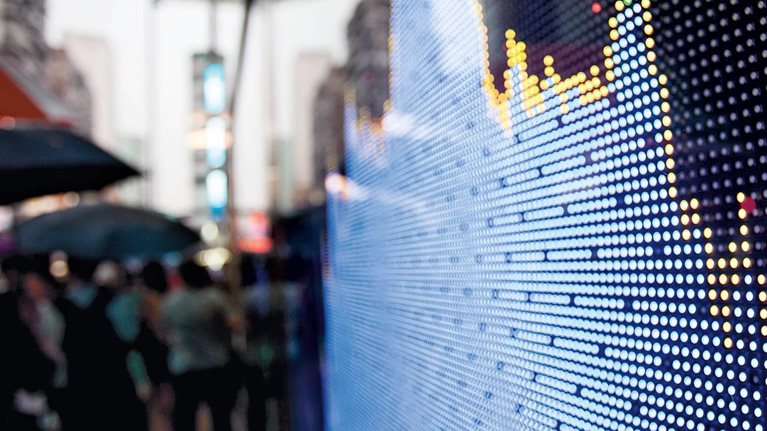
The value in digitally transforming credit risk management
- Search Search Please fill out this field.
- Fundamental Analysis
Model Risk: Definition, Management, and Examples
:max_bytes(150000):strip_icc():format(webp)/wk_headshot_aug_2018_02__william_kenton-5bfc261446e0fb005118afc9.jpg)
Gordon Scott has been an active investor and technical analyst or 20+ years. He is a Chartered Market Technician (CMT).
:max_bytes(150000):strip_icc():format(webp)/gordonscottphoto-5bfc26c446e0fb00265b0ed4.jpg)
What Is Model Risk?
Model risk is a type of risk that occurs when a financial model is used to measure quantitative information such as a firm's market risks or value transactions, and the model fails or performs inadequately and leads to adverse outcomes for the firm.
A model is a system, quantitative method, or approach that relies on assumptions and economic, statistical, mathematical, or financial theories and techniques. The model processes data inputs into a quantitative-estimate type of output.
Financial institutions and investors use models to identify the theoretical value of stock prices and to pinpoint trading opportunities. While models can be useful tools in investment analysis , they can also be prone to various risks that can occur from the usage of inaccurate data, programming errors, technical errors, and misinterpretation of the model's outputs.
Key Takeaways
- In finance, models are used extensively to identify potential future stock values, pinpoint trading opportunities, and help company managers make business decisions.
- Model risk is present whenever an insufficiently accurate model is used to make decisions.
- Model risk can stem from using a model with bad specifications, programming or technical errors, or data or calibration errors.
- Model risk can be reduced with model management such as testing, governance policies, and independent review.
Understanding Model Risk
Model risk is considered a subset of operational risk , as model risk mostly affects the firm that creates and uses the model. Traders or other investors who use a given model may not completely understand its assumptions and limitations, which limits the usefulness and application of the model itself.
In financial companies, model risk can affect the outcome of financial securities valuations , but it's also a factor in other industries. A model can incorrectly predict the probability of an airline passenger being a terrorist or the probability or a fraudulent credit card transaction. This can be due to incorrect assumptions, programming or technical errors, and other factors that increase the risk of a poor outcome.

What Does the Concept of Model Risk Tell You?
Any model is a simplified version of reality, and with any simplification, there is the risk that something will fail to be accounted for. Assumptions made to develop a model and inputs into the model can vary widely. The use of financial models has become very prevalent in the past decades, in step with advances in computing power, software applications, and new types of financial securities. Before developing a financial model, companies will often conduct a financial forecast , which is the process by which it determines the expectations of future results.
Some companies, such as banks, employ a model risk officer to establish a financial model risk management program aimed at reducing the likelihood of the bank suffering financial losses due to model risk issues. Components of the program include establishing model governance and policies. It also involves assigning roles and responsibilities to individuals who will develop, test, implement, and manage the financial models on an ongoing basis.
Real World Examples of Model Risk
Long-term capital management.
The Long-Term Capital Management (LTCM) debacle in 1998 was attributed to model risk. In this case, a small error in the firm's computer models was made larger by several orders of magnitude because of the highly leveraged trading strategy LTCM employed.
At its height, the hedge fund managed over $100 billion in assets and reported annual returns of over 40%. LTCM famously had two Nobel Prize winners in economics as principal shareholders, but the firm imploded due to its financial model that failed in that particular market environment.
JPMorgan Chase
Almost 15 years later, JPMorgan Chase (JPM) suffered massive trading losses from a value at risk (VaR) model that contained formula and operational errors. Risk managers use VaR models to estimate the future losses a portfolio could potentially incur. In 2012, CEO Jamie Dimon's proclaimed "tempest in a teapot" turned out to be a $6.2 billion loss resulting from trades gone wrong in its synthetic credit portfolio (SCP).
A trader had established large derivative positions that were flagged by the VaR model that existed at the time. In response, the bank's chief investment officer made adjustments to the VaR model, but due to a spreadsheet error in the model, trading losses were allowed to pile up without warning signals from the model.
This was not the first time that VaR models have failed. In 2007 and 2008, VaR models were criticized for failing to predict the extensive losses many banks suffered during the global financial crisis .
Roger Lowenstein. "When Genius Failed: The Rise and Fall of Long-Term Capital Management." Random House Trade Paperbacks, 2000.
Government Publishing Office. " JPMorgan Chase Whale Trades: A Case History of Derivatives Risks and Abuses ," Page 8. Accessed Sept. 7, 2020.
Government Publishing Office. " The Risks of Financial Modeling: VAR and the Economic Meltdown ," Page 3. Accessed Sept. 7, 2020.
:max_bytes(150000):strip_icc():format(webp)/Conditional_value_at_risk_final-6dc889ec0f2c4fc6802eafe69102698d.png)
- Terms of Service
- Editorial Policy
- Privacy Policy
- Your Privacy Choices
How to Make Risk Management Presentations Engaging and Actionable Across Your Organization
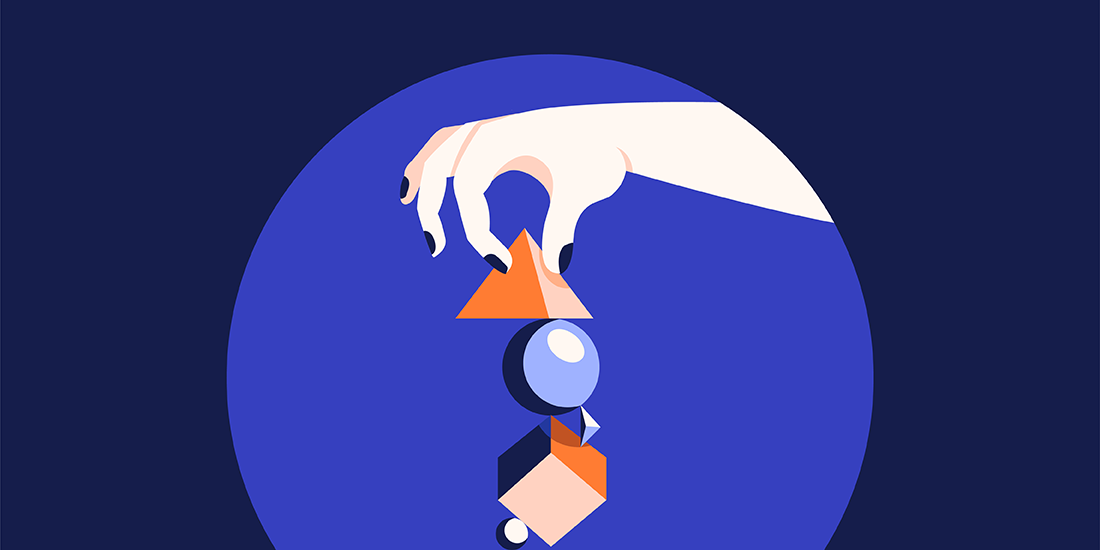
Life is full of risk. We face risks from the moment we wake up in the morning until we fall asleep at night. Will the alarm fail to sound? Will I get into a car accident on my way to work? Will I catch a virus when I go to dinner? Heck, there’s a risk— no matter how small— that we will die in our sleep during each night.
