HIGH SCHOOL
- ACT Tutoring
- SAT Tutoring
- PSAT Tutoring
- ASPIRE Tutoring
- SHSAT Tutoring
- STAAR Tutoring

GRADUATE SCHOOL
- MCAT Tutoring
- GRE Tutoring
- LSAT Tutoring
- GMAT Tutoring
- AIMS Tutoring
- HSPT Tutoring
- ISAT Tutoring
- SSAT Tutoring
Search 50+ Tests
Loading Page
math tutoring
- Elementary Math
- Pre-Calculus
- Trigonometry
science tutoring
Foreign languages.
- Mandarin Chinese
elementary tutoring
- Computer Science
Search 350+ Subjects
- Video Overview
- Tutor Selection Process
- Online Tutoring
- Mobile Tutoring
- Instant Tutoring
- How We Operate
- Our Guarantee
- Impact of Tutoring
- Reviews & Testimonials
- Media Coverage
- About Varsity Tutors
FREE Genetics Practice Tests
All genetics resources, free genetics diagnostic tests, genetics diagnostic test 1, free genetics practice tests, practice tests by concept, genetics practice test, cell division practice test, central dogma of biology practice test, dna mutations, damage, and repair mechanisms practice test, genetic engineering practice test, inheritance patterns, pedigrees, and genetic disorders practice test, laboratory procedures and important historical experiments practice test, mendelian genetics and the hardy-weinberg principle practice test, properties of nucleic acids practice test, regulation of gene expression in prokaryotes and eukaryotes practice test, viral genetics practice test, practice quizzes, genetics problem set 20, genetics problem set 19, genetics problem set 18, genetics problem set 17, genetics problem set 16, genetics problem set 15, genetics problem set 14, genetics problem set 13, genetics problem set 12, genetics problem set 11, genetics problem set 10, genetics problem set 9, genetics problem set 6, genetics problem set 5, genetics problem set 4, genetics problem set 3, genetics problem set 2, genetics problem set 1.

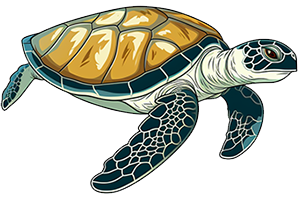
The Biology Corner
Biology Teaching Resources

Resources for Teaching Genetics
Genetics includes the study of heredity, or how traits are passed from parents to offspring. The topics of genetics vary and are constantly changing as we learn more about the genome and how we are influenced by our genes.
Inheritance Patterns and Punnett Squares
Notes and Slides on Mendelian Genetics – basic lesson to introduce genetics and probability
Simple Genetics Practice – using mendelian genetics and Punnett squares
Peas, Please – practice setting up squares for basic Mendelian traits in pea plants ( Key, TpT )
How to Solve Dihybrid Crosses – step by step guide on setting up 4×4 squares and determining ratios
Advanced Bunny Genetic Crosses with two traits – also includes fruit fly genetics Bunny Genetics 2 – basic crosses, rabbits have gray or white hair, black or red eyes
Practice Punnett Squares with Skinny Pigs – hairless guinea pigs are the result of a recessive gene ( Key, TpT )
Horse Genetics – basic practice worksheet on monohybrid and dihybrid crosses, using a gait trait found in horses
Fruit Fly Genetics (Vg) – practice worksheet on vestigial wing flies and eye color, which is sex-linked ( Key, TpT )
Genetics Practice Problems – crosses involving guinea pigs, goats, and pea plants; monohybrid and dihybrid crosses
Explore the Genetics of Corn Snakes – dihybrid crosses with corn snakes, color is polygenic
Blood Disorder Genetics – a worksheet with genetics problems that relate to specific disorders: sickle cell anemia, hemophilia, and Von Willebrand disease.
Heredity Wordsearch – fill in the blank, find the words on a puzzle, basic vocabulary
Genetics Review Guide – focus on vocabulary, Mendel’s crosses, and practice genetics with Punnett squares
Simulations and Hands-on Activities
Penny Genetics – flip a coin to compare actual outcomes versus predicted outcomes from a Punnett square
Heredity Simulation – use popsicle sticks to show how alleles are inherited
Variations on a Human Face – toss a penny to determine the features of a face, such as freckles, dimples; then draw that face.
Paper Pets – another simulation using paper models with traits for eyes, nose, mouth, and hair.
Codominance, Multiple Alleles, and Sex-Linkage
Codominance and Incomplete Dominance – practice genetics on roan cows and pink flowers
Cow Genetics – roan coloration and horns, also includes a two-trait cross
Slides and Notes on Sex-Linked Genes
Fruit Flies and X-Linked Traits – practice crosses that involve sex-linkage; fruit flies and calico cats
Sex Linked Traits – students practice doing crosses; tortoiseshell cats, colorblindness, and eye color in fruit flies
Drosophila (Fruit fly) Simulation – use a simulator to run crosses with flies to determine phenotype ratios and perform chi square analysis
Calico Cats and X Linked Traits – more practice with sex-linked traits, see also advanced version that goes into tortoiseshell vs calico
Why Are There No Male Calico Cats – case study and slide presentation that explore X inactivation and coat color in cats
Epistasis in Labrador Retrievers – explore the black (B_E_) , yellow (B_ee) , and brown (bbE_ ) color patterns in dogs
Sexy Chickens – inheritance patterns in birds, using the ZW sex determination concept
Blood Type Genetics – practice with blood type crosses and other ABO type alleles, multiple allele traits
Multiple Allele Traits in Chickens – shows how combs are inherited (rrpp x RRpp)
Frizzle Frazzled Chickens – incomplete dominance in feather types, with double dominant being unhealthy
How Do Genes Determine Skin Color? – this case study uses presentation slides to explore a real-life scenario where a Nigerian mother has two very fair skinned children.
Design-a-Species – using the rules of inheritance (Mendel), create an organism; with dominant and recessive traits, multiple allele traits, and codominance
Genetics Project – Create a species and show how its traits are influenced by genetics
Genetics Overview for AP Biology – summarizes the various types of crosses students may encounter on the AP test
Genetics and Statistics
Hardy-Weinberg Problem Set – statistical analysis, using HW equation and some dragons
Hardy Weinberg Simulation – track an allele in population by simulating how parents pass alleles to offspring
Corn Genetics and Chi Square – statistical analysis, using preserved corn and counting kernels
Albino Corn Genetics – grow corn, 3:1 albino ratio, lab report analyzes F1, F2 crosses
Chi Square Modeling Using Candy – count the number of each color in a bag to determine if they occur in equal proportions
Drosophila Virtual Lab – choose fruit fly parents and analyze offspring in this virtual lab
Genetics of Wisconsin Fast Plants – grow plants from seeds and analyze phenotype; perform chi square analysis
Human Genetics
Case Study – Cystic Fibrosis Mutations
Cystic Fibrosis and Cell Membrane Transport
SRY not SRY – case study on the sex determining region of the Y chromosomes
Case Study – How Do Genes Determine Skin Color
Analyzing Human Pedigrees
- Explore Inheritance Patterns of Sickle Cell Anemia
- Practice Pedigrees on Human Genetic Disorders
- Analyzing Human Pedigrees for AP Biology (includes sex linked traits)
- Exploring Autosomal Dominant Traits
Human Genetics Overview
Your Genes Your Choices – this is a more involved group assignment where groups read scenarios about genetic testing and ethics involved.
Genetic Science Ethics – survey as a group ethical questions involved genetics (cloning, gene therapy..)
Name the Gene – Explore Genes with BLAST – submit gene sequences to determine the related human trait

An official website of the United States government
The .gov means it's official. Federal government websites often end in .gov or .mil. Before sharing sensitive information, make sure you're on a federal government site.
The site is secure. The https:// ensures that you are connecting to the official website and that any information you provide is encrypted and transmitted securely.
- Publications
- Account settings
- Browse Titles
NCBI Bookshelf. A service of the National Library of Medicine, National Institutes of Health.
WormBook: The Online Review of C. elegans Biology [Internet]. Pasadena (CA): WormBook; 2005-2018.
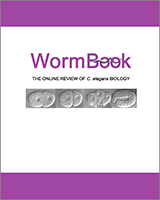
WormBook: The Online Review of C. elegans Biology [Internet].
Complementation *.
Karen Yook .
Affiliations
Created: October 6, 2005 .
Mutations in many genes can result in a similar phenotype. Finding a number of mutants with the same phenotype tells you little about how many genes you are dealing with, and how mutable those genes are until you can assign those mutations to genetic loci. The genetic assay for gene assignment is called the complementation test. The simplicity and robustness of this test makes it a fundamental genetic tool for gene assignment. However, there are occasional unexpected outcomes from this test that bear explanation. This chapter reviews the complementation test and its various outcomes, highlighting relatively rare but nonetheless interesting exceptions such as intragenic complementation and non-allelic non-complementation.
- 1. A simple test for assigning a mutation to a genetic locus
During the complementation test, if a phenotype is observed when a recessive mutation is combined in trans with another recessive mutation that has been mapped to the same area, it is concluded that these mutations are alleles of the same gene; neither allele produces a product that can restore wild-type function ( Figure 1 ). However, if the double heterozygote exhibits a wild-type phenotype, it is concluded that the two mutations are alleles of different genes; each mutant has a functional version of the other gene. This assay requires that the mutation in question is genetically linked to the test mutation and that both mutations are recessive. The usefulness of this test can be most appreciated after large mutagenesis experiments, as demonstrated by Sydney Brenner in his pioneering mutagenesis screen in C. elegans . Through mapping and complementation tests, he was able to place 256 autosomal ethylmethane sulphonate (EMS) induced mutations into 77 complementation groups, making the genetic analysis of C. elegans much easier to approach ( Brenner, 1974 ).
The complementation test. m1 and m2 are fictional alleles representing recessive mutations. Historical Note: The cis - trans test. In a more rigorous application of the complementation test; if the mutations do not complement one another when they are present (more...)
In essence, the complementation test is a very simple test and can quite satisfyingly give you an idea of the number of loci involved in a process after a successful mutagenesis experiment. Having said that, there are four considerations to keep in mind ( Hawley and Walker, 2003 ). First, both mutations must be recessive. Second, the mutations can cause different homozygous phenotypes. Third, the trans-heterozygote can exhibit a more severe phenotype than either homozygote alone. Fourth, a mutation can affect more than one gene product in a complex locus.
- Both mutations must be recessive. The complementation test will not work if either mutation exhibits dominance over the wild-type allele. In this case the phenotype of the dominant mutation will be exhibited whether or not the other allele is a mutation in the same gene; the mutations will appear to not complement. However, semi-dominant mutations can be put into complementation groups if the heterozygous phenotype is noticeably different from the homozygous phenotype (for examples see unc-58 and unc-93 ; Brenner, 1974 ; Greenwald and Horvitz, 1980 ; Park and Horvitz, 1986 ). Assigning a dominant mutation to a locus requires other methods (see Genetic suppression ).
- Mutations in the same gene can cause different homozygous phenotypes. If a gene functions in many processes, then mutations in this gene may impair each function independently. lin-3 is such a pleiotropic gene. lin-3 encodes the C. elegans member of the epidermal growth factor family and is an essential gene that is required in at least three different processes; vulval induction, male spicule development and hermaphrodite fertility ( Ferguson and Horvitz, 1985 ; Hill and Sternberg, 1992 ; Liu et al., 1999 ). Mutations in lin-3 disrupt these functions to different degrees; homozygotes of one allele, e1417 , are Vul whereas homozygotes of another allele, n1058 , exhibit defects in male spicule development, hermaphrodite fertility, viability, and only very weakly, vulval induction ( Figure 2A ). Thus initially, it would appear that e1417 and n1058 are alleles of different genes. To further complicate the issue, these alleles exhibit complex complementation interactions (see Intragenic complementation below).
- The trans-heterozygote can exhibit a phenotype, that is more severe than either homozygote alone. This is also termed "negative complementation". This result contrasts with the expected phenotype of the trans-heterozygote having the phenotype of the weaker allele. Although rare, negative complementation has been noted for the sqt-1 locus. In this case, animals homozygous for sc100 or sc103 are wild-type, but sc101 / sc103 male progeny have a weak Dumpy (Dpy) phenotype. Additionally, when sc100 is put in trans to a deficiency, the trans-heterozygotes have a weak Dpy phenotype ( Kusch and Edgar, 1986 ). In general, mutations in many of the collagen genes result in interesting allelic interactions as discussed below.
- A mutation can affect more than one gene product if the genes are in a complex locus. A classic example of this type of genetic relationship is the cha-1 / unc-17 locus ( Figure 3 ). cha-1 and unc-17 are tightly linked and mutations in cha-1 and unc-17 result in very similar, severe behavioral and developmental defects ( Rand and Russell, 1984 ; Rand, 1989 ). Although most alleles of unc-17 complemented cha-1 , three hypomorphic unc-17 alleles failed to complement cha-1 . Molecular characterization finally demonstrated that unc-17 and cha-1 encode independent mature transcripts produced by alternative splicing ( Alfonso et al., 1993 ; Alfonso et al., 1994 ). The non-complementing mutations fall into two groups, α and β. The one α mutation is a deletion of the non-coding region between cha-1 and unc-17 that removes sequences required for unc-17 RNA processing and most likely for cha-1 expression and/or splicing. The two β mutations have yet to be identified, but they have been mapped to the shared 5' untranslated region of the locus and thus probably disrupt transcriptional regulation of both unc-17 and cha-1 ( Alfonso et al., 1994 ). Thus the non-complementing alleles are actually alleles of both genes.
(A) LIN-3 is required in at least four processes, viability, vulval development, male spicule development and hermaphrodite fertility. lin-3 alleles exhibit a different allelic series for each process, suggesting that each process has a different, independently (more...)
cha-1 and unc-17 form a complex locus. (A) The complementation matrix of alleles of cha-1 and unc-17 . "+" indicates complementation, "—" indicates non-complementation. p1156 , e113 and e876 do not complement alleles of cha-1 or unc-17 (boxed in (more...)
