- Our Mission

How a Simple Presentation Framework Helps Students Learn
Explaining concepts to their peers helps students shore up their content knowledge and improve their communication skills.
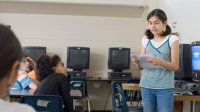
A few years ago, my colleague and I were awarded a Hawai‘i Innovation Fund Grant. The joy of being awarded the grant was met with dread and despair when we were informed that we would have to deliver a 15-minute presentation on our grant write-up to a room full of educational leaders. If that wasn’t intimidating enough, my colleague informed me that he was not going to be in Hawai‘i at the time of the presentation. I had “one shot,” just a 15-minute presentation to encapsulate all of the 17 pages of the grant I had cowritten, but how?
I worked hard to construct and deliver a presentation that was concise yet explicit. I was clear on the big picture of what the grant was composed of and provided a visual of it in practice. I made sure the audience understood the “why” behind the grant. I showed how it worked, the concrete elements of it, and how they made it successful. I finished with a scaffold that would help others know how to initiate it within their context, giving them the freedom to make it authentically their own.
I received good feedback from the presentation, and more important, what was shared positively impacted student learning in other classrooms across the state.
A Simple Framework for Presentations
That first presentation took me over a month to prepare, but afterward I noticed that my prep time for presentations shrank exponentially from a few months to a few (uninterrupted) days. Interestingly enough, as a by-product of creating the original presentation, I created an abstract framework that I have used for every professional learning presentation I have delivered since then. The “What, Why, How, and How-To” framework goes as follows:
- What? What can the audience easily connect to and know as a bridge to the unknown for the rest of the experience?
- Why? Why should they care to listen to (and learn from) the rest of the presentation? What’s in it for them to shift from passive listeners to actively engaged? The audience needs to know why you believe in this so much that you are compelled to share it.
- How? What are the key elements that make it unique? How is it effective in doing what it does? What are the intricacies of how it works?
- How-to? How could they start doing this on their own? How could this knowledge serve as a foundational springboard? Connect it to “why.”
Benefits for Students
One of the best parts of presentations is that they help the presenter to improve their communication skills. The presenter is learning how to give a presentation by doing it. To prepare a presentation, the presenter must know the intricate elements of what they are presenting and the rationale for their importance. In the presentation delivery, the presenter must be articulate and meticulous to ensure that everyone in the audience is able (and willing) to process the information provided.
It didn’t take long for me to realize that preparing and delivering presentations could provide a valuable learning opportunity for my students.
I recall teaching mathematical concepts whereby students would immediately apply knowledge learned to accomplish the task in silence and without any deeper questioning. Only after I asked them to provide presentations on these concepts did they regularly ask me, “Why is this important, again?” or “What makes this so special?” My students’ mathematical literacy grew through preparing presentations with the “What, Why, How, and How-To” framework, which supported them in their ability to demonstrate content knowledge through mathematical rigor (balancing conceptual understanding, skills and procedural fluency, and real-world application).
- The “what” served as the mathematical concept.
- The “why” demonstrated the real-world application of the concept.
- “The “how” demonstrated conceptual understanding of the concept.
- The “how-to” demonstrated skills and procedures of the concept.
In addition to content knowledge, the sequential competencies of clarity, cohesiveness, and captivation ensured that the presenter could successfully share the information with their audience. When combined, these framed a rubric that supported students in optimizing their presentation deliveries. The competencies are as follows:
1. Content knowledge. The presenter must display a deep understanding of what they are delivering in order to share the “what, why, how, and how-to” of the topic.
2. Clarity. The presenter must be clear with precise, academic language. As the content they deliver may be new to the audience, any lack of clarity will alienate the audience. Providing multiple modes of representation greatly addresses a variety of processing needs of a diverse audience.
3. Cohesiveness. When making clear connections, the presenter bridges gaps between each discrete component in how they all work together as integral elements of the topic. Any gaps too large may make the elements look disjointed or, worse, the audience feel lost.
4. Captivation. The presenter must captivate the audience through any combination of audience engagement or storytelling . They make the presentation flow with the energy of a song , and in the end, they leave the audience with a delicate balance of feeling fulfilled and inspired to learn more.
Anyone can build an effective presentation with the “What, Why, How, and How-To” framework, along with competencies of content knowledge, clarity, cohesiveness, and captivation. The better we teach and coach others on how to create and deliver presentations, the more we learn from these individuals through their work.
In my class, one multilingual learner responded to the prompt “What are the non-math (life lessons) you have found valuable from this class?” with “I learn what is learning and teaching... I truly understood how teaching is actually learning when I had presentation. I found a bit of desire to being a teacher. I hope you also learned something from this class.” I always learn from my students when they present.
- Center for Innovative Teaching and Learning
- Instructional Guide
Teaching with PowerPoint
When effectively planned and used, PowerPoint (or similar tools, like Google Slides) can enhance instruction. People are divided on the effectiveness of this ubiquitous presentation program—some say that PowerPoint is wonderful while others bemoan its pervasiveness. No matter which side you take, PowerPoint does offer effective ways to enhance instruction when used and designed appropriately.
PowerPoint can be an effective tool to present material in the classroom and encourage student learning. You can use PowerPoint to project visuals that would otherwise be difficult to bring to class. For example, in an anthropology class, a single PowerPoint presentation could project images of an anthropological dig from a remote area, questions asking students about the topic, a chart of related statistics, and a mini quiz about what was just discussed that provides students with information that is visual, challenging, and engaging.
PowerPoint can be an effective tool to present material in the classroom and encourage student learning.
This section is organized in three major segments: Part I will help faculty identify and use basic but important design elements, Part II will cover ways to enhance teaching and learning with PowerPoint, and Part III will list ways to engage students with PowerPoint.
PART I: Designing the PowerPoint Presentation
Accessibility.
- Student accessibility—students with visual or hearing impairments may not be able to fully access a PowerPoint presentation, especially those with graphics, images, and sound.
- Use an accessible layout. Built-in slide template layouts were designed to be accessible: “the reading order is the same for people with vision and for people who use assistive technology such as screen readers” (University of Washington, n.d.). If you want to alter the layout of a theme, use the Slide Master; this will ensure your slides will retain accessibility.
- Use unique and specific slide titles so students can access the material they need.
- Consider how you display hyperlinks. Since screen readers read what is on the page, you may want to consider creating a hyperlink using a descriptive title instead of displaying the URL.
- All visuals and tables should include alt text. Alt text should describe the visual or table in detail so that students with visual impairments can “read” the images with their screen readers. Avoid using too many decorative visuals.
- All video and audio content should be captioned for students with hearing impairments. Transcripts can also be useful as an additional resource, but captioning ensures students can follow along with what is on the screen in real-time.
- Simplify your tables. If you use tables on your slides, ensure they are not overly complex and do not include blank cells. Screen readers may have difficulty providing information about the table if there are too many columns and rows, and they may “think” the table is complete if they come to a blank cell.
- Set a reading order for text on your slides. The order that text appears on the slide may not be the reading order of the text. Check that your reading order is correct by using the Selection Pane (organized bottom-up).
- Use Microsoft’s Accessibility Checker to identify potential accessibility issues in your completed PowerPoint. Use the feedback to improve your PowerPoint’s accessibility. You could also send your file to the Disability Resource Center to have them assess its accessibility (send it far in advance of when you will need to use it).
- Save your PowerPoint presentation as a PDF file to distribute to students with visual impairments.
Preparing for the presentation
- Consider time and effort in preparing a PowerPoint presentation; give yourself plenty of lead time for design and development.
- PowerPoint is especially useful when providing course material online. Consider student technology compatibility with PowerPoint material put on the web; ensure images and graphics have been compressed for access by computers using dial-up connection.
PowerPoint is especially useful when providing course material online.
- Be aware of copyright law when displaying course materials, and properly cite source material. This is especially important when using visuals obtained from the internet or other sources. This also models proper citation for your students.
- Think about message interpretation for PowerPoint use online: will students be able to understand material in a PowerPoint presentation outside of the classroom? Will you need to provide notes and/or other material to help students understand complex information, data, or graphics?
- If you will be using your own laptop, make sure the classroom is equipped with the proper cables, drivers, and other means to display your presentation the way you have intended.
Slide content
- Avoid text-dense slides. It’s better to have more slides than trying to place too much text on one slide. Use brief points instead of long sentences or paragraphs and outline key points rather than transcribing your lecture. Use PowerPoint to cue and guide the presentation.
- Use the Notes feature to add content to your presentation that the audience will not see. You can access the Notes section for each slide by sliding the bottom of the slide window up to reveal the notes section or by clicking “View” and choosing “Notes Page” from the Presentation Views options.
- Relate PowerPoint material to course objectives to reinforce their purpose for students.
Number of slides
- As a rule of thumb, plan to show one slide per minute to account for discussion and time and for students to absorb the material.
- Reduce redundant or text-heavy sentences or bullets to ensure a more professional appearance.
- Incorporate active learning throughout the presentation to hold students’ interest and reinforce learning.
Emphasizing content
- Use italics, bold, and color for emphasizing content.
- Use of a light background (white, beige, yellow) with dark typeface or a dark background (blue, purple, brown) with a light typeface is easy to read in a large room.
- Avoid using too many colors or shifting colors too many times within the presentation, which can be distracting to students.
- Avoid using underlines for emphasis; underlining typically signifies hypertext in digital media.
Use of a light background with dark typeface or a dark background with a light typeface is easy to read in a large room.
- Limit the number of typeface styles to no more than two per slide. Try to keep typeface consistent throughout your presentation so it does not become a distraction.
- Avoid overly ornate or specialty fonts that may be harder for students to read. Stick to basic fonts so as not to distract students from the content.
- Ensure the typeface is large enough to read from anywhere in the room: titles and headings should be no less than 36-40-point font. The subtext should be no less than 32-point font.
Clip art and graphics
- Use clip art and graphics sparingly. Research shows that it’s best to use graphics only when they support the content. Irrelevant graphics and images have been proven to hinder student learning.
- Photographs can be used to add realism. Again, only use photographs that are relevant to the content and serve a pedagogical purpose. Images for decorative purposes are distracting.
- Size and place graphics appropriately on the slide—consider wrapping text around a graphic.
- Use two-dimensional pie and bar graphs rather than 3D styles which can interfere with the intended message.
Use clip art and graphics sparingly. Research shows that it’s best to use graphics only when they support the content.
Animation and sound
- Add motion, sound, or music only when necessary. When in doubt, do without.
- Avoid distracting animations and transitions. Excessive movement within or between slides can interfere with the message and students find them distracting. Avoid them or use only simple screen transitions.
Final check
- Check for spelling, correct word usage, flow of material, and overall appearance of the presentation.
- Colleagues can be helpful to check your presentation for accuracy and appeal. Note: Errors are more obvious when they are projected.
- Schedule at least one practice session to check for timing and flow.
- PowerPoint’s Slide Sorter View is especially helpful to check slides for proper sequencing as well as information gaps and redundancy. You can also use the preview pane on the left of the screen when you are editing the PowerPoint in “Normal” view.
- Prepare for plan “B” in case you have trouble with the technology in the classroom: how will you provide material located on your flash drive or computer? Have an alternate method of instruction ready (printing a copy of your PowerPoint with notes is one idea).
PowerPoint’s Slide Sorter View is especially helpful to check slides for proper sequencing and information gaps and redundancy.
PowerPoint Handouts
PowerPoint provides multiple options for print-based handouts that can be distributed at various points in the class.
Before class: students might like having materials available to help them prepare and formulate questions before the class period.
During class: you could distribute a handout with three slides and lines for notes to encourage students to take notes on the details of your lecture so they have notes alongside the slide material (and aren’t just taking notes on the slide content).
After class: some instructors wait to make the presentation available after the class period so that students concentrate on the presentation rather than reading ahead on the handout.
Never: Some instructors do not distribute the PowerPoint to students so that students don’t rely on access to the presentation and neglect to pay attention in class as a result.
- PowerPoint slides can be printed in the form of handouts—with one, two, three, four, six, or nine slides on a page—that can be given to students for reference during and after the presentation. The three-slides-per-page handout includes lined space to assist in note-taking.
- Notes Pages. Detailed notes can be printed and used during the presentation, or if they are notes intended for students, they can be distributed before the presentation.
- Outline View. PowerPoint presentations can be printed as an outline, which provides all the text from each slide. Outlines offer a welcome alternative to slide handouts and can be modified from the original presentation to provide more or less information than the projected presentation.
The Presentation
Alley, Schreiber, Ramsdell, and Muffo (2006) suggest that PowerPoint slide headline design “affects audience retention,” and they conclude that “succinct sentence headlines are more effective” in information recall than headlines of short phrases or single words (p. 233). In other words, create slide titles with as much information as is used for newspapers and journals to help students better understand the content of the slide.
- PowerPoint should provide key words, concepts, and images to enhance your presentation (but PowerPoint should not replace you as the presenter).
- Avoid reading from the slide—reading the material can be perceived as though you don’t know the material. If you must read the material, provide it in a handout instead of a projected PowerPoint slide.
- Avoid moving a laser pointer across the slide rapidly. If using a laser pointer, use one with a dot large enough to be seen from all areas of the room and move it slowly and intentionally.
Avoid reading from the slide—reading the material can be perceived as though you don’t know the material.
- Use a blank screen to allow students to reflect on what has just been discussed or to gain their attention (Press B for a black screen or W for a white screen while delivering your slide show; press these keys again to return to the live presentation). This pause can also be used for a break period or when transitioning to new content.
- Stand to one side of the screen and face the audience while presenting. Using Presenter View will display your slide notes to you on the computer monitor while projecting only the slides to students on the projector screen.
- Leave classroom lights on and turn off lights directly over the projection screen if possible. A completely dark or dim classroom will impede notetaking (and may encourage nap-taking).
- Learn to use PowerPoint efficiently and have a back-up plan in case of technical failure.
- Give yourself enough time to finish the presentation. Trying to rush through slides can give the impression of an unorganized presentation and may be difficult for students to follow or learn.
PART II: Enhancing Teaching and Learning with PowerPoint
Class preparation.
PowerPoint can be used to prepare lectures and presentations by helping instructors refine their material to salient points and content. Class lectures can be typed in outline format, which can then be refined as slides. Lecture notes can be printed as notes pages (notes pages: Printed pages that display author notes beneath the slide that the notes accompany.) and could also be given as handouts to accompany the presentation.
Multimodal Learning
Using PowerPoint can help you present information in multiple ways (a multimodal approach) through the projection of color, images, and video for the visual mode; sound and music for the auditory mode; text and writing prompts for the reading/writing mode; and interactive slides that ask students to do something, e.g. a group or class activity in which students practice concepts, for the kinesthetic mode (see Part III: Engaging Students with PowerPoint for more details). Providing information in multiple modalities helps improve comprehension and recall for all students.
Providing information in multiple modalities helps improve comprehension and recall for all students.
Type-on Live Slides
PowerPoint allows users to type directly during the slide show, which provides another form of interaction. These write-on slides can be used to project students’ comments and ideas for the entire class to see. When the presentation is over, the new material can be saved to the original file and posted electronically. This feature requires advanced preparation in the PowerPoint file while creating your presentation. For instructions on how to set up your type-on slide text box, visit this tutorial from AddictiveTips .
Write or Highlight on Slides
PowerPoint also allows users to use tools to highlight or write directly onto a presentation while it is live. When you are presenting your PowerPoint, move your cursor over the slide to reveal tools in the lower-left corner. One of the tools is a pen icon. Click this icon to choose either a laser pointer, pen, or highlighter. You can use your cursor for these options, or you can use the stylus for your smart podium computer monitor or touch-screen laptop monitor (if applicable).
Just-In-Time Course Material
You can make your PowerPoint slides, outline, and/or notes pages available online 24/7 through Blackboard, OneDrive, other websites. Students can review the material before class, bring printouts to class, and better prepare themselves for listening rather than taking a lot of notes during the class period. They can also come to class prepared with questions about the material so you can address their comprehension of the concepts.
PART III: Engaging Students with PowerPoint
The following techniques can be incorporated into PowerPoint presentations to increase interactivity and engagement between students and between students and the instructor. Each technique can be projected as a separate PowerPoint slide.
Running Slide Show as Students Arrive in the Classroom
This technique provides visual interest and can include a series of questions for students to answer as they sit waiting for class to begin. These questions could be on future texts or quizzes.
- Opening Question : project an opening question, e.g. “Take a moment to reflect on ___.”
- Think of what you know about ___.
- Turn to a partner and share your knowledge about ___.
- Share with the class what you have discussed with your partner.
- Focused Listing helps with recall of pertinent information, e.g. “list as many characteristics of ___, or write down as many words related to ___ as you can think of.”
- Brainstorming stretches the mind and promotes deep thinking and recall of prior knowledge, e.g. “What do you know about ___? Start with your clearest thoughts and then move on to those what are kind of ‘out there.’”
- Questions : ask students if they have any questions roughly every 15 minutes. This technique provides time for students to reflect and is also a good time for a scheduled break or for the instructor to interact with students.
- Note Check : ask students to “take a few minutes to compare notes with a partner,” or “…summarize the most important information,” or “…identify and clarify any sticking points,” etc.
- Questions and Answer Pairs : have students “take a minute to come with one question then see if you can stump your partner!”
- The Two-Minute Paper allows the instructor to check the class progress, e.g. “summarize the most important points of today’s lecture.” Have students submit the paper at the end of class.
- “If You Could Ask One Last Question—What Would It Be?” This technique allows for students to think more deeply about the topic and apply what they have learned in a question format.
- A Classroom Opinion Poll provides a sense of where students stand on certain topics, e.g. “do you believe in ___,” or “what are your thoughts on ___?”
- Muddiest Point allows anonymous feedback to inform the instructor if changes and or additions need to be made to the class, e.g. “What parts of today’s material still confuse you?”
- Most Useful Point can tell the instructor where the course is on track, e.g. “What is the most useful point in today’s material, and how can you illustrate its use in a practical setting?”
Positive Features of PowerPoint
- PowerPoint saves time and energy—once the presentation has been created, it is easy to update or modify for other courses.
- PowerPoint is portable and can be shared easily with students and colleagues.
- PowerPoint supports multimedia, such as video, audio, images, and
PowerPoint supports multimedia, such as video, audio, images, and animation.
Potential Drawbacks of PowerPoint
- PowerPoint could reduce the opportunity for classroom interaction by being the primary method of information dissemination or designed without built-in opportunities for interaction.
- PowerPoint could lead to information overload, especially with the inclusion of long sentences and paragraphs or lecture-heavy presentations with little opportunity for practical application or active learning.
- PowerPoint could “drive” the instruction and minimize the opportunity for spontaneity and creative teaching unless the instructor incorporates the potential for ingenuity into the presentation.
As with any technology, the way PowerPoint is used will determine its pedagogical effectiveness. By strategically using the points described above, PowerPoint can be used to enhance instruction and engage students.
Alley, M., Schreiber, M., Ramsdell, K., & Muffo, J. (2006). How the design of headlines in presentation slides affects audience retention. Technical Communication, 53 (2), 225-234. Retrieved from https://www.jstor.org/stable/43090718
University of Washington, Accessible Technology. (n.d.). Creating accessible presentations in Microsoft PowerPoint. Retrieved from https://www.washington.edu/accessibility/documents/powerpoint/
Selected Resources
Brill, F. (2016). PowerPoint for teachers: Creating interactive lessons. LinkedIn Learning . Retrieved from https://www.lynda.com/PowerPoint-tutorials/PowerPoint-Teachers-Create-Interactive-Lessons/472427-2.html
Huston, S. (2011). Active learning with PowerPoint [PDF file]. DE Oracle @ UMUC . Retrieved from http://contentdm.umuc.edu/digital/api/collection/p16240coll5/id/78/download
Microsoft Office Support. (n.d.). Make your PowerPoint presentations accessible to people with disabilities. Retrieved from https://support.office.com/en-us/article/make-your-powerpoint-presentations-accessible-to-people-with-disabilities-6f7772b2-2f33-4bd2-8ca7-ae3b2b3ef25
Tufte, E. R. (2006). The cognitive style of PowerPoint: Pitching out corrupts within. Cheshire, CT: Graphics Press LLC.
University of Nebraska Medical Center, College of Medicine. (n.d.). Active Learning with a PowerPoint. Retrieved from https://www.unmc.edu/com/_documents/active-learning-ppt.pdf
University of Washington, Department of English. (n.d.). Teaching with PowerPoint. Retrieved from https://english.washington.edu/teaching/teaching-powerpoint
Vanderbilt University, Center for Teaching. (n.d.). Making better PowerPoint presentations. Retrieved from https://cft.vanderbilt.edu/guides-sub-pages/making-better-powerpoint-presentations/

Suggested citation
Northern Illinois University Center for Innovative Teaching and Learning. (2020). Teaching with PowerPoint. In Instructional guide for university faculty and teaching assistants. Retrieved from https://www.niu.edu/citl/resources/guides/instructional-guide
Phone: 815-753-0595 Email: [email protected]
Connect with us on
Facebook page Twitter page YouTube page Instagram page LinkedIn page
Home Blog Presentation Ideas How to Present Complex Concepts: A Guide for Effective Communication
How to Present Complex Concepts: A Guide for Effective Communication
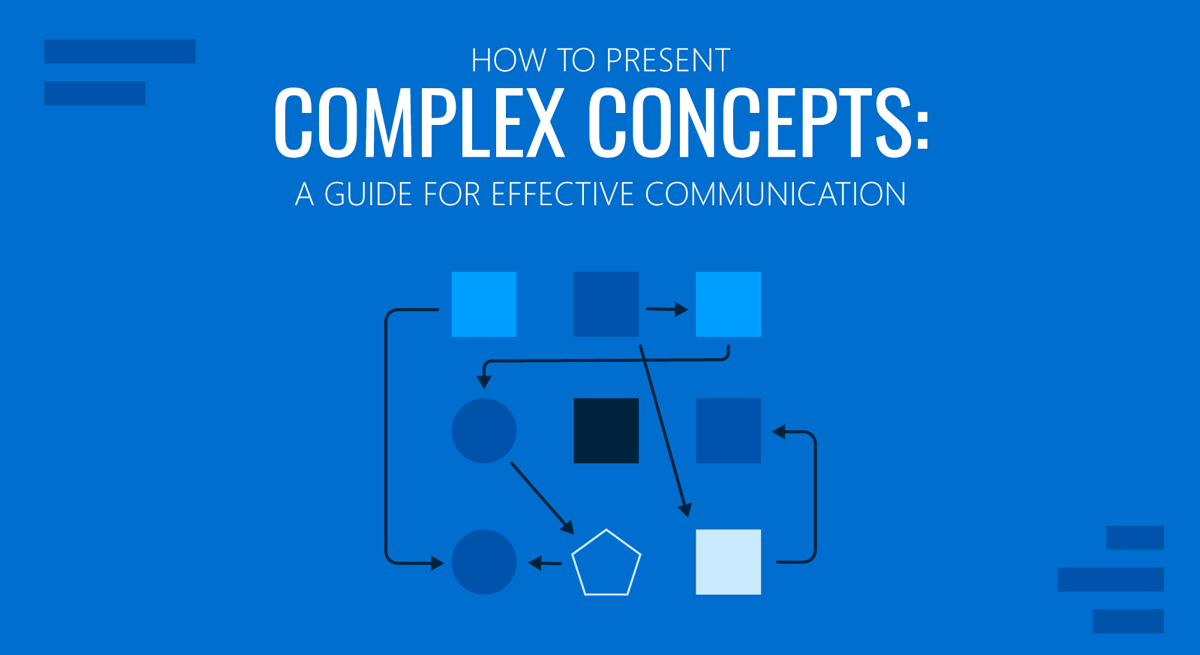
Have you ever been in front of an audience presenting a complex concept only to see in their faces that they’re not grasping what you’re saying? Not everyone has the same knowledge levels, much less about the same topics.
When you find yourself in this situation, it can be tough to bounce back and find another way to explain the complex concept to your audience. If not prepared, the panic might ruin your presentation.
So, how do you explain a complex topic to someone unfamiliar with it?
How can you communicate complex information so that anyone will understand?
Those are the questions we’ll be answering in this guide. We’ve collected our favorite techniques and tools from different corners of the web to give you a varied look at how anybody can explain anything to anyone.
Table of Contents
What is a Complex Concept?
Why are complex concepts difficult to communicate.
- Mind Mapping
- Storytelling and Analogies
- Four-Step Formula from How Communication Works
- Visual Aids and Interactivity
- Matt Abrahams’s 6 Tools
4 Examples of Effective Communication of Complex Concepts
Case study: explaining the cloud, mistakes to avoid when presenting complex ideas.
First up, let’s get the big words out of the way.
Do you know the difference between complex and complicated? Knowing the distinction will help you learn to present, explain, and articulate concepts to audiences of varying backgrounds.
The main difference between complex and complicated is that complicated refers to the level of detail, while complex refers to the difficulty level. Both consist of many interconnected parts, but the difference lies in how the parts affect the outcome.
Complicated concepts have a cause and effect, an expected result. A complicated concept can be explained and shared using formulas and systems. Regardless if a person is familiar with a topic, they’ll have an easier time understanding a set of rules or steps to get through a complicated concept. Complex topics are less predictable and difficult to understand.
For example, the construction of a car is complicated. But the traffic flow of a city is complex. Cooking and serving a fancy dish in a restaurant might be complicated, but the effects on the diners in the room are complex.
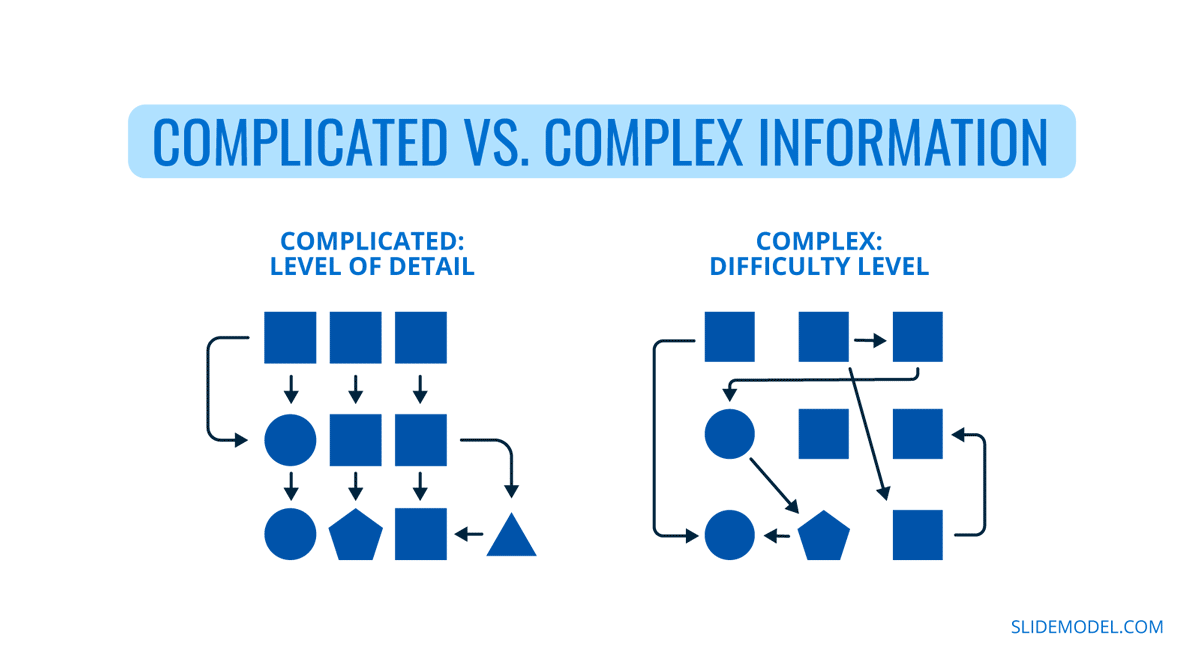
Most complex concepts exist in mathematics and science, topics only a few people are fluent in. It might be easy and straightforward for a Ph.D. candidate with a thesis idea about a complex science concept to explain to peers. Still, it will be incomprehensible to people in other fields or academic backgrounds.