Risk is simply an inherent element of everything we do, and business is no exception. Will a vital employee quit, or will there be a labor shortage? What will happen in the stock market, and how will it impact the economy? What if there is an accident or a lawsuit involving the company? What happens if a new product fails? What actions will be taken in the event of a security breach or equipment failure?
We might not be able to prevent risk, but we can manage it. Managing business risk requires identifying and understanding risks while seeking ways to reduce risk in a way that also supports other business goals.
Companies heavily invest every year in ways to mitigate and respond to risk. But how do they make sure everyone is on board?
There might be a variety of ways to communicate a risk management plan to all the relevant players, but a visual presentation can be effective in not only presenting the risk management plan, but also ensuring that it is engaging and actionable across your organization.
What to include when you prepare a risk management plan:
A written risk management plan for business should not only include a listing of possible risks, but it also should feature plans to manage risk and respond to incidents.
- Identify risks
Risk management refers to a variety of business aspects, both internal weaknesses, and external threats. Like much in life, knowing is half the battle, and therefore identifying risks is key in addressing them.
Risk management should be considered before embarking on any new task or project, and everyone connected to a business should be encouraged to identify additional risks. Not only should the risk itself be considered, but companies also should identify possible consequences to better prepare to address each one.
- Minimize risks
A variety of strategies are available to manage and minimize risks once they are identified. One popular method of mitigating risk involves the 4Ts:
- Transfer risk by assigning a responsible team or party to each identified risk.
- Tolerate risk by monitoring it before taking further action.
- Treat risk by taking actions that reduce the likelihood that it will occur.
- Terminate risk by adopting or amending processes that eliminate it.
- Assign roles
Staff members should be assigned to each potential risk or risk category. These individuals will be responsible for mitigating their assigned risks, as well as reporting and responding to applicable incidents. A list of these roles should be included in the risk management plan.
- Plan recovery
Each risk included in the management plan must be followed by a strategy for preventing and addressing issues. An effective risk management plan will include a compilation of business projects, the risk applicable to each and an operational plan to respond and recover from incidents. Part of that plan also should include updating mitigation efforts following an incident to prevent it from repeating.
- Communicate plan
A risk management plan can’t be effective unless everyone within a company is on board. In addition to presenting the plan to principle players, be sure that it is also published somewhere that the full risk management plan can be accessed and understood by anyone within the company at any time.
- Rinse and repeat
The most effective risk management plans are living documents, continually updated with new or changed risks and new strategies to address them. Each risk outlined in the plan should be periodically reevaluated and new risks identified. The plan also should be monitored along with staff turnover to ensure no tasks fall through the cracks.
Tips to make risk management presentations engaging and actionable across your organization:
Audience engagement is vital to a successful risk management training presentation. After all, if staff and executives are asleep they will hardly become familiar with the plan and their assigned roles.
- Include visual assets
About 90 percent of human thought is visually-based. Therefore, it’s no shocker that including visual assets within a presentation is one of the most effective strategies for engaging all types of audiences .
Releasing the risk management plan through a visual presentation is a great start, but the content within the slide deck is just as important. After all, the average PowerPoint slide includes 40 words , which is entirely too many. Instead, include more images, videos and animations within a financial risk management presentation or any other risk management training presentations.
- Illustrate data
Data is one of the most convincing sorts of content that can be presented to an audience. As anyone can attest— at least in most cases— numbers don’t lie. In fact, they can tell their own stories. A crowded slide full of stats and figures is a quick way to send your audience off to Dreamland.
Instead, illustrate your data through infographics. Beautiful.ai offers a host of various infographics through our smart slide templates. Just input your data and watch our artificial intelligence-powered presentation software design the infographic accordingly. Choose from infographics like scattergraphs , process diagrams , pie charts and bar graphs to tell the story of different risks and strategies to address them.
- Tell a story
According to the 2018 State of Attention survey, almost 90 percent of respondents said a strong narrative or story backing a presentation is critical in maintaining audience engagement. Sure, facts and data can persuade audiences and get them on board, but only if people are paying attention.
Stories have kept audiences engaged since before recorded history. Tell the story of your risk management plan by including real-life examples or by creating a character for hypothetical scenarios. Those unsure how to incorporate a story into the structure of their presentation can look to Beautiful.ai’s various presentation templates for inspiration.
- Include your audience
If you really want to keep your audience engaged with your risk management presentation slides, be sure you talk with people, not at them. Include your audience in your presentation by asking questions, taking surveys or presenting group activities. Of course, the first step is identifying who makes up that audience. You won’t necessarily present the same content to an executive board as to a room full of new hires.
One effective way to engage an audience with a risk management plan presentation from the very start is through a pre-presentation quiz or survey that gauges how much participants already know about risk management, like this example from the U.S. Small Business Association. Not only will the activity engage the audience, but it will alert participants to what they don’t know from the very start. Other engagement tools include Q&A sessions, humor and gamification.
As mentioned, the average PowerPoint slide consists of 40 words… way too many to keep audiences engaged. Remember, your presentation should be based on an outline of your plan, not a verbatim recitation of it.
Not only are uncluttered slides more effective, but shorter presentations also are more effective than longer ones, based on both audience attention and respect for time. Especially when delivering a risk management board presentation, it’s vital to respect your audience’s time. Beautiful.ai’s library of presentation templates can serve as a guideline to effective presentation lengths for a variety of topics.
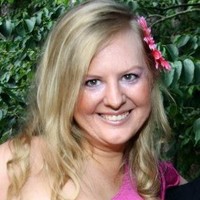
Samantha Pratt Lile
Samantha is an independent journalist, editor, blogger and content manager. Examples of her published work can be found at sites including the Huffington Post, Thrive Global, and Buzzfeed.
Recommended Articles
The ultimate guide to a pitch deck, what is generative ai and how your business can use it , 9 best free powerpoint alternatives, 10 best productivity apps of 2021: a solution for every problem.
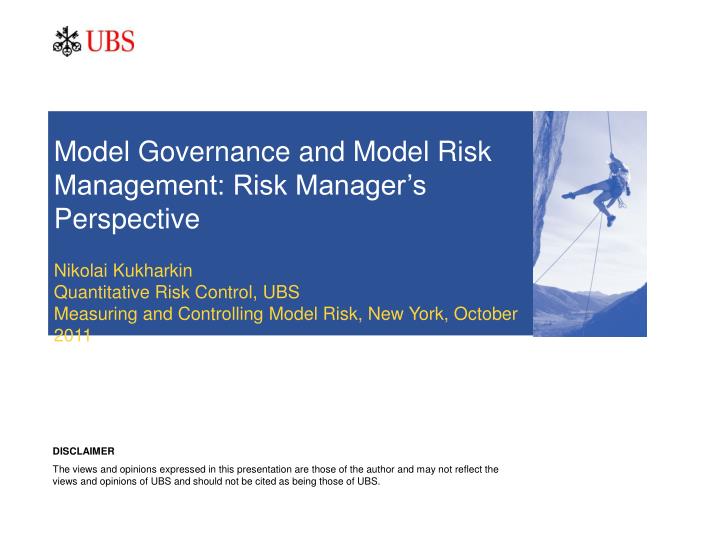
Model Governance and Model Risk Management: Risk Manager’s Perspective
Aug 16, 2014
460 likes | 926 Views
Model Governance and Model Risk Management: Risk Manager’s Perspective. Nikolai Kukharkin Quantitative Risk Control, UBS Measuring and Controlling Model Risk, New York, October 2011. DISCLAIMER
Share Presentation
- simplified description
- potential action plan items
- risk manager
- organizational changes
- effective model validation
- overall valuation risk
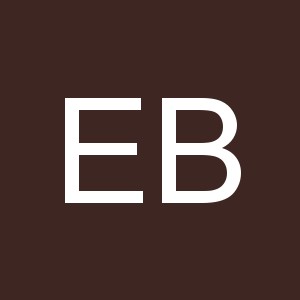
Presentation Transcript
Model Governance and Model Risk Management: Risk Manager’s Perspective Nikolai Kukharkin Quantitative Risk Control, UBS Measuring and Controlling Model Risk, New York, October 2011 DISCLAIMER The views and opinions expressed in this presentation are those of the author and may not reflect the views and opinions of UBS and should not be cited as being those of UBS.
What Can Go Wrong With Models? • More extensive policies, stricter regulations, and more comprehensive model risk management programs.