In summary, the complementation test is used to assign mutant alleles to specific genetic loci. Mutant alleles of the same gene fail to complement one another, while alleles of different genes do complement each other. In general, keeping in mind the considerations above, the interpretation of complementation test is quite straightforward. However, we are occasionally faced with complex patterns of complementation.
- 2. When the complementation test is not so simple
There are two ways the complementation test can mislead you. First, alleles of the same gene can sometimes complement each other, termed "intragenic complementation". Second, mutations of two separate genes can sometimes not complement one another, termed "non-allelic non-complementation". In both instances, complex allelic interactions are observed that can lead to problems knowing when and how to assign the mutant alleles to complementation groups. Although these allelic conundrums are found in many organisms, this discussion is focused on examples found in C. elegans .
2.1. Intragenic complementation
During intragenic complementation, alleles of the same gene complement one another, even though both alleles produce a faulty gene product. There are different means by which mutant alleles of the same gene can mutually correct one another. First, one mutant gene product may reduce the dosage of the other mutant product. Second, a faulty complex formed by one mutant gene product may be stabilized by the presence of an alternatively mutant gene product. Third, a gene product containing a mutation that affects one function may provide the function missing from an alternatively altered gene product.
2.1.1. Reducing dosage of a mutant product
Alleles of genes required for cuticle formation exhibit intragenic complementation ( De Melo et al., 2002 ; Kramer et al., 1988 ; Kusch and Edgar, 1986 ). In the case of the cuticle collagen sqt-1 , the recessive missense mutation, sc101 , is alleviated in trans by a null mutation, sc103 ( Table 2 and Table 3 ; Kramer and Johnson, 1993 ). Although both sc101 and sc103 homozygotes have tail defects, and sc101 / sc101 is a long weak left roller, the trans-heterozygotes are wild-type ( Kusch and Edgar, 1986 ; Kramer and Johnson, 1993 ). This result suggests that the presence of the sc101 product has a negative effect on normal collagen associations, since decreasing the amount of the mutant product (e.g. replacing one allele with an allele that produces no product) restores a wild-type phenotype. The poisoning effect of the Gly-X-Y mutations seems to be especially detrimental to collagen proteins and cuticle formation as they are often found in alleles that exhibit non-allelic non-complementation with other collagen genes (see Non-allelic non-complementation).
Mutated domains of the non-allelic, non-complementing SQT gene products.
Complex interactions are observed between collagen genes, sqt-1, 2, 3 and rol-8 .
2.1.2. Stabilizing a complex
In 1964, Crick and Orgel proposed that inter-allelic complementation may be due to a "good corrects bad" mechanism ( Crick and Orgel, 1964 ). In this scenario, a mutation in a subunit of a homomer or multimer would result in the mis-folding of the monomer subunit and disrupt the activity of the whole complex. Likewise, a subunit with a different mutation would also produce a faulty complex. However, a functional complex can be restored if one mutant subunit is able to structurally compensate for the other mutant subunit.
One example of this type of mutual correction may be demonstrated by alleles of let-2 . The initial identification of let-2 occurred in a screen for X-linked lethals and steriles ( Meneely and Herman, 1979 ). In this screen, four alleles of let-2 were identified and were shown to exhibit a complex complementation pattern. In fact, every allele complemented at least one other allele, including two alleles identified independently in other labs. A second screen published a few years later identified another eight alleles, which also exhibited the same complex pattern, giving rise to a complicated web of allelic interactions ( Figure 4A ; Meneely and Herman, 1981 ). These alleles also complement at least one other allele (see Table 4 ; Meneeley and Herman, 1981). The strongest allele, mn153 , is a mutation in an N-terminal splice site and does not complement any other allele except b246 . b246 exhibits the least severe phenotype of all the alleles; it is embryonic lethal only at non-permissive temperatures. Unexpectedly, b246 complements all other let-2 alleles (see Table 4 ). let-2 encodes an α2(IV) collagen chain, which is a major component of basement membrane (see Basement membranes ; Sibley et al., 1993 ). Like other collagen molecules, LET-2 has an extensive Gly-X-Y repeat domains, making up about three-quarters of the molecule, and conserved non-collagenous regions ( Figure 4B ). Type IV collagen molecules are made up of heterotrimers of one α2(IV) chain and 2 α1(IV) chains ( Figure 4C ). These heterotrimers dimerize via their conserved C-terminal NC1 domains and can tetramerize via their C-termini to form a collagen lattice that provides structural support for basement membranes. The strong mutations in the Gly-X-Y regions can disrupt trimer formation and cause the accumulation of misfolded heterotrimers in the cell in a temperature dependent manner ( Gupta et al., 1997 ). The b246 mutation occurs in a region of relatively high thermal stability and is therefore unlikely to have as drastic an effect on triple helical formation as mutations in regions of lower thermal stability (such as the mutations noted in bold in Figure 4B ) in subsequent complex formations ( Sibley et al., 1993 ). The presence of the b246 mutant product in the type IV collagen heterotrimers may help to stabilize the presence of other more severely altered heterotrimers in trans-heterozygotes.
Intragenic complementation of let-2 alleles. (A) let-2 alleles exhibit a very complex pattern of complementation. Lines between alleles indicate non-complementation (the expected outcome). Alleles that are not joined by a line complement one another to (more...)
Complementation tests among let-2 allele*.
2.1.3. Providing the missing function
Many examples of intragenic complementation have been reported for genes that encode products with independently functioning domains, or loci that are involved in independently regulated functions. In these cases, a mutation can result in a gene product that specifically disrupts one process while functioning relatively normally in others. Trans-heterozygotes made with such alleles exhibit intragenic complementation. This appears to be the most frequently reported type of intragenic complementation in C. elegans , perhaps since it results in some very interesting interactions. This type of intragenic complementation has been observed for a number of genes, including lin-3 , gld-1 , unc-5 , unc-84 , and bli-4 .
As mentioned before, lin-3 is required in different developmental processes. lin-3 is also independently mutable, such that a mutation in one part of the gene can disrupt one process while having minimal effects on other LIN-3 requiring processes. For example, the e1417 mutation only disrupts vulval induction, having no effect on male spicule development, while the n1058 mutation only weakly disrupts vulval induction, but strongly disrupts male spicule development ( Liu et al., 1999 ). These homozygous phenotypes suggest that each allele has residual function in certain tissues. Furthermore, the n1058 mutation can complement two other lin-3 alleles, e1417 and n378 , for vulval induction (see Table 1 and Table 3 in Liu et al., 1999 ). The intragenic complementation in this case suggests that residual function from each allele is enough to complement the missing tissue specific function of the other allele, especially since the null mutation, n1059 , does not complement any of these alleles ( Table 1 ). Molecular analysis of the lin-3 mutations supports these genetic observations. Specifically, e1417 has a mutation in an anchor-cell specific enhancer, which is required for LIN-3 function in vulval development, but would not affect LIN-3 function in other tissues ( Hwang and Sternberg, 2004 ; Figure 2B ). Additionally, e378 has a missense mutation resulting in reduction-of-function and n1058 has a splice site mutation, which may cause a truncated LIN-3 product or lowered amount of the LIN-3 product to be made ( Ferguson and Horvitz, 1985 ; Hwang and Sternberg, 2004 ; Liu et al., 1999 ). Neither of these mutations would completely remove LIN-3 function.
Phenotypes of lin-3 homozygous and heteroallelic strains.
Such functional compensation has also been observed for alleles of gld-1 , unc-5 and unc-84 ( Francis et al., 1995 ; Merz et al., 2001 ; Malone et al., 1999 ). For example, gld-1 encodes an RNA binding protein required for several aspects of cell cycle progression during gametogenesis ( Jones and Schedl, 1995 ). GLD-1 is required in a temporally and spatially regulated manner during germline development, and these requirements are reflected in the range of mutant phenotypes exhibited by gld-1 mutants. gld-1 mutations fall into five classes, A-E ( Francis et al., 1995 ). Class C mutations result in the masculinization of the germline so that only sperm and no oocytes are produced. gld-1 class D mutations result in the opposite phenotype of feminization of the germline so that only oocytes and no sperm are produced. Trans-heterozygotes between class C and D alleles produce a wild-type phenotype presumably because gene products carrying the class C mutation can provide GLD-1 function during spermatogenesis while products carrying the class D mutation provide GLD-1 function during oogenesis.
Finally, if a mutation results in the lack of expression of gene product in a subset of cells, a compensating mutation can be one that restores that expression pattern. One example of compensation by expression is illustrated by mutations of bli-4 . bli-4 encodes an essential Kex2/substilin-like protease that cleaves the N-terminus of pre-pro-peptides ( Thacker et al., 1995 ). Two lethal alleles of bli-4 , sy90 and h754 , which do not complement other lethal alleles of bli-4 , were found to complement the viable Blister allele, e937 ( Peters et al., 1991 ; Rose and Baillie, 1980 ). Other lethal alleles exacerbate the Blister phenotype of e937 . bli-4 encodes nine splice variants of the Kex2/substilin-like protease. All variants share the first 12 exons, including the coding region for the protease domain. Mutations in the protease domain affect all nine isoforms and result in embryonic arrest. e937 is a deletion that affects 5 of the 9 isoforms ( Thacker et al., 2000 ). The sy90 and h754 mutations have not been identified, suggesting that these mutations may lie in regulatory elements. In addition, sy90 and h754 homozygous mutants arrest development during L1, which is later than the other lethal mutants suggesting that these mutations alter expression or function of BLI-4 after the embryonic period. Thus in the e937 / sy90 or e937 / h754 trans-heterozygote it appears that enough BLI-4 is supplied by the e937 allele to proceed past L1 development while the sy90 or h754 alleles may provide enough BLI-4 in later stages to abrogate the Blister phenotype.
2.1.4. Other examples
One last example of intragenic complementation, which could occur through stabilizing a complex, or by providing a compensatory function, is exhibited by eat-2 alleles. eat-2 encodes a subunit of the nicotinic acetylcholine gated ion channel required for pharyngeal pumping ( Raizen et al., 1995 ). Nicotinic acetylcholine gated ion channels are hetero-pentamers that are activated by endogenous acetylcholine or drugs such as nicotine. The extracellular ligand binding sites are formed at the interfaces between two different subunits ( Changeux and Edelstein, 1998 ). Alleles of eat-2 fall into five classes, A-E. Although class A alleles do not complement alleles in any class, alleles in class B-E exhibit intragenic complementation ( Figure 5A ). The eat-2 mutations were sequenced and complementing mutations of class B-E were located in the extracellular domain of the subunit ( Figure 5B ; McKay et al., 2004 ). In general, disruptions in extracellular domains would be expected to alter ligand-binding sites. One can imagine then that a channel composed of either faulty subunit would not form any stable ligand binding sites; however, two differently altered subunits might stabilize the channel and the ligand binding sites to allow some increase in channel activity. Alternatively, in an eat-2 trans-heterozygote with two faulty EAT-2 subunits, two different channels might be made with compensatory ligand specificities, thus restoring the functions of the EAT-2 nicotinic acetylcholine receptor.
(A) Intragenic complementation of eat-2 alleles. The number of alleles in each class is noted in parentheses below the class letter along the bottom row. Intragenic complementation results are highlighted in yellow. *These trans-heterozygotes are not (more...)
2.2. Non-allelic non-complementation
Non-allelic non-complementation, also referred to as second-site non-complementation † , intergenic non-complementation, unlinked non-complementation, or second-site dominant enhancer, occurs when alleles of two different loci behave as if they are alleles of the same locus, e.g. the double heterozygote m1/+; +/m2 looks like either homozygote m1 +/m1 + or + m2/+ m2 . Such interactions are not routinely encountered during complementation testing, because such assays are usually done between mutations that have been mapped to the same region and thus have a physical connection on the chromosome. By contrast, this interaction between mutations does not result from their physical location in the genome, but instead reflects a functional connection between different gene products, and often implies that the gene products physically interact. Other experimental approaches may however reveal that this effect is not uncommon. Specifically, screens for new alleles of a gene have the potential to uncover non-allelic non-complementing mutations ( Hays et al., 1989 ). Hawley and Walker extensively review second-site non-complementation giving in depth examples of this event found in Drosophila and other organisms ( Hawley and Walker, 2003 ). The following examples from C. elegans complement their review. Non-allelic non-complementation can be due to two situations: First, one or both mutations act as poisons to protein complexes, thus functional protein complexes become a limiting factor in a process. Second, both mutations reduce a threshold level of gene product, thus the dosage of the individual gene product becomes a limiting factor in a process. These situations, referred to as The Poison and Dosage Models, were put forth by M. Fuller, T. Stearns and D. Botstein and are discussed in more detail below ( Fuller et al., 1989 ; Stearns and Botstein, 1988 ).
2.2.1. Non-allelic non-complementation by poison
In the Poison Model of non-allelic non-complementation, an altered gene product impairs the protein complex with which it normally associates. Although the complex-poisoning effect of this mutation does not result in a visible phenotype on its own in heterozygotes, a simultaneous mutation in another member of the protein complex can reveal a visible defect. Such interactions have been observed between α and β tubulin genes in Drosophila and yeast, where altered a tubulin act as poisons by either sequestering β tubulin or by disrupting the polymerization of the microtubule ( Fuller et al., 1989 ; Hays et al., 1989 ; Stearns and Botstein, 1988 ).
Such poisonous interactions have also been observed in C. elegans ; most notably, Kusch and Edgar observed many instances of unconventional allelic interactions in their study of mutations affecting body shape and morphology ( Kusch and Edgar, 1986 ). Specifically, it was noted that sqt-1 alleles did not complement certain sqt-3 or rol-8 mutations ( Table 3 ). sqt-1 , sqt-3 and rol-8 all encode cuticle collagens ( Cox et al., 1989 ; Kramer et al., 1988 ; Novelli et al., 2004 ; van der Keyl et al., 1994 ). Collagens undergo significant amounts of homo- and heteromeric interactions, propeptide processing and crosslinking that result in permanent associations ( Figure 6B ; Johnstone, 2000 ; Myllyharju and Kivirikko, 2004 ; Page and Winter, 2003 ). Thus, an aberrant collagen monomer can potentially disrupt the cuticle matrix at many stages of cuticle assembly. The prevalence of non-allelic non-complementation among alleles of cuticle collagen genes supports this notion. In addition, mutations among collagen alleles that exhibit non-allelic, non-complementation interactions tend to disrupt a Gly-X-Y repeat domain, which is proposed to be required for monomer associations ( Table 2 , Figure 6 ; Kramer and Johnson, 1993 ; Novelli et al., 2004 ; van der Keyl et al., 1994 ). This suggests that mutations in the Gly-X-Y repeats act as poisons by causing disruptions in trimer formation and by sequestering wild-type monomers in non-functional protein interactions thereby decreasing the amount of functional trimers.
