
Extract insights from Interviews. At Scale.
Artificial intelligence abstract for ppt: key points to cover.
Home » Artificial Intelligence Abstract for PPT: Key Points to Cover
Artificial Intelligence (AI) has revolutionized numerous industries, transforming the way we work, live, and interact. As we delve into an AI overview, several key highlights emerge that showcase its profound impact and potential. From machine learning algorithms to natural language processing, AI technologies continue to evolve at a rapid pace, offering unprecedented opportunities for innovation and efficiency.
In this presentation, we'll explore the fundamental concepts of AI, its current applications, and future prospects. We'll examine how AI is reshaping various sectors, including healthcare, finance, and manufacturing, while also addressing the ethical considerations and challenges that come with its widespread adoption. By understanding these AI overview highlights, we can better prepare for a future where intelligent machines play an increasingly significant role in our daily lives and business operations.
Core Components of an AI Overview Highlights
When crafting an AI overview for a presentation, it's crucial to highlight key components that provide a comprehensive understanding. Begin by introducing the fundamental concept of AI as a branch of computer science focused on creating intelligent machines. Explain how AI systems simulate human intelligence processes, including learning, reasoning, and self-correction.
Next, outline the core types of AI: narrow or weak AI, general AI, and superintelligent AI. Discuss machine learning as a subset of AI, emphasizing its ability to improve performance through experience. Highlight deep learning and neural networks as advanced techniques within machine learning. Address the ethical considerations surrounding AI development and implementation, including privacy concerns and potential job displacement. Finally, showcase real-world applications of AI across various industries, such as healthcare, finance, and transportation, to illustrate its transformative potential and current impact on society.
Defining Artificial Intelligence
Artificial Intelligence (AI) has emerged as a transformative force across industries, revolutionizing how we approach complex tasks and decision-making processes. At its core, AI refers to computer systems designed to mimic human intelligence, capable of learning, reasoning, and self-correction. These systems utilize advanced algorithms and vast amounts of data to perform tasks that typically require human cognition.
The field of AI encompasses various subsets, including machine learning, natural language processing, and computer vision. Machine learning enables systems to improve their performance over time without explicit programming. Natural language processing allows computers to understand, interpret, and generate human language. Computer vision empowers machines to analyze and interpret visual information from the world around them. Together, these components form the foundation of AI's capabilities, driving innovation and efficiency across diverse sectors.
Key Applications in Various Industries
Artificial Intelligence (AI) has become a transformative force across various industries, revolutionizing processes and unlocking new possibilities. In healthcare, AI-powered diagnostic tools assist medical professionals in detecting diseases earlier and with greater accuracy. Financial institutions harness AI algorithms for fraud detection and risk assessment, enhancing security and decision-making capabilities.
Manufacturing benefits from AI through predictive maintenance and quality control, optimizing production lines and reducing downtime. In retail, AI-driven personalization engines analyze customer data to deliver tailored product recommendations and improve shopping experiences. The transportation sector employs AI for route optimization and autonomous vehicle development, paving the way for safer and more efficient travel. As AI continues to evolve, its applications expand, promising innovative solutions to complex challenges across diverse fields.
Structuring Your PPT for an AI Overview Highlights
When crafting a PowerPoint presentation on AI, structuring your content effectively is crucial for engaging your audience. Begin by outlining the core components of artificial intelligence, such as machine learning, natural language processing, and computer vision. Next, highlight the transformative impact of AI across various industries, including healthcare, finance, and manufacturing.
Consider dedicating slides to real-world AI applications, showcasing how these technologies are solving complex problems and improving efficiency. Address both the benefits and potential challenges associated with AI adoption, such as ethical considerations and job market disruptions. Conclude your presentation with a forward-looking perspective, discussing emerging AI trends and their potential to shape our future. By organizing your PPT in this manner, you'll provide a comprehensive AI overview that captivates and informs your audience.
Essential Slides to Include
When crafting an AI overview for your presentation, it's crucial to highlight key points that capture the essence of artificial intelligence. Start with a slide defining AI and its core principles, emphasizing machine learning and neural networks. Follow this with a timeline showcasing AI's evolution, from early rule-based systems to today's deep learning models.
Next, dedicate a slide to AI's current applications across various industries, such as healthcare, finance, and transportation. Highlight how AI is transforming these sectors with concrete examples. Include a slide on the ethical considerations surrounding AI, touching on topics like bias in algorithms and data privacy concerns. Finally, conclude with a forward-looking slide discussing potential future developments in AI and their implications for society and business. This structure ensures a comprehensive yet concise AI overview that engages your audience and sparks meaningful discussions.
Best Practices for Presentation Design
When crafting an AI overview for a PowerPoint presentation, it's crucial to highlight key aspects that capture the essence of artificial intelligence. Begin by defining AI in clear, accessible terms, emphasizing its ability to mimic human intelligence and learn from data. Next, outline the main types of AI, such as narrow AI and general AI, to provide context for the audience.
Delve into the core components that power AI systems, including machine learning algorithms, neural networks, and deep learning. Illustrate these concepts with real-world applications, such as virtual assistants, autonomous vehicles, or predictive analytics in business. Address both the potential benefits and ethical considerations of AI adoption, touching on topics like improved efficiency, job displacement, and data privacy. Conclude your overview by discussing future trends and the potential impact of AI on various industries, encouraging viewers to consider its role in shaping our technological landscape.
Conclusion: Summarizing AI Overview Highlights
In summarizing the key points of our AI overview, several crucial aspects stand out. The potential of artificial intelligence to revolutionize various industries is evident, from healthcare to finance and beyond. However, it's essential to approach AI implementation with caution and ethical considerations.
As we've explored, AI's ability to process vast amounts of data and identify patterns offers unprecedented opportunities for innovation. Yet, challenges such as data privacy, algorithmic bias, and the need for human oversight remain significant concerns. Moving forward, striking a balance between harnessing AI's power and maintaining human-centric values will be paramount in shaping the future of this transformative technology.
Turn interviews into actionable insights
On this Page
Halo-Halo Business: Crafting a Unique Executive Summary
You may also like, generative ai consulting market: key insights.
Generative AI for product development: Top trends
Generative ai for hr: best tools to consider.
Unlock Insights from Interviews 10x faster
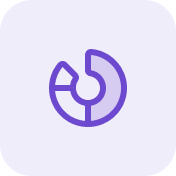
- Request demo
- Get started for free

- Conferences
- Last Updated: August 18, 2024
- In Top AI Tools
14 Best Presentations On Artificial Intelligence And Machine Learning in 2024

- by Jeevan Biswas
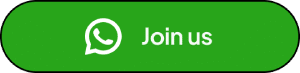
For a quick overview of a subject or a breakdown of concepts, SlideShare serves as a go-to platform for many. The recapitulations found in many of the presentations are both concise and informative.
The most popular presentations topics are the ones that have received the most number of likes and have been viewed more than the other presentations in a particular category.
AIM brings you the 14 most popular ppt topics on Artificial Intelligence, Machine Learning. Deep Learning and everything else in between.
Find: Top AI PPT Maker Tools
1) Artificial Intelligence and Law Overview
People who are not aware of what artificial intelligence is will find the topic presented in a very simple manner here.
Along with the explanation of what AI is, the two major approaches towards AI are discussed– logic and rules-based approach, and machine learning approach. Special emphasis on the machine learning approach can be seen in the slides devoted to its detailed examination. The examination goes beyond the rudimentary explanation of what machine learning is and presents examples of proxies that seem like machine learning but are not.
The presentation lists examples of AI in the field of law and identifies some of the limitations of AI technology.
2) What is Artificial Intelligence – Artificial Intelligence Tutorial For Beginners
For the uninitiated, this presentation offers an ideal rundown of AI. The question of AI being a threat is raised at the very beginning. However, as the presentation progresses, it discusses the basics necessary for understanding AI. The most basic question of what is artificial intelligence is answered.
A brief history of AI and the discussion on recent advances in the field of AI is also found. The various areas where AI currently sees practical application have been listed. Fascinating uses that AI can be put to in the future are also found in the presentation. The two approaches of achieving AI, machine learning and deep learning, is touched upon.
All in all, this presentation serves as a simple introduction to AI.
3) Why Social Media Chat Bots Are the Future of Communication
An exciting application of AI can be found in chatbots. Here, the limitless scope of chatbots is explored. The various milestones reached by leading players in bot technology such as Facebook, Skype and KIK are enumerated.
The evolution of chatbots and its absorption of more AI in the future is also looked into. E-Commerce is touted as the biggest beneficiary of the advancement in chatbots and that bot technology will owe its rise to services and commerce.
Two tech giants, Facebook and Google, have been pitted against each other based on their ongoing developments in this area and the question of who will emerge as the best is raised.
4) AI and the Future of Work
This presentation talks about the far-fetching applicability of AI and ML,and the perils of that applicability. In order to derive a better understanding of this presentation, it is advisable to first watch the original talk.
During the course of the presentation, many examples of how machines can learn and perform any human task that is repetitive in nature are cited.
Other possibilities suggested include the creation of new unheard jobs for human beings as a result of aggressive use of AI and other allied technologies. Qualities that are characteristic only of human beings, may be the basis on which these jobs will be created is also suggested.
It concludes with a message- Ride the train, don’t jump in front of it.
5) AI and Machine Learning Demystified
In this presentation, Carol Smith establishes that AI cannot replace humans. Smith conveys that AI can serve the purpose of enabling human beings in making better decisions.
The slides talk about how the actions of AI are the result of the human inputs going into its programming. An AI’s bias is not its own, but the human bias with which it has been programmed, is emphasised on.
Other issues such as the need for regulations and other considerations within it that require deliberation are also touched upon. The presentation leaves you with a message – Don’t fear AI, Explore it.
6) Study: The Future of VR, AR and Self-Driving Car
Though no descriptive breakdown of topics related to AI is found, the presentation offers interesting numerical insights into many questions. Statistics on three main subjects – artificial intelligence, virtual reality and wearable technology, is provided here.
A variety of questions and the numerical representations of their responses are found under four main categories:
- Will you purchase a self-driving car when they become available?
- Are you concerned with the rise of Artificial Intelligence?
- Is wearable technology part of your daily life?
- Do you own or intend to purchase a Virtual Reality headset in the next twelve months?
From consumer opinions to overall consensus of countries, the numbers show current trends and the possible trends in the future based on increasing development in the mentioned technologies.
7) Artificial Intelligence
There are many who have been introduced to AI only recently due to the buzz surrounding it and may not be aware of the early developments that led to its current status.
This presentation from 2009 offers a simple yet informative introduction to the rudiments of AI. AI’s history and a timeline of all the significant milestones in AI up to 2009 can be found. The presentation also provides an introduction to AI programming languages such as LISP and PROLOG.
For those who would like to have a crash course on the basics of AI in order to catch up with it current trends, this presentation serves the purpose.
8) Solve for X with AI: a VC view of the Machine Learning & AI landscape
While the concepts of AI or ML are not spoken about, light is shed on other important aspects of it. The presentation discusses about how many known tech giants such as Google are bolstering their AI capabilities through mergers and acquisitions.
The role of venture capital(VC) in the landscape of AI and machine learning,and the involvement of VC in the firms that were acquired are mentioned.
Another point highlighted is how large companies are moving towards ML and re-configuring themselves around ML, and how it is not a US-centric phenomenon. Key points have been expressed in the form of self-explanatory graphical representations. Rounding off the presentation is the possible direction that ML can take and a few pointers on achieving success in ML.
9) Deep Learning – The Past, Present and Future of Artificial Intelligence
This presentation provides a comprehensive insight into deep learning. Beginning with a brief history of AI and introduction to basics of machine learning such as its classification, the focus shifts towards deep learning entirely.
Various kinds of networks such as recurrent neural nets and generative adversarial networks have been discussed at length. Emphasis has been given to important aspects of these networks and other mechanisms such as natural language processing ( NLP ).
Detailed examples of practical applications and the scope of deep learning are found throughout the presentation. However, this presentation may prove difficult for first time learner’s of AI to comprehend.
10) The Future Of Work & The Work Of The Future
The subject of self-learning of robots and machines is explored here. Talking about the fictional Babel fish, it is suggested that the advancements in technology leading to improved learning and translations by machines made the Babel fish a near-real entity.
New ‘power’ values such as speed, networked governance, collaboration and transparency, among others, have been put forth and juxtaposed against older ones that are not fully technology driven.
Going against the popular assumption that robots and machines will replace human beings, the presentation proposes that we are on the brink of the largest job creation period in humanity.
11) Asia’s Artificial Intelligence Agenda
This presentation is a briefing paper by the MIT Technological Review and talks about how the global adoption of AI is being sped up by Asian countries. It suggests that Asia will not only benefit greatly from the rise in AI technology, but will also define it.
The data collected for the review has been summarized in the form of simple info-graphics. They are a numerical reflection of the mood surrounding the adoption of AI across different industries and how it could possibly impact human capital. The review also suggests that while there is awareness about AI in Asia, only a small percentage of companies are investing in it.
Pointers for business leaders in Asia to capitalize on AI is offered in the end along presentation with an info-graphic timeline of the history of AI.
Download review report in pdf
12) 10 Lessons Learned from Building Machine Learning Systems
While they are two separate presentations, they talk about the same subject- machine learning. The presentations are a summary of the analysis of machine learning adopted by two platforms, Netflix and Quora.
In case of Netflix, emphasis has been given to the choice of the right metric and the type of data used for testing and training. It also emphasises the need to understand the dependence between the data used and the models employed. The advice to optimize only areas that matter is offered.
The second presentation on Quora, talks about teaching machines only what is necessary. It stresses on the need the to focus on feature engineering and being thoughtful about the ML infrastructure. Another point it highlights is the combination of supervised and unsupervised being the key in ML application.
13) Design Ethics for Artificial Intelligence
With 135 slides, this presentation provides an exhaustive insight into the creation of an ethically sound AI. An introduction to the subject of User Experience(UX) design is followed by the rules that have to be considered during the designing process.
The chronological progression of UX, beginning with experience design and ending with intelligence design, and the direction in which this process is headed is also discussed.
Supported by powerful visuals, the presentation touches upon many essential considerations such as nature of intelligence, purpose of existence, awareness of self and the need for which the AI is created.
It raises a pertinent point that while creating AI, human beings are creating something that embodies qualities that they lack.
14) Artificial Intelligence
Made for a school competition in 2009, it provides many examples of cutting-edge applications of AI at the time.
Many of the examples, such as mind controlled prosthetic limbs, Ultra Hal Assistant and Dexter- the robot provide a trip down the AI memory lane where the applications of AI seemed like a page out of a sci-fi novel. It presents a list of areas where AI can assist human beings.
It concludes with a series of questions, some of which, are still being debated. Such as machines replacing human beings’ and human unemployment due to the use of machines.
📣 Want to advertise in AIM? Book here

Subscribe to The Belamy: Our Weekly Newsletter
Biggest ai stories, delivered to your inbox every week..
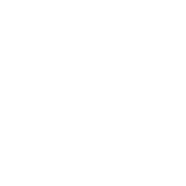
Discover how Cypher 2024 expands to the USA, bridging AI innovation gaps and tackling the challenges of enterprise AI adoption
© Analytics India Magazine Pvt Ltd & AIM Media House LLC 2024
- Terms of use
- Privacy Policy
Subscribe to Our Newsletter
The Belamy, our weekly Newsletter is a rage. Just enter your email below.
Subscribe to Our Youtube channel

AI Presentation Generator
AI Presentation Maker
AI Image Generator
WORD to PPT
Reports to PPT
Outline to PPT
Research Papers to PPT
AI PDF Summarizer
AI WORD Summarizer
AI File Summarizer
AI Document Summarizer
Convert to PPT
AI Summarizer
Convert Research Papers to PPT with AI
Summarize a Research Paper into a PowerPoint Presentation for quick understanding
Select and upload a Research Paper that needs to be summarized for a presentation.
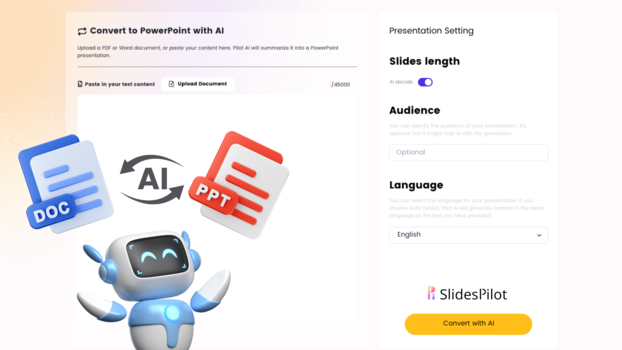
Choose from a variety of presentation template styles and select the one that best represents your content.
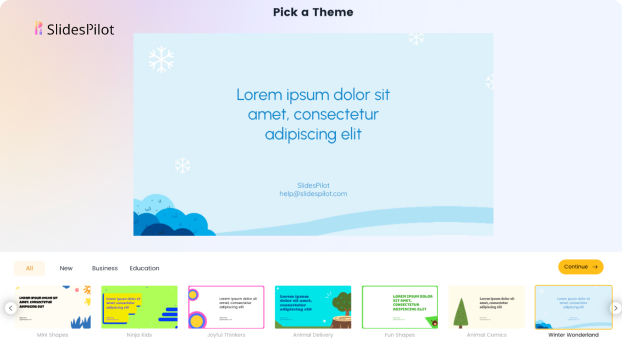
Relax and Watch the Magic Happen. Sit back and let AI do the heavy lifting for you! Get a customized design and stunning presentation filled with informative and professional content.
