A Systems View Across Time and Space
- Open access
- Published: 06 July 2021

Determinants of household electricity consumption in Greece: a statistical analysis
- Dimitra Kotsila 1 &
- Persefoni Polychronidou ORCID: orcid.org/0000-0003-1517-011X 2 , 3
Journal of Innovation and Entrepreneurship volume 10 , Article number: 19 ( 2021 ) Cite this article
12k Accesses
Metrics details
Over the last decades, the contemporary way of living, as well as the technology development, has increased the household electricity consumption. The excessive use of electricity has a negative impact on the environment, increasing the carbon footprint and contributing to the climate change. Governments are more and more concerned about the way our societies consume energy and are committed to reduce the greenhouse emissions. As the residential sector contributes significantly to energy use, it is crucial to investigate the socio-economic parameters, dwellings’ characteristics, and climate conditions that determine the electricity consumption in households. The data of this study are collected from 1801 dwellings from all regions of Greece and two statistical models are built. Both of them conclude that the most significant determinants influencing the electricity consumption are the number of occupants, the size of the dwelling, the heating type, the heating and cooling hours, and the weather conditions.
Introduction
In the last decades due to the increased demand and the improved lifestyle, energy demand in the residential sector has increased rapidly causing the policymakers’ concern. The international climate negotiation is an opportunity for decision-makers to promote enhancement of energy efficiency and reduction of greenhouse emissions and set commitments for renewable energy consumption. The recent “2030 Energy Strategy” and the Paris climate conference (COP21) focus on the previous objectives to keep a global temperature rise this century below 2 °C. According to IEA ( 2020a ), based on the most recent data of 2018 in Greece, the CO2 emissions from fuel combustion only come from electricity and heat producers (46.8%), transport (27.4%), industry (8.1%), other energy industries (8.1%), residential (6.5%), commercial and public services (1.6%), and other (1.6%).
In the last two decades, the Greek governments have given a high priority to the environmental protection; thus, they promoted the Renewable Energy Sources (RES). The main aim concerning the RES is to be able to participate in the electricity consumption for at least 40% by the end of 2020. Specifically, under the European Renewable Energy Directive 2009/28/EC (European Parliament and Council, 2009 ) and the Law 3851/2010 (Official Government Gazette, 2018 ), Greece needs to meet the following targets for RES contribution:
20% of the final energy consumption for heating and cooling
40% of the final electricity consumption
10% of the final energy consumption in transportation
According to the most recent provided data by IEA ( 2017 ), Greece has achieved its goal in the sector of heating and cooling (58.90%), but is far from its aim in the other two sectors (22.09% and 1.43%, respectively). However, Greece has made an impressive progress in the electricity sector, thanks to the rapid growth in installed wind and solar parks and the decrease in the total electricity demand.
In 2019, RES and gas are the primary fuels of electricity production in Greece (IEA, 2020b ). In particular, the total electricity production is based on RES (35.2%), gas (35.2%), coal (22.2%), and oil (9.2%), while in 2017 the corresponding percentages were the following: RES (25.1%), gas (31%), coal (34%), and oil (10%).
Policymakers must focus on the distribution of the electricity consumption, in order to detect and improve the most inefficient sectors. In 2018, the distribution of electricity consumption per sector has been the following: commercial and public services (36%), residential (33.9%), industry (25.1%), agriculture/foster (4.6%), and transport (0.6%); it must be noted that this is similar in the last ten years (IEA, 2020c ).
In the residential sector, the final energy consumption is used to heat the space (57%), for lighting and appliances (20%), for heater heating (12%), for cooking (7%), and for space cooling (4%) (Eurostat, 2018 ).
Consumption per capita is an important indicator to observe the tendency of electricity consumption through the years, as it offers a clear view of the electricity that every individual consumes. In Greece, in 2014 the per capita electricity consumption was 5062.61 kWh/capita while in 2010 was 5333.44 kWh/capita and in 2008 was 5805.19 kWh/capita (DataBank, 2020 ). The economic crisis in Greece since 2008–2009 influenced the electricity consumption as in 2013 the average consumption fell to 5029 kWh/capita. Consumption per household is another indicator to monitor the electricity consumption in the residential sector. In 2000, the electricity per household (hh) was 3717 kWh/hh, while in 2010 it was 4023 kWh/hh (World Energy Council, 2016 ).
The increasing trend of electricity consumption is a reality. Since the residential sector is one of the most demanding sectors regarding electricity consumption, it should be studied. Unfortunately, in Greece, the socio-economic parameters that influence the electricity consumption have never been extensively examined. From the previous studies, that are outlined in the “Literature review” section, the electricity consumption is relevant with demographic data, dwelling characteristics and geographical and climate conditions. This study analyzes the aforementioned parameters in Greece and it is based on real data from 1801 customers of a Greece’s electricity provider. The variables and the methodology that are used in the current analysis are in line with the deep literature review that was conducted by Jones et al. ( 2015 ).
This paper is organized as follows: in the “Literature review” section, an overview of the literature review relevant for this study is included. A description of the regression models and the data sources is given in the “Method” section. In the “Results and discussion” section, the main results are summarized. Finally, the conclusions are presented in the “Conclusions” section.
Literature review
On the international stage, residential electricity consumption is a considerably studied subject. Relevant studies are sequenced with the oldest first and the most recent last.
Studies regarding European countries
Halicioglu ( 2007 ) examines how the energy demand in residential sector in Turkey was influenced from the price and income. The income positively influences the electricity demand and accelerates purchases of electrical goods and services. The price of electricity negatively influences the electricity demand, while the urbanization positively influences the electricity demand, as it provides greater access to electricity.
Gram-Hanssen ( 2011 ) points out that the user’s practices influence the energy consumption. He examines 8500 detached houses in Denmark and he finds that the income, the size of the house, and the presence of teenagers (13–19 years old) all have a positive effect on electricity consumption.
Wiesmann et al. ( 2011 ) examine the relationship between the per capita electricity consumption and dwelling characteristics in Portuguese consumers. They conclude that the income, the appliance ownership, and the floor area have a positive influence on per capita electricity consumption. People who live in single-family houses and/or in urban households consume more electricity than those living in a block of flats and/or rural households. People per household, dwellings per building and more heating degree-days negatively influence the consumption per capita, since in colder regions households consume less electricity per capita than those in more moderate climates.
McLoughlin et al. ( 2012 ) examine the influence of dwelling and occupant characteristics on electricity consumption of 3941 Irish dwellings. Dwelling type, number of bedrooms, age of the head of household, and electrical appliances used for water heating and cooking have a positive effect on electricity consumption.
Bedir et al. ( 2013 ) point out that in Netherlands the household size, the dwelling type, and the duration of electrical appliances’ use (such as dryers and washing machines) have significant effect on the electricity consumption. Also, in the Netherlands, Brounen et al. ( 2012 ) analyze data of 300,000 dwellings. The dwelling type affects electricity consumption as the detached and semi-detached houses consume more electricity per capita than row houses or apartments. Houses with children and especially those that have teenagers are found to have a positive effect on per capita electricity consumption. The income has positive influence whereas the number of persons in household has a negative effect on per capita electricity consumption.
Studies regarding non-European countries
Ndiaye and Gabriel ( 2011 ) analyze electricity consumption of 62 dwellings of Oshawa (Ontario, Canada). Number of residents, house status, type of fuel used to heat the pool, type of fuel used in the heating system, type of fuel used in the domestic hot water heater, type of air-conditioning, and number of air changes per hour at 50 Pa are found to have a positive effect on the electricity consumption. On the other hand, the average number of weeks that the family leaves for vacation and the existence or not of an air conditioning system have a negative effect on the electricity.
Sanquist et al. ( 2012 ) base their research on data from the Residential Energy Consumption Survey (RECS) conducted on 2005 in the USA. The air-conditioning, laundry usage, personal computers, climate zone of dwelling, and TV use significantly influence the electricity consumption. Kavousian et al. ( 2013 ) examine the residential electricity consumption of 952 US dwellings in a view of daily maximum and minimum. Daily minimum consumption is influenced by weather, location, dwelling size, and the number of refrigerators when daily maximum consumption is influenced by the use of appliances that consume a lot and the number of residents. In the summer model, the primary factor that influences the electricity consumption is the cooling degrees days.
Tewathia ( 2014 ) conducts a survey in Delhi to find the determinants of electricity consumption. The household income, the number and the usage of electrical appliances, the size of the house, the family size, time spent out of the house, and the higher educational level influence the monthly electricity consumption through all the seasons. The educational level has a negative relationship as the higher educated families tend to consume less electricity. Filippini and Pachauri ( 2004 ) analyze the electricity demand in urban Indian households. The price is inelastic in electricity demand, so the price is not an inhibiting factor in residential electricity consumption. The income, the size, the regions, and the degrees of urbanity have a significant influence into the electricity consumption. Dwellings with more residents and younger households head had the tendency to consume less electricity from those that had less elder people.
Jones et al. ( 2015 ) conduct a broad literature review to investigate the factors that influence or not the domestic electricity consumption. They study 62 factors as potential factors that determine the electricity consumption. In relation to socio-economic factors, the higher household and disposable income, the more occupants and presence of teenagers have a positive effect on electricity consumption. In relation to dwelling factors, the dwelling age, the number of rooms, the number of bedrooms, and the floor area influence the electricity consumption. Regarding appliance factors, the following ones have a positive effect: more appliances, the existence of desktop computer, television, electric oven, refrigerator, dishwasher, tumble dryer, and higher use of washing machines and tumble dryer.
Esmaeilimoakher et al. ( 2016 ) accomplish an introduction to the factors that influence the electricity consumption. Their analysis is based on a survey conducted in nine households of Perth of Western Australia. The main results are that the average annual electricity consumption per person per m 2 floor area (AAEC/P m 2 ) has a negative correlation with the number of occupants and the dwellings size.
Studies regarding Greece
The research in the field of socio-economic determinants that influence electricity consumption in Greece is poor. There are a lot of works focused on macroeconomic factors that determine energy consumption. Only the study of Sardianou ( 2007 ) provides research that includes demographic data and examines electricity conservation behavior.
Donatos and Mergos ( 1991 ) examine the residential electricity demand in Greece during the period 1961–1986. Data are collected from a public database. It is found that the electricity demand is price inelastic and income elastic. The sales of appliances, as well as the heating degrees-days, are found to have an insignificant effect on electricity demand in contrast with the number of consumers that has a significant effect.
Hondroyiannis ( 2004 ) examines the elasticity of price and income in long-run and short-run demand for residential electricity. The examined period is 1986–1999 employing monthly data. In the short-run, the electricity demand is income inelastic and independent of the price, while in the long-run period, all variables, income, price, and weighted average temperature are found to affect electricity demand.
Polemis and Dagoumas ( 2013 ) conduct a similar with Hondroyiannis ( 2004 ) research. They use cointegration techniques and the vector error correction model to observe the long-run and short-run electricity demand. The data that are taken into account are for a longer period, from 1970 to 2011. In the long-run, the electricity demand is price inelastic and income elastic, while in the short-run the relevant elasticities are inelastic.
Sardianou ( 2007 ) investigates the determinants of household energy conservation. The analysis is based on a survey that has been conducted in 586 households of five main Athens’ regions. One of the findings is that people with higher income that own their houses, and have a large family are more willing to conserve energy. Also, the number of rooms, the dwelling’s size, sex, educational level, and marital status are found that do not have a significant influence in energy conservation. However, it is found that the larger electricity expenditures negatively influence the energy conservation behaviors and the older people are more energy-intensive users than the younger ones.
All variables that have been studied in the above papers are indicated in Tables 6 and 7 in the Appendix section. Tables 6 and 7 also present which of these variables have been studied in this paper.
Model specification
The ordinary least squares (OLS) regression is used to estimate the determinants that affect the electricity consumption in Greek households. A variety of studies that examined the determinants of electricity consumption have been conducted using OLS regression (Bedir et al., 2013 ; Brounen et al., 2012 ; Filippini & Pachauri, 2004 ; Gram-Hanssen, 2011 ; Halicioglu, 2007 ; Kavousian et al., 2013 ; McLoughlin et al., 2012 ; Ndiaye & Gabriel, 2011 ; Sanquist et al., 2012 ; Sardianou, 2007 ; Wiesmann et al., 2011 ). Two different models are employed to determine the electricity consumption. More specifically, a simple OLS regression model and a log-linear regression model are used to build the aforementioned models.
Data sources and description
Data are collected from a Greek electricity provider and refer only to residential dwellings. Data for the consumption and square meters area have been provided through the actual bills. The period of actual bills is not the same between bills and differs among dwellings. Thus, in order to calculate the consumption with accuracy, the consumption per day is calculated at first. Afterwards, consumption per month is calculated and only dwellings that have consumption 2017 are selected.
Demographics and behavior data are retrieved from a questionnaire that was provided through the electricity provider’s online platform. The questionnaire was answered from every individual that covers the bill. Only dwellings that already had a monthly consumption for 2017 and have also answered all the questions are selected. The dwellings with invalid values have been excluded from the data, so the final number of dwellings that are included in the analysis is 1801. Table 1 illustrates all available variables, as well as the type and the units of the variables of both models. Table 2 illustrates the summary of statistics of the variables of both models and it shows the levels of ordinal variables and their correspondence to the converted numbers. In addition, the factor type of each variable is indicated.
In the literature review, we have found out that many studies (Donatos & Mergos, 1991 ; Kavousian et al., 2013 ; Ndiaye & Gabriel, 2011 ; Sanquist et al., 2012 ; Wiesmann et al., 2011 ) include in their models the weather factors of heating degrees-days (HDD) and cooling degrees-days (CDD). HDD and CDD is the difference, in degrees, of outside temperature and base (18.3 °C ) temperature. HDD and CDD data for each prefecture and for 2017 have been downloaded from the weather stations of National Observatory of Athens (NOA). Data have been available in the weather website ( www.meteo.gr ) and have been recorded at a daily basis (Petrou, 2018 ). The yearly HDD and CDD have been calculated per prefecture and the weather data are then connected to the consumption data based on prefecture. Units of HDD and CDD are in 1000 °C days (the values of these variables are big numbers and they should be fitted in the model as in Wiesmann et al., 2011 ).
The data preparation, manipulation, visualization, and the regression analysis is conducted using programming language R through RStudio program.
Before conducting a regression analysis, an advanced statistical analysis is conducted to observe any associations or correlation between variables. Thus, pairwise comparisons and a correlation analysis (correlation matrix can be found in Table 8 in the Appendix section) are used. The insights of the analysis show a very high negative correlation between HDD and CDD, approximately −0.809 (see Table 8 ), so HDD is removed from the model. Furthermore, a high correlation between family type and number of occupants is observed, so family type is removed from the model.
In the sample, the final independent variables along with the dummy variables count to thirty three (33). It is essential to determine whether the subset of all independent variables yields to an adequate and appropriate model. Stepwise regression is a method that attempts to find the best regression model, without examining all the possible models (Berenson et al., 2014 ). There are two approaches of stepwise regression, the “forward selection” and the “backward elimination”. Both approaches use the Akaike Information Criterion (AIC) to find the best combination of variables based on balancing the model’s complexity and accuracy. The best model is the one with minimum AIC value. The regression model is built using the forward selection, where an initial model is defined that contains only the constant and each independent variable is retained in the model only if it improves the ability of the model to predict the dependent variable.
Results and discussion
The ols regression method.
The results of the OLS regressions are presented in Table 3 . In general, the results from both models are in agreement with the literature, as it will be indicated in this section. Most of the significant variables are common in both models, but there are variables that influence one model and not the other. Τhe log-linear model (model 2) has better goodness of fit from the linear model, in terms of the R 2 . Both models explain approximately 30% of the variance in electricity consumption. Even if this value does not seem satisfactory for a regression analysis, when comparing to the literature the R 2 is within the range published. Indeed, in Sardianou ( 2007 ), R 2 is approximately 10%; in Wiesmann et al. ( 2011 ), R 2 is approximately 33%; in Bedir et al. ( 2013 ), R 2 is approximately 50%; and in most other papers presented in the literature review, the R 2 value has not been given. One basic reason for this low value is that even though we have studied thirty-three variables (most given by the electricity provider and others found by the authors), more parameters need to be studied.
Square meters area has a significant effect on the average monthly electricity consumption in both models. To be more specific, if the square meters area increases by 1m 2 then an increase of 1.389 kWh/month according to model 1 and an increase on average yearly consumption by 0.3% according to model 2 are expected. These results are in line with previous studies (Bedir et al., 2013 ; Filippini & Pachauri, 2004 ; Gram-Hanssen, 2011 ; Jones et al., 2015 ; Kavousian et al., 2013 ; Tewathia, 2014 ; Wiesmann et al., 2011 ).