Cuticle collagens have a conserved domain structure. (A) Collagens in vertebrates have been demonstrated to form propeptide homo- or heteromeric helical trimers. Trimer formation is preceded by the association of the cysteine containing domains that (more...)
Non-allelic non-complementation has also been observed among genes required for synaptic vesicle fusion ( Yook et al., 2001 ). In particular, a hypomorphic allele of unc-13 , n2813 , acts as a poison in synaptic transmission. unc-13 ( n2813 ) is a recessive allele that causes a jerky, Unc phenotype due to decreased synaptic transmission. However, n2813 as a trans-heterozygote with mutations in other synaptic function loci exhibits an Unc phenotype even when wild-type copies of both loci are present (E. Jorgensen, pers. comm.). Furthermore, in a sensitive drug assay, n2813 exhibits a poisonous effect on synaptic transmission. UNC-13 is a diacylglycerol binding protein with multiple C2 Ca binding domains that physically interact with UNC-64 /syntaxin to prime vesicles for fusion to the plasma membrane ( Ahmed et al., 1992 ; Maruyama and Brenner, 1991 ; Richmond and Broadie, 2002 ). It was demonstrated that unc-13 ( n2813 ) did not complement a null or hypomorphic allele of unc-64 , although a null allele of unc-13 fully complemented the unc-64 null allele. These results suggest that synaptic transmission is not sensitive to the amount of its individual UNC-13 or UNC-64 protein molecules; however, the number of functional UNC-13 / UNC-64 protein complexes is a limiting factor. It is possible that UNC-13 ( n2813 ) acts as a poison to synaptic transmission by sequestering wild-type UNC-64 into non-functional complexes, thus making the amount of UNC-64 a limiting factor to synaptic transmission, following the Poison Model of non-allelic non-complementation ( Figure 7A ).
The Poison and Dosage Models of vesicle priming at the synapse. (A) A schematic of primed vesicles along the active zone at a wild-type synapse. In The Dosage Model, the unc-13(null)/+ ; unc-64(null)/+ trans-heterozygote would have half the amount of (more...)
These results support the view that non-allelic non-complementation signifies a physical interaction between the mutant gene products; however, in the same study it was demonstrated that this is not always the case. Specifically, unc-13 ( n2813 ) failed to complement mutations in other genes required for synaptic vesicle dynamics ( Figure 7B ). Whereas the greatest degree of non-complementation occurred between mutations in genes whose products are known to have a physical interaction (i.e. unc-13 and unc-64 , unc-13 and unc-18 , unc-64 and snb-1 ), non-complementation was also observed between genes whose products are not known to have a physical interaction (i.e. unc-13 and snb-1 , unc-13 and dpy-23 , unc-13 and unc-104 ). These observations suggest that the unc-13 ( n2813 ) aberrant gene product makes synaptic transmission sensitive to perturbations in other synaptic function loci. In particular, the effects of the UNC-13 poison extends to those loci that affect the concentration of synaptic components at the synapse, such as unc-104 and dpy-23 . However, there is a limit to this interaction as non-complementation was not observed for unc-13 and cha-1 , unc-13 and unc-17 or unc-13 and syd-1 trans-heterozygotes, as cha-1 , unc-17 and syd-1 do not play a direct role in the synaptic vesicle cycle (see references in Yook et al., 2001 ).
2.2.2. Non-allelic non-complementation by dosage (i.e. combined-haplo-insufficiency)
In the Dosage Model, the limiting factor in a process is the total amount of gene product such that a simultaneous decrease in the levels of expression of both genes results in a mutant phenotype. Dosage-sensitive processes have been reported for developmental pathways where events are controlled by protein gradients, such as observed in Drosophila and vertebrates ( Jackson and Berg, 1999 ; Kidd et al., 1999 ; Rancourt et al., 1995 ). Few examples of dosage sensitive processes have been reported in C. elegans ; however, combined-haplo-insufficiency has been reported among ram genes required in male tail ray morphogenesis ( Baird and Emmons, 1990 ). Baird and Emmons demonstrated that null-like mutations in ram-4 do not complement presumed null mutations in any of the other ram loci. Specifically, ram-4 (bx25ts) behaves like the deficiency, mDf9 , in its trans-heterozygous interactions with mutations at other ram loci. Unfortunately, these studies were limited to assaying gene interactions between ram alleles that were not necessarily nulls. Putative null alleles of the ram genes have since been obtained and verified and combined-haplo-insufficiency has been observed for some but not all of the ram loci (K.L. Chow, pers. comm.). Specifically, ram-1 (wx71) , ram-2 ( bx76 ) , and ram-4 (bx48) exhibit non-allelic non-complementation with one another, but not with ram-6(wx66) (K.L. Chow, pers. comm.). Further work has demonstrated that ram-1 , ram-2 / ram-3 and ram-4 encode cuticular collagens (Tam et al., International C. elegans Meeting 2003, 81; Tam et al., in prep.; Yu and Chow, International C. elegans Meeting 2001, 424; Yu et al., in prep.).
2.2.3. Other examples of non-allelic non-complementation
Non-allelic non-complementation has been noted in other processes. In particular, in a screen for suppressors of glp-1 , sog-1 alleles were demonstrated to not complement alleles of five other sog loci for glp-1 suppression ( Maine and Kimble, 1993 ). In addition, in a screen for suppressors of rol-3 lethality, srl-2 ( s2506 ) was demonstrated to not complement mutations in srl-1 ( Barbazuk et al., 1994 ). Unfortunately, molecular information is currently unavailable for these genes and mutations.
More recently, Chang et al. have reported non-allelic non-complementation between genes required for establishing left-right asymmetry of the ASE chemosensory neurons ( Chang et al., 2003 ). These researchers identified a number of lsy ( lim-6 symmetry) mutants that had altered asymmetric expression of ASEL or ASER specific reporter constructs. Mutations in one class were identified as alleles of unc-37 and cog-1 . Mutations in this class exhibited ectopic expression of an ASEL specific reporter, gcy-7 ::gfp , in ASER . These researchers also observed ectopic expression of gcy-7 ::gfp in ASER in an unc-37 ( e262 )/+;+/ cog-1 ( ot28 ) trans-heterozygote whereas there is only ASEL expression in either heterozygote alone. unc-37 encodes the C. elegans ortholog of the Groucho transcription co-factor and cog-1 encodes the ortholog of vertebrate Nkx6 type homeobox genes ( Palmer et al., 2002 ; Pflugrad et al., 1997 ). Biochemical studies have demonstrated that vertebrate COG-1 ortholog interacts with Drosophila Groucho through a conserved engrailed homolog (eh1) domain, thus it is likely that UNC-37 and COG-1 physically interact ( Muhr et al., 2001 ). Other lsy mutants in the same class exhibit non-allelic non-complementation with unc-37 and cog-1 ; however, these have yet to be characterized (Oliver Hobert pers. comm.).
- 3. Conclusion
Complementation occurs when two mutations together result in a wild-type phenotype. Non-complementation occurs when two mutations together result in a mutant phenotype. The complementation test is a simple and fundamental assay in genetics used to assign a mutation to a gene. However, if this test does not give a straightforward answer, valuable inferences about gene function and interaction may still be obtained if the situation is properly understood.
- 4. Acknowledgements
This chapter was completed after valuable feedback from members of the Hodgkin Lab, D. Williams, M. Best, K. L. Chow and an anonymous reviewer, all to whom I am very appreciative. I am indebted to O. Hobert, K. L. Chow and J. Kimble for sharing unpublished data. KJY was supported by a Ruth L. Kirchstein NRSA (F32A1050333) from NIH.
- 5. References
- Ahmed S. , Maruyama I.N. , Kozma R. , Lee J. , Brenner S. , Lim L. The Caenorhabditis elegans unc-13 gene product is a phospholipid-dependent high-affinity phorbol ester receptor. Biochem. J. (1992); 287 (Pt 3):995–999. [ PMC free article : PMC1133105 ] [ PubMed : 1445255 ]
- Alfonso A. , Grundahl K. , Duerr J.S. , Han H.P. , Rand J.B. The Caenorhabditis elegans unc-17 gene: a putative vesicular acetylcholine transporter. Science. (1993); 261 :617–619. [ PubMed : 8342028 ]
- Alfonso A. , Grundahl K. , McManus J.R. , Asbury J.M. , Rand J.B. Alternative splicing leads to two cholinergic proteins in Caenorhabditis elegans . J. Mol. Biol. (1994); 241 :627–630. [ PubMed : 8057385 ]
- Baird S.E. , Emmons S.W. Properties of a class of genes required for ray morphogenesis in Caenorhabditis elegans . Genetics. (1990); 126 :335–344. [ PMC free article : PMC1204188 ] [ PubMed : 2245913 ]
- Barbazuk W.B. , Johnsen R.C. , Baillie D.L. The generation and genetic analysis of suppressors of lethal mutations in the Caenorhabditis elegans rol-3(V) gene. Genetics. (1994); 136 :129–143. [ PMC free article : PMC1205765 ] [ PubMed : 8138151 ]
- Brenner S. The genetics of Caenorhabditis elegans . Genetics. (1974); 77 :71–94. [ PMC free article : PMC1213120 ] [ PubMed : 4366476 ]
- Chang S. , Johnston RJ Jr, Hobert O. A transcriptional regulatory cascade that controls left/right asymmetry in chemosensory neurons of C. elegans . Genes Dev. (2003); 17 :2123–2137. [ PMC free article : PMC196454 ] [ PubMed : 12952888 ]
- Changeux J.P. , Edelstein S.J. Allosteric receptors after 30 years. Neuron. (1998); 21 :959–980. [ PubMed : 9856454 ]
- Cox G.N. , Fields C. , Kramer J.M. , Rosenzweig B. , Hirsh D. Sequence comparisons of developmentally regulated collagen genes of Caenorhabditis elegans . Gene. (1989); 76 :331–344. [ PubMed : 2753356 ]
- Crick F.H. , Orgel L.E. The theory of inter-allelic complementation. J. Mol. Biol. (1964); 8 :161–165. [ PubMed : 14149958 ]
- De Melo J.V. , De Souza W. , Peixoto C.A. Ultrastructural analyses of the Caenorhabditis elegans DR 847 bli-1(n361) mutant which produces abnormal cuticle blisters. J. Submicrosc. Cytol. Pathol. (2002); 34 :291–297. [ PubMed : 12408362 ]
- Ferguson E.L. , Horvitz H.R. Identification and characterization of 22 genes that affect the vulval cell lineages of the nematode Caenorhabditis elegans . Genetics. (1985); 110 :17–72. [ PMC free article : PMC1202554 ] [ PubMed : 3996896 ]
- Francis R. , Barton M.K. , Kimble J. , Schedl T. gld-1 , a tumor suppressor gene required for oocyte development in Caenorhabditis elegans . Genetics. (1995); 139 :579–606. [ PMC free article : PMC1206368 ] [ PubMed : 7713419 ]
- Fuller M.T. , Regan C.L. , Green L.L. , Robertson B. , Deuring R. , Hays T.S. Interacting genes identify interacting proteins involved in microtubule function in Drosophila . Cell Motil Cytoskeleton. (1989); 14 :128–135. [ PubMed : 2684419 ]
- Greenwald I.S. , Horvitz H.R. unc-93(e1500) : a behavioral mutant of Caenorhabditis elegans that defines a gene with a wild-type null phenotype. Genetics. (1980); 96 :147–164. [ PMC free article : PMC1214286 ] [ PubMed : 6894129 ]
- Gupta M.C. , Graham P.L. , Kramer J.M. Characterization of alpha1(IV) collagen mutations in Caenorhabditis elegans and the effects of alpha1 and alpha2(IV) mutations on type IV collagen distribution. J. Cell. Biol. (1997); 137 :1185–1196. [ PMC free article : PMC2136222 ] [ PubMed : 9166417 ]
- Hawley, R.S., and Walker, M.Y. (2003). Advanced genetic analysis: finding meaning in a genome (Malden, Massachusetts: Oxford, Blackwell).
