Have a language expert improve your writing
Run a free plagiarism check in 10 minutes, generate accurate citations for free.
- Knowledge Base
- Null and Alternative Hypotheses | Definitions & Examples

Null & Alternative Hypotheses | Definitions, Templates & Examples
Published on May 6, 2022 by Shaun Turney . Revised on June 22, 2023.
The null and alternative hypotheses are two competing claims that researchers weigh evidence for and against using a statistical test :
- Null hypothesis ( H 0 ): There’s no effect in the population .
- Alternative hypothesis ( H a or H 1 ) : There’s an effect in the population.
Table of contents
Answering your research question with hypotheses, what is a null hypothesis, what is an alternative hypothesis, similarities and differences between null and alternative hypotheses, how to write null and alternative hypotheses, other interesting articles, frequently asked questions.
The null and alternative hypotheses offer competing answers to your research question . When the research question asks “Does the independent variable affect the dependent variable?”:
- The null hypothesis ( H 0 ) answers “No, there’s no effect in the population.”
- The alternative hypothesis ( H a ) answers “Yes, there is an effect in the population.”
The null and alternative are always claims about the population. That’s because the goal of hypothesis testing is to make inferences about a population based on a sample . Often, we infer whether there’s an effect in the population by looking at differences between groups or relationships between variables in the sample. It’s critical for your research to write strong hypotheses .
You can use a statistical test to decide whether the evidence favors the null or alternative hypothesis. Each type of statistical test comes with a specific way of phrasing the null and alternative hypothesis. However, the hypotheses can also be phrased in a general way that applies to any test.
Here's why students love Scribbr's proofreading services
Discover proofreading & editing
The null hypothesis is the claim that there’s no effect in the population.
If the sample provides enough evidence against the claim that there’s no effect in the population ( p ≤ α), then we can reject the null hypothesis . Otherwise, we fail to reject the null hypothesis.
Although “fail to reject” may sound awkward, it’s the only wording that statisticians accept . Be careful not to say you “prove” or “accept” the null hypothesis.
Null hypotheses often include phrases such as “no effect,” “no difference,” or “no relationship.” When written in mathematical terms, they always include an equality (usually =, but sometimes ≥ or ≤).
You can never know with complete certainty whether there is an effect in the population. Some percentage of the time, your inference about the population will be incorrect. When you incorrectly reject the null hypothesis, it’s called a type I error . When you incorrectly fail to reject it, it’s a type II error.
Examples of null hypotheses
The table below gives examples of research questions and null hypotheses. There’s always more than one way to answer a research question, but these null hypotheses can help you get started.
( ) | ||
Does tooth flossing affect the number of cavities? | Tooth flossing has on the number of cavities. | test: The mean number of cavities per person does not differ between the flossing group (µ ) and the non-flossing group (µ ) in the population; µ = µ . |
Does the amount of text highlighted in the textbook affect exam scores? | The amount of text highlighted in the textbook has on exam scores. | : There is no relationship between the amount of text highlighted and exam scores in the population; β = 0. |
Does daily meditation decrease the incidence of depression? | Daily meditation the incidence of depression.* | test: The proportion of people with depression in the daily-meditation group ( ) is greater than or equal to the no-meditation group ( ) in the population; ≥ . |
*Note that some researchers prefer to always write the null hypothesis in terms of “no effect” and “=”. It would be fine to say that daily meditation has no effect on the incidence of depression and p 1 = p 2 .
The alternative hypothesis ( H a ) is the other answer to your research question . It claims that there’s an effect in the population.
Often, your alternative hypothesis is the same as your research hypothesis. In other words, it’s the claim that you expect or hope will be true.
The alternative hypothesis is the complement to the null hypothesis. Null and alternative hypotheses are exhaustive, meaning that together they cover every possible outcome. They are also mutually exclusive, meaning that only one can be true at a time.
Alternative hypotheses often include phrases such as “an effect,” “a difference,” or “a relationship.” When alternative hypotheses are written in mathematical terms, they always include an inequality (usually ≠, but sometimes < or >). As with null hypotheses, there are many acceptable ways to phrase an alternative hypothesis.
Examples of alternative hypotheses
The table below gives examples of research questions and alternative hypotheses to help you get started with formulating your own.
Does tooth flossing affect the number of cavities? | Tooth flossing has an on the number of cavities. | test: The mean number of cavities per person differs between the flossing group (µ ) and the non-flossing group (µ ) in the population; µ ≠ µ . |
Does the amount of text highlighted in a textbook affect exam scores? | The amount of text highlighted in the textbook has an on exam scores. | : There is a relationship between the amount of text highlighted and exam scores in the population; β ≠ 0. |
Does daily meditation decrease the incidence of depression? | Daily meditation the incidence of depression. | test: The proportion of people with depression in the daily-meditation group ( ) is less than the no-meditation group ( ) in the population; < . |
Null and alternative hypotheses are similar in some ways:
- They’re both answers to the research question.
- They both make claims about the population.
- They’re both evaluated by statistical tests.
However, there are important differences between the two types of hypotheses, summarized in the following table.
A claim that there is in the population. | A claim that there is in the population. | |
| ||
Equality symbol (=, ≥, or ≤) | Inequality symbol (≠, <, or >) | |
Rejected | Supported | |
Failed to reject | Not supported |
Prevent plagiarism. Run a free check.
To help you write your hypotheses, you can use the template sentences below. If you know which statistical test you’re going to use, you can use the test-specific template sentences. Otherwise, you can use the general template sentences.
General template sentences
The only thing you need to know to use these general template sentences are your dependent and independent variables. To write your research question, null hypothesis, and alternative hypothesis, fill in the following sentences with your variables:
Does independent variable affect dependent variable ?
- Null hypothesis ( H 0 ): Independent variable does not affect dependent variable.
- Alternative hypothesis ( H a ): Independent variable affects dependent variable.
Test-specific template sentences
Once you know the statistical test you’ll be using, you can write your hypotheses in a more precise and mathematical way specific to the test you chose. The table below provides template sentences for common statistical tests.
( ) | ||
test
with two groups | The mean dependent variable does not differ between group 1 (µ ) and group 2 (µ ) in the population; µ = µ . | The mean dependent variable differs between group 1 (µ ) and group 2 (µ ) in the population; µ ≠ µ . |
with three groups | The mean dependent variable does not differ between group 1 (µ ), group 2 (µ ), and group 3 (µ ) in the population; µ = µ = µ . | The mean dependent variable of group 1 (µ ), group 2 (µ ), and group 3 (µ ) are not all equal in the population. |
There is no correlation between independent variable and dependent variable in the population; ρ = 0. | There is a correlation between independent variable and dependent variable in the population; ρ ≠ 0. | |
There is no relationship between independent variable and dependent variable in the population; β = 0. | There is a relationship between independent variable and dependent variable in the population; β ≠ 0. | |
Two-proportions test | The dependent variable expressed as a proportion does not differ between group 1 ( ) and group 2 ( ) in the population; = . | The dependent variable expressed as a proportion differs between group 1 ( ) and group 2 ( ) in the population; ≠ . |
Note: The template sentences above assume that you’re performing one-tailed tests . One-tailed tests are appropriate for most studies.
If you want to know more about statistics , methodology , or research bias , make sure to check out some of our other articles with explanations and examples.
- Normal distribution
- Descriptive statistics
- Measures of central tendency
- Correlation coefficient
Methodology
- Cluster sampling
- Stratified sampling
- Types of interviews
- Cohort study
- Thematic analysis
Research bias
- Implicit bias
- Cognitive bias
- Survivorship bias
- Availability heuristic
- Nonresponse bias
- Regression to the mean
Hypothesis testing is a formal procedure for investigating our ideas about the world using statistics. It is used by scientists to test specific predictions, called hypotheses , by calculating how likely it is that a pattern or relationship between variables could have arisen by chance.
Null and alternative hypotheses are used in statistical hypothesis testing . The null hypothesis of a test always predicts no effect or no relationship between variables, while the alternative hypothesis states your research prediction of an effect or relationship.
The null hypothesis is often abbreviated as H 0 . When the null hypothesis is written using mathematical symbols, it always includes an equality symbol (usually =, but sometimes ≥ or ≤).
The alternative hypothesis is often abbreviated as H a or H 1 . When the alternative hypothesis is written using mathematical symbols, it always includes an inequality symbol (usually ≠, but sometimes < or >).
A research hypothesis is your proposed answer to your research question. The research hypothesis usually includes an explanation (“ x affects y because …”).
A statistical hypothesis, on the other hand, is a mathematical statement about a population parameter. Statistical hypotheses always come in pairs: the null and alternative hypotheses . In a well-designed study , the statistical hypotheses correspond logically to the research hypothesis.
Cite this Scribbr article
If you want to cite this source, you can copy and paste the citation or click the “Cite this Scribbr article” button to automatically add the citation to our free Citation Generator.
Turney, S. (2023, June 22). Null & Alternative Hypotheses | Definitions, Templates & Examples. Scribbr. Retrieved August 21, 2024, from https://www.scribbr.com/statistics/null-and-alternative-hypotheses/
Is this article helpful?
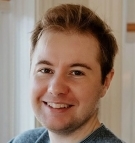
Shaun Turney
Other students also liked, inferential statistics | an easy introduction & examples, hypothesis testing | a step-by-step guide with easy examples, type i & type ii errors | differences, examples, visualizations, what is your plagiarism score.
9.1 Null and Alternative Hypotheses
The actual test begins by considering two hypotheses . They are called the null hypothesis and the alternative hypothesis . These hypotheses contain opposing viewpoints.
H 0 , the — null hypothesis: a statement of no difference between sample means or proportions or no difference between a sample mean or proportion and a population mean or proportion. In other words, the difference equals 0.
H a —, the alternative hypothesis: a claim about the population that is contradictory to H 0 and what we conclude when we reject H 0 .
Since the null and alternative hypotheses are contradictory, you must examine evidence to decide if you have enough evidence to reject the null hypothesis or not. The evidence is in the form of sample data.
After you have determined which hypothesis the sample supports, you make a decision. There are two options for a decision. They are reject H 0 if the sample information favors the alternative hypothesis or do not reject H 0 or decline to reject H 0 if the sample information is insufficient to reject the null hypothesis.
Mathematical Symbols Used in H 0 and H a :
equal (=) | not equal (≠) greater than (>) less than (<) |
greater than or equal to (≥) | less than (<) |
less than or equal to (≤) | more than (>) |
H 0 always has a symbol with an equal in it. H a never has a symbol with an equal in it. The choice of symbol depends on the wording of the hypothesis test. However, be aware that many researchers use = in the null hypothesis, even with > or < as the symbol in the alternative hypothesis. This practice is acceptable because we only make the decision to reject or not reject the null hypothesis.
Example 9.1
H 0 : No more than 30 percent of the registered voters in Santa Clara County voted in the primary election. p ≤ 30 H a : More than 30 percent of the registered voters in Santa Clara County voted in the primary election. p > 30
A medical trial is conducted to test whether or not a new medicine reduces cholesterol by 25 percent. State the null and alternative hypotheses.
Example 9.2
We want to test whether the mean GPA of students in American colleges is different from 2.0 (out of 4.0). The null and alternative hypotheses are the following: H 0 : μ = 2.0 H a : μ ≠ 2.0
We want to test whether the mean height of eighth graders is 66 inches. State the null and alternative hypotheses. Fill in the correct symbol (=, ≠, ≥, <, ≤, >) for the null and alternative hypotheses.
- H 0 : μ __ 66
- H a : μ __ 66
Example 9.3
We want to test if college students take fewer than five years to graduate from college, on the average. The null and alternative hypotheses are the following: H 0 : μ ≥ 5 H a : μ < 5
We want to test if it takes fewer than 45 minutes to teach a lesson plan. State the null and alternative hypotheses. Fill in the correct symbol ( =, ≠, ≥, <, ≤, >) for the null and alternative hypotheses.
- H 0 : μ __ 45
- H a : μ __ 45
Example 9.4
An article on school standards stated that about half of all students in France, Germany, and Israel take advanced placement exams and a third of the students pass. The same article stated that 6.6 percent of U.S. students take advanced placement exams and 4.4 percent pass. Test if the percentage of U.S. students who take advanced placement exams is more than 6.6 percent. State the null and alternative hypotheses. H 0 : p ≤ 0.066 H a : p > 0.066
On a state driver’s test, about 40 percent pass the test on the first try. We want to test if more than 40 percent pass on the first try. Fill in the correct symbol (=, ≠, ≥, <, ≤, >) for the null and alternative hypotheses.
- H 0 : p __ 0.40
- H a : p __ 0.40
Collaborative Exercise
Bring to class a newspaper, some news magazines, and some internet articles. In groups, find articles from which your group can write null and alternative hypotheses. Discuss your hypotheses with the rest of the class.
This book may not be used in the training of large language models or otherwise be ingested into large language models or generative AI offerings without OpenStax's permission.
Want to cite, share, or modify this book? This book uses the Creative Commons Attribution License and you must attribute Texas Education Agency (TEA). The original material is available at: https://www.texasgateway.org/book/tea-statistics . Changes were made to the original material, including updates to art, structure, and other content updates.
Access for free at https://openstax.org/books/statistics/pages/1-introduction
- Authors: Barbara Illowsky, Susan Dean
- Publisher/website: OpenStax
- Book title: Statistics
- Publication date: Mar 27, 2020
- Location: Houston, Texas
- Book URL: https://openstax.org/books/statistics/pages/1-introduction
- Section URL: https://openstax.org/books/statistics/pages/9-1-null-and-alternative-hypotheses
© Apr 16, 2024 Texas Education Agency (TEA). The OpenStax name, OpenStax logo, OpenStax book covers, OpenStax CNX name, and OpenStax CNX logo are not subject to the Creative Commons license and may not be reproduced without the prior and express written consent of Rice University.
- Skip to secondary menu
- Skip to main content
- Skip to primary sidebar
Statistics By Jim
Making statistics intuitive
Hypothesis Testing: Uses, Steps & Example
By Jim Frost 4 Comments
What is Hypothesis Testing?
Hypothesis testing in statistics uses sample data to infer the properties of a whole population . These tests determine whether a random sample provides sufficient evidence to conclude an effect or relationship exists in the population. Researchers use them to help separate genuine population-level effects from false effects that random chance can create in samples. These methods are also known as significance testing.

For example, researchers are testing a new medication to see if it lowers blood pressure. They compare a group taking the drug to a control group taking a placebo. If their hypothesis test results are statistically significant, the medication’s effect of lowering blood pressure likely exists in the broader population, not just the sample studied.
Using Hypothesis Tests
A hypothesis test evaluates two mutually exclusive statements about a population to determine which statement the sample data best supports. These two statements are called the null hypothesis and the alternative hypothesis . The following are typical examples:
- Null Hypothesis : The effect does not exist in the population.
- Alternative Hypothesis : The effect does exist in the population.
Hypothesis testing accounts for the inherent uncertainty of using a sample to draw conclusions about a population, which reduces the chances of false discoveries. These procedures determine whether the sample data are sufficiently inconsistent with the null hypothesis that you can reject it. If you can reject the null, your data favor the alternative statement that an effect exists in the population.
Statistical significance in hypothesis testing indicates that an effect you see in sample data also likely exists in the population after accounting for random sampling error , variability, and sample size. Your results are statistically significant when the p-value is less than your significance level or, equivalently, when your confidence interval excludes the null hypothesis value.
Conversely, non-significant results indicate that despite an apparent sample effect, you can’t be sure it exists in the population. It could be chance variation in the sample and not a genuine effect.
Learn more about Failing to Reject the Null .
5 Steps of Significance Testing
Hypothesis testing involves five key steps, each critical to validating a research hypothesis using statistical methods:
- Formulate the Hypotheses : Write your research hypotheses as a null hypothesis (H 0 ) and an alternative hypothesis (H A ).
- Data Collection : Gather data specifically aimed at testing the hypothesis.
- Conduct A Test : Use a suitable statistical test to analyze your data.
- Make a Decision : Based on the statistical test results, decide whether to reject the null hypothesis or fail to reject it.
- Report the Results : Summarize and present the outcomes in your report’s results and discussion sections.
While the specifics of these steps can vary depending on the research context and the data type, the fundamental process of hypothesis testing remains consistent across different studies.
Let’s work through these steps in an example!
Hypothesis Testing Example
Researchers want to determine if a new educational program improves student performance on standardized tests. They randomly assign 30 students to a control group , which follows the standard curriculum, and another 30 students to a treatment group, which participates in the new educational program. After a semester, they compare the test scores of both groups.
Download the CSV data file to perform the hypothesis testing yourself: Hypothesis_Testing .
The researchers write their hypotheses. These statements apply to the population, so they use the mu (μ) symbol for the population mean parameter .
- Null Hypothesis (H 0 ) : The population means of the test scores for the two groups are equal (μ 1 = μ 2 ).
- Alternative Hypothesis (H A ) : The population means of the test scores for the two groups are unequal (μ 1 ≠ μ 2 ).
Choosing the correct hypothesis test depends on attributes such as data type and number of groups. Because they’re using continuous data and comparing two means, the researchers use a 2-sample t-test .
Here are the results.

The treatment group’s mean is 58.70, compared to the control group’s mean of 48.12. The mean difference is 10.67 points. Use the test’s p-value and significance level to determine whether this difference is likely a product of random fluctuation in the sample or a genuine population effect.
Because the p-value (0.000) is less than the standard significance level of 0.05, the results are statistically significant, and we can reject the null hypothesis. The sample data provides sufficient evidence to conclude that the new program’s effect exists in the population.
Limitations
Hypothesis testing improves your effectiveness in making data-driven decisions. However, it is not 100% accurate because random samples occasionally produce fluky results. Hypothesis tests have two types of errors, both relating to drawing incorrect conclusions.
- Type I error: The test rejects a true null hypothesis—a false positive.
- Type II error: The test fails to reject a false null hypothesis—a false negative.
Learn more about Type I and Type II Errors .
Our exploration of hypothesis testing using a practical example of an educational program reveals its powerful ability to guide decisions based on statistical evidence. Whether you’re a student, researcher, or professional, understanding and applying these procedures can open new doors to discovering insights and making informed decisions. Let this tool empower your analytical endeavors as you navigate through the vast seas of data.
Learn more about the Hypothesis Tests for Various Data Types .
Share this:

Reader Interactions
June 10, 2024 at 10:51 am
Thank you, Jim, for another helpful article; timely too since I have started reading your new book on hypothesis testing and, now that we are at the end of the school year, my district is asking me to perform a number of evaluations on instructional programs. This is where my question/concern comes in. You mention that hypothesis testing is all about testing samples. However, I use all the students in my district when I make these comparisons. Since I am using the entire “population” in my evaluations (I don’t select a sample of third grade students, for example, but I use all 700 third graders), am I somehow misusing the tests? Or can I rest assured that my district’s student population is only a sample of the universal population of students?
June 10, 2024 at 1:50 pm
I hope you are finding the book helpful!
Yes, the purpose of hypothesis testing is to infer the properties of a population while accounting for random sampling error.
In your case, it comes down to how you want to use the results. Who do you want the results to apply to?
If you’re summarizing the sample, looking for trends and patterns, or evaluating those students and don’t plan to apply those results to other students, you don’t need hypothesis testing because there is no sampling error. They are the population and you can just use descriptive statistics. In this case, you’d only need to focus on the practical significance of the effect sizes.
On the other hand, if you want to apply the results from this group to other students, you’ll need hypothesis testing. However, there is the complicating issue of what population your sample of students represent. I’m sure your district has its own unique characteristics, demographics, etc. Your district’s students probably don’t adequately represent a universal population. At the very least, you’d need to recognize any special attributes of your district and how they could bias the results when trying to apply them outside the district. Or they might apply to similar districts in your region.
