- Business Essentials
- Leadership & Management
- Credential of Leadership, Impact, and Management in Business (CLIMB)
- Entrepreneurship & Innovation
- Digital Transformation
- Finance & Accounting
- Business in Society
- For Organizations
- Support Portal
- Media Coverage
- Founding Donors
- Leadership Team
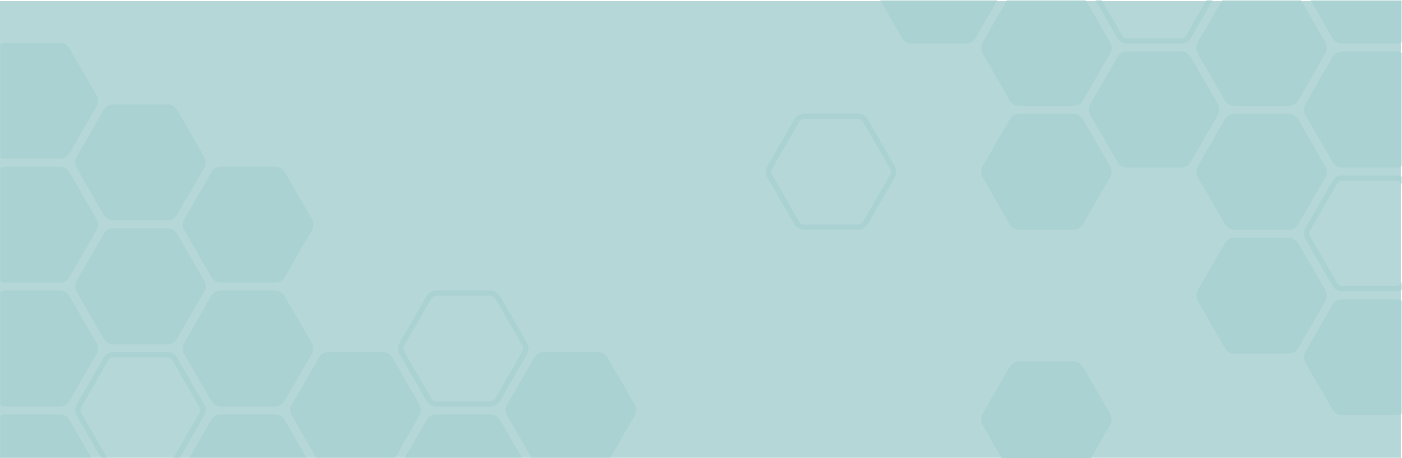
- Harvard Business School →
- HBS Online →
- Business Insights →

Business Insights
Harvard Business School Online's Business Insights Blog provides the career insights you need to achieve your goals and gain confidence in your business skills.
- Career Development
- Communication
- Decision-Making
- Earning Your MBA
- Negotiation
- News & Events
- Productivity
- Staff Spotlight
- Student Profiles
- Work-Life Balance
- AI Essentials for Business
- Alternative Investments
- Business Analytics
- Business Strategy
- Business and Climate Change
- Design Thinking and Innovation
- Digital Marketing Strategy
- Disruptive Strategy
- Economics for Managers
- Entrepreneurship Essentials
- Financial Accounting
- Global Business
- Launching Tech Ventures
- Leadership Principles
- Leadership, Ethics, and Corporate Accountability
- Leading with Finance
- Management Essentials
- Negotiation Mastery
- Organizational Leadership
- Power and Influence for Positive Impact
- Strategy Execution
- Sustainable Business Strategy
- Sustainable Investing
- Winning with Digital Platforms
17 Data Visualization Techniques All Professionals Should Know
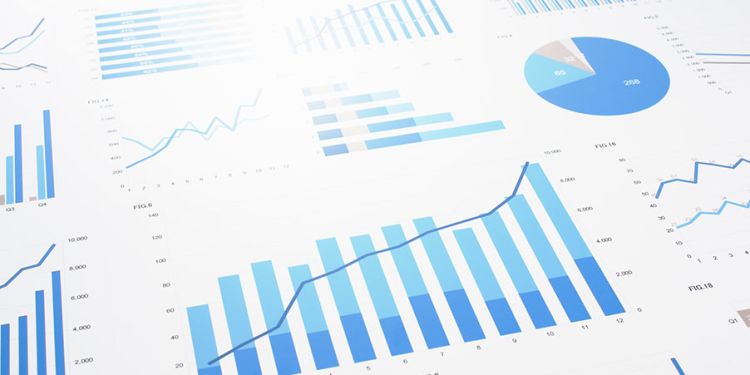
- 17 Sep 2019
There’s a growing demand for business analytics and data expertise in the workforce. But you don’t need to be a professional analyst to benefit from data-related skills.
Becoming skilled at common data visualization techniques can help you reap the rewards of data-driven decision-making , including increased confidence and potential cost savings. Learning how to effectively visualize data could be the first step toward using data analytics and data science to your advantage to add value to your organization.
Several data visualization techniques can help you become more effective in your role. Here are 17 essential data visualization techniques all professionals should know, as well as tips to help you effectively present your data.
Access your free e-book today.
What Is Data Visualization?
Data visualization is the process of creating graphical representations of information. This process helps the presenter communicate data in a way that’s easy for the viewer to interpret and draw conclusions.
There are many different techniques and tools you can leverage to visualize data, so you want to know which ones to use and when. Here are some of the most important data visualization techniques all professionals should know.
Data Visualization Techniques
The type of data visualization technique you leverage will vary based on the type of data you’re working with, in addition to the story you’re telling with your data .
Here are some important data visualization techniques to know:
- Gantt Chart
- Box and Whisker Plot
- Waterfall Chart
- Scatter Plot
- Pictogram Chart
- Highlight Table
- Bullet Graph
- Choropleth Map
- Network Diagram
- Correlation Matrices
1. Pie Chart

Pie charts are one of the most common and basic data visualization techniques, used across a wide range of applications. Pie charts are ideal for illustrating proportions, or part-to-whole comparisons.
Because pie charts are relatively simple and easy to read, they’re best suited for audiences who might be unfamiliar with the information or are only interested in the key takeaways. For viewers who require a more thorough explanation of the data, pie charts fall short in their ability to display complex information.
2. Bar Chart

The classic bar chart , or bar graph, is another common and easy-to-use method of data visualization. In this type of visualization, one axis of the chart shows the categories being compared, and the other, a measured value. The length of the bar indicates how each group measures according to the value.
One drawback is that labeling and clarity can become problematic when there are too many categories included. Like pie charts, they can also be too simple for more complex data sets.
3. Histogram

Unlike bar charts, histograms illustrate the distribution of data over a continuous interval or defined period. These visualizations are helpful in identifying where values are concentrated, as well as where there are gaps or unusual values.
Histograms are especially useful for showing the frequency of a particular occurrence. For instance, if you’d like to show how many clicks your website received each day over the last week, you can use a histogram. From this visualization, you can quickly determine which days your website saw the greatest and fewest number of clicks.
4. Gantt Chart

Gantt charts are particularly common in project management, as they’re useful in illustrating a project timeline or progression of tasks. In this type of chart, tasks to be performed are listed on the vertical axis and time intervals on the horizontal axis. Horizontal bars in the body of the chart represent the duration of each activity.
Utilizing Gantt charts to display timelines can be incredibly helpful, and enable team members to keep track of every aspect of a project. Even if you’re not a project management professional, familiarizing yourself with Gantt charts can help you stay organized.
5. Heat Map
A heat map is a type of visualization used to show differences in data through variations in color. These charts use color to communicate values in a way that makes it easy for the viewer to quickly identify trends. Having a clear legend is necessary in order for a user to successfully read and interpret a heatmap.
There are many possible applications of heat maps. For example, if you want to analyze which time of day a retail store makes the most sales, you can use a heat map that shows the day of the week on the vertical axis and time of day on the horizontal axis. Then, by shading in the matrix with colors that correspond to the number of sales at each time of day, you can identify trends in the data that allow you to determine the exact times your store experiences the most sales.
6. A Box and Whisker Plot

A box and whisker plot , or box plot, provides a visual summary of data through its quartiles. First, a box is drawn from the first quartile to the third of the data set. A line within the box represents the median. “Whiskers,” or lines, are then drawn extending from the box to the minimum (lower extreme) and maximum (upper extreme). Outliers are represented by individual points that are in-line with the whiskers.
This type of chart is helpful in quickly identifying whether or not the data is symmetrical or skewed, as well as providing a visual summary of the data set that can be easily interpreted.
7. Waterfall Chart

A waterfall chart is a visual representation that illustrates how a value changes as it’s influenced by different factors, such as time. The main goal of this chart is to show the viewer how a value has grown or declined over a defined period. For example, waterfall charts are popular for showing spending or earnings over time.
8. Area Chart

An area chart , or area graph, is a variation on a basic line graph in which the area underneath the line is shaded to represent the total value of each data point. When several data series must be compared on the same graph, stacked area charts are used.
This method of data visualization is useful for showing changes in one or more quantities over time, as well as showing how each quantity combines to make up the whole. Stacked area charts are effective in showing part-to-whole comparisons.
9. Scatter Plot

Another technique commonly used to display data is a scatter plot . A scatter plot displays data for two variables as represented by points plotted against the horizontal and vertical axis. This type of data visualization is useful in illustrating the relationships that exist between variables and can be used to identify trends or correlations in data.
Scatter plots are most effective for fairly large data sets, since it’s often easier to identify trends when there are more data points present. Additionally, the closer the data points are grouped together, the stronger the correlation or trend tends to be.
10. Pictogram Chart
Pictogram charts , or pictograph charts, are particularly useful for presenting simple data in a more visual and engaging way. These charts use icons to visualize data, with each icon representing a different value or category. For example, data about time might be represented by icons of clocks or watches. Each icon can correspond to either a single unit or a set number of units (for example, each icon represents 100 units).
In addition to making the data more engaging, pictogram charts are helpful in situations where language or cultural differences might be a barrier to the audience’s understanding of the data.
11. Timeline

Timelines are the most effective way to visualize a sequence of events in chronological order. They’re typically linear, with key events outlined along the axis. Timelines are used to communicate time-related information and display historical data.
Timelines allow you to highlight the most important events that occurred, or need to occur in the future, and make it easy for the viewer to identify any patterns appearing within the selected time period. While timelines are often relatively simple linear visualizations, they can be made more visually appealing by adding images, colors, fonts, and decorative shapes.
12. Highlight Table

A highlight table is a more engaging alternative to traditional tables. By highlighting cells in the table with color, you can make it easier for viewers to quickly spot trends and patterns in the data. These visualizations are useful for comparing categorical data.
Depending on the data visualization tool you’re using, you may be able to add conditional formatting rules to the table that automatically color cells that meet specified conditions. For instance, when using a highlight table to visualize a company’s sales data, you may color cells red if the sales data is below the goal, or green if sales were above the goal. Unlike a heat map, the colors in a highlight table are discrete and represent a single meaning or value.
13. Bullet Graph

A bullet graph is a variation of a bar graph that can act as an alternative to dashboard gauges to represent performance data. The main use for a bullet graph is to inform the viewer of how a business is performing in comparison to benchmarks that are in place for key business metrics.
In a bullet graph, the darker horizontal bar in the middle of the chart represents the actual value, while the vertical line represents a comparative value, or target. If the horizontal bar passes the vertical line, the target for that metric has been surpassed. Additionally, the segmented colored sections behind the horizontal bar represent range scores, such as “poor,” “fair,” or “good.”
14. Choropleth Maps

A choropleth map uses color, shading, and other patterns to visualize numerical values across geographic regions. These visualizations use a progression of color (or shading) on a spectrum to distinguish high values from low.
Choropleth maps allow viewers to see how a variable changes from one region to the next. A potential downside to this type of visualization is that the exact numerical values aren’t easily accessible because the colors represent a range of values. Some data visualization tools, however, allow you to add interactivity to your map so the exact values are accessible.
15. Word Cloud

A word cloud , or tag cloud, is a visual representation of text data in which the size of the word is proportional to its frequency. The more often a specific word appears in a dataset, the larger it appears in the visualization. In addition to size, words often appear bolder or follow a specific color scheme depending on their frequency.
Word clouds are often used on websites and blogs to identify significant keywords and compare differences in textual data between two sources. They are also useful when analyzing qualitative datasets, such as the specific words consumers used to describe a product.
16. Network Diagram

Network diagrams are a type of data visualization that represent relationships between qualitative data points. These visualizations are composed of nodes and links, also called edges. Nodes are singular data points that are connected to other nodes through edges, which show the relationship between multiple nodes.
There are many use cases for network diagrams, including depicting social networks, highlighting the relationships between employees at an organization, or visualizing product sales across geographic regions.
17. Correlation Matrix

A correlation matrix is a table that shows correlation coefficients between variables. Each cell represents the relationship between two variables, and a color scale is used to communicate whether the variables are correlated and to what extent.
Correlation matrices are useful to summarize and find patterns in large data sets. In business, a correlation matrix might be used to analyze how different data points about a specific product might be related, such as price, advertising spend, launch date, etc.
Other Data Visualization Options
While the examples listed above are some of the most commonly used techniques, there are many other ways you can visualize data to become a more effective communicator. Some other data visualization options include:
- Bubble clouds
- Circle views
- Dendrograms
- Dot distribution maps
- Open-high-low-close charts
- Polar areas
- Radial trees
- Ring Charts
- Sankey diagram
- Span charts
- Streamgraphs
- Wedge stack graphs
- Violin plots

Tips For Creating Effective Visualizations
Creating effective data visualizations requires more than just knowing how to choose the best technique for your needs. There are several considerations you should take into account to maximize your effectiveness when it comes to presenting data.
Related : What to Keep in Mind When Creating Data Visualizations in Excel
One of the most important steps is to evaluate your audience. For example, if you’re presenting financial data to a team that works in an unrelated department, you’ll want to choose a fairly simple illustration. On the other hand, if you’re presenting financial data to a team of finance experts, it’s likely you can safely include more complex information.
Another helpful tip is to avoid unnecessary distractions. Although visual elements like animation can be a great way to add interest, they can also distract from the key points the illustration is trying to convey and hinder the viewer’s ability to quickly understand the information.
Finally, be mindful of the colors you utilize, as well as your overall design. While it’s important that your graphs or charts are visually appealing, there are more practical reasons you might choose one color palette over another. For instance, using low contrast colors can make it difficult for your audience to discern differences between data points. Using colors that are too bold, however, can make the illustration overwhelming or distracting for the viewer.
Related : Bad Data Visualization: 5 Examples of Misleading Data
Visuals to Interpret and Share Information
No matter your role or title within an organization, data visualization is a skill that’s important for all professionals. Being able to effectively present complex data through easy-to-understand visual representations is invaluable when it comes to communicating information with members both inside and outside your business.
There’s no shortage in how data visualization can be applied in the real world. Data is playing an increasingly important role in the marketplace today, and data literacy is the first step in understanding how analytics can be used in business.
Are you interested in improving your analytical skills? Learn more about Business Analytics , our eight-week online course that can help you use data to generate insights and tackle business decisions.
This post was updated on January 20, 2022. It was originally published on September 17, 2019.