Unlike complicated concepts that can be explained with processes, many complex ideas are abstract or theoretical, making them difficult to convey with concrete examples or practical applications. For example, the concept of time is more challenging to explain than how to operate complicated machinery.
Complex concepts need to be studied and analyzed to get a full grasp of their scope. Experts on complex topics dedicate their lives to understanding and researching the material. Not everyone has that kind of time!
Likewise, the skill for communicating complex concepts doesn’t come quickly to everyone. It’s an ability that needs nurturing with practice and dedication. Even people who study complex concepts like quantum mechanics need to learn how to explain aspects of their work to unfamiliar people.
5 Proven Formulas For Communicating Complex Concepts so Anyone Can Understand
Now we’re getting to the good stuff.
Effectively explaining and presenting complex concepts is complicated yet not complex. Therefore you can use processes, structures, and systems to help you communicate any complex concept so anyone can understand. It’s a skill you can learn, practice, and master.
Not only scientists and researchers need to practice this skill, but also startup founders, executives, politicians, and community leaders. It’s such a critical skill that professionals worldwide have created systems and formulas for communicating complex concepts.
We’ve compiled a list of our favorite strategies that have helped us and can also help you improve your communication skills or complex concepts. As you go through the list, you’ll find similarities between strategies. Mainly, to communicate an unfamiliar concept, you need to:
- Meet the audience at their knowledge level
- Simplify
- Use analogies
Let’s dive in.
1. Mind Mapping
The concept of mind mapping is to visualize information that’s difficult to grasp and needs development with visual tools. First, you select the essential data about the concept; it can be the name or title. This preliminary information is the mind map’s center—or top—. From there, branch out secondary information that supports the first. Continue in this manner until you’ve developed the concept enough.
Mind mapping has many names; brain mapping, thinking maps, and thought organizing. But it comes down to simplifying a concept’s entirety by separating the pieces and examining connections and relations between them.
A mind map can help communicate your complex concept in two ways. First, it can help you strategize the text, presentation, and speech. A mind map or thought organizer can be the foundation for developing the communication of a complex topic.
Secondly, include a summarized mind map as a presentation slide. Not only is it a tool to develop explanations, but it’s also a visual tool to explain the concept to an audience. This final mindmap shouldn’t be precisely like the foundational mind map. It must be simplified and designed in a way that helps explain with engaging visual design .
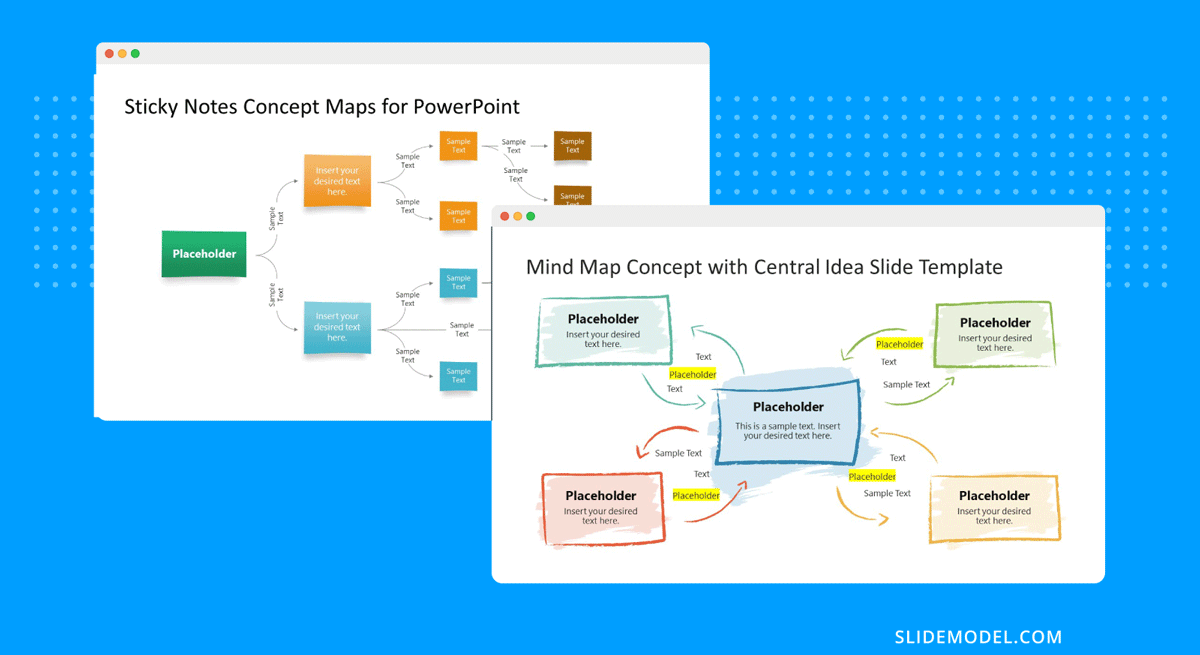
2. Storytelling and Analogies
Storytelling techniques are another proven method for sharing complex information. Using a story to explain a complex concept can be done in several ways. Stories can be used as an example or a way to share some background about the concept you’re discussing. The stories can be real-life examples or case studies that relate to your topic closely.
For stories to be compelling, they need to follow a literary structure. These are the four structures that best fit a presentation or a speech.
- The Hero’s Journey: Use the 12-step literary structure and apply it to science communication with a bit of creativity.
- Fact and Story: Share one fact and one story to support it. Then share another fact and a story to support it. Do this up to three times.
- What-How-Why: Explain the What, the How and Why in that order. Use a story as an example of each.
Nested Loops: Teel three different stories and then loop them in with similarities that refer to your topic.
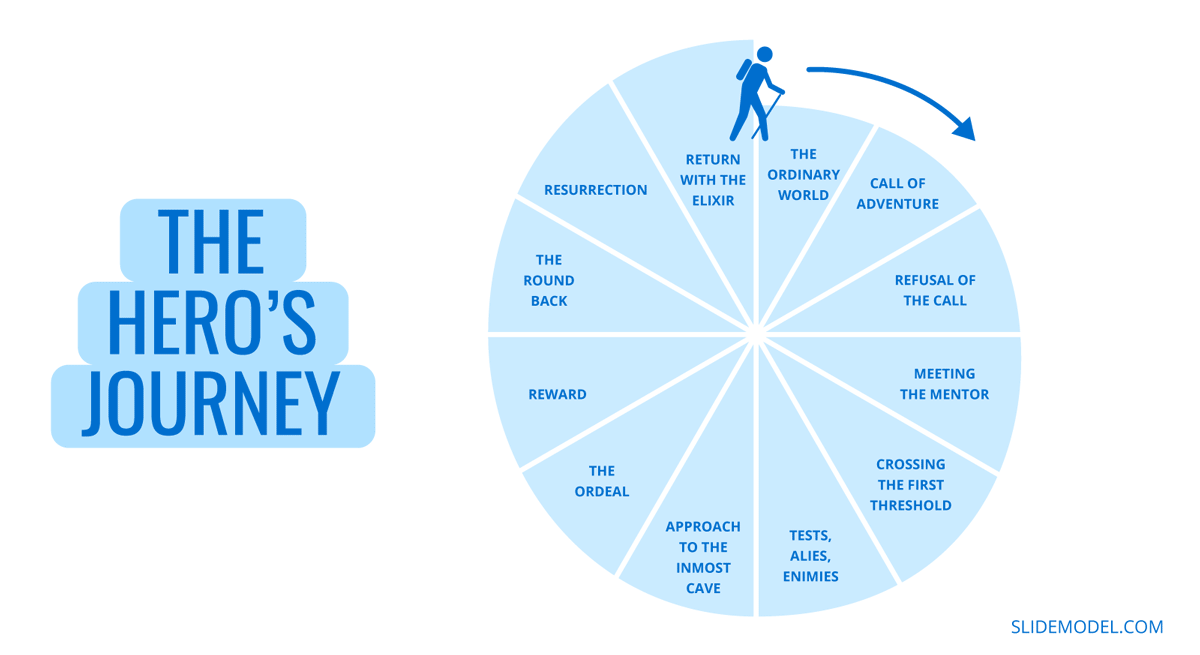
Another storytelling technique that’s particularly helpful for complex concepts is analogies. Analogies are so versatile that any concept—no matter how complex—can be explained using a story that’s completely different but similar in some way.
One of the most famous analogies of our time is the original iPod motto, “1,000 songs in your pocket.” Songs aren’t material things that can fit in a pocket like coins. But the digital data they take up, known by the developers and engineers as 5 GB, was too complex to explain to the general public in 2001.
3. Four-Step Formula from How Communication Works
In a video by How Communication Works , Bruce Lambert shares his four-step formula for effectively communicating a complex concept. They are:
- Focus on Problems
- Identify benefits or solutions
The main idea of this formula is to concentrate on the benefits and solutions the complex concept offers about a specific problem. Consider the concept of time and how we measure it. Even though we know that the measurement of time is a human construct, it helps us live our lives in order and with stability. There’s no need to talk about how an hour has 60 minutes or a minute has 60 seconds.
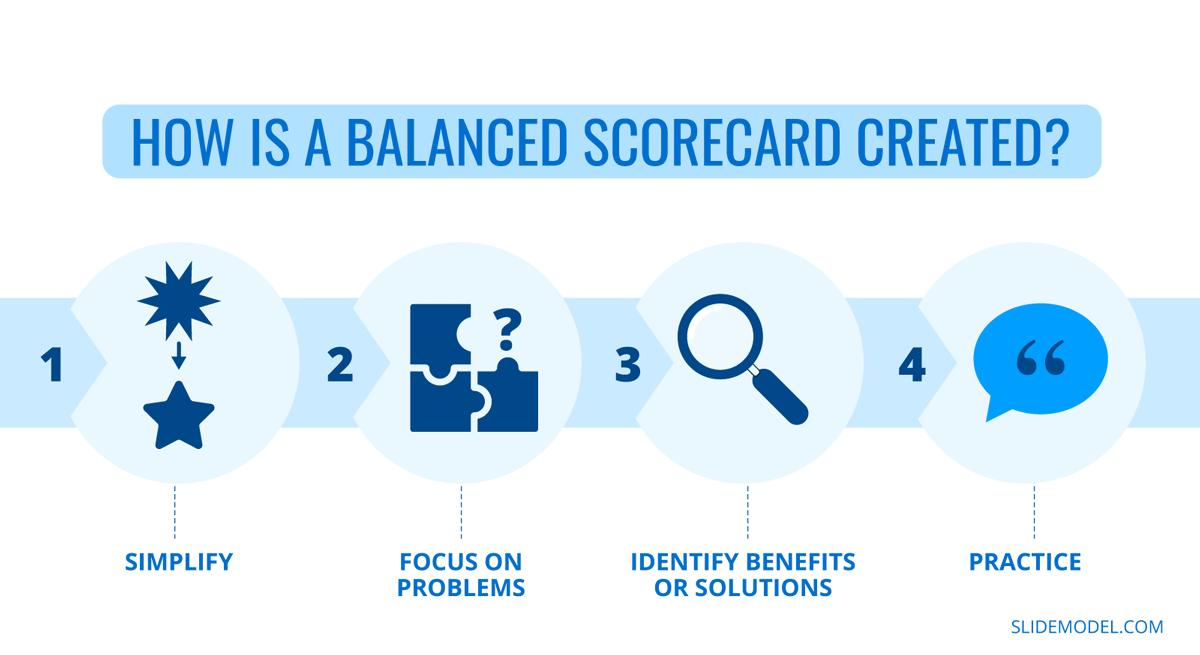
Simplify and practice are extremely important to this formula. You need to simplify the concept according to your audience, and you also need to practice your presentation at different levels. This way, you will always be prepared to share your complex concept.
4. Visual Aids and Interactivity
Visual aids can be a big help in explaining complex concepts. Although if your storytelling skills are well-developed, you don’t need visual aids at all. Or maybe just a couple.
Infographics and videos are the most common visual for explaining complex concepts. These visual types take a cue from storytelling and perform best when created with visual metaphors . Dry data and information on a canvas are no better than a boring speech.
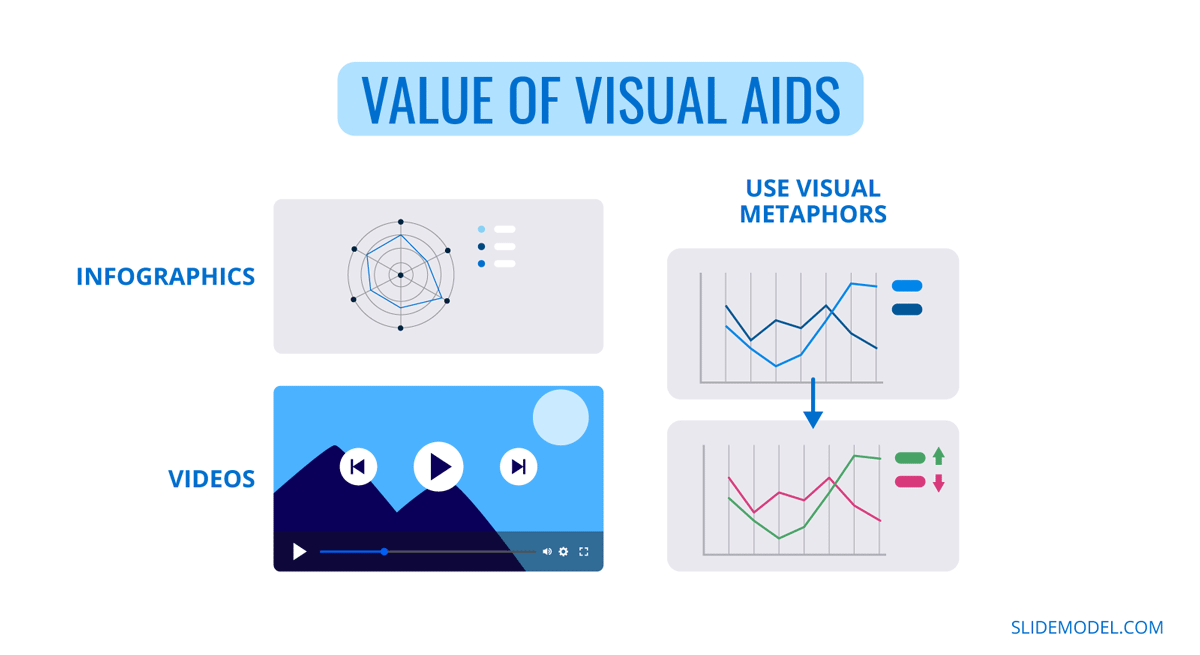
Interactivity makes your complex topic more accessible to anyone interested in learning about it. Interactive techniques include quizzes, hands-on activities, roleplaying, and conversation. Interactive science museums are one of the most engaging educational formulas .
After simplifying and visually representing complex data, the next step is to compile your insights into presentation-ready reports. These reports serve as the bridge between your deep understanding of a complex subject and the clear, accessible format needed for diverse audiences.
5. Matt Abrahams’s 6 Tools
In the Stanford Business blog , Matt Abrahams shares six tools to help explain complex concepts. Using the example of “Shooting a Bow and Arrow,” his team created this infographic to demonstrate each technique.
Take a look at Matt Abrahams’ tools:
- Diagram: Display the concept visually. We talk about this in the mind-mapping technique above.
- Deconstruct: Simplify. Dissect and take apart the concept into smaller parts.
- Compare: Use analogies to explain the complex concept using simple words and stories.
- Picture: Create and use visual aids to help develop and explain the concept.
- Backward Map: Start from the end and work your way back to the beginning.
- Chunk: Separate and categorize the concept into parts of similar subparts.
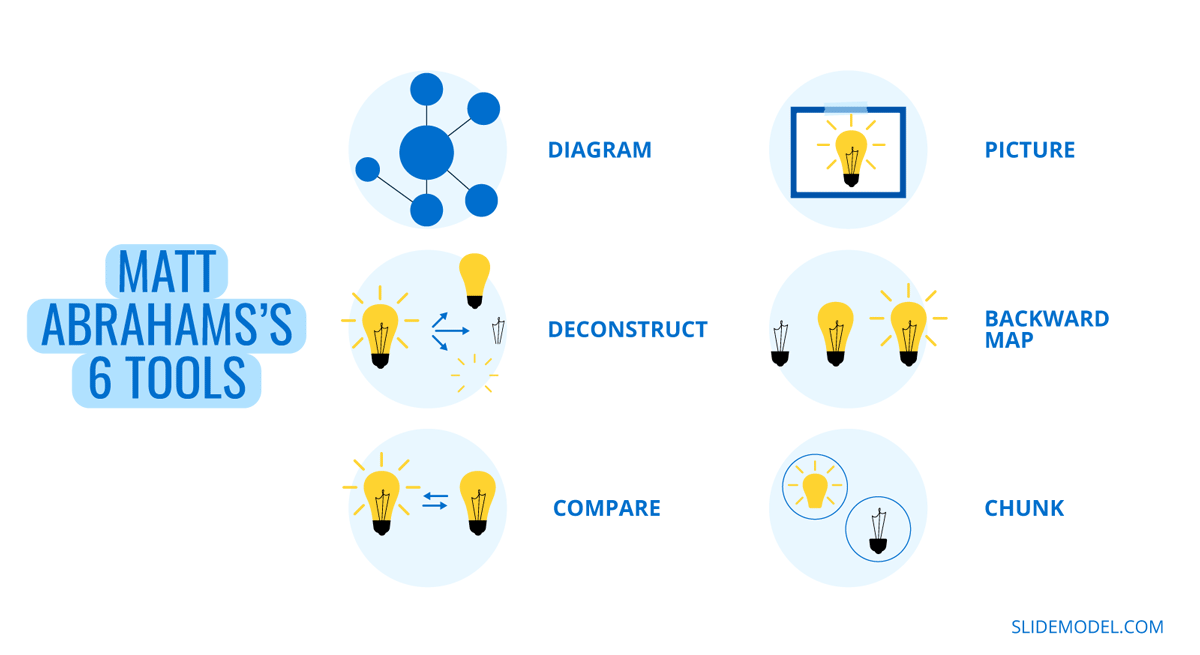
Pro Tip: Enhance your explanation strategy by using a comparison slide template . These slide templates can be effective for juxtaposing different concepts, illustrating contrasts or similarities with clarity. Whether you’re comparing two parts of a concept, different approaches, or contrasting theories, one of our slide comparison template can visually organize the information, making it more digestible for your audience.
Like learning any skill, communicating complex concepts must be learned. One of the best ways to acquire and increase your knowledge is to look at real-life examples. Inspiration is a conduit to action; it makes you feel like what you seek is an attainable skill.
For Dummies – Book and Content Series
When DOS for Dummies launched in 1991, the publishing market was full of beginners’ computer books that no one wanted to read. The author of DOS for Dummies, Dan Gookin, had the idea to create a beginner’s book that was fun and entertaining for people that wanted to learn to use computers but were overwhelmed. After all, in the early ’90s, computers were a novel complex concept!
The “For Dummies” book and digital series uses humor to make learning lighthearted and easy.
There’s a formula.
The central concept is separated into snackable sections of related topics. Each section has several chapters that relate to that topic. All the content is searchable with an index, meaning that people can choose to read from beginning to end or look up what they want to know on the spot.
Interactive Science Museums for Children
Another excellent example of how to communicate complex concepts to an audience with little or no knowledge of the subject is interactive science museums. The primary audience of these museums is school-age children, but their parents can also learn something new if they come along.
Are you looking for inspiration to explain complex information to anyone? Visit an interactive science museum and pay attention to their techniques. Try the activities and analyze your grasp of what the experience is teaching. How can you incorporate these ideas into your communication of complex concepts?
5 Levels Video Series
In Wired’s YouTube Channel, look for the 5 Levels Video Series . In these videos, experts from various fields explain a topic in five increasing levels of complexity. The five levels are; child, teen, college student, grad student, and expert.
Some of the complex concepts they have tackled in this series are astrophysics, music theory, quantum computing, and blockchain. Watch these videos to learn how to tailor how you explain the concept according to your audience’s communication level.
Infinity Ted Talk
Few things are more difficult to explain than the complex concept of infinity in mathematics. This whiteboard-style TED Talk explains the infinity of infinities with vibrant visual illustrations. Even if you’re not much into mathematics, you’ll be able to understand at least 80% of it.
Matt Abraham’s analysis and infographic on explaining complex concepts using 6 tools inspired this article. We appreciate how he shares actionable formulas to explain concepts, all wrapped up in one main formula you might not have noticed.
Create an overarching categorization of terms that make up your concept. Everything else grows from that. We recommend using this top-level formula as a foundation when preparing and presenting information about a complex concept.
Let’s consider the challenge of presenting what The Cloud is. To explain The Cloud efficiently and without confusing anyone, we can separate it into four main categories.
- What is The Cloud?
- Cloud Types
- Cloud Services
- Cloud Benefits & Risks
This categorization formula is the same formula writers, and content managers use for outlining articles and guides. It’s the same for web designers and e-commerce sites; categories are essential for creating a thread of understanding in any concept; simple, complex, or complicated. You’ll be surprised at how much easier everything becomes when using a formula as a guide.
As an example and in the spirit of Matt Abraham’s infographic, we’ll explain how to apply a formula to each category in The Cloud.
Mind-mapping
Using the “Diagraming” concept, and the mind-mapping technique explained earlier, we can try to explain what The Cloud is.
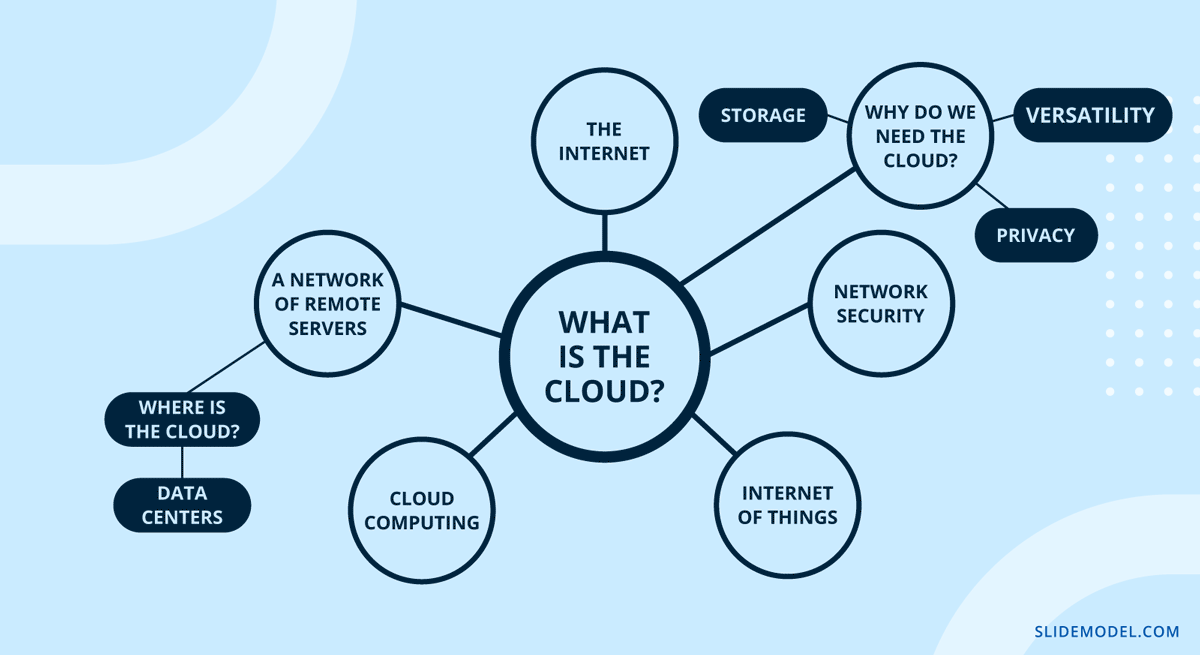
We start with the initial question: “What is The Cloud?”. Then, the second iteration of associated concepts answers the question by presenting a network of servers, introducing the internet, raising a question on its utility, and linking cloud computing, the Internet of Things (IoT), and network security.
By deepening into what remote servers are, we can ask ourselves, “Where is the Cloud?”, which the answer goes one indentation deeper by answering Data Centers. The same order logic is applied to reply: “Why do we need The Cloud?”. Then, we reply by highlighting Storage, Privacy, and Versatility as the main perks of working with The Cloud.
The mind mapping technique quickly helps us to identify concepts linked to the main idea we want to explain, boosting a creative thinking process.
Using the “Picture” and “Compare” Techniques, you can present analogies to known concepts, that can explain the difference between Public, Private, and Hybrid Clouds.