However, I’d imagine your 3rd graders probably adequately represent future classes of 3rd graders in your district. You need to be alert to changing demographics. At least in the short run I’d imagine they’d be representative of future classes.
Think about how these results will be used. Do they just apply to the students you measured? Then you don’t need hypothesis tests. However, if the results are being used to infer things about other students outside of the sample, you’ll need hypothesis testing along with considering how well your students represent the other students and how they differ.
I hope that helps!
June 10, 2024 at 3:21 pm
Thank you so much, Jim, for the suggestions in terms of what I need to think about and consider! You are always so clear in your explanations!!!!
June 10, 2024 at 3:22 pm
You’re very welcome! Best of luck with your evaluations!
Comments and Questions Cancel reply
What is The Null Hypothesis & When Do You Reject The Null Hypothesis
Julia Simkus
Editor at Simply Psychology
BA (Hons) Psychology, Princeton University
Julia Simkus is a graduate of Princeton University with a Bachelor of Arts in Psychology. She is currently studying for a Master's Degree in Counseling for Mental Health and Wellness in September 2023. Julia's research has been published in peer reviewed journals.
Learn about our Editorial Process
Saul McLeod, PhD
Editor-in-Chief for Simply Psychology
BSc (Hons) Psychology, MRes, PhD, University of Manchester
Saul McLeod, PhD., is a qualified psychology teacher with over 18 years of experience in further and higher education. He has been published in peer-reviewed journals, including the Journal of Clinical Psychology.
Olivia Guy-Evans, MSc
Associate Editor for Simply Psychology
BSc (Hons) Psychology, MSc Psychology of Education
Olivia Guy-Evans is a writer and associate editor for Simply Psychology. She has previously worked in healthcare and educational sectors.
On This Page:
A null hypothesis is a statistical concept suggesting no significant difference or relationship between measured variables. It’s the default assumption unless empirical evidence proves otherwise.
The null hypothesis states no relationship exists between the two variables being studied (i.e., one variable does not affect the other).
The null hypothesis is the statement that a researcher or an investigator wants to disprove.
Testing the null hypothesis can tell you whether your results are due to the effects of manipulating the dependent variable or due to random chance.
How to Write a Null Hypothesis
Null hypotheses (H0) start as research questions that the investigator rephrases as statements indicating no effect or relationship between the independent and dependent variables.
It is a default position that your research aims to challenge or confirm.
For example, if studying the impact of exercise on weight loss, your null hypothesis might be:
There is no significant difference in weight loss between individuals who exercise daily and those who do not.
Examples of Null Hypotheses
Research Question | Null Hypothesis |
---|---|
Do teenagers use cell phones more than adults? | Teenagers and adults use cell phones the same amount. |
Do tomato plants exhibit a higher rate of growth when planted in compost rather than in soil? | Tomato plants show no difference in growth rates when planted in compost rather than soil. |
Does daily meditation decrease the incidence of depression? | Daily meditation does not decrease the incidence of depression. |
Does daily exercise increase test performance? | There is no relationship between daily exercise time and test performance. |
Does the new vaccine prevent infections? | The vaccine does not affect the infection rate. |
Does flossing your teeth affect the number of cavities? | Flossing your teeth has no effect on the number of cavities. |
When Do We Reject The Null Hypothesis?
We reject the null hypothesis when the data provide strong enough evidence to conclude that it is likely incorrect. This often occurs when the p-value (probability of observing the data given the null hypothesis is true) is below a predetermined significance level.
If the collected data does not meet the expectation of the null hypothesis, a researcher can conclude that the data lacks sufficient evidence to back up the null hypothesis, and thus the null hypothesis is rejected.
Rejecting the null hypothesis means that a relationship does exist between a set of variables and the effect is statistically significant ( p > 0.05).
If the data collected from the random sample is not statistically significance , then the null hypothesis will be accepted, and the researchers can conclude that there is no relationship between the variables.
You need to perform a statistical test on your data in order to evaluate how consistent it is with the null hypothesis. A p-value is one statistical measurement used to validate a hypothesis against observed data.
Calculating the p-value is a critical part of null-hypothesis significance testing because it quantifies how strongly the sample data contradicts the null hypothesis.
The level of statistical significance is often expressed as a p -value between 0 and 1. The smaller the p-value, the stronger the evidence that you should reject the null hypothesis.
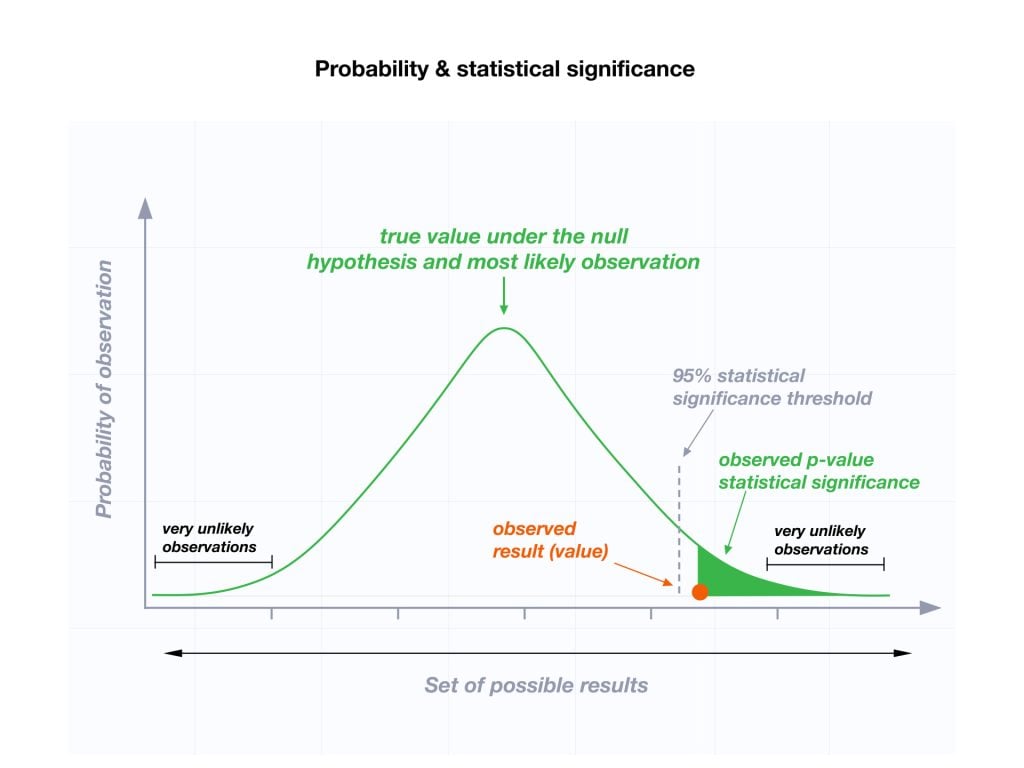
Usually, a researcher uses a confidence level of 95% or 99% (p-value of 0.05 or 0.01) as general guidelines to decide if you should reject or keep the null.
When your p-value is less than or equal to your significance level, you reject the null hypothesis.
In other words, smaller p-values are taken as stronger evidence against the null hypothesis. Conversely, when the p-value is greater than your significance level, you fail to reject the null hypothesis.
In this case, the sample data provides insufficient data to conclude that the effect exists in the population.
Because you can never know with complete certainty whether there is an effect in the population, your inferences about a population will sometimes be incorrect.
When you incorrectly reject the null hypothesis, it’s called a type I error. When you incorrectly fail to reject it, it’s called a type II error.
Why Do We Never Accept The Null Hypothesis?
The reason we do not say “accept the null” is because we are always assuming the null hypothesis is true and then conducting a study to see if there is evidence against it. And, even if we don’t find evidence against it, a null hypothesis is not accepted.
A lack of evidence only means that you haven’t proven that something exists. It does not prove that something doesn’t exist.
It is risky to conclude that the null hypothesis is true merely because we did not find evidence to reject it. It is always possible that researchers elsewhere have disproved the null hypothesis, so we cannot accept it as true, but instead, we state that we failed to reject the null.
One can either reject the null hypothesis, or fail to reject it, but can never accept it.
Why Do We Use The Null Hypothesis?
We can never prove with 100% certainty that a hypothesis is true; We can only collect evidence that supports a theory. However, testing a hypothesis can set the stage for rejecting or accepting this hypothesis within a certain confidence level.
The null hypothesis is useful because it can tell us whether the results of our study are due to random chance or the manipulation of a variable (with a certain level of confidence).
A null hypothesis is rejected if the measured data is significantly unlikely to have occurred and a null hypothesis is accepted if the observed outcome is consistent with the position held by the null hypothesis.
Rejecting the null hypothesis sets the stage for further experimentation to see if a relationship between two variables exists.
Hypothesis testing is a critical part of the scientific method as it helps decide whether the results of a research study support a particular theory about a given population. Hypothesis testing is a systematic way of backing up researchers’ predictions with statistical analysis.
It helps provide sufficient statistical evidence that either favors or rejects a certain hypothesis about the population parameter.
Purpose of a Null Hypothesis
- The primary purpose of the null hypothesis is to disprove an assumption.
- Whether rejected or accepted, the null hypothesis can help further progress a theory in many scientific cases.
- A null hypothesis can be used to ascertain how consistent the outcomes of multiple studies are.
Do you always need both a Null Hypothesis and an Alternative Hypothesis?
The null (H0) and alternative (Ha or H1) hypotheses are two competing claims that describe the effect of the independent variable on the dependent variable. They are mutually exclusive, which means that only one of the two hypotheses can be true.
While the null hypothesis states that there is no effect in the population, an alternative hypothesis states that there is statistical significance between two variables.
The goal of hypothesis testing is to make inferences about a population based on a sample. In order to undertake hypothesis testing, you must express your research hypothesis as a null and alternative hypothesis. Both hypotheses are required to cover every possible outcome of the study.
What is the difference between a null hypothesis and an alternative hypothesis?
The alternative hypothesis is the complement to the null hypothesis. The null hypothesis states that there is no effect or no relationship between variables, while the alternative hypothesis claims that there is an effect or relationship in the population.
It is the claim that you expect or hope will be true. The null hypothesis and the alternative hypothesis are always mutually exclusive, meaning that only one can be true at a time.
What are some problems with the null hypothesis?
One major problem with the null hypothesis is that researchers typically will assume that accepting the null is a failure of the experiment. However, accepting or rejecting any hypothesis is a positive result. Even if the null is not refuted, the researchers will still learn something new.
Why can a null hypothesis not be accepted?
We can either reject or fail to reject a null hypothesis, but never accept it. If your test fails to detect an effect, this is not proof that the effect doesn’t exist. It just means that your sample did not have enough evidence to conclude that it exists.
We can’t accept a null hypothesis because a lack of evidence does not prove something that does not exist. Instead, we fail to reject it.
Failing to reject the null indicates that the sample did not provide sufficient enough evidence to conclude that an effect exists.
If the p-value is greater than the significance level, then you fail to reject the null hypothesis.
Is a null hypothesis directional or non-directional?
A hypothesis test can either contain an alternative directional hypothesis or a non-directional alternative hypothesis. A directional hypothesis is one that contains the less than (“<“) or greater than (“>”) sign.
A nondirectional hypothesis contains the not equal sign (“≠”). However, a null hypothesis is neither directional nor non-directional.
A null hypothesis is a prediction that there will be no change, relationship, or difference between two variables.
The directional hypothesis or nondirectional hypothesis would then be considered alternative hypotheses to the null hypothesis.
Gill, J. (1999). The insignificance of null hypothesis significance testing. Political research quarterly , 52 (3), 647-674.
Krueger, J. (2001). Null hypothesis significance testing: On the survival of a flawed method. American Psychologist , 56 (1), 16.
Masson, M. E. (2011). A tutorial on a practical Bayesian alternative to null-hypothesis significance testing. Behavior research methods , 43 , 679-690.
Nickerson, R. S. (2000). Null hypothesis significance testing: a review of an old and continuing controversy. Psychological methods , 5 (2), 241.
Rozeboom, W. W. (1960). The fallacy of the null-hypothesis significance test. Psychological bulletin , 57 (5), 416.

13.1 Understanding Null Hypothesis Testing
Learning objectives.
- Explain the purpose of null hypothesis testing, including the role of sampling error.
- Describe the basic logic of null hypothesis testing.
- Describe the role of relationship strength and sample size in determining statistical significance and make reasonable judgments about statistical significance based on these two factors.
The Purpose of Null Hypothesis Testing
As we have seen, psychological research typically involves measuring one or more variables in a sample and computing descriptive statistics for that sample. In general, however, the researcher’s goal is not to draw conclusions about that sample but to draw conclusions about the population that the sample was selected from. Thus researchers must use sample statistics to draw conclusions about the corresponding values in the population. These corresponding values in the population are called parameters . Imagine, for example, that a researcher measures the number of depressive symptoms exhibited by each of 50 adults with clinical depression and computes the mean number of symptoms. The researcher probably wants to use this sample statistic (the mean number of symptoms for the sample) to draw conclusions about the corresponding population parameter (the mean number of symptoms for adults with clinical depression).
Unfortunately, sample statistics are not perfect estimates of their corresponding population parameters. This is because there is a certain amount of random variability in any statistic from sample to sample. The mean number of depressive symptoms might be 8.73 in one sample of adults with clinical depression, 6.45 in a second sample, and 9.44 in a third—even though these samples are selected randomly from the same population. Similarly, the correlation (Pearson’s r ) between two variables might be +.24 in one sample, −.04 in a second sample, and +.15 in a third—again, even though these samples are selected randomly from the same population. This random variability in a statistic from sample to sample is called sampling error . (Note that the term error here refers to random variability and does not imply that anyone has made a mistake. No one “commits a sampling error.”)
One implication of this is that when there is a statistical relationship in a sample, it is not always clear that there is a statistical relationship in the population. A small difference between two group means in a sample might indicate that there is a small difference between the two group means in the population. But it could also be that there is no difference between the means in the population and that the difference in the sample is just a matter of sampling error. Similarly, a Pearson’s r value of −.29 in a sample might mean that there is a negative relationship in the population. But it could also be that there is no relationship in the population and that the relationship in the sample is just a matter of sampling error.
In fact, any statistical relationship in a sample can be interpreted in two ways:
- There is a relationship in the population, and the relationship in the sample reflects this.
- There is no relationship in the population, and the relationship in the sample reflects only sampling error.
The purpose of null hypothesis testing is simply to help researchers decide between these two interpretations.
The Logic of Null Hypothesis Testing
Null hypothesis testing is a formal approach to deciding between two interpretations of a statistical relationship in a sample. One interpretation is called the null hypothesis (often symbolized H 0 and read as “H-naught”). This is the idea that there is no relationship in the population and that the relationship in the sample reflects only sampling error. Informally, the null hypothesis is that the sample relationship “occurred by chance.” The other interpretation is called the alternative hypothesis (often symbolized as H 1 ). This is the idea that there is a relationship in the population and that the relationship in the sample reflects this relationship in the population.
Again, every statistical relationship in a sample can be interpreted in either of these two ways: It might have occurred by chance, or it might reflect a relationship in the population. So researchers need a way to decide between them. Although there are many specific null hypothesis testing techniques, they are all based on the same general logic. The steps are as follows:
- Assume for the moment that the null hypothesis is true. There is no relationship between the variables in the population.
- Determine how likely the sample relationship would be if the null hypothesis were true.
- If the sample relationship would be extremely unlikely, then reject the null hypothesis in favor of the alternative hypothesis. If it would not be extremely unlikely, then retain the null hypothesis .
Following this logic, we can begin to understand why Mehl and his colleagues concluded that there is no difference in talkativeness between women and men in the population. In essence, they asked the following question: “If there were no difference in the population, how likely is it that we would find a small difference of d = 0.06 in our sample?” Their answer to this question was that this sample relationship would be fairly likely if the null hypothesis were true. Therefore, they retained the null hypothesis—concluding that there is no evidence of a sex difference in the population. We can also see why Kanner and his colleagues concluded that there is a correlation between hassles and symptoms in the population. They asked, “If the null hypothesis were true, how likely is it that we would find a strong correlation of +.60 in our sample?” Their answer to this question was that this sample relationship would be fairly unlikely if the null hypothesis were true. Therefore, they rejected the null hypothesis in favor of the alternative hypothesis—concluding that there is a positive correlation between these variables in the population.
A crucial step in null hypothesis testing is finding the likelihood of the sample result if the null hypothesis were true. This probability is called the p value . A low p value means that the sample result would be unlikely if the null hypothesis were true and leads to the rejection of the null hypothesis. A p value that is not low means that the sample result would be likely if the null hypothesis were true and leads to the retention of the null hypothesis. But how low must the p value be before the sample result is considered unlikely enough to reject the null hypothesis? In null hypothesis testing, this criterion is called α (alpha) and is almost always set to .05. If there is a 5% chance or less of a result as extreme as the sample result if the null hypothesis were true, then the null hypothesis is rejected. When this happens, the result is said to be statistically significant . If there is greater than a 5% chance of a result as extreme as the sample result when the null hypothesis is true, then the null hypothesis is retained. This does not necessarily mean that the researcher accepts the null hypothesis as true—only that there is not currently enough evidence to reject it. Researchers often use the expression “fail to reject the null hypothesis” rather than “retain the null hypothesis,” but they never use the expression “accept the null hypothesis.”
The Misunderstood p Value
The p value is one of the most misunderstood quantities in psychological research (Cohen, 1994) [1] . Even professional researchers misinterpret it, and it is not unusual for such misinterpretations to appear in statistics textbooks!
The most common misinterpretation is that the p value is the probability that the null hypothesis is true—that the sample result occurred by chance. For example, a misguided researcher might say that because the p value is .02, there is only a 2% chance that the result is due to chance and a 98% chance that it reflects a real relationship in the population. But this is incorrect . The p value is really the probability of a result at least as extreme as the sample result if the null hypothesis were true. So a p value of .02 means that if the null hypothesis were true, a sample result this extreme would occur only 2% of the time.
You can avoid this misunderstanding by remembering that the p value is not the probability that any particular hypothesis is true or false. Instead, it is the probability of obtaining the sample result if the null hypothesis were true.

“Null Hypothesis” retrieved from http://imgs.xkcd.com/comics/null_hypothesis.png (CC-BY-NC 2.5)
Role of Sample Size and Relationship Strength
Recall that null hypothesis testing involves answering the question, “If the null hypothesis were true, what is the probability of a sample result as extreme as this one?” In other words, “What is the p value?” It can be helpful to see that the answer to this question depends on just two considerations: the strength of the relationship and the size of the sample. Specifically, the stronger the sample relationship and the larger the sample, the less likely the result would be if the null hypothesis were true. That is, the lower the p value. This should make sense. Imagine a study in which a sample of 500 women is compared with a sample of 500 men in terms of some psychological characteristic, and Cohen’s d is a strong 0.50. If there were really no sex difference in the population, then a result this strong based on such a large sample should seem highly unlikely. Now imagine a similar study in which a sample of three women is compared with a sample of three men, and Cohen’s d is a weak 0.10. If there were no sex difference in the population, then a relationship this weak based on such a small sample should seem likely. And this is precisely why the null hypothesis would be rejected in the first example and retained in the second.