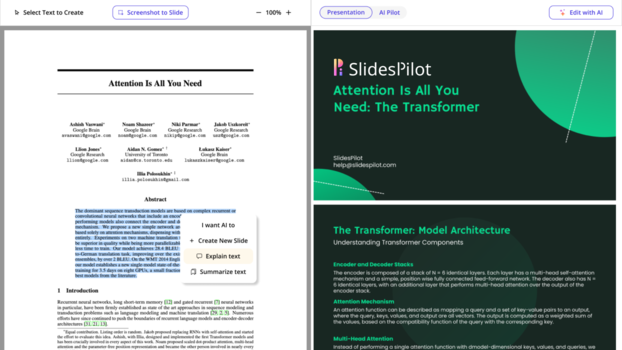
You can then edit the presentation using your preferred application, such as MS PowerPoint or Google Slides, or with our online AI Presentation Maker.
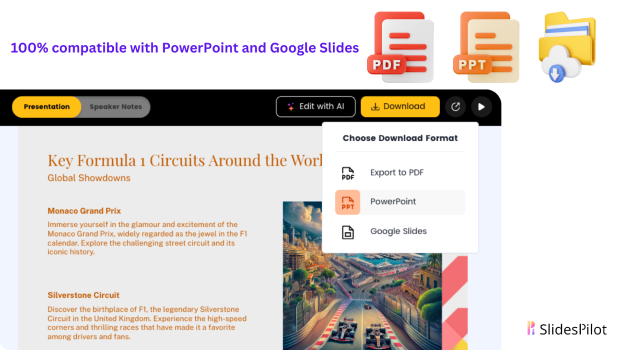
Superfast presentation creation
Join 1 million professionals, students, and educators
✓ Create with AI ✓ Convert to PPT with AI ✓ Compatible with PowerPoint ✓ Built in templates ✓ Auto Layout


An official website of the United States government
The .gov means it’s official. Federal government websites often end in .gov or .mil. Before sharing sensitive information, make sure you’re on a federal government site.
The site is secure. The https:// ensures that you are connecting to the official website and that any information you provide is encrypted and transmitted securely.
- Publications
- Account settings
Preview improvements coming to the PMC website in October 2024. Learn More or Try it out now .
- Advanced Search
- Journal List
- Digit Health
- v.9; Jan-Dec 2023
- PMC10328007
Improving accessibility of scientific research by artificial intelligence—An example for lay abstract generation
Boris schmitz.
1 Department of Rehabilitation Sciences, Faculty of Health, University of Witten/Herdecke, Witten, Germany
2 DRV Clinic Königsfeld, Center for Medical Rehabilitation, Ennepetal, Germany
The role of scientific research in modern society is essential for driving innovation, informing policy decisions, and shaping public opinion. However, communicating scientific findings to the general public can be challenging due to the technical and complex nature of scientific research. Lay abstracts are written summaries of scientific research that are designed to be easily understandable and provide a concise and clear overview of key findings and implications. Artificial intelligence language models have the potential to generate lay abstracts that are consistent and accurate while reducing the potential for misinterpretation or bias. This study presents examples of artificial intelligence-generated lay abstracts of recently published articles, which were produced using different currently available artificial intelligence tools. The generated abstracts were of high linguistic quality and accurately represented the findings of the original articles. Adopting lay summaries can increase the visibility, impact, and transparency of scientific research, and enhance scientists’ reputation among peers, while currently, available artificial intelligence models offer solutions to produce lay abstracts. However, the coherence and accuracy of artificial intelligence language models must be validated before they can be used for this purpose without restrictions.
Introduction
Scientific research plays a crucial role in modern society, driving innovation, informing policy decisions, and shaping public opinion. However, communicating scientific findings to the general population can be challenging, as scientific research is often highly technical and complex, requiring a deep understanding of specialized terminology and concepts. To bridge this gap and make scientific research more accessible to the general public, the concept of “lay abstracts” has been suggested as a powerful tool for communicating scientific work and findings.
Lay abstracts are written summaries of scientific research that are intended for a general audience with little or no background in the specific field. 1 , 2 They are designed to be easily understandable and should provide a concise and clear overview of the key findings and implications of the research. The concept of lay abstracts is not new, but it has gained increasing prominence in recent years as scientists and policymakers recognize the importance of engaging the public in scientific research and promoting scientific literacy. This is also of relevance, since Open Access Publishing is driven by the idea to make scientific research more accessible in general but has not yet overcome the hurdle of making the content of research papers more understandable to lay people including patients and patients’ representatives. 3 However, writing effective lay abstracts can be challenging. 4 Scientists must balance the need for accuracy and precision with the need for clarity and accessibility, and must find ways to explain complex scientific concepts in simple, easy-to-understand terms. Additionally, they must be aware of the potential for misinterpretation and ensure that their lay abstracts are clear and unambiguous. To write effective lay abstracts, scientists must first identify the key findings and implications of their research, and then distill this information into a concise, clear, and accessible format. Use of technical jargon and complex terminology should be avoided, and instead simple, everyday language that is easily understandable by the general public should be used. 1 , 2
Artificial intelligence (AI) is an emerging tool in multiple research areas including healthcare and has been suggested to assist communication also with patients. 5 – 8 Using AI language models to generate lay abstracts for scientific publications has the potential to ensure consistency and accuracy in the language used to describe scientific concepts, as well as reduce the potential for misinterpretation or bias. This can be done by the researchers themselves when producing a scientific report, but also by any interested reader after publication in almost any selected language. Additionally, AI language models can be trained on vast amounts of data, making them capable of generating lay abstracts that are tailored to specific audiences or fields of research, further improving accessibility and engagement. Furthermore, the use of AI will have an immense impact on healthcare education, offering the potential to generate summaries adapted to the knowledge level of student groups enabling them to access also advanced scientific content. 9
Here, examples of AI-generated lay abstracts of recently published articles are presented which were produced using currently available AI tools.
Using AI to generate lay abstracts
On 1 May 2023, three recent open-access original research articles from high-impact journals were randomly selected from Pubmed. 10 – 12 Articles and addressed research topics were selected by their potential public interest including patients, and an overall interested readership. Articles were then processed using the Google Chrome (Google, USA) extensions “Copilot” (1.0.5, SciSpace, India) using the “results of the paper” and “explain practical implications” options, and “Wiseone” (0.14.0, WiseOne, France) using the summarize option, as well as ChatGPT (Mar 23 release [free version, GPT-3.5 architecture], OpenAI OpCo, USA) using the prompt “Summarize the following text for a lay person” followed by pasting the introduction, results and discussion sections of the respective publication omitting tables, figures, references, and additional information.
Generated abstracts were between 115 and 200 words in length depending on the submitted article’s complexity, which is an appropriate length for a lay abstract. All applications produced summaries of high linguistic quality ( Table 1 ) within < 15 seconds. The user-friendliness of CoPilot and Wiseone was high since articles could be analyzed directly on the journal's website. The free version of ChatGPT is currently limited by the maximum number of tokens that can be used per query, affecting the input and output of text, which will not allow to summarize articles that exceed a certain word limit. While ChatGPT would require some knowledge about prompts to further extract information from the submitted text, CoPilot and Wiseone offer some additional options to select from. ChatGPT would translate into common languages upon prompt, while CoPilot offers a language selection which is missing in the current version of Wiseone. Wiseone also offers three bullet points (“key summaries”) ( Table 1 ) which support the summary of the text. Of note, generated abstracts were accurate in terms of study findings and highly congruent with the reported findings of the original articles. CoPilot and Wiseone made use of clear and easy-to-understand sentences from the original articles’ abstracts, adding some information from the full text. ChatGPT was able to produce lay abstracts from the provided full text without access to the abstract of the original article.
Table 1.
Lay abstracts produced by three different artificial intelligence (AI)-based language models.
Example 2 and 3: Iversen et al., Partial Oral Versus Intravenous Antibiotic Treatment of Endocarditis. |
The article discusses a study on patients with infective endocarditis on the left side of the heart. This condition is typically treated with intravenous antibiotics for up to 6 weeks, and patients require close monitoring during this time. The study looked at whether oral antibiotics could be a safe and efficient alternative to intravenous treatment for stable patients. The study enrolled 400 patients with endocarditis on the left side of the heart and randomly assigned them to receive either continued intravenous treatment or a shift to oral treatment. The study found that the two treatments had similar efficacy and safety, suggesting that oral antibiotics could be a viable alternative to intravenous treatment for certain patients. |
(1) A randomized, noninferiority, multicenter trial was conducted to determine if a partial oral antibiotic treatment for endocarditis was as effective and safe as continued intravenous treatment. The study found that partial oral treatment was noninferior to intravenous treatment in terms of efficacy and safety. (2) Partial oral treatment may offer benefits such as shorter hospital stays, reduced healthcare costs, and improved quality of life for patients. (3) The article describes a randomized, noninferiority, multicenter trial that investigated whether patients with infective endocarditis on the left side of the heart could be treated with oral antibiotics once they were in stable condition, instead of continuing intravenous treatment for up to 6 weeks. The study included 400 adults, and the results showed that partial oral antibiotic treatment was noninferior to intravenous treatment in terms of efficacy and safety. These findings suggest that a shift to oral antibiotics may be a viable option for treating infective endocarditis. |
The paper is about a study that compared the effectiveness and safety of continuing intravenous antibiotic treatment versus switching to oral antibiotic treatment in stable patients with infective endocarditis on the left side of the heart. The study found that switching to oral antibiotics was not associated with an increased risk of mortality in non-severely ill patients. Therefore, the results suggest that a shift from intravenous to oral antibiotics may be a safe and effective treatment option for stable patients with infective endocarditis on the left side of the heart. (1) It provides evidence that switching from intravenous to oral antibiotics may be a safe and effective treatment option for stable patients with infective endocarditis on the left side of the heart. (2) It helps to reduce the burden of intravenous treatment on patients and healthcare systems. (3) It adds to the existing knowledge about the treatment of infective endocarditis and may inform future clinical practice guidelines. |
Example 2: Nørgaard et al., Effect of Treadmill Perturbation-Based Balance Training on Fall Rates in Community-Dwelling Older Adults. |
This article reports on a study which investigated the effectiveness of treadmill perturbation-based balance training (PBT) in preventing falls among older adults living in the community. PBT is an exercise modality in which individuals are exposed to external disturbances in a safe environment with the goal of improving their reactive balance control. While previous studies have shown that PBT can produce substantial improvements in reactive balance control, the evidence regarding its effects on daily-life falls is inconsistent. In this study, participants in the PBT group completed four training sessions on a treadmill, while the control group performed regular treadmill walking. The study found that while there was no significant difference in daily-life fall rates between the two groups, the PBT group had significantly lower laboratory fall rates compared to the control group at the post-training assessment, 6-month follow-up, and 12-month follow-up. |
The paper found that a 4-session treadmill PBT intervention did not have a significant effect on daily-life fall rates among community-dwelling older adults. However, the study found a significant reduction in laboratory fall rates. The study suggests that an 80-minute PBT intervention may lead to a statistically nonsignificant 22% reduction in daily-life fall rates. These findings may have implications for fall prevention programs for older adults and may help guide the development of future interventions |
(1) Treadmill PBT did not significantly reduce daily-life fall rates in community-dwelling older adults, but did result in a significant decrease in falls in the laboratory setting. (2) PBT involves exposure to repeated external disturbances in a safe environment to improve reactive responses after slips and trips. (3) While PBT is considered a promising task-specific exercise modality for fall prevention, further research is needed to determine the optimal dose for retention and generalization of adaptations. A randomized clinical trial was conducted to evaluate the efficacy of a treadmill PBT intervention compared with regular treadmill walking in reducing daily-life fall rates among community-dwelling older adults. The study involved 140 participants aged 65 years or older who were able to walk without a walking aid. The results showed that the 4-session PBT intervention did not have a statistically significant effect on daily-life fall rates over a 12-month period. However, there was a significant reduction in laboratory fall rates. The study suggests the need for future research to investigate the possible effects of current treadmill PBT on daily-life falls. |
Example 3: McInnes et al., Bimekizumab in patients with psoriatic arthritis, naive to biologic treatment: a randomized, double-blind, placebo-controlled, phase 3 trial (BE OPTIMAL). |
Psoriatic arthritis is an immune-mediated inflammatory disease affecting the joints, skin, and nails. Patients usually take conventional drugs to alleviate symptoms, but if they are not effective, biological drugs may be prescribed. Bimekizumab is a new drug that can block interleukin (IL)-17A and IL-17F, which have been linked to the disease. Recent studies found that bimekizumab was effective in treating moderate-to-severe plaque psoriasis and psoriatic arthritis, with improvements lasting up to three years. The drug has been tested in two clinical trials, which have shown that it is superior to a placebo in treating psoriatic arthritis. Patients who took bimekizumab had a greater proportion of ACR50 and ACR70 responses, indicating significant improvement in joint and skin symptoms. Patients who switched from placebo to bimekizumab also showed improvement in their responses. The drug is considered safe and well-tolerated. |
The paper reports the results of a phase 3 clinical trial called BE OPTIMAL, which evaluated the effectiveness and safety of a drug called bimekizumab in treating psoriatic arthritis in patients who had not previously received biologic treatment. The study found that bimekizumab was more effective than placebo in reducing disease activity and improving symptoms, as well as inhibiting radiographic progression. The drug was generally well-tolerated, although Candida infections were commonly reported. The study was sponsored by UCB Pharma and registered with ClinicalTrials.gov. The practical implications of this paper are that bimekizumab may be an effective and safe treatment option for patients with psoriatic arthritis who have not previously received biologic therapy. The results of this study suggest that bimekizumab can reduce disease activity, improve symptoms, and inhibit radiographic progression. However, further research is needed to confirm these findings and to determine the long-term safety and efficacy of bimekizumab. |
(1) Bimekizumab, a monoclonal IgG1 antibody that selectively inhibits interleukin (IL)-17A and IL-17F, has shown superior improvements in joint, skin, and radiographic efficacy outcomes at week 16 compared with placebo in patients with psoriatic arthritis who were naive to biologic DMARDs. (2) The safety profile of bimekizumab, including the occurrence of fungal infections, was consistent with previous phase 3 studies in patients with plaque psoriasis, and with IL-17A inhibitors. (3) Bimekizumab could be a potential additional treatment option with long-term, sustained efficacy and a tolerable safety profile for patients with psoriatic arthritis who do not respond to conventional or biologic DMARDs. A study published in The Lancet Rheumatology reports that a drug called bimekizumab has shown significant improvement in joint, skin, and radiographic efficacy outcomes in patients with psoriatic arthritis who were naive to biologic disease-modifying antirheumatic drugs (DMARDs). Bimekizumab is a monoclonal antibody that selectively inhibits interleukin (IL)-17A and IL-17F, which have been implicated in the pathogenesis of psoriatic arthritis. The study found that significantly more patients receiving bimekizumab reached 50% or greater improvement in American College of Rheumatology criteria at week 16 compared to those receiving a placebo, and the safety profile was consistent with previous phase 3 studies. |
Adopting lay summaries can increase the visibility, impact, and transparency of scientific research, and enhance scientists’ reputation among peers. In the context of a changing science media landscape, lay summaries can create reliable, direct pathways between scientists and different audiences, including the general public, journalists, and decision-makers. Currently, available AI models already offer solutions to produce lay abstracts. However, it is crucial to validate the coherence and accuracy of AI-generated abstracts before their unrestricted use. Future research should focus on comparative studies, human-AI collaboration, expert evaluation, and broader user testing to ensure the reliability and credibility of AI-generated texts in the field of health research.
Acknowledgements
This article has been produced with the help of ChatGPT and Wiseone.
Author contributions: BS wrote and revised the manuscript and approved the final version of the manuscript.
The author(s) declared no potential conflicts of interest with respect to the research, authorship, and/or publication of this article.
Ethical approval: Not applicable.
Funding: The author(s) disclosed receipt of the following financial support for the research, authorship, and/or publication of this article: Boris Schmitz is supported by the European Commission within the Horizon 2020 framework program (grant number: 101017424).
Guarantor: BS.
ORCID iD: Boris Schmitz https://orcid.org/0000-0001-7041-7424
Patient consent: Not applicable.
Applications and Advances of Artificial Intelligence in Music Generation:A Review
In recent years, artificial intelligence (AI) has made significant progress in the field of music generation, driving innovation in music creation and applications. This paper provides a systematic review of the latest research advancements in AI music generation, covering key technologies, models, datasets, evaluation methods, and their practical applications across various fields. The main contributions of this review include: (1) presenting a comprehensive summary framework that systematically categorizes and compares different technological approaches, including symbolic generation, audio generation, and hybrid models, helping readers better understand the full spectrum of technologies in the field; (2) offering an extensive survey of current literature, covering emerging topics such as multimodal datasets and emotion expression evaluation, providing a broad reference for related research; (3) conducting a detailed analysis of the practical impact of AI music generation in various application domains, particularly in real-time interaction and interdisciplinary applications, offering new perspectives and insights; (4) summarizing the existing challenges and limitations of music quality evaluation methods and proposing potential future research directions, aiming to promote the standardization and broader adoption of evaluation techniques. Through these innovative summaries and analyses, this paper serves as a comprehensive reference tool for researchers and practitioners in AI music generation, while also outlining future directions for the field.
