- Utility Menu


GA4 Tracking Code

fa51e2b1dc8cca8f7467da564e77b5ea
- Make a Gift
- Join Our Email List
- Problem Solving in STEM
Solving problems is a key component of many science, math, and engineering classes. If a goal of a class is for students to emerge with the ability to solve new kinds of problems or to use new problem-solving techniques, then students need numerous opportunities to develop the skills necessary to approach and answer different types of problems. Problem solving during section or class allows students to develop their confidence in these skills under your guidance, better preparing them to succeed on their homework and exams. This page offers advice about strategies for facilitating problem solving during class.
How do I decide which problems to cover in section or class?
In-class problem solving should reinforce the major concepts from the class and provide the opportunity for theoretical concepts to become more concrete. If students have a problem set for homework, then in-class problem solving should prepare students for the types of problems that they will see on their homework. You may wish to include some simpler problems both in the interest of time and to help students gain confidence, but it is ideal if the complexity of at least some of the in-class problems mirrors the level of difficulty of the homework. You may also want to ask your students ahead of time which skills or concepts they find confusing, and include some problems that are directly targeted to their concerns.
You have given your students a problem to solve in class. What are some strategies to work through it?
- Try to give your students a chance to grapple with the problems as much as possible. Offering them the chance to do the problem themselves allows them to learn from their mistakes in the presence of your expertise as their teacher. (If time is limited, they may not be able to get all the way through multi-step problems, in which case it can help to prioritize giving them a chance to tackle the most challenging steps.)
- When you do want to teach by solving the problem yourself at the board, talk through the logic of how you choose to apply certain approaches to solve certain problems. This way you can externalize the type of thinking you hope your students internalize when they solve similar problems themselves.
- Start by setting up the problem on the board (e.g you might write down key variables and equations; draw a figure illustrating the question). Ask students to start solving the problem, either independently or in small groups. As they are working on the problem, walk around to hear what they are saying and see what they are writing down. If several students seem stuck, it might be a good to collect the whole class again to clarify any confusion. After students have made progress, bring the everyone back together and have students guide you as to what to write on the board.
- It can help to first ask students to work on the problem by themselves for a minute, and then get into small groups to work on the problem collaboratively.
- If you have ample board space, have students work in small groups at the board while solving the problem. That way you can monitor their progress by standing back and watching what they put up on the board.
- If you have several problems you would like to have the students practice, but not enough time for everyone to do all of them, you can assign different groups of students to work on different – but related - problems.
When do you want students to work in groups to solve problems?
- Don’t ask students to work in groups for straightforward problems that most students could solve independently in a short amount of time.
- Do have students work in groups for thought-provoking problems, where students will benefit from meaningful collaboration.
- Even in cases where you plan to have students work in groups, it can be useful to give students some time to work on their own before collaborating with others. This ensures that every student engages with the problem and is ready to contribute to a discussion.
What are some benefits of having students work in groups?
- Students bring different strengths, different knowledge, and different ideas for how to solve a problem; collaboration can help students work through problems that are more challenging than they might be able to tackle on their own.
- In working in a group, students might consider multiple ways to approach a problem, thus enriching their repertoire of strategies.
- Students who think they understand the material will gain a deeper understanding by explaining concepts to their peers.
What are some strategies for helping students to form groups?
- Instruct students to work with the person (or people) sitting next to them.
- Count off. (e.g. 1, 2, 3, 4; all the 1’s find each other and form a group, etc)
- Hand out playing cards; students need to find the person with the same number card. (There are many variants to this. For example, you can print pictures of images that go together [rain and umbrella]; each person gets a card and needs to find their partner[s].)
- Based on what you know about the students, assign groups in advance. List the groups on the board.
- Note: Always have students take the time to introduce themselves to each other in a new group.
What should you do while your students are working on problems?
- Walk around and talk to students. Observing their work gives you a sense of what people understand and what they are struggling with. Answer students’ questions, and ask them questions that lead in a productive direction if they are stuck.
- If you discover that many people have the same question—or that someone has a misunderstanding that others might have—you might stop everyone and discuss a key idea with the entire class.
After students work on a problem during class, what are strategies to have them share their answers and their thinking?
- Ask for volunteers to share answers. Depending on the nature of the problem, student might provide answers verbally or by writing on the board. As a variant, for questions where a variety of answers are relevant, ask for at least three volunteers before anyone shares their ideas.
- Use online polling software for students to respond to a multiple-choice question anonymously.
- If students are working in groups, assign reporters ahead of time. For example, the person with the next birthday could be responsible for sharing their group’s work with the class.
- Cold call. To reduce student anxiety about cold calling, it can help to identify students who seem to have the correct answer as you were walking around the class and checking in on their progress solving the assigned problem. You may even want to warn the student ahead of time: "This is a great answer! Do you mind if I call on you when we come back together as a class?"
- Have students write an answer on a notecard that they turn in to you. If your goal is to understand whether students in general solved a problem correctly, the notecards could be submitted anonymously; if you wish to assess individual students’ work, you would want to ask students to put their names on their notecard.
- Use a jigsaw strategy, where you rearrange groups such that each new group is comprised of people who came from different initial groups and had solved different problems. Students now are responsible for teaching the other students in their new group how to solve their problem.
- Have a representative from each group explain their problem to the class.
- Have a representative from each group draw or write the answer on the board.
What happens if a student gives a wrong answer?
- Ask for their reasoning so that you can understand where they went wrong.
- Ask if anyone else has other ideas. You can also ask this sometimes when an answer is right.
- Cultivate an environment where it’s okay to be wrong. Emphasize that you are all learning together, and that you learn through making mistakes.
- Do make sure that you clarify what the correct answer is before moving on.
- Once the correct answer is given, go through some answer-checking techniques that can distinguish between correct and incorrect answers. This can help prepare students to verify their future work.
How can you make your classroom inclusive?
- The goal is that everyone is thinking, talking, and sharing their ideas, and that everyone feels valued and respected. Use a variety of teaching strategies (independent work and group work; allow students to talk to each other before they talk to the class). Create an environment where it is normal to struggle and make mistakes.
- See Kimberly Tanner’s article on strategies to promoste student engagement and cultivate classroom equity.
A few final notes…
- Make sure that you have worked all of the problems and also thought about alternative approaches to solving them.
- Board work matters. You should have a plan beforehand of what you will write on the board, where, when, what needs to be added, and what can be erased when. If students are going to write their answers on the board, you need to also have a plan for making sure that everyone gets to the correct answer. Students will copy what is on the board and use it as their notes for later study, so correct and logical information must be written there.
For more information...
Tipsheet: Problem Solving in STEM Sections
Tanner, K. D. (2013). Structure matters: twenty-one teaching strategies to promote student engagement and cultivate classroom equity . CBE-Life Sciences Education, 12(3), 322-331.
- Designing Your Course
- A Teaching Timeline: From Pre-Term Planning to the Final Exam
- The First Day of Class
- Group Agreements
- Classroom Debate
- Flipped Classrooms
- Leading Discussions
- Polling & Clickers
- Teaching with Cases
- Engaged Scholarship
- Devices in the Classroom
- Beyond the Classroom
- On Professionalism
- Getting Feedback
- Equitable & Inclusive Teaching
- Artificial Intelligence
- Advising and Mentoring
- Teaching and Your Career
- Teaching Remotely
- Tools and Platforms
- The Science of Learning
- Bok Publications
- Other Resources Around Campus
Learn by .css-1v0lc0l{color:var(--chakra-colors-blue-500);} doing
Guided interactive problem solving that’s effective and fun. Master concepts in 15 minutes a day.
Data Analysis
Computer Science
Programming & AI
Science & Engineering
Join over 10 million people learning on Brilliant
Master concepts in 15 minutes a day.
Whether you’re a complete beginner or ready to dive into machine learning and beyond, Brilliant makes it easy to level up fast with fun, bite-sized lessons.
Effective, hands-on learning
Visual, interactive lessons make concepts feel intuitive — so even complex ideas just click. Our real-time feedback and simple explanations make learning efficient.
Learn at your level
Students and professionals alike can hone dormant skills or learn new ones. Progress through lessons and challenges tailored to your level. Designed for ages 13 to 113.
Guided bite-sized lessons
We make it easy to stay on track, see your progress, and build your problem-solving skills one concept at a time.
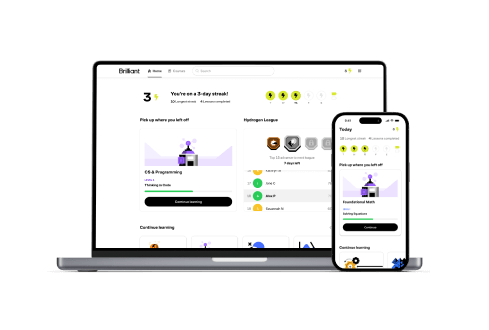
Stay motivated
Form a real learning habit with fun content that’s always well-paced, game-like progress tracking, and friendly reminders.
© 2024 Brilliant Worldwide, Inc., Brilliant and the Brilliant Logo are trademarks of Brilliant Worldwide, Inc.

Problem-Solving in Science and Technology Education
- First Online: 25 February 2023
Cite this chapter
- Bulent Çavaş 13 ,
- Pınar Çavaş 14 &
- Yasemin Özdem Yılmaz 15
Part of the book series: Contemporary Trends and Issues in Science Education ((CTISE,volume 56))
552 Accesses
1 Citations
This chapter focuses on problem-solving, which involves describing a problem, figuring out its root cause, locating, ranking and choosing potential solutions, as well as putting those solutions into action in science and technology education. This chapter covers (1) what problem-solving means for science and technology education; (2) what the problem-solving processes are and how these processes can be used step-by-step for effective problem-solving and (3) the use of problem-solving in citizen science projects supported by the European Union. The chapter also includes discussion of and recommendations for future scientific research in the field of science and technology education.
This is a preview of subscription content, log in via an institution to check access.
Access this chapter
Subscribe and save.
- Get 10 units per month
- Download Article/Chapter or eBook
- 1 Unit = 1 Article or 1 Chapter
- Cancel anytime
- Available as PDF
- Read on any device
- Instant download
- Own it forever
- Available as EPUB and PDF
- Compact, lightweight edition
- Dispatched in 3 to 5 business days
- Free shipping worldwide - see info
- Durable hardcover edition
Tax calculation will be finalised at checkout
Purchases are for personal use only
Institutional subscriptions
Similar content being viewed by others
Designing Problem-Solving for Meaningful Learning: A Discussion of Asia-Pacific Research
The Place of Problems in Problem Based Learning: A Case of Mathematics and Teacher Education
An Alternative Method to Promote Pupils’ Mathematical Understanding via Problem Solving
Anderson, H. O. (1967). Problem-solving and science teaching. School Science and Mathematics, 67 (3), 243–251. https://doi.org/10.1111/j.1949-8594.1967.tb15151.x
Article Google Scholar
Ausubel, D. P. (1968). Educational psychology: A cognitive view . Holt, Rinehart and Winston.
Google Scholar
Binkley, M., Erstad, O., Herman, J., Raizen, S., Ripley, M., Miller-Ricci, M., & Rumble, M. (2012). Defining twentyfirst century skills. In P. Griffin, B. McGaw, & E. Care (Eds.), Assessment and teaching of 21st century skills (pp. 17–66). Springer.
Bransford, J. D., & Stein, B. S. (1984). The IDEAL problem solver: A guide to improving thinking . W.H. Freeman & Co.
Chi, M. T. H., Feltovich, P. J., & Glaser, R. (1981). Categorization and representation of physics problems by experts and novices. Cognitive Science, 5 , 121–152.
Chin, C., & Chia, L. G. (2006). Problem-based learning: Using ill-structured problems in biology project work. Science Education, 90 (1), 44–67.
Egger, A. E., & Carpi, A. (2008). Data analysis and interpretation. Visionlearning, POS-1 , (1).
Gallagher, S. A., Stepien, W. J., & Rosenthal, H. (1992). The effects of problem-based learning on problem solving. Gifted Child Quarterly, 36 (4), 195–200.
Gallagher, S. A., Sher, B. T., Stepien, W. J., & Workman, D. (1995). Implementing problem-based learning in science classrooms. School Science and Mathematics, 95 (3), 136–146.
Garrett, R. M. (1986). Problem-solving in science education. Studies in Science Education, 13 , 70–95.
Glaser, R. (1992). Expert knowledge and processes of thinking. In D. F. Halpern (Ed.), Enhancing thinking skills in the sciences and mathematics (pp. 63–76). Erlbaum.
Greenwald, N. L. (2000). Learning from problems. The Science Teacher, 67 (4), 28–32.
Hobden, P. (1998). The role of routine problem tasks in science teaching. In B. J. Fraser & K. G. Tobin (Eds.), International handbook of science education, Vol. 1 (pp. 219–231).
Chapter Google Scholar
Ioannidou, O., & Erduran, S. (2021). Beyond hypothesis testing. Science & Education, 30 , 345–364. https://doi.org/10.1007/s11191-020-00185-9
Jonassen, D. H. (1997). Instructional design models for well-structured and ill-structured problem-solving learning outcomes. Educational Technology Research and Development, 45 (1), 65–94.
Koberg, D., & Bagnall, J. (1981). The design process is a problem-solving journey. In D. Koberg & J. Bagnall (Eds.), The all new universal Traveler: A soft-systems guide to creativity, problem-solving, and the process of reaching goals (pp. 16–17). William Kaufmann Inc.
Lawson, M. J. (2003). Problem solving. In J. P. Keeves et al. (Eds.), International handbook of educational research in the Asia-Pacific region ( Springer International Handbooks of Education, vol 11 ). Springer. https://doi.org/10.1007/978-94-017-3368-7_35
Mahanal, S., Zubaidah, S., Setiawan, D., Maghfiroh, H., & Muhaimin, F. G. (2022). ‘Empowering college students’ Problem-solving skills through RICOSRE’. Education Sciences, 12 (3), 196.
McComas, W. F. (1998). The principal elements of the nature of science: Dispelling the myths. In W. F. McComas (Ed.), The nature of science in science education (pp. 53–70). Springer.
Milopoulos, G., & Cerri, L. (2020). Recommendation for future use . EPINOIA S.A.
Murphy, P., & McCormick, R. (1997). Problem solving in science and technology education. Research in Science Education, 27 (3), 461–481.
Nezu, A. M. (2004). Problem solving and behavior therapy revisited. Behavior Therapy, 35 (1), 1–33. https://doi.org/10.1016/s0005-7894(04)80002-9
OECD. (2013). PISA 2012 assessment and analytical framework: Mathematics, Reading, science, problem solving and financial literacy . OECD. https://doi.org/10.1787/9789264190511-en
Book Google Scholar
Osborn, A. (1953). Applied imagination . Charles Scribner.
Osborne, J., & Dillon, J. (2008). Science education in Europe: Critical reflections . Nuffield Foundation.
Pérez, D. G., & Torregrosa, J. M. (1983). A model for problem-solving in accordance with scientific methodology. European Journal of Science Education, 5 (4), 447–455. https://doi.org/10.1080/0140528830050408
Pizzini, E. L. (1989). A rationale for and the development of a problem-solving model of instruction in science education. Science Education, 73 (5), 523–534.
Presseisen, B. Z. (1985). Thinking skills throughout the curriculum: A conceptual design . Research for Better Schools, Inc.
Sampson, V., Enderle, P., & Grooms, J. (2013). Argumentation in science education. The Science Teacher, 80 (5), 30.
Simon, H. A. (1973). The structure of ill-structured problems. Artificial Intelligence, 4 (3–4), 181–201.
Taconis, R. (1995). Understanding based problem solving . [Unpuplished PhD thesis],. University of Eindhoven.
Taconis, R., Ferguson-Hessler, M. G. M., & Broekkamp, H. (2001). Teaching science problem solving: An overview of experimental work. Journal of Research in Science Teaching, 38 (4), 442–468.
von Hippel, E., & von Kroch, G. (2016). Identifying viable “need-solution pairs”: Problem solving without problem formulation. Organization Science, 27 (1), 207–221. https://doi.org/10.1287/orsc.2015.1023
Woods, D. R. (1987). How might I teach problem solving? New Directions for Teaching and Learning, 30 , 55–71.
Download references
Author information
Authors and affiliations.
Faculty of Education, Dokuz Eylül University, Buca, Izmir, Türkiye
Bulent Çavaş
Faculty of Education, Ege University, Bornova, Izmir, Türkiye
Pınar Çavaş
Muğla Sıtkı Koçman University, Faculty of Education, Muğla, Türkiye
Yasemin Özdem Yılmaz
You can also search for this author in PubMed Google Scholar
Corresponding author
Correspondence to Bulent Çavaş .
Editor information
Editors and affiliations.
The STAN Place, Science Teachers Association of Nigeria, Abuja, Nigeria
Faculty of Education, Dokuz Eylul Universitesi, Buca, Turkey
Bulent Cavas
SOE, BEP 229F, University of Texas at Tyler, Tyler, TX, USA
Teresa Kennedy
Rights and permissions
Reprints and permissions
Copyright information
© 2023 The Author(s), under exclusive license to Springer Nature Switzerland AG
About this chapter
Çavaş, B., Çavaş, P., Yılmaz, Y.Ö. (2023). Problem-Solving in Science and Technology Education. In: Akpan, B., Cavas, B., Kennedy, T. (eds) Contemporary Issues in Science and Technology Education. Contemporary Trends and Issues in Science Education, vol 56. Springer, Cham. https://doi.org/10.1007/978-3-031-24259-5_18
Download citation
DOI : https://doi.org/10.1007/978-3-031-24259-5_18
Published : 25 February 2023
Publisher Name : Springer, Cham
Print ISBN : 978-3-031-24258-8
Online ISBN : 978-3-031-24259-5
eBook Packages : Education Education (R0)
Share this chapter
Anyone you share the following link with will be able to read this content:
Sorry, a shareable link is not currently available for this article.
Provided by the Springer Nature SharedIt content-sharing initiative
- Publish with us
Policies and ethics
- Find a journal
- Track your research

Change Password
Your password must have 8 characters or more and contain 3 of the following:.
- a lower case character,
- an upper case character,
- a special character
Password Changed Successfully
Your password has been changed
- Sign in / Register
Request Username
Can't sign in? Forgot your username?
Enter your email address below and we will send you your username
If the address matches an existing account you will receive an email with instructions to retrieve your username
A Detailed Characterization of the Expert Problem-Solving Process in Science and Engineering: Guidance for Teaching and Assessment
- Argenta M. Price
- Candice J. Kim
- Eric W. Burkholder
- Amy V. Fritz
- Carl E. Wieman
*Address correspondence to: Argenta M. Price ( E-mail Address: [email protected] ).
Department of Physics, Stanford University, Stanford, CA 94305
Search for more papers by this author
Graduate School of Education, Stanford University, Stanford, CA 94305
School of Medicine, Stanford University, Stanford, CA 94305
Department of Electrical Engineering, Stanford University, Stanford, CA 94305
A primary goal of science and engineering (S&E) education is to produce good problem solvers, but how to best teach and measure the quality of problem solving remains unclear. The process is complex, multifaceted, and not fully characterized. Here, we present a detailed characterization of the S&E problem-solving process as a set of specific interlinked decisions. This framework of decisions is empirically grounded and describes the entire process. To develop this, we interviewed 52 successful scientists and engineers (“experts”) spanning different disciplines, including biology and medicine. They described how they solved a typical but important problem in their work, and we analyzed the interviews in terms of decisions made. Surprisingly, we found that across all experts and fields, the solution process was framed around making a set of just 29 specific decisions. We also found that the process of making those discipline-general decisions (selecting between alternative actions) relied heavily on domain-specific predictive models that embodied the relevant disciplinary knowledge. This set of decisions provides a guide for the detailed measurement and teaching of S&E problem solving. This decision framework also provides a more specific, complete, and empirically based description of the “practices” of science.
INTRODUCTION
Many faculty members with new graduate students and many managers with employees who are recent college graduates have had similar experiences. Their advisees/employees have just completed a program of rigorous course work, often with distinction, but they seem unable to solve the real-world problems they encounter. The supervisor struggles to figure out exactly what the problem is and how they can guide the person in overcoming it. This paper is providing a way to answer those questions in the context of science and engineering (S&E). By characterizing the problem-solving process of experts, this paper investigates the “mastery” performance level and specifies an overarching learning goal for S&E students, which can be taught and measured to improve teaching.
