- Search Search Please fill out this field.

What Is a One-Tailed Test?
- Determining Significance
- One-Tailed Test FAQs
- Corporate Finance
- Financial Analysis
One-Tailed Test Explained: Definition and Example
:max_bytes(150000):strip_icc():format(webp)/wk_headshot_aug_2018_02__william_kenton-5bfc261446e0fb005118afc9.jpg)
Investopedia / Xiaojie Liu
A one-tailed test is a statistical test in which the critical area of a distribution is one-sided so that it is either greater than or less than a certain value, but not both. If the sample being tested falls into the one-sided critical area, the alternative hypothesis will be accepted instead of the null hypothesis.
Financial analysts use the one-tailed test to test an investment or portfolio hypothesis.
Key Takeaways
- A one-tailed test is a statistical hypothesis test set up to show that the sample mean would be higher or lower than the population mean, but not both.
- When using a one-tailed test, the analyst is testing for the possibility of the relationship in one direction of interest and completely disregarding the possibility of a relationship in another direction.
- Before running a one-tailed test, the analyst must set up a null and alternative hypothesis and establish a probability value (p-value).
A basic concept in inferential statistics is hypothesis testing . Hypothesis testing is run to determine whether a claim is true or not, given a population parameter. A test that is conducted to show whether the mean of the sample is significantly greater than and significantly less than the mean of a population is considered a two-tailed test . When the testing is set up to show that the sample mean would be higher or lower than the population mean, it is referred to as a one-tailed test. The one-tailed test gets its name from testing the area under one of the tails (sides) of a normal distribution , although the test can be used in other non-normal distributions.
Before the one-tailed test can be performed, null and alternative hypotheses must be established. A null hypothesis is a claim that the researcher hopes to reject. An alternative hypothesis is the claim supported by rejecting the null hypothesis.
A one-tailed test is also known as a directional hypothesis or directional test.
Example of the One-Tailed Test
Let's say an analyst wants to prove that a portfolio manager outperformed the S&P 500 index in a given year by 16.91%. They may set up the null (H 0 ) and alternative (H a ) hypotheses as:
H 0 : μ ≤ 16.91
H a : μ > 16.91
The null hypothesis is the measurement that the analyst hopes to reject. The alternative hypothesis is the claim made by the analyst that the portfolio manager performed better than the S&P 500. If the outcome of the one-tailed test results in rejecting the null, the alternative hypothesis will be supported. On the other hand, if the outcome of the test fails to reject the null, the analyst may carry out further analysis and investigation into the portfolio manager’s performance.
The region of rejection is on only one side of the sampling distribution in a one-tailed test. To determine how the portfolio’s return on investment compares to the market index, the analyst must run an upper-tailed significance test in which extreme values fall in the upper tail (right side) of the normal distribution curve. The one-tailed test conducted in the upper or right tail area of the curve will show the analyst how much higher the portfolio return is than the index return and whether the difference is significant.
1%, 5% or 10%
The most common significance levels (p-values) used in a one-tailed test.
Determining Significance in a One-Tailed Test
To determine how significant the difference in returns is, a significance level must be specified. The significance level is almost always represented by the letter p, which stands for probability. The level of significance is the probability of incorrectly concluding that the null hypothesis is false. The significance value used in a one-tailed test is either 1%, 5%, or 10%, although any other probability measurement can be used at the discretion of the analyst or statistician. The probability value is calculated with the assumption that the null hypothesis is true. The lower the p-value , the stronger the evidence that the null hypothesis is false.
If the resulting p-value is less than 5%, the difference between both observations is statistically significant, and the null hypothesis is rejected. Following our example above, if the p-value = 0.03, or 3%, then the analyst can be 97% confident that the portfolio returns did not equal or fall below the return of the market for the year. They will, therefore, reject H 0 and support the claim that the portfolio manager outperformed the index. The probability calculated in only one tail of a distribution is half the probability of a two-tailed distribution if similar measurements were tested using both hypothesis testing tools.
When using a one-tailed test, the analyst is testing for the possibility of the relationship in one direction of interest and completely disregarding the possibility of a relationship in another direction. Using our example above, the analyst is interested in whether a portfolio’s return is greater than the market’s. In this case, they do not need to statistically account for a situation in which the portfolio manager underperformed the S&P 500 index. For this reason, a one-tailed test is only appropriate when it is not important to test the outcome at the other end of a distribution.
How Do You Determine If It Is a One-Tailed or Two-Tailed Test?
A one-tailed test looks for an increase or decrease in a parameter. A two-tailed test looks for change, which could be a decrease or an increase.
What Is a One-Tailed T Test Used for?
A one-tailed T-test checks for the possibility of a one-direction relationship but does not consider a directional relationship in another direction.
When Should a Two-Tailed Test Be Used?
You would use a two-tailed test when you want to test your hypothesis in both directions.
University of Southern California. " FAQ: What Are the Differences Between One-Tailed and Two-Tailed Tests? "
:max_bytes(150000):strip_icc():format(webp)/GettyImages-927418170-696ccbe1ac5446bcb94cfe782635401b.jpg)
- Terms of Service
- Editorial Policy
- Privacy Policy
One-Tailed Hypothesis Tests: 3 Example Problems
In statistics, we use hypothesis tests to determine whether some claim about a population parameter is true or not.
Whenever we perform a hypothesis test, we always write a null hypothesis and an alternative hypothesis, which take the following forms:
H 0 (Null Hypothesis): Population parameter = ≤, ≥ some value
H A (Alternative Hypothesis): Population parameter , ≠ some value
There are two types of hypothesis tests:
- Two-tailed test : Alternative hypothesis contains the ≠ sign
- One-tailed test : Alternative hypothesis contains either or > sign
In a one-tailed test , the alternative hypothesis contains the less than (“”) sign. This indicates that we’re testing whether or not there is a positive or negative effect.
Check out the following example problems to gain a better understanding of one-tailed tests.
Example 1: Factory Widgets
Suppose it’s assumed that the average weight of a certain widget produced at a factory is 20 grams. However, one engineer believes that a new method produces widgets that weigh less than 20 grams.
To test this, he can perform a one-tailed hypothesis test with the following null and alternative hypotheses:
- H 0 (Null Hypothesis): μ ≥ 20 grams
- H A (Alternative Hypothesis): μ
Note : We can tell this is a one-tailed test because the alternative hypothesis contains the less than ( ) sign. Specifically, we would call this a left-tailed test because we’re testing if some population parameter is less than a specific value.
To test this, he uses the new method to produce 20 widgets and obtains the following information:
- n = 20 widgets
- x = 19.8 grams
- s = 3.1 grams
Plugging these values into the One Sample t-test Calculator , we obtain the following results:
- t-test statistic: -0.288525
- one-tailed p-value: 0.388
Since the p-value is not less than .05, the engineer fails to reject the null hypothesis.
He does not have sufficient evidence to say that the true mean weight of widgets produced by the new method is less than 20 grams.
Example 2: Plant Growth
Suppose a standard fertilizer has been shown to cause a species of plants to grow by an average of 10 inches. However, one botanist believes a new fertilizer can cause this species of plants to grow by an average of greater than 10 inches.
To test this, she can perform a one-tailed hypothesis test with the following null and alternative hypotheses:
- H 0 (Null Hypothesis): μ ≤ 10 inches
- H A (Alternative Hypothesis): μ > 10 inches
Note : We can tell this is a one-tailed test because the alternative hypothesis contains the greater than ( > ) sign. Specifically, we would call this a right-tailed test because we’re testing if some population parameter is greater than a specific value.
To test this claim, she applies the new fertilizer to a simple random sample of 15 plants and obtains the following information:
- n = 15 plants
- x = 11.4 inches
- s = 2.5 inches
- t-test statistic: 2.1689
- one-tailed p-value: 0.0239
Since the p-value is less than .05, the botanist rejects the null hypothesis.
She has sufficient evidence to conclude that the new fertilizer causes an average increase of greater than 10 inches.
Example 3: Studying Method
A professor currently teaches students to use a studying method that results in an average exam score of 82. However, he believes a new studying method can produce exam scores with an average value greater than 82.
To test this, he can perform a one-tailed hypothesis test with the following null and alternative hypotheses:
- H 0 (Null Hypothesis): μ ≤ 82
- H A (Alternative Hypothesis): μ > 82
To test this claim, the professor has 25 students use the new studying method and then take the exam. He collects the following data on the exam scores for this sample of students:
- t-test statistic: 3.6586
- one-tailed p-value: 0.0006
Since the p-value is less than .05, the professor rejects the null hypothesis.
He has sufficient evidence to conclude that the new studying method produces exam scores with an average score greater than 82.
Additional Resources
The following tutorials provide additional information about hypothesis testing:
Introduction to Hypothesis Testing What is a Directional Hypothesis? When Do You Reject the Null Hypothesis?
Statistics vs. Probability: What’s the Difference?
One sample z-test calculator, related posts, how to normalize data between -1 and 1, how to interpret f-values in a two-way anova, how to create a vector of ones in..., vba: how to check if string contains another..., how to determine if a probability distribution is..., what is a symmetric histogram (definition & examples), how to find the mode of a histogram..., how to find quartiles in even and odd..., how to calculate sxy in statistics (with example), how to calculate sxx in statistics (with example).
- Skip to secondary menu
- Skip to main content
- Skip to primary sidebar
Statistics By Jim
Making statistics intuitive
When Can I Use One-Tailed Hypothesis Tests?
By Jim Frost 16 Comments
One-tailed hypothesis tests offer the promise of more statistical power compared to an equivalent two-tailed design. While there is some debate about when you can use a one-tailed test, the general consensus among statisticians is that you should use two-tailed tests unless you have concrete reasons for using a one-tailed test.
In this post, I discuss when you should and should not use one-tailed tests. I’ll cover the different schools of thought and offer my own opinion.
If you need to learn the basics about these two types of test, please read my previous post: One-Tailed and Two-Tailed Hypothesis Tests Explained .
Two-Tailed Tests are the Default Choice
The vast majority of hypothesis tests that analysts perform are two-tailed because they can detect effects in both directions. This fact is generally the clincher. In most studies, you are interested in determining whether there is a positive effect or a negative effect. In other words, results in either direction provide essential information. If this statement describes your study, you must use a two-tailed test. There’s no need to read any further. Typically, you need a strong reason to move away from using two-tailed tests.
On the other hand, there are some cases where one-tailed tests are not only a valid option, but truly are a requirement.
Consequently, there is a spectrum that ranges from cases where one-tailed tests are definitely not appropriate to cases where they are required. In the middle of this spectrum, there are cases where analysts might disagree. The breadth of opinions extends from those who believe you should use one-tailed tests for only a few specific situations when they are required to those who are more lenient about their usage.
A Concrete Rule about Choosing Between One- and Two-Tailed Tests
Despite this disagreement, there is a hard and fast rule about the decision process itself upon which all statisticians agree. You must decide whether you will use a one-tailed or two-tailed test at the beginning of your study before you look at your data. You must not perform a two-tailed analysis, obtain non-significant results, and then try a one-tailed test to see if that is statistically significant. If you plan to use a one-tailed test, make this decision at the beginning of the study and explain why it is the proper choice.
The approach I take is to assume you’ll use a two-tailed test and then move away from that only after carefully determining that a one-tailed test is appropriate for your study. The following are potential reasons for why you might use a one-tailed hypothesis test.
Related post : 5 Steps for Conducting Scientific Studies with Statistical Analyses
One-Tailed Tests Can Be the Only Option
For some hypothesis tests, the mechanics of how a test functions dictate using a one-tailed methodology. Chi-squared tests and F-tests and are often one-tailed for this reason.
Chi-squared tests
Analysts often use chi-squared tests to determine whether data fit a theoretical distribution and whether categorical variables are independent . For these tests, when the chi-squared value exceeds the critical threshold, you have sufficient evidence to conclude that the data do not follow the distribution or that the categorical variables are dependent. The chi-squared value either reaches this threshold or it does not. For all values below the threshold, you fail to reject the null hypothesis. There is no other interpretation for very low chi-squared values. Hence, these tests are one-tailed by their nature.

