Advanced Synthesis and Catalysis
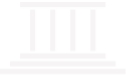

Subject Area and Category
- Organic Chemistry
Wiley-VCH Verlag
Publication type
16154150, 16154169
Information
How to publish in this journal
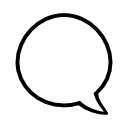
The set of journals have been ranked according to their SJR and divided into four equal groups, four quartiles. Q1 (green) comprises the quarter of the journals with the highest values, Q2 (yellow) the second highest values, Q3 (orange) the third highest values and Q4 (red) the lowest values.
The SJR is a size-independent prestige indicator that ranks journals by their 'average prestige per article'. It is based on the idea that 'all citations are not created equal'. SJR is a measure of scientific influence of journals that accounts for both the number of citations received by a journal and the importance or prestige of the journals where such citations come from It measures the scientific influence of the average article in a journal, it expresses how central to the global scientific discussion an average article of the journal is.
Evolution of the number of published documents. All types of documents are considered, including citable and non citable documents.
This indicator counts the number of citations received by documents from a journal and divides them by the total number of documents published in that journal. The chart shows the evolution of the average number of times documents published in a journal in the past two, three and four years have been cited in the current year. The two years line is equivalent to journal impact factor ™ (Thomson Reuters) metric.
Evolution of the total number of citations and journal's self-citations received by a journal's published documents during the three previous years. Journal Self-citation is defined as the number of citation from a journal citing article to articles published by the same journal.
Evolution of the number of total citation per document and external citation per document (i.e. journal self-citations removed) received by a journal's published documents during the three previous years. External citations are calculated by subtracting the number of self-citations from the total number of citations received by the journal’s documents.
International Collaboration accounts for the articles that have been produced by researchers from several countries. The chart shows the ratio of a journal's documents signed by researchers from more than one country; that is including more than one country address.
Not every article in a journal is considered primary research and therefore "citable", this chart shows the ratio of a journal's articles including substantial research (research articles, conference papers and reviews) in three year windows vs. those documents other than research articles, reviews and conference papers.
Ratio of a journal's items, grouped in three years windows, that have been cited at least once vs. those not cited during the following year.
Leave a comment
Name * Required
Email (will not be published) * Required
* Required Cancel
The users of Scimago Journal & Country Rank have the possibility to dialogue through comments linked to a specific journal. The purpose is to have a forum in which general doubts about the processes of publication in the journal, experiences and other issues derived from the publication of papers are resolved. For topics on particular articles, maintain the dialogue through the usual channels with your editor.
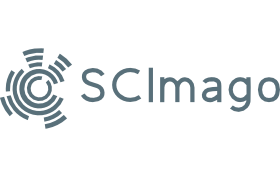
Follow us on @ScimagoJR Scimago Lab , Copyright 2007-2024. Data Source: Scopus®

Cookie settings
Cookie Policy
Legal Notice
Privacy Policy
Thank you for visiting nature.com. You are using a browser version with limited support for CSS. To obtain the best experience, we recommend you use a more up to date browser (or turn off compatibility mode in Internet Explorer). In the meantime, to ensure continued support, we are displaying the site without styles and JavaScript.
- View all journals
- Explore content
- About the journal
- Publish with us
- Sign up for alerts
- Review Article
- Published: 23 April 2024
Embracing data science in catalysis research
- Manu Suvarna ORCID: orcid.org/0000-0003-0927-0579 1 &
- Javier Pérez-Ramírez ORCID: orcid.org/0000-0002-5805-7355 1
Nature Catalysis ( 2024 ) Cite this article
1116 Accesses
16 Altmetric
Metrics details
- Biocatalysis
- Cheminformatics
- Computational science
- Heterogeneous catalysis
- Homogeneous catalysis
Accelerating catalyst discovery and development is of paramount importance in addressing the global energy, sustainability and healthcare demands. The past decade has witnessed significant momentum in harnessing data science concepts in catalysis research to aid the aforementioned cause. Here we comprehensively review how catalysis practitioners have leveraged data-driven strategies to solve complex challenges across heterogeneous, homogeneous and enzymatic catalysis. We delineate all studies into deductive or inductive modes, and statistically infer the prevalence of catalytic tasks, model reactions, data representations and choice of algorithms. We highlight frontiers in the field and knowledge transfer opportunities among the catalysis subdisciplines. Our critical assessment reveals a glaring gap in data science exploration in experimental catalysis, which we bridge by elaborating on four pillars of data science, namely descriptive, predictive, causal and prescriptive analytics. We advocate their adoption into routine experimental workflows and underscore the importance of data standardization to spur future research in digital catalysis.
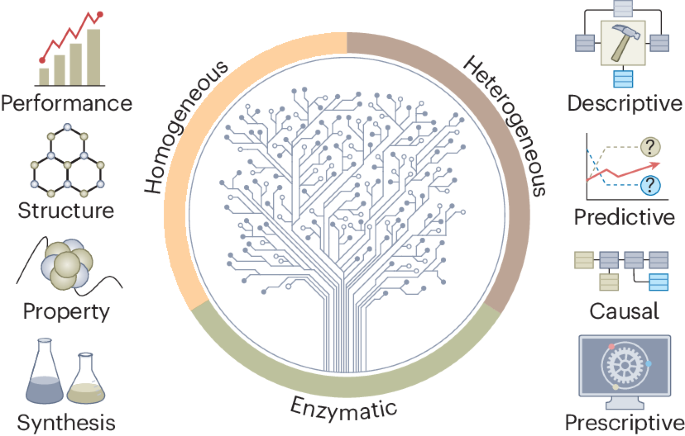
This is a preview of subscription content, access via your institution
Access options
Access Nature and 54 other Nature Portfolio journals
Get Nature+, our best-value online-access subscription
24,99 € / 30 days
cancel any time
Subscribe to this journal
Receive 12 digital issues and online access to articles
111,21 € per year
only 9,27 € per issue
Buy this article
- Purchase on Springer Link
- Instant access to full article PDF
Prices may be subject to local taxes which are calculated during checkout
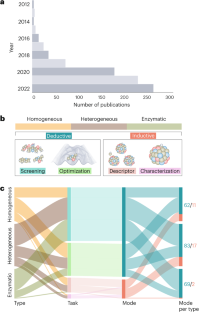
Similar content being viewed by others
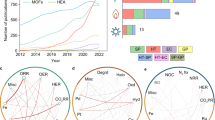
Language models and protocol standardization guidelines for accelerating synthesis planning in heterogeneous catalysis
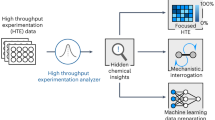
Probing the chemical ‘reactome’ with high-throughput experimentation data
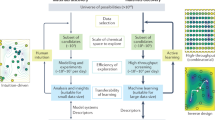
Human- and machine-centred designs of molecules and materials for sustainability and decarbonization
Vogt, C. & Weckhuysen, B. M. The concept of active site in heterogeneous catalysis. Nat. Rev. Chem. 6 , 89–111 (2022).