Kill All the Quants?... * • Risky Business on Wall Street: High-tech supernerds are playing dangerous games with your money TIME magazine, April 11, 1994 • Recipe for Disaster: The Formula That Killed Wall Street Wired Magazine, Feb 23, 2009 • The Minds Behind the Meltdown: How a swashbuckling breed of mathematicians and computer scientists nearly destroyed Wall Street WSJ, Jan 22, 2010 • Financial Crisis Can Be Traced to “the Quants” The Kansas City Star, Feb 22, 2010 *) Andrew W. Lo, “Kill All the Quants?: Models vs. Mania in the Current Financial Crisis”, 2009.
…Before They Are Born… Too large a proportion of recent "mathematical“ economics are mere concoctions, as imprecise as the initial assumptions they rest on, which allow the author to lose sight of the complexities and interdependencies of the real world in a maze of pretentious and unhelpful symbols. John Maynard Keynes, The General Theory of Employment, Interest and Money, 1935
…Or May Be Not? Myron Scholes, Risk Magazine, September 2011 • It should be a golden age for risk modeling and management • “One thing about a crisis is that it shakes old opinions and you start learning new things. I hope we do – I am very bullish on the future for quants.” • He warns against overreliance on models, and concedes they had a role in the crisis. But the common-sense reaction – embracing intuition, and rejecting the use of modeling and quantitative techniques – is also flawed, he argues… • Presumably you used your intuition in picking the model, and intuition can fail, too.
New FED/OCC Supervisory Guidance on Model Risk Management • Expands on existing regulatory guidance by broadening the scope beyond model validation to include all aspects of model risk management at all stages: model development, implementation, and use • Revises and expands model and model risk definition • Establishes comprehensive model risk management program requirements • More formalized and expanded model governance and controls • Increased standing of model risk management function: needs to be influential; have explicit authority to challenge model developers and users • Model validation • Introduces “effective challenge” standard • Key elements of comprehensive validation : • Evaluation of conceptual soundness • On-going monitoring • Outcomes analysis
Comprehensive Model Risk Management Program Requirements • The bar has been raised significantly with respect to the scope, formality, rigor, and prominence expected of banks’ model risk management programs. • “Model risk should be managed like other types of risk.” • Life-cycle view of model risk - model risk management framework is expected to include standards for model development, use, and maintenance to which all model owners, users, and other stakeholders will be held. • Broader roles and responsibilities – it is not just the responsibility of the model validation unit: model developers / owners, users, validators, senior management, internal audit • Model risk management is an on-going, continuous, process – not a periodic activity: • Monitoring model risks and limitations identified during development and validation • Monitoring and on-going validation of changes (i.e., products, exposures, activities, clients, or market conditions) that may impact model risks • Regular model performance monitoring (i.e., back-testing, benchmarking, sensitivity analysis, and stress testing) • Model risk reporting to senior management and the board of directors.
How Should Banks Respond? • Examiners expect a bank to perform a self-assessment against new regulatory guidance, and have a clear action plan for closing identified gaps. • Potential action plan items may include the following: • Revisions to policies and procedures • Revisions to roles and responsibilities • Organizational changes • Development of new standards and guidelines for model development, implementation, and use • Revised model inventories (including an inventory of model-specific risks and limitations) • Mappings of model risk mitigation controls against existing inventory of model risks and limitations • Creation / enhancement of on-going model monitoring processes • Creation / enhancement of model risk reporting • Additional model validation testing (e.g., vendor models) • Creation of annual model review process
Model Validation: What’s Next? • Financial industry obtains a significant share of revenue from products valued by mathematical models • Models are here to stay and reliance on them will only grow • Consequently, model risk is a topic of great, and growing, interest in the risk management arena • How to define it • How to measure it • How to manage it • Qualitatively reasonably well defined • Much less successfully quantified and even less successfully managed • What is expected from model validators and how is the role changing? • What are the key priorities for model validators?
Model Risk: Define and Manage A model can be defined as: A simplified description or representation of an entity or process, property, characteristic or behavior which cannot be represented or predicted with complete certainty.The output of a model is therefore an estimate or approximation. 1. Model risk is the potential for adverse consequences from decisions based on incorrect or misused model outputs and reports. 2. Model risk is the risk of error due to a deficiency in design or implementation of a pricing model. In other words, model risk is the risk of occurrence of a significant difference between the mark-to-model value of a product and its fair value. Or more flexible definition by R. Rebonato 3. Model Risk is the risk of occurrence of a significant difference between the mark-to-model value of a complex and/or illiquid instrument, and the price at which the same instrument is revealed to have traded in the market. Note that neither “true” nor “fair” value is mentioned, i.e. ->>> Market is the king More sophisticated / realistic / correct model is not necessarily the best
Model Governance Process • Regulators on Model Risk: FSA “Model Risk contributes to overall valuation risk. Model validation and model risk management processes are important elements of any valuation control framework. Whilst effective model validation is fundamental, model validation, however good, does not remove model risk. Few firms have sufficiently well developed frameworks for articulating model risk tolerance, and measuring and controlling model risks within that tolerance. We believe a better defined and implemented model risk management framework could therefore feed into a better defined and implemented valuation risk-management framework.” - from FSA’s “Dear CEO” letter on Valuation and Product Control principles, August 2008 • Regulators on Model Risk: FED and OCC Supervisory Guidance on Model Risk Management, April 2011 Model risk should be managed like other types of risk. Banks should identify the sources of risk and assess the magnitude. Model risk increases with greater model complexity, higher uncertainty about inputs and assumptions, broader use, and larger potential impact. …With an understanding of the source and magnitude of model risk in place, the next step is to manage it properly.
Model Risk Management Framework Goal is to set up a framework to explicitly, fully, and dynamically account for model risk • Partially accounted for by: • Qualitative capture • Models certification • Periodic model risk review • Quantitative capture • Model reserves • Sensitivity analysis • Portfolio reviews
Model Risk Management Framework Governance: Independent Verification Unit (IVU) with the mandate for: - independent review and certification of valuation models - independent risk-based review of model-related risks, i.e., the risk that the model either through a deficiency in design or implementation produces faulty output. The aim of the certification process is to obtain the required level of comfort that the model in question is functioning in an accurate and appropriate way. Model performance monitoring process: - Full review of potential model risks and existing control processes across systems and product areas taking into account factors including materiality, model choice, model applicability - This is independent from running certification processes, it challenges existing model issues and includes actions that are or should be taken Frequency and depth of reviews: Regulators requested model verification updates of the “high-risk” to be done more frequently, annually, compared to previous 5-y cycle for all certifications.
