- Open access
- Published: 06 April 2023

How photo editing in social media shapes self-perceived attractiveness and self-esteem via self-objectification and physical appearance comparisons
- Phillip Ozimek 1 ,
- Semina Lainas 2 ,
- Hans-Werner Bierhoff 2 &
- Elke Rohmann 2
BMC Psychology volume 11 , Article number: 99 ( 2023 ) Cite this article
22k Accesses
5 Citations
134 Altmetric
Metrics details
As photo editing behavior to enhance one?s appearance in photos becomes more and more prevalent on social network sites (SNSs), potential risks are increasingly discussed as well. The purpose of this study is to examine the relationship between photo editing behavior, self-objectification, physical appearance comparisons, self-perceived attractiveness, and self-esteem.
403 participants completed self-report questionnaires measuring the aformentioned constructs. A parallel-sequential multiple mediation model was conducted to examine the relationship between photo editing behavior and self-esteem considering multiple mediators.
The results indicate that photo editing behavior is negatively related to self-perceived attractiveness and self-esteem mediated via self-objectification and physical appearance comparisons.
Conclusions
The postulated mediation model was justified by our data. Thus, SNS users should be aware of potential negative consequences when using photo editing applications or filters.
Peer Review reports
Introduction
Sometimes I forget that I am human with a body, not a playdough that can be pressed and squeezed until it fits the predetermined mould this society has deemed “beautiful”. - Anonymous
Social media represent digital platforms based on new communication technologies fostering new possibilities for carrying out social interaction and communication [ 1 ]. They are based on Web 2.0 technology which allows the sharing of information among a large number of persons. Social media provide computer-mediated communication channels enabling users to communicate with each other.
An example of social media are social network sites which are defined by the use of profiles, the embeddedness in networks, and by the use of streams [ 2 ]. Profiles contain personal attributes related to the users which enable them to present themselves positively. Therefore, users are not necessarily obliged to include only true information in their profiles. Instead, deceptive self-presentation is a viable alternative. An example is research on dating platforms showing that the information provided is not always sincere [ 3 ]. With respect to height, weight, and age, 81% of profiles were not accurate because underreporting (especially with respect to weight) and overreporting (especially with respect to height) occurred. In addition, profile photographs also were inaccurate to some extent. This was less the case the more friends and family members were aware if the online dating profile.
Obviously, temptations to whitewash the profile are weighted against reality anchors and issues of credibility. Another feature of social network sites is their embeddedness in smaller or larger networks of users. Furthermore, social network sites comprise user-generated messages which are encoded in streams.
Social media including social media sites constitute a new context of social interaction which is contrasted with face-to-face interaction and digital communication media like email and video conferencing [ 1 ]. Social media differ from face-to-face interaction and digital communication media with respect to a plethora of communication variables including accessibility, latency, physicality, interdependence, synchronicity, permanence, verifiability and anonymity resulting in the reduction of time and distance barriers. For example, physicality contrasts face-to-face interactions taking place in a material context, which is tangible and perceptible, with an artificial environment based on digitalization. In general, the artificial environment facilitates communication. In addition, latency is reduced on the internet because it takes less time to share content within the social network site in comparison to face-to-face communication. Furthermore, the truth of messages is easier to verify on social media sites (e.g., by background checks). In general, social media facilitate communication processes considerably by offering the sender more channels to share information with many recipients.
An important domain of profile information refers to the use of profile photographs, which are more or less accurate [ 3 ]. Photo editing behavior tends to improve the impression conveyed by the profile photo at the cost of deception. In this context, social media communication reduces the verifiability of the accuracy of photographs with the consequence that the accuracy of the photograph is hard to verify. This contradicts the general trend [ 1 ], that social media enhance the likelihood of genuine communication and that the truth of messages is relatively easy to verify.
Photo editing behavior increases the options available for self-presentation on social network sites and constitutes a significant restriction on verifiability of the accuracy of profile photos. In addition, it is likely to be negatively correlated with self-perceived attractiveness. Therefore, the importance of photo editing behavior in the context of social network sites is high.
Photo editing behavior represents an emerging trend. According to a survey of the Renfrew Center Foundation [ 4 ], 50% of SNS users edit their photos before posting them to Social Network Sites (SNSs). Still, the effects of these new photo editing applications on the individual are largely unknown.
Compared to past decades, people are nowadays constantly confronted with highly edited beauty pictures on SNSs, which could significantly change the perception of beauty by raising beauty standards. Accordingly, individuals of average attractiveness may perceive themselves as less attractive when evaluated in comparison with photos of more attractive individuals which were edited by photo editing behavior. Analogous contrast effects have been found in a field study in which a moderately attractive woman was evaluated less positively following exposure to highly attractive actresses [ 5 , 6 ]. In addition, social comparisons on social media are likely to impair self-esteem [ 7 ].
What happens when the comparison is made with a more beautiful and optimized version of oneself? Numerous studies indicate a negative association between photo editing behavior on SNSs and body satisfaction [ 8 - 13 ]. Furthermore, users who retouched their pictures reported feeling less attractive, poorer self-esteem [ 12 ], and increased negative mood ([ 13 ]. Photo editing behavior may also encourage individuals to view their body as an object [ 14 ] reinforcing associated risks such as body shame, depression, and eating disorder [ 15 ].
The aim of this research is to reveal risks of the engagement in photo editing behavior. For this purpose, various factors identified in previous studies were incorporated into a parallel-sequential mediation model with multiple mediators. From a theoretical point of view, we integrate selfie editing, social comparisons, self-objectification, and well-being which is captured by self-esteem.
Whereas almost all previous studies have investigated the impact of photo editing behavior solely on body image, this study refers to self-perceived attractiveness in general by including the body and face as part of self-perceived attractiveness. One reason is that, so far, filters focus on the face and not on the body. Also, users post more pictures of their face on SNSs than full body pictures [ 16 ]. The face is usually more salient in pictures of oneself [ 13 ]. Thus, photo editing may lead individuals to pay closer attention to their facial attractiveness. Accordingly, photo editing behavior can have a significant effect on facial dissatisfaction, but not on body dissatisfaction [ 13 ].
Additionally, both men and women are included in this study. To date, almost all studies on photo editing have only included women, as they are more likely to engage in photo editing behavior [ 17 ] and experience higher pressure to conform to the cultural beauty ideal [ 18 ]. Yet, [ 10 ] found that photo editing behavior was positively associated with body dissatisfaction for both genders. The effects of photo editing behavior for men are nevertheless nearly unexplored.
Theoretical background
Photo editing behavior.
Photo editing behavior refers to the use of filters as well as various photo editing applications. While filtering options within Instagram change the face using a template with features, such as makeup, enlarged eyes, fuller lips, and narrower noses, photo editing applications provide more specific options. Thus, users can specifically select which parts of their face and body they want to edit. The functions range from changing skin tones, removing blemishes, slimming faces, making body parts slimmer, making body parts appear bigger, changing the shapes of noses, lips, cheeks, chins and eyes, and various makeup options.
Moreover, there are photo editing applications that use artificial intelligence (AI) to fully reconfigure the face [ 19 ]. While the use of photo editing options is mostly self-determined, as the user consciously decides which physical features should be changed, the use of filters or AI provides less self-determination. In this case, it is not the user but the technology that determines which of the photo’s physical features require modification. This could cause users to discover flaws in themselves that they would not have noticed without using the photo editing application.
As the physical appearance of users plays an important role in impression management on SNSs, photo editing behavior serves as an impression management strategy of online self-presentation [ 20 ] besides, for example, selecting one’s best photo. Regarding self-presentation, users can manage the impressions they have on others by minimizing perceived flaws or imperfections to get more favorable attention from others [ 3 ]. However, the use of photo editing applications can create an unrealistic expectation of one’s own attractiveness [ 21 ].
Self-perceived attractiveness
Self-perceived attractiveness refers to people’s beliefs about the quality of their physical appearance [ 22 ]. In contrast to body image, self-perceived attractiveness involves not only the perception of one’s own body but also of one’s face.
Several studies revealed a positive correlation between photo editing behavior and body dissatisfaction [ 8 - 13 ], whereas others found no significant association [ 8 , 11 ]. Overall, research has suggested that photo editing behavior may represent a risky behavior in terms of its potential to negatively impact body image [ 12 ] and facial satisfaction [ 13 ]. In addition, higher involvement in photo editing behavior, but not higher media exposure, is associated with higher body dissatisfaction [ 11 ]. Therefore, the importance of the general level of media exposure as a potential confounding variable is likely to by small. A plausible explanation of these results is that photo editing makes users think more about their flaws and imperfections [ 12 ]. Thus, individuals who engage in photo editing behavior are unfortunately more likely to notice a gap between their actual and ideal appearance [ 23 ]. This is likely to diminish self-perceived attractiveness in terms of appearance. Conversely, it can be argued that low self-perceived attractiveness tends to elicit photo editing behavior [ 24 ]. Accordingly, the first hypothesis states:
Photo editing behavior is negatively correlated with self-perceived attractiveness in terms of appearance.
- Self-objectification
As individuals engaging in photo editing behavior focus more on their appearance [ 16 ], it is tempting for them to anticipate the reactions of other users to the edited photo and look at themselves from an outside viewers’ perspective. Since the focus on many SNSs is on the user’s appearance, SNS users tend to expect to be evaluated based on their appearance [ 25 ]. Both conditions are risk factors for self-objectification.
Self-objectification is defined as the act of “[internalizing] an observer’s perspective on self” ([ 15 ] pp. 179 f.). The difference between self-objectification and body dissatisfaction is that self-objectification is a perspective toward the body, whereas body dissatisfaction involves negative feelings about one’s body [ 26 ]. As the objectification theory originally included only women, it was argued that women frequently experience sexual objectification by being valued for their appearance or by being regarded as objects. This regular experience of sexual objectification, such as exposure to objectifying media, socializes women to internalize an outside viewers’ perspective on their appearance [ 27 ]. Consequently, when a woman self-objectifies, she thinks about how her body might look to others [ 15 ]. The negative consequences of such an approach may include, among others, body dissatisfaction, body shame, disordered eating [ 28 ], depression [ 26 ], and lower well-being [ 29 ]. Meanwhile, it is proven that also men experience self-objectification and are therefore equally exposed to these risks [ 27 ].
In general, the nature of photo editing behavior activates feelings of self-objectification [12; 14] and physical appearance comparisons. Taking an outsider’s perspective makes users focus on their appearance rather than unobservable attributes such as abilities [ 16 , 27 , 30 ]. Additionally, photo editing behavior reinforces the evaluation of their appearance [ 31 ]. [ 32 ] argued that self-objectification can be triggered when people spend time editing their own photos because they view themselves in photos as manipulated objects. Furthermore, [ 33 ] proposed the circle of objectification, which suggests that individuals who self-objectify seek out more appearance comparisons, which in turn acerbate tendencies of self-objectification, as appearance comparisons increase the salience of one’s appearance [ 34 ]. Therefore, the positive association between photo editing behavior and self-objectivation may also be triggered by physical appearance comparisons resulting from self-objectification (cf., Sect. 2.4 ). Based on former studies, we derived as replication hypothesis:
Photo editing behavior is positively correlated with self-objectification.
- Physical appearance comparisons
In general, self-objectification is closely linked to appearance comparisons because both constructs share the perspective toward the body. According to social comparison theory, humans have an innate drive to compare themselves with others as a source for self-evaluation [ 35 ]. This happens relatively automatically. While social comparisons include abilities, affect, self-esteem, performance satisfaction, and other personal characteristics [ 36 ], physical appearance comparisons focus on physical characteristics [ 37 ]. In upward comparisons, the individual evaluates her- or himself relative to someone who is considered more attractive. [ 36 ].
During photo editing, users compare their own appearance to sociocultural beauty standards and might think about the required modification through photo editing to get closer to this ideal [ 38 ]. Therefore, photo editing behavior is likely to be positively associated with physical appearance comparisons.
Photo editing behavior is positively correlated with physical appearance comparisons.
In general, social comparisons tend to elicit contrast effects [ 36 ]. Therefore, upward comparisons are likely to reduce appearance evaluation. Individuals of average attractiveness may be perceived as less attractive when evaluated in comparison with more attractive individuals. Therefore, one’s tendency to engage in physical appearance comparisons is likely to be associated with body dissatisfaction [ 39 ], internalization of appearance ideals, low self-esteem, sexual objectification, body surveillance, and body shame [ 37 ]. Similar contrast effects have been demonstrated when a moderately attractive individual is evaluated following exposure to highly attractive media stimuli [ 40 ]. Moreover, individuals who perceive themselves as less attractive are more likely to engage in physical appearance comparisons and upward comparisons (Patrick et al., 2004). Although in theory individuals who are satisfied with their body may engage frequently in physical appearance comparisons, the empirical evidence reveals that individuals who perceive themselves as less attractive primarily engage in physical appearance comparisons, more than individuals who perceive themselves as attractive [ 41 ]. Therefore, empirical results and theoretical considerations lead to the following hypothesis:
Physical appearance comparisons are negatively correlated with self-perceived attractiveness in terms of appearance.
According to the self-discrepancy theory, individuals compare one self-state to another self-state and find that a discrepancy exists [ 42 ]. This discrepancy in turn triggers dissatisfaction. Therefore, the negative effects of photo editing behavior are likely to occur when SNS users perceive high discrepancy between their edited self (i.e., the idealized self) and real self [13; 42]. It is quite likely that the individual will fall short of the unrealistic beauty ideal promoted by filters and photo editing applications, resulting in a body-related self-discrepancy [ 43 ].
- Self-esteem
Individuals who express low self-esteem more often gravitate to physical appearance comparisons, seeking reassurance and validation compared with individuals high on self-esteem. However, they also more often engage in upward comparisons [ 44 ], which in turn is associated to a decrease in self-esteem [ 37 ]. Such upward comparisons may serve as a reminder of the beauty ideal they do not meet [ 41 ] eliciting a contrast effect.
Self-esteem represents an important part of subjective well-being. It is defined as the affective-evaluative facet of the self and includes cognitive-knowledge-based, affective-evaluative, and action guiding facets [ 45 ]. Moreover, self-perceived attractiveness is an important component of self-esteem. Numerous studies indicated a positive correlation between self-perceived attractiveness and self-esteem [ 44 , 46 - 49 ], as it is an important source of power and social status [ 50 ] Also, attractive individuals develop and internalize more positive self-views than less attractive people [ 51 ]. Several researchers have argued that it is not attractiveness itself that is associated with self-esteem, but individuals’ evaluation of their own attractiveness [ 52 ]. In conclusion, self-perceived attractiveness is likely to play an important role in determining self-esteem, possibly more important than objective attractiveness. This conclusion does not imply that individuals have only a vague idea of their objective attractiveness or no idea at all. There is definitely objectivity regarding self-perceived facial attractiveness [ 53 ]. Therefore, objective facial features affect self-perceived facial attractiveness. The fact that objective facial attractiveness is registered by individuals suggests that objective facial attractiveness may constitute a confounding factor with respect to self- esteem that could influence the results of the study (cf., the Discussion section). Nevertheless, self-perceived attractiveness is associated with self-esteem beyond objective facial attractiveness having a positive impact on self-confidence [ 54 ]. Moreover, individuals with high self-esteem are more likely to accept their physical appearance [ 52 ]. This reasoning leads to the following hypotheses:
Self-perceived attractiveness in terms of appearance is positively correlated with self-esteem.
In sum, photo editing behavior leads to self-objectification [12; 14; 20; 32] and physical appearance comparisons [ 24 ]. A possible explanation is that photo editing behavior makes users focus more on their appearance, which consequently increases physical appearance comparisons [ 16 ]. In turn, physical appearance comparisons are likely to trigger photo editing behavior to compensate for one’s optical flaws (cf., the occurrence of objective facial attractiveness; [ 53 ]). Since physical appearance comparisons [ 39 , 55 ] and self-objectification [ 28 ] are negatively correlated with body image and self-perceived attractiveness, it is reasonable to assume that the two constructs are mediators of the relationship between photo editing behavior and self-perceived attractiveness. When individuals self-objectify and compare themselves regarding their physical appearance, they may pay more attention to their physical appearance and more easily find a gap between their physical appearance and their beauty ideal. As a result, they presumably feel dissatisfied with their own appearance [ 30 ].
Finally, in H5 a parallel-sequential multiple mediation model is proposed, which is based on previous results in general and H1 to H4 in particular. The model connects photo editing behavior with self-esteem via three mediators.
Photo editing behavior is associated with higher self-objectification and more physical appearance comparisons, that result in lower self-perceived attractiveness, which, in turn, implies lower self-esteem.
An overview of the research plan including the hypotheses Footnote 1 is shown in Fig. 1 .
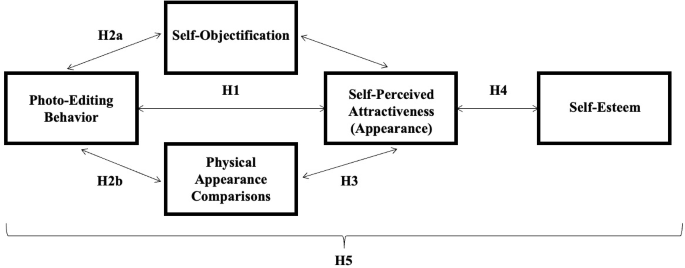
Overview of the research plan including hypotheses
The program G*Power (version 3.1.9.7; [ 56 ]) was used to calculate in advance how many participants constitute a sufficient sample size for a mediation model (i.e., multiple regression with 5 predictors). The significance level was set at 5%, Power (1-ß) at 95%. Furthermore, small to medium effect sizes were assumed, f 2 = 0.15 since in social psychological research these effect sizes are to be expected [ 57 ]. The appropriate sample size turned out to be N = 138. 403 participants met the inclusion criteria and provided complete data sets. 316 (78.4%) were female, 85 (21.1%) male and 2 (0.5%) identified as neither female nor male. The average age was 27.6 years ( SD = 8.3) ranging from 18 up to 61 years. 42.2% held an academic degree and 53.3% were at least high school graduates. 86.6% of the participants were students and most of them studied psychology (59.1%).
An online survey was conducted via Unipark ( https://www.unipark.de ). Participation was dependent on being active on SNSs in general. More specifically, the sample is based on Instagram members because Instagram is the most widely used SNS [ 44 ], primarily image-based compared to other SNSs [ 13 ], and has its own photo editing features. To confirm the inclusion criteria, participants were asked if they had an Instagram account. Therefore, the likelihood of engaging in photo editing behavior was expected to be relatively high for Instagram users. Furthermore, participants had to be at least 18 years old. They were recruited via social media posts following a snowball-sampling technique.
Questionnaires
Demographic variables, photo editing behavior, self-objectification, physical appearance comparison, self-perceived attractiveness and self-esteem, and Instagram activity were obtained. In general, higher scores indicate higher levels of the corresponding variable.
Photo editing scale
The Photo Editing Scale (PES) was newly developed to measure photo editing behavior related to the use of SNSs. It provides a brief measure and consists of five items, each of which are answered on a 5-point Likert scale with a response format ranging from 1 = “never” to 5 = “always”. To assess different types of photo editing behavior, participants were asked about their use of filters (e.g., “I use filters that beautify my facial features.”) and photo editing applications (e.g., “I edit my facial features before uploading a photo (using apps such as Facetune or Faceapp, for example”). One item was included focusing on body features (e.g., “I edit my figure before I upload a photo (e.g., with apps like Facetune or Faceapp)”). Two further items referred in general to using photo editing or not. Reliability analysis indicated a satisfactory internal consistency, α = 0.75, given the small number of items. The response scale of one item had to be inverted. Higher scores represent more photo editing behavior. On average, participants exhibited relatively low photo editing behavior-scores, M = 1.89, SD = 0.79. Note that the content validity of the items is high. The scale items are included in OSF (with respect to the double-blinded review process, the link will be added after acceptance of the paper).