F-tests are highly flexible tests that analysts use in a wide variety of scenarios. Some of these scenarios exclude the possibility of a two-tailed test. For instance, F-tests in ANOVA and the overall test of significance for linear models are similar to the chi-squared example. The F ratio can increase to the significance threshold or it does not. In one-way ANOVA, if the F-value surpasses the threshold, you can conclude that not all group means are equal. On the other hand, all F-values below the threshold yield the same interpretation—the sample provides insufficient evidence to conclude that the group means are unequal. No other effect or interpretation exists for very low F-values.

When a one-tailed version of the test is the only meaningful possibility, statistical software won’t ask you to make a choose. That’s why you’ll never need to choose between a one or two-tailed ANOVA F-test or chi-square tests.
In some cases, the nature of the test itself requires using a one-sided methodology, and it does not depend on the study area.
Effects can Occur in Only One Direction
On the other hand, other hypothesis tests can legitimately have one and two-tailed versions, and you need to choose between them based on the study area. Tests that fall in this category include t-tests , proportion tests, Poisson rate tests, variance tests, and some nonparametric tests for the median. In these cases, base the decision on subject-area knowledge about the possible effects.
For some study areas, the effect can exist in only one direction. It simply can’t exist in the other direction. To make this determination, you need to use your subject-area knowledge and understanding of physical limitations. In this case, if there were a difference in the untested direction, you would attribute it to random error regardless of how large it is. In other words, only chance can produce an observed effect in the other direction. If you have even the smallest notion that an observed effect in the other direction could be a real effect rather than random error, use a two-tailed test.
For example, imagine we are comparing an herbicide’s ability to kill weeds to no treatment. We randomly apply the herbicide to some patches of grass and no herbicide to other patches. It is inconceivable that the herbicide can promote weed growth. In the worst-case scenario, it is entirely ineffective, and the herbicide patches should be equivalent to the control group. If the herbicide patches ultimately have more weeds than the control group, we’ll chalk that up to random error regardless of the difference—even if it’s substantial. In this case, we are wholly justified using a one-tailed test to determine whether the herbicide is better than no treatment.
No Controversy So Far!
So far, the preceding two reasons fall entirely on safe ground. Using one-tailed tests because of its mechanics or because an effect can occur in only one direction should be acceptable to all statisticians. In fact, some statisticians believe that these are the only valid reasons for using one-tailed hypothesis tests. I happen to fall within this school of thought myself.
In the next section, I’ll discuss a scenario where some analysts believe you can choose between one and two-tailed tests, but others disagree with that notion.
You Only Need to Know About Effects in One Direction
In this scenario, effects can exist in both directions, but you only care about detecting an effect in one direction. Analysts use the one-tailed approach in this situation to boost the statistical power of the hypothesis test .
To even consider using a one-tailed test for this reason, you must be entirely sure there is no need to detect an effect in the other direction. While you gain more statistical power in one direction, the test has absolutely no power in the other direction.
Suppose you are testing a new vaccine and want to determine whether it’s better than the current vaccine. You use a one-tailed test to improve the test’s ability to learn whether the new vaccine is better. However, that’s unethical because the test cannot determine whether it is less effective. You risk missing valuable information by testing in only one direction.
However, there might be occasions where you, or science, genuinely don’t need to detect an effect in the untested direction. For example, suppose you are considering a new part that is cheaper than the current part. Your primary motivation for switching is the price reduction. The new part doesn’t have to be better than the current part, but it cannot be worse. In this case, it might be appropriate to perform a one-tailed test that determines whether the new part is worse than the old part. You won’t know if it is better, but you don’t need to know that.
As I mentioned, many statisticians don’t think you should use a one-tailed test for this type of scenario. My position is that you should set up a two-tailed test that produces the same power benefits as a one-tailed test because that approach will accurately capture the underlying fact that effects can occur in both directions.
However, before explaining this alternate approach, I need to describe an additional problem with the above scenario.
Beware of the Power that One-Tailed Tests Provide
The promise of extra statistical power in the direction of interest is tempting. After all, if you don’t care about effects in the opposite direction, what’s the problem? It turns out there is an additional penalty that comes with the extra power.
First, let’s see why one-tailed tests are more powerful than two-tailed tests with the same significance level . The graphs below display the t-distributions for two t-tests with the same sample size. I show the critical t-values for both tests. As you can see, the one-tailed test requires a less extreme t-value (1.725) to produce a statistically significant result in the right tail than the two-tailed test (2.086). In other words, a smaller effect is statistically significant in the one-tailed test.

Both tests have the same Type I error rate because we defined the significance level as 0.05. This type of error occurs when the test rejects a true null hypothesis—a false positive. This error rate corresponds to the total percentage of the shaded areas under the curve. While both tests have the same overall Type I error rate, the distribution of these errors is different.
To understand why, keep in mind that the critical regions also represent where the Type I errors occur. For a two-tailed test, these errors are split equally between the left and right tails. However, for a one-tailed test, all of these errors arise specifically in the one direction that you are interested in. Unfortunately, the error rate doubles in that direction compared to a two-tailed test. In the graphs above, the right tail has an error rate of 5% in the one-tailed test compared to 2.5% in the two-tailed test.
Related Post : Types of Errors in Hypothesis Tests
You Haven’t Changed Anything of Substance
By switching to a one-tailed test, you haven’t changed anything of substance to gain this extra power. All you’ve done is to redraw the critical region so that a smaller effect in the direction of interest is statistically significant. In this light, it’s not surprising that merely labeling smaller effects as being statistically significant also produces more false positives in that direction! And, the graphs reflect that fact.
If you want to increase the test’s power without increasing the Type I error rate, you’ll need to make a more fundamental change to your study’s design, such as increasing your sample size or more effectively controlling the variability.
Is the Higher False Positive Rate Worthwhile?
To use a one-tailed test to gain more power, you can’t care about detecting an effect in the other direction, and you have to be willing to accept twice the false positives in the direction you are interested. Remember, a false positive means that you will not obtain the benefits you expect.
Should you accept double the false positives in the direction of interest? Answering that question depends on the actions that a significant result will prompt. If you’re considering changing to a new production line, that’s a very costly decision. Doubling the false positives is problematic. Your company will spend a lot of money for a new manufacturing line, but it might not produce better products. However, if you’re changing suppliers for a part based on the test result, and their parts don’t cost more, a false positive isn’t an expensive problem.
Think carefully about whether the additional power is worth the extra false positives in your direction of interest! If you decide that the added power is worth the risk, consider my alternative approach below. It produces an equivalent amount of statistical power as the one-tailed approach. However, it uses a methodology that more accurately reflects the underlying reality of the study area and the goals of the analyst.
Alternative: Use a Two-Tailed Test with a Higher Significance Level
In my view, determining the possible directions of an effect and the statistical power of the analysis are two independent issues. Using a one-tailed test to boost power can obscure these matters and their ramifications. My recommendation is to use the following process:
- Identify the directions that an effect can occur, and then choose a one-tailed or two-tailed test accordingly.
- Choose the significance level to correctly set the sensitivity and false-positive rate based on your specific requirements.
This process breaks down the questions you need to answer into two separate issues, which allows you to consider each more carefully.
Now, let’s apply this process to the scenario where you’re studying an effect that can occur in both directions, but the following are both true:
- You care about effects in only one direction.
- Increasing the power of the test is worth a higher risk of false positives in that direction.
In this situation, using a one-tailed test to gain extra power seems like an acceptable solution. However, that approach attempts to solve the right problem by using the wrong methodology. Here’s my alternative method.
Instead of using a one-tailed test, consider using a two-tailed test and doubling the significance level, such as from 0.05 to 0.10. This approach increases your power while allowing the test methodology to match the reality of the situation better. It also increases the transparency of your goals as the analyst.
Related Post : Significance Levels and P-values
How the Two-Tailed Approach with a Higher Significance Level Works
To understand this approach, compare the graphs below. The top graph is one-sided and uses a significance level of 0.05. The bottom graph is two-sided and uses a significance level of 0.10.

As you can see in the graphs, the critical region on the right side of both distributions starts at the same critical t-value (1.725). Consequently, both the one- and two-tailed tests provide the same power in that direction. Additionally, there is a critical region in the other tail, which means that the test can detect effects in the opposite direction as well.
The end result is that the two-tailed test has the same power and an equal probability of a Type I error in the direction of interest. Great! And, you can detect effects in the other direction even though you might not need to know about them. Okay, that’s not a bad thing.
This Approach Is More Transparent
What’s so great about this approach? It makes your methodology choices more explicit while accurately reflecting a study area where effects can occur in both directions. Here’s how.
The significance level is an evidentiary standard for the amount of sample evidence required to reject the null hypothesis. By increasing the significance level from 0.05 to 0.10, you’re explicitly stating that you are lowering the amount of evidence necessary to reject the null, which logically increases the power of the test. Additionally, as you raise the significance level, the Type I error rate also increases by definition. This approach produces the same power gains as a one-tailed test. However, it more clearly indicates how the analyst set up a more sensitive test in exchange for a higher risk of false positives.
The problem with gaining the additional power by switching to a one-tailed test is that it obscures the fact that you’re weakening the evidentiary standard. After all, you’re not explicitly changing the significance level. That’s why the increase in the Type I error rate in the direction of interest can be surprising!
Decision Guidelines
We covered a lot in this post. Here’s a brief recap of when to use each type of test. For some tests, you don’t have to worry about this choice. However, if you do need to decide between using a one-tailed and a two-tailed test, follow these guidelines. If the effect can occur in:
- One direction: Use a one-tailed test and choose the correct alternative hypothesis .
- Both directions: Use a two-tailed test.
- Both directions, but you care about only one direction and you need the higher statistical power: Use a two-tailed test and double the significance level. Be aware that you are doubling the probability of a false positive.
Share this:

Reader Interactions
April 13, 2021 at 10:02 am
Thanks Jim!
April 12, 2021 at 1:57 pm
Another great post.
If my hypothesis was say, that intelligence overall will be greater for first group that took the study in 2010 than the second group that took the same test in 2020. Would this be one tailed because I have made a specific prediction about the direction of intelligence over time?
Thanks again, Grace
April 13, 2021 at 12:22 am
I think you’d have a stronger case for a one-tailed test if the studies were closer together in time. When they’re so far apart, it’s possible that intelligence could decline over the years. (I’ve seen it happen!) But, if the studies were say a month apart, you’d have a stronger case for saying that intelligence wouldn’t decline over such a short span of time and, therefore, a one-tailed test might be called for. Whenever you can say that an effect is only possible in one direction, that’s the strongest case for a one-tailed test where you won’t get any debate.
It sounds like you’re asking about a one-tailed test based on a prediction about the hypothesis. That’s not usually a good enough reason to use a one-tailed test by itself. Of course, as I mention, there is some debate about when it’s ok. At the very least, it could be based on your prediction and the fact that you don’t care about results in the other direction. If you wanted to get published in a journal, that wouldn’t fly. Outside the academic context, you’d probably get some analysts to agree with that case and others wouldn’t.
Just be aware of the drawbacks that I mention. By going to a one-tailed tests, you’re doubling the false positives in the hypothesis direction in which you’re interested. I only recommend one-tailed tests for cases where the effect can only possibly exist in one direction.
November 24, 2020 at 12:33 pm
Brilliant post, Jim! I use hypothesis tests all the time (always two-tailed), but with the explanation you provided here, I can raise the significance if more false positives (i.e., Type I errors) are not a problem. With that said, this approach would still have to get past reviewers in a manuscript submission, which is no sure thing. I’ll play with the numbers if this comes up again in my work — and I will read this post at least once more, too. Thanks for the insight.
November 24, 2020 at 10:51 pm
Great to hear from you again! I’m glad this post was helpful. I think typically you wouldn’t want to raise the significance level higher that 0.05. However, for those who change to a one-sided test and leave the significance level at 0.05, they’re doing that in effect.
Best wishes and Happy Thanksgiving!
November 18, 2020 at 6:19 am
November 8, 2020 at 12:49 am
By “not all the data falls within a particular region”, do you mean that some of the data collected fall in the region and others don’t , BUT the mean of all data in this particular sample do, which is the whole point of hypothesis testing? As to the curve, I think that is the hypothesized sampling distribution of the sample mean, with the sample collected being a member of the overall set. please advise whether this is right, if not , then, there really is something wrong with the understanding and I will go back to the text book : ), otherwise, my question regarding one tailed test remains, thank you so much Jim.
November 8, 2020 at 3:35 pm
I do highly recommend that you read the post I link for you. It’ll help!
There seems a crucial piece that you’re missing. Again, it’s totally understandable because it’s not obvious.
These tests don’t assess where individual data points fall in a distribution.
Instead, these tests assess one estimate of a population parameter and compare it to the null hypothesis value.
Let’s look at that in the context of a 1-sample t-test. In this case, you’re comparing the sample mean (which estimates the population mean) and comparing it to the null hypothesis value. So, it’s just one value (the sample mean), not all the data points. And, you’re looking to see where that value falls in relation to the null hypothesis value. In the graphs, the null hypothesis value is the peak. And, you’re looking to see how far out the mean is. And because the mean is only one value, it’ll fall only at one point on the graphs.
Again, read the other post. It’ll answer your questions. It doesn’t make sense for me retype what I wrote in that post here in the comments. If after reading that post you have more questions, I’ll be happy to answer! 🙂
November 7, 2020 at 12:42 am
Use your illustration “Null: The effect is less than or equal to zero. Alternative: The effect is greater than zero.” Say if the significance level is 0.05, all on the left side, we are saying that 5% of the data are in the region, and if we observe this unlikely event, then it’s unlikely that the hypothesized mean is the true mean, depending how rare you want your criteria to be. If say the alpha level is 1%, where the critical value is even further from the mean, and if the p value is still in there, then we can be even more confident.I hope I am right about the above, but even if I am, I am still not as comfortable with “less than” as with “ not equal to”, even though I can work through the mechanics and get most my practice questions right. Is it ok to say that, at most 1% of data is in there, given the distribution, because any means greater will have a lower percentage, so 0.9%, 0.8%, 0.7% etc as you shift your means to the right with no boundaries, so you can shift infinitely, therefore we can be 99% confident that it is less than, please? Or if not, what’s the logic in words please? Thank you.
November 8, 2020 at 12:15 am
That’s not what the significance level indicates. The significance level doesn’t indicate where the data fall. If you’re performing a one-tailed test and get significant results, it doesn’t mean all the data falls in a particular region of the curve. It means that the sample statistic, such as the mean effect, falls far enough away from zero in a particular direction such that the test statistic falls in the corresponding critical region. The curves you’re seeing in the graphs are not data distributions. They’re sampling distributions for the test statistic, which is an entirely different thing.
I think before trying to understand one-tailed tests, you should read more about how hypothesis tests work in general. Click that link to learn more about how they work, sampling distributions, and what significance levels and p-values actually mean. I can tell you have a few misconceptions about them. That’s ok because they’re tricky concepts. But, it’ll be difficult to understand one-tailed tests without fully understanding how hypothesis tests work.
November 5, 2020 at 9:19 am
I can tell that in a two tailed test, the rejection regions are such that only a certain percentage of data points falls within that range and if you happen to observe a data point within that range, then it’s ok to conclude that the hypothesized mean is unlikely the true mean. However, if I shift all the rejection region to one side, knowing how unlikely I will find something in there, and then somehow observe a data within the range, how does it lead to a conclusion that the true mean is greater or smaller than the hypothesized value please? How can I draw any conclusion from this observation ? If the true mean is to either the left or right of the hypothesized value, it will have its own distribution , rendering the existing distribution irrelevant for drawing conclusion about a different mean?
November 6, 2020 at 9:23 pm
To be technically correct, you’re not looking for data points to fall in the critical regions. Instead, you’re looking for sample statistics that fall in those regions. You don’t need to worry about the distribution changing based on whether the mean is greater than or less than. It all works on the same distribution, which is a sampling distribution for the test statistic.
Read my post about one-tailed and two-tailed tests . It’ll show you how they work and I believe will answer your questions. I show the distributions for both types. If you do have more questions after reading that, don’t hesitate to ask!
May 10, 2020 at 5:32 am
Nice article, thank you Mr. Frost. I am a statistician and I run in this problem regularly and I am still not clear with it. With “Both directions but you care about only one direction” I use the approach that I do 2-tail test on 5 % sig. level and if this is significant and my client is interested only in one direction, then I interpret that the one-sided effect is significant at 5 % level. Which may look weird, but it is a correct statement. Basically, I avoid stating that one sided effect is significant at 5 % level in the situation where the 2-sided p-value is e.g. 0.07 and 1-sided is 0.035. This I don’t interpret as significant on 5 % even if my client is interested only in one direction.
December 26, 2018 at 1:20 am
Ye ! This Is A Good Blog!
November 12, 2018 at 10:00 am
Its a good article Mr. Jim. It gives clarication to the one tailed and two tailed tests that we commonly use in research.
November 12, 2018 at 11:00 am
Thanks, Sreekumar! I’m glad it was helpful!
Comments and Questions Cancel reply
MA121: Introduction to Statistics
Setting Up Hypotheses
One- and two-tailed tests, learning objectives.
- Define Type I and Type II errors
- Interpret significant and non-significant differences
- Explain why the null hypothesis should not be accepted when the effect is not significant
In the James Bond case study, Mr. Bond was given 16 trials on which he judged whether a martini had been shaken or stirred. He was correct on 13 of the trials. From the binomial distribution , we know that the probability of being correct 13 or more times out of 16 if one is only guessing is 0.0106. Figure 1 shows a graph of the binomial distribution. The red bars show the values greater than or equal to 13. As you can see in the figure, the probabilities are calculated for the upper tail of the distribution. A probability calculated in only one tail of the distribution is called a " one-tailed probability ".

Figure 1. The binomial distribution. The upper (right-hand) tail is red.

Figure 2. The binomial distribution. Both tails are red.
Should the one-tailed or the two-tailed probability be used to assess Mr. Bond's performance? That depends on the way the question is posed. If we are asking whether Mr. Bond can tell the difference between shaken or stirred martinis, then we would conclude he could if he performed either much better than chance or much worse than chance. If he performed much worse than chance, we would conclude that he can tell the difference, but he does not know which is which. Therefore, since we are going to reject the null hypothesis if Mr. Bond does either very well or very poorly, we will use a two-tailed probability.
On the other hand, if our question is whether Mr. Bond is better than chance at determining whether a martini is shaken or stirred, we would use a one-tailed probability. What would the one-tailed probability be if Mr. Bond were correct on only 3 of the 16 trials? Since the one-tailed probability is the probability of the right-hand tail, it would be the probability of getting 3 or more correct out of 16. This is a very high probability and the null hypothesis would not be rejected.
You should always decide whether you are going to use a one-tailed or a two-tailed probability before looking at the data. Statistical tests that compute one-tailed probabilities are called one-tailed tests ; those that compute two-tailed probabilities are called two-tailed tests . Two-tailed tests are much more common than one-tailed tests in scientific research because an outcome signifying that something other than chance is operating is usually worth noting. One-tailed tests are appropriate when it is not important to distinguish between no effect and an effect in the unexpected direction. For example, consider an experiment designed to test the efficacy of a treatment for the common cold. The researcher would only be interested in whether the treatment was better than a placebo control. It would not be worth distinguishing between the case in which the treatment was worse than a placebo and the case in which it was the same because in both cases the drug would be worthless.
Some have argued that a one-tailed test is justified whenever the researcher predicts the direction of an effect. The problem with this argument is that if the effect comes out strongly in the non-predicted direction, the researcher is not justified in concluding that the effect is not zero. Since this is unrealistic, one-tailed tests are usually viewed skeptically if justified on this basis alone.
One Tailed Test or Two in Hypothesis Testing; One Tailed Distribution Area
Contents (Click to slip to that section):
- Alpha levels
- When should you use either test?
- One tailed distribution (how to find the area)
One tailed test or two in Hypothesis Testing: Overview
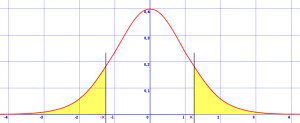
In hypothesis testing , you are asked to decide if a claim is true or not. For example, if someone says “all Floridian’s have a 50% increased chance of melanoma”, it’s up to you to decide if this claim holds merit. One of the first steps is to look up a z-score , and in order to do that , you need to know if it’s a one tailed test or two . You can figure this out in just a couple of steps. Back to top
One tailed test or two in Hypothesis Testing: Steps
If you’re lucky enough to be given a picture, you’ll be able to tell if your test is one-tailed or two-tailed by comparing it to the image above. However, most of the time you’re given questions, not pictures. So it’s a matter of deciphering the problem and picking out the important piece of information. You’re basically looking for keywords like equals , more than , or less than .
Example question #1: A government official claims that the dropout rate for local schools is 25% . Last year, 190 out of 603 students dropped out. Is there enough evidence to reject the government official’s claim?
Example question #2: A government official claims that the dropout rate for local schools is less than 25%. Last year, 190 out of 603 students dropped out. Is there enough evidence to reject the government official’s claim?
Example question #3: A government official claims that the dropout rate for local schools is greater than 25%. Last year, 190 out of 603 students dropped out. Is there enough evidence to reject the government official’s claim?
Step 1: Read the question.
Step 2: Rephrase the claim in the question with an equation.
- In example question #1, Drop out rate = 25%
- In example question #2, Drop out rate < 25%
- In example question #3, Drop out rate > 25%.
Step 3: If step 2 has an equals sign in it, this is a two-tailed test. If it has > or < it is a one-tailed test.
Like the explanation? Check out the Statistics How To Handbook , which has hundreds of easy to understand definitions and examples, just like this one!
Back to top
One Tailed Test or Two: Onto some more technical stuff
The above should have given you a brief overview of the differences between one-tailed tests and two-tailed tests. For the very beginning of your stats class, that’s probably all the information you need to get by. But once you hit ANOVA and regression analysis , things get a little more challenging.
1. Alpha levels
Alpha levels (sometimes just called “significance levels”) are used in hypothesis tests ; it is the probability of making the wrong decision when the null hypothesis is true. A one-tailed test has the entire 5% of the alpha level in one tail (in either the left, or the right tail). A two-tailed test splits your alpha level in half (as in the image to the left).
Let’s say you’re working with the standard alpha level of 0.5 (5%). A two tailed test will have half of this (2.5%) in each tail. Very simply, the hypothesis test might go like this:
- A null hypothesis might state that the mean = x . You’re testing if the mean is way above this or way below.
- You run a t-test , which churns out a t-statistic .
- If this test statistic falls in the top 2.5% or bottom 2.5% of its probability distribution (in this case, the t-distribution ), you would reject the null hypothesis .
The “cut off” areas created by your alpha levels are called rejection regions . It’s where you would reject the null hypothesis, if your test statistic happens to fall into one of those rejection areas. The terms “one tailed” and “two tailed” can more precisely be defined as referring to where your rejection regions are located. Back to top
A one-tailed test is where you are only interested in one direction. If a mean is x, you might want to know if a set of results is more than x or less than x. A one-tailed test is more powerful than a two-tailed test, as you aren’t considering an effect in the opposite direction.
Next : Left tailed test or right tailed test? Back to top