Number of occupants is strongly related with the electricity consumption (because of high beta coefficient and p -value < 0.01). In both models, more occupants consume more electricity. Studies of Gram-Hanssen ( 2011 ), Jones et al. ( 2015 ), Kavousian et al. ( 2013 ), and Ndiaye and Gabriel ( 2011 ) reach the same conclusion.
Heating hours, heating type, and the presence of secondary heaters have a significant positive effect on electricity consumption in both models. That is, heating hours influence the electricity consumption regardless the heating type. On the other hand, heating type of local units significantly influences the electricity consumption. Dwellings that use local units for heating the space seem to consume more electricity. The results reveal that houses with heating type of local units use possibly electrical appliances to heat their space which has an impact on their total consumption. The presence of secondary heaters has also a significant positive effect on the electricity consumption. Those results enhance the perspective that the electrical appliances used to heat the space have an impact on electricity consumption. Jones et al. ( 2015 ) mention that there are eight studies that found a positive effect of presence of electric space heating system on electricity consumption.
Cooling hours is also related with the electricity consumption. Both models agree to the positive relationship between cooling hours and electricity consumption, as expected. CDD also is found that has a positive effect on electricity consumption in both models. The presence of air-conditioning to cool the space has a significant effect in model 2 while in model 1 it is not found that influences the electricity consumption. This result is aligned with the literature review presented by Jones et al. ( 2015 ), since they present studies with both results.
According to model 1, the dwelling type has a significant effect on electricity consumption, as the single family houses seem to consume more electricity. This result is aligned with past studies of Bedir et al. ( 2013 ), Brounen et al. ( 2012 ), McLoughlin et al. ( 2012 ), and Wiesmann et al. ( 2011 ).
Both models reveal that the age of inhabitants positively influences the electricity consumption. So, as long as the occupants that cover the bill are elderly, the more electricity is consumed. This result is aligned with and also mentioned that there are eight studies that marked the positive effect of age of head of the household on the electricity consumption.
According to model 2, dwellings with occupants that spent more time in home are found to have a positive significant effect on electricity consumption, as it is expected and it is aligned with Ndiaye and Gabriel ( 2011 ) and Tewathia ( 2014 ), respectively.
Many variables of the collected data are found that have no significant influence on the electricity consumption. Those variables are gender, educational level, house status, marital status, dwelling floor, income, light behavior, and summer holidays.
As mentioned above, most of the variables that are found significant are common in both models, but there are variables that influence one model and not the other. This happens because in model 2 a non-linear relationship exists between the independent and dependent variables.
Two-stage least squares method (2SLS)
It is possible that a two-way causation exists between variables of the above models; thus, there is the need to test for endogeneity. Variables that are tested for endogeneity are those of square meter area, number of occupants, and heating hours. Square meter area is found as significant at the level of 10%; thus, 2SLS is conducted with endogenous variable the square meters area. Number of bedrooms and number of rooms have been inserted in the model as instrumental variables. Tables 4 and 5 illustrate the results of OLS, first stage of 2SLS and second stage of 2SLS for model 1 and model 2, respectively. The coefficients of square meter area and other variables have been fitted and the significant variables are the same with the OLS method.
Conclusions
This study focuses on the investigation of the socio-economic determinants, the dwellings characteristics, and the climatic conditions that influence the household electricity consumption. Two regression models, one linear and one log-linear, are built and the results have been presented in the previous section. The two-stage last squares method (TSLS) is used to explore the two-way causation of squared meter area. Most significant variables are the number of occupants, the size of the dwelling, the heating type, the heating and cooling hours, and the weather conditions. The results of this study are aligned with the ones presented in the literature review.
When acknowledging the conclusions of this analysis, policy makers could use suitable incentives to motivate customers to reduce their electricity consumption. The variables regarding heating and cooling the space are found as significant in both models. A good practice would be for the government to motivate people to renovate their houses in order to maintain the houses’ temperature and as a consequence it could reduce the cooling and heating electricity demand. As it is shown from this study, in Greece, houses use electricity to heat their spaces and in many cases they use secondary heaters. Thus, another measure could be to give a subsidy to urge consumers to change their heating systems to more efficient and cheaper ones, such as natural gas boilers.
Another strategy that policy makers could follow is to incentivize electricity providers for the electricity per hh reduction. The utilities then could provide a discount to those households that achieve an electricity reduction year by year. Thus, both utilities and consumers will win from this strategy. The customers will have the incentive of lower cost and will try to reduce their consumption.
Simultaneously, government and utilities could enhance and promote the usage of new technology electricity appliances, such as smart appliances and appliances with A++ energy label, that consume less electricity. By large campaigns, the consumers could be informed about how they can save if they change their old wasteful appliances.
To conclude, this research shows that the dwelling and household characteristics, as well as the climatic conditions, are essential predictors in models of electricity consumption. Further research on occupants’ behavior and on presence and functions of appliances will enhance the determinants of electricity consumption in housing.
Availability of data and materials
Data of this study were collected from the database of a Greek electricity provider. The research data cannot be shared publicly, because the individual privacy could be compromised. The authors after the approval of the Greek electricity provider could provide the final data set to a third researcher.
Bedir, M., Hasselaar, E., & Itard, L. (2013). Determinants of electricity consumption in Dutch dwellings. Energy and Buildings , 58 , 194–207. https://doi.org/10.1016/j.enbuild.2012.10.016 .
Article Google Scholar
Berenson, M. L., Levine, D. M., & Szabat, K. A. (2014). Basic business statistics, student value edition , (13th ed., ). Pearson.
Brounen, D., Kok, N., & Quigley, J. M. (2012). Residential energy use and conservation: Economics and demographics. Green Building, the Economy, and Public Policy , 56 (5), 931–945.
Google Scholar
DataBank (2020). World development indicators . DataBank Available at: https://data.worldbank.org/indicator/EG.USE.ELEC.KH.PC?locations=GR (Accessed 20 Oct 2020).
Donatos, G. S., & Mergos, G. J. (1991). Residential demand for electricity: The case of Greece. Energy Economics , 13 (1), 41–47. https://doi.org/10.1016/0140-9883(91)90054-4 .
Esmaeilimoakher, P., Urmee, T., Pryor, T., & Baverstock, G. (2016). Identifying the determinants of residential electricity consumption for social housing in Perth, Western Australia. Energy and Buildings , 133 , 403–413. https://doi.org/10.1016/j.enbuild.2016.09.063 .
European Parliament and Council (2009). Directive 2009/28/EC of the European Parliament and of the Council of 23 April 2009 on the promotion of the use of energy from renewable sources and amending and subsequently repealing Directives 2001/77/EC and 2003/30/EC. Official Journal of the European Union , 52 (L140), 16–62.
Eurostat. (2018). Energy consumption in households - statistics explained , available at: http://ec.europa.eu/eurostat/statistics-explained/images/1/16/Energy_consumption_households_final.xlsx (Accessed 26 Apr 2018).
Filippini, M., & Pachauri, S. (2004). Elasticities of electricity demand in urban Indian households. Energy Policy , 32 (3), 429–436. https://doi.org/10.1016/S0301-4215(02)00314-2 .
Gram-Hanssen, K. (2011). Households’ energy use - which is the more important: efficient technologies or user practices?, Energy End-Use Efficiency Issues (EEE) , (pp. 992–999).
Halicioglu, F. (2007). Residential electricity demand dynamics in Turkey. Energy Economics , 29 (2), 199–210. https://doi.org/10.1016/j.eneco.2006.11.007 .
Hondroyiannis, G. (2004). Estimating residential demand for electricity in Greece. Energy Economics , 26 (3), 319–334. https://doi.org/10.1016/j.eneco.2004.04.001 .
International Energy Agency (2017). Energy policies of IEA Countries, Greece 2017 review , available at: https://euagenda.eu/upload/publications/untitled-110952-ea.pdf (accessed 19 October 2020).
International Energy Agency (2020a). CO2 emissions by sector, Greece 1990-2018 , available at: https://www.iea.org/data-and-statistics?country=GREECE&fuel=CO2%20emissions&indicator=CO2BySector (accessed 19 October 2020).
International Energy Agency (2020b). Electricity generation by source, Greece 1990-2019 , available at: https://www.iea.org/data-and-statistics?country=GREECE&fuel=Electricity%20and%20heat&indicator=ElecGenByFuel (Accessed 19 Oct 2020).
International Energy Agency (2020c). Electricity final consumption by sector, Greece 1990-2018 , available at: https://www.iea.org/data-and-statistics?country=GREECE&fuel=Electricity%20and%20heat&indicator=ElecConsBySector (Accessed 19 Oct 2020).
Jones, R. V., Fuertes, A., & Lomas, K. J. (2015). The socio-economic, dwelling and appliance related factors affecting electricity consumption in domestic buildings. Renewable and Sustainable Energy Reviews , 43 , 901–917. https://doi.org/10.1016/j.rser.2014.11.084 .
Kavousian, A., Rajagopal, R., & Fischer, M. (2013). Determinants of residential electricity consumption: Using smart meter data to examine the effect of climate, building characteristics, appliance stock, and occupants’ behavior. Energy , 55 , 184–194. https://doi.org/10.1016/j.energy.2013.03.086 .
McLoughlin, F., Duffy, A., & Conlon, M. (2012). Characterising domestic electricity consumption patterns by dwelling and occupant socio-economic variables: an Irish case study. Energy and Buildings , 48 , 240–248. https://doi.org/10.1016/j.enbuild.2012.01.037 .
Ndiaye, D., & Gabriel, K. (2011). Principal component analysis of the electricity consumption in residential dwellings. Energy and Buildings , 43 (2), 446–453. https://doi.org/10.1016/j.enbuild.2010.10.008 .
Official Government Gazette. (2018). Accelerating the development of renewable energy sources to deal with climate change and other regulations addressing issues under the authority of the Ministry of Environment, Energy and Climate Change, 3851/2010 , 1753–1779.
Petrou A.M. J. (2018). “meteo.gr”, meteo.gr - weather forecasts for Greece , available at: http://www.meteo.gr/ (Accessed 10 Mar 2018).
Polemis, M. L., & Dagoumas, A. S. (2013). The electricity consumption and economic growth nexus: Evidence from Greece. Energy Policy , 62 , 798–808. https://doi.org/10.1016/j.enpol.2013.06.086 .
Sanquist, T. F., Orr, H., Shui, B., & Bittner, A. C. (2012). Lifestyle factors in U.S. residential electricity consumption. Energy Policy , 42 , 354–364. https://doi.org/10.1016/j.enpol.2011.11.092 .
Sardianou, E. (2007). Estimating energy conservation patterns of Greek households. Energy Policy , 35 (7), 3778–3791. https://doi.org/10.1016/j.enpol.2007.01.020 .
Tewathia, N. (2014). Determinants of the household electricity consumption: a case study of Delhi. International Journal of Energy Economics and Policy , 4 (3), 337–348.
Wiesmann, D., Lima Azevedo, I., Ferrão, P., & Fernández, J. E. (2011). Residential electricity consumption in Portugal: findings from top-down and bottom-up models. Energy Policy , 39 (5), 2772–2779. https://doi.org/10.1016/j.enpol.2011.02.047 .
World Energy Council. (2016). Electricity use per household | Electricity Consumption Efficiency| WEC, available at: https://wec-indicators.enerdata.net/xls/household-electricity-use.xls (Accessed 26 Feb 2018).
Download references
Acknowledgements
Not applicable.
The authors declare that they have no funding for their research.
Author information
Authors and affiliations.
Master in Business Administration (MBA), Hellenic Open University, Patras, Greece
Dimitra Kotsila
Department of Economics, International Hellenic University, Serres, Greece
Persefoni Polychronidou
Hellenic Open University, Patras, Greece
You can also search for this author in PubMed Google Scholar
Contributions
The paper was jointly conceived, developed, and written by both authors. The authors read and approved the final manuscript.
Corresponding author
Correspondence to Persefoni Polychronidou .
Ethics declarations
Competing interests.
The authors declare that they have no competing interests.
Additional information
Publisher’s note.
Springer Nature remains neutral with regard to jurisdictional claims in published maps and institutional affiliations.
In the following table, the correlation matrix between types of variables is presented. Due to the varying type of variables, a compass correlation matrix is created as follows:
Continuous/discrete/ordinal pair: Pearson correlation
Continuous/discrete/categorical pair: correlation coefficient or squared root of R 2 coefficient of linear regression of integer/numeric variable over factor/categorical variable. The value lies between 0 and 1.
Categorical pair: Cramer’s V value is computed based on Chi-squared test using. The value lies between 0 and 1.
The cutoff point is set 0.6. So, the variables of Family type and HDD are omitted from the analysis.
Rights and permissions
Open Access This article is licensed under a Creative Commons Attribution 4.0 International License, which permits use, sharing, adaptation, distribution and reproduction in any medium or format, as long as you give appropriate credit to the original author(s) and the source, provide a link to the Creative Commons licence, and indicate if changes were made. The images or other third party material in this article are included in the article's Creative Commons licence, unless indicated otherwise in a credit line to the material. If material is not included in the article's Creative Commons licence and your intended use is not permitted by statutory regulation or exceeds the permitted use, you will need to obtain permission directly from the copyright holder. To view a copy of this licence, visit http://creativecommons.org/licenses/by/4.0/ .
Reprints and permissions
About this article
Cite this article.
Kotsila, D., Polychronidou, P. Determinants of household electricity consumption in Greece: a statistical analysis. J Innov Entrep 10 , 19 (2021). https://doi.org/10.1186/s13731-021-00161-9
Download citation
Received : 27 January 2020
Accepted : 04 April 2021
Published : 06 July 2021
DOI : https://doi.org/10.1186/s13731-021-00161-9
Share this article
Anyone you share the following link with will be able to read this content:
Sorry, a shareable link is not currently available for this article.
Provided by the Springer Nature SharedIt content-sharing initiative
- Electricity
- Consumption
- Determinants
- Socio-economic
- Statistical analysis
Thank you for visiting nature.com. You are using a browser version with limited support for CSS. To obtain the best experience, we recommend you use a more up to date browser (or turn off compatibility mode in Internet Explorer). In the meantime, to ensure continued support, we are displaying the site without styles and JavaScript.
- View all journals
- Explore content
- About the journal
- Publish with us
- Sign up for alerts
- Published: 21 September 2020
Increase in domestic electricity consumption from particulate air pollution
- Pan He ORCID: orcid.org/0000-0003-1088-6290 1 , 2 na1 ,
- Jing Liang 3 na1 ,
- Yueming (Lucy) Qiu ORCID: orcid.org/0000-0001-9233-4996 3 na1 ,
- Qingran Li 4 &
- Bo Xing 5
Nature Energy volume 5 , pages 985–995 ( 2020 ) Cite this article
15k Accesses
109 Altmetric
Metrics details
- Energy and behaviour
- Environmental impact
Accurate assessment of environmental externalities of particulate air pollution is crucial to the design and evaluation of environmental policies. Current evaluations mainly focus on direct damages resulting from exposure, missing indirect co-damages that occur through interactions among the externalities, human behaviours and technologies. Our study provides an empirical assessment of such co-damages using customer-level daily and hourly electricity data of a large sample of residential and commercial consumers in Arizona, United States. We use an instrumental variable panel regression approach and find that particulate matter air pollution increases electricity consumption in residential buildings as well as in retail and recreation service industries. Air pollution also reduces the actual electricity generated by distributed-solar panels. Lower-income and minority ethnic groups are disproportionally impacted by air pollution and pay higher electricity bills associated with pollution avoidance, stressing the importance of incorporating the consideration of environmental justice in energy policy-making.