Model Governance Process – High-Risk Models Definition of High-Risk Models Define dimensions and criteria to categorize models as “high risk” Definition is altered based on new information / past actions Capture of High-Risk Models Agree on review types Run / participate in the reviews throughout the year Review Filter all models according to preliminary criteria Working Groups of IVU product specialists adjust filtered product list and define necessary actions Actions based on Reviews Consolidate results of reviews already undertaken Take further actions if necessary
Definition - Model Risk Types The following major model risks types were defined 1. Model inconsistencies or approximations Inconsistencies in mathematical assumptions of the model or its implementation Model assumed fit for purpose, although does not fully capture some features The model may be used outside of its range of applicability 2. Model choice Model choice uncertainty - several models are available (and one is being used) Many solutions could satisfy the same constraints 3. Calibration, model parameters, and input data issues Multiple sets of parameters can satisfy market; multiple sets of calibration instruments available, sometimes model cannot fit all of them simultaneously; uncertainty in model input parameters 4. Controls (booking approximations + level of oversight) The control environment into which the model is being released. Level of oversight by other control groups and therefore the probability of an error being detected 5. Complexity Exotic features, number of inputs, and the importance of inter-relationships between them models assumptions/conditions 6. Model/Product maturity and level of standardization Maturity, liquidity and rate of change of the market
Capture – Model Risk Scores Model risk scoring process Start with the certified product list and link and rank it according to the risk scores in several dimensions as well as materiality of positions: • Each product is described in terms of “High=3”, “Medium=2” or “Low=1” Risk Scores for each of the following six risk factors (combined Risk Score is between 6 and 18): 1. Model inconsistencies or approximations 2. Model Choice 3. Calibration, model parameters, and input data issues 4. Controls (booking approximations + level of oversight) 5. Complexity (exotic features, number of inputs, models assumptions/conditions) 6. Model/product maturity and level of standardization • Capability to compare model risk between models / products across all areas • This list is used as a starting point to identify models/products which will be subject to the annual review • Additional win – not just a formal more frequent re-certification, but rather a review targeting specific features which make this product/model high-risk
Is Theoretical Value Fair? • Most of the issues listed in the previous section can also give rise to model fair value adjustments Fair value is the price that would be received to sell an asset or paid to transfer a liability in an orderly transaction between market participants at the measurement date (FAS 157) • Accounting standards demand that if the value of asset or liability is not directly observable but rather obtained from a model (“marked-to-model"), it needs to be further adjusted to bring it into closer alignment with the market fair value. Why is such adjustment needed? • Model can have a known bias/deficiency • There can be an uncertainty around the model generated value due to: • existence of the alternative models (i.e., no industry standard) • non-uniqueness of calibration • uncertainty around (unobservable) model inputs • Terms “Model Fair Value adjustments” and “Model reserves” are often used interchangeably, but they mean different things and should not be mixed up
What Do Accounting Standards Say About Model Reserves? They say… NOTHING Model reserves may serve as a proxy, intuitive way to account for perceived model risk Model FV adjustments aim at “fine-tuning” model generated number to bring it into a better alignment with the market price. FV adjustments make the “best educated guess” of where the market is Model risk attempts to assess the tails of the theoretical price distribution, expresses how far off our “best guess” might be from the realized price • Model risk arises from the uncertainty in model specification, be it the model parameters and/or inputs (i.e., function arguments), or the model (function) itself. When not observable, FV of an asset is a variable characterized by some probability distribution. While model FV adjustments attempt to pinpoint the center of such distribution, its higher moments are the domain of model risk. • NOTE: Frequent practice of using “parameter uncertainty” and sometimes “alternative model” reserves to create a “conservative cushion” roughly the size of perceived model risk, contradicts the accounting standards which concentrate on fair value.
Inherent Model Risk • Valuation uncertainty beyond model FV adjustments is the domain of (inherent) model risk • Can be viewed as “residual” model risk. With proper model validation in place, inherent model risk can be minimized but never eliminated • Needs to be measured, monitored, and managed • Deserves a place in overall risk management framework on a par with market and credit risk • Should be considered alongside the market and credit risks in allocating capital, making business decisions, and managing the trading positions
Final Observations • There is always a risk that a model can be “wrong” • In part model risk is a variety of operational risk, i.e. the possibility of a human error • However, there is also an inherent uncertainty due to the very nature of financial modeling • The purpose of model governance is to set up policies and procedures that: • 1) minimize operational risk - Achieved through model validation, periodic reviews, model change management, back-testing, etc. • 2) provide for measurement, monitoring, and management of inherent model risk (model uncertainty) - Requires recognition of model uncertainty’s role alongside market and credit risk, and devising and implementing methods, processes and systems for measuring capturing reporting and managing model risk • Therefore, model risk deserves a place alongside market and credit risk in making business decisions (e.g., in capital allocation) as well as in the risk management and reporting process.
- More by User
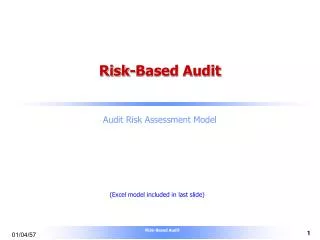
Risk-Based Audit
Risk-Based Audit. Audit Risk Assessment Model. (Excel model included in last slide). Audit Risk Model. AUDIT RISK MODEL Purpose to prioritize audit schedule for creation of audit plan. All risks are relative but can be compared by combining three key factors with equal overall weighting :
1.84k views • 31 slides
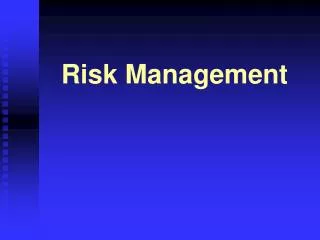
Risk Management
Risk Management. Risk Definitions. Risk Management The practice of dealing with project risk. It includes planning for risk, assessing risk, developing risk response strategies, and monitoring risk throughout the project life cycle. 35. Risk Definitions. Risk
1.24k views • 55 slides
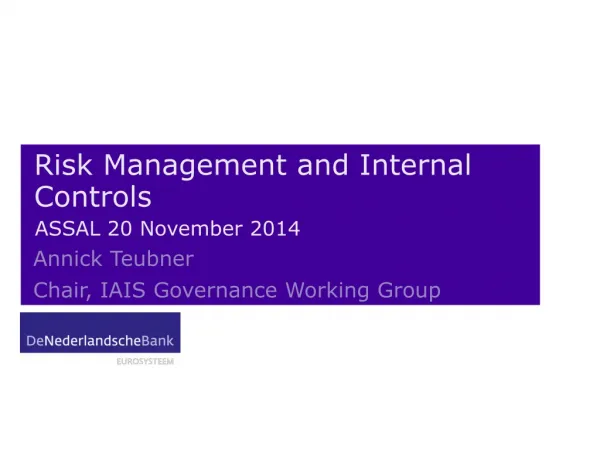
Risk Management and Internal Controls
Risk Management and Internal Controls. ASSAL 20 November 2014. Annick Teubner Chair, IAIS Governance Working Group. Agenda. Introduction Risk Management and Controls Why Risk governance and controls matter… Revision ICPs ’: topics 2014
429 views • 23 slides
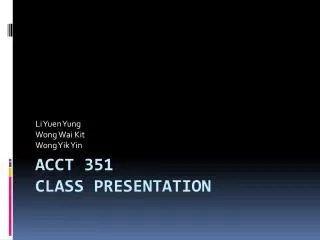
Acct 351 Class Presentation
Li Yuen Yung Wong Wai Kit Wong Yik Yin. Acct 351 Class Presentation . Agenda. Audit Risk Model Reasons for high audit risk in China Inherent Risk (IR) Control Risk (CR) Detection Risk (DR) Conclusion Recommendation . Audit Risk Model. Audit Risk Model. IR. CR. DR.
556 views • 27 slides
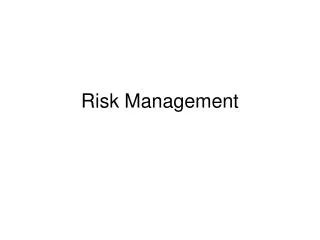
Risk Management. Objectives. Define risk management, risk identification, and risk control Understand how risk is identified and assessed Assess risk based on probability of occurrence and impact on an organization. Introduction.
725 views • 32 slides
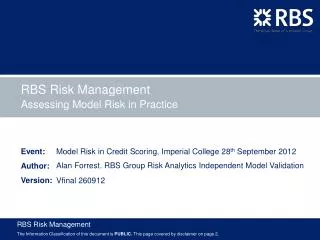
Assessing Model Risk in Practice
Assessing Model Risk in Practice. Model Risk in Credit Scoring, Imperial College 28 th September 2012. Alan Forrest. RBS Group Risk Analytics Independent Model Validation. Vfinal 260912. Assessing Model Risk in Practice. Disclaimer. Disclaimer
413 views • 15 slides
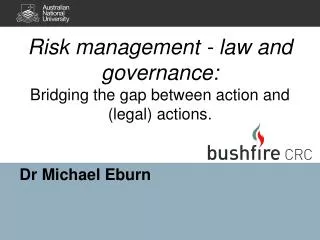
Risk management - law and governance: Bridging the gap between action and (legal) actions.
Risk management - law and governance: Bridging the gap between action and (legal) actions. Dr Michael Eburn. “The Risk Society”. Beck , Ulrich (1992), Risk Society; towards a new modernity ( Sage, London). Sees everything in terms of risk.
289 views • 12 slides
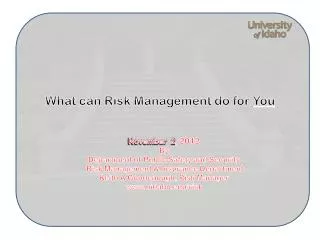
What can Risk Management do for You
What can Risk Management do for You. November 2 , 2012 By Department of Public Safety and Security Risk Management & Insurance Department Keith A Goodenough , Risk Manager www.uidaho.edu/risk. Identify exposures relevant to all U of I operations .