Instagram activity questionnaire
To measure Instagram activity, the Instagram Activity Questionnaire (IAQ; [ 58 ]) was used. The questionnaire consists of 38 items rated on a 5-point Likert scale from 1 = “never” to 5 = ”very often”, based on the two factors: Active (27 items; e.g., “I post pictures.”) and Passive (11 items; e.g., “I look at the photos of other users.”). Reliability analyses indicated very good internal consistencies (α IAQ = 0.91, α Active = 0.88, α Passive = 0.82). Higher scores indicate higher Instagram activity. Participants exhibited moderately high ratings of Instagram activity, i.e., M IAQTotal = 2.64, SD IAQ Total = 0.57, M Active = 2.52, SD Active = 0.64, M Passive = 2.91, SD Passive = 0.67, with highest ratings for passive Instagram use.
Self-objectification beliefs and behaviors scale
For measuring self-objectification, the Self-Objectification Beliefs and Behaviors Scale (SOBBS; [ 26 ]) was used. This is a relatively new measure that addresses the primary limitations of existing measures. Participants were asked to rate their level of agreement with each item using a 5-point Likert scale from 1 = “strongly disagree” to 5 = “strongly agree”. The 14 items are based on two factors: (1) internalizing an observer’s perspective of the body (7 items; e.g., “I try to imagine what my body looks like to others (i.e., like I am looking at myself from the outside)” and (2) equating the body to who one is as a person and valuing physical appearance above other attributes (7 items; e.g., “How I look is more important to me than how I think or feel.”). For the present German sample, the SOBBS was translated and validated by using the back-translation procedure (see Appendix A). The measure demonstrated very good internal consistencies in the original study [ 26 ] as well as in the current sample, α SOBBS_Total = 0.89, α Factor_1 = 0.89, α Factor_2 = 0.84. Higher scores indicate more self-objectification. In general, participants exhibited moderately high ratings of self-objectification, M Factor_1 = 2.92, SD Factor_1 = 0.88, M Factor_2 = 1.70, SD Factor_2 = 0.62, M SOBBS_Total = 2.31, SD SOBBS_Total = 0.66, with highest ratings on the first factor (internalizing an observer’s perspective of the body) and comparatively low ratings on the second factor (equating the body to who one is as a person and valuing physical appearance above other attributes).
Physical appearance comparison scale
The German version [ 59 ] of the Physical Appearance Comparison Scale (PACS; [ 60 ]) was used to assess an overall tendency to compare one’s own appearance with others (e.g., ‘‘In social situations, I sometimes compare my figure to the figures of other people’’). With only 5 items, the PACS is a very economical measure. Items are rated on a 5-point Likert scale (1 = “never” to 4 = “always”). Higher mean scores indicate higher frequency of physical appearance comparisons. As the most widely used measure for physical appearance comparisons, the PACS has demonstrated good reliability and validity (Schaefer & Thompson, 2014). Accordingly, [ 59 ] have found that the internal consistency of the scale based on a German sample is within an acceptable range. The current investigation revealed moderately high levels of physical appearance comparisons, M = 2.73, SD = 0.79 and a satisfactory internal consistency, α = 0.73.
Body-esteem scale
Self-perceived attractiveness was measured with the Body-Esteem Scale (BES) [ 61 ] that consists of 23 items rated on a 5-point Likert scale ranging from 1 = “never” to 5 = “always”. [ 61 ] suggested that feelings about one’s weight can be differentiated from feelings about one’s general appearance. Also, one’s own opinions may be differentiated from the opinions attributed to others. Therefore, the BES contains three subscales: (a) Appearance (6 items; e.g., “I wish I looked better”), (b) Weight (4 items; e.g., “I really like what I weigh”) and (c) Attribution (4 items; e.g., “People of my own age like my looks”). These subscales measure (a) general feelings about one’s own appearance, (b) weight satisfaction, and (c) evaluations attributed to others about one’s appearance [ 46 ]. For the present research, only the subscale on Appearance was used. Hence, 6 of the 14 items of the questionnaire were included and translated into German. The translation was verified through back-translation procedure (see Appendix B). There is good evidence that the original scale is valid and reliable over a wide age range [ 61 ]. Reliability analyses in the current sample indicated that internal consistency was excellent, α Appearance = 0.91. On average, participants ratings regarding their self-perceived attractiveness were moderately high, M Appearance = 2.72, SD Appearance = 0.74.
Rosenberg self-esteem scale
For measuring self-esteem, the German version of the Rosenberg Self-Esteem Scale (RSES) [ 62 ] was used, which showed good reliability and validity [ 63 ]. With respect to construct validity, strong positive correlations between self-esteem and both self-satisfaction and self-efficacy and a substantial negative correlation between self-esteem and self-derogation were obtained. The RSES includes six items rated on a 4-point Likert scale from 1 = “strongly disagree” to 4 = “strongly agree ” . Positive and negative feelings about the self were both assessed. The response scales of five of the ten items had to be inverted (e.g., “Every now and then I think I’m no good at all.“). Higher scores indicate higher self-esteem. The RSES is characterized by easy and quick use and high face validity [ 64 ]. [ 65 ] reported a satisfactory internal consistency for the German version of the RSES. In the current sample, reliability analyses indicated a very good internal consistency, α = 0.90. On average, participants reported relatively high self-esteem, M = 3.21, SD = 0.61.
Preliminary analysis
As indicated by Kolmogorov–Smirnov test and Q–Q plots, some of the scales (PES, BES, RSES) were not normally distributed. Consequently, Spearman’s rank correlation (Rho), which presupposes an ordinal association between variables, was used for all computations of their interrelations.
Validity checks
In general, the content validity of the employed scales is high. The construct validity of each of the scales included in hypothesis tests was scrutinized thoroughly except for the self-esteem scale whose construct validity was comprehensively demonstrated by [ 63 ]. For checking construct validity of the PES, it was correlated with the IAQ because Instagram use is likely to promote appearance concerns [ 12 ] and in this respect corresponds with the PES because of its visual, photo-oriented features. Therefore, a positive correlation between the IAQ and PES was expected. This expectation was confirmed because the IAQ was positively correlated with the PES, r s (401) = 0.358, p < .001, and its subscales, both Active, r s (401) = 0.207, p < .001, and Passive, r s (401) = 0.223, p < .001. Note that the highest correlation was displayed between PES and the total Instagram Activity Questionnaire accounting for 12.8% of common variance.
Subsequently, the construct validity of the German version of the Appearance subscale of the Body Esteem Scale was examined by correlating it with the passive subscale of the Instagram Activity Scale. High exposure to Instagram content in a passive mode is likely to undermine positive attitudes toward own appearance because of the elicitation of social comparisons which lead to body dissatisfaction [ 18 ]. Results correspond with the assumption of construct validity of the Appearance subscale, r s (401) = − 0.135 p < .01, indicating that higher passive Instagram consumption is negatively associated with positive attitudes toward own appearance.
Additionally, the construct validity of the Self-objectification Beliefs and Behaviors Scale (SOBBS) was examined by correlating it with the Body Esteem Scale (BES) because higher self-objectification seems to imply lower self-perceived attractiveness [ 66 ]. In correspondence with expectations significant negative correlations were found between self-perceived attractiveness and self-objectification, r s (401) = − 0.569 p < .001, as well as on corresponding subscales, referring to internalizing an observer’s perspective of the body , r s (401) = − 0.462, p < .001, and referring to equating the body to who one is as a person and valuing physical appearance above other attributes ), r s (401) = − 0.477, p < .001), respectively. The highest correlation was exhibited between the total SOBBS and perceived attractiveness. indicating 23.9% of common variance.
Finally, the construct validity of the Physical Appearance Comparison Scale was examined. In accordance with results by [ 7 , 67 ] indicating that social comparison orientation is positively associated with Facebook activity it was assumed that the PACS is positively linked with Instagram activity substituting comparison orientation by the Physical Appearance Comparison Scale and Facebook activity by Instagram activity. Note that comparison orientation and PACS both represent individual-difference measures of the readiness to perform social comparisons and that the Instagram activity questionnaire was developed analogously with the Facebook activity questionnaire. Both instruments capture behavioral reports of activities on SNSs. In correspondence with expectations and corroborating the construct validity of the PACS results indicated that total Instagram activity and PACS are correlated positively, r s (401) = 0.264, p < .01. In addition, the results for both active and passive Instagram activity measures correspond with the results for the overall activity measure.
Testing hypotheses
The hypotheses pertain to correlational relationships. The complete intercorrelation matrix is summarized in Appendix C. In H1 it was hypothesized that photo editing behavior is negatively correlated with self-perceived attractiveness. This hypothesis was confirmed because a significant negative correlation was found between photo editing behavior and self-perceived attractiveness in terms of appearance, r s (401) = − 0.146, p < .01.
As hypothesized in H2a , photo editing behavior displayed a significantly positive correlation with the SOBBS measuring self-objectification, r s (401) = 0.221, p < .001. This correlation represents a moderate effect. Moreover, with respect to the SOBBS-subscales, substantial negative correlations were found between photo editing behavior and internalizing an observer’s perspective of the body , r s (401) = 0.227, p < .001, and equating the body to who one is as a person and valuing physical appearance above other attributes , r s (401) = 0.124, p < .001. Therefore, H2 was confirmed.
Furthermore, the proposition ( H2b ) was investigated that photo editing behavior is positively associated with physical appearance comparisons. The results corresponded with H3 , r s (401) = 0.238, p < .01. More specifically, participants who were more active in terms of photo editing behavior exhibited more physical appearance comparisons.
H3 postulated a negative association between physical appearance comparisons and self-perceived attractiveness in terms of appearance. It was corroborated by a significant negative correlation between the subscale Appearance of the BES and physical appearance comparisons, r s (401) = − 0.536, p < .001, Therefore, H4 was confirmed.
H4 refers to the association between self-perceived attractiveness and self-esteem. Previous research demonstrated that self-perceived attractiveness and self-esteem are positively linked [44; 49]. Supporting previous results, self-perceived attractiveness and self-esteem were correlated positively. Specifically, the BES-subscale Appearance was positively associated with self-esteem, r s (401) = 0.604, p < .001, representing a strong effect. Thus, H5 was confirmed by the results.
The statistical mediation model summarized in H5 proposed that photo editing behavior is associated with higher self-objectification and more physical appearance comparisons, and that both mediators are associated with a lower self-perceived attractiveness, which, in turn, is associated with lower self-esteem. Note that the mediators self-objectification and physical appearance comparisons display a high positive correlation which is taken into account by applying a path-analytic model. In overview, the corresponding path analysis summarized in Fig. 2 , revealed a significant parallel-sequential multiple mediation in the expected direction, total indirect effect: β = − 0.020, BC 95% CI [-0.0377; − 0.0063]; overall model: F 4,398 = 7.12, p < .001, adj R 2 = 0.067. While the direct effect of photo editing behavior on self-esteem was not significant, β = 0.012, BC 95% CI [-0.0300; 0.0538], the indirect effect was mediated via self-objectification, physical appearance comparisons, and self-perceived attractiveness. Therefore, H5 was confirmed. Photo editing behavior significantly predicted more self-objectification as well as more physical appearance comparisons which both predicted lower self-perceived attractiveness and lower self-esteem. In addition, self-objectification directly predicted lower self-esteem.
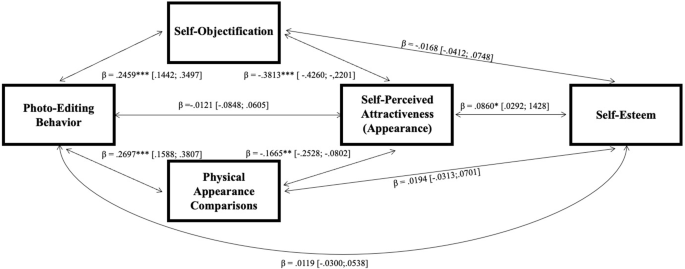
Mediation model. Note: m = 10,000; bootstrapping intervals in brackets; Age (ß = 0.015, p > .05; CI [-0.0445; 0.0679]) and gender (ß = 0.029, p > .05; CI [-0.0015; 0.0036]) as covariates show no significant effect on the statistical mediation model, all p s > 0.05. * p < .05, ** p < .01. *** p < .001
Post-hoc tests of observed power and replicability
We used the test of excessive significance (TES) [ 68 ] to calculate the success rate, median observed power, the inflation rate, the replicability index, and a test of insufficient variance (TIVA) based on 6 hypotheses-oriented effects (i.e., 5 t- statistics and 1 F -statistics based on intergroup deviations and mediation models). Therefore, we used the p-checker-app (see http://shinyapps.org/apps/p-checker/ ).
The TES revealed a success rate of 1, which indicates that 100% of our predictions were confirmed, and a median observed power of 99.6. In addition, the TES revealed a minimal inflation rate 0.004, which indicates that no more hypotheses have been confirmed than possible under consideration of the power. The r-index = 0.99 indicates that our findings can be (theoretically) replicated in X * 0.99 follow-up studies.
At last, the TIVA, X 2 (5) = 98.311, p = 1, var = 19.66, indicated that no bias was present confirming that all entered test statistics and p-values are in the expected direction.
The hypotheses connected the construct of photo editing with social comparison, self-objectification, and self-esteem as an indicator of well-being. Photo editing includes selfie editing as a special case. Whereas H1 to H4 postulated associations between two constructs, H5 combined five constructs based on a path-analytic model. In general, our findings support the hypotheses.
Consistent with other studies [ 8 - 13 ], the results regarding H1 indicate that photo editing behavior is associated with lower self-perceived attractiveness in terms of appearance. Although the explained variance is rather small, the corresponding correlation is highly significant. One explanation is that individuals who often edit their pictures create an idealized virtual self-image which enhances the discrepancy between the real and ideal self [ 24 ]. Furthermore, even people satisfied with their appearance presumably want to look even better and edit their selfies to post perfect ones which maximize ideal online self-presentation [ 24 , 53 ].
As expected in H2a , a significant positive correlation between photo editing behavior and self-objectification was found. On the one hand, self-objectification may predispose individuals to engage in photo editing behavior. On the other hand, photo editing behavior is likely to enhance feelings of self-objectification [ 12 , 14 ], as the individual simultaneously becomes the editor and the object of photo editing in general and selfie editing in particular. Self-objectification may foster an individual’s need to constantly present and improve his or her physical appearance to please others [ 14 ]. Therefore, people with a higher degree of self-objectification may place a higher value on posting photos that reflect the societal beauty ideal. Individuals who self-objectify are more likely to experience body shame and body dissatisfaction [ 28 ], Editing specific body parts may reduce the body to its component parts rather than viewing it as a fully functioning whole. As filters and photo editing applications tend to convey beauty ideals, the internalization of these messages may guide the perception of one’s appearance, leading to a more objectified view. [ 69 ] reported that time spent on SNSs was associated with higher self-objectification. Therefore, the correlation between photo editing behavior and self-objectification may be intensified by higher length of SNS use.
H2b postulated a positive association between photo editing behavior and physical appearance comparisons. The confirmation of H2b indicates that higher sores on the photo editing scale are associated with more intense immersion in physical appearance comparisons including the construction of self-other contrasts with respect to good looks. Note that the confirmation of both H2a and H2b in combination emphasizes that self-objectification and physical appearance comparisons are closely linked with each other. The correlation between both scales is substantial, r (401) = − 0.599, p > .001. Therefore, the confirmation of H2 taken together supports the notion that photo editing behavior is associated with change in the perspective toward the body.
The findings generally supported hypothesis H3 that physical appearance comparisons are negatively associated with self-perceived attractiveness in terms of the subscale Appearance of the BES. To explain these findings, it should be noted that such comparisons may serve as a reminder of beauty ideals that one does not meet [ 41 ]. This correlation should be particularly pronounced for SNS users, as upward physical appearance comparisons are likely to occur frequently on SNSs due to a general tendency of users to exaggerate their positive characteristics striving for positive self-presentation [ 70 ]. Intriguingly, physical appearance comparisons with peers may actually impair self-perceived attractiveness more than comparisons with fashion models, because the latter are perceived as less similar to oneself and, as a consequence, represent a less diagnostic comparison group [ 71 ]. Due to the high similarity of an optimized version of oneself to one’s real self, physical appearance comparisons with one’s artificially optimized self could have a negative effect on self-perceived attractiveness. These comparisons reveal what needs to be optimized to achieve the ideal. For example, lip injections, nose surgery, anti-wrinkle cream or weight loss could presumably make the edited selfie self-achievable, while in comparison, the appearance of a celebrity appears to be unachievable. People don’t recognize that the appearance of celebrities is usually artificially enhanced using make-up and software like Adobe Photoshop .
Individual differences in responses to physical appearance comparisons are likely. Specifically, upward comparisons could inspire some individuals, whereas others may feel discouraged. In accordance, [ 41 ] argued that reactions to physical appearance comparisons are largely a function of two individual differences: The extent to which one’s self-esteem is contingency based and one’s self-perceived attractiveness.
Several studies have already shown a positive association between self-perceived attractiveness and self-esteem [ 44 , 46 - 49 ]. The confirmation of H4 which states that self-perceived attractiveness is positively associated with self-esteem is in line with previous results. Highly attractive individuals are likely to internalize more positive self-views than less attractive people [ 51 ]. Interindividual differences should also be considered, as some people are more likely to define their self-esteem on the basis of meeting expectations such as societal beauty ideals [ 72 ]. This refers to the contingent self-esteem, which is based on the approval of others or on social comparisons [ 73 ]. Individuals who are more dependent on contingent self-esteem may be more concerned with attractiveness than others who, for example, rely more on academic success or social acceptance [ 41 ].
Self-esteem is likely to be influenced by both self-perceived attractiveness and objective attractiveness [ 53 ]. Therefore, objective attractiveness may constitute a confounding factor with respect to the link between self-perceived attractiveness and self-esteem because higher objective attractiveness could be associated both with both self-perceived attractiveness and self-esteem. Future studies, which should include a measure of objective attractiveness, could clarify this issue. Nevertheless, stereotype research indicates [ 74 ] that cultural reference systems and subjective impressions represent powerful determinants of self-esteem.
In general, the confirmation of H1 to H4 represents initial evidence for the mediation model postulated in H5 . But H5 goes beyond the other hypotheses by specifying specific paths which connect photo editing behavior with self-esteem. The employment of the sequential multiple mediator model in testing H5 allows to discover these paths. The results indicate that mediation via self-objectification and via physical appearance comparisons occupy central switching points in the model which are both associated with self-perceived attractiveness. Therefore, the link between photo editing behavior and self-esteem was sequentially mediated via self-objectification, physical appearance comparisons, and self-perceived attractiveness. Individuals who engage in photo editing behavior more often perform physical attractiveness comparisons with others sand self-objectify more frequently. Whereas self-objectification relates to self-esteem both indirectly via self-perceived attractiveness and directly, physical appearance comparisons are only indirectly connected with self-esteem via self-perceived attractiveness.
