- Open access
- Published: 09 September 2020

Electricity load forecasting: a systematic review
- Isaac Kofi Nti ORCID: orcid.org/0000-0001-9257-4295 1 ,
- Moses Teimeh 2 ,
- Owusu Nyarko-Boateng ORCID: orcid.org/0000-0003-0300-2469 3 &
- Adebayo Felix Adekoya ORCID: orcid.org/0000-0002-5029-2393 3
Journal of Electrical Systems and Information Technology volume 7 , Article number: 13 ( 2020 ) Cite this article
29k Accesses
118 Citations
Metrics details
The economic growth of every nation is highly related to its electricity infrastructure, network, and availability since electricity has become the central part of everyday life in this modern world. Hence, the global demand for electricity for residential and commercial purposes has seen an incredible increase. On the other side, electricity prices keep fluctuating over the past years and not mentioning the inadequacy in electricity generation to meet global demand. As a solution to this, numerous studies aimed at estimating future electrical energy demand for residential and commercial purposes to enable electricity generators, distributors, and suppliers to plan effectively ahead and promote energy conservation among the users. Notwithstanding, load forecasting is one of the major problems facing the power industry since the inception of electric power. The current study tried to undertake a systematic and critical review of about seventy-seven (77) relevant previous works reported in academic journals over nine years (2010–2020) in electricity demand forecasting. Specifically, attention was given to the following themes: (i) The forecasting algorithms used and their fitting ability in this field, (ii) the theories and factors affecting electricity consumption and the origin of research work, (iii) the relevant accuracy and error metrics applied in electricity load forecasting, and (iv) the forecasting period. The results revealed that 90% out of the top nine models used in electricity forecasting was artificial intelligence based, with artificial neural network (ANN) representing 28%. In this scope, ANN models were primarily used for short-term electricity forecasting where electrical energy consumption patterns are complicated. Concerning the accuracy metrics used, it was observed that root-mean-square error (RMSE) (38%) was the most used error metric among electricity forecasters, followed by mean absolute percentage error MAPE (35%). The study further revealed that 50% of electricity demand forecasting was based on weather and economic parameters, 8.33% on household lifestyle, 38.33% on historical energy consumption, and 3.33% on stock indices. Finally, we recap the challenges and opportunities for further research in electricity load forecasting locally and globally.
Electricity is the pivot in upholding highly technologically advanced industrialisation in every economy [ 1 , 2 , 3 ]. Almost every activity done in this modern era hinges on electricity. The demand and usage of electric energy increase globally as the years past [ 4 ]; however, the process of generating, transmitting, and distributing electrical energy remains complicated and costly. Hence, effective grid management is an essential role in reducing the cost of energy production and increased in generating the capacity to meet the growing demand in electric energy [ 5 ].
Accordingly, effective grid management involves proper load demand planning, adequate maintenance schedule for generating, transmission and distribution lines, and efficient load distribution through the supply lines. Therefore, an accurate load forecasting will go a long way to maximise the efficiency of the planning process in the power generation industries [ 5 , 6 ]. As a means to improve the accuracy of Electrical Energy Demand (EED) forecasting, several computational and statistical techniques have been applied to enhance forecast models [ 7 ].
EED forecasting techniques can be clustered into three (3), namely correlation, extrapolation, and a combination of both. The Extrapolation techniques (Trend analysis) involve fitting trend curves to primary historical data of electrical energy demand in a way to mirror the growth trend itself [ 7 , 8 ]. Here, the future value of electricity demand is obtained from estimating the trend curve function at the preferred future point. Despite its simplicity, its results are very realistic in some instances [ 8 ].
On the other hand, correlation techniques (End-use and Economic models) involve relating the system load to several economic and demographic factors [ 7 , 8 ]. Thus, the techniques ensure that the analysts capture the association existing between load increase patterns and other measurable factors. However, the disadvantage lies in the forecasting of economic and demographic factors, which is more complicated than the load forecast itself [ 7 , 8 ]. Usually, economic and demographic factors such as population, building permits, heating, employment, ventilation, air conditioning system information, weather data, building structure, and business are used in correlation techniques [ 7 , 8 , 9 ]. Nevertheless, some researchers group EED forecasting models into two, viz. data-driven (artificial intelligence) methods (same as the extrapolation techniques) and engineering methods (same as correlation the techniques) [ 9 ]. All the same, no single method is accepted scientifically superior in all situations.
Also, proper planning and useful applications of electric load forecasting require particular “forecasting intervals,” also referred to as “lead time”. Based on the lead time, load forecasting can be grouped into four (4), namely: very short-term load forecasting (VSTLF), short-term load forecasting (STLF), medium-term load forecasting (MTLF) and long-term load forecasting (LTLF) [ 6 , 7 , 10 ]. The VSTLF is applicable in real-time control, and its predicting period is within minutes to 1 h ahead. The STLF is for making forecasting within 1 h to 7 days or month ahead [ 11 ]. It is usually used for the day-to-day operations of the utility industry, such as scheduling the generation and transmission of electric energy. The MTLF is used for forecasting of fuel purchase, maintenance, utility assessments. Its forecasting period ranges from 1 week to 1 year. While the LTLF is for making forecasting beyond a year to 20 years ahead, it is suitable for forecasting the construction of new generations, strategic planning, and changes in the electric energy supply and delivery system [ 10 ].
Notwithstanding the above-mentioned techniques and approaches available, EED forecasting is seen to be complicated and cannot easily be solved with simple mathematical formulas [ 2 ]. Also, Hong and Fan [ 12 ] pointed out that electric load forecasting has been a primary problem for the electric power industries, since the inception of the electric power. Regardless of the difficulty in electric load forecasting, the optimal and proficient economic set-up of electric power systems has continually occupied a vital position in the electric power industries [ 13 ]. This exercise permits the utility industries to examine the dynamic growth in load demand patterns to facilitate continuity planning for a better and accurate power system expansion. Consequently, inaccurate prediction leads to power shortage, which can lead to “dumsor” and unneeded development in the power system leading to unwanted expenditure [ 7 , 14 ]. Besides, a robust EED forecasting is essential in developing countries having a low rate of electrification to facilitate a way for supporting the active development of the power systems [ 15 ].
Based on the sensitive nature of electricity demand forecasting in the power industries, there is a need for researchers and professionals to identify the challenges and opportunities in this area. Besides, as argued by Moher et al. [ 16 ], systematic reviews are the established reference for generating evidence in any research field for further studies. Our partial search of literature resulted in the following [ 10 , 12 , 17 , 18 , 19 , 20 , 21 ] papers that focused on comprehensive systematic review concerning the methods, models, and several methodologies used in electric load forecasting. Hammad et al. [ 10 ] compared forty-five (45) academic papers on electric load forecasting based on inputs, outputs, time frame, the scale of the project, and value. They revealed that despite the simplicity of regression models, they are mostly useful for long-term load forecasting compared with AI-based models such as ANN, Fuzzy logic, and SVM, which are appropriate for short-term forecasting.
Similarly, Hong and Fan [ 12 ] carried out a tutorial review of probabilistic EED forecasting. The paper focused on EED forecasting methodologies, special techniques, common misunderstandings and evaluation methods. Wang et al. [ 19 ] presented a comprehensive review of factors that affects EED forecasting, such as forecast model, evaluation metric, and input parameters. The paper reported that the commonly used evaluation metrics were the mean absolute error, MAPE, and RMSE. Likewise, Kuster et al. [ 22 ] presented a systematic review of 113 studies in electricity forecasting. The paper examined the timeframe, inputs, outputs, data sample size, scale, error type as criteria for comparing models aimed at identifying which model best suited for a case or scenario.
Also, Zhou et al. [ 17 ] presented a review of electric load classification in the smart-grid environment. The paper focused on the commonly used clustering techniques and well-known evaluation methods for EED forecasting. Another study in [ 21 ] presented a review of short-term EED forecasting based on artificial intelligence. Mele [ 20 ] presented an overview of the primary machine learning techniques used for furcating short-term EED. Gonzalez-Briones et al. [ 18 ] examined the critical machine learning models for EED forecasting using a 1-year dataset of a shoe store. Panda et al. [ 23 ] presented a comprehensive review of LTLF studies examining the various techniques and approaches adopted in LTLF.
The above-discussed works of literature show that two studies [ 20 , 21 ] address a comprehensive review on STLF, [ 23 ] addresses forecasting models based on LTLF. The study in [ 24 ] was entirely dedicated to STLF. Only a fraction (10%) of above systematic review studies included STLF, MTLF and LTLF papers in their review; however, as argued in [ 10 ], the lead time (forecasting interval) is a factor that positively influences the performance of a chosen model for EED forecasting studies. Again, a high percentage of these studies [ 10 , 12 , 17 , 18 , 19 , 20 , 21 , 22 , 24 ] concentrated on the methods (models), input parameter, and timeframe. Nevertheless, Wang et al. [ 19 ] revealed that the primary factors that influence EED forecasting models are property (characteristic) parameters of the building and weather parameters include. Besides, these parameters are territorial dependant and cultural bond. Thus, the weather pattern is not the same world-wide neither do we use the same building architecture and materials globally.
Notwithstanding, a higher percentage of previous systematic review studies overlooked the origin of studies and dataset of EED forecasting paper. Also, only a few studies [ 12 , 17 , 19 ] that examined the evaluation metrics used in EED forecasting models. However, as pointed out in [ 17 ], there is no single validity index that can correctly deal with any dataset and offer better performance always.
Despite all these review studies [ 10 , 12 , 17 , 18 , 19 , 20 , 21 , 22 , 24 ] on electricity load forecasting, a comprehensive systematic review of electricity load forecasting that takes into account all possible factors, such as the forecasting load (commercial, residential and combined), the forecast model (conventional and AI), model evaluation metrics and forecasting type (STLF, MTLF, and LTLF) that influences EED forecast models is still an open gate for research. Hence, to fill in the gap, this study presents an extensive systematic review of state-of-the-art literature based on electrical energy demand forecasting. The current review is classified according to the forecasting load (commercial, residential, and combined), the forecast model (conventional, AI and hybrids), model evaluation metrics, and forecasting type (STLF, MTLF, and LTLF). The Preferred-Reporting Items for Systematic-Review and Meta-Analysis (PRISMA) flow diagram was adopted for this study based on its ability to advance the value and quality of the systematic review as compared with other guidelines [ 16 , 25 , 26 ]. The current study contributes to knowledge as follows:
A comprehensive and detailed assessment of previous state-of-the-art studies on electricity demand forecasting; based on used methods, timeframe, the train and test split of data, error, and accuracy metrics applied to forecast.
We present a concise summary of the useful characteristics of compared techniques in electric load forecasting.
We identified the challenges and opportunities for further studies in electric load forecasting.
The remaining sections of the current paper are structured as follows. “ Methodology ” section presents the methods and materials used in the current study. “ Data collection ” section presents the results and a detailed discussion of the outcomes, and “ Study framework ” section presents the summary of findings and direction for future studies.
Methodology
The current study presents a systematic review of pertinent literature on electrical energy forecasting.
Data collection
A total of eighty-one (81) state-of-the-art research works published in journals, conferences, and magazines, and student’s thesis relevant to the scope of the current study were downloaded from the internet, thus using keywords and terms which included AI, Electricity Prediction (EP), Energy Forecasting (EF), Machine Learning (ML), and combination of AI and EP, AI and EF, ML and EP, ML and EF. Each downloaded literature was then carefully studied and categorised into the two methods of electrical load forecasting data-driven (artificial intelligence) methods and engineering methods.
Study framework
According to Moher et al. [ 16 ], the quality of every systematic review is based on building protocol, which outlines the justification, hypothesis, and planned methods of the investigation. However, only a few systematic review study reports of their framework. A detailed, well-described structure for systematic reviews facilitates the understanding and evaluation of the methods adopted. Hence, the PRISMA model [ 26 ] was adopted in this study (Fig. 1 ). As shown in Fig. 1 , the PRISMA presents the flow of information from one stage to another in a systematic review of the literature and gives the total number of the research identified, excluded, and included and the reasons for inclusion and exclusions.
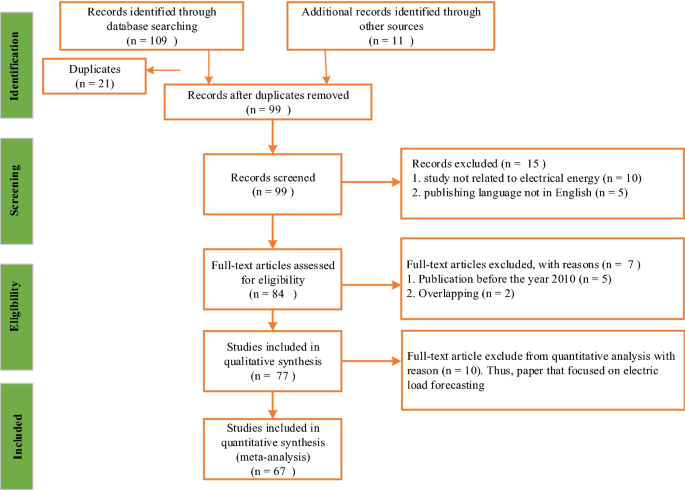
Source : Moher et al. [ 26 ]
The adopted PRISMA flow diagram.
The PRISMA flow diagram involved five (5) phases, as shown in Fig. 1 . Phase 1 consists of outlining the review scope, developing questions, and inclusion or exclusion. Phase 2 searches the literature with keywords to identify potential studies. Phase 3 includes determining the addition of a paper by screening its abstracts if it meets inclusion criteria. While phase 4 includes characterisation of paper for mapping by keywords. This review aimed to document an overview of research in the field of electric load forecasting to make way for future studies. As a result, a fifth (5) step offers an in-depth quantitative synthesis (meta-analysis) of studies included in the review.
Our search of literature retrieved one hundred and one (109) papers from online journals and eleven (11) from under sources, making one hundred and twelve (120) records in all (see Fig. 1 ). Of the 120 records, 21 were duplicates, hence, removed leaving ninety-one (99) record shortlisted for the screening stage. At the screening stage, fifteen (15) records were removed; thus, studies that were not related to electrical energy, and those that the primary publication language was not in English. Leaving eighty-four (84) records, of the 84 records, we further remove five (5) more records that were published before the year 2010, two (2) record omitted due to overlapping, this reduced eligible papers for analysis to seventy-seven (77). The 77 papers were used for the qualitative analysis. Ten (10) records that presented a review of electric load forecasting were also removed, and the remaining (67) were used for the quantitative analysis, as shown in Fig. 1 .
Results and discussion
Electrical energy consumption can be classified as residential (domestic), commercial (non-residential), or industrial. Residential or domestic refers to the home or a dwelling where people globally live from day-to-day. At the same time, commercial consumers are the business and industries that require massive supply than residential users for their businesses [ 27 ]. Selected literature was on electric load forecasting was classified into two main categories AI methods and engineering methods. However, each category was further grouped into residential and commercial or combined (residential and commercial), and the outcomes are presented. A total of seventy-seven (77) papers were eligible for the qualitative analysis, while sixty-seven (67) were included in the quantitative analysis, as already discussed above in this study.
AI methods used in electrical energy demand forecasting
This section presents the studies that were based on AI techniques.
Combined (commercial and residential)
Most works on EED forecasting sought to forecast the total load (residential and commercial) demand on the supplying authority. This section presents the selected studies that fell in this category of electric load forecasting.
Hybrid models
In a way to harness the strength in different machine learning techniques, some researchers sought to hybrid two or more ML techniques to improve the forecasting accuracy of their models.
A short-term (next-day) EED forecasting model based on the historical meteorological parameter to forecast the future load on the Greek Electric Network Grid using Support Vector Machine (SVM), ensemble XGBoost, Random Forest (RF), k-Nearest Neighbours (KNN), Neural Networks (NN) and Decision Trees (DT) was proposed by [ 28 ]. The mean absolute percentage error (MAPE) was used as a performance metric for comparison among selected models by the author. The study achieved a reduction in prediction error or + 4.74% compared with the Operator of the Electricity Market in Greece predictions. In other studies [ 29 ], long-term (10 years) EED forecasting using NN and Autoregressive Integrated Moving Average (ARIMA) was proposed to forecast the EED of Kuwait. Weather temperature and humidity, average salary, gross domestic, oil price, population, residence, passengers, currency earning rate, and economic factors like (total import and export in USD) were used as independent variables. The study concluded that NN outperformed ARIMA and weather parameters were found to be more significant than average salary, gross domestic and oil price.
A Particle Swarm Optimisation (PSO) and Differential Evolution (DE) for forecasting the Andhra Pradesh Grid using weather parameters were presented [ 6 ]. The reported concluded that better prediction accuracy was achieved with PSO and DE than the conventional time series forecast model. In another study, a Curve Fitting Algorithm (CFA) was proposed for forecasting electricity power demand for an hour/day/week/month [ 30 ]. Their study shows that future electricity demand can be effectively forecasted based on past demand. In a process to increase forecasting accuracy, historical electricity data combined with Twitter data was used as an input variable to hybrid ANN and SVM forecast model to forecast the electricity consumption in Dutch [ 14 ]. The authors compared the performance of ANN to SVM and concluded that the ANN outperformed the SVM. On the other side, the SVM performance in accuracy increases in long-term forecasting. The authors again admit that inclusion of weather data as input could not increase model performance. Similarly, in Ghana, an attempt to predict the 30-day ahead EED demand of the Bono region using a hybrid ML (MLP, SVM, and DT) based on historical weather and electricity demand was made [ 7 ]. The authors achieved 95% prediction accuracy; however, it affirms that the inclusion of household lifestyle as an input variable will improve prediction accuracy.
The hybrid of ML (SVM and RF) and time-series models Generalized Linear Model (GLM) and ARIMA forecast model was proposed for predict electricity consumption in South Africa based on the historical electricity price, load demand, and weather parameter [ 31 ]. The outcome of the study showed that the ARIMA-GLM combination performs better for long-term forecasting. Similarly, a combination of quantum search with SVM (quantum computing and the chaotic mechanics) for forecasting yearly EED in Taiwan was presented [ 32 ]. The empirical analysis revealed that the proposed model exhibits considerably enhanced forecasting performance than other SVM-based forecasting models. A hybrid of mode decomposition (EMD), PSO, and SVM model was present for forecasting short-term EED demand of the Australian electricity market [ 33 ]. Sulandari et al. [ 34 ] proposed a hybrid of ANN and Fuzzy algorithm and a recurrent formula (LRF) to predict electricity demand in Indonesian. The study results showed that the hybrid model performed well with low values of RMSE. Likewise, in [ 35 ], a hybrid of clustering technique (K-means) and ARIMA forecasting model was presented to forecast university buildings electricity demand. Paper revealed that the hybrid model outperformed the ARIMA model alone as a forecast model.
An artificial neural network (ANN)-based forecast model for short-term forecasting of Chhattisgarh State electricity demand was proposed [ 36 ], using historical weather data as input variables. The results conclude that ANN can efficiently forecast electricity demand. Likewise, an ANN model was applied to forecast the short-term electricity demand of the Iraqi National Grid [ 37 ]. The authors achieved high accuracy and a reasonable error margin. In a similar study, a DT algorithm forecast model was proposed for forecasting future EED on the Yola/Jimeta power transmission company using weather parameters. Again, a short to medium term EED forecasting using deep machine learning (ML) algorithm long short-term memory (LSTM)-based neural network enhanced with genetic algorithm (GA) for feature selection was proposed [ 9 ], to forecast France metropolitan’s electricity consumption. The mean absolute error (MAE) and root-mean-square error (RMSE) were used as the performance metrics, and the weather parameter was used in the independent variable. Their results affirm that weather parament is very useful in forecasting future electricity demand.
An enhanced Convolutional Neural Network (CNN) and enhanced SVM-based forecast model was presented for forecasting electricity price and load forecasting using [ 38 ]. Despite the enhancement made by authors, they recommended additional enhancement of classifiers to improve prediction. In a similar study, an ANN model to forecast consumer demand in North Cyprus was proposed [ 39 ]. Their results affirm the ability for ANN effective automatic modelling of electricity; however, the study concluded this could be achieved when the training and testing datasets are meaningful. A forecast model using a deep belief network using historical EED of Macedonian (2008–2014) was proposed to forecast a short-term (1 day) EED. The outcome of the forecast shows a reduction in MAPE by 8.6% by the proposed model compared with traditional techniques [ 2 ]. In Young et al. [ 40 ], an ANN with Bayesian regularisation algorithm-based model for short-term load forecasting of commercial building electricity usage was carried out. An ANN and hybrid methods to forecast electricity consumption of Turkey were proposed Aydogdu et al. [ 41 ]. The proposed model gave an average absolute prediction error of 2.25%.
Khwaja et al. [ 11 ] presented an ensemble ANN predictive model to enhance short-term electricity load forecasting. Different from existing studies, the authors combined both bagging and boosting techniques to train bagged-boosted ANNs. The study results showed that the proposed ensemble technique offered a reduction of both variance and bias compared to a bagged ANN, single ANN, and boosted ANN. Also, Ahmad et al. [ 42 ] combined Extreme Learning Machine and an enhanced Support Vector Machine to forecast short-term electricity demand. The study outcome showed that the proposed hybrid model outperformed other state-of-the-art predictive models in terms of performance and accuracy. Atef and Eltawil [ 43 ] proposed a deep-stacked LSTM forecasting model to forecast electricity demand. The paper reported that bidirectional (Bi-LSTM) networks outperformed unidirectional (Uni-LSTM) in terms of forecasting accuracy. Also, a generalised regression Neural Network (GRNN) predictive model was proposed in [ 44 ] to predict short-term electricity demand. The study results showed that a GRNN of 30 neurons offered better prediction accuracy in terms of MAPE and MAE than a GRNN of 10 neurons.
Fuzzy logic models
A Fuzzy Logic (FL)-based forecasting model for the next-day electricity demand in Albania was presented [ 45 ]. The time, the historical and forecasting value of the temperature and the previous day load (L) served as the independent variables for the forecast of the next-day consumption. The outcome of the study yielded accurate forecasting by the FL model. Motepe et al. [ 46 ] proposed an adaptive neuro-fuzzy inference system (ANFIS) model for forecasting South African electricity demand. The author concluded that adding temperature as an input parameter to the proposed model did not enhance forecast accuracy, as typically expected.
A new economy (stock indices) reflecting the STLF model for electricity demand forecasting was proposed [ 47 ]. The authors attempted to forecast the future demand for electricity based on the Taiwan Stock Exchange Capitalization Weighted Stock Index (TAIEX) data. The study revealed a significant relationship between stock market function and energy demand, which was helpful to financial analysis wanting to do reverse engineering.
Residential load forecasting
A survey in 2019 shows that EED forecasting, especially short-term load forecasting for individual (residential) electricity customers, plays an increasingly essential role in the future grid planning and operation Kong et al. [ 51 ]. Similarly, the outcome of Leahy and Lyons [ 48 ] affirms that water heating styled used by a household is even more essential than the number of electrical appliances when explaining domestic electrical energy usage. Furthermore, a study in Portugal shows that residential electricity consumption since 1990 has been increasing more rapidly than the Gross Domestic Product (GDP) per capita [ 49 ]. Therefore, studies that focused on forecasting residential EED is of necessity. However, the results revealed that eight (8) out of the sixty-seven (67) representing (11.94%) reviewed works focused on residential electricity forecasting, and a section of these studies is presented.
An hourly prediction of residential energy consumption using RF and SVM was proposed by Hedén [ 50 ], using 187 households in Austin. Error metrics are mean bias (MB), coefficient of variance (CV), and MAPE. The study achieved better forecasting accuracy compared with traditional time series models. Again, an LTSM for short-term residential load forecasting based on residential meter reading [ 51 ] and resident behaviour learning [ 52 ] was studied. The outcomes of these works showed the effectiveness of the LTSM in electricity demand forecasting.
In the same way, linear regression, RF, and SVM predictive model (data-driven) for estimation of city-scale energy use in buildings were proposed by Kontokosta and Tull [ 53 ]. The outcome of the study revealed that adequate electricity consumption in a building could be predicted using actual data from a moderately small subset of buildings. Likewise, unsupervised ML algorithms such as Self-Organising Maps (SOM), k-means, and k-medoid were used to cluster residential electricity based on their trend of electricity use within the day [ 54 ]. The study found that households and how they use electricity in the home can be categorised based on specific customer characteristics. Mcloughlin et al. [ 55 ] examined residential electricity consumption patterns in Irish based on occupant socio-economic and dwelling variables. The variable examined includes the number of bedrooms, dwelling type, age of household head, social class, household composition, and water heating.
The study outcome showed a positive association between maximum demand periods and several household appliances, especially dishwashers, electric cookers tumble dryers, with electric cook topping. The study further found that the time of use of electrical appliances was dependent on occupant characteristics, and younger occupants of a household tend to use more electricity than the older. Alike, an ARIMA, NN, and exponential smoothening forecast model were proposed for forecasting household electricity demand [ 56 ]. The authors concluded that forecasting accuracy varies considerably depending on the choice of forecasting techniques/tactic and configuration/selection of input parameters. Again, a neural network model for predicting residential building energy consumption was proposed by Biswas et al. [ 57 ]. The outcome of the study showed that models based on OWO-Newton algorithms and Levenberg–Marquardt outperformed previous literature.
Engineering and traditional electrical energy demand forecasting
This section presents the electrical energy demand forecasting study based on the traditional time-series and engineering models.
A predictive model for the prediction of medium-term (1-year) electricity consumption of general households based on the lifestyle of the household using Lasso and Group Lasso was proposed [ 58 ]. Their results showed that household lifestyle such as family composition, age, and house-type is good predictors of electricity consumption in a home. Similarly, in Nigeria, an attempt was made to estimate the electricity demand of residential users to support energy transitions using the engineering approach, such as calculating the total power consumed in a household based on the power rating of appliance and their duration of use [ 59 ]. The study concludes that the proposed system can serve policymaking in Nigeria to improve the financial sustainability of the energy supply structure. An extended Autoregressive Distributed Lag (ARDL) model was proposed to estimate residential electricity consumption per capita demand function, which depends on the GDP per capita in Algeria [ 60 ]. The study concluded that promoting financial growth among citizens of Algeria would reduce electricity consumption, since wealthier people (higher income earners) mighty use of more efficient appliances.
In Bogomolov et al. [ 61 ], authors used general public dynamics derived data from cellular network and energy consumption dataset to predict the next-day energy demand. The study could serve a model to enhance the energy meter to promote energy conservation. Likewise, the historical data of on–off times of residential appliances were used to predict the next-day electricity demand using Bayesian inference [ 62 ]. The study concluded that historical electricity consumption data only is not adequate for a decent eminence hourly forecast.
Also, an ARIMA and Holt-Winter model was proposed for forecasting the national electricity consumption of Pakistan from 1980 to 2011 [ 3 ]. The study revealed that the demand in household energy consumption would higher as compare with all other sectors. Jain et al. [ 63 ] proposed an ARIMA forecaster for forecasting electricity consumption. The proposed model achieved a MAPE of 6.63%. The authors concluded that the ARIMA model has the potential of computing in EED forecasting with other forecast techniques. Integration of three (3) forecasting model, long-range energy alternative planning (LEAP), ARIMA, and Holt-Winter, was proposed for forecasting long-term energy demand in Pakistan [ 64 ]. The study would be valid for energy supplies for accurate estimation of users’ demand in the future. The combination of Extreme Learning Machine and Multiple Regression for forecasting China’s electricity demand was proposed [ 65 ]. A quantile regression (QR) model for long-term electricity demand forecasting in South Africa within 2012 and 2015 was presented by Mokilane et al. [ 66 ]. The model was helpful to power distribution industries in the country.
An attempt was made to forecast the electricity consumption of Ghana by 2030 using ARIMA-based model. The study outcome projected that Ghana’s electricity consumption would grow from 8.5210 billion kWh in 2012 to 9.5597 billion kWh in 2030 [ 67 ]. However, in 2017, a report by the energy commission revealed that electricity consumption was 14,247 GWh [ 68 ]. A generalised additive model was adopted for forecasting medium-term electric energy demand in a South African power supply system [ 69 ]. The outcome of the study revealed a useful application of the proposed model in the power generation and distribution industries in the country. An investigation between the association of causal nexus and (environmental pollution, energy use, GDP per capita, and urbanisation) in an attempt to forecast Nigeria’s energy use by 2030 was carried out using the ARIMA and ETS models [ 70 ]. The study outcome showed better forecasting accuracy by both models, and a high rise in energy demand was observed.
Multiple regression analysis approaches for forecasting the yearly electricity demand in commercial sectors and electricity access rates in rural and urban households in some selected West African countries, which included Ghana, were carried out by Adeoye and Spataru [ 71 ]. The study showed that there is a very high variation in hourly electricity demand in the dry seasons. Their results affirm Nti et al. [ 7 ] report that the demand pattern of electricity in Ghana is highly dependent on the month of the year. In a way, one can say there is a partial agreement in these two studies. A time-series regression model for forecasting South African’s peak load demand was presented [ 72 ]. Experimental fallouts indicated that when the temperature is included as an input parameter, improvement in accuracy by the forecast model was realised. ARIMA-based predictive model for predicting both sectoral and total electrical energy consumption of Turkey for the next 15 years was proposed [ 73 ]. The study points out that the demand for electrical energy in agriculture sectors, transport, public service, residential, and commercial will keep increasing.
Similarly, partially linear additive quantile regression models for forecasting short-term electricity demand during the peak-demand periods (i.e., from 6:00 to 8:00 pm) were carried out in South African [ 74 ]. The authors found out that the use of the proposed system in power utility industries for the planning, scheduling, and dispatching of electricity activities will result in a minimal cost principally during the peak-period hours. Caro et al. [ 75 ] predicted the Spanish electricity demand using the ARIMA model. The study achieved an improvement in the short-term predictions of electricity demand with less computational time. A short-term electricity load forecasting model based on dynamic mode decomposition was proposed in [ 76 ], the proposed model showed better stability and accuracy compared with other predictive models.
Quantitative analysis of findings
The descriptive statistics of the study outcome is presented under this section with tables and charts.
Algorithms used for forecast models
As part of the aim of the review, we sought to find out the most used algorithms in electricity forecasting models. The most top nine (9) used algorithms found in the sixty-seven (67) article are presented (Fig. 2 ); this includes only algorithms that were used in more than a single paper. The study outcome revealed that 90% out of the top nine algorithms were AI-based, with ANN representing 28% of AI models used in electricity forecasting. Besides, the ANN models were primarily used for STLF where electrical energy consumption patterns are more intricate than LTLF. The traditional AFRIMA recorded 17.5% due to its efficiency in LTLF, where load fluctuations and periodicity are less critical.
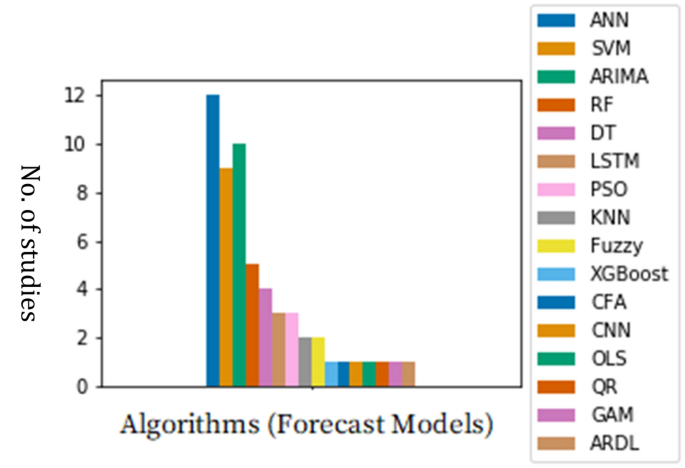
Top nine (9) most used algorithms for electricity forecasting
Additionally, a high percentage of regression models is used for LTLF prediction. The study outcome shows how AI is applied in various sectors of the economy to improve efficiency and profitability. Also, we observed that the SVM, PSO and Fuzzy are gaining more popularity in recent studies, a sign of increasing attention of researchers on these algorithms for EED forecasting.
Study origin
Table 1 shows the studies and their origin (countries). The origin of surveyed studies was examined, in order to find the linkage between the power crisis in the continent and studies on electrical energy demand forecasting. Concerning geographical coverage, it was found that a high number of studies (31.34%) were carried out in Europe, 17.91% in Africa and 19.40% in Asia. Making Africa the third-highest, however, interestingly, most of the studies in Africa were carried in South Africa (five representing 41.67%), three (3) representing 25% in Ghana, with the rest from Nigeria and other African countries. The energy crisis that hit Europe in 2008, reported by [ 77 ], can be attributed to the numerous studies in electricity forecast, as shown in Table 1 . It was further observed that 2 out of 3 studies in Ghana were based on national or regional electricity demand forecasting (one long-term, one short-term (1 month)). While the third study aimed at identifying the relationship between electricity demand and economic growth. Thus, it suggests that the limited number of studies in electricity demand forecasting by both academicians and professionals in this field might have partially contributed to the power shortages facing the nation, which in 2015 resulted in “Domsur”.
Used evaluation metrics
The performance of every forecasting model is examined based on the difference in error between the actual value \(\left( y \right)\) and the predicted value ( \(\hat{y}\) ). Several of these metrics were identified in the literature. However, we examined the most used in electrical energy demand forecasting to enable new beginners in the field of electricity demand forecasting to have a firm grip on which to apply in their study. Figure 3 shows the top four (4) most used error metrics in two or more studies. The results revealed that root-mean-square error RMSE (38%) was the most used error metric among EED forecasters, followed by mean absolute percentage error MAPE (35%). Due to the effectiveness in measure predictive model performance and their usefulness for short-term prediction, it was also observed that the MAPE is a standard primary metric as it is easy to both calculate and understand. The results affirm the findings in [ 12 , 19 , 22 ] that MAE, MAPE, and RMSE are the most commonly used evaluation metrics in EED forecasting model.
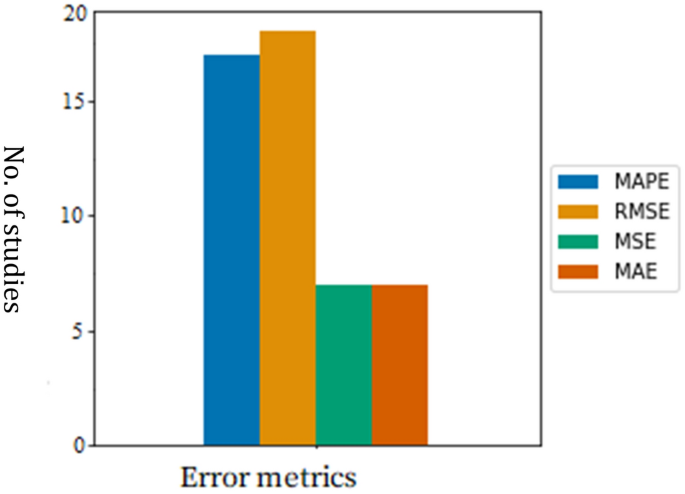
Most used error metrics in electricity load forecasting
Forecast type
Based on short-term load forecasting (STLF), medium-term load forecasting (MTLF), and long-term load forecasting (LTLF), it was observed that 80%, 15%, and 5% of the electrical energy demand forecasting were STLF, MTLF, and LTLF, respectively. The massive number of studies is based on STLF as compared with LTLF and MTLF call for further studies into the challenges associated with LTLF and MTLF electricity load forecasting. Again, 80% STLF forecasting affirms the 38% use of RMSE error metric, since it is for STLF forecasting. The results affirm the report in [ 22 ] that 43.6% of electricity forecasting are short-term prediction.
Model input parameters
The efficiency of every predictive model is believed to be partially dependent on the independent (input) variable [ 7 ]. At this level, the focus was to examine the different input variables used for electricity load forecasting. The type of independent variables (input features) used by electricity load forecasters was also examined. The current study observed that sixty (60) out of the sixty-seven (67) papers made known the input parameter to the proposed model. Table 2 presents the type of input variables and the percentage of studies that utilised it. It was observed that a high percentage (50%) of electricity demand forecasting was based on weather parameters; next was the historical electricity consumption pattern. The outcome exposed that little attention is given to household lifestyle in electricity demand forecasting. However, Nishida et al. [ 58 ] argue that residential (domestic) energy consumption differs depending on the lifestyle of the family. Family lifestyle, according to [ 56 ], cannot be undermined in electrical load forecasting. According to these studies, these factors include the life stage family composition, house type, age, home appliances possessed and their usage, family income, cultural background, social life, and lifestyle habits, which include how long to stay at home and how to spend holidays. The observations open the opportunity for further studies on the association between EED and household lifestyle.