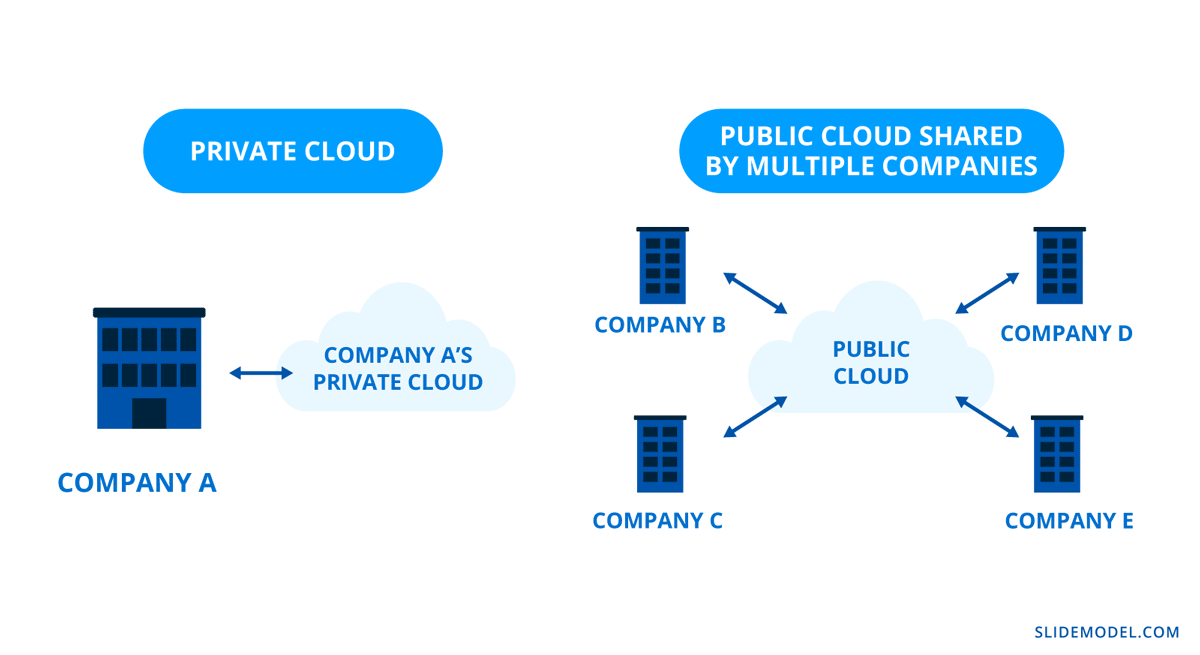
The image above explains the access options in Private vs. Public Cloud, referred to at an organizational level. To explain it in simpler words, a Public Cloud can be accessed from the Internet. The immediate model we can think of is a subscription service available for all customers with the same interests. On the other hand, the Private Cloud is restricted by an organization’s Intranet, and not shared publically.
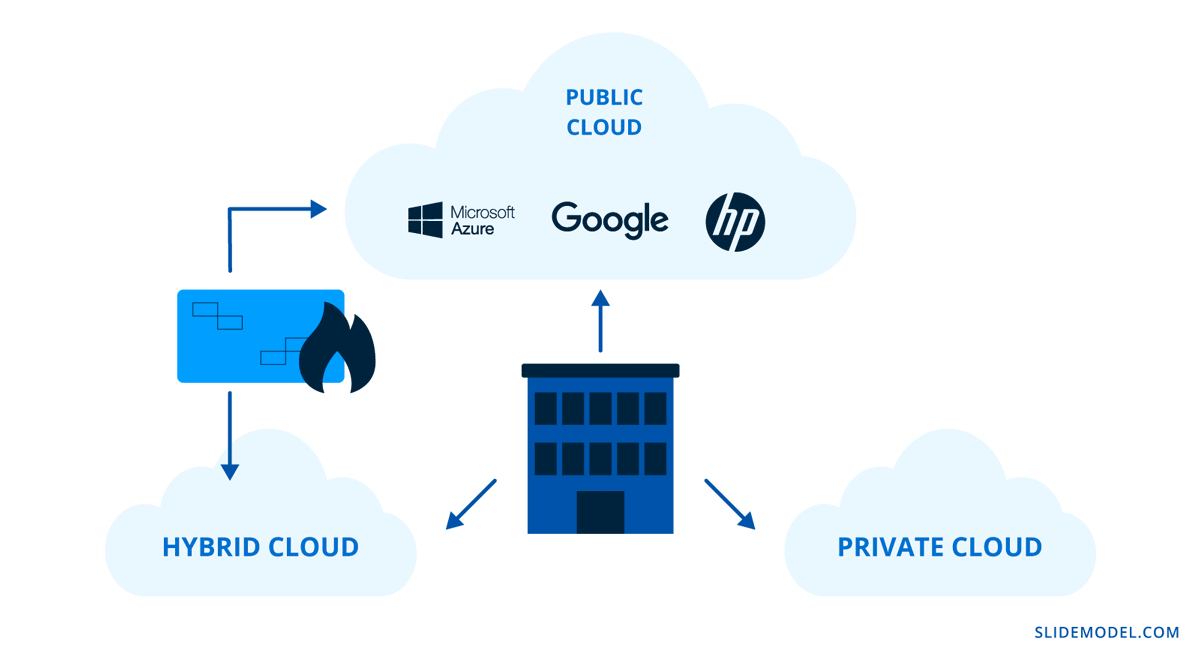
In the image above, it is clearly understood how the center of the definition is based on the “organization” and the owner of the resources. In the Public Cloud, the organization “hires” the services from a third-party provider (SaaS, PaaS, IaaS). In the Private Cloud, the organization hosts its own services, and in the hybrid scenario, the organization combines on-premise and off-premise resources to use services.
Four Step Formula
To use the “Chunk” method, we can apply the Four Step Formula for the next category, Cloud Services. Imagine you need to prepare and present a slide presentation and speech about services in The Cloud. The Four Step formula for explaining complex concepts is your ideal partner.
Let’s follow the formula.
- Simplify: Define Cloud services in as few words and sentences as possible.
- Focus on Problems (Pain points): Prepare talking points about topics like security concerns, compliance, data migration.
- Identify benefits or solutions: Explain how cloud services fix those problems and how.
- Practice: Prepare the slides and presentation speech. Practice the talk many times, using different audience types as inspiration. Try a variety of talking styles until you feel confident.
Explaining Benefits vs. Risks is a process easily understood when using a visual model such as a Comparison Chart . In this case, we shall present the list of Cloud Benefits & Risks by constructing that comparison in a visual format, helping users quickly spot whether a feature can be considered a benefit or a risk.
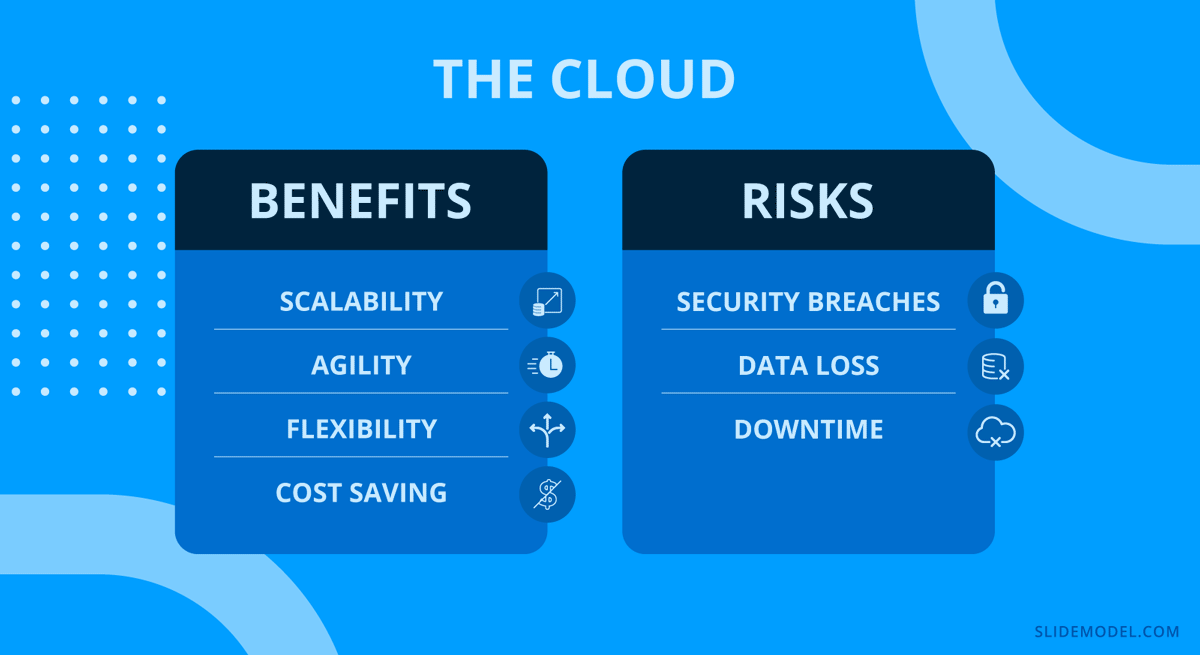
Learning many ways to communicate complex concepts efficiently is excellent. But better than that is knowing what NOT to do. Here are the biggest mistakes to avoid when communicating topics you want the audience to understand.
Not Paying Attention to The Audience
When presenting a complex concept, the first mistake is not paying attention to your audience. Being disconnected from the audience doesn’t help your cause; you need to make a connection from the start.
For that, you need to know the audience’s knowledge level of your topic. This information will help you decide the difficulty level you’ll use while presenting and how much industry jargon they’ll understand.
Find out as much as you can about the audience before presenting. Preferably before making the presentation . Ask these questions:
- What is the average age of the audience?
- What’s their average level of study?
- If adults, what average level of academic studies do they have, and in what fields?
The second instance where you can’t afford not to pay attention to the audience is when you’re presenting to them. Connecting with the audience will help them understand your complex topic and make you feel better as you talk, consequently improving the learning experience for everyone.
Mastering the art of connection with the audience using body language, compelling conversation rhythms, and making an impact aren’t innate skills for everyone. Most of you will have to learn, practice, get feedback, and practice some more.
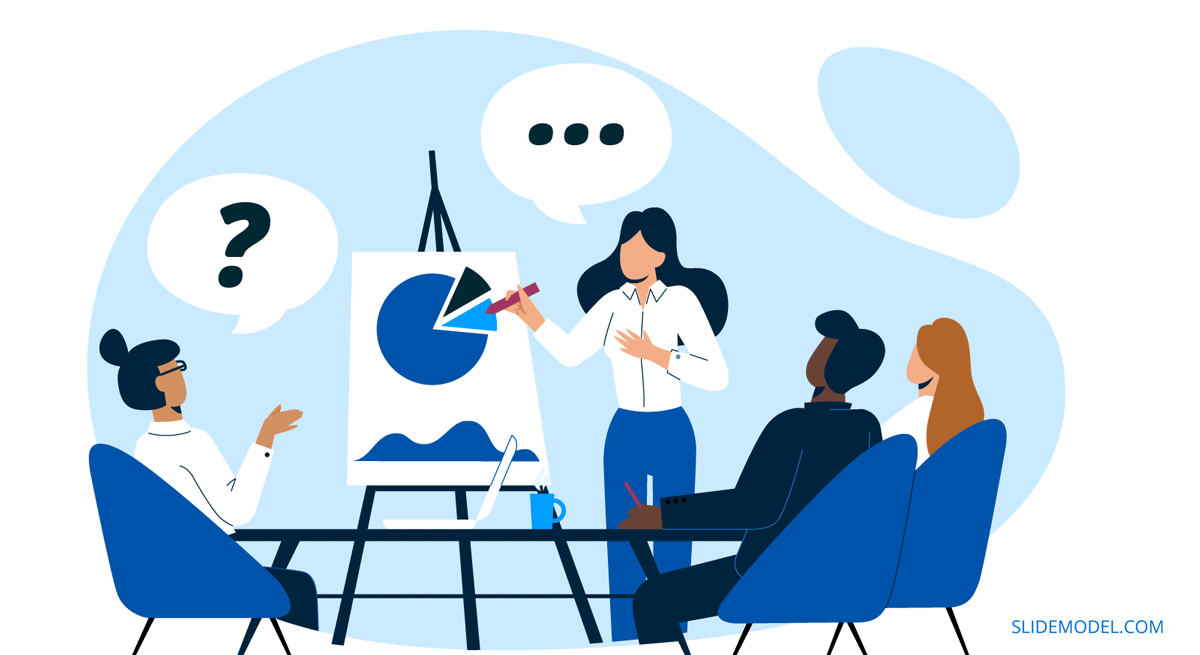
Not Being Ready for a Q&A Session
Questions are a tricky topic concerning presentations like keynote speeches and TED Talks. Some keynote speeches will have a Q&A Session, while no TED Talk will ever have one. Allowing questions in the middle of a presentation is detrimental to the session. They can take you off on tangents that will be difficult to come back from.
On the other hand, Q&A sessions at the end of a presentation can dilute the message or the impact the speaker has on the audience. TED Talks don’t have Q&A Sessions for this reason, to not take away from the presenter’s idea worth spreading.
Nevertheless, many presentations of complex concepts are educational and need a Q&A session to be comprehensive and of value to the audience. As a presenter, you need to be ready to answer some questions. You obviously won’t always know what the audience will ask, but you can deduce some questions by practicing with colleagues and peers and asking for feedback.
Unclear Slide Decks
This mistake refers to your visual presentation slides . Regardless if you’ll distribute the slide deck on its own or only use it as support when presenting, your slides can’t be communication disasters. Use PowerPoint templates to help with a graphic design foundation and adjust them with your information.
Be mindful of data visualization, mind maps, and visual metaphors. Ensure that your visualizations correctly represent your information and that nothing gets lost in translation. Remember to be clear and concise with infographics and widgets.
Get rid of clutter, use consistent colors, don’t mix too many fonts, and keep elements aligned. Browse the SlideModel Blog and improve your presentation skills with our design guides.
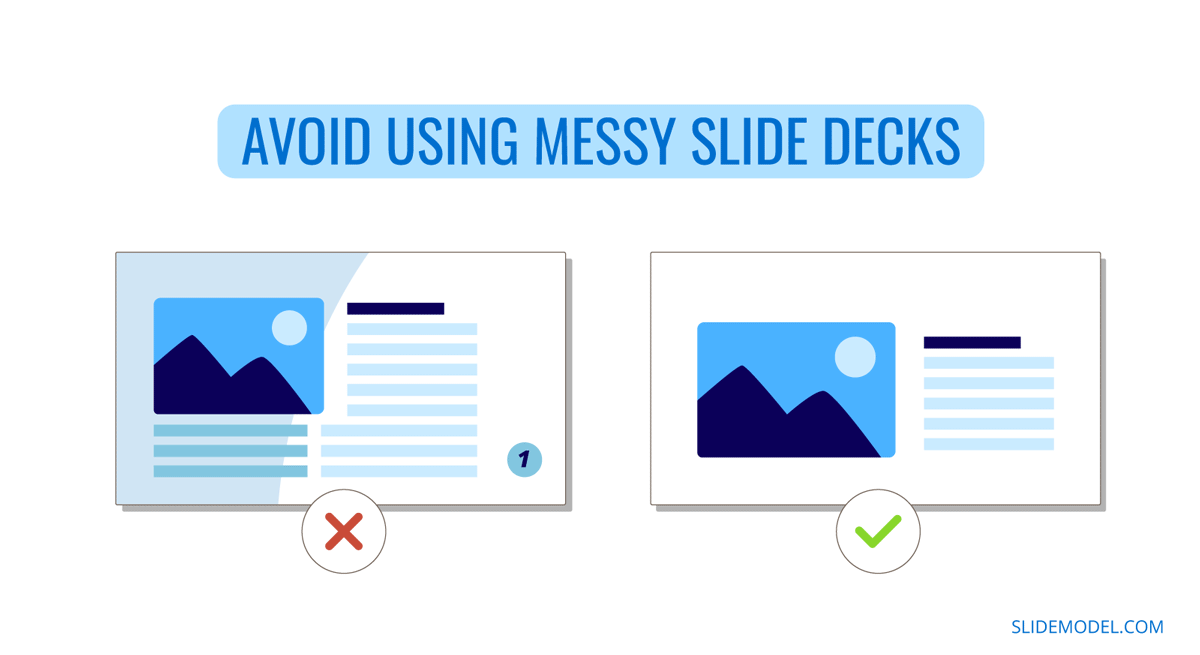
Not Practicing
Finally, a huge mistake to avoid is not practicing. Complex concepts are naturally difficult to understand; it’s up to you, the experts, to make them accessible. It’s not only about practicing a speech about a complex concept; it’s also about developing a strategy for sharing complex information.
If you want to be a master at communicating complex concepts to anyone, write your speech or main presentation points in different tones and for different audience levels. Practice them all and feel confident at every level. You’ll be so prepared that the audience will be smiling and nodding as you look at them and connect.
Do some research on other speakers and take some notes. For example, in the 5 Levels Video Series from Wired, you can watch and listen for similarities and differences at each level. Does the speaker change their body language? Are there props involved? Notice how the vocabulary and technical jargon increase as the levels go up.
And, of course, practice your articulation and speech cadence to be compelling and exciting to listen to.
Are you feeling the pressure? Communicating complex concepts isn’t easy. If it were, everyone would do it, and guides like this wouldn’t exist.
Thankfully, since the concept of explaining complex concepts is complicated and not complex, you can follow formulas, systems, and processes. Practice using mindmaps and visualizations as you develop the concept before preparing a presentation. Before you start to consider how to start a presentation , give yourself the space and time to try different techniques and practice explaining your concept at different levels.
Use SlideModel templates to build your presentation slides on PowerPoint and practice presenting with them. Become one with your slides, let the speech flow naturally, and let the words articulate well from your mouth. You’ll be surprised at how well audiences understand your complex concept next time you’re up on a stage or as the speaker in a virtual meeting or summit.
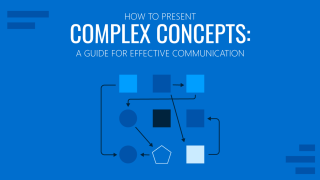
Like this article? Please share
Presentation Approaches, Presentation Ideas Filed under Presentation Ideas
Related Articles
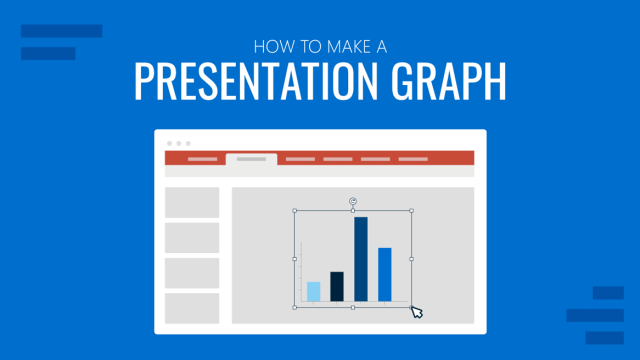
Filed under Design • March 27th, 2024
How to Make a Presentation Graph
Detailed step-by-step instructions to master the art of how to make a presentation graph in PowerPoint and Google Slides. Check it out!
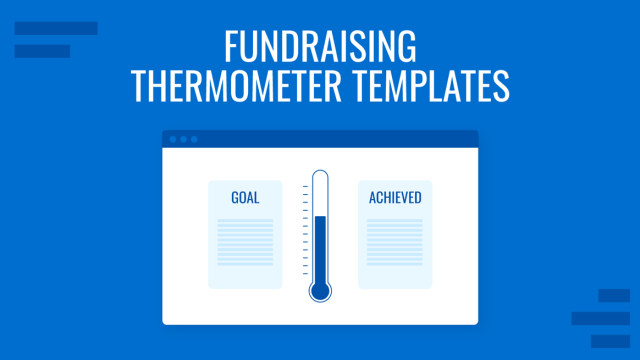
Filed under Presentation Ideas • February 29th, 2024
How to Make a Fundraising Presentation (with Thermometer Templates & Slides)
Meet a new framework to design fundraising presentations by harnessing the power of fundraising thermometer templates. Detailed guide with examples.
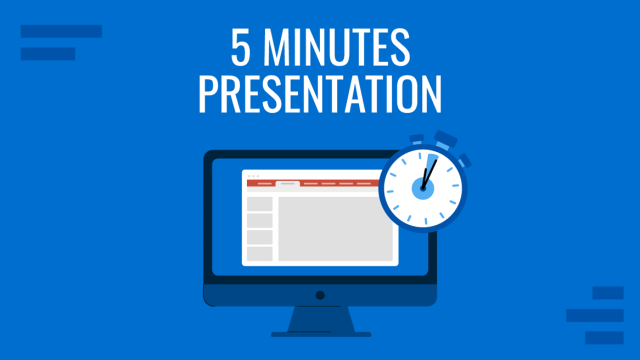
Filed under Presentation Ideas • February 15th, 2024
How to Create a 5 Minutes Presentation
Master the art of short-format speeches like the 5 minutes presentation with this article. Insights on content structure, audience engagement and more.
Leave a Reply
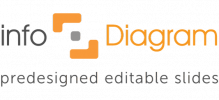
Blog – Creative Presentations Ideas
infoDiagram visual slide examples, PowerPoint diagrams & icons , PPT tricks & guides
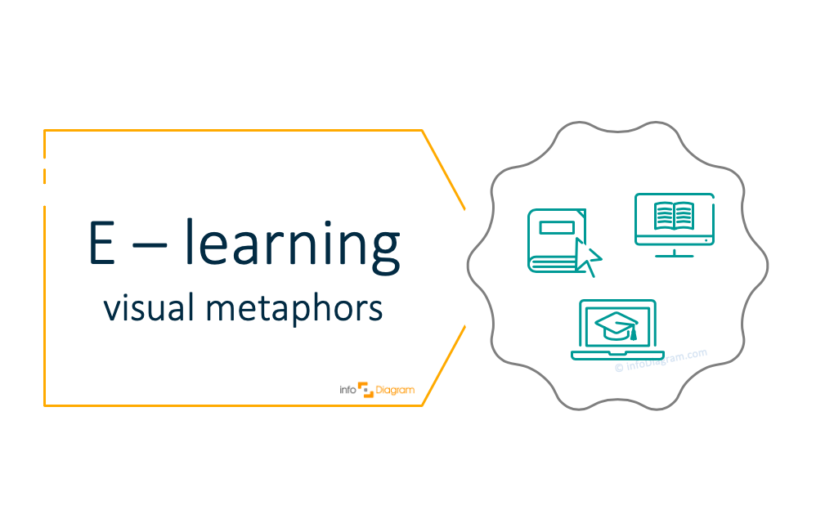
Illustrating E-learning in a Presentation [concept visualization]
Last Updated on September 28, 2020 by Anastasia
With the development of technologies, e-learning and remote education became usual. If you need to present the E-learning concept, get inspired by our icon ideas.
The challenge is how to illustrate the concept properly in a PowerPoint presentation so the slides look professional and clear. Icons are a way of communication and, due to their simplified graphical form, they may even be the only language around that everybody understands.
Modern elegant outline symbols for presenting e-learning concept
Below there are icon examples of style modern outline icons style. It features a variety of different approaches for representing E-learning. Book and speech bubbles with microphone symbols is a great way to demonstrate the classic tools in the learning process , and groups of people having online calls, or notes and laptop screens as a new method in E-education.
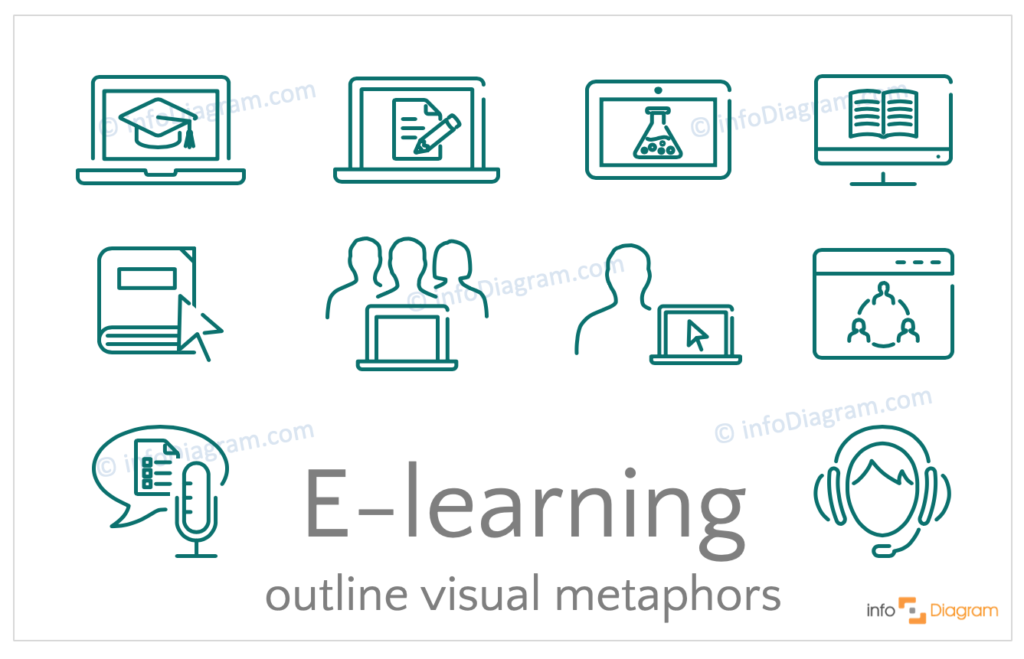
Use these symbol to make your product presentation more visual:
- combined symbol of a group of people with laptop presenting class or team colleagues
- group of people in a browser having an online call
- computer screen with a chemical probe illustrating the scientific process
- book icon as a universal symbol for the education process
- combination of notes and l aptop screen icons as a new method of taking notes
- female/male figure with computer icon working in IT branch
- speech bubble with a microphone as a new tool for taking notes
Design-neutral flat concept icons
This flat style of icons is more simple than outline one. It fits any presentation style and icons are recognizable even if you make them small. Click to see collection of such symbols we designed.
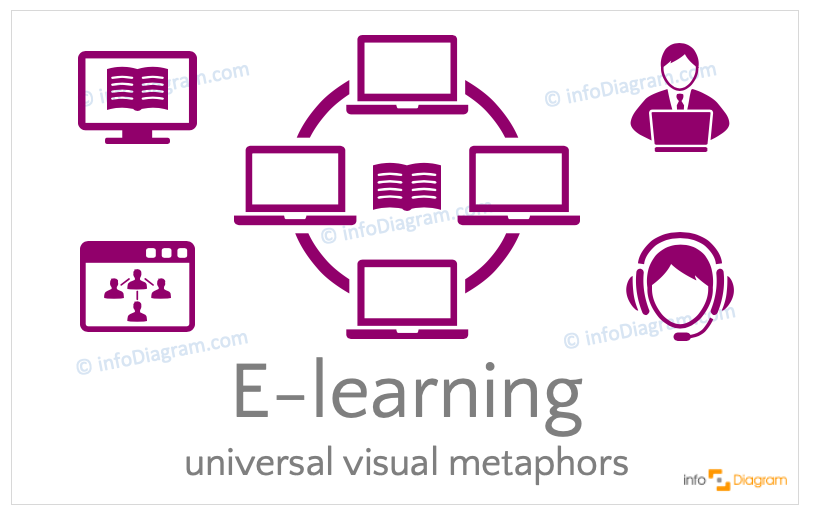
Here are some ideas of pictures representing e-learning:
- combined symbol of team members with a tablet having an online scheduled meeting
- book pictogram combined with the computer showing E-learning process
- general symbol of a person with headphones – e.g. e-course participant
- the person behind the computer – showing e-learning users
Creative unique hand-drawn icons collection
If you prefer a more creative look to your visual metaphors for presenting E-Learning ideas consider using handdrawn-like symbols. The scribbled book icon, chemical probe, or speech bubble with microphones are great symbols for depicting E-Learning presentation.