Similar content being viewed by others
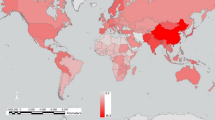
Global impacts of heat and water stress on food production and severe food insecurity
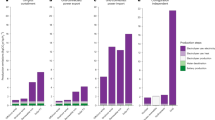
Worldwide greenhouse gas emissions of green hydrogen production and transport
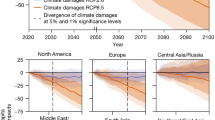
The economic commitment of climate change
Air pollution has resulted in many types of negative externalities, a situation that calls for policy intervention to address the associated damages. Policymakers and research are widely concerned with increases in mortality risk, which are direct damages induced by pollution, as well as co-damages in terms of other welfare losses. These damages are generated via different channels including the physical and mental health impact on human beings, decreases in labour productivity 1 , 2 , the decline in subjective well-being 3 , the harm of cognitive competence 4 , 5 , disturbances in ecosystem health 6 , a diminished value of local environmental amenities and properties 7 , increases in household medical expenditure and so on. Accurate assessment of such externalities is crucial to estimating the social cost of pollution for the design and evaluation of policies such as a Pigouvian tax imposed on polluters for such external costs or for pollution control, or a cap-and-trade programme that establishes a market issuing allowances to internalize such cost 8 . While direct pollution damages are often measured in existing studies, there are not many discussions in the literature about the magnitude of the co-damages. A key challenge to quantify these co-damages, however, is to understand the interactions among pollution, human behaviours 9 and technologies. People can mitigate exposure to environmental risks by taking various avoidance behaviours, such as adjusting outdoor activities 10 , 11 and purchasing face masks and air purification systems in the short term 9 , 12 , and migrating to new living locations in the longer term 13 . Avoidance behaviours alleviate the negative health impact of pollution 14 but come at a cost, for example, spending less time doing outdoor activities 10 , 15 , and may lead to further impacts such as increased energy consumption due to a shift from natural to mechanical ventilation 16 , and increased need for heating or air-conditioning or for other activities such as watching television 17 , 18 in residential buildings. Commercial buildings may also be affected via further complexities if individuals choose to work remotely due to air pollution to avoid exposure during commuting 19 . However, commercial buildings might have better indoor air quality due to better ventilation 20 so that people can stay in commercial buildings for longer period of time. These two effects can cancel out, and thus we hypothesize that air pollution does not have a statistically significant impact on commercial buildings as a whole. Such effects and the consequential extra environmental damage are, however, hardly addressed explicitly and quantitatively in current studies, which leads to biases in damage evaluation. Our paper fills in this gap in the literature.
While electricity demand is driven up by pollution-averting behaviours, air pollution can also reduce electricity supply. High concentrations of particulate matter reduce solar electricity generation due to the changed solar irradiance. The emission of aerosols can attenuate solar radiation by scattering and absorbing sunlight before it reaches the solar panel 21 , and thus reduces photovoltaic (PV) performance 22 , 23 . Large particles in particulate matter can also generate dust on top of solar panels. In areas with severe air pollution such as China, the potential of solar PV generation decreased on average by 11–15% between 1960 and 2015 (ref. 22 ); the decrease of point-of-array irradiance can even reach 35% in the most polluted areas 23 . Such interaction adds another dimension to the complexity of assessing pollution externalities. Existing studies take a predominantly engineering perspective that relies on computer simulations to calculate the change in solar irradiance due to air pollution or on field experiments to measure the changes in electricity generation of a few solar panels in response to air pollution. While providing critical estimation on the relationship between particulate pollution and solar electricity generation in certain refined meteorological and geographical conditions, these studies fall short in evaluating how much actual solar generation is affected at a large scale. Our paper contributes on empirical grounds and serves as a crucial reference for policy-making.
As pollution co-damages are closely related to both demand-side human behaviours and supply-side solar power generation, the distribution of these co-damages raises environmental justice concerns. Lower-income households or minority ethnic groups can be more vulnerable to the impact of air pollution. Individuals from these groups usually reside in locations with higher air pollution levels 24 . Moreover, they may live in affordable housing and buildings that are old, not well insulated and equipped with fewer energy-efficient appliances, all of which leads to higher energy-related expenditures 25 , 26 . Increased electricity bills due to more indoor hours, therefore, translates into a larger proportion of the household income for these groups, compared to their higher-income or non-minority counterparts. This constrains other essential expenditures such as on medical services by these lower-income and minority households, thus leading to further adverse health impacts 27 . Our analyses incorporate the equity aspects of pollution co-damages to provide necessary implications for policy design towards environmental justice.
This article demonstrates how the interactions among air pollution, human defensive behaviour and the energy supply system can influence the estimates of negative externalities caused by air pollution. Using consumer-level daily and hourly electricity consumption data and solar panel generation records in the city of Phoenix, Arizona, during the period 2013–2018, we show how particulate air pollution, indicated by concentrations of both PM10 and PM2.5 (that is, particulate matter 10 micrometres or less in diameter, and 2.5 micrometres or less, respectively), triggers consumer avoidance behaviours as well as lowers the generation of solar energy. Our sample covers 4,313 residential buildings and 17,422 commercial buildings. A variety of demographic and socio-economic characteristics are associated with the consumer dataset, based on which we further explore the heterogeneity of the co-damages associated with income and ethnicity. Estimates can be biased by endogeneity issues due to reverse causality (that is, air pollution induces changes in energy consumption as well as solar electricity generation, which in turn also affects the air quality) and missing variables (for example, unobservable characteristics of the local economy and physical environment can affect the air quality and energy consumption simultaneously). To address the endogenous biases, we use wind direction as an instrumental variable (IV) for pollution concentration. This IV has a direct impact on concentrations of pollutants but not on energy consumption, which creates variation in air quality that is exogenous to consumption, thus leading to an unbiased estimation of the pollutant coefficient. Our main results are based on daily average data. We also analyse hourly data to examine the intra-day heterogeneity in the impact on electricity usage. The study area of our analysis is the fifth-most populated city in the United States 28 and ranks among the top five most-polluted cities in the country 29 . This suggests that, even though they are based on a region of a developed country, our results can provide valuable insights and benchmark statistics when compared with studies in developing countries, with dense populations and low-ranked air quality.
Effect of air pollution on the demand sectors
Through an IV fixed-effects panel regression, we regress the individual household’s daily electricity consumption on air pollution level, while controlling for other confounding variables. Detailed modelling can be found in the Methods section. The validity of the IV estimation is also supported by the first-stage regression, which shows a significant positive correlation between the daily average cosine of the prevailing hourly wind direction angle and the concentration of air pollution, meaning that wind in the upwind direction of pollution sources would bring higher particulate concentration (columns 1 and 3 in Table 1 ). The considerable F statistics of far more than ten for testing the statistical significance of the excluded instrument indicate a strong IV in both the regressions for PM10 and PM2.5. We find that a higher concentration of particulate pollutant results in a statistically significant increase in residential electricity consumption. An increase of 1 µg m –3 in PM10 concentration raises the daily residential electricity consumption by 0.020 kW h (column 2 in Table 1 ). Residents are more sensitive to a change in PM2.5 concentration, as a 1 µg m –3 rise in PM2.5 concentration causes an 0.145 kW h (column 4 in Table 1 ) increase in daily electricity consumption. In this way, one more standard deviation of PM10 and PM2.5 would increase the daily residential electricity consumption by 0.85% and 1.74%, respectively, from the mean, based on the descriptive statistics in Supplementary Table 1 . Such effects are also seasonally heterogeneous (Supplementary Table 12 ) as the increased electricity consumption has a larger magnitude in the peak of summer (July and August), while the significance diminishes during the winter (November to April).
To validate our hypothesis that the increased electricity consumption is caused by averting behaviours that shift outdoor activities indoors, we next examine the pollution–kilowatt-hour relationship on an hourly basis. Results using hourly data confirm that air pollution increases residential electricity consumption and imply a possible reallocation of time due to air pollution. As shown in Fig. 1 , residential electricity consumption increases considerably during the daytime but decreases slightly during evenings when affected by air pollution. While both are statistically significant, the summed change (the area above the horizontal line of zero minus the area below) still shows an overall increase of daily electricity consumption aligning with the findings based on Table 1 . This possibly indicates a change of activities during the day: as air quality deteriorates, residents tend to participate in indoor energy-dependent activities such as watching television and turning on the heating or cooling system. They may also move activities usually conducted in the evenings, for example, doing the laundry, ahead to the daytime, so electricity consumption during the night-time drops. The drop in consumption during the evening might also be due to the effect of pre-cooling or pre-heating, from turning on the heating or cooling system during the daytime.
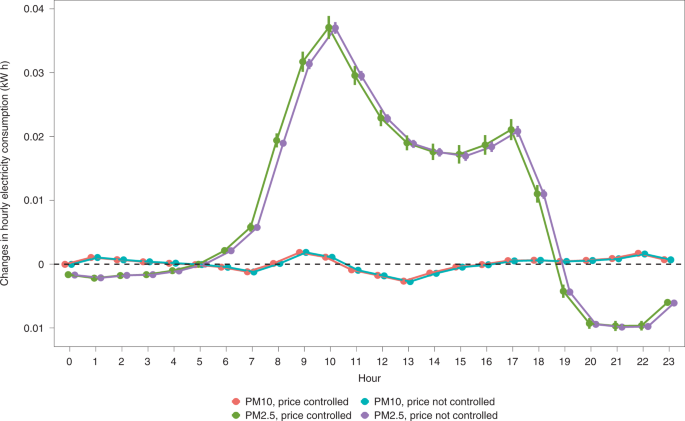
The coloured dots show the changes in hourly electricity consumption, obtained from panel regression at the hourly level. The coloured vertical lines show the 95% confidence intervals. As the information on hourly electricity price is available only for a small part of the residential and commercial samples, we conduct the analysis both with and without the regressor of price as a control variable.
Source data
To further support our findings, we test whether individuals tend to reduce outdoor trips, using a daily county-level dataset of mobility nationwide in the United States (details are included in the Methods ). As shown in Supplementary Table 13 , the number of trips per person decreases as the concentration of air pollution increases, implying that people are staying home for more hours due to air pollution.
We next discern the effects of air pollution among residential consumers with different socio-economic characteristics. The potential heterogeneous effects can be caused by different aspects of environmental injustice. On the one hand, as consumers of disadvantaged socio-economic status can be exposed to higher levels of pollution 8 and can live in houses that are less energy efficient 25 , 26 , their pollution-induced increase in electricity demand can be larger than that of their advantaged counterparts. On the other hand, their ability to self-protect against air pollution is likely to be restricted by their limited disposable income, or they may be simply less attentive to air pollution. If the effect of these constraints dominates their behavioural responses to pollution, then we may observe a smaller change in electricity demand for disadvantaged households. As a result, whether and how the effect of air pollution on electricity consumption differs across socio-economic status becomes an empirical question. Our summary statistics show that lower-income and non-white consumers are associated with higher particulate matter (PM) concentrations and lower baseline electricity consumption (Supplementary Table 2 ), implying a possible heterogeneous effect. Thus, we test such heterogeneity for different income and ethnic groups. Using the available data on household characteristics, the sample is divided into three levels of per capita income: low, medium and high (see Methods for details). The sample is also divided into four ethnic groups (White, Asian, Hispanic and other) to conduct the regression analysis separately.
The results show that lower-income and Hispanic consumers have a larger increase in electricity consumption in response to a unit increase in PM pollution. The IV estimates in Fig. 2 illustrate that the marginal effect of pollution on electricity demand is the highest for the low-income group. For ethnic groups, Hispanic consumers increase their electricity consumption more than white consumers. The empirical estimates for heterogeneous groups imply that the effect of low energy efficiency and high exposure possibly overrides the constraint of disposable income. By contrast, a previous study found that higher-income consumers need to use more energy in response to changing weather conditions in China 30 . Existing studies have found that lower-income consumers tend to live in homes that are not energy efficient 25 , 26 , which can lead to a higher increase in electricity consumption due to air pollution. Two studies 31 , 32 find that Hispanic households have higher energy use intensity due to residing in less energy-efficient homes. These findings of Hispanic households help justify our results because when air pollution increases and people need to spend more time indoors, inefficient homes (such as Hispanic homes) will increase their electricity consumption more compared to an efficient home. The medium-income group shows less of an electricity increase compared to both the low-income and high-income groups, which might result from low-income households having inefficient homes 25 , 26 and high-income households needing more energy in response to changing weather conditions 30 . The socio-economic heterogeneity embedded in air pollution issues requires more subtle investigations and tests given the multiple mechanisms that can balance the effects of each other. We also reran the model separately for each residential building to get the unique estimated impact for the individual consumer. The results show similar heterogeneity. As shown in Supplementary Fig. 1 , air pollution demonstrates a different marginal effect for each building, and the summary statistics in Supplementary Table 5 show a similar pattern as that observed in Fig. 2 .
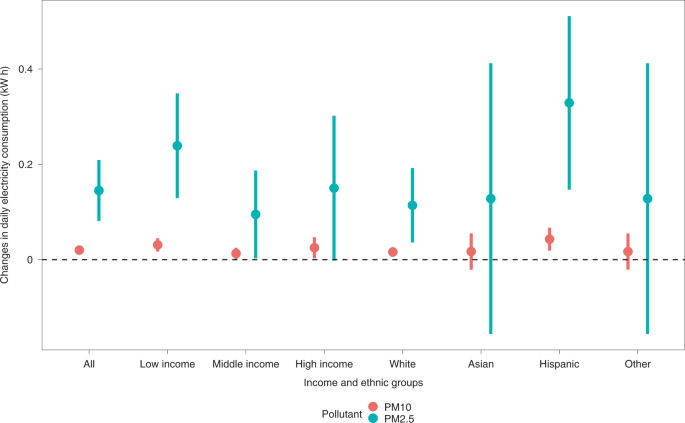
Results are based on IV methods. The solid dots represent the values of the coefficients that measure the change in daily electricity consumption in response to a 1 µg m –3 increase in PM concentration. The vertical lines represent 95% confidence intervals.
Our results show that contrary to findings in the residential sector, electricity usage in commercial buildings as a whole sample is not significantly affected by air pollution in general, although the usage in individual industries shows statistically significant changes. The results in Table 2 show that although the IV is still valid and strong (the coefficients of Wind cosine are positively significant in the first-stage results in columns 1 and 3, and the F statistics are considerable), IV estimates indicate no statistically significant effects (columns 2 and 4 in Table 2 ). In this way, the hypothesis that particulate pollution has no effect on energy use in commercial buildings as a whole cannot be rejected. We then examine if the hourly estimates could imply any indoor–outdoor activity shifts. There is not sufficient evidence to show that air pollution affects electricity usage in commercial buildings (Fig. 3 ). Although the results show a similar pattern of electricity consumption in commercial buildings as in residential buildings, the coefficients of hourly pollution concentrations are barely statistically significant.
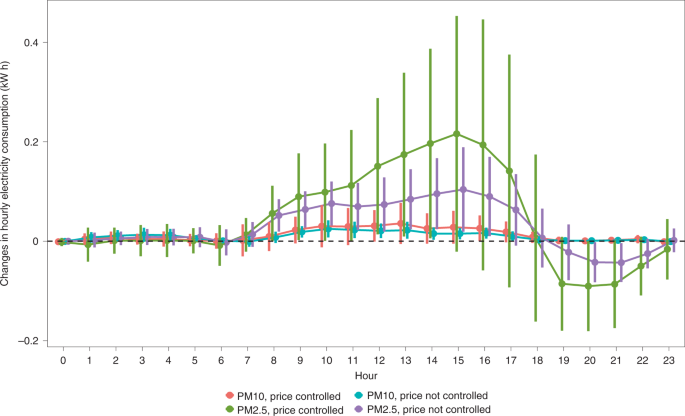
The coloured dots show the changes in hourly electricity consumption, obtained from panel regression at the hourly level. The coloured vertical lines show the 95% confidence intervals.
Such an insignificant effect on commercial buildings overall is likely a result of mixed effects by air pollution that cancel each other out. On one hand, when estimating the micro-environment exposure, incorporating work activities will induce higher exposure to air pollution compared to home-only activities, partially due to higher pollution exposure during transit or commuting 19 . This implies that workers have the incentive to stay at home or to work from home to avoid a higher average pollution exposure, which lowers the energy consumption of the commercial buildings. We further test this hypothesis by our analysis of the effect of air pollution on personal trips. With a daily county-level dataset of mobility nationwide in the United States, we test whether individuals tend to reduce outdoor trips (details are included in the Methods ). As shown in Supplementary Table 13 , the number of trips per person decreases as the concentration of air pollution increases. The same conclusion holds for both work trips (Supplementary Table 14 ) and non-work trips (Supplementary Table 15 ). On the other hand, commercial buildings on average might have a different building envelope or better building management system 20 that can lead to a better indoor environment 33 compared to residential buildings, so that when ambient air pollution increases, some people might want to stay inside commercial buildings for a longer period time, potentially increasing electricity in these buildings. Building occupants may also use less natural ventilation in polluted weather, and thus can increase the energy consumption of buildings due to increased mechanical ventilation 16 . These effects may cancel out so that we are not observing a statistically significant effect of air pollution on average for all commercial buildings in our sample.