The path-model specifies links from photo editing behavior to restricted self-esteem by focusing on unintended side-effects of photo editing behavior which is performed mainly to achieve positive consequences (e.g., improved self-presentation). From an applied viewpoint it would be desirable to inform users about the danger that such side-effects may occur. Such a cautionary note might include a broader concern related to the improvement of appearance in public. For example, people don’t recognize that the appearance of celebrities is usually artificially enhanced using make-up and software like Adobe Photoshop . Therefore, photo editing of selfies on SNSs is only one instance of a general trend to edit pictures. Reality is more elusive as it appears on the surface. The depiction of reality is a constructive endeavor which is subject to concealed issues of the editors. The depiction of reality is usually not a documentary but part of a narrative which the photo editor intends to project on the public screen. By understanding the underlying narrative, the contrast between natural appearance and edited photo of it is getting transparent. Because photo editing is likely to prevail in the future, the focus of psychoeducation as part of a psychological intervention technique should be a sensibilization for the wide spread of use of corresponding techniques.
Limitations and future research
This study is subject to several limitations. Firstly, the sample is not representative. For example, the data was obtained within an online context. But research indicates that differences in results occur between offline and online contexts. Specifically, the occurrence of gender differences in personality depended on the context of measurement [ 83 , 84 ]. Therefore, in future studies the results of online and offline measurement of the assessment of photo editing variables including personality variables like self-esteem should be compared with each other in order to increase the generalizabilitv of results.
In addition, young German participants were overrepresented. But the sample comprises individuals within a large age range and from different socioeconomic and academic backgrounds. Note that age plays a major role in the perception of facial attractiveness and self-esteem[ 75 ]. The present sample includes a range of individuals between 18 and 61 years old, with 86.65% being students, and thus more likely in their twenties. To account for doubts with respect to its representativity regarding the general population we added subanalyses including age as covariate in our mediation model. However, the results confirmed our previous results.
Furthermore, 59% of the participants were psychology students and only 21% of the participants were male. the high proportion of females in the sample could mean that the results are more typical for females than for males. In fact, it was found that women are more involved in photo editing behavior than men [16; 17], are more preoccupied with appearance than men [ 76 ], and experience higher pressure to conform to the societal beauty ideal [ 18 ]. Note that we added gender as covariate in our mediation model. No significant effects were found.
Another issue that goes beyond sample characteristics is that most filters focus primarily on realizing the female beauty ideal.
The variables measured in this study are based on self-report. Therefore, they may be influences by response biases. For example, it is important to note that participants may have underreported their photo editing behavior because they may have perceived this behavior as socially undesirable. In support of this argument, previous research found that 12% of photos posted under the #nofilter tag on Instagram did in fact include filters [ 77 ]. Therefore, future research could benefit from inclusion of a measure of social desirability. In defense of the data quality of the self-report scales, we investigated the construct validity of the scales. Results indicated that each of the variables which were represented in the hypotheses exhibit substantial construct validity. In addition, the content validity of all scales is high.
Additionally, the Body Esteem [ 61 ] is validated for individuals between 12 and 25 years of age. We suggest that the scale is validated in older populations. This is in line with our results showing the same effects with respect to our hypotheses regardless of adding age as covariate.
Please note that participation was based on having an Instagram account. There are reports in the literature regarding the percentage of individuals who edit their photos before publishing them on Instagram. These range between 30 and 90%. It can by assumed that the sample also included individuals who have no experience in photo editing, although they have an Instagram account. This was also evident by the low photo editing behavior score of the participants in this study. Nonetheless, although we determined a low score on photo editing behavior in our study, we found robust results confirming our hypotheses.
Based on sample characteristics (i.e., age, gender, and participants’s photo editing behavior), some points of criticism with respect to the generalizability of our data arise. However, according to our mediation analyses including age and gender as covariates these variables had no significant confounding effect on our results. Additionally, we calculated further post-hoc analyses with respect to replicability, post-hoc power as well as insufficient variance showing that our data seem to be replicable, unbiased, and generalizable. However, future studies with a more balanced sample are necessary to confirm our findings.
In addition, the statistical analyses in this study are correlational, meaning that no causal conclusions are warranted. Given the early phase of research on photo editing, this restriction may be acceptable. Furthermore, significant mediation does not imply true mediation but only that the data fits with the proposed mediation model [ 78 ]. Future studies are needed to examine causal inferences. For example, is photo editing behavior the cause of more self-objectification or vice versa? Such questions might be tackled by experimental studies [ 79 ], with respect to the negative impact of social comparisons on self-esteem) or longitudinal research design [ 80 ], with respect to effects of social media use on mental health).
Finally, the list of potential mediators between photo editing behavior and self-esteem includes variables that were not considered in our research design. It may include appearance contingent self-esteem [ 81 ], upward and downward social comparisons [ 24 ], and narcissism [ 82 ] Time spent on SNSs should be included as a possible confounding variable in future research. Furthermore, research should be conducted to determine the extent to which reactions to edited photos in the form of likes, comments, or compliments reinforce photo editing behavior.
Future research might investigate the outcome of photo editing behavior in contexts like dating platforms. As especially adolescents are vulnerable in terms of self-esteem and appearance-based self-worth, further research should also be conducted on the impact of photo editing behavior on this vulnerable target group. Future research might also explore more systematically reasons why users of SNSs edit their selfies and what motivates them to engage in photo editing behavior.
Data and materials availability
The datasets and materials used and/or analysed during the current study are available online at: https://osf.io/kz3gb/?view_only=02591d9f59544570853fa7d394c2bfc5 .
The hypotheses were not preregistered.
McFarland LA, Ployhart RE. Social media: a contextual framework to guide research and practice. J Appl Psychol. 2015;100(6):1653–77.
Article PubMed Google Scholar
Bayer JB, Triệu P, Ellison NB. Social media elements, ecologies, and effects. Ann Rev Psychol. 2020;71:471–97.
Article Google Scholar
Toma CT, Hancock JT, Ellison NB. Separating fact from fiction: an examination of deceptive self-presentation in online dating profiles. Pers Soc Psychol Bull. 2008;34(8):1023–36. https://doi.org/10.1177/0146167208318067 .
Renfrew Center Foundation. (2014). Afraid to be your selfie? Survey reveals most people photoshop their images Retrieved March 13, 2022 from http://renfrewcenter.com/news/afraid-be-your-selfie-survey-reveals-most-people-photoshop-their-images .
Kenrick DT, Guiterres SE. Contrast effects and judgments of physical attractiveness: when beauty becomes a social problem. J Personal Soc Psychol. 1980;38:131–40.
Vogel EA, Rose JP, Okdie BM, Eckles K, Franz B. Who compares and despairs? The effect of social comparison orientation on social media use and its outcomes. Pers Indiv Differ. 2015;86:249–56.
Ozimek P, Bierhoff HW. All my online-friends are better than me – three studies about ability-based comparative social media use, self-esteem, and depressive tendencies. Behav Inform Technol. 2020;39:1110–23.
Cohen R, Newton-John T, Slater A. The case for body positivity on social media: perspectives on current advances and future directions. J Health Psychol. 2021;26(13):2365–73.
Fardouly J, Vartanian LR. Social media and body image concerns: current research and future directions. Curr Opin Psychol. 2016;9:1–5.
Lonergan AR, Bussey K, Fardouly J, Griffiths S, Murray SB, Hay P, et al. Protect me from my selfie: examining the association between photo-based social media behaviors and self‐reported eating disorders in adolescence. Int J Eat Disord. 2020;53(5):755–66.
McLean SA, Paxton SJ, Wertheim EH, Masters J. Selfies and social media. J Eat Disorders. 2015;3(1):021.
Mills JS, Musto S, Williams L, Tiggermann M. Selfie” harm: Effects on mood and body image in young women. Body Image. 2018;27:86–92.
Tiggemann M, Anderberg I, Brown Z. Uploading your best self: selfie editing and body dissatisfaction. Body Image. 2020;33:175–82.
Lamp SJ, Cugle A, Silverman AL, Thomas MT, Liss M, Erchull MJ. Picture perfect: the relationship between selfie behaviors, self-objectification, and depressive symptoms. Sex Roles. 2019;81(11):704–12.
Fredrickson BL, Roberts TA. Objectification theory: toward understanding women’s lived experiences and mental health risks. Psychol Women Q. 1997;21(2):173–206.
Haferkamp N, Krämer NC. Social comparison 2.0: examining the effects of online profiles on social-networking sites. Cyberpsychology Behav Social Netw. 2011;14(5):309–14.
Othman S, Lyons T, Cohn JE, Shokri T, Bloom JD. The influence of photo editing applications on patients seeking facial plastic surgery services. Aesthetic Surg J. 2021;41(3):101–10.
Myers TA, Crowther JH. Social comparison as a predictor of body dissatisfaction: a meta-analytic review. J Abnorm Psychol. 2009;118(4):683–98.
Lavrence C, Cambre C. (2020). “Do I look like my selfie?”: Filters and the digital-forensic gaze.Social Media + Society, 6 (4).
Boursier V, Gioia F, Griffiths MD. Objectified body consciousness, body image control in photos, and problematic social networking: the role of appearance control beliefs. Front Psychol. 2020;11. https://doi.org/10.33897/fpsyg.2020.00147 .
Rajanala S, Maymone MB, Vashi NA. Selfies—living in the era of filtered photographs. JAMA Facial Plastic Surgery. 2018;20(6):443–4.
Belmi P, Neale M. Mirror, mirror on the wall, who’s the fairest of them all? Thinking that one is attractive increases the tendency to support inequality. Organ Behav Hum Decis Process. 2014;124(2):133–49.
Ahadzadeh AS, Sharif SP, Ong FS. Self-schema and self-discrepancy mediate the influence of Instagram usage on body image satisfaction among youth. Comput Hum Behav. 2017;68:8–16.
Chae J. Virtual makeover: Selfie-taking and social media use increase selfie-editing frequency through social comparison. Comput Hum Behav. 2017;66:370–6.
Siibak A. Constructing the self through the Photo selection - visual impression management on Social networking websites. Cyberpsychology: J Psychosocial Res Cyberspace. 2009;3(1):1.
Google Scholar
Lindner D, Tantleff-Dunn S. The development and psychometric evaluation of the Self-Objectification Beliefs and Behaviors Scale. Psychol Women Q. 2017;41(2):254–72.
Aubrey JS. Exposure to sexually objectifying media and body self-perceptions among college women: an examination of the selective exposure hypothesis and the role of moderating variables. Sex Roles. 2006;55(3):159–72.
Meier EP, Gray J. Facebook photo activity associated with body image disturbance in adolescent girls. Cyberpsychology Behav Social Netw. 2014;17(4):199–206.
Moradi B, Huang YP. Objectification theory and psychology of women: a decade of advances and future directions. Psychol Women Q. 2008;32(4):377–98.
Sun Q. Selfie editing and consideration of cosmetic surgery among young chinese women: the role of self-objectification and facial dissatisfaction. Sex Roles. 2021;84(11):670–9.
Zheng L, Zhang Y, Thing VL. A survey on image tampering and its detection in real-world photos. J Vis Commun Image Represent. 2019;58:380–99.
Fox J, Rooney MC. The dark triad and trait objectification as predictors of men’s use and self-presentation on social network sites. Pers Indiv Differ. 2015;76:161–5.
Strelan P, Hargreaves D. Women who objectify other women: the vicious circle of objectification. Sex Roles. 2005;52(9):707–12.
Tylka TL, Sabik NJ. Integrating social comparison theory and self-esteem within objectification theory to predict women’s disordered eating. Sex Roles. 2010;63(1):18–31.
Festinger L. A theory of social comparison processes. Hum Relat. 1954;7(2):117–40.
Gerber JP, Wheeler L, Suls J. A social comparison theory meta-analysis 60 + years on. Psychol Bull. 2018;144:177–97.
Schaefer LM, Thompson JK. The development and validation of the physical appearance comparison scale-revised (PACS-R). Eat Behav. 2014;15(2):209–17.
Grogan S, Rothery L, Cole J, Hall M. Posting selfies and body image in young adult women: the selfie paradox. J Social Media Soc. 2018;7(1):15–36.
Lee M, Lee HH. Social media photo activity, internalization, appearance comparison, and body satisfaction: the moderating role of photo-editing behavior. Comput Hum Behav. 2021;114:106–579.
Thornton B, Moore S. Physical attractiveness contrast effect: implications for self-esteem and evaluations of the social self. Pers Soc Psychol Bull. 1993;19:874–80.
Patrick H, Neighbors C, Knee CR. Appearance-related social comparisons: the role of contingent self-esteem and self-perceptions of attractiveness. Pers Soc Psychol Bull. 2004;30(4):501–14.
Higgins ET, Klein R, Strauman T. Self-concept discrepancy theory: a psychological model for distinguishing among different aspects of depression and anxiety. Soc Cogn. 1985;3(1):51–76.
Vartanian LR. Self-discrepancy theory and body image. Encyclopedia of Body Image and Human Appearance. 2012;2(1):711–7.
Perloff RM. Social media effects on young women’s body image concerns: theoretical perspectives and an agenda for research. Sex Roles. 2014;71(11):363–77.
Staudinger UM, Greve W. Das Selbst im Lebenslauf: Brückenschläge und Perspektivenwechsel zwischen Entwicklungs-und Sozialpsychologischen Zugängen [The self in lifecourse: conjunction and change in perspective between developmental and social psychological approaches]. Z für Sozialpsychologie. 1997;28:3–18.
Mendelson BK, White DR, Mendelson MJ. Self-esteem and body esteem: Effects of gender, age, and weight. J Appl Dev Psychol. 1996;17(3):321–46.
Feingold A. Good-looking people are not what we think. Psychol Bull. 1992;111(2):304–41.
Kim YJ, Hong EJ. Double mediating effects of child relationship satisfaction and self-esteem on the relationship between satisfaction with income and life satisfaction by divorced korean elderly. Rev Int Geographical Educ Online. 2021;11(8):675–89.
Wade TJ. Evolutionary theory and self-perception: sex differences in body esteem predictors of self‐perceived physical and sexual attractiveness and self‐esteem. Int J Psychol. 2000;35(1):36–45.
Anderson SL, Adams G, Plaut VC. The cultural grounding of personal relationship: the importance of attractiveness in everyday life. J Personal Soc Psychol. 2008;95(2):352–68.
Darley JM, Fazio RH. Expectancy confirmation processes arising in the social interaction sequence. Am Psychol. 1980;35(10):867–81.
Graber LW. (1981). Psychological considerations of orthodontic treatment. In: Lucker, G. W., Ribbens, K. A. & McNamara, J. A, editors Psychological Aspects of Facial Form , pp. 81– 118.
Kanavakis G, Halazonetis D, Katsaros C, Gkantidis N. (2021) Facial shape affects self- perceived facial attractiveness. PLoS ONE 16(2) : e0245557. https://doi.org/10.1371/journal
Jiang Z, Xu J, Gorlin M, Dhar R. Beautiful and confident: how boosting self-perceived attractiveness reduces preference uncertainty in context-dependent choices. J Mark Res. 2021;58(5):908–24.
O’Brien KS, Caputi P, Minto R, Peoples G, Hooper C, Kell S, et al. Upward and downward physical appearance comparisons: development of scales and examination of predictive qualities. Body Image. 2009;6(3):201–6.
Faul F, Erdfelder E, Lang A-G, Buchner A. G*power 3: a flexible statistical power analysis program for the social, behavioral, and biomedical sciences. Behav Res Methods. 2007;39:175–91.
Schäfer T, Schwarz M. The meaningfulness of effect sizes in psychological research: differences between subdisciplines and the impact of potential biases. Front Psychol. 2019. https://doi.org/10.3389/fpsyg.2019.00813 .
Article PubMed PubMed Central Google Scholar
Reimann LE, Ozimek P, Rohmann E, Bierhoff HW. Post more! The mediating role of social capital between Instagram use and satisfaction with life. Curr Psychol. 2021. https://doi.org/10.1007/s12144-021-02579-6 .
Mölbert C, Hautzinger M, Karnath HO, Zipfel S, Giel K. Validation of the physical appearance comparison scale (PACS) in a german sample: psychometric properties and association with eating behavior, body image and self-esteem. Psychother Psychosom Med Psychol. 2017;67(2):91–7.
Thompson JK, Heinberg LJ, Altabe M, Tantleff-Dunn S. The physical appearance comparison scale (PACS). Behav Therapist. 1991;14:174.
Mendelson BK, Mendelson MJ, White DR. Body-esteem scale for adolescents and adults. J Pers Assess. 2001;76(1):90–106.
Rosenberg M. Society and the adolescent self-image. Princeton, NJ: Princeton University Press; 1965.
Book Google Scholar
Von Collani G, Herzberg PY. Eine revidierte Fassung der deutschsprachigen Skala zum Selbstwertgefühl von Rosenberg. Z für Differentielle und Diagnostische Psychologie. 2003;24:3–7.
Blascovich J, Tomaka J. (1991) Measures of self-esteem. In J.P. Robinson, P.R. Shaver, & L.S. Wrightsman, editors. Measures of personality and social psychological attitudes (pp. 115–128) . San Diego: Academic Press.
Roth M, Decker O, Herzberg PY, Brähler E. Dimensionality and norms of the Rosenberg Self-Esteem Scale in a german general population sample. Eur J Psychol Assess. 2008;24(3):190–7.
Calogero RM, Tantleff-Dunn S, Thompson JK. Self-objectification in women: causes, consequences, and counteractions. Washington, DC: American Psychological Association; 2011.
Ozimek P, Bierhoff HW. Facebook use depending on age: the influence of social comparisons. Comput Hum Behav. 2016;61:271–9.
Schimmack U. (2018). The replicability revolution.Behavioral and Brain Sciences, 41 .
Slater A, Tiggemann M. Media exposure, extracurricular activities, and appearance-related comments as predictors of female adolescents’ self-objectification. Psychol Women Q. 2015;39(3):375–89.
Ozimek P, Förster J. The social online self-regulation theory. A review of self-regulation in social media. J Media Psychol. 2021;33:181–90.
Personality and Social Psychology Bulletin , 9 (3), 351–358.
Caso D, Schettino G, Fabbricatore R, Conner M. Change my selfie”: Relationships between self-objectification and selfie‐behavior in young italian women. J Appl Soc Psychol. 2020;50(9):538–49.
Kernis MH, Goldman BM. Assessing stability of self-esteem and contingent self-esteem. In: Kernis MH, editor. Self-esteem. Issues and answers. Hove: Psychology Press; 2006. pp. 77–85.
Bierhoff HW, Rohmann E, Ozimek P. Schubladendenken überwinden: Stereotype-Funktion, Wirkug, Reduktion. Weiterbildung. 2020;2020/1:12–5. ISSN 1861 – 0501.
Foos PW, Clark MC. Adult age and gender differences in perceptions of facial attractiveness: beauty is in the eye of the older beholder. J Genet Psychol. 2011;172(2):162–75.
Woodside DB. Assessing and treating men with eating disorders. Psychiatric Times. 2004;21(3):85–5.
Santarossa S, Woodruff SJ. #SocialMedia: exploring the relationship of social networking sites on body image, self-esteem, and eating disorders. Social Media + Society. 2017;3(2):2056305117704407.
Hayes AF, Scharkow M. The relative trustworthiness of inferential tests of the indirect effect in statistical mediation analysis: does method really matter? Psychol Sci. 2013;24(10):1918–27.
Ozimek P, Bierhoff HW. All my online-friends are better than me–three studies about ability-based comparative social media use, self-esteem, and depressive tendencies. Behav Inform Technol. 2020;39(10):1110–23.
Brailovskaia J, Rohmann E, Bierhoff HW, Margraf J, Köllner V. Relationships between addictive Facebook use, depressiveness, insomnia, and positive mental health in an inpatient sample: a german longitudinal study. J Behav Addictions. 2019;8:703–13.
Crocker J, Luhtanen RK, Cooper ML, Bouvrette A. Contingencies of self-worth in college students: theory and measurement. J Personal Soc Psychol. 2003;85(5):894–908.