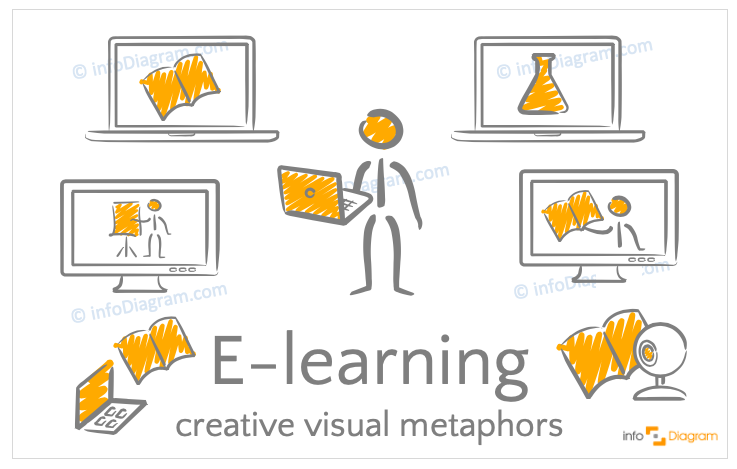
What topics you can use to express remote learning process:
- laptop and book icons as a classical symbol for E-learning
- combined icon computer screen with a chemical probe illustrating teaching some scientific or university or school content
- a male figure holding computer during E-studying
- male/female figures combined with computer symbol doing different learning activities: reading or presenting
- the camera as a tool for organizing online conferences or meetings
Multicolor flat e-learning concept icons collection
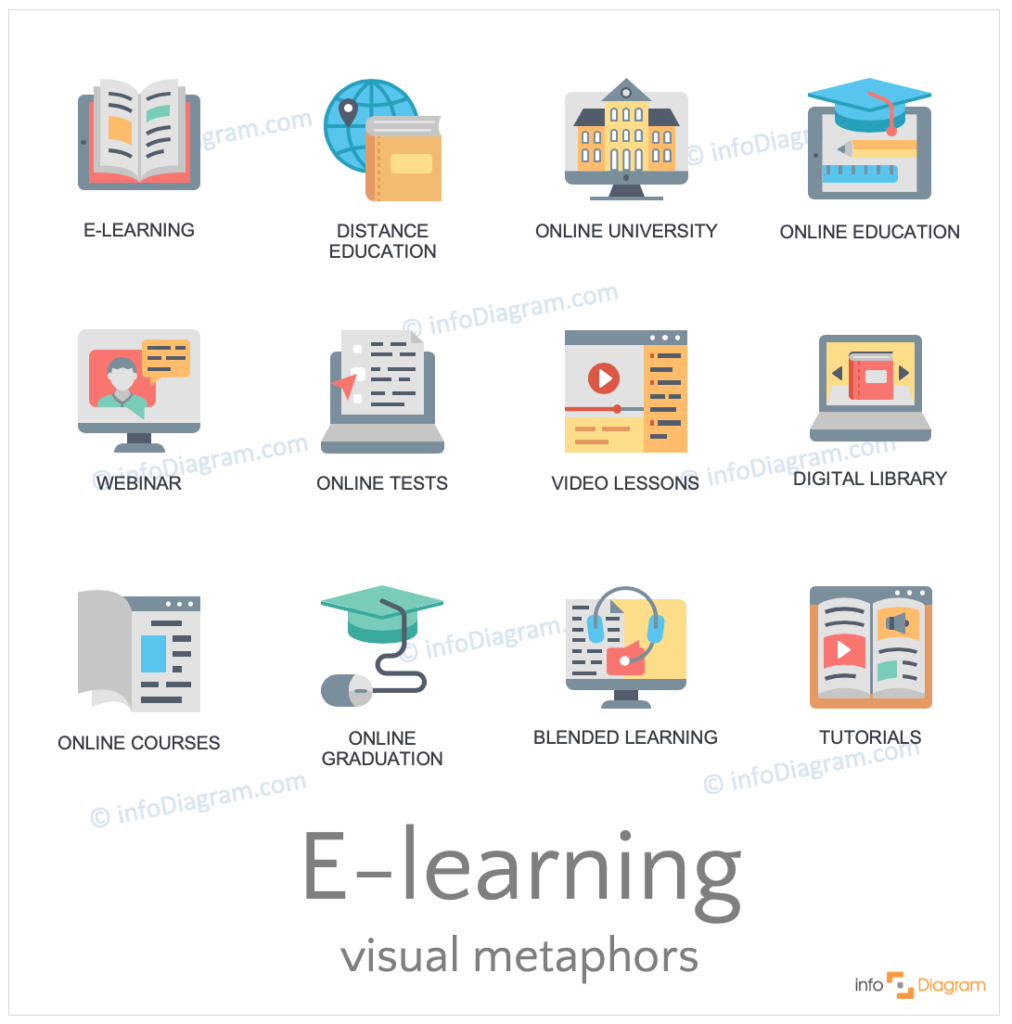
Those colorful icons will suit a presentation slide where you want to attract more attention to symbols. Here’s how you can illustrate the E-learning concept using those kinds of icons:
- the general collection of E-learning icon presenting the main idea of E-studying: distance education , online university, online education
- new online teaching methods showed with webinars icon, online test, video lesson or digital learning
- icons showing nowadays possibilities online courses , online graduation, tutorials
We hope these e-learning graphics will help you design a nice looking infographic that will make your presentation more engaging and easy to read.
If you like the suggested icons, you can get them from infoDiagram library. The best way to get them is by joining subscription access to PPT graphics here . It will allow you to download these symbols, and graphics from any presentation deck you find on the website.
More concept icons ideas
Need to show another concept in a presentation? Crisis, Leadership, Engagement, Growth you name it. Check our blog Ultimate List of Business Concepts Visualization to get inspired.
Leave a Reply Cancel reply
Your email address will not be published. Required fields are marked *
Save my name, email, and website in this browser for the next time I comment.
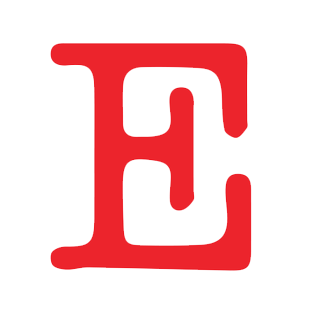
- Scholarly Community Encyclopedia
- Log in/Sign up


Video Upload Options
- MDPI and ACS Style
- Chicago Style
Concept learning, also known as category learning, concept attainment, and concept formation, is defined by Bruner, Goodnow, & Austin (1967) as "the search for and listing of attributes that can be used to distinguish exemplars from non exemplars of various categories". More simply put, concepts are the mental categories that help us classify objects, events, or ideas, building on the understanding that each object, event, or idea has a set of common relevant features. Thus, concept learning is a strategy which requires a learner to compare and contrast groups or categories that contain concept-relevant features with groups or categories that do not contain concept-relevant features. In a concept learning task, a human or machine learner is trained to classify objects by being shown a set of example objects along with their class labels. The learner simplifies what has been observed by condensing it in the form of an example. This simplified version of what has been learned is then applied to future examples. Concept learning may be simple or complex because learning takes place over many areas. When a concept is difficult, it is less likely that the learner will be able to simplify, and therefore will be less likely to learn. Colloquially, the task is known as learning from examples. Most theories of concept learning are based on the storage of exemplars and avoid summarization or overt abstraction of any kind.
1. Types of Concepts
Concept learning must be distinguished from learning by reciting something from memory (recall) or discriminating between two things that differ (discrimination). However, these issues are closely related, since memory recall of facts could be considered a "trivial" conceptual process where prior exemplars representing the concept are invariant. Similarly, while discrimination is not the same as initial concept learning, discrimination processes are involved in refining concepts by means of the repeated presentation of exemplars.
Concrete or Perceptual Concepts vs Abstract Concepts
Concrete concepts are objects that can be perceived by personal sensations and perceptions. These are objects like chairs and dogs where personal interactions occur with them and create a concept. [ 1 ] Concepts become more concrete as the word we use to associate with it has a perceivable entity. [ 2 ] According to Paivio’s dual -coding theory, concrete concepts are the one that is remembered easier from their perceptual memory codes. [ 3 ] Evidence has shown that when words are heard they are associated with a concrete concept and are re-enact any previous interaction with the word within the sensorimotor system. [ 4 ] Examples of concrete concepts in learning are early educational math concepts like adding and subtracting.
Abstract concepts are words and ideas that deal with emotions, personality traits and events. [ 5 ] Terms like "fantasy" or "cold" have a more abstract concept within them. Every person has their personal definition, which is ever changing and comparing, of abstract concepts. For example, cold could mean the physical temperature of the surrounding area or it could define the action and personality of another person. While within concrete concepts there is still a level of abstractness, concrete and abstract concepts can be seen on a scale. Some ideas like chair and dog are more cut and dry in their perceptions but concepts like cold and fantasy can be seen in a more obscure way. Examples of abstract concept learning are topics like religion and ethics. Abstract-concept learning is seeing the comparison of the stimuli based on a rule (e.g., identity, difference, oddity, greater than, addition, subtraction) and when it is a novel stimulus. [ 6 ] With abstract-concept learning have three criteria’s to rule out any alternative explanations to define the novelty of the stimuli. One transfer stimuli has to be novel to the individual. This means it needs to be a new stimulus to the individual. Two, there is no replication of the transfer stimuli. Third and lastly, to have a full abstract learning experience there has to be an equal amount of baseline performance and transfer performance. [ 6 ]
Binder, Westbury, McKiernan, Possing, and Medler (2005) [ 7 ] used fMRI to scan individuals' brains as they made lexical decisions on abstract and concrete concepts. Abstract concepts elicited greater activation in the left precentral gyrus, left inferior frontal gyrus and sulcus, and left superior temporal gyrus, whereas concrete concepts elicited greater activation in bilateral angular gyri, the right middle temporal gyrus, the left middle frontal gyrus, bilateral posterior cingulate gyri, and bilateral precunei.
In 1986 Allan Paivio [ 8 ] hypothesized the Dual Coding Theory, which states that both verbal and visual information is used to represent information. When thinking of the concept “dog” thoughts of both the word dog and an image of a dog occur. Dual Coding Theory assumes that abstract concepts involve the verbal semantic system and concrete concepts are additionally involved with the visual imaginary system.
Defined (or Relational) and Associated Concepts
Relational and associated concepts are words, ideas and thoughts that are connected in some form. For relational concepts they are connected in a universal definition. Common relational terms are up-down, left-right, and food-dinner. These ideas are learned in our early childhood and are important for children to understand. [ 9 ] These concepts are integral within our understanding and reasoning in conservation tasks. [ 10 ] Relational terms that are verbs and prepositions have a large influence on how objects are understood. These terms are more likely to create a larger understanding of the object and they are able to cross over to other languages. [ 11 ]
Associated concepts are connected by the individual’s past and own perception. Associative concept learning (also called functional concept learning) involves categorizing stimuli based on a common response or outcome regardless of perceptual similarity into appropriate categories. [ 12 ] This is associating these thoughts and ideas with other thoughts and ideas that are understood by a few or the individual. An example of this is in elementary school when learning the direction of the compass North, East, South and West. Teacher have used “Never Eat Soggy Waffles”, “Never Eat Sour Worms” and students were able to create their own version to help them learn the directions. [ 13 ]
Complex Concepts . Constructs such as a schema and a script are examples of complex concepts. A schema is an organization of smaller concepts (or features) and is revised by situational information to assist in comprehension. A script on the other hand is a list of actions that a person follows in order to complete a desired goal. An example of a script would be the process of buying a CD. There are several actions that must occur before the actual act of purchasing the CD and a script provides a sequence of the necessary actions and proper order of these actions in order to be successful in purchasing the CD.
2. Methods of Learning a Concept
Discovery – Every baby discovers concepts for itself, such as discovering that each of its fingers can be individually controlled or that care givers are individuals. Although this is perception driven, formation of the concept is more than memorizing perceptions.
Examples – Supervised or unsupervised generalizing from examples may lead to learning a new concept, but concept formation is more than generalizing from examples.
Words – Hearing or reading new words leads to learning new concepts, but forming a new concept is more than learning a dictionary definition. A person may have previously formed a new concept before encountering the word or phrase for it.
Exemplars comparison and contrast – An efficient way to learn new categories and to induce new categorization rules is by comparing a few example objects while being informed about their categorical relation. Comparing two exemplars while being informed that the two are from the same category allows identifying the attributes shared by the category members, as it exemplifies variability within this category. On the other hand, contrasting two exemplars while being informed that the two are from different categories may allow identifying attributes with diagnostic value. Within category comparison and between categories contrast are not similarly useful for category learning (Hammer et al., 2008), and the capacity to use these two forms of comparison-based learning changes at childhood (Hammer et al., 2009).
Invention – When prehistoric people who lacked tools used their fingernails to scrape food from killed animals or smashed melons, they noticed that a broken stone sometimes had a sharp edge like a fingernail and was therefore suitable for scraping food. Inventing a stone tool to avoid broken fingernails was a new concept.
3. Theoretical Issues
In general, the theoretical issues underlying concept learning are those underlying induction. These issues are addressed in many diverse publications, including literature on subjects like Version Spaces, Statistical Learning Theory, PAC Learning, Information Theory, and Algorithmic Information Theory. Some of the broad theoretical ideas are also discussed by Watanabe (1969,1985), Solomonoff (1964a,1964b), and Rendell (1986); see the reference list below.
4. Modern Psychological Theories
It is difficult to make any general statements about human (or animal) concept learning without already assuming a particular psychological theory of concept learning. Although the classical views of concepts and concept learning in philosophy speak of a process of abstraction, data compression, simplification, and summarization, currently popular psychological theories of concept learning diverge on all these basic points. The history of psychology has seen the rise and fall of many theories about concept learning. Classical conditioning (as defined by Pavlov) created the earliest experimental technique. Reinforcement learning as described by Watson and elaborated by Clark Hull created a lasting paradigm in behavioral psychology. Cognitive psychology emphasized a computer and information flow metaphor for concept formation. Neural network models of concept formation and the structure of knowledge have opened powerful hierarchical models of knowledge organization such as George Miller's Wordnet. Neural networks are based on computational models of learning using factor analysis or convolution. Neural networks also are open to neuroscience and psychophysiological models of learning following Karl Lashley and Donald Hebb.
4.1. Rule-Based
Rule-based theories of concept learning began with cognitive psychology and early computer models of learning that might be implemented in a high level computer language with computational statements such as if:then production rules. They take classification data and a rule-based theory as input which are the result of a rule-based learner with the hopes of producing a more accurate model of the data (Hekenaho 1997). The majority of rule-based models that have been developed are heuristic, meaning that rational analyses have not been provided and the models are not related to statistical approaches to induction. A rational analysis for rule-based models could presume that concepts are represented as rules, and would then ask to what degree of belief a rational agent should be in agreement with each rule, with some observed examples provided (Goodman, Griffiths, Feldman, and Tenenbaum). Rule-based theories of concept learning are focused more so on perceptual learning and less on definition learning. Rules can be used in learning when the stimuli are confusable, as opposed to simple. When rules are used in learning, decisions are made based on properties alone and rely on simple criteria that do not require a lot of memory ( Rouder and Ratcliff, 2006).
Example of rule-based theory:
"A radiologist using rule-based categorization would observe whether specific properties of an X-ray image meet certain criteria; for example, is there an extreme difference in brightness in a suspicious region relative to other regions? A decision is then based on this property alone." (see Rouder and Ratcliff 2006)
4.2. Prototype
The prototype view of concept learning holds that people abstract out the central tendency (or prototype) of the examples experienced and use this as a basis for their categorization decisions.
The prototype view of concept learning holds that people categorize based on one or more central examples of a given category followed by a penumbra of decreasingly typical examples. This implies that people do not categorize based on a list of things that all correspond to a definition, but rather on a hierarchical inventory based on semantic similarity to the central example(s).
4.3. Exemplar
Exemplar theory is the storage of specific instances (exemplars), with new objects evaluated only with respect to how closely they resemble specific known members (and nonmembers) of the category. This theory hypothesizes that learners store examples verbatim . This theory views concept learning as highly simplistic. Only individual properties are represented. These individual properties are not abstract and they do not create rules. An example of what exemplar theory might look like is, "water is wet". It is simply known that some (or one, or all) stored examples of water have the property wet. Exemplar based theories have become more empirically popular over the years with some evidence suggesting that human learners use exemplar based strategies only in early learning, forming prototypes and generalizations later in life. An important result of exemplar models in psychology literature has been a de-emphasis of complexity in concept learning. One of the best known exemplar theories of concept learning is the Generalized Context Model (GCM).
A problem with exemplar theory is that exemplar models critically depend on two measures: similarity between exemplars, and having a rule to determine group membership. Sometimes it is difficult to attain or distinguish these measures.
4.4. Multiple-Prototype
More recently, cognitive psychologists have begun to explore the idea that the prototype and exemplar models form two extremes. It has been suggested that people are able to form a multiple prototype representation, besides the two extreme representations. For example, consider the category 'spoon'. There are two distinct subgroups or conceptual clusters: spoons tend to be either large and wooden, or small and made of metal. The prototypical spoon would then be a medium-size object made of a mixture of metal and wood, which is clearly an unrealistic proposal. A more natural representation of the category 'spoon' would instead consist of multiple (at least two) prototypes, one for each cluster. A number of different proposals have been made in this regard (Anderson, 1991; Griffiths, Canini, Sanborn & Navarro, 2007; Love, Medin & Gureckis, 2004; Vanpaemel & Storms, 2008). These models can be regarded as providing a compromise between exemplar and prototype models.
4.5. Explanation-Based
The basic idea of explanation-based learning suggests that a new concept is acquired by experiencing examples of it and forming a basic outline. 1 Put simply, by observing or receiving the qualities of a thing the mind forms a concept which possesses and is identified by those qualities.
The original theory, proposed by Mitchell, Keller, and Kedar-Cabelli in 1986 and called explanation-based generalization, is that learning occurs through progressive generalizing. 2 This theory was first developed to program machines to learn. When applied to human cognition, it translates as follows: the mind actively separates information that applies to more than one thing and enters it into a broader description of a category of things. This is done by identifying sufficient conditions for something to fit in a category, similar to schematizing.
The revised model revolves around the integration of four mental processes – generalization, chunking, operationalization, and analogy 3 .
- Generalization is the process by which the characteristics of a concept which are fundamental to it are recognized and labeled. For example, birds have feathers and wings. Anything with feathers and wings will be identified as ‘bird’.
- When information is grouped mentally, whether by similarity or relatedness, the group is called a chunk. Chunks can vary in size from a single item with parts or many items with many parts. 4
- A concept is operationalized when the mind is able to actively recognize examples of it by characteristics and label it appropriately. 5
- Analogy is the recognition of similarities among potential examples. 6
This particular theory of concept learning is relatively new and more research is being conducted to test it.
4.6. Bayesian
Taking a mathematical approach to concept learning, Bayesian theories propose that the human mind produces probabilities for a certain concept definition, based on examples it has seen of that concept. [ 14 ] The Bayesian concept of Prior Probability stops learners' hypotheses being overly specific, while the likelihood of a hypothesis ensures the definition is not too broad.
For example- say a child is shown three horses by a parent and told these are called "horses"- she needs to work out exactly what the adult means by this word. She is much more likely to define the word "horses" as referring to either this type of animal or all animals , rather than an oddly specific example like "all horses except Clydedales" , which would be an unnatural concept. Meanwhile, the likelihood of 'horses' meaning 'all animals' when the three animals shown are all very similar is low. The hypothesis that the word "horse" refers to all animals of this species is most likely of the three possible definitions, as it has both a reasonable prior probability and likelihood given examples.
Bayes' theorem is important because it provides a powerful tool for understanding, manipulating and controlling data 5 that takes a larger view that is not limited to data analysis alone 6 . The approach is subjective, and this requires the assessment of prior probabilities 6 , making it also very complex. However, if Bayesians show that the accumulated evidence and the application of Bayes' law are sufficient, the work will overcome the subjectivity of the inputs involved 7 . Bayesian inference can be used for any honestly collected data and has a major advantage because of its scientific focus 6 .
One model that incorporates the Bayesian theory of concept learning is the ACT-R model, developed by John R. Anderson. The ACT-R model is a programming language that defines the basic cognitive and perceptual operations that enable the human mind by producing a step-by-step simulation of human behavior. This theory exploits the idea that each task humans perform consists of a series of discrete operations. The model has been applied to learning and memory, higher level cognition, natural language, perception and attention, human-computer interaction, education, and computer generated forces.
In addition to John R. Anderson, Joshua Tenenbaum has been a contributor to the field of concept learning; he studied the computational basis of human learning and inference using behavioral testing of adults, children, and machines from Bayesian statistics and probability theory, but also from geometry, graph theory, and linear algebra. Tenenbaum is working to achieve a better understanding of human learning in computational terms and trying to build computational systems that come closer to the capacities of human learners.
4.7. Component Display Theory
M. D. Merrill's component display theory (CDT) is a cognitive matrix that focuses on the interaction between two dimensions: the level of performance expected from the learner and the types of content of the material to be learned. Merrill classifies a learner's level of performance as: find, use, remember, and material content as: facts, concepts, procedures, and principles. The theory also calls upon four primary presentation forms and several other secondary presentation forms. The primary presentation forms include: rules, examples, recall, and practice. Secondary presentation forms include: prerequisites, objectives, helps, mnemonics, and feedback. A complete lesson includes a combination of primary and secondary presentation forms, but the most effective combination varies from learner to learner and also from concept to concept. Another significant aspect of the CDT model is that it allows for the learner to control the instructional strategies used and adapt them to meet his or her own learning style and preference. A major goal of this model was to reduce three common errors in concept formation: over-generalization, under-generalization and misconception.
- Paivio, Allan. (2014). Mind and Its Evolution : a Dual Coding Theoretical Approach.. Taylor and Francis. ISBN 978-1-317-71690-7. OCLC 868489792. http://www.worldcat.org/oclc/868489792
- Binder, J. R., Westbury, C. F., McKiernan, K. A., Possing, E. T., & Medler, D. A. (2005). Distinct brain systems for processing concrete and abstract words. Journal of Cognitive Neuroscience, 17, 905–917.
- Paivio, Allan (2014-01-14) (in en). Mind and Its Evolution: A Dual Coding Theoretical Approach (1 ed.). Psychology Press. doi:10.4324/9781315785233. ISBN 978-1-315-78523-3. https://www.taylorfrancis.com/books/9781315785233.
- Cappa, Stefano F.; Pulvermüller, Friedemann (July 2012). "Cortex special issue: Language and the motor system". Cortex 48 (7): 785–787. doi:10.1016/j.cortex.2012.04.010. ISSN 0010-9452. PMID 22579224. https://dx.doi.org/10.1016%2Fj.cortex.2012.04.010
- Katja Wiemer-Hastings, Katja; Xu, Xu (2005-09-10). "Content Differences for Abstract and Concrete Concepts". Cognitive Science 29 (5): 719–736. doi:10.1207/s15516709cog0000_33. ISSN 0364-0213. PMID 21702791. https://dx.doi.org/10.1207%2Fs15516709cog0000_33
- Katz, J. S., Wright, A. A., & Bodily, K. D. (2007). Issues in the Comparative Cognition of Abstract-Concept Learning. Comparative Cognition & Behavior Reviews, 2, 79–92.
- Binder, J. R.; Westbury, C. F.; McKiernan, K. A.; Possing, E. T.; Medler, D. A. (June 2005). "Distinct Brain Systems for Processing Concrete and Abstract Concepts". Journal of Cognitive Neuroscience 17 (6): 905–917. doi:10.1162/0898929054021102. ISSN 0898-929X. PMID 16021798. https://dx.doi.org/10.1162%2F0898929054021102
- Paivio, A. (1986). Mental representations: A dual coding approach. New York: Oxford University Press.
- Boehm, Ann (2004). The Psychoeducational Assessment of Preschool Children. London: Lawrence Erlbaum associates. pp. 186–203.
- Walker, Alice A. (1979-09). "The Development of Relational Concepts in Three-and Four-Year-Olds". The Journal of Educational Research. 73 (1): 37–40. doi:10.1080/00220671.1979.10885201. ISSN 0022-0671.
- J. Loewenstein, D. Gentner Relational language and the development of relational mapping Cognitive Psychology, 50 (2005), pp. 315-353
- Urcuioli, Peter J. (2009-04-08). "Responses and Acquired Equivalence Classes". Comparative Cognition Experimental Explorations of Animal Intelligence. Oxford University Press. pp. 405–422. doi:10.1093/acprof:oso/9780195377804.003.0022. ISBN 978-0-19-537780-4. https://dx.doi.org/10.1093%2Facprof%3Aoso%2F9780195377804.003.0022
- "Wind Directions:North, East, South, West". https://www.mnemonic-device.com/geography/wind-directions-north-east-south-west/.
- Tenenbaum, Joshua B. (1999). "Bayesian modeling of human concept learning". Advances in Neural Information Processing Systems 11 (12): 59–65. http://web.mit.edu/cocosci/Papers/bayes.pdf. Retrieved 30 January 2018.
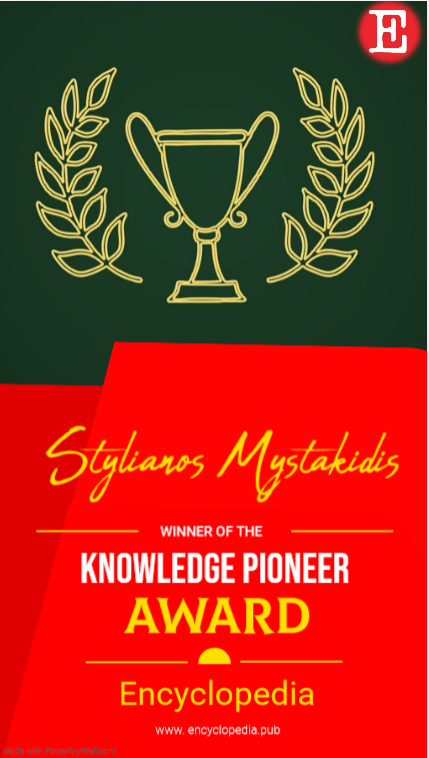
- Terms and Conditions
- Privacy Policy
- Advisory Board
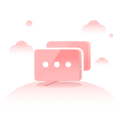
Got any suggestions?