Introduction
Music, as a universal and profound art form, transcends cultural and geographical boundaries, playing an unparalleled role in emotional expression (Juslin and Sloboda 2011 ) . With the rapid advancement of technology, music creation has evolved from the manual operations of the early 20th century, relying on analog devices and tape recordings, to today’s fully digital production environment (Katz 2010 ; Pinch and Bijsterveld 2012 ; Deruty et al. 2022 ; Oliver and Lalchev 2022 ) . In this evolution, the introduction of Artificial Intelligence (AI) has injected new vitality into music creation, driving the rapid development of automatic music generation technologies and bringing unprecedented opportunities for innovation (Briot, Hadjeres, and Pachet 2020 ; Zhang, Yan, and Briot 2023 ) .
Research Background and Current Status: The research on automatic music generation dates back more than 60 years, with the earliest attempts primarily based on grammatical rules and probabilistic models (Hiller and Isaacson 1979 ; Dash and Agres 2023 ) . However, with the rise of deep learning technologies, the field of AI music generation has entered an unprecedented period of prosperity (Goodfellow 2016 ; Moysis et al. 2023 ) . Modern AI technologies can not only handle symbolic music data but also generate high-fidelity audio content directly, with applications ranging from traditional instrument simulation to entirely new sound design (Oord et al. 2016 ; Lei et al. 2024 ) . Symbolic music generation relies on representations such as piano rolls and MIDI, enabling the creation of complex structured musical compositions; meanwhile, audio generation models deal directly with continuous audio signals, producing realistic and layered sounds (Dong et al. 2018 ; Ji, Yang, and Luo 2023 ) .
In recent years, AI music generation technologies have made remarkable progress, especially in the areas of model architecture and generation quality (Huang et al. 2018a ; Agostinelli et al. 2023 ) . The application of Generative Adversarial Networks (GANs), Transformer architectures, and the latest diffusion models has provided strong support for the diversity, structure, and expressiveness of generated music (Goodfellow et al. 2014 ; Vaswani 2017 ; Ho, Jain, and Abbeel 2020 ; Kong et al. 2020b ; Shahriar 2022 ) . Additionally, new hybrid model frameworks that combine the strengths of symbolic and audio generation further enhance the structural integrity and timbral expressiveness of generated music (Huang et al. 2018a ; Wang, Min, and Xia 2024 ; Qian et al. 2024 ) . These advancements have not only expanded the technical boundaries of AI music generation but also opened up new possibilities for music creation (Wang et al. 2024 ) .
Research Motivation: Despite significant advances in AI music generation, numerous challenges remain. Enhancing the originality and diversity of generated music, capturing long-term dependencies and complex structures in music, and developing more standardized evaluation methods are core issues that the field urgently needs to address. Furthermore, as the application areas of AI-generated music continue to expand—such as healthcare, content creation, and education—the demands for quality and control of generated music are also increasing. These challenges provide a broad space for future research and technological innovation.
Research Objectives: This paper aims to systematically review the latest research progress in symbolic and audio music generation, explore their potential and challenges in various application scenarios, and forecast future development directions. Through a comprehensive analysis of existing technologies and methods, this paper seeks to provide valuable references for researchers and practitioners in the AI music generation field and inspire further innovation and exploration. We hope that this research will promote the continuous innovation of AI in music creation, making it a core tool in music production in the future.The core logic of this review paper is illustrated in Figure 1 .

History of Music Production
Early Stages of Music Production
In the early 20th century, music production mainly relied on analog equipment and tape recording technology. Sound engineers and producers used large analog consoles for recording, mixing, and mastering. This period emphasized the craftsmanship and artistry of live performances, with the constraints of recording technology and equipment making the process of capturing each note filled with uncertainty and randomness. (Zak III 2001 ; Horning 2013 ) The introduction of synthesizers brought revolutionary changes to music creation, particularly in electronic music. In the 1970s, synthesizers became increasingly popular, with brands like Moog and Roland symbolizing the era of electronic music. Synthesizers generated various sounds by modulating waveforms (such as sine and triangle waves), allowing music producers to create a wide range of tones and effects on a single instrument, thereby greatly expanding the possibilities for musical expression (Pinch and Trocco 2004 ; Holmes 2012 ) .
The Rise of Digital Audio Workstations (DAWs)
With advances in digital technology, Digital Audio Workstations (DAWs) began to rise in the late 1980s and early 1990s. The advent of DAWs marked the transition of music production into the digital era, integrating recording, mixing, editing, and composition into a single software platform, making the music production process more efficient and convenient (Hracs, Seman, and Virani 2016 ; Danielsen 2018 ; Théberge 2021 ; Cross 2023 ) . The widespread application of MIDI (Musical Instrument Digital Interface) further propelled the development of digital music production. MIDI facilitated communication between digital instruments and computers, becoming a critical tool in modern music production. Renowned DAWs like Logic Pro, Ableton Live, and FL Studio provided producers with integrated working environments, streamlining the music creation process and democratizing music production (D’Errico 2016 ; Reuter 2022 ) .
Expansion of Plugins and Virtual Instruments
The popularity of DAWs fueled the development of plugins and virtual instruments. Plugins, as software extensions, added new functionalities or sound effects to DAWs, vastly expanding the creative potential of music production. Platforms like Kontakt offered various high-quality virtual instruments, while synthesizer plugins such as Serum and Phase Plant, utilizing advanced wavetable synthesis, provided producers with extensive sound design possibilities. The diversity and flexibility of plugins greatly broadened the creative space of music production, enabling producers to modulate, edit, and layer various sound effects within a single software environment (Tanev and Božinovski 2013 ; Wang 2017 ; Rambarran 2021 ) .
Application of Artificial Intelligence in Music Production
With technological advancement, Artificial Intelligence (AI) has gradually entered the field of music production. AI technologies can analyze large volumes of music data, extract patterns and features, and generate new music compositions. Max/MSP, an early interactive audio programming environment, allowed users to create their own sound effects and instruments through coding, marking the initial application of AI technology in music production (Tan and Li 2021 ; Hernandez-Olivan and Beltran 2022 ; Ford et al. 2024 ; Marschall 2007 ; Privato, Rampado, and Novello 2022 ) .
As AI technologies matured, machine learning-based tools emerged, capable of generating music based on given datasets and automating tasks such as mixing and mastering. Modern AI music generation technologies can not only simulate existing styles but also create entirely new musical forms, opening up new possibilities for music creation (Taylor, Ardeliya, and Wolfson 2024 ) .
Trends in Modern Music Production
Today’s music production is fully digital, with producers able to complete every step from composition to mastering within a DAW. The diversity and complexity of plugins continue to grow, including vocoders, resonators, and convolution reverbs, bringing infinite possibilities to music creation. The introduction of AI has further pushed the boundaries of music creation, making automation and intelligent production a reality (Briot, Hadjeres, and Pachet 2020 ; Agostinelli et al. 2023 ) . Modern music production is not only the result of technological accumulation but also a model of the fusion of art and technology. The incorporation of AI technologies has enriched the music creation toolbox and spurred the emergence of new musical styles, making music creation more diverse and dynamic (Deruty et al. 2022 ; Tao 2022 ; Goswami 2023 ) .
Music Representation
The representation of music data is a core component of AI music generation systems, directly influencing the quality and diversity of the generated results. Different music representation methods capture distinct characteristics of music, significantly affecting the input and output of AI models. Below are some commonly used music representation methods and their application scenarios:
3.1 Piano Roll
A piano roll is a two-dimensional matrix that visually represents the notes and timing of music, making it particularly suitable for capturing melody and chord structures. The rows of the matrix represent pitch, columns represent time, and the values indicate whether a particular pitch is activated at a given time point. This representation is widely used in deep learning models as it directly maps to the input and output layers of neural networks, facilitating the processing and generation of complex musical structures. For example, MuseGAN (Dong et al. 2018 ) uses piano roll representation for multi-part music, generating harmonically rich compositions through Generative Adversarial Networks (GANs).
3.2 MIDI (Musical Instrument Digital Interface)
MIDI is a digital protocol used to describe various musical parameters such as notes, pitch, velocity, tempo, and chords. MIDI files do not record actual audio data but rather instructions that control audio, making them highly flexible and allowing playback in various styles on different synthesizers and virtual instruments. MIDI is extensively used in music creation, arrangement, and AI music generation, especially in symbolic music generation, where it serves as a crucial format for input and output data. Its advantages lie in cross-platform and cross-device compatibility and the precise control of musical parameters. MusicVAE (Brunner et al. 2018 ) utilizes MIDI to represent symbolic music, where notes and timing are discrete, enabling the model to better capture structural features and generate music with complex harmony and melody.
3.3 Mel Frequency Cepstral Coefficients (MFCCs)
MFCCs are a compact representation of the spectral characteristics of audio signals, widely used in speech and music processing, particularly effective in capturing subtle differences in music. By decomposing audio signals into short-time frames and applying the Mel frequency scale, MFCCs capture audio features perceived by the human ear. Although primarily used in speech recognition, MFCCs also find extensive applications in music emotion analysis, style classification, and audio signal processing. For example, Google’s NSynth project uses MFCCs (Engel et al. 2017 ) for generating and classifying different timbres.
3.4 Sheet Music
Sheet music is a traditional form of music representation that records musical information through staff notation and various musical symbols. It includes not only pitch and rhythm but also dynamics, expressive marks, and other performance instructions. In AI music generation, sheet music representation is also employed, particularly for generating readable compositions that adhere to music theory. Models using sheet music as input, such as Music Transformer (Huang et al. 2018b ) , can generate compositions with complex structure and coherence.
3.5 Audio Waveform
The audio waveform directly represents the time-domain waveform of audio signals, suitable for generating and processing actual audio data. Although waveform representation involves large data volumes and complex processing, it provides the most raw and detailed audio information, crucial in audio synthesis and sound design. For instance, the WaveNet (van den Oord et al. 2016 ) model uses waveforms directly to generate highly realistic speech and music.
3.6 Spectrogram
A spectrogram converts audio signals into a frequency domain representation, showing how the spectrum of frequencies evolves over time. Common spectrograms include Short-Time Fourier Transform (STFT) spectrograms, Mel spectrograms, and Constant-Q transform spectrograms. Spectrograms are highly useful in music analysis, classification, and generation, as they capture both the frequency structure and temporal characteristics of audio signals. The Tacotron 2 (Wang et al. 2017 ) model uses spectrograms as intermediate representations for generating audio from text, transforming text input into Mel spectrograms and then using WaveNet to generate the final waveform audio. The DDSP model (Engel et al. 2020 ) employs spectrograms as intermediate representations to generate high-quality audio by manipulating frequency domain signals. It combines traditional Digital Signal Processing (DSP) techniques with deep learning models to generate realistic instrument timbres and complex audio effects, making it highly effective in music generation and sound design.
3.7 Chord Progressions
Chord progressions are sequences of chords that represent changes over time and are crucial in popular, jazz, and classical music. AI music generation systems can learn patterns of chord progressions to generate harmonious and structured music. For example, the ChordGAN model (Lu and Dubnov 2021 ) generates chord progressions for background harmonies in popular music.
3.8 Pitch Contour
Pitch contour represents the variation of pitch over time, particularly useful for analyzing and generating melodic lines. Pitch contours capture subtle pitch changes in music, aiding in generating smooth and natural melodies. OpenAI’s Jukebox model (Dhariwal et al. 2020 ) uses pitch contours to generate complete songs with coordinated melodies and background accompaniment.

Generative Models
The field of AI music generation can be divided into two main directions: symbolic music generation and audio music generation. These two approaches correspond to different levels and forms of music creation.

4.1 Symbolic Music Generation
Symbolic music generation uses AI technologies to create symbolic representations of music, such as MIDI files, sheet music, or piano rolls. The core of this approach lies in learning the structures of music, chord progressions, melodies, and rhythmic patterns to generate compositions with logical and structured music. These models typically handle discrete note data, and the generated results can be directly played or further converted into audio. In symbolic music generation, LSTM models have shown strong capabilities. For instance, DeepBach (Hadjeres, Pachet, and Nielsen 2017a ) uses LSTMs to generate Bach-style harmonies, producing harmonious chord progressions based on given musical fragments. However, symbolic music generation faces challenges in capturing long-term dependencies and complex structures, particularly when generating music on the scale of entire movements or songs, where maintaining long-range musical dependencies can be difficult.
Recently, Transformer-based symbolic music generation models have demonstrated more efficient capabilities in capturing long-term dependencies. For example, the Pop Music Transformer (Huang and Yang 2020 ) combines self-attention mechanisms and Transformer architecture to achieve significant improvements in generating pop music. Additionally, MuseGAN, a GAN-based multi-track symbolic music generation system, can generate multi-part music suitable for creating compositions with rich layers and complex harmonies. The MuseCoco model (Lu et al. 2023 ) combines natural language processing with music creation, generating symbolic music from text descriptions and allowing precise control over musical elements, making it ideal for creating complex symbolic music works. However, symbolic music generation mainly focuses on notes and structure, with limited control over timbre and expressiveness, highlighting its limitations.
4.2 Audio Music Generation
Audio music generation directly generates the audio signal of music, including waveforms and spectrograms, handling continuous audio signals that can be played back directly or used for audio processing. This approach is closer to the recording and mixing stages in music production, capable of producing music content with complex timbres and realism.
WaveNet (van den Oord et al. 2016 ) , a deep learning-based generative model, captures subtle variations in audio signals to generate expressive music audio, widely used in speech synthesis and music generation. Jukebox (Dhariwal et al. 2020 ) , developed by OpenAI, combines VQ-VAE and autoregressive models to generate complete songs with lyrics and complex structures, with sound quality and expressiveness approaching real recordings. However, audio music generation typically requires substantial computational resources, especially when handling large amounts of audio data. Additionally, audio generation models face challenges in controlling the structure and logic of music over extended durations.
Recent research on diffusion models has made significant progress, initially used for image generation but now extended to audio. For example, DiffWave (Kong et al. 2020b ) and WaveGrad (Chen et al. 2020b ) are two representative audio generation models; the former generates high-fidelity audio through a progressive denoising process, and the latter produces detailed audio through a similar diffusion process. The MeLoDy model (Stefani 1987 ) combines language models (LMs) and diffusion probability models (DPMs), reducing the number of forward passes while maintaining high audio quality, addressing computational efficiency issues. Noise2Music (Huang et al. 2023a ) , based on diffusion models, focuses on the correlation between text prompts and generated music, demonstrating the ability to generate music closely related to input text descriptions.
Overall, symbolic music generation and audio music generation represent the two primary directions of AI music generation. Symbolic music generation is suited for handling and generating structured, interpretable music, while audio music generation focuses more on the details and expressiveness of audio signals. Future research could combine these two methods to enhance the expressiveness and practicality of AI music generation, achieving seamless transitions from symbolic to audio, and providing more comprehensive technical support for music creation.
4.3 Current Major Types of Generative Models
The core of AI music generation lies in using different generative models to simulate and create music. Each model has its unique strengths and application scenarios. Below are some major generative models and their applications:
Long Short-Term Memory Networks (LSTM): LSTM excels in handling sequential data with temporal dependencies, effectively capturing long-term dependencies in music and generating coherent and expressive music sequences. Models like BachBot (Liang 2016 ) and DeepBach (Hadjeres, Pachet, and Nielsen 2017b ) utilize LSTMs to generate Bach-style music, demonstrating LSTM’s strong capabilities in music generation. However, LSTM models often require large amounts of data for training and have relatively high computational costs, limiting their application in resource-constrained environments.
Generative Adversarial Networks (GAN): GANs generate high-quality, realistic music content through adversarial training between a generator and a discriminator, making them particularly suitable for generating complex and diverse audio. For instance, DCGAN (Radford, Metz, and Chintala 2016 ) excels in generating high-fidelity audio. Models like WaveGAN (Donahue, McAuley, and Puckette 2019 ) and MuseGAN (Ji, Yang, and Luo 2023 ) have made significant progress in single-part and multi-part music generation, respectively. MusicGen (Copet et al. 2024 ) , developed by Meta, is a deep learning-based music generation model capable of producing high-quality, diverse music fragments from noise or specific input conditions. However, GANs can have unstable training processes and may suffer from mode collapse, leading to a lack of diversity in the generated music.
Transformer Architecture: Transformers leverage self-attention mechanisms to efficiently process sequential data, particularly adept at capturing long-range dependencies and complex structures in music compositions. Notable work includes the Music Transformer (Huang et al. 2018a ) , which uses self-attention to generate structured music segments, effectively capturing motifs and repetitive structures across multiple time scales. This results in music that is structurally coherent and closer to human compositional styles. MusicLM (Agostinelli et al. 2023 ) combines Transformer-based language models with audio generation, offering innovation in generating high-fidelity music audio from text descriptions. However, Transformer models require substantial computational resources for training and generation.
Variational Autoencoders (VAE): VAEs generate new data points by learning latent representations, suitable for tasks involving diversity and creativity in music generation. The MIDI-VAE model (Brunner et al. 2018 ) uses VAE for music style transfer, demonstrating the potential of VAE in generating diverse music. The Conditional VAE (CVAE) enhances diversity by introducing conditional information, reducing mode collapse risks. OpenAI’s Jukebox (Dhariwal et al. 2020 ) combines Vector Quantized VAE (VQ-VAE-2) with autoregressive models to generate complete songs with lyrics and complex structures. Compared to GANs or Transformers, VAE-generated music may lack musicality and coherence.
Diffusion Models: Diffusion models generate high-quality audio content by gradually removing noise, making them suitable for high-fidelity music generation. Recent research includes the Riffusion model (Forsgren and Martiros 2022 ) , utilizing the Stable Diffusion model for real-time music generation, producing music in various styles from text prompts or image conditions; Moûsai (Schneider et al. 2024 ) , a diffusion-based music generation system, generates persistent, high-quality music from text prompts in real time. The lengthy training and generation processes of diffusion models can limit their application in real-time music generation scenarios.