The importance of problem solving as an educational outcome has long been recognized, but too often postsecondary S&E graduates have serious difficulties when confronted with real-world problems ( Quacquarelli Symonds, 2018 ). This reflects two long-standing educational problems with regard to problem solving: how to properly measure it, and how to effectively teach it. We theorize that the root of these difficulties is that good “problem solving” is a complex multifaceted process, and the details of that process have not been sufficiently characterized. Better characterization of the problem-solving process is necessary to allow problem solving, and more particularly, the complex set of skills and knowledge it entails, to be measured and taught more effectively. We sought to create an empirically grounded conceptual framework that would characterize the detailed structure of the full problem-solving process used by skilled practitioners when solving problems as part of their work. We also wanted a framework that would allow use and comparison across S&E disciplines. To create such a framework, we examined the operational decisions (choices among alternatives that result in subsequent actions) that these practitioners make when solving problems in their discipline.
Various aspects of problem solving have been studied across multiple domains, using a variety of methods (e.g., Newell and Simon, 1972 ; Dunbar, 2000 ; National Research Council [NRC], 2012b ; Lintern et al. , 2018 ). These ranged from expert self-reflections (e.g., Polya, 1945 ), to studies on knowledge lean tasks to discover general problem-solving heuristics (e.g., Egan and Greeno, 1974 ), to comparisons of expert and novice performances on simplified problems across a variety of disciplines (e.g., Chase and Simon, 1973 ; Chi et al. , 1981 ; Larkin and Reif, 1979 ; Ericsson et al. , 2006 , 2018 ). These studies revealed important novice–expert differences—notably, that experts are better at identifying important features and have knowledge structures that allow them to reduce demands on working memory. Studies that specifically gave the experts unfamiliar problems in their disciplines also found that, relative to novices, they had more deliberate and reflective strategies, including more extensive planning and managing of their own behavior, and they could use their knowledge base to better define the problem ( Schoenfeld, 1985 ; Wineburg, 1998 ; Singh, 2002 ). While these studies focused on discrete cognitive steps of the individual, an alternative framing of problem solving has been in terms of “ecological psychology” of “situativity,” looking at how the problem solver views and interacts with the environment in terms of affordances and constraints ( Greeno, 1994 ). “Naturalistic decision making” is a related framework that specifically examines how experts make decisions in complex, real-world, settings, with an emphasis on the importance of assessing the situation surrounding the problem at hand ( Klein, 2008 ; Mosier et al. , 2018 ).
While this work on expertise has provided important insights into the problem-solving process, its focus has been limited. Most has focused on looking for cognitive differences between experts and novices using limited and targeted tasks, such as remembering the pieces on a chessboard ( Chase and Simon, 1973 ) or identifying the important concepts represented in an introductory physics textbook problem ( Chi et al. , 1981 ). It did not attempt to explore the full process of solving, particularly for solving the type of complex problem that a scientist or engineer encounters as a member of the workforce (“authentic problems”).
There have also been many theoretical proposals as to expert problem-solving practices, but with little empirical evidence as to their completeness or accuracy (e.g., Polya, 1945 ; Heller and Reif, 1984 ; Organisation for Economic Cooperation and Development [OECD], 2019 ). The work of Dunbar (2000) is a notable exception to the lack of empirical work, as his group did examine how biologists solved problems in their work by analyzing lab meetings held by eight molecular biology research groups. His groundbreaking work focused on creativity and discovery in the research process, and he identified the importance of analogical reasoning and distributed reasoning by scientists in answering research questions and gaining new insights. Kozma et al. (2000) studied professional chemists solving problems, but their work focused only on the use of specialized representations.
The “cognitive systems engineering” approach ( Lintern et al. , 2018 ) takes a more empirically based approach looking at experts solving problems in their work, and as such tends to span aspects of both the purely cognitive and the ecological psychological theories. It uses both observations of experts in authentic work settings and retrospective interviews about how experts carried out particular work tasks. This theoretical framing and the experimental methods are similar to what we use, particularly in the “naturalistic decision making” area of research ( Mosier et al. , 2018 ). That work looks at how critical decisions are made in solving specific problems in their real-world setting. The decision process is studied primarily through retrospective interviews about challenging cases faced by experts. As described below, our methods are adapted from that work ( Crandall et al. , 2006 ), though there are some notable differences in focus and field. A particular difference is that we focused on identifying what are decisions to be made, which are more straight-forward to identify from retrospective interviews than how those decisions are made. We all have the same ultimate goal, however, to improve the training/teaching of the respective expertise.
Problem solving is central to the processes of science, engineering, and medicine, so research and educational standards about scientific thinking and the process and practices of science are also relevant to this discussion. Work by Osborne and colleagues describes six styles of scientific reasoning that can be used to explain how scientists and students approach different problems ( Kind and Osborne, 2016 ). There are also numerous educational standards and frameworks that, based on theory, lay out the skills or practices that science and engineering students are expected to master (e.g., American Association for the Advancement of Science [AAAS], 2011 ; Next Generation Science Standards Lead States, 2013 ; OECD, 2019 ; ABET, 2020 ). More specifically related to the training of problem solving, Priemer et al. (2020) synthesizes literature on problem solving and scientific reasoning to create a “STEM [science, technology, engineering, and mathematics] and computer science framework for problem solving” that lays out steps that could be involved in a students’ problem-solving efforts across STEM fields. These frameworks provide a rich groundwork, but they have several limitations: 1) They are based on theoretical ideas of the practice of science, not empirical evidence, so while each framework contains overlapping elements of the problem-solving process, it is unclear whether they capture the complete process. 2) They are focused on school science, rather than the actual problem solving that practitioners carry out and that students will need to carry out in future STEM careers. 3) They are typically underspecified, so that the steps or practices apply generally, but it is difficult to translate them into measurable learning goals for students to practice. Working to address that, Clemmons et al. (2020) recently sought to operationalize the core competencies from the Vision and Change report ( AAAS, 2011 ), establishing a set of skills that biology students should be able to master.
Our work seeks to augment this prior work by building a conceptual framework that is empirically based, grounded in how scientists and engineers solve problems in practice instead of in school. We base our framework on the decisions that need to be made during problem solving, which makes each item clearly defined for practice and assessment. In our analysis of expert problem solving, we empirically identified the entire problem-solving process. We found this includes deciding when and how to use the steps and skills defined in the work described previously but also includes additional elements. There are also questions in the literature about how generalizable across fields a particular set of practices may be. Here, we present the first empirical examination of the entire problem-solving process, and we compare that process across many different S&E disciplines.
A variety of instructional methods have been used to try and teach science and engineering problem solving, but there has been little evidence of their efficacy at improving problem solving (for a review, see NRC, 2012b ). Research explicitly on teaching problem solving has primarily focused on textbook-type exercises and utilized step-by-step strategies or heuristics. These studies have shown limited success, often getting students to follow specific procedural steps but with little gain in actually solving problems and showing some potential drawbacks ( Heller and Reif, 1984 ; Heller et al. , 1992 ; Huffman, 1997 ; Heckler, 2010 ; Kuo et al. , 2017 ). As discussed later, the framework presented here offers guidance for different and potentially more effective approaches to teaching problem solving.
These challenges can be illustrated by considering three different problems taken from courses in mechanical engineering, physics, and biology, respectively ( Figure 1 ). All of these problems are challenging, requiring considerable knowledge and effort by the student to solve correctly. Problems such as these are routinely used to both assess students’ problem-solving skills, and students are expected to learn such skills by practicing doing such problems. However, it is obvious to any expert in the respective fields, that, while these problems might be complicated and difficult to answer, they are vastly different from solving authentic problems in that field. They all have well-defined answers that can be reached by straightforward solution paths. More specifically, they do not involve needing to use judgment to make any decisions based on limited information (e.g., insufficient to specify a correct decision with certainty). The relevant concepts and information and assumptions are all stated or obvious. The failure of problems like these to capture the complexity of authentic problem solving underlies the failure of efforts to measure and teach problem solving. Recognizing this failure motivated our efforts to more completely characterize the problem-solving process of practicing scientists, engineers, and doctors.
FIGURE 1. Example problems from courses or textbooks in mechanical engineering, physics and biology. Problems from: Mechanical engineering: Wayne State mechanical engineering sample exam problems (Wayne State, n.d.), Physics: A standard physics problem in nearly every advanced quantum mechanics course, Biology: Molecular Biology of the Cell 6th edition, Chapter 7 end of chapter problems ( Alberts et al ., 2014 ).
We are building on the previous work studying expert–novice differences and problem solving but taking a different direction. We sought to create an empirically grounded framework that would characterize the detailed structure of the full problem-solving process by focusing on the operational decisions that skilled practitioners make when successfully solving authentic problems in their scientific, engineering, or medical work. We chose to identify the decisions that S&E practitioners made, because, unlike potentially nebulous skills or general problem-solving steps that might change with the discipline, decisions are sufficiently specified that they can be individually practiced by students and measured by instructors or departments. The authentic problems that we analyzed are typical problems practitioners encounter in “doing” the science or engineering entailed in their jobs. In the language of traditional problem- solving and expertise research, such authentic problems are “ill-structured” ( Simon, 1973 ) and require “adaptive expertise” ( Hatano and Inagaki, 1986 ) to solve. However, our authentic problems are considerably more complex and unstructured than what is normally considered in those literatures, because not only do they lack a clear solution path, but in many cases, it is not clear a priori that they have any solution at all. Determining that, and whether the problem needs to be redefined to be soluble, is part of the successful expert solution process. Another way in which our set of decisions goes beyond the characterization of what is involved in adaptive expertise is the prominent role of making judgments with limited information.
A common reaction of scientists and engineers to seeing the list of decisions we obtain as our primary result is, “Oh, yes, these are things I always do in solving problems. There is nothing new here.” It is comforting that these decisions all look familiar; that supports their validity. However, what is new is not that experts are making such decisions, but rather that there is a relatively small but complete set of decisions that has now been explicitly identified and that applies so generally.
We have used a much larger and broader sample of experts in this work than used in prior expert–novice studies, and we used a more stringent selection criterion. Previous empirical work has typically involved just a few experts, almost always in a single domain, and included graduate students as “experts” in some cases. Our semistructured interview sample was 31 experienced practitioners from 10 different disciplines of science, engineering, and medicine, with demonstrated competence and accomplishments well beyond those of most graduate students. Also, approximately 25 additional experts from across science, engineering, and medicine served as consultants during the planning and execution of this work.
Our research question was: What are the decisions experts make in solving authentic problems, and to what extent is this set of decisions to be made consistent both within and across disciplines?
Our approach was designed to identify the level of consistency and unique differences across disciplines. Our hypothesis was that there would be a manageable number (20–50) of decisions to be made, with a large amount of overlap of decisions made between experts within each discipline and a substantial but smaller overlap across disciplines. We believed that if we had found that every expert and/or discipline used a large and completely unique set of decisions, it would have been an interesting research result but of little further use. If our hypothesis turned out to be correct, we expected that the set of decisions obtained would have useful applications in guiding teaching and assessment, as they would show how experts in the respective disciplines applied their content knowledge to solve problems and hence provide a model for what to teach. We were not expecting to find the nearly complete degree of overlap in the decisions made across all the experts.
We first conducted 22 relatively unstructured interviews with a range of S&E experts, in which we asked about problem-solving expertise in their fields. From these interviews, we developed an initial list of decisions to be made in S&E problem solving. To refine and validate the list, we then carried out a set of 31 semistructured interviews in which S&E experts chose a specific problem from their work and described the solution process in detail. The semistructured interviews were coded for the decisions represented, either explicitly stated or implied by a choice of action. This provided a framework of decisions that characterize the problem-solving process across S&E disciplines. The research was approved by the Stanford Institutional Review Board (IRB no. 48785), and informed consent was obtained from all the participants.
This work involved interviewing many experts across different fields. We defined experts as practicing scientists, engineers, or physicians with considerable experience working as faculty at highly rated universities or having several years of experience working in moderately high-level technical positions at successful companies. We also included a few longtime postdocs and research staff in biosciences to capture more details of experimental decisions from which faculty members in those fields often were more removed. This definition of expert allows us to identify the practices of skilled professionals; we are not studying what makes only the most exceptional experts unique.
Experts were volunteers recruited through direct contact via the research team's personal and professional networks and referrals from experts in our networks. This recruitment method likely biased our sample toward people who experienced relatively similar training (most were trained in STEM disciplines at U.S. universities within the last 15–50 years). Within this limitation, we attempted to get a large range of experts by field and experience. This included people from 10 different fields (including molecular biology/biochemistry, ecology, and medicine), 11 U.S. universities, and nine different companies or government labs, and the sample was 33% female (though our engineering sample only included one female). The medical experts were volunteers from a select group of medical school faculty chosen to serve as clinical reasoning mentors for medical students at a prestigious university. We only contacted people who met our criteria for being an “expert,” and everyone who volunteered was included in the study. Most of the people who were contacted volunteered, and the only reason given for not volunteering was insufficient time. Other than their disciplinary expertise, there was little to distinguish these experts beyond the fact they were acquaintances with members of the team or acquaintances of acquaintances of team or project advisory board members. The precise number from each field was determined largely by availability of suitable experts.
We defined an “authentic problem” to be one that these experts solve in their actual jobs. Generally, this meant research projects for the science and engineering faculty, design problems for the industry engineers, and patient diagnoses for the medical doctors. Such problems are characterized by complexity, with many factors involved and no obvious solution process, and involve substantial time, effort, and resources. Such problems involve far more complexity and many more decisions, particularly decisions with limited information, than the typical problems used in previous problem-solving research or used with students in instructional settings.
Creating an Initial List of Problem-Solving Decisions
We first interviewed 22 experts ( Table 1 ), most of whom were faculty at a prestigious university, in which we asked them to discuss expertise and problem solving in their fields as it related to their own experiences. This usually resulted in their discussing examples of one or more problems they had solved. Based on the first seven interviews, plus reflections on personal experience from the research team and review of the literature on expert problem solving and teaching of scientific practices ( Ericsson et al. , 2006 ; NRC, 2012a ; Wieman, 2015 ), we created a generic list of decisions that were made in S&E problem solving. In the rest of the unstructured interviews (15), we also provided the experts with our list and asked them to comment on any additions or deletions they would suggest. Faculty who had close supervision of graduate students and industry experts who had extensively supervised inexperienced staff were particularly informative. Their observations of the way inexperienced people could fail made them sensitive to the different elements of expertise and where incorrect decisions could be made. Although we initially expected to find substantial differences across disciplines, from early in the process, we noted a high degree of overlap across the interviews in the decisions that were described.
Discipline | Informal interviews (creation of initial list) | Structured interviews (validation/refinement) | Notes |
---|---|---|---|
Biology (5 biochem/molecular bio, 2 cell bio, 1 plant bio, 1 immunology, 1 ecology) | 2 | 8 | Female: 6, URM: 2 5 faculty, 2 industry 3 acad staff/postdoc (year 5+) |
Medicine (6 internal med or pediatrics, 1 oncology, 2 surgery) | 4 | 6 | Female: 4, URM: 1 All medical faculty |
Physics (4 experiment, 3 theory) | 2 | 5 | Female: 1, URM: 1 All faculty |
Electrical Engineering | 4 | 3 | 2 faculty, 4 industry, 1 acad. staff |
Chemical Engineering | 2 | 2 | Female: 1 3 industry, 1 acad. staff |
Mechanical Engineering | 2 | 2 | URM: 1, 2 faculty, 2 industry |
Earth Science | 1 | 2 | Female: 2, 2 faculty, 1 industry |
Chemistry | 1 | 2 | Female: 2, all faculty |
Computer Science | 2 | 1 | Female: 1, 2 faculty, 1 industry |
Biological Engineering | 2 | – | All faculty or acad. staff |
Total | 22 | 31 | Female: 17, URM: 5 |
URM (under-represented minority) included 3 African American and 2 Hispanic/Latinx. One medical faculty member was interviewed twice – in both informal and structure interviews, for a total of 53 interviews with 52 experts.
Refinement and Validation of the List of Decisions
After creating the preliminary list of decisions from the informal interviews, we conducted a separate set of more structured interviews to test and refine the list. Semistructured interviews were conducted with 31 experts from across science, engineering, and medical fields ( Table 1 ). For these interviews, we recruited experts from a range of universities and companies, though the range of institutions is still limited, given the sample size. Interviews were conducted in person or over video chat and were transcribed for analysis. In the semistructured interviews, experts were asked to choose a problem or two from their work that they could recall the details of solving and then describe the process, including all the steps and decisions they made. So that we could get a full picture of the successful problem-solving process, we decided to focus the interviews on problems that they had eventually solved successfully, though their processes inherently involved paths that needed to be revised and reconsidered. Transcripts from interviewees who agreed to have their interview transcript published are available in the supplemental data set.
Our interview protocol (see Supplemental Text) was inspired in part by the critical decision method of cognitive task analysis ( Crandall et al. , 2006 ; Lintern et al. , 2018 ), which was created for research in cognitive systems engineering and naturalistic decision making. There are some notable differences between our work and theirs, both in research goal and method. First, their goal is to improve training in specific fields by focusing on how critical decisions are made in that field during an unusual or important event; the analysis seeks to identify factors involved in making those critical decisions. We are focusing on the overall problem solving and how it compares across many different fields, which quickly led to attention on what decisions are to be made, rather than how a limited set of those decisions are made. We asked experts to describe a specific, but not necessarily unusual, problem in their work, and focused our analysis on identifying all decisions made, not reasons for making them or identifying which were most critical. The specific order of problem-solving steps was also less important to us, in part because it was clear that there was no consistent order that was followed. Second, we are looking at different types of work. Cognitive systems engineering work has primarily focused on performance in professions like firefighters, power plant operators, military technicians, and nurses. These tend to require time-sensitive critical skills that are taught with modest amounts of formal training. We are studying scientists, engineers, and doctors solving problems that require much longer and less time-critical solutions and for which the formal training occupies many years.
Given our different focus, we made several adaptations to eliminate some of the more time-consuming steps from the interview protocol, allowing us to limit the interview time to approximately 1 hour. Both protocols seek to elicit an accurate and complete reporting of the steps taken and decisions made in the process of solving a problem. Our general strategy was: 1) Have the expert explain the problem and talk step by step through the decisions involved in solving it, with relatively few interruptions from the interviewer except to keep the discussion focused on the specific problem and occasionally to ask for clarifications. 2) Ask follow-up questions to probe for more detail about particular steps and aspects of the problem-solving process. 3) Occasionally ask for general thoughts on how a novice's process might differ.
While some have questioned the reliability of information from retrospective interviews ( Nisbett and Wilson, 1977 ), we believe we avoid these concerns, because we are only identifying a decision to be made, which in this case, means identifying a well-defined action that was chosen from alternatives. This is less subjective and much more likely to be accurately recalled than is the rationale behind such a decision. See Ericsson and Simon (1980) . However, the decisions identified may still be somewhat limited—the process of deciding among possible actions might involve additional decisions in the moment, when the solution is still unknown, that we are unable to capture in the retrospective context. For the decisions we can identify, we are able to check their accuracy and completeness by comparing them with the actions taken in the conduct of the research/design. For example, consider this quote from a physician who had to re-evaluate a diagnosis, “And, in my very subjective sense, he seemed like he was being forthcoming and honest. Granted people can fool you, but he seemed like he was being forthcoming. So we had to reevaluate.” The physician then considered alternative diagnoses that could explain a test result that at first had indicated an incorrect diagnosis. While this quote does describe the (retrospective) reasoning behind a decision, we do not need to know whether that reasoning is accurately recalled. We can simply code this as “decision 18, how believable is info?” The physician followed up by considering alternative diagnoses, which in this context was coded as “26, how good is solution?” and “8, potential solutions?” This was followed by the description of the literature and additional tests conducted. These indicated actions taken that confirm the physician made a decision about the reliability of the information given by the patient.