We want to hear from you! Send us a message and help improve Slidesgo
Top searches
Trending searches
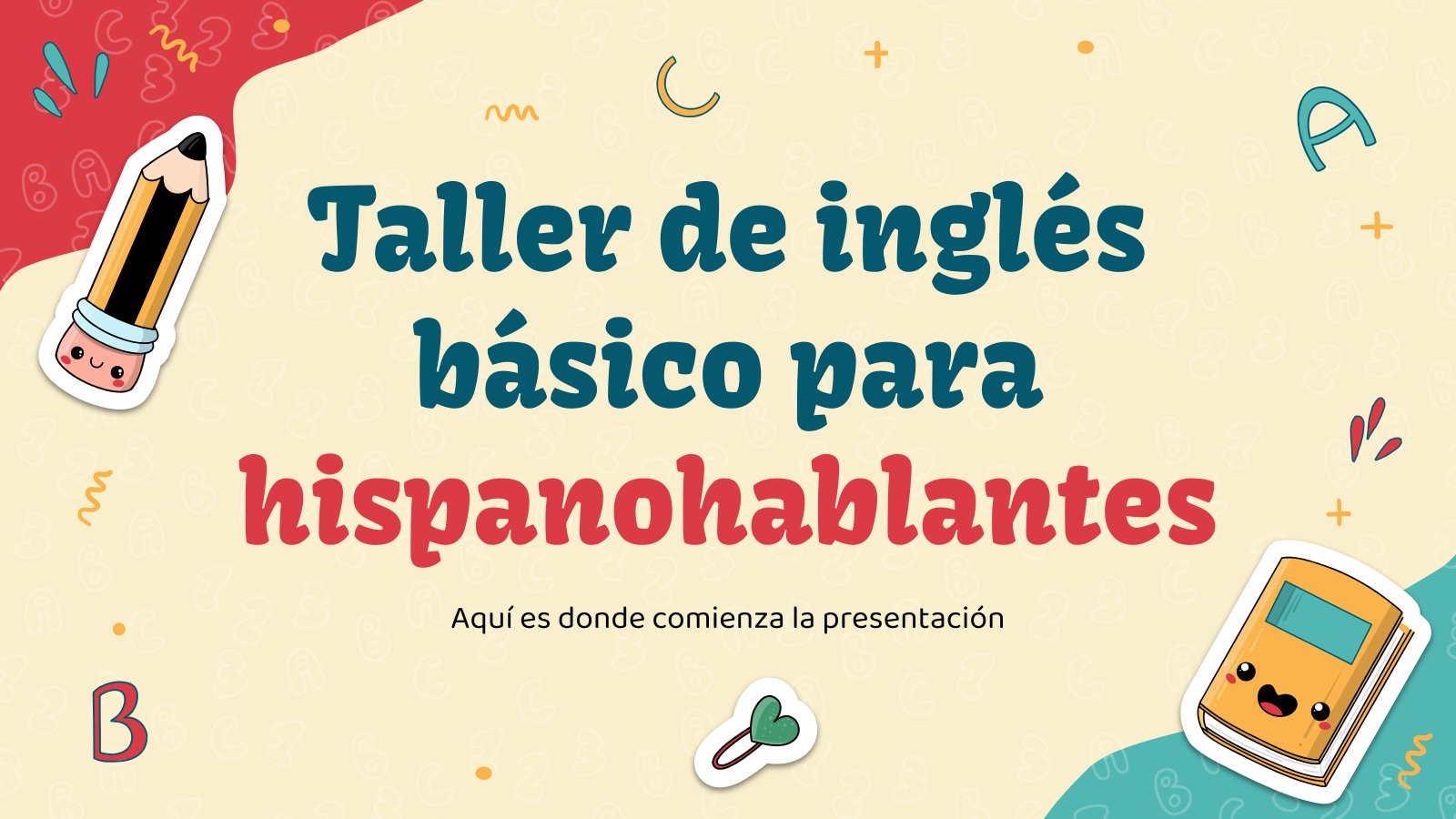
49 templates
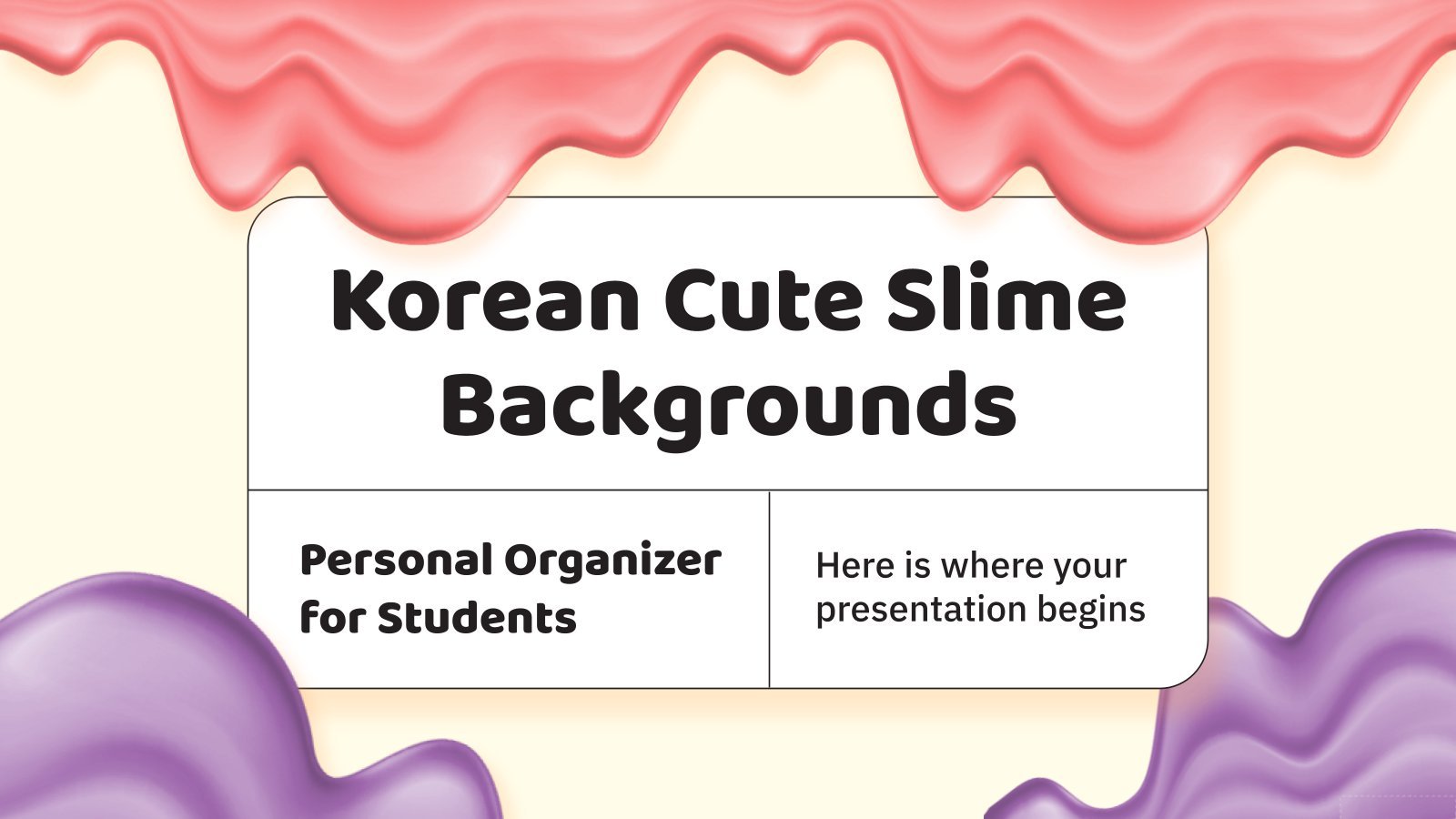
18 templates
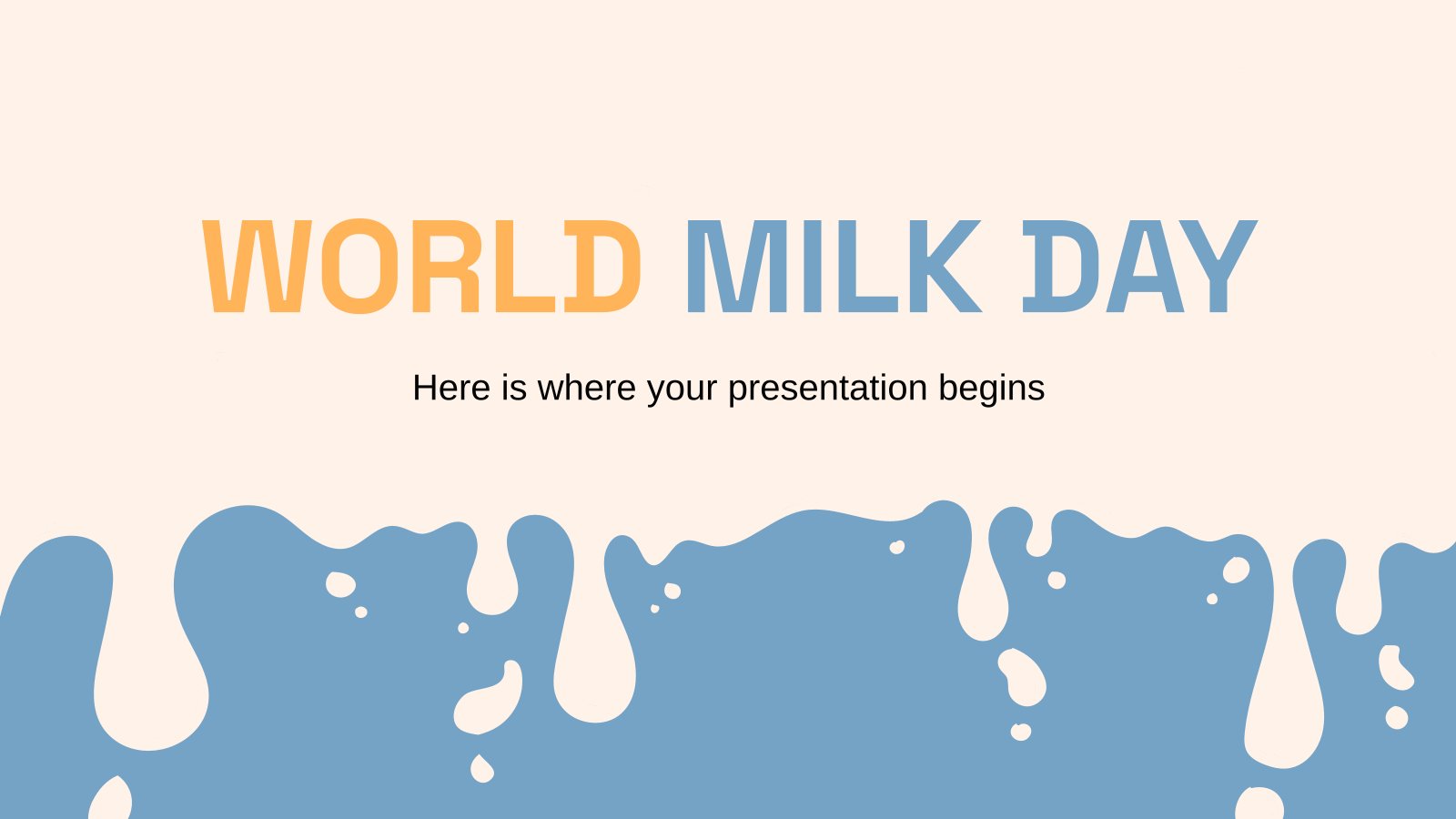
40 templates
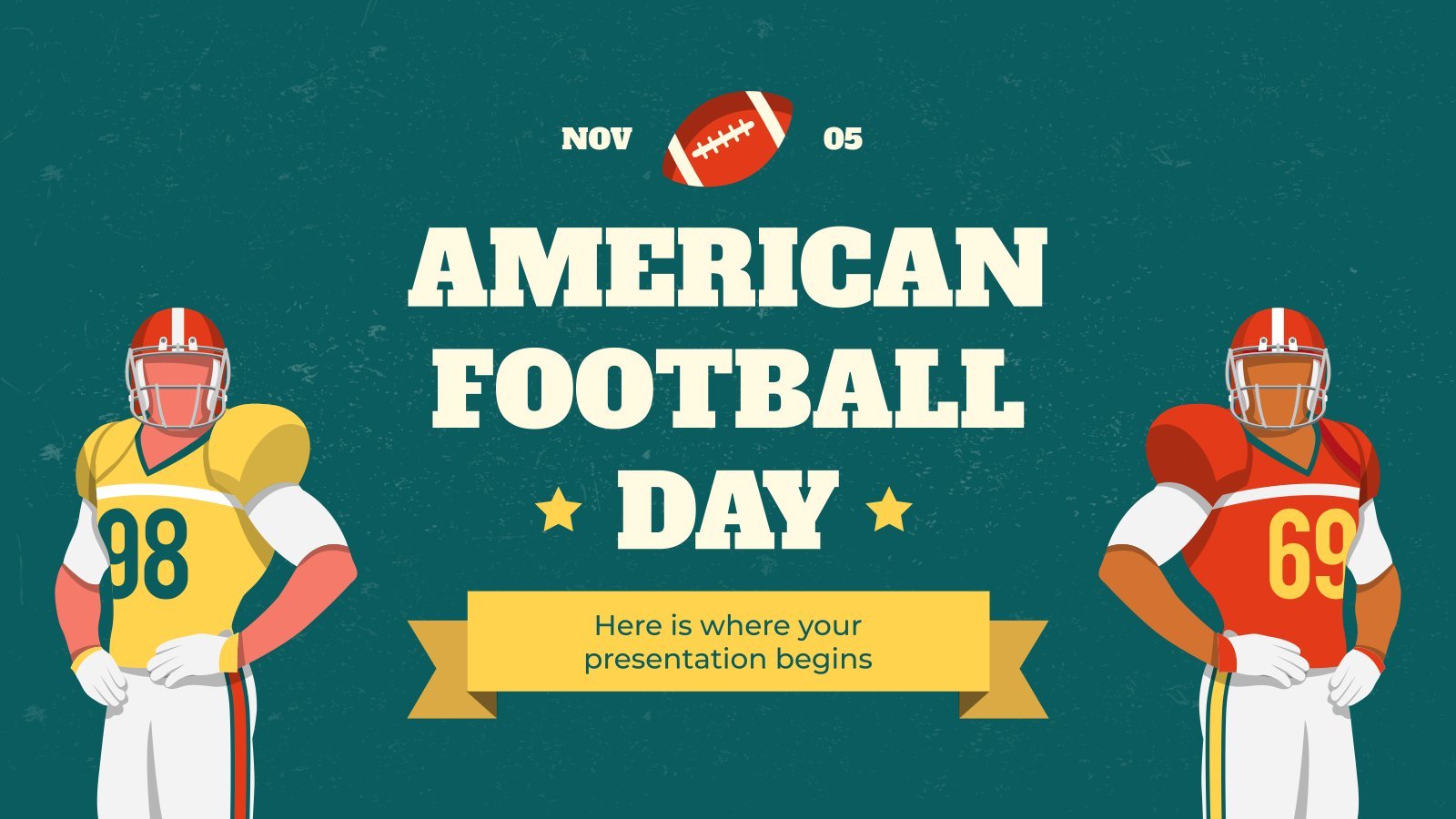
american football
16 templates
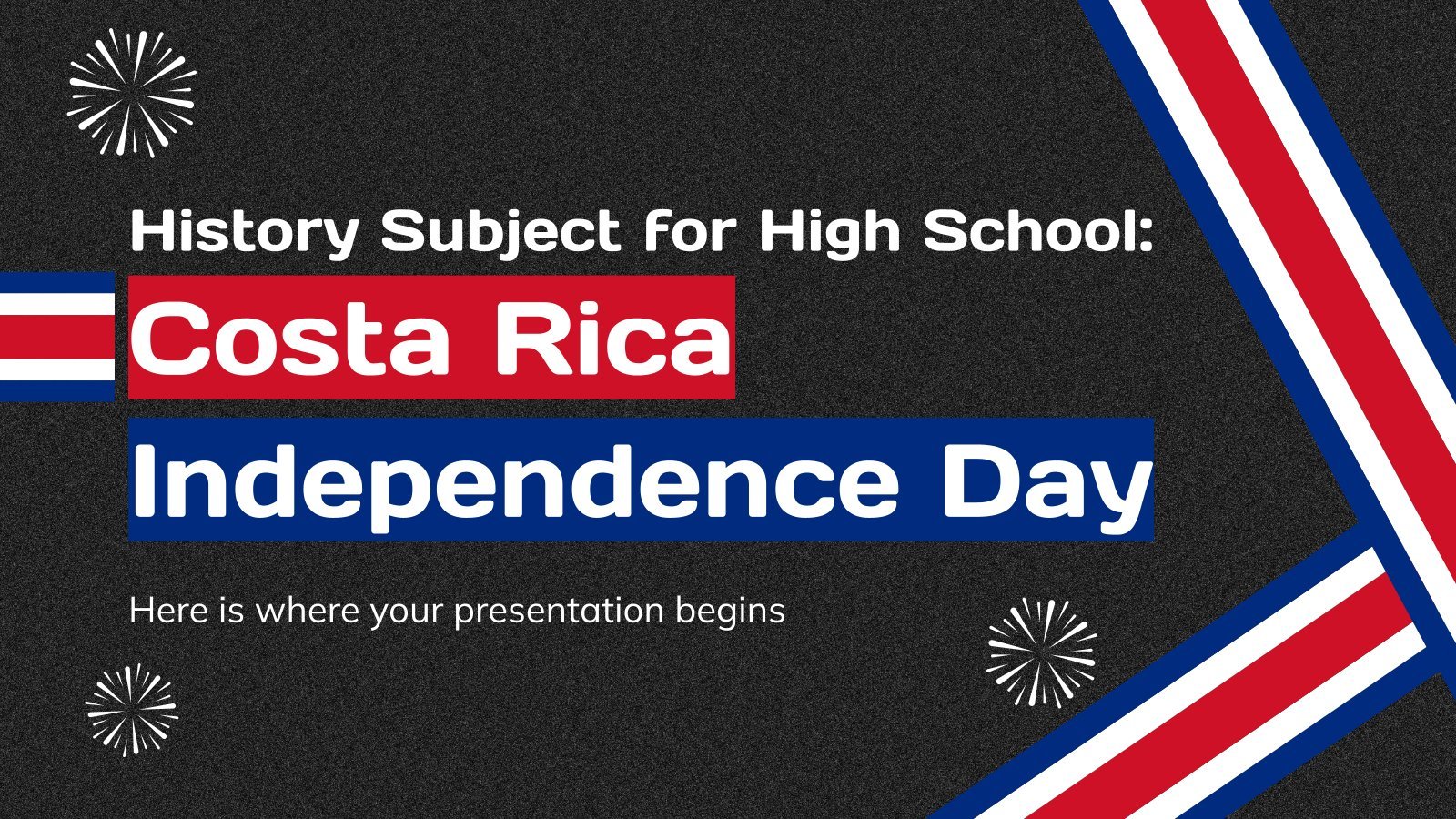
41 templates
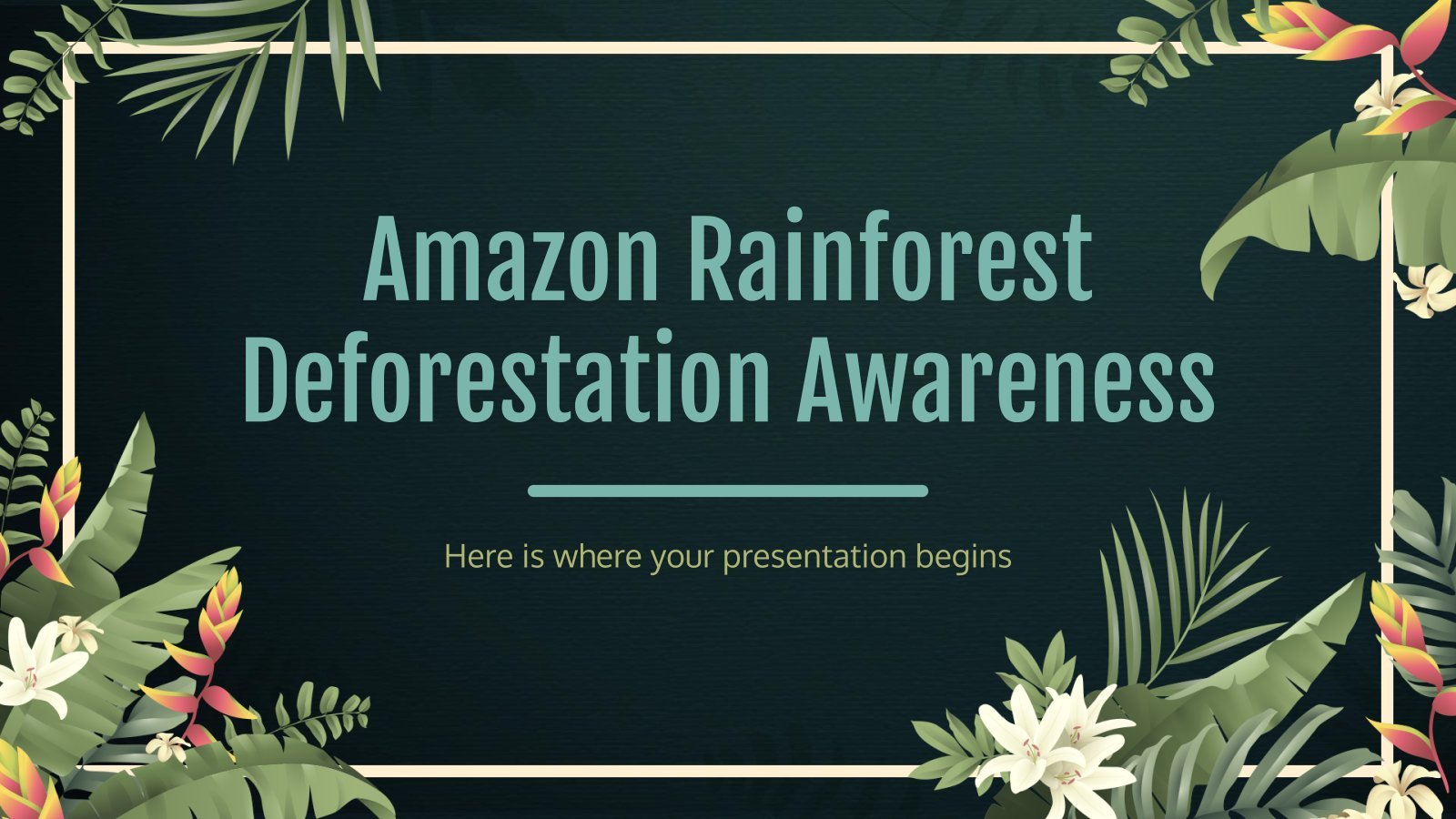
tropical rainforest
29 templates
E-Learning Presentation
Premium google slides theme and powerpoint template.
New and interesting technologies are being used for teaching. This means that you don’t need to be at school or at the university to take courses. If you need to prepare a webinar or you must give a presentation to talk about e-learning and these kind of advances in education, this new template by Slidesgo is the way to go!
If you’re a teacher, connecting with your students is what’s important, and you can achieve this with a professional design. To begin with, you’ll find some flat linear bicolor illustrations with wavy backgrounds, a style that is always trendy in design. As pictures and images are worth a thousand words, try to make the most of them. However, text is also essential, so let’s talk about the typography. Titles use a sans-serif typeface that works very well for digital screens. Body text also uses a sans-serif font, but this one has a modern look, which is a good added value for your presentation. But that’s not all. We know that numeric data is also key to stating your point, and that’s why we’ve decided to add graphs, timelines, maps and icons. Everything in this template is editable, making it the perfect choice for a custom presentation to suit your needs. What are you waiting for to download it?
Features of this template
- 100% editable and easy to modify
- 17 different slides to impress your audience
- Available in five colors: orange, blue, yellow, purple, and green
- Contains easy-to-edit graphics and maps
- Includes 1000+ icons and Flaticon's extension for customizing your slides
- Uses illustrated concepts from Storyset : editable color, different backgrounds, animated illustrations
- Designed to be used in Google Slides and Microsoft PowerPoint
- 16:9 widescreen format suitable for all types of screens
- Includes information about fonts, colors, and credits of the free and premium resources used
What are the benefits of having a Premium account?
What Premium plans do you have?
What can I do to have unlimited downloads?
Combines with:
This template can be combined with this other one to create the perfect presentation:
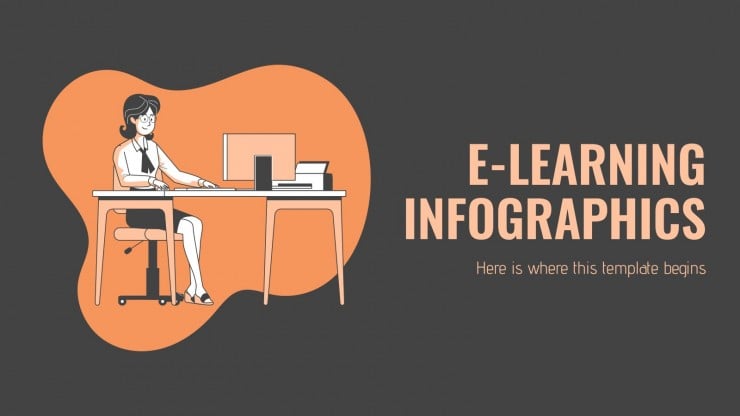
Don’t want to attribute Slidesgo?
Gain access to over 22600 templates & presentations with premium from 1.67€/month.