227 views • 6 slides
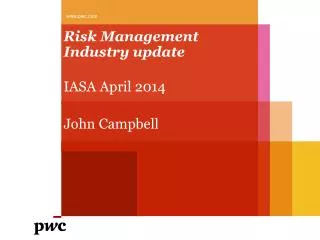
Risk Management Industry update
www.pwc.com. Risk Management Industry update. IASA April 2014. John Campbell. Agenda. Introduction Risk Management ORSA Model Risk Management Risk Appetite. Risk Management ORSA update. The Own Risk & Solvency Assessment.
833 views • 30 slides
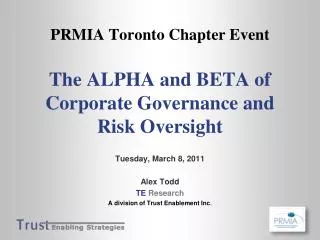
PRMIA Toronto Chapter Event The ALPHA and BETA of Corporate Governance and Risk Oversight
PRMIA Toronto Chapter Event The ALPHA and BETA of Corporate Governance and Risk Oversight. Tuesday, March 8, 2011 Alex Todd TE Research A division of Trust Enablement Inc . Understanding Systemic Risk. Risk Management & Systemic Risk. Risk Management in a Complex World. Risk Management.
630 views • 44 slides
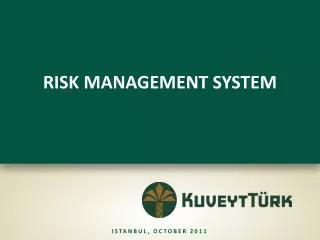
RISK MANAGEMENT SYSTEM
RISK MANAGEMENT SYSTEM. ISTANBUL, OCTOBER 2011. CALIBRI BOLD 42 pt. AGENDA . RISK MANAGEMENT IN BRIEF FUNCTION ORGANIZATION RESPONSIBILITIES ACTIVITIES REPORTS WORKS Risk Management Policies CREDIT RISK MARKET RISK OPERATIONAL RISK. RISK MANAGEMENT SYSTEM. RISK MANAGEMENT IN BRIEF.
1k views • 23 slides
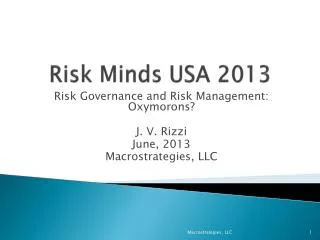
Risk Minds USA 2013
Risk Minds USA 2013. Risk Governance and Risk Management: Oxymorons ? J. V. Rizzi June, 2013 Macrostrategies , LLC. Table of Contents. Introduction Decisions Governance Why Change Conclusion. Introduction. Introduction. Risk governance worked, except for “rare” exceptions…
392 views • 24 slides
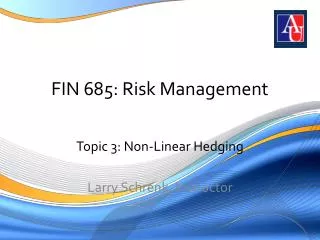
FIN 685: Risk Management
FIN 685: Risk Management. Topic 3: Non-Linear Hedging Larry Schrenk, Instructor. Topics. Black- Scholes Model Greeks Hedging An Extended Example. The Black- Scholes Model. Black- Scholes Formula. Variables. S = Spot Price X = Exercise Price r = Risk Free Rate
502 views • 41 slides
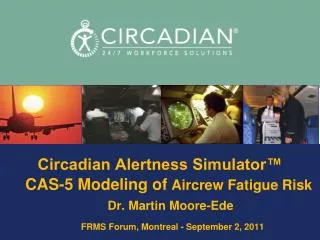
CAS-5 FATIGUE MODEL FOR AVIATION FRMS
Circadian Alertness Simulator™ CAS-5 Modeling of Aircrew Fatigue Risk Dr. Martin Moore-Ede FRMS Forum, Montreal - September 2, 2011. CAS-5 FATIGUE MODEL FOR AVIATION FRMS. A scientifically-validated fatigue risk model is a vital tool in aviation Fatigue Risk Management Systems (FRMS).
1.12k views • 63 slides
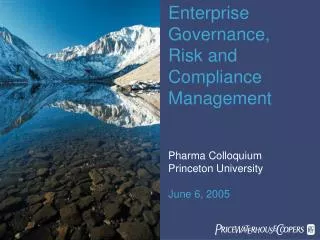
Enterprise Governance, Risk and Compliance Management Pharma Colloquium
Enterprise Governance, Risk and Compliance Management Pharma Colloquium Princeton University June 6, 2005 . PwC. Agenda. PwC Global CEO Survey on Governance, Risk and Compliance Regulatory Expectations COSO Enterprise Risk Management Open Compliance and Ethics Guidelines.
590 views • 27 slides
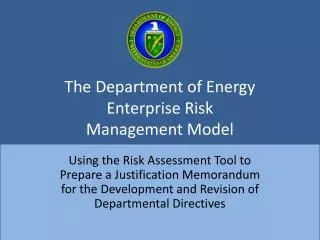
The Department of Energy Enterprise Risk Management Model
The Department of Energy Enterprise Risk Management Model. Using the Risk Assessment Tool to Prepare a Justification Memorandum for the Development and Revision of Departmental Directives. Enterprise Risk Management (ERM) Model - Background.
386 views • 10 slides
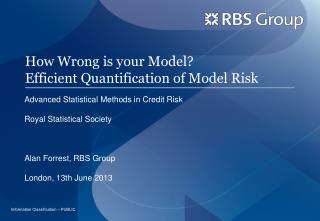
How Wrong is your Model? Efficient Quantification of Model Risk
How Wrong is your Model? Efficient Quantification of Model Risk. Advanced Statistical Methods in Credit Risk Royal Statistical Society Alan Forrest, RBS Group London, 13th June 2013. Information Classification – PUBLIC. Disclaimer. Thanks
394 views • 23 slides
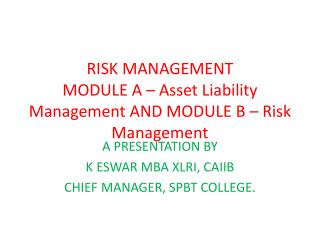
RISK MANAGEMENT MODULE A – Asset Liability Management AND MODULE B – Risk Management
RISK MANAGEMENT MODULE A – Asset Liability Management AND MODULE B – Risk Management. A PRESENTATION BY K ESWAR MBA XLRI, CAIIB CHIEF MANAGER, SPBT COLLEGE. BANKS TYPICALLY FACE THREE KINDS OF RISK. Type of Risk. Example.
1.05k views • 75 slides
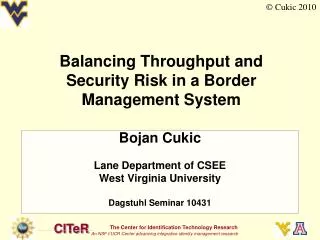
Balancing Throughput and Security Risk in a Border Management System
Balancing Throughput and Security Risk in a Border Management System. Bojan Cukic Lane Department of CSEE West Virginia University Dagstuhl Seminar 10431. UML Model with performance annotations. Performance Model. Risk Model. Application’s Performance/risk feedback. Framework.
216 views • 10 slides
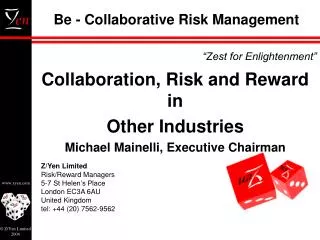
Be - Collaborative Risk Management
Be - Collaborative Risk Management. Collaboration, Risk and Reward in Other Industries Michael Mainelli, Executive Chairman. Agenda. Governance and the risk society Mutuals et al Enterprise risk/reward management systems Challenges for Property organisations Information sharing.
513 views • 23 slides
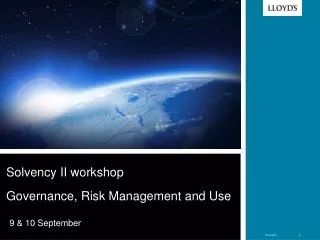
Solvency II workshop Governance, Risk Management and Use
Solvency II workshop Governance, Risk Management and Use. 9 & 10 September. Agenda. Introduction Model Change Themes from submissions Feedback from recent reviews Evidence Templates Planned Use Test Review Activity in Q4 Table discussions Minimum Standards update
493 views • 39 slides
What is Model Risk?
