- Flashes Safe Seven
- FlashLine Login
- Faculty & Staff Phone Directory
- Emeriti or Retiree
- All Departments
- Maps & Directions
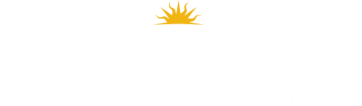
- Building Guide
- Departments
- Directions & Parking
- Faculty & Staff
- Give to University Libraries
- Library Instructional Spaces
- Mission & Vision
- Newsletters
- Circulation
- Course Reserves / Core Textbooks
- Equipment for Checkout
- Interlibrary Loan
- Library Instruction
- Library Tutorials
- My Library Account
- Open Access Kent State
- Research Support Services
- Statistical Consulting
- Student Multimedia Studio
- Citation Tools
- Databases A-to-Z
- Databases By Subject
- Digital Collections
- Discovery@Kent State
- Government Information
- Journal Finder
- Library Guides
- Connect from Off-Campus
- Library Workshops
- Subject Librarians Directory
- Suggestions/Feedback
- Writing Commons
- Academic Integrity
- Jobs for Students
- International Students
- Meet with a Librarian
- Study Spaces
- University Libraries Student Scholarship
- Affordable Course Materials
- Copyright Services
- Selection Manager
- Suggest a Purchase

Library Locations at the Kent Campus
- Architecture Library
- Fashion Library
- Map Library
- Performing Arts Library
- Special Collections and Archives
Regional Campus Libraries
- East Liverpool
- College of Podiatric Medicine

- Kent State University
- SPSS Tutorials
Pearson Correlation
Spss tutorials: pearson correlation.
- The SPSS Environment
- The Data View Window
- Using SPSS Syntax
- Data Creation in SPSS
- Importing Data into SPSS
- Variable Types
- Date-Time Variables in SPSS
- Defining Variables
- Creating a Codebook
- Computing Variables
- Computing Variables: Mean Centering
- Computing Variables: Recoding Categorical Variables
- Computing Variables: Recoding String Variables into Coded Categories (Automatic Recode)
- rank transform converts a set of data values by ordering them from smallest to largest, and then assigning a rank to each value. In SPSS, the Rank Cases procedure can be used to compute the rank transform of a variable." href="https://libguides.library.kent.edu/SPSS/RankCases" style="" >Computing Variables: Rank Transforms (Rank Cases)
- Weighting Cases
- Sorting Data
- Grouping Data
- Descriptive Stats for One Numeric Variable (Explore)
- Descriptive Stats for One Numeric Variable (Frequencies)
- Descriptive Stats for Many Numeric Variables (Descriptives)
- Descriptive Stats by Group (Compare Means)
- Frequency Tables
- Working with "Check All That Apply" Survey Data (Multiple Response Sets)
- Chi-Square Test of Independence
- One Sample t Test
- Paired Samples t Test
- Independent Samples t Test
- One-Way ANOVA
- How to Cite the Tutorials
Sample Data Files
Our tutorials reference a dataset called "sample" in many examples. If you'd like to download the sample dataset to work through the examples, choose one of the files below:
- Data definitions (*.pdf)
- Data - Comma delimited (*.csv)
- Data - Tab delimited (*.txt)
- Data - Excel format (*.xlsx)
- Data - SAS format (*.sas7bdat)
- Data - SPSS format (*.sav)
The bivariate Pearson Correlation produces a sample correlation coefficient, r , which measures the strength and direction of linear relationships between pairs of continuous variables. By extension, the Pearson Correlation evaluates whether there is statistical evidence for a linear relationship among the same pairs of variables in the population, represented by a population correlation coefficient, ρ (“rho”). The Pearson Correlation is a parametric measure.
This measure is also known as:
- Pearson’s correlation
- Pearson product-moment correlation (PPMC)
Common Uses
The bivariate Pearson Correlation is commonly used to measure the following:
- Correlations among pairs of variables
- Correlations within and between sets of variables
The bivariate Pearson correlation indicates the following:
- Whether a statistically significant linear relationship exists between two continuous variables
- The strength of a linear relationship (i.e., how close the relationship is to being a perfectly straight line)
- The direction of a linear relationship (increasing or decreasing)
Note: The bivariate Pearson Correlation cannot address non-linear relationships or relationships among categorical variables. If you wish to understand relationships that involve categorical variables and/or non-linear relationships, you will need to choose another measure of association.
Note: The bivariate Pearson Correlation only reveals associations among continuous variables. The bivariate Pearson Correlation does not provide any inferences about causation, no matter how large the correlation coefficient is.
Data Requirements
To use Pearson correlation, your data must meet the following requirements:
- Two or more continuous variables (i.e., interval or ratio level)
- Cases must have non-missing values on both variables
- Linear relationship between the variables
- the values for all variables across cases are unrelated
- for any case, the value for any variable cannot influence the value of any variable for other cases
- no case can influence another case on any variable
- The biviariate Pearson correlation coefficient and corresponding significance test are not robust when independence is violated.
- Each pair of variables is bivariately normally distributed
- Each pair of variables is bivariately normally distributed at all levels of the other variable(s)
- This assumption ensures that the variables are linearly related; violations of this assumption may indicate that non-linear relationships among variables exist. Linearity can be assessed visually using a scatterplot of the data.
- Random sample of data from the population
- No outliers
The null hypothesis ( H 0 ) and alternative hypothesis ( H 1 ) of the significance test for correlation can be expressed in the following ways, depending on whether a one-tailed or two-tailed test is requested:
Two-tailed significance test:
H 0 : ρ = 0 ("the population correlation coefficient is 0; there is no association") H 1 : ρ ≠ 0 ("the population correlation coefficient is not 0; a nonzero correlation could exist")
One-tailed significance test:
H 0 : ρ = 0 ("the population correlation coefficient is 0; there is no association") H 1 : ρ > 0 ("the population correlation coefficient is greater than 0; a positive correlation could exist") OR H 1 : ρ < 0 ("the population correlation coefficient is less than 0; a negative correlation could exist")
where ρ is the population correlation coefficient.
Test Statistic
The sample correlation coefficient between two variables x and y is denoted r or r xy , and can be computed as: $$ r_{xy} = \frac{\mathrm{cov}(x,y)}{\sqrt{\mathrm{var}(x)} \dot{} \sqrt{\mathrm{var}(y)}} $$
where cov( x , y ) is the sample covariance of x and y ; var( x ) is the sample variance of x ; and var( y ) is the sample variance of y .
Correlation can take on any value in the range [-1, 1]. The sign of the correlation coefficient indicates the direction of the relationship, while the magnitude of the correlation (how close it is to -1 or +1) indicates the strength of the relationship.
- -1 : perfectly negative linear relationship
- 0 : no relationship
- +1 : perfectly positive linear relationship
The strength can be assessed by these general guidelines [1] (which may vary by discipline):
- .1 < | r | < .3 … small / weak correlation
- .3 < | r | < .5 … medium / moderate correlation
- .5 < | r | ……… large / strong correlation
Note: The direction and strength of a correlation are two distinct properties. The scatterplots below [2] show correlations that are r = +0.90, r = 0.00, and r = -0.90, respectively. The strength of the nonzero correlations are the same: 0.90. But the direction of the correlations is different: a negative correlation corresponds to a decreasing relationship, while and a positive correlation corresponds to an increasing relationship.

Note that the r = 0.00 correlation has no discernable increasing or decreasing linear pattern in this particular graph. However, keep in mind that Pearson correlation is only capable of detecting linear associations, so it is possible to have a pair of variables with a strong nonlinear relationship and a small Pearson correlation coefficient. It is good practice to create scatterplots of your variables to corroborate your correlation coefficients.
[1] Cohen, J. (1988). Statistical power analysis for the behavioral sciences (2nd ed.). Hillsdale, NJ: Lawrence Erlbaum Associates.
[2] Scatterplots created in R using ggplot2 , ggthemes::theme_tufte() , and MASS::mvrnorm() .
Data Set-Up
Your dataset should include two or more continuous numeric variables, each defined as scale, which will be used in the analysis.
Each row in the dataset should represent one unique subject, person, or unit. All of the measurements taken on that person or unit should appear in that row. If measurements for one subject appear on multiple rows -- for example, if you have measurements from different time points on separate rows -- you should reshape your data to "wide" format before you compute the correlations.
Run a Bivariate Pearson Correlation
To run a bivariate Pearson Correlation in SPSS, click Analyze > Correlate > Bivariate .

The Bivariate Correlations window opens, where you will specify the variables to be used in the analysis. All of the variables in your dataset appear in the list on the left side. To select variables for the analysis, select the variables in the list on the left and click the blue arrow button to move them to the right, in the Variables field.

A Variables : The variables to be used in the bivariate Pearson Correlation. You must select at least two continuous variables, but may select more than two. The test will produce correlation coefficients for each pair of variables in this list.
B Correlation Coefficients: There are multiple types of correlation coefficients. By default, Pearson is selected. Selecting Pearson will produce the test statistics for a bivariate Pearson Correlation.
C Test of Significance: Click Two-tailed or One-tailed , depending on your desired significance test. SPSS uses a two-tailed test by default.
D Flag significant correlations: Checking this option will include asterisks (**) next to statistically significant correlations in the output. By default, SPSS marks statistical significance at the alpha = 0.05 and alpha = 0.01 levels, but not at the alpha = 0.001 level (which is treated as alpha = 0.01)
E Options : Clicking Options will open a window where you can specify which Statistics to include (i.e., Means and standard deviations , Cross-product deviations and covariances ) and how to address Missing Values (i.e., Exclude cases pairwise or Exclude cases listwise ). Note that the pairwise/listwise setting does not affect your computations if you are only entering two variable, but can make a very large difference if you are entering three or more variables into the correlation procedure.

Example: Understanding the linear association between weight and height
Problem statement.
Perhaps you would like to test whether there is a statistically significant linear relationship between two continuous variables, weight and height (and by extension, infer whether the association is significant in the population). You can use a bivariate Pearson Correlation to test whether there is a statistically significant linear relationship between height and weight, and to determine the strength and direction of the association.
Before the Test
In the sample data, we will use two variables: “Height” and “Weight.” The variable “Height” is a continuous measure of height in inches and exhibits a range of values from 55.00 to 84.41 ( Analyze > Descriptive Statistics > Descriptives ). The variable “Weight” is a continuous measure of weight in pounds and exhibits a range of values from 101.71 to 350.07.
Before we look at the Pearson correlations, we should look at the scatterplots of our variables to get an idea of what to expect. In particular, we need to determine if it's reasonable to assume that our variables have linear relationships. Click Graphs > Legacy Dialogs > Scatter/Dot . In the Scatter/Dot window, click Simple Scatter , then click Define . Move variable Height to the X Axis box, and move variable Weight to the Y Axis box. When finished, click OK .
To add a linear fit like the one depicted, double-click on the plot in the Output Viewer to open the Chart Editor. Click Elements > Fit Line at Total . In the Properties window, make sure the Fit Method is set to Linear , then click Apply . (Notice that adding the linear regression trend line will also add the R-squared value in the margin of the plot. If we take the square root of this number, it should match the value of the Pearson correlation we obtain.)
From the scatterplot, we can see that as height increases, weight also tends to increase. There does appear to be some linear relationship.
Running the Test
To run the bivariate Pearson Correlation, click Analyze > Correlate > Bivariate . Select the variables Height and Weight and move them to the Variables box. In the Correlation Coefficients area, select Pearson . In the Test of Significance area, select your desired significance test, two-tailed or one-tailed. We will select a two-tailed significance test in this example. Check the box next to Flag significant correlations .
Click OK to run the bivariate Pearson Correlation. Output for the analysis will display in the Output Viewer.
The results will display the correlations in a table, labeled Correlations .

A Correlation of Height with itself (r=1), and the number of nonmissing observations for height (n=408).
B Correlation of height and weight (r=0.513), based on n=354 observations with pairwise nonmissing values.
C Correlation of height and weight (r=0.513), based on n=354 observations with pairwise nonmissing values.
D Correlation of weight with itself (r=1), and the number of nonmissing observations for weight (n=376).
The important cells we want to look at are either B or C. (Cells B and C are identical, because they include information about the same pair of variables.) Cells B and C contain the correlation coefficient for the correlation between height and weight, its p-value, and the number of complete pairwise observations that the calculation was based on.
The correlations in the main diagonal (cells A and D) are all equal to 1. This is because a variable is always perfectly correlated with itself. Notice, however, that the sample sizes are different in cell A ( n =408) versus cell D ( n =376). This is because of missing data -- there are more missing observations for variable Weight than there are for variable Height.
If you have opted to flag significant correlations, SPSS will mark a 0.05 significance level with one asterisk (*) and a 0.01 significance level with two asterisks (0.01). In cell B (repeated in cell C), we can see that the Pearson correlation coefficient for height and weight is .513, which is significant ( p < .001 for a two-tailed test), based on 354 complete observations (i.e., cases with nonmissing values for both height and weight).
Decision and Conclusions
Based on the results, we can state the following:
- Weight and height have a statistically significant linear relationship ( r =.513, p < .001).
- The direction of the relationship is positive (i.e., height and weight are positively correlated), meaning that these variables tend to increase together (i.e., greater height is associated with greater weight).
- The magnitude, or strength, of the association is approximately moderate (.3 < | r | < .5).
- << Previous: Chi-Square Test of Independence
- Next: One Sample t Test >>
- Last Updated: Jul 10, 2024 11:08 AM
- URL: https://libguides.library.kent.edu/SPSS
Street Address
Mailing address, quick links.
- How Are We Doing?
- Student Jobs
Information
- Accessibility
- Emergency Information
- For Our Alumni
- For the Media
- Jobs & Employment
- Life at KSU
- Privacy Statement
- Technology Support
- Website Feedback
Bivariate Data: Examples, Definition and Analysis
On this page:
- What is bivariate data? Definition.
- Examples of bivariate data: with table.
- Bivariate data analysis examples: including linear regression analysis, correlation (relationship), distribution, and scatter plot.
Let’s define bivariate data:
We have bivariate data when we studying two variables . These variables are changing and are compared to find the relationships between them.
For example, if you are studying a group of students to find out their average math score and their age, you have two variables (math score and age).
If you are studying only one variable, for example only math score for these students, then we have univariate data .
When we are examining bivariate data, the two variables could depend on each other. One variable could influence another. In this case, we say that the bivariate data has:
- an independent variable and
- a dependent variable .
A classical example of dependent and independent variables are age and heights of the babies and toddlers. When age increases, the height also increases.
Let’s move on to some real-life and practical bivariate data examples.
Look at the following bivariate data table. It represents the age and average height of a group of babies and kids.
3 months | 58.5 |
6 months | 64 |
9 months | 68.5 |
1 years | 74 |
2 years | 81.2 |
3 years | 89.1 |
4 years | 95 |
5 years | 102.5 |
Commonly, bivariate data is stored in a table with two columns.
There are 2 types of relationship between the dependent and independent variable:
- A positive relationship (also called positive correlation) – that means if the independent variable increases, then the dependent variable would also increase and vice versa. The above example about the kids’ age and height is a classical positive relationship.
- A negative relationship (negative correlation) – when the independent variable increases and the dependent variable decrease and vice versa. Example: when the car age increases, the car price decreases.
So, we use bivariate data to compare two sets of data and to discover any relationships between them.
Bivariate Data Analysis
Bivariate analysis allows you to study the relationship between 2 variables and has many practical uses in the real life. It aims to find out whether there exists an association between the variables and what is its strength.
Bivariate analysis also allows you to test a hypothesis of association and causality. It also helps you to predict the values of a dependent variable based on the changes of an independent variable.
Let’s see how the bivariate data work with linear regression models .
Let’s say you have to study the relationship between the age and the systolic blood pressure in a company. You have a sample of 10 workers aged thirty to fifty-five years. The results are presented in the following bivariate data table.
1 | 37 | 130 |
2 | 38 | 140 |
3 | 40 | 132 |
4 | 42 | 149 |
5 | 45 | 144 |
6 | 48 | 157 |
7 | 50 | 161 |
8 | 52 | 145 |
9 | 53 | 165 |
10 | 55 | 162 |
Now, we need to display this table graphically to be able to make some conclusions.
Bivariate data is most often displayed using a scatter plot. This is a plot on a grid paper of y (y-axis) against x (x-axis) and indicates the behavior of given data sets.
Scatter plot is one of the popular types of graphs that give us a much more clear picture of a possible relationship between the variables.
Let’s build our Scatter Plot based on the table above:
The above scatter plot illustrates that the values seem to group around a straight line i.e it shows that there is a possible linear relationship between the age and systolic blood pressure.
You can create scatter plots very easily with a variety of free graphing software available online.
What does this graph show us?
It is obvious that there is a relationship between age and blood pressure and moreover this relationship is positive (i.e. we have positive correlation). The older the age, the higher the systolic blood pressure.
The line that you see in the graph is called “line of best fit” (or the regression line). The line of best fit aims to answer the question whether these two variables correlate. It can be used to help you determine trends within the data sets.
Furthermore, the line of best fit illustrates the strength of the correlation .
Let’s investigate further.
We constated that in our example, there is a positive and strong linear relationship between age and blood pressure. However, how strong is that relationship? What is its strength?
This is where the correlation coefficient comes to answer this question.
The correlation coefficient (R) is a numerical value measured between -1 and 1 . It indicates the strength of the linear relationship between two given variables. For describing a linear regression, the coefficient is called Pearson’s correlation coefficient.
When the correlation coefficient is closer to 1 it shows a strong positive relationship. When it is close to -1, there is a strong negative relationship. A value of 0 tells us that there is no relationship.
We need to calculate our correlation coefficient between age and blood pressure. There is a long formula (for Pearson’s correlation coefficient) for this but you don’t need to remember it.
All you need to do is to use a free or premium calculator such as those on www.socscistatistics.com . When we put our bivariate data on this calculator we got the following result:
The value of the correlation coefficient (R) is 0.8435. It shows a strong positive correlation.
Now, let’s calculate the equation of the regression line (the best fit line) to find out the slope of the line.
For that purpose let’s remind the simple linear regression equation :
Y = Β 0 + Β 1 X
X – the value of the independent variable, Y – the value of the dependent variable. Β 0 – is a constant (shows the value of Y when the value of X=0) Β 1 – the regression coefficient (shows how much Y changes for each unit change in X)
Again, we will use the same online software ( socscistatistics.com ) to calculate the linear regression equation. The result is:
Y = 1.612*X + 74.35
More on linear regression equation and explanation, you can see in our post for linear regression examples .
So, from the above bivariate data analysis example that includes workers of the company, we can say that blood pressure increased as the age increased. This indicates that age is a significant factor that influences the change of blood pressure.
Other popular positive bivariate data correlation examples are: temperature and the amount of the ice cream sales, alcohol consumption and cholesterol levels, weights and heights of college students, and etc.
Let’s see bivariate data analysis examples for a negative correlation.
The below bivariate data table shows the number of student absences and their final grades in a class.
1 | 0 | 90 |
2 | 1 | 85 |
3 | 1 | 88 |
4 | 2 | 84 |
5 | 3 | 82 |
6 | 3 | 80 |
7 | 4 | 75 |
8 | 5 | 60 |
9 | 6 | 72 |
10 | 7 | 64 |
It is quite obvious that these two variables have a negative correlation between them.
When the number of student absences increases, the final grades decrease.
Now, let’s plot the bivariate data from the table on a scatter plot and to create the best-fit line:
Note how the regression line looks – it has a downward slope.
This downward slope indicates there is a negative linear association.
We can calculate the correlation coefficient and linear regression equation. Here are the results:
- The value of the correlation coefficient (R) is -0.9061 . This is a strong negative correlation.
- The linear regression equation is Y = -3.971*X + 90.71.
We can conclude that the least number of lessons the students skip, the higher grade could be reached.
Conclusion:
The above bivariate data examples aim to help you understand better how does the bivariate analysis work.
Analyzing two variables is a common part of the inferential statistics types and calculations. Many business and scientific investigations include only two continuous variables.
The main questions that bivariate analysis has to answer are:
- Is there a correlation between 2 given variables?
- Is the relationship positive or negative?
- What is the degree of the correlation? Is it strong or weak?
If you need other practical examples in the area of management and analysis, our posts Venn diagram examples and decision tree examples might be helpful for you.
About The Author

Silvia Valcheva
Silvia Valcheva is a digital marketer with over a decade of experience creating content for the tech industry. She has a strong passion for writing about emerging software and technologies such as big data, AI (Artificial Intelligence), IoT (Internet of Things), process automation, etc.
EXCELLENT and illustrative presentation
Excellent. Simple and effective explanations

Thanks. Happy to help!
Clears the Whole concept in very simple and easy way,thanks a lot
Leave a Reply Cancel Reply
This site uses Akismet to reduce spam. Learn how your comment data is processed .
Bivariate Analysis Definition & Example
What is bivariate data.
Data in statistics is sometimes classified according to how many variables are in a particular study. For example, “height” might be one variable and “weight” might be another variable. Depending on the number of variables being looked at, the data might be univariate, or it might be bivariate.
When you conduct a study that looks at a single variable, that study involves univariate data. For example, you might study a group of college students to find out their average SAT scores or you might study a group of diabetic patients to find their weights. Bivariate data is when you are studying two variables . For example, if you are studying a group of college students to find out their average SAT score and their age , you have two pieces of the puzzle to find (SAT score and age). Or if you want to find out the weights and heights of diabetic patients, then you also have bivariate data. Bivariate data could also be two sets of items that are dependent on each other. For example:
- Ice cream sales compared to the temperature that day.
- Traffic accidents along with the weather on a particular day.
Bivariate data has many practical uses in real life. For example, it is pretty useful to be able to predict when a natural event might occur. One tool in the statistician’s toolbox is bivariate data analysis. Sometimes, something as simple as plotting one variable against another on a Cartesian plane can give you a clear picture of what the data is trying to tell you. For example, the scatterplot below shows the relationship between the time between eruptions at Old Faithful vs. the duration of the eruption.
Watch the video below for an overview on what bivariate data analysis is:

Can’t see the video? Click here to watch it on YouTube.
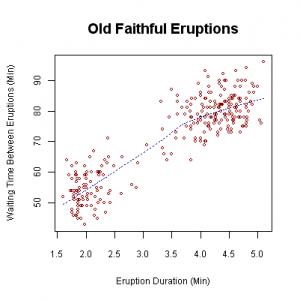
What is Bivariate Analysis?
Bivariate analysis means the analysis of bivariate data. It is one of the simplest forms of statistical analysis, used to find out if there is a relationship between two sets of values. It usually involves the variables X and Y.
- Univariate analysis is the analysis of one (“uni”) variable.
- Bivariate analysis is the analysis of exactly two variables.
- Multivariate analysis is the analysis of more than two variables.
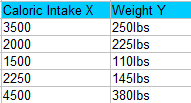
Types of Bivariate Analysis
Common types of bivariate analysis include:
1. Scatter plots ,
These give you a visual idea of the pattern that your variables follow.
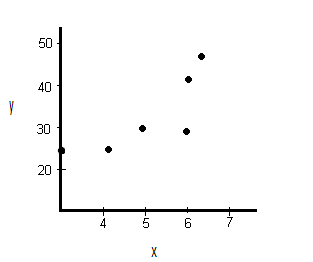
2. Regression Analysis
Regression analysis is a catch all term for a wide variety of tools that you can use to determine how your data points might be related. In the image above, the points look like they could follow an exponential curve (as opposed to a straight line). Regression analysis can give you the equation for that curve or line. It can also give you the correlation coefficient .
3. Correlation Coefficients
Calculating values for correlation coefficients are usually performed on a computer, although you can find the steps to find the correlation coefficient by hand here . This coefficient tells you if the variables are related. Basically, a zero means they aren’t correlated (i.e. related in some way), while a 1 (either positive or negative) means that the variables are perfectly correlated (i.e. they are perfectly in sync with each other).
Beyer, W. H. CRC Standard Mathematical Tables, 31st ed. Boca Raton, FL: CRC Press, pp. 536 and 571, 2002. Gonick, L. (1993). The Cartoon Guide to Statistics . HarperPerennial. Klein, G. (2013). The Cartoon Introduction to Statistics. Hill & Wamg. Vogt, W.P. (2005). Dictionary of Statistics & Methodology: A Nontechnical Guide for the Social Sciences . SAGE.
Conduct and Interpret a (Pearson) Bivariate Correlation
What is a Bivariate (Pearson) Correlation?
Bivariate Correlation is a widely used term in statistics. In fact, it entered the English language in 1561, 200 years before most of the modern statistic tests were discovered. It is derived from the Latin word correlation , which means relation. Correlation generally describes the effect that two or more phenomena occur together and therefore they are linked. Many academic questions and theories investigate these relationships. Is the time and intensity of exposure to sunlight related the likelihood of getting skin cancer? Are people more likely to repeat a visit to a museum the more satisfied they are? Do older people earn more money? Are wages linked to inflation? Do higher oil prices increase the cost of shipping? It is very important, however, to stress that correlation does not imply causation.
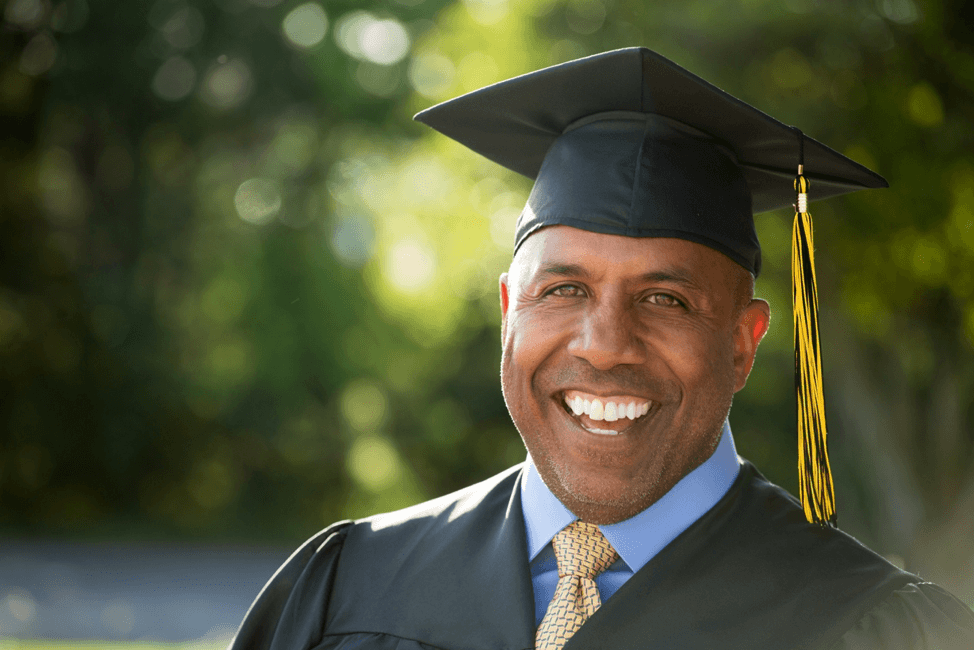
Discover How We Assist to Edit Your Dissertation Chapters
Aligning theoretical framework, gathering articles, synthesizing gaps, articulating a clear methodology and data plan, and writing about the theoretical and practical implications of your research are part of our comprehensive dissertation editing services.
- Bring dissertation editing expertise to chapters 1-5 in timely manner.
- Track all changes, then work with you to bring about scholarly writing.
- Ongoing support to address committee feedback, reducing revisions.
A correlation expresses the strength of linkage or co-occurrence between two variables in a single value between -1 and +1. This value that measures the strength of linkage is called correlation coefficient , which is represented typically as the letter r .
The correlation coefficient between two continuous-level variables is also called Pearson’s r or Pearson product-moment correlation coefficient. A positive r value expresses a positive relationship between the two variables (the larger A, the larger B) while a negative r value indicates a negative relationship (the larger A, the smaller B). A correlation coefficient of zero indicates no relationship between the variables at all. However correlations are limited to linear relationships between variables. Even if the correlation coefficient is zero, a non-linear relationship might exist.
Bivariate (Pearson) Correlation in SPSS
At this point it would be beneficial to create a scatter plot to visualize the relationship between our two test scores in reading and writing. The purpose of the scatter plot is to verify that the variables have a linear relationship. Other forms of relationship (circle, square) will not be detected when running Pearson’s Correlation Analysis. This would create a type II error because it would not reject the null hypothesis of the test of independence (‘the two variables are independent and not correlated in the universe’) although the variables are in reality dependent, just not linearly.
The scatter plot can either be found in Graphs/Chart Builder… or in Graphs/Legacy Dialog/Scatter Dot…
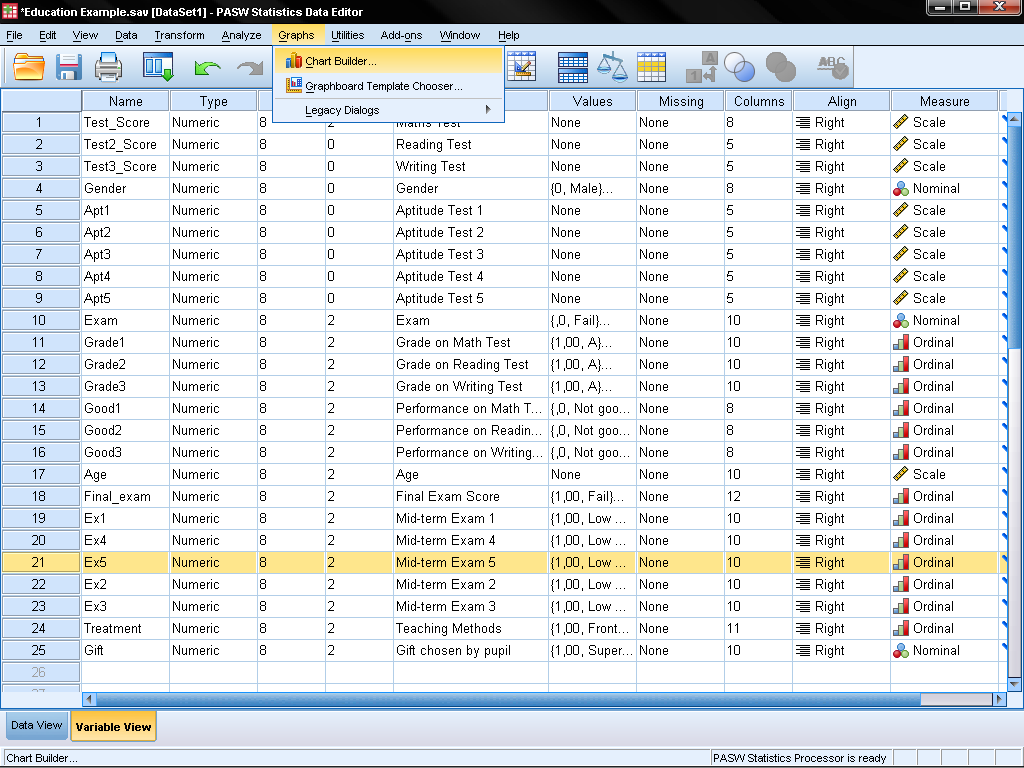
In the Chart Builder we simply choose in the Gallery tab the Scatter/Dot group of charts and drag the ‘Simple Scatter’ diagram (the first one) on the chart canvas. Next we drag variable Test_Score on the y-axis and variable Test2_Score on the x-Axis.
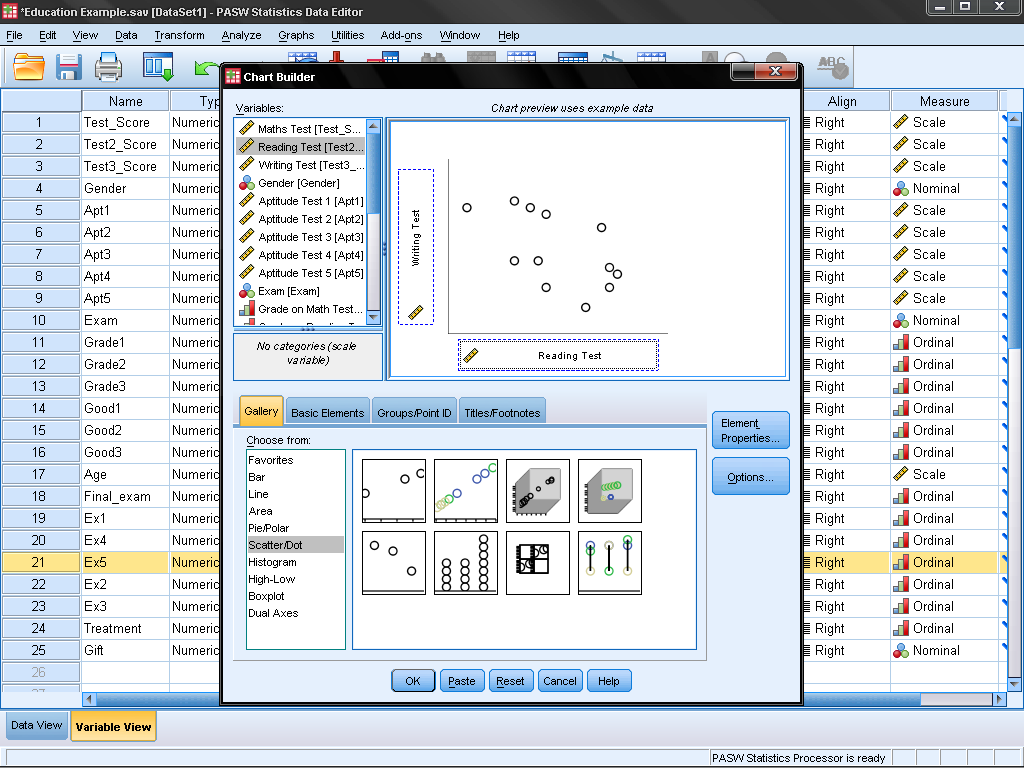
SPSS generates the scatter plot for the two variables. A double click on the output diagram opens the chart editor and a click on ‘Add Fit Line’ adds a linearly fitted line that represents the linear association that is represented by Pearson’s bivariate correlation.
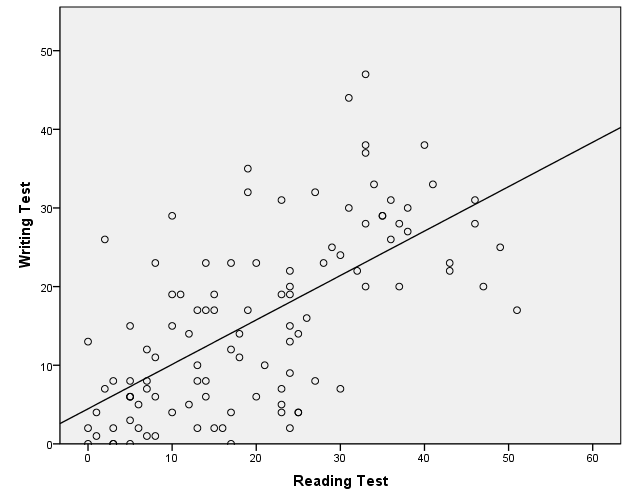
To calculate Pearson’s bivariate correlation coefficient in SPSS we have to open the dialog in Analyze/Correlation/Bivariate…
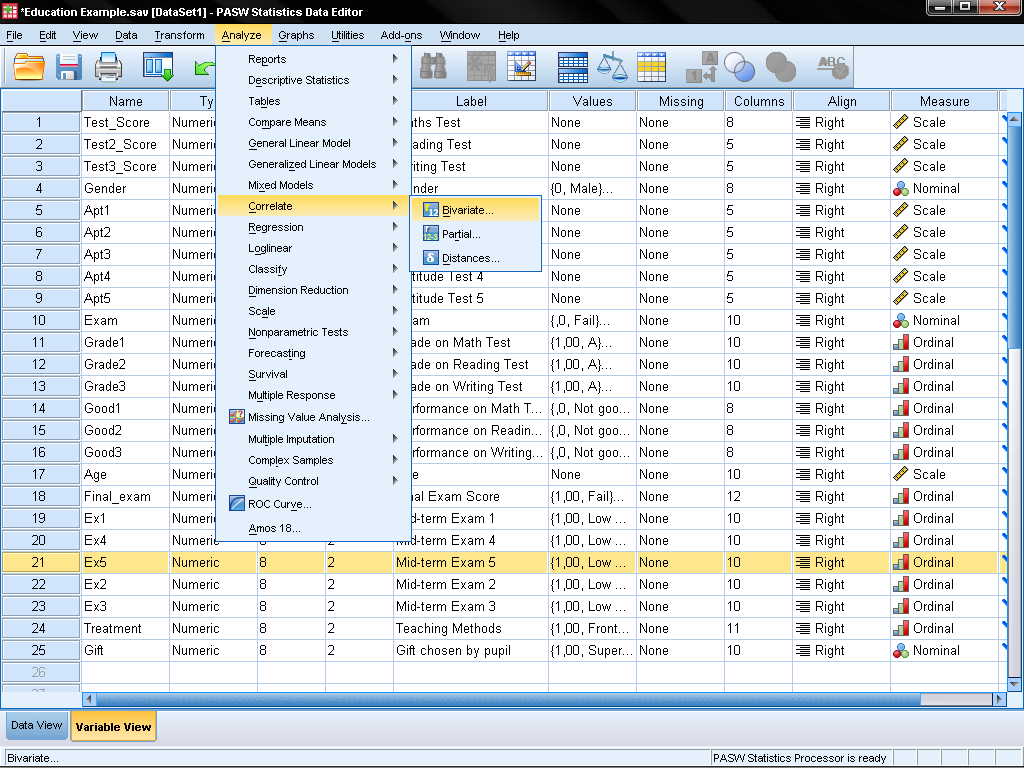
This opens the dialog box for all bivariate correlations (Pearson’s, Kendall’s, Spearman). Simply select the variables you want to calculate the bivariate correlation for and add them with the arrow.
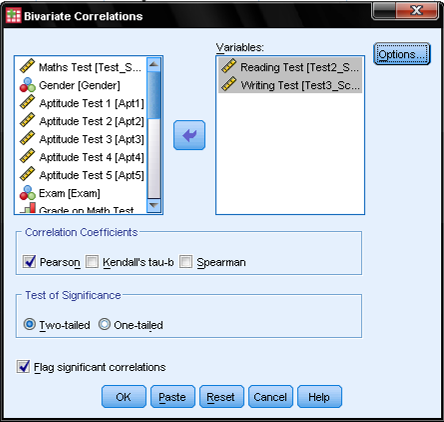
Select the bivariate correlation coefficient you need, in this case Pearson’s. For the Test of Significance we select the two-tailed test of significance, because we do not have an assumption whether it is a positive or negative correlation between the two variables Reading and Writing . We also leave the default tick mark at flag significant correlations which will add a little asterisk to all correlation coefficients with p<0.05 in the SPSS output.
Output, syntax, and interpretation can be found in our downloadable manual: Statistical Analysis: A Manual on Dissertation Statistics in SPSS (included in our member resources). Click here to download .
A correlation expresses the strength of linkage or co-occurrence between to variables in a single value between -1 and +1. This value that measures the strength of linkage is called correlation coefficient , which is represented typically as the letter r .
User Preferences
Content preview.
Arcu felis bibendum ut tristique et egestas quis:
- Ut enim ad minim veniam, quis nostrud exercitation ullamco laboris
- Duis aute irure dolor in reprehenderit in voluptate
- Excepteur sint occaecat cupidatat non proident
Keyboard Shortcuts
5.3 - inferences for correlations.
Let us consider testing the null hypothesis that there is zero correlation between two variables \(X_{j}\) and \(X_{k}\). Mathematically we write this as shown below:
\(H_0\colon \rho_{jk}=0\) against \(H_a\colon \rho_{jk} \ne 0 \)
Recall that the correlation is estimated by sample correlation \(r_{jk}\) given in the expression below:
\(r_{jk} = \dfrac{s_{jk}}{\sqrt{s^2_js^2_k}}\)
Here we have the sample covariance between the two variables divided by the square root of the product of the individual variances.
We shall assume that the pair of variables \(X_{j}\)and \(X_{k}\) are independently sampled from a bivariate normal distribution throughout this discussion; that is:
\(\left(\begin{array}{c}X_{1j}\\X_{1k} \end{array}\right)\), \(\left(\begin{array}{c}X_{2j}\\X_{2k} \end{array}\right)\), \(\dots\), \(\left(\begin{array}{c}X_{nj}\\X_{nk} \end{array}\right)\)
are independently sampled from a bivariate normal distribution.
To test the null hypothesis, we form the test statistic, t as below
\(t = r_{jk}\sqrt{\frac{n-2}{1-r^2_{jk}}}\) \(\dot{\sim}\) \( t_{n-2}\)
Under the null hypothesis, \(H_{o}\), this test statistic will be approximately distributed as t with n - 2 degrees of freedom.
Note! This approximation holds for larger samples. We will reject the null hypothesis, \(H_{o}\), at level \(α\) if the absolute value of the test statistic, t , is greater than the critical value from the t -table with n - 2 degrees of freedom; that is if:
\(|t| > t_{n-2, \alpha/2}\)
To illustrate these concepts let's return to our example dataset, the Wechsler Adult Intelligence Scale.
Example 5-5: Wechsler Adult Intelligence Scale Section
- Example
This data was analyzed using the SAS program in our last lesson, (Multivariate Normal Distribution), which yielded the computer output below.
Download the:
Dataset: wechsler.csv
SAS program: wechsler.sas
SAS Output: wechsler.lst
Find the Total Variance of the Wechsler Adult Intelligence Scale Data
To find the correlation matrix:
- Open the ‘wechsler’ data set in a new worksheet
- Stat > Basic Statistics > Correlation
- Highlight and select ‘info’, ‘sim’, ‘arith’, and ‘pict’ to move them into the variables window
- Select ‘ OK ’. The matrix of correlations, along with scatterplots, is displayed in the results area
Recall that these are data on n = 37 subjects taking the Wechsler Adult Intelligence Test. This test was broken up into four components:
- Information
- Similarities
- Picture Completion
Looking at the computer output we have summarized the correlations among variables in the table below:
Information | ||||
---|---|---|---|---|
Similarities | ||||
Arithmetic | ||||
Picture |
For example, the correlation between Similarities and Information is 0.77153.
Let's consider testing the null hypothesis that there is no correlation between Information and Similarities. This would be written mathematically as shown below:
\(H_0\colon \rho_{12}=0\)
We can then substitute values into the formula to compute the test statistic using the values from this example:
\begin{align} t &= r_{jk}\sqrt{\frac{n-2}{1-r^2_{jk}}}\\[10pt] &= 0.77153 \sqrt{\frac{37-2}{1-0.77153^2}}\\[10pt] &= 7.175 \end{align}
Looking at our t -table for 35 degrees of freedom and an \(\alpha\) level of .005, we get a critical value of \(t _ { ( d f , 1 - \alpha / 2 ) } = t _ { 35,0.9975 } = 3.030\). Therefore, we are going to look at the critical value under 0.0025 in the table (since 35 does not appear to use the closest df that does not exceed 35 which is 30) and in this case it is 3.030, meaning that \(t _ { ( d f , 1 - \alpha / 2 ) } = t _ { 35,0.9975 } = 3.030\) is close to 3.030.
Note! Some text tables provide the right tail probability (the graph at the top will have the area in the right tail shaded in) while other texts will provide a table with the cumulative probability - the graph will be shaded into the left. The concept is the same. For example, if the alpha was 0.01 then using the first text you would look under 0.005, and in the second text look under 0.995.
Because
\(7.175 > 3.030 = t_{35, 0.9975}\),
we can reject the null hypothesis that Information and Similarities scores are uncorrelated at the \(\alpha\) < 0.005 level.
Our conclusion is that Similarity scores increase with increasing Information scores ( t = 7.175; d.f . = 35; p < 0.0001). You will note here that we are not simply concluding that the results are significant. When drawing conclusions it is never adequate to simply state that the results are significant. In all cases, you should seek to describe what the results tell you about this data. In this case, because we rejected the null hypothesis we can conclude that the correlation is not equal to zero. Furthermore, because the actual sample correlation is greater than zero and our p-value is so small, we can conclude that there is a positive association between the two variables. Hence, our conclusion is that Similarity scores tend to increase with increasing values of Information scores.
You will also note that the conclusion includes information from the test. You should always back up your findings with the appropriate evidence: the test statistic, degrees of freedom (if appropriate), and p -value. Here the appropriate evidence is given by the test statistic t = 7.175; the degrees of freedom for the test, 35, and the p -value, less than 0.0001 as indicated by the computer printout. The p -value appears below each correlation coefficient in the SAS output.
Confidence Interval for \(p_{jk}\) Section
Once we conclude that there is a positive or negative correlation between two variables the next thing we might want to do is compute a confidence interval for the correlation. This confidence interval will give us a range of reasonable values for the correlation itself. The sample correlation, because it is bounded between -1 and 1 is typically not normally distributed or even approximately so. If the population correlation is near zero, the distribution of sample correlations may be approximately bell-shaped in distribution around zero. However, if the population correlation is near +1 or -1, the distribution of sample correlations will be skewed. For example, if \(p_{jk}= .9\), the distribution of sample correlations will be more concentrated near .9. Because they cannot exceed 1, they have more room to spread out to the left of .9, which causes a left-skewed shape. To adjust for this asymmetry or the skewness of distribution, we apply a transformation of the correlation coefficients. In particular, we are going to apply Fisher's transformation which is given in the expression below in Step 1 of our procedure for computing confidence intervals for the correlation coefficient.
\(z_{jk}=\frac{1}{2}\log\dfrac{1+r_{jk}}{1-r_{jk}}\)
Here we have one-half of the natural log of 1 plus the correlation, divided by one minus the correlation.
Note! In this course, whenever log is mentioned, unless specified otherwise, log stands for the natural log.
For large samples, this transform correlation coefficient z is going to be approximately normally distributed with the mean equal to the same transformation of the population correlation, as shown below, and a variance of 1 over the sample size minus 3.
\(z_{jk}\) \(\dot{\sim}\) \(N\left(\dfrac{1}{2}\log\dfrac{1+\rho_{jk}}{1-\rho_{jk}}, \dfrac{1}{n-3}\right)\)
Compute a (1 - \(\alpha\)) x 100% confidence interval for the Fisher transform of the population correlation.
\(\dfrac{1}{2}\log \dfrac{1+\rho_{jk}}{1-\rho_{jk}}\)
That is one-half log of 1 plus the correlation divided by 1 minus the correlation. In other words, this confidence interval is given by the expression below:
\(\left(\underset{Z_l}{\underbrace{Z_{jk}-\frac{Z_{\alpha/2}}{\sqrt{n-3}}}}, \underset{Z_U}{\underbrace{Z_{jk}+\frac{Z_{\alpha/2}}{\sqrt{n-3}}}}\right)\)
Here we take the value of Fisher's transform Z , plus and minus the critical value from the z table, divided by the square root of n - 3. The lower bound we will call the \(Z_{1}\) and the upper bound we will call the \(Z_{u}\).
Back transform the confidence values to obtain the desired confidence interval for \(\rho_{jk}\) This is given in the expression below:
\(\left(\dfrac{e^{2Z_l}-1}{e^{2Z_l}+1},\dfrac{e^{2Z_U}-1}{e^{2Z_U}+1}\right)\)
The first term we see is a function of the lower bound, the \(Z_{1}\). The second term is a function of the upper bound or \(Z_{u}\).
Let's return to the Wechsler Adult Intelligence Data to see how these procedures are carried out.
Example 5-6: Wechsler Adult Intelligence Data Section
Recall that the sample correlation between Similarities and Information was \(r_{12} = 0.77153\).
Step 1 : Compute the Fisher transform:
\begin{align} Z_{12} &= \frac{1}{2}\log \frac{1+r_{12}}{1-r_{12}}\\[5pt] &= \frac{1}{2}\log\frac{1+0.77153}{1-0.77153}\\[5pt] &= 1.024 \end{align}
You should confirm this value on your own.
Step 2 : Next, compute the 95% confidence interval for the Fisher transform, \(\frac{1}{2}\log \frac{1+\rho_{12}}{1-\rho_{12}}\) :
\begin{align} Z_l &= Z_{12}-Z_{0.025}/\sqrt{n-3} \\ &= 1.024 - \frac{1.96}{\sqrt{37-3}} \\ &= 0.6880 \end{align}
\begin{align} Z_U &= Z_{12}+Z_{0.025}/\sqrt{n-3} \\&= 1.024 + \frac{1.96}{\sqrt{37-3}} \\&= 1.3602 \end{align}
In other words, the value 1.024 plus or minus the critical value from the normal table, at \(α/2 = 0.025\), which in this case is 1.96. Divide by the square root of n minus 3. Subtracting the result from 1.024 yields a lower bound of 0.6880. Adding the result to 1.024 yields the upper bound of 1.3602.
Step 3 : Carry out the back-transform to obtain the 95% confidence interval for ρ 12 . This is shown in the expression below:
\(\left(\dfrac{\exp\{2Z_l\}-1}{\exp\{2Z_l\}+1},\dfrac{\exp\{2Z_U\}-1}{\exp\{2Z_U\}+1}\right)\)
\(\left(\dfrac{\exp\{2 \times 0.6880\}-1}{\exp\{2 \times 0.6880\}+1},\dfrac{\exp\{2\times 1.3602\}-1}{\exp\{2\times 1.3602\}+1}\right)\)
\((0.5967,0.8764)\)
This yields the interval from 0.5967 to 0.8764 .
Conclusion : In this case, we can conclude that we are 95% confident that the interval (0.5967, 0.8764) contains the correlation between Information and Similarities scores.
- Skip to main content
- Skip to primary sidebar
- Skip to footer
- QuestionPro

- Solutions Industries Gaming Automotive Sports and events Education Government Travel & Hospitality Financial Services Healthcare Cannabis Technology Use Case AskWhy Communities Audience Contactless surveys Mobile LivePolls Member Experience GDPR Positive People Science 360 Feedback Surveys
- Resources Blog eBooks Survey Templates Case Studies Training Help center