- Hays T.S. , Deuring R. , Robertson B. , Prout M. , Fuller M.T. Interacting proteins identified by genetic interactions: a missense mutation in alpha-tubulin fails to complement alleles of the testis-specific beta-tubulin gene of Drosophila melanogaster . Mol. Cell Biol. (1989); 9 :875–884. [ PMC free article : PMC362676 ] [ PubMed : 2498648 ]
- Hill R.J. , Sternberg P.W. The gene lin-3 encodes an inductive signal for vulval development in C. elegans . Nature. (1992); 358 :470–476. [ PubMed : 1641037 ]
- Hwang B.J. , Sternberg P.W. A cell-specific enhancer that specifies lin-3 expression in the C. elegans anchor cell for vulval development. Development. (2004); 131 :143–151. [ PubMed : 14660442 ]
- Jackson S.M. , Berg C.A. Soma-to-germline interactions during Drosophila oogenesis are influenced by dose-sensitive interactions between cut and the genes cappuccino , ovarian tumor and agnostic . Genetics. (1999); 153 :289–303. [ PMC free article : PMC1460760 ] [ PubMed : 10471713 ]
- Johnstone I.L. Cuticle collagen genes. Expression in Caenorhabditis elegans. Trends Genet. (2000); 16 :21–27. [ PubMed : 10637627 ]
- Jones A.R. , Schedl T. Mutations in gld-1 , a female germ cell-specific tumor suppressor gene in Caenorhabditis elegans , affect a conserved domain also found in Src-associated protein Sam68. Genes Dev. (1995); 9 :1491–1504. [ PubMed : 7601353 ]
- Kidd T. , Bland K.S. , Goodman C.S. Slit is the midline repellent for the robo receptor in Drosophila . Cell. (1999); 96 :785–794. [ PubMed : 10102267 ]
- Killeen M. , Tong J. , Krizus A. , Steven R. , Scott I. , Pawson T. , Culotti J. UNC-5 function requires phosphorylation of cytoplasmic tyrosine 482, but its UNC-40-independent functions also require a region between the ZU-5 and death domains. Dev. Biol. (2002); 251 :348–366. [ PubMed : 12435363 ]
- Kramer J.M. , Johnson J.J. Analysis of mutations in the sqt-1 and rol-6 collagen genes of Caenorhabditis elegans . Genetics. (1993); 135 :1035–1045. [ PMC free article : PMC1205736 ] [ PubMed : 8307321 ]
- Kramer J.M. , Johnson J.J. , Edgar R.S. , Basch C. , Roberts S. The sqt-1 gene of C. elegans encodes a collagen critical for organismal morphogenesis. Cell. (1988); 55 :555–565. [ PubMed : 3180220 ]
- Kusch M. , Edgar R.S. Genetic studies of unusual loci that affect body shape of the nematode Caenorhabditis elegans and may code for cuticle structural proteins. Genetics. (1986); 113 :621–639. [ PMC free article : PMC1202859 ] [ PubMed : 3732788 ]
- Liu J. , Tzou P. , Hill R.J. , Sternberg P.W. Structural requirements for the tissue-specific and tissue-general functions of the Caenorhabditis elegans epidermal growth factor LIN-3. Genetics. (1999); 153 :1257–1269. [ PMC free article : PMC1460807 ] [ PubMed : 10545457 ]
- Maine E.M. , Kimble J. Suppressors of glp-1 , a gene required for cell communication during development in Caenorhabditis elegans , define a set of interacting genes. Genetics. (1993); 135 :1011–1022. [ PMC free article : PMC1205734 ] [ PubMed : 8307319 ]
- Malone C.J. , Fixsen W.D. , Horvitz H.R. , Han M. UNC-84 localizes to the nuclear envelope and is required for nuclear migration and anchoring during C. elegans development. Development. (1999); 126 :3171–3181. [ PubMed : 10375507 ]
- Maruyama I.N. , Brenner S. A phorbol ester/diacylglycerol-binding protein encoded by the unc-13 gene of Caenorhabditis elegans . Proc. Natl. Acad. Sci. USA. (1991); 88 :5729–5733. [ PMC free article : PMC51951 ] [ PubMed : 2062851 ]
- McKay J.P. , Raizen D.M. , Gottschalk A. , Schafer W.R. , Avery L. eat-2 and eat-18 are required for nicotinic neurotransmission in the Caenorhabditis elegans pharynx. Genetics. (2004); 166 :161–169. [ PMC free article : PMC1470703 ] [ PubMed : 15020415 ]
- Meneely P.M. , Herman R.K. Lethals, steriles and deficiencies in a region of the X chromosome of Caenorhabditis elegans . Genetics. (1979); 92 :99–115. [ PMC free article : PMC1213963 ] [ PubMed : 574105 ]
- Meneely P.M. , Herman R.K. Suppression and function of X-linked lethal and sterile mutations in Caenorhabditis elegans . Genetics. (1981); 97 :65–84. [ PMC free article : PMC1214388 ] [ PubMed : 7196363 ]
- Merz D.C. , Zheng H. , Killeen M.T. , Krizus A. , Culotti J.G. Multiple signaling mechanisms of the UNC-6/netrin receptors UNC-5 and UNC-40/DCC in vivo . Genetics. (2001); 158 :1071–1080. [ PMC free article : PMC1461735 ] [ PubMed : 11454756 ]
- Muhr J. , Andersson E. , Persson M. , Jessell T.M. , Ericson J. Groucho-mediated transcriptional repression establishes progenitor cell pattern and neuronal fate in the ventral neural tube. Cell. (2001); 104 :861–873. [ PubMed : 11290324 ]
- Myllyharju J. , Kivirikko K.I. Collagens, modifying enzymes and their mutations in humans, flies and worms. Trends Genet. (2004); 20 :33–43. [ PubMed : 14698617 ]
- Novelli J. , Ahmed S. , Hodgkin J. Gene interactions in Caenorhabditis elegans define DPY-31 as a candidate procollagen C-proteinase and SQT-3/ROL-4 as its predicted major target. Genetics. (2004); 168 :1259–1273. [ PMC free article : PMC1448789 ] [ PubMed : 15579684 ]
- Page A.P. , Winter A.D. Enzymes involved in the biogenesis of the nematode cuticle. Adv. Parasitol. (2003); 53 :85–148. [ PubMed : 14587697 ]
- Palmer R.E. , Inoue T. , Sherwood D.R. , Jiang L.I. , Sternberg P.W. Caenorhabditis elegans cog-1 locus encodes GTX/Nkx6.1 homeodomain proteins and regulates multiple aspects of reproductive system development. Dev. Biol. (2002); 252 :202–213. [ PubMed : 12482710 ]
- Park E.C. , Horvitz H.R. C. elegans unc-105 mutations affect muscle and are suppressed by other mutations that affect muscle. Genetics. (1986); 113 :853–867. [ PMC free article : PMC1202916 ] [ PubMed : 3744029 ]
- Peters K. , McDowall J. , Rose A.M. Mutations in the bli-4 (I) locus of Caenorhabditis elegans disrupt both adult cuticle and early larval development. Genetics. (1991); 129 :95–102. [ PMC free article : PMC1204585 ] [ PubMed : 1936966 ]
- Pflugrad A. , Meir J.Y. , Barnes T.M. , Miller D.M. III. The Groucho-like transcription factor UNC-37 functions with the neural specificity gene unc-4 to govern motor neuron identity in C. elegans . Development. (1997); 124 :1699–1709. [ PubMed : 9165118 ]
- Pontecorvo, G. (1958). Trends in Genetic Analysis. (New York: Columbia University Press).
- Raizen D.M. , Lee R.Y. , Avery L. Interacting genes required for pharyngeal excitation by motor neuron MC in Caenorhabditis elegans . Genetics. (1995); 141 :1365–1382. [ PMC free article : PMC1206873 ] [ PubMed : 8601480 ]
- Rancourt D.E. , Tsuzuki T. , Capecchi M.R. Genetic interaction between hoxb-5 and hoxb-6 is revealed by nonallelic noncomplementation. Genes Dev. (1995); 9 :108–122. [ PubMed : 7828847 ]
- Rand J.B. Genetic analysis of the cha-1-unc-17 gene complex in Caenorhabditis . Genetics. (1989); 122 :73–80. [ PMC free article : PMC1203695 ] [ PubMed : 2731735 ]
- Rand J.B. , Russell R.L. Choline acetyltransferase-deficient mutants of the nematode Caenorhabditis elegans . Genetics. (1984); 106 :227–248. [ PMC free article : PMC1202253 ] [ PubMed : 6698395 ]
- Richmond J.E. , Broadie K.S. The synaptic vesicle cycle: exocytosis and endocytosis in Drosophila and C. elegans . Curr. Opin. Neurobiol. (2002); 12 :499–507. [ PubMed : 12367628 ]
- Rose A.M. , Baillie D.L. Genetic organization of the region around UNC-15 (I), a gene affecting paramyosin in Caenorhabditis elegans . Genetics. (1980); 96 :639–648. [ PMC free article : PMC1214366 ] [ PubMed : 7262541 ]
- Sibley M.H. , Johnson J.J. , Mello C.C. , Kramer J.M. Genetic identification, sequence, and alternative splicing of the Caenorhabditis elegans alpha 2(IV) collagen gene. J. Cell Biol. (1993); 123 :255–264. [ PMC free article : PMC2119826 ] [ PubMed : 7691828 ]
- Stearns T. , Botstein D. Unlinked noncomplementation: isolation of new conditional-lethal mutations in each of the tubulin genes of Saccharomyces cerevisiae . Genetics. (1988); 119 :249–260. [ PMC free article : PMC1203409 ] [ PubMed : 3294100 ]
- Thacker C. , Peters K. , Srayko M. , Rose A.M. The bli-4 locus of Caenorhabditis elegans encodes structurally distinct kex2/subtilisin-like endoproteases essential for early development and adult morphology. Genes Dev. (1995); 9 :956–971. [ PubMed : 7774813 ]
- Thacker C. , Srayko M. , Rose A.M. Mutational analysis of bli-4/kpc-4 reveals critical residues required for proprotein convertase function in C. elegans . Gene. (2000); 252 :15–25. [ PubMed : 10903434 ]
- van der Keyl H. , Kim H. , Espey R. , Oke C.V. , Edwards M.K. Caenorhabditis elegans sqt-3 mutants have mutations in the col-1 collagen gene. Dev. Dyn. (1994); 201 :86–94. [ PubMed : 7803850 ]
- Wood, W.B. (1988). The nematode Caenorhabditis elegans , (Cold Spring Harbor, N.Y.: Cold Spring Harbor Laboratory).
- Yook K.J. , Proulx S.R. , Jorgensen E.M. Rules of nonallelic noncomplementation at the synapse in Caenorhabditis elegans . Genetics. (2001); 158 :209–220. [ PMC free article : PMC1461624 ] [ PubMed : 11333231 ]
Edited by Jonathan Hodgkin and Philip Anderson. Last revised October 05, 2005. Published October 06, 2005. This chapter should be cited as: Yook, K. Complementation (October 06, 2005), WormBook , ed. The C. elegans Research Community, WormBook, doi/10.1895/wormbook.1.24.1, http://www .wormbook.org [ PMC free article : PMC4781631 ] [ PubMed : 18023121 ].
In Drosophila , "second-site non-complementation" or SSNC is the predominant title for this interaction, however to avoid confusion with intragenic mutations that modify allelic mutations as in the case of "second-site suppressors", we have opted to stay with the "nonallelic non-complementation" title.
To whom correspondence should be addressed. E-mail: [email protected]
- Entrez Gene
All WormBook content, except where otherwise noted, is licensed under a Creative Commons Attribution License, which permits unrestricted use, distribution, and reproduction in any medium, provided the original work is properly cited.
- Cite this Page Yook K. Complementation. 2005 Oct 6. In: WormBook: The Online Review of C. elegans Biology [Internet]. Pasadena (CA): WormBook; 2005-2018.
In this Page
Related information.
- PMC PubMed Central citations
- PubMed Links to PubMed
- Gene Locus Links
Similar articles in PubMed
- Genetic analysis of sterile mutants in the dpy-5 unc-13 (I) genomic region of Caenorhabditis elegans. [Mol Gen Genet. 1997] Genetic analysis of sterile mutants in the dpy-5 unc-13 (I) genomic region of Caenorhabditis elegans. McDowall JS, Rose AM. Mol Gen Genet. 1997 Jun; 255(1):60-77.
- Rules of nonallelic noncomplementation at the synapse in Caenorhabditis elegans. [Genetics. 2001] Rules of nonallelic noncomplementation at the synapse in Caenorhabditis elegans. Yook KJ, Proulx SR, Jorgensen EM. Genetics. 2001 May; 158(1):209-20.
- Mutations in the sup-38 gene of Caenorhabditis elegans suppress muscle-attachment defects in unc-52 mutants. [Genetics. 1992] Mutations in the sup-38 gene of Caenorhabditis elegans suppress muscle-attachment defects in unc-52 mutants. Gilchrist EJ, Moerman DG. Genetics. 1992 Oct; 132(2):431-42.
- Review Sometimes the result is not the answer: the truths and the lies that come from using the complementation test. [Genetics. 2006] Review Sometimes the result is not the answer: the truths and the lies that come from using the complementation test. Hawley RS, Gilliland WD. Genetics. 2006 Sep; 174(1):5-15.
- Review Caenorhabditis elegans ubiquinone biosynthesis genes. [Biofactors. 2003] Review Caenorhabditis elegans ubiquinone biosynthesis genes. Rodríguez-Aguilera JC, Asencio C, Ruiz-Ferrer M, Vela J, Navas P. Biofactors. 2003; 18(1-4):237-44.
Recent Activity
- Complementation - WormBook Complementation - WormBook
Your browsing activity is empty.
Activity recording is turned off.
Turn recording back on
Connect with NLM
National Library of Medicine 8600 Rockville Pike Bethesda, MD 20894
Web Policies FOIA HHS Vulnerability Disclosure
Help Accessibility Careers
Assignment tests (Go to assignment test web calculator [old, unmaintained version])
The idea behind assignment tests is to use individual genotypes to assign individuals to populations or clusters. Paetkau et al. (1995) developed the first assignment test approach for use on bears. The idea was fairly simple. Given a set of populations, and the allele frequencies of those populations, what is the likelihood of a given individualÔøΩs genotype in the population in which it was sampled versus its likelihood in the other populations in the set? An individual is assigned to the population for which it has the highest likelihood.
LetÔøΩs take a simple example with four alleles at one locus ( a, b, c , and d ) and three alleles at a second locus ( k, l, and m ):
Now, say we have an individual with the genotype abll . Its probability in Pop 1 is (2*0.2*0.1)*0.3 2 = 0.0036. Its probability in Pop 2 is (2*0.5*0.3)*0.6 2 = 0.108. The likelihood in Pop 2 is considerably higher than the likelihood in Pop 1. We ÔøΩassignÔøΩ it to Pop 2. Conversely, an individual with the genotype cdkl would be much more likely in Pop 1 (0.072) than in Pop 2 (0.0024). [ We need to calculate heterozygote probabilities as 2 times the product of the gene frequencies; remember the Punnett square idea that it could get A from Dad and a from Mom OR a from Dad and A from Mom ]. With a large number of loci, and the presence of rare alleles, we use logarithms to make the numbers easier to assess.