Wang D. A study of the relationship between narcissism, extraversion, body-esteem, social comparison orientation and selfie-editing behavior on social networking sites. Pers Indiv Differ. 2019;146:127–9.
Bunker CJ, Kwan VS. (2021). Do the offline and social media Big Five have the same dimensional structure, mean levels, and predictive validity of social media outcomes? Cyberpsychology: Journal of Psychosocial Research on Cyberspace, 15(4) .
Bunker CJ, Saysavanh SE, Kwan VS. Are gender differences in the big five the same on social media as offline? Computers in Human Behavior Reports. 2021;3:100085.
Download references
Acknowledgements
We acknowledge support by the Open Access Publication Funds of the Ruhr-Universität Bochum.
Open Access funding enabled and organized by Projekt DEAL.
Author information
Authors and affiliations.
Mental Health Research and Treatment Center, Department of Psychology, Ruhr-Universität Bochum, Bochum, Germany
Phillip Ozimek
Department of Social Psychology, Ruhr-Universität Bochum, Bochum, Germany
Semina Lainas, Hans-Werner Bierhoff & Elke Rohmann
You can also search for this author in PubMed Google Scholar
Contributions
P.O., S.L. developed the research idea, P.O., S.L. conducted the research, S.L. prepared all figures and the APPENDIX, P.O.: supervision, all authors wrote and reviewed the main manuscript.
Corresponding author
Correspondence to Phillip Ozimek .
Ethics declarations
Ethical approval and consent to participate.
We declare that our study was approved by the local ethical committee of the Ruhr University Bochum (protocol code 495). All the steps/ methods were performed in accordance with the relevant guidelines and regulations. Informed consent was given by all participants. The present manuscript has not been previously published or simultaneously submitted elsewhere.
Competing interests
Additional information, publisher’s note.
Springer Nature remains neutral with regard to jurisdictional claims in published maps and institutional affiliations.
Electronic supplementary material
Below is the link to the electronic supplementary material.
Supplementary Material 1
Supplementary material 2, supplementary material 3, rights and permissions.
Open Access This article is licensed under a Creative Commons Attribution 4.0 International License, which permits use, sharing, adaptation, distribution and reproduction in any medium or format, as long as you give appropriate credit to the original author(s) and the source, provide a link to the Creative Commons licence, and indicate if changes were made. The images or other third party material in this article are included in the article’s Creative Commons licence, unless indicated otherwise in a credit line to the material. If material is not included in the article’s Creative Commons licence and your intended use is not permitted by statutory regulation or exceeds the permitted use, you will need to obtain permission directly from the copyright holder. To view a copy of this licence, visit http://creativecommons.org/licenses/by/4.0/ . The Creative Commons Public Domain Dedication waiver ( http://creativecommons.org/publicdomain/zero/1.0/ ) applies to the data made available in this article, unless otherwise stated in a credit line to the data.
Reprints and permissions
About this article
Cite this article.
Ozimek, P., Lainas, S., Bierhoff, HW. et al. How photo editing in social media shapes self-perceived attractiveness and self-esteem via self-objectification and physical appearance comparisons. BMC Psychol 11 , 99 (2023). https://doi.org/10.1186/s40359-023-01143-0
Download citation
Received : 06 December 2022
Accepted : 25 March 2023
Published : 06 April 2023
DOI : https://doi.org/10.1186/s40359-023-01143-0
Share this article
Anyone you share the following link with will be able to read this content:
Sorry, a shareable link is not currently available for this article.
Provided by the Springer Nature SharedIt content-sharing initiative
- Photo editing
- Social media
- attractiveness
BMC Psychology
ISSN: 2050-7283
- General enquiries: [email protected]
materialmodifier: An R package of photo editing effects for material perception research
- Open access
- Published: 10 May 2023
- Volume 56 , pages 2657–2674, ( 2024 )
Cite this article
You have full access to this open access article
- Hiroyuki Tsuda ORCID: orcid.org/0000-0001-9396-5327 1 &
- Hideaki Kawabata 2
1383 Accesses
1 Altmetric
Explore all metrics
In this paper, we introduce an R package that performs automated photo editing effects. Specifically, it is an R implementation of an image-processing algorithm proposed by Boyadzhiev et al. ( 2015 ). The software allows the user to manipulate the appearance of objects in photographs, such as emphasizing facial blemishes and wrinkles, smoothing the skin, or enhancing the gloss of fruit. It provides a reproducible method to quantitatively control specific surface properties of objects (e.g., gloss and roughness), which is useful for researchers interested in topics related to material perception, from basic mechanisms of perception to the aesthetic evaluation of faces and objects. We describe the functionality, usage, and algorithm of the method, report on the findings of a behavioral evaluation experiment, and discuss its usefulness and limitations for psychological research. The package can be installed via CRAN, and documentation and source code are available at https://github.com/tsuda16k/materialmodifier .
Avoid common mistakes on your manuscript.
Introduction
Material perception is a rapidly growing research area in vision science today (Fleming, 2017 ; Komatsu & Goda, 2018 ; Spence, 2020 ) and it is relevant to a wide range of human cognition and behaviors (as described below). To study material perception, we need a set of controlled images for stimuli, such as images with high and low roughness. However, unlike basic visual features such as color and lightness, controlling specific material properties of objects in photographs is an intricate endeavor. To alleviate this situation, we created an R package called materialmodifier that can be used to modify the surface properties of objects such as gloss and roughness (Fig. 1 ). This method was proposed by Boyadzhiev et al. ( 2015 ), and we implemented it in the R package to make it accessible to psychologists. Before going into the details of the package, we briefly describe recent research trends in material perception to provide some background on our contribution.
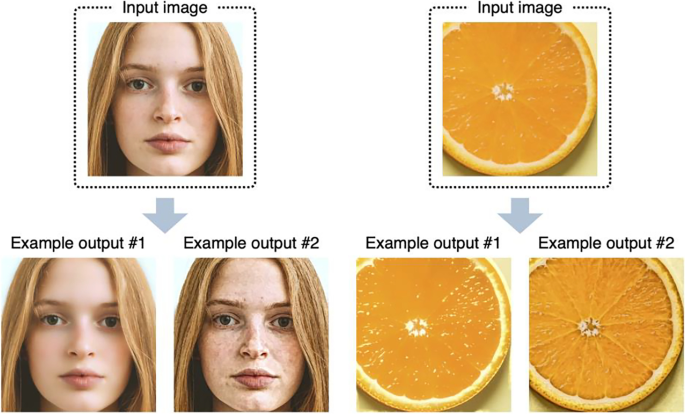
By using the materialmodifier package in R, the user can modify the appearance of objects in photographs. For example, they can make skin smoother or make marks or blemishes more visible; enhance the gloss of food or make it look wilted
People easily perceive and recognize materials in their daily lives and can identify categories of materials quickly and reliably (Fleming et al., 2013 ; Sharan et al., 2014 ). People can also distinguish subtle differences in certain material properties, such as the degree of surface roughness and gloss (Fleming, 2017 ). This visual ability is important for diagnosing the freshness of food or the health of a person based on the condition of their skin. Despite the subjective ease of material perception, achieving stability therein is a computationally challenging problem because retinal input for objects of the same material can vary greatly depending on illumination and the surface shape of the object (Anderson, 2020 ; Chadwick & Kentridge, 2015 ; Fleming, 2014 ). Recent theories suggest that the brain achieves material perception not through inverse-optics computation but through statistical inference based on internal image models (Fleming, 2014 ; Fleming & Storrs, 2019 ). From this perspective, systematic manipulation of image features and examining their effects on perception is an effective approach to understanding the mechanisms of material perception (Nishida, 2019 ).
Material perception is interesting because of its relevance to a wide range of human cognition and behaviors. For instance, material perception has been related to the perception of the freshness of foods (Arce-Lopera et al., 2013 ; Péneau et al., 2007 ), judgments of facial impressions from skin conditions (Fink et al., 2006 ; Fink & Matts, 2008 ; Jaeger et al., 2018 ; Nkengne et al., 2008 ; Stephen et al., 2009 ), action planning for touching objects and walking on slippery floors (Adams et al., 2016 ; Joh et al., 2006 ; Lesch et al., 2008 ), pathogen detection (Iwasa et al., 2020 ), product packaging design (Di Cicco et al., 2021 ), and aesthetic appreciation of textures (Stephens & Hoffman, 2016 ), paintings (Di Cicco et al., 2020 ), and sculptures (Schmidt, 2019 ). Furthermore, studies have explored how material perception contributes to other cognitive domains, such as memory (Tagai et al., 2016 ; Tsuda et al., 2020 ) and multisensory perception (Fujisaki, 2020 ; Spence, 2020 ).
Despite its wide importance in cognition and behavior, studies on material perception are relatively limited in size and scope. One of the reasons for this may be the difficulty of creating a set of controlled stimuli that differ in certain material properties. There are image databases of materials and textures that are useful for psychological research (Lagunas et al., 2019 ; Sawayama et al., 2019 ; Serrano et al., 2021 ; Sharan et al., 2014 ; van Zuijlen et al., 2021 ). However, we often need a new image set tailored for specific research purposes. In such cases, images are created either by manual photo editing, using software such as Photoshop and GIMP (Fink & Matts, 2008 ; Jaeger et al., 2018 ), taking photographs of objects (Motoyoshi et al., 2007 ), or using computer graphics rendering (Fleming et al., 2003 ). In any of these circumstances, a decent amount of time, effort, or technical expertise is required. Moreover, the manual editing of photographs suffers from the low reproducibility of the image production process.
In this study, we implemented the image processing algorithm proposed by Boyadzhiev et al. ( 2015 ) as an R package. It is one of the image-based material editing methods: a heuristic method that manipulates image features that are associated with human material perception. Specifically, it decomposes an image into spatial-frequency subbands (i.e., images representing specific spatial frequency information of the input image) and changes the input image’s appearance by manipulating (boosting/reducing) the energy of specific subbands therein (details are given in the Algorithm section). Although the algorithm for this method is simple and heuristic, it is effective and compelling for the following reasons. The human early visual system represents visual information in spatial frequency and orientation selective channels (Blakemore & Campbell, 1969 ), and a computational model of early vision based on spatial frequency decomposition explains human contrast detection/discrimination well (Schütt & Wichmann, 2017 ). Spatial frequency subband statistics are associated with the perception of the material properties of objects, e.g., gloss (Dror et al., 2004 ; Kiyokawa et al., 2021 ; Motoyoshi & Matoba, 2012 ). Manipulating the energy of specific spatial frequency subbands and their correlations can effectively modify the perceptual attributes of textures (Giesel & Zaidi, 2013 ; Portilla & Simoncelli, 2000 ). Therefore, image-editing methods based on the manipulation of an image’s spatial frequency characteristics can be thought of as effectively exploiting the mechanism by which the human visual system encodes information about the external world. In this regard, Boyadzhiev et al.’s (2015) method is interesting not only as an image-editing tool, but also as a model of vision.
In the following sections, we describe the functionality and usage of the package, illustrate the behavior of the algorithm with a number of image examples, and explain how the algorithm works. We also report on an experiment that examined how face and food images edited using this method affect viewers’ perception of the material properties of said faces and food, and discuss its usefulness and limitations in psychological research.
Functionality and usage of the package
This section describes the features of the package and how to use them. Note that detailed instructions and practical tips for using the package, as well as the source code, are provided on our GitHub page ( https://github.com/tsuda16k/materialmodifier ). The basic procedure to use this package is as follows.
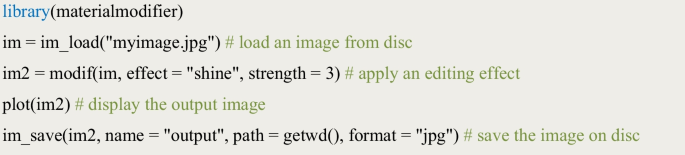
You can load an image from the disk with the im_load() function, and apply a material editing effect with the modif() function. The effect argument of the modif function specifies the type of material editing effect applied (explained below), and the strength argument determines the strength of the effect. The plot() function can be used to display an image. To save an image on disk, use the im_save() function, specifying the name of the output image file and the path where the image will be saved. You can load/save images in jpg/png/bmp format.
Figure 2 shows example outputs of the shine and aging effects. The shine effect manipulates very bright elements in the high spatial frequency bands in the input image (e.g., highlights and gloss), and the aging effect manipulates local dark elements (e.g., stains and blemishes). The higher the value of the strength parameter, the stronger the editing effect (the features are emphasized). If the value of the strength parameter is less than 1, the opposite effect will occur, e.g., the gloss will be weakened or blemishes will be reduced.
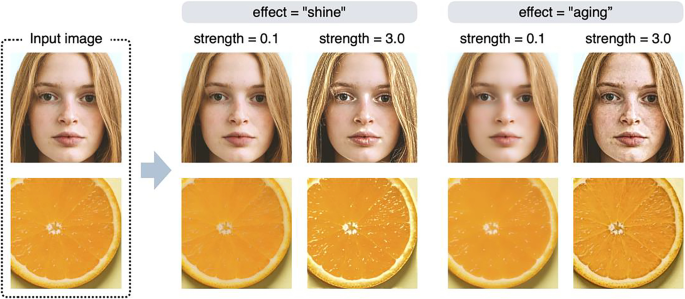
Example outputs of the shine and aging effects. The strength parameter controls the strength of the editing effect
Using the aging effect as an example, the effect of the strength parameter is examined in more detail in Fig. 3 . If the value of the strength parameter is greater than 1, a boosting effect that increases the stains/blemishes occurs; if it is less than 1, a reducing effect that decreases the stains/blemishes occurs. To achieve a boosting effect, a strength value of 1.5 to 4 usually yields reasonable results. The strength parameter can be a negative value, but in most cases, setting a negative value will produce unrealistic results (e.g., contrast reversal; see also Fig. 5 ). Note that if the strength parameter is 1, no effect occurs, and the input image is returned unchanged. This is because this parameter is a multiplication factor for the image feature being manipulated (a detailed description of this parameter is given in the Algorithm section).
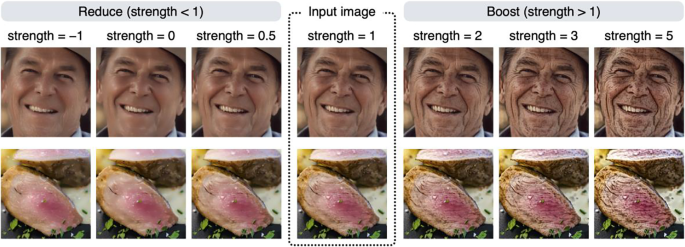
The effect of the strength parameter is examined using the aging effect. If the value of the strength parameter is greater than 1, a boosting effect occurs; if it is less than 1, a reducing effect occurs
You can also apply multiple editing effects simultaneously. For example, you can simultaneously apply the shine and the aging effects as follows.

This command simultaneously applies a shine effect of strength = 0.2 and an aging effect of strength = 3, resulting in a less shiny and more blemished image. This procedure is the same as the one used to create output example #2 in Fig. 1 . Although you can obtain almost the same result (but not identical, because the first process changes the input image for the second process) by applying each effect in turn (e.g., applying an aging effect to the output of a shine effect), we recommend doing them in a single line, as in the example above, because it saves time needed for image processing. The order of effect names specified in the effect argument does not affect the result; effect = c("shine", "aging") and effect = c("aging", "shine") produce identical results.
This package has several other effects in addition to the shine and aging effects. The available effects are shine, spots, rough, stain, blemish, shadow, and aging. A visual summary of these effects is shown in Fig. 4 . The first column of the figure shows the name of each effect, and the second column shows the perceptual features controlled by that effect. The third and subsequent columns show the input and output images.
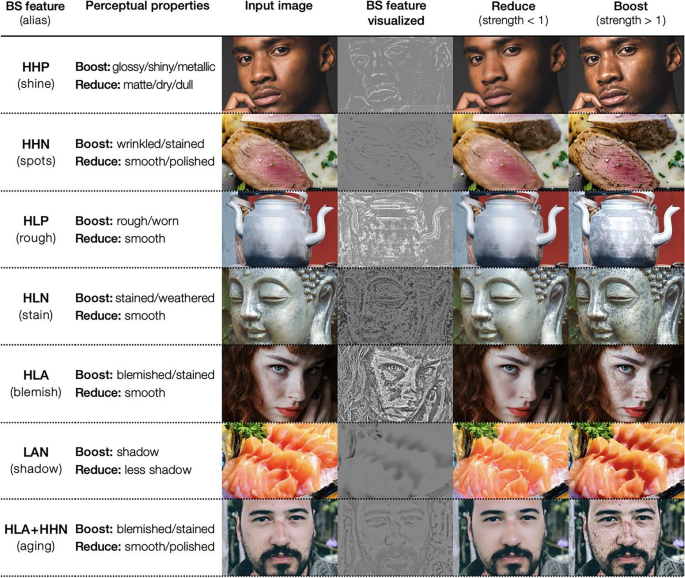
Visual summary of image editing effects. By specifying the name of an effect (or BS feature), the algorithm detects that feature in the input image and modifies the appearance of the input image by reducing or boosting the feature. Note that the aging effect controls both HLA and HHN features. See the main text for the definition of BS features
Figure 4 contains a column labeled “BS (band-sifting) feature”; this is an important term related to the image processing algorithm (briefly, an image component to be manipulated, extracted from the input image based on a certain criterion). The algorithm achieves image editing effects by decreasing or increasing the weights of the BS features in the input image. The effect names, such as shine and spots, are aliases for these BS features. The input to the effect argument of the modif function can also be the BS feature names.

Since it is easier to know what kind of editing effect will be achieved if there is an alias, our implementation allows the user to specify image editing by alias as well as by BS feature name.
To understand the nature of each image editing effect better, it is helpful to compare the results of all editing effects on a single image. Figure 5 summarizes the results of the editing effects on face and food images (note that the image in the row with a strength value of 1 is the input image). By comparing the images in the rows with large values of the strength parameter, it is easier to see the characteristics of each effect.
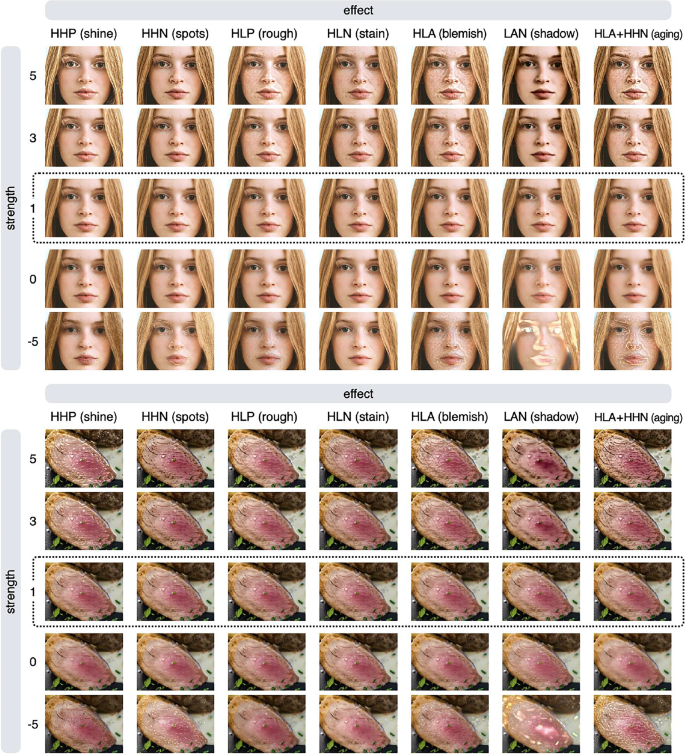
Summary of image processing results with different strength values for each effect. The image inside the dotted line is the input image. Each image in the row with a strength value of −5 has an unnatural appearance (contrast reversal) and is not suitable for use as a stimulus, but it is useful to visualize what feature in the input image is manipulated by each effect
Setting a negative value for the strength parameter often results in an unnatural image (see images in the bottom rows of Fig. 5 ), but using a large negative value for the strength parameter makes it easier to compare which areas are affected by each effect. For example, the rough effect and the blemish effect produce similar results, but if you compare the images in the row with a strength value of −5, you can clearly see that they are not identical. Technically, the blemish effect is equivalent to giving both the rough and stain effects at the same time. To acquire a more formal understanding of these properties, we need to know more about the specifics of how image processing algorithms work.