Other Models and Methods: Besides the models mentioned above, Convolutional Neural Networks (CNNs), other types of Recurrent Neural Networks (RNNs), and methods combining multiple models have also been applied in music generation. Additionally, rule-based methods and evolutionary algorithms offer diverse technical and creative approaches for music generation. For example, WaveNet (Oord et al. 2016 ) , a CNN-based model, is innovative in directly modeling audio signals. MelGAN (Kumar et al. 2019 ) uses efficient convolutional architectures to generate detailed audio.
4.4 Hybrid Model Framework:Integrating Symbolic and Audio Music Generation
Recently, researchers have recognized that combining the strengths of symbolic and audio music generation can significantly enhance the overall quality of generated music. Symbolic music generation models (e.g., MIDI or sheet music generation models) excel at capturing musical structure and logic, while audio generation models (e.g., WaveNet (Oord et al. 2016 ) or Jukebox (Dhariwal et al. 2020 ) ) focus on generating high-fidelity and complex timbre audio signals. However, each model type has distinct limitations: symbolic generation models often lack expressiveness in timbre, and audio generation models struggle with long-range structural modeling. To address these challenges, recent studies have proposed hybrid model frameworks that combine the advantages of symbolic and audio generation. A common strategy is to use methods that jointly employ Variational Autoencoders (VAE) and Transformers. For example, in models like MuseNet (Topirceanu, Barina, and Udrescu 2014 ) and MusicVAE (Yang et al. 2019 ) , symbolic music is first generated by a Transformer and then converted into audio signals. These models typically use VAE to capture latent representations of music and employ Transformers to generate sequential symbolic representations. Self-supervised learning methods have gained increasing attention in symbolic music generation. These approaches often involve pre-training models to capture structural information in music, which are then applied to downstream tasks. Models like Jukebox (Dhariwal et al. 2020 ) use self-supervised learning to enhance the generalization and robustness of generative models.
Additionally, combining hierarchical symbolic music generation with cascaded diffusion models has proven effective (Wang, Min, and Xia 2024 ) . This approach defines a hierarchical music language to capture semantic and contextual dependencies at different levels. The high-level language handles the overall structure of a song, such as paragraphs and phrases, while the low-level language focuses on notes, chords, and local patterns. Cascaded diffusion models train at each level, with each layer’s output conditioned on the preceding layer, enabling control over both the global structure and local details of the generated music.
The fusion of symbolic and audio generation frameworks combines symbolic representations with audio signals, resulting in music that is not only structurally coherent but also rich in timbre and detailed expression. The symbolic generation part ensures harmony and logic, while the audio generation part adds complex timbre and dynamic changes, paving the way for creating high-quality and multi-layered music.Examples of related work for different foundational models are shown in Table 1 . The development trajectory of AI music generation technology can be seen in Figure 2 .
Model Type Related Research Strengths Challenges Suitable Scenarios LSTM DeepBach, BachBot Good at capturing temporal dependencies and sequential data High computational cost, training requires large datasets, struggles with long-term dependencies Suitable for sequential music generation tasks, such as harmonization and melody generation GAN MuseGAN, WaveGAN High-quality, realistic generation, suitable for complex and diverse audio Training can be unstable, prone to mode collapse, limited in capturing structure and long-term dependencies Ideal for generating complex audio content like multi-instrument music or diverse sound effects Transformer Music Transformer, MusicLM Excellent at capturing long-range dependencies and complex structures High computational demand, requires large amounts of data for training Best for generating music with complex structures, long sequences, and coherent compositions VAE MIDI-VAE, Jukebox Encourages diversity and creativity, suitable for style transfer Generated music can lack musical coherence and expressiveness compared to GANs or Transformers Best for tasks requiring high variability and creativity, such as style transfer and music exploration Diffusion Models DiffWave,WaveGrad, Noise2Music High-quality audio generation, excels in producing high-fidelity music Training and generation time can be long, challenging in real-time scenarios Suitable for generating high-quality audio and sound effects, particularly in media production Hybrid Models MuseNet, MusicVAE Combines strengths of symbolic and audio models, controls structure and timbre Complexity in integrating different model types, requires more sophisticated tuning Ideal for creating music that requires both structural coherence and rich audio expressiveness, useful in advanced music composition
Model Name Base Architecture Dataset Used Data Representation Loss Function Year WaveNet CNN VCTK Corpus, YouTube Data Waveform L1 Loss 2016 BachBot LSTM Bach Chorale Dataset Symbolic Data Cross-Entropy Loss 2016 DCGAN CNN Lakh MIDI Dataset (LMD) Audio Waveform Binary Cross-Entropy Loss 2016 DeepBach LSTM Bach Chorale Dataset MIDI File Cross-Entropy Loss 2017 MuseGAN GAN Lakh MIDI Dataset (LMD) Multi-track MIDI Binary Cross-Entropy Loss 2018 MIDI-VAE VAE MIDI files (Classic, Jazz, Pop, Bach, Mozart) Pitch roll, Velocity roll, Instrument roll Cross Entropy, MSE, KL Divergence 2018 Music Transformer Transformer Lakh MIDI Dataset (LMD) MIDI File Cross-Entropy Loss 2019 WaveGAN GAN Speech Commands, AudioSet Audio Waveform GAN Loss (Wasserstein Distance) 2019 Jukebox VQ-VAE + Autoregressive 1.2 million songs (LyricWiki) Audio Waveform Reconstruction Loss, Perceptual Loss 2019 MelGAN GAN-based VCTK, LJSpeech Audio Waveform GAN Loss (Multi-Scale Discriminator) 2019 Pop Music Transformer Transformer-XL Custom Dataset (Pop piano music) REMI (Rhythm-Event-Metric Information) Cross-Entropy Loss 2020 DiffWave Diffusion Model VCTK, LJSpeech Waveform L1 loss, GAN loss 2020 Riffusion Diffusion + CLIP Large-Scale Popular Music Dataset (Custom) Spectrogram Image Diffusion Loss, Reconstruction Loss 2022 MusicLM Transformer + AudioLDM Free Music Archive (FMA) Audio Waveform Cross-Entropy Loss, Contrastive Loss 2023 MusicGen Transformer Shutterstock, Pond5 Audio Waveform Cross-Entropy Loss, Perceptual Loss 2023 Music ControlNet Diffusion Model MusicCaps ( 1800 hours) Audio Waveform Diffusion Loss 2023 Moûsai Diffusion Model Moûsai-2023 Mel-spectrogram Spectral loss, GAN loss 2023 MeLoDy LM-guided Diffusion 257k hours of non-vocal music Audio Waveform Cross-Entropy Loss, Diffusion Loss 2023 MuseCoco GAN-based Multiple MIDI datasets including Lakh MIDI and MetaMIDI Multi-track MIDI Binary Cross-Entropy Loss 2023 Noise2Music Diffusion Model MusicCaps, MTAT, Audioset Audio Waveform Diffusion Loss 2023
In the field of AI music generation, the choice and use of datasets profoundly impact model performance and the quality of generated results. Datasets not only provide the foundation for model training but also play a key role in enhancing the diversity, style, and expressiveness of generated music. This section introduces commonly used datasets in AI music generation and discusses their characteristics and application scenarios.
5.1 Commonly Used Open-Source Datasets for Music Generation
In the music generation domain, the following datasets are widely used resources that cover various research directions, from emotion recognition to audio synthesis. This section introduces these datasets, including their developers or owners, and briefly describes their specific applications.
• CAL500 (2007)
The CAL500 dataset (Turnbull et al. 2007 ) , developed by Gert Lanckriet and his team at the University of California, San Diego, contains 500 MP3 songs, each with detailed emotion tags. These tags are collected through subjective evaluations by listeners, covering various emotional categories. The dataset is highly valuable for static emotion recognition and emotion analysis research.
• MagnaTagATune (MTAT) (2008)
Developed by Edith Law, Kris West, Michael Mandel, Mert Bay, and J. Stephen Downie, the MagnaTagATune dataset (Law et al. 2009 ) uses an online game called ”TagATune” to collect data. It contains approximately 25,863 audio clips, each 29 seconds long, sourced from Magnatune.com songs. Each clip is associated with a binary vector of 188 tags, independently annotated by multiple players. This dataset is widely used in automatic music annotation, emotion recognition, and instrument classification research.
• Nottingham Music Dataset (2009)
The Nottingham Music Dataset (Boulanger-Lewandowski, Bengio, and Vincent 2012 ) was originally developed by Eric Foxley at the University of Nottingham and released on SourceForge. It includes over 1,000 traditional folk tunes suitable for ABC notation. The dataset has been widely used in traditional music generation, music style analysis, and symbolic music research.
• Million Song Dataset (MSD) (2011)
The Million Song Dataset (Bertin-Mahieux et al. 2011 ) is a benchmark dataset designed for large-scale music information retrieval research, providing a wealth of processed music features without including original audio or lyrics. It is commonly used in music recommendation systems and feature extraction algorithms.
• MediaEval Emotion in Music (2013)
The MediaEval Emotion in Music dataset (Soleymani et al. 2013 ) contains 1,000 MP3 songs specifically for music emotion recognition research. The emotion tags were obtained through subjective evaluations by a group of annotators, making it useful for developing and validating music emotion recognition models.
• AMG1608 (2015)
The AMG1608 dataset (Penha and Cozman 2015 ) , developed by Carmen Penha, Fabio G. Cozman, and researchers from the University of São Paulo, contains 1,608 music clips, each 30 seconds long, annotated for emotions by 665 subjects. The dataset is particularly suitable for personalized music emotion recognition research due to its detailed emotional annotations, especially those provided by 46 subjects who annotated over 150 songs.
• VCTK Corpus (2016)
Developed by the CSTR laboratory at the University of Edinburgh, the VCTK Corpus (Christophe Veaux 2017 ) contains speech data recorded by 110 native English speakers with different accents. Each speaker read about 400 sentences, including texts from news articles, rainbow passages, and accent archives. This dataset is widely used in Automatic Speech Recognition (ASR) and Text-to-Speech (TTS) model development.
• Lakh MIDI (2017)
The Lakh MIDI dataset (Raffel 2016 ) is a collection of 176,581 unique MIDI files, with 45,129 files matched and aligned with entries from the Million Song Dataset. It is designed to facilitate large-scale music information retrieval, including symbolic (using MIDI files only) and audio-based (using information extracted from MIDI files as annotations for matching audio files) research.
• NSynth (2017)
NSynth (Engel et al. 2017 ) , developed by Google’s Magenta team, is a large-scale audio dataset containing over 300,000 monophonic sound samples generated using instruments from commercial sample libraries. Each note has unique pitch, timbre, and envelope characteristics, sampled at 16 kHz and lasting 4 seconds. The dataset includes notes from various instruments sampled at different pitches and velocities.
• DEAM (2017)
The DEAM dataset (Aljanaki, Yang, and Soleymani 2017 ) , developed by a research team at the University of Geneva, is specifically designed for dynamic emotion recognition in music. It contains 1,802 musical pieces, including 1,744 45-second music clips and 58 full songs, covering genres such as rock, pop, electronic, country, and jazz. The songs are annotated with dynamic valence and arousal values over time, providing insights into the dynamic changes in musical emotion.
• LJSpeech (2017)
The LJSpeech dataset (Ito and Johnson 2017 ) is a public domain speech dataset consisting of 13,100 short audio clips of a single speaker reading from seven non-fiction books. Each clip has a corresponding transcription, with lengths ranging from 1 to 10 seconds and totaling about 24 hours. The texts were published between 1884 and 1964 and are in the public domain.
• Free Music Archive (FMA) (2017)
FMA (Defferrard et al. 2017 ) , developed by Michaël Defferrard and others from Ecole Polytechnique Fédérale de Lausanne (EPFL), is a large-scale music dataset sourced from the Free Music Archive (FMA). It contains 106,574 music tracks spanning 161 different genres, with high-quality full-length audio, rich metadata, precomputed audio features, and hierarchical genre labels. FMA is widely used in music classification, retrieval, style recognition, and audio feature extraction research.
• AudioSet (2017)
AudioSet (Gemmeke et al. 2017 ) , developed by Google, is a large-scale audio dataset containing over 2 million labeled 10-second audio clips collected from YouTube videos. The dataset uses a hierarchical ontology of 635 audio categories, covering various everyday sound events. Due to its broad audio categories and high-quality annotations, AudioSet is an important benchmark for audio event detection, classification, and multimodal learning.
• CH818 (2017)
The CH818 dataset (Hu and Yang 2017 ) contains 818 Chinese pop music clips annotated with emotion labels, mainly used for emotion-driven music generation and pop music style analysis. Despite challenges in annotation consistency, the dataset offers valuable resources for music generation and emotion recognition research in Chinese contexts.
• URMP Dataset (2018)
The URMP dataset (Li et al. 2018 ) is designed to facilitate audio-visual analysis of music performance. It includes 44 multi-instrument music pieces composed of individually recorded tracks synchronized for ensemble performance. The dataset provides MIDI scores, high-quality individual instrument recordings, and ensemble performance videos.
• MAESTRO (2018)
MAESTRO (MIDI and Audio Edited for Synchronous Tracks and Organization) (Hawthorne et al. 2018 ) is a dataset developed by Google AI, containing over 200 hours of aligned MIDI and audio recordings primarily sourced from international piano competitions. The MIDI data includes details like velocity and pedal controls, precisely aligned ( 3 ms) with high-quality audio (44.1–48 kHz 16-bit PCM stereo), making it an essential resource for music generation and automatic piano transcription research.
• Groove MIDI Dataset (GMD) (2019)
The Groove MIDI Dataset (Gillick et al. 2019 ) contains 13.6 hours of MIDI and audio data recording human-performed drum performances. Recorded with a Roland TD-11 V-Drum electronic drum kit, it includes 1,150 MIDI files and over 22,000 measures of drum grooves played by 10 drummers, including professionals and amateurs.
• GiantMIDI-Piano (2020)
The GiantMIDI-Piano dataset (Kong et al. 2020a ) comprises 10,855 solo piano pieces’ MIDI files, automatically transcribed from real recordings using a high-resolution piano transcription system. The dataset covers a rich repertoire from 2,786 composers and accurately captures musical details like pitch, onset, offset, and dynamics, making it a valuable resource for piano music generation, transcription, and music analysis.
• LakhNES (2019)
Developed by Chris Donahue, the LakhNES dataset (Donahue et al. 2019 ) is a large MIDI dataset focused on pre-training language models for multi-instrument music generation. It combines data from the Lakh MIDI and NES-MDB datasets, providing diverse and unique training material suitable for complex Transformer architectures in cross-domain multi-instrument music generation tasks.
• Slakh2100 (2019)
The Slakh2100 dataset (Manilow et al. 2019 ) consists of MIDI compositions and synthesized high-quality audio files, including 2,100 multi-track music pieces. Designed for audio source separation and multi-track audio modeling research, it provides rich multi-instrument training material for music information retrieval, audio separation, and music generation.
• MG-VAE (2020)
The MG-VAE dataset (Luo et al. 2020 ) , developed by a research team from Xi’an Jiaotong University, includes over 2,000 MIDI-formatted Chinese folk songs representing both Han and minority regions. It employs Variational Autoencoder (VAE) methods to separate pitch and rhythm into distinct latent spaces of style and content, supporting music style transfer and cross-cultural music generation research.
• Groove2Groove (2020)
The Groove2Groove dataset (Cífka, Şimşekli, and Richard 2020 ) is developed for music style transfer research, containing thousands of music audio clips with various styles and rhythms. It includes recordings of real instruments and synthesized audio, widely used in style transfer, music accompaniment generation, and automated arrangement studies.
• Hi-Fi Singer (2020)
Developed by the HiFiSinger project team, this dataset focuses on high-fidelity singing voice synthesis research (Chen et al. 2020a ) . It contains over 11 hours of high-quality singing recordings with a 48kHz sampling rate, addressing the challenges of high sampling rate modeling and fine acoustic details. It is widely used in high-quality singing voice synthesis, singing separation, and audio restoration research.
• MIDI-DDSP (2021)
The MIDI-DDSP dataset (Wu et al. 2021 ) combines MIDI files and synthesized high-quality audio using Differentiable Digital Signal Processing (DDSP) technology. It is used in research on physically modeled music generation and synthesis, supporting applications in instrument modeling and audio generation requiring detailed control over musical expression.
• Singing Voice Conversion (2023)
The Singing Voice Conversion dataset originates from the Singing Voice Conversion Challenge (SVCC 2023), derived from a subset of the NUS-HLT Speak-Sing dataset (Huang et al. 2023b ) . It includes singing and speech data from multiple singers, used for singing voice conversion and style transfer research, supporting the development of systems that can convert one singer’s vocal style to another, essential for singing synthesis and imitation studies.
Please refer to Table 3 for a comparison of the basic information of these datasets.