Fig. 1. Assignment graph for individuals sampled in two populations. Individuals in red were sampled in Pop 1, those in blue in Pop 2. Above the line are individuals ÔøΩassignedÔøΩ to Pop 2, below the line individuals assigned to Pop 1. Note that, for this example, one Pop1 individual is assigned to Pop 2, and one Pop 2 individual is assigned to Pop 1. Such ÔøΩmisassignedÔøΩ individuals might represent immigrants from the other population, or the descendants of such immigrants (or it could occur on the wrong side of the line by chance; the further from the line, the less likely the deviation occurs by chance; the more highly polymorphic loci we have, the stronger the evidence).
The problem of zeros . If an allele does not occur in a population ( p x = 0) then the assignment probability of an individual to that population will be 0. Several methods (described on the now-unsupported assignment calculator web site http://www2.biology.ualberta.ca/jbrzusto/Doh.php ) exist for adjusting the allele frequencies in the event of zeros.
Several alternatives now exist for assignment tests. One of these is to use Bayesian methods for deciding on the likelihood of assignment. J.-M. Cornuet has a package of such approaches at his web site
http://www.montpellier.inra.fr/URLB/geneclass/geneclass.html
The program runs in Windows, and has a range of options for dealing with the zero problem.
A variant on the assignment approach is to allow the data themselves to determine the population has subpopulations and, if so, how many. The program Structure uses Hardy-Weinberg equilibrium (HWE) assumptions to create clusters. That is, it sequentially assesses the fit of portions of the data to a set of k clusters, with each cluster maximally obeying HWE structure. Because an algorithm/equation underlies the expectation, maximum likelihood estimation (MLE) is suitable for the decisions about the k clusters.
The program Structurama by Huelsenbeck (http://www.structurama.org/), also calculates the algorithms of Pritchard (2000) with a few extra twists.

Fig. 2. Assignment graphic for a three cluster ( k = 3) assessment of Burrowing Owl populations, using the program Structure . Each of the three vertices represents exclusive assignment to one of the three clusters; points more toward the center have affinities with all three clusters. The square symbols are Florida owls (note that their centroid is very close to the Florida vertex), the triangles are CA populations (centroid basically right in the middle of the line between CA and MW, meaning we really can't tell much about owls sampled in California -- they could just as well belong to the MW cluster) and the stars are Rocky Mt. region individuals. Many of the California and Rocky Mt. region individuals were ÔøΩmisassignedÔøΩ.
References:
Paetkau, D, W. Calvert, I. Stirling, and C. Strobeck. 1995. Microsatellite analysis of population structure in Canadian polar bears. Mol. Ecol. 4: 347-354.
Pritchard, J.K., M. Stephens, and P. Donnelly. 2000. Inference of population structure using multilocus genotype data. Genetics 155: 945-959.
If you're seeing this message, it means we're having trouble loading external resources on our website.
If you're behind a web filter, please make sure that the domains *.kastatic.org and *.kasandbox.org are unblocked.
To log in and use all the features of Khan Academy, please enable JavaScript in your browser.
AP®︎/College Biology
Course: ap®︎/college biology > unit 5.
- Introduction to heredity
- Fertilization terminology: gametes, zygotes, haploid, diploid
- Alleles and genes
- Worked example: Punnett squares
- Mendel and his peas
- The law of segregation
- The law of independent assortment
- Probabilities in genetics
Mendelian genetics
- (Choice A) A
- (Choice B) B
- (Choice C) C
- (Choice D) D
- Patient Care & Health Information
- Tests & Procedures
- Genetic testing
Genetic testing involves examining your DNA, the chemical database that carries instructions for your body's functions. Genetic testing can reveal changes (mutations) in your genes that may cause illness or disease.
Although genetic testing can provide important information for diagnosing, treating and preventing illness, there are limitations. For example, if you're a healthy person, a positive result from genetic testing doesn't always mean you will develop a disease. On the other hand, in some situations, a negative result doesn't guarantee that you won't have a certain disorder.
Talking to your doctor, a medical geneticist or a genetic counselor about what you will do with the results is an important step in the process of genetic testing.
Genome sequencing
When genetic testing doesn't lead to a diagnosis but a genetic cause is still suspected, some facilities offer genome sequencing — a process for analyzing a sample of DNA taken from your blood.
Everyone has a unique genome, made up of the DNA in all of a person's genes. This complex testing can help identify genetic variants that may relate to your health. This testing is usually limited to just looking at the protein-encoding parts of DNA called the exome.
Products & Services
- A Book: Mayo Clinic Family Health Book, 5th Edition
- A Book: Mayo Clinic Guide to a Healthy Pregnancy
- Newsletter: Mayo Clinic Health Letter — Digital Edition
Why it's done
Genetic testing plays a vital role in determining the risk of developing certain diseases as well as screening and sometimes medical treatment. Different types of genetic testing are done for different reasons:
- Diagnostic testing. If you have symptoms of a disease that may be caused by genetic changes, sometimes called mutated genes, genetic testing can reveal if you have the suspected disorder. For example, genetic testing may be used to confirm a diagnosis of cystic fibrosis or Huntington's disease.
- Presymptomatic and predictive testing. If you have a family history of a genetic condition, getting genetic testing before you have symptoms may show if you're at risk of developing that condition. For example, this type of test may be useful for identifying your risk of certain types of colorectal cancer.
- Carrier testing. If you have a family history of a genetic disorder — such as sickle cell anemia or cystic fibrosis — or you're in an ethnic group that has a high risk of a specific genetic disorder, you may choose to have genetic testing before having children. An expanded carrier screening test can detect genes associated with a wide variety of genetic diseases and mutations and can identify if you and your partner are carriers for the same conditions.
- Pharmacogenetics. If you have a particular health condition or disease, this type of genetic testing may help determine what medication and dosage will be most effective and beneficial for you.
- Prenatal testing. If you're pregnant, tests can detect some types of abnormalities in your baby's genes. Down syndrome and trisomy 18 syndrome are two genetic disorders that are often screened for as part of prenatal genetic testing. Traditionally this is done looking at markers in blood or by invasive testing such as amniocentesis. Newer testing called cell-free DNA testing looks at a baby's DNA via a blood test done on the mother.
- Newborn screening. This is the most common type of genetic testing. In the United States, all states require that newborns be tested for certain genetic and metabolic abnormalities that cause specific conditions. This type of genetic testing is important because if results show there's a disorder such as congenital hypothyroidism, sickle cell disease or phenylketonuria (PKU), care and treatment can begin right away.
- Preimplantation testing. Also called preimplantation genetic diagnosis, this test may be used when you attempt to conceive a child through in vitro fertilization. The embryos are screened for genetic abnormalities. Embryos without abnormalities are implanted in the uterus in hopes of achieving pregnancy
More Information
Genetic testing care at Mayo Clinic
- Ambiguous genitalia
- Angelman syndrome
- Brugada syndrome
- Cavernous malformations
- Cerebral palsy
- Charcot-Marie-Tooth disease
- Choroid plexus carcinoma
- Colon polyps
- Congenital adrenal hyperplasia
- Craniosynostosis
- Cystic fibrosis
- Diabetes insipidus
- DiGeorge syndrome (22q11.2 deletion syndrome)
- Dilated cardiomyopathy
- Down syndrome
- Ehlers-Danlos syndrome
- Epidermolysis bullosa
- Factor V Leiden
- Gaucher disease
- Hemochromatosis
- Huntington's disease
- Hypertrophic cardiomyopathy
- Infertility
- Klinefelter syndrome
- Long QT syndrome
- Low sperm count
- Lynch syndrome
- Male infertility
- Marfan syndrome
- Muscular dystrophy
- Myelofibrosis
- Neurofibromatosis
- Noonan syndrome
- Paraganglioma
- Phenylketonuria (PKU)
- Pheochromocytoma
- Polycythemia vera
- Prader-Willi syndrome
- Pulmonary hypertension
- Rett syndrome
- Thalassemia
- Thoracic aortic aneurysm
- Triple X syndrome
- Turner syndrome
- Wilson's disease
Generally genetic tests have little physical risk. Blood and cheek swab tests have almost no risk. However, prenatal testing such as amniocentesis or chorionic villus sampling has a small risk of pregnancy loss (miscarriage).
Genetic testing can have emotional, social and financial risks as well. Discuss all risks and benefits of genetic testing with your doctor, a medical geneticist or a genetic counselor before you have a genetic test.
How you prepare
Before you have genetic testing, gather as much information as you can about your family's medical history. Then, talk with your doctor or a genetic counselor about your personal and family medical history to better understand your risk. Ask questions and discuss any concerns about genetic testing at that meeting. Also, talk about your options, depending on the test results.
If you're being tested for a genetic disorder that runs in families, you may want to consider discussing your decision to have genetic testing with your family. Having these conversations before testing can give you a sense of how your family might respond to your test results and how it may affect them.
Not all health insurance policies pay for genetic testing. So, before you have a genetic test, check with your insurance provider to see what will be covered.
In the United States, the federal Genetic Information Nondiscrimination Act of 2008 (GINA) helps prevent health insurers or employers from discriminating against you based on test results. Under GINA, employment discrimination based on genetic risk also is illegal. However, this act does not cover life, long-term care or disability insurance. Most states offer additional protection.
What you can expect
Depending on the type of test, a sample of your blood, skin, amniotic fluid or other tissue will be collected and sent to a lab for analysis.
- Blood sample. A member of your health care team takes the sample by inserting a needle into a vein in your arm. For newborn screening tests, a blood sample is taken by pricking your baby's heel.
- Cheek swab. For some tests, a swab sample from the inside of your cheek is collected for genetic testing.
- Amniocentesis. In this prenatal genetic test, your doctor inserts a thin, hollow needle through your abdominal wall and into your uterus to collect a small amount of amniotic fluid for testing.
- Chorionic villus sampling. For this prenatal genetic test, your doctor takes a tissue sample from the placenta. Depending on your situation, the sample may be taken with a tube (catheter) through your cervix or through your abdominal wall and uterus using a thin needle.
The amount of time it takes for you to receive your genetic test results depends on the type of test and your health care facility. Talk to your doctor, medical geneticist or genetic counselor before the test about when you can expect the results and have a discussion about them.
Positive results
If the genetic test result is positive, that means the genetic change that was being tested for was detected. The steps you take after you receive a positive result will depend on the reason you had genetic testing.
If the purpose is to:
- Diagnose a specific disease or condition, a positive result will help you and your doctor determine the right treatment and management plan.
- Find out if you are carrying a gene that could cause disease in your child, and the test is positive, your doctor, medical geneticist or a genetic counselor can help you determine your child's risk of actually developing the disease. The test results can also provide information to consider as you and your partner make family planning decisions.
- Determine if you might develop a certain disease, a positive test doesn't necessarily mean you'll get that disorder. For example, having a breast cancer gene ( BRCA1 or BRCA2 ) means you're at high risk of developing breast cancer at some point in your life, but it doesn't indicate with certainty that you'll get breast cancer. However, with some conditions, such as Huntington's disease, having the altered gene does indicate that the disease will eventually develop.
Talk to your doctor about what a positive result means for you. In some cases, you can make lifestyle changes that may reduce your risk of developing a disease, even if you have a gene that makes you more susceptible to a disorder. Results may also help you make choices related to treatment, family planning, careers and insurance coverage.
In addition, you may choose to participate in research or registries related to your genetic disorder or condition. These options may help you stay updated with new developments in prevention or treatment.

Negative results
A negative result means a mutated gene was not detected by the test, which can be reassuring, but it's not a 100 percent guarantee that you don't have the disorder. The accuracy of genetic tests to detect mutated genes varies, depending on the condition being tested for and whether or not the gene mutation was previously identified in a family member.
Even if you don't have the mutated gene, that doesn't necessarily mean you'll never get the disease. For example, the majority of people who develop breast cancer don't have a breast cancer gene ( BRCA1 or BRCA2 ). Also, genetic testing may not be able to detect all genetic defects.
Inconclusive results
In some cases, a genetic test may not provide helpful information about the gene in question. Everyone has variations in the way genes appear, and often these variations don't affect your health. But sometimes it can be difficult to distinguish between a disease-causing gene and a harmless gene variation. These changes are called variants of uncertain significance. In these situations, follow-up testing or periodic reviews of the gene over time may be necessary.
Genetic counseling
No matter what the results of your genetic testing, talk with your doctor, medical geneticist or genetic counselor about questions or concerns you may have. This will help you understand what the results mean for you and your family.
Clinical trials
Explore Mayo Clinic studies of tests and procedures to help prevent, detect, treat or manage conditions.
- National Library of Medicine. Help me understand genetics. Genetics Home Reference. https://ghr.nlm.nih.gov/primer. Accessed Feb. 13, 2017.
- Frequently asked questions about genetic testing. National Human Genome Research Institute. https://www.genome.gov/19516567/faq-about-genetic-testing/. Accessed Feb. 13, 2017.
- Genetic testing for hereditary cancer syndromes. National Cancer Institute. https://www.cancer.gov/about-cancer/causes-prevention/genetics/genetic-testing-fact-sheet. Accessed Feb. 13, 2017.
- Raby BA, et al. Genetic testing. http://www.uptodate.com/home. Accessed Feb. 13, 2017.
- Grody WW, et al. ACMG position statement on prenatal/preconception expanded carrier screening. Genetics in Medicine. 2013;15:482.
- Genetic testing. American Medical Association. https://www.ama-assn.org/content/genetic-testing. Accessed Feb. 13, 2017.
- Frequently asked questions. Prenatal genetic diagnostic tests. FAQ164. Pregnancy. American College of Obstetricians and Gynecologists. https://www.acog.org/-/media/For-Patients/faq164.pdf?dmc=1&ts=20161216T1208042192. Accessed Feb. 13, 2017.
- Breast cancer risk factors you cannot change. American Cancer Society. https://www.cancer.org/cancer/breast-cancer/risk-and-prevention/breast-cancer-risk-factors-you-cannot-change.html. Accessed Feb. 21, 2017.
- Atwal PS (expert opinion). Mayo Clinic, Jacksonville, Fla. Feb. 24, 2017.