January 8, 2020
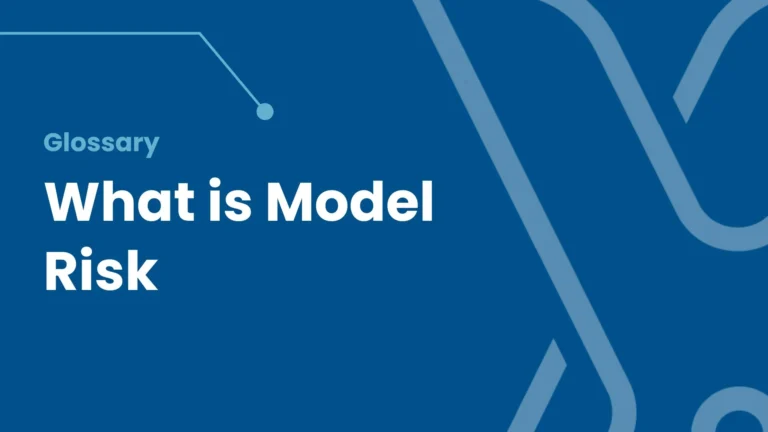
In recent years, more and more organisations are rapidly integrating predictive models across different business functions. Model use offer many benefits, but it also exposes organisations to greater operational risk.
Model risk is the risk that arises when a model fails or does not perform according to expectations.
When we use mathematical models to support decision making, we face model risk since mathematical models can produce incorrect outcomes.
Models can fail due to technical mistakes, such as lack of mathematical rigour, data issues, implementation bugs, and other issues and these types of model risk incidents can be considered part of operational risk. However, models produce incorrect results as well simply as a consequence of the inherent uncertainty related to mathematical modelling. This in itself is a characteristic feature of modelling but a failure to recognize this uncertainty properly constitutes a key element of model risk.
A final category of model risk accidents is related to using models that are not well understood, i.e. in situations when we are unable to properly explain why a model has come to a certain conclusion. In the latter case, there is a link with operational risk.
Model failure can lead to financial losses, regulatory or legal penalties, or damage to a bank’s reputation, resulting from the use of a model that contains data or assumptions that were not appropriate for the model.
In order to manage model risk, governance policies and frameworks should be applied.
The cornerstone of model risk governance is the implementation of three lines of defence:
- first line (the model developers) is responsible to persist in a structured document all assumptions, both on the data and on the actual model. Once a model is used in production, it is most often a first line’s responsibility as well to monitor the model to detect issues as quickly as possible.
- second line (the model validators) has to independently review this document to ensure that it is self-contained, that the results are reproducible and that the limitations of the models are well understood by the decision-makers, the users and the developers. This independent review consists of model validation .
- third line (audit) is responsible for verifying that the processes between first and second line are effectively implemented.
Two examples of model risk are the Credit Crisis of 2008 and the London Whale Trading Incident in 2012.
In the Credit Crisis, banks were relying on very simple models to estimate mortgage risk. Although the used models were mathematically correct, they were too simple to deal with the highly complex derivatives that were traded at that time.
In the London Whale Trading Incident, JPMorgan lost over $6 billion due to a mistake in a credit risk model.
In both cases, the root cause was a lack of proper governance that should have detected the flaws in the models that were being used.
Scale your model risk management
Learn how technology can transform how you and your team manage model risk.
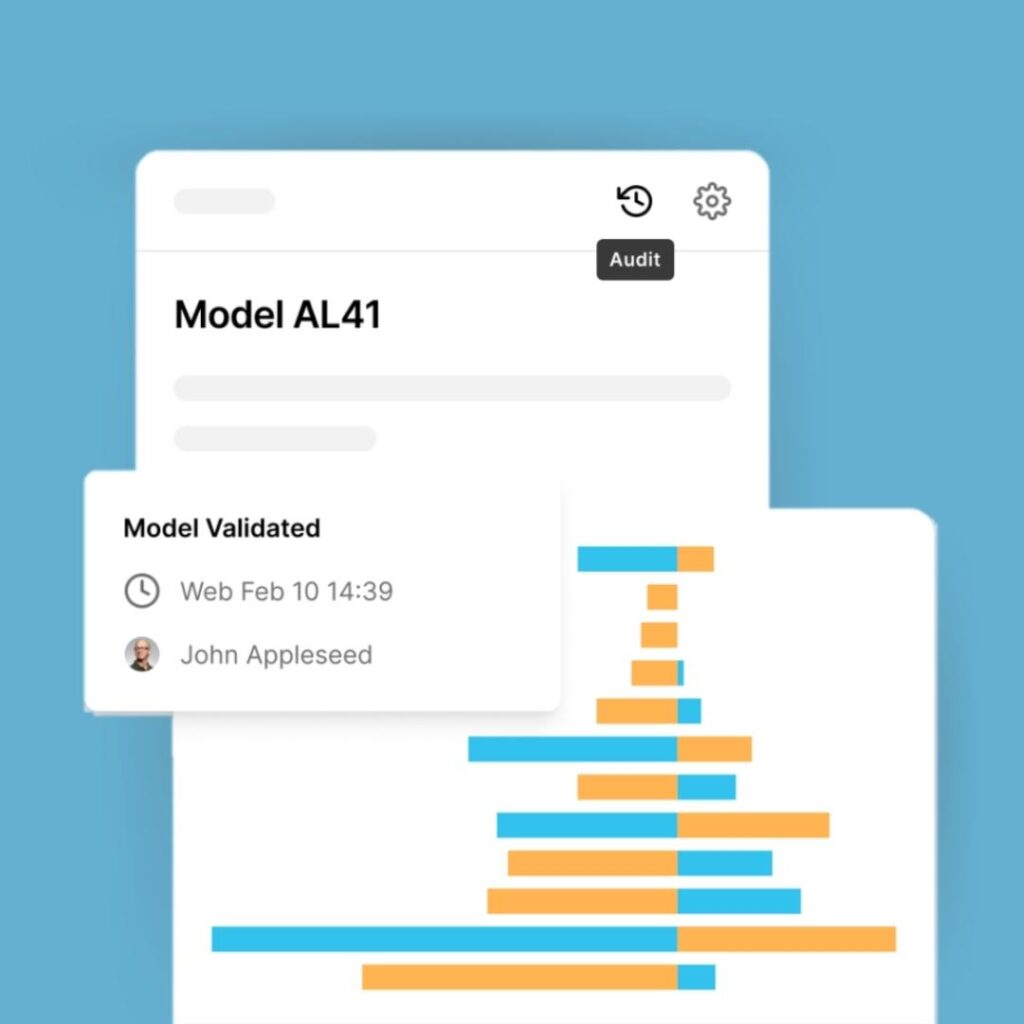
Automating ModelValidation at a Tier 1 Bank
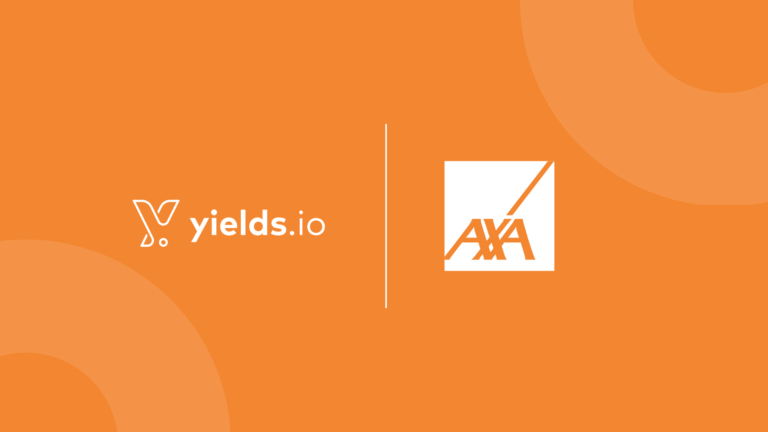
Automated VaR Calculation & Reporting at AXA Bank Belgium (part of Crelan Group)
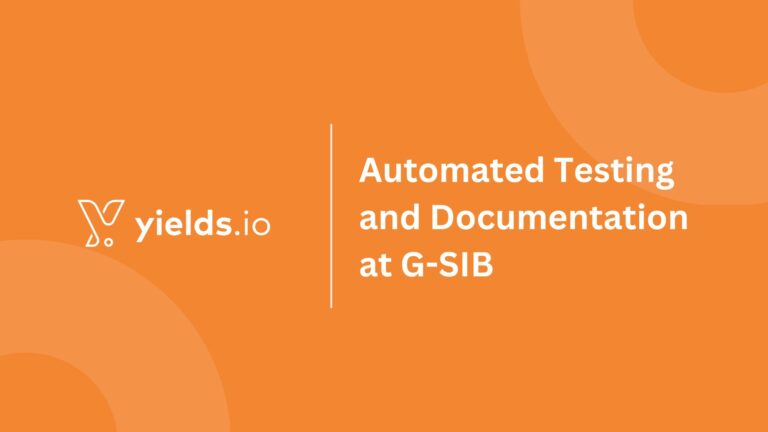
Automated Testing and Documentation at G-SIB
Home PowerPoint Templates Diagrams Three Lines of Defense PowerPoint Template
Three Lines of Defense PowerPoint Template
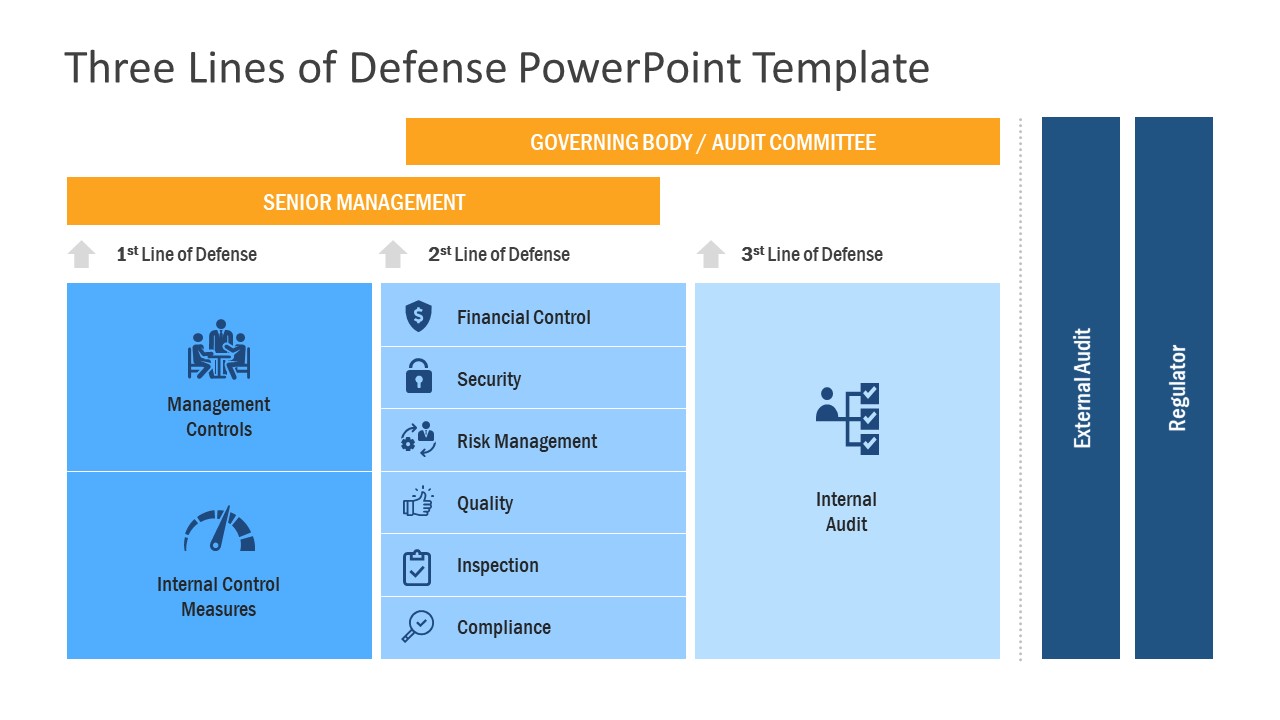