The insignificant effect of air pollution on commercial buildings as a whole actually validates our residential electricity consumption result. There could be a concern that our regression model still fails to capture some physical relationship between electricity consumption and other unmeasured meteorological variables, which correlates with air pollution. Or there could be a concern about an incorrectly specified functional form (an incorrect description of the relationship between our independent and dependent variables). As a result, the positive impact of air pollution on residential electricity consumption could be purely due to these physical relationships, and not due to consumer behavioural change. The insignificant result in the commercial sector actually implies that our regression model can capture those physical relationships well, so that our estimated increase in residential electricity consumption is indeed due to consumers’ behavioural changes.
Such statistically insignificant results of commercial buildings can, however, conceal the sectoral heterogeneity as air pollution can substantially affect the commercial sectors that are closely related to indoor activities. Due to the nature of different industries, each commercial building serves a specific purpose, with some sectors more likely to be affected by air pollution. Sectors such as retail trade, recreation and service can have increased electricity consumption where more of their customers spend more time inside the buildings to avoid being exposed to outdoor pollution. Thus, we separate the effect by sector as shown in Fig. 4 . With a similar averaged pollution concentration across all sectors (Supplementary Table 4 ), the retail sector responds most intensely to an increase in air pollution concentration (0.086 kW h increase in electricity consumption per µg m –3 increase of PM10 concentration, and 0.560 kW h increase per µg m –3 increase of PM2.5 concentration), followed by the recreation and service sector (0.026 kW h per µg m –3 and 0.167 kW h per µg m –3 , respectively). By contrast, the other sectors reduce their electricity consumption, also as expected (0.028 kW h per µg m –3 and 0.178 kW h per µg m –3 for PM10 and PM2.5, respectively; both significant at a 90% confidence level). As a result, one standard deviation increase of PM10 and PM2.5 would lead to a 1.82% and 3.34% increase, respectively, in the retail trading sector; 1.13% and 2.00% increase, respectively, in the recreation and service sector; and 0.79% and 1.39% reduction, respectively, of electricity consumption in the other sectors. These effects with opposite directions in different sectors balance each other out when summed, and thus lead to an insignificant change in energy consumption for the whole sample. Taken together with our analysis above, these results show that individuals are more likely to reduce outdoor trips in general and particularly those related to work. However, the final destinations for the remaining trips may shift at least partially from open spaces to sheltered areas, and thus lead to more energy consumption in malls, recreation centres and so on. This distributional result stresses the importance of looking into sectoral nuance based on understandings of how consumer behaviours differ by industry as a response to varying air quality.
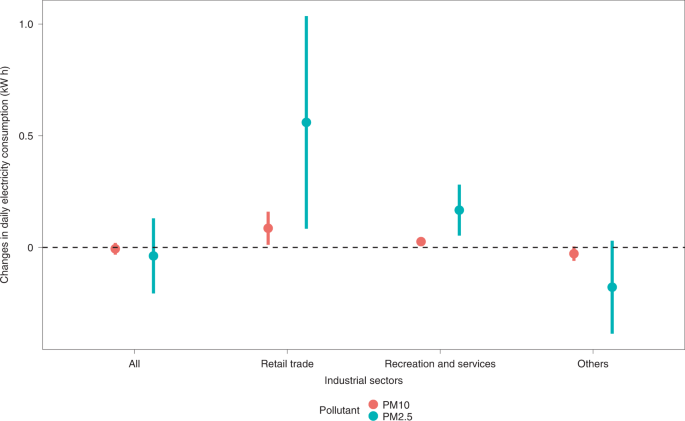
Effect of air pollution on the supply sector
We then used a similar panel IV regression to regress individual consumers’ daily solar electricity generation on air pollution level, while controlling for confounding variables ( Methods ). The IV of wind direction again proves powerful in explaining the variation of PM10 and PM2.5 with its positive significance in columns 1 and 3 in both Tables 3 and 4 , while the F statistics continue to verify it as strong. We find that particulate pollution also reduces the electricity generation of distributed-solar panels in both residential and commercial buildings. IV estimation shows that a 1 µg m –3 increase in PM10 concentration significantly reduces the electricity generated by solar panels by 0.435 kW h in residential buildings (column 2 in Table 3 ) and by 0.022 kW h in commercial buildings (column 2 in Table 4 ). PM2.5 has an even larger effect—a 1.888 kW h reduction per µg m –3 increase for residential buildings (column 4 in Table 3 ) and 0.093 kW h reduction per µg m –3 increase for commercial buildings (column 4 in Table 4 ). In terms of percentage change, one standard deviation increase of PM10 and PM2.5 would result in a 25.01% and 30.64% reduction, respectively, of solar electricity generation for residential buildings with solar panels from the mean solar electricity generation, and 0.13% and 0.15% reduction, respectively, for commercial buildings. The comparison also indicates that commercial buildings are much less affected if considering that the power of solar panels is on average larger in commercial buildings, referring to the descriptive statistics in Supplementary Tables 1 and 3 . A possible reason is that the solar panels in commercial buildings are better maintained, with dust cleaned off them in a timely manner.
This study explores the co-damage of air quality degradation via human defensive behaviour on the demand side and the performance of clean energy techniques on the supply side, respectively. Our results show that particulate pollution, while exposing individuals to health risks with direct emissions, can further add to their loss with regenerative feedback, which boosts energy consumption due to longer times spent indoors and the downgraded performance of solar panels. While previous studies predominantly focus on the positive consequences of the defensive behaviours in alleviating health impacts 10 , 12 , this research shows the possible pathways in which air pollution generates extra damage by interacting with such defensive behaviours 9 . Our analysis also shows that residents from low-income or Hispanic groups are more heavily affected, highlighting the vulnerability of those of specific socio-economic status in responding to environmental change and the potential environmental justice issues that should be addressed by policy design 24 , 27 .
Several limitations should be noted. First, our analysis addresses the situation in the city of Phoenix, Arizona. In spite of its top rank for air pollution levels in US cities, the concentration of PM is still far less than that in many developing countries such as Mexico or China 34 , 35 . Meanwhile, response levels can also differ due to cultural differences. Therefore, our results should be extrapolated with caution. In addition, our dataset lacks information on specific household end-use activities (for example, heating and cooling, or air purification). Thus, we are not able to pinpoint exactly what appliance or appliances are more intensively used against higher particulate concentrations, for further details on the mechanisms that we discuss. We leave these for future research that draws on high-resolution data in various geographical areas.
Several critical policy implications stem from the findings of this research. First, when calculating the marginal damage factors from air pollution, policymakers need to explicitly consider the co-damages generated from the feedback of consumer behaviours and clean technology performance, which is insufficiently discussed in the current literature, as well as policy analysis and evaluation. Lack of consideration of these pollution co-damages will lead to an under-estimation of the welfare gains from pollution control policies. Our results also stress the necessity to investigate comprehensively the consequences of air quality alerting systems, for example, alleviated health risks 36 , changed automobile traffic flows as individuals endeavour to escape from pollution as a response 37 , decreased outdoor recreation 10 , 38 and so on. Second, the fact that air pollution disproportionally affects those of low socio-economic status threatens energy and environmental justice, and again stresses that air pollution control can not only result in health benefits as a whole, but also contribute to an equitable distribution of such benefit. The disproportional impact also highlights the importance of energy policies that can improve the home energy efficiency of lower-income and ethnic minority groups to accelerate the achievement of fairness and equity. Third, our findings provide one more justification for the need to clean the electricity grid and improve the efficiency of renewable energy generation techniques. In addition, the expansion of solar power should consider the effect of air pollution when setting reasonable development targets. The results comparing the impacts on commercial PV units and residential PV units suggest that there should be clear messages or incentives to communicate the importance of the cleaning and maintenance of PV units to residential consumers.
The data were provided by Salt River Project, one of the two largest utility companies in Arizona. Hourly electricity consumption in kilowatt-hours was available for 4,313 residential units (spanning from May 2013 to April 2017) and 17,422 commercial units (spanning from May 2013 to April 2018). For the residential units in the sample, a Residential Equipment and Technology Survey was also conducted in 2014, which asked about detailed sociodemographic information, building characteristics, appliance and other energy technology attributes, and energy consumption behaviours. For the commercial units, a six-digit code in the North American Industry Classification System is available to identify the sector type of the building. We aggregate the electricity consumption to the daily level for analysis. The daily electricity price is constructed by taking the average of the hourly prices. For commercial consumers, both the electricity charge and demand charge are included as price variables. The zip code zone of each building is also available in the dataset, which enables a spatial match with the air quality and meteorological variables.
Salt River Project also has distributed-solar consumers in its service territory. These solar panels can be installed on the rooftop of buildings or can be ground-mounted. For each distributed-solar consumer, our dataset has information on the hourly electricity generated by the consumer’s solar panels, along with the installation dates of the solar panels. There are 260 residential distributed-solar consumers (6.03% of the residential sample) and 330 commercial distributed-solar consumers (1.89% of the commercial sample) in our dataset.
We combine meteorological observations from multiple sources. Records of air quality, including daily average concentrations of PM2.5 and PM10, are retrieved from pre-generated data files of the United States Environmental Protection Agency 39 . Climate factors including the daily average temperature, total precipitation and average wind speed are obtained from Global Surface Summary of the Day 40 . The hourly wind direction data come from the Environmental Protection Agency’s pre-generated data files. We obtain the solar irradiance data from the National Renewable Energy Laboratory’s National Solar Radiation Database 41 . For missing solar irradiance data for a given location in a given time period, we use the simulated solar irradiance by the National Renewable Energy Laboratory for a given day in that location in a typical meteorological year.
We adopt an inverse distance weighting interpolation that has commonly been used in previous literature 42 , 43 to match the air quality and meteorological records with the zip code zone of each building. First, the distance between each air quality monitoring station and the geometric centre of the zip code zone is calculated. Next, the daily records of all the stations less than 50 km away from the geometric centre are averaged together and weighted by their inversed distance to the centre. This weighted average is used as the matched air quality record for all the buildings within the zip code zone. The climate records are matched in a similar way. The inverse distance weighting is conducted in Stata 14.0 using the wtmean command with 34 meteorological stations and 67 air pollution monitoring stations. To test whether our analysis is sensitive to the radius of the inverse distance weighting procedure, we change the caliper to 10 km and 20 km and rerun the analysis, as shown in Supplementary Tables 16 – 19 (for 10 km) and Supplementary Tables 20 – 23 (for 20 km). The coefficients change only slightly in magnitude but their signs and statistical significance remain, indicating the robustness of our results.
Since datasets addressing individual travelling behaviour are rarely publicly available at the localized level for the study area, we resort to the COVID-19 Impact Analysis Platform by the University of Maryland 44 , 45 for a national-level exploration. Established for studies on COVID-19’s impact, this dataset includes the daily number of trips per person at the county level starting from 1 January 2020, which is further broken down into work and non-work trips. The information on trips comes from mobile device location data. Since the massive outbreak of COVID-19 in the United States took place no earlier than March, we adopt the records in January and February and match them with the air pollution and climate data from the above sources using a similar method.
Empirical strategies
We first estimate a generalized linear squared model on the panel dataset of residential and commercial units separately with the equation
where i indexes an individual residential or commercial consumer and t indexes the day of the sample. Elec_Con it refers to the daily electricity consumption of consumer i on day t . Pollution it is the daily average concentration of either PM10 or PM2.5. X it is a vector of control variables, including cooling degree days and heating degree days (estimated using daily average temperature), daily total precipitation, wind speed and electricity price (average daily electricity price for the residential consumers, and demand charge and energy charge for the commercial units). We also control for the concentration of ozone as another major pollutant that affects air quality and thus the outdoor activities of consumers. The variable α i is customer fixed effect, and it controls for the time-invariant attributes of the consumer such as square footage and the number of stories as well as environmental awareness of building occupants. The time fixed effects τ y and δ m include the year fixed effect and the month-of-year fixed effect. The time fixed effects capture the time-varying factors across years and seasons, such as economic development and change in local energy policies. Weekend and Holiday are dummy variables for holidays and weekends, respectively. The Holiday dummy is equal to one if the day belongs to the following US federal holidays: New Year’s Day, Martin Luther King Jr Day, Presidents’ Day, Memorial Day, Independence Day, Labor Day, Columbus Day, Veterans Day, Thanksgiving and Christmas. The ε i,t is the error term. Standard errors are clustered at the building level. We are interested in β 1 , which indicates the electricity use increase per µg m –3 increase of particulate concentration, ceteris paribus.
We analyse how the impact of air pollution differs by different income groups. Using the available data on household characteristics, the sample is divided into three levels of per capita income: low, medium and high. The division, provided by the Pew Research Center, is based on the minimum household income level of different household sizes varying from one to five (US$24,042/34,000/41,641/48,083/53,759 for middle income, and US$72,126/102,001/124,925/144,251/161,277 for upper income in 2014 (ref. 46 )). Since the household size is recorded as 1.5, 3.5 and 5 persons, we take an average of the two adjacent minimum household income levels for the 1.5- and 3.5-person households.
We test whether and by how much the particulate pollution affects solar energy generation with,
where Elec_Solar it refers to the daily electricity generated by solar for consumer i on day t , and the other terms are the same as in equation ( 1 ). The X it is modified to adapt to factors that can affect the power generation of solar panels, including climate factors that can affect the performance of solar power (temperature, precipitation, wind speed and surface albedo) and electricity prices, which can affect the motivation of consumers to actively maintain solar panels in good condition (consumers are encouraged to do so if the price is higher). The distributed-solar consumers in our sample were on a net-metering plan under which they could sell excessive solar electricity at retail electricity prices.
The naïve general least squares (GLS) estimation (results shown in Supplementary Tables 6 – 9 ) suffers from endogeneity issues due to reverse causality and missing variables 47 , 48 . As air pollution changes the behaviour patterns and increases the energy consumption of consumers, the latter can result in more electricity generation and thus pollution emissions. Meanwhile, if consumers spend more time indoors, the demand for vehicle travel may also decrease and lead to reduced emissions from transportation 48 . Omitting such pathways would lead to a biased estimation of the effect of air pollution. Besides, air quality and individual socio-economic activities can be jointly affected by the same factors, such as the local economy and physical environment 47 . Since all such factors cannot be observed in our datasets, these missing variables could bias the estimation.
To address these issues, we resort to using wind direction for an IV estimation. Its validity has been verified by multiple existing air pollution studies 47 , 49 , 50 . The idea is that wind direction affects regional air quality as it transports pollutants in specific directions. As the wind direction fluctuates on a daily or even hourly basis, it can convert the study area upwind or downwind of the pollution. Other than this pathway, wind direction (while controlling for wind speed) can hardly affect electricity consumption or solar electricity generation, and thus can meet the exclusive restriction for a valid IV.
We use the daily average cosine of the angle between the prevailing wind direction and the hourly wind direction as our IV following the previous studies 47 , 51 with modifications to adapt to our daily-level data. We first plot the distribution of the hourly wind direction of all the climate stations to obtain the prevailing wind direction, which turns out to be 180°. We then calculate the cosine of the angle between each hourly wind direction observation and this prevailing direction, and finally obtain the daily average for each climate station, which matches with different zip code zones. In this way, we can conduct the first-stage regression before running equation ( 1 ) or ( 2 ) as
where Wind_dir it indicates the daily wind direction variable, e it is the error term and other terms are the same as in equations ( 1 ) and ( 2 ). The coefficient γ 1 , after we run the first-stage model, is statistically significant with an F value larger than ten, implying that the IV is relevant and strong. We then use the predicted values of pollution from equation ( 3 ) in the second-stage model when we run equation ( 1 ) or ( 2 ).
It should be noted that the maximum value of the electricity consumption of the commercial buildings in our sample is extraordinarily large (Supplementary Table 3 ). However, there is no way for us to rule out the possibility that this value is reasonable given the decent variation of daily electricity consumption in the commercial buildings that this value belongs to. Therefore, we keep these potential outliers for the main analysis but also rerun the regressions, dropping commercial buildings with a maximum daily electricity consumption over 500 kW h and 1,000 kW h. The results provided in Supplementary Tables 10 and 11 show that our key results remain robust after the change. Also, about 10% of the buildings have a constant daily electricity consumption of zero in the raw data. We regard them as shut-down buildings and remove them from our sample.