About the Author
- Chapter 7: End-to-End Data »
- 7.2 Multimedia Data
- View page source
7.2 Multimedia Data
Multimedia data, comprised of audio, video, and still images, now makes up the majority of traffic on the Internet. Part of what has made the widespread transmission of multimedia across networks possible is advances in compression technology. Because multimedia data is consumed mostly by humans using their senses—vision and hearing—and processed by the human brain, there are unique challenges to compressing it. You want to try to keep the information that is most important to a human, while getting rid of anything that doesn’t improve the human’s perception of the visual or auditory experience. Hence, both computer science and the study of human perception come into play. In this section, we’ll look at some of the major efforts in representing and compressing multimedia data.
The uses of compression are not limited to multimedia data of course—for example, you may well have used a utility like zip or compress to compress files before sending them over a network, or to uncompress a data file after downloading. It turns out that the techniques used for compressing data—which are typically lossless , because most people don’t like to lose data from a file—also show up as part of the solution for multimedia compression. In contrast, lossy compression , commonly used for multimedia data, does not promise that the data received is exactly the same as the data sent. As noted above, this is because multimedia data often contains information that is of little utility to the human who receives it. Our senses and brains can only perceive so much detail. They are also very good at filling in missing pieces and even correcting some errors in what we see or hear. And, lossy algorithms typically achieve much better compression ratios than do their lossless counterparts; they can be an order of magnitude better or more.
To get a sense of how important compression has been to the spread of networked multimedia, consider the following example. A high-definition TV screen has something like 1080 × 1920 pixels, each of which has 24 bits of color information, so each frame is
1080 × 1920 × 24 = 50 Mb
so if you want to send 24 frames per second, that would be over 1 Gbps. That’s more than most Internet users have access to. By contrast, modern compression techniques can get a reasonably high-quality HDTV signal down to the range of 10 Mbps, a two order of magnitude reduction and well within the reach of most broadband users. Similar compression gains apply to lower quality video such as YouTube clips—Web video could never have reached its current popularity without compression to make all those entertaining videos fit within the bandwidth of today’s networks.
Compression techniques as applied to multimedia have been an area of great innovation, particularly lossy compression. Lossless techniques also have an important role to play, however. Indeed, most of the lossy techniques include some steps that are lossless, so we begin our discussion with an overview of lossless compression.
7.2.1 Lossless Compression Techniques
In many ways, compression is inseparable from data encoding. When thinking about how to encode a piece of data in a set of bits, we might just as well think about how to encode the data in the smallest set of bits possible. For example, if you have a block of data that is made up of the 26 symbols A through Z, and if all of these symbols have an equal chance of occurring in the data block you are encoding, then encoding each symbol in 5 bits is the best you can do (since 2 5 = 32 is the lowest power of 2 above 26). If, however, the symbol R occurs 50% of the time, then it would be a good idea to use fewer bits to encode the R than any of the other symbols. In general, if you know the relative probability that each symbol will occur in the data, then you can assign a different number of bits to each possible symbol in a way that minimizes the number of bits it takes to encode a given block of data. This is the essential idea of Huffman codes , one of the important early developments in data compression.
Run Length Encoding
Run length encoding (RLE) is a compression technique with a brute-force simplicity. The idea is to replace consecutive occurrences of a given symbol with only one copy of the symbol, plus a count of how many times that symbol occurs—hence, the name run length . For example, the string AAABBCDDDD would be encoded as 3A2B1C4D .
RLE turns out to be useful for compressing some classes of images. It can be used in this context by comparing adjacent pixel values and then encoding only the changes. For images that have large homogeneous regions, this technique is quite effective. For example, it is not uncommon that RLE can achieve compression ratios on the order of 8-to-1 for scanned text images. RLE works well on such files because they often contain a large amount of white space that can be removed. For those old enough to remember the technology, RLE was the key compression algorithm used to transmit faxes. However, for images with even a small degree of local variation, it is not uncommon for compression to actually increase the image byte size, since it takes 2 bytes to represent a single symbol when that symbol is not repeated.
Differential Pulse Code Modulation
Another simple lossless compression algorithm is Differential Pulse Code Modulation (DPCM). The idea here is to first output a reference symbol and then, for each symbol in the data, to output the difference between that symbol and the reference symbol. For example, using symbol A as the reference symbol, the string AAABBCDDDD would be encoded as A0001123333 because A is the same as the reference symbol, B has a difference of 1 from the reference symbol, and so on. Note that this simple example does not illustrate the real benefit of DPCM, which is that when the differences are small they can be encoded with fewer bits than the symbol itself. In this example, the range of differences, 0-3, can be represented with 2 bits each, rather than the 7 or 8 bits required by the full character. As soon as the difference becomes too large, a new reference symbol is selected.
DPCM works better than RLE for most digital imagery, since it takes advantage of the fact that adjacent pixels are usually similar. Due to this correlation, the dynamic range of the differences between the adjacent pixel values can be significantly less than the dynamic range of the original image, and this range can therefore be represented using fewer bits. Using DPCM, we have measured compression ratios of 1.5-to-1 on digital images. DPCM also works on audio, because adjacent samples of an audio waveform are likely to be close in value.
A slightly different approach, called delta encoding , simply encodes a symbol as the difference from the previous one. Thus, for example, AAABBCDDDD would be represented as A001011000 . Note that delta encoding is likely to work well for encoding images where adjacent pixels are similar. It is also possible to perform RLE after delta encoding, since we might find long strings of 0s if there are many similar symbols next to each other.
Dictionary-Based Methods
The final lossless compression method we consider is the dictionary-based approach, of which the Lempel-Ziv (LZ) compression algorithm is the best known. The Unix compress and gzip commands use variants of the LZ algorithm.
The idea of a dictionary-based compression algorithm is to build a dictionary (table) of variable-length strings (think of them as common phrases) that you expect to find in the data and then to replace each of these strings when it appears in the data with the corresponding index to the dictionary. For example, instead of working with individual characters in text data, you could treat each word as a string and output the index in the dictionary for that word. To further elaborate on this example, the word compression has the index 4978 in one particular dictionary; it is the 4978th word in /usr/share/dict/words . To compress a body of text, each time the string “compression” appears, it would be replaced by 4978. Since this particular dictionary has just over 25,000 words in it, it would take 15 bits to encode the index, meaning that the string “compression” could be represented in 15 bits rather than the 77 bits required by 7-bit ASCII. This is a compression ratio of 5-to-1! At another data point, we were able to get a 2-to-1 compression ratio when we applied the compress command to the source code for the protocols described in this book.
Of course, this leaves the question of where the dictionary comes from. One option is to define a static dictionary, preferably one that is tailored for the data being compressed. A more general solution, and the one used by LZ compression, is to adaptively define the dictionary based on the contents of the data being compressed. In this case, however, the dictionary constructed during compression has to be sent along with the data so that the decompression half of the algorithm can do its job. Exactly how you build an adaptive dictionary has been a subject of extensive research.
7.2.2 Image Representation and Compression (GIF, JPEG)
Given the ubiquitous use of digital imagery—this use was spawned by the invention of graphical displays, not high-speed networks—the need for standard representation formats and compression algorithms for digital imagery data has become essential. In response to this need, the ISO defined a digital image format known as JPEG , named after the Joint Photographic Experts Group that designed it. (The “Joint” in JPEG stands for a joint ISO/ITU effort.) JPEG is the most widely used format for still images in use today. At the heart of the definition of the format is a compression algorithm, which we describe below. Many techniques used in JPEG also appear in MPEG, the set of standards for video compression and transmission created by the Moving Picture Experts Group.
Before delving into the details of JPEG, we observe that there are quite a few steps to get from a digital image to a compressed representation of that image that can be transmitted, decompressed, and displayed correctly by a receiver. You probably know that digital images are made up of pixels (hence, the megapixels quoted in smartphone camera advertisements). Each pixel represents one location in the two-dimensional grid that makes up the image, and for color images each pixel has some numerical value representing a color. There are lots of ways to represent colors, referred to as color spaces ; the one most people are familiar with is RGB (red, green, blue). You can think of color as being a three dimensional quantity—you can make any color out of red, green, and blue light in different amounts. In a three-dimensional space, there are lots of different, valid ways to describe a given point (consider Cartesian and polar coordinates, for example). Similarly, there are various ways to describe a color using three quantities, and the most common alternative to RGB is YUV. The Y is luminance, roughly the overall brightness of the pixel, and U and V contain chrominance, or color information. Confoundingly, there are a few different variants of the YUV color space as well. More on this in a moment.
The significance of this discussion is that the encoding and transmission of color images (either still or moving) requires agreement between the two ends on the color space. Otherwise, of course, you’d end up with different colors being displayed by the receiver than were captured by the sender. Hence, agreeing on a color space definition (and perhaps a way to communicate which particular space is in use) is part of the definition of any image or video format.
Let’s look at the example of the Graphical Interchange Format (GIF). GIF uses the RGB color space and starts out with 8 bits to represent each of the three dimensions of color for a total of 24 bits. Rather than sending those 24 bits per pixel, however, GIF first reduces 24-bit color images to 8-bit color images. This is done by identifying the colors used in the picture, of which there will typically be considerably fewer than 2 24 , and then picking the 256 colors that most closely approximate the colors used in the picture. There might be more than 256 colors, however, so the trick is to try not to distort the color too much by picking 256 colors such that no pixel has its color changed too much.
The 256 colors are stored in a table, which can be indexed with an 8-bit number, and the value for each pixel is replaced by the appropriate index. Note that this is an example of lossy compression for any picture with more than 256 colors. GIF then runs an LZ variant over the result, treating common sequences of pixels as the strings that make up the dictionary—a lossless operation. Using this approach, GIF is sometimes able to achieve compression ratios on the order of 10:1, but only when the image consists of a relatively small number of discrete colors. Graphical logos, for example, are handled well by GIF. Images of natural scenes, which often include a more continuous spectrum of colors, cannot be compressed at this ratio using GIF. It is also not too hard for a human eye to detect the distortion caused by the lossy color reduction of GIF in some cases.
The JPEG format is considerably more well suited to photographic images, as you would hope given the name of the group that created it. JPEG does not reduce the number of colors like GIF. Instead, JPEG starts off by transforming the RGB colors (which are what you usually get out of a digital camera) to the YUV space. The reason for this has to do with the way the eye perceives images. There are receptors in the eye for brightness, and separate receptors for color. Because we’re very good at perceiving variations in brightness, it makes sense to spend more bits on transmitting brightness information. Since the Y component of YUV is, roughly, the brightness of the pixel, we can compress that component separately, and less aggressively, from the other two (chrominance) components.
As noted above, YUV and RGB are alternative ways to describe a point in a 3-dimensional space, and it’s possible to convert from one color space to another using linear equations. For one YUV space that is commonly used to represent digital images, the equations are:
The exact values of the constants here are not important, as long as the encoder and decoder agree on what they are. (The decoder will have to apply the inverse transformations to recover the RGB components needed to drive a display.) The constants are, however, carefully chosen based on the human perception of color. You can see that Y, the luminance, is a sum of the red, green, and blue components, while U and V are color difference components. U represents the difference between the luminance and blue, and V the difference between luminance and red. You may notice that setting R, G, and B to their maximum values (which would be 255 for 8-bit representations) will also produce a value of Y=255 while U and V in this case would be zero. That is, a fully white pixel is (255,255,255) in RGB space and (255,0,0) in YUV space.
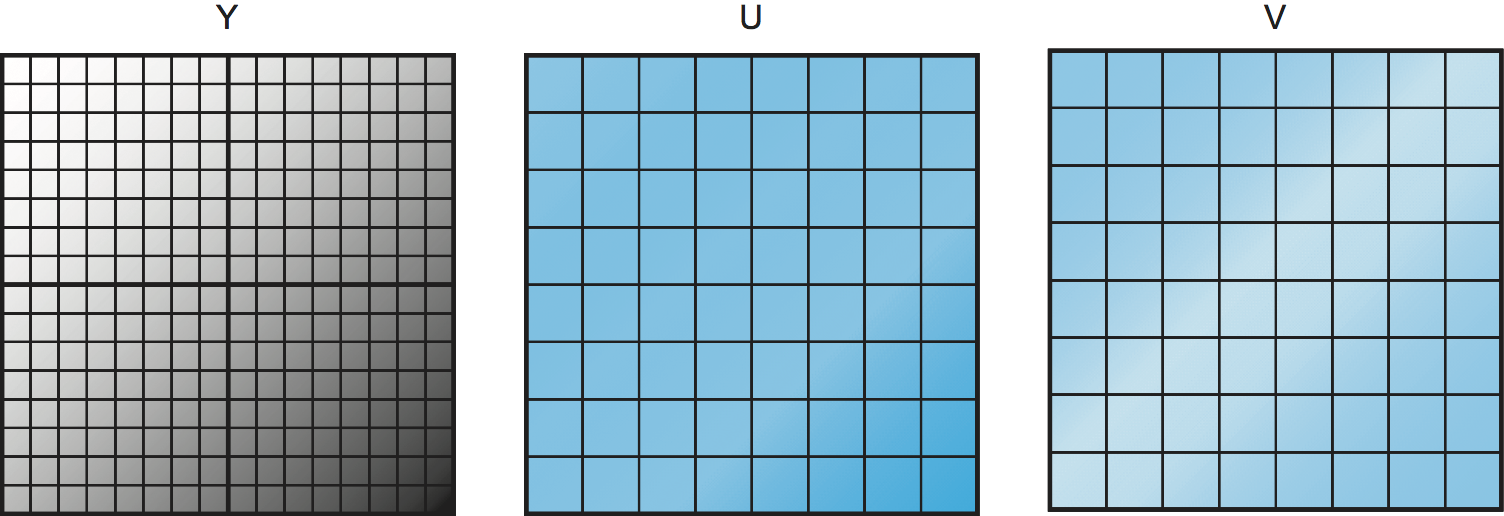
Figure 189. Subsampling the U and V components of an image.
Once the image has been transformed into YUV space, we can now think about compressing each of the three components separately. We want to be more aggressive in compressing the U and V components, to which human eyes are less sensitive. One way to compress the U and V components is to subsample them. The basic idea of subsampling is to take a number of adjacent pixels, calculate the average U or V value for that group of pixels, and transmit that, rather than sending the value for every pixel. Figure 189 illustrates the point. The luminance (Y) component is not subsampled, so the Y value of all the pixels will be transmitted, as indicated by the 16 × 16 grid of pixels on the left. In the case of U and V, we treat each group of four adjacent pixels as a group, calculate the average of the U or V value for that group, and transmit that. Hence, we end up with an 8 × 8 grid of U and V values to transmit. Thus, in this example, for every four pixels, we transmit six values (four Y and one each of U and V) rather than the original 12 values (four each for all three components), for a 50% reduction in information.
It’s worth noting that you could be either more or less aggressive in the subsampling, with corresponding increases in compression and decreases in quality. The subsampling approach shown here, in which chrominance is subsampled by a factor of two in both horizontal and vertical directions (and which goes by the identification 4:2:0), happens to match the most common approach used for both JPEG and MPEG.