- Genetic Testing for Breast Cancer
- Genetics and Cardiomyopathy
- Male hypogonadism
- Mapping the Genome
- Pregnancy after miscarriage
- Primary progressive aphasia
News from Mayo Clinic
- Genomics study enrolls 100,000 participants March 07, 2024, 03:30 p.m. CDT
- Comprehensive testing helps diagnose and manage rare genetic disorder Feb. 25, 2024, 12:00 p.m. CDT
- A silent tumor, precancerous polyps and the power of genetic screening Feb. 11, 2024, 12:00 p.m. CDT
- Understanding triple-negative breast cancer and its treatment Jan. 04, 2024, 04:00 p.m. CDT
- Mayo Clinic's DNA study reveals BRCA1 mutations in 3 sisters, prompts life-changing decisions Nov. 04, 2023, 11:00 a.m. CDT
- Beyond BRCA1/2: Pinpointing the risk of inherited breast cancer genes Oct. 28, 2023, 11:00 a.m. CDT
- Mayo Clinic and Oxford Nanopore announce collaboration to advance precision medicine for cancer and genetic disorders Oct. 19, 2023, 02:07 p.m. CDT
- Mayo Clinic Q and A: Understanding carrier screening for family planning Oct. 17, 2023, 02:40 p.m. CDT
- Mayo Clinic study sheds light on rare genetic disorder and blood cancers Oct. 11, 2023, 04:30 p.m. CDT
- New Mayo Clinic individualized medicine leader on a quest to bring cutting-edge medicine to all Sept. 23, 2023, 11:00 a.m. CDT
- Mayo Clinic cancer researchers targeting racial disparities in genomic data Sept. 02, 2023, 11:00 a.m. CDT
- Science Saturday: Mayo Clinic researchers use new innovative technology to diagnose teen brothers with ultra-rare genetic disorder Aug. 19, 2023, 11:00 a.m. CDT
- Science Saturday: A novel testing approach for newly identified VEXAS syndrome July 08, 2023, 11:00 a.m. CDT
- Mayo Clinic uses genomic testing broadly for rare diseases, improves patient care June 26, 2023, 09:02 p.m. CDT
- Science Saturday: Mayo Clinic doctor visits of the future could be powered by 'omics' research May 27, 2023, 11:00 a.m. CDT
- Researchers test DNA editing, recommend steps to improve accuracy May 02, 2023, 03:00 p.m. CDT
- Mayo Clinic in a new era of discoveries with rapid genome sequencing April 25, 2023, 03:00 p.m. CDT
- Science Saturday: A big step forward, bringing DNA sequencing data to routine patient care April 08, 2023, 11:00 a.m. CDT
- 9 common questions about genetic testing for cancer Feb. 06, 2023, 03:00 p.m. CDT
- Science Saturday: Mayo researchers streamline genetic testing in heart failure clinic, improve clinical care Jan. 28, 2023, 12:00 p.m. CDT
- Identifying inherited gene mutations in pancreatic cancer can lead to targeted therapies, better survival Nov. 24, 2022, 03:30 p.m. CDT
- Gather your family health history this Thanksgiving; it could save your life Nov. 22, 2022, 03:30 p.m. CDT
- Mayo Clinic Q and A: Genetic counseling after cancer diagnosis Nov. 14, 2022, 01:37 p.m. CDT
- A rare genetic cause for a common medical condition Nov. 09, 2022, 04:12 p.m. CDT
- Science Saturday: Mayo Clinic patient grateful after genetic test leads to unexpected, early detection of colon cancer Sept. 03, 2022, 11:00 a.m. CDT
- 9 common questions about genetic testing for cancer July 27, 2022, 01:03 p.m. CDT
- Science Saturday: The art and science of never giving up June 25, 2022, 11:00 a.m. CDT
- Scientists unravel genetic mystery of rare neurodevelopmental disorder, provide definitive diagnoses to 21 families worldwide June 21, 2022, 03:30 p.m. CDT
- Mayo Clinic Q and A: What is the benefit of visiting a genetic counselor? June 12, 2022, 11:00 a.m. CDT
- Science Saturday: What you should know about genetics, colorectal cancer May 07, 2022, 11:00 a.m. CDT
- Doctors & Departments
- Care at Mayo Clinic
Mayo Clinic does not endorse companies or products. Advertising revenue supports our not-for-profit mission.
- Opportunities
Mayo Clinic Press
Check out these best-sellers and special offers on books and newsletters from Mayo Clinic Press .
- Mayo Clinic on Incontinence - Mayo Clinic Press Mayo Clinic on Incontinence
- The Essential Diabetes Book - Mayo Clinic Press The Essential Diabetes Book
- Mayo Clinic on Hearing and Balance - Mayo Clinic Press Mayo Clinic on Hearing and Balance
- FREE Mayo Clinic Diet Assessment - Mayo Clinic Press FREE Mayo Clinic Diet Assessment
- Mayo Clinic Health Letter - FREE book - Mayo Clinic Press Mayo Clinic Health Letter - FREE book
Make twice the impact
Your gift can go twice as far to advance cancer research and care!
- Get Started
Analyze Data
- Visualize Results
Here, we demonstrate how to prepare, import, and analyze data. To evaluate baseline data, we use simulated genetic and non-genetic datasets to demonstrate how integrated data could outperform each data type used independently. The graphical results are shown on Visualize Results page. In this page, we focus on how and which functions are used to import data, reduce low variacne loci, integrate data, perform resampling cross-validations, calculate assignment accuracy, and identify informative loci. We also demonstrate how to perform a one-time assignment test on individuals of unknown origins using baseline data.
Evaluate baseline data
Baseline data are individuals and their features (e.g., genetic loci, non-genetic measurements) collected from known or source populations. We will demonstrate how to prepare and import three different data types: genetic, integrated, and non-genetic, but will only use the genetic data to show the scripts of performing resampling cross-validation.
Prepare and import genetic data
assignPOP accepts genetic data in GENEPOP 1 and STRUCTURE 2 formats. Both formats can be either diploid or haploid data.
When importing a GENEPOP file, two forms of GENEPOP can be read into R using the function read.Genepop() . Populatino names can be specified using the argument pop.names , and the order of population names should follow the group order in your GENEPOP file 3 . These population names can be used to customize the assignment accuracy plot later on. Use the argument haploid to specify your data type (e.g., use haploid=T for haploid data).
The example input file, simGenepop.txt , contains three simulated populations (or subpopulations) and each population has 30 individuals by 1,000 SNP loci. Depending on the data size, the importing process may take a few secnods to minutes. A progress bar will be running while importing the data. After importing the file, you should see the following message printed in your R console. Use the information to double check whether your data were correctly imported.
When importing a STRUCTURE file, use the function read.Structure() , and specify the data type using the argument haploid . Your Structure file should include locus names (each name separated by whitespace or tab) in the first row as the column name (header). The first and second column should be your sample ID and population label, respectively. No whitespace should be used within the sample ID and population label. The rest of columns are genetic data. It does not matter whether your file has column names for sample ID and/or population label.
After importing your STRUCTURE file, you will also see the printed message in the console. For the sake of simplicity, we only use the GENEPOP dataset to demonstrate the rest of analyses.
Missing data and homozygosity. If an locus has missing alleles or is homomzygous across all individuals in the dataset, the locus will be automatically removed.
Remove low variance loci (optional)
When analyzing genetic data, you can remove low variance loci 4 across the dataset for further analyses. The default of variance threshold is 0.95, meaning that a locus will be removed from the dataset if its major allele occurs in over 95% of individuals across the populations.
To remove low variance loci, use the following function and provide a new return object name ( YourGenepopRd in this case) :
After entering the code, R console should print the following message.
In our example, 307 low variance loci 5 were removed, leaving 693 loci in the new data set, which will be used in further analyses.
Concatenate genetic and non-genetic data
Concatenating genetic and non-genetic data is one of the novel features in this package. After importing your GENEPOP file, you can use the function compile.data() to concatenate a non-genetic dataset and the genetic matrix returned from either read.Genepop() , read.Structure() , or reduce.allele() . Your non-genetic data should be saved in a .csv file (elements separated by commas) or table-like text file (elements separated by spaces) in which the first column must be sample IDs that match the IDs in your GENEPOP or STRUCTURE file, and the rest of columns are non-genetic data, whether it is numeric or categorical. If a sample ID exists in only one of the data types, the individual will be ignored in the new integrated data.
To concatenate datasets, use the following function and provide a new return object name ( YourIntegrateData in this case):
The above morphData.csv file has the same individuals as the simGenepop.txt and it contains 4 numeric variables (morphometric measurements) for each individual. After executing the function, it will prompt the following message and wait for your answer to verify the data type (numeric or categorical).
If a variable is categorical data, it will automatically convert it to new dummy variables using model.matrix() so that the categorical data can be quantified for further analyses. If your data type was incorrectly identified, simply enter N and then follow the prompted dialogue to change your data type. If your data type is correctly identified (entry is Y in this example), then it will print the following message.
Now the integrated data (693 SNP loci plus 4 morphometric measurements) matrix is saved in the return object named comin .
Prepare and import non-genetic data
In addition to analyzing genetic-only or integrated data, you can perform the same analysis on non-genetic data so that you could compare the assignment results between data types. A non-genetic data set (collected from source populations) should include 1) sample IDs in the first column, 2) population label 6 in the last column, and 3) non-genetic data in columns between the sample ID and population label columns. The file should be saved in a .csv (comma delimited) or table-like text file that can be read into R using R basic functions read.csv() or read.table() . For example, if you are going to perform assignment test using the sample morphometric data ( morphData.csv ) provided in the pakcage, you would need to add a population column after the existing last column. It can be edited manually (e.g., using Excel) or by R scripts (see below). If your sample IDs and population names are numeric, make sure to convert them to factor data type using as.factor() function.
Now the non-genetic (morphometric) data with its population label is saved in the object morphdf_pop .
Perform resampling cross-validation
We provide two resampling cross-validation methods, Monte-Carlo and K -fold cross-validations , to evaluate baseline data. Monte-Carlo cross-validation helps estimate the mean and variance of assignment accuracy through resampling random training individuals; K -fold cross-validation helps determine membership probability across all individuals through using one group as test individuals and the remaining K-1 groups as training individuals (hence every individual is tested once).
When analyzing genetic data (genetic-only or integrated), users can specify what proportions of loci should be used as training loci, and determine whether the proportion of loci is chosen either randomly or based on locus F ST estimated within training individuals. This helps evaluate whether using a subset of loci performs as well as using all loci. Most informative loci also can be identified via the function check.loci (see Identify informative loci )
Monte-Carlo cross-validation
When performing Monte-Carlo cross-validation ( assign.MC() ), users can specify multiple proportions or multiple numbers of individuals from each population to be used as training individuals. Each combination of training individuals and features (e.g., loci or non-genetic measurements) can be resampled multiple times. For example, the code below performs Monte-Carlo cross-validation, with using 50%, 70%, and 90% of random individuals from each population (arg. train.inds ) crossed by top 10%, 25%, 50% of high F ST loci, and all loci (arg. train.loci , loci.sample="fst" ) as training data. Each combination of training data is resampled 30 times (arg. iterations ). As a result, it performs a total of 360 assignment tests (3 levels of training individuals by 4 levels of training loci by 30 iterations).
To use certain numbers, rather than proportions, of individuals from each population as training data, enter positive integers in the argument train.inds (e.g., train.inds = c(10, 20, 30) ). The reason of using equal number of traininig individuals from each population is that it can avoid biases due to unbalanced population sample size 7 , 8 .
If you are analyzing non-genetic data alone, the arguments, train.loci and loci.sample , will be automatically ignored.
Results of assignment tests will be saved in text files under a folder created via the argument dir . The above example creates a folder named “Result-folder.” And be sure to include a forward slash ( / ) at the end of the folder name.
In addition, you can use the argument model to select a classification method for prediction. In the above example, the Support Vector Machine (SVM) algorithm is used. Other SVM related arguments, svm.kernel and svm.cost , can be specified to fine tune the predictive model. See e1071 package for details about SVM. Other classification methods, including LDA , naive Bayes , decision tree , and random forest , can be specified to build predictive models. Type ?assign.MC to see more details.
K -fold cross validation
Because Monte-Carlo cross-validation that repeats assignment tests through resampling random individuals does not guarantee every individual was tested, estimating membership probability of all individuals in this way could be problematic. To estimate membership probability, we introduce K -fold cross-validation ( assign.kfold() ) in which individuals from each population are divided into K groups. One of the K groups is tested by the predictive model built based on the remaining K-1 groups. Such an assignment test repeats until every group was tested. Multiple K values can be specified (arg. k.fold ) in one analysis as follows.
The above example divides individuals from each population into 3, 4, or 5 groups (or folds). In each fold, 10%, 25%, and 50% of random loci ( loci.sample = "random" ) as well as all loci were sampled as training data. As a result, the K -fold cross-validation performs a total of 48 assignment tests (48 = (3+4+5) folds * 4 levels of training loci).
Similarly, one of the classification methods can be specified to build the predictive models. Linear Discriminant Funtion ( model="lda" ) was used in the above example.
Parallel computing. When performing resampling cross-validation, by default, it uses your available CPU cores/threads (N) minus one for parallel analyses such that N-1 assignment tests are performed simultaneously (assuming your computer’s CPU is multi-core). To change the number of cores/threads to be used, simply specify a number for the argument processors (e.g., processors = 2 for using two cores/threads).
Depending on the size of your dataset, running assign.MC() may take a few minutes to hours, whereas assign.kfold() should spend less time because it usually performs fewer tests. You can monitor the analysis status by counting the output files in the result folder.
Calculate assignment accuracy
When resampling cross-validations are done, you can calculate assignment accuracies using the following functions.
The functions read through each assignment result in the designated folder (arg. dir ) and calculate the assignment accuracies for overall and individual populations. The results will be saved in a text file named Rate_of…txt , and a return object ( accuMC and accuKF in the above examples). The output text file also can be read into R using the basic function read.table() as follows.
Compared with K -fold cross-validation, the results of Monte-Carlo cross-validation, with reasonable iterations (e.g., resample > 30 times), are more suitable for estimating mean and variance of assignment accuracy. As such, using the function accuracy.MC() for Monte-Carlo results is more useful than using accuracy.kfold() . The results generated by accuracy.MC() (or accuracy.kfold() ) can be visualized in an assignment accuracy plot; the results generated by the K -fold cross-validation ( assign.kfold() ) can be directly used to make a membership probability plot. See Visualize Results page for more details.