Three Lines of Defense PowerPoint Template provides 2 layout designs for a comprehensive risk management model. This template contains is graphics of model that is widely recognized as an important part of organizational risk management. The model itself helps organizations to allocate roles and responsibilities in such a way that they are not risk-driven. It further enables risk management professionals to avoid overlapping whiling assigning roles to employees. Therefore, the strategic model diagram in this PowerPoint template demonstrates management controls and risk mitigation measurements. The three lines of defense classify organizational processes and define activities as described below:
- First line of defense: It includes management controls and internal control measures. The management is responsible for ensuring that company is operating at acceptable risk mitigation levels.
- Second line of defense : This include all functional processes of business such as financial control, security, quality, inspection, and compliance. This facilitates the organization to ensure proper protocols are being followed.
- Third line of defense: It includes internal audit which provides assessment and effectiveness of the first two lines of defense to management.
The risk management Lines of Defense model assists professionals to measure various business activities. For example, skill training, policy documents, or communicating management frameworks. Therefore, Three Lines of Defense PowerPoint Template could assist business professionals to demonstrate organizational risk management strategies. The graphic model of risk management will help users to provide an overview of management strategies in a single slide view.
There are 4 slides of Three Lines of Defense PowerPoint Templates containing 2 versions of model design. The users can copy desired layout design according to presentation needs and color theme. The template also provides clipart icons as graphic representation of functions or processes.
You must be logged in to download this file.
Favorite Add to Collection
Details (4 slides)

Supported Versions:
Subscribe today and get immediate access to download our PowerPoint templates.
Related PowerPoint Templates
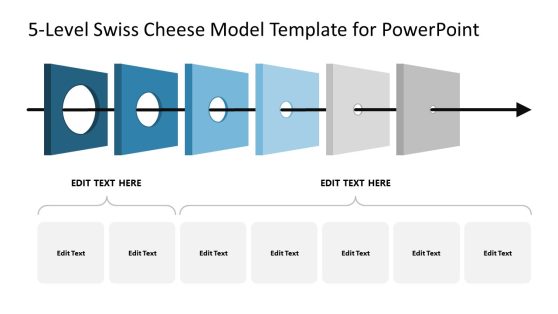
5-Level Swiss Cheese Model PowerPoint Template
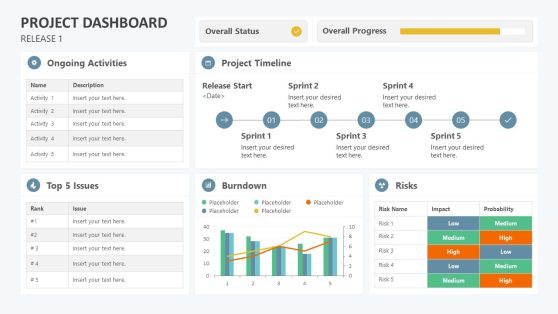
Project Management Dashboard PowerPoint Template
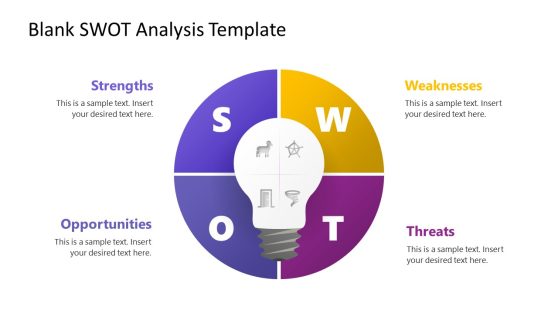
Blank SWOT Analysis PowerPoint Template
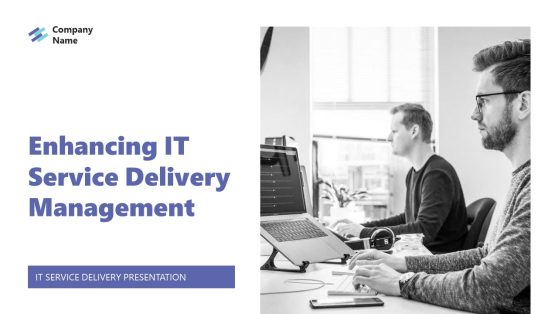
IT Service Delivery Management PowerPoint Template
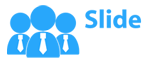
- Customer Favourites
Powerpoint Templates
Icon Bundle
Kpi Dashboard
Professional
Business Plans
Swot Analysis
Gantt Chart
Business Proposal
Marketing Plan
Project Management
Business Case
Business Model
Cyber Security
Business PPT
Digital Marketing
Digital Transformation
Human Resources
Product Management
Artificial Intelligence
Company Profile
Acknowledgement PPT
PPT Presentation
Reports Brochures
One Page Pitch
Interview PPT
All Categories
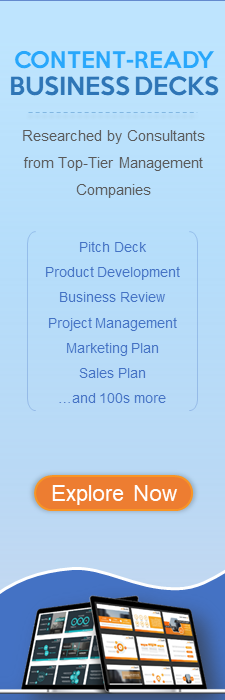
- You're currently reading page 1

Stages // require(['jquery'], function ($) { $(document).ready(function () { //removes paginator if items are less than selected items per page var paginator = $("#limiter :selected").text(); var itemsPerPage = parseInt(paginator); var itemsCount = $(".products.list.items.product-items.sli_container").children().length; if (itemsCount ? ’Stages’ here means the number of divisions or graphic elements in the slide. For example, if you want a 4 piece puzzle slide, you can search for the word ‘puzzles’ and then select 4 ‘Stages’ here. We have categorized all our content according to the number of ‘Stages’ to make it easier for you to refine the results.