Figure 190. Block diagram of JPEG compression.
Once subsampling is done, we now have three grids of pixels to deal with, and each one is dealt with separately. JPEG compression of each component takes place in three phases, as illustrated in Figure 190 . On the compression side, the image is fed through these three phases one 8 × 8 block at a time. The first phase applies the discrete cosine transform (DCT) to the block. If you think of the image as a signal in the spatial domain, then DCT transforms this signal into an equivalent signal in the spatial frequency domain. This is a lossless operation but a necessary precursor to the next, lossy step. After the DCT, the second phase applies a quantization to the resulting signal and, in so doing, loses the least significant information contained in that signal. The third phase encodes the final result, but in so doing also adds an element of lossless compression to the lossy compression achieved by the first two phases. Decompression follows these same three phases, but in reverse order.
DCT Phase
DCT is a transformation closely related to the fast Fourier transform (FFT). It takes an 8 × 8 matrix of pixel values as input and outputs an 8 × 8 matrix of frequency coefficients. You can think of the input matrix as a 64-point signal that is defined in two spatial dimensions ( x and y ); DCT breaks this signal into 64 spatial frequencies. To get an intuitive feel for spatial frequency, imagine yourself moving across a picture in, say, the x direction. You would see the value of each pixel varying as some function of x . If this value changes slowly with increasing x , then it has a low spatial frequency; if it changes rapidly, it has a high spatial frequency. So the low frequencies correspond to the gross features of the picture, while the high frequencies correspond to fine detail. The idea behind the DCT is to separate the gross features, which are essential to viewing the image, from the fine detail, which is less essential and, in some cases, might be barely perceived by the eye.
DCT, along with its inverse, which recovers the original pixels and during decompression, are defined by the following formulas:
where \(C(x) = 1/\sqrt{2}\) when \(x=0\) and \(1\) when \(x>0\) , and \(pixel(x,y)\) is the grayscale value of the pixel at position (x,y) in the 8 × 8 block being compressed; N = 8 in this case.
The first frequency coefficient, at location (0,0) in the output matrix, is called the DC coefficient . Intuitively, we can see that the DC coefficient is a measure of the average value of the 64 input pixels. The other 63 elements of the output matrix are called the AC coefficients . They add the higher-spatial-frequency information to this average value. Thus, as you go from the first frequency coefficient toward the 64th frequency coefficient, you are moving from low-frequency information to high-frequency information, from the broad strokes of the image to finer and finer detail. These higher-frequency coefficients are increasingly unimportant to the perceived quality of the image. It is the second phase of JPEG that decides which portion of which coefficients to throw away.

