- PRO Courses Guides New Tech Help Pro Expert Videos About wikiHow Pro Upgrade Sign In
- EDIT Edit this Article
- EXPLORE Tech Help Pro About Us Random Article Quizzes Request a New Article Community Dashboard This Or That Game Happiness Hub Popular Categories Arts and Entertainment Artwork Books Movies Computers and Electronics Computers Phone Skills Technology Hacks Health Men's Health Mental Health Women's Health Relationships Dating Love Relationship Issues Hobbies and Crafts Crafts Drawing Games Education & Communication Communication Skills Personal Development Studying Personal Care and Style Fashion Hair Care Personal Hygiene Youth Personal Care School Stuff Dating All Categories Arts and Entertainment Finance and Business Home and Garden Relationship Quizzes Cars & Other Vehicles Food and Entertaining Personal Care and Style Sports and Fitness Computers and Electronics Health Pets and Animals Travel Education & Communication Hobbies and Crafts Philosophy and Religion Work World Family Life Holidays and Traditions Relationships Youth
- Browse Articles
- Learn Something New
- Quizzes Hot
- Happiness Hub
- This Or That Game
- Train Your Brain
- Explore More
- Support wikiHow
- About wikiHow
- Log in / Sign up
- Education and Communications
- Mathematics
- Probability and Statistics

How to Find the Perfect Sample Size for Your Research Study
Last Updated: April 26, 2024 Fact Checked
Determining Key Values
- Standard Sample Size Formula
- Large or Unknown Population Formula
- Slovin's Formula
Calculator, Practice Problems, and Answers
This article was reviewed by Joseph Meyer . Joseph Meyer is a High School Math Teacher based in Pittsburgh, Pennsylvania. He is an educator at City Charter High School, where he has been teaching for over 7 years. Joseph is also the founder of Sandbox Math, an online learning community dedicated to helping students succeed in Algebra. His site is set apart by its focus on fostering genuine comprehension through step-by-step understanding (instead of just getting the correct final answer), enabling learners to identify and overcome misunderstandings and confidently take on any test they face. He received his MA in Physics from Case Western Reserve University and his BA in Physics from Baldwin Wallace University. There are 7 references cited in this article, which can be found at the bottom of the page. This article has been fact-checked, ensuring the accuracy of any cited facts and confirming the authority of its sources. This article has been viewed 452,456 times.
Scientific studies often rely on surveys distributed among a sample of some total population. Your sample will need to include a certain number of people, however, if you want it to accurately reflect the conditions of the overall population it's meant to represent. To calculate your necessary sample size, you'll need to determine several set values and plug them into an appropriate formula.

- Precision has a greater statistical impact when you work with a smaller group. For instance, if you wish to perform a survey among members of a local organization or employees of a small business, the population size should be accurate within a dozen or so people. [1] X Research source
- Larger surveys allow for a greater deviance in the actual population. For example, if your demographic includes everyone living in the United States, you could estimate the size to roughly 320 million people, even though the actual value may vary by hundreds of thousands.

- The margin of error is a percentage the indicates how close your sample results will be to the true value of the overall population discussed in your study.
- Smaller margin of errors will result in more accurate answers, but choosing a smaller margin of error will also require a larger sample.
- In this example, the margin of error essentially indicates that, if the entire population were asked the same poll question, you are “confident” that somewhere between 30% (35 - 5) and 40% (35 + 5) would agree with option A .

- In other words, choosing a confidence level of 95% allows you to claim that you 95% certain that your results accurately fall within your chosen margin of error.
- A larger confidence level indicates a greater degree of accuracy, but it will also require a larger sample. The most common confidence levels are 90% confident, 95% confident, and 99% confident.
- Setting a confidence level of 95% for the example stated in the margin of error step would mean that you are 95% certain that 30% to 40% of the total concerned population would agree with option A of your survey.

- Plainly stated, if 99% of your survey responses answer "Yes" and only 1% answer "No," the sample probably represents the overall population very accurately.
- On the other hand, if 45% answer "Yes" and 55% answer "No," there is a greater chance of error.
- Since this value is difficult to determine you give the actual survey, most researchers set this value at 0.5 (50%). This is the worst case scenario percentage, so sticking with this value will guarantee that your calculated sample size is large enough to accurately represent the overall population within your confidence interval and confidence level.

- You can calculate z-scores by hand, look for an online calculator, or find your z-score on a z-score table. Each of these methods can be fairly complex, however.
- 80% confidence => 1.28 z-score
- 85% confidence => 1.44 z-score
- 90% confidence => 1.65 z-score
- 95% confidence => 1.96 z-score
- 99% confidence => 2.58 z-score
Using the Standard Formula

- N = population size
- z = z-score
- e = margin of error
- p = standard of deviation

- Example: Determine the ideal survey size for a population size of 425 people. Use a 99% confidence level, a 50% standard of deviation, and a 5% margin of error.
- For 99% confidence, you would have a z-score of 2.58.

- = [2.58 2 * 0.5(1-0.5)] / 0.05 2 / 1 + [2.58 2 * 0.5(1-0.5)] / 0.05 2 * 425 ]
- = [6.6564 * 0.25] / 0.0025 / 1 + [6.6564 * 0.25] / 1.0625 ]
- = 665 / 2.5663
- = 259.39 (final answer)
Creating a Formula for Unknown or Very Large Populations

- Note that this equation is merely the top half of the full formula.

- Example: Determine the necessary survey size for an unknown population with a 90% confidence level, 50% standard of deviation, a 3% margin of error.
- For 90% confidence, use the z-score would be 1.65.

- = [1.65 2 * 0.5(1-0.5)] / 0.03 2
- = [2.7225 * 0.25] / 0.0009
- = 0.6806 / 0.0009
- = 756.22 (final answer)
Using Slovin's Formula

- Note that this is the least accurate formula and, as such, the least ideal. You should only use this if circumstances prevent you from determining an appropriate standard of deviation and/or confidence level (thereby preventing you from determining your z-score, as well).

- Example: Calculate the necessary survey size for a population of 240, allowing for a 4% margin of error.

- = 240 / (1 + 240 * 0.04 2 )
- = 240 / (1 + 240 * 0.0016)
- = 240 / (1 + 0.384}
- = 240 / (1.384)
- = 173.41 (final answer)

Community Q&A

You Might Also Like

- ↑ https://www.khanacademy.org/math/ap-statistics/gathering-data-ap/sampling-observational-studies/e/identifying-population-sample
- ↑ https://www.khanacademy.org/math/statistics-probability/confidence-intervals-one-sample/estimating-population-proportion/v/margin-of-error-1
- ↑ https://www.khanacademy.org/math/ap-statistics/xfb5d8e68:inference-categorical-proportions/introduction-confidence-intervals/a/interpreting-confidence-levels-and-confidence-intervals
- ↑ https://www.mathsisfun.com/data/standard-deviation-formulas.html
- ↑ https://www.mathsisfun.com/data/standard-deviation.html
- ↑ https://prudencexd.weebly.com/
- ↑ https://www.mathsisfun.com/data/sampling.html
About This Article

To calculate sample size, first find the population size, or number of people taking your study, and margin of error, which is the amount of error you'll allow in your results. Then, calculate your confidence level, which is how confident you are in percentage terms that your results will fall within your margin of error, and z-score, a constant value linked to your confidence level. Next, specify your standard of deviation, which is the amount of variation you expect in your results. Finally, plug your variables into the standard formula to figure out the sample size. To learn how to create a formula for unknown populations, read on! Did this summary help you? Yes No
- Send fan mail to authors
Reader Success Stories

Muhammad Abu-Salih
Apr 5, 2016
Did this article help you?

Akaninyene Akpan
Sep 9, 2017

Aug 19, 2017

Mar 28, 2023

Takele Teklu
Jun 29, 2020

Featured Articles

Trending Articles

Watch Articles

- Terms of Use
- Privacy Policy
- Do Not Sell or Share My Info
- Not Selling Info
Get all the best how-tos!
Sign up for wikiHow's weekly email newsletter
Sample Size Calculator
Find out the sample size.
This calculator computes the minimum number of necessary samples to meet the desired statistical constraints.
Confidence Level: | ||
Margin of Error: | ||
Population Proportion: | Use 50% if not sure | |
Population Size: | Leave blank if unlimited population size. | |
Find Out the Margin of Error
This calculator gives out the margin of error or confidence interval of observation or survey.
Confidence Level: | ||
Sample Size: | ||
Population Proportion: | ||
Population Size: | Leave blank if unlimited population size. | |
Related Standard Deviation Calculator | Probability Calculator
In statistics, information is often inferred about a population by studying a finite number of individuals from that population, i.e. the population is sampled, and it is assumed that characteristics of the sample are representative of the overall population. For the following, it is assumed that there is a population of individuals where some proportion, p , of the population is distinguishable from the other 1-p in some way; e.g., p may be the proportion of individuals who have brown hair, while the remaining 1-p have black, blond, red, etc. Thus, to estimate p in the population, a sample of n individuals could be taken from the population, and the sample proportion, p̂ , calculated for sampled individuals who have brown hair. Unfortunately, unless the full population is sampled, the estimate p̂ most likely won't equal the true value p , since p̂ suffers from sampling noise, i.e. it depends on the particular individuals that were sampled. However, sampling statistics can be used to calculate what are called confidence intervals, which are an indication of how close the estimate p̂ is to the true value p .
Statistics of a Random Sample
The uncertainty in a given random sample (namely that is expected that the proportion estimate, p̂ , is a good, but not perfect, approximation for the true proportion p ) can be summarized by saying that the estimate p̂ is normally distributed with mean p and variance p(1-p)/n . For an explanation of why the sample estimate is normally distributed, study the Central Limit Theorem . As defined below, confidence level, confidence intervals, and sample sizes are all calculated with respect to this sampling distribution. In short, the confidence interval gives an interval around p in which an estimate p̂ is "likely" to be. The confidence level gives just how "likely" this is – e.g., a 95% confidence level indicates that it is expected that an estimate p̂ lies in the confidence interval for 95% of the random samples that could be taken. The confidence interval depends on the sample size, n (the variance of the sample distribution is inversely proportional to n , meaning that the estimate gets closer to the true proportion as n increases); thus, an acceptable error rate in the estimate can also be set, called the margin of error, ε , and solved for the sample size required for the chosen confidence interval to be smaller than e ; a calculation known as "sample size calculation."
Confidence Level
The confidence level is a measure of certainty regarding how accurately a sample reflects the population being studied within a chosen confidence interval. The most commonly used confidence levels are 90%, 95%, and 99%, which each have their own corresponding z-scores (which can be found using an equation or widely available tables like the one provided below) based on the chosen confidence level. Note that using z-scores assumes that the sampling distribution is normally distributed, as described above in "Statistics of a Random Sample." Given that an experiment or survey is repeated many times, the confidence level essentially indicates the percentage of the time that the resulting interval found from repeated tests will contain the true result.
Confidence Level | z-score (±) |
0.70 | 1.04 |
0.75 | 1.15 |
0.80 | 1.28 |
0.85 | 1.44 |
0.92 | 1.75 |
0.95 | 1.96 |
0.96 | 2.05 |
0.98 | 2.33 |
0.99 | 2.58 |
0.999 | 3.29 |
0.9999 | 3.89 |
0.99999 | 4.42 |
Confidence Interval
In statistics, a confidence interval is an estimated range of likely values for a population parameter, for example, 40 ± 2 or 40 ± 5%. Taking the commonly used 95% confidence level as an example, if the same population were sampled multiple times, and interval estimates made on each occasion, in approximately 95% of the cases, the true population parameter would be contained within the interval. Note that the 95% probability refers to the reliability of the estimation procedure and not to a specific interval. Once an interval is calculated, it either contains or does not contain the population parameter of interest. Some factors that affect the width of a confidence interval include: size of the sample, confidence level, and variability within the sample.
There are different equations that can be used to calculate confidence intervals depending on factors such as whether the standard deviation is known or smaller samples (n<30) are involved, among others. The calculator provided on this page calculates the confidence interval for a proportion and uses the following equations:
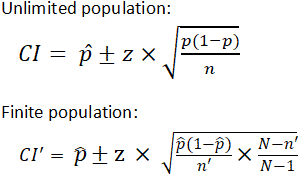
where is z score is the population proportion and are sample size is the population size |
Within statistics, a population is a set of events or elements that have some relevance regarding a given question or experiment. It can refer to an existing group of objects, systems, or even a hypothetical group of objects. Most commonly, however, population is used to refer to a group of people, whether they are the number of employees in a company, number of people within a certain age group of some geographic area, or number of students in a university's library at any given time.
It is important to note that the equation needs to be adjusted when considering a finite population, as shown above. The (N-n)/(N-1) term in the finite population equation is referred to as the finite population correction factor, and is necessary because it cannot be assumed that all individuals in a sample are independent. For example, if the study population involves 10 people in a room with ages ranging from 1 to 100, and one of those chosen has an age of 100, the next person chosen is more likely to have a lower age. The finite population correction factor accounts for factors such as these. Refer below for an example of calculating a confidence interval with an unlimited population.
EX: Given that 120 people work at Company Q, 85 of which drink coffee daily, find the 99% confidence interval of the true proportion of people who drink coffee at Company Q on a daily basis.
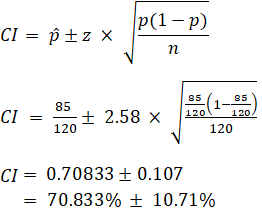
Sample Size Calculation
Sample size is a statistical concept that involves determining the number of observations or replicates (the repetition of an experimental condition used to estimate the variability of a phenomenon) that should be included in a statistical sample. It is an important aspect of any empirical study requiring that inferences be made about a population based on a sample. Essentially, sample sizes are used to represent parts of a population chosen for any given survey or experiment. To carry out this calculation, set the margin of error, ε , or the maximum distance desired for the sample estimate to deviate from the true value. To do this, use the confidence interval equation above, but set the term to the right of the ± sign equal to the margin of error, and solve for the resulting equation for sample size, n . The equation for calculating sample size is shown below.
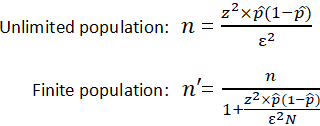
where is the z score is the margin of error is the population size is the population proportion |
EX: Determine the sample size necessary to estimate the proportion of people shopping at a supermarket in the U.S. that identify as vegan with 95% confidence, and a margin of error of 5%. Assume a population proportion of 0.5, and unlimited population size. Remember that z for a 95% confidence level is 1.96. Refer to the table provided in the confidence level section for z scores of a range of confidence levels.
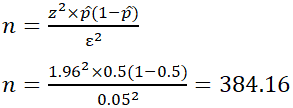
Thus, for the case above, a sample size of at least 385 people would be necessary. In the above example, some studies estimate that approximately 6% of the U.S. population identify as vegan, so rather than assuming 0.5 for p̂ , 0.06 would be used. If it was known that 40 out of 500 people that entered a particular supermarket on a given day were vegan, p̂ would then be 0.08.
Search | |
.png)
How AI is Redefining the Use of Audience Data

Read Article

Team Remesh
August 9, 2024
Market Research

Research 101
Want to Fix Things Fast? Take a Rapid Research Approach
Patrick Hyland
August 8, 2024
Employee Research

For Researchers, by Researchers: How Remesh Implements a Customer Feedback Loop
August 6, 2024
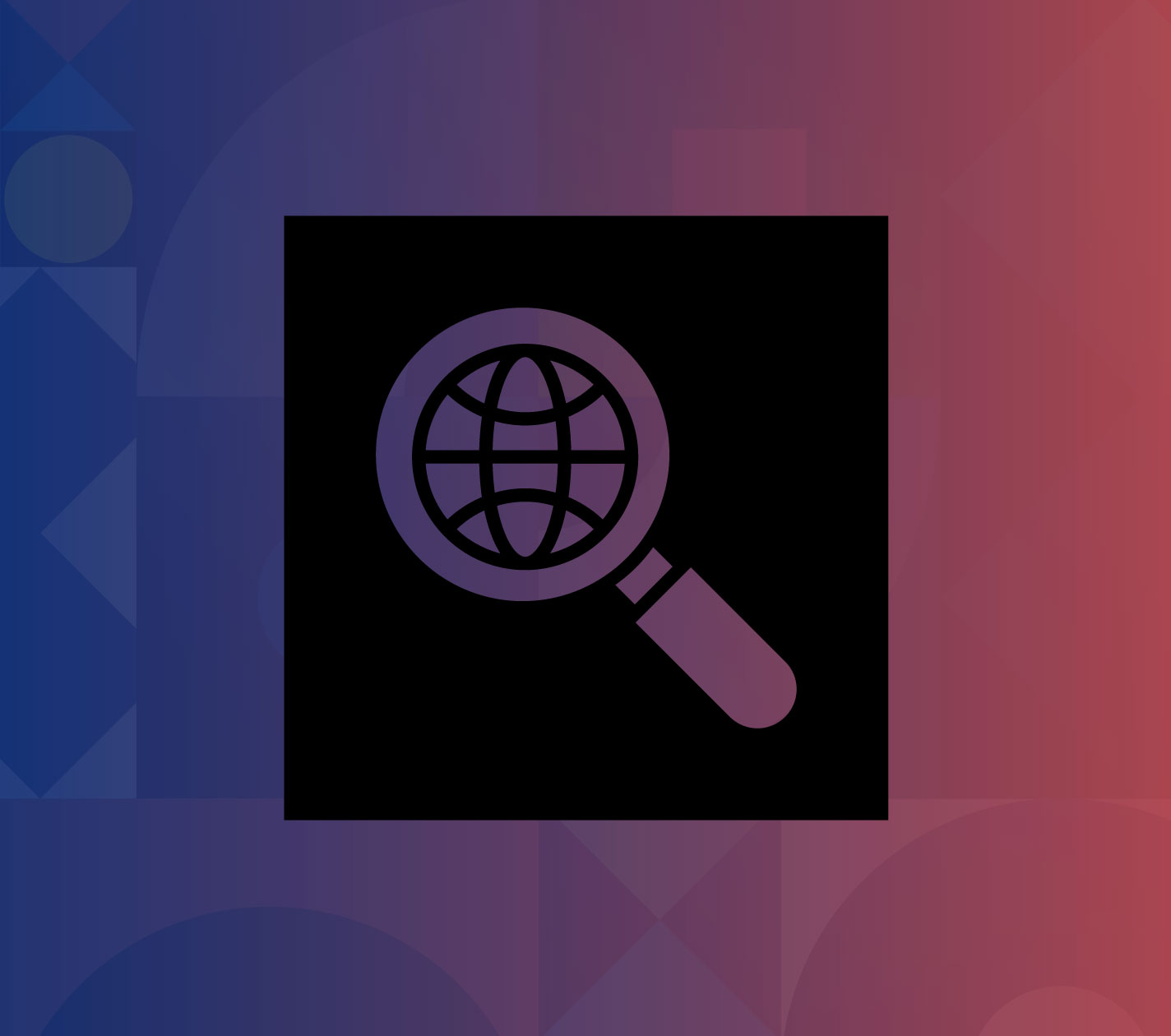
Advanced Research
The Global Research Revolution: From Clipboards to Clicks
August 1, 2024
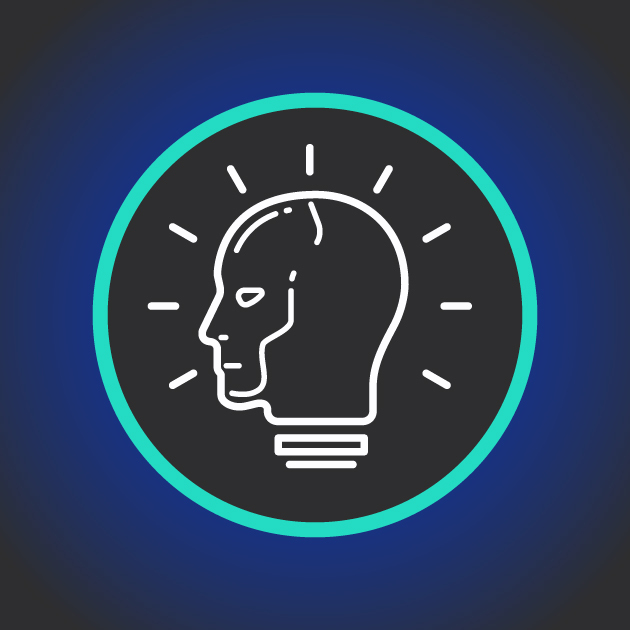
How and Where Remesh Uses AI in Market Research
July 19, 2024

How to Avoid Confirmation Bias in Research
June 25, 2024

Compressing Research Timelines Without Sacrificing Quality
June 21, 2024
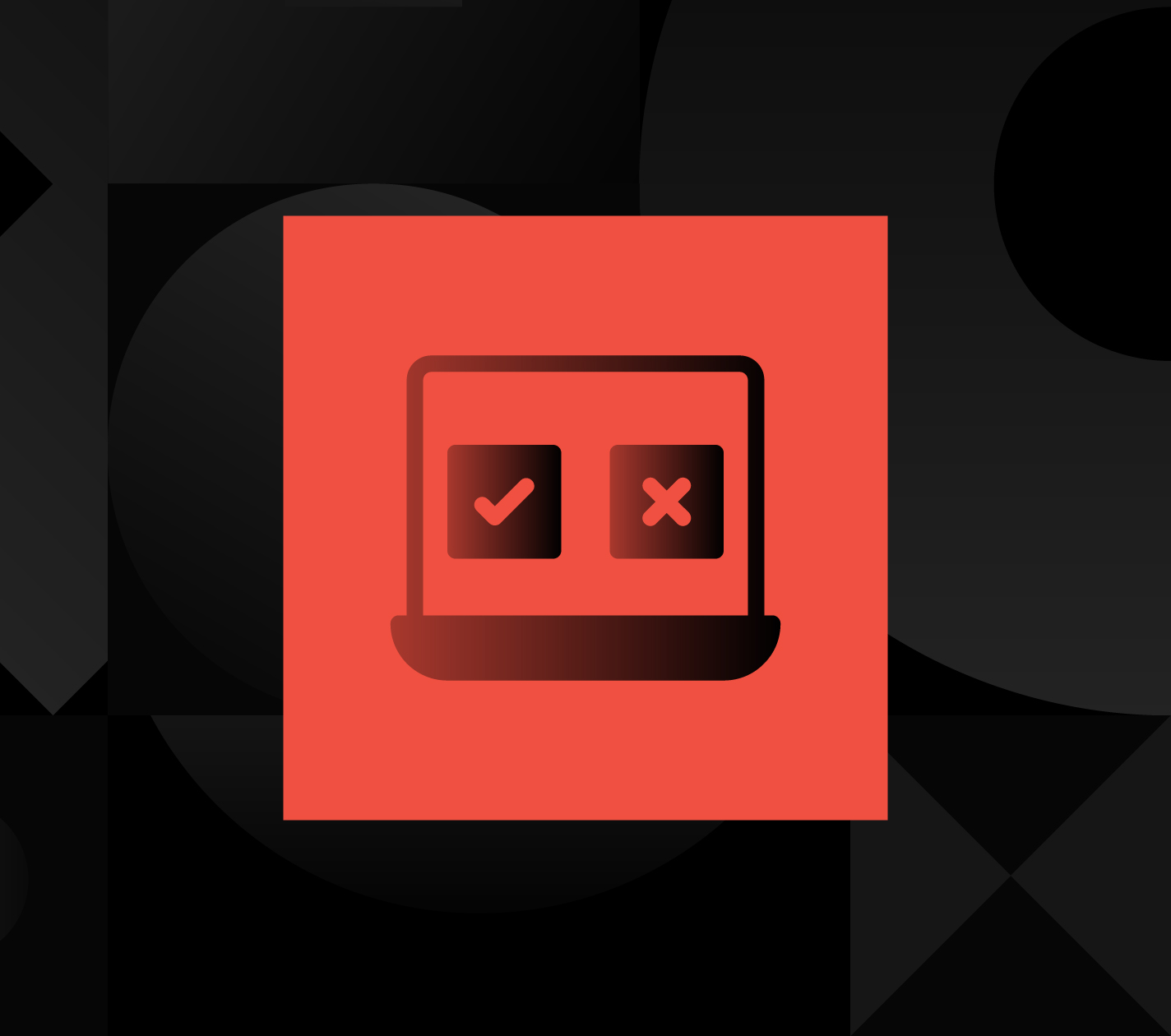
Hidden Insights: The Power of Participant Voting in Market Research

Want to Prevent Culture Drift? Take a Temporal Perspective
June 7, 2024
How To Calculate Sample Size Using a Sample Size Formula
Learn how to calculate sample size with a margin of error using these simple sample size formulas for your market research.