Of course, sometimes the result can be weak and the sample large, or the result can be strong and the sample small. In these cases, the two considerations trade off against each other so that a weak result can be statistically significant if the sample is large enough and a strong relationship can be statistically significant even if the sample is small. Table 13.1 shows roughly how relationship strength and sample size combine to determine whether a sample result is statistically significant. The columns of the table represent the three levels of relationship strength: weak, medium, and strong. The rows represent four sample sizes that can be considered small, medium, large, and extra large in the context of psychological research. Thus each cell in the table represents a combination of relationship strength and sample size. If a cell contains the word Yes , then this combination would be statistically significant for both Cohen’s d and Pearson’s r . If it contains the word No , then it would not be statistically significant for either. There is one cell where the decision for d and r would be different and another where it might be different depending on some additional considerations, which are discussed in Section 13.2 “Some Basic Null Hypothesis Tests”
Sample Size | Weak | Medium | Strong |
Small ( = 20) | No | No | = Maybe = Yes |
Medium ( = 50) | No | Yes | Yes |
Large ( = 100) | = Yes = No | Yes | Yes |
Extra large ( = 500) | Yes | Yes | Yes |
Although Table 13.1 provides only a rough guideline, it shows very clearly that weak relationships based on medium or small samples are never statistically significant and that strong relationships based on medium or larger samples are always statistically significant. If you keep this lesson in mind, you will often know whether a result is statistically significant based on the descriptive statistics alone. It is extremely useful to be able to develop this kind of intuitive judgment. One reason is that it allows you to develop expectations about how your formal null hypothesis tests are going to come out, which in turn allows you to detect problems in your analyses. For example, if your sample relationship is strong and your sample is medium, then you would expect to reject the null hypothesis. If for some reason your formal null hypothesis test indicates otherwise, then you need to double-check your computations and interpretations. A second reason is that the ability to make this kind of intuitive judgment is an indication that you understand the basic logic of this approach in addition to being able to do the computations.
Statistical Significance Versus Practical Significance
Table 13.1 illustrates another extremely important point. A statistically significant result is not necessarily a strong one. Even a very weak result can be statistically significant if it is based on a large enough sample. This is closely related to Janet Shibley Hyde’s argument about sex differences (Hyde, 2007) [2] . The differences between women and men in mathematical problem solving and leadership ability are statistically significant. But the word significant can cause people to interpret these differences as strong and important—perhaps even important enough to influence the college courses they take or even who they vote for. As we have seen, however, these statistically significant differences are actually quite weak—perhaps even “trivial.”
This is why it is important to distinguish between the statistical significance of a result and the practical significance of that result. Practical significance refers to the importance or usefulness of the result in some real-world context. Many sex differences are statistically significant—and may even be interesting for purely scientific reasons—but they are not practically significant. In clinical practice, this same concept is often referred to as “clinical significance.” For example, a study on a new treatment for social phobia might show that it produces a statistically significant positive effect. Yet this effect still might not be strong enough to justify the time, effort, and other costs of putting it into practice—especially if easier and cheaper treatments that work almost as well already exist. Although statistically significant, this result would be said to lack practical or clinical significance.

“Conditional Risk” retrieved from http://imgs.xkcd.com/comics/conditional_risk.png (CC-BY-NC 2.5)
Key Takeaways
- Null hypothesis testing is a formal approach to deciding whether a statistical relationship in a sample reflects a real relationship in the population or is just due to chance.
- The logic of null hypothesis testing involves assuming that the null hypothesis is true, finding how likely the sample result would be if this assumption were correct, and then making a decision. If the sample result would be unlikely if the null hypothesis were true, then it is rejected in favor of the alternative hypothesis. If it would not be unlikely, then the null hypothesis is retained.
- The probability of obtaining the sample result if the null hypothesis were true (the p value) is based on two considerations: relationship strength and sample size. Reasonable judgments about whether a sample relationship is statistically significant can often be made by quickly considering these two factors.
- Statistical significance is not the same as relationship strength or importance. Even weak relationships can be statistically significant if the sample size is large enough. It is important to consider relationship strength and the practical significance of a result in addition to its statistical significance.
- Discussion: Imagine a study showing that people who eat more broccoli tend to be happier. Explain for someone who knows nothing about statistics why the researchers would conduct a null hypothesis test.
- The correlation between two variables is r = −.78 based on a sample size of 137.
- The mean score on a psychological characteristic for women is 25 ( SD = 5) and the mean score for men is 24 ( SD = 5). There were 12 women and 10 men in this study.
- In a memory experiment, the mean number of items recalled by the 40 participants in Condition A was 0.50 standard deviations greater than the mean number recalled by the 40 participants in Condition B.
- In another memory experiment, the mean scores for participants in Condition A and Condition B came out exactly the same!
- A student finds a correlation of r = .04 between the number of units the students in his research methods class are taking and the students’ level of stress.
- Cohen, J. (1994). The world is round: p < .05. American Psychologist, 49 , 997–1003. ↵
- Hyde, J. S. (2007). New directions in the study of gender similarities and differences. Current Directions in Psychological Science, 16 , 259–263. ↵

Share This Book
- Increase Font Size
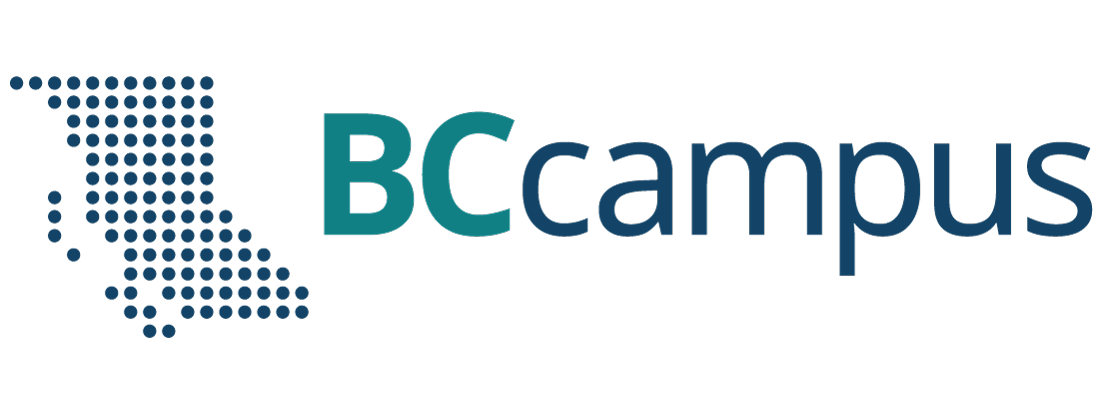
Want to create or adapt books like this? Learn more about how Pressbooks supports open publishing practices.
Chapter 13: Inferential Statistics
Understanding Null Hypothesis Testing
Learning Objectives
- Explain the purpose of null hypothesis testing, including the role of sampling error.
- Describe the basic logic of null hypothesis testing.
- Describe the role of relationship strength and sample size in determining statistical significance and make reasonable judgments about statistical significance based on these two factors.
The Purpose of Null Hypothesis Testing
As we have seen, psychological research typically involves measuring one or more variables for a sample and computing descriptive statistics for that sample. In general, however, the researcher’s goal is not to draw conclusions about that sample but to draw conclusions about the population that the sample was selected from. Thus researchers must use sample statistics to draw conclusions about the corresponding values in the population. These corresponding values in the population are called parameters . Imagine, for example, that a researcher measures the number of depressive symptoms exhibited by each of 50 clinically depressed adults and computes the mean number of symptoms. The researcher probably wants to use this sample statistic (the mean number of symptoms for the sample) to draw conclusions about the corresponding population parameter (the mean number of symptoms for clinically depressed adults).
Unfortunately, sample statistics are not perfect estimates of their corresponding population parameters. This is because there is a certain amount of random variability in any statistic from sample to sample. The mean number of depressive symptoms might be 8.73 in one sample of clinically depressed adults, 6.45 in a second sample, and 9.44 in a third—even though these samples are selected randomly from the same population. Similarly, the correlation (Pearson’s r ) between two variables might be +.24 in one sample, −.04 in a second sample, and +.15 in a third—again, even though these samples are selected randomly from the same population. This random variability in a statistic from sample to sample is called sampling error . (Note that the term error here refers to random variability and does not imply that anyone has made a mistake. No one “commits a sampling error.”)
One implication of this is that when there is a statistical relationship in a sample, it is not always clear that there is a statistical relationship in the population. A small difference between two group means in a sample might indicate that there is a small difference between the two group means in the population. But it could also be that there is no difference between the means in the population and that the difference in the sample is just a matter of sampling error. Similarly, a Pearson’s r value of −.29 in a sample might mean that there is a negative relationship in the population. But it could also be that there is no relationship in the population and that the relationship in the sample is just a matter of sampling error.
In fact, any statistical relationship in a sample can be interpreted in two ways:
- There is a relationship in the population, and the relationship in the sample reflects this.
- There is no relationship in the population, and the relationship in the sample reflects only sampling error.
The purpose of null hypothesis testing is simply to help researchers decide between these two interpretations.
The Logic of Null Hypothesis Testing
Null hypothesis testing is a formal approach to deciding between two interpretations of a statistical relationship in a sample. One interpretation is called the null hypothesis (often symbolized H 0 and read as “H-naught”). This is the idea that there is no relationship in the population and that the relationship in the sample reflects only sampling error. Informally, the null hypothesis is that the sample relationship “occurred by chance.” The other interpretation is called the alternative hypothesis (often symbolized as H 1 ). This is the idea that there is a relationship in the population and that the relationship in the sample reflects this relationship in the population.
Again, every statistical relationship in a sample can be interpreted in either of these two ways: It might have occurred by chance, or it might reflect a relationship in the population. So researchers need a way to decide between them. Although there are many specific null hypothesis testing techniques, they are all based on the same general logic. The steps are as follows:
- Assume for the moment that the null hypothesis is true. There is no relationship between the variables in the population.
- Determine how likely the sample relationship would be if the null hypothesis were true.
- If the sample relationship would be extremely unlikely, then reject the null hypothesis in favour of the alternative hypothesis. If it would not be extremely unlikely, then retain the null hypothesis .
Following this logic, we can begin to understand why Mehl and his colleagues concluded that there is no difference in talkativeness between women and men in the population. In essence, they asked the following question: “If there were no difference in the population, how likely is it that we would find a small difference of d = 0.06 in our sample?” Their answer to this question was that this sample relationship would be fairly likely if the null hypothesis were true. Therefore, they retained the null hypothesis—concluding that there is no evidence of a sex difference in the population. We can also see why Kanner and his colleagues concluded that there is a correlation between hassles and symptoms in the population. They asked, “If the null hypothesis were true, how likely is it that we would find a strong correlation of +.60 in our sample?” Their answer to this question was that this sample relationship would be fairly unlikely if the null hypothesis were true. Therefore, they rejected the null hypothesis in favour of the alternative hypothesis—concluding that there is a positive correlation between these variables in the population.
A crucial step in null hypothesis testing is finding the likelihood of the sample result if the null hypothesis were true. This probability is called the p value . A low p value means that the sample result would be unlikely if the null hypothesis were true and leads to the rejection of the null hypothesis. A high p value means that the sample result would be likely if the null hypothesis were true and leads to the retention of the null hypothesis. But how low must the p value be before the sample result is considered unlikely enough to reject the null hypothesis? In null hypothesis testing, this criterion is called α (alpha) and is almost always set to .05. If there is less than a 5% chance of a result as extreme as the sample result if the null hypothesis were true, then the null hypothesis is rejected. When this happens, the result is said to be statistically significant . If there is greater than a 5% chance of a result as extreme as the sample result when the null hypothesis is true, then the null hypothesis is retained. This does not necessarily mean that the researcher accepts the null hypothesis as true—only that there is not currently enough evidence to conclude that it is true. Researchers often use the expression “fail to reject the null hypothesis” rather than “retain the null hypothesis,” but they never use the expression “accept the null hypothesis.”
The Misunderstood p Value
The p value is one of the most misunderstood quantities in psychological research (Cohen, 1994) [1] . Even professional researchers misinterpret it, and it is not unusual for such misinterpretations to appear in statistics textbooks!
The most common misinterpretation is that the p value is the probability that the null hypothesis is true—that the sample result occurred by chance. For example, a misguided researcher might say that because the p value is .02, there is only a 2% chance that the result is due to chance and a 98% chance that it reflects a real relationship in the population. But this is incorrect . The p value is really the probability of a result at least as extreme as the sample result if the null hypothesis were true. So a p value of .02 means that if the null hypothesis were true, a sample result this extreme would occur only 2% of the time.
You can avoid this misunderstanding by remembering that the p value is not the probability that any particular hypothesis is true or false. Instead, it is the probability of obtaining the sample result if the null hypothesis were true.
Role of Sample Size and Relationship Strength
Recall that null hypothesis testing involves answering the question, “If the null hypothesis were true, what is the probability of a sample result as extreme as this one?” In other words, “What is the p value?” It can be helpful to see that the answer to this question depends on just two considerations: the strength of the relationship and the size of the sample. Specifically, the stronger the sample relationship and the larger the sample, the less likely the result would be if the null hypothesis were true. That is, the lower the p value. This should make sense. Imagine a study in which a sample of 500 women is compared with a sample of 500 men in terms of some psychological characteristic, and Cohen’s d is a strong 0.50. If there were really no sex difference in the population, then a result this strong based on such a large sample should seem highly unlikely. Now imagine a similar study in which a sample of three women is compared with a sample of three men, and Cohen’s d is a weak 0.10. If there were no sex difference in the population, then a relationship this weak based on such a small sample should seem likely. And this is precisely why the null hypothesis would be rejected in the first example and retained in the second.
Of course, sometimes the result can be weak and the sample large, or the result can be strong and the sample small. In these cases, the two considerations trade off against each other so that a weak result can be statistically significant if the sample is large enough and a strong relationship can be statistically significant even if the sample is small. Table 13.1 shows roughly how relationship strength and sample size combine to determine whether a sample result is statistically significant. The columns of the table represent the three levels of relationship strength: weak, medium, and strong. The rows represent four sample sizes that can be considered small, medium, large, and extra large in the context of psychological research. Thus each cell in the table represents a combination of relationship strength and sample size. If a cell contains the word Yes , then this combination would be statistically significant for both Cohen’s d and Pearson’s r . If it contains the word No , then it would not be statistically significant for either. There is one cell where the decision for d and r would be different and another where it might be different depending on some additional considerations, which are discussed in Section 13.2 “Some Basic Null Hypothesis Tests”
Sample Size | Weak relationship | Medium-strength relationship | Strong relationship |
---|---|---|---|
Small ( = 20) | No | No | = Maybe = Yes |
Medium ( = 50) | No | Yes | Yes |
Large ( = 100) | = Yes = No | Yes | Yes |
Extra large ( = 500) | Yes | Yes | Yes |
Although Table 13.1 provides only a rough guideline, it shows very clearly that weak relationships based on medium or small samples are never statistically significant and that strong relationships based on medium or larger samples are always statistically significant. If you keep this lesson in mind, you will often know whether a result is statistically significant based on the descriptive statistics alone. It is extremely useful to be able to develop this kind of intuitive judgment. One reason is that it allows you to develop expectations about how your formal null hypothesis tests are going to come out, which in turn allows you to detect problems in your analyses. For example, if your sample relationship is strong and your sample is medium, then you would expect to reject the null hypothesis. If for some reason your formal null hypothesis test indicates otherwise, then you need to double-check your computations and interpretations. A second reason is that the ability to make this kind of intuitive judgment is an indication that you understand the basic logic of this approach in addition to being able to do the computations.
Statistical Significance Versus Practical Significance
Table 13.1 illustrates another extremely important point. A statistically significant result is not necessarily a strong one. Even a very weak result can be statistically significant if it is based on a large enough sample. This is closely related to Janet Shibley Hyde’s argument about sex differences (Hyde, 2007) [2] . The differences between women and men in mathematical problem solving and leadership ability are statistically significant. But the word significant can cause people to interpret these differences as strong and important—perhaps even important enough to influence the college courses they take or even who they vote for. As we have seen, however, these statistically significant differences are actually quite weak—perhaps even “trivial.”
This is why it is important to distinguish between the statistical significance of a result and the practical significance of that result. Practical significance refers to the importance or usefulness of the result in some real-world context. Many sex differences are statistically significant—and may even be interesting for purely scientific reasons—but they are not practically significant. In clinical practice, this same concept is often referred to as “clinical significance.” For example, a study on a new treatment for social phobia might show that it produces a statistically significant positive effect. Yet this effect still might not be strong enough to justify the time, effort, and other costs of putting it into practice—especially if easier and cheaper treatments that work almost as well already exist. Although statistically significant, this result would be said to lack practical or clinical significance.
Key Takeaways
- Null hypothesis testing is a formal approach to deciding whether a statistical relationship in a sample reflects a real relationship in the population or is just due to chance.
- The logic of null hypothesis testing involves assuming that the null hypothesis is true, finding how likely the sample result would be if this assumption were correct, and then making a decision. If the sample result would be unlikely if the null hypothesis were true, then it is rejected in favour of the alternative hypothesis. If it would not be unlikely, then the null hypothesis is retained.
- The probability of obtaining the sample result if the null hypothesis were true (the p value) is based on two considerations: relationship strength and sample size. Reasonable judgments about whether a sample relationship is statistically significant can often be made by quickly considering these two factors.
- Statistical significance is not the same as relationship strength or importance. Even weak relationships can be statistically significant if the sample size is large enough. It is important to consider relationship strength and the practical significance of a result in addition to its statistical significance.
- Discussion: Imagine a study showing that people who eat more broccoli tend to be happier. Explain for someone who knows nothing about statistics why the researchers would conduct a null hypothesis test.
- The correlation between two variables is r = −.78 based on a sample size of 137.
- The mean score on a psychological characteristic for women is 25 ( SD = 5) and the mean score for men is 24 ( SD = 5). There were 12 women and 10 men in this study.
- In a memory experiment, the mean number of items recalled by the 40 participants in Condition A was 0.50 standard deviations greater than the mean number recalled by the 40 participants in Condition B.
- In another memory experiment, the mean scores for participants in Condition A and Condition B came out exactly the same!
- A student finds a correlation of r = .04 between the number of units the students in his research methods class are taking and the students’ level of stress.
Long Descriptions
“Null Hypothesis” long description: A comic depicting a man and a woman talking in the foreground. In the background is a child working at a desk. The man says to the woman, “I can’t believe schools are still teaching kids about the null hypothesis. I remember reading a big study that conclusively disproved it years ago.” [Return to “Null Hypothesis”]
“Conditional Risk” long description: A comic depicting two hikers beside a tree during a thunderstorm. A bolt of lightning goes “crack” in the dark sky as thunder booms. One of the hikers says, “Whoa! We should get inside!” The other hiker says, “It’s okay! Lightning only kills about 45 Americans a year, so the chances of dying are only one in 7,000,000. Let’s go on!” The comic’s caption says, “The annual death rate among people who know that statistic is one in six.” [Return to “Conditional Risk”]
Media Attributions
- Null Hypothesis by XKCD CC BY-NC (Attribution NonCommercial)
- Conditional Risk by XKCD CC BY-NC (Attribution NonCommercial)
- Cohen, J. (1994). The world is round: p < .05. American Psychologist, 49 , 997–1003. ↵
- Hyde, J. S. (2007). New directions in the study of gender similarities and differences. Current Directions in Psychological Science, 16 , 259–263. ↵
Values in a population that correspond to variables measured in a study.
The random variability in a statistic from sample to sample.
A formal approach to deciding between two interpretations of a statistical relationship in a sample.
The idea that there is no relationship in the population and that the relationship in the sample reflects only sampling error.
The idea that there is a relationship in the population and that the relationship in the sample reflects this relationship in the population.
When the relationship found in the sample would be extremely unlikely, the idea that the relationship occurred “by chance” is rejected.
When the relationship found in the sample is likely to have occurred by chance, the null hypothesis is not rejected.
The probability that, if the null hypothesis were true, the result found in the sample would occur.
How low the p value must be before the sample result is considered unlikely in null hypothesis testing.
When there is less than a 5% chance of a result as extreme as the sample result occurring and the null hypothesis is rejected.
Research Methods in Psychology - 2nd Canadian Edition Copyright © 2015 by Paul C. Price, Rajiv Jhangiani, & I-Chant A. Chiang is licensed under a Creative Commons Attribution-NonCommercial-ShareAlike 4.0 International License , except where otherwise noted.
