

Change Password
Your password must have 8 characters or more and contain 3 of the following:.
- a lower case character,
- an upper case character,
- a special character
Password Changed Successfully
Your password has been changed
- Sign in / Register
Request Username
Can't sign in? Forgot your username?
Enter your email address below and we will send you your username
If the address matches an existing account you will receive an email with instructions to retrieve your username
Design-Based Research: A Methodology to Extend and Enrich Biology Education Research
- Emily E. Scott
- Mary Pat Wenderoth
- Jennifer H. Doherty
*Address correspondence to: Emily E. Scott ( E-mail Address: [email protected] ).
Department of Biology, University of Washington, Seattle, WA 98195
Search for more papers by this author
Recent calls in biology education research (BER) have recommended that researchers leverage learning theories and methodologies from other disciplines to investigate the mechanisms by which students to develop sophisticated ideas. We suggest design-based research from the learning sciences is a compelling methodology for achieving this aim. Design-based research investigates the “learning ecologies” that move student thinking toward mastery. These “learning ecologies” are grounded in theories of learning, produce measurable changes in student learning, generate design principles that guide the development of instructional tools, and are enacted using extended, iterative teaching experiments. In this essay, we introduce readers to the key elements of design-based research, using our own research into student learning in undergraduate physiology as an example of design-based research in BER. Then, we discuss how design-based research can extend work already done in BER and foster interdisciplinary collaborations among cognitive and learning scientists, biology education researchers, and instructors. We also explore some of the challenges associated with this methodological approach.
INTRODUCTION
There have been recent calls for biology education researchers to look toward other fields of educational inquiry for theories and methodologies to advance, and expand, our understanding of what helps students learn to think like biologists ( Coley and Tanner, 2012 ; Dolan, 2015 ; Peffer and Renken, 2016 ; Lo et al. , 2019 ). These calls include the recommendations that biology education researchers ground their work in learning theories from the cognitive and learning sciences ( Coley and Tanner, 2012 ) and begin investigating the underlying mechanisms by which students to develop sophisticated biology ideas ( Dolan, 2015 ; Lo et al. , 2019 ). Design-based research from the learning sciences is one methodology that seeks to do both by using theories of learning to investigate how “learning ecologies”—that is, complex systems of interactions among instructors, students, and environmental components—support the process of student learning ( Brown, 1992 ; Cobb et al. , 2003 ; Collins et al. , 2004 ; Peffer and Renken, 2016 ).
The purpose of this essay is twofold. First, we want to introduce readers to the key elements of design-based research, using our research into student learning in undergraduate physiology as an example of design-based research in biology education research (BER). Second, we will discuss how design-based research can extend work already done in BER and explore some of the challenges of its implementation. For a more in-depth review of design-based research, we direct readers to the following references: Brown (1992) , Barab and Squire (2004) , and Collins et al. (2004) , as well as commentaries by Anderson and Shattuck (2012) and McKenney and Reeves (2013) .
WHAT IS DESIGN-BASED RESEARCH?
Design-based research is a methodological approach that aligns with research methods from the fields of engineering or applied physics, where products are designed for specific purposes ( Brown, 1992 ; Joseph, 2004 ; Middleton et al. , 2008 ; Kelly, 2014 ). Consequently, investigators using design-based research approach educational inquiry much as an engineer develops a new product: First, the researchers identify a problem that needs to be addressed (e.g., a particular learning challenge that students face). Next, they design a potential “solution” to the problem in the form of instructional tools (e.g., reasoning strategies, worksheets; e.g., Reiser et al. , 2001 ) that theory and previous research suggest will address the problem. Then, the researchers test the instructional tools in a real-world setting (i.e., the classroom) to see if the tools positively impact student learning. As testing proceeds, researchers evaluate the instructional tools with emerging evidence of their effectiveness (or lack thereof) and progressively revise the tools— in real time —as necessary ( Collins et al. , 2004 ). Finally, the researchers reflect on the outcomes of the experiment, identifying the features of the instructional tools that were successful at addressing the initial learning problem, revising those aspects that were not helpful to learning, and determining how the research informed the theory underlying the experiment. This leads to another research cycle of designing, testing, evaluating, and reflecting to refine the instructional tools in support of student learning. We have characterized this iterative process in Figure 1 after Sandoval (2014) . Though we have portrayed four discrete phases to design-based research, there is often overlap of the phases as the research progresses (e.g., testing and evaluating can occur simultaneously).
FIGURE 1. The four phases of design-based research experienced in an iterative cycle (A). We also highlight the main features of each phase of our design-based research project investigating students’ use of flux in physiology (B).
Design-based research has no specific requirements for the form that instructional tools must take or the manner in which the tools are evaluated ( Bell, 2004 ; Anderson and Shattuck, 2012 ). Instead, design-based research has what Sandoval (2014) calls “epistemic commitments” 1 that inform the major goals of a design-based research project as well as how it is implemented. These epistemic commitments are: 1) Design based research should be grounded in theories of learning (e.g., constructivism, knowledge-in-pieces, conceptual change) that both inform the design of the instructional tools and are improved upon by the research ( Cobb et al. , 2003 ; Barab and Squire, 2004 ). This makes design-based research more than a method for testing whether or not an instructional tool works; it also investigates why the design worked and how it can be generalized to other learning environments ( Cobb et al. , 2003 ). 2) Design-based research should aim to produce measurable changes in student learning in classrooms around a particular learning problem ( Anderson and Shattuck, 2012 ; McKenney and Reeves, 2013 ). This requirement ensures that theoretical research into student learning is directly applicable, and impactful, to students and instructors in classroom settings ( Hoadley, 2004 ). 3) Design-based research should generate design principles that guide the development and implementation of future instructional tools ( Edelson, 2002 ). This commitment makes the research findings broadly applicable for use in a variety of classroom environments. 4) Design-based research should be enacted using extended, iterative teaching experiments in classrooms. By observing student learning over an extended period of time (e.g., throughout an entire term or across terms), researchers are more likely to observe the full effects of how the instructional tools impact student learning compared with short-term experiments ( Brown, 1992 ; Barab and Squire, 2004 ; Sandoval and Bell, 2004 ).
HOW IS DESIGN-BASED RESEARCH DIFFERENT FROM AN EXPERIMENTAL APPROACH?
Many BER studies employ experimental approaches that align with traditional scientific methods of experimentation, such as using treatment versus control groups, randomly assigning treatments to different groups, replicating interventions across multiple spatial or temporal periods, and using statistical methods to guide the kinds of inferences that arise from an experiment. While design-based research can similarly employ these strategies for educational inquiry, there are also some notable differences in its approach to experimentation ( Collins et al. , 2004 ; Hoadley, 2004 ). In this section, we contrast the differences between design-based research and what we call “experimental approaches,” although both paradigms represent a form of experimentation.
The first difference between an experimental approach and design-based research regards the role participants play in the experiment. In an experimental approach, the researcher is responsible for making all the decisions about how the experiment will be implemented and analyzed, while the instructor facilitates the experimental treatments. In design-based research, both researchers and instructors are engaged in all stages of the research from conception to reflection ( Collins et al. , 2004 ). In BER, a third condition frequently arises wherein the researcher is also the instructor. In this case, if the research questions being investigated produce generalizable results that have the potential to impact teaching broadly, then this is consistent with a design-based research approach ( Cobb et al. , 2003 ). However, when the research questions are self-reflective about how a researcher/instructor can improve his or her own classroom practices, this aligns more closely with “action research,” which is another methodology used in education research (see Stringer, 2013 ).
A second difference between experimental research and design-based research is the form that hypotheses take and the manner in which they are investigated ( Collins et al. , 2004 ; Sandoval, 2014 ). In experimental approaches, researchers develop a hypothesis about how a specific instructional intervention will impact student learning. The intervention is then tested in the classroom(s) while controlling for other variables that are not part of the study in order to isolate the effects of the intervention. Sometimes, researchers designate a “control” situation that serves as a comparison group that does not experience the intervention. For example, Jackson et al. (2018) were interested in comparing peer- and self-grading of weekly practice exams to if they were equally effective forms of deliberate practice for students in a large-enrollment class. To test this, the authors (including authors of this essay J.H.D., M.P.W.) designed an experiment in which lab sections of students in a large lecture course were randomly assigned to either a peer-grading or self-grading treatment so they could isolate the effects of each intervention. In design-based research, a hypothesis is conceptualized as the “design solution” rather than a specific intervention; that is, design-based researchers hypothesize that the designed instructional tools, when implemented in the classroom, will create a learning ecology that improves student learning around the identified learning problem ( Edelson, 2002 ; Bell, 2004 ). For example, Zagallo et al. (2016) developed a laboratory curriculum (i.e., the hypothesized “design solution”) for molecular and cellular biology majors to address the learning problem that students often struggle to connect scientific models and empirical data. This curriculum entailed: focusing instruction around a set of target biological models; developing small-group activities in which students interacted with the models by analyzing data from scientific papers; using formative assessment tools for student feedback; and providing students with a set of learning objectives they could use as study tools. They tested their curriculum in a novel, large-enrollment course of upper-division students over several years, making iterative changes to the curriculum as the study progressed.
By framing the research approach as an iterative endeavor of progressive refinement rather than a test of a particular intervention when all other variables are controlled, design-based researchers recognize that: 1) classrooms, and classroom experiences, are unique at any given time, making it difficult to truly “control” the environment in which an intervention occurs or establish a “control group” that differs only in the features of an intervention; and 2) many aspects of a classroom experience may influence the effectiveness of an intervention, often in unanticipated ways, which should be included in the research team’s analysis of an intervention’s success. Consequently, the research team is less concerned with controlling the research conditions—as in an experimental approach—and instead focuses on characterizing the learning environment ( Barab and Squire, 2004 ). This involves collecting data from multiple sources as the research progresses, including how the instructional tools were implemented, aspects of the implementation process that failed to go as planned, and how the instructional tools or implementation process was modified. These characterizations can provide important insights into what specific features of the instructional tools, or the learning environment, were most impactful to learning ( DBR Collective, 2003 ).
A third difference between experimental approaches and design-based research is when the instructional interventions can be modified. In experimental research, the intervention is fixed throughout the experimental period, with any revisions occurring only after the experiment has concluded. This is critical for ensuring that the results of the study provide evidence of the efficacy of a specific intervention. By contrast, design-based research takes a more flexible approach that allows instructional tools to be modified in situ as they are being implemented ( Hoadley, 2004 ; Barab, 2014 ). This flexibility allows the research team to modify instructional tools or strategies that prove inadequate for collecting the evidence necessary to evaluate the underlying theory and ensures a tight connection between interventions and a specific learning problem ( Collins et al. , 2004 ; Hoadley, 2004 ).
Finally, and importantly, experimental approaches and design-based research differ in the kinds of conclusions they draw from their data. Experimental research can “identify that something meaningful happened; but [it is] not able to articulate what about the intervention caused that story to unfold” ( Barab, 2014 , p. 162). In other words, experimental methods are robust for identifying where differences in learning occur, such as between groups of students experiencing peer- or self-grading of practice exams ( Jackson et al. , 2018 ) or receiving different curricula (e.g., Chi et al. , 2012 ). However, these methods are not able to characterize the underlying learning process or mechanism involved in the different learning outcomes. By contrast, design-based research has the potential to uncover mechanisms of learning, because it investigates how the nature of student thinking changes as students experience instructional interventions ( Shavelson et al. , 2003 ; Barab, 2014 ). According to Sandoval (2014) , “Design research, as a means of uncovering causal processes, is oriented not to finding effects but to finding functions , to understanding how desired (and undesired) effects arise through interactions in a designed environment” (p. 30). In Zagallo et al. (2016) , the authors found that their curriculum supported students’ data-interpretation skills, because it stimulated students’ spontaneous use of argumentation during which group members coconstructed evidence-based claims from the data provided. Students also worked collaboratively to decode figures and identify data patterns. These strategies were identified from the researchers’ qualitative data analysis of in-class recordings of small-group discussions, which allowed them to observe what students were doing to support their learning. Because design-based research is focused on characterizing how learning occurs in classrooms, it can begin to answer the kinds of mechanistic questions others have identified as central to advancing BER ( National Research Council [NRC], 2012 ; Dolan, 2015 ; Lo et al. , 2019 ).
DESIGN-BASED RESEARCH IN ACTION: AN EXAMPLE FROM UNDERGRADUATE PHYSIOLOGY
To illustrate how design-based research could be employed in BER, we draw on our own research that investigates how students learn physiology. We will characterize one iteration of our design-based research cycle ( Figure 1 ), emphasizing how our project uses Sandoval’s four epistemic commitments (i.e., theory driven, practically applied, generating design principles, implemented in an iterative manner) to guide our implementation.
Identifying the Learning Problem
Understanding physiological phenomena is challenging for students, given the wide variety of contexts (e.g., cardiovascular, neuromuscular, respiratory; animal vs. plant) and scales involved (e.g., using molecular-level interactions to explain organism functioning; Wang, 2004 ; Michael, 2007 ; Badenhorst et al. , 2016 ). To address these learning challenges, Modell (2000) identified seven “general models” that undergird most physiology phenomena (i.e., control systems, conservation of mass, mass and heat flow, elastic properties of tissues, transport across membranes, cell-to-cell communication, molecular interactions). Instructors can use these models as a “conceptual framework” to help students build intellectual coherence across phenomena and develop a deeper understanding of physiology ( Modell, 2000 ; Michael et al. , 2009 ). This approach aligns with theoretical work in the learning sciences that indicates that providing students with conceptual frameworks improves their ability to integrate and retrieve knowledge ( National Academies of Sciences, Engineering, and Medicine, 2018 ).
Before the start of our design-based project, we had been using Modell’s (2000) general models to guide our instruction. In this essay, we will focus on how we used the general models of mass and heat flow and transport across membranes in our instruction. These two models together describe how materials flow down gradients (e.g., pressure gradients, electrochemical gradients) against sources of resistance (e.g., tube diameter, channel frequency). We call this flux reasoning. We emphasized the fundamental nature and broad utility of flux reasoning in lecture and lab and frequently highlighted when it could be applied to explain a phenomenon. We also developed a conceptual scaffold (the Flux Reasoning Tool) that students could use to reason about physiological processes involving flux.
Although these instructional approaches had improved students’ understanding of flux phenomena, we found that students often demonstrated little commitment to using flux broadly across physiological contexts. Instead, they considered flux to be just another fact to memorize and applied it to narrow circumstances (e.g., they would use flux to reason about ions flowing across membranes—the context where flux was first introduced—but not the bulk flow of blood in a vessel). Students also struggled to integrate the various components of flux (e.g., balancing chemical and electrical gradients, accounting for variable resistance). We saw these issues reflected in students’ lower than hoped for exam scores on the cumulative final of the course. From these experiences, and from conversations with other physiology instructors, we identified a learning problem to address through design-based research: How do students learn to use flux reasoning to explain material flows in multiple physiology contexts?
The process of identifying a learning problem usually emerges from a researcher’s own experiences (in or outside a classroom) or from previous research that has been described in the literature ( Cobb et al. , 2003 ). To remain true to Sandoval’s first epistemic commitment, a learning problem must advance a theory of learning ( Edelson, 2002 ; McKenney and Reeves, 2013 ). In our work, we investigated how conceptual frameworks based on fundamental scientific concepts (i.e., Modell’s general models) could help students reason productively about physiology phenomena (National Academies of Sciences, Engineering, and Medicine, 2018; Modell, 2000 ). Our specific theoretical question was: Can we characterize how students’ conceptual frameworks around flux change as they work toward robust ideas? Sandoval’s second epistemic commitment stated that a learning problem must aim to improve student learning outcomes. The practical significance of our learning problem was: Does using the concept of flux as a foundational idea for instructional tools increase students’ learning of physiological phenomena?
We investigated our learning problem in an introductory biology course at a large R1 institution. The introductory course is the third in a biology sequence that focuses on plant and animal physiology. The course typically serves between 250 and 600 students in their sophomore or junior years each term. Classes have the following average demographics: 68% male, 21% from lower-income situations, 12% from an underrepresented minority, and 26% first-generation college students.
Design-Based Research Cycle 1, Phase 1: Designing Instructional Tools
The first phase of design-based research involves developing instructional tools that address both the theoretical and practical concerns of the learning problem ( Edelson, 2002 ; Wang and Hannafin, 2005 ). These instructional tools can take many forms, such as specific instructional strategies, classroom worksheets and practices, or technological software, as long as they embody the underlying learning theory being investigated. They must also produce classroom experiences or materials that can be evaluated to determine whether learning outcomes were met ( Sandoval, 2014 ). Indeed, this alignment between theory, the nature of the instructional tools, and the ways students are assessed is central to ensuring rigorous design-based research ( Hoadley, 2004 ; Sandoval, 2014 ). Taken together, the instructional tools instantiate a hypothesized learning environment that will advance both the theoretical and practical questions driving the research ( Barab, 2014 ).
In our work, the theoretical claim that instruction based on fundamental scientific concepts would support students’ flux reasoning was embodied in our instructional approach by being the central focus of all instructional materials, which included: a revised version of the Flux Reasoning Tool ( Figure 2 ); case study–based units in lecture that explicitly emphasized flux phenomena in real-world contexts ( Windschitl et al. , 2012 ; Scott et al. , 2018 ; Figure 3 ); classroom activities in which students practiced using flux to address physiological scenarios; links to online videos describing key flux-related concepts; constructed-response assessment items that cued students to use flux reasoning in their thinking; and pretest/posttest formative assessment questions that tracked student learning ( Figure 4 ).
FIGURE 2. The Flux Reasoning Tool given to students at the beginning of the quarter.
FIGURE 3. An example flux case study that is presented to students at the beginning of the neurophysiology unit. Throughout the unit, students learn how ion flows into and out of cells, as mediated by chemical and electrical gradients and various ion/molecular channels, sends signals throughout the body. They use this information to better understand why Jaime experiences persistent neuropathy. Images from: uz.wikipedia.org/wiki/Fayl:Blausen_0822_SpinalCord.png and commons.wikimedia.org/wiki/File:Figure_38_01_07.jpg.
FIGURE 4. An example flux assessment question about ion flows given in a pre-unit/post-unit formative assessment in the neurophysiology unit.
Phase 2: Testing the Instructional Tools
In the second phase of design-based research, the instructional tools are tested by implementing them in classrooms. During this phase, the instructional tools are placed “in harm’s way … in order to expose the details of the process to scrutiny” ( Cobb et al. , 2003 , p. 10). In this way, researchers and instructors test how the tools perform in real-world settings, which may differ considerably from the design team’s initial expectations ( Hoadley, 2004 ). During this phase, if necessary, the design team may make adjustments to the tools as they are being used to account for these unanticipated conditions ( Collins et al. , 2004 ).
We implemented the instructional tools during the Autumn and Spring quarters of the 2016–2017 academic year. Students were taught to use the Flux Reasoning Tool at the beginning of the term in the context of the first case study unit focused on neurophysiology. Each physiology unit throughout the term was associated with a new concept-based case study (usually about flux) that framed the context of the teaching. Embedded within the daily lectures were classroom activities in which students could practice using flux. Students were also assigned readings from the textbook and videos related to flux to watch during each unit. Throughout the term, students took five exams that each contained some flux questions as well as some pre- and post-unit formative assessment questions. During Winter quarter, we conducted clinical interviews with students who would take our course in the Spring term (i.e., “pre” data) as well as students who had just completed our course in Autumn (i.e., “post” data).
Phase 3: Evaluating the Instructional Tools
The third phase of a design-based research cycle involves evaluating the effectiveness of instructional tools using evidence of student learning ( Barab and Squire, 2004 ; Anderson and Shattuck, 2012 ). This can be done using products produced by students (e.g., homework, lab reports), attitudinal gains measured with surveys, participation rates in activities, interview testimonials, classroom discourse practices, and formative assessment or exam data (e.g., Reiser et al. , 2001 ; Cobb et al. , 2003 ; Barab and Squire, 2004 ; Mohan et al. , 2009 ). Regardless of the source, evidence must be in a form that supports a systematic analysis that could be scrutinized by other researchers ( Cobb et al. , 2003 ; Barab, 2014 ). Also, because design-based research often involves multiple data streams, researchers may need to use both quantitative and qualitative analytical methods to produce a rich picture of how the instructional tools affected student learning ( Collins et al. , 2004 ; Anderson and Shattuck, 2012 ).
In our work, we used the quality of students’ written responses on exams and formative assessment questions to determine whether students improved their understanding of physiological phenomena involving flux. For each assessment question, we analyzed a subset of student’s pretest answers to identify overarching patterns in students’ reasoning about flux, characterized these overarching patterns, then ordinated the patterns into different levels of sophistication. These became our scoring rubrics, which identified five different levels of student reasoning about flux. We used the rubrics to code the remainder of students’ responses, with a code designating the level of student reasoning associated with a particular reasoning pattern. We used this ordinal rubric format because it would later inform our theoretical understanding of how students build flux conceptual frameworks (see phase 4). This also allowed us to both characterize the ideas students held about flux phenomena and identify the frequency distribution of those ideas in a class.
By analyzing changes in the frequency distributions of students’ ideas across the rubric levels at different time points in the term (e.g., pre-unit vs. post-unit), we could track both the number of students who gained more sophisticated ideas about flux as the term progressed and the quality of those ideas. If the frequency of students reasoning at higher levels increased from pre-unit to post-unit assessments, we could conclude that our instructional tools as a whole were supporting students’ development of sophisticated flux ideas. For example, on one neuromuscular ion flux assessment question in the Spring of 2017, we found that relatively more students were reasoning at the highest levels of our rubric (i.e., levels 4 and 5) on the post-unit test compared with the pre-unit test. This meant that more students were beginning to integrate sophisticated ideas about flux (i.e., they were balancing concentration and electrical gradients) in their reasoning about ion movement.