Home Market Research
Bivariate Analysis: What is it, Types + Examples
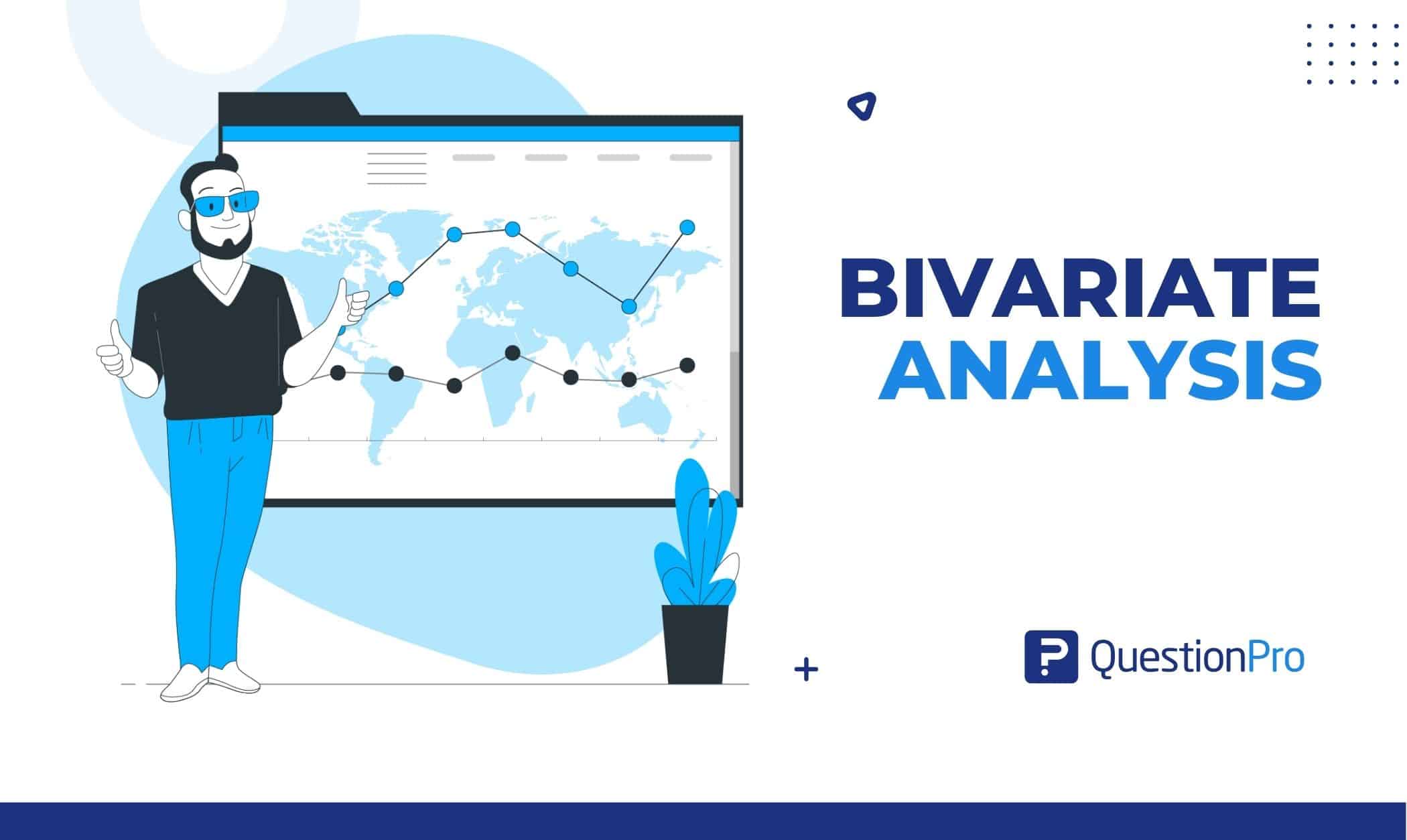
The bivariate analysis allows you to investigate the relationship between two variables. It is useful to determine whether there is a correlation between the variables and, if so, how strong the connection is. For researchers conducting a study, this is incredibly helpful.
This analysis verifies or refutes the causality and association hypothesis. It is useful in making predictions about the value of a dependent variable based on changes to the value of an independent variable.
In this blog, we will look at what bivariate analysis is, its types, and some examples.
What is bivariate analysis?
Bivariate analysis is a statistical method examining how two different things are related. The bivariate analysis aims to determine if there is a statistical link between the two variables and, if so, how strong and in which direction that link is.
It is a helpful technique for determining how two variables are connected and finding trends and patterns in the data. In statistical analysis , distinguishing between categorical data and numerical data is essential, as categorical data involves distinct categories or labels, while numerical data consists of measurable quantities.
Recognizing bivariate data is a prerequisite for analysis. Data analytics and data analysis are closely related processes that involve extracting insights from data to make informed decisions. Typically, X and Y are two of the measures included. The bivariate data can be understood as a pair (X, Y ).
LEARN ABOUT: Level of Analysis
Importance of bivariate analysis
Bivariate analysis is an important statistical method because it lets researchers look at the relationship between two variables and determine their relationship. This can be helpful in many different kinds of research, such as social science, medicine, marketing, and more.
Here are some reasons why bivariate analysis is important:
- Bivariate analysis helps identify trends and patterns: It can reveal hidden data trends and patterns by evaluating the relationship between two variables.
- Bivariate analysis helps identify cause and effect relationships: It can assess if two variables are statistically associated, assisting researchers in establishing which variable causes the other.
- It helps researchers make predictions: It allows researchers to predict future results by modeling the link between two variables.
- It helps inform decision-making: Business, public policy, and healthcare decision-making can benefit from bivariate analysis.
The ability to analyze the correlation between two variables is crucial for making sound judgments, and this analysis serves this purpose admirably.
Types of bivariate analysis
Many kinds of bivariate analysis can be used to determine how two variables are related. Here are some of the most common types.
1. Scatterplots
A scatterplot is a graph that shows how two variables are related to each other. It shows the values of one variable on the x-axis and the values of the other variable on the y-axis.
The pattern shows what kind of relationship there is between the two variables and how strong it is.
2. Correlation
Correlation is a statistical measure that shows how strong and in what direction two variables are linked.
A positive correlation means that when one variable goes up, so does the other. A negative correlation shows that when one variable goes up, the other one goes down.
3. Regression
This kind of analysis gives you access to all terms for various instruments that can be used to identify potential relationships between your data points.
The equation for that curve or line can also be provided to you using regression analysis . Additionally, it may show you the correlation coefficient.
4. Chi-square test
The chi-square test is a statistical method for identifying disparities in one or more categories between what was expected and what was observed. The test’s primary premise is to assess the actual data values to see what would be expected if the null hypothesis was valid.
Researchers use this statistical test to compare categorical variables within the same sample group. It also helps to validate or offer context for frequency counts.
A t-test is a statistical test that compares the means of two groups to see if they have a big difference. This analysis is appropriate when comparing the averages of two categories of a categorical variable.
6. ANOVA (Analysis of Variance)
The ANOVA test determines whether the averages of more than two groups differ from one another statistically. This comparison of averages of a numerical variable for more than two categories of a categorical variable is appropriate.
Example of bivariate analysis
Some examples of bivariate analysis are listed below:
Investigating the connection between education and income
In this case, one of the variables could be the level of education (e.g., high school, college, graduate school), and the other could be income.
A bivariate analysis could be used to determine if there is a significant relationship between these two variables and, if so, how strong and in what direction that relationship is.
Investigating the connection between aging and blood pressure
Here, age is one variable and blood pressure is another (systolic and diastolic).
It is possible to conduct an analysis of bivariate analysis to determine if and how strongly these two factors are related by testing for statistical significance.
These are just a few ways this analysis can be used to determine how two variables are related. The type of data and the research question will determine which techniques and statistical tests are used in the analysis.
LEARN ABOUT: Causal Research
The primary topic addressed by bivariate analysis is whether or not the two variables are correlated, and if so, whether or not the relationship is negative and to what degree. Typical research used in inferential statistics and calculations analyzes two variables. Numerous scientific and commercial projects focus on understanding the link between two continuous variables.
The QuestionPro research suite is an industry-leading package of enterprise-grade research tools for uncovering brand insights. It is a collection of tools for leveraging research and transforming ideas.
Bivariate analysis is a statistical analysis plan that compares two variables to understand their relationship better, and QuestionPro survey software can be used to collect data for this purpose.
Try QuestionPro Today!
LEARN MORE FREE TRIAL
MORE LIKE THIS
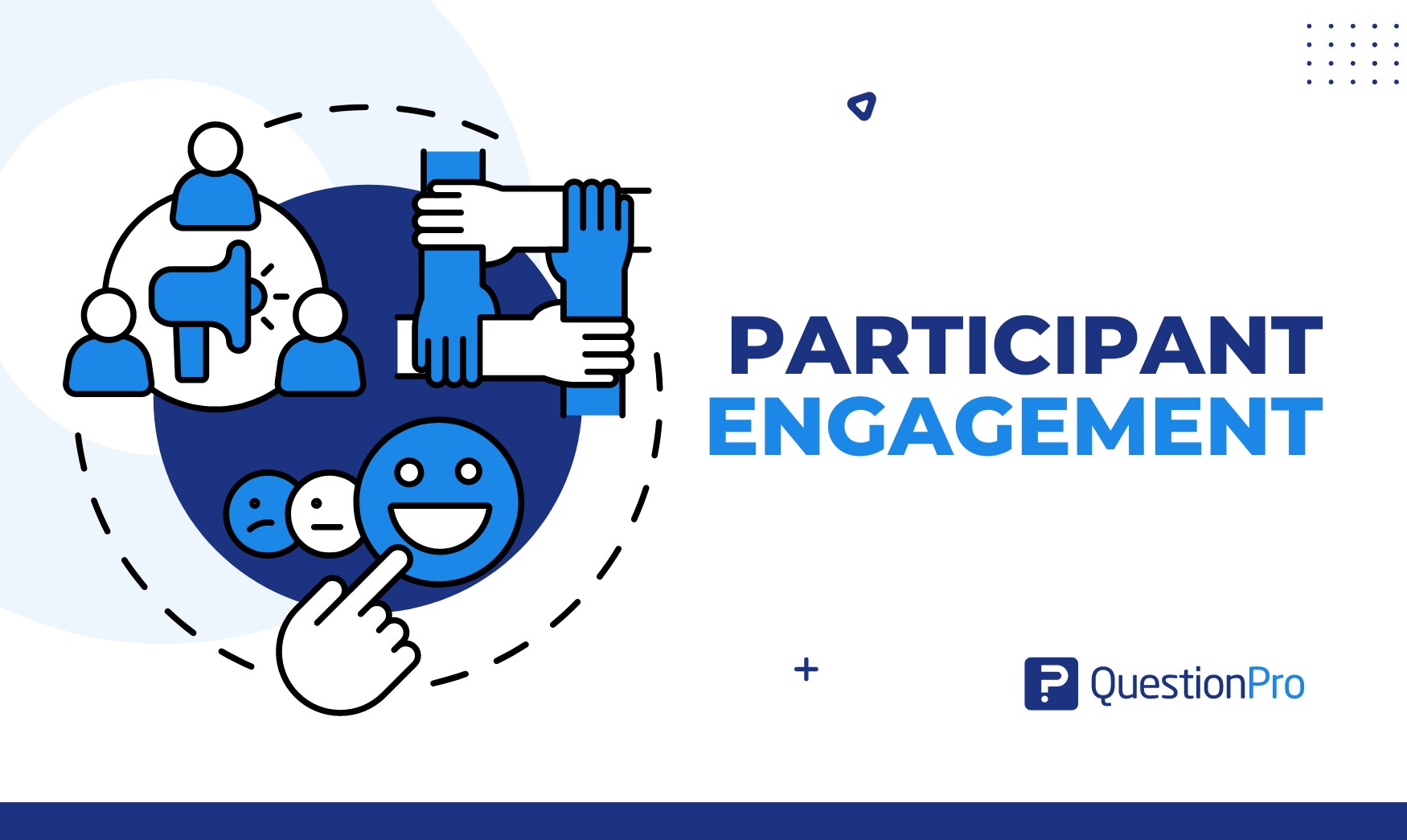
Participant Engagement: Strategies + Improving Interaction
Sep 12, 2024
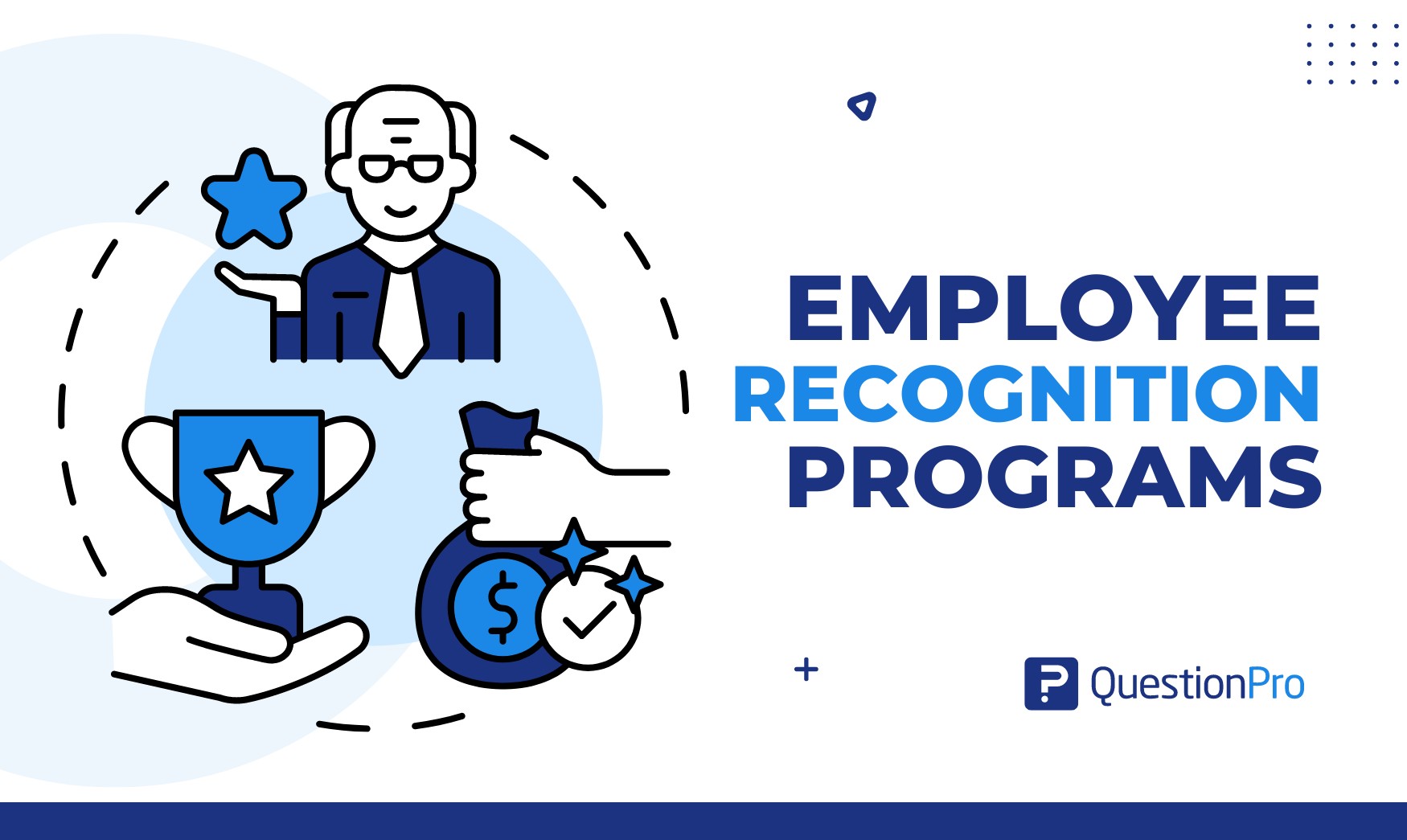
Employee Recognition Programs: A Complete Guide
Sep 11, 2024
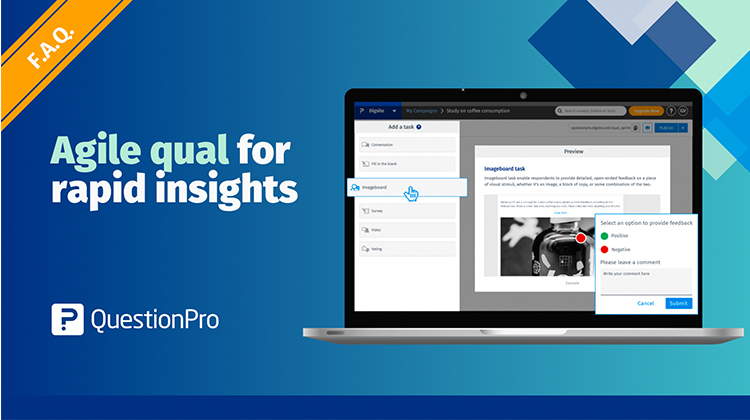
A guide to conducting agile qualitative research for rapid insights with Digsite
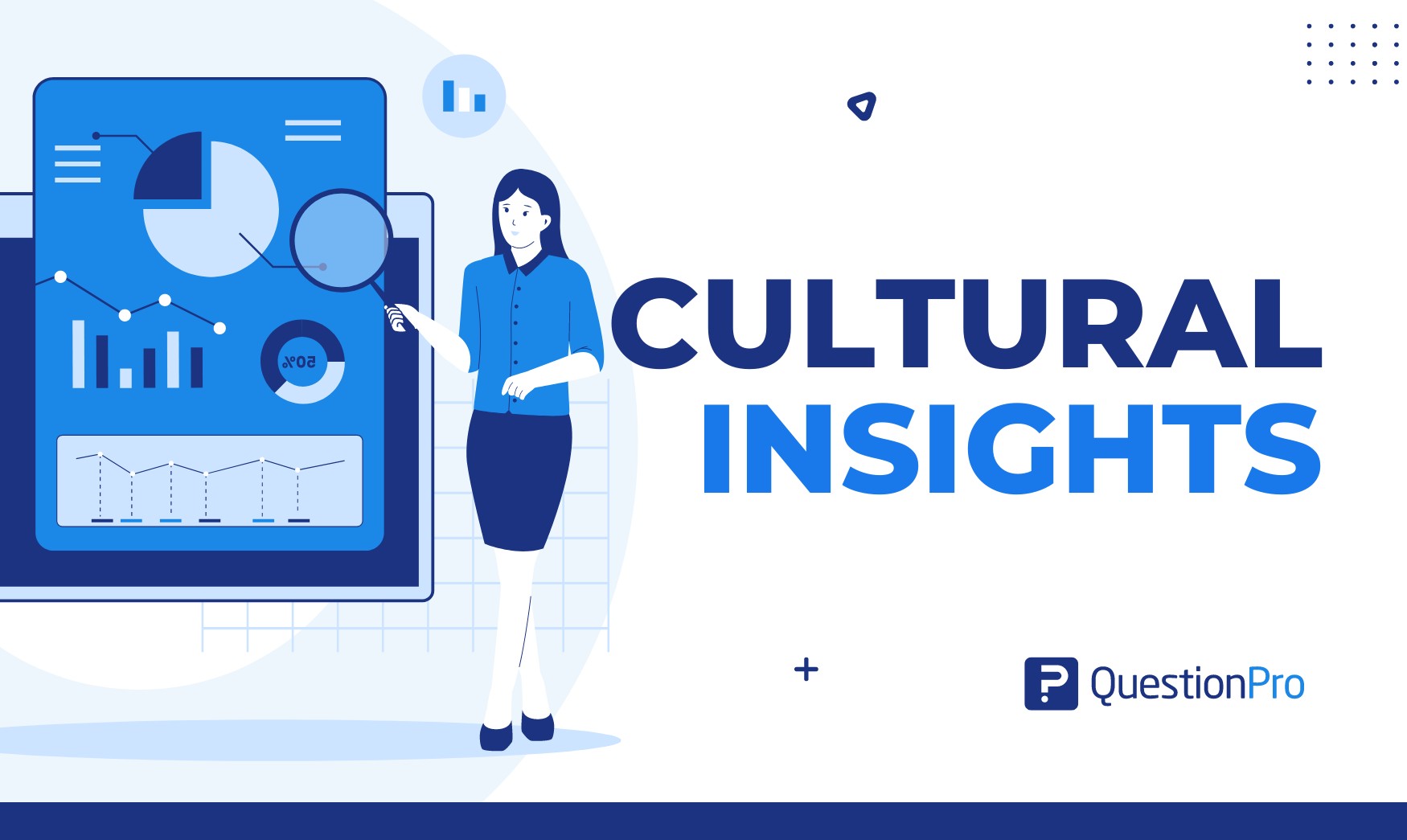
Cultural Insights: What it is, Importance + How to Collect?
Sep 10, 2024
Other categories
- Academic Research
- Artificial Intelligence
- Assessments
- Brand Awareness
- Case Studies
- Communities
- Consumer Insights
- Customer effort score
- Customer Engagement
- Customer Experience
- Customer Loyalty
- Customer Research
- Customer Satisfaction
- Employee Benefits
- Employee Engagement
- Employee Retention
- Friday Five
- General Data Protection Regulation
- Insights Hub
- Life@QuestionPro
- Market Research
- Mobile diaries
- Mobile Surveys
- New Features
- Online Communities
- Question Types
- Questionnaire
- QuestionPro Products
- Release Notes
- Research Tools and Apps
- Revenue at Risk
- Survey Templates
- Training Tips
- Tuesday CX Thoughts (TCXT)
- Uncategorized
- What’s Coming Up
- Workforce Intelligence