Quantization Phase
The second phase of JPEG is where the compression becomes lossy. DCT does not itself lose information; it just transforms the image into a form that makes it easier to know what information to remove. (Although not lossy, per se , there is of course some loss of precision during the DCT phase because of the use of fixed-point arithmetic.) Quantization is easy to understand—it’s simply a matter of dropping the insignificant bits of the frequency coefficients.
To see how the quantization phase works, imagine that you want to compress some whole numbers less than 100, such as 45, 98, 23, 66, and 7. If you decided that knowing these numbers truncated to the nearest multiple of 10 is sufficient for your purposes, then you could divide each number by the quantum 10 using integer arithmetic, yielding 4, 9, 2, 6, and 0. These numbers can each be encoded in 4 bits rather than the 7 bits needed to encode the original numbers.
Rather than using the same quantum for all 64 coefficients, JPEG uses a quantization table that gives the quantum to use for each of the coefficients, as specified in the formula given below. You can think of this table ( Quantum ) as a parameter that can be set to control how much information is lost and, correspondingly, how much compression is achieved. In practice, the JPEG standard specifies a set of quantization tables that have proven effective in compressing digital images; an example quantization table is given in Table 24 . In tables like this one, the low coefficients have a quantum close to 1 (meaning that little low-frequency information is lost) and the high coefficients have larger values (meaning that more high-frequency information is lost). Notice that as a result of such quantization tables many of the high-frequency coefficients end up being set to 0 after quantization, making them ripe for further compression in the third phase.
The basic quantization equation is
Decompression is then simply defined as
For example, if the DC coefficient (i.e., DCT(0,0)) for a particular block was equal to 25, then the quantization of this value using Table 24 would result in
During decompression, this coefficient would then be restored as 8 × 3 = 24.
Encoding Phase
The final phase of JPEG encodes the quantized frequency coefficients in a compact form. This results in additional compression, but this compression is lossless. Starting with the DC coefficient in position (0,0), the coefficients are processed in the zigzag sequence shown in Figure 191 . Along this zigzag, a form of run length encoding is used—RLE is applied to only the 0 coefficients, which is significant because many of the later coefficients are 0. The individual coefficient values are then encoded using a Huffman code. (The JPEG standard allows the implementer to use an arithmetic coding instead of the Huffman code.)
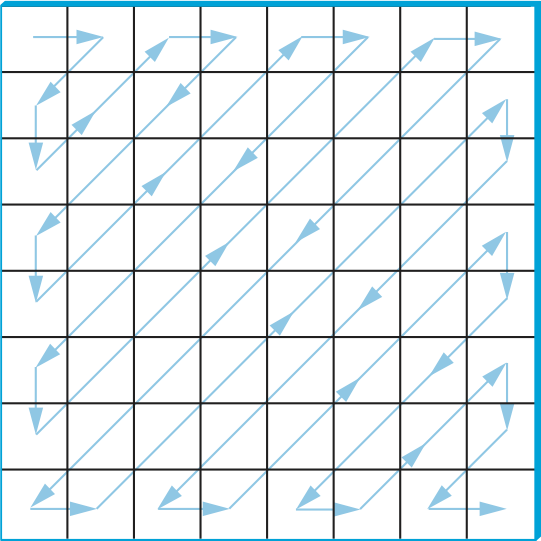
Figure 191. Zigzag traversal of quantized frequency coefficients.
In addition, because the DC coefficient contains a large percentage of the information about the 8 × 8 block from the source image, and images typically change slowly from block to block, each DC coefficient is encoded as the difference from the previous DC coefficient. This is the delta encoding approach described in a later section.
JPEG includes a number of variations that control how much compression you achieve versus the fidelity of the image. This can be done, for example, by using different quantization tables. These variations, plus the fact that different images have different characteristics, make it impossible to say with any precision the compression ratios that can be achieved with JPEG. Ratios of 30:1 are common, and higher ratios are certainly possible, but artifacts (noticeable distortion due to compression) become more severe at higher ratios.
7.2.3 Video Compression (MPEG)
We now turn our attention to the MPEG format, named after the Moving Picture Experts Group that defined it. To a first approximation, a moving picture (i.e., video) is simply a succession of still images—also called frames or pictures —displayed at some video rate. Each of these frames can be compressed using the same DCT-based technique used in JPEG. Stopping at this point would be a mistake, however, because it fails to remove the interframe redundancy present in a video sequence. For example, two successive frames of video will contain almost identical information if there is not much motion in the scene, so it would be unnecessary to send the same information twice. Even when there is motion, there may be plenty of redundancy since a moving object may not change from one frame to the next; in some cases, only its position changes. MPEG takes this interframe redundancy into consideration. MPEG also defines a mechanism for encoding an audio signal with the video, but we consider only the video aspect of MPEG in this section.
Frame Types
MPEG takes a sequence of video frames as input and compresses them into three types of frames, called I frames (intrapicture), P frames (predicted picture), and B frames (bidirectional predicted picture). Each frame of input is compressed into one of these three frame types. I frames can be thought of as reference frames; they are self-contained, depending on neither earlier frames nor later frames. To a first approximation, an I frame is simply the JPEG compressed version of the corresponding frame in the video source. P and B frames are not self-contained; they specify relative differences from some reference frame. More specifically, a P frame specifies the differences from the previous I frame, while a B frame gives an interpolation between the previous and subsequent I or P frames.
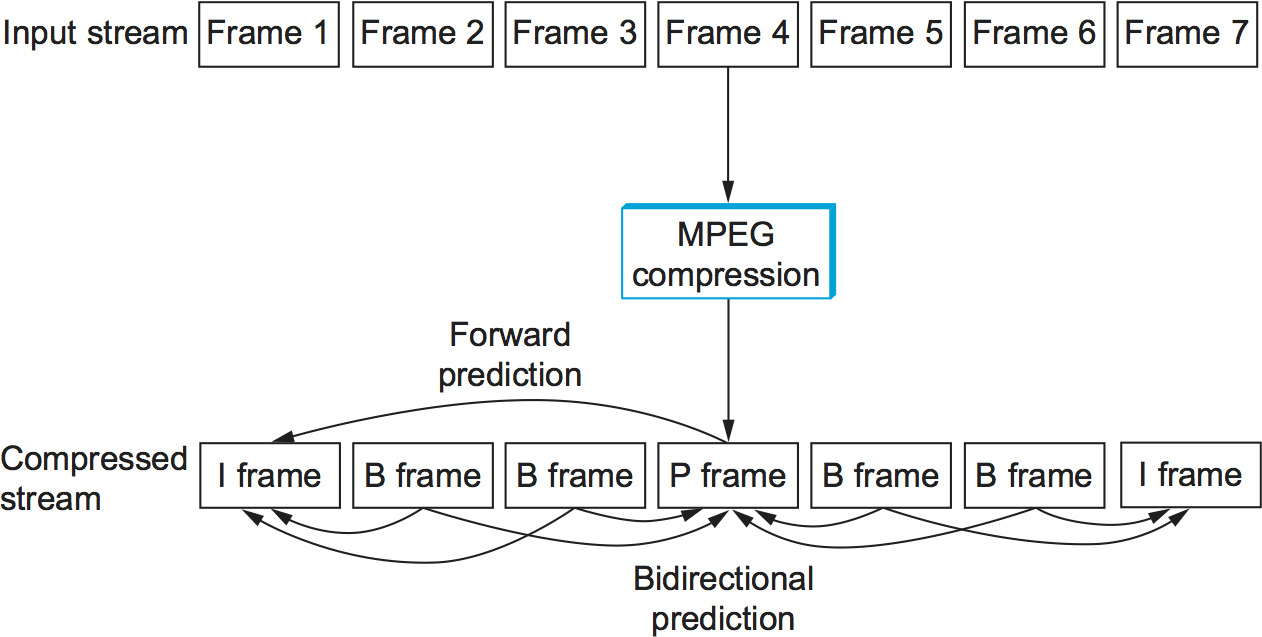
Figure 192. Sequence of I, P, and B frames generated by MPEG.
Figure 192 illustrates a sequence of seven video frames that, after being compressed by MPEG, result in a sequence of I, P, and B frames. The two I frames stand alone; each can be decompressed at the receiver independently of any other frames. The P frame depends on the preceding I frame; it can be decompressed at the receiver only if the preceding I frame also arrives. Each of the B frames depends on both the preceding I or P frame and the subsequent I or P frame. Both of these reference frames must arrive at the receiver before MPEG can decompress the B frame to reproduce the original video frame.
Note that, because each B frame depends on a later frame in the sequence, the compressed frames are not transmitted in sequential order. Instead, the sequence I B B P B B I shown in Figure 192 is transmitted as I P B B I B B. Also, MPEG does not define the ratio of I frames to P and B frames; this ratio may vary depending on the required compression and picture quality. For example, it is permissible to transmit only I frames. This would be similar to using JPEG to compress the video.
In contrast to the preceding discussion of JPEG, the following focuses on the decoding of an MPEG stream. It is a little easier to describe, and it is the operation that is more often implemented in networking systems today, since MPEG coding is so expensive that it is frequently done offline (i.e., not in real time). For example, in a video-on-demand system, the video would be encoded and stored on disk ahead of time. When a viewer wanted to watch the video, the MPEG stream would then be transmitted to the viewer’s machine, which would decode and display the stream in real time.
Let’s look more closely at the three frame types. As mentioned above, I frames are approximately equal to the JPEG compressed version of the source frame. The main difference is that MPEG works in units of 16 × 16 macroblocks. For a color video represented in YUV, the U and V components in each macroblock are subsampled into an 8 × 8 block, as we discussed above in the context of JPEG. Each 2 × 2 subblock in the macroblock is given by one U value and one V value—the average of the four pixel values. The subblock still has four Y values. The relationship between a frame and the corresponding macroblocks is given in Figure 193 .
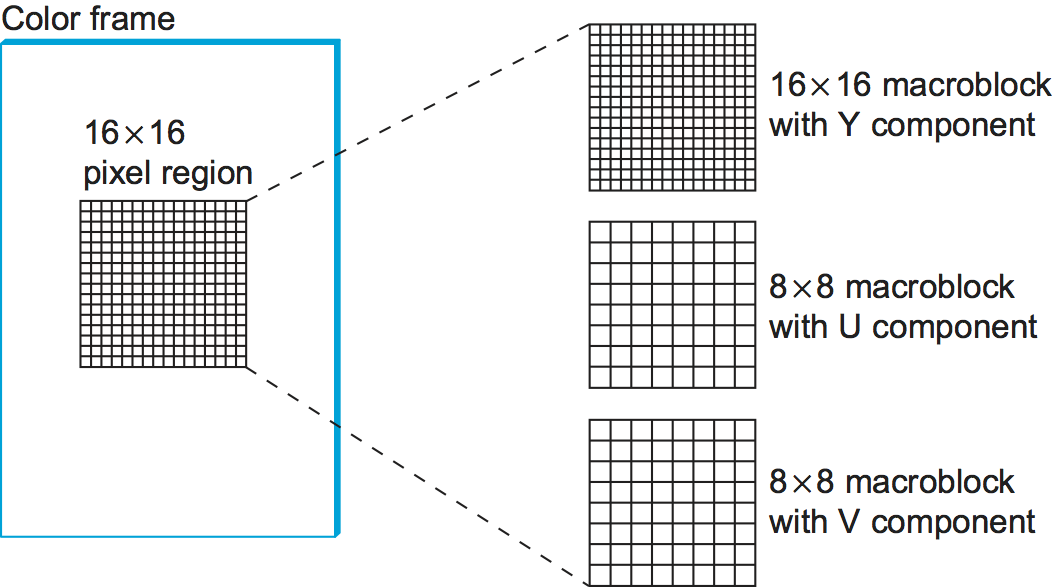
Figure 193. Each frame as a collection of macroblocks.
The P and B frames are also processed in units of macroblocks. Intuitively, we can see that the information they carry for each macroblock captures the motion in the video; that is, it shows in what direction and how far the macroblock moved relative to the reference frame(s). The following describes how a B frame is used to reconstruct a frame during decompression; P frames are handled in a similar manner, except that they depend on only one reference frame instead of two.
Before getting to the details of how a B frame is decompressed, we first note that each macroblock in a B frame is not necessarily defined relative to both an earlier and a later frame, as suggested above, but may instead simply be specified relative to just one or the other. In fact, a given macroblock in a B frame can use the same intracoding as is used in an I frame. This flexibility exists because if the motion picture is changing too rapidly then it sometimes makes sense to give the intrapicture encoding rather than a forward- or backward-predicted encoding. Thus, each macroblock in a B frame includes a type field that indicates which encoding is used for that macroblock. In the following discussion, however, we consider only the general case in which the macroblock uses bidirectional predictive encoding.
In such a case, each macroblock in a B frame is represented with a 4-tuple: (1) a coordinate for the macroblock in the frame, (2) a motion vector relative to the previous reference frame, (3) a motion vector relative to the subsequent reference frame, and (4) a delta ( \(\delta\) ) for each pixel in the macroblock (i.e., how much each pixel has changed relative to the two reference pixels). For each pixel in the macroblock, the first task is to find the corresponding reference pixel in the past and future reference frames. This is done using the two motion vectors associated with the macroblock. Then, the delta for the pixel is added to the average of these two reference pixels. Stated more precisely, if we let F p and F f denote the past and future reference frames, respectively, and the past/future motion vectors are given by (x p , y p ) and (x f , y f ), then the pixel at coordinate (x,y) in the current frame (denoted F c ) is computed as
where \(\delta\) is the delta for the pixel as specified in the B frame. These deltas are encoded in the same way as pixels in I frames; that is, they are run through DCT and then quantized. Since the deltas are typically small, most of the DCT coefficients are 0 after quantization; hence, they can be effectively compressed.
It should be fairly clear from the preceding discussion how encoding would be performed, with one exception. When generating a B or P frame during compression, MPEG must decide where to place the macroblocks. Recall that each macroblock in a P frame, for example, is defined relative to a macroblock in an I frame, but that the macroblock in the P frame need not be in the same part of the frame as the corresponding macroblock in the I frame—the difference in position is given by the motion vector. You would like to pick a motion vector that makes the macroblock in the P frame as similar as possible to the corresponding macroblock in the I frame, so that the deltas for that macroblock can be as small as possible. This means that you need to figure out where objects in the picture moved from one frame to the next. This is the problem of motion estimation , and several techniques (heuristics) for solving this problem are known. (We discuss papers that consider this problem at the end of this chapter.) The difficulty of this problem is one of the reasons why MPEG encoding takes longer than decoding on equivalent hardware. MPEG does not specify any particular technique; it only defines the format for encoding this information in B and P frames and the algorithm for reconstructing the pixel during decompression, as given above.
Effectiveness and Performance
MPEG typically achieves a compression ratio of 90:1, although ratios as high as 150:1 are not unheard of. In terms of the individual frame types, we can expect a compression ratio of approximately 30:1 for the I frames (this is consistent with the ratios achieved using JPEG when 24-bit color is first reduced to 8-bit color), while P and B frame compression ratios are typically three to five times smaller than the rates for the I frame. Without first reducing the 24 bits of color to 8 bits, the achievable compression with MPEG is typically between 30:1 and 50:1.
MPEG involves an expensive computation. On the compression side, it is typically done offline, which is not a problem for preparing movies for a video-on-demand service. Video can be compressed in real time using hardware today, but software implementations are quickly closing the gap. On the decompression side, low-cost MPEG video boards are available, but they do little more than YUV color lookup, which fortunately is the most expensive step. Most of the actual MPEG decoding is done in software. In recent years, processors have become fast enough to keep pace with 30-frames-per-second video rates when decoding MPEG streams purely in software—modern processors can even decode MPEG streams of high definition video (HDTV).
Video Encoding Standards
We conclude by noting that MPEG is an evolving standard of significant complexity. This complexity comes from a desire to give the encoding algorithm every possible degree of freedom in how it encodes a given video stream, resulting in different video transmission rates. It also comes from the evolution of the standard over time, with the Moving Picture Experts Group working hard to retain backwards compatibility (e.g., MPEG-1, MPEG-2, MPEG-4). What we describe in this book is the essential ideas underlying MPEG-based compression, but certainly not all the intricacies involved in an international standard.