To help validate this finding, we drew on three additional data streams: 1) from in-class group recordings of students working with flux items, we noted that students increasingly incorporated ideas about gradients and resistance when constructing their explanations as the term progressed; 2) from plant assessment items in the latter part of the term, we began to see students using flux ideas unprompted; and 3) from interviews, we observed that students who had already taken the course used flux ideas in their reasoning.
Through these analyses, we also noticed an interesting pattern in the pre-unit test data for Spring 2017 when compared with the frequency distribution of students’ responses with a previous term (Autumn 2016). In Spring 2017, 42% of students reasoned at level 4 or 5 on the pre-unit test, indicating these students already had sophisticated ideas about ion flux before they took the pre-unit assessment. This was surprising, considering only 2% of students reasoned at these levels for this item on the Autumn 2016 pre-unit test.
Phase 4: Reflecting on the Instructional Tools and Their Implementation
The final phase of a design-based research cycle involves a retrospective analysis that addresses the epistemic commitments of this methodology: How was the theory underpinning the research advanced by the research endeavor (theoretical outcome)? Did the instructional tools support student learning about the learning problem (practical outcome)? What were the critical features of the design solution that supported student learning (design principles)? ( Cobb et al. , 2003 ; Barab and Squire, 2004 ).
Theoretical Outcome (Epistemic Commitment 1).
Reflecting on how a design-based research experiment advances theory is critical to our understanding of how students learn in educational settings ( Barab and Squire, 2004 ; Mohan et al. , 2009 ). In our work, we aimed to characterize how students’ conceptual frameworks around flux change as they work toward robust ideas. To do this, we drew on learning progression research as our theoretical framing ( NRC, 2007 ; Corcoran et al. , 2009 ; Duschl et al. , 2011 ; Scott et al. , 2019 ). Learning progression frameworks describe empirically derived patterns in student thinking that are ordered into levels representing cognitive shifts in the ways students conceive a topic as they work toward mastery ( Gunckel et al. , 2012 ). We used our ion flux scoring rubrics to create a preliminary five-level learning progression framework ( Table 1 ). The framework describes how students’ ideas about flux often start with teleological-driven accounts at the lowest level (i.e., level 1), shift to focusing on driving forces (e.g., concentration gradients, electrical gradients) in the middle levels, and arrive at complex ideas that integrate multiple interacting forces at the higher levels. We further validated these reasoning patterns with our student interviews. However, our flux conceptual framework was largely based on student responses to our ion flux assessment items. Therefore, to further validate our learning progression framework, we needed a greater diversity of flux assessment items that investigated student thinking more broadly (i.e., about bulk flow, water movement) across physiological systems.
Level | Level descriptions | Student exemplars |
---|---|---|
5 | Principle-based reasoning with full consideration of interacting components | Change the membrane potential to −100mV/The in the cell will put for the positively charged potassium than the . |
4 | Emergent principle-based reasoning using individual components | Decrease the more positive/the concentration gradient and electrical gradient control the motion of charged particles. |
3 | Students use fragments of the principle to reason | Change concentration of outside K/If the , more K will rush into the cell. |
2 | Students provide storytelling explanations that are nonmechanistic | Close voltage-gated potassium channels/When the are closed then we will move back toward meaning that K+ ions will move into the cell causing the mV to go from −90 mV (K+ electrical potential) to −70 mV (RMP). |
1 | Students provide nonmechanistic (e.g., teleological) explanations | Transport proteins/ to cross membrane because it wouldn’t do it readily since it’s charged. |
Practical Outcome (Epistemic Commitment 2).
In design-based research, learning theories must “do real work” by improving student learning in real-world settings ( DBR Collective, 2003 ). Therefore, design-based researchers must reflect on whether or not the data they collected show evidence that the instructional tools improved student learning ( Cobb et al. , 2003 ; Sharma and McShane, 2008 ). We determined whether our flux-based instructional approach aided student learning by analyzing the kinds of answers students provided to our assessment questions. Specifically, we considered students who reasoned at level 4 or above as demonstrating productive flux reasoning. Because almost half of students were reasoning at level 4 or 5 on the post-unit assessment after experiencing the instructional tools in the neurophysiology unit (in Spring 2017), we concluded that our tools supported student learning in physiology. Additionally, we noticed that students used language in their explanations that directly tied to the Flux Reasoning Tool ( Figure 2 ), which instructed them to use arrows to indicate the magnitude and direction of gradient-driving forces. For example, in a posttest response to our ion flux item ( Figure 4 ), one student wrote:
Ion movement is a function of concentration and electrical gradients . Which arrow is stronger determines the movement of K+. We can make the electrical arrow bigger and pointing in by making the membrane potential more negative than Ek [i.e., potassium’s equilibrium potential]. We can make the concentration arrow bigger and pointing in by making a very strong concentration gradient pointing in.
Given that almost half of students reasoned at level 4 or above, and that students used language from the Flux Reasoning Tool, we concluded that using fundamental concepts was a productive instructional approach for improving student learning in physiology and that our instructional tools aided student learning. However, some students in the 2016–2017 academic year continued to apply flux ideas more narrowly than intended (i.e., for ion and simple diffusion cases, but not water flux or bulk flow). This suggested that students had developed nascent flux conceptual frameworks after experiencing the instructional tools but could use more support to realize the broad applicability of this principle. Also, although our cross-sectional interview approach demonstrated how students’ ideas, overall, could change after experiencing the instructional tools, it did not provide information about how a student developed flux reasoning.
Reflecting on practical outcomes also means interpreting any learning gains in the context of the learning ecology. This reflection allowed us to identify whether there were particular aspects of the instructional tools that were better at supporting learning than others ( DBR Collective, 2003 ). Indeed, this was critical for our understanding why 42% of students scored at level 3 and above on the pre-unit ion assessment in the Spring of 2017, while only 2% of students scored level 3 and above in Autumn of 2016. When we reviewed notes of the Spring 2017 implementation scheme, we saw that the pretest was due at the end of the first day of class after students had been exposed to ion flux ideas in class and in a reading/video assignment about ion flow, which may be one reason for the students’ high performance on the pretest. Consequently, we could not tell whether students’ initial high performance was due to their learning from the activities in the first day of class or for other reasons we did not measure. It also indicated we needed to close pretests before the first day of class for a more accurate measure of students’ incoming ideas and the effectiveness of the instructional tools employed at the beginning of the unit.
Design Principles (Epistemic Commitment 3).
Although design-based research is enacted in local contexts (i.e., a particular classroom), its purpose is to inform learning ecologies that have broad applications to improve learning and teaching ( Edelson, 2002 ; Cobb et al. , 2003 ). Therefore, design-based research should produce design principles that describe characteristics of learning environments that researchers and instructors can use to develop instructional tools specific to their local contexts (e.g., Edelson, 2002 ; Subramaniam et al. , 2015 ). Consequently, the design principles must balance specificity with adaptability so they can be used broadly to inform instruction ( Collins et al. , 2004 ; Barab, 2014 ).
From our first cycle of design-based research, we developed the following design principles: 1) Key scientific concepts should provide an overarching framework for course organization. This way, the individual components that make up a course, like instructional units, activities, practice problems, and assessments, all reinforce the centrality of the key concept. 2) Instructional tools should explicitly articulate the principle of interest, with specific guidance on how that principle is applied in context. This stresses the applied nature of the principle and that it is more than a fact to be memorized. 3) Instructional tools need to show specific instances of how the principle is applied in multiple contexts to combat students’ narrow application of the principle to a limited number of contexts.
Design-Based Research Cycle 2, Phase 1: Redesign and Refine the Experiment
The last “epistemic commitment” Sandoval (2014) articulated was that design-based research be an iterative process with an eye toward continually refining the instructional tools, based on evidence of student learning, to produce more robust learning environments. By viewing educational inquiry as formative research, design-based researchers recognize the difficulty in accounting for all variables that could impact student learning, or the implementation of the instructional tools, a priori ( Collins et al. , 2004 ). Robust instructional designs are the products of trial and error, which are strengthened by a systematic analysis of how they perform in real-world settings.
To continue to advance our work investigating student thinking using the principle of flux, we began a second cycle of design-based research that continued to address the learning problem of helping students reason with fundamental scientific concepts. In this cycle, we largely focused on broadening the number of physiological systems that had accompanying formative assessment questions (i.e., beyond ion flux), collecting student reasoning from a more diverse population of students (e.g., upper division, allied heath, community college), and refining and validating the flux learning progression with both written and interview data in a student through time. We developed a suite of constructed-response flux assessment questions that spanned neuromuscular, cardiovascular, respiratory, renal, and plant physiological contexts and asked students about several kinds of flux: ion movement, diffusion, water movement, and bulk flow (29 total questions; available at beyondmultiplechoice.org). This would provide us with rich qualitative data that we could use to refine the learning progression. We decided to administer written assessments and conduct interviews in a pretest/posttest manner at the beginning and end of each unit both as a way to increase our data about student reasoning and to provide students with additional practice using flux reasoning across contexts.
From this second round of designing instructional tools (i.e., broader range of assessment items), testing them in the classroom (i.e., administering the assessment items to diverse student populations), evaluating the tools (i.e., developing learning progression–aligned rubrics across phenomena from student data, tracking changes in the frequency distribution of students across levels through time), and reflecting on the tools’ success, we would develop a more thorough and robust characterization of how students use flux across systems that could better inform our creation of new instructional tools to support student learning.
HOW CAN DESIGN-BASED RESEARCH EXTEND AND ENRICH BER?
While design-based research has primarily been used in educational inquiry at the K–12 level (see Reiser et al. , 2001 ; Mohan et al. , 2009 ; Jin and Anderson, 2012 ), other science disciplines at undergraduate institutions have begun to employ this methodology to create robust instructional approaches (e.g., Szteinberg et al. , 2014 in chemistry; Hake, 2007 , and Sharma and McShane, 2008 , in physics; Kelly, 2014 , in engineering). Our own work, as well as that by Zagallo et al. (2016) , provides two examples of how design-based research could be implemented in BER. Below, we articulate some of the ways incorporating design-based research into BER could extend and enrich this field of educational inquiry.
Design-Based Research Connects Theory with Practice
One critique of BER is that it does not draw heavily enough on learning theories from other disciplines like cognitive psychology or the learning sciences to inform its research ( Coley and Tanner, 2012 ; Dolan, 2015 ; Peffer and Renken, 2016 ; Davidesco and Milne, 2019 ). For example, there has been considerable work in BER developing concept inventories as formative assessment tools that identify concepts students often struggle to learn (e.g., Marbach-Ad et al. , 2009 ; McFarland et al. , 2017 ; Summers et al. , 2018 ). However, much of this work is detached from a theoretical understanding of why students hold misconceptions in the first place, what the nature of their thinking is, and the learning mechanisms that would move students to a more productive understanding of domain ideas ( Alonzo, 2011 ). Using design-based research to understand the basis of students’ misconceptions would ground these practical learning problems in a theoretical understanding of the nature of student thinking (e.g., see Coley and Tanner, 2012 , 2015 ; Gouvea and Simon, 2018 ) and the kinds of instructional tools that would best support the learning process.
Design-Based Research Fosters Collaborations across Disciplines
Recently, there have been multiple calls across science, technology, engineering, and mathematics education fields to increase collaborations between BER and other disciplines so as to increase the robustness of science education research at the collegiate level ( Coley and Tanner, 2012 ; NRC, 2012 ; Talanquer, 2014 ; Dolan, 2015 ; Peffer and Renken, 2016 ; Mestre et al. , 2018 ; Davidesco and Milne, 2019 ). Engaging in design-based research provides both a mechanism and a motivation for fostering interdisciplinary collaborations, as it requires the design team to have theoretical knowledge of how students learn, domain knowledge of practical learning problems, and instructional knowledge for how to implement instructional tools in the classroom ( Edelson, 2002 ; Hoadley, 2004 ; Wang and Hannafin, 2005 ; Anderson and Shattuck, 2012 ). For example, in our current work, our research team consists of two discipline-based education learning scientists from an R1 institution, two physiology education researchers/instructors (one from an R1 institution the other from a community college), several physiology disciplinary experts/instructors, and a K–12 science education expert.
Design-based research collaborations have several distinct benefits for BER: first, learning or cognitive scientists could provide theoretical and methodological expertise that may be unfamiliar to biology education researchers with traditional science backgrounds ( Lo et al. , 2019 ). This would both improve the rigor of the research project and provide biology education researchers with the opportunity to explore ideas and methods from other disciplines. Second, collaborations between researchers and instructors could help increase the implementation of evidence-based teaching practices by instructors/faculty who are not education researchers and would benefit from support while shifting their instructional approaches ( Eddy et al. , 2015 ). This may be especially true for community college and primarily undergraduate institution faculty who often do not have access to the same kinds of resources that researchers and instructors at research-intensive institutions do ( Schinske et al. , 2017 ). Third, making instructors an integral part of a design-based research project ensures they are well versed in the theory and learning objectives underlying the instructional tools they are implementing in the classroom. This can improve the fidelity of implementation of the instructional tools, because the instructors understand the tools’ theoretical and practical purposes, which has been cited as one reason there have been mixed results on the impact of active learning across biology classes ( Andrews et al. , 2011 ; Borrego et al. , 2013 ; Lee et al. , 2018 ; Offerdahl et al. , 2018 ). It also gives instructors agency to make informed adjustments to the instructional tools during implementation that improve their practical applications while remaining true to the goals of the research ( Hoadley, 2004 ).
Design-Based Research Invites Using Mixed Methods to Analyze Data
The diverse nature of the data that are often collected in design-based research can require both qualitative and quantitative methodologies to produce a rich picture of how the instructional tools and their implementation influenced student learning ( Anderson and Shattuck, 2012 ). Using mixed methods may be less familiar to biology education researchers who were primarily trained in quantitative methods as biologists ( Lo et al. , 2019 ). However, according to Warfa (2016 , p. 2), “Integration of research findings from quantitative and qualitative inquiries in the same study or across studies maximizes the affordances of each approach and can provide better understanding of biology teaching and learning than either approach alone.” Although the number of BER studies using mixed methods has increased over the past decade ( Lo et al. , 2019 ), engaging in design-based research could further this trend through its collaborative nature of bringing social scientists together with biology education researchers to share research methodologies from different fields. By leveraging qualitative and quantitative methods, design-based researchers unpack “mechanism and process” by characterizing the nature of student thinking rather than “simply reporting that differences did or did not occur” ( Barab, 2014 , p. 158), which is important for continuing to advance our understanding of student learning in BER ( Dolan, 2015 ; Lo et al. , 2019 ).
CHALLENGES TO IMPLEMENTING DESIGN-BASED RESEARCH IN BER
As with any methodological approach, there can be challenges to implementing design-based research. Here, we highlight three that may be relevant to BER.
Collaborations Can Be Difficult to Maintain
While collaborations between researchers and instructors offer many affordances (as discussed earlier), the reality of connecting researchers across departments and institutions can be challenging. For example, Peffer and Renken (2016) noted that different traditions of scholarship can present barriers to collaboration where there is not mutual respect for the methods and ideas that are part and parcel to each discipline. Additionally, Schinske et al. (2017) identified several constraints that community college faculty face for engaging in BER, such as limited time or support (e.g., infrastructural, administrative, and peer support), which could also impact their ability to form the kinds of collaborations inherent in design-based research. Moreover, the iterative nature of design-based research requires these collaborations to persist for an extended period of time. Attending to these challenges is an important part of forming the design team and identifying the different roles researchers and instructors will play in the research.
Design-Based Research Experiments Are Resource Intensive
The focus of design-based research on studying learning ecologies to uncover mechanisms of learning requires that researchers collect multiple data streams through time, which often necessitates significant temporal and financial resources ( Collins et al., 2004 ; O’Donnell, 2004 ). Consequently, researchers must weigh both practical as well as methodological considerations when formulating their experimental design. For example, investigating learning mechanisms requires that researchers collect data at a frequency that will capture changes in student thinking ( Siegler, 2006 ). However, researchers may be constrained in the number of data-collection events they can anticipate depending on: the instructor’s ability to facilitate in-class collection events or solicit student participation in extracurricular activities (e.g., interviews); the cost of technological devices to record student conversations; the time and logistical considerations needed to schedule and conduct student interviews; the financial resources available to compensate student participants; the financial and temporal costs associated with analyzing large amounts of data.
Identifying learning mechanisms also requires in-depth analyses of qualitative data as students experience various instructional tools (e.g., microgenetic methods; Flynn et al. , 2006 ; Siegler, 2006 ). The high intensity of these in-depth analyses often limits the number of students who can be evaluated in this way, which must be balanced with the kinds of generalizations researchers wish to make about the effectiveness of the instructional tools ( O’Donnell, 2004 ). Because of the large variety of data streams that could be collected in a design-based research experiment—and the resources required to collect and analyze them—it is critical that the research team identify a priori how specific data streams, and the methods of their analysis, will provide the evidence necessary to address the theoretical and practical objectives of the research (see the following section on experimental rigor; Sandoval, 2014 ). These are critical management decisions because of the need for a transparent, systematic analysis of the data that others can scrutinize to evaluate the validity of the claims being made ( Cobb et al. , 2003 ).
Concerns with Experimental Rigor
The nature of design-based research, with its use of narrative to characterize versus control experimental environments, has drawn concerns about the rigor of this methodological approach. Some have challenged its ability to produce evidence-based warrants to support its claims of learning that can be replicated and critiqued by others ( Shavelson et al. , 2003 ; Hoadley, 2004 ). This is a valid concern that design-based researchers, and indeed all education researchers, must address to ensure their research meets established standards for education research ( NRC, 2002 ).
One way design-based researchers address this concern is by “specifying theoretically salient features of a learning environment design and mapping out how they are predicted to work together to produce desired outcomes” ( Sandoval, 2014 , p. 19). Through this process, researchers explicitly show before they begin the work how their theory of learning is embodied in the instructional tools to be tested, the specific data the tools will produce for analysis, and what outcomes will be taken as evidence for success. Moreover, by allowing instructional tools to be modified during the testing phase as needed, design-based researchers acknowledge that it is impossible to anticipate all aspects of the classroom environment that might impact the implementation of instructional tools, “as dozens (if not millions) of factors interact to produce the measureable outcomes related to learning” ( Hoadley, 2004 , p. 204; DBR Collective, 2003 ). Consequently, modifying instructional tools midstream to account for these unanticipated factors can ensure they retain their methodological alignment with the underlying theory and predicted learning outcomes so that inferences drawn from the design experiment accurately reflect what was being tested ( Edelson, 2002 ; Hoadley, 2004 ). Indeed, Barab (2014) states, “the messiness of real-world practice must be recognized, understood, and integrated as part of the theoretical claims if the claims are to have real-world explanatory value” (p. 153).
CONCLUSIONS
providing a methodology that integrates theories of learning with practical experiences in classrooms,
using a range of analytical approaches that allow for researchers to uncover the underlying mechanisms of student thinking and learning,
fostering interdisciplinary collaborations among researchers and instructors, and
characterizing learning ecologies that account for the complexity involved in student learning
By employing this methodology from the learning sciences, biology education researchers can enrich our current understanding of what is required to help biology students achieve their personal and professional aims during their college experience. It can also stimulate new ideas for biology education that can be discussed and debated in our research community as we continue to explore and refine how best to serve the students who pass through our classroom doors.
1 “Epistemic commitment” is defined as engaging in certain practices that generate knowledge in an agreed-upon way.
ACKNOWLEDGMENTS
We thank the UW Biology Education Research Group’s (BERG) feedback on drafts of this essay as well as Dr. L. Jescovich for last-minute analyses. This work was supported by a National Science Foundation award (NSF DUE 1661263/1660643). Any opinions, findings, and conclusions or recommendations expressed in this material are those of the author(s) and do not necessarily reflect the views of the NSF. All procedures were conducted in accordance with approval from the Institutional Review Board at the University of Washington (52146) and the New England Independent Review Board (120160152).