Article PubMed Google Scholar
Ye, R., Zhao, J., Wickemeyer, B. B., Toste, F. D. & Somorjai, G. A. Foundations and strategies of the construction of hybrid catalysts for optimized performances. Nat. Catal. 1 , 318–325 (2018).
Article Google Scholar
Copéret, C., Chabanas, M., Petroff Saint-Arroman, R. & Basset, J. M. Homogeneous and heterogeneous catalysis: bridging the gap through surface organometallic chemistry. Angew. Chem. Int. Ed. 42 , 156–181 (2003).
Ye, R., Hurlburt, T. J., Sabyrov, K., Alayoglu, S. & Somorjai, G. A. Molecular catalysis science: perspective on unifying the fields of catalysis. Proc. Natl Acad. Sci. USA 113 , 5159–5166 (2016).
Article CAS PubMed PubMed Central Google Scholar
Zhao, B., Han, Z. & Ding, K. The N-H functional group in organometallic catalysis. Angew. Chem. Int. Ed. 52 , 4744–4788 (2013).
Article CAS Google Scholar
Sheldon, R. A. & Woodley, J. M. Role of biocatalysis in sustainable chemistry. Chem. Rev. 118 , 801–838 (2018).
Article CAS PubMed Google Scholar
Munnik, P., de Jongh, P. E. & de Jong, K. P. Recent developments in the synthesis of supported catalysts. Chem. Rev. 115 , 6687–6718 (2015).
Bornscheuer, U. T. et al. Engineering the third wave of biocatalysis. Nature 485 , 185–194 (2012).
Grunwaldt, J.-D. & Schroer, C. G. Hard and soft X-ray microscopy and tomography in catalysis: bridging the different time and length scales. Chem. Soc. Rev. 39 , 4741–4753 (2010).
Meirer, F. & Weckhuysen, B. M. Spatial and temporal exploration of heterogeneous catalysts with synchrotron radiation. Nat. Rev. Mater. 3 , 324–340 (2018).
Chen, B. W. J., Xu, L. & Mavrikakis, M. Computational methods in heterogeneous catalysis. Chem. Rev. 121 , 1007–1048 (2021).
Durand, D. J. & Fey, N. Computational ligand descriptors for catalyst design. Chem. Rev. 119 , 6561–6594 (2019).
Wang, H. et al. Scientific discovery in the age of artificial intelligence. Nature 620 , 47–60 (2023).
LeCun, Y., Bengio, Y. & Hinton, G. Deep learning. Nature 521 , 436–444 (2015).
Kitchin, J. R. Machine learning in catalysis. Nat. Catal. 1 , 230–232 (2018).
Toyao, T. et al. Machine learning for catalysis informatics: recent applications and prospects. ACS Catal. 10 , 2260–2297 (2020).
Ma, X., Li, Z., Achenie, L. E. K. & Xin, H. Machine-learning-augmented chemisorption model for CO 2 electroreduction catalyst screening. J. Phys. Chem. Lett. 6 , 3528–3533 (2015).
Ahneman, D. T., Estrada, J. G., Lin, S., Dreher, S. D. & Doyle, A. G. Predicting reaction performance in C-N cross-coupling using machine learning. Science 360 , 186–190 (2018). The application of interpretable machine learning on a high-throughput Buchwald–Hartwig dataset to predict high-performing palladium catalysts and unravel their inhibition mechanism .
Kim, M. et al. Searching for an optimal multi-metallic alloy catalyst by active learning combined with experiments. Adv. Mater. 34 , 2108900 (2022).
Shields, B. J. et al. Bayesian reaction optimization as a tool for chemical synthesis. Nature 590 , 89–96 (2021). Development of Bayesian optimization on palladium-catalysed Mitsunobu and deoxyfluorination reactions where the algorithm consistently outperformed human decision-making in terms number of experiments and actual yields to optimize the process .
Wu, Z., Kan, S. B. J., Lewis, R. D., Wittmann, B. J. & Arnold, F. H. Machine learning-assisted directed protein evolution with combinatorial libraries. Proc. Natl Acad. Sci. USA 116 , 8852–8858 (2019).
Lu, H. et al. Machine learning-aided engineering of hydrolases for PET depolymerization. Nature 604 , 662–667 (2022).
Ulissi, Z. W., Medford, A. J., Bligaard, T. & Nørskov, J. K. To address surface reaction network complexity using scaling relations machine learning and DFT calculations. Nat. Commun. 8 , 14621 (2017).
Article PubMed PubMed Central Google Scholar
Li, F. et al. Deep learning-based kcat prediction enables improved enzyme-constrained model reconstruction. Nat. Catal. 5 , 662–672 (2022). A deep learning methodology to predict enzyme turnover numbers of metabolic enzymes from any organism merely from substrate structures and protein sequences .
Holeňa, M. & Baerns, M. Feedforward neural networks in catalysis: a tool for the approximation of the dependency of yield on catalyst composition and for knowledge extraction. Catal. Today 81 , 485–494 (2003). Amongst the earliest reports on applied machine learning in catalysis, wherein a feedforward neural network was used to predict propene yield based on the catalyst composition .
Baumes, L., Farrusseng, D., Lengliz, M. & Mirodatos, C. Using artificial neural networks to boost high-throughput discovery in heterogeneous catalysis. QSAR Comb. Sci. 23 , 767–778 (2004).
Burello, E., Farrusseng, D. & Rothenberg, G. Combinatorial explosion in homogeneous catalysis: screening 60,000 cross-coupling reactions. Adv. Synth. Catal. 346 , 1844–1853 (2004).
Corma, A. et al. Optimisation of olefin epoxidation catalysts with the application of high-throughput and genetic algorithms assisted by artificial neural networks (softcomputing techniques). J. Catal. 229 , 513–524 (2005).
Venkatasubramanian, V. The promise of artificial intelligence in chemical engineering: is it here, finally? AIChE J. 65 , 466–478 (2019).
Pyzer-Knapp, E. O. et al. Accelerating materials discovery using artificial intelligence, high performance computing and robotics. NPJ Comput. Mater. 8 , 84 (2022).
Pedregosa, F. et al. Scikit-learn: machine learning in Python. J. Mach. Learn. Res. 12 , 2825–2830 (2011).
Google Scholar
RDKit; https://www.rdkit.org/
Chanussot, L. et al. Open catalyst 2020 (OC20) dataset and community challenges. ACS Catal. 11 , 6059–6072 (2021). The most extensive database consisting of close to 1.3 million density DFT relaxations across a wide swath of materials, surfaces and adsorbates (nitrogen, carbon and oxygen chemistries) for application in heterogeneous catalysis .
Kearnes, S. M. et al. The open reaction database. J. Am. Chem. Soc. 143 , 18820–18826 (2021).
Yano, J. et al. The case for data science in experimental chemistry: examples and recommendations. Nat. Rev. Chem. 6 , 357–370 (2022).