Interview Coding
We coded the semistructured interviews in terms of decisions made, through iterative rounds of coding ( Chi, 1997 ), following a “directed content analysis approach,” which involves coding according to predefined theoretical categories and updating the codes as needed based on the data ( Hsieh and Shannon, 2005 ). Our predefined categories were the list of decisions we had developed during the informal interviews. This approach means that we limited the focus of our qualitative analysis—we were able to test and refine the list of decisions, but we did not seek to identify all possible categories of approach to selecting and solving problems. The goals of each iterative round of coding are described in the next three paragraphs. To code for decisions in general, we matched decisions from the list to statements in each interview, based on the following criteria: 1) there was an explicit statement of a decision or choice made or needing to be made; 2) there was the description of the outcome of a decision, such as listing important features of the problem (that had been decided on) or conclusions arrived at; or 3) there was a statement of actions taken that indicated a decision about the appropriate action had been made, usually from a set of alternatives. Two examples illustrate the types of comments we identified as decisions: A molecular biologist explicitly stated the decisions required to decompose a problem into subproblems (decision 11), “Which cell do we use? The gene. Which gene do we edit? Which part of that gene do we edit? How do we build the enzyme that is going to do the cutting? … And how do we read out that it worked?” An ecologist made a statement that was also coded as a decomposition decision, because it described the action taken: “So I analyze the bird data first on its own, rather than trying to smash all the taxonomic groups together because they seem really apples and oranges. And just did two kinds of analysis, one was just sort of across all of these cases, around the world.” A single statement could be coded as multiple decisions if they were occurring simultaneously in the story being recalled or were intimately interconnected in the context of that interview, as with the ecology quote, in which the last sentence leads into deciding what data analysis is needed. Inherent in nearly every one of these decisions was that there was insufficient information to know the answer with certainty, so judgment was required.
Our primary goal for the first iterative round of coding was to check whether our list was complete by checking for any decisions that were missing, as indicated by either an action taken or a stated decision that was not clearly connected to a decision on our initial list. In this round, we also clarified wording and combined decisions that we were consistently unable to differentiate during the coding. A sample of three interviews (from biology, medicine, and electrical engineering) were first coded independently by four coders (AP, EB, CK, and AF), then discussed. The decision list was modified to add decisions and update wording based on that discussion. Then the interviews were recoded with the new list and rediscussed, leading to more refinements to the list. Two additional interviews (from physics and chemical engineering) were then coded by three coders (AP, EB, and CK) and further similar refinements were made. Throughout the subsequent rounds of coding, we continued to check for missing decisions, but after the additions and adjustments made based on these five interviews, we did not identify any more missing decisions.
In our next round of coding, we focused on condensing overlapping decisions and refining wording to improve the clarity of descriptions as they applied across different disciplinary contexts and to ensure consistent interpretation by different coders. Two or three coders independently coded an additional 11 interviews, iteratively meeting to discuss codes identified in the interviews, refining wording and condensing the list to improve agreement and combine overlapping codes, and then using the updated list to code subsequent interviews. We condensed the list by combining decisions that represented the same cognitive process taking place at different times, that were discipline-specific variations on the same decision, or that were substeps involved in making a larger decision. We noticed that some decisions were frequently co-coded with others, particularly in some disciplines. But if they were identified as distinct a reasonable fraction of the time in any discipline, we listed them as separate. This provided us with a list, condensed from 42 to 29 discrete decisions (plus five additional non-decision themes that were so prevalent that they are important to describe), that gave good consistency between coders.
Finally, we used the resulting codes to tabulate which decisions occurred in each interview, simplifying our coding process to focus on deciding whether or not each decision had occurred, with an example if it did occur to back up the “yes” code, but no longer attempting to capture every time each decision was mentioned. Individual coders identified decisions mentioned in the remaining 15 interviews. Interviews that had been coded with the early versions of the list were also recoded to ensure consistency. Coders flagged any decisions they were unsure about occurring in a particular interview, and two to four coders (AP, EB, CK, and CW) met to discuss those debated codes, with most uncertainties being resolved by explanations from a team member who had more technical expertise in the field of the interview. Minor wording changes were made during this process to ensure that each description of a decision captured all instantiations of the decision across disciplines, but no significant changes to the list were needed or made.
Coding an interview in terms of decisions made and actions taken in the research often required a high level of expertise in the discipline in question. The coder had to be familiar with the conduct of research in the field in order to recognize which actions corresponded to a decision between alternatives, but our team was assembled with this requirement in mind. It included high-level expertise across five different fields of science, engineering, and medicine and substantial familiarity with several other fields.
Supplemental Table S1 shows the final tabulation of decisions identified in each interview. In the tabulation, most decisions were marked as either “yes” or “no” for each interview, though 65 out of 1054 total were marked as “implied,” for one of the following reasons: 1) for 40/65, based on the coder's knowledge of the field, it was clear that a step must have been taken to achieve an outcome or action, even though that decision was not explicitly mentioned (e.g., interviewees describe collecting certain raw data and then coming to a specific conclusion, so they must have decided how to analyze the data, even if they did not mention the analysis explicitly); 2) for 15/65, the interview context was important, in that multiple statements from different parts of the interview taken together were sufficient to conclude that the decision must have happened, though no single statement described that decision explicitly; 3) 10/65 involved a decision that was explicitly discussed as an important step in problem solving, but they did not directly state how it was related to the problem at hand, or it was stated only in response to a direct prompt from the interviewer. The proportion of decisions identified in each interview, broken down by either explicit or explicit + implied, is presented in Supplemental Tables S1 and S2. Table 2 and Figure 2 of the main text show explicit + implied decision numbers.
A. Selection and goals (Occur in 100% ) | B. Frame problem (100%) | C. Plan process for solving (100%) | D. Interpret info and choose solutions (100%) | E. Reflect (100%) | F. Implications and communicate results (84%) |
---|---|---|---|---|---|
1. (61%) What is important in field? | 4. (100%) Important features and info? | 10. (100%) Approximations and simplifications to make? | 16. (81%) Which calculations and data analysis? | 23. (77%) Assumptions and simplifications appropriate? | 27. (65%) Broader implications? |
2. (77%) Opportunity fits solver’s expertise? | 5. (100%) What predictive framework? | 11. (68%) How to decompose into sub-problems? | 17. (68%) How to represent and organize information? | 24. (84%) Additional knowledge needed? | 28. (55%) Audience for communication? |
3. (100%) Goals, criteria, constraints? | 6. (97%) How to narrow down problem? | 12. (90%) Most difficult or uncertain areas? | 18. (77%) How believable is information? | 25. (94%) How well is solving approach working? | 29. (68%) Best way to present work? |
7. (97%) Related problems? | 13. (100%) What info needed? | 19. (100%) How does info compare to predictions? | 26. (100%) How good is solution? | ||
8. (100%) Potential Solutions? | 14. (87%) Priorities? | 20. (71%) Any significant anomalies? | |||
9. (74%) Is problem solvable? | 15. (100%) Specific plan for getting information? | 21. (97%) Appropriate conclusions? | |||
22. (97%) What is best solution? |
a See supplementary text and Table S2 for full description and examples of each decision. A set of other non-decision knowledge and skill development themes were also frequently mentioned as important to professional success: Staying up to date in the field (84%), intuition and experience (77%), interpersonal and teamwork (100%), efficiency (32%), and attitude (68%).
b Percentage of interviews in which category or decision was mentioned.
c Numbering is for reference. In practice ordering is fluid – involves extensive iteration with other possible starting points.
d Chosen predictive framework(s) will inform all other decisions.
e Reflection occurs throughout process, and often leads to iteration. Reflection on solution occurs at the end as well.
FIGURE 2. Proportion of decisions coded in interviews by field. This tabulation includes decisions 1–29, not the additional themes. Error bars represent standard deviations. Number of interviews: total = 31; physical science = 9; biological science = 8; engineering = 8; medicine = 6. Compared with the sciences, slightly fewer decisions overall were identified in the coding of engineering and medicine interviews, largely for discipline-specific reasons. See Supplemental Table S2 and associated discussion.
Two of the interviews that had not been discussed during earlier rounds of coding (one physics [AP and EB], one medicine [AP and CK]) were independently coded by two coders to check interrater reliability using the final list of decisions. The goal of our final coding was to tabulate whether or not each expert described making each decision at any point in the problem-solving process, so the level of detail we chose for coding and interrater reliability was whether or not a decision was present in the entire interview. The decisions identified in each interview were compared for the two coders. For both interviews, the raters disagreed on whether or not only one of the 29 decisions occurred. Codes of “implied” were counted as agreement if the other coder selected either “yes” or “implied.” This equates to a percent agreement of 97% for each interview (28 agree/29 total decisions per interview = 97%). As a side note, there was also one disagreement per interview on the coding of the five other themes, but those themes were not a focus of this work nor the interviews.
We identified a total set of 29 decisions to be made (plus five other themes), all of which were identified in a large fraction of the interviews across all disciplines ( Table 2 and Figure 2 ). There was a surprising degree of overlap across the different fields with all the experts mentioning similar decisions to be made. All 29 were evident by the fifth semistructured interview, and on average, each interview revealed 85% of the 29 decisions. Many decisions occurred multiple times in an interview, with the number of times varying widely, depending on the length and complexity of the problem-solving process discussed.
We focused our analysis on what decisions needed to be made, not on the experts’ processes for making those decisions: noting that a choice happened, not how they selected and chose among different alternatives. This is because, while the decisions to be made were the same across disciplines, how the experts made those decisions varied greatly by discipline and individual. The process of making the decisions relied on specialized disciplinary knowledge and experience and may vary depending on demographics or other factors that our study design (both our sample and nature of retrospective interviews) did not allow us to investigate. However, while that knowledge was distinct and specialized, we could tell that it was consistently organized according to a common structure we call a “predictive framework,” as discussed in the “ Predictive Framework ” section below. Also, while every “decision” reflected a step in the problem solving involved in the work, and the expert being interviewed was involved in making or approving the decision, that does not mean the decision process was carried out only by that individual. In many cases, the experts described the decisions made in terms of ideas and results of their teams, and the importance of interpersonal skills and teamwork was an important non-decision theme raised in all interviews.
We were particularly concerned with the correctness and completeness of the set of decisions. Although the correctness was largely established by the statements in the interviews, we also showed the list of decisions to these experts at the end of the interviews as well as to about a dozen other experts. In all cases, they all agreed that these decisions were ones they and others in their field made when solving problems. The completeness of the list of decisions was confirmed by: 1) looking carefully at all specific actions taken in the described problem-solving process and checking that each action matched a corresponding decision from the list; and 2) the high degree of consistency in the set of decisions across all the interviews and disciplines. This implies that it is unlikely that there are important decisions that we are missing, because that would require any such missing decisions to be consistently unspoken by all 31 interviewees as well as consistently unrecognized by us from the actions that were taken in the problem-solving process.
In focusing on experts’ recollections of their successful solving of problems, our study design may have missed decisions that experts only made during failed problem-solving attempts. However, almost all interviews described solution paths that were not smooth and continuous, but rather involved going down numerous dead ends. There were approaches that were tried and failed, data that turned out to be ambiguous and worthless, and so on. Identifying the failed path involved reflection decisions (23–26). Often decision 9 (is problem solvable?) would be mentioned, because it described a path that was determined to be not solvable. For example, a biologist explained, “And then I ended up just switching to a different strain that did it [crawling off the plate] less. Because it was just … hard to really get them to behave themselves. I suppose if I really needed to rely on that very particular one, I probably would have exhausted the possibilities a bit more.” Thus, we expect unsuccessful problem solving would entail a smaller subset of decisions being made, particularly lack of reflection decisions, or poor choices on the decisions, rather than making a different set of decisions.
The set of decisions represent a remarkably consistent structure underlying S&E problem solving. For the purposes of presentation, we have categorized the decisions as shown in Figure 3 , roughly based on the purposes they achieve. However, the process is far less orderly and sequential than implied by this diagram, or in fact any characterization of an orderly “scientific method.” We were struck by how variable the sequence of decisions was in the descriptions provided. For example, experts who described how they began work on a problem sometimes discussed importance and goals (1–3, what is important in field?; opportunity fits solver’s expertise?; and goals, criteria, constraints?), but others mentioned a curious observation (20, any significant anomalies?), important features of their system that led them to questions (4, important features and info?, 6, how to narrow down problem?), or other starting points. We also saw that there were flexible connections between decisions and repeated iterations—jumping back to the same type of decision multiple times in the solution process, often prompted by reflection as new information and insights were developed. The sequence and number of iterations described varied dramatically by interview, and we cannot determine to what extent this was due to legitimate differences in the problem-solving process or to how the expert recalled and chose to describe the process. This lack of a consistent starting point, with jumping and iterating between decisions, has also been identified in the naturalistic decision-making literature ( Mosier et al. , 2018 ). Finally, the experts also often described considering multiple decisions simultaneously. In some interviews, a few decisions were always described together, while in others, they were clearly separate decisions. In summary, while the specific decisions themselves are fully grounded in expert practice, the categories and order shown here are artificial simplifications for presentation purposes.
FIGURE 3. Representation of problem-solving decisions by categories. The black arrows represent a hypothetical but unrealistic order of operations, the blue arrows represent more realistic iteration paths. The decisions are grouped into categories for presentation purposes; numbers indicate the number of decisions in each category. Knowledge and skill development were commonly mentioned themes but are not decisions.
The decisions contained in the seven categories are summarized here. See Supplemental Table S2 for specific examples of each decision across multiple disciplines.
Category A. Selection and Goals of the Problem
This category involves deciding on the importance of the problem, what criteria a solution must meet, and how well it matches the capabilities, resources, and priorities of the expert. As an example, an earth scientist described the goal of her project (decision 3, goals, criteria, constraints?) to map and date the earliest volcanic rocks associated with what is now Yellowstone and explained why the project was a good fit for her group (2, opportunity fits solver’s expertise?) and her decision to pursue the project in light of the significance of this type of eruption in major extinction events (1, what is important in field?). In many cases, decisions related to framing (see category B) were mentioned before decisions in this category or were an integral part of the process for developing goals.
1. What is important in the field?
What are important questions or problems? Where is the field heading? Are there advances in the field that open new possibilities?
2. Opportunity fits solver's expertise?
If and where are there gaps/opportunities to solve in field? Given experts’ unique perspectives and capabilities, are there opportunities particularly accessible to them? (This could involve challenging the status quo, questioning assumptions in the field.)
3. Goals, criteria, constraints?
a. What are the goals, design criteria, or requirements of the problem or its solution?
b. What is the scope of the problem?
c. What constraints are there on the solution?
d. What will be the criteria on which the solution is evaluated?
Category B. Frame Problem
These decisions lead to a more concrete formulation of the solution process and potential solutions. This involves identifying the key features of the problem and deciding on predictive frameworks to use (see “ Predictive Framework ” section below), as well as narrowing down the problem, often forming specific questions or hypotheses. Many of these decisions are guided by past problem solutions with which the expert is familiar and sees as relevant. The framing decisions of a physician can be seen in his discussion of a patient with liver failure who had previously been diagnosed with HIV but had features (4, important features and info?; 5, what predictive framework?) that made the physician question the HIV diagnosis (5, what predictive framework?; 26, how good is solution?). His team then searched for possible diagnoses that could explain liver failure and lead to a false-positive HIV test (7, related problems?; 8, potential solutions?), which led to their hypothesis the patient might have Q fever (6, how to narrow down problem?; 13, what info needed?; 15, specific plan for getting info?). While each individual decision is strongly supported by the data, the categories are groupings for presentation purposes. In particular, framing (category B) and planning (see category C) decisions often blended together in interviews.
a. Which available information is relevant to problem solving and why?
b. (When appropriate) Create/find a suitable abstract representation of core ideas and information Examples: physics, equation representing process involved; chemistry, bond diagrams/potential energy surfaces; biology, diagram of pathway steps.
5. What predictive framework?
Which potential predictive frameworks to use? (Decide among possible predictive frameworks or create framework.) This includes deciding on the appropriate level of mechanism and structure that the framework needs to embody to be most useful for the problem at hand.
6. How to narrow down the problem?
How to narrow down the problem? Often involves formulating specific questions and hypotheses.
7. Related problems?
What are related problems or work seen before, and what aspects of their problem-solving process and solutions might be useful in the present context? (This may involve reviewing literature and/or reflecting on experience.)
8. Potential solutions?
What are potential solutions? (This is based on experience and fitting some criteria for solution they have for a problem having general key features identified.)
9. Is problem solvable?
Is the problem plausibly solvable and is the solution worth pursuing given the difficulties, constraints, risks, and uncertainties?
Category C. Plan the Process for Solving
These decisions establish the specifics needed to solve the problem and include: how to simplify the problem and decompose it into pieces, what specific information is needed, how to obtain that information, and what are the resources needed and priorities? Planning by an ecologist can be seen in her extensive discussion of her process of simplifying (10, approximations/simplifications to make?) a meta-analysis project about changes in migration behavior, which included deciding what types of data she needed (13, what info needed?), planning how to conduct her literature search (15, specific plan for getting info?), difficulties in analyzing the data (12, most difficult/uncertain areas?; 16, which calculations and data analysis?), and deciding to analyze different taxonomic groups separately (11, how to decompose into subproblems?). In general, decomposition often resulted in multiple iterations through the problem-solving decisions, as subsets of decisions need to be made about each decomposed aspect of a problem. Framing (category B) and planning (category C) decisions occupied much of the interviews, indicating their importance.
10. Approximations and simplifications to make?
What approximations or simplifications are appropriate? How to simplify the problem to make it easier to solve? Test possible simplifications/approximations against established criteria.
11. How to decompose into subproblems?
How to decompose the problem into more tractable subproblems? (Subproblems are independently solvable pieces with their own subgoals.)
12. Most difficult or uncertain areas?
a. What are acceptable levels of uncertainty with which to proceed at various stages?
13. What info needed?
a. What will be sufficient to test and distinguish between potential solutions?
14. Priorities?
What to prioritize among many competing considerations? What to do first and how to obtain necessary resources?
Considerations could include: What's most important? Most difficult? Addressing uncertainties? Easiest? Constraints (time, materials, etc.)? Cost? Optimization and trade-offs? Availability of resources? (facilities/materials, funding sources, personnel)
15. Specific plan for getting information?
a. What are the general requirements of a problem-solving approach, and what general approach will they pursue? (These decisions are often made early in the problem-solving process as part of framing.)
b. How to obtain needed information? Then carry out those plans. (This could involve many discipline- and problem-specific investigation possibilities such as: designing and conducting experiments, making observations, talking to experts, consulting the literature, doing calculations, building models, or using simulations.)
c. What are achievable milestones, and what are metrics for evaluating progress?
d. What are possible alternative outcomes and paths that may arise during the problem-solving process, both consistent with predictive framework and not, and what would be paths to follow for the different outcomes?
Category D. Interpret Information and Choose Solution(s)
This category includes deciding how to analyze, organize, and draw conclusions from available information, reacting to unexpected information, and deciding upon a solution. A biologist studying aging in worms described how she analyzed results from her experiments, which included representing her results in survival curves and conducting statistical analyses (16, which calculations and data analysis?; 17, how to represent and organize info?), as well as setting up blind experiments (15, specific plan for getting info?) so that she could make unbiased interpretations (18, how believable is info?) of whether a worm was alive or dead. She also described comparing results with predictions to justify the conclusion that worm aging was related to fertility (19, how does info compare to predictions?; 21, appropriate conclusions?; 22, what is best solution?). Deciding how results compared with expectations based on a predictive framework was a key decision that often preceded several other decisions.
16. Which calculations and data analysis?
What calculations and data analysis are needed? Once determined, these must then be carried out.
17. How to represent and organize information?
What is the best way to represent and organize available information to provide clarity and insights? (Usually this will involve specialized and technical representations related to key features of predictive framework.)
18. How believable is the information?
Is information valid, reliable, and believable (includes recognizing potential biases)?
19. How does information compare to predictions?
As new information comes in, particularly from experiments or calculations, how does it compare with expected results (based on the predictive framework)?
20. Any significant anomalies?
a. Does potential anomaly fit within acceptable range of predictive framework(s) (given limitations of predictive framework and underlying assumptions and approximations)?
b. Is potential anomaly an unusual statistical variation or relevant data? Is it within acceptable levels of uncertainty?
21. Appropriate conclusions?
What are appropriate conclusions based on the data? (This involves making conclusions and deciding if they are justified.)