Share This Book
- School Guide
- Mathematics
- Number System and Arithmetic
- Trigonometry
- Probability
- Mensuration
- Maths Formulas
- Integration Formulas
- Differentiation Formulas
- Trigonometry Formulas
- Algebra Formulas
- Mensuration Formula
- Statistics Formulas
- Trigonometric Table
Null Hypothesis
Null Hypothesis , often denoted as H 0, is a foundational concept in statistical hypothesis testing. It represents an assumption that no significant difference, effect, or relationship exists between variables within a population. It serves as a baseline assumption, positing no observed change or effect occurring. The null is t he truth or falsity of an idea in analysis.
In this article, we will discuss the null hypothesis in detail, along with some solved examples and questions on the null hypothesis.
Table of Content
What is Null Hypothesis?
Null hypothesis symbol, formula of null hypothesis, types of null hypothesis, null hypothesis examples, principle of null hypothesis, how do you find null hypothesis, null hypothesis in statistics, null hypothesis and alternative hypothesis, null hypothesis and alternative hypothesis examples, null hypothesis – practice problems.
Null Hypothesis in statistical analysis suggests the absence of statistical significance within a specific set of observed data. Hypothesis testing, using sample data, evaluates the validity of this hypothesis. Commonly denoted as H 0 or simply “null,” it plays an important role in quantitative analysis, examining theories related to markets, investment strategies, or economies to determine their validity.
Null Hypothesis Meaning
Null Hypothesis represents a default position, often suggesting no effect or difference, against which researchers compare their experimental results. The Null Hypothesis, often denoted as H 0 asserts a default assumption in statistical analysis. It posits no significant difference or effect, serving as a baseline for comparison in hypothesis testing.
The null Hypothesis is represented as H 0 , the Null Hypothesis symbolizes the absence of a measurable effect or difference in the variables under examination.
Certainly, a simple example would be asserting that the mean score of a group is equal to a specified value like stating that the average IQ of a population is 100.
The Null Hypothesis is typically formulated as a statement of equality or absence of a specific parameter in the population being studied. It provides a clear and testable prediction for comparison with the alternative hypothesis. The formulation of the Null Hypothesis typically follows a concise structure, stating the equality or absence of a specific parameter in the population.
Mean Comparison (Two-sample t-test)
H 0 : μ 1 = μ 2
This asserts that there is no significant difference between the means of two populations or groups.
Proportion Comparison
H 0 : p 1 − p 2 = 0
This suggests no significant difference in proportions between two populations or conditions.
Equality in Variance (F-test in ANOVA)
H 0 : σ 1 = σ 2
This states that there’s no significant difference in variances between groups or populations.
Independence (Chi-square Test of Independence):
H 0 : Variables are independent
This asserts that there’s no association or relationship between categorical variables.
Null Hypotheses vary including simple and composite forms, each tailored to the complexity of the research question. Understanding these types is pivotal for effective hypothesis testing.
Equality Null Hypothesis (Simple Null Hypothesis)
The Equality Null Hypothesis, also known as the Simple Null Hypothesis, is a fundamental concept in statistical hypothesis testing that assumes no difference, effect or relationship between groups, conditions or populations being compared.
Non-Inferiority Null Hypothesis
In some studies, the focus might be on demonstrating that a new treatment or method is not significantly worse than the standard or existing one.
Superiority Null Hypothesis
The concept of a superiority null hypothesis comes into play when a study aims to demonstrate that a new treatment, method, or intervention is significantly better than an existing or standard one.
Independence Null Hypothesis
In certain statistical tests, such as chi-square tests for independence, the null hypothesis assumes no association or independence between categorical variables.
Homogeneity Null Hypothesis
In tests like ANOVA (Analysis of Variance), the null hypothesis suggests that there’s no difference in population means across different groups.
- Medicine: Null Hypothesis: “No significant difference exists in blood pressure levels between patients given the experimental drug versus those given a placebo.”
- Education: Null Hypothesis: “There’s no significant variation in test scores between students using a new teaching method and those using traditional teaching.”
- Economics: Null Hypothesis: “There’s no significant change in consumer spending pre- and post-implementation of a new taxation policy.”
- Environmental Science: Null Hypothesis: “There’s no substantial difference in pollution levels before and after a water treatment plant’s establishment.”
The principle of the null hypothesis is a fundamental concept in statistical hypothesis testing. It involves making an assumption about the population parameter or the absence of an effect or relationship between variables.
In essence, the null hypothesis (H 0 ) proposes that there is no significant difference, effect, or relationship between variables. It serves as a starting point or a default assumption that there is no real change, no effect or no difference between groups or conditions.
The null hypothesis is usually formulated to be tested against an alternative hypothesis (H 1 or H [Tex]\alpha [/Tex] ) which suggests that there is an effect, difference or relationship present in the population.
Null Hypothesis Rejection
Rejecting the Null Hypothesis occurs when statistical evidence suggests a significant departure from the assumed baseline. It implies that there is enough evidence to support the alternative hypothesis, indicating a meaningful effect or difference. Null Hypothesis rejection occurs when statistical evidence suggests a deviation from the assumed baseline, prompting a reconsideration of the initial hypothesis.
Identifying the Null Hypothesis involves defining the status quotient, asserting no effect and formulating a statement suitable for statistical analysis.
When is Null Hypothesis Rejected?
The Null Hypothesis is rejected when statistical tests indicate a significant departure from the expected outcome, leading to the consideration of alternative hypotheses. It occurs when statistical evidence suggests a deviation from the assumed baseline, prompting a reconsideration of the initial hypothesis.
In statistical hypothesis testing, researchers begin by stating the null hypothesis, often based on theoretical considerations or previous research. The null hypothesis is then tested against an alternative hypothesis (Ha), which represents the researcher’s claim or the hypothesis they seek to support.
The process of hypothesis testing involves collecting sample data and using statistical methods to assess the likelihood of observing the data if the null hypothesis were true. This assessment is typically done by calculating a test statistic, which measures the difference between the observed data and what would be expected under the null hypothesis.
In the realm of hypothesis testing, the null hypothesis (H 0 ) and alternative hypothesis (H₁ or Ha) play critical roles. The null hypothesis generally assumes no difference, effect, or relationship between variables, suggesting that any observed change or effect is due to random chance. Its counterpart, the alternative hypothesis, asserts the presence of a significant difference, effect, or relationship between variables, challenging the null hypothesis. These hypotheses are formulated based on the research question and guide statistical analyses.
Difference Between Null Hypothesis and Alternative Hypothesis
The null hypothesis (H 0 ) serves as the baseline assumption in statistical testing, suggesting no significant effect, relationship, or difference within the data. It often proposes that any observed change or correlation is merely due to chance or random variation. Conversely, the alternative hypothesis (H 1 or Ha) contradicts the null hypothesis, positing the existence of a genuine effect, relationship or difference in the data. It represents the researcher’s intended focus, seeking to provide evidence against the null hypothesis and support for a specific outcome or theory. These hypotheses form the crux of hypothesis testing, guiding the assessment of data to draw conclusions about the population being studied.
Criteria | Null Hypothesis | Alternative Hypothesis |
---|---|---|
Definition | Assumes no effect or difference | Asserts a specific effect or difference |
Symbol | H | H (or Ha) |
Formulation | States equality or absence of parameter | States a specific value or relationship |
Testing Outcome | Rejected if evidence of a significant effect | Accepted if evidence supports the hypothesis |
Let’s envision a scenario where a researcher aims to examine the impact of a new medication on reducing blood pressure among patients. In this context:
Null Hypothesis (H 0 ): “The new medication does not produce a significant effect in reducing blood pressure levels among patients.”
Alternative Hypothesis (H 1 or Ha): “The new medication yields a significant effect in reducing blood pressure levels among patients.”
The null hypothesis implies that any observed alterations in blood pressure subsequent to the medication’s administration are a result of random fluctuations rather than a consequence of the medication itself. Conversely, the alternative hypothesis contends that the medication does indeed generate a meaningful alteration in blood pressure levels, distinct from what might naturally occur or by random chance.
People Also Read:
Mathematics Maths Formulas Probability and Statistics
Example 1: A researcher claims that the average time students spend on homework is 2 hours per night.
Null Hypothesis (H 0 ): The average time students spend on homework is equal to 2 hours per night. Data: A random sample of 30 students has an average homework time of 1.8 hours with a standard deviation of 0.5 hours. Test Statistic and Decision: Using a t-test, if the calculated t-statistic falls within the acceptance region, we fail to reject the null hypothesis. If it falls in the rejection region, we reject the null hypothesis. Conclusion: Based on the statistical analysis, we fail to reject the null hypothesis, suggesting that there is not enough evidence to dispute the claim of the average homework time being 2 hours per night.
Example 2: A company asserts that the error rate in its production process is less than 1%.
Null Hypothesis (H 0 ): The error rate in the production process is 1% or higher. Data: A sample of 500 products shows an error rate of 0.8%. Test Statistic and Decision: Using a z-test, if the calculated z-statistic falls within the acceptance region, we fail to reject the null hypothesis. If it falls in the rejection region, we reject the null hypothesis. Conclusion: The statistical analysis supports rejecting the null hypothesis, indicating that there is enough evidence to dispute the company’s claim of an error rate of 1% or higher.
Q1. A researcher claims that the average time spent by students on homework is less than 2 hours per day. Formulate the null hypothesis for this claim?
Q2. A manufacturing company states that their new machine produces widgets with a defect rate of less than 5%. Write the null hypothesis to test this claim?
Q3. An educational institute believes that their online course completion rate is at least 60%. Develop the null hypothesis to validate this assertion?
Q4. A restaurant claims that the waiting time for customers during peak hours is not more than 15 minutes. Formulate the null hypothesis for this claim?
Q5. A study suggests that the mean weight loss after following a specific diet plan for a month is more than 8 pounds. Construct the null hypothesis to evaluate this statement?
Summary – Null Hypothesis and Alternative Hypothesis
The null hypothesis (H 0 ) and alternative hypothesis (H a ) are fundamental concepts in statistical hypothesis testing. The null hypothesis represents the default assumption, stating that there is no significant effect, difference, or relationship between variables. It serves as the baseline against which the alternative hypothesis is tested. In contrast, the alternative hypothesis represents the researcher’s hypothesis or the claim to be tested, suggesting that there is a significant effect, difference, or relationship between variables. The relationship between the null and alternative hypotheses is such that they are complementary, and statistical tests are conducted to determine whether the evidence from the data is strong enough to reject the null hypothesis in favor of the alternative hypothesis. This decision is based on the strength of the evidence and the chosen level of significance. Ultimately, the choice between the null and alternative hypotheses depends on the specific research question and the direction of the effect being investigated.

FAQs on Null Hypothesis
What does null hypothesis stands for.
The null hypothesis, denoted as H 0 , is a fundamental concept in statistics used for hypothesis testing. It represents the statement that there is no effect or no difference, and it is the hypothesis that the researcher typically aims to provide evidence against.
How to Form a Null Hypothesis?
A null hypothesis is formed based on the assumption that there is no significant difference or effect between the groups being compared or no association between variables being tested. It often involves stating that there is no relationship, no change, or no effect in the population being studied.
When Do we reject the Null Hypothesis?
In statistical hypothesis testing, if the p-value (the probability of obtaining the observed results) is lower than the chosen significance level (commonly 0.05), we reject the null hypothesis. This suggests that the data provides enough evidence to refute the assumption made in the null hypothesis.
What is a Null Hypothesis in Research?
In research, the null hypothesis represents the default assumption or position that there is no significant difference or effect. Researchers often try to test this hypothesis by collecting data and performing statistical analyses to see if the observed results contradict the assumption.
What Are Alternative and Null Hypotheses?
The null hypothesis (H0) is the default assumption that there is no significant difference or effect. The alternative hypothesis (H1 or Ha) is the opposite, suggesting there is a significant difference, effect or relationship.
What Does it Mean to Reject the Null Hypothesis?
Rejecting the null hypothesis implies that there is enough evidence in the data to support the alternative hypothesis. In simpler terms, it suggests that there might be a significant difference, effect or relationship between the groups or variables being studied.
How to Find Null Hypothesis?
Formulating a null hypothesis often involves considering the research question and assuming that no difference or effect exists. It should be a statement that can be tested through data collection and statistical analysis, typically stating no relationship or no change between variables or groups.
How is Null Hypothesis denoted?
The null hypothesis is commonly symbolized as H 0 in statistical notation.
What is the Purpose of the Null hypothesis in Statistical Analysis?
The null hypothesis serves as a starting point for hypothesis testing, enabling researchers to assess if there’s enough evidence to reject it in favor of an alternative hypothesis.
What happens if we Reject the Null hypothesis?
Rejecting the null hypothesis implies that there is sufficient evidence to support an alternative hypothesis, suggesting a significant effect or relationship between variables.
What are Test for Null Hypothesis?
Various statistical tests, such as t-tests or chi-square tests, are employed to evaluate the validity of the Null Hypothesis in different scenarios.
Please Login to comment...
Similar reads.
- Geeks Premier League
- School Learning
- Geeks Premier League 2023
- Math-Concepts
Improve your Coding Skills with Practice
What kind of Experience do you want to share?
User Preferences
Content preview.
Arcu felis bibendum ut tristique et egestas quis:
- Ut enim ad minim veniam, quis nostrud exercitation ullamco laboris
- Duis aute irure dolor in reprehenderit in voluptate
- Excepteur sint occaecat cupidatat non proident
Keyboard Shortcuts
Hypothesis testing.
Key Topics:
- Basic approach
- Null and alternative hypothesis
- Decision making and the p -value
- Z-test & Nonparametric alternative
Basic approach to hypothesis testing
- State a model describing the relationship between the explanatory variables and the outcome variable(s) in the population and the nature of the variability. State all of your assumptions .
- Specify the null and alternative hypotheses in terms of the parameters of the model.
- Invent a test statistic that will tend to be different under the null and alternative hypotheses.
- Using the assumptions of step 1, find the theoretical sampling distribution of the statistic under the null hypothesis of step 2. Ideally the form of the sampling distribution should be one of the “standard distributions”(e.g. normal, t , binomial..)
- Calculate a p -value , as the area under the sampling distribution more extreme than your statistic. Depends on the form of the alternative hypothesis.
- Choose your acceptable type 1 error rate (alpha) and apply the decision rule : reject the null hypothesis if the p-value is less than alpha, otherwise do not reject.
sampled from a with unknown mean μ and known variance σ . : μ = μ H : μ ≤ μ H : μ ≥ μ | : μ ≠ μ H : μ > μ H : μ < μ |
- \(\frac{\bar{X}-\mu_0}{\sigma / \sqrt{n}}\)
- general form is: (estimate - value we are testing)/(st.dev of the estimate)
- z-statistic follows N(0,1) distribution
- 2 × the area above |z|, area above z,or area below z, or
- compare the statistic to a critical value, |z| ≥ z α/2 , z ≥ z α , or z ≤ - z α
- Choose the acceptable level of Alpha = 0.05, we conclude …. ?
Making the Decision
It is either likely or unlikely that we would collect the evidence we did given the initial assumption. (Note: “likely” or “unlikely” is measured by calculating a probability!)
If it is likely , then we “ do not reject ” our initial assumption. There is not enough evidence to do otherwise.
If it is unlikely , then:
- either our initial assumption is correct and we experienced an unusual event or,
- our initial assumption is incorrect
In statistics, if it is unlikely, we decide to “ reject ” our initial assumption.
Example: Criminal Trial Analogy
First, state 2 hypotheses, the null hypothesis (“H 0 ”) and the alternative hypothesis (“H A ”)
- H 0 : Defendant is not guilty.
- H A : Defendant is guilty.
Usually the H 0 is a statement of “no effect”, or “no change”, or “chance only” about a population parameter.
While the H A , depending on the situation, is that there is a difference, trend, effect, or a relationship with respect to a population parameter.
- It can one-sided and two-sided.
- In two-sided we only care there is a difference, but not the direction of it. In one-sided we care about a particular direction of the relationship. We want to know if the value is strictly larger or smaller.
Then, collect evidence, such as finger prints, blood spots, hair samples, carpet fibers, shoe prints, ransom notes, handwriting samples, etc. (In statistics, the data are the evidence.)
Next, you make your initial assumption.
- Defendant is innocent until proven guilty.
In statistics, we always assume the null hypothesis is true .
Then, make a decision based on the available evidence.
- If there is sufficient evidence (“beyond a reasonable doubt”), reject the null hypothesis . (Behave as if defendant is guilty.)
- If there is not enough evidence, do not reject the null hypothesis . (Behave as if defendant is not guilty.)
If the observed outcome, e.g., a sample statistic, is surprising under the assumption that the null hypothesis is true, but more probable if the alternative is true, then this outcome is evidence against H 0 and in favor of H A .
An observed effect so large that it would rarely occur by chance is called statistically significant (i.e., not likely to happen by chance).
Using the p -value to make the decision
The p -value represents how likely we would be to observe such an extreme sample if the null hypothesis were true. The p -value is a probability computed assuming the null hypothesis is true, that the test statistic would take a value as extreme or more extreme than that actually observed. Since it's a probability, it is a number between 0 and 1. The closer the number is to 0 means the event is “unlikely.” So if p -value is “small,” (typically, less than 0.05), we can then reject the null hypothesis.
Significance level and p -value
Significance level, α, is a decisive value for p -value. In this context, significant does not mean “important”, but it means “not likely to happened just by chance”.
α is the maximum probability of rejecting the null hypothesis when the null hypothesis is true. If α = 1 we always reject the null, if α = 0 we never reject the null hypothesis. In articles, journals, etc… you may read: “The results were significant ( p <0.05).” So if p =0.03, it's significant at the level of α = 0.05 but not at the level of α = 0.01. If we reject the H 0 at the level of α = 0.05 (which corresponds to 95% CI), we are saying that if H 0 is true, the observed phenomenon would happen no more than 5% of the time (that is 1 in 20). If we choose to compare the p -value to α = 0.01, we are insisting on a stronger evidence!
Neither decision of rejecting or not rejecting the H entails proving the null hypothesis or the alternative hypothesis. We merely state there is enough evidence to behave one way or the other. This is also always true in statistics! |
So, what kind of error could we make? No matter what decision we make, there is always a chance we made an error.
Errors in Criminal Trial:
Errors in Hypothesis Testing
Type I error (False positive): The null hypothesis is rejected when it is true.
- α is the maximum probability of making a Type I error.
Type II error (False negative): The null hypothesis is not rejected when it is false.
- β is the probability of making a Type II error
There is always a chance of making one of these errors. But, a good scientific study will minimize the chance of doing so!
The power of a statistical test is its probability of rejecting the null hypothesis if the null hypothesis is false. That is, power is the ability to correctly reject H 0 and detect a significant effect. In other words, power is one minus the type II error risk.
\(\text{Power }=1-\beta = P\left(\text{reject} H_0 | H_0 \text{is false } \right)\)
Which error is worse?
Type I = you are innocent, yet accused of cheating on the test. Type II = you cheated on the test, but you are found innocent.
This depends on the context of the problem too. But in most cases scientists are trying to be “conservative”; it's worse to make a spurious discovery than to fail to make a good one. Our goal it to increase the power of the test that is to minimize the length of the CI.
We need to keep in mind:
- the effect of the sample size,
- the correctness of the underlying assumptions about the population,
- statistical vs. practical significance, etc…
(see the handout). To study the tradeoffs between the sample size, α, and Type II error we can use power and operating characteristic curves.
Assume data are independently sampled from a normal distribution with unknown mean μ and known variance σ = 9. Make an initial assumption that μ = 65. Specify the hypothesis: H : μ = 65 H : μ ≠ 65 z-statistic: 3.58 z-statistic follow N(0,1) distribution
The -value, < 0.0001, indicates that, if the average height in the population is 65 inches, it is unlikely that a sample of 54 students would have an average height of 66.4630. Alpha = 0.05. Decision: -value < alpha, thus Conclude that the average height is not equal to 65. |
What type of error might we have made?