- Alonzo, A. C. ( 2011 ). Learning progressions that support formative assessment practices . Measurement , 9 (2/3), 124–129. Google Scholar
- Anderson, T., & Shattuck, J. ( 2012 ). Design-based research: A decade of progress in education research? Educational Researcher , 41 (1), 16–25. Google Scholar
- Andrews, T. M., Leonard, M. J., Colgrove, C. A., & Kalinowski, S. T. ( 2011 ). Active learning not associated with student learning in a random sample of college biology courses . CBE—Life Sciences Education , 10 (4), 394–405. Link , Google Scholar
- Badenhorst, E., Hartman, N., & Mamede, S. ( 2016 ). How biomedical misconceptions may arise and affect medical students’ learning: A review of theoretical perspectives and empirical evidence . Health Professions Education , 2 (1), 10–17. Google Scholar
- Barab, S. ( 2014 ). Design-based research: A methodological toolkit for engineering change . In The Cambridge handbook of the learning sciences (2nd ed., pp. 151–170). Cambridge University Press. https://doi.org/10.1017/CBO9781139519526.011 Google Scholar
- Barab, S., & Squire, K. ( 2004 ). Design-based research: Putting a stake in the ground . Journal of the Learning Sciences , 13 (1), 1–14. Google Scholar
- Bell, P. ( 2004 ). On the theoretical breadth of design-based research in education . Educational Psychologist , 39 (4), 243–253. Google Scholar
- Borrego, M., Cutler, S., Prince, M., Henderson, C., & Froyd, J. E. ( 2013 ). Fidelity of implementation of research-based instructional strategies (RBIS) in engineering science courses . Journal of Engineering Education , 102 (3), 394–425. Google Scholar
- Brown, A. L. ( 1992 ). Design experiments: Theoretical and methodological challenges in creating complex interventions in classroom settings . Journal of the Learning Sciences , 2 (2), 141–178. Google Scholar
- Chi, M. T. H., Roscoe, R. D., Slotta, J. D., Roy, M., & Chase, C. C. ( 2012 ). Misconceived causal explanations for emergent processes . Cognitive Science , 36 (1), 1–61. Medline , Google Scholar
- Cobb, P., Confrey, J., diSessa, A., Lehrer, R., & Schauble, L. ( 2003 ). Design experiments in educational research . Educational Researcher , 32 (1), 9–13. Google Scholar
- Coley, J. D., & Tanner, K. D. ( 2012 ). Common origins of diverse misconceptions: Cognitive principles and the development of biology thinking . CBE—Life Sciences Education , 11 (3), 209–215. Link , Google Scholar
- Coley, J. D., & Tanner, K. ( 2015 ). Relations between intuitive biological thinking and biological misconceptions in biology majors and nonmajors . CBE—Life Sciences Education , 14 (1). https://doi.org/10.1187/cbe.14-06-0094 Medline , Google Scholar
- Collins, A., Joseph, D., & Bielaczyc, K. ( 2004 ). Design research: Theoretical and methodological issues . Journal of the Learning Sciences , 13 (1), 15–42. Google Scholar
- Corcoran, T., Mosher, F. A., & Rogat, A. D. ( 2009 ). Learning progressions in science: An evidence-based approach to reform (CPRE Research Report No. RR-63) . Philadelphia, PA: Consortium for Policy Research in Education. Google Scholar
- Davidesco, I., & Milne, C. ( 2019 ). Implementing cognitive science and discipline-based education research in the undergraduate science classroom . CBE—Life Sciences Education , 18 (3), es4. Link , Google Scholar
- Design-Based Research Collective . ( 2003 ). Design-based research: An emerging paradigm for educational inquiry . Educational Researcher , 32 (1), 5–8. Google Scholar
- Dolan, E. L. ( 2015 ). Biology education research 2.0 . CBE—Life Sciences Education , 14 (4), ed1. Link , Google Scholar
- Duschl, R., Maeng, S., & Sezen, A. ( 2011 ). Learning progressions and teaching sequences: A review and analysis . Studies in Science Education , 47 (2), 123–182. Google Scholar
- Eddy, S. L., Converse, M., & Wenderoth, M. P. ( 2015 ). PORTAAL: A classroom observation tool assessing evidence-based teaching practices for active learning in large science, technology, engineering, and mathematics classes . CBE—Life Sciences Education , 14 (2), ar23. Link , Google Scholar
- Edelson, D. C. ( 2002 ). Design research: What we learn when we engage in design . Journal of the Learning Sciences , 11 (1), 105–121. Google Scholar
- Flynn, E., Pine, K., & Lewis, C. ( 2006 ). The microgenetic method—Time for change? The Psychologist , 19 (3), 152–155. Google Scholar
- Gouvea, J. S., & Simon, M. R. ( 2018 ). Challenging cognitive construals: A dynamic alternative to stable misconceptions . CBE—Life Sciences Education , 17 (2), ar34. Link , Google Scholar
- Gunckel, K. L., Mohan, L., Covitt, B. A., & Anderson, C. W. ( 2012 ). Addressing challenges in developing learning progressions for environmental science literacy . In Alonzo, A. C.Gotwals, A. W. (Eds.), Learning progressions in science: Current challenges and future directions (pp. 39–75). Rotterdam: SensePublishers. https://doi.org/10.1007/978-94-6091-824-7_4 Google Scholar
- Hake, R. R. ( 2007 ). Design-based research in physics education research: A review . In Kelly, A. E.Lesh, R. A.Baek, J. Y. (Eds.), Handbook of design research methods in mathematics, science, and technology education (p. 24). New York: Routledge. Google Scholar
- Hoadley, C. M. ( 2004 ). Methodological alignment in design-based research . Educational Psychologist , 39 (4), 203–212. Google Scholar
- Jackson, M., Tran, A., Wenderoth, M. P., & Doherty, J. H. ( 2018 ). Peer vs. self-grading of practice exams: Which is better? CBE—Life Sciences Education , 17 (3), es44. https://doi.org/10.1187/cbe.18-04-0052 Link , Google Scholar
- Jin, H., & Anderson, C. W. ( 2012 ). A learning progression for energy in socio-ecological systems . Journal of Research in Science Teaching , 49 (9), 1149–1180. Google Scholar
- Joseph, D. ( 2004 ). The practice of design-based research: Uncovering the interplay between design, research, and the real-world context . Educational Psychologist , 39 (4), 235–242. Google Scholar
- Kelly, A. E. ( 2014 ). Design-based research in engineering education . In Cambridge handbook of engineering education research (pp. 497–518). New York, NY: Cambridge University Press. https://doi.org/10.1017/CBO9781139013451.032 Google Scholar
- Lee, C. J., Toven-Lindsey, B., Shapiro, C., Soh, M., Mazrouee, S., Levis-Fitzgerald, M., & Sanders, E. R. ( 2018 ). Error-discovery learning boosts student engagement and performance, while reducing student attrition in a bioinformatics course . CBE—Life Sciences Education , 17 (3), ar40. https://doi.org/10.1187/cbe.17-04-0061 Link , Google Scholar
- Lo, S. M., Gardner, G. E., Reid, J., Napoleon-Fanis, V., Carroll, P., Smith, E., & Sato, B. K. ( 2019 ). Prevailing questions and methodologies in biology education research: A longitudinal analysis of research in CBE — life sciences education and at the society for the advancement of biology education research . CBE—Life Sciences Education , 18 (1), ar9. Link , Google Scholar
- Marbach-Ad, G., Briken, V., El-Sayed, N. M., Frauwirth, K., Fredericksen, B., Hutcheson, S., … & Smith, A. C. ( 2009 ). Assessing student understanding of host pathogen interactions using a concept inventory . Journal of Microbiology & Biology Education , 10 (1), 43–50. Medline , Google Scholar
- McFarland, J. L., Price, R. M., Wenderoth, M. P., Martinková, P., Cliff, W., Michael, J. , … & Wright, A. ( 2017 ). Development and validation of the homeostasis concept inventory . CBE—Life Sciences Education , 16 (2), ar35. Link , Google Scholar
- McKenney, S., & Reeves, T. C. ( 2013 ). Systematic review of design-based research progress: Is a little knowledge a dangerous thing? Educational Researcher , 42 (2), 97–100. Google Scholar
- Mestre, J. P., Cheville, A., & Herman, G. L. ( 2018 ). Promoting DBER-cognitive psychology collaborations in STEM education . Journal of Engineering Education , 107 (1), 5–10. Google Scholar
- Michael, J. A. ( 2007 ). What makes physiology hard for students to learn? Results of a faculty survey . AJP: Advances in Physiology Education , 31 (1), 34–40. Medline , Google Scholar
- Michael, J. A., Modell, H., McFarland, J., & Cliff, W. ( 2009 ). The “core principles” of physiology: What should students understand? Advances in Physiology Education , 33 (1), 10–16. Medline , Google Scholar
- Middleton, J., Gorard, S., Taylor, C., & Bannan-Ritland, B. ( 2008 ). The “compleat” design experiment: From soup to nuts . In Kelly, A. E.Lesh, R. A.Baek, J. Y. (Eds.), Handbook of design research methods in education: Innovations in science, technology, engineering, and mathematics learning and teaching (pp. 21–46). New York, NY: Routledge. Google Scholar
- Modell, H. I. ( 2000 ). How to help students understand physiology? Emphasize general models . Advances in Physiology Education , 23 (1), S101–S107. Medline , Google Scholar
- Mohan, L., Chen, J., & Anderson, C. W. ( 2009 ). Developing a multi-year learning progression for carbon cycling in socio-ecological systems . Journal of Research in Science Teaching , 46 (6), 675–698. Google Scholar
- National Academies of Sciences, Engineering, and Medicine . ( 2018 ). How People Learn II: Learners, Contexts, and Cultures . Washington, DC: National Academies Press. Retrieved June 24, 2019, from https://doi.org/10.17226/24783 Google Scholar
- National Research Council (NRC) . ( 2002 ). Scientific research in education . Washington, DC: National Academies Press. Retrieved January 31, 2019, from https://doi.org/10.17226/10236 Google Scholar
- NRC . ( 2007 ). Taking science to school: Learning and teaching science in grades K–8 . Washington, DC: National Academies Press. Retrieved March 22, 2019, from www.nap.edu/catalog/11625/taking-science-to-school-learning-and-teaching-science-in-grades . https://doi.org/10.17226/11625 Google Scholar
- NRC . ( 2012 ). Discipline-based education research: Understanding and improving learning in undergraduate science and engineering . Washington, DC: National Academies Press. Retrieved from www.nap.edu/catalog/13362/discipline-based-education-research-understanding-and-improving-learning-in-undergraduate . https://doi.org/10.17226/13362 Google Scholar
- NRC . ( 2018 ). How people learn II: Learners, contexts, and cultures . Washington, DC: National Academies Press. Retrieved from www.nap.edu/read/24783/chapter/7 . https://doi.org/10.17226/24783 Google Scholar
- O’Donnell, A. M. ( 2004 ). A commentary on design research . Educational Psychologist , 39 (4), 255–260. Google Scholar
- Offerdahl, E. G., McConnell, M., & Boyer, J. ( 2018 ). Can I have your recipe? Using a fidelity of implementation (FOI) framework to identify the key ingredients of formative assessment for learning . CBE—Life Sciences Education , 17 (4), es16. Link , Google Scholar
- Peffer, M., & Renken, M. ( 2016 ). Practical strategies for collaboration across discipline-based education research and the learning sciences . CBE—Life Sciences Education , 15 (4), es11. Link , Google Scholar
- Reiser, B. J., Smith, B. K., Tabak, I., Steinmuller, F., Sandoval, W. A., & Leone, A. J. ( 2001 ). BGuILE: Strategic and conceptual scaffolds for scientific inquiry in biology classrooms . In Carver, S. M.Klahr, D. (Eds.), Cognition and instruction: Twenty-five years of progress (pp. 263–305). Mahwah, NJ: Lawrence Erlbaum Associates, Inc. Google Scholar
- Sandoval, W. ( 2014 ). Conjecture mapping: An approach to systematic educational design research . Journal of the Learning Sciences , 23 (1), 18–36. Google Scholar
- Sandoval, W. A., & Bell, P. ( 2004 ). Design-based research methods for studying learning in context: Introduction . Educational Psychologist , 39 (4), 199–201. Google Scholar
- Schinske, J. N., Balke, V. L., Bangera, M. G., Bonney, K. M., Brownell, S. E., Carter, R. S. , … & Corwin, L. A. ( 2017 ). Broadening participation in biology education research: Engaging community college students and faculty . CBE—Life Sciences Education , 16 (2), mr1. Link , Google Scholar
- Scott, E., Anderson, C. W., Mashood, K. K., Matz, R. L., Underwood, S. M., & Sawtelle, V. ( 2018 ). Developing an analytical framework to characterize student reasoning about complex processes . CBE—Life Sciences Education , 17 (3), ar49. https://doi.org/10.1187/cbe.17-10-0225 Link , Google Scholar
- Scott, E., Wenderoth, M. P., & Doherty, J. H. ( 2019 ). Learning progressions: An empirically grounded, learner-centered framework to guide biology instruction . CBE—Life Sciences Education , 18 (4), es5. https://doi.org/10.1187/cbe.19-03-0059 Link , Google Scholar
- Sharma, M. D., & McShane, K. ( 2008 ). A methodological framework for understanding and describing discipline-based scholarship of teaching in higher education through design-based research . Higher Education Research & Development , 27 (3), 257–270. Google Scholar
- Shavelson, R. J., Phillips, D. C., Towne, L., & Feuer, M. J. ( 2003 ). On the science of education design studies . Educational Researcher , 32 (1), 25–28. Google Scholar
- Siegler, R. S. ( 2006 ). Microgenetic analyses of learning . In Damon, W.Lerner, R. M. (Eds.), Handbook of child psychology (pp. 464–510). Hoboken, NJ: John Wiley & Sons, Inc. https://doi.org/10.1002/9780470147658.chpsy0211 Google Scholar
- Stringer, E. T. ( 2013 ). Action research . Thousand Oaks, CA: Sage Publications, Inc. Google Scholar
- Subramaniam, M., Jean, B. S., Taylor, N. G., Kodama, C., Follman, R., & Casciotti, D. ( 2015 ). Bit by bit: Using design-based research to improve the health literacy of adolescents . JMIR Research Protocols , 4 (2), e62. Medline , Google Scholar
- Summers, M. M., Couch, B. A., Knight, J. K., Brownell, S. E., Crowe, A. J., Semsar, K. , … & Batzli, J. ( 2018 ). EcoEvo-MAPS: An ecology and evolution assessment for introductory through advanced undergraduates . CBE—Life Sciences Education , 17 (2), ar18. Link , Google Scholar
- Szteinberg, G., Balicki, S., Banks, G., Clinchot, M., Cullipher, S., Huie, R. , … & Sevian, H. ( 2014 ). Collaborative professional development in chemistry education research: Bridging the gap between research and practice . Journal of Chemical Education , 91 (9), 1401–1408. Google Scholar
- Talanquer, V. ( 2014 ). DBER and STEM education reform: Are we up to the challenge? Journal of Research in Science Teaching , 51 (6), 809–819. Google Scholar
- Wang, F., & Hannafin, M. J. ( 2005 ). Design-based research and technology-enhanced learning environments . Educational Technology Research and Development , 53 (4), 5–23. Google Scholar
- Wang, J.-R. ( 2004 ). Development and validation of a Two-tier instrument to examine understanding of internal transport in plants and the human circulatory system . International Journal of Science and Mathematics Education , 2 (2), 131–157. Google Scholar
- Warfa, A.-R. M. ( 2016 ). Mixed-methods design in biology education research: Approach and uses . CBE—Life Sciences Education , 15 (4), rm5. Link , Google Scholar
- Windschitl, M., Thompson, J., Braaten, M., & Stroupe, D. ( 2012 ). Proposing a core set of instructional practices and tools for teachers of science . Science Education , 96 (5), 878–903. Google Scholar
- Zagallo, P., Meddleton, S., & Bolger, M. S. ( 2016 ). Teaching real data interpretation with models (TRIM): Analysis of student dialogue in a large-enrollment cell and developmental biology course . CBE—Life Sciences Education , 15 (2), ar17. Link , Google Scholar
- Codéveloppement d’un programme d’autogestion de la douleur chronique en ligne: un projet de recherche basé sur la conception et axé sur l’engagement des patients 12 March 2024 | Canadian Journal of Pain, Vol. 8, No. 1
- Behavioral assessment of soft skill development in a highly structured pre-health biology course for undergraduates 29 Aug 2024 | Journal of Microbiology & Biology Education, Vol. 25, No. 2
- Developing and Validating the Preschool Nutrition Education Practices Survey 1 Aug 2024 | Journal of Nutrition Education and Behavior, Vol. 56, No. 8
- Immediate Versus Delayed Low-Stakes Questioning: Encouraging the Testing Effect Through Embedded Video Questions to Support Students’ Knowledge Outcomes, Self-Regulation, and Critical Thinking 30 July 2024 | Technology, Knowledge and Learning, Vol. 87
- Innovative strategies to strengthen teaching-researching skills in chemistry and biology education: a systematic literature review 10 July 2024 | Frontiers in Education, Vol. 9
- Enhancing undergraduates’ engagement in a learning community by including their voices in the technological and instructional design 1 Jun 2024 | Computers & Education, Vol. 214
- Practice-Based Teacher Education Benefits Graduate Trainees and Their Students Through Inclusive and Active Teaching Methods 16 October 2023 | Journal for STEM Education Research, Vol. 7, No. 1
- Designing and Evaluating Generative AI-Based Voice-Interaction Agents for Improving L2 Learners’ Oral Communication Competence 2 July 2024
- A Research-Led Contribution of Engineering Education for a Sustainable Future 1 June 2024
- Development of Inclusive Tools Through Digital Fabrication for Chemistry Learning in Students with and Without Visual Impairment 5 August 2024
- Leveraging learning experience design: digital media approaches to influence motivational traits that support student learning behaviors in undergraduate online courses 11 October 2022 | Journal of Computing in Higher Education, Vol. 35, No. 3
- Investigating an Assessment Design that Prevents Students from Using ChatGPT as the Sole Basis to Pass Assessment at the Tertiary Level 30 November 2023 | E-Journal of Humanities, Arts and Social Sciences
- Spatial Variations in Aquatic Insect Community Structure in the Winam Gulf of Lake Victoria, Kenya 8 Sep 2023 | International Journal of Ecology, Vol. 2023
- The Perceived Effectiveness of Various Forms of Feedback on the Acquisition of Technical Skills by Advanced Learners in Simulation-Based Health Professions Education 28 Aug 2023 | Cureus, Vol. 44
- Occupational therapists' acceptance of 3D printing 22 August 2023 | South African Journal of Occupational Therapy, Vol. 53, No. 2
- An app by students for students – the DPaCK-model for a digital collaborative teamwork project to identify butterflies 4 August 2023 | Frontiers in Education, Vol. 8
- Applying DBR to design protocols for synchronous online Chinese learning: An activity theoretic perspective 1 Aug 2023 | System, Vol. 116
- Defining the Nature of Augmented Feedback for Learning Intraosseous Access Skills in Simulation-Based Health Professions Education 14 Jul 2023 | Cureus, Vol. 86
- Practice-based 21st-century teacher education: Design principles for adaptive expertise 1 Jul 2023 | Teaching and Teacher Education, Vol. 128
- Undergraduate students’ neurophysiological reasoning: what we learn from the attractive distractors students select 1 Jun 2023 | Advances in Physiology Education, Vol. 47, No. 2
- Oaks to arteries: the Physiology Core Concept of flow down gradients supports transfer of student reasoning 1 Jun 2023 | Advances in Physiology Education, Vol. 47, No. 2
- Audrey Chen ,
- Kimberley A. Phillips ,
- Jennifer E. Schaefer , and
- Patrick M. Sonner
- Kyle Frantz,, Monitoring Editor
- Optimizing the Learner’s Role in Feedback: Development of a Feedback-Preparedness Online Application for Medical Students in the Clinical Setting 8 May 2023 | Cureus, Vol. 42
- History, Status, and Development of AI-Based Learning Science 8 April 2023 | SN Computer Science, Vol. 4, No. 3
- An Analytical Dashboard of Collaborative Activities for the Knowledge Building 4 March 2023 | Technology, Knowledge and Learning, Vol. 29
- The Application of a Design-Based Research Framework for Simulation-Based Education 22 Nov 2022 | Cureus, Vol. 22
- Erin Stanfield ,
- Corin D. Slown ,
- Quentin Sedlacek , and
- Suzanne E. Worcester
- James Hewlett, Monitoring Editor
- 2022 | , Vol. 511
- The effect of the e-mentoring-based education program on professional development of preschool teachers 3 July 2021 | Education and Information Technologies, Vol. 27, No. 1
- 2022 | Education Sciences, Vol. 12, No. 8
- Training Digital Competences of Educators in Continuing Education: A Three-Level Approach 27 October 2022
- Design-based research as a framework for developing and deploying augmented reality applications and scenarios for intercultural exchange 13 December 2021
- Repetition Is Important to Students and Their Understanding during Laboratory Courses That Include Research 10 Sep 2021 | Journal of Microbiology & Biology Education, Vol. 22, No. 2
- Another look at the core concepts of physiology: revisions and resources 1 Dec 2020 | Advances in Physiology Education, Vol. 44, No. 4
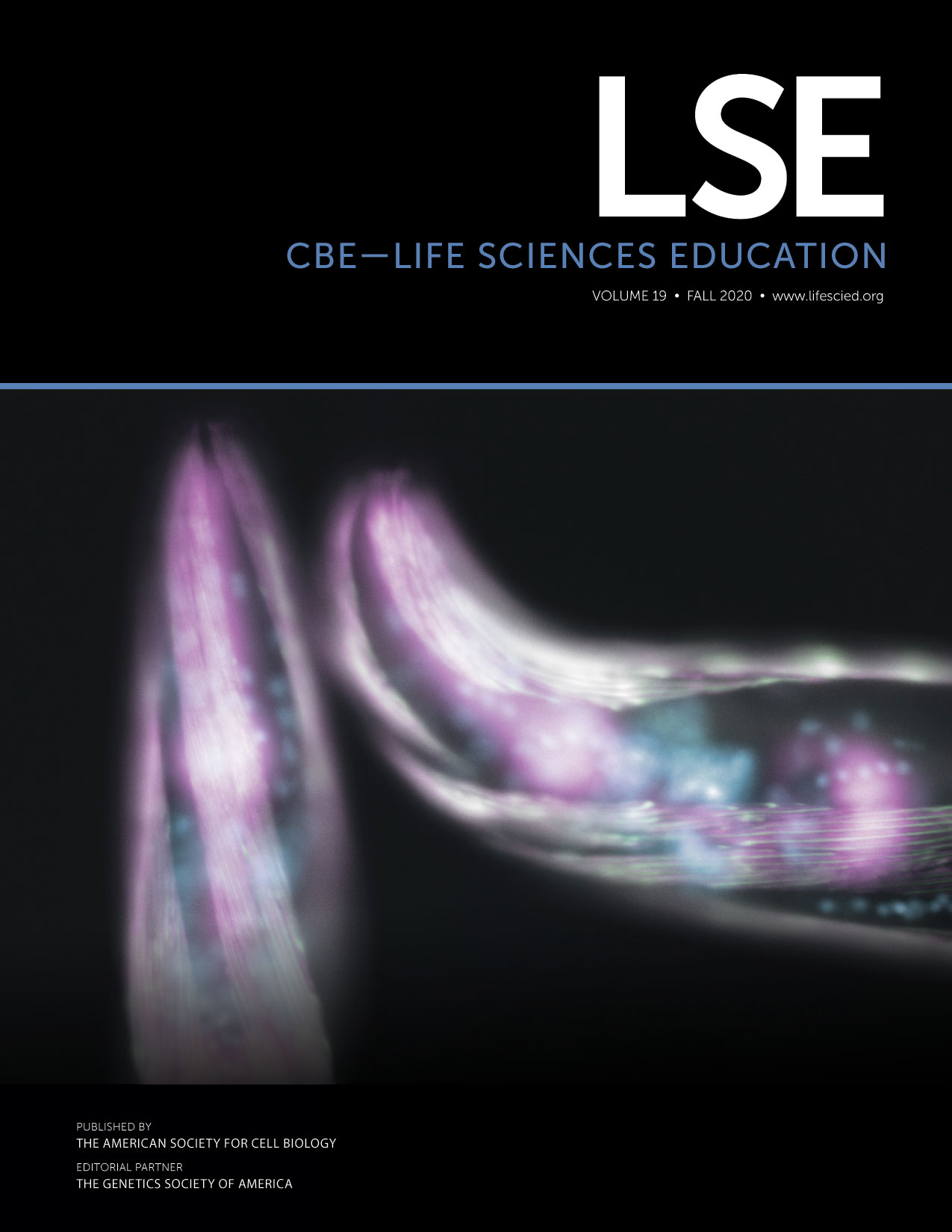
Submitted: 18 November 2019 Revised: 3 March 2020 Accepted: 25 March 2020
© 2020 E. E. Scott et al. CBE—Life Sciences Education © 2020 The American Society for Cell Biology. This article is distributed by The American Society for Cell Biology under license from the author(s). It is available to the public under an Attribution–Noncommercial–Share Alike 3.0 Unported Creative Commons License (http://creativecommons.org/licenses/by-nc-sa/3.0).