Are you already Premium? Log in
Available colors
Original Color
Related posts on our blog
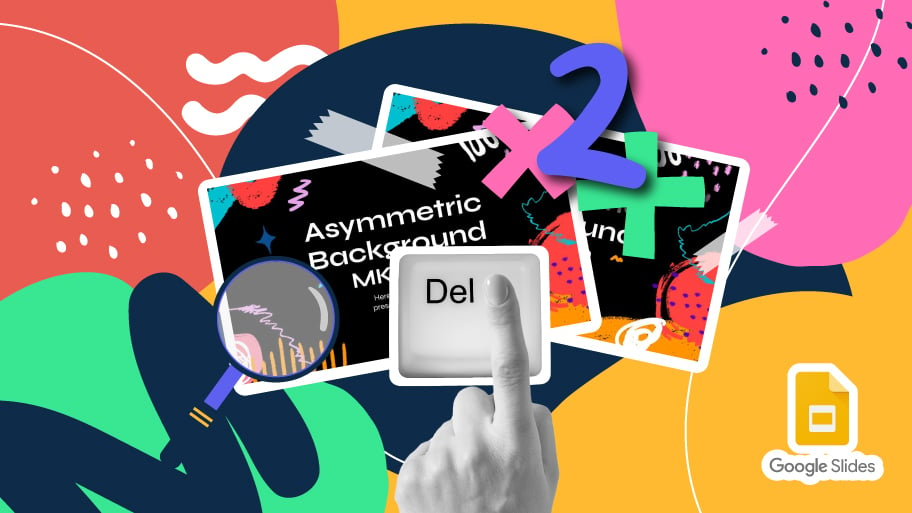
How to Add, Duplicate, Move, Delete or Hide Slides in Google Slides
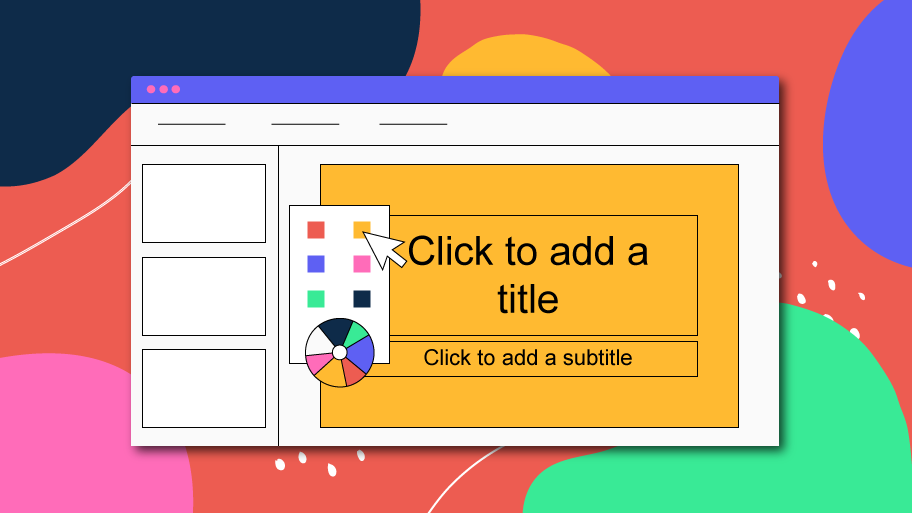
How to Change Layouts in PowerPoint
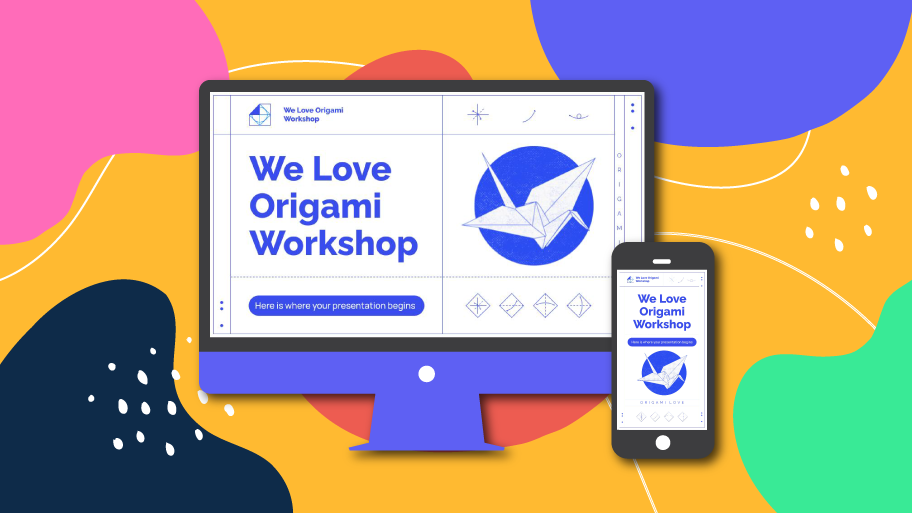
How to Change the Slide Size in Google Slides
Related presentations.
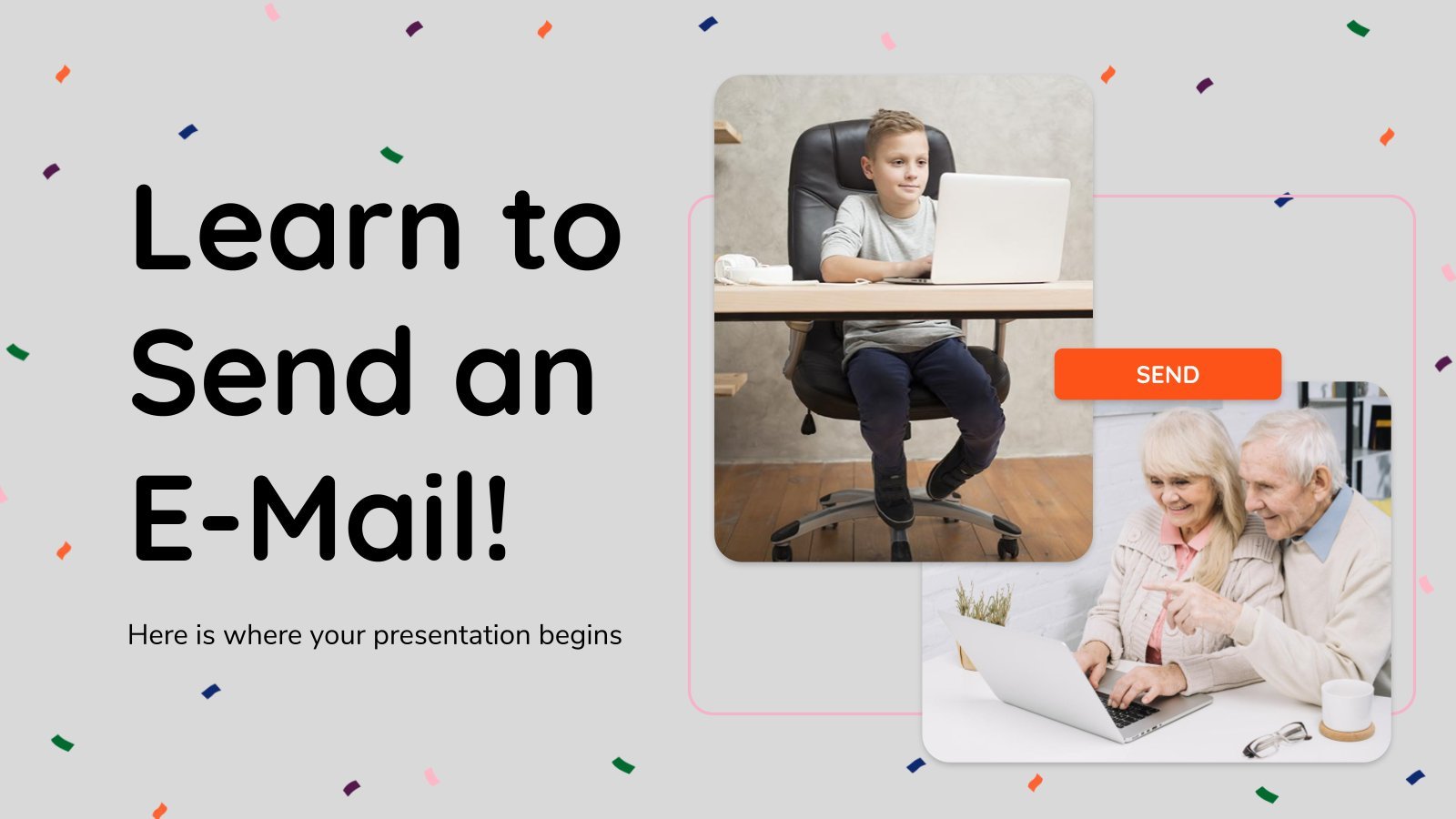
Premium template
Unlock this template and gain unlimited access
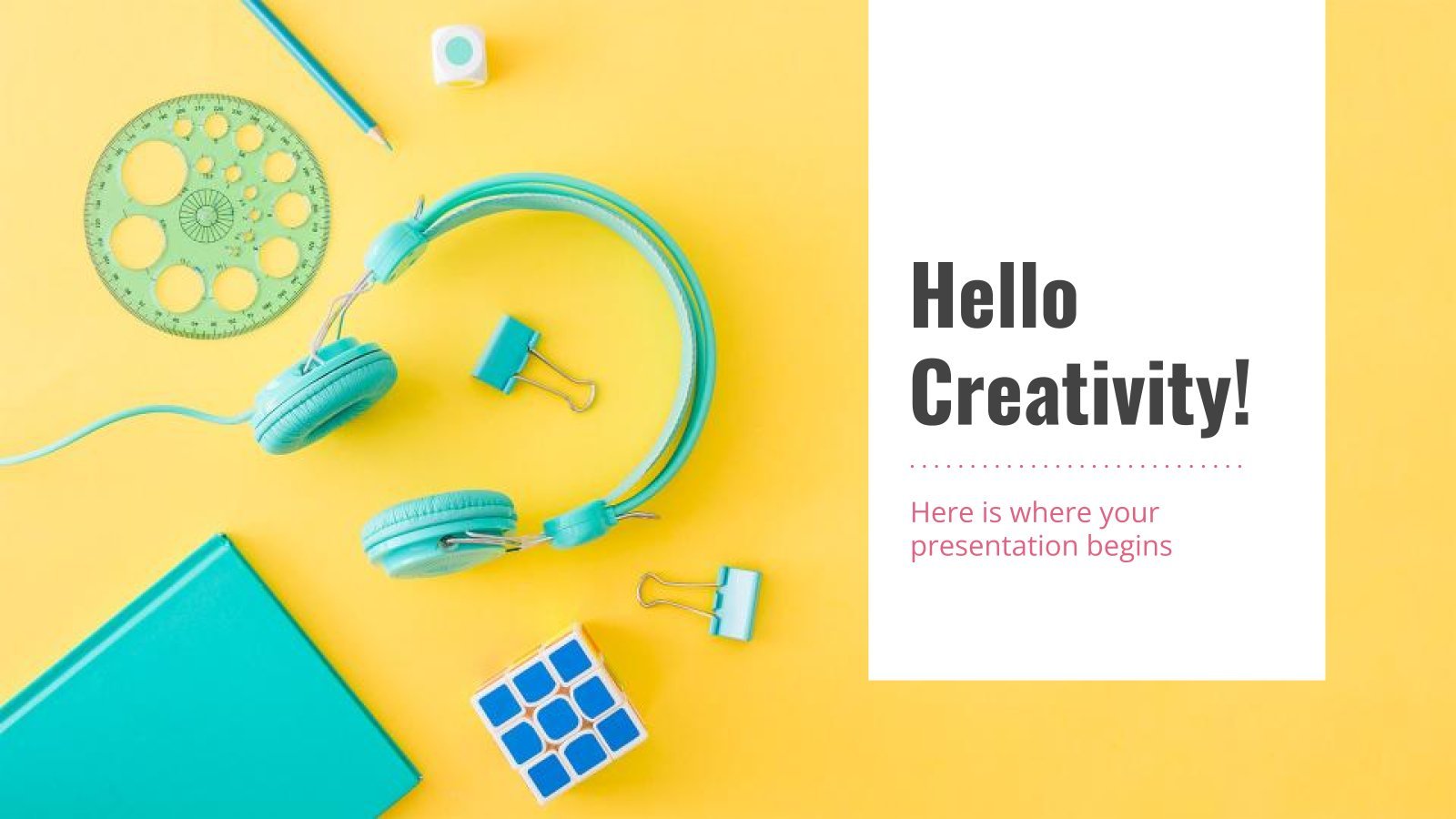
Register for free and start editing online
Concept Learning Strategies for Instruction
This document discusses strategies for teaching concepts. It defines concepts as categories of stimuli that share common features. There are two main types of concepts: concrete concepts, which involve physical characteristics like sight and touch, and abstract concepts, which are defined but not physical, like profit or norms. The document outlines two main strategies for teaching concepts: inquiry, which uses examples and non-examples, and expository, which presents the concept label and criteria. It notes both strengths, like helping with complex topics and building learning skills, and challenges of concept learning, such as overgeneralization, under-generalization, and misconceptions. Read less
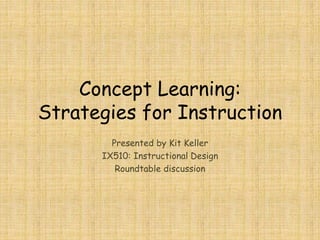
Recommended
More related content, what's hot, what's hot ( 20 ), viewers also liked, viewers also liked ( 20 ), similar to concept learning strategies for instruction, similar to concept learning strategies for instruction ( 20 ), recently uploaded, recently uploaded ( 20 ).
- 1. Concept Learning: Strategies for Instruction Presented by Kit Keller IX510: Instructional Design Roundtable discussion
- 2. Concept Learning Definitions: “Ability to apply knowledge across a variety of instances or circumstances” (Smith & Ragan, 2005, p. 172). “Concepts are categories of stimuli that have certain features in common” (UCLA-Fullerton, n.d., slide 1)
- 3. What do the shapes have in common?
- 4. “Concepts are categories of stimuli that have certain features in common” (UCLA-Fullerton, n.d., slide 1) Shapes all have: • Four straight lines • Four right angles • Four corners
- 5. What is a concept? “Concepts are mental categories for objects, events, or ideas that have a common set of features” (EduTech Wiki, 2005, para. 1) Characteristics: • Learning from examples • General-to specific ordering • Reductive and inductive reasoning “Concepts are categories of stimuli that have certain features in common” (UCLA- Fullerton, n.d., slide 1) Types of concepts: • Concrete • Abstract (Smith & Ragan, p. 172)
- 6. Strategy Selection Teaching Abstract Concepts • Known by specific definitions such as profit, norm, i.e., ideas Teaching Concrete Concepts • Physical characteristics such as sight, smell, taste, touch (Smith & Ragan, 2005, p. 172-173) Some- times overlap Two cognitive processes involved: • Generalization • Discrimination
- 7. Concept Instruction • Inquiry strategy: Presents examples and non- examples • Expository strategy: Presents the concept, label and major criteria ≠
- 8. Strengths & Challenges Strengths • Helps with complex topics • Builds learning skills Challenges • Overgeneralization • Under-generalization • Misconception
- 9. Gagne’ Hierarchy
- 10. References EduTech Wiki. (2010). Concept learning. Retrieved from http://edutechwiki.unige.ch/en/Concept_learning EduTech Wiki. (201e). Learning level. Retrieved from http://edutechwiki.unige.ch/en/Learning_level Smith, P. L., & Ragan, T. J. (2005). Instructional design (3rd ed.). Hoboken, NJ: John Wiley & Sons, Inc. UCLA-Fullerton. (n.d.). Concept learning. Retrieved from http://psych.fullerton.edu/navarick/concept.ppt

- Preferences
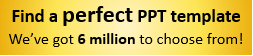
Concept Learning - PowerPoint PPT Presentation

Something went wrong! Please try again and reload the page.
Concept Learning
Concept learning inducing general functions from specific training examples is a main issue of machine learning. concept learning: acquiring the definition of a ... – powerpoint ppt presentation.
- Inducing general functions from specific training examples is a main issue of machine learning.
- Concept Learning Acquiring the definition of a general category from given sample positive and negative training examples of the category.
- Concept Learning can seen as a problem of searching through a predefined space of potential hypotheses for the hypothesis that best fits the training examples.
- The hypothesis space has a general-to-specific ordering of hypotheses, and the search can be efficiently organized by taking advantage of a naturally occurring structure over the hypothesis space.
- A Formal Definition for Concept Learning
- Inferring a boolean-valued function from training examples of
- its input and output.
- An example for concept-learning is the learning of bird-concept from the given examples of birds (positive examples) and non-birds (negative examples).
- We are trying to learn the definition of a concept from given examples.
- A set of example days, and each is described by six attributes.
- The task is to learn to predict the value of EnjoySport for arbitrary day,
- based on the values of its attribute values.
- Each hypothesis consists of a conjuction of constraints on the instance attributes.
- Each hypothesis will be a vector of six constraints, specifying the values of the six attributes
- (Sky, AirTemp, Humidity, Wind, Water, and Forecast).
- Each attribute will be
- ? - indicating any value is acceptable for the attribute (dont care)
- single value specifying a single required value (ex. Warm) (specific)
- 0 - indicating no value is acceptable for the attribute (no value)
- A hypothesis
- Sky AirTemp Humidity Wind Water Forecast
- lt Sunny, ? , ? , Strong , ? , Same gt
- The most general hypothesis that every day is a positive example
- lt?, ?, ?, ?, ?, ?gt
- The most specific hypothesis that no day is a positive example
- lt0, 0, 0, 0, 0, 0gt
- EnjoySport concept learning task requires learning the sets of days for which EnjoySportyes, describing this set by a conjunction of constraints over the instance attributes.
- Instances X set of all possible days, each described by the attributes
- Sky (values Sunny, Cloudy, Rainy)
- AirTemp (values Warm, Cold)
- Humidity (values Normal, High)
- Wind (values Strong, Weak)
- Water (values Warm, Cold)
- Forecast (values Same, Change)
- Target Concept (Function) c EnjoySport X ? 0,1
- Hypotheses H Each hypothesis is described by a conjunction of constraints on the attributes.
- Training Examples D positive and negative examples of the target function
- A hypothesis h in H such that h(x) c(x) for all x in D.
- Although the learning task is to determine a hypothesis h identical to the target concept cover the entire set of instances X, the only information available about c is its value over the training examples.
- Inductive learning algorithms can at best guarantee that the output hypothesis fits the target concept over the training data.
- Lacking any further information, our assumption is that the best hypothesis regarding unseen instances is the hypothesis that best fits the observed training data. This is the fundamental assumption of inductive learning.
- The Inductive Learning Hypothesis - Any hypothesis found to approximate the target function well over a sufficiently large set of training examples will also approximate the target function well over other unobserved examples.
- Concept learning can be viewed as the task of searching through a large space of hypotheses implicitly defined by the hypothesis representation.
- The goal of this search is to find the hypothesis that best fits the training examples.
- By selecting a hypothesis representation, the designer of the learning algorithm implicitly defines the space of all hypotheses that the program can ever represent and therefore can ever learn.
- Sky has 3 possible values, and other 5 attributes have 2 possible values.
- There are 96 ( 3.2.2.2.2.2) distinct instances in X.
- There are 5120 (5.4.4.4.4.4) syntactically distinct hypotheses in H.
- Two more values for attributes ? and 0
- Every hypothesis containing one or more 0 symbols represents the empty set of instances that is, it classifies every instance as negative.
- There are 973 ( 1 4.3.3.3.3.3) semantically distinct hypotheses in H.
- Only one more value for attributes ?, and one hypothesis representing empty set of instances.
- Although EnjoySport has small, finite hypothesis space, most learning tasks have much larger (even infinite) hypothesis spaces.
- We need efficient search algorithms on the hypothesis spaces.
- Many algorithms for concept learning organize the search through the hypothesis space by relying on a general-to-specific ordering of hypotheses.
- By taking advantage of this naturally occurring structure over the hypothesis space, we can design learning algorithms that exhaustively search even infinite hypothesis spaces without explicitly enumerating every hypothesis.
- Consider two hypotheses
- h1 (Sunny, ?, ?, Strong, ?, ?)
- h2 (Sunny, ?, ?, ?, ?, ?)
- Now consider the sets of instances that are classified positive by hl and by h2.
- Because h2 imposes fewer constraints on the instance, it classifies more instancesas positive.
- In fact, any instance classified positive by hl will also be classified positive by h2.
- Therefore, we say that h2 is more general than hl.
- For any instance x in X and hypothesis h in H, we say that x satisfies h if and only if h(x) 1.
- More-General-Than-Or-Equal Relation
- Let h1 and h2 be two boolean-valued functions defined over X.
- Then h1 is more-general-than-or-equal-to h2 (written h1 h2)
- if and only if any instance that satisfies h2 also satisfies h1.
- h1 is more-general-than h2 ( h1 gt h2) if and only if h1h2 is true and h2h1 is false. We also say h2 is more-specific-than h1.
- h2 gt h1 and h2 gt h3
- But there is no more-general relation between h1 and h3
- FIND-S Algorithm starts from the most specific hypothesis and generalize it by considering only positive examples.
- FIND-S algorithm ignores negative examples.
- As long as the hypothesis space contains a hypothesis that describes the true target concept, and the training data contains no errors, ignoring negative examples does not cause to any problem.
- FIND-S algorithm finds the most specific hypothesis within H that is consistent with the positive training examples.
- The final hypothesis will also be consistent with negative examples if the correct target concept is in H, and the training examples are correct.
- Initialize h to the most specific hypothesis in H
- 2. For each positive training instance x
- For each attribute constraint a, in h
- If the constraint a, is satisfied by x
- Then do nothing
- Else replace a, in h by the next more general constraint that is satisfied by x
- 3. Output hypothesis h
- Has FIND-S converged to the correct target concept?
- Although FIND-S will find a hypothesis consistent with the training data, it has no way to determine whether it has found the only hypothesis in H consistent with the data (i.e., the correct target concept), or whether there are many other consistent hypotheses as well.
- We would prefer a learning algorithm that could determine whether it had converged and, if not, at least characterize its uncertainty regarding the true identity of the target concept.
- Why prefer the most specific hypothesis?
- In case there are multiple hypotheses consistent with the training examples, FIND-S will find the most specific.
- It is unclear whether we should prefer this hypothesis over, say, the most general, or some other hypothesis of intermediate generality.
- Are the training examples consistent?
- In most practical learning problems there is some chance that the training examples will contain at least some errors or noise.
- Such inconsistent sets of training examples can severely mislead FIND-S, given the fact that it ignores negative examples.
- We would prefer an algorithm that could at least detect when the training data is inconsistent and, preferably, accommodate such errors.
- What if there are several maximally specific consistent hypotheses?
- In the hypothesis language H for the EnjoySport task, there is always a unique, most specific hypothesis consistent with any set of positive examples.
- However, for other hypothesis spaces there can be several maximally specific hypotheses consistent with the data.
- In this case, FIND-S must be extended to allow it to backtrack on its choices of how to generalize the hypothesis, to accommodate the possibility that the target concept lies along a different branch of the partial ordering than the branch it has selected.
- FIND-S outputs a hypothesis from H, that is consistent with the training examples, this is just one of many hypotheses from H that might fit the training data equally well.
- The key idea in the Candidate-Elimination algorithm is to output a description of the set of all hypotheses consistent with the training examples.
- Candidate-Elimination algorithm computes the description of this set without explicitly enumerating all of its members.
- This is accomplished by using the more-general-than partial ordering and maintaining a compact representation of the set of consistent hypotheses.
- The key difference between this definition of consistent and satisfies.
- An example x is said to satisfy hypothesis h when h(x) 1,
- regardless of whether x is a positive or negative example of
- the target concept.
- However, whether such an example is consistent with h depends
- on the target concept, and in particular, whether h(x) c(x).
- The Candidate-Elimination algorithm represents the set of
- all hypotheses consistent with the observed training examples.
- This subset of all hypotheses is called the version space with
- respect to the hypothesis space H and the training examples D,
- because it contains all plausible versions of the target concept.
- List-Then-Eliminate algorithm initializes the version space to contain all hypotheses in H, then eliminates any hypothesis found inconsistent with any training example.
- The version space of candidate hypotheses thus shrinks as more examples are observed, until ideally just one hypothesis remains that is consistent with all the observed examples.
- Presumably, this is the desired target concept.
- If insufficient data is available to narrow the version space to a single hypothesis, then the algorithm can output the entire set of hypotheses consistent with the observed data.
- List-Then-Eliminate algorithm can be applied whenever the hypothesis space H is finite.
- It has many advantages, including the fact that it is guaranteed to output all hypotheses consistent with the training data.
- Unfortunately, it requires exhaustively enumerating all hypotheses in H - an unrealistic requirement for all but the most trivial hypothesis spaces.
- A version space can be represented with its general and specific boundary sets.
- The Candidate-Elimination algorithm represents the version space by storing only its most general members G and its most specific members S.
- Given only these two sets S and G, it is possible to enumerate all members of a version space by generating hypotheses that lie between these two sets in general-to-specific partial ordering over hypotheses.
- Every member of the version space lies between these boundaries
- where x y means x is more general or equal to y.
- A version space with its general and specific boundary sets.
- The version space includes all six hypotheses shown here,
- but can be represented more simply by S and G.
- The Candidate-Elimination algorithm computes the version space containing all hypotheses from H that are consistent with an observed sequence of training examples.
- It begins by initializing the version space to the set of all hypotheses in H that is, by initializing the G boundary set to contain the most general hypothesis in H
- G0 ? lt?, ?, ?, ?, ?, ?gt
- and initializing the S boundary set to contain the most specific hypothesis
- S0 ? lt0, 0, 0, 0, 0, 0gt
- These two boundary sets delimit the entire hypothesis space, because every other hypothesis in H is both more general than S0 and more specific than G0.
- As each training example is considered, the S and G boundary sets are generalized and specialized, respectively, to eliminate from the version space any hypotheses found inconsistent with the new training example.
- After all examples have been processed, the computed version space contains all the hypotheses consistent with these examples and only these hypotheses.
- Initialize G to the set of maximally general hypotheses in H
- Initialize S to the set of maximally specific hypotheses in H
- For each training example d, do
- If d is a positive example
- Remove from G any hypothesis inconsistent with d ,
- For each hypothesis s in S that is not consistent with d ,-
- Remove s from S
- Add to S all minimal generalizations h of s such that
- h is consistent with d, and some member of G is more general than h
- Remove from S any hypothesis that is more general than another hypothesis in S
- If d is a negative example
- Remove from S any hypothesis inconsistent with d
- For each hypothesis g in G that is not consistent with d
- Remove g from G
- Add to G all minimal specializations h of g such that
- h is consistent with d, and some member of S is more specific than h
- Remove from G any hypothesis that is less general than another hypothesis in G
- S0 and G0 are the initial boundary sets corresponding to the most specific and most general hypotheses.
- Training examples 1 and 2 force the S boundary to become
- more general.
- They have no effect on the G boundary
- Given that there are six attributes that could be specified to specialize G2, why are there only three new hypotheses in G3?
- For example, the hypothesis h lt?, ?, Normal, ?, ?, ?gt is a minimal specialization of G2 that correctly labels the new example as a negative example, but it is not included in G3.
- The reason this hypothesis is excluded is that it is inconsistent with S2.
- The algorithm determines this simply by noting that h is not more general than the current specific boundary, S2.
- In fact, the S boundary of the version space forms a summary of the previously encountered positive examples that can be used to determine whether any given hypothesis is consistent with these examples.
- The G boundary summarizes the information from previously encountered negative examples. Any hypothesis more specific than G is assured to be consistent with past negative examples
- The fourth training example further generalizes the S boundary of the version space.
- It also results in removing one member of the G boundary, because this member fails to cover the new positive example.
- To understand the rationale for this step, it is useful to consider why the offending hypothesis must be removed from G.
- Notice it cannot be specialized, because specializing it would not make it cover the new example.
- It also cannot be generalized, because by the definition of G, any more general hypothesis will cover at least one negative training example.