What’s more, MPEG is not the only standard available for encoding video. For example, the ITU-T has also defined the H series for encoding real-time multimedia data. Generally, the H series includes standards for video, audio, control, and multiplexing (e.g., mixing audio, video, and data onto a single bit stream). Within the series, H.261 and H.263 were the first- and second-generation video encoding standards. In principle, both H.261 and H.263 look a lot like MPEG: They use DCT, quantization, and interframe compression. The differences between H.261/H.263 and MPEG are in the details.
Today, a partnership between the ITU-T and the MPEG group has lead to the joint H.264/MPEG-4 standard, which is used for both Blu-ray Discs and by many popular streaming sources (e.g., YouTube, Vimeo).
7.2.4 Transmitting MPEG over a Network
As we’ve noted, MPEG and JPEG are not just compression standards but also definitions of the format of video and images, respectively. Focusing on MPEG, the first thing to keep in mind is that it defines the format of a video stream ; it does not specify how this stream is broken into network packets. Thus, MPEG can be used for videos stored on disk, as well as videos transmitted over a stream-oriented network connection, like that provided by TCP.
What we describe below is called the main profile of an MPEG video stream that is being sent over a network. You can think of an MPEG profile as being analogous to a “version,” except the profile is not explicitly specified in an MPEG header; the receiver has to deduce the profile from the combination of header fields it sees.
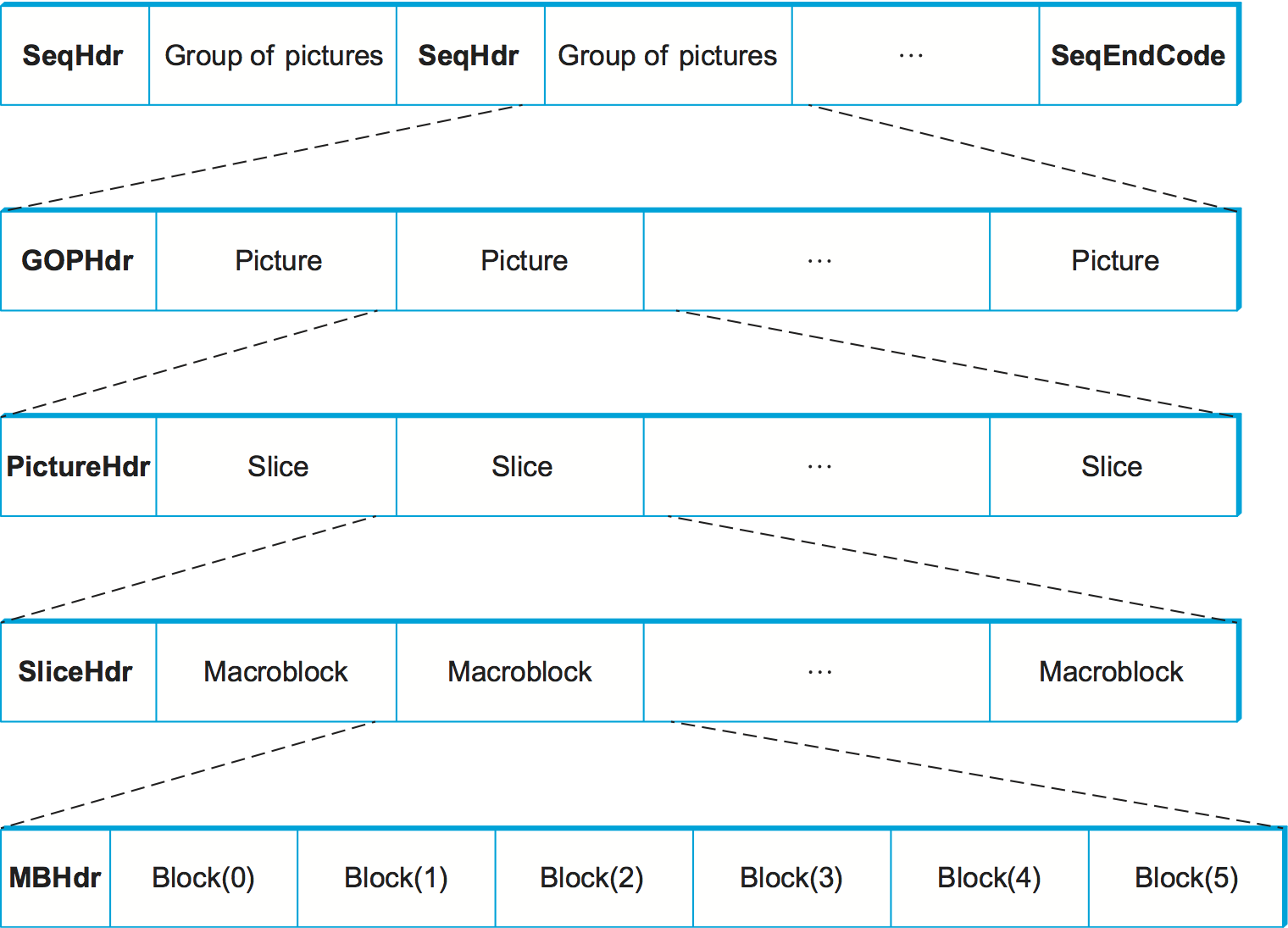
Figure 194. Format of an MPEG-compressed video stream.
A main profile MPEG stream has a nested structure, as illustrated in Figure 194 . (Keep in mind that this figure hides a lot of messy details.) At the outermost level, the video contains a sequence of groups of pictures (GOP) separated by a SeqHdr . The sequence is terminated by a SeqEndCode ( 0xb7 ). The SeqHdr that precedes every GOP specifies—among other things—the size of each picture (frame) in the GOP (measured in both pixels and macroblocks), the interpicture period (measured in μs), and two quantization matrices for the macroblocks within this GOP: one for intracoded macroblocks (I blocks) and one for intercoded macroblocks (B and P blocks). Since this information is given for each GOP—rather than once for the entire video stream, as you might expect—it is possible to change the quantization table and frame rate at GOP boundaries throughout the video. This makes it possible to adapt the video stream over time, as we discuss below.
Each GOP is given by a GOPHdr , followed by the set of pictures that make up the GOP. The GOPHdr specifies the number of pictures in the GOP, as well as synchronization information for the GOP (i.e., when the GOP should play, relative to the beginning of the video). Each picture, in turn, is given by a PictureHdr and a set of slices that make up the picture. (A slice is a region of the picture, such as one horizontal line.) The PictureHdr identifies the type of the picture (I, B, or P) and defines a picture-specific quantization table. The SliceHdr gives the vertical position of the slice, plus another opportunity to change the quantization table—this time by a constant scaling factor rather than by giving a whole new table. Next, the SliceHdr is followed by a sequence of macroblocks. Finally, each macroblock includes a header that specifies the block address within the picture, along with data for the six blocks within the macroblock: one for the U component, one for the V component, and four for the Y component. (Recall that the Y component is 16 × 16, while the U and V components are 8 × 8.)
It should be clear that one of the powers of the MPEG format is that it gives the encoder an opportunity to change the encoding over time. It can change the frame rate, the resolution, the mix of frame types that define a GOP, the quantization table, and the encoding used for individual macroblocks. As a consequence, it is possible to adapt the rate at which a video is transmitted over a network by trading picture quality for network bandwidth. Exactly how a network protocol might exploit this adaptability is currently a subject of research (see sidebar).
Another interesting aspect of sending an MPEG stream over the network is exactly how the stream is broken into packets. If sent over a TCP connection, packetization is not an issue; TCP decides when it has enough bytes to send the next IP datagram. When using video interactively, however, it is rare to transmit it over TCP, since TCP has several features that are ill suited to highly latency-sensitive applications (such as abrupt rate changes after a packet loss and retransmission of lost packets). If we are transmitting video using UDP, say, then it makes sense to break the stream at carefully selected points, such as at macroblock boundaries. This is because we would like to confine the effects of a lost packet to a single macroblock, rather than damaging several macroblocks with a single loss. This is an example of Application Level Framing, which was discussed in an earlier chapter.
Packetizing the stream is only the first problem in sending MPEG-compressed video over a network. The next complication is dealing with packet loss. On the one hand, if a B frame is dropped by the network, then it is possible to simply replay the previous frame without seriously compromising the video; 1 frame out of 30 is no big deal. On the other hand, a lost I frame has serious consequences—none of the subsequent B and P frames can be processed without it. Thus, losing an I frame would result in losing multiple frames of the video. While you could retransmit the missing I frame, the resulting delay would probably not be acceptable in a real-time videoconference. One solution to this problem would be to use the Differentiated Services techniques described in the previous chapter to mark the packets containing I frames with a lower drop probability than other packets.
One final observation is that how you choose to encode video depends on more than just the available network bandwidth. It also depends on the application’s latency constraints. Once again, an interactive application like videoconferencing needs small latencies. The critical factor is the combination of I, P, and B frames in the GOP. Consider the following GOP:
I B B B B P B B B B I
The problem this GOP causes a videoconferencing application is that the sender has to delay the transmission of the four B frames until the P or I that follows them is available. This is because each B frame depends on the subsequent P or I frame. If the video is playing at 15 frames per second (i.e., one frame every 67 ms), this means the first B frame is delayed 4 × 67 ms, which is more than a quarter of a second. This delay is in addition to any propagation delay imposed by the network. A quarter of a second is far greater than the 100-ms threshold that humans are able to perceive. It is for this reason that many videoconference applications encode video using JPEG, which is often called motion-JPEG. (Motion-JPEG also addresses the problem of dropping a reference frame since all frames are able to stand alone.) Notice, however, that an interframe encoding that depends upon only prior frames rather than later frames is not a problem. Thus, a GOP of
I P P P P I
would work just fine for interactive videoconferencing.
Adaptive Streaming
Because encoding schemes like MPEG allow for a trade-off between the bandwidth consumed and the quality of the image, there is an opportunity to adapt a video stream to match the available network bandwidth. This is effectively what video streaming services like Netflix do today.
For starters, let’s assume that we have some way to measure the amount of free capacity and level of congestion along a path, for example, by observing the rate at which packets are successfully arriving at the destination. As the available bandwidth fluctuates, we can feed that information back to the codec so that it adjusts its coding parameters to back off during congestion and to send more aggressively (with a higher picture quality) when the network is idle. This is analogous to the behavior of TCP, except in the video case we are actually modifying the total amount of data sent rather than how long we take to send a fixed amount of data, since we don’t want to introduce delay into a video application.
In the case of video-on-demand services like Netflix, we don’t adapt the encoding on the fly, but instead we encode a handful of video quality levels ahead of time, and save them to files named accordingly. The receiver simply changes the file name it requests to match the quality its measurements indicate the network will be able to deliver. The receiver watches its playback queue, and asks for a higher quality encoding when the queue becomes too full and a lower quality encoding when the queue becomes too empty.
How does this approach know where in the movie to jump to should the requested quality change? In effect, the receiver never asks the sender to stream the whole movie, but instead it requests a sequence of short movie segments, typically a few seconds long (and always on GOP boundary). Each segment is an opportunity to change the quality level to match what the network is able to deliver. (It turns out that requesting movie chunks also makes it easier to implement trick play , jumping around from one place to another in the movie.) In other words, a movie is typically stored as a set of N × M chunks (files): N quality levels for each of M segments.
There’s one last detail. Since the receiver is effectively requesting a sequence of discrete video chunks by name, the most common approach for issuing these requests is to use HTTP. Each chuck is a separate HTTP GET request with the URL identifying the specific chunk the receiver wants next. When you start downloading a movie, your video player first downloads a manifest file that contains nothing more than the URLs for the N × M chunks in the movie, and then it issues a sequence of HTTP requests using the appropriate URL for the situation. This general approach is called HTTP adaptive streaming , although it has been standardized in slightly different ways by various organizations, most notably MPEG’s DASH ( Dynamic Adaptive Streaming over HTTP ) and Apple’s HLS ( HTTP Live Streaming ).
7.2.5 Audio Compression (MP3)
Not only does MPEG define how video is compressed, but it also defines a standard for compressing audio. This standard can be used to compress the audio portion of a movie (in which case the MPEG standard defines how the compressed audio is interleaved with the compressed video in a single MPEG stream) or it can be used to compress stand-alone audio (for example, an audio CD). The MPEG audio compression standard is just one of many for audio compression, but the pivotal role it played means that MP3 (which stands for MPEG Layer III—see below) has become almost synonymous with audio compression.
To understand audio compression, we need to begin with the data. CD-quality audio, which is the de facto digital representation for high-quality audio, is sampled at a rate of 44.1 KHz (i.e., a sample is collected approximately once every 23 μs). Each sample is 16 bits, which means that a stereo (2-channel) audio stream results in a bit rate of
2 × 44.1 × 1000 × 16 = 1.41 Mbps
By comparison, traditional telephone-quality voice is sampled at a rate of 8 KHz, with 8-bit samples, resulting in a bit rate of 64 kbps.
Clearly, some amount of compression is going to be required to transmit CD-quality audio over a network of limited bandwidth. (Consider the fact that MP3 audio streaming became popular in an era when 1.5Mbps home Internet connections were a novelty). To make matters worse, synchronization and error correction overheads inflated the number of bits stored on a CD by a factor of three, so if you just read the data from the CD and sent it over the network, you would need 4.32 Mbps.
Just like video, there is lots of redundancy in audio, and compression takes advantage of this. The MPEG standards define three levels of compression, as enumerated in Table 25 . Of these, Layer III, which is more widely known as MP3, was for many years the most commonly used. In recent years, higher bandwidth codecs have proliferated as streaming audio has become the dominant way many people consume music.
To achieve these compression ratios, MP3 uses techniques that are similar to those used by MPEG to compress video. First, it splits the audio stream into some number of frequency subbands, loosely analogous to the way MPEG processes the Y, U, and V components of a video stream separately. Second, each subband is broken into a sequence of blocks, which are similar to MPEG’s macroblocks except they can vary in length from 64 to 1024 samples. (The encoding algorithm can vary the block size depending on certain distortion effects that are beyond our discussion.) Finally, each block is transformed using a modified DCT algorithm, quantized, and Huffman encoded, just as for MPEG video.
The trick to MP3 is how many subbands it elects to use and how many bits it allocates to each subband, keeping in mind that it is trying to produce the highest-quality audio possible for the target bit rate. Exactly how this allocation is made is governed by psychoacoustic models that are beyond the scope of this book, but to illustrate the idea consider that it makes sense to allocate more bits to low-frequency subbands when compressing a male voice and more bits to high-frequency subbands when compressing a female voice. Operationally, MP3 dynamically changes the quantization tables used for each subband to achieve the desired effect.
Once compressed, the subbands are packaged into fixed-size frames, and a header is attached. This header includes synchronization information, as well as the bit allocation information needed by the decoder to determine how many bits are used to encode each subband. As mentioned above, these audio frames can then be interleaved with video frames to form a complete MPEG stream. One interesting side note is that, while it might work to drop B frames in the network should congestion occur, experience teaches us that it is not a good idea to drop audio frames since users are better able to tolerate bad video than bad audio.