- Help & FAQ
Design-based research: What it is and why it matters to studying online learning
- Administration, Leadership & Technology
Research output : Contribution to journal › Article › peer-review
The ever-changing nature of online learning foregrounds the limits of separating research from design. In this article, we take the difficulty of making generalizable conclusions about designed environments as a core challenge of studying the educational psychology of online learning environments. We argue that both research and design can independently produce empirically derived knowledge, and we examine some of the configurations that allow us to simultaneously invent and study designed online learning environments. We revisit design-based research (DBR) methods and their epistemology, and discuss how they contribute various types of usable knowledge. Rather than compromising objectivity, we argue for how design researchers can acknowledge their intent and, in so doing, promote ways in which research and design can not only produce better interventions but also transform people and systems.
Original language | English (US) |
---|---|
Pages (from-to) | 207-220 |
Number of pages | 14 |
Journal | |
Volume | 57 |
Issue number | 3 |
DOIs | |
State | Published - 2022 |
ASJC Scopus subject areas
- Developmental and Educational Psychology
Access to Document
- 10.1080/00461520.2022.2079128
Other files and links
- Link to publication in Scopus
- Link to the citations in Scopus
Fingerprint
- Online Learning Keyphrases 100%
- Online Learning Environment Keyphrases 100%
- Design Research Keyphrases 100%
- Electronic Learning Social Sciences 100%
- Epistemology Keyphrases 50%
- Design Researchers Keyphrases 50%
- Educational Psychology Keyphrases 50%
- Usable Knowledge Keyphrases 50%
T1 - Design-based research
T2 - What it is and why it matters to studying online learning
AU - Hoadley, Christopher
AU - Campos, Fabio C.
N1 - Publisher Copyright: © 2022 The Author(s). Published with license by Taylor & Francis Group, LLC.
N2 - The ever-changing nature of online learning foregrounds the limits of separating research from design. In this article, we take the difficulty of making generalizable conclusions about designed environments as a core challenge of studying the educational psychology of online learning environments. We argue that both research and design can independently produce empirically derived knowledge, and we examine some of the configurations that allow us to simultaneously invent and study designed online learning environments. We revisit design-based research (DBR) methods and their epistemology, and discuss how they contribute various types of usable knowledge. Rather than compromising objectivity, we argue for how design researchers can acknowledge their intent and, in so doing, promote ways in which research and design can not only produce better interventions but also transform people and systems.
AB - The ever-changing nature of online learning foregrounds the limits of separating research from design. In this article, we take the difficulty of making generalizable conclusions about designed environments as a core challenge of studying the educational psychology of online learning environments. We argue that both research and design can independently produce empirically derived knowledge, and we examine some of the configurations that allow us to simultaneously invent and study designed online learning environments. We revisit design-based research (DBR) methods and their epistemology, and discuss how they contribute various types of usable knowledge. Rather than compromising objectivity, we argue for how design researchers can acknowledge their intent and, in so doing, promote ways in which research and design can not only produce better interventions but also transform people and systems.
UR - http://www.scopus.com/inward/record.url?scp=85133246220&partnerID=8YFLogxK
UR - http://www.scopus.com/inward/citedby.url?scp=85133246220&partnerID=8YFLogxK
U2 - 10.1080/00461520.2022.2079128
DO - 10.1080/00461520.2022.2079128
M3 - Article
AN - SCOPUS:85133246220
SN - 0046-1520
JO - Educational Psychologist
JF - Educational Psychologist
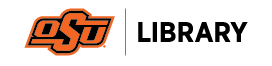
3 Design-Based Research and Interventions
Design-Based Research (DBR) is a research methodology used by researchers in the learning sciences. DBR is a concentrated, collaborative and participatory approach to educational inquiry. The basic process of DBR involves developing solutions or interventions to problems (Anderson & Shattuck, 2012). An “Intervention” is any interference that would modify a process or situation. Interventions are thus intentionally implemented change strategies (Sundell & Olsson, 2017). Data analysis takes the form of iterative comparisons. The purpose of this research perspective is to generate new theories and frameworks for conceptualising learning and instruction.
One positive aspect of DBR is that it can be employed to bring researchers and practitioners together to design context-based solutions to educational problems, which have deep-rooted meaning for practitioners about the relationship between educational theory and practice. DBR assumes a timeframe which allows for several rounds of review and iteration. It might be seen as a long-term and intensive approach to educational inquiry which is not really suitable for doctoral work, but increasingly there are examples of this approach being used (Goff & Getenet, 2017).
DBR provides a significant methodological approach for understanding and addressing problems of practice, particularly in the educational context, where a long criticism of educational research is that it is often divorced from the reality of the everyday (Design-Based Research Collective, 2003). DBR is about balancing practice and theory, meaning the researcher must act both as a practitioner and a researcher. DBR allows the collection of data in multiple ways and encourages the development of meaningful relationships with the data and the participants. DBR can also be used as a practical way to engage with real-life issues in education.
DBR & Interventions: GO-GN Insights
Roberts (2019) used a design-based research (DBR) approach to examine how secondary students expanded their learning from formal to informal learning environments using the open learning design intervention (OLDI) framework to support the development of open educational practices (OEP).
“We took some methods and research classes in my EdD program. I took Design-based research (DBR) and found it confusing and overwhelming. As such, I decided to take an extra course on case study research because it seemed to speak to me the most. In my mind I thought I could compare and contrast a variety of secondary school teachers integrating open ed practices. Through my initial exploration, I discovered that in my school district (30,000 + students), there are many teachers using OEP, but they were not interested in working “with” me, they wanted me to watch and observe them teach – then write about it. I began to understand that not only did I want to consider focusing my research on an emerging pedagogy (OEP) I also realized that I wanted to consider newer participatory methods. I did notmthink of DBR in this context when I took the initial course. “I knew I wanted to work with a teacher and complete some kind of intervention in order to support them in thinking about and actually integrating OEP. DBR was suggested to me multiple times, but I kept pushing it away. At the same time many of my supervisory committee and my peers did not think I should even consider DBR. I discovered that many researchers don’t know about it and are fearful of it. As I learned, when you do choose DBR, it is kind of like being an open learner in that you believe in the philosophy behind the DBR process. You just “are” a DBR researcher and educator. “It took many hours of reflection, reading about different examples of DBR, going to workshops and webinars about DBR in order to really see the possible benefits of DBR (collaborative, iterative, responsive, flexibility, balance between theory/ practice and relationships based) to get me to take the plunge…” (Verena Roberts)
Useful references for Design-Based Research: Anderson & Shattuck (2012);Design-Based Research Collective (2003); Goff & Getenet (2017); Sundell & Olsson(2017)
Research Methods Handbook Copyright © 2020 by Rob Farrow; Francisco Iniesto; Martin Weller; and Rebecca Pitt is licensed under a Creative Commons Attribution 4.0 International License , except where otherwise noted.
Share This Book

1st Edition
Design Research in Social Studies Education Critical Lessons from an Emerging Field
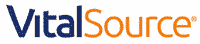
- Taylor & Francis eBooks (Institutional Purchase) Opens in new tab or window
Description
This edited volume showcases work from the emerging field of design-based research (DBR) within social studies education and explores the unique challenges and opportunities that arise when applying the approach in classrooms. Usually associated with STEM fields, DBR’s unique ability to generate practical theories of learning and to engineer theory-driven improvements to practice holds meaningful potential for the social studies. Each chapter describes a different DBR study, exploring the affordances and dilemmas of the approach. Chapters cover such topics as iterative design, using and producing theory, collaborating with educators, and the ways that DBR attends to historical, political, and social context.
Table of Contents
Beth C. Rubin is Professor of Education at the Graduate School of Education, Rutgers University, USA. Eric B. Freedman is Assistant Professor of Teacher Education and Secondary Social Studies at Sacred Heart University, USA. Jongsung Kim is Assistant Professor of Social Studies Education at the Graduate School of Education, Hiroshima University, Japan.
About VitalSource eBooks
VitalSource is a leading provider of eBooks.
- Access your materials anywhere, at anytime.
- Customer preferences like text size, font type, page color and more.
- Take annotations in line as you read.
Multiple eBook Copies
This eBook is already in your shopping cart. If you would like to replace it with a different purchasing option please remove the current eBook option from your cart.
Book Preview

The country you have selected will result in the following:
- Product pricing will be adjusted to match the corresponding currency.
- The title Perception will be removed from your cart because it is not available in this region.
Breadcrumbs Section. Click here to navigate to respective pages.

Design Research in Social Studies Education
DOI link for Design Research in Social Studies Education
Get Citation
This edited volume showcases work from the emerging field of design-based research (DBR) within social studies education and explores the unique challenges and opportunities that arise when applying the approach in classrooms. Usually associated with STEM fields, DBR’s unique ability to generate practical theories of learning and to engineer theory-driven improvements to practice holds meaningful potential for the social studies. Each chapter describes a different DBR study, exploring the affordances and dilemmas of the approach. Chapters cover such topics as iterative design, using and producing theory, collaborating with educators, and the ways that DBR attends to historical, political, and social context.
TABLE OF CONTENTS
Part | 27 pages, introduction, chapter 1 | 25 pages, design research in the social studies, part i | 78 pages, improving practice through iterative design, chapter 2 | 27 pages, from form to function, chapter 3 | 26 pages, using iterative design to improve student access and engagement in an online political communications simulation 1, chapter 4 | 23 pages, developing authentic performance assessments in a classroom mini-economy, part ii | 48 pages, using and producing theory, chapter 5 | 18 pages, applying theory to problematic practice, chapter 6 | 28 pages, from practice to theory, part iii | 48 pages, collaborating with educators, chapter 7 | 22 pages, intersecting goals in an elementary social studies design project, chapter 8 | 24 pages, design-based implementation research in a government classroom, part iv | 44 pages, contextualizing dbr historically, socially, and politically, chapter 9 | 20 pages, theorizing context in dbr, chapter 10 | 22 pages, beyond national discourses, part | 12 pages, chapter 11 | 10 pages, toward socially transformative design research for social studies.
- Privacy Policy
- Terms & Conditions
- Cookie Policy
- Taylor & Francis Online
- Taylor & Francis Group
- Students/Researchers
- Librarians/Institutions
Connect with us
Registered in England & Wales No. 3099067 5 Howick Place | London | SW1P 1WG © 2024 Informa UK Limited
Thank you for visiting nature.com. You are using a browser version with limited support for CSS. To obtain the best experience, we recommend you use a more up to date browser (or turn off compatibility mode in Internet Explorer). In the meantime, to ensure continued support, we are displaying the site without styles and JavaScript.
- View all journals
- Explore content
- About the journal
- Publish with us
- Sign up for alerts
- Open access
- Published: 05 February 2024
Research on the development of principles for designing elementary English speaking lessons using artificial intelligence chatbots
- Jihee Han 1 &
- Dongyub Lee 2
Humanities and Social Sciences Communications volume 11 , Article number: 212 ( 2024 ) Cite this article
2071 Accesses
1 Citations
1 Altmetric
Metrics details
- Science, technology and society
The present study was conducted with the aim of developing principles for designing elementary English speaking lessons using artificial intelligence chatbots. To achieve this, design and development research methods were applied, and initial design principles and detailed guidelines were developed through a review of relevant literature. Subsequently, the design principles were modified and refined through two rounds of expert validation and usability evaluation. The research results yielded a total of 10 principles for designing elementary English speaking lessons using artificial intelligence chatbots, including: 1) principle of media selection, 2) principle of creating a learning environment, 3) principle of content restructuring, 4) principle of stimulating and sustaining interest and motivation, 5) principle of providing guidance, 6) principle of scaffolded learning support, 7) principle of individualized feedback provision, 8) principle of fostering a learning environment that supports growth and development, 9) principle of communication and collaboration, and 10) principle of learning management. Additionally, a set of 24 detailed guidelines necessary for implementing each lesson design principle was developed. Based on the research findings, the principles for designing elementary English speaking lessons using artificial intelligence chatbots, as well as the theoretical and practical implications of the study, were discussed. Finally, the limitations of the research were identified, and suggestions for future research were proposed.
Similar content being viewed by others
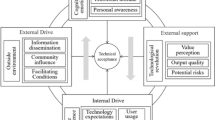
A qualitative analysis of Chinese higher education students’ intentions and influencing factors in using ChatGPT: a grounded theory approach
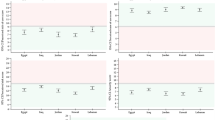
A multinational study on the factors influencing university students’ attitudes and usage of ChatGPT
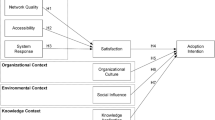
Analyzing ChatGPT adoption drivers with the TOEK framework
Introduction.
We are currently living in the era of the Fourth Industrial Revolution. With the rapid advancement of digital technologies such as artificial intelligence, big data, and the Internet of Things, fundamental innovations have occurred in various fields, leading to extensive changes throughout society. Education in schools is no exception. There is a growing trend of actively integrating these cutting-edge technologies into classrooms. Furthermore, with the widespread adoption of online education and remote learning due to the COVID-19 pandemic that began in 2020, there has been increased interest in utilizing various educational technologies (Edu-tech) for teaching and learning. The development of technology plays a catalytic role in changing the paradigm of the education system, and we are entering an era of a major transformation in education. In recent years, there have been various movements reflecting the current trends in English education in South Korea. In an English as a Foreign Language (EFL) environment like ours, students have very limited opportunities to use English in their daily lives. Therefore, it is important to maximize students’ speaking opportunities within the regular curriculum time to enable them to naturally acquire English. However, it is currently challenging to achieve this in Korean classrooms. Students only have 2 h per week (for grades 3–4) or 3 h per week (for grades 5–6) dedicated to learning English, which is insufficient for practice. Additionally, with an average class size of 23 students (OECD, 2019 ), it becomes difficult to provide appropriate feedback for individual speaking practice. Furthermore, there is a wide range of English proficiency levels among students in the classroom. However, assignments are uniformly provided, making it too easy for proficient students to practice the target language in the textbook, resulting in a lack of motivation to participate in the learning process. On the other hand, struggling students find it too difficult to even speak the target language and therefore refrain from verbal participation. Therefore, teachers need to explore the use of various Edu-tech tools in line with the current trends to address these issues and provide personalized lessons tailored to students’ levels, while offering effective feedback.
With the recent advancements in machine learning and deep learning, which are key technologies in artificial intelligence, learners now have access to various English programs. Artificial intelligence technologies are considered as alternatives to overcome the physical limitations of the EFL education environment, and there is a growing interest in the potential use of AI chatbots. Various interactive AI English education programs have been developed, and attempts are being made to integrate them into school education. Due to the high interest in AI chatbots, diverse research studies on AI chatbots in English education, both domestic and international, are underway. These studies include analyses of the characteristics of AI chatbots (Coniam D, 2014 ; Kim et al., 2022 ; Haristiani N, 2019 ; Huang et al., 2019 ; Kılıçkaya F, 2020 ; Dokukina I and Gumanova J, 2020 ; Pérez JQ et al., 2020 ; Yin Q and Satar M, 2020 ; Yoon and Park, 2020 ), research on developing AI chatbots (Mondal et al., 2018 ; Lee, 2018 ; Muhammad AF et al., 2020 ; Sung, 2022 ), and research on the use of AI chatbots in teaching and learning in school settings (Gayathri AN and Rajendran VV, 2021 ; Lin CJ and Mubarok H, 2021 ; Jeon, 2022 ; Yoo, 2021 ; Wu, 2022 ; Yang J, 2022 ; Mendoza S et al., 2022 ; Abidin et al., 2022 ). Although many research results have emerged, particularly in 2021, regarding the use of AI chatbots in teaching and learning in school settings, there is still a significant lack of related research. This is because there have not been many cases of utilizing AI chatbots in school settings, and research on the role of teachers and principles of lesson design in AI chatbot-assisted classes has been insufficient. In particular, it has been challenging to find research targeting elementary school students, likely due to their lower vocabulary level and limited proficiency in using diverse sentence structures, as the regular English curriculum is introduced from the third grade of elementary school in South Korea. However, it is expected that developing and implementing AI chatbots that incorporate vocabulary levels and sentence structures suitable for elementary English education, while stimulating learners’ interest, would yield significant effects (Kim and Lee, 2020 ; Chu and Min, 2019 ; Xia Q et al., 2023 ). Therefore, there is a strong demand for research on developing AI chatbots for use in elementary English classes and the principles of lesson design for AI chatbot-assisted classes.
The aim of this study is to develop principles for designing elementary English speaking lessons using AI chatbots and validate their effectiveness. Through this research, the developed principles for designing elementary English speaking lessons using AI chatbots will guide teachers in effectively incorporating AI chatbots into their English classes at the elementary school level, enabling students to achieve cognitive and affective goals in the English subject. In other words, the objective of this study is to develop design principles that guide the instructional design of elementary English speaking lessons using AI chatbots from an educational technology perspective. The specific research questions set to achieve these objectives are as follows:
What are the principles for designing elementary English speaking lessons using AI chatbots?
Are the principles for designing elementary English speaking lessons using AI chatbots valid?
Theoretical background
Elementary english curriculum in south korea.
In Korean elementary schools, English education was introduced as a new subject in the 6th national curriculum (1992–1997) and became an official subject in 1997. Since the 7th national curriculum (1997–2007) until the present, the overarching goal of the English curriculum has been to enhance English communication skills. In the revised curriculum announced by the Ministry of Education in December 2022, fostering communicative competence was presented as the comprehensive core competency of the English subject. It explicitly stated the intention to maximize the efficiency of learning by utilizing various media, information and communication technologies in line with the digital and AI educational environment in order to adapt to the changing times (Ministry of Education, 2022 , p.6).
There is increasing interest among researchers in exploring teaching methods that provide learners in the EFL context, such as in South Korea, with opportunities to understand discourse or writing and express their own thoughts and emotions. One of the prominent approaches is Communicative Language Teaching (CLT), which has been widely utilized (Richards, 2005 ). Within the CLT framework, various teaching methods have emerged, including the Natural Approach, Content-Based Instruction, and Task-Based Learning. Among them, Task-Based Learning has garnered significant attention from domestic researchers, particularly since Prabhu ( 1987 ) first proposed it in 1987. Task-Based Learning involves providing tasks that allow learners to naturally acquire and use the language while performing the tasks. Learners engage in interaction with their peers during task performance, using and acquiring the target language more efficiently (Nunan, 1999 ). In this study, we aim to develop principles for designing elementary English speaking lessons using AI chatbots, with a focus on Task-Based Learning as the underlying teaching approach.
A study on the use of chatbots in English education
Since 2019, there has been active research both domestically and internationally on the potential of using AI chatbots in foreign language education. These studies have primarily focused on examining the effects of using chatbots in English classes, particularly in terms of cognitive and affective aspects. Many studies have investigated the effects of chatbots on speaking skills, and most of them have shown statistically significant positive effects. Specifically, AI chatbots have been found to increase learners’ exposure to English language environments, provide more opportunities for English language use, and enhance their communication abilities (Yang, 2022 ). Learners have also benefited from immediate and effective spelling and grammar feedback from AI chatbots, leading to improved fluency (Haristiani, 2019 ), and the authenticity and accuracy of the English provided by chatbots have been effective in enhancing learners’ conversational skills (El Shazly, 2021 ; Muhammad et al., 2020 ).