Conclusions
The current study sought to reviewed state-of-the-art literature on electricity load forecasting to identify the challenges and opportunities for future studies. The outcome of the study revealed that electricity load forecasting is seen to be complicated for both engineers and academician and is still an ongoing area of research. The key findings are summarised as follows.
Several studies (90%) have applied AI in electrical energy demand forecasting as compared with traditional engineering and statistical method (10%) to address energy prediction problems; however, there are not enough studies benchmarking the performance of these methods.
There are few studies on EED forecasting in Africa countries (12 out of 67). Though the continent has progressive achievement in the creation of Regional Power Pools (PPP) over the last two decades, the continent still suffers from a lousy power network in most of its countries, leaving millions of people in Africa without electricity.
Temperature and rainfall as an input parameter to the EED forecasting model are seen to have a divergent view. At the same time, some sections of research recorded an improvement in accuracy and reported no improvement in accuracy when introduced and input. However, the current study attributes this to the difference in automorphic temperature globally and the different economic status among countries. An additional investigation will bring more clarity to the literature.
This study revealed that EED forecasting in the residential sector had seen little attention. On the other hand, Guo et al. [ 78 ] argue that the basic unit of electricity consumption is home.
It was observed that there had been a global increase in residential electricity demand, this according to the report in [ 49 ] can be attributed to the growing rate of buying electrical equipment and appliances of low quality due to higher living standards. However, a further probe into Soares et al. [ 49 ] assertion will bring clarity to literature because of the discrepancy in opinions in literature.
Lastly, the study revealed that there is a limited number of studies on load forecasting studies in Ghana. We, therefore, recommend rigorous researchers in this field in the country to enhance the economic growth of the country.
Our future study will focus on identifying the relationship between household lifestyle factors and electricity consumption in Ghana and predict load consumption based on identified factors since it is an area that has seen little or no attention in Ghana.
Availability of data and materials
All data generated or analysed during this study are included in this published article.
Abbreviations
- Electrical energy demand
- Artificial intelligence
Artificial neural network
Root-mean-square error
Mean absolute percentage error
Very short-term load forecasting
Short-term load forecasting
Medium-term load forecasting
Long-term load forecasting
Preferred-reporting items for systematic-review and meta-analysis
Electricity prediction
Energy forecasting
- Machine learning
Support vector machine
Random forest
k-nearest neighbours
Neural networks
Decision trees
Autoregressive integrated moving average
Particle swarm optimisation
Differential evolution
Curve fitting algorithm
Generalised linear model
Long short-term memory
Genetic algorithm
Mean absolute error
Convolutional neural network
Fuzzy logic
Adaptive neuro-fuzzy inference system
Generalised regression neural network
Taiwan stock exchange capitalisation-weighted stock index
Gross domestic product
Coefficient of variance
Self-organising maps
Autoregressive distributed lag
Long-range energy alternative planning
Quantile regression
Aung SS (2015) Electric power is the main driving force for industrialization. http://www.globalnewlightofmyanmar.com/electric-power-is-the-main-driving-force-for-industrialization/ . Accessed 2 Apr 2018
Dedinec A, Filiposka S, Dedinec A, Kocarev L (2016) Deep belief network based electricity load forecasting: an analysis of Macedonian case. Energy 115:1688–1700. https://doi.org/10.1016/j.energy.2016.07.090
Article Google Scholar
Hussain A, Rahman M, Memon JA (2016) Forecasting electricity consumption in Pakistan: the way forward. Energy Policy 90:73–80. https://doi.org/10.1016/j.enpol.2015.11.028
Jevgenijs S, Joeri deW, Kochnakyan A, Vivien F (2017) Forecasting electricity demand: an aid for practitioners. http://www.worldbank.org/energy/livewire . Accessed 15 Jun 2019
Zaman MU, Islam A, Sultana N (2018) Short term load forecasting based on internet of things (IoT). BRAC University, Dhaka
Google Scholar
Kumar CHJ, Veerakumari M (2012) Load forecasting of Andhra Pradesh grid using PSO, DE algorithms. Int J Adv Res Comput Eng Technol 1:179–184
Nti IK, Asafo-Adjei S, Agyemang M (2019) Predicting monthly electricity demand using soft-computing technique. Int Res J Eng Technol 06:1967–1973
Eeeguide.com (2014) Forecasting methodology. http://www.eeeguide.com/forecasting-methodology/ . Accessed 4 Jan 2019
Bouktif S, Fiaz A, Ouni A, Serhani MA (2018) Optimal deep learning LSTM model for electric load forecasting using feature selection and genetic algorithm: comparison with machine learning approaches †. Energies (MDPI) 11:1–20. https://doi.org/10.3390/en11071636
Hammad MA, Jereb B, Rosi B, Dragan D (2020) Methods and models for electric load forecasting: a comprehensive review. Logist Sustain Transp 11:51–76. https://doi.org/10.2478/jlst-2020-0004
Khwaja AS, Anpalagan A, Naeem M, Venkatesh B (2020) Joint bagged-boosted artificial neural networks: using ensemble machine learning to improve short-term electricity load forecasting. Electr Power Syst Res 179:106080. https://doi.org/10.1016/j.epsr.2019.106080
Hong T, Fan S (2016) Probabilistic electric load forecasting: a tutorial review. Int J Forecast 32:914–938. https://doi.org/10.1016/j.ijforecast.2015.11.011
Selvaraj KR, Sundararaj S, Ravi T (2013) Artificial neutral network based load forecasting and economic dispatch with particle swarm optimization. Int J Sci Eng Res 4:139–145
Stavast P (2014) Prediction of energy consumption using historical data and twitter. The University of Groningen, Groningen
Ouedraogo NS (2017) Modeling sustainable long-term electricity supply-demand in Africa. Appl Energy 190:1047–1067. https://doi.org/10.1016/j.apenergy.2016.12.162
Moher D, Shamseer L, Clarke M, Ghersi D, Liberati A, Petticrew M, Shekelle P, Stewart LA, Group P-P (2015) Preferred reporting items for systematic review and and explanation meta-analysis protocols (PRISMA-P) 2015: elaboration. Syst Rev 4:1–25
Zhou K, Yang S, Shen C (2013) A review of electric load classification in smart grid environment. Renew Sustain Energy Rev 24:103–110. https://doi.org/10.1016/j.rser.2013.03.023
Gonzalez-Briones A, Hernandez G, Corchado JM, Omatu S, Mohamad MS (2019) Machine learning models for electricity consumption forecasting: a review. In: 2019 2nd international conference on computer applications and information security, IEEE, pp 1–6. https://doi.org/10.1109/cais.2019.8769508
Wang Z, Li J, Zhu S, Zhao J, Deng S, Zhong S, Yin H, Li H, Qi Y, Gan Z (2019) A review of load forecasting of the distributed energy system. IOP Conf Ser Earth Environ Sci 237:042019. https://doi.org/10.1088/1755-1315/237/4/042019
Mele E (2019) A review of machine learning algorithms used for load forecasting at microgrid level. In: Sinteza 2019-International scientific conference on information technology and data related research, Singidunum University, pp 452–458. https://doi.org/10.15308/sinteza-2019-452-458
Shah RB (2019) A technological literature review on load forecasting in power system using artificial intelligence. Paripex-Indian J Res 8:14–15
Kuster C, Rezgui Y, Mourshed M (2017) Electrical load forecasting models: a critical systematic review. Sustain Cities Soc 35:257–270. https://doi.org/10.1016/j.scs.2017.08.009
Panda SK, Mohanty SN, Jagadev AK (2017) Long term electrical load forecasting: an empirical study across techniques and domains. Indian J Sci Technol 10:1–16
Jacob M, Neves C, Vukadinović-Greetham D (2020) Short term load forecasting. Forecast Assess Risk Individ Electr Peaks Math Planet Earth. https://doi.org/10.1007/978-3-030-28669-9_2
Article MATH Google Scholar
Appiahene P, Ussiph N, Missah YM (2018) Information technology impact on productivity. In: International conference on applied science and technology, Kumasi Technical University, Kumasi, Ghana, pp 122–133
Moher D, Liberati A, Tetzlaff J, Altman DG (2009) PRISMA Group, preferred reporting items for systematic reviews and meta-analyses: the PRISMA statement. Ann Intern Med 151:264–269. https://doi.org/10.7326/0003-4819-151-4-200908180-00135
Electricchoice.com (2016) Residential electricity for houses, apartments, condos, trailers, etc. https://www.electricchoice.com/residential-electricity . Accessed 10 Aug 2019
Kouroupetroglou P-N (2017) Machine learning techniques for short-term electric load forecasting. Aristotle University of Thessaloniki, Thessaloniki
Zakarya S, Abbas H, Belal M (2017) Long-term deep learning load forecasting based on social and economic factors in the Kuwait region. J Theor Appl Inf Technol 95:1524–1535
Soni A, Sharma AK (2013) Electricity load forecast for power system planning. Int Ref J Eng Sci 2:52–57
Angamuthu CR, Mukherjee A, Campion M, Salehfar H, Hansen T, Lin J, Ranganathan P (2018) A multi-stage price forecasting model for day-ahead electricity markets. Forecasting 1:3. https://doi.org/10.3390/forecast1010003
Lee CW, Lin BY (2016) Application of hybrid quantum tabu search with support vector regression (SVR) for load forecasting. Energies 9:1–16. https://doi.org/10.3390/en9110873
Hong WC, Fan GF (2019) Hybrid empirical mode decomposition with support vector regression model for short term load forecasting. Energies 12:1–16. https://doi.org/10.3390/en12061093
Sulandari W, Subanar MH, Lee PC (2020) Rodrigues, Indonesian electricity load forecasting using singular spectrum analysis, fuzzy systems and neural networks. Energy 190:116408. https://doi.org/10.1016/j.energy.2019.116408
Nepal B, Yamaha M, Yokoe A, Yamaji T (2020) Electricity load forecasting using clustering and ARIMA model for energy management in buildings. Jpn Archit Rev 3:62–76. https://doi.org/10.1002/2475-8876.12135
Ghore S, Goswami A (2015) Short term load forecasting of Chhattisgarh grid using artificial neural network. Int J Eng Dev Res 3:391–397
Kuhba H, Al-tamemi HAH (2016) Power system short-term load forecasting using artificial neural networks. Int J Eng Dev Res 4:78–87
Zahid M, Ahmed F, Javaid N, Abbasi R, Zainab KH, Javaid A, Bilal M, Akbar M, Ilahi M (2019) Electricity price and load forecasting using enhanced convolutional neural network and enhanced support vector regression in smart grids. Electronics 8:122. https://doi.org/10.3390/electronics8020122
Nwulu NI, Agboola OP (2012) Modelling and predicting electricity consumption using artificial neural networks. In: 2012 11th international conference on environmental and electrical engineering, pp 1059–1063
Young TC, Raya H, Youngdeok H, Young ML, (2016) Artificial neural network model for forecasting sub-hourly electricity usage in commercial buildings. Energy Buildings 111:184–194. https://doi.org/10.1016/j.enbuild.2015.11.045
Aydodu G, Yildiz O (2017) Forecasting the annual electricity consumption of Turkey using a hybrid model. In: 2017 25th signal processing and communication application conference, Antalya, Turkey, pp 1–4. https://doi.org/10.1109/SIU.2017.7960283
Ahmad W, Ayub N, Ali T, Irfan M, Awais M, Shiraz M, Glowacz A (2020) Towards short term electricity load forecasting using improved support vector machine and extreme learning machine. Energies 13:1–17. https://doi.org/10.3390/en13112907
Atef S, Eltawil AB (2020) Assessment of stacked unidirectional and bidirectional long short-term memory networks for electricity load forecasting. Electr Power Syst Res 187:106489. https://doi.org/10.1016/j.epsr.2020.106489
Azar AT, Khamis A, Kamal NA, Galli B (2020) Short term electricity load forecasting through machine learning. In: Hassanien A, Azar A, Gaber T, Oliva D, Tolba F (eds) International conference on artificial intelligence computer vision (AICV2020). AICV 2020. Advances in intelligent systems and computing, vol 1153, Springer, Cham. https://link.springer.com/chapter/10.1007/978-3-030-44289-7_40 . Accessed 5 July 2020
Konica JA, Hanelli L (2016) Forecasting next-day the electricity demand based on fuzzy logic method case for. J Multidiscip Eng Sci Technol 3:6172–6180
Motepe S, Hassan AN, Stopforth R (2019) South African distribution networks load forecasting using ANFIS. In: Proceedings of 2018 IEEE international conference power electronic drives energy system PEDES 2018, IEEE, Chennai, India, pp 1–6. https://doi.org/10.1109/pedes.2018.8707876
Lin CT, Der Chou L (2013) A novel economy reflecting short-term load forecasting approach. Energy Convers Manag 65:331–342. https://doi.org/10.1016/j.enconman.2012.08.001
Leahy E, Lyons S (2010) Energy use and appliance ownership in Ireland. Energy Policy 38:4265–4279. https://doi.org/10.1016/j.enpol.2010.03.056
Soares A, Gomes Á, Antunes CH (2014) Categorisation of residential electricity consumption as a basis for the assessment of the impacts of demand response actions. Renew Sustain Energy Rev 30:490–503. https://doi.org/10.1016/j.rser.2013.10.019
Hedén W (2016) Predicting hourly residential energy consumption using random forest and support vector regression an analysis of the impact of household clustering on the performance accuracy. KTH Royal Institute of Technology, Stockholm
Kong W, Dong ZY, Jia Y, Hill DJ, Xu Y, Zhang Y (2019) Short-term residential load forecasting based on LSTM recurrent neural network. IEEE Trans Smart Grid 10:841–851. https://doi.org/10.1109/TSG.2017.2753802
Kong W, Dong ZY, Hill DJ, Luo F, Xu Y (2018) Short-term residential load forecasting based on resident behaviour learning. IEEE Trans Power Syst 33:2016–2017. https://doi.org/10.1109/TPWRS.2017.2688178
Kontokosta CE, Tull C (2017) A data-driven predictive model of city-scale energy use in buildings. Appl Energy 197:303–317. https://doi.org/10.1016/j.apenergy.2017.04.005
McLoughlin F, Duffy A, Conlon M (2015) A clustering approach to domestic electricity load profile characterisation using smart metering data. Appl Energy 141:190–199. https://doi.org/10.1016/j.apenergy.2014.12.039
Mcloughlin F, Duffy A, Conlon M (2012) Characterising domestic electricity consumption patterns by dwelling and occupant socio-economic variables: an Irish case study. Energy Build 48:240–248. https://doi.org/10.1016/j.enbuild.2012.01.037
Veit A, Goebel C, Tidke R, Doblander C, Jacobsen H (2014) Household electricity demand forecasting-benchmarking state-of-the-art methods. arXiv:1603.00751 , pp 1–10
Biswas MAR, Robinson MD, Fumo N (2016) Prediction of residential building energy consumption: a neural network approach. Energy 117:84–92. https://doi.org/10.1016/j.energy.2016.10.066
Nishida K, Takeda A, Iwata S, Kiho M, Nakayama I (2017) Household energy consumption prediction by feature selection of lifestyle data. In: International conference on smart grid communication, Dresden, Germany, pp 235–240
Olaniyan K, Mclellan BC (2018) Estimating residential electricity consumption in Nigeria to support energy transitions. Sustain 10:1–22. https://doi.org/10.3390/su10051440
Bouznit M, Pablo-Romero MP (2018) Residential electricity consumption and economic growth in Algeria. Energies (MDPI). https://doi.org/10.3390/en11071656
Bogomolov A, Lepri B, Larcher R, Antonelli F, Pianesi F, Pentland A (2016) Energy consumption prediction using people dynamics derived from cellular network data. EPJ Data Sci. https://doi.org/10.1140/epjds/s13688-016-0075-3
Huber P, Gerber M, Rumsch A, Paice A (2018) Prediction of domestic appliances usage based on electrical consumption. Energy Inform 1:265–428
Jain PK, Quamer W, Pamula R (2018) Electricity consumption forecasting using time series analysis. In: Singh M, Gupta P, Tyagi V, Flusser J, Ören T (eds), Advanced computer data science ICACDS 2018. Communications in computer and information science, Springer, Singapore, Singapore. https://doi.org/10.1007/978-981-13-1813-9_33
Rehman USA, Cai Y, Fazal R, Das Walasai G, Mirjat NH (2017) An integrated modeling approach for forecasting long-term energy demand in Pakistan. Energies 10:1–23. https://doi.org/10.3390/en10111868
Liang Y, Niu D, Cao Y, Hong WC (2016) Analysis and modeling for China’s electricity demand forecasting using a hybrid method based on multiple regression and extreme learning machine: a view from carbon emission. Energies 9:1–22. https://doi.org/10.3390/en9110941
Mokilane P, Galpin J, Yadavalli VSS, Debba P, Koen R, Sibiya S (2018) Density forecasting for long-term electricity demand in South Africa using quantile regression. S Afr J Econ Manag Sci 21:1–14. https://doi.org/10.4102/sajems.v21i1.1757
Sarkodie SA (2017) Estimating Ghana’s electricity consumption by 2030: an ARIMA forecast, energy sources. Part B Econ Plan Policy 12:936–944. https://doi.org/10.1080/15567249.2017.1327993
Energy Commission, Energy Commission, Ghana 2018 Energy (Supply and Demand) Outlook for Ghana, Accra, 2018. http://www.energycom.gov.gh/planning/data-center/energy-outlook-for-ghana . Accessed 1 Feb 2020
Sigauke C (2017) Forecasting medium-term electricity demand in a South African electric power supply system. J Energy S Afr. https://doi.org/10.17159/2413-3051/2017/v28i4a2428
Asumadu-Sarkodie S, Owusu PA (2016) Forecasting Nigeria’s energy use by 2030, an econometric approach, Energy Sources. Part B Econ Plan Policy 11:990–997. https://doi.org/10.1080/15567249.2016.1217287
Adeoye O, Spataru C (2019) Modelling and forecasting hourly electricity demand in West African countries. Appl Energy 242:311–333. https://doi.org/10.1016/j.apenergy.2019.03.057
Sigauke C, Chikobvu D (2016) Peak electricity demand forecasting using time series regression models: an application to South African data. J Stat Manag Syst 19:567–586. https://doi.org/10.1080/09720510.2015.1086146
Ozturk S, Ozturk F (2018) Prediction of energy consumption of Turkey on sectoral bases by Arima model. Energy Econ Lett 5:23–30. https://doi.org/10.18488/journal.82.2018.51.23.30
Lebotsa ME, Sigauke C, Bere A, Fildes R, Boylan JE (2018) Short term electricity demand forecasting using partially linear additive quantile regression with an application to the unit commitment problem. Appl Energy 222:104–118. https://doi.org/10.1016/j.apenergy.2018.03.155
Caro E, Juan J, Cara J (2020) Periodically correlated models for short-term electricity load forecasting. Appl Math Comput 364:124642. https://doi.org/10.1016/j.amc.2019.124642
Article MathSciNet MATH Google Scholar
Kong X, Li C, Wang C, Zhang Y, Zhang J (2020) Short-term electrical load forecasting based on error correction using dynamic mode decomposition. Appl Energy 261:114368. https://doi.org/10.1016/j.apenergy.2019.114368
Auverlot D, Beeker É, Hossie G, Oriol L, Rigard-Cerison A (2014) The crisis of the european electricity system: diagnosis and possible ways forward. https://www.strategie.gouv.fr/sites/strategie.gouv.fr/files/archives/CGSP_Report_European_Electricity_System_030220141.pdf . Accessed 18 Apr 2019
Guo Z, Zhou K, Zhang C, Lu X, Chen W, Yang S (2018) Residential electricity consumption behavior: influencing factors, related theories and intervention strategies. Renew Sustain Energy Rev 81:399–412. https://doi.org/10.1016/j.rser.2017.07.046
Download references
Acknowledgements
Not applicable.
The authors declare that they did not receive any funding for this research.
Author information
Authors and affiliations.
Department of Computer Science, Sunyani Technical University, Box 206, Sunyani, Ghana
Isaac Kofi Nti
Department of Electrical and Electronic Engineering, Sunyani Technical University, Sunyani, Ghana
Moses Teimeh
Department of Computer Science and Informatics, University of Energy and Natural Resources, Sunyani, Ghana
Owusu Nyarko-Boateng & Adebayo Felix Adekoya
You can also search for this author in PubMed Google Scholar
Contributions
We, at this moment, declare that IKN and MT contributed to the design and implementation of the research. MT, IKN ON-B and AFA contributed to the analysis of the results and the writing of the manuscript. The manuscript has been read and approved by all named authors, and there are no other persons who satisfied the criteria for authorship but are not listed. All authors read and approved the final manuscript.
Corresponding author
Correspondence to Isaac Kofi Nti .
Ethics declarations
Competing interests.
The authors of the current study declare that they have no competing interests.
Additional information
Publisher's note.
Springer Nature remains neutral with regard to jurisdictional claims in published maps and institutional affiliations.
Rights and permissions
Open Access This article is licensed under a Creative Commons Attribution 4.0 International License, which permits use, sharing, adaptation, distribution and reproduction in any medium or format, as long as you give appropriate credit to the original author(s) and the source, provide a link to the Creative Commons licence, and indicate if changes were made. The images or other third party material in this article are included in the article's Creative Commons licence, unless indicated otherwise in a credit line to the material. If material is not included in the article's Creative Commons licence and your intended use is not permitted by statutory regulation or exceeds the permitted use, you will need to obtain permission directly from the copyright holder. To view a copy of this licence, visit http://creativecommons.org/licenses/by/4.0/ .
Reprints and permissions
About this article
Cite this article.
Nti, I.K., Teimeh, M., Nyarko-Boateng, O. et al. Electricity load forecasting: a systematic review. Journal of Electrical Systems and Inf Technol 7 , 13 (2020). https://doi.org/10.1186/s43067-020-00021-8
Download citation
Received : 06 April 2020
Accepted : 30 August 2020
Published : 09 September 2020
DOI : https://doi.org/10.1186/s43067-020-00021-8
Share this article
Anyone you share the following link with will be able to read this content:
Sorry, a shareable link is not currently available for this article.
Provided by the Springer Nature SharedIt content-sharing initiative
- Electricity load forecasting
Towards Sustainable Energy: A Systematic Review of Renewable Energy Sources, Technologies, and Public Opinions
Ieee account.
- Change Username/Password
- Update Address
Purchase Details
- Payment Options
- Order History
- View Purchased Documents
Profile Information
- Communications Preferences
- Profession and Education
- Technical Interests
- US & Canada: +1 800 678 4333
- Worldwide: +1 732 981 0060
- Contact & Support
- About IEEE Xplore
- Accessibility
- Terms of Use
- Nondiscrimination Policy
- Privacy & Opting Out of Cookies
A not-for-profit organization, IEEE is the world's largest technical professional organization dedicated to advancing technology for the benefit of humanity. © Copyright 2024 IEEE - All rights reserved. Use of this web site signifies your agreement to the terms and conditions.
- Original article
- Open access
- Published: 09 January 2018
A real options approach to renewable electricity generation in the Philippines
- Casper Boongaling Agaton ORCID: orcid.org/0000-0003-1153-262X 1 &
- Helmut Karl 2
Energy, Sustainability and Society volume 8 , Article number: 1 ( 2018 ) Cite this article
30k Accesses
20 Citations
5 Altmetric
Metrics details
The Philippines is making a significant stride to become energy independent by developing more sustainable sources of energy. However, investment in renewable energy is challenged by competitive oil prices, very high investment cost for renewable energy, and high local electricity prices. This paper evaluates the attractiveness of investing in renewable energy sources over continue using oil for electricity generation.
This paper uses the real options approach to analyze how the timing of investment in renewable energy depends on volatility of diesel price, electricity price, and externality for using oil.
The result presents a positive net present value for renewable energy investment. Under uncertainty in oil prices, dynamic optimization describes how waiting or delaying investment in renewables incurs loses. Decreasing the local electricity price and incorporating negative externality favor investment in renewable energy over continuing the use of oil for electricity generation.
Conclusions
Real options approach highlights the flexibility in the timing of making investment decisions. At the current energy regime in the Philippines, substituting renewable energy is a better option than continue importing oil for electricity generation. Policies should aim at supporting investment in more sustainable sources of energy by imposing externality for using oil or decreasing the price of electricity.
Environmental problems associated with emissions from fossil fuel, along with limited supply, volatile prices, and energy security, prompted developed and developing countries to find more reliable and sustainable sources of energy. Renewable energy (RE) sources, being abundant, inexhaustible, cleaner, and readily available, emerge as a promising alternative energy source. According to International Energy Agency (IEA), RE accounted to 13.7% of the world energy generation mix in 2015 [ 1 ]. With a rapid decline in RE costs, this percentage mix is expected to double by 2040 [ 2 ]. In the Philippines, the development and optimal use of RE resources is an essential part of the country’s low emission strategy and is vital to addressing climate change, energy security, and access to energy [ 3 ]. In 2015, renewable energy accounts to 25% of the country’s total electricity generation mix, only 1% from wind and solar energy [ 4 ]. Since the country is highly dependent on imported fossil fuels, sudden changes in the price of fuels in the world market may eventually affect the country’s energy security. Renewable energy serves as a long-term solution by introducing localized RE sources. However, despite the country’s huge potential for RE generation, investments in RE projects are challenged by competitive prices of fossil fuels, more mature technology for fossil fuels, and very high investment cost for renewable energy. These give us the motivation to make a study that analyzes the attractiveness of RE investments to address the country’s concern on energy sufficiency and sustainability.
One of the most common techniques in analyzing investment projects is the net present value (NPV). This technique is widely used by developers, financial institutions, and government agencies under the condition of definite cash flow. Since RE investment in emerging economies involves high risk from volatile energy prices and changing RE technologies, NPV undervalues investment opportunities and thus considered inappropriate for assessing RE projects in developing countries including the Philippines [ 5 ]. Real options approach (ROA) overcomes this limitation as it combines risks and uncertainties with flexibility in the timing of investment as a potential factor that gives additional value to the project [ 6 ]. Recent studies use ROA renewable energy investment particularly for wind, solar photovoltaic (PV), hydropower, concentrated solar power (CSP), and combination (hybrid) of RE with uncertainties in non-RE cost, certified emission reduction (CER), feed-in tariff (FIT), energy production, operations and maintenance (O&M) cost, research and development (R&D) grants, production tax credit (PTC), RE credit (REC), among others (see Table 1 ).
This paper contributes to the existing literature by proposing a ROA framework for analyzing RE projects for developing countries, particularly, island countries that are highly dependent on imported oil for electricity generation. While previous studies proposed a full system switch to RE [ 7 ] or applied the ROA model to large-scale RE projects [ 8 , 9 , 10 , 11 ], this study takes the case of Palawan island in the Philippines and focuses on a smaller scale project which is particularly more realistic to developing countries. Whereas previous works’ approaches used coal and gas for fuel price uncertainty [ 7 , 9 , 10 , 12 ], this work uses uncertainty in oil prices as the world energy mix is dominated by liquid fuel, more developing countries are dependent on imported oil, and that investments in renewable energy is affected more by volatility in oil prices than coal prices. Finally, this paper proposes an externality tax for using fossil fuels as it more applicable in developing countries than introducing CER price, PTC, REC, CO 2 price, and emission/externality cost as proposed in previous works [ 7 , 9 , 10 , 13 , 14 ].
Applying ROA, this study aims to evaluate whether investing in RE is a better option than continue using diesel for electricity generation by considering various uncertainties in diesel fuel price, local electricity prices, and imposing externality tax for using diesel. This finally aims to recommend various government actions to address environmental problem, supply chain, and national security regarding energy.
Real options approach
Myers [ 15 ] first referred ROA or real options valuation as the application of option pricing theory to valuate non-financial or “real” assets. Real option itself is “as the right, but not the obligation, to take an action (e.g., deferring, expanding, contracting or abandoning) at a predetermined cost, called exercise price, for a predetermined period of time – the life of the option” [ 16 ]. Investment decisions, in the real world, have main characteristics: irreversible, high risk and uncertain, and flexible [ 17 ]. These characteristics are not captured by traditional methods of valuation, such as NPV, discounted cash flow (DCF), internal rate of return (IRR), and return on investment (ROI) leading to poor policy and investment decisions. ROA, on the other hand, combines uncertainty and option flexibility which characterize many investment decisions in the energy sector.
This research applies ROA to analyze investment decisions whether to continue using diesel for electricity generation or invest in RE. We use the uncertainty in diesel prices as a main factor that affects investment decisions. Using dynamic optimization, we evaluate the maximized value of investment at each price of diesel, identify the trigger price for shifting technology from diesel-based electricity to RE, and analyze the value of waiting or delaying to invest in RE. Finally, we incorporate sensitivity analyses with respect to electricity prices and externality tax for using diesel.
- Dynamic optimization
We follow the method described by Dixit and Pindyck [ 18 ] and adopt the work of Detert and Kotani [ 7 ] on optimizing investment decision under uncertainty using dynamic programming. In this research, we describe a model of an investor that identifies the optimal value of either investing in RE or continue using diesel for electricity generation as shown in Eq. 1 (see Table 2 for the list of variables and parameters).
Using this model, we determine the option value, V D , t , by maximizing the investment at each price of diesel, D , from 0 to US$1000/barrel, for each investment period, t . We set the dynamic optimization process to 40 years which represent a situation where an investor is given a period to make an investment decision. After that period, he has no other option but to continue using diesel for electricity generation. The choice is valued for another 25 years to represent the lifetime of power plant using diesel. We set the value of T R to 25 years to represent the number of years of electricity generation using RE. Finally, we solve the problem backwards using dynamic programming from terminal period [ 7 , 19 ]. The uncertainty in diesel prices in Eqs. 2 and 3 as well as the Monte Carlo simulation in the dynamic optimization process is discussed in the next subsection.
Stochastic prices and Monte Carlo simulation
In line with the previous studies, we assume that the price of diesel is stochastic and follow geometric Brownian motion (GBM) [ 20 , 21 , 22 ]. Dixit and Pindyck [ 18 ] present the stochastic price process as
where α and σ represent the mean and volatility of diesel price, dt is the time increment, and dz is the increment of Wiener process equal to \( {\varepsilon}_t\sqrt{dt} \) such that ε t ~ N (0, 1). Using Ito’s lemma, we arrive at
We approximate Eq. 6 in discrete time as
To determine the drift and variance of P , we use the Augmented Dickey-Fuller (ADF) unit root test using the following regression equation
where \( c(1)=\left(\alpha -\frac{1}{2}{\sigma}^2\right)\Delta t \) and \( {e}_t=\sigma {\varepsilon}_t\sqrt{\Delta t} \) . We then estimate the maximum likelihood of the drift \( \alpha =\mu +\frac{1}{2}{s}^2 \) and variance σ = s , where α is the mean and s is the standard deviation of the series p t − p t + 1 [ 23 ].
In this research, we use the annual prices of diesel from 1980 to 2016. The result of ADF test as shown in Table 2 implies that the null hypothesis that p t has a unit root at all significant levels cannot be rejected. Therefore, P conforms GBM. We estimate the parameters α = 0.007614 and σ = 0.358889 and use in identifying stochastic prices of diesel under GBM (Table 3 ).
We use the Monte Carlo simulation to compute the expected net present value of electricity generation using diesel in Eqs. 2 and 3 . First, we approximate a vector of potential prices of diesel using the stochastic prices of GBM as follows:
This equation illustrates that the previous price affects the current price of diesel. Second, from the initial price of diesel, P D , 0 , we estimate the succeeding prices of diesel in each period using Eq. 9 . We incorporate these prices in Eq. 2 and calculate the present values of using diesel for electricity generation. Finally, we estimate the expected net present value at each initial price node i and repeat the whole process in a sufficiently large number of J = 10000 times and take the average as given by the equation
Trigger price of diesel
Dynamic optimization process in the previous sections generates the maximized option values of investment. From these simulation results, we identify the trigger price of diesel for switching to RE as follows
where \( {\widehat{P}}_D \) is the trigger price of diesel or the minimum price where the option value in the initial period V 0 ( P D , t ) is equal to the option value in the terminal period of investment \( {V}_{{\mathrm{T}}_R}\left({P}_{D,\mathrm{t}}\right) \) [ 7 , 18 , 24 ]. From the given equation, we define trigger price as the minimum price of diesel that maximizes the profit of shifting the source of electricity from diesel power plant to RE.
Data and scenarios
To determine a suitable set of parameter values for the baseline scenario, we use data from various sources that nearly reflects the investment environment for renewable energy project in Palawan. This is the largest island province in the Philippines composed of 1780 islands and islets that are currently not connected to the national grid and only depend on imported diesel and bunker fuel. The recent Calatagan Solar Farm project in Batangas is set as a benchmark of the data for investment in RE, as this project is the latest RE project in the Philippines and has similar geographic features with Palawan; hence, investment cost estimations are up-to-date and relatively comparable [ 25 ]. This 63.3 MW solar farm, covering a total area of 160 ha, projects to generate 88,620 MWh of electricity per year. It costs US$120 million and will operate for at least 25 years. We use the data from Palawan Electric Cooperative (PALECO) [ 26 ] to approximate the local electricity price and the quantity and costs of generating electricity from diesel.
Electricity prices in the Philippines varies from island to island depending on the source of energy, as well as various charges including the generation, transmission, distribution, metering, and loss. In Palawan, effective power rates also vary across different municipalities [ 26 ]. We employ these variations in the electricity price scenario by changing the electricity price in the baseline model. In this scenario, we aim to describe how policy in imposing electricity price ceiling or price floor affects the investment decisions particularly in introducing RE as a source for electricity generation.
Lastly, we consider the externality tax of electricity generation from diesel. This value represents the negative externality including, but not limiting to, health and environmental problems associated with combustion of diesel. We use the data of the estimated average external costs for electricity generation technologies from European Environmental Agency (EEA) [ 27 ]. For this scenario, we include externality costs, tax for estimating the net present value of using diesel in Eqs. 2 and 3 . We arbitrarily assign values, between 0 (for baseline) to US$ 80/MWh, which are lower than those reported in literature to describe a more realistic condition. We assume that RE source, particularly solar PV, produces minimal or nearly no externality.
Results and discussion
Baseline scenario.
Figure 1 and Table 4 show the result of dynamic optimization at the baseline scenario. The first point of interest is the positive net present value of RE. This implies that, using the traditional valuation method, renewable project is a good investment in the island of Palawan. This result is evident as the installation of solar energy projects grows rapidly in the recent years. In 2016, there are already 538.45 MW installed capacity of solar projects from the 4399.71 potential capacity in the whole country [ 25 ]. Caution must be applied as net present value is not the sole determinant of investment in ROA. The optimal timing that maximizes the value of investment opportunity under uncertainty must also be accounted for [ 18 ].
Option values at the baseline scenario. Legend: base_0: option values of energy investment at the initial period; base_T: option values of energy investment at the terminal period
Figure 1 shows the dynamics of the option values at different initial prices of diesel. Result shows that the option values decrease over diesel price as the cost of generating electricity increases with fuel price. The trigger price as indicated by the intersection of option value curves indicates the minimum price of diesel that maximizes the decision of shifting from diesel based to RE generation. The result in the baseline scenario at US$168/barrel is higher than the current price at US$101.6/barrel. Intuitively, this implies that waiting to invest in RE is a better option than investing at the current price of diesel. However, the value of waiting to invest as describe by the distance between option value curves from initial to terminal period is negative. As seen in Table 4 , the option value at the current price of diesel at the initial period of investment is US$141.38 million and decreases to 104.97 million at the terminal period. This results to a US$36.41 million loss from delaying or waiting to invest. This implies that waiting to invest in RE incurs losses.
Electricity price scenario
This scenario describes how adjusting the local electricity price affects the option values and the trigger price. Figures 2 and 3 show the dynamics of option values with increasing and decreasing electricity prices decreasing electricity prices (see Additional file 1 Table S2 for dynamic optimization result). Result shows that the option values shift upwards with increasing electricity prices. This shows that at higher electricity prices, the value of either renewable energy or diesel-based electricity both increases. However, the trigger prices of diesel also increase to US$172/barrel at US$220/MWh and US$185/barrel at US$250/MWh from the baseline electricity price of US$202/MWh. This suggests that increasing the electricity price encourages waiting or delaying to invest in RE.