Anika Nishat
March 22, 2024
What is sample size determination and why is it important
Finding an appropriate sample size, otherwise known as sample size determination, is a crucial first step in market research. Understanding why sample size is important is equally crucial. The answer: it ensures the robustness, reliability, and believability of your research findings. But how is sample size determined?
Calculating your sample size
During the course of your market research , you may be unable to reach the entire population you want to gather data about. While larger sample sizes bring you closer to a 1:1 representation of your target population, working with them can be time-consuming, expensive, and inconvenient. However, small samples risk yielding results that aren’t representative of the target population. It can be tricky because determining the ideal sample size for statistical significance ensures your research yields reliable and actionable insights.
Luckily, you can easily identify an ideal subset that represents the population and produces strong, statistically significant results that don’t gobble up all of your resources. In this article, we’ll teach you how to calculate sample size with a margin of error to identify that subset.
Five steps to finding your sample size
- Define population size or number of people
Designate your margin of error
- Determine your confidence level
- Predict expected variance
- Finalize your sample size
What is a good statistical sample size can vary depending on your research goals. But by following these five steps, you'll ensure you get the right selection size for your research needs.
Download: The State of AI in Market Research
Define the size of your population.
Your sample size needs will differ depending on the true population size or the total number of people you're looking to conclude on. That's why determining the minimum sample size for statistical significance is an important first step.
Defining the size of your population can be easier said than done. While there is a lot of population data available, you may be targeting a complex population or for which no reliable data currently exists.
Knowing the size of your population is more important when dealing with relatively small, easy-to-measure groups of people. If you're dealing with a larger population, take your best estimate, and roll with it.
This is the first step in a sample size formula, yielding more accurate results than a simple estimate – and accurately reflecting the population.
Random sample errors are inevitable whenever you're using a subset of your total population. Be confident that your results are accurate by designating how much error you intend to permit: that's your margin of error.
Sometimes called a "confidence interval," a margin of error indicates how much you're willing for your sample mean to differ from your population mean . It's often expressed alongside statistics as a plus-minus (±) figure, indicating a range which you can be relatively certain about.
For example, say you take a sample proportion of your colleagues with a designated 3% margin of error and find that 65% of your office uses some form of voice recognition technology at home. If you were to ask your entire office, you could be sure that in reality, as low as 62% and as high as 68% might use some form of voice recognition technology at home.
Determine how confident you can be
Your confidence level reveals how certain you can be that the true proportion of the total population would pick an answer within a particular range. The most common confidence levels are 90%, 95%, and 99%. Researchers most often employ a 95% confidence level.
Don't confuse confidence levels for confidence intervals (i.e., mean of error). Remember the distinction by thinking about how the concepts relate to each other to sample more confidently.
In our example from the previous step, when you put confidence levels and intervals together, you can say you're 95% certain that the true percentage of your colleagues who use voice recognition technology at home is within ± three percentage points from the sample mean of 65%, or between 62% and 68%.
Your confidence level corresponds to something called a "z-score." A z-score is a value that indicates the placement of your raw score (meaning the percent of your confidence level) in any number of standard deviations below or above the population mean.
Z-scores for the most common confidence intervals are:
- 90% = 2.576
- 99% = 2.576
While not as commonly used, the z-score for an 80% confidence interval is approximately 1.28. If you're using a different confidence interval, use this z-score table . A z-score sample calculator like this will quickly determine the appropriate value for your chosen confidence level.
Predict variance by calculating standard deviation in a sample
The last thing you'll want to consider when calculating your sample size is the amount of variance you expect to see among participant responses.
The standard deviation in a sample measures how much individual sample data points deviate from the average population.
Don't know how much variance to expect? Use the standard deviation of 0.5 to make sure your group is large enough.
Read: Best Practices for Writing Discussion Guides (eBook)
Finding your ideal sample size.
Now that you know what goes into determining sample size, you can easily calculate sample size online. Consider using a sample size calculator to ensure accuracy. Or, calculate it the old-fashioned way: by hand.
Below, find two sample size calculations - one for the known population proportion and one for the unknown population.
Sample size for known population
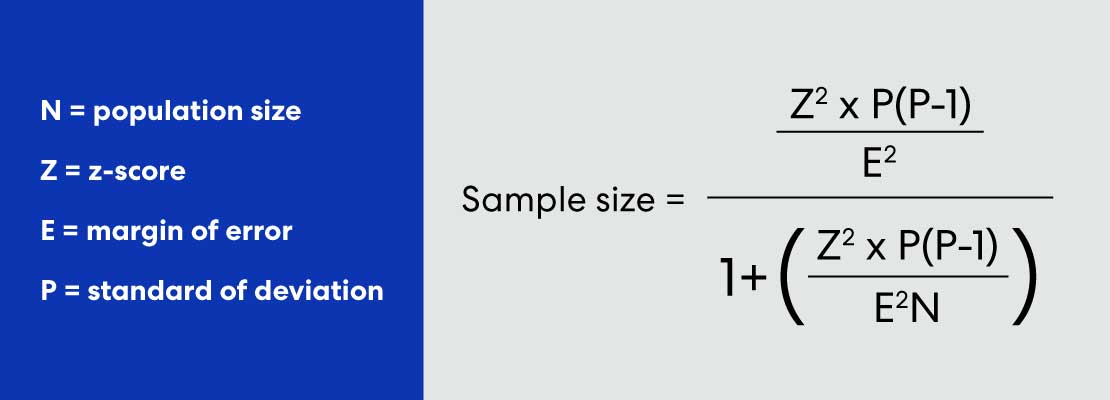
Sample size for unknown population
.jpeg)
Here’s how the calculations work out for our voice recognition technology example in an office of 500 people, with a 95% confidence level and 5% margin of error:

There you have it! 197 respondents are needed.
You can tweak some things if that number is too big to swallow.
Try increasing your margin of error or decreasing your confidence level. This will reduce the number of respondents necessary but, unfortunately, increase the chances of errors. Even so, understanding why trade-offs are necessary in sample size determination can help you make informed decisions.
Summing Up Sample Size
Calculating sample size sounds complicated - but, utilizing an easy sample size formula and even calculators are now available to make this tedious part of market research faster!
Once you've determined your sample size, you're ready to create and distribute your sample market research survey. This can be done through methods like running a focus group or even a customer satisfaction survey . Whatever you decide, you now have the information needed to make decisions with confidence.
Want to whip your research skills into shape? Check out our go-to eBook on writing discussion guides !
sample case study

Three Ways to Energize Your Employee Listening Program
Stay up-to date..
Stay ahead of the curve. Get it all. Or get what suits you. Our 101 material is great if you’re used to working with an agency. Are you a seasoned pro? Sign up to receive just our advanced materials.

Get insights in your inbox . Sign up to stay up to date on the latest research tactics and breakthroughs.

©All Rights Reserved 2024. Read our Privacy policy
No data capture tricks. No bull SEO content for ranking. Just good, solid, honest, research ed.
By entering your email address, you agree to receive marketing communications in accordance with our privacy policy.