Studies comparing and analyzing the interactions between chatbots and high- and low-achieving learners have shown some differences. It has been found that proficient learners are more engaged in conversations with chatbots and tend to have higher satisfaction, while struggling learners may discontinue the conversation prematurely (Xia et al., 2023 ). Similarly, according to Chiu et al. ( 2023 ), beginner-level students require teacher support for effective motivation, whereas advanced learners may be hindered by teacher intervention. According to Shin ( 2019 ), lower-achieving students tend to produce more utterances when sentences are shorter, while higher-achieving students engage in more extensive conversations and use verb phrases more diversely when presented with less challenging texts. These findings highlight the importance of considering learners’ English proficiency levels when designing English classes that incorporate chatbot interactions.
Learners have also shown a high interest in AI chatbot-assisted lessons in the affective domain, experiencing a sense of comfort. Particularly in speaking skills development, the anxiety often associated with traditional language learning methods has been reduced (Kılıçkaya, 2020 ; Mageira et al., 2022 ), and students have demonstrated a high level of interest and engagement with AI chatbots. These emotional stability, high interest, and attention have been found to enhance learners’ confidence and improve their learning immersion (Huang et al., 2019 ). However, learners’ motivation can decline over time, so it is necessary to design lessons that incorporate specific learning tasks to maintain consistent motivation (Yin and Satar, 2020 ).
Teaching and design of English speaking using artificial intelligence chatbots
As the results of utilizing AI chatbots in classroom settings have shown positive effects in cognitive and affective domains, the need for systematic principles in designing lessons using AI chatbots has been emphasized. To develop principles for designing elementary English speaking lessons using AI chatbots, it is necessary to analyze previous research related to principles and guidelines for designing English speaking lessons using AI chatbots, both domestically and internationally.
First, it is necessary to consider the selection of appropriate media. Teachers should choose diverse and multidimensional media, taking into account the learning conditions and content (Yu, 2022 ). Selecting a medium that allows learners and the chatbot to engage in conversations by changing the order of questions and answers can encourage learners to produce more utterances. Therefore, it is important to select a medium that is suitable for learners’ proficiency levels and enables meaningful interaction (Chapelle, 2001 ). In the context of designing English speaking lessons using AI chatbots, the medium refers to the selection of a chatbot builder.
Second, it is important to design the learning content and assign tasks considering the learners’ proficiency levels and specific situations. It is crucial to construct the content that is appropriate for learners’ levels and personal characteristics (Lin and Mubarok, 2021 ). Careful consideration should be given to various factors such as family structure, social norms, and financial circumstances when designing activities to ensure meaningful engagement for students (Vazhayil et al., 2019 ). A systematic approach is needed to provide learners with a meaningful and accessible learning experience (Woolf et al., 2013 ; El Shazly, 2021 ; Yang, 2022 ).
Third, it is essential to provide an optimized learning environment when conducting speaking lessons using AI chatbots. The technical infrastructure for utilizing AI chatbots should be prioritized and established (Vazhayil et al., 2019 ; Li, 2022 ). Issues such as external noise interfering with the recognition of learners’ voices should be minimized (Kim et al., 2022 ), and support should be provided to create an environment that is conducive to optimal performance (Bii et al., 2018 ). Additionally, it is important to encourage learners and reassure them when they encounter difficulties during interactions with AI chatbots to prevent them from feeling overwhelmed.
Fourth, detailed guidance on the usage and task activities of AI chatbots is necessary. Since learners are encountering AI chatbots for the first time, instructors should provide thorough instructions on how to use them (Mendoza et al., 2022 ). Introduction to educational objectives (Kılıçkaya, 2020 ) and specific language learning tasks (Yin and Satar, 2020 ) should also be included to enhance the efficiency of the learning process.
Fifth, it is necessary to provide learners with pre-learning opportunities. If learners pre-learn relevant vocabulary, sentence patterns, and other aspects before using the chatbot (Vazhayil et al., 2019 ), it can minimize the burden of using the target language.
Sixth, it is important to generate and maintain students’ interest. While the introduction of artificial intelligence technology through the use of chatbots can initially capture students’ interest and attention, strategies are needed to sustain their interest throughout the class (Coniam, 2014 ; Yin and Satar, 2020 ; Pérez et al., 2020 ). Therefore, it is crucial to develop various teaching and learning methods that appeal to students, such as incorporating quizzes, graphics, and animations that facilitate easy understanding by learners (Gayathri and Rajendran, 2021 ).
Seventh, teachers need to provide appropriate scaffolding to learners. When learners encounter difficulties in interacting with the chatbot, offering necessary visual or additional materials can facilitate continuous conversations among students (Mendoza et al., 2022 ). It should be noted that while scaffolding is effective for novice learners, it can hinder support from the teacher for advanced learners, as suggested by research findings (Kalyuga, 2007 ; Williamson and Eynon, 2020 ; Chiu et al., 2023 ). Hence, teachers should provide scaffolding tailored to the learners’ levels and characteristics, enabling them to engage in smooth interaction.
Eighth, strategies for promoting meaningful negotiation of meaning are needed to elicit additional utterances from learners. Utilizing strategies that encourage meaningful negotiation of meaning, teachers can specifically prompt learners’ speech (Bygate, 1987 ; Lin and Mubarok, 2021 ). Additionally, for words or expressions that the chatbot does not immediately understand, strategies such as “requesting repetition,” “eliciting clarification,” and “eliciting inference” have been proposed (Chu and Min, 2019 ).
Ninth, it is necessary to provide immediate and personalized feedback to learners’ utterances (Haristiani, 2019 ; Kılıçkaya, 2020 ; Dokukina and Gumanova, 2020 ; Xia et al., 2023 ). There are two ways to provide feedback: one is through an AI chatbot that recognizes learners’ utterances and provides immediate feedback, and the other is for teachers to provide feedback to learners who have difficulties in conversing with the chatbot.
Last, it is important to have learning management that allows students to appropriately review and evaluate their learning process (Mendoza et al., 2022 ). Providing reflection journals can facilitate students’ reflection on their tasks and presentations (Kong, 2020 ), and enabling learners to manage their learning materials and progress is also suggested (El Shazly, 2021 ; Xia et al., 2023 ). Learners should have the means to plan, review, and evaluate their learning process effectively.
Methodology
This study applied the Design and Development research methodology to develop principles for designing elementary English speaking classes using AI chatbots. Design and Development research is a systematic approach that aims to establish empirical foundations for creating new models, instructional or non-instructional products, and tools, as well as the development, evaluation, and validation processes associated with them (Richey and Klein, 2014 , p. 6). It serves the purpose of generating new knowledge and validating existing practices.
According to Richey and Klein ( 2014 ), there are two types of research in the field of design and development: “Products and tools research” and “Model research”(Table 1 ). “Products and tools research” describes and analyzes the design and development processes used in specific projects, making it context-dependent. On the other hand, “Model research” aims to provide a general analysis of new design and development processes and can be somewhat more generalized compared to “Products and tools research.” Model research is utilized in developing design models, and further, design principles, strategies, and guidelines (Richey and Klein, 2014 ).
In this study, we utilized “Model research” among these two types. Model research allows us to analyze the effectiveness and validity of existing or newly created models in the context of model development and the development process. Exploring model research involves three main topics: model development research, model validation research, and model use research.
First, “model development research” aims to develop comprehensive models and the processes associated with their components. Second, during the “model validation” phase, the validation of the model’s components is carried out. Lastly, in the “model use” phase, the conditions that affect the model’s use are studied, including research on the characteristics and expertise of designers and their decision-making processes.
In this particular study, which focuses on developing and validating a new instructional design model for elementary English speaking courses using AI chatbots, I performed model development research and model validation research. The specific procedures are outlined as follows.
First, the initial design principles were derived through a review of domestic and international literature related to using AI chatbots in classroom settings. The literature review encompassed academic papers, conference proceedings, institutional research reports, articles, and books. The main topics were AI chatbots and English-speaking classes, while subtopics were categorized into principles for designing classes using AI chatbots and models for designing classes using AI chatbots.
Second, to validate the viability of the initial design principles, an expert validation review was conducted. The expert panel consisted of individuals who held master’s or doctoral degrees in the relevant field and had published papers or presented on topics related to the research (Table 2 ). The validity assessment questionnaire for the design principles was adapted from Kim S ( 2016 a, 2016 b) to suit the present study. The questionnaire utilized a 4-point scale (4: strongly agree, 3: agree, 2: disagree, 1: strongly disagree) for closed-ended items and included open-ended items to allow experts to provide additional comments and opinion.
Third, a usability evaluation was conducted to determine if the developed instructional design principles utilizing the AI-based chatbot for elementary English-speaking classes were helpful for elementary school teachers in the field. Three elementary school teachers participated in the evaluation, selected based on their interest in AI chatbots or prior experience using them during class. The participants had a range of teaching experience, from 7 to 20 years, to ensure that the application of the developed design principles was feasible across different levels of teaching experience (Table 3 ).
During the usability evaluation, the participating teachers had one-on-one discussions with the researchers to receive explanations about the instructional design principles and discuss any areas of misunderstanding. Next, the teachers designed lessons based on the provided instructional design principles and, upon completing the lesson designs, responded to a usability evaluation questionnaire. The usability evaluation items were designed on a 4-point scale to assess the teachers’ understanding of the instructional design principles for AI-based elementary English-speaking classes and the practical assistance provided by the design principles in their actual lesson planning. The final section of the questionnaire allowed the teachers to freely provide their opinions on strengths, weaknesses, and suggestions for improvement.
The responses from the expert validation and usability evaluation were analyzed for validity and reliability using the Content Validity Index (CVI) and Inter-Rater Agreement (IRA) among the evaluators. Based on the input from experts and users, the final instructional design principles were developed.
The specific procedure of the study is as depicted in Fig. 1 .
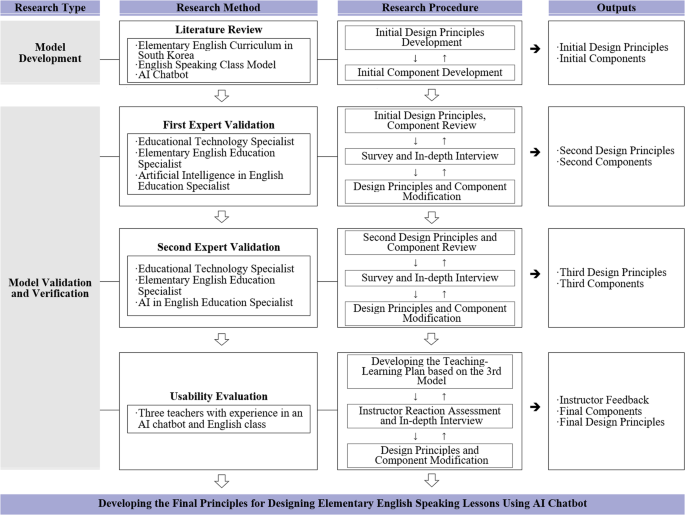
It indicates the research type, research methods, procedural steps, and the flow of outputs for developing the final model of instructional design.

Derivation of the initial design principles and components of the model
Through a review of existing literature, elements applicable to designing elementary English speaking classes using AI chatbots and general design principles were identified. Based on commonalities among the findings, the components were derived through an iterative process. As a result, five initial components of the model for designing elementary English speaking classes using AI chatbots were identified: AI chatbot learning tool, AI chatbot utilization curriculum, AI chatbot learning support, AI chatbot utilization activities, and AI chatbot learning outcomes and evaluation, as presented in the Table 4 .
Expert Validation results regarding the initial Components
The expert validation of the components of the principles for designing elementary English language classes using AI chatbots was conducted in two phases (Table 5 ). In the first phase of expert validation, the average score for the “level of components” was the highest at 3.60, while the other items ranged between 3.00 and 3.40. The IRA among the experts was 0.11, indicating a need for modifications in the overall design principles. IRA stands for the Index of IRA, which is an index representing the reliability of evaluations among experts. In this paper, it is calculated by dividing the number of items on which experts unanimously agreed by the total number of items (Rubio et al., 2003 ). In the primary expert validation, among the total of 9 domains, an IRA of 1.00 was observed, as one item received a score of 1. This is due to the fact that one of the five experts assigned a score of 2 to one or more items. However, in the second phase of expert validation, the revised components based on the converging opinions from the first phase were evaluated by the experts. The CVI was 1.00, indicating that the experts considered all items to be valid. The IRA was also 1.00, indicating high agreement among the experts and ensuring the reliability of their evaluations.
The expert reviews on the components conducted in the second phase are summarized in the below Table 6 . First, there were opinions from experts indicating that some components have incorrect hierarchy, and some sub-components are overlapping, suggesting the need to reorganize the components and sub-components and derive the upper-level components again. For example, the provision of individual feedback was considered more suitable for the sub-component of “AI Chatbot Utilization Activities”, according to one expert. Additionally, there were opinions suggesting that the components, “AI Chatbot Learning Tool”, “AI Chatbot Utilization Curriculum” and “AI Chatbot Learning Support” all seemed to be included in “AI Chatbot Utilization Activities”, making it difficult to distinguish each item effectively. Second, it was recommended that the descriptions of the components should be distinct and clearly presented, highlighting the differentiation between “AI Chatbot Utilization Activities” and “AI Chatbot Learning Support.” Third, since some sub-components are not well-differentiated within each component, there is a need to modify the names of the components to align with the corresponding sub-components.
Some of the initial components have a broader scope and lack clear explanations, thus requiring modification in response to the expert reviews. Fourth, there is a need to add certain sub-components to each component and provide clear explanations for them. For instance, one expert suggested adding the principle of sharing and reflecting on opinions with group members when utilizing the new technology of AI chatbots in certain activities. These expert reviews have been taken into account to make improvements.
Expert validation results for the initial design principles
The expert validation for the overall design principles was conducted, considering the criteria of validity, explanatory power, usefulness, universality, and comprehensibility. Expert opinions were examined and provided for the items of validity, explanatory power, usefulness, universality, and comprehensibility for the overall design principles in two rounds of validation. The summarized results of the expert validation for the overall design principles, conducted in the 1st and 2nd rounds, are presented in the Table 7 .
The results of the first expert validation review on the overall design principles showed generally high scores, with an average of 3.60 or above in all categories. The CVI was above 0.80 for all items, indicating that the participating experts found the design principles to be valid. The IRA was 0.80, indicating a reasonable level of consistency among the experts’ evaluations and establishing their reliability. However, one expert suggested that adding explanations and examples would facilitate teachers’ ability to design lessons according to the derived principles. In the second expert validation, explanations and examples were added, and a design principle and detailed guidelines related to communication and collaboration in group activities were included. The revised components were restructured and organized according to the design principles. In the second validation, all categories of the design principles, including validity, clarity, usefulness, universality, and comprehensibility, received the highest score of 4.00. The CVI was 1.00 for all items, indicating that all participating experts found the design principles to be valid. The IRA was also 1.00, suggesting a high level of consistency and reliability among the evaluators’ ratings.
The initial principles and detailed guidelines were restructured, revised, deleted, integrated, and refined based on the input from primary experts. As a result, a set of second-stage design principles and detailed guidelines was derived, consisting of a total of 10 principles and 24 detailed guidelines. The expert validation opinions and modifications incorporated during this process are summarized in Table 8 .
Firstly, the components were restructured as a result of the overall restructuring based on the expert feedback, addressing the unclear inclusion relationship between principles and detailed guidelines and eliminating any duplication or overlap with previously mentioned principles and detailed guidelines. Secondly, areas with low validity scores and suggestions for modifications based on the expert validation feedback were either removed or integrated, while essential principles and detailed guidelines representing the core aspects of the study were added. Any content that resembled or duplicated existing information was removed during this process. Thirdly, due to changes in some components and the addition and removal of principles, the overall positioning and restructuring of the framework were readjusted. Fourthly, the content was elaborated by providing more specific and actionable statements, modifying abstract and ambiguous descriptions into concrete statements that represent specific actions or behaviors. Lastly, examples and explanations were added to the detailed guidelines to facilitate understanding and provide references for designing English speaking courses using AI chatbots. These additions aimed to assist in comprehending the detailed guidelines and their practical application. The third-round design principles were improved based on these experts’ feedback and recommendations.
Usability evaluation results
The usability evaluation was conducted to assess the suitability of the developed 2nd iteration instructional design principles for actual classroom use by teachers. Three elementary school teachers working in schools in Seoul and Gyeongsangnam-do, South Korea participated in the usability evaluation. They were given an explanation of the developed instructional design principles by the researcher and were asked to imagine themselves designing an elementary English-speaking class using an AI chatbot. Based on this, they were requested to create a teaching and learning guide. Subsequently, a usability evaluation questionnaire was provided to assess the extent to which the instructional design principles were helpful in lesson planning.
The usability evaluation results for the two questions indicate an average score of 4.00, with both CVI and IRA showing a score of 1.00 (Table 9 ). All three teachers who participated in the usability evaluation provided positive responses, stating that design principles and detailed guidelines are helpful in designing English speaking lessons using AI chatbots. Their opinions on the strengths, weaknesses, and areas for improvement of each principle and model, as presented in open-ended questions, are summarized in Table 10 as follows.
The feedback gathered from Elementary school teachers through the usability evaluation questionnaire yielded the following results. The design principles were found to be helpful in the instructional design process, as they were accompanied by detailed explanations and examples. However, some examples were deemed insufficiently specific, and it was suggested that they should be presented more concretely using terminology familiar to classroom teachers.
The opinions of Elementary school teachers, obtained through usability evaluation, were incorporated into the final model development alongside the results of the secondary expert validation. The final model underwent improvements mainly at the level of terminology and relationships between terms, with no significant structural changes.
Final instructional design principles and guidelines
The final instructional design principles and guidelines derived from expert validation and usability evaluation are presented in the following Table 11 . The components include “Creating AI Chatbot Learning Environment,” “AI Chatbot Utilization Curriculum,” “AI Chatbot Teaching and Learning Activities,” and “Evaluation of AI Chatbot Learning”. A total of 10 instructional design principles and 24 detailed guidelines can be applied.
In this study, we aimed to develop instructional design principles and guidelines to support the design of elementary English speaking classes utilizing AI chatbots. Based on the results of the research, we can discuss the theoretical and practical aspects as follows:
First, through the development of instructional design principles and guidelines, we have enabled teachers to systematically design English speaking classes using AI chatbots. Unlike previous studies that only focus on measuring the cognitive and definitional effects of using AI chatbots in instruction (Kim, 2016 a, 2016 b; Han, 2020 ; Kılıçkaya, 2020 ) or provide instructional guidelines and models (Lin and Mubarok, 2021 ; Mendoza et al., 2022 ), our study includes design principles and guidelines that teachers need to consider during the instructional design process. In particular, there has been a growing interest in utilizing various Edu-tech in public elementary schools in South Korea since the outbreak of the COVID-19 pandemic in 2020. Teachers who are incorporating various edu-tech tools into their lessons might find it confusing, given the vast amount of new edu-tech resources being introduced. At this time, referencing the instructional design principles and guidelines for elementary English speaking classes using AI chatbots can be a valuable resource. Designing their own lessons with the guidance of these principles, especially those incorporating artificial intelligence chatbots, can undoubtedly reduce trial and error and provide useful materials for systematic implementation.
Second, we have applied a research methodology that integrates theoretical and practical aspects based on a review of relevant literature on AI chatbots in English language instruction. While previous studies have focused on developing instructional models based on students’ and teachers’ needs or addressing specific challenges in AI chatbot-assisted instruction (Mendoza et al., 2022 ), our study contributes to the field by providing a logical process for developing instructional designs. Through a comprehensive review of theories and literature related to AI chatbots, English speaking skills, and instructional design, we derived instructional design principles and guidelines, and further validated them through expert review. The research findings hold significance in guiding instructors to have a systematic and comprehensive perspective when designing their classes.
Third, our study offers ideas that extend beyond the application of AI chatbots in English language instruction alone. It provides insights into utilizing AI chatbots for various languages such as Korean, Chinese, and Japanese. When designing language-specific instruction, teachers can refer to the foundational design principles and guidelines that are essential for incorporating AI chatbots as a learning tool. Furthermore, although our study focuses on instructional design principles for elementary English-speaking classes, the principles and guidelines can be applied to middle school, high school, and university-level English speaking classes with appropriate modifications. By considering the target learners’ proficiency levels and corresponding curricula, our developed design principles and guidelines can be adapted for other levels of English-speaking instruction.
In conclusion, our research contributes to the field by developing instructional design principles and guidelines to support the design of elementary English speaking classes using AI chatbots. These guidelines provide teachers with a systematic and comprehensive approach to instructional design and can be applied not only in English language instruction but also in other languages. Additionally, the principles and guidelines can be extended to different educational levels, offering valuable insights for designing English speaking classes across various learner groups.
Based on the research results, the following conclusions can be drawn:
First, the instructional design of elementary English speaking classes using AI chatbots follows a structure where the activities revolve around the “AI chatbot teaching and learning activities” and conclude with reflection and evaluation of the learning process. Teachers have the flexibility to adapt and customize the process based on their specific contexts. This instructional design process relies on the underlying support of the learning tool called Dialogflow and the technical infrastructure required to manage it. Teachers need to align their instructional design with the available software and hardware resources. For example, if there are AI speakers available in the classroom, tasks can be assigned to the whole class or to small groups. Similarly, if there is a limited number of tablet PCs, tasks can be assigned to small groups or rotated among students.