Identify informative loci
In some cases, using a subset of high F ST loci may produce similar assignment accuracy with using all available loci. Identification of these loci not only could help reduce time and cost in preparing samples in the future but also could help identify loci that might be associated with functional genes.
The function check.loci() reads through each training locus file associated with the assignment test, counts the frequency of occurrence of each locus, and writes the results to a text file.
This function should only be used for the cross-validation results based on resampling high F ST training loci ( loci.sample = "fst" ) in the assign.MC() analysis.
By default, this function outputs top 20 loci - most frequently used high F ST training loci across your assignment tests, but an arbitrary number can be specified in the argument top.loci .
When the function is executed, as shown below, you will be prompted to choose which assignment results you would like to check. The options are the groups of different proportions or numbers of training individuals that you specified in the argument train.inds of the function assign.MC() .
Because a locus F ST value estimated based on a training set likely varies among subsets of training individuals, we recommend running the function check.loci() across all levels of proportions or numbers of training individuals to evaluate if the loci consistently are those high F ST loci. If so, then they could be recognized as truly informative loci (high F ST is not due to sampling artifacts).
The output file ( High_Fst_Locus_Freq.txt ) includes a list of locus names ordered by F ST value (highest F ST in the top row). The number in parentheses right after each locus name indicates the number of tests that locus appeared in that rank. A snapshot of output content is shown below.
The above results show that the top.1-top.11 loci are the top 11 highest F ST loci are across all 360 tests. Five loci ( top.12(5): Locus_113, Locus_114, Locus_115, Locus_320, and Locus_139 ) appear to be the 12th highest F ST locus across the tests, and Locus_113 occurs most frequently (248 out of 360 tests) in this rank.
Predict sources of unknown individuals
After baseline data were evaluated through resampling cross-validation and the assignment results are satisfactory, you could use the entire baseline data to build a predictive model and perform assignment tests on individuals of unknown origins to predict their source populations. Here we describe how to prepare and import files for different data types and perform the assignment test using the function assign.X() .
Prepare and import data of unknown individuals
To predict sources of unknown individuals, users should save the data of unknown individuals in another GENEPOP or STRUCTURE file, and then import it into R using the same functions as we used for the baseline data.
For the GENEPOP format, data should be saved with only one group - only one ‘pop’ label in the file (e.g., simGenepopX.txt ). For the STRUCTURE format, the column of population label should also be included, but the label can be anything (this column will be ignored when performing the assignment test).
You can also concatenate genetic and non-genetic data of unknown individuals using the function compile.data as follows.
The non-genetic ( morphDataX.csv ) and genetic ( simGenepopX.txt ) data should have identical sample IDs. In your non-genetic data, sample IDs should be saved in the first column, and there would be no population label in the last column.
If you are analyzing non-genetic data alone, use R basic functions, read.csv() or read.table() , to import your file.
Perform assignment test on unknown individuals
After importing your baseline data and unknown individuals (test data), the assignment test can be performed using the function assign.X . Make sure your training and test data have the same data type and feature names. Use the arguments x1 and x2 to specify the baseline and test data,respectively.
The assignment result will be saved in a text file named AssignmentResult.txt under the designated folder. This file includes sample IDs of your unknown individuals, predicted populations and the probabilities. When the assignment is done, it will prompt a message asking you whether you want to make a membership probability plot. Simply enter Y to visualize the results right away.
Handling unmatched alleles between baseline and test data. In some cases, alleles found in test data may not exist in baseline data, or vice versa. The function assign.X will check if alleles of a locus are identical between your baseline and test data. If they are different, it will ask you if you want to use the loci in common to continue the analysis. If answer ‘ Y ’, the assignment test will ignore the loci that have different alleles between baseline and test data.
Data process
Pca for data transformation.
The assignment functions, assign.MC() , assign.kfold() and assign.X() , use PCA for dimensionality reduction. It converts your independent variables to Principle Components and retain the PCs that have eigenvalues greater than 1 (the Kaiser-Guttman criterion) as new features (arg. pca.PCs="kaiser-guttman" ). Or, you can specify an integer in the argument pca.PCs (e.g., pca.PCs = 10 ) to choose a specific number of PCs to be retained.
The PCA will always perform on the genetic data, whether you are analyzing genetic-only or integrated data. When analyzing integrated data, you have three options to perform PCA on the data. First, you can perform PCA across all features (set arg. pca.method="mixed" ) - each PC includes information of genetic and non-genetic data. This is default setting. Second, you can perform PCA on genetic and non-genetic data independently (set arg. pca.method="independent" ) - transformed data includes genetic PCs and non-genetic PCs. Third, you can choose to use original non-genetic data as features without performing PCA on them (set. arg. pca.method="original" ). This option uses genetic PCs and original non-genetic data to build predictive models.
When analyzing non-genetic data alone, you can determine whether PCA is performed on the data or not. You set the argument pca.method=TRUE to perform PCA, or pca.method=FALSE to not perform PCA.
Data standardization
When analyzing non-genetic or integrated data, you have options to standardize (zero mean and unit variance) the independent variables or to keep the original data. If non-genetic data has varying ranges of values and is not standardized, it may be problematic when one feature has a variance that is orders of magnitude larger than others, as it might make the classifier unable to learn from other features correctly. To center and scale the data, set the argument scaled=TRUE in the function assign.MC() , assign.kfold() or assign.X() . If you are analyzing genetic data, then standardization is not required because all the loci (alleles) have been converted to a binary-like value 0, 0.5, or 1.
Reference and footnotes
Rousset, F. (2008). genepop’007: A Complete Re-Implementation of the Genepop Software for Windows and Linux. Molecular Ecology Resources 8(1): 103–106. ↩
Pritchard, J.K., Stephens, M. & Donnelly, P. (2000). Inference of Population Structure Using Multilocus Genotype Data. Genetics , 155, 945–959. ↩
The name order in the argument pop.names should match the group order in your GENEPOP file. For example, the first name, pop_A , in pop.names=c("pop_A","pop_B","pop_C") is the first group (pop) of individuals in the GENEPOP. If pop.names is not provided, pop. NUMBER (e.g., pop.1, pop.2) will be used as the population name. ↩
A low variance locus - which has a major allele in most individuals and a minor allele in very few individuals - is unlikely useful because if an allele only occurs in the training or test data, it will not help ascertain population membership of test individuals. ↩
In this case, we have 614 alleles across 307 loci, meaning that every locus is biallelic (307 loci x 2 alleles = 614 alleles) ↩
A column includes population names for each individual. Also be sure to include a column name in table header. ↩
Puechmaille, S.J. (2016). The program structure does not reliably recover the correct population structure when sampling is uneven: subsampling and new estimators alleviate the problem. Molecular Ecology Resources , 16, 608–627 ↩
Wang, J. (2016). The computer program Structure for assigning individuals to populations: easy to use but easier to misuse. Molecular Ecology Resources . ↩
Browse Course Material
Course info, instructors.
- Prof. David Housman
- Prof. Anne Giersch
Departments
- Health Sciences and Technology
As Taught In
- Biological Engineering
- Biomedicine
- Functional Genomics
- Developmental Biology
- Molecular Biology
Molecular Biology and Genetics in Modern Medicine
Assignments, problem sets.
Karyotyping assignment ( PDF )
Reading Assignments
Reading Assignment 1 ( PDF )
Associated Readings Allamand, Valerie, et al. “A Single Homozygous Point Mutation in a 3’ Untranslated Region Motif of Selenoprotein N mRNA Causes SEPN1-related Myopathy.” EMBO Reports 7 (2006): 450-454. Hatfield, Dolph L., and Vadim N. Gladyshev. “How Selenium Has Altered Our Understanding of the Genetic Code.” Molec and Cell Bio 22 (2002): 3565-3576.
Reading Assignment 2 ( PDF )
Associated Readings Watkins, Hugh, Ludwig Thierfelder, Ryuichiro Anan, John Jarcho, Akira Matsumori, William McKenna, J. G. Seidman, and Christine E. Seidman. “Independent Origin of Identical? Cardiac Myosin Heavy-Chain Mutations in Hypertrophic Cardiomyopathy.” Am J Hum Genetics 53 (1993): 1180-1185. Koenig, M., et al. “The Molecular Basis for Duchenne versus Becker Muscular Dystrophy: Correlation of Severity with Type of Deletion.” Am J Hum Genet 45 (1989): 498-506. Liebhaber, S. A., E.-U. Griese, I. Weiss, F. E. Cash, H. Ayyub, D. R. Higgs, and J. Horst. “Inactivation of Human α-globin Gene Expression by a de novo Deletion Located Upstream of the α-globin Gene Cluster.” PNAS 87 (1990): 9431-9435.
Reading Assignment 3 ( PDF )
Associated Readings Stamm, Christof, Ingeborg Friehs, Siew Yen Ho, Adrian M. Moran, Richard A. Jonas, and Pedro J. del Nido. “Review: Congenital Supravalvar Aortic Stenosis: A Simple Lesion?” European Journal of Cardio-thoracic Surgery 19 (2001): 195-202. Meyer-Lindenberg, Andreas, Carolyn B. Mervis, and Karen Faith Berman. “Neural Mechanisms in Williams Syndrome: A Unique Window to Genetic Influences on Cognition and Behaviour.” Nature Reviews Neuroscience 7 (2006): 380-393.
Reading Assignment 4 ( PDF )
Associated Readings Orr, Harry T., and Huda Y. Zoghbi. “Trinucleotide Repeat Disorders.” Annu Rev Neurosci 30 (2007): 575-621.
Reading Assignment 5 ( PDF )
Associated Readings Buiting, Karin, et al. “Epimutations in Prader-Willi and Angelman Syndromes: A Molecular Study of 136 Patients with an Imprinting Defect.” Am J Hum Genet 72 (2003): 571-577. Horsthemke, B., and K. Buiting. “Imprinting Defects on Human Chromosome 15.” Cytogenet Genome Res 113 (2006): 292-299. Williamson, Sarah L., and John Christodoulou. “Rett Syndrome: New Clinical and Molecular Insights.” Euro J Hum Genet 14 (2006): 896-903. Reik, Wolf, and Annabelle Lewis. “Co-evolution of X-chromosome Inactivation and Imprinting in Mammals.” Nature Rev Genetics 1, AOP (2005): 1-8. Robertson, Keith D. “DNA Methylation and Human Disease.” Nature Rev Genetics 6 (2005): 597-610.
Final Paper
Guidelines for writing the final paper ( PDF )

You are leaving MIT OpenCourseWare
You are using an outdated browser. Please upgrade your browser or activate Google Chrome Frame to improve your experience.
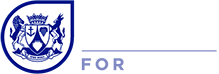
2022 DBE Self-study Guides Gr. 12 Life Sciences: Genetics and Inheritance
This study guide is intended to serve as a resource for teachers and learners. It provides notes, examples, problem-solving exercises with solutions and examples of practical activities.
Do you have an educational app, video, ebook, course or eResource?
Contribute to the Western Cape Education Department's ePortal to make a difference.
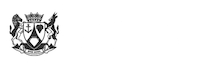
Home Contact us Terms of Use Privacy Policy Western Cape Government © 2024. All rights reserved.

Advertisement
Supported by
One in Five Milk Samples Nationwide Shows Genetic Traces of Bird Flu
There is no evidence that the milk is unsafe to drink, scientists say. But the survey result strongly hints that the outbreak may be widespread.
- Share full article

By Emily Anthes and Noah Weiland
Federal regulators have discovered fragments of bird flu virus in roughly 20 percent of retail milk samples tested in a nationally representative study, the Food and Drug Administration said in an online update on Thursday.
Samples from parts of the country that are known to have dairy herds infected with the virus were more likely to test positive, the agency said. Regulators said that there is no evidence that this milk poses a danger to consumers or that live virus is present in the milk on store shelves, an assessment public health experts have agreed with.
But finding traces of the virus in such a high share of samples from around the country is the strongest signal yet that the bird flu outbreak in dairy cows is more extensive than the official tally of 33 infected herds across eight states.
“It suggests that there is a whole lot of this virus out there,” said Richard Webby, a virologist and influenza expert at St. Jude Children’s Research Hospital.
Dr. Webby said that he believed it was still possible to eradicate the virus, which is known as H5N1, from the nation’s dairy farms. But it will be difficult to design effective control measures without knowing the scope of the outbreak, he said.
The findings also raise questions about how the virus has evaded detection and where else it might be silently spreading. Some scientists have criticized the federal testing strategy as too limited to reveal the true extent of viral spread.
Until Wednesday, when the Department of Agriculture announced mandatory testing of dairy cows moving across state lines, testing of cows had been voluntary and primarily focused on cows with obvious symptoms.
As of Wednesday, just 23 people had been tested for the virus, while 44 people were being monitored after exposure to it, according to the Centers for Disease Control and Prevention.
A widespread outbreak in cows would pose a greater risk to farm workers, the dairy industry and public health more broadly. Sustained spread among cows would give the virus more opportunities to acquire mutations that make it more transmissible among humans.
The F.D.A. did not provide details on Thursday regarding the number or sources of the samples.
“You’d want to go not just to the places you knew there was activity and cows — you want to go to places where at least there’s no reported” bird flu, Dr. Webby said.
Experts believe that the process of pasteurization, in which milk is briefly heated, should inactivate this bird flu virus, which known as H5N1.
“And when you destroy the virus, it’s going to release genetic material,” said Samuel Alcaine, a microbiologist and food scientist at Cornell University. The genetic fragments left behind are not capable of causing infection.
“It’s not surprising” to find them in milk, he added. “It doesn’t mean that the milk is not safe.”
Federal officials are still conducting the time-intensive tests required to determine whether any viable virus remains in the milk after pasteurization. Scientists have said that prospect is very unlikely.
Dr. Jeanne Marrazzo, the director of the National Institute of Allergy and Infectious Diseases, said at a news briefing Wednesday that some federally sponsored researchers had tested for live virus in retail milk but had not found any, a sign that pasteurization had killed the virus before the milk reached grocery shelves.