Option values at increasing electricity price scenario. Legend: base_0: option values of energy investment at the initial period; base_T: option values of energy investment at the terminal period; elec+1_0: option values at 10% higher electricity price than the base at the initial period; elec+1_T: option values at 10% higher electricity price than the base at the terminal period; elec+2_0: option values at 25% higher electricity price than the base at the initial period; elec+2_T: option values at 25% higher electricity price than the base at the terminal period
Option values at decreasing electricity price scenario. Legend: base_0: option values of energy investment at the initial period; base_T: option values of energy investment at the terminal period; elec−1_0: option values at 10% lower electricity price than the base at the initial period; elec−1_T: option values at 10% lower electricity price than the base at the terminal period; elec−2_0: option values at 25% lower electricity price than the base at the initial period; elec−2_T: option values at 25% lower electricity price than the base at the terminal period; elec−3_0: option values at 40% lower electricity price than the base at the initial period; elec−3_T: option values at 40% lower electricity price than the base at the terminal period
On the other hand, decreasing electricity prices shifts the option value curves downwards and decreasing the trigger price of diesel. This result is apparent as decreasing electricity price results to a lower revenue and thus lower profit for both options. The trigger prices of diesel decrease to US$160/barrel at US$180/MWh, US$150/barrel at US$150/MWh, and US$139/barrel at US$120/MWh price of electricity (Figs. 3 and 4 ). This suggests that lowering the electricity price decreases the timing to invest in renewable energy. Further, the option values become negative at electricity price below US$120/MWh. This implies that policy makers or power producers must not set an electricity price below US$120/MWh, as this will result to a loss for producing electricity from diesel as well as a negative investment for RE.
Trigger prices of diesel over electricity price
Externality scenario
This scenario describes how inclusion of externality tax from combustion of diesel affects the option values and triggers prices in investment in RE projects. The result in Fig. 5 (see Additional file 1 Table S3 for dynamic optimization result) shows that option values shift to the left. First, this implies that imposing externality tax decreases the revenue from electricity generation using diesel and thus decreasing the option values. Second, the unchanged lower boundary of the curves implies externality does not affect the value of investment in renewable energy. This is due to our assumption that electricity generation from RE produces no externality.
Option values at negative externality scenario. Legend: base_0: option values of energy investment with no externality at the initial period; base_T: option values of energy investment with no externality at the terminal period; ex1_0: option values at 20$/MWh externality cost at the initial period; ex1_T: option values at 20$/MWh externality cost at the terminal period; ex2_0: option values at 40$/MWh externality cost at the initial period; ex2_T: option values at 40$/MWh externality cost at the terminal period; ex3_0: option values at 60$/MWh externality cost at the initial period; ex3_T: option values at 60$/MWh externality cost at the terminal period; ex3_0: option values at 80$/MWh externality cost at the initial period; ex4_T: option values at 80$/MWh externality cost at the terminal period
With externality, the trigger prices of diesel decrease to US$140/barrel at US$20/MWh, US$111/barrel at US$40/MWh, US$82/barrel at US$60/MWh, and US$54/barrel at US$80/MWh externality cost (Figs. 5 and 6 ). This implies that imposing externality tax for diesel makes investment in RE more optimal than continue using diesel. Finally, the threshold of externality cost is US$46.55/MWh at the current diesel price of US$101.64/barrel. This is the minimum externality cost that favors immediate investment in RE than continue using diesel.
Trigger prices of diesel over negative externality
We evaluate investment environments and decision-making process for substituting diesel power plant with RE for electricity generation in the Philippines. Using real options approach under uncertainty in diesel prices, we identify the option values, trigger prices of diesel, and value of waiting to invest in RE. We analyze the sensitivity of investment decisions with respect to various electricity prices and addition of externality tax for using diesel.
ROA highlights the flexibility in the timing of making investment decisions. Our analyses conclude that for a developing country that is highly dependent on imported fuel, shifting to RE is a better option than continue using imported diesel. Policies should aim at supporting investment in more sustainable sources of energy by imposing externality for using fossil-based fuel or decreasing the price of electricity. This may negatively affect the power producers but encourage them to shift from diesel to renewable energy.
We summarized a unique approach to energy investment by replacing diesel with RE for electricity generation. We believe that the ROA framework introduced in this research is a good benchmark for further application. First, ROA may take account of environmental and social costs. This may include the cost of deforestation for solar farm, wildlife and habitat loss, air and water pollution, damage to public health, and loss of jobs. Finally, analyzing investment decisions with several RE resources includes dynamic optimization with different scenarios of generation mix from various RE sources. We are optimistic that this research becomes one-step forward for further analysis of investment in more sustainable sources of energy.
Abbreviations
Augmented Dickey-Fuller
Certified emission reduction
Concentrated solar power
Discounted cash flow
European Environmental Agency
Feed-in tariff
Geometric Brownian motion
International Energy Agency
Internal rate of return
Net present value
Operations and maintenance
Palawan Electric Cooperative
Production tax credit
Solar photovoltaic
Research and development
- Renewable energy
Renewable energy credit
Return on investment
IEA (2017) Key world energy statistics. International Energy Agency. https://www.iea.org/publications/freepublications/publication/KeyWorld2017.pdf Accessed 12 Oct 2017
BNEF (2017) New energy outlook 2017. Bloomberg New Energy Finance. https://data.bloomberglp.com/bnef/sites/14/2017/06/BNEF_NEO2017_ExecutiveSummary.pdf?elqTrackId=431b316cc3734996abdb55ddbbca0249&elq=0714ab8b3c51467a8b29e864d6fff67a&elqaid=7785&elqat=1&elqCampaignId = Accessed 12 Oct 2017
DOE (2012) Philippine Energy Plan 2012-2030. Philippines’ Department of Energy. https://www.doe.gov.ph/sites/default/files/pdf/pep/2012-2030_pep.pdf Accessed 09 Sept 2017
DOE (2016) Philippine Power Statistics 2015. Philippines’ Department of Energy. https://www.doe.gov.ph/sites/default/files/pdf/energy_statistics/power_statistics_2015_summary.pdf Accessed 01 Jan 2017
Kim K, Park H, Kim H (2017) Real options analysis for renewable energy investment decisions in developing countries. Renew Sust Energ Rev 75:918–926. https://doi.org/10.1016/j.rser.2016.11.073
Article Google Scholar
Brach MA (2003) Real options in practice. John Wiley & Sons, Inc., Hoboken, New Jersey
Google Scholar
Detert N, Kotani K (2013) A real options approach to energy investments in Mongolia. Energy Policy 56:136–150. https://doi.org/10.1016/j.enpol.2012.12.003
Weibel S, Madlener R (2015) Cost-effective design of ringwall storage hybrid power plants: a real options analysis. Energy Convers Manag 103:871–885. https://doi.org/10.1016/j.enconman.2015.06.043
Wesseh PK Jr, Lin B (2015) Renewable energy technologies as beacon of cleaner production: a real options valuation analysis for Liberia. J Clean Prod 90:300–310. https://doi.org/10.1016/j.jclepro.2014.11.062
Zhang MM, Zhou P, Zhou DQ (2016) A real options model for renewable energy investment with application to solar photovoltaic power generation in China. Energy Econ 59:213–226. https://doi.org/10.1016/j.eneco.2016.07.028
Kitzing L, Juul N, Drud N, Boomsma TK (2017) A real options approach to analyse wind energy investments under different support schemes. Appl Energy 188:83–96. https://doi.org/10.1016/j.apenergy.2016.11.104
Kim KT, Lee DJ, Park SJ (2014) Evaluation of R&D investments in wind power in Korea using real option. Renew Sust Energ Rev 40:335–347. https://doi.org/10.1016/j.rser.2014.07.165
Lee H, Park T, Kim B, Kim K, Kim H (2013) A real option-based model for promoting sustainable energy projects under the clean development mechanism. Energy Policy 54:360–368. https://doi.org/10.1016/j.rser.2014.07.165
Tian et al. (2017). The valuation of photovoltaic power generation under carbon market linkage based on real options. Appl Energy, 201:354-362. doi: https://doi.org/10.1016/j.apenergy.2016.12.092
Myers SC (1977) The determinants of corporate borrowing. J Financ Econ 5:147–175. https://doi.org/10.1016/0304-405X(77)90015-0
Copeland T, Antikarov V (2003) Real options: a practitioner’s guide. Cen gage Learning, New York
Baecker PN (2007) Real options and intellectual property: capital budgeting under imperfect patent protection. Springer Berlin Heidelberg
Bertsekas DP (2012) Dynamic programming and optimal control, Vol. 2, fourth ed. Athena Scientific.
Dixit AK, Pindyck RS (1994) Investment under uncertainty. Princeton University Press, New Jersey
Fonseca MN et al (2017) Oil price volatility: a real option valuation approach in an African oil field. J Pet Sci Eng 150:297–304. https://doi.org/10.1016/j.petrol.2016.12.024
Guedes J, Santos P (2016) Valuing an offshore oil exploration and production project through real options analysis. Energy Econ 60:377–386. https://doi.org/10.1016/j.eneco.2016.09.024
Postali FAS, Picchetti P (2006) Geometric Brownian motion and structural breaks in oil prices: a quantitative analysis. Energy Econ 28(4):506–522. https://doi.org/10.1016/j.eneco.2006.02.011
Insley M (2002) A real options approach to the valuation of a forestry investment. J Environ Econ Manag 44(3):471–492. https://doi.org/10.1006/jeem.2001.1209
Article MATH Google Scholar
Davis GA, Cairns RD (2012) Good timing: the economics of optimal stopping. J Econ Dyn Control 36(2):255–265. https://doi.org/10.1016/j.jedc.2011.09.008 .
DOE (2016) Awarded Solar Grid 2016. Philippines’ Department of Energy https://www.doe.gov.ph/sites/default/files/pdf/renewable_energy/awarded_solar_grid_20160630.pdf Accessed: 16 Jan 2017
Paleco (2016) Status of electrification. Palawan Electric Cooperative Accessed: 16 Jan 2017
EEA (2010). Estimated average EU external costs for electricity generation technologies in 2005. European Environmental Agency. http://www.eea.europa.eu/data-and-maps/figures/estimated-average-eu-external-costs Accessed 20 March 2017
Abadie LM, Chamorro JM (2014) Valuation of wind energy projects: a real options approach. Energies 7:3218–3255. https://doi.org/10.3390/en7053218
Jeon C, Lee J, Shin J (2015) Optimal subsidy estimation method using system dynamics and the real option model: photovoltaic technology case. Appl Energy 142:33–43. https://doi.org/10.1016/j.apenergy.2014.12.067
Barrera GM, Ramírez CZ, González JMG (2016) Application of real options valuation for analysing the impact of public R&D financing on renewable energy projects: a company’s perspective. Renew Sust Energ Rev 63:292–301. https://doi.org/10.1016/j.rser.2016.05.073
Eryilmaz D, Homans R (2016) How does uncertainty in renewable energy policy affect decisions to invest in wind energy? Electr J 29(3):64–71. https://doi.org/10.1016/j.tej.2015.12.002
Ritzenhofen I, Spinler S (2016) Optimal design of feed-in-tariffs to stimulate renewable energy investments under regulatory uncertainty—a real options analysis. Energy Econ 53:76–89. https://doi.org/10.1016/j.eneco.2014.12.008
Download references
Acknowledgements
We acknowledge the support by the DFG Open Access Publication Funds of the Ruhr-Universität Bochum.
Author information
Authors and affiliations.
Institute of Development Research and Development Policy, Ruhr University of Bochum, Universitaetsstr. 105, 44789, Bochum, Germany
Casper Boongaling Agaton
Faculty of Management and Economics, Ruhr University of Bochum, Universitaetsstr. 150, 44801, Bochum, Germany
Helmut Karl
You can also search for this author in PubMed Google Scholar
Contributions
CA conceptualized the research objectives and modeling scenarios. All authors contributed to the data analysis and writing of the final manuscript. All authors read and approved the manuscript.
Corresponding author
Correspondence to Casper Boongaling Agaton .
Ethics declarations
Competing interests.
The authors declare that they have no competing interests.
Publisher’s Note
Springer Nature remains neutral with regard to jurisdictional claims in published maps and institutional affiliations.
Additional file
Additional file 1:.
Table S1. ADF unit root test result of oil prices from 1981-2016. Table S2. Note: elec+2_0: option values at 25% higher electricity price than the base at the initial period; elec+2_T: option values at 25% higher electricity price than the base at the terminal period elec+1_0: option values at 10% higher electricity price than the base at the initial period; elec+1_T: option values at 10% higher electricity price than the base at the terminal period; base_0: option values of energy investment at the initial period; base_T: option values of energy investment at the terminal period; elec-1_0: option values at 10% lower electricity price than the base at the initial period; elec-1_T: option values at 10% lower electricity price than the base at the terminal period; elec-2_0: option values at 25% lower electricity price than the base at the initial period; elec-2_T: option values at 25% lower electricity price than the base at the terminal period; elec-3_0: option values at 40% lower electricity price than the base at the initial period; elec-3_T: option values at 40% lower electricity price than the base at the terminal period. Table S3. base_0: option values of energy investment with no externality at the initial period; base_T: option values of energy investment with no externality at the terminal period; ex1_0: option values at 20/ MWhexternalitycosttheinitialperiod; ex 1 T : optionvaluesat20/MWh externality cost at the terminal period; ex2_0: option values at 40/ MWhexternalitycosttheinitialperiod; ex 2 T : optionvaluesat40/MWh externality cost at the terminal period; ex3_0: option values at 60/ MWhexternalitycosttheinitialperiod;ex 3 T : optionvaluesat60/MWh externality cost at the terminal period; ex3_0: option values at 80/ MWhexternalitycosttheinitialperiod; ex 4 T : optionvaluesat80/MWh externality cost at the terminal period. (DOCX 95 kb)
Rights and permissions
Open Access This article is distributed under the terms of the Creative Commons Attribution 4.0 International License ( http://creativecommons.org/licenses/by/4.0/ ), which permits unrestricted use, distribution, and reproduction in any medium, provided you give appropriate credit to the original author(s) and the source, provide a link to the Creative Commons license, and indicate if changes were made.
Reprints and permissions
About this article
Cite this article.
Agaton, C.B., Karl, H. A real options approach to renewable electricity generation in the Philippines. Energ Sustain Soc 8 , 1 (2018). https://doi.org/10.1186/s13705-017-0143-y
Download citation
Received : 26 June 2017
Accepted : 14 December 2017
Published : 09 January 2018
DOI : https://doi.org/10.1186/s13705-017-0143-y
Share this article
Anyone you share the following link with will be able to read this content:
Sorry, a shareable link is not currently available for this article.
Provided by the Springer Nature SharedIt content-sharing initiative
- Price uncertainty
- Externality tax
Energy, Sustainability and Society
ISSN: 2192-0567
- General enquiries: [email protected]
Thank you for visiting nature.com. You are using a browser version with limited support for CSS. To obtain the best experience, we recommend you use a more up to date browser (or turn off compatibility mode in Internet Explorer). In the meantime, to ensure continued support, we are displaying the site without styles and JavaScript.
- View all journals
- My Account Login
- Explore content
- About the journal
- Publish with us
- Sign up for alerts
- Open access
- Published: 25 January 2023
Household energy-saving behavior, its consumption, and life satisfaction in 37 countries
- Xiangdan Piao 1 &
- Shunsuke Managi 2
Scientific Reports volume 13 , Article number: 1382 ( 2023 ) Cite this article
9073 Accesses
12 Citations
8 Altmetric
Metrics details
- Environmental social sciences
Since energy consumption became an important contributor to climate change owing to carbon emissions, energy-saving behavior and expenditure at the household level have been attracting scholars’ and policymakers’ attention. This study identified whether greenhouse gas emissions at the household level can be reduced through purchase of energy-saving goods and whether the energy-saving behavior enhanced with household income increase. We conducted a large-scale survey across 37 nations using internet-based and face-to-face approaches, collecting 100,956 observations. The wealth effect on energy consumption expenditure at the household level was found to be positive across countries, confirming that energy consumption increases with household wealth improvement. Furthermore, households show a positive association between household energy expenditure and life satisfaction in 27 out of 37 countries, including China, India, the United States, and Germany. Additionally, the favorable effects of household energy-saving behavior are confirmed. However, purchase of household energy-saving products has a limited effect on energy consumption expenditure, compared with that of energy-curtailment behavior. In conclusion, achieving a carbon–neutral household by reducing energy consumption expenditure at the household level is challenging; thus, along with the use of energy-saving goods, alternative energy sources, such as renewable energies, are recommended.
Similar content being viewed by others
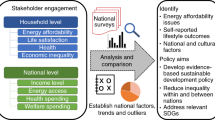
Multinational life satisfaction, perceived inequality and energy affordability
Andrew Chapman, Hidemichi Fujii & Shunsuke Managi
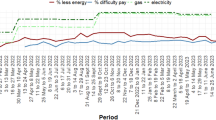
Self-reported energy use behaviour changed significantly during the cost-of-living crisis in winter 2022/23: insights from cross-sectional and longitudinal surveys in Great Britain
Gesche M. Huebner, Clare Hanmer, … Tadj Oreszczyn
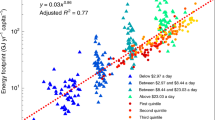
Large inequality in international and intranational energy footprints between income groups and across consumption categories
Yannick Oswald, Anne Owen & Julia K. Steinberger
Introduction
Energy consumption is closely related to global climate change through greenhouse gas emissions. Hence, enhancing humanity’s well-being via sustainable energy consumption and environmental conservation is crucial. In this study, we aim to identify whether greenhouse gas emissions at the household level can be reduced by reducing the energy consumption expenditure of households globally. In 2015, the United Nations proposed the sustainable development goals for sustaining humanity’s well-being, encompassing 17 multidimensional goals related to environment preservation, economics, and society. Subjective well-being is assumed to be a proxy for humanity’s well-being both in sociological and other psychological and economic aspects 1 , 2 , 3 .
Since the Industrial Revolution, fossil fuels, which include natural gas, coal, and oil, have become a crucial energy source for modern industries. As fossil fuel consumption is associated with greenhouse gas emissions, including carbon dioxide (CO2) emissions, global CO2 emissions from fossil fuels increased from 14 billion tons in 1971 to 34 billion tons in 2016 4 . The Fifth Assessment Report of climate change released in 2013 concludes that global warming is undoubtedly caused by human activities. The Paris agreement sets a clear goal to “limit global warming to well below 2, preferably to 1.5 degrees Celsius compared to pre-industrial levels” 5 . To achieve this goal, policies to reduce CO2 emissions were introduced across the globe. For example, according to the IEA 6 , in-building light, space heating, and water heating increased to 83%, 43%, and 39%, respectively, in 2018. Furthermore, the transition to zero-emission vehicles was announced in Europe, Asia, and the Americas 7 . Additionally, efficiency stars were initiated for electronic products to meet the energy efficiency standards of the United States Environmental Protection Agency and Department of Energy.
Numerous studies have examined the association between well-being and energy consumption, with inconclusive results 8 , 9 , 10 , 11 , 12 , 13 , 14 , 15 , 16 , 17 , 18 . Chapman et al. 13 used individual-level micro-cross-sectional data from 37 nations to demonstrate that households often have difficulties in terms of being able to afford the costs of energy consumption and that individuals from such households are more likely to experience a lower quality of life. Niu et al. 9 used country-level panel data from 50 countries to describe the positive causal effect of energy consumption and human development in these countries; the authors also encouraged governments to provide low-income residential electricity as public services. By contrast, using country-level panel data, Mazur 8 argued that the associations between energy, electricity consumption, and quality of life improvements are not significant. The author also stated that the significant association between these variables may originate from analyses of cross-sectional data at the country level. Jorgenson et al. 11 also discussed the relationship between energy intensity and human well-being, particularly within the context of central and eastern European nations; these authors found that the relationship between these two variables in these two contexts is rather complex and is undergoing dramatic changes.
Energy-saving behavior belongs to the category of pro-environmental behavior, with the latter being defined as altruistic, friendly, and contributive behavior toward environmental conservation 19 , 20 , 21 , 22 , 23 , 24 , 25 , 26 , 27 , 28 , 29 , 30 , 31 , 32 , 33 , 34 , 35 , 36 , 37 , 38 , 39 . In this study, energy-saving behaviors refer to those that reduce overall energy usage, including electricity and fuels 21 , 22 . To quantify the different types of energy-saving behavior, we adopt energy curtailment behavior and purchasing energy-saving goods as well as household energy efficiency behavior. Energy curtailment behavior is the low financial cost of energy consumption reduction behaviors, such as turning off power to appliances when not in use. Purchase of energy-saving goods reflect household energy efficiency as it reduces the high cost of energy consumption. Examples of variables used as proxies of energy-saving behavior are recycling, reuse, and energy-saving behavior in selection of the means of transportation 27 , 28 , 29 , 30 , 31 , 32 , 33 , 34 , 35 , 36 , 37 , 38 , 39 . Substantial literature has investigated the determinants of pro-environmental behavior and found the following key factors: knowledge of environmental issues, environmental experiences at a young age, culture, consumption beliefs, and psychological factors 29 , 30 , 31 , 32 , 33 , 34 , 35 , 36 , 37 , 38 , 39 , 40 .
Various studies show that unbridled energy consumption can be a threat to the environment 14 , 16 , 17 , 18 , 19 , 20 . Moreover, scholars and policymakers have been focusing their attention on the impact of household consumption. For example, the Japanese government has set up a goal for the household sector to reduce 66% of its CO2 emissions by 2030 to ensure the achievement of the nation’s greenhouse gas emissions reduction goals. Nonetheless, according to traditional economic theories and the subjective well-being framework, households consume energy within the context of their wealth constraints and aim to maximize the utility of the consumed energy. Subjective well-being has been described in past research as a useful measurement for assessing people’s well-being. Theoretical and empirical findings provide conflicting evidence on the association between environmental conservation goals and hedonic goals 32 , 33 , 35 , 41 , 42 , 43 .
When energy is seen as a consumption good, energy consumption expenditure may increase as household income increases, indicating a positive relationship between household income and energy consumption expenditure. The key energy consumption metric is the quantity of energy consumed (e.g., kWh) across the targeted households. Since price information is limited, transforming consumption expenditure into a quantity (e.g., kWh) is problematic. According to the theory, measurement issues are common in that household expenditure measurement is based on the expenditure amount rather than the quantity consumed. Theoretically and empirically, studies have tried to address this measurement issue by estimating the demand system 44 , 45 , 46 , 47 , 48 . Although the information relates to quantity, the observable measurement is the expenditure. Recently, Du et al. 47 estimated the energy demand function based on the demand system model. Thus, following previous research, the present study adopted this method of estimating using the energy demand equation. However, the goal framing theory presents the individual’s involvement in pro-environment behavior, that is, energy-saving behavior, under the normative goal, as practicing energy curtailment or energy efficiency behavior is the right thing to do 41 , 49 .
Moreover, if people are satisfied with their energy consumption, it might be difficult for households to reduce their energy consumption expenditure. In this context, policymakers should consider alternative tools to reduce greenhouse gas emissions at the household level. Investigations into the relationship between energy consumption at the household level and subjective well-being may provide insights as to whether households might be capable of cutting their energy consumption to help reduce greenhouse gas emissions. When the climate change situation is exacerbating, energy saving behavior is expected to sustain the environment. The fossil sourced energy, for example, electricity, curtailment behavior or energy efficiency behavior is proceeded 49 , 50 , 51 , 52 , 53 , 54 . However, research focusing on energy consumption, subjective well-being, and environmental-friendly energy consumption outcomes from a multi-national level perspective remain scarce. This study aims to address this knowledge gap.
This study contributes to the literature in the following aspects. First, the survey encompasses 37 nations, accounting for approximately 73% of the world’s population, providing data that serve to illustrate the effect of energy consumption expenditure on subjective well-being. The wealth effect is also examined within this context. The results are expected to highlight whether an increase in energy consumption leads to economic development. Second, this study lists the key determinants of energy consumption expenditure in households, providing important data that may have policy implications, such as being used in the simulation of energy consumption at the household level.
The remainder of this paper is structured as follows. Section " Methods " offers the study data and outlines the methodology, Section " Results " reports the results, and Section " Discussion " presents the discussion them. Section " Conclusion " concludes the paper.
Data collection
To explore the relationships between subjective well-being and energy consumption expenditure at the household level, this study conducted a large-scale, original, cross-sectional survey with samples from 37 nations using internet-based and face-to-face approaches. The data collection process was as follows. First, the random sampling process was applied to match the population age and gender characteristics. To do this, based on the gender and age distribution in each nation, the population was divided into numerous groups. Among all age and gender groups, restricted panels of women older than 60 years of age are scarce; therefore, an age group closest to it, that is, 55–59 years of age, was selected to avoid sample selection bias.
Second, the targeted respondents were randomly selected through a reputed company and the questionnaire was distributed to them via the internet. The company has comprehensive registered panels that enhance the collected samples to match the country's specified gender and age distributions. Moreover, the sample collection is conducted among countries separately, and to enhance the reliable of the empirical regression results, the sample size for each country is greater than 500. For each country, the number of observations ranged from 500–20,744, with the total number of observations being 100,956 over 2015–2017 (see Table 1 ).
Third, because internet users tend to be younger and more well-educated than non-internet users, the internet survey was likely to select individuals with better wealth status and good education level 55 . To counter this potential sample collection issue, the internet-based survey covered 32 nations, and the face-to-face survey was conducted in Mongolia, Myanmar, Egypt, Kazakhstan, and Sri Lanka, wherein the application of an internet-based survey was considered. Both types of surveys were conducted in Indonesia, India, and Vietnam. When conducting the face-to-face survey, survey agents visited the targeted area to collect data directly in the field along with the coauthor of this study. The agents were given extensive training. Although in the face-to-face survey, the random sampling process was not followed, the sample is valuable to present households’ energy consumption situation among rural or slum areas. Furthermore, the questionnaire was translated and repeatedly checked by professional translators to enhance accuracy. The internet-based survey covered 32 nations. The targeted countries were selected based on their regional representative population size, development representative economies, as well as cultural representativeness, that is, China and Japan are highly influenced by Confucianism, whereas Western countries share individualism, religion, and social norms.
The survey was designed to collect individuals’ perceived satisfaction in their lives, concerns about the environment, cooperation in energy usage that can be seen as energy-saving behavior, household income, energy expenditure, and other households’ demographics and economic background. In the choice items’ design for sensitive questions (e.g., household income), the exclusive items or “do not know” or unlikely-to-answer items were added to avoid dropout by respondents and improve the accuracy of the data. The survey type and number of observations in 37 nations are displayed in Table 1 .
Variable setting
Life satisfaction is a dependent variable based on the Organization of Economic Cooperation and Development guidelines 56 . When policymakers aim to improve citizens’ well-being, the individual well-being level is unobservable. Therefore, subjective well-being is adopted to reflect citizens’ well-being. In measuring subjective well-being, life satisfaction and happiness are utilized in the literature, and the Cantrill ladder that measure the overall satisfaction is widely adopted 57 , 58 , 59 . The robustness check is applied for happiness, a way to measure individual subjective well-being.
To measure life satisfaction, we asked respondents to answer the following question: “Please imagine a ladder with steps numbered 0–10. The top and bottom of the ladder represent the best and worst possible lives for you, respectively. On which step of the ladder would you say you personally feel you currently stand? (10 = Best possible life; and 0 = Worst possible life).” Regarding happiness levels, the respondents were asked, “Overall, how happy are you with your life?” The response scale ranged from 1–5 (1, unhappy ; 2, slightly unhappy ; 3, neither ; 4, slightly happy ; 5, very happy ).
Energy consumption expenditure at the household level was converted into US dollars (USD) for all countries and categorized as the energy-consumption share of the monthly income and household income (the exchange rate is of January 7, 2021). In particular, for energy consumption measurement, the respondents were asked, “What is the average share of the energy bill (including a charge for electricity/ gas/ water/ kerosene/ gasoline) out of your monthly income?” The choices were: do not use at all = 1, 1–9% = 2, 10–19% = 3, 20–29% = 4, 30–39% = 5, 40–49% = 6, 50% and above = 6. To reduce missing value observations, we added the “do not know” choice. The household energy consumption expenditure was converted based on the categorized energy consumption and monthly household income. The subjective price of energy was measured as follows: we asked respondents the following question: “How do you feel about electricity/gas/water/kerosene/gasoline bills? Please select an item that best describes your thoughts.” The response scale ranged from 1–6 (6, very expensive ; 5, slightly expensive ; 4, just right ; 3, slightly cheap ; 2, very cheap ; 1, do not care ; 0, do not use at all ). The subjective price was calculated equally for all energy categories (i.e., electricity, gas, water, kerosene, and gasoline bills).
The dummy variables of energy-saving behaviors include (1) energy-curtailment behaviors (e.g., saving electricity, fuel, etc.); and (2) purchasing energy-saving household products. Other control variables include household income, educational attainment, age, occupational status, household status, number of children, and gender dummy.
Methodology
To investigate the relationship between household energy consumption, subjective well-being, and the determinants of energy consumption expenditure at the household level, both Eqs. ( 1 ) and ( 2 ) were estimated using the ordered logit model 60 , 61 . The ordered probit, ordered logit, and ordinary least square (OLS) models are considered appropriate when the independent variable is ordinal 60 , 61 ; therefore, the ordered probit and OLS models were used as robustness check. The relationship between household energy consumption and subjective well-being is demonstrated in Eq. ( 1 ):
where \({L}_{iC}\) denotes the subjective well-being indices (e.g., life satisfaction and happiness levels) of individual \(i\) from country \(C\) . The independent variable, \({K}_{iC}\) , denotes household energy consumption and is a continuous variable. \(X\) is a set of exogenous variables, including the following socioeconomic factors: household income, education attainment, age, occupational status, household status, number of children, and gender dummy. While \({D}_{C}\) is the country dummy in country \(C\) used to capture country-level heterogeneity, \({\varepsilon }_{iC}\) is the error term . \(\alpha\) , \(\theta\) , \(\beta\) , and \(\delta\) are parameters estimated using an ordered logit regression model with Stata 16.
The Likelihood Ratio ( LR ) Chi-Square test and Pseudo R-squared for the ordered logistic regression model and the ordered probit model were applied to measure the goodness of the fit, whereas F-statistics and adjusted R-squared were used for the OLS model.
In Eq. ( 2 ), the association of life satisfaction and energy consumption expenditure at the household level for each country was estimated using an ordered logit model, as follows:
where life satisfaction is the dependent variable (ranging from 0–10, with 0 being the worst and 10 the best possible life). \(K\) is a continuous variable for energy consumption expenditure at the household level. Moreover, \(X\) denotes socioeconomic and demographic factors. \({\varepsilon }_{i}\) is the error term, and \(d\) , \(f\) , and \(m\) are the estimated parameters in the ordered logit regression model. All estimations were conducted using Stata 16.
The types of socioeconomic and demographic factors influencing the energy consumption expenditure of households were investigated using Eq. ( 3 ) based on energy demand equation and an OLS model 44 , 62 , 63 , 64 :
where the dependent variable, \({K}_{i}\) , is energy consumption expenditure at the household level and a continuous variable indicating a larger energy consumption as it increases. \(Z\) denotes household income, \(M\) denotes energy saving behavior, socioeconomic, demographic factors and other control variables, including subjective price of electricity/gas/water/kerosene/gasoline, household status, age, education attainment, and occupational status. The estimated parameters are \(a,b\) and \(c\) . All estimations were conducted using Stata 16. \({\varepsilon }_{i}\) is the error term. The robustness check was confirmed based on the two-stage least square estimation.
According to household consumption theory, measurement issues are common in that household expenditure measurement is based on the amount (e.g., USD) rather than the quantity consumed (e.g., kWh). Theoretically and empirically, previous studies aimed to address this measurement issue by estimating the demand system 44 , 45 , 46 , 47 , 48 . Following previous research, this study adopted the energy demand equation method. Consider that the parameter is positive values and statistically significant. In that case, the results indicate that energy is the normal good and that demand for energy consumption increases when household income does. As a robustness check, the different types of the energy demand equation were applied according to previous studies 49 , 50 , 51 .
Ethics approval and consent to participate
For the original cross-sectional survey conducted by a company (Nikkei Research Company) between 2015 and 2017, the study design was approved by the appropriate legal and ethics review board of Kyushu University. The data were collected with informed consent from participants, following legal and ethical guidelines. All the methods were carried out in accordance with relevant guidelines and regulations of Kyushu University.
Figure 1 presents the average monthly energy expenditure at the household level based on USD across the 37 surveyed nations. The households in Singapore expend the most amount of energy, that is, 748 USD each month on average. The energy consumption appears positively associated with the economic development level; for example, households from high-income countries, including France, Italy, Japan and the US, tend to consume more energy than those from low-income countries (e.g., Kazakhstan, Myanmar, and Mongolia). In India, Indonesia, and Vietnam, households with higher income expend more on energy than rural/slum households. For the energy expenditure to household income ratio, strong trends were not found between developing and developed countries. Notably, middle-income countries (e.g., Greece, Chile, Brazil, Egypt) spend a relatively higher share of total income on energy.
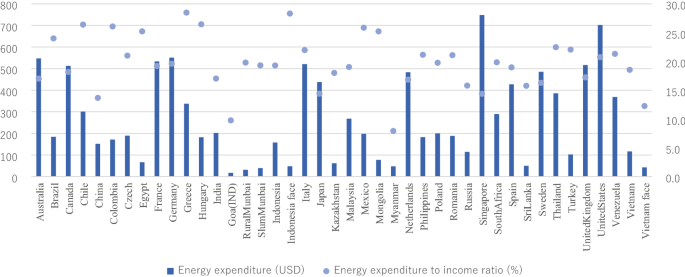
Average monthly energy expenditure at the household level across the 37 surveyed nations. Data source : Original survey.
The relationship between subjective well-being and energy consumption expenditure based on the ordered logit, ordered probit, and OLS models is shown in Table 2 , panel A. The LR Chi-Square test and Pseudo R-squared for the ordered logistic regression model and the ordered probit model were applied to measure the goodness of the fit, whereas F-statistics and adjusted R-squared were used for the OLS model. For the validation of the measurement of subjective well-being, life satisfaction and happiness measures were used. Importantly, the results from variated regression models are consistent, indicating a positive relationship between household energy consumption expenditure and the improvement of individuals’ subjective well-being. Regarding the model’s goodness of fit, the LR Chi-Square test with ordered logit and probit models, and the F-statistic in the OLS model are all statistically significant at 0.1%, which validates the regression model. As the consistency of the robustness results is derived from different models, the ordered logit model is applied in Table 2 (Panel B).
With the control variables being constant, energy consumption expenditure improves subjective well-being, including life satisfaction and happiness. The coefficients for the relationship of energy consumption with life satisfaction and with happiness are 0.018 and 0.008, respectively, and they are statistically significant at the 1% level; in other words, there is increased energy consumption for people who are satisfied with their lives and are happier. This is because electricity, water, gas, or gasoline are indispensable consumption goods in daily life. The results suggest that when policies lead to a reduction in the consumption of these goods at the household level, the life satisfaction of citizens is likely to decrease. When reducing energy consumption at the household level to reduce the emission of greenhouse gases, the conflicts of interest of individuals in these households (given that they derive life satisfaction from energy consumption) pose a challenge to policymakers; therefore, policymakers should devise strategies to improve both citizens’ living standards and environmental preservation.
Referring to the criteria developed by the World Bank, the standard classification of high-income nations and non-high-income nations is as follows. Based on the 2017 gross national income (GNI) per capita, the World Bank List of Economies (June 2018) presented the following criteria for nations to be classified as high-income and non-high-income nations, respectively: a GNI per capita of $12,056 or higher, and less than $12,056. According to this standard of classification, in this study, high-income nations comprise Japan, Singapore, Chile, Australia, the United States, Germany, the United Kingdom, France, Spain, Italy, Sweden, Canada, Netherlands, Greece, Hungary, Poland, and the Czech Republic, whereas non-high-income nations comprise Thailand, Malaysia, Indonesia, Vietnam, Philippines, Mexico, Venezuela, Brazil, Colombia, South Africa, India, Myanmar, Kazakhstan, Mongolia, Egypt, Russia, China, Turkey, Romania, and Sri Lanka.
Regarding the comparison of high- and non-high-income countries, energy consumption at the household level is more likely to lead to life satisfaction in non-high-income than in high-income countries. In high-income countries, the coefficients for the relationship of energy consumption with life satisfaction and with happiness are 0.010 and 0.003, respectively; these coefficients are 0.035 and 0.015, respectively, among non-high-income countries. Hence, in both high-income and non-high-income countries, an increase in energy consumption leads to an increase in life satisfaction; nonetheless, energy consumption is more crucial for households in non-high-income countries. Compared to the effect of energy consumption on satisfaction in high-income countries and non-high-income countries, individuals living in less urbanized countries appear more satisfied with energy consumption.