Schlexer Lamoureux, P. et al. Machine learning for computational heterogeneous catalysis. ChemCatChem 11 , 3581–3601 (2019).
Medford, A. J., Kunz, M. R., Ewing, S. M., Borders, T. & Fushimi, R. Extracting knowledge from data through catalysis informatics. ACS Catal. 8 , 7403–7429 (2018).
Maldonado, A. G. & Rothenberg, G. Predictive modeling in homogeneous catalysis: a tutorial. Chem. Soc. Rev. 39 , 1891–1902 (2010).
Mazurenko, S., Prokop, Z. & Damborsky, J. Machine learning in enzyme engineering. ACS Catal. 10 , 1210–1223 (2020).
Suvarna, M. & Pérez-Ramírez, J. Dataset: Embracing Data Science in Catalysis Research (Zenodo, 2024); https://doi.org/10.5281/zenodo.10640876
Zahrt, A. F. et al. Prediction of higher-selectivity catalysts by computer-driven workflow and machine learning. Science 363 , eaau5631 (2019). The study models multiple conformations of more than 800 prospective catalysts for the coupling reaction of imines and thiols, and trained machine learning algorithms on a subset of experimental results, to achieve highly accurate predictions of enantioselectivities .
Nguyen, T. N. et al. High-throughput experimentation and catalyst informatics for oxidative coupling of methane. ACS Catal. 10 , 921–932 (2020).
Tran, K. & Ulissi, Z. W. Active learning across intermetallics to guide discovery of electrocatalysts for CO 2 reduction and H 2 evolution. Nat. Catal. 1 , 696–703 (2018). A fully automated screening method developed by integrating machine learning and optimization algorithms to guide DFT calculations, for in silico prediction of electrocatalyst performance for CO 2 reduction and H 2 evolution .
Wang, G. et al. Accelerated discovery of multi-elemental reverse water–gas shift catalysts using extrapolative machine learning approach. Nat. Commun. 14 , 5861 (2023).
Amar, Y., Schweidtmann, A. M., Deutsch, P., Cao, L. & Lapkin, A. Machine learning and molecular descriptors enable rational solvent selection in asymmetric catalysis. Chem. Sci. 10 , 6697–6706 (2019).
Rinehart, N. I. et al. A machine-learning tool to predict substrate-adaptive conditions for Pd-catalyzed C-N couplings. Science 381 , 965–972 (2023).
Schweidtmann, A. M. et al. Machine learning meets continuous flow chemistry: automated optimization towards the pareto front of multiple objectives. Chem. Eng. J. 352 , 277–282 (2018).
O’Connor, N. J., Jonayat, A. S. M., Janik, M. J. & Senftle, T. P. Interaction trends between single metal atoms and oxide supports identified with density functional theory and statistical learning. Nat. Catal. 1 , 531–539 (2018).
Foppa, L. et al. Materials genes of heterogeneous catalysis from clean experiments and artificial intelligence. MRS Bull. 46 , 1016–1026 (2021).
Zhao, S. et al. Enantiodivergent Pd-catalyzed C-C bond formation enabled through ligand parameterization. Science 362 , 670–674 (2018).
Timoshenko, J., Lu, D., Lin, Y. & Frenkel, A. I. Supervised machine-learning-based determination of three-dimensional structure of metallic nanoparticles. J. Phys. Chem. Lett. 8 , 5091–5098 (2017). Application of deep learning to solve metal catalyst from XANES, broadly applicable to the determination of nanoparticle structures in operando studies and generalizable to other nanoscale systems .
Zheng, C. et al. Automated generation and ensemble-learned matching of X-ray absorption spectra. NPJ Comput. Mater. 4 , 12 (2018).
Mitchell, S. et al. Automated image analysis for single-atom detection in catalytic materials by transmission electron microscopy. J. Am. Chem. Soc. 144 , 8018–8029 (2022).
Büchler, J. et al. Algorithm-aided engineering of aliphatic halogenase WelO5* for the asymmetric late-stage functionalization of soraphens. Nat. Commun. 13 , 371 (2022).
Wulf, C. et al. A unified research data infrastructure for catalysis research - challenges and concepts. ChemCatChem 13 , 3223–3236 (2021).
Mendes, P. S. F., Siradze, S., Pirro, L. & Thybaut, J. W. Open data in catalysis: from today’s big picture to the future of small data. ChemCatChem 13 , 836–850 (2021).
Marshall, C. P., Schumann, J. & Trunschke, A. Achieving digital catalysis: strategies for data acquisition, storage and use. Angew. Chem. Int. Ed. 62 , e202302971 (2023).
Zavyalova, U., Holena, M., Schlögl, R. & Baerns, M. Statistical analysis of past catalytic data on oxidative methane coupling for new insights into the composition of high-performance catalysts. ChemCatChem 3 , 1935–1947 (2011).
Odabasi, C., Gunay, M. E. & Yildrim, R. Knowledge extraction for water gas shift reaction over noble metal catalysts from publications in the literature between 2002 and 2012. Int. J. Hydrog. Energy 39 , 5733–5746 (2014).
Suvarna, M., Araújo, T. P. & Pérez-Ramírez, J. A generalized machine learning framework to predict the space-time yield of methanol from thermocatalytic CO 2 hydrogenation. Appl. Catal. B Environ. 315 , 121530 (2022).
Mamun, O., Winther, K. T., Boes, J. R. & Bligaard, T. High-throughput calculations of catalytic properties of bimetallic alloy surfaces. Sci. Data 6 , 76 (2019).
Jinnouchi, R. & Asahi, R. Predicting catalytic activity of nanoparticles by a DFT-aided machine-learning algorithm. J. Phys. Chem. Lett. 8 , 4279–4283 (2017).
Berman, H. M. et al. The protein data bank. Nucleic Acids Res. 28 , 235–242 (2000).
Mitchell, A. L. et al. InterPro in 2019: improving coverage, classification and access to protein sequence annotations. Nucleic Acids Res. 47 , D351–D360 (2019).
UniProt Consortium. UniProt: a worldwide hub of protein knowledge. Nucleic Acids Res. 47 , D506–D515 (2019).
Schomburg, I., Chang, A. & Schomburg, D. BRENDA, enzyme data and metabolic information. Nucleic Acids Res. 30 , 47–49 (2002).
Nagano, N. EzCatDB: the enzyme catalytic-mechanism database. Nucleic Acids Res. 33 , D407–D412 (2005).
Finnigan, W. et al. RetroBioCat as a computer-aided synthesis planning tool for biocatalytic reactions and cascades. Nat. Catal. 4 , 98–104 (2021).
Winther, K. T. et al. Catalysis-Hub.org, an open electronic structure database for surface reactions. Sci. Data 6 , 75 (2019).
Álvarez-Moreno, M. et al. Managing the computational chemistry big data problem: the ioChem-BD platform. J. Chem. Inf. Model. 55 , 95–103 (2015).