- Therefore, the hypothesis must be dropped from the G boundary, thereby removing an entire branch of the partial ordering from the version space of hypotheses remaining under consideration
- After processing these four examples, the boundary sets S4 and G4 delimit the version space of all hypotheses consistent with the set of incrementally observed training examples.
- This learned version space is independent of the sequence in which the training examples are presented (because in the end it contains all hypotheses consistent with the set of examples).
- As further training data is encountered, the S and G boundaries will move monotonically closer to each other, delimiting a smaller and smaller version space of candidate hypotheses.
- The version space learned by the Candidate-Elimination Algorithm will converge toward the hypothesis that correctly describes the target concept, provided
- There are no errors in the training examples, and
- there is some hypothesis in H that correctly describes the target concept.
- What will happen if the training data contains errors?
- The algorithm removes the correct target concept from the version space.
- S and G boundary sets eventually converge to an empty version space if sufficient additional training data is available.
- Such an empty version space indicates that there is no hypothesis in H consistent with all observed training examples.
- A similar symptom will appear when the training examples are correct, but the target concept cannot be described in the hypothesis representation.
- e.g., if the target concept is a disjunction of feature attributes and the hypothesis space supports only conjunctive descriptions
- We have assumed that training examples are provided to the learner by some external teacher.
- Suppose instead that the learner is allowed to conduct experiments in which it chooses the next instance, then obtains the correct classification for this instance from an external oracle (e.g., nature or a teacher).
- This scenario covers situations in which the learner may conduct experiments in nature or in which a teacher is available to provide the correct classification.
- We use the term query to refer to such instances constructed by the learner, which are then classified by an external oracle.
- Considering the version space learned from the four training examples of the EnjoySport concept.
- What would be a good query for the learner to pose at this point?
- What is a good query strategy in general?
- The learner should attempt to discriminate among the alternative competing hypotheses in its current version space.
- Therefore, it should choose an instance that would be classified positive by some of these hypotheses, but negative by others.
- One such instance is ltSunny, Warm, Normal, Light, Warm, Samegt
- This instance satisfies three of the six hypotheses in the current version space.
- If the trainer classifies this instance as a positive example, the S boundary of the version space can then be generalized.
- Alternatively, if the trainer indicates that this is a negative example, the G boundary can then be specialized.
- In general, the optimal query strategy for a concept learner is to generate instances that satisfy exactly half the hypotheses in the current version space.
- When this is possible, the size of the version space is reduced by half with each new example, and the correct target concept can therefore be found with only ?log2 VS ? experiments.
- Even though the learned version space still contains multiple hypotheses, indicating that the target concept has not yet been fully learned, it is possible to classify certain examples with the same degree of confidence as if the target concept had been uniquely identified.
- Let us assume that the followings are new instances to be classified
- Instance A was is classified as a positive instance by every hypothesis in the current version space.
- Because the hypotheses in the version space unanimously agree that this is a positive instance, the learner can classify instance A as positive with the same confidence it would have if it had already converged to the single, correct target concept.
- Regardless of which hypothesis in the version space is eventually found to be the correct target concept, it is already clear that it will classify instance A as a positive example.
- Notice furthermore that we need not enumerate every hypothesis in the version space in order to test whether each classifies the instance as positive.
- This condition will be met if and only if the instance satisfies every member of S.
- The reason is that every other hypothesis in the version space is at least as general as some member of S.
- By our definition of more-general-than, if the new instance satisfies all members of S it must also satisfy each of these more general hypotheses.
- Instance B is classified as a negative instance by every hypothesis in the version space.
- This instance can therefore be safely classified as negative, given the partially learned concept.
- An efficient test for this condition is that the instance satisfies none of the members of G.
- Half of the version space hypotheses classify instance C as positive and half classify it as negative.
- Thus, the learner cannot classify this example with confidence until further training examples are available.
- Instance D is classified as positive by two of the version space hypotheses and negative by the other four hypotheses.
- In this case we have less confidence in the classification than in the unambiguous cases of instances A and B.
- Still, the vote is in favor of a negative classification, and one approach we could take would be to output the majority vote, perhaps with a confidence rating indicating how close the vote was.
- The Candidate-Elimination Algorithm will converge toward the true target concept provided it is given accurate training examples and provided its initial hypothesis space contains the target concept.
- What if the target concept is not contained in the hypothesis space?
- Can we avoid this difficulty by using a hypothesis space that includes every possible hypothesis?
- How does the size of this hypothesis space influence the ability of the algorithm to generalize to unobserved instances?
- How does the size of the hypothesis space influence the number of training examples that must be observed?
- In EnjoySpor t example, we restricted the hypothesis space to include only conjunctions of attribute values.
- Because of this restriction, the hypothesis space is unable to represent even simple disjunctive target concepts such as "Sky Sunny or Sky Cloudy."
- From first two examples ? S2 lt?, Warm, Normal, Strong, Cool, Changegt
- This is inconsistent with third examples, and there are no hypotheses consistent with these three examples
- PROBLEM We have biased the learner to consider only conjunctive hypotheses.
- ? We require a more expressive hypothesis space.
- The obvious solution to the problem of assuring that the target concept is in the hypothesis space H is to provide a hypothesis space capable of representing every teachable concept.
- Every possible subset of the instances X ? the power set of X.
- What is the size of the hypothesis space H (the power set of X) ?
- In EnjoySport, the size of the instance space X is 96.
- The size of the power set of X is 2X ? The size of H is 296
- Our conjunctive hypothesis space is able to represent only 973of these hypotheses.
- ? a very biased hypothesis space
- Let the hypothesis space H to be the power set of X.
- A hypothesis can be represented with disjunctions, conjunctions, and negations of our earlier hypotheses.
- The target concept "Sky Sunny or Sky Cloudy" could then be described as
- ltSunny, ?, ?, ?, ?, ?gt ? ltCloudy, ?, ?, ?, ?, ?gt
- NEW PROBLEM our concept learning algorithm is now completely unable to generalize beyond the observed examples.
- three positive examples (xl,x2,x3) and two negative examples (x4,x5) to the learner.
- S x1 ? x2 ? x3 and G ? (x4 ? x5) ? NO GENERALIZATION
- Therefore, the only examples that will be unambiguously classified by S and G are the observed training examples themselves.
- A learner that makes no a priori assumptions regarding the identity of the target concept has no rational basis for classifying any unseen instances.
- Inductive Leap A learner should be able to generalize training data using prior assumptions in order to classify unseen instances.
- The generalization is known as inductive leap and our prior assumptions are the inductive bias of the learner.
- Inductive Bias (prior assumptions) of Candidate-Elimination Algorithm is that the target concept can be represented by a conjunction of attribute values, the target concept is contained in the hypothesis space and training examples are correct.
- Inductive Bias
- Consider a concept learning algorithm L for the set of instances X. Let c be an arbitrary concept defined over X, and
- let Dc ltx , c(x)gt be an arbitrary set of training examples of c.
- Let L(xi, Dc) denote the classification assigned to the instance xi by L after training on the data Dc.
- The inductive bias of L is any minimal set of assertions B such that for any target concept c and corresponding training examples Dc the following formula holds.
- ROTE-LEARNER Learning corresponds simply to storing each observed training example in memory. Subsequent instances are classified by looking them up in memory. If the instance is found in memory, the stored classification is returned. Otherwise, the system refuses to classify the new instance.
- Inductive Bias No inductive bias
- CANDIDATE-ELIMINATION New instances are classified only in the case where all members of the current version space agree on the classification. Otherwise, the system refuses to classify the new instance.
- Inductive Bias the target concept can be represented in its hypothesis space.
- FIND-S This algorithm, described earlier, finds the most specific hypothesis consistent with the training examples. It then uses this hypothesis to classify all subsequent instances.
- Inductive Bias the target concept can be represented in its hypothesis space, and all instances are negative instances unless the opposite is entailed by its other know1edge.
- Concept learning can be seen as a problem of searching through a large predefined space of potential hypotheses.
- The general-to-specific partial ordering of hypotheses provides a useful structure for organizing the search through the hypothesis space.
- The FIND-S algorithm utilizes this general-to-specific ordering, performing a specific-to-general search through the hypothesis space along one branch of the partial ordering, to find the most specific hypothesis consistent with the training examples.
- The CANDIDATE-ELIMINATION algorithm utilizes this general-to-specific ordering to compute the version space (the set of all hypotheses consistent with the training data) by incrementally computing the sets of maximally specific (S) and maximally general (G) hypotheses.
- Because the S and G sets delimit the entire set of hypotheses consistent with the data, they provide the learner with a description of its uncertainty regarding the exact identity of the target concept. This version space of alternative hypotheses can be examined
- to determine whether the learner has converged to the target concept,
- to determine when the training data are inconsistent,
- to generate informative queries to further refine the version space, and
- to determine which unseen instances can be unambiguously classified based on the partially learned concept.
- The CANDIDATE-ELIMINATION algorithm is not robust to noisy data or to situations in which the unknown target concept is not expressible in the provided hypothesis space.
- Inductive learning algorithms are able to classify unseen examples only because of their implicit inductive bias for selecting one consistent hypothesis over another.
- If the hypothesis space is enriched to the point where there is a hypothesis corresponding to every possible subset of instances (the power set of the instances), this will remove any inductive bias from the CANDIDATE-ELIMINATION algorithm .
- Unfortunately, this also removes the ability to classify any instance beyond the observed training examples.
- An unbiased learner cannot make inductive leaps to classify unseen examples.
PowerShow.com is a leading presentation sharing website. It has millions of presentations already uploaded and available with 1,000s more being uploaded by its users every day. Whatever your area of interest, here you’ll be able to find and view presentations you’ll love and possibly download. And, best of all, it is completely free and easy to use.
You might even have a presentation you’d like to share with others. If so, just upload it to PowerShow.com. We’ll convert it to an HTML5 slideshow that includes all the media types you’ve already added: audio, video, music, pictures, animations and transition effects. Then you can share it with your target audience as well as PowerShow.com’s millions of monthly visitors. And, again, it’s all free.
About the Developers
PowerShow.com is brought to you by CrystalGraphics , the award-winning developer and market-leading publisher of rich-media enhancement products for presentations. Our product offerings include millions of PowerPoint templates, diagrams, animated 3D characters and more.


- SUGGESTED TOPICS
- The Magazine
- Newsletters
- Managing Yourself
- Managing Teams
- Work-life Balance
- The Big Idea
- Data & Visuals
- Reading Lists
- Case Selections
- HBR Learning
- Topic Feeds
- Account Settings
- Email Preferences
How to Make a “Good” Presentation “Great”
- Guy Kawasaki

Remember: Less is more.
A strong presentation is so much more than information pasted onto a series of slides with fancy backgrounds. Whether you’re pitching an idea, reporting market research, or sharing something else, a great presentation can give you a competitive advantage, and be a powerful tool when aiming to persuade, educate, or inspire others. Here are some unique elements that make a presentation stand out.
- Fonts: Sans Serif fonts such as Helvetica or Arial are preferred for their clean lines, which make them easy to digest at various sizes and distances. Limit the number of font styles to two: one for headings and another for body text, to avoid visual confusion or distractions.
- Colors: Colors can evoke emotions and highlight critical points, but their overuse can lead to a cluttered and confusing presentation. A limited palette of two to three main colors, complemented by a simple background, can help you draw attention to key elements without overwhelming the audience.
- Pictures: Pictures can communicate complex ideas quickly and memorably but choosing the right images is key. Images or pictures should be big (perhaps 20-25% of the page), bold, and have a clear purpose that complements the slide’s text.
- Layout: Don’t overcrowd your slides with too much information. When in doubt, adhere to the principle of simplicity, and aim for a clean and uncluttered layout with plenty of white space around text and images. Think phrases and bullets, not sentences.
As an intern or early career professional, chances are that you’ll be tasked with making or giving a presentation in the near future. Whether you’re pitching an idea, reporting market research, or sharing something else, a great presentation can give you a competitive advantage, and be a powerful tool when aiming to persuade, educate, or inspire others.

- Guy Kawasaki is the chief evangelist at Canva and was the former chief evangelist at Apple. Guy is the author of 16 books including Think Remarkable : 9 Paths to Transform Your Life and Make a Difference.
Partner Center
- MyU : For Students, Faculty, and Staff
Fall 2024 CSCI Special Topics Courses
Cloud computing.
Meeting Time: 09:45 AM‑11:00 AM TTh Instructor: Ali Anwar Course Description: Cloud computing serves many large-scale applications ranging from search engines like Google to social networking websites like Facebook to online stores like Amazon. More recently, cloud computing has emerged as an essential technology to enable emerging fields such as Artificial Intelligence (AI), the Internet of Things (IoT), and Machine Learning. The exponential growth of data availability and demands for security and speed has made the cloud computing paradigm necessary for reliable, financially economical, and scalable computation. The dynamicity and flexibility of Cloud computing have opened up many new forms of deploying applications on infrastructure that cloud service providers offer, such as renting of computation resources and serverless computing. This course will cover the fundamentals of cloud services management and cloud software development, including but not limited to design patterns, application programming interfaces, and underlying middleware technologies. More specifically, we will cover the topics of cloud computing service models, data centers resource management, task scheduling, resource virtualization, SLAs, cloud security, software defined networks and storage, cloud storage, and programming models. We will also discuss data center design and management strategies, which enable the economic and technological benefits of cloud computing. Lastly, we will study cloud storage concepts like data distribution, durability, consistency, and redundancy. Registration Prerequisites: CS upper div, CompE upper div., EE upper div., EE grad, ITI upper div., Univ. honors student, or dept. permission; no cr for grads in CSci. Complete the following Google form to request a permission number from the instructor ( https://forms.gle/6BvbUwEkBK41tPJ17 ).
CSCI 5980/8980
Machine learning for healthcare: concepts and applications.
Meeting Time: 11:15 AM‑12:30 PM TTh Instructor: Yogatheesan Varatharajah Course Description: Machine Learning is transforming healthcare. This course will introduce students to a range of healthcare problems that can be tackled using machine learning, different health data modalities, relevant machine learning paradigms, and the unique challenges presented by healthcare applications. Applications we will cover include risk stratification, disease progression modeling, precision medicine, diagnosis, prognosis, subtype discovery, and improving clinical workflows. We will also cover research topics such as explainability, causality, trust, robustness, and fairness.
Registration Prerequisites: CSCI 5521 or equivalent. Complete the following Google form to request a permission number from the instructor ( https://forms.gle/z8X9pVZfCWMpQQ6o6 ).
Visualization with AI
Meeting Time: 04:00 PM‑05:15 PM TTh Instructor: Qianwen Wang Course Description: This course aims to investigate how visualization techniques and AI technologies work together to enhance understanding, insights, or outcomes.
This is a seminar style course consisting of lectures, paper presentation, and interactive discussion of the selected papers. Students will also work on a group project where they propose a research idea, survey related studies, and present initial results.
This course will cover the application of visualization to better understand AI models and data, and the use of AI to improve visualization processes. Readings for the course cover papers from the top venues of AI, Visualization, and HCI, topics including AI explainability, reliability, and Human-AI collaboration. This course is designed for PhD students, Masters students, and advanced undergraduates who want to dig into research.
Registration Prerequisites: Complete the following Google form to request a permission number from the instructor ( https://forms.gle/YTF5EZFUbQRJhHBYA ). Although the class is primarily intended for PhD students, motivated juniors/seniors and MS students who are interested in this topic are welcome to apply, ensuring they detail their qualifications for the course.
Visualizations for Intelligent AR Systems
Meeting Time: 04:00 PM‑05:15 PM MW Instructor: Zhu-Tian Chen Course Description: This course aims to explore the role of Data Visualization as a pivotal interface for enhancing human-data and human-AI interactions within Augmented Reality (AR) systems, thereby transforming a broad spectrum of activities in both professional and daily contexts. Structured as a seminar, the course consists of two main components: the theoretical and conceptual foundations delivered through lectures, paper readings, and discussions; and the hands-on experience gained through small assignments and group projects. This class is designed to be highly interactive, and AR devices will be provided to facilitate hands-on learning. Participants will have the opportunity to experience AR systems, develop cutting-edge AR interfaces, explore AI integration, and apply human-centric design principles. The course is designed to advance students' technical skills in AR and AI, as well as their understanding of how these technologies can be leveraged to enrich human experiences across various domains. Students will be encouraged to create innovative projects with the potential for submission to research conferences.
Registration Prerequisites: Complete the following Google form to request a permission number from the instructor ( https://forms.gle/Y81FGaJivoqMQYtq5 ). Students are expected to have a solid foundation in either data visualization, computer graphics, computer vision, or HCI. Having expertise in all would be perfect! However, a robust interest and eagerness to delve into these subjects can be equally valuable, even though it means you need to learn some basic concepts independently.
Sustainable Computing: A Systems View
Meeting Time: 09:45 AM‑11:00 AM Instructor: Abhishek Chandra Course Description: In recent years, there has been a dramatic increase in the pervasiveness, scale, and distribution of computing infrastructure: ranging from cloud, HPC systems, and data centers to edge computing and pervasive computing in the form of micro-data centers, mobile phones, sensors, and IoT devices embedded in the environment around us. The growing amount of computing, storage, and networking demand leads to increased energy usage, carbon emissions, and natural resource consumption. To reduce their environmental impact, there is a growing need to make computing systems sustainable. In this course, we will examine sustainable computing from a systems perspective. We will examine a number of questions: • How can we design and build sustainable computing systems? • How can we manage resources efficiently? • What system software and algorithms can reduce computational needs? Topics of interest would include: • Sustainable system design and architectures • Sustainability-aware systems software and management • Sustainability in large-scale distributed computing (clouds, data centers, HPC) • Sustainability in dispersed computing (edge, mobile computing, sensors/IoT)
Registration Prerequisites: This course is targeted towards students with a strong interest in computer systems (Operating Systems, Distributed Systems, Networking, Databases, etc.). Background in Operating Systems (Equivalent of CSCI 5103) and basic understanding of Computer Networking (Equivalent of CSCI 4211) is required.
- Future undergraduate students
- Future transfer students
- Future graduate students
- Future international students
- Diversity and Inclusion Opportunities
- Learn abroad
- Living Learning Communities
- Mentor programs
- Programs for women
- Student groups
- Visit, Apply & Next Steps
- Information for current students
- Departments and majors overview
- Departments
- Undergraduate majors
- Graduate programs
- Integrated Degree Programs
- Additional degree-granting programs
- Online learning
- Academic Advising overview
- Academic Advising FAQ
- Academic Advising Blog
- Appointments and drop-ins
- Academic support
- Commencement
- Four-year plans
- Honors advising
- Policies, procedures, and forms
- Career Services overview
- Resumes and cover letters
- Jobs and internships
- Interviews and job offers
- CSE Career Fair
- Major and career exploration
- Graduate school
- Collegiate Life overview
- Scholarships
- Diversity & Inclusivity Alliance
- Anderson Student Innovation Labs
- Information for alumni
- Get engaged with CSE
- Upcoming events
- CSE Alumni Society Board
- Alumni volunteer interest form
- Golden Medallion Society Reunion
- 50-Year Reunion
- Alumni honors and awards
- Outstanding Achievement
- Alumni Service
- Distinguished Leadership
- Honorary Doctorate Degrees
- Nobel Laureates
- Alumni resources
- Alumni career resources
- Alumni news outlets
- CSE branded clothing
- International alumni resources
- Inventing Tomorrow magazine
- Update your info
- CSE giving overview
- Why give to CSE?