Dataset Name Year Type Scale Main Application Areas CAL500 2007 Audio 500 songs Emotion Recognition MagnaTagATune 2008 Audio 25,863 clips Music Annotation, Emotion Recognition Nottingham Music Dataset 2009 MIDI 1000 tunes Symbolic Music Analysis Million Song Dataset 2011 Audio 1,000,000 songs Music Information Retrieval MediaEval Emotion in Music 2013 Audio 1000 songs Emotion Recognition AMG1608 2015 Audio 1608 clips Emotion Recognition VCTK Corpus 2016 Audio 110 speakers Speech Recognition, TTS Lakh MIDI 2017 MIDI 176,581 files Music Information Retrieval NSynth 2017 Audio 300,000 samples Music Synthesis DEAM 2017 Audio 1802 songs Emotion Recognition LJSpeech 2017 Audio 13,100 clips Speech Synthesis Free Music Archive (FMA) 2017 Audio 106,574 songs Music Classification AudioSet 2017 Audio 2,000,000 clips Audio Event Detection CH818 2017 Audio 818 clips Emotion Recognition URMP 2018 Audio, Video, MIDI 44 performances Audio-Visual Analysis MAESTRO 2018 MIDI, Audio 200 hours Music Generation, Piano Transcription Groove MIDI Dataset 2019 MIDI, Audio 13.6 hours Rhythm Generation GiantMIDI-Piano 2020 MIDI 10,855 songs Music Transcription, Analysis LakhNES 2019 MIDI 775,000 multi-instrument examples Music Generation Slakh2100 2019 MIDI, Audio 2100 tracks Source Separation MG-VAE 2020 MIDI 2000 songs Style Transfer Groove2Groove 2020 Audio thousands of clips Style Transfer Hi-Fi Singer 2021 Audio 11 hours Singing Voice Synthesis MIDI-DDSP 2022 MIDI, Audio varied Music Generation, Synthesis Singing Voice Conversion 2023 Audio subset of NHSS Voice Conversion
5.2 Importance of Dataset Selection
High-quality datasets not only provide rich training material but also significantly enhance the performance of generative models across different musical styles and complex structures. Therefore, careful consideration of the following key factors is essential when selecting and constructing datasets:
• Diversity: A diverse dataset that covers a wide range of musical styles, structures, and expressions helps generative models learn different types of musical features. Diversity prevents models from overfitting to specific styles or structures, enhancing their creativity and adaptability in music generation. For example, the Lakh MIDI Dataset (Raffel 2016 ) and NSynth Dataset (Engel et al. 2017 ) are popular among researchers due to their diversity, encompassing a broad repertoire from classical to pop music.
• Scale: The scale of a dataset directly impacts a model’s generalization ability. Especially in deep learning models, large-scale datasets provide more training samples, enabling the model to better capture and learn complex musical patterns. This principle has been validated in many fields, such as Google Magenta’s use of large-scale datasets to train its generative models with significant results. For AI music generation, scale not only implies a large number of samples but also encompasses a broad range of musical styles and forms.
• Quality: The quality of a dataset largely determines the effectiveness of music generation. High-quality datasets typically include professionally recorded and annotated music, providing accurate and high-fidelity training material for models. For example, datasets like MUSDB18 (Stöter, Liutkus, and Ito 2018 ) and DAMP (Digital Archive of Mobile Performances) (Smule 2018 ) offer high-quality audio and detailed annotations, supporting precise training of music generation models.
• Label Information: Rich label information (e.g., pitch, dynamics, instrument type, emotion tags) provides generative models with more precise contextual information, enhancing expressiveness and accuracy in generated music. Datasets with detailed labels, such as The GiantMIDI Dataset (Kong et al. 2020a ) , include not only MIDI data but also detailed annotations of pitch, chords, and melody, allowing models to generate more expressive musical works.
5.3 Challenges Faced by Datasets Despite their critical role in AI music generation, datasets face several challenges that limit current model performance and further research advancement:
• Dataset Availability: High-quality and diverse music datasets are scarce, especially for tasks involving specific styles or high-fidelity audio generation. Publicly available datasets like the Lakh MIDI Dataset (Raffel 2016 ) , while extensive, still lack data in certain specific music styles or high-fidelity audio domains. This scarcity limits model performance on specific tasks and hinders research progress in diverse music generation.
• Copyright Issues: Copyright restrictions on music are a major barrier. Due to copyright protection, many high-quality music datasets cannot be publicly released, and researchers often have access only to limited datasets. This restriction not only limits data sources but also results in a lack of certain music styles in research. Copyright issues also affect the training and evaluation of music generation models, making it challenging to generalize research findings to broader musical domains.
• Dataset Bias: Music styles and structures within datasets often have biases, which can result in generative models producing less diverse outputs or favoring certain styles. For example, if a dataset is dominated by pop music, the model may be biased toward generating pop-style music, overlooking other types of music. This bias not only affects the model’s generalization ability but also limits its performance in diverse music generation.
5.4 Future Dataset Needs With the development of AI music generation technologies, the demand for larger, higher-quality, and more diverse datasets continues to grow. To drive progress in this field, future dataset development should focus on the following directions:
• Multimodal Datasets: Future research will increasingly focus on the use of multimodal data. Datasets containing audio, MIDI, lyrics, video, and other modalities will provide critical support for research on multimodal generative models. For example, the AudioSet Dataset (Gemmeke et al. 2017 ) , as a multimodal audio dataset, has already demonstrated potential in multimodal learning. By integrating various data forms, researchers can develop more complex and precise generative models, enhancing the expressiveness of music generation.
• Domain-Specific Datasets: As AI music generation technology becomes more prevalent across different application scenarios, developing datasets targeted at specific music styles or applications is increasingly important. For instance, datasets focused on therapeutic music or game music will aid in advancing research on specific tasks within these fields. The DAMP Dataset (Smule 2018 ) , which focuses on recordings from mobile devices, provides a foundation for developing domain-specific music generation models.
• Open Datasets: Encouraging more music copyright holders and research institutions to release high-quality datasets will be crucial for driving innovation and development in AI music generation. Open datasets not only increase data availability but also foster collaboration among researchers, accelerating technological advancement. Projects like Common Voice (Ardila et al. 2019 ) and Freesound (Fonseca et al. 2017 ) have significantly promoted research in speech and sound recognition through open data policies. Similar approaches in the music domain will undoubtedly lead to more innovative outcomes.
By making progress in these areas, the AI music generation field will gain access to richer and more representative data resources, driving continuous improvements in music generation technology. These datasets will not only support more efficient and innovative model development but also open up new possibilities for the practical application of AI in music creation.
Evaluation Methods
Evaluating the quality of AI-generated music has always been a focus of researchers. Since the early days of computer-generated music, assessing the quality of these works has been a key issue. Below are the significant research achievements at different stages.
6.1 Overview of Evaluation Methods
In terms of subjective evaluation, early research relied heavily on auditory judgments by human experts, a tradition dating back to the 1970s to 1990s. For example, (Loy and Abbott 1985 ) evaluated computer-generated music clips through listening tests. By the 2000s, subjective evaluation methods became more systematic. (Cuthbert and Ariza 2010 ) proposed a survey-based evaluation framework to study the emotional and aesthetic values of AI-generated music. With the advancement of deep learning technologies, the complexity of subjective evaluation further increased. (Papadopoulos, Roy, and Pachet 2016 ) and (Yang, Chou, and Yang 2017 ) introduced multidimensional emotional rating systems and evaluation models combining user experience, marking a milestone in subjective evaluation research. Recently, (Agarwal and Om 2021 ) proposed a multi-level evaluation framework based on emotion recognition, and (Chu et al. 2022 ) developed a user satisfaction measurement tool, which more accurately captures complex emotional responses and cultural relevance, making subjective evaluation methods more systematic and detailed.
Objective evaluation dates back to the 1980s when the quality of computer-generated music was assessed mainly through a combination of audio analysis and music theory. Cope (Cope 1996 ) pioneered the use of music theory rules for structured evaluation. Subsequently, Huron (Huron 2008 ) introduced a statistical analysis-based model for evaluating musical complexity and innovation, quantifying structural and harmonic features of music, thus providing important tools for objective evaluation. With the advent of machine learning, Conklin (Conklin 2003 ) and Briot et al. (Briot, Hadjeres, and Pachet 2017 ) developed more sophisticated objective evaluation systems using probabilistic models and deep learning techniques to analyze musical innovation and emotional expression.
6.2 Evaluation of Originality and Emotional Expression
The evaluation of originality became an important research direction in the 1990s. (Miranda 1995 ) and (Toiviainen and Eerola 2006 ) introduced early mechanisms for originality scoring through genetic algorithms and computational models. As AI technology advanced, researchers such as (Herremans, Chuan, and Chew 2017 ) combined Markov chains and style transfer techniques, further enhancing the systematic and diverse evaluation of originality. The evaluation of emotional expression began with audio signal processing. (Sloboda 1991 ) and (Picard 2000 ) laid the foundation for assessing emotional expression in music through the analysis of pitch, rhythm, and physiological signals. With the rise of multimodal analysis, (Kim et al. 2010 ) and (Yang and Chen 2012 ) developed emotion analysis models that combine audio and visual signals, significantly improving the accuracy and diversity of emotional expression evaluation.
6.3 Implementation Strategies of Evaluation Frameworks
The implementation strategies of evaluation frameworks have evolved from simple to complex. The combined use of qualitative and quantitative analysis was first proposed by Reimer (Reimer 1991 ) in the field of music education and later widely applied in the evaluation of AI-generated music. Modern evaluation frameworks, such as those by Lim et al. (2017), integrate statistical analysis with user feedback, offering new approaches for comprehensive evaluation of AI-generated music. Multidimensional rating systems originated from automated scoring in films and video content, with (Hastie et al. 2009 ) laying the groundwork for multidimensional rating models in music evaluation. (Herremans, Chew et al. 2016 ) further extended this concept to the evaluation of music creation quality. Interdisciplinary collaboration and customized evaluation tools have become increasingly important in recent AI music evaluation. Research by (Gabrielsson 2001 ) emphasized the significance of cross-disciplinary collaboration in developing evaluation tools tailored to different styles and cultures. Finally, automated evaluation and real-time feedback, as key directions in modern music evaluation, have significantly enhanced the efficiency and accuracy of music generation quality assessment through machine learning and real-time analysis technologies.
6.4 Conclusion
By integrating subjective and objective evaluation methods and considering originality and emotional expressiveness, a comprehensive quality evaluation framework can be constructed. The early research laid the foundation for current evaluation methods, and recent advancements, particularly in evaluating originality and emotional expression, have achieved notable success. This comprehensive evaluation approach helps to more accurately measure the performance of AI music generation systems and provides guidance for future research and development, advancing AI music generation technology toward the complexity and richness of human music creation.
Application Areas
AI music generation technology has broad and diverse applications, from healthcare to the creative industries, gradually permeating various sectors and demonstrating immense potential. Based on its development history, the following is a detailed description of various application areas and the historical development of relevant research.
7.1 Healthcare
AI music generation technology has gained widespread attention in healthcare, particularly in emotional regulation and rehabilitation therapy. In the 1990s, music therapy was widely used to alleviate stress and anxiety. (Standley 1986 ) studied the effect of music on anxiety symptoms and highlighted the potential of music as a non-pharmacological treatment method. Although the focus was mainly on natural music at the time, (Sacks 2008 ) , in his book Musicophilia, further explored the impact of music on the nervous system, indirectly pointing to the potential of customized music in neurological rehabilitation. With advancements in AI technology, generated music began to be applied in specific therapeutic scenarios. (Aalbers et al. 2017 ) demonstrated the positive impact of music therapy on emotional regulation and proposed personalized therapy through AI-generated music.
7.2 Content Creation
Content creation is one of the earliest fields where AI music generation technology was applied, evolving from experimental uses to mainstream creative tools. In the 1990s, David Cope’s Experiments (Cope 1996 ) in Musical Intelligence (EMI) (1996) was an early attempt at using AI-generated music for content creation. EMI could simulate various compositional styles, and its generated music was used in experimental works. Although the technology was still relatively basic, this pioneering research laid the foundation for future applications. In the 2000s, AI-generated music began to be widely used in creative industries like film and advertising. Startups such as Jukedeck developed music generation platforms using Generative Adversarial Networks (GANs) and Recurrent Neural Networks (RNNs) to create customized background music for short videos and ads. Briot et al. found that AI-generated music had approached human-created music in quality and complexity, highlighting AI’s potential to improve content creation efficiency (Briot, Hadjeres, and Pachet 2020 ) . Recently, AI music generation technology has been applied even more widely in content creation. OpenAI’s MuseNet (Payne 2019 ) and Google’s Magenta project (Magenta Team 2023 ) demonstrated the ability to generate complex, multi-style music, providing highly context-appropriate background music for films, games, and advertisements.
7.3 Education
AI music generation technology has revolutionized music education, becoming an important tool for understanding music theory and practical composition. In the early 21st century, AI began to be applied in music education. Pachet explored the potential of automatic composition software in education, generating simple exercises to help students understand music structures and harmonies (Pachet 2003 ) . These early systems aimed to assist rather than replace traditional teaching methods. As technology advanced, AI music generation systems became more intelligent and interactive. Platforms such as MusEDLab’s AI Duet and Soundtrap’s AI Music Tutor (MusedLab Team 2023 ) provide interactive educational experiences, listening to users’ performances, interpreting inputs, and offering instant feedback or real-time performance to help improve skills and understand musical nuances.
7.4 Social Media and Personalized Content
AI-generated music significantly enriches user experiences in social media and personalized content, with personalized recommendations and automated content generation becoming key trends. In the 2000s, social platforms like MySpace first introduced simple music generation algorithms to create background music for user profiles. Although technically basic, these early attempts laid the groundwork for personalized content generation. As social media platforms diversified, personalized content generation became mainstream. Music streaming platforms like Spotify and Pandora use AI to generate personalized playlists by analyzing user listening history and preferences, providing highly customized music experiences. AI-generated music is also used on short video platforms to enhance content appeal. Recently, AI-generated music has become an essential part of social media, with platforms like TikTok using AI to generate background music that quickly matches video content, significantly enhancing user experience. The personalized capabilities of AI-generated music greatly enhance user engagement and interaction on social media (Singh 2024 ) . Furthermore, its applications in virtual reality (VR) and augmented reality (AR) elevate immersive experiences, offering users novel sensory enjoyment.
7.5 Gaming and Interactive Entertainment
In gaming and interactive entertainment, AI music generation technology not only improves music creation efficiency but also enhances player immersion. Game developers began exploring algorithmic background music generation in the 1990s. For instance, The Sims series used procedural music generation that dynamically adjusted background music based on player actions and emotional states, laying the foundation for later game music generation. As games became more complex, AI music generation found broader applications in gaming. The concept of procedural audio was introduced into games, with Björk et al. exploring music generation in interactive environments (Bjork and Holopainen 2005 ) . By the 2010s, AI technology had evolved to enable dynamic music generation that could adapt in real-time to game environments and player interactions, particularly in open-world and massively multiplayer online games (MMORPGs). Recent studies, such as those by Foley et al. (2023), highlight AI-generated music’s role in dynamically creating appropriate background music based on player behavior and emotions, enhancing player immersion and interaction. AI-generated music and sound effects in games not only improve the gaming experience but also reduce development time and costs (Beatoven Team 2023 ) .
7.6 Creative Arts and Cultural Industries
AI-generated music has shown unique potential in the creative arts and cultural industries, pushing the boundaries of artistic creation. Xenakis combined algorithms with music composition (Xenakis 1992 ) , ushering in a new era of computer-assisted creativity, providing theoretical foundations and practical experience for AI’s application in the arts. Briot et al. discussed AI’s potential in generating complex musical forms (Briot, Hadjeres, and Pachet 2020 ) , applied in modern art and experimental music creation, showcasing AI-generated music’s broad applications in creative arts. Recently, AI-generated music has reached new heights in creative arts. Modern artists use AI technology to produce experimental music, breaking traditional boundaries of music composition. AI-generated music is also applied in dance choreography and theater scoring, enhancing the expressiveness of performing arts. In NFT (Non-Fungible Token) artworks, AI-generated music is part of the creation and sales process, driving new forms of digital art.
7.7 Broadcasting and Streaming
The application of AI-generated music in broadcasting and streaming services is expanding, significantly enhancing content richness and personalization. Early streaming platforms like Pandora and Last.fm used simple algorithms to generate recommended playlists based on user listening history, laying the foundation for later AI-generated music in streaming. By the 2010s, streaming services like Spotify began using deep learning and machine learning technologies to generate personalized music recommendations. Spotify’s Discover Weekly feature, a prime example, combines AI-generated music with recommendation systems to deliver highly customized music experiences. Recently, AI-generated music’s application in broadcasting and streaming has become more complex and diverse. For instance, AI-generated background music is used in news broadcasts and podcasts, enhancing the emotional expression of content. Streaming platforms also use AI-generated music to create seamless playlists tailored to different user contexts, such as fitness, relaxation, or work settings. AI-generated new music styles and experimental music offer users unprecedented auditory experiences.
7.8 Marketing and Brand Building
AI-generated music has unique applications in marketing and brand building, enhancing brand impact through customized music. In early brand marketing, background music was typically chosen by human planners, but with the development of AI technology, companies began exploring AI-generated music to enhance advertising impact. Initial applications focused on generating background music for ads to increase brand appeal. By the 2010s, AI-generated music became more common in advertising. Startups like Amper Music developed AI music generation platforms that help companies generate music aligned with their brand identity, strengthening emotional connections with audiences. Recently, the application of AI-generated music in brand building has deepened. Brands can use AI-generated music to create unique audio identities, enhancing brand recognition. AI-generated music is also widely used in cross-media marketing campaigns, seamlessly integrating with video, images, and text content, offering new ways to tell brand stories. Moreover, AI-generated music is used in interactive ads to create real-time background music that interacts with consumers, further strengthening brand-consumer connections.