Type I error is claiming that average student height is not 65 inches, when it really is. Type II error is failing to claim that the average student height is not 65in when it is.
We rejected the null hypothesis, i.e., claimed that the height is not 65, thus making potentially a Type I error. But sometimes the p -value is too low because of the large sample size, and we may have statistical significance but not really practical significance! That's why most statisticians are much more comfortable with using CI than tests.
Based on the CI only, how do you know that you should reject the null hypothesis? The 95% CI is (65.6628,67.2631) ... What about practical and statistical significance now? Is there another reason to suspect this test, and the -value calculations? |
There is a need for a further generalization. What if we can't assume that σ is known? In this case we would use s (the sample standard deviation) to estimate σ.
If the sample is very large, we can treat σ as known by assuming that σ = s . According to the law of large numbers, this is not too bad a thing to do. But if the sample is small, the fact that we have to estimate both the standard deviation and the mean adds extra uncertainty to our inference. In practice this means that we need a larger multiplier for the standard error.
We need one-sample t -test.
One sample t -test
- Assume data are independently sampled from a normal distribution with unknown mean μ and variance σ 2 . Make an initial assumption, μ 0 .
: μ = μ H : μ ≤ μ H : μ ≥ μ | : μ ≠ μ H : μ > μ H : μ < μ |
- t-statistic: \(\frac{\bar{X}-\mu_0}{s / \sqrt{n}}\) where s is a sample st.dev.
- t-statistic follows t -distribution with df = n - 1
- Alpha = 0.05, we conclude ….
Testing for the population proportion
Let's go back to our CNN poll. Assume we have a SRS of 1,017 adults.
We are interested in testing the following hypothesis: H 0 : p = 0.50 vs. p > 0.50
What is the test statistic?
If alpha = 0.05, what do we conclude?
We will see more details in the next lesson on proportions, then distributions, and possible tests.
- Search Search Please fill out this field.
What Is a Null Hypothesis?
The alternative hypothesis.
- Additional Examples
- Null Hypothesis and Investments
The Bottom Line
- Corporate Finance
- Financial Ratios
Null Hypothesis: What Is It, and How Is It Used in Investing?
Adam Hayes, Ph.D., CFA, is a financial writer with 15+ years Wall Street experience as a derivatives trader. Besides his extensive derivative trading expertise, Adam is an expert in economics and behavioral finance. Adam received his master's in economics from The New School for Social Research and his Ph.D. from the University of Wisconsin-Madison in sociology. He is a CFA charterholder as well as holding FINRA Series 7, 55 & 63 licenses. He currently researches and teaches economic sociology and the social studies of finance at the Hebrew University in Jerusalem.
:max_bytes(150000):strip_icc():format(webp)/adam_hayes-5bfc262a46e0fb005118b414.jpg)
A null hypothesis is a type of statistical hypothesis that proposes that no statistical significance exists in a set of given observations. Hypothesis testing is used to assess the credibility of a hypothesis by using sample data. Sometimes referred to simply as the “null,” it is represented as H 0 .
The null hypothesis, also known as “the conjecture,” is used in quantitative analysis to test theories about markets, investing strategies, and economies to decide if an idea is true or false.
Key Takeaways
- A null hypothesis is a type of conjecture in statistics that proposes that there is no difference between certain characteristics of a population or data-generating process.
- The alternative hypothesis proposes that there is a difference.
- Hypothesis testing provides a method to reject a null hypothesis within a certain confidence level.
- If you can reject the null hypothesis, it provides support for the alternative hypothesis.
- Null hypothesis testing is the basis of the principle of falsification in science.
Alex Dos Diaz / Investopedia
Understanding a Null Hypothesis
A gambler may be interested in whether a game of chance is fair. If it is, then the expected earnings per play come to zero for both players. If it is not, then the expected earnings are positive for one player and negative for the other.
To test whether the game is fair, the gambler collects earnings data from many repetitions of the game, calculates the average earnings from these data, then tests the null hypothesis that the expected earnings are not different from zero.
If the average earnings from the sample data are sufficiently far from zero, then the gambler will reject the null hypothesis and conclude the alternative hypothesis—namely, that the expected earnings per play are different from zero. If the average earnings from the sample data are near zero, then the gambler will not reject the null hypothesis, concluding instead that the difference between the average from the data and zero is explainable by chance alone.
A null hypothesis can only be rejected, not proven.
The null hypothesis assumes that any kind of difference between the chosen characteristics that you see in a set of data is due to chance. For example, if the expected earnings for the gambling game are truly equal to zero, then any difference between the average earnings in the data and zero is due to chance.
Analysts look to reject the null hypothesis because doing so is a strong conclusion. This requires evidence in the form of an observed difference that is too large to be explained solely by chance. Failing to reject the null hypothesis—that the results are explainable by chance alone—is a weak conclusion because it allows that while factors other than chance may be at work, they may not be strong enough for the statistical test to detect them.
An important point to note is that we are testing the null hypothesis because there is an element of doubt about its validity. Whatever information that is against the stated null hypothesis is captured in the alternative (alternate) hypothesis (H 1 ).
For the examples below, the alternative hypothesis would be:
- Students score an average that is not equal to seven.
- The mean annual return of a mutual fund is not equal to 8% per year.
In other words, the alternative hypothesis is a direct contradiction of the null hypothesis.
Null Hypothesis Examples
Here is a simple example: A school principal claims that students in her school score an average of seven out of 10 in exams. The null hypothesis is that the population mean is not 7.0. To test this null hypothesis, we record marks of, say, 30 students ( sample ) from the entire student population of the school (say, 300) and calculate the mean of that sample.
We can then compare the (calculated) sample mean to the (hypothesized) population mean of 7.0 and attempt to reject the null hypothesis. (The null hypothesis here—that the population mean is not 7.0—cannot be proved using the sample data. It can only be rejected.)
Take another example: The annual return of a particular mutual fund is claimed to be 8%. Assume that the mutual fund has been in existence for 20 years. The null hypothesis is that the mean return is not 8% for the mutual fund. We take a random sample of annual returns of the mutual fund for, say, five years (sample) and calculate the sample mean. We then compare the (calculated) sample mean to the (claimed) population mean (8%) to test the null hypothesis.
For the above examples, null hypotheses are:
- Example A: Students in the school don’t score an average of seven out of 10 in exams.
- Example B: The mean annual return of the mutual fund is not 8% per year.
For the purposes of determining whether to reject the null hypothesis (abbreviated H0), said hypothesis is assumed, for the sake of argument, to be true. Then the likely range of possible values of the calculated statistic (e.g., the average score on 30 students’ tests) is determined under this presumption (e.g., the range of plausible averages might range from 6.2 to 7.8 if the population mean is 7.0).
If the sample average is outside of this range, the null hypothesis is rejected. Otherwise, the difference is said to be “explainable by chance alone,” being within the range that is determined by chance alone.
How Null Hypothesis Testing Is Used in Investments
As an example related to financial markets, assume Alice sees that her investment strategy produces higher average returns than simply buying and holding a stock . The null hypothesis states that there is no difference between the two average returns, and Alice is inclined to believe this until she can conclude contradictory results.
Refuting the null hypothesis would require showing statistical significance, which can be found by a variety of tests. The alternative hypothesis would state that the investment strategy has a higher average return than a traditional buy-and-hold strategy.
One tool that can determine the statistical significance of the results is the p-value. A p-value represents the probability that a difference as large or larger than the observed difference between the two average returns could occur solely by chance.
A p-value that is less than or equal to 0.05 often indicates whether there is evidence against the null hypothesis. If Alice conducts one of these tests, such as a test using the normal model, resulting in a significant difference between her returns and the buy-and-hold returns (the p-value is less than or equal to 0.05), she can then reject the null hypothesis and conclude the alternative hypothesis.
How Is the Null Hypothesis Identified?
The analyst or researcher establishes a null hypothesis based on the research question or problem they are trying to answer. Depending on the question, the null may be identified differently. For example, if the question is simply whether an effect exists (e.g., does X influence Y?), the null hypothesis could be H 0 : X = 0. If the question is instead, is X the same as Y, the H 0 would be X = Y. If it is that the effect of X on Y is positive, H 0 would be X > 0. If the resulting analysis shows an effect that is statistically significantly different from zero, the null can be rejected.
How Is Null Hypothesis Used in Finance?
In finance , a null hypothesis is used in quantitative analysis. It tests the premise of an investing strategy, the markets, or an economy to determine if it is true or false.
For instance, an analyst may want to see if two stocks, ABC and XYZ, are closely correlated. The null hypothesis would be ABC ≠ XYZ.
How Are Statistical Hypotheses Tested?
Statistical hypotheses are tested by a four-step process . The first is for the analyst to state the two hypotheses so that only one can be right. The second is to formulate an analysis plan, which outlines how the data will be evaluated. The third is to carry out the plan and physically analyze the sample data. The fourth and final step is to analyze the results and either reject the null hypothesis or claim that the observed differences are explainable by chance alone.
What Is an Alternative Hypothesis?
An alternative hypothesis is a direct contradiction of a null hypothesis. This means that if one of the two hypotheses is true, the other is false.
A null hypothesis states there is no difference between groups or relationship between variables. It is a type of statistical hypothesis and proposes that no statistical significance exists in a set of given observations. “Null” means nothing.
The null hypothesis is used in quantitative analysis to test theories about economies, investing strategies, and markets to decide if an idea is true or false. Hypothesis testing assesses the credibility of a hypothesis by using sample data. It is represented as H 0 and is sometimes simply known as “the null.”
Sage Publishing. “ Chapter 8: Introduction to Hypothesis Testing ,” Page 4.
Sage Publishing. “ Chapter 8: Introduction to Hypothesis Testing ,” Pages 4 to 7.
Sage Publishing. “ Chapter 8: Introduction to Hypothesis Testing ,” Page 7.
:max_bytes(150000):strip_icc():format(webp)/Term-Definitions_p-value-61f80de6ec3f4bc481ce22dac498109c.png)
- Terms of Service
- Editorial Policy
- Privacy Policy
Thank you for visiting nature.com. You are using a browser version with limited support for CSS. To obtain the best experience, we recommend you use a more up to date browser (or turn off compatibility mode in Internet Explorer). In the meantime, to ensure continued support, we are displaying the site without styles and JavaScript.
- View all journals
- Explore content
- About the journal
- Publish with us
- Sign up for alerts
- Open access
- Published: 21 August 2024
Multi-habitat landscapes are more diverse and stable with improved function
- Talya D. Hackett ORCID: orcid.org/0000-0001-7727-8842 1 , 2 ,
- Alix M. C. Sauve 1 , 3 , 4 ,
- Kate P. Maia 1 , 5 ,
- Daniel Montoya ORCID: orcid.org/0000-0002-5521-5282 1 , 6 , 7 ,
- Nancy Davies 1 ,
- Rose Archer 1 ,
- Simon G. Potts ORCID: orcid.org/0000-0002-2045-980X 8 ,
- Jason M. Tylianakis ORCID: orcid.org/0000-0001-7402-5620 9 ,
- Ian P. Vaughan ORCID: orcid.org/0000-0002-7263-3822 10 &
- Jane Memmott 1
Nature ( 2024 ) Cite this article
Metrics details
- Ecological networks
- Ecosystem services
Conservation, restoration and land management are increasingly implemented at landscape scales 1 , 2 . However, because species interaction data are typically habitat- and/or guild-specific, exactly how those interactions connect habitats and affect the stability and function of communities at landscape scales remains poorly understood. We combine multi-guild species interaction data (plant–pollinator and three plant–herbivore–parasitoid communities, collected from landscapes with one, two or three habitats), a field experiment and a modelling approach to show that multi-habitat landscapes support higher species and interaction evenness, more complementary species interactions and more consistent robustness to species loss. These emergent network properties drive improved pollination success in landscapes with more habitats and are not explained by simply summing component habitat webs. Linking landscape composition, through community structure, to ecosystem function, highlights mechanisms by which several contiguous habitats can support landscape-scale ecosystem services.
Conservation policy and landscape management have moved from the historic protection of species and their habitats to ecosystem and landscape-level approaches 1 , 2 . Habitat heterogeneity 3 , 4 and the number of habitats in a landscape 5 , 6 contribute to species richness and ecosystem functioning, especially in agricultural landscapes 7 , 8 . At present, we lack a mechanistic understanding of how the number of habitats contributes to community structure and function. This understanding is key to the landscape-scale management of ecosystem services that depend on species interactions, such as pollination and pest control and to maintaining functioning ecosystems more generally. Ecological networks of species’ interactions provide a route to understanding functional responses to biodiversity changes 9 , 10 . Although communities host several guilds and transcend different habitats, network datasets encompassing both these characteristics remain scarce, meaning we might be missing important cross-habitat or guild cascades or functional effects. Researchers have recently started linking several networks, of one interaction type across habitats 11 , several interaction types across habitats 12 or several interactions at replicated sites of similar habitat composition 8 . However, a lack of independently derived measures of function has prevented these network changes from being linked mechanistically to functional outcomes.
Both structure and function of local communities can be affected by organisms dispersing between habitats 13 . Immigrating individuals may have a similar role to local species (that is, redundancy) or fill an empty ecological niche (that is, complementarity). If immigrating and local species respond differently to disturbances, this can reduce functional variability by ensuring that overall functionality is maintained 14 , 15 , 16 , 17 . Community impacts of dispersal may differ across trophic groups 18 , making the combination of habitat and guild replication critical. Despite the importance to both pure and applied ecology, it remains unknown whether landscapes are simply the sum of their habitat parts, in terms of both interaction structure and community function, or whether there are emergent properties, such as increased stability or functioning, that cannot be explained by their component habitats alone.
Here we evaluate how the number of habitats in a given area influences biodiversity, network structure, community stability and function across several interaction types (plant–pollinator and three types of plant–herbivore–parasitoid networks) in replicate landscapes. Using 30 independent field sites in southwest United Kingdom (Fig. 1a ), we test how landscapes with more habitats affect the plant and insect communities in them: specifically, we quantify the effects on species’ abundances, species richness, evenness (both in terms of insect species and the degree to which interactions are uniformly distributed among species) and robustness to species loss. Using a manipulative field experiment, we then test whether the number of habitats affects the ecological function of insect pollination. Finally, we develop a modelling approach to investigate if landscape-scale networks have emergent properties which cannot be explained by their component habitat networks.
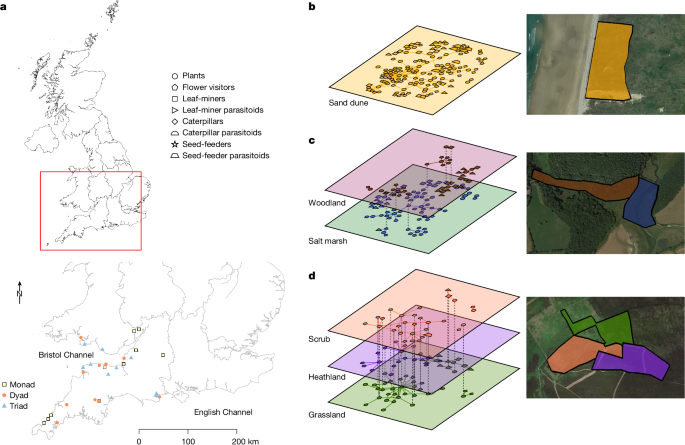
a , Map of sites for monads of one 9 ha habitat, dyads of two 4.5 ha habitats and triads of three 3 ha habitats in southwest United Kingdom. b – d , Visualization of the plant–insect interactions as multilayer networks and satellite images of Penhale Sands, a sand dune monad ( b ), Seet Bridge, a woodland and salt marsh dyad ( c ) and Hangman’s Hill, a scrub and heathland and grassland triad ( d ). Each layer corresponds to one habitat with nodes as species and shapes coding for the species type. Interspecific interactions in each habitat (layer) are represented with solid lines. Dashed lines connect nodes between layers representing the same species in different habitats. Map data sources: Office for National Statistics licensed under the Open Government Licence v.3.0; contains OS data © Crown copyright and database right 2021. Google Earth Pro Image © 2024 (CNES/Airbus ( b ) and Landsat/Copernicus ( c and d )).
We standardized field site size at 9 ha and varied the number of constituent habitats from one to three while selecting sites to allow a balanced replication of multi-habitat landscape types: thus, ten sites contained a single habitat (9 ha ‘monads’), ten sites contained two habitats (‘dyads’ with two 4.5 ha habitats) and ten sites contained three habitats (‘triads’ with three 3 ha habitats). Each habitat was selected from a pool of six habitats: grassland, heathland, woodland, salt marsh, sand dune and scrub (Fig. 1b–d and Supplementary Table 3 ) to avoid a habitat identity effect confounding that of the number of habitats, while also avoiding the issue of triads always having the same composition whereas monads and dyads differ. Over 2 years, we collected data on 11,482 interactions among 154 plant species and 954 insect species (5,729 flower–visitor interactions, 2,345 plant–leaf miner interactions, 697 plant–caterpillar interactions, 1,240 plant–seed feeding interactions and 1,471 herbivore–parasitoid interactions; see Fig. 1b–d for example networks, whereby species are depicted as nodes connected by interactions as links).
Community diversity and structure
There was a significant difference in community composition and network structure among monads, dyads and triads. Thus, insect species richness and abundance, plant species richness, floral abundance, insect species evenness and interaction evenness in 9 ha landscapes were higher when there were more habitats (multiple analysis of variance (MANOVA), F 1,28 = 5.366; P = 0.001; Fig. 2 and Supplementary Table 2 ). Specifically, pairwise MANOVAs demonstrated significant increases from monads to triads ( F 1,18 = 5.552; P = 0.005; Fig. 2 ). To better understand the specific aspects driving the MANOVA results, generalized linear models showed that more habitats in the landscape supported non-significant increases of plant species richness (mean ± s.d. for monads, 41.4 ± 20.77; dyads, 43.3 ± 22.5; triads, 59.1 ± 22.99; F 1,28 = 3.244; P = 0.082; Fig. 2b ) and interaction evenness (monads, 0.48 ± 0.07; dyads, 0.52 ± 0.06; triads, 0.52 ± 0.03; F 1,28 = 3.767; P = 0.062; Fig. 2e ) and a significant increase in insect species evenness (monads, 0.71 ± 0.11; dyads, 0.81 ± 0.06; triads, 0.83 ± 0.04; F 1,28 = 14.92; P < 0.001; Fig. 2f ); all other factors showed no significant difference when considered independently (floral abundance, insect species richness and insect abundance all F 1,28 < 0.714; P > 0.405; Fig. 2a,c,d ).