Category // require(['jquery'], function ($) { $(document).ready(function () { //removes paginator if items are less than selected items per page var paginator = $("#limiter :selected").text(); var itemsperpage = parseint(paginator); var itemscount = $(".products.list.items.product-items.sli_container").children().length; if (itemscount.
- 3D Man (24)
- Anatomy (2)
- Block Chain (3)
- Business Cards (14)
- Business Plan Word (2)
- Business Plans (89)
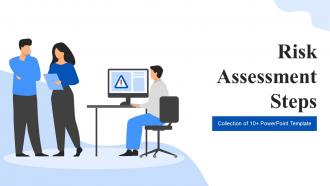

IMAGES
VIDEO
COMMENTS
Model Risk Management function and framework within a financial entity. The guidance aims to help banks understand the importance of model risk, how it may affect the P&L and capital and the most important steps to develop a model risk framework. Contents 5 3. MRM Framework 6 4. Model documentation and inventory 8 5. Model Life-Cycle 9 6 ...
Model Risk Management : Best Practices. Model risk and the importance of model risk management has gotten significant attention in the last few years. As financial companies increase their reliance on quants and quantitative models for decision making, they are increasingly exposed to model risk and are looking for ways to mitigate it. The ...
Definitions of roles and responsibilities for an effective Model Risk Management: board or "management body", senior management, model developers, model validators (2nd and 3rd lines of defence), model owners, model risk manager. Organisation charts including external resources involved in the modelling and validation process.
Model risk management. 1. Background and Motivation. The global financial crisis showed that controls and governance frameworks associated with valuation, risk and other operating models can be fragmented, incom-plete or unreliable. Therefore, regulators have increased scrutiny to ensure that financial institutions maintain effective and ...
the same quantification of model risk is difficult both for individual models and portfolios. This intractability can limit how compelling model risk management seems in the context of bank-wide risk management. 2. Quantification and reporting of model risk continue to be a challenge 8 Model Risk Management lobal Update 2019
Model risk management can be interpreted as the identification, assessment, monitoring, and mitigation of risks that arise from model deployment. While MRM had started to gain momentum following the global financial crisis, regulatory principles and guidance have been in effect since the early 2000s.
Model risk is the potential loss an institution may incur as a consequence of decisions that are principally based on the output of internal models as a result of errors in the development, implementation, or use of models. ... finance, Excel, business valuation, budgeting/forecasting, PowerPoint presentations, accounting and business strategy ...
Model risk arises when a financial model measures any company's quantitative information, such as its market risk or Value of transactions. As a result, the model fails or performs poorly, resulting in adverse effects for the company. A model is a system, quantitative method, or approach based on. Economic. Statistical.
The stakes in managing model risk have never been higher. When things go wrong, consequences can be severe. With digitization and automation, more models are being integrated into business processes, exposing institutions to greater model risk and consequent operational losses. The risk lies equally in defective models and model misuse.
Model Risk Management (MRM) Quality and Continuous Improvement: MRM provides actionable, timely, and value-added model validation activities. Through process improvement, automation, and training, MRM has improved quality and efficiency of validations. Timely and Complete: On track to complete 95%+ scheduled validation activities by 12/31/2021.
Model risk is a type of risk that occurs when a financial model used to measure a firm's market risks or value transactions fails or performs inadequately.
Tips to make risk management presentations engaging and actionable across your organization: Audience engagement is vital to a successful risk management training presentation. After all, if staff and executives are asleep they will hardly become familiar with the plan and their assigned roles. Include visual assets
Download risk diagrams and PowerPoint templates for project risk management. Under this category you can find affordable business diagrams and slide designs for Risk PPT presentations or Risk Management including awesome illustrations and Risk PowerPoint Templates with editable text that you can use to present a risk scenario or uncertainty.
* Source: Bank Risk Conference presentation in April 2015 by Konstantina Armata (Head of Global Model Validation & Governance at Deutsche Bank) = Process area. Process Design Organisation ... 2015 Aggregated Model Risk Score = 60 2015 Average Model's Risk Score = 12. THANK YOU. Title: Prezentacja programu PowerPoint
If you need to create a PowerPoint presentation about risk management, you can use a number of handy templates and techniques listed below. Risk Matrix. ... RAIDAR model is a risk management model used to assess risks, assumptions, issues, dependencies, action, and repairs. The model can be useful for assessing project risks and for their ...
Prepare the best presentation using our model risk management presentation templates and Google slides. Toggle Nav. Search. Search. Search . 5. Notifications 5. SlideGeeks added 303 new products (e.g. Completely ...
Use the parameters present in the risk analysis table and analyze the risk level. Demonstrate the steps to calculate the risk and its certainty utilizing the business risk PPT slides. Mention how to respond to the risk by highlighting positive and negative risk factors. Reduce the effects of the risks by incorporating risk management strategies ...
Model Governance and Model Risk Management: Risk Manager's Perspective Nikolai Kukharkin Quantitative Risk Control, UBS Measuring and Controlling Model Risk, New York, October 2011 DISCLAIMER The views and opinions expressed in this presentation are those of the author and may not reflect the views and opinions of UBS and should not be cited as being those of UBS.
This is a enterprise risk management analysis ppt model. This is a four stage process. The stages in this process are life insurance and risk management activities, input and risk identification, policy development, risk, based monitoring, financial reporting, contract and acquisition, operational. Slide 1 of 7.
For educational purposes, teachers can visually present risk analysis through this slide, and there are two background color variations available for this slide template. This PPT Template is 100% editable using PowerPoint, Google Slides, or Keynote. The Risk Analysis Diagram PowerPoint Template presents a complete process of risk analysis ...
Add or remove content, if needed. Grab this easy-to-understand risk assessment PowerPoint templates to figure out what could cause harm to the project, whether the hazards could be eliminated or not, what preventive measures should be taken to control the risks. Download risk assessment PPT slides now to execute the project easily.
Model risk is the risk that arises when a model fails or does not perform according to expectations. When we use mathematical models to support decision making, we face model risk since mathematical models can produce incorrect outcomes. Models can fail due to technical mistakes, such as lack of mathematical rigour, data issues, implementation ...
The graphic model of risk management will help users to provide an overview of management strategies in a single slide view. There are 4 slides of Three Lines of Defense PowerPoint Templates containing 2 versions of model design. The users can copy desired layout design according to presentation needs and color theme.
Risk Assessment Steps Powerpoint Ppt Template Bundles. Slide 1 of 7. Risk decision making model ppt sample download. Slide 1 of 6. Enterprise risk management categories with control model. Slide 1 of 5. Compliance risk and governance model. Slide 1 of 5. Risk response matrix ppt model icon.