22. What is the best solution?
a. Which of multiple candidate solutions are consistent with all available information and which can be rejected? (This could be based on comparing data with predicted results.)
b. What refinements need to be made to candidate solutions?
Category E. Reflect
Reflection decisions occur throughout the process and include deciding whether assumptions are justified, whether additional knowledge or information is needed, how well the solution approach is working, and whether potential and then final solutions are adequate. These decisions match the categories of reflection identified by Salehi (2018) . A mechanical engineer described developing a model (to inform surgical decisions) of which muscles allow the thumb to function in the most useful manner (22, what is best solution?), including reflecting on how well engineering approximations applied in the biological context (23, assumptions and simplifications appropriate?). He also described reflecting on his approach, that is, why he chose to use cadaveric models instead of mathematical models (25, how well is solving approach working?), and the limitations of his findings in that the “best” muscle identified was difficult to access surgically (26, how good is solution?; 27, broader implications?). Reflection decisions are made throughout the problem-solving process, often lead to reconsidering other decisions, and are critical for success.
23. Assumptions and simplifications appropriate?
a. Do the assumptions and simplifications made previously still look appropriate considering new information?
b Does predictive framework need to be modified?
24. Additional knowledge needed?
a. Is solver's relevant knowledge sufficient?
b. Is more information needed and, if so, what?
c. Does some information need to be checked? (Is there a need to repeat experiment or check a different source?)
25. How well is the problem-solving approach working?
How well is the problem-solving approach working, and does it need to be modified? This includes possibly modifying the goals. (One needs to reflect on one's strategy by evaluating progress toward the solution.) and reflecting on one’s strategy by evaluating progress toward the solution.
26. How good is the solution?
a. Decide by exploring possible failure modes and limitations—“try to break” solution.
b. Does it “make sense” and pass discipline-specific tests for solutions of this type of problem?
c. Does it completely meet the goals/criteria?
Category F. Implications and Communication of Results
These are decisions about the broader implications of the work, and how to communicate results most effectively. For example, a theoretical physicist developing a method to calculate the magnetic moment of the muon decided on who would be interested in his work (28, audience for communication?) and what would be the best way to present it (29, best way to present work?). He also discussed the implications of preliminary work on a simplified aspect of the problem (10, approximations and simplifications to make?) in terms of evaluating its impact on the scientific community and deciding on next steps (27, broader implications?; 29, best way to present work?). Many interviewees described that making decisions in this category affected their decisions in other categories.
27. Broader implications?
What are the broader implications of the results, including over what range of contexts does the solution apply? What outstanding problems in the field might it solve? What novel predictions can it enable? How and why might this be seen as interesting to a broader community?
28. Audience for communication?
What is the audience for communication of work, and what are their important characteristics?
29. Best way to present work?
What is the best way to present the work to have it understood, and its correctness and importance appreciated? How to make a compelling story of the work?
Category G. Ongoing Skill and Knowledge Development
Although we focused on decisions in the problem-solving process, the experts volunteered general skills and knowledge they saw as important elements of problem-solving expertise in their fields. These included teamwork and interpersonal skills (strongly emphasized), acquiring experience and intuition, and keeping abreast of new developments in their fields.
30. Stay up to date in field
a. Reviewing literature, which does involve making decisions as to which is important.
b. Learning relevant new knowledge (ideas and technology from literature, conferences, colleagues, etc.)
31. Intuition and experience
Acquiring experience and associated intuition to improve problem solving.
32. Interpersonal, teamwork
Includes navigating collaborations, team management, patient interactions, communication skills, etc., particularly as how these apply in the context of the various types of problem-solving processes.
33. Efficiency
Time management including learning to complete certain common tasks efficiently and accurately.
34. Attitude
Motivation and attitude toward the task. Factors such as interest, perseverance, dealing with stress, and confidence in decisions.
Predictive Framework
How the decisions were made was highly dependent on the discipline and problem. However, there was one element that was fundamental and common across all interviews: the early adoption of a “predictive framework” that the experts used throughout the problem-solving process. We define this framework as “a mental model of key features of the problem and the relationships between the features.” All the predictive frameworks involved some degree of simplification and approximation and an underlying level of mechanism that established the relationships between key features. The frameworks provided a structure of knowledge and facilitated the application of that knowledge to the problem at hand, allowing experts to repeatedly run “mental simulations” to make predictions for dependencies and observables and to interpret new information.
As an example, an ecologist described her predictive framework for migration, which incorporated important features such as environmental conditions and genetic differences between species and the mechanisms by which these interacted to impact the migration patterns for a species. She used this framework to guide her meta-analysis of changes in migration patterns, affecting everything from her choice of data sets to include to her interpretation of why migration patterns changed for different species. In many interviews, the frameworks used evolved as additional information was obtained, with additional features being added or underlying assumptions modified. For some problems, the relevant framework was well established and used with confidence, while for other problems, there was considerable uncertainty as to a suitable framework, so developing and testing the framework was a substantial part of the solution process.
A predictive framework contains the expert knowledge organization that has been observed in previous studies of expertise ( Egan and Greeno, 1974 ) but goes further, as here it serves as an explicit tool that guides most decisions and actions during the solving of complex problems. Mental models and mental simulations that are described in the naturalistic decision-making literature are similar, in that they are used to understand the problem and guide decisions ( Klein, 2008 ; Mosier et al. , 2018 ), but they do not necessarily contain the same level of mechanistic understanding of relationships that underlies the predictive frameworks used in science and engineering problem solving. While the use of predictive frameworks was universal, the individual frameworks themselves explicitly reflected the relevant specialized knowledge, structure, and standards of the discipline, and arguably largely define a discipline ( Wieman, 2019 ).
Discipline-Specific Variation
While the set of decisions to be made was highly consistent across disciplines, there were extensive differences within and across disciplines and work contexts, which reflected the differences in perspectives and experiences. These differences were usually evident in how experts made each of the specific decisions, but not in the choice of which decisions needed to be made. In other words, the solution methods, which included following standard accepted procedures in each field, were very different. For example, planning in some experimental sciences may involve formulating a multiyear construction and data-collection effort, while in medicine it may be deciding on a simple blood test. Some decisions, notably in categories A, D, and F, were less likely to be mentioned in particular disciplines, because of the nature of the problems. Specifically, decisions 1 (what is important in field?), 2 (opportunity fits solver’s expertise?), 27 (broader implications?), 28 (audience for communication?), and 29 (best way to present work?) were dependent on the scope of the problem being described and the expert's specific role in it. These were mentioned less frequently in interviews where the problem was assigned to the expert (most often engineering or industry) or where the importance or audience was implicit (most often in medicine). Decisions 16 (which calculations and data analysis?) and 17 (how to represent and organize info?) were particularly unlikely to be mentioned in medicine, because test results are typically provided to doctors not in the form or raw data, but rather already analyzed by a lab or other medical technology professional, so the doctors we interviewed did not need to make decisions themselves about how to analyze or represent the data. Qualitatively, we also noticed some differences between disciplines in the patterns of connections between decisions. When the problem involved development of a tool or product, most commonly the case in engineering, the interview indicated relatively rapid cycles between goals (3), framing problem/potential solutions (8), and reflection on the potential solution (26), before going through the other decisions. Biology, the experimental science most represented in our interviews, had strong links between planning (15), deciding on appropriate conclusions (21), and reflection on the solution (26). This is likely because the respective problems involved complex systems with many unknowns, so careful planning was unusually important for achieving definitive conclusions. See Supplemental Text and Supplemental Table S2 for additional notes on decisions that were mentioned at lower frequency and decisions that were likely to be interconnected, regardless of field.
This work has created a framework of decisions to characterize problem solving in science and engineering. This framework is empirically based and captures the successful problem-solving process of all experts interviewed. We see that several dozen experts across many different fields all make a common set of decisions when solving authentic problems. There are flexible linkages between decisions that are guided by reflection in a continually evolving process. We have also identified the nature of the “predictive frameworks” that S&E experts consistently use in problem solving. These predictive frameworks reveal how these experts organize their disciplinary knowledge to facilitate making decisions. Many of the decisions we identified are reflected in previous work on expertise and scientific problem solving. This is particularly true for those listed in the planning and interpreting information categories ( Egan and Greeno, 1974 ). The priority experts give to framing and planning decisions over execution compared with novices has been noted repeatedly (e.g., Chi et al. , 1988 ). Expert reflection has been discussed, but less extensively ( Chase and Simon, 1973 ), and elements of the selection and implications and communication categories have been included in policy and standards reports (e.g., AAAS, 2011 ). Thus, our framework of decisions is consistent with previous work on scientific practices and expertise, but it is more complete, specific, empirically based, and generalizable across S&E disciplines.
A limitation of this study is the small number of experts we have in total, from each discipline, and from underrepresented groups (especially lack of female representation in engineering). The lack of randomized selection of participants may also bias the sample toward experts who experienced similar academic training (STEM disciplines at U.S. universities). This means we cannot prove that there are not some experts who follow other paths in problem solving. As with any scientific model, the framework described here should be subjected to further tests and modifications as necessary. However, to our knowledge, this is a far larger sample than used in any previous study of expert problem solving. Although we see a large amount of variation both within and across disciplines in the problem-solving process, this is reflected in how experts make decisions, not in what decisions they make. The very high degree of consistency in the decisions made across the entire sample strongly suggests that we are capturing elements that are common to all experts across science and engineering. A second limitation is that decisions often overlap and co-occur in an interview, so the division between decision items is often somewhat ambiguous and could be defined somewhat differently. As noted, a number of these decisions can be interconnected, and in some fields are nearly always interconnected.
The set of decisions we have observed provides a general framework for characterizing, analyzing, and teaching S&E problem solving. These decisions likely define much of the set of cognitive skills a student needs to practice and master to perform as a skilled practitioner in S&E. This framework of decisions provides a detailed and structured way to approach the teaching and measurement of problem solving at the undergraduate, graduate, and professional training levels. For teaching, we propose using the process of “deliberate practice” ( Ericsson, 2018 ) to help students learn problem solving. Deliberate practice of problem solving would involve effective scaffolding and concentrated practice, with feedback, at making the specific decisions identified here in relevant contexts. In a course, this would likely involve only an appropriately selected set of the decisions, but a good research mentor would ensure that trainees have opportunities to practice and receive feedback on their performance on each of these 29 decisions. Future work is needed to determine whether there are additional decisions that were not identified in experts but are productive components of student problem solving and should also be practiced. Measurements of individual problem-solving expertise based on our decision list and the associated discipline-specific predictive frameworks will allow a detailed measure of an individual's discipline-specific problem-solving strengths and weaknesses relative to an established expert. This can be used to provide targeted feedback to the learner, and when aggregated across students in a program, feedback on the educational quality of the program. We are currently working on the implementation of these ideas in a variety of instructional settings and will report on that work in future publications.
As discussed in the Introduction , typical science and engineering problems fail to engage students in the complete problem-solving process. By considering which of the 29 decisions are required to answer the problem, we can more clearly articulate why. The biology problem, for example, requires students to decide on a predictive framework and access the necessary content knowledge, and they need to decide which information they need to answer the problem. However, other decisions are not required or are already made for them, such as deciding on important features and identifying anomalies. We propose that different problems, designed specifically to require students to make sets of the problem-solving decisions from our framework, will provide more effective tools for measuring, practicing, and ultimately mastering the full S&E problem-solving process.
Our preliminary work with the use of such decision-based problems for assessing problem-solving expertise is showing great promise. For several different disciplines, we have given test subjects a relevant context, requiring content knowledge covered in courses they have taken, and asked them to make decisions from the list presented here. Skilled practitioners in the relevant discipline respond in very consistent ways, while students respond very differently and show large differences that typically correlate with their different educational experiences. What apparently matters is not what content they have seen, but rather what decisions they have had practice making. Our approach was to identify the decisions made by experts, this being the task that educators want students to master. Our data do not exclude the possibility that students engage in and/or should learn other decisions as a productive part of the problem-solving process while they are learning. Future work would seek to identify decisions made at intermediate levels during the development of expertise, to identify potential learning progressions that could be used to teach problem solving more efficiently. What we have seen is consistent with previous work identifying expert–novice differences but provides a much more extensive and detailed picture of a student's strengths and weaknesses and the impacts of particular educational experiences. We have also carried out preliminary development of courses that explicitly involve students making and justifying many of these decisions in relevant contexts, followed by feedback on their decisions. Preliminary results from these courses are also encouraging. Future work will involve the more extensive development and application of decision-based measurement and teaching of problem solving.
ACKNOWLEDGMENTS
We acknowledge the many experts who agreed to be interviewed for this work, M. Flynn for contributions on expertise in mechanical engineering, and Shima Salehi for useful discussions. This work was funded by the Howard Hughes Medical Institute through an HHMI Professor grant to C.E.W.
- ABET . ( 2020 ). Criteria for accrediting engineering programs, 2020–2021 . Retrieved November 23, 2020, from www.abet.org/accreditation/accreditation-criteria/criteria-for-accrediting-engineering-programs-2020-2021 Google Scholar
- Alberts, B., Johnson, A., Lewis, J., Morgan, D., Raff, M., Roberts, K., & Walter, P. ( 2014 ). Control of gene expression . In Molecular Biology of the Cell (6th ed., pp. 436–437). New York: Garland Science. Retrieved November 12, 2020, from https://books.google.com/books?id=2xIwDwAAQBAJ Google Scholar
- American Association for the Advancement of Science . ( 2011 ). Vision and change in undergraduate biology education: A call to action . Washington, DC. Retrieved February 12, 2021, from https://visionandchange.org/finalreport Google Scholar
- Chi, M. T. H., Glaser, R., & Farr, M. J.( ( 1988 ). The nature of expertise . Hillsdale, NJ: Erlbaum. Google Scholar
- Crandall, B., Klein, G. A., & Hoffman, R. R. ( 2006 ). Working minds: A practitioner's guide to cognitive task analysis . Cambridge, MA: MIT Press. Google Scholar
- Egan, D. E., & Greeno, J. G. ( 1974 ). Theory of rule induction: Knowledge acquired in concept learning, serial pattern learning, and problem solving in L . In Gregg, W. (Ed.), Knowledge and cognition . Potomac, MD: Erlbaum. Google Scholar
- Ericsson, K. A., Charness, N., Feltovich, P. J., & Hoffman, R. R. , (Eds.) ( 2006 ). The Cambridge handbook of expertise and expert performance . Cambridge, United Kingdom: Cambridge University Press. Google Scholar
- Ericsson, K. A., Hoffman, R. R., Kozbelt, A., & Williams, A. A. , (Eds.) ( 2018 ). The Cambridge handbook of expertise and expert performance (2nd ed.). Cambridge, United Kingdom: Cambridge University Press. Google Scholar
- Hatano, G., & Inagaki, K. ( 1986 ). Two courses of expertise . In Stevenson, H. W.Azuma, H.Hakuta, K. (Eds.), A series of books in psychology. Child development and education in Japan (pp. 262–272). New York: Freeman/Times Books/Henry Holt. Google Scholar
- Klein, G. ( 2008 ). Naturalistic decision making . Human Factors , 50 (3), 456–460. Medline , Google Scholar
- Kozma, R., Chin, E., Russell, J., & Marx, N. ( 2000 ). The roles of representations and tools in the chemistry laboratory and their implications for chemistry learning . Journal of the Learning Sciences , 9 (2), 105–143. Google Scholar
- Lintern, G., Moon, B., Klein, G., & Hoffman, R. ( 2018 ). Eliciting and representing the knowledge of experts . In Ericcson, K. A.Hoffman, R. R.Kozbelt, A.Williams, A. M. (Eds.), The Cambridge handbook of expertise and expert performance (2nd ed). (pp. 165–191). Cambridge, United Kingdom: Cambridge University Press. Google Scholar
- Mosier, K., Fischer, U., Hoffman, R. R., & Klein, G. ( 2018 ). Expert professional judgments and “naturalistic decision making.” In Ericcson, K. A.Hoffman, R. R.Kozbelt, A.Williams, A. M. (Eds.), The Cambridge handbook of expertise and expert performance (2nd ed). (pp. 453–475). Cambridge, United Kingdom: Cambridge University Press. Google Scholar
- National Research Council (NRC) . ( 2012a ). A framework for K–12 science education: Practices, crosscutting concepts, and core ideas . Washington, DC: National Academies Press. Google Scholar
- Newell, A., & Simon, H. A. ( 1972 ). Human problem solving . Prentice-Hall. Google Scholar
- Next Generation Science Standards Lead States . ( 2013 ). Next Generation Science Standards: For states, by states . Washington, DC: National Academies Press. Google Scholar
- Polya, G. ( 1945 ). How to solve it: A new aspect of mathematical method . Princeton, NJ: Princeton University Press. Google Scholar
- Quacquarelli Symonds . ( 2018 ). The global skills gap in the 21st century . Retrieved July 20, 2021, from www.qs.com/portfolio-items/the-global-skills-gap-in-the-21st-century/ Google Scholar
- Salehi, S. ( 2018 ). Improving problem-solving through reflection (Doctoral dissertation) . Stanford Digital Repository, Stanford University. Retrieved February 18, 2021, from https://purl.stanford.edu/gc847wj5876 Google Scholar
- Schoenfeld, A. H. ( 1985 ). Mathematical problem solving . Orlando, FL: Academic Press. Google Scholar
- Wayne State University . ( n.d ). Mechanical engineering practice qualifying exams. Wayne State University Mechanical Engineering department . Retrieved February 23, 2021, from https://engineering.wayne.edu/me/exams/mechanics_of_materials_-_sample_pqe_problems_.pdf Google Scholar
- Wineburg, S. ( 1998 ). Reading Abraham Lincoln: An expert/expert study in the interpretation of historical texts . Cognitive Science , 22 (3), 319–346. https://doi.org/10.1016/S0364-0213(99)80043-3 Google Scholar
- Uncovering students’ problem-solving processes in game-based learning environments 1 Jun 2022 | Computers & Education, Vol. 182
- Student understanding of kinematics: a qualitative assessment 9 May 2022 | European Journal of Engineering Education, Vol. 5
- What decisions do experts make when doing back-of-the-envelope calculations? 5 April 2022 | Physical Review Physics Education Research, Vol. 18, No. 1
- Simulation led optical design assessments: Emphasizing practical and computational considerations in an upper division physics lecture course 1 Apr 2022 | American Journal of Physics, Vol. 90, No. 4
- Evidence-Based Principles for Worksheet Design 1 Sep 2021 | The Physics Teacher, Vol. 59, No. 6
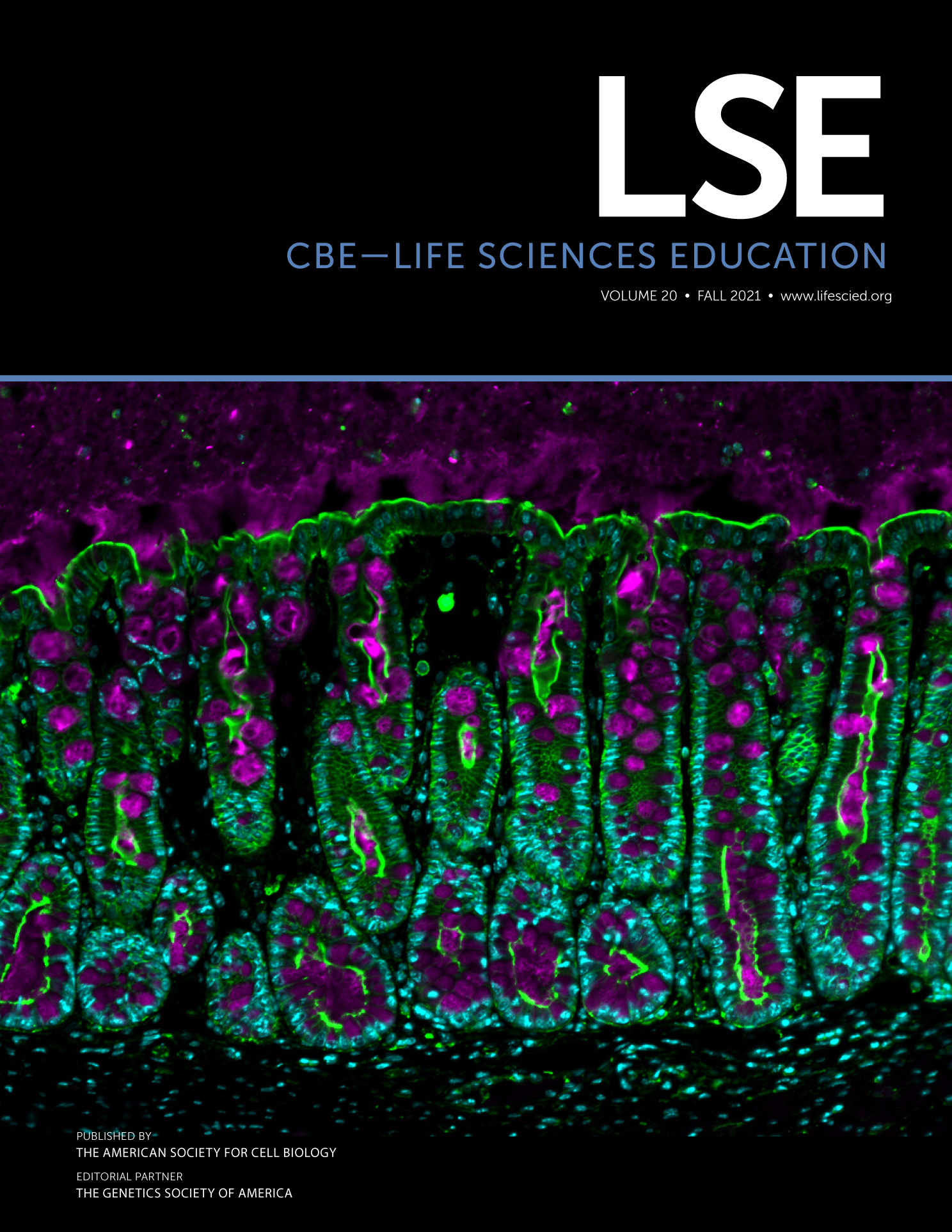
Submitted: 2 December 2020 Revised: 11 June 2021 Accepted: 23 June 2021
© 2021 A. M. Price et al. CBE—Life Sciences Education © 2021 The American Society for Cell Biology. This article is distributed by The American Society for Cell Biology under license from the author(s). It is available to the public under an Attribution–Noncommercial–Share Alike 3.0 Unported Creative Commons License (http://creativecommons.org/licenses/by-nc-sa/3.0).