Have a language expert improve your writing
Run a free plagiarism check in 10 minutes, generate accurate citations for free.
- Knowledge Base
- Correlation Coefficient | Types, Formulas & Examples
Correlation Coefficient | Types, Formulas & Examples
Published on August 2, 2021 by Pritha Bhandari . Revised on June 22, 2023.
A correlation coefficient is a number between -1 and 1 that tells you the strength and direction of a relationship between variables .
In other words, it reflects how similar the measurements of two or more variables are across a dataset.
Correlation coefficient value | Correlation type | Meaning |
---|---|---|
1 | Perfect positive correlation | When one variable changes, the other variables change in the same direction. |
0 | Zero correlation | There is no relationship between the variables. |
-1 | Perfect negative correlation | When one variable changes, the other variables change in the opposite direction. |
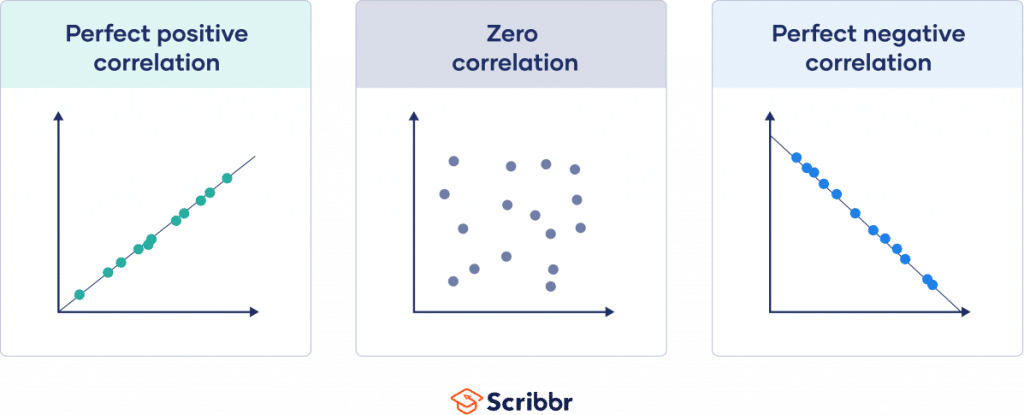
Table of contents
What does a correlation coefficient tell you, using a correlation coefficient, interpreting a correlation coefficient, visualizing linear correlations, types of correlation coefficients, pearson’s r, spearman’s rho, other coefficients, other interesting articles, frequently asked questions about correlation coefficients.
Correlation coefficients summarize data and help you compare results between studies.
Summarizing data
A correlation coefficient is a descriptive statistic . That means that it summarizes sample data without letting you infer anything about the population. A correlation coefficient is a bivariate statistic when it summarizes the relationship between two variables, and it’s a multivariate statistic when you have more than two variables.
If your correlation coefficient is based on sample data, you’ll need an inferential statistic if you want to generalize your results to the population. You can use an F test or a t test to calculate a test statistic that tells you the statistical significance of your finding.
Comparing studies
A correlation coefficient is also an effect size measure, which tells you the practical significance of a result.
Correlation coefficients are unit-free, which makes it possible to directly compare coefficients between studies.
Prevent plagiarism. Run a free check.
In correlational research , you investigate whether changes in one variable are associated with changes in other variables.
After data collection , you can visualize your data with a scatterplot by plotting one variable on the x-axis and the other on the y-axis. It doesn’t matter which variable you place on either axis.
Visually inspect your plot for a pattern and decide whether there is a linear or non-linear pattern between variables. A linear pattern means you can fit a straight line of best fit between the data points, while a non-linear or curvilinear pattern can take all sorts of different shapes, such as a U-shape or a line with a curve.
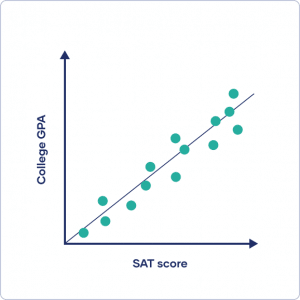
There are many different correlation coefficients that you can calculate. After removing any outliers , select a correlation coefficient that’s appropriate based on the general shape of the scatter plot pattern. Then you can perform a correlation analysis to find the correlation coefficient for your data.
You calculate a correlation coefficient to summarize the relationship between variables without drawing any conclusions about causation .
Both variables are quantitative and normally distributed with no outliers, so you calculate a Pearson’s r correlation coefficient .
The value of the correlation coefficient always ranges between 1 and -1, and you treat it as a general indicator of the strength of the relationship between variables.
The sign of the coefficient reflects whether the variables change in the same or opposite directions: a positive value means the variables change together in the same direction, while a negative value means they change together in opposite directions.
The absolute value of a number is equal to the number without its sign. The absolute value of a correlation coefficient tells you the magnitude of the correlation: the greater the absolute value, the stronger the correlation.
There are many different guidelines for interpreting the correlation coefficient because findings can vary a lot between study fields. You can use the table below as a general guideline for interpreting correlation strength from the value of the correlation coefficient.
While this guideline is helpful in a pinch, it’s much more important to take your research context and purpose into account when forming conclusions. For example, if most studies in your field have correlation coefficients nearing .9, a correlation coefficient of .58 may be low in that context.
Correlation coefficient | Correlation strength | Correlation type |
---|---|---|
-.7 to -1 | Very strong | Negative |
-.5 to -.7 | Strong | Negative |
-.3 to -.5 | Moderate | Negative |
0 to -.3 | Weak | Negative |
0 | None | Zero |
0 to .3 | Weak | Positive |
.3 to .5 | Moderate | Positive |
.5 to .7 | Strong | Positive |
.7 to 1 | Very strong | Positive |
The correlation coefficient tells you how closely your data fit on a line. If you have a linear relationship, you’ll draw a straight line of best fit that takes all of your data points into account on a scatter plot.
The closer your points are to this line, the higher the absolute value of the correlation coefficient and the stronger your linear correlation.
If all points are perfectly on this line, you have a perfect correlation.
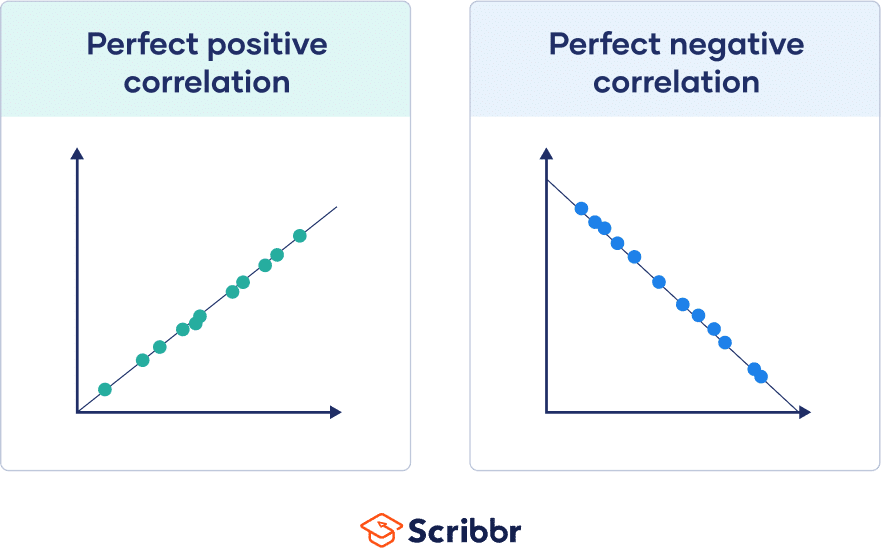
If all points are close to this line, the absolute value of your correlation coefficient is high .
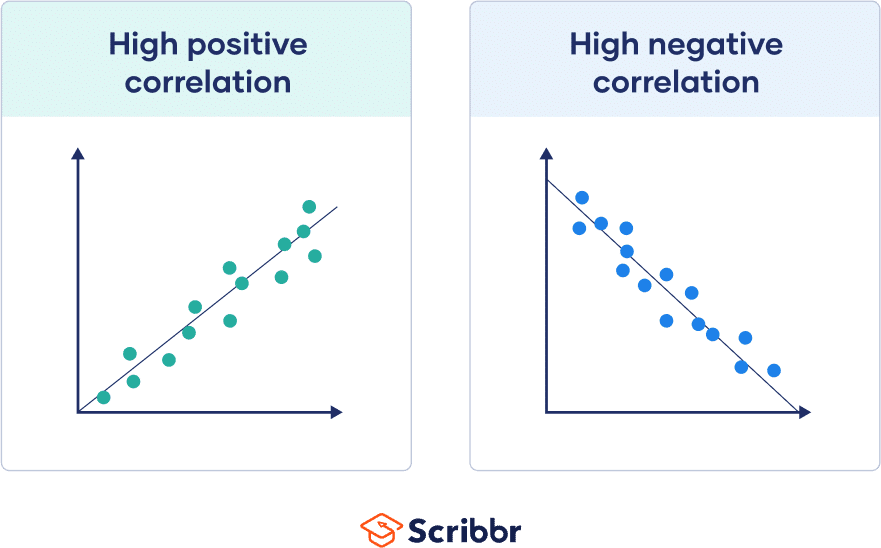
If these points are spread far from this line, the absolute value of your correlation coefficient is low .
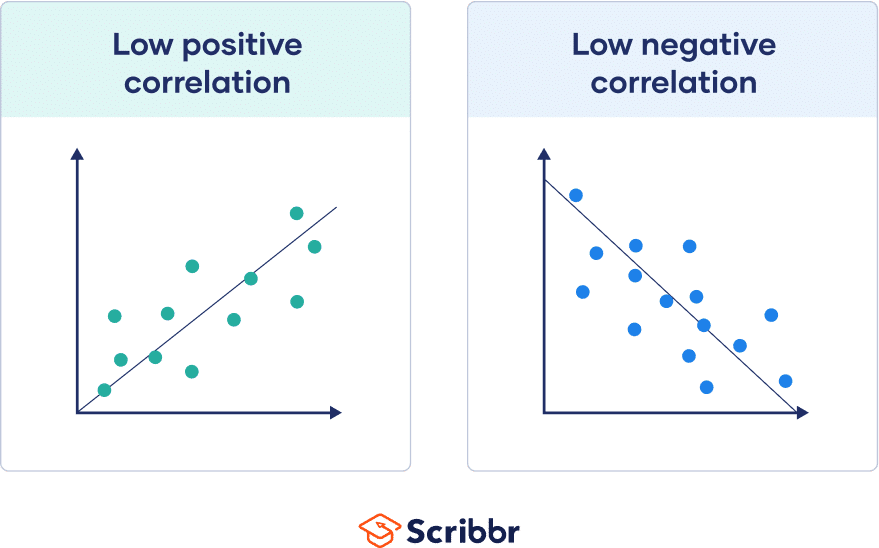
Note that the steepness or slope of the line isn’t related to the correlation coefficient value. The correlation coefficient doesn’t help you predict how much one variable will change based on a given change in the other, because two datasets with the same correlation coefficient value can have lines with very different slopes.
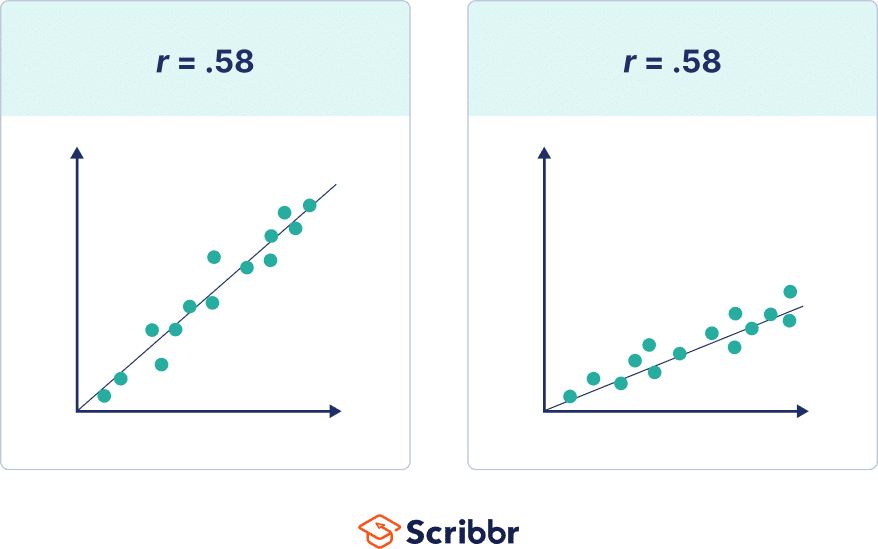
Receive feedback on language, structure, and formatting
Professional editors proofread and edit your paper by focusing on:
- Academic style
- Vague sentences
- Style consistency
See an example
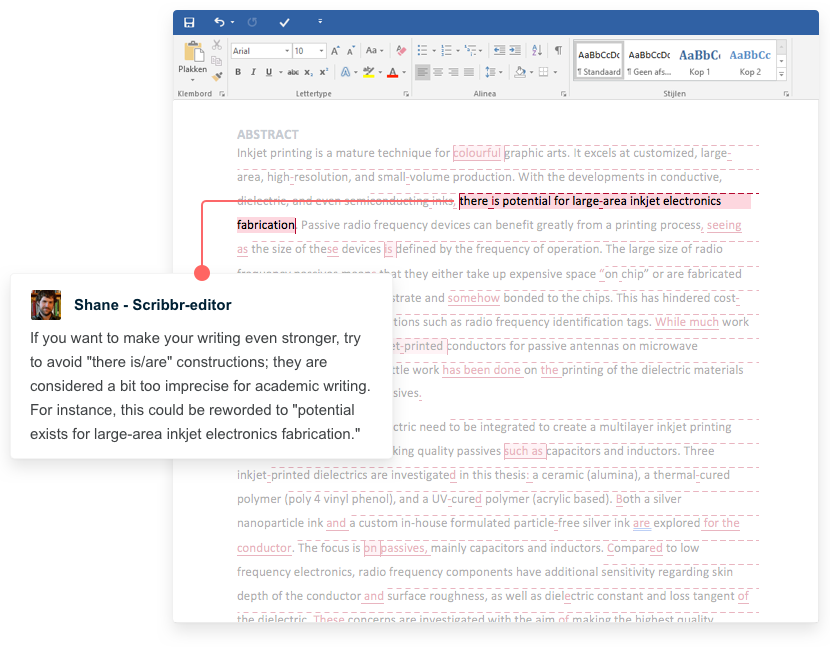
You can choose from many different correlation coefficients based on the linearity of the relationship, the level of measurement of your variables, and the distribution of your data.
For high statistical power and accuracy, it’s best to use the correlation coefficient that’s most appropriate for your data.
The most commonly used correlation coefficient is Pearson’s r because it allows for strong inferences. It’s parametric and measures linear relationships. But if your data do not meet all assumptions for this test, you’ll need to use a non-parametric test instead.
Non-parametric tests of rank correlation coefficients summarize non-linear relationships between variables. The Spearman’s rho and Kendall’s tau have the same conditions for use, but Kendall’s tau is generally preferred for smaller samples whereas Spearman’s rho is more widely used.
The table below is a selection of commonly used correlation coefficients, and we’ll cover the two most widely used coefficients in detail in this article.
Correlation coefficient | Type of relationship | Levels of measurement | Data distribution |
---|---|---|---|
Pearson’s r | Linear | Two quantitative (interval or ratio) variables | Normal distribution |
Spearman’s rho | Non-linear | Two , interval or ratio variables | Any distribution |
Point-biserial | Linear | One dichotomous (binary) variable and one quantitative ( or ratio) variable | Normal distribution |
Cramér’s V (Cramér’s φ) | Non-linear | Two | Any distribution |
Kendall’s tau | Non-linear | Two ordinal, interval or | Any distribution |
The Pearson’s product-moment correlation coefficient, also known as Pearson’s r, describes the linear relationship between two quantitative variables.
These are the assumptions your data must meet if you want to use Pearson’s r:
- Both variables are on an interval or ratio level of measurement
- Data from both variables follow normal distributions
- Your data have no outliers
- Your data is from a random or representative sample
- You expect a linear relationship between the two variables
The Pearson’s r is a parametric test, so it has high power. But it’s not a good measure of correlation if your variables have a nonlinear relationship, or if your data have outliers, skewed distributions, or come from categorical variables. If any of these assumptions are violated, you should consider a rank correlation measure.
The formula for the Pearson’s r is complicated, but most computer programs can quickly churn out the correlation coefficient from your data. In a simpler form, the formula divides the covariance between the variables by the product of their standard deviations .
Formula | Explanation |
---|---|
| = strength of the correlation between variables x and y = sample size = sum of what follows… = every x-variable value = every y-variable value = the product of each x-variable score and the corresponding y-variable score |
Pearson sample vs population correlation coefficient formula
When using the Pearson correlation coefficient formula, you’ll need to consider whether you’re dealing with data from a sample or the whole population.
The sample and population formulas differ in their symbols and inputs. A sample correlation coefficient is called r , while a population correlation coefficient is called rho, the Greek letter ρ.
The sample correlation coefficient uses the sample covariance between variables and their sample standard deviations.
Sample correlation coefficient formula | Explanation |
---|---|
| = strength of the correlation between variables x and y ( , ) = covariance of x and y = sample standard deviation of x = sample standard deviation of y |
The population correlation coefficient uses the population covariance between variables and their population standard deviations.
Population correlation coefficient formula | Explanation |
---|---|
| = strength of the correlation between variables X and Y ( , ) = covariance of X and Y = population standard deviation of X = population standard deviation of Y |
Spearman’s rho, or Spearman’s rank correlation coefficient, is the most common alternative to Pearson’s r . It’s a rank correlation coefficient because it uses the rankings of data from each variable (e.g., from lowest to highest) rather than the raw data itself.
You should use Spearman’s rho when your data fail to meet the assumptions of Pearson’s r . This happens when at least one of your variables is on an ordinal level of measurement or when the data from one or both variables do not follow normal distributions.
While the Pearson correlation coefficient measures the linearity of relationships, the Spearman correlation coefficient measures the monotonicity of relationships.
In a linear relationship, each variable changes in one direction at the same rate throughout the data range. In a monotonic relationship, each variable also always changes in only one direction but not necessarily at the same rate.
- Positive monotonic: when one variable increases, the other also increases.
- Negative monotonic: when one variable increases, the other decreases.
Monotonic relationships are less restrictive than linear relationships.
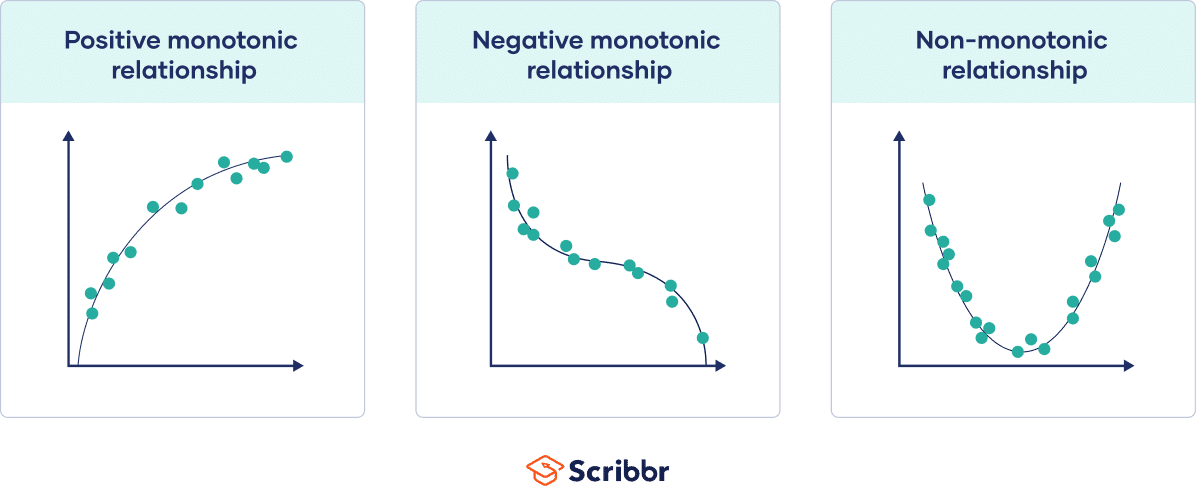
Spearman’s rank correlation coefficient formula
The symbols for Spearman’s rho are ρ for the population coefficient and r s for the sample coefficient. The formula calculates the Pearson’s r correlation coefficient between the rankings of the variable data.
To use this formula, you’ll first rank the data from each variable separately from low to high: every datapoint gets a rank from first, second, or third, etc.
Then, you’ll find the differences (d i ) between the ranks of your variables for each data pair and take that as the main input for the formula.
Spearman’s rank correlation coefficient formula | Explanation |
---|---|
| = strength of the rank correlation between variables = the difference between the x-variable rank and the y-variable rank for each pair of data = sum of the squared differences between x- and y-variable ranks = sample size |
If you have a correlation coefficient of 1, all of the rankings for each variable match up for every data pair. If you have a correlation coefficient of -1, the rankings for one variable are the exact opposite of the ranking of the other variable. A correlation coefficient near zero means that there’s no monotonic relationship between the variable rankings.
The correlation coefficient is related to two other coefficients, and these give you more information about the relationship between variables.
Coefficient of determination
When you square the correlation coefficient, you end up with the correlation of determination ( r 2 ). This is the proportion of common variance between the variables. The coefficient of determination is always between 0 and 1, and it’s often expressed as a percentage.
Coefficient of determination | Explanation |
---|---|
The correlation coefficient multiplied by itself |
The coefficient of determination is used in regression models to measure how much of the variance of one variable is explained by the variance of the other variable.
A regression analysis helps you find the equation for the line of best fit, and you can use it to predict the value of one variable given the value for the other variable.
A high r 2 means that a large amount of variability in one variable is determined by its relationship to the other variable. A low r 2 means that only a small portion of the variability of one variable is explained by its relationship to the other variable; relationships with other variables are more likely to account for the variance in the variable.
The correlation coefficient can often overestimate the relationship between variables, especially in small samples, so the coefficient of determination is often a better indicator of the relationship.
Coefficient of alienation
When you take away the coefficient of determination from unity (one), you’ll get the coefficient of alienation. This is the proportion of common variance not shared between the variables, the unexplained variance between the variables.
Coefficient of alienation | Explanation |
---|---|
1 – | One minus the coefficient of determination |
A high coefficient of alienation indicates that the two variables share very little variance in common. A low coefficient of alienation means that a large amount of variance is accounted for by the relationship between the variables.
If you want to know more about statistics , methodology , or research bias , make sure to check out some of our other articles with explanations and examples.
- Chi square test of independence
- Statistical power
- Descriptive statistics
- Degrees of freedom
- Pearson correlation
- Null hypothesis
Methodology
- Double-blind study
- Case-control study
- Research ethics
- Data collection
- Hypothesis testing
- Structured interviews
Research bias
- Hawthorne effect
- Unconscious bias
- Recall bias
- Halo effect
- Self-serving bias
- Information bias
A correlation reflects the strength and/or direction of the association between two or more variables.
- A positive correlation means that both variables change in the same direction.
- A negative correlation means that the variables change in opposite directions.
- A zero correlation means there’s no relationship between the variables.
A correlation is usually tested for two variables at a time, but you can test correlations between three or more variables.
A correlation coefficient is a single number that describes the strength and direction of the relationship between your variables.
Different types of correlation coefficients might be appropriate for your data based on their levels of measurement and distributions . The Pearson product-moment correlation coefficient (Pearson’s r ) is commonly used to assess a linear relationship between two quantitative variables.
These are the assumptions your data must meet if you want to use Pearson’s r :
Correlation coefficients always range between -1 and 1.
The sign of the coefficient tells you the direction of the relationship: a positive value means the variables change together in the same direction, while a negative value means they change together in opposite directions.
No, the steepness or slope of the line isn’t related to the correlation coefficient value. The correlation coefficient only tells you how closely your data fit on a line, so two datasets with the same correlation coefficient can have very different slopes.
To find the slope of the line, you’ll need to perform a regression analysis .
Cite this Scribbr article
If you want to cite this source, you can copy and paste the citation or click the “Cite this Scribbr article” button to automatically add the citation to our free Citation Generator.
Bhandari, P. (2023, June 22). Correlation Coefficient | Types, Formulas & Examples. Scribbr. Retrieved September 9, 2024, from https://www.scribbr.com/statistics/correlation-coefficient/
Is this article helpful?
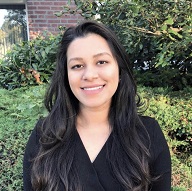
Pritha Bhandari
Other students also liked, correlational research | when & how to use, correlation vs. causation | difference, designs & examples, simple linear regression | an easy introduction & examples, what is your plagiarism score.

An official website of the United States government
The .gov means it’s official. Federal government websites often end in .gov or .mil. Before sharing sensitive information, make sure you’re on a federal government site.
The site is secure. The https:// ensures that you are connecting to the official website and that any information you provide is encrypted and transmitted securely.
- Publications
- Account settings
Preview improvements coming to the PMC website in October 2024. Learn More or Try it out now .
- Advanced Search
- Journal List
- HHS Author Manuscripts

Estimating the Correlation in Bivariate Normal Data with Known Variances and Small Sample Sizes 1
We consider the problem of estimating the correlation in bivariate normal data when the means and variances are assumed known, with emphasis on the small sample case. We consider eight different estimators, several of them considered here for the first time in the literature. In a simulation study, we found that Bayesian estimators using the uniform and arc-sine priors outperformed several empirical and exact or approximate maximum likelihood estimators in small samples. The arc-sine prior did better for large values of the correlation. For testing whether the correlation is zero, we found that Bayesian hypothesis tests outperformed significance tests based on the empirical and exact or approximate maximum likelihood estimators considered in small samples, but that all tests performed similarly for sample size 50. These results lead us to suggest using the posterior mean with the arc-sine prior to estimate the correlation in small samples when the variances are assumed known.
1 INTRODUCTION
Sir Francis Galton defined the theoretical concept of bivariate correlation in 1885, and a decade later Karl Pearson published the formula for the sample correlation coefficient, also known as Pearson’s r ( Rodgers and Nicewander, 1988 ). The sample correlation coefficient is still the most commonly used measure of correlation today as it assumes no knowledge of the means or variances of the individual groups and is the maximum likelihood estimator for the correlation coefficient in the bivariate normal distribution when the means and variances are unknown.
In the event that the variances are known, information is lost by using the sample correlation coefficient. We cannot simply substitute the known variance quantities into the denominator of the sample correlation coefficient since that results in an estimator that is not the maximum likelihood estimator and has the potential to fall outside the interval [−1, 1]. When the variances are known, we seek an estimator that takes advantage of this information.
Kendall and Stuart (1979) noted that conditional on the variances, the maximum likelihood estimator of the correlation is the solution of a cubic equation. Sampson (1978) proposed a consistent, asymptotically efficient estimator based on the cubic equation that avoided the need to solve the equation directly. In a simulation study, we found that when the true correlation is zero and the sample size is small, the variances of these estimators are undesirably large. This led us to search for more stable estimates of the correlation, which condition on the known variances and perform well when sample sizes are small.
Our interest in this problem arose in the context of probabilistic population projections. Alkema et al. (2011) developed a Bayesian hierarchical model for projecting the total fertility rate (TFR) in all countries. This model works well for projecting the TFR in individual countries. However, for creating aggregated regional projections, there was concern that excess correlation existed between the country fertility rates that was not accounted for in the model. To investigate this we considered correlations between the normalized forecast errors in different countries, conditional on the model parameters. Often there were as few as five to ten data points to estimate the correlation. For each pair of countries, these errors were treated as samples from a bivariate normal distribution with means equal to zero and variances equal to one. Determining whether the correlations between the countries are nonzero, and if so estimating them, is necessary to assess the predictive distribution of aggregated projections.
In Section 2 we describe the estimators we consider, in Section 3 we give the results of our simulation study, and in Section 4 we discuss alternative approaches.
2 ESTIMATORS OF CORRELATION
Let ( X i , Y i ), i = 1, …, n be independent and identically distributed observations from a bivariate normal distribution with means equal to zero, variances equal to one, and correlation unknown. We let SSx = ∑ i = 1 n X i 2 , SSy = ∑ i = 1 n Y i 2 , and SSxy = ∑ i = 1 n X i Y i and consider eight estimators of the correlation.
The first estimator is the maximum likelihood estimator for bivariate normal data when the variances are unknown. We refer to this as the sample correlation coefficient even though we have conditioned on the means being zero. This estimator is defined as follows:
The second estimator is a modification of the first estimator, where we assume the variances are known to be equal to one. We name this estimator the empirical estimator with known variances and define it as:
This estimator is unbiased yet is not guaranteed to fall in [−1, 1], especially for small samples. This unappealing property motivated us to define the third estimator called the truncated empirical estimator with known variances , ρ ̂ (3) , where the second estimator is truncated at −1 if it falls below −1 and at 1 if it falls above 1.
The maximum likelihood estimator (MLE) when the means are known to be zero and variances are known to be one is the fourth estimator. This estimator is found by solving the cubic equation
which results from setting the derivative of the log-likelihood equal to zero. If we define
then the three roots of this equation can be written fairly compactly, as follows:
Kendall and Stuart (1979) noted that at least one of the roots above is real and lies in the interval [−1, 1]. However, it is possible that all three roots are real and in the admissible interval, in which case the likelihood can be evaluated at each root to determine the true maximum likelihood estimate. Based on whether ( SSxy / n ) 2 is bigger than 3( SSx / n + SSy / n −1), and whether γ /(2 ψ ) is bigger than 1, Madansky (1958) specified conditions under which each of the three roots is the maximum likelihood estimate.
Sampson (1978) acknowledged the effort involved in computing the maximum likelihood estimate when the variances are known and proposed an asymptotically efficient estimator of the correlation based solely on the coefficients in the cubic equation (1) . Sampson’s estimator does not necessarily fall in the interval [−1, 1] so he suggested truncating the estimate to lie in the interval, as was done with the empirical estimator with known variances. This less computationally intensive estimator is referred to as Sampson’s truncated MLE approximation , ρ ̂ (5) , and is the fifth estimator we consider.
The remaining three estimators are Bayesian. Our sixth estimator is the posterior mean assuming a uniform prior , which has the form:
where X = ( X 1 , …, X n ) and Y = ( Y 1 , …, Y n ). The denominator is the integral of the likelihood of the bivariate normal data multiplied by 1/2, representing the Uniform(−1, 1) prior, while the numerator is the same but with the integrand multiplied by ρ for the expectation.
Jeffreys (1961) described the improper prior, conditional on the variances, as:
This prior was the basis for the seventh estimator: the posterior mean assuming a Jeffreys prior , ρ ̂ (7) .
Finally, Jeffreys (1961) noted that the arc-sine prior,
is similar to the Jeffreys prior, but integrable on [−1, 1]. The posterior mean assuming an arc-sine prior , ρ ̂ (8) , represents the eighth, and final, estimator investigated.
Each of these priors is shown in Figure 1 . The curve for the Jeffreys prior is an approximation since it is not integrable on [−1, 1]. Note that the arc-sine distribution on ρ is equivalent to placing a generalized beta (2, 1, 0.5, 0.5) of the first kind on | ρ | ( McDonald (1984) ). Similarly, the uniform prior corresponds to a generalized beta (1, 1, 1, 1) of the first kind on | ρ |. Of these estimators, the empirical estimator with known variances and truncated empirical estimator with known variances are, to our knowledge, proposed here for the first time.