Dr. Marrazzo cautioned that while the results were a small sample, the findings were “welcome news.”
“To really understand the scope here, we need to wait for the F.D.A. efforts,” she said.
Finding traces of the virus in 20 percent of commercial milk samples does not mean that 20 percent of the nation’s dairy herds are infected, experts cautioned. “It’s too early to try to do that back-of-the-napkin kind of calculation,” Dr. Alcaine said.
Milk from several farms is typically pooled. If the virus turns up in lots of milk samples drawn from one pool, it could mean that many cows are infected — or that a smaller number of infected cows are shedding large quantities of virus, Dr. Alcaine said.
Even in the latter case, however, a 20 percent positivity rate would suggest far more than 33 herds are infected, he noted.
In the Wednesday news briefing, Dr. Donald A. Prater, the acting director of the F.D.A.’s Center for Food Safety and Applied Nutrition, pointed to the novelty of the research effort. No studies have ever been completed on the effects of pasteurization on the bird flu virus in milk, he said.
Regulators were examining milk at various points in the commercial supply chain, he added, including milk on grocery shelves, as well as studying potential differences among milk products, such as those between whole milk and cream, Dr. Prater said.
Emily Anthes is a science reporter, writing primarily about animal health and science. She also covered the coronavirus pandemic. More about Emily Anthes
Noah Weiland writes about health care for The Times. More about Noah Weiland
An AI blood test purports to diagnose postpartum depression
Companies are using artificial intelligence to take aim at a host of health issues including this common pregnancy complication.

A previous version of this article incorrectly stated that Dionysus Digital Health received a $6 billion grant from the Department of Defense. It received $6 million.
Postpartum depression is a leading cause of maternal death, but its diagnosis and treatment is spotty at best, negligent at worst.
Now San Diego-based start-up Dionysus Digital Health is pitching a blood test to check for the condition, even before symptoms appear. The company says it has pinpointed a gene linking a person’s moods more closely to hormonal changes. The test uses machine learning to compare epigenetics — how genes are expressed — in a blood sample with benchmarks developed during a decade of research into pregnant people who did and didn’t develop postpartum depression.
Researchers at Dionysus’s academic partners, the Royal’s Institute of Mental Health Research and UVA Health, have published peer-reviewed papers affirming their findings, and the company is partnering with the Department of Defense and the National Institutes of Health for clinical trials, with the eventual goal of making the $250 test widely available and covered by insurance. But women’s health experts say better diagnostics for postpartum depression may not help if mothers can’t access treatment and support.
One in 7 mothers experience postpartum depression. When doctors screen for the condition, they typically use a questionnaire that asks patients how much they identify with statements such as “I have looked forward with enjoyment to things as much as I ever did” and “I have blamed myself unnecessarily when things went wrong.”
If properly diagnosed, mothers rarely receive the care they need. In one widely cited study, just one-third of pregnant patients who showed signs of mental disorders received treatment — which most often consisted of verbal “reassurance” from their providers.
“Our aspiration is you can be in treatment before you ever even experience a symptom,” Dionysus co-founder and chief scientist Vivienne Ming said in an interview with The Washington Post. “Now we can show it’s not just in your head.”
Ming is one of many researchers using artificial intelligence to hunt for new approaches to complicated health concerns. Palo Alto, Calif.-based Delfi Diagnostics has a test that uses artificial intelligence to detect signs of lung cancer. Researchers at Children’s National Hospital in Washington built an AI tool to diagnose rheumatic heart disease in children.
But AI systems can easily exacerbate existing bias or inequity in health care. A 2019 study found that an algorithm making recommendations for C-sections wrongly flagged Black women as high risk. Another algorithm, tasked with predicting health-care needs for a large diverse group of patients, consistently recommended less care for Black patients, another study showed.
Ming acknowledged concerns about bias, cost and effectiveness. It would probably take years for Dionysus to gain approval from the Food and Drug Administration or to get insurers and employers to agree to cover the cost of the test, Ming says. In the meantime, the company says it has received a $6 million grant from the Department of Defense to validate its test in more environments. The Department of Defense didn’t respond to a request for comment.
What could change
Dionysus imagines a world where providers administer a blood test between the second and third trimesters of pregnancy that flags women at higher risk of postpartum depression and other perinatal mood disorders . This, combined with other diagnostic methods, could allow health-care systems to funnel vulnerable mothers toward treatment — and even preventive care.
The American College of Obstetricians and Gynecologists recommends providers screen patients for postpartum depression multiple times during and after pregnancy, but that doesn’t always happen, said Elizabeth LaRusso, a psychiatrist specializing in women’s health. Some people make it all the way through their pre- and postnatal checkups without a provider ever mentioning depression . Low-income women and women of color are less likely to be screened than White mothers, LaRusso’s research has found .
LaRusso said she’d welcome any tool that makes it easier to catch postpartum depression before it leads to hospitalizations, job loss or suicide. But identifying at-risk mothers is only the first step: More screening won’t make a difference if patients can’t access the care they need, such as therapy or medication, she said.
How impactful the Dionysus test proves to be will depend in part on its affordability and whether insurance companies are willing to cover its cost. Perinatal mood and anxiety disorders cost $14 billion each year in lost wages and extra expenses, researchers estimate . If flagging more depression cases could reduce subsequent medical spending, insurers might be motivated to pay for the test, Ming said.
But insurers could also view depression diagnostics as a pathway to more medical spending, as patients seek treatment they otherwise wouldn’t have pursued, said Wendell Potter, a former insurance executive who advocates for industry reform. Ultimately, insurers and employers will decide individually what new medical technology to cover. If patients end up paying out of pocket for postpartum depression screening, tests like Dionysus’s could end up exacerbating existing inequities in maternal care, Potter said.
“I doubt the majority of Americans would be able to fork out of their own bank accounts how much [the test] would cost,” he said.
Is this a safe use of AI?
As companies and researchers propose uses for AI in health care, it will be essential to audit those systems for bias, AI experts say. Since machine learning systems are trained to recognize patterns, it’s easy for them to regurgitate any biases that show up in their training data, said Mark Sendak, a data scientist at the Duke Institute for Health Innovation (DIHI).
Critically, Sendak said, an AI model’s training data should reflect the population it’s meant to serve. Dionysus, for its part, says it first validated its test with a cohort of largely White patients at Johns Hopkins Hospital in Baltimore. Its partnerships with Emory University Hospital and the Department of Defense will help it further validate its model with more diverse groups of patients, Ming said.
Without recent advancements in machine learning, Dionysus would never have been able to link a particular gene to postpartum depression, Ming said. Similar discoveries may be close behind as companies rush to apply AI to medical challenges.
But progress could come with drawbacks, said Suresh Balu, program director at DIHI. If only people with disposable income can afford early screening and preventive care, existing gaps in health-care access will get worse. Finding out you’re at risk for an illness you may never get could come with anxiety — even people with a genetic predisposition to postpartum depression may never develop symptoms if that gene isn’t activated by environmental factors, according to Ming.
Ming said Dionysus’s eventual goal is to sell the postpartum depression test directly to consumers, letting people assess their risk years before they even become pregnant. It could change the lives of mothers and children for the better, she said — if mothers can access the care they need.
Help Desk: Making tech work for you
Help Desk is a destination built for readers looking to better understand and take control of the technology used in everyday life.
Take control: Sign up for The Tech Friend newsletter to get straight talk and advice on how to make your tech a force for good.
Tech tips to make your life easier: 10 tips and tricks to customize iOS 16 | 5 tips to make your gadget batteries last longer | How to get back control of a hacked social media account | How to avoid falling for and spreading misinformation online
Data and Privacy: A guide to every privacy setting you should change now . We have gone through the settings for the most popular (and problematic) services to give you recommendations. Google | Amazon | Facebook | Venmo | Apple | Android
Ask a question: Send the Help Desk your personal technology questions .
- When you should (and shouldn’t) rely on AI tools February 28, 2024 When you should (and shouldn’t) rely on AI tools February 28, 2024
- An AI blood test purports to diagnose postpartum depression April 29, 2024 An AI blood test purports to diagnose postpartum depression April 29, 2024
- How to leave a bad online review without being a jerk April 15, 2024 How to leave a bad online review without being a jerk April 15, 2024


IMAGES
VIDEO
COMMENTS
THINGS TO INCLUDE: ~DNA is responsible for traits. ~A section of DNA that codes for a specific trait is called a gene. ~Half of your DNA comes from each parent. ~Your traits are a combination of your parents' traits. ~DNA is the blueprint for proteins, which play many roles in the body. Plz don't copy, its just an example!!
Free Genetics Practice Tests. Our completely free Genetics practice tests are the perfect way to brush up your skills. Take one of our many Genetics practice tests for a run-through of commonly asked questions. You will receive incredibly detailed scoring results at the end of your Genetics practice test to help you identify your strengths and ...
Genetics Overview for AP Biology - summarizes the various types of crosses students may encounter on the AP test. Genetics and Statistics. ... this is a more involved group assignment where groups read scenarios about genetic testing and ethics involved. Genetic Science Ethics - survey as a group ethical questions involved genetics ...
25-30. Problem Set 6 ( PDF ) ( PDF ) 31-35. Problem Set 7 ( PDF ) ( PDF ) The teaching staff also recommends that students practice on problem sets and exams from previous years. An archive of past assignments and exams can be found in the study materials section of this course site. Problem Sets and Solutions.
The genetic assay for gene assignment is called the complementation test. The simplicity and robustness of this test makes it a fundamental genetic tool for gene assignment. However, there are occasional unexpected outcomes from this test that bear explanation. This chapter reviews the complementation test and its various outcomes, highlighting ...
Learn for free about math, art, computer programming, economics, physics, chemistry, biology, medicine, finance, history, and more. Khan Academy is a nonprofit with the mission of providing a free, world-class education for anyone, anywhere.
You will predict the outcome of genetic crosses involving one, two or three unlinked genes, as well as one, two, or three linked genes. In addition, you will design genetic crosses that can determine the genotype of an individual, and whether a trait is dominant or recessive. You will then draw a simple genetic map based upon data from test ...
Genetic assignment methods. Genetic assignment methods are a set of powerful statistical methods that are used to determine the relationship between individuals and populations. [1] The general principle behind them is to use multilocus genotypes to assign reference populations as origins of the individuals. [2]
Assignment tests. Assignment tests (Go to assignment test web calculator [old, unmaintained version]) The idea behind assignment tests is to use individual genotypes to assign individuals to populations or clusters. Paetkau et al. (1995) developed the first assignment test approach for use on bears. The idea was fairly simple.
Assignment Tests Geneclass II Cornuet et al. 1999, Genetics 153: 1989-2000 STRUCTURE Pritchard et al. 2000. Genetics 155: 945-959 CERVUS Marshall et al. 1998. Mol. Ecol. 7: 639-655 Potential methods: • Gene frequency estimates • Genetic distances (genetic correlations) - Cavalli-Sforza and Edwards chord distance - Nei's genetic distances
Mendelian genetics. Cystic fibrosis is an autosomal recessive disease caused by mutations in the cystic fibrosis transmembrane conductance regulator ( CFTR) gene. Individuals with cystic fibrosis have sticky mucus in their lungs and other organs. This mucus can lead to infections and additional complications.
Genetic testing involves examining your DNA, the chemical database that carries instructions for your body's functions. Genetic testing can reveal changes (mutations) in your genes that may cause illness or disease. Although genetic testing can provide important information for diagnosing, treating and preventing illness, there are limitations.
Perform assignment test on unknown individuals. After importing your baseline data and unknown individuals (test data), the assignment test can be performed using the function assign.X. Make sure your training and test data have the same data type and feature names. Use the arguments x1 and x2 to specify the baseline and test data,respectively.
Karyotyping assignment . Reading Assignments. Reading Assignment 1 . Associated Readings. Allamand, Valerie, et al. "A Single Homozygous Point Mutation in a 3' Untranslated Region Motif of Selenoprotein N mRNA Causes SEPN1-related Myopathy." EMBO Reports 7 (2006): 450-454. Hatfield, Dolph L., and Vadim N. Gladyshev.
By using genetic assignment methods, individuals with unknown genetic origin can be assigned to source populations. This knowledge is necessary in studying many key questions in ecology, evolution and conservation. ... To test this, we dropped out mixture individuals from the outside population and ran BONE analysis multiple times with ...
Various combinations of training individuals and features can be used to build predictive models and test on test individuals. When analysing genetic or genomic data, data dimensionality is reduced using principal component analysis. ... (Step 1-5), the function assign.X is used to perform a one-time, final assignment test for the unknown ...
This study guide is intended to serve as a resource for teachers and learners. It provides notes, examples, problem-solving exercises with solutions and examples of practical activities. Language: English. Curriculum Alignment: CAPS aligned. Publication Date: 2022-02-09. Grade:
Studying BIOL 3311 Genetics Laboratory at University of Houston? On Studocu you will find practice materials, lecture notes, coursework, assignments, essays and. Skip to main content. University; High School. Books; Discovery. Sign in. ... Test 1 review. 14 pages 2023/2024 None. 2023/2024 None. Save. WA4 - WA4. 1 page 2022/2023 None. 2022/2023 ...
Federal officials conducting a national survey of milk samples have found a high percentage carry genetic traces of bird flu virus. Robert F. Bukaty/Associated Press. By Emily Anthes and Noah ...
(SACRAMENTO) The Food and Drug Administration announced Tuesday that samples of pasteurized milk taken from grocery store shelves had tested positive for bird flu, also known as Highly Pathogenic Avian Influenza (HPAI) or H5N1.On Thursday, the agency announced that one in five milk samples nationwide showed genetic traces of the virus. Milk samples from areas with infected herds were more ...
It received $6 million. Postpartum depression is a leading cause of maternal death, but its diagnosis and treatment is spotty at best, negligent at worst. Now San Diego-based start-up Dionysus ...
Study with Quizlet and memorize flashcards containing terms like The hypothesis would not have been supported by the data if, Notice that the predicted percentages and laboratory percentages are not the same. These differences most likely result from a computation errora strong linkage between the genesexpected randomnessan unusual sample., A cross was performed, and the offspring shown to the ...