Request a demo
Dive Deeper
Sample Size Calculator
Table of contents
If you're conducting research and wonder how many measurements you need so that it is statistically significant , this sample size calculator is here to help you. All you need to do is ask yourself these three questions before you use it:
- How accurate should your result be? (margin of error)
- What level of confidence do you need? (confidence level)
- What is your initial estimate ? (proportion estimate)
Read on to learn how to calculate the sample size using this tool, and what do all the variables in the sample size calculation formula mean.
Sample size calculation formula
The equation that our sample size calculator uses is:
Z Z Z — The z-score associated with the confidence level you chose. Our statistical significance calculator calculates this value automatically, but if you want to learn how to calculate it by hand, take a look at the instructions of our confidence interval calculator .
M E \mathrm{ME} ME — Margin of error: it tells you that you can be sure (with a given confidence level, for example, 95%) that the real value doesn't differ from the one that you obtained by more than this margin of error. You can learn more about it at our margin of error calculator .
p p p — Your initial proportion estimate. For example, if you are conducting a survey among students trying to find out how many of them read more than 5 books last year, you may know a result of a previous survey — 40%. If you have no such estimate, use the conservative value of 50%.
n 1 n_1 n 1 — Required sample size.
If your population is finite — for example, you are conducting a survey among students of only one faculty — you need to include a correction in the following form:
- N N N — Total population size.
- n 2 n_2 n 2 — Size of the sample taken from the whole population that will make your research statistically significant.
How to calculate sample size: an example
We will analyze a survey case step-by-step so you can get a clear picture of how to use our sample size calculator . You are planning to conduct a survey to find out what is the proportion of students on your campus who regularly eat their lunch at the campus canteen.
Decide how accurate you want your result to be. Let's say that it is important for the canteen to know the result, with a margin of error of 2 % 2\% 2% maximum.
Decide on your confidence level . We can assume you want to be 99 % 99\% 99% sure that your result is correct.
Do you have an initial proportion guess ? Let's say you accessed a similar survey from 10 years ago, and the proportion was equal to 30 % 30\% 30% . You can assume it as your initial estimate.
Is the total population of students so high that you can assume it's infinite ? Probably not. You need to find the current data for the number of students on the campus — let's assume it is 25 , 000 25,000 25 , 000 .
All you need to do now is input all this data into our sample size calculator. It finds the sample size required for the result to be statistically significant is 3 , 051 3,051 3 , 051 . You need to ask that many students the same question… Are you sure you can't settle for a 95 % 95\% 95% confidence level? 😀
Other useful tools beyond the sample size calculator
Now that you know how to calculate sample size, you can go beyond and use it to calculate other statistics of interest in your research:
Sampling error calculator : sample size is the most influential feature when predicting the sampling error. Use it to calculate the error of your sample.
Normal probability calculator for sampling distributions : use your sample size, along with the population mean and standard deviation, to find the probability that your sample mean falls within a specific range.
Sampling distribution of the sample proportion calculator : use your sample size and the population proportion to find the probability that your sample proportion falls within a specific range.
.css-m482sy.css-m482sy{color:#2B3148;background-color:transparent;font-family:var(--calculator-ui-font-family),Verdana,sans-serif;font-size:20px;line-height:24px;overflow:visible;padding-top:0px;position:relative;}.css-m482sy.css-m482sy:after{content:'';-webkit-transform:scale(0);-moz-transform:scale(0);-ms-transform:scale(0);transform:scale(0);position:absolute;border:2px solid #EA9430;border-radius:2px;inset:-8px;z-index:1;}.css-m482sy .js-external-link-button.link-like,.css-m482sy .js-external-link-anchor{color:inherit;border-radius:1px;-webkit-text-decoration:underline;text-decoration:underline;}.css-m482sy .js-external-link-button.link-like:hover,.css-m482sy .js-external-link-anchor:hover,.css-m482sy .js-external-link-button.link-like:active,.css-m482sy .js-external-link-anchor:active{text-decoration-thickness:2px;text-shadow:1px 0 0;}.css-m482sy .js-external-link-button.link-like:focus-visible,.css-m482sy .js-external-link-anchor:focus-visible{outline:transparent 2px dotted;box-shadow:0 0 0 2px #6314E6;}.css-m482sy p,.css-m482sy div{margin:0;display:block;}.css-m482sy pre{margin:0;display:block;}.css-m482sy pre code{display:block;width:-webkit-fit-content;width:-moz-fit-content;width:fit-content;}.css-m482sy pre:not(:first-child){padding-top:8px;}.css-m482sy ul,.css-m482sy ol{display:block margin:0;padding-left:20px;}.css-m482sy ul li,.css-m482sy ol li{padding-top:8px;}.css-m482sy ul ul,.css-m482sy ol ul,.css-m482sy ul ol,.css-m482sy ol ol{padding-top:0;}.css-m482sy ul:not(:first-child),.css-m482sy ol:not(:first-child){padding-top:4px;} .css-63uqft{margin:auto;background-color:white;overflow:auto;overflow-wrap:break-word;word-break:break-word;}.css-63uqft code,.css-63uqft kbd,.css-63uqft pre,.css-63uqft samp{font-family:monospace;}.css-63uqft code{padding:2px 4px;color:#444;background:#ddd;border-radius:4px;}.css-63uqft figcaption,.css-63uqft caption{text-align:center;}.css-63uqft figcaption{font-size:12px;font-style:italic;overflow:hidden;}.css-63uqft h3{font-size:1.75rem;}.css-63uqft h4{font-size:1.5rem;}.css-63uqft .mathBlock{font-size:24px;-webkit-padding-start:4px;padding-inline-start:4px;}.css-63uqft .mathBlock .katex{font-size:24px;text-align:left;}.css-63uqft .math-inline{background-color:#f0f0f0;display:inline-block;font-size:inherit;padding:0 3px;}.css-63uqft .videoBlock,.css-63uqft .imageBlock{margin-bottom:16px;}.css-63uqft .imageBlock__image-align--left,.css-63uqft .videoBlock__video-align--left{float:left;}.css-63uqft .imageBlock__image-align--right,.css-63uqft .videoBlock__video-align--right{float:right;}.css-63uqft .imageBlock__image-align--center,.css-63uqft .videoBlock__video-align--center{display:block;margin-left:auto;margin-right:auto;clear:both;}.css-63uqft .imageBlock__image-align--none,.css-63uqft .videoBlock__video-align--none{clear:both;margin-left:0;margin-right:0;}.css-63uqft .videoBlock__video--wrapper{position:relative;padding-bottom:56.25%;height:0;}.css-63uqft .videoBlock__video--wrapper iframe{position:absolute;top:0;left:0;width:100%;height:100%;}.css-63uqft .videoBlock__caption{text-align:left;}@font-face{font-family:'KaTeX_AMS';src:url(/katex-fonts/KaTeX_AMS-Regular.woff2) format('woff2'),url(/katex-fonts/KaTeX_AMS-Regular.woff) format('woff'),url(/katex-fonts/KaTeX_AMS-Regular.ttf) format('truetype');font-weight:normal;font-style:normal;}@font-face{font-family:'KaTeX_Caligraphic';src:url(/katex-fonts/KaTeX_Caligraphic-Bold.woff2) format('woff2'),url(/katex-fonts/KaTeX_Caligraphic-Bold.woff) format('woff'),url(/katex-fonts/KaTeX_Caligraphic-Bold.ttf) format('truetype');font-weight:bold;font-style:normal;}@font-face{font-family:'KaTeX_Caligraphic';src:url(/katex-fonts/KaTeX_Caligraphic-Regular.woff2) format('woff2'),url(/katex-fonts/KaTeX_Caligraphic-Regular.woff) format('woff'),url(/katex-fonts/KaTeX_Caligraphic-Regular.ttf) format('truetype');font-weight:normal;font-style:normal;}@font-face{font-family:'KaTeX_Fraktur';src:url(/katex-fonts/KaTeX_Fraktur-Bold.woff2) format('woff2'),url(/katex-fonts/KaTeX_Fraktur-Bold.woff) format('woff'),url(/katex-fonts/KaTeX_Fraktur-Bold.ttf) format('truetype');font-weight:bold;font-style:normal;}@font-face{font-family:'KaTeX_Fraktur';src:url(/katex-fonts/KaTeX_Fraktur-Regular.woff2) format('woff2'),url(/katex-fonts/KaTeX_Fraktur-Regular.woff) format('woff'),url(/katex-fonts/KaTeX_Fraktur-Regular.ttf) format('truetype');font-weight:normal;font-style:normal;}@font-face{font-family:'KaTeX_Main';src:url(/katex-fonts/KaTeX_Main-Bold.woff2) format('woff2'),url(/katex-fonts/KaTeX_Main-Bold.woff) format('woff'),url(/katex-fonts/KaTeX_Main-Bold.ttf) format('truetype');font-weight:bold;font-style:normal;}@font-face{font-family:'KaTeX_Main';src:url(/katex-fonts/KaTeX_Main-BoldItalic.woff2) format('woff2'),url(/katex-fonts/KaTeX_Main-BoldItalic.woff) format('woff'),url(/katex-fonts/KaTeX_Main-BoldItalic.ttf) format('truetype');font-weight:bold;font-style:italic;}@font-face{font-family:'KaTeX_Main';src:url(/katex-fonts/KaTeX_Main-Italic.woff2) format('woff2'),url(/katex-fonts/KaTeX_Main-Italic.woff) format('woff'),url(/katex-fonts/KaTeX_Main-Italic.ttf) format('truetype');font-weight:normal;font-style:italic;}@font-face{font-family:'KaTeX_Main';src:url(/katex-fonts/KaTeX_Main-Regular.woff2) format('woff2'),url(/katex-fonts/KaTeX_Main-Regular.woff) format('woff'),url(/katex-fonts/KaTeX_Main-Regular.ttf) format('truetype');font-weight:normal;font-style:normal;}@font-face{font-family:'KaTeX_Math';src:url(/katex-fonts/KaTeX_Math-BoldItalic.woff2) format('woff2'),url(/katex-fonts/KaTeX_Math-BoldItalic.woff) format('woff'),url(/katex-fonts/KaTeX_Math-BoldItalic.ttf) format('truetype');font-weight:bold;font-style:italic;}@font-face{font-family:'KaTeX_Math';src:url(/katex-fonts/KaTeX_Math-Italic.woff2) format('woff2'),url(/katex-fonts/KaTeX_Math-Italic.woff) format('woff'),url(/katex-fonts/KaTeX_Math-Italic.ttf) format('truetype');font-weight:normal;font-style:italic;}@font-face{font-family:'KaTeX_SansSerif';src:url(/katex-fonts/KaTeX_SansSerif-Bold.woff2) format('woff2'),url(/katex-fonts/KaTeX_SansSerif-Bold.woff) format('woff'),url(/katex-fonts/KaTeX_SansSerif-Bold.ttf) format('truetype');font-weight:bold;font-style:normal;}@font-face{font-family:'KaTeX_SansSerif';src:url(/katex-fonts/KaTeX_SansSerif-Italic.woff2) format('woff2'),url(/katex-fonts/KaTeX_SansSerif-Italic.woff) format('woff'),url(/katex-fonts/KaTeX_SansSerif-Italic.ttf) format('truetype');font-weight:normal;font-style:italic;}@font-face{font-family:'KaTeX_SansSerif';src:url(/katex-fonts/KaTeX_SansSerif-Regular.woff2) format('woff2'),url(/katex-fonts/KaTeX_SansSerif-Regular.woff) format('woff'),url(/katex-fonts/KaTeX_SansSerif-Regular.ttf) format('truetype');font-weight:normal;font-style:normal;}@font-face{font-family:'KaTeX_Script';src:url(/katex-fonts/KaTeX_Script-Regular.woff2) format('woff2'),url(/katex-fonts/KaTeX_Script-Regular.woff) format('woff'),url(/katex-fonts/KaTeX_Script-Regular.ttf) format('truetype');font-weight:normal;font-style:normal;}@font-face{font-family:'KaTeX_Size1';src:url(/katex-fonts/KaTeX_Size1-Regular.woff2) format('woff2'),url(/katex-fonts/KaTeX_Size1-Regular.woff) format('woff'),url(/katex-fonts/KaTeX_Size1-Regular.ttf) format('truetype');font-weight:normal;font-style:normal;}@font-face{font-family:'KaTeX_Size2';src:url(/katex-fonts/KaTeX_Size2-Regular.woff2) format('woff2'),url(/katex-fonts/KaTeX_Size2-Regular.woff) format('woff'),url(/katex-fonts/KaTeX_Size2-Regular.ttf) format('truetype');font-weight:normal;font-style:normal;}@font-face{font-family:'KaTeX_Size3';src:url(/katex-fonts/KaTeX_Size3-Regular.woff2) format('woff2'),url(/katex-fonts/KaTeX_Size3-Regular.woff) format('woff'),url(/katex-fonts/KaTeX_Size3-Regular.ttf) format('truetype');font-weight:normal;font-style:normal;}@font-face{font-family:'KaTeX_Size4';src:url(/katex-fonts/KaTeX_Size4-Regular.woff2) format('woff2'),url(/katex-fonts/KaTeX_Size4-Regular.woff) format('woff'),url(/katex-fonts/KaTeX_Size4-Regular.ttf) format('truetype');font-weight:normal;font-style:normal;}@font-face{font-family:'KaTeX_Typewriter';src:url(/katex-fonts/KaTeX_Typewriter-Regular.woff2) format('woff2'),url(/katex-fonts/KaTeX_Typewriter-Regular.woff) format('woff'),url(/katex-fonts/KaTeX_Typewriter-Regular.ttf) format('truetype');font-weight:normal;font-style:normal;}.css-63uqft .katex{font:normal 1.21em KaTeX_Main,Times New Roman,serif;line-height:1.2;text-indent:0;text-rendering:auto;}.css-63uqft .katex *{-ms-high-contrast-adjust:none!important;border-color:currentColor;}.css-63uqft .katex .katex-version::after{content:'0.13.13';}.css-63uqft .katex .katex-mathml{position:absolute;clip:rect(1px,1px,1px,1px);padding:0;border:0;height:1px;width:1px;overflow:hidden;}.css-63uqft .katex .katex-html>.newline{display:block;}.css-63uqft .katex .base{position:relative;display:inline-block;white-space:nowrap;width:-webkit-min-content;width:-moz-min-content;width:-webkit-min-content;width:-moz-min-content;width:min-content;}.css-63uqft .katex .strut{display:inline-block;}.css-63uqft .katex .textbf{font-weight:bold;}.css-63uqft .katex .textit{font-style:italic;}.css-63uqft .katex .textrm{font-family:KaTeX_Main;}.css-63uqft .katex .textsf{font-family:KaTeX_SansSerif;}.css-63uqft .katex .texttt{font-family:KaTeX_Typewriter;}.css-63uqft .katex .mathnormal{font-family:KaTeX_Math;font-style:italic;}.css-63uqft .katex .mathit{font-family:KaTeX_Main;font-style:italic;}.css-63uqft .katex .mathrm{font-style:normal;}.css-63uqft .katex .mathbf{font-family:KaTeX_Main;font-weight:bold;}.css-63uqft .katex .boldsymbol{font-family:KaTeX_Math;font-weight:bold;font-style:italic;}.css-63uqft .katex .amsrm{font-family:KaTeX_AMS;}.css-63uqft .katex .mathbb,.css-63uqft .katex .textbb{font-family:KaTeX_AMS;}.css-63uqft .katex .mathcal{font-family:KaTeX_Caligraphic;}.css-63uqft .katex .mathfrak,.css-63uqft .katex .textfrak{font-family:KaTeX_Fraktur;}.css-63uqft .katex .mathtt{font-family:KaTeX_Typewriter;}.css-63uqft .katex .mathscr,.css-63uqft .katex .textscr{font-family:KaTeX_Script;}.css-63uqft .katex .mathsf,.css-63uqft .katex .textsf{font-family:KaTeX_SansSerif;}.css-63uqft .katex .mathboldsf,.css-63uqft .katex .textboldsf{font-family:KaTeX_SansSerif;font-weight:bold;}.css-63uqft .katex .mathitsf,.css-63uqft .katex .textitsf{font-family:KaTeX_SansSerif;font-style:italic;}.css-63uqft .katex .mainrm{font-family:KaTeX_Main;font-style:normal;}.css-63uqft .katex .vlist-t{display:inline-table;table-layout:fixed;border-collapse:collapse;}.css-63uqft .katex .vlist-r{display:table-row;}.css-63uqft .katex .vlist{display:table-cell;vertical-align:bottom;position:relative;}.css-63uqft .katex .vlist>span{display:block;height:0;position:relative;}.css-63uqft .katex .vlist>span>span{display:inline-block;}.css-63uqft .katex .vlist>span>.pstrut{overflow:hidden;width:0;}.css-63uqft .katex .vlist-t2{margin-right:-2px;}.css-63uqft .katex .vlist-s{display:table-cell;vertical-align:bottom;font-size:1px;width:2px;min-width:2px;}.css-63uqft .katex .vbox{display:-webkit-inline-box;display:-webkit-inline-flex;display:-ms-inline-flexbox;display:inline-flex;-webkit-flex-direction:column;-ms-flex-direction:column;flex-direction:column;-webkit-align-items:baseline;-webkit-box-align:baseline;-ms-flex-align:baseline;align-items:baseline;}.css-63uqft .katex .hbox{display:-webkit-inline-box;display:-webkit-inline-flex;display:-ms-inline-flexbox;display:inline-flex;-webkit-flex-direction:row;-ms-flex-direction:row;flex-direction:row;width:100%;}.css-63uqft .katex .thinbox{display:-webkit-inline-box;display:-webkit-inline-flex;display:-ms-inline-flexbox;display:inline-flex;-webkit-flex-direction:row;-ms-flex-direction:row;flex-direction:row;width:0;max-width:0;}.css-63uqft .katex .msupsub{text-align:left;}.css-63uqft .katex .mfrac>span>span{text-align:center;}.css-63uqft .katex .mfrac .frac-line{display:inline-block;width:100%;border-bottom-style:solid;}.css-63uqft .katex .mfrac .frac-line,.css-63uqft .katex .overline .overline-line,.css-63uqft .katex .underline .underline-line,.css-63uqft .katex .hline,.css-63uqft .katex .hdashline,.css-63uqft .katex .rule{min-height:1px;}.css-63uqft .katex .mspace{display:inline-block;}.css-63uqft .katex .llap,.css-63uqft .katex .rlap,.css-63uqft .katex .clap{width:0;position:relative;}.css-63uqft .katex .llap>.inner,.css-63uqft .katex .rlap>.inner,.css-63uqft .katex .clap>.inner{position:absolute;}.css-63uqft .katex .llap>.fix,.css-63uqft .katex .rlap>.fix,.css-63uqft .katex .clap>.fix{display:inline-block;}.css-63uqft .katex .llap>.inner{right:0;}.css-63uqft .katex .rlap>.inner,.css-63uqft .katex .clap>.inner{left:0;}.css-63uqft .katex .clap>.inner>span{margin-left:-50%;margin-right:50%;}.css-63uqft .katex .rule{display:inline-block;border:solid 0;position:relative;}.css-63uqft .katex .overline .overline-line,.css-63uqft .katex .underline .underline-line,.css-63uqft .katex .hline{display:inline-block;width:100%;border-bottom-style:solid;}.css-63uqft .katex .hdashline{display:inline-block;width:100%;border-bottom-style:dashed;}.css-63uqft .katex .sqrt>.root{margin-left:0.27777778em;margin-right:-0.55555556em;}.css-63uqft .katex .sizing.reset-size1.size1,.css-63uqft .katex .fontsize-ensurer.reset-size1.size1{font-size:1em;}.css-63uqft .katex .sizing.reset-size1.size2,.css-63uqft .katex .fontsize-ensurer.reset-size1.size2{font-size:1.2em;}.css-63uqft .katex .sizing.reset-size1.size3,.css-63uqft .katex .fontsize-ensurer.reset-size1.size3{font-size:1.4em;}.css-63uqft .katex .sizing.reset-size1.size4,.css-63uqft .katex .fontsize-ensurer.reset-size1.size4{font-size:1.6em;}.css-63uqft .katex .sizing.reset-size1.size5,.css-63uqft .katex .fontsize-ensurer.reset-size1.size5{font-size:1.8em;}.css-63uqft .katex .sizing.reset-size1.size6,.css-63uqft .katex .fontsize-ensurer.reset-size1.size6{font-size:2em;}.css-63uqft .katex .sizing.reset-size1.size7,.css-63uqft .katex .fontsize-ensurer.reset-size1.size7{font-size:2.4em;}.css-63uqft .katex .sizing.reset-size1.size8,.css-63uqft .katex .fontsize-ensurer.reset-size1.size8{font-size:2.88em;}.css-63uqft .katex .sizing.reset-size1.size9,.css-63uqft .katex .fontsize-ensurer.reset-size1.size9{font-size:3.456em;}.css-63uqft .katex .sizing.reset-size1.size10,.css-63uqft .katex .fontsize-ensurer.reset-size1.size10{font-size:4.148em;}.css-63uqft .katex .sizing.reset-size1.size11,.css-63uqft .katex .fontsize-ensurer.reset-size1.size11{font-size:4.976em;}.css-63uqft .katex .sizing.reset-size2.size1,.css-63uqft .katex .fontsize-ensurer.reset-size2.size1{font-size:0.83333333em;}.css-63uqft .katex .sizing.reset-size2.size2,.css-63uqft .katex .fontsize-ensurer.reset-size2.size2{font-size:1em;}.css-63uqft .katex .sizing.reset-size2.size3,.css-63uqft .katex .fontsize-ensurer.reset-size2.size3{font-size:1.16666667em;}.css-63uqft .katex .sizing.reset-size2.size4,.css-63uqft .katex .fontsize-ensurer.reset-size2.size4{font-size:1.33333333em;}.css-63uqft .katex .sizing.reset-size2.size5,.css-63uqft .katex .fontsize-ensurer.reset-size2.size5{font-size:1.5em;}.css-63uqft .katex .sizing.reset-size2.size6,.css-63uqft .katex .fontsize-ensurer.reset-size2.size6{font-size:1.66666667em;}.css-63uqft .katex .sizing.reset-size2.size7,.css-63uqft .katex .fontsize-ensurer.reset-size2.size7{font-size:2em;}.css-63uqft .katex .sizing.reset-size2.size8,.css-63uqft .katex .fontsize-ensurer.reset-size2.size8{font-size:2.4em;}.css-63uqft .katex .sizing.reset-size2.size9,.css-63uqft .katex .fontsize-ensurer.reset-size2.size9{font-size:2.88em;}.css-63uqft .katex .sizing.reset-size2.size10,.css-63uqft .katex .fontsize-ensurer.reset-size2.size10{font-size:3.45666667em;}.css-63uqft .katex .sizing.reset-size2.size11,.css-63uqft .katex .fontsize-ensurer.reset-size2.size11{font-size:4.14666667em;}.css-63uqft .katex .sizing.reset-size3.size1,.css-63uqft .katex .fontsize-ensurer.reset-size3.size1{font-size:0.71428571em;}.css-63uqft .katex .sizing.reset-size3.size2,.css-63uqft .katex .fontsize-ensurer.reset-size3.size2{font-size:0.85714286em;}.css-63uqft .katex .sizing.reset-size3.size3,.css-63uqft .katex .fontsize-ensurer.reset-size3.size3{font-size:1em;}.css-63uqft .katex .sizing.reset-size3.size4,.css-63uqft .katex .fontsize-ensurer.reset-size3.size4{font-size:1.14285714em;}.css-63uqft .katex .sizing.reset-size3.size5,.css-63uqft .katex .fontsize-ensurer.reset-size3.size5{font-size:1.28571429em;}.css-63uqft .katex .sizing.reset-size3.size6,.css-63uqft .katex .fontsize-ensurer.reset-size3.size6{font-size:1.42857143em;}.css-63uqft .katex .sizing.reset-size3.size7,.css-63uqft .katex .fontsize-ensurer.reset-size3.size7{font-size:1.71428571em;}.css-63uqft .katex .sizing.reset-size3.size8,.css-63uqft .katex .fontsize-ensurer.reset-size3.size8{font-size:2.05714286em;}.css-63uqft .katex .sizing.reset-size3.size9,.css-63uqft .katex .fontsize-ensurer.reset-size3.size9{font-size:2.46857143em;}.css-63uqft .katex .sizing.reset-size3.size10,.css-63uqft .katex .fontsize-ensurer.reset-size3.size10{font-size:2.96285714em;}.css-63uqft .katex .sizing.reset-size3.size11,.css-63uqft .katex .fontsize-ensurer.reset-size3.size11{font-size:3.55428571em;}.css-63uqft .katex .sizing.reset-size4.size1,.css-63uqft .katex .fontsize-ensurer.reset-size4.size1{font-size:0.625em;}.css-63uqft .katex .sizing.reset-size4.size2,.css-63uqft .katex .fontsize-ensurer.reset-size4.size2{font-size:0.75em;}.css-63uqft .katex .sizing.reset-size4.size3,.css-63uqft .katex .fontsize-ensurer.reset-size4.size3{font-size:0.875em;}.css-63uqft .katex .sizing.reset-size4.size4,.css-63uqft .katex .fontsize-ensurer.reset-size4.size4{font-size:1em;}.css-63uqft .katex .sizing.reset-size4.size5,.css-63uqft .katex .fontsize-ensurer.reset-size4.size5{font-size:1.125em;}.css-63uqft .katex .sizing.reset-size4.size6,.css-63uqft .katex .fontsize-ensurer.reset-size4.size6{font-size:1.25em;}.css-63uqft .katex .sizing.reset-size4.size7,.css-63uqft .katex .fontsize-ensurer.reset-size4.size7{font-size:1.5em;}.css-63uqft .katex .sizing.reset-size4.size8,.css-63uqft .katex .fontsize-ensurer.reset-size4.size8{font-size:1.8em;}.css-63uqft .katex .sizing.reset-size4.size9,.css-63uqft .katex .fontsize-ensurer.reset-size4.size9{font-size:2.16em;}.css-63uqft .katex .sizing.reset-size4.size10,.css-63uqft .katex .fontsize-ensurer.reset-size4.size10{font-size:2.5925em;}.css-63uqft .katex .sizing.reset-size4.size11,.css-63uqft .katex .fontsize-ensurer.reset-size4.size11{font-size:3.11em;}.css-63uqft .katex .sizing.reset-size5.size1,.css-63uqft .katex .fontsize-ensurer.reset-size5.size1{font-size:0.55555556em;}.css-63uqft .katex .sizing.reset-size5.size2,.css-63uqft .katex .fontsize-ensurer.reset-size5.size2{font-size:0.66666667em;}.css-63uqft .katex .sizing.reset-size5.size3,.css-63uqft .katex .fontsize-ensurer.reset-size5.size3{font-size:0.77777778em;}.css-63uqft .katex .sizing.reset-size5.size4,.css-63uqft .katex .fontsize-ensurer.reset-size5.size4{font-size:0.88888889em;}.css-63uqft .katex .sizing.reset-size5.size5,.css-63uqft .katex .fontsize-ensurer.reset-size5.size5{font-size:1em;}.css-63uqft .katex .sizing.reset-size5.size6,.css-63uqft .katex .fontsize-ensurer.reset-size5.size6{font-size:1.11111111em;}.css-63uqft .katex .sizing.reset-size5.size7,.css-63uqft .katex .fontsize-ensurer.reset-size5.size7{font-size:1.33333333em;}.css-63uqft .katex .sizing.reset-size5.size8,.css-63uqft .katex .fontsize-ensurer.reset-size5.size8{font-size:1.6em;}.css-63uqft .katex .sizing.reset-size5.size9,.css-63uqft .katex .fontsize-ensurer.reset-size5.size9{font-size:1.92em;}.css-63uqft .katex .sizing.reset-size5.size10,.css-63uqft .katex .fontsize-ensurer.reset-size5.size10{font-size:2.30444444em;}.css-63uqft .katex .sizing.reset-size5.size11,.css-63uqft .katex .fontsize-ensurer.reset-size5.size11{font-size:2.76444444em;}.css-63uqft .katex .sizing.reset-size6.size1,.css-63uqft .katex .fontsize-ensurer.reset-size6.size1{font-size:0.5em;}.css-63uqft .katex .sizing.reset-size6.size2,.css-63uqft .katex .fontsize-ensurer.reset-size6.size2{font-size:0.6em;}.css-63uqft .katex .sizing.reset-size6.size3,.css-63uqft .katex .fontsize-ensurer.reset-size6.size3{font-size:0.7em;}.css-63uqft .katex .sizing.reset-size6.size4,.css-63uqft .katex .fontsize-ensurer.reset-size6.size4{font-size:0.8em;}.css-63uqft .katex .sizing.reset-size6.size5,.css-63uqft .katex .fontsize-ensurer.reset-size6.size5{font-size:0.9em;}.css-63uqft .katex .sizing.reset-size6.size6,.css-63uqft .katex .fontsize-ensurer.reset-size6.size6{font-size:1em;}.css-63uqft .katex .sizing.reset-size6.size7,.css-63uqft .katex .fontsize-ensurer.reset-size6.size7{font-size:1.2em;}.css-63uqft .katex .sizing.reset-size6.size8,.css-63uqft .katex .fontsize-ensurer.reset-size6.size8{font-size:1.44em;}.css-63uqft .katex .sizing.reset-size6.size9,.css-63uqft .katex .fontsize-ensurer.reset-size6.size9{font-size:1.728em;}.css-63uqft .katex .sizing.reset-size6.size10,.css-63uqft .katex .fontsize-ensurer.reset-size6.size10{font-size:2.074em;}.css-63uqft .katex .sizing.reset-size6.size11,.css-63uqft .katex .fontsize-ensurer.reset-size6.size11{font-size:2.488em;}.css-63uqft .katex .sizing.reset-size7.size1,.css-63uqft .katex .fontsize-ensurer.reset-size7.size1{font-size:0.41666667em;}.css-63uqft .katex .sizing.reset-size7.size2,.css-63uqft .katex .fontsize-ensurer.reset-size7.size2{font-size:0.5em;}.css-63uqft .katex .sizing.reset-size7.size3,.css-63uqft .katex .fontsize-ensurer.reset-size7.size3{font-size:0.58333333em;}.css-63uqft .katex .sizing.reset-size7.size4,.css-63uqft .katex .fontsize-ensurer.reset-size7.size4{font-size:0.66666667em;}.css-63uqft .katex .sizing.reset-size7.size5,.css-63uqft .katex .fontsize-ensurer.reset-size7.size5{font-size:0.75em;}.css-63uqft .katex .sizing.reset-size7.size6,.css-63uqft .katex .fontsize-ensurer.reset-size7.size6{font-size:0.83333333em;}.css-63uqft .katex .sizing.reset-size7.size7,.css-63uqft .katex .fontsize-ensurer.reset-size7.size7{font-size:1em;}.css-63uqft .katex .sizing.reset-size7.size8,.css-63uqft .katex .fontsize-ensurer.reset-size7.size8{font-size:1.2em;}.css-63uqft .katex .sizing.reset-size7.size9,.css-63uqft .katex .fontsize-ensurer.reset-size7.size9{font-size:1.44em;}.css-63uqft .katex .sizing.reset-size7.size10,.css-63uqft .katex .fontsize-ensurer.reset-size7.size10{font-size:1.72833333em;}.css-63uqft .katex .sizing.reset-size7.size11,.css-63uqft .katex .fontsize-ensurer.reset-size7.size11{font-size:2.07333333em;}.css-63uqft .katex .sizing.reset-size8.size1,.css-63uqft .katex .fontsize-ensurer.reset-size8.size1{font-size:0.34722222em;}.css-63uqft .katex .sizing.reset-size8.size2,.css-63uqft .katex .fontsize-ensurer.reset-size8.size2{font-size:0.41666667em;}.css-63uqft .katex .sizing.reset-size8.size3,.css-63uqft .katex .fontsize-ensurer.reset-size8.size3{font-size:0.48611111em;}.css-63uqft .katex .sizing.reset-size8.size4,.css-63uqft .katex .fontsize-ensurer.reset-size8.size4{font-size:0.55555556em;}.css-63uqft .katex .sizing.reset-size8.size5,.css-63uqft .katex .fontsize-ensurer.reset-size8.size5{font-size:0.625em;}.css-63uqft .katex .sizing.reset-size8.size6,.css-63uqft .katex .fontsize-ensurer.reset-size8.size6{font-size:0.69444444em;}.css-63uqft .katex .sizing.reset-size8.size7,.css-63uqft .katex .fontsize-ensurer.reset-size8.size7{font-size:0.83333333em;}.css-63uqft .katex .sizing.reset-size8.size8,.css-63uqft .katex .fontsize-ensurer.reset-size8.size8{font-size:1em;}.css-63uqft .katex .sizing.reset-size8.size9,.css-63uqft .katex .fontsize-ensurer.reset-size8.size9{font-size:1.2em;}.css-63uqft .katex .sizing.reset-size8.size10,.css-63uqft .katex .fontsize-ensurer.reset-size8.size10{font-size:1.44027778em;}.css-63uqft .katex .sizing.reset-size8.size11,.css-63uqft .katex .fontsize-ensurer.reset-size8.size11{font-size:1.72777778em;}.css-63uqft .katex .sizing.reset-size9.size1,.css-63uqft .katex .fontsize-ensurer.reset-size9.size1{font-size:0.28935185em;}.css-63uqft .katex .sizing.reset-size9.size2,.css-63uqft .katex .fontsize-ensurer.reset-size9.size2{font-size:0.34722222em;}.css-63uqft .katex .sizing.reset-size9.size3,.css-63uqft .katex .fontsize-ensurer.reset-size9.size3{font-size:0.40509259em;}.css-63uqft .katex .sizing.reset-size9.size4,.css-63uqft .katex .fontsize-ensurer.reset-size9.size4{font-size:0.46296296em;}.css-63uqft .katex .sizing.reset-size9.size5,.css-63uqft .katex .fontsize-ensurer.reset-size9.size5{font-size:0.52083333em;}.css-63uqft .katex .sizing.reset-size9.size6,.css-63uqft .katex .fontsize-ensurer.reset-size9.size6{font-size:0.5787037em;}.css-63uqft .katex .sizing.reset-size9.size7,.css-63uqft .katex .fontsize-ensurer.reset-size9.size7{font-size:0.69444444em;}.css-63uqft .katex .sizing.reset-size9.size8,.css-63uqft .katex .fontsize-ensurer.reset-size9.size8{font-size:0.83333333em;}.css-63uqft .katex .sizing.reset-size9.size9,.css-63uqft .katex .fontsize-ensurer.reset-size9.size9{font-size:1em;}.css-63uqft .katex .sizing.reset-size9.size10,.css-63uqft .katex .fontsize-ensurer.reset-size9.size10{font-size:1.20023148em;}.css-63uqft .katex .sizing.reset-size9.size11,.css-63uqft .katex .fontsize-ensurer.reset-size9.size11{font-size:1.43981481em;}.css-63uqft .katex .sizing.reset-size10.size1,.css-63uqft .katex .fontsize-ensurer.reset-size10.size1{font-size:0.24108004em;}.css-63uqft .katex .sizing.reset-size10.size2,.css-63uqft .katex .fontsize-ensurer.reset-size10.size2{font-size:0.28929605em;}.css-63uqft .katex .sizing.reset-size10.size3,.css-63uqft .katex .fontsize-ensurer.reset-size10.size3{font-size:0.33751205em;}.css-63uqft .katex .sizing.reset-size10.size4,.css-63uqft .katex .fontsize-ensurer.reset-size10.size4{font-size:0.38572806em;}.css-63uqft .katex .sizing.reset-size10.size5,.css-63uqft .katex .fontsize-ensurer.reset-size10.size5{font-size:0.43394407em;}.css-63uqft .katex .sizing.reset-size10.size6,.css-63uqft .katex .fontsize-ensurer.reset-size10.size6{font-size:0.48216008em;}.css-63uqft .katex .sizing.reset-size10.size7,.css-63uqft .katex .fontsize-ensurer.reset-size10.size7{font-size:0.57859209em;}.css-63uqft .katex .sizing.reset-size10.size8,.css-63uqft .katex .fontsize-ensurer.reset-size10.size8{font-size:0.69431051em;}.css-63uqft .katex .sizing.reset-size10.size9,.css-63uqft .katex .fontsize-ensurer.reset-size10.size9{font-size:0.83317261em;}.css-63uqft .katex .sizing.reset-size10.size10,.css-63uqft .katex .fontsize-ensurer.reset-size10.size10{font-size:1em;}.css-63uqft .katex .sizing.reset-size10.size11,.css-63uqft .katex .fontsize-ensurer.reset-size10.size11{font-size:1.19961427em;}.css-63uqft .katex .sizing.reset-size11.size1,.css-63uqft .katex .fontsize-ensurer.reset-size11.size1{font-size:0.20096463em;}.css-63uqft .katex .sizing.reset-size11.size2,.css-63uqft .katex .fontsize-ensurer.reset-size11.size2{font-size:0.24115756em;}.css-63uqft .katex .sizing.reset-size11.size3,.css-63uqft .katex .fontsize-ensurer.reset-size11.size3{font-size:0.28135048em;}.css-63uqft .katex .sizing.reset-size11.size4,.css-63uqft .katex .fontsize-ensurer.reset-size11.size4{font-size:0.32154341em;}.css-63uqft .katex .sizing.reset-size11.size5,.css-63uqft .katex .fontsize-ensurer.reset-size11.size5{font-size:0.36173633em;}.css-63uqft .katex .sizing.reset-size11.size6,.css-63uqft .katex .fontsize-ensurer.reset-size11.size6{font-size:0.40192926em;}.css-63uqft .katex .sizing.reset-size11.size7,.css-63uqft .katex .fontsize-ensurer.reset-size11.size7{font-size:0.48231511em;}.css-63uqft .katex .sizing.reset-size11.size8,.css-63uqft .katex .fontsize-ensurer.reset-size11.size8{font-size:0.57877814em;}.css-63uqft .katex .sizing.reset-size11.size9,.css-63uqft .katex .fontsize-ensurer.reset-size11.size9{font-size:0.69453376em;}.css-63uqft .katex .sizing.reset-size11.size10,.css-63uqft .katex .fontsize-ensurer.reset-size11.size10{font-size:0.83360129em;}.css-63uqft .katex .sizing.reset-size11.size11,.css-63uqft .katex .fontsize-ensurer.reset-size11.size11{font-size:1em;}.css-63uqft .katex .delimsizing.size1{font-family:KaTeX_Size1;}.css-63uqft .katex .delimsizing.size2{font-family:KaTeX_Size2;}.css-63uqft .katex .delimsizing.size3{font-family:KaTeX_Size3;}.css-63uqft .katex .delimsizing.size4{font-family:KaTeX_Size4;}.css-63uqft .katex .delimsizing.mult .delim-size1>span{font-family:KaTeX_Size1;}.css-63uqft .katex .delimsizing.mult .delim-size4>span{font-family:KaTeX_Size4;}.css-63uqft .katex .nulldelimiter{display:inline-block;width:0.12em;}.css-63uqft .katex .delimcenter{position:relative;}.css-63uqft .katex .op-symbol{position:relative;}.css-63uqft .katex .op-symbol.small-op{font-family:KaTeX_Size1;}.css-63uqft .katex .op-symbol.large-op{font-family:KaTeX_Size2;}.css-63uqft .katex .op-limits>.vlist-t{text-align:center;}.css-63uqft .katex .accent>.vlist-t{text-align:center;}.css-63uqft .katex .accent .accent-body{position:relative;}.css-63uqft .katex .accent .accent-body:not(.accent-full){width:0;}.css-63uqft .katex .overlay{display:block;}.css-63uqft .katex .mtable .vertical-separator{display:inline-block;min-width:1px;}.css-63uqft .katex .mtable .arraycolsep{display:inline-block;}.css-63uqft .katex .mtable .col-align-c>.vlist-t{text-align:center;}.css-63uqft .katex .mtable .col-align-l>.vlist-t{text-align:left;}.css-63uqft .katex .mtable .col-align-r>.vlist-t{text-align:right;}.css-63uqft .katex .svg-align{text-align:left;}.css-63uqft .katex svg{display:block;position:absolute;width:100%;height:inherit;fill:currentColor;stroke:currentColor;fill-rule:nonzero;fill-opacity:1;stroke-width:1;stroke-linecap:butt;stroke-linejoin:miter;stroke-miterlimit:4;stroke-dasharray:none;stroke-dashoffset:0;stroke-opacity:1;}.css-63uqft .katex svg path{stroke:none;}.css-63uqft .katex img{border-style:none;min-width:0;min-height:0;max-width:none;max-height:none;}.css-63uqft .katex .stretchy{width:100%;display:block;position:relative;overflow:hidden;}.css-63uqft .katex .stretchy::before,.css-63uqft .katex .stretchy::after{content:'';}.css-63uqft .katex .hide-tail{width:100%;position:relative;overflow:hidden;}.css-63uqft .katex .halfarrow-left{position:absolute;left:0;width:50.2%;overflow:hidden;}.css-63uqft .katex .halfarrow-right{position:absolute;right:0;width:50.2%;overflow:hidden;}.css-63uqft .katex .brace-left{position:absolute;left:0;width:25.1%;overflow:hidden;}.css-63uqft .katex .brace-center{position:absolute;left:25%;width:50%;overflow:hidden;}.css-63uqft .katex .brace-right{position:absolute;right:0;width:25.1%;overflow:hidden;}.css-63uqft .katex .x-arrow-pad{padding:0 0.5em;}.css-63uqft .katex .cd-arrow-pad{padding:0 0.55556em 0 0.27778em;}.css-63uqft .katex .x-arrow,.css-63uqft .katex .mover,.css-63uqft .katex .munder{text-align:center;}.css-63uqft .katex .boxpad{padding:0 0.3em 0 0.3em;}.css-63uqft .katex .fbox,.css-63uqft .katex .fcolorbox{box-sizing:border-box;border:0.04em solid;}.css-63uqft .katex .cancel-pad{padding:0 0.2em 0 0.2em;}.css-63uqft .katex .cancel-lap{margin-left:-0.2em;margin-right:-0.2em;}.css-63uqft .katex .sout{border-bottom-style:solid;border-bottom-width:0.08em;}.css-63uqft .katex .angl{box-sizing:border-box;border-top:0.049em solid;border-right:0.049em solid;margin-right:0.03889em;}.css-63uqft .katex .anglpad{padding:0 0.03889em 0 0.03889em;}.css-63uqft .katex .eqn-num::before{counter-increment:katexEqnNo;content:'(' counter(katexEqnNo) ')';}.css-63uqft .katex .mml-eqn-num::before{counter-increment:mmlEqnNo;content:'(' counter(mmlEqnNo) ')';}.css-63uqft .katex .mtr-glue{width:50%;}.css-63uqft .katex .cd-vert-arrow{display:inline-block;position:relative;}.css-63uqft .katex .cd-label-left{display:inline-block;position:absolute;right:calc(50% + 0.3em);text-align:left;}.css-63uqft .katex .cd-label-right{display:inline-block;position:absolute;left:calc(50% + 0.3em);text-align:right;}.css-63uqft .katex-display{display:block;margin:1em 0;text-align:center;}.css-63uqft .katex-display>.katex{display:block;white-space:nowrap;}.css-63uqft .katex-display>.katex>.katex-html{display:block;position:relative;}.css-63uqft .katex-display>.katex>.katex-html>.tag{position:absolute;right:0;}.css-63uqft .katex-display.leqno>.katex>.katex-html>.tag{left:0;right:auto;}.css-63uqft .katex-display.fleqn>.katex{text-align:left;padding-left:2em;}.css-63uqft body{counter-reset:katexEqnNo mmlEqnNo;}.css-63uqft table{width:-webkit-max-content;width:-moz-max-content;width:max-content;}.css-63uqft .tableBlock{max-width:100%;margin-bottom:1rem;overflow-y:scroll;}.css-63uqft .tableBlock thead,.css-63uqft .tableBlock thead th{border-bottom:1px solid #333!important;}.css-63uqft .tableBlock th,.css-63uqft .tableBlock td{padding:10px;text-align:left;}.css-63uqft .tableBlock th{font-weight:bold!important;}.css-63uqft .tableBlock caption{caption-side:bottom;color:#555;font-size:12px;font-style:italic;text-align:center;}.css-63uqft .tableBlock caption>p{margin:0;}.css-63uqft .tableBlock th>p,.css-63uqft .tableBlock td>p{margin:0;}.css-63uqft .tableBlock [data-background-color='aliceblue']{background-color:#f0f8ff;color:#000;}.css-63uqft .tableBlock [data-background-color='black']{background-color:#000;color:#fff;}.css-63uqft .tableBlock [data-background-color='chocolate']{background-color:#d2691e;color:#fff;}.css-63uqft .tableBlock [data-background-color='cornflowerblue']{background-color:#6495ed;color:#fff;}.css-63uqft .tableBlock [data-background-color='crimson']{background-color:#dc143c;color:#fff;}.css-63uqft .tableBlock [data-background-color='darkblue']{background-color:#00008b;color:#fff;}.css-63uqft .tableBlock [data-background-color='darkseagreen']{background-color:#8fbc8f;color:#000;}.css-63uqft .tableBlock [data-background-color='deepskyblue']{background-color:#00bfff;color:#000;}.css-63uqft .tableBlock [data-background-color='gainsboro']{background-color:#dcdcdc;color:#000;}.css-63uqft .tableBlock [data-background-color='grey']{background-color:#808080;color:#fff;}.css-63uqft .tableBlock [data-background-color='lemonchiffon']{background-color:#fffacd;color:#000;}.css-63uqft .tableBlock [data-background-color='lightpink']{background-color:#ffb6c1;color:#000;}.css-63uqft .tableBlock [data-background-color='lightsalmon']{background-color:#ffa07a;color:#000;}.css-63uqft .tableBlock [data-background-color='lightskyblue']{background-color:#87cefa;color:#000;}.css-63uqft .tableBlock [data-background-color='mediumblue']{background-color:#0000cd;color:#fff;}.css-63uqft .tableBlock [data-background-color='omnigrey']{background-color:#f0f0f0;color:#000;}.css-63uqft .tableBlock [data-background-color='white']{background-color:#fff;color:#000;}.css-63uqft .tableBlock [data-text-align='center']{text-align:center;}.css-63uqft .tableBlock [data-text-align='left']{text-align:left;}.css-63uqft .tableBlock [data-text-align='right']{text-align:right;}.css-63uqft .tableBlock [data-vertical-align='bottom']{vertical-align:bottom;}.css-63uqft .tableBlock [data-vertical-align='middle']{vertical-align:middle;}.css-63uqft .tableBlock [data-vertical-align='top']{vertical-align:top;}.css-63uqft .tableBlock__font-size--xxsmall{font-size:10px;}.css-63uqft .tableBlock__font-size--xsmall{font-size:12px;}.css-63uqft .tableBlock__font-size--small{font-size:14px;}.css-63uqft .tableBlock__font-size--large{font-size:18px;}.css-63uqft .tableBlock__border--some tbody tr:not(:last-child){border-bottom:1px solid #e2e5e7;}.css-63uqft .tableBlock__border--bordered td,.css-63uqft .tableBlock__border--bordered th{border:1px solid #e2e5e7;}.css-63uqft .tableBlock__border--borderless tbody+tbody,.css-63uqft .tableBlock__border--borderless td,.css-63uqft .tableBlock__border--borderless th,.css-63uqft .tableBlock__border--borderless tr,.css-63uqft .tableBlock__border--borderless thead,.css-63uqft .tableBlock__border--borderless thead th{border:0!important;}.css-63uqft .tableBlock:not(.tableBlock__table-striped) tbody tr{background-color:unset!important;}.css-63uqft .tableBlock__table-striped tbody tr:nth-of-type(odd){background-color:#f9fafc!important;}.css-63uqft .tableBlock__table-compactl th,.css-63uqft .tableBlock__table-compact td{padding:3px!important;}.css-63uqft .tableBlock__full-size{width:100%;}.css-63uqft .textBlock{margin-bottom:16px;}.css-63uqft .textBlock__text-formatting--finePrint{font-size:12px;}.css-63uqft .textBlock__text-infoBox{padding:0.75rem 1.25rem;margin-bottom:1rem;border:1px solid transparent;border-radius:0.25rem;}.css-63uqft .textBlock__text-infoBox p{margin:0;}.css-63uqft .textBlock__text-infoBox--primary{background-color:#cce5ff;border-color:#b8daff;color:#004085;}.css-63uqft .textBlock__text-infoBox--secondary{background-color:#e2e3e5;border-color:#d6d8db;color:#383d41;}.css-63uqft .textBlock__text-infoBox--success{background-color:#d4edda;border-color:#c3e6cb;color:#155724;}.css-63uqft .textBlock__text-infoBox--danger{background-color:#f8d7da;border-color:#f5c6cb;color:#721c24;}.css-63uqft .textBlock__text-infoBox--warning{background-color:#fff3cd;border-color:#ffeeba;color:#856404;}.css-63uqft .textBlock__text-infoBox--info{background-color:#d1ecf1;border-color:#bee5eb;color:#0c5460;}.css-63uqft .textBlock__text-infoBox--dark{background-color:#d6d8d9;border-color:#c6c8ca;color:#1b1e21;}.css-63uqft .text-overline{-webkit-text-decoration:overline;text-decoration:overline;}.css-63uqft.css-63uqft{color:#2B3148;background-color:transparent;font-family:var(--calculator-ui-font-family),Verdana,sans-serif;font-size:20px;line-height:24px;overflow:visible;padding-top:0px;position:relative;}.css-63uqft.css-63uqft:after{content:'';-webkit-transform:scale(0);-moz-transform:scale(0);-ms-transform:scale(0);transform:scale(0);position:absolute;border:2px solid #EA9430;border-radius:2px;inset:-8px;z-index:1;}.css-63uqft .js-external-link-button.link-like,.css-63uqft .js-external-link-anchor{color:inherit;border-radius:1px;-webkit-text-decoration:underline;text-decoration:underline;}.css-63uqft .js-external-link-button.link-like:hover,.css-63uqft .js-external-link-anchor:hover,.css-63uqft .js-external-link-button.link-like:active,.css-63uqft .js-external-link-anchor:active{text-decoration-thickness:2px;text-shadow:1px 0 0;}.css-63uqft .js-external-link-button.link-like:focus-visible,.css-63uqft .js-external-link-anchor:focus-visible{outline:transparent 2px dotted;box-shadow:0 0 0 2px #6314E6;}.css-63uqft p,.css-63uqft div{margin:0;display:block;}.css-63uqft pre{margin:0;display:block;}.css-63uqft pre code{display:block;width:-webkit-fit-content;width:-moz-fit-content;width:fit-content;}.css-63uqft pre:not(:first-child){padding-top:8px;}.css-63uqft ul,.css-63uqft ol{display:block margin:0;padding-left:20px;}.css-63uqft ul li,.css-63uqft ol li{padding-top:8px;}.css-63uqft ul ul,.css-63uqft ol ul,.css-63uqft ul ol,.css-63uqft ol ol{padding-top:0;}.css-63uqft ul:not(:first-child),.css-63uqft ol:not(:first-child){padding-top:4px;} Statistical parameters
Confidence level
Margin of error
Proportion estimate
Your initial estimate of the result you will obtain.
Sample size
Correction for finite population
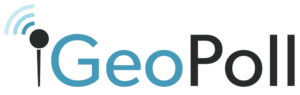
How to Determine Sample Size for a Research Study
Frankline kibuacha | apr. 06, 2021 | 3 min. read.
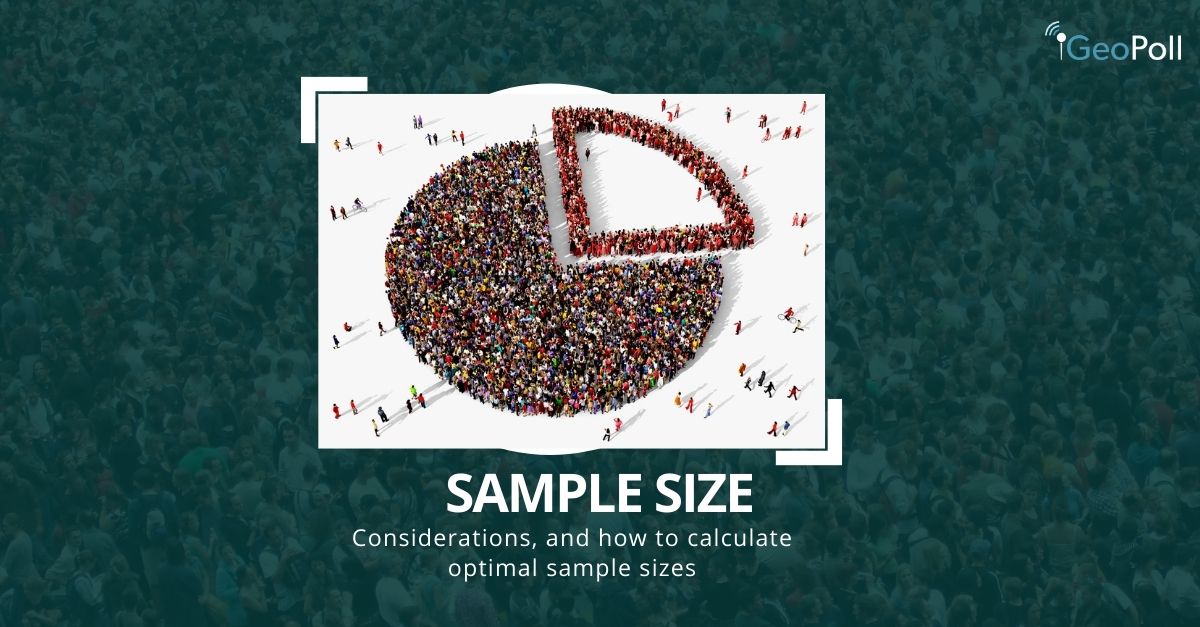
This article will discuss considerations to put in place when determining your sample size and how to calculate the sample size.
Confidence Interval and Confidence Level
As we have noted before, when selecting a sample there are multiple factors that can impact the reliability and validity of results, including sampling and non-sampling errors . When thinking about sample size, the two measures of error that are almost always synonymous with sample sizes are the confidence interval and the confidence level.
Confidence Interval (Margin of Error)
Confidence intervals measure the degree of uncertainty or certainty in a sampling method and how much uncertainty there is with any particular statistic. In simple terms, the confidence interval tells you how confident you can be that the results from a study reflect what you would expect to find if it were possible to survey the entire population being studied. The confidence interval is usually a plus or minus (±) figure. For example, if your confidence interval is 6 and 60% percent of your sample picks an answer, you can be confident that if you had asked the entire population, between 54% (60-6) and 66% (60+6) would have picked that answer.
Confidence Level
The confidence level refers to the percentage of probability, or certainty that the confidence interval would contain the true population parameter when you draw a random sample many times. It is expressed as a percentage and represents how often the percentage of the population who would pick an answer lies within the confidence interval. For example, a 99% confidence level means that should you repeat an experiment or survey over and over again, 99 percent of the time, your results will match the results you get from a population.
The larger your sample size, the more confident you can be that their answers truly reflect the population. In other words, the larger your sample for a given confidence level, the smaller your confidence interval.
Standard Deviation
Another critical measure when determining the sample size is the standard deviation, which measures a data set’s distribution from its mean. In calculating the sample size, the standard deviation is useful in estimating how much the responses you receive will vary from each other and from the mean number, and the standard deviation of a sample can be used to approximate the standard deviation of a population.
The higher the distribution or variability, the greater the standard deviation and the greater the magnitude of the deviation. For example, once you have already sent out your survey, how much variance do you expect in your responses? That variation in responses is the standard deviation.
Population Size
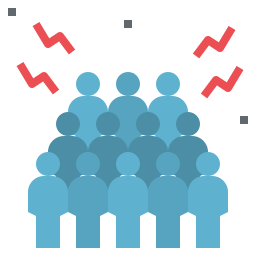
As demonstrated through the calculation below, a sample size of about 385 will give you a sufficient sample size to draw assumptions of nearly any population size at the 95% confidence level with a 5% margin of error, which is why samples of 400 and 500 are often used in research. However, if you are looking to draw comparisons between different sub-groups, for example, provinces within a country, a larger sample size is required. GeoPoll typically recommends a sample size of 400 per country as the minimum viable sample for a research project, 800 per country for conducting a study with analysis by a second-level breakdown such as females versus males, and 1200+ per country for doing third-level breakdowns such as males aged 18-24 in Nairobi.
How to Calculate Sample Size
As we have defined all the necessary terms, let us briefly learn how to determine the sample size using a sample calculation formula known as Andrew Fisher’s Formula.
- Determine the population size (if known).
- Determine the confidence interval.
- Determine the confidence level.
- Determine the standard deviation ( a standard deviation of 0.5 is a safe choice where the figure is unknown )
- Convert the confidence level into a Z-Score. This table shows the z-scores for the most common confidence levels:
80% | 1.28 | |
85% | 1.44 | |
90% | 1.65 | |
95% | 1.96 | |
99% | 2.58 |
- Put these figures into the sample size formula to get your sample size.
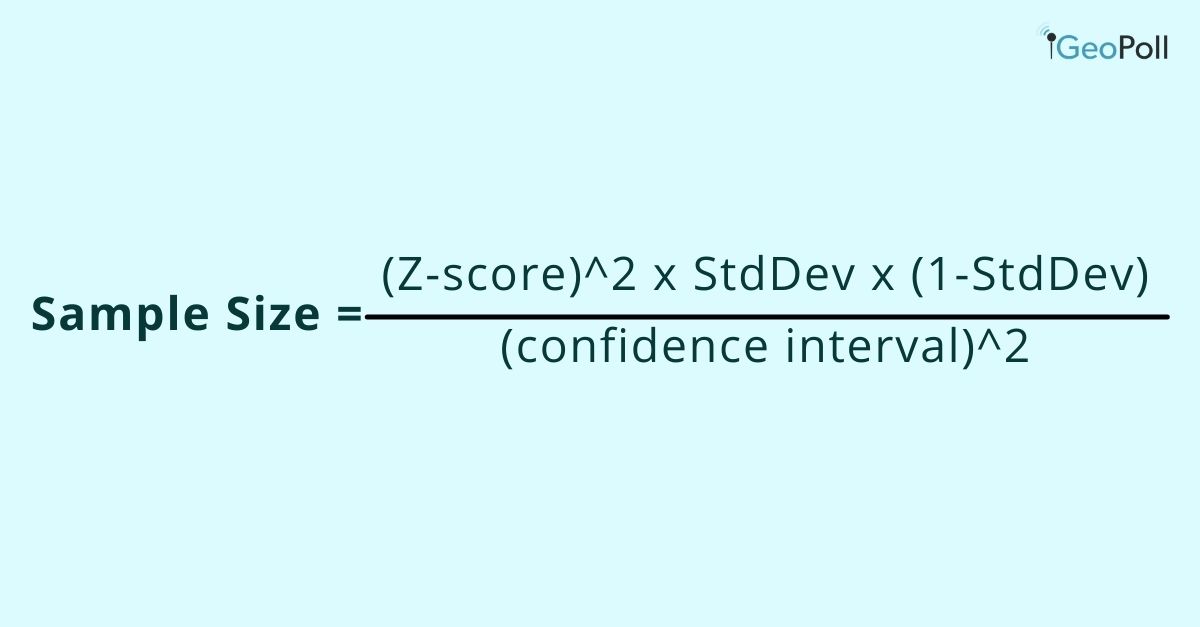
Here is an example calculation:
Say you choose to work with a 95% confidence level, a standard deviation of 0.5, and a confidence interval (margin of error) of ± 5%, you just need to substitute the values in the formula:
((1.96)2 x .5(.5)) / (.05)2
(3.8416 x .25) / .0025
.9604 / .0025
Your sample size should be 385.
Fortunately, there are several available online tools to help you with this calculation. Here’s an online sample calculator from Easy Calculation. Just put in the confidence level, population size, the confidence interval, and the perfect sample size is calculated for you.
GeoPoll’s Sampling Techniques
With the largest mobile panel in Africa, Asia, and Latin America, and reliable mobile technologies, GeoPoll develops unique samples that accurately represent any population. See our country coverage here , or contact our team to discuss your upcoming project.
Related Posts
Sample Frame and Sample Error
Probability and Non-Probability Samples
How GeoPoll Conducts Nationally Representative Surveys
- Tags market research , Market Research Methods , sample size , survey methodology
Calculate your ideal sample size
Last updated
11 May 2023
Reviewed by
Miroslav Damyanov
Whether you're conducting market research , medical trials, or social science studies, understanding how to calculate your ideal sample size is essential for a successful research project .
Make research less tedious
Dovetail streamlines research to help you uncover and share actionable insights
- What is a sample size?
The sample size is the number of participants or data points a researcher needs to collect to make inferences about a larger population. Researchers use sample size to conclude a population.
For example, if a researcher wants to know the average height of adult males in the United States, the population would be all adult males in the US. The researcher would collect a sample of adult males, measure their height, and use this information to estimate the average size of all adult males in the US.
- Understanding sample size
Comprehending sample size is vital for conducting meaningful research that provides reliable and accurate results. Here are some key concepts that can help you better understand this critical aspect of research:
Representativeness
A sample is considered emblematic if it accurately reflects the population characteristics from which it’s drawn. To ensure representativeness, researchers should use appropriate sampling methods , such as random or stratified sampling .
Confidence level
This is the degree of certainty the results obtained from a sample accurately represent the population. A confidence level of 95% means there is a 95% chance the population parameter falls within the confidence interval .
The margin of error
The margin of error is the sampling error expected in the results due to using a cross-section instead of the entire population.
This refers to the ability of a study to detect an actual effect—if it exists. A study with high power has a greater chance of detecting a significant impact, while a study with low power may fail to see the effect, even if it exists.
Effect size
This is the magnitude of the difference between groups or the strength of the relationship between variables. The larger the effect size, the stronger the relationship.
By selecting an appropriate sample size, researchers can ensure their findings represent the population being studied and have the necessary level of precision and confidence.
Does having a statistically significant sample size matter?
A statistically significant sample size is the minimum number of participants required to detect a meaningful difference in the studied population. Statistical significance doesn't guarantee the validity or importance of the study results.
However, it provides evidence that the observed differences in the sample are unlikely coincidental. Therefore, a statistically significant sample size is vital in drawing valid conclusions and making informed decisions based on the study results.
- How to calculate sample size
Quantifying the ideal sample size requires careful consideration of several factors, including the research question , the desired accuracy, and the confidence level. Here's a general overview of how to calculate sample size:
Power analysis is a statistical method used to determine the ideal sample size based on the effect size, the significance level, and the study's desired power.
Confidence intervals are an analytical method used to estimate the range of values probable to contain the actual population variable with a certain confidence level.
The margin of error is a demographic method used to gauge the scope of values likely to have the true population parameter with a certain precision level.
The sample size calculation may differ depending on the statistical method and study design.
Sample size calculator .css-5oqtrw{background:transparent;border:0;color:#0C0020;cursor:pointer;display:-webkit-inline-box;display:-webkit-inline-flex;display:-ms-inline-flexbox;display:inline-flex;font-size:18px;font-weight:600;line-height:40px;outline:0;padding:0;} .css-17ofuq7{-webkit-align-items:center;-webkit-box-align:center;-ms-flex-align:center;align-items:center;background:transparent;border:0;color:inherit;cursor:pointer;-webkit-flex-shrink:0;-ms-flex-negative:0;flex-shrink:0;background:transparent;border:0;color:#0C0020;cursor:pointer;display:-webkit-inline-box;display:-webkit-inline-flex;display:-ms-inline-flexbox;display:inline-flex;font-size:18px;font-weight:600;line-height:40px;outline:0;padding:0;}.css-17ofuq7:disabled{opacity:0.6;pointer-events:none;} .css-7jswzl{-webkit-align-items:center;-webkit-box-align:center;-ms-flex-align:center;align-items:center;display:inline-block;height:28px;-webkit-box-pack:center;-ms-flex-pack:center;-webkit-justify-content:center;justify-content:center;width:28px;-webkit-text-decoration:none;text-decoration:none;}.css-7jswzl svg{height:100%;width:100%;margin-bottom:-4px;}
Maximize your research’s impact with the right sample size and get an accurate representation of your target audience.
Sample size
The total number of people whose opinion or behavior your sample will represent.
The probability that your sample accurately reflects the attitudes of your population. The industry standard is 95%.
The range (measured as a percentage) that your population’s responses may deviate from your sample’s.
Sample size formula
The formulas for calculating sample size depend on the statistical method used. Here are the commonly used formulas:
Power analysis : N = [(Zα/2 + Zβ) / ES] ^ 2
N = sample size
Zα/2 = the critical value of the standard normal distribution for a specified level of significance
Zβ = the critical value of the standard normal distribution for a particular power of the study
ES = the effect size, representing the magnitude of the difference or effect the study tries to detect
Confidence intervals : n = [(z * σ) / E] ^ 2
z = the critical value of the standard normal distribution for a specified level of confidence
σ = the standard deviation of the population
E = the desired margin of error
The margin of error : MOE = Z * (σ / √n)
Z = the z-score associated with the desired level of confidence (e.g., for 95% confidence, Z = 1.96)
n = the sample size
Margin of error calculator
Optimize your research’s impact when you improve the margin of error.
Margin of error
The number of people who took your survey.
These formulas are general guidelines, and researchers should consult with a statistician or use statistical software to ensure the sample size calculation is appropriate for their particular research question and study design.
Is a larger sample size better?
Yes, a larger sample size is better. A larger sample size increases the statistical power of a study, meaning it's more likely to detect actual effects or differences between groups.
Why use a sample size calculator?
A sample size calculator is used to determine the optimal sample size needed to obtain reliable and valid results in a study. It considers several factors, including the desired level of statistical power, the expected effect size, and the significance level.
Should you be using a customer insights hub?
Do you want to discover previous research faster?
Do you share your research findings with others?
Do you analyze research data?
Start for free today, add your research, and get to key insights faster
Editor’s picks
Last updated: 18 April 2023
Last updated: 27 February 2023
Last updated: 6 February 2023
Last updated: 5 February 2023
Last updated: 16 April 2023
Last updated: 9 March 2023
Last updated: 30 April 2024
Last updated: 12 December 2023
Last updated: 11 March 2024
Last updated: 4 July 2024
Last updated: 6 March 2024
Last updated: 5 March 2024
Last updated: 13 May 2024
Latest articles
Related topics, .css-je19u9{-webkit-align-items:flex-end;-webkit-box-align:flex-end;-ms-flex-align:flex-end;align-items:flex-end;display:-webkit-box;display:-webkit-flex;display:-ms-flexbox;display:flex;-webkit-flex-direction:row;-ms-flex-direction:row;flex-direction:row;-webkit-box-flex-wrap:wrap;-webkit-flex-wrap:wrap;-ms-flex-wrap:wrap;flex-wrap:wrap;-webkit-box-pack:center;-ms-flex-pack:center;-webkit-justify-content:center;justify-content:center;row-gap:0;text-align:center;max-width:671px;}@media (max-width: 1079px){.css-je19u9{max-width:400px;}.css-je19u9>span{white-space:pre;}}@media (max-width: 799px){.css-je19u9{max-width:400px;}.css-je19u9>span{white-space:pre;}} decide what to .css-1kiodld{max-height:56px;display:-webkit-box;display:-webkit-flex;display:-ms-flexbox;display:flex;-webkit-align-items:center;-webkit-box-align:center;-ms-flex-align:center;align-items:center;}@media (max-width: 1079px){.css-1kiodld{display:none;}} build next, decide what to build next, log in or sign up.
Get started for free
Root out friction in every digital experience, super-charge conversion rates, and optimise digital self-service
Uncover insights from any interaction, deliver AI-powered agent coaching, and reduce cost to serve
Increase revenue and loyalty with real-time insights and recommendations delivered straight to teams on the ground
Know how your people feel and empower managers to improve employee engagement, productivity, and retention
Take action in the moments that matter most along the employee journey and drive bottom line growth
Whatever they’re are saying, wherever they’re saying it, know exactly what’s going on with your people
Get faster, richer insights with qual and quant tools that make powerful market research available to everyone
Run concept tests, pricing studies, prototyping + more with fast, powerful studies designed by UX research experts
Track your brand performance 24/7 and act quickly to respond to opportunities and challenges in your market
Meet the operating system for experience management
- Free Account
- Product Demos
- For Digital
- For Customer Care
- For Human Resources
- For Researchers
- Financial Services
- All Industries
Popular Use Cases
- Customer Experience
- Employee Experience
- Employee Exit Interviews
- Net Promoter Score
- Voice of Customer
- Customer Success Hub
- Product Documentation
- Training & Certification
- XM Institute
- Popular Resources
- Customer Stories
- Artificial Intelligence
- Market Research
- Partnerships
- Marketplace
The annual gathering of the experience leaders at the world’s iconic brands building breakthrough business results.
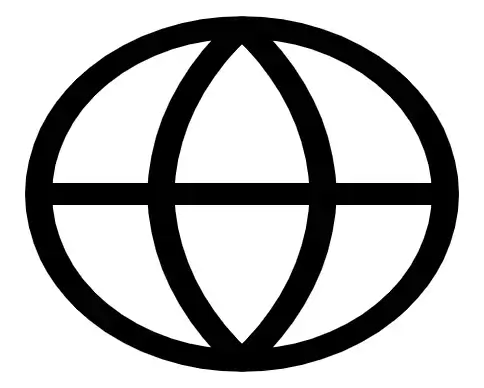
- English/AU & NZ
- Español/Europa
- Español/América Latina
- Português Brasileiro
- REQUEST DEMO
- Experience Management
- Sampling Methods
- How To determine Sample Size
Try Qualtrics for free
Determining sample size: how to make sure you get the correct sample size.
16 min read Sample size can make or break your research project. Here’s how to master the delicate art of choosing the right sample size.
What is sample size?
Sample size is the beating heart of any research project. It’s the invisible force that gives life to your data, making your findings robust, reliable and believable.
Sample size is what determines if you see a broad view or a focus on minute details; the art and science of correctly determining it involves a careful balancing act. Finding an appropriate sample size demands a clear understanding of the level of detail you wish to see in your data and the constraints you might encounter along the way.
Remember, whether you’re studying a small group or an entire population, your findings are only ever as good as the sample you choose.
Free eBook: Empower your market research efforts today
Let’s delve into the world of sampling and uncover the best practices for determining sample size for your research.
How to determine sample size
“How much sample do we need?” is one of the most commonly-asked questions and stumbling points in the early stages of research design . Finding the right answer to it requires first understanding and answering two other questions:
How important is statistical significance to you and your stakeholders?
What are your real-world constraints.
At the heart of this question is the goal to confidently differentiate between groups, by describing meaningful differences as statistically significant. Statistical significance isn’t a difficult concept, but it needs to be considered within the unique context of your research and your measures.
First, you should consider when you deem a difference to be meaningful in your area of research. While the standards for statistical significance are universal, the standards for “meaningful difference” are highly contextual.
For example, a 10% difference between groups might not be enough to merit a change in a marketing campaign for a breakfast cereal, but a 10% difference in efficacy of breast cancer treatments might quite literally be the difference between life and death for hundreds of patients. The exact same magnitude of difference has very little meaning in one context, but has extraordinary meaning in another. You ultimately need to determine the level of precision that will help you make your decision.
Within sampling, the lowest amount of magnification – or smallest sample size – could make the most sense, given the level of precision needed, as well as timeline and budgetary constraints.
If you’re able to detect statistical significance at a difference of 10%, and 10% is a meaningful difference, there is no need for a larger sample size, or higher magnification. However, if the study will only be useful if a significant difference is detected for smaller differences – say, a difference of 5% — the sample size must be larger to accommodate this needed precision. Similarly, if 5% is enough, and 3% is unnecessary, there is no need for a larger statistically significant sample size.
You should also consider how much you expect your responses to vary. When there isn’t a lot of variability in response, it takes a lot more sample to be confident that there are statistically significant differences between groups.
For instance, it will take a lot more sample to find statistically significant differences between groups if you are asking, “What month do you think Christmas is in?” than if you are asking, “How many miles are there between the Earth and the moon?”. In the former, nearly everybody is going to give the exact same answer, while the latter will give a lot of variation in responses. Simply put, when your variables do not have a lot of variance, larger sample sizes make sense.
Statistical significance
The likelihood that the results of a study or experiment did not occur randomly or by chance, but are meaningful and indicate a genuine effect or relationship between variables.
Magnitude of difference
The size or extent of the difference between two or more groups or variables, providing a measure of the effect size or practical significance of the results.
Actionable insights
Valuable findings or conclusions drawn from data analysis that can be directly applied or implemented in decision-making processes or strategies to achieve a particular goal or outcome.
It’s crucial to understand the differences between the concepts of “statistical significance”, “magnitude of difference” and “actionable insights” – and how they can influence each other:
- Even if there is a statistically significant difference, it doesn’t mean the magnitude of the difference is large: with a large enough sample, a 3% difference could be statistically significant
- Even if the magnitude of the difference is large, it doesn’t guarantee that this difference is statistically significant: with a small enough sample, an 18% difference might not be statistically significant
- Even if there is a large, statistically significant difference, it doesn’t mean there is a story, or that there are actionable insights
There is no way to guarantee statistically significant differences at the outset of a study – and that is a good thing.
Even with a sample size of a million, there simply may not be any differences – at least, any that could be described as statistically significant. And there are times when a lack of significance is positive.
Imagine if your main competitor ran a multi-million dollar ad campaign in a major city and a huge pre-post study to detect campaign effects, only to discover that there were no statistically significant differences in brand awareness . This may be terrible news for your competitor, but it would be great news for you.
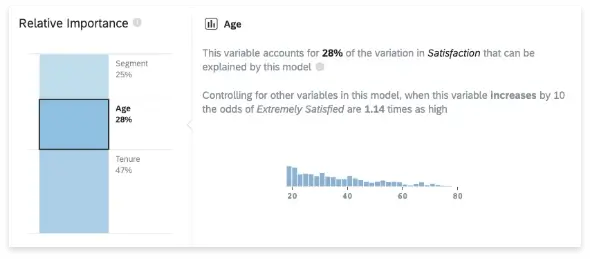
With Stats iQ™ you can analyze your research results and conduct significance testing
As you determine your sample size, you should consider the real-world constraints to your research.
Factors revolving around timings, budget and target population are among the most common constraints, impacting virtually every study. But by understanding and acknowledging them, you can definitely navigate the practical constraints of your research when pulling together your sample.
Timeline constraints
Gathering a larger sample size naturally requires more time. This is particularly true for elusive audiences, those hard-to-reach groups that require special effort to engage. Your timeline could become an obstacle if it is particularly tight, causing you to rethink your sample size to meet your deadline.
Budgetary constraints
Every sample, whether large or small, inexpensive or costly, signifies a portion of your budget. Samples could be like an open market; some are inexpensive, others are pricey, but all have a price tag attached to them.
Population constraints
Sometimes the individuals or groups you’re interested in are difficult to reach; other times, they’re a part of an extremely small population. These factors can limit your sample size even further.
What’s a good sample size?
A good sample size really depends on the context and goals of the research. In general, a good sample size is one that accurately represents the population and allows for reliable statistical analysis.
Larger sample sizes are typically better because they reduce the likelihood of sampling errors and provide a more accurate representation of the population. However, larger sample sizes often increase the impact of practical considerations, like time, budget and the availability of your audience. Ultimately, you should be aiming for a sample size that provides a balance between statistical validity and practical feasibility.
4 tips for choosing the right sample size
Choosing the right sample size is an intricate balancing act, but following these four tips can take away a lot of the complexity.
1) Start with your goal
The foundation of your research is a clearly defined goal. You need to determine what you’re trying to understand or discover, and use your goal to guide your research methods – including your sample size.
If your aim is to get a broad overview of a topic, a larger, more diverse sample may be appropriate. However, if your goal is to explore a niche aspect of your subject, a smaller, more targeted sample might serve you better. You should always align your sample size with the objectives of your research.
2) Know that you can’t predict everything
Research is a journey into the unknown. While you may have hypotheses and predictions, it’s important to remember that you can’t foresee every outcome – and this uncertainty should be considered when choosing your sample size.
A larger sample size can help to mitigate some of the risks of unpredictability, providing a more diverse range of data and potentially more accurate results. However, you shouldn’t let the fear of the unknown push you into choosing an impractically large sample size.
3) Plan for a sample that meets your needs and considers your real-life constraints
Every research project operates within certain boundaries – commonly budget, timeline and the nature of the sample itself. When deciding on your sample size, these factors need to be taken into consideration.
Be realistic about what you can achieve with your available resources and time, and always tailor your sample size to fit your constraints – not the other way around.
4) Use best practice guidelines to calculate sample size
There are many established guidelines and formulas that can help you in determining the right sample size.
The easiest way to define your sample size is using a sample size calculator , or you can use a manual sample size calculation if you want to test your math skills. Cochran’s formula is perhaps the most well known equation for calculating sample size, and widely used when the population is large or unknown.
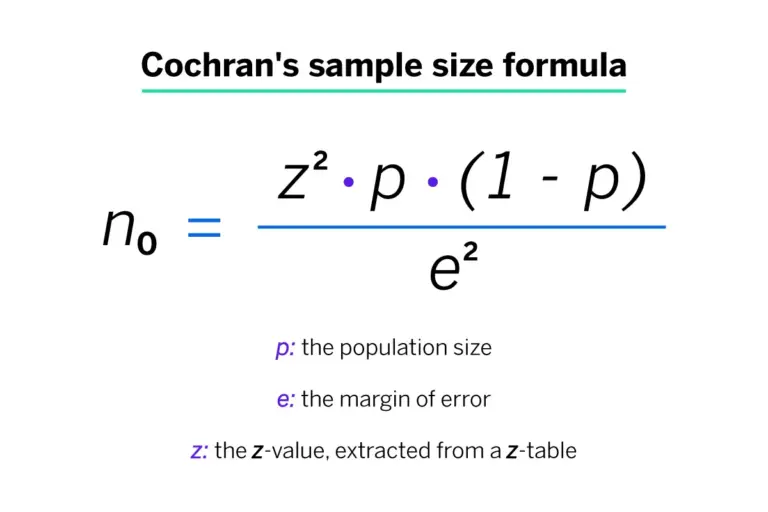
Beyond the formula, it’s vital to consider the confidence interval, which plays a significant role in determining the appropriate sample size – especially when working with a random sample – and the sample proportion. This represents the expected ratio of the target population that has the characteristic or response you’re interested in, and therefore has a big impact on your correct sample size.
If your population is small, or its variance is unknown, there are steps you can still take to determine the right sample size. Common approaches here include conducting a small pilot study to gain initial estimates of the population variance, and taking a conservative approach by assuming a larger variance to ensure a more representative sample size.
Empower your market research
Conducting meaningful research and extracting actionable intelligence are priceless skills in today’s ultra competitive business landscape. It’s never been more crucial to stay ahead of the curve by leveraging the power of market research to identify opportunities, mitigate risks and make informed decisions.
Equip yourself with the tools for success with our essential eBook, “The ultimate guide to conducting market research” .
With this front-to-back guide, you’ll discover the latest strategies and best practices that are defining effective market research. Learn about practical insights and real-world applications that are demonstrating the value of research in driving business growth and innovation.