Table 3 presents the association between life satisfaction and energy consumption expenditure at the household level in each country by estimating Eq. ( 2 ) based on the ordered logit model for each country. There is a positive relationship between energy consumption expenditure and life satisfaction in 27 out of the 37 nations. For example, the coefficient of this relationship is 0.062 in Brazil, and is statistically significant at the 1% level. An increase in energy consumption expenditure positively impacts the life satisfaction of households in Brazil, meaning that individuals with greater energy expenditure tend to be satisfied with their lives. Similar results are found in other countries: Canada, Chile, China, Egypt, France, Germany, Greece, India, Indonesia, Italy, and Japan. As life satisfaction is a proxy of well-being, energy consumption is expected to increase when households can afford more energy to obtain higher life satisfaction. These results indicate that most of the developed and developing countries analyzed face a conflict of interest in addressing individuals’ life satisfaction and environment conservation goals; these countries include China and India that are home to large populations that have a positive desire for energy consumption.
However, the association between life satisfaction and energy consumption expenditure at the household level was non-significant across some countries. In Australia, the coefficient of this association is positive but not statistically significant; hence, an increase in energy expenditure is not completely associated with life satisfaction at the household level here. Similar results are found in the Netherlands, Hungary, Sweden, Singapore, Poland, the Czech Republic, and Colombia. In these countries, energy consumption is at an adequate level, and additional energy consumption does not lead to higher life satisfaction. It may be that households consume an adequate amount of energy with their income and energy price.
Tables 4 , 5 , 6 , and 7 display the determinant factors of household energy consumption in 37 nations by estimating the energy demand equation for each country using Eq. ( 3 ). The key energy consumption metric is the quantity of energy consumed (e.g., kWh) across the targeted households. Since price information is limited, transforming consumption expenditure into a quantity (e.g., kWh) is problematic. As explained earlier, this study adopted the energy demand equation.
There are positive relationships between energy consumption expenditure at the household level and household income across countries. If the coefficients for household income are positive and statistically significant, this means that energy consumption expenditure at the household level would increase with an increase in household income ensuing from economic development in the country, ceteris paribus. The positive coefficients for the association between energy consumption expenditure and household income range from 0.756 (Japan) to 3.613 (the Philippines) in our sample, indicating that an additional 10,000 USD would lead to an additional energy consumption expenditure at the household level of approximately 17.3% (Japan) – 445% (Mongolia). The number is calculated using the magnitude of the coefficient/energy consumption expenditure. The results also show that homeowners tend to consume more energy than renters in Australia, Brazil, Canada, Chile, China, Colombia, Germany, India, Italy, Japan, Malaysia, Mexico, Russia, the United States, and Vietnam. This indicates that if individuals live in their own houses, the household energy consumption expenditure tends to be higher owing to the wealth effect, as energy is a normal consumption good. Overall, the wealth effect on energy consumption expenditure at the household level is increasing in our sample, and with economic development, energy consumption may increase.
The following factors are confirmed to reduce energy consumption at the household level: (1) energy-curtailment behavior regarding electricity, (2) higher education, and (3) age. The energy-saving effect is confirmed in households. In Canada, the coefficient of energy-saving behaviors is -0.642, indicating that households consume 12.5% less energy when they adopt both energy curtailment behavior and non-saving groups (64.2/513). The Canadian household average energy consumption is 513 USD. Similar results are seen in Colombia, Germany, India, Indonesia, Italy, Japan, the Netherlands, Poland, Russia, Turkey, the United Kingdom, and the United States. The magnitude of the effect of energy curtailment behavior ranged from 6.4% (Russia) to 32% (India) less energy consumption expenditure. Hence, energy-saving behaviors have a favorable effect on environmentally preferable outcomes. By contrast, households in Indonesia save electricity as they tend to spend more on purchasing energy.
Individuals with higher education tend to save energy in 23 out of the 37 nations. For instance, the coefficient for individuals with university-level education is -2.292 and statistically significant at the 1% level. This suggests that households with individuals who have university-level education have less energy consumption expenditure than households with individuals with junior high school or lower levels of education. Similar results are seen in Brazil, Canada, Chile, Colombia, the Czech Republic, France, Germany, Hungary, India, Indonesia, Japan, Malaysia, the Netherlands, the Philippines, Poland, Russia, Singapore, South Africa, Spain, Sweden, Turkey, the United Kingdom, and the United States. Encouraging households to engage in energy curtailment behaviors and higher educational attainment may lead to environment-friendly outcomes.
Surprisingly, purchasing energy-saving household products has a limited effect on reducing energy consumption expenditure at the household level. The coefficients for purchasing energy-saving household products are negative, ranging between -0.044 and -0.763, and are statistically significant in Australia, Canada, the Czech Republic, Italy, and Kazakhstan. Hence, the purchase of these products in these five countries decreases energy expenditure from 2.9% (China) to 14% (Australia). However, the relationship between energy consumption expenditure at the household level and purchasing energy-saving household products is non-significant in the other countries. Moreover, in Poland and Turkey, households that purchase these products consume more energy than those that do not. Therefore, purchasing energy-saving household products has a limited contribution to energy saving at the household level.
The findings also show that older individuals tend to have lower energy consumption. The coefficients for the age variable are negative and statistically significant in 30 countries (out of 37). The effect of age on energy consumption expenditure ranges between -0.003 and -0.148, indicating that as the average age of individuals increases by one year, their monthly energy consumption expenditure reduces from 0.3–14.8 USD. This may be because older individuals are more likely to live frugally.
In this study, we identify whether greenhouse gas emissions at the household level can be reduced by reducing energy consumption expenditure at the household level. To confirm this, (1) we investigate the relationship between energy consumption expenditure at the household level and life satisfaction, and (2) examine the effect of energy-saving behavior on reducing energy consumption expenditure at the household level, and find the following trends.
First, the evidence shows a positive association between energy consumption expenditure at the household level and subjective well-being; specifically, an increase in the former is expected to increase the likelihood of people being satisfied with their lives in 27 (out of 37) of the surveyed countries, including China and the United States. These results corroborate the evidence in prior research 32 , 33 , 35 , 41 , 42 , 43 , and that endeavoring to support the achievement of environmental conservation goals through reducing energy-related greenhouse gas emissions at the household level is likely to pose many challenges. Compared to the effect of energy consumption on satisfaction in high-income countries and non-high-income countries, individuals living in less urbanized countries appear more satisfied with energy consumption.
Second, (1) energy curtailment behaviors, (2) higher education, and (3) age show environmentally favorable effects on energy consumption expenditure at the household level, consistent with prior studies 29 , 30 , 31 , 32 , 33 , 34 , 35 , 36 , 37 , 38 , 39 , 40 . A policy implication of this finding is that encouraging energy-saving behaviors, reducing population, promoting higher educational attainment, and adopting frugal lifestyles (often related to older adults) may facilitate sustainable energy consumption and general well-being. However, the purchase of energy-saving products has a limited effect on energy consumption expenditure, and policymakers and corporations may need to make greater efforts in the research and development of energy-saving electronic products.
Globally, both energy prices and household income have been increasing in recent decades. Increasing energy prices are believed to reduce household energy consumption. However, increased household wealth allows households to consume more energy. In recent decades, owing to economic growth, a standard household can afford greater amounts of energy. Moreover, when household income increases, the household income spent on energy increases.
Based on previous studies on household energy consumption, energy is confirmed as a normal good 44 , 62 , 63 , 64 . Since energy is a normal good, an increase in energy consumption is more likely to lead to greater well-being. When household income increases, the share of normal goods (energy in this case) also increases in the overall household budget. If energy were an inferior good, then as household income increases, the expenditure on energy would decrease under the new optimized consumption choices. The results are consistent with previous studies showing that wealthy households consume more energy 65 , 66 , whereas prior studies investigated the link between economic growth and CO2 at the country level. Therefore, achieving a reduction in energy consumption, and simultaneously, carbon emissions, might be difficult under a household’s rational decision-making.
At the household level, energy-saving behavior and purchasing energy-saving products will reduce energy consumption expenditure. We found that either energy curtailment behavior or purchasing energy-saving electronic products will have a limited effect when household energy is mainly sourced from fossil fuels; relying on energy-saving goods will have a limited effect when pursuing the goal of carbon emission reduction. As the carbon neutral goal should be achieved until the 2050s, household energy sourced from renewable energy or other non-fossil fuels might be a good alternative to reduce the household sector’s carbon emissions. To help address the climate change issue caused by emissions at the household consumer level, various governments are enacting legislation to reduce such emissions.
However, according to this study’s results, when fossil fuels are the primary energy source, reducing greenhouse gas emissions by decreasing energy consumption at the household level might prove difficult. Fortunately, the renewable energy sector is developing rapidly. This study encourages stakeholders to seek alternatives, such as nuclear power or preferably renewable energy, to reduce greenhouse gas emissions at the household level.
The study has the following limitations. First, the sample selection approach could result in selection bias in the survey. We primarily relied on an internet survey approach, which might have skewed the sample toward wealthy and well-educated households. To address this issue, face-to-face surveys were conducted in Mongolia, Myanmar, Egypt, Kazakhstan, Sri Lanka, Indonesia, India, and Malaysia to confirm the robustness of the main results. However, sample selection bias might still exist. Future studies should use comprehensive datasets to investigate household energy consumption, well-being, and environmental sustainability. Second, as the information on energy prices is limited, households’ expenditure on energy is used to measure household energy consumption in each country. We assume that the greater the amount spent on energy, the greater the amount of energy consumed by households. This assumption could potentially cause bias in the results. Therefore, future studies should use comprehensive price information with accurate household energy consumption information.
Energy consumption is considered a major contributor to climate change due to CO2 emissions. Therefore, energy consumption at the household level has caught the attention of numerous researchers and policymakers. Our study raises two questions: how does energy consumption influence life satisfaction? How can energy consumption at the household level be reduced? By conducting a large-scale survey using both internet-based and face-to-face approaches across 37 nations on 6 continents (comprising approximately 73% of the world population), this study demonstrates the relationship between life satisfaction and energy consumption expenditure at the household level, as well as the determinants of energy consumption behavior in households.
Data availability
The datasets used and analyzed during the current study are available from the corresponding author on reasonable request. The questionnaire and data are available upon reasonable request to the authors.
Hamermesh, D. Economic aspects of job satisfaction in Essays of Labor Market Analysis (eds. Ashenfelter, O. & Oates, W.) (Wiley, 1977).
Diener, E., Oishi, S. & Tay, L. Advances in subjective well-being research. Nat. Hum. Behav. 2 , 253–260 (2018).
Article Google Scholar
Clark, W. A. V., Yi, D. & Huang, Y. Subjective well-being in China’s changing society. Proc. Natl. Acad. Sci. 116 , 16799–16804 (2019).
Article ADS CAS Google Scholar
IEA. World CO2 emissions from fuel combustion by fuel, 1971–2019 https://www.iea.org/data-and-statistics/charts/world-co2-emissions-from-fuel-combustion-by-fuel-1971-2019 (2021b).
UNFCCC. IEA coverage electric vehicle. The Paris Agreement https://unfccc.int/process-and-meetings/the-paris-agreement/the-paris-agreement (2022).
IEA. Policy coverage of total final energy consumption in buildings, 2000–2018 https://www.iea.org/data-and-statistics/charts/policy-coverage-of-total-final-energy-consumption-in-buildings-2000-2018 (2021a).
IEA. Global EV policy explorer https://www.iea.org/data-and-statistics/data-tools/global-ev-policy-explorer (2022).
Mazur, A. Does increasing energy or electricity consumption improve quality of life in industrial nations?. Energy Policy 39 , 2568–2572 (2011).
Niu, S. et al. Electricity consumption and human development level: A comparative analysis based on panel data for 50 countries. Int. J. Electr. Power Energy Syst. 53 , 338–347 (2013).
Ouedraogo, N. S. Energy consumption and human development: evidence from a panel cointegration and error correction model. Energy 63 , 28–41 (2013).
Jorgenson, A. K., Alekseyko, A. & Giedraitis, V. Energy consumption, human well-being and economic development in central and eastern European nations: A cautionary tale of sustainability. Energy Policy 66 , 419–427 (2014).
Schmitt, M. T., Aknin, L. B., Axsen, J. & Shwom, R. L. Unpacking the relationships between pro-environmental behavior, life satisfaction, and perceived ecological threat. Ecol. Econ. 143 , 130–140 (2018).
Chapman, A., Fujii, H. & Managi, S. Multinational life satisfaction, perceived inequality and energy affordability. Nat. Sustain. 2 , 508–514 (2019).
Soytas, U., Sari, R. & Ewing, B. T. Energy consumption, income, and carbon emissions in the United States. Ecol. Econ. 62 , 482–489 (2017).
Zhang, X. P. & Cheng, X. M. Energy consumption, carbon emissions, and economic growth in China. Ecol. Econ. 68 , 2706–2712 (2009).
Zhao, H. X. & Magoulès, F. A review on the prediction of building energy consumption. Renew. Sust. Energ. Rev. 16 , 3586–3592 (2012).
Andersson, D., Nässén, J., Larsson, J. & Holmberg, J. Greenhouse gas emissions and subjective well-being: an analysis of Swedish households. Ecol. Econ. 102 , 75–82 (2014).
Islam, M. & Managi, S. Green growth and pro-environmental behavior: sustainable resource management using natural capital accounting in India. Resour. Conserv. Recycl. 145 , 126–138 (2019).
Stern, P. C. New environmental theories: toward a coherent theory of environmentally significant behavior. J. Soc. Issues. 56 , 407–424 (2000).
Aizawa, H., Yoshida, H. & Sakai, S. Current results and future perspectives for Japanese recycling of home electrical appliances. Resour. Conserv. Recycl. 52 , 1399–1410. https://doi.org/10.1016/j.resconrec.2008.07.013 (2008).
Berardi, U. A cross-country comparison of the building energy consumptions and their trends. Resour. Conserv. Recycl. 123 , 230–241. https://doi.org/10.1016/j.resconrec.2016.03.014 (2017).
Byrne, S. & O’Regan, B. Attitudes and actions towards recycling behaviours in the Limerick, Ireland region. Resour. Conserv. Recycl. 87 , 89–96. https://doi.org/10.1016/j.resconrec.2014.03.001 (2014).
Eriksson, L., Garvill, J. & Nordlund, A. M. Acceptability of single and combined transport policy measures: The importance of environmental and policy specific beliefs. Transp. Res. Part A Policy Pract. 42 , 1117–1128 (2008).
Hansmann, R., Bernasconi, P., Smieszek, T., Loukopoulos, P. & Scholz, R. W. Justifications and self-organization as determinants of recycling behavior: The case of used batteries. Resour. Conserv. Recycl. 47 , 33–159. https://doi.org/10.1016/j.resconrec.2005.10.006 (2006).
Sweeney, J. C., Kresling, J., Webb, D., Soutar, G. N. & Mazzarol, T. Energy saving behaviours: Development of a practice-based model. Energy Policy 61 , 371–381 (2013).
Gardner, G. T. & Stern, P. C. Environmental Problems and Human Behavior (Pearson Custom Publishing, 2002).
Google Scholar
Klöckner, C. A. & Oppedal, I. O. General vs. Domain specific recycling behaviour—applying a multilevel comprehensive action determination model to recycling in Norwegian student homes. Resour. Conserv. Recycl. 55 , 463–471. https://doi.org/10.1016/j.resconrec.2010.12.009 (2011).
Diener, E. Subjective well-being. Psychol. Bull. 95 , 542–575 (1984).
Article CAS Google Scholar
Kahneman, D., Krueger, A. B., Schkade, D. A., Schwarz, N. & Stone, A. A. A survey method for characterizing daily life experience: The day reconstruction method. Science 306 , 1776–1780 (2004).
Biddle, S. & Mutrie, N. Psychology of Physical Activity: Determinants, Well-being and Interventions (Routledge, 2007).
Welsch, H., Binder, M. & Blankenberg, A-K. Green behavior, green self-image, and subjective well-being: Separating affective and cognitive relationships. Ecol. Econ. 179 , 106854 (2021) . doi: https://doi.org/10.1016/j.ecolecon.2020.106854
Brown, K. W. & Kasser, T. Are psychological and ecological well-being compatible? The role of values, mindfulness, and lifestyle. Soc. Indic. Res. 74 , 349–368 (2005).
Welsch, H. Happiness and green lifestyle in Handbook on Wellbeing, Happiness and the Environment (eds. Rehdanz, K., Maddison, D. & Welsch, H.) 449–366 (Elgar, 2020).
Binder, M., Blankenberg, A.-K. & Welsch, H. Pro-environmental norms and subjective well-being: Panel evidence from the UK. Soc. Ind. Res. https://doi.org/10.1007/s11205-020-02426-4 (2020).
Suárez-Varela, M., Guardiola, J. & González-Gómez, F. Do pro-environmental behaviors and awareness contribute to improve subjective well-being?. Appl. Res. Qual. Life. 11 , 429–444 (2016).
Kasser, T. Living both well and sustainably: A review of the literature, with some reflections on future research, interventions, and policy. Phil. Trans. R. Soc. A. https://doi.org/10.1098/rsta.2016.0369 (2017).
De Groot, J. I. M. & Steg, L. Mean or green: Which values can promote stable pro-environmental behavior?. Conserv. Lett. 2 , 61–66 (2009).
Binder, M., Blankenberg, A. K. & Guardiola, J. Does it have to be a sacrifice? Different notions of the good life, pro-environmental behavior and their heterogeneous impact on well-being. Ecol. Econ. 167 , 106448 (2020).
Li, D., Zhao, L., Ma, S., Shao, S. & Zhang, L. What influences an individual’s pro-environmental behavior? A literature review. Res. Cons. Recycl. 146 , 28–34 (2019).
Besagni, G. & Borgarello, M. The determinants of residential energy expenditure in Italy. Energy 165 , 369–386 (2018).
Steg, L., Bolderdijk, J. W., Keizer, K. & Perlaviciute, G. An integrated framework for encouraging pro-environmental behaviour: The role of values, situational factors and goals. J. Environ. Psychol. 38 , 104–115 (2014).
Binder, M., Blankenberg, A.-K. & Welsch, H. Pro-environmental norms and subjective well-being: Panel evidence from the UK. Soc. Indic. Res. https://doi.org/10.1007/s11205-020-02426-4.(inpress) (2020).
Deaton, A. & Muellbauer, J. Economics and Consumer Behavior (Cambridge University Press, 1980).
Deaton, A. & Muellbauer, J. An almost ideal demand system. Am. Econ. Rev. 70 , 312–326. https://doi.org/10.2307/1924200 (1980).
Cherchye, L., De Rock, B. & Vermeulen, F. Married with children: A collective labor supply model with detailed time use and intrahousehold expenditure information. Am. Econ. Rev. 102 , 3377–3405. https://doi.org/10.1257/aer.102.7.3377 (2012).
Du, Q. et al. The energy rebound effect of residential buildings: evidence from urban and rural areas in China. Energy Policy 153 , 112235. https://doi.org/10.1016/j.enpol.2021.112235 (2021).
Piao, X. Sweets or alcohol? The gender battle within Japanese families. Econ. Bull. 37 , 190–203 (2017).
Lindenberg, S. & Steg, L. Normative, gain and hedonic goal frames guiding environmental behavior. J. Soc. Issues 63 , 117–137 (2007).
Umit, R., Poortinga, W., Jokinen, P. & Pohjolainen, P. The role of income in energy efficiency and curtailment behaviours: Findings from 22 European countries. Energy Res. Soc. Sci. 53 , 206–214 (2019).
Trotta, G. Factors affecting energy-saving behaviours and energy efficiency investments in British households. Energy Policy 114 , 529–539 (2018).
Bastida, L., Cohen, J. J., Kollmann, A., Moya, A. & Reichl, J. Exploring the role of ICT on household behavioural energy efficiency to mitigate global warming. Renew. Sustain. Energy Rev. 103 , 455–462 (2019).
Lam, T. W. L. et al. The influences of emotional factors on householders’ decarbonizing cooling behaviour in a subtropical Metropolitan City: An application of the extended theory of planned behaviour. Sci. Total Environ. 807 , 150826 (2022).
Guo, Q., Wang, Y. & Dong, X. Effects of smart city construction on energy saving and CO2 emission reduction: Evidence from China. Appl. Energy 313 , 118879 (2022).
Pew Research Center (2015). Coverage in Internet Surveys, who web-only surveys miss and how that affects results. http://www.pewresearch.org/2015/09/22/coverage-error-in-internet-surveys/ . Accessed 25 December 2022.
OECD. Guidelines on Measuring Subjective Well-being (OECD Publishing, 2013).
Helliwell, J., Layard, R. & Sachs, J. World Happiness Report (Earth Institute, New York, 2012).
Kahneman, D. & Deaton, A. High income improves evaluation of life but not emotional well-being. Proc. Natl Acad. Sci. USA 107 , 16489–16493 (2010).
Piao, X., Ma, X., Tsurumi, T. & Managi, S. Social capital, negative event, life satisfaction and sustainable community: Evidence from 37 countries. Appl. Res. Qual. Life 17 , 1311–1330 (2022).
Wooldridge, J. Econometric Analysis of Cross Section and Panel Data 2nd edn. (The MIT Press, Cambridge, 2010).
MATH Google Scholar
Greene, W. H. Econometric Analysis 7th edn. (Pearson, New York, 2012).
Hayashi, F. Is the Japanese extended family altruistically linked - A test based on Engel curves. J. Polit. Econ. 103 , 661–674 (1995).
Browning, M., Chiappori, P.-A. & Lewbel, A. Estimating consumption economies of scale, adult equivalence scales, and household bargaining power. Rev. Econ. Stud. 80 , 1267–1303 (2013).
Article MATH Google Scholar
Piao, X. Marriage stability and private versus shared expenditures within families: Evidence from Japanese families. Soc. Indic. Res. 153 , 533–559 (2012).
Gorus, M. S. & Aydin, M. The relationship between energy consumption, economic growth, and CO2 emission in MENA countries: Causality analysis in the frequency domain. Energy 168 , 815–822 (2019).
Sulaiman, C. & Abdul-Rahim, A. S. The relationship between CO 2 emission, energy consumption and economic growth in Malaysia: A three-way linkage approach. Environ Sci Pollut Res 24 , 25204–25220. https://doi.org/10.1007/s11356-017-0092-1 (2017).
Download references
This research was supported by the Environment Research and Technology Development Fund (JPMEERF20201001) of the Environmental Restoration and Conservation Agency of Japan and JSPS KAKENHI Grant Number JP20H00648, and this Research was supported by Takahashi Industrial and Economic Research Foundation, Grant number J220000023.
Author information
Authors and affiliations.
Faculty of Humanities and Social Science, Iwate University, 3-18-34 Ueda, Morioka, Iwate, 020-8550, Japan
Xiangdan Piao
Urban Institute & School of Engineering, Kyushu University, 744 Motooka Nishi-Ku, Fukuoka, 819-0395, Japan
Shunsuke Managi
You can also search for this author in PubMed Google Scholar

Contributions
X.P. conducted the analysis, prepared the first draft of the manuscript, and participated in the revision of the manuscript. S.M. supervised the manuscript.
Corresponding author
Correspondence to Xiangdan Piao .
Ethics declarations
Competing interests.
The authors declare no competing interests.
Additional information
Publisher's note.
Springer Nature remains neutral with regard to jurisdictional claims in published maps and institutional affiliations.
Supplementary Information
Supplementary information., rights and permissions.
Open Access This article is licensed under a Creative Commons Attribution 4.0 International License, which permits use, sharing, adaptation, distribution and reproduction in any medium or format, as long as you give appropriate credit to the original author(s) and the source, provide a link to the Creative Commons licence, and indicate if changes were made. The images or other third party material in this article are included in the article's Creative Commons licence, unless indicated otherwise in a credit line to the material. If material is not included in the article's Creative Commons licence and your intended use is not permitted by statutory regulation or exceeds the permitted use, you will need to obtain permission directly from the copyright holder. To view a copy of this licence, visit http://creativecommons.org/licenses/by/4.0/ .
Reprints and permissions
About this article
Cite this article.
Piao, X., Managi, S. Household energy-saving behavior, its consumption, and life satisfaction in 37 countries. Sci Rep 13 , 1382 (2023). https://doi.org/10.1038/s41598-023-28368-8
Download citation
Received : 09 August 2022
Accepted : 17 January 2023
Published : 25 January 2023
DOI : https://doi.org/10.1038/s41598-023-28368-8
Share this article
Anyone you share the following link with will be able to read this content:
Sorry, a shareable link is not currently available for this article.
Provided by the Springer Nature SharedIt content-sharing initiative
By submitting a comment you agree to abide by our Terms and Community Guidelines . If you find something abusive or that does not comply with our terms or guidelines please flag it as inappropriate.
Quick links
- Explore articles by subject
- Guide to authors
- Editorial policies
Sign up for the Nature Briefing newsletter — what matters in science, free to your inbox daily.

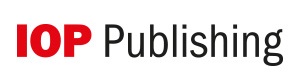
We apologize for the inconvenience...
To ensure we keep this website safe, please can you confirm you are a human by ticking the box below.
If you are unable to complete the above request please contact us using the below link, providing a screenshot of your experience.
https://ioppublishing.org/contacts/
Green building practices to integrate renewable energy in the construction sector: a review
- Review Article
- Open access
- Published: 15 December 2023
- Volume 22 , pages 751–784, ( 2024 )
Cite this article
You have full access to this open access article
- Lin Chen 1 , 2 ,
- Ying Hu 1 , 2 ,
- Ruiyi Wang 1 , 2 ,
- Xiang Li 1 , 2 ,
- Zhonghao Chen 3 ,
- Jianmin Hua 1 , 2 ,
- Ahmed I. Osman ORCID: orcid.org/0000-0003-2788-7839 4 ,
- Mohamed Farghali 5 , 6 ,
- Lepeng Huang 1 , 2 ,
- Jingjing Li 3 ,
- Liang Dong 7 , 8 , 9 ,
- David W. Rooney 4 &
- Pow-Seng Yap 3
6576 Accesses
3 Altmetric
Explore all metrics
The building sector is significantly contributing to climate change, pollution, and energy crises, thus requiring a rapid shift to more sustainable construction practices. Here, we review the emerging practices of integrating renewable energies in the construction sector, with a focus on energy types, policies, innovations, and perspectives. The energy sources include solar, wind, geothermal, and biomass fuels. Case studies in Seattle, USA, and Manama, Bahrain, are presented. Perspectives comprise self-sufficiency, microgrids, carbon neutrality, intelligent buildings, cost reduction, energy storage, policy support, and market recognition. Incorporating wind energy into buildings can fulfill about 15% of a building's energy requirements, while solar energy integration can elevate the renewable contribution to 83%. Financial incentives, such as a 30% subsidy for the adoption of renewable technologies, augment the appeal of these innovations.
Similar content being viewed by others
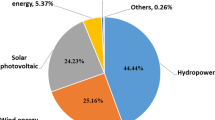
Cost, environmental impact, and resilience of renewable energy under a changing climate: a review
Ahmed I. Osman, Lin Chen, … Pow-Seng Yap
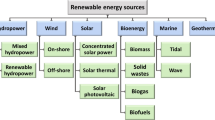
Social, environmental, and economic consequences of integrating renewable energies in the electricity sector: a review
Mohamed Farghali, Ahmed I. Osman, … David W. Rooney
Wind Power Generation
Avoid common mistakes on your manuscript.
Introduction
With the implementation of economic globalization and the expansion of economic regions, the global consumption of energy and resources is growing rapidly at an average annual rate of 2.2% (Chen et al. 2023a ; Salam et al. 2020 ). The construction industry, as the main sector of energy consumption, accounts for 36% of the total global energy consumption (Chen et al. 2022a ). The rapid growth of the global population will require more urban building capacity in the next 40 years than in the past 4000 years (Chen et al. 2022b ; Gottlieb et al. 2023 ), but traditional buildings rely heavily on coal, oil, natural gas, and other non-renewable energy sources, and excessive energy use causes energy depletion and high pollution. Environmental instability, such as the greenhouse effect and extreme weather caused by energy, have aroused widespread concern for green, low-carbon, sustainable, and other renewable energy. At the same time, international energy deployment has set a goal of near net-zero emissions by 2050, as the construction industry is under intense pressure from energy scarcity and fossil fuel depletion (Zhang et al. 2022 ). Europe and the USA have redefined regulations and policies related to the development of near-zero-energy buildings for the development of renewable energy (Liu and Rodriguez 2021 ; Yang et al. 2022b ), and China also committed to the international government's "dual-carbon" goal of reaching peak carbon by 2030 and carbon neutrality by 2060 (Osman et al. 2023 ; Paris Agreement 2015 ). The application of renewable energy in buildings has, therefore, become a major driver of the energy transition in conventional buildings and an important cornerstone of urban planning and development strategies to reduce the contribution of the building sector to climate change and energy use.
Renewable energy, as an innovative alternative energy, plays a leading role in getting rid of fossil fuel dependence and mitigating climate change. It is used to reduce greenhouse gas emissions stemming from energy consumption during construction projects (Ghaffarian Hoseini et al. 2013 ; Yang et al. 2022b ). This approach aims to create environmentally friendly, energy-efficient, and sustainable buildings. Moreover, it stands as a pivotal contributor to the evolving global energy landscape as governments worldwide commit to addressing climate change and advancing sustainable development goals. Renewable energy was first used in the European Union, where the main objective was to reduce greenhouse gas emissions, thus improving energy efficiency (Yang et al. 2022b ). In China, the initial application of renewable energy in building construction encompassed solar, wind, geothermal, and other sources. As technology in this field continues to mature, it plays a pivotal role in fostering the growth of a sustainable energy ecosystem. This is achieved by assessing how various technologies impact the enhancement of performance efficiency and the regulation of overall energy consumption levels (Zhang et al. 2015 ). Renewable energy is progressively becoming the energy strategy for numerous countries; for instance, the USA is investigating the economic feasibility of incorporating solar and geothermal technologies into heat pump systems (Kim and Junghans 2023 ), while Poland is employing wind and photovoltaic sources to facilitate its energy transition (Igliński et al. 2022 ). Therefore, the development and utilization of renewable energy plays a key role in building energy efficiency and emission reduction and promotes the sustainable development of buildings in the energy sector and even globally through existing natural resources and techno-economic measures.
This review systematically analyzes the current status and potential of renewable energy applications in the building sector. The review highlights the advantages of renewable energy applications in the building sector, such as solar, geothermal, wind, and biomass, as well as the challenges of technological innovation and development. It also provides examples of buildings in the construction sector that have successfully used renewable energy, describes the types of renewable energy used and the socio-economic benefits derived from their use, and analyzes the challenges and lessons learned during implementation. In addition, this review provides an in-depth look at the global policy and regulatory framework for renewable energy in buildings, considering the impact of policy on renewable energy adoption and, from there, analyzing the opportunities and barriers to policy implementation. The paper further explores the latest technological advancements like machine learning and Internet of Things technologies in renewable energy within the building sector and systematically evaluates their potential impact on renewable energy utilization for sustainable cities. Finally, the review concludes by discussing the prospects of renewable energy in the building sector, examining both the potential and challenges involved in promoting its widespread adoption.
Overview of renewable energies in the building sector
Renewable energy derived from natural resources, is less harmful to the environment than fossil fuels and serves as an alternative to traditional energy sources (Dey et al. 2022 ). Renewable energy in buildings refers to the integration of sustainable energy sources, such as solar, wind, geothermal, and biomass, into the full building life cycle of design, construction, operation, and maintenance to reduce dependence on fossil fuels and traditional energy sources, promoting environmental sustainability and mitigating climate change. The roots of renewable energy in architecture can be traced back to early experiments in passive solar design, maximizing the use of sunlight for heating and natural ventilation to design the orientation of buildings (Gong et al. 2022 ; Ionescu et al. 2015 ). With the increasing awareness of environmental protection, the application technology of renewable energy in modern buildings has also gained momentum for innovative development.
The application of renewable energy in buildings depends mainly on the characteristics of the energy required for the building and the type of different energy sources. Among the existing renewable energy sources, solar, wind, hydro, tidal, geothermal, biomass, and hydrogen are widely recognized as key and mature technologies in the renewable energy sector. However, solar, wind, geothermal, and biomass energy have a greater potential to fulfill the energy needs of buildings (Khan and Al-Ghamdi 2021 ; Wu and Skye 2021 ), as shown in Fig. 1 .
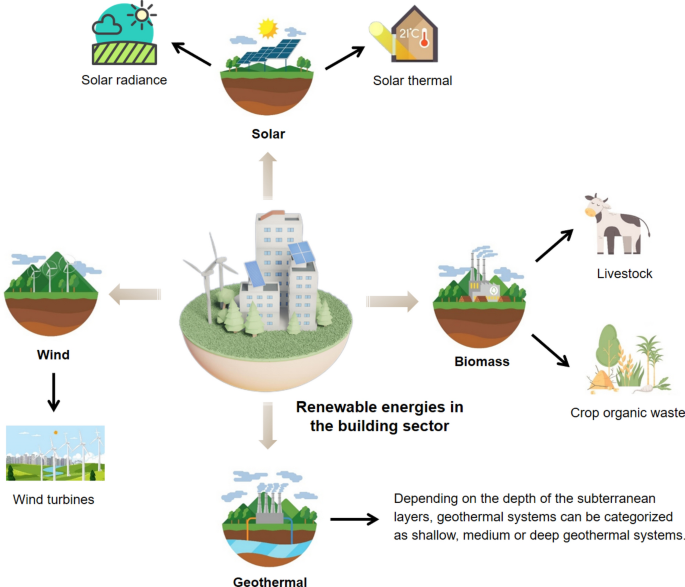
Types and sources of renewable energy in the building sector. Advancing the use of renewable energy within buildings is crucial for combatting climate change. The figure presented visually categorizes the types of renewable energy prevalent in the building sector. The dominant forms include solar energy, wind energy, geothermal energy, and biomass energy. Gaining a comprehensive understanding of these energy sources is pivotal. By integrating renewable installations with existing infrastructure and aligning them with energy demand patterns and environmental considerations, we can optimize overall efficiency
Solar energy
Solar energy stands as the most accessible and commonly adopted form of renewable energy, achieved by capturing the ionization of the sun's radiant energy. It is acclaimed for its limitless supply and eco-friendly attributes, positioning it as the leading-edge renewable energy technology poised to replace fossil fuels. Aldhshan et al. ( 2021 ) defined solar energy as one of the sustainable energy sources for generating electricity using photovoltaic systems. Building solar energy technology, the main source of energy from solar radiation and thermal energy in two aspects, photovoltaic technology and solar thermal energy for the integrated application of buildings is currently commonly used renewable energy technologies, and from the building characterization of the form can be divided into active solar energy and passive solar energy. Photovoltaic systems, solar power generation, and solar hot water are the main components of active solar systems, while building orientation, air circulation, and thermal biomass together constitute passive solar systems (Dey et al. 2022 ). Wu and Skye ( 2021 ) conducted statistics on the total amount of renewable energy utilized in residential buildings in the USA, where solar energy accounted for 31% of the total energy consumption.
The use of photovoltaic technology is critical to reducing building operating costs. Researchers use Geographic Information Systems to model photovoltaic systems and explore the economic and environmental benefits, showing that photovoltaic systems on rooftops could save the government an estimated $202 billion in costs while dramatically improving environmental performance (Asif et al. 2019 ). At the same time, solar distributed integration technology can meet the functional requirements of different components of the building according to specific requirements. The collected solar technology is applied to building windows to control the increase and decrease of building solar heat and thermal insulation, and the integrated application of distributed energy in building components greatly improves the comfort of occupants and climate energy-saving control (Vasiliev et al. 2019 ).
Improving the environmental performance of buildings and facilitating climate circulation is one of the characteristics of solar technology as a renewable energy source. For instance, Vassiliades et al. ( 2022 ) observed that active solar building integration systems can change the characteristics of buildings and reduce the negative climate impacts of building public spaces. In addition, the integrated application of solar energy and photovoltaic technology has a greater advantage in improving energy utilization efficiency and reducing energy demand. Research in Italy describes how a photovoltaic thermal solar-assisted heat pump system integrated with a photovoltaic thermal collector and a vapor-compression heat pump can be used to meet all of a building's thermal needs, increasing the efficiency of solar energy exploitation while reducing the consumption of ground-source heat pumps (Miglioli et al. 2023 ). Another study analyzed the capabilities of the solar cooling system by developing a dynamic calculation model compared to conventional systems, and the calculation results showed that the solar system can increase the renewable energy factor to 83% and reduce energy demand by 48% (Bilardo et al. 2020 ).