Gensch, T. et al. A comprehensive discovery platform for organophosphorus ligands for catalysis. J. Am. Chem. Soc. 144 , 1205–1217 (2022).
Bengio, Y., Courville, A. & Vincent, P. Representation learning: a review and new perspectives. IEEE Trans. Pattern Anal. Mach. Intell. 35 , 1798–1828 (2013).
Schmidt, J., Marques, M. R. G., Botti, S. & Marques, M. A. L. Recent advances and applications of machine learning in solid-state materials science. NPJ Comput. Mater. 5 , 83 (2019).
Sanchez-Lengeling, B. & Aspuru-Guzik, A. Inverse molecular design using machine learning: generative models for matter engineering. Science 361 , 360–365 (2018).
Mitchell, J. B. O. Machine learning methods in chemoinformatics. WIREs Comput. Mol. Sci. 4 , 468–481 (2014).
Wigh, D. S., Goodman, J. M. & Lapkin, A. A. A review of molecular representation in the age of machine learning. WIREs Comput. Mol. Sci. 12 , e1603 (2022).
Krenn, M., Häse, F., Nigam, A., Friederich, P. & Aspuru-Guzik, A. Self-referencing embedded strings (SELFIES): a 100% robust molecular string representation. Mach. Learn. Sci. Technol. 1 , 045024 (2020).
Kononova, O. et al. Text-mined dataset of inorganic materials synthesis recipes. Sci. Data 6 , 203 (2019).
Olivetti, E. A. et al. Data-driven materials research enabled by natural language processing and information extraction. Appl. Phys. Rev. 7 , 041317 (2020).
Kim, E. et al. Materials synthesis insights from scientific literature via text extraction and machine learning. Chem. Mater. 29 , 9436–9444 (2017).
Jensen, Z. et al. A machine learning approach to zeolite synthesis enabled by automatic literature data extraction. ACS Cent. Sci. 5 , 892–899 (2019).
Luo, Y. et al. MOF synthesis prediction enabled by automatic data mining and machine learning. Angew. Chem. Int. Ed. 61 , e202200242 (2022).
Zheng, Z., Zhang, O., Borgs, C., Chayes, J. T. & Yaghi, O. M. ChatGPT chemistry assistant for text mining and the prediction of MOF synthesis. J. Am. Chem. Soc. 145 , 18048–18062 (2023).
Suvarna, M., Vaucher, A. C., Mitchell, S., Laino, T. & Pérez-Ramírez, J. Language models and protocol standardization guidelines for accelerating synthesis planning in heterogeneous catalysis. Nat. Commun. 14 , 7964 (2023).
Lai, N. S. et al. Artificial intelligence (AI) workflow for catalyst design and optimization. Ind. Eng. Chem. Res. 62 , 17835–17848 (2023).
Probst, D. et al. Biocatalysed synthesis planning using data-driven learning. Nat. Commun. 13 , 964 (2022).
Moon, J. et al. Active learning guides discovery of a champion four-metal perovskite oxide for oxygen evolution electrocatalysis. Nat. Mater. 23 , 108–115 (2024).
Zhong, M. et al. Accelerated discovery of CO 2 electrocatalysts using active machine learning. Nature 581 , 178–183 (2020). Discovery of Cu-Al electrocatalysts, though DFT aided machine learning, to efficiently reduce CO 2 to ethylene with a Faradaic efficiency of 80% .
Torres, J. A. G. et al. A multi-objective active learning platform and web app for reaction optimization. J. Am. Chem. Soc. 144 , 19999–20007 (2022).
Greenhalgh, J. C., Fahlberg, S. A., Pfleger, B. F. & Romero, P. A. Machine learning-guided acyl-ACP reductase engineering for improved in vivo fatty alcohol production. Nat. Commun. 12 , 5825 (2021).
Tallorin, L. et al. Discovering de novo peptide substrates for enzymes using machine learning. Nat. Commun. 9 , 5253 (2018).
Schwaller, P. et al. Molecular transformer: a model for uncertainty-calibrated chemical reaction prediction. ACS Cent. Sci. 5 , 1572–1583 (2019).
Anstine, D. M. & Isayev, O. Generative models as an emerging paradigm in the chemical sciences. J. Am. Chem. Soc. 145 , 8736–8750 (2023).
Gómez-Bombarelli, R. et al. Automatic chemical design using a data-driven continuous representation of molecules. ACS Cent. Sci. 4 , 268–276 (2018). A method to convert discrete representations of molecules into multidimensional continuous representations for generating compounds in silico .
Repecka, D. et al. Expanding functional protein sequence spaces using generative adversarial networks. Nat. Mach. Intell. 3 , 324–333 (2021).
Hawkins-Hooker, A. et al. Generating functional protein variants with variational autoencoders. PLoS Comput. Biol. 17 , e1008736 (2021).
Johnson, S. R. et al. Computational scoring and experimental evaluation of enzymes generated by neural networks. Preprint at https://www.biorxiv.org/content/10.1101/2023.03.04.531015v1 (2023).
Schilter, O., Vaucher, A., Schwaller, P. & Laino, T. Designing catalysts with deep generative models and computational data. A case study for Suzuki cross coupling reactions. Digit. Discov. 2 , 728–735 (2023).
Kreutter, D., Schwaller, P. & Reymond, J.-L. Predicting enzymatic reactions with a molecular transformer. Chem. Sci. 12 , 8648–8659 (2021).
Popova, M., Isayev, O. & Tropsha, A. Deep reinforcement learning for de novo drug design. Sci. Adv. 4 , eaap7885 (2018).
Zhou, Z., Li, X. & Zare, R. N. Optimizing chemical reactions with deep reinforcement learning. ACS Cent. Sci. 3 , 1337–1344 (2017). A fully automated deep reinforcement learning to optimize chemical reactions where the model iteratively records the results of a chemical reaction and chooses new experimental conditions to improve the reaction outcome .
Lan, T. & An, Q. Discovering catalytic reaction networks using deep reinforcement learning from first-principles. J. Am. Chem. Soc. 143 , 16804–16812 (2021).
Song, Z. et al. Adaptive design of alloys for CO 2 activation and methanation via reinforcement learning Monte Carlo tree search algorithm. J. Phys. Chem. Lett. 14 , 3594–3601 (2023).
Suvarna, M., Preikschas, P. & Pérez-Ramírez, J. Identifying descriptors for promoted rhodium-based catalysts for higher alcohol synthesis via machine learning. ACS Catal. 12 , 15373–15385 (2022).
Smith, A., Keane, A., Dumesic, J. A., Huber, G. W. & Zavala, V. M. A machine learning framework for the analysis and prediction of catalytic activity from experimental data. Appl. Catal. B Environ. 263 , 118257 (2020).
Vellayappan, K. et al. Impacts of catalyst and process parameters on Ni-catalyzed methane dry reforming via interpretable machine learning. Appl. Catal. B Environ. 330 , 122593 (2023).