- Multimedia Tutorial
- Multimedia - Home
- Multimedia - Introduction
- Multimedia - Systems
- Multimedia - Authoring
- Multimedia - Images & Graphics
- Multimedia - Sound & Audio
- Multimedia - Digital Audio Coding
- Multimedia Useful Resources
- Multimedia - Quick Guide
- Multimedia - Useful Resources
- Selected Reading
- UPSC IAS Exams Notes
- Developer's Best Practices
- Questions and Answers
- Effective Resume Writing
- HR Interview Questions
- Computer Glossary
Multimedia Images & Graphics
An image consists of a rectangular array of dots called pixels. The size of the image is specified in terms of width X height, in numbers of the pixels. The physical size of the image, in inches or centimeters, depends on the resolution of the device on which the image is displayed. The resolution is usually measured in DPI (Dots Per Inch). An image will appear smaller on a device with a higher resolution than on one with a lower resolution. For color images, one needs enough bits per pixel to represent all the colors in the image. The number of the bits per pixel is called the depth of the image.
Image data types
Images can be created by using different techniques of representation of data called data type like monochrome and colored images. Monochrome image is created by using single color whereas colored image is created by using multiple colors. Some important data types of images are following:
1-bit images - An image is a set of pixels. Note that a pixel is a picture element in digital image. In 1-bit images, each pixel is stored as a single bit (0 or 1). A bit has only two states either on or off, white or black, true or false. Therefore, such an image is also referred to as a binary image, since only two states are available. 1-bit image is also known as 1-bit monochrome images because it contains one color that is black for off state and white for on state.
A 1-bit image with resolution 640*480 needs a storage space of 640*480 bits.
640 x 480 bits. = (640 x 480) / 8 bytes = (640 x 480) / (8 x 1024) KB= 37.5KB.
The clarity or quality of 1-bit image is very low.
8-bit Gray level images - Each pixel of 8-bit gray level image is represented by a single byte (8 bits). Therefore each pixel of such image can hold 2 8 =256 values between 0 and 255. Therefore each pixel has a brightness value on a scale from black (0 for no brightness or intensity) to white (255 for full brightness or intensity). For example, a dark pixel might have a value of 15 and a bright one might be 240.
A grayscale digital image is an image in which the value of each pixel is a single sample, which carries intensity information. Images are composed exclusively of gray shades, which vary from black being at the weakest intensity to white being at the strongest. Grayscale images carry many shades of gray from black to white. Grayscale images are also called monochromatic, denoting the presence of only one (mono) color (chrome). An image is represented by bitmap. A bitmap is a simple matrix of the tiny dots (pixels) that form an image and are displayed on a computer screen or printed.
A 8-bit image with resolution 640 x 480 needs a storage space of 640 x 480 bytes=(640 x 480)/1024 KB= 300KB. Therefore an 8-bit image needs 8 times more storage space than 1-bit image.
24-bit color images - In 24-bit color image, each pixel is represented by three bytes, usually representing RGB (Red, Green and Blue). Usually true color is defined to mean 256 shades of RGB (Red, Green and Blue) for a total of 16777216 color variations. It provides a method of representing and storing graphical image information an RGB color space such that a colors, shades and hues in large number of variations can be displayed in an image such as in high quality photo graphic images or complex graphics.
Many 24-bit color images are stored as 32-bit images, and an extra byte for each pixel used to store an alpha value representing special effect information.
A 24-bit color image with resolution 640 x 480 needs a storage space of 640 x 480 x 3 bytes = (640 x 480 x 3) / 1024=900KB without any compression. Also 32-bit color image with resolution 640 x 480 needs a storage space of 640 x 480 x 4 bytes= 1200KB without any compression.
Disadvantages
Require large storage space
Many monitors can display only 256 different colors at any one time. Therefore, in this case it is wasteful to store more than 256 different colors in an image.
8-bit color images - 8-bit color graphics is a method of storing image information in a computer's memory or in an image file, where one byte (8 bits) represents each pixel. The maximum number of colors that can be displayed at once is 256. 8-bit color graphics are of two forms. The first form is where the image stores not color but an 8-bit index into the color map for each pixel, instead of storing the full 24-bit color value. Therefore, 8-bit image formats consists of two parts: a color map describing what colors are present in the image and the array of index values for each pixel in the image. In most color maps each color is usually chosen from a palette of 16,777,216 colors (24 bits: 8 red, 8green, 8 blue).
The other form is where the 8-bits use 3 bits for red, 3 bits for green and 2 bits for blue. This second form is often called 8-bit true color as it does not use a palette at all. When a 24-bit full color image is turned into an 8-bit image, some of the colors have to be eliminated, known as color quantization process.
A 8-bit color image with resolution 640 x 480 needs a storage space of 640 x 480 bytes=(640 x 480) / 1024KB= 300KB without any compression.
Color lookup tables
A color loop-up table (LUT) is a mechanism used to transform a range of input colors into another range of colors. Color look-up table will convert the logical color numbers stored in each pixel of video memory into physical colors, represented as RGB triplets, which can be displayed on a computer monitor. Each pixel of image stores only index value or logical color number. For example if a pixel stores the value 30, the meaning is to go to row 30 in a color look-up table (LUT). The LUT is often called a Palette.
Characteristic of LUT are following:
The number of entries in the palette determines the maximum number of colors which can appear on screen simultaneously.
The width of each entry in the palette determines the number of colors which the wider full palette can represent.
A common example would be a palette of 256 colors that is the number of entries is 256 and thus each entry is addressed by an 8-bit pixel value. Each color can be chosen from a full palette, with a total of 16.7 million colors that is the each entry is of 24 bits and 8 bits per channel which sets the total combinations of 256 levels for each of the red, green and blue components 256 x 256 x 256 =16,777,216 colors.
Image file formats
GIF- Graphics Interchange Formats - The GIF format was created by Compuserve. It supports 256 colors. GIF format is the most popular on the Internet because of its compact size. It is ideal for small icons used for navigational purpose and simple diagrams. GIF creates a table of up to 256 colors from a pool of 16 million. If the image has less than 256 colors, GIF can easily render the image without any loss of quality. When the image contains more colors, GIF uses algorithms to match the colors of the image with the palette of optimum set of 256 colors available. Better algorithms search the image to find and the optimum set of 256 colors.
Thus GIF format is lossless only for the image with 256 colors or less. In case of a rich, true color image GIF may lose 99.998% of the colors. GIF files can be saved with a maximum of 256 colors. This makes it is a poor format for photographic images.
GIFs can be animated, which is another reason they became so successful. Most animated banner ads are GIFs. GIFs allow single bit transparency that is when you are creating your image, you can specify which color is to be transparent. This provision allows the background colors of the web page to be shown through the image.
JPEG- Joint Photographic Experts Group - The JPEG format was developed by the Joint Photographic Experts Group. JPEG files are bitmapped images. It store information as 24-bit color. This is the format of choice for nearly all photograph images on the internet. Digital cameras save images in a JPEG format by default. It has become the main graphics file format for the World Wide Web and any browser can support it without plug-ins. In order to make the file small, JPEG uses lossy compression. It works well on photographs, artwork and similar materials but not so well on lettering, simple cartoons or line drawings. JPEG images work much better than GIFs. Though JPEG can be interlaced, still this format lacks many of the other special abilities of GIFs, like animations and transparency, but they really are only for photos.
PNG- Portable Network Graphics - PNG is the only lossless format that web browsers support. PNG supports 8 bit, 24 bits, 32 bits and 48 bits data types. One version of the format PNG-8 is similar to the GIF format. But PNG is the superior to the GIF. It produces smaller files and with more options for colors. It supports partial transparency also. PNG-24 is another flavor of PNG, with 24-bit color supports, allowing ranges of color akin to high color JPEG. PNG-24 is in no way a replacement format for JPEG because it is a lossless compression format. This means that file size can be rather big against a comparable JPEG. Also PNG supports for up to 48 bits of color information.
TIFF- Tagged Image File Format - The TIFF format was developed by the Aldus Corporation in the 1980 and was later supported by Microsoft. TIFF file format is widely used bitmapped file format. It is supported by many image editing applications, software used by scanners and photo retouching programs.
TIFF can store many different types of image ranging from 1 bit image, grayscale image, 8 bit color image, 24 bit RGB image etc. TIFF files originally use lossless compression. Today TIFF files also use lossy compression according to the requirement. Therefore, it is a very flexible format. This file format is suitable when the output is printed. Multi-page documents can be stored as a single TIFF file and that is way this file format is so popular. The TIFF format is now used and controlled by Adobe.
BMP- Bitmap - The bitmap file format (BMP) is a very basic format supported by most Windows applications. BMP can store many different type of image: 1 bit image, grayscale image, 8 bit color image, 24 bit RGB image etc. BMP files are uncompressed. Therefore, these are not suitable for the internet. BMP files can be compressed using lossless data compression algorithms.
EPS- Encapsulated Postscript - The EPS format is a vector based graphic. EPS is popular for saving image files because it can be imported into nearly any kind of application. This file format is suitable for printed documents. Main disadvantage of this format is that it requires more storage as compare to other formats.
PDF- Portable Document Format - PDF format is vector graphics with embedded pixel graphics with many compression options. When your document is ready to be shared with others or for publication. This is only format that is platform independent. If you have Adobe Acrobat you can print from any document to a PDF file. From illustrator you can save as .PDF.
EXIF- Exchange Image File - Exif is an image format for digital cameras. A variety of tage are available to facilitate higher quality printing, since information about the camera and picture - taking condition can be stored and used by printers for possible color correction algorithms.it also includes specification of file format for audio that accompanies digital images.
WMF- Windows MetaFile - WMF is the vector file format for the MS-Windows operating environment. It consists of a collection of graphics device interface function calls to the MS-Windows graphice drawing library.Metafiles are both small and flexible, hese images can be displayed properly by their proprietary softwares only.
PICT - PICT images are useful in Macintosh software development, but you should avoid them in desktop publishing. Avoid using PICT format in electronic publishing-PICT images are prone to corruption.
Photoshop - This is the native Photoshop file format created by Adobe. You can import this format directly into most desktop publishing applications.
To Continue Learning Please Login
- School Guide
- Mathematics
- Number System and Arithmetic
- Trigonometry
- Probability
- Mensuration
- Maths Formulas
- Class 8 Maths Notes
- Class 9 Maths Notes
- Class 10 Maths Notes
- Class 11 Maths Notes
- Class 12 Maths Notes
What are the different ways of Data Representation?
- What are the Different Kinds of Data Scientists?
- Different types of Coding Schemes to represent data
- Graphical Representation of Data
- What are the types of statistics?
- Textual Presentation of Data: Meaning, Suitability, and Drawbacks
- Diagrammatic and Graphic Presentation of Data
- Different Types of Data in Data Mining
- Tabular Presentation of Data: Meaning, Objectives, Features and Merits
- What is a Dataset: Types, Features, and Examples
- Data Manipulation: Definition, Examples, and Uses
- Collection and Presentation of Data
- What is Data Organization?
- Different forms of data representation in today's world
- Difference Between Presentation and Representation
- What are the Basic Data Types in PHP ?
- Processing of Raw Data to Tidy Data in R
- Graph and its representations
- Difference between Information and Data
- Data Preprocessing and Its Types
The process of collecting the data and analyzing that data in large quantity is known as statistics. It is a branch of mathematics trading with the collection, analysis, interpretation, and presentation of numeral facts and figures.
It is a numerical statement that helps us to collect and analyze the data in large quantity the statistics are based on two of its concepts:
- Statistical Data
- Statistical Science
Statistics must be expressed numerically and should be collected systematically.
Data Representation
The word data refers to constituting people, things, events, ideas. It can be a title, an integer, or anycast. After collecting data the investigator has to condense them in tabular form to study their salient features. Such an arrangement is known as the presentation of data.
It refers to the process of condensing the collected data in a tabular form or graphically. This arrangement of data is known as Data Representation.
The row can be placed in different orders like it can be presented in ascending orders, descending order, or can be presented in alphabetical order.
Example: Let the marks obtained by 10 students of class V in a class test, out of 50 according to their roll numbers, be: 39, 44, 49, 40, 22, 10, 45, 38, 15, 50 The data in the given form is known as raw data. The above given data can be placed in the serial order as shown below: Roll No. Marks 1 39 2 44 3 49 4 40 5 22 6 10 7 45 8 38 9 14 10 50 Now, if you want to analyse the standard of achievement of the students. If you arrange them in ascending or descending order, it will give you a better picture. Ascending order: 10, 15, 22, 38, 39, 40, 44. 45, 49, 50 Descending order: 50, 49, 45, 44, 40, 39, 38, 22, 15, 10 When the row is placed in ascending or descending order is known as arrayed data.
Types of Graphical Data Representation
Bar chart helps us to represent the collected data visually. The collected data can be visualized horizontally or vertically in a bar chart like amounts and frequency. It can be grouped or single. It helps us in comparing different items. By looking at all the bars, it is easy to say which types in a group of data influence the other.
Now let us understand bar chart by taking this example Let the marks obtained by 5 students of class V in a class test, out of 10 according to their names, be: 7,8,4,9,6 The data in the given form is known as raw data. The above given data can be placed in the bar chart as shown below: Name Marks Akshay 7 Maya 8 Dhanvi 4 Jaslen 9 Muskan 6
A histogram is the graphical representation of data. It is similar to the appearance of a bar graph but there is a lot of difference between histogram and bar graph because a bar graph helps to measure the frequency of categorical data. A categorical data means it is based on two or more categories like gender, months, etc. Whereas histogram is used for quantitative data.
For example:
The graph which uses lines and points to present the change in time is known as a line graph. Line graphs can be based on the number of animals left on earth, the increasing population of the world day by day, or the increasing or decreasing the number of bitcoins day by day, etc. The line graphs tell us about the changes occurring across the world over time. In a line graph, we can tell about two or more types of changes occurring around the world.
For Example:
Pie chart is a type of graph that involves a structural graphic representation of numerical proportion. It can be replaced in most cases by other plots like a bar chart, box plot, dot plot, etc. As per the research, it is shown that it is difficult to compare the different sections of a given pie chart, or if it is to compare data across different pie charts.
Frequency Distribution Table
A frequency distribution table is a chart that helps us to summarise the value and the frequency of the chart. This frequency distribution table has two columns, The first column consist of the list of the various outcome in the data, While the second column list the frequency of each outcome of the data. By putting this kind of data into a table it helps us to make it easier to understand and analyze the data.
For Example: To create a frequency distribution table, we would first need to list all the outcomes in the data. In this example, the results are 0 runs, 1 run, 2 runs, and 3 runs. We would list these numerals in numerical ranking in the foremost queue. Subsequently, we ought to calculate how many times per result happened. They scored 0 runs in the 1st, 4th, 7th, and 8th innings, 1 run in the 2nd, 5th, and the 9th innings, 2 runs in the 6th inning, and 3 runs in the 3rd inning. We set the frequency of each result in the double queue. You can notice that the table is a vastly more useful method to show this data. Baseball Team Runs Per Inning Number of Runs Frequency 0 4 1 3 2 1 3 1
Sample Questions
Question 1: Considering the school fee submission of 10 students of class 10th is given below:
In order to draw the bar graph for the data above, we prepare the frequency table as given below. Fee submission No. of Students Paid 6 Not paid 4 Now we have to represent the data by using the bar graph. It can be drawn by following the steps given below: Step 1: firstly we have to draw the two axis of the graph X-axis and the Y-axis. The varieties of the data must be put on the X-axis (the horizontal line) and the frequencies of the data must be put on the Y-axis (the vertical line) of the graph. Step 2: After drawing both the axis now we have to give the numeric scale to the Y-axis (the vertical line) of the graph It should be started from zero and ends up with the highest value of the data. Step 3: After the decision of the range at the Y-axis now we have to give it a suitable difference of the numeric scale. Like it can be 0,1,2,3…….or 0,10,20,30 either we can give it a numeric scale like 0,20,40,60… Step 4: Now on the X-axis we have to label it appropriately. Step 5: Now we have to draw the bars according to the data but we have to keep in mind that all the bars should be of the same length and there should be the same distance between each graph
Question 2: Watch the subsequent pie chart that denotes the money spent by Megha at the funfair. The suggested colour indicates the quantity paid for each variety. The total value of the data is 15 and the amount paid on each variety is diagnosed as follows:
Chocolates – 3
Wafers – 3
Toys – 2
Rides – 7
To convert this into pie chart percentage, we apply the formula: (Frequency/Total Frequency) × 100 Let us convert the above data into a percentage: Amount paid on rides: (7/15) × 100 = 47% Amount paid on toys: (2/15) × 100 = 13% Amount paid on wafers: (3/15) × 100 = 20% Amount paid on chocolates: (3/15) × 100 = 20 %
Question 3: The line graph given below shows how Devdas’s height changes as he grows.
Given below is a line graph showing the height changes in Devdas’s as he grows. Observe the graph and answer the questions below.
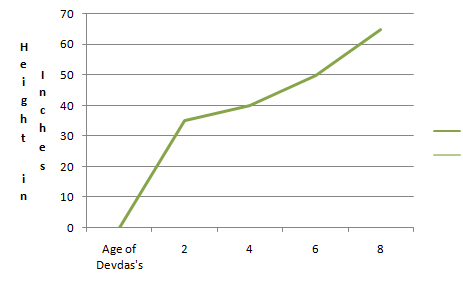
(i) What was the height of Devdas’s at 8 years? Answer: 65 inches (ii) What was the height of Devdas’s at 6 years? Answer: 50 inches (iii) What was the height of Devdas’s at 2 years? Answer: 35 inches (iv) How much has Devdas’s grown from 2 to 8 years? Answer: 30 inches (v) When was Devdas’s 35 inches tall? Answer: 2 years.
Please Login to comment...
Similar reads.
- School Learning
Improve your Coding Skills with Practice
What kind of Experience do you want to share?
Multimedia data mining: state of the art and challenges
- Published: 16 November 2010
- Volume 51 , pages 35–76, ( 2011 )
Cite this article
- Chidansh Amitkumar Bhatt 1 &
- Mohan S. Kankanhalli 1
2893 Accesses
64 Citations
Explore all metrics
Advances in multimedia data acquisition and storage technology have led to the growth of very large multimedia databases. Analyzing this huge amount of multimedia data to discover useful knowledge is a challenging problem. This challenge has opened the opportunity for research in Multimedia Data Mining (MDM). Multimedia data mining can be defined as the process of finding interesting patterns from media data such as audio, video, image and text that are not ordinarily accessible by basic queries and associated results. The motivation for doing MDM is to use the discovered patterns to improve decision making. MDM has therefore attracted significant research efforts in developing methods and tools to organize, manage, search and perform domain specific tasks for data from domains such as surveillance, meetings, broadcast news, sports, archives, movies, medical data, as well as personal and online media collections. This paper presents a survey on the problems and solutions in Multimedia Data Mining, approached from the following angles: feature extraction, transformation and representation techniques, data mining techniques, and current multimedia data mining systems in various application domains. We discuss main aspects of feature extraction, transformation and representation techniques. These aspects are: level of feature extraction, feature fusion, features synchronization, feature correlation discovery and accurate representation of multimedia data. Comparison of MDM techniques with state of the art video processing, audio processing and image processing techniques is also provided. Similarly, we compare MDM techniques with the state of the art data mining techniques involving clustering, classification, sequence pattern mining, association rule mining and visualization. We review current multimedia data mining systems in detail, grouping them according to problem formulations and approaches. The review includes supervised and unsupervised discovery of events and actions from one or more continuous sequences. We also do a detailed analysis to understand what has been achieved and what are the remaining gaps where future research efforts could be focussed. We then conclude this survey with a look at open research directions.
This is a preview of subscription content, log in via an institution to check access.
Access this article
Price includes VAT (Russian Federation)
Instant access to the full article PDF.
Rent this article via DeepDyve
Institutional subscriptions
Similar content being viewed by others
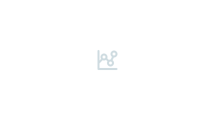
A Comprehensive Survey of Clustering Algorithms
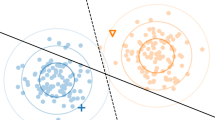
A survey on semi-supervised learning
A survey of transfer learning.
Agrawal R, Imielinski T, Swami AN (1993) Mining association rules between sets of items in large databases. In: ACM SIGMOD international conference on management of data, pp 207–216
Agrawal R, Srikant R (1995) Mining sequential patterns. In: International conference on data engineering
Ajmera J, McCowan I, Bourlard H (2002) Robust hmm-based speech/music segmentation. In: IEEE international conference on acoustics, speech and signal processing, pp 1746–1749
Aradhye H, Toderici G, Yagnik J (2009) Video2text: learning to annotate video content. In: International conference on data mining workshops, pp 144–151