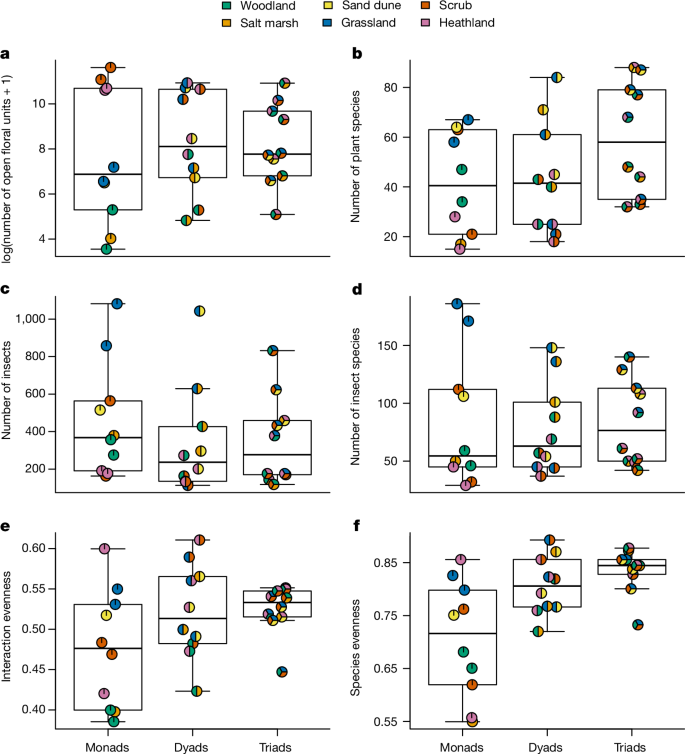
a – f , Differences among monads, dyads and triads in terms of floral abundance ( a ), plant species richness ( b ), insect abundance ( c ), insect species richness ( d ), interaction evenness ( e ) and species evenness ( f ). Circles indicate each site and the habitat combination therein, with a random horizontal jitter to reduce overlap. Data are from 30 independent field sites, 558,386 open floral units (154 plant species) and 11,482 interactions (954 insect species). Boxes represent the 25% (Q1) and 75% (Q3) quartiles around the median line and whiskers are Q1 − 1.5× IQR to Q1 and Q3 to Q3 + 1.5× IQR. See Extended Data Fig. 1 and Supplementary Information section 6 for subwebs.
Community robustness
We measured stability as community robustness, a network-level calculation of the resistance of a community to species loss through secondary extinction, including all interaction types. Although stability has several components, to address the scope of this study, our data sampling design focused on spatial variability across a landscape and did not consider temporal dynamics. Mimicking bottom-up habitat degradation, we simulated removal of plant species across the landscape from least to most abundant at a given site because rare species are more likely to go extinct first 15 . Our robustness analysis ( Methods ) allows for rewiring, whereby species reallocate their interactions following the loss of a resource 16 , 19 and accounts for shared species between interaction types resulting from ontogenetic diet shifts (for example, herbivorous caterpillar to pollinating butterfly); thus measuring the effects of species loss propagating through the multilayer network across different interaction types. There was no difference in mean robustness among monads, dyads and triads ( F 2,27 = 0.183; P = 0.83) but the variability of robustness decreased significantly as habitat number increased (interquartile range (IQR) of 0.105 in monads, 0.064 in dyads and 0.047 in triads; Brown–Forsythe’s test F 2,14997 = 1,272.9; P < 0.001; Fig. 3 ). More flexible rewiring rules make the effect on robustness variability more apparent (Fig. 3 and Extended Data Fig. 2 ). This variability trend is stronger with sequential removal of rare-to-common species than with random removal of species, indicating that secondary extinction trajectories are not purely a result of species loss but rather associated with the distribution of rare species across habitats (Fig. 3 and Extended Data Fig. 3 ). Plant beta-diversity was similar in the monad, dyad and triad sites, indicating that the variability trend in robustness is unlikely to be a consequence of sampling bias because of specific habitat combinations overlapping among triads (Supplementary Information section 1 ).
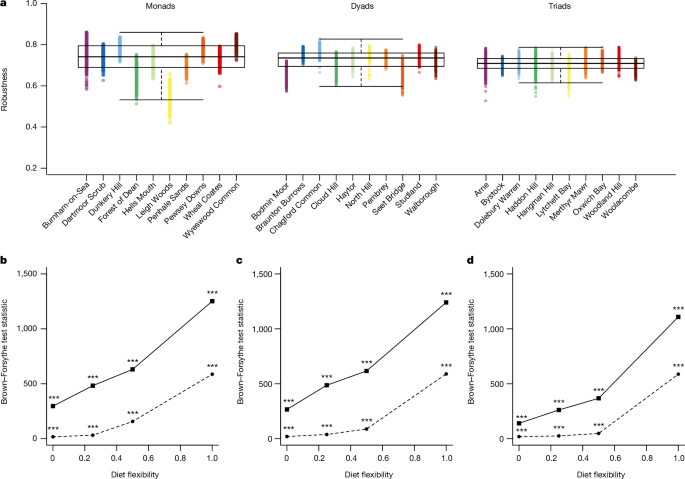
a , Robustness calculations for each site with 100% dietary flexibility for insects and 50% extinction threshold. Each point is a robustness calculation, colour-coded for each site within landscape type. Boxplots indicate the variation for all sites of a landscape type (monad, dyad or triad). b – d , Brown–Forsythe’s test statistic (two-sided), measuring the equality of group variance, for three extinction thresholds (25% ( b ); 50% ( c ); 75% ( d )) and various levels of dietary flexibility. Dashed lines correspond to the random extinction scenario, stars code for the significance level of the test. For each landscape type (monad, dyad and triad), n = 5,000 (ten sites × 500 replicates). Boxes represent the 25% (Q1) and 75% (Q3) quartiles around the median line and whiskers are Q1 − 1.5× IQR to Q1 and Q3 to Q3 + 1.5× IQR. Asterisks indicate significance (*** P < 0.001; all less than P = 7.46 × 10 −8 ).
Community function
We conducted a manipulative field experiment to test how several habitats affected pollination function, defined here as fruit weight and quality. At the centre of each monad and triad, we placed 20 potted wild-type strawberry plants, Fragaria vesca , grown under standardized conditions, as they began flowering (Extended Data Fig. 4 ). Strawberries are an excellent bioassay plant as pollination quality can be easily quantified 8 , 20 with several high-quality insect pollinator visits leading to larger and more symmetrical fruits (Class I fruits versus Class II fruits; Extended Data Fig. 5 ) 21 . Plants were left on site for 14 days to be pollinated and then kept in a pollinator-free greenhouse for 28 days. Ripe strawberries were weighed and graded as being Class I, if perfectly symmetrical, or Class II, if otherwise ( Methods ; Extended Data Fig. 5 ). Strawberries from triads were not heavier ( F 1,16 = 0.091; P = 0.122; Extended Data Fig. 6a ), but they were 30.3% more frequently Class I than those at monad sites ( t 11.3 = 3.263; P = 0.007; Extended Data Fig. 6b ), indicating that pollination was more effective at sites with more habitats. Triad sites were also more consistent in yielding high proportions of Class I fruits (Brown–Forsythe’s test F 1,11 = 10.65; P = 0.007; Extended Data Fig. 6b ).
Pollination is improved by several and varied pollinators 21 , 22 but, although we found overall community differences between monads, dyads and triads (Fig. 2 ), pollinators were not more abundant or more species-rich at triads (Extended Data Fig. 1a,b ). Therefore, to investigate if differences in pollination function could be explained by community interaction differences, we assessed the interaction complementarity of the pollinator community at each site by calculating the dietary dissimilarity of all recorded flower visitors, which has been shown experimentally to improve pollination success 23 . We then used a principal coordinate analysis and calculated the dispersion of the community to evaluate the breadth of dietary dissimilarity across all species at each site; thus sites with more dispersed diets will have higher interaction complementarity ( Methods ). Flower-visiting species at triads differed more in their diets (that is, had greater interaction complementarity) than those at monads ( t 10 = 8.42, P < 0.001; Extended Data Fig. 6c ). Thus, although triads do not support more abundant or rich insect communities, they do host sets of pollinator species with more complementary diets; this observation is robust to the removal of rare or under-sampled species (Supplementary Information section 2 ). Flower–visitor interaction complementarity predicted the proportion of Class I strawberries ( F 3,14 = 3.475; P = 0.045; Extended Data Fig. 6d ), which were higher with greater interaction complementarity but not fruit weight ( F 3,14 = 1.211; P = 0.342) which did not differ across monad and triad sites.
Additive effects versus emergent properties
Lastly, we sought to understand whether the observed patterns in community structure and interaction complementarity of triads were due to an additive effect of several habitats or whether habitat combinations in the landscape present emergent properties. We used a null model and focused on the largest of the component networks: the plant–pollinator network. For each triad site, we created 1,000 null triads from independent observations at the component habitats (monads), while preserving the number of sampled interactions. We then calculated interaction evenness and complementarity for each null triad and compared it to the corresponding empirical triad ( Methods ). Interaction evenness was typically higher in empirical triads than null counterparts (7 of 10 triads; Fig. 4a ) whereas interaction complementarity was lower (7 of 10 triads; Fig. 4b ). These differences weakened but did not reduce to zero when controlling for the number of plant species at the site, indicating that the greater plant species richness of triads (Fig. 2b ) only partially explains these emergent properties (Fig. 4c,d ). It is possible that interaction evenness and complementarity could be due to differences in the plant phylogenetic diversity at a site if distinct phylogenetic groups are associated with different habitats and thus establish distinct sets of interactions. Increased interaction evenness and decreased complementarity at empirical versus null triads could, therefore, be associated with differences in plant phylogenetic diversity, which is indeed positively correlated with interaction complementarity (repeated measures correlation test r 9,969 = 0.158, P < 0.001 and r 9,939 = 0.158, P < 0.001 in Extended Data Fig. 7b,d , respectively) and negatively correlated with interaction evenness ( r 9,969 = −0.238, P < 0.001 and r 9,939 = −0.201, P < 0.001 in Extended Data Fig. 7a,c , respectively). When constraining both models to include equal sampling completeness, the trend is weakened but broadly similar (Supplementary Information section 3 and Extended Data Fig. 8 ).
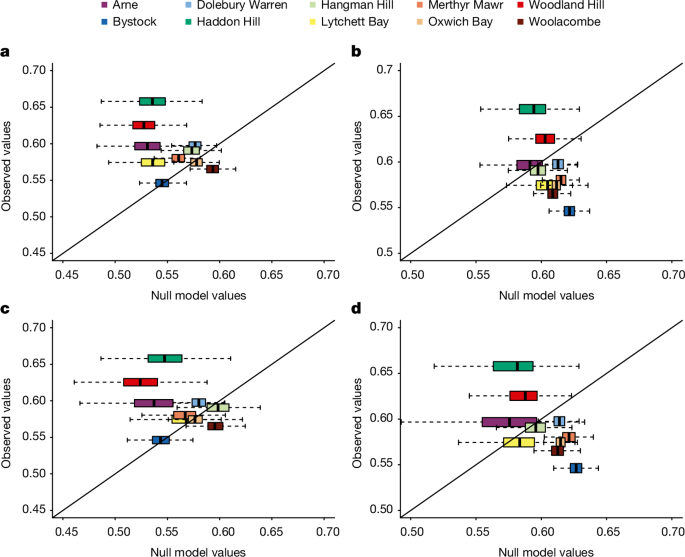
Two variants of the same null model examining the potential for emergent properties at real triad habitat combinations. a – d , Controlling only for the number of interactions ( a , b ); also controlling for the number of plant species ( c , d ). Boxplots of the null model values of the interaction evenness ( a , c ) and functional dispersion ( b , d ) are plotted against the observed value at each site. Interaction complementarity is calculated as the functional dispersion of species at the real or null triads. If the boxplot overlaps the 1:1, then the observed value falls within the null hypothesis; if it is below, the observed value is less than under the null hypothesis and, if above, more than under the null hypothesis. For each boxplot (one per site), n = 1,000 replicates. Boxes represent the 25% (Q1) and 75% (Q3) quartiles around the median line and whiskers are Q1 − 1.5× IQR to Q1 and Q3 to Q3 + 1.5× IQR.
Landscape-scale effects are various and likely to contain trade-offs. Our results show that landscapes comprising several habitats support higher species and interaction evenness, more functionally diverse communities, with more consistent stability and greater pollination function, probably due to increased environmental heterogeneity at the landscape scale. Indeed, at the habitat-scale, a heterogeneous habitat structure allows for more niches and therefore higher biodiversity 3 , 7 , 24 . Our conclusions are unlikely to be confounded by surrounding patch size (Supplementary Information section 4 ) or sampling completeness differences (Supplementary Information section 5 ). For practicality, many management plans are habitat specific and focus on protecting habitat patches that are large and connected to similar ones (for example, many of the Living Landscapes, United Kingdom 2 ; and Natura 2000 networks, European Union; along with prairie restoration projects, USA 25 ). If multi-habitat landscapes are supporting communities with improved structure and functionality and more consistent robustness to species loss, as shown here, then maintaining diverse connected natural habitats across the wider landscape is likely also to be important. This is key to species conservation, as some species may depend on several habitats for different life stages (for example, different habitat requirements for larval herbivory and adult floral resources), ecological needs (for example, nesting in one habitat but foraging in another) or maximizing of resource availability across seasons (for example, the phenology of flowering plants varies among habitats).
Landscape simplification and habitat loss are significant stressors on biodiversity, community structure and ecosystem function. We found that more natural habitats provide a greater consistency in robustness. The higher variability in single-habitat landscapes means that extremes of robustness are more common, putting communities at individual sites more frequently at risk of cascading effects of species loss. At the landscape scale, the benefits of multi-habitat configurations therefore allow for a buffering effect, even if there is no average loss of robustness in landscapes with fewer habitats; more robust habitats might compensate for lower robustness in contiguous habitats resulting in a landscape-level decrease in robustness variability. To explore the mechanistic path underlying this trend (for example, with path analysis) would require several replications for each habitat combination; however, our results point to potential effects of landscape heterogeneity on community stability being mediated through interaction evenness 26 (Fig. 2e ) and tuned by plant phylogenetic diversity 27 (Extended Data Fig. 7a,c ). Our model indicates that this relationship between several habitats and robustness variability is more pronounced when species have greater flexibility to switch resources. This rewiring flexibility might be an important community response to stressors such as climate change and biodiversity loss 28 , suggesting that multi-habitat landscape configurations could provide even greater protection against environmental change.
Robustness to species loss and the rewiring of interactions are both related to interaction generalism. A greater proportion of generalists in the empirical triads could explain lower interaction complementarity compared to the null triads; yet, empirically, triad interaction complementarity was still higher than in empirical monads. Our field experiment indicates that several habitats supported better pollination with better-quality fruit set, which is explained not by more flower-visiting species or increased visits but rather by this higher interaction complementarity of pollinators at triads. Interaction complementarity, which could reduce the effect of conspecific pollen deposition 29 , positively correlates with the phylogenetic diversity of the plants supporting the food webs, suggesting that multi-habitat landscapes might increase complementarity through an increase in plant phylogenetic (and presumably functional) diversity.
Several habitat landscapes may therefore support both more interaction complementarity (for successful plant reproduction 30 ) than single-habitat landscapes and greater redundancy through generalist species (which is important for robustness 31 ) than expected by compiling the interactions from several independent habitats. Collectively, this link from landscape composition, through the plant–insect community structure, to ecosystem function provides a mechanism through which several habitats across the landscape can support stability and better ecosystem services.
Field sites
We sampled 30 sites in southwest England and southern Wales, each containing one, two or three of six habitat types: woodland, heathland, grassland, salt marsh, sand dunes and scrub. We sampled at ten single-habitat sites, monads, ten two-habitat sites, dyads and ten three-habitat sites, triads. The site size sampled remained constant, thus monads were a single habitat 9 ha in size, dyads consisted of two adjacent 4.5 ha habitats and triads consisted of three adjacent 3 ha habitats. A 9 ha field site size was selected to capture the diversity of different taxa across monads, dyads and triads, while also allowing effective sampling of all taxa at each site (plants, herbivores, pollinators and parasitoids). All sites were surrounded by the same habitats as those in the field site or water, urban environment or farmland habitats and each field site was visited once in May–September 2014 and three times in April–September 2015. In 2015, all sites were visited before any site was repeated. In each sampling round, sites were visited in ten three-site cycles, each comprising one monad, dyad and triad. The order of visited sites was randomized within both cycle and round.
Potential sites were initially selected with Arc GIS v.10.1 using the 2007 Land Cover Map 32 . Three GIS models selected sites that were (1) single-habitat 9 ha sites with a 500 m buffer that did not include any other habitat of interest (for example, urban, farmland or water were allowed in the buffer); (2) two 4.5 ha contiguous habitats with a 500 m buffer that did not include any other habitat of interest; and (3) three 3 ha contiguous habitats with a 500 m buffer that did not include any other habitat of interest. This created a long list of potential sites that were then verified and narrowed down using satellite images (Google Earth 2013) and finally ground-truthed to confirm habitat types, make final selections and outline appropriate habitat plots. The final site list was based on ease of access, travel time, the need to avoid geographic clustering of any site (Fig. 1a ) or habitat type, along with our ability to secure permission to sample. We selected habitat combinations such that habitats were represented equally across monads, dyads and triads, while accounting for the restrictions of what was available in the southwest United Kingdom. Because some habitat combinations did not exist in accordance with our selection criteria and others consistently occur together (for example, sand dunes and salt marshes often border grasslands), across monads, dyads and triads, we sampled fewer sand dunes and salt marshes and more grassland and scrub. For a full list of sites see Supplementary Table 3 .
Data collection
At each site, on each visit, we sampled along six 35 m transects arranged as follows: six transects in the one monad habitat, three in each dyad habitat and two in each triad habitat. The transect start location and direction were randomly selected before arrival on site and changed on each of the four visits. Thus, in total we sampled along 24 transects at each site.
We designed our data collection under the assumption that sampling intensity is the main driver of species–area relationships, whereas the influence of patch size on per-unit-area (alpha) diversity is weak or absent 33 , 34 . Thus, larger patches typically have more species in total because they contain a variety of microhabitats. Repeated sampling across these larger patches would capture more microhabitats and therefore show high between-sample (beta) diversity 34 . Therefore, to control for these area effects on richness, the sampling effort was consistent at the site level 33 , 34 and thus we standardized the number of samples per site to avoid a patch size bias. Collection protocol closely followed ref. 12 and is described below.
Plant sampling
On each visit, a 0.5 × 0.5 m 2 gridded quadrat was placed on alternating sides of the transect every 10 m, resulting in four quadrats per transect. All plants were identified and given a vegetation abundance score (as in refs. 12 , 35 ). Category 1 species were rare, only present once to a few times (vegetation occupied 1–2% of the quadrat area), category 2 were present in high enough numbers to be seen easily (occupied less than 10% of the quadrat area), category 3 could be seen throughout the quadrat (less than 50% of the area) and category 4 were dominated by the given species (more than 50% of the area). Tree vegetation to a height of 2 m and grasses, the latter collectively pooled, were all classified on this 1–4 scale. All other flowering plant species were identified 36 and floral abundance was further classified with buds, open, wilted and seed-set floral units counted. We calculated these per floral unit for flowers arranged in umbels, heads, capitula and spikes. Vegetation cover for flowering species was determined by the number of times a plant touched one of the 36 cross points formed by the intersecting grids on the quadrat. Any plant species that did not fall within a quadrat but which occurred within 30 m of the transect, were recorded but not included in quantitative analysis.
Plant–flower visitor network
On each visit, between 09:00 and 17:30 in dry, warm (minimum 15 °C) conditions with little to no wind, flower visitors were sampled by haphazardly walking for 20 min, no more than 30 m from the transect. All insects found on a flower head were collected using a hand net. Visited flowers were identified to species in the field and flower visitors were identified to species by taxonomists (see ‘Acknowledgements’).
Plant–herbivore–parasitoid networks
On each visit, we collected leaf miners and caterpillars from 1 m 2 quadrats every 10 m on either side of the transect by visual searching of leaves to a height of 2 m. They were collected and stored individually and returned to the laboratory for rearing.
Leaf miners were initially identified from the leaf mine pattern 37 , 38 and caterpillar species were identified at larval stage 39 . Individual larvae from both groups were reared in separate pots and checked every 2–3 days for emergence. Emerged adults, either parasitoid or herbivore, were identified by taxonomists (see Acknowledgements). We used adult identification of surviving individuals to confirm larval identifications, where possible, to ensure accurate identification for herbivores that were either killed by a parasitoid or died during rearing.