Roh, J. et al. Interpretable machine learning framework for catalyst performance prediction and validation with dry reforming of methane. Appl. Catal. B Environ. 343 , 123454 (2024).
McCullough, K., Williams, T., Mingle, K., Jamshidi, P. & Lauterbach, J. High-throughput experimentation meets artificial intelligence: a new pathway to catalyst discovery. Phys. Chem. Chem. Phys. 22 , 11174–11196 (2020).
Suzuki, K. et al. Statistical analysis and discovery of heterogeneous catalysts based on machine learning from diverse published data. ChemCatChem 11 , 4537–4547 (2019).
Butler, K. T., Davies, D. W., Cartwright, H., Isayev, O. & Walsh, A. Machine learning for molecular and materials science. Nature 559 , 547–555 (2018).
Oviedo, F., Ferres, J. L., Buonassisi, T. & Butler, K. T. Interpretable and explainable machine learning for materials science and chemistry. Acc. Mater. Res. 3 , 597–607 (2022).
Esterhuizen, J. A., Goldsmith, B. R. & Linic, S. Interpretable machine learning for knowledge generation in heterogeneous catalysis. Nat. Catal. 5 , 175–184 (2022).
Wu, K. & Doyle, A. G. Parameterization of phosphine ligands demonstrates enhancement of nickel catalysis via remote steric effects. Nat. Chem. 9 , 779–784 (2017).
Weng, B. et al. Simple descriptor derived from symbolic regression accelerating the discovery of new perovskite catalysts. Nat. Commun. 11 , 3513 (2020).
Ouyang, R., Curtarolo, S., Ahmetcik, E., Scheffler, M. & Ghiringhelli, L. M. SISSO: a compressed-sensing method for identifying the best low-dimensional descriptor in an immensity of offered candidates. Phys. Rev. Mater. 2 , 083802 (2018).
Foppa, L. et al. Data-centric heterogeneous catalysis: identifying rules and materials genes of alkane selective oxidation. J. Am. Chem. Soc. 145 , 3427–3442 (2023).
Li, Z., Ma, X. & Xin, H. Feature engineering of machine-learning chemisorption models for catalyst design. Catal. Today 280 , 232–238 (2017).
Lundberg, S. M. et al. From local explanations to global understanding with explainable AI for trees. Nat. Mach. Intell. 2 , 56–67 (2020).
Timoshenko, J. et al. Linking the evolution of catalytic properties and structural changes in copper-zinc nanocatalysts using operando EXAFS and neural-networks. Chem. Sci. 11 , 3727–3736 (2020).
Wilkinson, M. D. et al. The FAIR guiding principles for scientific data management and stewardship. Sci. Data 3 , 160018 (2016).
Scheffler, M. et al. FAIR data enabling new horizons for materials research. Nature 604 , 635–642 (2022).
Abolhasani, M. & Kumacheva, E. The rise of self-driving labs in chemical and materials sciences. Nat. Synth. 2 , 483–492 (2023). A review of self-driving labs through the integration of machine learning, lab automation and robotics to accelerate digital data curation and enable data-driven discoveries in chemical sciences .
MacLeod, B. P. et al. Self-driving laboratory for accelerated discovery of thin-film materials. Sci. Adv. 6 , eaaz8867 (2020).
Download references
Acknowledgements
This study was created as part of NCCR Catalysis (grant no. 180544), a National Centre of Competence in Research funded by the Swiss National Science Foundation. We thank C. Ko, M. E. Usteri, T. Zou and P. Preikschas for fruitful discussions on the manuscript and help with illustrations.
Author information
Authors and affiliations.
Institute for Chemical and Bioengineering, Department of Chemistry and Applied Biosciences, ETH Zurich, Zurich, Switzerland
Manu Suvarna & Javier Pérez-Ramírez
You can also search for this author in PubMed Google Scholar
Contributions
M.S. and J.P.-R. conceived the project. M.S. led the data collection and analysis efforts, and wrote the manuscript. J.P.-R. supervised the project, wrote the manuscript, and managed resources and funding. Both authors provided input to the manuscript and approved the final version.
Corresponding author
Correspondence to Javier Pérez-Ramírez .
Ethics declarations
Competing interests.
The authors declare no competing interests.
Additional information
Publisher’s note Springer Nature remains neutral with regard to jurisdictional claims in published maps and institutional affiliations.
Supplementary information
Supplementary information.
Supplementary Notes 1 and 2, Tables 1–3 and Fig. 1.
Source data
41929_2024_1150_moesm2_esm.xlsx.
Source Data Fig. 1a Raw data for creating timeline plot of research trends in data-driven catalysis. Source Data Fig. 1c Raw data for creating alluvial plots linking catalysis subdisciplines with different tasks and modes. Source Data Fig. 2a Raw data for network plot mapping relations of deductive tasks based on catalysis type. Source Data Fig. 2b Raw data for network plot mapping relations of deductive tasks based on driving. Source Data Fig. 4 Raw data for establishing structure-property-performance relations through ML.
Rights and permissions
Springer Nature or its licensor (e.g. a society or other partner) holds exclusive rights to this article under a publishing agreement with the author(s) or other rightsholder(s); author self-archiving of the accepted manuscript version of this article is solely governed by the terms of such publishing agreement and applicable law.
Reprints and permissions
About this article
Cite this article.
Suvarna, M., Pérez-Ramírez, J. Embracing data science in catalysis research. Nat Catal (2024). https://doi.org/10.1038/s41929-024-01150-3
Download citation
Received : 08 December 2023
Accepted : 29 February 2024
Published : 23 April 2024
DOI : https://doi.org/10.1038/s41929-024-01150-3
Share this article
Anyone you share the following link with will be able to read this content:
Sorry, a shareable link is not currently available for this article.
Provided by the Springer Nature SharedIt content-sharing initiative

Quick links
- Explore articles by subject
- Guide to authors
- Editorial policies
Sign up for the Nature Briefing: AI and Robotics newsletter — what matters in AI and robotics research, free to your inbox weekly.


Maintenance work is planned for Wednesday 1st May 2024 from 9:00am to 11:00am (BST).
During this time, the performance of our website may be affected - searches may run slowly and some pages may be temporarily unavailable. If this happens, please try refreshing your web browser or try waiting two to three minutes before trying again.
We apologise for any inconvenience this might cause and thank you for your patience.
Catalysis Science & Technology
Synthesis of a sacubitril precursor via the construction of one-pot chemoenzymatic cascades †.