Artigan JA (1975) Clustering algorithms. Wiley, New York
Google Scholar
Baillie M, Jose JM (2004) An audio-based sports video segmentation and event detection algorithm. In: Workshop on event mining, detection and recognition of events in video
Barnard K, Duygulu P, Forsyth DA, de Freitas N, Blei DM, Jordan MI (2003) Matching words and pictures. J Mach Learn Res 3:1107–1135
Article MATH Google Scholar
Benitez AB, Smith JR, Chang SF (2000) A multimedia information network for knowledge representation. SPIE, Bellingham
Box G, Jenkins GM, Reinsel G (1994) Time series analysis: forecasting and control. Pearson Education, Paris
MATH Google Scholar
Briggs F, Raich R, Fern X (2009) Audio classification of bird species: a statistical manifold approach. In: IEEE international conference on data mining (ICDM), pp 51–60
Chang E, Goh K, Sychay G, Wu G (2002) Content-based annotation for multimodal image retrieval using bayes point machines. IEEE Trans Circuits Syst Video Technol 13(1):26–38
Article Google Scholar
Chang E, Li C, Wang J (1999) Searching near replicas of image via clustering. In: SPIE multimedia storage and archiving systems, vol 6
Chen M, Chen SC, Shyu ML (2007) Hierarchical temporal association mining for video event detection in video databases. In: Multimedia databases and data management
Chen M, Chen SC, Shyu ML, Wickramaratna K (2006) Semantic event detection via multimodal data mining. IEEE Signal Process Mag 23:38–46
Chen SC, Shyu ML, Zhang C, Strickrott J (2001) Multimedia data mininig for traffic video sequenices. In: ACM SIGKDD
Chen SC, Shyu ML, Chen M, Zhang C (2004) A decision tree-based multimodal data mining framework for soccer goal detection. In: IEEE international conference multimedia and expo, pp 265–268
Dai K, Zhang J, Li G (2006) Video mining: concepts, approaches and applications. In: Multi-media modelling
Darrell T, Pentland A (1993) Space-time gestures. In: IEEE Computing Society conference on computer vision and pattern recognition, pp 335–340
Davis SB, Mermelstein P (1980) Comparison of parametric representations for monosyllabic word recognition in continuously spoken sentences. IEEE Trans Acoust Speech Signal Process 28(4):357–366
Dhillon I (2001) Co-clustering documents and words using bipartite spectral graph partitioning. In: ACM SIGKDD
Dimitriadis D, Maragos P (2003) Robust energy demodulation based on continuous models with application to speech recognition. In: European conference on speech communication and technology
Duda R, Hart P, Stork D (2001) Pattern classification. Wiley, New York
El-Maleh K, Klein M, Petrucci G, Kabal P (2000) Speech/music discrimination for multimedia application. In: International conference on acoustics, speech and signal processing, pp 2445–2448
Ellom BL, Hansen JHL (1998) Automatic segmentation of speech recorded in uknown noisy channel characteristics. Speech Commun 25:97–116
Ester M, Kriegel HP, Sander J, Xu X (1996) A density-based algorithm for discovering clusters in large spatial databases with noise. In: International conference on knowledge discovery and data mining, pp 226–231
Fu CS, Chen W, Jianhao MH, Sundaram H, Zhong D (1998) A fully automated content based video search engine supporting spatio-temporal queries. IEEE Trans Circuits Syst Video Technol 8(5):602–615
Faloutsos C, Equitz W, Flickner M, Niblack W, Petkovic D, Barber R (1994) Efficient and effective querying by image content. Journal of Intelligent Information Systems 3:231–262
Fan J, Gao Y, Luo H (2007) Hierarchical classification for automatic image annotation. In: ACM SIGIR, pp 111–118
Fan J, Gao Y, Luo H, Jain R (2008) Mining multilevel image semantics via hierarchical classification. IEEE Trans Multimedia 10(2):167–187
Fan J, Gao Y, Luo H, Xu G (2005) Statistical modeling and conceptualization of natural scenes. Pattern Recogn 38(6):865–885
Fersini E, Messina E, Arosio G, Archetti F (2009) Audio-based emotion recognition in judicial domain: a multilayer support vector machines approach. In: Machine learning and data mining in pattern recognition (MLDM), pp 594–602
Foote JT (1997) Content-based retrieval of music and audio. SPIE 3229:138–147
Forsati R, Mahdavi M (2010) Web text mining using harmony search. In: Recent advances in harmony search algorithm, pp 51–64
Frakes WB, Baeza-Yates R (1992) Information retrieval: data structures and algorithms. Prentice-Hall, Englewood Cliffs
Frigui H, Caudill J (2007) Mining visual and textual data for constructing a multi-modal thesaurus. In: SIAM international conference on data mining
Furui S (1981) Cepstral analysis technique for automatic speaker verification. IEEE Trans Acoust Speech Signal Process 29(2):254–272
Gajic B, Paliwal KK (2001) Robust feature extraction using subband spectral centroid histograms. In: International conference on acoustics, speech and signal processing, vol 1, pp 85–88
Gao J, Sun Y, Suo H, Zhao Q, Yan Y (2009) Waps: an audio program surveillance system for large scale web data stream. In: International conference on web information systems and mining (WISM), pp 116–128
Gao Y, Fan J (2006) Incorporate concept ontology to enable probabilistic concept reasoning for multi-level image annotation. In: ACM MIR
Garner P, Fukadam T, Komori Y (2004) A differential spectral voice activity detector. In: International conference on acoustics, speech and signal processing, vol 1, pp 597–600
Ghitza O (1987) Auditory nerve representation as a front-end in a noisy environment. Comput Speech Lang 2(1):109–130
Goh KS, Miyahara K, Radhakrishan R, Xiong Z, Divakaran A (2004) Audio-visual event detection based on mining of semantic audio-visual labels. In: SPIE conference on storage and retrieval of multimedia databases, vol 5307, pp 292–299
Gold B, Morgan N (2000) Speech and audio signal processing: processing and perception of speech and music. Wiley, New York
Gool LV, Breitenstein MD, Gammeter S, Grabner H, Quack T (2009) Mining from large image sets. In: ACM international conference on image and video retrieval(CIVR), pp 1–8
Gorkani MM, Con R, Picard W (1994) Texture orientation for sorting photos at a glance. In: IEEE conference on pattern recognition
Guo GD, Li SZ (2003) Content-based audio classification and retrieval by support vector machines. IEEE Trans Neural Netw 14(1):209–215
Guo Z, Zhang Z, Xing EP, Faloutsos C (2007) Enhanced max margin learning on multimodal data mining in a multimedia database. In: ACM international conference knowledge discovery and data mining
Hall M, Frank E, Holmes G, Pfahringer B, Reutemann P, Ian H (2009) The Weka data mining software: an update. In: SIGKDD explorations, vol 11
Han J, Kamber M (2006) Data mining concepts and techniques. Morgan Kaufmann, San Mateo
Han J, Pei J (2000) Mining frequent patterns by pattern-growth: methodology and implications. ACM SIGKDD Explorations Newsletter 2(2):14–20
Harb H, Chen L, Auloge JY (2001) Speech/music/silence and gender detection algorithm. In: International conference on distributed multimedia systems, pp 257–262
He R, Xiong N, Yang L, Park J (2010) Using multi-modal semantic association rules to fuse keywords and visual features automatically for web image retrieval. In: International conference on information fusion
He R, Zhan W (2009) Multi-modal mining in web image retrieval. In: Asia-Pacific conference on computational intelligence and industrial applications
Hermansky H (1987) An efficient speaker independent automatic speech recognition by simulation of some properties of human auditory perception. In: International conference on acoustics, speech and signal processing, pp 1156–1162
Hermansky H (1987) An efficient speaker independent automatic speech recognition by simulation of some properties of human auditory perception. In: IEEE international conference on acoustics, speech and signal processing, pp 1156–1162
Hermansky H (1990) Perceptual linear predictive (plp) analysis of speech. J Acoust Soc Am 87(4):1738–1752
Hermansky H, Morgan N (1994) Rasta processing of speech. IEEE Trans Acoust Speech Signal Process 2(4):578–589
Hermansky H, Morgan N, Bayya A, Kohn, P (1991) Compensation for the effect of the communication channel in auditory-like analysis of speech. In: European conference on speech communication and technology pp, 578–589
Hermansky H, Sharma S (1998) Traps-classifiers of temporal patterns. In: International conference on speech and language processing
Hipp J, Güntzer U, Nakhaeizadeh G (2000) Algorithms for association rule mining a general survey and comparison. SIGKDD Explorations 2(2):1–58
Huang J, Kumar S, Zabih R (1998) An automatic hierarchical image classification scheme. In: ACM multimedia
Hwan OJ, Lee JK, Kote S (2003) Real time video data mining for surveillance video streams. In: Pacific-Asia conference on knowledge discovery and data mining
Jain AK, Murty MN, Flynn PJ (1999) Data clustering: a review. ACM Comput Surv (CSUR) 31(3):264–323
Jiang C, Coenena F, Sandersona R, Zito M (2010) Text classification using graph mining-based feature extraction. Knowl-based Syst 23(4):302–308
Jiang T (2009) Learning image text associations. IEEE Trans Knowl Data Eng 21(2):161–177
Juang BH, Rabiner L (1993) Fundamentals of speech recognition. Prentice-Hall, Englewood Cliffs
Kemp T, Schmidt M, Westphal M, Waibel A (2000) Strategies for automatic segmentation of audio data. In: International conference on acoustics, speech and signal processing
Kotsiantis S, Kanellopoulos D (2006) Association rules mining: a recent overview. Int Trans Comput Sci Eng 32(1):71–82
Kruskal JB (1983) An overview of sequence comparison: timewarps, string edits and macromolecules. SIAM Rev 25:201–237
Article MATH MathSciNet Google Scholar
Kubin G, Kleijn WB (1994) Time-scale modification of speech based on a nonlinear oscillator model. In: IEEE international conference on acoustics, speech and signal processing
Kurabayashi S, Kiyoki Y (2010) Mediamatrix: A video stream retrieval system with mechanisms for mining contexts of query examples. In: Database systems for advanced applications (DASFAA)
Leavitt N (2002) Let’s hear it for audio mining. Computer 35:23–25
Li D, Dimitrova N, Li M, Sethi KI (2003) Multimedia content processing through cross-modal association. In: ACM multimedia, pp 604–611
Li N, Wu DD (2010) Using text mining and sentiment analysis for online forums hotspot detection and forecast. Decis Support Syst 48:354–368
Li SZ (2000) Content-based audio classification and retrieval using the nearest feature line method. In: International conference on acoustics, speech and signal processing, vol 8(5), pp 619–625
Li Y, Shapiro LG, Bilmes JA (2005) A generative/discriminative learning algorithm for image classification. In: IEEE international conference of computer vision
Lilt D, Kubala F (2004) Online speaker clustering. In: IEEE international conference on acoustics, speech, and signal processing (ICASSP)
Lin L, Ravitz G, Shyu ML, Chen SC (2007) Video semantic concept discovery using multimodal-based association classification. In: IEEE international conference on multimedia and expo, pp 859–862
Lin L, Shyu ML (2009) Mining high-level features from video using associations and correlations. In: International conference on semantic computing, pp 137–144
Lin L, Shyu ML, Ravitz G, Chen SC (2009) Video semantic concept detection via associative classification. In: IEEE international conference on multimedia and expo, pp 418–421
Lin W, Jin R, Hauptmann AG (2002) Triggering memories of conversations using multimodal classifiers. In: Workshop on intelligent situation aware media and presentation
Lin WH, Hauptmann A (2003) Meta-classification: combining multimodal classifiers. Lect Notes Comput Sci 2797:217–231
Lin WH, Jin R, Hauptmann AG (2002) News video classification using svm-based multimodal classifiers and combination strategies. In: ACM multimedia
Liu J, Jiang L, Wu Z, Zheng Q, Qian Y (2010) Mining preorder relation between knowledge elements from text. In: ACM symposium on applied computing
Liu Q, Sung A, Qiao M (2009) Spectrum steganalysis of wav audio streams. In: International conference on machine learning and data mining in pattern recognition (MLDM), pp 582–593
Mannila H, Toivonen H, Verkamo AI (1997) Discovery of frequent episodes in event sequences. Data Mining Knowledge Discovery 1:259–289
Maragos P (1991) Fractal aspects of speech signals: dimension and interpolation. In: IEEE international conference on acoustics, speech and signal processing
Maragos P, Potamianos A (1999) Fractal dimensios of speech sounds: computation and application to automatic speech recognition. J Acoust Soc Am 105(3):1925–1932
Mase K, Sawamoto Y, Koyama Y, Suzuki T, Katsuyama K (2009) Interaction pattern and motif mining method for doctor-patient multi-modal dialog analysis. In: Multimodal sensor-based systems and mobile phones for social computing, pp 1–4
Matsuo Y, Shirahama K, Uehara K (2003) Video data mining: extracting cinematic rules from movies. In: International workshop on multimedia data mining, pp 18–27
Megalooikonomou V, Davataikos C, Herskovits EH (1999) Mining lesion-deficit associations in a brain image database. In: ACM SIGKDD
Meinedo H, Neto J (2005) A stream-based audio segmentation, classification and clustering pre-processing system for broadcast news using ann models. In: Interspeech—Eurospeech
Mesgarani N, Shamma S, Slaney M (2004) Speech discrimination based on multiscale spectrotemporal modulations. In: International conference on acoustics, speech and signal processing, vol 1, pp 601–604
Messina A, Montagnuolo M (2009) A generalised cross-modal clustering method applied to multimedia news semantic indexing and retrieval. In: International conference on world wide web (WWW), pp 321–330
Montagnuolo M, Messina A, Ferri M (2010) Hmnews: a multimodal news data association framework. In: Symposium on applied computing (SAC), pp 1823–1824
Moreno PJ, Rifkin R (2000) Using the fisher kernel method for web audio classification. In: IEEE international conference on acoustics, speech and signal processing
Nørvåg K, Øivind Eriksen T, Skogstad KI (2006) Mining association rules in temporal document collections. In: International symposium on methodologies for intelligent systems (ISMIS), pp 745–754
Nørvåg K, Fivelstad OK (2009) Semantic-based temporal text-rule mining. In: International conference on computational linguistics and intelligent text processing, pp 442–455
Oates T, Cohen P (1996) Searching for structure in multiplestreams of data. In: International conference of machine learning, pp 346–354
Oh J, Bandi B (2002) Multimedia data mining framework for raw video sequences. In: International workshop on multimedia data mining (MDM/KDD), pp 1–10
Ordonez C, Omiecinski E (1999) Discovering association rules based on image content. In: IEEE advances in digital libraries conference
Pan J, Faloutsos C (2002) Videocube: a novel tool for video mining and classification. In: International conference on Asian digital libraries (ICADL), pp 194–205
Pan JY, Yang HJ, Faloutsos C, Duygulu P (2004) Automatic multimedia cross-modal correlation discovery. In: ACM SIGKDD conference on knowledge discovery and data mining
Patel N, Sethi I (2007) Multimedia data mining: an overview. In: Multimedia data mining and knowledge discovery. Springer
Pentland A, Picard RW, Sclaroff S (1996) Photobook: content-based manipulation of image databases. Int J Comput Vis 18:233–254
Pfeiffer S, Fischer S, Effelsberg W (1996) Automatic audio content analysis. In: ACM multimedia, pp 21–30
Pinquier J, Rouas JL, Andre-Obrecht R (2002) Robust speech/music classification in audio documents. In: International conference on speech and language processing, vol 3, pp 2005–2008
Porter M (1980) An algorithm for suffix stripping. Program 14(3):130–137
Quatieri TF, Hofstetter EM (1990) Short-time signal representation by nonlinear difference equations. In: International conference on acoustics, speech and signal processing
Quinlan JR (1993) C4.5: programs for machine learning. Morgan Kaufmann, San Mateo
Rabiner LR (1989) A tutorial on hidden Markov models and selected applications in speech recognition. Proc IEEE 77(2):257–286
Rajendran P, Madheswaran M (2009) An improved image mining technique for brain tumour classification using efficient classifier. International Journal of Computer Science and Information Security (IJCSIS) 6(3):107–116
Ramachandran C, Malik R, Jin X, Gao J, Nahrstedt K, Han J (2009) Videomule: a consensus learning approach to multi-label classification from noisy user-generated videos. In: ACM international conference on multimedia, pp 721–724
Ribeiro MX, Balan AGR, Felipe JC, Traina AJM, Traina C (2009) Mining statistical association rules to select the most relevant medical image features. In: Mining complex data. Springer, pp 113–131
Rijsbergen CJV (1986) A non-classical logic for information retrieval. Comput J 29(6):481–485
Robertson SE (1977) The probability ranking principle. J Doc 33:294–304
Salton G, Wong A, Yang CS (1975) A vector space model for automatic indexing. Commun ACM 18(11):613–620
Saraceno C, Leonardi R (1997) Audio as a support to scene change detection and characterization of video sequences. In: IEEE international conference on acoustics, speech, and signal processing (ICASSP), vol 4, pp 2597–2600
Saunders J (1996) Real-time discrimination of broadcast speech/music. ICASSP 2:993–996
Sclaroff S, Kollios G, Betke M, Rosales R (2001) Motion mining. In: International workshop on multimedia databases and image communication
Seneff S (1984) Pitch and spectral estimation of speech based on an auditory synchrony model. In: IEEE international conference on acoustics, speech and signal processing, pp 3621–3624
Seneff S (1988) A joint synchrony/mean-rate model of auditory speech processing. J Phon 16(1):57–76
Shao X, Xu C, Kankanhalli MS (2003) Applying neural network on content based audio classification. In: IEEE Pacific-Rim conference on multimedia
Sheikholeslami G, Chatterjee S, Zhang A (1998) Wavecluster: a multi-resolution clustering approach for very large spatial databases. In: International conference on very large data bases (VLDB), pp 428–439
Shirahama K, Ideno K, Uehara K (2005) Video data mining: mining semantic patterns with temporal constraints from movies. In: IEEE international symposium on multimedia
Shirahama K, Ideno K, Uehara K (2008) A time constrained sequential pattern mining for extracting semantic events in videoss. In: Multimedia data mining. Springer Link
Shirahama K, Iwamoto K, Uehara K (2004) Video data mining: rhythms in a movie. In: International conference on multimedia and expo
Shirahama K, Sugihara C, Matsumura K, Matsuoka Y, Uehara K (2009) Mining event definitions from queries for video retrieval on the internet. In: International conference on data mining workshops, pp 176–183
Shyu ML, Xie Z, Chen M, Chen SC (2008) Video semantic event concept detection using a subspace based multimedia data mining framework. IEEE Trans Multimedia 10(2):252–259
Smith JR, Chang SF (1996) Local color and texture extraction and spatial query. IEEE Int Conf Image Proc 3:1011–1014
Sohn J, Kim NS, Sun W (1999) A statistical model-based voice activity detection. IEEE Signal Process Lett 6(1):1–3
Steinbach M, Karypis G, Kumar V (2000) A comparison of document clustering techniques. In: ACM SIGKDD world text mining conference
Stembridge B, Corish B (2004) Patent data mining and effective portfolio management. Intellect Asset Manage
Stricker M, Orengo M (1995) Similarity of color images. Storage retr image video databases (SPIE) 2420:381–392
Swain MJ, Ballard DH Color indexing. Int J Comput Vis 7(7):11–32
Tada T, Nagashima T, Okada Y (2009) Rule-based classification for audio data based on closed itemset mining. In: International multiconference of engineers and computer scientists (IMECS)
Tong S, Chang E (2001) Support vector machine active learning for image retrieval. In: ACM multimedia
Townshend B (1990) Nonlinear prediction of speech signals. In: IEEE international conference on acoustics, speech and signal processing
Trippe A (2003) Patinformatics: tasks to tools. World Pat Inf 25:211–221
Vailaya A, Figueiredo M, Jain AK, Zhang HJ (1998) A bayesian framework for semantic classification of outdoor vacation images. In: SPIE, vol 3656
Vapnik V (1995) The nature of statistical learning theory. Springer, Berlin
Victor SP, Peter SJ (2010) A novel minimum spanning tree based clustering algorithm for image mining. European Journal of Scientific Research (EJSR) 40(4):540–546
Wang JZ, Li J, Wiederhold G, Firschein O (2001) Classifying objectionable websites based on image content. In: Lecture notes in computer science, pp 232–242
Wei S, Zhao Y, Zhu Z, Liu N (2009) Multimodal fusion for video search reranking. IEEE Trans Knowl Data Eng 99(1):1191–1199
Williams G, Ellis D (1999) Speech/music discrimination based on posterior probability features. In: Eurospeech
Wu Y, Chang EY, Tseng BL (2005) Multimodal metadata fusion using causal strength. In: ACM multimedia, pp 872–881
Wynne H, Lee ML, Zhang J (2002) Image mining: trends and developments. J Intell Inf Syst 19(1):7–23
Xie L, Kennedy L, Chang SF, Lin CY, Divakaran A, Sun H (2004) Discover meaningful multimedia patterns with audio-visual concepts and associated text. In: IEEE international conference on image processing
Yamato J, Ohya J, Ishii K (1992) Recognizing human action in time-sequential images using hiddenmarkov model. In: IEEE Computing Society conference on computer vision and pattern recognition, pp 379–385
Yan R, Yang J, Hauptmann AG (2004) Learning query class dependent weights in automatic video retrieval. In: ACM multimedia, pp 548–555
Yang Y, Akers L, Klose T, Yang CB (2008) Text mining and visualization tools—impressions of emerging capabilities. World Pat Inf 30:280–293
Yeung M, Yeo BL, Liu B (2001) Extracting story units from long programs for video browsing and navigation. In: Readings in multimedia computing and networking. Morgan Kaufmann, San Mateo
Yeung MM, Yeo BL (1996) Time-constrained clustering for segmentation of video into story unites. Int Conf Pattern Recognit 3:375–380
Zaiane O, Han J, Li Z, Chee S, Chiang J (1998) Multimediaminer: a system prototype for multimedia data mining. In: ACM SIGMOD, pp 581–583
Zhang C, Chen WB, Chen X, Tiwari R, Yang L, Warner G (2009) A multimodal data mining framework for revealing common sources of spam images. J Multimedia 4(5):321–330
Zhang HJ, Zhong D (1995) A scheme for visual feature based image indexing. In: SPIE conference on storage and retrieval for image and video databases
Zhang R, Zhang Z, Li M, Ma WY, Zhang HJ (2005) A probabilistic semantic model for image annotation and multi-modal image retrieval. In: IEEE international conference of computer vision
Zhang T, Kuo CCJ (2001) Audio content analysis for online audiovisual data segmentation and classification. IEEE Trans Speech Audio Process 9(4):441–457
Zhang T, Ramakrishnan R, Livny M (1996) Birch: an efficient data clustering method for very large databases. In: SIGMOD conference, pp 103–114
Zhu R, Yao M, Liu Y (2009) Image classification approach based on manifold learning in web image mining. In: International conference on advanced data mining and applications (ADMA), pp 780–787
Zhu X, Wu X, Elmagarmid AK, Wu L (2005) Video data mining: semantic indexing and event detection from the association perspective. IEEE Trans Knowl Data Eng 17(5):665–677
Ziang J, Ward W, Pellom B (2002) Phone based voice activity detection using online bayesian adaptation with conjugate normal distributions. In: International conference on acoustics, speech and signal processing
Download references
Author information
Authors and affiliations.
School of Computing, National University of Singapore, Singapore, 117417, Singapore
Chidansh Amitkumar Bhatt & Mohan S. Kankanhalli
You can also search for this author in PubMed Google Scholar
Corresponding author
Correspondence to Chidansh Amitkumar Bhatt .
Rights and permissions
Reprints and permissions
About this article
Bhatt, C.A., Kankanhalli, M.S. Multimedia data mining: state of the art and challenges. Multimed Tools Appl 51 , 35–76 (2011). https://doi.org/10.1007/s11042-010-0645-5
Download citation
Published : 16 November 2010
Issue Date : January 2011
DOI : https://doi.org/10.1007/s11042-010-0645-5
Share this article
Anyone you share the following link with will be able to read this content:
Sorry, a shareable link is not currently available for this article.
Provided by the Springer Nature SharedIt content-sharing initiative
- Multimodal data mining
- Probabilistic temporal multimedia data mining
- Video mining
- Audio mining
- Image mining
- Text mining
- Find a journal
- Publish with us
- Track your research