Building structures and designs need to integrate the use of solar energy resources to maximize the use of solar energy, which is often overlooked in many existing buildings. The building-integrated photovoltaic thermal systems can meet the electrical and thermal energy requirements of a building's domestic use, but the inconsistent supply of solar energy makes it very difficult to integrate building-integrated photovoltaic thermal air collectors into the building structure, and the system design is strongly influenced by the structural load-bearing capacity of the building (Şirin et al. 2023 ). Building-integrated photovoltaics play an important role in promoting the design and implementation of zero-energy buildings, but economic and technical obstacles need to be overcome. Regular maintenance and replacement of photovoltaic system components and auxiliary equipment are issues that designers focus on. The resulting economic cost directly affects its policies and the proprietor's willingness to support (Maghrabie et al. 2021 ).
In conclusion, solar energy offers significant benefits for buildings by reducing operating costs, enhancing the functionality of building components, improving energy use efficiency, and diminishing energy demand. Nonetheless, challenges persist, including the need for technological advancements, high maintenance and renewal costs, and the intricacies of structural design tailored for building applications. Therefore, integrated consideration of the utilization of solar energy resources is essential to maximize their potential.
Wind energy
With the development of offshore wind energy, the application of wind energy in the building sector is gradually becoming widespread and is considered to be one of the most commercially promising renewable energy sources (Zhang et al. 2023 ). The wind energy system consists of wind turbines, which work on the principle of converting kinetic energy into electrical energy, mechanical energy, and other required energy using wind vortex machines. Similar to solar, wind systems can be categorized into active and passive systems based on the size of the turbine, with the biggest difference between the two being the type of power drive, with the active rotating with the motor and the passive rotating with the wind direction (Palraj and Rajamanickam 2020 ). Wind power generation using wind energy, development of natural ventilation systems, wind energy testing, and wind impedance design are now common technologies for renewable energy applications in buildings (Deymi-Dashtebayaz et al. 2022 ; Peng et al. 2020b ).
The most direct impact of wind power generation is to reduce carbon emissions and consumption of non-renewable energy. According to research statistics, as of 2017, the use of wind energy resources has avoided at least 600 million tons of greenhouse gas emissions (Yousefi et al. 2019 ). The integration of wind energy systems in buildings generates renewable energy on the construction site, which can provide around 15% of the building's energy needs (Kwok and Hu 2023 ). The building design adopts a natural ventilation system to achieve the effect of indoor and outdoor air circulation through natural wind power, which can reduce the degree of dependence on air conditioning and, to a certain extent, reduce energy consumption. In this context, Wang et al. ( 2021a ) developed an innovative energy-efficient turbine damper ventilator, which reduces unwanted exhaust airflow to provide a comfortable indoor environment. Also, it can stabilize the air exchange in the building to meet minimum air quality standards.
Compared with conventional energy sources, wind energy generation equipment requires a significant investment in manufacturing and installation, including maintenance costs at a later stage. The electricity converted by the wind turbine and then supplied by the heat pump was simulated using the Energy PLAN software, and the results exhibited that the total energy cost increases by 653.2% under this scenario (Noorollahi et al. 2021 ). Noise generated by the operation of wind turbines is a concern for nearby residents, which originates from the mutual collision of turbine components and noise generated by air vibration (Zhang et al. 2023 ). In urban environments, the height and density between buildings limit the utilization of wind energy. The wind speed and direction between elevated buildings may be affected by blocking and turbulence, reducing the efficiency of wind power generation (Kwok and Hu 2023 ). Also, due to the uncontrollable and uncertain characteristics of wind, wind power generation is intermittent, which also seriously affects the efficiency of energy use (Roga et al. 2022 ).
In conclusion, while wind energy substantially contributes to emission reductions and meets the energy demands of buildings, it comes with higher upfront costs. Its efficiency is heavily dependent on natural wind speeds and is significantly influenced by the building's layout. Therefore, there is an urgent need to explore smart or other new technologies to improve the efficiency of wind energy use.
Geothermal energy
Geothermal energy is derived from the Earth's internal heat (Osman et al. 2023 ). The constant heat flow within the Earth contributes to the storage of internal heat, while rainfall within the Earth's crust plays a crucial role in completing the water cycle (Palmero-Marrero et al. 2020 ). Therefore, geothermal energy is a non-intermittent renewable energy source that is not dependent on climate or time of day and can supply energy 24 hours a day independently of external conditions. In terms of usage, solar and wind energy are more used for power generation, while geothermal energy is mainly used for heat production and cooling. In addition, it can work in conjunction with other energy systems, such as solar energy, to add effective for improving industrial competitiveness and positively impact job creation and economic development in the medium to long term. Depending on the depth of the subterranean layers, they can be categorized as shallow, intermediate and deep geothermal systems, but there is no specific universal definition or classification (Romanov and Leiss 2022b ). Geothermal technology development can be utilized for power generation, direct use, and heat extraction through shallow ground-source heat pumps.
Compared to conventional heating and cooling systems, geothermal energy systems can improve energy efficiency while significantly reducing energy costs and greenhouse gas emissions. D'Agostino et al. ( 2022b ) conducted simulation modeling based on Energy Plus software to systematically analyze the energy retrofitting of existing buildings with two types of low-hale geothermal systems: ground-source heat pumps and geo-aerothermal heat exchangers. The scholar observed that the use of these systems significantly reduces primary energy demand, energy costs, and CO 2 emissions compared to conventional gas boilers, demonstrating their effectiveness in achieving the goal of net-zero-energy buildings. Geothermal systems operate quietly without the noise of traditional heating, ventilation, and air conditioning systems, improving building operating comfort and health (Shah et al. 2022 ). At the same time, geothermal systems require a relatively small land area, making them suitable for urban environments where space is limited. Studies have estimated the land use intensity of geothermal power plants in buildings to be 7.5 m 2 per MW per year, which is much smaller than other energy technologies (Tester et al. 2021 ). In addition, geothermal systems can be integrated with a variety of architectural styles and provide design flexibility for buildings by utilizing different sizes and configurations of ground-source heat pumps to meet specific heating and cooling needs based on building size, load requirements, and space availability.
Despite the low operating costs of geothermal energy technologies, the need to drill holes and install underground components results in high installation costs and significant upfront investment costs (Hu et al. 2021 ; Lizana et al. 2018 ). The geological characteristics of the building project site largely determine the success or failure of a geothermal system (Chen and Feng 2020 ). Therefore, an accurate assessment of subsurface conditions is essential to determine the feasibility and potential output of a geothermal system, and uncertainties in geology can increase the risk of drilling failures and lead to additional costs. The application of geothermal energy is site-specific, and locations with sufficient thermal potential have become challenging. Studies have calculated subsurface thermal storage capacities of 8,300–16,600 GJ to satisfy winter heating in buildings using finite element methods (Chen and Feng 2020 ). In addition, the establishment and operation of geothermal systems have potential impacts on the environment. The noise generated by drilling construction and the treatment of geothermal fluids are all challenges faced by geothermal technology.
In summary, geothermal energy offers substantial improvements in energy efficiency, reductions in energy costs, and decreases in greenhouse gas emissions. Its design flexibility for integration with buildings presents a solution for energy transitions in space-limited urban structures. However, its high installation costs and the necessity for thorough evaluations of geological conditions and environmental impacts remain challenges.
Biomass energy
Biomass derived from organic materials extracted from living or sentient organisms such as animals, plants, or microorganisms can be combusted through aerobic and anaerobic digestion to produce energy and is by far the longest renewable energy source used by humans (Yang et al. 2022a ). Biomass in the building sector is usually utilized in the form of biomaterials in structural or non-structural parts of buildings to reduce dependence on fossil fuels and lower emissions. In general, biomass energy predominantly relies on resources like wood, agroforestry residues, plant fibers, as well as various organic waste materials, encompassing human, animal, and plant wastes. Biogas and direct combustion techniques primarily find application in the context of building energy needs. Additionally, biomass, including materials such as construction waste and animal excreta, can be utilized to generate electricity through dedicated power plants (Khan and Al-Ghamdi 2021 ). For example, Rahman et al. ( 2015 ) investigated biomass energy by studying the peak load of a biomass-powered 115 kW power plant, which can meet the power demand of an entire residential building. Thus, biomass can be utilized in buildings in several areas, such as biomass gas, biomass fuel, biomass heat, and biomass power generation (Allouhi et al. 2021 ; Furubayashi and Nakata 2021 ; Wu and Skye 2021 ).
Biomass plays a vital role in advancing the objectives of the Europe 2020 climate and energy strategy (Farghali et al. 2023b ). This involves initiatives like replacing conventional boilers with more efficient models and emphasizing renewable energy sources. For example, Las-Heras-Casas et al. ( 2018 ) investigated the possibility of substituting central fossil fuel boilers with biomass alternatives across diverse climatic zones in the peninsular region during winter. Their findings indicated the potential for significant reductions in non-renewable energy consumption (up to 93%) and substantial decreases in carbon dioxide emissions (up to 94%). Biomass offers a low-carbon footprint, given its carbon–neutral nature (Wang et al. 2018 ). Comparatively, using wood chips and pellets as fuel for biomass boilers instead of diesel resulted in substantial greenhouse gas reductions of 40,000 tons of carbon dioxide over 30 years for 54,241 households (Rafique and Williams 2021 ). Biomass boilers are well-known for their heating efficiency. Solid biomass fuels with calorific values between 14 and 23 MJ/kg exhibit high combustion efficiencies, with peak mass collection efficiencies of around 98% for wood chip pellets powered by 50 kW boilers, though overall collection efficiencies typically range from 70% to 90% (Baumgarten et al. 2022 ; Wang et al. 2017 ). The efficiency is further enhanced when biomass wood is compressed into pellets under high pressure and temperature (Hartmann and Lenz 2019 ). However, it is important to note that biomass combustion can lead to corrosion on heating surfaces due to boiler deposits (Chen et al. 2021b ). Furthermore, Pognant et al. ( 2018 ) highlighted that woodchip boilers may not be as environmentally friendly as natural gas boilers on a local scale. Nonetheless, woodchip boilers do improve local air quality and significantly reduce local ground-level particulate matter concentrations.
Biomass has a high calorific value of chemical energy and can be used directly in technologies such as combustion to generate electricity or in the production of biofuels. These biofuels can be burned to produce high-quality and high-temperature heat applications for heating buildings (He et al. 2019 ; Khan and Al-Ghamdi 2021 ). In addition to energy applications, biomass itself can also be used as a construction material, providing an environmentally friendly alternative to building components such as structural elements or thermal insulation. Studies have observed that the use of phase change materials made of biomass-derived porous carbon in buildings has a positive impact in terms of improved building thermal performance and building energy efficiency (Jiang et al. 2022b ). Furthermore, the integration of biomass energy into the construction sector can diversify energy supply chains and enhance energy security by reducing dependence on imported fossil fuels. Smart building energy efficiency systems that mix solar photovoltaic thermal panels and biomass heaters improve energy reliability while meeting building energy efficiency, and the availability of biomass energy throughout the year makes the biomass heaters in the system promote energy security (Behzadi et al. 2023 ).
As biomass is mainly derived from cultivated products, wood, or other wastes, extensive extraction for energy can exacerbate the destruction of vegetation, and biomass materials often compete with other land uses such as agriculture and forestry for resources such as land and water, balancing competing demands is essential for sustainable biomass extraction (Bungau et al. 2022 ; Yana et al. 2022 ). Some biomass materials may be less durable and less resistant to environmental factors such as moisture, pests, and fire than traditional building materials, and ensuring the long-term performance and longevity of biomass-based systems may require additional treatment and protection measures (Liuzzi et al. 2020 ). In addition, biomass materials have limited availability and may vary in quality and performance depending on factors such as seasonal cycles, climatic conditions, and regional differences (Hiloidhari et al. 2023 ). Therefore, ensuring a continuous and reliable supply of biomass materials may be a challenge for large-scale construction projects.
In summary, biomass holds a pivotal position in Europe's climate and energy strategy. It notably curtails non-renewable energy consumption and diminishes greenhouse gas emissions. Nonetheless, to harness biomass sustainably in extensive construction projects, challenges like resource competition, material durability, and supply reliability must be tackled.
This section delves into the pros and cons of four renewable energy types for building applications. Renewable energies leverage the inherent benefits of natural resources, curbing our reliance on fossil fuels to elevate energy efficiency and cut down greenhouse gas emissions. Yet, they are often marred by substantial upfront infrastructure costs and hurdles in technological advancement and innovation.
Case studies of renewable energy use in the building sector
In order to further concretize the above viewpoint and more intuitively demonstrate the application of renewable energy in building practice, the following section will conduct in-depth case studies through two cases: the Bullitt Center and Bahrain World Trade Center. We will elaborate on the successful implementation of renewable energy in these buildings, analyze the benefits of using these energy sources, as well as the challenges and lessons learned during the implementation process. These two cases provide us with valuable insights on how to promote renewable energy more widely in the construction field.
The Bullitt Center, Seattle, USA
Completed in 2013, the Bullitt Center, situated in Seattle, Washington, goes beyond conventional boundaries in energy efficiency, environmental responsibility, and occupant comfort. It serves as a prominent model of sustainable architecture, showcasing the seamless integration of renewable energy. This groundbreaking commercial structure not only redefines eco-friendly buildings but also establishes fresh benchmarks for energy efficiency, earning global recognition as one of the greenest edifices worldwide.
The core of the sustainable development of the Bullitt Center is the reliance on solar panels as the main source of renewable energy. The roof of the building is decorated with many photovoltaic solar panels, which can capture sufficient sunlight in the Pacific Northwest and convert sunlight into clean, renewable electricity. Solar power generation is the core of the building's net-zero-energy goal, and the building also has renewable technologies such as rainwater collection, composting toilets, and ground-source heat pumps. Based on the work of multiple authors in this field, we can further emphasize the advantages of the Brett Center in renewable energy integration, energy efficiency, and sustainability.
The Bullitt Center has attained net-zero-energy status, a milestone emphasized by Caballero et al. ( 2023 ), who highlight the crucial role of photovoltaic systems in this achievement. The extensive array of solar panels installed at the Bullitt Center ensures that it generates more energy than it consumes, thus solidifying its net-zero-energy building designation. This is consistent with the global shift toward sustainable building practices driven by high electricity costs and renewable energy availability. Energy self-sufficiency can be achieved. On-site photovoltaic systems, such as those on the roof of the Bullitt Center, are crucial for energy self-sufficiency in urban areas with limited rooftop space (D'Agostino et al. 2022a ). Some researches highlight the importance of combining solar power generation with improved insulation during roof renovation (D'Agostino et al. 2022c ). The Bullitt Center utilizes rooftop installation of solar panels to ensure that a significant portion of its energy demand comes from on-site renewable energy, maximizing energy efficiency and renewable energy utilization. This intervention greatly reduces costs. In addition, it adopts an energy-saving design, which maximizes the use of natural lighting in the building and reduces the need for artificial lighting during the day. Efficient heating and cooling systems, as well as advanced insulation technology, ensure that the energy consumption of buildings remains extremely low, ultimately achieving energy self-sufficiency.
By collecting rainwater, the Bullitt Center has reduced its dependence on traditional water sources, saving water and energy required for water treatment and distribution. The framework of the rainwater harvesting system is shown in Fig. 2 , as demonstrated by the research of (Ali and Sang 2023 ); the rainwater harvesting system effectively addresses the water and energy shortages of sustainable urban development. If designed properly, rainwater harvesting is economically feasible (Almeida et al. 2021 ). At the same time, the performance of rainwater collection system is influenced by climate zones (Ali and Sang 2023 ), and the Bullitt Center experiences a mild marine climate and regular rainfall, which is conducive to the functioning of rainwater collection systems. To further reduce water waste, the Bullitt Center has adopted composting toilets. These innovative devices reduce water consumption and wastewater treatment requirements, helping to reduce the overall environmental impact of buildings.
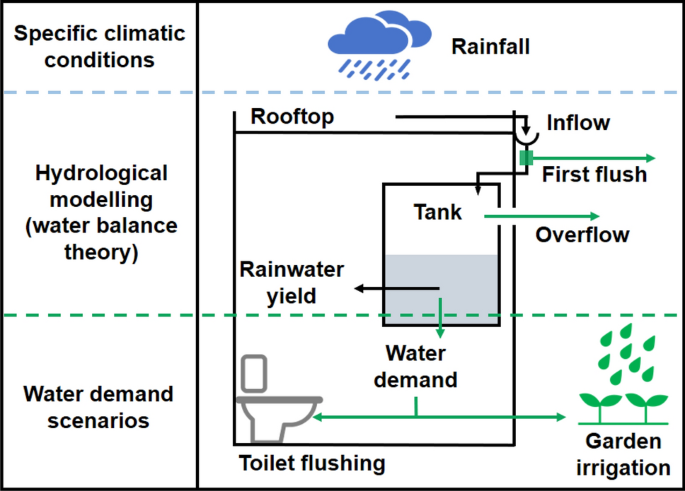
The framework of the rainwater collection system. This figure illustrates the operational mechanism of the rainwater harvesting system. The system captures rainwater via the roof, directs it through designated pipelines to a storage tank, and subsequently distributes it for toilet flushing and garden irrigation based on water requirements
The rainwater collection system at the Bullitt Center not only saves water but also helps reduce the risk of urban flooding. The research by Hdeib and Aouad ( 2023 ) suggested that rainwater harvesting systems can alleviate urban floods. Although their research focuses on arid areas, these principles apply to various climates. Effectively managing rainwater can help enhance its ability to withstand extreme weather events. In addition, the Bullitt Center also has certain economic benefits. Megahed and Radwan ( 2020 ) pointed out the economic advantages of using solar energy, including generating potential revenue by selling surplus energy to the grid. The model proposed by Ye et al. ( 2023 ) indicated that photovoltaic panel rainwater harvesting systems can allocate resources more effectively, increasing revenue while saving water and energy. This method is in line with the spirit of resource optimization and economic efficiency of the Bullitt Center.
Although these technologies bring many benefits to sustainability and energy efficiency, they also pose some challenges during implementation. In the case of the Bullitt Center, we examine the hurdles associated with various renewable technologies. Deploying multiple renewable energy sources frequently demands navigating intricate and continuously evolving regulatory frameworks. The Bullitt Center's commitment to renewable energy, such as solar panels, geothermal heating, and refrigeration systems, requires compliance with various federal, state, and local regulations related to renewable energy generation, grid interconnection, and building codes. Ensuring compliance with these regulations while breaking the boundaries of sustainable design is a daunting challenge. In addition, integrating various renewable energy sources into a building presents challenges related to system compatibility and coordination (Canale et al. 2021 ). Coordinating the operation of these systems, optimizing their performance, and ensuring their harmonious collaboration require high-level technical expertise and skilled technical personnel. Ensuring that the Bullitt Center has access to the necessary professional knowledge and resources is crucial for the success of these technologies.
The initial capital expenditures linked to the installation of multiple renewable technologies can be substantial. While these investments often result in long-term energy and water savings, securing the necessary upfront funding can be a hurdle, particularly for projects with limited budgets. Furthermore, all renewable technologies entail ongoing maintenance obligations to guarantee their sustained reliability and performance. Solar panels require regular cleaning and occasional maintenance, while geothermal systems require continuous monitoring. The continuous maintenance and monitoring of rainwater collection systems are crucial for preventing issues such as blockage, algae growth, or bacterial contamination (Clark et al. 2019 ), and key maintenance is needed to ensure water quality and system efficiency. Effectively managing these maintenance tasks and resolving unexpected failures can be resource-intensive and challenging.
The success of the Bullitt Center highlights the importance of adopting a holistic approach to sustainability, which focuses not only on energy efficiency but also on water conservation, material selection, and overall environmental impact. At the same time, the design and planning phase of the Bullitt Center is very detailed, with architects, engineers, and sustainable development experts involved. They need to make cautious, data-driven design decisions that consider local climate, energy, and resource availability. In addition, it is necessary to advocate and collaborate with local authorities to adjust building codes and regulations to adapt to innovative sustainability characteristics. Engaging with policy makers and regulatory agencies can promote the implementation of advanced green building practices. The Bullitt Center's success can be a model for similar projects in different regions and climates. Future endeavors should consider how lessons from the Bullitt Center can be adapted to their unique contexts.
This section delves into the Bullitt Center's accomplishments and challenges related to sustainability. The Bullitt Center achieved net-zero-energy status by leveraging photovoltaic systems, optimizing the use of solar energy and natural light. Furthermore, their efficient rainwater harvesting methods diminish reliance on conventional water sources, while also mitigating urban flooding. These sustainable practices not only benefit the environment but also present potential economic advantages, including the possibility of profit from excess energy. However, the adoption of such innovative technologies is not without its difficulties. Challenges include compliance with regulatory standards, harmonizing multiple renewable energy sources, and navigating both initial investment and recurring expenses. The Bullitt Center's achievements highlight the merit of a holistic approach to sustainability, considering local climatic conditions, available resources, and regulatory frameworks. Their success could pave the way for similar sustainable projects in future.
Bahrain World Trade Center, Manama, Bahrain
Bahrain is located in the southwest of the Persian Gulf, between Qatar and Saudi Arabia, with a tropical desert climate and hot and humid summers. The Bahrain World Trade Center is located on the Persian Gulf coast of the capital city of Manama, costs $96 million with a total construction area of 12,096 m 2 , and consists of two identical towers, each with a height of over 240 m and a total of 50 floors. Designers have set up a 75-ton bridge at the 16th, 25th, and 35th floors between the two towers and fix three horizontal axis wind turbines with a diameter of 29 m and their connected generators on these three bridges.
Due to the advantage of geographical location, the potential of wind energy resources has been explored. The study conducted by Adnan et al. ( 2021 ) in Pakistan emphasizes the importance of accurately evaluating wind energy resources to ensure efficient utilization of wind energy potential. This analysis includes average wind speed, Weibull parameters, as well as power and energy density, which helps determine suitable locations for wind energy production. In addition, reasonable building layout, height, and corner shape can also improve wind density and utilize urban wind energy (Juan et al. 2022 ). As shown in Fig. 3 , the wind power generation diagram of the Bahrain World Trade Center is located in a coastal urban area. By designing the building as a sail, sea wind convection is formed between the buildings, accelerating the wind speed, and making wind turbine power generation possible. In addition, batteries can be discharged in the event of insufficient wind power, assisting and stabilizing users' electricity usage by setting up to store excess electrical energy.
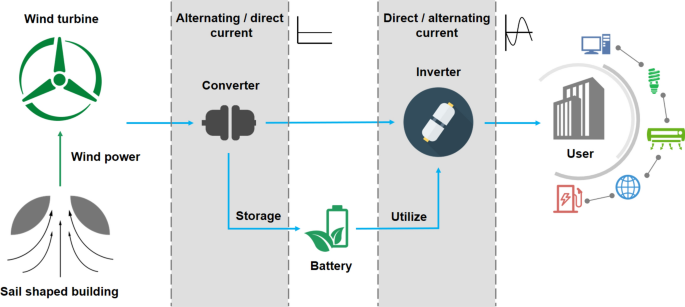
Schematic diagram of wind power generation for Bahrain World Trade Center. Incorporating an innovative design, the structure resembles a sail, strategically positioned to harness the prevailing sea winds. This unique design promotes wind convection between the buildings, channeling the flow efficiently. Positioned at an optimal height within the structure, a wind turbine captures this enhanced airflow, converting the kinetic energy of the wind into electrical power. This generated direct current is then processed through a converter, facilitating its efficient storage and transmission. In the final step, an inverter transforms the stored direct current back into alternating current to meet the building's electrical needs. This seamless integration of architecture and renewable energy technology not only serves the building's power requirements but also stands as a testament to sustainable and forward-thinking design
The Bahrain World Trade Center was a pioneer in incorporating wind power into its architectural design but faced several major challenges during its implementation. These challenges not only include technical and engineering aspects but also affect the functionality and overall sustainability of the building. The efficiency of wind turbines needs to be considered in urban environments characterized by turbulent wind patterns. Traditional wind farms are usually located in open areas with consistent wind flow to ensure optimal energy generation. In contrast, urban environments have complex wind patterns due to the presence of high-rise buildings and structures that disrupt and redirect airflow. To address this challenge, computational fluid dynamics simulations and wind tunnel tests can be used to evaluate the wind direction around buildings (Arteaga-López et al. 2019 ). These results can provide a basis for the design and layout of turbines, maximizing their exposure to the mainstream wind direction while reducing the impact of turbulence.
Maintaining wind turbines at considerable heights in coastal environments is a unique challenge. In this case, the standard maintenance procedures for ground turbines are not sufficient. Professional equipment, such as cranes or elevators, that can reach extreme heights is crucial for turbine maintenance. In addition, daily inspections and repairs need to consider harsh coastal environments, including exposure to salt water and humidity, which may accelerate wear and corrosion (Mourad et al. 2023 ). Developing maintenance plans for Bahrain World Trade Center turbines is crucial for their long-term reliability.
Managing the noise and vibration generated by wind turbines is crucial for creating a favorable working environment for the occupants of buildings. Excessive noise and vibration may disrupt office space, affect attention, and reduce the overall comfort of occupants (Karasmanaki 2022 ). Innovative solutions, such as sound barriers or damping mechanisms, may be incorporated into turbine design to mitigate these impacts. In addition, the layout and insulation of the building may have been optimized to reduce the spread of noise and vibration into the internal space.
Incorporating the relevant research findings into the context of the Bahrain World Trade Center, we can find that the wind turbine integration of the Bahrain World Trade Center is not only a symbol of architectural originality but also a practical model for sustainable urban development: by strategically placing wind turbines between the twin towers, Bahrain World Trade Center effectively utilizes wind energy, reduces dependence on traditional energy, and contributes to environmental sustainability. In addition, by incorporating wind energy, the Bahrain World Trade Center aligns with the broader concept of hybrid renewable systems and demonstrates how multiple renewable energy sources work together to improve energy efficiency. As emphasized by the research institute, the Bahrain World Trade Center reflects the importance attached to the utilization of wind energy resources and the assessment of wind energy potential in urban environments.
This section highlights the Bahrain World Trade Center's innovative approach to harnessing wind energy, using its unique sail-inspired design to optimize sea wind convection and turbine output. While integrated batteries ensure power during low-wind situations, challenges arise from urban wind dynamics, demanding sophisticated placement strategies. Additionally, maintaining turbines in coastal heights brings its own set of challenges, and addressing noise and vibration is crucial for ensuring comfort within the building.
- Policy and regulatory framework
Renewable energy policies and regulatory frameworks in the building sector
As a key sector of national economic growth, the construction industry has played an indispensable role in promoting China's urbanization process, but it has also had an irreversible impact on the global environment, the most direct impact on human survival being the series of chain reactions brought about by the greenhouse effect (Ahmed et al. 2021 ). The overexploitation and consumption of non-renewable energy sources, especially fossil fuels, is the main driver of anthropogenic greenhouse gas emissions. According to the Global Carbon Atlas, greenhouse gas emissions from fossil fuel combustion account for 28.9% of total global emissions in 2022 (Liu et al. 2023b ). However, primary energy sources, such as fossil fuels, have limited reserves globally, and the scarcity of resources is facing a serious challenge, and there is an urgent need for the global energy mix to transition to sustainable energy sources (Chen et al. 2023b ; Hoang et al. 2021b ).
Notably, the year-on-year decline in the cost of renewable power generation has contributed to a trend of continued growth in renewable energy sector applications, with approximately 77% of capacity additions to sustainable energy generation due to solar and wind energy in 2017 (Al-Shahri et al. 2021 ). Besides, according to the World Health Organization, 7 million people die each year due to air pollution, which mainly stems from the burning of fossil fuels (Arya 2022 ). The development and implementation of regulatory frameworks and policies aimed at accelerating the deployment of renewable energy are therefore critical for mitigating climate change, enhancing energy security, and promoting sustainable development (Lu et al. 2020 ).
In the construction industry, policies and regulatory frameworks influence the use of renewable energy (Gielen et al. 2019 ). Inês et al. ( 2020 ) reviewed the development of energy policies in five countries, namely the USA, Germany, the UK, Denmark, and China, to provide a comprehensive overview of sustainable energy policies that promote renewable energy. Meanwhile, the concept of biomaterials has been applied in the construction industry, where the use of biomaterials can reduce the carbon footprint of the construction process, improve sustainability, and reduce dependence on limited resources (Raza et al. 2023 ). However, the disposal of construction materials and waste management can be affected by waste regulations related to biomaterials (Philp 2018 ). Figure 4 shows the history of the evolution of policy and regulatory frameworks for renewable energy in construction. These policies and regulations will vary between countries and regions, but they are all guiding the construction industry to adopt renewable energy for more sustainable practices.
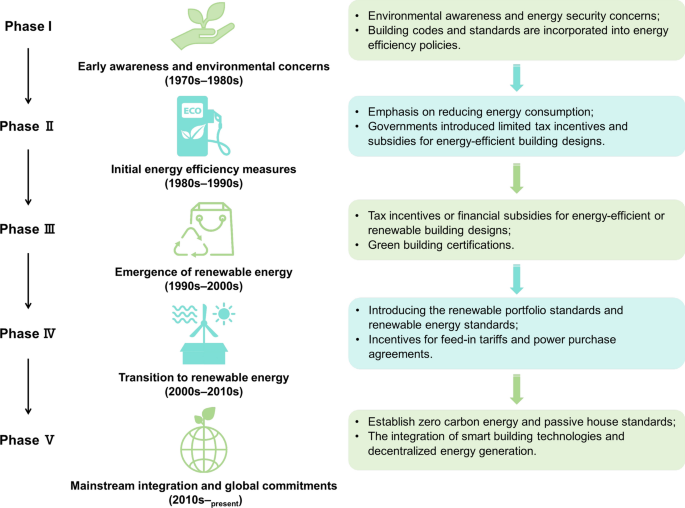
History of the evolution of renewable energy policies and regulatory frameworks in the building sector. The figure presented delineates a quintet of stages marking the evolution of renewable energy policies within the building sector. It chronicles a journey from nascent environmental consciousness to an unwavering global pledge, underscoring the pivotal influence of such policies on sustainable development and climate initiatives. Central to this narrative is the indispensable role of governmental regulations and policies in championing the emergence and growth of renewable energy technologies. In alignment with tenets of sustainable development, these policies foster the adoption of cleaner energy modalities, curtail ecological adversities, and enhance societal well-being
Since the onset of the oil crisis in the last century, growing environmental concerns and energy security issues have prompted the exploration of strategies related to renewable energy and sustainability in a variety of fields, including buildings (Economidou et al. 2020 ). Renewable energy and sustainability strategies have evolved from environmental awareness to a fully market-driven phase, where experimental initiatives in the 1990s to the development of mandatory standards in the 2000s became an important turning point, such as the Renewable Portfolio Standard and the Renewable Energy Standard, which marked a successful transition of renewable energy policies from theory to practice (Solangi et al. 2021 ; Tan et al. 2021 ). International agreements such as the Kyoto Protocol and the Paris Agreement have played a key role in the development of global sustainability strategies, emphasizing the importance of coordinated action and shared commitment in combating climate change (Cifuentes-Faura 2022 ; Ottonelli et al. 2023 ). In addition, technological advances have become an integral part of renewable energy strategies, with smart building technologies, decentralized energy production, and energy storage solutions increasing efficiency and resilience (Walker et al. 2021 ). Future strategies will emphasize circular economy principles, resilience, and the integration of multiple approaches to create holistic and adaptive solutions for sustainable development (Farghali et al. 2023a ; Hoang et al. 2021a ).
The strategic principles for the development and implementation of policy and regulatory frameworks in the field of renewable energy and sustainable development reflect a proactive, forward-looking approach to adapting to changing environmental challenges and technological advances (Jiang et al. 2022a ). As promised in the Paris Agreement, policymakers prioritize international cooperation to align national strategies with global sustainable development goals (Iacobuţă et al. 2022 ). Effective strategic markets include market-driven incentives that are predominantly government-led, using mainly economic instruments to promote the use of renewable energy and application practices in the building sector (Solaymani 2021 ). It also emphasizes the importance of circular economy principles and builds the capacity to cope with future uncertainties. Together, these principles guide policy development and implementation to support renewable energy adoption, sustainability, and resilience in response to the changing global landscape.
In summary, governments and international organizations play an indispensable role in the development and implementation of renewable energy policies and regulatory frameworks. The formulation of strategic policies plays a pivotal role in curbing energy consumption, mitigating environmental repercussions, and steering the building sector toward elevated sustainability benchmarks, aligning with the escalating imperative for sustainable development. Nevertheless, an inclusive consideration of stakeholders is paramount to guarantee that these policies are both robust and effectively enforceable.
Impact of policies and regulations on the adoption of renewable energy
In the context of addressing the global climate crisis, countries around the world have committed to autonomous greenhouse gas contributions at the United Nations Climate Change Conference (Meinshausen et al. 2022 ). The European Union is at the forefront of global carbon action, announcing the "European Green Deal" in 2019, which sets an ambitious goal of achieving net-zero greenhouse gases emissions by 2050 (Lu et al. 2020 ). The realization of the target is based on a comprehensive policy framework for the development and implementation of climate, energy, environmental, and economic policies and regulations to improve energy efficiency, reduce greenhouse gas emissions, and promote the sustainable development of buildings. Policies that encourage the adoption of renewable energy in buildings are the most effective and carbon-reducing actions (Topcu and Tugcu 2020 ). Policies to support the application of renewable energy are diverse, ranging from economic incentives such as financial subsidies and tax breaks to the setting of stringent energy efficiency standards and renewable energy portfolio mandates (Romano et al. 2017 ). Table 1 shows the variety of policies that have been adopted to promote renewable energy solutions in the built environment in major energy countries, both nationally and internationally.
Based on the analyses in Table 1 , the results of the study indicate that policies to promote the development of renewable energy in buildings fall into four main categories: policy regulation, economic incentives, market transformation, and building performance and quality assurance. Government documents are developed or revised to mandate minimum energy requirements for buildings, renewable energy development regimes, and, most commonly, feed-in tariff harmonization. Economic measures such as financial subsidies and tax breaks incentivize renewable energy applications and expand their reach, and this is because the financial viability of renewable energy projects in buildings is influenced by the incentives and feed-in tariff subsidy rate, which determines the attractiveness of the investment. The development and application of renewable energy technologies such as solar, wind, and geothermal energy in buildings are now maturing, and actively exploring innovative developments in new renewable energy technologies and looking for market transitions is a strong guarantee that renewable energy can be sustainable in the long term. In addition, energy-efficient design, construction, and operation of buildings are legally encouraged through the creation of independent performance certificates for building materials, quality, structural elements, or technologies, and certified buildings typically consume less energy, thereby reducing greenhouse gas emissions and overall energy demand.
Economic incentives, mainly tax credits and financial subsidies provide substantial benefits to stakeholders such as real estate developers, for whom the government clears financing barriers and increases the economic attractiveness of clean energy investments to investors (Zhang et al. 2021 ). Through a series of renewable energy policies such as tax credits to subsidize photovoltaic projects and high government prices for surplus solar power, the US has invested $250 million in encouraging the development of solar rooftops since 2010, and photovoltaic applications have exploded, with a 20-fold increase in new installations alone in six years (Song et al. 2016 ). The knock-on effect of this policy has also led to the increasing integration of solar panels, wind turbines, and energy efficiency systems into building projects, thus increasing renewable energy capacity.
Based on the linear relationship between financial investment and renewable energy development, it can be seen that the implementation of financial incentives expands the competitiveness of the renewable energy market and drives the application of renewable energy in the building sector toward more efficient and cost-effective solutions, thus contributing to the economic growth of the renewable energy building sector (Shahbaz et al. 2021 ). Policies such as Leadership in Energy and Environmental Design, which combines green building certification with renewable energy, provide a new direction for sustainable building practices and renewable energy integration, lowering the operating costs for owners and occupants and improving energy efficiency, thus stimulating renewable energy use (Abd Rahman et al. 2021 ).
In conclusion, policy frameworks and regulations have a direct impact on the adoption of renewable energy in the construction sector. The implementation of policies and regulations stimulates the development of renewable energy and leads to technological advances in the sustainable building-related chain. These strategic initiatives not only align with global sustainable development aspirations but also expedite the shift toward an environmentally friendly and sustainable architectural landscape.
Opportunities and obstacles to policy development
In the development of sustainable development policies, the circular economy has been favored by policymakers for its ability to promote economic growth while also reducing dependence on raw materials and energy (Knäble et al. 2022 ). Its principle is to advocate the reuse and recycling of materials, thus maximizing the use of waste recycling to promote sustainable practices from construction to deconstruction and to reduce resource consumption to achieve the goal of sustainable development, which is consistent with the concept of renewable energy (Hossain et al. 2020 ). Hoang et al. ( 2021a ) integrated renewable energy into a smart city energy system by integrating more than two renewable energy components into the building system, achieving good emission reduction benefits and laying the foundation for exploring more sustainable and cleaner energy production in future. Researchers have determined that 1112 million tons of standard coal were consumed in the building sector in 2018 (Guo et al. 2021 ). With the depletion of non-renewable energy resources globally, it is important to develop competing policy frameworks that are in line with circular economy practices in order to adapt to the rapidly changing trends of the energy revolution (Danish and Senjyu 2023b ).