By default, the modif function targets the entire image for editing. However, in some situations, you may want to edit only certain objects or areas of the image (for example, you may want to edit only the skin area of a portrait). By using a mask image, you limit editing to certain areas within an image. To use this feature, you need to prepare a mask image of the same size as the input image you wish to edit. The mask image contains the area to be edited—white in color—and the rest of the image—which is black. The mask image does not have to be a binary image; gray can be present (the intensity of the gray will be used to alpha blend the input image with the edited image). For example, the mask image representing the skin region of a face image is shown in Fig. 6 .
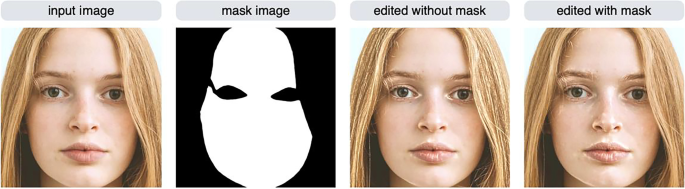
Editing only specific areas in an image using a mask image. The results of the HHP (shine) effect (strength = 3) with and without using a mask image are shown
The image edited without using a mask image has an increased gloss not only on the skin, but also on the hair and eyes. On the other hand, the image edited using a mask image has increased gloss only in the skin area. To use the masking feature, a mask image in the mask argument of the modif function must be specified.

In this section, we will describe the image processing algorithm in detail. First, there are two points to note. First, the user does not necessarily need to understand the details of the algorithms to use this package. In fact, as we have seen, it is possible to perform image processing by simply specifying the name of the effect and the strength parameter. However, by reading this section, users will have a better understanding of the behavior of this package and will be able to use it in an advanced way. Secondly, this paper will explain the algorithm at a conceptual level, which would be appropriate for the average psychologist. The technical and mathematical aspects are explained in the original paper (Boyadzhiev et al., 2015 ).
Boyadzhiev et al. ( 2015 ) proposed an image-based material editing method called “band-sifting decomposition.” It extracts and controls a variety of perceptual properties of images such as gloss, roughness, and blemishes based on a combination of image processing procedures. How this algorithm modifies the surface appearance of an input image is shown in Fig. 7 , using the control of blemishes as an example. Pixels corresponding to image features that are to be manipulated (e.g., blemishes) are extracted from the lightness channel of the input image based on specific criteria. By decreasing or increasing the lightness value of the pixels in the feature image (Fig. 7 , top right), the appearance of the object surface in the input image is controlled.
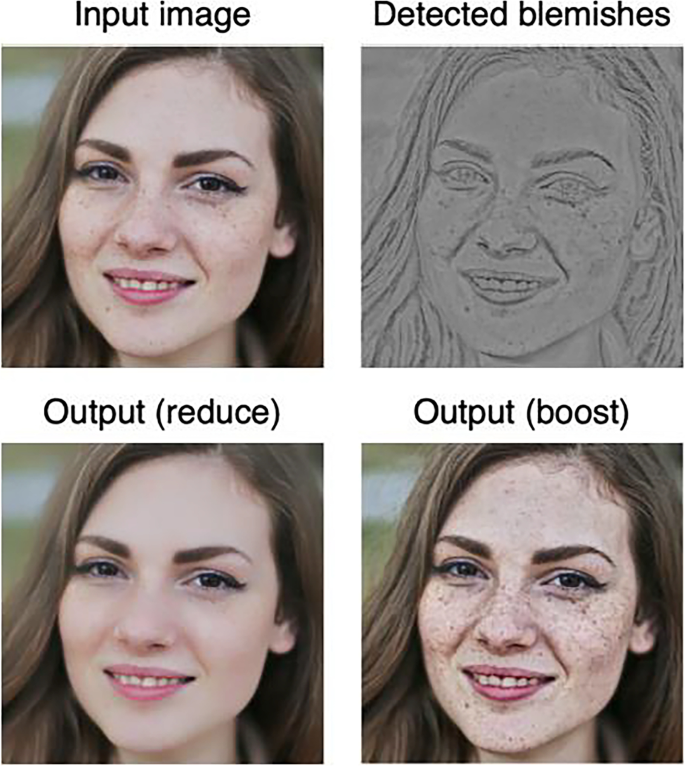
The central idea of the material editing algorithm. Pixels corresponding to image features that are to be manipulated (e.g., blemishes; top right) are extracted from the lightness channel of the input image based on specific criteria. By decreasing or increasing the lightness value of those pixels, the appearance of the object surface in the input image is controlled (bottom left and bottom right)
The overall flow of image processing is summarized in Fig. 8 . The input image is first converted to the CIELAB color space. We only process the lightness channel (L channel) and keep the color channels intact. The L channel is log-transformed and then decomposed into “scale subbands”; each subband image represents the lightness information at a given scale (or spatial frequency). As in Boyadzhiev et al. ( 2015 ), we employed the “guided filter” (He et al., 2010 ) to perform scale decomposition. This procedure is a type of band-pass filtering, which decomposes the image based on spatial frequency. This decomposition differentiates between small-scale elements, such as blobs and wrinkles, and large-scale gradients, such as shading and shadows. The number of subband images is determined by the resolution of the input image: if the shorter side of the input image has N pixels, then log 2 N − 1 subband images are produced (e.g., if N = 512, then 8 subband images are produced). The decomposition also produces a low-frequency residual.
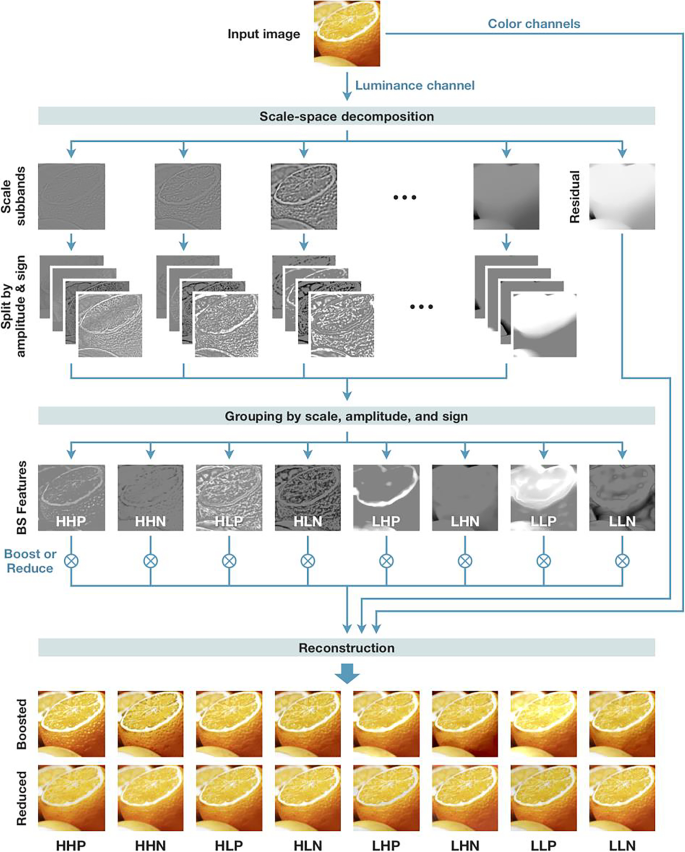
The image processing flow for material editing. The L channel of an input image is decomposed into multiple component images based on three criteria: scale (spatial frequency), amplitude (low or high contrast), and sign (sign of pixel value: positive or negative). The component images are assigned to eight groups based on scale, amplitude, and sign. The images are then combined (added together) within each group, resulting in eight images that we call BS (band-sift) features. These images represent different aspects of the perceptual quality of the input image, which can be used to control the appearance of objects. See the main text for a definition of each abbreviation (e.g., HHP)
Each subband image is further decomposed into four images based on the amplitude and sign of the pixels in that subband, and this process is the core idea of the algorithm. For amplitude, the standard deviation (1 SD) of pixel values in each subband is used as a threshold between high and low amplitude pixels to separate low- and high-contrast regions of the subband. Each (low/high) amplitude image is then separated by the sign of pixel values, positive or negative; all the negative-value pixels of an amplitude image are set to zero to produce a positive image, and all the positive-value pixels of an amplitude image are set to zero to produce a negative image. Thus, each scale subband is decomposed into four images (high/low amplitude × positive/negative sign; the images of the row labeled “split by amplitude & sign” in Fig. 8 ).
Next, all the component images (8 scale subbands × 2 amplitudes × 2 signs = 32 images in total, in this example) are grouped by the combination of scale (high or low), amplitude (high or low), and sign (positive or negative). Note that the scale subbands are classified as either high or low frequency (two categories, instead of eight in the original decomposition). If we have N scale subbands, then the first floor (N/2) images are categorized as high frequency and the remaining images as low frequency. This grouping assigns the component images into eight (and always eight, regardless of the resolution of the input image) groups. The images in each group are relatively similar to each other (because they have similar spatial frequencies and belong to the same amplitude and sign group). Finally, the images (pixel values) in each group are added together, resulting in eight images that we call BS features (the images of the row labeled “BS features” in Fig. 8 ). As in Boyadzhiev et al. ( 2015 ), we call each BS feature by the acronym of its grouping criterion. For example, HHP represents the grouping criterion (and the resultant image or BS feature) of the H igh spatial frequency, H igh amplitude, and P ositive sign.
The BS features represent distinct information associated with the perceived material properties of objects in the input image. For example, HHP represents bright (because of their high amplitude and positive sign) and small (because of their high spatial frequency) spots, typically found on wet and glossy surfaces, whereas the HHN (high frequency; high amplitude; negative sign) feature represents small dark blobs that are typical of wrinkles and blemishes in the skin. To amplify gloss, for example, we will boost the HHP feature (i.e., all the pixel values of the HHP image are multiplied by a coefficient greater than 1). To reduce wrinkles, we will reduce the HHN feature (i.e., multiply the HHN image by a coefficient less than 1). Subsequently, all the BS features and the residual image are added together and inverse-log transformed to reconstruct the L channel. The L channel is then combined with color channels and converted back to the standard RGB (red, green, blue) color space to produce the final output (Fig. 8 , images in the bottom two rows).
Depending on which BS feature is being manipulated (boosted/reduced), we obtain different material editing effects. The bottom rows of Fig. 8 show the effect of boosting or reducing each BS feature. For example, for the column labeled HHP, the upper image is the result of boosting the HHP feature, while the lower image is the result of reducing the HHP feature. Manipulating BS features with a positive sign (e.g., HH P and LL P ) adjusts the bright areas in the input image, resulting in the editing of features such as glossiness, while manipulating BS features with a negative sign (e.g., HH N and HL N ) adjusts the dark areas in the input image, resulting in the editing of features such as blemishes. Note that not all BS features produce perceptually meaningful changes in the input image. Based on Boyadzhiev et al. ( 2015 ) and our observations, we have given aliases (alternative names) to some of the BS features that can be used most effectively for perceptual editing effects. For example, HHP is called the “shine” feature because it can be used to manipulate gloss, and HHN is called the “spots” feature because it can be used to manipulate small stains and wrinkles (see Fig. 4 for the list of aliases).
Advanced usage of the package
This section describes how to use the package, based on an understanding of how the algorithm works. By using the modif2() function, image manipulation can be performed by specifying a BS feature in detail. There are two ways to do this: using acronyms, or specifying scale, amplitude, and sign separately.
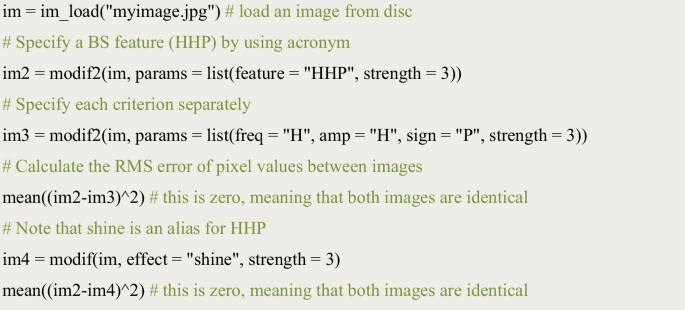
A list of parameters, which specifies a BS feature to be manipulated, is given to the params argument. A BS feature can be specified by the feature parameter using acronyms. A strength value must also be specified in the list. Instead of using acronyms, each criterion can be specified individually. The freq parameter specifies the spatial frequency (“H” for high spatial frequency, “L” for low spatial frequency, or “A” for all frequencies). The amp parameter specifies the amplitude (“H” for high amplitude, “L” for low amplitude, or “A” for all (both) amplitudes). The sign parameter specifies the sign (“P” for positive sign, “L” for negative sign, or “A” for both signs).
The advantage of specifying the features we want to manipulate using individual criteria is that we have more freedom to specify the scale. As mentioned in the algorithm section, the number of scale-subband images that the algorithm creates is determined by the resolution of the input image. The number of scale subband images for an image can be known using the modif_dim() function. In addition to the number of subband images, this function also outputs the indices of high- and low-scale images. An example is shown below, where the input image is 500 × 500 px in size.
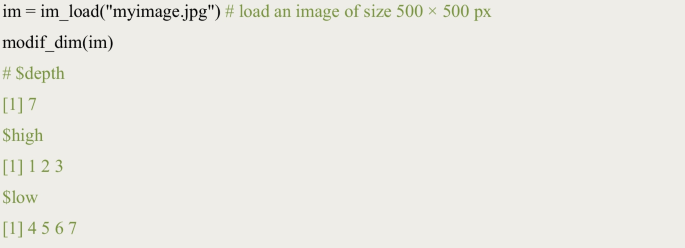
The output of the modif_dim() function shows that the number of subband images to be created from this image is 7; the indices for higher-scale (spatial frequency) images are 1, 2, and 3, and the indices for lower-scale images are 4, 5, 6, and 7. This shows that, in the case of this input image, freq = “H” is equivalent to setting freq = 1:3, and freq = “L” is equivalent to setting freq = 4:7. Therefore, each pair of commands below will produce the same output (but note that which freq corresponds to H/L depends on the resolution of the input image).
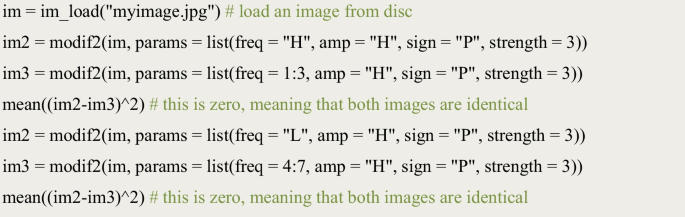
What is the advantage of being able to specify the scale to be manipulated in detail? We may want to manipulate only a specific scale, rather than as a high- or low-scale group. To understand this motivation, the result of manipulating individual scales is shown in the bottom row of Fig. 9 . When freq = 1, regions such as eyebrows, which are not considered to be skin gloss, are controlled. Image features with the highest spatial frequency (i.e., freq = 1) often reflect the high spatial frequency noise. Therefore, in some cases it is useful to exclude the highest spatial frequency subband when controlling images. The image in the top middle of Fig. 9 has scale = 1, 2, 3, which is identical to specifying freq = "H" (and therefore this command specifies the HHP feature and is equivalent to the shine effect). Some areas of the eyebrows are brighter in this image because the image subband with freq = 1 has been manipulated. On the other hand, the image in the top right of Fig. 9 does not show a manipulation of the freq = 1 region, so there is no change in the brightness of the eyebrows.
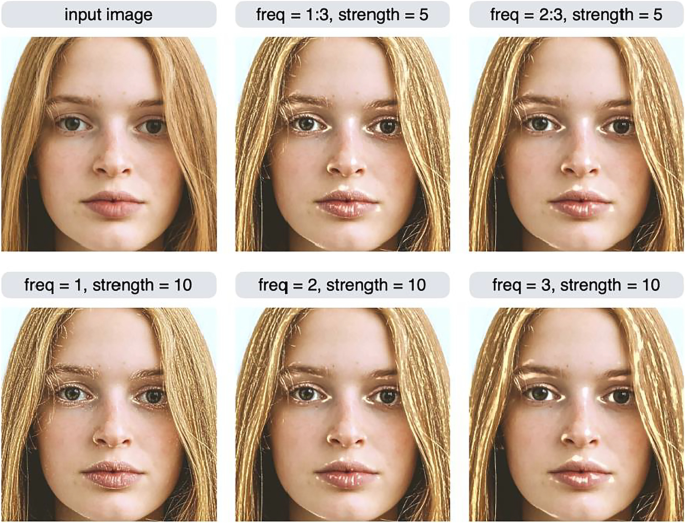
Variants of the HHP (or shine) effect. The modif2 function can be used to specify only one scale subband to be controlled (bottom row), or multiple scale subbands (top middle, top right). The argument of the function was params = list(freq = x, amp = "H", sign = "P", strength = y). The x and y values are shown in the label of each image
Another example of the effect of selecting a particular scale is shown in Fig. 10 . These are variants of the HLA (blemish) effect. Instead of specifying freq = "H" (or, equivalently, freq = 1:3), we can specify freq = 1:2 (excluding the third scale), which gives a somewhat different result (Fig. 10 , top right) than the normal blemish effect (Fig. 10 , top middle).
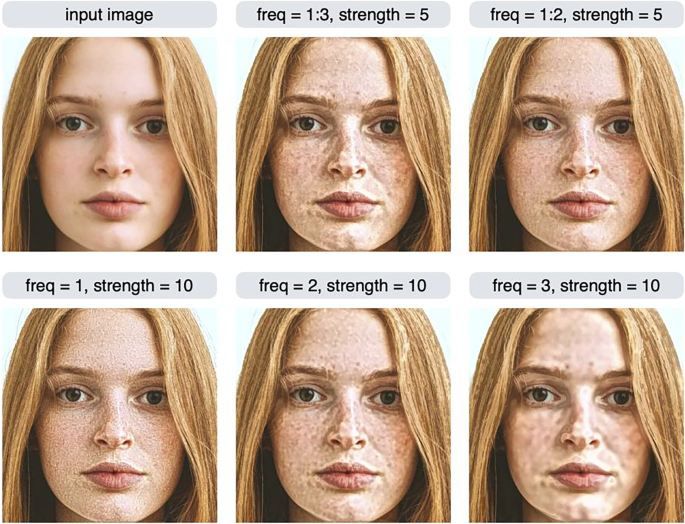
Variants of the HLA (or blemish) effect. The argument of the modif2 function was params = list(freq = x, amp = "L", sign = "A", strength = y). The x and y values are shown in the label of each image. Excluding the third scale resulted in a somewhat different output than the normal stain effect (top right)
Recall that in the case of the modif function, it is possible to apply multiple effects simultaneously. A similar approach can be taken with the modif2 function. The following script shows how to apply the spots/rough effect simultaneously using the modif2 function. A list containing several lists of parameters is given as the params argument to the function.