Seed herbivores and their parasitoids were collected in seeds in the first and fourth sampling rounds (that is, once in September 2014 and once in August–September 2015). Along each transect, we collected up to 50 seeds from plants expected to host seed feeders 12 , 40 . Seeds were collected from haphazardly sampled plants within 10 m of the transect and, where possible, from different plants, equally spaced along the transect. Each sample of up to 50 seeds was stored collectively in the same pot and checked weekly until adult herbivores and parasitoids emerged (up to 8 months). Each emerged insect, seed feeder or parasitoid, was collected, stored individually and identified by taxonomists. Insects were successfully reared from 23 plant species ( Anthyllis vulneraria, Aster tripolium, Centaurea nigra, Cirsium arvense, Cirsium eriophorum, Cirsium palustre, Cirsium vulgare, Crataegus monogyna, Lathyrus pratensis, Lotus corniculatus, Ornithopus perpusillus, Rhinanthus minor, Rosa rugose, Rubus fruticosus agg., Senecio jacobaea, Succisa pratensis, Trifolium arvense, Trifolium pratense, Trifolium repens, Ulex europaeus, Ulex galii, Viccia sativa and Vicia cracca ).
Pollination experiment
Between 4 and 14 July 2015, we placed 20 wild strawberry plants ( F. vesca ) in four 15 l buckets in the centre of each monad and triad; dyads were excluded to allow all plants to be placed and retrieved in the flowering time. Each bucket was surrounded by chicken wire to discourage disturbance by wildlife and livestock (Extended Data Fig. 4 ). Plants were at the point of flowering when put in the field, then left for 14 days to allow for natural pollination and then retrieved. It was not possible to collect data on strawberry visitation as the plants could only be left in position for a short period (as seed set occurs relatively quickly) and it was not feasible to simultaneously sample 20 geographically distant field sites (Fig. 1 ) in a meaningful fashion to record pollinator visitation during this period. F. vesca was selected as it grows naturally in the region, is visited by a wide range of pollinators 8 and, although partly wind pollinated, insects are crucial for its successful, uniform pollination 21 , leading to higher seed set and more symmetrical fruits. Moreover, in commercial varieties, better pollination is linked with increased shelf life and market value 13 . At the end of the field experiment, we removed all new flower buds and stored the plants in an insect-free greenhouse, watering daily. Strawberries were picked when ripe, weighed and graded according to commercial symmetry ratings 41 : fruit containing only mild defects in shape (Class I) and those with more severe defects (Class II) (see Extended Data Fig. 5 for example pictures); fruit symmetry significantly affects the market value of commercial strawberries, hence the existence of a grading system. Fruit classes were assigned blindly by an assessor with no knowledge of the field sites, to avoid assessment bias. We stopped fruit collection on greenhouse-stored plants after 28 days.
Data analysis
Data across all visits were summed to create one network per site with edges weighted by interaction frequency. All analyses were performed and graphs created in the R statistical environment (v.3.6.0) 42 . All data and code are available at Zenodo ( https://doi.org/10.5281/zenodo.11184586 ) 43 .
Community structure
We used a MANOVA to test for overall differences in community and network structure among monads, dyads and triads, based on plant species richness, floral abundance, insect species richness, insect abundance, Pielou’s species evenness and interaction evenness, which are expected to change according to land use 44 . Species evenness was calculated using vegan 45 and interaction evenness using bipartite 46 . To determine the factors contributing to the MANOVA results, we performed pairwise MANOVAs between landscape types and general linear models for each of the six structural aspects (response variables). All residuals were normally distributed and homoscedastic, except for floral abundance, which required a log-transformation: log( x + 1).
To determine the response of ecological communities to species extinction, we evaluated the robustness of plant–insect networks to extinction of plant species from the least to the most common (as in ref. 8 ). We evaluated how common a plant species is by its average proportion in the landscape; the commonness C is of plant species i in site s calculated as:
where H s is the number of habitats in site s , a ij / A j is the proportion of plant species i in habitat j , defining A j as the total abundance of plant species in habitat j and a ij being the abundance of plant species i in habitat j . To calculate this metric for a given plant species, we used its average number of quadrat cross points in each habitat for a given site as a proxy of its local relative abundance.
We modelled a flexible behavioural response of upper trophic levels to their host plant’s extinction. Specifically, the extinction of a plant species can generate cascading loss of species which then allows for rewiring of the network. Herein, we assume that insects are able to reallocate part of their diet on similar resources/hosts, which we determined by identifying the taxa on which species with a similar niche (that is, sharing part of their diet with the focal insect species) feed 16 . Following ref. 19 , we allowed species to reallocate lost interactions to alternative resources/hosts following a primary extinction and probability to interact with a given alternative resource/host was proportional to its abundance (approximated with interaction frequencies). We explored a range of species flexibility, from 0% flexibility (no rewiring allowed) to 100% flexibility (full reallocation of all lost interactions), including intermediate flexibility levels: 25% and 50%. We also explored the range of species’ sensitivity to interaction loss, expressed as a percentage of observed feeding interaction events below which a species is considered extinct: 25%, 50% and 75% of lost interactions (all cases are shown in Extended Data Figs. 2 and 3 and robustness to extinctions with full reallocation and 50% sensitivity level is shown in Fig. 3 ). We extend the approach of ref. 19 to multipartite networks with species interacting at different life stages, by assuming that if one life stage of one species goes extinct (for example, caterpillars), so do the others (for example, the corresponding adult butterfly in the flower–visitor network). Thus, species loss can propagate between two types of networks as a result of species being pollinators, herbivores or even parasitoids during different life stages or ecological requirements. Our approach allows rewiring alternatives and species extinction to be evaluated, respectively, for each interaction type and, for each life stage, when applicable.
We repeated this simulation 100 times, both under this scenario and under random removal. Whereas the former tests the response to plant species loss, the latter provides a control scenario accounting for the contribution of basic properties of the networks (size, number of links and so on) to community robustness.
The effect of habitat numbers (one or three) on fruit weight and the proportion of Class 1 strawberries (a measure of fruit quality that is determined by pollination success) was assessed using a mixed effect model with site as a random effect and the landscape type (monad or triad) as a fixed effect, using the package lme4 (ref. 47 ).
Interaction complementarity
Our field experiment supported the idea that pollination function is higher in sites with three habitats than in sites with a single habitat, even if pollinator richness and abundance were similar in sites with different numbers of habitats. Therefore, we asked whether the pollinator communities of sites with more habitats use floral resources in more complementary ways, compared with single-habitat sites, given that there is evidence that interaction complementarity can be associated with increased function 23 . Our measure of interaction complementarity was adapted from functional diversity analysis methods (for example, refs. 48 , 49 ) which measures, for instance, the breadth in species functional traits in ecological communities. Here we measure the breadth of pollinators’ use of flower resources; under the assumption that pollinator species with more dissimilar patterns of resource use complement the resource use of other pollinators, thereby increasing pollination function of the community 22 .
We started by computing the dissimilarity in resource use of pollinators from the plant–pollinator network data. To: (1) have a more complete picture of how complementary pollinator species interactions were in our communities and (2) create a multidimensional functional space which was comparable across sites, we computed a Bray–Curtis dissimilarity matrix from the complete plant–pollinator interaction network, that is all 30 sites pooled together in one interaction network. To control for a potential sampling completeness bias across pollinator species, we normalized pollinator interaction weights, so that interaction weights for each pollinator species summed to 1. We then performed a principal coordinate analysis (PCoA), to place pairwise dissimilarities into a multidimensional space, in which each species is represented by one point and Euclidean distances between species are proportional to their dissimilarities in resource use (Supplementary Fig. 1 ). We assessed the quality of the functional space using the mean squared deviation index 50 and with no obvious break point, selected the space with ten dimensions. Using the FD package 51 , we then calculated the functional dispersion of each monad and triad site, measured as the sum of the distances of all species in that site to the community centroid. We took this to represent the overall pollinator interaction complementarity at the site level.
Data were normally distributed and had unequal variance, thus we used a Welch’s two sample t -test to determine the difference between the interaction complementarity at monads and triads. Using a linear model, we then tested whether interaction complementarity at each site predicted fruit weight and the proportion of Class 1 strawberries.
Null model tests for additive effects versus emergent properties of several habitats
Using a null model approach 27 , 52 , we tested whether the network properties we measured on the sites with several habitats are different from those expected if landscape-scale food webs are simply the sum of their habitats (the null hypothesis). To do so, we first created null triads, that is landscape-scale networks constructed from data collected at monad sites randomly assembled to represent the empirical triad landscapes. Then, we quantified interaction evenness and interaction complementarity for null triads and compared these to empirical triads.
Similar to other measures of biodiversity, network properties are expected to be affected by the size of the sampling area 53 as in Supplementary Fig. 2 for three hypothetical habitats: H 1 , H 2 and H 3 . If landscape-scale food webs are simply the sum of their habitats, then the null hypothesis should translate as follows in terms of species richness and number of interspecific interactions: a triad made of { H 1 , H 2 , H 3 } (each of area Δ /3, Δ being the area of the sampled site) should have fewer or an equal number of links \({L}_{\left\{{H}_{1},{H}_{2},{H}_{3}\right\},\left\{\varDelta /3,\varDelta /3,\varDelta /3\right\}}\) than the sum of the links in each habitat of size Δ /3 (that is, \({L}_{{H}_{1},\varDelta /3}+{L}_{{H}_{2},\varDelta /3}+{L}_{{H}_{3},\varDelta /3}\) ). Indeed, a lower number of links would occur if interactions are shared across several habitats. The same rationale applies to the number of species S :
We created two nested null models that generate random plant–insect interaction networks to test emergent properties within triads.
Null model no. 1 . For an observed triad T k made of { H 1 , H 2 , H 3 }, we created three random networks for each habitat H i by subsampling the observed monad networks in the corresponding habitats. Thus, a null triad of woodland, heathland and grassland would be created by subsampling the equivalent number of interactions from woodland, heathland and grassland monads. A random network for the habitat H i in the triad T k was generated by subsampling \({{N}}_{{{T}}_{{k}},{{H}}_{{i}}}\) (the number of interaction events to consider for the {triad T k , habitat H i }-tuple) interactions among those observed in the monad with habitat type H i (hereafter, monad \({{M}}_{{{H}}_{{i}}}\) ). The probability of sampling one interaction was assumed to be proportional to the number of times it had been observed in the monad \({{M}}_{{{H}}_{{i}}}\) . For an interaction between species b and species a in monad \({{M}}_{{{H}}_{{i}}}\) , this probability is:
where \({{N}}_{{ab},{{M}}_{{{H}}_{{i}}}}\) is the number of individuals of species b recorded interacting with species a in monad \({{M}}_{{{H}}_{{i}}}\) and \({{N}}_{{{M}}_{{{H}}_{{i}}}}\) is the total number of insect individuals seen interacting within monad \({{M}}_{{{H}}_{{i}}}\) .
Null model no. 2 . In this null model, we also controlled the diversity of the plant community to test whether it contributes to the differences between the observed triads and their random counterparts generated with null model no. 1. To this end, we follow the same steps as for the null model no. 1 but sampled interactions within a subset of monad \({{M}}_{{{H}}_{{i}}}\) involving the same number of plant species as observed interacting in the habitat H i of the triad T k .
Evaluating emergent properties
Following the construction of each null triad, we calculated the network properties of interest (interaction evenness and interaction complementarity) and compared them to those of the observed triads. The boxplots in the insets of Fig. 4 and Extended Data Fig. 8 show the range of values for a given network value that we can reasonably expect for a given site under the null hypothesis. If the boxplot overlaps the 1:1 line (that is, x = y line), then the observed value falls within the null hypothesis. If it is below that line, the observed value is less than under the null hypothesis and, if above, more than under the null hypothesis.
Evaluating phylogenetic diversity
To account for the effect of plant phylogenetic diversity in the empirical and simulated triads, we constructed plant phylogenetic trees for each community and measured their phylogenetic diversity as the mean tree branch length. To build one tree per community, we cropped the Daphne phylogeny, a comprehensive dated phylogeny of the European flora 54 , to only include plant species available in each of our communities. Our visitation dataset contains 149 plant species, out of which 139 species (93%) can be found in the Daphne phylogenetic tree. We standardized synonyms for species that were separately identified using Global Biodiversity Information Facility (GBIF). The remaining ten plant species that are not recorded in the Daphne phylogeny can be divided into two groups as follows (Supplementary Table 1 ):
Plant species that are the only representatives of their genus in our pollination dataset ( n = 3).
Plant species that are not the only representatives of their genus in the dataset ( n = 7).
These plant species were assigned an alternative replacement species, in their genus, selected from the available species in the phylogeny dataset. The replacements for species in the first group were randomly chosen, as fine-scale phylogenetic distances will probably be less important when a genus is represented by a single species in the data. The replacements for species in the second group were chosen more carefully, as they co-occur with congeneric species, following three steps:
We selected the species from the focal genus available in Daphne phylogeny which were not already part of the interaction dataset.
In GBIF we obtained the coordinates of the occurrences—if any—of these possible species in the United Kingdom.
For each of the seven species in the second group, we ordered their alternative species from the most to least likely species (measured as number of occurrences in our sampling area). We then selected the top most likely species, which had at least twice as many occurrences as the next likely species. Therefore, the selected species had similarly high occurrences, whereas non-selected species had half or fewer occurrences than the selected species with least occurrences.
If a community included species from the second group and for these species more than one alternative was selected, alternative trees for that community were built and their mean phylogenetic diversity calculated.
We calculated repeated measures correlations between plant phylogenetic diversity and both interaction evenness and interaction complementarity using rmcorr 55 .
Reporting summary
Further information on research design is available in the Nature Portfolio Reporting Summary linked to this article.
Data availability
All data and code are available at Zenodo ( https://doi.org/10.5281/zenodo.11184586 ) 43 .
Mace, G. M. Whose conservation? Science 345 , 1558–1560 (2014).
ADS CAS PubMed Google Scholar
Lawton, J. H. et al. Making Space for Nature: A Review of England’s Wildlife Sites and Ecological Network (Department for Environment, Food and Rural Affairs, 2010).
Sirami, C. et al. Increasing crop heterogeneity enhances multitrophic diversity across agricultural regions. Proc. Natl Acad. Sci. USA 116 , 16442–16447 (2019).
ADS CAS PubMed PubMed Central Google Scholar
Ben-Hur, E. & Kadmon, R. An experimental test of the area–heterogeneity tradeoff. Proc. Natl Acad. Sci. USA 117 , 4815–4822 (2020).
Watling, J. I. et al. Support for the habitat amount hypothesis from a global synthesis of species density studies. Ecol. Lett. 23 , 674–681 (2020).
PubMed Google Scholar
Fahrig, L. Ecological responses to habitat fragmentation per se. Annu. Rev. Ecol. Evol. Syst. https://doi.org/10.1146/annurev-ecolsys-110316-022612 (2017).
Renard, D. & Tilman, D. National food production stabilized by crop diversity. Nature 571 , 257–260 (2019).
Morrison, B. M. L., Brosi, B. J. & Dirzo, R. Agricultural intensification drives changes in hybrid network robustness by modifying network structure. Ecol. Lett. 23 , 359–369 (2020).
Harvey, E., Gounand, I., Ward, C. L. & Altermatt, F. Bridging ecology and conservation: from ecological networks to ecosystem function. J. Appl. Ecol. 54 , 371–379 (2017).
Google Scholar
Dehling, D. M. & Stouffer, D. B. Bringing the Eltonian niche into functional diversity. Oikos https://doi.org/10.1111/oik.05415 (2018).
Timóteo, S., Correia, M., Rodríguez-Echeverría, S., Freitas, H. & Heleno, R. Multilayer networks reveal the spatial structure of seed-dispersal interactions across the Great Rift landscapes. Nat. Commun. 9 , 140 (2018).
ADS PubMed PubMed Central Google Scholar
Hackett, T. D. et al. Reshaping our understanding of species’ roles in landscape-scale networks. Ecol. Lett. https://doi.org/10.1111/ele.13292 (2019).
Tscharntke, T. et al. Landscape moderation of biodiversity patterns and processes—eight hypotheses. Biol. Rev. 87 , 661–685 (2012).
Blüthgen, N. & Klein, A. M. Functional complementarity and specialisation: the role of biodiversity in plant–pollinator interactions. Basic Appl. Ecol. 12 , 282–291 (2011).
Gámez-Virués, S. et al. Landscape simplification filters species traits and drives biotic homogenization. Nat. Commun. 6 , 8568 (2015).
Staniczenko, P. P. A., Lewis, O. T., Jones, N. S. & Reed-Tsochas, F. Structural dynamics and robustness of food webs. Ecol. Lett. 13 , 891–899 (2010).
Loreau, M., Mouquet, N. & Gonzalez, A. Biodiversity as spatial insurance in heterogeneous landscapes. Proc. Natl Acad. Sci. USA 100 , 12765–12770 (2003).
Limberger, R., Pitt, A., Hahn, M. W. & Wickham, S. A. Spatial insurance in multi-trophic metacommunities. Ecol. Lett. 22 , 1828–1837 (2019).
PubMed PubMed Central Google Scholar
Schleuning, M. et al. Ecological networks are more sensitive to plant than to animal extinction under climate change. Nat. Commun. 7 , 13965 (2016).
MacInnis, G. & Forrest, J. R. K. Pollination by wild bees yields larger strawberries than pollination by honey bees. J. Appl. Ecol. https://doi.org/10.1111/1365-2664.13344 (2019).
Klatt, B. K. et al. Bee pollination improves crop quality, shelf life and commercial value. Proc. R. Soc. B 281 , 20132440 (2014).
Fründ, J., Dormann, C. F., Holzschuh, A. & Tscharntke, T. Bee diversity effects on pollination depend on functional complementarity and niche shifts. Ecology https://doi.org/10.1890/12-1620.1 (2013).
Stavert, J. R., Bartomeus, I., Beggs, J. R., Gaskett, A. C. & Pattemore, D. E. Plant species dominance increases pollination complementarity and plant reproductive function. Ecology https://doi.org/10.1002/ecy.2749 (2019).
Zirbel, C. R., Grman, E., Bassett, T. & Brudvig, L. A. Landscape context explains ecosystem multifunctionality in restored grasslands better than plant diversity. Ecology https://doi.org/10.1002/ecy.2634 (2019).
Wachenheim, C. J., Lesch, W. C. & Dhingra, N. The Conservation Reserve Program: A Literature Review (Department of Agribusiness and Applied Economics, 2014).
Tylianakis, J. M., Laliberte, E., Nielsen, A. & Bascompte, J. Conservation of species interaction networks. Biol. Conserv. 143 , 2270–2279 (2010).
Peralta, G. Merging evolutionary history into species interaction networks. Funct. Ecol. 30 , 1917–1925 (2016).
Bartley, T. J. et al. Food web rewiring in a changing world. Nat. Ecol. Evol. 3 , 345–354 (2019).
Morales, C. L. & Traveset, A. Interspecific pollen transfer: magnitude, prevalence and consequences for plant fitness. Crit. Rev. Plant Sci. 27 , 221–238 (2008).
CAS Google Scholar
Magrach, A., Molina, F. P. & Bartomeus, I. Niche complementarity among pollinators increases community-level plant reproductive success. Peer Community J. 1 , 1 (2021).
Sheykhali, S. et al. Robustness to extinction and plasticity derived from mutualistic bipartite ecological networks. Sci. Rep. 10 , 9783 (2020).
Morton, D. et al. Final Report for LCM2007—the New UK Land Cover Map (Centre for Ecology & Hydrology, 2011).
Hill, J. L., Curran, P. J. & Foody, G. M. The effect of sampling on the species–area curve. Glob. Ecol. Biogeogr. Lett. 4 , 97–106 (1994).
Schoereder, J. H. et al. Should we use proportional sampling for species–area studies? J. Biogeogr. 31 , 1219–1226 (2004).
Gibson, R. H., Pearce, S., Morris, R. J., Symondson, W. O. C. & Memmott, J. Plant diversity and land use under organic and conventional agriculture: a whole-farm approach. J. Appl. Ecol. https://doi.org/10.1111/j.1365-2664.2007.01292.x (2007).