* Corresponding authors
a School of Pharmacy, Changzhou University, Changzhou 213164, Jiangsu, P. R. China E-mail: [email protected]
b Tianjin Institute of Industrial Biotechnology, Chinese Academy of Sciences, Tianjin 300308, P. R. China E-mail: [email protected]
c Key Laboratory of Engineering Biology for Low-Carbon Manufacturing, Chinese Academy of Sciences, 32 West 7th Avenue, Tianjin Airport Economic Area, Tianjin 300308, P. R. China
d National Innovation Center for Synthetic Biotechnology, 32 West 7th Avenue, Tianjin 300308, P. R. China
Enzymatic cascade synthesis of valuable chiral pharmaceutical intermediates has attracted attention owing to efficient and environmentally friendly routes. Currently, the synthesis of a key precursor of sacubitril valsartan sodium hydrate LCZ696 proceeds via separate steps of metal-catalyzed asymmetric hydrogenation and enzymatic transamination. Herein, we present a one-pot enzymatic cascade strategy for the construction of two chiral centers without the separation of intermediates. Upon enzyme discovery and identification, an ene-reductase Go ER from Gluconobacter oxydans was incorporated in one-pot cascades with enzymatic transamination. Three different biocatalytic cascades were developed, utilizing whole cells co-expressing two enzymes, a mixture of whole cells expressing a single enzyme, and purified enzymes, affording a key intermediate in up to 87% yield and 99% de. More importantly, the scale-up preparation afforded 68.5% isolated yield with excellent diastereoselectivity. This study not only offers a sustainable alternative but also paves the way for the scalable production of a key sacubitril precursor.
Supplementary files
- Supplementary information PDF (2717K)
- Crystal structure data CIF (863K)
Article information
Download citation, permissions.

Synthesis of a sacubitril precursor via the construction of one-pot chemoenzymatic cascades
L. Chen, G. Qu, Z. Cai, B. Yuan and Z. Sun, Catal. Sci. Technol. , 2024, Advance Article , DOI: 10.1039/D4CY00145A
To request permission to reproduce material from this article, please go to the Copyright Clearance Center request page .
If you are an author contributing to an RSC publication, you do not need to request permission provided correct acknowledgement is given.
If you are the author of this article, you do not need to request permission to reproduce figures and diagrams provided correct acknowledgement is given. If you want to reproduce the whole article in a third-party publication (excluding your thesis/dissertation for which permission is not required) please go to the Copyright Clearance Center request page .
Read more about how to correctly acknowledge RSC content .
Social activity
Search articles by author.
This article has not yet been cited.
Advertisements
Structure and Properties of Dysprosium Titanate Powder Produced by the Mechanochemical Method
- Production Processes and Properties of Powders
- Published: 19 June 2016
- Volume 59 , pages 304–310, ( 2018 )
Cite this article
- Zh. V. Eremeeva 1 ,
- V. S. Panov 1 ,
- L. V. Myakisheva 1 ,
- A. N. Lizunov 2 ,
- A. A. Nepapushev 1 ,
- D. A. Sidorenko 1 ,
- A. V. Pavlik 1 &
- E. V. Apostolova 1
54 Accesses
4 Citations
Explore all metrics
The structure and main physicochemical properties of dysprosium titanate powders prepared by mechanochemical synthesis from the low-temperature modification of titanium oxide and modification of dysprosium oxide are investigated applying X-ray phase analysis (XPA), scanning electron microscopy, Raman spectroscopy (Raman spectra), transmission electron microscopy, and chemical analysis. It is established based on XPA that the initial oxides completely transform into X-ray amorphous dysprosium titanate (Dy 2 TiO 5 ) during the mechanochemical treatment of a mixture for 30–60 min. A microelectron diffraction pattern of Dy 2 TiO 5 powders prepared by mechanosynthesis has a ring structure characteristic of the X-ray amorphous phase with a certain amount of inclusions of a crystalline phase. The dysprosium titanate powder fabricated by induction melting possesses the regular cubic crystalline lattice with a parameter of 3.4 Å.
This is a preview of subscription content, log in via an institution to check access.
Access this article
Price includes VAT (Russian Federation)
Instant access to the full article PDF.
Rent this article via DeepDyve
Institutional subscriptions
Similar content being viewed by others
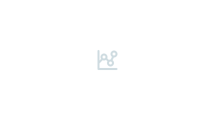
Mechanochemical Synthesis of Dy2TiO5 Single-Phase Crystalline Nanopowders and Investigation of Their Properties
Structural transformations of tio2 during mechanical activation and subsequent annealing.
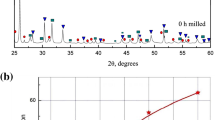
Mechanochemical Synthesis of PZT Powders and the Effects of Mechanical Activation on Solid-State Sintering Kinetics
Sickafus, K.E., Grimes, R.W., Valdez, J.A., Cleave, A., Ming, Tang., Ishimaru, Manabu., Corish Siobhan, M., Stanek, Ch.R., and Uberuaga, B.P., Radiationinduced amorphization resistance and radiation tolerance in structurally related oxides, Nature Mater. , 2007, no. 6, pp. 217–223.
Article Google Scholar
Risovanny, V.D., Varlashova, E.E., Fridman, S.R., Ponomarenko, V.B., and Shcheglov, A.V., Comparative characteristics of absorbing cluster assemblies WWER-1000 and PWR, Atom. Energ. , 1998, vol. 84, no. 6, pp. 508–513.
Google Scholar
Belash, N.N., Kushtym, A.V., Tatarinov, V.R., and Chernov, I.A., Analysis of developments of constructions and materials for CPS ARabsorber elements with the increased efficiency, Yadern. Radiats. Tekhnol. , 2007, vol. 7, nos. 3–4, pp. 18–28.
Risovanny, V.D., Zakharov, A.V., and Muraleva, E.M., New advanced absorbent materials for nuclear reactors on thermal neutrons, Vopr. Atom. Nauki Tekh. Ser: Fiz. Radiats. Povrezhd. Radiats. Materialoved. , 2005, no. 3, pp. 87–93.
Risovanny, V.D., Zakharov, A.V., Muraleva, E.M., Kosenkov, V.M., and Latypov, R.N., Dysprozium hafnate as absorbing material for control rods, J. Nucl. Mater. , 2006, vol. 355, pp. 163–170.
Fridman, S.R., Risovanny, V.D., Zakharov, A.V., and Toporova, V.G., Radiation stability of WWER-1000 CPS ARabsorber element with boron carbide, Vopr. Atom. Nauki Tekh. Ser: Fiz. Radiats. Povrezhd. Radiats. Materialoved. , 2001, no. 2, pp. 84–90.
Perova, E.B., Spiridonov, L.N., and Komisarova, L.N., Phase equilibria in the HfO2–Dy2O3 system, Izv. Akad. Nauk SSSR. Neorg. Mater. , 1982, vol. 8, no. 10, pp. 1878–1882.
Makhmudov, F.A., Shaimardanov, E.N., and Kabgov, Kh.B., Preparation and properties of nanostructured dysprosium oxides, Dokl. Akad. Nauk Resp. Tadzhikistan , 2013, vol. 56, no. 2, pp. 130–134.
Sinha, A. and Sharma, B.P., Development of dysprosium titanate based ceramics, J. Amer. Ceram. Soc. , 2005, no. 2, pp. 238–241.
Khalameida, S.V., Some new approaches to mechanochemical synthesis of nanodispersed barium titanate, Nanosist., Nanomater., Nanotechnol. (Ukraina) , 2009, vol. 7, no. 3, pp. 911–918.