We further test how air pollution affects residential and commercial electricity consumption at the hourly level. The identification is similar to equation ( 1 ) but using the matched hourly data of electricity use and air quality (lagged for one hour). The electricity consumption and solar electricity generation of one particular hour will not influence the air quality of the previous hour, and thus there is no reverse causality issue. In addition, such an immediate hourly reaction of building energy use will not lead to an immediate change (within the same hour) in local PM pollution levels for the following reason. The hourly change in building electricity consumption leads to an hourly change in electricity generated at power plants. The coal-fired power plants surrounding the Phoenix metropolitan area are all located at least 100 miles away. This implies that the transmission of the PM pollution from these power plants to Phoenix will take time (considering that the average wind speed in Arizona cities is less than 23 miles per hour and the average wind speed in our sample is 2.66 m s –1 or 6 miles per hour), and thus will not influence the local PM pollution within an hour. The notable hourly variation in local PM pollution (such as in morning hours and late afternoon hours) in Arizona mostly comes from other sources such as motor vehicles and road dust, instead of from power plants, based on the study by Clements et al. (ref. 52 ). As a result, the hourly change in building energy consumption will not alter local PM pollution in the Phoenix metropolitan area immediately.
To examine whether individuals stay at home instead of commuting to work on polluted days, we conduct a regression analysis on personal trips with,
where Trip jt indicates the trips per person in county j on day t ; π j and dow t denote the county and day-of-week fixed effects; and the other terms are similar to those in equations ( 1 ) and ( 2 ) but at the county level. On the basis of regressions using the total trips, we further test the effect of pollution concentration on the work and non-work trips. Due to a similar source of endogeneity, we are instrumenting the pollution using the wind direction with,
where Wind_dir jt indicates the daily wind direction variable for county j on day t , and the other terms are the same as in equation ( 4 ). We calculate the daily average cosine of the angle between the prevailing wind direction and the hourly wind direction as our IV in a similar way to that described above. The prevailing wind direction is retrieved from the median of the wind angle of each county during the study period.
Data availability
Records of air quality and hourly wind direction were retrieved from pre-generated data files of the United States Environmental Protection Agency at https://aqs.epa.gov/aqsweb/airdata/download_files.html . Climate factors were obtained from Global Surface Summary of the Day at ftp://ftp.ncdc.noaa.gov/pub/data/gsod/ . The solar irradiance data from the National Renewable Energy Laboratory’s National Solar Radiation Database is at https://maps.nrel.gov/nsrdb-viewer . The high-frequency electricity data are from the Salt River Project. As they are restricted by a non-disclosure agreement, they are available from the authors upon reasonable request and with permission from the SRP. The county-level trip data are available upon request from the COVID-19 Impact Analysis Platform of the University of Maryland at https://data.covid.umd.edu/about/index.html . Source data are provided with this paper.
Code availability
All data and models are processed in Stata 14.0. The figures are produced in R studio (based on R 3.6.1). All custom code is available on GitHub at https://github.com/hepannju/Increase-in-domestic-electricity-consumption-from-particulate-air-pollution .
Graff Zivin, J. & Neidell, M. The impact of pollution on worker productivity. Amer. Econ. Rev. 102 , 3652–3673 (2012).
Article Google Scholar
Chang, T., Graff Zivin, J., Gross, T. & Neidell, M. Particulate pollution and the productivity of pear packers. Am. Econ. J. Econ. Policy 8 , 141–169 (2016).
Levinson, A. Valuing public goods using happiness data: the case of air quality. J. Public Econ. 96 , 869–880 (2012).
Chen, X. Smog, cognition and real-world decision-making. Int. J. Health Policy Manag. 8 , 76–80 (2019).
Zhang, X., Chen, X. & Zhang, X. The impact of exposure to air pollution on cognitive performance. Proc. Natl Acad. Sci. USA 115 , 9193–9197 (2018).
de Marco, A. et al. Impacts of air pollution on human and ecosystem health, and implications for the National Emission Ceilings Directive: insights from Italy. Environ. Int. 125 , 320–333 (2019).
Chay, K. & Greenstone, M. Does air quality matter? Evidence from the housing market. J. Polit. Economy 113 , 376–424 (2005).
Goulder, L. & Schein, A. Carbon Taxes Vs. Cap and Trade: a Critical Review (National Bureau of Economic Research, 2013); https://doi.org/10.3386/w19338
Sun, C., Kahn, M. E. & Zheng, S. Self-protection investment exacerbates air pollution exposure inequality in urban China. Ecol. Econ. 131 , 468–474 (2017).
Zivin Graff, J. & Neidell, M. Days of haze: environmental information disclosure and intertemporal avoidance behavior. J. Environ. Econ. Manage. 58 , 119–128 (2009).
Ward, A. L. S. & Beatty, T. K. Who responds to air quality alerts? Environ. Resource Econ. 65 , 487–511 (2016).
Zhang, J. & Mu, Q. Air pollution and defensive expenditures: evidence from particulate-filtering facemasks. J. Environ. Econ. Manage. 92 , 517–536 (2018).
Chen, S., Oliva, P. & Zhang, P. The Effect of Air Pollution on Migration: Evidence from China (National Bureau of Economic Research, 2017); https://doi.org/10.3386/w24036
Graff Zivin, J. & Neidell, M. Environment, health, and human capital. J. Econ. Lit. 51 , 689–730 (2013).
Sexton, A. L. Health and Environmental Implications of Americans’ Time Use Responses to External Stimuli: Essays on Air-Quality Alerts and Daylight Savings Time. PhD thesis, Univ. of Minnesota (2012).
Tong, Z., Chen, Y., Malkawi, A., Liu, Z. & Freeman, R. B. Energy saving potential of natural ventilation in China: the impact of ambient air pollution. Appl. Energy 179 , 660–668 (2016).
Cao, X., Dai, X. & Liu, J. Building energy-consumption status worldwide and the state-of-the-art technologies for zero-energy buildings during the past decade. Energy Build. 128 , 198–213 (2016).
Hiller, C. Factors influencing residents’ energy use—a study of energy-related behaviour in 57 Swedish homes. Energy Build. 87 , 243–252 (2015).
de Nazelle, A. et al. Improving estimates of air pollution exposure through ubiquitous sensing technologies. Environ. Pollut. 176 , 92–99 (2013).
Kumar, P. et al. Indoor air quality and energy management through real-time sensing in commercial buildings. Energy Build. 111 , 145–153 (2016).
Streets, D. G., Wu, Y. & Chin, M. Two‐decadal aerosol trends as a likely explanation of the global dimming/brightening transition. Geophys. Res. Lett . https://doi.org/10.1029/2006GL026471 (2006).
Sweerts, B. et al. Estimation of losses in solar energy production from air pollution in China since 1960 using surface radiation data. Nat. Energy 4 , 657–663 (2019).
Li, X., Wagner, F., Peng, W., Yang, J. & Mauzerall, D. L. Reduction of solar photovoltaic resources due to air pollution in China. Proc. Natl Acad. Sci. USA 114 , 11867–11872 (2017).
Tessum, C. W. et al. Inequity in consumption of goods and services adds to racial–ethnic disparities in air pollution exposure. Proc. Natl Acad. Sci. USA 116 , 6001–6006 (2019).
Nair, G., Gustavsson, L. & Mahapatra, K. Factors influencing energy efficiency investments in existing Swedish residential buildings. Energy Policy 38 , 2956–2963 (2010).
Liang, J., Liu, P., Qiu, Y., Wang, Y. D. & Xing, B. Time-of-use electricity pricing and residential low-carbon energy technology adoption. Energy J. 41 , 1944–9089 (2020).
White, L. V. & Sintov, N. D. Health and financial impacts of demand-side response measures differ across sociodemographic groups. Nat. Energy 5 , 50–60 (2020).
Phoenix now the 5th-largest city in the US, census says. Fox News https://www.foxnews.com/us/phoenix-now-the-5th-largest-city-in-the-us-census-says (2017).
Ridlington, E. & Leavit, C. Trouble in the Air—Millions of Americans Breathe Polluted Air (Environment America Research & Policy Center, 2018); https://environmentarizonacenter.org/sites/environment/files/reports/Trouble%20in%20the%20Air%20vAZ.pdf
Li, Y., Pizer, W. A. & Wu, L. Climate change and residential electricity consumption in the Yangtze River Delta, China. Proc. Natl Acad. Sci. USA 116 , 472–477 (2019).
Reames, T. G. Targeting energy justice: exploring spatial, racial/ethnic and socioeconomic disparities in urban residential heating energy efficiency. Energy Policy 97 , 549–558 (2016).
Bednar, D. J., Reames, T. G. & Keoleian, G. A. The intersection of energy and justice: modeling the spatial, racial/ethnic and socioeconomic patterns of urban residential heating consumption and efficiency in Detroit, Michigan. Energy Build. 143 , 25–34 (2017).
Ng, L. C., Musser, A., Persily, A. K. & Emmerich, S. J. Indoor air quality analyses of commercial reference buildings. Build. Environ. 58 , 179–187 (2012).
Arceo, E., Hanna, R. & Oliva, P. Does the effect of pollution on infant mortality differ between developing and developed countries? Evidence from Mexico City. Econ. J. 126 , 257–280 (2016).
Freeman, R., Liang, W., Song, R. & Timmins, C. Willingness to pay for clean air in China. J. Environ. Econ. Manage. 94 , 188–216 (2019).
Janke, K. Air pollution, avoidance behaviour and children’s respiratory health: evidence from England. J. Health Econ. 38 , 23–42 (2014).
Tribby, C. P., Miller, H. J., Song, Y. & Smith, K. R. Do air quality alerts reduce traffic? An analysis of traffic data from the Salt Lake City metropolitan area, Utah, USA. Transp. Policy 30 , 173–185 (2013).
Noonan, D. S. Smoggy with a chance of altruism: the effects of ozone alerts on outdoor recreation and driving in Atlanta. Policy Stud. J. 42 , 122–145 (2014).
Air Quality Pre-generated Data Files (United States Environmental Protection Agency, 2013–2018); https://aqs.epa.gov/aqsweb/airdata/download_files.html#Raw
Global Summary of the Day (National Centers for Environmental Information, 2013–2018); ftp://ftp.ncdc.noaa.gov/pub/data/gsod/
National Solar Radiation Database (National Renewable Energy Laboratory, accessed 7 January 2019); https://maps.nrel.gov/nsrdb-viewer
Zhang, P., Deschenes, O., Meng, K. & Zhang, J. Temperature effects on productivity and factor reallocation: evidence from a half million Chinese manufacturing plants. J. Environ. Econ. Manage. 88 , 1–17 (2018).
Deschênes, O. & Greenstone, M. Climate change, mortality, and adaptation: evidence from annual fluctuations in weather in the US. Am. Econ. J. Appl. Econ. 3 , 152–185 (2011).
Zhang, L. et al. An interactive COVID-19 mobility impact and social distancing analysis platform. Preprint at https://www.medrxiv.org/content/10.1101/2020.04.29.20085472v1 (2020).
University of Maryland COVID-19 Impact Analysis Platform (Maryland Transportation Institute, 2020); https://data.covid.umd.edu
Who Is ‘Middle Income’ and ‘Upper Income’ in 2014? (Pew Research Center, 2016); https://www.pewsocialtrends.org/2016/05/11/americas-shrinking-middle-class-a-close-look-at-changes-within-metropolitan-areas/st_2016-05-12_middle-class-geo-03/
Barwick, P. J., Li, S., Deyu, R. & Zahur, N. B. Air Pollution, Health Spending and Willingness to Pay for Clean Air in China (Semantic Scholar, 2018); https://pdfs.semanticscholar.org/7819/8c81716d00638acd74fadf1c12a19202d7c1.pdf
Sager, L. Estimating the effect of air pollution on road safety using atmospheric temperature inversions. J. Environ. Econ. Manage. 98 , 102250 (2019).
Deryugina, T., Heutel, G., Miller, N. H., Molitor, D. & Reif, J. The mortality and medical costs of air pollution: evidence from changes in wind direction. Amer. Econ. Rev. 109 , 4178–4219 (2019).
Zheng, S., Cao, J., Kahn, M. E. & Sun, C. Real estate valuation and cross-boundary air pollution externalities: evidence from Chinese cities. J. Real Estate Finance Econ. 48 , 398–414 (2014).
Anderson, M. L. As the wind blows: the effects of long-term exposure to air pollution on mortality. J. Eur. Econ. Assoc. 18 , jvz051 (2019).
Google Scholar
Clements, A. L. et al. Source identification of coarse particles in the Desert Southwest, USA using Positive Matrix Factorization. AtmosPollut. Res. 8 , 873–884 (2017).
Download references
Acknowledgements
Funding for this research was provided by the National Science Foundation under grant no. 1757329. We thank J.H. Scofield, C. Canfield, Y. Li, H. Zhang and the seminar participants at the Center for Global Sustainability of University of Maryland, Division of Resource Economics and Management of University of West Virginia, and the Institute of Energy, Environment and Economy of Tsinghua University for their helpful comments during the preparation of this paper.
Author information
These authors contributed equally: Pan He, Jing Liang, Yueming (Lucy) Qiu.
Authors and Affiliations
Department of Earth System Science, Institute for Global Change Studies, Tsinghua University, Beijing, People’s Republic of China
School of Earth and Ocean Sciences, Cardiff University, Cardiff, UK
School of Public Policy, University of Maryland, College Park, MD, USA
Jing Liang & Yueming (Lucy) Qiu
Nicholas School of the Environment, Duke University, Durham, NC, USA
Department of Forecasting, Resource Planning and Development, Salt River Project, Tempe, AZ, USA
You can also search for this author in PubMed Google Scholar
Contributions
All the authors conceived the paper and designed the research. P.H., J.L. and Y.Q. designed the analysis methods, performed the analyses and wrote and revised the paper. B.X. processed the data. Q.L. reviewed several draughts and made revisions.
Corresponding authors
Correspondence to Pan He or Yueming (Lucy) Qiu .
Ethics declarations
Competing interests.
The authors declare no competing interests.

Additional information
Publisher’s note Springer Nature remains neutral with regard to jurisdictional claims in published maps and institutional affiliations.
Supplementary information
Supplementary information.
Supplementary Tables 1–23 and Fig. 1.
Source Data Fig. 1
Source data of Fig. 1.
Source Data Fig. 2
Source data of Fig. 2.
Source Data Fig. 3
Source data of Fig. 3.
Source Data Fig. 4
Source data of Fig. 4.
Rights and permissions
Reprints and permissions
About this article
Cite this article.
He, P., Liang, J., Qiu, Y.(. et al. Increase in domestic electricity consumption from particulate air pollution. Nat Energy 5 , 985–995 (2020). https://doi.org/10.1038/s41560-020-00699-0
Download citation
Received : 23 March 2020
Accepted : 23 August 2020
Published : 21 September 2020
Issue Date : December 2020
DOI : https://doi.org/10.1038/s41560-020-00699-0
Share this article
Anyone you share the following link with will be able to read this content:
Sorry, a shareable link is not currently available for this article.
Provided by the Springer Nature SharedIt content-sharing initiative
Quick links
- Explore articles by subject
- Guide to authors
- Editorial policies
Sign up for the Nature Briefing: Anthropocene newsletter — what matters in anthropocene research, free to your inbox weekly.

IEEE Account
- Change Username/Password
- Update Address
Purchase Details
- Payment Options
- Order History
- View Purchased Documents
Profile Information
- Communications Preferences
- Profession and Education
- Technical Interests
- US & Canada: +1 800 678 4333
- Worldwide: +1 732 981 0060
- Contact & Support
- About IEEE Xplore
- Accessibility
- Terms of Use
- Nondiscrimination Policy
- Privacy & Opting Out of Cookies
A not-for-profit organization, IEEE is the world's largest technical professional organization dedicated to advancing technology for the benefit of humanity. © Copyright 2024 IEEE - All rights reserved. Use of this web site signifies your agreement to the terms and conditions.
Research on energy storage capacity optimization of rural household photovoltaic system considering energy storage sharing
- Research Article
- Published: 10 July 2024
Cite this article
- Weijun Wang 1 &
- Keyi Kang 1
With the promotion of the photovoltaic (PV) industry throughout the county, the scale of rural household PV continues to expand. However, due to the randomness of PV power generation, large-scale household PV grid connection has a serious impact on the safe and stable operation of the distribution network. Based on this background, this paper considers three typical scenarios, including household PV without energy storage, household PV with distributed energy storage, and household PV with centralized energy storage. Then, a calculation model for PV local consumption rate and annual net cost under different scenarios is constructed. Combined with a natural village in Shandong Province, the PV local consumption rate and annual net cost under three scenarios are compared and analyzed, and the potential of energy storage sharing in reducing storage capacity and improving PV local consumption is explored. The results show that configuring energy storage for household PV can significantly improve the power self-balancing capability. When meeting the same PV local consumption, household PV centralized energy storage can achieve smaller energy storage configuration and lower cost compared to household PV distributed energy storage. Finally, suggestions are proposed to further promote the development of household PV energy storage system. The research results can provide reference for improving the local consumption of rural household PV and accelerating the application of household PV energy storage system.