AI music generation technology has shown significant value across multiple fields. From healthcare to content creation, education to social media, AI not only improves music generation efficiency but also greatly expands the scope of music applications. As technology continues to advance, AI music generation will play an increasingly important role in more fields, driving comprehensive innovation in music creation and application. These applications demonstrate AI’s innovative potential in music generation and highlight its importance in improving human quality of life, enhancing creative efficiency, and promoting cultural innovation.
Challenges and Future Directions
Despite significant progress in AI music generation technology, multiple challenges remain, providing rich avenues for future exploration. The current technological bottlenecks are primarily centered on the following key issues:
Firstly, the diversity and originality of generated music remain major concerns for researchers. Early generative systems, such as David Cope’s Experiments in Musical Intelligence (EMI) (Computer History Museum 2023 ) , were successful at mimicking existing styles but often produced music that was stylistically similar and lacked innovation. This limitation in diversity has persisted in later deep learning models. Although the introduction of Generative Adversarial Networks (GANs) and Recurrent Neural Networks (RNNs) improved diversity, the results still often suffer from “mode collapse”—where generated pieces across samples are too similar in style, lacking true originality. This phenomenon was extensively discussed in Briot et al., highlighting the potential limitations of deep learning models in music creation (Briot, Hadjeres, and Pachet 2020 ) .
Secondly, effectively capturing long-term dependencies and complex structures in music is a critical challenge in AI music generation (Briot, Hadjeres, and Pachet 2020 ) . As a time-based art form, music’s structure and emotional expression often rely on complex temporal spans and hierarchies (Hawthorne et al. 2018 ) . Current AI models struggle with this complexity, and although some studies have attempted to address this by increasing the number of layers in the model or introducing new architectures (such as Transformer models), results show that models still find it difficult to generate music with deep structural coherence and long-term dependencies. The core issue is how to enable models to maintain overall macro coherence while showcasing rich details and diversity at the micro level during music generation.
The standardization of evaluation methods has also been a persistent challenge in assessing the quality of AI-generated music. Traditional evaluation methods mainly rely on subjective assessments by human listeners, but these methods often lack consistency and objectivity (Yang and Chen 2012 ) . With the expanding applications of AI-generated music, the need for more objective and consistent evaluation standards has grown. Researchers have begun exploring quantitative evaluation methods based on statistical analysis and music theory (Herremans, Chew et al. 2016 ) however, effectively integrating these methods with subjective assessments remains an area needing further exploration (Engel et al. 2017 ) . The refinement of such evaluation systems is crucial for advancing the practical applications of AI music generation technology.
Facing these challenges, future research directions can focus on the following areas:
Exploring New Music Representations and Generation Methods: Introducing more flexible and diverse music representation forms can help generative models better capture the complexity and diversity of music. Research in this area can draw on recent findings in cognitive science and music theory to develop generation mechanisms that better reflect the human creative process.
Enhancing Control Capabilities of Hybrid Models: By incorporating more contextual information (such as emotion tags or style markers), AI-generated music can achieve greater progress in personalization and diversity. The control capabilities of hybrid models directly affect the expressiveness and user experience of generated music, making this a critical direction for future research.
Applying Interdisciplinary Approaches: Combining music theory, cognitive science, and deep learning will be key to advancing AI music generation. This approach can enhance the ability of generative models to capture complex musical structures and make AI-generated music more aligned with human aesthetic and emotional needs. Interdisciplinary collaboration can lead to the development of more intelligent and human-centered music generation systems.
Real-Time Generation and Interaction: Real-time generation and adjustment of music will bring unprecedented flexibility and creative space to music creation and performance. Particularly in interactive entertainment and live performances, real-time generation technology will significantly enhance user experience and artistic expressiveness.
By conducting in-depth research in these directions, AI music generation technology is expected to overcome existing limitations, achieving higher levels of structural coherence, expressiveness, and diversity, thus opening new possibilities for music creation and application. This will not only drive the intelligent evolution of music creation but also profoundly impact the development of human music culture.
This paper provides a comprehensive review of the key technologies, models, datasets, evaluation methods, and application scenarios in the field of AI music generation, offering a series of summaries and future directions based on the latest research findings. By reviewing and analyzing existing studies, this paper presents a new summarization framework that systematically categorizes and compares different technological approaches, including symbolic generation, audio generation, and hybrid models, thereby offering researchers a clear overview of the field. Through extensive research and analysis, this paper covers emerging topics such as multimodal datasets and emotional expression evaluation and reveals the potential impact of AI music generation across various application areas, including healthcare, education, and entertainment.
However, despite significant advances in the diversity, originality, and standardization of evaluation methods, AI music generation technology still faces numerous challenges. In particular, capturing complex musical structures, handling long-term dependencies, and ensuring the innovation of generated music remain pressing issues. Future research should focus more on the diversity and quality of datasets, explore new generation methods, and promote interdisciplinary collaboration to overcome the current limitations of the technology.
Overall, this paper provides a comprehensive knowledge framework for the field of AI music generation through systematic summaries and analyses, offering valuable references for future research directions and priorities. This not only contributes to the advancement of AI music generation technology but also lays the foundation for the intelligent and diverse development of music creation. As technology continues to evolve, the application prospects of AI in the music domain will become even broader. Future researchers can build upon this work to further expand the field, bringing more innovation and breakthroughs to music generation.
- Aalbers et al. (2017) Aalbers, S.; Fusar-Poli, L.; Freeman, R. E.; Spreen, M.; Ket, J. C.; Vink, A. C.; Maratos, A.; Crawford, M.; Chen, X.-J.; and Gold, C. 2017. Music therapy for depression. Cochrane database of systematic reviews , 1(11).
- Agarwal and Om (2021) Agarwal, G.; and Om, H. 2021. An efficient supervised framework for music mood recognition using autoencoder-based optimised support vector regression model. IET Signal Processing , 15(2): 98–121.
- Agostinelli et al. (2023) Agostinelli, A.; Denk, T. I.; Borsos, Z.; Engel, J.; Verzetti, M.; Caillon, A.; Huang, Q.; Jansen, A.; Roberts, A.; Tagliasacchi, M.; et al. 2023. Musiclm: Generating music from text. arXiv preprint arXiv:2301.11325 .
- Aljanaki, Yang, and Soleymani (2017) Aljanaki, A.; Yang, Y.-H.; and Soleymani, M. 2017. Developing a benchmark for emotional analysis of music. PloS one , 12(3): e0173392.
- Ardila et al. (2019) Ardila, R.; Branson, M.; Davis, K.; Henretty, M.; Kohler, M.; Meyer, J.; Morais, R.; Saunders, L.; Tyers, F. M.; and Weber, G. 2019. Common voice: A massively-multilingual speech corpus. arXiv preprint arXiv:1912.06670 .
- Beatoven Team (2023) Beatoven Team. 2023. AI-Generated Music for Games: What Game Developers Should Consider. https://www.beatoven.ai/blog/ai-generated-music-for-games-what-game-developers-should-consider/ . This blog discusses the considerations game developers should keep in mind when using AI-generated music, including the impact on player experience, the need for dynamic adaptability, and the balance between AI and human creativity in game soundtracks.
- Bertin-Mahieux et al. (2011) Bertin-Mahieux, T.; Ellis, D. P.; Whitman, B.; and Lamere, P. 2011. The million song dataset. No Journal Information Available .
- Bjork and Holopainen (2005) Bjork, S.; and Holopainen, J. 2005. Patterns in game design , volume 11. Charles River Media Hingham.
- Boulanger-Lewandowski, Bengio, and Vincent (2012) Boulanger-Lewandowski, N.; Bengio, Y.; and Vincent, P. 2012. Modeling temporal dependencies in high-dimensional sequences: Application to polyphonic music generation and transcription. arXiv preprint arXiv:1206.6392 .
- Briot, Hadjeres, and Pachet (2017) Briot, J.-P.; Hadjeres, G.; and Pachet, F.-D. 2017. Deep learning techniques for music generation–a survey. arXiv preprint arXiv:1709.01620 .
- Briot, Hadjeres, and Pachet (2020) Briot, J.-P.; Hadjeres, G.; and Pachet, F.-D. 2020. Deep learning techniques for music generation , volume 1. Springer.
- Brunner et al. (2018) Brunner, G.; Konrad, A.; Wang, Y.; and Wattenhofer, R. 2018. MIDI-VAE: Modeling Dynamics and Instrumentation of Music with Applications to Style Transfer. arXiv:1809.07600.
- Chen et al. (2020a) Chen, J.; Tan, X.; Luan, J.; Qin, T.; and Liu, T.-Y. 2020a. Hifisinger: Towards high-fidelity neural singing voice synthesis. arXiv preprint arXiv:2009.01776 .
- Chen et al. (2020b) Chen, N.; Zhang, Y.; Zen, H.; Weiss, R. J.; Norouzi, M.; and Chan, W. 2020b. Wavegrad: Estimating gradients for waveform generation. arXiv preprint arXiv:2009.00713 .
- Christophe Veaux (2017) Christophe Veaux, K. M., Junichi Yamagishi. 2017. CSTR VCTK Corpus: English Multi-speaker Corpus for CSTR Voice Cloning Toolkit. Dataset available from University of Edinburgh, The Centre for Speech Technology Research (CSTR). Date Available: 2017-04-04.
- Chu et al. (2022) Chu, H.; Kim, J.; Kim, S.; Lim, H.; Lee, H.; Jin, S.; Lee, J.; Kim, T.; and Ko, S. 2022. An empirical study on how people perceive AI-generated music. In Proceedings of the 31st ACM International Conference on Information & Knowledge Management , 304–314.
- Cífka, Şimşekli, and Richard (2020) Cífka, O.; Şimşekli, U.; and Richard, G. 2020. Groove2groove: One-shot music style transfer with supervision from synthetic data. IEEE/ACM Transactions on Audio, Speech, and Language Processing , 28: 2638–2650.
- Computer History Museum (2023) Computer History Museum. 2023. Algorithmic Music: David Cope and EMI. https://computerhistory.org/blog/algorithmic-music-david-cope-and-emi/ . This article explores the work of David Cope and his Experiments in Musical Intelligence (EMI), detailing how Cope developed algorithms to compose music in the style of famous composers, blending creativity with technology and sparking debates about the role of AI in art.
- Conklin (2003) Conklin, D. 2003. Music generation from statistical models. In Proceedings of the AISB 2003 Symposium on Artificial Intelligence and Creativity in the Arts and Sciences , 30–35. Citeseer.
- Cope (1996) Cope, D. 1996. Experiments in musical intelligence , volume 12. AR editions Madison, WI.
- Copet et al. (2024) Copet, J.; Kreuk, F.; Gat, I.; Remez, T.; Kant, D.; Synnaeve, G.; Adi, Y.; and Défossez, A. 2024. Simple and Controllable Music Generation. arXiv:2306.05284.
- Cross (2023) Cross, I. 2023. Music in the digital age: commodity, community, communion. AI & Society , 38: 2387–2400. Received: 10 October 2022; Accepted: 11 April 2023; Published: 28 April 2023; Issue Date: December 2023.
- Cuthbert and Ariza (2010) Cuthbert, M. S.; and Ariza, C. 2010. music21: A toolkit for computer-aided musicology and symbolic music data. No Journal Information Available .
- Danielsen (2018) Danielsen, A. 2018. Music, Media and Technological Creativity in the Digital Age . No Publisher Information Available.
- Dash and Agres (2023) Dash, A.; and Agres, K. 2023. AI-Based Affective Music Generation Systems: A Review of Methods and Challenges. ACM Computing Surveys .
- Defferrard et al. (2017) Defferrard, M.; Benzi, K.; Vandergheynst, P.; and Bresson, X. 2017. FMA: A Dataset For Music Analysis. arXiv:1612.01840.
- D’Errico (2016) D’Errico, M. A. 2016. Interface Aesthetics: Sound, Software, and the Ecology of Digital Audio Production . Ph.d. dissertation, University of California, Los Angeles, Los Angeles.
- Deruty et al. (2022) Deruty, E.; Grachten, M.; Lattner, S.; Nistal, J.; and Aouameur, C. 2022. On the development and practice of ai technology for contemporary popular music production. Transactions of the International Society for Music Information Retrieval , 5(1): 35–50.
- Dhariwal et al. (2020) Dhariwal, P.; Jun, H.; Payne, C.; Kim, J. W.; Radford, A.; and Sutskever, I. 2020. Jukebox: A Generative Model for Music. arXiv preprint .
- Donahue et al. (2019) Donahue, C.; Mao, H. H.; Li, Y. E.; Cottrell, G. W.; and McAuley, J. 2019. LakhNES: Improving multi-instrumental music generation with cross-domain pre-training. arXiv preprint arXiv:1907.04868 .
- Donahue, McAuley, and Puckette (2019) Donahue, C.; McAuley, J.; and Puckette, M. 2019. Adversarial Audio Synthesis. arXiv:1802.04208.
- Dong et al. (2018) Dong, H.-W.; Hsiao, W.-Y.; Yang, L.-C.; and Yang, Y.-H. 2018. Musegan: Multi-track sequential generative adversarial networks for symbolic music generation and accompaniment. In Proceedings of the AAAI Conference on Artificial Intelligence , volume 32.
- Engel et al. (2020) Engel, J.; Hantrakul, L.; Gu, C.; and Roberts, A. 2020. DDSP: Differentiable digital signal processing. arXiv preprint arXiv:2001.04643 .
- Engel et al. (2017) Engel, J.; Resnick, C.; Roberts, A.; Dieleman, S.; Norouzi, M.; Eck, D.; and Simonyan, K. 2017. Neural audio synthesis of musical notes with wavenet autoencoders. In International Conference on Machine Learning , 1068–1077. PMLR.
- Fonseca et al. (2017) Fonseca, E.; Pons Puig, J.; Favory, X.; Font Corbera, F.; Bogdanov, D.; Ferraro, A.; Oramas, S.; Porter, A.; and Serra, X. 2017. Freesound datasets: a platform for the creation of open audio datasets. In Hu X, Cunningham SJ, Turnbull D, Duan Z, editors. Proceedings of the 18th ISMIR Conference; 2017 oct 23-27; Suzhou, China.[Canada]: International Society for Music Information Retrieval; 2017. p. 486-93. International Society for Music Information Retrieval (ISMIR).
- Ford et al. (2024) Ford, C.; Noel-Hirst, A.; Cardinale, S.; Loth, J.; Sarmento, P.; Wilson, E.; others; and Bryan-Kinns, N. 2024. Reflection Across AI-based Music Composition. No Journal Information Available .
- Forsgren and Martiros (2022) Forsgren, S.; and Martiros, H. 2022. Riffusion - Stable diffusion for real-time music generation. No Journal Information Available .
- Gabrielsson (2001) Gabrielsson, A. 2001. Emotion perceived and emotion felt: Same or different? Musicae scientiae , 5(1_suppl): 123–147.
- Gemmeke et al. (2017) Gemmeke, J. F.; Ellis, D. P.; Freedman, D.; Jansen, A.; Lawrence, W.; Moore, R. C.; Plakal, M.; and Ritter, M. 2017. Audio set: An ontology and human-labeled dataset for audio events. In 2017 IEEE international conference on acoustics, speech and signal processing (ICASSP) , 776–780. IEEE.
- Gillick et al. (2019) Gillick, J.; Roberts, A.; Engel, J.; Eck, D.; and Bamman, D. 2019. Learning to groove with inverse sequence transformations. In International conference on machine learning , 2269–2279. PMLR.
- Goodfellow (2016) Goodfellow, I. 2016. Deep learning , volume 196. MIT press.
- Goodfellow et al. (2014) Goodfellow, I.; Pouget-Abadie, J.; Mirza, M.; Xu, B.; Warde-Farley, D.; Ozair, S.; Courville, A.; and Bengio, Y. 2014. Generative adversarial nets. Advances in neural information processing systems , 27.
- Goswami (2023) Goswami, A. 2023. Music and Artificial Intelligence: Exploring the Intersection of Creativity and Technology. Sangeet Galaxy , 12(2).
- Hadjeres, Pachet, and Nielsen (2017a) Hadjeres, G.; Pachet, F.; and Nielsen, F. 2017a. DeepBach: a Steerable Model for Bach Chorales Generation. In Precup, D.; and Teh, Y. W., eds., Proceedings of the 34th International Conference on Machine Learning , volume 70 of Proceedings of Machine Learning Research , 1362–1371. PMLR.
- Hadjeres, Pachet, and Nielsen (2017b) Hadjeres, G.; Pachet, F.; and Nielsen, F. 2017b. Deepbach: a steerable model for bach chorales generation. In International conference on machine learning , 1362–1371. PMLR.
- Hastie et al. (2009) Hastie, T.; Tibshirani, R.; Friedman, J. H.; and Friedman, J. H. 2009. The elements of statistical learning: data mining, inference, and prediction , volume 2. Springer.
- Hawthorne et al. (2018) Hawthorne, C.; Stasyuk, A.; Roberts, A.; Simon, I.; Huang, C.-Z. A.; Dieleman, S.; Elsen, E.; Engel, J.; and Eck, D. 2018. Enabling factorized piano music modeling and generation with the MAESTRO dataset. arXiv preprint arXiv:1810.12247 .
- Hernandez-Olivan and Beltran (2022) Hernandez-Olivan, C.; and Beltran, J. R. 2022. Music Composition with Deep Learning: A Review. In Advances in Speech and Music Technology: Computational Aspects and Applications , 25–50. No Publisher Information Available.
- Herremans, Chew et al. (2016) Herremans, D.; Chew, E.; et al. 2016. Tension ribbons: Quantifying and visualising tonal tension. No Journal Information Available .