IMAGES
VIDEO
COMMENTS
Here are some important data visualization techniques to know: 1. Pie Chart. Pie charts are one of the most common and basic data visualization techniques, used across a wide range of applications. Pie charts are ideal for illustrating proportions, or part-to-whole comparisons.
The Power of Good Data Visualization. Data visualization involves the use of graphical representations of data, such as graphs, charts, and maps. Compared to descriptive statistics or tables, visuals provide a more effective way to analyze data, including identifying patterns, distributions, and correlations and spotting outliers in complex ...
7.2 Multimedia Data ... which we describe below. Many techniques used in JPEG also appear in MPEG, the set of standards for video compression and transmission created by the Moving Picture Experts Group. ... CD-quality audio, which is the de facto digital representation for high-quality audio, is sampled at a rate of 44.1 KHz (i.e., a sample is ...
Multimedia Data Representations. In this chapter we focus on the underlying representations of common forms of media Audio, Graphics and Video. In the next chapter we focus on compression techniques as we shortly understand why these data can be large in storage size (even when compressed). The topics we consider here are specifically:
A typical multimedia data mining system, or framework, or method always consists of the following three key components. Given the raw multimedia data, the very first component for mining the multimedia data is to convert a specific raw data collection into a representation in an abstract space which is called the feature space.This process is called feature extraction or feature learning.
Summary. Most features can be represented in the form of one (or more) of the four common base models: vectors, strings, graphs/trees, and fuzzy/probabilistic logic-based representations. Many features, such as colors, textures, and shapes, are commonly represented in the form of histograms that quantify the contribution of each individual ...
Authored or synthesized multimedia objects on the other hand have explicit authoring structures. Multimedia data models thus need to represent the visual features of the data as well as the underlying structures. In order to associate multimedia data with information about their content, a conceptual representation needs to be given for every ...
In this context, a MultiMedia DataBase Management System (MMDBMS) is the system that provides support for multimedia data types, adding the usual facilities for the creation, storage, access, query, and control of the multimedia database. Consequently, the different data types involved in multimedia databases require special methods for optimal ...
1 Introduction: Multimedia Applications and Data Manageme nt Requirements 1 1.1 Heterogeneity 1 1.2 Imprecision and Subjectivity 8 1.3 Components of a Multimedia Database Management System 12 1.4 Summary 19 2 Models for Multimedia Data 20 2.1 Overview of Traditional Data Models 21 2.2 Multimedia Data Modeling 32 2.3 Models of Media Features 34
The authors also describe an effective solution to large-scale video search, along with an application of audio data classification and categorization. This novel, self-contained book examines how the merging of multimedia and data mining research can promote the understanding and advance the development of knowledge discovery in multimedia data.
26.4.2 Multimedia data mining. Multimedia data mining can be defined as a process that finds patterns in various types of data, including images, audio, video, and animation. Text mining and hypertext/hypermedia data mining are closely related to multimedia data mining because most of the information that is described in these fields are also ...
Therefore, developing optimal feature representation for multimedia data is the crucial step for the multimedia data understanding. This special issue serves as a forum for researchers all over the world to discuss their works and recent advances in representation learning methods and its applications in multimedia analysis. Both state-of- the ...
Fig. 1 Multimedia data mining state of the art review scheme - Identifying specific problems encountered during data mining of multimedia data from feature extraction, transformation, representation and data mining techniques perspective. - Discuss the current approaches to solve the identified problems and their limitations.
Media types (2) Represented in term of the dimensions of the space the data are in: 0-dimensional data: this type of data is the regular, alphanumeric data (e.g., text) 1-dimensional data: this type of data has one dimension (i.e., time) of the space imposed into them (e.g., audio) 2-dimensional data: this type of data has two dimensions (i.e., x, y) of the
Multimedia in principle means data of more than one medium. It usually refers to data representing multiple types of medium to capture information and experiences related to objects and events. Commonly used forms of data are numbers, alphanumeric, text, images, audio, and video. In common usage, people refer a data set as multimedia only when ...
At the fundamental level, the transceiver is how the computer interprets anything (this is where you can find binary). A wire can either be sent electrical signals, or it cannot (there is no in between for on and off after all). This means that the representation for when a wire is sent an electrical signal has to be of 2 possible values.
MCS communicates media and multimedia objects between the various components of a multimedia information system, providing appropriate quality of service (QoS) guarantees. Since row, sensed data is often not directly usable, MPS processes media objects for the benefit of the other components. For example, multiple media objects may be fused to ...
Image data types. Images can be created by using different techniques of representation of data called data type like monochrome and colored images. Monochrome image is created by using single color whereas colored image is created by using multiple colors. Some important data types of images are following: 1-bit images- An image is a set of ...
The audio data at the click may be displayed and the offending audio sample deleted or modified. Figure 13 illustrates a visual representation of audio data before and after the click's removal. MULTIMEDIA PRESENTATION TECHNIQUES AND TECHNOLOGY 317 Audio Waveform with "Click" «* = Actual Audio Sample Figure 13.
Publisher Summary. This chapter focuses on multimedia presentation techniques and technology. Multimedia may be considered as the integration of the human senses into a computer environment for the purpose of improving communication between the computer and its user, and among the users. Computer based multimedia affects all three major ...
Abstract. The rapid development of digital communication technology in the areas of diversity and performance is ongoing—with no end in sight. The trend toward the integration of classic media continues. Verbal communication and data transmission have now become inseparable in modern mobile networks. The debut of digital technology has made ...
It can be drawn by following the steps given below: Step 1: firstly we have to draw the two axis of the graph X-axis and the Y-axis. The varieties of the data must be put on the X-axis (the horizontal line) and the frequencies of the data must be put on the Y-axis (the vertical line) of the graph.
Advances in multimedia data acquisition and storage technology have led to the growth of very large multimedia databases. Analyzing this huge amount of multimedia data to discover useful knowledge is a challenging problem. This challenge has opened the opportunity for research in Multimedia Data Mining (MDM). Multimedia data mining can be defined as the process of finding interesting patterns ...