Learn how to determine sample size
To choose the correct sample size, you need to consider a few different factors that affect your research, and gain a basic understanding of the statistics involved. You’ll then be able to use a sample size formula to bring everything together and sample confidently, knowing that there is a high probability that your survey is statistically accurate.
The steps that follow are suitable for finding a sample size for continuous data – i.e. data that is counted numerically. It doesn’t apply to categorical data – i.e. put into categories like green, blue, male, female etc.
Stage 1: Consider your sample size variables
Before you can calculate a sample size, you need to determine a few things about the target population and the level of accuracy you need:
1. Population size
How many people are you talking about in total? To find this out, you need to be clear about who does and doesn’t fit into your group. For example, if you want to know about dog owners, you’ll include everyone who has at some point owned at least one dog. (You may include or exclude those who owned a dog in the past, depending on your research goals.) Don’t worry if you’re unable to calculate the exact number. It’s common to have an unknown number or an estimated range.
2. Margin of error (confidence interval)
Errors are inevitable – the question is how much error you’ll allow. The margin of error , AKA confidence interval, is expressed in terms of mean numbers. You can set how much difference you’ll allow between the mean number of your sample and the mean number of your population. If you’ve ever seen a political poll on the news, you’ve seen a confidence interval and how it’s expressed. It will look something like this: “68% of voters said yes to Proposition Z, with a margin of error of +/- 5%.”
3. Confidence level
This is a separate step to the similarly-named confidence interval in step 2. It deals with how confident you want to be that the actual mean falls within your margin of error. The most common confidence intervals are 90% confident, 95% confident, and 99% confident.
4. Standard deviation
This step asks you to estimate how much the responses you receive will vary from each other and from the mean number. A low standard deviation means that all the values will be clustered around the mean number, whereas a high standard deviation means they are spread out across a much wider range with very small and very large outlying figures. Since you haven’t yet run your survey, a safe choice is a standard deviation of .5 which will help make sure your sample size is large enough.
Stage 2: Calculate sample size
Now that you’ve got answers for steps 1 – 4, you’re ready to calculate the sample size you need. This can be done using an online sample size calculator or with paper and pencil.
1. Find your Z-score
Next, you need to turn your confidence level into a Z-score. Here are the Z-scores for the most common confidence levels:
- 90% – Z Score = 1.645
- 95% – Z Score = 1.96
- 99% – Z Score = 2.576
If you chose a different confidence level, use this Z-score table (a resource owned and hosted by SJSU.edu) to find your score.
2. Use the sample size formula
Plug in your Z-score, standard of deviation, and confidence interval into the sample size calculator or use this sample size formula to work it out yourself:
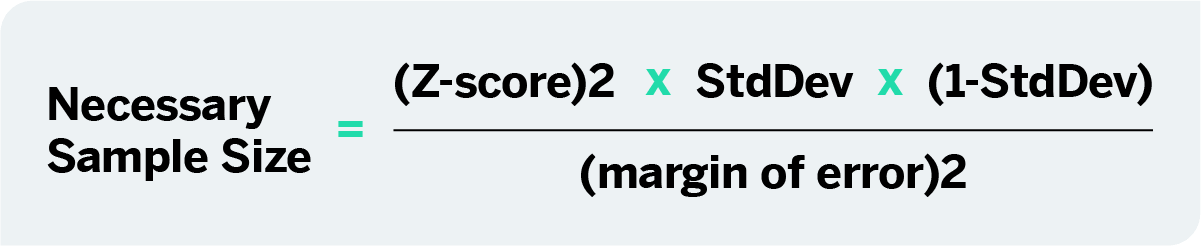
This equation is for an unknown population size or a very large population size. If your population is smaller and known, just use the sample size calculator.
What does that look like in practice?
Here’s a worked example, assuming you chose a 95% confidence level, .5 standard deviation, and a margin of error (confidence interval) of +/- 5%.
((1.96)2 x .5(.5)) / (.05)2
(3.8416 x .25) / .0025
.9604 / .0025
385 respondents are needed
Voila! You’ve just determined your sample size.
eBook: 2022 Market Research Global Trends Report
Related resources
Convenience sampling 15 min read, non-probability sampling 17 min read, probability sampling 8 min read, stratified random sampling 13 min read, simple random sampling 10 min read, sampling methods 15 min read, sampling and non-sampling errors 10 min read, request demo.
Ready to learn more about Qualtrics?
- Search Menu
- Sign in through your institution
- Advance Articles
- Editor's Choice
- Cover Archive
- Author videos
- Supplements
- Anniversary Collection
- Cover Images
- Author Guidelines
- Submission Site
- Open Access Options
- Why publish with NDT?
- About the ERA
- Editorial Board
- Advertising and Corporate Services
- Journals Career Network
- Self-Archiving Policy
- Dispatch Dates
- Terms and Conditions
- Editorial Fellowship
- The ERA Journals
- Journals on Oxford Academic
- Books on Oxford Academic
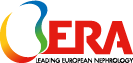
Article Contents
Introduction, conclusions.
- < Previous
Sample size calculations: basic principles and common pitfalls
- Article contents
- Figures & tables
- Supplementary Data
Marlies Noordzij, Giovanni Tripepi, Friedo W Dekker, Carmine Zoccali, Michael W Tanck, Kitty J Jager, Sample size calculations: basic principles and common pitfalls, Nephrology Dialysis Transplantation , Volume 25, Issue 5, May 2010, Pages 1388–1393, https://doi.org/10.1093/ndt/gfp732
- Permissions Icon Permissions
One of the most common requests that statisticians get from investigators are sample size calculations or sample size justifications. The sample size is the number of patients or other experimental units included in a study, and determining the sample size required to answer the research question is one of the first steps in designing a study.
Although most statistical textbooks describe techniques for sample size calculation, it is often difficult for investigators to decide which method to use. There are many formulas available which can be applied for different types of data and study designs. However, all of these formulas should be used with caution since they are sensitive to errors, and small differences in selected parameters can lead to large differences in the sample size. In this paper, we discuss the basic principles of sample size calculations, the most common pitfalls and the reporting of these calculations.
The sample size is the number of patients or other experimental units included in a study, and one of the first practical steps in designing a trial is the choice of the sample size needed to answer the research question. Also in the critical appraisal of the results of published trials, evaluating the sample size required to answer the research question is an important step in interpreting the relevance of these results. It is therefore not surprising that one of the most frequent requests that statistical consultants get from investigators are sample size calculations or sample size justifications.
Techniques for sample size calculations are described in most conventional statistical textbooks. However, the wide range of formulas that can be used for specific situations and study designs makes it difficult for most investigators to decide which method to use. Moreover, these calculations are sensitive to errors because small differences in selected parameters can lead to large differences in the sample size.
In this paper, we explain the basic principles of sample size calculations by means of examples from the nephrology literature. In addition, we discuss the most common pitfalls in sample size calculations and comment on how to report these calculations.
Why sample size calculations?
The main aim of a sample size calculation is to determine the number of participants needed to detect a clinically relevant treatment effect. Pre-study calculation of the required sample size is warranted in the majority of quantitative studies. Usually, the number of patients in a study is restricted because of ethical, cost and time considerations. However, if the sample size is too small, one may not be able to detect an important existing effect, whereas samples that are too large may waste time, resources and money. It is therefore important to optimize the sample size. Moreover, calculating the sample size in the design stage of the study is increasingly becoming a requirement when seeking ethical committee approval for a research project.
Components of sample size calculations
In order to calculate the sample size, it is required to have some idea of the results expected in a study. In general, the greater the variability in the outcome variable, the larger the sample size required to assess whether an observed effect is a true effect. On the other hand, the more effective (or harmful!) a tested treatment is, the smaller the sample size needed to detect this positive or negative effect. Calculating the sample size for a trial requires four basic components:
1. The type I error (alpha). Clinical studies are usually performed in a sample from a population rather than in the whole study population. In research, we are testing hypotheses to determine whether (results in) particular samples differ from each other. On the one hand, the null hypothesis (H 0 ) hypothesizes that the groups of subjects (samples) that are being compared are not different, that is they come from the same source population. The alternative hypothesis (H 1 ), on the other hand, hypothesizes that these groups are different and that therefore they seem to be drawn from different source populations. Sample size calculations are needed to define at what number of subjects it becomes quite unlikely that adding more subjects will change the conclusion.
In the process of hypothesis–testing, two fundamental errors can occur. These errors are called type I and type II errors, and an overview of these errors is presented in Table 1 .
Overview of errors in clinical research
. | Population . | |
---|---|---|
Difference does not exist . | ||
Difference does not exist | False negative result | |
Type II error (beta) | ||
Difference exists | False positive result | Power (1-beta) |
Type I error (alpha) |
. | Population . | |
---|---|---|
Difference does not exist . | ||
Difference does not exist | False negative result | |
Type II error (beta) | ||
Difference exists | False positive result | Power (1-beta) |
Type I error (alpha) |
The type I error (alpha) measures the probability that, given the H 0 that the samples come from the same source population, the differences found are likely to happen. In other words, the alpha represents the chance of a falsely rejecting H 0 and picking up a false-positive effect. The alpha is most commonly fixed at 0.05, which means that the researcher desires a <5% chance of drawing a false-positive conclusion.
2. Power. Instead of a false-positive conclusion, investigators can also draw a false-negative conclusion. In such cases, they conclude that there is no difference between two groups or treatments when in reality there is, or in other words, they falsely accept the H 0 that the compared samples come from the same source population. This is called a type II error (beta). Conventionally, the beta is set at a level of 0.20, meaning that the researcher desires a <20% chance of a false-negative conclusion. For the calculation of the sample size, one needs to know the power of a study. The power reflects the ability to pick up an effect that is present in a population using a test based on a sample from that population (true positive). The power is the complement of beta: 1-beta. So, in case of a beta of 0.20, the power would be 0.80 or 80%, representing the probability of avoiding a false-negative conclusion, or the chance of correctly rejecting a null hypothesis.
3. The smallest effect of interest. The smallest effect of interest is the minimal difference between the studied groups that the investigator wishes to detect and is often referred to as the minimal clinically relevant difference, sometimes abbreviated as MCRD. This should be a difference that the investigator believes to be clinically relevant and biologically plausible. For continuous outcome variables, the minimal clinically relevant difference is a numerical difference. For example, if body weight is the outcome of a trial, an investigator could choose a difference of 5 kg as the minimal clinically relevant difference. In a trial with a binary outcome, for example the effect of a drug on the development of a myocardial infarction (yes/no), an investigator should estimate a relevant difference between the event rates in both treatment groups and could choose, for instance, a difference of 10% between the treatment group and the control group as minimal clinically relevant difference. Even a small change in the expected difference with treatment has a major effect on the estimated sample size, as the sample size is inversely proportional to the square of the difference. For instance, if one would need 1000 subjects to detect an absolute difference of 4.8%, 4000 subjects per treatment group would be required to detect a 2.4% difference.
4. The variability. Finally, the sample size calculation is based on using the population variance of a given outcome variable that is estimated by means of the standard deviation (SD) in case of a continuous outcome. Because the variance is usually an unknown quantity, investigators often use an estimate obtained from a pilot study or use information from a previously performed study. For example, in an echocardiography substudy of the Australian Initiating Dialysis Early And Late (IDEAL) Study, Cooper et al. aim to determine whether the timing of dialysis initiation has an effect on left ventricular mass. For their sample size calculation, the investigators used recent data from another laboratory indicating that the mean left ventricular mass in renal failure patients in Australia is 140 g/m 2 with an SD of 60 g/m 2 .
A summary of all components of sample size calculations is presented in Table 2 .
Summary of the components for sample size calculations
Component . | Definition . |
---|---|
Alpha (type I error) | The probability of falsely rejecting H and detecting a statistically significant difference when the groups in reality are not different, i.e. the chance of a false-positive result. |
Beta (type II error) | The probability of falsely accepting H and not detecting a statistically significant difference when a specified difference between the groups in reality exists, i.e. the chance of a false-negative result. |
Power (1-beta) | The probability of correctly rejecting H and detecting a statistically significant difference when a specified difference between the groups in reality exists. |
Minimal clinically relevant difference | The minimal difference between the groups that the investigator considers biologically plausible and clinically relevant. |
Variance | The variability of the outcome measure, expressed as the SD in case of a continuous outcome. |
Component . | Definition . |
---|---|
Alpha (type I error) | The probability of falsely rejecting H and detecting a statistically significant difference when the groups in reality are not different, i.e. the chance of a false-positive result. |
Beta (type II error) | The probability of falsely accepting H and not detecting a statistically significant difference when a specified difference between the groups in reality exists, i.e. the chance of a false-negative result. |
Power (1-beta) | The probability of correctly rejecting H and detecting a statistically significant difference when a specified difference between the groups in reality exists. |
Minimal clinically relevant difference | The minimal difference between the groups that the investigator considers biologically plausible and clinically relevant. |
Variance | The variability of the outcome measure, expressed as the SD in case of a continuous outcome. |
Abbreviations: H 0 , null hypothesis; i.e. the compared samples come from the same source population (the compared groups are not different from each other); SD, standard deviation.
How to calculate the sample size for randomized controlled trials
Formulas for sample size calculation differ depending on the type of study design and the studies outcome(s). These calculations are particularly of interest in the design of randomized controlled trials (RCTs). In RCTs, a lot of money is invested, and it is therefore important to be sufficiently sure that enough patients are included in the study arms in order to find as statistically significant a difference that we assume there is in the population. In general, sample size calculations are performed based on the primary outcome of the study.
Based on two examples, we will now demonstrate how to calculate sample size using the simplest formulas for an RCT comparing two groups of equal size. Suppose one wished to study the effect of a new hypertensive drug on (i) systolic blood pressure (SBP) as a continuous outcome and (ii) SBP as a binary outcome, i.e. below or above 140 mmHg (hypertension yes/no). These situations are illustrated in Box 1 and Box 2 , respectively [ 1 ].
SBP as a continuous outcome
n = the sample size in each of the groups
μ 1 = population mean in treatment Group 1
μ 2 = population mean in treatment Group 2
μ 1 − μ 2 = the difference the investigator wishes to detect
σ 2 = population variance (SD)
a = conventional multiplier for alpha = 0.05
When the significance level alpha is chosen at 0.05, like in these examples, one should enter the value 1.96 for a in the formula. Similarly, when beta is chosen at 0.20, the value 0.842 should be filled in for b in the formula. These multipliers for conventional values of alpha and beta can be found in Table 3 .
Multipliers for conventional values of alpha and beta
Multipliers for conventional values of alpha . | |
---|---|
Alpha | Multiplier |
0.05 | 1.96 |
0.01 | 2.58 |
Multipliers for conventional values of beta | |
Beta | Multiplier |
0.20 | 0.842 |
0.10 | 1.28 |
0.05 | 1.64 |
0.01 | 2.33 |
Multipliers for conventional values of alpha . | |
---|---|
Alpha | Multiplier |
0.05 | 1.96 |
0.01 | 2.58 |
Multipliers for conventional values of beta | |
Beta | Multiplier |
0.20 | 0.842 |
0.10 | 1.28 |
0.05 | 1.64 |
0.01 | 2.33 |
Suppose the investigators consider a difference in SBP of 15 mmHg between the treated and the control group (μ 1 – μ 2 ) as clinically relevant and specified such an effect to be detected with 80% power (0.80) and a significance level alpha of 0.05. Past experience with similar experiments, with similar measuring methods and similar subjects, suggests that the data will be approximately normally distributed with an SD of 20 mmHg. Now we have all of the specifications needed for determining sample size using the approach as summarized in Box 1 . Entering the values in the formula yields: 2 × [(1.96 + 0.842) 2 × 20 2 ] / 15 2 = 27.9, this means that a sample size of 28 subjects per group is needed to answer the research question.
SBP as a binary outcome
For a study with a binary outcome, calculating the required sample size is slightly more complicated. It should be calculated based on the number of events rather than on the number of people in the study (Box 2 ). The number of events can be increased either by choosing higher risk patients, by increasing the follow-up time, or by increasing the sample size.
p 1 = proportion of subjects with hypertension in treatment Group 1
q 1 = proportion of subjects without hypertension in treatment Group 1 (= 1− p 1 )
p 2 = proportion of with hypertension in treatment Group 2
q 2 = proportion of subjects without hypertension in treatment Group 2 (= 1− p 2 )
x = the difference the investigator wishes to detect
In this case, we suppose that the investigators consider a difference in event rate of 10% (0.10) as clinically relevant. Based on recently published findings from studies with a similar design, they expect that the proportion of subjects with hypertension in the treated group will be ∼20% ( p 1 = 0.20) and in the control group, ∼30% ( p 2 = 0.30). This automatically means that q 1 and q 2 are 0.80 and 0.70, respectively. Again, the investigators assume a power of 80% (0.80) and an alpha of 0.05, which means that the value 1.96 should be filled in for a and the value 0.842 should be filled in for b . We can now enter all values in the formula presented in Box 2 : [(1.96+0.842) 2 × (0.20 × 0.80 + 0.30 × 0.70)] / 0.10 2 = 290.5, this means that a sample size of 291 subjects per group is needed to answer the research question.
Many of the formulas to calculate sample size are not straightforward, and it is recommendable to ask for the help of a statistician in all but the most basic studies. For some simple clinical trials, nomograms or graphs can be used to estimate the sample size required for the study. An example of such a nomogram published by Altman is presented in Figure 1 [ 2 ]. However, one should keep in mind that, although these graphical methods work well, they often make assumptions about the type of data and statistical tests to be used.
Nomogram for the calculation of sample size or power (adapted from Altman 1982) [ 2 ].
Other outcome types
In many trials, the outcomes may not be continuous or binary as above, but instead may be survival (e.g. time to event). In these cases, the details of calculation differ, but using the four aforementioned components, persist through calculations with other types of outcomes. However, other assumptions can be necessary.
Other study designs than RCTs
In this paper, we focus on sample size calculations for RCTs, but also for studies with another design such as case-control or cohort studies, sample size calculations are sometimes required. Although the calculation of sample size is based on the same principles for all parallel study designs, the formulas for sample size calculations for other study designs than RCTs often need some adaptations. For example, published formulas for case-control designs provide sample sizes required to determine that an odds ratio is significantly different from one, after adjustment for potential confounders [ 3 ]. Also, sample size calculations for special types of RCTs, like cluster-randomized trials, in which health interventions are allocated randomly to intact clusters, communities, health centres or practices rather than to individual subjects, need an alternative approach [ 4 ]. The same holds true for trials with a crossover design, because these studies compare the results of two treatments on the same group of patients. The sample size calculated for a crossover study can also be used for a study that compares the value of a variable after treatment with its value before treatment [ 5 ]. Finally, sample size calculations for clinical trials testing the equivalence rather than the superiority between two treatments need another approach. These equivalence or non-inferiority trials usually demand higher sample sizes [ 6 ]. The aforementioned alternative calculations are less common and more complicated and will in most cases require statistical assistance.
Common pitfalls
The calculation of the sample size is troubled by a large amount of imprecision, because investigators rarely have good estimates of the parameters necessary for the calculation. Unfortunately, the required sample size is very sensitive to the choice of these parameters.
The effects of selecting alpha and the power
In most cases, the conventional choices of an alpha of 0.05 and a power of 0.80 are adequate. However, dependent on the topic studied, other assumptions can be made. Different assumptions of alpha and the power will directly influence the sample size, as is illustrated by Table 4 . A lower alpha and a higher power will both lead to a larger sample size and as a result to higher costs. To be aware of the influence of changes in these parameters, it can be helpful to perform sample size calculations for different values of the parameters (sensitivity analyses). In case of doubt, one should generally choose the largest sample size.
Approximate relative sample size for different levels of alpha and power
. | Alpha (type I error) . | ||
---|---|---|---|
0.05 . | 0.01 . | 0.001 . | |
Power (1-beta) | |||
0.80 | 100 | 149 | 218 |
0.90 | 134 | 190 | 266 |
0.99 | 234 | 306 | 402 |
. | Alpha (type I error) . | ||
---|---|---|---|
0.05 . | 0.01 . | 0.001 . | |
Power (1-beta) | |||
0.80 | 100 | 149 | 218 |
0.90 | 134 | 190 | 266 |
0.99 | 234 | 306 | 402 |
Estimating the difference and SD
Studies often aim to determine parameters like event rates in the treated group and the control group. Needing to estimate these parameters before the start of the study therefore seems strange for many investigators. It is, however, important to realize that the parameters they are estimating in order to calculate the required sample size are not the population parameters as such, but the treatment effects they consider biologically plausible and clinically relevant.
In most studies, investigators estimate the difference of interest and the standard deviation based on results from a pilot study, published data or on their own knowledge and opinion. This means that the calculation of an appropriate sample size partly relies on subjective choices or crude estimates of certain factors which may seem rather artificial to some. Unless the pilot study was large, using information from a pilot study often results in unreliable estimates of the variability and the minimal clinically relevant difference. By definition, pilot studies are underpowered, and the observed difference in a pilot study is therefore an imprecise estimate of the difference in the population. Not accounting for this sampling error will lead to underpowered studies [ 7 ]. Also published reports could provide an estimate of the outcome in the control group. Although they often incorporate a lot of differences with the study one aims to perform, such as dissimilar eligibility criteria, endpoints and treatments, some information on the control group usually exists [ 8 ]. Finally, another approach is to survey experts in the field to determine what difference would need to be demonstrated for them to adapt a new treatment in terms of costs and risks [ 9 ].
After mentioning these pitfalls, it may seem useless to perform a sample size calculation. However, even if based on estimates and assumptions, a sample size calculation is considerably more useful than a completely arbitrary choice.
Post hoc sample calculations
Sometimes, published studies wrongfully report their ‘power’ instead of 95% confidence intervals (CIs). The power should always be calculated prior to a study to determine the required sample size, since it is the pre-study probability that the study will detect a minimum effect regarded as clinically significant. After the study is conducted, one should not perform any ‘ post hoc ’ power calculations. Once the effect of the study is known, investigators should use the 95% CI to express the amount of uncertainty around the effect estimate.
Reporting of sample size calculations
According to the CONSORT statement, sample size calculations should be reported and justified in all published RCTs [ 10 ]. These calculations provide important information. Firstly, they specify the primary endpoint, which safeguards against changing the planned outcome and claiming a large effect on an outcome which was not the original primary outcome. Secondly, knowing the planned size alerts readers to potential problems, like problems with the recruitment or an early stop of the trial [ 8 ]. Readers of a published trial should be able to find all assumptions underlying the sample size calculation; the alpha, the power, the event rate in the control group and the treatment effect of interest (or the event rate in the treated group). Many studies only include statements like ‘we calculated that the sample size in each treatment group should be 250 at an alpha of 0.05 and a power of 0.80’. However, such a statement is almost meaningless because it neglects the estimates for the effect of interest and the variability. Based on an example from the IDEAL Study, we can illustrate that a better way to report these calculations would be: ‘A clinically significant effect of 10% or more over the 3 years would be of interest. Assuming 3-year survival rates in the control group and the intervention group of 64% and 74% respectively, with a two-sided significance of 0.05 and a power of 0.8, a total of 800–1000 patients will be required’ [ 11 ].
Although, ideally, all four components conventionally required for sample size calculation should be published, Charles et al. [ 12 ] recently showed that of 215 published reports of RCTs, 10 (5%) did not report any sample size calculation, and 92 (43%) did not report all the required parameters. Moreover, a Danish study by Chan et al. [ 13 ] demonstrated that, also in study protocols, sample size calculations are often poorly reported, and that explicit discrepancies between protocols and published papers are common. They found that only 11 of 62 (18%) identified studies described existing sample size calculations fully and consistently in both the protocol and the publication.
Further reading
Methods for sample size calculations are described in several general statistics textbooks, such as Altman (1991) [ 14 ] or Bland (2000) [ 15 ]. Specialized books which discuss sample size determination in many situations were published by Machin et al. (1997) [ 16 ] and Lemeshow et al. (1990) [ 17 ].
In addition, there are different software programs that can assist in sample size calculations. Examples of validated and user-friendly programmes that can be applied to calculate the sample size for several types of data and study designs are nQuery Advisor, PASS and ‘Power and Precision’. For those programmes, a paid license is required. There are also a number of websites that allow free sample size calculations. However, those programmes are not always reliable. An example of a reliable, freely available website is: http://www.stat.uiowa.edu/∼rlenth/Power/index.html [ 18 ].
In conclusion, the calculation of the sample size is one of the first and most important steps in designing a study. Although techniques for sample size calculations are described in many statistical textbooks, performing these calculations can be complicated. Because sample size calculations are sensitive to errors and because of the high costs related to performing an RCT, we recommend to perform the calculations with caution or to ask for statistical advice during the designing phase of the study.
Conflict of interest statement. The results presented in this paper have not been published previously in whole or part, except in abstract format.
Google Scholar
Google Preview
- hypertension
- type i errors
- type ii errors
- false-positive results
- false-negative results
- outcome variable
Month: | Total Views: |
---|---|
December 2016 | 1 |
January 2017 | 321 |
February 2017 | 915 |
March 2017 | 614 |
April 2017 | 93 |
May 2017 | 554 |
June 2017 | 809 |
July 2017 | 599 |
August 2017 | 879 |
September 2017 | 749 |
October 2017 | 689 |
November 2017 | 886 |
December 2017 | 3,717 |
January 2018 | 4,611 |
February 2018 | 4,399 |
March 2018 | 5,236 |
April 2018 | 5,116 |
May 2018 | 4,446 |
June 2018 | 3,893 |
July 2018 | 3,818 |
August 2018 | 4,413 |
September 2018 | 4,016 |
October 2018 | 4,481 |
November 2018 | 4,978 |
December 2018 | 3,689 |
January 2019 | 3,598 |
February 2019 | 3,870 |
March 2019 | 5,266 |
April 2019 | 5,063 |
May 2019 | 6,292 |
June 2019 | 4,914 |
July 2019 | 4,394 |
August 2019 | 4,006 |
September 2019 | 4,211 |
October 2019 | 4,380 |
November 2019 | 3,751 |
December 2019 | 3,139 |
January 2020 | 3,255 |
February 2020 | 3,544 |
March 2020 | 3,196 |
April 2020 | 3,936 |
May 2020 | 2,438 |
June 2020 | 3,467 |
July 2020 | 2,293 |
August 2020 | 2,181 |
September 2020 | 2,657 |
October 2020 | 2,903 |
November 2020 | 2,732 |
December 2020 | 2,575 |
January 2021 | 2,181 |
February 2021 | 2,688 |
March 2021 | 3,434 |
April 2021 | 2,939 |
May 2021 | 2,494 |
June 2021 | 2,107 |
July 2021 | 2,057 |
August 2021 | 2,092 |
September 2021 | 2,376 |
October 2021 | 2,599 |
November 2021 | 2,549 |
December 2021 | 2,239 |
January 2022 | 2,137 |
February 2022 | 2,393 |
March 2022 | 2,768 |
April 2022 | 2,578 |
May 2022 | 2,288 |
June 2022 | 1,975 |
July 2022 | 1,667 |
August 2022 | 1,614 |
September 2022 | 1,755 |
October 2022 | 1,795 |
November 2022 | 1,906 |
December 2022 | 1,756 |
January 2023 | 1,716 |
February 2023 | 1,432 |
March 2023 | 2,180 |
April 2023 | 1,989 |
May 2023 | 1,843 |
June 2023 | 1,526 |
July 2023 | 1,632 |
August 2023 | 1,320 |
September 2023 | 1,554 |
October 2023 | 1,825 |
November 2023 | 1,890 |
December 2023 | 1,706 |
January 2024 | 1,908 |
February 2024 | 1,905 |
March 2024 | 2,191 |
April 2024 | 1,905 |
May 2024 | 1,636 |
June 2024 | 986 |
July 2024 | 922 |
August 2024 | 340 |
Email alerts
Citing articles via.
- ndt Twitter
- ERA Twitter
- ERA Facebook
- ERA Instagram
- ERA LinkedIn
- Recommend to Your Librarian
Affiliations
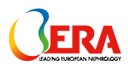
- Online ISSN 1460-2385
- Print ISSN 0931-0509
- Copyright © 2024 European Renal Association
- About Oxford Academic
- Publish journals with us
- University press partners
- What we publish
- New features
- Open access
- Institutional account management
- Rights and permissions
- Get help with access
- Accessibility
- Advertising
- Media enquiries
- Oxford University Press
- Oxford Languages
- University of Oxford
Oxford University Press is a department of the University of Oxford. It furthers the University's objective of excellence in research, scholarship, and education by publishing worldwide
- Copyright © 2024 Oxford University Press
- Cookie settings
- Cookie policy
- Privacy policy
- Legal notice
This Feature Is Available To Subscribers Only
Sign In or Create an Account
This PDF is available to Subscribers Only
For full access to this pdf, sign in to an existing account, or purchase an annual subscription.