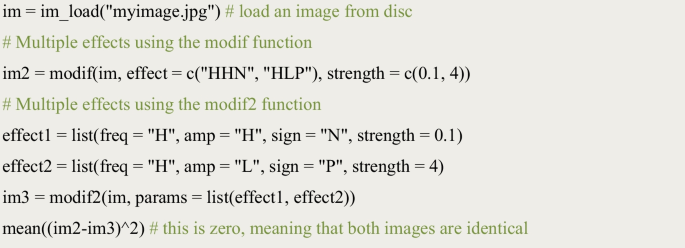
In summary, the modif2 function allows the user to specify the scale subband (i.e., spatial frequency) to be manipulated in more detail than the modif function, and provides greater flexibility in controlling the appearance of images.

Evaluation experiment
In this section, we report on an online experiment that evaluated how material editing effects can produce perceptual changes in an input image. We created a series of images with different strength parameter values for each editing effect (HHP, HHN, etc.), and conducted an experiment in which we asked participants to rate the perceived material properties of objects, such as gloss and roughness, as well as the naturalness of the images. The material, data, and R scripts for this experiment are available on OSF: https://osf.io/72dqz/ .
Participants
To detect an effect size of Cohen’s d = 0.55 (a difference of 0.5 on a six-point scale; the standard deviation was based on our pilot study) with 90% power (alpha = .05, two-tailed), G*Power (Faul et al., 2007 ) suggested that we needed 37 participants. In total, 48 participants (female=24, male=24) took part in the experiment. Participants were recruited from psychology classes at Keio University and Doshisha University (M age = 21.1, SD age = 3.8), and they provided informed consent to take part in the study. The study was approved by the Ethics Committees of Keio University (#21026) and Doshisha University (#22070).
Two human face images and two food images were used in the experiment. The face images were of an Asian male and a Caucasian female, selected from the Chicago Face Database (Ma et al., 2015 ; image names: CFD-AM-215-120-N and CFD-WF-003-003-N). The food images were photographs of meat and of a sliced orange; they were selected from public domain licensed images at a stock photos website. Each image was edited with six editing effects (HHP, HHN, HLP, HLN, HLA, and HLA+HHN), and seven strength values (0, 0.25, 0.5, 1, 1.5, 2, and 2.5) were set for face images and 0.25, 0.5, 1, 2, 3, and 4 for the food images. The shadow effect was not included in the experiment because we assumed that shadows are a less important feature in the perception of material attributes tested in the experiment. The reason for varying the range of the strength parameter for the face and food images is that people are more perceptually sensitive to editing manipulations of faces than of non-face objects (Boyadzhiev et al., 2015 ). For each original image, 42 image variations (6 editing effects × 7 strength values) were created, resulting in 168 stimulus images. Note that images with a strength value of 1 are identical to the original (unedited) image. All images were 500 × 500 px in size and presented at that size on the display.
Design and procedure
For each stimulus, participants rated six attributes (five material properties and naturalness) on a six-point scale. The material attributes were matte–gloss, dry–wet, opaque–translucent, rough–smooth, and old–young/fresh. These attributes are representative dimensions in skin perception (Otaka et al., 2019 ) and food perception (Hanada, 2020 ; Spence et al., 2022 ). Participants were instructed to rate the naturalness of the images, that is, photorealism. An image was presented in the center of the display and participants responded by pressing a key (there was no time limit on the response). Each image set (42 variations of an original image) and attribute was rated in blocks and in random order. Within each block, images of varying editing effects and strength values were presented in random order. Images with a strength value of 1 were presented twice, while images with other strength values were presented once. The total number of trials was 1152 (4 image contents × 6 rating dimensions × 6 editing effects × (7+1) strength values). Participants completed practice trials prior to the experimental session (a face image and a food image were used in this trial, but not in the experimental session).
The data of five participants were not recorded (possibly owing to network errors). Thus, 43 participants (20 male and 23 female) were included in the analysis.
Figure 11 shows the averaged standardized rating values for each condition (standardization was calculated by subtracting the mean rating value for images with a strength value of 1 from each rating value) and their 95% confidence intervals. The results of the face images are shown in the top row and those of the food images are shown in the bottom row. Each column shows the results for each rating attribute. Each image editing effect is indicated by the color of the line and the shape of the symbol. As an example of how to interpret this graph, editing a face image with a strength value of 0 with the HLA+HHN (aging) effect increases the gloss rating by two points on a six-point scale compared to the unedited image (see the red line in the upper leftmost column in Fig. 11 ).
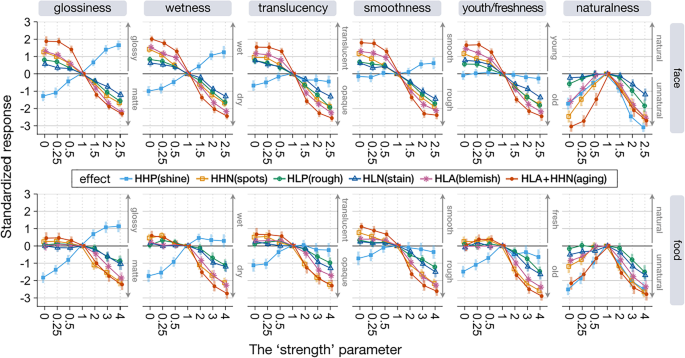
Results of the evaluation experiment. The results of each rating attribute (column) are shown for each image category (face and food). The ratings are standardized with respect to the ratings for unedited images (i.e., images with a strength parameter value of 1). Error bars indicate 95% CI
To test each material editing effect per rating attribute, the Friedman test (Myles & Douglas, 1973 ) was conducted for each experimental condition using the stats::friedman.test function in R. As responses for the unedited stimulus (strength parameter = 1) were collected twice in each condition, we averaged them before performing the statistical tests. In two conditions, the effect of image editing was not significant (χ 2 (6) = 10.1, p = 0.12 for HHP on old–young rating for face, and χ 2 (6) = 9.8, p = 0.14 for HHP on rough–smooth rating for food). In all other conditions, each editing effect influenced each rating value (all p s < 0.05, corrected for each rating attribute and editing effect with the Bonferroni procedure).
The results show that each editing effect changed the perceived material properties in the expected directions. For example, the HHP effect increased or reduced glossiness and wetness ratings, and reduced the translucency rating. The HHP effect on the smoothness rating was statistically significant only for face stimuli, and its effect on the freshness rating was statistically significant only for food stimuli. The aging (HLA+HHN) effect changed the skin youthfulness rating to old or young depending on the strength parameter value, and changed the food freshness rating to old (but did not increase freshness). The aging effect also affected the other rating dimensions in the same way as observed for the HLA and HHN effects. The HHN (spots), HLP (rough), HLN (stain), and HLA (blemish) are features related to surface roughness and uniformity, all of which affected the smoothness rating. That is, when these features were boosted, the stimulus was rated as rough, and when they were reduced, the stimulus was rated as smooth.
We also found that the roughness-related editing effects (HHN/HLP/HLN/HLA) were also associated with ratings of material properties other than roughness. That is, facial stimuli rated as rough skin were rated as matte/dry/opaque, and facial stimuli rated as smooth skin were rated as glossy/wet/translucent. These results suggest that the HHN/HLP/HLN/HLA effects manipulate image features that are commonly used as perceptual cues across these ratings. Effects such as HLP (rough) and HLN (stain) were rated very similarly (Fig. 11 ). However, this result does not imply that these editing effects create similar images. In fact, the HLP and HLN effects give different textures to the input image (Fig. 12 ). Both are similar in that they increase surface roughness, but HLP produces unevenness across the entire surface of the skin, while HLN emphasizes localized dark areas such as stains and blemishes. We believe that the rating scales used in this experiment did not reflect these subtle differences in perceptual experience.
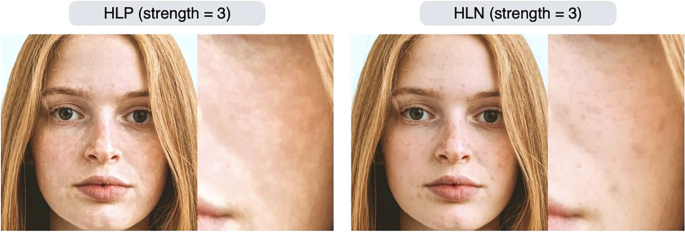
Difference between HLP (rough) and HLN (stain) effects. The image to the right of the output image is a magnified view of the woman’s left cheek
In general, when comparing the results for faces with those for food, the effect of reduce edits (strength parameter value less than 1) on perception was small for food. This may be due to the nature of the image (food) objects used in the experiments: raw meat and oranges, which tend to have a high degree of gloss and translucency. In fact, the glossiness, wetness, translucency, smoothness, and youthfulness/freshness ratings for the unedited (strength = 1) images were all higher for food (4.1 vs. 3.7 for glossiness, 4.3 vs. 3.6 for wetness, 4.0 vs. 3.7 for translucency, 4.2 vs. 3.8 for smoothness, and 4.2 vs. 3.7 for youthfulness/freshness, two sample t -test, two sided, all p s < 0.05). Thus, perceptual effects of reduce editing on the food images used in the experiment would have been difficult to observe. These results are not considered a procedural artifact (ceiling effect). This is because ratings for stimuli with an intensity parameter of 1 were approximately 4, and there was room for higher ratings.
The results of the naturalness rating showed that the further away from 1 the strength parameter value was, the more unnatural the image was rated (although for some editing effects, such as HLP and HLN, image naturalness was preserved for reducing effects). Therefore, if the naturalness of the image is important, the strength parameter should not be set to an extreme value.
Limitations
This section describes some of the limitations of this package. First, depending on the input image, the material editing effect may not work as intended. If the feature of interest is weak or absent in the input image, the algorithm cannot control the image in that dimension. For example, if a photo of a woman’s face with smooth skin due to heavy makeup or strong lighting is used as an input image, the algorithm cannot increase skin blemishes because it cannot add features that are not present in the input image (Fig. 13 ). The average face, which is often used in face perception research, also has this limitation because average faces tend to have smooth facial textures.
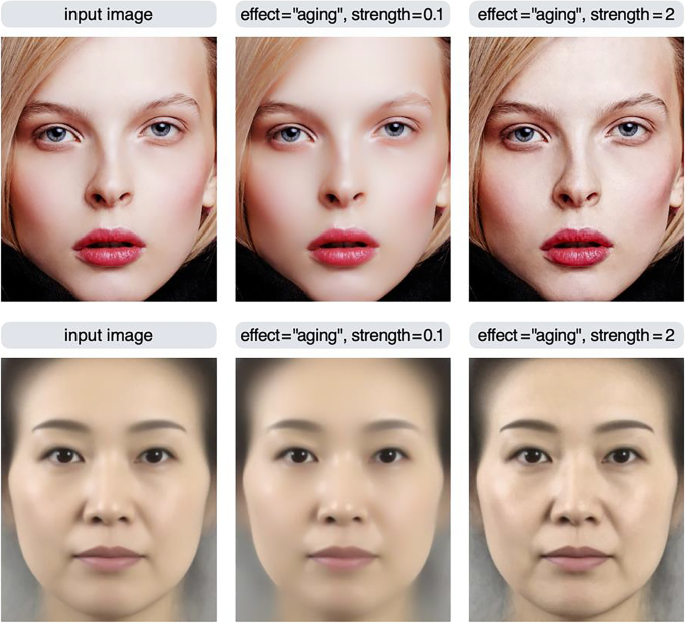
Results of applying the aging effect to a photo of a face (top) and an average face of Japanese females (bottom; adapted from Nakamura et al., 2020 ). When the skin of the input image is smooth, the aging effect does not work well
Figure 14 shows another example where the editing effect is less apparent: the HHN effect is applied to make the food look wilted (middle row), but it does not look wilted enough. One way to deal with this is to increase the strength value, but the larger the value, the more unnatural the image will look. Another way is to apply multiple editing effects (right column). This will increase the spots while concurrently eliminating the gloss, giving the image a more wilted appearance.
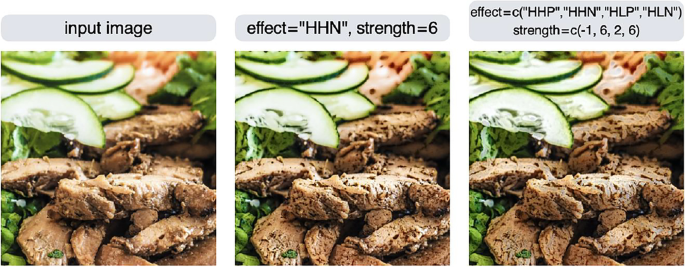
By combining multiple editing effects, it may be possible to create a desired change in appearance. Observe the image at a larger size to see the changes in the image
Second, the names of effects such as shine and blemish do not necessarily correspond to the appearance of the output image. For example, the effect named “stain” is just an alias for the image feature selection criterion HLN, and the manipulation of the HLN feature is just an adjustment to the lightness information of high spatial frequency, low amplitude, and minus sign in the input image. Therefore, when you want to manipulate stains in an image, it could be more effective to use other effects such as the spots (HHN) effect rather than the stain (HLN) effect. The parameter settings to achieve the desired effect will likely vary depending on the scale of the object and the lighting of the scene, which will be a practical difficulty when using this technique.
Third, the psychophysical properties of the material editing effects are not clear. That is, doubling the strength parameter does not necessarily mean that the perceptual effect will be doubled. It is not a trivial problem to formalize the relationship between the strength parameters and perception, because it will be specific to each material dimension, and possibly to each image category. For example, Boyadzhiev et al. ( 2015 ) compared naturalness ratings for face and non-face images and reported that face images are more likely to look unnatural with small image changes. Material perception is subject to complex nonlinearities. As a result, in studies that require a series of controlled images that are perceptually equidistant across each condition, researchers may need to conduct psychophysics experiments to assess the psychophysical properties of the image set.
Finally, it should be noted that the processing time required for image conversion depends on the resolution of the input image. The larger the input image, the more processing time required (Fig. 15 ). If the resolution of the input image is equal to or smaller than 1024 × 1024 px, the process will be completed in a relatively short time. However, if the resolution of the input image is 2048 × 2048 px, the processing time will be more than a minute (these numbers will also depend on the machine specs). Therefore, the resolution of the input image should be as small as possible, especially when a large number of images need to be prepared. The modif and modif2 functions have an argument named max_size. If the shorter side of the input image is larger than max_size, the image will be automatically scaled down so that the shorter side of the input image matches max_size. The default value of max_size is set to 1280. Thus, if an image with a resolution of 2000 × 3000 px is used as the input image, the output image will have a resolution of 1280 × 1920 px. If you do not want to change the resolution of the image, you can enter a larger value for max_size, or set max_size = NA. This feature was provided to avoid the extremely long execution time when a high-resolution image such as 4K is accidentally used as input.
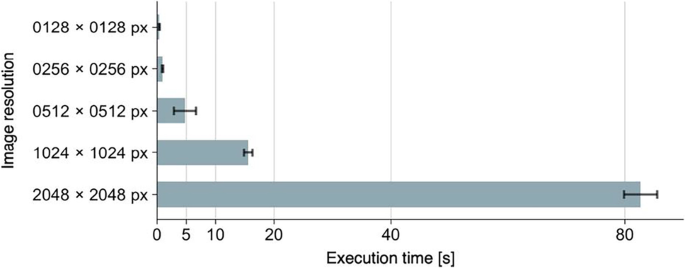
Execution time of the modif function. Ten measurements were taken for each resolution, and the mean and 95% CI of the execution time were calculated. Tested on a MacBook Air (M1, 2020, 8 GB). The type of effect (shine, blemish, etc.) has no effect on the execution time
In this paper, we presented materialmodifier, an R package for photo editing effects. The software uses image processing techniques to parametrically manipulate the surface properties of objects (e.g., gloss/roughness) in photographs, providing an automatic and reproducible method to create a set of image stimuli. We have confirmed that this software can be used effectively to control the appearance of faces, foods, and objects. We believe that this software will be useful for researchers interested in topics related to material perception, such as face perception and aesthetic evaluation of objects. The package can be installed via CRAN, and documentation and source code are available at https://github.com/tsuda16k/materialmodifier .