This is a preview of subscription content, log in via an institution to check access.
Access this article
Subscribe and save.
- Get 10 units per month
- Download Article/Chapter or Ebook
- 1 Unit = 1 Article or 1 Chapter
- Cancel anytime
Price includes VAT (Russian Federation)
Instant access to the full article PDF.
Rent this article via DeepDyve
Institutional subscriptions
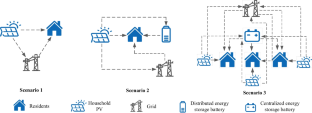
Data availability
The datasets used or analyzed during the current study are available from the corresponding author on reasonable request.
Abbreviations
The amount of rural households
Household PV power generation of the i -th household at the j -th hour (kWh)
Load demand of the i -th household at the j -th hour (kWh)
The amount of power mismatch for the i -th household at the j -th hour (kWh)
Household PV direct consumption of the i -th household at the j -th hour (kWh)
PV local consumption rate in Scenario 1 (%)
PV local consumption rate in Scenario 2 (%)
PV local consumption rate in Scenario 3 (%)
Charging power of the energy storage battery of the i -th household at the j -th hour in Scenario 2 (kWh)
Discharging power of the energy storage battery of the i -th household at the j -th hour in Scenario 2 (kWh)
Charging limit of the energy storage battery (%)
Discharging limit of the energy storage battery (%)
Capacity of the distributed energy storage battery of the i -th household in Scenario 2 (kWh)
Energy storage battery charging efficiency (%)
Energy storage battery discharging efficiency (%)
The amount of electricity stored in the energy storage battery of the i -th household at the j -th hour in Scenario 2 (kWh)
Energy storage battery maximum state of charge (%)
Energy storage battery minimum state of charge (%)
The amount of electricity sold or purchased by the i -th household from the power grid at the j -th hour in Scenario 2 (kWh)
Charging power of the energy storage battery of the i -th household at the j -th hour in Scenario 3 (kWh)
Discharging power of the energy storage battery of the i -th household at the j -th hour in Scenario 3 (kWh)
Capacity of centralized energy storage in Scenario 3 (kWh)
The amount of electricity stored by the centralized energy storage at the j -th hour in Scenario 3 (kWh)
Total charging capacity of the centralized energy storage battery in the j -th hour (kWh)
Total discharging capacity of the centralized energy storage battery in the j -th hour (kWh)
The amount of electricity sold or purchased from the power grid at the j -th hour in Scenario 3 (kWh)
Total amount of power mismatch at the j -th hour (kWh)
Annual PV grid-connected income in Scenario 1 (CNY)
Annual PV grid-connected income in Scenario 2 (CNY)
Annual PV grid-connected income in Scenario 3 (CNY)
Electricity price of PV grid connection (CNY/kWh)
Sales electricity price of the power grid (CNY/kWh)
Annual power purchase cost in Scenario 1 (CNY)
Annual power purchase cost in Scenario 2 (CNY)
Annual power purchase cost in Scenario 3 (CNY)
Subsidy income of energy storage in Scenario 2 (CNY)
Subsidy income of energy storage in Scenario 3 (CNY)
Subsidy electricity price of energy storage (CNY/kWh)
Subsidy period of energy storage (Year)
Energy storage unit capacity cost (CNY/kWh)
Energy storage investment cost in Scenario 2 (CNY)
Annual operation and maintenance cost in Scenario 2 (CNY)
The discount rate (%)
Energy storage investment cost in Scenario 3 (CNY)
Annual operation and maintenance cost in Scenario 3 (CNY)
Operating cycle of energy storage (Year)
Operating cost per unit capacity (CNY/kWh)
Annual net cost of household PV system in Scenario 1 (CNY)
Annual net cost of household PV energy storage system in Scenario 2 (CNY)
Annual net cost of household PV energy storage system in Scenario 3 (CNY)
Power of solar PV panels (kW)
Solar radiation intensity (W/m 2 )
Incidence angle efficiency (%)
PV panel area (m 2 )
Solar transmittance (%)
Efficiency of PV conversion to electricity (%)
Chen C, Hong L, Chen Y, Tan Q, Li L, Wang W (2024) Optimal configuration of distributed energy storage considering intending island recovery in faulty distribution networks. Int J Electr Power Energy Syst 158. https://doi.org/10.1016/j.ijepes.2024.109982
China Power (2022) Summary of national photovoltaic subsidy policies in 2022, http://www.chinapower.com.cn/tynfd/hyyw/20220415/144303.html . Accessed 31 March 2024
Dong Y, Zhang Y, Liu S (2024) The impacts and instruments of energy transition regulations on environmental pollution. Environ Impact Assess Rev 105. https://doi.org/10.1016/j.eiar.2024.107448
Gao Y, Cai Y, Liu C (2022): Annual operating characteristics analysis of photovoltaic-energy storage microgrid based on retired lithium iron phosphate batteries. J Energy Storage 45. https://doi.org/10.1016/j.est.2021.103769
Guo J, Liu Y, Guo Y, Xu L (2020) Configuration evaluation and operation optimization model of energy storage in different typical user-side. Power Syst Technol 44:4245–4254. https://doi.org/10.13335/j.1000-3673.pst.2020.0181
Article Google Scholar
Guo X, Gao Y, Zheng D, Ning Y, Zhao Q (2020b) Study on short-term photovoltaic power prediction model based on the Stacking ensemble learning. Energy Rep 6:1424–1431. https://doi.org/10.1016/j.egyr.2020.11.006
Hanfang L, Lin H, Tan Q, Wu P, Wang C, Gejirifu D, Huang L (2020) Research on the policy route of China’s distributed photovoltaic power generation. Energy Rep 6:254–263. https://doi.org/10.1016/j.egyr.2019.12.027
Henni S, Staudt P, Weinhardt C (2021) A sharing economy for residential communities with PV-coupled battery storage: benefits, pricing and participant matching. Appl Energy 301. https://doi.org/10.1016/j.apenergy.2021.117351
Huang P, Sun Y, Lovati M, Zhang X (2021) Solar-photovoltaic-power-sharing-based design optimization of distributed energy storage systems for performance improvements. Energy 222. https://doi.org/10.1016/j.energy.2021.119931
Li D, Cai W (2021) Optimal configuration of photovoltaic energy storage capacity for large power users. Energy Rep 7:468–478. https://doi.org/10.1016/j.egyr.2021.10.015
Li R, Jin X, Yang P, Zheng M, Zheng Y, Cai C, Sun X, Luo Z, Zhao L, Huang Z, Yang W (2024) Capacity optimization of a wind-photovoltaic-electrolysis-battery (WPEB) hybrid energy system for power and hydrogen generation. Int J Hydrogen Energy 52:311–333. https://doi.org/10.1016/j.ijhydene.2023.08.029
Article CAS Google Scholar
Li Y, Qian F, Gao W, Fukuda H, Wang Y (2022) Techno-economic performance of battery energy storage system in an energy sharing community. J Energy Storage 50. https://doi.org/10.1016/j.est.2022.104247
Lu Q, Zhang Z, Lü S (2020) Home energy management in smart households: optimal appliance scheduling model with photovoltaic energy storage system. Energy Rep 6:2450–2462. https://doi.org/10.1016/j.egyr.2020.09.001
Lu J, Zhou Q, Qi W, Qu S, Bi J (2023) The heterogeneous effects of Chinese industrial parks on environmental pollution. Sci Total Environ 896:165279. https://doi.org/10.1016/j.scitotenv.2023.165279
Ma J, Zhu L, Shen Y, Rong X, Zhang H, Yang H (2021) Demand response-based commercial mode and operation strategy of customer-side energy storage system. Energy Rep 7:1390–1399. https://doi.org/10.1016/j.egry.2021.09.123
National Energy Administration (2023) China’s household photovoltaic installed capacity has exceeded 100 million kW, covering over 5 million households. https://www.nea.gov.cn/2023-11/14/c_1310750360.htm . Accessed 10 March, 2024
National Energy Administration (2024) Photovoltaic power generation construction status in 2023. https://www.nea.gov.cn/2024-02/28/c_1310765696.htm . Accessed 2 March, 2024
National Power Energy Storage Standardization Technical Committee (SAC/TC 550) general technical requirements for electrochemical energy storage system in power system, GB/T 36558–2018. https://www.doc88.com/p-79739957206425.html . Accessed 31 Mar 2024
Parra D, Norman SA, Walker GS, Gillott M (2016) Optimum community energy storage system for demand load shifting. Appl Energy 174:130–143. https://doi.org/10.1016/j.apenergy.2016.04.082
Pires ALG, Rotella Junior P, Rocha LCS, Peruchi RS, Janda K, Miranda RdC (2023) Environmental and financial multi-objective optimization: hybrid wind-photovoltaic generation with battery energy storage systems. J Energy Storage 66. https://doi.org/10.1016/j.est.2023.107425
Sardi J, Mithulananthan N, Islam MM, Gan CK (2021) Framework of virtual microgrids formation using community energy storage in residential networks with rooftop photovoltaic units. J Energy Storage 35. https://doi.org/10.1016/j.est.2021.102250
Sun T, Shan M, Rong X, Yang X (2022) Estimating the spatial distribution of solar photovoltaic power generation potential on different types of rural rooftops using a deep learning network applied to satellite images. Appl Energy 315. https://doi.org/10.1016/j.apenergy.2022.119025
Walker A, Kwon S (2021) Analysis on impact of shared energy storage in residential community: individual versus shared energy storage. Appl Energy 282. https://doi.org/10.1016/j.apenergy.2020.116172
Wang C, Zhang L, Zhang K, Song S, Liu Y (2022a) Distributed energy storage planning considering reactive power output of energy storage and photovoltaic. Energy Rep 8:562–569. https://doi.org/10.1016/j.egyr.2022.05.155
Wang Z, Wang Y, Huang F, Shuai C, Li J, Ding L, Cheng X (2022c) The environmental impact of household domestic energy consumption in rural areas: empirical evidence from China’s photovoltaic poverty alleviation regions. Sustain Prod Consum 30:1019–1031. https://doi.org/10.1016/j.spc.2022.01.022
Wang R, Wen X, Wang X, Fu Y, Zhang Y (2022b) Low carbon optimal operation of integrated energy system based on carbon capture technology, LCA carbon emissions and ladder-type carbon trading. Appl Energy 311. https://doi.org/10.1016/j.apenergy.2022.118664
Wang W, Kang K, Sun G, Xiao L (2023) Configuration optimization of energy storage and economic improvement for household photovoltaic system considering multiple scenarios. J Energy Storage 67. https://doi.org/10.1016/j.est.2023.107631
Wu F, Zhang H (2018) Study on optimized configuration of energy storage for photovoltaic power generation. Smart Power 46:1–6. https://doi.org/10.3969/j.issn.1673-7598.2018.07.001
Xu X, Guan C, Jin J (2018) Valuing the carbon assets of distributed photovoltaic generation in China. Energy Policy 121:374–382. https://doi.org/10.1016/j.enpol.2018.06.046
Yamamoto Y (2017) Feed-in tariffs combined with capital subsidies for promoting the adoption of residential photovoltaic systems. Energy Policy 111:312–320. https://doi.org/10.1016/j.enpol.2017.09.041
Yu S, Han R, Zhang J (2023) Reassessment of the potential for centralized and distributed photovoltaic power generation in China: on a prefecture-level city scale. Energy 262. https://doi.org/10.1016/j.energy.2022.125436
Zhang J, Zhang X, Wu J, Huang C, Dai Y, Ma G (2020a) Study on household energy management methods considering household photovoltaic power generation. Power Syst Clean Energy 36:117–123. https://doi.org/10.3969/j.issn.1674-3814.2020.02.016
Zhang M, Zhang K, Hu W, Zhu B, Wang P, Wei Y-M (2020) Exploring the climatic impacts on residential electricity consumption in Jiangsu, China. Energy Policy 140. https://doi.org/10.1016/j.enpol.2020.111398
Zhao X, Zeng Y, Zhao D (2015) Distributed solar photovoltaics in China: policies and economic performance. Energy 88:572–583. https://doi.org/10.1016/j.energy.2015.05.084
Zheng L, Su R, Sun X, Guo S (2023) Historical PV-output characteristic extraction based weather-type classification strategy and its forecasting method for the day-ahead prediction of PV output. Energy 271. https://doi.org/10.1016/j.energy.2023.127009
Zhou HS, Passey R, Bruce A, Sproul AB (2022) Impact of residential battery energy storage systems on the peak reverse power flows from distributed photovoltaic systems. J Energy Storage 52. https://doi.org/10.1016/j.est.2022.104817
Download references
This research is supported by the National Social Science Foundation of China (Grant No. 23BGL024).
Author information
Authors and affiliations.
Department of Economic Management, North China Electric Power University, No.689, Huadian Road, Baoding, 071000, China
Weijun Wang & Keyi Kang
You can also search for this author in PubMed Google Scholar
Contributions
Weijun Wang: conceptualization, methodology, supervision, visualization, investigation, and validation. Keyi Kang: data curation, writing—original draft, software, and writing—review and editing.
Corresponding author
Correspondence to Keyi Kang .
Ethics declarations
Ethics approval and consent to participate.
Not applicable.
Consent for publication
Competing interests.
The authors declare no competing interests.
Additional information
Responsible Editor: Philippe Garrigues
Publisher's Note
Springer Nature remains neutral with regard to jurisdictional claims in published maps and institutional affiliations.
Rights and permissions
Springer Nature or its licensor (e.g. a society or other partner) holds exclusive rights to this article under a publishing agreement with the author(s) or other rightsholder(s); author self-archiving of the accepted manuscript version of this article is solely governed by the terms of such publishing agreement and applicable law.
Reprints and permissions
About this article
Wang, W., Kang, K. Research on energy storage capacity optimization of rural household photovoltaic system considering energy storage sharing. Environ Sci Pollut Res (2024). https://doi.org/10.1007/s11356-024-34206-9
Download citation
Received : 16 May 2023
Accepted : 29 June 2024
Published : 10 July 2024
DOI : https://doi.org/10.1007/s11356-024-34206-9
Share this article
Anyone you share the following link with will be able to read this content:
Sorry, a shareable link is not currently available for this article.
Provided by the Springer Nature SharedIt content-sharing initiative
- Household PV
- Centralized energy storage
- Distributed energy storage
- Energy storage sharing
- PV local consumption
- Power self-balancing capability
- Annual net cost
- Find a journal
- Publish with us
- Track your research
Information
- Author Services
Initiatives
You are accessing a machine-readable page. In order to be human-readable, please install an RSS reader.
All articles published by MDPI are made immediately available worldwide under an open access license. No special permission is required to reuse all or part of the article published by MDPI, including figures and tables. For articles published under an open access Creative Common CC BY license, any part of the article may be reused without permission provided that the original article is clearly cited. For more information, please refer to https://www.mdpi.com/openaccess .
Feature papers represent the most advanced research with significant potential for high impact in the field. A Feature Paper should be a substantial original Article that involves several techniques or approaches, provides an outlook for future research directions and describes possible research applications.
Feature papers are submitted upon individual invitation or recommendation by the scientific editors and must receive positive feedback from the reviewers.
Editor’s Choice articles are based on recommendations by the scientific editors of MDPI journals from around the world. Editors select a small number of articles recently published in the journal that they believe will be particularly interesting to readers, or important in the respective research area. The aim is to provide a snapshot of some of the most exciting work published in the various research areas of the journal.
Original Submission Date Received: .