Second, the instructional design principles developed in this study for English speaking classes using AI chatbots can contribute to increasing the attainability of English language goals and standards within the curriculum. The design principles offer options for teachers to choose between repetitive or question-and-answer-based chatbots according to the students’ proficiency levels, enabling personalized instruction. Traditional teacher-centered lecture-style instruction has limitations in achieving personalized instruction, but AI chatbot-assisted instruction can provide an alternative to overcome the physical constraints of dense classroom environments and limited English instruction time. Furthermore, considering the English education environment in South Korean elementary schools, we are exploring methods to replace native English teachers currently placed in elementary schools. Using AI chatbots for English speaking lessons could potentially serve as an alternative to substitute native English teachers. Therefore, in South Korea’s English education environment, it is expected that using AI chatbots for English speaking lessons will have a more significant impact.
Third, AI chatbot-assisted English speaking classes have the potential to reduce the proficiency gap caused by socioeconomic disparities. English language education in Korea heavily relies on private tutoring, and improving speaking skills, one of the four language skills, requires significant investment of time and effort. Utilizing an AI chatbot for English speaking classes allows learners to practice their English speaking skills not only during regular class hours but also after school, enhancing their communication abilities. However, to achieve this, it is crucial to provide each student with a tablet PC or Chromebook and establish wireless internet environments in students’ homes.
Some limitations and suggestions for future research based on the research process and results are as follows:
First, this study focused on elementary school students in South Korea, and the application of the developed instructional design was limited to elementary schools. Therefore, it is necessary to compare the differences and effectiveness of applying the instructional design model to middle school and high school students. To generalize the instructional design model to different educational levels, it is important to analyze which aspects of the design principles and guidelines need to be modified and improved when applying them to middle school and high school students.
Second, the process of planning and implementing AI chatbot-assisted English speaking classes requires more time and effort compared to traditional lecture-style instruction. It requires knowledge of tools like Dialogflow for AI chatbot development and practical experience in creating AI chatbots and learning materials. Additionally, to implement these classes during instructional time, securing tablet PCs, establishing wireless internet environments, and technical preparations like logging into the Google Assistant app on all devices using the teacher’s Google account are necessary. Given these challenges, there is a possibility that teachers might feel burdened and hesitate to implement AI chatbot-assisted instruction. Therefore, educational institutions should establish the necessary technological infrastructure to support teachers in utilizing various AI learning tools, reducing the time and cost burden associated with instructional design.
Third, as AI chatbots are capable of various forms of input and output, including text and speech, it is essential to develop instructional design models not only for English speaking but also for listening, reading, and writing in the field of English education. This would provide guidelines for teachers to conduct interactive English language classes in all four language skills. Further research is needed to explore the ways in which AI chatbots can be utilized in English language instruction across these four areas.
In conclusion, the research has developed instructional design principles and guidelines to support the design of elementary English speaking classes using AI chatbots. These guidelines provide a systematic and comprehensive approach to instructional design, not only for English language instruction but also for other languages. They can be extended to different educational levels, offering valuable insights for designing English speaking classes for diverse learner groups. However, further research is required to address limitations and explore the application of the instructional design model to different educational levels and language skills.
Data availability
All data generated or analyzed during this study are included in this published article.
Abidin TNE, Azam NHN, Yusof MAM (2022) Telegram bot usage as an instructional design method. Int J Account 7:42
Google Scholar
Bii PK, Too JK, Mukwa CW (2018) Teacher attitude towards use of chatbots in routine teaching.Univers J Educ Res 6(7):1586–1597
Article Google Scholar
Bygate M (1987) Speaking. Oxford university press
Chapelle CA (2001) Computer applications in second language acquisition. Cambridge University Press
Chang J, Park J, Park J (2021) An analysis on the trends of education research related to artificial intelligence chatbot Korea: focusing on implications for use in science education. J Learner Centered Curric Instr 21(13):729–743
Chiu TK, Moorhouse BL, Chai CS, Ismailov M (2023) Teacher support and student motivation to learn with Artificial Intelligence (AI) based chatbot. Interact Learn Environ 1–17. https://doi.org/10.1080/10494820.2023.2172044
Chu SY, Min DG (2019) A study of using task-based artificial intelligence (AI) chatbot for further interaction in English and the analysis of students’ production. Prim Engl Educ 25(2):27–52
Coniam D (2014) The linguistic accuracy of chatbots: usability from an ESL perspective. Text Talk 34(5):545–567
Dokukina I, Gumanova J (2020) The rise of chatbots—new personal assistants in foreign language learning. Procedia Comput Sci 169:542–546
El Shazly R (2021) Effects of artificial intelligence on English speaking anxiety and speaking performance: A case study. Expert Syst 38(3):e12667
Gayathri AN, Rajendran VV (2021) English Master AMMU: Chatbot for English Learning. In 2021 IEEE Mysore Sub Section International Conference (MysuruCon). IEEE, pp 618–623
Han DE (2020) The effects of voice-based AI chatbots on Korean EFL middle school students’ speaking competence and affective domains. Asia-Pac J Converg Res Interchange 6(7):71–80
Article ADS Google Scholar
Haristiani N (2019) Artificial Intelligence (AI) chatbot as language learning medium: an inquiry. J Phys Conf Ser 1387:012020
Hong SH, Yoon T, Lee S, Oh EJ (2021) An analysis of using dialogue based chatbot in elementary English education. J Korea Elem Educ 31(5):31–55
Huang W, Hew KF, Gonda DE (2019) Designing and evaluating three chatbot-enhanced activities for a flipped graduate course. Int J Mech Eng Robot Res 8(5):813–818
Hwang J (2020) A study on the development of interaction English learning model for children at home using artificial intelligence (AI) speech recognition speakers (Unpublished master’s thesis). Korea University, Seoul, Korea
Jeon J (2022) Exploring AI chatbot affordances in the EFL classroom: Young learners’ experiences and perspectives. Comput Assist Lang Learn, 1–26. https://doi.org/10.1080/09588221.2021.2021241
Kalyuga S (2007) Expertise reversal effect and its implications for learner-tailored instruction. Educ Psychol Rev 19:509–539
Kılıçkaya F (2020) Using a chatbot, replika, to practice writing through conversations in L2 English: a case study. In New Technological applications for foreign and second language learning and teaching. IGI Global, pp 221–238
Kim NY (2016a) Effects of voice chat on EFL learners’ speaking ability according to proficiency levels. Multimed-Assist Lang Learn 19:4
Kim H, Yang H, Shin D, Lee JH (2022) Design principles and architecture of a second language learning chatbot. Lang Learn Technol 26(1):1–18
CAS Google Scholar
Kim S (2016b) A study on development of a instructional design model for mobile inquiry learning. Seoul National University, Seoul, Korea
Kim S, Lee S (2020) An analysis of a primary school English speaking lesson using an AI-powered conversational English learning application: A focus on student engagement as well as learners’ and teachers’ perceptions of the class. Prim Engl Educ 26(3):177–202
Kong YJ (2020) Development of instructional strategies for English speaking in class using artificial intelligence speaker. Seoul National University
Lee D, Park S (2019) A developmental plan for an English conversation learning chatbot through the application of elementary school English textbooks. Prim Engl Educ 25(4):79–100
Lee DH (2018) A study for the development of an English learning chatbot system based on artificial intelligence. Second Engl Educ 11(1):45–68
Lee SM (2002) Task-based development of students’ meaning negotiation skills in English classes. Unpublished master’s thesis. The University of Birmingham, Birmingham
Li J (2022) Research on the influence of internalization of extrinsic motivation in english learning based on artificial intelligence assisted technology. In 2022 International Conference on Information System, Computing and Educational Technology (ICISCET). IEEE, pp 12–14
Lin CJ, Mubarok H (2021) Learning analytics for investigating the mind map-guided AI chatbot approach in an EFL flipped speaking classroom. Educ Technol Soc 24(4):16–35
Mageira K, Pittou D, Papasalouros A, Kotis K, Zangogianni P, Daradoumis A (2022) Educational AI chatbots for content and language integrated learning. Appl Sci 12(7):3239
Article CAS Google Scholar
Mendoza S, Sánchez-Adame LM, Urquiza-Yllescas JF, González-Beltrán BA, Decouchant D (2022) A model to develop chatbots for assisting the teaching and learning process. Sensors 22(15):5532
Article ADS PubMed PubMed Central Google Scholar
Ministry of Education (2022) English education curriculum. Ministry of Education, Sejong
Mondal A, Dey M, Das D, Nagpal S, Garda K (2018) Chatbot: An automated conversation system for the educational domain. In: International joint symposium on artificial intelligence and natural language processing (iSAI-NLP). IEEE, pp 1–5
Muhammad AF, Susanto D, Alimudin A, Adila F, Assidiqi MH, Nabhan S (2020) Developing English conversation chatbot using dialogflow. In: International electronics symposium (IES). IEEE, pp 468–475
Nunan D (1999) Second language teaching & learning. Heinle & Heinle Publishers, 7625 Empire Dr., Florence, KY, p 41042–2978
OECD (2019) Education at a Glance
Penny UR (1996) A course in language teaching: practice and theory. Cambridge University Press, pp 120–133
Pérez JQ, Daradoumis T, Puig JMM (2020) Rediscovering the use of chatbots in education: a systematic literature review. Comput Appl Eng Educ 28(6):1549–1565
Prabhu NS (1987) Second language pedagogy (Vol. 20). Oxford university press, Oxford
Richards JC (2005) Communicative language teaching today. SEAMEO Regional Language Centre, Singapore
Richey RC, Klein JD (2014) Design and development research: Methods, strategies, and issues. Routledge
Rubio DM, Berg-Weger M, Tebb SS, Lee ES, Rauch S (2003) Objectifying content validity: conducting a content validity study in social work research. Soc Work Res 27(2):94–104
Shin D (2019) Feasibility and constraints in applying an ai chatbot to English education. Brain, Digital, Learn 9(2):29–40
Sung MC (2022) Development of prospective teachers’ adaptive expertise in AI Chatbots: comparative analyses of google dialogflow ES and CX. Multimed-Assist Lang Learn 25(2):132–151
Vazhayil A, Shetty R, Bhavani RR, Akshay N (2019) Focusing on teacher education to introduce AI in schools: Perspectives and illustrative findings. In 2019 IEEE tenth international conference on technology for education (T4E). IEEE, pp 71–77
Williamson B, Eynon R (2020) Historical threads, missing links, and future directions in AI in education. Learn, Media Technol 45(3):223–235
Woolf BP, Lane HC, Chaudhri VK, Kolodner JL (2013) AI grand challenges for education. AI Mag 34(4):66–84
Wu L (2022) Case Study on Application of Artificial Intelligence to Oral English Teaching in Vocational Colleges. In: International Conference on Computation, Big-Data and Engineering (ICCBE). IEEE, pp 71–74
Xia Q, Chiu TK, Chai CS, Xie K (2023) The mediating effects of needs satisfaction on the relationships between prior knowledge and self-regulated learning through artificial intelligence chatbot. Br J Educ Technol 54(4):967–986
Yang J (2022) Perceptions of preservice teachers on AI chatbots in English education. International Journal of Internet. Broadcast Commun 14(1):44–52
MathSciNet Google Scholar
Yin Q, Satar M (2020) English as a foreign language learner interactions with chatbots: Negotiation for meaning. Int Online J Educ Teach 7(2):390–410
Yoo YJ (2021) Design based research on learning environment using artificial intelligence chatbot: Focus on English speaking class in elementary school. Unpublished master’s thesis]. Korea National University of Education
Yoon YB, Park MA (2020) Artificial intelligence and primary English education: with special reference to chatbots. J Korea Elem Educ 31:77–90
Yu M (2022) Research on construction of college english education mode based on artificial intelligence. In: International Conference on Computation, Big-Data and Engineering (ICCBE). IEEE, pp 195–198
Download references
Acknowledgements
This research is based on the first author’s doctoral dissertation.
Author information
Authors and affiliations.
Nammyeong Elementary School, Namhae, Republic of Korea
Department of Education, Gyeongsang National University, Jinju, Republic of Korea
Dongyub Lee
You can also search for this author in PubMed Google Scholar
Contributions
JH contributed to the research design, data acquisition, data analysis, and writing of the original draft of this paper. DL contributed to the conceptualization, supervision, writing up, and editing of the original draft of this paper.
Corresponding author
Correspondence to Dongyub Lee .
Ethics declarations
Competing interests.
The authors declare no competing interests.
Ethical approval
This article does not contain any studies with human participants performed by any of the authors. Therefore, this article does not require ethical approval.
Informed consent
There are no human participants in this article and informed consent is not applicable.
Additional information
Publisher’s note Springer Nature remains neutral with regard to jurisdictional claims in published maps and institutional affiliations.
Rights and permissions
Open Access This article is licensed under a Creative Commons Attribution 4.0 International License, which permits use, sharing, adaptation, distribution and reproduction in any medium or format, as long as you give appropriate credit to the original author(s) and the source, provide a link to the Creative Commons license, and indicate if changes were made. The images or other third party material in this article are included in the article’s Creative Commons license, unless indicated otherwise in a credit line to the material. If material is not included in the article’s Creative Commons license and your intended use is not permitted by statutory regulation or exceeds the permitted use, you will need to obtain permission directly from the copyright holder. To view a copy of this license, visit http://creativecommons.org/licenses/by/4.0/ .
Reprints and permissions
About this article
Cite this article.
Han, J., Lee, D. Research on the development of principles for designing elementary English speaking lessons using artificial intelligence chatbots. Humanit Soc Sci Commun 11 , 212 (2024). https://doi.org/10.1057/s41599-024-02646-w
Download citation
Received : 28 July 2023
Accepted : 08 January 2024
Published : 05 February 2024
DOI : https://doi.org/10.1057/s41599-024-02646-w
Share this article
Anyone you share the following link with will be able to read this content:
Sorry, a shareable link is not currently available for this article.
Provided by the Springer Nature SharedIt content-sharing initiative
Quick links
- Explore articles by subject
- Guide to authors
- Editorial policies

Information
- Author Services
Initiatives
You are accessing a machine-readable page. In order to be human-readable, please install an RSS reader.
All articles published by MDPI are made immediately available worldwide under an open access license. No special permission is required to reuse all or part of the article published by MDPI, including figures and tables. For articles published under an open access Creative Common CC BY license, any part of the article may be reused without permission provided that the original article is clearly cited. For more information, please refer to https://www.mdpi.com/openaccess .
Feature papers represent the most advanced research with significant potential for high impact in the field. A Feature Paper should be a substantial original Article that involves several techniques or approaches, provides an outlook for future research directions and describes possible research applications.
Feature papers are submitted upon individual invitation or recommendation by the scientific editors and must receive positive feedback from the reviewers.
Editor’s Choice articles are based on recommendations by the scientific editors of MDPI journals from around the world. Editors select a small number of articles recently published in the journal that they believe will be particularly interesting to readers, or important in the respective research area. The aim is to provide a snapshot of some of the most exciting work published in the various research areas of the journal.
Original Submission Date Received: .
- Active Journals
- Find a Journal
- Proceedings Series
- For Authors
- For Reviewers
- For Editors
- For Librarians
- For Publishers
- For Societies
- For Conference Organizers
- Open Access Policy
- Institutional Open Access Program
- Special Issues Guidelines
- Editorial Process
- Research and Publication Ethics
- Article Processing Charges
- Testimonials
- Preprints.org
- SciProfiles
- Encyclopedia
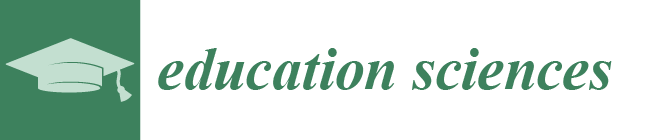
Article Menu
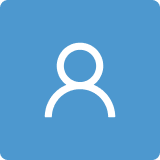
- Subscribe SciFeed
- Recommended Articles
- Author Biographies
- Google Scholar
- on Google Scholar
- Table of Contents
Find support for a specific problem in the support section of our website.
Please let us know what you think of our products and services.
Visit our dedicated information section to learn more about MDPI.
JSmol Viewer
Designing and situating text to promote textual dexterity in the context of project-based science instruction.
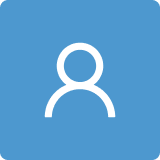
1. Designing and Situating Text to Promote Textual Dexterity in the Context of Project-Based Science Instruction
2. why text in science instruction, 3. theories in support of the integration of text and science, 4. empirical support for advancing the integration of literacy and science instruction, 5. text and national standards, 6. project-based learning and project-based science instruction, 7. what roles have texts played in investigation- and project-based science teaching, 8. how should texts be designed to optimize their use.
Think about all that you have learned about how organisms survive in their environment. Look closely at the pictures of the koala and its habitat. What do you think could explain how the koala survives? Why would it be the only species to survive from that time period? What happened to the rest of the species?
You probably hear the word “energy” used a lot. Maybe an adult has asked you, “Where do you get all that energy?”. You may have eaten something called “an energy bar”. You may hear an advertisement for “energy-saving light bulbs”. Now that you are studying erosion, you have been learning about the energy that moving water can transfer to the material with which it collides. How can this word, “energy” be used to describe so many different things? What do scientists mean when they use the word, energy? In this text, we will learn about what scientists mean when they use the word energy, and we will learn about two kinds of energy.
First, there is the question: Are birds really navigating? That is, are they figuring out how to get from one place to another, or are they just following some instinct that tells them where to go? Think about that question for a few minutes. If you wanted to learn the answer to this question: Are birds really navigating? What would you do? [Wouldn’t it be cool if we could just interview the birds?]
9. The Design of Tasks to Support the Productive Use of Texts in Science
- What are some of the questions that scientists have about snowy owls?
- Why do scientists think that owls might stop at places like airports that are wide open spaces?
- How did this “owl sleuth” conduct his research?
To check your understanding of how humans gather and interpret sound energy, turn to your partner and, using the diagram above, trace the path of sound energy to and through the ear to the brain.
10. The Role of the Teacher in Supporting Student Sensemaking with Text
- The koala’s traits and how those traits support its survival;
- The unique habitat of the koala (the eucalyptus tree) and the key role the eucalyptus tree has played in the survival story;
- How scientists have used what evidence there is from 45,000 years ago to think through what might have happened to the other 23 large species that lived at the same time as the koala and yet did not survive the major changes to their habitat;
- Current threats to the survival of the koala.
11. Episode 1: “We’re Not Just Dropping All of What We’ve Learned”
12. episode 2: but, why, 13. episode 3: “think about what you have learned…”, 14. episode 4: a missed opportunity, 15. discussion, author contributions, institutional review board statement, informed consent statement, data availability statement, acknowledgments, conflicts of interest.