- Publications
- Conferences & Events
- Professional Learning
- Science Standards
- Awards & Competitions
- Instructional Materials
- Free Resources
- For Preservice Teachers
- NCCSTS Case Collection
- Science and STEM Education Jobs
- Interactive eBooks+
- Digital Catalog
- Regional Product Representatives
- e-Newsletters
- Browse All Titles
- Bestselling Books
- Latest Books
- Popular Book Series
- Submit Book Proposal
- Web Seminars
- National Conference • New Orleans 24
- Leaders Institute • New Orleans 24
- National Conference • Philadelphia 25
- Exhibits & Sponsorship
- Submit a Proposal
- Conference Reviewers
- Past Conferences
- Latest Resources
- Professional Learning Units & Courses
- For Districts
- Online Course Providers
- Schools & Districts
- College Professors & Students
- The Standards
- Teachers and Admin
- eCYBERMISSION
- Toshiba/NSTA ExploraVision
- Junior Science & Humanities Symposium
- Teaching Awards
- Climate Change
- Earth & Space Science
- New Science Teachers
- Early Childhood
- Middle School
- High School
- Postsecondary
- Informal Education
- Journal Articles
- Lesson Plans
- e-newsletters
- Science & Children
- Science Scope
- The Science Teacher
- Journal of College Sci. Teaching
- Connected Science Learning
- NSTA Reports
- Next-Gen Navigator
- Science Update
- Teacher Tip Tuesday
- Trans. Sci. Learning
MyNSTA Community
- My Collections
A Problem-Solving Experiment
Using Beer’s Law to Find the Concentration of Tartrazine
The Science Teacher—January/February 2022 (Volume 89, Issue 3)
By Kevin Mason, Steve Schieffer, Tara Rose, and Greg Matthias
Share Start a Discussion
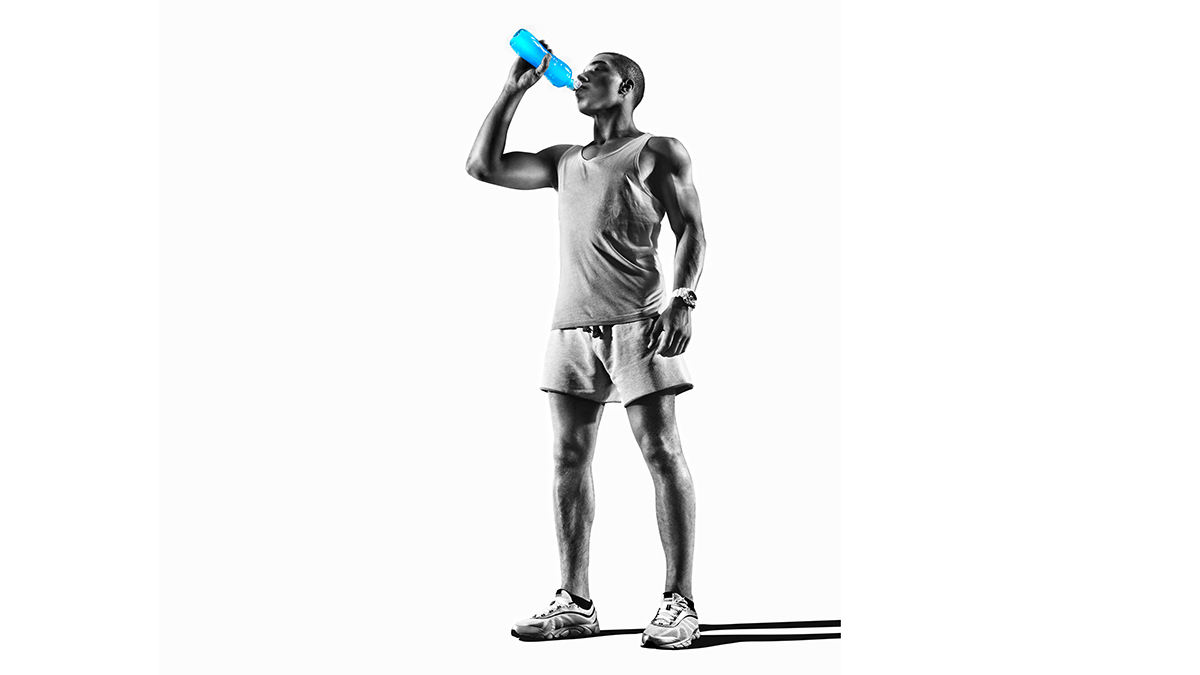
A problem-solving experiment is a learning activity that uses experimental design to solve an authentic problem. It combines two evidence-based teaching strategies: problem-based learning and inquiry-based learning. The use of problem-based learning and scientific inquiry as an effective pedagogical tool in the science classroom has been well established and strongly supported by research ( Akinoglu and Tandogan 2007 ; Areepattamannil 2012 ; Furtak, Seidel, and Iverson 2012 ; Inel and Balim 2010 ; Merritt et al. 2017 ; Panasan and Nuangchalerm 2010 ; Wilson, Taylor, and Kowalski 2010 ).
Floyd James Rutherford, the founder of the American Association for the Advancement of Science (AAAS) Project 2061 once stated, “To separate conceptually scientific content from scientific inquiry,” he underscored, “is to make it highly probable that the student will properly understand neither” (1964, p. 84). A more recent study using randomized control trials showed that teachers that used an inquiry and problem-based pedagogy for seven months improved student performance in math and science ( Bando, Nashlund-Hadley, and Gertler 2019 ). A problem-solving experiment uses problem-based learning by posing an authentic or meaningful problem for students to solve and inquiry-based learning by requiring students to design an experiment to collect and analyze data to solve the problem.
In the problem-solving experiment described in this article, students used Beer’s Law to collect and analyze data to determine if a person consumed a hazardous amount of tartrazine (Yellow Dye #5) for their body weight. The students used their knowledge of solutions, molarity, dilutions, and Beer’s Law to design their own experiment and calculate the amount of tartrazine in a yellow sports drink (or citrus-flavored soda).
According to the Next Generation Science Standards, energy is defined as “a quantitative property of a system that depends on the motion and interactions of matter and radiation with that system” ( NGSS Lead States 2013 ). Interactions of matter and radiation can be some of the most challenging for students to observe, investigate, and conceptually understand. As a result, students need opportunities to observe and investigate the interactions of matter and radiation. Light is one example of radiation that interacts with matter.
Light is electromagnetic radiation that is detectable to the human eye and exhibits properties of both a wave and a particle. When light interacts with matter, light can be reflected at the surface, absorbed by the matter, or transmitted through the matter ( Figure 1 ). When a single beam of light enters a substance at a perpendicularly (at a 90 ° angle to the surface), the amount of reflection is minimal. Therefore, the light will either be absorbed by the substance or be transmitted through the substance. When a given wavelength of light shines into a solution, the amount of light that is absorbed will depend on the identity of the substance, the thickness of the container, and the concentration of the solution.
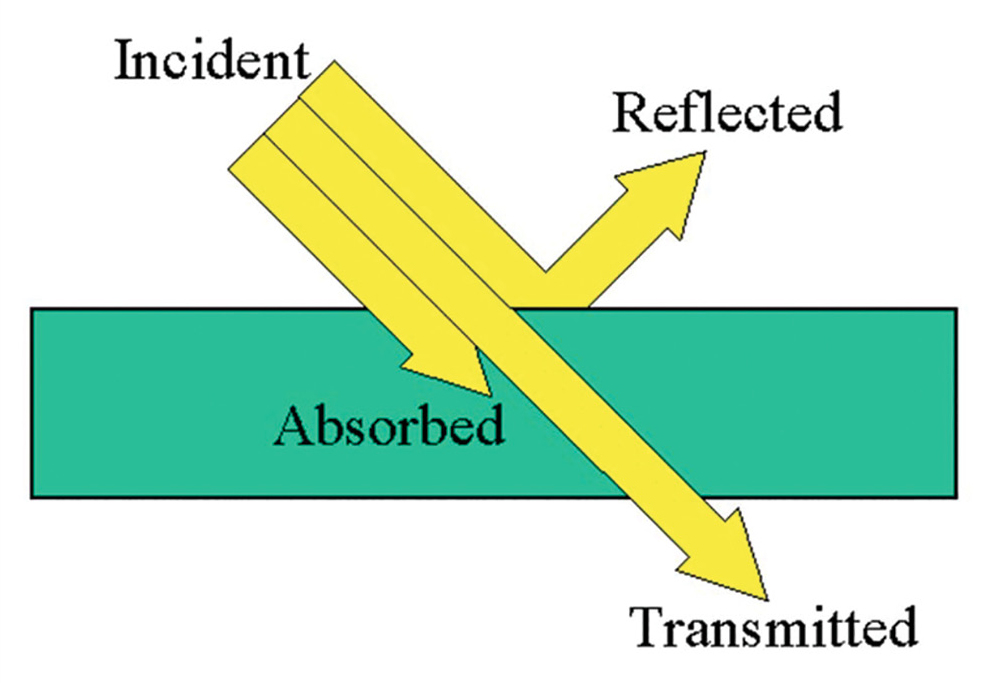
Light interacting with matter.
(Retrieved from https://etorgerson.files.wordpress.com/2011/05/light-reflect-refract-absorb-label.jpg ).
Beer’s Law states the amount of light absorbed is directly proportional to the thickness and concentration of a solution. Beer’s Law is also sometimes known as the Beer-Lambert Law. A solution of a higher concentration will absorb more light and transmit less light ( Figure 2 ). Similarly, if the solution is placed in a thicker container that requires the light to pass through a greater distance, then the solution will absorb more light and transmit less light.
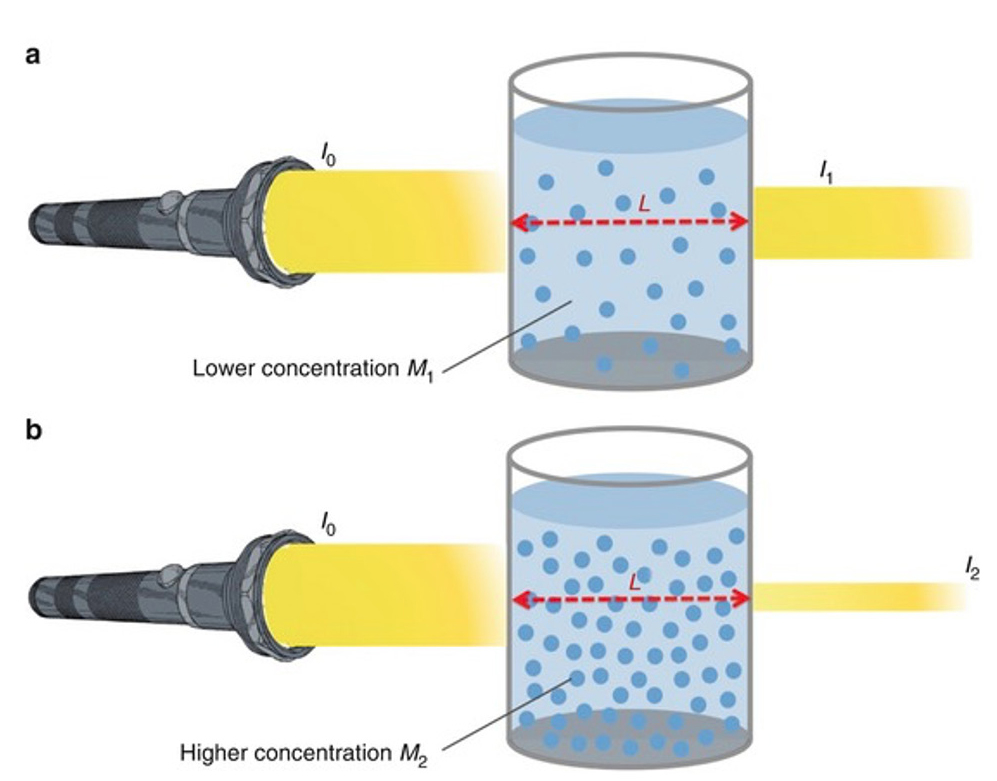
Light transmitted through a solution.
(Retrieved from https://media.springernature.com/original/springer-static/image/chp%3A10.1007%2F978-3-319-57330-4_13/MediaObjects/432946_1_En_13_Fig4_HTML.jpg ).
Definitions of key terms.
Absorbance (A) – the process of light energy being captured by a substance
Beer’s Law (Beer-Lambert Law) – the absorbance (A) of light is directly proportional to the molar absorptivity (ε), thickness (b), and concentration (C) of the solution (A = εbC)
Concentration (C) – the amount of solute dissolved per amount of solution
Cuvette – a container used to hold a sample to be tested in a spectrophotometer
Energy (E) – a quantitative property of a system that depends on motion and interactions of matter and radiation with that system (NGSS Lead States 2013).
Intensity (I) – the amount or brightness of light
Light – electromagnetic radiation that is detectable to the human eye and exhibits properties of both a wave and a particle
Molar Absorptivity (ε) – a property that represents the amount of light absorbed by a given substance per molarity of the solution and per centimeter of thickness (M-1 cm-1)
Molarity (M) – the number of moles of solute per liters of solution (Mol/L)
Reflection – the process of light energy bouncing off the surface of a substance
Spectrophotometer – a device used to measure the absorbance of light by a substance
Tartrazine – widely used food and liquid dye
Transmittance (T) – the process of light energy passing through a substance
The amount of light absorbed by a solution can be measured using a spectrophotometer. The solution of a given concentration is placed in a small container called a cuvette. The cuvette has a known thickness that can be held constant during the experiment. It is also possible to obtain cuvettes of different thicknesses to study the effect of thickness on the absorption of light. The key definitions of the terms related to Beer’s Law and the learning activity presented in this article are provided in Figure 3 .
Overview of the problem-solving experiment
In the problem presented to students, a 140-pound athlete drinks two bottles of yellow sports drink every day ( Figure 4 ; see Online Connections). When she starts to notice a rash on her skin, she reads the label of the sports drink and notices that it contains a yellow dye known as tartrazine. While tartrazine is safe to drink, it may produce some potential side effects in large amounts, including rashes, hives, or swelling. The students must design an experiment to determine the concentration of tartrazine in the yellow sports drink and the number of milligrams of tartrazine in two bottles of the sports drink.
While a sports drink may have many ingredients, the vast majority of ingredients—such as sugar or electrolytes—are colorless when dissolved in water solution. The dyes added to the sports drink are responsible for the color of the sports drink. Food manufacturers may use different dyes to color sports drinks to the desired color. Red dye #40 (allura red), blue dye #1 (brilliant blue), yellow dye #5 (tartrazine), and yellow dye #6 (sunset yellow) are the four most common dyes or colorants in sports drinks and many other commercial food products ( Stevens et al. 2015 ). The concentration of the dye in the sports drink affects the amount of light absorbed.
In this problem-solving experiment, the students used the previously studied concept of Beer’s Law—using serial dilutions and absorbance—to find the concentration (molarity) of tartrazine in the sports drink. Based on the evidence, the students then determined if the person had exceeded the maximum recommended daily allowance of tartrazine, given in mg/kg of body mass. The learning targets for this problem-solving experiment are shown in Figure 5 (see Online Connections).
Pre-laboratory experiences
A problem-solving experiment is a form of guided inquiry, which will generally require some prerequisite knowledge and experience. In this activity, the students needed prior knowledge and experience with Beer’s Law and the techniques in using Beer’s Law to determine an unknown concentration. Prior to the activity, students learned how Beer’s Law is used to relate absorbance to concentration as well as how to use the equation M 1 V 1 = M 2 V 2 to determine concentrations of dilutions. The students had a general understanding of molarity and using dimensional analysis to change units in measurements.
The techniques for using Beer’s Law were introduced in part through a laboratory experiment using various concentrations of copper sulfate. A known concentration of copper sulfate was provided and the students followed a procedure to prepare dilutions. Students learned the technique for choosing the wavelength that provided the maximum absorbance for the solution to be tested ( λ max ), which is important for Beer’s Law to create a linear relationship between absorbance and solution concentration. Students graphed the absorbance of each concentration in a spreadsheet as a scatterplot and added a linear trend line. Through class discussion, the teacher checked for understanding in using the equation of the line to determine the concentration of an unknown copper sulfate solution.
After the students graphed the data, they discussed how the R2 value related to the data set used to construct the graph. After completing this experiment, the students were comfortable making dilutions from a stock solution, calculating concentrations, and using the spectrophotometer to use Beer’s Law to determine an unknown concentration.
Introducing the problem
After the initial experiment on Beer’s Law, the problem-solving experiment was introduced. The problem presented to students is shown in Figure 4 (see Online Connections). A problem-solving experiment provides students with a valuable opportunity to collaborate with other students in designing an experiment and solving a problem. For this activity, the students were assigned to heterogeneous or mixed-ability laboratory groups. Groups should be diversified based on gender; research has shown that gender diversity among groups improves academic performance, while racial diversity has no significant effect ( Hansen, Owan, and Pan 2015 ). It is also important to support students with special needs when assigning groups. The mixed-ability groups were assigned intentionally to place students with special needs with a peer who has the academic ability and disposition to provide support. In addition, some students may need additional accommodations or modifications for this learning activity, such as an outlined lab report, a shortened lab report format, or extended time to complete the analysis. All students were required to wear chemical-splash goggles and gloves, and use caution when handling solutions and glass apparatuses.
Designing the experiment
During this activity, students worked in lab groups to design their own experiment to solve a problem. The teacher used small-group and whole-class discussions to help students understand the problem. Students discussed what information was provided and what they need to know and do to solve the problem. In planning the experiment, the teacher did not provide a procedure and intentionally provided only minimal support to the students as needed. The students designed their own experimental procedure, which encouraged critical thinking and problem solving. The students needed to be allowed to struggle to some extent. The teacher provided some direction and guidance by posing questions for students to consider and answer for themselves. Students were also frequently reminded to review their notes and the previous experiment on Beer’s Law to help them better use their resources to solve the problem. The use of heterogeneous or mixed-ability groups also helped each group be more self-sufficient and successful in designing and conducting the experiment.