- Active Journals
- Find a Journal
- Proceedings Series
- For Authors
- For Reviewers
- For Editors
- For Librarians
- For Publishers
- For Societies
- For Conference Organizers
- Open Access Policy
- Institutional Open Access Program
- Special Issues Guidelines
- Editorial Process
- Research and Publication Ethics
- Article Processing Charges
- Testimonials
- Preprints.org
- SciProfiles
- Encyclopedia
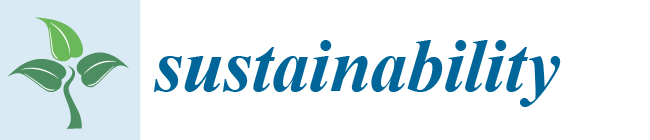
Article Menu
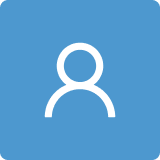
- Subscribe SciFeed
- Recommended Articles
- Google Scholar
- on Google Scholar
- Table of Contents
Find support for a specific problem in the support section of our website.
Please let us know what you think of our products and services.
Visit our dedicated information section to learn more about MDPI.
JSmol Viewer
Impact of urban morphology on high-density commercial block energy consumption in severe cold regions.
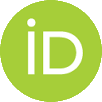
1. Introduction
1.1. background, 1.2. literature review, 1.2.1. research on context of form–energy relationship, 1.2.2. research on mechanisms of form–energy relationship, 1.3. research question framing, 2. materials and methods, 2.1. case study, 2.1.1. study area, 2.1.2. selection of measurement points, 2.2. prototype analysis of commercial block morphology, 2.2.1. classification of basic types, 2.2.2. morphological analysis based on block morphology parameters, 2.3. simulation workflow, 2.3.1. generation of simulation models, 2.3.2. parameter setting for simulation, 2.3.3. simulation of block energy consumption, 2.4. correlation analysis, 3.1. energy performance analysis of 40 high-density commercial blocks, 3.1.1. overall energy consumption statistical analysis, 3.1.2. impact of basic layout types on eui, 3.2. correlation between morphological parameters and eui, 3.2.1. correlation analysis, 3.2.2. impact of morphological parameters on eui, 3.3. analysis of effect of single morphological parameter on eui, 3.3.1. simulation of bd interference with eui, 3.3.2. simulation of far interference with eui, 3.3.3. simulation of bhsd interference with eui, 4. discussion, 4.1. the main findings of this study, 4.2. implications of this study, 4.3. limitations and future work, 5. conclusions, author contributions, institutional review board statement, informed consent statement, data availability statement, conflicts of interest.
- IEA. World Energy Outlook 2023 ; IEA: Paris, France, 2023; Licence: CC BY 4.0 (report); CC BY NC SA 4.0 (Annex A); Available online: https://www.iea.org/reports/world-energy-outlook-2023 (accessed on 24 October 2023).
- Friedlingstein, P.; Osullivan, M.; Jones, M.W.; Andrew, R.M.; Gregor, L.; Hauck, J.; Le Quere, C.; Peters, W. Global Carbon Budget 2022. EarthSystem Sci. Data 2022 , 14 , 4811–4900. [ Google Scholar ] [ CrossRef ]
- Building Energy Efficiency Research Center, Tsinghua University. China Building Energy Efficiency Year-end Development Research Report 2022 (Public Construction Special Topic) ; China Construction Industry Publishing House: Beijing, China, 2022. [ Google Scholar ]
- Mei, H.; Chen, Y.; Shi, X.; Gao, F.; Fei, T. Barriers and Strategies of Building Carbon Neutral in Cold Regions. China Sci. Found. 2023 , 37 , 364–371. [ Google Scholar ] [ CrossRef ]
- GB 50176-2016 ; Code for Thermal Design of Civil Buildings. Ministry of Housing and Urban-Rural Development: Beijing, China, 2016.
- Dong, C.F. Density and urban form. J. Archit. 2012 , 07 , 22–27. [ Google Scholar ]
- Martin, L.; March, L. Urban Space and Structures ; Cambridge University Press: London, UK, 1972. [ Google Scholar ]
- Xu, X.; Ou, J.; Liu, P.; Liu, X.; Zhang, H. Investigating the impacts of three-dimensional spatial structures on CO2 emissions at the urban scale. Sci. Total Environ. 2021 , 762 , 143096. [ Google Scholar ] [ CrossRef ] [ PubMed ]
- Wong, N.H.; Jusuf, S.K.; Syafii, N.I.; Chen, Y.; Hajadi, N.; Sathyanarayanan, H.; Manickavasagam, Y.V. Evaluation of the impact of the surrounding urban morphology on building energy consumption. Sol. Energy 2011 , 85 , 57–71. [ Google Scholar ] [ CrossRef ]
- Feng, G.; Sha, S.; Xu, X. Analysis of the building envelope influence to building energy consumption in the cold regions. Procedia Eng. 2016 , 146 , 244–250. [ Google Scholar ] [ CrossRef ]
- Arboit, M.; Diblasi, A.; Llano, J.F.; de Rosa, C. Assessing the solar potential of low-density urban environments in Andean cities with desert climates: The case of the city of Mendoza, in Argentina. Renew. Energy 2008 , 33 , 1733–1748. [ Google Scholar ] [ CrossRef ]
- You, Y.; Kim, S. Revealing the mechanism of urban morphology affecting residential energy efficiency in Seoul, Korea. Sustain. Cities Soc. 2018 , 43 , 176–190. [ Google Scholar ] [ CrossRef ]
- Leng, H.; Chen, X.; Ma, Y.; Wong, N.H.; Ming, T. Urban morphology and building heating energy consumption: Evidence from Harbin, a severe cold region city. Energy Build. 2020 , 224 , 110143. [ Google Scholar ] [ CrossRef ]
- Zinzi, M.; Carnielo, E.; Mattoni, B. On the relation between urban climate and energy performance of buildings. A three-years experience in Rome, Italy. Appl. Energy 2018 , 221 , 148–160. [ Google Scholar ] [ CrossRef ]
- Zhu, S.; Li, Y.; Wei, S.; Wang, C.; Zhang, X.; Jin, X.; Zhou, X.; Shi, X. The impact of urban vegetation morphology on urban building energy consumption during summer and winter seasons in Nanjing, China. Landsc. Urban Plan 2022 , 228 , 104576. [ Google Scholar ] [ CrossRef ]
- Xie, M.; Wang, M.; Zhong, H.; Li, X.; Li, B.; Mendis, T.; Xu, S. The impact of urban morphology on the building energy consumption and solar energy generation potential of university dormitory blocks. Sustain. Cities Soc. 2023 , 96 , 104644. [ Google Scholar ] [ CrossRef ]
- Quan, S.J.; Li, C. Urban form and building energy use: A systematic review of measures, mechanisms, and methodologies. Renew. Sustain. Energy Rev. 2021 , 139 , 110662. [ Google Scholar ] [ CrossRef ]
- Xia, B.; Li, Z. Optimized methods for morphological design of mesoscale cities based on performance analysis: Taking the residential urban blocks as examples. Sustain. Cities Soc. 2021 , 64 , 102489. [ Google Scholar ] [ CrossRef ]
- Tian, J.; Xu, S. A morphology-based evaluation on block-scale solar potential for residential area in central China. Sol. Energy 2021 , 221 , 332–347. [ Google Scholar ] [ CrossRef ]
- Song, S.; Leng, H.; Xu, H.; Guo, R.; Zhao, Y. Impact of Urban Morphology and Climate on Heating Energy Consumption of Buildings in Severe Cold Regions. Int. J. Environ. Res. Public Health 2020 , 17 , 8354. [ Google Scholar ] [ CrossRef ]
- Ratti, C.; Baker, N.; Steemers, K. Energy consumption and urban texture. Energy Build. 2005 , 37 , 762–776. [ Google Scholar ] [ CrossRef ]
- Oh, M.; Jang, K.M.; Kim, Y. Empirical analysis of building energy consumption and urban form in a large city: A case of Seoul, South Korea. energy Build. Energy Build. 2021 , 245 , 111046. [ Google Scholar ] [ CrossRef ]
- Steemers, K. Energy and the city: Density, buildings and transportation. Energy Build. 2003 , 35 , 3–14. [ Google Scholar ] [ CrossRef ]
- Strømann-Andersen, J.; Sattrup, P.A. The urban canyon and building energy use: Urban density versus daylight and passive solar gains. Energy Build. 2011 , 43 , 2011–2020. [ Google Scholar ] [ CrossRef ]
- Shareef, S.; Altan, H. Urban block configuration and the impact on energy consumption: A case study of sinuous morphology. Renew. Sustain. Energy Rev. 2022 , 163 , 112507. [ Google Scholar ] [ CrossRef ]
- El Bat, S.; Romani, Z.; Bozonnet, E.; Draoui, A.; Allard, F. Optimizing urban courtyard form through the coupling of outdoor zonal approach and building energy modeling. Energy 2023 , 264 , 126176. [ Google Scholar ] [ CrossRef ]
- Zhang, J.; Xu, L.; Shabunko, V.; Tay, S.E.R.; Sun, H.; Lau, S.S.Y.; Reindl, T. Impact of urban block types on solar potential and energy use efficiency of tropical high-density urban buildings. Appl. Energy 2018 , 240 , 513–533. [ Google Scholar ] [ CrossRef ]
- Wang, J.; Liu, W.; Sha, C.; Zhang, W.; Liu, Z.; Wang, Z.; Wang, R.; Du, X. Evaluation of the impact of urban morphology on commercial building carbon emissions at the block scale—A study of commercial buildings in Beijing. J. Clean. Prod. 2023 , 408 , 137191. [ Google Scholar ] [ CrossRef ]
- Vartholomaios, A. A parametric sensitivity analysis of the influence of urban form on domestic energy consumption for heating and cooling in a Mediterranean city. Sustain. Cities Soc. 2017 , 28 , 135–145. [ Google Scholar ] [ CrossRef ]
- Berghauser Pont, M.Y.; Haupt, P.A. Space, Density and Urban Form ; Technische Universiteit Delft: Delft, Netherlands, 2009. [ Google Scholar ]
- de Lemos Martins, T.A.; Faraut, S.; Adolphe, L. Influence of context-sensitive urban and architectural design factors on the energy demand of buildings in Toulouse. France. Energy Build 2019 , 190 , 262–278. [ Google Scholar ] [ CrossRef ]
- Pears, A. Does higher density really reduce household energy requirements? It depends. Urban Pol. Res. 2005 , 23 , 367–369. [ Google Scholar ] [ CrossRef ]
- Holden, E.; Norland, I.T. Three challenges for the compact city as a sustainable urban form: Household consumption of energy and transport in eight. Urban Stud. 2005 , 42 , 2145–2166. [ Google Scholar ] [ CrossRef ]
- Quan, S.J.; Economou, A.; Grasl, T.; Yang, P.P.J. Computing energy performance of building density, shape and typology in urban context. Energy Procedia 2014 , 61 , 1602–1605. [ Google Scholar ] [ CrossRef ]
- Rode, P.; Keim, C.; Robazza, G.; Viejo, P.; Schofield, J. Cities and energy: Urban morphology and residential heat-energy demand. Environ. Plan. B Plan. Des. 2013 , 40 , 138–162. [ Google Scholar ] [ CrossRef ]
- D′Acci, L.; Batty, M. (Eds.) The Mathematics of Urban Morphology ; Springer International Publishing: Basel, Switzerland, 2019. [ Google Scholar ]
- GB 50189-2015 ; Design standard for energy efficiency of public buildings. Ministry of Housing and Urban-Rural Development: Beijing, China, 2015.
- Hourly Observation Data of China Surface Weather Stations. National Meteorological Information Center of China. Available online: https://data.cma.cn/en/?r=data/detail&dataCode=F.0003.0007.S002 (accessed on 1 April 2020).
- Kavgic, M.; Mavrogianni, A.; Mumovic, D.; Summerfield, A.; Stevanovic, Z.; Djurovic-Petrovic, M. A review of bottom-up building stock models for energy consumption in the residential sector. Build Environ. 2010 , 45 , 1683–1697. [ Google Scholar ] [ CrossRef ]
- Energy, Office of the Department of Energy. What is OpenStudio. Available online: https://www.openstudio.net/ (accessed on 6 October 2015).