3. When Should You Use a One-Tailed Test?
In the above examples, you were given specific wording like “greater than” or “less than.” Sometimes you, the researcher, do not have this information and you have to choose the test.
For example, you develop a drug which you think is just as effective as a drug already on the market (it also happens to be cheaper). You could run a two-tailed test (to test that it is more effective and to also check that it is less effective). But you don’t really care about it being more effective, just that it isn’t any less effective (after all, your drug is cheaper). You can run a one-tailed test to check that your drug is at least as effective as the existing drug.
On the other hand, it would be inappropriate (and perhaps, unethical) to run a one-tailed test for this scenario in the opposite direction (i.e. to show the drug is more effective). This sounds reasonable until you consider there may be certain circumstances where the drug is less effective. If you fail to test for that, your research will be useless.
Consider both directions when deciding if you should run a one tailed test or two. If you can skip one tail and it’s not irresponsible or unethical to do so, then you can run a one-tailed test. Back to top
One tailed Test or Two: How to find the area of a one-tailed distribution: Steps
There are a few ways to find the area under a one tailed distribution curve. The easiest, by far, is looking up the value in a table like the z-table . A z-table gives you percentages, which represent the area under a curve . For example, a table value of 0.5000 is 50% of the area and 0.2000 is 20% of the area.
If you are looking for other area problems*, see the normal distribution curve index . The index lists seven possible types of area, including two tailed, one tailed, and areas to the left and right of z.
*You can also calculate areas with integral calculus . See The Area Problem .
Note : In order to use a z-table , you need to split your z-value up into decimal places (e.g. tenths and hundredths). For example, if you are asked to find the area in a one tailed distribution with a z-value of 0.21, split this into tenths (0.2) and hundredths (0.01).
One tailed distribution: Steps for finding the area in a z-table
Step 1: Look up your z-score in the z-table . Looking up the value means finding the intersection of your two decimals (see note above). For example, if you are asked to find the area in a one tailed distribution to the left of z = -0.46, look up 0.46 in the table (note: ignore negative values. If you have a negative value, use its absolute value ). The table below shows that the value in the intersection for 0.46 is .1772. This figure was obtained by looking up 0.4 in the left hand column and 0.06 in the top row.
z | 0.00 | 0.01 | 0.02 | 0.03 | 0.04 | 0.05 | 0.06 | 0.07 | 0.08 | 0.09 |
---|---|---|---|---|---|---|---|---|---|---|
0.0 | 0.0000 | 0.0040 | 0.0080 | 0.0120 | 0.0160 | 0.0199 | 0.0239 | 0.0279 | 0.0319 | 0.0359 |
0.1 | 0.0398 | 0.0438 | 0.0478 | 0.0517 | 0.0557 | 0.0596 | 0.0636 | 0.0675 | 0.0714 | 0.0753 |
0.2 | 0.0793 | 0.0832 | 0.0871 | 0.0910 | 0.0948 | 0.0987 | 0.1026 | 0.1064 | 0.1103 | 0.1141 |
0.3 | 0.1179 | 0.1217 | 0.1255 | 0.1293 | 0.1331 | 0.1368 | 0.1406 | 0.1443 | 0.1480 | 0.1517 |
0.4 | 0.1554 | 0.1591 | 0.1628 | 0.1664 | 0.1700 | 0.1736 | 0.1772 | 0.1808 | 0.1844 | 0.1879 |
0.5 | 0.1915 | 0.1950 | 0.1985 | 0.2019 | 0.2054 | 0.2088 | 0.2123 | 0.2157 | 0.2190 | 0.2224 |
Step 2: Take the area you just found in step 2 and add .500. That’s because the area in the right-hand z-table is the area between the mean and the z-score. You want the entire area up to that point, so: .5000 + .1772 = .6772.
Step 3: Subtract from 1 to get the tail area: 1 – .6772 = 0.3228.
That’s it!
One Tailed Test or Two: References
Gonick, L. (1993). The Cartoon Guide to Statistics . HarperPerennial. Heath, D. (2002). An Introduction to Experimental Design and Statistics for Biology. CRC Press. IDRE: FAQ: What are the differences between one-tailed and two-tailed tests? Retrieved May 27, 2018 from: https://stats.idre.ucla.edu/other/mult-pkg/faq/general/faq-what-are-the-differences-between-one-tailed-and-two-tailed-tests/
- The Open University
- Accessibility hub
- Guest user / Sign out
- Study with The Open University
My OpenLearn Profile
Personalise your OpenLearn profile, save your favourite content and get recognition for your learning
About this free course
Become an ou student, download this course, share this free course.
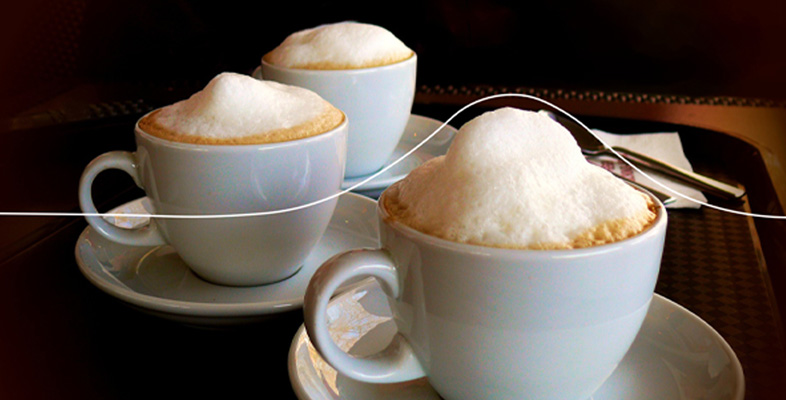
Start this free course now. Just create an account and sign in. Enrol and complete the course for a free statement of participation or digital badge if available.
4 One-tailed vs two-tailed test
To gain a deeper understanding of how to conduct a hypothesis test, this section will delve into the concepts of one-tailed and two-tailed tests. These tests are vital tools in statistical hypothesis testing, and the decision of which test to employ depends on the research question and hypothesis under examination. It is crucial to give careful thought to the suitable type of test to ensure that the hypothesis is thoroughly tested and precise conclusions are derived from the data. This section will elaborate on this topic in greater detail.
To commence, complete the following activity pertaining to the formulation of null and alternative hypotheses. This exercise may be somewhat challenging, but it serves as an excellent introduction to upcoming discussions – don’t be concerned if you find it difficult!
Activity 3 Hypotheses setting
Read the following statements and then develop a null hypothesis and an alternative hypothesis.
‘It is believed that OU students need to set aside no longer than, on average, 15 hours to study an entire session of an OU course. However, a researcher believes that OU students spend longer studying an entire session of an OU course.’
H 0 : OU students spend, on average, no more than 15 hours studying an entire session of OU course.
H a : OU students spend, on average, more than 15 hours studying an entire session of OU course.
They can also be written as:
H 0 : µ ≤ 15 hours studies
H a : µ > 15 hours studies
µ is a symbol for a population mean. Remember, H 0 and H a are always opposites.
Did you identify any differences between the hypotheses you developed in Activity 1 and Activity 3? The set of hypotheses in Activity 1 has an equal (=) or not equal (≠) supposition (sign) in the statement. However, in Activity 3, the set of hypotheses has less than or equal to (≤) and greater than (>) supposition (sign) in the statement. This creates different conditions that lead to acceptance or rejection of the null hypothesis.

Module 7 - Comparing Continuous Outcomes
- Page:
- 1
- | 2
- | 3
- | 4
- | 5
- | 6
- | 7
- | 8
- | 9

One Sample t-test
One-tailed and two-tailed tests of significance, a one-tailed test of hypothesis, a two-tailed test of hypothesis, one sample t-test using r.

Investigators wanted to determine whether children who were born very prematurely have poorer cognitive ability than children born at full term. To investigate they enrolled 100 children who had been born prematurely and measured their IQ in order to compare the sample mean to a normative IQ of 100. In essence, the normative IQ is a historical or external (μ 0 ). In the sample of 100 children who had been born prematurely the data for IQ were as follows: X̄ = 95.8, SD = 17.5" . The investigators wish to use a one-tailed test of significance.
A research hypothesis that states that two groups differ without specifying direction, i.e., which is greater, is a two-tailed hypothes is . In contrast, a research hypothesis that specifies direction, e.g., that the mean in a sample group will be less than the mean in a historic comparison group is a one-tailed hypothesis . Similarly, a hypothesis that a mean in a sample group will be greater than the mean in a historic comparison group is also a one-tailed hypothesis .
Suppose we conduct a test of significance to compare two groups (call them A and B) using large samples. The test statistic could be either a t score or a Z score, depending on the test we choose, but if the sample is very large the t or Z scores will be similar. Suppose also that we have specified an "alpha" level of 0.05, i.e., an error rate of 5% for concluding that the groups differ when they really don't. In other words, we will use p≤0.05 as the criterion for statistical significance.
Two-tailed test: The first figure below shows that with a two-tailed test in which we acknowledge that one group's mean could be either above or below the other, the alpha error rate has to be split into the upper and lower tails, i.e., with half of our alpha (0.025) in each tail. Therefore, we need to achieve a test statistic that is either less than -1.96 or greater than +1.96.
One-tailed test (lower tail): In the middle figure the hypothesis is that group A has a mean less than group B, perhaps because it is unreasonable to think the mean IQ in group A would be greater than that in group B. If so, all of the 5% alpha is in the lower tail, and we only need to achieve a test statistic less than 1.645 to achieve "statistical significance."
One-tailed test (upper tail): The third image shows a one-tailed test in which the hypothesis is that group A has a mean value greater than that of group B, so all of the alpha is in the upper tail, meaning that we need a test statistic greater than +1.645 to achieve statistical significance.
Clearly, the two-tailed test is more conservative because, regardless of direction, the test statistic has to be more than 1.96 units away from the null. The vast majority of tests that are reported are two-tailed tests. However, there are occasionally situations in which a one-tailed test hypothesis can be justified.
A one-tailed test could be justified in the study examining whether children who had been born very prematurely have lower IQ scores than children who had a normal, full term gestation, since there is no reason to believe that those born prematurely would have higher IQs.
First, we set up the hypotheses: Null hypothesis : H 0 : μ prem = 100 (i.e., that there is no difference or association) Children born prematurely have mean IQs that are not different from those of the general population (μ=100)
Alternative hypothesis (i.e., the research hypothesis): H 0 :μ prem < 100) Children born prematurely have lower mean IQ than the general population (μ<100).
The t statistic= -2.4. We can look up the corresponding p-value with df=99, or we can use R to compute the probability.
> pt(-2.4,99) [1] 0.009132283
Since p=0.009, we reject the null hypothesis and accept the alternative hypothesis. We conclude that children born prematurely have lower mean IQ scores than children in the general population who had a full term gestation.
What if we had performed a two-tailed test of hypothesis on the same data?
The null hypothesis would be the same, but the alternative hypothesis would be:
Alternative hypothesis (research hypothesis: H A : μ prem ≠100 Children born prematurely have IQ scores that are different (either lower or higher) from children from the general population who had full term gestation.
The calculation of the t statistic would also be the same, but the p-value will be different.
> 2*pt(-2.4,99) [1] 0.01826457
The probability is the area under the standard normal distribution that is either lower than -2.40 or greater than +2.40. So the probability is 0.009+0.009 = 0.018, i.e., a probability of 0.009 in both the lower and upper tail. We still conclude that children born prematurely have a significantly lower mean IQ than the general population (mean=95.8, s=17.5, p=0.018).
When performing two-tailed tests, direction is not specified in the hypothesis, but one should report the direction in any report, publication, or presentation, e.g., "Children who had been born prematurely had lower mean IQ scores than in the general population."
The t-statistic was developed and published in 1908 by William Gosset, a chemist/statistician working for the Guinness brewery in Dublin. Guinness employees were not allowed to publish under their own name, so Gosset published under the pseudonym "Student". |
In the example for IQ tests above we were given the mean IQ and standard deviation for the children who had been born prematurely. However, suppose we were given an Excel spreadsheet with the raw data listed in a column for "iq"?
In Excel we could save this data as a .CSV file using the "Save as" function. We could then import the data in the .CSV file into R and analyze the data as follows:
[Note that R defaults to performing the more conservative two-tailed test unless a one-tailed test is specified as we will describe below.]
> t.test(iq,mu=100)
One Sample t-test data: iq t = -2.3801, df=99, p-value = 0.01922 alternative hypothesis: true mean is not equal to 100 95 percent confidence interval: 92.35365 99.30635 Sample estimates mean of x 95.83
In order to perform a one-tailed test, you need to specify the alternative hypothesis. For example:
> t.test(iq,mu=100, alternative="less")
> t.test(iq,mu=100, alternative="greater")
return to top | previous page | next page
Content ©2021. Some Rights Reserved. Date last modified: October 13, 2021. Wayne W. LaMorte, MD, PhD, MPH