- College priorities
- Give online now
- External relations
- Giving priorities
- Donor stories
- Impact of giving
- Ways to give to CSE
- Matching gifts
- CSE directories
- Invest in your company and the future
- Recruit our students
- Connect with researchers
- K-12 initiatives
- Diversity initiatives
- Research news
- Give to CSE
- CSE priorities
- Corporate relations
- Information for faculty and staff
- Administrative offices overview
- Office of the Dean
- Academic affairs
- Finance and Operations
- Communications
- Human resources
- Undergraduate programs and student services
- CSE Committees
- CSE policies overview
- Academic policies
- Faculty hiring and tenure policies
- Finance policies and information
- Graduate education policies
- Human resources policies
- Research policies
- Research overview
- Research centers and facilities
- Research proposal submission process
- Research safety
- Award-winning CSE faculty
- National academies
- University awards
- Honorary professorships
- Collegiate awards
- Other CSE honors and awards
- Staff awards
- Performance Management Process
- Work. With Flexibility in CSE
- K-12 outreach overview
- Summer camps
- Outreach events
- Enrichment programs
- Field trips and tours
- CSE K-12 Virtual Classroom Resources
- Educator development
- Sponsor an event
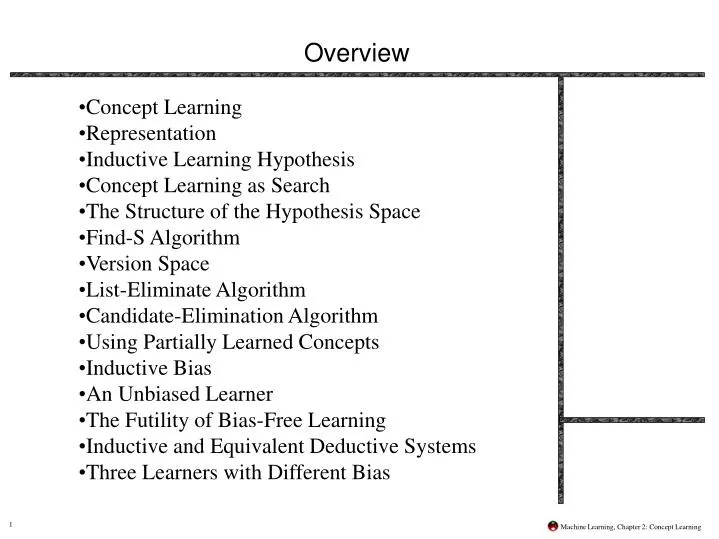
Machine Learning, Chapter 2: Concept Learning
Nov 01, 2014
920 likes | 1.68k Views
Overview. Concept Learning Representation Inductive Learning Hypothesis Concept Learning as Search The Structure of the Hypothesis Space Find-S Algorithm Version Space List-Eliminate Algorithm Candidate-Elimination Algorithm Using Partially Learned Concepts Inductive Bias
Share Presentation
- concept learning
- specific hypothesis
- candidate elimination
- maximally specific hypothesis
- candidate elimination algorithm detailed
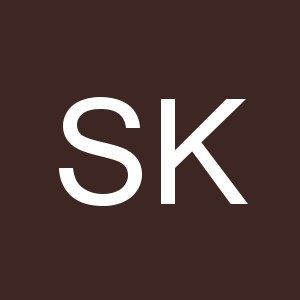
Presentation Transcript
Overview • Concept Learning • Representation • Inductive Learning Hypothesis • Concept Learning as Search • The Structure of the Hypothesis Space • Find-S Algorithm • Version Space • List-Eliminate Algorithm • Candidate-Elimination Algorithm • Using Partially Learned Concepts • Inductive Bias • An Unbiased Learner • The Futility of Bias-Free Learning • Inductive and Equivalent Deductive Systems • Three Learners with Different Bias 1 Machine Learning, Chapter 2: Concept Learning
The Example Windsurfing can be fun, but this depends on the weather. The following data is collected: Sky AirTemp Humidity Wind Water Forecast EnjoySport Sunny Warm Normal Strong Warm Same Yes Sunny Warm High Strong Warm Same Yes Rainy Cold High Strong Warm Change No Sunny Warm High Strong Cool Change Yes Is there a pattern here - what is the general concept? Concept Learning Representation Inductive Learning... 2 Machine Learning, Chapter 2: Concept Learning
Concept Learning Concept = boolean-valued function defined over a large set of objects or events. Concept learning = Inferring a boolean-valued function from training examples of input and output of the function. How can we represent a concept? Concept Learning Representation Inductive Learning... 3 Machine Learning, Chapter 2: Concept Learning
Representation (1/2) Ø = empty set Many possible representations, but here: • Instance x: A collection of attributes (Sky, AirTemp, Humidity, etc.) • Target function c: • Hypothesis h: A conjunction of constraints on the attributes. • A constraint can be: • a specific value (e.g. Water = Warm) • don’t care (e.g. Water = ?) • no value allowed (e.g. Water = Ø) • Training example d: An instance xi paired with the • target function c, Concept Learning Representation Inductive Learning... Concept Learning as... negative example positive example 4 Machine Learning, Chapter 2: Concept Learning
Representation (2/2) Instances X: The set of possible instances x. Hypotheses H: The set of possible hypotheses h. Training examples D: The set of possible training examples d. x = instance h = hypothesis d = training example Concept Learning Representation Inductive Learning... Concept Learning as... 5 Machine Learning, Chapter 2: Concept Learning
Inductive Learning Hypothesis Concept learning = Inferring a boolean-valued function from training examples of input and output of the function. - ”member of” x = instance h = hypothesis d = training example c = target function Thus, concept learning is to find a hH so that h(x)=c(x) for all xX. Problem:We might not have access to allx! Solution:Find a h that fits the examples, and take the achieved function as an approximation of the true c(x). This principle is called induction. Inductive learning hypothesis: Any hypothesis found to approximate the target function well over a sufficiently large set of training examples will also approximate the target function well over other unobserved examples. Concept Learning Representation Inductive Learning... Concept Learning as... The structure of H 6 Machine Learning, Chapter 2: Concept Learning
Concept Learning as Search Concept learning = Inferring a boolean-valued function from training examples of input and output of the function. - ”member of” x = instance h = hypothesis d = training example c = target function Concept learning is to find a hH so that h(x)=c(x) for all xX. A search problem: search through the hypothesis space H after the best h. Representation Inductive Learning... Concept Learning as... The Structure of H Find-S Algorithm 7 Machine Learning, Chapter 2: Concept Learning
The Structure of H x = instance h = hypothesis d = training example c = target function Many algorithms for concept learning organize the search by using a useful structure in the hypothesis space: general-to-specific ordering! An example: h1 = <Sunny, ?, ?, Strong, ?, ?> h2 = <Sunny, ?, ?, ?, ?, ?> h3 = <Sunny, ?, ?, ?, Cool, ?> Because h2 imposes fewer constraints, it will cover more instances x in X than both h1 and h3. h2 is more general than h1 and h3. In a similar manner, we can define more-specific-than. Instance space Hypothesis space Inductive Learning... Concept Learning as... The Structure of H Find-S Algorithm Version Space 8 Machine Learning, Chapter 2: Concept Learning
Find-S Algorithm x = instance h = hypothesis d = training example c = target function Finds the most specific hypothesis matching the training example (hence the name). 1. Initialize h to the most specific hypothesis in H 2. For each positive training instance x For each attribute constraint ai in h If the constraint ai in h is satisfied by x Then do nothing Else replace ai in h by the next more general constraint that is satisfied by x 3. Output hypothesis h Concept Learning as... The Structure of H Find-S Algorithm Version Space List-Eliminate... 9 Machine Learning, Chapter 2: Concept Learning
Find-S algorithm example x = instance h = hypothesis d = training example c = target function Concept Learning as... The Structure of H Find-S Algorithm Version Space List-Eliminate... 10 Machine Learning, Chapter 2: Concept Learning
Problems with Find-S There are several problems with the Find-S algorithm: It cannot determine if it has learnt the concept. There might be several other hypotheses that match as well – has it found the only one? It cannot detect when training data is inconsistent. We would like to detect and be tolerant to errors and noise. Why do we want the most specific hypothesis? Some other hypothesis might be more useful. Depending on H, there might be several maximally consistent hypotheses, and there is no way for Find-S to find them. All of them are equally likely. Concept Learning as... The Structure of H Find-S Algorithm Version Space List-Eliminate... 11 Machine Learning, Chapter 2: Concept Learning
Version Space x = instance h = hypothesis d = training example c = target function Definition: A hypothesis h is consistent with a set of training examples D if and only if h(x)=c(x) for each example in D. Version space = the subset of all hypotheses in H consistent with the training examples D. Definition: The version space, denoted VSH,D, with respect to hypothesis space H and training examples D, is the subset of hypotheses from H consistent with the training examples in D. The Structure of H Find-S Algorithm Version Space List-Eliminate... Candidate-Elimination... 12 Machine Learning, Chapter 2: Concept Learning
Representation of Version Space x = instance h = hypothesis d = training example c = target function VSH,D=version space Option 1: List all of the members in the version space. Works only when the hypothesis space H is finite! Option 2: Store only the set of most general members G and the set of most specific members S. Given these two sets, it is possible to generate any member of the version space as needed. The Structure of H Find-S Algorithm Version Space List-Eliminate... Candidate-Elimination... 13 Machine Learning, Chapter 2: Concept Learning
List-Eliminate Algorithm x = instance h = hypothesis d = training example c = target function VSH,D=version space 1. VSH,D a list containing every hypothesis in H 2. For each training example, remove from VSH,D any hypothesis that is inconsistent with the training example h(x)≠c(x) 3. Output the list of hypotheses in VSH,D Advantage: Guaranteed to output all hypotheses consistent with the training examples. But inefficient! Even in this simple example, there are 1+4·3·3·3·3 = 973 semantically distinct hypotheses. Find-S Algorithm Version Space List-Eliminate... Candidate-Elimination... Using Partially... 14 Machine Learning, Chapter 2: Concept Learning
Candidate-Elimination Algorithm x = instance h = hypothesis d = training example c = target function VSH,D=version space G maximally general hypothesis in H S maximally specific hypothesis in H For each training example modify G and S so that G and S are consistent with d Version Space List-Eliminate... Candidate-Elimination... Using Partially... Inductive Bias 15 Machine Learning, Chapter 2: Concept Learning
For each training example Candidate-Elimination Algorithm (detailed) x = instance h = hypothesis d = training example c = target function VSH,D=version space G maximally general hypotheses in H S maximally specific hypotheses in H • Ifd is a positive example • Remove from G any hypothesis that is inconsistent with d • For each hypothesis s in S that is not consistent with d • Remove s from S. • Add to S all minimal generalizations h of s such that • h consistent with d • Some member of G is more general than h • Remove from S any hypothesis that is more general than another hypothesis in S • Ifd is a negative example • Remove from S any hypothesis that is inconsistent with d • For each hypothesis g in G that is not consistent with d • Remove g from G. • Add to G all minimal specializations h of g such that • h consistent with d • Some member of S is more specific than h • Remove from G any hypothesis that is less general than another hypothesis in G 16 Machine Learning, Chapter 2: Concept Learning
Example of Candidate-Elimination x = instance h = hypothesis d = training example c = target function VSH,D=version space G = set of most general members in H S = set of most specific members in H Version Space List-Eliminate... Candidate-Elimination... Using Partially... Inductive Bias 17 Machine Learning, Chapter 2: Concept Learning
Properties of Candidate-Elimination x = instance h = hypothesis d = training example c = target function VSH,D=version space G = set of most general members in H S = set of most specific members in H • Converges to target concept when • No error in training examples • Target concept is in H • Converges to an empty version space when • Inconsistency in training data • Target concept cannot be described by hypothesis representation Version Space List-Eliminate... Candidate-Elimination... Using Partially... Inductive Bias 18 Machine Learning, Chapter 2: Concept Learning
Using Partially Learned Concepts x = instance h = hypothesis d = training example c = target function VSH,D=version space G = set of most general members in H S = set of most specific members in H New instance Votes: Pos Neg 6 0 0 6 3 3 2 4 List-Eliminate... Candidate-Elimination... Using Partially... Inductive Bias An Unbiased Learner 19 Machine Learning, Chapter 2: Concept Learning
xc 1 1 2 0 3 1 Inductive Bias x = instance h = hypothesis d = training example c = target function VSH,D=version space G = set of most general members in H S = set of most specific members in H New example: Each instance is a single integer [1,4] Training examples: There is no way to represent this simple example by using our representation. Why? The hypotheses space H is biased, since it only allow conjunctions of attribute values. Candidate-Elimination... Using Partially... Inductive Bias An Unbiased Learner The Futility of... 20 Machine Learning, Chapter 2: Concept Learning
An Unbiased Learner x = instance h = hypothesis d = training example c = target function VSH,D=version space G = set of most general members in H S = set of most specific members in H Solution(?): Let H be the set of all possible subsets of X – the power set of X – denoted as P(X). It contains 2|X| hypotheses, where, |X| is the number of distinct instances. For our example, where each instance is a single integer [1,4] : P(X)= Using Partially... Inductive Bias An Unbiased Learner The Futility of... Inductive Bias Revisited 21 Machine Learning, Chapter 2: Concept Learning
xc 1 1 2 0 3 1 The Futility of Bias-free Learning x = instance h = hypothesis d = training example c = target function VSH,D=version space G = set of most general members in H S = set of most specific members in H For our example, we get after three training examples: How do we classify a new instance x=4? The only examples that are classified are the training examples themselves. In order to learn the target concept one would have to present every single instance in X as a training example! Each unobserved instance will be classified positive by precisely half the hypotheses in VSH,D and negative by the other half. Inductive Bias An Unbiased Learner The Futility of... Inductive Bias Revisited Inductive and ... 22 Machine Learning, Chapter 2: Concept Learning
Inductive Bias Revisited x = instance h = hypothesis d = training example c = target function VSH,D=version space G = set of most general members in H S = set of most specific members in H =“for all” =“member of” =“logical and” =“implies” Inductive bias is necessary in order to be able to generalize! Definition: The inductive bias of L is any minimal set of assertions B such that for any target concept c and corresponding training data D Where yz means that z deductively follows from y. An Unbiased Learner The Futility of... Inductive Bias Revisited Inductive and ... Three Learners... 23 Machine Learning, Chapter 2: Concept Learning
Inductive and equivalent deductive systems x = instance h = hypothesis d = training example c = target function VSH,D=version space G = set of most general members in H S = set of most specific members in H The Futility of... Inductive Bias Revisited Inductive and ... Three Learners... Summary 24 Machine Learning, Chapter 2: Concept Learning
Three Learners with different Biases x = instance h = hypothesis d = training example c = target function VSH,D=version space G = set of most general members in H S = set of most specific members in H 1. Rote learner – simply stores instances No bias – unable to generalize 2. Candidate-Elimination Bias: Target concept is in hypothesis space H 3. Find-S Bias: Target concept is in hypothesis space H + All instances are negative unless taught otherwise Inductive Bias Revisited Inductive and ... Three Learners... Summary 25 Machine Learning, Chapter 2: Concept Learning
Summary • Concept learning as search through H • Many algorithms use general-to-specific ordering of H • Find-S algorithm • Version space and version space representations • List-Eliminate algorithm • Candidate-Elimination algorithm • Inductive leaps possible only if learner is biased • Inductive learners can be modelled by equivalent deductive systems Inductive and ... Three Learners... Summary 26 Machine Learning, Chapter 2: Concept Learning
- More by User
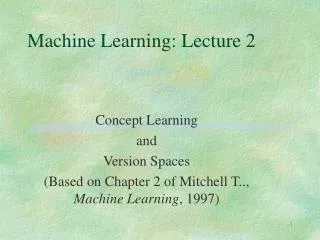
Machine Learning: Lecture 2
Machine Learning: Lecture 2. Concept Learning and Version Spaces (Based on Chapter 2 of Mitchell T.., Machine Learning , 1997). Birds. Things. Animals. Cars. What is a Concept?.
518 views • 20 slides
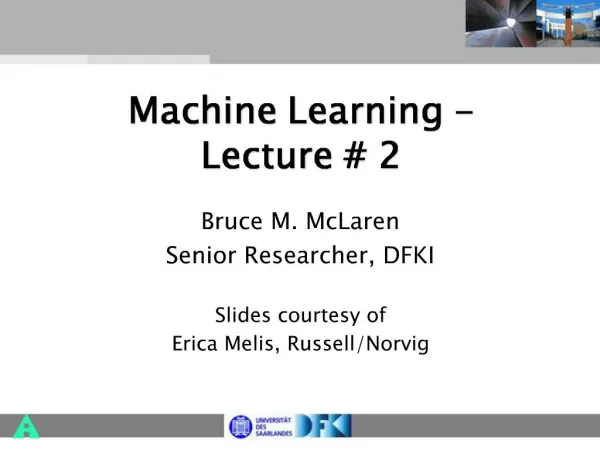
Machine Learning - Lecture 2
Overview. Ensemble Learning. Ensemble Learning. Overview of a DFKI Machine Learning Project. Neural NetworksBrainsNeural NetworksPerceptronsMulti-layer PerceptronsSupport Vector MachinesApplications of Neural Networks. Learning from Text. Ensemble Learning. Last lecture: We chose a single hypo
552 views • 51 slides
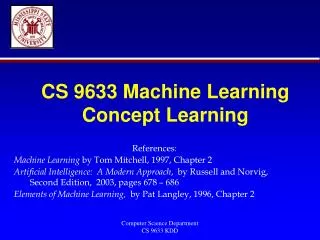
CS 9633 Machine Learning Concept Learning
CS 9633 Machine Learning Concept Learning. References: Machine Learning by Tom Mitchell, 1997, Chapter 2 Artificial Intelligence: A Modern Approach , by Russell and Norvig, Second Edition, 2003, pages 678 – 686 Elements of Machine Learning, by Pat Langley, 1996, Chapter 2.
930 views • 62 slides
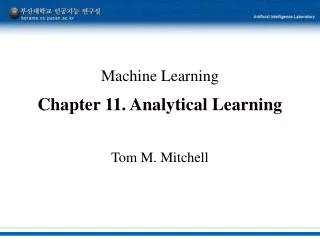
Machine Learning Chapter 11. Analytical Learning
Machine Learning Chapter 11. Analytical Learning. Tom M. Mitchell. Outline. Two formulations for learning: Inductive and Analytical Perfect domain theories and Prolog-EBG. A Positive Example. The Inductive Generalization Problem. Given: Instances Hypotheses Target Concept
1.5k views • 13 slides
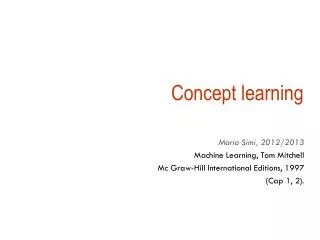
Concept learning
Concept learning. Maria Simi, 2012/2013 Machine Learning, Tom Mitchell Mc Graw -Hill International Editions, 1997 ( Cap 1, 2). Introduction to machine learning. Introduction to machine learning When appropriate and when not appropriate Task definition
1.22k views • 50 slides
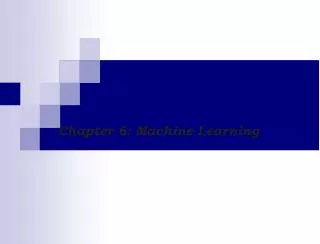
Chapter 6: Machine Learning
Chapter 6: Machine Learning. Objectives. At the end of the chapter, you should be able to:. Describe how each method can be used to perform classification tasks – given some input features you should be able to describe how the out classification is determined.
520 views • 31 slides
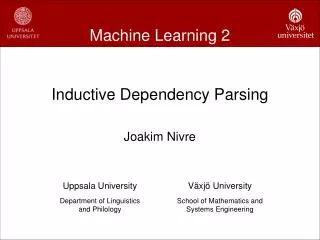
Machine Learning 2
Machine Learning 2. Inductive Dependency Parsing Joakim Nivre. Inductive Dependency Parsing. Dependency-based representations … have restricted expressivity but provide a transparent encoding of semantic structure. have restricted complexity in parsing. Inductive machine learning …
335 views • 15 slides
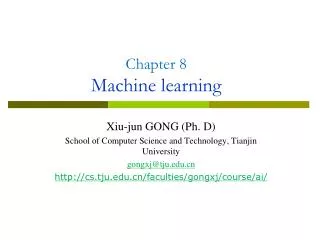
Chapter 8 Machine learning
Chapter 8 Machine learning. Xiu-jun GONG (Ph. D) School of Computer Science and Technology, Tianjin University [email protected] http:// cs.tju.edu.cn/faculties/gongxj/course/ai /. Outline. What is machine learning Tasks of Machine Learning The Types of Machine Learning
582 views • 29 slides
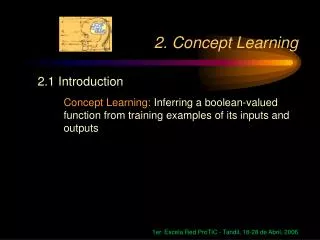
2. Concept Learning
2. Concept Learning. 2.1 Introduction Concept Learning: Inferring a boolean-valued function from training examples of its inputs and outputs. 2. Concept Learning. 2.2 A Concept Learning Task: “Days in which Aldo enjoys his favorite water sport”. 2. Concept Learning.
1.2k views • 31 slides
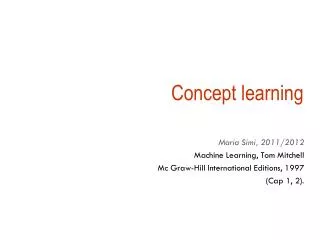
Concept learning. Maria Simi, 2011/2012 Machine Learning, Tom Mitchell Mc Graw -Hill International Editions, 1997 ( Cap 1, 2). Introduction to machine learning. Introduction to machine learning When appropriate and when not appropriate Task definition
875 views • 50 slides
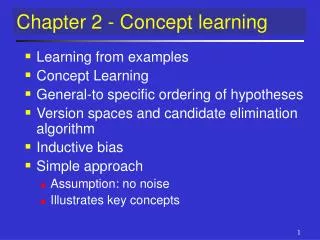
Chapter 2 - Concept learning
Chapter 2 - Concept learning. Learning from examples Concept Learning General-to specific ordering of hypotheses Version spaces and candidate elimination algorithm Inductive bias Simple approach Assumption: no noise Illustrates key concepts. Concept learning task.
916 views • 48 slides
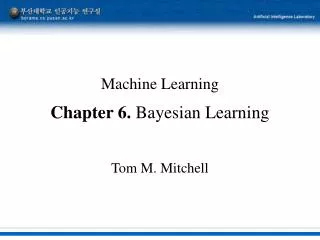
Machine Learning Chapter 6. Bayesian Learning
Machine Learning Chapter 6. Bayesian Learning. Tom M. Mitchell. Bayesian Learning. Bayes Theorem MAP, ML hypotheses MAP learners Minimum description length principle Bayes optimal classifier Naive Bayes learner Example: Learning over text data Bayesian belief networks
1.27k views • 49 slides
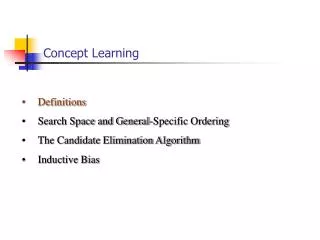
Concept Learning
Concept Learning. Definitions Search Space and General-Specific Ordering The Candidate Elimination Algorithm Inductive Bias. Definition. The problem is to learn a function mapping examples into two classes: positive and negative.
395 views • 22 slides
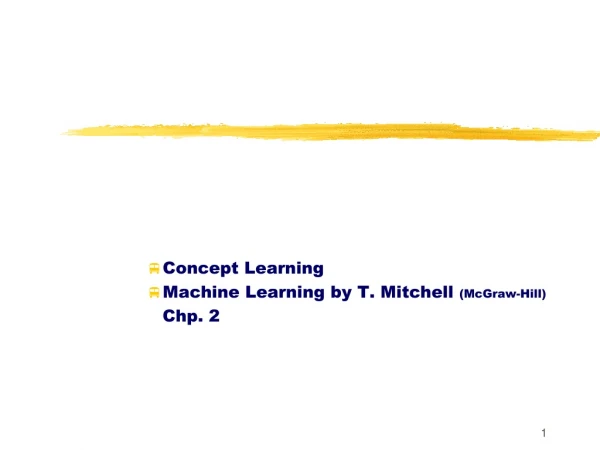
Concept Learning Machine Learning by T. Mitchell (McGraw-Hill) Chp. 2
Concept Learning Machine Learning by T. Mitchell (McGraw-Hill) Chp. 2. Much of learning involves acquiring general concepts from specific training examples e.g. what is a bird? what is a chair?
195 views • 16 slides
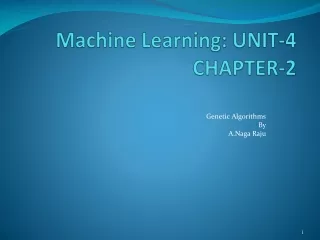
Machine Learning: UNIT-4 CHAPTER-2
Machine Learning: UNIT-4 CHAPTER-2. Genetic Algorithms By A.Naga Raju. Overview of Genetic Algorithms (GAs). GA is a learning method motivated by analogy to biological evolution.
135 views • 13 slides
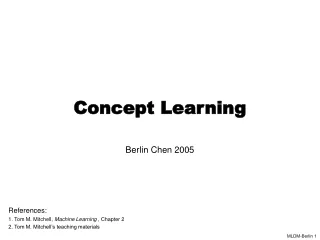
Concept Learning. Berlin Chen 2005. References: 1. Tom M. Mitchell, Machine Learning , Chapter 2 2. Tom M. Mitchell’s teaching materials. What is a Concept ?. Concept of Dogs. Concept of Cats. Concept of Horses. Concept of Birds. Concept of Fishes.
410 views • 34 slides
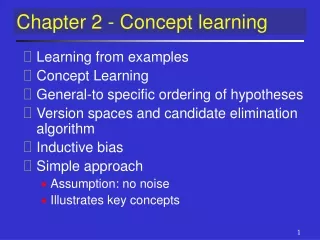
561 views • 48 slides
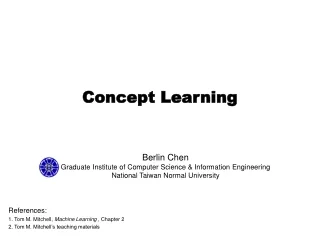
Concept Learning. Berlin Chen Graduate Institute of Computer Science & Information Engineering National Taiwan Normal University. References: 1. Tom M. Mitchell, Machine Learning , Chapter 2 2. Tom M. Mitchell’s teaching materials. What is a Concept ?. Concept of Dogs. Concept of
367 views • 34 slides
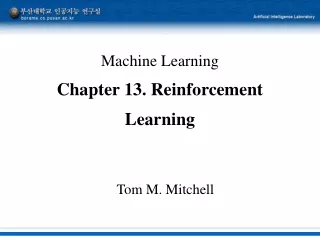
Machine Learning Chapter 13. Reinforcement Learning
Machine Learning Chapter 13. Reinforcement Learning. Tom M. Mitchell. Control Learning. Consider learning to choose actions, e.g., Robot learning to dock on battery charger Learning to choose actions to optimize factory output Learning to play Backgammon
248 views • 19 slides
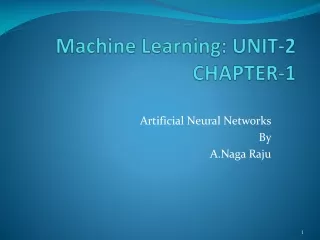
Machine Learning: UNIT-2 CHAPTER-1
Machine Learning: UNIT-2 CHAPTER-1. Artificial Neural Networks By A.Naga Raju. What is an Artificial Neural Network?. It is a formalism for representing functions inspired from biological systems and composed of parallel computing units which each compute a simple function.
141 views • 14 slides
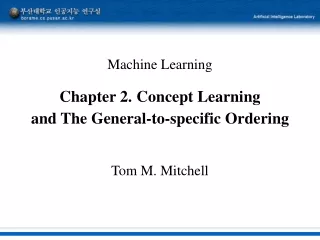
Machine Learning Chapter 2. Concept Learning and The General-to-specific Ordering
Machine Learning Chapter 2. Concept Learning and The General-to-specific Ordering. Tom M. Mitchell. Outline. Learning from examples General-to-specific ordering over hypotheses Version spaces and candidate elimination algorithm Picking new examples The need for inductive bias
481 views • 25 slides
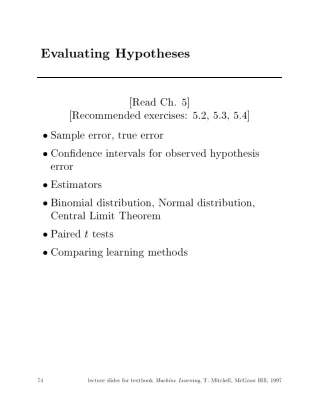
Machine Learning -Chapter -5
Sample error, true error Confidence intervals for observed hypothesis error Estimators Binomial distribution, Normal distribution, Central Limit Theorem Paired t tests Comparing learning methods
210 views • 20 slides

IMAGES
VIDEO
COMMENTS
2. BASICS OF LEARNING • Learning is defined as "the acquisition of knowledge or skills through study, experience, or being taught". 3. • Learning style refers to the preferred way that one acquires, processes, and retains information at the best. 4. Basically there are three dimensions of learning.
When combined, these framed a rubric that supported students in optimizing their presentation deliveries. The competencies are as follows: 1. Content knowledge. The presenter must display a deep understanding of what they are delivering in order to share the "what, why, how, and how-to" of the topic. 2.
Presentation Transcript. Analyzing Concepts Concept Learning Concepts are categories of stimuli that have certain features in common. The shapes on the left are all members of a conceptual category: rectangle. Their common features are (1) 4 lines; (2) opposite lines parallel; (3) lines connected at ends; (4) lines form 4 right angles.
Concept learning, also known as category learning, concept attainment, and concept formation, is defined by Bruner, Goodnow, ... Secondary presentation forms include: prerequisites, objectives, helps, mnemonics, and feedback. A complete lesson includes a combination of primary and secondary presentation forms, but the most effective combination ...
Trying to rush through slides can give the impression of an unorganized presentation and may be difficult for students to follow or learn. PART II: Enhancing Teaching and Learning with PowerPoint Class Preparation. PowerPoint can be used to prepare lectures and presentations by helping instructors refine their material to salient points and ...
A mind map can help communicate your complex concept in two ways. First, it can help you strategize the text, presentation, and speech. A mind map or thought organizer can be the foundation for developing the communication of a complex topic. Secondly, include a summarized mind map as a presentation slide.
In partic- ular, the concept model we address is the set of assumptions, data, and inferences used in. instructional design for concept learning. Components of this design model include as- sumptions about: * the nature of concepts, * the way concepts are learned, * how concept learning is measured, and. * how conditions should be designed for.
CONCEPT LEARNING: How To Make It Happen In The Classroom SYDELLE D. EHRENBERG Despite much talk about con cept-centered curricu'um, too many students still just learn facts. Teachers report, and tests show, that even those students who seem to have learned concepts often fail to apply them to new out similar situa ...
Learning - an active, social process • Learning involves a social process of constructing meaning. • The role of educators • Organize for learning rather than plan for teaching • Focus on what students will do to learn and what the educator will do to inspire and support learning (Gagnon & Collay, 2006)
Free Google Slides theme and PowerPoint template. Project based learning helps children understand concepts better because it makes them put in use all their skills: planning, collaboration, critical thinking, idea planning…. If you're interested in giving your lessons a new approach, this template with real content curated by a pedagogist ...
With the development of technologies, e-learning and remote education became usual. If you need to present the E-learning concept, get inspired by our icon ideas. The challenge is how to illustrate the concept properly in a PowerPoint presentation so the slides look professional and clear. Icons are a way of communication and, due to their
Concept learning, also known as category learning, concept attainment, and concept formation, is defined by Bruner, Goodnow, & Austin (1967) as "the search for and listing of attributes that can be used to distinguish exemplars from non exemplars of various categories". ... Secondary presentation forms include: prerequisites, objectives, helps ...
Presentation Transcript. 1. Concept-Based Teaching and Learning As educators, we are preparing our students for a world that is much different than the world we ourselves experienced. Therefore, it stands to reason that the education experienced today by our students must be different. For several years, education reform experts have advocated ...
Premium Google Slides theme and PowerPoint template. New and interesting technologies are being used for teaching. This means that you don't need to be at school or at the university to take courses. If you need to prepare a webinar or you must give a presentation to talk about e-learning and these kind of advances in education, this new ...
1. The effects of presentations on learning related concepts and other aspects of understanding. 2. How to characterize the ways presentations differ from each other. 3. The potential benefits of combining presentations to achieve the advantages of both. First, how do presentations of a concept affect learning of other concepts?
The document outlines two main strategies for teaching concepts: inquiry, which uses examples and non-examples, and expository, which presents the concept label and criteria. It notes both strengths, like helping with complex topics and building learning skills, and challenges of concept learning, such as overgeneralization, under ...
Title: Concept Learning. 1. Concept Learning. Inducing general functions from specific training. examples is a main issue of machine learning. Concept Learning Acquiring the definition of a. general category from given sample positive and. negative training examples of the category. Concept Learning can seen as a problem of.
When in doubt, adhere to the principle of simplicity, and aim for a clean and uncluttered layout with plenty of white space around text and images. Think phrases and bullets, not sentences. As an ...
Concept Learning and Version Spaces. Concept Learning and Version Spaces. Based Ch.2 of Tom Mitchell's Machine Learning and lecture slides by Uffe Kjaerulff. Presentation Overview. Concept learning as boolean function approximation Ordering of hypothesis Version spaces and candidate-elimination algorithm The role of bias. 763 views • 17 slides
Machine Learning for Healthcare: Concepts and Applications. ... This is a seminar style course consisting of lectures, paper presentation, and interactive discussion of the selected papers. Students will also work on a group project where they propose a research idea, survey related studies, and present initial results. ...
The Structure of H Find-S Algorithm 7 Machine Learning, Chapter 2: Concept Learning. The Structure of H x = instance h = hypothesis d = training example c = target function Many algorithms for concept learning organize the search by using a useful structure in the hypothesis space: general-to-specific ordering!