Adams, W. J., Kerrigan, I. S., & Graf, E. W. (2016). Touch influences perceived gloss. Scientific Reports, 6 (1), 21866. https://doi.org/10.1038/srep21866
Article PubMed PubMed Central Google Scholar
Anderson, B. L. (2020). Mid-level vision. Current Biology, 30 (3), R105–R109. https://doi.org/10.1016/j.cub.2019.11.088
Article PubMed Google Scholar
Arce-Lopera, C., Masuda, T., Kimura, A., Wada, Y., & Okajima, K. (2013). Luminance distribution as a determinant for visual freshness perception: Evidence from image analysis of a cabbage leaf. Food Quality and Preference, 27 (2), 202–207. https://doi.org/10.1016/j.foodqual.2012.03.005
Article Google Scholar
Blakemore, C., & Campbell, F. W. (1969). On the existence of neurons in the human visual system selectively sensitive to the orientation and size of retinal images. The Journal of Physiology, 203 (1), 237–260. https://doi.org/10.1113/jphysiol.1969.sp008862
Boyadzhiev, I., Bala, K., Paris, S., & Adelson, E. (2015). Band-sifting decomposition for image-based material editing. ACM Transactions on Graphics, 34 (5), 1–16. https://doi.org/10.1145/2809796
Chadwick, A. C., & Kentridge, R. W. (2015). The perception of gloss: A review. Vision Research, 109 , 221–235. https://doi.org/10.1016/j.visres.2014.10.026
Di Cicco, F., Wiersma, L., Wijntjes, M., & Pont, S. (2020). Material properties and image cues for convincing grapes: The know-how of the 17th-century pictorial recipe by Willem Beurs. Art & Perception, 8 (3–4), 337–362. https://doi.org/10.1163/22134913-bja10019
Di Cicco, F., Zhao, Y., Wijntjes, M. W. A., Pont, S. C., & Schifferstein, H. N. J. (2021). A juicy orange makes for a tastier juice: The neglected role of visual material perception in packaging design. Food Quality and Preference, 88 , 104086. https://doi.org/10.1016/j.foodqual.2020.104086
Dror, R. O., Willsky, A. S., & Adelson, E. H. (2004). Statistical characterization of real-world illumination. Journal of Vision, 4 (9), 11–11. https://doi.org/10.1167/4.9.11
Faul, F., Erdfelder, E., Lang, A.-G., & Buchner, A. (2007). G*Power 3: A flexible statistical power analysis program for the social, behavioral, and biomedical sciences. Behavior Research Methods, 39 , 175–191. https://doi.org/10.3758/bf03193146
Fink, B., & Matts, P. (2008). The effects of skin colour distribution and topography cues on the perception of female facial age and health. Journal of the European Academy of Dermatology and Venereology, 22 (4), 493–498. https://doi.org/10.1111/j.1468-3083.2007.02512.x
Fink, B., Grammer, K., & Matts, P. (2006). Visible skin color distribution plays a role in the perception of age, attractiveness, and health in female faces. Evolution and Human Behavior, 27 (6), 433–442. https://doi.org/10.1016/j.evolhumbehav.2006.08.007
Fleming, R. W. (2014). Visual perception of materials and their properties. Vision Research, 94 , 62–75. https://doi.org/10.1016/j.visres.2013.11.004
Fleming, R. W. (2017). Material perception. Annual Review of Vision Science, 3 (1), 365–388. https://doi.org/10.1146/annurev-vision-102016-061429
Fleming, R. W., & Storrs, K. R. (2019). Learning to see stuff. Current Opinion in Behavioral Sciences, 30 , 100–108. https://doi.org/10.1016/j.cobeha.2019.07.004
Fleming, R. W., Dror, R. O., & Adelson, E. H. (2003). Real-world illumination and the perception of surface reflectance properties. Journal of Vision, 3 (5), 3. https://doi.org/10.1167/3.5.3
Fleming, R. W., Wiebel, C., & Gegenfurtner, K. (2013). Perceptual qualities and material classes. Journal of Vision, 13 (8), 9–9. https://doi.org/10.1167/13.8.9
Fujisaki, W. (2020). Multisensory Shitsukan perception. Acoustical Science and Technology, 41 (1), 189–195. https://doi.org/10.1250/ast.41.189
Giesel, M., & Zaidi, Q. (2013). Frequency-based heuristics for material perception. Journal of Vision, 13 (14), 7–7. https://doi.org/10.1167/13.14.7
Hanada, M. (2020). Food-texture dimensions expressed by Japanese onomatopoeic words. Journal of Texture Studies, 51 (3), 398–411. https://doi.org/10.1111/jtxs.12499
He, K., Sun, J., & Tang, X. (2010). Guided image filtering. In K. Daniilidis, P. Maragos, & N. Paragios (Eds.), Computer vision – ECCV 2010. ECCV 2010. Lecture notes in computer science (pp. 1–14). Springer. https://doi.org/10.1007/978-3-642-15549-9_1
Chapter Google Scholar
Iwasa, K., Komatsu, T., Kitamura, A., & Sakamoto, Y. (2020). Visual perception of moisture is a pathogen detection mechanism of the behavioral immune system. Frontiers in Psychology, 11 , 170. https://doi.org/10.3389/fpsyg.2020.00170
Jaeger, B., Wagemans, F. M. A., Evans, A. M., & van Beest, I. (2018). Effects of facial skin smoothness and blemishes on trait impressions. Perception, 47 (6), 608–625. https://doi.org/10.1177/0301006618767258
Joh, A. S., Adolph, K. E., Campbell, M. R., & Eppler, M. A. (2006). Why walkers slip: Shine is not a reliable cue for slippery ground. Perception & Psychophysics, 68 (3), 339–352. https://doi.org/10.3758/bf03193681
Kiyokawa, H., Tashiro, T., Yamauchi, Y., & Nagai, T. (2021). Spatial frequency effective for increasing perceived glossiness by contrast enhancement. Frontiers in Psychology, 12 , 625135. https://doi.org/10.3389/fpsyg.2021.625135
Komatsu, H., & Goda, N. (2018). Neural mechanisms of material perception: Quest on Shitsukan. Neuroscience, 392 , 329–347. https://doi.org/10.1016/j.neuroscience.2018.09.001
Lagunas, M., Malpica, S., Serrano, A., Garces, E., Gutierrez, D., & Masia, B. (2019). A similarity measure for material appearance. ACM Transactions on Graphics (TOG), 38 (4), 1–12. https://doi.org/10.1145/3306346.3323036
Lesch, M. F., Chang, W.-R., & Chang, C.-C. (2008). Visually based perceptions of slipperiness: Underlying cues, consistency and relationship to coefficient of friction. Ergonomics, 51 (12), 1973–1983. https://doi.org/10.1080/00140130802558979
Ma, D. S., Correll, J., & Wittenbrink, B. (2015). The Chicago face database: A free stimulus set of faces and norming data. Behavior Research Methods, 47 (4), 1122–1135. https://doi.org/10.3758/s13428-014-0532-5
Motoyoshi, I., & Matoba, H. (2012). Variability in constancy of the perceived surface reflectance across different illumination statistics. Vision Research, 53 (1), 30–39. https://doi.org/10.1016/j.visres.2011.11.010
Motoyoshi, I., Nishida, S., Sharan, L., & Adelson, E. H. (2007). Image statistics and the perception of surface qualities. Nature, 447 (7141), 206–209. https://doi.org/10.1038/nature05724
Myles, H., & Douglas, A. W. (1973). Nonparametric Statistical Methods . John Wiley & Sons.
Google Scholar
Nakamura, K., Ohta, A., Uesaki, S., Maeda, M., & Kawabata, H. (2020). Geometric morphometric analysis of Japanese female facial shape in relation to psychological impression space. In Heliyon (Vol. 6, Issue 10, p. e05148). Elsevier BV. https://doi.org/10.1016/j.heliyon.2020.e05148
Nishida, S. (2019). Image statistics for material perception. Current Opinion in Behavioral Sciences, 30 , 94–99. https://doi.org/10.1016/j.cobeha.2019.07.003
Nkengne, A., Bertin, C., Stamatas, G., Giron, A., Rossi, A., Issachar, N., & Fertil, B. (2008). Influence of facial skin attributes on the perceived age of Caucasian women. Journal of the European Academy of Dermatology and Venereology, 22 (8), 982–991. https://doi.org/10.1111/j.1468-3083.2008.02698.x
Otaka, H., Shimakura, H., & Motoyoshi, I. (2019). Perception of human skin conditions and image statistics. JOSA A, 36 (9), 1609–1616. https://doi.org/10.1364/JOSAA.36.001609
Péneau, S., Brockhoff, P. B., Escher, F., & Nuessli, J. (2007). A comprehensive approach to evaluate the freshness of strawberries and carrots. Postharvest Biology and Technology, 45 (1), 20–29. https://doi.org/10.1016/j.postharvbio.2007.02.001
Portilla, J., & Simoncelli, E. P. (2000). A parametric texture model based on joint statistics of complex wavelet coefficients. International Journal of Computer Vision, 40 (1), 49–71.
Sawayama, M., Dobashi, Y., Okabe, M., Hosokawa, K., Koumura, T., Saarela, T., Olkkonen, M., & Nishida, S. (2019). Visual discrimination of optical material properties: A large-scale study. bioRxiv . Published online October 10, 2019. https://doi.org/10.1101/800870
Schmidt, F. (2019). The art of shaping materials. Art & Perception, 8 (3–4), 407–433. https://doi.org/10.1163/22134913-20191116
Schütt, H. H., & Wichmann, F. A. (2017). An image-computable psychophysical spatial vision model. Journal of Vision, 17 (12), 12–12. https://doi.org/10.1167/17.12.12
Serrano, A., Chen, B., Wang, C., Piovarči, M., Seidel, H.-P., Didyk, P., & Myszkowski, K. (2021). The effect of shape and illumination on material perception. ACM Transactions on Graphics, 40 (4), 1–16. https://doi.org/10.1145/3450626.3459813
Sharan, L., Rosenholtz, R., & Adelson, E. H. (2014). Accuracy and speed of material categorization in real-world images. Journal of Vision, 14 (9), 12. https://doi.org/10.1167/14.9.12
Spence, C. (2020). Shitsukan — the multisensory perception of quality. Multisensory Research, 33 (7), 737–775. https://doi.org/10.1163/22134808-bja10003
Spence, C., Motoki, K., & Petit, O. (2022). Factors influencing the visual deliciousness/eye-appeal of food. Food Quality and Preference, 102 , 104672. https://doi.org/10.1016/j.foodqual.2022.104672
Stephen, I. D., Law Smith, M. J., Stirrat, M. R., & Perrett, D. I. (2009). Facial skin coloration affects perceived health of human faces. International Journal of Primatology, 30 (6), 845–857. https://doi.org/10.1007/s10764-009-9380-z
Stephens, K. D., & Hoffman, D. D. (2016). On visual texture preference: Can an ecological model explain why people like some textures more than others? Perception, 45 (5), 527–551. https://doi.org/10.1177/0301006616629026
Tagai, K., Ohtaka, H., & Nittono, H. (2016). Faces with light makeup are better recognized than faces with heavy makeup. Frontiers in Psychology, 7 , 226. https://doi.org/10.3389/fpsyg.2016.00226
Tsuda, H., Fujimichi, M., Yokoyama, M., & Saiki, J. (2020). Material constancy in perception and working memory. Journal of Vision, 20 (10), 10. https://doi.org/10.1167/jov.20.10.10
Van Zuijlen, M. J., Lin, H., Bala, K., Pont, S. C., & Wijntjes, M. W. (2021). Materials In Paintings (MIP): An interdisciplinary dataset for perception, art history, and computer vision. Plos One, 16 (8), e0255109. https://doi.org/10.1371/journal.pone.0255109
Download references
Acknowledgments
This work was supported by JSPS KAKENHI, Grant Numbers JP19K23376 for HT, and JP19H05733 for HK.
Author information
Authors and affiliations.
Faculty of Psychology, Doshisha University, Kyoto, Japan
Hiroyuki Tsuda
Department of Psychology, Faculty of Letters, Keio University, Tokyo, Japan
Hideaki Kawabata
You can also search for this author in PubMed Google Scholar
Corresponding authors
Correspondence to Hiroyuki Tsuda or Hideaki Kawabata .
Ethics declarations
Commercial relationships, additional information, open practices statement.
The material, data, and R scripts for the evaluation experiment are available at https://osf.io/72dqz/ . The source code for the R package is available at https://github.com/tsuda16k/materialmodifier
Publisher’s note
Springer Nature remains neutral with regard to jurisdictional claims in published maps and institutional affiliations.
Rights and permissions
Open Access This article is licensed under a Creative Commons Attribution 4.0 International License, which permits use, sharing, adaptation, distribution and reproduction in any medium or format, as long as you give appropriate credit to the original author(s) and the source, provide a link to the Creative Commons licence, and indicate if changes were made. The images or other third party material in this article are included in the article's Creative Commons licence, unless indicated otherwise in a credit line to the material. If material is not included in the article's Creative Commons licence and your intended use is not permitted by statutory regulation or exceeds the permitted use, you will need to obtain permission directly from the copyright holder. To view a copy of this licence, visit http://creativecommons.org/licenses/by/4.0/ .
Reprints and permissions
About this article
Tsuda, H., Kawabata, H. materialmodifier: An R package of photo editing effects for material perception research. Behav Res 56 , 2657–2674 (2024). https://doi.org/10.3758/s13428-023-02116-2
Download citation
Accepted : 27 March 2023
Published : 10 May 2023
Issue Date : March 2024
DOI : https://doi.org/10.3758/s13428-023-02116-2
Share this article
Anyone you share the following link with will be able to read this content:
Sorry, a shareable link is not currently available for this article.
Provided by the Springer Nature SharedIt content-sharing initiative
- Material perception
- Image processing
- Open-source software
- Find a journal
- Publish with us
- Track your research
- Contents Contents
- Article Info Article Info
- Metrics Metrics
- Comment 1 Comment
- Related Related
- Cited Cited
- Article title
- Introduction
- Quick View of default Photoshop workspace
- The Options Bar or Control panel
- Adjustment Panels
- Photo editing
- Image cleaning
- Removing image background
- Preparation of the final photo
- Pointing structure
- Composing the figure
- Acknowledgements
This website uses cookies in order to improve your web experience. Read our Cookies Policy
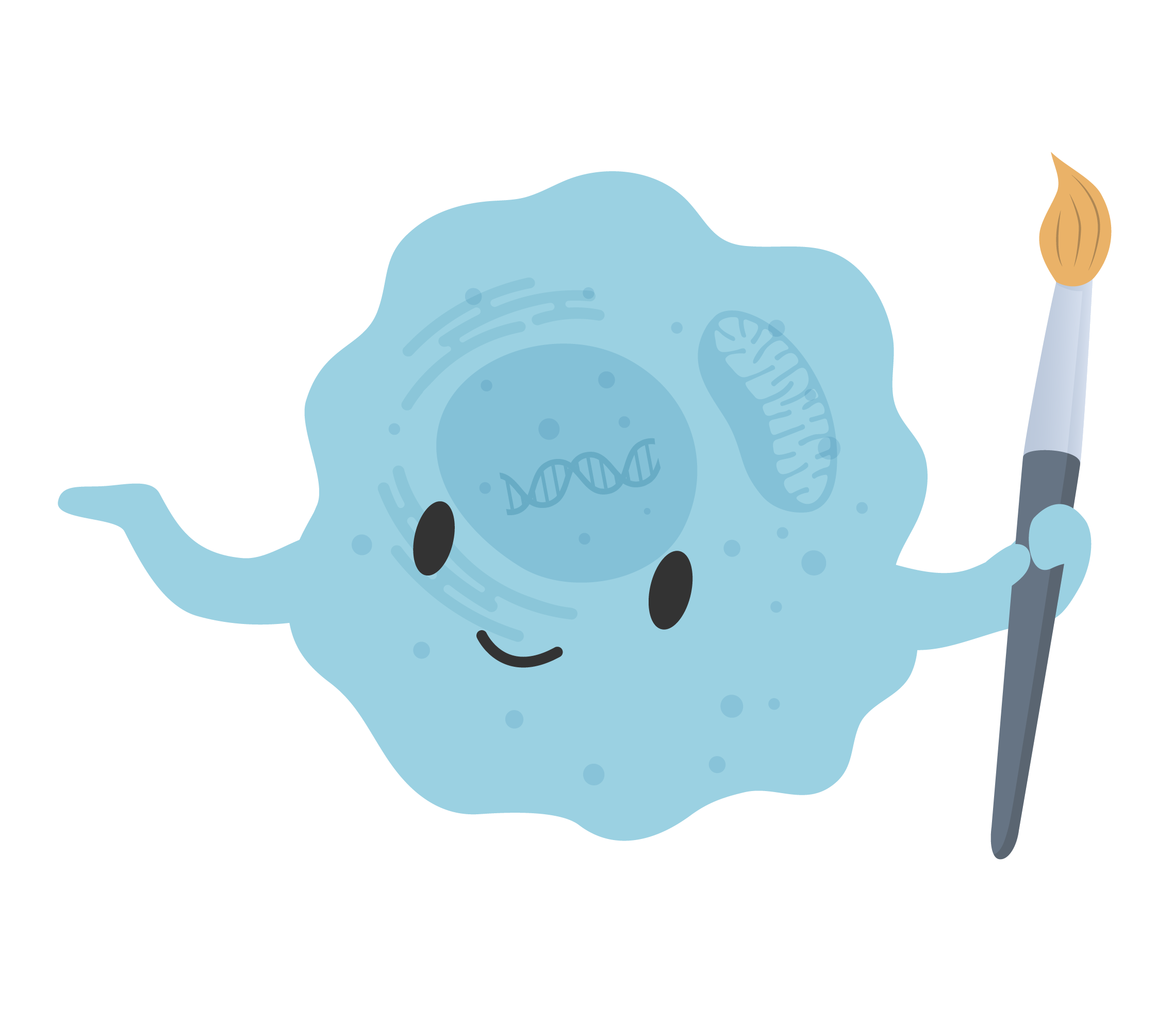
Create Professional Science Figures in Minutes
Join the largest science communication research community.
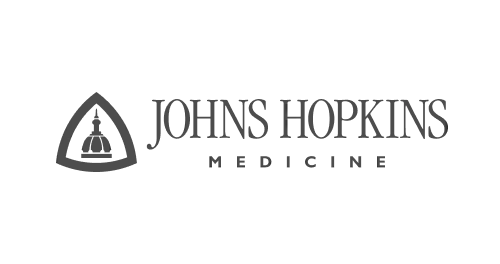
THOUSANDS OF ICONS
Browse over 50,000 icons and templates from over 30 fields of life science, curated and vetted by industry professionals.
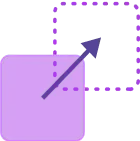
DRAG AND DROP
Simple drag-and-drop functionality enables you to create scientific figures up to 50 times faster.
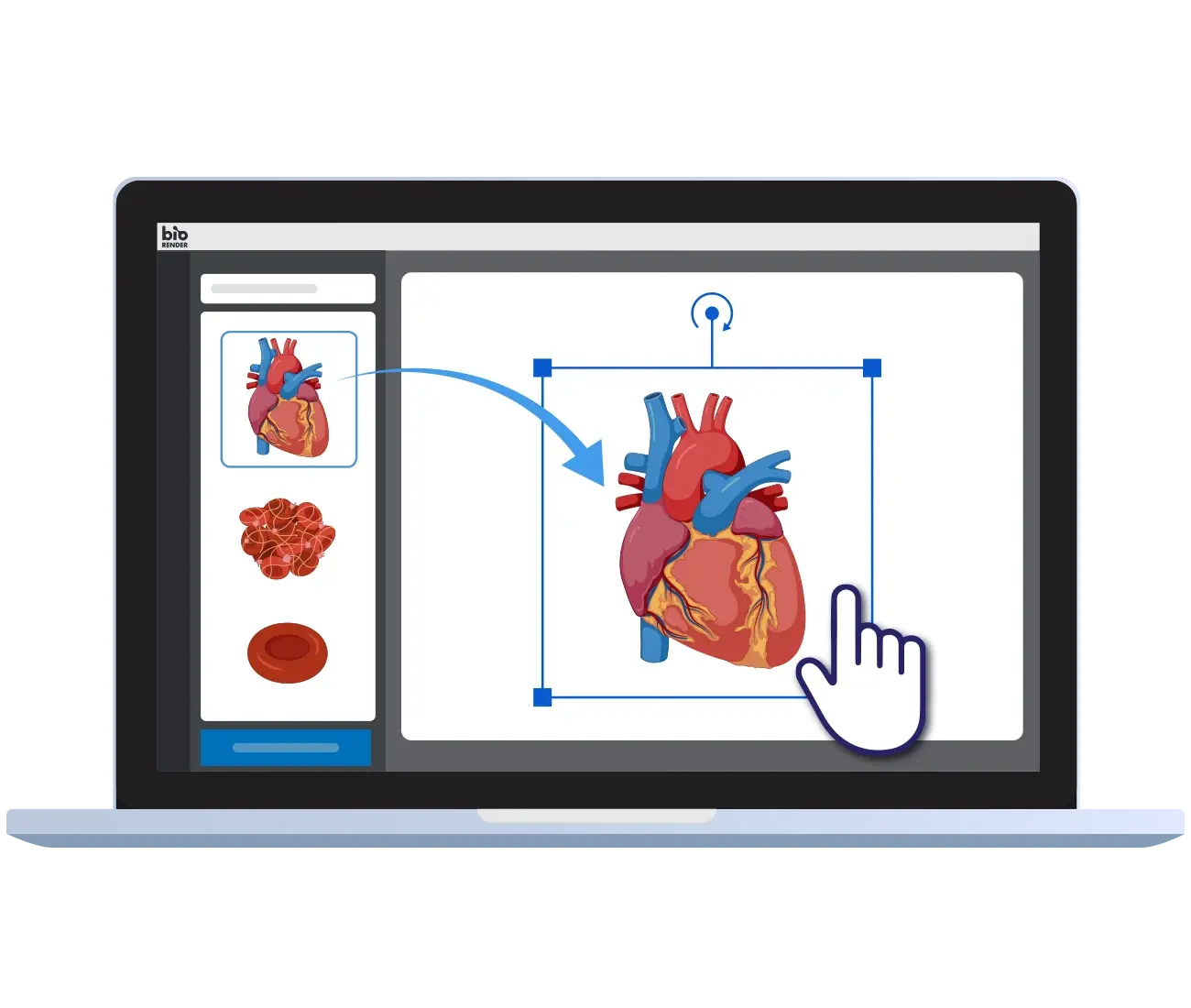
CUSTOM ICONS
Find icons you need for even the most specialized fields of life science, or we’ll create it in as little as 48 hours ( conditions apply ).