Research Hypothesis In Psychology: Types, & Examples
Saul McLeod, PhD
Editor-in-Chief for Simply Psychology
BSc (Hons) Psychology, MRes, PhD, University of Manchester
Saul McLeod, PhD., is a qualified psychology teacher with over 18 years of experience in further and higher education. He has been published in peer-reviewed journals, including the Journal of Clinical Psychology.
Learn about our Editorial Process
Olivia Guy-Evans, MSc
Associate Editor for Simply Psychology
BSc (Hons) Psychology, MSc Psychology of Education
Olivia Guy-Evans is a writer and associate editor for Simply Psychology. She has previously worked in healthcare and educational sectors.
On This Page:
A research hypothesis, in its plural form “hypotheses,” is a specific, testable prediction about the anticipated results of a study, established at its outset. It is a key component of the scientific method .
Hypotheses connect theory to data and guide the research process towards expanding scientific understanding
Some key points about hypotheses:
- A hypothesis expresses an expected pattern or relationship. It connects the variables under investigation.
- It is stated in clear, precise terms before any data collection or analysis occurs. This makes the hypothesis testable.
- A hypothesis must be falsifiable. It should be possible, even if unlikely in practice, to collect data that disconfirms rather than supports the hypothesis.
- Hypotheses guide research. Scientists design studies to explicitly evaluate hypotheses about how nature works.
- For a hypothesis to be valid, it must be testable against empirical evidence. The evidence can then confirm or disprove the testable predictions.
- Hypotheses are informed by background knowledge and observation, but go beyond what is already known to propose an explanation of how or why something occurs.
Predictions typically arise from a thorough knowledge of the research literature, curiosity about real-world problems or implications, and integrating this to advance theory. They build on existing literature while providing new insight.
Types of Research Hypotheses
Alternative hypothesis.
The research hypothesis is often called the alternative or experimental hypothesis in experimental research.
It typically suggests a potential relationship between two key variables: the independent variable, which the researcher manipulates, and the dependent variable, which is measured based on those changes.
The alternative hypothesis states a relationship exists between the two variables being studied (one variable affects the other).
A hypothesis is a testable statement or prediction about the relationship between two or more variables. It is a key component of the scientific method. Some key points about hypotheses:
- Important hypotheses lead to predictions that can be tested empirically. The evidence can then confirm or disprove the testable predictions.
In summary, a hypothesis is a precise, testable statement of what researchers expect to happen in a study and why. Hypotheses connect theory to data and guide the research process towards expanding scientific understanding.
An experimental hypothesis predicts what change(s) will occur in the dependent variable when the independent variable is manipulated.
It states that the results are not due to chance and are significant in supporting the theory being investigated.
The alternative hypothesis can be directional, indicating a specific direction of the effect, or non-directional, suggesting a difference without specifying its nature. It’s what researchers aim to support or demonstrate through their study.
Null Hypothesis
The null hypothesis states no relationship exists between the two variables being studied (one variable does not affect the other). There will be no changes in the dependent variable due to manipulating the independent variable.
It states results are due to chance and are not significant in supporting the idea being investigated.
The null hypothesis, positing no effect or relationship, is a foundational contrast to the research hypothesis in scientific inquiry. It establishes a baseline for statistical testing, promoting objectivity by initiating research from a neutral stance.
Many statistical methods are tailored to test the null hypothesis, determining the likelihood of observed results if no true effect exists.
This dual-hypothesis approach provides clarity, ensuring that research intentions are explicit, and fosters consistency across scientific studies, enhancing the standardization and interpretability of research outcomes.
Nondirectional Hypothesis
A non-directional hypothesis, also known as a two-tailed hypothesis, predicts that there is a difference or relationship between two variables but does not specify the direction of this relationship.
It merely indicates that a change or effect will occur without predicting which group will have higher or lower values.
For example, “There is a difference in performance between Group A and Group B” is a non-directional hypothesis.
Directional Hypothesis
A directional (one-tailed) hypothesis predicts the nature of the effect of the independent variable on the dependent variable. It predicts in which direction the change will take place. (i.e., greater, smaller, less, more)
It specifies whether one variable is greater, lesser, or different from another, rather than just indicating that there’s a difference without specifying its nature.
For example, “Exercise increases weight loss” is a directional hypothesis.
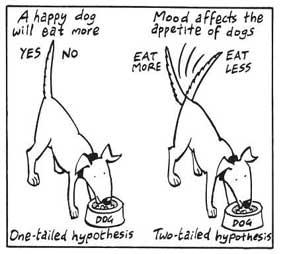
Falsifiability
The Falsification Principle, proposed by Karl Popper , is a way of demarcating science from non-science. It suggests that for a theory or hypothesis to be considered scientific, it must be testable and irrefutable.
Falsifiability emphasizes that scientific claims shouldn’t just be confirmable but should also have the potential to be proven wrong.
It means that there should exist some potential evidence or experiment that could prove the proposition false.
However many confirming instances exist for a theory, it only takes one counter observation to falsify it. For example, the hypothesis that “all swans are white,” can be falsified by observing a black swan.
For Popper, science should attempt to disprove a theory rather than attempt to continually provide evidence to support a research hypothesis.
Can a Hypothesis be Proven?
Hypotheses make probabilistic predictions. They state the expected outcome if a particular relationship exists. However, a study result supporting a hypothesis does not definitively prove it is true.
All studies have limitations. There may be unknown confounding factors or issues that limit the certainty of conclusions. Additional studies may yield different results.
In science, hypotheses can realistically only be supported with some degree of confidence, not proven. The process of science is to incrementally accumulate evidence for and against hypothesized relationships in an ongoing pursuit of better models and explanations that best fit the empirical data. But hypotheses remain open to revision and rejection if that is where the evidence leads.
- Disproving a hypothesis is definitive. Solid disconfirmatory evidence will falsify a hypothesis and require altering or discarding it based on the evidence.
- However, confirming evidence is always open to revision. Other explanations may account for the same results, and additional or contradictory evidence may emerge over time.
We can never 100% prove the alternative hypothesis. Instead, we see if we can disprove, or reject the null hypothesis.
If we reject the null hypothesis, this doesn’t mean that our alternative hypothesis is correct but does support the alternative/experimental hypothesis.
Upon analysis of the results, an alternative hypothesis can be rejected or supported, but it can never be proven to be correct. We must avoid any reference to results proving a theory as this implies 100% certainty, and there is always a chance that evidence may exist which could refute a theory.
How to Write a Hypothesis
- Identify variables . The researcher manipulates the independent variable and the dependent variable is the measured outcome.
- Operationalized the variables being investigated . Operationalization of a hypothesis refers to the process of making the variables physically measurable or testable, e.g. if you are about to study aggression, you might count the number of punches given by participants.
- Decide on a direction for your prediction . If there is evidence in the literature to support a specific effect of the independent variable on the dependent variable, write a directional (one-tailed) hypothesis. If there are limited or ambiguous findings in the literature regarding the effect of the independent variable on the dependent variable, write a non-directional (two-tailed) hypothesis.
- Make it Testable : Ensure your hypothesis can be tested through experimentation or observation. It should be possible to prove it false (principle of falsifiability).
- Clear & concise language . A strong hypothesis is concise (typically one to two sentences long), and formulated using clear and straightforward language, ensuring it’s easily understood and testable.
Consider a hypothesis many teachers might subscribe to: students work better on Monday morning than on Friday afternoon (IV=Day, DV= Standard of work).
Now, if we decide to study this by giving the same group of students a lesson on a Monday morning and a Friday afternoon and then measuring their immediate recall of the material covered in each session, we would end up with the following:
- The alternative hypothesis states that students will recall significantly more information on a Monday morning than on a Friday afternoon.
- The null hypothesis states that there will be no significant difference in the amount recalled on a Monday morning compared to a Friday afternoon. Any difference will be due to chance or confounding factors.
More Examples
- Memory : Participants exposed to classical music during study sessions will recall more items from a list than those who studied in silence.
- Social Psychology : Individuals who frequently engage in social media use will report higher levels of perceived social isolation compared to those who use it infrequently.
- Developmental Psychology : Children who engage in regular imaginative play have better problem-solving skills than those who don’t.
- Clinical Psychology : Cognitive-behavioral therapy will be more effective in reducing symptoms of anxiety over a 6-month period compared to traditional talk therapy.
- Cognitive Psychology : Individuals who multitask between various electronic devices will have shorter attention spans on focused tasks than those who single-task.
- Health Psychology : Patients who practice mindfulness meditation will experience lower levels of chronic pain compared to those who don’t meditate.
- Organizational Psychology : Employees in open-plan offices will report higher levels of stress than those in private offices.
- Behavioral Psychology : Rats rewarded with food after pressing a lever will press it more frequently than rats who receive no reward.