- Herremans, Chuan, and Chew (2017) Herremans, D.; Chuan, C.-H.; and Chew, E. 2017. A functional taxonomy of music generation systems. ACM Computing Surveys (CSUR) , 50(5): 1–30.
- Hiller and Isaacson (1979) Hiller, L. A.; and Isaacson, L. M. 1979. Experimental Music; Composition with an electronic computer . Greenwood Publishing Group Inc.
- Ho, Jain, and Abbeel (2020) Ho, J.; Jain, A.; and Abbeel, P. 2020. Denoising diffusion probabilistic models. Advances in neural information processing systems , 33: 6840–6851.
- Holmes (2012) Holmes, T. 2012. Electronic and Experimental Music: Technology, Music, and Culture . New York: Routledge, 4th edition. ISBN 9780203128428.
- Horning (2013) Horning, S. S. 2013. Chasing Sound: Technology, Culture, and the Art of Studio Recording from Edison to the LP . Baltimore: Johns Hopkins University Press. ISBN 9781421410234.
- Hracs, Seman, and Virani (2016) Hracs, B. J.; Seman, M.; and Virani, T. E., eds. 2016. The Production and Consumption of Music in the Digital Age , volume 5. New York: Routledge.
- Hu and Yang (2017) Hu, X.; and Yang, Y.-H. 2017. Cross-dataset and cross-cultural music mood prediction: A case on western and chinese pop songs. IEEE Transactions on Affective Computing , 8(2): 228–240.
- Huang et al. (2018a) Huang, C.-Z. A.; Vaswani, A.; Uszkoreit, J.; Shazeer, N.; Simon, I.; Hawthorne, C.; Dai, A. M.; Hoffman, M. D.; Dinculescu, M.; and Eck, D. 2018a. Music transformer. arXiv preprint arXiv:1809.04281 .
- Huang et al. (2018b) Huang, C.-Z. A.; Vaswani, A.; Uszkoreit, J.; Shazeer, N.; Simon, I.; Hawthorne, C.; Dai, A. M.; Hoffman, M. D.; Dinculescu, M.; and Eck, D. 2018b. Music Transformer. arXiv:1809.04281.
- Huang et al. (2023a) Huang, Q.; Park, D. S.; Wang, T.; Denk, T. I.; Ly, A.; Chen, N.; Zhang, Z.; Zhang, Z.; Yu, J.; Frank, C.; et al. 2023a. Noise2music: Text-conditioned music generation with diffusion models. arXiv preprint arXiv:2302.03917 .
- Huang et al. (2023b) Huang, W.-C.; Violeta, L. P.; Liu, S.; Shi, J.; and Toda, T. 2023b. The singing voice conversion challenge 2023. In 2023 IEEE Automatic Speech Recognition and Understanding Workshop (ASRU) , 1–8. IEEE.
- Huang and Yang (2020) Huang, Y.-S.; and Yang, Y.-H. 2020. Pop music transformer: Beat-based modeling and generation of expressive pop piano compositions. In Proceedings of the 28th ACM international conference on multimedia , 1180–1188.
- Huron (2008) Huron, D. 2008. Sweet anticipation: Music and the psychology of expectation . MIT press.
- Ito and Johnson (2017) Ito, K.; and Johnson, L. 2017. The LJ Speech Dataset. https://keithito.com/LJ-Speech-Dataset/ .
- Ji, Yang, and Luo (2023) Ji, S.; Yang, X.; and Luo, J. 2023. A survey on deep learning for symbolic music generation: Representations, algorithms, evaluations, and challenges. ACM Computing Surveys , 56(1): 1–39.
- Juslin and Sloboda (2011) Juslin, P. N.; and Sloboda, J. 2011. Handbook of music and emotion: Theory, research, applications . Oxford University Press.
- Katz (2010) Katz, M. 2010. Capturing sound: How technology has changed music . Univ of California Press.
- Kim et al. (2010) Kim, Y. E.; Schmidt, E. M.; Migneco, R.; Morton, B. G.; Richardson, P.; Scott, J.; Speck, J. A.; and Turnbull, D. 2010. Music emotion recognition: A state of the art review. In Proc. ismir , volume 86, 937–952.
- Kong et al. (2020a) Kong, Q.; Li, B.; Chen, J.; and Wang, Y. 2020a. Giantmidi-piano: A large-scale midi dataset for classical piano music. arXiv preprint arXiv:2010.07061 .
- Kong et al. (2020b) Kong, Z.; Ping, W.; Huang, J.; Zhao, K.; and Catanzaro, B. 2020b. Diffwave: A versatile diffusion model for audio synthesis. arXiv preprint arXiv:2009.09761 .
- Kumar et al. (2019) Kumar, K.; Kumar, R.; De Boissiere, T.; Gestin, L.; Teoh, W. Z.; Sotelo, J.; De Brebisson, A.; Bengio, Y.; and Courville, A. C. 2019. Melgan: Generative adversarial networks for conditional waveform synthesis. Advances in neural information processing systems , 32.
- Law et al. (2009) Law, E.; West, K.; Mandel, M. I.; Bay, M.; and Downie, J. S. 2009. Evaluation of algorithms using games: The case of music tagging. In ISMIR , 387–392. Citeseer.
- Lei et al. (2024) Lei, W.; Wang, J.; Ma, F.; Huang, G.; and Liu, L. 2024. A Comprehensive Survey on Human Video Generation: Challenges, Methods, and Insights. arXiv preprint arXiv:2407.08428 .
- Li et al. (2018) Li, B.; Liu, X.; Dinesh, K.; Duan, Z.; and Sharma, G. 2018. Creating a multitrack classical music performance dataset for multimodal music analysis: Challenges, insights, and applications. IEEE Transactions on Multimedia , 21(2): 522–535.
- Liang (2016) Liang, F. 2016. Bachbot: Automatic Composition in the Style of Bach Chorales. University of Cambridge , 8(3.1): 19–48.
- Loy and Abbott (1985) Loy, G.; and Abbott, C. 1985. Programming languages for computer music synthesis, performance, and composition. ACM Computing Surveys (CSUR) , 17(2): 235–265.
- Lu and Dubnov (2021) Lu, C.; and Dubnov, S. 2021. ChordGAN: Symbolic music style transfer with chroma feature extraction. In Proceedings of the 2nd Conference on AI Music Creativity (AIMC), Online , 18–22.
- Lu et al. (2023) Lu, P.; Xu, X.; Kang, C.; Yu, B.; Xing, C.; Tan, X.; and Bian, J. 2023. Musecoco: Generating symbolic music from text. arXiv preprint arXiv:2306.00110 .
- Luo et al. (2020) Luo, J.; Yang, X.; Ji, S.; and Li, J. 2020. MG-VAE: deep Chinese folk songs generation with specific regional styles. In Proceedings of the 7th Conference on Sound and Music Technology (CSMT) Revised Selected Papers , 93–106. Springer.
- Magenta Team (2023) Magenta Team. 2023. Magenta: Exploring Machine Learning in Art and Music Creation. https://magenta.tensorflow.org/ . Magenta is a research project exploring the role of machine learning in art and music creation. Started by researchers and engineers from the Google Brain team, Magenta focuses on developing deep learning and reinforcement learning algorithms, and building tools to extend artists’ processes.
- Manilow et al. (2019) Manilow, E.; Wichern, G.; Seetharaman, P.; and Le Roux, J. 2019. Cutting music source separation some Slakh: A dataset to study the impact of training data quality and quantity. In 2019 IEEE Workshop on Applications of Signal Processing to Audio and Acoustics (WASPAA) , 45–49. IEEE.
- Marschall (2007) Marschall, O. A. 2007. Machine Composition-Between Lisp and Max: Between AI and Music . Master’s thesis, Lisp, Max, maxlisp and other recombinations. Master’s thesis.
- Miranda (1995) Miranda, E. R. 1995. An artificial intelligence approach to sound design. Computer Music Journal , 19(2): 59–75.
- Moysis et al. (2023) Moysis, L.; Iliadis, L. A.; Sotiroudis, S. P.; Boursianis, A. D.; Papadopoulou, M. S.; Kokkinidis, K.-I. D.; Volos, C.; Sarigiannidis, P.; Nikolaidis, S.; and Goudos, S. K. 2023. Music deep learning: deep learning methods for music signal processing—a review of the state-of-the-art. Ieee Access , 11: 17031–17052.
- MusedLab Team (2023) MusedLab Team. 2023. MusedLab: Music Experience Design Lab. https://musedlab.org/ . MusedLab is dedicated to exploring and creating new ways to engage people with music through technology, designing tools, instruments, and experiences that make music creation accessible to everyone. The lab combines research in music, education, and technology to develop innovative solutions for music learning and interaction.
- Oliver and Lalchev (2022) Oliver, P. G.; and Lalchev, S. 2022. Digital transformation in the music industry: how the COVID-19 pandemic has accelerated new business opportunities. In Rethinking the Music Business: Music Contexts, Rights, Data, and COVID-19 , 55–72. Springer.
- Oord et al. (2016) Oord, A. v. d.; Dieleman, S.; Zen, H.; Simonyan, K.; Vinyals, O.; Graves, A.; Kalchbrenner, N.; Senior, A.; and Kavukcuoglu, K. 2016. Wavenet: A generative model for raw audio. arXiv preprint arXiv:1609.03499 .
- Pachet (2003) Pachet, F. 2003. The continuator: Musical interaction with style. Journal of New Music Research , 32(3): 333–341.
- Papadopoulos, Roy, and Pachet (2016) Papadopoulos, A.; Roy, P.; and Pachet, F. 2016. Assisted lead sheet composition using flowcomposer. In Principles and Practice of Constraint Programming: 22nd International Conference, CP 2016, Toulouse, France, September 5-9, 2016, Proceedings 22 , 769–785. Springer.
- Payne (2019) Payne, C. 2019. MuseNet. https://openai.com/blog/musenet . OpenAI, 25 Apr. 2019.
- Penha and Cozman (2015) Penha, C.; and Cozman, F. G. 2015. The AMG1608 dataset for music emotion recognition. In 2015 IEEE International Conference on Acoustics, Speech and Signal Processing (ICASSP) , 717–721. Brisbane, Australia: IEEE.
- Picard (2000) Picard, R. W. 2000. Affective computing . MIT press.
- Pinch and Bijsterveld (2012) Pinch, T.; and Bijsterveld, K. 2012. The Oxford handbook of sound studies . OUP USA.
- Pinch and Trocco (2004) Pinch, T.; and Trocco, F. 2004. The Invention and Impact of the Moog Synthesizer . Cambridge, MA and London, England: Harvard University Press. ISBN 9780674042162.
- Privato, Rampado, and Novello (2022) Privato, N.; Rampado, O.; and Novello, A. 2022. A Creative Tool for the Musician Combining LSTM and Markov Chains in Max/MSP. In International Conference on Computational Intelligence in Music, Sound, Art and Design (Part of EvoStar) , 228–242. Cham: Springer International Publishing.
- Qian et al. (2024) Qian, Y.; Wang, T.; Tong, X.; Jin, X.; Xu, D.; Zheng, B.; Ge, T.; Yu, F.; and Zhu, S.-C. 2024. MusicAOG: an Energy-Based Model for Learning and Sampling a Hierarchical Representation of Symbolic Music. arXiv preprint arXiv:2401.02678 .
- Radford, Metz, and Chintala (2016) Radford, A.; Metz, L.; and Chintala, S. 2016. Unsupervised Representation Learning with Deep Convolutional Generative Adversarial Networks. arXiv:1511.06434.
- Raffel (2016) Raffel, C. 2016. Learning-based methods for comparing sequences, with applications to audio-to-midi alignment and matching . Columbia University.
- Rambarran (2021) Rambarran, S. 2021. Virtual Music: Sound, Music, and Image in the Digital Era . Bloomsbury Publishing USA.
- Reimer (1991) Reimer, B. 1991. A philosophy of music education. Journal of Aesthetics and Art Criticism , 49(3).
- Reuter (2022) Reuter, A. 2022. Who let the DAWs out? The digital in a new generation of the digital audio workstation. Popular Music and Society , 45(2): 113–128.
- Sacks (2008) Sacks, O. 2008. Musicophilia: Tales of music and the brain . Vintage.
- Schneider et al. (2024) Schneider, F.; Kamal, O.; Jin, Z.; and Schölkopf, B. 2024. Moûsai: Efficient Text-to-Music Diffusion Models. In Ku, L.-W.; Martins, A.; and Srikumar, V., eds., Proceedings of the 62nd Annual Meeting of the Association for Computational Linguistics (Volume 1: Long Papers) , 8050–8068. Bangkok, Thailand: Association for Computational Linguistics.
- Shahriar (2022) Shahriar, S. 2022. GAN computers generate arts? A survey on visual arts, music, and literary text generation using generative adversarial network. Displays , 73: 102237.
- Singh (2024) Singh, P. 2024. Media 2.0: A Journey through AI-Enhanced Communication and Content. Media and Al: Navigating , 127.
- Sloboda (1991) Sloboda, J. A. 1991. Music structure and emotional response: Some empirical findings. Psychology of music , 19(2): 110–120.
- Smule (2018) Smule. 2018. Digital Archive of Mobile Performances (DAMP). https://www.smule.com/songs . [Online; accessed 15-March-2018].
- Soleymani et al. (2013) Soleymani, M.; Caro, M. N.; Schmidt, E. M.; Sha, C.-Y.; and Yang, Y.-H. 2013. 1000 songs for emotional analysis of music. In Proceedings of the 2nd ACM international workshop on Crowdsourcing for multimedia , 1–6.
- Standley (1986) Standley, J. M. 1986. Music research in medical/dental treatment: meta-analysis and clinical applications. Journal of music therapy , 23(2): 56–122.
- Stefani (1987) Stefani, G. 1987. Melody: a popular perspective. Popular Music , 6(1): 21–35.
- Stöter, Liutkus, and Ito (2018) Stöter, F.-R.; Liutkus, A.; and Ito, N. 2018. The 2018 signal separation evaluation campaign. In Latent Variable Analysis and Signal Separation: 14th International Conference, LVA/ICA 2018, Guildford, UK, July 2–5, 2018, Proceedings 14 , 293–305. Springer.
- Tan and Li (2021) Tan, X.; and Li, X. 2021. A Tutorial on AI Music Composition. In Proceedings of the 29th ACM International Conference on Multimedia , 5678–5680.
- Tanev and Božinovski (2013) Tanev, G.; and Božinovski, A. 2013. Virtual Studio Technology Inside Music Production. In International Conference on ICT Innovations , 231–241. Heidelberg: Springer International Publishing.
- Tao (2022) Tao, F. 2022. A New Harmonisation of Art and Technology: Philosophic Interpretations of Artificial Intelligence Art. Critical Arts , 36(1-2): 110–125.
- Taylor, Ardeliya, and Wolfson (2024) Taylor, J.; Ardeliya, V. E.; and Wolfson, J. 2024. Exploration of Artificial Intelligence in Creative Fields: Generative Art, Music, and Design. International Journal of Cyber and IT Service Management , 4(1): 39–45.
- Théberge (2021) Théberge, P. 2021. Any Sound You Can Imagine: Making Music/Consuming Technology . Wesleyan University Press.
- Toiviainen and Eerola (2006) Toiviainen, P.; and Eerola, T. 2006. Autocorrelation in meter induction: The role of accent structure. The Journal of the Acoustical Society of America , 119(2): 1164–1170.
- Topirceanu, Barina, and Udrescu (2014) Topirceanu, A.; Barina, G.; and Udrescu, M. 2014. Musenet: Collaboration in the music artists industry. In 2014 European Network Intelligence Conference , 89–94. IEEE.
- Turnbull et al. (2007) Turnbull, D.; Barrington, L.; Torres, D.; and Lanckriet, G. 2007. Towards musical query-by-semantic-description using the cal500 data set. In Proceedings of the 30th annual international ACM SIGIR conference on Research and development in information retrieval , 439–446.
- van den Oord et al. (2016) van den Oord, A.; Dieleman, S.; Zen, H.; Simonyan, K.; Vinyals, O.; Graves, A.; Kalchbrenner, N.; Senior, A.; and Kavukcuoglu, K. 2016. WaveNet: A Generative Model for Raw Audio. arXiv:1609.03499.
- Vaswani (2017) Vaswani, A. 2017. Attention is all you need. arXiv preprint arXiv:1706.03762 .
- Wang et al. (2024) Wang, L.; Zhao, Z.; Liu, H.; Pang, J.; Qin, Y.; and Wu, Q. 2024. A review of intelligent music generation systems. Neural Computing and Applications , 36(12): 6381–6401.
- Wang (2017) Wang, Y. 2017. The Design and Study of Virtual Sound Field in Music Production. Journal of The Korea Society of Computer and Information , 22(7): 83–91.
- Wang et al. (2017) Wang, Y.; Skerry-Ryan, R.; Stanton, D.; Wu, Y.; Weiss, R. J.; Jaitly, N.; Yang, Z.; Xiao, Y.; Chen, Z.; Bengio, S.; et al. 2017. Tacotron: A fully end-to-end text-to-speech synthesis model. arXiv preprint arXiv:1703.10135 , 164.
- Wang, Min, and Xia (2024) Wang, Z.; Min, L.; and Xia, G. 2024. Whole-song hierarchical generation of symbolic music using cascaded diffusion models. arXiv preprint arXiv:2405.09901 .
- Wu et al. (2021) Wu, Y.; Manilow, E.; Deng, Y.; Swavely, R.; Kastner, K.; Cooijmans, T.; Courville, A.; Huang, C.-Z. A.; and Engel, J. 2021. MIDI-DDSP: Detailed control of musical performance via hierarchical modeling. arXiv preprint arXiv:2112.09312 .