The density of each of the priors for the Bayesian estimators are shown. The Jeffreys curve is an approximation since it is not integrable on [−1, 1]. Observe that the arc-sine and Jeffreys priors are very similar, but the Jeffreys puts more weight on extreme values.
3 SIMULATION STUDY
3.1 estimating the correlation.
Samples of sizes 5, 10, and 50 were generated from a bivariate normal distribution with means equal to zero, variances equal to one, and a specified correlation value. The estimators were first evaluated for positive and negative values of the correlation and were all found to be symmetric. Thus values of the correlation were sampled uniformly from symmetric intervals on [−1, 1] to analyze how the estimators performed for different magnitudes of correlation. The estimators were compared based on root mean squared error using one million samples. The results are shown in Table 1 .
Root mean squared errors multiplied by 1000 are shown for each estimator based on one million simulated data sets ( n =sample size). The estimators with the smallest root mean squared error are shown in bold for each sample size and each true correlation interval.
Estimator | | | | |||||
---|---|---|---|---|---|---|
[0,1] | [0,.25] | [.25,.50] | [.50,.75] | [.75,1] | ||
5 | Sample Correlation Coeff | 352 | 442 | 406 | 326 | 172 |
Emp w/ Known Var | 516 | 452 | 479 | 529 | 595 | |
Trunc Emp w/ Known Var | 387 | 419 | 399 | 369 | 358 | |
MLE | 373 | 464 | 437 | 352 | ||
Sampson’s MLE Approx | 382 | 462 | 435 | 357 | 232 | |
Mean w/ Uniform Prior | 332 | 244 | ||||
Mean w/ Jeffreys Prior | 311 | 358 | 354 | 182 | ||
Mean w/ Arc-sine Prior | 299 | 316 | 330 | 325 | 213 | |
10 | Sample Correlation Coeff | 240 | 311 | 280 | 213 | 101 |
Emp w/ Known Var | 365 | 319 | 338 | 373 | 421 | |
Trunc Emp w/ Known Var | 299 | 314 | 312 | 295 | 274 | |
MLE | 248 | 334 | 295 | |||
Sampson’s MLE Approx | 249 | 333 | 295 | 206 | 90 | |
Mean w/ Uniform Prior | 227 | 124 | ||||
Mean w/ Jeffreys Prior | 222 | 277 | 261 | 208 | 92 | |
Mean w/ Arc-sine Prior | 217 | 254 | 251 | 219 | 109 | |
50 | Sample Correlation Coeff | 104 | 139 | 122 | 88 | 39 |
Emp w/ Known Var | 163 | 143 | 151 | 167 | 188 | |
Trunc Emp w/ Known Var | 150 | 143 | 151 | 161 | 145 | |
MLE | 100 | 142 | 117 | |||
Sampson’s MLE Approx | 100 | 142 | 117 | |||
Mean w/ Uniform Prior | 116 | 82 | 33 | |||
Mean w/ Jeffreys Prior | 98 | 135 | 78 | 30 | ||
Mean w/ Arc-sine Prior | 98 | 131 | 116 | 80 | 32 |
Numerical issues arose when computing the integrals involved in the posterior mean estimators in cases where the true correlation value was extremely close to one in magnitude. To handle this, a tolerance of 10 −6 × n was put on the value of | SSx + SSy ± 2 SSxy | since SSx + SSy ± 2 SSxy = 0 signifies a correlation of ∓1, respectively. When this tolerance was satisfied, the correlation estimate was given the appropriate value of 1 or −1. This approximation was used about ten times out of one million in the [0, 1] interval and thirty times out of one million in the [0.75, 1] interval for each sample size.
For the first column, since the correlations were drawn uniformly from the interval [−1, 1], the Bayesian estimator assuming a uniform prior will have the lowest mean squared error according to theory. In samples of size 5, the uniform and arc-sine priors had superior performance over the entire [−1, 1] interval compared to the other estimators, with a root mean squared error of about 0.3. The empirical estimator with known variances performed least well, whereas the maximum likelihood estimator and sample correlation coefficient performed similarly, with the sample correlation coefficient doing slightly better. This suggests that in small sample sizes, knowing the variances yields no improvement when using the maximum likelihood estimator.
However, when the correlations are decomposed by magnitude, a different story is told. For extreme correlation values, the sample correlation coefficient, maximum likelihood estimator and posterior mean assuming a Jeffreys prior had the smallest root mean squared errors. The Jeffreys prior is highly concentrated at extreme correlation values so we would expect it to outperform the other Bayesian estimators in the last interval. The posterior mean assuming an arc-sine prior and that assuming a uniform prior had root mean squared errors 1.3 and 1.5 times as large as that for the MLE, or best estimator. Conversely, at low values of correlation, the uniform and arc-sine posterior mean estimates had significantly lower root mean squared error than all other estimators. The posterior median estimators for each of the priors was also considered. Overall they performed very similar to the posterior mean estimates and hence are not included here.
In general, one does not know the magnitude of the correlation to be estimated, so an estimator that performs well for all levels of correlation is desired. Both the posterior mean assuming an arc-sine prior and that assuming a uniform prior had routinely low root mean squared error values when compared to the other estimators and were fairly consistent across the different correlation magnitudes. Therefore, we concluded that these should be the methods of choice for small sample sizes. One might argue that if estimating large correlations accurately is of greater interest then the posterior mean assuming the arc-sine prior should be used since it outperforms that with a uniform prior at extreme correlations.
As the sample size increased from 5 to 10 and from 10 to 50, the root mean squared errors decreased for all estimators, as expected. For samples of size 50, the root mean squared errors for correlations on the entire interval [−1, 1] were low and effectively the same for all estimators except the empirical estimators when the variances are known. However, the estimators’ performances by magnitude of the correlation still varied as in the case of samples of size 5.
Sampson’s truncated approximation of the maximum likelihood estimator performed similarly to the maximum likelihood estimator for smaller sample sizes and almost identically for the larger sample sizes. This is because, as the sample size increases, the probability of the cubic equation having more than one real root goes to zero. Thus, large samples make it easier to use properties of cubic equations to pinpoint the correct MLE root.
Figure 2 shows the first 5,000 samples of each estimator’s correlation estimates and the true correlation values for samples of size 5. Notice that the empirical estimate with known variances often lay outside the range [−1, 1]. In addition, for small values of the correlation, the empirical estimates, maximum likelihood estimates and Sampson’s estimates were extremely variable, spanning most of the interval [−1, 1]. The Bayesian estimates showed a closer association overall between the true correlation value and the estimates, especially when the true correlation was small. However, there was some curvature in the tails of the plots for the Bayesian estimators, suggesting that the estimators typically underestimate the magnitude of the correlation when the true correlation is high. This is to be expected, as the Bayesian approach shrinks estimators away from the extremes.

For samples of size 5, the true and estimated correlation values for each estimator is shown above for the first 5,000 samples. The dotted lines in the empirical with known variances plot mark the admissible interval [−1, 1].
3.2 Hypothesis Tests
Estimating the value of the correlation is important, but often with small sample sizes our interest is not in its actual value but simply in whether or not it is non-zero. We often have knowledge about the sign of the correlation between two variables. Here we consider the case when we are interested in testing if the correlation is positive.
One way of testing this is to look at the confidence bounds of the estimators. A level 0.05 test of whether the true correlation is positive can be derived by generating numerous samples of independent bivariate normal random variables with means equal to zero and variances equal to one, calculating a correlation estimate for each sample, and determining the sample 95% quantile of the correlations. A level 0.05 test then rejects the hypothesis that the correlation is zero in favor of the alternative that it is positive if the estimate obtained is greater than the 95% quantile, i.e. the significance test bound. Table 2 shows the 95% significance test bounds for all non-Bayesian estimators based on one million simulations with ρ = 0. For example, for the sample correlation coefficient, the significance test bound for samples of size 5 is 0.73, indicating that about 5% of the samples resulted in an estimated correlation value greater than 0.73.
95% Significance Test bounds for testing if ρ > 0 for the non-Bayesian estimators when ρ = 0 based on one million simulated data sets.
Sample Size | 5 | 10 | 50 |
---|---|---|---|
Sample Correlation Coeff | 0.729 | 0.522 | 0.233 |
Emp w/ Known Var | 0.731 | 0.518 | 0.232 |
Truncated Emp w/ Known Var | 0.731 | 0.518 | 0.232 |
MLE | 0.754 | 0.565 | 0.241 |
Sampson’s MLE Approx | 0.756 | 0.566 | 0.241 |
For Bayesian tests, Jeffreys (1935 , 1961 ) developed ideas based on Bayes factors for testing/deciding between two models; see also Kass and Raftery (1995) . A Bayes factor, B 10 , is the ratio of the probability of the data under the alternative model to the probability of the data under the null model. Equivalently, it is the ratio of the posterior odds for the alternative against the null model, to its prior odds. A test that rejects the null hypothesis when B 10 > 1 minimizes the sum of the probabilities of Type I and Type II errors if the prior odds between the models are equal to one.
However, if we wish to fix the probability of a Type I error at 0.05 for example, we can generate data under the null model and determine the value c such that the probability under the null model that the Bayes factor is greater than c is 0.05. A level 0.05 test is then carried out for the null model against the alternative model by rejecting the null model if the Bayes factor is greater than c . This method was used with ρ = 0 as the null hypothesis and ρ > 0 as the alternative hypothesis to compare the performance of the Bayesian and non-Bayesian methods when the Type I error is fixed at 0.05. Note that the Bayes factor is
where p ( ρ ) is one of the three prior distributions for ρ and the denominator is the product of the marginal probabilities assuming ρ = 0, or independence. The factor of two in equation (2) is due to the fact all prior distributions are centered at zero. The Bayes factor is undefined for the Jeffreys prior so we do not consider it here forward.
Table 3 shows the values of c obtained for the various prior distributions and sample sizes. We see that as sample size increased, the values of c decreased since the amount of evidence for the null increased. Also, the values of c for the arc-sine prior were much greater than those for the uniform prior, reflecting the fact that the arc-sine prior places more weight on extreme correlation values.
Value of c such that the Bayes factor has 5% probability of exceeding c if the true value of ρ is 0 (i.e. P( B 10 > c | ρ = 0) = 0.05) based on one million simulated data sets.
Sample Size | 5 | 10 | 50 |
---|---|---|---|
Uniform Prior | 2.701 | 2.304 | 1.238 |
Arc-sine Prior | 2.275 | 1.715 | 0.817 |
Table 4 shows the power when the true correlation was uniformly generated from various intervals for each of the non-Bayesian significance tests and the tests based on Bayes factors. In samples of size 5 the Bayesian tests had the greatest power over the entire [0, 1] interval and for the most extreme correlation values. For the smaller correlation values, all tests, except possibly those based on the MLE and Sampson’s MLE, performed about the same. The tests based on the arc-sine prior and uniform prior performed similarly for all correlation values and sample sizes. As sample size increased, the difference between the powers of the tests based on the MLE and Sampson’s MLE and all others decreased.
Average power multiplied by 1000 over intervals for ρ when testing ρ = 0 vs ρ > 0 at the 0.05 significance level based on one million simulated data sets. For the non-Bayesian estimators, the significance test bounds found in Table 2 were used. The Bayesian tests were based on the Bayes factors using the value of c listed in Table 3 . The tests with the largest power are shown in bold for each sample size and each correlation interval.
Test Based on | ||||||
---|---|---|---|---|---|---|
[0,1] | [0,.25] | [.25,.50] | [.50,.75] | [.75,1] | ||
5 | Sample Correlation Coeff | 383 | 81 | 187 | 423 | 839 |
Emp w/ Known Var | 288 | 348 | 517 | |||
Trunc Emp w/ Known Var | 288 | 348 | 517 | |||
MLE | 356 | 69 | 141 | 352 | 862 | |
Sampson’s MLE Approx | 355 | 69 | 140 | 350 | 861 | |
Uniform Prior | 82 | 196 | 869 | |||
Arc-sine Prior | 81 | 192 | 440 | |||
10 | Sample Correlation Coeff | 529 | 106 | 708 | 977 | |
Emp w/ Known Var | 441 | 302 | 562 | 793 | ||
Trunc Emp w/ Known Var | 441 | 302 | 562 | 793 | ||
MLE | 505 | 88 | 262 | 682 | ||
Sampson’s MLE Approx | 505 | 88 | 260 | 680 | ||
Uniform Prior | 105 | 325 | 722 | 985 | ||
Arc-sine Prior | 104 | 323 | 987 | |||
50 | Sample Correlation Coeff | 770 | 833 | 998 | ||
Emp w/ Known Var | 759 | 245 | 800 | 993 | ||
Trunc Emp w/ Known Var | 759 | 245 | 800 | 993 | ||
MLE | 768 | 238 | 834 | |||
Sampson’s MLE Approx | 768 | 238 | 834 | |||
Uniform Prior | 998 | |||||
Arc-sine Prior |
As mentioned, tests based on the Bayes factor are optimal in that they minimize the sum of the probabilities of Type I and Type II errors when simulating from the prior. For this reason the uniform prior performs best over the entire interval [0,1] for all sample sizes. Table 5 shows the average value of the Type I and Type II error probabilities when the standard rule of rejecting the null hypothesis when the Bayes factor is greater than one is used. This optimal Bayesian method is compared with the significance test bound procedure for the non-Bayesian estimators via this average error measure. The Bayesian tests had the smallest average error for samples of size 5. The MLE and Sampson’s MLE approximation performed very similarly to the Bayesian tests at the extreme correlation values.
Average error probability, [Type I + Type II]/2, when testing if ρ = 0 versus ρ > 0, multiplied by 1000, based on one million simulated data sets. The error probabilities for the non-Bayesian tests are based on 0.05 level significance tests and the Bayesian test error probabilities are based on rejecting the null hypothesis that ρ = 0 if the Bayes factor is greater than 1. The tests with the smallest average error are shown in bold for each sample size and each correlation interval.
Test Based on | ||||||
---|---|---|---|---|---|---|
[0,1] | [0,.25] | [.25,.50] | [.50,.75] | [.75,1] | ||
5 | Sample Correlation Coeff | 333 | 485 | 431 | 313 | 106 |
Emp w/ Known Var | 381 | 480 | 426 | 351 | 267 | |
Trunc Emp w/ Known Var | 381 | 480 | 426 | 351 | 267 | |
MLE | 347 | 490 | 455 | 349 | 94 | |
Sampson’s MLE Approx | 348 | 491 | 455 | 350 | 95 | |
Uniform Prior | 113 | |||||
Arc-sine Prior | 289 | 469 | 374 | 225 | ||
10 | Sample Correlation Coeff | 261 | 472 | 362 | 171 | 37 |
Emp w/ Known Var | 304 | 470 | 374 | 244 | 129 | |
Trunc Emp w/ Known Var | 304 | 470 | 374 | 244 | 129 | |
MLE | 272 | 481 | 394 | 184 | ||
Sampson’s MLE Approx | 273 | 481 | 395 | 185 | ||
Uniform Prior | 76 | |||||
Arc-sine Prior | 240 | 458 | 319 | 132 | 51 | |
50 | Sample Correlation Coeff | 140 | 400 | 109 | 26 | 25 |
Emp w/ Known Var | 145 | 402 | 125 | 29 | 25 | |
Trunc Emp w/ Known Var | 145 | 402 | 125 | 29 | 25 | |
MLE | 141 | 406 | 108 | 25 | 25 | |
Sampson’s MLE Approx | 141 | 406 | 108 | 25 | 25 | |
Uniform Prior | 33 | 32 | ||||
Arc-sine Prior | 142 | 411 | 114 |
At larger sample sizes, the tests performed effectively equally well. For the extreme correlation values with samples of size 50, all tests have essentially 100% power so their average error achieves its lower bound at one-half the Type I error rate. Notice again that the tests based on the arc-sine prior had slightly smaller average error than that assuming a uniform prior at extreme correlation values and that its performance on the entire interval [0, 1] was close to the uniform, which was best.
4 DISCUSSION
We have considered the estimation of the correlation in bivariate normal data when the means and variances are assumed known, with emphasis on the small sample situation. Using simulation, we found that the posterior mean using a uniform prior or an arc-sine prior consistently outperformed several previously proposed empirical and exact and approximate maximum likelihood estimators for small samples. The arc-sine prior performed similarly to the uniform prior for small values of ρ , and better for large values of ρ in small samples. This suggests using the posterior mean with the arc-sine prior for estimation when it is important to identify extreme correlations.
For testing whether the correlation is zero, we carried out a simulation for positive values of ρ within specified intervals, and found that Bayesian tests had smaller average error than the non-Bayesian tests when n = 5. With n = 50, however, all the tests performed similarly.
Spruill and Gastwirth (1982) derived estimators of the correlation when the data are normal but the variables are contained in separate locations and cannot be combined. Their work combines the data into groups based on the value of one variable to obtain an estimate of the correlation. This differs from the more usual situation considered here where both variables are available in their sampled pairs.
Estimation of the sample correlation coefficient with truncation was investigated by Gajjar and Subrahmaniam (1978) . However, it is the underlying distribution that is assumed to be truncated instead of the estimator as here.
Data sets and distributions for which use of the sample correlation coefficient is inappropriate were investigated by Carroll (1961) . Norris and Hjelm (1961) considered estimation of correlation when the underlying distribution is not normal, and Farlie (1960) considered it for general bivariate distribution functions. Since we limit ourselves to the bivariate normal distribution, we did not consider these estimators.
Olkin and Pratt (1958) derived unbiased estimates of the correlation in the case when the means are known and the case when all parameters are unknown. This addresses different situations to the one we have considered, where the variances are also assumed known.
Others have considered estimating the correlation in a Bayesian framework for the bivariate normal setting. Berger and Sun (2008) addressed this problem using objective priors whose posterior quantiles match up with the corresponding frequentist quantiles. Ghosh et al. (2010) extended these results by considering a probability matching criterion based on highest posterior density regions and the inversion of test statistics. However, in both cases the focus was on matching frequentist probabilities rather than estimation accuracy.
Much of the other Bayesian correlation work relates to estimation of covariance matrices. Barnard et al. (2000) discussed prior distributions on covariance matrices by decomposing the covariance matrix into Σ = SRS where S = diag ( s ) is a diagonal matrix of standard deviations and R is the correlation matrix. With this, one can use the prior factorization p ( σ , R ) = p ( σ ) p ( R | σ ) to specify a prior on the covariance matrix. Barnard et al. (2000) suggest some default choices for the prior distribution on R that are independent of σ . Specifically they mention the possibility of placing a uniform distribution on R , p ( R ) ∝ 1, where R must be positive definite. The marginal distributions of the individual correlations are then not uniform. Alternatively, for a ( d × d ) matrix R one can specify
where R ii is the ith principal submatrix of R . This is the marginal distribution of R when Σ has a standard inverse-Wishart distribution with ν degrees of freedom and results in the following marginal distribution on the pairwise correlations
Uniform marginal distributions for all pairwise correlations comes from the choice ν = d + 1. Note that for ν = 2 and d = 2, this prior reduces to the arc-sine prior. This is the boundary case that is the most diffuse prior in the class. Barnard et al. (2000) discussed using these priors for shrinkage estimation of regression coefficients and a general location-scale model for both categorical and continuous variables. Zhang et al. (2006) focused on methods for sampling such correlation matrices.
Liechty et al. (2004) considered a model where all correlations have a common truncated normal prior distribution under the constraint that the resulting correlation matrix be positive definite. They also considered the model where the correlations or observed variables are clustered into groups that share a common mean and variance. Chib and Greenberg (1998) assumed a multivariate truncated normal prior in the context of a multivariate probit model, and Liu and Sun (2000) and Liu (2001) assumed a Jeffreys’ prior on R in the context of a multivariate probit and multivariate multiple regression model.
A number of advances have been made with respect to estimation of the covariance matrix treating the variances as unknown, unlike here. Geisser and Cornfield (1963) developed posterior distributions for multivariate normal parameters with an objective prior, and Yang and Berger (1994) focused on estimation with reference priors. Geisser (1965) , Tiwari et al. (1989) , and Press and Zellner (1978) derived posterior distributions of the multiple correlation coefficient using the prior from Geisser and Cornfield, an informative beta distribution, and diffuse and natural conjugate priors assuming fixed regressors, respectively. It is possible that some of these ideas regarding prior specification of covariance matrices could be applied to the present setting or be used to extend this work to the multivariate setting.
1 This work was supported by NICHD grant R01 HD54511. Raftery’s research was also partially supported by NIH grant R01 GM084163, and NSF grants ATM0724721 and IIS0534094. The authors thank Sam Clark, Jon Wellner and the Probabilistic Population Projections Group at the University of Washington for helpful comments and discussion.
- Alkema L, Raftery AE, Gerland P, Clark SJ, Pelletier F, Buettner T, Heilig G. Probabilistic Projections of the Total Fertility Rate for All Countries. Demography. 2011; 48 (3):815–839. [ PMC free article ] [ PubMed ] [ Google Scholar ]
- Barnard J, McCulloch R, Meng X. Modeling Covariance Matrices in Terms of Standard Deviations and Correlations, With Application to Shrinkage. Statistics Sinica. 2000; 10 :1281–1311. [ Google Scholar ]
- Berger JO, Sun D. Objective Priors for the Bivariate Normal Model. Annals of Statistics. 2008; 36 :963–982. [ Google Scholar ]
- Carroll JB. The Nature of the Data, or How to Choose a Correlation Coefficient. Psychometrika. 1961; 26 :347–372. [ Google Scholar ]
- Chib S, Greenberg E. Analysis of Multivariate Probit Models. Biometrika. 1998; 85 :347–361. [ Google Scholar ]
- Farlie DJG. The Performance of Some Correlation Coefficients for a General Bivariate Distribution. Biometrika. 1960; 47 :307–323. [ Google Scholar ]
- Gajjar AV, Subrahmaniam K. On the Sample Correlation Coefficient in the Truncated Bivariate Normal Population. Communications in Statistics - Simulation and Computation. 1978; 7 :455–477. [ Google Scholar ]
- Geisser S. Bayesian Estimation in Multivariate Analysis. The Annals of Mathematical Statistics. 1965; 36 :150–159. [ Google Scholar ]
- Geisser S, Cornfield J. Posterior Distributions for Multivariate Normal Parameters. Journal of the Royal Statistical Society Series B (Methodological) 1963; 25 :368–376. [ Google Scholar ]
- Ghosh M, Mrkherjee B, Santra U, Kim D. Bayesian and Likelihood-based Inference for the Bivariate Normal Correlation Coefficient. Journal of Statistical Planning and Inference. 2010; 140 :1410–1416. [ Google Scholar ]
- Jeffreys H. Some Tests of Significance, Treated by the Theory of Probability. Proceedings of the Cambridge Philosophy Society. 1935; 31 :203–222. [ Google Scholar ]
- Jeffreys H. Theory of Probability. Oxford University Press; 1961. [ Google Scholar ]
- Kass RE, Raftery AE. Bayes Factors. Journal of the American Statistical Association. 1995; 90 :773–795. [ Google Scholar ]
- Kendall SM, Stuart A. The Advanced Theory of Statistics. 4. Vol. 2. MacMillan Publishing Co., Inc; 1979. [ Google Scholar ]
- Liechty JC, Liechty M, Muller P. Bayesian Correlation Estimation. Biometrika. 2004; 91 :1–14. [ Google Scholar ]
- Liu C. Discussion: Bayesian Analysis of Multivariate Probit Model. Journal of Computational and Graphical Statistics. 2001; 10 :75–81. [ Google Scholar ]
- Liu C, Sun DX. Analysis of Interval Censored Data from Fractionated Experiments using Covariance Adjustments. Technometrics. 2000; 42 :353–365. [ Google Scholar ]
- Madansky A. On the Maximum Likelihood Estimate of the Correlation Coefficient. Rand Corporation; Santa Monica, Calif: 1958. Report No. P-1355. [ Google Scholar ]
- McDonald JB. Some Generalized Functions for the Size Distribution of Income. Econometrica. 1984; 52 (3):647–665. [ Google Scholar ]
- Norris RC, Hjelm HF. Nonnormality and Product Moment Correlation. The Journal of Experimental Education. 1961; 29 :261–270. [ Google Scholar ]
- Olkin I, Pratt JW. Unbiased Estimation of Certain Correlation Coefficients. The Annals of Mathematical Statistics. 1958; 29 :201–211. [ Google Scholar ]
- Press SJ, Zellner A. Posterior Distribution for the Multiple Correlation Coefficient with Fixed Regressors. Journal of Econometrics. 1978; 8 :307–321. [ Google Scholar ]
- Rodgers JL, Nicewander WA. Thirteen Ways to Look at the Correlation Coefficient. The American Statistician. 1988; 42 :59–66. [ Google Scholar ]
- Sampson AR. Simple BAN Estimators of Correlations for Certain Multivariate Normal Models with Known Variances. Journal of the American Statistical Association. 1978; 73 :859–862. [ Google Scholar ]
- Spruill NL, Gastwirth JL. On the Estimation of the Correlation Coefficient from Grouped Data. Journal of the American Statistical Association. 1982; 77 :614–620. [ Google Scholar ]
- Tiwari RC, Chib S, Jammalamadaka SR. Bayes Estimation of the Multiple Correlation Coefficient. Communications in Statistics - Theory and Methods. 1989; 18 :1401–1413. [ Google Scholar ]
- Yang R, Berger JO. Posterior Distributions for Multivariate Normal Parameters. Annals of Statistics. 1994; 22 :1195–1211. [ Google Scholar ]
- Zhang X, Boscardin WJ, Belin TR. Sampling Correlation Matrices in Bayesian Models With Correlated Latent Variables. Journal of Computational and Graphical Statistics. 2006; 15 :880–896. [ Google Scholar ]