- Skip to primary navigation
- Skip to main content
- Skip to primary sidebar
Statistical Methods and Data Analytics
FAQ: What are the differences between one-tailed and two-tailed tests?
When you conduct a test of statistical significance, whether it is from a correlation, an ANOVA, a regression or some other kind of test, you are given a p-value somewhere in the output. If your test statistic is symmetrically distributed, you can select one of three alternative hypotheses. Two of these correspond to one-tailed tests and one corresponds to a two-tailed test. However, the p-value presented is (almost always) for a two-tailed test. But how do you choose which test? Is the p-value appropriate for your test? And, if it is not, how can you calculate the correct p-value for your test given the p-value in your output?
What is a two-tailed test?
First let’s start with the meaning of a two-tailed test. If you are using a significance level of 0.05, a two-tailed test allots half of your alpha to testing the statistical significance in one direction and half of your alpha to testing statistical significance in the other direction. This means that .025 is in each tail of the distribution of your test statistic. When using a two-tailed test, regardless of the direction of the relationship you hypothesize, you are testing for the possibility of the relationship in both directions. For example, we may wish to compare the mean of a sample to a given value x using a t-test. Our null hypothesis is that the mean is equal to x . A two-tailed test will test both if the mean is significantly greater than x and if the mean significantly less than x . The mean is considered significantly different from x if the test statistic is in the top 2.5% or bottom 2.5% of its probability distribution, resulting in a p-value less than 0.05.
What is a one-tailed test?
Next, let’s discuss the meaning of a one-tailed test. If you are using a significance level of .05, a one-tailed test allots all of your alpha to testing the statistical significance in the one direction of interest. This means that .05 is in one tail of the distribution of your test statistic. When using a one-tailed test, you are testing for the possibility of the relationship in one direction and completely disregarding the possibility of a relationship in the other direction. Let’s return to our example comparing the mean of a sample to a given value x using a t-test. Our null hypothesis is that the mean is equal to x . A one-tailed test will test either if the mean is significantly greater than x or if the mean is significantly less than x , but not both. Then, depending on the chosen tail, the mean is significantly greater than or less than x if the test statistic is in the top 5% of its probability distribution or bottom 5% of its probability distribution, resulting in a p-value less than 0.05. The one-tailed test provides more power to detect an effect in one direction by not testing the effect in the other direction. A discussion of when this is an appropriate option follows.
When is a one-tailed test appropriate?
Because the one-tailed test provides more power to detect an effect, you may be tempted to use a one-tailed test whenever you have a hypothesis about the direction of an effect. Before doing so, consider the consequences of missing an effect in the other direction. Imagine you have developed a new drug that you believe is an improvement over an existing drug. You wish to maximize your ability to detect the improvement, so you opt for a one-tailed test. In doing so, you fail to test for the possibility that the new drug is less effective than the existing drug. The consequences in this example are extreme, but they illustrate a danger of inappropriate use of a one-tailed test.
So when is a one-tailed test appropriate? If you consider the consequences of missing an effect in the untested direction and conclude that they are negligible and in no way irresponsible or unethical, then you can proceed with a one-tailed test. For example, imagine again that you have developed a new drug. It is cheaper than the existing drug and, you believe, no less effective. In testing this drug, you are only interested in testing if it less effective than the existing drug. You do not care if it is significantly more effective. You only wish to show that it is not less effective. In this scenario, a one-tailed test would be appropriate.
When is a one-tailed test NOT appropriate?
Choosing a one-tailed test for the sole purpose of attaining significance is not appropriate. Choosing a one-tailed test after running a two-tailed test that failed to reject the null hypothesis is not appropriate, no matter how "close" to significant the two-tailed test was. Using statistical tests inappropriately can lead to invalid results that are not replicable and highly questionable–a steep price to pay for a significance star in your results table!
Deriving a one-tailed test from two-tailed output
The default among statistical packages performing tests is to report two-tailed p-values. Because the most commonly used test statistic distributions (standard normal, Student’s t) are symmetric about zero, most one-tailed p-values can be derived from the two-tailed p-values.
Below, we have the output from a two-sample t-test in Stata. The test is comparing the mean male score to the mean female score. The null hypothesis is that the difference in means is zero. The two-sided alternative is that the difference in means is not zero. There are two one-sided alternatives that one could opt to test instead: that the male score is higher than the female score (diff > 0) or that the female score is higher than the male score (diff < 0). In this instance, Stata presents results for all three alternatives. Under the headings Ha: diff < 0 and Ha: diff > 0 are the results for the one-tailed tests. In the middle, under the heading Ha: diff != 0 (which means that the difference is not equal to 0), are the results for the two-tailed test.
Two-sample t test with equal variances ------------------------------------------------------------------------------ Group | Obs Mean Std. Err. Std. Dev. [95% Conf. Interval] ---------+-------------------------------------------------------------------- male | 91 50.12088 1.080274 10.30516 47.97473 52.26703 female | 109 54.99083 .7790686 8.133715 53.44658 56.53507 ---------+-------------------------------------------------------------------- combined | 200 52.775 .6702372 9.478586 51.45332 54.09668 ---------+-------------------------------------------------------------------- diff | -4.869947 1.304191 -7.441835 -2.298059 ------------------------------------------------------------------------------ Degrees of freedom: 198 Ho: mean(male) - mean(female) = diff = 0 Ha: diff < 0 Ha: diff != 0 Ha: diff > 0 t = -3.7341 t = -3.7341 t = -3.7341 P < t = 0.0001 P > |t| = 0.0002 P > t = 0.9999
Note that the test statistic, -3.7341, is the same for all of these tests. The two-tailed p-value is P > |t|. This can be rewritten as P(>3.7341) + P(< -3.7341). Because the t-distribution is symmetric about zero, these two probabilities are equal: P > |t| = 2 * P(< -3.7341). Thus, we can see that the two-tailed p-value is twice the one-tailed p-value for the alternative hypothesis that (diff < 0). The other one-tailed alternative hypothesis has a p-value of P(>-3.7341) = 1-(P<-3.7341) = 1-0.0001 = 0.9999. So, depending on the direction of the one-tailed hypothesis, its p-value is either 0.5*(two-tailed p-value) or 1-0.5*(two-tailed p-value) if the test statistic symmetrically distributed about zero.
In this example, the two-tailed p-value suggests rejecting the null hypothesis of no difference. Had we opted for the one-tailed test of (diff > 0), we would fail to reject the null because of our choice of tails.
The output below is from a regression analysis in Stata. Unlike the example above, only the two-sided p-values are presented in this output.
Source | SS df MS Number of obs = 200 -------------+------------------------------ F( 2, 197) = 46.58 Model | 7363.62077 2 3681.81039 Prob > F = 0.0000 Residual | 15572.5742 197 79.0486001 R-squared = 0.3210 -------------+------------------------------ Adj R-squared = 0.3142 Total | 22936.195 199 115.257261 Root MSE = 8.8909 ------------------------------------------------------------------------------ socst | Coef. Std. Err. t P>|t| [95% Conf. Interval] -------------+---------------------------------------------------------------- science | .2191144 .0820323 2.67 0.008 .0573403 .3808885 math | .4778911 .0866945 5.51 0.000 .3069228 .6488594 _cons | 15.88534 3.850786 4.13 0.000 8.291287 23.47939 ------------------------------------------------------------------------------
For each regression coefficient, the tested null hypothesis is that the coefficient is equal to zero. Thus, the one-tailed alternatives are that the coefficient is greater than zero and that the coefficient is less than zero. To get the p-value for the one-tailed test of the variable science having a coefficient greater than zero, you would divide the .008 by 2, yielding .004 because the effect is going in the predicted direction. This is P(>2.67). If you had made your prediction in the other direction (the opposite direction of the model effect), the p-value would have been 1 – .004 = .996. This is P(<2.67). For all three p-values, the test statistic is 2.67.
Your Name (required)
Your Email (must be a valid email for us to receive the report!)
Comment/Error Report (required)
How to cite this page
- © 2024 UC REGENTS