- Aukerman, M.; Chambers Schuldt, L. What matters most? Toward a robust and socially just science of reading. Read. Res. Q. 2021 , 56 , S85–S103. [ Google Scholar ] [ CrossRef ]
- Norris, S.P.; Phillips, L.M. How literacy in its fundamental sense is central to scientific literacy. Sci. Educ. 2003 , 87 , 224–240. [ Google Scholar ] [ CrossRef ]
- Moje, E.B. Doing and teaching disciplinary literacy with adolescent learners: A social and cultural enterprise. Harv. Educ. Rev. 2015 , 85 , 254–278. [ Google Scholar ] [ CrossRef ]
- Lemke, J.L. Textual Politics: Discourse and Social Dynamics ; Taylor & Francis: London, UK, 2005. [ Google Scholar ]
- Brown, A.L.; Campione, J.C. Communities of learning and thinking, or a context by any other name. In Contemporary Issues in Teaching and Learning ; Karger: Basel, Switzerland, 2002; pp. 120–126. [ Google Scholar ]
- Polman, J.L.; Gebre, E.H. Towards critical appraisal of infographics as scientific inscriptions. J. Res. Sci. Teach. 2015 , 52 , 868–893. [ Google Scholar ] [ CrossRef ]
- Metz, K.E.; Cardace, A.; Berson, E.; Ly, U.; Wong, N.; Disk-Hilton, S.; Metz, S.E.; Wilson, M. Primary grade children’s capacity to understand microevolution: The power of leveraging their fruitful intuitions and engagement in scientific practices. J. Learn. Sci. 2019 , 28 , 556–615. [ Google Scholar ] [ CrossRef ]
- Fitzgerald, M.S.; Palincsar, A.S. Teaching practices that support student sensemaking across grades and disciplines: A conceptual review. Review of Research in Education. 2019 , 42 , 227–248. [ Google Scholar ] [ CrossRef ]
- Kintsch, W. An overview of top-down and bottom-up effects in comprehension: The CI perspective. Discourse Process. 1998 , 39 , 125–128. [ Google Scholar ]
- Duke, N.K.; Pearson, P.D.; Strachan, S.L.; Billman, A.K. Essential elements of fostering and teaching reading comprehension. In What Reseaarch Has to Say about Reading Instruction , 4th ed.; Samuels, S.J., Farstrup, A.E., Eds.; International Reading Association: Newark, DE, USA, 2011; pp. 51–93. [ Google Scholar ]
- Freebody, P.; Luke, A. Literacies programs: Debates and demands in cultural context. Prospect. Aust. J. TESOL 1990 , 5 , 7–16. [ Google Scholar ]
- Wertsch, J.B. Voices of the Mind: A Sociocultural Approach to Mediated Action ; Harvard University Press: Cambridge, UK, 1991. [ Google Scholar ]
- Cartwright, K.B.; Duke, N.K. The DRIVE model of reading: Making the complexity of reading accessible. Read. Teach. 2019 , 73 , 7–15. [ Google Scholar ] [ CrossRef ]
- Cooper, G.; Thomas, D.P.; Prain, V.; Fraser, S. Associations between Australian students’ literacy achievement in early secondary school and senior secondary participation in science: Accessing cultural and science capital. Int. J. Sci. Educ. 2022 , 44 , 1549–1564. [ Google Scholar ] [ CrossRef ]
- Zhu, Y. Reading matters more than mathematics in science learning: An analysis of the relationship between student achievement in reading, mathematics, and science. Int. J. Sci. Educ. 2022 , 44 , 1–17. [ Google Scholar ] [ CrossRef ]
- Blank, R.K. What Is the Impact of Decline in Science Instructional Time in Elementary School? Noyce Foundation: Palo Alto, CA, USA, 2012. [ Google Scholar ]
- Guthrie, J.T.; McRae, A.; Coddington, C.S.; Klauda, S.L.; Wigfield, A.; Barbosa, P. Impacts of comprehensive reading instruction on diverse outcomes of low- and high-achieving readers. J. Learn. Disabil. 2009 , 4 , 195–214. [ Google Scholar ] [ CrossRef ] [ PubMed ]
- Purcell-Gates, V.; Duke, N.K.; Martineau, J.A. Learning to read and write genre-specific text: Roles of authentic experience and explicit teaching. Read. Res. Q. 2007 , 42 , 8–45. [ Google Scholar ] [ CrossRef ]
- Hynes, M.; Swenson, J. The humanistic side of engineering: Considering social science and humanities dimensions of engineering in education and research. J. Pre-Coll. Eng. Educ. Res. 2013 , 3 , 31–42. [ Google Scholar ] [ CrossRef ]
- Kähler, J.; Hahn, I.; Köller, O. The development of early scientific literacy gaps in kindergarten children. Int. J. Sci. Educ. 2020 , 42 , 1988–2007. [ Google Scholar ] [ CrossRef ]
- Lennox, R.; Hepburn, K.; Leaman, E.; van Houten, N. ‘I’m probably just gonna skim’: An assessment of undergraduate students’ primary scientific literature reading approaches. Int. J. Sci. Educ. 2020 , 42 , 1409–1429. [ Google Scholar ] [ CrossRef ]
- Kim, J.S.; Gilbert, J.B.; Relyea, J.E.; Rich, P.; Scherer, E.; Burkhauser, M.A.; Tvedt, J.N. Time to transfer: Long-term effects of a sustained and spiraled content literacy intervention in the elementary grades. Dev. Psychol. 2024 , 60 , 1279–1297. [ Google Scholar ] [ CrossRef ]
- Connor, C.M.; Dombek, J.; Crowe, E.C.; Spencer, M.; Tighe, E.L.; Coffinger, S.; Zargar, E.; Wood, T.; Petscher, Y. Acquiring science and social studies knowledge in kindergarten through fourth grade: Conceptualization, design, implementation, and efficacy testing of content-area literacy instruction (CALI). J. Educ. Psychol. 2017 , 109 , 301–320. [ Google Scholar ] [ CrossRef ]
- Cervetti, G.N.; Barber, J.; Dorph, R.; Pearson, P.D.; Goldschmidt, P.G. The impact of an integrated approach to science and literacy in elementary school classrooms. J. Res. Sci. Teach. 2012 , 49 , 631–658. [ Google Scholar ] [ CrossRef ]
- Fitzgerald, M.S. Facilitating the interplay of text and experience in scientific inquiry. Language Arts. 2023 , 100 , 282–294. [ Google Scholar ] [ CrossRef ]
- Romance, N.R.; Vitale, M.R. A curriculum strategy that expands time for in-depth elementary science instruction by using science-based reading strategies: Effects of a year-long study in grade four. J. Res. Sci. Teach. 1992 , 29 , 545–554. [ Google Scholar ] [ CrossRef ]
- Wright, T.S.; Gotwals, A.W. Supporting kindergartners’ science talk in the context of an integrated science and disciplinary literacy curriculum. Elem. Sch. J. 2017 , 117 , 513–537. [ Google Scholar ] [ CrossRef ]
- National Governors Association Center for Best Practices, Council of Chief State School Officers. Common Core State Standards for English Language Arts. 2010. Available online: http://www.corestandards.org/ELA-Literacy (accessed on 29 August 2024).
- NGSS Lead State Partners. Next Generation Science Standards: For States, by States ; The National Academies Press: Washington, DC, USA, 2013. [ Google Scholar ]
- Fang, Z. The language demands of science reading in middle school. Int. J. Sci. Educ. 2006 , 28 , 491–520. [ Google Scholar ] [ CrossRef ]
- O’Hallaron, C.L.; Palincsar, A.S.; Schleppegrell, M.J. Reading science: Using systemic functional linguistics to support critical language awareness. Linguist. Educ. 2015 , 32 , 55–67. [ Google Scholar ] [ CrossRef ]
- Zembal Saul, C. Learning to teach elementary school science as argument. Sci. Educ. 2009 , 93 , 687–719. [ Google Scholar ] [ CrossRef ]
- Baines, A.; DeBarger, A.; DeVivo, K.; Warner, N.; Santos, S.; Brinkman, J.; Udall, D.; Zuckerbrod, N.; Felsen, K.; Urban, R. Key Principles for Project-Based Learning ; Lucas Education Research: San Rafael, CA, USA, 2021. [ Google Scholar ]
- Chen, C.H.; Yang, Y.C. Revisiting the effects of project-based learning on students’ academic achievement: A meta-analysis investigating moderators. Educ. Res. Rev. 2019 , 26 , 71–81. [ Google Scholar ] [ CrossRef ]
- Miller, E.; Krajcik, J. Promoting deep learning through project-based learning: A design problem. Discip. Interdiscip. Sci. Educ. Res. 2019 , 1 , 1–10. [ Google Scholar ] [ CrossRef ]
- Anholt. Stone Girl, Bone Girl: The Story of Mary Anning ; Frances Lincoln Children’s Books: London, UK, 2006. [ Google Scholar ]
- Cervetti, G.N.; Barber, J. Text in hands-on science. In Finding the Right Texts: What Works for Beginning and Struggling Readers ; Hiebert, E.H., Sailors, M., Eds.; Guilford: New York, NY, USA, 2008; pp. 89–108. [ Google Scholar ]
- Butterworth, C.; Gaggioti, L. Lunchbox: The Story of Your Food ; Candlewick Press: Somerville, MA, USA, 2013. [ Google Scholar ]
- Aston, D.H.; Long, S. A Seed Is Sleepy ; Chronicle Books: San Francisco, CA, USA, 2007. [ Google Scholar ]
- Peterson, R. Field Guide to Birds of North America ; Houghton Mifflin: Boston, MA, USA, 2012. [ Google Scholar ]
- Hegarty, M. Dynamic visualizations and learning: Getting to the difficult questions. Learn. Instr. 2004 , 14 , 343–351. [ Google Scholar ] [ CrossRef ]
- Chambliss, M.J.; Torney-Purta, J.; Richardson, W.K. The effects of reading well-written passages on students’ civic understanding and engagement. Citizsh. Teach. Learn. 2015 , 11 , 49–67. [ Google Scholar ] [ CrossRef ]
- Manderino, M. Disciplinary literacy in new literacies environments: Expanding the intersections of literate practice for adolescents. In 61st Yearbook of the Literacy Research Association ; Dunston, P.J., Fullerton, S.K., Bates, C.C., Headley, K., Stecker, P.M., Eds.; Literacy Research Association: Oak Creek, WI, USA, 2012; pp. 119–133. [ Google Scholar ]
- Valencia, S.W.; Wixson, K.K.; Pearson, P.D. Putting text complexity in context: Refocusing on comprehension of complex text. Elem. Sch. J. 2014 , 115 , 270–289. [ Google Scholar ] [ CrossRef ]
- Aronson, E. The Jigsaw Classroom ; Sage: Thousand Oaks, CA, USA, 1978. [ Google Scholar ]
- Cervetti, G.N.; DiPardo, A.L.; Staley, S.J. Entering the conversation: Exploratory talk in middle school science. Elem. Sch. J. 2014 , 114 , 547–572. [ Google Scholar ] [ CrossRef ]
- Hogan, K.; Nastasi, B.K.; Pressley, M. Discourse patterns and collaborative scientific reasoning in peer and teacher-guided discussions. Cogn. Instr. 1999 , 17 , 379–432. [ Google Scholar ] [ CrossRef ]
- Puntambeker, S.; Stylianou, A.; Goldstein, J. Comparing classroom enactments of an inquiry curriculum: Lessons learned from two teachers. J. Learn. Sci. 2007 , 16 , 81–130. [ Google Scholar ] [ CrossRef ]
- Thompson, J.; Hagenah, S.; Kang, H.; Stroupe, D.; Braaten, M.; Colley, C.; Windschitl, M. Rigor and responsiveness in classroom activity. Teach. Coll. Rec. 2016 , 118 , 1–58. [ Google Scholar ] [ CrossRef ]
- McNeill, K.L.; Pimentel, D.S. Scientific discourse in three urban classrooms: The role of the teacher in engaging high school students in argumentation. Sci. Educ. 2009 , 94 , 203–229. [ Google Scholar ] [ CrossRef ]
- Lampert, M. Learning teaching in, from, and for practice: What do we mean? J. Teach. Educ. 2010 , 61 , 21–34. [ Google Scholar ] [ CrossRef ]
- Fitzgerald, M.S. Overlapping opportunities for literacy learning and social-emotional learning in elementary-grade project-based instruction. Am. J. Educ. 2020 , 126 , 573–601. [ Google Scholar ] [ CrossRef ]
- National Academies of Sciences, Engineering, and Medicine. Science and Engineering in Preschool through Elementary Grades: The Brilliance of Children and the Strengths of Educators ; The National Academies Press: Washington, DC, USA, 2022. [ Google Scholar ] [ CrossRef ]
- National Academies of Sciences, Engineering, and Medicine. Equity in K-12 STEM Education: Framing Decisions for the Future ; The National Academies Press: Washington, DC, USA, 2024. [ Google Scholar ] [ CrossRef ]
- Davis, N.R.; Schaeffer, J. Troubling troubled waters in elementary science education: Politics, ethics and black children’s conceptions of water [justice] in the era of Flint. Cogn. Instr. 2019 , 37 , 367–389. [ Google Scholar ] [ CrossRef ]
- Smith, B.E.; Carlone, H.B.; Ziegler, H.; Janumyan, Y.; Conley, Z.; Chen, J.; Jen, T. Youths’ investigations of critical urban forestry through multimodal sensemaking. J. Sci. Educ. Technol. 2024 . [ Google Scholar ] [ CrossRef ]
Learning Set Questions | Sample Disciplinary Core Ideas | Samples Science Practices | Sample Crosscutting Concepts | Example Texts (Example Roles) |
---|---|---|---|---|
What do squirrels need to survive? | Evidence of common ancestry and diversity Adaptation | Developing and using models Obtain, evaluate, and communicate info | Cause and effect Systems and system models | Squirrel Survival Texts (Provide information to supplement first-hand evidence) |
How are the squirrel’s structures unique and important? | Natural selection Inheritance of traits Variation of traits | Planning and carrying out investigations Constructing explanations | Structure and function Patterns Cause and effect | For Squirrels, It’s Headfirst and Down! (Everyday experiences shown in new way) |
What other organisms live around here, and does the squirrel need them to survive? | Biodiversity and humans Ecosystem dynamics, functioning, and resilience | Asking questions and defining problems Engaging in argument from evidence | Systems and system models Structure and function | Organism Texts (Establish connections between investigations and crosscutting concepts) |
How do scientists use evidence to find out about prehistoric organisms? | Adaptation Weather and climate Evidence of common ancestry and diversity | Analyzing and interpreting data Constructing explanations | Scale, proportion, and quantity Stability and change Structure and function | Animals from Today and Long Ago (Aspects of natural world unlikely to be familiar) |
How do we use fossils to figure out how organisms changed over time? | Evidence of common ancestry and diversity Adaptation Biodiversity and humans | Planning and carrying out investigations Analyzing and interpreting data | Stability and change Systems and system models Structure and function | Stone Girl Bone Girl: The Story of Mary Anning [ ] (Connect investigations to work of scientists) |
Why did some animals die out and some live? | Adaptation Biodiversity and humans Evidence of common ancestry and diversity | Developing and using models Obtaining, evaluating, and communicating info. | Systems and system models Cause and effect | The Koala: A Success Story! (Illustrating scientific practices) |
Example Text | Example Role | Placement | Task |
---|---|---|---|
Squirrel Survival Texts (e.g., Escaping Predators, Finding Food, Raising Young, Surviving Winter) | Provide information to supplement first-hand evidence | Students read these texts after using photographs and videos to make observations and draw initial models to describe how squirrels survive | After reading, students revisit and revise models describing how squirrels survive in their habitats, based on new information from the texts. |
For Squirrels, It’s Headfirst and Down! | Show everyday experiences in a new way | Students read this text after making video observations and conducting investigations of how squirrels eat and jump compared to humans | After reading, students revisit and revise models describing how squirrels survive in their habitats, based on new information from the text. |
Organism Structure Function Texts (e.g., ants, cottontail rabbits, coyotes, earthworks, white pine trees, red-tailed hawks, garden spiders) | Establish connections between investigations and crosscutting concepts | Students read this text after activating their prior knowledge related to observing organisms around their community and brainstorming how organisms interact with one another within a system | After reading one or more of the texts, students collaborate to construct a class model that describes interactions among the organisms, including squirrels. |
Animals from Today and Long Ago | Illustrate aspects of the natural world unlikely to be familiar to students | Students read this text after examining and comparing diagrams and skeletons of the Juramaia (a prehistoric mammal) and squirrels and making claims about how the organism’s structures helped them survive in their habitats | After reading this text, students revisit and make additions to a class model that describes interactions of organisms that lived in a prehistoric environment. |
The Koala: A Success Story! | Illustrate scientific practices | Students read this text at the end of the unit as an opportunity to apply what they learned about organisms’ survival in different habitats | After reading, students compare the koala to squirrels and the stegosaurus, and make claims about why some organisms survive changes in their environment and others die out. |
The statements, opinions and data contained in all publications are solely those of the individual author(s) and contributor(s) and not of MDPI and/or the editor(s). MDPI and/or the editor(s) disclaim responsibility for any injury to people or property resulting from any ideas, methods, instructions or products referred to in the content. |
Share and Cite
Fitzgerald, M.S.; Palincsar, A.S. Designing and Situating Text to Promote Textual Dexterity in the Context of Project-Based Science Instruction. Educ. Sci. 2024 , 14 , 960. https://doi.org/10.3390/educsci14090960
Fitzgerald MS, Palincsar AS. Designing and Situating Text to Promote Textual Dexterity in the Context of Project-Based Science Instruction. Education Sciences . 2024; 14(9):960. https://doi.org/10.3390/educsci14090960
Fitzgerald, Miranda S., and Annemarie Sullivan Palincsar. 2024. "Designing and Situating Text to Promote Textual Dexterity in the Context of Project-Based Science Instruction" Education Sciences 14, no. 9: 960. https://doi.org/10.3390/educsci14090960
Article Metrics
Further information, mdpi initiatives, follow mdpi.
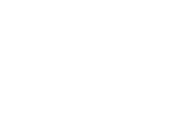
Subscribe to receive issue release notifications and newsletters from MDPI journals
Notice: You are viewing an unstyled version of this page. Are you using a very old browser? If so, please consider upgrading
Skip to content Skip to navigation Skip to footer
Saving Lives Improving Mothers' Care 2022 - Lessons learned to inform maternity care from the UK and Ireland Confidential Enquiries into Maternal Deaths and Morbidity 2018-20
Menu | In this section
This page contains the compiled materials for the 2022 MBRRACE-UK Saving Lives, Improving Mothers' Care annual report published on the 10th November 2022.
The full compiled 2022 report including all supplementary material is available below. Alongside the full report are a shorter 'Core Report' containing limited surveillance and confidential enquiry results, a lay summary and infographic.
Full compiled report

ERRATUM: Typographical error in Table 2.3 corrected in updated version above.
Core Report

Other report materials

arXiv's Accessibility Forum starts next month!
Help | Advanced Search
Computer Science > Networking and Internet Architecture
Title: residual-based adaptive huber loss (rahl) -- design of an improved huber loss for cqi prediction in 5g networks.
Abstract: The Channel Quality Indicator (CQI) plays a pivotal role in 5G networks, optimizing infrastructure dynamically to ensure high Quality of Service (QoS). Recent research has focused on improving CQI estimation in 5G networks using machine learning. In this field, the selection of the proper loss function is critical for training an accurate model. Two commonly used loss functions are Mean Squared Error (MSE) and Mean Absolute Error (MAE). Roughly speaking, MSE put more weight on outliers, MAE on the majority. Here, we argue that the Huber loss function is more suitable for CQI prediction, since it combines the benefits of both MSE and MAE. To achieve this, the Huber loss transitions smoothly between MSE and MAE, controlled by a user-defined hyperparameter called delta. However, finding the right balance between sensitivity to small errors (MAE) and robustness to outliers (MSE) by manually choosing the optimal delta is challenging. To address this issue, we propose a novel loss function, named Residual-based Adaptive Huber Loss (RAHL). In RAHL, a learnable residual is added to the delta, enabling the model to adapt based on the distribution of errors in the data. Our approach effectively balances model robustness against outliers while preserving inlier data precision. The widely recognized Long Short-Term Memory (LSTM) model is employed in conjunction with RAHL, showcasing significantly improved results compared to the aforementioned loss functions. The obtained results affirm the superiority of RAHL, offering a promising avenue for enhanced CQI prediction in 5G networks.
Comments: | |
Subjects: | Networking and Internet Architecture (cs.NI); Artificial Intelligence (cs.AI) |
Cite as: | [cs.NI] |
(or [cs.NI] for this version) | |
Focus to learn more arXiv-issued DOI via DataCite (pending registration) |
Submission history
Access paper:.
- HTML (experimental)
- Other Formats

References & Citations
- Google Scholar
- Semantic Scholar
BibTeX formatted citation

Bibliographic and Citation Tools
Code, data and media associated with this article, recommenders and search tools.
- Institution
arXivLabs: experimental projects with community collaborators
arXivLabs is a framework that allows collaborators to develop and share new arXiv features directly on our website.
Both individuals and organizations that work with arXivLabs have embraced and accepted our values of openness, community, excellence, and user data privacy. arXiv is committed to these values and only works with partners that adhere to them.
Have an idea for a project that will add value for arXiv's community? Learn more about arXivLabs .
Integrating AI chatbots into the metaverse: Pre-service English teachers’ design works and perceptions
- Published: 27 August 2024
Cite this article
- Yohan Hwang ORCID: orcid.org/0000-0003-3688-4779 1 ,
- Seongyong Lee ORCID: orcid.org/0000-0002-9436-4272 2 &
- Jaeho Jeon ORCID: orcid.org/0000-0002-1161-3676 3
64 Accesses
1 Altmetric
Explore all metrics
Alongside technological advances, the educational potential of artificial intelligence (AI) chatbots and the metaverse has generated significant interest in the field of computer-assisted language learning (CALL). However, despite this heightened interest, there have been no studies that have delved into the effective integration of these two technologies into educational contexts. In response to this concern, this research examined a teacher training course where pre-service teachers designed and used their customized chatbots within the context of the metaverse space. Fifty-five pre-service English teachers were assigned to the chatbot-only group (COG) ( n = 31) and the chatbot-metaverse group (CMG) ( n = 24). We first explored the CMG’s chatbot design works and teaching demonstrations in metaverse spaces and compared them to those of the COG, who developed and utilized chatbots in a physical classroom setting. We further compared their perceptions related to experiences with chatbot-based lesson designing and teaching demonstrations, using a survey and reflection papers. The comparison of design works and teaching demonstrations revealed that while both groups recognized the value and effectiveness of AI chatbots in the language classroom, the participants in the CMG tended to develop more authentic, immersive, and interactive learning tasks, with the metaverse space playing a crucial role as a context. Analysis of a survey and reflection papers indicated that the CMG reported more positive perceptions than the COG. We discussed how the metaverse space might have influenced the way teachers developed and integrated chatbots into their educational contexts. Pedagogical and theoretical implications regarding the combined use of AI chatbot and metaverse technologies were also provided.
This is a preview of subscription content, log in via an institution to check access.
Access this article
Subscribe and save.
- Get 10 units per month
- Download Article/Chapter or eBook
- 1 Unit = 1 Article or 1 Chapter
- Cancel anytime
Price includes VAT (Russian Federation)
Instant access to the full article PDF.
Rent this article via DeepDyve
Institutional subscriptions
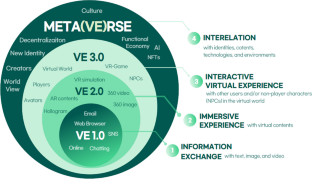
Similar content being viewed by others
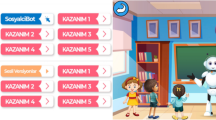
The effect of AI-powered chatbots in social studies education
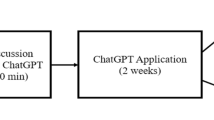
Large language models in education: A focus on the complementary relationship between human teachers and ChatGPT
Envisioned Pedagogical Uses of Chatbots in Higher Education and Perceived Benefits and Challenges
Explore related subjects.