Xue, J., Wang, J., and Wan, D., Nanosized barium titanate powder by mechanical activation, J. Amer. Ceram. Soc. , 2000, vol. 83, no. 1, pp. 232–234.
Lyashenko, L.P., Shcherbakova, L.G., Kolbanev, I.V., Knerel’man, E.I., and Davydova, G.I., Mechanism of structure formation in samarium and holmium titanates prepared from mechanically activated oxides, Inorg. Mater. , 2007, vol. 43, no. 1, pp. 46–54.
Szafraniak-Wiza, I., Hilczer, B., Talik, E., Pietraszko, A., and Malic, B., Ferroelectric perovskite nanopowders obtained by mechanochemical synthesis, Process. Appl. Ceram. , 2010, vol. 4, no. 3, pp. 99–106.
Anokhin, A.S., Lyanguzov, N.V., Roshal’, S.B., Yuzyuk, Yu.I., and Wen Wang, Raman spectra of polycrystalline bismuth titanate nanotubes, Phys. Solid State , 2011, vol. 53, no. 9, pp. 1867–1871.
Shindo, D. and Oikawa, T., Analytical Electron Microscopy for Materials Science , Tokyo: Springer, 2002.
Book Google Scholar
Brandon, D. and Kaplan, W.D., Microstructural Characterization of Materials, Wiley , 2002, 2nd ed.
Sidorova, O.V., Aleshina, L.A., and Kalinkin, A.M., Influence of mechanical activation of the structural state of strontium titanate, Fundam. Issled. , 2014, no. 12–2, pp. 280–288. https://doi.org/www.fundamentalresearch.ru/ru/article/view?id=36218 (accessed: May 17, 2016).
Download references
Author information
Authors and affiliations.
National University of Science and Technology “MISiS”, Moscow, 119049, Russia
Zh. V. Eremeeva, V. S. Panov, L. V. Myakisheva, A. A. Nepapushev, D. A. Sidorenko, A. V. Pavlik & E. V. Apostolova
PAO ELEMASh, Elektrostal’, Moscow oblast, 144001, Russia
A. N. Lizunov
You can also search for this author in PubMed Google Scholar
Corresponding author
Correspondence to Zh. V. Eremeeva .
Additional information
Original Russian Text © Zh.V. Eremeeva, V.S. Panov, L.V. Myakisheva, A.N. Lizunov, A.A. Nepapushev, D.A. Sidorenko, A.V. Pavlik, E.V. Apostolova, 2017, published in Izvestiya Vysshikh Uchebnykh Zavedenii, Poroshkovaya Metallurgiya i Funktsional’nye Pokrytiya, 2017, No. 1, pp. 11–19.
About this article
Eremeeva, Z.V., Panov, V.S., Myakisheva, L.V. et al. Structure and Properties of Dysprosium Titanate Powder Produced by the Mechanochemical Method. Russ. J. Non-ferrous Metals 59 , 304–310 (2018). https://doi.org/10.3103/S1067821218030045
Download citation
Received : 01 June 2016
Accepted : 15 June 2016
Published : 19 June 2016
Issue Date : May 2018
DOI : https://doi.org/10.3103/S1067821218030045
Share this article
Anyone you share the following link with will be able to read this content:
Sorry, a shareable link is not currently available for this article.
Provided by the Springer Nature SharedIt content-sharing initiative
- titanium and dysprosium oxides
- mechanochemical synthesis
- X-ray amorphous dysprosium titanate
- absorbing elements
- electron microscopy
- specific surface area
- bulk density
- Find a journal
- Publish with us
- Track your research
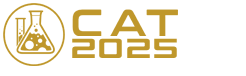
Victor Mukhin
- Scientific Program
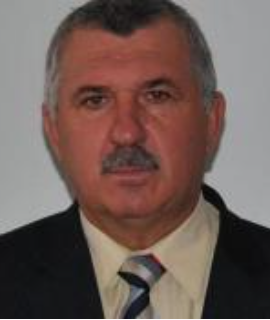
Title : Active carbons as nanoporous materials for solving of environmental problems
However, up to now, the main carriers of catalytic additives have been mineral sorbents: silica gels, alumogels. This is obviously due to the fact that they consist of pure homogeneous components SiO2 and Al2O3, respectively. It is generally known that impurities, especially the ash elements, are catalytic poisons that reduce the effectiveness of the catalyst. Therefore, carbon sorbents with 5-15% by weight of ash elements in their composition are not used in the above mentioned technologies. However, in such an important field as a gas-mask technique, carbon sorbents (active carbons) are carriers of catalytic additives, providing effective protection of a person against any types of potent poisonous substances (PPS). In ESPE “JSC "Neorganika" there has been developed the technology of unique ashless spherical carbon carrier-catalysts by the method of liquid forming of furfural copolymers with subsequent gas-vapor activation, brand PAC. Active carbons PAC have 100% qualitative characteristics of the three main properties of carbon sorbents: strength - 100%, the proportion of sorbing pores in the pore space – 100%, purity - 100% (ash content is close to zero). A particularly outstanding feature of active PAC carbons is their uniquely high mechanical compressive strength of 740 ± 40 MPa, which is 3-7 times larger than that of such materials as granite, quartzite, electric coal, and is comparable to the value for cast iron - 400-1000 MPa. This allows the PAC to operate under severe conditions in moving and fluidized beds. Obviously, it is time to actively develop catalysts based on PAC sorbents for oil refining, petrochemicals, gas processing and various technologies of organic synthesis.
Victor M. Mukhin was born in 1946 in the town of Orsk, Russia. In 1970 he graduated the Technological Institute in Leningrad. Victor M. Mukhin was directed to work to the scientific-industrial organization "Neorganika" (Elektrostal, Moscow region) where he is working during 47 years, at present as the head of the laboratory of carbon sorbents. Victor M. Mukhin defended a Ph. D. thesis and a doctoral thesis at the Mendeleev University of Chemical Technology of Russia (in 1979 and 1997 accordingly). Professor of Mendeleev University of Chemical Technology of Russia. Scientific interests: production, investigation and application of active carbons, technological and ecological carbon-adsorptive processes, environmental protection, production of ecologically clean food.
Quick Links
- Conference Brochure
- Tentative Program


IMAGES
VIDEO
COMMENTS
Catal. 2024, 366, 1331-1340; DOI: 10.1002/adsc.202301037) The front cover picture, made by the graphic designer Isabela Guzman (São Paulo - Brazil) and used with permission, illustrates a Δ 9 -tetrahydrocannabinol (THC) molecule surrounded by a pair of hands and by leaves from Cannabis sativa, the plant from which THC originates.
A leading organic chemistry and catalysis journal that publishes original research and reviews. It aims to inspire a new era of chemical science and interdisciplinary collaboration for a better quality of life.