One-Tailed Hypothesis
Ai generator.
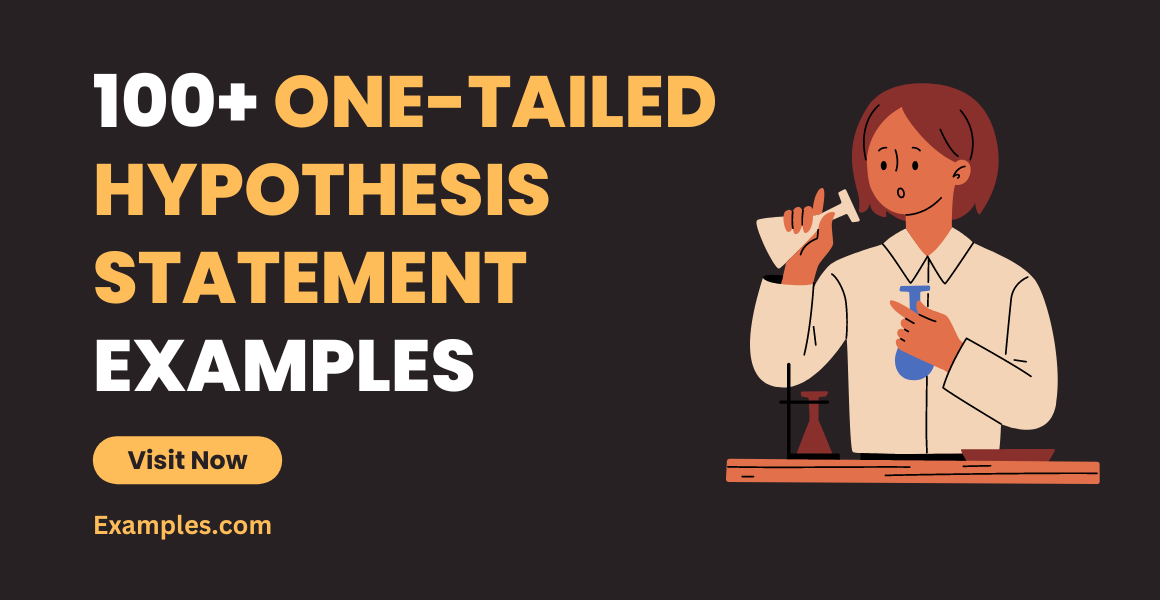
The one-tailed hypothesis, a specific avenue in scientific inquiry, allows researchers to predict the direction of an outcome with precision. By focusing on one end of a spectrum, this approach can sharpen the lens of investigation, making results more interpretable. Whether you’re new to the concept or seeking a refresher, this guide will walk you through thesis statement examples, crafting methods, and indispensable tips to enhance your research journey. Dive in!
What is One Tailed Hypothesis?
A one-tailed hypothesis, also known as a directional hypothesis , predicts the specific direction of the relationship between variables. It suggests that one variable will be greater than, less than, or different from another variable, but not both. In contrast to a two-tailed hypothesis, which tests for a relationship in both directions, a one-tailed hypothesis tests for a relationship in a single direction.
In statistical terms, a one-tailed test looks for the possibility of the relationship in one direction of interest, and it will reject the null hypothesis only if the result is in that specified direction. This is used when researchers have a specific prediction about the direction of their results.
What is an example of a Simple hypothesis statement?
A simple hypothesis statement presents a suggested relationship between two variables – one being the cause and the other the effect. Here’s an example:
Increased physical activity leads to a decrease in body weight.
In this simple hypothesis:
- Independent Variable (cause) : Increased physical activity
- Dependent Variable (effect) : Decrease in body weight
100 One Tailed Hypothesis Statement Examples
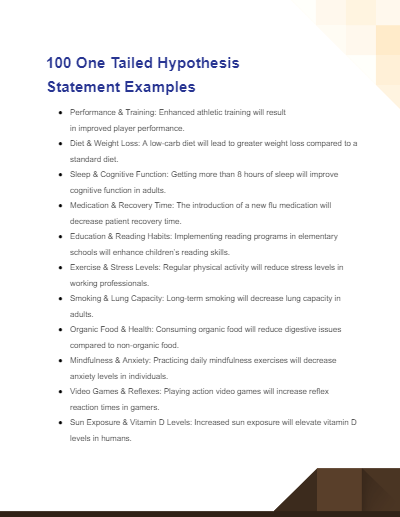
Size: 205 KB
Delve into the intriguing world of one-tailed hypotheses, where researchers anticipate outcomes in a distinct direction. By zooming into one side of the possibility spectrum, these statements allow a sharp focus on anticipated results. From the myriad domains of science to everyday observations, one-tailed hypotheses inform and inspire. Discover this refined facet of research through illustrative examples. You should also take a look at our pyschology hypothesis .
- Performance & Training : Enhanced athletic training will result in improved player performance.
- Diet & Weight Loss : A low-carb diet will lead to greater weight loss compared to a standard diet.
- Sleep & Cognitive Function : Getting more than 8 hours of sleep will improve cognitive function in adults.
- Medication & Recovery Time : The introduction of a new flu medication will decrease patient recovery time.
- Education & Reading Habits : Implementing reading programs in elementary schools will enhance children’s reading skills.
- Exercise & Stress Levels : Regular physical activity will reduce stress levels in working professionals.
- Smoking & Lung Capacity : Long-term smoking will decrease lung capacity in adults.
- Organic Food & Health : Consuming organic food will reduce digestive issues compared to non-organic food.
- Mindfulness & Anxiety : Practicing daily mindfulness exercises will decrease anxiety levels in individuals.
- Video Games & Reflexes : Playing action video games will increase reflex reaction times in gamers.
- Sun Exposure & Vitamin D Levels : Increased sun exposure will elevate vitamin D levels in humans.
- Alcohol Consumption & Reaction Time : Higher alcohol intake will lead to slower reaction times.
- Plant Growth & Light Color : Blue light will promote faster plant growth compared to green light.
- Music & Concentration : Listening to classical music will enhance concentration during study sessions.
- Sugar Intake & Energy Levels : High sugar consumption will lead to subsequent decreased energy levels.
- Online Classes & Student Engagement : Physical classroom sessions will yield higher student engagement than online classes.
- Pet Ownership & Happiness Levels : Owning a pet will increase happiness levels in elderly individuals.
- Yoga & Flexibility : Regular yoga practice will increase flexibility in participants.
- Mountain Altitude & Breathing Difficulties : Residing at higher altitudes will lead to more frequent breathing difficulties.
- Artificial Sweeteners & Appetite : Consuming artificial sweeteners will increase appetite in individuals.
- Protein Intake & Muscle Growth : Consuming higher protein amounts post-exercise will foster greater muscle development.
- Social Media Usage & Loneliness : Increased hours on social media will elevate feelings of loneliness in teenagers.
- Watering Frequency & Plant Height : Watering plants twice a day will result in taller plant growth.
- Chocolate Consumption & Mood : Eating dark chocolate will improve mood in individuals.
- Homework & Academic Achievement : Assigning more homework will boost academic scores in middle school students.
- Coffee Intake & Alertness : Drinking coffee will increase alertness in sleepy individuals.
- Vitamin C & Cold Duration : Consuming vitamin C will shorten the duration of common colds.
- Noise Levels & Concentration : Higher noise levels will decrease concentration in students studying.
- Fish Oil & Heart Health : Daily intake of fish oil supplements will enhance heart health in adults.
- Air Pollution & Respiratory Issues : Living in areas with high air pollution will increase respiratory problems in inhabitants.
- Outdoor Activities & Vision : Spending more time outdoors will reduce the likelihood of developing myopia in children.
- Meditation & Blood Pressure : Regular meditation will lower blood pressure in hypertensive patients.
- Reading Print vs Digital : Reading printed books will lead to better content retention compared to digital versions.
- Exposure to Nature & Mental Well-being : Spending time in natural settings will boost mental well-being.
- E-books & Eye Strain : Prolonged reading on digital devices will increase eye strain.
- Milk Consumption & Bone Density : Higher milk consumption during childhood will result in increased bone density in adulthood.
- Bilingualism & Cognitive Flexibility : Being bilingual will enhance cognitive flexibility in individuals.
- Fast Food Intake & Cholesterol Levels : Consuming fast food more than thrice a week will raise cholesterol levels.
- Aerobic Exercise & Endurance : Engaging in aerobic exercises will heighten endurance levels in athletes.
- Mentoring & Career Advancement : Having a mentor will accelerate career advancement in professionals.
- Spicy Food & Metabolism : Consuming spicy food will boost metabolism rates in individuals.
- Group Study & Learning : Studying in groups will enhance understanding and retention of academic content.
- Gaming & Hand-Eye Coordination : Engaging in video gaming will improve hand-eye coordination.
- Soft Drink Consumption & Tooth Decay : Frequent consumption of soft drinks will increase the risk of tooth decay.
- Public Speaking Training & Confidence : Undergoing public speaking training will boost confidence levels in individuals.
- Vegetarian Diet & Energy Levels : Adopting a vegetarian diet will increase energy levels.
- Music Lessons & Mathematical Ability : Taking music lessons will enhance mathematical abilities in children.
- Dairy Intake & Skin Health : Consuming dairy frequently will lead to more skin issues like acne.
- Weight Training & Bone Density : Regular weight training will increase bone density in women.
- Puzzles & Cognitive Sharpness : Engaging in puzzles will enhance cognitive sharpness in the elderly.
- Green Tea & Metabolism : Regular consumption of green tea will elevate metabolic rates.
- Ergonomic Chairs & Back Pain : Using ergonomic chairs will reduce back pain incidences among office workers.
- Organic Farming & Crop Yield : Organic farming methods will produce lower crop yields compared to conventional farming.
- Distance Running & Joint Health : Engaging in distance running will heighten the risk of joint problems.
- Learning Styles & Information Retention : Auditory learners will retain more information from podcasts than from reading.
- Cold Showers & Alertness : Taking cold showers in the morning will increase alertness levels.
- Red Wine & Heart Health : Moderate consumption of red wine will benefit heart health.
- Pet Therapy & Stress Levels : Interacting with pets will decrease stress levels in hospital patients.
- Gardening & Mental Wellbeing : Engaging in gardening activities will improve mental well-being in adults.
- Night-time Screen Exposure & Sleep Quality : Exposure to screens before bedtime will reduce sleep quality.
- Carbohydrate Timing & Muscle Recovery : Consuming carbohydrates post-exercise will accelerate muscle recovery.
- Fermented Foods & Gut Health : Regular consumption of fermented foods will promote a healthier gut.
- Multivitamins & Immunity : Daily intake of multivitamins will boost the immune system.
- Breakfast & Academic Performance : Eating a balanced breakfast will improve academic performance in students.
- Lectures & Student Engagement : Interactive lectures will result in higher student engagement than traditional lectures.
- Olive Oil & Heart Diseases : Consuming olive oil regularly will reduce the risk of heart diseases.
- Fasting & Body Detoxification : Engaging in periodic fasting will enhance body detoxification.
- Mobile Usage & Attention Span : Prolonged mobile usage will reduce attention spans in young adults.
- Swimming & Lung Capacity : Regular swimming will improve lung capacity and efficiency.
- Singing & Lung Health : Engaging in singing exercises will enhance lung health.
- Local Produce & Nutrient Density : Consuming locally sourced produce will offer higher nutrient density than imported goods.
- Travel & Cultural Sensitivity : Individuals who travel internationally will display higher cultural sensitivity.
- Art Classes & Creativity : Participating in art classes will boost creativity levels in children.
- Solar Energy & Carbon Footprint : Adopting solar energy for homes will reduce the carbon footprint.
- Work Breaks & Productivity : Taking regular short breaks during work will enhance overall productivity.
- Barefoot Running & Foot Health : Engaging in barefoot running will strengthen foot muscles.
- High Heels & Posture : Wearing high heels frequently will negatively impact posture.
- Laughter & Immunity : Engaging in frequent laughter will strengthen the immune response.
- Playtime & Child Development : Increased playtime will foster better cognitive development in children.
- Agriculture & Soil Quality : Using natural fertilizers will improve long-term soil quality.
- Vaccination & Disease Immunity : Regular vaccination will decrease the susceptibility to certain diseases in populations.
- Language Acquisition & Brain Plasticity : Learning a new language after the age of 30 will boost brain plasticity.
- Urban Living & Mental Stress : Residing in urban areas will increase levels of mental stress compared to rural settings.
- Tree Planting & Air Quality : Increasing the number of trees in an urban environment will enhance air quality.
- Active Commuting & Fitness Level : People who commute by walking or cycling will have better fitness levels than those who use motorized transport.
- Therapeutic Techniques & Trauma Recovery : Applying cognitive-behavioral therapy will hasten the trauma recovery process in victims.
- Robotics Learning & Problem-Solving Skills : Engaging in robotics classes will foster advanced problem-solving skills in students.
- Acoustic Music & Sleep Quality : Listening to acoustic music before bedtime will improve sleep quality.
- Home Cooking & Nutrient Intake : Preparing meals at home will lead to a higher nutrient intake than eating out.
- Nature Walks & Creativity : Going on regular nature walks will spur creativity in individuals.
- Caffeine & Short-Term Memory : Intake of caffeine will enhance short-term memory retention.
- Aloe Vera & Skin Hydration : Applying aloe vera will improve skin hydration more effectively than regular creams.
- Aquatic Plants & Water Quality : Introducing aquatic plants to polluted water bodies will aid in improving water quality.
- Classical Training & Music Comprehension : Undergoing classical music training will amplify music comprehension skills.
- Digital Detox & Well-being : Engaging in a weekly digital detox will elevate feelings of well-being.
- Aeroponics & Plant Growth Rate : Employing aeroponics will foster faster plant growth compared to traditional soil methods.
- Memory Training & Dementia Prevention : Engaging in regular memory training exercises will reduce the risk of dementia in the elderly.
- Natural Light Exposure & Work Productivity : Work environments with more natural light will boost employee productivity.
- Manual Labor & Muscle Development : Engaging in manual labor will lead to enhanced muscle development over time.
- Charity Work & Life Satisfaction : Individuals involved in regular charity work will report higher levels of life satisfaction.
One Tailed Alternative Hypothesis Statement Examples
The alternative hypothesis is a statement that contradicts the null hypothesis, suggesting a particular direction or effect. The following examples reflect this directionality in their assertions.
- Dark Chocolate & Mood Enhancement : Consuming dark chocolate will lead to mood enhancement.
- Music Therapy & Pain Reduction : Music therapy sessions will decrease patients’ pain perception.
- Meditation & Stress Levels : Regular meditation sessions will lower individuals’ reported stress levels.
- Mountain Climbing & Lung Efficiency : Engaging in mountain climbing will improve lung efficiency.
- Yoga & Flexibility : Regular yoga practice will increase overall body flexibility.
- Organic Food & Health Benefits : Consuming organic foods will provide more health benefits than non-organic foods.
- Virtual Reality & Spatial Awareness : Virtual reality training will enhance spatial awareness.
- E-books & Reading Speed : Reading e-books will increase reading speed compared to traditional books.
- Grape Seed Extract & Blood Pressure : Consuming grape seed extract will lower blood pressure.
- Natural Cosmetics & Skin Health : Using natural cosmetics will result in healthier skin.
One Tailed Null Hypothesis Statement Examples
The null hypothesis asserts no significant difference or effect, and it is what researchers aim to disprove. Here, the examples display predictions of no specific directional effect.
- Decaffeinated Coffee & Energy Levels : Consuming decaffeinated coffee will have no impact on energy levels.
- White Noise & Sleep Quality : Playing white noise during sleep will not affect sleep quality.
- Artificial Sweeteners & Weight Gain : Consuming artificial sweeteners will have no effect on weight gain.
- Aerobic Exercises & Bone Density : Engaging in aerobic exercises will not change bone density.
- Remote Work & Job Satisfaction : Working remotely will have no difference in job satisfaction compared to in-office work.
- Video Games & Hand-Eye Coordination : Playing video games will not affect hand-eye coordination.
- Herbal Tea & Digestion : Drinking herbal tea will have no significant impact on digestion.
- Online Learning & Information Retention : Online learning will not influence the rate of information retention.
- Cold Pressed Oils & Nutrient Value : Using cold-pressed oils will not change nutrient value compared to other oils.
- Minimalist Living & Life Satisfaction : Adopting a minimalist lifestyle will not affect life satisfaction.
One Tailed T Test Hypothesis Statement Examples
T-tests compare the means of two groups to ascertain if the observed differences are statistically significant. The one-tailed T-test looks for a difference in one specific direction.
- Math Tutors & Grade Improvement : Students receiving extra math tuition will score significantly higher on math tests.
- Interval Training & Calorie Burn : Interval training sessions will result in a significantly higher calorie burn than steady-state exercises.
- Dietary Fiber & Cholesterol Levels : Diets high in fiber will significantly lower cholesterol levels.
- Journaling & Emotional Well-being : Individuals who journal regularly will report significantly higher emotional well-being scores.
- Mentoring & Career Advancement : Employees with mentors will have a significantly faster career advancement trajectory.
- Language Immersion & Fluency : Language immersion programs will lead to significantly better fluency than traditional language courses.
- Stand-up Desks & Energy Levels : Using stand-up desks will result in significantly higher energy levels throughout the workday.
- Thermal Insulation & Energy Consumption : Homes with thermal insulation will use significantly less energy for heating.
- Bike Lanes & Urban Cycling : Cities with dedicated bike lanes will witness a significant increase in urban cycling.
- Recycling Education & Waste Reduction : Communities with recycling education programs will show a significant reduction in waste.
One Tailed Research Hypothesis Statement Examples
Research hypotheses are used to predict a relationship between variables or an outcome. These examples are focused on particular research outcomes.
- Probiotics & Gut Health : Regular consumption of probiotics will significantly improve gut health.
- Sunscreen & Skin Aging : Daily application of sunscreen will significantly slow down skin aging.
- Vitamin D & Bone Health : A higher intake of vitamin D will significantly boost bone health.
- Brain Games & Cognitive Function : Playing brain games will significantly enhance cognitive function.
- Plant-based Diet & Heart Disease : Following a plant-based diet will significantly reduce the risk of heart disease.
- Interactive Teaching & Student Engagement : Interactive teaching methods will result in significantly higher student engagement.
- Online Therapy & Mental Health : Using online therapy platforms will bring about significant improvements in mental health.
- Acupressure & Pain Management : Acupressure treatments will lead to significant reductions in chronic pain.
- Hydrotherapy & Muscle Recovery : Engaging in hydrotherapy will speed up muscle recovery significantly.
- High-Intensity Training & Fat Loss : Undertaking high-intensity training sessions will lead to significant fat loss.
One Tailed Correlation Hypothesis Statement Examples
Correlation hypothesis assert a relationship between two variables. The following examples indicate a predicted correlation in one direction.
- Parental Involvement & Academic Success : A positive correlation exists between parental involvement and students’ academic success.
- Exercise Frequency & Mood Improvement : There’s a positive correlation between exercise frequency and mood improvement.
- Financial Literacy & Savings Rate : A positive correlation is present between financial literacy and personal savings rate.
- Public Transport Availability & Urban Air Quality : Increased public transport availability correlates with better urban air quality.
- Nutritional Education & Healthy Eating Habits : There’s a positive correlation between nutritional education and adoption of healthy eating habits.
- Social Media Use & Loneliness : Increased social media use correlates with feelings of loneliness.
- Reading Habits & Vocabulary Size : A positive correlation exists between regular reading habits and vocabulary size.
- Professional Development & Job Satisfaction : Professional development opportunities correlate positively with job satisfaction.
- Outdoor Activities & Physical Health : Engaging in outdoor activities correlates positively with improved physical health.
- Public Libraries & Community Engagement : The presence of public libraries correlates with increased community engagement.
Directional One Tailed Hypothesis Statement Examples
Directional hypotheses specifically indicate the expected direction of a relationship or effect.
- Plant Diet & Cholesterol : Transitioning to a plant-based diet will reduce cholesterol levels.
- Regular Exercise & Heart Rate : Engaging in regular exercise will lower resting heart rate.
- Sugar Intake & Dental Health : Increased sugar intake will deteriorate dental health.
- Stretching & Muscle Injury : Regular stretching before workouts will decrease the risk of muscle injuries.
- Water Intake & Skin Hydration : Drinking more water daily will improve skin hydration.
- Red Meat & Inflammation : Consuming red meat frequently will increase bodily inflammation.
- Mindfulness Practices & Anxiety : Engaging in mindfulness practices will decrease levels of anxiety.
- Homework & Academic Performance : Assigning more homework will improve students’ academic performance.
- Nature Exposure & Mental Well-being : Spending more time in nature will enhance mental well-being.
- Public Speaking Training & Confidence : Engaging in public speaking training will boost individual confidence.
One Tailed Hypothesis Statement Examples on Psychology
Focused on psychological phenomena, these hypotheses predict behavior, cognition, emotion, or other psychological outcomes.
- Cognitive Behavioral Therapy & Depression : Cognitive behavioral therapy will reduce symptoms of depression.
- Positive Reinforcement & Child Behavior : Using positive reinforcement will improve child behavior.
- Sleep Deprivation & Cognitive Performance : Sleep deprivation will lower cognitive performance.
- Group Therapy & Social Anxiety : Participating in group therapy will reduce symptoms of social anxiety.
- Mindfulness Meditation & Attention Span : Practicing mindfulness meditation will enhance attention span.
- Stress & Immune System Function : Chronic stress will weaken immune system function.
- Art Therapy & Emotional Expression : Engaging in art therapy will improve emotional expression.
- Parenting Styles & Child Self-esteem : Authoritative parenting styles will boost child self-esteem.
- Peer Pressure & Teen Decision Making : Peer pressure will negatively influence teen decision-making.
- Dream Analysis & Emotional Insight : Engaging in dream analysis will provide deeper emotional insight.
One Tailed Hypothesis Statement Examples for Research Paper
Suited for academic contexts, these hypotheses are tailored to address specific research inquiries.
- Organic Farming & Soil Health : Organic farming practices will lead to improved soil health.
- LED Lighting & Energy Consumption : Transitioning to LED lighting will reduce overall energy consumption.
- Aquaponics & Crop Yield : Implementing aquaponics will result in a higher crop yield.
- Green Roofing & Urban Temperature : Installing green roofs will lower urban temperature.
- Bilingual Education & Cognitive Flexibility : Adopting bilingual education will improve students’ cognitive flexibility.
- Noise Pollution & Bird Migration : Increasing noise pollution will disrupt bird migration patterns.
- Urbanization & Freshwater Availability : Rapid urbanization will decrease freshwater availability.
- Agroforestry & Biodiversity : Implementing agroforestry practices will increase local biodiversity.
- Remote Sensing & Forest Conservation : Using remote sensing tools will enhance forest conservation efforts.
- Virtual Reality & Surgical Training : Integrating virtual reality into surgical training will enhance surgeons’ skills.
How do you write a hypothesis for a one-tailed test? – A Step by Step Guide
Understanding how to articulate a one-tailed hypothesis is crucial for proper statistical testing. The specificity of direction in a one-tailed hypothesis allows for precise predictions. Here’s a step-by-step guide to help you formulate a one-tailed hypothesis:
- Identify the Research Question Start by pinpointing what you aim to explore. Your research question will guide the hypothesis formulation. Example: “Does practicing mindfulness reduce anxiety levels?”
- Select Relevant Variables Determine the dependent and independent variables. The dependent variable is what you’re measuring (e.g., anxiety levels), and the independent variable is the factor you believe will influence it (e.g., mindfulness practice).
- Determine the Direction A one-tailed test hypothesizes a specific direction. Decide if you’re suggesting an increase, decrease, or any specific directional effect between variables. Example: “Mindfulness reduces anxiety” implies a decrease in anxiety due to mindfulness.
- Formulate the Null Hypothesis (H?) The null hypothesis posits no effect or difference. Given the above, the null hypothesis would state: “Mindfulness does not reduce anxiety levels.”
- State the Alternative Hypothesis (H? or Ha) This is your actual prediction. For our example, it would be: “Mindfulness reduces anxiety levels.”
- Ensure Clarity and Specificity Your hypothesis should be clear and concise, free from ambiguity. Avoid vague terms and ensure it’s testable.
- Review Existing Literature Before finalizing, glance through existing research. Make sure your hypothesis is rooted in established theory or previous findings but brings something new to the table.
- Validate with Peers or Mentors Share your formulated hypothesis with colleagues or mentors for feedback. They might offer insights you hadn’t considered.
- Finalize and Proceed Once you’re satisfied with the clarity, direction, and foundation of your hypothesis, you can proceed to the research phase.
Tips for Writing One Tailed Hypothesis
- Simplicity is Key: Make your hypothesis concise. Avoid jargon unless writing for a specialized audience.
- Be Directional: The essence of a one-tailed hypothesis is its direction. Ensure you’re indicating a specific increase or decrease.
- Root it in Research: Your hypothesis should have a basis in existing literature or observations. This adds credibility.
- Avoid Absolutes: Steer clear of words like “always” or “never”. In research, there are often exceptions.
- Stay Testable: Ensure your hypothesis can be empirically tested using available tools and within a reasonable timeframe.
- Use Clear Variables: Clearly identify and define all variables you’re examining. Ambiguity can lead to confusion later.
- Consider Potential Biases: Ensure your hypothesis isn’t influenced by personal beliefs or biases.
- Stay Open to Being Wrong: The purpose of research is discovery. A disproven hypothesis is as valuable as a confirmed one.
- Iterate: If initial tests or feedback suggest issues with your hypothesis, don’t be afraid to revise and refine.
- Practice Makes Perfect: The more hypotheses you write, the more adept you’ll become at crafting precise and testable predictions.
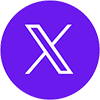
Text prompt
- Instructive
- Professional
10 Examples of Public speaking
20 Examples of Gas lighting