Rose, F. & O’Reilly, C. The Wild Flower Key: How to Identify Wild Flowers, Trees and Shrubs in Britain and Ireland (Frederick Warne, 2006).
Dickerson, B. The Identification of Leaf-mining Lepidoptera (British Leafminers, 2007).
Pitkin, B., Ellis, W., Plant, C. & Edmunds, R. The leaf and stem miners of British flies and other insects . UK Flymines www.ukflymines.co.uk/index.php (2007).
Porter, J. The Colour Identification Guide to Caterpillars of the British Isles (Macrolepidoptera) (Apollo Books, 1997).
Evans, D. M., Pocock, M. J. O. & Memmott, J. The robustness of a network of ecological networks to habitat loss. Ecol. Lett. 16 , 844–852 (2013).
Commission Delegated Regulation (EU) 2019/428—of 12 July 2018—Amending Implementing Regulation (EU) No 543/2011 as Regards Marketing Standards in the Fruit and Vegetables Sector (EU, 2018).
R Core Team. R: A Language and Environment for Statistical Computing (R Foundation for Statistical Computing, 2020).
Hackett, T. D., Sauve, A., Maia, K. P., Montoya, D., Davies, N., Archer, R., Potts, S. G., Tylianakis, J. M., Vaughan, I. P., & Memmott, J. Multi-habitat landscapes. Zenodo 10.5281/zenodo.11184586 (2024).
Tylianakis, J. M., Tscharntke, T. & Lewis, O. T. Habitat modification alters the structure of tropical host–parasitoid food webs. Nature 445 , 202–205 (2007).
Oksanen, J. et al. vegan: Community ecology. R package v.2.5-7 (CRAN, 2020).
Dormann C., Fruend J., Bluethgen N. & Gruber B. Indices, graphs and null models: analyzing bipartite ecological networks. Open Ecol. J. 2 , 7−24 (2009).
Bates, D., Mächler, M., Bolker, B. M. & Walker, S. C. Fitting linear mixed-effects models using lme4. J. Stat. Softw. 67 , 1–48 (2015).
Laliberté, E. & Legendre, P. A distance-based framework for measuring functional diversity from multiple traits. Ecology 91 , 299–305 (2010).
Villéger, S., Mason, N. W. H. & Mouillot, D. New multidimensional functional diversity indices for a multifaceted framework in functional ecology. Ecology https://doi.org/10.1890/07-1206.1 (2008).
Maire, E., Grenouillet, G., Brosse, S. & Villéger, S. How many dimensions are needed to accurately assess functional diversity? A pragmatic approach for assessing the quality of functional spaces. Glob. Ecol. Biogeogr. https://doi.org/10.1111/geb.12299 (2015).
Laliberté, E., Legendre, P. & Shipley, B. FD: measuring functional diversity from multiple traits, and other tools for functional ecology. R package v.1.0-12.3 (CRAN, 2014).
Bennett, A. B. & Gratton, C. Floral diversity increases beneficial arthropod richness and decreases variability in arthropod community composition. Ecol. Appl. 23 , 86–95 (2013).
Galiana, N. et al. The spatial scaling of species interaction networks. Nat. Ecol. Evol. 2 , 782–790 (2018).
Durka, W. & Michalski, S. G. Daphne: a dated phylogeny of a large European flora for phylogenetically informed ecological analyses. Ecology 93 , 2297–2297 (2012).
Bakdash, J. Z., Maintainer, L. R. M. & Marusich, L. R. rmcorr: repeated measures correlation. Front. Psychol. https://doi.org/10.3389/fpsyg.2017.00456 (2017).
Download references
Acknowledgements
We thank field and laboratory assistants, K. White, J. Morton, M. Broyles, H. Morse, C. Doran and S. Sanghera; taxonomists, M. Wilson, J. Deeming, B. Levey, A. Polaszek, P. M. Pavett and R. Barnett, who were essential in identifying the insects; and greenhouse manager, T. Pitman, for help with strawberry plant care. A. Scott and G. Rowlands provided GIS support for site selection and habitat patch calculations, respectively. The Wildlife Trust, National Trust and private landowners allowed us access to field sites. K. Baldock and members of Community Ecology Research Oxford group provided valuable feedback and discussions. This research was funded by NERC (NE/K006568/1). J.M.T. was funded by the Marsden Fund (UOC1705).
Author information
Authors and affiliations.
School of Biological Sciences, University of Bristol, Bristol, UK
Talya D. Hackett, Alix M. C. Sauve, Kate P. Maia, Daniel Montoya, Nancy Davies, Rose Archer & Jane Memmott
Department of Biology, University of Oxford, Oxford, UK
Talya D. Hackett
Department of Computer Science, University of Bristol, Bristol, UK
Alix M. C. Sauve
University of Bordeaux, Integrative and Theoretical Ecology group, LabEx COTE, Pessac, France
Institute of Biosciences Institute, University of São Paulo, São Paulo, Brazil
Kate P. Maia
Basque Centre for Climate Change (BC3), Parque Científico UPV-EHU, Leioa, Spain
Daniel Montoya
IKERBASQUE, Basque Foundation for Science, Bilbao, Spain
Centre for Agri-Environmental Research, School of Agriculture, Policy and Development, University of Reading, Reading, UK
Simon G. Potts
Bioprotection Aotearoa and Centre for Integrative Ecology, School of Biological Sciences, University of Canterbury, Christchurch, New Zealand
Jason M. Tylianakis
School of Biosciences, Cardiff University, Sir Martin Evans Building, Cardiff, UK
Ian P. Vaughan
You can also search for this author in PubMed Google Scholar
Contributions
The study was conceived by J.M. and D.M. and designed by T.D.H., J.M., D.M. and A.M.C.S. with input from all authors. Fieldwork was carried out by T.D.H., N.D. and R.A. A.M.C.S. led on development of the robustness model. T.D.H., K.P.M. and I.P.V. carried out the interaction complementarity analysis. T.D.H., A.M.C.S. and K.P.M. developed the null model analyses. All further analyses were completed by T.D.H., A.M.C.S., K.P.M. and I.P.V. with input from all authors. T.D.H. led the writing of the manuscript, all authors contributed to drafts of the manuscript and gave final approval for publication.
Corresponding authors
Correspondence to Talya D. Hackett or Jane Memmott .
Ethics declarations
Competing interests.
The authors declare no competing interests.
Peer review
Peer review information.
Nature thanks Ingo Grass and the other, anonymous, reviewer(s) for their contribution to the peer review of this work. Peer reviewer reports are available.
Additional information
Publisher’s note Springer Nature remains neutral with regard to jurisdictional claims in published maps and institutional affiliations.
Extended data figures and tables
Extended data fig. 1 flower visitors structural box plots..
Differences among flower-visitors at monads, dyads and triads in terms of: A. abundance B. species richness, C. interaction evenness and D. species evenness. Circles indicate each site and the habitat combination therein with a random horizontal jitter to reduce overlap. Data are from 30 independent field sites and 5,729 flower-visitor interactions (526 species). Boxes represent the 25% (Q1) and 75% (Q3) quartiles around the median line, and whiskers are Q1 − 1.5xIQR to Q1 and Q3 to Q3 + 1.5xIQR.
Extended Data Fig. 2 Least-to-most abundant scenario.
Robustness calculations for each site under multiple extinction scenarios and with different levels of dietary flexibility. Dietary flexibility for insects was set at 0% (A, E, I), 25% (B, F, J), 75% (C, G, K) and 100% (D, H, L) dietary flexibility. Species’ sensitivity to interaction loss was set at: 25% (A-D), 50% (E-H) and 75% (I-L) of interactions. Plant species were removed from least to most abundant. For each landscape type (monad, dyad and triad), n = 5,000 (10 sites x 500 replicates). Boxes represent the 25% (Q1) and 75% (Q3) quartiles around the median line, and whiskers are Q1 − 1.5xIQR to Q1 and Q3 to Q3 + 1.5xIQR.
Extended Data Fig. 3 Random removal scenario.
Robustness calculations for each site under multiple extinction scenarios and with different levels of dietary flexibility. Dietary flexibility for insects was set at 0% (A, E, I), 25% (B, F, J), 75% (C, G, K) and 100% (D, H, L) dietary flexibility. Species’ sensitivity to interaction loss was set at: 25% (A-D), 50% (E-H) and 75% (I-L) of interactions. Plant species were removed randomly. For each landscape type (monad, dyad and triad), n = 5,000 (10 sites x 500 replicates). Boxes represent the 25% (Q1) and 75% (Q3) quartiles around the median line, and whiskers are Q1 − 1.5xIQR to Q1 and Q3 to Q3 + 1.5xIQR.
Extended Data Fig. 4 Photograph of pollination strawberry bioassay experiment.
Pollination experiment set-up at a monad (left) and triad (right). Five wild-type strawberry ( Fragaria vesca) plants were placed in each of the four buckets (total 20 plants) and surrounded with chicken wire to prevent grazing. Plants in flower bud were placed at habitat boundaries, where relevant, and as close to the centre of the plot as possible, left for two weeks and then retrieved and kept in a pollinator-free greenhouse.
Extended Data Fig. 5 Example of a Class I and Class II strawberry.
Perfect pollination allows all achenes to be pollinated evenly and fully and the surrounding tissue will swell to form fleshy fruit. This results in a symmetrical, Class I fruit (left). Imperfect pollination leads to an asymmetric, Class II (right), fruit as flesh only forms around fully pollinated achenes. In the same mechanism, better pollination also leads to larger fruit. While the Class I and II terminology was developed for commercial strawberries, it can be applied to wild-type strawberries too.
Extended Data Fig. 6 Strawberry fruit weight and quality and interaction complementarity.
Difference in (A) Fruit weight, (B) Fruit quality and (C) Interaction complementarity at Monad and Triad sites. (D) The relationship between Interaction complementarity and the proportion of class 1 strawberries. Yellow squares are Monad sites and blue triangles are triads. Interaction complementarity is calculated as the functional dispersion of the species at each site and measured as the sum of the distances of all species in that site to the community centroid. Twenty strawberry plants were placed at 20 independent field sites, yielding a total of 144 strawberries. Boxes represent the 25% (Q1) and 75% (Q3) quartiles around the median line, and whiskers are Q1 − 1.5xIQR to Q1 and Q3 to Q3 + 1.5xIQR.
Extended Data Fig. 7 Null model 1&2: Effect of phylogenetic diversity.
Interaction evenness (A&C) and interaction complementarity (B&D) as a function of plant phylogenetic diversity in random triads (coloured points) and observed triads (circles with coloured fill). Interaction complementarity is calculated as the functional dispersion of species at the real or null triads. Two variants of the same null model are displayed here, one controlling only for the number of interactions (A&B), and the other (C&D) also controlling also for the number of plant species.
Extended Data Fig. 8 Null model 3: Preserving sampling completeness.
Null Model results while additionally constraining for equal sampling completeness: Interaction evenness (A and E) and functional dispersion (C and G) as a function of plant phylogenetic diversity in random triads (coloured points) and observed triads (circles with coloured fill). Two variants of the same null model are displayed here, one only controlling only for the number of interactions (first row), and the other controlling also for the number of plant species. Within each plot, insets (B, D, F, H) compare the expected value of interaction evenness and functional dispersion against the observed values. For each boxplot (1 per site), n = 1,000 replicates. Boxes represent the 25% (Q1) and 75% (Q3) quartiles around the median line, and whiskers are Q1 − 1.5xIQR to Q1 and Q3 to Q3 + 1.5xIQR.
Supplementary information
Supplementary information.
Supplementary Information sections 1–7, including Figs. 1–14, Tables 1–3 and references.
Reporting Summary
Peer review file, rights and permissions.
Open Access This article is licensed under a Creative Commons Attribution 4.0 International License, which permits use, sharing, adaptation, distribution and reproduction in any medium or format, as long as you give appropriate credit to the original author(s) and the source, provide a link to the Creative Commons licence, and indicate if changes were made. The images or other third party material in this article are included in the article’s Creative Commons licence, unless indicated otherwise in a credit line to the material. If material is not included in the article’s Creative Commons licence and your intended use is not permitted by statutory regulation or exceeds the permitted use, you will need to obtain permission directly from the copyright holder. To view a copy of this licence, visit http://creativecommons.org/licenses/by/4.0/ .
Reprints and permissions
About this article
Cite this article.
Hackett, T.D., Sauve, A.M.C., Maia, K.P. et al. Multi-habitat landscapes are more diverse and stable with improved function. Nature (2024). https://doi.org/10.1038/s41586-024-07825-y
Download citation
Received : 06 September 2022
Accepted : 12 July 2024
Published : 21 August 2024
DOI : https://doi.org/10.1038/s41586-024-07825-y
Share this article
Anyone you share the following link with will be able to read this content:
Sorry, a shareable link is not currently available for this article.
Provided by the Springer Nature SharedIt content-sharing initiative
By submitting a comment you agree to abide by our Terms and Community Guidelines . If you find something abusive or that does not comply with our terms or guidelines please flag it as inappropriate.
Quick links
- Explore articles by subject
- Guide to authors
- Editorial policies
Sign up for the Nature Briefing newsletter — what matters in science, free to your inbox daily.


IMAGES
COMMENTS
It is one of two mutually exclusive hypotheses about a population in a hypothesis test. When your sample contains sufficient evidence, you can reject the null and conclude that the effect is statistically significant. Statisticians often denote the null hypothesis as H 0 or H A. Null Hypothesis H0: No effect exists in the population.
Example 1: Weight of Turtles. A biologist wants to test whether or not the true mean weight of a certain species of turtles is 300 pounds. To test this, he goes out and measures the weight of a random sample of 40 turtles. Here is how to write the null and alternative hypotheses for this scenario: H0: μ = 300 (the true mean weight is equal to ...
The null hypothesis and the alternative hypothesis are types of conjectures used in statistical tests to make statistical inferences, which are formal methods of reaching conclusions and separating scientific claims from statistical noise.. The statement being tested in a test of statistical significance is called the null hypothesis. The test of significance is designed to assess the strength ...
Table of contents. Step 1: State your null and alternate hypothesis. Step 2: Collect data. Step 3: Perform a statistical test. Step 4: Decide whether to reject or fail to reject your null hypothesis. Step 5: Present your findings. Other interesting articles. Frequently asked questions about hypothesis testing.
The null and alternative hypotheses are two competing claims that researchers weigh evidence for and against using a statistical test: Null hypothesis (H 0): There's no effect in the population. Alternative hypothesis (H a or H 1): There's an effect in the population. The effect is usually the effect of the independent variable on the ...
The actual test begins by considering two hypotheses.They are called the null hypothesis and the alternative hypothesis.These hypotheses contain opposing viewpoints. H 0, the —null hypothesis: a statement of no difference between sample means or proportions or no difference between a sample mean or proportion and a population mean or proportion. In other words, the difference equals 0.
The first step in hypothesis testing is to set up two competing hypotheses. The hypotheses are the most important aspect. If the hypotheses are incorrect, your conclusion will also be incorrect. The two hypotheses are named the null hypothesis and the alternative hypothesis. The null hypothesis is typically denoted as H 0.
Every hypothesis test — regardless of the population parameter involved — requires the above three steps. ... In statistics, we always assume the null hypothesis is true. That is, the null hypothesis is always our initial assumption. The prosecution team then collects evidence — such as finger prints, blood spots, hair samples, carpet ...
No. Remember that in null hypothesis testing, the p-value is the probability of the data given the null hypothesis. For those who think in formula, p-value is calculated as P(data|H 0). It does not warrant conclusions about the probability of the null hypothesis given the data - this suggestsP(H0|data).
The researchers write their hypotheses. These statements apply to the population, so they use the mu (μ) symbol for the population mean parameter.. Null Hypothesis (H 0): The population means of the test scores for the two groups are equal (μ 1 = μ 2).; Alternative Hypothesis (H A): The population means of the test scores for the two groups are unequal (μ 1 ≠ μ 2).
The actual test begins by considering two hypotheses.They are called the null hypothesis and the alternative hypothesis.These hypotheses contain opposing viewpoints. \(H_0\): The null hypothesis: It is a statement of no difference between the variables—they are not related. This can often be considered the status quo and as a result if you cannot accept the null it requires some action.
A hypothesis test consists of five steps: 1. State the hypotheses. State the null and alternative hypotheses. These two hypotheses need to be mutually exclusive, so if one is true then the other must be false. 2. Determine a significance level to use for the hypothesis. Decide on a significance level.
Null hypothesis significance testing: On the survival of a flawed method. American Psychologist, 56(1), 16. Masson, M. E. (2011). A tutorial on a practical Bayesian alternative to null-hypothesis significance testing. Behavior research methods, 43, 679-690. Nickerson, R. S. (2000). Null hypothesis significance testing: a review of an old and ...
Null and Alternative Hypotheses. The actual test begins by considering two hypotheses.They are called the null hypothesis and the alternative hypothesis.These hypotheses contain opposing viewpoints. \(H_0\): The null hypothesis: It is a statement of no difference between the variables—they are not related. This can often be considered the status quo and as a result if you cannot accept the ...
Step 7: Based on Steps 5 and 6, draw a conclusion about H 0. If F calculated is larger than F α, then you are in the rejection region and you can reject the null hypothesis with ( 1 − α) level of confidence. Note that modern statistical software condenses Steps 6 and 7 by providing a p -value. The p -value here is the probability of getting ...
Region of rejection / Critical region: The set of values of the test statistic for which the null hypothesis is rejected. Power of a test (1 − β) Size: For simple hypotheses, this is the test's probability of incorrectly rejecting the null hypothesis. The false positive rate. For composite hypotheses this is the supremum of the probability ...
Null hypothesis testing is a formal approach to deciding whether a statistical relationship in a sample reflects a real relationship in the population or is just due to chance. The logic of null hypothesis testing involves assuming that the null hypothesis is true, finding how likely the sample result would be if this assumption were correct ...
Null hypothesis testing is a formal approach to deciding whether a statistical relationship in a sample reflects a real relationship in the population or is just due to chance. The logic of null hypothesis testing involves assuming that the null hypothesis is true, finding how likely the sample result would be if this assumption were correct ...
Below these are summarized into six such steps to conducting a test of a hypothesis. Set up the hypotheses and check conditions: Each hypothesis test includes two hypotheses about the population. One is the null hypothesis, notated as H 0, which is a statement of a particular parameter value. This hypothesis is assumed to be true until there is ...
A hypothesis test is a formal statistical test we use to reject or fail to reject a statistical hypothesis. We always use the following steps to perform a hypothesis test: Step 1: State the null and alternative hypotheses. The null hypothesis, denoted as H0, is the hypothesis that the sample data occurs purely from chance.
Hypothesis A premise or claim that we want to test. Null Hypothesis: H 0 Currently accepted value for a parameter (middle of the distribution). Is assumed true for the purpose of carrying out the hypothesis test. • Always contains an "=" {=, , } • The null value implies a specific sampling distribution for the test statistic • H 0
Null hypothesis, often denoted as H0, is a foundational concept in statistical hypothesis testing. It represents an assumption that no significant difference, effect, or relationship exists between variables within a population. Learn more about Null Hypothesis, its formula, symbol and example in this article
Specify the null and alternative hypotheses in terms of the parameters of the model. Invent a test statistic that will tend to be different under the null and alternative hypotheses. Using the assumptions of step 1, find the theoretical sampling distribution of the statistic under the null hypothesis of step 2.
Null Hypothesis: A null hypothesis is a type of hypothesis used in statistics that proposes that no statistical significance exists in a set of given observations. The null hypothesis attempts to ...
If the boxplot overlaps the 1:1, then the observed value falls within the null hypothesis; if it is below, the observed value is less than under the null hypothesis and, if above, more than under ...