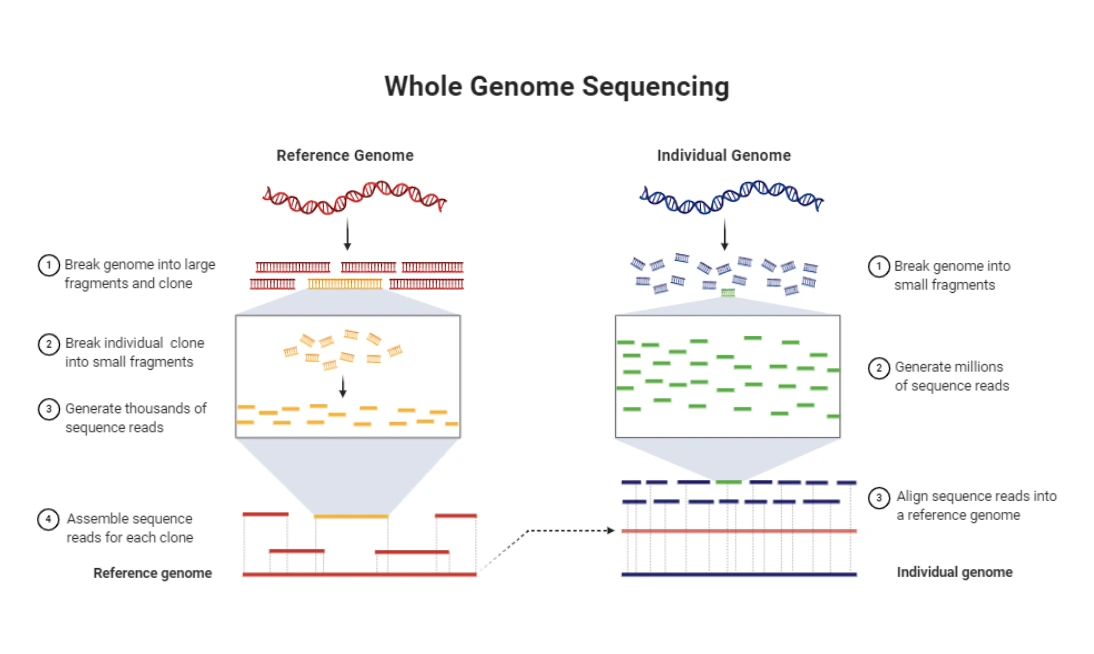
Figure: During HSV infection, memory CD4-T-cells respond to antigens in neural tissue by secreting IFN-γ and loosening tight junctions between endothelial cells, allowing antibodies to cross the blood-brain-barrier.
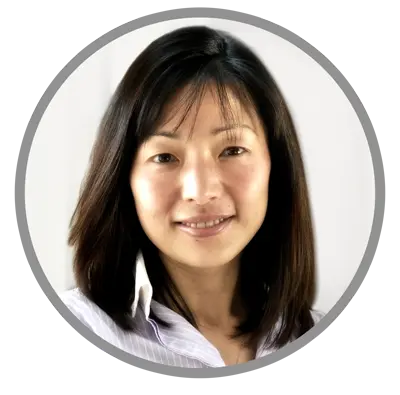
Ready to get started?
Thank you for visiting nature.com. You are using a browser version with limited support for CSS. To obtain the best experience, we recommend you use a more up to date browser (or turn off compatibility mode in Internet Explorer). In the meantime, to ensure continued support, we are displaying the site without styles and JavaScript.
- View all journals
- Explore content
- About the journal
- Publish with us
- Sign up for alerts
- NATURE INDEX
- 01 May 2024
Plagiarism in peer-review reports could be the ‘tip of the iceberg’
- Jackson Ryan 0
Jackson Ryan is a freelance science journalist in Sydney, Australia.
You can also search for this author in PubMed Google Scholar
Time pressures and a lack of confidence could be prompting reviewers to plagiarize text in their reports. Credit: Thomas Reimer/Zoonar via Alamy
Mikołaj Piniewski is a researcher to whom PhD students and collaborators turn when they need to revise or refine a manuscript. The hydrologist, at the Warsaw University of Life Sciences, has a keen eye for problems in text — a skill that came in handy last year when he encountered some suspicious writing in peer-review reports of his own paper.
Last May, when Piniewski was reading the peer-review feedback that he and his co-authors had received for a manuscript they’d submitted to an environmental-science journal, alarm bells started ringing in his head. Comments by two of the three reviewers were vague and lacked substance, so Piniewski decided to run a Google search, looking at specific phrases and quotes the reviewers had used.
To his surprise, he found the comments were identical to those that were already available on the Internet, in multiple open-access review reports from publishers such as MDPI and PLOS. “I was speechless,” says Piniewski. The revelation caused him to go back to another manuscript that he had submitted a few months earlier, and dig out the peer-review reports he received for that. He found more plagiarized text. After e-mailing several collaborators, he assembled a team to dig deeper.
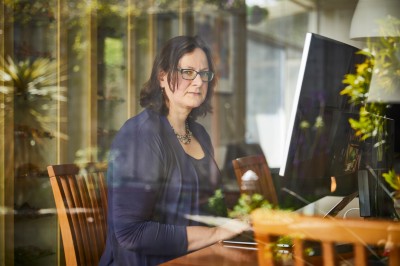
Meet this super-spotter of duplicated images in science papers
The team published the results of its investigation in Scientometrics in February 1 , examining dozens of cases of apparent plagiarism in peer-review reports, identifying the use of identical phrases across reports prepared for 19 journals. The team discovered exact quotes duplicated across 50 publications, saying that the findings are just “the tip of the iceberg” when it comes to misconduct in the peer-review system.
Dorothy Bishop, a former neuroscientist at the University of Oxford, UK, who has turned her attention to investigating research misconduct, was “favourably impressed” by the team’s analysis. “I felt the way they approached it was quite useful and might be a guide for other people trying to pin this stuff down,” she says.
Peer review under review
Piniewski and his colleagues conducted three analyses. First, they uploaded five peer-review reports from the two manuscripts that his laboratory had submitted to a rudimentary online plagiarism-detection tool . The reports had 44–100% similarity to previously published online content. Links were provided to the sources in which duplications were found.
The researchers drilled down further. They broke one of the suspicious peer-review reports down to fragments of one to three sentences each and searched for them on Google. In seconds, the search engine returned a number of hits: the exact phrases appeared in 22 open peer-review reports, published between 2021 and 2023.
The final analysis provided the most worrying results. They took a single quote — 43 words long and featuring multiple language errors, including incorrect capitalization — and pasted it into Google. The search revealed that the quote, or variants of it, had been used in 50 peer-review reports.
Predominantly, these reports were from journals published by MDPI, PLOS and Elsevier, and the team found that the amount of duplication increased year-on-year between 2021 and 2023. Whether this is because of an increase in the number of open-access peer-review reports during this time or an indication of a growing problem is unclear — but Piniewski thinks that it could be a little bit of both.
Why would a peer reviewer use plagiarized text in their report? The team says that some might be attempting to save time , whereas others could be motivated by a lack of confidence in their writing ability, for example, if they aren’t fluent in English.
The team notes that there are instances that might not represent misconduct. “A tolerable rephrasing of your own words from a different review? I think that’s fine,” says Piniewski. “But I imagine that most of these cases we found are actually something else.”
The source of the problem
Duplication and manipulation of peer-review reports is not a new phenomenon. “I think it’s now increasingly recognized that the manipulation of the peer-review process, which was recognized around 2010, was probably an indication of paper mills operating at that point,” says Jennifer Byrne, director of biobanking at New South Wales Health in Sydney, Australia, who also studies research integrity in scientific literature.
Paper mills — organizations that churn out fake research papers and sell authorships to turn a profit — have been known to tamper with reviews to push manuscripts through to publication, says Byrne.
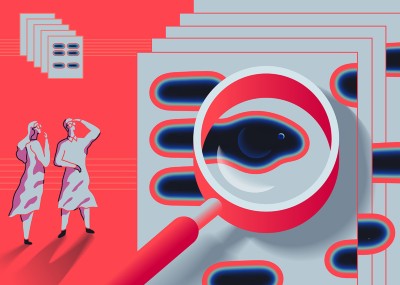
The fight against fake-paper factories that churn out sham science
However, when Bishop looked at Piniewski’s case, she could not find any overt evidence of paper-mill activity. Rather, she suspects that journal editors might be involved in cases of peer-review-report duplication and suggests studying the track records of those who’ve allowed inadequate or plagiarized reports to proliferate.
Piniewski’s team is also concerned about the rise of duplications as generative artificial intelligence (AI) becomes easier to access . Although his team didn’t look for signs of AI use, its ability to quickly ingest and rephrase large swathes of text is seen as an emerging issue.
A preprint posted in March 2 showed evidence of researchers using AI chatbots to assist with peer review, identifying specific adjectives that could be hallmarks of AI-written text in peer-review reports .
Bishop isn’t as concerned as Piniewski about AI-generated reports, saying that it’s easy to distinguish between AI-generated text and legitimate reviewer commentary. “The beautiful thing about peer review,” she says, is that it is “one thing you couldn’t do a credible job with AI”.
Preventing plagiarism
Publishers seem to be taking action. Bethany Baker, a media-relations manager at PLOS, who is based in Cambridge, UK, told Nature Index that the PLOS Publication Ethics team “is investigating the concerns raised in the Scientometrics article about potential plagiarism in peer reviews”.
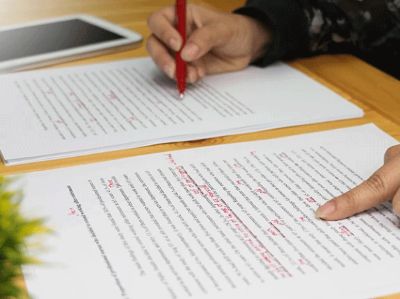
How big is science’s fake-paper problem?
An Elsevier representative told Nature Index that the publisher “can confirm that this matter has been brought to our attention and we are conducting an investigation”.
In a statement, the MDPI Research Integrity and Publication Ethics Team said that it has been made aware of potential misconduct by reviewers in its journals and is “actively addressing and investigating this issue”. It did not confirm whether this was related to the Scientometrics article.
One proposed solution to the problem is ensuring that all submitted reviews are checked using plagiarism-detection software. In 2022, exploratory work by Adam Day, a data scientist at Sage Publications, based in Thousand Oaks, California, identified duplicated text in peer-review reports that might be suggestive of paper-mill activity. Day offered a similar solution of using anti-plagiarism software , such as Turnitin.
Piniewski expects the problem to get worse in the coming years, but he hasn’t received any unusual peer-review reports since those that originally sparked his research. Still, he says that he’s now even more vigilant. “If something unusual occurs, I will spot it.”
doi: https://doi.org/10.1038/d41586-024-01312-0
Piniewski, M., Jarić, I., Koutsoyiannis, D. & Kundzewicz, Z. W. Scientometrics https://doi.org/10.1007/s11192-024-04960-1 (2024).
Article Google Scholar
Liang, W. et al. Preprint at arXiv https://doi.org/10.48550/arXiv.2403.07183 (2024).
Download references
Related Articles
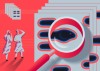
- Peer review
- Research management
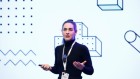
Illuminating ‘the ugly side of science’: fresh incentives for reporting negative results
Career Feature 08 MAY 24
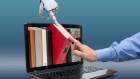
Algorithm ranks peer reviewers by reputation — but critics warn of bias
Nature Index 25 APR 24
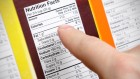
Researchers want a ‘nutrition label’ for academic-paper facts
Nature Index 17 APR 24
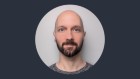
Structure peer review to make it more robust
World View 16 APR 24
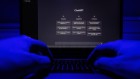
Is ChatGPT corrupting peer review? Telltale words hint at AI use
News 10 APR 24
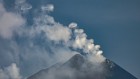
Mount Etna’s spectacular smoke rings and more — April’s best science images
News 03 MAY 24
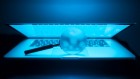
How reliable is this research? Tool flags papers discussed on PubPeer
News 29 APR 24
Clinician Researcher/Group Leader in Cancer Cell Therapies
An excellent opportunity is available for a Group Leader with expertise in cellular therapies to join the Cancer Research program at QIMR Berghofer.
Herston, Brisbane (AU)
QIMR Berghofer
Faculty Positions at the Center for Machine Learning Research (CMLR), Peking University
CMLR's goal is to advance machine learning-related research across a wide range of disciplines.
Beijing, China
Center for Machine Learning Research (CMLR), Peking University
Faculty Positions at SUSTech Department of Biomedical Engineering
We seek outstanding applicants for full-time tenure-track/tenured faculty positions. Positions are available for both junior and senior-level.
Shenzhen, Guangdong, China
Southern University of Science and Technology (Biomedical Engineering)
Southeast University Future Technology Institute Recruitment Notice
Professor openings in mechanical engineering, control science and engineering, and integrating emerging interdisciplinary majors
Nanjing, Jiangsu (CN)
Southeast University
Staff Scientist
A Staff Scientist position is available in the laboratory of Drs. Elliot and Glassberg to study translational aspects of lung injury, repair and fibro
Maywood, Illinois
Loyola University Chicago - Department of Medicine
Sign up for the Nature Briefing newsletter — what matters in science, free to your inbox daily.
Quick links
- Explore articles by subject
- Guide to authors
- Editorial policies
Help | Advanced Search
Computer Science > Computer Vision and Pattern Recognition
Title: rethinking image editing detection in the era of generative ai revolution.
Abstract: The accelerated advancement of generative AI significantly enhance the viability and effectiveness of generative regional editing methods. This evolution render the image manipulation more accessible, thereby intensifying the risk of altering the conveyed information within original images and even propagating misinformation. Consequently, there exists a critical demand for robust capable of detecting the edited images. However, the lack of comprehensive dataset containing images edited with abundant and advanced generative regional editing methods poses a substantial obstacle to the advancement of corresponding detection methods. We endeavor to fill the vacancy by constructing the GRE dataset, a large-scale generative regional editing dataset with the following advantages: 1) Collection of real-world original images, focusing on two frequently edited scenarios. 2) Integration of a logical and simulated editing pipeline, leveraging multiple large models in various modalities. 3) Inclusion of various editing approaches with distinct architectures. 4) Provision of comprehensive analysis tasks. We perform comprehensive experiments with proposed three tasks: edited image classification, edited method attribution and edited region localization, providing analysis of distinct editing methods and evaluation of detection methods in related fields. We expect that the GRE dataset can promote further research and exploration in the field of generative region editing detection.
Submission history
Access paper:.
- Other Formats
References & Citations
- Google Scholar
- Semantic Scholar
BibTeX formatted citation

Bibliographic and Citation Tools
Code, data and media associated with this article, recommenders and search tools.
- Institution
arXivLabs: experimental projects with community collaborators
arXivLabs is a framework that allows collaborators to develop and share new arXiv features directly on our website.
Both individuals and organizations that work with arXivLabs have embraced and accepted our values of openness, community, excellence, and user data privacy. arXiv is committed to these values and only works with partners that adhere to them.
Have an idea for a project that will add value for arXiv's community? Learn more about arXivLabs .

IMAGES
VIDEO
COMMENTS
The guide's focus is on digital photo editing and the production of figures using Adobe Photoshop to produce publication-quality figures for scientific publications.
As photo editing behavior to enhance one?s appearance in photos becomes more and more prevalent on social network sites (SNSs), potential risks are increasingly discussed as well. The purpose of this study is to examine the relationship between photo editing behavior, self-objectification, physical appearance comparisons, self-perceived attractiveness, and self-esteem. 403 participants ...
However, based on the research highlighted above, photo-editing as a behaviour has been associated with body concerns including body dissatisfaction and greater consideration of cosmetic surgery (Lonergan et al., 2019, Othman et al., 2021). With this in mind, it is possible that there is a "feedback loop" within the model such that photo ...
View a PDF of the paper titled GLIDE: Towards Photorealistic Image Generation and Editing with Text-Guided Diffusion Models, by Alex Nichol and 7 other authors View PDF Abstract: Diffusion models have recently been shown to generate high-quality synthetic images, especially when paired with a guidance technique to trade off diversity for fidelity.
Around 99% of the participants posted <5 photos per week and 81% of them had edited a photo before posting. The average time spent by a majority of the participants to edit one photo was less than 5 minutes. About 25% of the participants edited more than 40% of the total photos posted on social media.
The structural equation model for photo editing did not have adequate power to determine an overall significant association between photo editing investment and ACSS scores. One explanation for this result may be that, in contrast to social media use, photo editing application use does not affect self-esteem enough to drive interest in cosmetic ...
In this paper, we introduce an R package that performs automated photo editing effects. Specifically, it is an R implementation of an image-processing algorithm proposed by Boyadzhiev et al. (2015). The software allows the user to manipulate the appearance of objects in photographs, such as emphasizing facial blemishes and wrinkles, smoothing the skin, or enhancing the gloss of fruit. It ...
Figures for scientific publications go through various stages from the planning, to the capturing of images, to the production of finished figures for publication. This guide is meant to familiarise the reader with the main image-editing software used by professional photographers. The guide's focus is on digital photo editing and the production of figures using Adobe Photoshop to produce ...
Start making professional scientific figures today. Browse 1000s of icons & templates from many fields of life sciences. Create science figures in minutes with BioRender scientific illustration software!
Text-guided image editing can have a transformative impact in supporting creative applications. A key challenge is to generate edits that are faithful to input text prompts, while consistent with input images. We present Imagen Editor, a cascaded diffusion model built, by fine-tuning Imagen on text-guided image inpainting. Imagen Editor's edits are faithful to the text prompts, which is ...
Adobe Illustrator is another popular image editing software. It is a vector-based drawing program that allows the user to import images, create drawings, and align multiple images into one figure. The figure that is generated can be exported as a high-resolution image that is ready for publication. Illustrator allows the user to fully customize ...
ImageMagick is another tool that can be used to read and write images in many commonly used formats (e.g., PNG, JPEG, FIG, TIFF, PDF, etc). For this reason, it can modify images in nearly any manner. It allows users to composite images, animate, manage color, decorate, draw, and delineate image features (e.g., edges of colors).
However, there are two basic categories of image manipulation or image editing in scientific research articles: 1) Clarification. 2) Deception. In clarification, an author may, quite ethically, attempt to "clean up" an image in order for it to be more readable. Image editing software programs, such as Adobe Photoshop, allow for the digital ...
EdiBERT, a generative model for image editing. Advances in computer vision are pushing the limits of im-age manipulation, with generative models sampling detailed images on various tasks. However, a specialized model is often developed and trained for each specific task, even though many image edition tasks share similarities.
Revising isn't the first step in the process of writing a research paper, but it is perhaps the most important. Many students skip the revision process, mistaking editing for revision. While editing is also very important, revision is an integral part of any good writing process. During revision, you should try to see your work from different ...
Paper mills — organizations that churn out fake research papers and sell authorships to turn a profit — have been known to tamper with ... Research data; Language editing; Scientific editing;
We propose a method for editing images from human instructions: given an input image and a written instruction that tells the model what to do, our model follows these instructions to edit the image. To obtain training data for this problem, we combine the knowledge of two large pretrained models -- a language model (GPT-3) and a text-to-image model (Stable Diffusion) -- to generate a large ...
The new paper describes the study's findings through February 2023 and details how the trial's 14 participants -- 12 adults and two children -- responded to receiving EDIT-101 in one eye. Key ...
The accelerated advancement of generative AI significantly enhance the viability and effectiveness of generative regional editing methods. This evolution render the image manipulation more accessible, thereby intensifying the risk of altering the conveyed information within original images and even propagating misinformation. Consequently, there exists a critical demand for robust capable of ...
On the basis of real-time RT-PCR and sequencing, the Centers for Disease Control and Prevention confirmed HPAI A(H5N1) virus infection in the conjunctival and nasopharyngeal swab specimens ...
open source image editing software General Image Manipulation Program or. GIMP was launched in 1995, making image editing accessible to everyone. T oday various software such as Darktable, Digikam ...