Want to create or adapt books like this? Learn more about how Pressbooks supports open publishing practices.
Quantitative Data Analysis With SPSS
16 Quantitative Analysis with SPSS: Correlation
Mikaila Mariel Lemonik Arthur
So far in this text, we have only looked at relationships involving at least one discrete variable. But what if we want to explore relationships between two continuous variables? Correlation is a tool that lets us do just that. [1] The way correlation works is detailed in the chapter on Correlation and Regression ; this chapter, then, will focus on how to produce scatterplots (the graphical representations of the data upon which correlation procedures are based); bivariate correlations and correlation matrices (which can look at many variables, but only two at a time); and partial correlations (which enable the analyst to examine a bivariate correlation while controlling for a third variable).
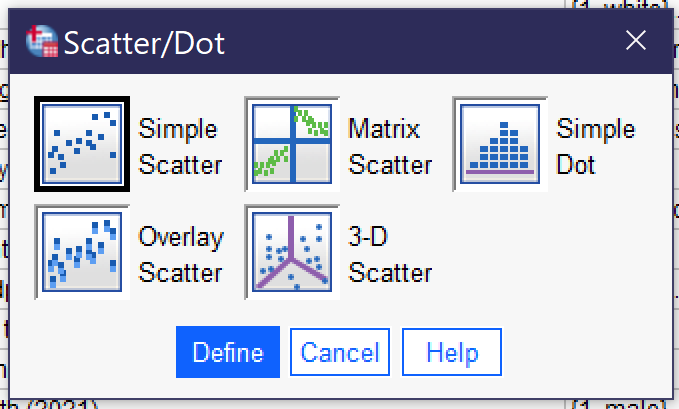
Scatterplots
To produce a scatterplot, go to Graphs → Legacy Dialogs → Scatter/Dot (Alt+G, Alt+L, Alt+S), as shown in Figure 13 in the chapter on Quantitative Analysis with SPSS: Univariate Analysis . Choose “Simple Scatter” for a scatterplot with two variables, as shown in Figure 1.
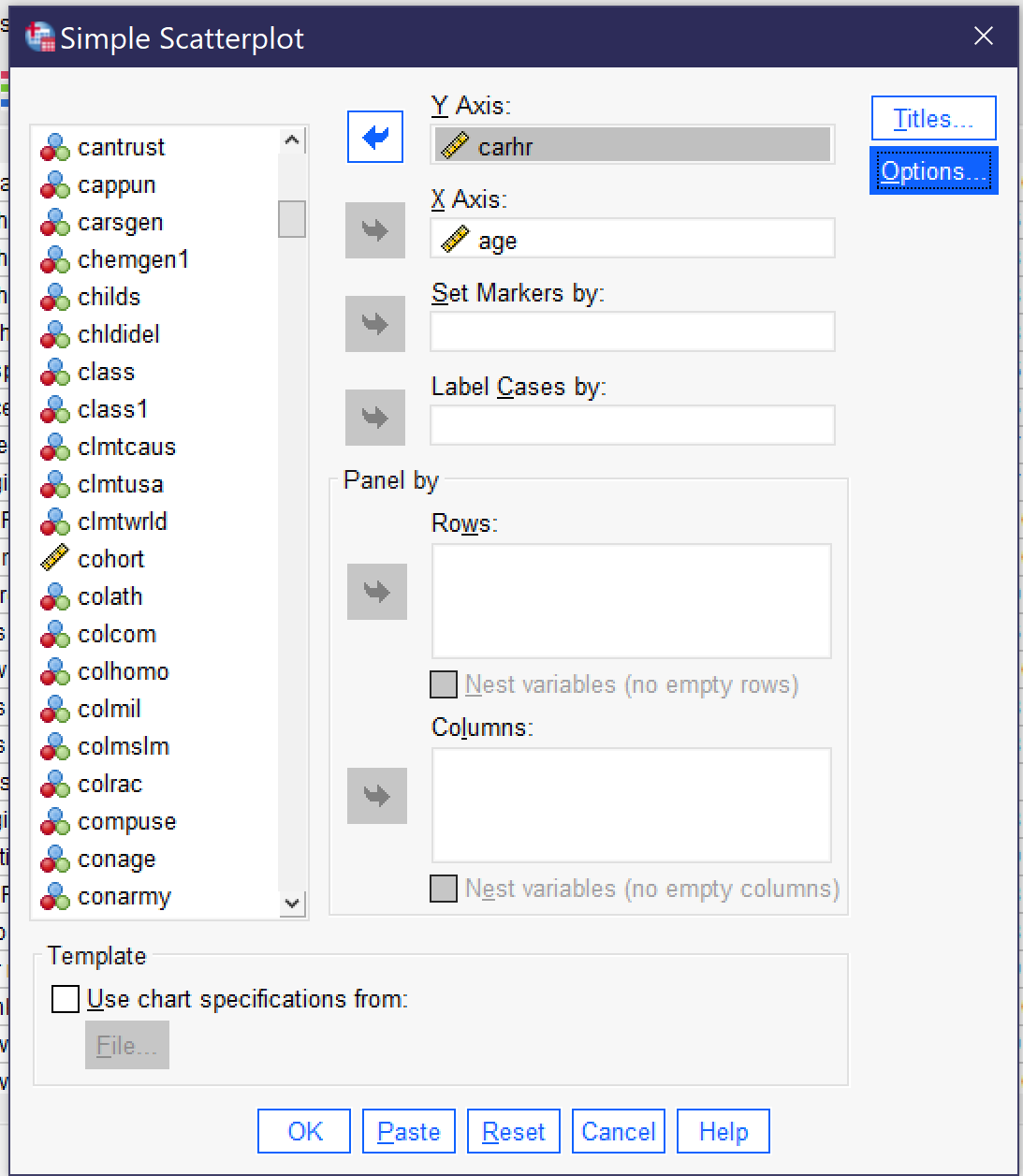
This brings up the dialog for creating a scatterplot, as shown in Figure 2. The independent variable is placed in the X Axis box, as it is a graphing convention to always put the independent variable on the X axis (you can remember this because X comes before Y, therefore X is the independent variable and Y is the dependent variable, and X goes on the X axis while Y goes on the Y axis). Then the dependent variable is placed in the Y Axis box.
There are a variety of other options in the simple scatter dialog, but most are rarely used. In a small dataset, Label Cases by allows you to specify a variable that will be used to label the dots in the scatterplot (for instance, in a database of states you could label the dots with the 2-letter state code).
Once the scatterplot is set up with the independent and dependent variables, click OK to continue. The scatterplot will then appear in the output. In this case, we have used the independent variable AGE and the dependent variable CARHR to look at whether there is a relationship between the respondent’s age and how many hours they spend in a car per week. The resulting scatterplot is shown in Figure 3.
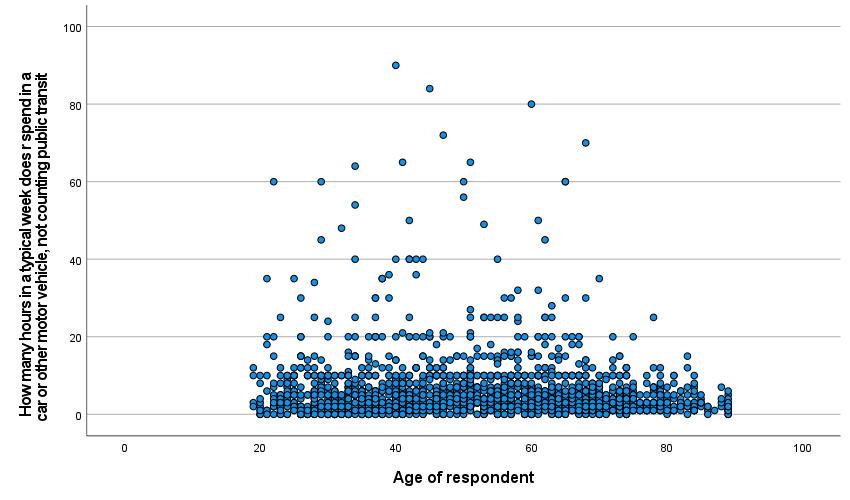
In some scatterplots, it is easy to observe the relationship between the variables. In others, like the one in Figure 3, the pattern of dots is too complex to make it possible to really see the relationship. A tool to help analysts visualize the relationship is the line of best fit , as discussed in the chapter on Correlation and Regression . This line is the line mathematically calculated to be the closest possible to the greatest number of dots. To add the line of best fit, sometimes called the regression line or the fit line, to your scatterplot, go to the scatterplot in the output window and double-click on it. This will open up the Chart Editor window. Then go to Elements → Fit Line at Total, as shown in Figure 4. This will bring up the Properties window. Under the Fit Line tab, be sure the Linear button is selected; click apply if needed and close out.
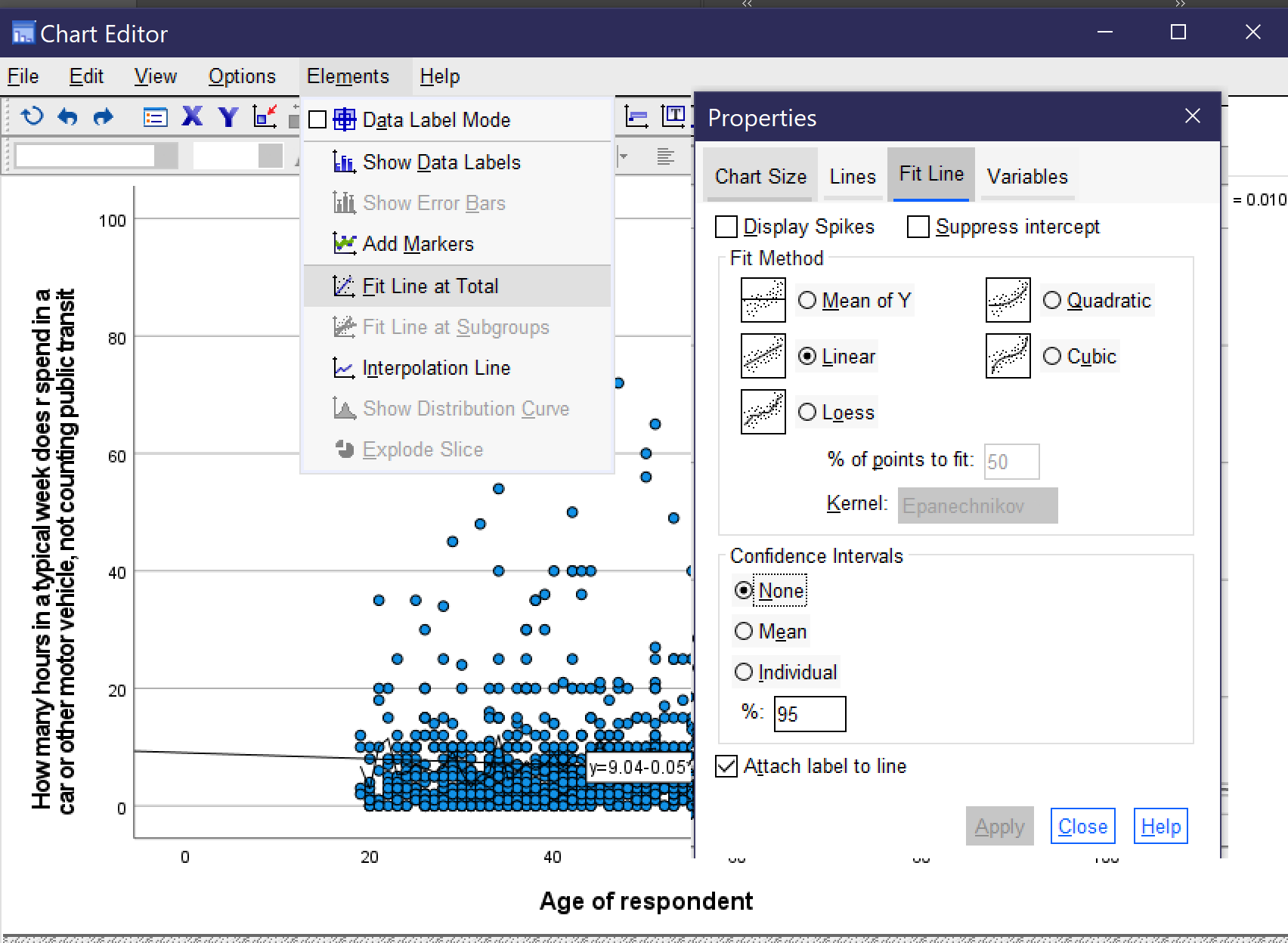
Doing so will add a line with an equation to the scatterplot, as shown in Figure 5. [2] From looking at the line, we can see that age age goes up, time spent in the car per week goes down, but only slightly. The equation confirms this. As shown in the graph, the equation for this line is [latex]y=9.04-0.05x[/latex]. This equation tells us that the line crosses the y axis at 9.04 and that the line goes down 0.05 hours per week in the car for every one year that age goes up (that’s about 3 minutes).
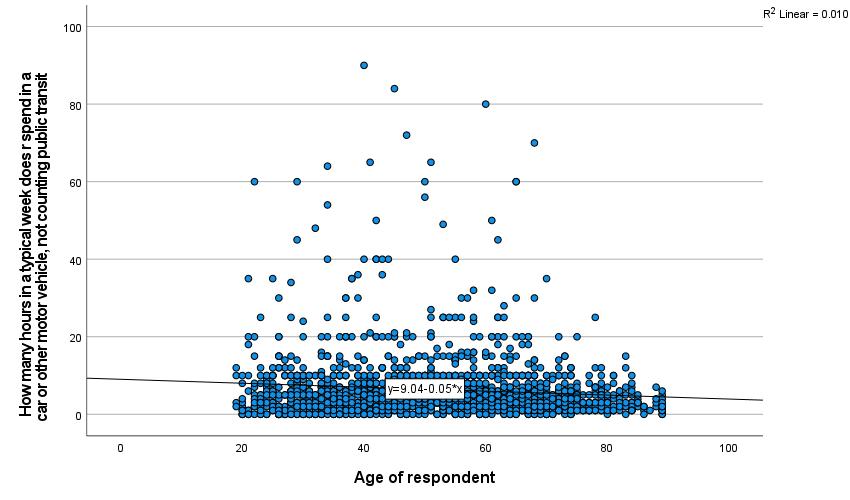
What if we are interested in a whole bunch of different variables? It would take a while to produce scatterplots for each pair of variables. But there is an option for producing them all at once, if smaller and a bit harder to read. This is a scatterplot matrix. To produce a scatterplot matrix, go to Graphs → Legacy Dialogs → Scatter/Dot (Alt+G, Alt+L, Alt+S), as in Figure 1. But this time, choose Matrix from the dialog that appears.
In the Scatterplot Matrix dialog, select all of the variables you are interested in and put them in the Matrix Variables box, and then click OK. The many other options here, as in the case of the simple scatterplot, are rarely used.
The scatterplot matrix will then be produced. As you can see in Figure 7, the scatterplot matrix involves a series of smaller scatterplots, one for each pair of variables specified. Here we specified CARHR and AGE, the two variables we were already using, and added REALINC, the respondent’s family’s income in real (inflation-adjusted) dollars. It is possible, using the same instructions detailed above, to add lines of best fit to the little scatterplots in the scatterplot matrix. Note that each little scatterplot appears twice, once with the variable on the x-axis and once with the variable on the y-axis. You only need to pay attention to one version of each pair of scatterplots.
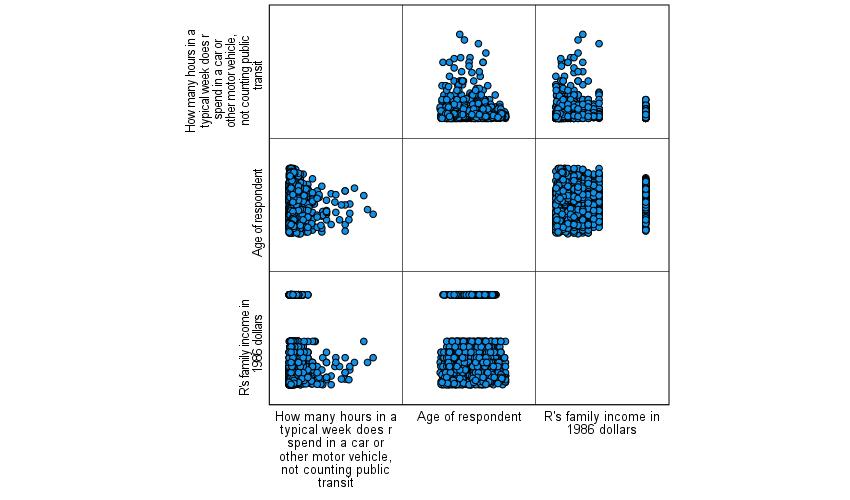
Keep in mind that while you can include discrete variables in a scatterplot, the resulting scatterplot will be very hard to read as most of the dots will just be stacked on top of each other. See Figure 8 for an example of a scatterplot matrix that uses some binary and ordinal variables so you are aware of what to expect in such circumstances. Here, we are looking at the relationships between pairs of the three variables real family income, whether the respondent works for themselves or someone else, and how they would rate their family income from the time that they were 16 in comparison to that of others. As you can see, including discrete variables in a scatterplot produces a series of stripes which are not very useful for analytical purposes.
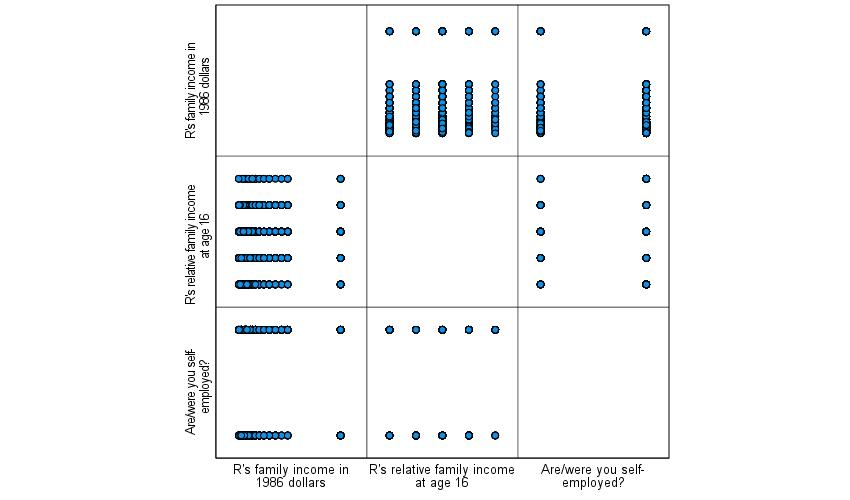
Correlation
Scatterplots can help us visualize the relationships between our variables. But they cannot tell us whether the patterns we observe are statistically significant—or how strong the relationships are. For this, we turn to correlation, as discussed in the chapter on Correlation and Regression . Correlations are bivariate in nature—in other words, each correlation looks at the relationship between two variables. However, like in the case of the scatterplot matrix discussed above, we can produce a correlation matrix with results for a series of pairs of variables all shown in one table.
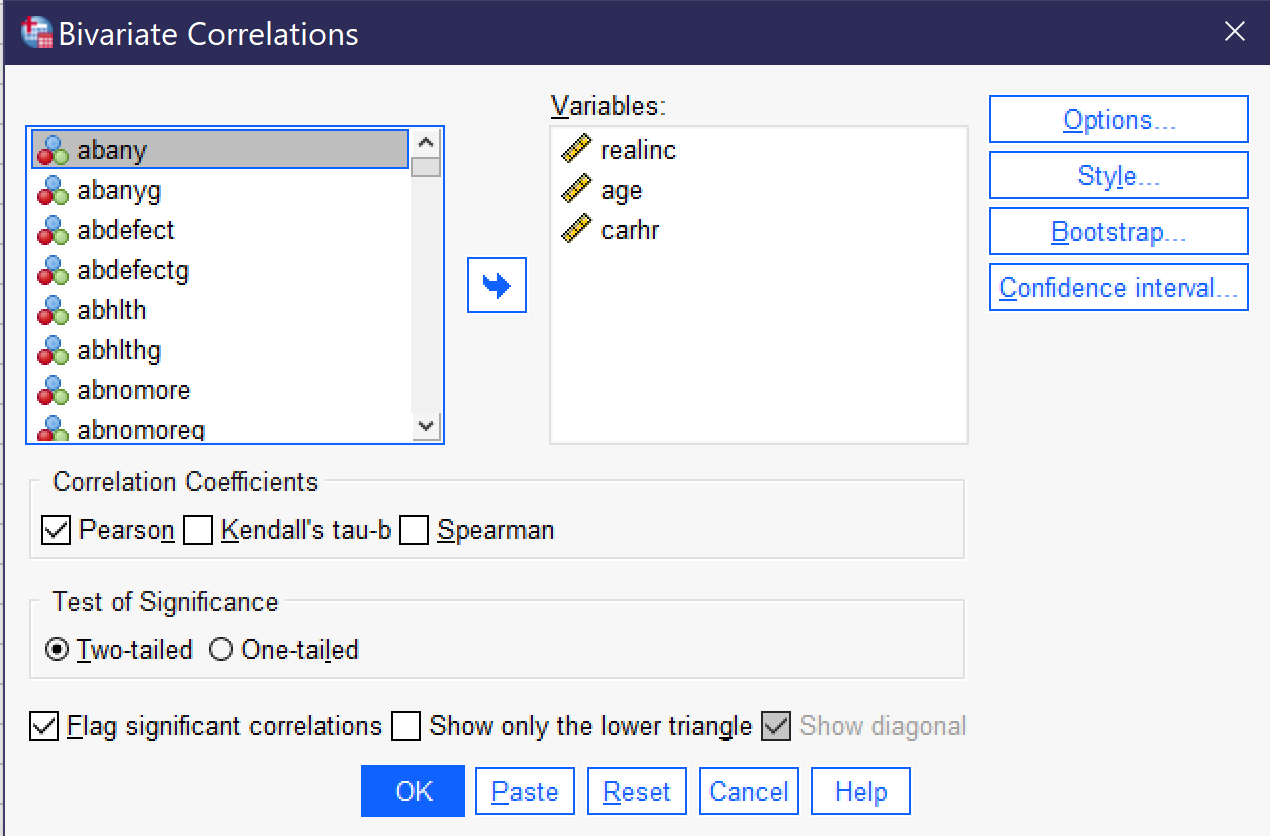
To produce a correlation matrix, go to Analyze → Correlate → Bivariate (Alt+A, Alt+C, Alt+B). Put all of the variables of interest in the Variables box. Be sure Flag significant correlations is checked and select your correlation coefficient. Note that the dialog provides the option of three different correlation coefficients, Pearson, Kendall’s tau-b, and Spearman. The first, Pearson, is used when looking at the relationship between two continuous variables; the other two are used when looking at the relationship between two ordinal variables. [3] In most cases, you will want the two-tailed test of significance. Under options, you can request that means and standard deviations are also produced. When your correlation is set up, as shown in Figure 8, click OK to produce it. The results will be as shown in Table 1 (the order of variables in the table is determined by the order in which they were entered into the bivariate correlation dialog).
R’s family income in 1986 dollars | Age of respondent | How many hours in a typical week does r spend in a car or other motor vehicle, not counting public transit | ||
---|---|---|---|---|
R’s family income in 1986 dollars | Pearson Correlation | 1 | .017 | -.062 |
Sig. (2-tailed) | .314 | .013 | ||
N | 3509 | 3336 | 1613 | |
Age of respondent | Pearson Correlation | .017 | 1 | -.100 |
Sig. (2-tailed) | .314 | <.001 | ||
N | 3336 | 3699 | 1710 | |
How many hours in a typical week does r spend in a car or other motor vehicle, not counting public transit | Pearson Correlation | -.062 | -.100 | 1 |
Sig. (2-tailed) | .013 | <.001 | ||
N | 1613 | 1710 | 1800 | |
*. Correlation is significant at the 0.05 level (2-tailed). | ||||
**. Correlation is significant at the 0.01 level (2-tailed). |
As in the scatterplot matrix above, each correlation appears twice, so you only need to look at half of the table—above or below the diagonal. Note that in the diagonal, you are seeing the correlation of each variable with itself, so a perfect 1 for complete agreement and the number of cases with valid responses on that variable. For each pair of variables, the correlation matrix includes the N, or number of respondents included in the analysis; the Sig. (2-tailed), or the p value of the correlation; and the Pearson Correlation, which is the measure of association in this analysis. It is starred to further indicate the significance level. The direction, indicated by a + or – sign, tells us whether the relationship is direct or inverse. Therefore, for each pair of variables, you can determine the significance, strength, and direction of the relationship. Taking the results in Table 1 one variable pair at a time, we can thus conclude that:
- The relationship between age and family income is not significant. (We could say there is a weak positive association, but since this association is not significant, we often do not comment on it.)
- The relationship between time spent in a car per week and family income is significant at the p<0.05 level. It is a weak negative relationship—in other words, as family income goes up, time spent in a car each week goes down, but only a little bit.
- The relationship between time spent in a car per week and age is significant at the p<0.001 level. It is a moderate negative relationship—in other words, as age goes up, time spent in a car each week goes down.
Partial Correlation
Partial correlation analysis is an analytical procedure designed to allow you to examine the association between two continuous variables while controlling for a third variable. Remember that when we control for a variable, what we are doing is holding that variable constant so we can see what the relationship between our independent and dependent variables would look like without the influence of the third variable on that relationship.
Once you’ve developed a hypothesis about the relationship between the independent, dependent, and control or intervening variable and run appropriate descriptive statistics, the first step in partial correlation analysis is to run a regular bivariate correlation with all of your variables, as shown above, and interpret your results.
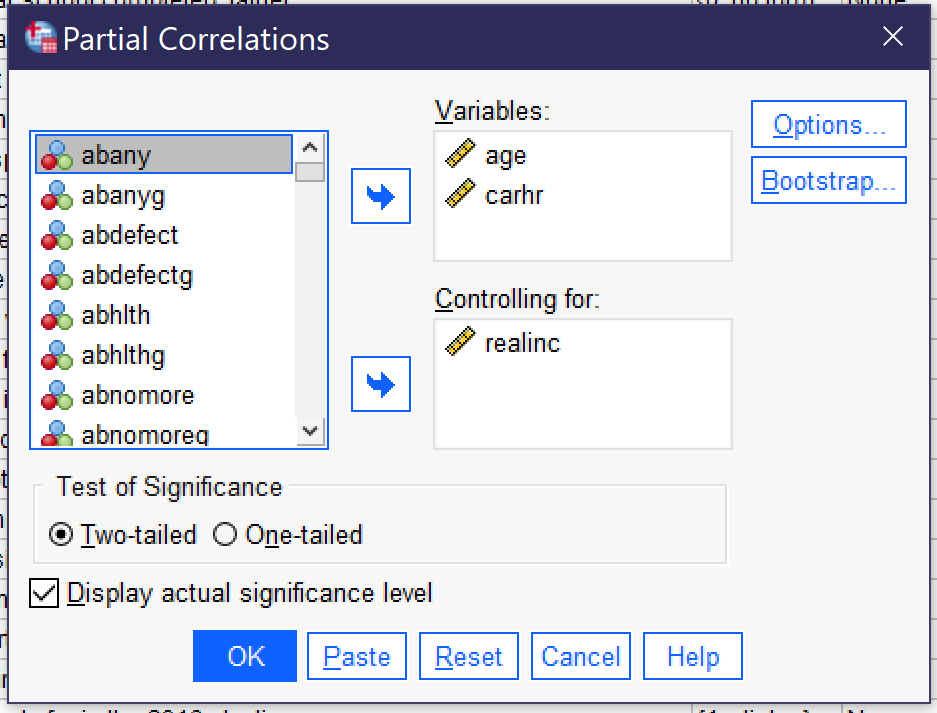
After running and interpreted the results of your bivariate correlation matrix, the next step is to produce the partial correlation by going to Analyze → Correlate → Partial (Alt+A, Alt+C, Alt+R). Place the independent and dependent variables in the Variables box, and the control variable in the Controlling for box, as shown in Figure 9. Note that the partial correlation assumes continuous variables and will only produce the Pearson correlation. The resulting partial correlation Table 2 will look much like the original bivariate correlation, but will show that the third variable has been controlled for, as shown in Table 2. To interpret the results of the partial correlation, begin by looking at the significance and association displayed and interpret them as usual.
Control Variables | Age of respondent | How many hours in a typical week does r spend in a car or other motor vehicle, not counting public transit | ||
---|---|---|---|---|
R’s family income in 1986 dollars | Age of respondent | Correlation | 1.000 | -.106 |
Significance (2-tailed) | . | <.001 | ||
df | 0 | 1547 | ||
How many hours in a typical week does r spend in a car or other motor vehicle, not counting public transit | Correlation | -.106 | 1.000 | |
Significance (2-tailed) | <.001 | . | ||
df | 1547 | 0 |
To interpret the results, we again look at significance, strength, and direction. Here, we find that the relationship is significant at the p<0.001 level and it is a weak negative relationship. As age goes up, time spent in a car each week goes down.
After interpreting the results of the bivariate correlation, compare the value of the measure of association in the correlation to that in the partial correlation to see how they differ. Keep in mind that we ignore the + or – sign when we do this, just considering the actual number (the absolute value). In this case, then, we would be comparing 0.100 from the bivariate correlation to 0.106 from the partial correlation. The number in the partial correlation is just a little bit higher. So what does this mean?
Interpreting Partial Correlation Coefficients
To determine how to interpret the results of your partial correlation, figure out which of the following criteria applies:
- If the correlation between x and y is smaller in the bivariate correlation than in the partial correlation: the third variable is a suppressor variable. This means that when we don’t control for the third variable, the relationship between x and y seems smaller than it really is. So, for example, if I give you an exam with a very strict time limit to see if how much time you spend in class predicts your exam score, the exam time limit might suppress the relationship between class time and exam scores. In other words, if we control for the time limit on the exam, your time in class might better predict your exam score.
- If the correlation between x and y is bigger in the bivariate correlation than in the partial correlation, this means that the third variable is a mediating variable. This is another way of saying that it is an intervening variable —in other words, the relationship between x and y seems larger than it really is because some other variable z intervenes in the relationship between x and y to change the nature of that relationship. So, for example, if we are interested in the relationship between how tall you are and how good you are at basketball, we might find a strong relationship. However, if we added the additional variable of how many hours a week you practice shooting hoops, we might find the relationship between height and basketball skill is much diminished.
- It is additionally possible for the direction of the relationship to change. So, for example, we might find that there is a direct relationship between miles run and marathon performance, but if we add frequency of injuries, then running more miles might reduce your marathon performance.
- If the value of Pearson’s r is the same or very similar in the bivariate and partial correlations, the third variable has little or no effect. In other words, the relationship between x and y is basically the same regardless of whether we consider the influence of the third variable, and thus we can conclude that the third variable does not really matter much and the relationship of interest remains the one between our independent and dependent variables.
Finally, remember that significance still matters ! If neither the bivariate correlation nor the partial correlation is significant, we cannot reject our null hypothesis and thus we cannot conclude that there is anything happening amongst our variables. If both the bivariate correlation and the partial correlation are significant, we can reject the null hypothesis and proceed according to the instructions for interpretation as discussed above. If the original bivariate correlation was not significant but the partial correlation was significant, we cannot reject the null hypothesis in regards to the relationship between our independent and dependent variables alone. However, we can reject the null hypothesis that there is no relationship between the variables as long as we are controlling for the third variable! If the original bivariate correlation was significant but the partial correlation was not significant, we can reject the null hypothesis in regards to the relationship between our independent and dependent variables, but we cannot reject the null hypothesis when considering the role of our third variable. While we can’t be sure what is going on in such a circumstance, the analyst should conduct more analysis to try to see what the relationship between the control variable and the other variables of interest might be.
So, what about our example above? Well, the number in our partial correlation was higher, even if just a little bit, than the number in our bivariate correlation. This means that family income is a suppressor variable. In other words, when we do not control for family income, the relationship between age and time spent in the car seems smaller than it really is. But here is where we find the limits of what the computer can do to help us with our analysis—the computer cannot explain why controlling for income makes the relationship between age and time spent in the car larger. We have to figure that out ourselves. What do you think is going on here?
- Choose two continuous variables of interest. Produce a scatterplot with regression line and describe what you see.
- Choose three continuous variables of interest. Produce a scatterplot matrix for the three variables and describe what you see.
- Using the same three continuous variables, produce a bivariate correlation matrix. Interpret your results, paying attention to statistical significance, direction, and strength.
- Choose one of your three variables to use as a control variable. Write a hypothesis about how controlling for this variable will impact the relationship between the other two variables.
- Produce a partial correlation. Interpret your results, paying attention to statistical significance, direction, and strength.
- Compare the results of your partial correlation to the results from the correlation of those same two variables in Question 3 (when the other variable is not controlled for). How have the results changed? What does that tell you about the impact of the control variable?
Media Attributions
- scatter dot dialog © IBM SPSS is licensed under a All Rights Reserved license
- simple scatter dialog © IBM SPSS is licensed under a All Rights Reserved license
- scatter of carhrs and age © Mikaila Mariel Lemonik Arthur is licensed under a CC BY-NC-ND (Attribution NonCommercial NoDerivatives) license
- scatter fit line © IBM SPSS is licensed under a All Rights Reserved license
- scatter with line © Mikaila Mariel Lemonik Arthur is licensed under a CC BY-NC-ND (Attribution NonCommercial NoDerivatives) license
- scatterplot matrix dialog © IBM SPSS is licensed under a All Rights Reserved license
- matrix scatter © Mikaila Mariel Lemonik Arthur is licensed under a CC BY-NC-ND (Attribution NonCommercial NoDerivatives) license
- scatter binary ordinal © Mikaila Mariel Lemonik Arthur is licensed under a All Rights Reserved license
- bivariate correlation dialog © IBM SPSS is licensed under a All Rights Reserved license
- partial correlation dialog © IBM SPSS is licensed under a All Rights Reserved license
- Note that the bivariate correlation procedures discussed in this chapter can also be used with ordinal variables when appropriate options are selected, as will be detailed below. ↵
- It will also add the R 2 ; see the chapter on Correlation and Regression for more on how to interpret this. ↵
- A detailed explanation of each of these measures of association is found in the chapter An In-Depth Look At Measures of Association . ↵
The line that best minimizes the distance between itself and all of the points in a scatterplot.
A variable hypothesized to intervene in the relationship between an independent and a dependent variable; in other words, a variable that is affected by the independent variable and in turn affects the dependent variable.
Social Data Analysis Copyright © 2021 by Mikaila Mariel Lemonik Arthur is licensed under a Creative Commons Attribution-NonCommercial-ShareAlike 4.0 International License , except where otherwise noted.