An official website of the United States government
The .gov means it’s official. Federal government websites often end in .gov or .mil. Before sharing sensitive information, make sure you’re on a federal government site.
The site is secure. The https:// ensures that you are connecting to the official website and that any information you provide is encrypted and transmitted securely.
- Publications
- Account settings
Preview improvements coming to the PMC website in October 2024. Learn More or Try it out now .
- Advanced Search
- Journal List
- Malays J Med Sci
- v.28(2); 2021 Apr
A Step-by-Step Process on Sample Size Determination for Medical Research
Determination of a minimum sample size required for a study is a major consideration which all researchers are confronted with at the early stage of developing a research protocol. This is because the researcher will need to have a sound prerequisite knowledge of inferential statistics in order to enable him/her to acquire a thorough understanding of the overall concept of a minimum sample size requirement and its estimation. Besides type I error and power of the study, some estimates for effect sizes will also need to be determined in the process to calculate or estimate the sample size. The appropriateness in calculating or estimating the sample size will enable the researchers to better plan their study especially pertaining to recruitment of subjects. To facilitate a researcher in estimating the appropriate sample size for their study, this article provides some recommendations for researchers on how to determine the appropriate sample size for their studies. In addition, several issues related to sample size determination were also discussed.
Introduction
Sample size calculation or estimation is an important consideration which necessitate all researchers to pay close attention to when planning a study, which has also become a compulsory consideration for all experimental studies ( 1 ). Moreover, nowadays, the selection of an appropriate sample size is also drawing much attention from researchers who are involved in observational studies when they are developing research proposals as this is now one of the factors that provides a valid justification for the application of a research grant ( 2 ). Sample size must be estimated before a study is conducted because the number of subjects to be recruited for a study will definitely have a bearing on the availability of vital resources such as manpower, time and financial allocation for the study. Nevertheless, a thorough understanding of the need to estimate or calculate an appropriate sample size for a study is crucial for a researcher to appreciate the effort expended in it.
Ideally, one can determine the parameter of a variable from a population through a census study. A census study recruits each and every subject in a population and an analysis is conducted to determine the parameter or in other words, the true value of a specific variable will be calculated in a targeted population. This approach of analysis is known as descriptive analysis. On the other hand, the estimate that is derived from a sample study is termed as a ‘statistic’ because it analyses sample data and subsequently makes inferences and conclusions from the results. This approach of analysis is known as inferential analysis, which is also the most preferred approach in research because drawing a conclusion from the sample data is much easier than performing a census study, due to various constraints especially in terms of cost, time and manpower.
In a census study, the accuracy of the parameters cannot be disputed because the parameters are derived from all subjects in the population. However, when statistics are derived from a sample, it is possible for readers to query to what extent these statistics are representative of the true values in the population. Thus, researchers will need to provide an additional piece of evidence besides the statistics, which is the P -value. The statistical significance or usually termed as ‘ P -value less than 0.05’, and it shall stand as an evidence or justification that the statistics derived from the sample can be inferred to the larger population. Some scholars may argue over the utility and versatility of P -value but it is nevertheless still applicable and acceptable until now ( 3 – 5 ).
Why It is Necessary to Perform a Sample Size Calculation or Estimation?
In order for the analysis to be conducted for addressing a specific objective of a study to be able to generate a statistically-significant result, a particular study must be conducted using a sufficiently large sample size that can detect the target effect sizes with an acceptable margin of error. In brief, a sample size is determined by three elements: i) type I error (alpha); ii) power of the study (1-type II error) and iii) effect size. A proper understanding of the concept of type I error and type II error will require a lengthy discussion. The prerequisite knowledge of statistical inference, probability and distribution function is also required to understand the overall concept ( 6 – 7 ). However, in sample size calculation, the values of both type I and type II errors are usually fixed. Type I error is usually fixed at 0.05 and sometimes 0.01 or 0.10, depending on the researcher. Meanwhile, power is usually set at 80% or 90% indicating 20% or 10% type II error, respectively. Hence, the only one factor that remains unspecified in the calculation of a sample size is the effect size of a study.
Effect size measures the ‘magnitude of effect’ of a test and it is independent of influences by the sample size ( 8 ). In other words, effect size measures the real effect of a test irrespective of its sample size. With reference to statistical tests, it is an expected parameter of a particular association (or correlation or relationship) with other tests in a targeted population. In a real setting, the parameter of a variable in a targeted population is usually unknown and therefore a study will be conducted to test and confirm these effect sizes. However, for the purpose of sample size calculation, it is still necessary to estimate the target effect sizes. By the same token, Cohen ( 9 ) presented in his article that a larger sample size is necessary to estimate small effect sizes and vice versa.
The main advantage of estimating the minimum sample size required is for planning purposes. For example, if the minimum sample size required for a particular study is estimated to be 300 subjects and a researcher already knows that he/she can only recruit 15 subjects in a month from a single centre. Thus, the researchers will need at least 20 months for data collection if there is only one study site. If the plan for data collection period is shorter than 20 months, then the researchers may consider to recruit subjects in more than one centre. In case where the researchers will not be able to recruit 300 subjects within the planned data collection period, the researchers may need to revisit the study objective or plan for a totally different study instead. If the researcher still wishes to pursue the study but is unable to meet the minimum required sample size; then it is likely that the study may not be able to reach a valid conclusion at the end, which will result in a waste of resources because it does not add any scientific contributions.
How to Calculate or Estimate Sample Size?
Sample size calculation serves two important functions. First, it aims to estimate a minimum sample size that can be sufficient for achieving a target level of accuracy in an estimate for a specific population parameter. In this instance, the researcher aims to produce an estimate that is expected to be equally accurate as an actual parameter in the target population. Second, it also aims to determine the level of statistical significance (i.e. P -value < 0.05) attained by these desired effect sizes. In other words, a researcher aims to infer the statistics derived from the sample to that of the larger population. In this case, a specific statistical test will be applied and the P -value will be calculated by using the statistical test (which will determine the level of statistical significance).
For univariate statistical test such as independent sample t -test or Pearson’s chi-square test, these sample size calculations can be done manually using a rather simple formula. However, the manual calculation can still be difficult for researchers who are non-statisticians. Various sample size software have now been introduced which make these sample size calculation easier. Nevertheless, a researcher may still experience some difficulty in using the software if he/she is not familiar with the concept of sample size calculation and the statistical tests. Therefore, various scholars have expended some effort to assist the researchers in the determination of sample sizes for various purposes by publishing sample size tables for various statistical tests ( 10 – 12 ). These sample sizes tables can be used to estimate the minimum sample size that is required for a study. Although such tables may have only a limited capacity for the selection of various effect sizes, and their corresponding sample size requirements; it is nonetheless much more practical and easier to use.
For some study objectives, it is often much easier to estimate the sample size based on a rule-of-thumb instead of manual calculation or sample size software. Taking an example of an objective of a study that needs to be answered using multivariate analysis, the estimation of an association between a set of predictors and an outcome can be very complicated if it involves many independent variables. In addition, the actual ‘effect size’ can range from low to high, which renders it even more difficult to be estimated. Therefore, it is recommended to adopt the conventional rule-of-thumb for estimating these sample sizes in these circumstances. Although some scholars have initially thought that the concept of rule-of-thumb may not be as scientifically robust when compared to actual calculations, it is still considered to be an acceptable approach ( 13 – 15 ). Table 1 illustrates some published articles for various sample size determinations for descriptive studies and statistical tests.
Summary of published articles related to sample size determination for various statistical tests
Published articles | ||
---|---|---|
a. | To estimate parameters for population | Krejcie and Morgan ( ), Lachin ( ), Campbell et al. ( ), Bartlett et al. ( ), Israel ( ), Naing et al. ( ). |
b. | To infer the results for larger population | |
Correlation | Cohen ( ), Algina and Olejnik ( ), Bujang and Nurakmal ( ). | |
Intra-class correlation | Fleiss and Cohen ( ), Bonett ( ), Zou ( ), Bujang and Baharum ( ). | |
Kappa agreement test | Cicchetti ( ), Flack et al. ( ), Cantor ( ), Sim and Wright ( ), Bujang and Baharum ( ). | |
Independent sample -test and paired -test | Lachin ( ), Cohen ( ), Dupont and Plummer ( ). | |
One-way ANOVA | Cohen ( ), Jan and Shieh ( ). | |
Pearson’s chi-square | Lachin ( ), Cohen ( ), Dupont and Plummer ( ). | |
Cronbach’s alpha | Bonett ( ), Bonett ( ), Bonett and Wright ( ), Bujang et al. ( ). | |
Sensitivity and specificity | Buderer ( ), Malhotra and Indrayan ( ), Bujang and Adnan ( ). | |
Linear regression or Multiple linear regression | Cohen ( ), Dupont and Plummer ( ), Hsieh et al. ( ), Knofczynski and Mundfrom ( ), Tabachnick and Fidell ( ), Bujang et al. ( ). | |
Analysis of covariance | Borm et al. ( ), Bujang et al. ( ). | |
Logistic regression | Peduzzi et al. ( ), Hsieh et al. ( ), Bujang et al. ( ). | |
Survival analysis | Lachin ( ), Lachin and Foulkes ( ), Dupont and Plummer ( ). | |
Cox regression | Peduzzi et al. ( ), Hsieh and Lavori ( ), Schmoor et al. ( ). | |
Exploratory factor analysis | Barrett and Kline ( ), Osborne and Costello ( ), Bujang et al. ( ), Bujang et al. ( ). |
In brief, the present paper will be proposing five main steps for sample size determination as shown in Figure 1 . The following provides an initial description and then a discussion of each of these five steps:

Recommended steps in sample size determination
Step 1: To Understand the Objective of the Study
The objective of a study has to be measurable or in other words, can be determined by using statistical analysis. Sometimes, a single study may have several objectives. One of the common approaches to achieve this is to estimate the sample size required for every single objective and then the minimum required sample size for the study will be selected to be the highest number of all sample sizes calculated. However, this paper recommends that the minimum sample size be calculated only for the primary objective, which will remain valid as long as the primary objective is more important than all the other objectives. This also means that the calculation of minimum sample size for any other objectives (apart from the primary objective) will only be considered unless they are considered to be equally important as the primary objective. For the development of a research proposal, different institutions may apply different approaches for sample size determinations and hence, it is mandatory to adhere to their specific requirements for sample size determinations.
However, the estimation or calculation of sample size for every study objective can be further complicated by the fact that some of the secondary objectives may require a larger sample size than the primary objective. If the recruitment of a larger number of subjects is not an issue, then it will always be viable to obtain a larger sample size in order to accommodate the sample size requirements for each and every objective of the study. Otherwise, it may be advisable for a researcher to forgo some of the secondary objectives so that they will not be too burdensome for the him/her.
Step 2: To Select the Appropriate Statistical Analysis
Researchers have to decide the appropriate analysis or statistical test to be used to answer the study objective; regardless of whether the aim is to determine a single mean, or a prevalence, or correlation, or association, just to name a few. The formula that will be used to estimate or calculate the sample size will be the same as the formula for performing the statistical test that will be used to answer the objective of study. For example, if an independent sample t -test has to be used for analysis, then its sample size formula should be based on an independent sample t -test. Hence, there is no a single formula for sample size calculation or estimation which can apply universally to all situations and circumstances.
Step 3: To Calculate or Estimate the Sample Size
Estimating or calculating the sample size can be done either by using manual calculation, sample size software, sample size tables from scientific published articles, or by adopting various acceptable rule-of-thumbs. Since both the type I and type II errors are already pre-specified and fixed, hence only the effect size remains to be specified in order for the determination of an appropriate sample size. To illustrate this point, it will be easier to demonstrate by using a case scenario as an example. Say a researcher would like to study an effectiveness of a new diet programme to reduce weight. The researcher believes the new diet programme is better than the conventional diet programme. It was found that the conventional diet programme can reduce on average 1 kg in 1 month. How many subjects are required to prove that the new diet programme is better than the conventional diet programme?
Based on Step 1 and Step 2, a researcher has decided to apply the independent sample t -test to answer the objective of study. Next, the researcher will need to specify the effect size after having both type I error and power set at 0.05% and 80%, respectively (type II error = 20%). What margin of effect size will be appropriate? This shall depend on the condition itself or the underlying research rationale which can then be further classified into two categories. In the first category, the research rationale is to prove that the new diet programme (for reducing weight) is superior to the conventional diet programme. In this case, the researcher should aim for sizeably large effect size. In other words, the difference between means of the weight reduction (which constitutes part of the effect size for independent sample t -test) should be sufficiently large to demonstrate the superiority of the new diet programme over the conventional diet programme.
In the second category, the research rationale is to measure accurately the effectiveness of the new diet programme to reduce weight in comparison with conventional diet programme, irrespective of whether the difference between both programmes is large or small. In this situation, the difference does not matter since the researcher aims to measure an exact difference between them, which means that it can only tolerate a very low margin of difference. In this circumstance, the researcher will therefore only be able to accept the smaller effect sizes. The estimate of effect sizes in this instance can be reviewed either from literatures, pilot study, historical data and rarely by using an educated guess.
The acceptable or desirable effect size that can be found from the literature can vary over a wide range. Thus, one of the better options is to seek for the relevant information from published articles of recent studies (within 5 years) that applied almost similar research design such as used the same treatments and had reported about similar patient characteristics. If none of these published articles can provide a rough estimate of the desired effect size, then the researcher may have to consider conducting a pilot study to obtain a rough estimate of the closest approximation to the actual desired effect size. Besides, historical data or secondary data can also be used to estimate the desired effect size, provided that the researcher has access to the secondary data of the two diet programmes. However, it must be emphasised that deriving the effect size from secondary data may not always be feasible since the performance of the new intervention may still not yet have been assessed.
The last option is to estimate the desired effect size based on a scientifically or a clinically meaningful effect. This means the researcher, through his or her own knowledge and experience, is able to determine an expectation of the difference in effect, and then to set a target difference (namely, effect size) to be achieved. For example, a researcher makes an educated guess about the new diet programme, and requires it to achieve a minimum difference of 3 kg in weight reduction per month in order for it to demonstrate superiority over the conventional diet programme. Although it is always feasible to set a large effect size especially if the new diet programme has proven to be a more rigorous intervention and probably also costlier; however, there is also a risk for the study to might have possibly failed to report a statistically significant result if it has subsequently been found that the actual effect size is much smaller than that adopted by the study, after the analysis has been completed. Therefore, it is usually quite a challenging task to estimate an accurate effect size since the exact value of the effect size is not known until the study is completed. However, the researcher will still have to set the value of effect size for the purpose of sample size calculation or estimation.
Next is to calculate or estimate sample size either based on manual calculation, sample size software, sample size tables or by adopting a conventional rule of thumb. Referring to the example for illustration purposes, the sample size calculation was calculated by using the sample size software as follows; with a study setting of equal sample size for both groups, the mean reduction is set at only 1 kg with within group standard deviation estimated at 0.8 (derived from literature, pilot study or based on a reliable source), type I error at 0.05 and 80% power, a minimum sample size of 11 subjects are required for each group (both for new diet programme and conventional diet programme). The sample size was calculated using Power and Sample Size (PS) software (by William D Dupont and W Dale Plummer, Jr. is licensed under a Creative Commons Attribution-NonCommercial- NoDerivs 3.0 United States License).
Step 4: To Provide an Additional Allowance During Subject Recruitment to Cater for a Certain Proportion of Non-Response
After the minimum required sample size has been identified, it is necessary to provide additional allowances to cater for potential non-response subjects. A minimum required sample size simply means the minimum number of subjects a study must have after recruitment is completed. Thus, researchers must ideally be able to recruit subjects at least beyond the minimum required sample size. To avoid underestimation of sample size, researchers will need to anticipate the problem of non-response and then to make up for it by recruiting more subjects on top of the minimum sample size, usually by 20% to 30%. If, for example, the researcher is expecting a high non-response rate in a self-administered survey, then he/she should provide an allowance for it by adding more than 30% such as 40% to 50%. The occurrence of non-response could also happen in various other scenarios such as dropping out or loss to follow-up in a cohort study and experimental studies. Besides that, missing data or loss of records are also potential problems that can result in attrition in observational studies.
Referring to previous example as an illustration, by adding 20% of non-response rate in each group, 14 subjects are required in each group. The calculation should be done as follow:
Likewise, for a 30% non-response rate, the sample size required in each group will then be increased to 16 subjects (11/0.7 = 15.7 ≈ 16).
Step 5: To Write a Sample Size Statement
The sample size statement is important and it is usually included in the protocol or manuscript. In the existing research literatures, the sample size statement is written in various styles. This paper recommends for the sample size statement to start by reminding the readers or reviewers about the main objective of study. Hence, this paper recommends all the elements from Step 1 until Step 4 (study objective, appropriate statistical analysis, sample size estimation/calculation and non-response rate) should be fully stated in the sample size statement. Therefore, a proposed outline of this sample size statement of the previous example for two weight-losing diet programmes is as follows:
“This study hypothesised that the new diet programme is better than conventional diet programme in terms of weight reduction at a 1-month follow-up. Therefore, the sample size formula is derived from the independent sample t -test. Based on the results of a previous study (cite the appropriate reference), all the response within each subject group are assumed to be normally distributed with a within-group standard deviation (SD) of 0.80 kg. If the true mean difference of the new diet programme versus the conventional diet programme is estimated at 1.0 kg, the study will need to recruit 11 subjects in each group to be able to reject the null hypothesis that the population means of the new diet programme and conventional diet programme are found to be equal with a type I error of 0.05 and with at least 80% power of this study. By providing an additional allowance of 20% in sample recruitment due to possible non-response rate, the required sample size has been increased to 14 subjects in each group. The formula of sample size calculation is based on a study reported by Dupont and Plummer ( 31 ).”
Discussion on Effect Size Planning
Sample size is just an estimate indicating a minimum required number of sample data that is necessary to be collected to derive an accurate estimate for the target population or to obtain statistically significant results based on the desired effect sizes. In order to calculate or estimate sample size, researchers will need to provide some initial estimates for effect sizes. It is usually quite challenging to provide a reasonably accurate value of the effect size because the exact values of these effect sizes are usually not known and can only be derived from the study after the analysis is completed. Hence, the discrepancies of the effect sizes are commonly expected where the researchers will usually either overestimate or underestimate them.
A major problem often arises when the researchers overestimate the effect sizes during sample size estimation, which can lead to a failure of a study to detect a statistically significant result. To avoid such a problem, the researchers are encouraged to recruit more subjects than the minimum required sample size of the study. By referring to the same example previously (new diet programme versus conventional diet programme), if the required sample size is 11 subjects in each group, then researchers may consider recruiting more than 11 subjects such as 18 to 20 subjects in each group. This is possible if the researchers have the capability in terms of manpower and research grant to recruit more subjects and also if there are adequate number of subjects available to be recruited.
After the study is completed, if the difference in mean reduction was found not at least 1 kg after 1 month, then the result might not be statistically significant (depending on the actual value of the within-group SD) for a sample size of 11 subjects in each group. However, if the researchers had recruited 18 subjects in each group, the study will still obtain significant results even though the difference of mean reduction was 0.8 kg (if the within-group SD is estimated to be 0.8, and an equal sample size is planned for both groups, with type I error set at 0.05 and power of at least 80%). In this situation, researcher would still be able to draw a conclusion that the difference in mean reduction after one month was 0.8 kg, and this result was statistically significant. Such a conclusion is perhaps more meaningful than stating a non-significant result ( P > 0.05) for another study with only 11 subjects in each group.
However, it is necessary to always bear in mind that obtaining a larger sample size merely to show that P -value is less than 0.05 is not the right thing to do and it can also result in a waste of resources. Hence, the purpose of increasing the size of the sample from 11 to 18 per group is not merely for obtaining a P -value of less than 0.05; but more importantly, it is now able to draw a valid and clinically-significant conclusion from the smallest acceptable value of the effect size. In other words, the researcher is now able to tolerate a smaller effect size by stating that the difference in mean reduction of 0.8 kg is also considered to be a sizeable effect size because it is clinically significant. However, if the researcher insists that the difference in mean reduction should be at least 1.0 kg, then it will be necessary to maintain a minimum sample of only 11 subjects per group. It is now clear that such a subjective variation in the overall consideration of the magnitude of this effect size sometimes depends on the effectiveness and the cost of the new diet programme and hence, this will always require some degree of clinical judgement.
The concept of setting a desired value of the effect size is almost identical for all types of statistical test. The above example is only describing an analysis using the independent sample t -test. Since each statistical test may require a different effect size in its calculation or estimation of the sample size; thus, it is necessary for the researchers to be familiarised with each of these statistical tests in order to be able to set the desired values of the effect sizes for the study. In addition, further assistance may be sought from statisticians or biostatisticians for the determination of an adequate minimum sample size required for these studies.
Another Example of Sample Size Estimation Using General Rule of Thumb
Say a study aims to determine the association of factors with optimal HbA1c level as determined by its cut-off point of < 6.5% among patients with type 2 diabetes mellitus (T2DM). Previous study had already estimated that several significant factors were identified, and then included as three to four variables in the final model consisting of parameters that were selected from demographic profile of patients and clinical parameters (cite the appropriate reference). Now, the question is: How many T2DM patients should the study recruit in order to answer the study objective?
Step 1: To Understand the Objective of Study
The study aims to determine a set of independent variables that show a significant association with optimal HbA1c level (as determined by its cut-off point of < 6.5%) among T2DM patients.
Step 2: To Decide the Appropriate Statistical Analysis
In this example, the outcome variable is in the categorical and binary form, such as HbA1c level of < 6.5% versus ≥ 6.5%. On the other hand, there are about 3 to 4 independent variables, which can be expressed in both the categorical and numerical form. Therefore, an appropriate statistical analysis shall be logistic regression.
Step 3: To Estimate or Calculate the Sample Size Required
Since this study will require a multivariate regression analysis, thus it is recommended to estimate sample size based on the general rule of thumb. This is because the calculation of sample size for a multivariate regression analysis can be very complicated as the analysis will involve many variables and effect sizes. There are several general rules of thumb available for estimating the sample size for multivariate logistic regression. One of the latest rule of thumb is proposed by Bujang et al. ( 44 ). Two approaches are introduced here, namely: i) sample size estimation based on concept of event per variable (EPV) and ii) sample size estimation based on a simple formula.
- i) Sample size estimation based on a concept EPV 50
For EPV 50, the researcher will need to know the prevalence of the ‘good’ outcome category and the number of subjects in the ‘good’ outcome category to fit the rule of EPV 50 ( 14 , 44 ). Say, the prevalence of ‘good’ outcome category is reported at 70% (cite the appropriate reference). Then, with a total of four independent variables, the minimum sample size required in the ‘poor’ outcome category will be at least 200 subjects in order to fulfil the condition for EPV 50 (i.e. 200/4 = 50). On the other hand, by estimating the prevalence of ‘good’ outcome at 70.0%, this study will therefore need to recruit at least 290 subjects in order to ensure that a minimum 200 subjects will be obtained in the ‘poor’ outcome category (70/100 x 290 = 203, and 203 > 200).
- ii) Sample size estimation based on a formula of n = 100 + 50i (where i represents number of independent variable in the final model)
When using this formula, the researcher will first need to set the total number of independent variables in the final model ( 44 ). As stated in the example, the total number of independent variables were estimated to be about three to four (cite the appropriate reference). Then, with a total of four independent variables, the minimum required sample size will be 300 patients [(i.e. 100 + 50 ( 4 ) = 300].
Step 4: To Provide Additional Allowance for a Certain Proportion of Non-Response Rate
In order to make up for a rough estimate of 20.0% of non-response rate, the minimum sample size requirement is calculated to be 254 patients (i.e. 203/0.8) by estimating the sample size based on the EPV 50, and is calculated to be 375 patients (i.e. 300/0.8) by estimating the sample size based on the formula n = 100 + 50 i.
There were previously two approaches that were introduced to estimate sample size for logistic regression. Say, if the researcher chooses to apply the formula n = 100 + 50 i. Therefore, the sample size statement will be written as follows:
“The main objective of this study is to determine the association of factors with optimal HbA1c level as determined by its cut-off point of < 6.5% among patients with type 2 diabetes mellitus (T2DM). The sample size estimation is derived from the general rule of thumb for logistic regression proposed by Bujang et al. ( 44 ), which had established a simple guideline of sample size determination for logistic regression. In this study, Bujang et al. ( 44 ) suggested to calculate the sample size by basing on a formula n = 100 + 50 i. The estimated total number of independent variables was about three to four (cite the appropriate reference). Thus, with a total of four independent variables, the minimum required sample size will be 300 patients (i.e. 100 + 50 ( 4 ) = 300). By providing an additional allowance to cater for a possible dropout rate of 20%, this study will therefore need at least a sample size of 300/0.8 = 375 patients.”
Other Issues
Previously, there are four different approaches to estimate an effect size such as: i) by deriving it from the literature; ii) by using historical data or secondary data to estimate it; iii) by determining the clinical meaningful effect and last but not least and iv) by deriving it from the results of a pilot study. It is a controversial practice to estimate the effect size from a pilot study because it may not be accurate since the effect size has been derived from a small sample provided by a pilot study ( 52 – 55 ). In reality, many researchers often encounter great difficulties in the estimation of sample size either i) when the required effect size is not reported by the existing literature; or ii) if some new, innovative research proposals which may pose pioneering research questions that have never been addressed; or iii) if the research is examining a new intervention or exploring a new research area in where no similar studies have previously been conducted. Although there are many concerns about validity of using pilot studies for power calculation, further research is still being conducted in pilot studies in order to apply more scientifically robust approaches for using pilot studies in gathering preliminary support for subsequent research. For example, there are now many published studies regarding guidelines for estimating sample size requirements in pilot studies ( 54 – 61 ).
This article has sought to provide a brief but clear guidance on how to determine the minimum sample size requirements for all researchers. Sample size calculation can be a difficult task, especially for the junior researcher. However, the availability of sample size software, and sample size tables for sample size determination based on various statistical tests, and several recommended rules of thumb which can be helpful for guiding the researchers in the determination of an adequate sample size for their studies. For the sake of brevity and convenience, this paper hereby proposes a useful checklist that is presented in Table 2 , which aims to guide and assist all researchers to determine an adequate sample size for their studies.
A step-by-step guide for sample size determination
Steps | Processes | Checklist |
---|---|---|
Step 1 | To understand the objective of study | |
a. The objective of study can be addressed by statistical analysis. | ( ) | |
Step 2 | To decide the appropriate statistical analysis | |
a. The appropriate statistical test to answer the objective of study has been selected. | ( ) | |
Step 3 | To estimate or calculate the sample size | |
a. It is necessary to ensure that the basis for which the determination of the effect sizes and/or conditions and assumptions for the use of a rule of thumb are robust and appropriate. | ( ) | |
b. It is necessary to state clearly the planned effect sizes for the statistical test/ the conditions and assumptions for the use of a rule of thumb for sample size estimation. | ( ) | |
c. Sample size is estimated by either i) using a manual calculation; ii) using a sample size software; iii) referring to a sample size table or iv) using a wellrecognised rule of thumb. | ( ) | |
d. It is necessary to ensure that the estimated sample size is feasible to be recruited within the allocated time period for recruitment. | ( ) | |
Step 4 | To provide additional allowance to cater for the possibility of non-response rate | |
a. It is necessary to decide whether the total non-response rate is acceptable (or not). | ( ) | |
b. It is necessary to adjust the estimated sample size by incorporating an additional allowance to cater for a certain percentage of non-response rate. | ( ) | |
Step 5 | To write a sample size statement | |
The sample size statement should include the following details: | ||
a. The study objective or its hypothesis. | ( ) | |
b. The choice of the statistical test to address the study objective. | ( ) | |
c. It is necessary to state clearly the effect sizes for the statistical test/the conditions and assumptions for the use of a rule of thumb for sample size estimation. | ( ) | |
d. It is necessary to cite all relevant reference(s) or justification(s) supporting the planned effect sizes/condition(s) and assumption(s) for the use of a rule of thumb for sample size estimation. | ( ) | |
e. It is necessary to state clearly the cut-off values for type I error and power, except when the sample size estimation is based on a rule of thumb (then it will become unnecessary to do so). | ( ) | |
f. It is necessary to state clearly the possibility of non-response rate, and to provide an additional allowance to cater for it by recruiting more than the minimum sample size. | ( ) | |
g. To state the sample size to be recruited. | ( ) |
Acknowledgements
I would like to thank the Director General of Health, Ministry of Health Malaysia for his permission to publish this article. I would also thank Dr Ang Swee Hung and Mr Hoon Yon Khee for proofreading this article.
Conflict of Interest