- Zhu, D.D.; Yan, D.; Wang, C.; Hong, T. Comparison of building energy simulation software: DeST. EnergyPlus DOE-2 Build. Sci. 2012 , 28 , 213–222. [ Google Scholar ] [ CrossRef ]
- Howard, B.; Parshall, L.; Thompson, J.; Hammer, S.; Dickinson, J.; Modi, V. Spatial distribution of urban building energy consumption by end use. Energy Build. 2012 , 45 , 141–151. [ Google Scholar ] [ CrossRef ]
- Wang, P.; Liu, Z.; Zhang, L. Sustainability of compact cities: A review of InterBuilding Effect on building energy and solar energy use. Sustain. Cities Soc. 2021 , 72 , 103035. [ Google Scholar ] [ CrossRef ]
- Natanian, J.; Wortmann, T. Simplified evaluation metrics for generative energydriven urban design: A morphological study of residential blocks in Tel Aviv. Energy Build. 2021 , 240 , 110916. [ Google Scholar ] [ CrossRef ]
- Pan, W.; Mei, H. Exploring the clustering nature of morphological patterns of building clusters in cold regions. J. Archit. 2020 , 78–82. [ Google Scholar ]
- Xie, X.; Sahin, O.; Luo, Z.; Yao, R. Impact of neighbourhood-scale climate characteristics on building heating demand and night ventilation cooling potential. Renew. Energy 2020 , 150 , 943–956. [ Google Scholar ] [ CrossRef ]
- Xu, X.; Liu, Y.; Wang, W.; Xu, N.; Liu, K.; Yu, G. Urban Layout Optimization Based on Genetic Algorithm for Microclimate Performance in the Cold Region of China. Appl. Sci. 2019 , 9 , 4747. [ Google Scholar ] [ CrossRef ]
Click here to enlarge figure
1. Central Mall | 2. Biyot Plaza | 3. Renhe Spring | 4. Global Power Mall | 5. McKellar General Store | 6. Songlei Daoli Store | 7. Zhuozhan Shopping Center | 8. Hart Shopping Mall |
9. Shangri-La Hotel | 10. 14th Avenue West, Central Street | 11. Turbot International Commodity City | 12. Zhenlong Commercial Building | 13. Xiu Long Commercial Building | 14. Manhattan Commercial Building | 15. Xin Sheng Long Commercial Building | 16. Tian Di Metal Materials |
17 Pacific Place | 18. Jingyang Pedestrian Street | 19. International Home Appliances City | 20. Central Street Chinese Medicine Street | 21. Harajuku Spring | 22. Changyun Building | 23. Central Classic | 24. Life Insurance Company |
25. Harvey Plaza | 26. Gourmet Baroque | 27. Hei Long Jiang Daily Group | 28. Song Lei Nangang Store | 29. Yuanda Shopping Center | 30. Great World Mall | 31. Provincial Finance Building | 32. Green Sea Building |
33. Orvis Business Plaza | 34. Qiulin Company | 35. Gangnam International Building | 36. Xiangfang Wanda Store | 37. Xiangfang Wanda 2 | 38. Songshan Chuangzhi Building | 39. Pufa Building | 40. Red Brick Street |
Courtyard | Courtyard-T | Slab | Slab-T | Point | Point-T | |
---|---|---|---|---|---|---|
Basic type | ||||||
Commercial block | 1, 10, 13, 17, 20, 21, 27, 34, 38 | 8, 14, 15,16, 22, 24, 31, 32, 35 | 3, 13,19, 20, 37 | 6, 9, 22, 23, 28, 36, 39 | 2, 7, 11, 26, 29 | 4, 5, 12, 15,18, 25, 30, 31, 33 |
Name | Abbreviation | Formula | Schematic |
---|---|---|---|
Building density | BD | BD = | |
Floor area ratio | FAR | FAR = | |
Building height standard deviation | BHSD | BHSD = |
Block Code | BD | FAR | BHSD | Block Code | BD | FAR | BHSD |
---|---|---|---|---|---|---|---|
1 | 0.56 | 1.58 | 4.5 | 21 | 0.44 | 2.19 | 7.5 |
2 | 0.53 | 2.13 | 0.0 | 22 | 0.6 | 1.69 | 4.4 |
3 | 0.41 | 1.73 | 6.0 | 23 | 0.47 | 3.37 | 17.2 |
4 | 0.37 | 6.84 | 32.7 | 24 | 0.36 | 4.45 | 27.0 |
5 | 0.48 | 4.03 | 30.0 | 25 | 0.45 | 5.83 | 38.3 |
6 | 0.58 | 3.11 | 14.1 | 26 | 0.45 | 4.24 | 21.0 |
7 | 0.61 | 1.69 | 8.2 | 27 | 0.31 | 1.45 | 3.7 |
8 | 0.39 | 3.12 | 15.9 | 28 | 0.2 | 3.26 | 41.3 |
9 | 0.35 | 3.63 | 21.7 | 29 | 0.7 | 2.34 | 6.0 |
10 | 0.49 | 2.54 | 21.0 | 30 | 0.66 | 5.57 | 31.9 |
11 | 0.56 | 2.58 | 8.9 | 31 | 0.46 | 2.87 | 21.6 |
12 | 0.42 | 4.29 | 18.1 | 32 | 0.3 | 1.78 | 13.0 |
13 | 0.47 | 2.3 | 5.1 | 33 | 0.38 | 5.78 | 36.9 |
14 | 0.49 | 3.85 | 17.9 | 34 | 0.46 | 2.39 | 20.8 |
15 | 0.47 | 2.86 | 34.0 | 35 | 0.45 | 4.25 | 22.0 |
16 | 0.45 | 2.3 | 14.4 | 36 | 0.47 | 1.87 | 21.2 |
17 | 0.35 | 2.09 | 27.2 | 37 | 0.32 | 1.79 | 7.5 |
18 | 0.39 | 4.73 | 31.2 | 38 | 0.39 | 2.05 | 3.4 |
19 | 0.45 | 1.53 | 2.4 | 39 | 0.37 | 5.63 | 32.9 |
20 | 0.48 | 1.05 | 7.5 | 40 | 0.55 | 1.11 | 0.0 |
Basic Type | Spatial Types | Basic Type | Spatial Types | ||||||
---|---|---|---|---|---|---|---|---|---|
Group 1. Changes in BD, FAR = 4 | Group 2. Changes in FAR, BD = 0.5 | Group 3. Changes in BHSD, ABH = 20 m | Group 4. Changes in BHSD, ABH = 30 m | ||||||
BD | Block Number | FAR | Block Number | BHSD (m) | Block Number | BHSD (m) | Block Number | ||
Courtyard | 0.4–0.6 | C1–C9 | 3–7 | C10–C18 | 0, 4, 8, 12 | C19–C22 | Courtyard-T | 24, 28, 32, 36 | C23–C26 |
Slab | 0.4–0.75 | S1–S15 | 3–7 | S16–S24 | 0, 4, 8, 12 | S25–S28 | Slab-T | 24, 28, 32, 36 | S29–S32 |
Point | 0.4–0.75 | P1–P15 | 3–7 | P16–P24 | 0, 4, 8, 12 | P25–P28 | Point-T | 24, 28, 32, 36 | P29–P32 |
Computational Moment | 1 | 2 | 3 | 4 | 5 | 6 | 7 | 8 | 9 | 10 | 11 | 12 |
---|---|---|---|---|---|---|---|---|---|---|---|---|
Room occupancy rate (%) | 0 | 0 | 0 | 0 | 0 | 20 | 0 | 20 | 50 | 80 | 80 | 80 |
Computational moment | 13 | 14 | 15 | 16 | 17 | 18 | 19 | 20 | 21 | 22 | 23 | 24 |
Room occupancy rate (%) | 80 | 80 | 80 | 80 | 80 | 80 | 80 | 70 | 50 | 0 | 0 | 0 |
Parameters | Values for Commercial Buildings in Severe Cold Regions | |
---|---|---|
Window-to-wall ratio | South 0.3, east 0.2, west 0.2, north 0.1 | |
Heat transfer coefficient of construction W/(m ·K) | Roof | 0.35 |
Walls | 0.45 | |
Transparent material (window) | 2.5 | |
Person-related parameters | Heat dissipation (W) | Apparent heat 75, latent heat 106 |
Dissipation of moisture (g/h) | 158 | |
Floor space occupied per capita (m ) | 8 | |
HVAC system | Heating period | 20 October–20 April |
Cooling period | 15 June–15 July | |
Simulation period | Annual energy consumption simulation | 1 January–31 December |
Daily energy consumption simulation | Winter/summer peak energy use day |
BD | FAR | BHSD | ||
---|---|---|---|---|
Winter Daily EUI | Pearson’s r | −0.135 | 0.370 | 0.036 |
p-value | 0.406 | 0.010 * | 0.826 | |
Summer Daily EUI | Pearson’s r | −0.234 | 0.444 | 0.120 |
p-value | 0.146 | 0.004 ** | 0.459 |
The statements, opinions and data contained in all publications are solely those of the individual author(s) and contributor(s) and not of MDPI and/or the editor(s). MDPI and/or the editor(s) disclaim responsibility for any injury to people or property resulting from any ideas, methods, instructions or products referred to in the content. |
Share and Cite
Wang, Y.; Pan, W.; Liao, Z. Impact of Urban Morphology on High-Density Commercial Block Energy Consumption in Severe Cold Regions. Sustainability 2024 , 16 , 5795. https://doi.org/10.3390/su16135795
Wang Y, Pan W, Liao Z. Impact of Urban Morphology on High-Density Commercial Block Energy Consumption in Severe Cold Regions. Sustainability . 2024; 16(13):5795. https://doi.org/10.3390/su16135795
Wang, Yueran, Wente Pan, and Ziyan Liao. 2024. "Impact of Urban Morphology on High-Density Commercial Block Energy Consumption in Severe Cold Regions" Sustainability 16, no. 13: 5795. https://doi.org/10.3390/su16135795
Article Metrics
Article access statistics, further information, mdpi initiatives, follow mdpi.
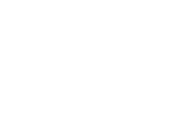
Subscribe to receive issue release notifications and newsletters from MDPI journals
- DOI: 10.46914/1562-2959-2024-1-2-139-154
- Corpus ID: 271031757
14 References
A comprehensive multi-criteria assessment of solar-driven refrigeration systems for fish preservation in africa based on energy, economic, environmental and social dimensions, investigating factors to promote energy: socio-political barriers to renewable energy sector in india, charting a sustainable future: transformative policies for india's energy, agriculture, and transport sectors, information communication technology, economic growth, natural resources, and renewable energy production: evaluating the asymmetric and symmetric impacts of artificial intelligence in robotics and innovative economies, does energy consumption play a key role re-evaluating the energy consumption-economic growth nexus from gdp growth rates forecasting, examining the multiple impacts of renewable energy development on redefined energy security in china: a panel quantile regression approach, public-private partnerships investment in energy as new determinant of renewable energy: the role of political cooperation in china and india, forecasting electricity production from various energy sources in türkiye: a predictive analysis of time series, deep learning, and hybrid models, optimization of energy consumption in industrial robots, a review, exploring the influencing factors of urban residential electricity consumption in china, related papers.
Showing 1 through 3 of 0 Related Papers
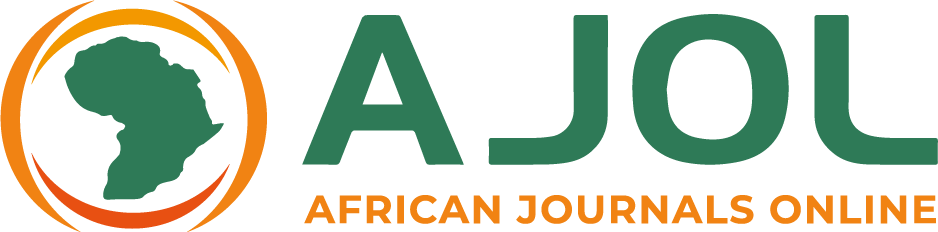
Africa Insight Journal / Africa Insight / Vol. 53 No. 1 (2023) / Articles (function() { function async_load(){ var s = document.createElement('script'); s.type = 'text/javascript'; s.async = true; var theUrl = 'https://www.journalquality.info/journalquality/ratings/2407-www-ajol-info-ai'; s.src = theUrl + ( theUrl.indexOf("?") >= 0 ? "&" : "?") + 'ref=' + encodeURIComponent(window.location.href); var embedder = document.getElementById('jpps-embedder-ajol-ai'); embedder.parentNode.insertBefore(s, embedder); } if (window.attachEvent) window.attachEvent('onload', async_load); else window.addEventListener('load', async_load, false); })();
Article sidebar, article details, main article content, harnessing ghana’s soft power for global influence, emmanuel amoah-darkwah, daniel dramani kipo-sunyehzi.
This paper aims to add to the field of foreign policy in general and, specifically, make an insightful contribution to Ghana’s use of soft power in projecting her image internationally. The research involved largely secondary data sources and a few primary pieces of information that is experts’ opinions. Foreign policy formulation and implementation by states is underpinned by exerting both hard and soft power in the international system. Ghana was the first country in Sub-Saharan Africa to gain independence from colonial rule as far back as 1957 and has made a tremendous contribution to achieving Pan-Africanism. It enjoys an enviable position as a bastion of peace in Africa. In contemporary times, states are investing in soft power strategies, policies, and programmes to grow their influence globally. Soft power is the ability to persuade state and non-state actors to attain goals through attraction rather than force. A state’s soft power emanates from its political values, its culture, and its foreign policies. Ghana's political values (example, a haven of democracy), rich cultural heritage, economic diplomacy and good neighbourliness foreign policy has positioned the country as a soft power state. The country’s involvement in peacekeeping missions globally has engendered admiration in the international system. This paper emanates from the examination of the strategies, policies, and programmes that Ghana is using to cement its soft power position internationally. Three major themes emerged from the data that were gathered and analysed, namely: soft power, foreign policy, and gastro diplomacy.
AJOL is a Non Profit Organisation that cannot function without donations. AJOL and the millions of African and international researchers who rely on our free services are deeply grateful for your contribution. AJOL is annually audited and was also independently assessed in 2019 by E&Y.
Your donation is guaranteed to directly contribute to Africans sharing their research output with a global readership.
- For annual AJOL Supporter contributions, please view our Supporters page.
Journal Identifiers
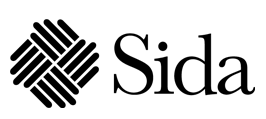

IMAGES
VIDEO
COMMENTS
Research Question: This paper presents a review of empirical methods used by authors to determine the influence of different groups of factors that influence households' electricity consumption ...
Data collection. To explore the relationships between subjective well-being and energy consumption expenditure at the household level, this study conducted a large-scale, original, cross-sectional ...
3.1 Statistics on journals and fields. Table 1 reveals that a total of 1134 papers were published in 70 journals, of which about 75% (833) were published in 10 journals (Table 2).The more striking revelation from Table 2 is that approximately half of these studies are from 3 major journals: Energy Policy, Energy, and Energy Economics.Table 2 also reveals an interesting trend in terms of ...
The overview of this research demonstrates that numerous prediction approaches and notable influential elements have been investigated to provide a comprehensive picture of energy-related aspects on the scale of building energy consumption. Specific research on home energy lifestyles, on the other hand, is inevitable and prospective in emerging ...
Feature papers represent the most advanced research with significant potential for high impact in the field. ... As energy label system is found to have a significant impact on residential electricity consumption, this paper suggests that the government may consider to promote the uses of labeling systems for more electrical appliances without ...
Abstract. This study is a meta-analytic investigation of energy consumption and economic growth. It makes a literature survey on the dynamic causal relationship between broad variables of energy and economic growth for the period 1974-2021. The objective of this study is to investigate stationarity, cointegration and direction of causality ...
Furthermore, there is a dearth of research estimating city-level final energy consumption, which hampers investigations into other crucial aspects such as the energy structure of city-level ...
Over time, several relationships have been defined between electricity consumption and a region's social and economic variables, with income as the main factor. This paper uses multiple correspondence analysis to identify the categories of dwellings and, from a graphical point of view (positioning maps), the effects of the different characteristics that influence the electricity consumption ...
1. Introduction. World energy demand in a large number of contexts, including the current state-of-the-art, allowing the devastating impact of global warming on the different situations where countries and people work together to reach the Paris agreement target well below temperature 2.0 °C (Kona et al., 2018, IEA, 2017).In recent decades, the worldwide use of energy has risen exponentially ...
Methods. We divide useful energy into the following types: consumption patterns for (1) space heating, (2) hot water, (3) process heat, (4) space cooling, (5) process cooling, (6) mechanical ...
Energy transformation and sustainability is a global challenge, for many developing countries, facing energy-related issues like wide demand-supply consumption pattern by shifting or reducing ...
Additionally, what also made this research interesting was the data sources used, wherein he stated that one of the data sources used in the research came from the household energy consumption ...
The variables considered were urban growth and energy consumption. The result revealed that ecological footprint is enhanced by urban growth and extensive consumption of energy. The research advised non-retardation of economic growth of the NEXT-11 countries with the release of less or no harmful substances into the environment.
As previously mentioned papers were excluded for a wide variety of reasons. Every paper remotely relevant to research on electricity consumption classification using smart meter data is included for further screening. After the initial search and screening of the 2099 unique articles, 552 were deemed related and relevant.
The growth rate of electricity consumption has important implications for business and public policy. This article describes altered trends in the relationship between growth in economic hypotheses to explain them. utility system stakeholders to rethink old assumptions and prepare for a new reality of lower growth rates in electricity consumption.
Consumption per capita is an important indicator to observe the tendency of electricity consumption through the years, as it offers a clear view of the electricity that every individual consumes. In Greece, in 2014 the per capita electricity consumption was 5062.61 kWh/capita while in 2010 was 5333.44 kWh/capita and in 2008 was 5805.19 kWh ...
The total electricity end-use is of interest for the purpose of this paper, and other terms, e.g., renewable electricity generation or use (Azam et al., 2021), are not covered in this review. 2 For example, the heterogeneous Dumitrescu-Hurlin ( Dumitrescu and Hurlin, 2012 ) causality method is a procedure proposed as an extension of the Granger ...
We find that a higher concentration of particulate pollutant results in a statistically significant increase in residential electricity consumption. An increase of 1 µg m -3 in PM10 ...
In this paper, we present a new method for forecasting power consumption. Household power consumption prediction is essential to manage and plan energy utilization. This study proposes a new technique using machine learning models based on the stationary wavelet transform (SWT) and transformers to forecast household power consumption in different resolutions. This approach works by leveraging ...
The purpose of this study is to analyse the long-run causal relationship among the per capita electricity consumption (PCEC) and per capita gross domestic product (PCGDP), urban population (UP) and employment (EMP) pattern in India over the period of 1991-2018.To analyse the long-run association Johansen co-integration test has been used.
This Paper reviews about the Conventional Machine Learning Models as well as the recent models, allowing predicting electricity consumption. A number of research works are concerned with the set ...
This study examines the effects of renewable and non-renewable energy consumption on environmental sustainability. We employed the fixed-effects regression model with Driscoll-Kraay robust standard errors for a global panel of 173 countries over the 1996-2020 period and several results are revealed. First renewable energy consumption ...
With the promotion of the photovoltaic (PV) industry throughout the county, the scale of rural household PV continues to expand. However, due to the randomness of PV power generation, large-scale household PV grid connection has a serious impact on the safe and stable operation of the distribution network. Based on this background, this paper considers three typical scenarios, including ...
Carbon neutrality has become a common goal for all humanity. The total energy consumption of the building sector has grown by an average of 1% per year over the past 10 years, reaching 133 EJ (exajoules) in 2022, accounting for 30% of the world's total energy consumption [].According to the Global Carbon Project platform, China is the world's largest CO 2 emitter, accounting for 30.68% of ...
The results of the research are: the current state of Kazakhstani energy sector is revealed against the background of anti-Russian sanctions, increased energy prices and global uncertainty; a comparative analysis of electricity production is carried out; an economic assessment of primary and final energy consumption from 2015 to 2023 was ...
We quantitatively capture the big picture of Data Analytics research in the electricity sector, thus displaying the high-level status quo of research activity. 2. ... Most studies in our pool focus on electricity consumption. A few papers also forecast other energy sources in addition to electricity, such as petroleum [191] or natural gas ...
electricity consumption of Delhi has gone up from 23 % to 25.2% 10. Over the period of 2006 to 2012, the annual p er capita electricity consumption has increased from 671.9 kWh t o 879.22 kWh 11 ...
This paper aims to add to the field of foreign policy in general and, specifically, make an insightful contribution to Ghana's use of soft power in projecting her image internationally. The research involved largely secondary data sources and a few primary pieces of information that is experts' opinions. Foreign policy formulation and implementation by states is underpinned by exerting ...
Using the energy time shift characteristics of the electric energy storage system, it can reasonably transfer the electric energy, stabilize the fluctuation of wind power output, and then improve the wind power consumption level of the system [23]. In this paper, lead-acid batteries suitable for large-scale power systems are selected.