Want to create or adapt books like this? Learn more about how Pressbooks supports open publishing practices.
24 15. Bivariate analysis
Chapter outline.
- What is bivariate data analysis? (5 minute read time)
- Chi-square (4 minute read time)
- Correlations (5 minute read time)
- T-tests (5-minute read time)
- ANOVA (6-minute read time)
Content warning: examples include discussions of anxiety symptoms.
So now we get to the math! Just kidding. Mostly. In this chapter, you are going to learn more about bivariate analysis , or analyzing the relationship between two variables. I don’t expect you to finish this chapter and be able to execute everything you just read about – instead, the big goal here is for you to be able to understand what bivariate analysis is, what kinds of analyses are available, and how you can use them in your research.
Take a deep breath, and let’s look at some numbers!
15.1 What is bivariate analysis?
Learning objectives.
Learners will be able to…
- Define bivariate analysis
- Explain when we might use bivariate analysis in social work research
Did you know that ice cream causes shark attacks? It’s true! When ice cream sales go up in the summer, so does the rate of shark attacks. So you’d better put down that ice cream cone, unless you want to make yourself look more delicious to a shark.
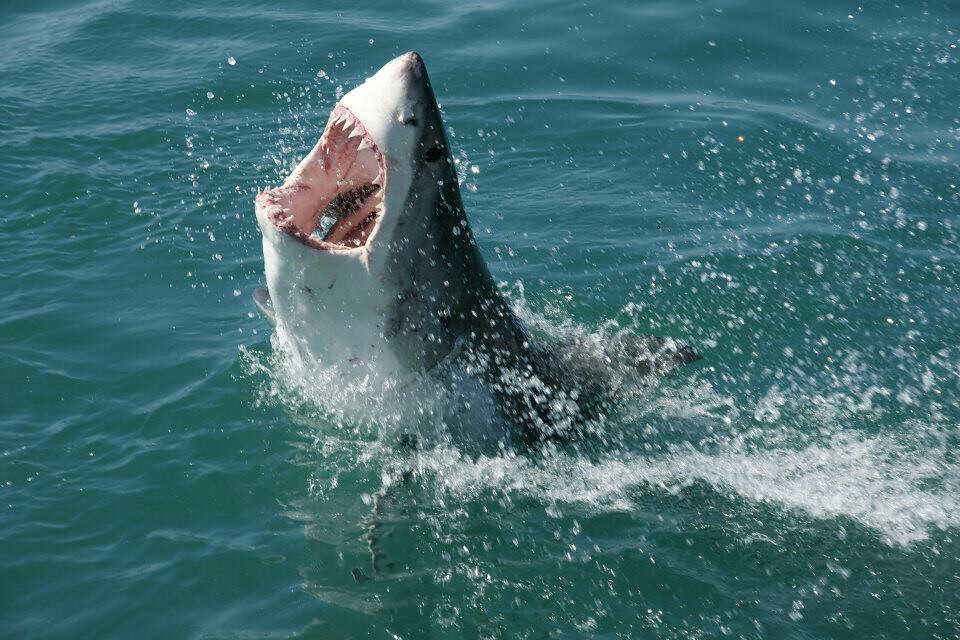
Ok, so it’s quite obviously not true that ice cream causes shark attacks. But if you looked at these two variables and how they’re related, you’d notice that during times of the year with high ice cream sales, there are also the most shark attacks. Despite the fact that the conclusion we drew about the relationship was wrong, it’s nonetheless true that these two variables appear related, and researchers figured that out through the use of bivariate analysis. (For a refresher on correlation versus causation, head back to Chapter 8 .)
Bivariate analysis consists of a group of statistical techniques that examine the relationship between two variables. We could look at how anti-depressant medications and appetite are related, whether there is a relationship between having a pet and emotional well-being, or if a policy-maker’s level of education is related to how they vote on bills related to environmental issues.
Bivariate analysis forms the foundation of multivariate analysis, which we don’t get to in this book. All you really need to know here is that there are steps beyond bivariate analysis, which you’ve undoubtedly seen in scholarly literature already! But before we can move forward with multivariate analysis, we need to understand whether there are any relationships between our variables that are worth testing.
A study from Kwate, Loh, White, and Saldana (2012) illustrates this point. These researchers were interested in whether the lack of retail stores in predominantly Black neighborhoods in New York City could be attributed to the racial differences of those neighborhoods. Their hypothesis was that race had a significant effect on the presence of retail stores in a neighborhood, and that Black neighborhoods experience “retail redlining” – when a retailer decides not to put a store somewhere because the area is predominantly Black.
The researchers needed to know if the predominant race of a neighborhood’s residents was even related to the number of retail stores. With bivariate analysis, they found that “predominantly Black areas faced greater distances to retail outlets; percent Black was positively associated with distance to nearest store for 65 % (13 out of 20) stores” (p. 640). With this information in hand, the researchers moved on to multivariate analysis to complete their research.
Statistical significance
Before we dive into analyses, let’s talk about statistical significance. Statistical significance is the extent to which our statistical analysis has produced a result that is likely to represent a real relationship instead of some random occurrence. But just because a relationship isn’t random doesn’t mean it’s useful for drawing a sound conclusion.
We went into detail about statistical significance in Chapter 5 . You’ll hopefully remember that there, we laid out some key principles from the American Statistical Association for understanding and using p-values in social science:
- P-values can indicate how incompatible the data are with a specified statistical model. P-values can provide evidence against the null hypothesis or the underlying assumptions of the statistical model the researchers used.
- P-values do not measure the probability that the studied hypothesis is true, or the probability that the data were produced by random chance alone. Both are inaccurate, though common, misconceptions about statistical significance.
- Scientific conclusions and business or policy decisions should not be based only on whether a p-value passes a specific threshold. More nuance is needed to interpret scientific findings, as a conclusion does not become true or false when it passes from p=0.051 to p=0.049.
- Proper inference requires full reporting and transparency, rather than cherry-picking promising findings or conducting multiple analyses and only reporting those with significant findings. For the authors of this textbook, we believe the best response to this issue is for researchers make their data openly available to reviewers and general public and register their hypotheses in a public database prior to conducting analyses.
- A p-value, or statistical significance, does not measure the size of an effect or the importance of a result. In our culture, to call something significant is to say it is larger or more important, but any effect, no matter how tiny, can produce a small p-value if the study is rigorous enough. Statistical significance is not equivalent to scientific, human, or economic significance.
- By itself, a p-value does not provide a good measure of evidence regarding a model or hypothesis. For example, a p-value near 0.05 taken by itself offers only weak evidence against the null hypothesis. Likewise, a relatively large p-value does not imply evidence in favor of the null hypothesis; many other hypotheses may be equally or more consistent with the observed data. (adapted from Wasserstein & Lazar, 2016, p. 131-132). [1]
A statistically significant result is not necessarily a strong one. Even a very weak result can be statistically significant if it is based on a large enough sample. The word significant can cause people to interpret these differences as strong and important, to the extent that they might even affect someone’s behavior. As we have seen however, these statistically significant differences are actually quite weak—perhaps even “trivial.” The correlation between ice cream sales and shark attacks is statistically significant, but practically speaking, it’s meaningless.
There is debate about acceptable p- values in some disciplines. In medical sciences, a p- value even smaller than 0.05 is often favored, given the stakes of biomedical research. Some researchers in social sciences and economics argue that a higher p -value of up to 0.10 still constitutes strong evidence. Other researchers think that p -values are entirely overemphasized and that there are better measures of statistical significance. At this point in your research career, it’s probably best to stick with 0.05 because you’re learning a lot at once, but it’s important to know that there is some debate about p- values and that you shouldn’t automatically discount relationships with a p -value of 0.06.
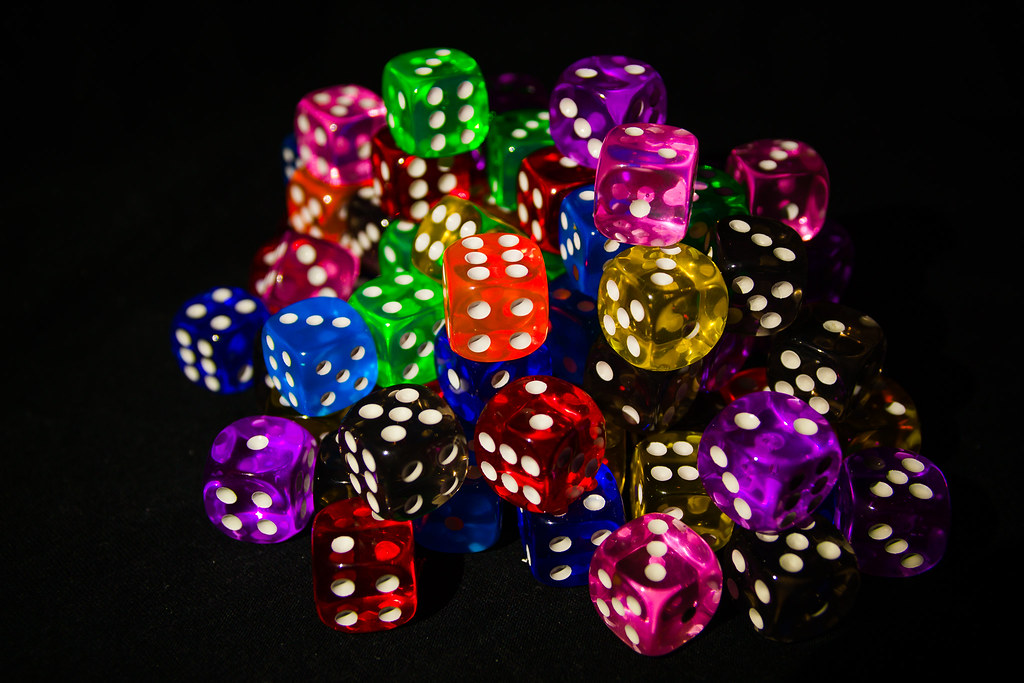
A note about “assumptions”
For certain types of bivariate, and in general for multivariate, analysis, we assume a few things about our data and the way it’s distributed. The characteristics we assume about our data that makes it suitable for certain types of statistical tests are called assumptions . For instance, we assume that our data has a normal distribution. While I’m not going to go into detail about these assumptions because it’s beyond the scope of the book, I want to point out that it is important to check these assumptions before your analysis.
Something else that’s important to note is that going through this chapter, the data analyses presented are merely for illustrative purposes – the necessary assumptions have not been checked. So don’t draw any conclusions based on the results shared.
For this chapter, I’m going to use a data set from IPUMS USA , where you can get individual-level, de-identified U.S. Census and American Community Survey data. The data are clean and the data sets are large, so it can be a good place to get data you can use for practice.
Key Takeaways
- Bivariate analysis is a group of statistical techniques that examine the relationship between two variables.
- You need to conduct bivariate analyses before you can begin to draw conclusions from your data, including in future multivariate analyses.
- Statistical significance and p-values help us understand the extent to which the relationships we see in our analyses are real relationships, and not just random or spurious .
- Find a study from your literature review that uses quantitative analyses. What kind of bivariate analyses did the authors use? You don’t have to understand everything about these analyses yet!
- What do the p -values of their analyses tell you?
15.2 Chi-square
- Explain the uses of Chi-square test for independence
- Explain what kind of variables are appropriate for a Chi-square test
- Interpret results of a Chi-square test and draw a conclusion about a hypothesis from the results
The first test we’re going to introduce you to is known as a Chi-square test (sometimes denoted as χ 2 ) and is foundational to analyzing relationships between nominal or ordinal variables. A Chi-square test for independence (Chi-square for short) is a statistical test to determine whether there is a significant relationship between two nominal or ordinal variables. The “test for independence” refers to the null hypothesis of our comparison – that the two variables are independent and have no relationship.
A Chi-square can only be used for the relationship between two nominal or ordinal variables – there are other tests for relationships between other types of variables that we’ll talk about later in this chapter. For instance, you could use a Chi-square to determine whether there is a significant relationship between a person’s self-reported race and whether they have health insurance through their employer. (We will actually take a look at this a little later.)
Chi-square tests the hypothesis that there is a relationship between two categorical variables by comparing the values we actually observed and the value we would expect to occur based on our null hypothesis. T he expected value is a calculation based on your data when it’s in a summarized form called a contingency table , which is a visual representation of a cross-tabulation of categorical variables to demonstrate all the possible occurrences of your categories. I know that sounds complex, so let’s look at an example.
Earlier, we talked about looking at the relationship between a person’s race and whether they have health insurance through an employer. Based on 2017 American Community Survey data from IPUMS, this is what a contingency table for these two variables would look like.
1,037,071 | 1,401,453 | 2,438,524 | |
177,648 | 177,648 | 317,308 | |
24,123 | 12,142 | 36,265 | |
71,155 | 105,596 | 176,751 | |
75,117 | 46,699 | 121,816 | |
46,107 | 53,269 | 87,384 | |
So now we know what our observed values for these categories are. Next, let’s think about our expected values. We don’t need to get so far into it as to put actual numbers to it, but we can come up with a hypothesis based on some common knowledge about racial differences in employment. (We’re going to be making some generalizations here, so remember that there can be exceptions.)
An applied example
Let’s say research shows that people who identify as black, indigenous, and people of color ( BIPOC ) tend to hold multiple part-time jobs and have a higher unemployment rate in general. Given that, our hypothesis based on this data could be that BIPOC people are less likely to have employer-provided health insurance. Before we can assess a likelihood, we need to know if these to variables are even significantly related. Here’s where our Chi-square test comes in!
I’ve used SPSS to run these tests, so depending on what statistical program you use, your outputs might look a little different.
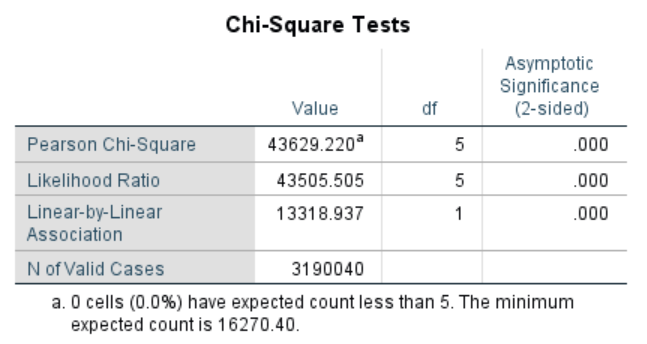
There are a number of different statistics reported here. What I want you to focus on is the first line, the Pearson Chi-Square, which is the most commonly used statistic for larger samples that have more than two categories each. (The other two lines are alternatives to Pearson that SPSS puts out automatically, but they are appropriate for data that is different from ours, so you can ignore them. You can also ignore the “df” column for now, as it’s a little advanced for what’s in this chapter.)
The last column gives us our statistical significance level, which in this case is 0.00. So what conclusion can we draw here? The significant Chi-square statistic means we can reject the null hypothesis (which is that our two variables are not related). There is likely a strong relationship between our two variables that is probably not random, meaning that we should further explore the relationship between a person’s race and whether they have employer-provided health insurance. Are there other factors that affect the relationship between these two variables? That seems likely. (One thing to keep in mind is that this is a large data set, which can inflate statistical significance levels. However, for the purposes of our exercises, we’ll ignore that for now.)
What we cannot conclude is that these two variables are causally related. That is, someone’s race doesn’t cause them to have employer-provided health insurance or not. It just appears to be a contributing factor, but we are not accounting for the effect of other variables on the relationship we observe (yet).
- The Chi-square test is designed to test the null hypothesis that our two variables are not related to each other.
- The Chi-square test is only appropriate for nominal and/or ordinal variables.
- A statistically significant Chi-square statistic means we can reject the null hypothesis and assume our two variables are, in fact, related.
- A Chi-square test doesn’t let us draw any conclusions about causality because it does not account for the influence of other variables on the relationship we observe.
- Which two variables would you most like to use in the analysis?
- What about the relationship between these two variables interests you in light of what your literature review has shown so far?
15.3 Correlations
- Define correlation and understand how to use it in quantitative analysis
- Explain what kind of variables are appropriate for a correlation
- Interpret a correlation coefficient
- Define the different types of correlation – positive and negative
- Interpret results of a correlation and draw a conclusion about a hypothesis from the results
A correlation is a relationship between two variables in which their values change together. For instance, we might expect education and income to be correlated – as a person’s educational attainment (how much schooling they have completed) goes up, so does their income. What about minutes of exercise each week and blood pressure? We would probably expect those who exercise more have lower blood pressures than those who don’t. We can test these relationships using correlation analyses. Correlations are appropriate only for two interval/ratio variables.
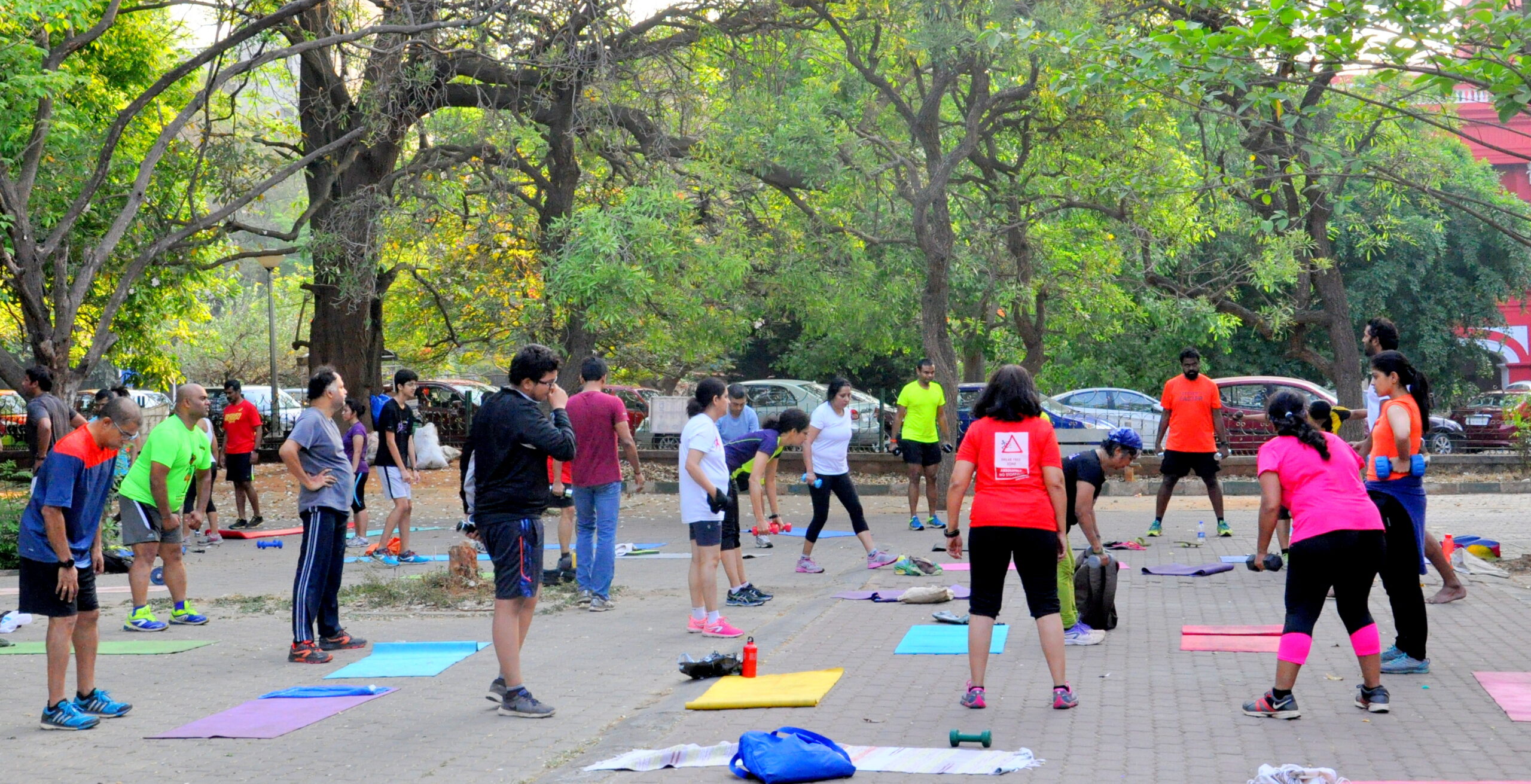
It’s very important to understand that correlations can tell you about relationships, but not causes – as you’ve probably already heard, correlation is not causation! Go back to our example about shark attacks and ice cream sales from the beginning of the chapter. Clearly, ice cream sales don’t cause shark attacks, but the two are strongly correlated (most likely because both increase in the summer for other reasons). This relationship is an example of a spurious relationship , or a relationship that appears to exist between to variables, but in fact does not and is caused by other factors. We hear about these all the time in the news and correlation analyses are often misrepresented. As we talked about in Chapter 4 when discussing critical information literacy, your job as a researcher and informed social worker is to make sure people aren’t misstating what these analyses actually mean, especially when they are being used to harm vulnerable populations.
Let’s say we’re looking at the relationship between age and income among indigenous people in the United States. In the data set we’ve been using so far, these folks generally fall into the racial category of American Indian/Alaska native, so we’ll use that category because it’s the best we can do. Using SPSS, this is the output you’d get with these two variables for this group. We’ll also limit the analysis to people age 18 and over since children are unlikely to report an individual income.
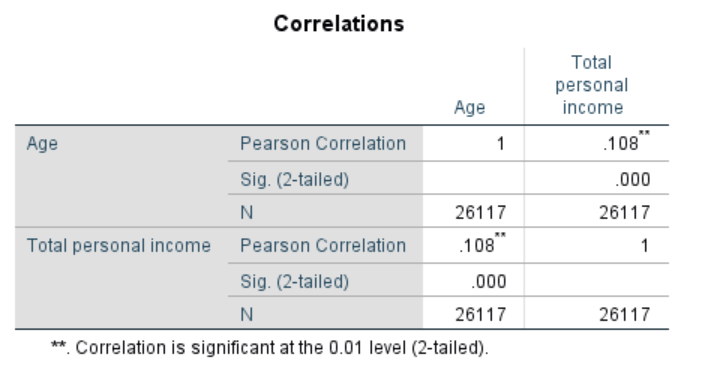
Here’s Pearson again, but don’t be confused – this is not the same test as the Chi-square, it just happens to be named after the same person. First, let’s talk about the number next to Pearson Correlation, which is the correlation coefficient. The c orrelation coefficient is a statistically derived value between -1 and 1 that tells us the magnitude and direction of the relationship between two variables. A statistically significant correlation coefficient like the one in this table (denoted by a p -value of 0.01) means the relationship is not random.
The magnitude of the relationship is how strong the relationship is and can be determined by the absolute value of the coefficient. In the case of our analysis in the table above, the correlation coefficient is 0.108, which denotes a pretty weak relationship. This means that, among the population in our sample, age and income don’t have much of an effect on each other. (If the correlation coefficient were -0.108, the conclusion about its strength would be the same.)
In general, you can say that a correlation coefficient with an absolute value below 0.5 represents a weak correlation. Between 0.5 and 0.75 represents a moderate correlation, and above 0.75 represents a strong correlation. Although the relationship between age and income in our population is statistically significant, it’s also very weak.
The sign on your correlation coefficient tells you the direction of your relationship. A p ositive correlation or direct relationship occurs w hen two variables move together in the same direction – as one increases, so does the other, or, as one decreases, so does the other. Correlation coefficients will be positive, so that means the correlation we calculated is a positive correlation and the two variables have a direct, though very weak, relationship. For instance, in our example about shark attacks and ice cream, the number of both shark attacks and pints of ice cream sold would go up, meaning there is a direct relationship between the two.
A negative correlation or inverse relationship occurs w hen two variables change in opposite directions – one goes up, the other goes down and vice versa. The correlation coefficient will be negative. For example, if you were studying social media use and found that time spent on social media corresponded to lower scores on self-esteem scales, this would represent an inverse relationship.
Correlations are important to run at the outset of your analyses so you can start thinking about how variables relate to each other and whether you might want to include them in future multivariate analyses. For instance, if you’re trying to understand the relationship between receipt of an intervention and a particular outcome, you might want to test whether client characteristics like race or gender are correlated with your outcome; if they are, they should be plugged into subsequent multivariate models. If not, you might want to consider whether to include them in multivariate models.
A final note
Just because the correlation between your dependent variable and your primary independent variable is weak or not statistically significant doesn’t mean you should stop your work. For one thing, disproving your hypothesis is important for knowledge-building. For another, the relationship can change when you consider other variables in multivariate analysis, as they could mediate or moderate the relationships.
- Correlations are a basic measure of the strength of the relationship between two interval/ratio variables.
- A correlation between two variables does not mean one variable causes the other one to change. Drawing conclusions about causality from a simple correlation is likely to lead to you to describing a spurious relationship, or one that exists at face value, but doesn’t hold up when more factors are considered.
- Correlations are a useful starting point for almost all data analysis projects.
- The magnitude of a correlation describes its strength and is indicated by the correlation coefficient, which can range from -1 to 1.
- A positive correlation, or direct relationship, occurs when the values of two variables move together in the same direction.
- A negative correlation, or inverse relationship, occurs when the value of one variable moves one direction, while the value of the other variable moves the opposite direction.
15.4 T-tests
- Describe the three different types of t-tests and when to use them.
- Explain what kind of variables are appropriate for t-tests.
At a very basic level, t-tests compare the means between two groups, the same group at two points in time, or a group and a hypothetical mean. By doing so using this set of statistical analyses, you can learn whether these differences are reflective of a real relationship or not (whether they are statistically significant).
Say you’ve got a data set that includes information about marital status and personal income (which we do!). You want to know if married people have higher personal (not family) incomes than non-married people, and whether the difference is statistically significant. Essentially, you want to see if the difference in average income between these two groups is down to chance or if it warrants further exploration. What analysis would you run to find this information? A t-test!
A lot of social work research focuses on the effect of interventions and programs, so t-tests can be particularly useful. Say you were studying the effect of a smoking cessation hotline on the number of days participants went without smoking a cigarette. You might want to compare the effect for men and women, in which case you’d use an independent samples t-test. If you wanted to compare the effect of your smoking cessation hotline to others in the country and knew the results of those, you would use a one-sample t-test. And if you wanted to compare the average number of cigarettes per day for your participants before they started a tobacco education group and then again when they finished, you’d use a paired-samples t-test. Don’t worry – we’re going into each of these in detail below.
So why are they called t-tests? Basically, when you conduct a t-test, you’re comparing your data to a theoretical distribution of data known as the t distribution to get the t statistic. The t distribution is normal, so when your data are not normally distributed, a t distribution can approximate a normal distribution well enough for you to test some hypotheses. (Remember our discussion of assumptions in section 15.1 – one of them is that data be normally distributed.) Ultimately, the t statistic that the test produces allows you to determine if any differences are statistically significant.
For t-tests, you need to have an interval/ratio dependent variable and a nominal or ordinal independent variable. Basically, you need an average (using an interval or ratio variable) to compare across mutually exclusive groups (using a nominal or ordinal variable).
Let’s jump into the three different types of t- tests.
Paired samples t- test
The paired samples t -test is used to compare two means for the same sample tested at two different times or under two different conditions. This comparison is appropriate for pretest-post-test designs or within-subjects experiments. The null hypothesis is that the means at the two times or under the two conditions are the same in the population. The alternative hypothesis is that they are not the same.
For example, say you are testing the effect of pet ownership on anxiety symptoms. You have access to a group of people who have the same diagnosis involving anxiety who do not have pets, and you give them a standardized anxiety inventory questionnaire. Then, each of these participants gets some kind of pet and after 6 months, you give them the same standardized anxiety questionnaire.
To compare their scores on the questionnaire at the beginning of the study and after 6 months of pet ownership, you would use paired samples t-test. Since the sample includes the same people, the samples are “paired” (hence the name of the test). If the t-statistic is statistically significant, there is evidence that owning a pet has an effect on scores on your anxiety questionnaire.
Independent samples/two samples t-test
An independent/two samples t-test is used to compare the means of two separate samples. The two samples might have been tested under different conditions in a between-subjects experiment, or they could be pre-existing groups in a cross-sectional design (e.g., women and men, extroverts and introverts). The null hypothesis is that the means of the two populations are the same. The alternative hypothesis is that they are not the same.
Let’s go back to our example related to anxiety diagnoses and pet ownership. Say you want to know if people who own pets have different scores on certain elements of your standard anxiety questionnaire than people who don’t own pets.
You have access to two groups of participants: pet owners and non-pet owners. These groups both fit your other study criteria. You give both groups the same questionnaire at one point in time. You are interested in two questions, one about self-worth and one about feelings of loneliness. You can calculate mean scores for the questions you’re interested in and then compare them across two groups. If the t-statistic is statistically significant, then there is evidence of a difference in these scores that may be due to pet ownership.
One-sample t-test
Finally, let’s talk about a one sample t-test. This t-test is appropriate when there is an external benchmark to use for your comparison mean, either known or hypothesized. The null hypothesis for this kind of test is that the mean in your sample is different from the mean of the population. The alternative hypothesis is that the means are different.
Let’s say you know the average years of post-high school education for Black women, and you’re interested in learning whether the Black women in your study are on par with the average. You could use a one-sample t-test to determine how your sample’s average years of post-high school education compares to the known value in the population. This kind of t-test is useful when a phenomenon or intervention has already been studied, or to see how your sample compares to your larger population.
- There are three types of t- tests that are each appropriate for different situations. T-tests can only be used with an interval/ratio dependent variable and a nominal/ordinal independent variable.
- T-tests in general compare the means of one variable between either two points in time or conditions for one group, two different groups, or one group to an external benchmark variable..
- In a paired-samples t-test , you are comparing the means of one variable in your data for the same group , either at two different times or under two different conditions, and testing whether the difference is statistically significant.
- In an independent samples t-test , you are comparing the means of one variable in your data for two different groups to determine if any difference is statistically significant.
- In a one-sample t-test , you are comparing the mean of one variable in your data to an external benchmark, either observed or hypothetical.
- Which t-test makes the most sense for your data and research design? Why?
- Which variable would be an appropriate dependent variable? Why?
- Which variable would be an interesting independent variable? Why?
15.5 ANOVA ( AN alysis O f VA riance)
- Explain what kind of variables are appropriate for ANOVA
- Explain the difference between one-way and two-way ANOVA
- Come up with an example of when each type of ANOVA is appropriate
Analysis of variance , generally abbreviated to ANOVA for short, is a statistical method to examine how a dependent variable changes as the value of a categorical independent variable changes. It serves the same purpose as the t-tests we learned in 15.4: it tests for differences in group means. ANOVA is more flexible in that it can handle any number of groups, unlike t-tests, which are limited to two groups (independent samples) or two time points (dependent samples). Thus, the purpose and interpretation of ANOVA will be the same as it was for t-tests.
There are two types of ANOVA: a one-way ANOVA and a two-way ANOVA. One-way ANOVAs are far more common than two-way ANOVAs.
One-way ANOVA
The most common type of ANOVA that researchers use is the one-way ANOVA , which is a statistical procedure to compare the means of a variable across three or more groups of an independent variable. Let’s take a look at some data about income of different racial and ethnic groups in the United States. The data in Table 15.2 below comes from the US Census Bureau’s 2018 American Community Survey [2] . The racial and ethnic designations in the table reflect what’s reported by the Census Bureau, which is not fully representative of how people identify racially.
American Indian and Alaska Native | $20,709 |
Asian | $40,878 |
Black/African American | $23,303 |
Native Hawaiian or Other Pacific Islander | $25,304 |
White | $36,962 |
Two or more races | $19,162 |
Another race | $20,482 |
Off the bat, of course, we can see a difference in the average income between these groups. Now, we want to know if the difference between average income of these racial and ethnic groups is statistically significant, which is the perfect situation to use one-way ANOVA. To conduct this analysis, we need the person-level data that underlies this table, which I was able to download from IPUMS. For this analysis, race is the independent variable (nominal) and total income is the dependent variable (interval/ratio). Let’s assume for this exercise that we have no other data about the people in our data set besides their race and income. (If we did, we’d probably do another type of analysis.)
I used SPSS to run a one-way ANOVA using this data. With the basic analysis, the first table in the output was the following.
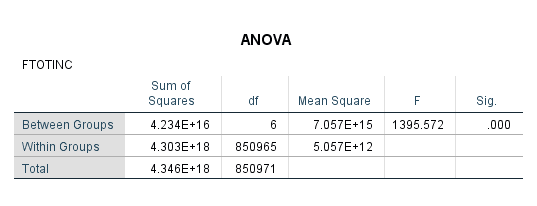
Without going deep into the statistics, the column labeled “F” represents our F statistic, which is similar to the T statistic in a t-test in that it gives a statistical point of comparison for our analysis. The important thing to noticed here, however, is our significance level, which is .000. Sounds great! But we actually get very little information here – all we know is that the between-group differences are statistically significant as a whole, but not anything about the individual groups.
This is where post hoc tests come into the picture. Because we are comparing each race to each other race, that adds up to a lot of comparisons, and statistically, this increases the likelihood of a type I error. A post hoc test in ANOVA is a way to correct and reduce this error after the fact (hence “post hoc”). I’m only going to talk about one type – the Bonferroni correction – because it’s commonly used. However, there are other types of post hoc tests you may encounter.
When I tell SPSS to run the ANOVA with a Bonferroni correction, in addition to the table above, I get a very large table that runs through every single comparison I asked it to make among the groups in my independent variable – in this case, the different races. Figure 15.4 below is the first grouping in that table – they will all give the same conceptual information, though some of the signs on the mean difference and, consequently the confidence intervals, will vary.
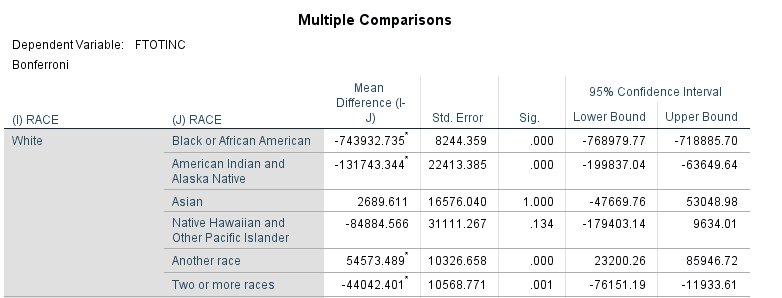
Now we see some points of interest. As you’d expect knowing what we know from prior research, race seems to have a pretty strong influence on a person’s income. (Notice I didn’t say “effect” – we don’t have enough information to establish causality!) The significance levels for the mean of White people’s incomes compared to the mean of several races are .000. Interestingly, for Asian people in the US, race appears to have no influence on their income compared to White people in the US. The significance level for Native Hawaiians and Pacific Islanders is also relatively high.
So what does this mean? We can say with some confidence that, overall, race seems to influence a person’s income. In our hypothetical data set, since we only have race and income, this is a great analysis to conduct. But do we think that’s the only thing that influences a person’s income? Probably not. To look at other factors if we have them, we can use a two-way ANOVA.
Two-way ANOVA and n- way ANOVA
A two-way ANOVA is a statistical procedure to compare the means of a variable across groups using multiple independent variables to distinguish among groups. For instance, we might want to examine income by both race and gender, in which case, we would use a two-way ANOVA. Fundamentally, the procedures and outputs for two-way ANOVA are almost identical to one-way ANOVA, just with more cross-group comparisons, so I am not going to run through an example in SPSS for you.
You may also see textbooks or scholarly articles refer to n- way ANOVAs. Essentially, just like you’ve seen throughout this book, the n can equal just about any number. However, going far beyond a two-way ANOVA increases your likelihood of a type I error, for the reasons discussed in the previous section.
You may notice that this book doesn’t get into multivariate analysis at all. Regression analysis, which you’ve no doubt seen in many academic articles you’ve read, is an incredibly complex topic. There are entire courses and textbooks on the multiple different types of regression analysis, and we did not think we could adequately cover regression analysis at this level. Don’t let that scare you away from learning about it – just understand that we don’t expect you to know about it at this point in your research learning.
- One-way ANOVA is a statistical procedure to compare the means of a variable across three or more categories of an independent variable. This analysis can help you understand whether there are meaningful differences in your sample based on different categories like race, geography, gender, or many others.
- Two-way ANOVA is almost identical to one-way ANOVA, except that you can compare the means of a variable across multiple independent variables.
- Would you want to conduct a two-way or n -way ANOVA? If so, what other independent variables would you use, and why?
- Wasserstein, R. L., & Lazar, N. A. (2016). The ASA statement on p-values: context, process, and purpose. The American Statistician, 70 , p. 129-133. ↵
- Steven Ruggles, Sarah Flood, Ronald Goeken, Josiah Grover, Erin Meyer, Jose Pacas and Matthew Sobek. IPUMS USA: Version 10.0 [dataset]. Minneapolis, MN: IPUMS, 2020. https://doi.org/10.18128/D010.V10.0 ↵
a group of statistical techniques that examines the relationship between two variables
"Assuming that the null hypothesis is true and the study is repeated an infinite number times by drawing random samples from the same populations(s), less than 5% of these results will be more extreme than the current result" (Cassidy et al., 2019, p. 233).
The characteristics we assume about our data, like that it is normally distributed, that makes it suitable for certain types of statistical tests
A relationship where it appears that two variables are related BUT they aren't. Another variable is actually influencing the relationship.
a statistical test to determine whether there is a significant relationship between two categorical variables
variables whose values are organized into mutually exclusive groups but whose numerical values cannot be used in mathematical operations.
a visual representation of across-tabulation of categorical variables to demonstrate all the possible occurrences of categories
a relationship between two variables in which their values change together.
when a relationship between two variables appears to be causal but can in fact be explained by influence of a third variable
Graduate research methods in social work Copyright © 2020 by Matthew DeCarlo, Cory Cummings, Kate Agnelli is licensed under a Creative Commons Attribution-NonCommercial-ShareAlike 4.0 International License , except where otherwise noted.
Share This Book
Bivariate Correlation and Regression
- First Online: 09 November 2016
Cite this chapter
- Abdulkader Aljandali 6
Part of the book series: Statistics and Econometrics for Finance ((SEFF))
4399 Accesses
An essential part of managing any organisation be it governmental, commercial, industrial or social, is planning for the future by generating adequate forecasts of factors that are central to that organisation’s successful operation. Methods of forecasting fall into two groups; qualitative and quantitative. Among the former fall expert judgment and intuitive approaches. Such methods are particularly used by management when conditions in the past are unlikely to hold in the future. This and the next chapter develop the quantitative regression approach to forecasting.
This is a preview of subscription content, log in via an institution to check access.
Access this chapter
Subscribe and save.
- Get 10 units per month
- Download Article/Chapter or eBook
- 1 Unit = 1 Article or 1 Chapter
- Cancel anytime
- Available as PDF
- Read on any device
- Instant download
- Own it forever
- Available as EPUB and PDF
- Compact, lightweight edition
- Dispatched in 3 to 5 business days
- Free shipping worldwide - see info
- Durable hardcover edition
Tax calculation will be finalised at checkout
Purchases are for personal use only
Institutional subscriptions
Author information
Authors and affiliations.
Accounting, Finance and Economics Department, Regent’s University, London, UK
Abdulkader Aljandali
You can also search for this author in PubMed Google Scholar
Rights and permissions
Reprints and permissions
Copyright information
© 2016 Springer International Publishing Switzerland
About this chapter
Aljandali, A. (2016). Bivariate Correlation and Regression. In: Quantitative Analysis and IBM® SPSS® Statistics. Statistics and Econometrics for Finance. Springer, Cham. https://doi.org/10.1007/978-3-319-45528-0_7
Download citation
DOI : https://doi.org/10.1007/978-3-319-45528-0_7
Published : 09 November 2016
Publisher Name : Springer, Cham
Print ISBN : 978-3-319-45527-3
Online ISBN : 978-3-319-45528-0
eBook Packages : Mathematics and Statistics Mathematics and Statistics (R0)
Share this chapter
Anyone you share the following link with will be able to read this content:
Sorry, a shareable link is not currently available for this article.
Provided by the Springer Nature SharedIt content-sharing initiative
- Publish with us
Policies and ethics
- Find a journal
- Track your research