Students created a procedure for their experiment with the teacher providing suggestions or posing questions to enhance the experimental design, if needed. Safety was addressed during this consultation to correct safety concerns in the experimental design or provide safety precautions for the experiment. Students needed to wear splash-proof goggles and gloves throughout the experiment. In a few cases, students realized some opportunities to improve their experimental design during the experiment. This was allowed with the teacher’s approval, and the changes to the procedure were documented for the final lab report.
Conducting the experiment
A sample of the sports drink and a stock solution of 0.01 M stock solution of tartrazine were provided to the students. There are many choices of sports drinks available, but it is recommended that the ingredients are checked to verify that tartrazine (yellow dye #5) is the only colorant added. This will prevent other colorants from affecting the spectroscopy results in the experiment. A citrus-flavored soda could also be used as an alternative because many sodas have tartrazine added as well. It is important to note that tartrazine is considered safe to drink, but it may produce some potential side effects in large amounts, including rashes, hives, or swelling. A list of the materials needed for this problem-solving experiment is shown in Figure 6 (see Online Connections).
This problem-solving experiment required students to create dilutions of known concentrations of tartrazine as a reference to determine the unknown concentration of tartrazine in a sports drink. To create the dilutions, the students were provided with a 0.01 M stock solution of tartrazine. The teacher purchased powdered tartrazine, available from numerous vendors, to create the stock solution. The 0.01 M stock solution was prepared by weighing 0.534 g of tartrazine and dissolving it in enough distilled water to make a 100 ml solution. Yellow food coloring could be used as an alternative, but it would take some research to determine its concentration. Since students have previously explored the experimental techniques, they should know to prepare dilutions that are somewhat darker and somewhat lighter in color than the yellow sports drink sample. Students should use five dilutions for best results.
Typically, a good range for the yellow sports drink is standard dilutions ranging from 1 × 10-3 M to 1 × 10-5 M. The teacher may need to caution the students that if a dilution is too dark, it will not yield good results and lower the R2 value. Students that used very dark dilutions often realized that eliminating that data point created a better linear trendline, as long as it didn’t reduce the number of data points to fewer than four data points. Some students even tried to use the 0.01 M stock solution without any dilution. This was much too dark. The students needed to do substantial dilutions to get the solutions in the range of the sports drink.
After the dilutions are created, the absorbance of each dilution was measured using a spectrophotometer. A Vernier SpectroVis (~$400) spectrophotometer was used to measure the absorbance of the prepared dilutions with known concentrations. The students adjusted the spectrophotometer to use different wavelengths of light and selected the wavelength with the highest absorbance reading. The same wavelength was then used for each measurement of absorbance. A wavelength of 650 nanometers (nm) provided an accurate measurement and good linear relationship. After measuring the absorbance of the dilutions of known concentrations, the students measured the absorbance of the sports drink with an unknown concentration of tartrazine using the spectrophotometer at the same wavelength. If a spectrophotometer is not available, a color comparison can be used as a low-cost alternative for completing this problem-solving experiment ( Figure 7 ; see Online Connections).
Analyzing the results
After completing the experiment, the students graphed the absorbance and known tartrazine concentrations of the dilutions on a scatter-plot to create a linear trendline. In this experiment, absorbance was the dependent variable, which should be graphed on the y -axis. Some students mistakenly reversed the axes on the scatter-plot. Next, the students used the graph to find the equation for the line. Then, the students solve for the unknown concentration (molarity) of tartrazine in the sports drink given the linear equation and the absorbance of the sports drink measured experimentally.
To answer the question posed in the problem, the students also calculated the maximum amount of tartrazine that could be safely consumed by a 140 lb. person, using the information given in the problem. A common error in solving the problem was not converting the units of volume given in the problem from ounces to liters. With the molarity and volume in liters, the students then calculated the mass of tartrazine consumed per day in milligrams. A sample of the graph and calculations from one student group are shown in Figure 8 . Finally, based on their calculations, the students answered the question posed in the original problem and determined if the person’s daily consumption of tartrazine exceeded the threshold for safe consumption. In this case, the students concluded that the person did NOT consume more than the allowable daily limit of tartrazine.
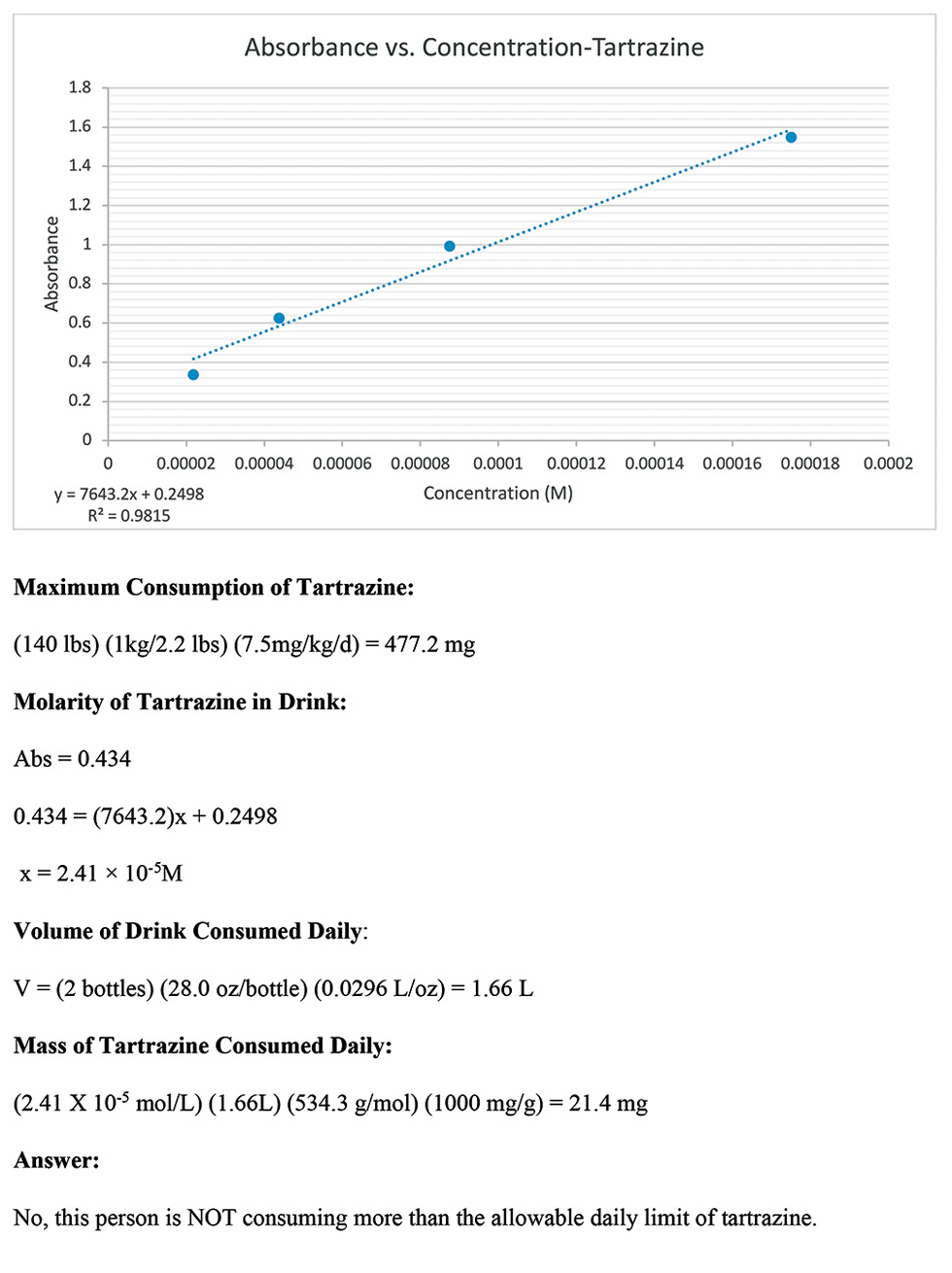
Sample graph and calculations from a student group.
Communicating the results
After conducting the experiment, students reported their results in a written laboratory report that included the following sections: title, purpose, introduction, hypothesis, materials and methods, data and calculations, conclusion, and discussion. The laboratory report was assessed using the scoring rubric shown in Figure 9 (see Online Connections). In general, the students did very well on this problem-solving experiment. Students typically scored a three or higher on each criteria of the rubric. Throughout the activity, the students successfully demonstrated their ability to design an experiment, collect data, perform calculations, solve a problem, and effectively communicate those results.
This activity is authentic problem-based learning in science as the true concentration of tartrazine in the sports drink was not provided by the teacher or known by the students. The students were generally somewhat biased as they assumed the experiment would result in exceeding the recommended maximum consumption of tartrazine. Some students struggled with reporting that the recommended limit was far higher than the two sports drinks consumed by the person each day. This allows for a great discussion about the use of scientific methods and evidence to provide unbiased answers to meaningful questions and problems.
The most common errors in this problem-solving experiment were calculation errors, with the most common being calculating the concentrations of the dilutions (perhaps due to the use of very small concentrations). There were also several common errors in communicating the results in the laboratory report. In some cases, students did not provide enough background information in the introduction of the report. When the students communicated the results, some students also failed to reference specific data from the experiment. Finally, in the discussion section, some students expressed concern or doubts in the results, not because there was an obvious error, but because they did not believe the level consumed could be so much less than the recommended consumption limit of tartrazine.
The scientific study and investigation of energy and matter are salient topics addressed in the Next Generation Science Standards ( Figure 10 ; see Online Connections). In a chemistry classroom, students should have multiple opportunities to observe and investigate the interaction of energy and matter. In this problem-solving experiment students used Beer’s Law to collect and analyze data to determine if a person consumed an amount of tartrazine that exceeded the maximum recommended daily allowance. The students correctly concluded that the person in the problem did not consume more than the recommended daily amount of tartrazine for their body weight.
In this activity students learned to work collaboratively to design an experiment, collect and analyze data, and solve a problem. These skills extend beyond any one science subject or class. Through this activity, students had the opportunity to do real-world science to solve a problem without a previously known result. The process of designing an experiment may be difficult for some students that are often accustomed to being given an experimental procedure in their previous science classroom experiences. However, because students sometimes struggled to design their own experiment and perform the calculations, students also learned to persevere in collecting and analyzing data to solve a problem, which is a valuable life lesson for all students. ■

Online Connections
The Beer-Lambert Law at Chemistry LibreTexts: https://bit.ly/3lNpPEi
Beer’s Law – Theoretical Principles: https://teaching.shu.ac.uk/hwb/chemistry/tutorials/molspec/beers1.htm
Beer’s Law at Illustrated Glossary of Organic Chemistry: http://www.chem.ucla.edu/~harding/IGOC/B/beers_law.html
Beer Lambert Law at Edinburgh Instruments: https://www.edinst.com/blog/the-beer-lambert-law/
Beer’s Law Lab at PhET Interactive Simulations: https://phet.colorado.edu/en/simulation/beers-law-lab
Figure 4. Problem-solving experiment problem statement: https://bit.ly/3pAYHtj
Figure 5. Learning targets: https://bit.ly/307BHtb
Figure 6. Materials list: https://bit.ly/308a57h
Figure 7. The use of color comparison as a low-cost alternative: https://bit.ly/3du1uyO
Figure 9. Summative performance-based assessment rubric: https://bit.ly/31KoZRj
Figure 10. Connecting to the Next Generation Science Standards : https://bit.ly/3GlJnY0
Kevin Mason ( [email protected] ) is Professor of Education at the University of Wisconsin–Stout, Menomonie, WI; Steve Schieffer is a chemistry teacher at Amery High School, Amery, WI; Tara Rose is a chemistry teacher at Amery High School, Amery, WI; and Greg Matthias is Assistant Professor of Education at the University of Wisconsin–Stout, Menomonie, WI.
Akinoglu, O., and R. Tandogan. 2007. The effects of problem-based active learning in science education on students’ academic achievement, attitude and concept learning. Eurasia Journal of Mathematics, Science, and Technology Education 3 (1): 77–81.
Areepattamannil, S. 2012. Effects of inquiry-based science instruction on science achievement and interest in science: Evidence from Qatar. The Journal of Educational Research 105 (2): 134–146.
Bando R., E. Nashlund-Hadley, and P. Gertler. 2019. Effect of inquiry and problem-based pedagogy on learning: Evidence from 10 field experiments in four countries. The National Bureau of Economic Research 26280.
Furtak, E., T. Seidel, and H. Iverson. 2012. Experimental and quasi-experimental studies of inquiry-based science teaching: A meta-analysis. Review of Educational Research 82 (3): 300–329.
Hansen, Z., H. Owan, and J. Pan. 2015. The impact of group diversity on class performance. Education Economics 23 (2): 238–258.
Inel, D., and A. Balim. 2010. The effects of using problem-based learning in science and technology teaching upon students’ academic achievement and levels of structuring concepts. Pacific Forum on Science Learning and Teaching 11 (2): 1–23.
Merritt, J., M. Lee, P. Rillero, and B. Kinach. 2017. Problem-based learning in K–8 mathematics and science education: A literature review. The Interdisciplinary Journal of Problem-based Learning 11 (2).
NGSS Lead States. 2013. Next Generation Science Standards: For states, by states. Washington, DC: National Academies Press.
Panasan, M., and P. Nuangchalerm. 2010. Learning outcomes of project-based and inquiry-based learning activities. Journal of Social Sciences 6 (2): 252–255.
Rutherford, F.J. 1964. The role of inquiry in science teaching. Journal of Research in Science Teaching 2 (2): 80–84.
Stevens, L.J., J.R. Burgess, M.A. Stochelski, and T. Kuczek. 2015. Amounts of artificial food dyes and added sugars in foods and sweets commonly consumed by children. Clinical Pediatrics 54 (4): 309–321.
Wilson, C., J. Taylor, and S. Kowalski. 2010. The relative effects and equity of inquiry-based and commonplace science teaching on students’ knowledge, reasoning, and argumentation. Journal of Research in Science Teaching 47 (3): 276–301.
Chemistry Crosscutting Concepts Curriculum Disciplinary Core Ideas General Science Inquiry Instructional Materials Labs Lesson Plans Mathematics NGSS Pedagogy Science and Engineering Practices STEM Teaching Strategies Technology Three-Dimensional Learning High School
You may also like
Reports Article
Web Seminar
Join us on Thursday, November 21, 2024, from 7:00 PM to 8:00 PM ET, to learn about strategies educators can use when applying for a science teaching p...
NSTA Press Book
Here’s a fresh way to help your students learn life science by determining how you can help them learn best. Uncovering Student Ideas in Life Scienc...

What is Problem Solving? (Steps, Techniques, Examples)
What is problem solving, definition and importance.
Problem solving is the process of finding solutions to obstacles or challenges you encounter in your life or work. It is a crucial skill that allows you to tackle complex situations, adapt to changes, and overcome difficulties with ease. Mastering this ability will contribute to both your personal and professional growth, leading to more successful outcomes and better decision-making.
Problem-Solving Steps
The problem-solving process typically includes the following steps:
- Identify the issue : Recognize the problem that needs to be solved.
- Analyze the situation : Examine the issue in depth, gather all relevant information, and consider any limitations or constraints that may be present.
- Generate potential solutions : Brainstorm a list of possible solutions to the issue, without immediately judging or evaluating them.
- Evaluate options : Weigh the pros and cons of each potential solution, considering factors such as feasibility, effectiveness, and potential risks.
- Select the best solution : Choose the option that best addresses the problem and aligns with your objectives.
- Implement the solution : Put the selected solution into action and monitor the results to ensure it resolves the issue.
- Review and learn : Reflect on the problem-solving process, identify any improvements or adjustments that can be made, and apply these learnings to future situations.
Defining the Problem
To start tackling a problem, first, identify and understand it. Analyzing the issue thoroughly helps to clarify its scope and nature. Ask questions to gather information and consider the problem from various angles. Some strategies to define the problem include:
- Brainstorming with others
- Asking the 5 Ws and 1 H (Who, What, When, Where, Why, and How)
- Analyzing cause and effect
- Creating a problem statement
Generating Solutions
Once the problem is clearly understood, brainstorm possible solutions. Think creatively and keep an open mind, as well as considering lessons from past experiences. Consider:
- Creating a list of potential ideas to solve the problem
- Grouping and categorizing similar solutions
- Prioritizing potential solutions based on feasibility, cost, and resources required
- Involving others to share diverse opinions and inputs
Evaluating and Selecting Solutions
Evaluate each potential solution, weighing its pros and cons. To facilitate decision-making, use techniques such as:
- SWOT analysis (Strengths, Weaknesses, Opportunities, Threats)
- Decision-making matrices
- Pros and cons lists
- Risk assessments
After evaluating, choose the most suitable solution based on effectiveness, cost, and time constraints.
Implementing and Monitoring the Solution
Implement the chosen solution and monitor its progress. Key actions include:
- Communicating the solution to relevant parties
- Setting timelines and milestones
- Assigning tasks and responsibilities
- Monitoring the solution and making adjustments as necessary
- Evaluating the effectiveness of the solution after implementation
Utilize feedback from stakeholders and consider potential improvements. Remember that problem-solving is an ongoing process that can always be refined and enhanced.
Problem-Solving Techniques
During each step, you may find it helpful to utilize various problem-solving techniques, such as:
- Brainstorming : A free-flowing, open-minded session where ideas are generated and listed without judgment, to encourage creativity and innovative thinking.
- Root cause analysis : A method that explores the underlying causes of a problem to find the most effective solution rather than addressing superficial symptoms.
- SWOT analysis : A tool used to evaluate the strengths, weaknesses, opportunities, and threats related to a problem or decision, providing a comprehensive view of the situation.
- Mind mapping : A visual technique that uses diagrams to organize and connect ideas, helping to identify patterns, relationships, and possible solutions.
Brainstorming
When facing a problem, start by conducting a brainstorming session. Gather your team and encourage an open discussion where everyone contributes ideas, no matter how outlandish they may seem. This helps you:
- Generate a diverse range of solutions
- Encourage all team members to participate
- Foster creative thinking
When brainstorming, remember to:
- Reserve judgment until the session is over
- Encourage wild ideas
- Combine and improve upon ideas
Root Cause Analysis
For effective problem-solving, identifying the root cause of the issue at hand is crucial. Try these methods:
- 5 Whys : Ask “why” five times to get to the underlying cause.
- Fishbone Diagram : Create a diagram representing the problem and break it down into categories of potential causes.
- Pareto Analysis : Determine the few most significant causes underlying the majority of problems.
SWOT Analysis
SWOT analysis helps you examine the Strengths, Weaknesses, Opportunities, and Threats related to your problem. To perform a SWOT analysis:
- List your problem’s strengths, such as relevant resources or strong partnerships.
- Identify its weaknesses, such as knowledge gaps or limited resources.
- Explore opportunities, like trends or new technologies, that could help solve the problem.
- Recognize potential threats, like competition or regulatory barriers.
SWOT analysis aids in understanding the internal and external factors affecting the problem, which can help guide your solution.
Mind Mapping
A mind map is a visual representation of your problem and potential solutions. It enables you to organize information in a structured and intuitive manner. To create a mind map:
- Write the problem in the center of a blank page.
- Draw branches from the central problem to related sub-problems or contributing factors.
- Add more branches to represent potential solutions or further ideas.
Mind mapping allows you to visually see connections between ideas and promotes creativity in problem-solving.
Examples of Problem Solving in Various Contexts
In the business world, you might encounter problems related to finances, operations, or communication. Applying problem-solving skills in these situations could look like:
- Identifying areas of improvement in your company’s financial performance and implementing cost-saving measures
- Resolving internal conflicts among team members by listening and understanding different perspectives, then proposing and negotiating solutions
- Streamlining a process for better productivity by removing redundancies, automating tasks, or re-allocating resources
In educational contexts, problem-solving can be seen in various aspects, such as:
- Addressing a gap in students’ understanding by employing diverse teaching methods to cater to different learning styles
- Developing a strategy for successful time management to balance academic responsibilities and extracurricular activities
- Seeking resources and support to provide equal opportunities for learners with special needs or disabilities
Everyday life is full of challenges that require problem-solving skills. Some examples include:
- Overcoming a personal obstacle, such as improving your fitness level, by establishing achievable goals, measuring progress, and adjusting your approach accordingly
- Navigating a new environment or city by researching your surroundings, asking for directions, or using technology like GPS to guide you
- Dealing with a sudden change, like a change in your work schedule, by assessing the situation, identifying potential impacts, and adapting your plans to accommodate the change.