The development of renewable energy policies based on the combination of circular economy principles and smart renewable energy systems has great potential for expanding the use of renewable energy in the construction sector. Nag et al. ( 2022 ) applied circular economy principles to develop a wind turbine-based renewable energy system, whereby the refurbishment and remanufacturing of wind turbines achieves an extension of the life of the wind turbine. This synergy is aligned with the broader Sustainable Development Goals to minimize energy demand through resource optimization and material reuse. Smart city energy, on the other hand, relies on smart technological resources to improve energy efficiency in a high-quality manner, mainly by intelligently managing heating, cooling, and lighting systems, among others, to facilitate energy sharing within the community (Wang et al. 2021b ). In essence, the harmonious combination of circular economy and smart renewable energy systems will provide for a more sustainable, efficient, and resilient adoption of renewable energy in the building sector. The organic combination of financial incentives, energy efficiency, and market transformation is achieved for policymakers, symbolizing the transition of renewable energy policy toward a greener, more resource-based future.
However, the process of promoting and developing renewable energy in the building sector is not without obstacles, and Fig. 5 shows the challenges and practical implications of implementing renewable energy policies in the building sector. Although many governments have provided financial support for the development of renewable energy, the cost of renewable energy development is much higher than conventional energy sources compared to the high initial cost of renewable energy technologies, and the amount of government subsidies does not solve the fundamental problem, which poses a significant barrier for building owners (Chen et al. 2021a ). Frequent policy changes and regulatory uncertainty also make building investors and developers hesitant, and research has found that policy stability determines the long-term planning of renewable energy projects (Alola and Saint Akadiri 2021 ). The intermittent nature of solar and wind energy poses a challenge to grid integration and stability, as renewable energy access to building community grids is subject to both national regulations and policy regulations. At the same time, complex permitting procedures and technical requirements for grid integration further add to the complexity of implementing renewable energy systems in buildings (Wainer et al. 2022 ). Besides, the extent to which policies on renewable energy in buildings are developed and implemented at the national and regional levels is an important indication of the effectiveness of ensuring policy regulation (Busch et al. 2021 ).
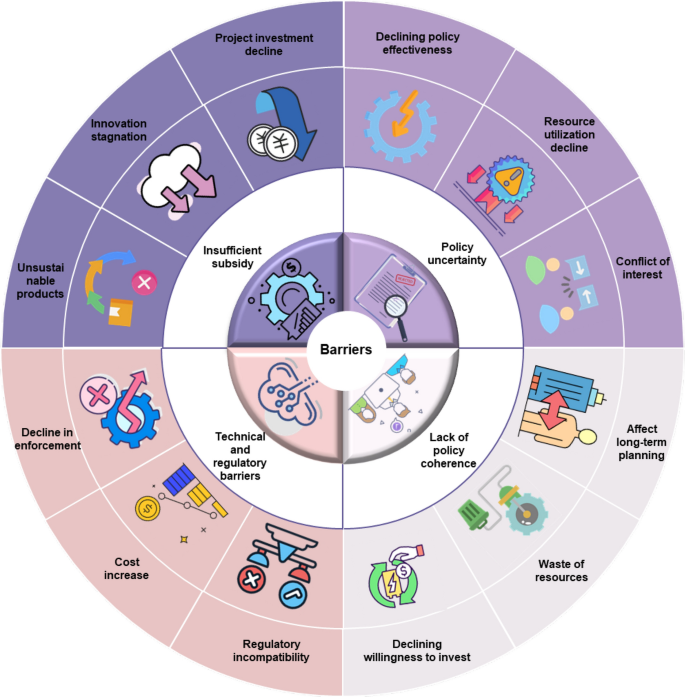
Barriers to and impacts of renewable energy policies in the building sector. The figure delineates the predominant obstacles hindering the extensive integration of renewable energy within the building sector. These encompass limited governmental financial support, ambiguity in policy direction, intricate regulatory systems, a dearth of expertise, and the efficacy of policy execution. Moreover, the figure also illustrates the actual impacts and consequences of these barriers, providing a comprehensive overview of the challenges faced by various stakeholders and emphasizing the multifaceted nature of these barriers and their potential impacts. Overcoming these challenges is pivotal for enhancing renewable energy assimilation, realizing sustainable development objectives, and cultivating a robustly built milieu
Lessons learned from the implementation of renewable energy policies emphasize the flexibility of policy formulation and the need to continuously adapt to regional differences and new technologies that are continuously evolving. A sound assessment of the underlying national circumstances to develop renewable energy policies that are appropriate to the changing environment and regular policy reviews determine the longevity of policy implementation (Agyekum et al. 2021 ). At the same time, the adoption of different stakeholders' opinions ensures the policy's relevance and breadth (Neij et al. 2021 ). A study by Scheller et al. ( 2021 ) suggested that stakeholders influence the entire process of solar photovoltaic residential decision-making, and the shift in the role of participants from passive engagement to active information searching averts potential risks and challenges for policy makers and ensures that policies take into account a wide range of interests and needs. In addition, renewable energy portfolio standards, energy efficiency standards for appliances and buildings, are tailored to the specific needs of the local building sector for renewable energy to maximize the effect and impact of policy development (Danish and Senjyu 2023a ).
In conclusion, while potential avenues exist for renewable energy policies, especially at the nexus of smart and circular economic synergies, challenges such as high upfront investments, grid instability, and policy inconsistencies persist. This section delves into the policy and regulatory landscapes that bolster renewable energy adoption in buildings. An in-depth analysis of the policies and regulations championed by nations to facilitate renewable energy integration is presented, considering economic, social, and institutional dimensions. The resultant effects on sustainability and energy efficiency are also dissected. For effective execution of these renewable energy strategies, it is crucial to harness potential opportunities like smart and circular economy synergies, while concurrently navigating impediments like hefty initial investments, scarcity of expertise, and policy ambiguity, all within a cohesive and sustainable framework.
Innovations in renewable energy for building sustainability
Recent technological advancements in building-based renewable energy.
In recent years, as the construction industry gradually gets rid of its dependence on fossil fuels and countries to reduce carbon emissions to achieve sustainable development requirements, the use of solar energy, wind energy, geothermal energy, and other renewable energy has received widespread attention. Renewable energy technologies can address issues such as the global energy crisis, food insecurity, and climate change by providing environmentally friendly clean energy and are expected to make a significant contribution to the sustainable development of the construction industry (Izam et al. 2022 ). At present, renewable energy is not only a resource but has become a viable alternative to major transformational technologies. To this end, countries around the world are trying to innovate and improve renewable energy technologies and methods to promote the application of mature renewable energy technologies in the field of construction to achieve the best use of renewable energy in the construction industry.
Solar energy is one of the most environmentally friendly and sustainable renewable energy sources available today (Dehghani Madvar et al. 2018 ; Kannan and Vakeesan 2016 ). Research has shown that solar energy is the most abundant source of renewable energy, easy to obtain and low-cost, and has shown great potential to meet world energy needs in future (Adenle 2020 ). Due to its reliability and efficient performance, solar energy has become one of the most popular energy sources and will play an important role in future of energy. At present, the application of solar technology in the construction field mainly includes solar photovoltaic power generation, concentrated solar power generation, solar hot water systems, and solar air conditioning refrigeration technology. The main contribution of the above technology in the building is to save energy for heating (namely space heating and water heating), refrigeration, ventilation, electricity, and lighting (Bosu et al. 2023 ).
At present, the global installed capacity of solar photovoltaic power generation continues to increase and has become a rapidly developing industry (Diwania et al. 2020 ). However, the low conversion efficiency, high price, and large impact of climatic conditions of photovoltaic cell energy storage are the main obstacles to the promotion and stable development of this technology in the construction industry (Durganjali et al. 2020 ). As Table 2 shows, improvements in solar systems and innovative applications for integration with other energy sources or materials have led to significant advances in many aspects, including power generation and heating efficiency. The application of information technology, such as the Internet of Things and artificial intelligence, to solar photovoltaic systems has become one of the feasible solutions to the above problems. In addition, machine learning technologies such as artificial neural networks and support vector machines have great potential in predicting solar radiation intensity and power generation, and applying them to solar photovoltaic systems can also significantly improve the efficiency of photovoltaic power generation (Mellit and Kalogirou 2014 ; Sobri et al. 2018 ). In addition to improving solar technology, the integrated application of multiple energy sources or materials, as well as the development of various new materials of solar cells, have also contributed to the innovation and technological progress of solar power generation in the construction field. For the solar heat collection/refrigeration system using solar heat for building space heating, energy-saving technologies such as roof pool heat storage, phase change material heat storage, and new materials are directly or indirectly applied to the building design to maximize the use of solar energy provides an effective solution (Peng et al. 2020a ).
In addition to solar energy, other renewable energy technologies such as wind and geothermal energy, are also widely used in the construction sector. The most important part of any wind energy system is the wind turbine, which converts wind energy into mechanical energy that can be used for a variety of applications (Kumar et al. 2016 ). However, most of the early wind turbines installed in urban environments were restricted by limited land resources and distance from buildings. As a result, the design and performance of wind energy systems are increasingly advanced and innovative, especially in building-integrated wind energy systems (Rezaeiha et al. 2020 ; Stathopoulos et al. 2018 ), including Savonius-Darrieus hybrid rotors, piezoelectric generators, flag type triboelectric nano-generators, bladeless turbines, and more (Acarer et al. 2020 ; Bagheri et al. 2019 ).
As the demand for green and low-carbon technologies continues to rise, biomass applications in the building sector are poised to play an increasingly crucial role in promoting energy conservation, emission reduction, and sustainable development. For instance, Ebrahimi-Moghadam and Farzaneh-Gord ( 2023 ) devised an eco-friendly tri-generation system driven by an externally-fired gas turbine cycle, utilizing municipal solid waste biomass. This innovative system incorporates a double-effect absorption chiller/heater and undergoes a comprehensive evaluation based on energy, eco-exergy, and environmental analyses to gauge its reliability. The research employs a pioneering optimization strategy, combining Artificial Neural Networks and a multi-criteria Salp Swarm Algorithm to determine the optimal system size and operational parameters. Practical application is demonstrated through a case study, where the developed models are used to meet the electrical, heating, and cooling requirements of a selected building using real data and advanced energy architecture software. The findings underscore the significance of factors like municipal solid mass flow rate and compressor pressure ratio in shaping system performance. Additionally, eco-exergy analysis reveals that a substantial portion of the total cost is attributed to specific system components, particularly the gas turbine and gasifier (40% and 23%, respectively). At peak efficiency, the system can generate 541 kW of electricity, produce 2052 kW of heat, and provide 2650 kW of cooling. Remarkably, the levelized cost of electricity generation stands at $0.083/kWh, with an associated environmental factor of 1.3 kgCO 2 /kWh, showcasing the potential of biomass-fired gas turbine cycles to satisfy building energy demands efficiently.
Integrating wind energy and biomass integration represents another promising and sustainable energy option. In this context, Liu et al. ( 2023a ) aimed to create a near-zero-energy neighborhood in an industrial city to reduce greenhouse gas emissions. They utilized biomass waste for energy production and incorporated a battery pack system for energy storage. The Fanger model was employed to assess occupants' thermal comfort, and hot water production was detailed. Using TRNSYS software, the building's transient performance was simulated for one year. Wind turbines were employed as electricity generators, with excess energy stored in batteries for use during low-wind conditions. Hot water was generated through a biomass waste system and stored in a tank, while a humidifier provided ventilation, and a heat pump addressed heating and cooling needs. The findings indicated that a 6-kW wind turbine could supply the building's power needs and charge the batteries, resulting in a zero-energy building. Biomass fuel was used to maintain hot water, with an average consumption of 200 g per hour.
In addition, geothermal energy, as a non-intermittent and potentially inexhaustible energy source, can be divided into shallow, medium, and deep geothermal energy technologies according to depth, which can meet the heating and cooling needs of building groups with different energy efficiency levels and has great potential in space regulation in buildings (Romanov and Leiss 2022a ). Recent technological innovations and advances in geothermal systems in the building factor have focused on the optimal design of shallow geothermal systems to improve their efficiency and the application of new materials and integration with other technologies. In pursuit of achieving clean heating in northern rural regions, a novel cooperative heating system, comprising a biomass boiler and a multi-source heat pump, has been introduced. This system is designed based on building heat load requirements and available resources, seamlessly integrating biomass energy, geothermal energy, and air energy sources. A dynamic simulation model, facilitated by TRNSYS software, has been developed to maintain energy balance, and an optimization model is proposed to minimize annual costs. The effectiveness of this model is demonstrated through its application in the Miaofuan rural community in Linzi Town, Linyi County, Dezhou City, Shandong Province, China. The optimized cooperative system exhibits significant cost reductions of 9.6%, 14.2%, and 11.7%, respectively, compared to the individual operation of geothermal heat pumps, air source heat pumps, and biomass boilers. Consequently, this cooperative heating system emerges as a highly suitable solution for rural areas seeking efficient and sustainable heating solutions (Hou et al. 2023 ). As shown in Table 3 , the main recent findings of various researchers on the technological advances and integrated innovative applications of wind and geothermal energy systems in buildings are summarized.
In summary, the latest technological advancements in the application of renewable energy in the construction field include the application of new materials, improvements, and new designs to the structure of renewable energy systems and integrated applications with other technologies or multiple energy sources. The continuous improvement and innovation of renewable energy technology have enabled it to overcome key technical shortcomings and fully leverage the advantages of renewable energy. It not only provides more environmentally friendly and sustainable choices for the construction industry but also provides greater creative space for architects and designers. With the continuous development and innovation of renewable energy technology in future, the construction industry is expected to bring more innovation, support the better integration of renewable energy systems into buildings, and create more opportunities for the construction industry to achieve significant decarburization and cost savings.
Impact of technological advancements on renewable energy adoption in buildings
As shown in Table 4 , technological progress has significantly influenced the application of renewable energy in the construction field. In recent years, the pursuit of higher efficiency has been the main driving force for innovation. Efficiency is also very important at the level of renewable energy systems, and various variables stimulate people's desire for more efficient technologies (Rathore and Panwar 2022 ). The floating photovoltaic technology is considered to have good development prospects due to its high power generation efficiency and no need to occupy land resources. The system is being developed based on new cell technologies, biodegradable materials, and advanced tracking mechanisms. However, the ecological impact, economic benefits, and optimization of size and system used are still challenges that need to be further addressed (Gorjian et al. 2021 ).
The modern technological development of wind power systems and their components, as well as reasonable architectural design optimization, have also made significant progress in generating power output and efficiency. Secondly, although nuclear energy has broad application prospects, natural disasters, and human hazards have always threatened this technology. Therefore, the development and application of nuclear energy are prudent, and the scale of utilization is still relatively low (Wei et al. 2023 ). Technological progress and innovation can not only improve the conversion and output efficiency of renewable energy systems but also reduce costs, thereby improving their performance and durability, making them more suitable for different regions and types of building applications. In future, the growth of the renewable energy industry mainly depends on reducing system costs and government policy support (Buonomano et al. 2023 ).
Energy storage technology can quickly and flexibly adjust the power of the power system, and the application of various energy storage devices to wind and solar power generation systems can provide an effective means to solve the problem of unstable renewable energy generation (Infield and Freris 2020 ). Giving full play to the advantages of various artificial intelligence technologies and cooperating with the energy storage system in the power system can improve the service life of the energy storage system and realize the optimal control of the multi-objective power system, which is the research direction of the integrated application of energy storage system and renewable energy in future (Abdalla et al. 2021 ). Chen et al. ( 2021b ) proposed an artificial intelligence-based useful evaluation model to predict the impact of renewable energy and energy efficiency on the economy. This model can help enhance energy efficiency to 97% and improve the utilization rate of renewable energy. Another study applied artificial neural networks and statistical analysis to create decision support systems and evaluated the solar energy potential of Mashhad City, Iran, using photovoltaic system simulation tools. The results show that the artificial neural network model can successfully predict electricity consumption in summer and winter, with an accuracy of 99% (Ghadami et al. 2021 ). Overall, machine learning technologies such as artificial neural networks and artificial intelligence have brought enormous potential for renewable energy applications in the construction industry, which can improve energy efficiency, reduce energy costs, reduce carbon emissions, and promote the development of the construction industry toward a more sustainable and environmentally friendly direction. The continuous development of these technologies will help create a smarter and greener building environment.
In conclusion, technological advancements offer promising prospects for integrating renewable energy into the construction sector. These advances encompass innovative design potential, superior system performance and resilience, heightened environmental benefits, augmented socio-economic returns, and data-driven innovations. Additionally, the strategic optimization of building layouts combined with the adoption of novel materials and technologies can further decrease the operational costs of renewable energy systems.
Emerging trends in renewable energy technology
The limitations of standalone renewable energy systems, like wind and solar power, characterized by unpredictability and uncertainty, lead to reduced utilization rates and increased construction expenses associated with these sources. In order to overcome these problems, hybrid renewable energy systems are receiving increasing attention from scholars and are widely used to address the challenges mentioned above (Farghali et al. 2023a ; Hajiaghasi et al. 2019 ). The use of machine learning and artificial intelligence technology for design optimization and cost control of hybrid renewable energy systems is an emerging trend, including support vector machines, genetic algorithms, and particle swarm optimization algorithms. Wen et al. ( 2019 ) proposed a deep recursive neural network model for aggregating power loads and predicting photovoltaic power generation and optimized the load scheduling of grid-connected community microgrids using particle swarm optimization. The results indicated that the community microgrid based on deep learning for solar power generation and load forecasting has achieved a reduction in total cost and an improvement in system reliability. Ramli et al. ( 2018 ) used a multi-objective adaptive differential evolution algorithm to optimize the design of a hybrid photovoltaic/wind/diesel microgrid system with battery storage. In addition, new algorithms such as the ant colony algorithm, bacterial foraging algorithm, and artificial bee colony algorithm are gradually being applied in the prediction and optimization research of hybrid renewable energy (Wei et al. 2023 ).
In recent years, there has been increasing research on the thermal storage performance and application prospects of phase change materials, among which the application of phase change materials in solar energy, architecture, and automobiles is prominent (Sikiru et al. 2022 ). The system combining solar collectors with suitable phase change materials has been proven through experiments to have better overall performance than traditional flat panel solar collectors (Palacio et al. 2020 ). As a commonly used thermal storage material, phase change materials have the disadvantage of low thermal conductivity. Therefore, Abuşka et al. ( 2019 ) developed a new type of solar air collector that combined phase change material Rubitherm RT54HC with aluminum honeycomb and studied the effect of using honeycomb cores on the thermal performance of phase change material thermal storage collectors under natural convection conditions. The results showed that the honeycomb core can effectively improve the thermal conductivity of phase change materials and is a promising thermal conductivity-enhancing material, especially during discharge. In addition, recent breakthroughs in nanomaterials, including quantum dots, nanoparticles, nanotubes, and nanowires, have significant implications for creating the next generation of efficient and low-cost solar cells. The safe and easy solution bonding of non-aggregated, monodisperse, passivated semiconductor nanoparticles with good photoelectric properties opens a new door for photovoltaic devices currently under study (Baviskar and Sankapal 2021 ).
In summary, technological advancements in renewable energy open new avenues for sustainable building and eco-friendly design. With science and technology's relentless evolution, the incorporation of renewable energy within the construction realm is poised to embrace intelligence, diversity, and digitization. At the same time, technological progress can also help solve the challenges of some renewable energy systems in practical applications, promoting their wider application in the building factor.
This section delves into the latest innovations concerning four prevalent renewable energy types used in buildings, scrutinizing the prospective influence of these breakthroughs in construction. Continuous refinement and inventive strategies in renewable energy systems, coupled with the amalgamation of diverse technologies, materials, and energy forms, bolster a building's environmental, energy, and economic advantages, thus championing a broader adoption of renewable methodologies in construction. Finally, the recent research hotspots and emerging development trends in the field of renewable energy are presented.
Perspective
With the increasing population and density in urban areas, having low-energy buildings with the least greenhouse gas emissions has become more important (Shirinbakhsh and Harvey 2023 ). In future, the vibrant prospects of renewable energy in the construction industry will be influenced by a series of complex and closely interrelated factors. This section will provide an in-depth outlook on the development of renewable energy in the construction industry from two main perspectives, namely prospects and potential challenges.
Energiewende
Energiewende is becoming a booster for the application of renewable energy in the construction industry. In the context of energiewende, renewable energy is widely regarded as the core element driving the development of building energy. In future, the construction industry will increasingly rely on the application of renewable energy, especially solar, wind, and geothermal energy. Besides, solar energy, as one of the most common and renewable energy sources, will play an important role in buildings. Photovoltaic power generation systems will be widely used on roofs, walls, and windows of buildings, turning them into distributed power producers. This distributed power generation model helps to reduce dependence on traditional energy and achieve a greener and more sustainable energy supply.
Additionally, wind energy is also an important part of the transformation of building energy. Wind power generation devices will not only be limited to traditional wind farms but will also be integrated into high-rise buildings, bridges, and other building structures. The widespread application of this type of wind energy will effectively utilize the wind energy resources in cities, provide clean electricity for buildings, and reduce environmental loads. In addition, geothermal energy, as a stable and reliable form of energy, will also be widely used in the construction field. Geothermal energy can be used in heating and cooling systems of buildings, reducing reliance on traditional energy and improving energy efficiency. Through underground heat exchange technology, buildings can achieve efficient energy conversion and reduce energy consumption in different seasons.
Biomass holds promising prospects for expanding renewable energy adoption in the building sector. As a versatile and sustainable energy source, biomass can be used for various applications, such as heating, cooling, and electricity generation, making it a valuable addition to the renewable energy mix. Additionally, biomass offers the advantage of energy storage through technologies like phase change materials, enhancing its suitability for meeting variable energy demands in buildings. Furthermore, the utilization of agricultural and forestry residues as biomass feedstock can contribute to waste reduction and resource optimization, aligning with sustainable building practices. As efforts to decarbonize the building sector intensify, biomass's potential to provide renewable, locally sourced energy while reducing greenhouse gas emissions positions it as a compelling option for advancing the adoption of renewable energy in buildings.
In summary, the introduction of renewable energy to the construction realm offers significant technological advancement. This shift also ensures a move toward greater sustainability. The synergy of architects, engineers, energy specialists, and other experts fosters the seamless integration and innovation of renewable energy solutions within architectural designs.
Energy self-sufficiency and microgrid technology
Energy self-sufficiency and microgrid technology are becoming leaders in the construction field. With the continuous innovation of technology, buildings are gradually moving toward the goal of energy self-sufficiency. By integrating solar power generation, energy storage systems, and intelligent energy management technologies, buildings are expected to achieve a certain degree of separation from traditional power networks and achieve the goal of self-power supply. This concept of energy self-sufficiency will further reduce reliance on traditional energy, enabling buildings to meet energy needs more independently.
The rise of microgrid technology will bring significant changes to building energy management. A microgrid is a small energy network composed of multiple energy components (such as solar cells, energy storage devices, and gas generators), which can achieve efficient utilization and sharing of local energy. Microgrid technology utilizes renewable resources to ensure the stability and sustainability of buildings or cities based on artificial intelligence, such as metaheuristics (Evolutionary, Swarm, Physically based, Human based, hybrid, and other standalone algorithms), and machine learning (Model-based Control, Reinforcement Learning, Fuzzy Logic), which helps better cope with energy fluctuations and intermittency (Tajjour and Singh Chandel 2023 ). The development of microgrid technology will also enhance the reliability of building energy. In traditional central power systems, once a fault or interruption occurs, the entire area may be affected. Additionally, microgrid technology allows the energy system inside the building to automatically switch to backup energy in the event of a power outage, ensuring the continuous operation of key equipment and improving the reliability and stability of energy supply.
Carbon neutrality and sustainable development
The United Nations General Assembly, with sustainable development as its core, has formulated the 2030 Agenda for Sustainable Development, aimed at addressing environmental, economic, and social challenges in the process of human development. This agenda is an action plan for humanity, the Earth, and prosperity (Woon et al. 2023 ). Meanwhile, carbon neutrality and sustainable development have become important issues that cannot be ignored in the construction industry. With increasing global attention to climate change and environmental issues, the construction industry is actively responding to carbon neutrality goals and striving to reduce carbon emissions. In this context, renewable energy is seen as a crucial solution for achieving carbon neutrality goals.
Renewable energy, as a non-emission energy source, has obvious advantages. Renewable energy sources such as solar energy, wind energy, and hydropower not only do not produce harmful gases such as carbon dioxide in the energy production process, but their sustainability enables them to provide clean energy for buildings in the long term. This makes renewable energy one of the important means to achieve carbon neutrality goals.
In future, the construction industry will gradually reduce its dependence on high-carbon-emitting energy sources such as traditional coal and oil and turn its attention to renewable energy. Photovoltaic power generation systems will be more widely installed on roofs, walls, and even windows of buildings, and wind power plants may become a part of high-rise buildings. Geothermal energy technology will play a greater role in heating and cooling. Biomass systems, including biomass boilers and biogas generators, can be integrated into buildings to provide reliable and carbon–neutral energy. These changes will not only bring significant reductions in carbon emissions but also provide greater security and reliability in the energy supply. In addition to direct carbon emissions reduction, the promotion of renewable energy will also stimulate innovation and technological progress. While seeking higher energy efficiency and lower carbon emissions, the construction industry will face pressure from technological upgrading and innovation, which will promote the development of new materials, new equipment, and intelligent energy management technologies, thereby further promoting the application and development of renewable energy.
Intelligent building and energy internet
Intelligent buildings and the energy internet have been widely recognized as important directions for the future development of the construction industry. With the continuous progress of technology, buildings will gradually become intelligent and digitized, creating more intelligent conditions for efficient energy utilization. In future, intelligent building technology will play an important role in enabling buildings to achieve intelligent regulation and optimize energy use. Through the application of sensors, data analysis, and automatic control systems, buildings can collect environmental information such as energy consumption, temperature, humidity, brightness, and room occupancy, allowing for energy decomposition and equipment identification and generating timely and personalized recommendations to achieve efficient energy utilization (Alsalemi et al. 2022 ). For example, in cold winter, the system can automatically adjust the temperature and time of the heating system to ensure a comfortable indoor environment while minimizing energy waste.
The integration of architecture and energy internet will further enhance the effective utilization rate of energy. By connecting the building energy system to the energy internet, buildings can achieve precise matching with energy supply. The energy internet will allow buildings to flexibly adjust according to actual demand and energy supply, thereby maximizing energy utilization. For example, when there is sufficient energy supply, buildings can store excess energy, and when there is a shortage of energy supply, priority can be given to utilizing reserve energy to ensure the normal operation of the building.
This section delves into the envisioned future of the construction sector, with a spotlight on the transformative influence of renewable energy. It forecasts the industry's trajectory toward energy autonomy, underscores the advantages of adopting microgrid systems, and highlights the worldwide momentum toward carbon–neutral commitments. Furthermore, it explores the synergistic melding of smart buildings with the energy internet to enhance energy consumption efficiency.
Technological innovation and cost reduction
Technological innovation is the core driving force behind the application of renewable energy in the construction industry. With the continuous progress of technology, renewable energy technologies are also constantly innovating and evolving. However, despite significant progress, further efforts are still needed to reduce costs and improve efficiency to better meet the needs of the construction industry. In the field of renewable energy, cost has always been considered one of the key factors restricting its widespread application. To achieve the large-scale application of renewable energy technology in buildings, it is necessary to find ways to reduce the costs of production, installation, and maintenance. Especially for some emerging technologies, such as solar thin films and wind energy storage, their research and manufacturing costs are relatively high, requiring continuous investment and efforts to achieve cost reduction.
Energy storage technology
The role of energy storage technology in the field of renewable energy is becoming increasingly prominent, especially in the face of the intermittency and volatility of renewable energy. These characteristics make energy storage a key factor in achieving a stable supply of renewable energy. However, current energy storage technologies still face a series of challenges in terms of cost, efficiency, and reliability, requiring continuous improvement and innovation.
With the continuous growth of renewable energy sources such as solar and wind energy, the demand for energy storage technology is becoming increasingly urgent. Photovoltaic and wind power generation systems have fluctuating production capacity due to weather and other factors, while energy demand is all-weather. Therefore, efficient energy storage technology can store excess energy for release when needed, thereby ensuring the stability of the energy supply. However, there are still some limitations to current energy storage technologies. On the one hand, cost issues have limited the popularization of energy storage technology. Currently, some commonly used energy storage technologies, such as lithium-ion batteries, have superior performance but high manufacturing costs, especially for large-scale applications. On the other hand, the efficiency and reliability of some energy storage technologies also need to be improved. For example, some energy storage systems may experience certain losses during the energy conversion and storage process, which reduces the overall efficiency of the system.
In the future, the improvement and innovation of energy storage technology will be a hot topic in the field of renewable energy. Scientists and engineers are exploring new energy storage materials and technologies to reduce costs, improve efficiency, and extend the lifespan of systems. The research on new battery technologies and energy storage materials will provide new possibilities for addressing the challenges posed by the volatility of renewable energy.
Integrated design and multidisciplinary cooperation
In the context of the increasingly urgent global energy situation, achieving the maximum potential of renewable energy has become an urgent task in the construction field. In order to achieve this goal, the energy system, building structure, and function in architectural design need to achieve close integration, which can maximize the application effect of renewable energy. Therefore, interdisciplinary cooperation has become crucial, and cross-border cooperation in professional fields such as architects, engineers, and energy experts will help find the best technology integration solutions to create more efficient and reliable renewable energy applications.
Implementing integrated architectural design requires close cooperation from experts in various fields. Architects need to integrate the needs of energy systems into their architectural design, considering how to maximize the utilization of renewable energy sources such as solar and wind energy without affecting the appearance and functionality of the building. Engineers need to ensure the coordination between the building structure and the energy system, optimize the layout, and ensure the efficient operation of the energy system. Energy experts need to provide energy analysis and technical support for the entire system to ensure the rational application of renewable energy.
Based on interdisciplinary cooperation, innovative technology integration solutions will be born. This may include integrating photovoltaic power generation systems into the exterior walls of buildings, utilizing the exterior scenery of buildings to enhance wind power generation, or organically integrating ground-source heat pump systems with building structures. By integrating multidisciplinary expertise, the most suitable and innovative renewable energy solutions can be found for each construction project. This integrated design and multidisciplinary collaboration can help solve the problem of energy waste in traditional architectural design. Traditional buildings usually consider energy systems and building design separately, resulting in inefficient energy utilization. By implementing integrated design, buildings can respond more intelligently to energy needs and reduce unnecessary waste.
Policy support and market recognition
In the context of global energy issues gradually heating up, policy support and market recognition have become the two pillars to promote the application of renewable energy in the construction industry. The government's policy support not only provides important guarantees for the development of renewable energy but also stimulates the enthusiasm of building owners and developers to adopt these technologies at the policy level. The government can effectively promote the transformation and upgrading of the industry by focusing on investment in the field of new technology research and development, formulating incentive policies to promote technological progress and innovative technology pilot diffusion, increasing research and development investment, guiding social capital participation, and fully leveraging the lateral incentive effect of policies (Xie et al. 2023 ).
At the same time, market recognition is also crucial for the promotion and application of renewable energy. The realization of market recognition requires people to deeply recognize the enormous economic and environmental benefits that renewable energy can bring in long-term operations. This not only involves the return on initial investment but also relates to the savings in energy costs and the reduction of environmental burden in long-term operations. With the increasing awareness of environmental protection in society, people's demand for green performance in buildings is also increasing, which further strengthens the market's demand for renewable energy.
Policy support and market recognition have jointly built the foundation for the development of renewable energy in the construction industry. The guiding role of government policies enables renewable energy technologies to quickly enter the market, while market recognition ensures the stability and sustainability of these technologies in practical applications. This dual support not only promotes technological innovation in renewable energy but also provides a solid guarantee for the sustainable development of the construction industry.
Upgrade of energy infrastructure
To achieve the large-scale application of renewable energy in the construction industry, it is necessary to focus on upgrading energy infrastructure. Nowadays, the demand for energy in the construction industry is increasing, and traditional energy infrastructure often finds it difficult to meet the effective transmission and utilization needs of renewable energy. Therefore, by upgrading our existing energy infrastructure, we can create more favorable conditions for the integration and application of renewable energy. Upgrading energy infrastructure is not only about technological progress but also about optimizing and innovating existing systems. The introduction of intelligent technology is crucial in this process. By introducing technologies such as intelligent monitoring, data analysis, and remote control, energy infrastructure can more efficiently manage and control renewable energy. This will help address the challenges of intermittency and volatility in renewable energy, ensuring a stable supply of energy.
On the other hand, upgrading energy infrastructure also requires attention to equipment updates and renovations. The new generation of equipment and technologies, such as advanced transmission lines and efficient energy storage devices, will provide more reliable and efficient support for the transmission and utilization of renewable energy. By integrating these innovative devices with existing energy systems, we can achieve the upgrading and modernization of energy infrastructure. In addition, upgrading energy infrastructure also requires cooperation with multiple parties. Building owners, energy suppliers, technology providers, and other parties need to work closely together to promote the upgrading process of energy infrastructure. The government's support will also play a crucial role in promoting the smooth upgrading of energy infrastructure through policy guidance and financial support.
In summary, renewable energy in the construction industry will play an increasingly important role in achieving energiewende, reducing carbon emissions, and promoting sustainable development. Although facing multiple challenges such as technology, costs, and policies, these challenges will gradually be overcome with the continuous innovation of technology and the gradual recognition of the market. Cross-disciplinary cooperation, policy support, and market guidance will be key to achieving the development of renewable energy in the construction industry. Through continuous efforts, the construction industry is expected to achieve a green transformation of energy and create a more sustainable and environmentally friendly building environment for humanity.
This section expounds upon the prospects and obstacles of weaving renewable energy into the construction landscape. It accentuates the imperative of technological breakthroughs and cost-cutting measures and underscores energy storage's role in offsetting renewable energy's intermittency. The section also champions interdisciplinary teamwork for optimal design solutions and underscores how regulatory backing and market acknowledgment can bolster renewable energy adoption. Furthermore, overhauling the existing energy framework and embracing contemporary technologies and systems emerge as quintessential to meet the requisites of seamless renewable energy integration.
Renewable energy, known for its environmental benefits, is crucial in addressing growing energy demand and mitigating global warming. This review assesses its application in construction, covering technologies like solar, wind, biomass, and geothermal energy. While offering eco-friendliness, renewables enhance building energy efficiency and cut operational costs. In addition, this work also introduces successful application cases of renewable energy technology in the construction field, which can often achieve multifunctional sustainable development through the adoption of renewable energy technologies, and finally analyzes the difficulties and challenges faced in the application process. From a policy perspective, governments and international organizations play a crucial role in formulating and implementing renewable energy-related policy standards. However, problems such as high initial investment, unstable power grids, and inconsistent policies may be encountered when implementing policies. The implementation of the above policies and regulations will stimulate the sustainable development of renewable energy in the construction industry while promoting innovation and progress in related technological research. This paper presents the latest technological advances and innovative designs for various types of renewable energy systems. The results show that the application of advanced artificial intelligence technologies, such as machine learning, plays an important role in the optimization and improvement of various renewable energy systems. Secondly, the application of hybrid renewable energy systems and innovative building design and layout are also effective ways to enhance the advantages of renewable energy and achieve multi-purpose. In the future, the improvement and innovation of energy storage technology will be a research hotspot in the field of renewable energy, providing new possibilities for addressing the challenges brought by renewable energy fluctuations. Meanwhile, policy support and market guidance will be key to achieving the development of renewable energy in the construction industry.