- Artificial Intelligence
- Digital Education and Educational Technology
Data availability
The datasets generated during and/or analyzed during the current study are available from the corresponding author upon reasonable request.
Al-Furaih, S. A. A. (2017). Perceptions of pre-service teachers on the design of a learning environment based on the seven principles of good practice. Education and Information Technologies, 22 (6), 3187–3205. https://doi.org/10.1007/s10639-017-9580-7
Article Google Scholar
Barrette, C. M. (2015). Usefulness of technology adoption research in introducing an online workbook. System, 49 , 133–144. https://doi.org/10.1016/j.system.2015.01.005
Brown, H. D., & Lee, H. (2015). Teaching by principles: An interactive approach to language pedagogy . Pearson.
Google Scholar
Campbell, L. O., Heller, S., & Pulse, L. (2022). Student-created video: An active learning approach in online environments. Interactive Learning Environments, 30 (6), 1145–1154. https://doi.org/10.1080/10494820.2020.1711777
Chen, Z. (2022). Exploring the application scenarios and issues facing Metaverse technology in education. Interactive Learning Environments . https://doi.org/10.1080/10494820.2022.2133148
Cheng, J., & Chen, C. (2016). The crossroads of English language learners, task-based instruction, and 3D multi-user virtual learning in Second Life. Computers & Education, 102 , 152–171.
Choudhury, N. (2014). World wide web and its journey from Web 1.0 to Web 4.0. International Journal of Computer Science and Information Technologies, 5 (6), 8096–8100.
Crosthwaite, P., & Luciana, & Wijaya, D. (2023). Exploring language teachers’ lesson planning for corpus-based language teaching: A focus on developing TPACK for corpora and DDL. Computer Assisted Language Learning, 37 (7), 1392–1420. https://doi.org/10.1080/09588221.2021.1995001
Dale, E. (1969). Audio-visual methods in teaching . Dryden Press.
Davis, F. D. (1989). Perceived usefulness, perceived ease of use, and user acceptance of information technology. MIS Quarterly, 13 (3), 319–340.
Deutschmann, M., & Panichi, L. (2009). Instructional design, learner modeling, and teacher practice in Second Life. In J. Molka-Danielsen & M. Deutschmann (Eds.), Learning and teaching in the virtual world of Second Life (pp. 27–44). Tapir Academic Press.
Dörnyei, Z. (2007). Research methods in applied linguistics . Oxford University Press.
Gurer, M. D. (2021). Examining technology acceptance of pre-service mathematics teachers in Turkey: A structural equation modeling approach. Education and Information Technologies, 26 (4), 4709–4729. https://doi.org/10.1007/s10639-021-10493-4
Hae, J., Suk, T., Hauck, T., & Laine, H. (2023). Influence of avatar facial appearance on users’ perceived embodiment and presence in immersive virtual reality. Electronics . https://doi.org/10.3390/electronics12030583
Hew, K. F., Huang, W., Du, J., & Jia, C. (2023). Using chatbots to support student goal setting and social presence in fully online activities: Learner engagement and perceptions. Journal of Computing in Higher Education, 35 (1), 40–68. https://doi.org/10.1007/s12528-022-09338-x
Huang, Y. C., Backman, S. J., Backman, K. F., McGuire, F. A., & Moore, D. W. (2019). An investigation of motivation and experience in virtual learning environments: A self-determination theory. Education and Information Technologies, 24 (1), 591–611. https://doi.org/10.1007/s10639-018-9784-5
Huang, W., Hew, K. F., & Fryer, L. K. (2022). Chatbots for language learning—Are they really useful? A systematic review of chatbot-supported language learning. Journal of Computer Assisted Learning, 38 (1), 237–257. https://doi.org/10.1111/jcal.12610
Hwang, Y. (2023). When makers meet the metaverse: Effects of creating NFT metaverse exhibition in maker education. Computers & Education, 194 , 104693. https://doi.org/10.1016/j.compedu.2022.104693
Hwang, G. J., & Chein, S. Y. (2022). Definition, roles, and potential research issues of the metaverse in education: An artificial intelligence perspective. Computers and Education: Artificial Intelligence, 3 , 100082. https://doi.org/10.1016/j.caeai.2022.100082
Hwang, G. J., Tu, Y. F., & Chu, H. C. (2023a). Conceptions of the metaverse in higher education: A draw-a-picture analysis and surveys to investigate the perceptions of students with different motivation levels. Computers & Education, 203 , 104868. https://doi.org/10.1016/j.compedu.2023.104868
Hwang, Y., Shin, D., & Lee, H. (2023b). Students’ perception on immersive learning through 2D and 3D metaverse platforms. Educational Technology Research and Development, 71 , 1687–1708. https://doi.org/10.1007/s11423-023-10238-9
Jennett, C., Cox, A. L., Cairns, P., Dhoparee, S., Epps, A., Tijs, T., & Walton, A. (2008). Measuring and defining the experience of immersion in games. International Journal of Human-Computer Studies, 66 (9), 641–661. https://doi.org/10.1016/j.ijhcs.2008.04.004
Jeon, J. (2022). Exploring AI chatbot affordances in the EFL classroom: Young learners’ experiences and perspectives. Computer Assisted Language Learning , 1–26. https://doi.org/10.1080/09588221.2021.2021241
Jeon, J., Lee, S., & Choe, H. (2022). Enhancing EFL pre-service teachers’ affordance noticing and utilizing with the Synthesis of Qualitative Evidence strategies: An exploratory study of a customizable virtual environment platform. Computers & Education, 190 , 104620. https://doi.org/10.1016/j.compedu.2022.104620
Jeong, K. O. (2017). Preparing EFL student teachers with new technologies in the Korean context. Computer Assisted Language Learning, 30 (6), 488–509. https://doi.org/10.1080/09588221.2017.1321554
Ji, H., Han, I., & Ko, Y. (2023). A systematic review of conversational AI in language education: Focusing on the collaboration with human teachers. Journal of Research on Technology in Education, 55 (1), 48–63. https://doi.org/10.1080/15391523.2022.2142873
Kasneci, E., Sessler, K., Küchemann, S., Bannert, M., Dementieva, D., Fischer, F., Gasser, U., Groh, G., Günnemann, S., Hüllermeier, E., Krusche, S., Kutyniok, G., Michaeli, T., Nerdel, C., Pfeffer, J., Poquet, O., Sailer, M., Schmidt, A., Seidel, T., & Kasneci, G. (2023). ChatGPT for good? On opportunities and challenges of large language models for education. Learning and Individual Differences, 103 , 102274. https://doi.org/10.1016/j.lindif.2023.102274
Kim, H., & Lee, J. H. (2022). SMART Teacher Lab: A learning platform for the professional development of EFL teachers. Language Learning, 26 (2), 25–37.
Kim, H., Yang, H., & Lee, J. H. (2022). Design principles and architecture of a second language learning chatbot. Language Learning & Technology, 26 (1), 1–18.
Kuznetcova, I., Glassman, M., & Lin, T.-J. (2019). Multi-user virtual environments as a pathway to distributed social networks in the classroom. Computers & Education, 130 , 26–39. https://doi.org/10.1016/j.compedu.2018.11.004
Lan, Y. J., Hsiao, I. Y. T., & Shih, M. F. (2018). Effective learning design of game-based 3D virtual language learning environments for special education students. Educational Technology and Society, 21 (3), 213–227.
Lave, J., & Wenger, E. (1991). Situated learning: Legitimate peripheral participation . Cambridge University Press.
Book Google Scholar
Lee, H., & Hwang, Y. (2022). Technology-enhanced education through VR-making and metaverse-linking to foster teacher readiness and sustainable learning. Sustainability, 14 (8), 4786. https://doi.org/10.3390/su14084786
Lee, S., & Jeon, J. (2024). Visualizing a disembodied agent: Young EFL learners’ perceptions of voice-controlled conversational agents as language partners. Computer Assisted Language Learning, 37 (5–6), 1048–1073. https://doi.org/10.1080/09588221.2022.2067182
Lee, S. M., & Wu, J. G. (2023). Teaching with immersive virtual reality: Perceptions of Korean trainee teachers. International Journal of Computer-Assisted Language Learning and Teaching, 13 (1), 1–14. https://doi.org/10.4018/IJCALLT.334362
Lee, J. H., Yang, H., Shin, D., & Kim, H. (2020). Chatbots. ELT Journal, 74 (3), 338–344. https://doi.org/10.1093/elt/ccaa035
Lee, S. M., Yang, Z., & Wu, J. G. (2023). Live, play, and learn: Language learner engagement in the immersive VR environment. Education and Information Technologies . https://doi.org/10.1007/s10639-023-12215-4
Lee, S., Jeon, J., & Choe, H. (2024a). Enhancing pre-service teachers' global Englishes awareness with technology: A focus on AI chatbots in 3D metaverse environments. TESOL Quarterly . https://doi.org/10.1002/tesq.3300
Lee, J. H., Shin, D., & Hwang, Y. (2024b). Exploring the potential of large language model-based task-oriented dialogue chatbots from learner perspectives. SSRN . https://doi.org/10.2139/ssrn.4644306
Li, M., & Yu, Z. (2022). A systematic review on the metaverse-based blended English learning. Frontiers in Psychology, 13 , 1087508. https://doi.org/10.3389/fpsyg.2022.1087508
Long, M. H. (1985). Input and second language acquisition theory. In S. Gass & C. Madden (Eds.), Input in second language acquisition (pp. 377–393). Newbury House.
Lv, Z. (2023). Generative artificial intelligence in the metaverse era. Cognitive Robotics, 3 , 208–217. https://doi.org/10.1016/j.cogr.2023.06.001
Moore, G. C., & Benbasat, I. (2001). Development of an instrument to measure the perceptions of adopting an information technology innovation. Information Systems Research, 2 (3), 192–222.
Nami, F. (2022). Developing in-service teachers’ pedagogical knowledge of CALL through project-oriented tasks: The case of an online professional development course. ReCALL, 34 (1), 110–125. https://doi.org/10.1017/S0958344021000148
Nishino, T. (2012). Modeling teacher beliefs and practices in context: A multimethods approach. The Modern Language Journal, 96 (3), 380–399. https://doi.org/10.1111/j.1540-4781.2012.01364.x
Pica, T. (1996). Second language learning through interaction Multiple perspectives. Working Paper in Educational Linguistics, 12 (1), 1–22.
MathSciNet Google Scholar
Rama, P. S., Black, R. W., Van Es, E., & Warschauer, M. (2012). Affordances for second language learning in World of Warcraft. ReCALL, 24 (3), 322–338. https://doi.org/10.1017/S0958344012000171
Reeve, J., & Tseng, C.-M. (2011). Agency as a fourth aspect of students’ engagement during learning activities. Contemporary Educational Psychology, 36 (4), 257–267. https://doi.org/10.1016/j.cedpsych.2011.05.002
Rospigliosi, P. (2023). Artificial intelligence in teaching and learning: What questions should we ask of ChatGPT? Interactive Learning Environments, 31 (1), 1–3. https://doi.org/10.1080/10494820.2023.2180191
Serafini, F., & Reid, S. (2019). Multimodal content analysis: Expanding analytical approaches to content analysis. Visual Communication . https://doi.org/10.1177/1470357219864133
Sra, M., & Pattanaik, S. (2023). Enhancing the sense of presence in virtual reality. IEEE Computer Graphics and Applications . https://doi.org/10.1109/MCG.2023.3252182
Teo, T. (2015). Comparing pre-service and in-service teachers’ acceptance of technology: Assessment of measurement invariance and latent mean differences. Computers & Education, 83 , 22–31. https://doi.org/10.1016/j.compedu.2014.11.015
Tondeur, J., Van Braak, J., Siddiq, F., & Scherer, R. (2016). Time for a new approach to prepare future teachers for educational technology use: Its meaning and measurement. Computers & Education, 94 , 134–150. https://doi.org/10.1016/j.compedu.2015.11.009
Venkatesh, V., & Davis, F. D. (2000). A theoretical extension of the technology acceptance model: Four longitudinal field studies. Management Science, 46 , 186–204. https://doi.org/10.1287/mnsc.46.2.186.11926
Wang, C., Lan, Y. J., Tseng, W. T., Lin, Y. T. R., & Gupta, K. C. L. (2020). On the effects of 3D virtual worlds in language learning–a meta-analysis. Computer Assisted Language Learning, 33 (8), 891–915. https://doi.org/10.1080/09588221.2019.1598444
Wu, J. G., Zhang, D., & Lee, S. M. (2023). Into the brave new metaverse: Envisaging future language teaching and learning. IEEE Transactions on Learning Technologies, 17 , 1–11. https://doi.org/10.1109/TLT.2023.3259470
Yang, T. C., & Chen, J.-H. (2023). Pre-service teachers’ perceptions and intentions regarding the use of chatbots through statistical and lag sequential analysis. Computers and Education: Artificial Intelligence, 4 , 100119. https://doi.org/10.1016/j.caeai.2022.100119
Zhang, X., Chen, Y., Hu, L., & Wang, Y. (2022). The metaverse in education: Definition, framework, features, potential applications, challenges, and future research topics. Frontiers in Psychology, 13 , 1016300. https://doi.org/10.3389/fpsyg.2022.1016300
Download references
Author information
Authors and affiliations.
Department of English Language and Literature, Jeonbuk National University, 567 Baekje-daero, Deokjin-gu, Jeonju, Jeollabuk-Do, 54896, Republic of Korea
Yohan Hwang
School of Education and English, University of Nottingham Ningbo China, 199 Taikang East Road, Ningbo, Zhejiang, 315100, China
Seongyong Lee
Department of Curriculum and Instruction, Indiana University Bloomington, 201 N. Rose Avenue, Bloomington, IN, 47405-1006, USA
You can also search for this author in PubMed Google Scholar
Corresponding author
Correspondence to Seongyong Lee .
Ethics declarations
Conflict of interest.
No potential conflict of interest was reported by the authors.
Additional information
Publisher's note.
Springer Nature remains neutral with regard to jurisdictional claims in published maps and institutional affiliations.
Appendix 3. Transcription convention
Tr = teacher; S = student
((transcriber’s note))
? rising intonation
[ overlapped utterance
(3) pauses in seconds
Rights and permissions
Springer Nature or its licensor (e.g. a society or other partner) holds exclusive rights to this article under a publishing agreement with the author(s) or other rightsholder(s); author self-archiving of the accepted manuscript version of this article is solely governed by the terms of such publishing agreement and applicable law.
Reprints and permissions
About this article
Hwang, Y., Lee, S. & Jeon, J. Integrating AI chatbots into the metaverse: Pre-service English teachers’ design works and perceptions. Educ Inf Technol (2024). https://doi.org/10.1007/s10639-024-12924-4
Download citation
Received : 14 August 2023
Accepted : 25 July 2024
Published : 27 August 2024
DOI : https://doi.org/10.1007/s10639-024-12924-4
Share this article
Anyone you share the following link with will be able to read this content:
Sorry, a shareable link is not currently available for this article.
Provided by the Springer Nature SharedIt content-sharing initiative
- Pre-service teacher
- Artificial intelligence
- Find a journal
- Publish with us
- Track your research

IMAGES
VIDEO
COMMENTS
Conclusion. Design-based research methods are a thirty-year old tradition from the learning sciences that have been taken up in many domains as a way to study designed interventions that challenge the traditional relationship between research and design, as is the case with online learning.
Educational design-based research (DBR) can be characterized as research in which the design of educational materials (e.g., computer tools, learning activities, or a professional development program) is a crucial part of the research. That is, the design of learning environments is interwoven with the testing or developing of theory.
Design research is defined as "the systematic study of designing, developing and evaluating educational interventions (such as programs, teaching-learning strategies and materials, products and systems) as solutions for complex problems in educational practice, which also aims at advancing our knowledge about the characteristics of these interventions and the processes of designing and ...
Recent calls in biology education research (BER) have recommended that researchers leverage learning theories and methodologies from other disciplines to investigate the mechanisms by which students to develop sophisticated ideas. We suggest design-based research from the learning sciences is a compelling methodology for achieving this aim. Design-based research investigates the "learning ...
Design-based research (DBR) evolved near the beginning of the 21st century and was heralded as a practical research methodology that could effectively bridge the chasm between research and practice in formal education.
lead to the development of "usable knowledge" (Lagemann, 2002). Design-based research (Brown, 1992; Collins, 1992) is an emerging paradigm for the study of learning in context through th. systematic design and study of instructional strategies and tools. We argue that design-based research can help create and extend knowledge about dev.
Against the backdrop of the expansion of the literacy curriculum to include multiliteracies in education systems around the world, we discuss how a design-based research approach can contribute to practical outcomes in building the participating teachers' confidence and competence in their pedagogical practices, developing scalable lesson resources for other teachers to use and adapt, and ...
Design-based research methods are a thirty-year old trad-ition from the learning sciences that have been taken up in many domains as a way to study designed interventions that challenge the traditional relationship between research and design, as is the case with online learning.
We argue that both research and design can independently produce empirically derived knowledge, and we examine some of the configurations that allow us to simultaneously invent and study designed online learning environments. We revisit design-based research (DBR) methods and their epistemology, and discuss how they contribute various types of ...
To cite this article: Christopher Hoadley & Fabio C. Campos (2022): Design-based research: What it is and why it matters to studying online learning, Educational Psychologist, DOI: 10.1080 ...
1. Overview of Design-Based Research Design-Based Research (DBR) is a core methodology of the learning sciences. Begun as a movement away from experimental psychology, DBR was proposed as means to study learning amidst the ―blooming, buzzing confusion‖ of classrooms (Brown, 1992, p. 141). It is a way to develop theory that takes
the impact of the DBR-led lesson study approach, as well as the benefitsgenerally of applying DBR methods when attempting to connect research to practice. Design-based research DBR is an approach specificallydeveloped as a means to connect educational research to practice (Penuel et al., 2011; Coburn et al., 2013).
This paper describes aspirations and contributions that grew out of a developmental cycle of design-based research (DBR) implementation conducted over a three-year project. DBR engineers new learning environments and improves learning in context whilst communicating usable knowledge for learning and teaching in complex settings.
Design-Based Research and Interventions. Design-Based Research (DBR) is a research methodology used by researchers in the learning sciences. DBR is a concentrated, collaborative and participatory approach to educational inquiry. The basic process of DBR involves developing solutions or interventions to problems (Anderson & Shattuck, 2012).
Critical Lessons from an Emerging Field. This edited volume showcases work from the emerging field of design-based research (DBR) within social studies education and explores the unique challenges and opportunities that arise when applying the approach in classrooms. Usually associated with STEM fields, DBR's unique ability to generate ...
Lessons learned from a design-based research implementation: a researc... Go to citation Crossref Google Scholar Teachers' noticing and interpretations of students' responses to silen...
This is a design-based research project, oriented towards the creation of products and to educational innovation (Štemberger and Cencič 2016). This section details the four phases of the model ...
According to the Design-Based Research Collective (2003): "First, the central goals of designing learning environments and developing theories or "prototheories" of learning are intertwined. Second, development and research take place through continuous cycles of design, enactment, analysis, and redesign.
This paper describes the outcomes and lessons learned from an application of design-based research (DBR) in the implementation and refinement of a teacher professional development (PD) program that is a key component of the overall project. ... A design-based research approach (DBR) employing mixed methods was utilized over the four years of ...
Learner Experiences During the Design-Based Research Process for a Problem-Based Instructional Design Course. Yun Claire Park https ... A., Zech L., Bransford J., & The Cognition and Technology Group at Vanderbilt. (1998). Doing with understanding: lessons from research on problem- and project-based learning. The Journal of the Learning ...
This edited volume showcases work from the emerging field of design-based research (DBR) within social studies education and explores the unique challenges and opportunities that arise when applying the approach in classrooms. Usually associated with STEM fields, DBR's unique ability to generate practical theories of learning and to engineer ...
Next, the teachers designed lessons based on the provided instructional design principles and, upon completing the lesson designs, responded to a usability evaluation questionnaire.
Specifically, we share lessons learned from years of designing texts that (a) advance knowledge-building in the context of project-based science teaching and (b) advance readers' textual dexterity. Our research is conducted in the context of project-based learning in science, and we approach our inquiry from multiple theoretical perspectives.
Design-based research. DBR, specifically the Integrated Learning Design Framework (ILDF, Bannan-Ritland, Citation 2009), was adopted for this study as it provided guidance in the form of iterative phases for collaboratively designing an educational product.Previously, this framework has been leveraged for collaborative learning with teachers by Bannan (Citation 2013) and Bannan et al ...
The National Perinatal Epidemiology Unit (NPEU) is a multidisciplinary research unit based at the University of Oxford. Our work involves running randomised con. The National Perinatal Epidemiology Unit (NPEU) is a multidisciplinary research unit based at the University of Oxford. ... Lessons learned to inform maternity care from the UK and ...
The Channel Quality Indicator (CQI) plays a pivotal role in 5G networks, optimizing infrastructure dynamically to ensure high Quality of Service (QoS). Recent research has focused on improving CQI estimation in 5G networks using machine learning. In this field, the selection of the proper loss function is critical for training an accurate model. Two commonly used loss functions are Mean ...
Research also notes that design-based training modules are pivotal for the success of teacher preparation programs, as pre-service teachers, who do not have classroom-based teaching experiences, can be active learners of both technology and pedagogy (Campbell et al., 2022; Nami, 2022; Tondeur et al., 2016). However, the integration of these two ...
Presently he is a professor in electrical engineering section, Department of Applied Physics, University of Calcutta, India.His research interests include fuzzy control system design, stochastic optimization applications, robotics, etc. He has published more than 35 research articles in international and national journals or conferences.