IMAGES
VIDEO
COMMENTS
Let's perform the hypothesis test on the husband's age and wife's age data in which the sample correlation based on n = 170 couples is r = 0.939. To test H 0: ρ = 0 against the alternative H A: ρ ≠ 0, we obtain the following test statistic: t ∗ = r n − 2 1 − R 2 = 0.939 170 − 2 1 − 0.939 2 = 35.39. To obtain the P -value, we need ...
The bivariate Pearson Correlation produces a sample correlation coefficient, r, which measures the strength and direction of linear relationships between pairs of continuous variables.By extension, the Pearson Correlation evaluates whether there is statistical evidence for a linear relationship among the same pairs of variables in the population, represented by a population correlation ...
Bivariate analysis also allows you to test a hypothesis of association and causality. It also helps you to predict the values of a dependent variable based on the changes of an independent variable. ... Let's see bivariate data analysis examples for a negative correlation. Example 3: The below bivariate data table shows the number of student ...
Bivariate analysis means the analysis of bivariate data. It is one of the simplest forms of statistical analysis, used to find out if there is a relationship between two sets of values. It usually involves the variables X and Y. Univariate analysis is the analysis of one ("uni") variable. Bivariate analysis is the analysis of exactly two ...
Bivariate correlation; Pearson product-moment correlation coefficient (PPMCC) ... The data doesn't allow you to reject the null hypothesis and doesn't provide support for the alternative hypothesis. Example: Deciding whether to reject the null hypothesis For the correlation between weight and height in a sample of 10 newborns, ...
A significant result indicates only that the correlation is not 0, it does not indicate the direction of the correlation. The logic behind this hypothesis test is based on the assumption the null hypothesis is true which means there is no correlation in the population. An example is shown in the scatter plot on the left.
Select the bivariate correlation coefficient you need, in this case Pearson's. For the Test of Significance we select the two-tailed test of significance, because we do not have an assumption whether it is a positive or negative correlation between the two variables Reading and Writing.We also leave the default tick mark at flag significant correlations which will add a little asterisk to ...
The p-value is calculated using a t -distribution with n − 2 degrees of freedom. The formula for the test statistic is t = r n−2√ 1−r2√. The value of the test statistic, t, is shown in the computer or calculator output along with the p-value. The test statistic t has the same sign as the correlation coefficient r.
A typical null hypothesis about the population regression slope is that the independent variable (X) has no linear relation with the dependent variable (Y). H 0: YX 0 Its paired research hypothesis is nondirectional (a two-tailed test): H1: YX 0 Other hypothesis pairs are directional (one-tailed tests): H : 0 H : 0 H : 0 or H : 0 1 YX 1 YX
Pearson Correlation Sig. (2-tailed) N Pearson Correlation Sig. (2-tailed) N weight height weight height Correlation is significant at the 0.01 level (2 il d) **. Pearson's Correlation Coefficient Example 1: SPSS Output Conclusion: At significance level of 0.05, we reject null hypothesis and conclude that in the population there is
Example 5: Biology. Biologists often collect bivariate data to understand how two variables are related among plants or animals. For example, a biologist may collect data on total rainfall and total number of plants in different regions: The biologist may then decide to calculate the correlation between the two variables and find it to be 0.926.
5.3 - Inferences for Correlations. Let us consider testing the null hypothesis that there is zero correlation between two variables X j and X k. Mathematically we write this as shown below: H 0: ρ j k = 0 against H a: ρ j k ≠ 0. Recall that the correlation is estimated by sample correlation r j k given in the expression below: r j k = s j k ...
The bivariate analysis allows you to investigate the relationship between two variables. It is useful to determine whether there is a correlation between the variables and, if so, how strong the connection is. For researchers conducting a study, this is incredibly helpful. This analysis verifies or refutes the causality and association hypothesis.
A correlation coefficient offers another way to perform bivariate analysis. The most common type of correlation coefficient is the Pearson Correlation Coefficient, which is a measure of the linear association between two variables. It has a value between -1 and 1 where:-1 indicates a perfectly negative linear correlation between two variables
What does a correlation coefficient tell you? Correlation coefficients summarize data and help you compare results between studies.. Summarizing data. A correlation coefficient is a descriptive statistic.That means that it summarizes sample data without letting you infer anything about the population. A correlation coefficient is a bivariate statistic when it summarizes the relationship ...
Pearson's correlation coefficient, when applied to a population, is commonly represented by the Greek letter ρ (rho) and may be referred to as the population correlation coefficient or the population Pearson correlation coefficient.Given a pair of random variables (,) (for example, Height and Weight), the formula for ρ [10] is [11], = (,) where is the covariance
1 INTRODUCTION. Sir Francis Galton defined the theoretical concept of bivariate correlation in 1885, and a decade later Karl Pearson published the formula for the sample correlation coefficient, also known as Pearson's r (Rodgers and Nicewander, 1988).The sample correlation coefficient is still the most commonly used measure of correlation today as it assumes no knowledge of the means or ...
Once you've developed a hypothesis about the relationship between the independent, dependent, and control or intervening variable and run appropriate descriptive statistics, the first step in partial correlation analysis is to run a regular bivariate correlation with all of your variables, as shown above, and interpret your results. Figure 9.
A bivariate correlation is a measure of whether and how two variables covary linearly, that is, whether the variance of one changes in a linear fashion as the variance of the other changes. ... Examples are Spearman's correlation coefficient, Kendall's tau, Biserial correlation, and Chi-square analysis. Pearson correlation coefficient ...
Analysis of variance, generally abbreviated to ANOVA for short, is a statistical method to examine how a dependent variable changes as the value of a categorical independent variable changes. It serves the same purpose as the t-tests we learned in 15.4: it tests for differences in group means.
The study, published in the journal Annals of Internal Medicine, analyzed Medicare claims data from 2016 to 2019 for over 700,000 patients. They found that the mortality rate for female patients was 8.15 percent when receiving care from female physicians, compared to 8.38 percent when the physician was male.
The finding is consistent with the causal story that the hypothesis represents, and to that extent, it offers support for this story. Nevertheless, there are many reasons why an observed statistically significant relationship might be spurious. The correlation might, for example, reflect the influence of one or more other and uncontrolled ...
Spearman's rank correlation by its very title is a bivariate measure of association when the data comprise ranks, for example, two consumers might rank five competing brands from 1 ("most preferred") to 5 ("least preferred"). Spearman's ρ measures the degree of similarity between the consumers' rank scores.