Browse the latest issues of Advanced Synthesis and Catalysis, a peer-reviewed journal covering all aspects of organic synthesis and catalysis. Find articles on topics such as green chemistry, natural products, drug discovery, and more.
Advanced Synthesis & Catalysis. Download Product Flyer; Permissions; Advanced Synthesis & Catalysis. Edited By:Joe P. Richmond, Chairman of the Editorial Board Eric N. Jacobsen. Online ISSN: 1615-4169. Journals Journal. View on Wiley Online Library. Download Product Flyer ...
Photoredox‐Catalyzed Carbon Radical Generation from α‐Keto‐N,O‐acetals: Synthesis of Functionalized Azepino[1,2‐a]indoles and Azepino[1,2‐a]furo[3,2‐b]indoles Paul Seefeldt, Alexander Villinger, Malte Brasholz
Advanced Synthesis & Catalysis (ASC) is the leading primary journal in organic, organometallic, and applied chemistry. The high impact of ASC can be attributed to the unique focus of the journal, which publishes exciting new results from academic and industrial labs on efficient, practical, and environmentally friendly organic synthesis. While ...
Advanced Synthesis & Catalysis (ASC) is the leading primary journal in organic, organometallic, and applied chemistry. The high impact of ASC can be attributed to the unique focus of the journal, which publishes exciting new results from academic and industrial labs on efficient, practical, and environmentally friendly organic synthesis. ...
A peer-reviewed journal that publishes research on efficient and practical organic synthesis and catalysis for sustainable development. It covers topics such as organometallic, polymer, asymmetric, biocatalytic, and applied synthesis, as well as process research and biotechnology.
Language models based on transformer architectures (such as GPT-3.5 or GPT-4) are expected to play a crucial role in expediting chemistry and catalysis research. Their advanced natural language ...
A peer-reviewed journal that publishes original research articles on various topics of organic synthesis and catalysis. The latest issue covers topics such as hetero- and hydro-trifluoromethylations, stereoselective three-component construction, deoxygenative hydrogenation, dihydropyrazolo synthesis, alkynylation, cycloaddition, alkylation, alkynylation, alkylation, alkynylation, alkylation, alkynylation, alkylation, alkynylation, alkylation, alkynylation, alkylation, alkynylation, alkylation, alkynylation, alkylation, alkynylation, alkylation, alkynylation, alkylation, alkynylation, alkylation, alkynylation, alkylation, alkynylation, alkylation, alkynylation, alkylation, alkynylation, alkylation, alkynyl
The unique properties of carbon nanotubes, a new class of nanomaterials, make them usable as catalyst supports for various reactions. A pilot reactor has been constructed for producing nanotubes. The nanotubes obtained in this reactor have displayed high performance in a number of catalytic processes. A continuous laboratory-scale reactor for the synthesis of binary and mixed oxide nanosized ...
Catal. 2024, 366, 544-547; DOI: 10.1002/adsc.202400048) The front cover illustrates the streamlined synthesis of representative non-alternant polycyclic aromatic hydrocarbons through Lewis acid-catalyzed Prins-type cycloaromatization (on the earth).
On the Cover. The front cover image illustrates the oxidation of primary aromatic and aliphatic allylic alcohols to valuable aldehydes catalyzed by a recombinant aryl-alcohol oxidase of Pleurotus eryngii P34. This versatile enzyme demonstrates high stability against the by-product of the reaction, H 2 O 2, and in the presence of organic solvents.
XXII International Symposium on Homogeneous Catalysis; Quantum Bioinorganic Chemistry (QBIC) Celebrating 140 Years of the Swedish Chemical Society; ... An advanced insulin synthesis is presented that utilizes one-pot/stepwise disulfide bond formation enabled by acid-activated S-protected cysteine sulfoxides in the presence of chloride anion. S ...
Currently, the synthesis of a key precursor of sacubitril valsartan sodium hydrate LCZ696 proceeds via separate steps of metal-catalyzed asymmetric hydrogenatio Jump to main content . ... Catalysis Science & Technology. ... 2024, Advance Article , DOI: 10.1039/D4CY00145A To request permission to reproduce material from this article, ...
Advanced Synthesis and Catalysis. Articles & Issues. Menu. Articles & Issues. Latest issue; All issues; Articles in press; Submit search. Volume 362, Issue 1 January 2020. ... select article Transition‐Metal‐Free Three‐Component Synthesis of Tertiary Aryl Amines from Nitro Compounds, Boronic Acids, and Trialkyl Phosphites.
The possibility of using furfurol for the production of ash-free high-strength active carbons with spheroidal particles as adsorbents and catalyst supports is substantiated. A single-stage process that incorporates the resinification of furfurol, the molding of a spherical product, and its hardening while allowing the process cycle time and the cost of equipment to be reduced is developed ...
Advanced Synthesis & Catalysis is an international journal dedicated to the advancement of efficient and practical synthesis, which is a joint effort by academic and industrial chemists to meet the global and societal challenges with which chemistry is faced in the 21st Century. The journal brings together chemists from various areas of ...
The structure and main physicochemical properties of dysprosium titanate powders prepared by mechanochemical synthesis from the low-temperature modification of titanium oxide and modification of dysprosium oxide are investigated applying X-ray phase analysis (XPA), scanning electron microscopy, Raman spectroscopy (Raman spectra), transmission electron microscopy, and chemical analysis. It is ...
Advanced Synthesis and Catalysis. Articles & Issues. Menu. Articles & Issues. Latest issue; All issues; Articles in press; Submit search. Volume 365, Issue 18 19 September 2023. Download full issue. Previous vol/issue. Next vol/issue. Actions for selected articles. ... select article Synthesis of Bicyclo[2.1.1]hexan‐5‐ones via a Sequential ...
Rhodium‐Catalyzed Chemo‐ and Enantioselective Hydrogenation of Alkynyl‐Aryl Hydrazones. Dongyang Fan. Yanhua Hu. Feng Jiang. Zhenfeng Zhang. Wanbin Zhang. Advanced Synthesis & Catalysis. First published: 31 March 2018. Abstract.
Catalysis Conference is a networking event covering all topics in catalysis, chemistry, chemical engineering and technology during October 19-21, 2017 in Las Vegas, USA. Well noted as well attended meeting among all other annual catalysis conferences 2018, chemical engineering conferences 2018 and chemistry webinars.
Advanced Synthesis and Catalysis. Articles & Issues. Menu. Articles & Issues. Latest issue; All issues; Articles in press; Submit search. ... Show all article previews Show all article previews. select article Palladium Catalysis: Dependence of the Efficiency of C−C Bond Formation on Carboxylate Ligand and Alkali Metal Carboxylate or ...
Advanced Synthesis & Catalysis. Volume 366, Issue 8 p. 1669-1669. Cover. Free Access. Front Cover Picture: Ru(II) Catalyzed Oxidative Dehydrogenative Annulation and Spirocyclization of Isoquinolones with N-Substituted Maleimides (Adv. Synth. Catal. 8/2024) Kaushik Seal, Kaushik Seal.