Abd Rahman NM, Lim CH, Fazlizan A (2021) Optimizing the energy saving potential of public hospital through a systematic approach for green building certification in Malaysia. J Build Eng 43:103088. https://doi.org/10.1016/j.jobe.2021.103088
Article Google Scholar
Abdalla AN, Nazir MS, Tao H, Cao SQ, Ji RD, Jiang MX, Yao L (2021) Integration of energy storage system and renewable energy sources based on artificial intelligence: an overview. J Energy Storage 40:102811. https://doi.org/10.1016/j.est.2021.102811
Abuşka M, Şevik S, Kayapunar A (2019) Experimental analysis of solar air collector with PCM-honeycomb combination under the natural convection. Sol Energy Mater Sol Cells 195:299–308. https://doi.org/10.1016/j.solmat.2019.02.040
Article CAS Google Scholar
Acarer S, Uyulan C, Karadeniz ZH (2020) Optimization of radial inflow wind turbines for urban wind energy harvesting. Energy 202:117772. https://doi.org/10.1016/j.energy.2020.117772
Adenle AA (2020) Assessment of solar energy technologies in Africa-opportunities and challenges in meeting the 2030 agenda and sustainable development goals. Energy Policy 137:111180. https://doi.org/10.1016/j.enpol.2019.111180
Adnan M, Ahmad J, Ali SF, Imran M (2021) A techno-economic analysis for power generation through wind energy: a case study of Pakistan. Energy Rep 7:1424–1443. https://doi.org/10.1016/j.egyr.2021.02.068
Agyekum EB, Amjad F, Mohsin M, Ansah MNS (2021) A bird’s eye view of Ghana’s renewable energy sector environment: a multi-criteria decision-making approach. Util Policy 70:101219. https://doi.org/10.1016/j.jup.2021.101219
Ahmed N, Abdel-Hamid M, Abd El-Razik MM, El-Dash KM (2021) Impact of sustainable design in the construction sector on climate change. Ain Shams Eng J 12:1375–1383. https://doi.org/10.1016/j.asej.2020.11.002
Al-Shahri OA, Ismail FB, Hannan MA, Lipu MSH, Al-Shetwi AQ, Begum RA, Al-Muhsen NFO, Soujeri E (2021) Solar photovoltaic energy optimization methods, challenges and issues: a comprehensive review. J Clean Prod 284:125465. https://doi.org/10.1016/j.jclepro.2020.125465
Alanis Ruiz C, Kalkman I, Blocken B (2021) Aerodynamic design optimization of ducted openings through high-rise buildings for wind energy harvesting. Build Environ 202:108028. https://doi.org/10.1016/j.buildenv.2021.108028
Aldhshan SRS, Maulud KNA, Jaafar WSWM, Karim OA, Pradhan B (2021) Energy consumption and spatial assessment of renewable energy penetration and building energy efficiency in Malaysia: a review. Sustainability 13:9244. https://doi.org/10.3390/su13169244
Ali S, Sang Y-F (2023) Implementing rainwater harvesting systems as a novel approach for saving water and energy in flat urban areas. Sustain Cities Soc 89:104304. https://doi.org/10.1016/j.scs.2022.104304
Allouhi A, Rehman S, Krarti M (2021) Role of energy efficiency measures and hybrid PV/biomass power generation in designing 100% electric rural houses: a case study in Morocco. Energy Build 236:110770. https://doi.org/10.1016/j.enbuild.2021.110770
Almeida AP, Liberalesso T, Silva CM, Sousa V (2021) Dynamic modelling of rainwater harvesting with green roofs in university buildings. J Clean Prod 312:127655. https://doi.org/10.1016/j.jclepro.2021.127655
Alola AA, Saint Akadiri S (2021) Clean energy development in the United States amidst augmented socioeconomic aspects and country-specific policies. Renew Energy 169:221–230. https://doi.org/10.1016/j.renene.2021.01.022
Alsalemi A, Himeur Y, Bensaali F, Amira A (2022) An innovative edge-based Internet of Energy solution for promoting energy saving in buildings. Sustain Cities Soc 78:103571. https://doi.org/10.1016/j.scs.2021.103571
Arteaga-López E, Ángeles-Camacho C, Bañuelos-Ruedas F (2019) Advanced methodology for feasibility studies on building-mounted wind turbines installation in urban environment: applying CFD analysis. Energy 167:181–188. https://doi.org/10.1016/j.energy.2018.10.191
Arya A (2022) Problems of increasing air pollution and certain management strategies. In: Arora S, Kumar A, Ogita S, Yau Y-Y (ed) Chapter 19 Springer Nature Singapore, Singapore, pp 457–486. https://doi.org/10.1007/978-981-16-4445-0_19
Asif M, Hassanain MA, Nahiduzzaman KM, Sawalha H (2019) Techno-economic assessment of application of solar PV in building sector A case study from Saudi Arabia. Smart Sustain Built Environ 8:34–52. https://doi.org/10.1108/Sasbe-06-2018-0032
Badenes B, Sanner B, Pla MAM, Cuevas JM, Bartoli F, Ciardelli F, Gonzalez RM, Ghafar AN, Fontana P, Zuniga LL, Urchueguia JF (2020) Development of advanced materials guided by numerical simulations to improve performance and cost-efficiency of borehole heat exchangers (BHEs). Energy. https://doi.org/10.1016/j.energy.2020.117628
Bagheri M, Delbari SH, Pakzadmanesh M, Kennedy CA (2019) City-integrated renewable energy design for low-carbon and climate resilient communities. Appl Energy 239:1212–1225. https://doi.org/10.1016/j.apenergy.2019.02.031
Balaban O, de Oliveira JAP (2017) Sustainable buildings for healthier cities: assessing the co-benefits of green buildings in Japan. J Clean Prod 163:S68–S78. https://doi.org/10.1016/j.jclepro.2016.01.086
Baumgarten B, Grammer P, Ehard F, Winkel O, Vogt U, Baumbach G, Scheffknecht G, Thorwarth H (2022) Novel metal mesh filter using water-based regeneration for small-scale biomass boilers. Biomass Convers Biorefin 12:3183–3195. https://doi.org/10.1007/s13399-020-00959-9
Baviskar PK, Sankapal BR (2021) Dye-sensitized solar cells. In: Dhoble SJ, Kalyani NT, Vengadaesvaran B, Kariem Arof A (ed) Elsevier, pp 179–211. https://doi.org/10.1016/b978-0-12-823710-6.00020-0
Behzadi A, Thorin E, Duwig C, Sadrizadeh S (2023) Supply-demand side management of a building energy system driven by solar and biomass in Stockholm: a smart integration with minimal cost and emission. Energy Convers Manag 292:117420. https://doi.org/10.1016/j.enconman.2023.117420
Bianco V, Cascetta F, Nardini S (2021) Analysis of technology diffusion policies for renewable energy. The case of the Italian solar photovoltaic sector. Sustain Energy Technol Assess 46:101250. https://doi.org/10.1016/j.seta.2021.101250
Bilardo M, Ferrara M, Fabrizio E (2020) Performance assessment and optimization of a solar cooling system to satisfy renewable energy ratio (RER) requirements in multi-family buildings. Renew Energy 155:990–1008. https://doi.org/10.1016/j.renene.2020.03.044
Bosu I, Mahmoud H, Ookawara S, Hassan H (2023) Applied single and hybrid solar energy techniques for building energy consumption and thermal comfort: a comprehensive review. Sol Energy 259:188–228. https://doi.org/10.1016/j.solener.2023.05.006
Bungau CC, Bungau T, Prada IF, Prada MF (2022) Green buildings as a necessity for sustainable environment development: dilemmas and challenges. Sustainability 14:13121. https://doi.org/10.3390/su142013121
Buonomano A, Barone G, Forzano C (2023) Latest advancements and challenges of technologies and methods for accelerating the sustainable energy transition. Energy Rep 9:3343–3355. https://doi.org/10.1016/j.egyr.2023.02.015
Busch H, Ruggiero S, Isakovic A, Hansen T (2021) Policy challenges to community energy in the EU: a systematic review of the scientific literature. Renew Sustain Energy Rev 151:111535. https://doi.org/10.1016/j.rser.2021.111535
Caballero V, Briones A, Coca-Ortegon A, Perez A, Barrios B, de la Mano M (2023) Analysis and simulation of an urban-industrial sustainable energy community: a use case in San Juan de Mozarrifar using photovoltaic energy. Energy Rep 9:1589–1605. https://doi.org/10.1016/j.egyr.2022.12.059
Canale L, Di Fazio AR, Russo M, Frattolillo A, Dell’Isola M (2021) An overview on functional integration of hybrid renewable energy systems in multi-energy buildings. Energies 14:1078. https://doi.org/10.3390/en14041078
Chen C, Cao X, Zhang S, Lei Z, Zhao K (2022) Dynamic characteristic and decoupling relationship of energy consumption on China’s construction industry. Buildings. https://doi.org/10.3390/buildings12101745
Chen JP, Feng SH (2020) Evaluating a large geothermal absorber’s energy extraction and storage performance in a common geological condition. Appl Energy 279:115793. https://doi.org/10.1016/j.apenergy.2020.115793
Chen L, Chen Z, Zhang Y, Liu Y, Osman AI, Farghali M, Hua J, Al-Fatesh A, Ihara I, Rooney DW, Yap P-S (2023a) Artificial intelligence-based solutions for climate change: a review. Environ Chem Lett. https://doi.org/10.1007/s10311-023-01617-y
Article PubMed PubMed Central Google Scholar
Chen L, Huang LP, Hua JM, Chen ZH, Wei LL, Osman AI, Fawzy S, Rooney DW, Dong L, Yap PS (2023b) Green construction for low-carbon cities: a review. Environ Chem Lett 21:1627–1657. https://doi.org/10.1007/s10311-022-01544-4
Chen L, Msigwa G, Yang M, Osman AI, Fawzy S, Rooney DW, Yap P-S (2022b) Strategies to achieve a carbon neutral society: a review. Environ Chem Lett 20:2277–2310. https://doi.org/10.1007/s10311-022-01435-8
Article CAS PubMed PubMed Central Google Scholar
Chen LY, Gao X, Hua CX, Gong ST, Yue AB (2021a) Evolutionary process of promoting green building technologies adoption in China: a perspective of government. J Clean Prod 279:123607. https://doi.org/10.1016/j.jclepro.2020.123607
Chen S, Li Z, Li W (2021b) Integrating high share of renewable energy into power system using customer-sited energy storage. Renew Sustain Energy Rev 143:110893. https://doi.org/10.1016/j.rser.2021.110893
Cifuentes-Faura J (2022) European Union policies and their role in combating climate change over the years. Air Qual Atmos Health 15:1333–1340. https://doi.org/10.1007/s11869-022-01156-5
Clark GG, Jamal R, Weidhaas J (2019) Roofing material and irrigation frequency influence microbial risk from consuming homegrown lettuce irrigated with harvested rainwater. Sci Total Environ 651:1011–1019. https://doi.org/10.1016/j.scitotenv.2018.09.277
Article CAS PubMed Google Scholar
D’Agostino D, Mazzella S, Minelli F, Minichiello F (2022a) Obtaining the NZEB target by using photovoltaic systems on the roof for multi-storey buildings. Energy Build 267:112147. https://doi.org/10.1016/j.enbuild.2022.112147
D’Agostino D, Minichiello F, Petito F, Renno C, Valentino A (2022b) Retrofit strategies to obtain a NZEB using low enthalpy geothermal energy systems. Energy 239:122307. https://doi.org/10.1016/j.energy.2021.122307
D’Agostino D, Parker D, Melia P, Dotelli G (2022c) Optimizing photovoltaic electric generation and roof insulation in existing residential buildings. Energy Build 255:111652. https://doi.org/10.1016/j.enbuild.2021.111652
Danish MSS, Senjyu T (2023a) AI-enabled energy policy for a sustainable future. Sustainability 15:7643. https://doi.org/10.3390/su15097643
Danish MSS, Senjyu T (2023b) Shaping the future of sustainable energy through AI-enabled circular economy policies. Circ Econ 2:100040. https://doi.org/10.1016/j.cec.2023.100040
de Gracia A (2019) Dynamic building envelope with PCM for cooling purposes—proof of concept. Appl Energy 235:1245–1253. https://doi.org/10.1016/j.apenergy.2018.11.061
De Masi RF, Festa V, Gigante A, Ruggiero S, Vanoli GP (2022) Analysis of COVID-19 crisis-related building energy retrofit incentives in Italy. Energy Rep 8:378–383. https://doi.org/10.1016/j.egyr.2022.10.220
Dehghani Madvar M, Alhuyi Nazari M, Tabe Arjmand J, Aslani A, Ghasempour R, Ahmadi MH (2018) Analysis of stakeholder roles and the challenges of solar energy utilization in Iran. Int J Low-Carbon Technol 13:438–451. https://doi.org/10.1093/ijlct/cty044
Dey S, Sreenivasulu A, Veerendra GTN, Rao KV, Babu PSSA (2022) Renewable energy present status and future potentials in India: an overview. Innov Green Dev 1:100006. https://doi.org/10.1016/j.igd.2022.100006
Deymi-Dashtebayaz M, Baranov IV, Nikitin A, Davoodi V, Sulin A, Norani M, Nikitina V (2022) An investigation of a hybrid wind-solar integrated energy system with heat and power energy storage system in a near-zero energy building-a dynamic study. Energy Convers Manag 269:116085. https://doi.org/10.1016/j.enconman.2022.116085
Diwania S, Agrawal S, Siddiqui AS, Singh S (2020) Photovoltaic–thermal (PV/T) technology: a comprehensive review on applications and its advancement. Int J Energy Environ Eng 11:33–54. https://doi.org/10.1007/s40095-019-00327-y
Durganjali CS, Bethanabhotla S, Kasina S, Radhika DS (2020) Recent developments and future advancements in solar panels technology. J Phys Conf Ser 1495:012018. https://doi.org/10.1088/1742-6596/1495/1/012018
Ebrahimi-Moghadam A, Farzaneh-Gord M (2023) A sustainable optimal biomass waste-driven CCHP system to boost the nearly zero energy building concept. Energy Convers Manag 277:116669. https://doi.org/10.1016/j.enconman.2023.116669
Economidou M, Todeschi V, Bertoldi P, D’Agostino D, Zangheri P, Castellazzi L (2020) Review of 50 years of EU energy efficiency policies for buildings. Energy Build 225:110322. https://doi.org/10.1016/j.enbuild.2020.110322
Farghali M, Osman AI, Chen Z, Abdelhaleem A, Ihara I, Mohamed IMA, Yap P-S, Rooney DW (2023a) Social, environmental, and economic consequences of integrating renewable energies in the electricity sector: a review. Environ Chem Lett 21:1381–1418. https://doi.org/10.1007/s10311-023-01587-1
Farghali M, Osman AI, Mohamed IMA, Chen Z, Chen L, Ihara I, Yap P-S, Rooney DW (2023) Strategies to save energy in the context of the energy crisis: a review. Environ Chem Lett. https://doi.org/10.1007/s10311-023-01591-5
Franco MAJQ, Pawar P, Wu XY (2021) Green building policies in cities: a comparative assessment and analysis. Energy Build 231:110561. https://doi.org/10.1016/j.enbuild.2020.110561
Furubayashi T, Nakata T (2021) Analysis of woody biomass utilization for heat, electricity, and CHP in a regional city of Japan. J Clean Prod 290:125665. https://doi.org/10.1016/j.jclepro.2020.125665
Gad R, Mahmoud H, Ookawara S, Hassan H (2022) Energy, exergy, and economic assessment of thermal regulation of PV panel using hybrid heat pipe-phase change material cooling system. J Clean Prod 364:132489. https://doi.org/10.1016/j.jclepro.2022.132489
Garcia-Saez I, Mendez J, Ortiz C, Loncar D, Becerra JA, Chacartegui R (2019) Energy and economic assessment of solar organic rankine cycle for combined heat and power generation in residential applications. Renew Energy 140:461–476. https://doi.org/10.1016/j.renene.2019.03.033
Ghadami N, Gheibi M, Kian Z, Faramarz MG, Naghedi R, Eftekhari M, Fathollahi-Fard AM, Dulebenets MA, Tian GD (2021) Implementation of solar energy in smart cities using an integration of artificial neural network, photovoltaic system and classical Delphi methods. Sustain Cities Soc 74:103149. https://doi.org/10.1016/j.scs.2021.103149
Ghaffarian Hoseini A, Dahlan ND, Berardi U, GhaffarianHoseini A, Makaremi N, GhaffarianHoseini M (2013) Sustainable energy performances of green buildings: a review of current theories, implementations and challenges. Renew Sustain Energy Rev 25:1–17. https://doi.org/10.1016/j.rser.2013.01.010
Gielen D, Boshell F, Saygin D, Bazilian MD, Wagner N, Gorini R (2019) The role of renewable energy in the global energy transformation. Energy Strategy Rev 24:38–50. https://doi.org/10.1016/j.esr.2019.01.006
Gold R (2021) Status report on electrification policy: where to next? Curr Sustain Renew Energy Rep 8:114–122. https://doi.org/10.1007/s40518-021-00180-w
Gong Q, Kou F, Sun X, Zou Y, Mo J, Wang X (2022) Towards zero energy buildings: a novel passive solar house integrated with flat gravity-assisted heat pipes. Appl Energy 306:117981. https://doi.org/10.1016/j.apenergy.2021.117981
Gorjian S, Sharon H, Ebadi H, Kant K, Scavo FB, Tina GM (2021) Recent technical advancements, economics and environmental impacts of floating photovoltaic solar energy conversion systems. J Clean Prod 278:124285. https://doi.org/10.1016/j.jclepro.2020.124285
Gottlieb SC, Frederiksen N, Molby LF, Fredslund L, Primdahl MB, Rasmussen TV (2023) Roadmap for the transition to biogenic building materials: a socio-technical analysis of barriers and drivers in the Danish construction industry. J Clean Prod 414:137554. https://doi.org/10.1016/j.jclepro.2023.137554
Guo SY, Yan D, Hu S, Zhang Y (2021) Modelling building energy consumption in China under different future scenarios. Energy 214:119063. https://doi.org/10.1016/j.energy.2020.119063
Hajiaghasi S, Salemnia A, Hamzeh M (2019) Hybrid energy storage system for microgrids applications: a review. J Energy Storage 21:543–570. https://doi.org/10.1016/j.est.2018.12.017
Hartmann H, Lenz V (2019) Biomass energy heat provision in modern small-scale systems. In: Kaltschmitt M (ed) Springer New York, New York, NY, pp 533–586. https://doi.org/10.1007/978-1-4939-7813-7_248
Hdeib R, Aouad M (2023) Rainwater harvesting systems: an urban flood risk mitigation measure in arid areas. Water Sci Eng 16:219–225. https://doi.org/10.1016/j.wse.2023.04.0041674-2370
He JX, Zhu RQ, Lin BQ (2019) Prospects, obstacles and solutions of biomass power industry in China. J Clean Prod 237:117783. https://doi.org/10.1016/j.jclepro.2019.117783
Hiloidhari M, Sharno MA, Baruah DC, Bezbaruah AN (2023) Green and sustainable biomass supply chain for environmental, social and economic benefits. Biomass Bioenerg 175:106893. https://doi.org/10.1016/j.biombioe.2023.106893
Hoang AT, Pham VV, Nguyen XP (2021a) Integrating renewable sources into energy system for smart city as a sagacious strategy towards clean and sustainable process. J Clean Prod 305:127161. https://doi.org/10.1016/j.jclepro.2021.127161
Hoang AT, Sandro N, Olcer AI, Ong HC, Chen WH, Chong CT, Thomas S, Bandh SA, Nguyen XP (2021b) Impacts of COVID-19 pandemic on the global energy system and the shift progress to renewable energy: opportunities, challenges, and policy implications. Energy Policy 154:112322. https://doi.org/10.1016/j.enpol.2021.112322
Hossain MU, Ng ST, Antwi-Afari P, Amor B (2020) Circular economy and the construction industry: existing trends, challenges and prospective framework for sustainable construction. Renew Sustain Energy Rev 130:109948. https://doi.org/10.1016/j.rser.2020.109948
Hou S, Guo J, Yu Z, Zhang L, Sun J (2023) Optimization of biomass energy coupled multi-source heat pumps cooperative heating system: a case study. Energy Sources Part a Recovery Util Environ Eff 45:8153–8166. https://doi.org/10.1080/15567036.2023.2223179
Hu G, Liu LB, Tao DC, Song J, Tse KT, Kwok KCS (2020) Deep learning -based investigation of wind pressures on tall building under interference effects. J Wind Eng Ind Aerodyn 201:104138. https://doi.org/10.1016/j.jweia.2020.104138
Hu Y, Cheng H, Tao S (2021) Opportunity and challenges in large-scale geothermal energy exploitation in China. Crit Rev Environ Sci Technol 52:3813–3834. https://doi.org/10.1080/10643389.2021.1971004
Iacobuţă GI, Brandi C, Dzebo A, Elizalde Duron SD (2022) Aligning climate and sustainable development finance through an SDG lens. The role of development assistance in implementing the Paris Agreement. Glob Environ Chang 74:102509. https://doi.org/10.1016/j.gloenvcha.2022.102509
Igliński B, Pietrzak MB, Kiełkowska U, Skrzatek M, Kumar G, Piechota G (2022) The assessment of renewable energy in Poland on the background of the world renewable energy sector. Energy 261:125319. https://doi.org/10.1016/j.energy.2022.125319
Inês C, Guilherme PL, Esther M-G, Swantje G, Stephen H, Lars H (2020) Regulatory challenges and opportunities for collective renewable energy prosumers in the EU. Energy Policy 138:111212. https://doi.org/10.1016/j.enpol.2019.111212
Infield D, Freris L (2020) Renewable energy in power systems. https://www.wiley.com/en-us/Renewable+Energy+in+Power+Systems-p-9780470017494
Ionescu C, Baracu T, Vlad GE, Necula H, Badea A (2015) The historical evolution of the energy efficient buildings. Renew Sustain Energy Rev 49:243–253. https://doi.org/10.1016/j.rser.2015.04.062
Izam NSMN, Itam Z, Sing WL, Syamsir A (2022) Sustainable development perspectives of solar energy technologies with focus on solar photovoltaic-a review. Energies 15:2790. https://doi.org/10.3390/en15082790
Jafari SAH, Kwok KCS, Safaei F, Kosasih B, Zhao M (2019) Aerodynamic analysis of a stator-augmented linear cascade wind turbine. Wind Energy 22:1148–1163. https://doi.org/10.1002/we.2346
Jiang S, Wei XK, Jia JY, Ma GF (2022a) Toward sustaining the development of green residential buildings in China: a tripartite evolutionary game analysis. Build Environ 223:109466. https://doi.org/10.1016/j.buildenv.2022.109466
Jiang T, Zhang Y, Olayiwola S, Lau C, Fan M, Ng K, Tan G (2022b) Biomass-derived porous carbons support in phase change materials for building energy efficiency: a review. Mater Today Energy 23:100905. https://doi.org/10.1016/j.mtener.2021.100905
Juan YH, Rezaeiha A, Montazeri H, Blocken B, Wen CY, Yang AS (2022) CFD assessment of wind energy potential for generic high-rise buildings in close proximity: impact of building arrangement and height. Appl Energy 321:119328. https://doi.org/10.1016/j.apenergy.2022.119328
Kannan N, Vakeesan D (2016) Solar energy for future world: - a review. Renew Sustain Energy Rev 62:1092–1105. https://doi.org/10.1016/j.rser.2016.05.022
Karasmanaki E (2022) Is it safe to live near wind turbines? reviewing the impacts of wind turbine noise. Energy Sustain Dev 69:87–102. https://doi.org/10.1016/j.esd.2022.05.012
Khan SA, Al-Ghamdi SG (2021) Renewable and integrated renewable energy systems for buildings and their environmental and socio-economic sustainability assessment. In: (ed) Springer, pp 127–144. https://doi.org/10.1007/978-3-030-67529-5_6
Kılıç U, Kekezoğlu B (2022) A review of solar photovoltaic incentives and policy: selected countries and Turkey. Ain Shams Eng J 13:101669. https://doi.org/10.1016/j.asej.2021.101669
Kim C (2021) A review of the deployment programs, impact, and barriers of renewable energy policies in Korea. Renew Sustain Energy Rev 144:110870. https://doi.org/10.1016/j.rser.2021.110870
Kim H, Junghans L (2023) Economic feasibility of achieving net-zero emission building (NZEB) by applying solar and geothermal energy sources to heat pump systems: a case in the United States residential sector. J Clean Prod 416:137822. https://doi.org/10.1016/j.jclepro.2023.137822
Knäble D, de Quevedo PE, Pérez-Cornejo C, Baumgärtler T (2022) The impact of the circular economy on sustainable development: a European panel data approach. Sustain Prod Consum 34:233–243. https://doi.org/10.1016/j.spc.2022.09.016
Kumar Y, Ringenberg J, Depuru SS, Devabhaktuni VK, Lee JW, Nikolaidis E, Andersen B, Afjeh A (2016) Wind energy: trends and enabling technologies. Renew Sustain Energy Rev 53:209–224. https://doi.org/10.1016/j.rser.2015.07.200
Kumar JCR, Majid MA (2020) Renewable energy for sustainable development in India: current status, future prospects, challenges, employment, and investment opportunities. Energy, Sustain Soc 10:2. https://doi.org/10.1186/s13705-019-0232-1
Kwok KCS, Hu G (2023) Wind energy system for buildings in an urban environment. J Wind Eng Ind Aerodyn 234:105349. https://doi.org/10.1016/j.jweia.2023.105349
Las-Heras-Casas J, López-Ochoa LM, Paredes-Sánchez JP, López-González LM (2018) Implementation of biomass boilers for heating and domestic hot water in multi-family buildings in Spain: energy, environmental, and economic assessment. J Clean Prod 176:590–603. https://doi.org/10.1016/j.jclepro.2017.12.061
Li H, Ni L, Yao Y, Sun C (2020) Annual performance experiments of an earth-air heat exchanger fresh air-handling unit in severe cold regions: operation, economic and greenhouse gas emission analyses. Renew Energy 146:25–37. https://doi.org/10.1016/j.renene.2019.06.058
Lin P, Ding F, Hu G, Li C, Xiao Y, Tse KT, Kwok KCS, Kareem A (2022) Machine learning-enabled estimation of crosswind load effect on tall buildings. J Wind Eng Ind Aerodyn. https://doi.org/10.1016/j.jweia.2021.104860
Liu B, Rodriguez D (2021) Renewable energy systems optimization by a new multi-objective optimization technique: a residential building. J Build Eng 35:102094. https://doi.org/10.1016/j.jobe.2020.102094
Liu Y, Yan G, Albdeiri MS, Singh Chauhan B, Salah B, Saleem W, A. El-Sayed T, Li J (2023) Techno-economic analysis and optimization of near-zero energy and emission neighborhoods using biomass waste. Chemosphere 334:138978. https://doi.org/10.1016/j.chemosphere.2023.138978
Liu Z, Deng Z, Davis S, Ciais P (2023b) Monitoring global carbon emissions in 2022. Nat Rev Earth Environ 4:205–206. https://doi.org/10.1038/s43017-023-00406-z
Liuzzi S, Rubino C, Martellotta F, Stefanizzi P, Casavola C, Pappalettera G (2020) Characterization of biomass-based materials for building applications: the case of straw and olive tree waste. Ind Crop Prod 147:112229. https://doi.org/10.1016/j.indcrop.2020.112229
Lizana J, Chacartegui R, Barrios-Padura A, Ortiz C (2018) Advanced low-carbon energy measures based on thermal energy storage in buildings: a review. Renew Sustain Energy Rev 82:3705–3749. https://doi.org/10.1016/j.rser.2017.10.093
Lu YH, Khan ZA, Alvarez-Alvarado MS, Zhang Y, Huang ZJ, Imran M (2020) A critical review of sustainable energy policies for the promotion of renewable energy sources. Sustainability 12:5078. https://doi.org/10.3390/su12125078
Maghrabie MH, Elsaid K, Sayed ET, Abdelkareem MA, Wilberforce T, Olabi AG (2021) Building-integrated photovoltaic/thermal (BIPVT) systems: applications and challenges. Sustain Energy Technol Assess 45:101151. https://doi.org/10.1016/j.seta.2021.101151
Megahed TF, Radwan A (2020) Performance investigation of zero-building-integrated photovoltaic roof system: a case study in Egypt. Alex Eng J 59:5053–5067. https://doi.org/10.1016/j.aej.2020.09.031
Meinshausen M, Lewis J, McGlade C, Gutschow J, Nicholls Z, Burdon R, Cozzi L, Hackmann B (2022) Realization of Paris Agreement pledges may limit warming just below 2 degrees C. Nature 604:304–309. https://doi.org/10.1038/s41586-022-04553-z
Mellit A, Kalogirou SA (2014) MPPT-based artificial intelligence techniques for photovoltaic systems and its implementation into field programmable gate array chips: review of current status and future perspectives. Energy 70:1–21. https://doi.org/10.1016/j.energy.2014.03.102
Miglioli A, Aste N, Del Pero C, Leonforte F (2023) Photovoltaic-thermal solar-assisted heat pump systems for building applications: integration and design methods. Energy Built Environ 4:39–56. https://doi.org/10.1016/j.enbenv.2021.07.002
Mourad AHI, Almomani A, Sheikh IA, Elsheikh AH (2023) Failure analysis of gas and wind turbine blades: a review. Eng Fail Anal 146:107107. https://doi.org/10.1016/j.engfailanal.2023.107107
Nag U, Sharma SK, Padhi SS (2022) Evaluating value requirement for industrial product-service system in circular economy for wind power-based renewable energy firms. J Clean Prod 340:130689. https://doi.org/10.1016/j.jclepro.2022.130689
Nardecchia F, Groppi D, Garcia DA, Bisegna F, de Santoli L (2021) A new concept for a mini ducted wind turbine system. Renew Energy 175:610–624. https://doi.org/10.1016/j.renene.2021.04.097
Neij L, Sandin S, Benner M, Johansson M, Mickwitz P (2021) Bolstering a transition for a more sustainable energy system: a transformative approach to evaluations of energy efficiency in buildings. Energy Res Soc Sci 72:101864. https://doi.org/10.1016/j.erss.2020.101864
Nie YZ, Deng MS, Shan M, Yang XD (2023) Clean and low-carbon heating in the building sector of China: 10-year development review and policy implications. Energy Policy 179:113659. https://doi.org/10.1016/j.enpol.2023.113659
Noorollahi Y, Khatibi A, Eslami S (2021) Replacing natural gas with solar and wind energy to supply the thermal demand of buildings in Iran: a simulation approach. Sustain Energy Technol Assess 44:101047. https://doi.org/10.1016/j.seta.2021.101047
Osman AI, Chen L, Yang M, Msigwa G, Farghali M, Fawzy S, Rooney DW, Yap P-S (2023) Cost, environmental impact, and resilience of renewable energy under a changing climate: a review. Environ Chem Lett 21:741–764. https://doi.org/10.1007/s10311-022-01532-8
Ottonelli J, Lazaro LLB, Andrade JCS, Abram S (2023) Do solar photovoltaic clean development mechanism projects contribute to sustainable development in Latin America? prospects for the Paris Agreement. Energy Policy 174:113428. https://doi.org/10.1016/j.enpol.2023.113428
Palacio M, Rincon A, Carmona M (2020) Experimental comparative analysis of a flat plate solar collector with and without PCM. Sol Energy 206:708–721. https://doi.org/10.1016/j.solener.2020.06.047
Palmero-Marrero AI, Gomes F, Sousa J, Oliveira AC (2020) Energetic analysis of a thermal building using geothermal and solar energy sources. Energy Rep 6:201–206. https://doi.org/10.1016/j.egyr.2020.11.268
Palraj M, Rajamanickam P (2020) Motion control of a barge for offshore wind turbine (OWT) using gyrostabilizer. Ocean Eng 209:107500. https://doi.org/10.1016/j.oceaneng.2020.107500
Pan S, Kong YL, Chen CF, Pang ZH, Wang JY (2020) Optimization of the utilization of deep borehole heat exchangers. Geotherm Energy 8:6. https://doi.org/10.1186/s40517-020-0161-4
Paris Agreement (2015) Paris Agreement. https://unfccc.int/process-and-meetings/the-paris-agreement
Peng J, Yan J, Zhai Z, Markides CN, Lee ES, Eicker U, Zhao X, Kuhn TE, Sengupta M, Taylor RA (2020a) Solar energy integration in buildings. Appl Energy 264:114740. https://doi.org/10.1016/j.apenergy.2020.114740
Peng Z, Deng W, Tenorio R (2020b) An integrated low-energy ventilation system to improve indoor environment performance of school buildings in the cold climate zone of China. Build Environ 182:107153. https://doi.org/10.1016/j.buildenv.2020.107153
Philp J (2018) The bioeconomy, the challenge of the century for policy makers. New Biotechnol 40:11–19. https://doi.org/10.1016/j.nbt.2017.04.004
Pognant F, Bo M, Nguyen CV, Salizzoni P, Clerico M (2018) Design, modelling and assessment of emission scenarios resulting from a network of wood biomass boilers. Environ Model Assess 23:157–164. https://doi.org/10.1007/s10666-017-9563-5
Rafique A, Williams AP (2021) Reducing household greenhouse gas emissions from space and water heating through low-carbon technology: identifying cost-effective approaches. Energy Build 248:111162. https://doi.org/10.1016/j.enbuild.2021.111162
Rahman H, Sharif MR, Ahmed R, Nijam T, Shoeb MA (2015) Designing of biomass based power plant for residential building energy system. In: 2015 International conference on electrical engineering and information communication technology (ICEEICT), pp 1–6. https://doi.org/10.1109/ICEEICT.2015.7307366
Ramli MAM, Bouchekara HREH, Alghamdi AS (2018) Optimal sizing of PV/wind/diesel hybrid microgrid system using multi-objective self-adaptive differential evolution algorithm. Renew Energy 121:400–411. https://doi.org/10.1016/j.renene.2018.01.058
Rathore N, Panwar NL (2022) Outline of solar energy in India: advancements, policies, barriers, socio-economic aspects and impacts of COVID on solar industries. Int J Ambient Energy 43:7630–7642. https://doi.org/10.1080/01430750.2022.2075925
Raza S, Orooji Y, Ghasali E, Hayat A, Karimi-Maleh H, Lin H (2023) Engineering approaches for CO 2 converting to biomass coupled with nanobiomaterials as biomediated towards circular bioeconomy. J CO2 Util 67:102295. https://doi.org/10.1016/j.jcou.2022.102295
Rezaeiha A, Montazeri H, Blocken B (2020) A framework for preliminary large-scale urban wind energy potential assessment: roof-mounted wind turbines. Energy Convers Manag 214:112770. https://doi.org/10.1016/j.enconman.2020.112770
Roga S, Bardhan S, Kumar Y, Dubey SK (2022) Recent technology and challenges of wind energy generation: a review. Sustain Energy Technol Assess 52:102239. https://doi.org/10.1016/j.seta.2022.102239
Romano AA, Scandurra G, Carfora A, Fodor M (2017) Renewable investments: the impact of green policies in developing and developed countries. Renew Sustain Energy Rev 68:738–747. https://doi.org/10.1016/j.rser.2016.10.024
Romanov D, Leiss B (2022) Geothermal energy at different depths for district heating and cooling of existing and future building stock. Renew Sustain Energy Rev. https://doi.org/10.1016/j.rser.2022.112727
Romanov D, Leiss B (2022b) Geothermal energy at different depths for district heating and cooling of existing and future building stock. Renew Sustain Energy Rev 167:112727. https://doi.org/10.1016/j.rser.2022.112727
Salam RA, Amber KP, Ratyal NI, Alam M, Akram N, Munoz CQG, Marquez FPG (2020) An overview on energy and development of energy integration in major South Asian Countries: the building sector. Energies 13:5776. https://doi.org/10.3390/en13215776
Scheller F, Doser I, Schulte E, Johanning S, McKenna R, Bruckner T (2021) Stakeholder dynamics in residential solar energy adoption: findings from focus group discussions in Germany. Energy Res Soc Sci 76:102065. https://doi.org/10.1016/j.erss.2021.102065
Shah AR, Krarti M, Huang J (2022) Energy performance evaluation of shallow ground source heat pumps for residential buildings. Energies 15:1025. https://doi.org/10.3390/en15031025
Shahbaz M, Topcu BA, Sarigul SS, Vo XV (2021) The effect of financial development on renewable energy demand: the case of developing countries. Renew Energy 178:1370–1380. https://doi.org/10.1016/j.renene.2021.06.121
Shi J, Kong D (2021) Floor heave mechanism and anti-slide piles control technology in deep and large-span chamber. Appl Sci 11:4576. https://doi.org/10.3390/app11104576
Shirinbakhsh M, Harvey LDD (2023) Feasibility of achieving net-zero energy performance in high-rise buildings using solar energy. Energy Built Environ. https://doi.org/10.1016/j.enbenv.2023.07.007
Shukla AK, Sudhakar K, Baredar P, Mamat R (2018) Solar PV and BIPV system: barrier, challenges and policy recommendation in India. Renew Sustain Energy Rev 82:3314–3322. https://doi.org/10.1016/j.rser.2017.10.013
Sikiru S, Oladosu TL, Amosa TI, Kolawole SY, Soleimani H (2022) Recent advances and impact of phase change materials on solar energy: a comprehensive review. J Energy Storage 53:105200. https://doi.org/10.1016/j.est.2022.105200
Sinha S, Chandel SS, Malik P (2021) Investigation of a building-integrated solar photovoltaic-wind-battery hybrid energy system: a case study. Int J Energy Res 45:21534–21539. https://doi.org/10.1002/er.7184
Şirin C, Goggins J, Hajdukiewicz M (2023) A review on building-integrated photovoltaic/thermal systems for green buildings. Appl Therm Eng 229:120607. https://doi.org/10.1016/j.applthermaleng.2023.120607
Sobri S, Koohi-Kamali S, Abd Rahim N (2018) Solar photovoltaic generation forecasting methods: a review. Energy Convers Manag 156:459–497. https://doi.org/10.1016/j.enconman.2017.11.019
Solangi YA, Cheng LS, Shah SAA (2021) Assessing and overcoming the renewable energy barriers for sustainable development in Pakistan: an integrated AHP and fuzzy TOPSIS approach. Renew Energy 173:209–222. https://doi.org/10.1016/j.renene.2021.03.141
Solaymani S (2021) A review on energy and renewable energy policies in Iran. Sustainability 13:7328. https://doi.org/10.3390/su13137328
Song AT, Lu L, Liu ZZ, Wong MS (2016) A study of incentive policies for building-integrated photovoltaic technology in Hong Kong. Sustainability 8:769. https://doi.org/10.3390/su8080769
Soto A, Martinez PJ, Martinez P, Tudela JA (2021) Simulation and experimental study of residential building with north side wind tower assisted by solar chimneys. J Build Eng 43:102562. https://doi.org/10.1016/j.jobe.2021.102562
Stathopoulos T, Alrawashdeh H, Al-Quraan A, Blocken B, Dilimulati A, Paraschivoiu M, Pilay P (2018) Urban wind energy: some views on potential and challenges. J Wind Eng Ind Aerodyn 179:146–157. https://doi.org/10.1016/j.jweia.2018.05.018
Taherahmadi J, Noorollahi Y, Panahi M (2021) Toward comprehensive zero energy building definitions: a literature review and recommendations. Int J Sustain Energ 40:120–148. https://doi.org/10.1080/14786451.2020.1796664
Tajjour S, Singh Chandel S (2023) A comprehensive review on sustainable energy management systems for optimal operation of future-generation of solar microgrids. Sustain Energy Technol Assess 58:103377. https://doi.org/10.1016/j.seta.2023.103377
Tan QL, Ding YH, Zheng J, Dai M, Zhang YM (2021) The effects of carbon emissions trading and renewable portfolio standards on the integrated wind-photovoltaic-thermal power-dispatching system: real case studies in China. Energy 222:119927. https://doi.org/10.1016/j.energy.2021.119927
Tester JW, Beckers KF, Hawkins AJ, Lukawski MZ (2021) The evolving role of geothermal energy for decarbonizing the United States. Energy Environ Sci 14:6211–6241. https://doi.org/10.1039/d1ee02309h
Topcu M, Tugcu CT (2020) The impact of renewable energy consumption on income inequality: evidence from developed countries. Renew Energy 151:1134–1140. https://doi.org/10.1016/j.renene.2019.11.103
Tori F, Bustamante W, Vera S (2022) Analysis of net zero energy buildings public policies at the residential building sector: a comparison between Chile and selected countries. Energy Policy 161:112707. https://doi.org/10.1016/j.enpol.2021.112707
Vasiliev M, Nur-E-Alam M, Alameh K (2019) Recent developments in solar energy-harvesting technologies for building integration and distributed energy generation. Energies 12:1080. https://doi.org/10.3390/en12061080
Vassiliades C, Savvides A, Buonomano A (2022) Building integration of active solar energy systems for facades renovation in the urban fabric: effects on the thermal comfort in outdoor public spaces in Naples and Thessaloniki. Renew Energy 190:30–47. https://doi.org/10.1016/j.renene.2022.03.094
Wainer A, Petrovics D, van der Grijp N (2022) The grid access of energy communities a comparison of power grid governance in France and Germany. Energy Policy 170:113159. https://doi.org/10.1016/j.enpol.2022.113159
Walker C, Devine-Wright P, Rohse M, Gooding L, Devine-Wright H, Gupta R (2021) What is ‘local’ about smart local energy systems? emerging stakeholder geographies of decentralised energy in the United Kingdom. Energy Res Soc Sci 80:102182. https://doi.org/10.1016/j.erss.2021.102182
Wang BH, Klemes JJ, Malasek J, Hemzal M, Liang YT, Zeng M (2021a) A novel turbine ventilator with a damper regulator to adjust exhausted air for energy-saving in buildings. J Build Eng 38:102141. https://doi.org/10.1016/j.jobe.2020.102141
Wang C, Gu J, Martinez OS, Crespo RG (2021b) Economic and environmental impacts of energy efficiency over smart cities and regulatory measures using a smart technological solution. Sustain Energy Technol Assess 47:101422. https://doi.org/10.1016/j.seta.2021.101422
Wang K, Masiol M, Thimmaiah D, Zhang Y, Hopke PK (2017) Performance evaluation of two 25 kW residential wood pellet boiler heating systems. Energy Fuels 31:12174–12182. https://doi.org/10.1021/acs.energyfuels.7b01868
Wang K, Satyro MA, Taylor R, Hopke PK (2018) Thermal energy storage tank sizing for biomass boiler heating systems using process dynamic simulation. Energy Build 175:199–207. https://doi.org/10.1016/j.enbuild.2018.07.023
Wei M, Lee SH, Hong TZ, Conlon B, McKenzie L, Hendron B, German A (2021) Approaches to cost-effective near-net zero energy new homes with time-of-use value of energy and battery storage. Adv Appl Energy 2:100018. https://doi.org/10.1016/j.adapen.2021.100018
Wei PY, Bamisile O, Adun H, Cai DS, Obiora S, Li J, Huang Q (2023) Bibliographical progress in hybrid renewable energy systems’ integration, modelling, optimization, and artificial intelligence applications: a critical review and future research perspective. Energy Sources Part a-Recovery Util Environ Eff 45:2058–2088. https://doi.org/10.1080/15567036.2023.2181888
Welsch B, Gollner-Volker L, Schulte DO, Bar K, Sass I, Schebek L (2018) Environmental and economic assessment of borehole thermal energy storage in district heating systems. Appl Energy 216:73–90. https://doi.org/10.1016/j.apenergy.2018.02.011
Wen LL, Zhou KL, Yang SL, Lu XH (2019) Optimal load dispatch of community microgrid with deep learning based solar power and load forecasting. Energy 171:1053–1065. https://doi.org/10.1016/j.energy.2019.01.075
Woon KS, Phuang ZX, Taler J, Varbanov PS, Chong CT, Klemes JJ, Lee CT (2023) Recent advances in urban green energy development towards carbon emissions neutrality. Energy 267:126502. https://doi.org/10.1016/j.energy.2022.126502
Wu W, Skye HM (2021) Residential net-zero energy buildings: review and perspective. Renew Sustain Energy Rev 142:110859. https://doi.org/10.1016/j.rser.2021.110859
Wu X, Yang C, Han W, Pan Z (2022) Integrated design of solar photovoltaic power generation technology and building construction based on the Internet of Things. Alex Eng J 61:2775–2786. https://doi.org/10.1016/j.aej.2021.08.003
Xie B-C, Zhang R-Y, Chen X-P (2023) China’s optimal development pathway of intermittent renewable power towards carbon neutrality. J Clean Prod 406:136903. https://doi.org/10.1016/j.jclepro.2023.136903
Yana S, Nizar M, Irhamni MD (2022) Biomass waste as a renewable energy in developing bio-based economies in Indonesia: a review. Renew Sustain Energy Rev 160:112268. https://doi.org/10.1016/j.rser.2022.112268
Yang CW, Kwon H, Bang B, Jeong S, Lee UD (2022a) Role of biomass as low-carbon energy source in the era of net zero emissions. Fuel 328:125206. https://doi.org/10.1016/j.fuel.2022.125206
Yang M, Chen L, Wang J, Msigwa G, Osman AI, Fawzy S, Rooney DW, Yap P-S (2022b) Circular economy strategies for combating climate change and other environmental issues. Environ Chem Lett. https://doi.org/10.1007/s10311-022-01499-6
Ye W, Ma E, Liao L, Hui Y, Liang S, Ji Y, Yu S (2023) Applicability of photovoltaic panel rainwater harvesting system in improving water-energy-food nexus performance in semi-arid areas. Sci Total Environ 896:164938. https://doi.org/10.1016/j.scitotenv.2023.164938
Yousefi H, Abbaspour A, Seraj H (2019) Worldwide development of wind energy and CO 2 emission reduction. Environ Energy Econ Res 3:1–9. https://doi.org/10.22097/eeer.2019.164295.1064
Yousefi Roshan M, Khaleghinia J, Eshagh Nimvari M, Salarian H (2021) Performance improvement of Darrieus wind turbine using different cavity layouts. Energy Convers Manag 246:114693. https://doi.org/10.1016/j.enconman.2021.114693
Zeh R, Ohlsen B, Philipp D, Bertermann D, Kotz T, Jocic N, Stockinger V (2021) Large-scale geothermal collector systems for 5th generation district heating and cooling networks. Sustainability. https://doi.org/10.3390/su13116035
Zhang S, Oclon P, Klemes JJ, Michorczyk P, Pielichowska K, Pielichowski K (2022) Renewable energy systems for building heating, cooling and electricity production with thermal energy storage. Renew Sustain Energy Rev 165:112560. https://doi.org/10.1016/j.rser.2022.112560
Zhang SC, Fu YJ, Yang XY, Xu W (2021) Assessment of mid-to-long term energy saving impacts of nearly zero energy building incentive policies in cold region of China. Energy Build 241:110938. https://doi.org/10.1016/j.enbuild.2021.110938
Zhang WJ, Liu S, Li NP, Xie H, Li XQ (2015) Development forecast and technology roadmap analysis of renewable energy in buildings in China. Renew Sustain Energy Rev 49:395–402. https://doi.org/10.1016/j.rser.2015.04.135
Zhang Z, Liu X, Zhao D, Post S, Chen J (2023) Overview of the development and application of wind energy in New Zealand. Energy Built Environ 4:725–742. https://doi.org/10.1016/j.enbenv.2022.06.009
Zhao XG, Wang W, Wu L (2021) A dynamic analysis of research and development incentive on China’s photovoltaic industry based on system dynamics model. Energy 233:121141. https://doi.org/10.1016/j.energy.2021.121141
Download references
Acknowledgements
Dr. Ahmed I. Osman and Prof. David W. Rooney wish to acknowledge the support of the Bryden Centre project (project ID VA5048), which was awarded by The European Union’s INTERREG VA Program, managed by the Special EU Programs Body (SEUPB), with match funding provided by the Department for the Economy in Northern Ireland and the Department of Business, Enterprise, and Innovation in the Republic of Ireland.