- Xenakis (1992) Xenakis, I. 1992. Formalized music: thought and mathematics in composition . 6. Pendragon Press.
- Yang, Chou, and Yang (2017) Yang, L.-C.; Chou, S.-Y.; and Yang, Y.-H. 2017. MidiNet: A convolutional generative adversarial network for symbolic-domain music generation. arXiv preprint arXiv:1703.10847 .
- Yang et al. (2019) Yang, R.; Chen, T.; Zhang, Y.; and Xia, G. 2019. Inspecting and interacting with meaningful music representations using VAE. arXiv preprint arXiv:1904.08842 .
- Yang and Chen (2012) Yang, Y.-H.; and Chen, H. H. 2012. Machine recognition of music emotion: A review. ACM Transactions on Intelligent Systems and Technology (TIST) , 3(3): 1–30.
- Zak III (2001) Zak III, A. J. 2001. The poetics of rock: Cutting tracks, making records . Univ of California Press.
- Zhang, Yan, and Briot (2023) Zhang, N.; Yan, J.; and Briot, J.-P. 2023. Artificial intelligence techniques for pop music creation: A real music production perspective. No Journal Information Available .
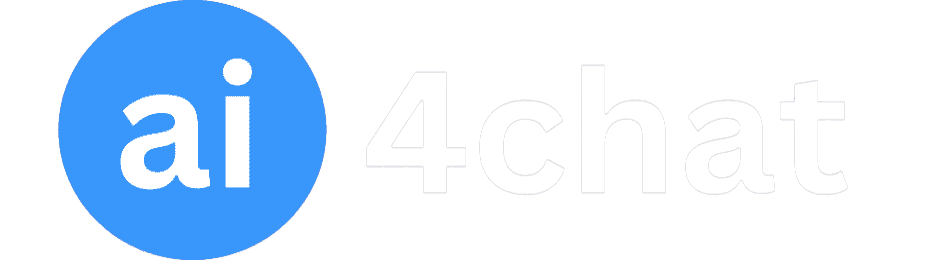
Abstract Generator - AI Tool for Academic Essay Abstracts
Introduction to abstract generator-free ai-powered abstract creation.
Welcome to Abstract Generator, your AI-driven solution for crafting precise and compelling academic essay abstracts with ease. Designed specifically for university students and researchers, this advanced tool leverages artificial intelligence to provide accurate, coherent, and concise abstracts that align with academic standards. Whether you're pressed for time or need assistance in summarizing complex essays, Abstract Generator ensures the production of high-quality abstracts while maintaining academic integrity and adherence to stylistic guidelines.
Use Cases of Abstract Generator
For university students.
University students often face tight deadlines and multiple assignments. Abstract Generator can alleviate some of this pressure by quickly producing coherent and academically styled abstracts for their research papers and essays. For instance, a student writing a paper on climate change can input the main points of their research, and the tool will generate a concise, professional abstract, saving them valuable time.
For Researchers
Researchers need to maintain high standards of academic writing while managing various projects. Abstract Generator aids researchers by offering an efficient way to generate abstracts that adhere to academic norms. Imagine a researcher working on a complex study about AI in healthcare; by summarizing their findings and inputting key information into the tool, they can receive a polished abstract that highlights the significance and methodology of their study, ready for journal submission.
Who Can Use Abstract Generator
University students.
University students often face the challenge of summarizing lengthy academic essays into concise abstracts. Abstract Generator can assist in reducing time spent on this task while maintaining high standards of academic integrity and style adherence. This AI-powered tool ensures that every generated abstract is both accurate and efficient, making it an indispensable resource for students across various disciplines.
Researchers
For researchers, producing precise and comprehensive abstracts is crucial for the dissemination of their work. Abstract Generator aids researchers by offering a reliable means to create abstracts that accurately reflect their papers' contents, ensuring clarity and professionalism. By leveraging this tool, researchers can focus more on their core activities, trusting the AI to handle abstract creation.
How to Use Abstract Generator
Step 1: enter the details.
Begin by entering the details of your academic essay into the text input field. This includes key points, main ideas, or any other relevant information you want included in the abstract.
Step 2: Send the Message
Once you've entered the necessary details, click the "Send Message" button. The AI bot will process your input and generate a comprehensive and accurate abstract based on the provided information.
Step 3: Review and Modify
After the AI generates the abstract, review the output. If you need any modifications, such as making the abstract shorter or more detailed, simply type your request in a follow-up message. The AI will adjust the abstract accordingly based on your instructions.
Frequently Asked Questions for Abstract Generator
How do i use the ai abstract generator.
Using the AI Abstract Generator is simple. Input the details of your academic essay or paper into the text input field and hit the 'Send Message' button. The AI will generate an abstract based on the provided information. If you need adjustments, you can provide further instructions in a follow-up message.
Can I modify the generated abstract?
Yes, you can. If you want the abstract to be shorter, more detailed, or adjusted in any other way, you can mention your specific requirements in a follow-up message. The AI will refine the abstract according to your directions.
Is the abstract generation instant?
The abstract generation process is designed to be quick and efficient. Once you submit your details, the AI will typically generate an abstract within a few moments.
Does the tool ensure academic integrity and style adherence?
Absolutely. The AI Abstract Generator is designed to create abstracts that adhere to academic integrity and style guidelines, ensuring that the generated content is appropriate for university students and researchers.
Is my data safe with the AI Abstract Generator?
We take data privacy seriously. The information you provide to the AI Abstract Generator is used solely for the purpose of generating the abstract. We do not store or share your data without your consent.
What types of academic documents can I use this tool for?
The AI Abstract Generator is versatile and can be used for a wide range of academic documents, including essays, research papers, thesis papers, and more. Its algorithm is equipped to handle various academic writing needs.
Questions about AI4Chat? We are here to help!
For any inquiries, drop us an email at [email protected] . We’re always eager to assist and provide more information.
What Is AI4Chat?
What features are available on ai4chat.
- 🔍 Google Search Results: Generate content that's current and fact-based using Google's search results.
- 📂 Categorizing Chats into Folders: Organize your chats for easy access and management.
- 🏷 Adding Labels: Tag your chats for quick identification and sorting.
- 📷 Custom Chat Images: Set a custom image for each chat, personalizing your chat interface.
- 🔢 Word Count: Monitor the length of your chats with a word count feature.
- 🎨 Tone Selection: Customize the tone of chatbot responses to suit the mood or context of the conversation.
- 📝 Chat Description: Add descriptions to your chats for context and clarity, making it easier to revisit and understand chat histories.
- 🔎 Search: Easily find past chats with a powerful search feature, improving your ability to recall information.
- 🔗 Sharable Chat Link: Generate a link to share your chat, allowing others to view the conversation.
- 🌍 Multilingual Chat in 75+ Languages: Communicate and generate content in over 75 languages, expanding your global reach.
- 💻 AI Code Assistance: Leverage AI to generate code in any programming language, debug errors, or ask any coding-related questions. Our AI models are specially trained to understand and provide solutions for coding queries, making it an invaluable tool for developers seeking to enhance productivity, learn new programming concepts, or solve complex coding challenges efficiently.
- 📁 AI Chat with Files and Images: Upload images or files and ask questions related to their content. AI automatically understands and answers questions based on the content or context of the uploaded files.
- 📷 AI Text to Image & Image to Image: Create stunning visuals with models like Stable Diffusion, Midjourney, DALLE v2, DALLE v3, and Leonardo AI.
- 🎙 AI Text to Voice/Speech: Transform text into engaging audio content.
- 🎵 AI Text to Music: Convert your text prompts into melodious music tracks. Leverage the power of AI to craft unique compositions based on the mood, genre, or theme you specify in your text.
- 🎥 AI Text to Video: Convert text scripts into captivating video content.
- 🔍 AI Image to Text with Context Understanding: Not only extract text from images but also understand the context of the visual content. For example, if a user uploads an image of a teddy bear, AI will recognize it as such.
- 🔀 AI Image to Video: Turn images into dynamic videos with contextual understanding.
- 📸 AI Professional Headshots: Generate professional-quality avatars or profile photos with AI.
- ✂ AI Image Editor, Resizer and Compressor, Upscale: Enhance, optimize, and upscale your images with AI-powered tools.
- 🎼 AI Music to Music: Enhance or transform existing music tracks by inputting an audio file. AI analyzes your music and generates a continuation or variation, offering a new twist on your original piece.
- 🗣 AI Voice Chat: Experience interactive voice responses with AI personalities.
- ☁ Cloud Storage: All content generated is saved to the cloud, ensuring you can access your creations from any device, anytime.
Which Languages Does AI4Chat Support?
How do i toggle between different ai models, can i personalize my chats, what is a credit, can i upgrade, downgrade, or cancel my current plan anytime, what happens if i run out of credits, do unused credits carry forward to the next month, is there an option for unlimited usage, do i need a credit card to get started, what is the refund policy for subscriptions and one-time credit purchases, are payments safe, do you offer team or volume discounts, do you offer api access, can i use generated content for commercial purposes, is it easy to cancel my membership, where can i download the ai4chat mobile app, can i use the content generated using ai4chat for commercial purposes, how do i contact support, more questions, all set to level up your content game.
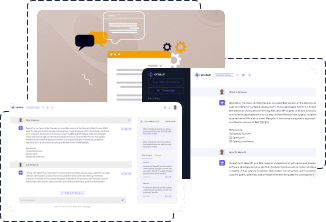
All in One AI platform for AI chat, image, video, music, and voice generatation. Create custom AI bots and workflows in minutes from any device, anywhere.
- AI Art & Images
- AI Music & Voice
AI4Chat © 2024. All Rights Reserved.
- Privacy Policy
Want some more features?
- - History to store generated content
- - Access to mobile apps for content generation on the go
- - Access to 500+ other AI tools and templates
IEEE Account
- Change Username/Password
- Update Address
Purchase Details
- Payment Options
- Order History
- View Purchased Documents
Profile Information
- Communications Preferences
- Profession and Education
- Technical Interests
- US & Canada: +1 800 678 4333
- Worldwide: +1 732 981 0060
- Contact & Support
- About IEEE Xplore
- Accessibility
- Terms of Use
- Nondiscrimination Policy
- Privacy & Opting Out of Cookies
A not-for-profit organization, IEEE is the world's largest technical professional organization dedicated to advancing technology for the benefit of humanity. © Copyright 2024 IEEE - All rights reserved. Use of this web site signifies your agreement to the terms and conditions.
A Self-Efficacy Theory-based Study on the Teachers’ Readiness to Teach Artificial Intelligence in Public Schools in Sri Lanka
New citation alert added.
This alert has been successfully added and will be sent to:
You will be notified whenever a record that you have chosen has been cited.
To manage your alert preferences, click on the button below.
New Citation Alert!
Please log in to your account
Information & Contributors
Bibliometrics & citations, view options, index terms.
Applied computing
Computer-managed instruction
Interactive learning environments
Learning management systems
Social and professional topics
Professional topics
Computing education
Adult education
Computational thinking
K-12 education
Student assessment
Recommendations
A survey of australian teachers' self-efficacy and assessment approaches for the k-12 digital technologies curriculum.
As K-12 computer science (CS) education has been introduced to a number of countries around the world, the CS education community has been busy working to understand the learning and teaching of computing at these year levels, as well as how to build ...
CS in Schools: Developing a sustainable Coding Programme in Australian Schools
Digital technology is compulsory in schools in most states at most year levels in Australia. However, a recent survey of over 400 Australian schools in 2019 found that 96% have had difficulty hiring qualified technology teachers and 39% of schools have ...
Developing K-8 Computer Science Teachers' Content Knowledge, Self-efficacy, and Attitudes through Evidence-based Professional Development
Broadening participation in computer science (CS) for primary/elementary students is a growing movement, spurred by computing workforce demands and the need for younger students to develop skills in problem solving and critical/computational thinking. ...
Information
Published in.

Association for Computing Machinery
New York, NY, United States
Publication History
Check for updates, author tags.
- Teaching Artificial Intelligence
- K-12 Education
- Self-Efficacy Theory
- Teacher Professional Development
- Research-article
Contributors
Other metrics, bibliometrics, article metrics.
- 0 Total Citations
- 0 Total Downloads
- Downloads (Last 12 months) 0
- Downloads (Last 6 weeks) 0
View options
View or Download as a PDF file.
View online with eReader .
Login options
Check if you have access through your login credentials or your institution to get full access on this article.
Full Access
Share this publication link.
Copying failed.
Share on social media
Affiliations, export citations.
- Please download or close your previous search result export first before starting a new bulk export. Preview is not available. By clicking download, a status dialog will open to start the export process. The process may take a few minutes but once it finishes a file will be downloadable from your browser. You may continue to browse the DL while the export process is in progress. Download
- Download citation
- Copy citation
We are preparing your search results for download ...
We will inform you here when the file is ready.
Your file of search results citations is now ready.
Your search export query has expired. Please try again.

IMAGES
VIDEO
COMMENTS
Together, these elements form the foundation of an effective AI paper abstract, guiding readers through the research's motivation and intended contributions to the field of artificial intelligence. Methods and Results in AI Paper Abstracts. When crafting an AI paper abstract, it's crucial to highlight the methods and results effectively.
A Brief Introduction To Artificial Intelligence
Best Practices for Presentation Design. When crafting an AI overview for a PowerPoint presentation, it's crucial to highlight key aspects that capture the essence of artificial intelligence. Begin by defining AI in clear, accessible terms, emphasizing its ability to mimic human intelligence and learn from data.
Generative AI: A Review on Models and Applications
The field of artificial intelligence (AI) has shown an upward trend of growth in the 21st century (from 2000 to 2015). The evolution in AI has advanced the development of human society in our own time, with dramatic revolutions shaped by both theories and techniques. However, the multidisciplinary and fast-growing features make AI a field in which it is difficult to be well understood. In this ...
derivative. Artificial Intelligence is an emerging technology. that started modifying educational tools and. institutions. Educatio n is a field where the p resence of. teachers is must w hich is ...
Artificial intelligence: A powerful paradigm for scientific ...
The document discusses various aspects and applications of artificial intelligence including knowledge representation, problem solving, learning, natural language processing, motion and manipulation, and perception. It describes how AI research aims to endow machines with various intelligent capabilities and traits displayed by humans such as reasoning, learning, and language understanding ...
14 Best Presentations On Artificial Intelligence ...
Abstract and Figures. This paper focus on the History of A.I. and how it begun as an idea and, the definition of artificial intelligence and gives a detailed description of Artificial Intelligence ...
Tsu-Jui Fu, William Yang Wang, Daniel McDuff, Yale Song. View a PDF of the paper titled DOC2PPT: Automatic Presentation Slides Generation from Scientific Documents, by Tsu-Jui Fu and 3 other authors. Creating presentation materials requires complex multimodal reasoning skills to summarize key concepts and arrange them in a logical and visually ...
The Impact of Artificial Intelligence on Innovation
The impact of artificial intelligence on human society and ...
Abstract: Advances in artificial intelligence and machine learning (AI/ML) algorithms are not only the fastest growing areas but also provide endless possibilities in many different science and engineering disciplines including computer communication networks. These technologies are used by billions of people. Any person who has a smartphone can tangibly experience advances in communication ...
"Best Paper Award Second Prize" ICGECD 2020 -2nd International Conference on General Education and Contemporary Development, October 23-24, 2020 with our research paper Artificial intelligence ...
754 Words. 4 Pages. Open Document. Abstract :Artificial intelligence (AI) is a science that involves simulation of intelligent behaviours in machineries, like visual perception, decision making, speech recognition and so on. While the rate of progress in AI has been patchy and unpredictable, there have been significant advances.
Convert Research Papers to PPT with AI
Artificial intelligence (AI) is an emerging tool in multiple research areas including healthcare and has been suggested to assist communication also with patients. 5 -8 Using AI language models to generate lay abstracts for scientific publications has the potential to ensure consistency and accuracy in the language used to describe scientific ...
Research Objectives: This paper aims to systematically review the latest research progress in symbolic and audio music generation, explore their potential and challenges in various application scenarios, and forecast future development directions. Through a comprehensive analysis of existing technologies and methods, this paper seeks to provide valuable references for researchers and ...
Using the AI Abstract Generator is simple. Input the details of your academic essay or paper into the text input field and hit the 'Send Message' button. The AI will generate an abstract based on the provided information. If you need adjustments, you can provide further instructions in a follow-up message.
Abstract: Generative artificial intelligence can make powerful artifacts when used at scale, but developing trust in these artifacts and controlling their creation are essential for user adoption. Published in: Computer ( Volume: 55 , Issue: 10 , October 2022 ) Article #: Page (s): 107 - 112. Date of Publication: 27 September 2022.
Introduction. The dawn of the 2020s has witnessed a major shakeup in digital writing technologies due to a sudden evolution in the field of artificial "intelligence" (AI), with generative AI (GAI) systems emerging as one of the most transformative and debated technologies.
Artificial intelligence and electrical automation control technologies are evolving and growing together with science and technological advancement. More and more artificial intelligence technology is being used in electrical automation control, which provides a good foundation for the development of automation control technology support. This paper introduces artificial intelligence and ...
Findings.This study identified several key findings: 1) Teachers generally reported low self-efficacy regarding their ability to teach AI, 2) Teachers' self-efficacy was most influenced by their emotional and physiological states, as well as their imaginary experiences related to teaching AI, 3) Surprisingly, mastery experiences had a lesser impact on their self-efficacy for teaching AI, and 4 ...