IMAGES
VIDEO
COMMENTS
One-tailed test: Alternative hypothesis contains either < or > sign; In a one-tailed test, the alternative hypothesis contains the less than ("<") or greater than (">") sign. This indicates that we're testing whether or not there is a positive or negative effect. Check out the following example problems to gain a better understanding ...
One-tailed hypothesis tests are also known as directional and one-sided tests because you can test for effects in only one direction. When you perform a one-tailed test, the entire significance level percentage goes into the extreme end of one tail of the distribution. In the examples below, I use an alpha of 5%.
One-Tailed Test: A one-tailed test is a statistical test in which the critical area of a distribution is one-sided so that it is either greater than or less than a certain value, but not both. If ...
To test this, she can perform a one-tailed hypothesis test with the following null and alternative hypotheses: H0 (Null Hypothesis): μ ≤ 10 inches. HA (Alternative Hypothesis): μ > 10 inches. Note: We can tell this is a one-tailed test because the alternative hypothesis contains the greater than (>) sign. Specifically, we would call this a ...
One-tailed hypothesis tests offer the promise of more statistical power compared to an equivalent two-tailed design. While there is some debate about when you can use a one-tailed test, the general consensus among statisticians is that you should use two-tailed tests unless you have concrete reasons for using a one-tailed test. In this post, I ...
The one-tailed hypothesis is rejected only if the sample proportion is much greater than \(0.5\). The alternative hypothesis in the two-tailed test is \(\pi \neq 0.5\). In the one-tailed test it is \(\pi > 0.5\). ... For example, consider an experiment designed to test the efficacy of a treatment for the common cold. The researcher would only ...
In a one-tailed hypothesis example, all significance will occur on only one side of the bell curve. It is useful to determine whether there is a statistically significant difference between groups ...
At this point, you might use a statistical test, like unpaired or 2-sample t-test, to see if there's a significant difference between the two groups' means. Typically, an unpaired t-test starts with two hypotheses. The first hypothesis is called the null hypothesis, and it basically says there's no difference in the means of the two groups.
The one-tailed hypothesis is rejected only if the sample proportion is much greater than 0.5 0.5. The alternative hypothesis in the two-tailed test is n ≠ 0.5 n ≠ 0.5. In the one-tailed test it is π > 0.5 π > 0.5. You should always decide whether you are going to use a one-tailed or a two-tailed probability before looking at the data.
Courses on Khan Academy are always 100% free. Start practicing—and saving your progress—now: https://www.khanacademy.org/math/statistics-probability/signifi...
Khanmigo is now free for all US educators! Plan lessons, develop exit tickets, and so much more with our AI teaching assistant.
This statistics video tutorial explains when you should use a one tailed test vs a two tailed test when solving problems associated with hypothesis testing. ...
In coin flipping, the null hypothesis is a sequence of Bernoulli trials with probability 0.5, yielding a random variable X which is 1 for heads and 0 for tails, and a common test statistic is the sample mean (of the number of heads) ¯. If testing for whether the coin is biased towards heads, a one-tailed test would be used - only large numbers of heads would be significant.
The two red tails are the alpha level, divided by two (i.e. α/2). Alpha levels (sometimes just called "significance levels") are used in hypothesis tests; it is the probability of making the wrong decision when the null hypothesis is true. A one-tailed test has the entire 5% of the alpha level in one tail (in either the left, or the right tail).
4 One-tailed vs two-tailed test. To gain a deeper understanding of how to conduct a hypothesis test, this section will delve into the concepts of one-tailed and two-tailed tests. These tests are vital tools in statistical hypothesis testing, and the decision of which test to employ depends on the research question and hypothesis under examination.
One Sample t-test data: iq t = -2.3801, df=99, p-value = 0.01922 alternative hypothesis: true mean is not equal to 100 95 percent confidence interval: 92.35365 99.30635 Sample estimates mean of x 95.83. In order to perform a one-tailed test, you need to specify the alternative hypothesis. For example: > t.test(iq,mu=100, alternative="less") or
A one-tailed test is used when a tested hypothesis looks for whether a value is notably higher or lower than expected by chance. Explore the definition and examples of one-tailed tests and ...
A directional (one-tailed) hypothesis predicts the nature of the effect of the independent variable on the dependent variable. It predicts in which direction the change will take place. ... For example, the hypothesis that "all swans are white," can be falsified by observing a black swan. For Popper, science should attempt to disprove a ...
So, depending on the direction of the one-tailed hypothesis, its p-value is either .5*(two-tailed p-value) or 1-.5*(two-tailed p-value) if the test statistic symmetrically distributed about zero. In this example, the two-tailed p-value suggests rejecting the null hypothesis of no difference. Had we opted for the one-tailed test of (diff > 0 ...
The one-tailed hypothesis is rejected only if the sample proportion is much greater than 0.5. The alternative hypothesis in the two-tailed test is π ≠ 0.5. ... For example, consider an experiment designed to test the efficacy of a treatment for the common cold. The researcher would only be interested in whether the treatment was better than ...
This video explains the differences between one-tailed (right and left) and two-tailed hypothesis tests (directional vs. non-directional)proportion (p) temp...
The one-tailed test is a statistical hypothesis testing method. To reject the null hypothesis sample mean should be either greater or less than the population mean. This test is also referred to as a directional test or directional hypothesis. The test is run to prove a claim either true or false. The determination of this test cannot be ...
One Tailed Research Hypothesis Statement Examples. Research hypotheses are used to predict a relationship between variables or an outcome. These examples are focused on particular research outcomes. Probiotics & Gut Health: Regular consumption of probiotics will significantly improve gut health.