- 8 Examples: Top Problem Solving Skills
- Problem Solving Skills: 25 Performance Review Phrases Examples
- How to Resolve Employee Conflict at Work [Steps, Tips, Examples]
- 30 Examples: Self Evaluation Comments for Problem Solving
- Effective Decision Making Process: 7 Steps with Examples
- 174 Performance Feedback Examples (Reliability, Integrity, Problem Solving)
Problem Solving
Handbooks, special collections, etc.
- Brown, S. I.: M.I. Walter (1990). The Art of Problem Posing . Hillsdale, New Jersey, Hove and London: Lawrence Erlbaum Associates, Publishers
- Karp, A., & Wasserman, N. (2015). Mathematics in Middle and Secondary School. A problem solving approach . Charlotte, NC: Information Age.
- Krulik, S. & Reys, R. E. (1980). Problem Solving in School Mathematics, 1980 Yearbook of the National Council of Teachers of Mathematics , Reston, VA: NCTM.
- Liljedahl. P., Santos-Trigo, M., Malaspina, U., Bruder, R. (2016). Problem Solving in Mathematics Education . Springer.
- Newell, A; H. Simon (1972). Human problem solving . Englewood Cliffs, NJ: Prentice Hall.
- Polya, G. (1973). How to solve it . Princeton, NJ: Princeton University Press.
- Polya, G. (1981). Mathematical discovery . New York: John Wiley & Sons.
- Polya, G. (1954). Mathematics and plausible reasoning; Vol. 1. Introduction and analogy in mathematics; Vol. 2. Patterns of plausible inference . Princeton, NJ: Princeton University Press.
- Schoenfeld, A. (1985). Mathematical problem solving . New York, NY: Academic Press.
- Schoenfeld, A. (1992). Learning to think mathematically: problem solving, metacognition, and sense-making in mathematics. In D. A. Grouws (Ed.), Handbook for research on mathematics teaching and learning (pp. 334-370). Reston, VA: NCTM.
- Watson, A., Mason, J. (2005). Mathematics as a constructive activity. Learners Generating examples. Mahwah, NJ – London: Lawrence Erlbaum Associates, Publishers.
- Watson, A., Ohtani, M. (2015). Task design in mathematics education . Springer.
Papers and chapters
- Bernardo, A. B. (2001). Analogical problem construction and transfer in mathematical problem solving. Educational Psychology , 21(2), 137-150.
- Cai, J., Hwang, S., Jiang, C., & Silber, S. (2015). Problem posing research in mathematics: Some answered and unanswered questions. In F.M. Singer, N. Ellerton, & J. Cai (Eds.), Mathematical problem posing: From research to effective practice (pp. 3–34). Springer.
- Cooney, Thomas J. (1985). A Beginning Teacher's View of Problem Solving. Journal for Research in Mathematics Education, Vol. 16, No. 5, 324-336.
- English, L. D. & Gainsburg, J. (2016). Problem solving in a 21st- Century mathematics education.In L. D. English & D. Kirshner (Eds.), Handbook of international research in mathematics education (pp. 313–335). NY: Routledge.
- Kilpatrick, J. (1978) Variables and methodologies in research on problem solving. In L. Hartfield (Ed.), Mathematical problem solving . Columbus, OH: ERIC, 7-20.
- Kilpatrick, J. (1985) A retrospective account of the past twenty-five years of research on teaching mathematical problem solving. In E.A.Silver (ed), Teaching and learning mathematical problem solving: Multiple research perspectives (1-16). Hillsdale, NJ: Lawrence Erlbaum.
- Kilpatrick, J. (1987). Problem formulating: Where do good problem come from? In A.H. Schoenfeld (Ed.), Cognitive science and mathematics education (pp. 123–147). Hillsdale,NJ: Erlbaum.
- Novick, L., & Holyoak, K. (1991). Mathematical problem solving by analogy. Journal of Experimental Psychology, 17(3), 398–415.
- Santos-Trigo, M. (2007). Mathematical problem solving: An evolving research and practice domain. ZDM—The International Journal on Mathematics Education , 39(5, 6): 523–536.
- Schoenfeld, A. H. (1982). Some thoughts on problem-solving research and mathematics education. In F. K. Lester & J. Garofalo (Eds.), Mathematical problem solving: Issues in research (pp. 27–37). Philadelphia: Franklin Institute Press.
- Schoenfeld, A. (1987). What’s all the fuss about metacognition. In Cognitive Science and Mathematics Education /edited by A.Schoenfeld. New Jersey-London: Lawrence Erlbaum Associates, Publishers.
- Schoenfeld, A. (1989).Explorations of Students' Mathematical Beliefs and Behavior Journal for Research in Mathematics Education , Vol. 20, No. 4, 338-355.
- Silver, E. (1982). Knowledge organization and mathematical problem solving. In F. K. Lester &J. Garofalo (Eds.), Mathematical problem solving: Issues in research (pp. 15–25). Philadelphia: Franklin Institute Press.
- Silver, E.; J. Mamona-Downs, S. Leung; P. Kenney (1996). Posing mathematical problems: an exploratory study. Journal for Research in Mathematics Education , 27 (3), 293-309.
- Singer, F., Ellerton, N., & Cai, J. (2013). Problem posing research in mathematics education: New questions and directions. Educational Studies in Mathematics, 83(1), 9–26.
- Stanic G; J. Kilpatrick (1989). Historical Perspectives on problem solving in the mathematics curriculum. In R. Charles & E. Silver (Eds.), The teaching and assessing of mathematical problem solving. Reston, VA: National Council of Teachers of Mathematics, 1-22.
Faculty publications
- Karp, A. (2002). Math problems in blocks: How to write them and why. PRIMUS , 12(4), 289-304.
- Karp, A. (2006). And now put aside your pens and calculators...: On mental problem solving in the high school mathematics lesson. Focus on Learning Problems in Mathematics , 28(1), 23-36.
- Karp, A., & Marcantonio, N. (2010). “The number which is always positive, even if it is negative” (On studying the concept of absolute value). Investigations in Mathematics Learning, 2(3), 43-68.
- Karp, A. (2015). Problems in old Russian textbooks: How they were selected In K. Bjarnadottir et al. (Eds.) “ Dig where you stand” 3 (pp. 203-218). Uppsala: Uppsala University
A few TC dissertations
- DeGraaf, E. (2015). What makes a good problem? Perspectives of students, teachers, and mathematicians.
- McCarron, Craig S. (2011). Comparing the Effects of Metacognitive Prompts and Contrasting Cases on Transfer in Solving Algebra Problems.
- Nahornick, Ashley. (2014). The effect of group dynamics on high-school students' creativity and problem-solving strategies with investigative open-ended non-routine problems.
- Smith, J.P. (1973). The effect of general versus specific heuristics in mathematical problem solving tasks .
- Tjoe, Hartono Hardi (2011). Which approaches do students prefer? Analyzing the mathematical problem solving behavior of mathematically gifted students .
Members of the TSG
Alexander Karp
Philip Smith
Program Director : Professor Alexander Karp
Teachers College, Columbia University 323 Thompson
Phone: (212) 678-3381 Fax: (212) 678-8319
Email: tcmath@tc.edu
- Starting a Business
- Growing a Business
- Small Business Guide
- Business News
- Science & Technology
- Money & Finance
- For Subscribers
- Write for Entrepreneur
- Tips White Papers
- Entrepreneur Store
- United States
- Asia Pacific
- Middle East
- United Kingdom
- South Africa
Copyright © 2024 Entrepreneur Media, LLC All rights reserved. Entrepreneur® and its related marks are registered trademarks of Entrepreneur Media LLC
Most Problems Fall Into 1 of 3 Layers — Here's How to Effectively Approach Each One In entrepreneurship, not all problems are created equal. I've found that there are three layers of problems, and each one requires its own type of solution — here's what they are and how to approach each one.
By Hope Horner Edited by Chelsea Brown Sep 13, 2024
Key Takeaways
- I have found that most problems fall into one of three layers, and each one calls for a different approach to solve.
- In this article, I break down these three layers and explain how each one should be approached.
Opinions expressed by Entrepreneur contributors are their own.
As business owners and leaders, we often encounter a variety of problems in our organizations, but not all problems are created equal.
I've found that most issues fall into one of three layers, each requiring a different approach to solve. Below, I'll break down the three layers so you can tailor your business's solutions to the right problem type.
Related: 2 Steps to Determine the Best Possible Solution to Any Problem
Layer 1: Simple mistakes
For Layer 1 problems, a process is in place, and the person involved knows exactly what they should be doing. The issue here is that they simply made a mistake . It happens to the best of us — sometimes, we just slip up.
When a Layer 1 problem pops up, your first move should be to remind the person of the correct process. A quick, gentle nudge is often all that's needed to get things back on track. These are the kinds of problems that can be fixed with a brief conversation or a simple reminder.
If this kind of mistake starts happening regularly, it's time to dig a little deeper. There may be something else going on — stress, disengagement or even burnout. In these cases, it's important to address the root cause rather than just the symptom. Consistent Layer 1 problems could signal that the employee needs support, whether that's through better time management, more frequent breaks or addressing any personal issues that might be affecting their work.
No matter what the specifics entail, it's best to address a Layer 1 problem quickly, ideally providing feedback within 24 hours. The sooner you address it, the easier it is to course-correct and prevent the mistake from becoming a recurring issue.
Layer 2: Lack of understanding
The second layer of problems is a bit more complex. For Layer 2 problems, a process is in place, but the person doesn't fully understand it. This could happen for several reasons — maybe they're new and still learning, or maybe their training wasn't as thorough as it should have been. Either way, the root of the problem is a lack of understanding, not just a simple mistake.
The solution for a Layer 2 problem is straightforward: training. Whether that involves a refresher course or sitting down one-on-one to go over the process again, the goal is to ensure the person fully understands what's expected of them. Training helps close the knowledge gap and equips the employee with the tools they need to succeed.
If a Layer 2 problem keeps happening, it's a sign that your training materials — or your training methods — might need an update. Take a look at what you're teaching compared to the outcomes you're seeing. Are there gaps in the training? Are there certain parts of the process that employees consistently struggle with? If so, it might be time to update your training to better meet the needs of your team.
When you're addressing a Level 2 problem, aim to share feedback within a week. This gives you enough time to reassess and retrain while keeping the issue fresh in the employee's mind. Also, consider including others who might also benefit from the refresher. This proactive approach can help prevent similar problems from arising with other team members.
Related: 5 Steps to Creatively Solving Business Problems
Layer 3: Lack of process
Finally, we have the third layer of problems, which occurs when there's no process in place at all. If there's no process, you can't expect your team to know what to do. Layer 3 problems often happen when your business has grown or changed, and you're facing new challenges that existing processes just don't cover. They're a great sign that it's time to create or overhaul some new processes.
Layer 3 problems are the most complex because they require you to build something from scratch. The first step is to assess the situation and define what needs to be done. Once you have a clear understanding of the problem, you can begin creating a process that addresses the issue. This might involve mapping out the steps, assigning responsibilities and ensuring that the process aligns with the overall goals of the organization.
Once the process is in place, it's also essential to train your team so they know how to execute it. You may need to hold workshops, provide ongoing support and be available to answer any questions as they arise.
If a Layer 3 problem keeps happening, it could mean that the process you created isn't quite right for the team's needs. In this case, you may need to tweak or update the process or create supplemental processes to cover other parts of the business.
Typically, it takes 2-4 weeks to properly assess a Layer 3 problem, define and document the solution and then train (and retrain) the relevant teams. This might seem like a long time, but it's worth it to ensure that the process is solid and that your team is prepared to follow it long-term.
Why it matters
Understanding the three layers of problems is crucial for effective problem-solving in any organization. You don't want your managers to overthink or waste too much time solving Layer 1 problems — these should be quick fixes. On the other hand, you don't want them to rush through solving Layer 3 problems, as these require more careful planning and execution.
It's also important to look for trends. For example, if you have a lot of Layer 2 problems, it might be a sign that your training methods need improvement. If you're seeing a lot of Layer 1 problems, it could be time to review your hiring practices or provide more support to your team.
Related: Facing a Tough Problem? Try These Hacks to Find the Solution You Need
By identifying the layer of the problem, you can set the right expectations around the amount of time and effort needed to find a solution. Next time you face a challenge, ask yourself: Which layer does this problem belong to? Approaching it with this framework will save you time, effort and maybe even a few headaches along the way.
Entrepreneur Leadership Network® Contributor
CEO of Lemonlight
Want to be an Entrepreneur Leadership Network contributor? Apply now to join.
Editor's Pick Red Arrow
- She Started a Business When She Couldn't Satisfy a European Craving in the U.S. — and It Made More Than $30 Million Last Year
- Lock Use This 'Simple Yet Timeless' Career Advice That Will Change Your Outlook on Career Advancement
- How to Overcome Imposter Syndrome and Start a Business, According to Gary Vee, a Serial Entrepreneur Worth Over $200 Million
- Lock Most People Hate This One Leadership Style — Here's How to Avoid It
- An Iconic McDonald's Treat Is About to Get a Makeover — Here's What to Expect
- Lock Is Your Co-Worker a 'Workplace Catfish'? An Expert Explains How to Uncover the Truth — Before You Pay the Price.
Most Popular Red Arrow
Hasbro's ceo saw a 'clear signal' that it was time to embrace ai for dungeons & dragons.
AI could generate story ideas for the game, he said.
6 Effective Tactics for Handling a Toxic Boss
Salvaging your dignity from an abusive boss is a job all its own.
Old Data Systems Are Holding Businesses Captive — Here are 7 Reasons to Embrace Modern Data Architectures
Discover why modern data architectures are essential for leveraging AI and big data. From scalability and real-time analytics to improved security and cost efficiency, explore the key benefits driving today's data strategies.
Rude, Uncivil Behavior Destroys Productivity and Drives Turnover — Here's How to Foster a Respectful Work Environment
Discussing the negative impact of uncivil behavior in the workplace and how to ensure civility on your team.
She Started a T-Shirt Side Hustle as a Recent Grad Working at 'People' Magazine. It Led to a DM From Levi's and $400 Million.
When Michelle Wahler, co-founder and former CEO of Beyond Yoga, moved to California, she went "full steam ahead" on a new venture.
ChatGPT's Sam Altman Says This Is the One Thing Keeps Him Up at Night
Altman sat down with Oprah Winfrey to talk about his hopes, dreams, and fears for AI.
Successfully copied link
- Data Science
- Data Analysis
- Data Visualization
- Machine Learning
- Deep Learning
- Computer Vision
- Artificial Intelligence
- AI ML DS Interview Series
- AI ML DS Projects series
- Data Engineering
- Web Scrapping
Genetic Algorithms and Genetic Programming for Advanced Problem Solving
Genetic algorithms (GAs) and genetic programming (GP) are branches of evolutionary computing, a subset of artificial intelligence where solutions evolve over time to fit a given set of parameters or solve specific problems. These techniques are inspired by the biological concepts of reproduction, mutation, and natural selection.
This article explores some intriguing and practical applications of genetic algorithms and genetic programming across various industries.
Table of Content
Introduction to Genetic Algorithms
Introduction to genetic programming, key principles of evolutionary algorithms, examples in optimization problems, real-world applications of genetic algorithms and genetic programing, tools and libraries for genetic algorithms in python.
Genetic Algorithms (GAs) are optimization techniques inspired by the principles of natural selection and genetics. They operate on a population of potential solutions, evolving these solutions through processes analogous to biological evolution, such as selection, crossover (recombination), and mutation.
Key Components of Genetic Algorithms
- Population: A set of candidate solutions.
- Chromosomes: Data structures representing solutions, typically encoded as strings of binary or other types of genes.
- Fitness Function: A function that evaluates how good a solution is with respect to the problem being solved.
- Selection: The process of choosing the fittest individuals to reproduce.
- Crossover: The process of combining two parent solutions to produce offspring.
- Mutation: The process of making random alterations to offspring to maintain genetic diversity.
Genetic Programming (GP) extends the concept of genetic algorithms to evolve programs or expressions. Instead of evolving a set of parameters or solutions, GP evolves entire programs or expressions that can perform a task or solve a problem.
Key Components of Genetic Programming
- Population: A set of programs or expressions.
- Fitness Function: A measure of how well a program or expression performs a given task.
- Selection, Crossover, and Mutation: Similar to GAs, but applied to program structures or expressions.
Evolutionary Algorithms (EAs), including GAs and GP, are based on several fundamental principles:
- Natural Selection: The idea that better solutions are more likely to be selected for reproduction.
- Genetic Variation: Diversity in the population is introduced through crossover and mutation to explore a wider solution space.
- Survival of the Fittest: Solutions are evaluated based on a fitness function, and the fittest solutions are more likely to be selected for the next generation.
These principles ensure that the algorithm explores a variety of solutions and converges towards optimal or near-optimal solutions.
1. Knapsack Problem
The Knapsack Problem is a classic optimization problem where the goal is to maximize the total value of items placed in a knapsack without exceeding its weight capacity.
GA Approach:
- Representation: Items are represented as binary strings, where each bit indicates whether an item is included.
- Fitness Function: Evaluates the total value of selected items while penalizing solutions that exceed the weight limit.
- Crossover and Mutation: Involve swapping items between solutions or flipping bits to introduce variation.
Example: Solving the 0/1 Knapsack Problem using GAs can yield good approximations even for large instances where exact methods become computationally infeasible.
2. Traveling Salesman Problem (TSP)
The Traveling Salesman Problem involves finding the shortest possible route that visits a set of cities and returns to the origin city.
- Representation: Routes are represented as permutations of city indices.
- Fitness Function: Measures the total distance of the route.
- Crossover and Mutation: Involves techniques like order crossover (OX) and swap mutation to preserve the feasibility of routes.
Example: GAs can effectively solve TSP instances with hundreds of cities, providing near-optimal solutions in a reasonable time frame.
3. Scheduling Problems
Scheduling Problems involve assigning resources to tasks over time, aiming to optimize certain criteria like minimizing completion time or maximizing resource utilization.
- Representation: Schedules are represented as sequences or matrices of tasks and resources.
- Fitness Function: Measures the quality of the schedule based on criteria like total completion time or resource conflicts.
- Crossover and Mutation: Involve swapping tasks or adjusting schedules to explore different configurations.
Example: GAs are used in job-shop scheduling to minimize makespan or in timetabling to ensure conflicts are resolved efficiently.
1. Optimizing Complex Systems
One of the classic applications of genetic algorithms is in optimizing complex systems where traditional approaches might fail due to the vastness of the solution space. For example, GAs have been effectively used in airline industry for scheduling flights and crew assignments, considering numerous constraints and objectives. Similarly, they have been applied to optimize the layout of components on a computer chip, which involves a highly complex configuration space.
2. Automated Design and Creativity
In the field of automated design, GAs can generate innovative solutions to engineering problems. For instance, NASA has used genetic algorithms to design antennae for spacecrafts. These algorithms generated unconventional, asymmetric designs that performed better than the traditional symmetrical ones. Similarly, in architecture, GAs have helped in creating novel building layouts that optimize space utilization and environmental factors like sunlight and airflow.
3. Financial Market Analysis
Genetic algorithms have found a niche in the financial sector for portfolio optimization and algorithmic trading. By simulating numerous investment scenarios, GAs help in identifying the best allocation of assets that maximizes returns and minimizes risks. Moreover, they are used in trading algorithms to predict market movements and execute trades at optimal times.
4. Game Development and AI
In game development, genetic programming has been employed to evolve behaviors for non-player characters (NPCs), making them more challenging and realistic opponents. For example, GAs have been used to develop strategic behaviors in games like chess and Go, where the vast number of possible moves makes brute force approaches impractical.