- Solutions Industry Gaming Automotive Sports and events Education Government Travel & Hospitality Financial Services Healthcare Member Experience Technology Use case AskWhy Communities Audience InsightsHub InstantAnswers Digsite LivePolls Journey Mapping GDPR Positive People Science 360 Feedback Surveys Research Edition
- Resources Blog eBooks Survey Templates Case Studies Training Webinars Help center
Sample Size Calculator
Do you know how many people have to answer your survey no problem. use our sample size calculator to know the exact number of surveys to run in your study..
Whenever you’re running and planning a research study, there’s nothing as important as knowing the exact number of surveys that you need for the study to be considered valid research material. This is when we need to know what’s the best way to you’ll be able to determine the sample size for your research.
We’re lovers of technology and automation here at QuesionPro. This is why we’ve created a simple sample calculator, so that just a few clicks away, determine the exact number of surveys to run.
If you’d like to know a bit more about how to use this sample calculator, please read the following part, and you’ll become an expert in Sample Calculation.
Sample size calculator for your research
With this calculator, you’ll be able to quickly and effectively measure the sample size of your next research. Surely, using it will allow you to save a lot of time. Take the most advantage out of it and use it whenever needed.
IMAGES
COMMENTS
If you have a small to moderate population and know all of the key values, you should use the standard formula. The standard formula for sample size is: Sample Size = [z 2 * p(1-p)] / e 2 / 1 + [z 2 * p(1-p)] / e 2 * N] N = population size; z = z-score; e = margin of error; p = standard of deviation
In this method a value E is calculated based on decided sample size. The value if E should lies within 10 to 20 for optimum sample size. If a value of E is less than 10 then more animal should be included and if it is more than 20 then sample size should be decreased. E = Total number of animals - Total number of groups.
Sample Size Calculation. Sample size is a statistical concept that involves determining the number of observations or replicates (the repetition of an experimental condition used to estimate the variability of a phenomenon) that should be included in a statistical sample. It is an important aspect of any empirical study requiring that ...
Sample size calculation is an essential methodological issue in clinical research. It is crucial to ensure that the study has sufficient participants in order to detect the expected effect estimate. ... Requirements for sample size calculation based on the prevalence rate. 1) Population size: this indicates an estimate of the number of ...
If a difference of 15 mmHg in MAP is considered between the phenylephrine and the placebo group as clinically significant (μ1− μ2) and be detected with 80% power and a significance level alpha of 0.05. [ 7] n = 2 × ( [1.96 + 0.842] 2 × 20 2 )/15 2 = 27.9. That means 28 subjects per group is the sample size.
PS Power and Sample Size Calculation 15 or Sample Size Calculator 16 are practical tools for power and sample size calculations in studies with dichotomous, continuous, or survival outcome measures. The support offered by these tools varies in terms of the type of interface and the mathematical formula or assumptions used for calculation. 17 - 20
Now that you know what goes into determining sample size, you can easily calculate sample size online. Consider using a sample size calculator to ensure accuracy. Or, calculate it the old-fashioned way: by hand. Below, find two sample size calculations - one for the known population proportion and one for the unknown population.
Sample size calculation formula. The equation that our sample size calculator uses is: n_1 = Z^2\cdot p \cdot \frac {1-p} {\mathrm {ME}^2} n1 = Z 2 ⋅ p ⋅ ME21 − p. where: Z Z — The z-score associated with the confidence level you chose. Our statistical significance calculator calculates this value automatically, but if you want to learn ...
Using the formula (or calculator above), you can calculate your sample size to 384. Example 2: Company-wide survey. In contrast, if you conducted a company-wide survey with 300 employees and wanted an 80% confidence level and a 10% margin of error, your sample size would be 37.
2. Use the sample size formula. Plug in your Z-score, standard of deviation, and confidence interval into the sample size calculator or use this sample size formula to work it out yourself: This equation is for an unknown population size or a very large population size. If your population is smaller and known, just use the sample size calculator.
In the initial stage of planning a research study, sample size calcu- ... sample size calculations in health research are summarized. The remainder of this paper is organized as follows. In Section 2, ... ematical formula or assumptions used for calculation. 17- 20 2.3 | Statistical analysis to be used is important in ...
Department of Quantitative Health Sciences, Lerner Research Institute. Cleveland Clinic, 9500 Euclid Ave./ JJN3-01, Cleveland, OH 44195. Email: [email protected]. We present the hypothesis tests and the corresponding sample size estimation formulas by. the type of study design and type of outcome. 1.
There are many formulas used for calculating sample size. One of the most common formulas used is Yamane's formula: n = N/ (1+N (e)2. The variables in this formula are: n = the sample size. N ...
2.58. Put these figures into the sample size formula to get your sample size. Here is an example calculation: Say you choose to work with a 95% confidence level, a standard deviation of 0.5, and a confidence interval (margin of error) of ± 5%, you just need to substitute the values in the formula: ( (1.96)2 x .5 (.5)) / (.05)2.
The formulas for calculating sample size depend on the statistical method used. Here are the commonly used formulas: Power analysis: N = [ (Zα/2 + Zβ) / ES] ^ 2. Where: N = sample size. Zα/2 = the critical value of the standard normal distribution for a specified level of significance.
This can be done using an online sample size calculator or with paper and pencil. 1. Find your Z-score. Next, you need to turn your confidence level into a Z-score. Here are the Z-scores for the most common confidence levels: 90% - Z Score = 1.645. 95% - Z Score = 1.96. 99% - Z Score = 2.576.
The sample size is the number of patients or other experimental units included in a study, and determining the sample size required to answer the research question is one of the first steps in designing a study. ... Formulas for sample size calculation differ depending on the type of study design and the studies outcome(s). These calculations ...
Sample size calculation formula - sample size determination. With all the necessary terms defined, it's time to learn how to determine sample size using a sample calculation formula. Your confidence level corresponds to a Z-score. This is a constant value needed for this equation. Here are the z-scores for the most common confidence levels:
The formula that will be used to estimate or calculate the sample size will be the same as the formula for performing the statistical test that will be used to answer the objective of study. For example, if an independent sample t-test has to be used for analysis, then its sample size formula should be based on an independent sample t-test ...
The resulting sample size formula, is often applied with a conservative estimate of p (e.g., 0.5): ... Qualitative research approaches sample size determination with a distinctive methodology that diverges from quantitative methods. Rather than relying on predetermined formulas or statistical calculations, it involves a subjective and iterative ...
or calculate the sample size will be the same formula for performing the statistical test that will be used to answer the objective of study. 3. Calculate or Estimate the Sample Size Estimating or calculating the sample size can be done either by using manual calculation, sample size software, sample size tables from scientific published
Use our sample size calculator to know the exact number of surveys to run in your study and learn the formula and steps to calculate your sample size easily. Products Survey Software Easy to use and accessible for everyone. Design, send and analyze online surveys. ... Sample size calculator for your research.
The following simple formula is used for calculating the minimum sample size in a cross-sectional study for estimating the prevalence or a proportion: $$ n\ge {Z}^2.p\left (1-p\right)/ {d}^2 $$. (9.1) where n is the sample size, Z is the value of standard normal deviation corresponding to the level of confidence.
The sample size is a crucial consideration in research because it directly impacts the reliability and extent to which you can generalise those findings to the larger population. ... This method considers the effect size, significance level, and power to calculate the sample size. Essentially, you will need to determine what level of confidence ...