This work was supported by the SEUPB, Bryden Centre project (project ID VA5048).
Author information
Authors and affiliations.
School of Civil Engineering, Chongqing University, Chongqing, 400045, China
Lin Chen, Ying Hu, Ruiyi Wang, Xiang Li, Jianmin Hua & Lepeng Huang
Key Laboratory of New Technology for Construction of Cities in Mountain Area, Ministry of Education, Chongqing University, Chongqing, 400045, China
Department of Civil Engineering, Xi’an Jiaotong-Liverpool University, Suzhou, 215123, China
Zhonghao Chen, Jingjing Li & Pow-Seng Yap
School of Chemistry and Chemical Engineering, Queen’s University Belfast, Belfast, Northern Ireland, BT9 5AG, UK
Ahmed I. Osman & David W. Rooney
Department of Agricultural Engineering and Socio-Economics, Kobe University, Kobe, 657-8501, Japan
Mohamed Farghali
Department of Animal and Poultry Hygiene and Environmental Sanitation, Faculty of Veterinary Medicine, Assiut University, Assiut, 71526, Egypt
Department of Public and International Affairs, City University of Hong Kong, Kowloon Tong, 999077, Hong Kong, Special Administrative Region of China
School of Energy and Environment, City University of Hong Kong, Kowloon Tong, 999077, Hong Kong, Special Administrative Region of China
Sustainable Development Lab, Centre for Public Affairs and Law, City University of Hong Kong, Kowloon Tong, 999077, Hong Kong, Special Administrative Region of China
You can also search for this author in PubMed Google Scholar
Contributions
LC was involved in conceptualization, writing, and review and editing. YH, RW, XL, ZC, and JH were involved in data gathering, writing, and review. AIO was involved in writing, review and editing, and supervision. MF was involved in writing, review and editing, and data editing. LH was involved in writing and review. JL and LD were involved in data gathering, writing, and review. DWR and PSY were involved in review and editing and supervision. LC, YH, RW, and XL jointly conceived the study and led the writing of the article with equal contributions. All other authors have contributed to data collection and analysis, interpretation of results, and writing of the article and are listed in alphabetical order.
Corresponding authors
Correspondence to Jianmin Hua , Ahmed I. Osman or Pow-Seng Yap .
Ethics declarations
Conflict of interest.
The authors declare that they have no known competing financial interests or personal relationships that could have appeared to influence the work reported in this paper.
Additional information
Publisher's note.
Springer Nature remains neutral with regard to jurisdictional claims in published maps and institutional affiliations.
Rights and permissions
Open Access This article is licensed under a Creative Commons Attribution 4.0 International License, which permits use, sharing, adaptation, distribution and reproduction in any medium or format, as long as you give appropriate credit to the original author(s) and the source, provide a link to the Creative Commons licence, and indicate if changes were made. The images or other third party material in this article are included in the article's Creative Commons licence, unless indicated otherwise in a credit line to the material. If material is not included in the article's Creative Commons licence and your intended use is not permitted by statutory regulation or exceeds the permitted use, you will need to obtain permission directly from the copyright holder. To view a copy of this licence, visit http://creativecommons.org/licenses/by/4.0/ .
Reprints and permissions
About this article
Chen, L., Hu, Y., Wang, R. et al. Green building practices to integrate renewable energy in the construction sector: a review. Environ Chem Lett 22 , 751–784 (2024). https://doi.org/10.1007/s10311-023-01675-2
Download citation
Received : 10 October 2023
Accepted : 16 November 2023
Published : 15 December 2023
Issue Date : April 2024
DOI : https://doi.org/10.1007/s10311-023-01675-2
Share this article
Anyone you share the following link with will be able to read this content:
Sorry, a shareable link is not currently available for this article.
Provided by the Springer Nature SharedIt content-sharing initiative
- Green building
- Carbon neutral construction
- Renewable energy
- Energy transition
- Energy self-sufficiency
- Find a journal
- Publish with us
- Track your research
The Rise of Electric Vehicles and Their Integration in the Energy Systems
- Center for Integrated Mobility Sciences
Research output : Contribution to conference › Paper
NREL Publication Number
- NREL/CP-5400-81402
- electric vehicles
- sustainable mobility
This output contributes to the following UN Sustainable Development Goals (SDGs)
Access to Document
- https://www.ier.unam.mx/~eogm/isres2020/assets/img/teachers/Muratori%20-ISRES2021.pdf
Fingerprint
- Energy Systems Engineering 100%
- Integration Engineering 100%
- Electric Vehicle Engineering 100%
- Crude Oil Material Science 100%
- Battery (Electrochemical Energy Engineering) Material Science 50%
- Fuel Material Science 50%
- Greenhouse Gas Material Science 50%
- Renewables Engineering 33%
T1 - The Rise of Electric Vehicles and Their Integration in the Energy Systems
AU - Muratori, Matteo
N2 - Transportation is currently the least-diversified energy demand sector, with over 90% of energy use coming from petroleum. As a result, transportation recently became the largest source of GHG emissions in the U.S. and mobility needs for passenger and freight are growing rapidly. However, after over a century of petroleum dominance, new disruptive technologies and business models offer a pathway to decarbonize the sector. Transportation is at a turning point. On the horizon lies a future where affordable and abundant renewable electricity can be used to power cost-competitive battery electric vehicles (EVs) and produce energy-dense low-carbon fuels enabling to fully decarbonize transportation systems across all modes. EVs are experiencing a rapid rise in popularity over the past few years as the technology has matured and costs have declined, and support for clean transportation has promoted awareness, increased charging opportunities, and facilitated EV adoption. Large-scale EV adoption would both disrupt the transportation sector and lead to far-reaching consequences for electricity systems including new opportunities for significant load growth. And the integration of EVs into power systems presents unique opportunities for synergistic improvement of the efficiency and economics of mobility and the power grid, especially complementing renewables. The demand-side flexibility provided by managed EV charging offers significant potential benefits for the grid over multiple timescales and applications. Managed charging can support power system planning and operations during normal and extreme conditions, benefitting EV owners and other electricity consumers alike. At NREL, we're laying the scientific groundwork to get there. Among many other things, our research is finding ways for EVs to support grid planning and operations across several timescales and to fully exploit the synergies between EVs and renewables to provide transformative sustainable solutions for the integrated energy systems of the future.
AB - Transportation is currently the least-diversified energy demand sector, with over 90% of energy use coming from petroleum. As a result, transportation recently became the largest source of GHG emissions in the U.S. and mobility needs for passenger and freight are growing rapidly. However, after over a century of petroleum dominance, new disruptive technologies and business models offer a pathway to decarbonize the sector. Transportation is at a turning point. On the horizon lies a future where affordable and abundant renewable electricity can be used to power cost-competitive battery electric vehicles (EVs) and produce energy-dense low-carbon fuels enabling to fully decarbonize transportation systems across all modes. EVs are experiencing a rapid rise in popularity over the past few years as the technology has matured and costs have declined, and support for clean transportation has promoted awareness, increased charging opportunities, and facilitated EV adoption. Large-scale EV adoption would both disrupt the transportation sector and lead to far-reaching consequences for electricity systems including new opportunities for significant load growth. And the integration of EVs into power systems presents unique opportunities for synergistic improvement of the efficiency and economics of mobility and the power grid, especially complementing renewables. The demand-side flexibility provided by managed EV charging offers significant potential benefits for the grid over multiple timescales and applications. Managed charging can support power system planning and operations during normal and extreme conditions, benefitting EV owners and other electricity consumers alike. At NREL, we're laying the scientific groundwork to get there. Among many other things, our research is finding ways for EVs to support grid planning and operations across several timescales and to fully exploit the synergies between EVs and renewables to provide transformative sustainable solutions for the integrated energy systems of the future.
KW - electric vehicles
KW - sustainable mobility
T2 - International Symposium on Renewable Energy and Sustainability 2020
Y2 - 8 November 2021 through 12 November 2021
- IPR Intranet
INSTITUTE FOR POLICY RESEARCH
- Working Papers
Energy Transitions in Regulated Markets (WP-24-12)
Gautam Gowrisankaran, Ashley Langer, and Mar Reguant
Gautam Gowrisankaran , Professor of Economics, Columbia University
Ashley Langer , Associate Professor of Economics, University of Arizona
Mar Reguant , Professor of Economics and IPR Associate, Northwestern University
Download PDF
ORIGINAL RESEARCH article
Dual-network fiber-hydrogel membrane for osmotic energy harvesting provisionally accepted.
- 1 Donghua University, China
The final, formatted version of the article will be published soon.
Osmotic energy harvesting was a promising way to alleviate energy crisis with reverse electrodialysis (RED) membrane-based technology. Charged hydrogel combined with other materials was an effective strategy to overcome problems, including restricted functional groups and complicated fabrication, but the effect of the respective charges of the two materials combined on the membrane properties has rarely been studied in depth. Herein, a new method was proposed that charged hydrogel was equipped with charged filter paper to form dual network fiber-hydrogel membrane for osmotic energy harvesting, which had excellent ion selectivity (beyond 0.9 under high concentration gradient), high ion transference number and energy conversion efficiency (beyond 32.5% under wide range concentration gradient), good property of osmotic energy conversion (~4.84 W/m2 under 50-fold KCl and ~6.75 W/m2 under simulated sea water and river water). Moreover, the power density was attributed to the surface-space charge synergistic effect from large amounts overlapping of electric double layer (EDL), so that the transmembrane ion transport was enhanced. It might be a valid mode to extensively develop the osmotic energy harvesting.
Keywords: Dual-network, Fiber-Hydrogel Membrane, osmotic, Energy Harvesting, membrane
Received: 16 Mar 2024; Accepted: 09 Apr 2024.
Copyright: © 2024 LiCheng and Huiqing. This is an open-access article distributed under the terms of the Creative Commons Attribution License (CC BY) . The use, distribution or reproduction in other forums is permitted, provided the original author(s) or licensor are credited and that the original publication in this journal is cited, in accordance with accepted academic practice. No use, distribution or reproduction is permitted which does not comply with these terms.
* Correspondence: Prof. Wu Huiqing, Donghua University, Shanghai, China
People also looked at
Print Preview
U.s. department of energy - energy efficiency and renewable energy, alternative fuels data center.
- Printable Version
- Production & Distribution
- Research & Development
- Benefits & Considerations
- Laws & Incentives
Electric Vehicle Research and Development
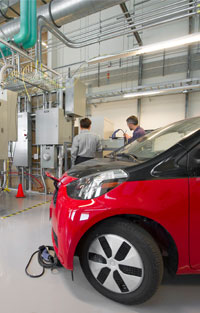
The U.S. Department of Energy (DOE) is working with its partners in the public and private sectors to research, develop, demonstrate, and deploy technologies that enhance the performance of electric-drive vehicles.
Environmental and Market Analysis
Electric vehicles (EVs) and hybrid electric vehicles (HEVs) offer reduced operating costs, fuel savings, and environmental benefits. For example, the National Renewable Energy Laboratory (NREL) and Idaho National Laboratory report, Levelized Cost of Charging Electric Vehicles in the United States , finds that driving an EV instead of a comparable conventional vehicle, can save a driver as much as $14,500 on fuel costs over 15 years. Additionally, Argonne National Laboratory’s (ANL) Autonomie model demonstrates that an all-electric vehicle is more than three times more efficient than its conventional counterpart. In Assessment of Light-Duty Plug-In Electric Vehicles in the United States, 2010-2019 , ANL estimates the electricity generation for the operation of an all-electric vehicle produces 53% less emissions than the tailpipe emissions from the operation of a gasoline vehicle.
Additionally, ANL’s An Extensive Study on Sizing, Energy Consumption, and Cost of Advanced Vehicle Technologies report demonstrates significant improvements in fuel economy over time. By 2045, HEVs could achieve a 43% to 81% improvement in fuel economy and PHEVs could achieve a 73% to 96% improvement in fuel economy. In its National Economic Value Assessment of Plug-In Electric Vehicles , NREL uses a scenario approach to estimate costs and benefits of increased EV market growth across the United States. Under various EV adoption scenarios, NREL estimates a 22% to 36% decrease in greenhouse gas emission reductions.
Further, the widespread adoption of EVs will require a robust network of charging stations, from home-based AC charging to DC powered extreme fast charging. Researchers are examining opportunities and impacts associated with a full range of charging technologies.
See the following resources for more information related to research in environmental and market analysis:
- Electric Vehicle Grid Integration
- Vehicle Electrification
- Transportation Energy Data Book: Edition 40
- National Economic Value Assessment of Plug-In Electric Vehicles
- An Extensive Study on Sizing, Energy Consumption, and Cost of Advanced Vehicle Technologies
- Cradle-to-Grave Lifecycle Analysis of U.S. Light-Duty Vehicle-Fuel Pathways: A Greenhouse Gas Emissions and Economic Assessment of Current (2015) and Future (2025-2030) Technologies
Maps & Data
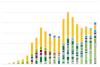
More Electricity Data | All Maps & Data
Case Studies
- Electric School Buses Clear the Air in the Mid-Atlantic
- Seattle Rideshare Fleet Adds EVs, Enjoys Success
- Massachusetts School Fleets Get Answers through Electric Bus Testing
More Electricity Case Studies | All Case Studies
Publications
- Electric Vehicles (Conceptos básicos sobre los vehículos eléctricos)
- Duluth Transit Authority Battery-Electric Bus Evaluation
- Foothill Transit Battery Electric Bus Demonstration Results: Second Report
More Electricity Publications | All Publications
- Laws and Incentives Search
- Electric Vehicle Charging Station Locations

Registration Opens for Energy & Power Research Day to Be Held on April 23, 2024
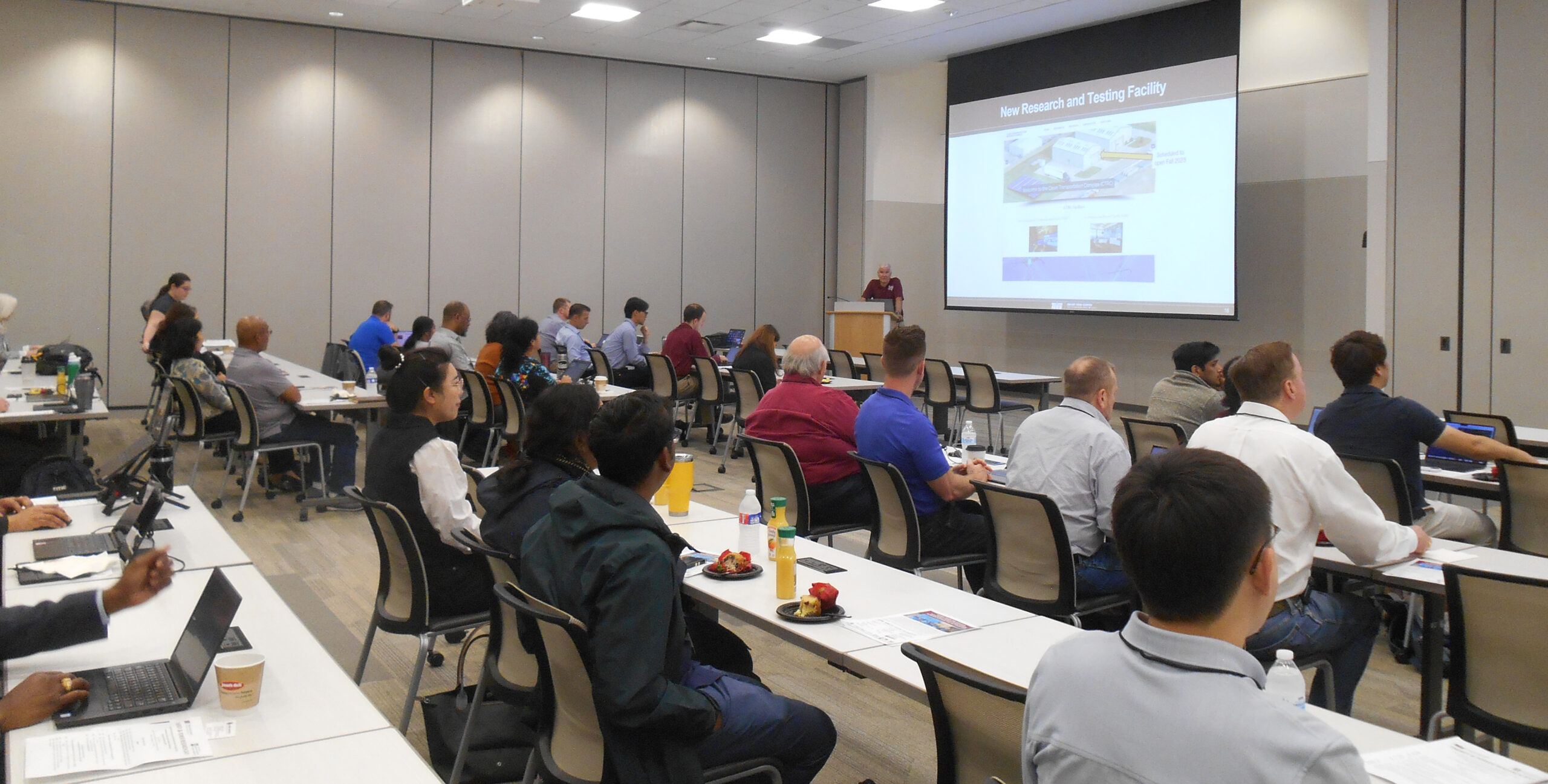
The next Energy & Power Research Day will be held in person at the Center for Infrastructure (CIR) on RELLIS campus on April 23, 2024. It will include presentations on current projects, demonstrations, and networking with industry. Sponsors of the event are the Smart Grid Center (SGC) of Texas Engineering Experiment Station (TEES) and the Energy and Power Group (EPG) of the Department of Electrical and Computer Engineering, Texas A&M University.
Regular registration costs $75 until April 16, 2024. Then, standard registration fee is $100/person until April 23, 2024. It is free for TEES Smart Grid Center member companies, TAMU faculty, and TAMU students. For relevant discount codes, please contact [email protected] .
REGISTER HERE!
Research session 1 (tom overbye, session chair) (10:35 to 11:50, room 1107-1108), lunch (pre-function area and room 1107-1108), control room hands-on activities and demos (rooms 3339 and 3350), research session 2 (jonathan snodgrass, session chair) (13:00 to 15:00, room 1107-1108), research session 3 (farnaz safdarian, session chair) (15:00 to 16:00, room 1107-1108), register now.
We have a room block reserved at rate of $94/night at Hampton Inn College Station (320 Texas Ave., S., College Station, TX 7784) for April 22, 2024. Booking your room is simple, just go here and select “Book a Room” to receive your group’s preferred rate.

IMAGES
VIDEO
COMMENTS
Electric Power Systems Research is an international medium for the publication of original papers concerned with the generation, transmission, distribution and utilization of electrical energy. The journal aims at presenting important results of work in this field, whether in the form of applied research, development of new procedures or ...
Forecasting has been an essential part of the power and energy industry. Researchers and practitioners have contributed thousands of papers on forecasting electricity demand and prices, and renewable generation (e.g., wind and solar power). This article offers a brief review of influential energy forecasting papers; summarizes research trends; discusses importance of reproducible research and ...
Wind energy is the second major preference of renewable energy for electricity generation after hydro power [103] due to its relatively simple/easy infrastructure, cost-effectiveness, and maturity ... paper, and food production [197]. Download : Download high-res image (229KB) Download : Download full-size image; Fig. 22. Hydrokinetic System ...
Energy Science & Engineering is a sustainable energy journal publishing high-impact fundamental and applied research that will help secure an ... attention and many studies have focused on using solar energy for electricity generation. ... and parabolic trough collectors. In this paper, solar thermal technologies including soar trough ...
The appraising emanation and extension of renewable energy in contemporary scenario spatulation of electricity through natural resources (wind, solar, hydropower) has proliferate. In 2022, 41.4% of energy proportions stationed is through renewable sources. In accordance with statistics only 3.2% of the summation electricity is being generated by nuclear sources in 2021. Because of ...
A total of eighty-one (81) state-of-the-art research works published in journals, conferences, and magazines, and student's thesis relevant to the scope of the current study were downloaded from the internet, thus using keywords and terms which included AI, Electricity Prediction (EP), Energy Forecasting (EF), Machine Learning (ML), and combination of AI and EP, AI and EF, ML and EP, ML and EF.
Since CO 2 emissions are the main cause of global warming, the best way to tackle it is to focus on the sectors that have contributed most to these emissions, namely transport and power generation. Switching to Renewable Energy Sources (RES) with the electric vehicles is apparently the best option toward a sustainable future. In addition, changing the traditional fuel based transports with ...
These challenges can be met by developing an efficient energy storage system and developing cheap, efficient, and abundant PV solar cells. This article discusses the solar energy system as a whole and provides a comprehensive review on the direct and the indirect ways to produce electricity from solar energy and the direct uses of solar energy.
Finally, research is generally focused on one narrow aspect of a larger problem; we argue that energy research needs a more integrated approach 156 (Fig. 3f). Energy policy is the manner in which ...
Abstract and Figures. This paper presents a discussion of the future of the electric energy system, addressing the entire spectrum from power generation, through substations, to distribution and ...
The aim of the paper is to ascertain if renewable energy sources are sustainable and examine how a shift from fossil fuel-based energy sources to renewable energy sources would help reduce climate change and its impact. A qualitative research was employed by reviewing peer-reviewed papers in the area of study.
The use of renewable energy resources, such as solar, wind, and biomass will not diminish their availability. Sunlight being a constant source of energy is used to meet the ever-increasing energy need. This review discusses the world's energy needs, renewable energy technologies for domestic use, and highlights public opinions on renewable energy. A systematic review of the literature was ...
In another research paper a new approach to integrate complementarity between RES in planning 100% renewable energy-based systems has been presented . A new objective function is proposed to optimize the combination of the output power of renewable resources and the water flow of hydropower reservoirs, considering daily and annual variability.
Hydro power is currently the largest renewable energy source for power generation around the world. Hydro electricity generation has had a strong increase over the past 50 years. It was 340 terawatt-hour (TWh) in 1950 and covered about one-third of the global electricity demand. It increased to 1,500 TWh in 1975 and further to 2,994 in 2005.
The Philippines is making a significant stride to become energy independent by developing more sustainable sources of energy. However, investment in renewable energy is challenged by competitive oil prices, very high investment cost for renewable energy, and high local electricity prices. This paper evaluates the attractiveness of investing in renewable energy sources over continue using oil ...
The fossil sourced energy, for example, electricity, curtailment behavior or energy efficiency behavior is proceeded 49,50,51,52,53,54. However, research focusing on energy consumption, subjective ...
To be specific, four papers discuss behavior interventions and energy saving, two papers explore rebound effects, and one paper deals with transportation; another big block is concerned with environmental impact, with five papers on climate and one paper on resources and biodiversity. ... the research on energy poverty and electricity ...
67. JRTE-2020. Solar Energy Technology. Sumedha R.G. Weliwaththage, Udara S.P.R. Arach chige. Faculty of Technology, University of Sri Jayewardenepura, Sri Lanka. Abstract Energy resources can ...
The major sources of renewable. energy include solar, wind, biomass, geothermal, hydropower. and tidal energy. Non renewable energy, such as coal, natural. gas and oil, require costly explorations ...
The use of fossil fuels in electricity generation is one of the major contributors to greenhouse gas emissions (GHG) worldwide (Intergovernmental Panel on Climate Change 2014).A large de-carbonization of the energy system is necessary to reduce and stabilize carbon dioxide (CO 2) and other GHG emissions in the atmosphere (IPCC 2014).A portfolio of de-carbonization strategies and technologies ...
The building sector is significantly contributing to climate change, pollution, and energy crises, thus requiring a rapid shift to more sustainable construction practices. Here, we review the emerging practices of integrating renewable energies in the construction sector, with a focus on energy types, policies, innovations, and perspectives. The energy sources include solar, wind, geothermal ...
Call for Papers Advanced Power Converters, Protection, and Control for Electric Systems. Submission deadline: Tuesday, 31 December 2024 Expected Publication Month: May 2025 . Advanced power converters, protection, and control are attracting increasing attention due to the rapid growth of renewable energy and electric vehicles in electric power ...
Transportation is at a turning point. On the horizon lies a future where affordable and abundant renewable electricity can be used to power cost-competitive battery electric vehicles (EVs) and produce energy-dense low-carbon fuels enabling to fully decarbonize transportation systems across all modes.
Working Papers; Energy Transitions in Regulated Markets (WP-24-12) Energy Transitions in Regulated Markets (WP-24-12) Gautam Gowrisankaran, Ashley Langer, and Mar Reguant. Natural gas has replaced coal as the dominant fuel for U.S. electricity generation. However, U.S. states that regulate electric utilities have retired coal more slowly than ...
More specifically, the research on Electrical engineering in the conference is related to Transformer. Concepts in Wind power, as well as related topics in Wind speed, are covered in the Electric power system research presented in IEEE International Conference on Power and Energy. What are the most cited papers published at the conference?
Osmotic energy harvesting was a promising way to alleviate energy crisis with reverse electrodialysis (RED) membrane-based technology. Charged hydrogel combined with other materials was an effective strategy to overcome problems, including restricted functional groups and complicated fabrication, but the effect of the respective charges of the two materials combined on the membrane properties ...
By 2045, HEVs could achieve a 43% to 81% improvement in fuel economy and PHEVs could achieve a 73% to 96% improvement in fuel economy. In its National Economic Value Assessment of Plug-In Electric Vehicles, NREL uses a scenario approach to estimate costs and benefits of increased EV market growth across the United States.
In order to reduce energy consumption, improve driving mileage, and make vehicles adopt driver styles, research on automatic optimization of control strategy based on driver style is conducted in this paper. According to the structure of the powertrain, the vehicle control strategy is designed and a driver-style recognition model based on fuzzy recognition is added to the rule-based control ...
Sponsors of the event are the Smart Grid Center (SGC) of Texas Engineering Experiment Station (TEES) and the Energy and Power Group (EPG) of the Department of Electrical and Computer Engineering. Regular registration costs $75 until April 16, 2024. Then, standard registration fee is $100/person until April 23, 2024.