5. Machine Learning and Data Mining
GAs are increasingly integrated into machine learning workflows, particularly in feature selection and model optimization. By selecting the most relevant features from large datasets, GAs improve the efficiency and accuracy of predictive models. Additionally, they are used to optimize neural network architectures in deep learning without human intervention.
6. Robotics and Autonomous Vehicles
In robotics, genetic programming can automate the design of control systems for autonomous robots, enabling them to adapt to new environments and perform complex tasks without explicit programming. This approach has been instrumental in developing autonomous vehicles, where GAs optimize driving strategies based on real-time data.
Python provides a variety of libraries and tools for implementing genetic algorithms. Here are some popular options:
- DEAP (Distributed Evolutionary Algorithms in Python): DEAP is a flexible and efficient library for evolutionary algorithms. It provides tools for defining custom genetic algorithms, including selection, crossover, and mutation operators. The features include customizable operators, easy integration with existing code, and extensive documentation.
- PyGAD: PyGAD is a library designed for creating and experimenting with genetic algorithms in Python. It offers an intuitive API and support for various optimization problems. The feature include simple syntax, built-in optimization functions, and support for multi-objective problems.
- Genetic Algorithm Library (GALib): GALib is a library specifically focused on genetic algorithms and provides a range of functionalities for implementing and experimenting with GAs. The feature include comprehensive GA components, support for custom fitness functions, and easy configuration.
- Scikit-Optimize: Scikit-Optimize extends the Scikit-learn library to include optimization algorithms, including evolutionary algorithms for hyperparameter tuning and other optimization tasks. The features include integration with Scikit-learn, support for various optimization techniques, and user-friendly interface.
Genetic Algorithms and Genetic Programming are powerful tools for solving a wide range of optimization and search problems. By mimicking natural evolution, these techniques can explore large and complex solution spaces to find effective solutions across various domains, including engineering, finance, and biomedicine. The availability of specialized libraries in Python further simplifies the implementation of these algorithms, making them accessible to a broader audience of researchers and practitioners. As technology advances, the integration of GAs and GP with fields like artificial intelligence and machine learning is expected to yield even more innovative solutions to complex challenges.
Please Login to comment...
Similar reads.
- PS5 Pro Launched: Controller, Price, Specs & Features, How to Pre-Order, and More
- How to Make Money on Twitch
- How to activate Twitch on smart TV
- 105 Funny Things to Do to Make Someone Laugh
- #geekstreak2024 – 21 Days POTD Challenge Powered By Deutsche Bank
Improve your Coding Skills with Practice
What kind of Experience do you want to share?
Researcher Highlight: Harrison Walker
Janell Lees
Sep 13, 2024, 3:55 PM
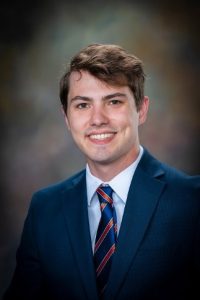
During my initial year at Vanderbilt, I participated in several research rotations, which provided me with exposure to various aspects of materials science and engineering. These rotations were crucial in helping me identify my specific research interests and in developing a multidisciplinary approach to problem-solving. This experience allowed me to collaborate with diverse teams of researchers, gain hands-on experience with advanced scientific equipment, and develop a broader perspective on the field of materials science. The rotations also helped me understand the interconnectedness of different research areas within materials science, which has proven invaluable in my current work combining computational and experimental methods.
Following my rotations at Vanderbilt, I have had the privilege of interning at Oak Ridge National Laboratory. This internship was a pivotal moment in my research journey, offering me the opportunity to work alongside leading scientists and utilize cutting-edge facilities. At Oak Ridge, I have deepened my understanding of advanced computational techniques and electron microscopy. This experience has not only enhanced my technical skills but also solidified my commitment to interdisciplinary research. It has underscored the importance of integrating various scientific approaches to tackle complex problems in materials science.
Overall, these experiences have been instrumental in shaping my research trajectory. They have equipped me with the tools and insights necessary to navigate the challenges of my current work, where I strive to bridge the gap between computational and experimental approaches to advance our understanding of vibrational properties in materials.
My research focuses on understanding how materials vibrate at the atomic level. I study “phonons,” which are quantum mechanical descriptions of vibrational waves in solid materials. Phonons can be thought of as quantized sound waves, just as photons are quanta of light.
I use a specialized instrument called a monochromated and aberration-corrected scanning transmission electron microscope (MACSTEM). This powerful tool allows me to perform electron energy loss spectroscopy (EELS) with high spatial and energy resolution. With this setup, I can precisely measure vibrational modes in materials and gain insights into how the local atomic environment influences material properties.
To complement my experiments, I employ several computational techniques. I use Density Functional Theory (DFT) to calculate the forces on atoms when they’re slightly displaced from their equilibrium positions. This method helps us determine the full range of vibrations (phonon dispersion) and the density of vibrational states in crystalline materials, which are crucial for understanding their vibrational and thermal behavior.
I also develop machine learning models based on DFT calculations. These models are trained on the energies and forces calculated by DFT, allowing us to bridge the gap between the accuracy of quantum mechanical calculations and the efficiency needed for larger-scale simulations. Using these machine learning-generated force fields, I perform Molecular Dynamics (MD) simulations. These simulations let us model how atoms and molecules move over time in systems that are much larger and more complex than what we can study with DFT alone.
To better compare our computational results with experiments, I simulate how the electron beam in our microscope interacts with the sample. I use a method called frequency-resolved frozen phonon multislice, which models how the electron beam travels through the sample, interacting with the vibrating atoms along the way. This allows me to generate theoretical electron energy loss spectra that can be directly compared with our experimental MACSTEM results.
By combining experimental techniques with advanced computer simulations, we are gaining deeper insights into the fundamental behavior of materials. At the nanoscale, heat dissipation becomes a critical factor in the performance and longevity of electronic components. By leveraging our understanding of phonon behavior, we can design novel materials with tailored thermal properties. These materials could revolutionize heat conduction in microprocessors and other high-performance computing components. For instance, by manipulating the phonon dispersion and scattering mechanisms, we might create materials that channel heat away from sensitive areas with unprecedented efficiency.
VINSE Experience
My first year at Vanderbilt University brought an unexpected but incredibly rewarding opportunity: serving as a teaching assistant at the Vanderbilt Institute of Nanoscale Science and Engineering (VINSE). This role turned out to be a perfect blend of my love for scientific research and my growing passion for education and outreach.
As a VINSE TA, I wore many hats. One day I’d be brainstorming new lab ideas, the next I’d be guiding students through experiments in VINSE’s cutting-edge cleanroom and analytical facilities. It was hands-on learning at its finest—not just for the students, but for me too. I got to train on an impressive array of advanced scientific instruments and learn fabrication techniques that significantly expanded my technical toolkit.
At VINSE we covered a wide spectrum of materials science and nanotechnology topics. Students got to fabricate and characterize solar cells, design, and produce microfluidic devices, synthesize, and analyze 2D materials, and even develop innovative new products. It was exciting to see their eyes light up as abstract concepts turned into tangible results right before their eyes.
Even after my official TA duties wrapped up, I couldn’t bring myself to fully step away from VINSE. I stayed involved with some of their outreach programs, which gave me the chance to introduce materials science to local middle and high school students. These young minds would come into our cleanroom, wide-eyed and curious, and leave with a newfound excitement for science.
As my own research has shifted more towards computational methods and simulations, these outreach activities became even more valuable to me. They kept me connected to the hands-on, experimental side of materials science. Plus, there’s something special about seeing a young student grasp a complex concept for the first time—it’s a reminder of why I fell in love with this field in the first place.
I often think about how much I would have loved this kind of exposure when I was their age. Being able to provide that introduction to materials science for these students has been incredibly fulfilling. It’s not just about sharing knowledge—it’s about sparking curiosity and showing them the exciting possibilities in science and engineering.
Explore Story Topics
- Uncategorized
- Harrison Walker
- Materials Science
- Oak Ridge National Laboratory
- VINSE General News

- Instructions for Authors
- Submit Log in/Register
Share this article with
Create alert.
Solving NP-Complete Problems Efficiently
How to cite: Vega, F. Solving NP-Complete Problems Efficiently. Preprints 2024 , 2024081631. https://doi.org/10.20944/preprints202408.1631.v2 Vega, F. Solving NP-Complete Problems Efficiently. Preprints 2024, 2024081631. https://doi.org/10.20944/preprints202408.1631.v2 Copy
Vega, F. Solving NP-Complete Problems Efficiently. Preprints 2024 , 2024081631. https://doi.org/10.20944/preprints202408.1631.v2
Vega, F. (2024). Solving NP-Complete Problems Efficiently. Preprints. https://doi.org/10.20944/preprints202408.1631.v2
Vega, F. 2024 "Solving NP-Complete Problems Efficiently" Preprints. https://doi.org/10.20944/preprints202408.1631.v2
Copyright: This is an open access article distributed under the Creative Commons Attribution License which permits unrestricted use, distribution, and reproduction in any medium, provided the original work is properly cited.
Comments (0)
Not displayed online.
Mathematical equations can be typed in either LaTeX formats \\[ ... \\] or $$ ... $$, or MathML format <math> ... </math>. Try the LaTeX or MathML example.
Type equation: | Preview: |
Please click a symbol to insert it into the message box below:
Please enter the link here:
Optionally, you can enter text that should appear as linked text:
Please enter or paste the URL to the image here (please only use links to jpg/jpeg, png and gif images):
Type author name or keywords to filter the list of references in this group (you can add a new citation under Bibliography): |
No existing citations in Discussion Group |
Wikify editor is a simple editor for wiki-style mark-up. It was written by MDPI for Sciforum in 2014. The rendering of the mark-up is based on Wiky.php with some tweaks. Rendering of mathematical equations is done with MathJax . Please send us a message for support or for reporting bugs.
Comments must follow the standards of professional discourse and should focus on the scientific content of the article. Insulting or offensive language, personal attacks and off-topic remarks will not be permitted. Comments must be written in English. Preprints reserves the right to remove comments without notice. Readers who post comments are obliged to declare any competing interests, financial or otherwise.
We encourage comments and feedback from a broad range of readers. See criteria for comments and our Diversity statement.
what’s this?
Add a record of this review to Publons to track and showcase your reviewing expertise across the world’s journals.
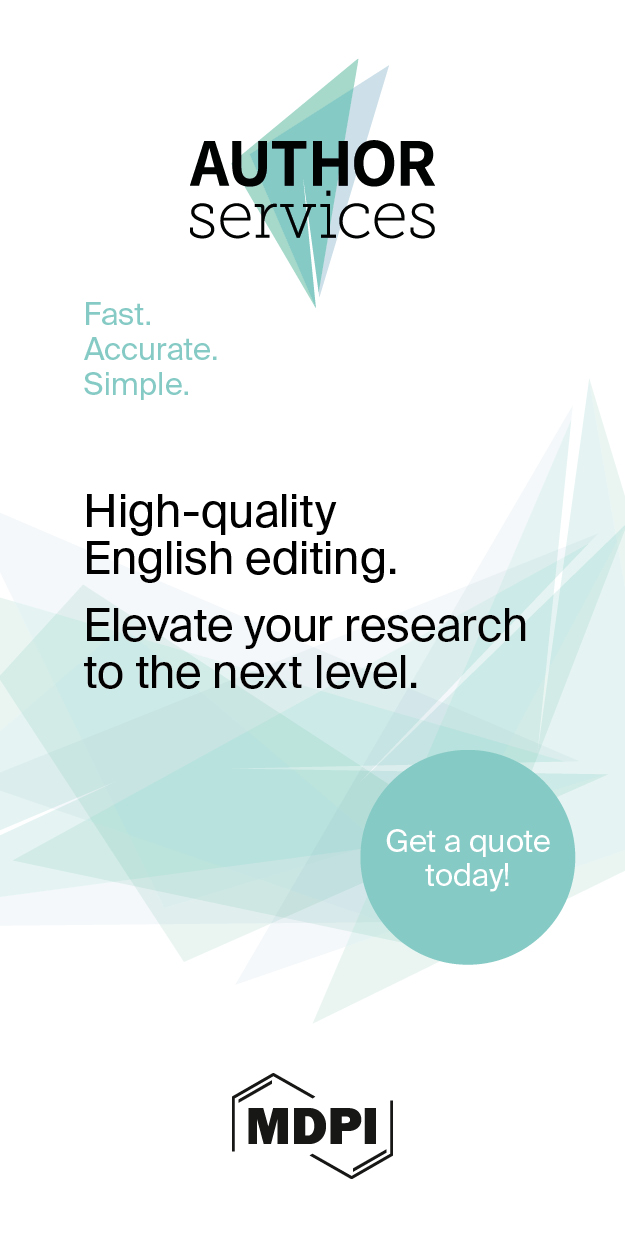

IMAGES
VIDEO
COMMENTS
Problem Solving in STEM. Solving problems is a key component of many science, math, and engineering classes. If a goal of a class is for students to emerge with the ability to solve new kinds of problems or to use new problem-solving techniques, then students need numerous opportunities to develop the skills necessary to approach and answer ...
ABSTRACT. Background: Recent developments in STEM and computer science education put a strong emphasis on twenty-first-century skills, such as solving authentic problems. These skills typically transcend single disciplines. Thus, problem-solving must be seen as a multidisciplinary challenge, and the corresponding practices and processes need to be described using an integrated framework.
If this problem persists, tell us. Our mission is to provide a free, world-class education to anyone, anywhere. Khan Academy is a 501(c)(3) nonprofit organization. ... This information might be about you, your preferences or your device and is mostly used to make the site work as you expect it to. The information does not usually directly ...
Brilliant - Build quantitative skills in math, science, and computer science with hands-on, interactive lessons. ... We make it easy to stay on track, see your progress, and build your problem-solving skills one concept at a time. Stay motivated. Form a real learning habit with fun content that's always well-paced, game-like progress tracking ...
The scientific method can be used to address any situation or problem where a theory can be developed. Although more often associated with natural sciences, it can also be used to develop theories in social sciences (such as psychology, sociology and linguistics), using both. information is information that can be measured, and tends to focus ...
Balancing disciplinary knowledge and practical reasoning in problem solving is needed for meaningful learning. In STEM problem solving, science subject matter with associated practices often appears distant to learners due to its abstract nature. Consequently, learners experience difficulties making meaningful connections between science and their daily experiences. Applying Dewey's idea of ...
As an instructional tool, problem solving attempts to situate the learning of scientific ideas and practices in an applicative context, thus providing an opportunity to transform science learning into an active, relevant, and motivating experience. Problem solving is also frequently a central strategy in the assessment of students ...
Scientists and engineers often spend days choosing a problem and years solving it. This imbalance limits impact. Here, we offer a framework for problem choice: prompts for ideation, guidelines for evaluating impact and likelihood of success, the importance of fixing one parameter at a time, and opportunities afforded by failure.
Abstract. This chapter focuses on problem-solving, which involves describing a problem, figuring out its root cause, locating, ranking and choosing potential solutions, as well as putting those solutions into action in science and technology education. This chapter covers (1) what problem-solving means for science and technology education; (2 ...
Engaging learners in the excitement of science, helping them discover the value of evidence-based reasoning and higher-order cognitive skills, and teaching them to become creative problem solvers have long been goals of science education reformers. But the means to achieve these goals, especially methods to promote creative thinking in scientific problem solving, have not become widely known ...
The traditional approach to teaching science problem solving is having the students work individually on a large number of problems. This approach has long been overtaken by research suggesting and testing other methods, which are expected to be more effective.
A primary goal of science and engineering (S&E) education is to produce good problem solvers, but how to best teach and measure the quality of problem solving remains unclear. The process is complex, multifaceted, and not fully characterized. Here, we present a detailed characterization of the S&E problem-solving process as a set of specific interlinked decisions. This framework of decisions ...
This paper presents a categorization of lab activities based on both their main educational goal and the way they deal with phenomena and models. Afterwards, it discusses the extent to which each type of lab activities fosters the development of problem-solving skills. Previous. laboratory activities. science education.
Examines effective and innovative teaching approaches for science problem solving by analyzing experimental research articles published between 1985 and 1995. Authors use a model of the cognitive capacities needed for effective science problem solving, composed of a knowledge base and a skills base. They also analyze learning conditions such as feedback and group work. Researchers identified ...
1. Identifying a problem and asking questions about that problem. 2. Selecting information to respond to the problem and evaluating it. 3. Drawing conclusions from the evidence. Critical thinking can be developed through focussed learning activities. Students not only need to receive information but also benefit from being encouraged to think ...
We would refer to these as 'complex puzzles,' as they test reasoning skills, but they do not test the appropriate application of specialised knowledge, as is extensively required in every authentic science and engineering problem. Recent work on the standardised assessment of problem-solving skills has recognised the importance of the ...
A problem-solving experiment is a learning activity that uses experimental design to solve an authentic problem. It combines two evidence-based teaching strategies: problem-based learning and inquiry-based learning. The use of problem-based learning and scientific inquiry as an effective pedagogical tool in the science classroom has been well established and strongly supported by research ...
With planning and hard work, the right science fair project might bump up a student's chances for a scholarship or a trip to one of the science competitions sponsored by the Society for Science. ... For more problem-solving science fair project ideas, follow the STEM-Inspirations Science Fair Projects board on Pinterest.
In military science, problem solving is linked to the concept of "end-states", ... In collaborative problem solving people work together to solve real-world problems. Members of problem-solving groups share a common concern, a similar passion, and/or a commitment to their work. Members can ask questions, wonder, and try to understand common issues.
The problem-solving process typically includes the following steps: Identify the issue: Recognize the problem that needs to be solved. Analyze the situation: Examine the issue in depth, gather all relevant information, and consider any limitations or constraints that may be present. Generate potential solutions: Brainstorm a list of possible ...
15K views. Science Problem Solving Activities. Egg Drop. Divide the class into groups, and give each group a basic set of supplies (this could include paper, tape, a paper towel roll, rubber bands ...
Regardless of topic, the students in a section of Solving Complex Problems all work together in the first few class sessions to predict what challenges will arise and to parse the overall problem into a series of 5 to 10 themes. For example, themes might include the environmental context of the problem, engineering challenges, public relations ...
Mathematical problem solving: An evolving research and practice domain. ZDM—The International Journal on Mathematics Education, 39(5, 6): 523-536. Schoenfeld, A. H. (1982). Some thoughts on problem-solving research and mathematics education. In F. K. Lester & J. Garofalo (Eds.), Mathematical problem solving: Issues in research (pp. 27-37 ...
Layer 1: Simple mistakes. For Layer 1 problems, a process is in place, and the person involved knows exactly what they should be doing. The issue here is that they simply made a mistake.It happens ...
I also began to discuss work-life balance with my colleagues—and found that many of them faced similar pressures. Some of us agreed to disconnect from work-related discussions after 6 p.m. One colleague even went as far as to purchase a separate work phone, which she would turn off in the evenings to maintain clear boundaries between work and ...
The reflex agent of AI directly maps states into action. Whenever these agents fail to operate in an environment where the state of mapping is too large and not easily performed by the agent, then the stated problem dissolves and sent to a problem-solving domain which breaks the large stored problem into the smaller storage area and resolves one by
During my initial year at Vanderbilt, I participated in several research rotations, which provided me with exposure to various aspects of materials science and engineering. These rotations were crucial in helping me identify my specific research interests and in developing a multidisciplinary approach to problem-solving. This experience allowed me to collaborate with diverse teams of […]
The P versus NP problem is a fundamental question in computer science. It asks whether problems whose solutions can be quickly verified can also be quickly solved. Here, "quickly" refers to computational time that grows proportionally to the size of the input (polynomial time). While the problem's roots trace back to a 1955 letter from John Nash, its formalization is attributed to Stephen Cook ...
A new series of reasoning models for solving hard problems. Available starting 9.12 ... They can reason through complex tasks and solve harder problems than previous models in science, coding, and math. ... To match the new capabilities of these models, we've bolstered our safety work, internal governance, and federal government collaboration
Making Difficult Quantum Many-Body Calculations Possible Solving quantum many-body problems with wavefunction matching. Image courtesy of Dean Lee Wavefunction matching replaces the short distance part of the two-body wavefunction for a realistic interaction with that of a simple easily computable interaction. The result is a new interaction that can be handled in quantum many-body calculations.